Get Your ALL ACCESS Shop Pass here →
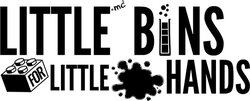

Drops Of Water On A Penny
Have you ever thought about how many drops of water can fit on a penny ? Try this fun science experiment with things found in your purse or pocket! Explore the surface tension of water with this fun and easy penny lab with the kids. We are always on the hunt for simple science experiments , and this one is just super fun and easy!
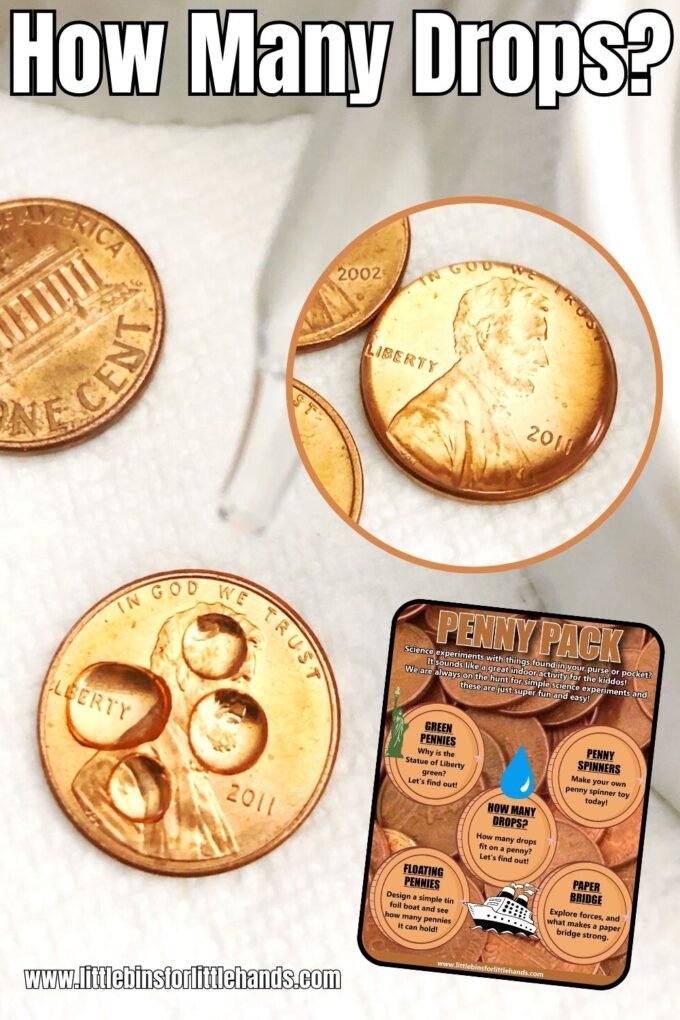
Explore How Many Water Drops On Penny
Add this simple penny lab to your science activities this season. If you want to learn about the surface tension of water, let’s dig in. While you’re at it, make sure to check out these other fun water science experiments.
Exploring water drops on a penny is a fantastic science experiment for kids because it’s simple and fascinating. By placing drops of water onto a penny, kids can observe how the water beads up and forms a dome-like shape, defying gravity.
This phenomenon occurs due to surface tension , the cohesive force that causes water molecules to stick together. Through this experiment, kids can learn about surface tension and how it affects the behavior of liquids. They can also experiment with variables such as the number of water drops or the penny’s cleanliness to see how it impacts the results. Additionally, this experiment can be efficiently conducted with everyday household items, making it accessible and engaging for scientists of all ages.
Water Drops On A Penny Project
You can also easily turn your penny experiment into a fantastic presentation along with your hypothesis. Check out the resources below to get started.
- Easy Science Fair Projects
- Science Project Tips From A Teacher
- Science Fair Board Ideas
Penny Lab Experiment In The Classroom
TIP: If you want to add a little variety to this experiment, swap out the pennies for nickels, dimes, and quarters. Ask your students to guess how many drops will fit on each coin. Record the date from the experiment and make a class graph chart with your results!
Additional ideas for penny lab hypotheses include using different liquids or clean vs. dirty pennies . What else can you think of when creating an experiment?
Get Your Free Printable Penny Lab Guide!
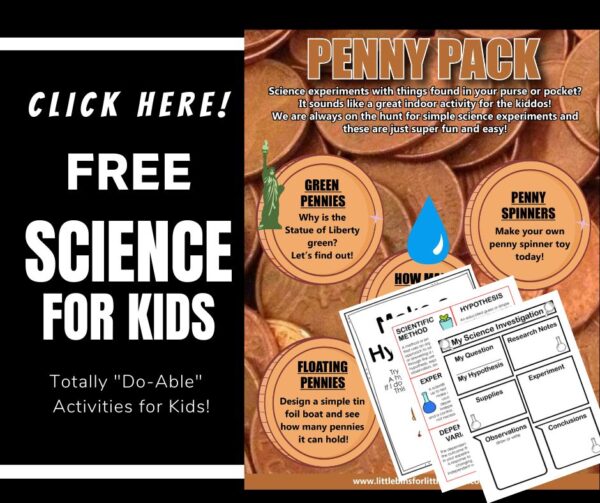
Penny Lab Experiment
Let’s investigate how many drops of water can fit on a penny. Grab your purse, turn over the couch cushions, or break out the piggy bank; it’s time to find some pennies to experiment with!
- Eyedropper or pipette
- Food coloring (makes seeing this in action MUCH easier, but is optional)
- Small bowls
Penny Experiment Set Up:
STEP 1: Add water to both of your bowls, and one of them, add green food coloring. This is optional if you want to see the drops a bit better.
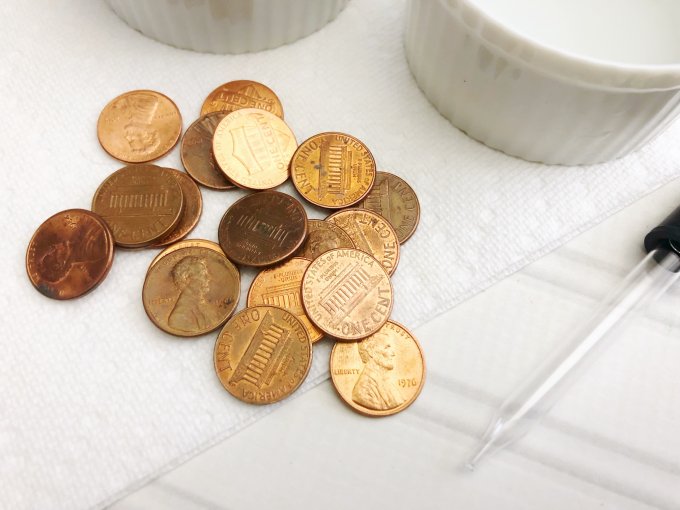
STEP 2: Use an eyedropper or pipette to pick up and carefully drip one drop of water at a time onto the penny.
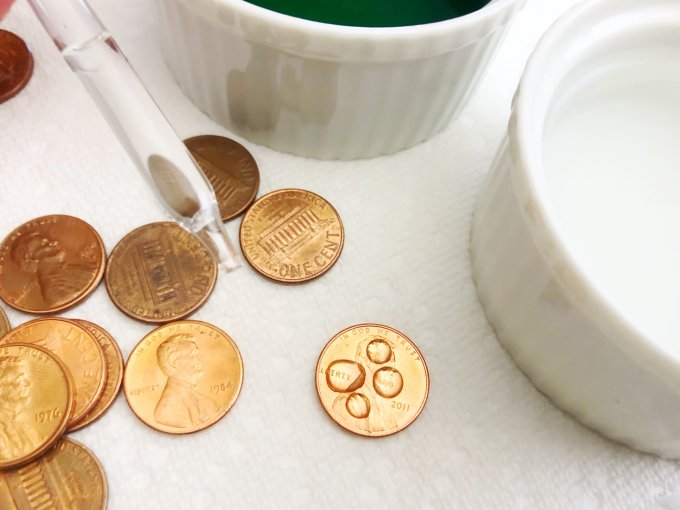
STEP 3: Count how many drops you can fit onto one penny until the water overflows.
We were able to get ours up to about 27! Go ahead and record the data for separate trials on the same coin. What can you conclude?
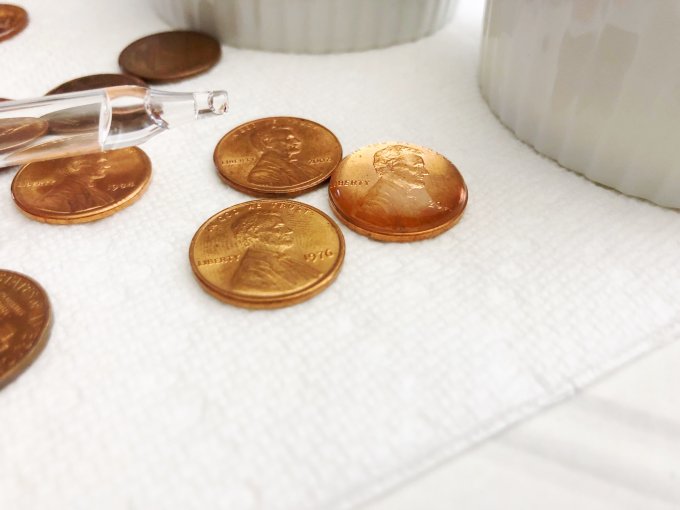
Why Do So Many Drops Of Water Fit On A Penny?
Were you surprised that a lot more drops of water fit on a penny than you predicted? We had 27 drops of water on ours!
What property of water allows the water to stick to the penny? Surface tension and cohesion are why you can get so many drops of water on a penny.
Cohesion is the “stickiness” of like molecules to one another. Water molecules love to stick together! Surface tension is the result of all the water molecules sticking together. Learn more about the surface tension of water !
Once the water has reached the edge of the penny, a dome shape begins to form. This is due to the surface tension forming a shape with the least amount of surface area possible (like bubbles )!
What happens if you use a different liquid like alcohol? Can you as many drops on a penny?
More Fun Penny Experiments
- Sink the boat challenge!
- Penny Spinners
- Green Pennies Experiment
- Paper Bridge STEM Challenge
- Lemon Battery STEM Project
Use the Scientific Method
This drops of water on a penny experiment is a fantastic opportunity to use the scientific method and record your experiment using the free mini worksheet pack below.
You can read about using the scientific method here , and find more information on the independent and dependent variables !
The first step in the scientific method is asking a question and developing a hypothesis.
Apply the scientific method to this drops-on-a-penny science activity and turn it into a surface tension experiment by choosing a question to investigate.
- How many drops do you think will fit on a penny? (PREDICTION)
- What happens when one drop of water meets another drop? (OBSERVATION)
- Which coin held the most water? (EXPLANATION)
- Can you think of everyday examples of surface tension? (APPLICATION)
Helpful Science Resources To Get You Started
Here are a few resources that will help you introduce science more effectively to your kiddos or students and feel confident yourself when presenting materials. You’ll find helpful free printables throughout.
- Best Science Practices (as it relates to the scientific method)
- Science Vocabulary
- 8 Science Books for Kids
- All About Scientists
- Science Supplies List
- Science Tools for Kids
More Science Experiments To Try
Check out our list of science experiments for Jr Scientists!
- Walking Water
- Rubber Egg Experiment
- Why Do Things Float In Salt Water?
- Water Density Experiment
Printable Science Projects For Kids
If you’re looking to grab all of our printable science projects in one convenient place plus exclusive worksheets and bonuses like a STEAM Project pack, our Science Project Pack is what you need! Over 300+ Pages!
- 90+ classic science activities with journal pages, supply lists, set up and process, and science information. NEW! Activity-specific observation pages!
- Best science practices posters and our original science method process folders for extra alternatives!
- Be a Collector activities pack introduces kids to the world of making collections through the eyes of a scientist. What will they collect first?
- Know the Words Science vocabulary pack includes flashcards, crosswords, and word searches that illuminate keywords in the experiments!
- My science journal writing prompts explore what it means to be a scientist!!
- Bonus STEAM Project Pack: Art meets science with doable projects!
- Bonus Quick Grab Packs for Biology, Earth Science, Chemistry, and Physics
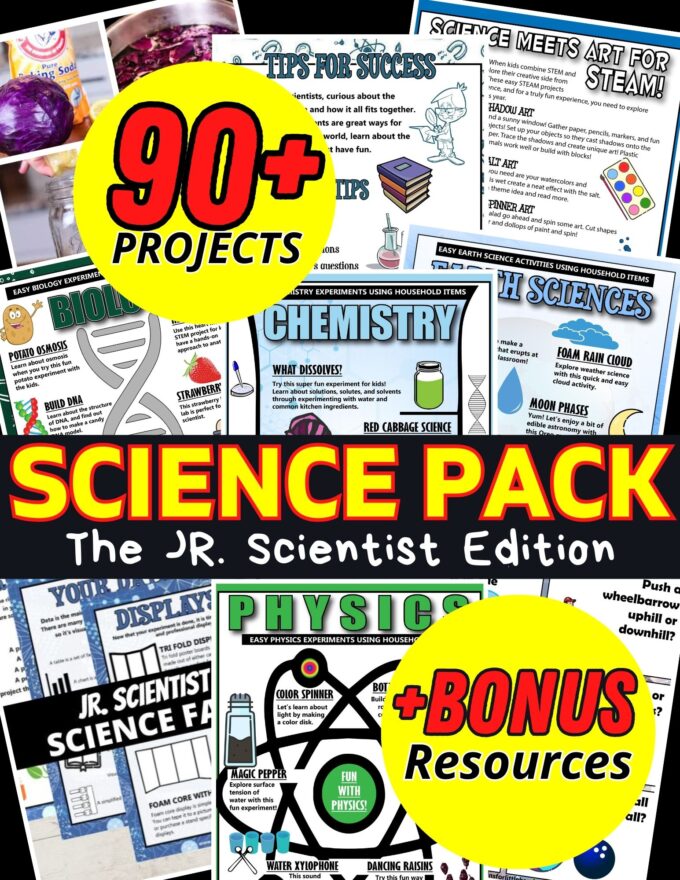
One Comment
- Pingback: Simple Science Experiments For Kids | Little Bins for Little Hands
Comments are closed.
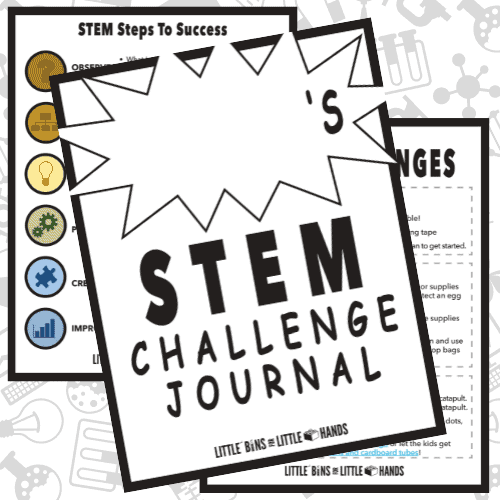
Subscribe to receive a free 5-Day STEM Challenge Guide
~ projects to try now ~.
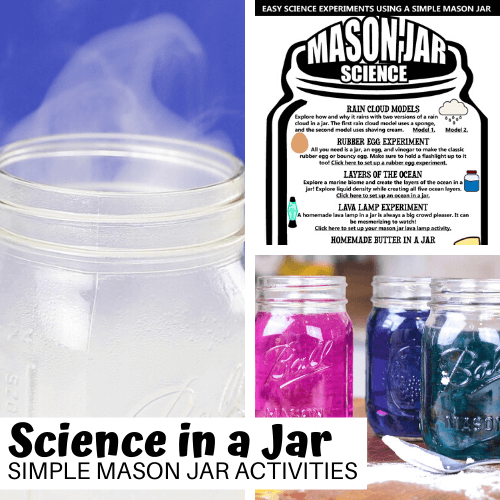
Drops on a Coin
Activity length, 10-15 mins., activity type, discrepant event (investigatable).
In this demonstration, students' assumptions are challenged as they observe the power of surface tension in water droplets.
Although a water molecule has an overall neutral charge, the actual structure of a water molecule makes it a polar molecule (it has a positive end and a negative end). The two hydrogen atoms are slightly positive, and the oxygen atom is slightly negative. A weak link occurs between the negatively charged oxygen atom of one water molecule and the positively charged hydrogen atoms of a neighbouring water molecule. This weak link is called a hydrogen bond . The polarity of the water molecule can also cause it to be attracted to molecules of other polar substances.
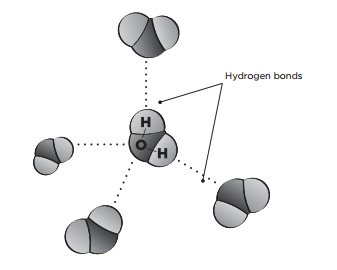
There are two types of "stickiness" in this demonstration: cohesion and adhesion.
The attraction between water molecules is called cohesion . The cohesive force that occurs between water molecules is so strong that, at the water's surface, it creates a "skin", which is known as surface tension . Surface tension is strong enough to support insects that crawl across the water's surface, like water striders (Gerridae).
The attraction of water molecules to other substances, like soil or glass, is called adhesion . As drops of water are added onto a penny, the adhesive force between the water and the penny keeps the water from falling off.
Cohesive forces are strong, but not unbreakable. As a water drop builds up and out, usually bulging over the sides of the penny, the cohesive forces will eventually be overcome by the force of gravity on the water molecules. The "skin" will burst, and all of the water will spill off.
The cohesive forces between polar molecules are stronger than those between non-polar molecules, such as those in oil or syrup. That's why you can make a bigger "pile" of water than of oil or syrup.
Describe the cohesive and adhesive properties of water.
Per Student or Pair: 5 cent coin or penny an eyedropper water mineral/baby/olive oil corn syrup
Key Questions
- How many drops of water they think a coin can hold? Were your predictions correct?
- Does it matter if the coin is heads or tails?
- Will syrup/oil hold more or less drops than water? Why?
- How is it possible to get so many water drops on a coin?
- What causes the ‘skin’ on the surface of the big droplet?
- Would a 10 cent coin hold more or less drops? Why?
- Teacher Tip: Younger students may believe a 10 cent coin holds more because it is worth more despite being smaller.
- Place a coin on a table or desk.
- Holding the eyedropper close to the surface of the coin, carefully squeeze water droplets onto the coin, one at a time. The droplets should pool up on the coin, creating a big droplet of water. Get the students to count the drops.
- Stop squeezing when the droplet on the coin breaks up and overflows. The count is the number of drops that the coin could hold before the one that caused the coin to overflow.
- Wipe off the coin or use a new one.
- As before, gradually add drops of oil or syrup to the surface of the coin using the eyedropper. Get the students to count the drops.
Teacher Tip: To make sure your count is accurate, hold the eyedropper far enough above the coin so that the drop has to fall a short distance before fusing with the droplet on the coin.
- Perform this as a student activity, rather than just a demo.
- Add a drop of soap/detergent to the water you use. It reduces the surface tension causing a dramatic reduction in the number of drops that will fit on the coin.
- Start with a full glass of plain water (with a dry rim to prevent the water from dripping down the side of the glass). How many coins can we add to the water without the glass overflowing?
- Gently add coins one by one. Because of surface tension, the water will rise above the rim of the glass before it spills (just like the initial experiment). Compare your original prediction with the number of coins you were able to add.
About the sticker
Artist: Jeff Kulak
Jeff is a senior graphic designer at Science World. His illustration work has been published in the Walrus, The National Post, Reader’s Digest and Chickadee Magazine. He loves to make music, ride bikes, and spend time in the forest.
Comet Crisp
T-Rex and Baby
Artist: Michelle Yong
Michelle is a designer with a focus on creating joyful digital experiences! She enjoys exploring the potential forms that an idea can express itself in and helping then take shape.
Buddy the T-Rex
Science Buddies
Artist: Ty Dale
From Canada, Ty was born in Vancouver, British Columbia in 1993. From his chaotic workspace he draws in several different illustrative styles with thick outlines, bold colours and quirky-child like drawings. Ty distils the world around him into its basic geometry, prompting us to look at the mundane in a different way.
Western Dinosaur
Time-Travel T-Rex
Related Resources
Wonderful water, what are the physical and chemical properties of water that make it so unique and necessary for living things…, we believe that now, more than ever, the world needs people who care about science. help us fund the future and next generation of problem solvers, wonder seekers, world changers and nerds..
Let’s simplify art for kids. Unlock your membership library today!🔑

- Make Learning Fun
Penny Experiment with Water Drops
A penny experiment that demonstrates how water tension works and water can hold together in a dome shape – a super simple science experiment for kids!
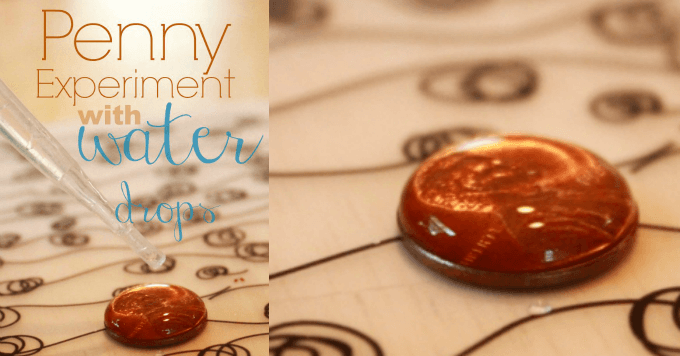
Drop water on a penny and it just runs over the side, right?
Maybe not…
Maia was excited to show us a penny experiment she learned at school that demonstrated how water can hold together in a dome shape. This super simple science experiment requires only a penny, some water, and a dropper.
The Penny Experiment in Action
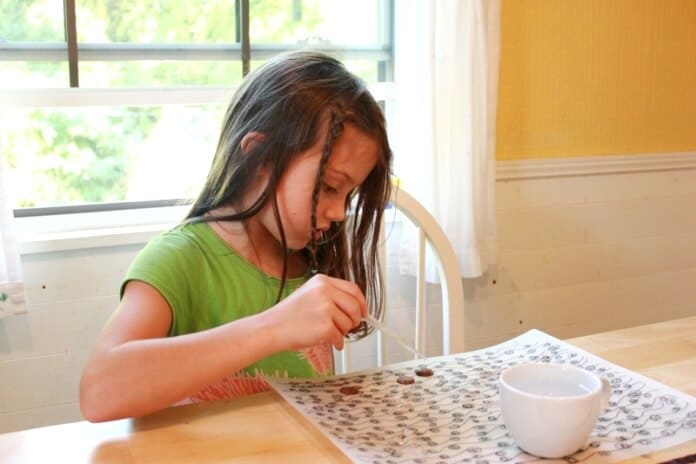
She showed us how we could carefully squeeze a drop of water at a time onto the top of the penny…
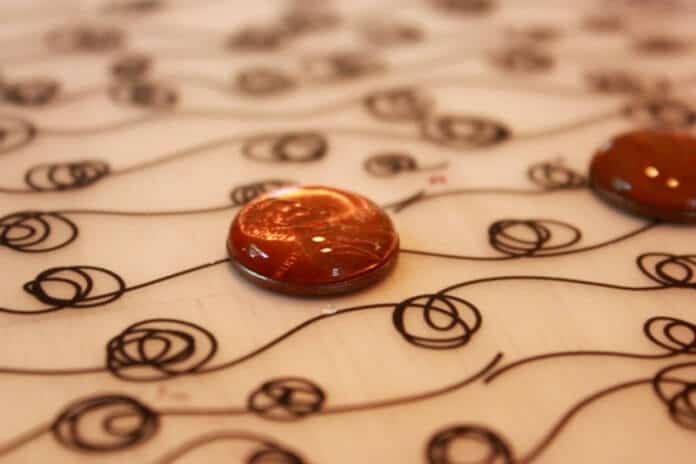
…and the droplets would combine to form a bubble of water.
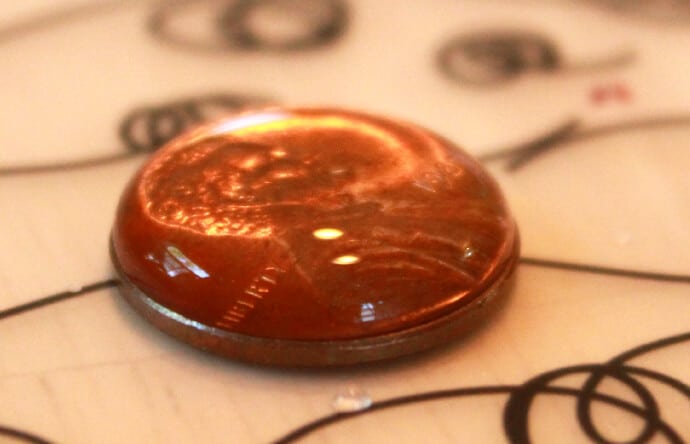
Maia thought it looked a little like a snow globe (one of her favorite things).
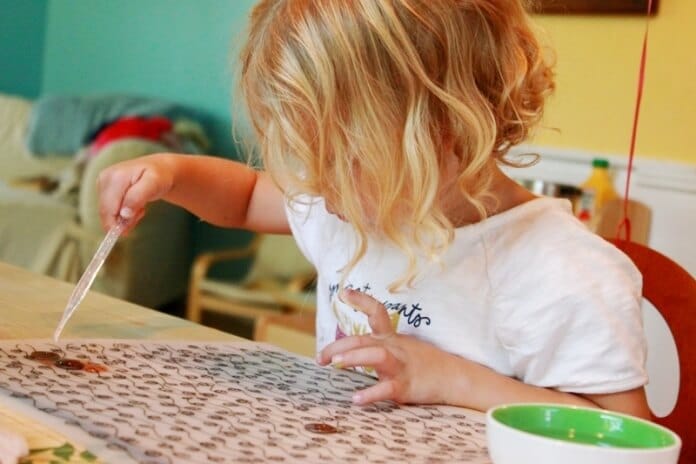
We each tried the penny experiment to see how many drops of water it would take before it reached its limit. Because once it did, the water bubble burst and flowed over the edge of the penny.
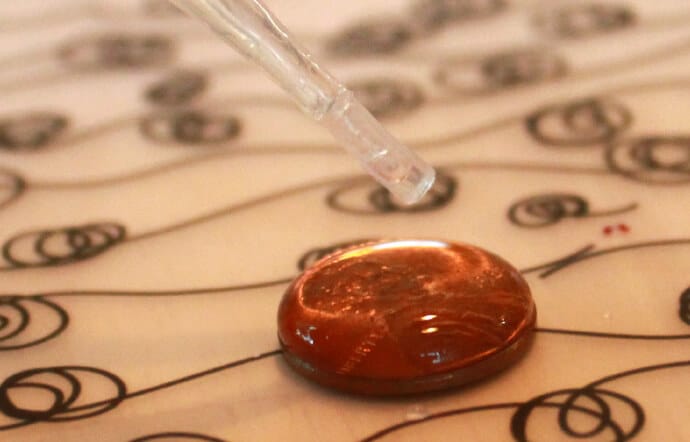
Pretty cool, eh? We all had fun with this one. I think it’s definitely worth keeping it in mind for a rainy afternoon. Or just when you need a quick and easy activity for the kiddos.
By the way, if you want the technical explanation for why this works and what’s going on with the water, check out Steve Spangler Science .
3 More Favorite Simple Science Experiments for Kids
- The Rainbow Milk Science Experiment
- Make Painted Daisies
- Make a Baking Soda Volcano
P.S. Want even more science? Here’s my collection of The Best Kids Science Experiments to Try at Home .
Pin It for Later
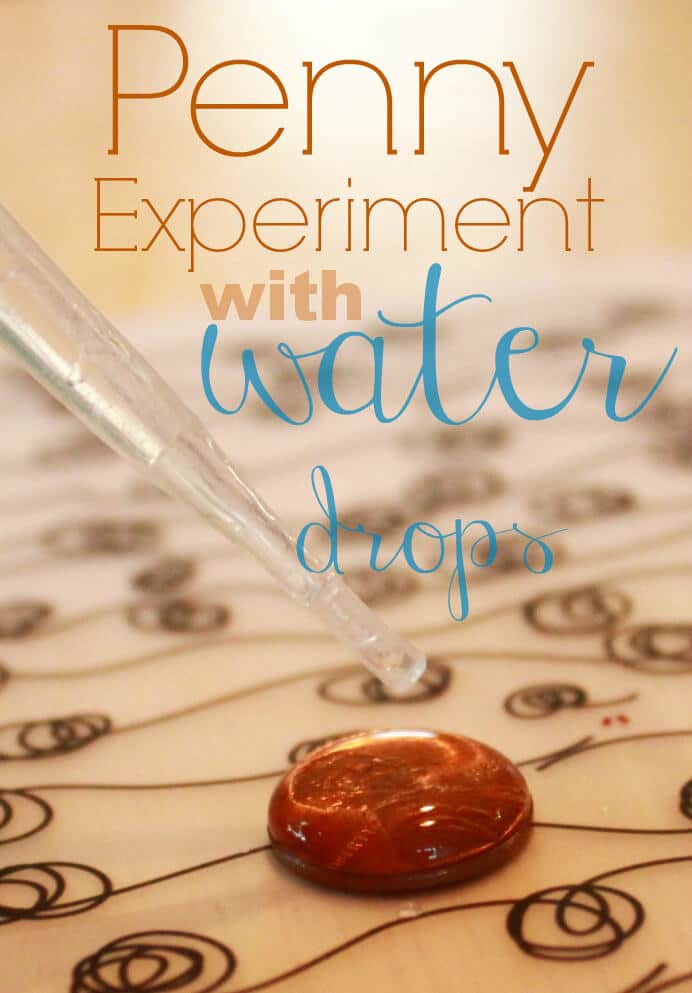
Related Posts
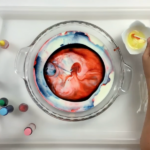
This magic milk science experiment is awesome. Super simple to try with materials you already…
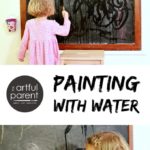
Do your kids like to paint with water? This easiest and least messy of kids'…
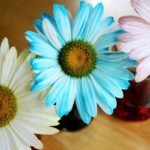
Painted daisies are one of our favorite simple science experiment for kids and are always…
- big kids , preschoolers
Jean Van't Hul
Leave a reply.
Your email address will not be published. Required fields are marked *
Save my name, email, and website in this browser for the next time I comment.

FREE EBOOK!
Subscribe to get your free copy of the 10 BEST Kids Art Ideas eBook!
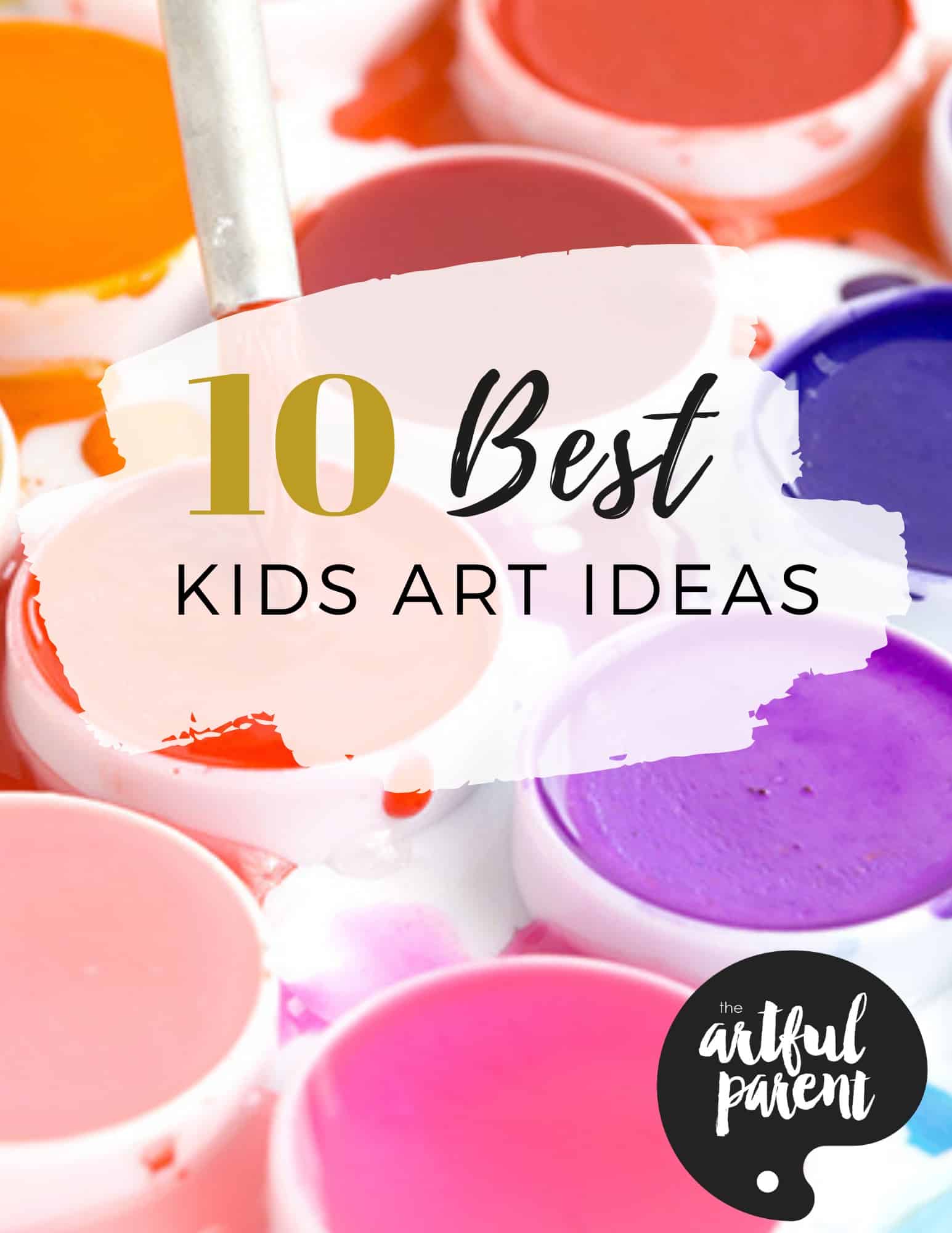
MOST VIEWED POSTS
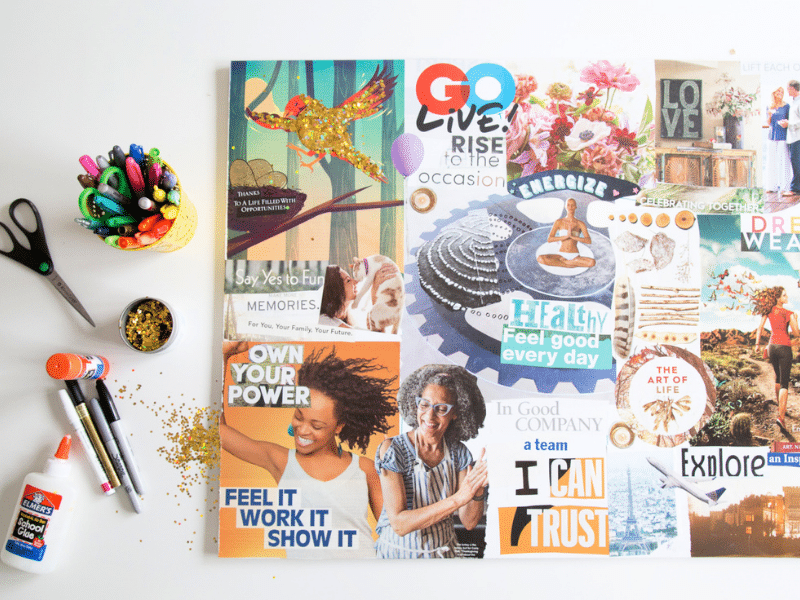
How to Make a Vision Board That Works in 9 Simple Steps
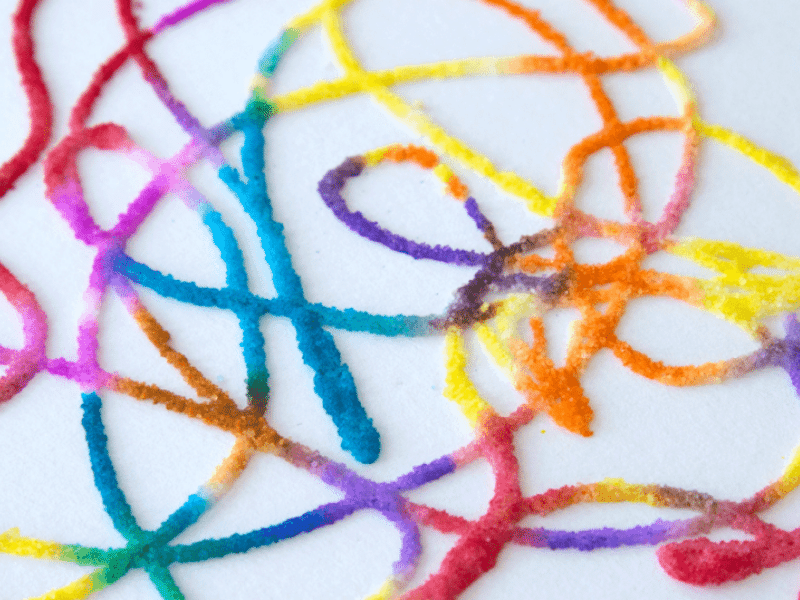
How to Make Raised Salt Paintings
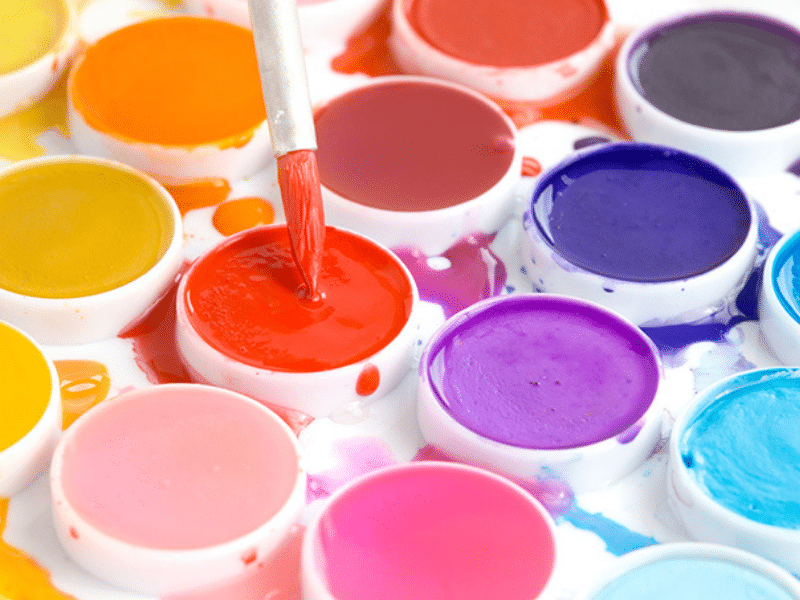
The Best Art Ideas for 2024
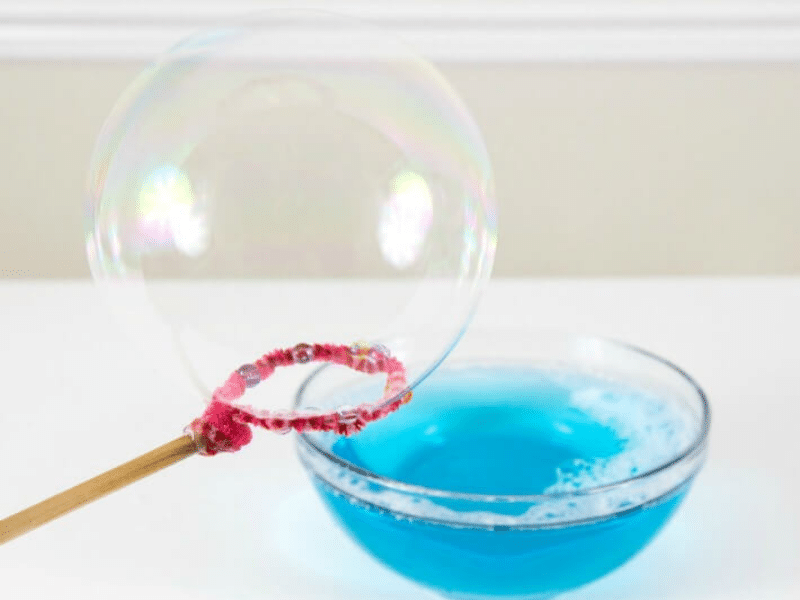
How to Make Homemade Bubbles
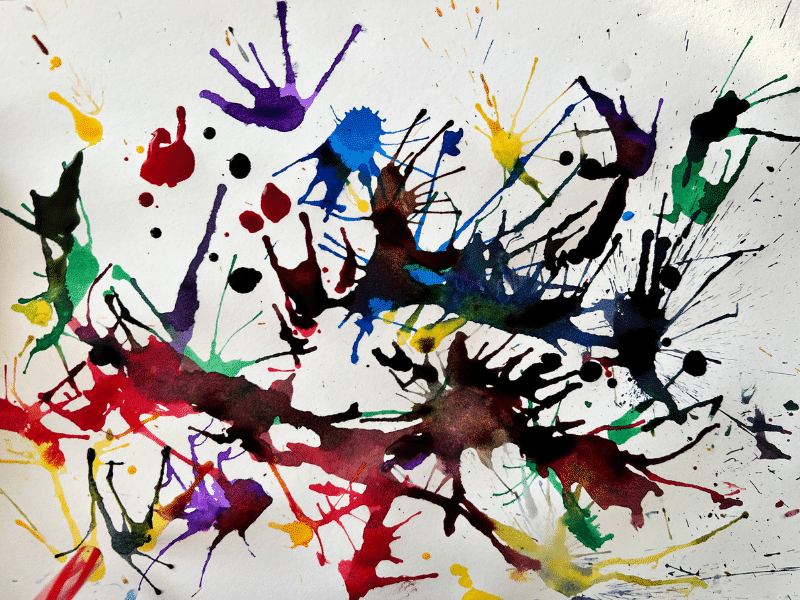
How to Do Blow Painting with Straws
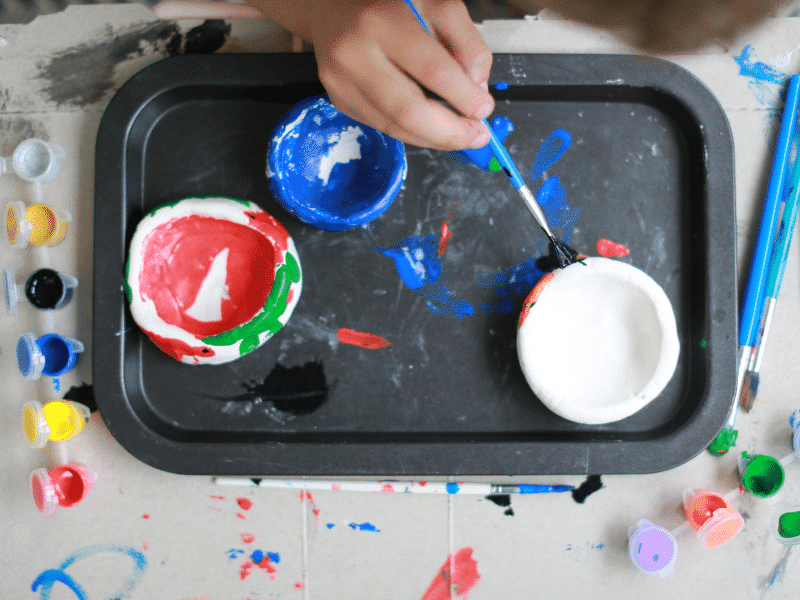
15 Amazing Clay Projects for Kids
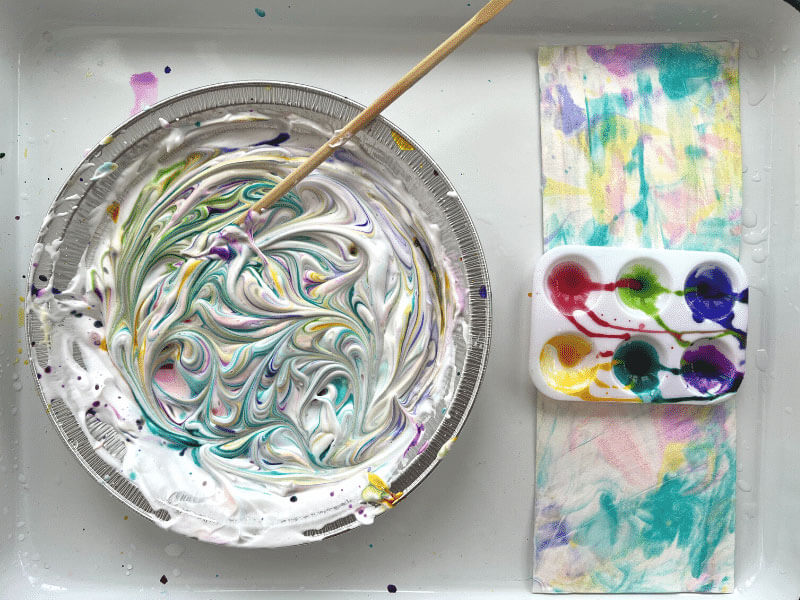
6 Easy Paper Marbling Techniques
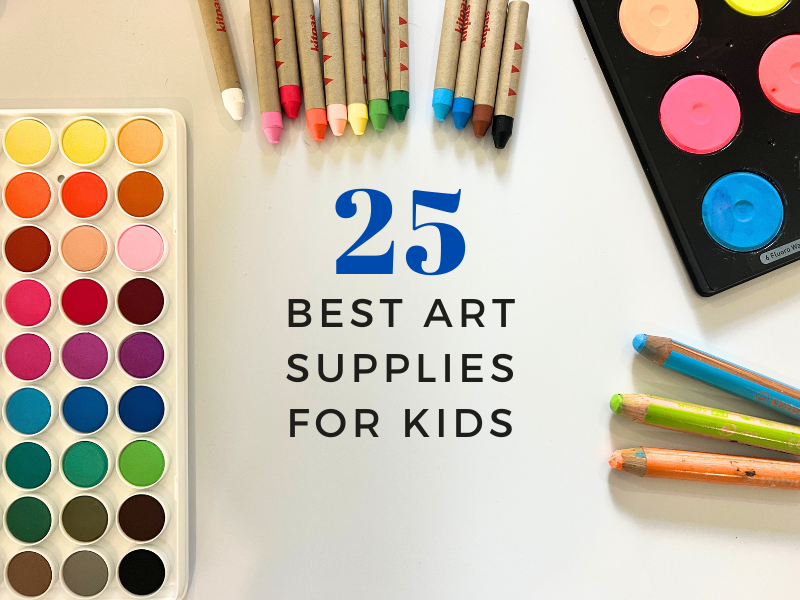
The Best Art Supplies for 2024
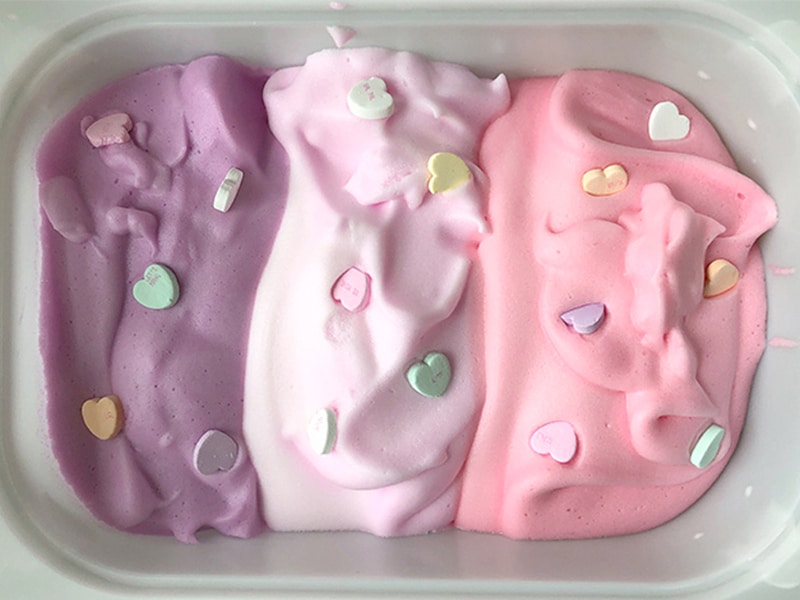
How to Make Aquafaba
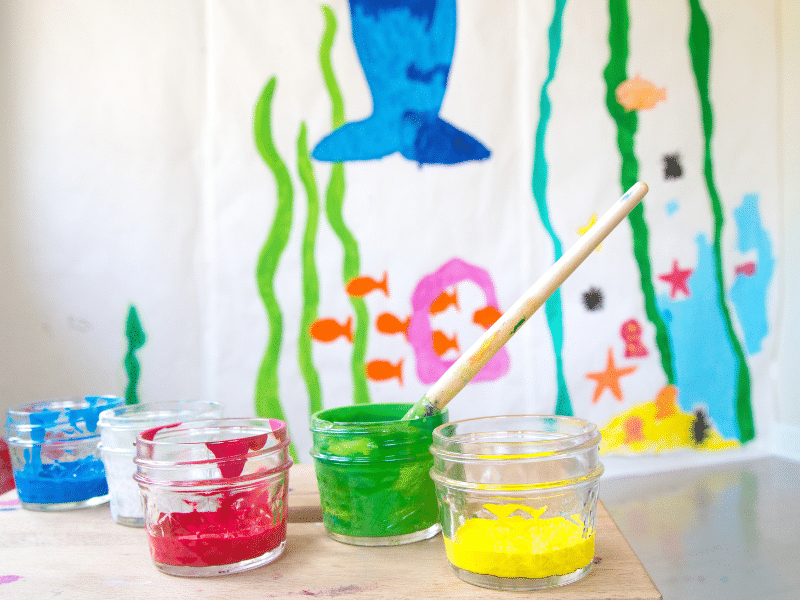
The Benefits of Arts for Kids
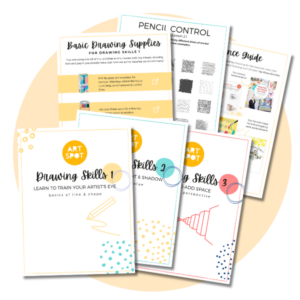
GET THE ARTFUL PARENT – BEST OF THE PAST 12 MONTHS EBOOK FOR FREE!
Just fill out your details below and we’ll send you the ebook containing the Artful Parent’s most visited activities from the past 12 months, for free!
Discover More
Art activities, art supplies, gifts guide, kids art spot, join our newsletter and get your free ebook with the artful parent's best art ideas for kids, join our newsletter and get a free ebook with the artful parent's 10 best kids art ideas, stay connected with us, quick links.
- Privacy Policy
June 25, 2015
Measure Surface Tension with a Penny
A soapy science activity from Science Buddies
By Science Buddies
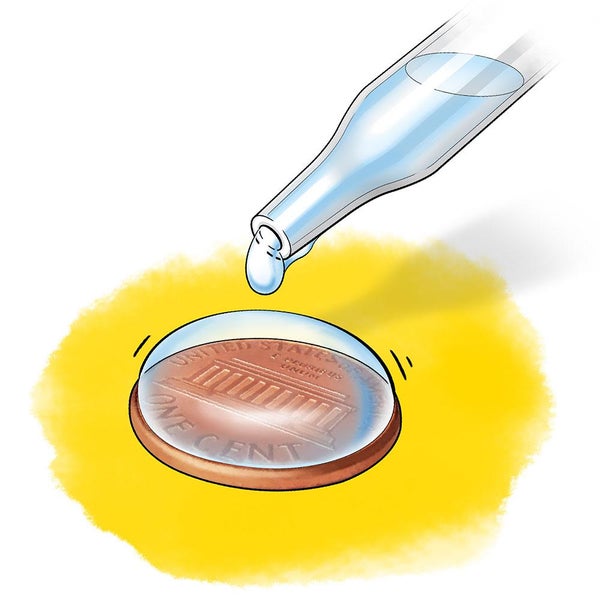
Learn about the secrets of soap in this surprising surface tension-testing activity!
George Retseck
Key concepts Chemistry Molecules Surface tension
Introduction Have you ever noticed on a rainy day how water forms droplets on a window? Why does it do that instead of spreading out evenly over the whole surface? You might not guess it but this property of water is also related to washing dishes and doing the laundry. How? It all has to do with something called surface tension. Try this activity to learn more!
Background You have probably noticed that if you look at a surface outside on a rainy day or spill some water inside, the liquid tends to form droplets that stick up from a surface instead of spreading out into an even sheet. This occurs because water is made up of many tiny molecules that are all attracted to one another. Molecules in the middle of a drop of water are pulled evenly in all directions by all the nearby molecules. Those near the droplet’s surface, however, are pulled mostly inward by the water molecules below them. This creates "surface tension." The surface of the water droplet is held together by the attraction between molecules.
On supporting science journalism
If you're enjoying this article, consider supporting our award-winning journalism by subscribing . By purchasing a subscription you are helping to ensure the future of impactful stories about the discoveries and ideas shaping our world today.
Now, think about washing dirty dishes or clothing. There will be lots of tiny little holes and cracks that water needs to get into to wash away dirt and grime, such as the spaces between the fibers of a shirt or between a plate and bits of dried food. In order for water to flow more easily into these small spaces, you need to decrease its surface tension. You can do this by adding soap, which is a surfactant (a material that decreases the surface tension of a liquid). In this activity you will see how soap decreases the surface tension of water by putting water droplets on top of a penny.
Medicine dropper or eyedropper
Glass, cup or small bowl
Dish towel or paper towel
Flat, level surface that can get wet, such as a kitchen counter
Paper and pencil or pen (optional)
Preparation
Place your penny on a flat, level surface that can get a little wet, such as a kitchen counter.
Fill a glass, cup or small bowl with tap water.
Fill the medicine dropper with water.
Now carefully add one drop of water at a time to the top of the penny. Hold the medicine dropper just above the top of the penny (not touching it) so each new drop has to fall a short distance before it merges with the drop on the penny. You can write down the number of drops you add if you like. How many drops of water do you think will fit on top of the penny? Watch the drop on top of the penny carefully as it grows. It should keep getting bigger and bigger until it touches the edges of the penny.
Keep adding drops (refill your medicine dropper as necessary) one at a time. How big does the drop on the penny get before it finally spills over the edges?
Once the drop spills over the penny’s edge, use a towel to completely dry off the penny and surrounding surface. How many drops of water were you able to add before the water ran over the penny’s sides?
Mix a small amount of dish soap with your tap water.
Now, repeat the experiment using soapy water. Do you think you will be able to add more drops or less before the liquid spills over the sides of the penny? Again, slowly add one drop at a time. How big does the drop of water on top of the penny get before it breaks and flows over the edges?
Extra : Try the experiment with different liquids or other things you can find in your kitchen. (Make sure you have an adult's approval to use any liquids before you handle them.) How do different soaps and detergents like hand soap or laundry detergent compare with one another? What about other liquids like milk or juice? Which ones make the biggest (or smallest) drops? With the most or least number of drops?
Extra : Try using something other than a penny to collect the droplets. What happens if you use different materials, such as the flat top of a small plastic bottle cap or a button?
[break] Observations and results You should find that plain tap water produces a much larger, stable drop of water on top of the penny than the soapy water does. This is because plain tap water has higher surface tension, so the surface is "stronger" and can hold together a larger drop. Adding soap lowers the water’s surface tension so the drop becomes weaker and breaks apart sooner. Making water molecules stick together less is what helps soaps clean dishes and clothes more easily.
More to explore Sticky Water , from Exploratorium Soap , from Exploratorium Measuring Surface Tension of Water with a Penny , from Science Buddies Surface Tension Science: Build a Raft Powered by Soap , from Scientific American Science Activities for All Ages! , from Science Buddies
This activity brought to you in partnership with Science Buddies
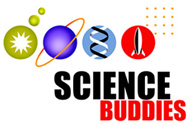
The Science Kiddo
Tuesday, january 20, 2015, surface tension | drops of water on a coin + free printable.

- Set the coin on a flat surface.
- Fill a plastic pipette with water.
- Carefully squeeze out water drop by drop from the pipette onto the coin. Count how many drops fit on the coin before the dome breaks and the water spills over.
- Keep track of your results on this free printable table ! There is room to do three trials for each coin so you can average the trials together to see which coin holds the most water!

32 comments:

This is fun! Going to do it with my kids later today! Thank you for sharing it with us #pintorials
AWESOME! Have so much fun :)

Wow! This looks like a pretty cool experiment!
Hey thanks!
This is an awesome experiment! What fun! I love fun and easy experiments. I am definitely sharing this on my STEM board. Thanks so much for sharing!
Thank you for stopping by and for sharing!!
Thanks for sharing your article on the Hip Homeschool Moms Hop. I chose it as my favorite article from last week's Hop, so you are featured on this week's Hop! Don't forget to stop by and grab your "I was featured on Hip Homeschool Moms" button! Your article has also been added to our Science Board on Pinterest :-) We are looking forward to seeing more articles from you in the future!
Thank you so much, I am so honored! And I was so touched by what you said on the featured article about hands-on activities and my website :) I love stopping by the Hip Homeschool Hop every week to see what is new.
Just popped over to this experiment after reading your Magic Milk......have to try this one too!!
Your work is very good and I appreciate you and hopping for some more informative posts. Thank you for sharing great information to us. Litecoin
Thanks for taking the time to discuss this, I feel strongly about it and love learning more on this topic. If possible, as you gain expertise, mayo school online would you mind updating your blog with extra information? It is extremely helpful for me.
Thanks For sharing this Superb article.I use this Article to show my assignment in college www.srichaitanyaschool.net holiday homework .it is useful For me Great Work
Does not apply to SBA loans taken out before the stimulus bill. As to non-SBA loans, they can be before or after the bill's enactment. The LoanRepublic
It’s appropriate time to make some plans for the future and it is time to be happy. I have read this post and if I could I wish to suggest you few interesting things or advice. Perhaps you could write next articles referring to this article. I desire to read even more things about it! hinada.com
When your website or blog goes live for the first time, it is exciting. That is until you realize no one but you and your. about Isro
Wow! Such an amazing and helpful post this is. I really really love it. It's so good and so awesome. I am just amazed. I hope that you continue to do your work like this in the future also https://aquafiltermag.com
I am looking for and I love to post a comment that "The content of your post is awesome" Great work! idealica
I am looking for and I love to post a comment that "The content of your post is awesome" Great work! NJ Parcels
Easily, the article is actually the best topic on this registry related issue. I fit in with your conclusions and will eagerly look forward to your next updates. NJ Parcels
Good to become visiting your weblog again, it has been months for me. Nicely this article that i've been waited for so long. I will need this post to total my assignment in the college, and it has exact same topic together with your write-up. Thanks, good share. NJ Parcels

I am looking for and I love to post a comment that "The content of your post is awesome" Great work! Laravel Project Management
I definitely enjoying every little bit of it and I have you bookmarked to check out new stuff you post. Hotel Management System In PHP MySqli With Free Source Code Download
I am looking for and I love to post a comment that "The content of your post is awesome" Great work! forni a legna
Easily, the article is actually the best topic on this registry related issue. I fit in with your conclusions and will eagerly look forward to your next updates. caminetti
Good to become visiting your weblog again, it has been months for me. Nicely this article that i've been waited for so long. I will need this post to total my assignment in the college, and it has exact same topic together with your write-up. Thanks, good share. stufe
I am looking for and I love to post a comment that "The content of your post is awesome" Great work! idealica tropfen apotheke
Easily, the article is actually the best topic on this registry related issue. I fit in with your conclusions and will eagerly look forward to your next updates. idealica tropfen test
Through this post, I know that your good knowledge in playing with all the pieces was very helpful. I notify that this is the first place where I find issues I've been searching for. You have a clever yet attractive way of writing. idealica tropfen test
Easily, the article is actually the best topic on this registry related issue. I fit in with your conclusions and will eagerly look forward to your next updates. idealica tropfen apotheke
Good to become visiting your weblog again, it has been months for me. Nicely this article that i've been waited for so long. I will need this post to total my assignment in the college, and it has exact same topic together with your write-up. Thanks, good share. idealica tropfen erfahrungen

Excellent Blog! I would like to thank for the efforts you have made in writing this post. I am hoping the same best work from you in the future as well. I wanted to thank you for this websites! Thanks for sharing. Great websites!very nice information very helpful php projects
Be awesome. Leave a Comment.
- World of Science
- Everything All At Once
- Unstoppable
- Jack and the Geniuses
- Documentary
- The End Is Nye
- Saves the World
- The Science Guy
- Science Rules!
- Episode Guide
- CEO The Planetary Society
Penny Droplets
What you need.
- an eye-dropper
- liquid dish-soap
- a glass of water
What You Do
- Guess how many water drops you can fit on the front of a penny.
- Try it. How many drops will stay on the face of a penny without spilling water off the sides?
- Repeat the experiment, say, three times. The number of drops will probably come out about the same each time.
- Each time you do it, write down the number of drops.
- Add a tablespoon of dish soap to your glass of water. Stir gently, don’t make too many bubbles.
- How many soapy drops can you fit on the front of a penny now?
- Try it again.
What's Happening?
You just saw three important forces tugging on the water: gravity, cohesion, and adhesion. Gravity flattens the droplets, cohesion holds the droplets together, and adhesion holds the drops on the surface of the coin.
The cohesive force is the pull of the water molecules on themselves. Each successive drop sticks to the water that’s already on the coin. We often call this cohesive force “surface tension”. It’s what makes water drops look like they’re wrapped in invisible skins. Soap reduces the cohesive force, and breaks the surface tension. Soapy water makes smaller drops than plain water. Since soapy drops are smaller, more soapy drops will fit on a penny than plain water drops. Count ‘em!

Article
- Volume 24, issue 18
- ACP, 24, 10833–10848, 2024
- Peer review
- Related articles
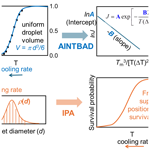
Modeling homogeneous ice nucleation from drop-freezing experiments: impact of droplet volume dispersion and cooling rates
Ravi kumar reddy addula, ingrid de almeida ribeiro, valeria molinero, baron peters.
Homogeneous nucleation is the prominent mechanism of glaciation in cirrus and other high-altitude clouds. Ice nucleation rates can be studied in laboratory assays that gradually lower the temperature of pure water droplets. These experiments can be performed with different cooling rates, with different droplet sizes, and often with a distribution of droplet sizes. We combine nucleation theory, survival probability analysis, and published data on the fraction of frozen droplets as a function of temperature to understand how the cooling rate, droplet size, and size dispersity influence the nucleation rates. The framework, implemented in the Python code AINTBAD (Analysis of Ice nucleation Temperature for B and A Determination), provides a temperature-dependent nucleation rate on a per volume basis, in terms of approximately temperature-independent prefactor ( A ) and barrier ( B ) parameters. We find that dispersion in droplet diameters of less than an order of magnitude, if not properly included in the analysis, can cause apparent nucleation barriers to be underestimated by 50 %. This result highlights the importance of droplet size dispersion in efforts to model glaciation in the polydisperse droplets of clouds. We also developed a theoretical framework, implemented in the Python code IPA (Inhomogeneous Poisson Analysis), to predict the fraction of frozen droplets at each temperature for arbitrary droplet size dispersions and cooling rates. Finally, we present a sensitivity analysis for the effect of temperature uncertainty on the nucleation spectrum. Our framework can improve models for ice nucleation in clouds by explicitly accounting for droplet polydispersity and cooling rates.
- Article (PDF, 3447 KB)
- Supplement (424 KB)
- Article (3447 KB)
- Full-text XML

Addula, R. K. R., de Almeida Ribeiro, I., Molinero, V., and Peters, B.: Modeling homogeneous ice nucleation from drop-freezing experiments: impact of droplet volume dispersion and cooling rates, Atmos. Chem. Phys., 24, 10833–10848, https://doi.org/10.5194/acp-24-10833-2024, 2024.
The thermodynamics and kinetics of ice formation from water are important for atmospheric science ( Koop et al. , 2000 ; Möhler et al. , 2007 ; DeMott et al. , 2010 ; Knopf and Alpert , 2023 ), preservation of biologically active substances ( Morris et al. , 2012 ; Zachariassen and Kristiansen , 2000 ), and storage of food products ( Goff , 1997 ; Li and Sun , 2002 ). Nucleation, the first step in ice formation, heralds the onset of important subsequent changes: rapid growth of ice domains ( Shultz , 2018 ; Barrett et al. , 2019 ; Sibley et al. , 2021 ), the release of latent heat ( Riechers et al. , 2013 ; Dobbie and Jonas , 2001 ), and the freeze concentration of impurities ( Deck et al. , 2022 ; Deville , 2017 ; Stoll et al. , 2021 ). A quantitative understanding of these processes requires models that accurately predict ice nucleation kinetics. In most applications, the primary source of nuclei is heterogeneous nucleation on various surfaces and impurities under mild supercooling ( Alpert and Knopf , 2016 ; Zhang and Maeda , 2022 ; Stan et al. , 2009 ; Kubota , 2019 ). However, homogeneous nucleation of ice occurs under deep supercooling for pure water droplets in the atmosphere ( Koop et al. , 2000 ; Knopf and Alpert , 2023 ; Herbert et al. , 2015 ; Heymsfield and Miloshevich , 1993 ; Spichtinger et al. , 2023 ) and in laboratory experiments ( Murray et al. , 2010 ; Atkinson et al. , 2016 ; Shardt et al. , 2022 ; Riechers et al. , 2013 ; Laksmono et al. , 2015 ).
Special assays have been developed to study ice nucleation kinetics by monitoring hundreds of small supercooled water droplets ( Laval et al. , 2009 ; Shardt et al. , 2022 ; Ando et al. , 2018 ; Tarn et al. , 2020 ). These experiments provide an independent realization of the nucleation time and/or temperature for each droplet ( Tarn et al. , 2020 ; Shardt et al. , 2022 ). Typically, the kinetics are studied via induction times in isothermal conditions (constant supercooling) ( Alpert and Knopf , 2016 ; Herbert et al. , 2014 ; Knopf et al. , 2020 ) or via the spectrum of ice nucleation temperatures at a constant cooling rate ( Zhang and Maeda , 2022 ; Ando et al. , 2018 ; Shardt et al. , 2022 ; Murray et al. , 2010 ). These two types of experiments have important similarities and differences.
For droplets subjected to constant supercooling, the induction time is exponentially distributed. Several analyses have modeled the exponential decay to understand how nucleation rates depend on supercooling ( Alpert and Knopf , 2016 ; Herbert et al. , 2014 ; Knopf et al. , 2020 ). In experiments where the supercooling is gradually increased, the distribution of nucleation times is more complicated ( Murray et al. , 2010 ; Riechers et al. , 2013 ). Typically, no nucleation events occur until the temperature drops below some critical temperature, and then the nucleation times and temperatures all occur within a focused range ( Murray et al. , 2010 ; Riechers et al. , 2013 ; Shardt et al. , 2022 ). The narrow range of ice nucleation temperatures has motivated the use of a single temperature cutoff for ice nucleation in cloud models ( Kärcher and Lohmann , 2002 ). This approach, however, cannot account for the known impact of the cooling rate – which spans about 0.01 to 1 K min −1 – in the formation of ice in clouds ( Stephens , 1978 ; Kärcher and Seifert , 2016 ; Shardt et al. , 2022 ). Likewise, cloud models typically assume a monodisperse distribution of droplet sizes, while the range of sizes of droplets in clouds typically spans 2 to 50 µ m ( Igel and van den Heever , 2017 ). The combined impact of the cooling rate and droplet size distribution on the analysis of droplet freezing experiments and the prediction of cloud properties has not been, to our knowledge, addressed to date.
To justify new elements of our approach to introduce the cooling rate and droplet polydispersity into the interpretation and prediction of experimental data, we briefly discuss the capabilities and gaps in existing models for analyzing the experiments with steadily cooled droplets. Analyses of drop-freezing experiments can be grouped according to two distinguishing criteria. The first distinction pertains to the models used for interpreting the nucleation rate. Kubota ( 2019 ) used empirical nucleation rate models, while others have used theoretically motivated rate expressions (often based on classical nucleation theory) ( Ickes et al. , 2017 ; Murray et al. , 2010 ; Riechers et al. , 2013 ). Empirical rate models can provide excellent fits to the nucleation rate data, and successful empirical approaches sometimes inspire new theoretical models. However, the fitted rate expressions for the nucleation rate from an empirical model lack the interpretability and generalizability afforded by a successful fit to theoretical rate models.
A second distinction pertains to the analysis and interpretation of the droplet nucleation data themselves. Some studies focus on the fraction of droplets that nucleate in a specific supercooling range, i.e., the nucleation spectrum ( Murray et al. , 2010 ; Shardt et al. , 2022 ; Ando et al. , 2018 ). The nucleation spectrum has sometimes been interpreted as an intrinsic property of supercooled water and/or the nucleants present in the system ( Zhang and Maeda , 2022 ; Alpert and Knopf , 2016 ; Knopf and Alpert , 2023 ). However, it also depends on variables beyond chemical or interfacial properties, e.g., the cooling rates and droplet diameters. An alternative explanation for the nucleation spectrum begins with the survival probability formalism. In survival probability analyses, the probability that a droplet remains liquid steadily declines with time in proportion to the changing rate of ice nucleation. The survival probability formalism is easily used in combination with theoretical models for the nucleation rate, but the combination remains rare in the ice nucleation literature. Indeed, prior combinations of survival probability and nucleation theory in the ice literature have focused on heterogeneous nucleation ( Wright and Petters , 2013 ; Marcolli et al. , 2007 ; Alpert and Knopf , 2016 ).
In this work, we combine survival probability analysis with classical nucleation theory to quantitatively predict the effects of different droplet volumes ( Atkinson et al. , 2016 ) and cooling rates ( Shardt et al. , 2022 ). The experiments that motivated our study observed homogeneous nucleation in narrowly selected droplet diameters, cooled at a steady rate deep into the metastable zone. We are inspired by the experiments to achieve precise control of droplet diameters, but the atmospheric clouds will naturally have a distribution of droplet diameters ( Painemal and Zuidema , 2011 ; Igel and van den Heever , 2017 ). We demonstrate a method to extract theoretically derived nucleation rate parameters from the experimental survival probability data of monodispersed droplets and droplets with a distribution of diameters. We find that the dispersion of droplet sizes typically found in clouds, if ignored in the data analysis, can cause serious errors in the predicted slopes of the nucleation rate vs. temperature. These nucleation rate slopes are known to significantly impact the prediction of cloud properties ( Herbert et al. , 2015 ; Spichtinger et al. , 2023 ). To address this, we develop a theoretical framework to predict the fraction of frozen droplets considering an arbitrary dispersion of droplet sizes at any specified cooling rate. We implement this framework in a Python code named IPA (Inhomogeneous Poisson Analysis), which predicts the fraction of frozen droplets as a function of temperature using the distribution of droplet sizes and cooling rates as input. We expect that this model and its implementation will help improve the accuracy of cloud microphysics predictions by accounting for the natural variability in droplet sizes and cooling rates observed in atmospheric conditions.
The probability that a single droplet of volume V is not frozen in a given time t can be modeled using the master equation ( Cox and Oakes , 1984 ):
Here P ( t | V ) is the probability, J is the nucleation rate on a per volume per time basis, and V is the droplet volume. For the nucleation rate on a per droplet per time basis, we multiply the nucleation rate ( J ) by the droplet volume ( V ). Note that J itself is independent of the droplet volume, and accordingly parameters that define J should also be independent of the volume. The temperature is constant, and the rate of nucleation in each liquid droplet also remains constant in induction time measurements. On integrating Eq. ( 1 ), the survival probability becomes P ( t | V ) = exp [ - J V t ] . This result has been used to analyze nucleation data in several crystallization studies, e.g., by plotting ln P ( t | V ) vs. t to estimate J and its supersaturation dependence ( Alpert and Knopf , 2016 ; Knopf and Alpert , 2023 ; Stöckel et al. , 2005 ; Kubota , 2019 ; Sear , 2014 ). In contrast, in experiments where the supercooling increases with time, the nucleation rate in each liquid droplet also increases with time ( Peters , 2011 ) . The survival probability can be obtained by integrating Eq. ( 1 ):
where P is a function of time. However, the data are usually reported as a function of temperature or supercooling ( Murray et al. , 2010 ; Shardt et al. , 2022 ). Since the experiments are conducted at a specific cooling rate R ( Murray et al. , 2010 ; Shardt et al. , 2022 ), we replace the time variable with temperature using the following relation:
where T m is the melting temperature. After variable transformation, the survival probability becomes
Equation ( 4 ) separates protocol-specific factors (droplet diameter and cooling rate) from intrinsic properties of the nucleation kinetics and their dependence on temperature.
Now we need theoretical models or experimental data for the nucleation rate to predict the survival probability. Classical nucleation theory gives the rate for homogeneous nucleation as ( Volmer and Weber , 1926 ; Becker and Döring , 1935 )
where A is the kinetic prefactor, γ is the interfacial free energy between the ice and water, λ f is the latent heat of freezing, k B is the Boltzmann constant, T m is the melting point of ice, v 0 is the molar volume of ice, and T is the absolute temperature.
The nucleation rate J is a product of the equilibrium concentration of clusters of a critical size and the non-equilibrium flux to post-critical sizes. Classical nucleation theory predicts prefactors and exponential terms with explicit temperature dependencies. The exponent in classical nucleation theory is interpreted as a Gibbs free-energy barrier: Δ G * / k B T . It depends explicitly on both the absolute temperature and the supercooling. To account for the temperature dependence of nucleation and the time-dependent temperature, we use δ T = ( T m - T ) / T m as the dimensionless temperature and rewrite the expression for J as
Here B = ( 16 π γ 3 v 0 2 ) / ( 3 λ f 2 k B T m ) contains shape factors, physical constants, the latent heat, and interfacial free energy. These quantities are nearly independent of temperature for the narrow temperature range where homogeneous nucleation is observed in the experiments ( Kashchiev , 2000 ; Sear , 2007 ; Koop et al. , 2000 ). Thus the parameter B should be a nearly temperature-independent parameter, while the barrier Δ G * / k B T is a strong function of temperature because of the 1 / Δ T 2 factor.
The prefactor is related to both the frequency at which water molecules at the ice–water interface attach to the critical nucleus and the number of ice molecules that must attach to surmount the barrier. The prefactor is proportional to the self-diffusivity of water, and therefore it depends on temperature. However, over the small range of nucleation temperatures in this study (ca. 2 K), we assume that the prefactor is temperature independent.
Note that both parameters, A and B , are assumed to be temperature-independent constants over the narrow range of ice nucleation temperatures. However, they may both differ from values that would be theoretically estimated using properties of ice and water at T m . The value of the interfacial free energy when calculated from the fitted values of B is 33 mN m −1 at 235.5 K, higher than the interfacial free energy reported by Ickes et al. ( 2015 ) (i.e., 29.0 mN m −1 ). We show in Sect. 9.2 that by assuming that A is independent of temperature, we transfer its temperature dependence to the effective nucleation barrier.
Using Eq. ( 6 ) in Eq. ( 4 ), the survival probability becomes
To our knowledge, Eq. ( 7 ) has not been used in previous studies of ice nucleation. It isolates parameter B , a property of nucleation kinetics, from the dimensionless group ( A V T m / R ) . The latter depends not only on intrinsic properties of ice and water ( A and T m ) but also on V and R , which may be experimental choices or cloud conditions. Equation ( 7 ) is valid for water droplets of volume V . In most experiments, there is a distribution of volumes which leads to a distribution of droplet nucleation rates. We consider a distribution of droplet sizes in Sect. 5 , but first, we demonstrate that the model can predict the effect of droplet volume for narrowly size-selected droplets.
Across the range of nucleation temperatures observed in experiments ( Atkinson et al. , 2016 ; Shardt et al. , 2022 ) for homogeneous ice nucleation (234–238 K), the factor (1− δ T ) in the rate expression is always near 0.9. Hence, the nucleation rate expression is approximately J = A exp ( B ′ / δ T 2 ) , where B ′ is approximately B ′ = B / ( 1 - δ T ) ≈ 1.1 B . With this approximation, we have an analytical solution for the survival probability as follows:
where “erfc” denotes the complementary error function. To illustrate the use of Eqs. ( 7 ) and ( 8 ), we analyze one of the survival probability data sets (droplet diameter corresponding to 3.8–6.2 µ m) obtained from Atkinson et al. ( 2016 ) . Optimized fits of the analytical solution (Eq. 8 , with A ′ = 1.76 × 10 39 cm −3 s −1 and B ′ = 1.3578 ) and the numerical integration (Eq. 7 , with A = 8.68 × 10 41 cm −3 s −1 and B =1.2722 ) are shown in Fig. 1 . Even though the fits show excellent agreement in both analytical and numerical approaches, we note that a 10 % error in the exponent (from approximating 1 - δ T ≈ 1.0 ) leads to a nearly 1000-fold error in A ′ and a 10 % error in B ′ relative to A and B . We conclude that precise A and B values require careful treatment of even weak temperature dependencies within J . Although the prefactor and barriers are different, the predicted nucleation rates are not. For example, at 234.9 K both approaches give an estimate of the nucleation rate of 1.44×10 9 cm −3 s −1 .
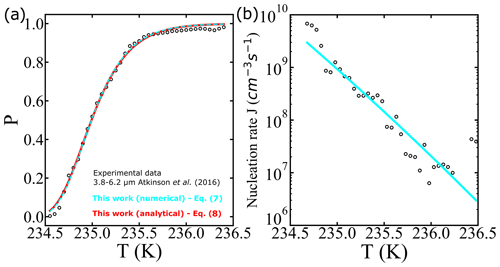
Figure 1 (a) Illustration of survival probability fits with analytical (continuous red line) and numerical (dashed cyan line) solutions for the integral in Eq. ( 7 ). The experimental data represented by the empty black circles used as a reference are from Atkinson et al. ( 2016 ) . (b) Comparison of estimated nucleation rates from experiments using survival probability data as described in Atkinson et al. ( 2016 ) (black circles), and the nucleation rate computed using Eq. ( 7 ) (cyan line).
The noisy estimates of J in Fig. 1 b have been obtained by a finite difference in the cumulative survival probability data. For the finite-difference procedure, large numbers of droplets are needed to obtain an estimate of J from the incremental nucleation events in each Δ T interval. As seen in Fig. 1 b, there is considerable noise in the J estimates even in an experiment with hundreds of droplets. Our data analysis approach directly fits a model to the cumulative fraction of frozen droplets. It should therefore remain accurate for data sets with smaller numbers of droplets.
We implemented the numerical integration in Eq. ( 7 ) and analytical model of Eq. ( 8 ) in a Python code to estimate A , B , and J from experimental drop-freezing data. The code outputs the parameters A and B from Eq. ( 7 ). These are used to compute the nucleation barriers Δ G , the temperature that corresponds to 50 % of frozen droplets T 50 , and the homogeneous-nucleation rate evaluated at T 50 using J hom model ( δ T ) = A exp ( - B / [ ( 1 - δ T ) δ T 2 ] ) . The AINTBAD (Analysis of Ice nucleation Temperature for B and A Determination) code is illustrated in Fig. 2 . The code is available at https://github.com/Molinero-Group/volume-dispersion ( Addula et al. , 2024 ).
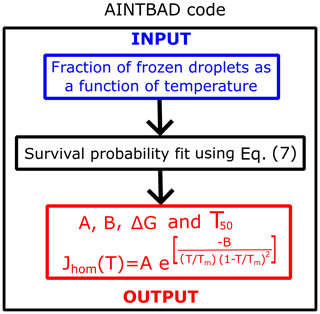
Figure 2 Flowchart of the AINTBAD (Analysis of Ice nucleation Temperature for B and A Determination) code.
We use the minimize function from the “scipy.optimize” module in Python to optimize the difference between the target survival probability and the predicted one by adjusting the parameters A and B . The chosen optimization method is the Nelder–Mead algorithm, suitable for functions without explicit derivatives. Optional settings include a convergence tolerance of 10 −4 and a maximum iteration limit of 1000.
Atkinson et al. ( 2016 ) monitored the freezing temperatures of narrowly size-selected droplets cooled to temperatures near 235 K at a steady rate of 1.0 K min −1 . A total of 581 droplets were used in the diameter range of 3.8–18.8 µ m, with an average of 96 droplets for each diameter. The range of droplet diameters in each experiment and the fraction of droplets that remain at each temperature can be seen as data points in Fig. 3 a. We have analyzed the data from Atkinson et al. ( 2016 ) in two ways. First, we separately fitted the data for each diameter range to Eq. ( 7 ). Because the range of diameters of each size-selected group is narrow, we have assumed that all droplets in each size range are spheres with the mean diameter for that range. These fits (not shown) result in independent estimates of the optimized nucleation prefactor A and the barrier parameter B from each of the six experiments. Table 1 shows the range of droplet diameters in each experiment, the independent log 10 A and B estimates, the predicted free-energy barrier β Δ G = B / [ ( 1 - δ T ) δ T 2 ] at 235.5 K, and the predicted nucleation rate (from J = A exp [ - B / ( ( 1 - δ T ) δ T 2 ) ] ) at 235.5 K. The separate A and B estimates vary considerably, but they are highly correlated to each other. Figure 3 b shows B vs. log 10 A for each of the independent estimates. When B is small (large), A is also small (large). The estimated parameters compensate for errors in each other such that all six data sets yield models that predict consistent nucleation rates. The predicted nucleation rates are shown in Table 1 for the temperature of 235.5 K.
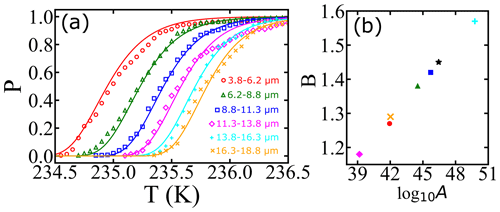
Figure 3 (a) Illustration of survival probability fits for homogeneous nucleation with varied droplet diameters at a cooling rate of 1 K min −1 . Open symbols represent experimental data from Atkinson et al. ( 2016 ) , and continuous lines represent model predictions from a global data fit. Colors of symbols indicate different droplet diameters. The individual fits are not shown because the curves overlap the data and globally fitted model predictions closely. (b) Illustration of the correlation between the log 10 A and B parameters obtained from individual fits. The black star indicates the estimation obtained by the global fit, and other symbols correspond to estimations for different diameters of droplets.
Table 1 Computed nucleation rate parameters A and B for various groups of droplet diameters using the volume corresponding to the mean diameter of the group. D is the mean diameter of the droplets in the group in micrometers ( µ m), β Δ G is the free-energy barrier for nucleation, and J is the nucleation rate computed using fit parameters in Eq. ( 6 ). Estimations of β Δ G and J correspond to a temperature of 235.5 K.
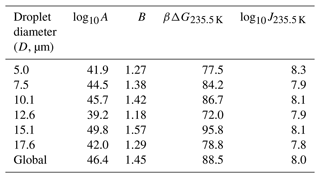
Download Print Version | Download XLSX
The measurements of Atkinson et al. ( 2016 ) were all made in the same way, so the same fundamental nucleation rate expression should describe all six size-selected data sets. Accordingly, we reanalyzed the data of Atkinson et al. ( 2016 ) with one global rate expression ( J = A exp [ - B / ( ( 1 - δ T ) δ T 2 ) ] ), keeping the same A and B values across all six data sets. The nucleation rate parameters obtained from the global fit are A = 2.79 × 10 46 (cm −3 s −1 ) and B =1.45 . Figure 3 a shows the experimental data for different droplet diameters along with model predictions from the global fit. We emphasize that these are six curves, accurately fitted with just two free parameters, and that both parameters have a clear physical and theoretical interpretation. However, we note that the theoretical relationship between B and β Δ G reflects only the reversible work to create a nucleus at equilibrium, but the parameter B as obtained from experimental data also reflects activation energy contributions from the prefactor. See Sect. 9.2 for more explanation about this point. Indeed, using the expression B = ( 16 π γ 3 v 0 2 ) / ( 3 λ f 2 k B T m ) and the global fit B =1.45 in Table 1 results in γ =33 mJ m −2 at 235.5 K, which is above the median value of the ice–liquid surface tension in the literature ( Ickes et al. , 2015 ) .
At a temperature of 235.5 K, the global fit yields a prediction of J =10 8 cm −3 s −1 for the nucleation rate, which is consistent with predictions from the independent fits. The free-energy barrier at 235.5 K from the global fit is 88.5 k B T . This is again similar to those values obtained from fits to the individual size-selected data sets (Table 1 ).
Although the rate predictions show remarkable internal consistency, the inferred barriers are scattered and larger than barriers which have been inferred from other data sets ( Murray et al. , 2010 ; Shardt et al. , 2022 ; Riechers et al. , 2013 ). The discrepancy may be a consequence of theoretically unaccounted for temperature dependencies within the prefactor. Note that the data sets in cyan and pink in Fig. 3 a actually cross over each other. The crossover indicates that small droplets are nucleating at warmer temperatures than the larger droplets, which should not occur according to nucleation theory. These two anomalous curves correspond to the two most extreme estimates of A and B (upper right and lower left in Fig. 3 b). Thus scatter in the A and B parameters seems to be a true reflection of experiment-to-experiment variation.
Section 5 explores how size dispersity, i.e., the distribution of droplet sizes around the mean diameter, influences the inferred rate parameters. Section 6 examines whether diameter dispersity within the narrow, but non-zero, diameter ranges of Atkinson et al. ( 2016 ) may still affect the inferred rate parameters.
Experiments that report on droplet diameter dispersity ( Murray et al. , 2010 ; Shardt et al. , 2022 ; Ando et al. , 2018 ) consistently report a broader range of diameters than the droplets reported on in Atkinson et al. ( 2016 ) . This section develops a superposition formula to predict the survival probability for experiments with a broad distribution of droplet diameters. We use the term superposition for a data analysis that retains the stratification in freezing temperature but otherwise pools droplets together regardless of their size. As seen from Fig. 3 and as predicted in Eq. ( 7 ), large droplets in a broad distribution will nucleate early (at milder supercooling levels), while small droplets will survive to deeper supercooling levels. If all temperature dependence comes from the free-energy barrier B / [ ( 1 - δ T ) δ T 2 ] , then large droplets that nucleate at milder supercooling levels will also nucleate with higher free-energy barriers.
The steep sigmoidal survival probabilities for droplets of a specific size, when superimposed, result in a more gradual sigmoid. The gradual sigmoid looks deceptively like the theoretical prediction in Eq. ( 7 ) but with artificially reduced barrier B and prefactor A parameters. The analysis here shows how a distribution of droplet diameters broadens the nucleation spectrum, decreasing the inferred nucleation rate barrier.
The joint survival probability distribution with volume and temperature variables is given by
where ρ ( V ) is the normalized distribution of droplet volumes and P ( δ T | V ) is the survival probability for droplets of a specific volume, i.e., Eq. ( 7 ). The survival probability in time and temperature is obtained by integrating over droplet volumes in the joint distribution:
Here we provide an example calculation to illustrate the effects of a broad droplet volume distribution. Let the normalized (gamma-type) distribution of droplet sizes be ρ ( V ) = 8 V 0 - 2 V exp ( - 2 V / V 0 ) . Here V 0 is the mean volume of the entire range of droplets. Let the survival probability for droplets of any specific diameter be given by P ( δ T | V ) in Eq. ( 7 ), with the global fit values of A and B , as reported in Table 1 . The survival probability for the distribution of droplet volumes can then be obtained using Eqs. ( 9 ) and ( 10 ).
We set V 0 =1057.1 µ m 3 in ρ ( V ) to obtain a distribution with droplets of diameters between 3.0 and 20 µ m. Note that the model volume distribution spans the range of sizes in the experiments of Atkinson et al. ( 2016 ) ; Fig. 4 shows the gradually decreasing survival probability from the superposition as a continuous black curve with more steeply changing diameter-selected P ( δ T | V ) data in the background.
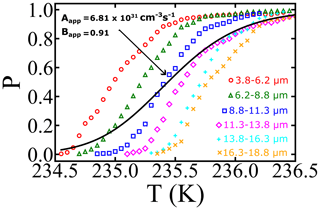
Figure 4 Illustrating the effects of the distribution of droplet diameter on survival probabilities. Open symbols are the experimental survival probabilities for different groups of size-selected droplets from Atkinson et al. ( 2016 ) . The continuous black line is the superposition survival probability of Eq. ( 10 ) from the same kinetics and for a distribution of droplets from 3.8–18.8 µ m.
If we had been unaware of the droplet polydispersity or had not accounted for it, we might have interpreted the black curve in Fig. 4 using a survival probability analysis with nucleation theory: for droplets corresponding to the mean diameter d , the volume is computed assuming spherical droplets; i.e., V 0 = ( π d 3 / 6 ) . Thus variations in diameter directly translate into changes in volume. To illustrate how droplet diameter dispersity influences the inferred nucleation rate parameters, we re-optimized A and B to minimize the residuals between the dispersity superposition result in P ( δ T ) and the naive specific-volume model P ( δ T | V 0 ) . The resulting A and B values are 6.81×10 31 cm −3 s −1 and 0.91, respectively. The inferred prefactor ( A apparent ) is 15 orders of magnitude smaller than that from the global fit of the sets with narrow volume distribution, and the inferred barrier parameter ( B apparent ) has been reduced by nearly 40 %. Moreover, the inferred free-energy barrier at 235.5 K is now estimated to be β Δ G =55.7 k B T , relative to a value of 88.5 k B T based on the global fit values to the diameter-selected droplet data. The calculation illustrates how a failure to account for diameter dispersity causes a spurious broadening of the nucleation spectrum and reduction in the inferred prefactor A , barrier parameter B , and free-energy barriers.
Once we know the variation in droplet diameters, resultant survival probabilities can easily be computed with the help of the Python code presented in Sect. 9 and available on GitHub ( https://github.com/Molinero-Group/volume-dispersion , Addula et al. , 2024 ). Here variation refers to the overall differences in droplet sizes within a sample, encompassing any deviation from the average droplet diameter. The inputs needed for the program are the proposed distribution of droplet diameters (Gaussian, uniform, gamma, etc.) and the variation in the nucleation rate with temperature (see Sect. 9.1 ). The output from the code is the effective survival probability.
First, we ask whether the range of droplet diameters in each experiment by Atkinson et al. ( 2016 ) , with each spanning a few micrometers ( µ m), is already broad enough to adversely impact the inferred nucleation parameters. We have considered two test cases for the analysis: one with a midpoint of each reported diameter range as the diameter of all droplets in that group (as shown in on the vertical axis of Fig. 5 ) and the second with a uniform distribution of droplet volumes over the corresponding diameter ranges (as shown on the horizontal axis of Fig. 5 ). If the ranges of size are sufficiently narrow relative to the mean, then the A and B parameters resulting from a fit to the superposition become identical to those from monodisperse droplets of the mean size. Figure 6 shows that the relative width of the volume distribution is sufficient to predict the superposition error. Specifically, for less than 1 % error in B ( B apparent / B actual > 0.99 ), we must have Δ V / V < 0.25 . The parity plots for the two values of A and B are presented in Fig. 5 . As all the data points are close to the x = y line, we conclude that the droplet diameter ranges in Atkinson et al. ( 2016 ) are sufficiently narrow to ignore diameter dispersion when inferring the nucleation kinetics.
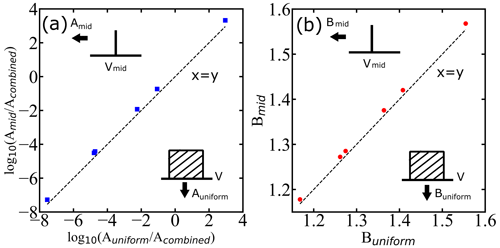
Figure 5 Parity plots showing how droplet diameter dispersion influences the inferred A and B parameters. Symbols are inferred A and B parameters with a sharp monodisperse droplet distribution (delta function) corresponding to mean diameter of droplets (mid) as shown by the drawing pointing at the vertical axis and with a uniform distribution of droplets (uniform) as shown by the drawing pointing at the horizontal axis. A combined is the prefactor estimated using the global fit. Intervals and midpoints for V correspond to the diameter window of Atkinson et al. ( 2016 ) . The dashed black lines represent x = y lines (parity).
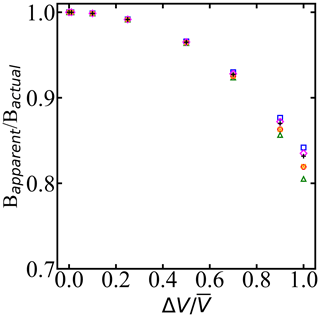
Figure 6 When droplet diameter dispersity is ignored, the inferred B apparent relative to B actual depends on the uniform spread in volume Δ V relative to the mean volume V ‾ . Δ V is the width of a uniform distribution of droplet volumes according to the uniform distribution. Symbols of the same color correspond to different widths of uniform distribution, and different colors correspond to different mean volumes of the droplets. B actual is the predicted B with monodisperse droplets, and B apparent is the computed B with dispersity in the droplet volume.
Figure 6 shows the ratio between the apparent B parameter from the superposition of survival probabilities of droplets with volumes V ‾ ± Δ V and the true B parameter. The analysis shows that the groups with 2 µ m variation in diameter resulted in the same nucleation rate parameters with approximately less than 1 % variation in estimated free-energy barriers. Our analysis in Fig. 6 quantifies the effect of the dispersion of droplets in the experiments on predicted B parameters, assuming droplets have uniform distribution. Given Δ V / V ‾ and B values from an analysis that imposes volume dispersity, Fig. 6 can be used to estimate the true value of B . The analysis shows that to obtain B within 1 % of the correct value, the volume dispersity should be no more than 25 % of the mean volume.
The combined survival probability and nucleation theory expression, as shown in Eq. ( 7 ), also predicts that the cooling rate will impact the nucleation spectrum. In this section, we analyze data from Shardt et al. ( 2022 ) , whose experiments are performed at two different cooling rates (0.1 and 1.0 K min −1 ) with diameter-selected droplets of 75 and 100 µ m. Shardt et al. ( 2022 ) report the uncertainty in the droplet diameters to be 5 µ m. We model their droplet diameter distribution using a Gaussian distribution with a mean of 75 (or 100) µ m and a standard deviation of 5 µ m. We have analyzed the survival probability data across the two droplet diameters and two cooling rates with one global fit. Global fits to the survival probability data across the cooling rates and droplet diameters are shown in Fig. 7 . The computed nucleation rate parameters from the global fit are A = 5.72 × 10 28 cm −3 s −1 and B =0.81 . The predictions of free-energy barriers across the cooling rates and droplet diameters are presented in Table 2 .
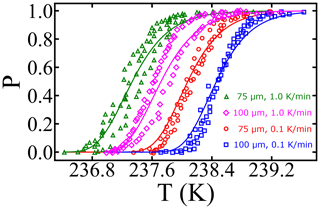
Figure 7 Survival probability fits for homogeneous-nucleation data with different droplet diameters and cooling rates. Open symbols represent experimental data from Shardt et al. ( 2022 ) , and the continuous lines represent model predictions.
The predictions of B have a similar order of magnitude but are approximately 25 % lower when compared to other estimates ( Riechers et al. , 2013 ). We suspect the variation may stem from the difficulties in measuring the precise temperatures of the droplets ( Shardt et al. , 2022 ; Tarn et al. , 2020 ; Atkinson et al. , 2016 ). We also note that the computed nucleation rate parameters A and B from Shardt et al. ( 2022 ) are lower than those from the study of Atkinson et al. ( 2016 ) . The difference may be due to the uncertainty in droplet temperature measurements; however the two experiments report similar uncertainties in droplet temperatures. Specifically, experiments by Shardt et al. ( 2022 ) indicated the uncertainty in temperature measurements to be ±0.2 K, and experiments by Atkinson et al. ( 2016 ) indicated uncertainty of ±0.3 K.
Table 2 The computed free-energy barriers for various droplet diameters across the cooling rates. T 50 is the temperature corresponding to a survival probability of 0.5.
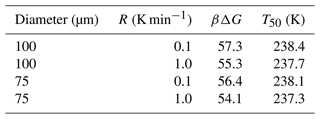
Figure 8 shows a comparison for the homogeneous-nucleation rates using experimental data from Shardt et al. ( 2022 ) (blue diamonds) and Atkinson et al. ( 2016 ) (green squares). Continuous lines indicate different parametrizations: the fit using the AINTBAD code J hom model ( T ) , where A = 2.79 × 10 46 cm −3 s −1 and B =1.45 for the temperature range of 234.8 to 236.8 K and A = 5.72 × 10 28 cm −3 s −1 and B =0.81 for 237.0 to 239.1 K (continuous red lines); the parametrization proposed by fitting multiple experimental data, J hom equation ( T ) = exp [ - 3.9126 T + 939.916 ] ( Atkinson et al. , 2016 ) (cyan line); and the parametrizations based on classical nucleation theory (CNT) from Qiu et al. ( 2019 ) (black line) and from Koop and Murray ( 2016 ) (magenta line). In a small temperature range, J hom model ( T ) captures the experimental data points well. The proposed model, J hom model ( T ) , which works well for micrometer-sized droplets at lower temperatures, may have limitations in accurately capturing the complex nucleation processes occurring in larger droplets at higher temperatures. Thus, J hom model ( T ) can be used only to predict the homogeneous-nucleation rate in the temperature range of the input data used to fit the model.
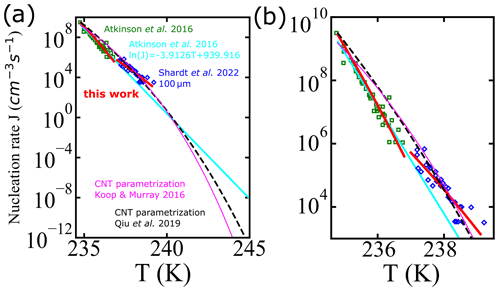
Figure 8 (a) Comparison of the nucleation rate vs. temperature from experiments of Shardt et al. ( 2022 ) (blue diamonds) and Atkinson et al. ( 2016 ) (green squares), with the empirical model proposed by Atkinson et al. ( 2016 ) (continuous cyan line), global fit of A and B in the model J = A exp [ - B / ( ( 1 - δ T ) δ T 2 ) ] fitted to Atkinson et al. ( 2016 ) and Shardt et al. ( 2022 ) (continuous red lines), and the CNT parametrizations from Qiu et al. ( 2019 ) (dashed black line) and Koop and Murray ( 2016 ) (continuous magenta line). The temperature axis extends to 245 K, which is the upper plausible limit of homogeneous-nucleation temperatures as defined in Herbert et al. ( 2015 ) . Panel (b) shows the nucleation rate in the region from 235 to 240 K only.
All parametrizations predict nucleation rates within an order of magnitude of each other and for experiments for temperatures between 235 and 240 K. However, there is a small gap in the data near 237 K. Figure 8 suggests a slight disagreement between experimental rates at temperatures below 237 K and those above 237 K. Models for ice nucleation in cloud droplets require nucleation rates that remain accurate over a broad range of temperatures and droplet diameters. Although it is not possible to discriminate between models based on the currently available data, physics-based models should help to build parametrizations that are internally consistent and valid over a broad temperature range.
We developed a versatile code capable of taking various parametrizations for the homogeneous-nucleation rate J hom ( T ) , the droplet diameter distributions (Gaussian, Gamma, uniform, exponential, etc.), and cooling rates to compute the survival probability or fraction of frozen droplets. The code IPA (Inhomogeneous Poisson Analysis) is illustrated in Fig. 9 . We use the nucleation rate data vs. temperature as the input to compute the survival probability using the following equation:
Equation ( 11 ) is a general representation for any given J value; i.e., it is a version of Eq. ( 7 ) that can be used with other nucleation rate models. We evaluate the integral numerically using the trapezoidal rule. Even though Eq. ( 11 ) is strictly valid only for a given constant volume of the droplets, we can use Eq. ( 11 ) in combination with Eq. ( 10 ) to account for the distribution of diameters. Our code includes diverse nucleation rate variations with temperature, including the local parametrization J hom model ( T ) discussed in the preceding section, the CNT parametrization from sources like Qiu et al. ( 2019 ) and Koop and Murray ( 2016 ) , and the empirical parametrization from Atkinson et al. ( 2016 ) . Additionally, users can integrate any other parametrization into the code. The code is publicly accessible at https://github.com/Molinero-Group/volume-dispersion ( Addula et al. , 2024 ).
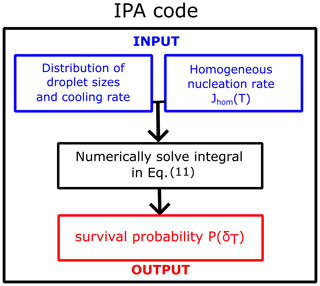
Figure 9 Flowchart of the IPA (Inhomogeneous Poisson Analysis) code to obtain the survival probability of water droplets as a function of temperature for any distribution of droplet diameters, cooling rate, and homogeneous-nucleation rate parametrization.
9.1 Survival probability predictions for cloud data using CNT parametrization
To extend J hom ( T ) to higher temperatures and predict freezing for any diameter distribution, we use J hom CNT ( T ) based on classical nucleation theory (CNT) parametrization of experimental properties of water as previously described in Qiu et al. ( 2017 , 2019 ) . According to CNT, the rate of nucleation is given by
where T is the absolute temperature, k B is the Boltzmann constant, A ( T ) is the prefactor, and Δ G hom is the free-energy barrier associated with the formation of a critical ice nucleus. The temperature dependence of the prefactor follows the one of the diffusion coefficient of liquid water using the Vogel–Fulcher–Tammann (VFT) model and was obtained from Koop and Murray ( 2016 ) . The free-energy barrier is formulated as
where Δ μ ( T ) is the excess chemical potential of the liquid with respect to the crystal, ρ is the density of the crystal, and γ ice-liq is the surface tension of the ice–liquid interface. We follow the procedure developed by Qiu et al. ( 2019 ) to compute the homogeneous-nucleation rate J hom ( T ) as a function of experimental properties of water and ice. In summary, the temperature dependence of the free-energy barriers is computed with Eq. ( 13 ). The ice–liquid surface tension at the melting point was selected to match γ ice-liq ( T m )=31.20 mJ m −2 , which in turn matches J hom at T hom =238 K for microliter-sized droplets cooled at 1 K min −1 following the experimental data of Atkinson et al. ( 2016 ) and Riechers et al. ( 2013 ) . We approximate the temperature dependence of the ice–liquid surface tension γ ice-liq ( T ) by Turnbull's relation ( Turnbull , 2004 ) , where γ ice-liq ( T ) / γ ice-liq ( T m ) = Δ H m ( T ) / Δ H m ( T m ) . This parametrization was previously used in Qiu et al. ( 2019 ) to study heterogeneous ice nucleation.
Utilizing the CNT parametrization from Qiu et al. ( 2019 ) as an input, we integrate it with diverse droplet diameter distributions and cooling rates. The distribution of water droplets in clouds has been examined through the gamma distribution function ( Liu et al. , 1995 ; Painemal and Zuidema , 2011 ; Igel and van den Heever , 2017 ). In Fig. 10 a, we showcase how different gamma diameter distributions, manipulated by altering the shape parameter as suggested in Igel and van den Heever ( 2017 ) , impact droplet diameters. Note that we did not fit a distribution to the data but assumed a distribution based on known properties of droplet sizes in clouds. Additionally, Fig. 10 b illustrates the survival probability computed via the IPA code using a fixed cooling rate, q c =1 K min −1 , that is typical in clouds ( Shardt et al. , 2022 ) . Notably, the inset reveals a correlation between the shape parameter and freezing temperature. We also include the survival probability for monodisperse droplets, using the most likely diameter from the distributions. Importantly, when the diameter distribution is broader, it has a significant impact on the freezing temperatures.
Typical rates of cooling in clouds span ∼0.01 to 1 K min −1 ( Stephens , 1978 ; Kärcher and Seifert , 2016 ; Shardt et al. , 2022 ). Furthermore, Fig. 10 c shows that by each 10-fold increase in the cooling rate, T 50 decreases by approximately 0.5 K. These analyses indicate that an explicit account of the cooling rate and droplet size distribution are important for accurate modeling of cloud microphysical properties.
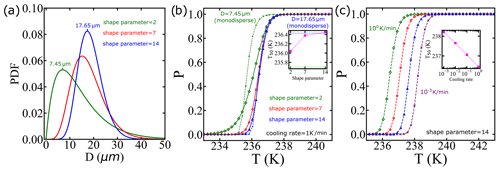
Figure 10 We use the CNT parametrization from Qiu et al. ( 2019 ) as an input, and we integrate it with diverse droplet diameter distributions and cooling rates. (a) We employ a gamma distribution characterized by different shape parameters as used in Igel and van den Heever ( 2017 ) , where the most likely diameter is indicated for shape parameters 2 and 14. (b) The survival probability predictions for the distributions shown in (a) and cooling rate of q c =1 K min −1 . The dashed lines represent the survival probability considering the case of droplets with a single diameter with the same values as the most likely values in (a) . The inset shows the temperature corresponding to a survival probability of 0.5 using the droplet diameter distribution with different shape parameters, with horizontal dotted lines indicating the results using monodisperse droplets. (c) The survival probability predictions using the same droplet diameter distribution but with varying cooling rates.
9.2 B as obtained from experiments reflects both diffusion and nucleation barriers
Each of the two data sets, those from Atkinson et al. ( 2016 ) and Shardt et al. ( 2022 ) shown in Figs. 3 and 7, respectively, follow the theoretically predicted trends in the cooling rate and droplet size dependence. When compared to each other, the larger droplets of Shardt et al. ( 2022 ) , as expected, nucleate at higher temperatures than those of Atkinson et al. ( 2016 ) . Therefore, there is no discrepancy between theoretical expectations and the directly monitored nucleation temperatures. However, the estimated Gibbs free-energy barriers from the data of Shardt et al. ( 2022 ) are smaller than those estimated from the data of Atkinson et al. ( 2016 ) . If Δ G * / k B T = B / ( T * Δ T 2 ) with a constant B value, then Δ G * should be larger for the droplets of Shardt et al. ( 2022 ) , which nucleate at higher temperatures. We have shown that size dispersion causes an underestimation of the B and A parameters. As detailed in Sect. 10, noise in the temperature measurements can also broaden the distribution of nucleation temperatures, causing a similar underestimation of B , A , and Δ G * . However, uncertainty in droplet temperatures cannot explain why the Δ G * values obtained from Shardt et al. ( 2022 ) are smaller, as that data set has lower uncertainty ( ±0.2 K) than the data set from Atkinson et al. ( 2016 ) ( ±0.3 K).
Alternatively, larger nucleation barriers at the lower temperatures (larger supercooling levels) may result from the combined effects of the nucleation barrier (estimated with the AINTBAD code) and diffusion barriers within the prefactor (not yet considered). We analyze this possibility by first predicting the survival probability of liquid droplets upon cooling for a proposed narrow distribution of droplet volumes using the IPA code with a cooling rate of 1 K min −1 and the J hom CNT ( T ) CNT parametrization from Qiu et al. ( 2019 ) (Fig. 11 b and c) and then analyzing these synthetic survival probabilities with the IPA code to extract the effective barrier from the B parameter. Figure 11 a shows that the values of Δ G at T 50 obtained from the AINTBAD code align closely with the sum of free-energy barriers for diffusion and homogeneous nucleation of the CNT parametrization of Qiu et al. ( 2019 ) . This suggests that the high effective barriers for the smaller droplets may originate in a steeply increasing barrier for diffusion. Such an increase is not represented in the parametrization of Qiu et al. ( 2019 ) or in Koop and Murray ( 2016 ) , who model the temperature dependence of the diffusion using the Vogel–Fulcher–Tammann (VFT) equation with T 0 =148 K, while recent experiments support a steeper decrease in the self-diffusion coefficient D ( T ) of water as it approaches its maximum in isobaric heat capacity at 229 K ( Pathak et al. , 2021 ). We interpret the increase in the steepness of D ( T ) on approaching the temperature of maximum heat capacity to be possibly responsible for the larger apparent barrier obtained from the AINTBAD fits to the experimental data. Our finding supports the need for a reassessment of the temperature dependence of the prefactor in the parametrizations of the homogeneous ice nucleation rates based on the most current experimental data.
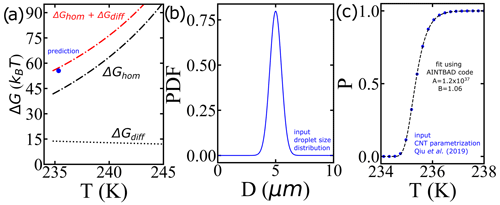
Figure 11 (a) Comparison between the nucleation barriers computed using the CNT parametrization from Qiu et al. ( 2019 ) and the predicted one from the model. (b) Distribution of droplet diameters, (c) survival probability prediction using the IPA code and the CNT parametrization from Qiu et al. ( 2019 ) (blue circles) and the fit using the AINTBAD code (dashed black line). The narrow distribution essentially behaves like a delta distribution.
Another important factor that has a significant effect on the measured nucleation spectrum is the measurement of droplet temperature. The estimations of the droplet temperatures in freezing experiments show large variability ( Tarn et al. , 2020 ; Shardt et al. , 2022 ). The highest level of accuracy in the temperature measurements is ±0.2 K ( Shardt et al. , 2022 ).
In this section, we conduct a sensitivity analysis using our model to quantify the impact of temperature measurement uncertainty on estimated free-energy barriers. To perform this analysis, we utilized data from frozen 75 µ m droplets, as presented in Shardt et al. ( 2022 ) , which were collected at a cooling rate of 0.1 K min −1 . Through the HUB-backward code from de Almeida Ribeiro et al. ( 2023 ) , we determined the optimized differential spectra denoted n m ( T ) based on the frozen fraction (represented by the continuous red line in Fig. 12 a). The resulting parameters derived from this analysis were T mode =238.2 K and s =0.33 , where T mode represents the most probable freezing temperature within the distribution and s characterizes the distribution's spread. Subsequently, we employed the original distribution (continuous red line in Fig. 12 b) to generate random temperature values, augmenting them with random values drawn from a uniform distribution within the range of −0.4 to +0.4 K (or −0.2 to 0.2 K). These additional values introduce noise into the data. We sampled a total of 100 temperature values, equivalent to simulating the behavior of 100 droplets in an experimental setup.
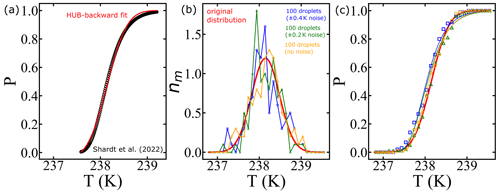
Figure 12 Illustration of the effect of temperature uncertainty on the nucleation spectrum. (a) We use the HUB-backward code ( de Almeida Ribeiro et al. , 2023 ) to find the best fit (continuous red line) of the experimental data ( Shardt et al. , 2022 ) (black circles). (b) The continuous red line shows the optimized differential spectra denoted n m ( T ) , with resulting parameters T mode =238.2 K and s =0.33 , where T mode represents the most probable freezing temperature within the distribution and s characterizes the distribution's spread. We use the continuous red line as the original distribution to generate 100 temperature values (orange circles). Random noise is introduced into the temperature ( ±0.4 K shown in blue squares; ±0.2 K shown in green triangles). (c) The survival probability fit (continuous lines) of the artificially generated data based on Eq. ( 8 ).
The resulting differential freezing spectra are illustrated by the blue squares and green triangles in Fig. 12 b. For each case, we calculated the survival probability and fitted the data using Eq. ( 7 ), resulting in the continuous lines depicted in Fig. 12 c. The effects of temperature variation on the nucleation spectrum are summarized in Table 3 . We conclude that measurements with ±0.2 and ±0.4 K variations resulted in 8 % and 14 % variation, respectively, in the computed free-energy barriers. Even though the predictions of free-energy barriers show a strong dependence on the uncertainty in temperature measurements, the nucleation rates are insensitive, as shown in Table 3 . However, the noise in the predicted rate data increases with the noise in temperature measurements.
Table 3 The computed free-energy barriers and nucleation rates for various uncertainties in the temperature measurements. The mean and standard deviation of B and β Δ G are computed from five different estimates. We consider T 50 to be 238.2 K for all the cases presented in this table.
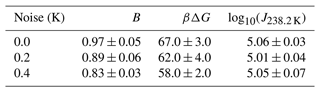
Homogeneous ice nucleation is the predominant mechanism of glaciation in cirrus and other high-altitude clouds, making the accurate representation of cloud microphysics highly dependent on the homogeneous-nucleation rates. It has been shown that the predictions of cloud models are sensitive to the rate of cooling and variations in the slope of the nucleation rate with temperature ( Herbert et al. , 2015 ). In this study, we demonstrate that the cooling rate and dispersion in droplet diameters can lead to substantial changes in the freezing temperature of droplets, which stresses the need to incorporate these variations into cloud models.
Homogeneous-nucleation rates can be obtained from experiments that record the freezing temperature while pure water droplets are gradually cooled to temperatures far below 0 °C. Prior studies have analyzed these experiments using Poisson statistics to infer rates at different temperatures, and then they have fitted the rate vs. temperature data to empirical or theoretical rate expressions. Here we directly analyze the fraction of frozen droplets vs. temperature data to estimate rate parameters within a stochastic survival probability framework. We implement our approach in a Python code, AINTBAD (Fig. 13 ), that extracts the prefactor A and barrier B parameters according to a CNT-type rate expression. An advantage of our method is that it does not require a large number of droplets to estimate accurate nucleation rates at each temperature. Although AINTBAD does not directly use nucleation rates in the optimization, it yields accurate estimates of the nucleation rate and avoids the noise associated with numerical differentiation.
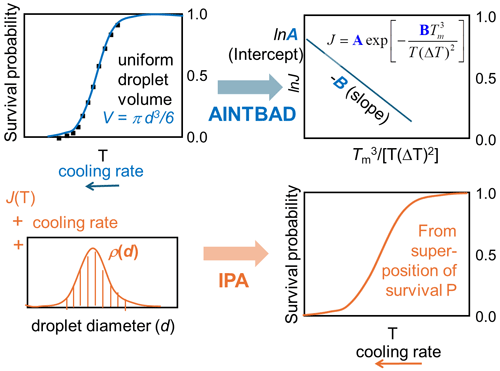
Figure 13 Diagram illustrating the usage of the AINTBAD and IPA codes.
We applied the AINTBAD code to analyze the homogeneous-nucleation data obtained from two different studies: Atkinson et al. ( 2016 ) and Shardt et al. ( 2022 ) . We first used the new framework to extract parameters and rate expressions from experiments on six groups of diameter-selected droplets, from 5.0±1.2 to 17.5±1.2 µ m. The analysis gave similar prefactors, barriers, and rates across all six experiments. We further showed that all six experiments can be fitted with just two parameters from one global parametrization. The results of Shardt et al. ( 2022 ) including four experiments with two droplet sizes and two cooling rates were similarly fitted with a single pair of A and B values.
We derived a superposition formula to predict how the distribution of droplet sizes causes a broadening in the distribution of nucleation temperatures. The broadening causes the AINTBAD analysis to underestimate the B parameter relative to that obtained from monodisperse droplets of the same mean size. For example, pooling droplets with a broad range of diameters from 4 to 19 µ m significantly reduces the slope of the fraction of frozen droplets with temperature and leads to a ∼40 % reduction in the inferred barrier. The erroneous parametrization would result in large errors for glaciation rates in cloud microphysics models. Accurate parametrizations can be obtained from experiments like those of Atkinson et al. ( 2016 ) and Shardt et al. ( 2022 ) , in which the droplet size distributions are sufficiently narrow to yield parameters that are indistinguishable from perfectly monodisperse droplets.
Our analysis suggests that the barrier obtained with AINTBAD is the sum of the nucleation and diffusion barriers. As the nucleation barriers decrease monotonously with temperature, we infer that the increase in the overall barrier for nucleation at 235.5 K originates in a steeper temperature dependence of the diffusion coefficient of water D that controls the temperature dependence of the prefactor. Our interpretation is consistent with the steep decrease in D ( T ) unveiled by experiments on water approaching the maximum in the isobaric heat capacity at 229 K ( Pathak et al. , 2021 ) and calls for a reassessment of the representation of D ( T ) in CNT parametrizations of ice nucleation rates.
While laboratory experiments strive to study nucleation in the narrowest possible distribution of droplet sizes to avoid spurious impacts on the parametrization of nucleation rates, clouds can have a relatively broad distribution in the size of water droplets. We developed the Python code IPA to predict the nucleation spectrum for any given distribution of droplet diameters at any cooling rate. As input, IPA uses the distribution of droplet diameters and a parametrization of the nucleation rate J ( T ) from the literature (Fig. 13 ). IPA includes various previously reported parametrizations and can be extended to use others introduced by users. We have demonstrated the application of IPA in predicting the impact of droplet diameter distributions typical of clouds on the evolution of the fraction of frozen droplets with temperature. By integrating the cooling rate and size dependence into the ice nucleation rates, the results and tools provided in this study could be used to improve and test approximations made in cloud models.
We restrict our discussion in this article to homogeneous nucleation, but it might be possible to develop similar methods for analysis of heterogeneous-nucleation data. A key challenge is that pure water droplets vary only in volume, while heterogeneous-nucleation sites may vary in the surface chemistry, pore geometry, and size (area) of the active region. These differences lead to sites with different barriers and also different prefactors. Except for special cases of highly regular surfaces, the estimated A and B parameters will then reflect a superposition of survival probabilities from many different types of sites. To illustrate this point, we analyze the data of the fraction of ice vs. temperature for ice nucleation by kaolinite from Zhang and Maeda ( 2022 ) using the AINTBAD code. The estimated barrier at T 50 =267.2 K is approximately 2 k B T (Fig. S1 in the Supplement), which is a low value indicative of a superposition with nucleation sites of many different barriers. Further developments are needed to disentangle the contributions of different sites to the heterogeneous nucleation of ice.
The codes and data that support the findings of this study are available in the GitHub repository at https://github.com/Molinero-Group/volume-dispersion (last access: 18 March 2024), as well as at https://doi.org/10.5281/zenodo.13770437 ( Addula et al. , 2024 ) .
The supplement related to this article is available online at: https://doi.org/10.5194/acp-24-10833-2024-supplement .
RKRA, IdAR, VM, and BP designed the project and prepared the manuscript. RKRA, IdAR, and BP developed the models and performed the analysis.
The contact author has declared that none of the authors has any competing interests.
Publisher’s note: Copernicus Publications remains neutral with regard to jurisdictional claims made in the text, published maps, institutional affiliations, or any other geographical representation in this paper. While Copernicus Publications makes every effort to include appropriate place names, the final responsibility lies with the authors.
We thank Geoffrey Poon, Max Flattery, and Conrad Morris for helpful discussions. This work was supported by the Air Force Office of Scientific Research through MURI award no. FA9550-20-1-0351.
This research has been supported by the Air Force Office of Scientific Research (MURI award no. FA9550-20-1-0351).
This paper was edited by Hinrich Grothe and reviewed by two anonymous referees.
Addula, R. K. R., de Almeida Ribeiro, I., Molinero, B., and Peters, B.: AINTBAD and IPA code and input data, Zenodo [code, data set], https://doi.org/10.5281/zenodo.13770437 , 2024. a , b , c , d
Alpert, P. A. and Knopf, D. A.: Analysis of isothermal and cooling-rate-dependent immersion freezing by a unifying stochastic ice nucleation model, Atmos. Chem. Phys., 16, 2083–2107, https://doi.org/10.5194/acp-16-2083-2016 , 2016. a , b , c , d , e , f
Ando, K., Arakawa, M., and Terasaki, A.: Freezing of micrometer-sized liquid droplets of pure water evaporatively cooled in a vacuum, Phys. Chem. Chem. Phys., 20, 28435–28444, 2018. a , b , c , d
Atkinson, J. D., Murray, B. J., and O'Sullivan, D.: Rate of Homogenous Nucleation of Ice in Supercooled Water, J. Phys. Chem. A, 120, 6513–6520, 2016. a , b , c , d , e , f , g , h , i , j , k , l , m , n , o , p , q , r , s , t , u , v , w , x , y , z , aa , ab , ac , ad , ae , af , ag , ah
Barrett, A. I., Westbrook, C. D., Nicol, J. C., and Stein, T. H. M.: Rapid ice aggregation process revealed through triple-wavelength Doppler spectrum radar analysis, Atmos. Chem. Phys., 19, 5753–5769, https://doi.org/10.5194/acp-19-5753-2019 , 2019. a
Becker, R. and Döring, W.: Kinetische Behandlung der Keimbildung in übersättigten Dämpfen, Ann. Physik, 416, 719–752, 1935. a
Cox, D. and Oakes, D.: Analysis of Survival Data, Chapman & Hall/CRC Monographs on Statistics & Applied Probability, Taylor & Francis, ISBN 9780412244902, https://books.google.com/books?id=Y4pdM2soP4IC (last access: 16 September 2024), 1984. a
de Almeida Ribeiro, I., Meister, K., and Molinero, V.: HUB: a method to model and extract the distribution of ice nucleation temperatures from drop-freezing experiments, Atmos. Chem. Phys., 23, 5623–5639, https://doi.org/10.5194/acp-23-5623-2023 , 2023. a , b
Deck, L. T., Ochsenbein, D. R., and Mazzotti, M.: Stochastic shelf-scale modeling framework for the freezing stage in freeze-drying processes, Int. J. Pharm., 613, 121276, https://doi.org/10.1016/j.ijpharm.2021.121276 , 2022. a
DeMott, P. J., Prenni, A. J., Liu, X., Kreidenweis, S. M., Petters, M. D., Twohy, C. H., Richardson, M. S., Eidhammer, T., and Rogers, D. C.: Predicting global atmospheric ice nuclei distributions and their impacts on climate, P. Natl. Acad. Sci. USA, 107, 11217–11222, 2010. a
Deville, S.: Ice-Templating: Processing Routes, Architectures, and Microstructures, 171–252, Springer, Cham, https://doi.org/10.1007/978-3-319-50515-2_4 , ISBN 978-3-319-50515-2, 2017. a
Dobbie, S. and Jonas, P.: Radiative influences on the structure and lifetime of cirrus clouds, Q. J. Roy. Meteor. Soc., 127, 2663–2682, 2001. a
Goff, H.: Colloidal aspects of ice cream – A review, Int. Dairy J., 7, 363–373, 1997. a
Herbert, R. J., Murray, B. J., Whale, T. F., Dobbie, S. J., and Atkinson, J. D.: Representing time-dependent freezing behaviour in immersion mode ice nucleation, Atmos. Chem. Phys., 14, 8501–8520, https://doi.org/10.5194/acp-14-8501-2014 , 2014. a , b
Herbert, R. J., Murray, B. J., Dobbie, S. J., and Koop, T.: Sensitivity of liquid clouds to homogenous freezing parameterizations, Geophys. Res. Lett., 42, 1599–1605, 2015. a , b , c , d
Heymsfield, A. J. and Miloshevich, L. M.: Homogeneous ice nucleation and supercooled liquid water in orographic wave clouds, J. Atmos. Sci., 50, 2335–2353, 1993. a
Ickes, L., Welti, A., Hoose, C., and Lohmann, U.: Classical nucleation theory of homogeneous freezing of water: thermodynamic and kinetic parameters, Phys. Chem. Chem. Phys., 17, 5514–5537, 2015. a , b
Ickes, L., Welti, A., and Lohmann, U.: Classical nucleation theory of immersion freezing: sensitivity of contact angle schemes to thermodynamic and kinetic parameters, Atmos. Chem. Phys., 17, 1713–1739, https://doi.org/10.5194/acp-17-1713-2017 , 2017. a
Igel, A. L. and van den Heever, S. C.: The importance of the shape of cloud droplet size distributions in shallow cumulus clouds. Part II: Bulk microphysics simulations, J. Atmos. Sci., 74, 259–273, 2017. a , b , c , d , e
Kärcher, B. and Lohmann, U.: A parameterization of cirrus cloud formation: Homogeneous freezing including effects of aerosol size, J. Geophys. Res.-Atmos., 107, 1–9, https://doi.org/10.1029/2001JD000470 , 2002. a
Kärcher, B. and Seifert, A.: On homogeneous ice formation in liquid clouds, Q. J. Roy. Meteor. Soc., 142, 1320–1334, 2016. a , b
Kashchiev, D.: Nucleation: basic theory with applications, Butterworth Heinemann, Oxford, ISBN 9780750646826, 2000. a
Knopf, D. A. and Alpert, P. A.: Atmospheric ice nucleation, Nat. Rev. Phys., 5, 203–217, 2023. a , b , c , d
Knopf, D. A., Alpert, P. A., Zipori, A., Reicher, N., and Rudich, Y.: Stochastic nucleation processes and substrate abundance explain time-dependent freezing in supercooled droplets, NPJ Clim. Atmos. Sci., 3, 1–9, https://doi.org/10.1038/s41612-020-0106-4 , 2020. a , b
Koop, T. and Murray, B. J.: A physically constrained classical description of the homogeneous nucleation of ice in water, J. Chem. Phys., 145, 211915, https://doi.org/10.1063/1.4962355 , 2016. a , b , c , d , e
Koop, T., Luo, B., Tsias, A., and Peter, T.: Water activity as the determinant for homogeneous ice nucleation in aqueous solutions, Nature, 406, 611–614, 2000. a , b , c
Kubota, N.: Random distribution active site model for ice nucleation in water droplets, Cryst. Eng. Comm., 21, 3810–3821, 2019. a , b , c
Laksmono, H., McQueen, T. A., Sellberg, J. A., Loh, N. D., Huang, C., Schlesinger, D., Sierra, R. G., Hampton, C. Y., Nordlund, D., Beye, M., Martin, A. V., Barty, A., Seibert, M. M., Messerschmidt, M., Williams, G. J., Boutet, S., Amann-Winkel, K., Loerting, T., Pettersson, L. G. M., Bogan, M. J., and Nilsson, A.: Anomalous Behavior of the Homogeneous Ice Nucleation Rate in “No-Man's Land”, J. Phys. Chem. Lett., 6, 2826–2832, 2015. a
Laval, P., Crombez, A., and Salmon, J. B.: Microfluidic Droplet Method for Nucleation Kinetics Measurements, Langmuir, 25, 1836–1841, 2009. a
Li, B. and Sun, D.: Novel methods for rapid freezing and thawing of foods – a review, J. Food Eng., 54, 175–182, 2002. a
Liu, Y., Laiguang, Y., Weinong, Y., and Feng, L.: On the size distribution of cloud droplets, Atmos. Res., 35, 201–216, https://doi.org/10.1016/0169-8095(94)00019-A , 1995. a
Marcolli, C., Gedamke, S., Peter, T., and Zobrist, B.: Efficiency of immersion mode ice nucleation on surrogates of mineral dust, Atmos. Chem. Phys., 7, 5081–5091, https://doi.org/10.5194/acp-7-5081-2007 , 2007. a
Möhler, O., DeMott, P. J., Vali, G., and Levin, Z.: Microbio logy and atmospheric processes: the role of biological particles in cloud physics, Biogeosciences, 4, 1059–1071, https://doi.org/10.5194/bg-4-1059-2007 , 2007. a
Morris, G. J., Acton, E., Murray, B. J., and Fonseca, F.: Freezing injury: The special case of the sperm cell, Cryobiology, 64, 71–80, https://doi.org/10.1016/j.cryobiol.2011.12.002 , 2012. a
Murray, B. J., Broadley, S. L., Wilson, T. W., Bull, S. J., Wills, R. H., Christenson, H. K., and Murray, E. J.: Kinetics of the homogeneous freezing of water, Phys. Chem. Chem. Phys., 12, 10380–10387, 2010. a , b , c , d , e , f , g , h , i , j
Painemal, D. and Zuidema, P.: Assessment of MODIS cloud effective radius and optical thickness retrievals over the Southeast Pacific with VOCALS-REx in situ measurements, J. Geophys. Res.-Atmos., 116, D24206, https://doi.org/10.1029/2011JD016155 , 2011. a , b
Pathak, H., Späh, A., Esmaeildoost, N., Sellberg, J. A., Kim, K. H., Perakis, F., Amann-Winkel, K., Ladd-Parada, M., Koliyadu, J., Lane, T. J., Yang, C., Lemke, H. T., Oggenfuss, A. R., Johnson, P. J. M., Deng, Y., Zerdane, S., Mankowsky, R., Beaud, P., and Nilsson, A.: Enhancement and maximum in the isobaric specific-heat capacity measurements of deeply supercooled water using ultrafast calorimetry, P. Natl. Acad. Sci. USA, 118, e2018379118, https://doi.org/10.1073/pnas.2018379118 , 2021. a , b
Peters, B.: Supersaturation rates and schedules: Nucleation kinetics from isothermal metastable zone widths, J. Cryst. Growth, 317, 79–83, 2011. a
Qiu, Y., Odendahl, N., Hudait, A., Mason, R. H., Bertram, A. K., Paesani, F., DeMott, P. J., and Molinero, V.: Ice Nucleation Efficiency of Hydroxylated Organic Surfaces Is Controlled by Their Structural Fluctuations and Mismatch to Ice, J. Am. Chem. Soc., 139, 3052–3064, 2017. a
Qiu, Y., Hudait, A., and Molinero, V.: How Size and Aggregation of Ice-Binding Proteins Control Their Ice Nucleation Efficiency, J. Am. Chem. Soc., 141, 7439–7452, 2019. a , b , c , d , e , f , g , h , i , j , k , l , m
Riechers, B., Wittbracht, F., Hütten, A., and Koop, T.: The homogeneous ice nucleation rate of water droplets produced in a microfluidic device and the role of temperature uncertainty, Phys. Chem. Chem. Phys., 15, 5873–5887, 2013. a , b , c , d , e , f , g , h
Sear, R. P.: Nucleation: theory and applications to protein solutions and colloidal suspensions, J. Phys. Condens. Matter., 19, 033101, https://doi.org/10.1088/0953-8984/19/3/033101 , 2007. a
Sear, R. P.: Quantitative studies of crystal nucleation at constant supersaturation: experimental data and models, Cryst. Eng. Comm., 16, 6506–6522, 2014. a
Shardt, N., Isenrich, F. N., Waser, B., Marcolli, C., Kanji, Z. A., deMello, A. J., and Lohmann, U.: Homogeneous freezing of water droplets for different volumes and cooling rates, Phys. Chem. Chem. Phys., 24, 28213–28221, 2022. a , b , c , d , e , f , g , h , i , j , k , l , m , n , o , p , q , r , s , t , u , v , w , x , y , z , aa , ab , ac , ad , ae , af , ag , ah , ai , aj
Shultz, M. J.: Crystal growth in ice and snow, Phys. Today, 71, 34–39, 2018. a
Sibley, D. N., Llombart, P., Noya, E. G., Archer, A. J., and MacDowell, L. G.: How ice grows from premelting films and water droplets, Nat. Commun., 12, 1–11, https://doi.org/10.1038/s41467-020-20318-6 , 2021. a
Spichtinger, P., Marschalik, P., and Baumgartner, M.: Impact of formulations of the homogeneous nucleation rate on ice nucleation events in cirrus, Atmos. Chem. Phys., 23, 2035–2060, https://doi.org/10.5194/acp-23-2035-2023 , 2023. a , b
Stan, C. A., Schneider, G. F., Shevkoplyas, S. S., Hashimoto, M., Ibanescu, M., Wiley, B. J., and Whitesides, G. M.: A microfluidic apparatus for the study of ice nucleation in supercooled water drops, Lab Chip, 9, 2293–2305, 2009. a
Stephens, G. L.: Radiation Profiles in Extended Water Clouds. II: Parameterization Schemes, J. Atmos. Sci., 35, 2123–2132, https://doi.org/10.1175/1520-0469(1978)035<2123:RPIEWC>2.0.CO;2 , 1978. a , b
Stoll, N., Eichler, J., Hörhold, M., Shigeyama, W., and Weikusat, I.: A Review of the Microstructural Location of Impurities in Polar Ice and Their Impacts on Deformation, Front. Earth Sci., 8, 615613, https://doi.org/10.3389/feart.2020.615613 , 2021. a
Stöckel, P., Weidinger, I. M., Baumgärtel, H., and Leisner, T.: Rates of Homogeneous Ice Nucleation in Levitated H2O and D2O Droplets, J. Phys. Chem. A, 109, 2540–2546, 2005. a
Tarn, M. D., Sikora, S. N. F., Porter, G. C. E., Wyld, B. V., Alayof, M., Reicher, N., Harrison, A. D., Rudich, Y., Shim, J. U., and Murray, B. J.: On-chip analysis of atmospheric ice-nucleating particles in continuous flow, Lab Chip, 20, 2889–2910, 2020. a , b , c , d
Turnbull, D.: Formation of Crystal Nuclei in Liquid Metals, J. Appl. Phys., 21, 1022–1028, 2004. a
Volmer, M. and Weber, A.: Keimbildung in übersättigten Gebilden, Z. Phys. Chem., 119, 277–301, https://doi.org/10.1515/zpch-1926-11927 , 1926. a
Wright, T. P. and Petters, M. D.: The role of time in heterogeneous freezing nucleation, J. Geophys. Res.-Atmos., 118, 3731–3743, 2013. a
Zachariassen, K. E. and Kristiansen, E.: Ice Nucleation and Antinucleation in Nature, Cryobiology, 41, 257–279, 2000. a
Zhang, X. and Maeda, N.: Nucleation curves of ice in the presence of nucleation promoters, Chem. Eng. Sci., 262, 118017, https://doi.org/10.1016/j.ces.2022.118017 , 2022. a , b , c , d
- Introduction
- Analytical model to analyze the nucleation of monodispersed droplets
- A computer code for analysis of drop-freezing experiments
- Analysis of nucleation spectrum in monodispersed droplets
- Droplets with distribution of volume
- How narrow should a droplet distribution be to safely assume a single volume?
- Effect of cooling rate on nucleation parameters
- Comparing homogeneous-nucleation-rate parametrizations
- A computer code to predict the survival probability using any droplet diameter distribution and cooling rate
- Impact of temperature uncertainty on the apparent nucleation barriers
- Conclusions
- Code and data availability
- Author contributions
- Competing interests
- Acknowledgements
- Financial support
- Review statement

COMMENTS
Wash and rinse a penny in tap water. Dry it completely with a paper towel. Place the penny on a flat surface. The flatter the surface, the better this experiment is going to go. Use an eyedropper or pipette to draw up water. Carefully, drop individual drops of water onto the flat surface of the penny. Keep track of the water drops as you add ...
Penny Experiment Set Up: STEP 1: Add water to both of your bowls, and one of them, add green food coloring. This is optional if you want to see the drops a bit better. STEP 2: Use an eyedropper or pipette to pick up and carefully drip one drop of water at a time onto the penny. STEP 3: Count how many drops you can fit onto one penny until the ...
In this activity, you'll experience surface tension and cohesion by testing how many drops of water you can you fit on a penny. You might be surprised considering pennies are so small! ... Drops on a Penny Experiment Environmental Science, Physical Science, Physics, Life Science, Earth Science, MS.PS1A.b, UE.PS1A.c,
This science experiment teaches kids about the concept of surface tension. They'll also be exploring how soap destroys surface tension by pushing the water molecules apart from each other. When you ask kids how many drops of water will fit on a penny, they'll probably guess 3 or 4 drops. Maybe 5. Everyone knows that drops of water aren't ...
Instruction for Penny Drop Experiment: 1. Place your penny on a flat surface. If you are worried about getting water on the table or the floor, you can place a paper towel underneath the penny. 2. Pour some water in a small container. 3. Ask your child to predict how many drops he or she thinks will fit on the penny.
Place a coin on a table or desk. Holding the eyedropper close to the surface of the coin, carefully squeeze water droplets onto the coin, one at a time. The droplets should pool up on the coin, creating a big droplet of water. Get the students to count the drops. Stop squeezing when the droplet on the coin breaks up and overflows.
Step 2: Place the penny on paper towel. Step 3: Use an eye dropper to place drops of WATER on the penny (one at a time) until ANY amount of water runs over the edge of the penny. Step 4: Record the number of drops for that trial in the table. Repeat Steps 1 - 4 three more times before calculating your average. Trial 1.
Coin and Water Experiment | Water Drops on a Penny • The Science Kiddo. Have you wondered how many drops of water can fit on a penny? Try this coin and water experiment and keep track of your results on a free printable chart!
How many drops of water can fit on a penny? 💧 Use pennies and a medicine dropper to learn all about water! Observe two fascinating properties of water, adhe...
Practice squeezing out only one drop at a time. • Pennies. • Cups with water. • Paper towels or wax paper. • Eyedroppers or pipettes. • Place a penny on a paper towel or piece of wax paper. Ask your child how many drops of water they think can fit on the surface of the penny. • Ask your child to begin adding drops of water to the ...
Slowly press down on the plunger, allowing one drop of water at a time to fall onto the penny. Watch the penny very carefully. The drop of water forming on top of the penny will gradually get larger. Stop pushing on the plunger as soon as the drop spills over the edge of the penny, as shown in Figure 4.
A penny experiment that demonstrates how water tension works and water can hold together in a dome shape - a super simple science experiment for kids! Drop water on a penny and it just runs over the side, right? Maybe not... Maia was excited to show us a penny experiment she learned at school that demonstrated how water can hold together in a dome shape. This super simple science experiment ...
Paper and pencil or pen (optional) Preparation. Place your penny on a flat, level surface that can get a little wet, such as a kitchen counter. Fill a glass, cup or small bowl with tap water ...
A cup of water. A variety of coins (we used a penny, a nickel, a dime, and a quarter) Plastic pipette. Free printable table to keep track of the results! (Remember to change the page orientation to landscape before printing.) Directions: Set the coin on a flat surface. Fill a plastic pipette with water. Carefully squeeze out water drop by drop ...
Guess how many water drops you can fit on the front of a penny. Try it. How many drops will stay on the face of a penny without spilling water off the sides? Repeat the experiment, say, three times. The number of drops will probably come out about the same each time. Each time you do it, write down the number of drops. Part 2:
Drops of water on a PENNY experiment / How many drops can fit on a penny?Made for parents and teachersScience Kits and morehttps://elementarysciencen.wixsite...
Place your penny on a flat, level surface that can get a little wet, like a kitchen counter. Fill a glass, cup, or small bowl with tap water. Fill the medicine dropper with water. Now, carefully add one drop of water at a time to the top of the penny. Hold the medicine dropper just above the top of the penny (not touching it), so each new drop ...
The alternate hypothesis is that the side of the penny does make a difference. H 0: The number of water drops a penny can hold is not influenced by "side of penny". H A: The number of water drops a penny can hold is influenced by "side of penny". 7) Design an experiment which tests your hypothesis. Describe it below.
When too much water is added, the drop becomes too heavy, and it falls. Draw three pictures of a penny at different points in the demonstration. Picture 1: Penny at the beginning with only a few drops of water on it. Picture 2: Penny holding the maximum number of drops on it.
1. Rinse a penny in tap water. Dry thoroughly with a paper towel. 2. Place the penny on a fresh paper towel. 3. Fill a beaker with 25 mL of tap water. 4. Using a pipet, slowly drop individual droplets of water onto the surface of the penny. 5. Count each drop until the water begins to spill over the sides of the penny.
Repeat the experiment (steps 2-6) for rubbing alcohol and then soapy water. Be sure to rinse and dry the penny thoroughly between experiments if reusing! You should also clean the droppers if you are reusing them. 7. Add the number of droplets of water the penny held for Run 1, Run 2, and Run 3 and
As drops of water are added onto a penny, the adhesive force between the water and the penny keeps the water from falling off. Cohesive forces are strong, but not unbreakable. As a water drop builds up and out, usually bulging over the sides of the penny, the cohesive forces will eventually be overcome by the force of gravity on the water ...
Place the penny, heads up, on top of a paper towel. 3. Hold your dropper about 1-inch above the penny and add drops of water to the surface of the penny until it overflows. 4. Record the number of drops of water the surface of the penny can hold in the table on the next page under the column labeled "Run 1.". 5.
Abstract. Homogeneous nucleation is the prominent mechanism of glaciation in cirrus and other high-altitude clouds. Ice nucleation rates can be studied in laboratory assays that gradually lower the temperature of pure water droplets. These experiments can be performed with different cooling rates, with different droplet sizes, and often with a distribution of droplet sizes. We combine ...