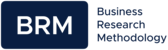

Regression Analysis
Regression analysis is a quantitative research method which is used when the study involves modelling and analysing several variables, where the relationship includes a dependent variable and one or more independent variables. In simple terms, regression analysis is a quantitative method used to test the nature of relationships between a dependent variable and one or more independent variables.
The basic form of regression models includes unknown parameters (β), independent variables (X), and the dependent variable (Y).
Regression model, basically, specifies the relation of dependent variable (Y) to a function combination of independent variables (X) and unknown parameters (β)
Y ≈ f (X, β)
Regression equation can be used to predict the values of ‘y’, if the value of ‘x’ is given, and both ‘y’ and ‘x’ are the two sets of measures of a sample size of ‘n’. The formulae for regression equation would be
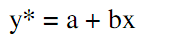
Do not be intimidated by visual complexity of correlation and regression formulae above. You don’t have to apply the formula manually, and correlation and regression analyses can be run with the application of popular analytical software such as Microsoft Excel, Microsoft Access, SPSS and others.
Linear regression analysis is based on the following set of assumptions:
1. Assumption of linearity . There is a linear relationship between dependent and independent variables.
2. Assumption of homoscedasticity . Data values for dependent and independent variables have equal variances.
3. Assumption of absence of collinearity or multicollinearity . There is no correlation between two or more independent variables.
4. Assumption of normal distribution . The data for the independent variables and dependent variable are normally distributed
My e-book, The Ultimate Guide to Writing a Dissertation in Business Studies: a step by step assistance offers practical assistance to complete a dissertation with minimum or no stress. The e-book covers all stages of writing a dissertation starting from the selection to the research area to submitting the completed version of the work within the deadline. John Dudovskiy
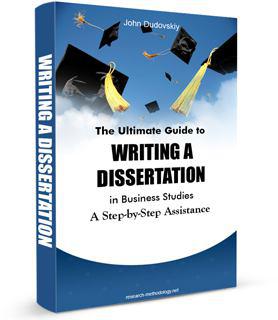
- Privacy Policy

Home » Regression Analysis – Methods, Types and Examples
Regression Analysis – Methods, Types and Examples
Table of Contents

Regression Analysis
Regression analysis is a set of statistical processes for estimating the relationships among variables . It includes many techniques for modeling and analyzing several variables when the focus is on the relationship between a dependent variable and one or more independent variables (or ‘predictors’).
Regression Analysis Methodology
Here is a general methodology for performing regression analysis:
- Define the research question: Clearly state the research question or hypothesis you want to investigate. Identify the dependent variable (also called the response variable or outcome variable) and the independent variables (also called predictor variables or explanatory variables) that you believe are related to the dependent variable.
- Collect data: Gather the data for the dependent variable and independent variables. Ensure that the data is relevant, accurate, and representative of the population or phenomenon you are studying.
- Explore the data: Perform exploratory data analysis to understand the characteristics of the data, identify any missing values or outliers, and assess the relationships between variables through scatter plots, histograms, or summary statistics.
- Choose the regression model: Select an appropriate regression model based on the nature of the variables and the research question. Common regression models include linear regression, multiple regression, logistic regression, polynomial regression, and time series regression, among others.
- Assess assumptions: Check the assumptions of the regression model. Some common assumptions include linearity (the relationship between variables is linear), independence of errors, homoscedasticity (constant variance of errors), and normality of errors. Violation of these assumptions may require additional steps or alternative models.
- Estimate the model: Use a suitable method to estimate the parameters of the regression model. The most common method is ordinary least squares (OLS), which minimizes the sum of squared differences between the observed and predicted values of the dependent variable.
- I nterpret the results: Analyze the estimated coefficients, p-values, confidence intervals, and goodness-of-fit measures (e.g., R-squared) to interpret the results. Determine the significance and direction of the relationships between the independent variables and the dependent variable.
- Evaluate model performance: Assess the overall performance of the regression model using appropriate measures, such as R-squared, adjusted R-squared, and root mean squared error (RMSE). These measures indicate how well the model fits the data and how much of the variation in the dependent variable is explained by the independent variables.
- Test assumptions and diagnose problems: Check the residuals (the differences between observed and predicted values) for any patterns or deviations from assumptions. Conduct diagnostic tests, such as examining residual plots, testing for multicollinearity among independent variables, and assessing heteroscedasticity or autocorrelation, if applicable.
- Make predictions and draw conclusions: Once you have a satisfactory model, use it to make predictions on new or unseen data. Draw conclusions based on the results of the analysis, considering the limitations and potential implications of the findings.
Types of Regression Analysis
Types of Regression Analysis are as follows:
Linear Regression
Linear regression is the most basic and widely used form of regression analysis. It models the linear relationship between a dependent variable and one or more independent variables. The goal is to find the best-fitting line that minimizes the sum of squared differences between observed and predicted values.
Multiple Regression
Multiple regression extends linear regression by incorporating two or more independent variables to predict the dependent variable. It allows for examining the simultaneous effects of multiple predictors on the outcome variable.
Polynomial Regression
Polynomial regression models non-linear relationships between variables by adding polynomial terms (e.g., squared or cubic terms) to the regression equation. It can capture curved or nonlinear patterns in the data.
Logistic Regression
Logistic regression is used when the dependent variable is binary or categorical. It models the probability of the occurrence of a certain event or outcome based on the independent variables. Logistic regression estimates the coefficients using the logistic function, which transforms the linear combination of predictors into a probability.
Ridge Regression and Lasso Regression
Ridge regression and Lasso regression are techniques used for addressing multicollinearity (high correlation between independent variables) and variable selection. Both methods introduce a penalty term to the regression equation to shrink or eliminate less important variables. Ridge regression uses L2 regularization, while Lasso regression uses L1 regularization.
Time Series Regression
Time series regression analyzes the relationship between a dependent variable and independent variables when the data is collected over time. It accounts for autocorrelation and trends in the data and is used in forecasting and studying temporal relationships.
Nonlinear Regression
Nonlinear regression models are used when the relationship between the dependent variable and independent variables is not linear. These models can take various functional forms and require estimation techniques different from those used in linear regression.
Poisson Regression
Poisson regression is employed when the dependent variable represents count data. It models the relationship between the independent variables and the expected count, assuming a Poisson distribution for the dependent variable.
Generalized Linear Models (GLM)
GLMs are a flexible class of regression models that extend the linear regression framework to handle different types of dependent variables, including binary, count, and continuous variables. GLMs incorporate various probability distributions and link functions.
Regression Analysis Formulas
Regression analysis involves estimating the parameters of a regression model to describe the relationship between the dependent variable (Y) and one or more independent variables (X). Here are the basic formulas for linear regression, multiple regression, and logistic regression:
Linear Regression:
Simple Linear Regression Model: Y = β0 + β1X + ε
Multiple Linear Regression Model: Y = β0 + β1X1 + β2X2 + … + βnXn + ε
In both formulas:
- Y represents the dependent variable (response variable).
- X represents the independent variable(s) (predictor variable(s)).
- β0, β1, β2, …, βn are the regression coefficients or parameters that need to be estimated.
- ε represents the error term or residual (the difference between the observed and predicted values).
Multiple Regression:
Multiple regression extends the concept of simple linear regression by including multiple independent variables.
Multiple Regression Model: Y = β0 + β1X1 + β2X2 + … + βnXn + ε
The formulas are similar to those in linear regression, with the addition of more independent variables.
Logistic Regression:
Logistic regression is used when the dependent variable is binary or categorical. The logistic regression model applies a logistic or sigmoid function to the linear combination of the independent variables.
Logistic Regression Model: p = 1 / (1 + e^-(β0 + β1X1 + β2X2 + … + βnXn))
In the formula:
- p represents the probability of the event occurring (e.g., the probability of success or belonging to a certain category).
- X1, X2, …, Xn represent the independent variables.
- e is the base of the natural logarithm.
The logistic function ensures that the predicted probabilities lie between 0 and 1, allowing for binary classification.
Regression Analysis Examples
Regression Analysis Examples are as follows:
- Stock Market Prediction: Regression analysis can be used to predict stock prices based on various factors such as historical prices, trading volume, news sentiment, and economic indicators. Traders and investors can use this analysis to make informed decisions about buying or selling stocks.
- Demand Forecasting: In retail and e-commerce, real-time It can help forecast demand for products. By analyzing historical sales data along with real-time data such as website traffic, promotional activities, and market trends, businesses can adjust their inventory levels and production schedules to meet customer demand more effectively.
- Energy Load Forecasting: Utility companies often use real-time regression analysis to forecast electricity demand. By analyzing historical energy consumption data, weather conditions, and other relevant factors, they can predict future energy loads. This information helps them optimize power generation and distribution, ensuring a stable and efficient energy supply.
- Online Advertising Performance: It can be used to assess the performance of online advertising campaigns. By analyzing real-time data on ad impressions, click-through rates, conversion rates, and other metrics, advertisers can adjust their targeting, messaging, and ad placement strategies to maximize their return on investment.
- Predictive Maintenance: Regression analysis can be applied to predict equipment failures or maintenance needs. By continuously monitoring sensor data from machines or vehicles, regression models can identify patterns or anomalies that indicate potential failures. This enables proactive maintenance, reducing downtime and optimizing maintenance schedules.
- Financial Risk Assessment: Real-time regression analysis can help financial institutions assess the risk associated with lending or investment decisions. By analyzing real-time data on factors such as borrower financials, market conditions, and macroeconomic indicators, regression models can estimate the likelihood of default or assess the risk-return tradeoff for investment portfolios.
Importance of Regression Analysis
Importance of Regression Analysis is as follows:
- Relationship Identification: Regression analysis helps in identifying and quantifying the relationship between a dependent variable and one or more independent variables. It allows us to determine how changes in independent variables impact the dependent variable. This information is crucial for decision-making, planning, and forecasting.
- Prediction and Forecasting: Regression analysis enables us to make predictions and forecasts based on the relationships identified. By estimating the values of the dependent variable using known values of independent variables, regression models can provide valuable insights into future outcomes. This is particularly useful in business, economics, finance, and other fields where forecasting is vital for planning and strategy development.
- Causality Assessment: While correlation does not imply causation, regression analysis provides a framework for assessing causality by considering the direction and strength of the relationship between variables. It allows researchers to control for other factors and assess the impact of a specific independent variable on the dependent variable. This helps in determining the causal effect and identifying significant factors that influence outcomes.
- Model Building and Variable Selection: Regression analysis aids in model building by determining the most appropriate functional form of the relationship between variables. It helps researchers select relevant independent variables and eliminate irrelevant ones, reducing complexity and improving model accuracy. This process is crucial for creating robust and interpretable models.
- Hypothesis Testing: Regression analysis provides a statistical framework for hypothesis testing. Researchers can test the significance of individual coefficients, assess the overall model fit, and determine if the relationship between variables is statistically significant. This allows for rigorous analysis and validation of research hypotheses.
- Policy Evaluation and Decision-Making: Regression analysis plays a vital role in policy evaluation and decision-making processes. By analyzing historical data, researchers can evaluate the effectiveness of policy interventions and identify the key factors contributing to certain outcomes. This information helps policymakers make informed decisions, allocate resources effectively, and optimize policy implementation.
- Risk Assessment and Control: Regression analysis can be used for risk assessment and control purposes. By analyzing historical data, organizations can identify risk factors and develop models that predict the likelihood of certain outcomes, such as defaults, accidents, or failures. This enables proactive risk management, allowing organizations to take preventive measures and mitigate potential risks.
When to Use Regression Analysis
- Prediction : Regression analysis is often employed to predict the value of the dependent variable based on the values of independent variables. For example, you might use regression to predict sales based on advertising expenditure, or to predict a student’s academic performance based on variables like study time, attendance, and previous grades.
- Relationship analysis: Regression can help determine the strength and direction of the relationship between variables. It can be used to examine whether there is a linear association between variables, identify which independent variables have a significant impact on the dependent variable, and quantify the magnitude of those effects.
- Causal inference: Regression analysis can be used to explore cause-and-effect relationships by controlling for other variables. For example, in a medical study, you might use regression to determine the impact of a specific treatment while accounting for other factors like age, gender, and lifestyle.
- Forecasting : Regression models can be utilized to forecast future trends or outcomes. By fitting a regression model to historical data, you can make predictions about future values of the dependent variable based on changes in the independent variables.
- Model evaluation: Regression analysis can be used to evaluate the performance of a model or test the significance of variables. You can assess how well the model fits the data, determine if additional variables improve the model’s predictive power, or test the statistical significance of coefficients.
- Data exploration : Regression analysis can help uncover patterns and insights in the data. By examining the relationships between variables, you can gain a deeper understanding of the data set and identify potential patterns, outliers, or influential observations.
Applications of Regression Analysis
Here are some common applications of regression analysis:
- Economic Forecasting: Regression analysis is frequently employed in economics to forecast variables such as GDP growth, inflation rates, or stock market performance. By analyzing historical data and identifying the underlying relationships, economists can make predictions about future economic conditions.
- Financial Analysis: Regression analysis plays a crucial role in financial analysis, such as predicting stock prices or evaluating the impact of financial factors on company performance. It helps analysts understand how variables like interest rates, company earnings, or market indices influence financial outcomes.
- Marketing Research: Regression analysis helps marketers understand consumer behavior and make data-driven decisions. It can be used to predict sales based on advertising expenditures, pricing strategies, or demographic variables. Regression models provide insights into which marketing efforts are most effective and help optimize marketing campaigns.
- Health Sciences: Regression analysis is extensively used in medical research and public health studies. It helps examine the relationship between risk factors and health outcomes, such as the impact of smoking on lung cancer or the relationship between diet and heart disease. Regression analysis also helps in predicting health outcomes based on various factors like age, genetic markers, or lifestyle choices.
- Social Sciences: Regression analysis is widely used in social sciences like sociology, psychology, and education research. Researchers can investigate the impact of variables like income, education level, or social factors on various outcomes such as crime rates, academic performance, or job satisfaction.
- Operations Research: Regression analysis is applied in operations research to optimize processes and improve efficiency. For example, it can be used to predict demand based on historical sales data, determine the factors influencing production output, or optimize supply chain logistics.
- Environmental Studies: Regression analysis helps in understanding and predicting environmental phenomena. It can be used to analyze the impact of factors like temperature, pollution levels, or land use patterns on phenomena such as species diversity, water quality, or climate change.
- Sports Analytics: Regression analysis is increasingly used in sports analytics to gain insights into player performance, team strategies, and game outcomes. It helps analyze the relationship between various factors like player statistics, coaching strategies, or environmental conditions and their impact on game outcomes.
Advantages and Disadvantages of Regression Analysis
Advantages of Regression Analysis | Disadvantages of Regression Analysis |
---|---|
Provides a quantitative measure of the relationship between variables | Assumes a linear relationship between variables, which may not always hold true |
Helps in predicting and forecasting outcomes based on historical data | Requires a large sample size to produce reliable results |
Identifies and measures the significance of independent variables on the dependent variable | Assumes no multicollinearity, meaning that independent variables should not be highly correlated with each other |
Provides estimates of the coefficients that represent the strength and direction of the relationship between variables | Assumes the absence of outliers or influential data points |
Allows for hypothesis testing to determine the statistical significance of the relationship | Can be sensitive to the inclusion or exclusion of certain variables, leading to different results |
Can handle both continuous and categorical variables | Assumes the independence of observations, which may not hold true in some cases |
Offers a visual representation of the relationship through the use of scatter plots and regression lines | May not capture complex non-linear relationships between variables without appropriate transformations |
Provides insights into the marginal effects of independent variables on the dependent variable | Requires the assumption of homoscedasticity, meaning that the variance of errors is constant across all levels of the independent variables |
About the author
Muhammad Hassan
Researcher, Academic Writer, Web developer
You may also like

Probability Histogram – Definition, Examples and...

Data Analysis – Process, Methods and Types

Textual Analysis – Types, Examples and Guide

Histogram – Types, Examples and Making Guide

Content Analysis – Methods, Types and Examples

Critical Analysis – Types, Examples and Writing...
- How it works
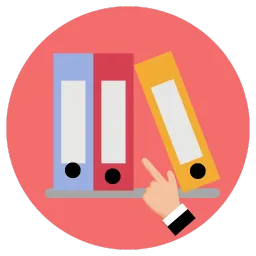
A Beginner’s Guide to Regression Analysis
Published by Owen Ingram at September 1st, 2021 , Revised On July 5, 2022
Are you good with data-driven decisions at work? If not, why? What is stopping you from getting on the crest of a wave? There could be just one answer to these questions, and that is “too much data getting in the way.” Do not worry; there is a solution to every problem in this world, and there is definitely one for parsing through tons of data.
Yes, you heard it right! You will not have to get in trouble with the number crunching and counting with this solution. What is the solution?
Well, without further ado, we would like to introduce you to “regression,” which precisely is allowing one to see into the future.
What is Regression Analysis?
Here is a scenario to help you understand what regression is and how it helps you make better strategic decisions in research.
Let’s say you are the CEO of a company and are trying to predict the profit margin for the next month. Now you might have a lot of factors in your mind that can affect the number. Be it the number of sales you get in the month, the number of employees not taking leaves, or the number of hours each worker gives daily. But what if things do not go as planned? The “what if” list here has no stop; it can go on forever. All these impacting factors here are variables, and regression analysis is the process of mathematically figuring out which of these variables actually have an impact and which are not plausible.
So, we can say that regression analysis helps you find the relationship between a set of dependent and independent variables. There are different ways to find this relationship between variables, which in statistics is named “ regression models .”
We will learn about each in the next heading.
Types of Regression Models
If you are not sure which type of regression model you should use for a particular study, this section might help you.
Though there are numerous types of regression models depending on the type of variables , these are the most common ones.
Linear Regression
Logistic regression, ridge regression, lasso regression, polynomial regression, bayesian linear regression.
Linear regression is the real workhorse of the industry and probably is the first type that comes to mind. It is often known as Linear Least Squares and Ordinary Least Squares . This model consists of a dependent variable and a predictable variable that align with each other. Hence, the name linear regression. If the data you are dealing with contains more than one independent variable , then the linear regression here would be Multi-Linear Regression .
Logistic Regression comes into play when the dependent variable is discrete. This means that the target value will only have one or two values. For instance, a true or false, a yes or no, a 0 or 1, and so on. In this case, a sigmoid curve describes the relationship between the independent and dependent variables .
When using this regression model for the data analysis process , two things should strictly be taken into consideration:
- Make sure there is no multi-linearity (like that in the linear regression model) or correlation between the two variables in the dataset
- Also, ensure that the size of data is big with the equal manifestation of values to come in targeted variables
When there is a high correlation between the independent and dependent variables, this type of regression is used. It is simply because, with multi collinear data, least-square estimates give impartial numbers. However, if the collinearity is high, there might be a slight chance of unfair judgment.
Thus, a bias matrix is brought to the surface in ridge regression. This powerful type of regression is less vulnerable to overfitting. Are you familiar with the ‘overfitting’ word?
Overfitting in statistics is a modeling error that one makes when the function is too closely brought into line with limited data points. When a model in research has been compromised with this error, it might lose its value all at once.
Lasso Regression is best suitable for performing regularization alongside feature selection. This type of regression hinders the absolute size of the regression coefficient. What happens next? The coefficient value will almost come nearer zero, which the complete opposite of what happened in Ridge Regression.
This is why feature selection utilizes this regression model that helps to select a set of features from the dataset. Only required and limited features are used in Lasso Regression, and all the other features are zero. Researchers get rid of the overfitting in the model by doing this. But what if the independent variables are highly collinear?
In that case, this model will only choose one variable and turn the others to zero. We can say that it is somewhat like the Ridge Regression but with variable selection.
This is another type of regression that is almost the same as Multi-Linear Regression but with some changes. In the Polynomial Regression Model, the relationship between the two variables, dependent and independent , is denoted by the nth degree. While in a Multi-Linear Regression Model, the line is linear, here it is the opposite. The best fit line in Polynomial Regression passing through all the points is curved. This curve either depends on the value of n or the value of X.
This model is also prone to overfitting. It is best to assess the curve towards the end as the higher polynomials might give strange and unexpected results on extrapolation.
The last type of regression model we are going to discuss is the Bayesian Linear Regression. Have you heard of the Bayes theorem? Well, this regression type basically uses that to figure out the value of regression coefficients.
It is a lot like both Ridge Regression and Linear Regression, but the stability here is much higher. In this model, we find the value of the posterior distribution of the features instead of working on the least squares.
FAQs About Regression Analysis
What is regression.
It is a technique to find out the relationship between the dependent and independent variables
What is a linear regression model?
Linear Regression Model helps determine the relationship between different continuous variables by fitting a linear equation for dealing with data.
What is the difference between multi-linear regression and polynomial regression?
The only difference between Multi-Linear Regression and polynomial repression is that in the latter relationship between ‘x’ and ‘y’ is denoted by the nth value, so the line here is a curve. While in Multi-Linear, the line is straight.
What is overfitting in statistics?
When a function in statistics corresponds too closely to a particular set of data, some modeling error is possible. This modeling error is called overfitting.
What is ridge regression?
It is a method of finding the coefficients of multiple regression models in which the independent variables are highly correlated. In other words, it is a method to develop a parsimonious model when the number of predictable variables is higher than the observations in a set.
You May Also Like
This introductory article aims to define, elaborate and exemplify transferability in qualitative research.
Nominal Data is a type of qualitative data that divides variables into groups and categories. Nominal data helps to find valuable information about a sample.
a multiple linear regression model is used when there are two or more independent variables—x1 and x2—that are predicted to change another variable, y.
USEFUL LINKS
LEARNING RESOURCES
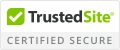
COMPANY DETAILS
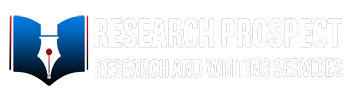
- How It Works
Have a language expert improve your writing
Run a free plagiarism check in 10 minutes, generate accurate citations for free.
- Knowledge Base
- Simple Linear Regression | An Easy Introduction & Examples
Simple Linear Regression | An Easy Introduction & Examples
Published on February 19, 2020 by Rebecca Bevans . Revised on June 22, 2023.
Simple linear regression is used to estimate the relationship between two quantitative variables . You can use simple linear regression when you want to know:
- How strong the relationship is between two variables (e.g., the relationship between rainfall and soil erosion).
- The value of the dependent variable at a certain value of the independent variable (e.g., the amount of soil erosion at a certain level of rainfall).
Regression models describe the relationship between variables by fitting a line to the observed data. Linear regression models use a straight line, while logistic and nonlinear regression models use a curved line. Regression allows you to estimate how a dependent variable changes as the independent variable(s) change.
If you have more than one independent variable, use multiple linear regression instead.
Table of contents
Assumptions of simple linear regression, how to perform a simple linear regression, interpreting the results, presenting the results, can you predict values outside the range of your data, other interesting articles, frequently asked questions about simple linear regression.
Simple linear regression is a parametric test , meaning that it makes certain assumptions about the data. These assumptions are:
- Homogeneity of variance (homoscedasticity) : the size of the error in our prediction doesn’t change significantly across the values of the independent variable.
- Independence of observations : the observations in the dataset were collected using statistically valid sampling methods , and there are no hidden relationships among observations.
- Normality : The data follows a normal distribution .
Linear regression makes one additional assumption:
- The relationship between the independent and dependent variable is linear : the line of best fit through the data points is a straight line (rather than a curve or some sort of grouping factor).
If your data do not meet the assumptions of homoscedasticity or normality, you may be able to use a nonparametric test instead, such as the Spearman rank test.
If your data violate the assumption of independence of observations (e.g., if observations are repeated over time), you may be able to perform a linear mixed-effects model that accounts for the additional structure in the data.
Here's why students love Scribbr's proofreading services
Discover proofreading & editing
Simple linear regression formula
The formula for a simple linear regression is:

- y is the predicted value of the dependent variable ( y ) for any given value of the independent variable ( x ).
- B 0 is the intercept , the predicted value of y when the x is 0.
- B 1 is the regression coefficient – how much we expect y to change as x increases.
- x is the independent variable ( the variable we expect is influencing y ).
- e is the error of the estimate, or how much variation there is in our estimate of the regression coefficient.
Linear regression finds the line of best fit line through your data by searching for the regression coefficient (B 1 ) that minimizes the total error (e) of the model.
While you can perform a linear regression by hand , this is a tedious process, so most people use statistical programs to help them quickly analyze the data.
Simple linear regression in R
R is a free, powerful, and widely-used statistical program. Download the dataset to try it yourself using our income and happiness example.
Dataset for simple linear regression (.csv)
Load the income.data dataset into your R environment, and then run the following command to generate a linear model describing the relationship between income and happiness:
This code takes the data you have collected data = income.data and calculates the effect that the independent variable income has on the dependent variable happiness using the equation for the linear model: lm() .
To learn more, follow our full step-by-step guide to linear regression in R .
To view the results of the model, you can use the summary() function in R:
This function takes the most important parameters from the linear model and puts them into a table, which looks like this:
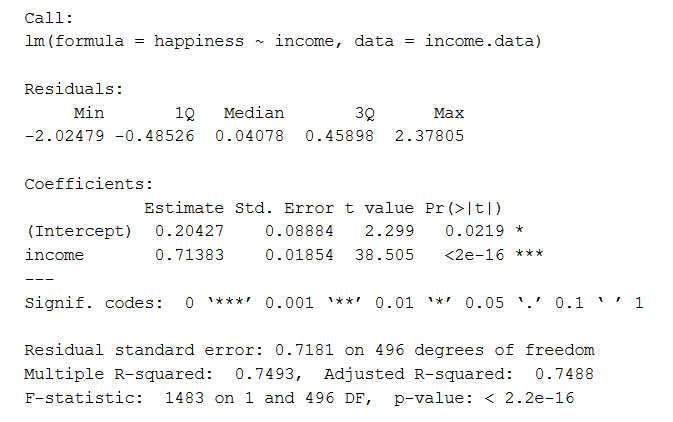
This output table first repeats the formula that was used to generate the results (‘Call’), then summarizes the model residuals (‘Residuals’), which give an idea of how well the model fits the real data.
Next is the ‘Coefficients’ table. The first row gives the estimates of the y-intercept, and the second row gives the regression coefficient of the model.
Row 1 of the table is labeled (Intercept) . This is the y-intercept of the regression equation, with a value of 0.20. You can plug this into your regression equation if you want to predict happiness values across the range of income that you have observed:
The next row in the ‘Coefficients’ table is income. This is the row that describes the estimated effect of income on reported happiness:
The Estimate column is the estimated effect , also called the regression coefficient or r 2 value. The number in the table (0.713) tells us that for every one unit increase in income (where one unit of income = 10,000) there is a corresponding 0.71-unit increase in reported happiness (where happiness is a scale of 1 to 10).
The Std. Error column displays the standard error of the estimate. This number shows how much variation there is in our estimate of the relationship between income and happiness.
The t value column displays the test statistic . Unless you specify otherwise, the test statistic used in linear regression is the t value from a two-sided t test . The larger the test statistic, the less likely it is that our results occurred by chance.
The Pr(>| t |) column shows the p value . This number tells us how likely we are to see the estimated effect of income on happiness if the null hypothesis of no effect were true.
Because the p value is so low ( p < 0.001), we can reject the null hypothesis and conclude that income has a statistically significant effect on happiness.
The last three lines of the model summary are statistics about the model as a whole. The most important thing to notice here is the p value of the model. Here it is significant ( p < 0.001), which means that this model is a good fit for the observed data.
When reporting your results, include the estimated effect (i.e. the regression coefficient), standard error of the estimate, and the p value. You should also interpret your numbers to make it clear to your readers what your regression coefficient means:
It can also be helpful to include a graph with your results. For a simple linear regression, you can simply plot the observations on the x and y axis and then include the regression line and regression function:
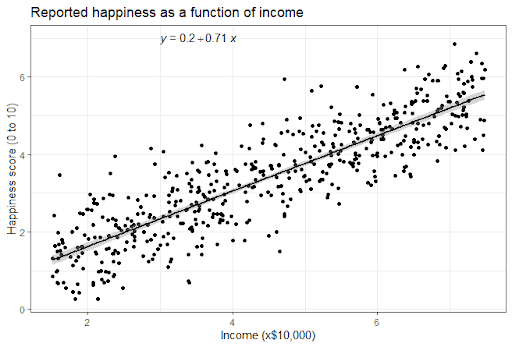
Receive feedback on language, structure, and formatting
Professional editors proofread and edit your paper by focusing on:
- Academic style
- Vague sentences
- Style consistency
See an example
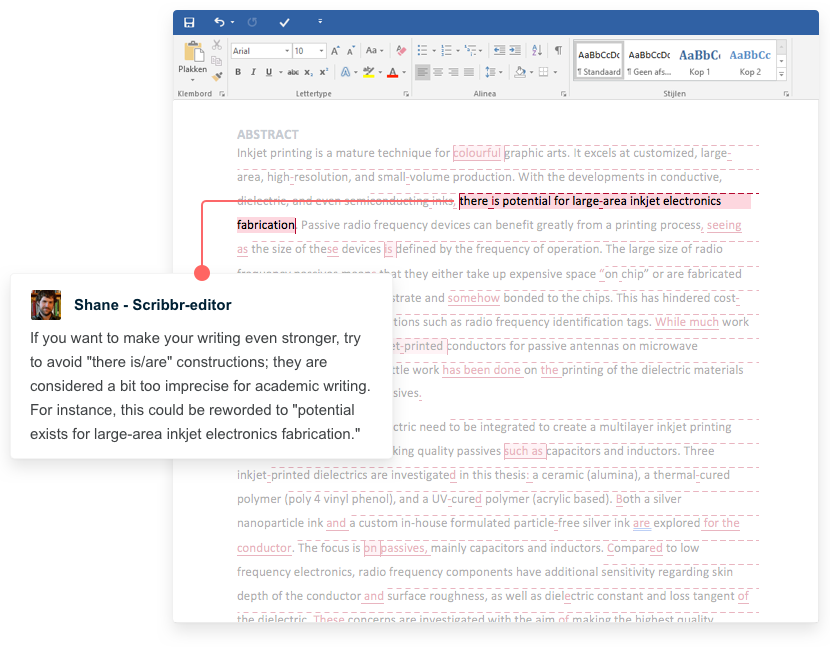
No! We often say that regression models can be used to predict the value of the dependent variable at certain values of the independent variable. However, this is only true for the range of values where we have actually measured the response.
We can use our income and happiness regression analysis as an example. Between 15,000 and 75,000, we found an r 2 of 0.73 ± 0.0193. But what if we did a second survey of people making between 75,000 and 150,000?
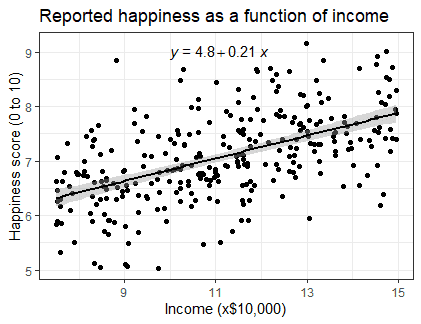
The r 2 for the relationship between income and happiness is now 0.21, or a 0.21-unit increase in reported happiness for every 10,000 increase in income. While the relationship is still statistically significant (p<0.001), the slope is much smaller than before.
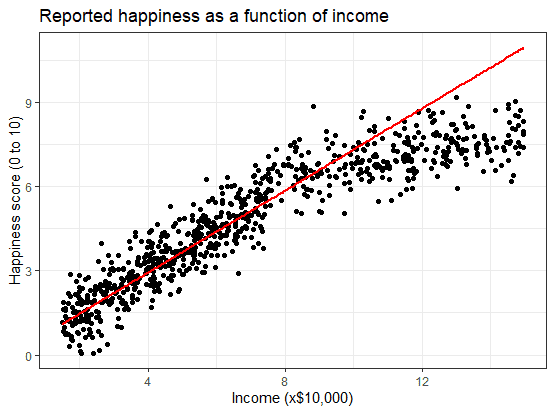
What if we hadn’t measured this group, and instead extrapolated the line from the 15–75k incomes to the 70–150k incomes?
You can see that if we simply extrapolated from the 15–75k income data, we would overestimate the happiness of people in the 75–150k income range.
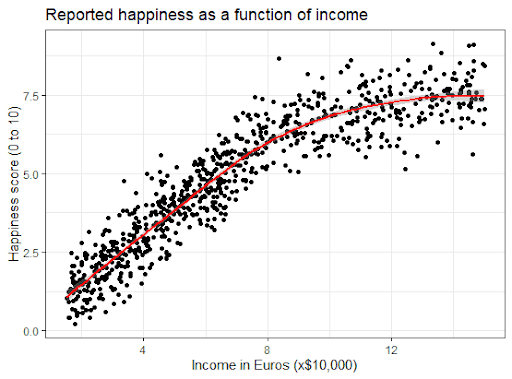
If we instead fit a curve to the data, it seems to fit the actual pattern much better.
It looks as though happiness actually levels off at higher incomes, so we can’t use the same regression line we calculated from our lower-income data to predict happiness at higher levels of income.
Even when you see a strong pattern in your data, you can’t know for certain whether that pattern continues beyond the range of values you have actually measured. Therefore, it’s important to avoid extrapolating beyond what the data actually tell you.
If you want to know more about statistics , methodology , or research bias , make sure to check out some of our other articles with explanations and examples.
- Chi square test of independence
- Statistical power
- Descriptive statistics
- Degrees of freedom
- Pearson correlation
- Null hypothesis
Methodology
- Double-blind study
- Case-control study
- Research ethics
- Data collection
- Hypothesis testing
- Structured interviews
Research bias
- Hawthorne effect
- Unconscious bias
- Recall bias
- Halo effect
- Self-serving bias
- Information bias
A regression model is a statistical model that estimates the relationship between one dependent variable and one or more independent variables using a line (or a plane in the case of two or more independent variables).
A regression model can be used when the dependent variable is quantitative, except in the case of logistic regression, where the dependent variable is binary.
Simple linear regression is a regression model that estimates the relationship between one independent variable and one dependent variable using a straight line. Both variables should be quantitative.
For example, the relationship between temperature and the expansion of mercury in a thermometer can be modeled using a straight line: as temperature increases, the mercury expands. This linear relationship is so certain that we can use mercury thermometers to measure temperature.
Linear regression most often uses mean-square error (MSE) to calculate the error of the model. MSE is calculated by:
- measuring the distance of the observed y-values from the predicted y-values at each value of x;
- squaring each of these distances;
- calculating the mean of each of the squared distances.
Linear regression fits a line to the data by finding the regression coefficient that results in the smallest MSE.
Cite this Scribbr article
If you want to cite this source, you can copy and paste the citation or click the “Cite this Scribbr article” button to automatically add the citation to our free Citation Generator.
Bevans, R. (2023, June 22). Simple Linear Regression | An Easy Introduction & Examples. Scribbr. Retrieved July 4, 2024, from https://www.scribbr.com/statistics/simple-linear-regression/
Is this article helpful?
Rebecca Bevans
Other students also liked, an introduction to t tests | definitions, formula and examples, multiple linear regression | a quick guide (examples), linear regression in r | a step-by-step guide & examples, what is your plagiarism score.
Regression Analysis
The estimation of relationships between a dependent variable and one or more independent variables
What is Regression Analysis?
Regression analysis is a set of statistical methods used for the estimation of relationships between a dependent variable and one or more independent variables . It can be utilized to assess the strength of the relationship between variables and for modeling the future relationship between them.
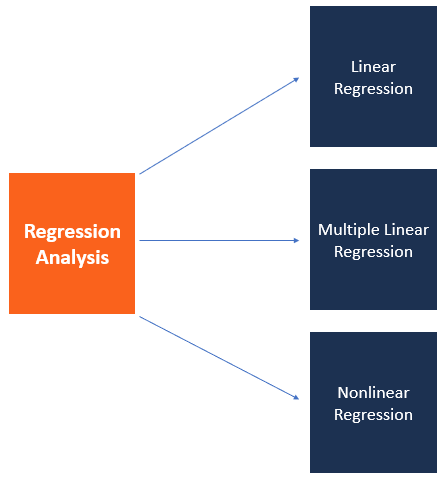
Regression analysis includes several variations, such as linear, multiple linear, and nonlinear. The most common models are simple linear and multiple linear. Nonlinear regression analysis is commonly used for more complicated data sets in which the dependent and independent variables show a nonlinear relationship.
Regression analysis offers numerous applications in various disciplines, including finance .
Regression Analysis – Linear Model Assumptions
Linear regression analysis is based on six fundamental assumptions:
- The dependent and independent variables show a linear relationship between the slope and the intercept.
- The independent variable is not random.
- The value of the residual (error) is zero.
- The value of the residual (error) is constant across all observations.
- The value of the residual (error) is not correlated across all observations.
- The residual (error) values follow the normal distribution.
Regression Analysis – Simple Linear Regression
Simple linear regression is a model that assesses the relationship between a dependent variable and an independent variable. The simple linear model is expressed using the following equation:
Y = a + bX + ϵ
- Y – Dependent variable
- X – Independent (explanatory) variable
- a – Intercept
- b – Slope
- ϵ – Residual (error)
Check out the following video to learn more about simple linear regression:
Regression Analysis – Multiple Linear Regression
Multiple linear regression analysis is essentially similar to the simple linear model, with the exception that multiple independent variables are used in the model. The mathematical representation of multiple linear regression is:
Y = a + b X 1 + c X 2 + d X 3 + ϵ
- X 1 , X 2 , X 3 – Independent (explanatory) variables
- b, c, d – Slopes
Multiple linear regression follows the same conditions as the simple linear model. However, since there are several independent variables in multiple linear analysis, there is another mandatory condition for the model:
- Non-collinearity: Independent variables should show a minimum correlation with each other. If the independent variables are highly correlated with each other, it will be difficult to assess the true relationships between the dependent and independent variables.
Regression Analysis in Finance
Regression analysis comes with several applications in finance. For example, the statistical method is fundamental to the Capital Asset Pricing Model (CAPM) . Essentially, the CAPM equation is a model that determines the relationship between the expected return of an asset and the market risk premium.
The analysis is also used to forecast the returns of securities, based on different factors, or to forecast the performance of a business. Learn more forecasting methods in CFI’s Budgeting and Forecasting Course !
1. Beta and CAPM
In finance, regression analysis is used to calculate the Beta (volatility of returns relative to the overall market) for a stock. It can be done in Excel using the Slope function .
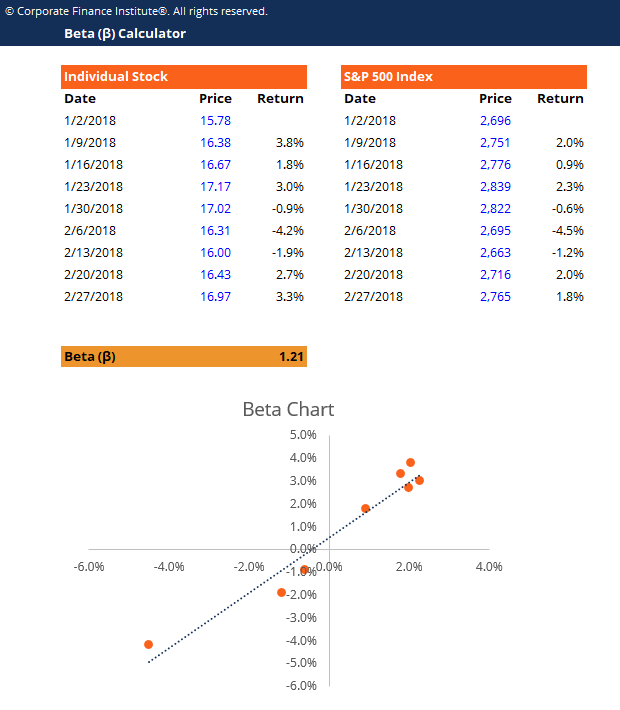
Download CFI’s free beta calculator !
2. Forecasting Revenues and Expenses
When forecasting financial statements for a company, it may be useful to do a multiple regression analysis to determine how changes in certain assumptions or drivers of the business will impact revenue or expenses in the future. For example, there may be a very high correlation between the number of salespeople employed by a company, the number of stores they operate, and the revenue the business generates.
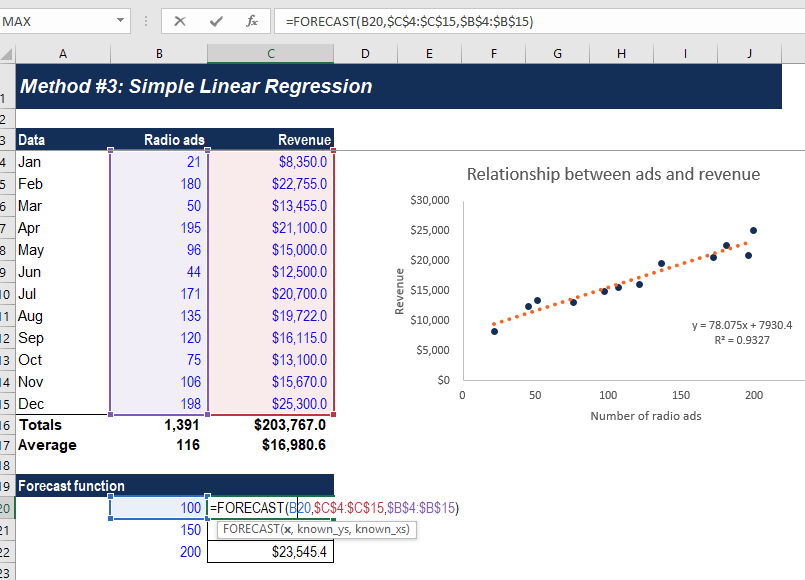
The above example shows how to use the Forecast function in Excel to calculate a company’s revenue, based on the number of ads it runs.
Learn more forecasting methods in CFI’s Budgeting and Forecasting Course !
Regression Tools
Excel remains a popular tool to conduct basic regression analysis in finance, however, there are many more advanced statistical tools that can be used.
Python and R are both powerful coding languages that have become popular for all types of financial modeling, including regression. These techniques form a core part of data science and machine learning where models are trained to detect these relationships in data.
Learn more about regression analysis, Python, and Machine Learning in CFI’s Business Intelligence & Data Analysis certification.
Additional Resources
To learn more about related topics, check out the following free CFI resources:
- Cost Behavior Analysis
- Forecasting Methods
- Joseph Effect
- Variance Inflation Factor (VIF)
- See all data science resources
- Share this article
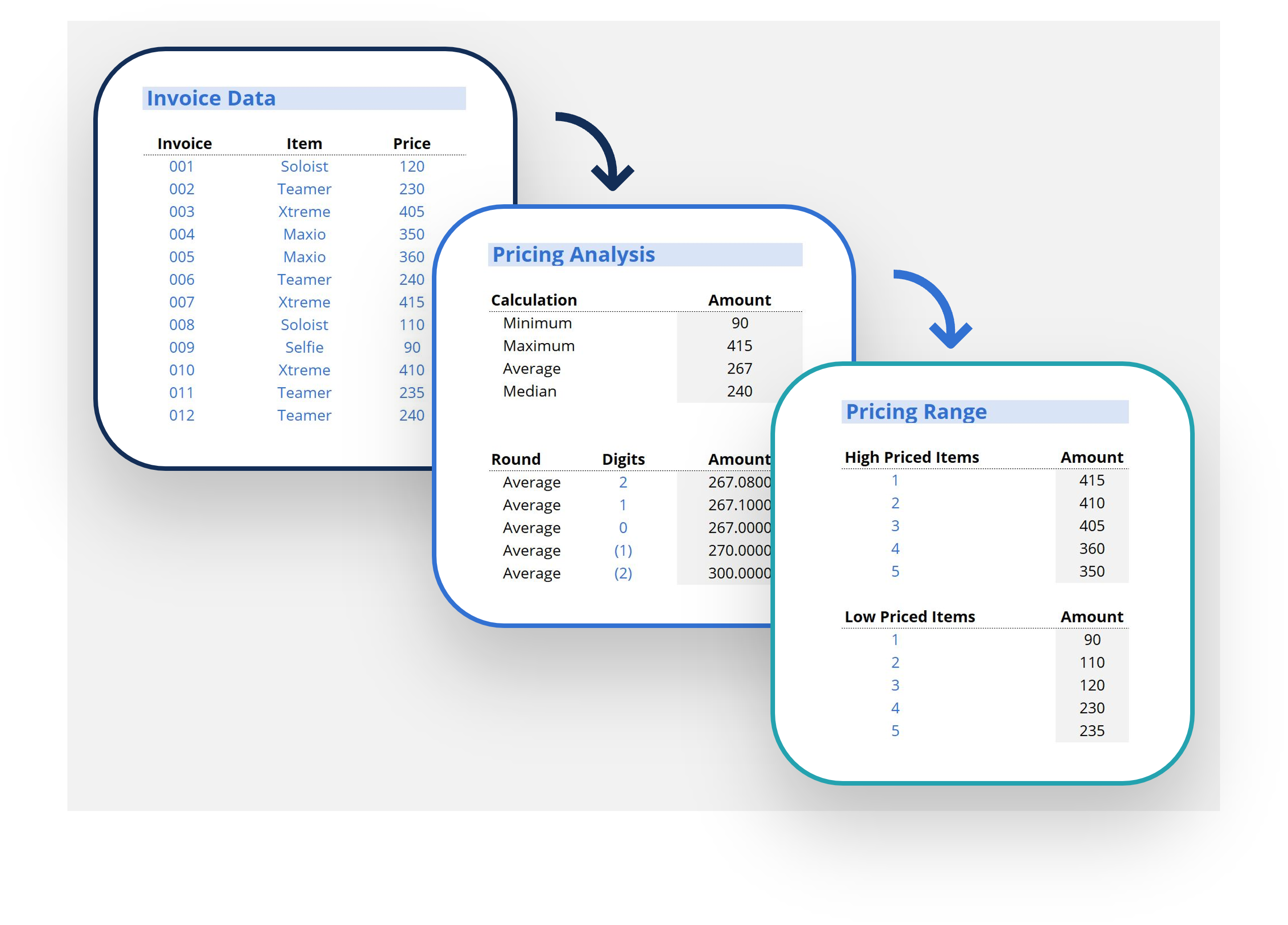
Create a free account to unlock this Template
Access and download collection of free Templates to help power your productivity and performance.
Already have an account? Log in
Supercharge your skills with Premium Templates
Take your learning and productivity to the next level with our Premium Templates.
Upgrading to a paid membership gives you access to our extensive collection of plug-and-play Templates designed to power your performance—as well as CFI's full course catalog and accredited Certification Programs.
Already have a Self-Study or Full-Immersion membership? Log in
Access Exclusive Templates
Gain unlimited access to more than 250 productivity Templates, CFI's full course catalog and accredited Certification Programs, hundreds of resources, expert reviews and support, the chance to work with real-world finance and research tools, and more.
Already have a Full-Immersion membership? Log in
Suggestions or feedback?
MIT News | Massachusetts Institute of Technology
- Machine learning
- Social justice
- Black holes
- Classes and programs
Departments
- Aeronautics and Astronautics
- Brain and Cognitive Sciences
- Architecture
- Political Science
- Mechanical Engineering
Centers, Labs, & Programs
- Abdul Latif Jameel Poverty Action Lab (J-PAL)
- Picower Institute for Learning and Memory
- Lincoln Laboratory
- School of Architecture + Planning
- School of Engineering
- School of Humanities, Arts, and Social Sciences
- Sloan School of Management
- School of Science
- MIT Schwarzman College of Computing
Explained: Regression analysis

Previous image Next image
Share this news article on:
Related links.
- Department of Economics
- Department of Mathematics
- Explained: "Linear and nonlinear systems"
Related Topics
- Mathematics
More MIT News
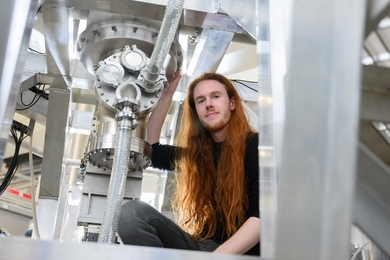
Studying astrophysically relevant plasma physics
Read full story →
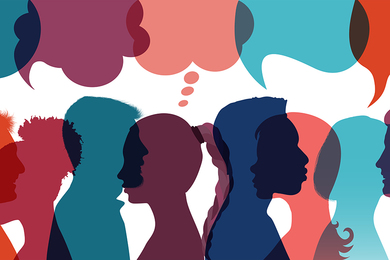
What is language for?
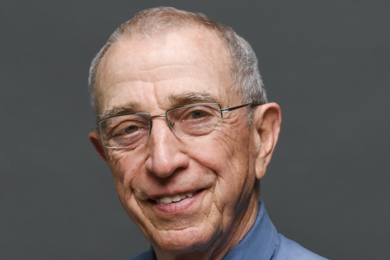
Signal processing: How did we get to where we’re going?
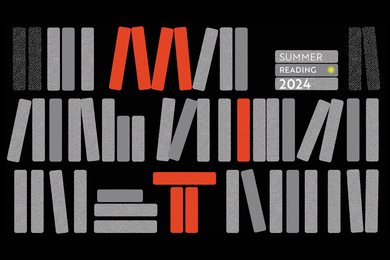
Summer 2024 reading from MIT
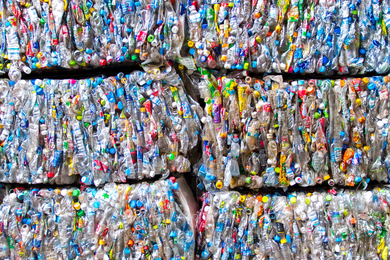
How to increase the rate of plastics recycling
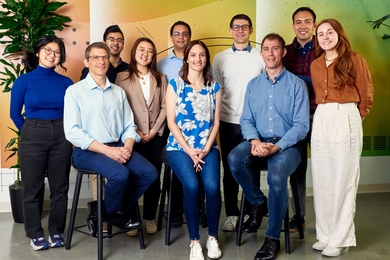
Pioneering the future of materials extraction
- More news on MIT News homepage →
Massachusetts Institute of Technology 77 Massachusetts Avenue, Cambridge, MA, USA
- Map (opens in new window)
- Events (opens in new window)
- People (opens in new window)
- Careers (opens in new window)
- Accessibility
- Social Media Hub
- MIT on Facebook
- MIT on YouTube
- MIT on Instagram
Thank you for visiting nature.com. You are using a browser version with limited support for CSS. To obtain the best experience, we recommend you use a more up to date browser (or turn off compatibility mode in Internet Explorer). In the meantime, to ensure continued support, we are displaying the site without styles and JavaScript.
- View all journals
- Explore content
- About the journal
- Publish with us
- Sign up for alerts
- Published: 31 January 2022
The clinician’s guide to interpreting a regression analysis
- Sofia Bzovsky 1 ,
- Mark R. Phillips ORCID: orcid.org/0000-0003-0923-261X 2 ,
- Robyn H. Guymer ORCID: orcid.org/0000-0002-9441-4356 3 , 4 ,
- Charles C. Wykoff 5 , 6 ,
- Lehana Thabane ORCID: orcid.org/0000-0003-0355-9734 2 , 7 ,
- Mohit Bhandari ORCID: orcid.org/0000-0001-9608-4808 1 , 2 &
- Varun Chaudhary ORCID: orcid.org/0000-0002-9988-4146 1 , 2
on behalf of the R.E.T.I.N.A. study group
Eye volume 36 , pages 1715–1717 ( 2022 ) Cite this article
21k Accesses
9 Citations
1 Altmetric
Metrics details
- Outcomes research
Introduction
When researchers are conducting clinical studies to investigate factors associated with, or treatments for disease and conditions to improve patient care and clinical practice, statistical evaluation of the data is often necessary. Regression analysis is an important statistical method that is commonly used to determine the relationship between several factors and disease outcomes or to identify relevant prognostic factors for diseases [ 1 ].
This editorial will acquaint readers with the basic principles of and an approach to interpreting results from two types of regression analyses widely used in ophthalmology: linear, and logistic regression.
Linear regression analysis
Linear regression is used to quantify a linear relationship or association between a continuous response/outcome variable or dependent variable with at least one independent or explanatory variable by fitting a linear equation to observed data [ 1 ]. The variable that the equation solves for, which is the outcome or response of interest, is called the dependent variable [ 1 ]. The variable that is used to explain the value of the dependent variable is called the predictor, explanatory, or independent variable [ 1 ].
In a linear regression model, the dependent variable must be continuous (e.g. intraocular pressure or visual acuity), whereas, the independent variable may be either continuous (e.g. age), binary (e.g. sex), categorical (e.g. age-related macular degeneration stage or diabetic retinopathy severity scale score), or a combination of these [ 1 ].
When investigating the effect or association of a single independent variable on a continuous dependent variable, this type of analysis is called a simple linear regression [ 2 ]. In many circumstances though, a single independent variable may not be enough to adequately explain the dependent variable. Often it is necessary to control for confounders and in these situations, one can perform a multivariable linear regression to study the effect or association with multiple independent variables on the dependent variable [ 1 , 2 ]. When incorporating numerous independent variables, the regression model estimates the effect or contribution of each independent variable while holding the values of all other independent variables constant [ 3 ].
When interpreting the results of a linear regression, there are a few key outputs for each independent variable included in the model:
Estimated regression coefficient—The estimated regression coefficient indicates the direction and strength of the relationship or association between the independent and dependent variables [ 4 ]. Specifically, the regression coefficient describes the change in the dependent variable for each one-unit change in the independent variable, if continuous [ 4 ]. For instance, if examining the relationship between a continuous predictor variable and intra-ocular pressure (dependent variable), a regression coefficient of 2 means that for every one-unit increase in the predictor, there is a two-unit increase in intra-ocular pressure. If the independent variable is binary or categorical, then the one-unit change represents switching from one category to the reference category [ 4 ]. For instance, if examining the relationship between a binary predictor variable, such as sex, where ‘female’ is set as the reference category, and intra-ocular pressure (dependent variable), a regression coefficient of 2 means that, on average, males have an intra-ocular pressure that is 2 mm Hg higher than females.
Confidence Interval (CI)—The CI, typically set at 95%, is a measure of the precision of the coefficient estimate of the independent variable [ 4 ]. A large CI indicates a low level of precision, whereas a small CI indicates a higher precision [ 5 ].
P value—The p value for the regression coefficient indicates whether the relationship between the independent and dependent variables is statistically significant [ 6 ].
Logistic regression analysis
As with linear regression, logistic regression is used to estimate the association between one or more independent variables with a dependent variable [ 7 ]. However, the distinguishing feature in logistic regression is that the dependent variable (outcome) must be binary (or dichotomous), meaning that the variable can only take two different values or levels, such as ‘1 versus 0’ or ‘yes versus no’ [ 2 , 7 ]. The effect size of predictor variables on the dependent variable is best explained using an odds ratio (OR) [ 2 ]. ORs are used to compare the relative odds of the occurrence of the outcome of interest, given exposure to the variable of interest [ 5 ]. An OR equal to 1 means that the odds of the event in one group are the same as the odds of the event in another group; there is no difference [ 8 ]. An OR > 1 implies that one group has a higher odds of having the event compared with the reference group, whereas an OR < 1 means that one group has a lower odds of having an event compared with the reference group [ 8 ]. When interpreting the results of a logistic regression, the key outputs include the OR, CI, and p-value for each independent variable included in the model.
Clinical example
Sen et al. investigated the association between several factors (independent variables) and visual acuity outcomes (dependent variable) in patients receiving anti-vascular endothelial growth factor therapy for macular oedema (DMO) by means of both linear and logistic regression [ 9 ]. Multivariable linear regression demonstrated that age (Estimate −0.33, 95% CI − 0.48 to −0.19, p < 0.001) was significantly associated with best-corrected visual acuity (BCVA) at 100 weeks at alpha = 0.05 significance level [ 9 ]. The regression coefficient of −0.33 means that the BCVA at 100 weeks decreases by 0.33 with each additional year of older age.
Multivariable logistic regression also demonstrated that age and ellipsoid zone status were statistically significant associated with achieving a BCVA letter score >70 letters at 100 weeks at the alpha = 0.05 significance level. Patients ≥75 years of age were at a decreased odds of achieving a BCVA letter score >70 letters at 100 weeks compared to those <50 years of age, since the OR is less than 1 (OR 0.96, 95% CI 0.94 to 0.98, p = 0.001) [ 9 ]. Similarly, patients between the ages of 50–74 years were also at a decreased odds of achieving a BCVA letter score >70 letters at 100 weeks compared to those <50 years of age, since the OR is less than 1 (OR 0.15, 95% CI 0.04 to 0.48, p = 0.001) [ 9 ]. As well, those with a not intact ellipsoid zone were at a decreased odds of achieving a BCVA letter score >70 letters at 100 weeks compared to those with an intact ellipsoid zone (OR 0.20, 95% CI 0.07 to 0.56; p = 0.002). On the other hand, patients with an ungradable/questionable ellipsoid zone were at an increased odds of achieving a BCVA letter score >70 letters at 100 weeks compared to those with an intact ellipsoid zone, since the OR is greater than 1 (OR 2.26, 95% CI 1.14 to 4.48; p = 0.02) [ 9 ].
The narrower the CI, the more precise the estimate is; and the smaller the p value (relative to alpha = 0.05), the greater the evidence against the null hypothesis of no effect or association.
Simply put, linear and logistic regression are useful tools for appreciating the relationship between predictor/explanatory and outcome variables for continuous and dichotomous outcomes, respectively, that can be applied in clinical practice, such as to gain an understanding of risk factors associated with a disease of interest.
Schneider A, Hommel G, Blettner M. Linear Regression. Anal Dtsch Ärztebl Int. 2010;107:776–82.
Google Scholar
Bender R. Introduction to the use of regression models in epidemiology. In: Verma M, editor. Cancer epidemiology. Methods in molecular biology. Humana Press; 2009:179–95.
Schober P, Vetter TR. Confounding in observational research. Anesth Analg. 2020;130:635.
Article Google Scholar
Schober P, Vetter TR. Linear regression in medical research. Anesth Analg. 2021;132:108–9.
Szumilas M. Explaining odds ratios. J Can Acad Child Adolesc Psychiatry. 2010;19:227–9.
Thiese MS, Ronna B, Ott U. P value interpretations and considerations. J Thorac Dis. 2016;8:E928–31.
Schober P, Vetter TR. Logistic regression in medical research. Anesth Analg. 2021;132:365–6.
Zabor EC, Reddy CA, Tendulkar RD, Patil S. Logistic regression in clinical studies. Int J Radiat Oncol Biol Phys. 2022;112:271–7.
Sen P, Gurudas S, Ramu J, Patrao N, Chandra S, Rasheed R, et al. Predictors of visual acuity outcomes after anti-vascular endothelial growth factor treatment for macular edema secondary to central retinal vein occlusion. Ophthalmol Retin. 2021;5:1115–24.
Download references
R.E.T.I.N.A. study group
Varun Chaudhary 1,2 , Mohit Bhandari 1,2 , Charles C. Wykoff 5,6 , Sobha Sivaprasad 8 , Lehana Thabane 2,7 , Peter Kaiser 9 , David Sarraf 10 , Sophie J. Bakri 11 , Sunir J. Garg 12 , Rishi P. Singh 13,14 , Frank G. Holz 15 , Tien Y. Wong 16,17 , and Robyn H. Guymer 3,4
Author information
Authors and affiliations.
Department of Surgery, McMaster University, Hamilton, ON, Canada
Sofia Bzovsky, Mohit Bhandari & Varun Chaudhary
Department of Health Research Methods, Evidence & Impact, McMaster University, Hamilton, ON, Canada
Mark R. Phillips, Lehana Thabane, Mohit Bhandari & Varun Chaudhary
Centre for Eye Research Australia, Royal Victorian Eye and Ear Hospital, East Melbourne, VIC, Australia
Robyn H. Guymer
Department of Surgery, (Ophthalmology), The University of Melbourne, Melbourne, VIC, Australia
Retina Consultants of Texas (Retina Consultants of America), Houston, TX, USA
Charles C. Wykoff
Blanton Eye Institute, Houston Methodist Hospital, Houston, TX, USA
Biostatistics Unit, St. Joseph’s Healthcare Hamilton, Hamilton, ON, Canada
Lehana Thabane
NIHR Moorfields Biomedical Research Centre, Moorfields Eye Hospital, London, UK
Sobha Sivaprasad
Cole Eye Institute, Cleveland Clinic, Cleveland, OH, USA
Peter Kaiser
Retinal Disorders and Ophthalmic Genetics, Stein Eye Institute, University of California, Los Angeles, CA, USA
David Sarraf
Department of Ophthalmology, Mayo Clinic, Rochester, MN, USA
Sophie J. Bakri
The Retina Service at Wills Eye Hospital, Philadelphia, PA, USA
Sunir J. Garg
Center for Ophthalmic Bioinformatics, Cole Eye Institute, Cleveland Clinic, Cleveland, OH, USA
Rishi P. Singh
Cleveland Clinic Lerner College of Medicine, Cleveland, OH, USA
Department of Ophthalmology, University of Bonn, Bonn, Germany
Frank G. Holz
Singapore Eye Research Institute, Singapore, Singapore
Tien Y. Wong
Singapore National Eye Centre, Duke-NUD Medical School, Singapore, Singapore
You can also search for this author in PubMed Google Scholar
- Varun Chaudhary
- , Mohit Bhandari
- , Charles C. Wykoff
- , Sobha Sivaprasad
- , Lehana Thabane
- , Peter Kaiser
- , David Sarraf
- , Sophie J. Bakri
- , Sunir J. Garg
- , Rishi P. Singh
- , Frank G. Holz
- , Tien Y. Wong
- & Robyn H. Guymer
Contributions
SB was responsible for writing, critical review and feedback on manuscript. MRP was responsible for conception of idea, critical review and feedback on manuscript. RHG was responsible for critical review and feedback on manuscript. CCW was responsible for critical review and feedback on manuscript. LT was responsible for critical review and feedback on manuscript. MB was responsible for conception of idea, critical review and feedback on manuscript. VC was responsible for conception of idea, critical review and feedback on manuscript.
Corresponding author
Correspondence to Varun Chaudhary .
Ethics declarations
Competing interests.
SB: Nothing to disclose. MRP: Nothing to disclose. RHG: Advisory boards: Bayer, Novartis, Apellis, Roche, Genentech Inc.—unrelated to this study. CCW: Consultant: Acuela, Adverum Biotechnologies, Inc, Aerpio, Alimera Sciences, Allegro Ophthalmics, LLC, Allergan, Apellis Pharmaceuticals, Bayer AG, Chengdu Kanghong Pharmaceuticals Group Co, Ltd, Clearside Biomedical, DORC (Dutch Ophthalmic Research Center), EyePoint Pharmaceuticals, Gentech/Roche, GyroscopeTx, IVERIC bio, Kodiak Sciences Inc, Novartis AG, ONL Therapeutics, Oxurion NV, PolyPhotonix, Recens Medical, Regeron Pharmaceuticals, Inc, REGENXBIO Inc, Santen Pharmaceutical Co, Ltd, and Takeda Pharmaceutical Company Limited; Research funds: Adverum Biotechnologies, Inc, Aerie Pharmaceuticals, Inc, Aerpio, Alimera Sciences, Allergan, Apellis Pharmaceuticals, Chengdu Kanghong Pharmaceutical Group Co, Ltd, Clearside Biomedical, Gemini Therapeutics, Genentech/Roche, Graybug Vision, Inc, GyroscopeTx, Ionis Pharmaceuticals, IVERIC bio, Kodiak Sciences Inc, Neurotech LLC, Novartis AG, Opthea, Outlook Therapeutics, Inc, Recens Medical, Regeneron Pharmaceuticals, Inc, REGENXBIO Inc, Samsung Pharm Co, Ltd, Santen Pharmaceutical Co, Ltd, and Xbrane Biopharma AB—unrelated to this study. LT: Nothing to disclose. MB: Research funds: Pendopharm, Bioventus, Acumed—unrelated to this study. VC: Advisory Board Member: Alcon, Roche, Bayer, Novartis; Grants: Bayer, Novartis—unrelated to this study.
Additional information
Publisher’s note Springer Nature remains neutral with regard to jurisdictional claims in published maps and institutional affiliations.
Rights and permissions
Reprints and permissions
About this article
Cite this article.
Bzovsky, S., Phillips, M.R., Guymer, R.H. et al. The clinician’s guide to interpreting a regression analysis. Eye 36 , 1715–1717 (2022). https://doi.org/10.1038/s41433-022-01949-z
Download citation
Received : 08 January 2022
Revised : 17 January 2022
Accepted : 18 January 2022
Published : 31 January 2022
Issue Date : September 2022
DOI : https://doi.org/10.1038/s41433-022-01949-z
Share this article
Anyone you share the following link with will be able to read this content:
Sorry, a shareable link is not currently available for this article.
Provided by the Springer Nature SharedIt content-sharing initiative
Quick links
- Explore articles by subject
- Guide to authors
- Editorial policies

When to Use Regression Analysis (With Examples)
Regression analysis can be used to:
- estimate the effect of an exposure on a given outcome
- predict an outcome using known factors
- balance dissimilar groups
- model and replace missing data
- detect unusual records
In the text below, we will go through these points in greater detail and provide a real-world example of each.
1. Estimate the effect of an exposure on a given outcome
Regression can model linear and non-linear associations between an exposure (or treatment) and an outcome of interest. It can also simultaneously model the relationship between more than 1 exposure and an outcome, even when these exposures interact with each other.
Example: Exploring the relationship between Body Mass Index (BMI) and all-cause mortality
De Gonzales et al. used a Cox regression model to estimate the association between BMI and mortality among 1.46 million white adults.
As expected, they found that the risk of mortality increases with progressively higher than normal levels of BMI.
The takeaway message is that regression analysis enabled them to quantify that association while adjusting for smoking, alcohol consumption, physical activity, educational level and marital status — all potential confounders of the relationship between BMI and mortality.
2. Predict an outcome using known factors
A regression model can also be used to predict things like stock prices, weather conditions, the risk of getting a disease, mortality, etc. based on a set of known predictors (also called independent variables).
Example: Predicting malaria in South Africa using seasonal climate data
Kim et al. used Poisson regression to develop a malaria prediction model using climate data such as temperature and precipitation in South Africa.
The model performed best with short-term predictions.
Anyway, the important thing to notice here is the amount of complexities that a regression model can handle. For instance in this example, the model had to be flexible enough to account for non-linear and delayed associations between malaria transmission and climate factors.
This is a recurrent theme with predictive models: We start with a simple model, then we keep adding complexities until we get a satisfying result — this is why we call it model building .
3. Balance dissimilar groups
Proving that a relationship exists between some independent variable X and an outcome Y does not mean much if this result cannot be generalized beyond your sample.
In order for your results to generalize well, the sample you’re working with has to resemble the population from which it was drawn. If it doesn’t, you can use regression to balance some important characteristics in the sample to make it representative of the population of interest.
Another case where you would want to balance dissimilar groups is in a randomized controlled trial, where the objective is to compare the outcome between the group who received the intervention and another one that serves as control/reference. But in order for the comparison to make sense, the 2 groups must have similar characteristics.
Example: Evaluating how sleep quality is affected by sleep hygiene education and behavioral therapy
Nishinoue et al. conducted a randomized controlled trial to compare sleep quality between 2 groups of participants:
- The treatment group: Participants received sleep hygiene education and behavioral therapy
- The control group: Participants received sleep hygiene education only
A generalized linear model (a generalized form of linear regression) was used to:
- Evaluate how sleep quality changed between groups
- Adjust for age, gender, job title, smoking and drinking habits, body-mass index, and mental health to make the groups more comparable
4. Model and replace missing data
Modeling missing data is an important part of data analysis, especially in cases where you have high non-response rates (so a high number of missing values) like in telephone surveys.
Before jumping into imputing missing data, first you must determine:
- How important the variables that have missing values are in your analysis
- The percentage of missing values
- If these values were missing at random or not
Based on this analysis, you can then choose to:
- Delete observations with missing values
- Replace missing data with the column’s mean or median
- Use a a regression model to replace missing data
Example: Using multiple imputation to replace missing data in a medical study
Beynon et al. studied the prognostic role of alcohol and smoking at diagnosis of head and neck cancer.
But before they built their statistical model, they noticed that 11 variables (including smoking status and alcohol intake and other covariates) had missing values, so they used a technique called MICE (Multiple Imputation by Chained Equations) which runs regression models under the hood to replace missing values.
5. Detect unusual records
Regression models alongside other statistical techniques can be used to model how “normal data” should look like, the purpose being to detect values that deviate from this norm. These are referred to as “anomalies” or “outliers” in the data.
Most applications of anomaly detection is outside the healthcare domain. It is typically used for detection of financial frauds, atypical online behavior of website visitors, detection of anomalies in machine performance in a factory, etc.
Example: Detecting critical cases of patients undergoing heart surgery
Presbitero et al. used a time-varying autoregressive model (along with other statistical measures) to flag abnormal cases of patients undergoing heart surgery using data on their blood measurements.
Their goal is to ultimately prevent patient death by allowing early intervention to take place through the use of this early warning detection algorithm.
Further reading
- Variables to Include in a Regression Model
- Understand Linear Regression Assumptions
- 7 Tricks to Get Statistically Significant p-Values
- How to Handle Missing Data in Practice: Guide for Beginners
- Skip to secondary menu
- Skip to main content
- Skip to primary sidebar
Statistics By Jim
Making statistics intuitive
Making Predictions with Regression Analysis
By Jim Frost 37 Comments
If you were able to make predictions about something important to you, you’d probably love that, right? It’s even better if you know that your predictions are sound. In this post, I show how to use regression analysis to make predictions and determine whether they are both unbiased and precise.
You can use regression equations to make predictions. Regression equations are a crucial part of the statistical output after you fit a model. The coefficients in the equation define the relationship between each independent variable and the dependent variable. However, you can also enter values for the independent variables into the equation to predict the mean value of the dependent variable.
Related post : When Should I Use Regression Analysis?
The Regression Approach for Predictions
Using regression to make predictions doesn’t necessarily involve predicting the future. Instead, you predict the mean of the dependent variable given specific values of the independent variable(s). For our example, we’ll use one independent variable to predict the dependent variable. I measured both of these variables at the same point in time.

The general procedure for using regression to make good predictions is the following:
- Research the subject-area so you can build on the work of others. This research helps with the subsequent steps.
- Collect data for the relevant variables.
- Specify and assess your regression model.
- If you have a model that adequately fits the data, use it to make predictions.
While this process involves more work than the psychic approach, it provides valuable benefits. With regression, we can evaluate the bias and precision of our predictions:
- Bias in a statistical model indicates that the predictions are systematically too high or too low.
- Precision represents how close the predictions are to the observed values.
When we use regression to make predictions, our goal is to produce predictions that are both correct on average and close to the real values. In other words, we need predictions that are both unbiased and precise.
Example Scenario for Regression Predictions
We’ll use a regression model to predict body fat percentage based on body mass index (BMI). I collected these data for a study with 92 middle school girls. The variables we measured include height, weight, and body fat measured by a Hologic DXA whole-body system. I’ve calculated the BMI using the height and weight measurements. DXA measurements of body fat percentage are considered to be among the best.
You can download the CSV data file: Predict_BMI .
Why might we want to use BMI to predict body fat percentage? It’s more expensive to obtain your body fat percentage through a direct measure like DXA. If you can use your BMI to predict your body fat percentage, that provides valuable information more easily and cheaply. Let’s see if BMI can produce good predictions!
Finding a Good Regression Model for Predictions
We have the data. Now, we need to determine whether there is a statistically significant relationship between the variables. Relationships, or correlations between variables, are crucial if we want to use the value of one variable to predict the value of another. We also need to evaluate the suitability of the regression model for making predictions.
We have only one independent variable (BMI), so we can use a fitted line plot to display its relationship with body fat percentage. The relationship between the variables is curvilinear. I’ll use a polynomial term to fit the curvature. In this case, I’ll include a quadratic (squared) term. The fitted line plot below suggests that this model fits the data.

Related post : Curve Fitting using Linear and Nonlinear Regression
This curvature is readily apparent because we have only one independent variable and we can graph the relationship. If your model has more than one independent variable, use separate scatterplots to display the association between each independent variable and the dependent variable so you can evaluate the nature of each relationship.
Assess the residual plots
You should also assess the residual plots . If you see patterns in the residual plots, you know that your model is incorrect and that you need to reevaluate it. Non-random residuals indicate that the predicted values are biased. You need to fix the model to produce unbiased predictions.
Learn how to choose the correct regression model .
The residual plots below also confirm the unbiased fit because the data points fall randomly around zero and follow a normal distribution.

Interpret the regression output
In the statistical output below, the p-values indicate that both the linear and squared terms are statistically significant. Based on all of this information, we have a model that provides a statistically significant and unbiased fit to these data. We have a valid regression model. However, there are additional issues we must consider before we can use this model to make predictions.

As an aside, the curved relationship is interesting. The flattening curve indicates that higher BMI values are associated with smaller increases in body fat percentage.
Other Considerations for Valid Predictions
Precision of the predictions.
Previously, we established that our regression model provides unbiased predictions of the observed values. That’s good. However, it doesn’t address the precision of those predictions. Precision measures how close the predictions are to the observed values. We want the predictions to be both unbiased and close to the actual values. Predictions are precise when the observed values cluster close to the predicted values.
Regression predictions are for the mean of the dependent variable. If you think of any mean, you know that there is variation around that mean. The same applies to the predicted mean of the dependent variable. In the fitted line plot, the regression line is nicely in the center of the data points. However, there is a spread of data points around the line. We need to quantify that spread to know how close the predictions are to the observed values. If the spread is too large, the predictions won’t provide useful information.
Later, I’ll generate predictions and show you how to assess the precision.
Related post : Understand Precision in Applied Regression to Avoid Costly Mistakes
Goodness-of-Fit Measures
Goodness-of-fit measures, like R-squared , assess the scatter of the data points around the fitted value. The R-squared for our model is 76.1%, which is good but not great. For a given dataset, higher R-squared values represent predictions that are more precise. However, R-squared doesn’t tell us directly how precise the predictions are in the units of the dependent variable. We can use the standard error of the regression (S) to assess the precision in this manner. However, for this post, I’ll use prediction intervals to evaluate precision.
Related post : Standard Error of the Regression vs. R-squared
New Observations versus Data Used to Fit the Model
R-squared and S indicate how well the model fits the observed data. We need predictions for new observations that the analysis did not use during the model estimation process. Assessing that type of fit requires a different goodness-of-fit measure, the predicted R-squared.
Predicted R-squared measures how well the model predicts the value of new observations. Statistical software packages calculate it by sequentially removing each observation, fitting the model, and determining how well the model predicts the removed observations.
If the predicted R-squared is much lower than the regular R-squared, you know that your regression model doesn’t predict new observations as well as it fits the current dataset. This situation should make you wary of the predictions.
The statistical output below shows that the predicted R-squared (74.14%) is nearly equal to the regular R-squared (76.06%) for our model. We have reason to believe that the model predicts new observations nearly as well as it fits the dataset.

Related post: How to Interpret Adjusted R-squared and Predicted R-squared
Make Predictions Only Within the Range of the Data
Regression predictions are valid only for the range of data used to estimate the model. The relationship between the independent variables and the dependent variable can change outside of that range. In other words, we don’t know whether the shape of the curve changes. If it does, our predictions will be invalid.
The graph shows that the observed BMI values range from 15-35. We should not make predictions outside of this range.
Make Predictions Only for the Population You Sampled
The relationships that a regression model estimates might be valid for only the specific population that you sampled. Our data were collected from middle school girls that are 12-14 years old. The relationship between BMI and body fat percentage might be different for males and different age groups.
Using our Regression Model to Make Predictions
We have a valid regression model that appears to produce unbiased predictions and can predict new observations nearly as well as it predicts the data used to fit the model. Let’s go ahead and use our model to make a prediction and assess the precision.
It is possible to use the regression equation and calculate the predicted values ourselves. However, I’ll use statistical software to do this for us. Not only is this approach easier and more accurate, but I’ll also have it calculate the prediction intervals so we can assess the precision.
I’ll use the software to predict the body fat percentage for a BMI of 18. The prediction output is below.

Interpreting the Regression Prediction Results
The output indicates that the mean value associated with a BMI of 18 is estimated to be ~23% body fat. Again, this mean applies to the population of middle school girls. Let’s assess the precision using the confidence interval (CI) and the prediction interval (PI).
The confidence interval is the range where the mean value for girls with a BMI of 18 is likely to fall. We can be 95% confident that this mean is between 22.1% and 23.9%. However, this confidence interval does not help us evaluate the precision of individual predictions.
A prediction interval is the range where a single new observation is likely to fall. Narrower prediction intervals represent more precise predictions. For an individual middle school girl with a BMI of 18, we can be 95% confident that her body fat percentage is between 16% and 30%.
The range of the prediction interval is always wider than the confidence interval due to the greater uncertainty of predicting an individual value rather than the mean.
Is this prediction sufficiently precise? To make this determination, we’ll need to use our subject-area knowledge in conjunction with any specific requirements we have. I’m not a medical expert, but I’d guess that the 14 point range of 16-30% is too imprecise to provide meaningful information. If this is true, our regression model is too imprecise to be useful.
Don’t Focus On Only the Fitted Values
As we saw in this post, using regression analysis to make predictions is a multi-step process. After collecting the data, you need to specify a valid model. The model must satisfy several conditions before you make predictions. Finally, be sure to assess the precision of the predictions. It’s all too easy to get lulled into a false sense of security by focusing on only the fitted value and not consider the prediction interval.
If you’re learning regression and like the approach I use in my blog, check out my eBook!


Share this:

Reader Interactions
December 30, 2023 at 9:37 pm
Hi Jim, your content is superb – thank you for the valuable resource you provide! I am working on a specific problem. I am attempting to predict the price of a security (actually 2 separate securities) using a number of independent variables, most of which are pricing or other related (forward-looking) securities pricing as well as general economic data. More specifically, I am hoping to predict the price of two separate securities based on the exact same set of independent variables – I am not sure but believe this to be generally known as multivariate multiple linear regression. I have continuous linear values for both dependent and independent variables. However, I’m unclear if there is a specific technique to regress two dependent variables simultaneously on the exact same set of independent variables, or, if I should just regress the dependent variables separately and interpret the results independently (I need both predictions). It should also be noted the dependent variables are inversely correlated with a pearson’s r of -0.610425241 (using about 1,000 datapoints). Also, if there is a good textbook or course you would recommend that covers how to conduct this type of regression, I would greatly appreciate it – I did a decent amount of regression in grad school many years ago but nothing horribly complex. Thank you!
Best, Jim .
May 6, 2023 at 6:56 am
God bless you. This would be perfect, if made in excel, so laymen could have more insight on what is happening. Thank you.
August 4, 2022 at 7:53 am
Thank you for the nice text. I have found in my job that academic research using observational datasets has surprisingly little focus on prediction accuracy of a model. Furthermore, the model selection process is often blurry and the final model might have been chosen quite haphazardly. Some model assumptions might be checked along with some goodness of fit -test, but usually nothing is mentioned about prediction accuracy.
Even the absolute correct model can have large (parameter/function) variance. For prediction, there is also the irreducible error. And even if one uses an unbiased model (unbiased parameter estimates), research shows (Harrell, Zhang, Chatfield, Faraway, Breiman etc.) that model bias will be present. Thus, we don’t even have unbiasedness. And on top of that, we have variance.
I am quite keen on machine learning, where the focus is on prediction accuracy. The approach is kind of like “the proof is in the pudding”. I find it not to be the case for “traditional statistics”, where the aim is more on interpretation (inference). Obviously, a machine learning model is not readily interpretable and it could even be impossible.
If a statistical model focused on inference (interpretation of the parameters) does not predict well, what is its use? If it’s a poor model, it most likely will predict poorly. So you should test that. Even if it’s the correct model, the predictions can be poor because of the variance. Even with an absolute correct unbiased model with large variance, your sample is probably way off from the truth. This leads to poor predictions. How happy can you really be if and when even with a correct model you predict poorly?
Having said all this, I’m leaning towards the opinion that every statistical model should incorporate prediction. Preferably to a new dataset (from the same phenomenon). I think this could help the reproducebility problem disrupting the academic research world.
Any thoughts on this?
October 6, 2021 at 5:49 am
Hello, I enjoy reading through your post. following from South Eastern Kenya University
October 7, 2021 at 11:43 pm
Hi Seku! Welcome to my website! I’m so glad that you’ve found it to be helpful! Happy reading!
September 28, 2021 at 4:14 am
Hello Jim, Thanks a lot for this great post and all sub-links which was really useful for me to understand all the aspects I need to build a regression model and to do forecast. My question is related to multiple regression, what if one important variable is categorical but has many values inside which are difficult to group them. How can I encode it without distorting my model with many numeric category. Thanks a lot
September 29, 2021 at 1:28 am
Coding your categorical variable is a very subject-area specific process. Consequently, I can’t give you a specific answer. However, you’ll need find a system of sorting all your observations into a set of categories. You must find a method so that all observations fall unambiguously into one, and only one, category. These categories must be mutually exclusive. All observations in your study must fall within one category.
November 23, 2020 at 4:27 pm
Hope you are doing well. If a researcher has constructed a new test and would like to investigate to what extent the new test is able to predict the subjects’ performance on an already established test, which test should be taken as a predictor and which one as the outcome measure in the regression analysis?
My intuition is that if the results of the new test can predict subjects’ scores on the old test, we have to consider the new test as the predictor as we are interested in finding out to what extent it can predict the unique variance of the old test.
Thanks in advance and
October 9, 2020 at 4:09 am
hi sir, i have a hypothesis where : amount customers have spent at a store in the last 12 months predicts likelihood they recommend the brand to others. which type of regression would this be and what are the measurements of scale for each IV and DV? thanks!
June 11, 2020 at 11:34 am
Hi Jim, very interesting read. I was wondering, I’ve read a little on Cox for prediction modelling (though not much I’ve found compared to logistic regression models). In prediction time is always important I suppose. Is there any benefit to using Cox over LR? I am looking at risk of developing a condition within 3 years based on certain subject characteristics. Many thanks for your help.
June 7, 2020 at 8:47 am
Hi Jim, an excellent and helpful read thanks. I was hoping you could help me confirm how I would apply the logistic regression equation to generate a risk score for participants to calculate a ROC curve? Thanks!
May 28, 2020 at 9:24 am
Nice explanation. It helped in my project.
May 13, 2020 at 7:08 pm
Hi professor,
I followed up your subjects, really they are valuable and appreciated. However, i have a question, if i have a dependent variable and 4 or 5 independent variables, what is the best method to develop a correct statistical equation which correlate all of them??
May 12, 2020 at 11:18 pm
Hello Sir. How can we predict final exam results from class assignments marks
May 13, 2020 at 3:52 pm
You’re in the right post for the answers you seek! I spell out the process here. If you have more specific questions, please post them after reading thoroughly.
May 9, 2020 at 7:48 am
Hello professor,
Your posts helped me a lot in reshaping my knowledge in regression models. I want to ask you how can we use time as a predictor along side other predictors to perform prediction. What I can’t undrestand is when plotting time against my dependant variable, I find no correlation. So how can I design my study using time?
I hope that I made myself clear.
Thank you again.
May 11, 2020 at 1:11 am
Using regression to analyze time series data is possible. However, it raises a number of other considerations. It’s far too complex to go into in the comments section. However, you should first determine whether time is related to your dependent variable. Instead of a correlation, try graphing it using a time series plot. You can then see if there’s any sort of relationship between time and your DV. Cyclical patterns might not show up as a correlation put would be visible on a time series plot. There’s a bunch of time series analysis methods that you can incorporate into regression analysis. At some point, I might write posts about that. However, it involves many details that I can’t quickly summarize. But, you can factor in the effect of time along with other factors that related to your DV.
I wish I could be more helpful. And perhaps down the road I’ll have something just perfect for you. But alas I don’t right now. I’m sure you could do a search and find more information though.
April 15, 2020 at 12:46 pm
Hello Professor Jim I am a profound admirer of your work and your posts has helped me very much.
When I read this post I thought you were going to mention and talk also about forecasts. But you were talking about regular regressions predictions. So I would like to ask you something important to the scientific investigation I am working on. Do you think that, if besides predict the impact of a IV on a DV, I decide to use the model that I will buld to forecast future values of my dependent variable. Do you think it would add a considerable amount of work? in terms of modelling and code building for the calculations?
Thank you very much.
April 16, 2020 at 11:05 pm
I’m so happy to hear that my posts have been helpful! 🙂
Forecasting in regression uses the same methodology as predictions. You’re still using the IVs to predict the DV. The difference, of course, is that you’re using past values of the IVs to predict future values of the DV. If you’re familiar with fitting regression models, fitting a forecasting model isn’t necessarily going to be more work than a regular regression model. You’ll still need to go through the process of determining which variables to include in your model. Given the forecast nature, you’ll need to think about the variables, the timing of the variables, and how they influence the DV. In addition to the more typical IVs, you’ll need to consider things such as seasonal patterns and other trends over time. Given that the model incorporates time, you will need to pay more attention to the potential problem of autocorrelation in the residuals, which I describe in my post about least squares assumptions . So, there are definitely some different considerations for a forecast model, but, again I wouldn’t say that it’s necessarily harder than a non-forecast model. As usual, it comes down to research, getting the right data, including the correct variables, and checking the assumptions.
I hope this helps!
January 24, 2020 at 1:21 am
I’m starting out in Predictive Analytics and found your article very useful and informative.
I’m currently working on a use case where the quality of a product is directly affected by a temperature parameter (which was found by root cause analysis). So our objective is to maintain the temperature at the nominal value and provide predictions on when the tempertaure may vary. But unfortunately quality data is not available. Hence we need to work with the temperature and additonal process parameters data available to us.
My queries are as follows:
Can I predict the temperature variance and assume that the quality of the product will be in sync to a certain extent ?
Is regression analysis the best methodology for my use case ?
Are there any open source tools available for doing this predictive analytics ?
June 28, 2019 at 9:48 am
Hello dear,
Thank you for all your interesting posts.
I’m beginner in regression and I would like to use logistic Model to predict surrenders in life insurance.
I would like to well understand the prediction probabilities.
In my model I us the age (in months) of the contract in the portefollio, the gender of Policy holder, …
when making prediction, for age 57, gender M for example, what’s does the predicted probability mean?
Does it mean that it’s the probability of the contract to be surrended at age 57 given the gender of the Policy holder?
June 30, 2019 at 8:48 pm
Hi N’Dah,
Yes, the prediction the probability of that a 57 year old male will surrender the policy. That assumes the model provides a good fit and satisfies the necessary assumptions. I write more about binary logistic regression . It’s a post that uses binary logistic regression to analyze a political group in the U.S. But, I do talk about interpreting the output, which might be helpful.
I hope that helps!
May 28, 2019 at 3:26 am
Why is the standard error of estimate or prediction higher when the predictive quality of variables is lower?
May 27, 2019 at 5:41 am
Good Read. Easy to understand keep it up.
May 9, 2019 at 3:31 am
I really appreciate your support in regression analysis. Actually i have data on milk yield of buffaloes. Different buffaloes yield milk in different number of days. in order to rank buffaloes i need to put milk to a standard milk period 305 days. Some buffaloes have lactation length higher than 305 days, other less than 305 days. How to develop factors for correction/prediction of milk of all buffaloes on one standard
May 10, 2019 at 2:09 pm
Hi Musarrat,
The process of identifying the correct variables to include in your model is a mix between subject area knowledge and statistics. To develop an initial list of potential factors, you’ll need to research the subject area and use your expertise to identify candidates. I don’t know the dairy industry so, unfortunately, I can’t help you there.
I suggest you read my post about choosing the correct regression model for some tips. Additionally, consider buying my ebook on regression analysis which can help you with the process.
May 8, 2019 at 2:36 am
Unlike Standard error of regression ( https://statisticsbyjim.com/regression/standard-error-regression-vs-r-squared/ ), the assessment by calculating prediction intervals in this article doesn’t seem to be comprehensive because with SE of regression, it is clear by the rule-of-thumb that a certain number of points must fall within the bounds based on the confidence level (95%, 99%) – this of course depends on how precise we want.
In the case of prediction intervals, the usage of subject matter expertise was mentioned and the calculations were based on every point (where the conditions of independent variables are given). Now, I wonder how to quantify and assess the precision of model based on a one-off calculation?
Considering such scenario, is SE of regression followed/ used typically unless one has a lot of subject expertise and ways to calculate PI for all the data points and subsequently assess the precision of the prediction precision?
Thanks Jim!
October 20, 2018 at 11:36 pm
I am one week before my thesis submission and wish I had found your site much earlier. Your explanations are so clear and concise. You are a great teacher Jim!
October 21, 2018 at 1:29 am
Thank you so much, Keryn! I strive to make these explanations as straightforward as possible, so I really appreciate your kind words!
September 21, 2018 at 10:30 am
Using the body mass index data set as an example. Suppose these results were gained from several different groups. For example one group worked out regularly, one group didn’t work out but maintained a healthy diet, one group didn’t work out and maintained a poor diet, etc. Can we use the group average differences between estimated results (based on the regression equation) and the actual results to determine of one group was significantly different from the others in terms of that group being consistently above or below the regression line?
September 10, 2018 at 12:22 am
Hello, how can I predict the dependent variable for a new case in spss?
April 19, 2018 at 12:36 am
Nice article. Very clear and easy to understand. Bravo.
April 19, 2018 at 2:16 am
Thank you, James!
December 14, 2017 at 2:27 pm
Oh my! I came across you during the final week of my stat class. You just enlightened me in this regression area. I wish I came upon you during my first week of class. It is easier to grasp stats when it is explained plainly and their correlation with whatever in life you will be doing. Safe to say I passed (barely) my stat basically with following step by step without understanding why I am doing it in such a way and why.
December 14, 2017 at 5:00 pm
Hi Ginalyn, thanks for taking the time to write such a nice comment! It made my day! I’m glad you found my blog to be helpful. I always try to explain statistics in the most straightforward, simple manner possible. I’m glad you passed!
September 27, 2017 at 8:46 am
Thanks for the deep insight; indeed your idea brings me back in trying to seek as much closer to reality predictions on our daily life phenomenal. As this universe in as much as the orderly chaotic manner, some predictions becomes erroneously to the extent that they are rendered uncertain for the decision making. In validation of a model in question, the uncertainty would be clarified by using a set of conditions for prediction and suitable intervals (limits).
May 4, 2017 at 11:25 pm
Comments and Questions Cancel reply
- Skip to main content
- Skip to primary sidebar
- Skip to footer
- QuestionPro

- Solutions Industries Gaming Automotive Sports and events Education Government Travel & Hospitality Financial Services Healthcare Cannabis Technology Use Case NPS+ Communities Audience Contactless surveys Mobile LivePolls Member Experience GDPR Positive People Science 360 Feedback Surveys
- Resources Blog eBooks Survey Templates Case Studies Training Help center

Home Market Research
Regression Analysis: Definition, Types, Usage & Advantages
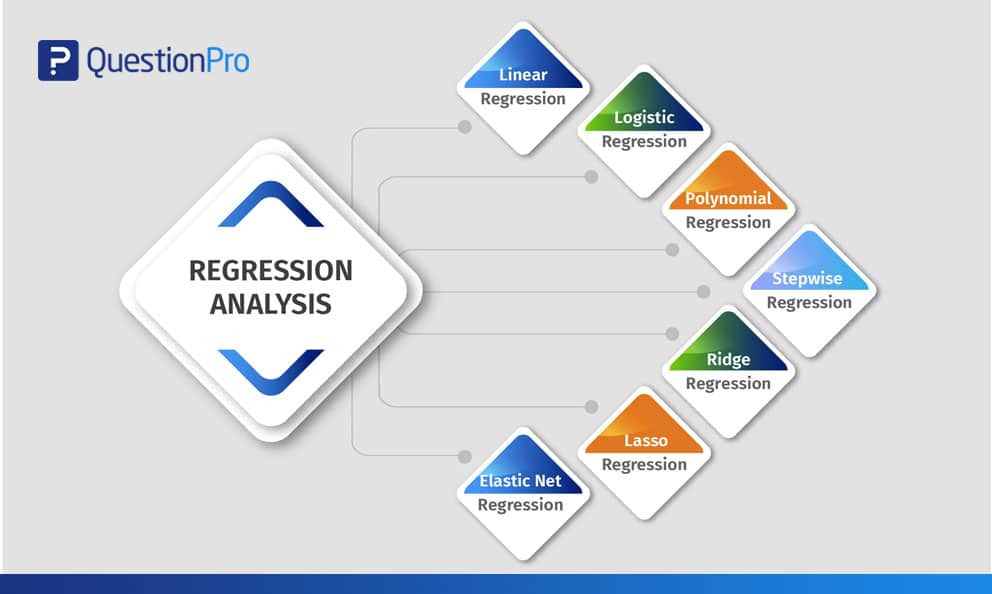
Regression analysis is perhaps one of the most widely used statistical methods for investigating or estimating the relationship between a set of independent and dependent variables. In statistical analysis , distinguishing between categorical data and numerical data is essential, as categorical data involves distinct categories or labels, while numerical data consists of measurable quantities.
It is also used as a blanket term for various data analysis techniques utilized in a qualitative research method for modeling and analyzing numerous variables. In the regression method, the dependent variable is a predictor or an explanatory element, and the dependent variable is the outcome or a response to a specific query.
LEARN ABOUT: Statistical Analysis Methods
Content Index
Definition of Regression Analysis
Types of regression analysis, regression analysis usage in market research, how regression analysis derives insights from surveys, advantages of using regression analysis in an online survey.
Regression analysis is often used to model or analyze data. Most survey analysts use it to understand the relationship between the variables, which can be further utilized to predict the precise outcome.
For Example – Suppose a soft drink company wants to expand its manufacturing unit to a newer location. Before moving forward, the company wants to analyze its revenue generation model and the various factors that might impact it. Hence, the company conducts an online survey with a specific questionnaire.
After using regression analysis, it becomes easier for the company to analyze the survey results and understand the relationship between different variables like electricity and revenue – here, revenue is the dependent variable.
LEARN ABOUT: Level of Analysis
In addition, understanding the relationship between different independent variables like pricing, number of workers, and logistics with the revenue helps the company estimate the impact of varied factors on sales and profits.
Survey researchers often use this technique to examine and find a correlation between different variables of interest. It provides an opportunity to gauge the influence of different independent variables on a dependent variable.
Overall, regression analysis saves the survey researchers’ additional efforts in arranging several independent variables in tables and testing or calculating their effect on a dependent variable. Different types of analytical research methods are widely used to evaluate new business ideas and make informed decisions.
Create a Free Account
Researchers usually start by learning linear and logistic regression first. Due to the widespread knowledge of these two methods and ease of application, many analysts think there are only two types of models. Each model has its own specialty and ability to perform if specific conditions are met.
This blog explains the commonly used seven types of multiple regression analysis methods that can be used to interpret the enumerated data in various formats.
01. Linear Regression Analysis
It is one of the most widely known modeling techniques, as it is amongst the first elite regression analysis methods picked up by people at the time of learning predictive modeling. Here, the dependent variable is continuous, and the independent variable is more often continuous or discreet with a linear regression line.
Please note that multiple linear regression has more than one independent variable than simple linear regression. Thus, linear regression is best to be used only when there is a linear relationship between the independent and a dependent variable.
A business can use linear regression to measure the effectiveness of the marketing campaigns, pricing, and promotions on sales of a product. Suppose a company selling sports equipment wants to understand if the funds they have invested in the marketing and branding of their products have given them substantial returns or not.
Linear regression is the best statistical method to interpret the results. The best thing about linear regression is it also helps in analyzing the obscure impact of each marketing and branding activity, yet controlling the constituent’s potential to regulate the sales.
If the company is running two or more advertising campaigns simultaneously, one on television and two on radio, then linear regression can easily analyze the independent and combined influence of running these advertisements together.
LEARN ABOUT: Data Analytics Projects
02. Logistic Regression Analysis
Logistic regression is commonly used to determine the probability of event success and event failure. Logistic regression is used whenever the dependent variable is binary, like 0/1, True/False, or Yes/No. Thus, it can be said that logistic regression is used to analyze either the close-ended questions in a survey or the questions demanding numeric responses in a survey.
Please note logistic regression does not need a linear relationship between a dependent and an independent variable, just like linear regression. Logistic regression applies a non-linear log transformation for predicting the odds ratio; therefore, it easily handles various types of relationships between a dependent and an independent variable.
Logistic regression is widely used to analyze categorical data, particularly for binary response data in business data modeling. More often, logistic regression is used when the dependent variable is categorical, like to predict whether the health claim made by a person is real(1) or fraudulent, to understand if the tumor is malignant(1) or not.
Businesses use logistic regression to predict whether the consumers in a particular demographic will purchase their product or will buy from the competitors based on age, income, gender, race, state of residence, previous purchase, etc.
03. Polynomial Regression Analysis
Polynomial regression is commonly used to analyze curvilinear data when an independent variable’s power is more than 1. In this regression analysis method, the best-fit line is never a ‘straight line’ but always a ‘curve line’ fitting into the data points.
Please note that polynomial regression is better to use when two or more variables have exponents and a few do not.
Additionally, it can model non-linearly separable data offering the liberty to choose the exact exponent for each variable, and that too with full control over the modeling features available.
When combined with response surface analysis, polynomial regression is considered one of the sophisticated statistical methods commonly used in multisource feedback research. Polynomial regression is used mostly in finance and insurance-related industries where the relationship between dependent and independent variables is curvilinear.
Suppose a person wants to budget expense planning by determining how long it would take to earn a definitive sum. Polynomial regression, by taking into account his/her income and predicting expenses, can easily determine the precise time he/she needs to work to earn that specific sum amount.
04. Stepwise Regression Analysis
This is a semi-automated process with which a statistical model is built either by adding or removing the dependent variable on the t-statistics of their estimated coefficients.
If used properly, the stepwise regression will provide you with more powerful data at your fingertips than any method. It works well when you are working with a large number of independent variables. It just fine-tunes the unit of analysis model by poking variables randomly.
Stepwise regression analysis is recommended to be used when there are multiple independent variables, wherein the selection of independent variables is done automatically without human intervention.
Please note, in stepwise regression modeling, the variable is added or subtracted from the set of explanatory variables. The set of added or removed variables is chosen depending on the test statistics of the estimated coefficient.
Suppose you have a set of independent variables like age, weight, body surface area, duration of hypertension, basal pulse, and stress index based on which you want to analyze its impact on the blood pressure.
In stepwise regression, the best subset of the independent variable is automatically chosen; it either starts by choosing no variable to proceed further (as it adds one variable at a time) or starts with all variables in the model and proceeds backward (removes one variable at a time).
Thus, using regression analysis, you can calculate the impact of each or a group of variables on blood pressure.
05. Ridge Regression Analysis
Ridge regression is based on an ordinary least square method which is used to analyze multicollinearity data (data where independent variables are highly correlated). Collinearity can be explained as a near-linear relationship between variables.
Whenever there is multicollinearity, the estimates of least squares will be unbiased, but if the difference between them is larger, then it may be far away from the true value. However, ridge regression eliminates the standard errors by appending some degree of bias to the regression estimates with a motive to provide more reliable estimates.
If you want, you can also learn about Selection Bias through our blog.
Please note, Assumptions derived through the ridge regression are similar to the least squared regression, the only difference being the normality. Although the value of the coefficient is constricted in the ridge regression, it never reaches zero suggesting the inability to select variables.
Suppose you are crazy about two guitarists performing live at an event near you, and you go to watch their performance with a motive to find out who is a better guitarist. But when the performance starts, you notice that both are playing black-and-blue notes at the same time.
Is it possible to find out the best guitarist having the biggest impact on sound among them when they are both playing loud and fast? As both of them are playing different notes, it is substantially difficult to differentiate them, making it the best case of multicollinearity, which tends to increase the standard errors of the coefficients.
Ridge regression addresses multicollinearity in cases like these and includes bias or a shrinkage estimation to derive results.
06. Lasso Regression Analysis
Lasso (Least Absolute Shrinkage and Selection Operator) is similar to ridge regression; however, it uses an absolute value bias instead of the square bias used in ridge regression.
It was developed way back in 1989 as an alternative to the traditional least-squares estimate with the intention to deduce the majority of problems related to overfitting when the data has a large number of independent variables.
Lasso has the capability to perform both – selecting variables and regularizing them along with a soft threshold. Applying lasso regression makes it easier to derive a subset of predictors from minimizing prediction errors while analyzing a quantitative response.
Please note that regression coefficients reaching zero value after shrinkage are excluded from the lasso model. On the contrary, regression coefficients having more value than zero are strongly associated with the response variables, wherein the explanatory variables can be either quantitative, categorical, or both.
Suppose an automobile company wants to perform a research analysis on average fuel consumption by cars in the US. For samples, they chose 32 models of car and 10 features of automobile design – Number of cylinders, Displacement, Gross horsepower, Rear axle ratio, Weight, ¼ mile time, v/s engine, transmission, number of gears, and number of carburetors.
As you can see a correlation between the response variable mpg (miles per gallon) is extremely correlated to some variables like weight, displacement, number of cylinders, and horsepower. The problem can be analyzed by using the glmnet package in R and lasso regression for feature selection.
07. Elastic Net Regression Analysis
It is a mixture of ridge and lasso regression models trained with L1 and L2 norms. The elastic net brings about a grouping effect wherein strongly correlated predictors tend to be in/out of the model together. Using the elastic net regression model is recommended when the number of predictors is far greater than the number of observations.
Please note that the elastic net regression model came into existence as an option to the lasso regression model as lasso’s variable section was too much dependent on data, making it unstable. By using elastic net regression, statisticians became capable of over-bridging the penalties of ridge and lasso regression only to get the best out of both models.
A clinical research team having access to a microarray data set on leukemia (LEU) was interested in constructing a diagnostic rule based on the expression level of presented gene samples for predicting the type of leukemia. The data set they had, consisted of a large number of genes and a few samples.
Apart from that, they were given a specific set of samples to be used as training samples, out of which some were infected with type 1 leukemia (acute lymphoblastic leukemia) and some with type 2 leukemia (acute myeloid leukemia).
Model fitting and tuning parameter selection by tenfold CV were carried out on the training data. Then they compared the performance of those methods by computing their prediction mean-squared error on the test data to get the necessary results.
A market research survey focuses on three major matrices; Customer Satisfaction , Customer Loyalty , and Customer Advocacy . Remember, although these matrices tell us about customer health and intentions, they fail to tell us ways of improving the position. Therefore, an in-depth survey questionnaire intended to ask consumers the reason behind their dissatisfaction is definitely a way to gain practical insights.
However, it has been found that people often struggle to put forth their motivation or demotivation or describe their satisfaction or dissatisfaction. In addition to that, people always give undue importance to some rational factors, such as price, packaging, etc. Overall, it acts as a predictive analytic and forecasting tool in market research.
When used as a forecasting tool, regression analysis can determine an organization’s sales figures by taking into account external market data. A multinational company conducts a market research survey to understand the impact of various factors such as GDP (Gross Domestic Product), CPI (Consumer Price Index), and other similar factors on its revenue generation model.
Obviously, regression analysis in consideration of forecasted marketing indicators was used to predict a tentative revenue that will be generated in future quarters and even in future years. However, the more forward you go in the future, the data will become more unreliable, leaving a wide margin of error .
Case study of using regression analysis
A water purifier company wanted to understand the factors leading to brand favorability. The survey was the best medium for reaching out to existing and prospective customers. A large-scale consumer survey was planned, and a discreet questionnaire was prepared using the best survey tool .
A number of questions related to the brand, favorability, satisfaction, and probable dissatisfaction were effectively asked in the survey. After getting optimum responses to the survey, regression analysis was used to narrow down the top ten factors responsible for driving brand favorability.
All the ten attributes derived (mentioned in the image below) in one or the other way highlighted their importance in impacting the favorability of that specific water purifier brand.
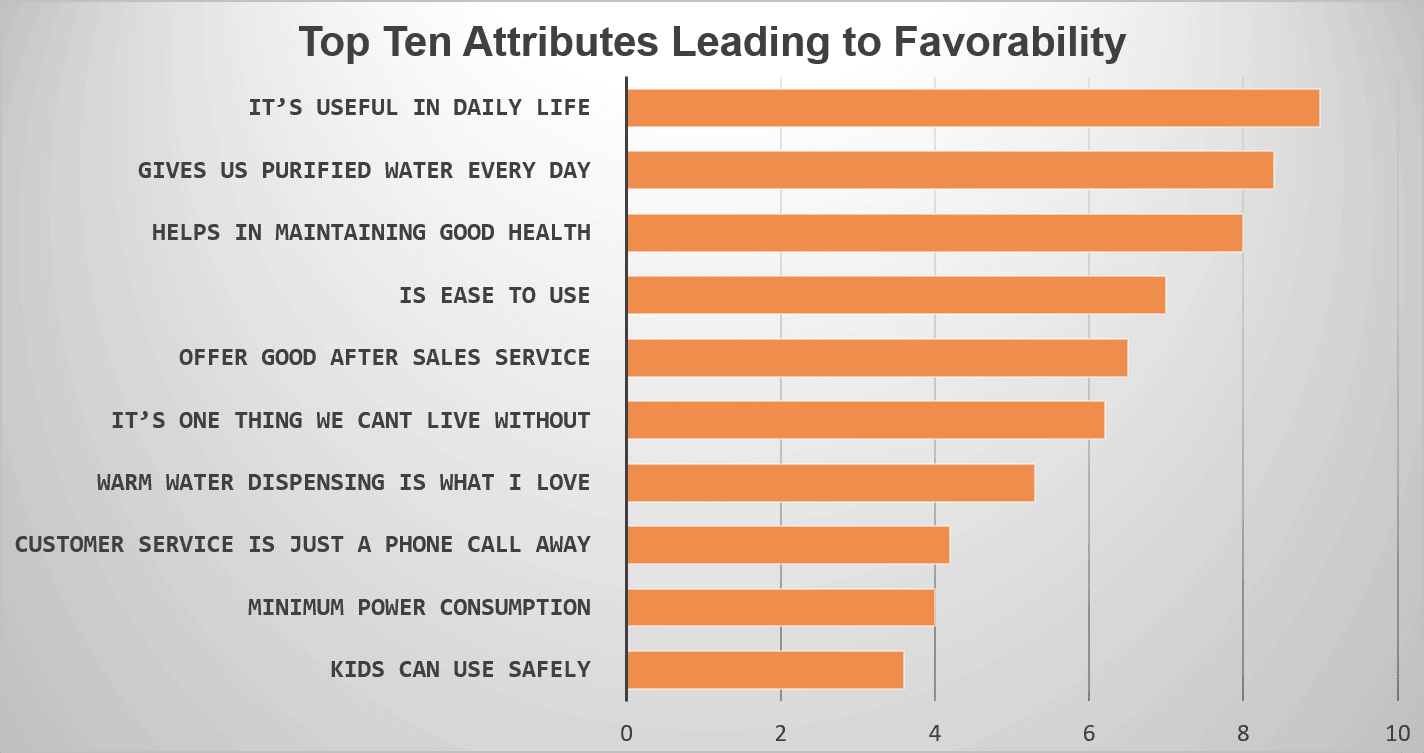
It is easy to run a regression analysis using Excel or SPSS, but while doing so, the importance of four numbers in interpreting the data must be understood.
The first two numbers out of the four numbers directly relate to the regression model itself.
- F-Value: It helps in measuring the statistical significance of the survey model. Remember, an F-Value significantly less than 0.05 is considered to be more meaningful. Less than 0.05 F-Value ensures survey analysis output is not by chance.
- R-Squared: This is the value wherein the independent variables try to explain the amount of movement by dependent variables. Considering the R-Squared value is 0.7, a tested independent variable can explain 70% of the dependent variable’s movement. It means the survey analysis output we will be getting is highly predictive in nature and can be considered accurate.
The other two numbers relate to each of the independent variables while interpreting regression analysis.
- P-Value: Like F-Value, even the P-Value is statistically significant. Moreover, here it indicates how relevant and statistically significant the independent variable’s effect is. Once again, we are looking for a value of less than 0.05.
- Interpretation: The fourth number relates to the coefficient achieved after measuring the impact of variables. For instance, we test multiple independent variables to get a coefficient. It tells us, ‘by what value the dependent variable is expected to increase when independent variables (which we are considering) increase by one when all other independent variables are stagnant at the same value.
In a few cases, the simple coefficient is replaced by a standardized coefficient demonstrating the contribution from each independent variable to move or bring about a change in the dependent variable.
01. Get access to predictive analytics
Do you know utilizing regression analysis to understand the outcome of a business survey is like having the power to unveil future opportunities and risks?
For example, after seeing a particular television advertisement slot, we can predict the exact number of businesses using that data to estimate a maximum bid for that slot. The finance and insurance industry as a whole depends a lot on regression analysis of survey data to identify trends and opportunities for more accurate planning and decision-making.
02. Enhance operational efficiency
Do you know businesses use regression analysis to optimize their business processes?
For example, before launching a new product line, businesses conduct consumer surveys to better understand the impact of various factors on the product’s production, packaging, distribution, and consumption.
A data-driven foresight helps eliminate the guesswork, hypothesis, and internal politics from decision-making. A deeper understanding of the areas impacting operational efficiencies and revenues leads to better business optimization.
03. Quantitative support for decision-making
Business surveys today generate a lot of data related to finance, revenue, operation, purchases, etc., and business owners are heavily dependent on various data analysis models to make informed business decisions.
For example, regression analysis helps enterprises to make informed strategic workforce decisions. Conducting and interpreting the outcome of employee surveys like Employee Engagement Surveys, Employee Satisfaction Surveys, Employer Improvement Surveys, Employee Exit Surveys, etc., boosts the understanding of the relationship between employees and the enterprise.
It also helps get a fair idea of certain issues impacting the organization’s working culture, working environment, and productivity. Furthermore, intelligent business-oriented interpretations reduce the huge pile of raw data into actionable information to make a more informed decision.
04. Prevent mistakes from happening due to intuitions
By knowing how to use regression analysis for interpreting survey results, one can easily provide factual support to management for making informed decisions. ; but do you know that it also helps in keeping out faults in the judgment?
For example, a mall manager thinks if he extends the closing time of the mall, then it will result in more sales. Regression analysis contradicts the belief that predicting increased revenue due to increased sales won’t support the increased operating expenses arising from longer working hours.
Regression analysis is a useful statistical method for modeling and comprehending the relationships between variables. It provides numerous advantages to various data types and interactions. Researchers and analysts may gain useful insights into the factors influencing a dependent variable and use the results to make informed decisions.
With QuestionPro Research, you can improve the efficiency and accuracy of regression analysis by streamlining the data gathering, analysis, and reporting processes. The platform’s user-friendly interface and wide range of features make it a valuable tool for researchers and analysts conducting regression analysis as part of their research projects.
Sign up for the free trial today and let your research dreams fly!
FREE TRIAL RICHIEDI DEMO
MORE LIKE THIS
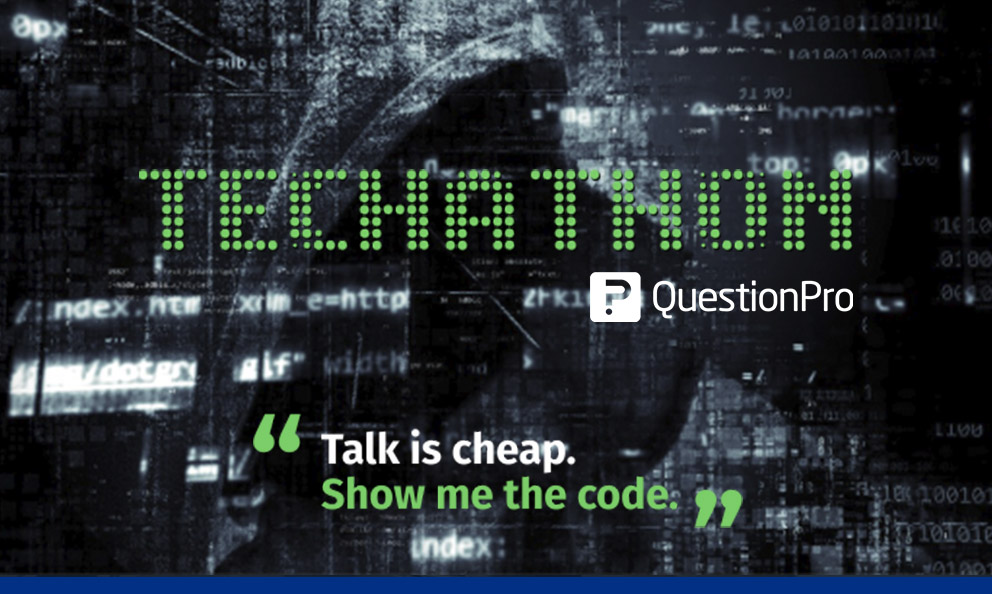
Techathon by QuestionPro: An Amazing Showcase of Tech Brilliance
Jul 3, 2024
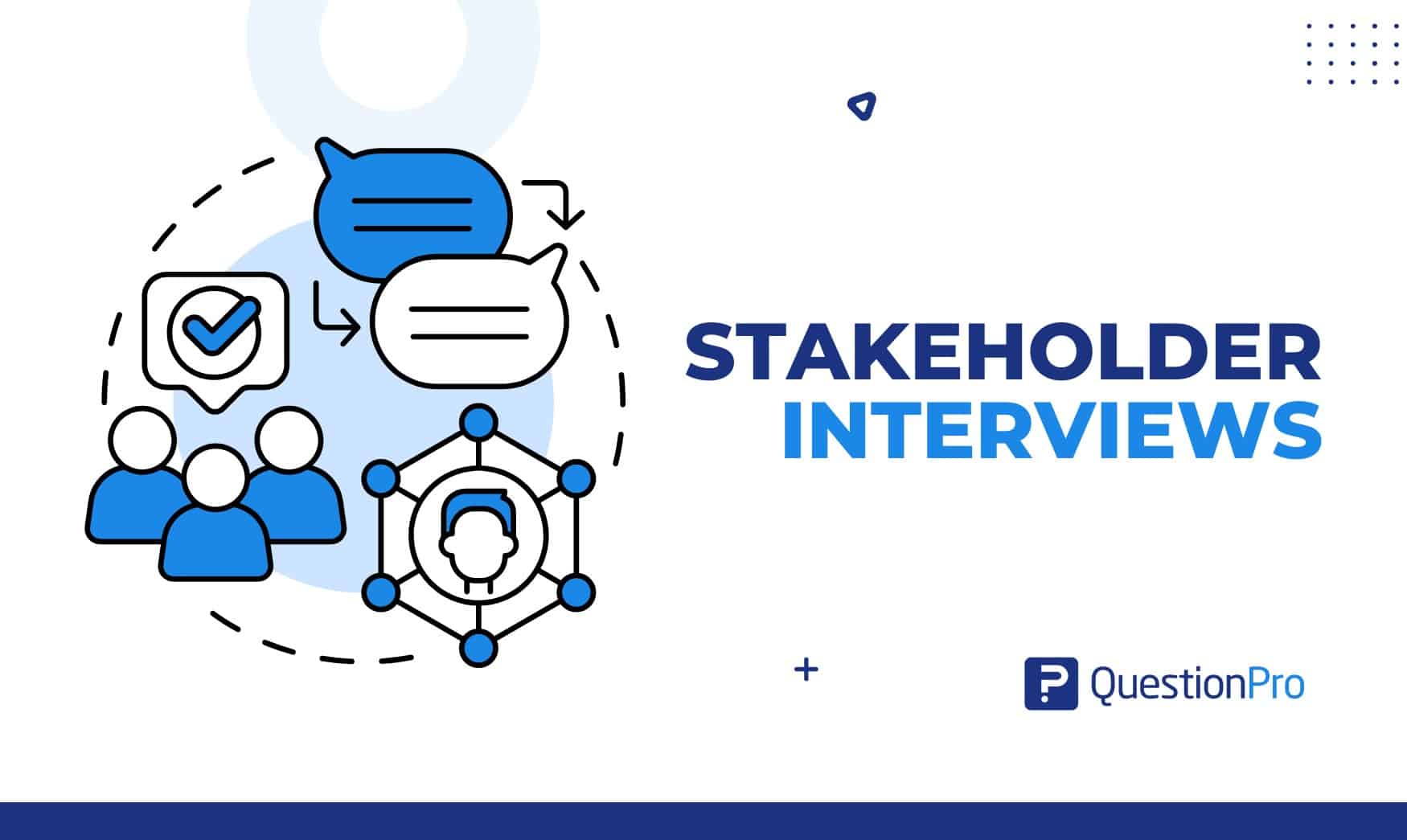
Stakeholder Interviews: A Guide to Effective Engagement
Jul 2, 2024
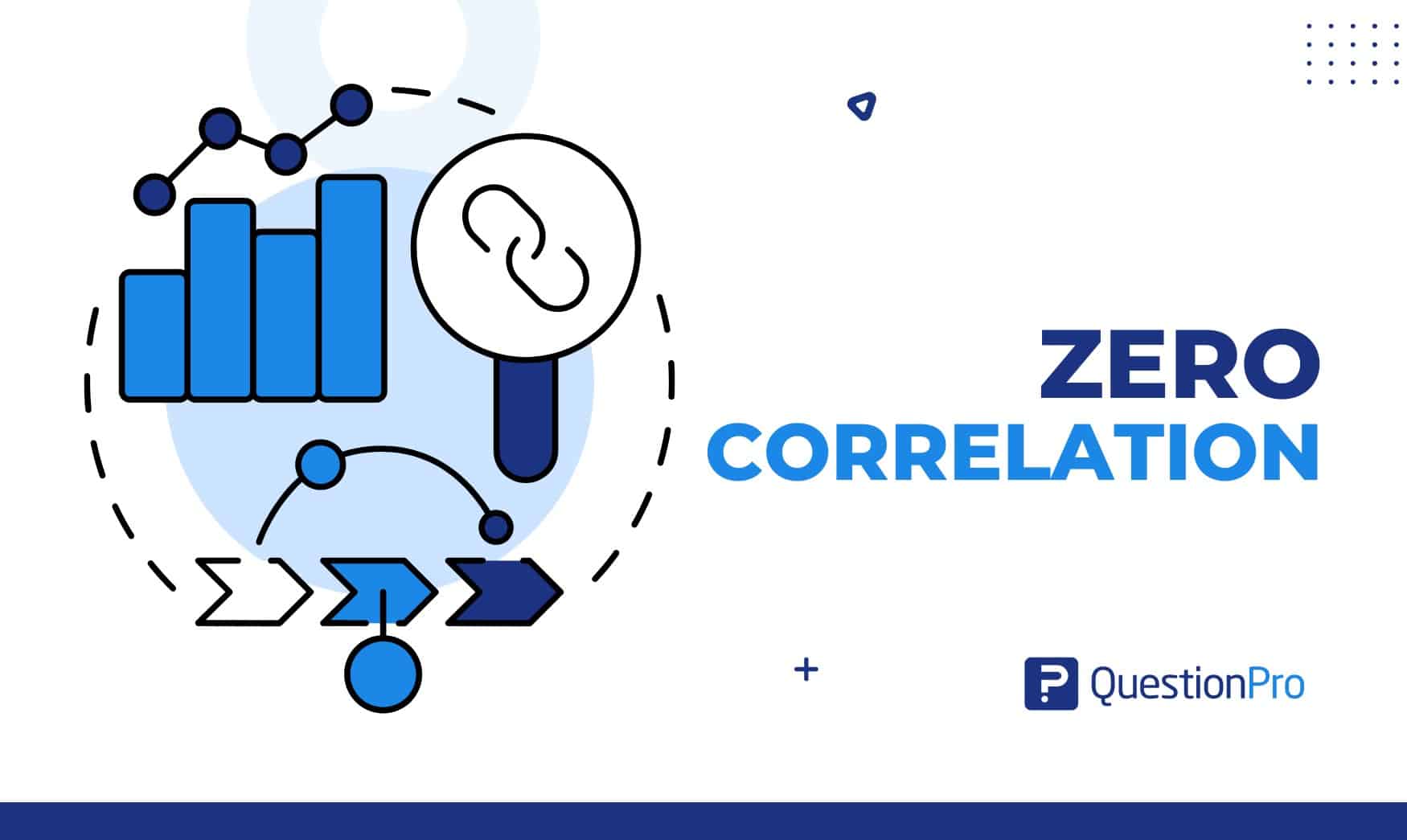
Zero Correlation: Definition, Examples + How to Determine It
Jul 1, 2024
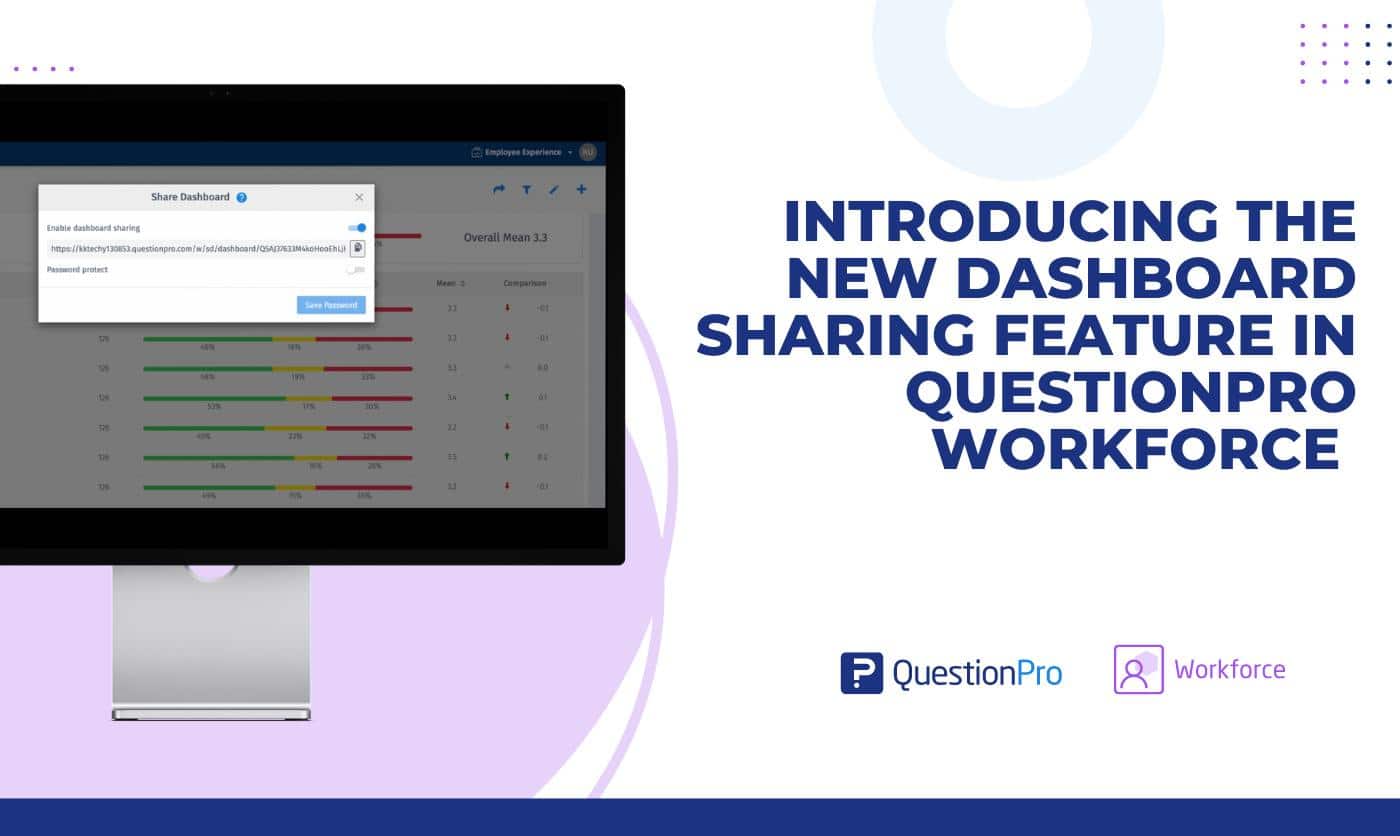
When You Have Something Important to Say, You want to Shout it From the Rooftops
Jun 28, 2024
Other categories
- Academic Research
- Artificial Intelligence
- Assessments
- Brand Awareness
- Case Studies
- Communities
- Consumer Insights
- Customer effort score
- Customer Engagement
- Customer Experience
- Customer Loyalty
- Customer Research
- Customer Satisfaction
- Employee Benefits
- Employee Engagement
- Employee Retention
- Friday Five
- General Data Protection Regulation
- Insights Hub
- Life@QuestionPro
- Market Research
- Mobile diaries
- Mobile Surveys
- New Features
- Online Communities
- Question Types
- Questionnaire
- QuestionPro Products
- Release Notes
- Research Tools and Apps
- Revenue at Risk
- Survey Templates
- Training Tips
- Tuesday CX Thoughts (TCXT)
- Uncategorized
- Video Learning Series
- What’s Coming Up
- Workforce Intelligence
A behind-the-scenes blog about research methods at Pew Research Center. For our latest findings, visit pewresearch.org .
- Survey Methods
A short intro to linear regression analysis using survey data
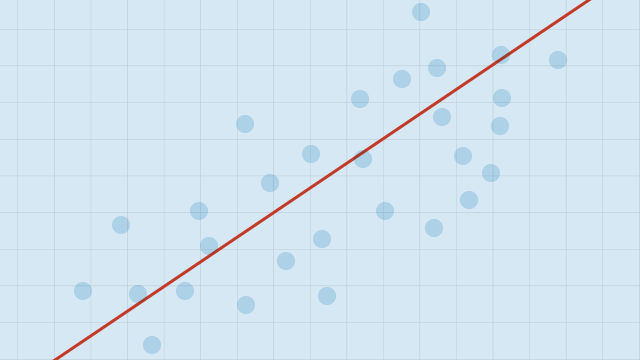
Many of Pew Research Center’s survey analyses show relationships between two variables. For example, our reports may explore how attitudes about one thing — such as views of the economy — are associated with attitudes about another thing — such as views of the president’s job performance. Or they might look at how different demographic groups respond to the same survey question.
But analysts are sometimes interested in understanding how multiple factors might contribute simultaneously to the same outcome. One useful tool to help us make sense of these kinds of problems is regression. Regression is a statistical method that allows us to look at the relationship between two variables, while holding other factors equal.
This post will show how to estimate and interpret linear regression models with survey data using R. We’ll use data taken from a Pew Research Center 2016 post-election survey, and you can download the dataset for your own use here . We’ll discuss both bivariate regression, which has one outcome variable and one explanatory variable, and multiple regression, which has one outcome variable and multiple explanatory variables.
This post is meant as a brief introduction to how to estimate a regression model in R. It also offers a brief explanation of some of the aspects that need to be accounted for in the process.
Bivariate regression models with survey data
In the Center’s 2016 post-election survey, respondents were asked to rate then President-elect Donald Trump on a 0–100 “feeling thermometer.” Respondents were told, “a rating of zero degrees means you feel as cold and negative as possible. A rating of 100 degrees means you feel as warm and positive as possible. You would rate the person at 50 degrees if you don’t feel particularly positive or negative toward the person.”
We can use R’s plot function to take a look at the answers people gave. The plot below shows the distribution of the ratings of Trump. Round numbers and increments of 5 typically received more responses than other numbers. For example, 50 had a larger number of responses than 49.
In most survey research we also want to represent a population (in this case, the adult population in the U.S.), which requires weighting the data to known national statistics. Weights are used to correct for under- and overrepresentation among different demographic groups in our sample (like age, gender, region, education, race). When working with weighted survey data, we need to account for these weights correctly. Otherwise, population estimates, standard errors and significance tests will be incorrect.
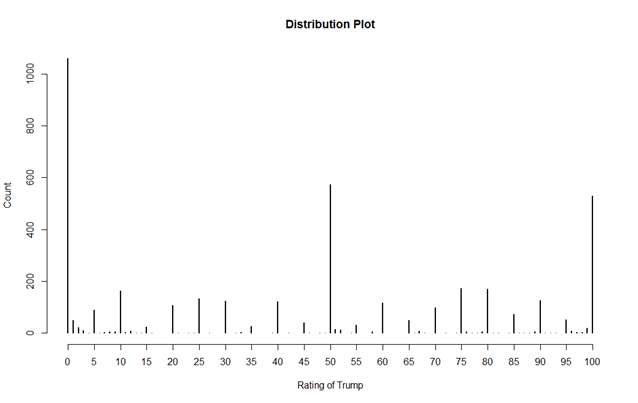
One option for working with survey data in R is to use the “survey” package. For an introduction on working with survey data in R, see our earlier blog post .
The first step involves creating a survey design object with our weights variable. Below, we define the “d_design” object with the corresponding weight from the WEIGHT_W23 variable. We can use this survey object to perform a wide variety of analyses included in the `survey` package. In this case, we’ll use it to calculate averages and run a regression.
The `svymean()` function lets us calculate Trump’s average thermometer rating and its standard error. Overall, the average rating of Trump among those who gave him a rating in this data is 43, but we know from existing research that public views of Trump differ substantially by race , among other things. We can see this by tabulating the average Trump thermometer score by the race/ethnicity variable in the dataset (“F_RACETHN_RECRUITMENT”). The `svyby()` function lets us do that separately for each race category:
We can see that there is a large difference between whites, blacks and Hispanics, with whites rating Trump at least 23 points higher than the other racial/ethnic groups do. (The “other” and “don’t know/refused” categories account for about 7% of the public.) However, since we know that there are large racial and ethnic differences in party identification , it may be that the racial divide in Trump ratings is a function of partisanship. This is where regression comes in.
By using the regression function `svyglm()` in R, we can conduct a regression analysis that includes party differences in the same model as race. Using `svyglm()` from the survey package (rather than `lm()` or `glm()`) is important because it accounts for the survey weights while estimating the model. The output from our `svyglm()` function will allow us to see whether a racial gap persists even after accounting for differences in partisanship between racial groups.
First, we can look at the results when we only include race in the regression:
When interpreting regression output, we want to examine the coefficients of the independent variables. These are given by the values in the “Estimate” column.
Notice that the estimate and standard error for the “(Intercept)” are identical to the values we calculated earlier for white non-Hispanics. By default, R treats the first category in an independent variable as the reference category. The coefficients for the other racial groups show how each group differs from whites in terms of the Trump thermometer score. Notice that the coefficients for blacks, Hispanics and those who identify with other racial groups are all negative. This means that, on average, the ratings of Trump are lower across each of these groups compared to whites. For example, the coefficient for blacks is -23.7. This can be interpreted as meaning that, on average, Trump’s thermometer rating is 23.7 points lower for blacks than for whites. If we think back to the overall averages, this makes sense because all the nonwhite racial/ethnic groups rated Trump lower than whites did. And, in fact, if you combine the intercept estimate with the estimate for non-Hispanic blacks, you get 49.3–23.7 = 25.6, exactly what we saw in the simple tabulation above.
Multiple regression models with survey data
Regression becomes a more useful tool when researchers want to look at multiple factors simultaneously. If we want to know whether the racial divide persists even after accounting for differences in party identification, we can enter partisanship into the regression equation. Note that the only difference here is one added explanatory variable (F_PARTYSUM_FINAL) which contains responses to questions about which political party the respondents identify with or lean toward. Since we have two independent variables now, the reference categories are now the group of people who are in the first level for the F_RACETHN_RECRUITMENT and F_PARTYSUM_FINAL variables. In this case, that means that the intercept is the expected average thermometer score among non-Hispanic whites who also identify as or lean Republican.
After including a new variable for partisanship, the racial and ethnic differences almost entirely disappear. The coefficients are quite small (none exceed 5) and are not statistically significant at p < 0.05. For blacks, we can interpret the coefficient of -2.1 as meaning that if we hold party constant, race does not explain differences in Trump’s rating. We would expect both black and white Republicans to give similar ratings of Trump. Likewise, we would expect only small differences between white and black Democrats. In contrast, party matters a lot: Democrats rate Trump about 51 points lower than Republicans on average. Those who don’t lean toward either party rate Trump about 39 points lower than Republicans.
Further analysis could be conducted to explore how other factors might account for variance in Trump thermometer ratings. Perhaps there are significant interactions that we haven’t accounted for (e.g., it might be the case that there is some kind of interaction between race and partisanship that isn’t accounted for in the simple additive model that we looked at above), and it is always important to remember that standard regression analysis of the kind presented in this post is not sufficient to show causal relationships. Regression allows us to sort out the relationships between many variables simultaneously, but we can’t say that just because a significant relationship was found between two variables, one caused the other. Regression is a useful tool for summarizing descriptive relationships, but it is not a silver bullet (see this post for more on where regression can go wrong).
Categories:
More from Decoded
How do people in the u.s. take pew research center surveys, anyway.
Here, we address some of the most common questions we receive about the nuts and bolts of taking a U.S.-focused Pew Research Center poll.
How Public Polling Has Changed in the 21st Century
A new study found that 61% of national pollsters used different methods in 2022 than in 2016. And last year, 17% of pollsters used multiple methods to sample or interview people – up from 2% in 2016.
What 2020’s Election Poll Errors Tell Us About the Accuracy of Issue Polling
Given the errors in 2016 and 2020 election polling, how much should we trust polls that attempt to measure opinions on issues?
A Field Guide to Polling: Election 2020 Edition
While survey research in the United States is a year-round undertaking, the public’s focus on polling is never more intense than during the run-up to a presidential election.
Methods 101: How is polling done around the world?
Polling in different parts of the world can be very challenging, because what works in one country may not work in a different country.
MORe from decoded
To browse all of Pew Research Center findings and data by topic, visit pewresearch.org
About Decoded
This is a blog about research methods and behind-the-scenes technical matters at Pew Research Center. To get our latest findings, visit pewresearch.org .
Copyright 2024 Pew Research Center
Root out friction in every digital experience, super-charge conversion rates, and optimize digital self-service
Uncover insights from any interaction, deliver AI-powered agent coaching, and reduce cost to serve
Increase revenue and loyalty with real-time insights and recommendations delivered to teams on the ground
Know how your people feel and empower managers to improve employee engagement, productivity, and retention
Take action in the moments that matter most along the employee journey and drive bottom line growth
Whatever they’re are saying, wherever they’re saying it, know exactly what’s going on with your people
Get faster, richer insights with qual and quant tools that make powerful market research available to everyone
Run concept tests, pricing studies, prototyping + more with fast, powerful studies designed by UX research experts
Track your brand performance 24/7 and act quickly to respond to opportunities and challenges in your market
Explore the platform powering Experience Management
- Free Account
- Product Demos
- For Digital
- For Customer Care
- For Human Resources
- For Researchers
- Financial Services
- All Industries
Popular Use Cases
- Customer Experience
- Employee Experience
- Net Promoter Score
- Voice of Customer
- Customer Success Hub
- Product Documentation
- Training & Certification
- XM Institute
- Popular Resources
- Customer Stories
- Artificial Intelligence
- Market Research
- Partnerships
- Marketplace
The annual gathering of the experience leaders at the world’s iconic brands building breakthrough business results, live in Salt Lake City.
- English/AU & NZ
- Español/Europa
- Español/América Latina
- Português Brasileiro
- REQUEST DEMO
- Experience Management
- Survey Data Analysis & Reporting
- Regression Analysis
Try Qualtrics for free
The complete guide to regression analysis.
19 min read What is regression analysis and why is it useful? While most of us have heard the term, understanding regression analysis in detail may be something you need to brush up on. Here’s what you need to know about this popular method of analysis.
When you rely on data to drive and guide business decisions, as well as predict market trends, just gathering and analyzing what you find isn’t enough — you need to ensure it’s relevant and valuable.
The challenge, however, is that so many variables can influence business data: market conditions, economic disruption, even the weather! As such, it’s essential you know which variables are affecting your data and forecasts, and what data you can discard.
And one of the most effective ways to determine data value and monitor trends (and the relationships between them) is to use regression analysis, a set of statistical methods used for the estimation of relationships between independent and dependent variables.
In this guide, we’ll cover the fundamentals of regression analysis, from what it is and how it works to its benefits and practical applications.
Free eBook: 2024 global market research trends report
What is regression analysis?
Regression analysis is a statistical method. It’s used for analyzing different factors that might influence an objective – such as the success of a product launch, business growth, a new marketing campaign – and determining which factors are important and which ones can be ignored.
Regression analysis can also help leaders understand how different variables impact each other and what the outcomes are. For example, when forecasting financial performance, regression analysis can help leaders determine how changes in the business can influence revenue or expenses in the future.
Running an analysis of this kind, you might find that there’s a high correlation between the number of marketers employed by the company, the leads generated, and the opportunities closed.
This seems to suggest that a high number of marketers and a high number of leads generated influences sales success. But do you need both factors to close those sales? By analyzing the effects of these variables on your outcome, you might learn that when leads increase but the number of marketers employed stays constant, there is no impact on the number of opportunities closed, but if the number of marketers increases, leads and closed opportunities both rise.
Regression analysis can help you tease out these complex relationships so you can determine which areas you need to focus on in order to get your desired results, and avoid wasting time with those that have little or no impact. In this example, that might mean hiring more marketers rather than trying to increase leads generated.
How does regression analysis work?
Regression analysis starts with variables that are categorized into two types: dependent and independent variables. The variables you select depend on the outcomes you’re analyzing.
Understanding variables:
1. dependent variable.
This is the main variable that you want to analyze and predict. For example, operational (O) data such as your quarterly or annual sales, or experience (X) data such as your net promoter score (NPS) or customer satisfaction score (CSAT) .
These variables are also called response variables, outcome variables, or left-hand-side variables (because they appear on the left-hand side of a regression equation).
There are three easy ways to identify them:
- Is the variable measured as an outcome of the study?
- Does the variable depend on another in the study?
- Do you measure the variable only after other variables are altered?
2. Independent variable
Independent variables are the factors that could affect your dependent variables. For example, a price rise in the second quarter could make an impact on your sales figures.
You can identify independent variables with the following list of questions:
- Is the variable manipulated, controlled, or used as a subject grouping method by the researcher?
- Does this variable come before the other variable in time?
- Are you trying to understand whether or how this variable affects another?
Independent variables are often referred to differently in regression depending on the purpose of the analysis. You might hear them called:
Explanatory variables
Explanatory variables are those which explain an event or an outcome in your study. For example, explaining why your sales dropped or increased.
Predictor variables
Predictor variables are used to predict the value of the dependent variable. For example, predicting how much sales will increase when new product features are rolled out .
Experimental variables
These are variables that can be manipulated or changed directly by researchers to assess the impact. For example, assessing how different product pricing ($10 vs $15 vs $20) will impact the likelihood to purchase.
Subject variables (also called fixed effects)
Subject variables can’t be changed directly, but vary across the sample. For example, age, gender, or income of consumers.
Unlike experimental variables, you can’t randomly assign or change subject variables, but you can design your regression analysis to determine the different outcomes of groups of participants with the same characteristics. For example, ‘how do price rises impact sales based on income?’
Carrying out regression analysis
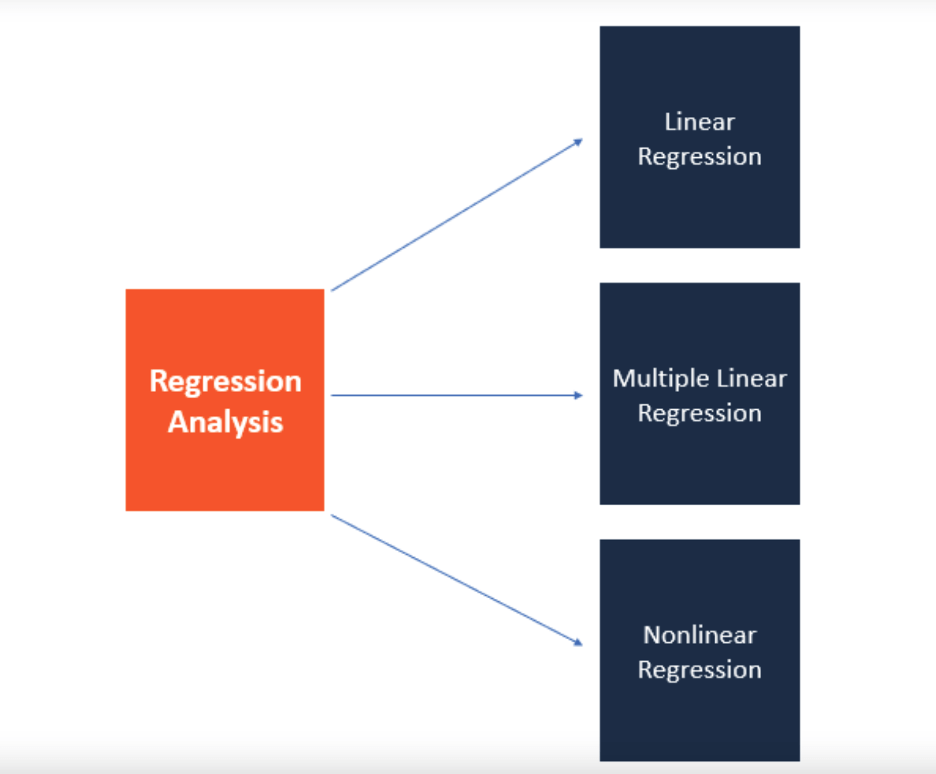
So regression is about the relationships between dependent and independent variables. But how exactly do you do it?
Assuming you have your data collection done already, the first and foremost thing you need to do is plot your results on a graph. Doing this makes interpreting regression analysis results much easier as you can clearly see the correlations between dependent and independent variables.
Let’s say you want to carry out a regression analysis to understand the relationship between the number of ads placed and revenue generated.
On the Y-axis, you place the revenue generated. On the X-axis, the number of digital ads. By plotting the information on the graph, and drawing a line (called the regression line) through the middle of the data, you can see the relationship between the number of digital ads placed and revenue generated.
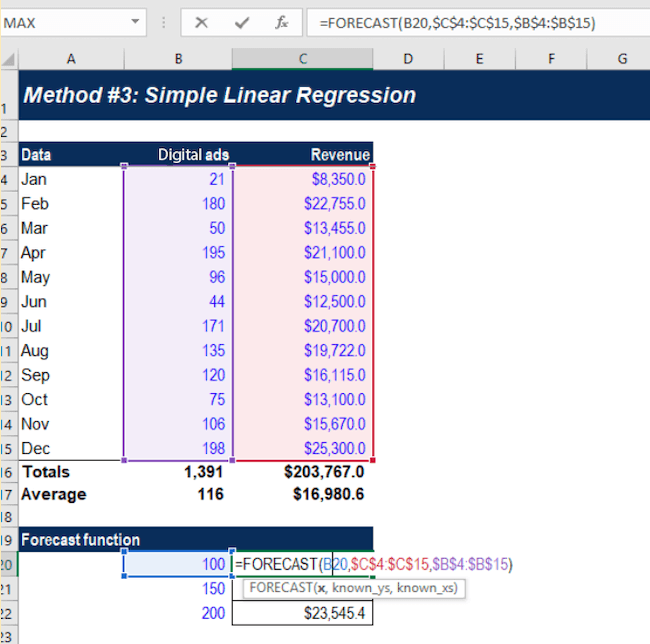
This regression line is the line that provides the best description of the relationship between your independent variables and your dependent variable. In this example, we’ve used a simple linear regression model.
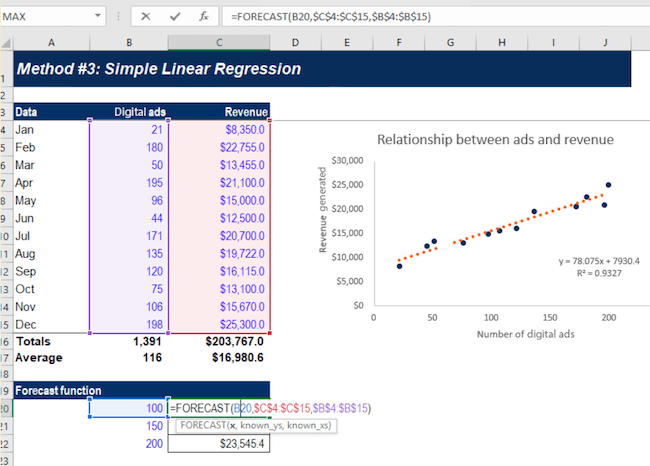
Statistical analysis software can draw this line for you and precisely calculate the regression line. The software then provides a formula for the slope of the line, adding further context to the relationship between your dependent and independent variables.
Simple linear regression analysis
A simple linear model uses a single straight line to determine the relationship between a single independent variable and a dependent variable.
This regression model is mostly used when you want to determine the relationship between two variables (like price increases and sales) or the value of the dependent variable at certain points of the independent variable (for example the sales levels at a certain price rise).
While linear regression is useful, it does require you to make some assumptions.
For example, it requires you to assume that:
- the data was collected using a statistically valid sample collection method that is representative of the target population
- The observed relationship between the variables can’t be explained by a ‘hidden’ third variable – in other words, there are no spurious correlations.
- the relationship between the independent variable and dependent variable is linear – meaning that the best fit along the data points is a straight line and not a curved one
Multiple regression analysis
As the name suggests, multiple regression analysis is a type of regression that uses multiple variables. It uses multiple independent variables to predict the outcome of a single dependent variable. Of the various kinds of multiple regression, multiple linear regression is one of the best-known.
Multiple linear regression is a close relative of the simple linear regression model in that it looks at the impact of several independent variables on one dependent variable. However, like simple linear regression, multiple regression analysis also requires you to make some basic assumptions.
For example, you will be assuming that:
- there is a linear relationship between the dependent and independent variables (it creates a straight line and not a curve through the data points)
- the independent variables aren’t highly correlated in their own right
An example of multiple linear regression would be an analysis of how marketing spend, revenue growth, and general market sentiment affect the share price of a company.
With multiple linear regression models you can estimate how these variables will influence the share price, and to what extent.
Multivariate linear regression
Multivariate linear regression involves more than one dependent variable as well as multiple independent variables, making it more complicated than linear or multiple linear regressions. However, this also makes it much more powerful and capable of making predictions about complex real-world situations.
For example, if an organization wants to establish or estimate how the COVID-19 pandemic has affected employees in its different markets, it can use multivariate linear regression, with the different geographical regions as dependent variables and the different facets of the pandemic as independent variables (such as mental health self-rating scores, proportion of employees working at home, lockdown durations and employee sick days).
Through multivariate linear regression, you can look at relationships between variables in a holistic way and quantify the relationships between them. As you can clearly visualize those relationships, you can make adjustments to dependent and independent variables to see which conditions influence them. Overall, multivariate linear regression provides a more realistic picture than looking at a single variable.
However, because multivariate techniques are complex, they involve high-level mathematics that require a statistical program to analyze the data.
Logistic regression
Logistic regression models the probability of a binary outcome based on independent variables.
So, what is a binary outcome? It’s when there are only two possible scenarios, either the event happens (1) or it doesn’t (0). e.g. yes/no outcomes, pass/fail outcomes, and so on. In other words, if the outcome can be described as being in either one of two categories.
Logistic regression makes predictions based on independent variables that are assumed or known to have an influence on the outcome. For example, the probability of a sports team winning their game might be affected by independent variables like weather, day of the week, whether they are playing at home or away and how they fared in previous matches.
What are some common mistakes with regression analysis?
Across the globe, businesses are increasingly relying on quality data and insights to drive decision-making — but to make accurate decisions, it’s important that the data collected and statistical methods used to analyze it are reliable and accurate.
Using the wrong data or the wrong assumptions can result in poor decision-making, lead to missed opportunities to improve efficiency and savings, and — ultimately — damage your business long term.
- Assumptions
When running regression analysis, be it a simple linear or multiple regression, it’s really important to check that the assumptions your chosen method requires have been met. If your data points don’t conform to a straight line of best fit, for example, you need to apply additional statistical modifications to accommodate the non-linear data. For example, if you are looking at income data, which scales on a logarithmic distribution, you should take the Natural Log of Income as your variable then adjust the outcome after the model is created.
- Correlation vs. causation
It’s a well-worn phrase that bears repeating – correlation does not equal causation. While variables that are linked by causality will always show correlation, the reverse is not always true. Moreover, there is no statistic that can determine causality (although the design of your study overall can).
If you observe a correlation in your results, such as in the first example we gave in this article where there was a correlation between leads and sales, you can’t assume that one thing has influenced the other. Instead, you should use it as a starting point for investigating the relationship between the variables in more depth.
- Choosing the wrong variables to analyze
Before you use any kind of statistical method, it’s important to understand the subject you’re researching in detail. Doing so means you’re making informed choices of variables and you’re not overlooking something important that might have a significant bearing on your dependent variable.
- Model building The variables you include in your analysis are just as important as the variables you choose to exclude. That’s because the strength of each independent variable is influenced by the other variables in the model. Other techniques, such as Key Drivers Analysis, are able to account for these variable interdependencies.
Benefits of using regression analysis
There are several benefits to using regression analysis to judge how changing variables will affect your business and to ensure you focus on the right things when forecasting.
Here are just a few of those benefits:
Make accurate predictions
Regression analysis is commonly used when forecasting and forward planning for a business. For example, when predicting sales for the year ahead, a number of different variables will come into play to determine the eventual result.
Regression analysis can help you determine which of these variables are likely to have the biggest impact based on previous events and help you make more accurate forecasts and predictions.
Identify inefficiencies
Using a regression equation a business can identify areas for improvement when it comes to efficiency, either in terms of people, processes, or equipment.
For example, regression analysis can help a car manufacturer determine order numbers based on external factors like the economy or environment.
Using the initial regression equation, they can use it to determine how many members of staff and how much equipment they need to meet orders.
Drive better decisions
Improving processes or business outcomes is always on the minds of owners and business leaders, but without actionable data, they’re simply relying on instinct, and this doesn’t always work out.
This is particularly true when it comes to issues of price. For example, to what extent will raising the price (and to what level) affect next quarter’s sales?
There’s no way to know this without data analysis. Regression analysis can help provide insights into the correlation between price rises and sales based on historical data.
How do businesses use regression? A real-life example
Marketing and advertising spending are common topics for regression analysis. Companies use regression when trying to assess the value of ad spend and marketing spend on revenue.
A typical example is using a regression equation to assess the correlation between ad costs and conversions of new customers. In this instance,
- our dependent variable (the factor we’re trying to assess the outcomes of) will be our conversions
- the independent variable (the factor we’ll change to assess how it changes the outcome) will be the daily ad spend
- the regression equation will try to determine whether an increase in ad spend has a direct correlation with the number of conversions we have
The analysis is relatively straightforward — using historical data from an ad account, we can use daily data to judge ad spend vs conversions and how changes to the spend alter the conversions.
By assessing this data over time, we can make predictions not only on whether increasing ad spend will lead to increased conversions but also what level of spending will lead to what increase in conversions. This can help to optimize campaign spend and ensure marketing delivers good ROI.
This is an example of a simple linear model. If you wanted to carry out a more complex regression equation, we could also factor in other independent variables such as seasonality, GDP, and the current reach of our chosen advertising networks.
By increasing the number of independent variables, we can get a better understanding of whether ad spend is resulting in an increase in conversions, whether it’s exerting an influence in combination with another set of variables, or if we’re dealing with a correlation with no causal impact – which might be useful for predictions anyway, but isn’t a lever we can use to increase sales.
Using this predicted value of each independent variable, we can more accurately predict how spend will change the conversion rate of advertising.
Regression analysis tools
Regression analysis is an important tool when it comes to better decision-making and improved business outcomes. To get the best out of it, you need to invest in the right kind of statistical analysis software.
The best option is likely to be one that sits at the intersection of powerful statistical analysis and intuitive ease of use, as this will empower everyone from beginners to expert analysts to uncover meaning from data, identify hidden trends and produce predictive models without statistical training being required.
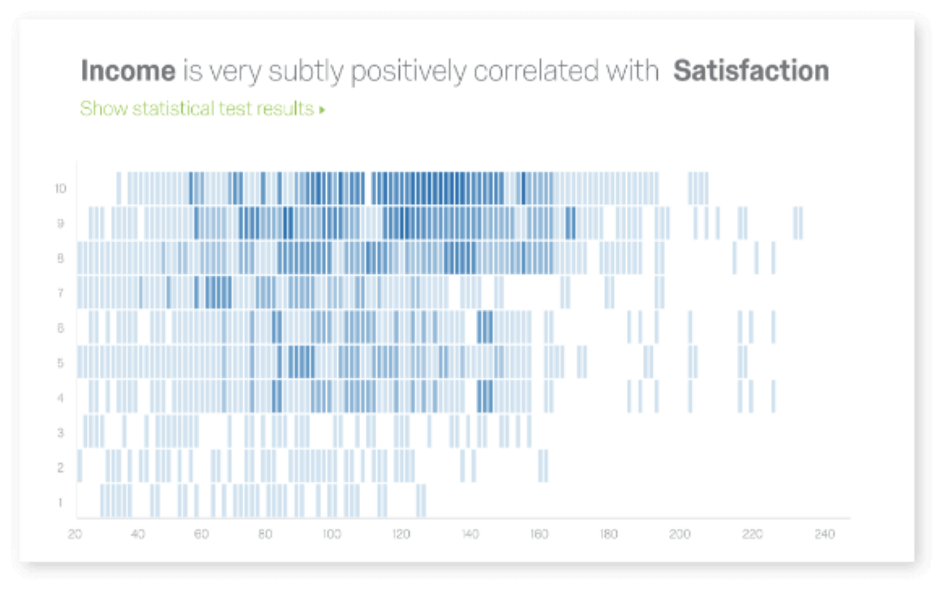
To help prevent costly errors, choose a tool that automatically runs the right statistical tests and visualizations and then translates the results into simple language that anyone can put into action.
With software that’s both powerful and user-friendly, you can isolate key experience drivers, understand what influences the business, apply the most appropriate regression methods, identify data issues, and much more.
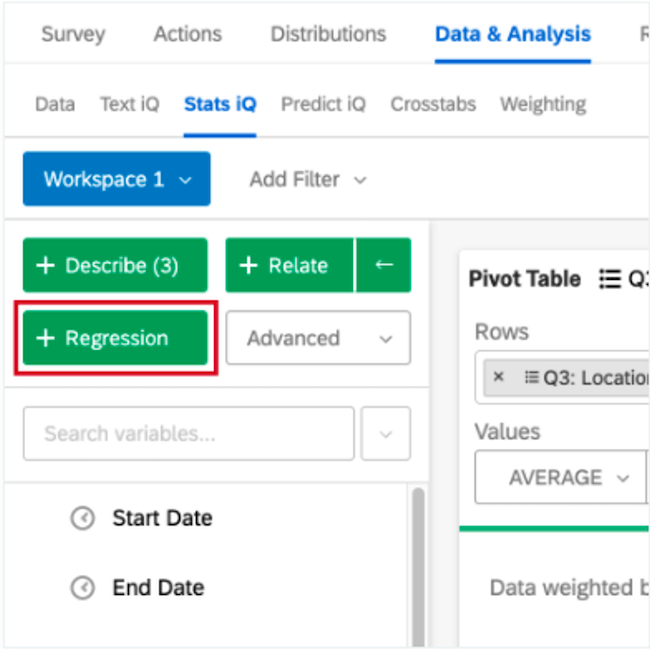
With Qualtrics’ Stats iQ™, you don’t have to worry about the regression equation because our statistical software will run the appropriate equation for you automatically based on the variable type you want to monitor. You can also use several equations, including linear regression and logistic regression, to gain deeper insights into business outcomes and make more accurate, data-driven decisions.
Related resources
Analysis & Reporting
Data Analysis 31 min read
Social media analytics 13 min read, kano analysis 21 min read, margin of error 11 min read, data saturation in qualitative research 8 min read, thematic analysis 11 min read, behavioral analytics 12 min read, request demo.
Ready to learn more about Qualtrics?

An official website of the United States government
The .gov means it’s official. Federal government websites often end in .gov or .mil. Before sharing sensitive information, make sure you’re on a federal government site.
The site is secure. The https:// ensures that you are connecting to the official website and that any information you provide is encrypted and transmitted securely.
- Publications
- Account settings
Preview improvements coming to the PMC website in October 2024. Learn More or Try it out now .
- Advanced Search
- Journal List
- Cardiopulm Phys Ther J
- v.20(3); 2009 Sep
Regression Analysis for Prediction: Understanding the Process
Phillip b palmer.
1 Hardin-Simmons University, Department of Physical Therapy, Abilene, TX
Dennis G O'Connell
2 Hardin-Simmons University, Department of Physical Therapy, Abilene, TX
Research related to cardiorespiratory fitness often uses regression analysis in order to predict cardiorespiratory status or future outcomes. Reading these studies can be tedious and difficult unless the reader has a thorough understanding of the processes used in the analysis. This feature seeks to “simplify” the process of regression analysis for prediction in order to help readers understand this type of study more easily. Examples of the use of this statistical technique are provided in order to facilitate better understanding.
INTRODUCTION
Graded, maximal exercise tests that directly measure maximum oxygen consumption (VO 2 max) are impractical in most physical therapy clinics because they require expensive equipment and personnel trained to administer the tests. Performing these tests in the clinic may also require medical supervision; as a result researchers have sought to develop exercise and non-exercise models that would allow clinicians to predict VO 2 max without having to perform direct measurement of oxygen uptake. In most cases, the investigators utilize regression analysis to develop their prediction models.
Regression analysis is a statistical technique for determining the relationship between a single dependent (criterion) variable and one or more independent (predictor) variables. The analysis yields a predicted value for the criterion resulting from a linear combination of the predictors. According to Pedhazur, 15 regression analysis has 2 uses in scientific literature: prediction, including classification, and explanation. The following provides a brief review of the use of regression analysis for prediction. Specific emphasis is given to the selection of the predictor variables (assessing model efficiency and accuracy) and cross-validation (assessing model stability). The discussion is not intended to be exhaustive. For a more thorough explanation of regression analysis, the reader is encouraged to consult one of many books written about this statistical technique (eg, Fox; 5 Kleinbaum, Kupper, & Muller; 12 Pedhazur; 15 and Weisberg 16 ). Examples of the use of regression analysis for prediction are drawn from a study by Bradshaw et al. 3 In this study, the researchers' stated purpose was to develop an equation for prediction of cardiorespiratory fitness (CRF) based on non-exercise (N-EX) data.
SELECTING THE CRITERION (OUTCOME MEASURE)
The first step in regression analysis is to determine the criterion variable. Pedhazur 15 suggests that the criterion have acceptable measurement qualities (ie, reliability and validity). Bradshaw et al 3 used VO 2 max as the criterion of choice for their model and measured it using a maximum graded exercise test (GXT) developed by George. 6 George 6 indicated that his protocol for testing compared favorably with the Bruce protocol in terms of predictive ability and had good test-retest reliability ( ICC = .98 –.99). The American College of Sports Medicine indicates that measurement of VO 2 max is the “gold standard” for measuring cardiorespiratory fitness. 1 These facts support that the criterion selected by Bradshaw et al 3 was appropriate and meets the requirements for acceptable reliability and validity.
SELECTING THE PREDICTORS: MODEL EFFICIENCY
Once the criterion has been selected, predictor variables should be identified (model selection). The aim of model selection is to minimize the number of predictors which account for the maximum variance in the criterion. 15 In other words, the most efficient model maximizes the value of the coefficient of determination ( R 2 ). This coefficient estimates the amount of variance in the criterion score accounted for by a linear combination of the predictor variables. The higher the value is for R 2 , the less error or unexplained variance and, therefore, the better prediction. R 2 is dependent on the multiple correlation coefficient ( R ), which describes the relationship between the observed and predicted criterion scores. If there is no difference between the predicted and observed scores, R equals 1.00. This represents a perfect prediction with no error and no unexplained variance ( R 2 = 1.00). When R equals 0.00, there is no relationship between the predictor(s) and the criterion and no variance in scores has been explained ( R 2 = 0.00). The chosen variables cannot predict the criterion. The goal of model selection is, as stated previously, to develop a model that results in the highest estimated value for R 2 .
According to Pedhazur, 15 the value of R is often overestimated. The reasons for this are beyond the scope of this discussion; however, the degree of overestimation is affected by sample size. The larger the ratio is between the number of predictors and subjects, the larger the overestimation. To account for this, sample sizes should be large and there should be 15 to 30 subjects per predictor. 11 , 15 Of course, the most effective way to determine optimal sample size is through statistical power analysis. 11 , 15
Another method of determining the best model for prediction is to test the significance of adding one or more variables to the model using the partial F-test . This process, which is further discussed by Kleinbaum, Kupper, and Muller, 12 allows for exclusion of predictors that do not contribute significantly to the prediction, allowing determination of the most efficient model of prediction. In general, the partial F-test is similar to the F-test used in analysis of variance. It assesses the statistical significance of the difference between values for R 2 derived from 2 or more prediction models using a subset of the variables from the original equation. For example, Bradshaw et al 3 indicated that all variables contributed significantly to their prediction. Though the researchers do not detail the procedure used, it is highly likely that different models were tested, excluding one or more variables, and the resulting values for R 2 assessed for statistical difference.
Although the techniques discussed above are useful in determining the most efficient model for prediction, theory must be considered in choosing the appropriate variables. Previous research should be examined and predictors selected for which a relationship between the criterion and predictors has been established. 12 , 15
It is clear that Bradshaw et al 3 relied on theory and previous research to determine the variables to use in their prediction equation. The 5 variables they chose for inclusion–gender, age, body mass index (BMI), perceived functional ability (PFA), and physical activity rating (PAR)–had been shown in previous studies to contribute to the prediction of VO 2 max (eg, Heil et al; 8 George, Stone, & Burkett 7 ). These 5 predictors accounted for 87% ( R = .93, R 2 = .87 ) of the variance in the predicted values for VO 2 max. Based on a ratio of 1:20 (predictor:sample size), this estimate of R , and thus R 2 , is not likely to be overestimated. The researchers used changes in the value of R 2 to determine whether to include or exclude these or other variables. They reported that removal of perceived functional ability (PFA) as a variable resulted in a decrease in R from .93 to .89. Without this variable, the remaining 4 predictors would account for only 79% of the variance in VO 2 max. The investigators did note that each predictor variable contributed significantly ( p < .05 ) to the prediction of VO 2 max (see above discussion related to the partial F-test).
ASSESSING ACCURACY OF THE PREDICTION
Assessing accuracy of the model is best accomplished by analyzing the standard error of estimate ( SEE ) and the percentage that the SEE represents of the predicted mean ( SEE % ). The SEE represents the degree to which the predicted scores vary from the observed scores on the criterion measure, similar to the standard deviation used in other statistical procedures. According to Jackson, 10 lower values of the SEE indicate greater accuracy in prediction. Comparison of the SEE for different models using the same sample allows for determination of the most accurate model to use for prediction. SEE % is calculated by dividing the SEE by the mean of the criterion ( SEE /mean criterion) and can be used to compare different models derived from different samples.
Bradshaw et al 3 report a SEE of 3.44 mL·kg −1 ·min −1 (approximately 1 MET) using all 5 variables in the equation (gender, age, BMI, PFA, PA-R). When the PFA variable is removed from the model, leaving only 4 variables for the prediction (gender, age, BMI, PA-R), the SEE increases to 4.20 mL·kg −1 ·min −1 . The increase in the error term indicates that the model excluding PFA is less accurate in predicting VO 2 max. This is confirmed by the decrease in the value for R (see discussion above). The researchers compare their model of prediction with that of George, Stone, and Burkett, 7 indicating that their model is as accurate. It is not advisable to compare models based on the SEE if the data were collected from different samples as they were in these 2 studies. That type of comparison should be made using SEE %. Bradshaw and colleagues 3 report SEE % for their model (8.62%), but do not report values from other models in making comparisons.
Some advocate the use of statistics derived from the predicted residual sum of squares ( PRESS ) as a means of selecting predictors. 2 , 4 , 16 These statistics are used more often in cross-validation of models and will be discussed in greater detail later.
ASSESSING STABILITY OF THE MODEL FOR PREDICTION
Once the most efficient and accurate model for prediction has been determined, it is prudent that the model be assessed for stability. A model, or equation, is said to be “stable” if it can be applied to different samples from the same population without losing the accuracy of the prediction. This is accomplished through cross-validation of the model. Cross-validation determines how well the prediction model developed using one sample performs in another sample from the same population. Several methods can be employed for cross-validation, including the use of 2 independent samples, split samples, and PRESS -related statistics developed from the same sample.
Using 2 independent samples involves random selection of 2 groups from the same population. One group becomes the “training” or “exploratory” group used for establishing the model of prediction. 5 The second group, the “confirmatory” or “validatory” group is used to assess the model for stability. The researcher compares R 2 values from the 2 groups and assessment of “shrinkage,” the difference between the two values for R 2 , is used as an indicator of model stability. There is no rule of thumb for interpreting the differences, but Kleinbaum, Kupper, and Muller 12 suggest that “shrinkage” values of less than 0.10 indicate a stable model. While preferable, the use of independent samples is rarely used due to cost considerations.
A similar technique of cross-validation uses split samples. Once the sample has been selected from the population, it is randomly divided into 2 subgroups. One subgroup becomes the “exploratory” group and the other is used as the “validatory” group. Again, values for R 2 are compared and model stability is assessed by calculating “shrinkage.”
Holiday, Ballard, and McKeown 9 advocate the use of PRESS-related statistics for cross-validation of regression models as a means of dealing with the problems of data-splitting. The PRESS method is a jackknife analysis that is used to address the issue of estimate bias associated with the use of small sample sizes. 13 In general, a jackknife analysis calculates the desired test statistic multiple times with individual cases omitted from the calculations. In the case of the PRESS method, residuals, or the differences between the actual values of the criterion for each individual and the predicted value using the formula derived with the individual's data removed from the prediction, are calculated. The PRESS statistic is the sum of the squares of the residuals derived from these calculations and is similar to the sum of squares for the error (SS error ) used in analysis of variance (ANOVA). Myers 14 discusses the use of the PRESS statistic and describes in detail how it is calculated. The reader is referred to this text and the article by Holiday, Ballard, and McKeown 9 for additional information.
Once determined, the PRESS statistic can be used to calculate a modified form of R 2 and the SEE . R 2 PRESS is calculated using the following formula: R 2 PRESS = 1 – [ PRESS / SS total ], where SS total equals the sum of squares for the original regression equation. 14 Standard error of the estimate for PRESS ( SEE PRESS ) is calculated as follows: SEE PRESS =, where n equals the number of individual cases. 14 The smaller the difference between the 2 values for R 2 and SEE , the more stable the model for prediction. Bradshaw et al 3 used this technique in their investigation. They reported a value for R 2 PRESS of .83, a decrease of .04 from R 2 for their prediction model. Using the standard set by Kleinbaum, Kupper, and Muller, 12 the model developed by these researchers would appear to have stability, meaning it could be used for prediction in samples from the same population. This is further supported by the small difference between the SEE and the SEE PRESS , 3.44 and 3.63 mL·kg −1 ·min −1 , respectively.
COMPARING TWO DIFFERENT PREDICTION MODELS
A comparison of 2 different models for prediction may help to clarify the use of regression analysis in prediction. Table Table1 1 presents data from 2 studies and will be used in the following discussion.
Comparison of Two Non-exercise Models for Predicting CRF
Variables | Heil et al = 374 | Bradshaw et al = 100 |
---|---|---|
Intercept | 36.580 | 48.073 |
Gender (male = 1, female = 0) | 3.706 | 6.178 |
Age (years) | 0.558 | −0.246 |
Age | −7.81 E-3 | |
Percent body fat | −0.541 | |
Body mass index (kg-m ) | −0.619 | |
Activity code (0-7) | 1.347 | |
Physical activity rating (0–10) | 0.671 | |
Perceived functional abilty | 0.712 | |
) | ||
.88 (.77) | .93 (.87) | |
4.90·mL–kg ·min | 3.44 mL·kg min | |
12.7% | 8.6% |
As noted above, the first step is to select an appropriate criterion, or outcome measure. Bradshaw et al 3 selected VO 2 max as their criterion for measuring cardiorespiratory fitness. Heil et al 8 used VO 2 peak. These 2 measures are often considered to be the same, however, VO 2 peak assumes that conditions for measuring maximum oxygen consumption were not met. 17 It would be optimal to compare models based on the same criterion, but that is not essential, especially since both criteria measure cardiorespiratory fitness in much the same way.
The second step involves selection of variables for prediction. As can be seen in Table Table1, 1 , both groups of investigators selected 5 variables to use in their model. The 5 variables selected by Bradshaw et al 3 provide a better prediction based on the values for R 2 (.87 and .77), indicating that their model accounts for more variance (87% versus 77%) in the prediction than the model of Heil et al. 8 It should also be noted that the SEE calculated in the Bradshaw 3 model (3.44 mL·kg −1 ·min −1 ) is less than that reported by Heil et al 8 (4.90 mL·kg −1 ·min −1 ). Remember, however, that comparison of the SEE should only be made when both models are developed using samples from the same population. Comparing predictions developed from different populations can be accomplished using the SEE% . Review of values for the SEE% in Table Table1 1 would seem to indicate that the model developed by Bradshaw et al 3 is more accurate because the percentage of the mean value for VO 2 max represented by error is less than that reported by Heil et al. 8 In summary, the Bradshaw 3 model would appear to be more efficient, accounting for more variance in the prediction using the same number of variables. It would also appear to be more accurate based on comparison of the SEE% .
The 2 models cannot be compared based on stability of the models. Each set of researchers used different methods for cross-validation. Both models, however, appear to be relatively stable based on the data presented. A clinician can assume that either model would perform fairly well when applied to samples from the same populations as those used by the investigators.
The purpose of this brief review has been to demystify regression analysis for prediction by explaining it in simple terms and to demonstrate its use. When reviewing research articles in which regression analysis has been used for prediction, physical therapists should ensure that the: (1) criterion chosen for the study is appropriate and meets the standards for reliability and validity, (2) processes used by the investigators to assess both model efficiency and accuracy are appropriate, 3) predictors selected for use in the model are reasonable based on theory or previous research, and 4) investigators assessed model stability through a process of cross-validation, providing the opportunity for others to utilize the prediction model in different samples drawn from the same population.
Log in using your username and password
- Search More Search for this keyword Advanced search
- Latest content
- Current issue
- Write for Us
- BMJ Journals
You are here
- Volume 24, Issue 4
- Understanding and interpreting regression analysis
- Article Text
- Article info
- Citation Tools
- Rapid Responses
- Article metrics

- http://orcid.org/0000-0002-7839-8130 Parveen Ali 1 , 2 ,
- http://orcid.org/0000-0003-0157-5319 Ahtisham Younas 3 , 4
- 1 School of Nursing and Midwifery , University of Sheffield , Sheffield , South Yorkshire , UK
- 2 Sheffiled University Interpersonal Violence Research Group , The University of Sheffiled SEAS , Sheffield , UK
- 3 Faculty of Nursing , Memorial University of Newfoundland , St. John's , Newfoundland and Labrador , Canada
- 4 Swat College of Nursing , Mingora, Swat , Pakistan
- Correspondence to Ahtisham Younas, Memorial University of Newfoundland, St. John's, NL A1C 5S7, Canada; ay6133{at}mun.ca
https://doi.org/10.1136/ebnurs-2021-103425
Statistics from Altmetric.com
Request permissions.
If you wish to reuse any or all of this article please use the link below which will take you to the Copyright Clearance Center’s RightsLink service. You will be able to get a quick price and instant permission to reuse the content in many different ways.
- statistics & research methods
Introduction
A nurse educator is interested in finding out the academic and non-academic predictors of success in nursing students. Given the complexity of educational and clinical learning environments, demographic, clinical and academic factors (age, gender, previous educational training, personal stressors, learning demands, motivation, assignment workload, etc) influencing nursing students’ success, she was able to list various potential factors contributing towards success relatively easily. Nevertheless, not all of the identified factors will be plausible predictors of increased success. Therefore, she could use a powerful statistical procedure called regression analysis to identify whether the likelihood of increased success is influenced by factors such as age, stressors, learning demands, motivation and education.
What is regression?
Purposes of regression analysis.
Regression analysis has four primary purposes: description, estimation, prediction and control. 1 , 2 By description, regression can explain the relationship between dependent and independent variables. Estimation means that by using the observed values of independent variables, the value of dependent variable can be estimated. 2 Regression analysis can be useful for predicting the outcomes and changes in dependent variables based on the relationships of dependent and independent variables. Finally, regression enables in controlling the effect of one or more independent variables while investigating the relationship of one independent variable with the dependent variable. 1
Types of regression analyses
There are commonly three types of regression analyses, namely, linear, logistic and multiple regression. The differences among these types are outlined in table 1 in terms of their purpose, nature of dependent and independent variables, underlying assumptions, and nature of curve. 1 , 3 However, more detailed discussion for linear regression is presented as follows.
- View inline
Comparison of linear, logistic and multiple regression
Linear regression and interpretation
Linear regression analysis involves examining the relationship between one independent and dependent variable. Statistically, the relationship between one independent variable (x) and a dependent variable (y) is expressed as: y= β 0 + β 1 x+ε. In this equation, β 0 is the y intercept and refers to the estimated value of y when x is equal to 0. The coefficient β 1 is the regression coefficient and denotes that the estimated increase in the dependent variable for every unit increase in the independent variable. The symbol ε is a random error component and signifies imprecision of regression indicating that, in actual practice, the independent variables are cannot perfectly predict the change in any dependent variable. 1 Multiple linear regression follows the same logic as univariate linear regression except (a) multiple regression, there are more than one independent variable and (b) there should be non-collinearity among the independent variables.
Factors affecting regression
Linear and multiple regression analyses are affected by factors, namely, sample size, missing data and the nature of sample. 2
Small sample size may only demonstrate connections among variables with strong relationship. Therefore, sample size must be chosen based on the number of independent variables and expect strength of relationship.
Many missing values in the data set may affect the sample size. Therefore, all the missing values should be adequately dealt with before conducting regression analyses.
The subsamples within the larger sample may mask the actual effect of independent and dependent variables. Therefore, if subsamples are predefined, a regression within the sample could be used to detect true relationships. Otherwise, the analysis should be undertaken on the whole sample.
Building on her research interest mentioned in the beginning, let us consider a study by Ali and Naylor. 4 They were interested in identifying the academic and non-academic factors which predict the academic success of nursing diploma students. This purpose is consistent with one of the above-mentioned purposes of regression analysis (ie, prediction). Ali and Naylor’s chosen academic independent variables were preadmission qualification, previous academic performance and school type and the non-academic variables were age, gender, marital status and time gap. To achieve their purpose, they collected data from 628 nursing students between the age range of 15–34 years. They used both linear and multiple regression analyses to identify the predictors of student success. For analysis, they examined the relationship of academic and non-academic variables across different years of study and noted that academic factors accounted for 36.6%, 44.3% and 50.4% variability in academic success of students in year 1, year 2 and year 3, respectively. 4
Ali and Naylor presented the relationship among these variables using scatter plots, which are commonly used graphs for data display in regression analysis—see examples of various scatter plots in figure 1 . 4 In a scatter plot, the clustering of the dots denoted the strength of relationship, whereas the direction indicates the nature of relationships among variables as positive (ie, increase in one variable results in an increase in the other) and negative (ie, increase in one variable results in decrease in the other).
- Download figure
- Open in new tab
- Download powerpoint
An Example of Scatter Plot for Regression.
Table 2 presents the results of regression analysis for academic and non-academic variables for year 4 students’ success. The significant predictors of student success are denoted with a significant p value. For every, significant predictor, the beta value indicates the percentage increase in students’ academic success with one unit increase in the variable.
Regression model for the final year students (N=343)
Conclusions
Regression analysis is a powerful and useful statistical procedure with many implications for nursing research. It enables researchers to describe, predict and estimate the relationships and draw plausible conclusions about the interrelated variables in relation to any studied phenomena. Regression also allows for controlling one or more variables when researchers are interested in examining the relationship among specific variables. Some of the key considerations are presented that may be useful for researchers undertaking regression analysis. While planning and conducting regression analysis, researchers should consider the type and number of dependent and independent variables as well as the nature and size of sample. Choosing a wrong type of regression analysis with small sample may result in erroneous conclusions about the studied phenomenon.
Ethics statements
Patient consent for publication.
Not required.
- Montgomery DC ,
- Schneider A ,
Twitter @parveenazamali, @@Ahtisham04
Funding The authors have not declared a specific grant for this research from any funding agency in the public, commercial or not-for-profit sectors.
Competing interests None declared.
Provenance and peer review Commissioned; internally peer reviewed.
Read the full text or download the PDF:
- Business Essentials
- Leadership & Management
- Credential of Leadership, Impact, and Management in Business (CLIMB)
- Entrepreneurship & Innovation
- Digital Transformation
- Finance & Accounting
- Business in Society
- For Organizations
- Support Portal
- Media Coverage
- Founding Donors
- Leadership Team
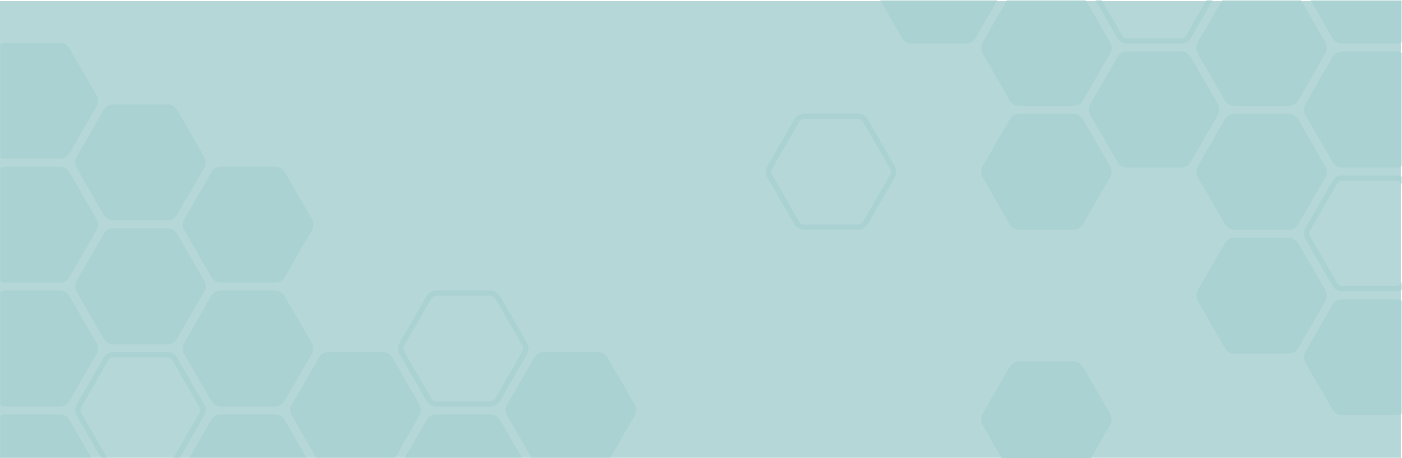
- Harvard Business School →
- HBS Online →
- Business Insights →
Business Insights
Harvard Business School Online's Business Insights Blog provides the career insights you need to achieve your goals and gain confidence in your business skills.
- Career Development
- Communication
- Decision-Making
- Earning Your MBA
- Negotiation
- News & Events
- Productivity
- Staff Spotlight
- Student Profiles
- Work-Life Balance
- AI Essentials for Business
- Alternative Investments
- Business Analytics
- Business Strategy
- Business and Climate Change
- Design Thinking and Innovation
- Digital Marketing Strategy
- Disruptive Strategy
- Economics for Managers
- Entrepreneurship Essentials
- Financial Accounting
- Global Business
- Launching Tech Ventures
- Leadership Principles
- Leadership, Ethics, and Corporate Accountability
- Leading Change and Organizational Renewal
- Leading with Finance
- Management Essentials
- Negotiation Mastery
- Organizational Leadership
- Power and Influence for Positive Impact
- Strategy Execution
- Sustainable Business Strategy
- Sustainable Investing
- Winning with Digital Platforms
What Is Regression Analysis in Business Analytics?
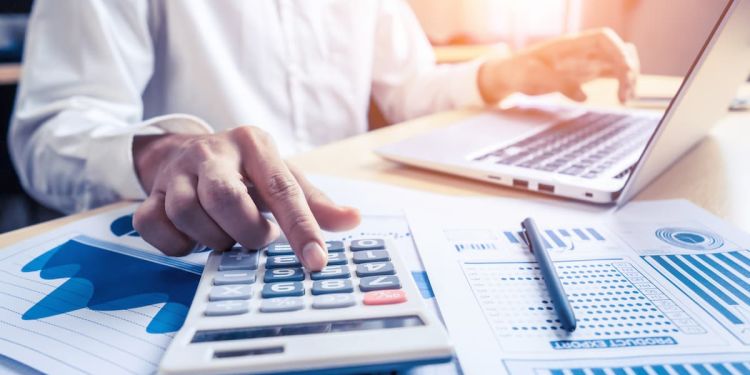
- 14 Dec 2021
Countless factors impact every facet of business. How can you consider those factors and know their true impact?
Imagine you seek to understand the factors that influence people’s decision to buy your company’s product. They range from customers’ physical locations to satisfaction levels among sales representatives to your competitors' Black Friday sales.
Understanding the relationships between each factor and product sales can enable you to pinpoint areas for improvement, helping you drive more sales.
To learn how each factor influences sales, you need to use a statistical analysis method called regression analysis .
If you aren’t a business or data analyst, you may not run regressions yourself, but knowing how analysis works can provide important insight into which factors impact product sales and, thus, which are worth improving.
Access your free e-book today.
Foundational Concepts for Regression Analysis
Before diving into regression analysis, you need to build foundational knowledge of statistical concepts and relationships.
Independent and Dependent Variables
Start with the basics. What relationship are you aiming to explore? Try formatting your answer like this: “I want to understand the impact of [the independent variable] on [the dependent variable].”
The independent variable is the factor that could impact the dependent variable . For example, “I want to understand the impact of employee satisfaction on product sales.”
In this case, employee satisfaction is the independent variable, and product sales is the dependent variable. Identifying the dependent and independent variables is the first step toward regression analysis.
Correlation vs. Causation
One of the cardinal rules of statistically exploring relationships is to never assume correlation implies causation. In other words, just because two variables move in the same direction doesn’t mean one caused the other to occur.
If two or more variables are correlated , their directional movements are related. If two variables are positively correlated , it means that as one goes up or down, so does the other. Alternatively, if two variables are negatively correlated , one goes up while the other goes down.
A correlation’s strength can be quantified by calculating the correlation coefficient , sometimes represented by r . The correlation coefficient falls between negative one and positive one.
r = -1 indicates a perfect negative correlation.
r = 1 indicates a perfect positive correlation.
r = 0 indicates no correlation.
Causation means that one variable caused the other to occur. Proving a causal relationship between variables requires a true experiment with a control group (which doesn’t receive the independent variable) and an experimental group (which receives the independent variable).
While regression analysis provides insights into relationships between variables, it doesn’t prove causation. It can be tempting to assume that one variable caused the other—especially if you want it to be true—which is why you need to keep this in mind any time you run regressions or analyze relationships between variables.
With the basics under your belt, here’s a deeper explanation of regression analysis so you can leverage it to drive strategic planning and decision-making.
Related: How to Learn Business Analytics without a Business Background
What Is Regression Analysis?
Regression analysis is the statistical method used to determine the structure of a relationship between two variables (single linear regression) or three or more variables (multiple regression).
According to the Harvard Business School Online course Business Analytics , regression is used for two primary purposes:
- To study the magnitude and structure of the relationship between variables
- To forecast a variable based on its relationship with another variable
Both of these insights can inform strategic business decisions.
“Regression allows us to gain insights into the structure of that relationship and provides measures of how well the data fit that relationship,” says HBS Professor Jan Hammond, who teaches Business Analytics, one of three courses that comprise the Credential of Readiness (CORe) program . “Such insights can prove extremely valuable for analyzing historical trends and developing forecasts.”
One way to think of regression is by visualizing a scatter plot of your data with the independent variable on the X-axis and the dependent variable on the Y-axis. The regression line is the line that best fits the scatter plot data. The regression equation represents the line’s slope and the relationship between the two variables, along with an estimation of error.
Physically creating this scatter plot can be a natural starting point for parsing out the relationships between variables.

Types of Regression Analysis
There are two types of regression analysis: single variable linear regression and multiple regression.
Single variable linear regression is used to determine the relationship between two variables: the independent and dependent. The equation for a single variable linear regression looks like this:

In the equation:
- ŷ is the expected value of Y (the dependent variable) for a given value of X (the independent variable).
- x is the independent variable.
- α is the Y-intercept, the point at which the regression line intersects with the vertical axis.
- β is the slope of the regression line, or the average change in the dependent variable as the independent variable increases by one.
- ε is the error term, equal to Y – ŷ, or the difference between the actual value of the dependent variable and its expected value.
Multiple regression , on the other hand, is used to determine the relationship between three or more variables: the dependent variable and at least two independent variables. The multiple regression equation looks complex but is similar to the single variable linear regression equation:

Each component of this equation represents the same thing as in the previous equation, with the addition of the subscript k, which is the total number of independent variables being examined. For each independent variable you include in the regression, multiply the slope of the regression line by the value of the independent variable, and add it to the rest of the equation.
How to Run Regressions
You can use a host of statistical programs—such as Microsoft Excel, SPSS, and STATA—to run both single variable linear and multiple regressions. If you’re interested in hands-on practice with this skill, Business Analytics teaches learners how to create scatter plots and run regressions in Microsoft Excel, as well as make sense of the output and use it to drive business decisions.
Calculating Confidence and Accounting for Error
It’s important to note: This overview of regression analysis is introductory and doesn’t delve into calculations of confidence level, significance, variance, and error. When working in a statistical program, these calculations may be provided or require that you implement a function. When conducting regression analysis, these metrics are important for gauging how significant your results are and how much importance to place on them.

Why Use Regression Analysis?
Once you’ve generated a regression equation for a set of variables, you effectively have a roadmap for the relationship between your independent and dependent variables. If you input a specific X value into the equation, you can see the expected Y value.
This can be critical for predicting the outcome of potential changes, allowing you to ask, “What would happen if this factor changed by a specific amount?”
Returning to the earlier example, running a regression analysis could allow you to find the equation representing the relationship between employee satisfaction and product sales. You could input a higher level of employee satisfaction and see how sales might change accordingly. This information could lead to improved working conditions for employees, backed by data that shows the tie between high employee satisfaction and sales.
Whether predicting future outcomes, determining areas for improvement, or identifying relationships between seemingly unconnected variables, understanding regression analysis can enable you to craft data-driven strategies and determine the best course of action with all factors in mind.
Do you want to become a data-driven professional? Explore our eight-week Business Analytics course and our three-course Credential of Readiness (CORe) program to deepen your analytical skills and apply them to real-world business problems.

About the Author
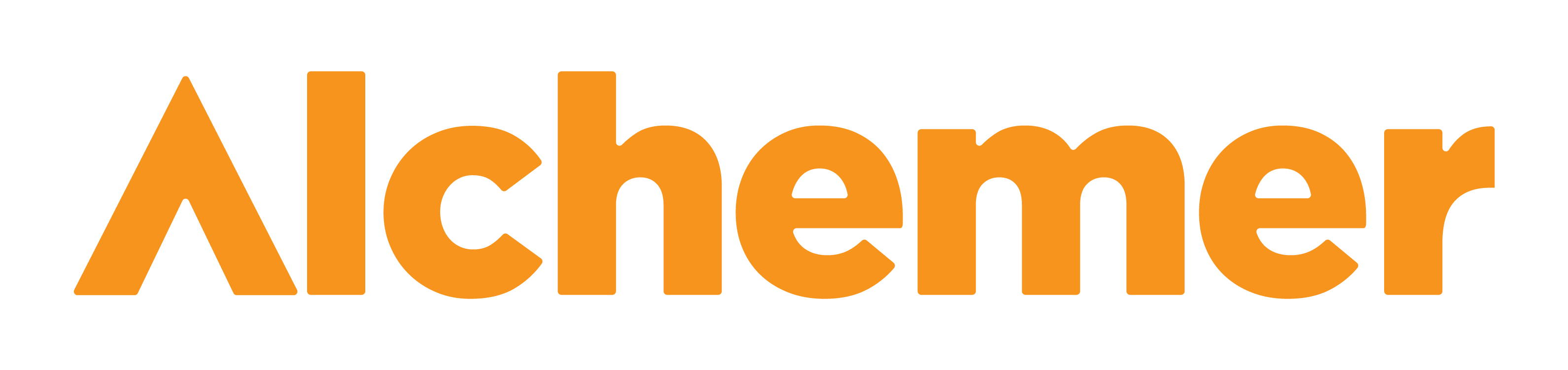
What is Regression Analysis and Why Should I Use It?
- Survey Tips
Alchemer is an incredibly robust online survey software platform. It’s continually voted one of the best survey tools available on G2, FinancesOnline, and others. To make it even easier, we’ve created a series of blogs to help you better understand how to get the most from your Alchemer account.
Regression analysis is a powerful statistical method that allows you to examine the relationship between two or more variables of interest.
While there are many types of regression analysis, at their core they all examine the influence of one or more independent variables on a dependent variable.
Regression analysis provides detailed insight that can be applied to further improve products and services.
Here at Alchemer, we offer hands-on application training events during which customers learn how to become super users of our software.
In order to understand the value being delivered at these training events, we distribute follow-up surveys to attendees with the goals of learning what they enjoyed, what they didn’t, and what we can improve on for future sessions.
The data collected from these feedback surveys allows us to measure the levels of satisfaction that our attendees associate with our events, and what variables influence those levels of satisfaction.
Could it be the topics covered in the individual sessions of the event? The length of the sessions? The food or catering services provided? The cost to attend? Any of these variables have the potential to impact an attendee’s level of satisfaction.
By performing a regression analysis on this survey data, we can determine whether or not these variables have impacted overall attendee satisfaction, and if so, to what extent.
This information then informs us about which elements of the sessions are being well received, and where we need to focus attention so that attendees are more satisfied in the future.
What is regression analysis and what does it mean to perform a regression?
Regression analysis is a reliable method of identifying which variables have impact on a topic of interest. The process of performing a regression allows you to confidently determine which factors matter most, which factors can be ignored, and how these factors influence each other.
In order to understand regression analysis fully, it’s essential to comprehend the following terms:
- Dependent Variable: This is the main factor that you’re trying to understand or predict.
- Independent Variables: These are the factors that you hypothesize have an impact on your dependent variable.
In our application training example above, attendees’ satisfaction with the event is our dependent variable. The topics covered, length of sessions, food provided, and the cost of a ticket are our independent variables.
How does regression analysis work?
In order to conduct a regression analysis, you’ll need to define a dependent variable that you hypothesize is being influenced by one or several independent variables.
You’ll then need to establish a comprehensive dataset to work with. Administering surveys to your audiences of interest is a terrific way to establish this dataset. Your survey should include questions addressing all of the independent variables that you are interested in.
Let’s continue using our application training example. In this case, we’d want to measure the historical levels of satisfaction with the events from the past three years or so (or however long you deem statistically significant), as well as any information possible in regards to the independent variables.
Perhaps we’re particularly curious about how the price of a ticket to the event has impacted levels of satisfaction.
To begin investigating whether or not there is a relationship between these two variables, we would begin by plotting these data points on a chart, which would look like the following theoretical example.
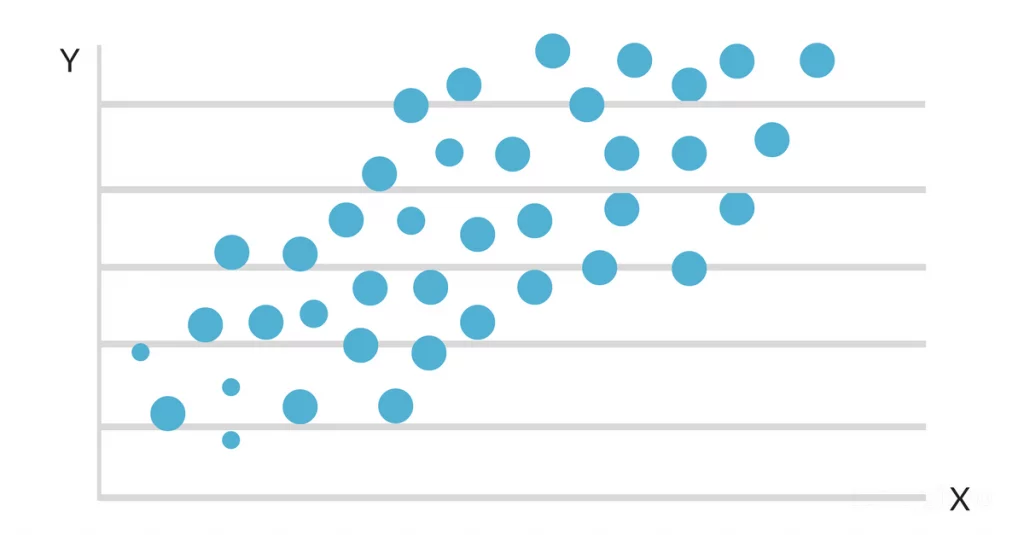
(Plotting your data is the first step in figuring out if there is a relationship between your independent and dependent variables)
Our dependent variable (in this case, the level of event satisfaction) should be plotted on the y-axis, while our independent variable (the price of the event ticket) should be plotted on the x-axis.
Once your data is plotted, you may begin to see correlations. If the theoretical chart above did indeed represent the impact of ticket prices on event satisfaction, then we’d be able to confidently say that the higher the ticket price, the higher the levels of event satisfaction.
But how can we tell the degree to which ticket price affects event satisfaction?
To begin answering this question, draw a line through the middle of all of the data points on the chart. This line is referred to as your regression line, and it can be precisely calculated using a standard statistics program like Excel.
We’ll use a theoretical chart once more to depict what a regression line should look like.
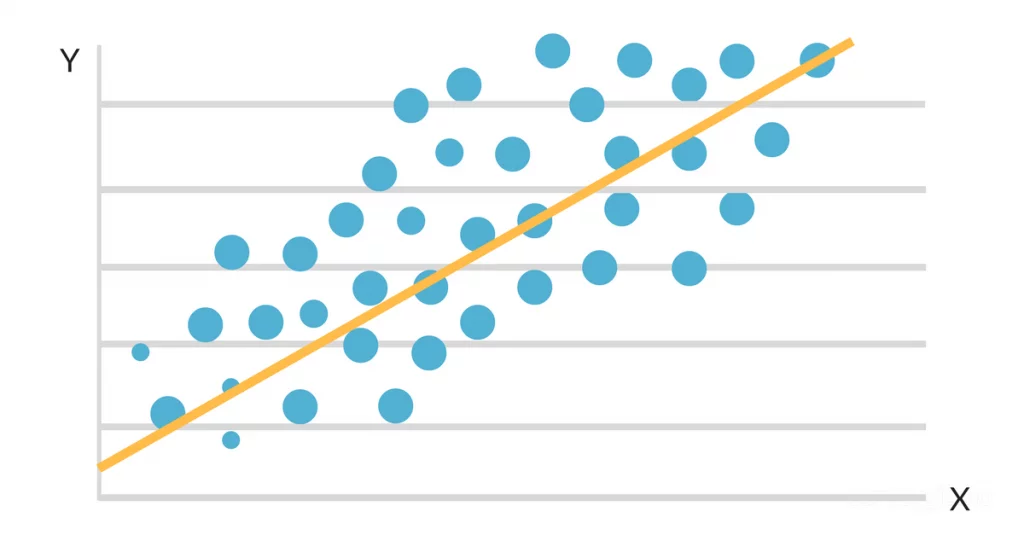
The regression line represents the relationship between your independent variable and your dependent variable.
Excel will even provide a formula for the slope of the line, which adds further context to the relationship between your independent and dependent variables.
The formula for a regression line might look something like Y = 100 + 7X + error term .
This tells you that if there is no “X”, then Y = 100. If X is our increase in ticket price, this informs us that if there is no increase in ticket price, event satisfaction will still increase by 100 points.
You’ll notice that the slope formula calculated by Excel includes an error term. Regression lines always consider an error term because in reality, independent variables are never precisely perfect predictors of dependent variables. This makes sense while looking at the impact of ticket prices on event satisfaction — there are clearly other variables that are contributing to event satisfaction outside of price.
Your regression line is simply an estimate based on the data available to you. So, the larger your error term, the less definitively certain your regression line is.
Why should your organization use regression analysis?
Regression analysis is helpful statistical method that can be leveraged across an organization to determine the degree to which particular independent variables are influencing dependent variables.
The possible scenarios for conducting regression analysis to yield valuable, actionable business insights are endless.
The next time someone in your business is proposing a hypothesis that states that one factor, whether you can control that factor or not, is impacting a portion of the business, suggest performing a regression analysis to determine just how confident you should be in that hypothesis! This will allow you to make more informed business decisions, allocate resources more efficiently, and ultimately boost your bottom line.
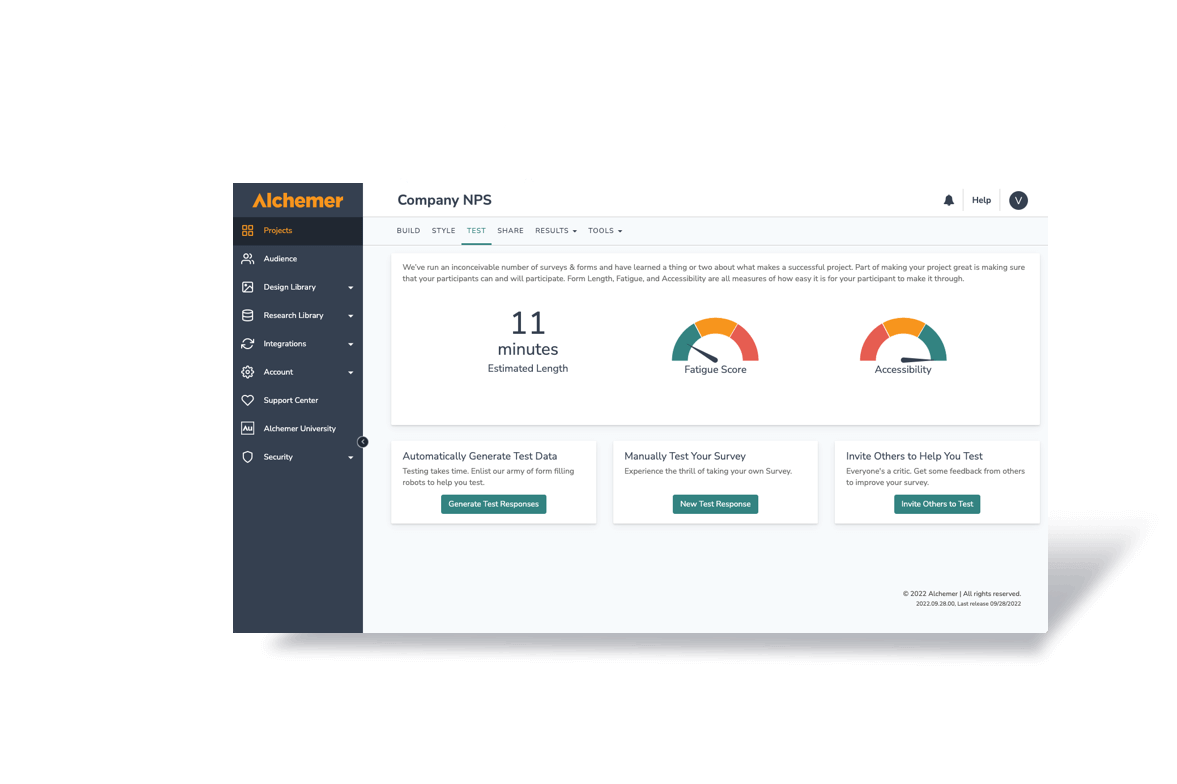
See all blog posts >
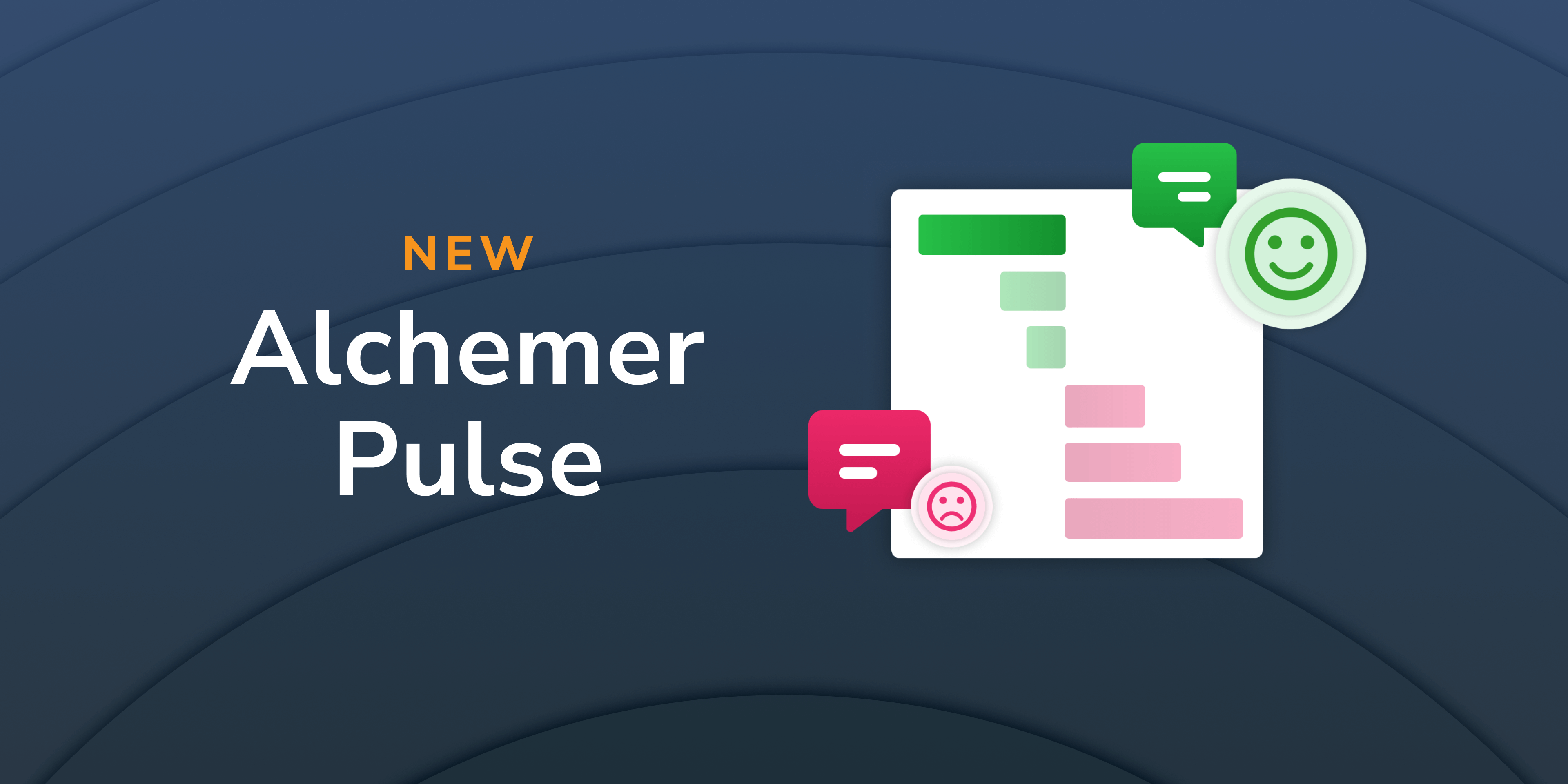
- AI , Alchemer Pulse , Customer Experience , Customer Feedback
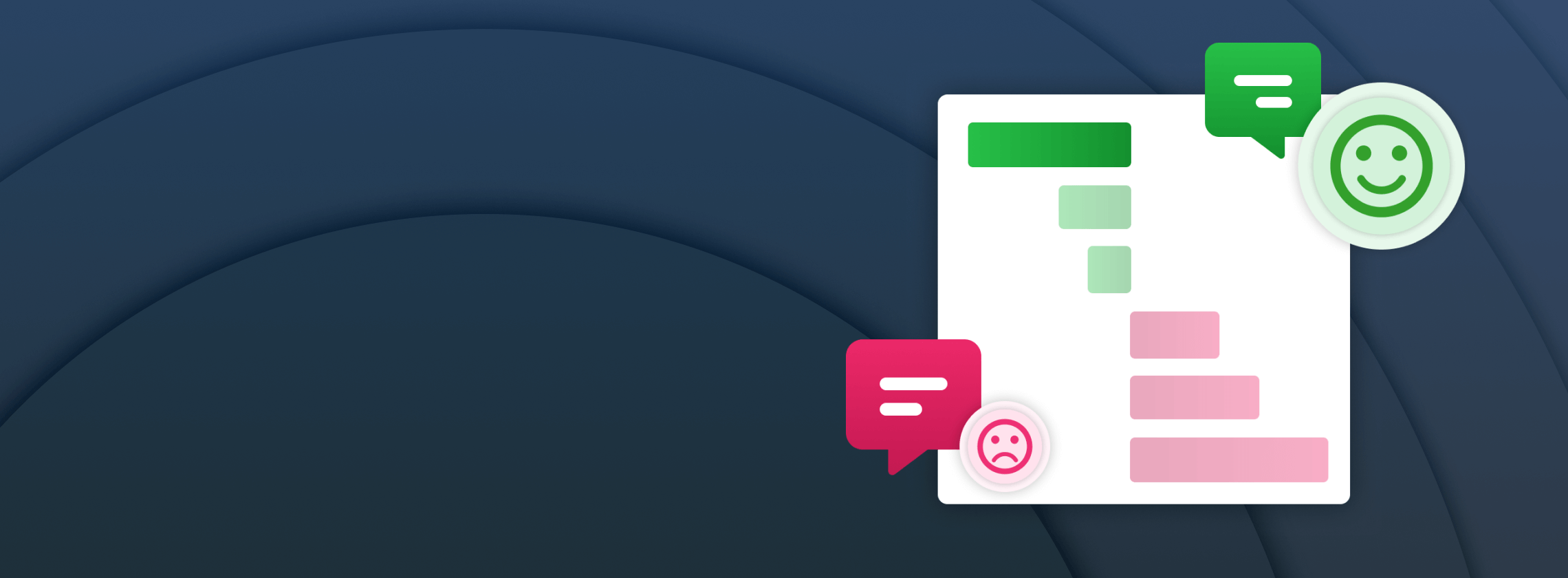
- AI , Alchemer Pulse , Press Release , Product News
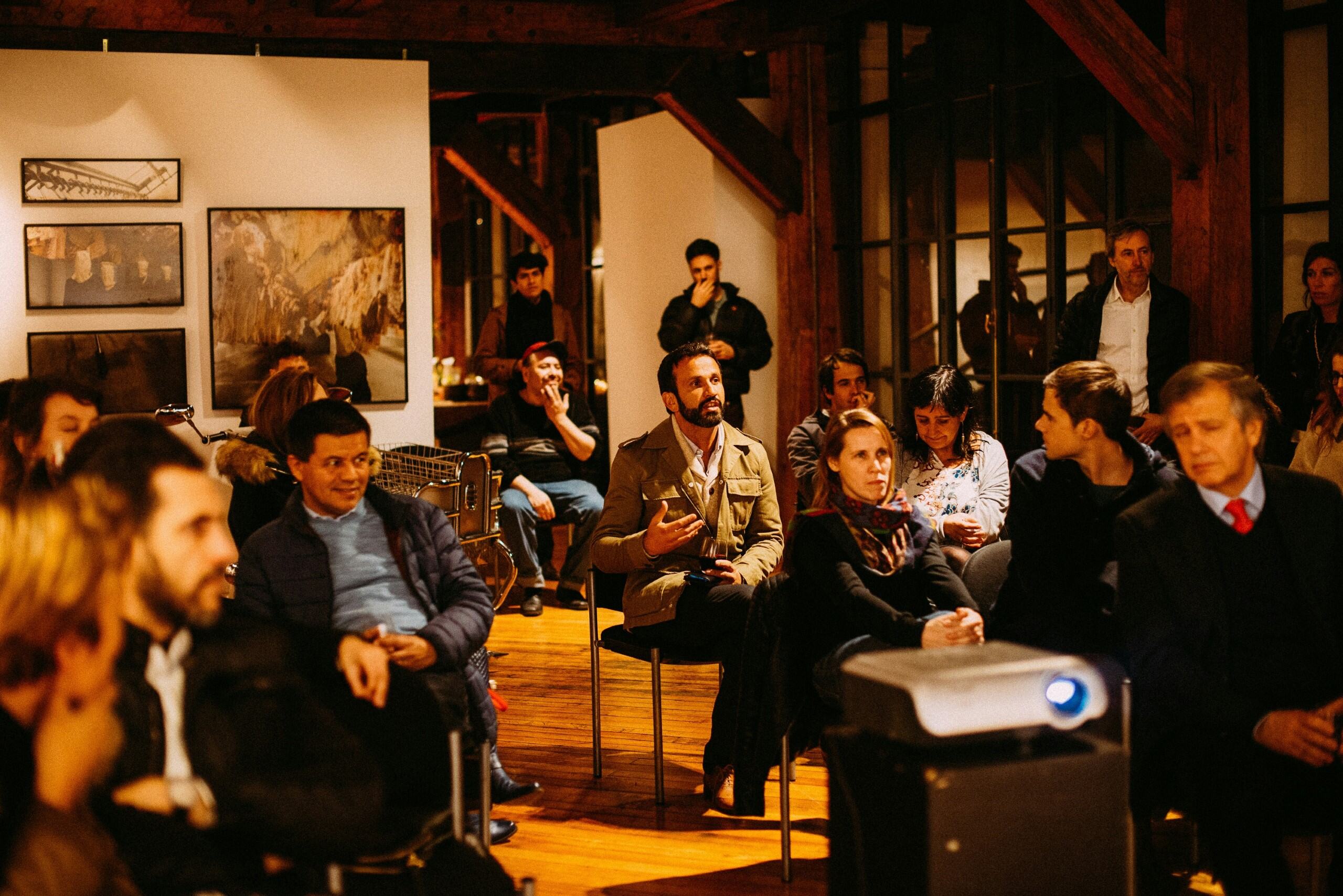
- Alchemer Survey , Citizen Feedback , Feedback Collection
See it in Action

- Privacy Overview
- Strictly Necessary Cookies
- 3rd Party Cookies
This website uses cookies so that we can provide you with the best user experience possible. Cookie information is stored in your browser and performs functions such as recognising you when you return to our website and helping our team to understand which sections of the website you find most interesting and useful.
Strictly Necessary Cookie should be enabled at all times so that we can save your preferences for cookie settings.
If you disable this cookie, we will not be able to save your preferences. This means that every time you visit this website you will need to enable or disable cookies again.
This website uses Google Analytics to collect anonymous information such as the number of visitors to the site, and the most popular pages.
Keeping this cookie enabled helps us to improve our website.
Please enable Strictly Necessary Cookies first so that we can save your preferences!

- SUGGESTED TOPICS
- The Magazine
- Newsletters
- Managing Yourself
- Managing Teams
- Work-life Balance
- The Big Idea
- Data & Visuals
- Reading Lists
- Case Selections
- HBR Learning
- Topic Feeds
- Account Settings
- Email Preferences
Research: Using AI at Work Makes Us Lonelier and Less Healthy
- David De Cremer
- Joel Koopman

Employees who use AI as a core part of their jobs report feeling more isolated, drinking more, and sleeping less than employees who don’t.
The promise of AI is alluring — optimized productivity, lightning-fast data analysis, and freedom from mundane tasks — and both companies and workers alike are fascinated (and more than a little dumbfounded) by how these tools allow them to do more and better work faster than ever before. Yet in fervor to keep pace with competitors and reap the efficiency gains associated with deploying AI, many organizations have lost sight of their most important asset: the humans whose jobs are being fragmented into tasks that are increasingly becoming automated. Across four studies, employees who use it as a core part of their jobs reported feeling lonelier, drinking more, and suffering from insomnia more than employees who don’t.
Imagine this: Jia, a marketing analyst, arrives at work, logs into her computer, and is greeted by an AI assistant that has already sorted through her emails, prioritized her tasks for the day, and generated first drafts of reports that used to take hours to write. Jia (like everyone who has spent time working with these tools) marvels at how much time she can save by using AI. Inspired by the efficiency-enhancing effects of AI, Jia feels that she can be so much more productive than before. As a result, she gets focused on completing as many tasks as possible in conjunction with her AI assistant.
- David De Cremer is a professor of management and technology at Northeastern University and the Dunton Family Dean of its D’Amore-McKim School of Business. His website is daviddecremer.com .
- JK Joel Koopman is the TJ Barlow Professor of Business Administration at the Mays Business School of Texas A&M University. His research interests include prosocial behavior, organizational justice, motivational processes, and research methodology. He has won multiple awards from Academy of Management’s HR Division (Early Career Achievement Award and David P. Lepak Service Award) along with the 2022 SIOP Distinguished Early Career Contributions award, and currently serves on the Leadership Committee for the HR Division of the Academy of Management .
Partner Center
A Modified Regression Model for Analysing the Performance of Metamaterial Antenna Using Machine Learning and Deep Learning
- Published: 02 July 2024
- Volume 136 , pages 1769–1789, ( 2024 )
Cite this article
- Rovin Tiwari 1 ,
- Raghavendra Sharma 1 &
- Rahul Dubey 2
Explore all metrics
Metamaterial (MM) is an artificial constituent, which as a distinct properties i.e., negative permittivity and refractive-index, which doesn’t subsists naturally in the environment. MM is widely used in the antenna application owing to their abundant advantages. Split Ring-Resonator configuration in MM antenna can enhance the performance of antenna. Therefore, the present study aims to develop an improved regression model, which evaluates the performance of MM antennas effectively. In that context, initially, the study performs, pre-processing by removing the unnecessary data and missing values. Then, the feature extraction is employed with Bi-LSTM, which extracts the efficient features. Followed by this, the training and testing split is performed as 80% training data and 20% of testing data. The modified regression model is constructed with empirical loss function and XG-Boost algorithms. By implementing the proposed model, the prediction phase is enhanced efficiently. The experimental evaluation of the proposed model is accomplished with Metamaterial antenna dataset (MM antenna dataset), which is openly available and the analysis is done in terms of error rate and accuracy. The proposed system attains 99.23% accuracy rate.
This is a preview of subscription content, log in via an institution to check access.
Access this article
Subscribe and save.
- Get 10 units per month
- Download Article/Chapter or Ebook
- 1 Unit = 1 Article or 1 Chapter
- Cancel anytime
Price includes VAT (Russian Federation)
Instant access to the full article PDF.
Rent this article via DeepDyve
Institutional subscriptions
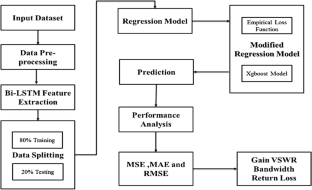
Similar content being viewed by others
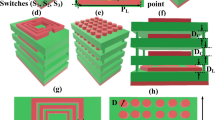
Machine learning assisted metamaterial-based reconfigurable antenna for low-cost portable electronic devices
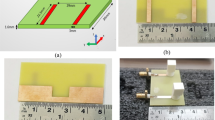
Design and Optimization of MIMO Dielectric Resonator Antenna Using Machine Learning for Sub-6 GHz based on 5G IoT Applications
Investigation and Optimization of Dielectric Resonator MIMO Antenna Using Machine Learning Approach
Data availability.
I checked data availability statement is correct.
Chettri, L., & Bera, R. (2019). A comprehensive survey on internet of things (IoT) toward 5G wireless systems. IEEE Internet of Things Journal, 7 , 16–32.
Article Google Scholar
Anab, M., Khattak, M. I., Owais, S. M., Khattak, A. A., & Sultan, A. (2020). Design and analysis of millimeter wave dielectric resonator antenna for 5G wireless communication systems. Progress In Electromagnetics Research C, 98 , 239–255.
Babu, K. V., Das, S., Sree, G. N. J., Patel, S. K., Saradhi, M. P., & Tagore, M. (2022). Design and development of miniaturized MIMO antenna using parasitic elements and machine learning (ML) technique for lower sub 6 GHz 5G applications. AEU-International Journal of Electronics and Communications, 153 , 154281.
Google Scholar
Ozpinar, H., Aksimsek, S., & Tokan, N. T. (2020). A novel compact, broadband, high gain millimeter-wave antenna for 5G beam steering applications. IEEE Transactions on Vehicular Technology, 69 , 2389–2397.
Ullah, H., & Tahir, F. A. (2020). A high gain and wideband narrow-beam antenna for 5G millimeter-wave applications. IEEE Access, 8 , 29430–29434.
Pan, M., Huang, H., Fan, B., Chen, W., Li, S., Xie, Q., et al. (2021). Theoretical design of a triple-band perfect metamaterial absorber based on graphene with wide-angle insensitivity. Results in Physics, 23 , 104037.
Patil, K. S., & Rufus, E. (2022). Design of bio-implantable antenna using metamaterial substrate. Wireless Personal Communications, 124 , 1443–1455.
Luo, S., Li, Y., Xia, Y., Yang, G., Sun, L., & Zhao, L. (2019). Mutual coupling reduction of a dual-band antenna array using dual-frequency metamaterial structure. The Applied Computational Electromagnetics Society Journal, 34 , 403–410.
Yilmaz, V. S., Bilgin, G., Aydin, E., & Kara, A. (2019). Miniaturised antenna at a sub-GHZ band for industrial remote controllers. IET Microwaves, Antennas & Propagation, 13 , 1408–1413.
Zhou, D., Wang, H., Deng, L., Qiu, L. L., & Huang, S. (2022). Metamaterial-based frequency reconfigurable microstrip antenna for wideband and improved gain performance. International Journal of RF and Microwave Computer-Aided Engineering, 32 , e22988.
T. Pavani, A. Naga Jyothi, A. Ushasree, Y. Rajasree Rao, and U. Kumari. (2021)."Design of Metamaterial Loaded Dipole Antenna for GPR", in Microelectronics, Electromagnetics and Telecommunications (pp. 71-77). Springer
Sharma, A., Singh, H., & Gupta, A. (2022). A review analysis of metamaterial-based absorbers and their applications. Journal of Superconductivity and Novel Magnetism, 35 , 1–17.
Hwang, S., Lee, B., Kim, D. H., & Park, J. Y. (2018). Design of S-band phased array antenna with high isolation using broadside coupled split ring resonator. Journal of electromagnetic engineering and science, 18 , 108–116.
Mathur, S., & Badone, A. (2019). A methodological study and analysis of machine learning algorithms. International Journal of Advanced Technology and Engineering Exploration, 6 , 45–49.
Albon, C. (2018). Machine learning with python cookbook: Practical solutions from preprocessing to deep learning . O’Reilly Media Inc.
El Sayed, M., Ibrahim, A., Mirjalili, S., Zhang, Y. D., Elnazer, S., & Zaki, R. M. (2022). Optimized ensemble algorithm for predicting metamaterial antenna parameters. Computers, Materials and Continua, 71 , 4989–5003.
Abdelhamid, A. A., & Alotaibi, S. R. (2022). Robust prediction of the bandwidth of metamaterial antenna using deep learning. CMC-Computers Materials & Continua, 72 , 2305–2321.
Manh, L. H., Grimaccia, F., Mussetta, M., & Zich, R. E. (2014). Optimization of a dual ring antenna by means of artificial neural network. Progress In Electromagnetics Research B, 58 , 59–69.
Xiao, L.-Y., Shao, W., Jin, F.-L., & Wang, B.-Z. (2018). Multiparameter modeling with ANN for antenna design. IEEE Transactions on Antennas and Propagation, 66 , 3718–3723.
Kurniawati, N., Arif, F., & Alam, S. (2021). Predicting rectangular patch microstrip antenna dimension using machine learning. The Journal of Communication, 16 , 394–399.
Liu, F., Zhang, W., Sun, Y., Liu, J., Miao, J., He, F., et al. (2020). Secure deep learning for intelligent terahertz metamaterial identification. Sensors, 20 , 5673.
Mukherjee, P., Mukherjee, A., & Chatterjee, K. (2022). Artificial neural network based dimension prediction of rectangular microstrip antenna. Journal of The Institution of Engineers, 103 (4), 1–7.
Sharma, K., & Pandey, G. P. (2020). Designing a Compact Microstrip Antenna Using the Machine Learning Approach. Journal of Telecommunications and Information Technology, 4 , 44–52.
SuriyaPrakashJambunatham, H. R. D., & Kumar, D. S. (2021). A machine learning-based approach for antenna design using class_reg algorithm optimized using genetic algorithm. International Journal for Research in Applied Science & Engineering Technology, 9 , 1682–1686.
Jin, J., Zhang, C., Feng, F., Na, W., Ma, J., & Zhang, Q.-J. (2019). Deep neural network technique for high-dimensional microwave modeling and applications to parameter extraction of microwave filters. IEEE Transactions on Microwave Theory and Techniques, 67 , 4140–4155.
El Misilmani, H. M., Naous, T., & Al Khatib, S. K. (2020). A review on the design and optimization of antennas using machine learning algorithms and techniques. International Journal of RF and Microwave Computer-Aided Engineering, 30 , e22356.
Wu, Q., Wang, H., & Hong, W. (2020). Multistage collaborative machine learning and its application to antenna modeling and optimization. IEEE Transactions on Antennas and Propagation, 68 , 3397–3409.
Sharma, K., & Pandey, G. P. (2021). Efficient modelling of compact microstrip antenna using machine learning. AEU-International Journal of Electronics and Communications, 135 , 153739.
Wu, Q., Cao, Y., Wang, H., & Hong, W. (2020). Machine-learning-assisted optimization and its application to antenna designs: Opportunities and challenges. China Communications, 17 , 152–164.
S. S. Yadav, S. Hiremath, P. Surisetti, V. Kumar, and S. K. Patra. (2022). "Application of machine learning framework for next‐generation wireless networks: Challenges and case studies", handbook of intelligent computing and optimization for sustainable development (pp.81–99)
Cui, L., Zhang, Y., Zhang, R., & Liu, Q. H. (2020). A modified efficient KNN method for antenna optimization and design. IEEE Transactions on Antennas and Propagation, 68 , 6858–6866.
Download references
Acknowledgements
I checked Funding and declaration statement is correct.
Author information
Authors and affiliations.
Department of Electronics & Communication Engineering, Amity University, Gwalior, Madhya Pradesh, India
Rovin Tiwari & Raghavendra Sharma
Department of Electronics Engineering, Madhav Institute of Technology and Science, Gwalior, Madhya Pradesh, India
Rahul Dubey
You can also search for this author in PubMed Google Scholar
Corresponding author
Correspondence to Rovin Tiwari .
Ethics declarations
Conflict of interest.
There is no conflict of interest.
Additional information
Publisher's note.
Springer Nature remains neutral with regard to jurisdictional claims in published maps and institutional affiliations.
Rights and permissions
Springer Nature or its licensor (e.g. a society or other partner) holds exclusive rights to this article under a publishing agreement with the author(s) or other rightsholder(s); author self-archiving of the accepted manuscript version of this article is solely governed by the terms of such publishing agreement and applicable law.
Reprints and permissions
About this article
Tiwari, R., Sharma, R. & Dubey, R. A Modified Regression Model for Analysing the Performance of Metamaterial Antenna Using Machine Learning and Deep Learning. Wireless Pers Commun 136 , 1769–1789 (2024). https://doi.org/10.1007/s11277-024-11359-x
Download citation
Accepted : 13 June 2024
Published : 02 July 2024
Issue Date : June 2024
DOI : https://doi.org/10.1007/s11277-024-11359-x
Share this article
Anyone you share the following link with will be able to read this content:
Sorry, a shareable link is not currently available for this article.
Provided by the Springer Nature SharedIt content-sharing initiative
- Metamaterial antenna
- Machine learning
- Deep Learning
- XG-Boost and Bi-LSTM
- Find a journal
- Publish with us
- Track your research
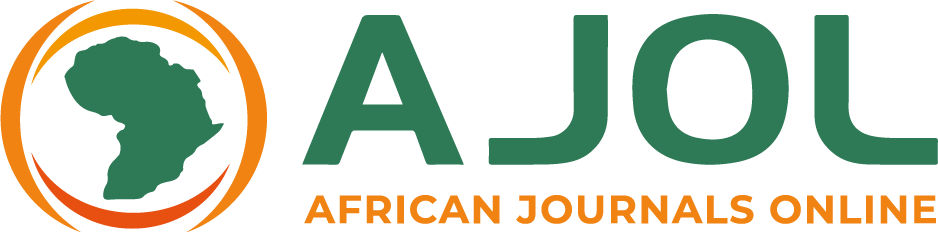
African Scientific Annual Review Journal / African Scientific Annual Review / Vol. 1 No. 1 (2024) / Articles (function() { function async_load(){ var s = document.createElement('script'); s.type = 'text/javascript'; s.async = true; var theUrl = 'https://www.journalquality.info/journalquality/ratings/2407-www-ajol-info-asarev'; s.src = theUrl + ( theUrl.indexOf("?") >= 0 ? "&" : "?") + 'ref=' + encodeURIComponent(window.location.href); var embedder = document.getElementById('jpps-embedder-ajol-asarev'); embedder.parentNode.insertBefore(s, embedder); } if (window.attachEvent) window.attachEvent('onload', async_load); else window.addEventListener('load', async_load, false); })();
Article sidebar.
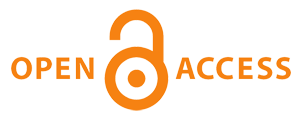
Article Details

This work is licensed under a Creative Commons Attribution-NonCommercial 4.0 International License .
Main Article Content
Analyzing the relationship between government revenue and economic growth in kenya from 2012-2022 using multiple linear regression, paul kinyua ngari, sharon atieno ooko, maryann wanjiku huho, chemos sammy kibet, allen nyachieo onchimbo.
The relationship between government revenue and economic growth is a debate that has existed for a long time in the living history.Government revenue impacts economic growth differently within different regions. Some researchers argue that government revenue positively affects economic growth while others argue that the relationship is negative. However, minimal literature exists exploring the relationship between the two variables at country specific level. The objective of this study was to determine the relationship between Government revenue and economic growth in Kenya. The research adopted the correlational study design. The study used secondary data collected from the Central Bank of Kenya, KNBS, and Government records such as the finance Act. We collected data on different sources of Government Revenue such income tax, Value Added Tax (VAT), excise duty, import duty, Other tax income. The study also included data on non-tax revenue. The set of data under the study was from the financial years 2011/2012 to 2022/2023. The analysis has been done by the use of R software. To identify the level of association of the study variables such as GDP,Income tax,VAT,excise tax,import duty,other tax and non-tax revenue, he study employs multiple linear regression analysis. To check on the level of significance, we tested at 5% significant levels. The p-value is 0.008462 which was less than 0.05 hence we reject the null hypothesis and conclude that there is significant positive relationship between Government Revenue and Economic growth in Kenya.
AJOL is a Non Profit Organisation that cannot function without donations. AJOL and the millions of African and international researchers who rely on our free services are deeply grateful for your contribution. AJOL is annually audited and was also independently assessed in 2019 by E&Y.
Your donation is guaranteed to directly contribute to Africans sharing their research output with a global readership.
- For annual AJOL Supporter contributions, please view our Supporters page.
Journal Identifiers
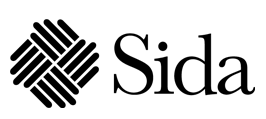
Scientists use gravitational wave research to shed light on 2000-year-old computer
Researchers used bayesian analysis and gravitational wave research to help identify the purpose of one of the computer’s mysterious ‘calendar rings’..
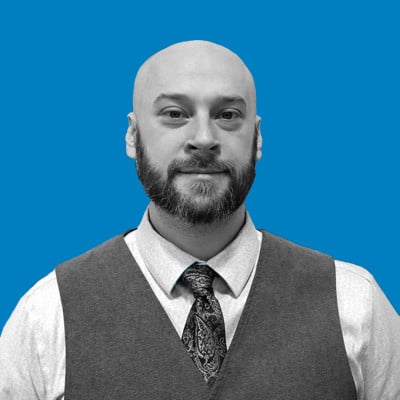
John Loeffler

Andy Montgomery / Flickr
Since its discovery over a hundred years ago, the Antikythera mechanism—a 2,000-year-old mechanical computer recovered from an ancient shipwreck off the coast of Greece—has been one of the most remarkable mysteries in archaeology, and new research may reveal further clues about its purpose.
A new study published last week in the Horological Journal reveals fresh details about the ancient Antikythera mechanism, a sophisticated hand-operated mechanical computer discovered in 1901 near the Greek island of Antikythera.
Divers exploring a sunken shipwreck found the shoebox-sized device, which dates back to the second century BCE. Although fragmented and heavily corroded, its intricate gears hinted at a complex mechanism that appears to predict eclipses and calculate the astronomical positions of planets.
Now, recent research by scientists at the University of Glasgow (UG) has provided new insights into the mechanism’s so-called ‘calendar ring’. Utilizing statistical analysis techniques, the researchers determined that the ring most likely contained 354 holes, aligning with the lunar calendar rather than the Egyptian or other 360-day calendars.
Graham Woan, a professor at UG’s School of Physics & Astronomy spearheaded the study after getting an unusual tip from a colleague. “Towards the end of last year, a colleague pointed to me to data acquired by YouTuber Chris Budiselic, who was looking to make a replica of the calendar ring and was investigating ways to determine just how many holes it contained,” Woan said in a UG statement .
“It struck me as an interesting problem,” Woan added. “I set about using some statistical techniques to answer the question.”
Woan applied Bayesian analysis, which quantifies uncertainty based on incomplete data, revealed that the ring likely had 354 or 355 holes, based on the positions of the surviving holes and the placement of the ring’s six remaining fragments.
Gravitational wave research helped identify lunar ‘calendar ring’
Dr. Joseph Bayley, a research associate at UG’s Institute for Gravitational Research and co-author of the paper, added to the study by applying techniques used in gravitational wave detection to further analyze the ring. By adapting methods from the LIGO detectors, which measure spacetime ripples caused by astronomical events, Bayley scrutinized the calendar ring using Markov Chain Monte Carlo and nested sampling methods.
The results confirmed the high probability of a 354-hole ring within a radius of 77.1mm, with a radial variation of just 0.028mm between each hole, showcasing the extraordinary precision of the ancient Greek artisans who crafted the device.
“The precision of the holes’ positioning would have required highly accurate measurement techniques and an incredibly steady hand to punch them,” Bayley said. This precision reinforces the notion that the Antikythera mechanism was used to track the lunar calendar.
“It’s a neat symmetry that we’ve adapted techniques we use to study the universe today to understand more about a mechanism that helped people keep track of the heavens nearly two millennia ago,” Woan said.
The study sheds light on the remarkable craftsmanship behind the Antikythera mechanism and its use in ancient Greece. “We hope that our findings, although less supernaturally spectacular than those made by Indiana Jones, will help deepen our understanding of how this remarkable device was made and used by the Greeks,” Woan added.
The Blueprint Daily
Stay up-to-date on engineering, tech, space, and science news with The Blueprint.
By clicking sign up, you confirm that you accept this site's Terms of Use and Privacy Policy
ABOUT THE EDITOR
John Loeffler John is a writer and programmer living in New York City. He writes about computers, gadgetry, gaming, VR/AR, and related consumer technologies. You can find him on Twitter @thisdotjohn
POPULAR ARTICLES
Windspider gets funding for possibly world’s tallest crane for turbines, bio-inspired hydrogel boosts nerve regrowth, heals spinal cord injury, new method recycles mixed fiber textiles; can reduce fast fashion waste, explosive fire extingushers, robotic frisbee throwers, the best of ie this week, related articles.

Beat the heat: White roofs can bring down city temperature by 34.16°F

Libya-bound Chinese war drones disguised as wind turbines seized by Italy

Fusion confusion: World’s largest nuclear reactor won’t power up for 15 years

World’s 1st anode-free solid-state battery is powerful, cheap, long-lasting
Human Subjects Office
Medical terms in lay language.
Please use these descriptions in place of medical jargon in consent documents, recruitment materials and other study documents. Note: These terms are not the only acceptable plain language alternatives for these vocabulary words.
This glossary of terms is derived from a list copyrighted by the University of Kentucky, Office of Research Integrity (1990).
For clinical research-specific definitions, see also the Clinical Research Glossary developed by the Multi-Regional Clinical Trials (MRCT) Center of Brigham and Women’s Hospital and Harvard and the Clinical Data Interchange Standards Consortium (CDISC) .
Alternative Lay Language for Medical Terms for use in Informed Consent Documents
A B C D E F G H I J K L M N O P Q R S T U V W X Y Z
ABDOMEN/ABDOMINAL body cavity below diaphragm that contains stomach, intestines, liver and other organs ABSORB take up fluids, take in ACIDOSIS condition when blood contains more acid than normal ACUITY clearness, keenness, esp. of vision and airways ACUTE new, recent, sudden, urgent ADENOPATHY swollen lymph nodes (glands) ADJUVANT helpful, assisting, aiding, supportive ADJUVANT TREATMENT added treatment (usually to a standard treatment) ANTIBIOTIC drug that kills bacteria and other germs ANTIMICROBIAL drug that kills bacteria and other germs ANTIRETROVIRAL drug that works against the growth of certain viruses ADVERSE EFFECT side effect, bad reaction, unwanted response ALLERGIC REACTION rash, hives, swelling, trouble breathing AMBULATE/AMBULATION/AMBULATORY walk, able to walk ANAPHYLAXIS serious, potentially life-threatening allergic reaction ANEMIA decreased red blood cells; low red cell blood count ANESTHETIC a drug or agent used to decrease the feeling of pain, or eliminate the feeling of pain by putting you to sleep ANGINA pain resulting from not enough blood flowing to the heart ANGINA PECTORIS pain resulting from not enough blood flowing to the heart ANOREXIA disorder in which person will not eat; lack of appetite ANTECUBITAL related to the inner side of the forearm ANTIBODY protein made in the body in response to foreign substance ANTICONVULSANT drug used to prevent seizures ANTILIPEMIC a drug that lowers fat levels in the blood ANTITUSSIVE a drug used to relieve coughing ARRHYTHMIA abnormal heartbeat; any change from the normal heartbeat ASPIRATION fluid entering the lungs, such as after vomiting ASSAY lab test ASSESS to learn about, measure, evaluate, look at ASTHMA lung disease associated with tightening of air passages, making breathing difficult ASYMPTOMATIC without symptoms AXILLA armpit
BENIGN not malignant, without serious consequences BID twice a day BINDING/BOUND carried by, to make stick together, transported BIOAVAILABILITY the extent to which a drug or other substance becomes available to the body BLOOD PROFILE series of blood tests BOLUS a large amount given all at once BONE MASS the amount of calcium and other minerals in a given amount of bone BRADYARRHYTHMIAS slow, irregular heartbeats BRADYCARDIA slow heartbeat BRONCHOSPASM breathing distress caused by narrowing of the airways
CARCINOGENIC cancer-causing CARCINOMA type of cancer CARDIAC related to the heart CARDIOVERSION return to normal heartbeat by electric shock CATHETER a tube for withdrawing or giving fluids CATHETER a tube placed near the spinal cord and used for anesthesia (indwelling epidural) during surgery CENTRAL NERVOUS SYSTEM (CNS) brain and spinal cord CEREBRAL TRAUMA damage to the brain CESSATION stopping CHD coronary heart disease CHEMOTHERAPY treatment of disease, usually cancer, by chemical agents CHRONIC continuing for a long time, ongoing CLINICAL pertaining to medical care CLINICAL TRIAL an experiment involving human subjects COMA unconscious state COMPLETE RESPONSE total disappearance of disease CONGENITAL present before birth CONJUNCTIVITIS redness and irritation of the thin membrane that covers the eye CONSOLIDATION PHASE treatment phase intended to make a remission permanent (follows induction phase) CONTROLLED TRIAL research study in which the experimental treatment or procedure is compared to a standard (control) treatment or procedure COOPERATIVE GROUP association of multiple institutions to perform clinical trials CORONARY related to the blood vessels that supply the heart, or to the heart itself CT SCAN (CAT) computerized series of x-rays (computerized tomography) CULTURE test for infection, or for organisms that could cause infection CUMULATIVE added together from the beginning CUTANEOUS relating to the skin CVA stroke (cerebrovascular accident)
DERMATOLOGIC pertaining to the skin DIASTOLIC lower number in a blood pressure reading DISTAL toward the end, away from the center of the body DIURETIC "water pill" or drug that causes increase in urination DOPPLER device using sound waves to diagnose or test DOUBLE BLIND study in which neither investigators nor subjects know what drug or treatment the subject is receiving DYSFUNCTION state of improper function DYSPLASIA abnormal cells
ECHOCARDIOGRAM sound wave test of the heart EDEMA excess fluid collecting in tissue EEG electric brain wave tracing (electroencephalogram) EFFICACY effectiveness ELECTROCARDIOGRAM electrical tracing of the heartbeat (ECG or EKG) ELECTROLYTE IMBALANCE an imbalance of minerals in the blood EMESIS vomiting EMPIRIC based on experience ENDOSCOPIC EXAMINATION viewing an internal part of the body with a lighted tube ENTERAL by way of the intestines EPIDURAL outside the spinal cord ERADICATE get rid of (such as disease) Page 2 of 7 EVALUATED, ASSESSED examined for a medical condition EXPEDITED REVIEW rapid review of a protocol by the IRB Chair without full committee approval, permitted with certain low-risk research studies EXTERNAL outside the body EXTRAVASATE to leak outside of a planned area, such as out of a blood vessel
FDA U.S. Food and Drug Administration, the branch of federal government that approves new drugs FIBROUS having many fibers, such as scar tissue FIBRILLATION irregular beat of the heart or other muscle
GENERAL ANESTHESIA pain prevention by giving drugs to cause loss of consciousness, as during surgery GESTATIONAL pertaining to pregnancy
HEMATOCRIT amount of red blood cells in the blood HEMATOMA a bruise, a black and blue mark HEMODYNAMIC MEASURING blood flow HEMOLYSIS breakdown in red blood cells HEPARIN LOCK needle placed in the arm with blood thinner to keep the blood from clotting HEPATOMA cancer or tumor of the liver HERITABLE DISEASE can be transmitted to one’s offspring, resulting in damage to future children HISTOPATHOLOGIC pertaining to the disease status of body tissues or cells HOLTER MONITOR a portable machine for recording heart beats HYPERCALCEMIA high blood calcium level HYPERKALEMIA high blood potassium level HYPERNATREMIA high blood sodium level HYPERTENSION high blood pressure HYPOCALCEMIA low blood calcium level HYPOKALEMIA low blood potassium level HYPONATREMIA low blood sodium level HYPOTENSION low blood pressure HYPOXEMIA a decrease of oxygen in the blood HYPOXIA a decrease of oxygen reaching body tissues HYSTERECTOMY surgical removal of the uterus, ovaries (female sex glands), or both uterus and ovaries
IATROGENIC caused by a physician or by treatment IDE investigational device exemption, the license to test an unapproved new medical device IDIOPATHIC of unknown cause IMMUNITY defense against, protection from IMMUNOGLOBIN a protein that makes antibodies IMMUNOSUPPRESSIVE drug which works against the body's immune (protective) response, often used in transplantation and diseases caused by immune system malfunction IMMUNOTHERAPY giving of drugs to help the body's immune (protective) system; usually used to destroy cancer cells IMPAIRED FUNCTION abnormal function IMPLANTED placed in the body IND investigational new drug, the license to test an unapproved new drug INDUCTION PHASE beginning phase or stage of a treatment INDURATION hardening INDWELLING remaining in a given location, such as a catheter INFARCT death of tissue due to lack of blood supply INFECTIOUS DISEASE transmitted from one person to the next INFLAMMATION swelling that is generally painful, red, and warm INFUSION slow injection of a substance into the body, usually into the blood by means of a catheter INGESTION eating; taking by mouth INTERFERON drug which acts against viruses; antiviral agent INTERMITTENT occurring (regularly or irregularly) between two time points; repeatedly stopping, then starting again INTERNAL within the body INTERIOR inside of the body INTRAMUSCULAR into the muscle; within the muscle INTRAPERITONEAL into the abdominal cavity INTRATHECAL into the spinal fluid INTRAVENOUS (IV) through the vein INTRAVESICAL in the bladder INTUBATE the placement of a tube into the airway INVASIVE PROCEDURE puncturing, opening, or cutting the skin INVESTIGATIONAL NEW DRUG (IND) a new drug that has not been approved by the FDA INVESTIGATIONAL METHOD a treatment method which has not been proven to be beneficial or has not been accepted as standard care ISCHEMIA decreased oxygen in a tissue (usually because of decreased blood flow)
LAPAROTOMY surgical procedure in which an incision is made in the abdominal wall to enable a doctor to look at the organs inside LESION wound or injury; a diseased patch of skin LETHARGY sleepiness, tiredness LEUKOPENIA low white blood cell count LIPID fat LIPID CONTENT fat content in the blood LIPID PROFILE (PANEL) fat and cholesterol levels in the blood LOCAL ANESTHESIA creation of insensitivity to pain in a small, local area of the body, usually by injection of numbing drugs LOCALIZED restricted to one area, limited to one area LUMEN the cavity of an organ or tube (e.g., blood vessel) LYMPHANGIOGRAPHY an x-ray of the lymph nodes or tissues after injecting dye into lymph vessels (e.g., in feet) LYMPHOCYTE a type of white blood cell important in immunity (protection) against infection LYMPHOMA a cancer of the lymph nodes (or tissues)
MALAISE a vague feeling of bodily discomfort, feeling badly MALFUNCTION condition in which something is not functioning properly MALIGNANCY cancer or other progressively enlarging and spreading tumor, usually fatal if not successfully treated MEDULLABLASTOMA a type of brain tumor MEGALOBLASTOSIS change in red blood cells METABOLIZE process of breaking down substances in the cells to obtain energy METASTASIS spread of cancer cells from one part of the body to another METRONIDAZOLE drug used to treat infections caused by parasites (invading organisms that take up living in the body) or other causes of anaerobic infection (not requiring oxygen to survive) MI myocardial infarction, heart attack MINIMAL slight MINIMIZE reduce as much as possible Page 4 of 7 MONITOR check on; keep track of; watch carefully MOBILITY ease of movement MORBIDITY undesired result or complication MORTALITY death MOTILITY the ability to move MRI magnetic resonance imaging, diagnostic pictures of the inside of the body, created using magnetic rather than x-ray energy MUCOSA, MUCOUS MEMBRANE moist lining of digestive, respiratory, reproductive, and urinary tracts MYALGIA muscle aches MYOCARDIAL pertaining to the heart muscle MYOCARDIAL INFARCTION heart attack
NASOGASTRIC TUBE placed in the nose, reaching to the stomach NCI the National Cancer Institute NECROSIS death of tissue NEOPLASIA/NEOPLASM tumor, may be benign or malignant NEUROBLASTOMA a cancer of nerve tissue NEUROLOGICAL pertaining to the nervous system NEUTROPENIA decrease in the main part of the white blood cells NIH the National Institutes of Health NONINVASIVE not breaking, cutting, or entering the skin NOSOCOMIAL acquired in the hospital
OCCLUSION closing; blockage; obstruction ONCOLOGY the study of tumors or cancer OPHTHALMIC pertaining to the eye OPTIMAL best, most favorable or desirable ORAL ADMINISTRATION by mouth ORTHOPEDIC pertaining to the bones OSTEOPETROSIS rare bone disorder characterized by dense bone OSTEOPOROSIS softening of the bones OVARIES female sex glands
PARENTERAL given by injection PATENCY condition of being open PATHOGENESIS development of a disease or unhealthy condition PERCUTANEOUS through the skin PERIPHERAL not central PER OS (PO) by mouth PHARMACOKINETICS the study of the way the body absorbs, distributes, and gets rid of a drug PHASE I first phase of study of a new drug in humans to determine action, safety, and proper dosing PHASE II second phase of study of a new drug in humans, intended to gather information about safety and effectiveness of the drug for certain uses PHASE III large-scale studies to confirm and expand information on safety and effectiveness of new drug for certain uses, and to study common side effects PHASE IV studies done after the drug is approved by the FDA, especially to compare it to standard care or to try it for new uses PHLEBITIS irritation or inflammation of the vein PLACEBO an inactive substance; a pill/liquid that contains no medicine PLACEBO EFFECT improvement seen with giving subjects a placebo, though it contains no active drug/treatment PLATELETS small particles in the blood that help with clotting POTENTIAL possible POTENTIATE increase or multiply the effect of a drug or toxin (poison) by giving another drug or toxin at the same time (sometimes an unintentional result) POTENTIATOR an agent that helps another agent work better PRENATAL before birth PROPHYLAXIS a drug given to prevent disease or infection PER OS (PO) by mouth PRN as needed PROGNOSIS outlook, probable outcomes PRONE lying on the stomach PROSPECTIVE STUDY following patients forward in time PROSTHESIS artificial part, most often limbs, such as arms or legs PROTOCOL plan of study PROXIMAL closer to the center of the body, away from the end PULMONARY pertaining to the lungs
QD every day; daily QID four times a day
RADIATION THERAPY x-ray or cobalt treatment RANDOM by chance (like the flip of a coin) RANDOMIZATION chance selection RBC red blood cell RECOMBINANT formation of new combinations of genes RECONSTITUTION putting back together the original parts or elements RECUR happen again REFRACTORY not responding to treatment REGENERATION re-growth of a structure or of lost tissue REGIMEN pattern of giving treatment RELAPSE the return of a disease REMISSION disappearance of evidence of cancer or other disease RENAL pertaining to the kidneys REPLICABLE possible to duplicate RESECT remove or cut out surgically RETROSPECTIVE STUDY looking back over past experience
SARCOMA a type of cancer SEDATIVE a drug to calm or make less anxious SEMINOMA a type of testicular cancer (found in the male sex glands) SEQUENTIALLY in a row, in order SOMNOLENCE sleepiness SPIROMETER an instrument to measure the amount of air taken into and exhaled from the lungs STAGING an evaluation of the extent of the disease STANDARD OF CARE a treatment plan that the majority of the medical community would accept as appropriate STENOSIS narrowing of a duct, tube, or one of the blood vessels in the heart STOMATITIS mouth sores, inflammation of the mouth STRATIFY arrange in groups for analysis of results (e.g., stratify by age, sex, etc.) STUPOR stunned state in which it is difficult to get a response or the attention of the subject SUBCLAVIAN under the collarbone SUBCUTANEOUS under the skin SUPINE lying on the back SUPPORTIVE CARE general medical care aimed at symptoms, not intended to improve or cure underlying disease SYMPTOMATIC having symptoms SYNDROME a condition characterized by a set of symptoms SYSTOLIC top number in blood pressure; pressure during active contraction of the heart
TERATOGENIC capable of causing malformations in a fetus (developing baby still inside the mother’s body) TESTES/TESTICLES male sex glands THROMBOSIS clotting THROMBUS blood clot TID three times a day TITRATION a method for deciding on the strength of a drug or solution; gradually increasing the dose T-LYMPHOCYTES type of white blood cells TOPICAL on the surface TOPICAL ANESTHETIC applied to a certain area of the skin and reducing pain only in the area to which applied TOXICITY side effects or undesirable effects of a drug or treatment TRANSDERMAL through the skin TRANSIENTLY temporarily TRAUMA injury; wound TREADMILL walking machine used to test heart function
UPTAKE absorbing and taking in of a substance by living tissue
VALVULOPLASTY plastic repair of a valve, especially a heart valve VARICES enlarged veins VASOSPASM narrowing of the blood vessels VECTOR a carrier that can transmit disease-causing microorganisms (germs and viruses) VENIPUNCTURE needle stick, blood draw, entering the skin with a needle VERTICAL TRANSMISSION spread of disease
WBC white blood cell

IMAGES
VIDEO
COMMENTS
Use regression analysis to describe the relationships between a set of independent variables and the dependent variable. Regression analysis produces a regression equation where the coefficients represent the relationship between each independent variable and the dependent variable. You can also use the equation to make predictions. As a statistician, I should probably tell you that I love all ...
Regression analysis is a quantitative research method which is used when the study involves modelling and analysing several variables, where the relationship includes a dependent variable and one or more independent variables. In simple terms, regression analysis is a quantitative method used to test the nature of relationships between a dependent variable and one or more independent variables.
Regression analysis also helps in predicting health outcomes based on various factors like age, genetic markers, or lifestyle choices. Social Sciences: Regression analysis is widely used in social sciences like sociology, psychology, and education research. Researchers can investigate the impact of variables like income, education level, or ...
This tutorial covers many facets of regression analysis including selecting the correct type of regression analysis, specifying the best model, interpreting the results, assessing the fit of the model, generating predictions, and checking the assumptions. I close the post with examples of different types of regression analyses.
Logistic Regression. Logistic Regression comes into play when the dependent variable is discrete. This means that the target value will only have one or two values. For instance, a true or false, a yes or no, a 0 or 1, and so on. In this case, a sigmoid curve describes the relationship between the independent and dependent variables.
Regression allows you to estimate how a dependent variable changes as the independent variable (s) change. Simple linear regression example. You are a social researcher interested in the relationship between income and happiness. You survey 500 people whose incomes range from 15k to 75k and ask them to rank their happiness on a scale from 1 to ...
Multiple linear regression analysis is essentially similar to the simple linear model, with the exception that multiple independent variables are used in the model. The mathematical representation of multiple linear regression is: Y = a + b X1 + c X2 + d X3 + ϵ. Where: Y - Dependent variable. X1, X2, X3 - Independent (explanatory) variables.
The regression analysis creates the single line that best summarizes the distribution of points. Mathematically, the line representing a simple linear regression is expressed through a basic equation: Y = a 0 + a 1 X. Here X is hours spent studying per week, the "independent variable.". Y is the exam scores, the "dependent variable ...
Regression analysis is an important statistical method that is commonly used to determine the relationship between several factors ... Logistic regression in medical research. Anesth Analg. 2021 ...
10.7 Poisson Regression and Weighted Least Squares 2og 10.7.1 Exampl — Internationae l Grosses of Movies (continued) 209 io.g Summary 211 11 Nonlinear Regression 2/5 11.1 Introduction 215 11.2 Concepts and Background Material 216 11.3 Methodology 21 g 11.3.1 Nonlinear Least Squares Estimation 21 g 11.3.2 Inference for Nonlinear Regression ...
The takeaway message is that regression analysis enabled them to quantify that association while adjusting for smoking, alcohol consumption, physical activity, educational level and marital status — all potential confounders of the relationship between BMI and mortality. 2. Predict an outcome using known factors.
The general procedure for using regression to make good predictions is the following: Research the subject-area so you can build on the work of others. This research helps with the subsequent steps. Collect data for the relevant variables. Specify and assess your regression model.
A Refresher on Regression Analysis. Understanding one of the most important types of data analysis. by. Amy Gallo. November 04, 2015. uptonpark/iStock/Getty Images. You probably know by now that ...
Understanding Regression Analysis: An Introductory Guide presents the fundamentals of regression analysis, from its meaning to uses, in a concise, easy-to-read, and non-technical style. It illustrates how regression coefficients are estimated, interpreted, and used in a variety of settings within the social sciences, business, law, and public ...
After using regression analysis, it becomes easier for the company to analyze the survey results and understand the relationship between different variables like electricity and revenue - here, revenue is the dependent variable. ... Regression analysis usage in market research. A market research survey focuses on three major matrices ...
Bivariate regression models with survey data. In the Center's 2016 post-election survey, respondents were asked to rate then President-elect Donald Trump on a 0-100 "feeling thermometer.". Respondents were told, "a rating of zero degrees means you feel as cold and negative as possible. A rating of 100 degrees means you feel as warm ...
Regression analysis is a statistical method. It's used for analyzing different factors that might influence an objective - such as the success of a product launch, business growth, a new marketing campaign - and determining which factors are important and which ones can be ignored.
Regression analysis is a statistical technique for determining the relationship between a single dependent (criterion) variable and one or more independent (predictor) variables. The analysis yields a predicted value for the criterion resulting from a linear combination of the predictors. According to Pedhazur, 15 regression analysis has 2 uses ...
Linear regression analysis involves examining the relationship between one independent and dependent variable. Statistically, the relationship between one independent variable (x) and a dependent variable (y) is expressed as: y= β 0 + β 1 x+ε. In this equation, β 0 is the y intercept and refers to the estimated value of y when x is equal to 0.
Regression analysis is a statistical method for analyzing a relationship between two or more variables in such a manner that one of the variables can be predicted or explained by the information on the other variables. The term "regression" was first introduced by Sir Francis Galton in the late 1800s to explain the relation between heights ...
Regression analysis is the statistical method used to determine the structure of a relationship between two variables (single linear regression) or three or more variables (multiple regression). According to the Harvard Business School Online course Business Analytics, regression is used for two primary purposes: To study the magnitude and ...
The primary purpose of regression analysis is to describe the relationship between variables, but it can also be used to: Estimate the value of one variable using the known values of other variables. Predict results and shifts in a variable based on its relationship with other variables.
Regression analysis is a reliable method of identifying which variables have impact on a topic of interest. The process of performing a regression allows you to confidently determine which factors matter most, which factors can be ignored, and how these factors influence each other. In order to understand regression analysis fully, it's ...
Indeed, the use of regression analysis was almost non- existent before the middle of the last century and did not really become a widely used tool until perhaps the late 1960's and early 1970's. Even then the computational ability of even the largest IBM machines is laughable by today's standards.
Research has suggested that recurrent maltreatment may be best predicted by a combination of factors that vary across families. The present study set out to determine whether a pattern-centered analytic approach would better predict families at high risk for recurrence when compared to logistic regression methods. Archival data from substantiated investigations during 2003 were collected from ...
Summary. The promise of AI is alluring — optimized productivity, lightning-fast data analysis, and freedom from mundane tasks — and both companies and workers alike are fascinated (and more ...
Regression algorithms have been the significant tools, which were required while employing ML in the antenna design, analysis and performance prediction. By utilizing these algorithms and considerable size dataset, a system which denotes the non-linear mapping function relationship among the geometrical parameters of antenna and characteristics ...
The set of data under the study was from the financial years 2011/2012 to 2022/2023. The analysis has been done by the use of R software. To identify the level of association of the study variables such as GDP,Income tax,VAT,excise tax,import duty,other tax and non-tax revenue, he study employs multiple linear regression analysis.
Scientists use gravitational wave research to shed light on 2000-year-old computer. Researchers used Bayesian analysis and gravitational wave research to help identify the purpose of one of the ...
For clinical research-specific definitions, see also the Clinical Research Glossary developed by the Multi-Regional Clinical Trials (MRCT) Center of Brigham and Women's Hospital and Harvard and the Clinical Data Interchange Standards Consortium (CDISC). Alternative Lay Language for Medical Terms for use in Informed Consent Documents