- Research article
- Open access
- Published: 06 February 2017

Blended learning effectiveness: the relationship between student characteristics, design features and outcomes
- Mugenyi Justice Kintu ORCID: orcid.org/0000-0002-4500-1168 1 , 2 ,
- Chang Zhu 2 &
- Edmond Kagambe 1
International Journal of Educational Technology in Higher Education volume 14 , Article number: 7 ( 2017 ) Cite this article
771k Accesses
237 Citations
36 Altmetric
Metrics details
This paper investigates the effectiveness of a blended learning environment through analyzing the relationship between student characteristics/background, design features and learning outcomes. It is aimed at determining the significant predictors of blended learning effectiveness taking student characteristics/background and design features as independent variables and learning outcomes as dependent variables. A survey was administered to 238 respondents to gather data on student characteristics/background, design features and learning outcomes. The final semester evaluation results were used as a measure for performance as an outcome. We applied the online self regulatory learning questionnaire for data on learner self regulation, the intrinsic motivation inventory for data on intrinsic motivation and other self-developed instruments for measuring the other constructs. Multiple regression analysis results showed that blended learning design features (technology quality, online tools and face-to-face support) and student characteristics (attitudes and self-regulation) predicted student satisfaction as an outcome. The results indicate that some of the student characteristics/backgrounds and design features are significant predictors for student learning outcomes in blended learning.
Introduction
The teaching and learning environment is embracing a number of innovations and some of these involve the use of technology through blended learning. This innovative pedagogical approach has been embraced rapidly though it goes through a process. The introduction of blended learning (combination of face-to-face and online teaching and learning) initiatives is part of these innovations but its uptake, especially in the developing world faces challenges for it to be an effective innovation in teaching and learning. Blended learning effectiveness has quite a number of underlying factors that pose challenges. One big challenge is about how users can successfully use the technology and ensuring participants’ commitment given the individual learner characteristics and encounters with technology (Hofmann, 2014 ). Hofmann adds that users getting into difficulties with technology may result into abandoning the learning and eventual failure of technological applications. In a report by Oxford Group ( 2013 ), some learners (16%) had negative attitudes to blended learning while 26% were concerned that learners would not complete study in blended learning. Learners are important partners in any learning process and therefore, their backgrounds and characteristics affect their ability to effectively carry on with learning and being in blended learning, the design tools to be used may impinge on the effectiveness in their learning.
This study tackles blended learning effectiveness which has been investigated in previous studies considering grades, course completion, retention and graduation rates but no studies regarding effectiveness in view of learner characteristics/background, design features and outcomes have been done in the Ugandan university context. No studies have also been done on how the characteristics of learners and design features are predictors of outcomes in the context of a planning evaluation research (Guskey, 2000 ) to establish the effectiveness of blended learning. Guskey ( 2000 ) noted that planning evaluation fits in well since it occurs before the implementation of any innovation as well as allowing planners to determine the needs, considering participant characteristics, analyzing contextual matters and gathering baseline information. This study is done in the context of a plan to undertake innovative pedagogy involving use of a learning management system (moodle) for the first time in teaching and learning in a Ugandan university. The learner characteristics/backgrounds being investigated for blended learning effectiveness include self-regulation, computer competence, workload management, social and family support, attitude to blended learning, gender and age. We investigate the blended learning design features of learner interactions, face-to-face support, learning management system tools and technology quality while the outcomes considered include satisfaction, performance, intrinsic motivation and knowledge construction. Establishing the significant predictors of outcomes in blended learning will help to inform planners of such learning environments in order to put in place necessary groundwork preparations for designing blended learning as an innovative pedagogical approach.
Kenney and Newcombe ( 2011 ) did their comparison to establish effectiveness in view of grades and found that blended learning had higher average score than the non-blended learning environment. Garrison and Kanuka ( 2004 ) examined the transformative potential of blended learning and reported an increase in course completion rates, improved retention and increased student satisfaction. Comparisons between blended learning environments have been done to establish the disparity between academic achievement, grade dispersions and gender performance differences and no significant differences were found between the groups (Demirkol & Kazu, 2014 ).
However, blended learning effectiveness may be dependent on many other factors and among them student characteristics, design features and learning outcomes. Research shows that the failure of learners to continue their online education in some cases has been due to family support or increased workload leading to learner dropout (Park & Choi, 2009 ) as well as little time for study. Additionally, it is dependent on learner interactions with instructors since failure to continue with online learning is attributed to this. In Greer, Hudson & Paugh’s study as cited in Park and Choi ( 2009 ), family and peer support for learners is important for success in online and face-to-face learning. Support is needed for learners from all areas in web-based courses and this may be from family, friends, co-workers as well as peers in class. Greer, Hudson and Paugh further noted that peer encouragement assisted new learners in computer use and applications. The authors also show that learners need time budgeting, appropriate technology tools and support from friends and family in web-based courses. Peer support is required by learners who have no or little knowledge of technology, especially computers, to help them overcome fears. Park and Choi, ( 2009 ) showed that organizational support significantly predicts learners’ stay and success in online courses because employers at times are willing to reduce learners’ workload during study as well as supervisors showing that they are interested in job-related learning for employees to advance and improve their skills.
The study by Kintu and Zhu ( 2016 ) investigated the possibility of blended learning in a Ugandan University and examined whether student characteristics (such as self-regulation, attitudes towards blended learning, computer competence) and student background (such as family support, social support and management of workload) were significant factors in learner outcomes (such as motivation, satisfaction, knowledge construction and performance). The characteristics and background factors were studied along with blended learning design features such as technology quality, learner interactions, and Moodle with its tools and resources. The findings from that study indicated that learner attitudes towards blended learning were significant factors to learner satisfaction and motivation while workload management was a significant factor to learner satisfaction and knowledge construction. Among the blended learning design features, only learner interaction was a significant factor to learner satisfaction and knowledge construction.
The focus of the present study is on examining the effectiveness of blended learning taking into consideration learner characteristics/background, blended learning design elements and learning outcomes and how the former are significant predictors of blended learning effectiveness.
Studies like that of Morris and Lim ( 2009 ) have investigated learner and instructional factors influencing learning outcomes in blended learning. They however do not deal with such variables in the contexts of blended learning design as an aspect of innovative pedagogy involving the use of technology in education. Apart from the learner variables such as gender, age, experience, study time as tackled before, this study considers social and background aspects of the learners such as family and social support, self-regulation, attitudes towards blended learning and management of workload to find out their relationship to blended learning effectiveness. Identifying the various types of learner variables with regard to their relationship to blended learning effectiveness is important in this study as we embark on innovative pedagogy with technology in teaching and learning.
Literature review
This review presents research about blended learning effectiveness from the perspective of learner characteristics/background, design features and learning outcomes. It also gives the factors that are considered to be significant for blended learning effectiveness. The selected elements are as a result of the researcher’s experiences at a Ugandan university where student learning faces challenges with regard to learner characteristics and blended learning features in adopting the use of technology in teaching and learning. We have made use of Loukis, Georgiou, and Pazalo ( 2007 ) value flow model for evaluating an e-learning and blended learning service specifically considering the effectiveness evaluation layer. This evaluates the extent of an e-learning system usage and the educational effectiveness. In addition, studies by Leidner, Jarvenpaa, Dillon and Gunawardena as cited in Selim ( 2007 ) have noted three main factors that affect e-learning and blended learning effectiveness as instructor characteristics, technology and student characteristics. Heinich, Molenda, Russell, and Smaldino ( 2001 ) showed the need for examining learner characteristics for effective instructional technology use and showed that user characteristics do impact on behavioral intention to use technology. Research has dealt with learner characteristics that contribute to learner performance outcomes. They have dealt with emotional intelligence, resilience, personality type and success in an online learning context (Berenson, Boyles, & Weaver, 2008 ). Dealing with the characteristics identified in this study will give another dimension, especially for blended learning in learning environment designs and add to specific debate on learning using technology. Lin and Vassar, ( 2009 ) indicated that learner success is dependent on ability to cope with technical difficulty as well as technical skills in computer operations and internet navigation. This justifies our approach in dealing with the design features of blended learning in this study.
Learner characteristics/background and blended learning effectiveness
Studies indicate that student characteristics such as gender play significant roles in academic achievement (Oxford Group, 2013 ), but no study examines performance of male and female as an important factor in blended learning effectiveness. It has again been noted that the success of e- and blended learning is highly dependent on experience in internet and computer applications (Picciano & Seaman, 2007 ). Rigorous discovery of such competences can finally lead to a confirmation of high possibilities of establishing blended learning. Research agrees that the success of e-learning and blended learning can largely depend on students as well as teachers gaining confidence and capability to participate in blended learning (Hadad, 2007 ). Shraim and Khlaif ( 2010 ) note in their research that 75% of students and 72% of teachers were lacking in skills to utilize ICT based learning components due to insufficient skills and experience in computer and internet applications and this may lead to failure in e-learning and blended learning. It is therefore pertinent that since the use of blended learning applies high usage of computers, computer competence is necessary (Abubakar & Adetimirin, 2015 ) to avoid failure in applying technology in education for learning effectiveness. Rovai, ( 2003 ) noted that learners’ computer literacy and time management are crucial in distance learning contexts and concluded that such factors are meaningful in online classes. This is supported by Selim ( 2007 ) that learners need to posses time management skills and computer skills necessary for effectiveness in e- learning and blended learning. Self-regulatory skills of time management lead to better performance and learners’ ability to structure the physical learning environment leads to efficiency in e-learning and blended learning environments. Learners need to seek helpful assistance from peers and teachers through chats, email and face-to-face meetings for effectiveness (Lynch & Dembo, 2004 ). Factors such as learners’ hours of employment and family responsibilities are known to impede learners’ process of learning, blended learning inclusive (Cohen, Stage, Hammack, & Marcus, 2012 ). It was also noted that a common factor in failure and learner drop-out is the time conflict which is compounded by issues of family , employment status as well as management support (Packham, Jones, Miller, & Thomas, 2004 ). A study by Thompson ( 2004 ) shows that work, family, insufficient time and study load made learners withdraw from online courses.
Learner attitudes to blended learning can result in its effectiveness and these shape behavioral intentions which usually lead to persistence in a learning environment, blended inclusive. Selim, ( 2007 ) noted that the learners’ attitude towards e-learning and blended learning are success factors for these learning environments. Learner performance by age and gender in e-learning and blended learning has been found to indicate no significant differences between male and female learners and different age groups (i.e. young, middle-aged and old above 45 years) (Coldwell, Craig, Paterson, & Mustard, 2008 ). This implies that the potential for blended learning to be effective exists and is unhampered by gender or age differences.
Blended learning design features
The design features under study here include interactions, technology with its quality, face-to-face support and learning management system tools and resources.
Research shows that absence of learner interaction causes failure and eventual drop-out in online courses (Willging & Johnson, 2009 ) and the lack of learner connectedness was noted as an internal factor leading to learner drop-out in online courses (Zielinski, 2000 ). It was also noted that learners may not continue in e- and blended learning if they are unable to make friends thereby being disconnected and developing feelings of isolation during their blended learning experiences (Willging & Johnson, 2009). Learners’ Interactions with teachers and peers can make blended learning effective as its absence makes learners withdraw (Astleitner, 2000 ). Loukis, Georgious and Pazalo (2007) noted that learners’ measuring of a system’s quality, reliability and ease of use leads to learning efficiency and can be so in blended learning. Learner success in blended learning may substantially be affected by system functionality (Pituch & Lee, 2006 ) and may lead to failure of such learning initiatives (Shrain, 2012 ). It is therefore important to examine technology quality for ensuring learning effectiveness in blended learning. Tselios, Daskalakis, and Papadopoulou ( 2011 ) investigated learner perceptions after a learning management system use and found out that the actual system use determines the usefulness among users. It is again noted that a system with poor response time cannot be taken to be useful for e-learning and blended learning especially in cases of limited bandwidth (Anderson, 2004 ). In this study, we investigate the use of Moodle and its tools as a function of potential effectiveness of blended learning.
The quality of learning management system content for learners can be a predictor of good performance in e-and blended learning environments and can lead to learner satisfaction. On the whole, poor quality technology yields no satisfaction by users and therefore the quality of technology significantly affects satisfaction (Piccoli, Ahmad, & Ives, 2001 ). Continued navigation through a learning management system increases use and is an indicator of success in blended learning (Delone & McLean, 2003 ). The efficient use of learning management system and its tools improves learning outcomes in e-learning and blended learning environments.
It is noted that learner satisfaction with a learning management system can be an antecedent factor for blended learning effectiveness. Goyal and Tambe ( 2015 ) noted that learners showed an appreciation to Moodle’s contribution in their learning. They showed positivity with it as it improved their understanding of course material (Ahmad & Al-Khanjari, 2011 ). The study by Goyal and Tambe ( 2015 ) used descriptive statistics to indicate improved learning by use of uploaded syllabus and session plans on Moodle. Improved learning is also noted through sharing study material, submitting assignments and using the calendar. Learners in the study found Moodle to be an effective educational tool.
In blended learning set ups, face-to-face experiences form part of the blend and learner positive attitudes to such sessions could mean blended learning effectiveness. A study by Marriot, Marriot, and Selwyn ( 2004 ) showed learners expressing their preference for face-to-face due to its facilitation of social interaction and communication skills acquired from classroom environment. Their preference for the online session was only in as far as it complemented the traditional face-to-face learning. Learners in a study by Osgerby ( 2013 ) had positive perceptions of blended learning but preferred face-to-face with its step-by-stem instruction. Beard, Harper and Riley ( 2004 ) shows that some learners are successful while in a personal interaction with teachers and peers thus prefer face-to-face in the blend. Beard however dealt with a comparison between online and on-campus learning while our study combines both, singling out the face-to-face part of the blend. The advantage found by Beard is all the same relevant here because learners in blended learning express attitude to both online and face-to-face for an effective blend. Researchers indicate that teacher presence in face-to-face sessions lessens psychological distance between them and the learners and leads to greater learning. This is because there are verbal aspects like giving praise, soliciting for viewpoints, humor, etc and non-verbal expressions like eye contact, facial expressions, gestures, etc which make teachers to be closer to learners psychologically (Kelley & Gorham, 2009 ).
Learner outcomes
The outcomes under scrutiny in this study include performance, motivation, satisfaction and knowledge construction. Motivation is seen here as an outcome because, much as cognitive factors such as course grades are used in measuring learning outcomes, affective factors like intrinsic motivation may also be used to indicate outcomes of learning (Kuo, Walker, Belland, & Schroder, 2013 ). Research shows that high motivation among online learners leads to persistence in their courses (Menager-Beeley, 2004 ). Sankaran and Bui ( 2001 ) indicated that less motivated learners performed poorly in knowledge tests while those with high learning motivation demonstrate high performance in academics (Green, Nelson, Martin, & Marsh, 2006 ). Lim and Kim, ( 2003 ) indicated that learner interest as a motivation factor promotes learner involvement in learning and this could lead to learning effectiveness in blended learning.
Learner satisfaction was noted as a strong factor for effectiveness of blended and online courses (Wilging & Johnson, 2009) and dissatisfaction may result from learners’ incompetence in the use of the learning management system as an effective learning tool since, as Islam ( 2014 ) puts it, users may be dissatisfied with an information system due to ease of use. A lack of prompt feedback for learners from course instructors was found to cause dissatisfaction in an online graduate course. In addition, dissatisfaction resulted from technical difficulties as well as ambiguous course instruction Hara and Kling ( 2001 ). These factors, once addressed, can lead to learner satisfaction in e-learning and blended learning and eventual effectiveness. A study by Blocker and Tucker ( 2001 ) also showed that learners had difficulties with technology and inadequate group participation by peers leading to dissatisfaction within these design features. Student-teacher interactions are known to bring satisfaction within online courses. Study results by Swan ( 2001 ) indicated that student-teacher interaction strongly related with student satisfaction and high learner-learner interaction resulted in higher levels of course satisfaction. Descriptive results by Naaj, Nachouki, and Ankit ( 2012 ) showed that learners were satisfied with technology which was a video-conferencing component of blended learning with a mean of 3.7. The same study indicated student satisfaction with instructors at a mean of 3.8. Askar and Altun, ( 2008 ) found that learners were satisfied with face-to-face sessions of the blend with t-tests and ANOVA results indicating female scores as higher than for males in the satisfaction with face-to-face environment of the blended learning.
Studies comparing blended learning with traditional face-to-face have indicated that learners perform equally well in blended learning and their performance is unaffected by the delivery method (Kwak, Menezes, & Sherwood, 2013 ). In another study, learning experience and performance are known to improve when traditional course delivery is integrated with online learning (Stacey & Gerbic, 2007 ). Such improvement as noted may be an indicator of blended learning effectiveness. Our study however, delves into improved performance but seeks to establish the potential of blended learning effectiveness by considering grades obtained in a blended learning experiment. Score 50 and above is considered a pass in this study’s setting and learners scoring this and above will be considered to have passed. This will make our conclusions about the potential of blended learning effectiveness.
Regarding knowledge construction, it has been noted that effective learning occurs where learners are actively involved (Nurmela, Palonen, Lehtinen & Hakkarainen, 2003 , cited in Zhu, 2012 ) and this may be an indicator of learning environment effectiveness. Effective blended learning would require that learners are able to initiate, discover and accomplish the processes of knowledge construction as antecedents of blended learning effectiveness. A study by Rahman, Yasin and Jusoff ( 2011 ) indicated that learners were able to use some steps to construct meaning through an online discussion process through assignments given. In the process of giving and receiving among themselves, the authors noted that learners learned by writing what they understood. From our perspective, this can be considered to be accomplishment in the knowledge construction process. Their study further shows that learners construct meaning individually from assignments and this stage is referred to as pre-construction which for our study, is an aspect of discovery in the knowledge construction process.
Predictors of blended learning effectiveness
Researchers have dealt with success factors for online learning or those for traditional face-to-face learning but little is known about factors that predict blended learning effectiveness in view of learner characteristics and blended learning design features. This part of our study seeks to establish the learner characteristics/backgrounds and design features that predict blended learning effectiveness with regard to satisfaction, outcomes, motivation and knowledge construction. Song, Singleton, Hill, and Koh ( 2004 ) examined online learning effectiveness factors and found out that time management (a self-regulatory factor) was crucial for successful online learning. Eom, Wen, and Ashill ( 2006 ) using a survey found out that interaction, among other factors, was significant for learner satisfaction. Technical problems with regard to instructional design were a challenge to online learners thus not indicating effectiveness (Song et al., 2004 ), though the authors also indicated that descriptive statistics to a tune of 75% and time management (62%) impact on success of online learning. Arbaugh ( 2000 ) and Swan ( 2001 ) indicated that high levels of learner-instructor interaction are associated with high levels of user satisfaction and learning outcomes. A study by Naaj et al. ( 2012 ) indicated that technology and learner interactions, among other factors, influenced learner satisfaction in blended learning.
Objective and research questions of the current study
The objective of the current study is to investigate the effectiveness of blended learning in view of student satisfaction, knowledge construction, performance and intrinsic motivation and how they are related to student characteristics and blended learning design features in a blended learning environment.
Research questions
What are the student characteristics and blended learning design features for an effective blended learning environment?
Which factors (among the learner characteristics and blended learning design features) predict student satisfaction, learning outcomes, intrinsic motivation and knowledge construction?
Conceptual model of the present study
The reviewed literature clearly shows learner characteristics/background and blended learning design features play a part in blended learning effectiveness and some of them are significant predictors of effectiveness. The conceptual model for our study is depicted as follows (Fig. 1 ):
Conceptual model of the current study
Research design
This research applies a quantitative design where descriptive statistics are used for the student characteristics and design features data, t-tests for the age and gender variables to determine if they are significant in blended learning effectiveness and regression for predictors of blended learning effectiveness.
This study is based on an experiment in which learners participated during their study using face-to-face sessions and an on-line session of a blended learning design. A learning management system (Moodle) was used and learner characteristics/background and blended learning design features were measured in relation to learning effectiveness. It is therefore a planning evaluation research design as noted by Guskey ( 2000 ) since the outcomes are aimed at blended learning implementation at MMU. The plan under which the various variables were tested involved face-to-face study at the beginning of a 17 week semester which was followed by online teaching and learning in the second half of the semester. The last part of the semester was for another face-to-face to review work done during the online sessions and final semester examinations. A questionnaire with items on student characteristics, design features and learning outcomes was distributed among students from three schools and one directorate of postgraduate studies.
Participants
Cluster sampling was used to select a total of 238 learners to participate in this study. Out of the whole university population of students, three schools and one directorate were used. From these, one course unit was selected from each school and all the learners following the course unit were surveyed. In the school of Education ( n = 70) and Business and Management Studies ( n = 133), sophomore students were involved due to the fact that they have been introduced to ICT basics during their first year of study. Students of the third year were used from the department of technology in the School of Applied Sciences and Technology ( n = 18) since most of the year two courses had a lot of practical aspects that could not be used for the online learning part. From the Postgraduate Directorate ( n = 17), first and second year students were selected because learners attend a face-to-face session before they are given paper modules to study away from campus.
The study population comprised of 139 male students representing 58.4% and 99 females representing 41.6% with an average age of 24 years.
Instruments
The end of semester results were used to measure learner performance. The online self-regulated learning questionnaire (Barnard, Lan, To, Paton, & Lai, 2009 ) and the intrinsic motivation inventory (Deci & Ryan, 1982 ) were applied to measure the constructs on self regulation in the student characteristics and motivation in the learning outcome constructs. Other self-developed instruments were used for the other remaining variables of attitudes, computer competence, workload management, social and family support, satisfaction, knowledge construction, technology quality, interactions, learning management system tools and resources and face-to-face support.
Instrument reliability
Cronbach’s alpha was used to test reliability and the table below gives the results. All the scales and sub-scales had acceptable internal consistency reliabilities as shown in Table 1 below:
Data analysis
First, descriptive statistics was conducted. Shapiro-Wilk test was done to test normality of the data for it to qualify for parametric tests. The test results for normality of our data before the t- test resulted into significant levels (Male = .003, female = .000) thereby violating the normality assumption. We therefore used the skewness and curtosis results which were between −1.0 and +1.0 and assumed distribution to be sufficiently normal to qualify the data for a parametric test, (Pallant, 2010 ). An independent samples t -test was done to find out the differences in male and female performance to explain the gender characteristics in blended learning effectiveness. A one-way ANOVA between subjects was conducted to establish the differences in performance between age groups. Finally, multiple regression analysis was done between student variables and design elements with learning outcomes to determine the significant predictors for blended learning effectiveness.
Student characteristics, blended learning design features and learning outcomes ( RQ1 )
A t- test was carried out to establish the performance of male and female learners in the blended learning set up. This was aimed at finding out if male and female learners do perform equally well in blended learning given their different roles and responsibilities in society. It was found that male learners performed slightly better ( M = 62.5) than their female counterparts ( M = 61.1). An independent t -test revealed that the difference between the performances was not statistically significant ( t = 1.569, df = 228, p = 0.05, one tailed). The magnitude of the differences in the means is small with effect size ( d = 0.18). A one way between subjects ANOVA was conducted on the performance of different age groups to establish the performance of learners of young and middle aged age groups (20–30, young & and 31–39, middle aged). This revealed a significant difference in performance (F(1,236 = 8.498, p < . 001).
Average percentages of the items making up the self regulated learning scale are used to report the findings about all the sub-scales in the learner characteristics/background scale. Results show that learner self-regulation was good enough at 72.3% in all the sub-scales of goal setting, environment structuring, task strategies, time management, help-seeking and self-evaluation among learners. The least in the scoring was task strategies at 67.7% and the highest was learner environment structuring at 76.3%. Learner attitude towards blended learning environment is at 76% in the sub-scales of learner autonomy, quality of instructional materials, course structure, course interface and interactions. The least scored here is attitude to course structure at 66% and their attitudes were high on learner autonomy and course interface both at 82%. Results on the learners’ computer competences are summarized in percentages in the table below (Table 2 ):
It can be seen that learners are skilled in word processing at 91%, email at 63.5%, spreadsheets at 68%, web browsers at 70.2% and html tools at 45.4%. They are therefore good enough in word processing and web browsing. Their computer confidence levels are reported at 75.3% and specifically feel very confident when it comes to working with a computer (85.7%). Levels of family and social support for learners during blended learning experiences are at 60.5 and 75% respectively. There is however a low score on learners being assisted by family members in situations of computer setbacks (33.2%) as 53.4% of the learners reported no assistance in this regard. A higher percentage (85.3%) is reported on learners getting support from family regarding provision of essentials for learning such as tuition. A big percentage of learners spend two hours on study while at home (35.3%) followed by one hour (28.2%) while only 9.7% spend more than three hours on study at home. Peers showed great care during the blended learning experience (81%) and their experiences were appreciated by the society (66%). Workload management by learners vis-à-vis studying is good at 60%. Learners reported that their workmates stand in for them at workplaces to enable them do their study in blended learning while 61% are encouraged by their bosses to go and improve their skills through further education and training. On the time spent on other activities not related to study, majority of the learners spend three hours (35%) while 19% spend 6 hours. Sixty percent of the learners have to answer to someone when they are not attending to other activities outside study compared to the 39.9% who do not and can therefore do study or those other activities.
The usability of the online system, tools and resources was below average as shown in the table below in percentages (Table 3 ):
However, learners became skilled at navigating around the learning management system (79%) and it was easy for them to locate course content, tools and resources needed such as course works, news, discussions and journal materials. They effectively used the communication tools (60%) and to work with peers by making posts (57%). They reported that online resources were well organized, user friendly and easy to access (71%) as well as well structured in a clear and understandable manner (72%). They therefore recommended the use of online resources for other course units in future (78%) because they were satisfied with them (64.3%). On the whole, the online resources were fine for the learners (67.2%) and useful as a learning resource (80%). The learners’ perceived usefulness/satisfaction with online system, tools, and resources was at 81% as the LMS tools helped them to communicate, work with peers and reflect on their learning (74%). They reported that using moodle helped them to learn new concepts, information and gaining skills (85.3%) as well as sharing what they knew or learned (76.4%). They enjoyed the course units (78%) and improved their skills with technology (89%).
Learner interactions were seen from three angles of cognitivism, collaborative learning and student-teacher interactions. Collaborative learning was average at 50% with low percentages in learners posting challenges to colleagues’ ideas online (34%) and posting ideas for colleagues to read online (37%). They however met oftentimes online (60%) and organized how they would work together in study during the face-to-face meetings (69%). The common form of communication medium frequently used by learners during the blended learning experience was by phone (34.5%) followed by whatsapp (21.8%), face book (21%), discussion board (11.8%) and email (10.9%). At the cognitive level, learners interacted with content at 72% by reading the posted content (81%), exchanging knowledge via the LMS (58.4%), participating in discussions on the forum (62%) and got course objectives and structure introduced during the face-to-face sessions (86%). Student-teacher interaction was reported at 71% through instructors individually working with them online (57.2%) and being well guided towards learning goals (81%). They did receive suggestions from instructors about resources to use in their learning (75.3%) and instructors provided learning input for them to come up with their own answers (71%).
The technology quality during the blended learning intervention was rated at 69% with availability of 72%, quality of the resources was at 68% with learners reporting that discussion boards gave right content necessary for study (71%) and the email exchanges containing relevant and much needed information (63.4%) as well as chats comprising of essential information to aid the learning (69%). Internet reliability was rated at 66% with a speed considered averagely good to facilitate online activities (63%). They however reported that there was intermittent breakdown during online study (67%) though they could complete their internet program during connection (63.4%). Learners eventually found it easy to download necessary materials for study in their blended learning experiences (71%).
Learner extent of use of the learning management system features was as shown in the table below in percentage (Table 4 ):
From the table, very rarely used features include the blog and wiki while very often used ones include the email, forum, chat and calendar.
The effectiveness of the LMS was rated at 79% by learners reporting that they found it useful (89%) and using it makes their learning activities much easier (75.2%). Moodle has helped learners to accomplish their learning tasks more quickly (74%) and that as a LMS, it is effective in teaching and learning (88%) with overall satisfaction levels at 68%. However, learners note challenges in the use of the LMS regarding its performance as having been problematic to them (57%) and only 8% of the learners reported navigation while 16% reported access as challenges.
Learner attitudes towards Face-to-face support were reported at 88% showing that the sessions were enjoyable experiences (89%) with high quality class discussions (86%) and therefore recommended that the sessions should continue in blended learning (89%). The frequency of the face-to-face sessions is shown in the table below as preferred by learners (Table 5 ).
Learners preferred face-to-face sessions after every month in the semester (33.6%) and at the beginning of the blended learning session only (27.7%).
Learners reported high intrinsic motivation levels with interest and enjoyment of tasks at 83.7%, perceived competence at 70.2%, effort/importance sub-scale at 80%, pressure/tension reported at 54%. The pressure percentage of 54% arises from learners feeling nervous (39.2%) and a lot of anxiety (53%) while 44% felt a lot of pressure during the blended learning experiences. Learners however reported the value/usefulness of blended learning at 91% with majority believing that studying online and face-to-face had value for them (93.3%) and were therefore willing to take part in blended learning (91.2%). They showed that it is beneficial for them (94%) and that it was an important way of studying (84.3%).
Learner satisfaction was reported at 81% especially with instructors (85%) high percentage reported on encouraging learner participation during the course of study 93%, course content (83%) with the highest being satisfaction with the good relationship between the objectives of the course units and the content (90%), technology (71%) with a high percentage on the fact that the platform was adequate for the online part of the learning (76%), interactions (75%) with participation in class at 79%, and face-to-face sessions (91%) with learner satisfaction high on face-to-face sessions being good enough for interaction and giving an overview of the courses when objectives were introduced at 92%.
Learners’ knowledge construction was reported at 78% with initiation and discovery scales scoring 84% with 88% specifically for discovering the learning points in the course units. The accomplishment scale in knowledge construction scored 71% and specifically the fact that learners were able to work together with group members to accomplish learning tasks throughout the study of the course units (79%). Learners developed reports from activities (67%), submitted solutions to discussion questions (68%) and did critique peer arguments (69%). Generally, learners performed well in blended learning in the final examination with an average pass of 62% and standard deviation of 7.5.
Significant predictors of blended learning effectiveness ( RQ 2)
A standard multiple regression analysis was done taking learner characteristics/background and design features as predictor variables and learning outcomes as criterion variables. The data was first tested to check if it met the linear regression test assumptions and results showed the correlations between the independent variables and each of the dependent variables (highest 0.62 and lowest 0.22) as not being too high, which indicated that multicollinearity was not a problem in our model. From the coefficients table, the VIF values ranged from 1.0 to 2.4, well below the cut off value of 10 and indicating no possibility of multicollinearity. The normal probability plot was seen to lie as a reasonably straight diagonal from bottom left to top right indicating normality of our data. Linearity was found suitable from the scatter plot of the standardized residuals and was rectangular in distribution. Outliers were no cause for concern in our data since we had only 1% of all cases falling outside 3.0 thus proving the data as a normally distributed sample. Our R -square values was at 0.525 meaning that the independent variables explained about 53% of the variance in overall satisfaction, motivation and knowledge construction of the learners. All the models explaining the three dependent variables of learner satisfaction, intrinsic motivation and knowledge construction were significant at the 0.000 probability level (Table 6 ).
From the table above, design features (technology quality and online tools and resources), and learner characteristics (attitudes to blended learning, self-regulation) were significant predictors of learner satisfaction in blended learning. This means that good technology with the features involved and the learner positive attitudes with capacity to do blended learning with self drive led to their satisfaction. The design features (technology quality, interactions) and learner characteristics (self regulation and social support), were found to be significant predictors of learner knowledge construction. This implies that learners’ capacity to go on their work by themselves supported by peers and high levels of interaction using the quality technology led them to construct their own ideas in blended learning. Design features (technology quality, online tools and resources as well as learner interactions) and learner characteristics (self regulation), significantly predicted the learners’ intrinsic motivation in blended learning suggesting that good technology, tools and high interaction levels with independence in learning led to learners being highly motivated. Finally, none of the independent variables considered under this study were predictors of learning outcomes (grade).
In this study we have investigated learning outcomes as dependent variables to establish if particular learner characteristics/backgrounds and design features are related to the outcomes for blended learning effectiveness and if they predict learning outcomes in blended learning. We took students from three schools out of five and one directorate of post-graduate studies at a Ugandan University. The study suggests that the characteristics and design features examined are good drivers towards an effective blended learning environment though a few of them predicted learning outcomes in blended learning.
Student characteristics/background, blended learning design features and learning outcomes
The learner characteristics, design features investigated are potentially important for an effective blended learning environment. Performance by gender shows a balance with no statistical differences between male and female. There are statistically significant differences ( p < .005) in the performance between age groups with means of 62% for age group 20–30 and 67% for age group 31 –39. The indicators of self regulation exist as well as positive attitudes towards blended learning. Learners do well with word processing, e-mail, spreadsheets and web browsers but still lag below average in html tools. They show computer confidence at 75.3%; which gives prospects for an effective blended learning environment in regard to their computer competence and confidence. The levels of family and social support for learners stand at 61 and 75% respectively, indicating potential for blended learning to be effective. The learners’ balance between study and work is a drive factor towards blended learning effectiveness since their management of their workload vis a vis study time is at 60 and 61% of the learners are encouraged to go for study by their bosses. Learner satisfaction with the online system and its tools shows prospect for blended learning effectiveness but there are challenges in regard to locating course content and assignments, submitting their work and staying on a task during online study. Average collaborative, cognitive learning as well as learner-teacher interactions exist as important factors. Technology quality for effective blended learning is a potential for effectiveness though features like the blog and wiki are rarely used by learners. Face-to-face support is satisfactory and it should be conducted every month. There is high intrinsic motivation, satisfaction and knowledge construction as well as good performance in examinations ( M = 62%, SD = 7.5); which indicates potentiality for blended learning effectiveness.
Significant predictors of blended learning effectiveness
Among the design features, technology quality, online tools and face-to-face support are predictors of learner satisfaction while learner characteristics of self regulation and attitudes to blended learning are predictors of satisfaction. Technology quality and interactions are the only design features predicting learner knowledge construction, while social support, among the learner backgrounds, is a predictor of knowledge construction. Self regulation as a learner characteristic is a predictor of knowledge construction. Self regulation is the only learner characteristic predicting intrinsic motivation in blended learning while technology quality, online tools and interactions are the design features predicting intrinsic motivation. However, all the independent variables are not significant predictors of learning performance in blended learning.
The high computer competences and confidence is an antecedent factor for blended learning effectiveness as noted by Hadad ( 2007 ) and this study finds learners confident and competent enough for the effectiveness of blended learning. A lack in computer skills causes failure in e-learning and blended learning as noted by Shraim and Khlaif ( 2010 ). From our study findings, this is no threat for blended learning our case as noted by our results. Contrary to Cohen et al. ( 2012 ) findings that learners’ family responsibilities and hours of employment can impede their process of learning, it is not the case here since they are drivers to the blended learning process. Time conflict, as compounded by family, employment status and management support (Packham et al., 2004 ) were noted as causes of learner failure and drop out of online courses. Our results show, on the contrary, that these factors are drivers for blended learning effectiveness because learners have a good balance between work and study and are supported by bosses to study. In agreement with Selim ( 2007 ), learner positive attitudes towards e-and blended learning environments are success factors. In line with Coldwell et al. ( 2008 ), no statistically significant differences exist between age groups. We however note that Coldwel, et al dealt with young, middle-aged and old above 45 years whereas we dealt with young and middle aged only.
Learner interactions at all levels are good enough and contrary to Astleitner, ( 2000 ) that their absence makes learners withdraw, they are a drive factor here. In line with Loukis (2007) the LMS quality, reliability and ease of use lead to learning efficiency as technology quality, online tools are predictors of learner satisfaction and intrinsic motivation. Face-to-face sessions should continue on a monthly basis as noted here and is in agreement with Marriot et al. ( 2004 ) who noted learner preference for it for facilitating social interaction and communication skills. High learner intrinsic motivation leads to persistence in online courses as noted by Menager-Beeley, ( 2004 ) and is high enough in our study. This implies a possibility of an effectiveness blended learning environment. The causes of learner dissatisfaction noted by Islam ( 2014 ) such as incompetence in the use of the LMS are contrary to our results in our study, while the one noted by Hara and Kling, ( 2001 ) as resulting from technical difficulties and ambiguous course instruction are no threat from our findings. Student-teacher interaction showed a relation with satisfaction according to Swan ( 2001 ) but is not a predictor in our study. Initiating knowledge construction by learners for blended learning effectiveness is exhibited in our findings and agrees with Rahman, Yasin and Jusof ( 2011 ). Our study has not agreed with Eom et al. ( 2006 ) who found learner interactions as predictors of learner satisfaction but agrees with Naaj et al. ( 2012 ) regarding technology as a predictor of learner satisfaction.
Conclusion and recommendations
An effective blended learning environment is necessary in undertaking innovative pedagogical approaches through the use of technology in teaching and learning. An examination of learner characteristics/background, design features and learning outcomes as factors for effectiveness can help to inform the design of effective learning environments that involve face-to-face sessions and online aspects. Most of the student characteristics and blended learning design features dealt with in this study are important factors for blended learning effectiveness. None of the independent variables were identified as significant predictors of student performance. These gaps are open for further investigation in order to understand if they can be significant predictors of blended learning effectiveness in a similar or different learning setting.
In planning to design and implement blended learning, we are mindful of the implications raised by this study which is a planning evaluation research for the design and eventual implementation of blended learning. Universities should be mindful of the interplay between the learner characteristics, design features and learning outcomes which are indicators of blended learning effectiveness. From this research, learners manifest high potential to take on blended learning more especially in regard to learner self-regulation exhibited. Blended learning is meant to increase learners’ levels of knowledge construction in order to create analytical skills in them. Learner ability to assess and critically evaluate knowledge sources is hereby established in our findings. This can go a long way in producing skilled learners who can be innovative graduates enough to satisfy employment demands through creativity and innovativeness. Technology being less of a shock to students gives potential for blended learning design. Universities and other institutions of learning should continue to emphasize blended learning approaches through installation of learning management systems along with strong internet to enable effective learning through technology especially in the developing world.
Abubakar, D. & Adetimirin. (2015). Influence of computer literacy on post-graduates’ use of e-resources in Nigerian University Libraries. Library Philosophy and Practice. From http://digitalcommons.unl.edu/libphilprac/ . Retrieved 18 Aug 2015.
Ahmad, N., & Al-Khanjari, Z. (2011). Effect of Moodle on learning: An Oman perception. International Journal of Digital Information and Wireless Communications (IJDIWC), 1 (4), 746–752.
Google Scholar
Anderson, T. (2004). Theory and Practice of Online Learning . Canada: AU Press, Athabasca University.
Arbaugh, J. B. (2000). How classroom environment and student engagement affect learning in internet-basedMBAcourses. Business Communication Quarterly, 63 (4), 9–18.
Article Google Scholar
Askar, P. & Altun, A. (2008). Learner satisfaction on blended learning. E-Leader Krakow , 2008.
Astleitner, H. (2000) Dropout and distance education. A review of motivational and emotional strategies to reduce dropout in web-based distance education. In Neuwe Medien in Unterricht, Aus-und Weiterbildung Waxmann Munster, New York.
Barnard, L., Lan, W. Y., To, Y. M., Paton, V. O., & Lai, S. (2009). Measuring self regulation in online and blended learning environments’. Internet and Higher Education, 12 (1), 1–6.
Beard, L. A., Harper, C., & Riley, G. (2004). Online versus on-campus instruction: student attitudes & perceptions. TechTrends, 48 (6), 29–31.
Berenson, R., Boyles, G., & Weaver, A. (2008). Emotional intelligence as a predictor for success in online learning. International Review of Research in open & Distance Learning, 9 (2), 1–16.
Blocker, J. M., & Tucker, G. (2001). Using constructivist principles in designing and integrating online collaborative interactions. In F. Fuller & R. McBride (Eds.), Distance education. Proceedings of the Society for Information Technology & Teacher Education International Conference (pp. 32–36). ERIC Document Reproduction Service No. ED 457 822.
Cohen, K. E., Stage, F. K., Hammack, F. M., & Marcus, A. (2012). Persistence of master’s students in the United States: Developing and testing of a conceptual model . USA: PhD Dissertation, New York University.
Coldwell, J., Craig, A., Paterson, T., & Mustard, J. (2008). Online students: Relationships between participation, demographics and academic performance. The Electronic Journal of e-learning, 6 (1), 19–30.
Deci, E. L., & Ryan, R. M. (1982). Intrinsic Motivation Inventory. Available from selfdeterminationtheory.org/intrinsic-motivation-inventory/ . Accessed 2 Aug 2016.
Delone, W. H., & McLean, E. R. (2003). The Delone and McLean model of information systems success: A Ten-year update. Journal of Management Information Systems, 19 (4), 9–30.
Demirkol, M., & Kazu, I. Y. (2014). Effect of blended environment model on high school students’ academic achievement. The Turkish Online Journal of Educational Technology, 13 (1), 78–87.
Eom, S., Wen, H., & Ashill, N. (2006). The determinants of students’ perceived learning outcomes and satisfaction in university online education: an empirical investigation’. Decision Sciences Journal of Innovative Education, 4 (2), 215–235.
Garrison, D. R., & Kanuka, H. (2004). Blended learning: Uncovering its transformative potential in higher education. Internet and Higher Education, 7 (2), 95–105.
Goyal, E., & Tambe, S. (2015). Effectiveness of Moodle-enabled blended learning in private Indian Business School teaching NICHE programs. The Online Journal of New Horizons in Education, 5 (2), 14–22.
Green, J., Nelson, G., Martin, A. J., & Marsh, H. (2006). The causal ordering of self-concept and academic motivation and its effect on academic achievement. International Education Journal, 7 (4), 534–546.
Guskey, T. R. (2000). Evaluating Professional Development . Thousands Oaks: Corwin Press.
Hadad, W. (2007). ICT-in-education toolkit reference handbook . InfoDev. from http://www.infodev.org/en/Publication.301.html . Retrieved 04 Aug 2015.
Hara, N. & Kling, R. (2001). Student distress in web-based distance education. Educause Quarterly. 3 (2001).
Heinich, R., Molenda, M., Russell, J. D., & Smaldino, S. E. (2001). Instructional Media and Technologies for Learning (7th ed.). Englewood Cliffs: Prentice-Hall.
Hofmann, J. (2014). Solutions to the top 10 challenges of blended learning. Top 10 challenges of blended learning. Available on cedma-europe.org .
Islam, A. K. M. N. (2014). Sources of satisfaction and dissatisfaction with a learning management system in post-adoption stage: A critical incident technique approach. Computers in Human Behaviour, 30 , 249–261.
Kelley, D. H. & Gorham, J. (2009) Effects of immediacy on recall of information. Communication Education, 37 (3), 198–207.
Kenney, J., & Newcombe, E. (2011). Adopting a blended learning approach: Challenges, encountered and lessons learned in an action research study. Journal of Asynchronous Learning Networks, 15 (1), 45–57.
Kintu, M. J., & Zhu, C. (2016). Student characteristics and learning outcomes in a blended learning environment intervention in a Ugandan University. Electronic Journal of e-Learning, 14 (3), 181–195.
Kuo, Y., Walker, A. E., Belland, B. R., & Schroder, L. E. E. (2013). A predictive study of student satisfaction in online education programs. International Review of Research in Open and Distributed Learning, 14 (1), 16–39.
Kwak, D. W., Menezes, F. M., & Sherwood, C. (2013). Assessing the impact of blended learning on student performance. Educational Technology & Society, 15 (1), 127–136.
Lim, D. H., & Kim, H. J. (2003). Motivation and learner characteristics affecting online learning and learning application. Journal of Educational Technology Systems, 31 (4), 423–439.
Lim, D. H., & Morris, M. L. (2009). Learner and instructional factors influencing learner outcomes within a blended learning environment. Educational Technology & Society, 12 (4), 282–293.
Lin, B., & Vassar, J. A. (2009). Determinants for success in online learning communities. International Journal of Web-based Communities, 5 (3), 340–350.
Loukis, E., Georgiou, S. & Pazalo, K. (2007). A value flow model for the evaluation of an e-learning service. ECIS, 2007 Proceedings, paper 175.
Lynch, R., & Dembo, M. (2004). The relationship between self regulation and online learning in a blended learning context. The International Review of Research in Open and Distributed Learning, 5 (2), 1–16.
Marriot, N., Marriot, P., & Selwyn. (2004). Accounting undergraduates’ changing use of ICT and their views on using the internet in higher education-A Research note. Accounting Education, 13 (4), 117–130.
Menager-Beeley, R. (2004). Web-based distance learning in a community college: The influence of task values on task choice, retention and commitment. (Doctoral dissertation, University of Southern California). Dissertation Abstracts International, 64 (9-A), 3191.
Naaj, M. A., Nachouki, M., & Ankit, A. (2012). Evaluating student satisfaction with blended learning in a gender-segregated environment. Journal of Information Technology Education: Research, 11 , 185–200.
Nurmela, K., Palonen, T., Lehtinen, E. & Hakkarainen, K. (2003). Developing tools for analysing CSCL process. In Wasson, B. Ludvigsen, S. & Hoppe, V. (eds), Designing for change in networked learning environments (pp 333–342). Dordrecht, The Netherlands, Kluwer.
Osgerby, J. (2013). Students’ perceptions of the introduction of a blended learning environment: An exploratory case study. Accounting Education, 22 (1), 85–99.
Oxford Group, (2013). Blended learning-current use, challenges and best practices. From http://www.kineo.com/m/0/blended-learning-report-202013.pdf . Accessed on 17 Mar 2016.
Packham, G., Jones, P., Miller, C., & Thomas, B. (2004). E-learning and retention key factors influencing student withdrawal. Education and Training, 46 (6–7), 335–342.
Pallant, J. (2010). SPSS Survival Mannual (4th ed.). Maidenhead: OUP McGraw-Hill.
Park, J.-H., & Choi, H. J. (2009). Factors influencing adult learners’ decision to drop out or persist in online learning. Educational Technology & Society, 12 (4), 207–217.
Picciano, A., & Seaman, J. (2007). K-12 online learning: A survey of U.S. school district administrators . New York, USA: Sloan-C.
Piccoli, G., Ahmad, R., & Ives, B. (2001). Web-based virtual learning environments: a research framework and a preliminary assessment of effectiveness in basic IT skill training. MIS Quarterly, 25 (4), 401–426.
Pituch, K. A., & Lee, Y. K. (2006). The influence of system characteristics on e-learning use. Computers & Education, 47 (2), 222–244.
Rahman, S. et al, (2011). Knowledge construction process in online learning. Middle East Journal of Scientific Research, 8 (2), 488–492.
Rovai, A. P. (2003). In search of higher persistence rates in distance education online programs. Computers & Education, 6 (1), 1–16.
Sankaran, S., & Bui, T. (2001). Impact of learning strategies and motivation on performance: A study in Web-based instruction. Journal of Instructional Psychology, 28 (3), 191–198.
Selim, H. M. (2007). Critical success factors for e-learning acceptance: Confirmatory factor models. Computers & Education, 49 (2), 396–413.
Shraim, K., & Khlaif, Z. N. (2010). An e-learning approach to secondary education in Palestine: opportunities and challenges. Information Technology for Development, 16 (3), 159–173.
Shrain, K. (2012). Moving towards e-learning paradigm: Readiness of higher education instructors in Palestine. International Journal on E-Learning, 11 (4), 441–463.
Song, L., Singleton, E. S., Hill, J. R., & Koh, M. H. (2004). Improving online learning: student perceptions of useful and challenging characteristics’. Internet and Higher Education, 7 (1), 59–70.
Stacey, E., & Gerbic, P. (2007). Teaching for blended learning: research perspectives from on-campus and distance students. Education and Information Technologies, 12 , 165–174.
Swan, K. (2001). Virtual interactivity: design factors affecting student satisfaction and perceived learning in asynchronous online courses. Distance Education, 22 (2), 306–331.
Article MathSciNet Google Scholar
Thompson, E. (2004). Distance education drop-out: What can we do? In R. Pospisil & L. Willcoxson (Eds.), Learning Through Teaching (Proceedings of the 6th Annual Teaching Learning Forum, pp. 324–332). Perth, Australia: Murdoch University.
Tselios, N., Daskalakis, S., & Papadopoulou, M. (2011). Assessing the acceptance of a blended learning university course. Educational Technology & Society, 14 (2), 224–235.
Willging, P. A., & Johnson, S. D. (2009). Factors that influence students’ decision to drop-out of online courses. Journal of Asynchronous Learning Networks, 13 (3), 115–127.
Zhu, C. (2012). Student satisfaction, performance and knowledge construction in online collaborative learning. Educational Technology & Society, 15 (1), 127–137.
Zielinski, D. (2000). Can you keep learners online? Training, 37 (3), 64–75.
Download references
Authors’ contribution
MJK conceived the study idea, developed the conceptual framework, collected the data, analyzed it and wrote the article. CZ gave the technical advice concerning the write-up and advised on relevant corrections to be made before final submission. EK did the proof-reading of the article as well as language editing. All authors read and approved the final manuscript.
Competing interests
The authors declare that they have no competing interests.
Author information
Authors and affiliations.
Mountains of the Moon University, P.O. Box 837, Fort Portal, Uganda
Mugenyi Justice Kintu & Edmond Kagambe
Vrije Universiteit Brussel, Pleinlaan 2, Brussels, 1050, Ixelles, Belgium
Mugenyi Justice Kintu & Chang Zhu
You can also search for this author in PubMed Google Scholar
Corresponding author
Correspondence to Mugenyi Justice Kintu .
Rights and permissions
Open Access This article is distributed under the terms of the Creative Commons Attribution 4.0 International License ( http://creativecommons.org/licenses/by/4.0/ ), which permits unrestricted use, distribution, and reproduction in any medium, provided you give appropriate credit to the original author(s) and the source, provide a link to the Creative Commons license, and indicate if changes were made.
Reprints and permissions
About this article
Cite this article.
Kintu, M.J., Zhu, C. & Kagambe, E. Blended learning effectiveness: the relationship between student characteristics, design features and outcomes. Int J Educ Technol High Educ 14 , 7 (2017). https://doi.org/10.1186/s41239-017-0043-4
Download citation
Received : 13 July 2016
Accepted : 23 November 2016
Published : 06 February 2017
DOI : https://doi.org/10.1186/s41239-017-0043-4
Share this article
Anyone you share the following link with will be able to read this content:
Sorry, a shareable link is not currently available for this article.
Provided by the Springer Nature SharedIt content-sharing initiative
- Blended learning effectiveness
- Learner characteristics
- Design features
- Learning outcomes and significant predictors

An official website of the United States government
The .gov means it’s official. Federal government websites often end in .gov or .mil. Before sharing sensitive information, make sure you’re on a federal government site.
The site is secure. The https:// ensures that you are connecting to the official website and that any information you provide is encrypted and transmitted securely.
- Publications
- Account settings
Preview improvements coming to the PMC website in October 2024. Learn More or Try it out now .
- Advanced Search
- Journal List
- Behav Sci (Basel)

Assessing Cognitive Factors of Modular Distance Learning of K-12 Students Amidst the COVID-19 Pandemic towards Academic Achievements and Satisfaction
Yung-tsan jou.
1 Department of Industrial and Systems Engineering, Chung Yuan Christian University, Taoyuan 320, Taiwan; wt.ude.ucyc@uojty (Y.-T.J.); moc.oohay@enimrahcrolfas (C.S.S.)
Klint Allen Mariñas
2 School of Industrial Engineering and Engineering Management, Mapua University, Manila 1002, Philippines
3 Department of Industrial Engineering, Occidental Mindoro State College, San Jose 5100, Philippines
Charmine Sheena Saflor
Associated data.
Not applicable.
The COVID-19 pandemic brought extraordinary challenges to K-12 students in using modular distance learning. According to Transactional Distance Theory (TDT), which is defined as understanding the effects of distance learning in the cognitive domain, the current study constructs a theoretical framework to measure student satisfaction and Bloom’s Taxonomy Theory (BTT) to measure students’ academic achievements. This study aims to evaluate and identify the possible cognitive capacity influencing K-12 students’ academic achievements and satisfaction with modular distance learning during this new phenomenon. A survey questionnaire was completed through an online form by 252 K-12 students from the different institutions of Occidental Mindoro. Using Structural Equation Modeling (SEM), the researcher analyses the relationship between the dependent and independent variables. The model used in this research illustrates cognitive factors associated with adopting modular distance learning based on students’ academic achievements and satisfaction. The study revealed that students’ background, experience, behavior, and instructor interaction positively affected their satisfaction. While the effects of the students’ performance, understanding, and perceived effectiveness were wholly aligned with their academic achievements. The findings of the model with solid support of the integrative association between TDT and BTT theories could guide decision-makers in institutions to implement, evaluate, and utilize modular distance learning in their education systems.
1. Introduction
The 2019 coronavirus is the latest infectious disease to develop rapidly worldwide [ 1 ], affecting economic stability, global health, and education. Most countries have suspended thee-to-face classes in order to curb the spread of the virus and reduce infections [ 2 ]. One of the sectors impacted has been education, resulting in the suspension of face-to-face classes to avoid spreading the virus. The Department of Education (DepEd) has introduced modular distance learning for K-12 students to ensure continuity of learning during the COVID-19 pandemic. According to Malipot (2020), modular learning is one of the most popular sorts of distance learning alternatives to traditional face-to-face learning [ 3 ]. As per DepEd’s Learner Enrolment and Survey Forms, 7.2 million enrollees preferred “modular” remote learning, TV and radio-based practice, and other modalities, while two million enrollees preferred online learning. It is a method of learning that is currently being used based on the preferred distance learning mode of the students and parents through the survey conducted by the Department of Education (DepEd); this learning method is mainly done through the use of printed and digital modules [ 4 ]. It also concerns first-year students in rural areas; the place net is no longer available for online learning. Supporting the findings of Ambayon (2020), modular teaching within the teach-learn method is more practical than traditional educational methods because students learn at their own pace during this modular approach. This educational platform allows K-12 students to interact in self-paced textual matter or digital copy modules. With these COVID-19 outbreaks, some issues concerned students’ academic, and the factors associated with students’ psychological status during the COVID-19 lockdown [ 5 ].
Additionally, this new learning platform, modular distance learning, seems to have impacted students’ ability to discover and challenged their learning skills. Scholars have also paid close attention to learner satisfaction and academic achievement when it involves distance learning studies and have used a spread of theoretical frameworks to assess learner satisfaction and educational outcomes [ 6 , 7 ]. Because this study aimed to boost academic achievement and satisfaction in K-12 students, the researcher thoroughly applied transactional distance theory (TDT) to understand the consequences of distance in relationships in education. The TDT was utilized since it has the capability to establish the psychological and communication factors between the learners and the instructors in distance education that could eventually help researchers in identifying the variables that might affect students’ academic achievement and satisfaction [ 8 ]. In this view, distance learning is primarily determined by the number of dialogues between student and teacher and the degree of structuring of the course design. It contributes to the core objective of the degree to boost students’ modular learning experiences in terms of satisfaction. On the other hand, Bloom’s Taxonomy Theory (BTT) was applied to investigate the students’ academic achievements through modular distance learning [ 6 ]. Bloom’s theory was employed in addition to TDT during this study to enhance students’ modular educational experiences. Moreover, TDT was utilized to check students’ modular learning experiences in conjuction with enhacing students’ achievements.
This study aimed to detect the impact of modular distance learning on K-12 students during the COVID-19 pandemic and assess the cognitive factors affecting academic achievement and student satisfaction. Despite the challenging status of the COVID-19 outbreak, the researcher anticipated a relevant result of modular distance learning and pedagogical changes in students, including the cognitive factors identified during this paper as latent variables as possible predictors for the utilization of K-12 student academic achievements and satisfaction.
1.1. Theoretical Research Framework
This study used TDT to assess student satisfaction and Bloom’s theory to quantify academic achievement. It aimed to assess the impact of modular distance learning on academic achievement and student satisfaction among K-12 students. The Transactional Distance Theory (TDT) was selected for this study since it refers to student-instructor distance learning. TDT Moore (1993) states that distance education is “the universe of teacher-learner connections when learners and teachers are separated by place and time.” Moore’s (1990) concept of ”Transactional Distance” adopts the distance that occurs in all linkages in education, according to TDT Moore (1993). Transactional distance theory is theoretically critical because it states that the most important distance is transactional in distance education, rather than geographical or temporal [ 9 , 10 ]. According to Garrison (2000), transactional distance theory is essential in directing the complicated experience of a cognitive process such as distance teaching and learning. TDT evaluates the role of each of these factors (student perception, discourse, and class organization), which can help with student satisfaction research [ 11 ]. Bloom’s Taxonomy is a theoretical framework for learning created by Benjamin Bloom that distinguishes three learning domains: Cognitive domain skills center on knowledge, comprehension, and critical thinking on a particular subject. Bloom recognized three components of educational activities: cognitive knowledge (or mental abilities), affective attitude (or emotions), and psychomotor skills (or physical skills), all of which can be used to assess K-12 students’ academic achievement. According to Jung (2001), “Transactional distance theory provides a significant conceptual framework for defining and comprehending distance education in general and a source of research hypotheses in particular,” shown in Figure 1 [ 12 ].

Theoretical Research Framework.
1.2. Hypothesis Developments and Literature Review
This section will discuss the study hypothesis and relate each hypothesis to its related studies from the literature.
There is a significant relationship between students’ background and students’ behavior .
The teacher’s guidance is essential for students’ preparedness and readiness to adapt to a new educational environment. Most students opt for the Department of Education’s “modular” distance learning options [ 3 ]. Analyzing students’ study time is critical for behavioral engagement because it establishes if academic performance is the product of student choice or historical factors [ 13 ].
There is a significant relationship between students’ background and students’ experience .
Modules provide goals, experiences, and educational activities that assist students in gaining self-sufficiency at their speed. It also boosts brain activity, encourages motivation, consolidates self-satisfaction, and enables students to remember what they have learned [ 14 ]. Despite its success, many families face difficulties due to their parents’ lack of skills and time [ 15 ].
There is a significant relationship between students’ behavior and students’ instructor interaction .
Students’ capacity to answer problems reflects their overall information awareness [ 5 ]. Learning outcomes can either cause or result in students and instructors behavior. Students’ reading issues are due to the success of online courses [ 16 ].
There is a significant relationship between students’ experience and students’ instructor interaction .
The words “student experience” relate to classroom participation. They establish a connection between students and their school, teachers, classmates, curriculum, and teaching methods [ 17 ]. The three types of student engagement are behavioral, emotional, and cognitive. Behavioral engagement refers to a student’s enthusiasm for academic and extracurricular activities. On the other hand, emotional participation is linked to how children react to their peers, teachers, and school. Motivational engagement refers to a learner’s desire to learn new abilities [ 18 ].
There is a significant relationship between students’ behavior and students’ understanding .
Individualized learning connections, outstanding training, and learning culture are all priorities at the Institute [ 19 , 20 ]. The modular technique of online learning offers additional flexibility. The use of modules allows students to investigate alternatives to the professor’s session [ 21 ].
There is a significant relationship between students’ experience and students’ performance .
Student conduct is also vital in academic accomplishment since it may affect a student’s capacity to study as well as the learning environment for other students. Students are self-assured because they understand what is expected [ 22 ]. They are more aware of their actions and take greater responsibility for their learning.
There is a significant relationship between students’ instructor interaction and students’ understanding .
Modular learning benefits students by enabling them to absorb and study material independently and on different courses. Students are more likely to give favorable reviews to courses and instructors if they believe their professors communicated effectively and facilitated or supported their learning [ 23 ].
There is a significant relationship between students’ instructor interaction and students’ performance.
Students are more engaged and active in their studies when they feel in command and protected in the classroom. Teachers play an essential role in influencing student academic motivation, school commitment, and disengagement. In studies on K-12 education, teacher-student relationships have been identified [ 24 ]. Positive teacher-student connections improve both teacher attitudes and academic performance.
There is a significant relationship between students’ understanding and students’ satisfaction .
Instructors must create well-structured courses, regularly present in their classes, and encourage student participation. When learning objectives are completed, students better understand the course’s success and learning expectations. “Constructing meaning from verbal, written, and graphic signals by interpreting, exemplifying, classifying, summarizing, inferring, comparing, and explaining” is how understanding is characterized [ 25 ].
There is a significant relationship between students’ performance and student’s academic achievement .
Academic emotions are linked to students’ performance, academic success, personality, and classroom background [ 26 ]. Understanding the elements that may influence student performance has long been a goal for educational institutions, students, and teachers.
There is a significant relationship between students’ understanding and students’ academic achievement .
Modular education views each student as an individual with distinct abilities and interests. To provide an excellent education, a teacher must adapt and individualize the educational curriculum for each student. Individual learning may aid in developing a variety of exceptional and self-reliant attributes [ 27 ]. Academic achievement is the current level of learning in the Philippines [ 28 ].
There is a significant relationship between students’ performance and students’ satisfaction .
Academic success is defined as a student’s intellectual development, including formative and summative assessment data, coursework, teacher observations, student interaction, and time on a task [ 29 ]. Students were happier with course technology, the promptness with which content was shared with the teacher, and their overall wellbeing [ 30 ].
There is a significant relationship between students’ academic achievement and students’ perceived effectiveness .
Student satisfaction is a short-term mindset based on assessing students’ educational experiences [ 29 ]. The link between student satisfaction and academic achievement is crucial in today’s higher education: we discovered that student satisfaction with course technical components was linked to a higher relative performance level [ 31 ].
There is a significant relationship between students’ satisfaction and students’ perceived effectiveness.
There is a strong link between student satisfaction and their overall perception of learning. A satisfied student is a direct effect of a positive learning experience. Perceived learning results had a favorable impact on student satisfaction in the classroom [ 32 ].
2. Materials and Methods
2.1. participants.
The principal area under study was San Jose, Occidental Mindoro, although other locations were also accepted. The survey took place between February and March 2022, with the target population of K-12 students in Junior and Senior High Schools from grades 7 to 12, aged 12 to 20, who are now implementing the Modular Approach in their studies during the COVID-19 pandemic. A 45-item questionnaire was created and circulated online to collect the information. A total of 300 online surveys was sent out and 252 online forms were received, a total of 84% response rate [ 33 ]. According to several experts, the sample size for Structural Equation Modeling (SEM) should be between 200 and 500 [ 34 ].
2.2. Questionnaire
The theoretical framework developed a self-administered test. The researcher created the questionnaire to examine and discover the probable cognitive capacity influencing K-12 students’ academic achievement in different parts of Occidental Mindoro during this pandemic as well as their satisfaction with modular distance learning. The questionnaire was designed through Google drive as people’s interactions are limited due to the effect of the COVID-19 pandemic. The questionnaire’s link was sent via email, Facebook, and other popular social media platforms.
The respondents had to complete two sections of the questionnaire. The first is their demographic information, including their age, gender, and grade level. The second is about their perceptions of modular learning. The questionnaire is divided into 12 variables: (1) Student’s Background, (2) Student’s Experience, (3) Student’s Behavior, (4) Student’s Instructor Interaction, (5) Student’s Performance, (6) Student’s Understanding, (7) Student’s Satisfaction, (8) Student’s Academic Achievement, and (9) Student’s Perceived Effectiveness. A 5-point Likert scale was used to assess all latent components contained in the SEM shown in Table 1 .
The construct and measurement items.
Construct | Items | Measures | Supporting Reference |
---|---|---|---|
Students’ Background (SB) | SB1 | Are you having difficulty with Modular Distance Learning. | Pe Dangle, Y.R. (2020) [ ] |
SB2 | I prefer Modular Distance Learning Rather than traditional face-to-face training, I prefer the Modular Distance Learning Approach. | Aksan, J.A. (2021) [ ] | |
SB3 | Modular learning aids students in increasing their productivity in education and learning while promoting flexibility in terms of content, time, and space. | Shuja, A. et al. (2019) [ ] | |
SB4 | I have a lot of time to answer the activities with a modular teaching technique. | Aksan, J.A. (2021) [ ] | |
SB5 | I acquire the same amount of learning from using the module as I do from learning in a face-to-face or classroom situation. | Natividad, E. (2021) [ ] | |
Students’ Behavior (SBE) | SBE1 | I feel confident in studying and performing well in the modular class. | Delfino, A.P. (2019) [ ] |
SBE2 | I employed rehearsing techniques like reviewing my notes over and over again. | Lowerison et al. (2006) [ ] | |
SBE3 | I can recall my understanding from the past and help me to understand words. | Santillan, S.C. et al. (2021) [ ] | |
SBE4 | I retain a critical mindset throughout my studies, considering before accepting or rejecting. | Bordeos (2021) [ ] | |
SBE5 | Usually I plan my weekly module work in advance. | Karababa et al. (2010) [ ] | |
Students’ Experience (SE) | SE1 | The way the module materials were presented helped to maintain my interest. | Allen et al. (2020) [ ] |
SE2 | I do not experience any problems during modular distance learning | Amir al (2020) [ ] | |
SE3 | The instructions for completing the assessed tasks were simple to understand. | Santillan et al. (2021) [ ] | |
SE4 | During distance learning, I am not stressed. | Amir et al. (2020) [ ] | |
SE5 | The study workload on this module fitted with my personal circumstances. | Allen et al. (2020) [ ] | |
Students’ Instructor Interaction (SI) | SI1 | The instructor updated me on my progress in the course regularly. | Gray & DiLoreto (2020) [ ] |
SI2 | On this subject, I was satisfied with my teacher’s assistance. | Allen et al. (2020) [ ] | |
SI3 | I kept in touch with the course’s instructor regularly. | Gray & DiLoreto (2020) [ ] | |
SI4 | The instructor was concerned about my performance in this class. | Gray & DiLoreto (2020) [ ] | |
SI5 | My teacher feedback on assessed tasks helped me prepare for the next assessment. | Allen et al. (2020) [ ] | |
Students’ Understanding (SAU) | SAU1 | Modular Distance Learning allows me to take my time to understand my school works. | Abuhassna et al. (2020) [ ] |
SAU2 | The distance learning program met my expectations in terms of quality. | Woolf et al. (2020) [ ] | |
SAU3 | Modular Distance Learning helps me to improve my understanding and skills and also helps to gather new knowledge. | Bordeos (2021) [ ] | |
SAU4 | Modular Distance Learning is a helpful tool to get so focused on activities in my classes. | Abuhassna et al. (2020) [ ] | |
SAU5 | Modular Distance Learning motivates me to study more about the course objectives. | Abuhassna et al. (2020) [ ] | |
Students’ Performance (SP) | SP1 | I can effectively manage my study time and complete assignments on schedule. | Richardson and Swan (2003) [ ] |
SP2 | When completing projects or participating in class discussions, combine ideas or concepts from several courses. | Delfino, A.P. (2019) [ ] | |
SP3 | I employed elaboration techniques like summarizing the material and relating it to previous knowledge. | Lowerison et al. (2006) [ ] | |
SP4 | In my studies, I am self-disciplined and find it easy to schedule reading and homework time. | Richardson and Swan (2003) [ ] | |
SP5 | I was confident in my capacity to learn and do well in class. | Delfino, A.P. (2019) [ ] | |
Student’s Academic Achievement (SAA) | SAA1 | I have more opportunities to reflect on what I’ve learned in modular classes. | Dziuban et al. (2015) [ ] |
SAA2 | I am committed to completing my homework (readings, assignments) on time and engaging fully in class discussions. | Mt. San Antonio College (2012) [ ] | |
SAA3 | My modular learning experience has increased my opportunity to access and use information. | Dziuban et al. (2015) [ ] | |
SAA4 | I employed assessment, evaluation, and criticizing procedures for assessing, evaluating, and critiquing the material. | Lowerison et al. (2006) [ ] | |
SAA5 | I am skilled at juggling many responsibilities while working under time constraints. | Estelami (2013) [ ] | |
Students’ Satisfaction (SS) | SS1 | I am always interested in learning about new things. | Abuhassna et al. (2020) [ ] |
SS2 | I study more efficiently with distance learning. | Amir (2020) [ ] | |
SS3 | Modular learning suits me better than face-to-face classes. | Abuhassna et al. (2020) [ ] | |
SS4 | I prefer distance learning to classroom learning. | Amir et al. (2020) [ ] | |
SS5 | Overall, I am pleased with the module’s quality. | Santillan, S.C. et al. (2021) [ ] | |
Students’ Perceived Effectiveness (SPE) | SPE1 | I made use of learning possibilities and resources in this modular distance learning. | Lowerison et al. (2006) [ ] |
SPE2 | I would recommend modular distance learning study to other students. | Abuhassna et al. (2020) [ ] | |
SPE3 | These classes also challenge me to conduct more independent research and not rely on a single source of information. | Mt. San Antonio College (2012) [ ] | |
SPE4 | Overall, this modular distance learning has been a good platform for studying during the pandemic. | Lowerison et al., 2006) [ ] | |
SPE5 | Overall, I am satisfied with this modular distance learning course. | Aman (2009) [ ] |
2.3. Structural Equation Modeling (SEM)
All the variables have been adapted from a variety of research in the literature. The observable factors were scored on a Likert scale of 1–5, with one indicating “strongly disagree” and five indicating “strongly agree”, and the data were analyzed using AMOS software. Theoretical model data were confirmed by Structural Equation Modeling (SEM). SEM is more suitable for testing the hypothesis than other methods [ 53 ]. There are many fit indices in the literature, of which the most commonly used are: CMIN/DF, Comparative Fit Index (CFI), AGFI, GFI, and Root Mean Square Error (RMSEA). Table 2 demonstrates the Good Fit Values and Acceptable Fit Values of the fit indices, respectively. AGFI and GFI are based on residuals; when sample size increases, the value of the AGFI also increase. It takes a value between 0 and 1. The fit is good if the value is more significant than 0.80. GFI is a model index that spans from 0 to 1, with values above 0.80 deemed acceptable. An RMSEA of 0.08 or less suggests a good fit [ 54 ], and a value of 0.05 to 0.08 indicates an adequate fit [ 55 ].
Acceptable Fit Values.
Fit Indices | Acceptable Range | Reference |
---|---|---|
CMIN/DF | <3.00 | Norberg et al., 2007 [ ]; Li et al., 2013 [ ] |
GFI | ≥0.80 | Doloi et al., 2012 [ ] |
CFI | >0.70 | Norberg et al., 2007 [ ]; Chen et al., 2012 [ ] |
RMSEA | ≤0.08 | Doloi et al., 2012 [ ] |
AGFI | >0.08 | Jaccard and Wan (1996) [ ] |
TLI | >0.08 | Jafari et al., 2021 [ ] |
IFI | >0.08 | Lee et al., 2015 [ ] |
3. Results and Discussion
Figure 2 demonstrates the initial SEM for the cognitive factors of Modular Distance learning towards academic achievements and satisfaction of K-12 students during the COVID-19 pandemic. According to the figure below, three hypotheses were not significant: Students’ Behavior to Students’ Instructor Interaction (Hypothesis 3), Students’ Understanding of Students’ Academic Achievement (Hypothesis 11), and Students’ Performance to Students’ Satisfaction (Hypothesis 12). Therefore, a revised SEM was derived by removing this hypothesis in Figure 3 . We modified some indices to enhance the model fit based on previous studies using the SEM approach [ 47 ]. Figure 3 demonstrates the final SEM for evaluating cognitive factors affecting academic achievements and satisfaction and the perceived effectiveness of K-12 students’ response to Modular Learning during COVID-19, shown in Table 3 . Moreover, Table 4 demonstrates the descriptive statistical results of each indicator.

Initial SEM with indicators for evaluating the cognitive factors of modular distance learning towards academic achievements and satisfaction of K-12 students during COVID-19 pandemic.

Revised SEM with indicators for evaluating the cognitive factors of modular distance learning towards academic achievements and satisfaction of K-12 students during the COVID-19 pandemic.
Summary of the Results.
Hypothesis | -Value | Interpretation | |
---|---|---|---|
H1 | There is a significant relationship between Students’ Background and Students’ Behavior | 0.001 | Significant |
H2 | There is a significant relationship between Students’ Background and Students’ Experiences. | 0.001 | Significant |
H3 | There is a significant relationship between Students’ Behavior and Students’ instructor Interaction. | 0.155 | Not Significant |
H4 | There is a significant relationship between Students’ experience and Students—Interaction | 0.020 | Significant |
H5 | There is a significant relationship between Students’ Behavior and Students’ Understanding | 0.212 | Not Significant |
H6 | There is a significant relationship between Students’ experience and Students’ Performance | 0.001 | Significant |
H7 | There is a significant relationship between Students’ instructor Interaction and Students’ Understanding | 0.008 | Significant |
H8 | There is a significant relationship between Students’ Instructor—Interaction and students’ Performance | 0.018 | Significant |
H9 | There is a significant relationship between students’ Understanding and Students’ Satisfaction | 0.001 | Significant |
H10 | There is a significant relationship between students’ Performance and Students’ Academic Achievement | 0.001 | Significant |
H11 | There is a significant relationship between students’ understanding and Students’ Academic Achievement | 0.001 | Significant |
H12 | There is a significant relationship between students’ Performance and Students Satisfaction | 0.602 | Not Significant |
H13 | There is a significant relationship between Students’ Academic Achievement and students’ Perceived Effectiveness | 0.001 | Significant |
H14 | There is a significant relationship between students’ Satisfaction and Students’ Perceived Effectiveness | 0.001 | Significant |
Descriptive statistic results.
Factor | Item | Mean | SD | Factor Loading | |
---|---|---|---|---|---|
Initial Model | Final Model | ||||
Students’ Background | SB1 | 3.437 | 0.9147 | 0.052 | - |
SB2 | 3.000 | 1.1707 | 0.480 | 0.562 | |
SB3 | 3.663 | 0.8042 | 0.551 | 0.551 | |
SB4 | 3.742 | 0.8797 | 0.381 | - | |
SB5 | 3.024 | 1.0746 | 0.557 | 0.629 | |
Students’ Behavior | SBE1 | 3.667 | 0.8468 | 0.551 | - |
SBE2 | 3.829 | 0.8075 | 0.463 | - | |
SBE3 | 3.873 | 0.7305 | 0.507 | - | |
SBE4 | 3.833 | 0.8157 | 0.437 | - | |
SBE 5 | 3.849 | 0.8188 | 0.585 | - | |
Students’ Experience | SE1 | 3.853 | 0.8458 | 0.669 | 0.686 |
SE2 | 2.825 | 1.0643 | 0.572 | 0.525 | |
SE3 | 3.591 | 0.9035 | 0.630 | 0.634 | |
SE4 | 2.758 | 1.1260 | 0.639 | 0.611 | |
SE5 | 3.615 | 0.8693 | 0.552 | 0.551 | |
Students’ Instructor Interaction | SI1 | 3.754 | 0.7698 | 0.689 | - |
SI2 | 3.817 | 0.9095 | 0.655 | 0.541 | |
SI3 | 3.730 | 0.9269 | 0.731 | 0.645 | |
SI4 | 3.929 | 0.7487 | 0.685 | 0.568 | |
SI5 | 3.909 | 0.7805 | 0.669 | 0.597 | |
Students’ Understanding | SAU1 | 4.028 | 0.8152 | 0.647 | 0.652 |
SAU2 | 3.464 | 0.8390 | 0.691 | 0.704 | |
SAU3 | 3.873 | 0.8325 | 0.658 | 0.620 | |
SAU4 | 3.750 | 0.8775 | 0.731 | 0.741 | |
SAU5 | 3.794 | 0.8591 | 0.740 | 0.717 | |
Students’ Performance | SP1 | 3.762 | 0.8602 | 0.640 | - |
SP2 | 3.881 | 0.7995 | 0.708 | 0.655 | |
SP3 | 3.778 | 0.8781 | 0.582 | 0.606 | |
SP4 | 3.905 | 0.7927 | 0.647 | 0.585 | |
SP5 | 3.976 | 0.8275 | 0.630 | 0.673 | |
Student’s Academic Achievement | SAA1 | 3.885 | 0.7976 | 0.696 | 0.713 |
SAA2 | 3.929 | 0.7749 | 0.653 | 0.658 | |
SAA3 | 3.762 | 0.8319 | 0.632 | 0.615 | |
SAA4 | 3.837 | 0.7790 | 0.612 | 0.597 | |
SAA5 | 3.694 | 0.8959 | 0.559 | - | |
Students’ Satisfaction | SS1 | 4.087 | 0.7835 | 0.189 | - |
SS2 | 3.361 | 0.9943 | 0.657 | 0.669 | |
SS3 | 2.960 | 1.1390 | 0.779 | 0.659 | |
SS4 | 2.889 | 1.1516 | 0.759 | 0.677 | |
SS5 | 3.377 | 1.0918 | 0.802 | 0.803 | |
Students’ Perceived Effectiveness | SPE1 | 3.829 | 0.7875 | 0.580 | 0.558 |
SPE2 | 3.405 | 1.0153 | 0.730 | 0.750 | |
PE3 | 3.790 | 0.8178 | 0.490 | - | |
PE4 | 3.813 | 0.9367 | 0.614 | - | |
PE5 | 3.492 | 1.0000 | 0.696 | 0.690 |
The current study was improved by Moore’s transactional distance theory (TDT) and Bloom’s taxonomy theory (BTT) to evaluate cognitive factors affecting academic achievements and satisfaction and the perceived effectiveness of K-12 students’ response toward modular learning during COVID-19. SEM was utilized to analyze the correlation between Student Background (SB), Student Experience (SE), Student Behavior (SBE), Student Instructor Interaction (SI), Student Performance (SP), Student Understanding (SAU), Student Satisfaction (SS), Student’s Academic achievement (SAA), and Student’s Perceived effectiveness (SPE). A total of 252 data samples were acquired through an online questionnaire.
According to the findings of the SEM, the students’ background in modular learning had a favorable and significant direct effect on SE (β: 0.848, p = 0.009). K-12 students should have a background and knowledge in modular systems to better experience this new education platform. Putting the students through such an experience would support them in overcoming all difficulties that arise due to the limitations of the modular platforms. Furthermore, SEM revealed that SE had a significant adverse impact on SI (β: 0.843, p = 0.009). The study shows that students who had previous experience with modular education had more positive perceptions of modular platforms. Additionally, students’ experience with modular distance learning offers various benefits to them and their instructors to enhance students’ learning experiences, particularly for isolated learners.
Regarding the Students’ Interaction—Instructor, it positively impacts SAU (β: 0.873, p = 0.007). Communication helps students experience positive emotions such as comfort, satisfaction, and excitement, which aim to enhance their understanding and help them attain their educational goals [ 62 ]. The results revealed that SP substantially impacted SI (β: 0.765; p = 0.005). A student becomes more academically motivated and engaged by creating and maintaining strong teacher-student connections, which leads to successful academic performance.
Regarding the Students’ Understanding Response, the results revealed that SAA (β: 0.307; p = 0.052) and SS (β: 0.699; p = 0.008) had a substantial impact on SAU. Modular teaching is concerned with each student as an individual and with their specific capability and interest to assist each K-12 student in learning and provide quality education by allowing individuality to each learner. According to the Department of Education, academic achievement is the new level for student learning [ 63 ]. Meanwhile, SAA was significantly affected by the Students’ Performance Response (β: 0.754; p = 0.014). It implies that a positive performance can give positive results in student’s academic achievement, and that a negative performance can also give negative results [ 64 ]. Pekrun et al. (2010) discovered that students’ academic emotions are linked to their performance, academic achievement, personality, and classroom circumstances [ 26 ].
Results showed that students’ academic achievement significantly positively affects SPE (β: 0.237; p = 0.024). Prior knowledge has had an indirect effect on academic accomplishment. It influences the amount and type of current learning system where students must obtain a high degree of mastery [ 65 ]. According to the student’s opinion, modular distance learning is an alternative solution for providing adequate education for all learners and at all levels in the current scenario under the new education policy [ 66 ]. However, the SEM revealed that SS significantly affected SPE (β: 0.868; p = 0.009). Students’ perceptions of learning and satisfaction, when combined, can provide a better knowledge of learning achievement [ 44 ]. Students’ perceptions of learning outcomes are an excellent predictor of student satisfaction.
Since p -values and the indicators in Students’ Behavior are below 0.5, therefore two paths connecting SBE to students’ interaction—instructor (0.155) and students’ understanding (0.212) are not significant; thus, the latent variable Students’ Behavior has no effect on the latent variable Students’ Satisfaction and academic achievement as well as perceived effectiveness on modular distance learning of K12 students. This result is supported by Samsen-Bronsveld et al. (2022), who revealed that the environment has no direct influence on the student’s satisfaction, behavior engagement, and motivation to study [ 67 ]. On the other hand, the results also showed no significant relationship between Students’ Performance and Students’ Satisfaction (0.602) because the correlation p -values are greater than 0.5. Interestingly, this result opposed the other related studies. According to Bossman & Agyei (2022), satisfaction significantly affects performance or learning outcomes [ 68 ]. In addition, it was discovered that the main drivers of the students’ performance are the students’ satisfaction [ 64 , 69 ].
The result of the study implies that the students’ satisfaction serves as the mediator between the students’ performance and the student-instructor interaction in modular distance learning for K-12 students [ 70 ].
Table 5 The reliabilities of the scales used, i.e., Cronbach’s alphas, ranged from 0.568 to 0.745, which were in line with those found in other studies [ 71 ]. As presented in Table 6 , the IFI, TLI, and CFI values were greater than the suggested cutoff of 0.80, indicating that the specified model’s hypothesized construct accurately represented the observed data. In addition, the GFI and AGFI values were 0.828 and 0.801, respectively, indicating that the model was also good. The RMSEA value was 0.074, lower than the recommended value. Finally, the direct, indirect, and total effects are presented in Table 7 .
Construct Validity Model.
Factor | Number of Items | Cronbach’s α |
---|---|---|
Students’ Background | 3 | 0.598 |
Students’ Behavior | 5 | 0.682 |
Students’ Experience | 5 | 0.761 |
Students’ Instructor Interaction | 5 | 0.817 |
Students’ Understanding | 5 | 0.825 |
Students’ Performance | 5 | 0.768 |
Students’ Academic Achievement | 5 | 0.770 |
Students’ Satisfaction | 5 | 0.777 |
Students’ Perceived Effectiveness | 5 | 0.772 |
Total | 0.752 |
Goodness of Fit Measures of SEM | Parameter Estimates | Minimum Cut-Off | Interpretation |
---|---|---|---|
CMIN/DF | 2.375 | <3.0 | Acceptable |
Comparative Fit Index (CFI) | 0.830 | >0.8 | Acceptable |
Incremental Fit Index (IFI) | 0.832 | >0.8 | Acceptable |
Tucker Lewis Index (TLI) | 0.812 | >0.8 | Acceptable |
Goodness of Fit Index (GFI) | 0.812 | >0.8 | Acceptable |
Adjusted Goodness of Fit Index (AGFI) | 0.803 | >0.8 | Acceptable |
Root Mean Square Error (RMSEA) | 0.074 | <0.08 | Acceptable |
Direct effect, indirect effect, and total effect.
No. | Variable | Direct Effects | -Value | Indirect Effects | -Value | Total Effects | -Value |
---|---|---|---|---|---|---|---|
1 | SB–SE | 0.848 | 0.009 | - | - | 0.848 | 0.009 |
2 | SB–SI | - | - | 0.715 | 0.006 | 0.715 | 0.006 |
3 | SB–SAU | - | - | 0.624 | 0.006 | 0.624 | 0.006 |
4 | SB–SP | - | - | 0.547 | 0.004 | 0.547 | 0.004 |
5 | SB–SAA | - | - | 0.604 | 0.006 | 0.604 | 0.006 |
6 | SB–SS | - | - | 0.436 | 0.006 | 0.436 | 0.006 |
7 | SB–SPE | - | - | 0.522 | 0.007 | 0.522 | 0.006 |
8 | SE–SI | 0.843 | 0.009 | - | - | 0.843 | 0.009 |
9 | SE–SAU | - | - | 0.736 | 0.010 | 0.736 | 0.010 |
10 | SE–SP | - | - | 0.645 | 0.005 | 0.645 | 0.005 |
11 | SE–SAA | - | - | 0.713 | 0.006 | 0.713 | 0.006 |
12 | SE–SS | - | - | 0.514 | 0.006 | 0.514 | 0.006 |
13 | SE–SPE | - | - | 0.615 | 0.006 | 0.615 | 0.006 |
14 | SI–SAU | 0.873 | 0.007 | - | - | 0.873 | 0.007 |
15 | SI–SP | 0.765 | 0.005 | - | - | 0.765 | 0.005 |
16 | SI–SAA | - | - | 0.845 | 0.004 | 0.845 | 0.004 |
17 | SI–SS | - | - | 0.610 | 0.004 | 0.610 | 0.004 |
18 | SI–SPE | - | - | 0.730 | 0.007 | 0.730 | 0.007 |
19 | SAU–SP | - | - | - | - | - | - |
20 | SAU–SAA | 0.307 | 0.052 | - | - | 0.307 | 0.052 |
21 | SAU–SS | 0.699 | 0.008 | - | - | 0.699 | 0.008 |
22 | SAU–SPE | - | - | 0.680 | 0.011 | 0.680 | 0.011 |
23 | SP–SAA | 0.754 | 0.014 | - | - | 0.754 | 0.014 |
24 | SP–SS | - | - | - | - | - | - |
25 | SP–SPE | - | - | 0.179 | 0.018 | 0.179 | 0.018 |
26 | SAA–SS | - | - | - | - | - | - |
27 | SAA–SPE | 0.237 | 0.024 | - | - | 0.237 | 0.024 |
28 | SS–SPE | 0.868 | 0.009 | - | - | 0.868 | 0.009 |
Table 6 shows that the five parameters, namely the Incremental Fit Index, Tucker Lewis Index, the Comparative Fit Index, Goodness of Fit Index, and Adjusted Goodness Fit Index, are all acceptable with parameter estimates greater than 0.8, whereas mean square error is excellent with parameter estimates less than 0.08.
4. Conclusions
The education system has been affected by the 2019 coronavirus disease; face-to-face classes are suspended to control and reduce the spread of the virus and infections [ 2 ]. The suspension of face-to-face classes results in the application of modular distance learning for K-12 students according to continuity of learning during the COVID-19 pandemic. With the outbreak of COVID-19, some issues concerning students’ academic Performance and factors associated with students’ psychological status are starting to emerge, which impacted the students’ ability to learn. This study aimed to perceive the impact of Modular Distance learning on the K-12 students amid the COVID-19 pandemic and assess cognitive factors affecting students’ academic achievement and satisfaction.
This study applied Transactional Distance Theory (TDT) and Bloom Taxonomy Theory (BTT) to evaluate cognitive factors affecting students’ academic achievements and satisfaction and evaluate the perceived effectiveness of K-12 students in response to modular learning. This study applied Structural Equation Modeling (SEM) to test hypotheses. The application of SEM analyzed the correlation among students’ background, experience, behavior, instructor interaction, performance, understanding, satisfaction, academic achievement, and student perceived effectiveness.
A total of 252 data samples were gathered through an online questionnaire. Based on findings, this study concludes that students’ background in modular distance learning affects their behavior and experience. Students’ experiences had significant effects on the performance and understanding of students in modular distance learning. Student instructor interaction had a substantial impact on performance and learning; it explains how vital interaction with the instructor is. The student interacting with the instructor shows that the student may receive feedback and guidance from the instructor. Understanding has a significant influence on students’ satisfaction and academic achievement. Student performance has a substantial impact on students’ academic achievement and satisfaction. Perceived effectiveness was significantly influenced by students’ academic achievement and student satisfaction. However, students’ behavior had no considerable effect on students’ instructor interaction, and students’ understanding while student performance equally had no significant impact on student satisfaction. From this study, students are likely to manifest good performance, behavior, and cognition when they have prior knowledge with regard to modular distance learning. This study will help the government, teachers, and students take the necessary steps to improve and enhance modular distance learning that will benefit students for effective learning.
The modular learning system has been in place since its inception. One of its founding metaphoric pillars is student satisfaction with modular learning. The organization demonstrated its dedication to the student’s voice as a component of understanding effective teaching and learning. Student satisfaction research has been transformed by modular learning. It has caused the education research community to rethink long-held assumptions that learning occurs primarily within a metaphorical container known as a “course.” When reviewing studies on student satisfaction from a factor analytic perspective, one thing becomes clear: this is a complex system with little consensus. Even the most recent factor analytical studies have done little to address the lack of understanding of the dimensions underlying satisfaction with modular learning. Items about student satisfaction with modular distance learning correspond to forming a psychological contract in factor analytic studies. The survey responses are reconfigured into a smaller number of latent (non-observable) dimensions that the students never really articulate but are fully expected to satisfy. Of course, instructors have contracts with their students. Studies such as this one identify the student’s psychological contact after the fact, rather than before the class. The most important aspect is the rapid adoption of this teaching and learning mode in Senior High School. Another balancing factor is the growing sense of student agency in the educational process. Students can express their opinions about their educational experiences in formats ranging from end-of-course evaluation protocols to various social networks, making their voices more critical.
Furthermore, they all agreed with latent trait theory, which holds that the critical dimensions that students differentiate when expressing their opinions about modular learning are formed by the combination of the original items that cannot be directly observed—which underpins student satisfaction. As stated in the literature, the relationship between student satisfaction and the characteristic of a psychological contract is illustrated. Each element is translated into how it might be expressed in the student’s voice, and then a contract feature and an assessment strategy are added. The most significant contributor to the factor pattern, engaged learning, indicates that students expect instructors to play a facilitative role in their teaching. This dimension corresponds to the relational contract, in which the learning environment is stable and well organized, with a clear path to success.
5. Limitations and Future Work
This study was focused on the cognitive capacity of modular distance learning towards academic achievements and satisfaction of K-12 students during the COVID-19 pandemic. The sample size in this study was small, at only 252. If this study is repeated with a larger sample size, it will improve the results. The study’s restriction was to the province of Occidental Mindoro; Structural Equation Modeling (SEM) was used to measure all the variables. Thus, this will give an adequate solution to the problem in the study.
The current study underlines that combining TDT and BTT can positively impact the research outcome. The contribution the current study might make to the field of modular distance learning has been discussed and explained. Based on this research model, the nine (9) factors could broadly clarify the students’ adoption of new learning environment platform features. Thus, the current research suggests that more investigation be carried out to examine relationships among the complexity of modular distance learning.
Funding Statement
This research received no external funding.
Author Contributions
Data collection, methodology, writing and editing, K.A.M.; data collection, writing—review and editing, Y.-T.J. and C.S.S. All authors have read and agreed to the published version of the manuscript.
Institutional Review Board Statement
Informed consent statement.
Informed consent was obtained from all subjects involved in the study.
Data Availability Statement
Conflicts of interest.
The authors declare no conflict of interest.
Publisher’s Note: MDPI stays neutral with regard to jurisdictional claims in published maps and institutional affiliations.
- Research article
- Open access
- Published: 03 March 2021
A comparative study regarding distance learning and the conventional face-to-face approach conducted problem-based learning tutorial during the COVID-19 pandemic
- Chi-chung Foo ORCID: orcid.org/0000-0001-8849-6597 1 ,
- Billy Cheung 2 &
- Kent-man Chu ORCID: orcid.org/0000-0002-0332-4321 1
BMC Medical Education volume 21 , Article number: 141 ( 2021 ) Cite this article
170k Accesses
77 Citations
3 Altmetric
Metrics details
Educational pedagogies were modified during the COVID-19 pandemic to minimise interruption to teaching. One approach has been the distance learning problem-based learning (PBL) tutorial utilising the online peer-to-peer platform. The aim of this study was to compare the performance of students using distance learning PBL tutorials using with that of students utilising the conventional face-to-face approach.
This retrospective study was conducted in a single academic institution. We compared two groups of fourth-year medical students from the same class: one group used distance learning (DL); the other, the face-to-face (FF) method. We used students’ baseline performance at the preceding block for one-to-one propensity score matching. Students utilising the PBL tutorial were given grades by their tutors according to a standardised scoring system encompassing five key areas (score range: 0–10). The main outcome was a student’s total score (i.e., the sum of the scores from the five key areas, ranging from 0 to 50).
We matched 62 students in each group. With four tutorials, there were 490 observations, with 245 in each group. The mean total score for the DL group was 37.5 ± 4.6, which was significantly lower than that of the FF group (39.0 ± 4.4, p < 0.001). We noted that students in the DL group had a significantly lower scores for all five areas of proficiency: participation, communication, preparation, critical thinking and group skills.
Findings of this study revealed that the performance of students utilising the DL PBL tutorials was lower than that of students participating in the conventional FF approach. Further studies are needed to ascertain the underlying cause.
Peer Review reports
During the first half of 2020, the world was challenged by the coronavirus pandemic on an unprecedented scale. In response, many people adopted the practice of social distancing, and schools suspended classes and activities. Medical students were devoid of opportunities to enter hospital premises because of tightened infection control measures. Educators adopted innovative measures to maintain learning opportunities for students who stayed at home [ 1 , 2 , 3 ]. Some of these measures, including online lectures or webinars, were in place before the COVID-19 outbreak [ 4 ]. Others were hastily put into place during the pandemic. Given its user-friendly design, online peer-to-peer platforms became extremely popular. Lectures, tutorials, skills demonstrations, and even bedside teaching for medical students can be conducted via this type of platform [ 5 , 6 ]. For example, at the University of Hong Kong Li Ka Shing Faculty of Medicine offered a FF PBL tutorial using online peer-to-peer platform software. To many, such adaptations served as a lifeline to continue medical education during the coronavirus outbreak. It was also envisaged that some of these educational adaptations would persist after the pandemic. How effective these adaptations have been and how they compare with the conventional teaching method should be evaluated. A study on surgical skills teaching reported that using Web-based DL was well-received by undergraduate students [ 6 ]. The aim of this study was to evaluate the proficiencies in five key areas of students who took PBL tutorials by DL, an adaptation during the COVID-19 pandemic, and to compare them with the proficiency levels of students who learned via the conventional FF method.
This was a retrospective study conducted in May 2020 at the Li Ka Shing Faculty of Medicine, University of Hong Kong; it was approved by the Institutional Review Board of the University of Hong Kong/Hospital Authority Hong Kong West Cluster (IRB reference number: UW 20–381). The subjects were medical students who were in their fourth year of their six-year medical curriculum. These students had been exposed to the PBL teaching approach since their first and second years and were familiar with the format. In their fourth year, students in this class were split into three groups, with each rotating through three Junior Clerkship (JC) rotation blocks-- Medicine, Surgery, and Multidisciplinary clerkship-- between November 2019 and April 2020. From February to May 2020, classes were suspended because of the outbreak of the severe acute respiratory syndrome coronavirus 2 (SARS-CoV-2). The conventional FF PBL in the Surgery block was replaced by DL, using the online peer-to-peer platform software ZOOM (Zoom Video Communications, San Jose, CA, USA). The tutors, content, group size, duration, and assessment criteria remained the same. All students from rotation one had participated in conventional PBL tutorials before the class suspension, whereas students from rotation three had engaged in DL (online) PBL exclusively after the outbreak.
Eight cases were presented for discussion in a total of four tutorials. We gave the paper-based case materials to students prior to the tutorials and encouraged pre-class preparation. The PBL scenarios included breast mass, neck swelling, rectal bleeding, abdominal distension, haematuria, acute retention of urine, abdominal pain in an adult patient and abdominal pain in a paediatric patient. Each tutorial lasted for two hours and was considered sufficient for students to go through two scenarios, discuss the relevant history and physical examination findings, decide on the suitable investigations, come up with working diagnosis and suggest the appropriate management. The group size was 11–12 students. Students were randomly allocated into groups; they remained in the same groups throughout the clerkship. Tutors were randomly assigned, and students had different tutors for the four tutorials. The scenarios were described over several pages and some leading questions were given. Students discussed approaches to the clinical problems and explored related issues. They addressed one or more learning objectives that were considered relevant. Tutors acted as facilitators and played minimal roles unless students strayed from a case. At the end of the session, tutors used a standardised form for evaluating the proficiency levels of students in five key areas: participation, communication, preparation, critical thinking and group skills. Tutors expected students to demonstrate adequate preparation on the applicable topic prior to each tutorial, active engagement in group discussions, adequate communication skills for expressing their viewpoints and raising relevant questions, the ability to manage controversies rationally, and attentiveness to other members without dominating the discussion. A score from 1 to 10 was given for each of these areas, with 10 being the highest. The total score represented the sum of the scores from all five key areas.
We compared the PBL performance of students in rotation three-- the DL group using the online platform – to that of students in rotation one, the conventional FF group; the latter functioned as the control group. We retrieved their PBL outcomes and overall assessments for the preceding Clinical Foundation Block (CFB), taken during the period August to October 2019, for baseline comparison. The CFB tutorials were all conducted using the conventional FF method; for these five PBL tutorials, students were assessed with the same evaluation form (scores ranging from 0 to 10). The overall assessment comprised the PBL assessment (20%), small group/bedside skills learning (60%), and a logbook (20%). Students in the FF group and DL groups were matched by propensity scores according to their performance (i.e., using PBL scores from the CFB). Matching was one to one, using the nearest neighbour method and tolerance of 0.5. Categorical variables were compared using the χ 2 test. Continuous variables were compared with the independent sample t -test. A p -value of < 0.05 was considered statistically significant. The statistical analysis was performed using IBM SPSS version 25 (IBM, USA).
There were 77 and 75 students in the FF and DL groups, respectively. After propensity score matching, 62 students remained in each group. Matching for the remaining 15 and 13 students in the FF and DL groups, respectively, were not possible; therefore, they were excluded. Twenty-nine tutors were involved. With four tutorials, there were a total of 496 observations (248 per group). However, there were three absentees in the FF and DL groups, respectively, resulting in 245 observations per group. Gender composition, age, ethnicity and overall assessments for the CFB of the two groups are shown in Table 1 , indicating comparability between the two groups. Their PBL performance in the preceding CFB was also comparable after propensity score matching (79.5 versus 79.9, p = 0.737).
The PBL performance of the two groups during JC is shown in Table 2 . Students in the FF group scored significantly higher. The mean total score for the DL group was 37.5, which was significantly lower than the score for the FF group (39.0, p < 0.001). Moreover, assessments regarding participation, communication, preparation, critical thinking and group skills were uniformly lower for the DL group compared to those for the control group.
A subgroup analysis was performed to evaluate the effect of different tutorials and tutors. Table 3 shows a comparison of students’ performance for the four different tutorials. The mean total score was higher for the four tutorials; the difference was only significant for the first and third tutorials. A comparison of the two groups was also performed for individual tutors. Of the 29 tutors involved, six were excluded because they taught students in either the FF or DL group exclusively. Among the remaining 23, eight (34.8%) rated the proficiencies of students in the FF group higher and two (8.7%) rated those of students in the DL group higher (Fig. 1 ). The difference was not significant for remaining 13 tutors (56.5%).
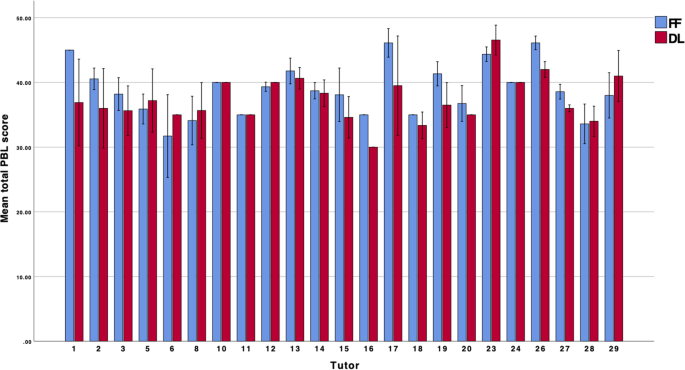
Mean PBL scores according to tutors
E-learning has been in place for some time [ 4 ]. Many have viewed it as the preferred mode of teaching for the future, as students are given more flexibility [ 7 , 8 ]. This type of learning has become indispensable during the COVID-19 pandemic when social contact is minimal. However, e-learning has certain limitations [ 9 ]. It is reasonable to believe that many educational adaptations adopted during the pandemic will persist. Indeed some of the novel ones may result in a better overall learning experience for students. Therefore, it is worthwhile to evaluate them.
PBL was first popularised at the McMaster University in Canada [ 10 , 11 ]. Contrary to traditional lecture-based teaching, PBL encourages active and student-directed learning. Students are trained in independent learning, teamwork, and communication skills [ 12 ]. Some have suggested that students who utilised PBL curricula have emerged as better problem solvers [ 13 ]. For a PBL tutorial group to be efficient, members’ initiation is crucial, with all striving to function as a productive members.
Findings of this study revealed that students using DL method performed at a significantly lower level than students learning via the conventional FF approach. One possible explanation was that students and tutors had to adapt a new way of conducting the PBL tutorial. Wilcha cited technical challenges like establishing a reliable internet connection, problems with hardware and software learning platforms, etc. as some of the weakness of online teaching in a systematic review [ 9 ]. However, the software was relatively user-friendly, and the format of the tutorials remained the same. The time needed for students and tutors to become familiar with the new ‘environment’ should have been minimal. Technical issues such as Internet connectivity and lag time did not seem to be major problems in this locality. The fact that lower performance was also observed at the third tutorial suggested there was more than a transitional issue.
Modern digital communication technology has allowed us to trump geographical barriers [ 14 , 15 ]. Online platforms provide opportunities to meet and discuss without being physically close to each other. However, this type of technology may not reproduce the same interpersonal distance as physical presence [ 16 ]. Students may feel distant and detached from the rest of the group despite being connected via the computer screen and audio. The perception of being an outsider may reduce one’s eagerness to participate and contribute. In this study, students were required to keep the audio and video on throughout the tutorials, but there were occasions in which students only revealed or unmuted themselves when they were prompted to do so. Most students participated in the PBL tutorials from their residences via video conferencing. The casual ambiance might have appeared ‘unreal’ for learning, requiring psychological adaptation. Students were also more prone to distractions from surrounding persons or events. Prior studies have shown that DL using online platforms is associated with reduced student engagement, reduced communication and poor motivation [ 17 , 18 , 19 ].
Tutors can be affected too. Although tutors played minimal roles in this study, apart from evaluating students, they might have been inclined to intervene when needed and prone to be distracted. Nevertheless, these are only postulations; further research is warranted. A survey should be conducted to ascertain the perceptions of students and tutors regarding online tutorials and ways to improve the overall learning and teaching experience.
There were several limitations to this study. There was no randomisation, and the comparison was subjected to bias. The chance of bias was minimised by matching student performance at baseline. The tutor effect was another confounding factor. Although we used a structured evaluation form with clear guidance regarding scoring, there was a possibility of variations among tutors, with some being more stringent than others. Tutors in this study were regularly involved in PBL teaching, but there was no prior training or standardisation in terms of scoring. For some tutors, there was little variation in scores between the five areas of proficiency, which indicated that the tutors were more inclined to give an overall impression of students’ performance. This situation limited the ability to single out specific areas. There were tutors (tutors 10, 11 and 24 in Fig. 1 ) that gave every students the same score. Again this reduced the sensitivity to detect a difference, if any, between the two groups. It was postulated that this was why a lower score was observed in the DL group in tutorial two and four but the difference was insignificant. Additionally, tutorials for the two groups were conducted at different times, and students in the DL group were learning during a pandemic, which was clearly a torment to some. Thus, the negative psychological impact on them might have affected their performance. Furthermore, some classes or bedside teachings were suspended at the time. It has had been a suggested that people working from home during the pandemic may be more prone to loneliness, and hence, decreased efficiency [ 20 ].
Innovative educational adaptations have been essential during the COVID-19 pandemic. However, further evaluation before permanent adoption is warranted. A direct transition from the conventional way of teaching into an online-based format may not have the same impact. This study showed that students who used DL PBL tutorials exhivited lower levels of proficiency in key area than students who utilised the conventional FF approach. Further studies are needed to ascertain the underlying cause.
Availability of data and materials
The datasets generated and / or analysed during the current study are available from the corresponding author on request.
Eva KW, Anderson MB. Medical education adaptations: really good stuff for educational transition during a pandemic. Med Educ. 2020;54(6):494.
Chick RC, Clifton GT, Peace KM, Propper BW, Hale DF, Alseidi AA, Vreeland TJ. Using technology to maintain the education of residents during the COVID-19 pandemic. J Surg Educ. 2020;77(4):729–32.
Liang ZC, Ooi SBS, Wang W. Pandemics and their impact on medical training: lessons from Singapore. Acad Med. 2020;95(9):1359–61.
Kim S. The future of E-learning in medical education: current trend and future opportunity. J Educ Eval Health Prof. 2006;3:3.
Article Google Scholar
Tsang ACO, Lee PP, Chen JY, Leung GKK. From bedside to Webside: a neurological clinical teaching experience. Med Educ. 2020;54(7):660.
Co M, Chu KM. Distant surgical teaching during COVID-19 - a pilot study on final year medical students. Surg Pract. 2020. https://doi.org/10.1111/1744-1633 .
Shah D. Online education: should we take it seriously? Climacteric. 2016;19(1):3–6.
Ruiz JG, Mintzer MJ, Leipzig RM. The impact of E-learning in medical education. Acad Med. 2006;81(3):207–12.
Wilcha RJ. Effectiveness of virtual medical teaching during the COVID-19 crisis: systematic review. JMIR Med Educ. 2020;6(2):e20963.
Neufeld VR, Barrows HS. The "McMaster philosophy": an approach to medical education. J Med Educ. 1974;49(11):1040–50.
Google Scholar
Neville AJ, Norman GR. PBL in the undergraduate MD program at McMaster University: three iterations in three decades. Acad Med. 2007;82(4):370–4.
Kamin CSDR, Wilson B, Armacost M, Breedon T. The development of a collaborative distance learning program to facilitate pediatric problem-based learning. Med Educ Online. 1999;4:2.
Distlehorst LH, Dawson E, Robbs RS, Barrows HS. Problem-based learning outcomes: the glass half-full. Acad Med. 2005;80(3):294–9.
Grange ES, Neil EJ, Stoffel M, Singh AP, Tseng E, Resco-Summers K, Fellner BJ, Lynch JB, Mathias PC, Mauritz-Miller K, et al. Responding to COVID-19: the UW medicine information technology services experience. Appl Clin Inform. 2020;11(2):265–75.
Lamba P. Teleconferencing in medical education: a useful tool. Australas Med J. 2011;4(8):442–7.
Norman ETH, Huegel D. The distance between us: using construal level theory to understand interpersonal distance in a digital age. Front Digit Humanit. 2016;3:5.
Lee ICJ, Koh H, Lai SH, Hwang NC. Academic coaching of medical students during the COVID-19 pandemic. Med Educ. 2020;54(12):1184–5.
Longhurst GJ, Stone DM, Dulohery K, Scully D, Campbell T, Smith CF. Strength, weakness, opportunity, threat (SWOT) analysis of the adaptations to anatomical education in the United Kingdom and Republic of Ireland in response to the Covid-19 pandemic. Anat Sci Educ. 2020;13(3):301–11.
Kaup S, Jain R, Shivalli S, Pandey S, Kaup S. Sustaining academics during COVID-19 pandemic: the role of online teaching-learning. Indian J Ophthalmol. 2020;68(6):1220–1.
Loneliness: The other side of working from home https://www.medicaldaily.com/loneliness-other-side-working-home-working-home-lonely-lifestyle-workplace-452535 . Accessed 1 June 2020.
Download references
Acknowledgements
Not applicable.
This study did not receive funding support.
Author information
Authors and affiliations.
Department of Surgery, Li Ka Shing Faculty of Medicine, University of Hong Kong, Hong Kong, SAR, China
Chi-chung Foo & Kent-man Chu
Department of Surgery, Queen Mary Hospital, Hong Kong, SAR, China
Billy Cheung
You can also search for this author in PubMed Google Scholar
Contributions
All authors contributed to the study conception and design. Chi-chung Foo: Conception of work, acquisition of data, data analysis, drafting of manuscript, final approval. Billy Cheung: Conception of work, acquisition of data, data analysis, final approval. Kent-man Chu:Conception of work, data analysis, final approval.
Corresponding author
Correspondence to Chi-chung Foo .
Ethics declarations
Ethics approval and consent to participate.
This study was performed in accordance with the ethical standards of the institutional and national research committee and with the 1964 Helsinki Declaration and its later amendments or comparable ethical standards. It was reviewed by the Institutional review board of the University of Hong Kong/Hospital Authority Hong Kong West Cluster (reference no: UW 20–381) and was approved WITHOUT the need of informed consent.
Competing interests
The authors declare no that they have no competing interests.

Additional information
Publisher’s note.
Springer Nature remains neutral with regard to jurisdictional claims in published maps and institutional affiliations.
Supplementary Information
Additional file 1..
The standardised form for tutors to evaluate students’ proficiency levels was attached as supplementary material.
Rights and permissions
Open Access This article is licensed under a Creative Commons Attribution 4.0 International License, which permits use, sharing, adaptation, distribution and reproduction in any medium or format, as long as you give appropriate credit to the original author(s) and the source, provide a link to the Creative Commons licence, and indicate if changes were made. The images or other third party material in this article are included in the article's Creative Commons licence, unless indicated otherwise in a credit line to the material. If material is not included in the article's Creative Commons licence and your intended use is not permitted by statutory regulation or exceeds the permitted use, you will need to obtain permission directly from the copyright holder. To view a copy of this licence, visit http://creativecommons.org/licenses/by/4.0/ . The Creative Commons Public Domain Dedication waiver ( http://creativecommons.org/publicdomain/zero/1.0/ ) applies to the data made available in this article, unless otherwise stated in a credit line to the data.
Reprints and permissions
About this article
Cite this article.
Foo, Cc., Cheung, B. & Chu, Km. A comparative study regarding distance learning and the conventional face-to-face approach conducted problem-based learning tutorial during the COVID-19 pandemic. BMC Med Educ 21 , 141 (2021). https://doi.org/10.1186/s12909-021-02575-1
Download citation
Received : 25 November 2020
Accepted : 16 February 2021
Published : 03 March 2021
DOI : https://doi.org/10.1186/s12909-021-02575-1
Share this article
Anyone you share the following link with will be able to read this content:
Sorry, a shareable link is not currently available for this article.
Provided by the Springer Nature SharedIt content-sharing initiative
- Problem-based learning
- Distance learning
- Online education
BMC Medical Education
ISSN: 1472-6920
- Submission enquiries: [email protected]
- General enquiries: [email protected]
Perceived Effects of Modular Distance Learning on the Students in Aumbay Elementary School
International Journal of Research and Innovation in Social Science (IJRISS) 2022, 6(9), 496-506
11 Pages Posted: 20 Jan 2023
Livelyn Argallon
Um peñaplata college, jaycee rylle loreño, honey jane paham, jovenil bacatan.
UM Peñaplata College; The Rizal Memorial Colleges; Holy Cross of Davao College
Date Written: September 2022
This research aimed to gather data on the perceived effects of modular distance learning on students. The following research objectives informed this research: 1) to determine the perceived effects of modular distance learning on the students and 2) to determine the significant difference in the perceived effects of modular distance learning on grades 4,5 and 6 students. This quantitative study employed a descriptive survey to determine and identify the perceived effects of modular distance learning on grades 4,5 and 6 students. The research instrument used in this study is a survey questionnaire, which is used to acquire the necessary data. Based on the result, the study revealed that students agreed on using modular distance learning as a teaching method. They agreed most with the statements and the benefits of this approach. The study also revealed no significant difference in the perceived effects of modular distance learning on students when categorized according to gender. This means that students had the same perceptions when categorized according to gender. However, the study also revealed a significant difference in the perceived effects of modular distance learning on the students when categorized according to their grade level. It means that students had a different perception when they were grouped according to their grade level. Therefore, researchers concluded that in terms of gender, modular distance learning was not affected by their perception of the modular approach. At the same time, the perception of the students had a difference in terms of their grade level. This study implies that this modular distance learning approach helps students improve their perception of modules. Therefore, a modular approach must be continued in face-to-face instruction even if the pandemic vanishes. Researchers may recommend to the faculty/teachers in Aumbay Elementary School to use modules so students can study in advance; teachers/faculty might monitor their student’s progress and be more flexible and even-minded in any situation to provide and help the students. Moreover, Schools may provide guidelines regarding the implementation of limited face-to-face and modular distance learning so that students can learn in different approaches; parents should also give attention to their children and support them in such activities. Furthermore, future researchers may incorporate other variables when they conduct a study related to our research.
Keywords: modular approach, perceived effects, modular distance learning, students
JEL Classification: Y80
Suggested Citation: Suggested Citation
Jovenil Bacatan (Contact Author)
Um peñaplata college ( email ).
Penaplata Island Garden City of Samal, Davao del Norte 8119 Philippines
The Rizal Memorial Colleges ( email )
Davao City, Davao del Sur 8000 Philippines
Holy Cross of Davao College ( email )
Sta. Ana Avenue Davao City, Davao del Sur 8000 Philippines
Do you have a job opening that you would like to promote on SSRN?
Paper statistics, related ejournals, pedagogy ejournal.
Subscribe to this fee journal for more curated articles on this topic
Cognitive Psychology eJournal
Developmental psychology ejournal.
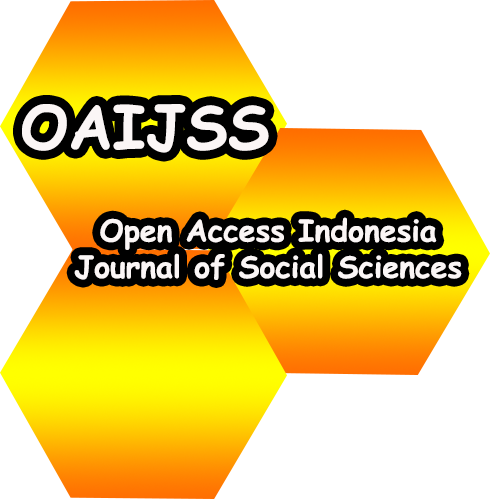
Effect of Modular Distance Learning Approach to Academic Performance in Mathematics of Students in Mindanao State University-Sulu Senior High School Amidst COVID-19 Pandemic
Article sidebar.
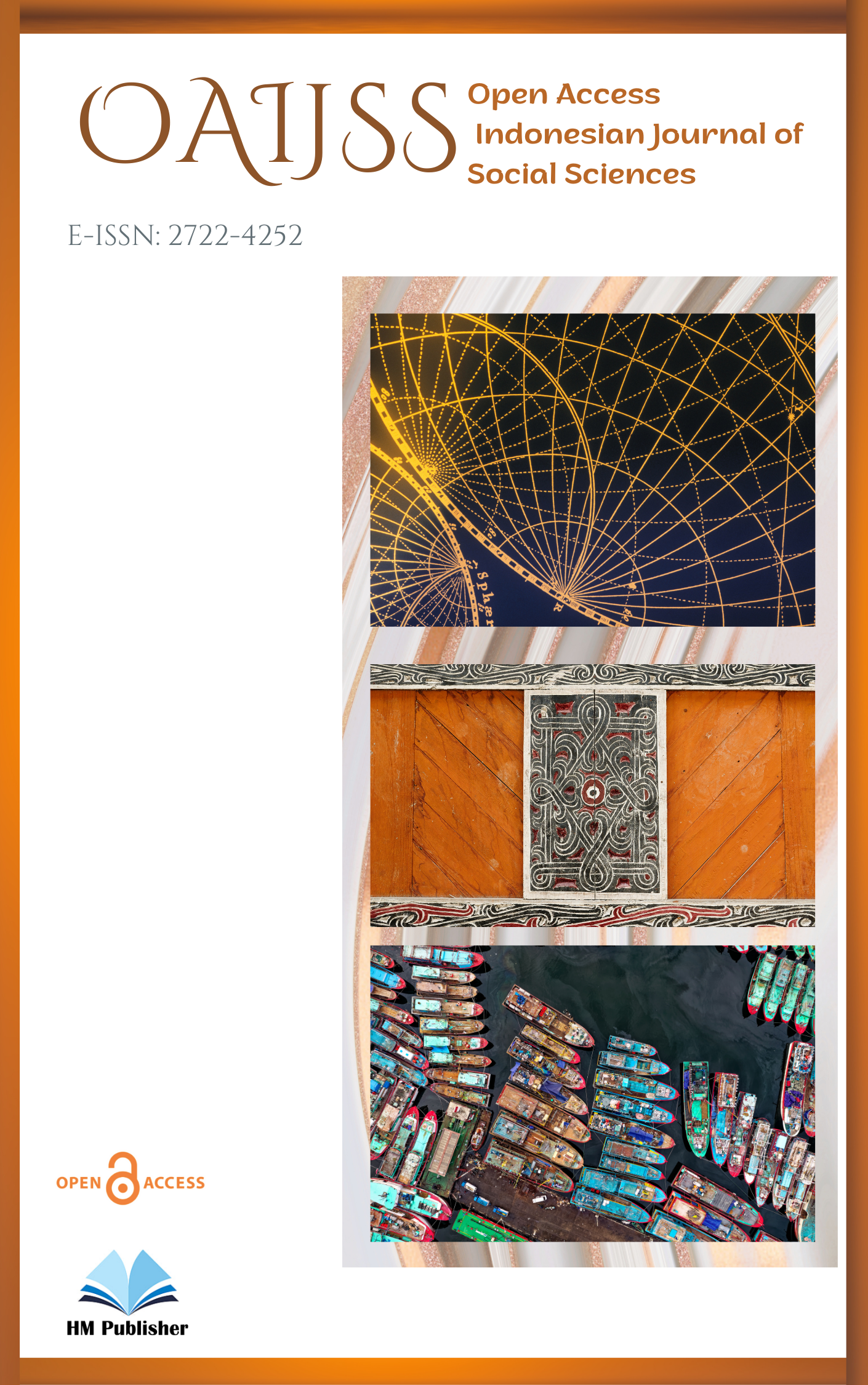
Main Article Content
This study investigated the instructional competencies of teachers in The existence of COVID-19 pandemic brought extraordinary challenges to the stakeholders, teachers, parents, and students. Thus, the researcher believed that there is an effect of teaching-learning process in new normal education to students’ performance most especially using modular type of learning in Mathematics. With this, the study sought to determine the perception of the students regarding modular distance learning approach (MDLA) in Mathematics, identify the challenges of the students, examine the effect of MDLA to academic performance of students in Mathematics, determine the level of academic performance of students, determine the significant difference on perceptions when they grouped according to their gender and age, and determine the relationship of students’ perceptions regarding MDLA to their academic performance in Mathematics. The descriptive research design was utilized in this study. The researcher gathered one hundred seventy eight (178) grade 11 STEM students currently enrolled in MSU-Sulu Senior High School through the use of purposive random sampling. The survey questionnaire was applied in the study. Mean, frequency counts and percentage, t-test for independent samples, one-way analysis of variance (ANOVA), and person product-moment correlation were used to analyze and interpret the data. Based on the result, the study revealed that students’ perceptions agreed on using modular distance learning approach (MDLA). It means the students had positive perceptions regarding MDLA in Mathematics. The study also revealed that students agreed on using modular distance learning approach (MDLA) in Math have little challenges. It had also a positive effect to students’ performance in which students performed very satisfactory in Mathematics which means they had good quality performance. However, the study also revealed that it has no significant difference on their perceptions when they are grouped by gender and age which means the students had the same perceptions.
Article Details
Open Access Indonesia Journal of Social Sciences (OAIJSS) allow the author(s) to hold the copyright without restrictions and allow the author(s) to retain publishing rights without restrictions, also the owner of the commercial rights to the article is the author.
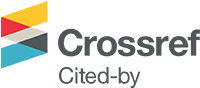
- Reference Manager
- Simple TEXT file
People also looked at
Original research article, a comparative analysis of student performance in an online vs. face-to-face environmental science course from 2009 to 2016.
- Department of Biology, Fort Valley State University, Fort Valley, GA, United States
A growing number of students are now opting for online classes. They find the traditional classroom modality restrictive, inflexible, and impractical. In this age of technological advancement, schools can now provide effective classroom teaching via the Web. This shift in pedagogical medium is forcing academic institutions to rethink how they want to deliver their course content. The overarching purpose of this research was to determine which teaching method proved more effective over the 8-year period. The scores of 548 students, 401 traditional students and 147 online students, in an environmental science class were used to determine which instructional modality generated better student performance. In addition to the overarching objective, we also examined score variabilities between genders and classifications to determine if teaching modality had a greater impact on specific groups. No significant difference in student performance between online and face-to-face (F2F) learners overall, with respect to gender, or with respect to class rank were found. These data demonstrate the ability to similarly translate environmental science concepts for non-STEM majors in both traditional and online platforms irrespective of gender or class rank. A potential exists for increasing the number of non-STEM majors engaged in citizen science using the flexibility of online learning to teach environmental science core concepts.
Introduction
The advent of online education has made it possible for students with busy lives and limited flexibility to obtain a quality education. As opposed to traditional classroom teaching, Web-based instruction has made it possible to offer classes worldwide through a single Internet connection. Although it boasts several advantages over traditional education, online instruction still has its drawbacks, including limited communal synergies. Still, online education seems to be the path many students are taking to secure a degree.
This study compared the effectiveness of online vs. traditional instruction in an environmental studies class. Using a single indicator, we attempted to see if student performance was effected by instructional medium. This study sought to compare online and F2F teaching on three levels—pure modality, gender, and class rank. Through these comparisons, we investigated whether one teaching modality was significantly more effective than the other. Although there were limitations to the study, this examination was conducted to provide us with additional measures to determine if students performed better in one environment over another ( Mozes-Carmel and Gold, 2009 ).
The methods, procedures, and operationalization tools used in this assessment can be expanded upon in future quantitative, qualitative, and mixed method designs to further analyze this topic. Moreover, the results of this study serve as a backbone for future meta-analytical studies.
Origins of Online Education
Computer-assisted instruction is changing the pedagogical landscape as an increasing number of students are seeking online education. Colleges and universities are now touting the efficiencies of Web-based education and are rapidly implementing online classes to meet student needs worldwide. One study reported “increases in the number of online courses given by universities have been quite dramatic over the last couple of years” ( Lundberg et al., 2008 ). Think tanks are also disseminating statistics on Web-based instruction. “In 2010, the Sloan Consortium found a 17% increase in online students from the years before, beating the 12% increase from the previous year” ( Keramidas, 2012 ).
Contrary to popular belief, online education is not a new phenomenon. The first correspondence and distance learning educational programs were initiated in the mid-1800s by the University of London. This model of educational learning was dependent on the postal service and therefore wasn't seen in American until the later Nineteenth century. It was in 1873 when what is considered the first official correspondence educational program was established in Boston, Massachusetts known as the “Society to Encourage Home Studies.” Since then, non-traditional study has grown into what it is today considered a more viable online instructional modality. Technological advancement indubitably helped improve the speed and accessibility of distance learning courses; now students worldwide could attend classes from the comfort of their own homes.
Qualities of Online and Traditional Face to Face (F2F) Classroom Education
Online and traditional education share many qualities. Students are still required to attend class, learn the material, submit assignments, and complete group projects. While teachers, still have to design curriculums, maximize instructional quality, answer class questions, motivate students to learn, and grade assignments. Despite these basic similarities, there are many differences between the two modalities. Traditionally, classroom instruction is known to be teacher-centered and requires passive learning by the student, while online instruction is often student-centered and requires active learning.
In teacher-centered, or passive learning, the instructor usually controls classroom dynamics. The teacher lectures and comments, while students listen, take notes, and ask questions. In student-centered, or active learning, the students usually determine classroom dynamics as they independently analyze the information, construct questions, and ask the instructor for clarification. In this scenario, the teacher, not the student, is listening, formulating, and responding ( Salcedo, 2010 ).
In education, change comes with questions. Despite all current reports championing online education, researchers are still questioning its efficacy. Research is still being conducted on the effectiveness of computer-assisted teaching. Cost-benefit analysis, student experience, and student performance are now being carefully considered when determining whether online education is a viable substitute for classroom teaching. This decision process will most probably carry into the future as technology improves and as students demand better learning experiences.
Thus far, “literature on the efficacy of online courses is expansive and divided” ( Driscoll et al., 2012 ). Some studies favor traditional classroom instruction, stating “online learners will quit more easily” and “online learning can lack feedback for both students and instructors” ( Atchley et al., 2013 ). Because of these shortcomings, student retention, satisfaction, and performance can be compromised. Like traditional teaching, distance learning also has its apologists who aver online education produces students who perform as well or better than their traditional classroom counterparts ( Westhuis et al., 2006 ).
The advantages and disadvantages of both instructional modalities need to be fully fleshed out and examined to truly determine which medium generates better student performance. Both modalities have been proven to be relatively effective, but, as mentioned earlier, the question to be asked is if one is truly better than the other.
Student Need for Online Education
With technological advancement, learners now want quality programs they can access from anywhere and at any time. Because of these demands, online education has become a viable, alluring option to business professionals, stay-at home-parents, and other similar populations. In addition to flexibility and access, multiple other face value benefits, including program choice and time efficiency, have increased the attractiveness of distance learning ( Wladis et al., 2015 ).
First, prospective students want to be able to receive a quality education without having to sacrifice work time, family time, and travel expense. Instead of having to be at a specific location at a specific time, online educational students have the freedom to communicate with instructors, address classmates, study materials, and complete assignments from any Internet-accessible point ( Richardson and Swan, 2003 ). This type of flexibility grants students much-needed mobility and, in turn, helps make the educational process more enticing. According to Lundberg et al. (2008) “the student may prefer to take an online course or a complete online-based degree program as online courses offer more flexible study hours; for example, a student who has a job could attend the virtual class watching instructional film and streaming videos of lectures after working hours.”
Moreover, more study time can lead to better class performance—more chapters read, better quality papers, and more group project time. Studies on the relationship between study time and performance are limited; however, it is often assumed the online student will use any surplus time to improve grades ( Bigelow, 2009 ). It is crucial to mention the link between flexibility and student performance as grades are the lone performance indicator of this research.
Second, online education also offers more program choices. With traditional classroom study, students are forced to take courses only at universities within feasible driving distance or move. Web-based instruction, on the other hand, grants students electronic access to multiple universities and course offerings ( Salcedo, 2010 ). Therefore, students who were once limited to a few colleges within their immediate area can now access several colleges worldwide from a single convenient location.
Third, with online teaching, students who usually don't participate in class may now voice their opinions and concerns. As they are not in a classroom setting, quieter students may feel more comfortable partaking in class dialogue without being recognized or judged. This, in turn, may increase average class scores ( Driscoll et al., 2012 ).
Benefits of Face-to-Face (F2F) Education via Traditional Classroom Instruction
The other modality, classroom teaching, is a well-established instructional medium in which teaching style and structure have been refined over several centuries. Face-to-face instruction has numerous benefits not found in its online counterpart ( Xu and Jaggars, 2016 ).
First and, perhaps most importantly, classroom instruction is extremely dynamic. Traditional classroom teaching provides real-time face-to-face instruction and sparks innovative questions. It also allows for immediate teacher response and more flexible content delivery. Online instruction dampens the learning process because students must limit their questions to blurbs, then grant the teacher and fellow classmates time to respond ( Salcedo, 2010 ). Over time, however, online teaching will probably improve, enhancing classroom dynamics and bringing students face-to face with their peers/instructors. However, for now, face-to-face instruction provides dynamic learning attributes not found in Web-based teaching ( Kemp and Grieve, 2014 ).
Second, traditional classroom learning is a well-established modality. Some students are opposed to change and view online instruction negatively. These students may be technophobes, more comfortable with sitting in a classroom taking notes than sitting at a computer absorbing data. Other students may value face-to-face interaction, pre and post-class discussions, communal learning, and organic student-teacher bonding ( Roval and Jordan, 2004 ). They may see the Internet as an impediment to learning. If not comfortable with the instructional medium, some students may shun classroom activities; their grades might slip and their educational interest might vanish. Students, however, may eventually adapt to online education. With more universities employing computer-based training, students may be forced to take only Web-based courses. Albeit true, this doesn't eliminate the fact some students prefer classroom intimacy.
Third, face-to-face instruction doesn't rely upon networked systems. In online learning, the student is dependent upon access to an unimpeded Internet connection. If technical problems occur, online students may not be able to communicate, submit assignments, or access study material. This problem, in turn, may frustrate the student, hinder performance, and discourage learning.
Fourth, campus education provides students with both accredited staff and research libraries. Students can rely upon administrators to aid in course selection and provide professorial recommendations. Library technicians can help learners edit their papers, locate valuable study material, and improve study habits. Research libraries may provide materials not accessible by computer. In all, the traditional classroom experience gives students important auxiliary tools to maximize classroom performance.
Fifth, traditional classroom degrees trump online educational degrees in terms of hiring preferences. Many academic and professional organizations do not consider online degrees on par with campus-based degrees ( Columbaro and Monaghan, 2009 ). Often, prospective hiring bodies think Web-based education is a watered-down, simpler means of attaining a degree, often citing poor curriculums, unsupervised exams, and lenient homework assignments as detriments to the learning process.
Finally, research shows online students are more likely to quit class if they do not like the instructor, the format, or the feedback. Because they work independently, relying almost wholly upon self-motivation and self-direction, online learners may be more inclined to withdraw from class if they do not get immediate results.
The classroom setting provides more motivation, encouragement, and direction. Even if a student wanted to quit during the first few weeks of class, he/she may be deterred by the instructor and fellow students. F2F instructors may be able to adjust the structure and teaching style of the class to improve student retention ( Kemp and Grieve, 2014 ). With online teaching, instructors are limited to electronic correspondence and may not pick-up on verbal and non-verbal cues.
Both F2F and online teaching have their pros and cons. More studies comparing the two modalities to achieve specific learning outcomes in participating learner populations are required before well-informed decisions can be made. This study examined the two modalities over eight (8) years on three different levels. Based on the aforementioned information, the following research questions resulted.
RQ1: Are there significant differences in academic performance between online and F2F students enrolled in an environmental science course?
RQ2: Are there gender differences between online and F2F student performance in an environmental science course?
RQ3: Are there significant differences between the performance of online and F2F students in an environmental science course with respect to class rank?
The results of this study are intended to edify teachers, administrators, and policymakers on which medium may work best.
Methodology
Participants.
The study sample consisted of 548 FVSU students who completed the Environmental Science class between 2009 and 2016. The final course grades of the participants served as the primary comparative factor in assessing performance differences between online and F2F instruction. Of the 548 total participants, 147 were online students while 401 were traditional students. This disparity was considered a limitation of the study. Of the 548 total students, 246 were male, while 302 were female. The study also used students from all four class ranks. There were 187 freshmen, 184 sophomores, 76 juniors, and 101 seniors. This was a convenience, non-probability sample so the composition of the study set was left to the discretion of the instructor. No special preferences or weights were given to students based upon gender or rank. Each student was considered a single, discrete entity or statistic.
All sections of the course were taught by a full-time biology professor at FVSU. The professor had over 10 years teaching experience in both classroom and F2F modalities. The professor was considered an outstanding tenured instructor with strong communication and management skills.
The F2F class met twice weekly in an on-campus classroom. Each class lasted 1 h and 15 min. The online class covered the same material as the F2F class, but was done wholly on-line using the Desire to Learn (D2L) e-learning system. Online students were expected to spend as much time studying as their F2F counterparts; however, no tracking measure was implemented to gauge e-learning study time. The professor combined textbook learning, lecture and class discussion, collaborative projects, and assessment tasks to engage students in the learning process.
This study did not differentiate between part-time and full-time students. Therefore, many part-time students may have been included in this study. This study also did not differentiate between students registered primarily at FVSU or at another institution. Therefore, many students included in this study may have used FVSU as an auxiliary institution to complete their environmental science class requirement.
Test Instruments
In this study, student performance was operationalized by final course grades. The final course grade was derived from test, homework, class participation, and research project scores. The four aforementioned assessments were valid and relevant; they were useful in gauging student ability and generating objective performance measurements. The final grades were converted from numerical scores to traditional GPA letters.
Data Collection Procedures
The sample 548 student grades were obtained from FVSU's Office of Institutional Research Planning and Effectiveness (OIRPE). The OIRPE released the grades to the instructor with the expectation the instructor would maintain confidentiality and not disclose said information to third parties. After the data was obtained, the instructor analyzed and processed the data though SPSS software to calculate specific values. These converted values were subsequently used to draw conclusions and validate the hypothesis.
Summary of the Results: The chi-square analysis showed no significant difference in student performance between online and face-to-face (F2F) learners [χ 2 (4, N = 548) = 6.531, p > 0.05]. The independent sample t -test showed no significant difference in student performance between online and F2F learners with respect to gender [ t (145) = 1.42, p = 0.122]. The 2-way ANOVA showed no significant difference in student performance between online and F2F learners with respect to class rank ( Girard et al., 2016 ).
Research question #1 was to determine if there was a statistically significant difference between the academic performance of online and F2F students.
Research Question 1
The first research question investigated if there was a difference in student performance between F2F and online learners.
To investigate the first research question, we used a traditional chi-square method to analyze the data. The chi-square analysis is particularly useful for this type of comparison because it allows us to determine if the relationship between teaching modality and performance in our sample set can be extended to the larger population. The chi-square method provides us with a numerical result which can be used to determine if there is a statistically significant difference between the two groups.
Table 1 shows us the mean and SD for modality and for gender. It is a general breakdown of numbers to visually elucidate any differences between scores and deviations. The mean GPA for both modalities is similar with F2F learners scoring a 69.35 and online learners scoring a 68.64. Both groups had fairly similar SDs. A stronger difference can be seen between the GPAs earned by men and women. Men had a 3.23 mean GPA while women had a 2.9 mean GPA. The SDs for both groups were almost identical. Even though the 0.33 numerical difference may look fairly insignificant, it must be noted that a 3.23 is approximately a B+ while a 2.9 is approximately a B. Given a categorical range of only A to F, a plus differential can be considered significant.
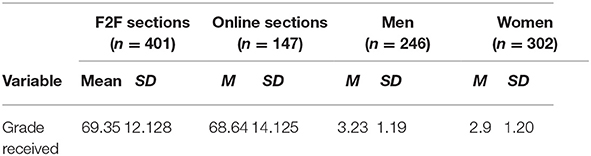
Table 1 . Means and standard deviations for 8 semester- “Environmental Science data set.”
The mean grade for men in the environmental online classes ( M = 3.23, N = 246, SD = 1.19) was higher than the mean grade for women in the classes ( M = 2.9, N = 302, SD = 1.20) (see Table 1 ).
First, a chi-square analysis was performed using SPSS to determine if there was a statistically significant difference in grade distribution between online and F2F students. Students enrolled in the F2F class had the highest percentage of A's (63.60%) as compared to online students (36.40%). Table 2 displays grade distribution by course delivery modality. The difference in student performance was statistically significant, χ 2 (4, N = 548) = 6.531, p > 0.05. Table 3 shows the gender difference on student performance between online and F2F students.
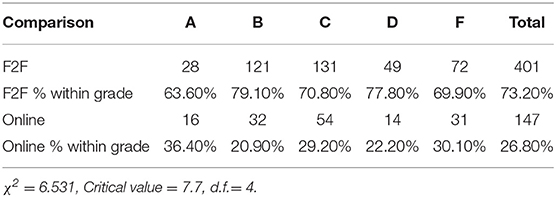
Table 2 . Contingency table for student's academic performance ( N = 548).
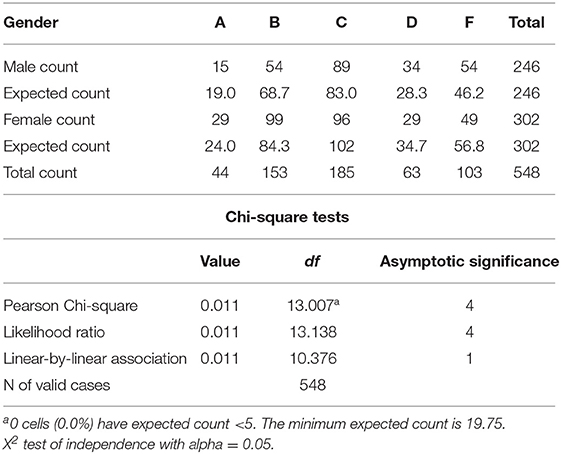
Table 3 . Gender * performance crosstabulation.
Table 2 shows us the performance measures of online and F2F students by grade category. As can be seen, F2F students generated the highest performance numbers for each grade category. However, this disparity was mostly due to a higher number of F2F students in the study. There were 401 F2F students as opposed to just 147 online students. When viewing grades with respect to modality, there are smaller percentage differences between respective learners ( Tanyel and Griffin, 2014 ). For example, F2F learners earned 28 As (63.60% of total A's earned) while online learners earned 16 As (36.40% of total A's earned). However, when viewing the A grade with respect to total learners in each modality, it can be seen that 28 of the 401 F2F students (6.9%) earned As as compared to 16 of 147 (10.9%) online learners. In this case, online learners scored relatively higher in this grade category. The latter measure (grade total as a percent of modality total) is a better reflection of respective performance levels.
Given a critical value of 7.7 and a d.f. of 4, we were able to generate a chi-squared measure of 6.531. The correlating p -value of 0.163 was greater than our p -value significance level of 0.05. We, therefore, had to accept the null hypothesis and reject the alternative hypothesis. There is no statistically significant difference between the two groups in terms of performance scores.
Research Question 2
The second research question was posed to evaluate if there was a difference between online and F2F varied with gender. Does online and F2F student performance vary with respect to gender? Table 3 shows the gender difference on student performance between online and face to face students. We used chi-square test to determine if there were differences in online and F2F student performance with respect to gender. The chi-square test with alpha equal to 0.05 as criterion for significance. The chi-square result shows that there is no statistically significant difference between men and women in terms of performance.
Research Question 3
The third research question tried to determine if there was a difference between online and F2F varied with respect to class rank. Does online and F2F student performance vary with respect to class rank?
Table 4 shows the mean scores and standard deviations of freshman, sophomore, and junior and senior students for both online and F2F student performance. To test the third hypothesis, we used a two-way ANOVA. The ANOVA is a useful appraisal tool for this particular hypothesis as it tests the differences between multiple means. Instead of testing specific differences, the ANOVA generates a much broader picture of average differences. As can be seen in Table 4 , the ANOVA test for this particular hypothesis states there is no significant difference between online and F2F learners with respect to class rank. Therefore, we must accept the null hypothesis and reject the alternative hypothesis.
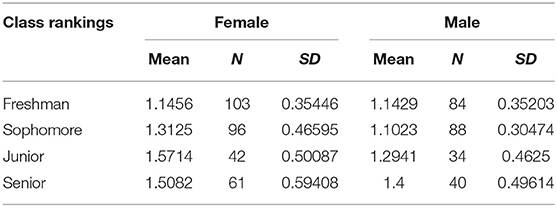
Table 4 . Descriptive analysis of student performance by class rankings gender.
The results of the ANOVA show there is no significant difference in performance between online and F2F students with respect to class rank. Results of ANOVA is presented in Table 5 .
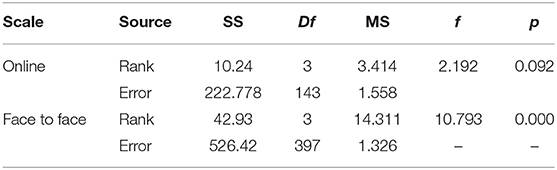
Table 5 . Analysis of variance (ANOVA) for online and F2F of class rankings.
As can be seen in Table 4 , the ANOVA test for this particular hypothesis states there is no significant difference between online and F2F learners with respect to class rank. Therefore, we must accept the null hypothesis and reject the alternative hypothesis.
Discussion and Social Implications
The results of the study show there is no significant difference in performance between online and traditional classroom students with respect to modality, gender, or class rank in a science concepts course for non-STEM majors. Although there were sample size issues and study limitations, this assessment shows both online learners and classroom learners perform at the same level. This conclusion indicates teaching modality may not matter as much as other factors. Given the relatively sparse data on pedagogical modality comparison given specific student population characteristics, this study could be considered innovative. In the current literature, we have not found a study of this nature comparing online and F2F non-STEM majors with respect to three separate factors—medium, gender, and class rank—and the ability to learn science concepts and achieve learning outcomes. Previous studies have compared traditional classroom learning vs. F2F learning for other factors (including specific courses, costs, qualitative analysis, etcetera, but rarely regarding outcomes relevant to population characteristics of learning for a specific science concepts course over many years) ( Liu, 2005 ).
In a study evaluating the transformation of a graduate level course for teachers, academic quality of the online course and learning outcomes were evaluated. The study evaluated the ability of course instructors to design the course for online delivery and develop various interactive multimedia models at a cost-savings to the respective university. The online learning platform proved effective in translating information where tested students successfully achieved learning outcomes comparable to students taking the F2F course ( Herman and Banister, 2007 ).
Another study evaluated the similarities and differences in F2F and online learning in a non-STEM course, “Foundations of American Education” and overall course satisfaction by students enrolled in either of the two modalities. F2F and online course satisfaction was qualitatively and quantitative analyzed. However, in analyzing online and F2F course feedback using quantitative feedback, online course satisfaction was less than F2F satisfaction. When qualitative data was used, course satisfaction was similar between modalities ( Werhner, 2010 ). The course satisfaction data and feedback was used to suggest a number of posits for effective online learning in the specific course. The researcher concluded that there was no difference in the learning success of students enrolled in the online vs. F2F course, stating that “in terms of learning, students who apply themselves diligently should be successful in either format” ( Dell et al., 2010 ). The author's conclusion presumes that the “issues surrounding class size are under control and that the instructor has a course load that makes the intensity of the online course workload feasible” where the authors conclude that the workload for online courses is more than for F2F courses ( Stern, 2004 ).
In “A Meta-Analysis of Three Types of Interaction Treatments in Distance Education,” Bernard et al. (2009) conducted a meta-analysis evaluating three types of instructional and/or media conditions designed into distance education (DE) courses known as interaction treatments (ITs)—student–student (SS), student–teacher (ST), or student–content (SC) interactions—to other DE instructional/interaction treatments. The researchers found that a strong association existed between the integration of these ITs into distance education courses and achievement compared with blended or F2F modalities of learning. The authors speculated that this was due to increased cognitive engagement based in these three interaction treatments ( Larson and Sung, 2009 ).
Other studies evaluating students' preferences (but not efficacy) for online vs. F2F learning found that students prefer online learning when it was offered, depending on course topic, and online course technology platform ( Ary and Brune, 2011 ). F2F learning was preferred when courses were offered late morning or early afternoon 2–3 days/week. A significant preference for online learning resulted across all undergraduate course topics (American history and government, humanities, natural sciences, social, and behavioral sciences, diversity, and international dimension) except English composition and oral communication. A preference for analytical and quantitative thought courses was also expressed by students, though not with statistically significant results ( Mann and Henneberry, 2014 ). In this research study, we looked at three hypothesis comparing online and F2F learning. In each case, the null hypothesis was accepted. Therefore, at no level of examination did we find a significant difference between online and F2F learners. This finding is important because it tells us traditional-style teaching with its heavy emphasis on interpersonal classroom dynamics may 1 day be replaced by online instruction. According to Daymont and Blau (2008) online learners, regardless of gender or class rank, learn as much from electronic interaction as they do from personal interaction. Kemp and Grieve (2014) also found that both online and F2F learning for psychology students led to similar academic performance. Given the cost efficiencies and flexibility of online education, Web-based instructional systems may rapidly rise.
A number of studies support the economic benefits of online vs. F2F learning, despite differences in social constructs and educational support provided by governments. In a study by Li and Chen (2012) higher education institutions benefit the most from two of four outputs—research outputs and distance education—with teaching via distance education at both the undergraduate and graduate levels more profitable than F2F teaching at higher education institutions in China. Zhang and Worthington (2017) reported an increasing cost benefit for the use of distance education over F2F instruction as seen at 37 Australian public universities over 9 years from 2003 to 2012. Maloney et al. (2015) and Kemp and Grieve (2014) also found significant savings in higher education when using online learning platforms vs. F2F learning. In the West, the cost efficiency of online learning has been demonstrated by several research studies ( Craig, 2015 ). Studies by Agasisti and Johnes (2015) and Bartley and Golek (2004) both found the cost benefits of online learning significantly greater than that of F2F learning at U.S. institutions.
Knowing there is no significant difference in student performance between the two mediums, institutions of higher education may make the gradual shift away from traditional instruction; they may implement Web-based teaching to capture a larger worldwide audience. If administered correctly, this shift to Web-based teaching could lead to a larger buyer population, more cost efficiencies, and more university revenue.
The social implications of this study should be touted; however, several concerns regarding generalizability need to be taken into account. First, this study focused solely on students from an environmental studies class for non-STEM majors. The ability to effectively prepare students for scientific professions without hands-on experimentation has been contended. As a course that functions to communicate scientific concepts, but does not require a laboratory based component, these results may not translate into similar performance of students in an online STEM course for STEM majors or an online course that has an online laboratory based co-requisite when compared to students taking traditional STEM courses for STEM majors. There are few studies that suggest the landscape may be changing with the ability to effectively train students in STEM core concepts via online learning. Biel and Brame (2016) reported successfully translating the academic success of F2F undergraduate biology courses to online biology courses. However, researchers reported that of the large-scale courses analyzed, two F2F sections outperformed students in online sections, and three found no significant difference. A study by Beale et al. (2014) comparing F2F learning with hybrid learning in an embryology course found no difference in overall student performance. Additionally, the bottom quartile of students showed no differential effect of the delivery method on examination scores. Further, a study from Lorenzo-Alvarez et al. (2019) found that radiology education in an online learning platform resulted in similar academic outcomes as F2F learning. Larger scale research is needed to determine the effectiveness of STEM online learning and outcomes assessments, including workforce development results.
In our research study, it is possible the study participants may have been more knowledgeable about environmental science than about other subjects. Therefore, it should be noted this study focused solely on students taking this one particular class. Given the results, this course presents a unique potential for increasing the number of non-STEM majors engaged in citizen science using the flexibility of online learning to teach environmental science core concepts.
Second, the operationalization measure of “grade” or “score” to determine performance level may be lacking in scope and depth. The grades received in a class may not necessarily show actual ability, especially if the weights were adjusted to heavily favor group tasks and writing projects. Other performance indicators may be better suited to properly access student performance. A single exam containing both multiple choice and essay questions may be a better operationalization indicator of student performance. This type of indicator will provide both a quantitative and qualitative measure of subject matter comprehension.
Third, the nature of the student sample must be further dissected. It is possible the online students in this study may have had more time than their counterparts to learn the material and generate better grades ( Summers et al., 2005 ). The inverse holds true, as well. Because this was a convenience non-probability sampling, the chances of actually getting a fair cross section of the student population were limited. In future studies, greater emphasis must be placed on selecting proper study participants, those who truly reflect proportions, types, and skill levels.
This study was relevant because it addressed an important educational topic; it compared two student groups on multiple levels using a single operationalized performance measure. More studies, however, of this nature need to be conducted before truly positing that online and F2F teaching generate the same results. Future studies need to eliminate spurious causal relationships and increase generalizability. This will maximize the chances of generating a definitive, untainted results. This scientific inquiry and comparison into online and traditional teaching will undoubtedly garner more attention in the coming years.
Our study compared learning via F2F vs. online learning modalities in teaching an environmental science course additionally evaluating factors of gender and class rank. These data demonstrate the ability to similarly translate environmental science concepts for non-STEM majors in both traditional and online platforms irrespective of gender or class rank. The social implications of this finding are important for advancing access to and learning of scientific concepts by the general population, as many institutions of higher education allow an online course to be taken without enrolling in a degree program. Thus, the potential exists for increasing the number of non-STEM majors engaged in citizen science using the flexibility of online learning to teach environmental science core concepts.
Limitations of the Study
The limitations of the study centered around the nature of the sample group, student skills/abilities, and student familiarity with online instruction. First, because this was a convenience, non-probability sample, the independent variables were not adjusted for real-world accuracy. Second, student intelligence and skill level were not taken into consideration when separating out comparison groups. There exists the possibility that the F2F learners in this study may have been more capable than the online students and vice versa. This limitation also applies to gender and class rank differences ( Friday et al., 2006 ). Finally, there may have been ease of familiarity issues between the two sets of learners. Experienced traditional classroom students now taking Web-based courses may be daunted by the technical aspect of the modality. They may not have had the necessary preparation or experience to efficiently e-learn, thus leading to lowered scores ( Helms, 2014 ). In addition to comparing online and F2F instructional efficacy, future research should also analyze blended teaching methods for the effectiveness of courses for non-STEM majors to impart basic STEM concepts and see if the blended style is more effective than any one pure style.
Data Availability Statement
The datasets generated for this study are available on request to the corresponding author.
Ethics Statement
The studies involving human participants were reviewed and approved by Fort Valley State University Human Subjects Institutional Review Board. Written informed consent for participation was not required for this study in accordance with the national legislation and the institutional requirements.
Author Contributions
JP provided substantial contributions to the conception of the work, acquisition and analysis of data for the work, and is the corresponding author on this paper who agrees to be accountable for all aspects of the work in ensuring that questions related to the accuracy or integrity of any part of the work are appropriately investigated and resolved. FJ provided substantial contributions to the design of the work, interpretation of the data for the work, and revised it critically for intellectual content.
This research was supported in part by funding from the National Science Foundation, Awards #1649717, 1842510, Ñ900572, and 1939739 to FJ.
Conflict of Interest
The authors declare that the research was conducted in the absence of any commercial or financial relationships that could be construed as a potential conflict of interest.
Acknowledgments
The authors would like to thank the reviewers for their detailed comments and feedback that assisted in the revising of our original manuscript.
Agasisti, T., and Johnes, G. (2015). Efficiency, costs, rankings and heterogeneity: the case of US higher education. Stud. High. Educ. 40, 60–82. doi: 10.1080/03075079.2013.818644
CrossRef Full Text | Google Scholar
Ary, E. J., and Brune, C. W. (2011). A comparison of student learning outcomes in traditional and online personal finance courses. MERLOT J. Online Learn. Teach. 7, 465–474.
Google Scholar
Atchley, W., Wingenbach, G., and Akers, C. (2013). Comparison of course completion and student performance through online and traditional courses. Int. Rev. Res. Open Dist. Learn. 14, 104–116. doi: 10.19173/irrodl.v14i4.1461
Bartley, S. J., and Golek, J. H. (2004). Evaluating the cost effectiveness of online and face-to-face instruction. Educ. Technol. Soc. 7, 167–175.
Beale, E. G., Tarwater, P. M., and Lee, V. H. (2014). A retrospective look at replacing face-to-face embryology instruction with online lectures in a human anatomy course. Am. Assoc. Anat. 7, 234–241. doi: 10.1002/ase.1396
PubMed Abstract | CrossRef Full Text | Google Scholar
Bernard, R. M., Abrami, P. C., Borokhovski, E., Wade, C. A., Tamim, R. M., Surkesh, M. A., et al. (2009). A meta-analysis of three types of interaction treatments in distance education. Rev. Educ. Res. 79, 1243–1289. doi: 10.3102/0034654309333844
Biel, R., and Brame, C. J. (2016). Traditional versus online biology courses: connecting course design and student learning in an online setting. J. Microbiol. Biol. Educ. 17, 417–422. doi: 10.1128/jmbe.v17i3.1157
Bigelow, C. A. (2009). Comparing student performance in an online versus a face to face introductory turfgrass science course-a case study. NACTA J. 53, 1–7.
Columbaro, N. L., and Monaghan, C. H. (2009). Employer perceptions of online degrees: a literature review. Online J. Dist. Learn. Administr. 12.
Craig, R. (2015). A Brief History (and Future) of Online Degrees. Forbes/Education . Available online at: https://www.forbes.com/sites/ryancraig/2015/06/23/a-brief-history-and-future-of-online-degrees/#e41a4448d9a8
Daymont, T., and Blau, G. (2008). Student performance in online and traditional sections of an undergraduate management course. J. Behav. Appl. Manag. 9, 275–294.
Dell, C. A., Low, C., and Wilker, J. F. (2010). Comparing student achievement in online and face-to-face class formats. J. Online Learn. Teach. Long Beach 6, 30–42.
Driscoll, A., Jicha, K., Hunt, A. N., Tichavsky, L., and Thompson, G. (2012). Can online courses deliver in-class results? A comparison of student performance and satisfaction in an online versus a face-to-face introductory sociology course. Am. Sociol. Assoc . 40, 312–313. doi: 10.1177/0092055X12446624
Friday, E., Shawnta, S., Green, A. L., and Hill, A. Y. (2006). A multi-semester comparison of student performance between multiple traditional and online sections of two management courses. J. Behav. Appl. Manag. 8, 66–81.
Girard, J. P., Yerby, J., and Floyd, K. (2016). Knowledge retention in capstone experiences: an analysis of online and face-to-face courses. Knowl. Manag. ELearn. 8, 528–539. doi: 10.34105/j.kmel.2016.08.033
Helms, J. L. (2014). Comparing student performance in online and face-to-face delivery modalities. J. Asynchr. Learn. Netw. 18, 1–14. doi: 10.24059/olj.v18i1.348
Herman, T., and Banister, S. (2007). Face-to-face versus online coursework: a comparison of costs and learning outcomes. Contemp. Issues Technol. Teach. Educ. 7, 318–326.
Kemp, N., and Grieve, R. (2014). Face-to-Face or face-to-screen? Undergraduates' opinions and test performance in classroom vs. online learning. Front. Psychol. 5:1278. doi: 10.3389/fpsyg.2014.01278
Keramidas, C. G. (2012). Are undergraduate students ready for online learning? A comparison of online and face-to-face sections of a course. Rural Special Educ. Q . 31, 25–39. doi: 10.1177/875687051203100405
Larson, D.K., and Sung, C. (2009). Comparing student performance: online versus blended versus face-to-face. J. Asynchr. Learn. Netw. 13, 31–42. doi: 10.24059/olj.v13i1.1675
Li, F., and Chen, X. (2012). Economies of scope in distance education: the case of Chinese Research Universities. Int. Rev. Res. Open Distrib. Learn. 13, 117–131.
Liu, Y. (2005). Effects of online instruction vs. traditional instruction on student's learning. Int. J. Instruct. Technol. Dist. Learn. 2, 57–64.
Lorenzo-Alvarez, R., Rudolphi-Solero, T., Ruiz-Gomez, M. J., and Sendra-Portero, F. (2019). Medical student education for abdominal radiographs in a 3D virtual classroom versus traditional classroom: a randomized controlled trial. Am. J. Roentgenol. 213, 644–650. doi: 10.2214/AJR.19.21131
Lundberg, J., Castillo-Merino, D., and Dahmani, M. (2008). Do online students perform better than face-to-face students? Reflections and a short review of some Empirical Findings. Rev. Univ. Soc. Conocim . 5, 35–44. doi: 10.7238/rusc.v5i1.326
Maloney, S., Nicklen, P., Rivers, G., Foo, J., Ooi, Y. Y., Reeves, S., et al. (2015). Cost-effectiveness analysis of blended versus face-to-face delivery of evidence-based medicine to medical students. J. Med. Internet Res. 17:e182. doi: 10.2196/jmir.4346
Mann, J. T., and Henneberry, S. R. (2014). Online versus face-to-face: students' preferences for college course attributes. J. Agric. Appl. Econ . 46, 1–19. doi: 10.1017/S1074070800000602
Mozes-Carmel, A., and Gold, S. S. (2009). A comparison of online vs proctored final exams in online classes. Imanagers J. Educ. Technol. 6, 76–81. doi: 10.26634/jet.6.1.212
Richardson, J. C., and Swan, K. (2003). Examining social presence in online courses in relation to student's perceived learning and satisfaction. J. Asynchr. Learn. 7, 68–88.
Roval, A. P., and Jordan, H. M. (2004). Blended learning and sense of community: a comparative analysis with traditional and fully online graduate courses. Int. Rev. Res. Open Dist. Learn. 5. doi: 10.19173/irrodl.v5i2.192
Salcedo, C. S. (2010). Comparative analysis of learning outcomes in face-to-face foreign language classes vs. language lab and online. J. Coll. Teach. Learn. 7, 43–54. doi: 10.19030/tlc.v7i2.88
Stern, B. S. (2004). A comparison of online and face-to-face instruction in an undergraduate foundations of american education course. Contemp. Issues Technol. Teach. Educ. J. 4, 196–213.
Summers, J. J., Waigandt, A., and Whittaker, T. A. (2005). A comparison of student achievement and satisfaction in an online versus a traditional face-to-face statistics class. Innov. High. Educ. 29, 233–250. doi: 10.1007/s10755-005-1938-x
Tanyel, F., and Griffin, J. (2014). A Ten-Year Comparison of Outcomes and Persistence Rates in Online versus Face-to-Face Courses . Retrieved from: https://www.westga.edu/~bquest/2014/onlinecourses2014.pdf
Werhner, M. J. (2010). A comparison of the performance of online versus traditional on-campus earth science students on identical exams. J. Geosci. Educ. 58, 310–312. doi: 10.5408/1.3559697
Westhuis, D., Ouellette, P. M., and Pfahler, C. L. (2006). A comparative analysis of on-line and classroom-based instructional formats for teaching social work research. Adv. Soc. Work 7, 74–88. doi: 10.18060/184
Wladis, C., Conway, K. M., and Hachey, A. C. (2015). The online STEM classroom-who succeeds? An exploration of the impact of ethnicity, gender, and non-traditional student characteristics in the community college context. Commun. Coll. Rev. 43, 142–164. doi: 10.1177/0091552115571729
Xu, D., and Jaggars, S. S. (2016). Performance gaps between online and face-to-face courses: differences across types of students and academic subject areas. J. Higher Educ. 85, 633–659. doi: 10.1353/jhe.2014.0028
Zhang, L.-C., and Worthington, A. C. (2017). Scale and scope economies of distance education in Australian universities. Stud. High. Educ. 42, 1785–1799. doi: 10.1080/03075079.2015.1126817
Keywords: face-to-face (F2F), traditional classroom teaching, web-based instructions, information and communication technology (ICT), online learning, desire to learn (D2L), passive learning, active learning
Citation: Paul J and Jefferson F (2019) A Comparative Analysis of Student Performance in an Online vs. Face-to-Face Environmental Science Course From 2009 to 2016. Front. Comput. Sci. 1:7. doi: 10.3389/fcomp.2019.00007
Received: 15 May 2019; Accepted: 15 October 2019; Published: 12 November 2019.
Reviewed by:
Copyright © 2019 Paul and Jefferson. This is an open-access article distributed under the terms of the Creative Commons Attribution License (CC BY) . The use, distribution or reproduction in other forums is permitted, provided the original author(s) and the copyright owner(s) are credited and that the original publication in this journal is cited, in accordance with accepted academic practice. No use, distribution or reproduction is permitted which does not comply with these terms.
*Correspondence: Jasmine Paul, paulj@fvsu.edu
Academia.edu no longer supports Internet Explorer.
To browse Academia.edu and the wider internet faster and more securely, please take a few seconds to upgrade your browser .
Enter the email address you signed up with and we'll email you a reset link.
- We're Hiring!
- Help Center
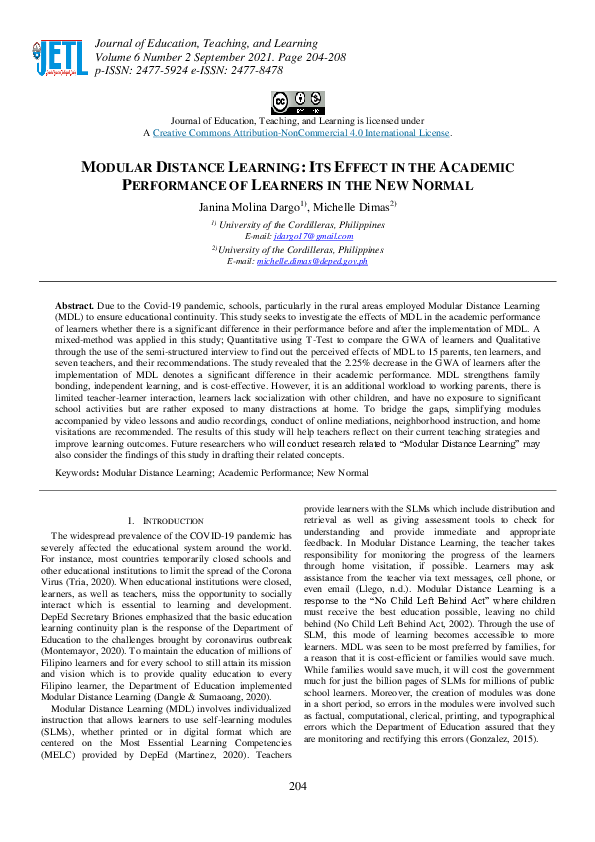
Modular Distance Learning: Its Effect in the Academic Performance of Learners in the New Normal

2021, JETL (Journal of Education, Teaching and Learning)
Due to Covid-19 pandemic, schools, particularly in the rural areas employed Modular Distance Learning (MDL) to ensure education continuity. This study seeks to investigate the effects of MDL in the academic performance of learners whether there is a significant difference in their performance before and after the implementation of MDL. Mixed method was applied in this study; Quantitative using T-Test to compare the GWA of learners and Qualitative through the use of semi-structured interview to find out the perceived effect of MDL to 15 parents, 10 learners, and 7 teachers and their recommendations. The study revealed that the 2.25% decrease in the GWA of learners after the implementation of MDL denotes a significant difference in their academic performance. MDL strengthens family bonding, independent learning, and is cost-effective. However, it is an additional workload to working parents, there is limited teacher-learner interaction, learners lack socialization with other children ...
Related Papers
Psychology and Education: A Multidisciplinary Journal
Psychology and Education , ROMEL LAGRIO
The education sector was greatly affected by the global health crisis of COVID-19, resulting in massive changes in our education setup , which contributed to various problems and challenges encountered during the implementation of the modular distance learning modality. This study aimed to determine the strategies and challenges encountered by teachers in implementing modular distance learning and its impact on students' academic performance. A descriptive research design was employed. The researchers utilized an online survey method for data gathering. A total of 60 teachers and 187 selected Grade 7 learners were the study's respondents utilizing total enumeration for teachers and stratified random sampling for learners.The study's findings show that teachers could employ strategies such as setting a submission schedule and creating a group chat with the learners. Moreover, establish the appropriate health and safety protocols and safety nets for learners against violence and abuse at home and in the community, and train school personnel for the Learning Delivery Modality (LMD).On the other hand, teachers professed that printing modules were time-consuming, the distance of the learner's home from the school hindered the teachers in providing technical assistance, and learners needed help following instructions. Parents answered the modules of the learners. The need for printing materials was a significant challenge.Most of the student's grades during the first quarter were within the range of 80-84, which was considered a satisfactory academic performance. Moreover, the results signified a negligible negative correlation between teachers' strategies in implementing modular distance learning and students' academic performance. The study suggests revisiting the school's plans for implementing modular distance learning and strengthening the partnership of the school, parents, and stakeholders.
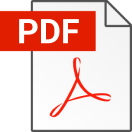
IJMRAP Editor
During this pandemic, several schools opted for modular remote education. One of the elementary schools that selected Modular Distance Learning (MDL) as their primary mode of instruction for various reasons is Antipuluan Elementary School, a public elementary school in the Municipality of Narra, Palawan, the Philippines. However, the usage of this modality, which is unknown to many, has presented difficulties for everyone-including school staff, students, and their parents. Hence the conduct of this study. This quantitative research employed a Descriptive-Correlational Approach and involved 15 elementary teachers, 141 pupils, and 141 parents as the main data sources. A researcher-made questionnaire was used to collect data, which was then analyzed using mean, standard deviation, and Pearson product-moment correlation. The study found that the extent of Modular Distance Learning modality implementation was High, teachers', pupils', and parents' degrees of acceptance of the MDL implementation were High, and there was a strong relationship between the teachers' degree of acceptance of MDL implementation and the degree of its implementation. The perceived effects of MDL implementation have a direct relationship with the degree of their acceptance by teachers and parents.
Psychology and Education
This study investigated the limitations experienced by students, parents, and teachers in the implementation of Modular Distance Learning in Lagundi-CCL National High School during the school year 2021-2022. The researcher utilized the combination of quantitative and qualitative methods of research. An online research questionnaire utilizing Google Form was used to gather necessary information from the eighty (80) students, eighty (80) parents, and thirty-one (31) teachers who served as the respondents of this paper. Based on the results, the three major limitations experienced by students were: 1) insufficient knowledge of parents/ family members; 2) unavailability of gadgets; and 3) too many activities. In addition, parents' three major limitations were: 1) insufficient knowledge about the lessons; 2) difficulties in schedule of distribution and retrieval of modules; and 3) working parents. Furthermore, the identified limitations of teachers were: 1) too many additional tasks for teachers; 2) unavailability of self-learning modules; and 3) students who were lagging behind. From these limitations the respondents had given their suggestions. The students suggested that: 1) lessen the activities that are given to them; 2) conduct an online class even once a week; and 3) give additional time to answer the learning tasks. Meanwhile, parents' suggestions were: 1) enough information and examples in the modules should be given; 2) lessen the learning tasks; and 3) guide the parents on how to assist their children. Lastly, teachers' suggestions include: 1) proper dissemination of program, projects, and activities related to modular distance learning; 2) capacitate parents and students on MDL; and 3) distribution and retrieval should be done every other two weeks. The researchers crafted a process framework which may serve as basis in the modification of the implementation of modular distance learning which included seven (7) strategic dimensions.
International Journal of Applied Research in Social Sciences
Gerald Malabarbas
Face-to-face classes were temporarily suspended and shifted to modular print learning modality due to the COVID-19 pandemic. The study aimed to determine if there significant difference and relationship between parents’ involvement in modular distance learning and the academic performance of the Grade 6 learners in a public elementary school. The results revealed that parents’ involvement in their child's MDL varies substantially according to their educational attainment and family monthly income. Similarly, the educational attainment of parents and their family's monthly income are predictors of their children's academic achievement. Furthermore, it was disclosed that fathers were more likely than mothers to be involved in the learners' MDL. The findings also revealed that there was a correlation between parents' involvement in modular learning and their children's academic performance. Furthermore, the study supports prior results that parental involvement ...
Indonesian Journal of Educational Research and Review
Leomarich Casinillo
Due to the COVID-19 pandemic, schools, particularly in rural areas, employed Modular Distance Learning (MDL) to ensure educational continuity. Modular distance learning is the current learning modality of primary education, where parents serve as parent-teachers to their children. This study seeks to evaluate the experiences of students and teachers of Elementary School, on modular distance learning during the pandemic. This study used the qualitative method of interviewing nine students and six teachers to learn about their MDL experiences. Data process involves combining related concepts and themes to produce a more structured and organized picture of the data. MDL strengthens family bonding, promotes independent learning, and economizes money and time. However, it is an additional workload for working parents; there needs to be more teacher-student interaction, preventing pupils from socializing and gadget distractions. The article revealed that MDL has positive and negative experiences for teachers and students. Therefore, the impact may vary depending on individual circumstances and adaptability. The study suggests that suitable strategies should address any challenges during implementation and evaluation. Furthermore, teachers must undergo training related to MDL to address existing problems in delivering their lessons.
AJHSSR Journal
The general purpose of this study was to find out the level of extent on the parental involvement in the implementation of modular distance learning approach in Botolan District, Division of Zambales, Philippines during school year 2020-2021. The study revealed that the parent-respondent is a typical female in her early adulthood, married, high school graduate with part-time work and meagre income whose children are at primary grade level. The academic performance of the parent-respondents' children was assessed-Very Satisfactory‖. Perceived-Highly Involved‖ on Parent as a Teacher and Acceptance of the Self-Learning Module while-Involved‖ on Submission of the Self-Learning Module. There is significant difference when grouped according to highest educational attainment towards Parent as a Teacher, Acceptance and Submission of the Self-learning module respectively; significant when grouped according to family income towards Parent as Teacher and Acceptance of the Self-Learning Module; while significant on number of children studying in the elementary level towards Parent as Teacher and Submission of the Self-Learning Module respectively. There is significant difference on the perception towards dimensions on the level ofextent on the parental involvement in the implementation of modular distance learning approach. There is negatively weak or little relationship between the level of academic performance and the level ofextent on the parental involvement in the implementation of modular distance learning approach. Based on the summary of the investigations conducted and the conclusions arrived at, the researcher recommended that the parents are encouraged to be given orientation to heighten awareness on their respective limited roles in the implementation of the self-learning modular approach; that parents are encouraged to help children developed with high levels of self-directed learning, to have strong for learning.\
COVID-19 Pandemics have an impact on many aspects of life, including education. The Department of Education developed the Basic Education Learning Continuity Plan in response to the outbreak. This plan outlines learning delivery techniques such as blended learning, which is a combination of face-to-face, modular distance learning, and TV/radio-based education based on the learners' context. As a result, the DepEd permits schools to select a learning mode depending on available resources and student requirements. Thus, this study investigates the primary teachers' readiness, parental support towards modular distance learning, and its effects to the learners' performance. Based on the findings drawn from the study, the following conclusion drawn: The levels of Teachers readiness were very much ready. While in the level of parental support were much supportive. Lastly, the learner's performance was satisfactory. Moreover, it is concluded that teacher's readiness and leaners performance had no bearing with the way learners performed in class while the parental challenges and learners performance were associated with the way learners performed in class.
Psychology and Education , Maritis Magallanes Cagas , Myra A. Ambalong
Parents' engagement played an important role in parent-teacher partnership in educating the children to have a harmonious collaboration in motivating the children's learning. Due to this pandemic, parents were appreciated as facilitators in the learning process of the learners since children were not allowed to go to school for face-to-face interaction with the teacher. Modular Distance learning modalities were implemented most of the schools especially Dalamas Integrated School wherein internet connection was not available in the said area. This study aimed to assess the parents' engagement in modular distance learning and the learners' academic performance in the school year 2021-2022. The study utilized the descriptive-correlational research design. Findings revealed that the respondents' engagement in modular distance learning helped them realize that education was very important to their children and that they encouraged their children to do their homework. However, the data revealed that engagement of parents in modular distance learning did not necessarily affect the academic performance of the learners and that their engagement was not differentiated based on their socio-demographic profile.
Psychology and Education , Ehlz Marie N. Sacnanas
As educational system was hit by a global catastrophe, the introduction of modular distance learning outspread to sustain the quality of education towards the learners. To make this work, parents forced to embrace the new system of learning. With this, the parents were having a hard time on scheduling between their work and children's learning, and on facilitating the learning from home scheme. This study dug into the parents' involvement and attitude towards the modular distance learning system. The data were evaluated by interpretation and the method used in gathering data is qualitative. Content analysis allows researchers identify and analyze the correct words, topics, or concepts. The researchers conducted interviews to six parents from Badian, Cebu. The parents' involvement in this study were determined by purposive sampling technique. From the responses of the parents, the researchers developed three essential themes: (1) The Challenges, (2) The Time, (3) The Rating, (4) The Improvement, and (5) The Advantages and Disadvantages. These themes emphasized the lived experiences and battles of the parents in the distance learning system during the pandemic. The researchers were able to extract problems and meaning of consequences for parents' lived experience of MDL. Parents' talent in shaping their children's learning is not an easy job, rather it was found to be difficult. But additional colors were added to help shape it and made the children's future more worthwhile.
EPRA International Journal of Research & Development (IJRD)
Emma Trovela
This research investigated the parents and learners’ perceptions on modular distance learning that they are experiencing during this time of pandemic as part contemporary new normal education setup. The main purpose of this study was to understand parents’ and learners’ perceptions on modular distance learning as contemporary teaching strategy and how they coped with the experiences and challenges of the new normal education settings. The participants of this study where five (5) senior high school learners and five (5) parents/guardians of senior high school learners of Sta. Catalina Integrated National High School. The research was conducted in Majayjay District from School Year 2020-2021. This study used Qualitative Research through Descriptive research where in-depth interviewing and storytelling was done to gather the narratives or accounts of the research participants. Using an interview protocol and with a strong collaboration with the participants, the researcher will manage...
Loading Preview
Sorry, preview is currently unavailable. You can download the paper by clicking the button above.
RELATED PAPERS
International Journal of Arts and Social Science
JOEL D POTANE , Keziah CanEte
Behavioral Sciences
Charmine Sheena Saflor
International Journal of Multidisciplinary: Applied Business and Education Research
Norvin De Ocampo
CERN European Organization for Nuclear Research - Zenodo
Alcher Arpilleda
Trans Stellar Journals
TJPRC Publication , FITZGERALD KINTANAR
Psychology and Education , Sammy Boy B. Guzman
Psychology and Education , Mayline Atienza
JPAIR Institutional Research
MARINO PAMOGAS
Challenges and Barriers Encountered by G10-Agoncillo Learners in the Implementation of Modular Distance Learning at Taal National High School
Waylie Niña De Claro
European Journal of Education Studies
Romel C . Mutya , Christine Cudillo
Psychology and Education , Harlyn L. Palmes , Harry Palmes
International Journal of Research Studies in Education
Baby Miranda
Zenodo (CERN European Organization for Nuclear Research)
Lucilyn Luis
Environment and Social Psychology
jason V chavez
Tazanna Sevilla
International Journal of Scientific & Research
Regie Bangoy
Sapienza: International Journal of Interdisciplinary Studies
Jimmy Rey Cabardo
Instabright International Journal of Multidisciplinary Research
Michael L . Bordeos
benjie morastil
RELATED TOPICS
- We're Hiring!
- Help Center
- Find new research papers in:
- Health Sciences
- Earth Sciences
- Cognitive Science
- Mathematics
- Computer Science
- Academia ©2024
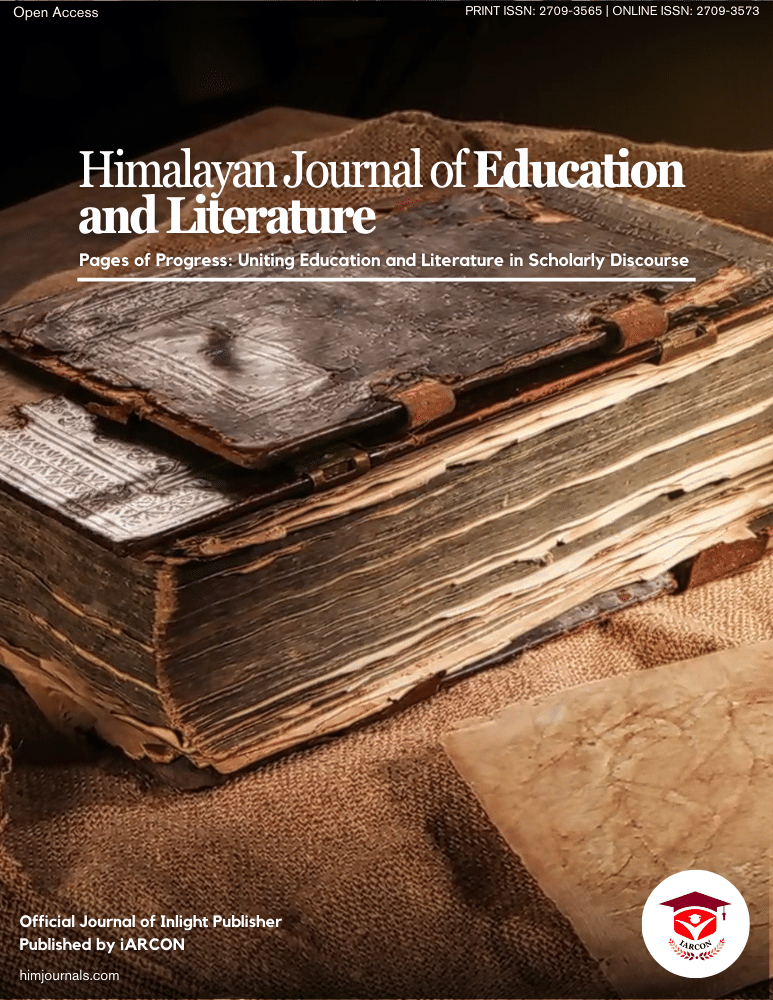
- All Archives
- Special Issue
- Aim and Scope
- Editorial Board
- Journal Insights
- Abstracting and Indexing
- Announcements
- For Authors
- For Reviewers
- Ethics and Policies
- Open Access Policies
- Plagiarism Policies
- Research Ethics Policy
- Authorship and Contributorship
- Informed Consent Policy
- Conflict of Interest
- Content Licensing
- Corrections and Retractions
- Complaints and Appeals
- Article Submission
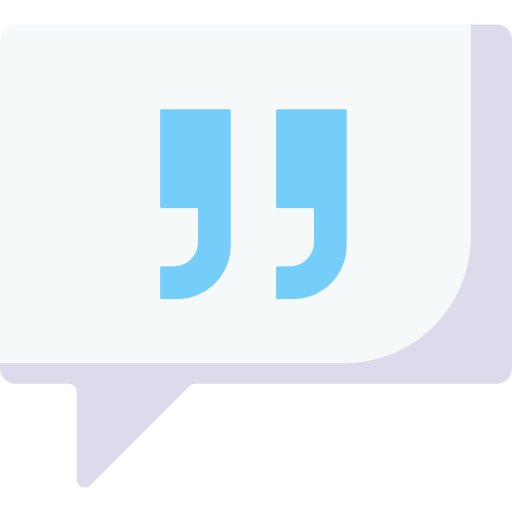
Information
- Author Services
Initiatives
You are accessing a machine-readable page. In order to be human-readable, please install an RSS reader.
All articles published by MDPI are made immediately available worldwide under an open access license. No special permission is required to reuse all or part of the article published by MDPI, including figures and tables. For articles published under an open access Creative Common CC BY license, any part of the article may be reused without permission provided that the original article is clearly cited. For more information, please refer to https://www.mdpi.com/openaccess .
Feature papers represent the most advanced research with significant potential for high impact in the field. A Feature Paper should be a substantial original Article that involves several techniques or approaches, provides an outlook for future research directions and describes possible research applications.
Feature papers are submitted upon individual invitation or recommendation by the scientific editors and must receive positive feedback from the reviewers.
Editor’s Choice articles are based on recommendations by the scientific editors of MDPI journals from around the world. Editors select a small number of articles recently published in the journal that they believe will be particularly interesting to readers, or important in the respective research area. The aim is to provide a snapshot of some of the most exciting work published in the various research areas of the journal.
Original Submission Date Received: .
- Active Journals
- Find a Journal
- Proceedings Series
- For Authors
- For Reviewers
- For Editors
- For Librarians
- For Publishers
- For Societies
- For Conference Organizers
- Open Access Policy
- Institutional Open Access Program
- Special Issues Guidelines
- Editorial Process
- Research and Publication Ethics
- Article Processing Charges
- Testimonials
- Preprints.org
- SciProfiles
- Encyclopedia
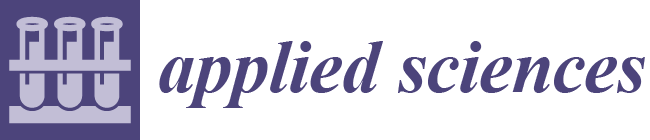
Article Menu
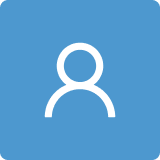
- Subscribe SciFeed
- Recommended Articles
- Google Scholar
- on Google Scholar
- Table of Contents
Find support for a specific problem in the support section of our website.
Please let us know what you think of our products and services.
Visit our dedicated information section to learn more about MDPI.
JSmol Viewer
Multi-agent simulation approach for modular integrated construction supply chain.
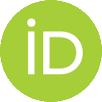
1. Introduction
2. related works, 2.1. overview of modular integrated construction (mic), 2.2. simulation applications in mic logistics.
- Discrete event simulation (DES): used to model the operation of a system as a discrete sequence of events in time, effective for systems where the state changes at specific points, such as the arrival of trucks or the completion of module assembly tasks [ 36 ].
- Agent-based simulation (ABS): models the individual behaviors of agents, representing various entities in the logistics network, such as trucks, warehouses, or modules. Each agent operates based on a set of rules, and their interactions can lead to complex system behavior. This paradigm is highly suitable for capturing the dynamic and stochastic nature of MiC supply chain [ 23 ].
- System dynamics (SD): focuses on the feedback loops and time delays affecting the entire system’s behavior, useful for strategic-level decision-making and long-term planning [ 37 ].
3. Proposed Agent-Based Model
3.1. from physical process to the multi-agent model.
- Inventory System: Tracks the availability and status of prefabricated components in stock at the supplier level.
- Geographic Information System (GIS) such as Global Positioning System (GPS) and Open Street Map (OSM) [ 47 ]: Provides real-time tracking of transport vehicles, ensuring efficient route planning and the timely delivery of components and modules.
- Manufacturing Execution System (MES) [ 48 ]: Manages the production process at MiC factories, integrating physical processes with automation and control systems to ensure high-quality module fabrication.
- Radio-Frequency Identification (RFID) [ 49 ]: Tracks the movement and status of components and modules throughout the supply chain.
- Building Information Modeling (BIM) [ 50 ]: Provides detailed digital representations of the construction project, enabling the precise planning and execution of the assembly operations for integrated modules.
3.2. Agent-Based Model Overview
- Suppliers ( S i ): Responsible for providing prefabricated components. Each supplier agent manages the production and dispatch of components to fabrication centers (i.e., MiC factories).
- Transporters ( T 1 , k : S i -> F j and T 2 , l : F j -> C S ): These agents simulate the transportation vehicles that move prefabricated components from suppliers to fabrication centers and then integrated modules from fabrication centers to construction sites.
- MiC factories ( F j ): These agents represent the fabrication centers where prefabricated components are assembled into integrated construction modules.
- Construction sites (CSs): The final destination agents where the integrated modules are assembled into the final construction.
3.3. Modeling of Interactions between Agents
3.4. model implementation using anylogic, 3.4.1. why anylogic, 3.4.2. model implementation, 3.4.3. model validation, 4. results and discussion, 4.1. study scenarios, 4.1.1. scenario 1: prefabricated component construction without mic factories.
- Number of suppliers: For a mid-sized project, we assume five suppliers to provide different components such as beams, columns, slabs, and exterior panels. These components are crucial for the structural integrity of the construction.
- Number of vehicles: Each supplier uses vehicles to deliver components to the construction site, based on the typical logistics capacity required to maintain a steady flow of components. We will conduct a sensitivity analysis by varying the number of vehicles per supplier from one to five to find the optimal number that minimizes the total cost.
- Demand generation: The demand for components is deterministic and based on project management derived from BIM, with an average of 60 orders per day. This demand is influenced by the construction schedule and project planning, ensuring that the supply chain remains responsive to the dynamic needs of the construction project.
- Initial components in storage: Each supplier starts with an initial inventory between 5 and 10 components, providing a buffer to meet the immediate demand.
- Production capacity/rate: Each supplier has a stochastic production capacity, with an average of 10–15 components per day depending on the complexity and type of component, with a production rate that ensures steady output to meet the ongoing demand.
- Cost Parameters: – Fixed cost per vehicle per day (=EUR 150):This cost per vehicle per day is a set amount that must be paid to maintain the vehicle in the fleet, regardless of whether it is actively used for transportation on that day. It is incurred regardless of the level of activity and the distance traveled. For examples, this includes costs such as vehicle leasing or rental fees, insurance, permits, and salaries for drivers and staff. – Variable cost per kilometer (=EUR 2): This cost reflects the additional expenses incurred for every kilometer the vehicle travels. This includes fuel consumption, wear and tear, and other distance-dependent costs. – Project delay penalty per day (=EUR 500): Refers to a financial penalty that is imposed for each day a project extends beyond its scheduled completion date. For instance, if a construction project is supposed to be completed in 120 days but takes 130 days to finish, a penalty of EUR 500 will be charged for each of the 10 extra days, resulting in a total penalty of EUR 5000. This penalty is intended to incentivize timely project completion and to compensate for the additional costs and potential losses incurred due to the delay.
- Vehicle speed: The speed of the vehicles is stochastic, with an average speed of 50–70 km/h.
- Geo-locations: – Construction site is located in Paris, France; – Supplier 1 is 85 km from the construction site; – Supplier 2 is 130 km from the construction site; – Supplier 3 is 145 km from the construction site; – Supplier 4 is 75 km from the construction site; – Supplier 5 is 135 km from the construction site.
- The normal project completion time for this scenario is set at 120 days. Any delays beyond this period will incur a project delay penalty as mentioned in the cost parameters.
4.1.2. Scenario 2: Fully MiC Process
- Number of Suppliers: Similar to Scenario 1, we assume five suppliers providing different components required for the modules. This allows for a diverse range of components, ensuring that all necessary parts are available for the integrated modules.
- Number of MiC Factories: We assume one MiC factory responsible for assembling the integrated modules from the supplied components. Centralized assembly at a single factory can improve efficiency and reduce complexity in logistics.
- Number of Vehicles: – Transporter 1 ( T 1 ) for components: Similar to Scenario 1, each supplier uses vehicles for transportation of prefabricated components to the factories. We will conduct a sensitivity analysis by varying the number of vehicles per supplier from one to five to find the optimal number that minimizes the total cost. – Transporter 2 ( T 2 ) for modules: The MiC factory uses one vehicle for module delivery to the construction site. This is based on the need to maintain a steady flow of modules while minimizing transportation costs.
- Demand generation: The demand for components and modules is deterministic and based on project management derived from BIM, with an average of 60 component requests per day by the MiC factory and three integrated module requests per day by the construction site.
- Initial components in storage: Each supplier starts with an initial inventory between 5 and 10 components, providing a buffer to meet the immediate demand for construction components.
- Production Capacity/Rate: – Each supplier has a stochastic production capacity, with an average of 10–15 components per day depending on the complexity and type of component. – Each factory has a production capacity of three modules per day. This rate ensures a continuous supply of modules to the construction site while maintaining high-quality standards.
- Cost Parameters: – Fixed cost per vehicle per day: EUR 150 for T 1 , EUR 200 for T 2 . The higher cost for T 2 reflects the more complex logistics and handling required for transporting integrated modules. – Variable cost per kilometer: EUR 2 for T 1 , EUR 3 for T 2 . Transporting integrated modules incurs higher variable costs due to their size and weight. – Project delay penalty per day: EUR 500. This penalty is consistent across both scenarios, incentivizing timely project completion and compensating for potential financial losses due to delays.
- Vehicle Speed: The speed of the vehicles is stochastic, with an average speed of 50–70 km/h for T 1 and 40–60 km/h for T 2 . The lower speed for T 2 reflects the additional care and slower speeds required for transporting larger, more delicate modules.
- Geo-locations: – Construction site is located in Paris, France; – MiC factory is 20 km from the construction site; – Supplier 1 is 70 km from the MiC factory; – Supplier 2 is 125 km from the MiC factory; – Supplier 3 is 155 km from the MiC factory; – Supplier 4 is 75 km from the MiC factory; – Supplier 5 is 125 km from the MiC factory.
- The normal project completion time for this scenario is set at 120 days. Any delays beyond this period will incur a project delay penalty.
4.2. Results
4.2.1. results of scenario 1, 4.2.2. results of scenario 2, 4.3. discussion, 4.4. limitations and future research directions, 5. conclusions, author contributions, institutional review board statement, informed consent statement, data availability statement, conflicts of interest, abbreviations.
CSC | Construction Supply Chain |
OSC | Off-Site Construction |
PC | Prefabricated Components |
MC | Modular Construction |
MiC | Modular Integrated Construction |
DES | Discrete Event Simulation |
SD | System Dynamics |
ABM | Agent-Based Modeling |
OSM | OpenStreetMap |
BIM | Building Information Modeling |
IoT | Internet of Things |
S | Set of suppliers to produce the prefabricated components of modules, indexed by i |
F | Set of MiC factories to fabricate the integrated modules, indexed by j |
Set of transporters from suppliers to MiC factories, indexed by k | |
Set of transporters from MiC factories to the construction site, indexed by l | |
Final construction site |
- Mofolasayo, A. A framework for the evaluation of the decision between onsite and offsite construction using life cycle analysis (LCA) concepts and system dynamics modeling. World J. Civ. Eng. Archit. 2023 , 2 , 1–31. [ Google Scholar ] [ CrossRef ]
- Hou, L.; Tan, Y.; Luo, W.; Xu, S.; Mao, C.; Moon, S. Towards a more extensive application of off-site construction: A technological review. Int. J. Constr. Manag. 2022 , 22 , 2154–2165. [ Google Scholar ] [ CrossRef ]
- Hussein, M.; Eltoukhy, A.E.; Karam, A.; Shaban, I.A.; Zayed, T. Modelling in off-site construction supply chain management: A review and future directions for sustainable modular integrated construction. J. Clean. Prod. 2021 , 310 , 127503. [ Google Scholar ] [ CrossRef ]
- Lopez, D.; Froese, T.M. Analysis of costs and benefits of panelized and modular prefabricated homes. Procedia Eng. 2016 , 145 , 1291–1297. [ Google Scholar ] [ CrossRef ]
- Lawson, M.; Ogden, R.; Goodier, C.I. Design in Modular Construction ; CRC Press: Boca Raton, FL, USA, 2014; Volume 476. [ Google Scholar ]
- Liao, L.; Yang, C.; Quan, L. Construction supply chain management: A systematic literature review and future development. J. Clean. Prod. 2023 , 382 , 135230. [ Google Scholar ] [ CrossRef ]
- Chen, Z.; Hammad, A.W. Mathematical modelling and simulation in construction supply chain management. Autom. Constr. 2023 , 156 , 105147. [ Google Scholar ] [ CrossRef ]
- Liu, Q.; Ma, Y.; Chen, L.; Pedrycz, W.; Skibniewski, M.J.; Chen, Z.S. Artificial intelligence for production, operations and logistics management in modular construction industry: A systematic literature review. Inf. Fusion 2024 , 109 , 102423. [ Google Scholar ] [ CrossRef ]
- Abdelmageed, S.; Zayed, T. A study of literature in modular integrated construction-Critical review and future directions. J. Clean. Prod. 2020 , 277 , 124044. [ Google Scholar ] [ CrossRef ]
- Attajer, A.; Mecheri, B. Framework for Modeling the Propagation of Disturbances in Smart Construction Sites. In Proceedings of the 21st International Conference on Smart Business Technologies, 2024; pp. 80–87. [ Google Scholar ]
- Baghalzadeh Shishehgarkhaneh, M.; Keivani, A.; Moehler, R.C.; Jelodari, N.; Roshdi Laleh, S. Internet of Things (IoT), Building Information Modeling (BIM), and Digital Twin (DT) in construction industry: A review, bibliometric, and network analysis. Buildings 2022 , 12 , 1503. [ Google Scholar ] [ CrossRef ]
- Ghaffarianhoseini, A.; Tookey, J.; Ghaffarianhoseini, A.; Naismith, N.; Azhar, S.; Efimova, O.; Raahemifar, K. Building Information Modelling (BIM) uptake: Clear benefits, understanding its implementation, risks and challenges. Renew. Sustain. Energy Rev. 2017 , 75 , 1046–1053. [ Google Scholar ] [ CrossRef ]
- Eneyew, D.D.; Capretz, M.A.; Bitsuamlak, G.T. Toward smart-building digital twins: BIM and IoT data integration. IEEE Access 2022 , 10 , 130487–130506. [ Google Scholar ] [ CrossRef ]
- Borkowski, A.S. Low-Cost Internet of Things Solution for Building Information Modeling Level 3B—Monitoring, Analysis and Management. J. Sens. Actuator Netw. 2024 , 13 , 19. [ Google Scholar ] [ CrossRef ]
- Zhai, Y.; Chen, K.; Zhou, J.X.; Cao, J.; Lyu, Z.; Jin, X.; Shen, G.Q.; Lu, W.; Huang, G.Q. An Internet of Things-enabled BIM platform for modular integrated construction: A case study in Hong Kong. Adv. Eng. Inform. 2019 , 42 , 100997. [ Google Scholar ] [ CrossRef ]
- Zhou, J.X.; Shen, G.Q.; Yoon, S.H.; Jin, X. Customization of on-site assembly services by integrating the internet of things and BIM technologies in modular integrated construction. Autom. Constr. 2021 , 126 , 103663. [ Google Scholar ] [ CrossRef ]
- Zhong, R.Y.; Peng, Y.; Fang, J.; Xu, G.; Xue, F.; Zou, W.; Huang, G.Q. Towards Physical Internet-enabled prefabricated housing construction in Hong Kong. IFAC-PapersOnLine 2015 , 48 , 1079–1086. [ Google Scholar ] [ CrossRef ]
- Zhong, R.Y.; Peng, Y.; Xue, F.; Fang, J.; Zou, W.; Luo, H.; Ng, S.T.; Lu, W.; Shen, G.Q.; Huang, G.Q. Prefabricated construction enabled by the Internet-of-Things. Autom. Constr. 2017 , 76 , 59–70. [ Google Scholar ] [ CrossRef ]
- Hossain, M.U.; Ng, S.T.; Antwi-Afari, P.; Amor, B. Circular economy and the construction industry: Existing trends, challenges and prospective framework for sustainable construction. Renew. Sustain. Energy Rev. 2020 , 130 , 109948. [ Google Scholar ] [ CrossRef ]
- Garusinghe, G.D.A.U.; Perera, B.A.K.S.; Weerapperuma, U.S. Integrating circular economy principles in modular construction to enhance sustainability. Sustainability 2023 , 15 , 11730. [ Google Scholar ] [ CrossRef ]
- Jayawardana, J.; Sandanayake, M.; Jayasinghe, J.; Kulatunga, A.K.; Zhang, G. A comparative life cycle assessment of prefabricated and traditional construction—A case of a developing country. J. Build. Eng. 2023 , 72 , 106550. [ Google Scholar ] [ CrossRef ]
- Adabre, M.A.; Chan, A.P.; Darko, A.; Hosseini, M.R. Facilitating a transition to a circular economy in construction projects: Intermediate theoretical models based on the theory of planned behaviour. Build. Res. Inf. 2023 , 51 , 85–104. [ Google Scholar ] [ CrossRef ]
- Hussein, M.; Karam, A.; Eltoukhy, A.E.; Darko, A.; Zayed, T. Optimized multimodal logistics planning of modular integrated construction using hybrid multi-agent and metamodeling. Autom. Constr. 2023 , 145 , 104637. [ Google Scholar ] [ CrossRef ]
- Goubran, S.; Walker, T.; Cucuzzella, C.; Schwartz, T. Green building standards and the united nations’ sustainable development goals. J. Environ. Manag. 2023 , 326 , 116552. [ Google Scholar ] [ CrossRef ]
- Nguyen, T.D.H.N.; Moon, H.; Ahn, Y. Critical review of trends in modular integrated construction research with a focus on sustainability. Sustainability 2022 , 14 , 12282. [ Google Scholar ] [ CrossRef ]
- Siebers, P.O.; Aickelin, U. Introduction to multi-agent simulation. In Encyclopedia of Decision Making and Decision Support Technologies ; IGI Global: Hershey, PA, USA, 2008; pp. 554–564. [ Google Scholar ]
- Attajer, A.; Darmoul, S.; Chaabane, S.; Riane, F.; Sallez, Y. Benchmarking simulation software capabilities against distributed control requirements: FlexSim vs AnyLogic. In Proceedings of the Service Oriented, Holonic and Multi-Agent Manufacturing Systems for Industry of the Future: Proceedings of SOHOMA 2020, Paris, France, 1–2 October 2020; Springer: Berlin/Heidelberg, Germany, 2021; pp. 520–531. [ Google Scholar ]
- Khan, A.; Yu, R.; Liu, T.; Guan, H.; Oh, E. Drivers towards adopting modular integrated construction for affordable sustainable housing: A total interpretive structural modelling (TISM) method. Buildings 2022 , 12 , 637. [ Google Scholar ] [ CrossRef ]
- Hyun, H.; Kim, H.; Lee, H.S.; Park, M.; Lee, J. Integrated design process for modular construction projects to reduce rework. Sustainability 2020 , 12 , 530. [ Google Scholar ] [ CrossRef ]
- Wuni, I.Y.; Shen, G.Q. Critical success factors for modular integrated construction projects: A review. Build. Res. Inf. 2020 , 48 , 763–784. [ Google Scholar ] [ CrossRef ]
- Wuni, I.Y.; Shen, G.Q.; Hwang, B.G. Risks of modular integrated construction: A review and future research directions. Front. Eng. Manag. 2020 , 7 , 63–80. [ Google Scholar ] [ CrossRef ]
- Wuni, I.Y.; Mazher, K.M. Ending the suitability quantification dilemma: Intelligent decision support system for modular integrated construction in a high-density metropolis. Constr. Innov. 2023 , 24 , 1026–1047. [ Google Scholar ] [ CrossRef ]
- Sharafi, P.; Rashidi, M.; Samali, B.; Ronagh, H.; Mortazavi, M. Identification of factors and decision analysis of the level of modularization in building construction. J. Archit. Eng. 2018 , 24 , 04018010. [ Google Scholar ] [ CrossRef ]
- Wuni, I.Y.; Shen, G.Q. Towards a decision support for modular integrated construction: An integrative review of the primary decision-making actors. Int. J. Constr. Manag. 2022 , 22 , 929–948. [ Google Scholar ] [ CrossRef ]
- Zhang, Z.; Pan, W. Multi-criteria decision analysis for tower crane layout planning in high-rise modular integrated construction. Autom. Constr. 2021 , 127 , 103709. [ Google Scholar ] [ CrossRef ]
- Taiwo, R.; Hussein, M.; Zayed, T. An integrated approach of simulation and regression analysis for assessing productivity in modular integrated construction projects. Buildings 2022 , 12 , 2018. [ Google Scholar ] [ CrossRef ]
- Kaya, H.D. Using System Dynamics to Support Strategic Decision-Making: The Case of Digitalization in Modular Construction Company. Master’s Thesis, Middle East Technical University, Ankara, Turkey, 2022. [ Google Scholar ]
- Jaśkowski, P.; Sobotka, A.; Czarnigowska, A. Decision model for planning material supply channels in construction. Autom. Constr. 2018 , 90 , 235–242. [ Google Scholar ] [ CrossRef ]
- Li, J.Q.; Han, Y.Q.; Duan, P.Y.; Han, Y.Y.; Niu, B.; Li, C.D.; Zheng, Z.X.; Liu, Y.P. Meta-heuristic algorithm for solving vehicle routing problems with time windows and synchronized visit constraints in prefabricated systems. J. Clean. Prod. 2020 , 250 , 119464. [ Google Scholar ] [ CrossRef ]
- Almashaqbeh, M.; El-Rayes, K. Minimizing transportation cost of prefabricated modules in modular construction projects. Eng. Constr. Archit. Manag. 2022 , 29 , 3847–3867. [ Google Scholar ] [ CrossRef ]
- Chen, J.H.; Yan, S.; Tai, H.W.; Chang, C.Y. Optimizing profit and logistics for precast concrete production. Can. J. Civ. Eng. 2017 , 44 , 393–406. [ Google Scholar ] [ CrossRef ]
- Liu, J.; Soleimanifar, M.; Lu, M. Resource-loaded piping spool fabrication scheduling: Material-supply-driven optimization. Vis. Eng. 2017 , 5 , 5. [ Google Scholar ] [ CrossRef ]
- Bamana, F.; Lehoux, N.; Cloutier, C. Simulation of a construction project: Assessing impact of just-in-time and lean principles. J. Constr. Eng. Manag. 2019 , 145 , 05019005. [ Google Scholar ] [ CrossRef ]
- Hussein, M.; Darko, A.; Eltoukhy, A.E.; Zayed, T. Sustainable logistics planning in modular integrated construction using multimethod simulation and Taguchi approach. J. Constr. Eng. Manag. 2022 , 148 , 04022022. [ Google Scholar ] [ CrossRef ]
- Tak, A.N.; Taghaddos, H.; Mousaei, A.; Hermann, U.R. Evaluating industrial modularization strategies: Local vs. overseas fabrication. Autom. Constr. 2020 , 114 , 103175. [ Google Scholar ]
- Zhang, H.; Yu, L. Dynamic transportation planning for prefabricated component supply chain. Eng. Constr. Archit. Manag. 2020 , 27 , 2553–2576. [ Google Scholar ] [ CrossRef ]
- Esch, T.; Zeidler, J.; Palacios-Lopez, D.; Marconcini, M.; Roth, A.; Mönks, M.; Leutner, B.; Brzoska, E.; Metz-Marconcini, A.; Bachofer, F.; et al. Towards a large-scale 3D modeling of the built environment—Joint analysis of TanDEM-X, Sentinel-2 and open street map data. Remote Sens. 2020 , 12 , 2391. [ Google Scholar ] [ CrossRef ]
- Peiris, A.; Hui, F.K.P.; Duffield, C.; Wang, J.; Garcia, M.G.; Chen, Y.; Ngo, T. Digitalising modular construction: Enhancement of off-site manufacturing productivity via a manufacturing execution & control (MEC) system. Comput. Ind. Eng. 2023 , 178 , 109117. [ Google Scholar ]
- Li, C.Z.; Zhong, R.Y.; Xue, F.; Xu, G.; Chen, K.; Huang, G.G.; Shen, G.Q. Integrating RFID and BIM technologies for mitigating risks and improving schedule performance of prefabricated house construction. J. Clean. Prod. 2017 , 165 , 1048–1062. [ Google Scholar ] [ CrossRef ]
- Darko, A.; Chan, A.P.; Yang, Y.; Tetteh, M.O. Building information modeling (BIM)-based modular integrated construction risk management–Critical survey and future needs. Comput. Ind. 2020 , 123 , 103327. [ Google Scholar ] [ CrossRef ]
- Jiang, Y.; Li, M.; Guo, D.; Wu, W.; Zhong, R.Y.; Huang, G.Q. Digital twin-enabled smart modular integrated construction system for on-site assembly. Comput. Ind. 2022 , 136 , 103594. [ Google Scholar ] [ CrossRef ]
- Yeung, T.; Martinez, J.; Sharoni, L.O.; Leao, J.; Sacks, R. Automatic Parametric Generation of Simulation Models from Project Information in Digital Twin Construction. In Proceedings of the International Conference on Computing in Civil and Building Engineering, Cape Town, South Africa, 26–28 October 2022; Springer: Berlin/Heidelberg, Germany, 2022; pp. 633–650. [ Google Scholar ]
- Abdelmageed, S.; Abdelkhalek, S.; Hussien, M.; Zayed, T. A hybrid simulation model for modules installation in modular integrated construction projects. Int. J. Constr. Manag. 2023 , 1–12. [ Google Scholar ] [ CrossRef ]
- Volneikina, E.; Kukartseva, O.; Menshenin, A.; Tynchenko, V.; Degtyareva, K. Simulation-Dynamic Modeling Of Supply Chains Based On Big Data. In Proceedings of the 2023 22nd International Symposium INFOTEH-JAHORINA (INFOTEH), East Sarajevo, Bosnia and Herzegovina, 15–17 March 2023; IEEE: Piscataway, NJ, USA, 2023; pp. 1–6. [ Google Scholar ]
- Haklay, M.; Weber, P. Openstreetmap: User-generated street maps. IEEE Pervasive Comput. 2008 , 7 , 12–18. [ Google Scholar ] [ CrossRef ]
- Ministère de la Transition Écologique. RE2020: Une Nouvellé Etape Vers une Future Règlementation Environnementale des Bâtiments Neufs Plus Ambitieuse Contre le Changement Climatique. 2020. Available online: https://dynalec.fr/re-2020-une-nouvelle-etape/ (accessed on 30 May 2024).
- Jang, J.Y.; Kim, J.I.; Koo, C.; Kim, T.W. Automated components—Vehicle allocation planning for precast concrete projects. J. Manag. Eng. 2022 , 38 , 04022059. [ Google Scholar ] [ CrossRef ]
- Subramanya, K.; Kermanshachi, S.; Rouhanizadeh, B. Modular construction vs. traditional construction: Advantages and limitations: A comparative study. In Proceedings of the Creative Construction e-Conference 2020, Opatija, Croatia, 28 June–1 July 2020; Budapest University of Technology and Economics: Budapest, Hungary, 2020; pp. 11–19. [ Google Scholar ]
- Wuni, I.Y.; Shen, G.Q.; Mahmud, A.T. Critical risk factors in the application of modular integrated construction: A systematic review. Int. J. Constr. Manag. 2022 , 22 , 133–147. [ Google Scholar ] [ CrossRef ]
- Loo, B.P.; Wong, R.W. Towards a conceptual framework of using technology to support smart construction: The case of modular integrated construction (MiC). Buildings 2023 , 13 , 372. [ Google Scholar ] [ CrossRef ]
Click here to enlarge figure
Study | Focus | Limitations Addressed |
---|---|---|
[ ] | Inventory management in OSC | Single supply chain stage, does not address dynamic interactions or uncertainties |
[ ] | Vehicle routing for OSC logistics | Single supply chain stage, lacks integration with other stages and consideration of real-world uncertainties |
[ ] | Integrated truck planning for OSC | Single supply chain stage, does not incorporate dynamic interactions between different supply chain stages |
[ ] | Combining production with logistics in OSC | Integrated approach, but overlooks uncertainties and dynamic interactions critical for real-world applications |
[ ] | Integrating logistics with installation in OSC | Holistic approach, but lacks consideration of uncertainties and stakeholder dynamics |
[ ] | DES simulation for OSC logistics | Models complex processes over time but does not fully integrate all supply chain stages or stakeholder dynamics |
[ , , ] | Multi-method simulation with optimization for MiC | Robust framework addressing dynamic interactions, but further research needed for holistic integration of all supply chain stages |
This Study | Multi-agent simulation for holistic MiC logistics | Comprehensive integration of all supply chain stages, addressing dynamic interactions, uncertainties, and real-time data integration |
Agent | Properties and Parameters | Behaviors and Functions |
---|---|---|
Construction Site | Demand generation event based on BIM and project planning. Parameter: MiC factory associated with the demand. | Function: GenerateDemand() for integrated modules. |
MiC Factory | Event to generate demand for prefabricated components from suppliers. Parameter: Supplier associated with the demand, number of vehicles assigned to transport the module. | Process: Fabrication of integrated modules (see a). Function: FabricationTime() for module fabrication duration, GenerateDemand() to generate demand for components, ManageVehicles() to manage vehicle assignments for transportation. |
Supplier | Parameter: Number of vehicles assigned, capacity, ComponentsInStorage, ProductionRate. | Process: Fabrication of prefabricated components (see b), Inventory modeling using system dynamics (see c). Function: ProductionTime() for component production duration, ProduceComponents() to manage the production of components, ManageInventory() to manage inventory levels and storage, ScheduleTransport() to schedule transport of components to factories. |
Transporter | Parameter: Associated supplier and factory. | Process: State chart (see d). Function: TransportComponents() to manage transport of components from suppliers to factories, UpdateStatus() to update transport status and availability. |
Transporter | Parameter: Associated factory and construction site. | Process: State chart (see e). Functions: TransportModules() to manage transport of modules from factories to construction sites, UpdateStatus() to update transport status and availability. |
Parameter | Scenario 1 | Scenario 2 | Unit |
---|---|---|---|
Number of Suppliers | 5 | 5 | - |
Number of MiC Factories | - | 1 | - |
Number of Vehicles ( ) per Supplier | [1, 2, 3, 4, 5] | [1, 2, 3, 4, 5] | - |
Number of Vehicles ( ) per Factory | - | 1 | - |
Average Requests per Day | 60 C by the construction site | 60 C by the MiC Factory, 3 M by the construction site | C: Components, M: Modules |
Fixed Cost per Vehicle per Day | 150 | 150 for , 200 for | EUR |
Variable Cost per km | 2 | 2 for , 3 for | EUR |
Project Delay Penalty per Day | 500 | 500 | EUR |
Initial Components In Storage per supplier | uniform(5, 10) | uniform(5, 10) | Components |
Production Capacity per Day | uniform(10, 15) C per supplier | uniform(10, 15) C per supplier, 3 M per factory | C: Components, M: Modules |
Vehicle Speed | uniform(50, 70) | uniform(50, 70) for , uniform(40, 60) for | km/h |
Supplier Locations (from the construction site) | Supplier 1: 85, Supplier 2: 130, Supplier 3: 145, Supplier 4: 75, Supplier 5: 135 | Supplier 1: 85, Supplier 2: 130, Supplier 3: 145, Supplier 4: 75, Supplier 5: 135 | km |
Supplier Locations (from the MiC factory) | - | Supplier 1: 70, Supplier 2: 125, Supplier 3: 155, Supplier 4: 75, Supplier 5: 125 | km |
MiC Factory Location (from the construction site) | - | 20 | km |
Construction Site Location | Paris, France | - | |
Normal Project Completion Time | 120 | days |
Number of Vehicles per Supplier | Total Variable Cost (EUR) | Total Fixed Cost (EUR) | Penalty (EUR) | Total Cost (EUR) |
---|---|---|---|---|
1 | 1,938,540 | 237,000 | 98,000 | 2,273,540 |
2 | 1,938,540 | 238,500 | 19,500 | 2,196,540 |
3 | 1,938,540 | 238,500 | 0 | 2,177,040 |
4 | 1,938,540 | 258,000 | 0 | 2,196,540 |
5 | 1,938,540 | 322,500 | 0 | 2,261,040 |
Number of Vehicles per Supplier | Total Variable Cost for (EUR) | Total Variable Cost for (EUR) | Total Fixed Cost for (EUR) | Total Fixed Cost for (EUR) | Penalty (EUR) | Total Cost (EUR) |
---|---|---|---|---|---|---|
1 | 1,870,500 | 43,320 | 220,500 | 58,800 | 87,000 | 2,280,120 |
2 | 1,870,500 | 43,320 | 220,500 | 29,400 | 13,500 | 2,177,220 |
3 | 1,870,500 | 43,320 | 220,500 | 19,600 | 0 | 2,153,920 |
4 | 1,870,500 | 43,320 | 258,000 | 17,200 | 0 | 2,189,020 |
5 | 1,870,500 | 43,320 | 322,500 | 17,200 | 0 | 2,253,520 |
The statements, opinions and data contained in all publications are solely those of the individual author(s) and contributor(s) and not of MDPI and/or the editor(s). MDPI and/or the editor(s) disclaim responsibility for any injury to people or property resulting from any ideas, methods, instructions or products referred to in the content. |
Share and Cite
Attajer, A.; Mecheri, B. Multi-Agent Simulation Approach for Modular Integrated Construction Supply Chain. Appl. Sci. 2024 , 14 , 5286. https://doi.org/10.3390/app14125286
Attajer A, Mecheri B. Multi-Agent Simulation Approach for Modular Integrated Construction Supply Chain. Applied Sciences . 2024; 14(12):5286. https://doi.org/10.3390/app14125286
Attajer, Ali, and Boubakeur Mecheri. 2024. "Multi-Agent Simulation Approach for Modular Integrated Construction Supply Chain" Applied Sciences 14, no. 12: 5286. https://doi.org/10.3390/app14125286
Article Metrics
Further information, mdpi initiatives, follow mdpi.
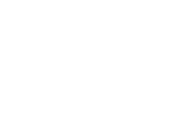
Subscribe to receive issue release notifications and newsletters from MDPI journals

IMAGES
VIDEO
COMMENTS
The term "modular approach" refers to learning that takes the form of individualized instruction and allows students to use Self-Learning Modules (SLMs) in the print or advanced format/electronic ...
The scales built from qualitative research will be retested through preliminary quantitative research. Preliminary quantitative research is conducted with a small sample (70 students) to test the reliability of the scale with Cronbach's Alpha coefficient and exploratory factor analysis for each scale. The purpose of preliminary quantitative ...
Research design. This research applies a quantitative design where descriptive statistics are used for the student characteristics and design features data, t-tests for the age and gender variables to determine if they are significant in blended learning effectiveness and regression for predictors of blended learning effectiveness.
This investigation's in-depth locus is to identify modular classes' impact on academic performance among Grade Six learners. The researchers employed quantitative research using cluster sampling - 50 participants among the sixth-grade learners. Using google forms, links were provided to gather data.
The purpose of this descriptive paper was to explore and synthesize literature related to understanding modular learning and how it can be implemented effectively so faculty members embrace its use. An in-depth review of literature addressed topics including, Educational Theories supporting modular learning, the development of modular learning,
The COVID-19 pandemic brought extraordinary challenges to K-12 students in using modular distance learning. According to Transactional Distance Theory (TDT), which is defined as understanding the effects of distance learning in the cognitive domain, the current study constructs a theoretical framework to measure student satisfaction and Bloom's Taxonomy Theory (BTT) to measure students ...
PDF | On Jun 25, 2021, Felicisimo Castroverde and others published Modular distance learning modality: Challenges of teachers in teaching amid the Covid-19 pandemic | Find, read and cite all the ...
Background Educational pedagogies were modified during the COVID-19 pandemic to minimise interruption to teaching. One approach has been the distance learning problem-based learning (PBL) tutorial utilising the online peer-to-peer platform. The aim of this study was to compare the performance of students using distance learning PBL tutorials using with that of students utilising the ...
However, the usage of this modality, which is unknown to many, has presented difficulties for everyone-including school staff, students, and their parents. Hence the conduct of this study. This quantitative research employed a Descriptive-Correlational Approach and involved 15 elementary teachers, 141 pupils, and 141 parents as the main data ...
This quantitative study employed a descriptive survey to determine and identify the perceived effects of modular distance learning on grades 4,5 and 6 students. The research instrument used in this study is a survey questionnaire, which is used to acquire the necessary data.
This study investigated the instructional competencies of teachers in The existence of COVID-19 pandemic brought extraordinary challenges to the stakeholders, teachers, parents, and students. Thus, the researcher believed that there is an effect of teaching-learning process in new normal education to students' performance most especially using modular type of learning in Mathematics.
The mean grade for men in the environmental online classes (M = 3.23, N = 246, SD = 1.19) was higher than the mean grade for women in the classes (M = 2.9, N = 302, SD = 1.20) (see Table 1).First, a chi-square analysis was performed using SPSS to determine if there was a statistically significant difference in grade distribution between online and F2F students.
It can also be noted that 44.14% stated that they were having difficulty adapting to the Modular (Printed) Distance Learning, 43.10% expressed difficulties in answering the Self-Learning Modules ...
Hence, this research has broader ramifications that can direct the Bureau of Learning Delivery to orient the module writers to further contextualize the learning materials that can compound learner's disposition and academic performance. Keywords: Attitude, Academic Performance, COVID-19, New Normal Education, Modular Learning INTRODUCTION
A Quantitative Study of an Online Learning Platform's Impact on High School Students' Engagement, Academic Achievement, and Student Satisfaction in a Mathematics Class Mariah Minkkinen [email protected] Follow this and additional works at: https://red.mnstate.edu/thesis Part of the Mathematics Commons
ABSTRACT. Education in the new normal is a challenging task in the Philippines in an attempt to push through education amidst. the deadly pandemic caused by covid-19. The Department of Education ...
This paper explores the student learning experience using technology as an e-learning tool during the COVID-19 pandemic. This article utilized qualitative methods to examine the quality of student learning using deep and surface approaches to understand what influences student engagement with technology. Interviews were conducted with 21 students from various academic majors using deductive ...
Due to Covid-19 pandemic, schools, particularly in the rural areas employed Modular Distance Learning (MDL) to ensure education continuity. This study seeks to investigate the effects of MDL in the academic performance of learners whether there is a significant difference in their performance before and after the implementation of MDL.
The Covid-19 pandemic has caused a historical disturbance in the delivery of education in the Philippines. The major purpose of this study was to determine the effectiveness of modular distance learning modality to the Academic Performance of Students on its more than a year implementation.
learning is effective; modular distance learning helps them to. build their c onfidence with a mean rating of 3.59 and a standard. deviation of 1.17 with a verbal description of high and ...
1. Introduction. These days, there is a move toward modular approach to curriculum implementation. The approach has drawn a special attention in most nations' education system particularly in technical and vocational education and higher education (Malik, Citation 2012).MoE [Ministry of Education] (Citation 2013) of Ethiopia asserted that there has been an increasing focus on modular ...
The shift from traditional on-site to off-site construction marks a significant evolution in the construction industry, characterized by increasing levels of prefabrication. These advancements enhance construction efficiency, reduce lead times, and mitigate environmental impacts, leading to modular integrated construction (MiC). However, MiC presents complex supply chain challenges ...
The purpose of this study was to examine the effectiveness of modular teaching approach on learning of. university students. In order to test the r elative effectiveness of independent variable, i ...
The key purpose of this research was to find out the effectiveness of modular approach in teaching in order to assess the student learning, performance and achievement and to determine whether the ...