- How it works

"Christmas Offer"
Terms & conditions.
As the Christmas season is upon us, we find ourselves reflecting on the past year and those who we have helped to shape their future. It’s been quite a year for us all! The end of the year brings no greater joy than the opportunity to express to you Christmas greetings and good wishes.
At this special time of year, Research Prospect brings joyful discount of 10% on all its services. May your Christmas and New Year be filled with joy.
We are looking back with appreciation for your loyalty and looking forward to moving into the New Year together.
"Claim this offer"
In unfamiliar and hard times, we have stuck by you. This Christmas, Research Prospect brings you all the joy with exciting discount of 10% on all its services.
Offer valid till 5-1-2024
We love being your partner in success. We know you have been working hard lately, take a break this holiday season to spend time with your loved ones while we make sure you succeed in your academics
Discount code: RP0996Y
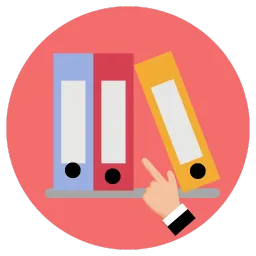
How to Write a Research Design – Guide with Examples
Published by Alaxendra Bets at August 14th, 2021 , Revised On June 24, 2024
A research design is a structure that combines different components of research. It involves the use of different data collection and data analysis techniques logically to answer the research questions .
It would be best to make some decisions about addressing the research questions adequately before starting the research process, which is achieved with the help of the research design.
Below are the key aspects of the decision-making process:
- Data type required for research
- Research resources
- Participants required for research
- Hypothesis based upon research question(s)
- Data analysis methodologies
- Variables (Independent, dependent, and confounding)
- The location and timescale for conducting the data
- The time period required for research
The research design provides the strategy of investigation for your project. Furthermore, it defines the parameters and criteria to compile the data to evaluate results and conclude.
Your project’s validity depends on the data collection and interpretation techniques. A strong research design reflects a strong dissertation , scientific paper, or research proposal .
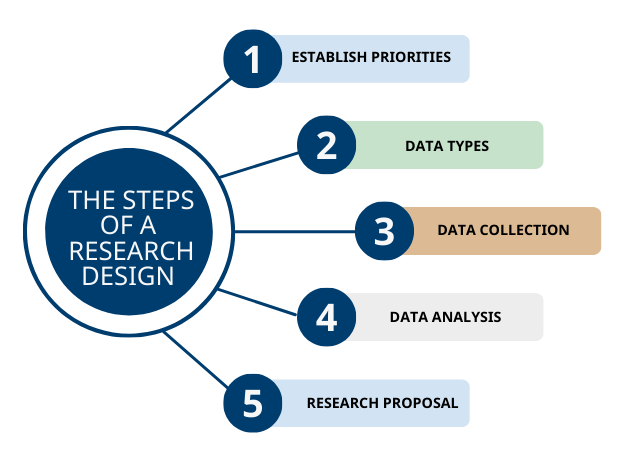
Step 1: Establish Priorities for Research Design
Before conducting any research study, you must address an important question: “how to create a research design.”
The research design depends on the researcher’s priorities and choices because every research has different priorities. For a complex research study involving multiple methods, you may choose to have more than one research design.
Multimethodology or multimethod research includes using more than one data collection method or research in a research study or set of related studies.
If one research design is weak in one area, then another research design can cover that weakness. For instance, a dissertation analyzing different situations or cases will have more than one research design.
For example:
- Experimental research involves experimental investigation and laboratory experience, but it does not accurately investigate the real world.
- Quantitative research is good for the statistical part of the project, but it may not provide an in-depth understanding of the topic .
- Also, correlational research will not provide experimental results because it is a technique that assesses the statistical relationship between two variables.
While scientific considerations are a fundamental aspect of the research design, It is equally important that the researcher think practically before deciding on its structure. Here are some questions that you should think of;
- Do you have enough time to gather data and complete the write-up?
- Will you be able to collect the necessary data by interviewing a specific person or visiting a specific location?
- Do you have in-depth knowledge about the different statistical analysis and data collection techniques to address the research questions or test the hypothesis ?
If you think that the chosen research design cannot answer the research questions properly, you can refine your research questions to gain better insight.
Step 2: Data Type you Need for Research
Decide on the type of data you need for your research. The type of data you need to collect depends on your research questions or research hypothesis. Two types of research data can be used to answer the research questions:
Primary Data Vs. Secondary Data
Qualitative vs. quantitative data.
Also, see; Research methods, design, and analysis .
Need help with a thesis chapter?
- Hire an expert from ResearchProspect today!
- Statistical analysis, research methodology, discussion of the results or conclusion – our experts can help you no matter how complex the requirements are.
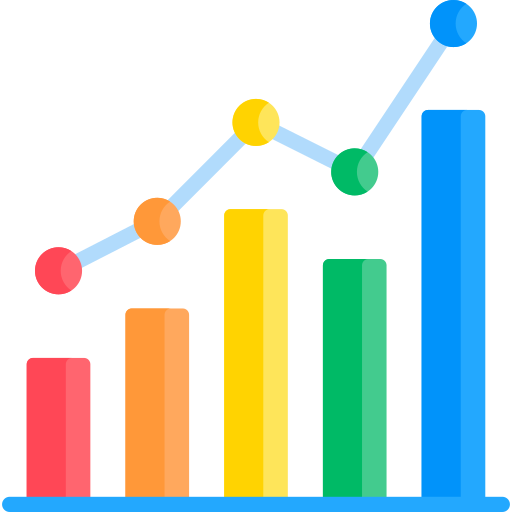
Step 3: Data Collection Techniques
Once you have selected the type of research to answer your research question, you need to decide where and how to collect the data.
It is time to determine your research method to address the research problem . Research methods involve procedures, techniques, materials, and tools used for the study.
For instance, a dissertation research design includes the different resources and data collection techniques and helps establish your dissertation’s structure .
The following table shows the characteristics of the most popularly employed research methods.
Research Methods
Step 4: Procedure of Data Analysis
Use of the correct data and statistical analysis technique is necessary for the validity of your research. Therefore, you need to be certain about the data type that would best address the research problem. Choosing an appropriate analysis method is the final step for the research design. It can be split into two main categories;
Quantitative Data Analysis
The quantitative data analysis technique involves analyzing the numerical data with the help of different applications such as; SPSS, STATA, Excel, origin lab, etc.
This data analysis strategy tests different variables such as spectrum, frequencies, averages, and more. The research question and the hypothesis must be established to identify the variables for testing.
Qualitative Data Analysis
Qualitative data analysis of figures, themes, and words allows for flexibility and the researcher’s subjective opinions. This means that the researcher’s primary focus will be interpreting patterns, tendencies, and accounts and understanding the implications and social framework.
You should be clear about your research objectives before starting to analyze the data. For example, you should ask yourself whether you need to explain respondents’ experiences and insights or do you also need to evaluate their responses with reference to a certain social framework.
Step 5: Write your Research Proposal
The research design is an important component of a research proposal because it plans the project’s execution. You can share it with the supervisor, who would evaluate the feasibility and capacity of the results and conclusion .
Read our guidelines to write a research proposal if you have already formulated your research design. The research proposal is written in the future tense because you are writing your proposal before conducting research.
The research methodology or research design, on the other hand, is generally written in the past tense.
How to Write a Research Design – Conclusion
A research design is the plan, structure, strategy of investigation conceived to answer the research question and test the hypothesis. The dissertation research design can be classified based on the type of data and the type of analysis.
Above mentioned five steps are the answer to how to write a research design. So, follow these steps to formulate the perfect research design for your dissertation .
ResearchProspect writers have years of experience creating research designs that align with the dissertation’s aim and objectives. If you are struggling with your dissertation methodology chapter, you might want to look at our dissertation part-writing service.
Our dissertation writers can also help you with the full dissertation paper . No matter how urgent or complex your need may be, ResearchProspect can help. We also offer PhD level research paper writing services.
Frequently Asked Questions
What is research design.
Research design is a systematic plan that guides the research process, outlining the methodology and procedures for collecting and analysing data. It determines the structure of the study, ensuring the research question is answered effectively, reliably, and validly. It serves as the blueprint for the entire research project.
How to write a research design?
To write a research design, define your research question, identify the research method (qualitative, quantitative, or mixed), choose data collection techniques (e.g., surveys, interviews), determine the sample size and sampling method, outline data analysis procedures, and highlight potential limitations and ethical considerations for the study.
How to write the design section of a research paper?
In the design section of a research paper, describe the research methodology chosen and justify its selection. Outline the data collection methods, participants or samples, instruments used, and procedures followed. Detail any experimental controls, if applicable. Ensure clarity and precision to enable replication of the study by other researchers.
How to write a research design in methodology?
To write a research design in methodology, clearly outline the research strategy (e.g., experimental, survey, case study). Describe the sampling technique, participants, and data collection methods. Detail the procedures for data collection and analysis. Justify choices by linking them to research objectives, addressing reliability and validity.
You May Also Like
Not sure how to approach a company for your primary research study? Don’t worry. Here we have some tips for you to successfully gather primary study.
To help students organise their dissertation proposal paper correctly, we have put together detailed guidelines on how to structure a dissertation proposal.
Struggling to find relevant and up-to-date topics for your dissertation? Here is all you need to know if unsure about how to choose dissertation topic.
As Featured On
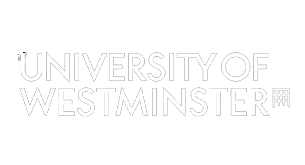
USEFUL LINKS
LEARNING RESOURCES
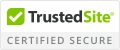
COMPANY DETAILS
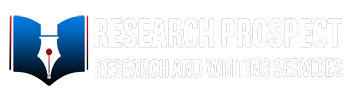
Splash Sol LLC

- How It Works
Have a language expert improve your writing
Run a free plagiarism check in 10 minutes, automatically generate references for free.
- Knowledge Base
- Methodology
Research Design | Step-by-Step Guide with Examples
Published on 5 May 2022 by Shona McCombes . Revised on 20 March 2023.
A research design is a strategy for answering your research question using empirical data. Creating a research design means making decisions about:
- Your overall aims and approach
- The type of research design you’ll use
- Your sampling methods or criteria for selecting subjects
- Your data collection methods
- The procedures you’ll follow to collect data
- Your data analysis methods
A well-planned research design helps ensure that your methods match your research aims and that you use the right kind of analysis for your data.
Table of contents
Step 1: consider your aims and approach, step 2: choose a type of research design, step 3: identify your population and sampling method, step 4: choose your data collection methods, step 5: plan your data collection procedures, step 6: decide on your data analysis strategies, frequently asked questions.
- Introduction
Before you can start designing your research, you should already have a clear idea of the research question you want to investigate.
There are many different ways you could go about answering this question. Your research design choices should be driven by your aims and priorities – start by thinking carefully about what you want to achieve.
The first choice you need to make is whether you’ll take a qualitative or quantitative approach.
Qualitative research designs tend to be more flexible and inductive , allowing you to adjust your approach based on what you find throughout the research process.
Quantitative research designs tend to be more fixed and deductive , with variables and hypotheses clearly defined in advance of data collection.
It’s also possible to use a mixed methods design that integrates aspects of both approaches. By combining qualitative and quantitative insights, you can gain a more complete picture of the problem you’re studying and strengthen the credibility of your conclusions.
Practical and ethical considerations when designing research
As well as scientific considerations, you need to think practically when designing your research. If your research involves people or animals, you also need to consider research ethics .
- How much time do you have to collect data and write up the research?
- Will you be able to gain access to the data you need (e.g., by travelling to a specific location or contacting specific people)?
- Do you have the necessary research skills (e.g., statistical analysis or interview techniques)?
- Will you need ethical approval ?
At each stage of the research design process, make sure that your choices are practically feasible.
Prevent plagiarism, run a free check.
Within both qualitative and quantitative approaches, there are several types of research design to choose from. Each type provides a framework for the overall shape of your research.
Types of quantitative research designs
Quantitative designs can be split into four main types. Experimental and quasi-experimental designs allow you to test cause-and-effect relationships, while descriptive and correlational designs allow you to measure variables and describe relationships between them.
With descriptive and correlational designs, you can get a clear picture of characteristics, trends, and relationships as they exist in the real world. However, you can’t draw conclusions about cause and effect (because correlation doesn’t imply causation ).
Experiments are the strongest way to test cause-and-effect relationships without the risk of other variables influencing the results. However, their controlled conditions may not always reflect how things work in the real world. They’re often also more difficult and expensive to implement.
Types of qualitative research designs
Qualitative designs are less strictly defined. This approach is about gaining a rich, detailed understanding of a specific context or phenomenon, and you can often be more creative and flexible in designing your research.
The table below shows some common types of qualitative design. They often have similar approaches in terms of data collection, but focus on different aspects when analysing the data.
Your research design should clearly define who or what your research will focus on, and how you’ll go about choosing your participants or subjects.
In research, a population is the entire group that you want to draw conclusions about, while a sample is the smaller group of individuals you’ll actually collect data from.
Defining the population
A population can be made up of anything you want to study – plants, animals, organisations, texts, countries, etc. In the social sciences, it most often refers to a group of people.
For example, will you focus on people from a specific demographic, region, or background? Are you interested in people with a certain job or medical condition, or users of a particular product?
The more precisely you define your population, the easier it will be to gather a representative sample.
Sampling methods
Even with a narrowly defined population, it’s rarely possible to collect data from every individual. Instead, you’ll collect data from a sample.
To select a sample, there are two main approaches: probability sampling and non-probability sampling . The sampling method you use affects how confidently you can generalise your results to the population as a whole.
Probability sampling is the most statistically valid option, but it’s often difficult to achieve unless you’re dealing with a very small and accessible population.
For practical reasons, many studies use non-probability sampling, but it’s important to be aware of the limitations and carefully consider potential biases. You should always make an effort to gather a sample that’s as representative as possible of the population.
Case selection in qualitative research
In some types of qualitative designs, sampling may not be relevant.
For example, in an ethnography or a case study, your aim is to deeply understand a specific context, not to generalise to a population. Instead of sampling, you may simply aim to collect as much data as possible about the context you are studying.
In these types of design, you still have to carefully consider your choice of case or community. You should have a clear rationale for why this particular case is suitable for answering your research question.
For example, you might choose a case study that reveals an unusual or neglected aspect of your research problem, or you might choose several very similar or very different cases in order to compare them.
Data collection methods are ways of directly measuring variables and gathering information. They allow you to gain first-hand knowledge and original insights into your research problem.
You can choose just one data collection method, or use several methods in the same study.
Survey methods
Surveys allow you to collect data about opinions, behaviours, experiences, and characteristics by asking people directly. There are two main survey methods to choose from: questionnaires and interviews.
Observation methods
Observations allow you to collect data unobtrusively, observing characteristics, behaviours, or social interactions without relying on self-reporting.
Observations may be conducted in real time, taking notes as you observe, or you might make audiovisual recordings for later analysis. They can be qualitative or quantitative.
Other methods of data collection
There are many other ways you might collect data depending on your field and topic.
If you’re not sure which methods will work best for your research design, try reading some papers in your field to see what data collection methods they used.
Secondary data
If you don’t have the time or resources to collect data from the population you’re interested in, you can also choose to use secondary data that other researchers already collected – for example, datasets from government surveys or previous studies on your topic.
With this raw data, you can do your own analysis to answer new research questions that weren’t addressed by the original study.
Using secondary data can expand the scope of your research, as you may be able to access much larger and more varied samples than you could collect yourself.
However, it also means you don’t have any control over which variables to measure or how to measure them, so the conclusions you can draw may be limited.
As well as deciding on your methods, you need to plan exactly how you’ll use these methods to collect data that’s consistent, accurate, and unbiased.
Planning systematic procedures is especially important in quantitative research, where you need to precisely define your variables and ensure your measurements are reliable and valid.
Operationalisation
Some variables, like height or age, are easily measured. But often you’ll be dealing with more abstract concepts, like satisfaction, anxiety, or competence. Operationalisation means turning these fuzzy ideas into measurable indicators.
If you’re using observations , which events or actions will you count?
If you’re using surveys , which questions will you ask and what range of responses will be offered?
You may also choose to use or adapt existing materials designed to measure the concept you’re interested in – for example, questionnaires or inventories whose reliability and validity has already been established.
Reliability and validity
Reliability means your results can be consistently reproduced , while validity means that you’re actually measuring the concept you’re interested in.
For valid and reliable results, your measurement materials should be thoroughly researched and carefully designed. Plan your procedures to make sure you carry out the same steps in the same way for each participant.
If you’re developing a new questionnaire or other instrument to measure a specific concept, running a pilot study allows you to check its validity and reliability in advance.
Sampling procedures
As well as choosing an appropriate sampling method, you need a concrete plan for how you’ll actually contact and recruit your selected sample.
That means making decisions about things like:
- How many participants do you need for an adequate sample size?
- What inclusion and exclusion criteria will you use to identify eligible participants?
- How will you contact your sample – by mail, online, by phone, or in person?
If you’re using a probability sampling method, it’s important that everyone who is randomly selected actually participates in the study. How will you ensure a high response rate?
If you’re using a non-probability method, how will you avoid bias and ensure a representative sample?
Data management
It’s also important to create a data management plan for organising and storing your data.
Will you need to transcribe interviews or perform data entry for observations? You should anonymise and safeguard any sensitive data, and make sure it’s backed up regularly.
Keeping your data well organised will save time when it comes to analysing them. It can also help other researchers validate and add to your findings.
On their own, raw data can’t answer your research question. The last step of designing your research is planning how you’ll analyse the data.
Quantitative data analysis
In quantitative research, you’ll most likely use some form of statistical analysis . With statistics, you can summarise your sample data, make estimates, and test hypotheses.
Using descriptive statistics , you can summarise your sample data in terms of:
- The distribution of the data (e.g., the frequency of each score on a test)
- The central tendency of the data (e.g., the mean to describe the average score)
- The variability of the data (e.g., the standard deviation to describe how spread out the scores are)
The specific calculations you can do depend on the level of measurement of your variables.
Using inferential statistics , you can:
- Make estimates about the population based on your sample data.
- Test hypotheses about a relationship between variables.
Regression and correlation tests look for associations between two or more variables, while comparison tests (such as t tests and ANOVAs ) look for differences in the outcomes of different groups.
Your choice of statistical test depends on various aspects of your research design, including the types of variables you’re dealing with and the distribution of your data.
Qualitative data analysis
In qualitative research, your data will usually be very dense with information and ideas. Instead of summing it up in numbers, you’ll need to comb through the data in detail, interpret its meanings, identify patterns, and extract the parts that are most relevant to your research question.
Two of the most common approaches to doing this are thematic analysis and discourse analysis .
There are many other ways of analysing qualitative data depending on the aims of your research. To get a sense of potential approaches, try reading some qualitative research papers in your field.
A sample is a subset of individuals from a larger population. Sampling means selecting the group that you will actually collect data from in your research.
For example, if you are researching the opinions of students in your university, you could survey a sample of 100 students.
Statistical sampling allows you to test a hypothesis about the characteristics of a population. There are various sampling methods you can use to ensure that your sample is representative of the population as a whole.
Operationalisation means turning abstract conceptual ideas into measurable observations.
For example, the concept of social anxiety isn’t directly observable, but it can be operationally defined in terms of self-rating scores, behavioural avoidance of crowded places, or physical anxiety symptoms in social situations.
Before collecting data , it’s important to consider how you will operationalise the variables that you want to measure.
The research methods you use depend on the type of data you need to answer your research question .
- If you want to measure something or test a hypothesis , use quantitative methods . If you want to explore ideas, thoughts, and meanings, use qualitative methods .
- If you want to analyse a large amount of readily available data, use secondary data. If you want data specific to your purposes with control over how they are generated, collect primary data.
- If you want to establish cause-and-effect relationships between variables , use experimental methods. If you want to understand the characteristics of a research subject, use descriptive methods.
Cite this Scribbr article
If you want to cite this source, you can copy and paste the citation or click the ‘Cite this Scribbr article’ button to automatically add the citation to our free Reference Generator.
McCombes, S. (2023, March 20). Research Design | Step-by-Step Guide with Examples. Scribbr. Retrieved 21 October 2024, from https://www.scribbr.co.uk/research-methods/research-design/
Is this article helpful?
Shona McCombes
- USC Libraries
- Research Guides
Organizing Your Social Sciences Research Paper
- Types of Research Designs
- Purpose of Guide
- Design Flaws to Avoid
- Independent and Dependent Variables
- Glossary of Research Terms
- Reading Research Effectively
- Narrowing a Topic Idea
- Broadening a Topic Idea
- Extending the Timeliness of a Topic Idea
- Academic Writing Style
- Applying Critical Thinking
- Choosing a Title
- Making an Outline
- Paragraph Development
- Research Process Video Series
- Executive Summary
- The C.A.R.S. Model
- Background Information
- The Research Problem/Question
- Theoretical Framework
- Citation Tracking
- Content Alert Services
- Evaluating Sources
- Primary Sources
- Secondary Sources
- Tiertiary Sources
- Scholarly vs. Popular Publications
- Qualitative Methods
- Quantitative Methods
- Insiderness
- Using Non-Textual Elements
- Limitations of the Study
- Common Grammar Mistakes
- Writing Concisely
- Avoiding Plagiarism
- Footnotes or Endnotes?
- Further Readings
- Generative AI and Writing
- USC Libraries Tutorials and Other Guides
- Bibliography
Introduction
Before beginning your paper, you need to decide how you plan to design the study .
The research design refers to the overall strategy and analytical approach that you have chosen in order to integrate, in a coherent and logical way, the different components of the study, thus ensuring that the research problem will be thoroughly investigated. It constitutes the blueprint for the collection, measurement, and interpretation of information and data. Note that the research problem determines the type of design you choose, not the other way around!
De Vaus, D. A. Research Design in Social Research . London: SAGE, 2001; Trochim, William M.K. Research Methods Knowledge Base. 2006.
General Structure and Writing Style
The function of a research design is to ensure that the evidence obtained enables you to effectively address the research problem logically and as unambiguously as possible . In social sciences research, obtaining information relevant to the research problem generally entails specifying the type of evidence needed to test the underlying assumptions of a theory, to evaluate a program, or to accurately describe and assess meaning related to an observable phenomenon.
With this in mind, a common mistake made by researchers is that they begin their investigations before they have thought critically about what information is required to address the research problem. Without attending to these design issues beforehand, the overall research problem will not be adequately addressed and any conclusions drawn will run the risk of being weak and unconvincing. As a consequence, the overall validity of the study will be undermined.
The length and complexity of describing the research design in your paper can vary considerably, but any well-developed description will achieve the following :
- Identify the research problem clearly and justify its selection, particularly in relation to any valid alternative designs that could have been used,
- Review and synthesize previously published literature associated with the research problem,
- Clearly and explicitly specify hypotheses [i.e., research questions] central to the problem,
- Effectively describe the information and/or data which will be necessary for an adequate testing of the hypotheses and explain how such information and/or data will be obtained, and
- Describe the methods of analysis to be applied to the data in determining whether or not the hypotheses are true or false.
The research design is usually incorporated into the introduction of your paper . You can obtain an overall sense of what to do by reviewing studies that have utilized the same research design [e.g., using a case study approach]. This can help you develop an outline to follow for your own paper.
NOTE: Use the SAGE Research Methods Online and Cases and the SAGE Research Methods Videos databases to search for scholarly resources on how to apply specific research designs and methods . The Research Methods Online database contains links to more than 175,000 pages of SAGE publisher's book, journal, and reference content on quantitative, qualitative, and mixed research methodologies. Also included is a collection of case studies of social research projects that can be used to help you better understand abstract or complex methodological concepts. The Research Methods Videos database contains hours of tutorials, interviews, video case studies, and mini-documentaries covering the entire research process.
Creswell, John W. and J. David Creswell. Research Design: Qualitative, Quantitative, and Mixed Methods Approaches . 5th edition. Thousand Oaks, CA: Sage, 2018; De Vaus, D. A. Research Design in Social Research . London: SAGE, 2001; Gorard, Stephen. Research Design: Creating Robust Approaches for the Social Sciences . Thousand Oaks, CA: Sage, 2013; Leedy, Paul D. and Jeanne Ellis Ormrod. Practical Research: Planning and Design . Tenth edition. Boston, MA: Pearson, 2013; Vogt, W. Paul, Dianna C. Gardner, and Lynne M. Haeffele. When to Use What Research Design . New York: Guilford, 2012.
Action Research Design
Definition and Purpose
The essentials of action research design follow a characteristic cycle whereby initially an exploratory stance is adopted, where an understanding of a problem is developed and plans are made for some form of interventionary strategy. Then the intervention is carried out [the "action" in action research] during which time, pertinent observations are collected in various forms. The new interventional strategies are carried out, and this cyclic process repeats, continuing until a sufficient understanding of [or a valid implementation solution for] the problem is achieved. The protocol is iterative or cyclical in nature and is intended to foster deeper understanding of a given situation, starting with conceptualizing and particularizing the problem and moving through several interventions and evaluations.
What do these studies tell you ?
- This is a collaborative and adaptive research design that lends itself to use in work or community situations.
- Design focuses on pragmatic and solution-driven research outcomes rather than testing theories.
- When practitioners use action research, it has the potential to increase the amount they learn consciously from their experience; the action research cycle can be regarded as a learning cycle.
- Action research studies often have direct and obvious relevance to improving practice and advocating for change.
- There are no hidden controls or preemption of direction by the researcher.
What these studies don't tell you ?
- It is harder to do than conducting conventional research because the researcher takes on responsibilities of advocating for change as well as for researching the topic.
- Action research is much harder to write up because it is less likely that you can use a standard format to report your findings effectively [i.e., data is often in the form of stories or observation].
- Personal over-involvement of the researcher may bias research results.
- The cyclic nature of action research to achieve its twin outcomes of action [e.g. change] and research [e.g. understanding] is time-consuming and complex to conduct.
- Advocating for change usually requires buy-in from study participants.
Coghlan, David and Mary Brydon-Miller. The Sage Encyclopedia of Action Research . Thousand Oaks, CA: Sage, 2014; Efron, Sara Efrat and Ruth Ravid. Action Research in Education: A Practical Guide . New York: Guilford, 2013; Gall, Meredith. Educational Research: An Introduction . Chapter 18, Action Research. 8th ed. Boston, MA: Pearson/Allyn and Bacon, 2007; Gorard, Stephen. Research Design: Creating Robust Approaches for the Social Sciences . Thousand Oaks, CA: Sage, 2013; Kemmis, Stephen and Robin McTaggart. “Participatory Action Research.” In Handbook of Qualitative Research . Norman Denzin and Yvonna S. Lincoln, eds. 2nd ed. (Thousand Oaks, CA: SAGE, 2000), pp. 567-605; McNiff, Jean. Writing and Doing Action Research . London: Sage, 2014; Reason, Peter and Hilary Bradbury. Handbook of Action Research: Participative Inquiry and Practice . Thousand Oaks, CA: SAGE, 2001.
Case Study Design
A case study is an in-depth study of a particular research problem rather than a sweeping statistical survey or comprehensive comparative inquiry. It is often used to narrow down a very broad field of research into one or a few easily researchable examples. The case study research design is also useful for testing whether a specific theory and model actually applies to phenomena in the real world. It is a useful design when not much is known about an issue or phenomenon.
- Approach excels at bringing us to an understanding of a complex issue through detailed contextual analysis of a limited number of events or conditions and their relationships.
- A researcher using a case study design can apply a variety of methodologies and rely on a variety of sources to investigate a research problem.
- Design can extend experience or add strength to what is already known through previous research.
- Social scientists, in particular, make wide use of this research design to examine contemporary real-life situations and provide the basis for the application of concepts and theories and the extension of methodologies.
- The design can provide detailed descriptions of specific and rare cases.
- A single or small number of cases offers little basis for establishing reliability or to generalize the findings to a wider population of people, places, or things.
- Intense exposure to the study of a case may bias a researcher's interpretation of the findings.
- Design does not facilitate assessment of cause and effect relationships.
- Vital information may be missing, making the case hard to interpret.
- The case may not be representative or typical of the larger problem being investigated.
- If the criteria for selecting a case is because it represents a very unusual or unique phenomenon or problem for study, then your interpretation of the findings can only apply to that particular case.
Case Studies. Writing@CSU. Colorado State University; Anastas, Jeane W. Research Design for Social Work and the Human Services . Chapter 4, Flexible Methods: Case Study Design. 2nd ed. New York: Columbia University Press, 1999; Gerring, John. “What Is a Case Study and What Is It Good for?” American Political Science Review 98 (May 2004): 341-354; Greenhalgh, Trisha, editor. Case Study Evaluation: Past, Present and Future Challenges . Bingley, UK: Emerald Group Publishing, 2015; Mills, Albert J. , Gabrielle Durepos, and Eiden Wiebe, editors. Encyclopedia of Case Study Research . Thousand Oaks, CA: SAGE Publications, 2010; Stake, Robert E. The Art of Case Study Research . Thousand Oaks, CA: SAGE, 1995; Yin, Robert K. Case Study Research: Design and Theory . Applied Social Research Methods Series, no. 5. 3rd ed. Thousand Oaks, CA: SAGE, 2003.
Causal Design
Causality studies may be thought of as understanding a phenomenon in terms of conditional statements in the form, “If X, then Y.” This type of research is used to measure what impact a specific change will have on existing norms and assumptions. Most social scientists seek causal explanations that reflect tests of hypotheses. Causal effect (nomothetic perspective) occurs when variation in one phenomenon, an independent variable, leads to or results, on average, in variation in another phenomenon, the dependent variable.
Conditions necessary for determining causality:
- Empirical association -- a valid conclusion is based on finding an association between the independent variable and the dependent variable.
- Appropriate time order -- to conclude that causation was involved, one must see that cases were exposed to variation in the independent variable before variation in the dependent variable.
- Nonspuriousness -- a relationship between two variables that is not due to variation in a third variable.
- Causality research designs assist researchers in understanding why the world works the way it does through the process of proving a causal link between variables and by the process of eliminating other possibilities.
- Replication is possible.
- There is greater confidence the study has internal validity due to the systematic subject selection and equity of groups being compared.
- Not all relationships are causal! The possibility always exists that, by sheer coincidence, two unrelated events appear to be related [e.g., Punxatawney Phil could accurately predict the duration of Winter for five consecutive years but, the fact remains, he's just a big, furry rodent].
- Conclusions about causal relationships are difficult to determine due to a variety of extraneous and confounding variables that exist in a social environment. This means causality can only be inferred, never proven.
- If two variables are correlated, the cause must come before the effect. However, even though two variables might be causally related, it can sometimes be difficult to determine which variable comes first and, therefore, to establish which variable is the actual cause and which is the actual effect.
Beach, Derek and Rasmus Brun Pedersen. Causal Case Study Methods: Foundations and Guidelines for Comparing, Matching, and Tracing . Ann Arbor, MI: University of Michigan Press, 2016; Bachman, Ronet. The Practice of Research in Criminology and Criminal Justice . Chapter 5, Causation and Research Designs. 3rd ed. Thousand Oaks, CA: Pine Forge Press, 2007; Brewer, Ernest W. and Jennifer Kubn. “Causal-Comparative Design.” In Encyclopedia of Research Design . Neil J. Salkind, editor. (Thousand Oaks, CA: Sage, 2010), pp. 125-132; Causal Research Design: Experimentation. Anonymous SlideShare Presentation; Gall, Meredith. Educational Research: An Introduction . Chapter 11, Nonexperimental Research: Correlational Designs. 8th ed. Boston, MA: Pearson/Allyn and Bacon, 2007; Trochim, William M.K. Research Methods Knowledge Base. 2006.
Cohort Design
Often used in the medical sciences, but also found in the applied social sciences, a cohort study generally refers to a study conducted over a period of time involving members of a population which the subject or representative member comes from, and who are united by some commonality or similarity. Using a quantitative framework, a cohort study makes note of statistical occurrence within a specialized subgroup, united by same or similar characteristics that are relevant to the research problem being investigated, rather than studying statistical occurrence within the general population. Using a qualitative framework, cohort studies generally gather data using methods of observation. Cohorts can be either "open" or "closed."
- Open Cohort Studies [dynamic populations, such as the population of Los Angeles] involve a population that is defined just by the state of being a part of the study in question (and being monitored for the outcome). Date of entry and exit from the study is individually defined, therefore, the size of the study population is not constant. In open cohort studies, researchers can only calculate rate based data, such as, incidence rates and variants thereof.
- Closed Cohort Studies [static populations, such as patients entered into a clinical trial] involve participants who enter into the study at one defining point in time and where it is presumed that no new participants can enter the cohort. Given this, the number of study participants remains constant (or can only decrease).
- The use of cohorts is often mandatory because a randomized control study may be unethical. For example, you cannot deliberately expose people to asbestos, you can only study its effects on those who have already been exposed. Research that measures risk factors often relies upon cohort designs.
- Because cohort studies measure potential causes before the outcome has occurred, they can demonstrate that these “causes” preceded the outcome, thereby avoiding the debate as to which is the cause and which is the effect.
- Cohort analysis is highly flexible and can provide insight into effects over time and related to a variety of different types of changes [e.g., social, cultural, political, economic, etc.].
- Either original data or secondary data can be used in this design.
- In cases where a comparative analysis of two cohorts is made [e.g., studying the effects of one group exposed to asbestos and one that has not], a researcher cannot control for all other factors that might differ between the two groups. These factors are known as confounding variables.
- Cohort studies can end up taking a long time to complete if the researcher must wait for the conditions of interest to develop within the group. This also increases the chance that key variables change during the course of the study, potentially impacting the validity of the findings.
- Due to the lack of randominization in the cohort design, its external validity is lower than that of study designs where the researcher randomly assigns participants.
Healy P, Devane D. “Methodological Considerations in Cohort Study Designs.” Nurse Researcher 18 (2011): 32-36; Glenn, Norval D, editor. Cohort Analysis . 2nd edition. Thousand Oaks, CA: Sage, 2005; Levin, Kate Ann. Study Design IV: Cohort Studies. Evidence-Based Dentistry 7 (2003): 51–52; Payne, Geoff. “Cohort Study.” In The SAGE Dictionary of Social Research Methods . Victor Jupp, editor. (Thousand Oaks, CA: Sage, 2006), pp. 31-33; Study Design 101. Himmelfarb Health Sciences Library. George Washington University, November 2011; Cohort Study. Wikipedia.
Cross-Sectional Design
Cross-sectional research designs have three distinctive features: no time dimension; a reliance on existing differences rather than change following intervention; and, groups are selected based on existing differences rather than random allocation. The cross-sectional design can only measure differences between or from among a variety of people, subjects, or phenomena rather than a process of change. As such, researchers using this design can only employ a relatively passive approach to making causal inferences based on findings.
- Cross-sectional studies provide a clear 'snapshot' of the outcome and the characteristics associated with it, at a specific point in time.
- Unlike an experimental design, where there is an active intervention by the researcher to produce and measure change or to create differences, cross-sectional designs focus on studying and drawing inferences from existing differences between people, subjects, or phenomena.
- Entails collecting data at and concerning one point in time. While longitudinal studies involve taking multiple measures over an extended period of time, cross-sectional research is focused on finding relationships between variables at one moment in time.
- Groups identified for study are purposely selected based upon existing differences in the sample rather than seeking random sampling.
- Cross-section studies are capable of using data from a large number of subjects and, unlike observational studies, is not geographically bound.
- Can estimate prevalence of an outcome of interest because the sample is usually taken from the whole population.
- Because cross-sectional designs generally use survey techniques to gather data, they are relatively inexpensive and take up little time to conduct.
- Finding people, subjects, or phenomena to study that are very similar except in one specific variable can be difficult.
- Results are static and time bound and, therefore, give no indication of a sequence of events or reveal historical or temporal contexts.
- Studies cannot be utilized to establish cause and effect relationships.
- This design only provides a snapshot of analysis so there is always the possibility that a study could have differing results if another time-frame had been chosen.
- There is no follow up to the findings.
Bethlehem, Jelke. "7: Cross-sectional Research." In Research Methodology in the Social, Behavioural and Life Sciences . Herman J Adèr and Gideon J Mellenbergh, editors. (London, England: Sage, 1999), pp. 110-43; Bourque, Linda B. “Cross-Sectional Design.” In The SAGE Encyclopedia of Social Science Research Methods . Michael S. Lewis-Beck, Alan Bryman, and Tim Futing Liao. (Thousand Oaks, CA: 2004), pp. 230-231; Hall, John. “Cross-Sectional Survey Design.” In Encyclopedia of Survey Research Methods . Paul J. Lavrakas, ed. (Thousand Oaks, CA: Sage, 2008), pp. 173-174; Helen Barratt, Maria Kirwan. Cross-Sectional Studies: Design Application, Strengths and Weaknesses of Cross-Sectional Studies. Healthknowledge, 2009. Cross-Sectional Study. Wikipedia.
Descriptive Design
Descriptive research designs help provide answers to the questions of who, what, when, where, and how associated with a particular research problem; a descriptive study cannot conclusively ascertain answers to why. Descriptive research is used to obtain information concerning the current status of the phenomena and to describe "what exists" with respect to variables or conditions in a situation.
- The subject is being observed in a completely natural and unchanged natural environment. True experiments, whilst giving analyzable data, often adversely influence the normal behavior of the subject [a.k.a., the Heisenberg effect whereby measurements of certain systems cannot be made without affecting the systems].
- Descriptive research is often used as a pre-cursor to more quantitative research designs with the general overview giving some valuable pointers as to what variables are worth testing quantitatively.
- If the limitations are understood, they can be a useful tool in developing a more focused study.
- Descriptive studies can yield rich data that lead to important recommendations in practice.
- Appoach collects a large amount of data for detailed analysis.
- The results from a descriptive research cannot be used to discover a definitive answer or to disprove a hypothesis.
- Because descriptive designs often utilize observational methods [as opposed to quantitative methods], the results cannot be replicated.
- The descriptive function of research is heavily dependent on instrumentation for measurement and observation.
Anastas, Jeane W. Research Design for Social Work and the Human Services . Chapter 5, Flexible Methods: Descriptive Research. 2nd ed. New York: Columbia University Press, 1999; Given, Lisa M. "Descriptive Research." In Encyclopedia of Measurement and Statistics . Neil J. Salkind and Kristin Rasmussen, editors. (Thousand Oaks, CA: Sage, 2007), pp. 251-254; McNabb, Connie. Descriptive Research Methodologies. Powerpoint Presentation; Shuttleworth, Martyn. Descriptive Research Design, September 26, 2008; Erickson, G. Scott. "Descriptive Research Design." In New Methods of Market Research and Analysis . (Northampton, MA: Edward Elgar Publishing, 2017), pp. 51-77; Sahin, Sagufta, and Jayanta Mete. "A Brief Study on Descriptive Research: Its Nature and Application in Social Science." International Journal of Research and Analysis in Humanities 1 (2021): 11; K. Swatzell and P. Jennings. “Descriptive Research: The Nuts and Bolts.” Journal of the American Academy of Physician Assistants 20 (2007), pp. 55-56; Kane, E. Doing Your Own Research: Basic Descriptive Research in the Social Sciences and Humanities . London: Marion Boyars, 1985.
Experimental Design
A blueprint of the procedure that enables the researcher to maintain control over all factors that may affect the result of an experiment. In doing this, the researcher attempts to determine or predict what may occur. Experimental research is often used where there is time priority in a causal relationship (cause precedes effect), there is consistency in a causal relationship (a cause will always lead to the same effect), and the magnitude of the correlation is great. The classic experimental design specifies an experimental group and a control group. The independent variable is administered to the experimental group and not to the control group, and both groups are measured on the same dependent variable. Subsequent experimental designs have used more groups and more measurements over longer periods. True experiments must have control, randomization, and manipulation.
- Experimental research allows the researcher to control the situation. In so doing, it allows researchers to answer the question, “What causes something to occur?”
- Permits the researcher to identify cause and effect relationships between variables and to distinguish placebo effects from treatment effects.
- Experimental research designs support the ability to limit alternative explanations and to infer direct causal relationships in the study.
- Approach provides the highest level of evidence for single studies.
- The design is artificial, and results may not generalize well to the real world.
- The artificial settings of experiments may alter the behaviors or responses of participants.
- Experimental designs can be costly if special equipment or facilities are needed.
- Some research problems cannot be studied using an experiment because of ethical or technical reasons.
- Difficult to apply ethnographic and other qualitative methods to experimentally designed studies.
Anastas, Jeane W. Research Design for Social Work and the Human Services . Chapter 7, Flexible Methods: Experimental Research. 2nd ed. New York: Columbia University Press, 1999; Chapter 2: Research Design, Experimental Designs. School of Psychology, University of New England, 2000; Chow, Siu L. "Experimental Design." In Encyclopedia of Research Design . Neil J. Salkind, editor. (Thousand Oaks, CA: Sage, 2010), pp. 448-453; "Experimental Design." In Social Research Methods . Nicholas Walliman, editor. (London, England: Sage, 2006), pp, 101-110; Experimental Research. Research Methods by Dummies. Department of Psychology. California State University, Fresno, 2006; Kirk, Roger E. Experimental Design: Procedures for the Behavioral Sciences . 4th edition. Thousand Oaks, CA: Sage, 2013; Trochim, William M.K. Experimental Design. Research Methods Knowledge Base. 2006; Rasool, Shafqat. Experimental Research. Slideshare presentation.
Exploratory Design
An exploratory design is conducted about a research problem when there are few or no earlier studies to refer to or rely upon to predict an outcome . The focus is on gaining insights and familiarity for later investigation or undertaken when research problems are in a preliminary stage of investigation. Exploratory designs are often used to establish an understanding of how best to proceed in studying an issue or what methodology would effectively apply to gathering information about the issue.
The goals of exploratory research are intended to produce the following possible insights:
- Familiarity with basic details, settings, and concerns.
- Well grounded picture of the situation being developed.
- Generation of new ideas and assumptions.
- Development of tentative theories or hypotheses.
- Determination about whether a study is feasible in the future.
- Issues get refined for more systematic investigation and formulation of new research questions.
- Direction for future research and techniques get developed.
- Design is a useful approach for gaining background information on a particular topic.
- Exploratory research is flexible and can address research questions of all types (what, why, how).
- Provides an opportunity to define new terms and clarify existing concepts.
- Exploratory research is often used to generate formal hypotheses and develop more precise research problems.
- In the policy arena or applied to practice, exploratory studies help establish research priorities and where resources should be allocated.
- Exploratory research generally utilizes small sample sizes and, thus, findings are typically not generalizable to the population at large.
- The exploratory nature of the research inhibits an ability to make definitive conclusions about the findings. They provide insight but not definitive conclusions.
- The research process underpinning exploratory studies is flexible but often unstructured, leading to only tentative results that have limited value to decision-makers.
- Design lacks rigorous standards applied to methods of data gathering and analysis because one of the areas for exploration could be to determine what method or methodologies could best fit the research problem.
Cuthill, Michael. “Exploratory Research: Citizen Participation, Local Government, and Sustainable Development in Australia.” Sustainable Development 10 (2002): 79-89; Streb, Christoph K. "Exploratory Case Study." In Encyclopedia of Case Study Research . Albert J. Mills, Gabrielle Durepos and Eiden Wiebe, editors. (Thousand Oaks, CA: Sage, 2010), pp. 372-374; Taylor, P. J., G. Catalano, and D.R.F. Walker. “Exploratory Analysis of the World City Network.” Urban Studies 39 (December 2002): 2377-2394; Exploratory Research. Wikipedia.
Field Research Design
Sometimes referred to as ethnography or participant observation, designs around field research encompass a variety of interpretative procedures [e.g., observation and interviews] rooted in qualitative approaches to studying people individually or in groups while inhabiting their natural environment as opposed to using survey instruments or other forms of impersonal methods of data gathering. Information acquired from observational research takes the form of “ field notes ” that involves documenting what the researcher actually sees and hears while in the field. Findings do not consist of conclusive statements derived from numbers and statistics because field research involves analysis of words and observations of behavior. Conclusions, therefore, are developed from an interpretation of findings that reveal overriding themes, concepts, and ideas. More information can be found HERE .
- Field research is often necessary to fill gaps in understanding the research problem applied to local conditions or to specific groups of people that cannot be ascertained from existing data.
- The research helps contextualize already known information about a research problem, thereby facilitating ways to assess the origins, scope, and scale of a problem and to gage the causes, consequences, and means to resolve an issue based on deliberate interaction with people in their natural inhabited spaces.
- Enables the researcher to corroborate or confirm data by gathering additional information that supports or refutes findings reported in prior studies of the topic.
- Because the researcher in embedded in the field, they are better able to make observations or ask questions that reflect the specific cultural context of the setting being investigated.
- Observing the local reality offers the opportunity to gain new perspectives or obtain unique data that challenges existing theoretical propositions or long-standing assumptions found in the literature.
What these studies don't tell you
- A field research study requires extensive time and resources to carry out the multiple steps involved with preparing for the gathering of information, including for example, examining background information about the study site, obtaining permission to access the study site, and building trust and rapport with subjects.
- Requires a commitment to staying engaged in the field to ensure that you can adequately document events and behaviors as they unfold.
- The unpredictable nature of fieldwork means that researchers can never fully control the process of data gathering. They must maintain a flexible approach to studying the setting because events and circumstances can change quickly or unexpectedly.
- Findings can be difficult to interpret and verify without access to documents and other source materials that help to enhance the credibility of information obtained from the field [i.e., the act of triangulating the data].
- Linking the research problem to the selection of study participants inhabiting their natural environment is critical. However, this specificity limits the ability to generalize findings to different situations or in other contexts or to infer courses of action applied to other settings or groups of people.
- The reporting of findings must take into account how the researcher themselves may have inadvertently affected respondents and their behaviors.
Historical Design
The purpose of a historical research design is to collect, verify, and synthesize evidence from the past to establish facts that defend or refute a hypothesis. It uses secondary sources and a variety of primary documentary evidence, such as, diaries, official records, reports, archives, and non-textual information [maps, pictures, audio and visual recordings]. The limitation is that the sources must be both authentic and valid.
- The historical research design is unobtrusive; the act of research does not affect the results of the study.
- The historical approach is well suited for trend analysis.
- Historical records can add important contextual background required to more fully understand and interpret a research problem.
- There is often no possibility of researcher-subject interaction that could affect the findings.
- Historical sources can be used over and over to study different research problems or to replicate a previous study.
- The ability to fulfill the aims of your research are directly related to the amount and quality of documentation available to understand the research problem.
- Since historical research relies on data from the past, there is no way to manipulate it to control for contemporary contexts.
- Interpreting historical sources can be very time consuming.
- The sources of historical materials must be archived consistently to ensure access. This may especially challenging for digital or online-only sources.
- Original authors bring their own perspectives and biases to the interpretation of past events and these biases are more difficult to ascertain in historical resources.
- Due to the lack of control over external variables, historical research is very weak with regard to the demands of internal validity.
- It is rare that the entirety of historical documentation needed to fully address a research problem is available for interpretation, therefore, gaps need to be acknowledged.
Howell, Martha C. and Walter Prevenier. From Reliable Sources: An Introduction to Historical Methods . Ithaca, NY: Cornell University Press, 2001; Lundy, Karen Saucier. "Historical Research." In The Sage Encyclopedia of Qualitative Research Methods . Lisa M. Given, editor. (Thousand Oaks, CA: Sage, 2008), pp. 396-400; Marius, Richard. and Melvin E. Page. A Short Guide to Writing about History . 9th edition. Boston, MA: Pearson, 2015; Savitt, Ronald. “Historical Research in Marketing.” Journal of Marketing 44 (Autumn, 1980): 52-58; Gall, Meredith. Educational Research: An Introduction . Chapter 16, Historical Research. 8th ed. Boston, MA: Pearson/Allyn and Bacon, 2007.
Longitudinal Design
A longitudinal study follows the same sample over time and makes repeated observations. For example, with longitudinal surveys, the same group of people is interviewed at regular intervals, enabling researchers to track changes over time and to relate them to variables that might explain why the changes occur. Longitudinal research designs describe patterns of change and help establish the direction and magnitude of causal relationships. Measurements are taken on each variable over two or more distinct time periods. This allows the researcher to measure change in variables over time. It is a type of observational study sometimes referred to as a panel study.
- Longitudinal data facilitate the analysis of the duration of a particular phenomenon.
- Enables survey researchers to get close to the kinds of causal explanations usually attainable only with experiments.
- The design permits the measurement of differences or change in a variable from one period to another [i.e., the description of patterns of change over time].
- Longitudinal studies facilitate the prediction of future outcomes based upon earlier factors.
- The data collection method may change over time.
- Maintaining the integrity of the original sample can be difficult over an extended period of time.
- It can be difficult to show more than one variable at a time.
- This design often needs qualitative research data to explain fluctuations in the results.
- A longitudinal research design assumes present trends will continue unchanged.
- It can take a long period of time to gather results.
- There is a need to have a large sample size and accurate sampling to reach representativness.
Anastas, Jeane W. Research Design for Social Work and the Human Services . Chapter 6, Flexible Methods: Relational and Longitudinal Research. 2nd ed. New York: Columbia University Press, 1999; Forgues, Bernard, and Isabelle Vandangeon-Derumez. "Longitudinal Analyses." In Doing Management Research . Raymond-Alain Thiétart and Samantha Wauchope, editors. (London, England: Sage, 2001), pp. 332-351; Kalaian, Sema A. and Rafa M. Kasim. "Longitudinal Studies." In Encyclopedia of Survey Research Methods . Paul J. Lavrakas, ed. (Thousand Oaks, CA: Sage, 2008), pp. 440-441; Menard, Scott, editor. Longitudinal Research . Thousand Oaks, CA: Sage, 2002; Ployhart, Robert E. and Robert J. Vandenberg. "Longitudinal Research: The Theory, Design, and Analysis of Change.” Journal of Management 36 (January 2010): 94-120; Longitudinal Study. Wikipedia.
Meta-Analysis Design
Meta-analysis is an analytical methodology designed to systematically evaluate and summarize the results from a number of individual studies, thereby, increasing the overall sample size and the ability of the researcher to study effects of interest. The purpose is to not simply summarize existing knowledge, but to develop a new understanding of a research problem using synoptic reasoning. The main objectives of meta-analysis include analyzing differences in the results among studies and increasing the precision by which effects are estimated. A well-designed meta-analysis depends upon strict adherence to the criteria used for selecting studies and the availability of information in each study to properly analyze their findings. Lack of information can severely limit the type of analyzes and conclusions that can be reached. In addition, the more dissimilarity there is in the results among individual studies [heterogeneity], the more difficult it is to justify interpretations that govern a valid synopsis of results. A meta-analysis needs to fulfill the following requirements to ensure the validity of your findings:
- Clearly defined description of objectives, including precise definitions of the variables and outcomes that are being evaluated;
- A well-reasoned and well-documented justification for identification and selection of the studies;
- Assessment and explicit acknowledgment of any researcher bias in the identification and selection of those studies;
- Description and evaluation of the degree of heterogeneity among the sample size of studies reviewed; and,
- Justification of the techniques used to evaluate the studies.
- Can be an effective strategy for determining gaps in the literature.
- Provides a means of reviewing research published about a particular topic over an extended period of time and from a variety of sources.
- Is useful in clarifying what policy or programmatic actions can be justified on the basis of analyzing research results from multiple studies.
- Provides a method for overcoming small sample sizes in individual studies that previously may have had little relationship to each other.
- Can be used to generate new hypotheses or highlight research problems for future studies.
- Small violations in defining the criteria used for content analysis can lead to difficult to interpret and/or meaningless findings.
- A large sample size can yield reliable, but not necessarily valid, results.
- A lack of uniformity regarding, for example, the type of literature reviewed, how methods are applied, and how findings are measured within the sample of studies you are analyzing, can make the process of synthesis difficult to perform.
- Depending on the sample size, the process of reviewing and synthesizing multiple studies can be very time consuming.
Beck, Lewis W. "The Synoptic Method." The Journal of Philosophy 36 (1939): 337-345; Cooper, Harris, Larry V. Hedges, and Jeffrey C. Valentine, eds. The Handbook of Research Synthesis and Meta-Analysis . 2nd edition. New York: Russell Sage Foundation, 2009; Guzzo, Richard A., Susan E. Jackson and Raymond A. Katzell. “Meta-Analysis Analysis.” In Research in Organizational Behavior , Volume 9. (Greenwich, CT: JAI Press, 1987), pp 407-442; Lipsey, Mark W. and David B. Wilson. Practical Meta-Analysis . Thousand Oaks, CA: Sage Publications, 2001; Study Design 101. Meta-Analysis. The Himmelfarb Health Sciences Library, George Washington University; Timulak, Ladislav. “Qualitative Meta-Analysis.” In The SAGE Handbook of Qualitative Data Analysis . Uwe Flick, editor. (Los Angeles, CA: Sage, 2013), pp. 481-495; Walker, Esteban, Adrian V. Hernandez, and Micheal W. Kattan. "Meta-Analysis: It's Strengths and Limitations." Cleveland Clinic Journal of Medicine 75 (June 2008): 431-439.
Mixed-Method Design
- Narrative and non-textual information can add meaning to numeric data, while numeric data can add precision to narrative and non-textual information.
- Can utilize existing data while at the same time generating and testing a grounded theory approach to describe and explain the phenomenon under study.
- A broader, more complex research problem can be investigated because the researcher is not constrained by using only one method.
- The strengths of one method can be used to overcome the inherent weaknesses of another method.
- Can provide stronger, more robust evidence to support a conclusion or set of recommendations.
- May generate new knowledge new insights or uncover hidden insights, patterns, or relationships that a single methodological approach might not reveal.
- Produces more complete knowledge and understanding of the research problem that can be used to increase the generalizability of findings applied to theory or practice.
- A researcher must be proficient in understanding how to apply multiple methods to investigating a research problem as well as be proficient in optimizing how to design a study that coherently melds them together.
- Can increase the likelihood of conflicting results or ambiguous findings that inhibit drawing a valid conclusion or setting forth a recommended course of action [e.g., sample interview responses do not support existing statistical data].
- Because the research design can be very complex, reporting the findings requires a well-organized narrative, clear writing style, and precise word choice.
- Design invites collaboration among experts. However, merging different investigative approaches and writing styles requires more attention to the overall research process than studies conducted using only one methodological paradigm.
- Concurrent merging of quantitative and qualitative research requires greater attention to having adequate sample sizes, using comparable samples, and applying a consistent unit of analysis. For sequential designs where one phase of qualitative research builds on the quantitative phase or vice versa, decisions about what results from the first phase to use in the next phase, the choice of samples and estimating reasonable sample sizes for both phases, and the interpretation of results from both phases can be difficult.
- Due to multiple forms of data being collected and analyzed, this design requires extensive time and resources to carry out the multiple steps involved in data gathering and interpretation.
Burch, Patricia and Carolyn J. Heinrich. Mixed Methods for Policy Research and Program Evaluation . Thousand Oaks, CA: Sage, 2016; Creswell, John w. et al. Best Practices for Mixed Methods Research in the Health Sciences . Bethesda, MD: Office of Behavioral and Social Sciences Research, National Institutes of Health, 2010Creswell, John W. Research Design: Qualitative, Quantitative, and Mixed Methods Approaches . 4th edition. Thousand Oaks, CA: Sage Publications, 2014; Domínguez, Silvia, editor. Mixed Methods Social Networks Research . Cambridge, UK: Cambridge University Press, 2014; Hesse-Biber, Sharlene Nagy. Mixed Methods Research: Merging Theory with Practice . New York: Guilford Press, 2010; Niglas, Katrin. “How the Novice Researcher Can Make Sense of Mixed Methods Designs.” International Journal of Multiple Research Approaches 3 (2009): 34-46; Onwuegbuzie, Anthony J. and Nancy L. Leech. “Linking Research Questions to Mixed Methods Data Analysis Procedures.” The Qualitative Report 11 (September 2006): 474-498; Tashakorri, Abbas and John W. Creswell. “The New Era of Mixed Methods.” Journal of Mixed Methods Research 1 (January 2007): 3-7; Zhanga, Wanqing. “Mixed Methods Application in Health Intervention Research: A Multiple Case Study.” International Journal of Multiple Research Approaches 8 (2014): 24-35 .
Observational Design
This type of research design draws a conclusion by comparing subjects against a control group, in cases where the researcher has no control over the experiment. There are two general types of observational designs. In direct observations, people know that you are watching them. Unobtrusive measures involve any method for studying behavior where individuals do not know they are being observed. An observational study allows a useful insight into a phenomenon and avoids the ethical and practical difficulties of setting up a large and cumbersome research project.
- Observational studies are usually flexible and do not necessarily need to be structured around a hypothesis about what you expect to observe [data is emergent rather than pre-existing].
- The researcher is able to collect in-depth information about a particular behavior.
- Can reveal interrelationships among multifaceted dimensions of group interactions.
- You can generalize your results to real life situations.
- Observational research is useful for discovering what variables may be important before applying other methods like experiments.
- Observation research designs account for the complexity of group behaviors.
- Reliability of data is low because seeing behaviors occur over and over again may be a time consuming task and are difficult to replicate.
- In observational research, findings may only reflect a unique sample population and, thus, cannot be generalized to other groups.
- There can be problems with bias as the researcher may only "see what they want to see."
- There is no possibility to determine "cause and effect" relationships since nothing is manipulated.
- Sources or subjects may not all be equally credible.
- Any group that is knowingly studied is altered to some degree by the presence of the researcher, therefore, potentially skewing any data collected.
Atkinson, Paul and Martyn Hammersley. “Ethnography and Participant Observation.” In Handbook of Qualitative Research . Norman K. Denzin and Yvonna S. Lincoln, eds. (Thousand Oaks, CA: Sage, 1994), pp. 248-261; Observational Research. Research Methods by Dummies. Department of Psychology. California State University, Fresno, 2006; Patton Michael Quinn. Qualitiative Research and Evaluation Methods . Chapter 6, Fieldwork Strategies and Observational Methods. 3rd ed. Thousand Oaks, CA: Sage, 2002; Payne, Geoff and Judy Payne. "Observation." In Key Concepts in Social Research . The SAGE Key Concepts series. (London, England: Sage, 2004), pp. 158-162; Rosenbaum, Paul R. Design of Observational Studies . New York: Springer, 2010;Williams, J. Patrick. "Nonparticipant Observation." In The Sage Encyclopedia of Qualitative Research Methods . Lisa M. Given, editor.(Thousand Oaks, CA: Sage, 2008), pp. 562-563.
Philosophical Design
Understood more as an broad approach to examining a research problem than a methodological design, philosophical analysis and argumentation is intended to challenge deeply embedded, often intractable, assumptions underpinning an area of study. This approach uses the tools of argumentation derived from philosophical traditions, concepts, models, and theories to critically explore and challenge, for example, the relevance of logic and evidence in academic debates, to analyze arguments about fundamental issues, or to discuss the root of existing discourse about a research problem. These overarching tools of analysis can be framed in three ways:
- Ontology -- the study that describes the nature of reality; for example, what is real and what is not, what is fundamental and what is derivative?
- Epistemology -- the study that explores the nature of knowledge; for example, by what means does knowledge and understanding depend upon and how can we be certain of what we know?
- Axiology -- the study of values; for example, what values does an individual or group hold and why? How are values related to interest, desire, will, experience, and means-to-end? And, what is the difference between a matter of fact and a matter of value?
- Can provide a basis for applying ethical decision-making to practice.
- Functions as a means of gaining greater self-understanding and self-knowledge about the purposes of research.
- Brings clarity to general guiding practices and principles of an individual or group.
- Philosophy informs methodology.
- Refine concepts and theories that are invoked in relatively unreflective modes of thought and discourse.
- Beyond methodology, philosophy also informs critical thinking about epistemology and the structure of reality (metaphysics).
- Offers clarity and definition to the practical and theoretical uses of terms, concepts, and ideas.
- Limited application to specific research problems [answering the "So What?" question in social science research].
- Analysis can be abstract, argumentative, and limited in its practical application to real-life issues.
- While a philosophical analysis may render problematic that which was once simple or taken-for-granted, the writing can be dense and subject to unnecessary jargon, overstatement, and/or excessive quotation and documentation.
- There are limitations in the use of metaphor as a vehicle of philosophical analysis.
- There can be analytical difficulties in moving from philosophy to advocacy and between abstract thought and application to the phenomenal world.
Burton, Dawn. "Part I, Philosophy of the Social Sciences." In Research Training for Social Scientists . (London, England: Sage, 2000), pp. 1-5; Chapter 4, Research Methodology and Design. Unisa Institutional Repository (UnisaIR), University of South Africa; Jarvie, Ian C., and Jesús Zamora-Bonilla, editors. The SAGE Handbook of the Philosophy of Social Sciences . London: Sage, 2011; Labaree, Robert V. and Ross Scimeca. “The Philosophical Problem of Truth in Librarianship.” The Library Quarterly 78 (January 2008): 43-70; Maykut, Pamela S. Beginning Qualitative Research: A Philosophic and Practical Guide . Washington, DC: Falmer Press, 1994; McLaughlin, Hugh. "The Philosophy of Social Research." In Understanding Social Work Research . 2nd edition. (London: SAGE Publications Ltd., 2012), pp. 24-47; Stanford Encyclopedia of Philosophy . Metaphysics Research Lab, CSLI, Stanford University, 2013.
Sequential Design
- The researcher has a limitless option when it comes to sample size and the sampling schedule.
- Due to the repetitive nature of this research design, minor changes and adjustments can be done during the initial parts of the study to correct and hone the research method.
- This is a useful design for exploratory studies.
- There is very little effort on the part of the researcher when performing this technique. It is generally not expensive, time consuming, or workforce intensive.
- Because the study is conducted serially, the results of one sample are known before the next sample is taken and analyzed. This provides opportunities for continuous improvement of sampling and methods of analysis.
- The sampling method is not representative of the entire population. The only possibility of approaching representativeness is when the researcher chooses to use a very large sample size significant enough to represent a significant portion of the entire population. In this case, moving on to study a second or more specific sample can be difficult.
- The design cannot be used to create conclusions and interpretations that pertain to an entire population because the sampling technique is not randomized. Generalizability from findings is, therefore, limited.
- Difficult to account for and interpret variation from one sample to another over time, particularly when using qualitative methods of data collection.
Betensky, Rebecca. Harvard University, Course Lecture Note slides; Bovaird, James A. and Kevin A. Kupzyk. "Sequential Design." In Encyclopedia of Research Design . Neil J. Salkind, editor. (Thousand Oaks, CA: Sage, 2010), pp. 1347-1352; Cresswell, John W. Et al. “Advanced Mixed-Methods Research Designs.” In Handbook of Mixed Methods in Social and Behavioral Research . Abbas Tashakkori and Charles Teddle, eds. (Thousand Oaks, CA: Sage, 2003), pp. 209-240; Henry, Gary T. "Sequential Sampling." In The SAGE Encyclopedia of Social Science Research Methods . Michael S. Lewis-Beck, Alan Bryman and Tim Futing Liao, editors. (Thousand Oaks, CA: Sage, 2004), pp. 1027-1028; Nataliya V. Ivankova. “Using Mixed-Methods Sequential Explanatory Design: From Theory to Practice.” Field Methods 18 (February 2006): 3-20; Bovaird, James A. and Kevin A. Kupzyk. “Sequential Design.” In Encyclopedia of Research Design . Neil J. Salkind, ed. Thousand Oaks, CA: Sage, 2010; Sequential Analysis. Wikipedia.
Systematic Review
- A systematic review synthesizes the findings of multiple studies related to each other by incorporating strategies of analysis and interpretation intended to reduce biases and random errors.
- The application of critical exploration, evaluation, and synthesis methods separates insignificant, unsound, or redundant research from the most salient and relevant studies worthy of reflection.
- They can be use to identify, justify, and refine hypotheses, recognize and avoid hidden problems in prior studies, and explain data inconsistencies and conflicts in data.
- Systematic reviews can be used to help policy makers formulate evidence-based guidelines and regulations.
- The use of strict, explicit, and pre-determined methods of synthesis, when applied appropriately, provide reliable estimates about the effects of interventions, evaluations, and effects related to the overarching research problem investigated by each study under review.
- Systematic reviews illuminate where knowledge or thorough understanding of a research problem is lacking and, therefore, can then be used to guide future research.
- The accepted inclusion of unpublished studies [i.e., grey literature] ensures the broadest possible way to analyze and interpret research on a topic.
- Results of the synthesis can be generalized and the findings extrapolated into the general population with more validity than most other types of studies .
- Systematic reviews do not create new knowledge per se; they are a method for synthesizing existing studies about a research problem in order to gain new insights and determine gaps in the literature.
- The way researchers have carried out their investigations [e.g., the period of time covered, number of participants, sources of data analyzed, etc.] can make it difficult to effectively synthesize studies.
- The inclusion of unpublished studies can introduce bias into the review because they may not have undergone a rigorous peer-review process prior to publication. Examples may include conference presentations or proceedings, publications from government agencies, white papers, working papers, and internal documents from organizations, and doctoral dissertations and Master's theses.
Denyer, David and David Tranfield. "Producing a Systematic Review." In The Sage Handbook of Organizational Research Methods . David A. Buchanan and Alan Bryman, editors. ( Thousand Oaks, CA: Sage Publications, 2009), pp. 671-689; Foster, Margaret J. and Sarah T. Jewell, editors. Assembling the Pieces of a Systematic Review: A Guide for Librarians . Lanham, MD: Rowman and Littlefield, 2017; Gough, David, Sandy Oliver, James Thomas, editors. Introduction to Systematic Reviews . 2nd edition. Los Angeles, CA: Sage Publications, 2017; Gopalakrishnan, S. and P. Ganeshkumar. “Systematic Reviews and Meta-analysis: Understanding the Best Evidence in Primary Healthcare.” Journal of Family Medicine and Primary Care 2 (2013): 9-14; Gough, David, James Thomas, and Sandy Oliver. "Clarifying Differences between Review Designs and Methods." Systematic Reviews 1 (2012): 1-9; Khan, Khalid S., Regina Kunz, Jos Kleijnen, and Gerd Antes. “Five Steps to Conducting a Systematic Review.” Journal of the Royal Society of Medicine 96 (2003): 118-121; Mulrow, C. D. “Systematic Reviews: Rationale for Systematic Reviews.” BMJ 309:597 (September 1994); O'Dwyer, Linda C., and Q. Eileen Wafford. "Addressing Challenges with Systematic Review Teams through Effective Communication: A Case Report." Journal of the Medical Library Association 109 (October 2021): 643-647; Okoli, Chitu, and Kira Schabram. "A Guide to Conducting a Systematic Literature Review of Information Systems Research." Sprouts: Working Papers on Information Systems 10 (2010); Siddaway, Andy P., Alex M. Wood, and Larry V. Hedges. "How to Do a Systematic Review: A Best Practice Guide for Conducting and Reporting Narrative Reviews, Meta-analyses, and Meta-syntheses." Annual Review of Psychology 70 (2019): 747-770; Torgerson, Carole J. “Publication Bias: The Achilles’ Heel of Systematic Reviews?” British Journal of Educational Studies 54 (March 2006): 89-102; Torgerson, Carole. Systematic Reviews . New York: Continuum, 2003.
- << Previous: Purpose of Guide
- Next: Design Flaws to Avoid >>
- Last Updated: Oct 24, 2024 10:02 AM
- URL: https://libguides.usc.edu/writingguide
An official website of the United States government
Official websites use .gov A .gov website belongs to an official government organization in the United States.
Secure .gov websites use HTTPS A lock ( Lock Locked padlock icon ) or https:// means you've safely connected to the .gov website. Share sensitive information only on official, secure websites.
- Publications
- Account settings
- Advanced Search
- Journal List

Ten Simple Rules for Writing Research Papers
Weixiong zhang.
- Author information
- Article notes
- Copyright and License information
* E-mail: [email protected]
The author has declared that no competing interests exist.
Collection date 2014 Jan.
This is an open-access article distributed under the terms of the Creative Commons Attribution License ., which permits unrestricted use, distribution, and reproduction in any medium, provided the original author and source are credited.
The importance of writing well can never be overstated for a successful professional career, and the ability to write solid papers is an essential trait of a productive researcher. Writing and publishing a paper has its own life cycle; properly following a course of action and avoiding missteps can be vital to the overall success not only of a paper but of the underlying research as well. Here, we offer ten simple rules for writing and publishing research papers.
As a caveat, this essay is not about the mechanics of composing a paper, much of which has been covered elsewhere, e.g., [1] , [2] . Rather, it is about the principles and attitude that can help guide the process of writing in particular and research in general. In this regard, some of the discussion will complement, extend, and refine some advice given in early articles of this Ten Simple Rules series of PLOS Computational Biology [3] – [8] .
Rule 1: Make It a Driving Force
Never separate writing a paper from the underlying research. After all, writing and research are integral parts of the overall enterprise. Therefore, design a project with an ultimate paper firmly in mind. Include an outline of the paper in the initial project design documents to help form the research objectives, determine the logical flow of the experiments, and organize the materials and data to be used. Furthermore, use writing as a tool to reassess the overall project, reevaluate the logic of the experiments, and examine the validity of the results during the research. As a result, the overall research may need to be adjusted, the project design may be revised, new methods may be devised, and new data may be collected. The process of research and writing may be repeated if necessary.
Rule 2: Less Is More
It is often the case that more than one hypothesis or objective may be tackled in one project. It is also not uncommon that the data and results gathered for one objective can serve additional purposes. A decision on having one or more papers needs to be made, and the decision will be affected by various factors. Regardless of the validity of these factors, the overriding consideration must be the potential impact that the paper may have on the research subject and field. Therefore, the significance, completeness, and coherence of the results presented as a whole should be the principal guide for selecting the story to tell, the hypothesis to focus upon, and materials to include in the paper, as well as the yardstick for measuring the quality of the paper. By this metric, less is more , i.e., fewer but more significant papers serve both the research community and one's career better than more papers of less significance.
Rule 3: Pick the Right Audience
Deciding on an angle of the story to focus upon is the next hurdle to jump at the initial stage of the writing. The results from a computational study of a biological problem can often be presented to biologists, computational scientists, or both; deciding what story to tell and from what angle to pitch the main idea is important. This issue translates to choosing a target audience, as well as an appropriate journal, to cast the main messages to. This is critical for determining the organization of the paper and the level of detail of the story, so as to write the paper with the audience in mind. Indeed, writing a paper for biologists in general is different from writing for specialists in computational biology.
Rule 4: Be Logical
The foundation of “lively” writing for smooth reading is a sound and clear logic underlying the story of the paper. Although experiments may be carried out independently, the result from one experiment may form premises and/or provide supporting data for the next experiment. The experiments and results, therefore, must be presented in a logical order. In order to make the writing an easy process to follow, this logical flow should be determined before any other writing strategy or tactic is exercised. This logical order can also help you avoid discussing the same issue or presenting the same argument in multiple places in the paper, which may dilute the readers' attention.
An effective tactic to help develop a sound logical flow is to imaginatively create a set of figures and tables, which will ultimately be developed from experimental results, and order them in a logical way based on the information flow through the experiments. In other words, the figures and tables alone can tell the story without consulting additional material. If all or some of these figures and tables are included in the final manuscript, make every effort to make them self-contained (see Rule 5 below), a favorable feature for the paper to have. In addition, these figures and tables, as well as the threading logical flow, may be used to direct or organize research activities, reinforcing Rule 1.
Rule 5: Be Thorough and Make It Complete
Completeness is a cornerstone for a research paper, following Rule 2. This cornerstone needs to be set in both content and presentation. First, important and relevant aspects of a hypothesis pursued in the research should be discussed with detailed supporting data. If the page limit is an issue, focus on one or two main aspects with sufficient details in the main text and leave the rest to online supporting materials. As a reminder, be sure to keep the details of all experiments (e.g., parameters of the experiments and versions of software) for revision, post-publication correspondence, or importantly, reproducibility of the results. Second, don't simply state what results are presented in figures and tables, which makes the writing repetitive because they are self-contained (see below), but rather, interpret them with insights to the underlying story to be told (typically in the results section) and discuss their implication (typically in the discussion section).
Third, make the whole paper self-contained. Introduce an adequate amount of background and introductory material for the right audience (following Rule 3). A statistical test, e.g., hypergeometric tests for enrichment of a subset of objects, may be obvious to statisticians or computational biologists but may be foreign to others, so providing a sufficient amount of background is the key for delivery of the material. When an uncommon term is used, give a definition besides a reference to it. Fourth, try to avoid “making your readers do the arithmetic” [9] , i.e., be clear enough so that the readers don't have to make any inference from the presented data. If such results need to be discussed, make them explicit even though they may be readily derived from other data. Fifth, figures and tables are essential components of a paper, each of which must be included for a good reason; make each of them self-contained with all required information clearly specified in the legend to guide interpretation of the data presented.
Rule 6: Be Concise
This is a caveat to Rule 5 and is singled out to emphasize its importance. Being thorough is not a license to writing that is unnecessarily descriptive, repetitive, or lengthy. Rather, on the contrary, “simplicity is the ultimate sophistication” [10] . Overly elaborate writing is distracting and boring and places a burden on the readers. In contrast, the delivery of a message is more rigorous if the writing is precise and concise. One excellent example is Watson and Crick's Nobel-Prize-winning paper on the DNA double helix structure [11] —it is only two pages long!
Rule 7: Be Artistic
A complete draft of a paper requires a lot of work, so it pays to go the extra mile to polish it to facilitate enjoyable reading. A paper presented as a piece of art will give referees a positive initial impression of your passion toward the research and the quality of the work, which will work in your favor in the reviewing process. Therefore, concentrate on spelling, grammar, usage, and a “lively” writing style that avoids successions of simple, boring, declarative sentences. Have an authoritative dictionary with a thesaurus and a style manual, e.g., [1] , handy and use them relentlessly. Also pay attention to small details in presentation, such as paragraph indentation, page margins, and fonts. If you are not a native speaker of the language the paper is written in, make sure to have a native speaker go over the final draft to ensure correctness and accuracy of the language used.
Rule 8: Be Your Own Judge
A complete manuscript typically requires many rounds of revision. Taking a correct attitude during revision is critical to the resolution of most problems in the writing. Be objective and honest about your work and do not exaggerate or belittle the significance of the results and the elegance of the methods developed. After working long and hard, you are an expert on the problem you studied, and you are the best referee of your own work, after all . Therefore, inspect the research and the paper in the context of the state of the art.
When revising a draft, purge yourself out of the picture and leave your passion for your work aside. To be concrete, put yourself completely in the shoes of a referee and scrutinize all the pieces—the significance of the work, the logic of the story, the correctness of the results and conclusions, the organization of the paper, and the presentation of the materials. In practice, you may put a draft aside for a day or two—try to forget about it completely—and then come back to it fresh, consider it as if it were someone else's writing, and read it through while trying to poke holes in the story and writing. In this process, extract the meaning literally from the language as written and do not try to use your own view to interpret or extrapolate from what was written. Don't be afraid to throw away pieces of your writing and start over from scratch if they do not pass this “not-yourself” test. This can be painful, but the final manuscript will be more logically sound and better organized.
Rule 9: Test the Water in Your Own Backyard
It is wise to anticipate the possible questions and critiques the referees may raise and preemptively address their concerns before submission. To do so, collect feedback and critiques from others, e.g., colleagues and collaborators. Discuss your work with them and get their opinions, suggestions, and comments. A talk at a lab meeting or a departmental seminar will also help rectify potential issues that need to be addressed. If you are a graduate student, running the paper and results through the thesis committee may be effective to iron out possible problems.

Rule 10: Build a Virtual Team of Collaborators
When a submission is rejected or poorly reviewed, don't be offended and don't take it personally. Be aware that the referees spent their time on the paper, which they might have otherwise devoted to their own research, so they are doing you a favor and helping you shape the paper to be more accessible to the targeted audience. Therefore, consider the referees as your collaborators and treat the reviews with respect. This attitude can improve the quality of your paper and research.
Read and examine the reviews objectively—the principles set in Rule 8 apply here as well. Often a criticism was raised because one of the aspects of a hypothesis was not adequately studied, or an important result from previous research was not mentioned or not consistent with yours. If a critique is about the robustness of a method used or the validity of a result, often the research needs to be redone or more data need to be collected. If you believe the referee has misunderstood a particular point, check the writing. It is often the case that improper wording or presentation misled the referee. If that's the case, revise the writing thoroughly. Don't argue without supporting data. Don't submit the paper elsewhere without additional work. This can only temporally mitigate the issue, you will not be happy with the paper in the long run, and this may hurt your reputation.
Finally, keep in mind that writing is personal, and it takes a lot of practice to find one's style. What works and what does not work vary from person to person. Undoubtedly, dedicated practice will help produce stronger papers with long-lasting impact.
Acknowledgments
Thanks to Sharlee Climer, Richard Korf, and Kevin Zhang for critical reading of the manuscript.
Funding Statement
The author received no specific funding for this article.
- 1. Strunk W Jr, White EB (1999) The Elements of Style. 4th edition. New York: Longman. [ Google Scholar ]
- 2. Zinsser W (2006) On Writing Well: The Classic Guide to Writing Nonfiction. 30th anniversary edition. New York: Harper Perennial. [ Google Scholar ]
- 3. Bourne PE (2005) Ten simple rules for getting published. PLOS Comput Biol 1: e57 doi: 10.1371/journal.pcbi.0010057 [ DOI ] [ PMC free article ] [ PubMed ] [ Google Scholar ]
- 4. Erren TC, Cullen P (2007) Ten simple rules for doing your best research, according to Hamming. PLOS Comput Biol 3: e213 doi: 10.1371/journal.pcbi.0030213 [ DOI ] [ PMC free article ] [ PubMed ] [ Google Scholar ]
- 5. Bourne PE (2007) Ten simple rules for making good oral presentations. PLOS Comput Biol 3: e77 doi: 10.1371/journal.pcbi.0030077 [ DOI ] [ PMC free article ] [ PubMed ] [ Google Scholar ]
- 6. Erren TC, Bourne PE (2007) Ten simple rules for a good poster presentation. PLOS Comput Biol 3: e102 doi: 10.1371/journal.pcbi.0030102 [ DOI ] [ PMC free article ] [ PubMed ] [ Google Scholar ]
- 7. Bourne PE, Korngreen A (2006) Ten simple rules for reviewers. PLOS Comput Biol 2: e110 doi: 10.1371/journal.pcbi.0020110 [ DOI ] [ PMC free article ] [ PubMed ] [ Google Scholar ]
- 8. Logan DW, Sandal M, Gardner PP, Manske M, Bateman A (2010) Ten simple rules for editing Wikipedia. PLOS Comput Biol 6: e1000941 doi: 10.1371/journal.pcbi.1000941 [ DOI ] [ PMC free article ] [ PubMed ] [ Google Scholar ]
- 9. Johnson DS (2002) A theoretician's guide to the experimental analysis of algorithms. In Goldwasser MH, Johnson DS, McGeoch CC, editors. Data Structures, Near Neighbor Searches, and Methodology: Fifth and Sixth DIMACS Implementation Challenges. Providence: American Mathematical Society. pp.215–250. [ Google Scholar ]
- 10. Wikiquote page on Leonardo Da Vinci. Available: http://en.wikiquote.org/wiki/Leonardo_da_Vinci#Quotes_about_Leonardo . Accessed 13 December 2013.
- 11. Watson JD, Crick FHC (1953) Molecular structure of nucleic acids. Nature 171: 737–738 Available: http://www.nature.com/nature/dna50/watsoncrick.pdf . Accessed 31 December 2013. [ DOI ] [ PubMed ] [ Google Scholar ]
- View on publisher site
- PDF (122.1 KB)
- Collections
Similar articles
Cited by other articles, links to ncbi databases.
- Download .nbib .nbib
- Format: AMA APA MLA NLM
Add to Collections
- Privacy Policy

Home » Research Design – Types, Methods and Examples
Research Design – Types, Methods and Examples
Table of Contents

Research Design
Definition:
Research design refers to the overall strategy or plan for conducting a research study. It outlines the methods and procedures that will be used to collect and analyze data, as well as the goals and objectives of the study. Research design is important because it guides the entire research process and ensures that the study is conducted in a systematic and rigorous manner.
Types of Research Design
Types of Research Design are as follows:
Descriptive Research Design
This type of research design is used to describe a phenomenon or situation. It involves collecting data through surveys, questionnaires, interviews, and observations. The aim of descriptive research is to provide an accurate and detailed portrayal of a particular group, event, or situation. It can be useful in identifying patterns, trends, and relationships in the data.
Correlational Research Design
Correlational research design is used to determine if there is a relationship between two or more variables. This type of research design involves collecting data from participants and analyzing the relationship between the variables using statistical methods. The aim of correlational research is to identify the strength and direction of the relationship between the variables.
Experimental Research Design
Experimental research design is used to investigate cause-and-effect relationships between variables. This type of research design involves manipulating one variable and measuring the effect on another variable. It usually involves randomly assigning participants to groups and manipulating an independent variable to determine its effect on a dependent variable. The aim of experimental research is to establish causality.
Quasi-experimental Research Design
Quasi-experimental research design is similar to experimental research design, but it lacks one or more of the features of a true experiment. For example, there may not be random assignment to groups or a control group. This type of research design is used when it is not feasible or ethical to conduct a true experiment.
Case Study Research Design
Case study research design is used to investigate a single case or a small number of cases in depth. It involves collecting data through various methods, such as interviews, observations, and document analysis. The aim of case study research is to provide an in-depth understanding of a particular case or situation.
Longitudinal Research Design
Longitudinal research design is used to study changes in a particular phenomenon over time. It involves collecting data at multiple time points and analyzing the changes that occur. The aim of longitudinal research is to provide insights into the development, growth, or decline of a particular phenomenon over time.
Structure of Research Design
The format of a research design typically includes the following sections:
- Introduction : This section provides an overview of the research problem, the research questions, and the importance of the study. It also includes a brief literature review that summarizes previous research on the topic and identifies gaps in the existing knowledge.
- Research Questions or Hypotheses: This section identifies the specific research questions or hypotheses that the study will address. These questions should be clear, specific, and testable.
- Research Methods : This section describes the methods that will be used to collect and analyze data. It includes details about the study design, the sampling strategy, the data collection instruments, and the data analysis techniques.
- Data Collection: This section describes how the data will be collected, including the sample size, data collection procedures, and any ethical considerations.
- Data Analysis: This section describes how the data will be analyzed, including the statistical techniques that will be used to test the research questions or hypotheses.
- Results : This section presents the findings of the study, including descriptive statistics and statistical tests.
- Discussion and Conclusion : This section summarizes the key findings of the study, interprets the results, and discusses the implications of the findings. It also includes recommendations for future research.
- References : This section lists the sources cited in the research design.
Example of Research Design
An Example of Research Design could be:
Research question: Does the use of social media affect the academic performance of high school students?
Research design:
- Research approach : The research approach will be quantitative as it involves collecting numerical data to test the hypothesis.
- Research design : The research design will be a quasi-experimental design, with a pretest-posttest control group design.
- Sample : The sample will be 200 high school students from two schools, with 100 students in the experimental group and 100 students in the control group.
- Data collection : The data will be collected through surveys administered to the students at the beginning and end of the academic year. The surveys will include questions about their social media usage and academic performance.
- Data analysis : The data collected will be analyzed using statistical software. The mean scores of the experimental and control groups will be compared to determine whether there is a significant difference in academic performance between the two groups.
- Limitations : The limitations of the study will be acknowledged, including the fact that social media usage can vary greatly among individuals, and the study only focuses on two schools, which may not be representative of the entire population.
- Ethical considerations: Ethical considerations will be taken into account, such as obtaining informed consent from the participants and ensuring their anonymity and confidentiality.
How to Write Research Design
Writing a research design involves planning and outlining the methodology and approach that will be used to answer a research question or hypothesis. Here are some steps to help you write a research design:
- Define the research question or hypothesis : Before beginning your research design, you should clearly define your research question or hypothesis. This will guide your research design and help you select appropriate methods.
- Select a research design: There are many different research designs to choose from, including experimental, survey, case study, and qualitative designs. Choose a design that best fits your research question and objectives.
- Develop a sampling plan : If your research involves collecting data from a sample, you will need to develop a sampling plan. This should outline how you will select participants and how many participants you will include.
- Define variables: Clearly define the variables you will be measuring or manipulating in your study. This will help ensure that your results are meaningful and relevant to your research question.
- Choose data collection methods : Decide on the data collection methods you will use to gather information. This may include surveys, interviews, observations, experiments, or secondary data sources.
- Create a data analysis plan: Develop a plan for analyzing your data, including the statistical or qualitative techniques you will use.
- Consider ethical concerns : Finally, be sure to consider any ethical concerns related to your research, such as participant confidentiality or potential harm.
When to Write Research Design
Research design should be written before conducting any research study. It is an important planning phase that outlines the research methodology, data collection methods, and data analysis techniques that will be used to investigate a research question or problem. The research design helps to ensure that the research is conducted in a systematic and logical manner, and that the data collected is relevant and reliable.
Ideally, the research design should be developed as early as possible in the research process, before any data is collected. This allows the researcher to carefully consider the research question, identify the most appropriate research methodology, and plan the data collection and analysis procedures in advance. By doing so, the research can be conducted in a more efficient and effective manner, and the results are more likely to be valid and reliable.
Purpose of Research Design
The purpose of research design is to plan and structure a research study in a way that enables the researcher to achieve the desired research goals with accuracy, validity, and reliability. Research design is the blueprint or the framework for conducting a study that outlines the methods, procedures, techniques, and tools for data collection and analysis.
Some of the key purposes of research design include:
- Providing a clear and concise plan of action for the research study.
- Ensuring that the research is conducted ethically and with rigor.
- Maximizing the accuracy and reliability of the research findings.
- Minimizing the possibility of errors, biases, or confounding variables.
- Ensuring that the research is feasible, practical, and cost-effective.
- Determining the appropriate research methodology to answer the research question(s).
- Identifying the sample size, sampling method, and data collection techniques.
- Determining the data analysis method and statistical tests to be used.
- Facilitating the replication of the study by other researchers.
- Enhancing the validity and generalizability of the research findings.
Applications of Research Design
There are numerous applications of research design in various fields, some of which are:
- Social sciences: In fields such as psychology, sociology, and anthropology, research design is used to investigate human behavior and social phenomena. Researchers use various research designs, such as experimental, quasi-experimental, and correlational designs, to study different aspects of social behavior.
- Education : Research design is essential in the field of education to investigate the effectiveness of different teaching methods and learning strategies. Researchers use various designs such as experimental, quasi-experimental, and case study designs to understand how students learn and how to improve teaching practices.
- Health sciences : In the health sciences, research design is used to investigate the causes, prevention, and treatment of diseases. Researchers use various designs, such as randomized controlled trials, cohort studies, and case-control studies, to study different aspects of health and healthcare.
- Business : Research design is used in the field of business to investigate consumer behavior, marketing strategies, and the impact of different business practices. Researchers use various designs, such as survey research, experimental research, and case studies, to study different aspects of the business world.
- Engineering : In the field of engineering, research design is used to investigate the development and implementation of new technologies. Researchers use various designs, such as experimental research and case studies, to study the effectiveness of new technologies and to identify areas for improvement.
Advantages of Research Design
Here are some advantages of research design:
- Systematic and organized approach : A well-designed research plan ensures that the research is conducted in a systematic and organized manner, which makes it easier to manage and analyze the data.
- Clear objectives: The research design helps to clarify the objectives of the study, which makes it easier to identify the variables that need to be measured, and the methods that need to be used to collect and analyze data.
- Minimizes bias: A well-designed research plan minimizes the chances of bias, by ensuring that the data is collected and analyzed objectively, and that the results are not influenced by the researcher’s personal biases or preferences.
- Efficient use of resources: A well-designed research plan helps to ensure that the resources (time, money, and personnel) are used efficiently and effectively, by focusing on the most important variables and methods.
- Replicability: A well-designed research plan makes it easier for other researchers to replicate the study, which enhances the credibility and reliability of the findings.
- Validity: A well-designed research plan helps to ensure that the findings are valid, by ensuring that the methods used to collect and analyze data are appropriate for the research question.
- Generalizability : A well-designed research plan helps to ensure that the findings can be generalized to other populations, settings, or situations, which increases the external validity of the study.
Research Design Vs Research Methodology
About the author.
Muhammad Hassan
Researcher, Academic Writer, Web developer
You may also like

Research Objectives – Types, Examples and...

Assignment – Types, Examples and Writing Guide

Appendix in Research Paper – Examples and...

Dissertation Methodology – Structure, Example...

Evaluating Research – Process, Examples and...

Thesis – Structure, Example and Writing Guide
Leave a comment x.
Save my name, email, and website in this browser for the next time I comment.
- How It Works
- PhD thesis writing
- Master thesis writing
- Bachelor thesis writing
- Dissertation writing service
- Dissertation abstract writing
- Thesis proposal writing
- Thesis editing service
- Thesis proofreading service
- Thesis formatting service
- Coursework writing service
- Research paper writing service
- Architecture thesis writing
- Computer science thesis writing
- Engineering thesis writing
- History thesis writing
- MBA thesis writing
- Nursing dissertation writing
- Psychology dissertation writing
- Sociology thesis writing
- Statistics dissertation writing
- Buy dissertation online
- Write my dissertation
- Cheap thesis
- Cheap dissertation
- Custom dissertation
- Dissertation help
- Pay for thesis
- Pay for dissertation
- Senior thesis
- Write my thesis
How To Write A Research Design Like A Pro
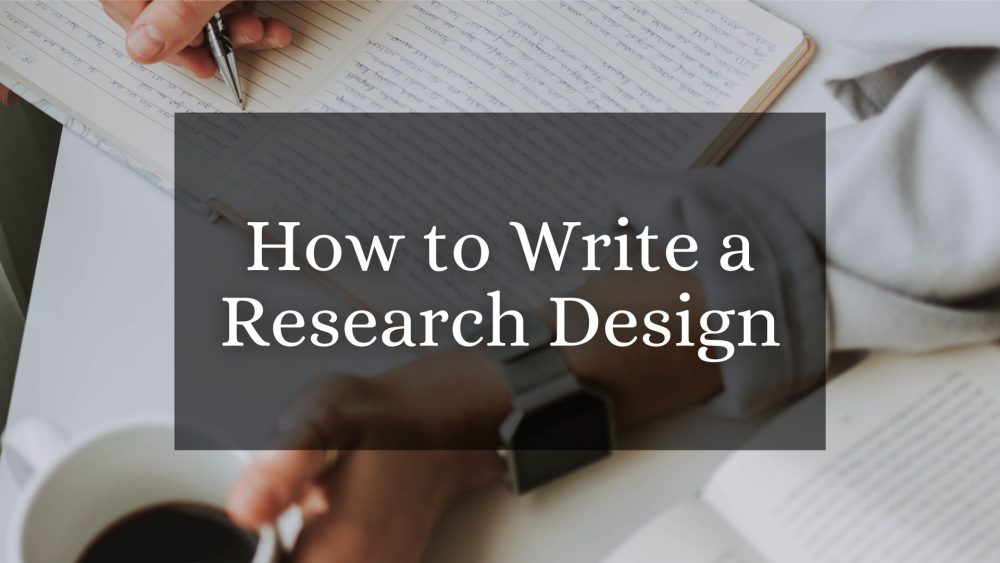
The overall strategy that a researcher chooses to address all the different parts of their study in a logical and clear manner is known as a research design.
So, what is research design in research paper? A research design is a general plan explaining what one looks to do so as to answer the research question. Generally, it is a detailed outline of how research or an investigation will take place including; how data will be collected, which tools will be employed and how they will be used, and the ways through with the data will be analyzed.
It lays out the method you use to collect, measure, and analyze information. It states that you do this logically and coherently to ensure that you thoroughly address the research problem with which you are dealing. There are numerous types of research design including:
Action Study Research Design Case Study Research Design Casual Research Design Cohort Research Design Cross-Sectional Research Design Correlational Research Design Descriptive Research Design Experimental Research Design Exploratory Research Design Historical Research Design Longitudinal Research Design Observational Research Design Philosophical Research Design Qualitative Research Design Quantitative Research Design Sequential Research Design
The research paper design you choose depends on the research problem. You should analyze the problem carefully and consider it from numerous perspectives. You may consider using a mixed methods research design which is a combination of any two designs listed above. But you must choose a type of research design that is strong and will make your project progress smoothly.
Example Of A Nursing Research Design
To assess the links between professional satisfaction, job satisfaction, and contributing factors using a quantitative approach, an appropriate method is to gain use questionnaires or surveys that provide numerical data from the sample. To achieve an appropriate sample, a sampling plan should be developed. In this case, the population of concern will be identified. This will be nursing staff members, possibly across a wide range of departments to gain a better insight into the links overall. A stratified sampling method would be appropriate here to ensure that the sample is made up of sub-populations that are in line with the sub-populations of the total: the strata should include gender, number of years in nursing, department, and any other factors that could be confounding variables. This will ensure that the sample is representative of the population of interest. In a population of 1000 nurses, a confidence interval of five, and a confidence level of 99%, the sample size needed is 400. Inclusion criteria will include: nursing staff working at the hospital, ability to speak the language that the survey is administered in, and those that have given informed consent. Exclusion criteria will be visiting nursing staff, staff who are not nurses, and those that do not hold relevant nursing criteria.
Sampling Plan: Qualitative
A more appropriate methodology for qualitative approaches is to use interviews or focus groups. This means that the sample size can be much smaller, often as low as ten. In this case, the sampling plan will have the same population of concern, but a different approach to sampling can be used. It may be more appropriate to use quota, self-selection sampling here, as nursing staff need to be willing to give up some time to respond. This has drawbacks, including self-selection bias, but it would be unethical to force nursing staff to participate in the project, especially considering interviews can take one hour or more. The inclusion and exclusion criteria are as above.
How to Write a Research Design Proposal
For most research problems, you will have to make some tradeoffs. One design can be strong in some areas and weak in other areas. This is the reason many students choose to select more than one design to gather all the information accurately and effectively they need to address the problem. This is one of the first things you should know about how to write research design and methods section.
- Consider Your Practicalities and Priorities
What do we mean when we say you need to think about practicalities and priorities? Another thing to know about how to write design and methodology of the research is asking several questions before settling on one or two methodologies. You will not have the time or resources to conduct tests using several research designs, so you need to write down and answer precisely what your priorities are and the practical nature of your study.
A good place to start is at the library where you have access to other academic studies in your field. You can find similar studies and look at published samples that have been approved by experts in the field. You can also get a sense of the number of resources you have available. Pre-planning is a great way of making sure your project stays on track.
- Determine the Kind of Information You Need
The next to know about how to write a qualitative research design is figuring out the kind of information or data you need to answer the research problem. There are two places where you get this: through primary and secondary data. In your research study, you get original data through experiments, interviews, and surveys. This is information you analyze and incorporate into your research finding.
Your study will also incorporate information gathered by someone else in previous studies. This type of data is available in libraries and online databases where you can look at national statistics, official records, and publications from academic and government sources.
- Identify How You Are Going to Collect Information
Once you know the kind of information you need to gather (qualitative and quantitative) you need to decide where, when, and how you will gather it. How to write a research design requires you to describe your research methods. This means putting in detail the materials, procedures, tools, and techniques you will use and apply. You also need to point out the criteria you will use to choose your participants and sources. (For example, how many participants will you need to fill out services to get a good method to sample).
- Decide How You Are Going to Analyze the Information
Another thing you need to know about how to write a research design relates to the way you are going to analyze the information you collect. The process of analysis is the last step you need to develop your research design. Numerous computer applications will sort through information and retrieve what you need to answer the research problem (For example, Access and Excel). Identify the ones you will use and state this in the research design.
- Draft Your Research Design as You Would Other Sections
Now you can start writing the first draft. You should approach this like you would other academic assignments. Use a draft that lists all the sub-sections you need to address in the research design. Be clear and concise. The research design should not include your opinions. It must show the reader an exact description of the way you conducted your study.
- Revise Your Research Design After Some Time Away
Hindsight is one of the best things that can come from separating yourself from your assignment for a few days. We recommend students remove themselves completely from their work to get a mental break. The distance will help them rethink their writing and make changes that improve the overall quality of an assignment.
The trick is to do stay away a few days instead of just a few hours. The time away from any piece of writing will allow for more self-evaluation that is objective. Many students will find ways to remove, add, or rearrange words, phrases, sentences, and paragraphs that make their assignments stronger.
- Edit and Proofread Your Research Design for Perfection
These two activities are not interchangeable. Editing focuses on deep issues like correcting sentence constructions and word choices. A thorough editing session will improve things like clarity, readability, and tone. Proofreading focuses on details like grammar, punctuation, and misspellings. It will also look at page numbering, formatting, alignment, and visual elements.
Both activities are important stages of the writing process. A great editor will begin his or her work during the revising process. A great proofread will also begin his or her work during the editing process. While they may overlap you should always treat them as two separate tasks and designate enough time to do each without distraction.
- Have a Colleague Review Your Work for Feedback
Having a colleague or peer review your work is an important step to the academic writing process. A person or a group of people that understands your field and the high standard of researching and writing that comes with putting together a great research paper can valuable toward your success at the collegiate and graduate levels. Even if you can only show your work to one person for a few hours, his or her feedback can help you make changes to improve the overall quality of your research design.
Here are some questions you should consider before asking someone to review your work:
Do they understand the research subject and/or topic? Do they know the professor or panel that will grade your work? Have they submitted research studies in the past? Do they have great to excellent grades when it comes to research? Are they committed to providing you constructive criticism and feedback?
What to Do If You Can’t Do the Research Design
You may not have enough time to create this section, especially when you have a short deadline. On these occasions, it is a good idea to find a template for a research design paper. You can find templates online or can refer to published research papers in academic journals. The formats are standard so as long as you apply your words to a template that matches your design approach.
If you need more information or assistance learning about how to write a research design section, our customer support team can point you to more resources or put you in contact with one of our academic writing and editing experts. Each expert has earned either a bachelor’s or master’s degree and specialize in specific disciplines. You can rest assured that you will be assigned someone that knows your field inside and outside and can give you the writing research design and methodology help that you need to excel academically.
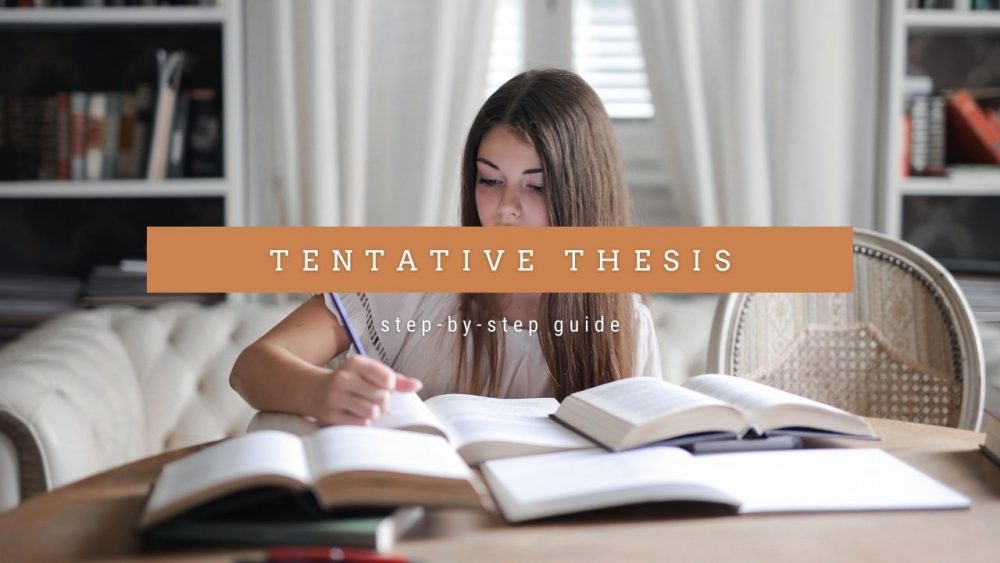
Leave a Reply Cancel reply
Your email address will not be published. Required fields are marked *
Comment * Error message
Name * Error message
Email * Error message
Save my name, email, and website in this browser for the next time I comment.
As Putin continues killing civilians, bombing kindergartens, and threatening WWIII, Ukraine fights for the world's peaceful future.
Ukraine Live Updates
Writing a Research Paper
This page lists some of the stages involved in writing a library-based research paper.
Although this list suggests that there is a simple, linear process to writing such a paper, the actual process of writing a research paper is often a messy and recursive one, so please use this outline as a flexible guide.
Discovering, Narrowing, and Focusing a Researchable Topic
- Try to find a topic that truly interests you
- Try writing your way to a topic
- Talk with your course instructor and classmates about your topic
- Pose your topic as a question to be answered or a problem to be solved
Finding, Selecting, and Reading Sources
You will need to look at the following types of sources:
- library catalog, periodical indexes, bibliographies, suggestions from your instructor
- primary vs. secondary sources
- journals, books, other documents
Grouping, Sequencing, and Documenting Information
The following systems will help keep you organized:
- a system for noting sources on bibliography cards
- a system for organizing material according to its relative importance
- a system for taking notes
Writing an Outline and a Prospectus for Yourself
Consider the following questions:
- What is the topic?
- Why is it significant?
- What background material is relevant?
- What is my thesis or purpose statement?
- What organizational plan will best support my purpose?
Writing the Introduction
In the introduction you will need to do the following things:
- present relevant background or contextual material
- define terms or concepts when necessary
- explain the focus of the paper and your specific purpose
- reveal your plan of organization
Writing the Body
- Use your outline and prospectus as flexible guides
- Build your essay around points you want to make (i.e., don’t let your sources organize your paper)
- Integrate your sources into your discussion
- Summarize, analyze, explain, and evaluate published work rather than merely reporting it
- Move up and down the “ladder of abstraction” from generalization to varying levels of detail back to generalization
Writing the Conclusion
- If the argument or point of your paper is complex, you may need to summarize the argument for your reader.
- If prior to your conclusion you have not yet explained the significance of your findings or if you are proceeding inductively, use the end of your paper to add your points up, to explain their significance.
- Move from a detailed to a general level of consideration that returns the topic to the context provided by the introduction.
- Perhaps suggest what about this topic needs further research.
Revising the Final Draft
- Check overall organization : logical flow of introduction, coherence and depth of discussion in body, effectiveness of conclusion.
- Paragraph level concerns : topic sentences, sequence of ideas within paragraphs, use of details to support generalizations, summary sentences where necessary, use of transitions within and between paragraphs.
- Sentence level concerns: sentence structure, word choices, punctuation, spelling.
- Documentation: consistent use of one system, citation of all material not considered common knowledge, appropriate use of endnotes or footnotes, accuracy of list of works cited.

Academic and Professional Writing
This is an accordion element with a series of buttons that open and close related content panels.
Analysis Papers
Reading Poetry
A Short Guide to Close Reading for Literary Analysis
Using Literary Quotations
Play Reviews
Writing a Rhetorical Précis to Analyze Nonfiction Texts
Incorporating Interview Data
Grant Proposals
Planning and Writing a Grant Proposal: The Basics
Additional Resources for Grants and Proposal Writing
Job Materials and Application Essays
Writing Personal Statements for Ph.D. Programs
- Before you begin: useful tips for writing your essay
- Guided brainstorming exercises
- Get more help with your essay
- Frequently Asked Questions
Resume Writing Tips
CV Writing Tips
Cover Letters
Business Letters
Proposals and Dissertations
Resources for Proposal Writers
Resources for Dissertators
Research Papers
Planning and Writing Research Papers
Quoting and Paraphrasing
Writing Annotated Bibliographies
Creating Poster Presentations
Writing an Abstract for Your Research Paper
Thank-You Notes
Advice for Students Writing Thank-You Notes to Donors
Reading for a Review
Critical Reviews
Writing a Review of Literature
Scientific Reports
Scientific Report Format
Sample Lab Assignment
Writing for the Web
Writing an Effective Blog Post
Writing for Social Media: A Guide for Academics
- Search Menu
- Sign in through your institution
- Advance articles
- Editor's Choice
- Supplements
- French Abstracts
- Portuguese Abstracts
- Spanish Abstracts
- Author Guidelines
- Submission Site
- Open Access
- About International Journal for Quality in Health Care
- About the International Society for Quality in Health Care
- Editorial Board
- Advertising and Corporate Services
- Journals Career Network
- Self-Archiving Policy
- Dispatch Dates
- Contact ISQua
- Journals on Oxford Academic
- Books on Oxford Academic
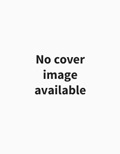
Article Contents
Primacy of the research question, structure of the paper, writing a research article: advice to beginners.
- Article contents
- Figures & tables
- Supplementary Data
Thomas V. Perneger, Patricia M. Hudelson, Writing a research article: advice to beginners, International Journal for Quality in Health Care , Volume 16, Issue 3, June 2004, Pages 191–192, https://doi.org/10.1093/intqhc/mzh053
- Permissions Icon Permissions
Writing research papers does not come naturally to most of us. The typical research paper is a highly codified rhetorical form [ 1 , 2 ]. Knowledge of the rules—some explicit, others implied—goes a long way toward writing a paper that will get accepted in a peer-reviewed journal.
A good research paper addresses a specific research question. The research question—or study objective or main research hypothesis—is the central organizing principle of the paper. Whatever relates to the research question belongs in the paper; the rest doesn’t. This is perhaps obvious when the paper reports on a well planned research project. However, in applied domains such as quality improvement, some papers are written based on projects that were undertaken for operational reasons, and not with the primary aim of producing new knowledge. In such cases, authors should define the main research question a posteriori and design the paper around it.
Generally, only one main research question should be addressed in a paper (secondary but related questions are allowed). If a project allows you to explore several distinct research questions, write several papers. For instance, if you measured the impact of obtaining written consent on patient satisfaction at a specialized clinic using a newly developed questionnaire, you may want to write one paper on the questionnaire development and validation, and another on the impact of the intervention. The idea is not to split results into ‘least publishable units’, a practice that is rightly decried, but rather into ‘optimally publishable units’.
What is a good research question? The key attributes are: (i) specificity; (ii) originality or novelty; and (iii) general relevance to a broad scientific community. The research question should be precise and not merely identify a general area of inquiry. It can often (but not always) be expressed in terms of a possible association between X and Y in a population Z, for example ‘we examined whether providing patients about to be discharged from the hospital with written information about their medications would improve their compliance with the treatment 1 month later’. A study does not necessarily have to break completely new ground, but it should extend previous knowledge in a useful way, or alternatively refute existing knowledge. Finally, the question should be of interest to others who work in the same scientific area. The latter requirement is more challenging for those who work in applied science than for basic scientists. While it may safely be assumed that the human genome is the same worldwide, whether the results of a local quality improvement project have wider relevance requires careful consideration and argument.
Once the research question is clearly defined, writing the paper becomes considerably easier. The paper will ask the question, then answer it. The key to successful scientific writing is getting the structure of the paper right. The basic structure of a typical research paper is the sequence of Introduction, Methods, Results, and Discussion (sometimes abbreviated as IMRAD). Each section addresses a different objective. The authors state: (i) the problem they intend to address—in other terms, the research question—in the Introduction; (ii) what they did to answer the question in the Methods section; (iii) what they observed in the Results section; and (iv) what they think the results mean in the Discussion.
In turn, each basic section addresses several topics, and may be divided into subsections (Table 1 ). In the Introduction, the authors should explain the rationale and background to the study. What is the research question, and why is it important to ask it? While it is neither necessary nor desirable to provide a full-blown review of the literature as a prelude to the study, it is helpful to situate the study within some larger field of enquiry. The research question should always be spelled out, and not merely left for the reader to guess.
Typical structure of a research paper
The Methods section should provide the readers with sufficient detail about the study methods to be able to reproduce the study if so desired. Thus, this section should be specific, concrete, technical, and fairly detailed. The study setting, the sampling strategy used, instruments, data collection methods, and analysis strategies should be described. In the case of qualitative research studies, it is also useful to tell the reader which research tradition the study utilizes and to link the choice of methodological strategies with the research goals [ 3 ].
The Results section is typically fairly straightforward and factual. All results that relate to the research question should be given in detail, including simple counts and percentages. Resist the temptation to demonstrate analytic ability and the richness of the dataset by providing numerous tables of non-essential results.
The Discussion section allows the most freedom. This is why the Discussion is the most difficult to write, and is often the weakest part of a paper. Structured Discussion sections have been proposed by some journal editors [ 4 ]. While strict adherence to such rules may not be necessary, following a plan such as that proposed in Table 1 may help the novice writer stay on track.
References should be used wisely. Key assertions should be referenced, as well as the methods and instruments used. However, unless the paper is a comprehensive review of a topic, there is no need to be exhaustive. Also, references to unpublished work, to documents in the grey literature (technical reports), or to any source that the reader will have difficulty finding or understanding should be avoided.
Having the structure of the paper in place is a good start. However, there are many details that have to be attended to while writing. An obvious recommendation is to read, and follow, the instructions to authors published by the journal (typically found on the journal’s website). Another concerns non-native writers of English: do have a native speaker edit the manuscript. A paper usually goes through several drafts before it is submitted. When revising a paper, it is useful to keep an eye out for the most common mistakes (Table 2 ). If you avoid all those, your paper should be in good shape.
Common mistakes seen in manuscripts submitted to this journal
Huth EJ . How to Write and Publish Papers in the Medical Sciences , 2nd edition. Baltimore, MD: Williams & Wilkins, 1990 .
Browner WS . Publishing and Presenting Clinical Research . Baltimore, MD: Lippincott, Williams & Wilkins, 1999 .
Devers KJ , Frankel RM. Getting qualitative research published. Educ Health 2001 ; 14 : 109 –117.
Docherty M , Smith R. The case for structuring the discussion of scientific papers. Br Med J 1999 ; 318 : 1224 –1225.
Email alerts
Citing articles via.
- X (formerly Twitter)
- Recommend to your Library
Affiliations
- Online ISSN 1464-3677
- Print ISSN 1353-4505
- Copyright © 2024 International Society for Quality in Health Care and Oxford University Press
- About Oxford Academic
- Publish journals with us
- University press partners
- What we publish
- New features
- Open access
- Institutional account management
- Rights and permissions
- Get help with access
- Accessibility
- Advertising
- Media enquiries
- Oxford University Press
- Oxford Languages
- University of Oxford
Oxford University Press is a department of the University of Oxford. It furthers the University's objective of excellence in research, scholarship, and education by publishing worldwide
- Copyright © 2024 Oxford University Press
- Cookie settings
- Cookie policy
- Privacy policy
- Legal notice
This Feature Is Available To Subscribers Only
Sign In or Create an Account
This PDF is available to Subscribers Only
For full access to this pdf, sign in to an existing account, or purchase an annual subscription.
Loading metrics
Open Access
Ten simple rules for good research practice
* E-mail: [email protected]
Affiliations Center for Reproducible Science, University of Zurich, Zurich, Switzerland, Epidemiology, Biostatistics and Prevention Institute, University of Zurich, Zurich, Switzerland

Affiliation Department of Clinical Research, University Hospital Basel, University of Basel, Basel, Switzerland
Affiliation Human Neuroscience Platform, Fondation Campus Biotech Geneva, Geneva, Switzerland
Affiliation Department of Environmental Sciences, Zoology, University of Basel, Basel, Switzerland
Affiliation Institute of Social and Preventive Medicine, University of Bern, Bern, Switzerland
Affiliation SIB Training Group, SIB Swiss Institute of Bioinformatics, Lausanne, Switzerland
Affiliations Department of Clinical Research, University Hospital Basel, University of Basel, Basel, Switzerland, Meta-Research Innovation Center at Stanford (METRICS), Stanford University, Stanford, California, United States of America, Meta-Research Innovation Center Berlin (METRIC-B), Berlin Institute of Health, Berlin, Germany
Affiliation Applied Face Cognition Lab, University of Lausanne, Lausanne, Switzerland
Affiliation Faculty of Psychology, UniDistance Suisse, Brig, Switzerland
Affiliation Statistical Consultant, Edinburgh, United Kingdom
- Simon Schwab,
- Perrine Janiaud,
- Michael Dayan,
- Valentin Amrhein,
- Radoslaw Panczak,
- Patricia M. Palagi,
- Lars G. Hemkens,
- Meike Ramon,
- Nicolas Rothen,
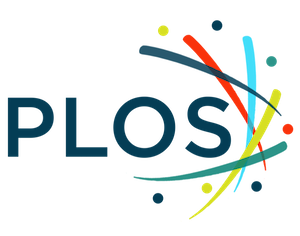
Published: June 23, 2022
- https://doi.org/10.1371/journal.pcbi.1010139
- Reader Comments
Citation: Schwab S, Janiaud P, Dayan M, Amrhein V, Panczak R, Palagi PM, et al. (2022) Ten simple rules for good research practice. PLoS Comput Biol 18(6): e1010139. https://doi.org/10.1371/journal.pcbi.1010139
Copyright: © 2022 Schwab et al. This is an open access article distributed under the terms of the Creative Commons Attribution License , which permits unrestricted use, distribution, and reproduction in any medium, provided the original author and source are credited.
Funding: S.S. received funding from SfwF (Stiftung für wissenschaftliche Forschung an der Universität Zürich; grant no. STWF-19-007). The funder had no role in study design, data collection and analysis, decision to publish, or preparation of the manuscript.
Competing interests: The authors have declared that no competing interests exist.
This is a PLOS Computational Biology Methods paper.
Introduction
The lack of research reproducibility has caused growing concern across various scientific fields [ 1 – 5 ]. Today, there is widespread agreement, within and outside academia, that scientific research is suffering from a reproducibility crisis [ 6 , 7 ]. Researchers reach different conclusions—even when the same data have been processed—simply due to varied analytical procedures [ 8 , 9 ]. As we continue to recognize this problematic situation, some major causes of irreproducible research have been identified. This, in turn, provides the foundation for improvement by identifying and advocating for good research practices (GRPs). Indeed, powerful solutions are available, for example, preregistration of study protocols and statistical analysis plans, sharing of data and analysis code, and adherence to reporting guidelines. Although these and other best practices may facilitate reproducible research and increase trust in science, it remains the responsibility of researchers themselves to actively integrate them into their everyday research practices.
Contrary to ubiquitous specialized training, cross-disciplinary courses focusing on best practices to enhance the quality of research are lacking at universities and are urgently needed. The intersections between disciplines offer a space for peer evaluation, mutual learning, and sharing of best practices. In medical research, interdisciplinary work is inevitable. For example, conducting clinical trials requires experts with diverse backgrounds, including clinical medicine, pharmacology, biostatistics, evidence synthesis, nursing, and implementation science. Bringing researchers with diverse backgrounds and levels of experience together to exchange knowledge and learn about problems and solutions adds value and improves the quality of research.
The present selection of rules was based on our experiences with teaching GRP courses at the University of Zurich, our course participants’ feedback, and the views of a cross-disciplinary group of experts from within the Swiss Reproducibility Network ( www.swissrn.org ). The list is neither exhaustive, nor does it aim to address and systematically summarize the wide spectrum of issues including research ethics and legal aspects (e.g., related to misconduct, conflicts of interests, and scientific integrity). Instead, we focused on practical advice at the different stages of everyday research: from planning and execution to reporting of research. For a more comprehensive overview on GRPs, we point to the United Kingdom’s Medical Research Council’s guidelines [ 10 ] and the Swedish Research Council’s report [ 11 ]. While the discussion of the rules may predominantly focus on clinical research, much applies, in principle, to basic biomedical research and research in other domains as well.
The 10 proposed rules can serve multiple purposes: an introduction for researchers to relevant concepts to improve research quality, a primer for early-career researchers who participate in our GRP courses, or a starting point for lecturers who plan a GRP course at their own institutions. The 10 rules are grouped according to planning (5 rules), execution (3 rules), and reporting of research (2 rules); see Fig 1 . These principles can (and should) be implemented as a habit in everyday research, just like toothbrushing.
- PPT PowerPoint slide
- PNG larger image
- TIFF original image
GRP, good research practices.
https://doi.org/10.1371/journal.pcbi.1010139.g001
Research planning
Rule 1: specify your research question.
Coming up with a research question is not always simple and may take time. A successful study requires a narrow and clear research question. In evidence-based research, prior studies are assessed in a systematic and transparent way to identify a research gap for a new study that answers a question that matters [ 12 ]. Papers that provide a comprehensive overview of the current state of research in the field are particularly helpful—for example, systematic reviews. Perspective papers may also be useful, for example, there is a paper with the title “SARS-CoV-2 and COVID-19: The most important research questions.” However, a systematic assessment of research gaps deserves more attention than opinion-based publications.
In the next step, a vague research question should be further developed and refined. In clinical research and evidence-based medicine, there is an approach called population, intervention, comparator, outcome, and time frame (PICOT) with a set of criteria that can help framing a research question [ 13 ]. From a well-developed research question, subsequent steps will follow, which may include the exact definition of the population, the outcome, the data to be collected, and the sample size that is required. It may be useful to find out if other researchers find the idea interesting as well and whether it might promise a valuable contribution to the field. However, actively involving the public or the patients can be a more effective way to determine what research questions matter.
The level of details in a research question also depends on whether the planned research is confirmatory or exploratory. In contrast to confirmatory research, exploratory research does not require a well-defined hypothesis from the start. Some examples of exploratory experiments are those based on omics and multi-omics experiments (genomics, bulk RNA-Seq, single-cell, etc.) in systems biology and connectomics and whole-brain analyses in brain imaging. Both exploration and confirmation are needed in science, and it is helpful to understand their strengths and limitations [ 14 , 15 ].
Rule 2: Write and register a study protocol
In clinical research, registration of clinical trials has become a standard since the late 1990 and is now a legal requirement in many countries. Such studies require a study protocol to be registered, for example, with ClinicalTrials.gov, the European Clinical Trials Register, or the World Health Organization’s International Clinical Trials Registry Platform. Similar effort has been implemented for registration of systematic reviews (PROSPERO). Study registration has also been proposed for observational studies [ 16 ] and more recently in preclinical animal research [ 17 ] and is now being advocated across disciplines under the term “preregistration” [ 18 , 19 ].
Study protocols typically document at minimum the research question and hypothesis, a description of the population, the targeted sample size, the inclusion/exclusion criteria, the study design, the data collection, the data processing and transformation, and the planned statistical analyses. The registration of study protocols reduces publication bias and hindsight bias and can safeguard honest research and minimize waste of research [ 20 – 22 ]. Registration ensures that studies can be scrutinized by comparing the reported research with what was actually planned and written in the protocol, and any discrepancies may indicate serious problems (e.g., outcome switching).
Note that registration does not mean that researchers have no flexibility to adapt the plan as needed. Indeed, new or more appropriate procedures may become available or known only after registration of a study. Therefore, a more detailed statistical analysis plan can be amended to the protocol before the data are observed or unblinded [ 23 , 24 ]. Likewise, registration does not exclude the possibility to conduct exploratory data analyses; however, they must be clearly reported as such.
To go even further, registered reports are a novel article type that incentivize high-quality research—irrespective of the ultimate study outcome [ 25 , 26 ]. With registered reports, peer-reviewers decide before anyone knows the results of the study, and they have a more active role in being able to influence the design and analysis of the study. Journals from various disciplines increasingly support registered reports [ 27 ].
Naturally, preregistration and registered reports also have their limitations and may not be appropriate in a purely hypothesis-generating (explorative) framework. Reports of exploratory studies should indeed not be molded into a confirmatory framework; appropriate rigorous reporting alternatives have been suggested and start to become implemented [ 28 , 29 ].
Rule 3: Justify your sample size
Early-career researchers in our GRP courses often identify sample size as an issue in their research. For example, they say that they work with a low number of samples due to slow growth of cells, or they have a limited number of patient tumor samples due to a rare disease. But if your sample size is too low, your study has a high risk of providing a false negative result (type II error). In other words, you are unlikely to find an effect even if there truly was an effect.
Unfortunately, there is more bad news with small studies. When an effect from a small study was selected for drawing conclusions because it was statistically significant, low power increases the probability that an effect size is overestimated [ 30 , 31 ]. The reason is that with low power, studies that due to sampling variation find larger (overestimated) effects are much more likely to be statistically significant than those that happen to find smaller (more realistic) effects [ 30 , 32 , 33 ]. Thus, in such situations, effect sizes are often overestimated. For the phenomenon that small studies often report more extreme results (in meta-analyses), the term “small-study effect” was introduced [ 34 ]. In any case, an underpowered study is a problematic study, no matter the outcome.
In conclusion, small sample sizes can undermine research, but when is a study too small? For one study, a total of 50 patients may be fine, but for another, 1,000 patients may be required. How large a study needs to be designed requires an appropriate sample size calculation. Appropriate sample size calculation ensures that enough data are collected to ensure sufficient statistical power (the probability to reject the null hypothesis when it is in fact false).
Low-powered studies can be avoided by performing a sample size calculation to find out the required sample size of the study. This requires specifying a primary outcome variable and the magnitude of effect you are interested in (among some other factors); in clinical research, this is often the minimal clinically relevant difference. The statistical power is often set at 80% or larger. A comprehensive list of packages for sample size calculation are available [ 35 ], among them the R package “pwr” [ 36 ]. There are also many online calculators available, for example, the University of Zurich’s “SampleSizeR” [ 37 ].
A worthwhile alternative for planning the sample size that puts less emphasis on null hypothesis testing is based on the desired precision of the study; for example, one can calculate the sample size that is necessary to obtain a desired width of a confidence interval for the targeted effect [ 38 – 40 ]. A general framework to sample size justification beyond a calculation-only approach has been proposed [ 41 ]. It is also worth mentioning that some study types have other requirements or need specific methods. In diagnostic testing, one would need to determine the anticipated minimal sensitivity or specificity; in prognostic research, the number of parameters that can be used to fit a prediction model given a fixed sample size should be specified. Designs can also be so complex that a simulation (Monte Carlo method) may be required.
Sample size calculations should be done under different assumptions, and the largest estimated sample size is often the safer bet than a best-case scenario. The calculated sample size should further be adjusted to allow for possible missing data. Due to the complexity of accurately calculating sample size, researchers should strongly consider consulting a statistician early in the study design process.
Rule 4: Write a data management plan
In 2020, 2 Coronavirus Disease 2019 (COVID-19) papers in leading medical journals were retracted after major concerns about the data were raised [ 42 ]. Today, raw data are more often recognized as a key outcome of research along with the paper. Therefore, it is important to develop a strategy for the life cycle of data, including suitable infrastructure for long-term storage.
The data life cycle is described in a data management plan: a document that describes what data will be collected and how the data will be organized, stored, handled, and protected during and after the end of the research project. Several funders require a data management plan in grant submissions, and publishers like PLOS encourage authors to do so as well. The Wellcome Trust provides guidance in the development of a data management plan, including real examples from neuroimaging, genomics, and social sciences [ 43 ]. However, projects do not always allocate funding and resources to the actual implementation of the data management plan.
The Findable, Accessible, Interoperable, and Reusable (FAIR) data principles promote maximal use of data and enable machines to access and reuse data with minimal human intervention [ 44 ]. FAIR principles require the data to be retained, preserved, and shared preferably with an immutable unique identifier and a clear usage license. Appropriate metadata will help other researchers (or machines) to discover, process, and understand the data. However, requesting researchers to fully comply with the FAIR data principles in every detail is an ambitious goal.
Multidisciplinary data repositories that support FAIR are, for example, Dryad (datadryad.org https://datadryad.org/ ), EUDAT ( www.eudat.eu ), OSF (osf.io https://osf.io/ ), and Zenodo (zenodo.org https://zenodo.org/ ). A number of institutional and field-specific repositories may also be suitable. However, sometimes, authors may not be able to make their data publicly available for legal or ethical reasons. In such cases, a data user agreement can indicate the conditions required to access the data. Journals highlight what are acceptable and what are unacceptable data access restrictions and often require a data availability statement.
Organizing the study artifacts in a structured way greatly facilitates the reuse of data and code within and outside the lab, enhancing collaborations and maximizing the research investment. Support and courses for data management plans are sometimes available at universities. Another 10 simple rules paper for creating a good data management plan is dedicated to this topic [ 45 ].
Rule 5: Reduce bias
Bias is a distorted view in favor of or against a particular idea. In statistics, bias is a systematic deviation of a statistical estimate from the (true) quantity it estimates. Bias can invalidate our conclusions, and the more bias there is, the less valid they are. For example, in clinical studies, bias may mislead us into reaching a causal conclusion that the difference in the outcomes was due to the intervention or the exposure. This is a big concern, and, therefore, the risk of bias is assessed in clinical trials [ 46 ] as well as in observational studies [ 47 , 48 ].
There are many different forms of bias that can occur in a study, and they may overlap (e.g., allocation bias and confounding bias) [ 49 ]. Bias can occur at different stages, for example, immortal time bias in the design of the study, information bias in the execution of the study, and publication bias in the reporting of research. Understanding bias allows us researchers to remain vigilant of potential sources of bias when peer-reviewing and designing own studies. We summarized some common types of bias and some preventive steps in Table 1 , but many other forms of bias exist; for a comprehensive overview, see the Oxford University’s Catalogue of Bias [ 50 ].
https://doi.org/10.1371/journal.pcbi.1010139.t001
Here are some noteworthy examples of study bias from the literature: An example of information bias was observed when in 1998 an alleged association between the measles, mumps, and rubella (MMR) vaccine and autism was reported. Recall bias (a subtype of information bias) emerged when parents of autistic children recalled the onset of autism after an MMR vaccination more often than parents of similar children who were diagnosed prior to the media coverage of that controversial and meanwhile retracted study [ 51 ]. A study from 2001 showed better survival for academy award-winning actors, but this was due to immortal time bias that favors the treatment or exposure group [ 52 , 53 ]. A study systematically investigated self-reports about musculoskeletal symptoms and found the presence of information bias. The reason was that participants with little computer-time overestimated, and participants with a lot of computer-time spent underestimated their computer usage [ 54 ].
Information bias can be mitigated by using objective rather than subjective measurements. Standardized operating procedures (SOP) and electronic lab notebooks additionally help to follow well-designed protocols for data collection and handling [ 55 ]. Despite the failure to mitigate bias in studies, complete descriptions of data and methods can at least allow the assessment of risk of bias.
Research execution
Rule 6: avoid questionable research practices.
Questionable research practices (QRPs) can lead to exaggerated findings and false conclusions and thus lead to irreproducible research. Often, QRPs are used with no bad intentions. This becomes evident when methods sections explicitly describe such procedures, for example, to increase the number of samples until statistical significance is reached that supports the hypothesis. Therefore, it is important that researchers know about QRPs in order to recognize and avoid them.
Several questionable QRPs have been named [ 56 , 57 ]. Among them are low statistical power, pseudoreplication, repeated inspection of data, p -hacking [ 58 ], selective reporting, and hypothesizing after the results are known (HARKing).
The first 2 QRPs, low statistical power and pseudoreplication, can be prevented by proper planning and designing of studies, including sample size calculation and appropriate statistical methodology to avoid treating data as independent when in fact they are not. Statistical power is not equal to reproducibility, but statistical power is a precondition of reproducibility as the lack thereof can result in false negative as well as false positive findings (see Rule 3 ).
In fact, a lot of QRP can be avoided with a study protocol and statistical analysis plan. Preregistration, as described in Rule 2, is considered best practice for this purpose. However, many of these issues can additionally be rooted in institutional incentives and rewards. Both funding and promotion are often tied to the quantity rather than the quality of the research output. At universities, still only few or no rewards are given for writing and registering protocols, sharing data, publishing negative findings, and conducting replication studies. Thus, a wider “culture change” is needed.
Rule 7: Be cautious with interpretations of statistical significance
It would help if more researchers were familiar with correct interpretations and possible misinterpretations of statistical tests, p -values, confidence intervals, and statistical power [ 59 , 60 ]. A statistically significant p -value does not necessarily mean that there is a clinically or biologically relevant effect. Specifically, the traditional dichotomization into statistically significant ( p < 0.05) versus statistically nonsignificant ( p ≥ 0.05) results is seldom appropriate, can lead to cherry-picking of results and may eventually corrupt science [ 61 ]. We instead recommend reporting exact p -values and interpreting them in a graded way in terms of the compatibility of the null hypothesis with the data [ 62 , 63 ]. Moreover, a p -value around 0.05 (e.g., 0.047 or 0.055) provides only little information, as is best illustrated by the associated replication power: The probability that a hypothetical replication study of the same design will lead to a statistically significant result is only 50% [ 64 ] and is even lower in the presence of publication bias and regression to the mean (the phenomenon that effect estimates in replication studies are often smaller than the estimates in the original study) [ 65 ]. Claims of novel discoveries should therefore be based on a smaller p -value threshold (e.g., p < 0.005) [ 66 ], but this really depends on the discipline (genome-wide screenings or studies in particle physics often apply much lower thresholds).
Generally, there is often too much emphasis on p -values. A statistical index such as the p -value is just the final product of an analysis, the tip of the iceberg [ 67 ]. Statistical analyses often include many complex stages, from data processing, cleaning, transformation, addressing missing data, modeling, to statistical inference. Errors and pitfalls can creep in at any stage, and even a tiny error can have a big impact on the result [ 68 ]. Also, when many hypothesis tests are conducted (multiple testing), false positive rates may need to be controlled to protect against wrong conclusions, although adjustments for multiple testing are debated [ 69 – 71 ].
Thus, a p -value alone is not a measure of how credible a scientific finding is [ 72 ]. Instead, the quality of the research must be considered, including the study design, the quality of the measurement, and the validity of the assumptions that underlie the data analysis [ 60 , 73 ]. Frameworks exist that help to systematically and transparently assess the certainty in evidence; the most established and widely used one is Grading of Recommendations, Assessment, Development and Evaluations (GRADE; www.gradeworkinggroup.org ) [ 74 ].
Training in basic statistics, statistical programming, and reproducible analyses and better involvement of data professionals in academia is necessary. University departments sometimes have statisticians that can support researchers. Importantly, statisticians need to be involved early in the process and on an equal footing and not just at the end of a project to perform the final data analysis.
Rule 8: Make your research open
In reality, science often lacks transparency. Open science makes the process of producing evidence and claims transparent and accessible to others [ 75 ]. Several universities and research funders have already implemented open science roadmaps to advocate free and public science as well as open access to scientific knowledge, with the aim of further developing the credibility of research. Open research allows more eyes to see it and critique it, a principle similar to the “Linus’s law” in software development, which says that if there are enough people to test a software, most bugs will be discovered.
As science often progresses incrementally, writing and sharing a study protocol and making data and methods readily available is crucial to facilitate knowledge building. The Open Science Framework (osf.io) is a free and open-source project management tool that supports researchers throughout the entire project life cycle. OSF enables preregistration of study protocols and sharing of documents, data, analysis code, supplementary materials, and preprints.
To facilitate reproducibility, a research paper can link to data and analysis code deposited on OSF. Computational notebooks are now readily available that unite data processing, data transformations, statistical analyses, figures and tables in a single document (e.g., R Markdown, Jupyter); see also the 10 simple rules for reproducible computational research [ 76 ]. Making both data and code open thus minimizes waste of funding resources and accelerates science.
Open science can also advance researchers’ careers, especially for early-career researchers. The increased visibility, retrievability, and citations of datasets can all help with career building [ 77 ]. Therefore, institutions should provide necessary training, and hiring committees and journals should align their core values with open science, to attract researchers who aim for transparent and credible research [ 78 ].
Research reporting
Rule 9: report all findings.
Publication bias occurs when the outcome of a study influences the decision whether to publish it. Researchers, reviewers, and publishers often find nonsignificant study results not interesting or worth publishing. As a consequence, outcomes and analyses are only selectively reported in the literature [ 79 ], also known as the file drawer effect [ 80 ].
The extent of publication bias in the literature is illustrated by the overwhelming frequency of statistically significant findings [ 81 ]. A study extracted p -values from MEDLINE and PubMed Central and showed that 96% of the records reported at least 1 statistically significant p -value [ 82 ], which seems implausible in the real world. Another study plotted the distribution of more than 1 million z -values from Medline, revealing a huge gap from −2 to 2 [ 83 ]. Positive studies (i.e., statistically significant, perceived as striking or showing a beneficial effect) were 4 times more likely to get published than negative studies [ 84 ].
Often a statistically nonsignificant result is interpreted as a “null” finding. But a nonsignificant finding does not necessarily mean a null effect; absence of evidence is not evidence of absence [ 85 ]. An individual study may be underpowered, resulting in a nonsignificant finding, but the cumulative evidence from multiple studies may indeed provide sufficient evidence in a meta-analysis. Another argument is that a confidence interval that contains the null value often also contains non-null values that may be of high practical importance. Only if all the values inside the interval are deemed unimportant from a practical perspective, then it may be fair to describe a result as a null finding [ 61 ]. We should thus never report “no difference” or “no association” just because a p -value is larger than 0.05 or, equivalently, because a confidence interval includes the “null” [ 61 ].
On the other hand, studies sometimes report statistically nonsignificant results with “spin” to claim that the experimental treatment is beneficial, often by focusing their conclusions on statistically significant differences on secondary outcomes despite a statistically nonsignificant difference for the primary outcome [ 86 , 87 ].
Findings that are not being published have a tremendous impact on the research ecosystem, distorting our knowledge of the scientific landscape by perpetuating misconceptions, and jeopardizing judgment of researchers and the public trust in science. In clinical research, publication bias can mislead care decisions and harm patients, for example, when treatments appear useful despite only minimal or even absent benefits reported in studies that were not published and thus are unknown to physicians [ 88 ]. Moreover, publication bias also directly affects the formulation and proliferation of scientific theories, which are taught to students and early-career researchers, thereby perpetuating biased research from the core. It has been shown in modeling studies that unless a sufficient proportion of negative studies are published, a false claim can become an accepted fact [ 89 ] and the false positive rates influence trustworthiness in a given field [ 90 ].
In sum, negative findings are undervalued. They need to be more consistently reported at the study level or be systematically investigated at the systematic review level. Researchers have their share of responsibilities, but there is clearly a lack of incentives from promotion and tenure committees, journals, and funders.
Rule 10: Follow reporting guidelines
Study reports need to faithfully describe the aim of the study and what was done, including potential deviations from the original protocol, as well as what was found. Yet, there is ample evidence of discrepancies between protocols and research reports, and of insufficient quality of reporting [ 79 , 91 – 95 ]. Reporting deficiencies threaten our ability to clearly communicate findings, replicate studies, make informed decisions, and build on existing evidence, wasting time and resources invested in the research [ 96 ].
Reporting guidelines aim to provide the minimum information needed on key design features and analysis decisions, ensuring that findings can be adequately used and studies replicated. In 2008, the Enhancing the QUAlity and Transparency Of Health Research (EQUATOR) network was initiated to provide reporting guidelines for a variety of study designs along with guidelines for education and training on how to enhance quality and transparency of health research. Currently, there are 468 reporting guidelines listed in the network; see the most prominent guidelines in Table 2 . Furthermore, following the ICMJE recommendations, medical journals are increasingly endorsing reporting guidelines [ 97 ], in some cases making it mandatory to submit the appropriate reporting checklist along with the manuscript.
https://doi.org/10.1371/journal.pcbi.1010139.t002
The use of reporting guidelines and journal endorsement has led to a positive impact on the quality and transparency of research reporting, but improvement is still needed to maximize the value of research [ 98 , 99 ].
Conclusions
Originally, this paper targeted early-career researchers; however, throughout the development of the rules, it became clear that the present recommendations can serve all researchers irrespective of their seniority. We focused on practical guidelines for planning, conducting, and reporting of research. Others have aligned GRP with similar topics [ 100 , 101 ]. Even though we provide 10 simple rules, the word “simple” should not be taken lightly. Putting the rules into practice usually requires effort and time, especially at the beginning of a research project. However, time can also be redeemed, for example, when certain choices can be justified to reviewers by providing a study protocol or when data can be quickly reanalyzed by using computational notebooks and dynamic reports.
Researchers have field-specific research skills, but sometimes are not aware of best practices in other fields that can be useful. Universities should offer cross-disciplinary GRP courses across faculties to train the next generation of scientists. Such courses are an important building block to improve the reproducibility of science.
Acknowledgments
This article was written along the Good Research Practice (GRP) courses at the University of Zurich provided by the Center of Reproducible Science ( www.crs.uzh.ch ). All materials from the course are available at https://osf.io/t9rqm/ . We appreciated the discussion, development, and refinement of this article within the working group “training” of the SwissRN ( www.swissrn.org ). We are grateful to Philip Bourne for a lot of valuable comments on the earlier versions of the manuscript.
- View Article
- PubMed/NCBI
- Google Scholar
- 35. H. G. Zhang EZ. CRAN task view: Clinical trial design, monitoring, and analysis. 20 Jun 2021 [cited 3 Mar 2022]. Available: https://CRAN.R-project.org/view=ClinicalTrials .
- 36. Champely S. pwr: Basic Functions for Power Analysis. 2020. Available: https://CRAN.R-project.org/package=pwr .
- 43. Outputs Management Plan—Grant Funding. In: Wellcome [Internet]. [cited 13 Feb 2022]. Available: https://wellcome.org/grant-funding/guidance/how-complete-outputs-management-plan .
- 62. Cox DR, Donnelly CA. Principles of Applied Statistics. Cambridge University Press; 2011. Available: https://play.google.com/store/books/details?id=BMLMGr4kbQYC .
- 74. GRADE approach. [cited 3 Mar 2022]. Available: https://training.cochrane.org/grade-approach .

COMMENTS
Step 2: Data Type you Need for Research. Decide on the type of data you need for your research. The type of data you need to collect depends on your research questions or research hypothesis. Two types of research data can be used to answer the research questions: Primary Data Vs. Secondary Data.
A research design is a strategy for answering your research question using empirical data. Creating a research design means making decisions about: Your overall research objectives and approach. Whether you'll rely on primary research or secondary research. Your sampling methods or criteria for selecting subjects. Your data collection methods.
Table of contents. Step 1: Consider your aims and approach. Step 2: Choose a type of research design. Step 3: Identify your population and sampling method. Step 4: Choose your data collection methods. Step 5: Plan your data collection procedures. Step 6: Decide on your data analysis strategies.
Designing and Proposing Your Research Project. Designing your own study and writing your research proposal takes time, often more so than conducting the study. This practical, accessible guide walks you through the entire process. You will learn to identify and narrow your research topic, develop your research question, design your study, and ...
Before beginning your paper, you need to decide how you plan to design the study.. The research design refers to the overall strategy and analytical approach that you have chosen in order to integrate, in a coherent and logical way, the different components of the study, thus ensuring that the research problem will be thoroughly investigated. It constitutes the blueprint for the collection ...
After all, writing and research are integral parts of the overall enterprise. Therefore, design a project with an ultimate paper firmly in mind. Include an outline of the paper in the initial project design documents to help form the research objectives, determine the logical flow of the experiments, and organize the materials and data to be used.
Table of contents. Step 1: Define your variables. Step 2: Write your hypothesis. Step 3: Design your experimental treatments. Step 4: Assign your subjects to treatment groups. Step 5: Measure your dependent variable. Other interesting articles. Frequently asked questions about experiments.
Formatting a Chicago paper. The main guidelines for writing a paper in Chicago style (also known as Turabian style) are: Use a standard font like 12 pt Times New Roman. Use 1 inch margins or larger. Apply double line spacing. Indent every new paragraph ½ inch. Place page numbers in the top right or bottom center.
When to Write Research Design. Research design should be written before conducting any research study. It is an important planning phase that outlines the research methodology, data collection methods, and data analysis techniques that will be used to investigate a research question or problem. The research design helps to ensure that the ...
Phone: (954)639-9261/ +7 (776) 1673319. email: [email protected]. email: [email protected]. Printing company «FLASH PRINT». Address: Saryarka avenue, 8. Astana, Kazakhstan. email ...
this Ten Simple Rules series of PLOS Computational Biology [3-8]. Rule 1: Make It a Driving Force Never separate writing a paper from the underlying research. After all, writing and research are integral parts of the overall enterprise. Therefore, design a project with an ultimate paper firmly in mind. Include an outline of the paper in the
Use a draft that lists all the sub-sections you need to address in the research design. Be clear and concise. The research design should not include your opinions. It must show the reader an exact description of the way you conducted your study. Revise Your Research Design After Some Time Away.
To structure your methods section, you can use the subheadings of "Participants," "Materials," and "Procedures.". These headings are not mandatory—aim to organize your methods section using subheadings that make sense for your specific study. Note that not all of these topics will necessarily be relevant for your study.
Writing a Research Paper. This page lists some of the stages involved in writing a library-based research paper. Although this list suggests that there is a simple, linear process to writing such a paper, the actual process of writing a research paper is often a messy and recursive one, so please use this outline as a flexible guide.
After all, writing and research are integral parts of the overall enterprise. Therefore, design a project with an ultimate paper firmly in mind. Include an outline of the paper in the initial project design documents to help form the research objectives, determine the logical flow of the experiments, and organize the materials and data to be used.
Reading Time: 13 minutes In this article I will show you how to write a research paper using the four LEAP writing steps. The LEAP academic writing approach is a step-by-step method for turning research results into a published paper.. The LEAP writing approach has been the cornerstone of the 70 + research papers that I have authored and the 3700+ citations these paper have accumulated within ...
The typical research paper is a highly codified rhetorical form [1, 2]. Knowledge of the rules—some explicit, others implied—goes a long way toward writing a paper that will get accepted in a peer-reviewed journal. Primacy of the research question. A good research paper addresses a specific research question.
Step 4: Create a research design. The research design is a practical framework for answering your research questions. It involves making decisions about the type of data you need, the methods you'll use to collect and analyze it, and the location and timescale of your research. There are often many possible paths you can take to answering ...
Rule 3: Justify your sample size. Early-career researchers in our GRP courses often identify sample size as an issue in their research. For example, they say that they work with a low number of samples due to slow growth of cells, or they have a limited number of patient tumor samples due to a rare disease.
Develop a thesis statement. Create a research paper outline. Write a first draft of the research paper. Write the introduction. Write a compelling body of text. Write the conclusion. The second draft. The revision process. Research paper checklist.