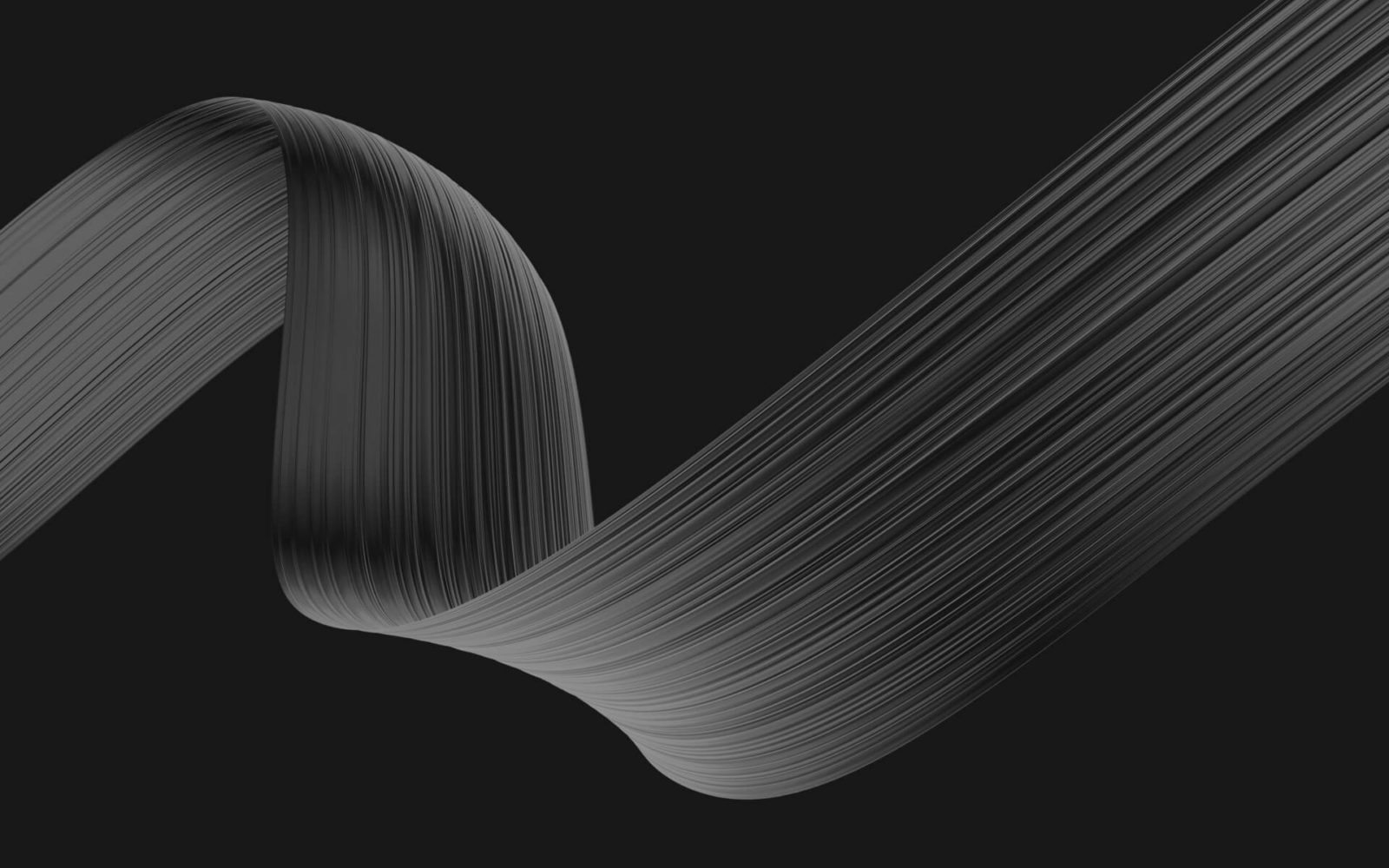

How to write a hypothesis for marketing experimentation
- Apr 11, 2021
- 5 minute read
- Creating your strongest marketing hypothesis
The potential for your marketing improvement depends on the strength of your testing hypotheses.
But where are you getting your test ideas from? Have you been scouring competitor sites, or perhaps pulling from previous designs on your site? The web is full of ideas and you’re full of ideas – there is no shortage of inspiration, that’s for sure.
Coming up with something you want to test isn’t hard to do. Coming up with something you should test can be hard to do.
Hard – yes. Impossible? No. Which is good news, because if you can’t create hypotheses for things that should be tested, your test results won’t mean mean much, and you probably shouldn’t be spending your time testing.
Taking the time to write your hypotheses correctly will help you structure your ideas, get better results, and avoid wasting traffic on poor test designs.
With this post, we’re getting advanced with marketing hypotheses, showing you how to write and structure your hypotheses to gain both business results and marketing insights!
By the time you finish reading, you’ll be able to:
- Distinguish a solid hypothesis from a time-waster, and
- Structure your solid hypothesis to get results and insights
To make this whole experience a bit more tangible, let’s track a sample idea from…well…idea to hypothesis.
Let’s say you identified a call-to-action (CTA)* while browsing the web, and you were inspired to test something similar on your own lead generation landing page. You think it might work for your users! Your idea is:
“My page needs a new CTA.”
*A call-to-action is the point where you, as a marketer, ask your prospect to do something on your page. It often includes a button or link to an action like “Buy”, “Sign up”, or “Request a quote”.
The basics: The correct marketing hypothesis format
Level up: moving from a good to great hypothesis, it’s based on a science, building marketing hypotheses to create insights, what makes a great hypothesis.
A well-structured hypothesis provides insights whether it is proved, disproved, or results are inconclusive.
You should never phrase a marketing hypothesis as a question. It should be written as a statement that can be rejected or confirmed.
Further, it should be a statement geared toward revealing insights – with this in mind, it helps to imagine each statement followed by a reason :
- Changing _______ into ______ will increase [conversion goal], because:
- Changing _______ into ______ will decrease [conversion goal], because:
- Changing _______ into ______ will not affect [conversion goal], because:
Each of the above sentences ends with ‘because’ to set the expectation that there will be an explanation behind the results of whatever you’re testing.
It’s important to remember to plan ahead when you create a test, and think about explaining why the test turned out the way it did when the results come in.
Understanding what makes an idea worth testing is necessary for your optimization team.
If your tests are based on random ideas you googled or were suggested by a consultant, your testing process still has its training wheels on. Great hypotheses aren’t random. They’re based on rationale and aim for learning.
Hypotheses should be based on themes and analysis that show potential conversion barriers.
At Conversion, we call this investigation phase the “Explore Phase” where we use frameworks like the LIFT Model to understand the prospect’s unique perspective. (You can read more on the the full optimization process here).
A well-founded marketing hypothesis should also provide you with new, testable clues about your users regardless of whether or not the test wins, loses or yields inconclusive results.
These new insights should inform future testing: a solid hypothesis can help you quickly separate worthwhile ideas from the rest when planning follow-up tests.
“Ultimately, what matters most is that you have a hypothesis going into each experiment and you design each experiment to address that hypothesis.” – Nick So, VP of Delivery
Here’s a quick tip :
If you’re about to run a test that isn’t going to tell you anything new about your users and their motivations, it’s probably not worth investing your time in.
Let’s take this opportunity to refer back to your original idea:
Ok, but what now ? To get actionable insights from ‘a new CTA’, you need to know why it behaved the way it did. You need to ask the right question.
To test the waters, maybe you changed the copy of the CTA button on your lead generation form from “Submit” to “Send demo request”. If this change leads to an increase in conversions, it could mean that your users require more clarity about what their information is being used for.
That’s a potential insight.
Based on this insight, you could follow up with another test that adds copy around the CTA about next steps: what the user should anticipate after they have submitted their information.
For example, will they be speaking to a specialist via email? Will something be waiting for them the next time they visit your site? You can test providing more information, and see if your users are interested in knowing it!
That’s the cool thing about a good hypothesis: the results of the test, while important (of course) aren’t the only component driving your future test ideas. The insights gleaned lead to further hypotheses and insights in a virtuous cycle.
The term “hypothesis” probably isn’t foreign to you. In fact, it may bring up memories of grade-school science class; it’s a critical part of the scientific method .
The scientific method in testing follows a systematic routine that sets ideation up to predict the results of experiments via:
- Collecting data and information through observation
- Creating tentative descriptions of what is being observed
- Forming hypotheses that predict different outcomes based on these observations
- Testing your hypotheses
- Analyzing the data, drawing conclusions and insights from the results
Don’t worry! Hypothesizing may seem ‘sciency’, but it doesn’t have to be complicated in practice.
Hypothesizing simply helps ensure the results from your tests are quantifiable, and is necessary if you want to understand how the results reflect the change made in your test.
A strong marketing hypothesis allows testers to use a structured approach in order to discover what works, why it works, how it works, where it works, and who it works on.
“My page needs a new CTA.” Is this idea in its current state clear enough to help you understand what works? Maybe. Why it works? No. Where it works? Maybe. Who it works on? No.
Your idea needs refining.
Let’s pull back and take a broader look at the lead generation landing page we want to test.
Imagine the situation: you’ve been diligent in your data collection and you notice several recurrences of Clarity pain points – meaning that there are many unclear instances throughout the page’s messaging.
Rather than focusing on the CTA right off the bat, it may be more beneficial to deal with the bigger clarity issue.
Now you’re starting to think about solving your prospects conversion barriers rather than just testing random ideas!
If you believe the overall page is unclear, your overarching theme of inquiry might be positioned as:
- “Improving the clarity of the page will reduce confusion and improve [conversion goal].”
By testing a hypothesis that supports this clarity theme, you can gain confidence in the validity of it as an actionable marketing insight over time.
If the test results are negative : It may not be worth investigating this motivational barrier any further on this page. In this case, you could return to the data and look at the other motivational barriers that might be affecting user behavior.
If the test results are positive : You might want to continue to refine the clarity of the page’s message with further testing.
Typically, a test will start with a broad idea — you identify the changes to make, predict how those changes will impact your conversion goal, and write it out as a broad theme as shown above. Then, repeated tests aimed at that theme will confirm or undermine the strength of the underlying insight.
You believe you’ve identified an overall problem on your landing page (there’s a problem with clarity). Now you want to understand how individual elements contribute to the problem, and the effect these individual elements have on your users.
It’s game time – now you can start designing a hypothesis that will generate insights.
You believe your users need more clarity. You’re ready to dig deeper to find out if that’s true!
If a specific question needs answering, you should structure your test to make a single change. This isolation might ask: “What element are users most sensitive to when it comes to the lack of clarity?” and “What changes do I believe will support increasing clarity?”
At this point, you’ll want to boil down your overarching theme…
- Improving the clarity of the page will reduce confusion and improve [conversion goal].
…into a quantifiable hypothesis that isolates key sections:
- Changing the wording of this CTA to set expectations for users (from “submit” to “send demo request”) will reduce confusion about the next steps in the funnel and improve order completions.
Does this answer what works? Yes: changing the wording on your CTA.
Does this answer why it works? Yes: reducing confusion about the next steps in the funnel.
Does this answer where it works? Yes: on this page, before the user enters this theoretical funnel.
Does this answer who it works on? No, this question demands another isolation. You might structure your hypothesis more like this:
- Changing the wording of the CTA to set expectations for users (from “submit” to “send demo request”) will reduce confusion for visitors coming from my email campaign about the next steps in the funnel and improve order completions.
Now we’ve got a clear hypothesis. And one worth testing!
1. It’s testable.
2. It addresses conversion barriers.
3. It aims at gaining marketing insights.
Let’s compare:
The original idea : “My page needs a new CTA.”
Following the hypothesis structure : “A new CTA on my page will increase [conversion goal]”
The first test implied a problem with clarity, provides a potential theme : “Improving the clarity of the page will reduce confusion and improve [conversion goal].”
The potential clarity theme leads to a new hypothesis : “Changing the wording of the CTA to set expectations for users (from “submit” to “send demo request”) will reduce confusion about the next steps in the funnel and improve order completions.”
Final refined hypothesis : “Changing the wording of the CTA to set expectations for users (from “submit” to “send demo request”) will reduce confusion for visitors coming from my email campaign about the next steps in the funnel and improve order completions.”
Which test would you rather your team invest in?
Before you start your next test, take the time to do a proper analysis of the page you want to focus on. Do preliminary testing to define bigger issues, and use that information to refine and pinpoint your marketing hypothesis to give you forward-looking insights.
Doing this will help you avoid time-wasting tests, and enable you to start getting some insights for your team to keep testing!
Share this post
Other articles you might like
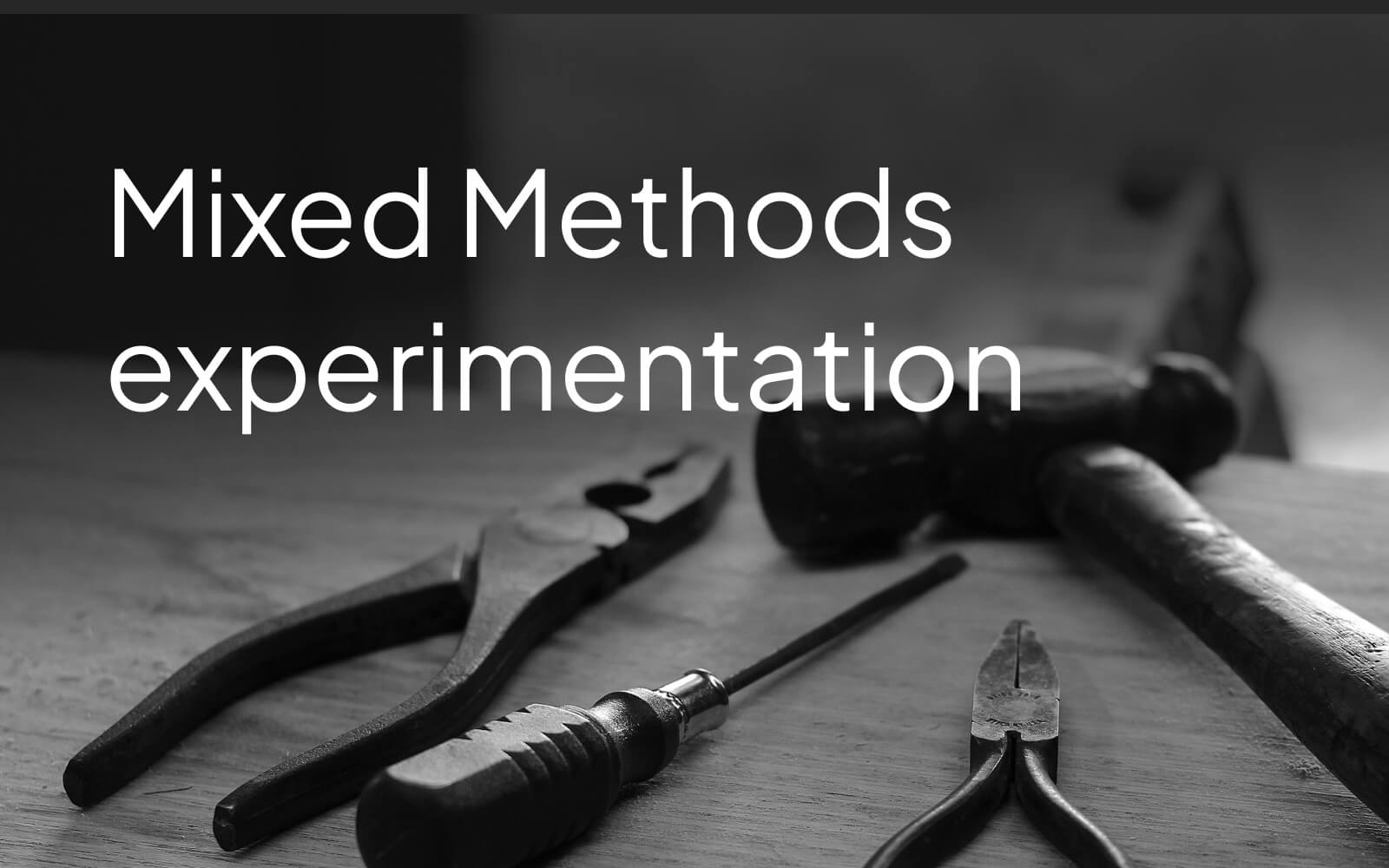
Mixed Methods Experimentation
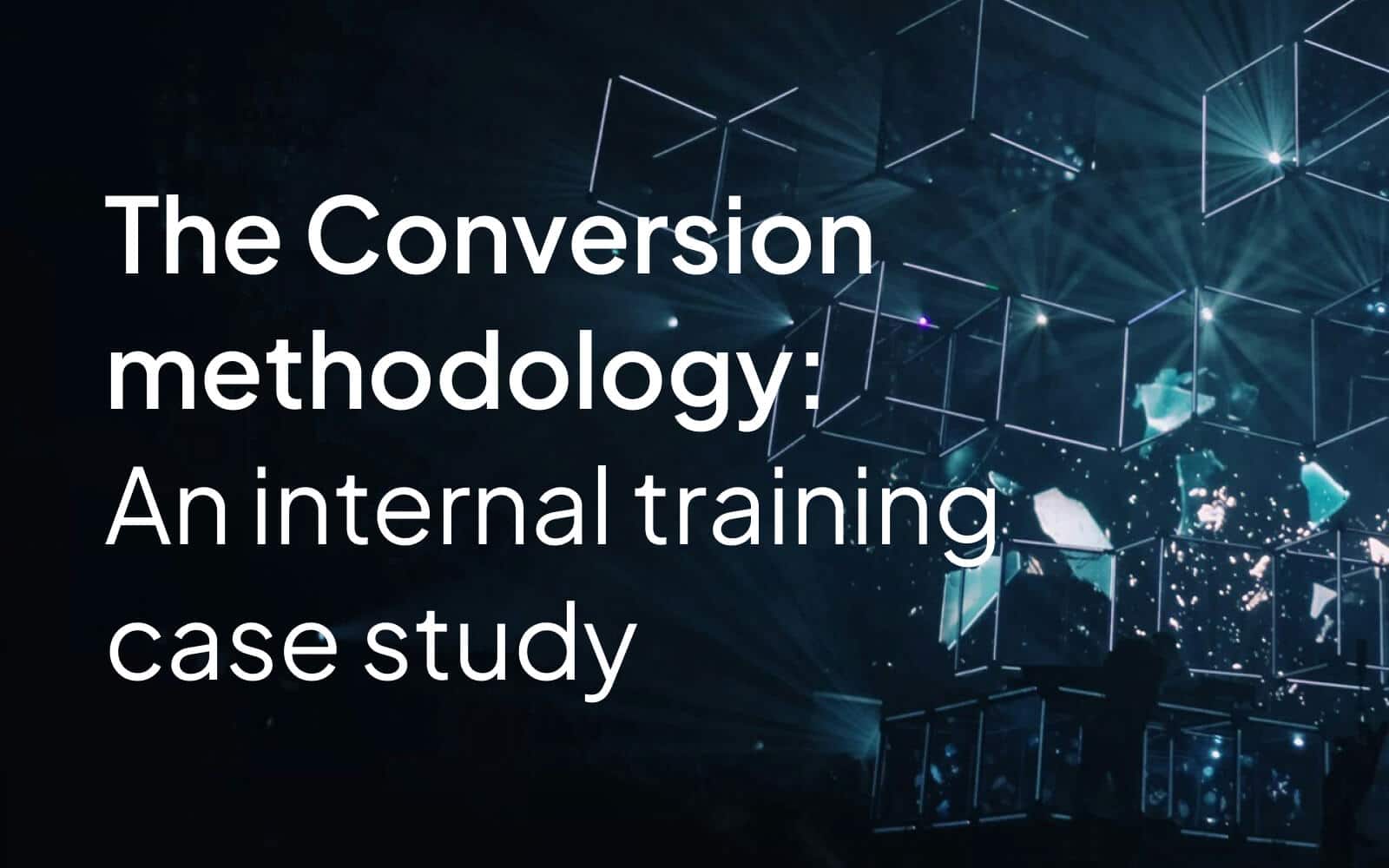
The Conversion Methodology: an internal training case study
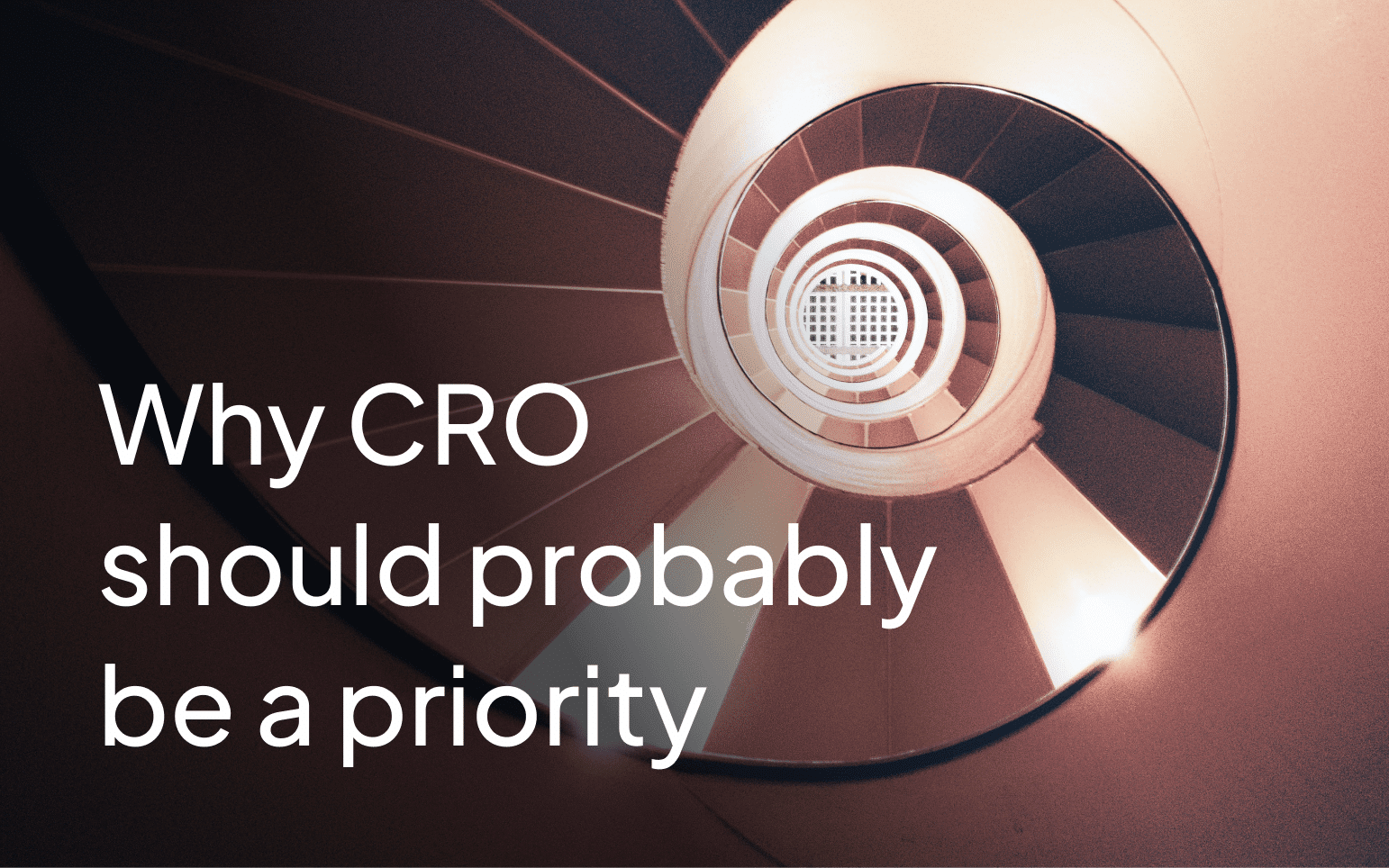
Why CRO should (probably) be a priority
- Business Essentials
- Leadership & Management
- Credential of Leadership, Impact, and Management in Business (CLIMB)
- Entrepreneurship & Innovation
- Digital Transformation
- Finance & Accounting
- Business in Society
- For Organizations
- Support Portal
- Media Coverage
- Founding Donors
- Leadership Team
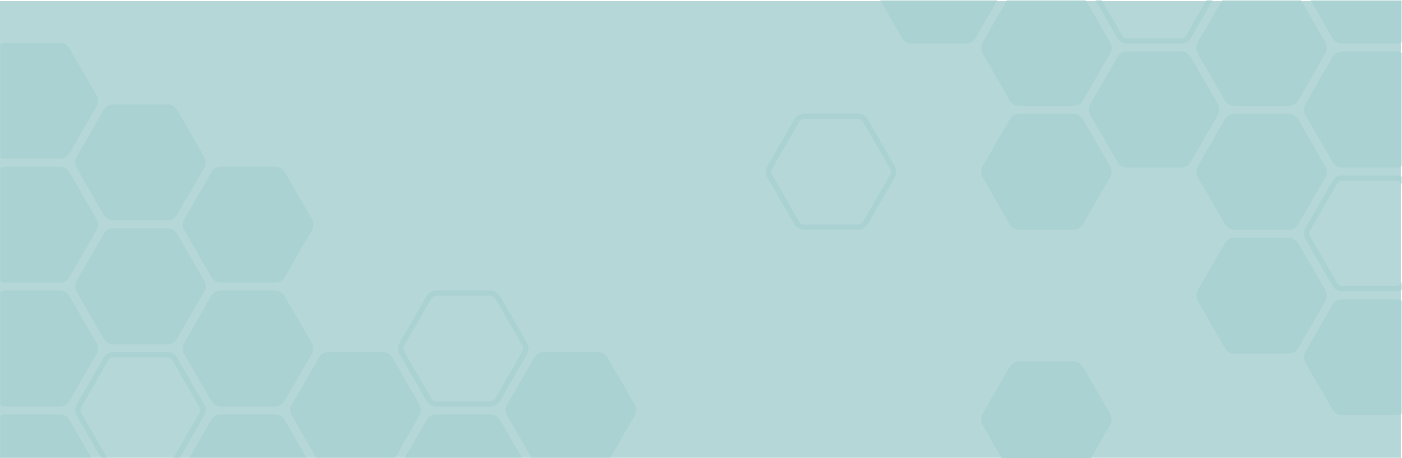
- Harvard Business School →
- HBS Online →
- Business Insights →
Business Insights
Harvard Business School Online's Business Insights Blog provides the career insights you need to achieve your goals and gain confidence in your business skills.
- Career Development
- Communication
- Decision-Making
- Earning Your MBA
- Negotiation
- News & Events
- Productivity
- Staff Spotlight
- Student Profiles
- Work-Life Balance
- AI Essentials for Business
- Alternative Investments
- Business Analytics
- Business Strategy
- Business and Climate Change
- Creating Brand Value
- Design Thinking and Innovation
- Digital Marketing Strategy
- Disruptive Strategy
- Economics for Managers
- Entrepreneurship Essentials
- Financial Accounting
- Global Business
- Launching Tech Ventures
- Leadership Principles
- Leadership, Ethics, and Corporate Accountability
- Leading Change and Organizational Renewal
- Leading with Finance
- Management Essentials
- Negotiation Mastery
- Organizational Leadership
- Power and Influence for Positive Impact
- Strategy Execution
- Sustainable Business Strategy
- Sustainable Investing
- Winning with Digital Platforms
A Beginner’s Guide to Hypothesis Testing in Business
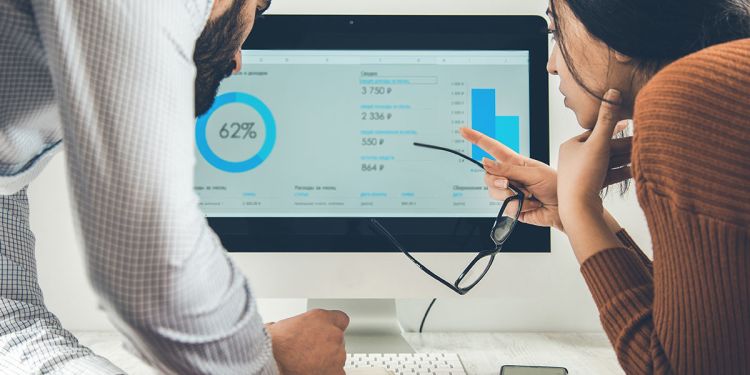
- 30 Mar 2021
Becoming a more data-driven decision-maker can bring several benefits to your organization, enabling you to identify new opportunities to pursue and threats to abate. Rather than allowing subjective thinking to guide your business strategy, backing your decisions with data can empower your company to become more innovative and, ultimately, profitable.
If you’re new to data-driven decision-making, you might be wondering how data translates into business strategy. The answer lies in generating a hypothesis and verifying or rejecting it based on what various forms of data tell you.
Below is a look at hypothesis testing and the role it plays in helping businesses become more data-driven.
Access your free e-book today.
What Is Hypothesis Testing?
To understand what hypothesis testing is, it’s important first to understand what a hypothesis is.
A hypothesis or hypothesis statement seeks to explain why something has happened, or what might happen, under certain conditions. It can also be used to understand how different variables relate to each other. Hypotheses are often written as if-then statements; for example, “If this happens, then this will happen.”
Hypothesis testing , then, is a statistical means of testing an assumption stated in a hypothesis. While the specific methodology leveraged depends on the nature of the hypothesis and data available, hypothesis testing typically uses sample data to extrapolate insights about a larger population.
Hypothesis Testing in Business
When it comes to data-driven decision-making, there’s a certain amount of risk that can mislead a professional. This could be due to flawed thinking or observations, incomplete or inaccurate data , or the presence of unknown variables. The danger in this is that, if major strategic decisions are made based on flawed insights, it can lead to wasted resources, missed opportunities, and catastrophic outcomes.
The real value of hypothesis testing in business is that it allows professionals to test their theories and assumptions before putting them into action. This essentially allows an organization to verify its analysis is correct before committing resources to implement a broader strategy.
As one example, consider a company that wishes to launch a new marketing campaign to revitalize sales during a slow period. Doing so could be an incredibly expensive endeavor, depending on the campaign’s size and complexity. The company, therefore, may wish to test the campaign on a smaller scale to understand how it will perform.
In this example, the hypothesis that’s being tested would fall along the lines of: “If the company launches a new marketing campaign, then it will translate into an increase in sales.” It may even be possible to quantify how much of a lift in sales the company expects to see from the effort. Pending the results of the pilot campaign, the business would then know whether it makes sense to roll it out more broadly.
Related: 9 Fundamental Data Science Skills for Business Professionals
Key Considerations for Hypothesis Testing
1. alternative hypothesis and null hypothesis.
In hypothesis testing, the hypothesis that’s being tested is known as the alternative hypothesis . Often, it’s expressed as a correlation or statistical relationship between variables. The null hypothesis , on the other hand, is a statement that’s meant to show there’s no statistical relationship between the variables being tested. It’s typically the exact opposite of whatever is stated in the alternative hypothesis.
For example, consider a company’s leadership team that historically and reliably sees $12 million in monthly revenue. They want to understand if reducing the price of their services will attract more customers and, in turn, increase revenue.
In this case, the alternative hypothesis may take the form of a statement such as: “If we reduce the price of our flagship service by five percent, then we’ll see an increase in sales and realize revenues greater than $12 million in the next month.”
The null hypothesis, on the other hand, would indicate that revenues wouldn’t increase from the base of $12 million, or might even decrease.
Check out the video below about the difference between an alternative and a null hypothesis, and subscribe to our YouTube channel for more explainer content.
2. Significance Level and P-Value
Statistically speaking, if you were to run the same scenario 100 times, you’d likely receive somewhat different results each time. If you were to plot these results in a distribution plot, you’d see the most likely outcome is at the tallest point in the graph, with less likely outcomes falling to the right and left of that point.

With this in mind, imagine you’ve completed your hypothesis test and have your results, which indicate there may be a correlation between the variables you were testing. To understand your results' significance, you’ll need to identify a p-value for the test, which helps note how confident you are in the test results.
In statistics, the p-value depicts the probability that, assuming the null hypothesis is correct, you might still observe results that are at least as extreme as the results of your hypothesis test. The smaller the p-value, the more likely the alternative hypothesis is correct, and the greater the significance of your results.
3. One-Sided vs. Two-Sided Testing
When it’s time to test your hypothesis, it’s important to leverage the correct testing method. The two most common hypothesis testing methods are one-sided and two-sided tests , or one-tailed and two-tailed tests, respectively.
Typically, you’d leverage a one-sided test when you have a strong conviction about the direction of change you expect to see due to your hypothesis test. You’d leverage a two-sided test when you’re less confident in the direction of change.

4. Sampling
To perform hypothesis testing in the first place, you need to collect a sample of data to be analyzed. Depending on the question you’re seeking to answer or investigate, you might collect samples through surveys, observational studies, or experiments.
A survey involves asking a series of questions to a random population sample and recording self-reported responses.
Observational studies involve a researcher observing a sample population and collecting data as it occurs naturally, without intervention.
Finally, an experiment involves dividing a sample into multiple groups, one of which acts as the control group. For each non-control group, the variable being studied is manipulated to determine how the data collected differs from that of the control group.

Learn How to Perform Hypothesis Testing
Hypothesis testing is a complex process involving different moving pieces that can allow an organization to effectively leverage its data and inform strategic decisions.
If you’re interested in better understanding hypothesis testing and the role it can play within your organization, one option is to complete a course that focuses on the process. Doing so can lay the statistical and analytical foundation you need to succeed.
Do you want to learn more about hypothesis testing? Explore Business Analytics —one of our online business essentials courses —and download our Beginner’s Guide to Data & Analytics .

About the Author
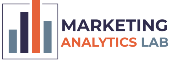
Marketing Analytics Lab
Hypothesis Testing in Marketing Research
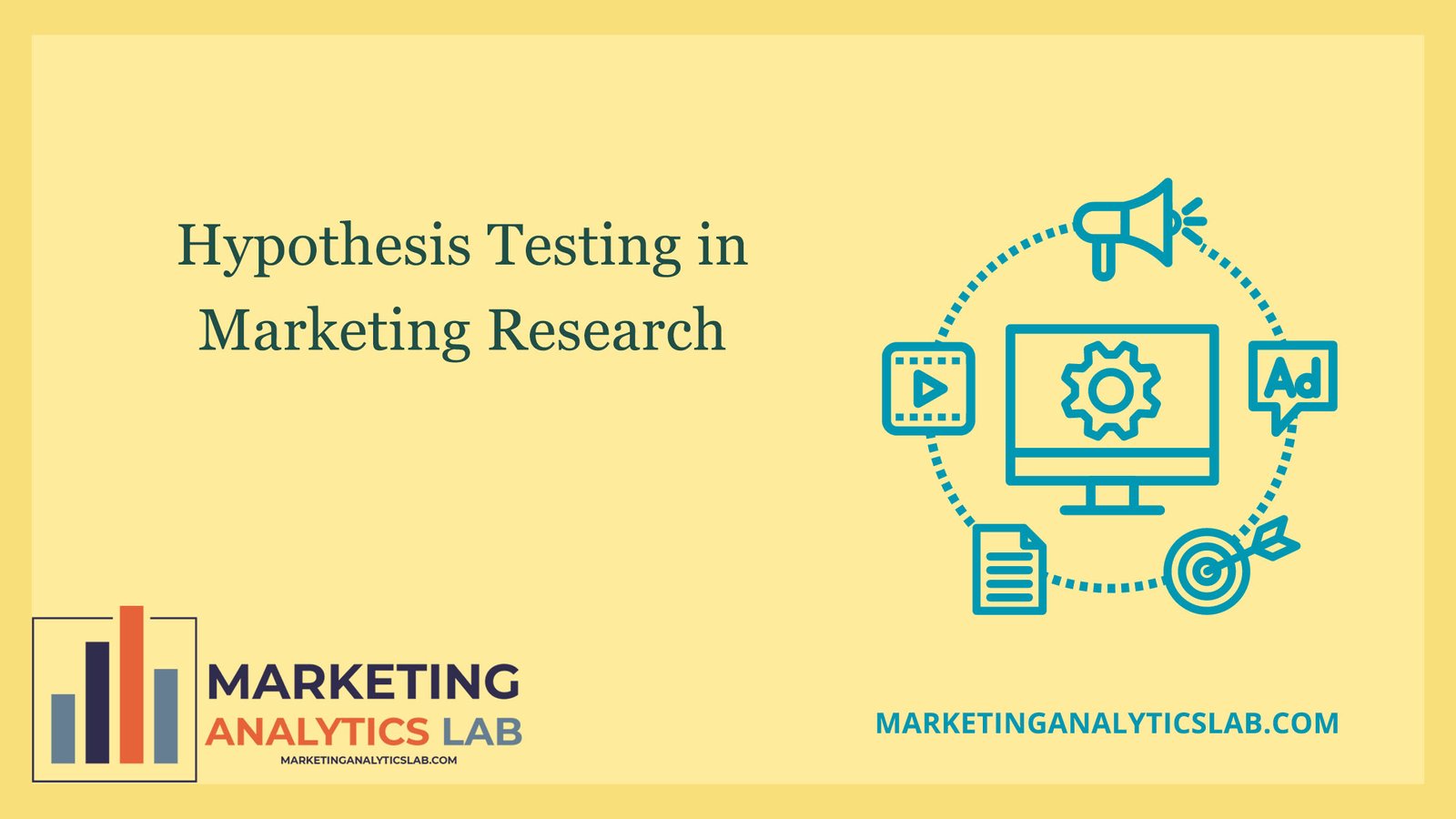
Hypothesis testing is a fundamental statistical method used in marketing research to make inferences about a population based on sample data. It helps researchers and marketers determine whether there is enough evidence to support a specific claim or hypothesis about consumer behavior, market trends, or the effectiveness of marketing strategies. This article explores the principles of hypothesis testing, its application in marketing research, and the key concepts and techniques involved.
1. What is Hypothesis Testing?
Overview : Hypothesis testing is a statistical procedure used to evaluate whether a hypothesis about a population parameter is supported by sample data. The process involves making a claim, testing it against observed data, and determining whether to accept or reject the claim based on statistical evidence.
Key Concepts :
- Null Hypothesis (H₀) : The null hypothesis represents the default assumption that there is no effect or difference. It is the hypothesis that researchers seek to test against.
- Alternative Hypothesis (H₁ or Ha) : The alternative hypothesis represents the claim that there is an effect or difference. It is what researchers aim to provide evidence for.
- Significance Level (α) : The significance level, often set at 0.05, is the probability of rejecting the null hypothesis when it is actually true. It defines the threshold for determining statistical significance.
- P-Value : The p-value is the probability of obtaining test results at least as extreme as the observed results, assuming the null hypothesis is true. A p-value less than the significance level indicates strong evidence against the null hypothesis.
- Test Statistic : The test statistic is a standardized value calculated from the sample data used to determine whether to reject the null hypothesis. Common test statistics include t-values, z-values, and F-values.
2. Steps in Hypothesis Testing
1. Formulate Hypotheses :
- Null Hypothesis (H₀) : State the default assumption, such as “There is no difference in customer satisfaction before and after implementing a new marketing campaign.”
- Alternative Hypothesis (H₁) : State the claim being tested, such as “There is a difference in customer satisfaction before and after implementing a new marketing campaign.”
2. Choose the Significance Level (α) :
- Typically set at 0.05, 0.01, or 0.10, depending on the research context and desired level of confidence.
3. Collect and Analyze Data :
- Gather data through surveys, experiments, or other methods and calculate the test statistic based on the sample data.
4. Determine the P-Value :
- Compare the p-value to the significance level to assess the strength of evidence against the null hypothesis.
5. Make a Decision :
- Reject H₀ : If the p-value is less than the significance level, reject the null hypothesis and accept the alternative hypothesis.
- Fail to Reject H₀ : If the p-value is greater than the significance level, fail to reject the null hypothesis.
6. Draw Conclusions :
- Interpret the results in the context of the research question and make recommendations based on the findings.
3. Applications in Marketing Research
A. evaluating marketing campaign effectiveness.
Overview : Hypothesis testing can assess whether a marketing campaign has significantly impacted consumer behavior or sales performance.
- Null Hypothesis (H₀) : “The new marketing campaign has no effect on sales.”
- Alternative Hypothesis (H₁) : “The new marketing campaign has a significant effect on sales.”
Application :
- Analyze pre- and post-campaign sales data to determine if there is a statistically significant increase in sales.
- Data-Driven Decisions : Make informed decisions about continuing, modifying, or discontinuing marketing campaigns based on statistical evidence.
b. Testing Product Preferences
Overview : Hypothesis testing can help understand consumer preferences and evaluate whether different product features or attributes influence purchasing decisions.
- Null Hypothesis (H₀) : “There is no difference in consumer preference between Product A and Product B.”
- Alternative Hypothesis (H₁) : “There is a significant difference in consumer preference between Product A and Product B.”
- Conduct surveys or experiments to compare consumer preferences and analyze the results to determine if there is a significant difference.
- Product Development : Use findings to guide product development and design strategies that align with consumer preferences.
c. Assessing Customer Satisfaction
Overview : Hypothesis testing can evaluate changes in customer satisfaction levels due to changes in products, services, or customer experience initiatives.
- Null Hypothesis (H₀) : “Customer satisfaction scores have not changed after implementing the new customer service strategy.”
- Alternative Hypothesis (H₁) : “Customer satisfaction scores have significantly changed after implementing the new customer service strategy.”
- Analyze customer satisfaction survey data before and after the implementation of the strategy to assess the impact.
- Improvement Strategies : Identify effective strategies for enhancing customer satisfaction and loyalty.
d. Market Segmentation Analysis
Overview : Hypothesis testing can be used to evaluate whether different market segments have distinct characteristics or responses to marketing efforts.
- Null Hypothesis (H₀) : “There is no difference in purchase behavior between different customer segments.”
- Alternative Hypothesis (H₁) : “There is a significant difference in purchase behavior between different customer segments.”
- Analyze purchase data from different segments to identify significant differences and tailor marketing strategies accordingly.
- Targeted Marketing : Develop targeted marketing strategies based on differences in behavior between market segments.
4. Common Hypothesis Tests in Marketing Research
Overview : The t-test is used to compare the means of two groups to determine if they are significantly different from each other.
- Independent Samples t-Test : Compares means between two independent groups (e.g., control vs. treatment groups).
- Paired Samples t-Test : Compares means from the same group at different times (e.g., pre- and post-campaign).
b. Chi-Square Test
Overview : The chi-square test assesses the association between categorical variables.
- Evaluate whether there is a significant relationship between categorical variables, such as product preference by demographic group.
c. ANOVA (Analysis of Variance)
Overview : ANOVA is used to compare means across three or more groups to determine if there are significant differences among them.
- Assess differences in consumer satisfaction or purchasing behavior across multiple product categories or market segments.
5. Challenges and Considerations
A. sample size.
Overview : The accuracy of hypothesis testing results depends on the sample size. Small sample sizes may lead to unreliable results.
Considerations :
- Power Analysis : Conduct power analysis to determine the appropriate sample size needed to detect meaningful differences.
b. Assumptions
Overview : Hypothesis tests rely on certain assumptions, such as normality and equal variances. Violations of these assumptions can affect test results.
- Test Assumptions : Check and address assumptions before conducting hypothesis tests.
c. Interpreting Results
Overview : Proper interpretation of results is crucial for making informed decisions. Avoid overinterpreting results based on statistical significance alone.
- Practical Significance : Consider the practical significance and relevance of findings in addition to statistical significance.
6. Conclusion: Utilizing Hypothesis Testing in Marketing Research
Hypothesis testing is a valuable tool in marketing research for making data-driven decisions and evaluating the effectiveness of marketing strategies. By formulating hypotheses, analyzing data, and interpreting results, businesses can gain insights into consumer behavior, assess marketing initiatives, and optimize strategies.
Despite challenges such as sample size and assumptions, hypothesis testing provides a structured approach to understanding and addressing marketing questions. By leveraging hypothesis testing, businesses can enhance their marketing efforts, improve decision-making, and achieve greater success in a competitive marketplace.
Leave a Reply Cancel reply
Your email address will not be published. Required fields are marked *
Save my name, email, and website in this browser for the next time I comment.
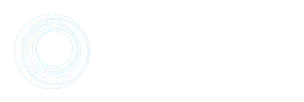
Expert Advice on Developing a Hypothesis for Marketing Experimentation
- Conversion Rate Optimization
Simbar Dube
Every marketing experimentation process has to have a solid hypothesis.
That’s a must – unless you want to be roaming in the dark and heading towards a dead-end in your experimentation program.
Hypothesizing is the second phase of our SHIP optimization process here at Invesp.
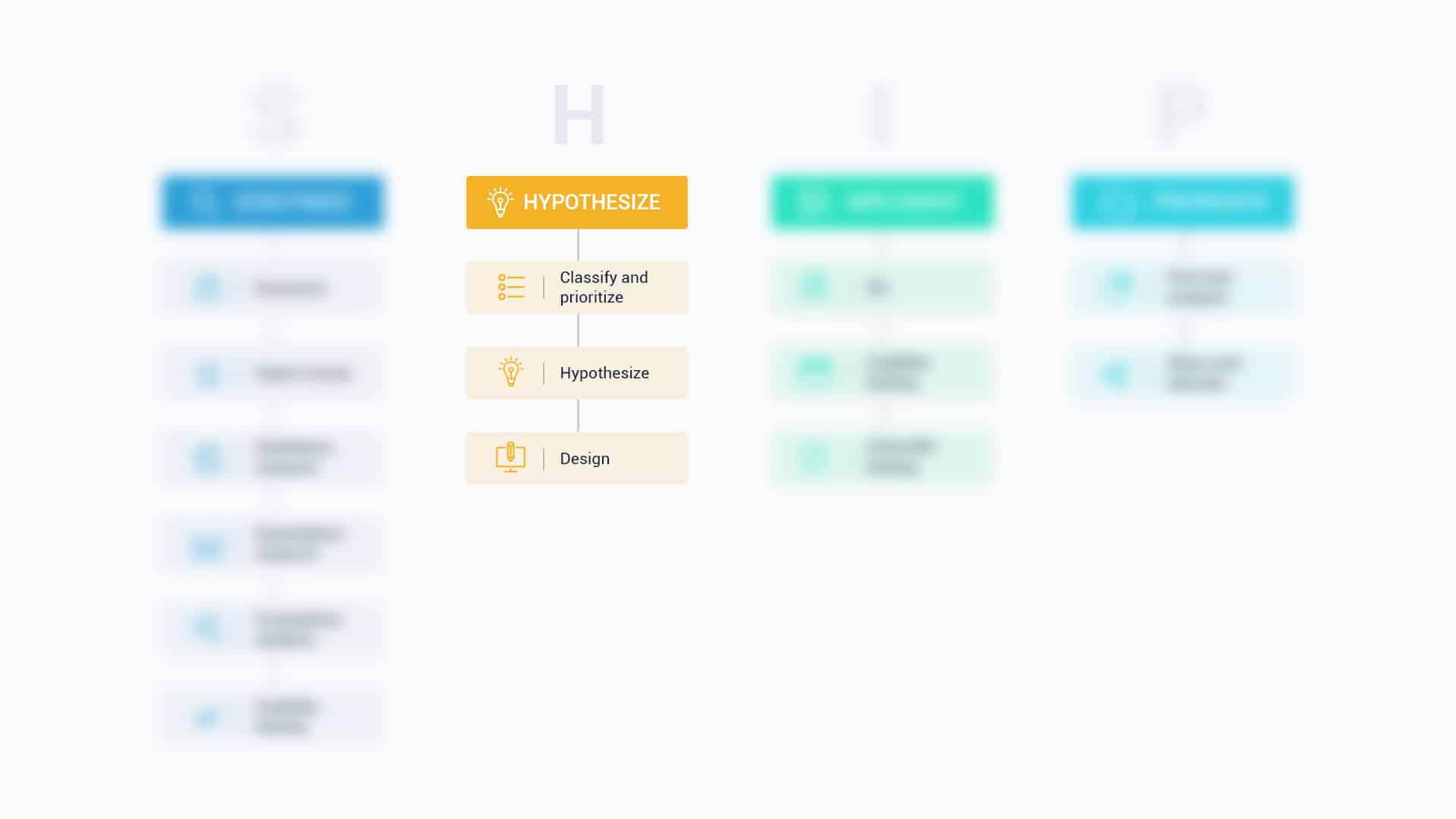
It comes after we have completed the research phase.
This is an indication that we don’t just pull a hypothesis out of thin air. We always make sure that it is based on research data.
But having a research-backed hypothesis doesn’t mean that the hypothesis will always be correct. In fact, tons of hypotheses bear inconclusive results or get disproved.
The main idea of having a hypothesis in marketing experimentation is to help you gain insights – regardless of the testing outcome.
By the time you finish reading this article, you’ll know:
- The essential tips on what to do when crafting a hypothesis for marketing experiments
- How a marketing experiment hypothesis works
How experts develop a solid hypothesis
The basics: marketing experimentation hypothesis.
A hypothesis is a research-based statement that aims to explain an observed trend and create a solution that will improve the result. This statement is an educated, testable prediction about what will happen.
It has to be stated in declarative form and not as a question.
“ If we add magnification info, product video and making virtual mirror buttons, will that improve engagement? ” is not declarative, but “ Improving the experience of product pages by adding magnification info, product video and making virtual mirror buttons will increase engagement ” is.
Here’s a quick example of how a hypothesis should be phrased:
- Replacing ___ with __ will increase [conversion goal] by [%], because:
- Removing ___ and __ will decrease [conversion goal] by [%], because:
- Changing ___ into __ will not affect [conversion goal], because:
- Improving ___ by ___will increase [conversion goal], because:
As you can see from the above sentences, a good hypothesis is written in clear and simple language. Reading your hypothesis should tell your team members exactly what you thought was going to happen in an experiment.
Another important element of a good hypothesis is that it defines the variables in easy-to-measure terms, like who the participants are, what changes during the testing, and what the effect of the changes will be:
Example : Let’s say this is our hypothesis:
Displaying full look items on every “continue shopping & view your bag” pop-up and highlighting the value of having a full look will improve the visibility of a full look, encourage visitors to add multiple items from the same look and that will increase the average order value, quantity with cross-selling by 3% .
Who are the participants :
Visitors.
What changes during the testing :
Displaying full look items on every “continue shopping & view your bag” pop-up and highlighting the value of having a full look…
What the effect of the changes will be:
Will improve the visibility of a full look, encourage visitors to add multiple items from the same look and that will increase the average order value, quantity with cross-selling by 3% .
Don’t bite off more than you can chew! Answering some scientific questions can involve more than one experiment, each with its own hypothesis. so, you have to make sure your hypothesis is a specific statement relating to a single experiment.
How a Marketing Experimentation Hypothesis Works
Assuming that you have done conversion research and you have identified a list of issues ( UX or conversion-related problems) and potential revenue opportunities on the site. The next thing you’d want to do is to prioritize the issues and determine which issues will most impact the bottom line.
Having ranked the issues you need to test them to determine which solution works best. At this point, you don’t have a clear solution for the problems identified. So, to get better results and avoid wasting traffic on poor test designs, you need to make sure that your testing plan is guided.
This is where a hypothesis comes into play.
For each and every problem you’re aiming to address, you need to craft a hypothesis for it – unless the problem is a technical issue that can be solved right away without the need to hypothesize or test.
One important thing you should note about an experimentation hypothesis is that it can be implemented in different ways.
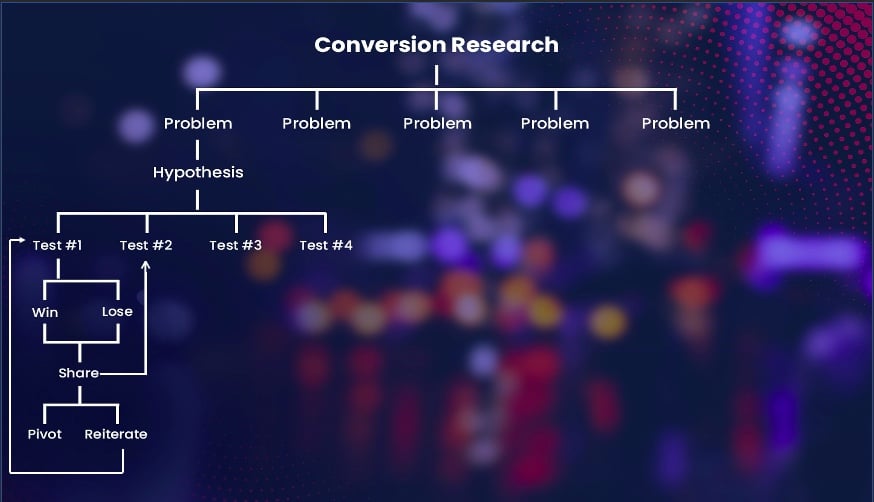
This means that one hypothesis can have four or five different tests as illustrated in the image above. Khalid Saleh , the Invesp CEO, explains:
“There are several ways that can be used to support one single hypothesis. Each and every way is a possible test scenario. And that means you also have to prioritize the test design you want to start with. Ultimately the name of the game is you want to find the idea that has the biggest possible impact on the bottom line with the least amount of effort. We use almost 18 different metrics to score all of those.”
In one of the recent tests we launched after watching video recordings, viewing heatmaps, and conducting expert reviews, we noticed that:
- Visitors were scrolling to the bottom of the page to fill out a calculator so as to get a free diet plan.
- Brand is missing
- Too many free diet plans – and this made it hard for visitors to choose and understand.
- No value proposition on the page
- The copy didn’t mention the benefits of the paid program
- There was no clear CTA for the next action
To help you understand, let’s have a look at how the original page looked like before we worked on it:
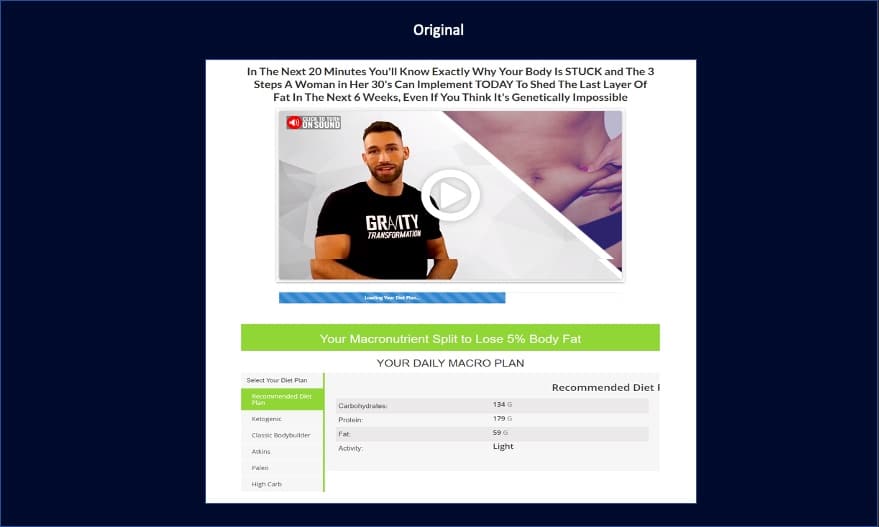
So our aim was to make the shopping experience seamless for visitors, make the page more appealing and not confusing. In order to do that, here is how we phrased the hypothesis for the page above:
Improving the experience of optin landing pages by making the free offer accessible above the fold and highlighting the next action with a clear CTA and will increase the engagement on the offer and increase the conversion rate by 1%.
For this particular hypothesis, we had two design variations aligned to it:
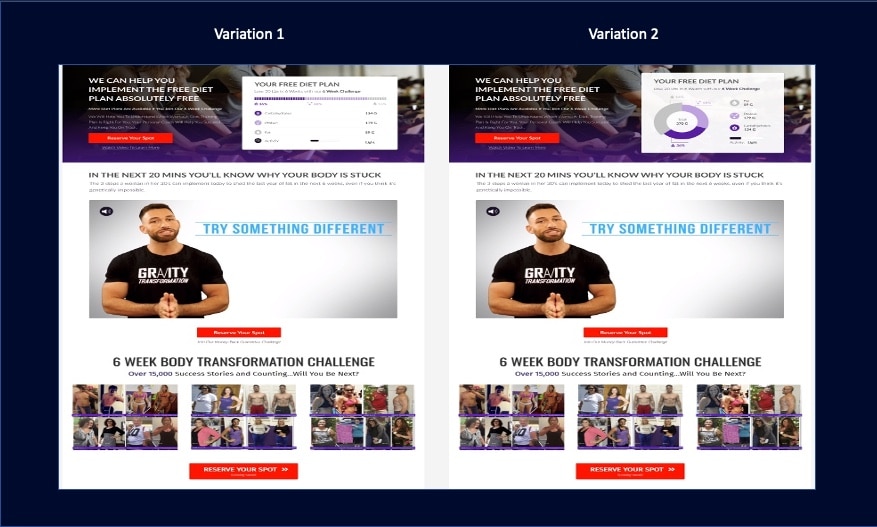
The two above designs are different, but they are aligned to one hypothesis. This goes on to show how one hypothesis can be implemented in different ways. Looking at the two variations above – which one do you think won?
Yes, you’re right, V2 was the winner.
Considering that there are many ways you can implement one hypothesis, so when you launch a test and it fails, it doesn’t necessarily mean that the hypothesis was wrong. Khalid adds:
“A single failure of a test doesn’t mean that the hypothesis is incorrect. Nine times out of ten it’s because of the way you’ve implemented the hypothesis. Look at the way you’ve coded and look at the copy you’ve used – you are more likely going to find something wrong with it. Always be open.”
So there are three things you should keep in mind when it comes to marketing experimentation hypotheses:
- It takes a while for this hypothesis to really fully test it.
- A single failure doesn’t necessarily mean that the hypothesis is incorrect.
- Whether a hypothesis is proved or disproved, you can still learn something about your users.
I know it’s never easy to develop a hypothesis that informs future testing – I mean it takes a lot of intense research behind the scenes, and tons of ideas to begin with. So, I reached out to six CRO experts for tips and advice to help you understand more about developing a solid hypothesis and what to include in it.
Maurice says that a solid hypothesis should have not more than one goal:
Maurice Beerthuyzen – CRO/CXO Lead at ClickValue “Creating a hypothesis doesn’t begin at the hypothesis itself. It starts with research. What do you notice in your data, customer surveys, and other sources? Do you understand what happens on your website? When you notice an opportunity it is tempting to base one single A/B test on one hypothesis. Create hypothesis A and run a single test, and then move forward to the next test. With another hypothesis. But it is very rare that you solve your problem with only one hypothesis. Often a test provides several other questions. Questions which you can solve with running other tests. But based on that same hypothesis! We should not come up with a new hypothesis for every test. Another mistake that often happens is that we fill the hypothesis with multiple goals. Then we expect that the hypothesis will work on conversion rate, average order value, and/or Click Through Ratio. Of course, this is possible, but when you run your test, your hypothesis can only have one goal at once. And what if you have two goals? Just split the hypothesis then create a secondary hypothesis for your second goal. Every test has one primary goal. What if you find a winner on your secondary hypothesis? Rerun the test with the second hypothesis as the primary one.”
Jon believes that a strong hypothesis is built upon three pillars:
Jon MacDonald – President and Founder of The Good Respond to an established challenge – The challenge must have a strong background based on data, and the background should state an established challenge that the test is looking to address. Example: “Sign up form lacks proof of value, incorrectly assuming if users are on the page, they already want the product.” Propose a specific solution – What is the one, the single thing that is believed will address the stated challenge? Example: “Adding an image of the dashboard as a background to the signup form…”. State the assumed impact – The assumed impact should reference one specific, measurable optimization goal that was established prior to forming a hypothesis. Example: “…will increase signups.” So, if your hypothesis doesn’t have a specific, measurable goal like “will increase signups,” you’re not really stating a test hypothesis!”
Matt uses his own hypothesis builder to collate important data points into a single hypothesis.
Matt Beischel – Founder of Corvus CRO Like Jon, Matt also breaks down his hypothesis writing process into three sections. Unlike Jon, Matt sections are: Comprehension Response Outcome I set it up so that the names neatly match the “CRO.” It’s a sort of “mad-libs” style fill-in-the-blank where each input is an important piece of information for building out a robust hypothesis. I consider these the minimum required data points for a good hypothesis; if you can’t completely fill out the form, then you don’t have a good hypothesis. Here’s a breakdown of each data point: Comprehension – Identifying something that can be improved upon Problem: “What is a problem we have?” Observation Method: “How did we identify the problem?” Response – Change that can cause improvement Variation: “What change do we think could solve the problem?” Location: “Where should the change occur?” Scope: “What are the conditions for the change?” Audience: “Who should the change affect?” Outcome – Measurable result of the change that determines the success Behavior Change : “What change in behavior are we trying to affect?” Primary KPI: “What is the important metric that determines business impact?” Secondary KPIs: “Other metrics that will help reinforce/refute the Primary KPI” Something else to consider is that I have a “user first” approach to formulating hypotheses. My process above is always considered within the context of how it would first benefit the user. Now, I do feel that a successful experiment should satisfy the needs of BOTH users and businesses, but always be in favor of the user. Notice that “Behavior Change” is the first thing listed in Outcome, not primary business KPI. Sure, at the end of the day you are working for the business’s best interests (both strategically and financially), but placing the user first will better inform your decision making and prioritization; there’s a reason that things like personas, user stories, surveys, session replays, reviews, etc. exist after all. A business-first ideology is how you end up with dark patterns and damaging brand credibility.”
One of the many mistakes that CROs make when writing a hypothesis is that they are focused on wins and not on insights. Shiva advises against this mindset:
Shiva Manjunath – Marketing Manager and CRO at Gartner “Test to learn, not test to win. It’s a very simple reframe of hypotheses but can have a magnitude of difference. Here’s an example: Test to Win Hypothesis: If I put a product video in the middle of the product page, I will improve add to cart rates and improve CVR. Test to Learn Hypothesis: If I put a product video on the product page, there will be high engagement with the video and it will positively influence traffic What you’re doing is framing your hypothesis, and test, in a particular way to learn as much as you can. That is where you gain marketing insights. The more you run ‘marketing insight’ tests, the more you will win. Why? The more you compound marketing insight learnings, your win velocity will start to increase as a proxy of the learnings you’ve achieved. Then, you’ll have a higher chance of winning in your tests – and the more you’ll be able to drive business results.”
Lorenzo says it’s okay to focus on achieving a certain result as long as you are also getting an answer to: “Why is this event happening or not happening?”
Lorenzo Carreri – CRO Consultant “When I come up with a hypothesis for a new or iterative experiment, I always try to find an answer to a question. It could be something related to a problem people have or an opportunity to achieve a result or a way to learn something. The main question I want to answer is “Why is this event happening or not happening?” The question is driven by data, both qualitative and quantitative. The structure I use for stating my hypothesis is: From [data source], I noticed [this problem/opportunity] among [this audience of users] on [this page or multiple pages]. So I believe that by [offering this experiment solution], [this KPI] will [increase/decrease/stay the same].
Jakub Linowski says that hypotheses are meant to hold researchers accountable:
Jakub Linowski – Chief Editor of GoodUI “They do this by making your change and prediction more explicit. A typical hypothesis may be expressed as: If we change (X), then it will have some measurable effect (A). Unfortunately, this oversimplified format can also become a heavy burden to your experiment design with its extreme reductionism. However you decide to format your hypotheses, here are three suggestions for more flexibility to avoid limiting yourself. One Or More Changes To break out of the first limitation, we have to admit that our experiments may contain a single or multiple changes. Whereas the classic hypothesis encourages a single change or isolated variable, it’s not the only way we can run experiments. In the real world, it’s quite normal to see multiple design changes inside a single variation. One valid reason for doing this is when wishing to optimize a section of a website while aiming for a greater effect. As more positive changes compound together, there are times when teams decide to run bigger experiments. An experiment design (along with your hypotheses) therefore should allow for both single or multiple changes. One Or More Metrics A second limitation of many hypotheses is that they often ask us to only make a single prediction at a time. There are times when we might like to make multiple guesses or predictions to a set of metrics. A simple example of this might be a trade-off experiment with a guess of increased sales but decreased trial signups. Being able to express single or multiple metrics in our experimental designs should therefore be possible. Estimates, Directional Predictions, Or Unknowns Finally, traditional hypotheses also tend to force very simple directional predictions by asking us to guess whether something will increase or decrease. In reality, however, the fidelity of predictions can be higher or lower. On one hand, I’ve seen and made experiment estimations that contain specific numbers from prior data (ex: increase sales by 14%). While at other times it should also be acceptable to admit the unknown and leave the prediction blank. One example of this is when we are testing a completely novel idea without any prior data in a highly exploratory type of experiment. In such cases, it might be dishonest to make any sort of predictions and we should allow ourselves to express the unknown comfortably.”
Conclusion
So there you have it! Before you jump on launching a test, start by making sure that your hypothesis is solid and backed by research. Ask yourself the questions below when crafting a hypothesis for marketing experimentation:
- Is the hypothesis backed by research?
- Can the hypothesis be tested?
- Does the hypothesis provide insights?
- Does the hypothesis set the expectation that there will be an explanation behind the results of whatever you’re testing?
Don’t worry! Hypothesizing may seem like a very complicated process, but it’s not complicated in practice especially when you have done proper research.
If you enjoyed reading this article and you’d love to get the best CRO content – delivered by the best experts in the industry – straight to your inbox, every week. Please subscribe here .
Share This Article
Join 25,000+ marketing professionals.
Subscribe to Invesp’s blog feed for future articles delivered to receive weekly updates by email.
Discover Similar Topics
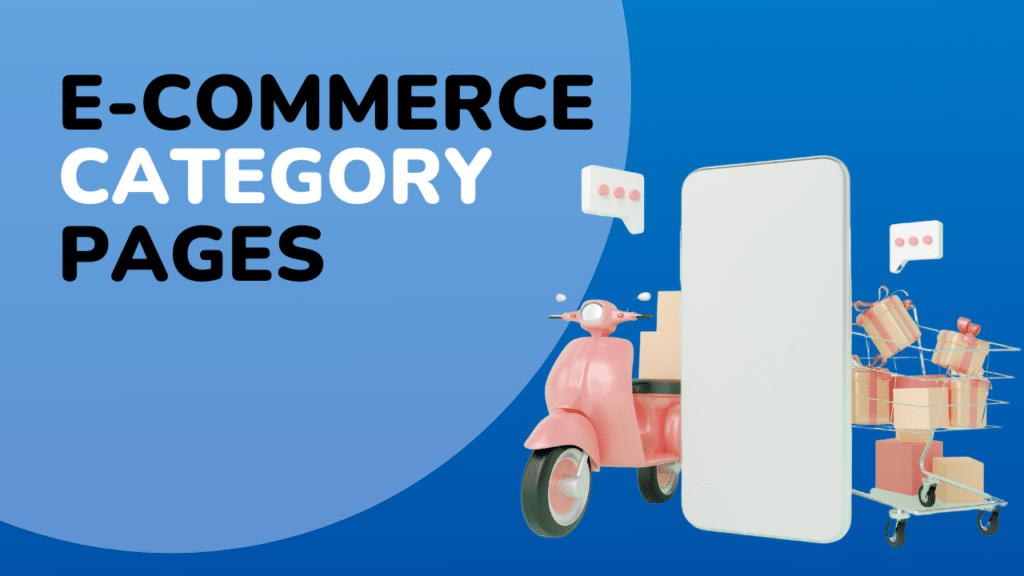
How to Create Effective E-commerce Category Pages
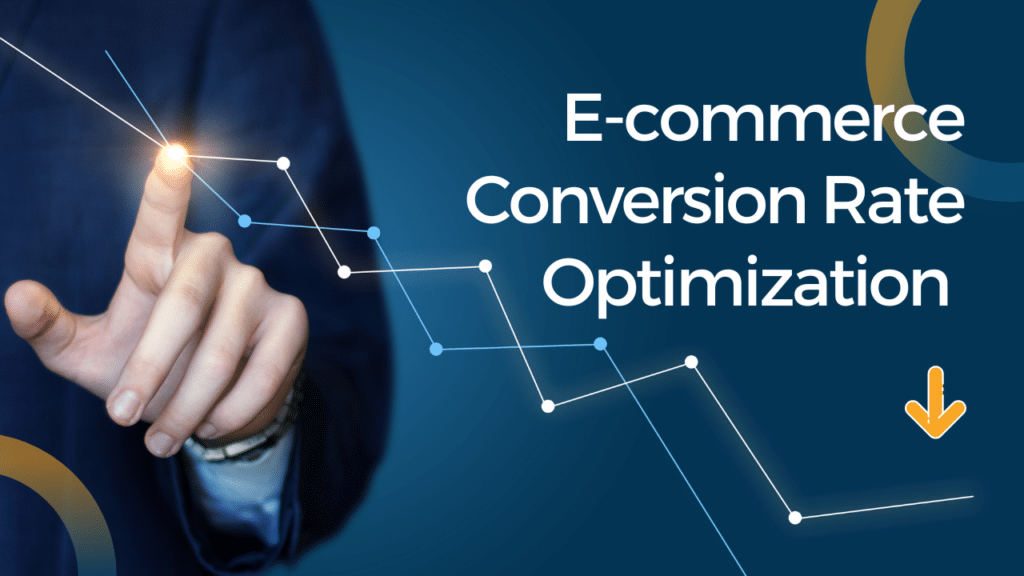
The Science Behind Successful Ecommerce Conversion Rate Optimization
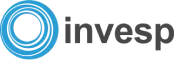
Our Services
- Conversion Optimization Training
- Conversion Rate Optimization Professional Services
- Landing Page Optimization
- Conversion Rate Audit
- Design for Growth
- Conversion Research & Discovery
- End to End Digital Optimization
By Industry
- E-commerce CRO Services
- Lead Generation CRO Services
- SaaS CRO Services
- Startup CRO Program
- Case Studies
- Privacy Policy
- © 2006-2020 All rights reserved. Invesp
Subscribe with us
- US office: Chicago, IL
- European office: Istanbul, Turkey
- +1.248.270.3325
- [email protected]
- Conversion Rate Optimization Services
- © 2006-2023 All rights reserved. Invesp
- Popular Topics
- A/B Testing
- Business & Growth
- Copywriting
- Infographics
- Landing Pages
- Sales & Marketing

- Free Resources
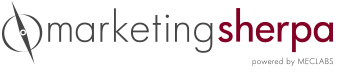
A/B Testing in Digital Marketing: Example of four-step hypothesis framework
The more accurate your customer insights, the more impressive your marketing results. We’ve written today’s MarketingSherpa article to help you improve those customers insights. Read on for a hypothesis example we created to answer a question a MarketingSherpa reader emailed us.
|
by Daniel Burstein , Senior Director, Content & Marketing, MarketingSherpa and MECLABS Institute
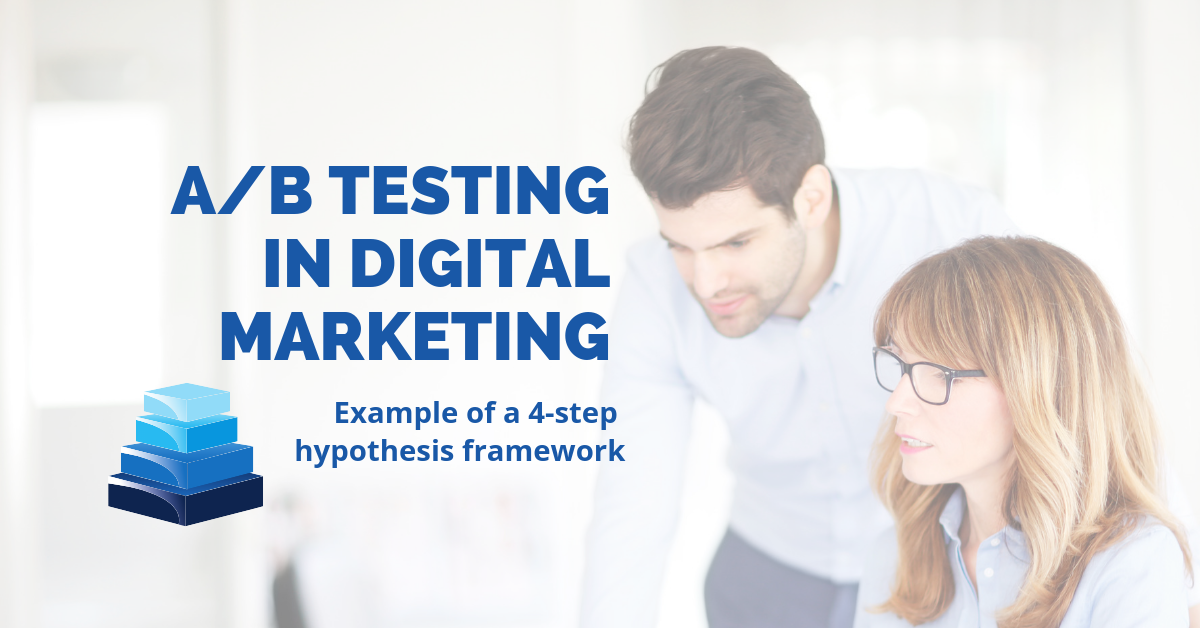
This article was originally published in the MarketingSherpa email newsletter .
If you are a marketing expert — whether in a brand’s marketing department or at an advertising agency — you may feel the need to be absolutely sure in an unsure world.
What should the headline be? What images should we use? Is this strategy correct? Will customers value this promo?
This is the stuff you’re paid to know. So you may feel like you must boldly proclaim your confident opinion.
But you can’t predict the future with 100% accuracy. You can’t know with absolute certainty how humans will behave. And let’s face it, even as marketing experts we’re occasionally wrong.
It’s not bad, it’s healthy. And the most effective way to overcome that doubt is by testing our marketing creative to see what really works.
Developing a hypothesis
After we published Value Sequencing: A step-by-step examination of a landing page that generated 638% more conversions , a MarketingSherpa reader emailed us and asked …
Great stuff Daniel. Much appreciated. I can see you addressing all the issues there.
I thought I saw one more opportunity to expand on what you made. Would you consider adding the IF, BY, WILL, BECAUSE to the control/treatment sections so we can see what psychology you were addressing so we know how to create the hypothesis to learn from what the customer is currently doing and why and then form a test to address that? The video today on customer theory was great (Editor’s Note: Part of the MarketingExperiments YouTube Live series ) . I think there is a way to incorporate that customer theory thinking into this article to take it even further.
Developing a hypothesis is an essential part of marketing experimentation. Qualitative-based research should inform hypotheses that you test with real-world behavior.
The hypotheses help you discover how accurate those insights from qualitative research are. If you engage in hypothesis-driven testing, then you ensure your tests are strategic (not just based on a random idea) and built in a way that enables you to learn more and more about the customer with each test.
And that methodology will ultimately lead to greater and greater lifts over time, instead of a scattershot approach where sometimes you get a lift and sometimes you don’t, but you never really know why.
Here is a handy tool to help you in developing hypotheses — the MECLABS Four-Step Hypothesis Framework.
As the reader suggests, I will use the landing page test referenced in the previous article as an example. ( Please note: While the experiment in that article was created with a hypothesis-driven approach, this specific four-step framework is fairly new and was not in common use by the MECLABS team at that time, so I have created this specific example after the test was developed based on what I see in the test).
Here is what the hypothesis would look like for that test, and then we’ll break down each part individually:
If we emphasize the process-level value by adding headlines, images and body copy, we will generate more leads because the value of a longer landing page in reducing the anxiety of calling a TeleAgent outweighs the additional friction of a longer page.
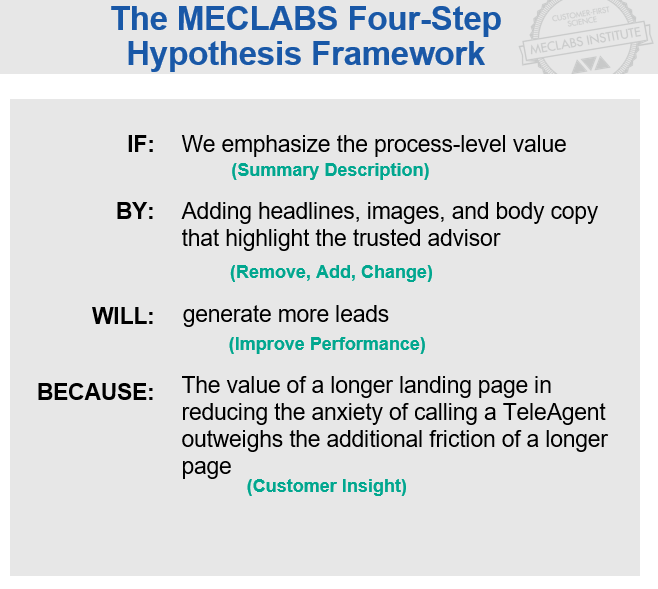
IF: Summary description
The hypothesis begins with an overall statement about what you are trying to do in the experiment. In this case, the experiment is trying to emphasize the process-level value proposition (one of the four essential levels of value proposition ) of having a phone call with a TeleAgent.
The control landing page was emphasizing the primary value proposition of the brand itself.
The treatment landing page is essentially trying to answer this value proposition question: If I am your ideal customer, why should I call a TeleAgent rather than take any other action to learn more about my Medicare options?
The control landing page was asking a much bigger question that customers weren’t ready to say “yes” to yet, and it was overlooking the anxiety inherent in getting on a phone call with someone who might try to sell you something: If I am your ideal customer, why should I buy from your company instead of any other company.
This step answers WHAT you are trying to do.
BY: Remove, add, change
The next step answers HOW you are going to do it.
As Flint McGlaughlin, CEO and Managing Director of MECLABS Institute teaches, there are only three ways to improve performance: removing, adding or changing .
In this case, the team focused mostly on adding — adding headlines, images and body copy that highlighted the TeleAgents as trusted advisors.
“Adding” can be counterintuitive for many marketers. The team’s original landing page was short. Conventional wisdom says customers won’t read long landing pages. When I’m presenting to a group of marketers, I’ll put a short and long landing page on a slide and ask which page they think achieved better results.
Invariably I will hear, “Oh, the shorter page. I would never read something that long.”
That first-person statement is a mistake. Your marketing creative should not be based on “I” — the marketer. It should be based on “they” — the customer.
Most importantly, you need to focus on the customer at a specific point in time — when he or she is in the mindspace of considering to take an action like purchase a product or in need of more information before they decide to download a whitepaper. And sometimes in these situations, longer landing pages perform better.
In the case of this landing page, even the customer may not necessarily favor a long landing page all the time. But in the real-world situation when they are considering whether to call a TeleAgent or not, the added value helps more customers decide to take the action.
WILL: Improve performance
This is your KPI (key performance indicator). This step answers another HOW question: How do you know your hypothesis has been supported or refuted?
You can choose secondary metrics to monitor during your test as well. This might help you interpret the customer behavior observed in the test.
But ultimately, the hypothesis should rest on a single metric.
For this test, the goal was to generate more leads. And the treatment did — 638% more leads.
BECAUSE: Customer insight
This last step answers a WHY question — why did the customers act this way?
This helps you determine what you can learn about customers based on the actions observed in the experiment.
This is ultimately why you test. To learn about the customer and continually refine your company’s customer theory .
In this case, the team theorized that the value of a longer landing page in reducing the anxiety of calling a TeleAgent outweighs the additional friction of a longer landing page.
And the test results support that hypothesis.
Related Resources
The Hypothesis and the Modern-Day Marketer
Boost your Conversion Rate with a MECLABS Quick Win Intensive
Designing Hypotheses that Win: A four-step framework for gaining customer wisdom and generating marketing results
Improve Your Marketing
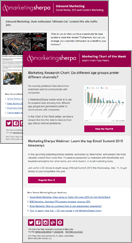
Join our thousands of weekly case study readers.
Enter your email below to receive MarketingSherpa news, updates, and promotions:
Note: Already a subscriber? Want to add a subscription? Click Here to Manage Subscriptions
Get Better Business Results With a Skillfully Applied Customer-first Marketing Strategy
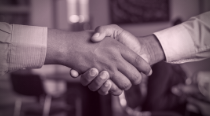
The customer-first approach of MarketingSherpa’s agency services can help you build the most effective strategy to serve customers and improve results, and then implement it across every customer touchpoint.
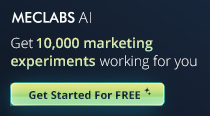
Get headlines, value prop, competitive analysis, and more.
Marketer Vs Machine
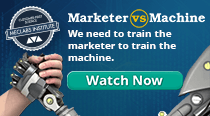
Marketer Vs Machine: We need to train the marketer to train the machine.
Free Marketing Course
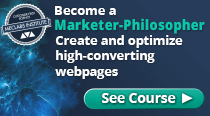
Become a Marketer-Philosopher: Create and optimize high-converting webpages (with this free online marketing course)
Project and Ideas Pitch Template
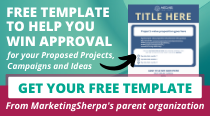
A free template to help you win approval for your proposed projects and campaigns
Six Quick CTA checklists
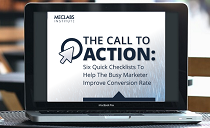
These CTA checklists are specifically designed for your team — something practical to hold up against your CTAs to help the time-pressed marketer quickly consider the customer psychology of your “asks” and how you can improve them.
Infographic: How to Create a Model of Your Customer’s Mind
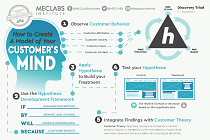
You need a repeatable methodology focused on building your organization’s customer wisdom throughout your campaigns and websites. This infographic can get you started.
Infographic: 21 Psychological Elements that Power Effective Web Design
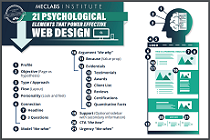
To build an effective page from scratch, you need to begin with the psychology of your customer. This infographic can get you started.
Receive the latest case studies and data on email, lead gen, and social media along with MarketingSherpa updates and promotions.
- Your Email Account
- Customer Service Q&A
- Search Library
- Content Directory:
Questions? Contact Customer Service at [email protected]
© 2000-2024 MarketingSherpa LLC, ISSN 1559-5137 Editorial HQ: MarketingSherpa LLC, PO Box 50032, Jacksonville Beach, FL 32240
The views and opinions expressed in the articles of this website are strictly those of the author and do not necessarily reflect in any way the views of MarketingSherpa, its affiliates, or its employees.
Hypotheses in Marketing Science: Literature Review and Publication Audit
- Published: May 2001
- Volume 12 , pages 171–187, ( 2001 )
Cite this article
- J. Scott Armstrong 1 ,
- Roderick J. Brodie 2 &
- Andrew G. Parsons 2
974 Accesses
91 Citations
3 Altmetric
Explore all metrics
We examined three approaches to research in marketing: exploratory hypotheses, dominant hypothesis, and competing hypotheses. Our review of empirical studies on scientific methodology suggests that the use of a single dominant hypothesis lacks objectivity relative to the use of exploratory and competing hypotheses approaches. We then conducted a publication audit of over 1,700 empirical papers in six leading marketing journals during 1984–1999. Of these, 74% used the dominant hypothesis approach, while 13% used multiple competing hypotheses, and 13% were exploratory. Competing hypotheses were more commonly used for studying methods (25%) than models (17%) and phenomena (7%). Changes in the approach to hypotheses since 1984 have been modest; there was a slight decrease in the percentage of competing hypotheses to 11%, which is explained primarily by an increasing proportion of papers on phenomena. Of the studies based on hypothesis testing, only 11% described the conditions under which the hypotheses would apply, and dominant hypotheses were below competing hypotheses in this regard. Marketing scientists differed substantially in their opinions about what types of studies should be published and what was published. On average, they did not think dominant hypotheses should be used as often as they were, and they underestimated their use.
This is a preview of subscription content, log in via an institution to check access.
Access this article
Subscribe and save.
- Get 10 units per month
- Download Article/Chapter or eBook
- 1 Unit = 1 Article or 1 Chapter
- Cancel anytime
Price includes VAT (Russian Federation)
Instant access to the full article PDF.
Rent this article via DeepDyve
Institutional subscriptions
Similar content being viewed by others
Normative Criteria for the Development and Appraisal of Marketing Theory
Research Method Topics and Issues that Reduce the Value of Reported Empirical Insights in the Marketing Literatures: An Abstract
Marketing Theory: The Present Stage Of Development
References for appendix 2.
Agarwal MK, and VR Rao. (1996). “An Empirical Comparison of Consumer-Based Measures of Brand Equity,” Marketing Letters , 7, 237–248.
Google Scholar
Bult JR, and T Wansbeek. (1995). “Optimal Selection for Direct Mail,” Marketing Science , 14(4) 378–394.
Foekens EW, PSH Leeflang, and DR Wittink. (1997). “Hierarchical Versus Other Market Share Models for Markets with Many Items,” International Journal of Research in Marketing , 14, 359–378.
Johnson MD, EW Anderson, and C Fornell. (1995). “Rational and Adaptive Performance Expectations in a Customer Satisfaction Framework,” Journal of Consumer Research , 21, 695–707.
Krafft M. (1999). “An empirical Investigation of the Antecedents of Sales Force Control Systems,” Journal of Marketing , 63, 120–134.
Mittal V, P Kumar, and M Tsiros. (1999). “Attribute-level Performance, Satisfaction, and Behavioural Intentions over Time: A Consumption System Approach,” Journal of Marketing , 63, 88–101.
Naik PA, MK Mantrala, and AG Sawyer. (1998). “Planning Media Schedules in the Presence of Dynamic Advertising Quality,” Marketing Science , 17, 214–235.
Pechmann C, and C Shih. (1999). “Smoking Scenes in Movies and Antismoking Advertisements before Movies: Effects on Youth,” Journal of Marketing , 63, 1–13.
Szymanski DM, LC Troy, and SG Bharadwaj. (1995). “Order of Entry and Business Performance: An Empirical Synthesis and Reexamination,” Journal of Marketing , 59, 17–33.
Abramowitz SI, B Gomes, and CV Abramowitz. (1975). “Publish or Politic: Referee Bias in Manuscript Review,” Journal of Applied Social Psychology , 5, 187–200.
AMA Task Force on the Development of Marketing Thought. (1988). “Developing, Disseminating, and Utilizing Marketing Knowledge,” Journal of Marketing , 52, 1–25.
Anderson LM. (1994). “Marketing Science: Where's the Beef?” Business Horizons , (Jan–Feb), 8–16.
Armstrong JS. (1979). “Advocacy and Objectivity in Science,” Management Science , 25, 423–428.
Armstrong JS. (1980). “Advocacy as a Scientific Strategy: The Mitroff Myth,” Academy of Management Review , 5, 509–511.
Armstrong JS. (1988). “Research Needs in Forecasting,” International Journal of Forecasting , 4, 449–465.
Armstrong JS. (1991). “Prediction of Consumer Behavior by Experts and Novices,” Journal of Consumer Research , 18, 251–256.
Armstrong JS, and R. Hubbard. (1991). “Does the Need for Agreement Among Reviewers Inhibit the Publication of Controversial Findings?” Behavioral and Brain Sciences , 14, 136–137.
Bass FM. (1993). “The Future of Research in Marketing: Marketing Science,” Journal of Marketing Research , 30, 1–6.
Begg CB, and JA Berlin. (1989). “Publication Bias and Dissemination of Clinical Research,” Journal of the National Cancer Institute , 81(2), 107–115.
Ben-Shakar G, M Bar-Hillel, Y Bilu, and G Shefler. (1998). “Seek and Ye Shall Find: Test Results Are What You Hypothesize They Are,” Journal of Behavioral Decision Making , 11, 235–249.
Bettman JR, N Capon, and RJ Lutz. (1975). “Cognitive Algebra in Multi-Attribute Attitude Models,” Journal of Marketing Research , 12, 151–164.
Bloom PN. (1987). Knowledge Development in Marketing . Lexington, MA: Lexington Books.
Burger JM, and R Petty. (1981). “The Low-Ball Compliance Technique: Task or Person Commitment?” Journal of Personality and Social Psychology , 40, 492–500.
Broad W, and N Wade. (1982). Betrayers of the Truth: Fraud and Deceit in the Halls of Science . New York: Simon and Schuster.
Bruner J, and MC Potter. (1964). “Interference in Visual Recognition,” Science , 144, 424–425.
Chamberlin TC. (1965). “The Method of Multiple Working Hypotheses,” Science , 148, 754–759. (Reprint of an 1890 paper).
Chapman LJ, and JP Chapman. (1969). “Illusory Correlation as an Obstacle to the Use of Valid Psychodiagnostic Signs,” Journal of Abnormal Psychology , 74, 271–280.
Cialdini RB, JT Cacioppo, R Bassett, and JA Miller. (1978). “Low-Ball Procedure for Producing Compliance: Commitment Then Cost,” Journal of Personality and Social Psychology , 36, 463–476.
Cohen J. (1994). “The Earth is Round (p < 0.05),” American Psychologist , 49, 997–1003.
Coursol A, and EE Wagner. (1986), “Effect of Positive Findings on Submission and Acceptance Rates: A Note on Meta-analysis Bias,” Professional Psychology: Research and Practice , 17,(No. 2), 137.
Demsetz H. (1974). “Two Systems of Belief About Monopoly”. In H J Goldschmid, H M Mann, and J F Weston (eds.), Industrial Concentration: The New Learning . Boston: Little, Brown, pp. 164–184.
Dunbar K. (1993). “Concept Discovery in a Scientific Domain,” Cognitive Science , 17, 397–434.
Dunbar K. (1995). “How Scientists Really Reason: Scientific Reasoning in Real-world Laboratories.” In R J Sternberg and J E Davidson (eds.), The Nature of Insight . Cambridge, MA: MIT Press, pp. 365–395.
Elaad E, A Ginton, and G Ben-Shakhar. (1994). “The Effects of Prior expectations and Outcome Knowledge on Polygraph Examiners' Decisions,” Journal of Behavioral Decision Making , 7, 279–292.
Farris H, and R Revlin. (1989). “The Discovery Process: A Counterfactual Strategy,” Social Studies of Science , 19, 497–513.
Goldfarb RS. (1995). “The Economist-as-audience Needs a Methodology of Plausible Inference,” Journal of Economic Methodology , 2, 201–222.
Goodstein LD, and KL Brazis. (1970). “Credibility of Psychologists: An Empirical Study,” Psychological Reports , 27, 835–838.
Gorman ME, and ME Gorman. (1984), “A Comparison of Disconfirmatory, Confirmatory and Control Strategies on Wason's 2–4–6 Task,” The Quarterly Journal of Experimental Psychology , 36A, 629–648.
Greenwald AG, AR Pratkanis, MR Leippe, and MH Baumgardner. (1986). “Under What Conditions Does Theory Obstruct Progress?” Psychological Review , 93, 216–229.
Hogarth RM. (1978). “A Note on Aggregating Opinions,” Organizational Behavior and Human Performance , 21, 40–46.
Hubbard R, and JS Armstrong. (1994). “Replications and Extensions in Marketing: Rarely Published but Quite Contrary,” International Journal of Research in Marketing , 11, 233–248.
Hubbard R, and JS Armstrong. (1992). “Are Null Results Becoming an Endangered Species?” Marketing Letters , 3, 127–136.
Jones WH, and D Russell. (1980). “The Selective Processing of Belief Disconfirming Information,” European Journal of Social Psychology , 10, 309–312.
Klayman J, and Y Ha. (1987). “Confirmation, Disconfirmation, and Information in Hypothesis Testing,” Psychological Review , 94, 211–228.
Klayman J, and Y Ha. (1989). “Hypothesis Testing in Rule Discovery: Strategy, Structure, and Content,” Journal of Experimental Psychology , 15, 596–604.
Koehler JJ. (1993). “The Influence of Prior Beliefs on Scientific Judgements of Evidence Quality,” Organizational Behavior and Human Decision Processes , 56, 28–55.
Leone RP, and R Schultz. (1980). “A Study of Marketing Generalizations,” Journal of Marketing , 44, 10–18.
Libby R, and RK Blashfield. (1978). “Performance of a Composite as a Function of the Number of Judges,” Organizational Behavior and Human Performance , 21, 121–129.
Lord CG, L Ross, and MR Lepper. (1979). “Biased Assimilation and Attitude Polarization: The Effects of Prior Theories on Subsequently Considered Evidence,” Journal of Personality and Social Psychology , 37, 2098–2109.
Mahoney MJ. (1977). “Publication Prejudices: An Experimental Study of Confirmatory Bias in the Peer Review System,” Cognitive Therapy and Research , 1, 161–175.
McCloskey DN, and ST Ziliak. (1996). “The Standard Error of Regressions,” Journal of Economic Literature , 34, 97–114.
McDonald J. (1992). “Is Strong Inference Really Superior to Simple Inference,” Synthese , 92, 261–282.
McKenzie CRM. (1998). “Taking into Account the Strength of an Alternative Hypothesis,” Journal of Experimental Psychology , 24, 771–792.
Mitroff I. (1972). “The Myth of Objectivity, or, Why Science Needs a New Psychology of Science,” Management Science , 18, B613–B618.
Mynatt C, ME Doherty, and RD Tweney. (1978). “Consequences of Confirmation and Disconfirmation in a Simulated Research Environment,” Quarterly Journal of Experimental Psychology , 30, 395–406.
Platt JR. (1964). “Strong Inference,” Science , 146, 347–353.
Pollay RW. (1984). “Lydiametrics: Applications of Econometrics to the History of Advertising,” Journal of Advertising History , 1(2), 3–15.
Rodgers R, and JE Hunter. (1994). “The Discard of Study Evidence by Literature Reviewers,” Journal of Applied Behavioral Science , 30, 329–345.
Rust RT, DR Lehmann, and JU Farley. (1990). “Estimating the Publication Bias of Meta-Analysis,” Journal of Marketing Research , 27, 220–226.
Sawyer AG, and JP Peter. (1983). “The Significance of Statistical Significance Tests in Marketing Research,” Journal of Marketing Research , 20, 122–133.
Wason PC. (1960). “On the Failure to Eliminate Hypotheses in a Conceptual Task,” Quarterly Journal of Experimental Psychology , 12, 129–140.
Wason PC. (1968). “Reasoning About a Rule,” Quarterly Journal of Experimental Psychology , 20, 273–281.
Wells WD. (1993). “Discovery-oriented Consumer Research,” Journal of Consumer Research , 19, 489–504.
Download references
Author information
Authors and affiliations.
Wharton School, University of Pennsylvania, Philadelphia, PA, 19104
J. Scott Armstrong
Department of Marketing, University of Auckland, Auckland, New Zealand
Roderick J. Brodie & Andrew G. Parsons
You can also search for this author in PubMed Google Scholar
Rights and permissions
Reprints and permissions
About this article
Armstrong, J.S., Brodie, R.J. & Parsons, A.G. Hypotheses in Marketing Science: Literature Review and Publication Audit. Marketing Letters 12 , 171–187 (2001). https://doi.org/10.1023/A:1011169104290
Download citation
Issue Date : May 2001
DOI : https://doi.org/10.1023/A:1011169104290
Share this article
Anyone you share the following link with will be able to read this content:
Sorry, a shareable link is not currently available for this article.
Provided by the Springer Nature SharedIt content-sharing initiative
- competing hypotheses
- dominant hypotheses
- exploratory studies
- marketing generalizations
- multiple hypotheses
- Find a journal
- Publish with us
- Track your research
How to Conduct the Perfect Marketing Experiment [+ Examples]

Updated: January 11, 2022
Published: August 30, 2021
After months of hard work, multiple coffee runs, and navigation of the latest industry changes, you've finally finished your next big marketing campaign.
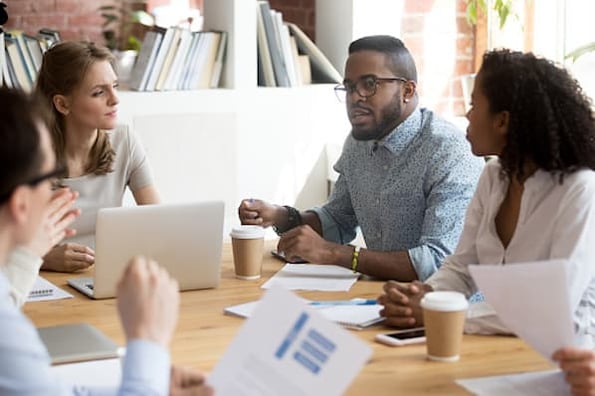
Complete with social media posts, PPC ads, and a sparkly new logo, it's the campaign of a lifetime.
But how do you know it will be effective?

While there's no sure way to know if your campaign will turn heads, there is a way to gauge whether those new aspects of your strategy will be effective.
If you want to know if certain components of your campaign are worth the effort, consider conducting a marketing experiment.
Marketing experiments give you a projection of how well marketing methods will perform before you implement them. Keep reading to learn how to conduct an experiment and discover the types of experiments you can run.
What are marketing experiments?
A marketing experiment is a form of market research in which your goal is to discover new strategies for future campaigns or validate existing ones.
For instance, a marketing team might create and send emails to a small segment of their readership to gauge engagement rates, before adding them to a campaign.
It's important to note that a marketing experiment isn't synonymous with a marketing test. Marketing experiments are done for discovery, while a test confirms theories.
Why should you run a marketing experiment?
Think of running a marketing experiment as taking out an insurance policy on future marketing efforts. It’s a way to minimize your risk and ensure that your efforts are in line with your desired results.
Imagine spending hours searching for the perfect gift. You think you’ve found the right one, only to realize later that it doesn’t align with your recipient’s taste or interests. Gifts come with receipts but there’s no money-back guarantee when it comes to marketing campaigns.
An experiment will help you better understand your audience, which in turn will enable you to optimize your strategy for a stronger performance.
How to Conduct Marketing Experiments
- Brainstorm and prioritize experiment ideas.
- Find one idea to focus on.
- Make a hypothesis.
- Collect research.
- Select your metrics.
- Execute the experiment.
- Analyze the results.
Performing a marketing experiment involves doing research, structuring the experiment, and analyzing the results. Let's go through the seven steps necessary to conduct a marketing experiment.
1. Brainstorm and prioritize experiment ideas.
The first thing you should do when running a marketing experiment is start with a list of ideas.
Don’t know where to start? Look at your current priorities. What goals are you focusing on for the next quarter or the next year?
From there, analyze historical data. Were your past strategies worked in the past and what were your low performers?
As you dig into your data, you may find that you still have unanswered questions about which strategies may be most effective. From there, you can identify potential reasons behind low performance and start brainstorming some ideas for future experiments.
Then, you can rank your ideas by relevance, timeliness, and return on investment so that you know which ones to tackle first.
Keep a log of your ideas online, like Google Sheets , for easy access and collaboration.
2. Find one idea to focus on.
Now that you have a log of ideas, you can pick one to focus on.
Ideally, you organize your list based on current priorities. As such, as the business evolves, your priorities may change and affect how you rank your ideas.
Say you want to increase your subscriber count by 1,000 over the next quarter. You’re several weeks away from the start of the quarter and after looking through your data, you notice that users don’t convert once they land on your landing page.
Your landing page would be a great place to start your experiment. It’s relevant to your current goals and will yield a large return on your investment.
Even unsuccessful experiments, meaning those that do not yield expected results, are incredibly valuable as they help you to better understand your audience.
3. Make a hypothesis.
Hypotheses aren't just for science projects. When conducting a marketing experiment, the first step is to make a hypothesis you're curious to test.
A good hypothesis for your landing page can be any of the following:
- Changing the CTA copy from "Get Started" to "Join Our Community" will increase sign-ups by 5%.
- Removing the phone number field from the landing page form will increase the form completion rate by 25%.
- Adding a security badge on the landing page will increase the conversion rate by 10%.
This is a good hypothesis because you can prove or disprove it, it isn't subjective, and has a clear measurement of achievement.
A not-so-good hypothesis will tackle several elements at once, be unspecific and difficult to measure. For example: "By updating the photos, CTA, and copy on the landing page, we should get more sign-ups.
Here’s why this doesn’t work: Testing several variables at once is a no-go when it comes to experimenting because it will be unclear which change(s) impacted the results. The hypothesis also doesn’t mention how the elements would be changed nor what would constitute a win.
Formulating a hypothesis takes some practice, but it’s the key to building a robust experiment.
4. Collect research.
After creating your hypothesis, begin to gather research. Doing this will give you background knowledge about experiments that have already been conducted and get an idea of possible outcomes.
Researching your experiment can help you modify your hypothesis if needed.
Say your hypothesis is, "Changing the CTA copy from "Get Started" to "Join Our Community" will increase sign-ups by 5%." You may conduct more market research to validate your ideas surrounding your user persona and if they will resonate better with a community-focused approach.
It would be helpful to look at your competitors’ landing pages and see which strategies they’re using during your research.
5. Select your metrics.
Once you've collected the research, you can choose which avenue you will take and what metrics to measure.
For instance, if you’re running an email subject line experiment, the open rate is the right metric to track.
For a landing page, you’ll likely be tracking the number of submissions during the testing period. If you’re experimenting on a blog, you might focus on the average time on page.
It all depends on what you’re tracking and the question you want to answer with your experiment.
6. Execute the experiment.
Now it's time to create and perform the experiment.
Depending on what you’re testing, this may be a cross-functional project that requires collaborating with other teams.
For instance, if you’re testing a new landing page CTA, you’ll likely need a copywriter or UX writer.
Everyone involved in this experiment should know:
- The hypothesis and goal of the experiment
- The timeline and duration
- The metrics you’ll track
7. Analyze the results.
Once you've run the experiment, collect and analyze the results.
You want to gather enough data for statistical significance .
Use the metrics you've decided upon in the second step and conclude if your hypothesis was correct or not.
The prime indicators for success will be the metrics you chose to focus on.
For instance, for the landing page example, did sign-ups increase as a result of the new copy? If the conversion rate met or went above the goal, the experiment would be considered successful and one you should implement.
If it’s unsuccessful, your team should discuss the potential reasons why and go back to the drawing board. This experiment may spark ideas of new elements to test.
Now that you know how to conduct a marketing experiment, let's go over a few different ways to run them.
Marketing Experiment Examples
There are many types of marketing experiments you can conduct with your team. These tests will help you determine how aspects of your campaign will perform before you roll out the campaign as a whole.
A/B testing is one of the popular ways to marketing in which two versions of a webpage, email, or social post are presented to an audience (randomly divided in half). This test determines which version performs better with your audience.
This method is useful because you can better understand the preferences of users who will be using your product.
Find below the types of experiments you can run.
Your website is arguably your most important digital asset. As such, you’ll want to make sure it’s performing well.
If your bounce rate is high, the average time on page is low, or your visitors aren’t navigating your site in the way you’d like, it may be time to run an experiment.
2. Landing Pages
Landing pages are used to convert visitors into leads. If your landing page is underperforming, running an experiment can yield high returns.
The great thing about running a test on a landing page is that there are typically only a few elements to test: your background image, your copy, form, and CTA .
Experimenting with different CTAs can improve the number of people who engage with your content.
For instance, instead of using "Buy Now!" to pull customers in, why not try, "Learn more."
You can also test different colors of CTAs as opposed to the copy.
4. Paid Media Campaigns
There are so many different ways to experiment with ads.
Not only can you test ads on various platforms to see which ones reach your audience the best, but you can also experiment with the type of ad you create.
As a big purveyor of GIFs in the workplace, animating ads are a great way to catch the attention of potential customers. Those may work great for your brand.
You may also find that short videos or static images work better.
Additionally, you might run different types of copy with your ads to see which language compels your audience to click.
To maximize your return on ad spend (ROAS), run experiments on your paid media campaigns.
4. Social Media Platforms
Is there a social media site you're not using? For instance, lifestyle brands might prioritize Twitter and Instagram, but implementing Pinterest opens the door for an untapped audience.
You might consider testing which hashtags or visuals you use on certain social media sites to see how well they perform.
The more you use certain social platforms, the more iterations you can create based on what your audience responds to.
You might even use your social media analytics to determine which countries or regions you should focus on — for instance, my Twitter Analytics , below, demonstrates where most of my audience resides.
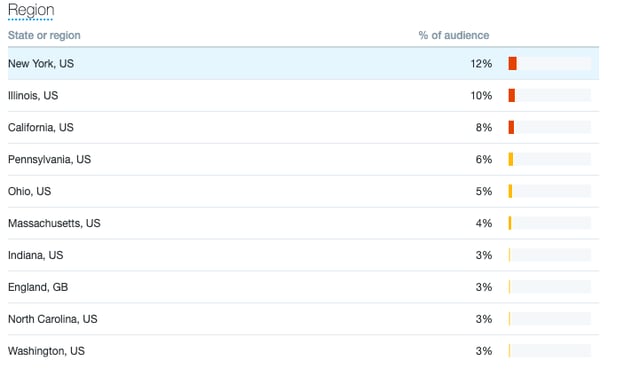
If alternatively, I saw most of my audience came from India, I might need to alter my social strategy to ensure I catered to India's time zone.
When experimenting with different time zones, consider making content specific to the audience you're trying to reach.
Your copy — the text used in marketing campaigns to persuade, inform, or entertain an audience — can make or break your marketing strategy .
If you’re not in touch with your audience, your message may not resonate. Perhaps you haven’t fleshed out your user persona or you’ve conducted limited research.
As such, it may be helpful to test what tone and concepts your audience enjoys. A/B testing is a great way to do this, you can also run surveys and focus groups to better understand your audience.
Email marketing continues to be one of the best digital channels to grow and nurture your leads.
If you have low open or high unsubscribe rates, it's worth running experiments to see what your audience will respond best to.
Perhaps your subject lines are too impersonal or unspecific. Or the content in your email is too long.
By playing around with various elements in your email, you can figure out the right strategy to reach your audience.
Ultimately, marketing experiments are a cost-effective way to get a picture of how new content ideas will work in your next campaign, which is critical for ensuring you continue to delight your audience.
Editor's Note: This post was originally published in December 2019 and has been updated for comprehensiveness.
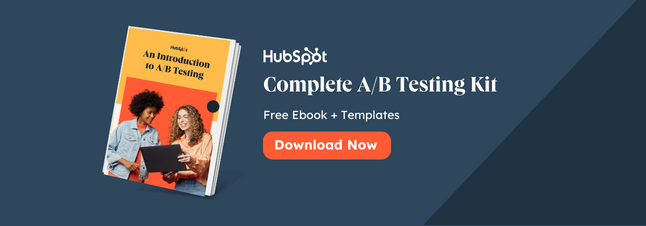
Don't forget to share this post!
Related articles.
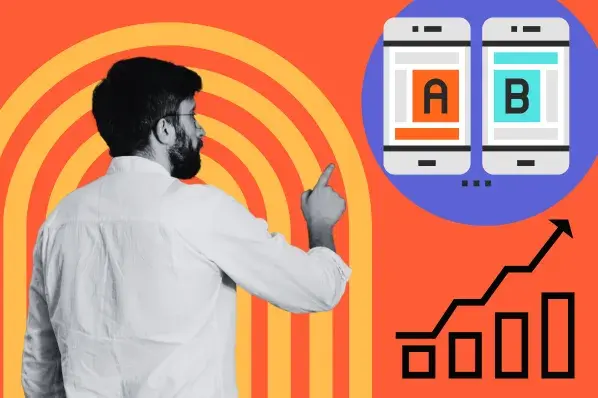
How to Determine Your A/B Testing Sample Size & Time Frame
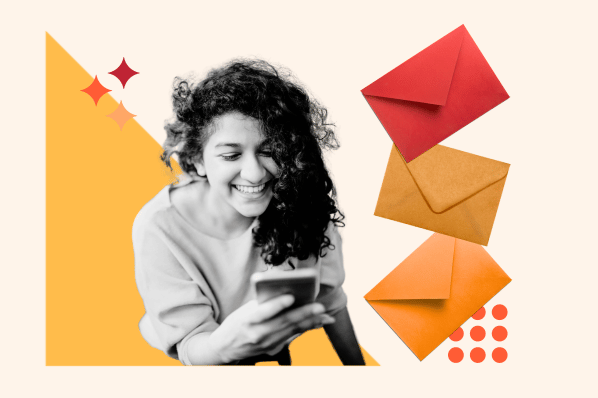
How The Hustle Got 43,876 More Clicks
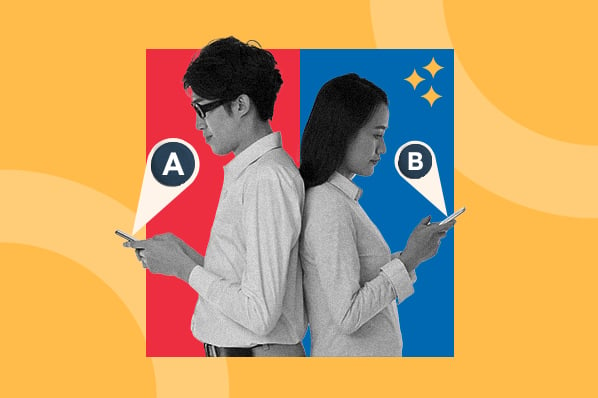
How to Do A/B Testing: 15 Steps for the Perfect Split Test
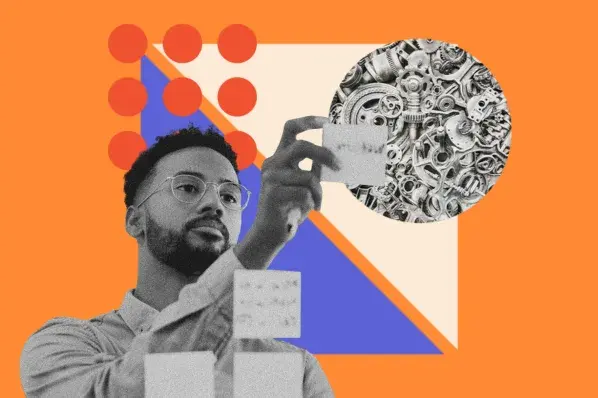
What Most Brands Miss With User Testing (That Costs Them Conversions)
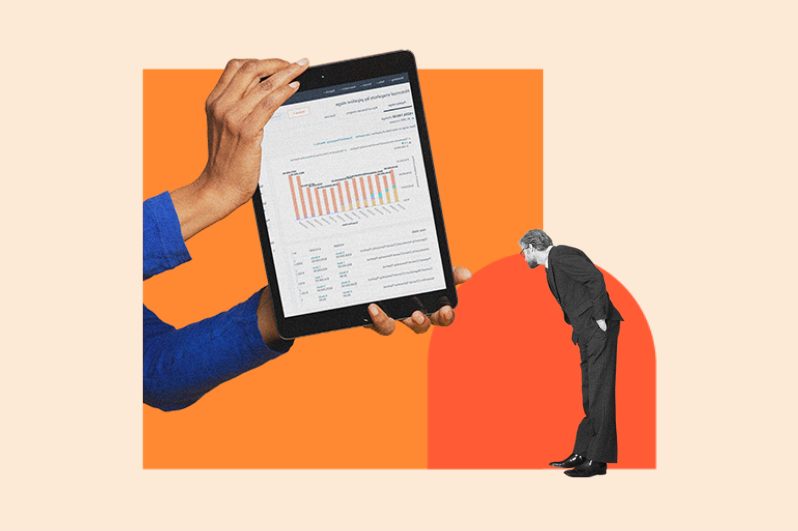
Multivariate Testing: How It Differs From A/B Testing
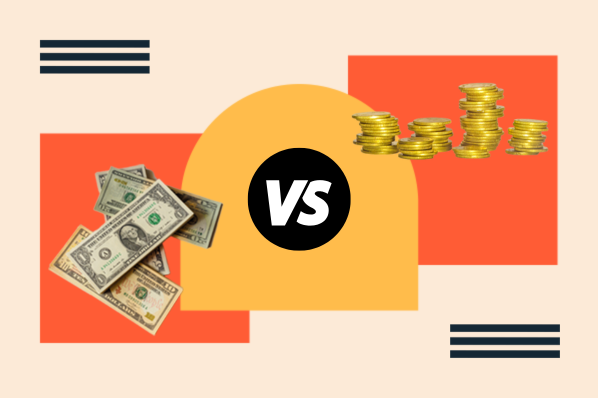
How to A/B Test Your Pricing (And Why It Might Be a Bad Idea)
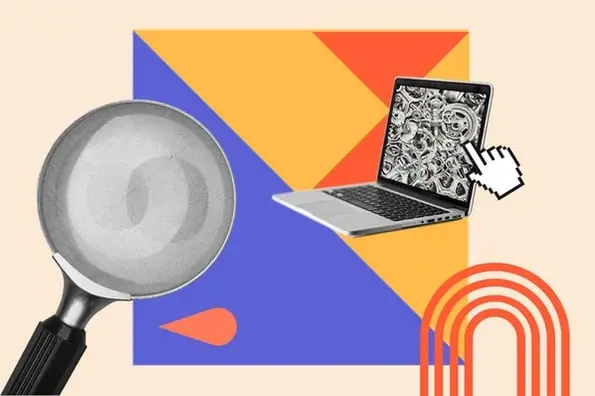
11 A/B Testing Examples From Real Businesses
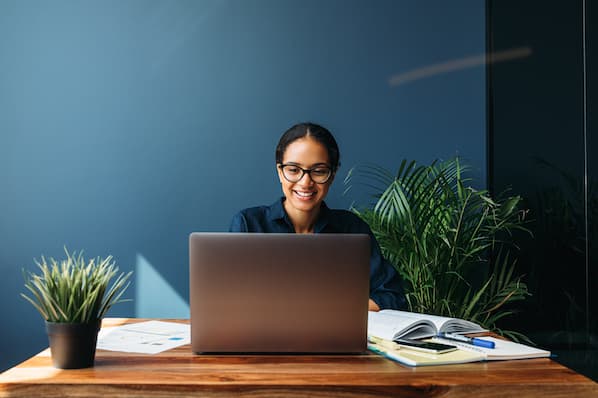
15 of the Best A/B Testing Tools for 2024
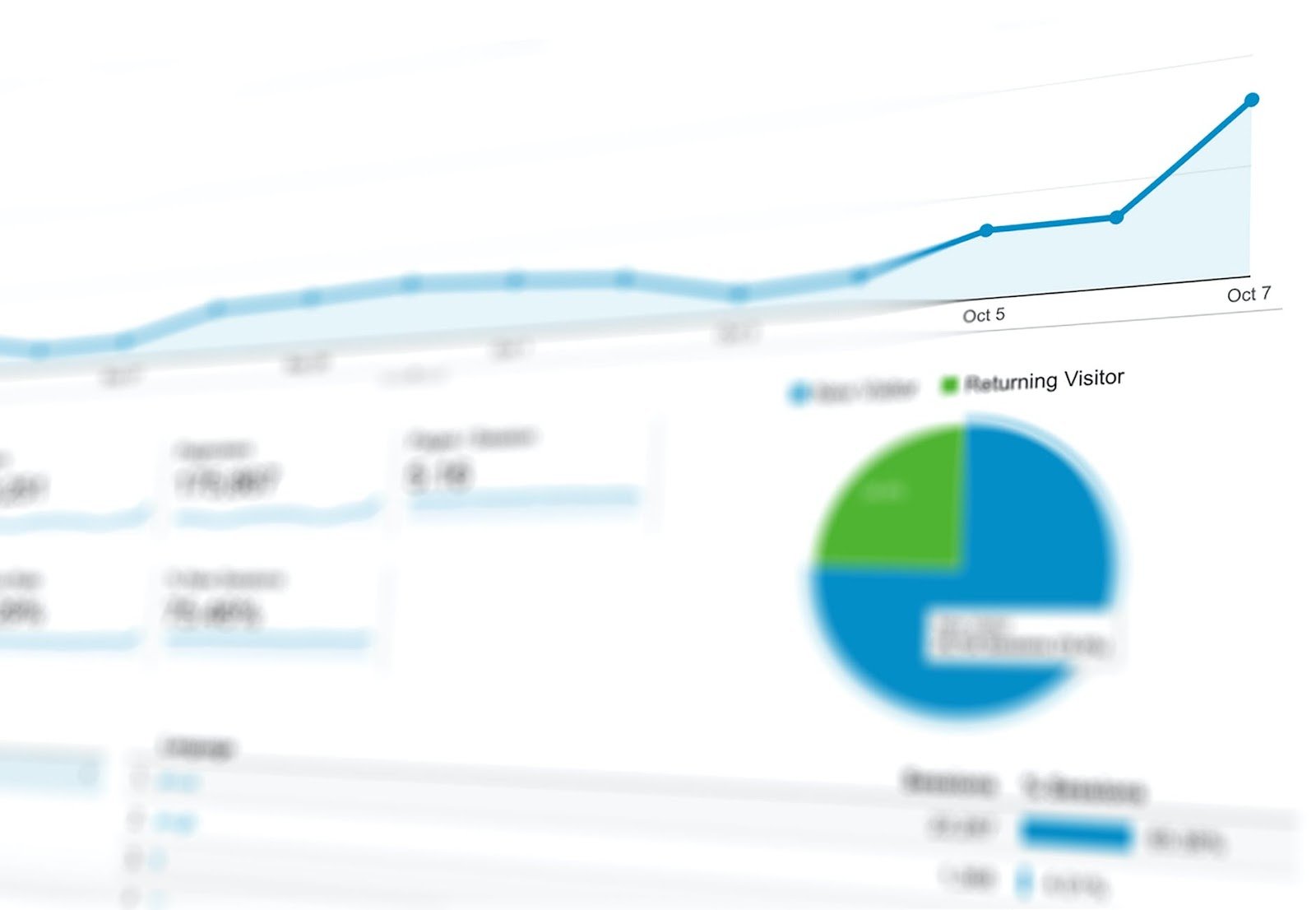
These 20 A/B Testing Variables Measure Successful Marketing Campaigns
![hypothesis in marketing How to Understand & Calculate Statistical Significance [Example]](https://www.hubspot.com/hubfs/FEATURED%20IMAGE-Nov-22-2022-06-25-18-4060-PM.png)
How to Understand & Calculate Statistical Significance [Example]
Learn more about A/B and how to run better tests.
Marketing software that helps you drive revenue, save time and resources, and measure and optimize your investments — all on one easy-to-use platform
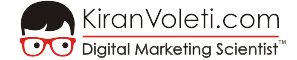
How to use a Hypothesis for Marketing Analytics
- September 5, 2023
- Digital Marketing , Social Media
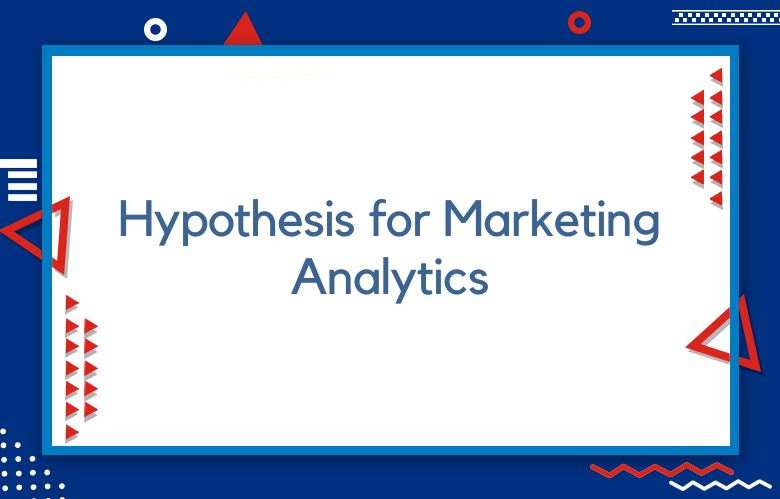
Hypothesis is a much-underused concept in marketing analytics that can yield significant results. It is a method of testing a marketing theory or proposition before investing substantial resources into implementation. Using this approach can ensure that resources are prioritized to achieve better outcomes. So, let’s dive into the hypothesis and how it can be used in marketing analytics.
What is a Hypothesis?
A hypothesis is a statement derived from a prospective marketing action or change to the existing strategy that might potentially ameliorate a current predicament.
For instance, your website’s CTA button’s lack of action may inflict a high bounce rate, which can draw up a hypothesis. “Adjusting the CTA button’s position and color will reduce the bounce rate.
“This proposition is the hypothesis, and the objective of the analysis is to either back it up with trustworthy data or conclude that it isn’t helpful or practical.
Why Use Hypothesis in Marketing Analytics?
The answer is simple: using a hypothesis can improve analytical accuracy, detect unexpected outcomes, and reduce unnecessary costs.
By conducting a hypothesis before implementing marketing campaigns , companies can save money and time by filtering out unpromising alternatives.
Understanding the Hypothesis for Marketing Analytics
Marketing has become one of the most important aspects of modern-day business operations. With the ever-increasing competition in the market, businesses need to leverage various technological tools and analytical techniques to understand consumer behavior and make informed decisions about their marketing strategies .
One such tool that has gained immense popularity in recent years is marketing analytics. Marketing analytics helps businesses gather, analyze, and interpret large data sets to draw meaningful insights about their target audience.
We will discuss the hypothesis for marketing analytics and how it can help businesses make data-driven decisions.
Understanding the hypothesis is crucial for any marketing analytics project. An idea is based on previous data, research, and assumptions about a problem or opportunity.
In other words, it is an assumption the analyst makes about the relationship between variables.
The hypothesis for marketing analytics should be created based on the analysis objectives and the available data. It can help businesses identify patterns, trends, and relationships between variables that can inform their marketing strategies.
Hypothesis-Driven Analysis Method
This analytical method follows a plan of action that starts with listing the presumptive reasons for the problem. In continuation, we write out the solution or change required, and it ends by stating the critical overviews and measurable factors held up by the assumption.
The next stage is to execute a hypothesis and determine whether a desired outcome is achievable. It can be done by testing the proposition on a subset of the audience, A/B testing , depending on available resources.
Key Hypothesis Components
A well-constructed hypothesis contains two vital components – the problem statement and the proposed solution.
The problem statement in hypothesis detection starts by examining an existing issue’s hypotheses to find a solution, e.g., low conversion rates on the landing page. When proposing a solution, retain prospects that are reachable and measurable.
A hypothesis’s remedy should also include a specific assumption, for example, “Changing the font’s color and size may increase transparency on the landing page and drive up conversion rates.”
How to Use a Hypothesis for Marketing Analytics: A Step-by-Step Guide
Are you tired of making major marketing decisions based solely on gut feelings? Do you wish there was a way to make data-driven decisions with confidence?
Look no further than hypothesis testing! Hypothesis testing is a statistical method that allows you to validate or reject assumptions about your data.
In marketing analytics, this method can help you identify the most effective strategies and make informed decisions about where to invest your resources. Below, we’ll explore the steps for using a hypothesis in your marketing analytics.
Decoding the Hypothesis for Marketing Analytics
As a marketer, you aim to build an effective strategy to reach your audience and increase conversions. But how do you identify the factors that contribute to your success?
Marketing analytics is the solution that helps you create data-backed decisions. However, without a hypothesis, analytics is just a bunch of numbers. It aims to help you understand how to define a marketing hypothesis, its components, and its advantages.
Firstly, let’s define a marketing hypothesis. It is a statement that predicts how a particular marketing strategy, tactic, or change in the marketing mix will affect your desired outcome. The idea usually starts with an ‘if-then’ statement.
For example, “If we increase our social media advertising budget, then we will increase website traffic by 20%.” This statement predicts that increasing social media advertising will cause website traffic to increase.
Identify Your Hypothesis
Before you start your analysis, you need to state what you think will happen. This is your hypothesis. Your hypothesis should be specific and measurable.
For example, if you want to test whether adding a phone number to your website leads to more leads, your hypothesis might be, “Adding a phone number to the website will increase lead generation by 20%.”
Choose a Statistical Test
Once you have your hypothesis, it’s time to choose a statistical test to validate it. The type of test you choose will depend on the kind of data you have and what you’re trying to prove.
For example, you might use a t-test comparing two groups. If you’re comparing multiple groups, you might use an ANOVA. You might use a Pearson’s r test if you’re looking for a correlation.
Many resources are available online to help you choose the proper test for your hypothesis.
Collect Your Data
Before you can run your statistical test, you need to collect data. Ensure you collect enough data to ensure your results are statistically significant.
You can use tools like Google Analytics, HubSpot, or Salesforce to collect data on website traffic, leads generated, and other vital metrics.
Run Your Test
Now, it’s time to put your hypothesis and data to the test! Run your chosen statistical test and compare the results to your idea. If the results match your vision, congratulations!
You’ve validated your assumption. If the results don’t match your hypothesis, don’t panic. Use the data to refine your idea and try again.
Draw Conclusions and Make Decisions
Once you’ve validated your hypothesis, it’s time to draw conclusions and make decisions. Use your data to determine whether your marketing efforts are working or changes are needed. This data-driven approach will help you make informed decisions and increase your chances of success.
Conclusion:
The marketing world is uncertain, and every marketer must make decisions based on approximations that may or may not bring success.
Using hypotheses in marketing analytics provides a reliable and cheaper way of testing speculation and determining more efficiently what works and what doesn’t. Particularly in handling intense competition and limited resources, marketing teams must maximize their investments.
Hypothesis-driven analysis ensures that marketing choices are driven by data and logic, reducing the probability of making poor judgments. So, start engaging with hypotheses and make informed decisions that benefit users and the business growth rate.
Call: +91 9848321284
Email: [email protected]
Kiran Voleti
Kiran Voleti is an Entrepreneur , Digital Marketing Consultant , Social Media Strategist , Internet Marketing Consultant, Creative Designer and Growth Hacker.
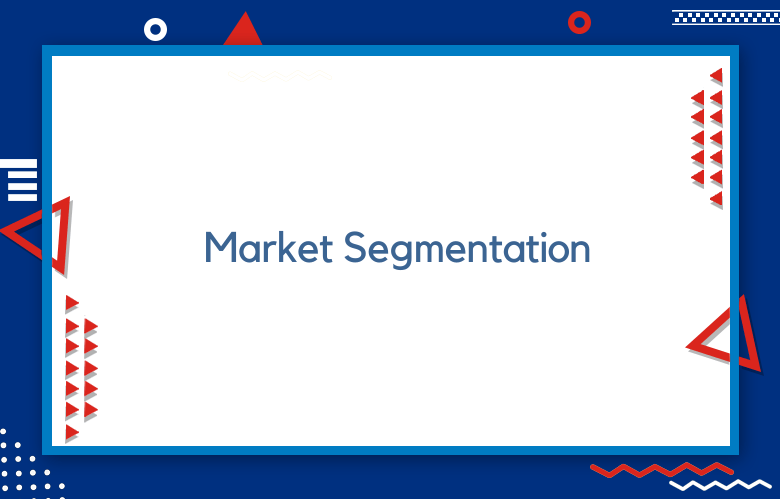
Market Segmentation: How Can You Use Market Segmentation To Grow Your Business
Market segmentation is a crucial part of effective marketing. It divides a more significant market…
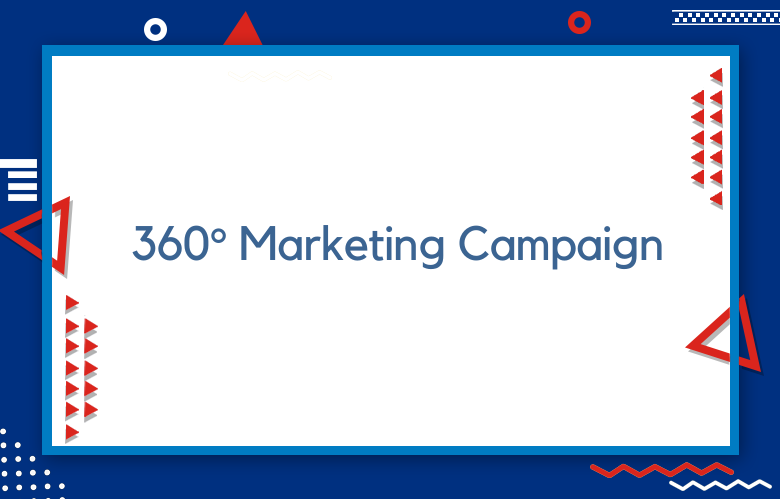
360° Marketing Campaign: How Can You Grow Your Business With 360-Degree Digital Marketing Strategy
A 360° marketing campaign considers your customer's entire experience with your brand. It reaches them…
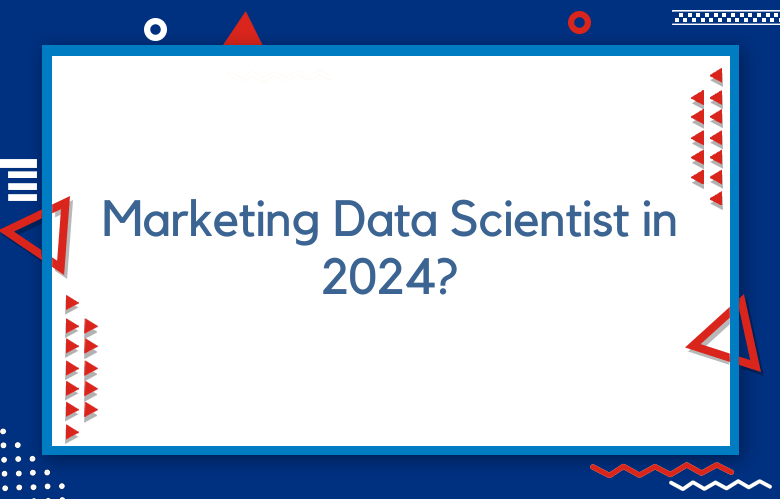
How do you become a Marketing Data Scientist in 2024?
The rise of Big Data has given birth to a new breed of professionals called…
Leave a Reply Cancel reply
Your email address will not be published. Required fields are marked *
- previous post: Deep Neural Networks for Marketing Analytics
- next post: No-Code AI for Marketing: Unlocking Automation and Growth

- What is Strategy?
- Business Models
- Developing a Strategy
- Strategic Planning
- Competitive Advantage
- Growth Strategy
- Market Strategy
- Customer Strategy
- Geographic Strategy
- Product Strategy
- Service Strategy
- Pricing Strategy
- Distribution Strategy
- Sales Strategy
- Marketing Strategy
- Digital Marketing Strategy
- Organizational Strategy
- HR Strategy – Organizational Design
- HR Strategy – Employee Journey & Culture
- Process Strategy
- Procurement Strategy
- Cost and Capital Strategy
- Business Value
- Market Analysis
- Problem Solving Skills
- Strategic Options
- Business Analytics
- Strategic Decision Making
- Process Improvement
- Project Planning
- Team Leadership
- Personal Development
- Leadership Maturity Model
- Leadership Team Strategy
- The Leadership Team
- Leadership Mindset
- Communication & Collaboration
- Problem Solving
- Decision Making
- People Leadership
- Strategic Execution
- Executive Coaching
- Strategy Coaching
- Business Transformation
- Strategy Workshops
- Leadership Strategy Survey
- Leadership Training
- Who’s Joe?
“A fact is a simple statement that everyone believes. It is innocent, unless found guilty. A hypothesis is a novel suggestion that no one wants to believe. It is guilty until found effective.”
– Edward Teller, Nuclear Physicist
During my first brainstorming meeting on my first project at McKinsey, this very serious partner, who had a PhD in Physics, looked at me and said, “So, Joe, what are your main hypotheses.” I looked back at him, perplexed, and said, “Ummm, my what?” I was used to people simply asking, “what are your best ideas, opinions, thoughts, etc.” Over time, I began to understand the importance of hypotheses and how it plays an important role in McKinsey’s problem solving of separating ideas and opinions from facts.
What is a Hypothesis?
“Hypothesis” is probably one of the top 5 words used by McKinsey consultants. And, being hypothesis-driven was required to have any success at McKinsey. A hypothesis is an idea or theory, often based on limited data, which is typically the beginning of a thread of further investigation to prove, disprove or improve the hypothesis through facts and empirical data.
The first step in being hypothesis-driven is to focus on the highest potential ideas and theories of how to solve a problem or realize an opportunity.
Let’s go over an example of being hypothesis-driven.
Let’s say you own a website, and you brainstorm ten ideas to improve web traffic, but you don’t have the budget to execute all ten ideas. The first step in being hypothesis-driven is to prioritize the ten ideas based on how much impact you hypothesize they will create.

The second step in being hypothesis-driven is to apply the scientific method to your hypotheses by creating the fact base to prove or disprove your hypothesis, which then allows you to turn your hypothesis into fact and knowledge. Running with our example, you could prove or disprove your hypothesis on the ideas you think will drive the most impact by executing:
1. An analysis of previous research and the performance of the different ideas 2. A survey where customers rank order the ideas 3. An actual test of the ten ideas to create a fact base on click-through rates and cost
While there are many other ways to validate the hypothesis on your prioritization , I find most people do not take this critical step in validating a hypothesis. Instead, they apply bad logic to many important decisions . An idea pops into their head, and then somehow it just becomes a fact.
One of my favorite lousy logic moments was a CEO who stated,
“I’ve never heard our customers talk about price, so the price doesn’t matter with our products , and I’ve decided we’re going to raise prices.”
Luckily, his management team was able to do a survey to dig deeper into the hypothesis that customers weren’t price-sensitive. Well, of course, they were and through the survey, they built a fantastic fact base that proved and disproved many other important hypotheses.

Why is being hypothesis-driven so important?
Imagine if medicine never actually used the scientific method. We would probably still be living in a world of lobotomies and bleeding people. Many organizations are still stuck in the dark ages, having built a house of cards on opinions disguised as facts, because they don’t prove or disprove their hypotheses. Decisions made on top of decisions, made on top of opinions, steer organizations clear of reality and the facts necessary to objectively evolve their strategic understanding and knowledge. I’ve seen too many leadership teams led solely by gut and opinion. The problem with intuition and gut is if you don’t ever prove or disprove if your gut is right or wrong, you’re never going to improve your intuition. There is a reason why being hypothesis-driven is the cornerstone of problem solving at McKinsey and every other top strategy consulting firm.
How do you become hypothesis-driven?
Most people are idea-driven, and constantly have hypotheses on how the world works and what they or their organization should do to improve. Though, there is often a fatal flaw in that many people turn their hypotheses into false facts, without actually finding or creating the facts to prove or disprove their hypotheses. These people aren’t hypothesis-driven; they are gut-driven.
The conversation typically goes something like “doing this discount promotion will increase our profits” or “our customers need to have this feature” or “morale is in the toilet because we don’t pay well, so we need to increase pay.” These should all be hypotheses that need the appropriate fact base, but instead, they become false facts, often leading to unintended results and consequences. In each of these cases, to become hypothesis-driven necessitates a different framing.
• Instead of “doing this discount promotion will increase our profits,” a hypothesis-driven approach is to ask “what are the best marketing ideas to increase our profits?” and then conduct a marketing experiment to see which ideas increase profits the most.
• Instead of “our customers need to have this feature,” ask the question, “what features would our customers value most?” And, then conduct a simple survey having customers rank order the features based on value to them.
• Instead of “morale is in the toilet because we don’t pay well, so we need to increase pay,” conduct a survey asking, “what is the level of morale?” what are potential issues affecting morale?” and what are the best ideas to improve morale?”
Beyond, watching out for just following your gut, here are some of the other best practices in being hypothesis-driven:
Listen to Your Intuition
Your mind has taken the collision of your experiences and everything you’ve learned over the years to create your intuition, which are those ideas that pop into your head and those hunches that come from your gut. Your intuition is your wellspring of hypotheses. So listen to your intuition, build hypotheses from it, and then prove or disprove those hypotheses, which will, in turn, improve your intuition. Intuition without feedback will over time typically evolve into poor intuition, which leads to poor judgment, thinking, and decisions.
Constantly Be Curious
I’m always curious about cause and effect. At Sports Authority, I had a hypothesis that customers that received service and assistance as they shopped, were worth more than customers who didn’t receive assistance from an associate. We figured out how to prove or disprove this hypothesis by tying surveys to transactional data of customers, and we found the hypothesis was true, which led us to a broad initiative around improving service. The key is you have to be always curious about what you think does or will drive value, create hypotheses and then prove or disprove those hypotheses.
Validate Hypotheses
You need to validate and prove or disprove hypotheses. Don’t just chalk up an idea as fact. In most cases, you’re going to have to create a fact base utilizing logic, observation, testing (see the section on Experimentation ), surveys, and analysis.
Be a Learning Organization
The foundation of learning organizations is the testing of and learning from hypotheses. I remember my first strategy internship at Mercer Management Consulting when I spent a good part of the summer combing through the results, findings, and insights of thousands of experiments that a banking client had conducted. It was fascinating to see the vastness and depth of their collective knowledge base. And, in today’s world of knowledge portals, it is so easy to disseminate, learn from, and build upon the knowledge created by companies.
NEXT SECTION: DISAGGREGATION
DOWNLOAD STRATEGY PRESENTATION TEMPLATES
THE $150 VALUE PACK - 600 SLIDES 168-PAGE COMPENDIUM OF STRATEGY FRAMEWORKS & TEMPLATES 186-PAGE HR & ORG STRATEGY PRESENTATION 100-PAGE SALES PLAN PRESENTATION 121-PAGE STRATEGIC PLAN & COMPANY OVERVIEW PRESENTATION 114-PAGE MARKET & COMPETITIVE ANALYSIS PRESENTATION 18-PAGE BUSINESS MODEL TEMPLATE
JOE NEWSUM COACHING

EXECUTIVE COACHING STRATEGY COACHING ELEVATE360 BUSINESS TRANSFORMATION STRATEGY WORKSHOPS LEADERSHIP STRATEGY SURVEY & WORKSHOP STRATEGY & LEADERSHIP TRAINING
THE LEADERSHIP MATURITY MODEL
Explore other types of strategy.
BIG PICTURE WHAT IS STRATEGY? BUSINESS MODEL COMP. ADVANTAGE GROWTH
TARGETS MARKET CUSTOMER GEOGRAPHIC
VALUE PROPOSITION PRODUCT SERVICE PRICING
GO TO MARKET DISTRIBUTION SALES MARKETING
ORGANIZATIONAL ORG DESIGN HR & CULTURE PROCESS PARTNER
EXPLORE THE TOP 100 STRATEGIC LEADERSHIP COMPETENCIES
TYPES OF VALUE MARKET ANALYSIS PROBLEM SOLVING
OPTION CREATION ANALYTICS DECISION MAKING PROCESS TOOLS
PLANNING & PROJECTS PEOPLE LEADERSHIP PERSONAL DEVELOPMENT


- Subscribers
- How To Use a New AI App and AI Agents To Build Your Best Landing Page
- The MECLABS AI Guild in Action: Teamwork in Crafting Their Optimal Landing Page
- How MECLABS AI Is Being Used To Build the AI Guild
- MECLABS AI’s Problem Solver in Action
- MECLABS AI: Harness AI With the Power of Your Voice
- Harnessing MECLABS AI: Transform Your Copywriting and Landing Pages
- MECLABS AI: Overcome the ‘Almost Trap’ and Get Real Answers
- MECLABS AI: A brief glimpse into what is coming!
- Transforming Marketing with MECLABS AI: A New Paradigm
- Creative AI Marketing: Escaping the ‘Vending Machine Mentality’

A/B Testing: Example of a good hypothesis
Want to know the secret to always running successful tests?
The answer is to formulate a hypothesis .
Now when I say it’s always successful, I’m not talking about always increasing your Key Performance Indicator (KPI). You can “lose” a test, but still be successful.
That sounds like an oxymoron, but it’s not. If you set up your test strategically, even if the test decreases your KPI, you gain a learning , which is a success! And, if you win, you simultaneously achieve a lift and a learning. Double win!
The way you ensure you have a strategic test that will produce a learning is by centering it around a strong hypothesis.
So, what is a hypothesis?
By definition, a hypothesis is a proposed statement made on the basis of limited evidence that can be proved or disproved and is used as a starting point for further investigation.
Let’s break that down:
It is a proposed statement.
- A hypothesis is not fact, and should not be argued as right or wrong until it is tested and proven one way or the other.
It is made on the basis of limited (but hopefully some ) evidence.
- Your hypothesis should be informed by as much knowledge as you have. This should include data that you have gathered, any research you have done, and the analysis of the current problems you have performed.
It can be proved or disproved.
- A hypothesis pretty much says, “I think by making this change , it will cause this effect .” So, based on your results, you should be able to say “this is true” or “this is false.”
It is used as a starting point for further investigation.
- The key word here is starting point . Your hypothesis should be formed and agreed upon before you make any wireframes or designs as it is what guides the design of your test. It helps you focus on what elements to change, how to change them, and which to leave alone.
How do I write a hypothesis?
The structure of your basic hypothesis follows a CHANGE: EFFECT framework.
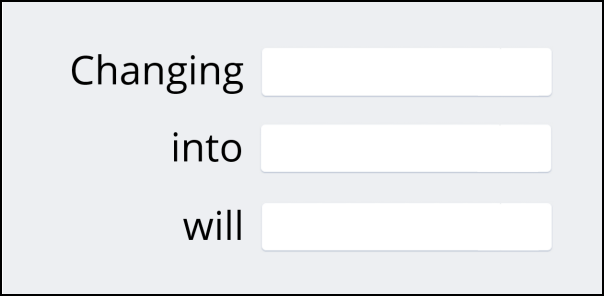
While this is a truly scientific and testable template, it is very open-ended. Even though this hypothesis, “Changing an English headline into a Spanish headline will increase clickthrough rate,” is perfectly valid and testable, if your visitors are English-speaking, it probably doesn’t make much sense.
So now the question is …
How do I write a GOOD hypothesis?
To quote my boss Tony Doty , “This isn’t Mad Libs.”
We can’t just start plugging in nouns and verbs and conclude that we have a good hypothesis. Your hypothesis needs to be backed by a strategy. And, your strategy needs to be rooted in a solution to a problem .
So, a more complete version of the above template would be something like this:
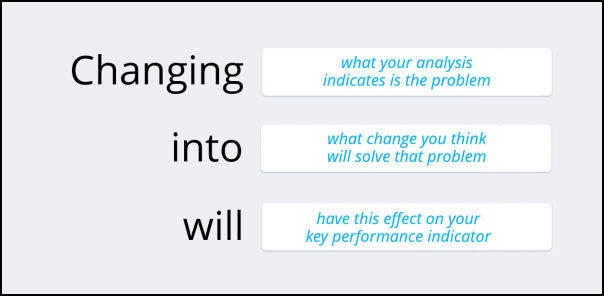
In order to have a good hypothesis, you don’t necessarily have to follow this exact sentence structure, as long as it is centered around three main things:
Presumed problem
Proposed solution
Anticipated result
After you’ve completed your analysis and research, identify the problem that you will address. While we need to be very clear about what we think the problem is, you should leave it out of the hypothesis since it is harder to prove or disprove. You may want to come up with both a problem statement and a hypothesis .
For example:
Problem Statement: “The lead generation form is too long, causing unnecessary friction .”
Hypothesis: “By changing the amount of form fields from 20 to 10, we will increase number of leads.”
When you are thinking about the solution you want to implement, you need to think about the psychology of the customer. What psychological impact is your proposed problem causing in the mind of the customer?
For example, if your proposed problem is “There is a lack of clarity in the sign-up process,” the psychological impact may be that the user is confused.
Now think about what solution is going to address the problem in the customer’s mind. If they are confused, we need to explain something better, or provide them with more information. For this example, we will say our proposed solution is to “Add a progress bar to the sign-up process.” This leads straight into the anticipated result.
If we reduce the confusion in the visitor’s mind (psychological impact) by adding the progress bar, what do we foresee to be the result? We are anticipating that it would be more people completing the sign-up process. Your proposed solution and your KPI need to be directly correlated.
Note: Some people will include the psychological impact in their hypothesis. This isn’t necessarily wrong, but we do have to be careful with assumptions. If we say that the effect will be “Reduced confusion and therefore increase in conversion rate,” we are assuming the reduced confusion is what made the impact. While this may be correct, it is not measureable and it is hard to prove or disprove.
To summarize, your hypothesis should follow a structure of: “If I change this, it will have this effect,” but should always be informed by an analysis of the problems and rooted in the solution you deemed appropriate.
Related Resources:
A/B Testing 101: How to get real results from optimization
The True Value of Data
15 Years of Marketing Research in 11 Minutes
Marketing Analytics: 6 simple steps for interpreting your data
Website A/B Testing: 4 tips to beat an unbeatable landing page
Online Cart: 6 ideas to test and optimize your checkout process
B2B Gamification: Autodesk’s two approaches to in-trial marketing [Video]
How to Discover Exactly What the Customer Wants to See on the Next Click: 3 critical…
The 21 Psychological Elements that Power Effective Web Design (Part 3)
The 21 Psychological Elements that Power Effective Web Design (Part 2)
The 21 Psychological Elements that Power Effective Web Design (Part 1)
Thanks for the article. I’ve been trying to wrap my head around this type of testing because I’d like to use it to see the effectiveness on some ads. This article really helped. Thanks Again!
Hey Lauren, I am just getting to the point that I have something to perform A-B testing on. This post led me to this site which will and already has become a help in what to test and how to test .
Again, thanks for getting me here .
Good article. I have been researching different approaches to writing testing hypotheses and this has been a help. The only thing I would add is that it can be useful to capture the insight/justification within the hypothesis statement. IF i do this, THEN I expect this result BECAUSE I have this insight.
@Kaya Great!
Good article – but technically you can never prove an hypothesis, according to the principle of falsification (Popper), only fail to disprove the null hypothesis.
Leave A Reply Cancel Reply
Your email address will not be published.
Save my name, email, and website in this browser for the next time I comment.
- Quick Win Clinics
- Research Briefs
- A/B Testing
- Conversion Marketing
- Copywriting
- Digital Advertising
- Digital Analytics
- Digital Subscriptions
- E-commerce Marketing
- Email Marketing
- Lead Generation
- Social Marketing
- Value Proposition
- Research Services
- Video – Transparent Marketing
- Video – 15 years of marketing research in 11 minutes
- Lecture – The Web as a Living Laboratory
- Featured Research
Welcome, Login to your account.
Recover your password.
A password will be e-mailed to you.
From Hypothesis to Results: Mastering the Art of Marketing Experiments
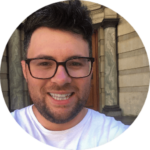
Max 16 min read
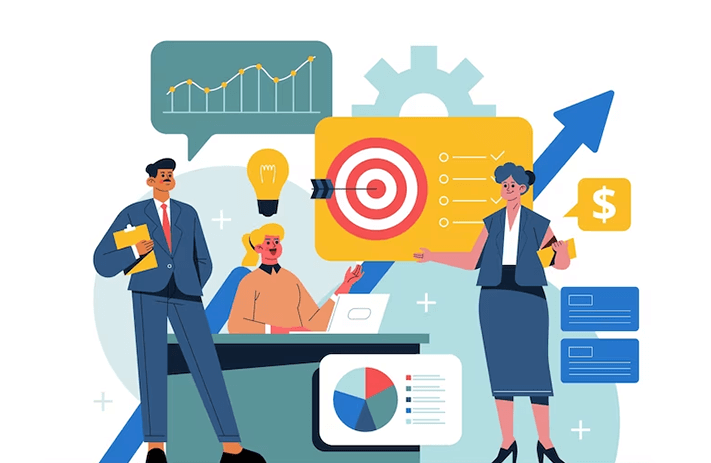
Click the button to start reading
Suppose you’re trying to convince your friend to watch your favorite movie. You could either tell them about the intriguing plot or show them the exciting trailer.
To find out which approach works best, you try both methods with different friends and see which one gets more people to watch the movie.
Marketing experiments work in much the same way, allowing businesses to test different marketing strategies, gather feedback from their target audience, and make data-driven decisions that lead to improved outcomes and growth.
By testing different approaches and measuring their outcomes, companies can identify what works best for their unique target audience and adapt their marketing strategies accordingly. This leads to more efficient use of marketing resources and results in higher conversion rates, increased customer satisfaction, and, ultimately, business growth.
Marketing experiments are the backbone of building an organization’s culture of learning and curiosity, encouraging employees to think outside the box and challenge the status quo.
In this article, we will delve into the fundamentals of marketing experiments, discussing their key elements and various types. By the end, you’ll be in a position to start running these tests and securing better marketing campaigns with explosive results.
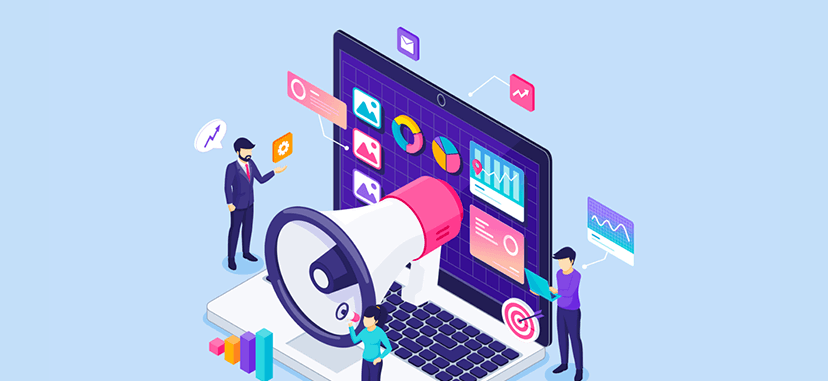
Why Digital Marketing Experiments Matter
One of the most effective ways to drive growth and optimize marketing strategies is through digital marketing experiments. These experiments provide invaluable insights into customer preferences, behaviors, and the overall effectiveness of marketing efforts, making them an essential component of any digital marketing strategy.
Digital marketing experiments matter for several reasons:
- Customer-centric approach: By conducting experiments, businesses can gain a deeper understanding of their target audience’s preferences and behaviors. This enables them to tailor their marketing efforts to better align with customer needs, resulting in more effective and engaging campaigns.
- Data-driven decision-making: Marketing experiments provide quantitative data on the performance of different marketing strategies and tactics. This empowers businesses to make informed decisions based on actual results rather than relying on intuition or guesswork. Ultimately, this data-driven approach leads to more efficient allocation of resources and improved marketing outcomes.
- Agility and adaptability: Businesses must be agile and adaptable to keep up with emerging trends and technologies. Digital marketing experiments allow businesses to test new ideas, platforms, and strategies in a controlled environment, helping them stay ahead of the curve and quickly respond to changing market conditions.
- Continuous improvement: Digital marketing experiments facilitate an iterative process of testing, learning, and refining marketing strategies. This ongoing cycle of improvement enables businesses to optimize their marketing efforts, drive better results, and maintain a competitive edge in the digital marketplace.
- ROI and profitability: By identifying which marketing tactics are most effective, businesses can allocate their marketing budget more efficiently and maximize their return on investment. This increased profitability can be reinvested into the business, fueling further growth and success.
Developing a culture of experimentation allows businesses to continuously improve their marketing strategies, maximize their ROI, and avoid being left behind by the competition.
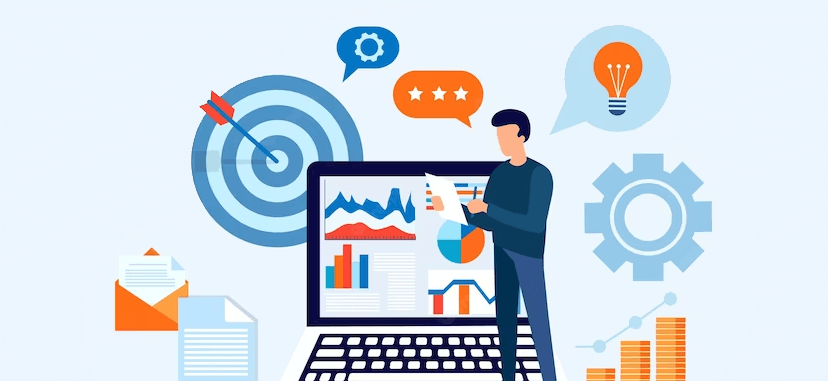
The Fundamentals of Digital Marketing Experiments
Marketing experiments are structured tests that compare different marketing strategies, tactics, or assets to determine which one performs better in achieving specific objectives.
These experiments use a scientific approach, which involves formulating hypotheses, controlling variables, gathering data, and analyzing the results to make informed decisions.
Marketing experiments provide valuable insights into customer preferences and behaviors, enabling businesses to optimize their marketing efforts and maximize returns on investment (ROI).
There are several types of marketing experiments that businesses can use, depending on their objectives and available resources.
The most common types include:
A/B testing
A/B testing, also known as split testing, is a simple yet powerful technique that compares two variations of a single variable to determine which one performs better.
In an A/B test, the target audience is randomly divided into two groups: one group is exposed to version A (the control). In contrast, the other group is exposed to version B (the treatment). The performance of both versions is then measured and compared to identify the one that yields better results.
A/B testing can be applied to various marketing elements, such as headlines, calls-to-action, email subject lines, landing page designs, and ad copy. The primary advantage of A/B testing is its simplicity, making it easy for businesses to implement and analyze.
Multivariate testing
Multivariate testing is a more advanced technique that allows businesses to test multiple variables simultaneously.
In a multivariate test, several elements of a marketing asset are modified and combined to create different versions. These versions are then shown to different segments of the target audience, and their performance is measured and compared to determine the most effective combination of variables.
Multivariate testing is beneficial when optimizing complex marketing assets, such as websites or email templates, with multiple elements that may interact with one another. However, this method requires a larger sample size and more advanced analytical tools compared to A/B testing.
Pre-post analysis
Pre-post analysis involves comparing the performance of a marketing strategy before and after implementing a change.
This type of experiment is often used when it is not feasible to conduct an A/B or multivariate test, such as when the change affects the entire customer base or when there are external factors that cannot be controlled.
While pre-post analysis can provide useful insights, it is less reliable than A/B or multivariate testing because it does not account for potential confounding factors. To obtain accurate results from a pre-post analysis, businesses must carefully control for external influences and ensure that the observed changes are indeed due to the implemented modifications.
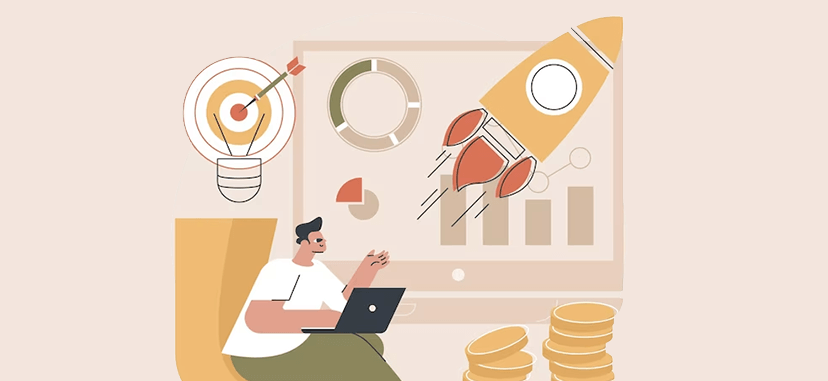
How To Start Growth Marketing Experiments
To conduct effective marketing experiments, businesses must pay attention to the following key elements:
Clear objectives
Having clear objectives is crucial for a successful marketing experiment. Before starting an experiment, businesses must identify the specific goals they want to achieve, such as increasing conversions, boosting engagement, or improving click-through rates. Clear objectives help guide the experimental design and ensure the results are relevant and actionable.
Hypothesis-driven approach
A marketing experiment should be based on a well-formulated hypothesis that predicts the expected outcome. A reasonable hypothesis is specific, testable, and grounded in existing knowledge or data. It serves as the foundation for experimental design and helps businesses focus on the most relevant variables and outcomes.
Proper experimental design
A marketing experiment requires a well-designed test that controls for potential confounding factors and ensures the reliability and validity of the results. This includes the random assignment of participants, controlling for external influences, and selecting appropriate variables to test. Proper experimental design increases the likelihood that observed differences are due to the tested variables and not other factors.
Adequate sample size
A successful marketing experiment requires an adequate sample size to ensure the results are statistically significant and generalizable to the broader target audience. The required sample size depends on the type of experiment, the expected effect size, and the desired level of confidence. In general, larger sample sizes provide more reliable and accurate results but may also require more resources to conduct the experiment.
Data-driven analysis
Marketing experiments rely on a data-driven analysis of the results. This involves using statistical techniques to determine whether the observed differences between the tested variations are significant and meaningful. Data-driven analysis helps businesses make informed decisions based on empirical evidence rather than intuition or gut feelings.
By understanding the fundamentals of marketing experiments and following best practices, businesses can gain valuable insights into customer preferences and behaviors, ultimately leading to improved outcomes and growth.
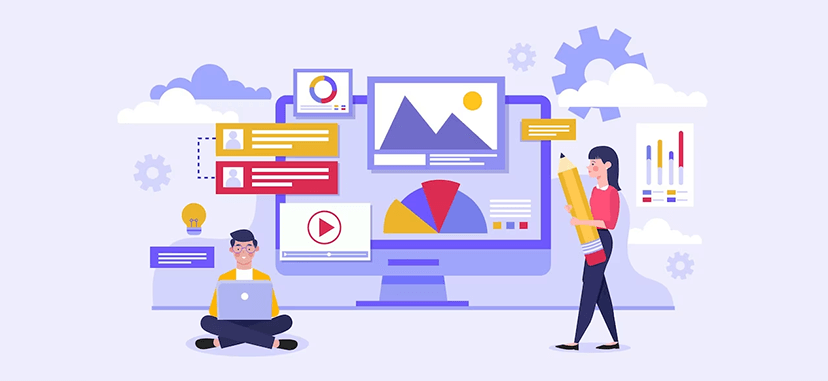
Setting up Your First Marketing Experiment
Embarking on your first marketing experiment can be both exciting and challenging. Following a systematic approach, you can set yourself up for success and gain valuable insights to improve your marketing efforts.
Here’s a step-by-step guide to help you set up your first marketing experiment.
Identifying your marketing objectives
Before diving into your experiment, it’s essential to establish clear marketing objectives. These objectives will guide your entire experiment, from hypothesis formulation to data analysis.
Consider what you want to achieve with your marketing efforts, such as increasing website conversions, improving open email rates, or boosting social media engagement.
Make sure your objectives are specific, measurable, achievable, relevant, and time-bound (SMART) to ensure that they are actionable and provide meaningful insights.
Formulating a hypothesis
With your marketing objectives in mind, the next step is formulating a hypothesis for your experiment. A hypothesis is a testable prediction that outlines the expected outcome of your experiment. It should be based on existing knowledge, data, or observations and provide a clear direction for your experimental design.
For example, suppose your objective is to increase email open rates. In that case, your hypothesis might be, “Adding the recipient’s first name to the email subject line will increase the open rate by 10%.” This hypothesis is specific, testable, and clearly linked to your marketing objective.
Designing the experiment
Once you have a hypothesis in place, you can move on to designing your experiment. This involves several key decisions:
Choosing the right testing method:
Select the most appropriate testing method for your experiment based on your objectives, hypothesis, and available resources.
As discussed earlier, common testing methods include A/B, multivariate, and pre-post analyses. Choose the method that best aligns with your goals and allows you to effectively test your hypothesis.
Selecting the variables to test:
Identify the specific variables you will test in your experiment. These should be directly related to your hypothesis and marketing objectives. In the email open rate example, the variable to test would be the subject line, specifically the presence or absence of the recipient’s first name.
When selecting variables, consider their potential impact on your marketing objectives and prioritize those with the greatest potential for improvement. Also, ensure that the variables are easily measurable and can be manipulated in your experiment.
Identifying the target audience:
Determine the target audience for your experiment, considering factors such as demographics, interests, and behaviors. Your target audience should be representative of the larger population you aim to reach with your marketing efforts.
When segmenting your audience for the experiment, ensure that the groups are as similar as possible to minimize potential confounding factors.
In A/B or multivariate testing, this can be achieved through random assignment, which helps control for external influences and ensures a fair comparison between the tested variations.
Executing the experiment
With your experiment designed, it’s time to put it into action.
This involves several key considerations:
Timing and duration:
Choose the right timing and duration for your experiment based on factors such as the marketing channel, target audience, and the nature of the tested variables.
The duration of the experiment should be long enough to gather a sufficient amount of data for meaningful analysis but not so long that it negatively affects your marketing efforts or causes fatigue among your target audience.
In general, aim for a duration that allows you to reach a predetermined sample size or achieve statistical significance. This may vary depending on the specific experiment and the desired level of confidence.
Monitoring the experiment:
During the experiment, monitor its progress and performance regularly to ensure that everything is running smoothly and according to plan. This includes checking for technical issues, tracking key metrics, and watching for any unexpected patterns or trends.
If any issues arise during the experiment, address them promptly to prevent potential biases or inaccuracies in the results. Additionally, avoid making changes to the experimental design or variables during the experiment, as this can compromise the integrity of the results.
Analyzing the results
Once your experiment has concluded, it’s time to analyze the data and draw conclusions.
This involves two key aspects:
Statistical significance:
Statistical significance is a measure of the likelihood that the observed differences between the tested variations are due to the variables being tested rather than random chance. To determine statistical significance, you will need to perform a statistical test, such as a t-test or chi-squared test, depending on the nature of your data.
Generally, a result is considered statistically significant if the probability of the observed difference occurring by chance (the p-value) is less than a predetermined threshold, often set at 0.05 or 5%. This means there is a 95% confidence level that the observed difference is due to the tested variables and not random chance.
Practical significance:
While statistical significance is crucial, it’s also essential to consider the practical significance of your results. This refers to the real-world impact of the observed differences on your marketing objectives and business goals.
To assess practical significance, consider the effect size of the observed difference (e.g., the percentage increase in email open rates) and the potential return on investment (ROI) of implementing the winning variation. This will help you determine whether the experiment results are worth acting upon and inform your marketing decisions moving forward.
A systematic approach to designing growth marketing experiments helps you to design, execute, and analyze your experiment effectively, ultimately leading to better marketing outcomes and business growth.
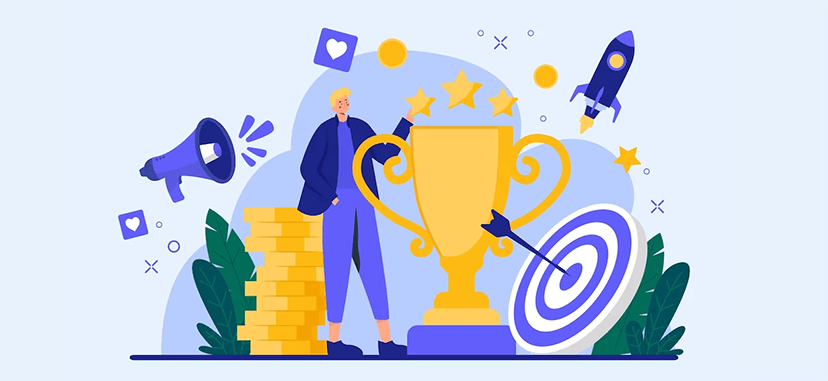
Examples of Successful Marketing Experiments
In this section, we will explore three fictional case studies of successful marketing experiments that led to improved marketing outcomes. These examples will demonstrate the practical application of marketing experiments across different channels and provide valuable lessons that can be applied to your own marketing efforts.
Example 1: Redesigning a website for increased conversions
AcmeWidgets, an online store selling innovative widgets, noticed that its website conversion rate had plateaued.
They conducted a marketing experiment to test whether a redesigned landing page could improve conversions. They hypothesized that a more visually appealing and user-friendly design would increase conversion rates by 15%.
AcmeWidgets used A/B testing to compare their existing landing page (the control) with a new, redesigned version (the treatment). They randomly assigned website visitors to one of the two landing pages. They tracked conversions over a period of four weeks.
At the end of the experiment, AcmeWidgets found that the redesigned landing page had a conversion rate 18% higher than the control. The results were statistically significant, and the company decided to implement the new design across its entire website.
As a result, AcmeWidgets experienced a substantial increase in sales and revenue.
Example 2: Optimizing email marketing campaigns
EcoTravel, a sustainable travel agency, wanted to improve the open rates of their monthly newsletter. They hypothesized that adding a sense of urgency to the subject line would increase open rates by 10%.
To test this hypothesis, EcoTravel used A/B testing to compare two different subject lines for their newsletter:
- “Discover the world’s most beautiful eco-friendly destinations” (control)
- “Last chance to book: Explore the world’s most beautiful eco-friendly destinations” (treatment)
EcoTravel sent the newsletter to a random sample of their subscribers. Half received the control subject line, and the other half received the treatment. They then tracked the open rates for both groups over one week.
The results of the experiment showed that the treatment subject line, which included a sense of urgency, led to a 12% increase in open rates compared to the control.
Based on these findings, EcoTravel incorporated a sense of urgency in their future email subject lines to boost newsletter engagement.
Example 3: Improving social media ad performance
FitFuel, a meal delivery service for fitness enthusiasts, was looking to improve its Facebook ad campaign’s click-through rate (CTR). They hypothesized that using an image of a satisfied customer enjoying a FitFuel meal would increase CTR by 8% compared to their current ad featuring a meal image alone.
FitFuel conducted an A/B test on their Facebook ad campaign, comparing the performance of the control ad (meal image only) with the treatment ad (customer enjoying a meal). They targeted a similar audience with both ad variations and measured the CTR over two weeks. The experiment revealed that the treatment ad, featuring the customer enjoying a meal, led to a 10% increase in CTR compared to the control ad. FitFuel decided to update its
Facebook ad campaign with the new image, resulting in a more cost-effective campaign and higher return on investment.
Lessons learned from these examples
These fictional examples of successful marketing experiments highlight several key takeaways:
- Clearly defined objectives and hypotheses: In each example, the companies had specific marketing objectives and well-formulated hypotheses, which helped guide their experiments and ensure relevant and actionable results.
- Proper experimental design: Each company used the appropriate testing method for their experiment and carefully controlled variables, ensuring accurate and reliable results.
- Data-driven decision-making: The companies analyzed the data from their experiments to make informed decisions about implementing changes to their marketing strategies, ultimately leading to improved outcomes.
- Continuous improvement: These examples demonstrate that marketing experiments can improve marketing efforts continuously. By regularly conducting experiments and applying the lessons learned, businesses can optimize their marketing strategies and stay ahead of the competition.
- Relevance across channels: Marketing experiments can be applied across various marketing channels, such as website design, email campaigns, and social media advertising. Regardless of the channel, the principles of marketing experimentation remain the same, making them a valuable tool for marketers in diverse industries.
By learning from these fictional examples and applying the principles of marketing experimentation to your own marketing efforts, you can unlock valuable insights, optimize your marketing strategies, and achieve better results for your business.
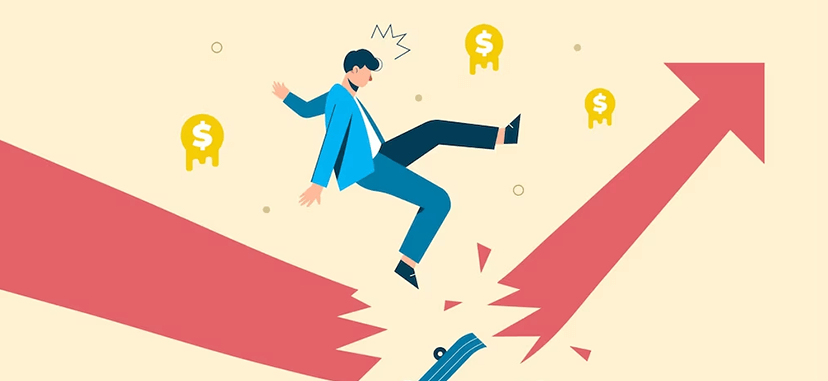
Common Pitfalls of Marketing Experiments and How to Avoid Them
Conducting marketing experiments can be a powerful way to optimize your marketing strategies and drive better results.
However, it’s important to be aware of common pitfalls that can undermine the effectiveness of your experiments. In this section, we will discuss some of these pitfalls and provide tips on how to avoid them.
Insufficient sample size
An insufficient sample size can lead to unreliable results and limit the generalizability of your findings. When your sample size is too small, you run the risk of not detecting meaningful differences between the tested variations or incorrectly attributing the observed differences to random chance.
To avoid this pitfall, calculate the required sample size for your experiment based on factors such as the expected effect size, the desired level of confidence, and the type of statistical test you will use.
In general, larger sample sizes provide more reliable and accurate results but may require more resources to conduct the experiment. Consider adjusting your experimental design or testing methods to accommodate a larger sample size if necessary.
Lack of clear objectives
Your marketing experiment may not provide meaningful or actionable insights without clear objectives. Unclear objectives can lead to poorly designed experiments, irrelevant variables, or difficulty interpreting the results.
To prevent this issue, establish specific, measurable, achievable, relevant, and time-bound (SMART) objectives before starting your experiment. These objectives should guide your entire experiment, from hypothesis formulation to data analysis, and ensure that your findings are relevant and useful for your marketing efforts.
Confirmation bias
Confirmation bias occurs when you interpret the results of your experiment in a way that supports your pre-existing beliefs or expectations. This can lead to inaccurate conclusions and suboptimal marketing decisions.
To minimize confirmation bias, approach your experiments with an open mind and be willing to accept results that challenge your assumptions.
Additionally, involve multiple team members in the data analysis process to ensure diverse perspectives and reduce the risk of individual biases influencing the interpretation of the results.
Overlooking external factors
External factors, such as changes in market conditions, seasonal fluctuations, or competitor actions, can influence the results of your marketing experiment and potentially confound your findings. Ignoring these factors may lead to inaccurate conclusions about the effectiveness of your marketing strategies.
To account for external factors, carefully control for potential confounding variables during the experimental design process. This might involve using random assignment, testing during stable periods, or controlling for known external influences.
Consider running follow-up experiments or analyzing historical data to confirm your findings and rule out the impact of external factors.
Tips for avoiding these pitfalls
By being aware of these common pitfalls and following best practices, you can ensure the success of your marketing experiments and obtain valuable insights for your marketing efforts. Here are some tips to help you avoid these pitfalls:
- Plan your experiment carefully: Invest time in the planning stage to establish clear objectives, calculate an adequate sample size, and design a robust experiment that controls for potential confounding factors.
- Use a hypothesis-driven approach: Formulate a specific, testable hypothesis based on existing knowledge or data to guide your experiment and focus on the most relevant variables and outcomes.
- Monitor your experiment closely: Regularly check the progress of your experiment, address any issues that arise, and ensure that your experiment is running smoothly and according to plan.
- Analyze your data objectively: Use statistical techniques to determine the significance of your results and consider the practical implications of your findings before making marketing decisions.
- Learn from your experiments: Apply the lessons learned from your experiments to continuously improve your marketing strategies and stay ahead of the competition.
By avoiding these common pitfalls and following best practices, you can increase the effectiveness of your marketing experiments, gain valuable insights into customer preferences and behaviors, and ultimately drive better results for your business.
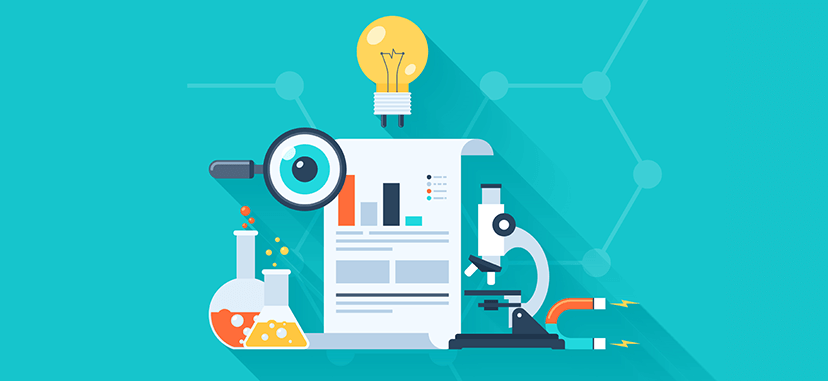
Building a Culture of Experimentation
To truly reap the benefits of marketing experiments, it’s essential to build a culture of experimentation within your organization. This means fostering an environment where curiosity, learning, data-driven decision-making, and collaboration are valued and encouraged.

Encouraging curiosity and learning within your organization
Cultivating curiosity and learning starts with leadership. Encourage your team to ask questions, explore new ideas, and embrace a growth mindset.
Promote ongoing learning by providing resources, such as training programs, workshops, or access to industry events, that help your team stay up-to-date with the latest marketing trends and techniques.
Create a safe environment where employees feel comfortable sharing their ideas and taking calculated risks. Emphasize the importance of learning from both successes and failures and treat every experiment as an opportunity to grow and improve.
Adopting a data-driven mindset
A data-driven mindset is crucial for successful marketing experimentation. Encourage your team to make decisions based on data rather than relying on intuition or guesswork. This means analyzing the results of your experiments objectively, using statistical techniques to determine the significance of your findings, and considering the practical implications of your results before making marketing decisions.
To foster a data-driven culture, invest in the necessary tools and technologies to collect, analyze, and visualize data effectively. Train your team on how to use these tools and interpret the data to make informed marketing decisions.
Regularly review your data-driven efforts and adjust your strategies as needed to continuously improve and optimize your marketing efforts.
Integrating experimentation into your marketing strategy
Establish a systematic approach to conducting marketing experiments to fully integrate experimentation into your marketing strategy. This might involve setting up a dedicated team or working group responsible for planning, executing, and analyzing experiments or incorporating experimentation as a standard part of your marketing processes.
Create a roadmap for your marketing experiments that outlines each project’s objectives, hypotheses, and experimental designs. Monitor the progress of your experiments and adjust your roadmap as needed based on the results and lessons learned.
Ensure that your marketing team has the necessary resources, such as time, budget, and tools, to conduct experiments effectively. Set clear expectations for the role of experimentation in your marketing efforts and emphasize its importance in driving better results and continuous improvement.
Collaborating across teams for a holistic approach
Marketing experiments often involve multiple teams within an organization, such as design, product, sales, and customer support. Encourage cross-functional collaboration to ensure a holistic approach to experimentation and leverage each team’s unique insights and expertise.
Establish clear communication channels and processes for sharing information and results from your experiments. This might involve regular meetings, shared documentation, or internal presentations to keep all stakeholders informed and engaged.
Collaboration also extends beyond your organization. Connect with other marketing professionals, industry experts, and thought leaders to learn from their experiences, share your own insights, and stay informed about the latest trends and best practices in marketing experimentation.
By building a culture of experimentation within your organization, you can unlock valuable insights, optimize your marketing strategies, and drive better results for your business.
Encourage curiosity and learning, adopt a data-driven mindset, integrate experimentation into your marketing strategy, and collaborate across teams to create a strong foundation for marketing success.
If you’re new to marketing experiments, don’t be intimidated—start small and gradually expand your efforts as your confidence grows. By embracing a curious and data-driven mindset, even small-scale experiments can lead to meaningful insights and improvements.
As you gain experience, you can tackle more complex experiments and further refine your marketing strategies.
Remember, continuous learning and improvement is the key to success in marketing experimentation. By regularly conducting experiments, analyzing the results, and applying the lessons learned, you can stay ahead of the competition and drive better results for your business.
So, take the plunge and start experimenting today—your marketing efforts will be all the better.
#ezw_tco-2 .ez-toc-title{ font-size: 120%; ; ; } #ezw_tco-2 .ez-toc-widget-container ul.ez-toc-list li.active{ background-color: #ededed; } Table of Contents
Manage your remote team with teamly. get your 100% free account today..
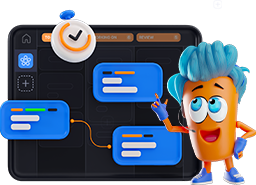
PC and Mac compatible
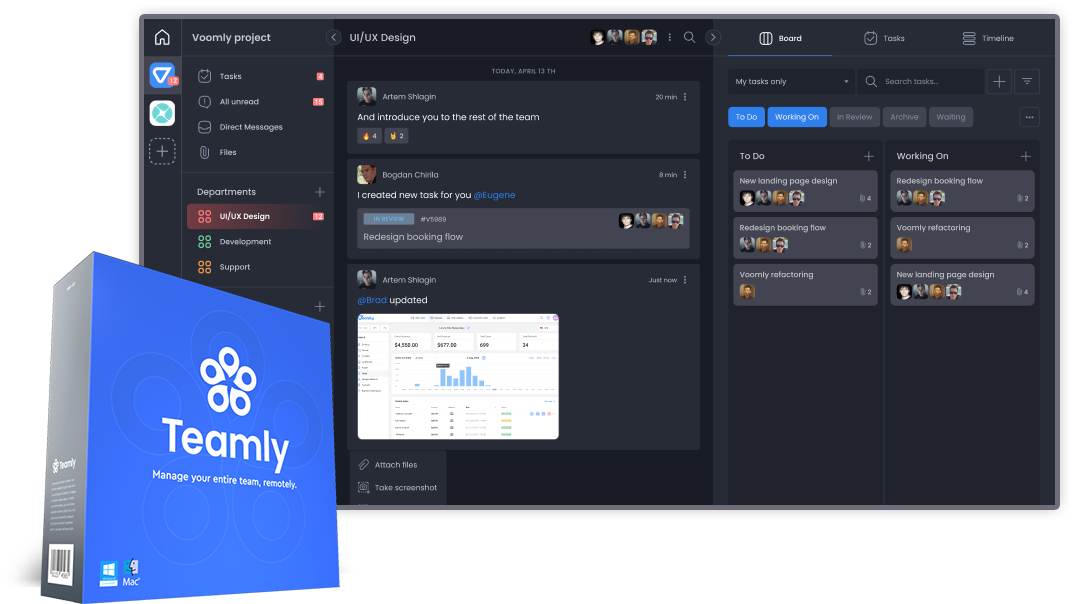
Teamly is everywhere you need it to be. Desktop download or web browser or IOS/Android app. Take your pick.
Get Teamly for FREE by clicking below.
No credit card required. completely free.

Teamly puts everything in one place, so you can start and finish projects quickly and efficiently.
Keep reading.
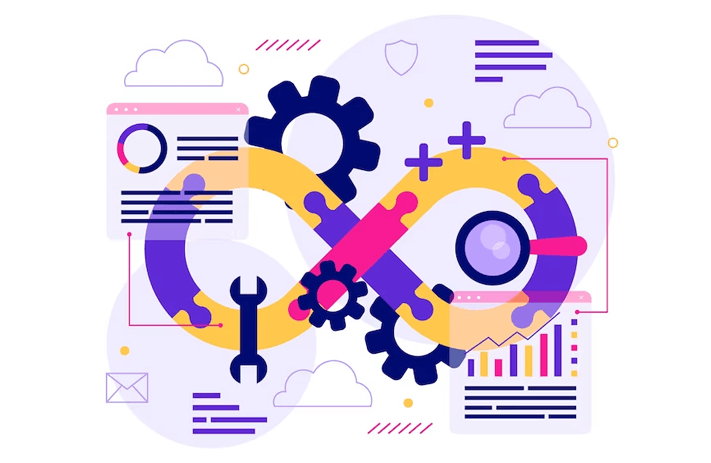
Agile Methodology
What Is Pi Planning in Agile? A Guide to Synchronized Success
What Is Pi Planning in Agile? A Guide to Synchronized SuccessBorn out of a need to break free from traditional, rigid project management methodologies, Agile offers a flexible, collaborative approach to the work environment. It’s not just a methodology, however; it’s a mindset that emphasizes rapid iterations, continuous feedback, and a relentless focus on delivering …
Continue reading “What Is Pi Planning in Agile? A Guide to Synchronized Success”
Max 12 min read
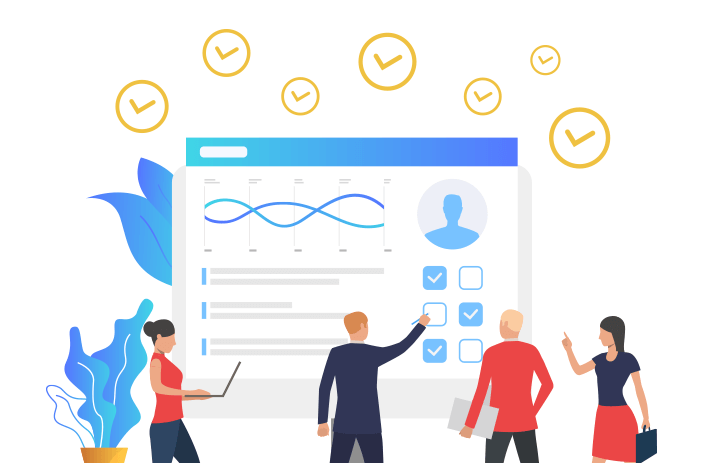
Project Management
Learning to Roll with It: Wave Planning in Project Management
Learning to Roll with It: Wave Planning in Project ManagementDo you ever decide to take a trip, and suddenly find yourself thinking through every detail, right down to where you’re going to eat meals and what toiletries to pack in your suitcase? It’s easy to get ahead of ourselves sometimes. As the Birds sang in …
Continue reading “Learning to Roll with It: Wave Planning in Project Management”
Max 9 min read
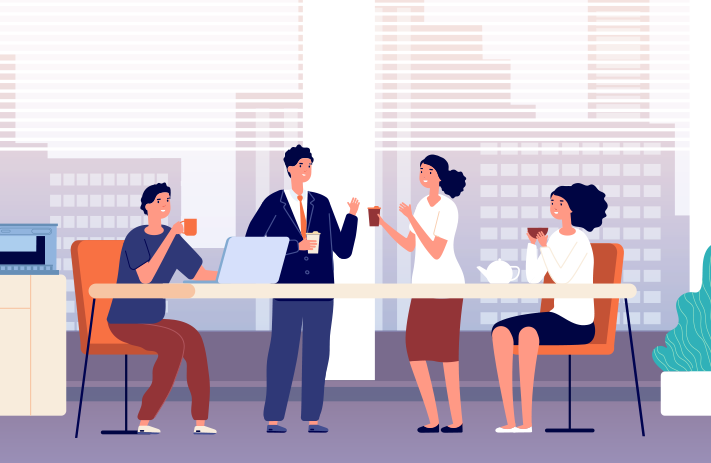
Best Practices
Tired of Slackers at Work? The Secrets Behind Social Loafing: and What to Do About it
Tired of Slackers at Work? The Secrets Behind Social Loafing: and What to Do About itHave you ever shown up to a potluck with a liter of soda and a bag of chips, then helped yourself to a plateful of baby back ribs? Or participated in a book group discussion without having read the book? …
Continue reading “Tired of Slackers at Work? The Secrets Behind Social Loafing: and What to Do About it”
Project Management Software Comparisons

Asana vs Wrike

Basecamp vs Slack

Smartsheet vs Airtable

Trello vs ClickUp

Monday.com vs Jira Work Management
Trello vs asana.
Get Teamly for FREE Enter your email and create your account today!
You must enter a valid email address
You must enter a valid email address!
- Privacy Policy

Home » What is a Hypothesis – Types, Examples and Writing Guide
What is a Hypothesis – Types, Examples and Writing Guide
Table of Contents

Definition:
Hypothesis is an educated guess or proposed explanation for a phenomenon, based on some initial observations or data. It is a tentative statement that can be tested and potentially proven or disproven through further investigation and experimentation.
Hypothesis is often used in scientific research to guide the design of experiments and the collection and analysis of data. It is an essential element of the scientific method, as it allows researchers to make predictions about the outcome of their experiments and to test those predictions to determine their accuracy.
Types of Hypothesis
Types of Hypothesis are as follows:
Research Hypothesis
A research hypothesis is a statement that predicts a relationship between variables. It is usually formulated as a specific statement that can be tested through research, and it is often used in scientific research to guide the design of experiments.
Null Hypothesis
The null hypothesis is a statement that assumes there is no significant difference or relationship between variables. It is often used as a starting point for testing the research hypothesis, and if the results of the study reject the null hypothesis, it suggests that there is a significant difference or relationship between variables.
Alternative Hypothesis
An alternative hypothesis is a statement that assumes there is a significant difference or relationship between variables. It is often used as an alternative to the null hypothesis and is tested against the null hypothesis to determine which statement is more accurate.
Directional Hypothesis
A directional hypothesis is a statement that predicts the direction of the relationship between variables. For example, a researcher might predict that increasing the amount of exercise will result in a decrease in body weight.
Non-directional Hypothesis
A non-directional hypothesis is a statement that predicts the relationship between variables but does not specify the direction. For example, a researcher might predict that there is a relationship between the amount of exercise and body weight, but they do not specify whether increasing or decreasing exercise will affect body weight.
Statistical Hypothesis
A statistical hypothesis is a statement that assumes a particular statistical model or distribution for the data. It is often used in statistical analysis to test the significance of a particular result.
Composite Hypothesis
A composite hypothesis is a statement that assumes more than one condition or outcome. It can be divided into several sub-hypotheses, each of which represents a different possible outcome.
Empirical Hypothesis
An empirical hypothesis is a statement that is based on observed phenomena or data. It is often used in scientific research to develop theories or models that explain the observed phenomena.
Simple Hypothesis
A simple hypothesis is a statement that assumes only one outcome or condition. It is often used in scientific research to test a single variable or factor.
Complex Hypothesis
A complex hypothesis is a statement that assumes multiple outcomes or conditions. It is often used in scientific research to test the effects of multiple variables or factors on a particular outcome.
Applications of Hypothesis
Hypotheses are used in various fields to guide research and make predictions about the outcomes of experiments or observations. Here are some examples of how hypotheses are applied in different fields:
- Science : In scientific research, hypotheses are used to test the validity of theories and models that explain natural phenomena. For example, a hypothesis might be formulated to test the effects of a particular variable on a natural system, such as the effects of climate change on an ecosystem.
- Medicine : In medical research, hypotheses are used to test the effectiveness of treatments and therapies for specific conditions. For example, a hypothesis might be formulated to test the effects of a new drug on a particular disease.
- Psychology : In psychology, hypotheses are used to test theories and models of human behavior and cognition. For example, a hypothesis might be formulated to test the effects of a particular stimulus on the brain or behavior.
- Sociology : In sociology, hypotheses are used to test theories and models of social phenomena, such as the effects of social structures or institutions on human behavior. For example, a hypothesis might be formulated to test the effects of income inequality on crime rates.
- Business : In business research, hypotheses are used to test the validity of theories and models that explain business phenomena, such as consumer behavior or market trends. For example, a hypothesis might be formulated to test the effects of a new marketing campaign on consumer buying behavior.
- Engineering : In engineering, hypotheses are used to test the effectiveness of new technologies or designs. For example, a hypothesis might be formulated to test the efficiency of a new solar panel design.
How to write a Hypothesis
Here are the steps to follow when writing a hypothesis:
Identify the Research Question
The first step is to identify the research question that you want to answer through your study. This question should be clear, specific, and focused. It should be something that can be investigated empirically and that has some relevance or significance in the field.
Conduct a Literature Review
Before writing your hypothesis, it’s essential to conduct a thorough literature review to understand what is already known about the topic. This will help you to identify the research gap and formulate a hypothesis that builds on existing knowledge.
Determine the Variables
The next step is to identify the variables involved in the research question. A variable is any characteristic or factor that can vary or change. There are two types of variables: independent and dependent. The independent variable is the one that is manipulated or changed by the researcher, while the dependent variable is the one that is measured or observed as a result of the independent variable.
Formulate the Hypothesis
Based on the research question and the variables involved, you can now formulate your hypothesis. A hypothesis should be a clear and concise statement that predicts the relationship between the variables. It should be testable through empirical research and based on existing theory or evidence.
Write the Null Hypothesis
The null hypothesis is the opposite of the alternative hypothesis, which is the hypothesis that you are testing. The null hypothesis states that there is no significant difference or relationship between the variables. It is important to write the null hypothesis because it allows you to compare your results with what would be expected by chance.
Refine the Hypothesis
After formulating the hypothesis, it’s important to refine it and make it more precise. This may involve clarifying the variables, specifying the direction of the relationship, or making the hypothesis more testable.
Examples of Hypothesis
Here are a few examples of hypotheses in different fields:
- Psychology : “Increased exposure to violent video games leads to increased aggressive behavior in adolescents.”
- Biology : “Higher levels of carbon dioxide in the atmosphere will lead to increased plant growth.”
- Sociology : “Individuals who grow up in households with higher socioeconomic status will have higher levels of education and income as adults.”
- Education : “Implementing a new teaching method will result in higher student achievement scores.”
- Marketing : “Customers who receive a personalized email will be more likely to make a purchase than those who receive a generic email.”
- Physics : “An increase in temperature will cause an increase in the volume of a gas, assuming all other variables remain constant.”
- Medicine : “Consuming a diet high in saturated fats will increase the risk of developing heart disease.”
Purpose of Hypothesis
The purpose of a hypothesis is to provide a testable explanation for an observed phenomenon or a prediction of a future outcome based on existing knowledge or theories. A hypothesis is an essential part of the scientific method and helps to guide the research process by providing a clear focus for investigation. It enables scientists to design experiments or studies to gather evidence and data that can support or refute the proposed explanation or prediction.
The formulation of a hypothesis is based on existing knowledge, observations, and theories, and it should be specific, testable, and falsifiable. A specific hypothesis helps to define the research question, which is important in the research process as it guides the selection of an appropriate research design and methodology. Testability of the hypothesis means that it can be proven or disproven through empirical data collection and analysis. Falsifiability means that the hypothesis should be formulated in such a way that it can be proven wrong if it is incorrect.
In addition to guiding the research process, the testing of hypotheses can lead to new discoveries and advancements in scientific knowledge. When a hypothesis is supported by the data, it can be used to develop new theories or models to explain the observed phenomenon. When a hypothesis is not supported by the data, it can help to refine existing theories or prompt the development of new hypotheses to explain the phenomenon.
When to use Hypothesis
Here are some common situations in which hypotheses are used:
- In scientific research , hypotheses are used to guide the design of experiments and to help researchers make predictions about the outcomes of those experiments.
- In social science research , hypotheses are used to test theories about human behavior, social relationships, and other phenomena.
- I n business , hypotheses can be used to guide decisions about marketing, product development, and other areas. For example, a hypothesis might be that a new product will sell well in a particular market, and this hypothesis can be tested through market research.
Characteristics of Hypothesis
Here are some common characteristics of a hypothesis:
- Testable : A hypothesis must be able to be tested through observation or experimentation. This means that it must be possible to collect data that will either support or refute the hypothesis.
- Falsifiable : A hypothesis must be able to be proven false if it is not supported by the data. If a hypothesis cannot be falsified, then it is not a scientific hypothesis.
- Clear and concise : A hypothesis should be stated in a clear and concise manner so that it can be easily understood and tested.
- Based on existing knowledge : A hypothesis should be based on existing knowledge and research in the field. It should not be based on personal beliefs or opinions.
- Specific : A hypothesis should be specific in terms of the variables being tested and the predicted outcome. This will help to ensure that the research is focused and well-designed.
- Tentative: A hypothesis is a tentative statement or assumption that requires further testing and evidence to be confirmed or refuted. It is not a final conclusion or assertion.
- Relevant : A hypothesis should be relevant to the research question or problem being studied. It should address a gap in knowledge or provide a new perspective on the issue.
Advantages of Hypothesis
Hypotheses have several advantages in scientific research and experimentation:
- Guides research: A hypothesis provides a clear and specific direction for research. It helps to focus the research question, select appropriate methods and variables, and interpret the results.
- Predictive powe r: A hypothesis makes predictions about the outcome of research, which can be tested through experimentation. This allows researchers to evaluate the validity of the hypothesis and make new discoveries.
- Facilitates communication: A hypothesis provides a common language and framework for scientists to communicate with one another about their research. This helps to facilitate the exchange of ideas and promotes collaboration.
- Efficient use of resources: A hypothesis helps researchers to use their time, resources, and funding efficiently by directing them towards specific research questions and methods that are most likely to yield results.
- Provides a basis for further research: A hypothesis that is supported by data provides a basis for further research and exploration. It can lead to new hypotheses, theories, and discoveries.
- Increases objectivity: A hypothesis can help to increase objectivity in research by providing a clear and specific framework for testing and interpreting results. This can reduce bias and increase the reliability of research findings.
Limitations of Hypothesis
Some Limitations of the Hypothesis are as follows:
- Limited to observable phenomena: Hypotheses are limited to observable phenomena and cannot account for unobservable or intangible factors. This means that some research questions may not be amenable to hypothesis testing.
- May be inaccurate or incomplete: Hypotheses are based on existing knowledge and research, which may be incomplete or inaccurate. This can lead to flawed hypotheses and erroneous conclusions.
- May be biased: Hypotheses may be biased by the researcher’s own beliefs, values, or assumptions. This can lead to selective interpretation of data and a lack of objectivity in research.
- Cannot prove causation: A hypothesis can only show a correlation between variables, but it cannot prove causation. This requires further experimentation and analysis.
- Limited to specific contexts: Hypotheses are limited to specific contexts and may not be generalizable to other situations or populations. This means that results may not be applicable in other contexts or may require further testing.
- May be affected by chance : Hypotheses may be affected by chance or random variation, which can obscure or distort the true relationship between variables.
About the author
Muhammad Hassan
Researcher, Academic Writer, Web developer
You may also like

Dissertation Methodology – Structure, Example...

APA Table of Contents – Format and Example

Research Design – Types, Methods and Examples

Research Questions – Types, Examples and Writing...

Research Objectives – Types, Examples and...

Future Research – Thesis Guide
How to Generate and Validate Product Hypotheses

Every product owner knows that it takes effort to build something that'll cater to user needs. You'll have to make many tough calls if you wish to grow the company and evolve the product so it delivers more value. But how do you decide what to change in the product, your marketing strategy, or the overall direction to succeed? And how do you make a product that truly resonates with your target audience?
There are many unknowns in business, so many fundamental decisions start from a simple "what if?". But they can't be based on guesses, as you need some proof to fill in the blanks reasonably.
Because there's no universal recipe for successfully building a product, teams collect data, do research, study the dynamics, and generate hypotheses according to the given facts. They then take corresponding actions to find out whether they were right or wrong, make conclusions, and most likely restart the process again.
On this page, we thoroughly inspect product hypotheses. We'll go over what they are, how to create hypothesis statements and validate them, and what goes after this step.
What Is a Hypothesis in Product Management?
A hypothesis in product development and product management is a statement or assumption about the product, planned feature, market, or customer (e.g., their needs, behavior, or expectations) that you can put to the test, evaluate, and base your further decisions on . This may, for instance, regard the upcoming product changes as well as the impact they can result in.
A hypothesis implies that there is limited knowledge. Hence, the teams need to undergo testing activities to validate their ideas and confirm whether they are true or false.
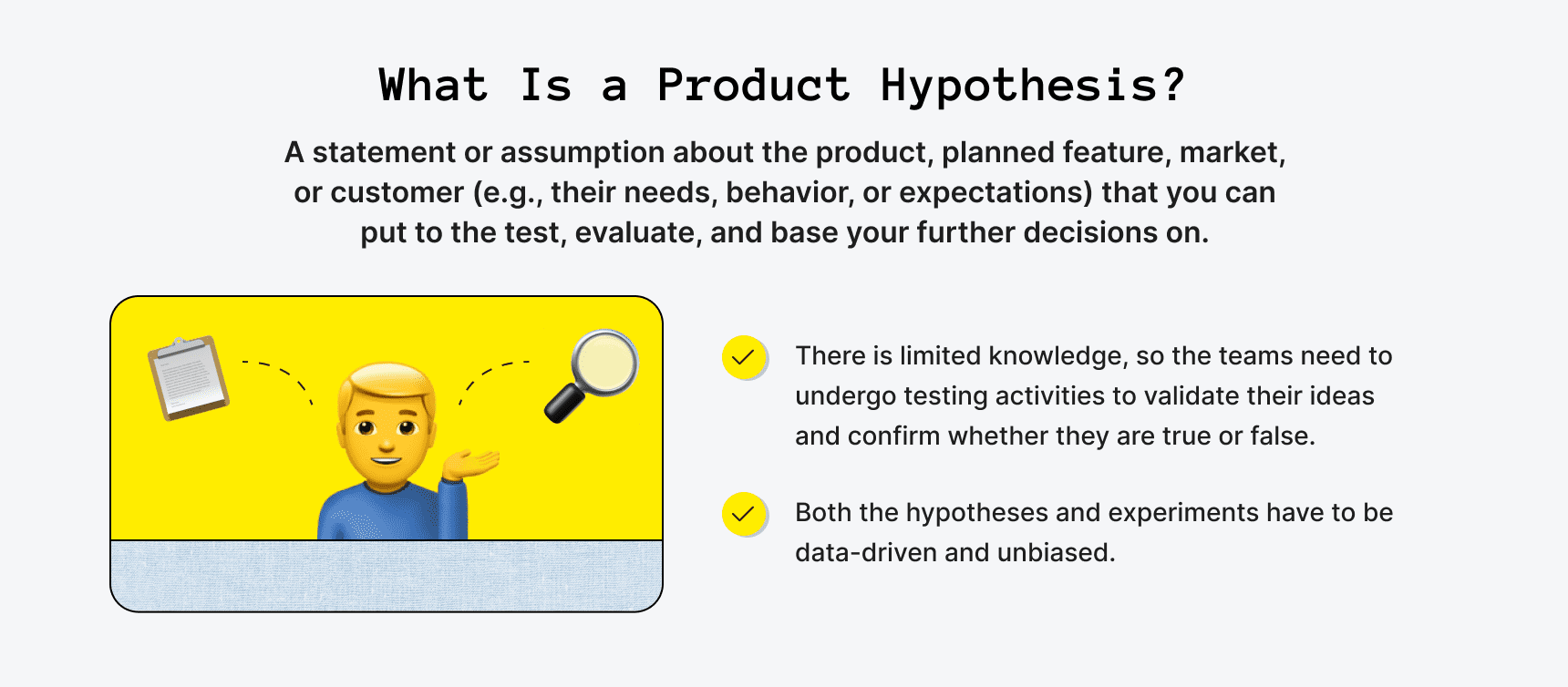
Hypotheses guide the product development process and may point at important findings to help build a better product that'll serve user needs. In essence, teams create hypothesis statements in an attempt to improve the offering, boost engagement, increase revenue, find product-market fit quicker, or for other business-related reasons.
It's sort of like an experiment with trial and error, yet, it is data-driven and should be unbiased . This means that teams don't make assumptions out of the blue. Instead, they turn to the collected data, conducted market research , and factual information, which helps avoid completely missing the mark. The obtained results are then carefully analyzed and may influence decision-making.
Such experiments backed by data and analysis are an integral aspect of successful product development and allow startups or businesses to dodge costly startup mistakes .
When do teams create hypothesis statements and validate them? To some extent, hypothesis testing is an ongoing process to work on constantly. It may occur during various product development life cycle stages, from early phases like initiation to late ones like scaling.
In any event, the key here is learning how to generate hypothesis statements and validate them effectively. We'll go over this in more detail later on.
Idea vs. Hypothesis Compared
You might be wondering whether ideas and hypotheses are the same thing. Well, there are a few distinctions.
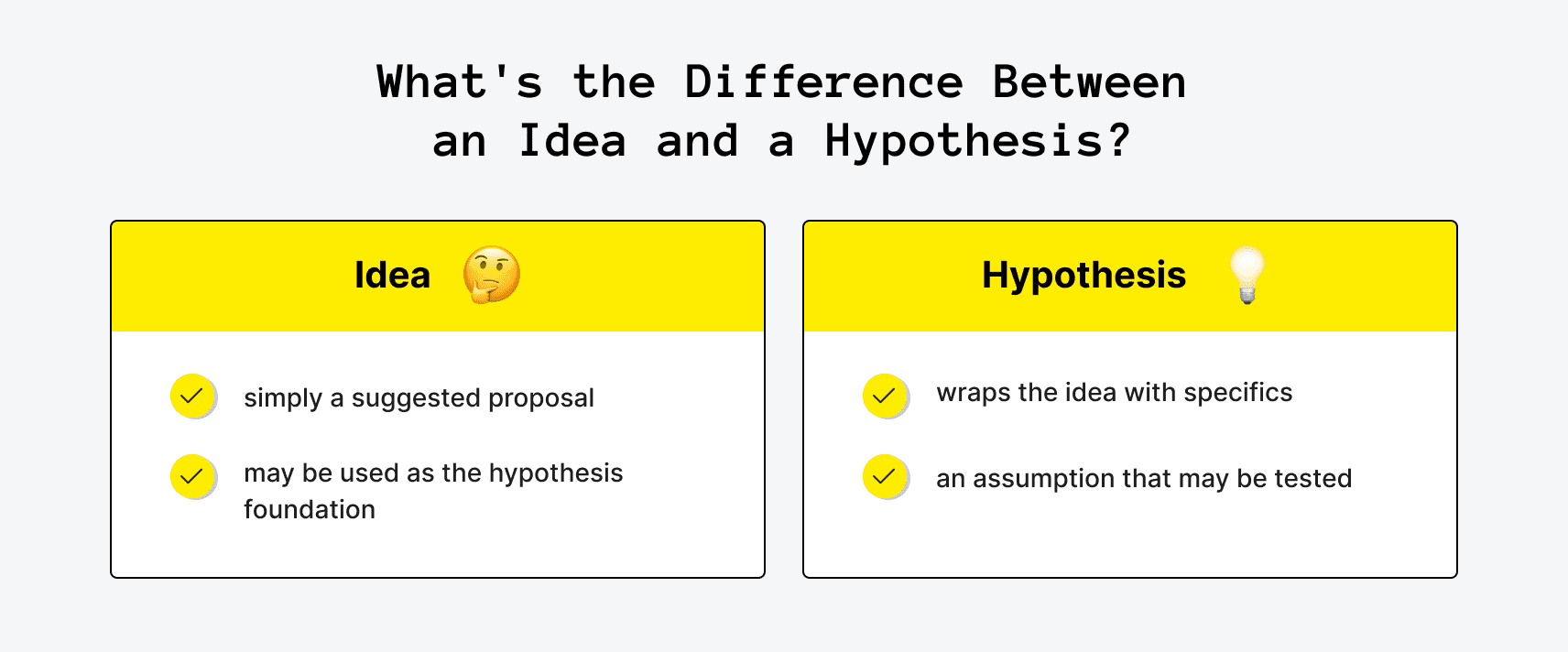
An idea is simply a suggested proposal. Say, a teammate comes up with something you can bring to life during a brainstorming session or pitches in a suggestion like "How about we shorten the checkout process?". You can jot down such ideas and then consider working on them if they'll truly make a difference and improve the product, strategy, or result in other business benefits. Ideas may thus be used as the hypothesis foundation when you decide to prove a concept.
A hypothesis is the next step, when an idea gets wrapped with specifics to become an assumption that may be tested. As such, you can refine the idea by adding details to it. The previously mentioned idea can be worded into a product hypothesis statement like: "The cart abandonment rate is high, and many users flee at checkout. But if we shorten the checkout process by cutting down the number of steps to only two and get rid of four excessive fields, we'll simplify the user journey, boost satisfaction, and may get up to 15% more completed orders".
A hypothesis is something you can test in an attempt to reach a certain goal. Testing isn't obligatory in this scenario, of course, but the idea may be tested if you weigh the pros and cons and decide that the required effort is worth a try. We'll explain how to create hypothesis statements next.
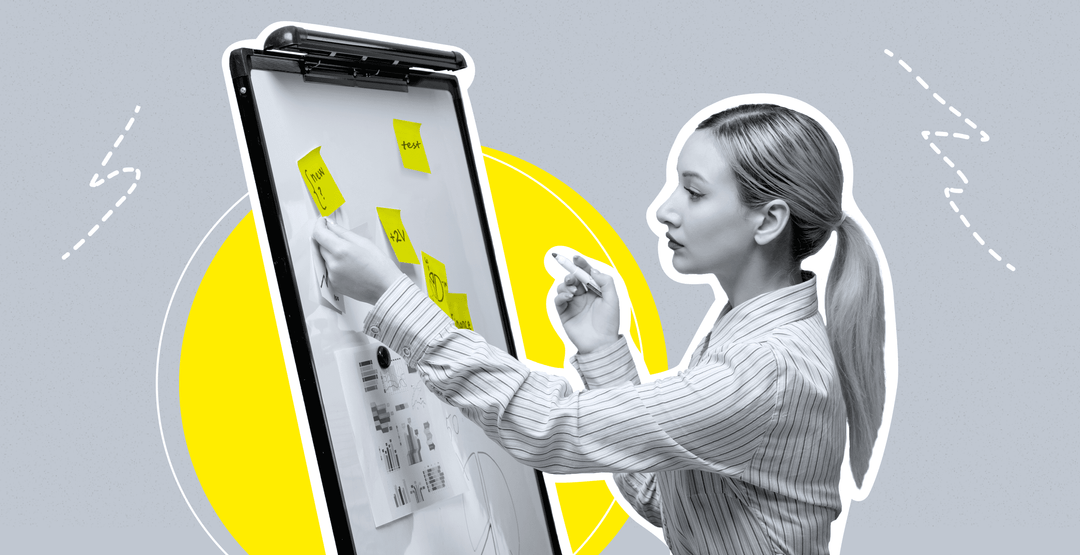
How to Generate a Hypothesis for a Product
The last thing those developing a product want is to invest time and effort into something that won't bring any visible results, fall short of customer expectations, or won't live up to their needs. Therefore, to increase the chances of achieving a successful outcome and product-led growth , teams may need to revisit their product development approach by optimizing one of the starting points of the process: learning to make reasonable product hypotheses.
If the entire procedure is structured, this may assist you during such stages as the discovery phase and raise the odds of reaching your product goals and setting your business up for success. Yet, what's the entire process like?
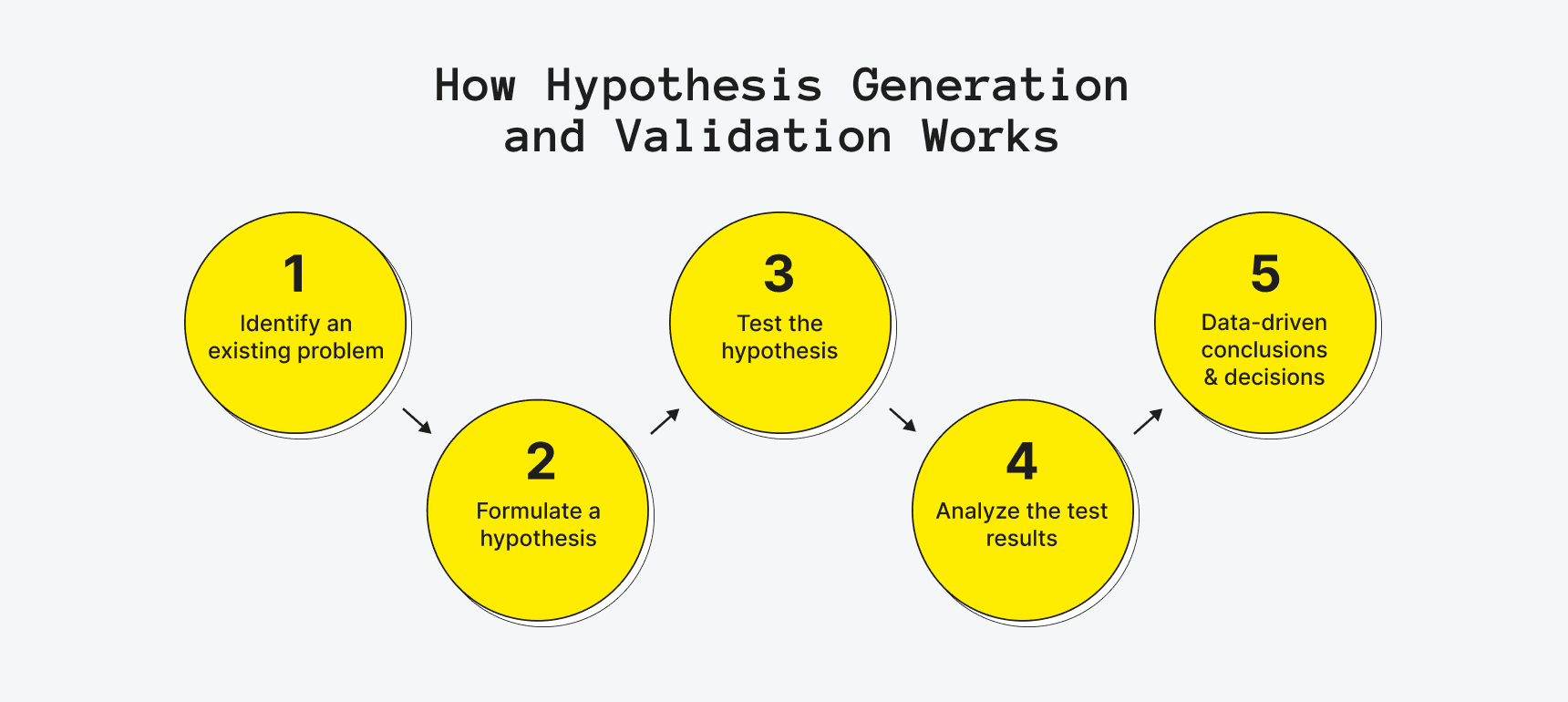
- It all starts with identifying an existing problem . Is there a product area that's experiencing a downfall, a visible trend, or a market gap? Are users often complaining about something in their feedback? Or is there something you're willing to change (say, if you aim to get more profit, increase engagement, optimize a process, expand to a new market, or reach your OKRs and KPIs faster)?
- Teams then need to work on formulating a hypothesis . They put the statement into concise and short wording that describes what is expected to achieve. Importantly, it has to be relevant, actionable, backed by data, and without generalizations.
- Next, they have to test the hypothesis by running experiments to validate it (for instance, via A/B or multivariate testing, prototyping, feedback collection, or other ways).
- Then, the obtained results of the test must be analyzed . Did one element or page version outperform the other? Depending on what you're testing, you can look into various merits or product performance metrics (such as the click rate, bounce rate, or the number of sign-ups) to assess whether your prediction was correct.
- Finally, the teams can make conclusions that could lead to data-driven decisions. For example, they can make corresponding changes or roll back a step.
How Else Can You Generate Product Hypotheses?
Such processes imply sharing ideas when a problem is spotted by digging deep into facts and studying the possible risks, goals, benefits, and outcomes. You may apply various MVP tools like (FigJam, Notion, or Miro) that were designed to simplify brainstorming sessions, systemize pitched suggestions, and keep everyone organized without losing any ideas.
Predictive product analysis can also be integrated into this process, leveraging data and insights to anticipate market trends and consumer preferences, thus enhancing decision-making and product development strategies. This approach fosters a more proactive and informed approach to innovation, ensuring products are not only relevant but also resonate with the target audience, ultimately increasing their chances of success in the market.
Besides, you can settle on one of the many frameworks that facilitate decision-making processes , ideation phases, or feature prioritization . Such frameworks are best applicable if you need to test your assumptions and structure the validation process. These are a few common ones if you're looking toward a systematic approach:
- Business Model Canvas (used to establish the foundation of the business model and helps find answers to vitals like your value proposition, finding the right customer segment, or the ways to make revenue);
- Lean Startup framework (the lean startup framework uses a diagram-like format for capturing major processes and can be handy for testing various hypotheses like how much value a product brings or assumptions on personas, the problem, growth, etc.);
- Design Thinking Process (is all about interactive learning and involves getting an in-depth understanding of the customer needs and pain points, which can be formulated into hypotheses followed by simple prototypes and tests).
Need a hand with product development?
Upsilon's team of pros is ready to share our expertise in building tech products.

How to Make a Hypothesis Statement for a Product
Once you've indicated the addressable problem or opportunity and broken down the issue in focus, you need to work on formulating the hypotheses and associated tasks. By the way, it works the same way if you want to prove that something will be false (a.k.a null hypothesis).
If you're unsure how to write a hypothesis statement, let's explore the essential steps that'll set you on the right track.
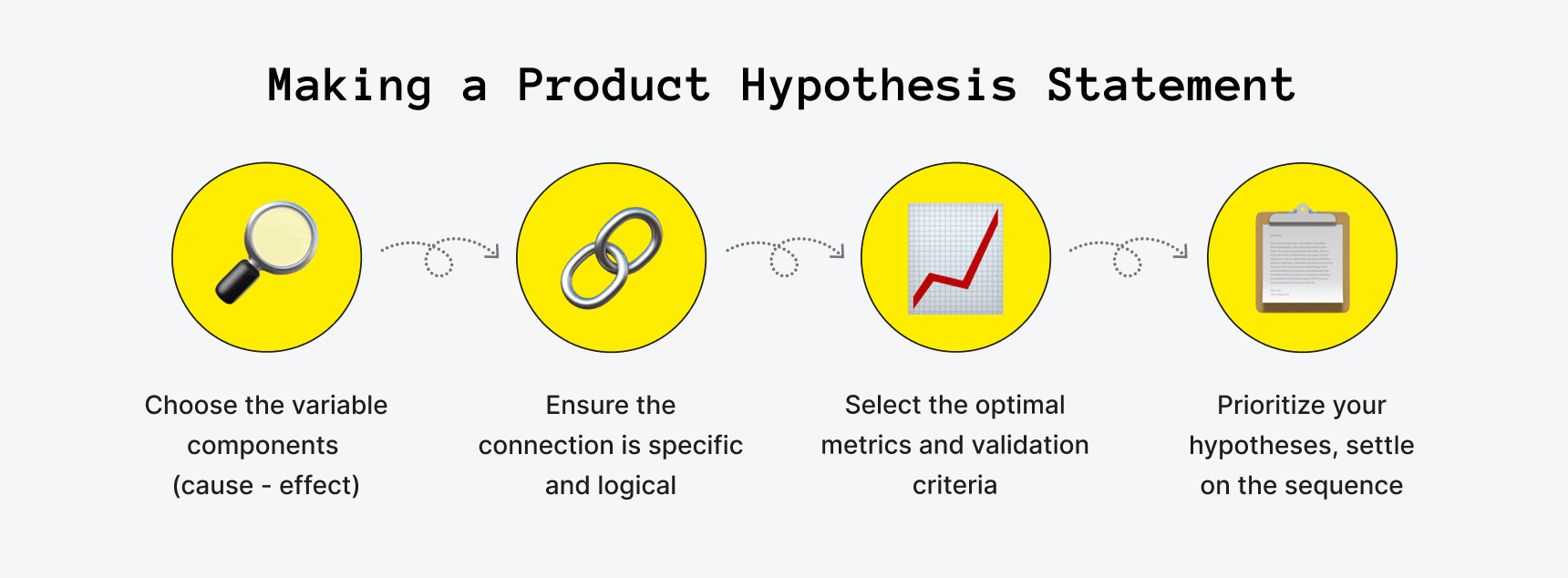
Step 1: Allocate the Variable Components
Product hypotheses are generally different for each case, so begin by pinpointing the major variables, i.e., the cause and effect . You'll need to outline what you think is supposed to happen if a change or action gets implemented.
Put simply, the "cause" is what you're planning to change, and the "effect" is what will indicate whether the change is bringing in the expected results. Falling back on the example we brought up earlier, the ineffective checkout process can be the cause, while the increased percentage of completed orders is the metric that'll show the effect.
Make sure to also note such vital points as:
- what the problem and solution are;
- what are the benefits or the expected impact/successful outcome;
- which user group is affected;
- what are the risks;
- what kind of experiments can help test the hypothesis;
- what can measure whether you were right or wrong.
Step 2: Ensure the Connection Is Specific and Logical
Mind that generic connections that lack specifics will get you nowhere. So if you're thinking about how to word a hypothesis statement, make sure that the cause and effect include clear reasons and a logical dependency .
Think about what can be the precise and link showing why A affects B. In our checkout example, it could be: fewer steps in the checkout and the removed excessive fields will speed up the process, help avoid confusion, irritate users less, and lead to more completed orders. That's much more explicit than just stating the fact that the checkout needs to be changed to get more completed orders.
Step 3: Decide on the Data You'll Collect
Certainly, multiple things can be used to measure the effect. Therefore, you need to choose the optimal metrics and validation criteria that'll best envision if you're moving in the right direction.
If you need a tip on how to create hypothesis statements that won't result in a waste of time, try to avoid vagueness and be as specific as you can when selecting what can best measure and assess the results of your hypothesis test. The criteria must be measurable and tied to the hypotheses . This can be a realistic percentage or number (say, you expect a 15% increase in completed orders or 2x fewer cart abandonment cases during the checkout phase).
Once again, if you're not realistic, then you might end up misinterpreting the results. Remember that sometimes an increase that's even as little as 2% can make a huge difference, so why make 50% the merit if it's not achievable in the first place?
Step 4: Settle on the Sequence
It's quite common that you'll end up with multiple product hypotheses. Some are more important than others, of course, and some will require more effort and input.
Therefore, just as with the features on your product development roadmap , prioritize your hypotheses according to their impact and importance. Then, group and order them, especially if the results of some hypotheses influence others on your list.
Product Hypothesis Examples
To demonstrate how to formulate your assumptions clearly, here are several more apart from the example of a hypothesis statement given above:
- Adding a wishlist feature to the cart with the possibility to send a gift hint to friends via email will increase the likelihood of making a sale and bring in additional sign-ups.
- Placing a limited-time promo code banner stripe on the home page will increase the number of sales in March.
- Moving up the call to action element on the landing page and changing the button text will increase the click-through rate twice.
- By highlighting a new way to use the product, we'll target a niche customer segment (i.e., single parents under 30) and acquire 5% more leads.

How to Validate Hypothesis Statements: The Process Explained
There are multiple options when it comes to validating hypothesis statements. To get appropriate results, you have to come up with the right experiment that'll help you test the hypothesis. You'll need a control group or people who represent your target audience segments or groups to participate (otherwise, your results might not be accurate).
What can serve as the experiment you may run? Experiments may take tons of different forms, and you'll need to choose the one that clicks best with your hypothesis goals (and your available resources, of course). The same goes for how long you'll have to carry out the test (say, a time period of two months or as little as two weeks). Here are several to get you started.

Feedback and User Testing
Talking to users, potential customers, or members of your own online startup community can be another way to test your hypotheses. You may use surveys, questionnaires, or opt for more extensive interviews to validate hypothesis statements and find out what people think. This assumption validation approach involves your existing or potential users and might require some additional time, but can bring you many insights.
Conduct A/B or Multivariate Tests
One of the experiments you may develop involves making more than one version of an element or page to see which option resonates with the users more. As such, you can have a call to action block with different wording or play around with the colors, imagery, visuals, and other things.
To run such split experiments, you can apply tools like VWO that allows to easily construct alternative designs and split what your users see (e.g., one half of the users will see version one, while the other half will see version two). You can track various metrics and apply heatmaps, click maps, and screen recordings to learn more about user response and behavior. Mind, though, that the key to such tests is to get as many users as you can give the tests time. Don't jump to conclusions too soon or if very few people participated in your experiment.
Build Prototypes and Fake Doors
Demos and clickable prototypes can be a great way to save time and money on costly feature or product development. A prototype also allows you to refine the design. However, they can also serve as experiments for validating hypotheses, collecting data, and getting feedback.
For instance, if you have a new feature in mind and want to ensure there is interest, you can utilize such MVP types as fake doors . Make a short demo recording of the feature and place it on your landing page to track interest or test how many people sign up.
Usability Testing
Similarly, you can run experiments to observe how users interact with the feature, page, product, etc. Usually, such experiments are held on prototype testing platforms with a focus group representing your target visitors. By showing a prototype or early version of the design to users, you can view how people use the solution, where they face problems, or what they don't understand. This may be very helpful if you have hypotheses regarding redesigns and user experience improvements before you move on from prototype to MVP development.
You can even take it a few steps further and build a barebone feature version that people can really interact with, yet you'll be the one behind the curtain to make it happen. There were many MVP examples when companies applied Wizard of Oz or concierge MVPs to validate their hypotheses.
Or you can actually develop some functionality but release it for only a limited number of people to see. This is referred to as a feature flag , which can show really specific results but is effort-intensive.
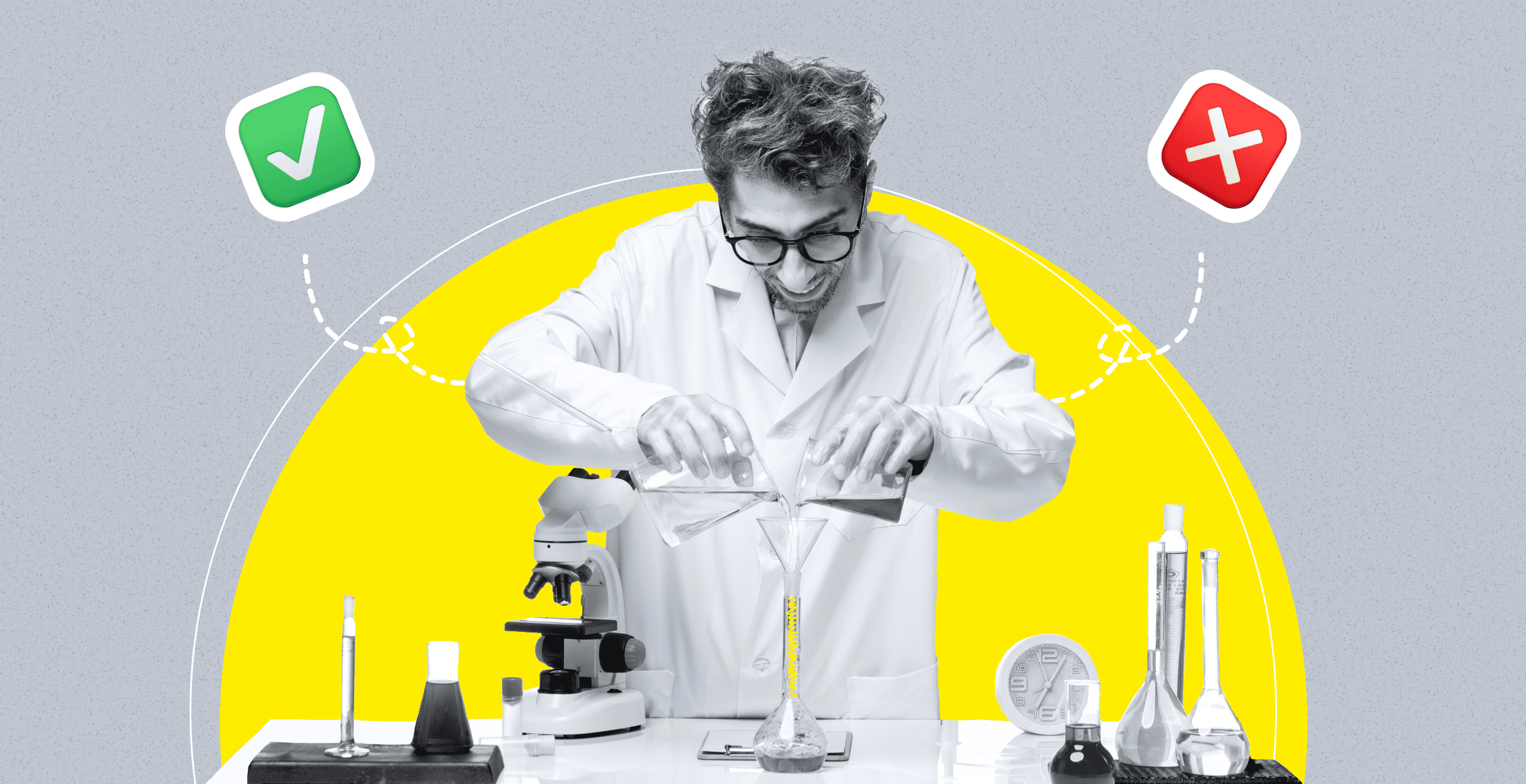
What Comes After Hypothesis Validation?
Analysis is what you move on to once you've run the experiment. This is the time to review the collected data, metrics, and feedback to validate (or invalidate) the hypothesis.
You have to evaluate the experiment's results to determine whether your product hypotheses were valid or not. For example, if you were testing two versions of an element design, color scheme, or copy, look into which one performed best.
It is crucial to be certain that you have enough data to draw conclusions, though, and that it's accurate and unbiased . Because if you don't, this may be a sign that your experiment needs to be run for some additional time, be altered, or held once again. You won't want to make a solid decision based on uncertain or misleading results, right?
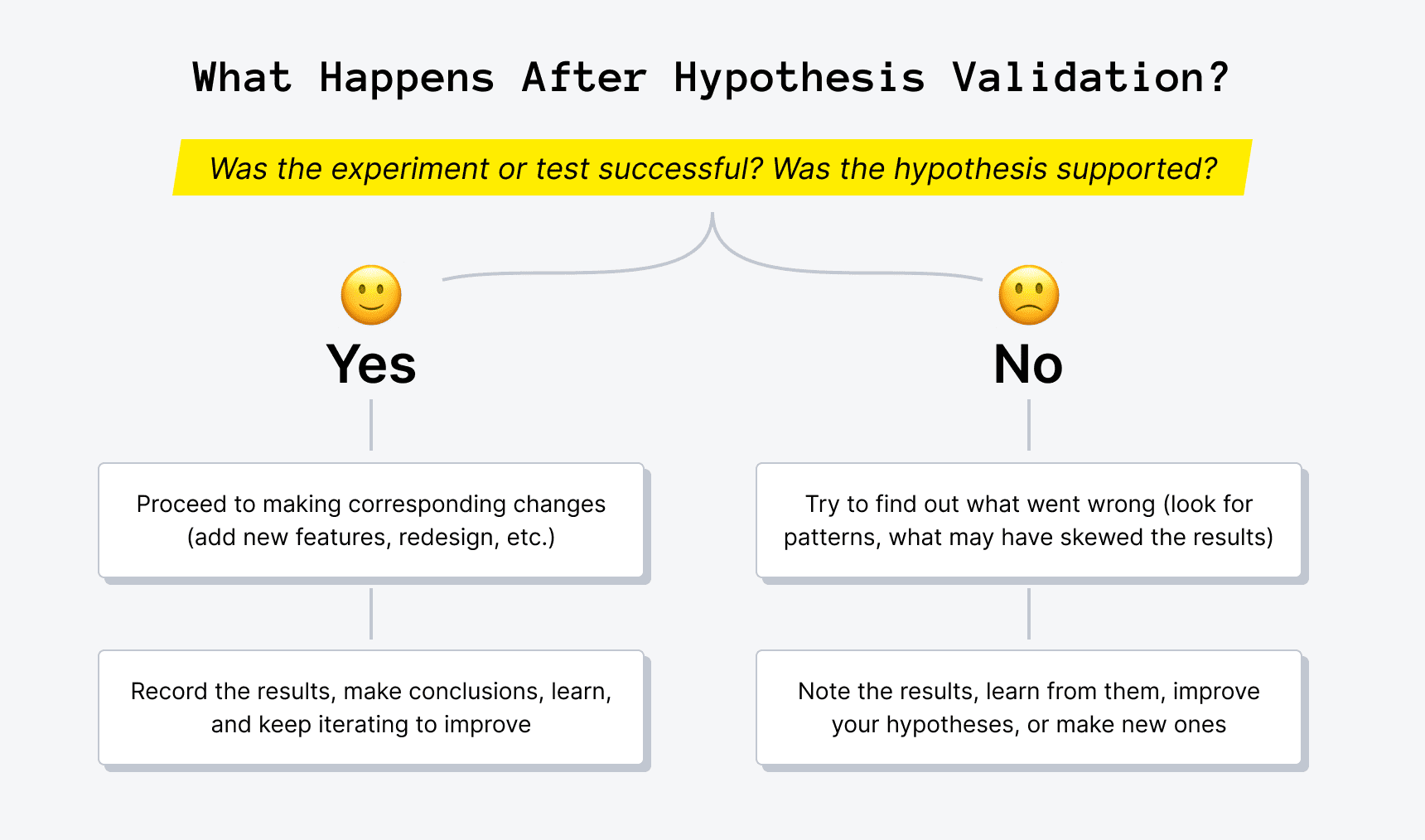
- If the hypothesis was supported , proceed to making corresponding changes (such as implementing a new feature, changing the design, rephrasing your copy, etc.). Remember that your aim was to learn and iterate to improve.
- If your hypothesis was proven false , think of it as a valuable learning experience. The main goal is to learn from the results and be able to adjust your processes accordingly. Dig deep to find out what went wrong, look for patterns and things that may have skewed the results. But if all signs show that you were wrong with your hypothesis, accept this outcome as a fact, and move on. This can help you make conclusions on how to better formulate your product hypotheses next time. Don't be too judgemental, though, as a failed experiment might only mean that you need to improve the current hypothesis, revise it, or create a new one based on the results of this experiment, and run the process once more.
On another note, make sure to record your hypotheses and experiment results . Some companies use CRMs to jot down the key findings, while others use something as simple as Google Docs. Either way, this can be your single source of truth that can help you avoid running the same experiments or allow you to compare results over time.
Have doubts about how to bring your product to life?
Upsilon's team of pros can help you build a product most optimally.
Final Thoughts on Product Hypotheses
The hypothesis-driven approach in product development is a great way to avoid uncalled-for risks and pricey mistakes. You can back up your assumptions with facts, observe your target audience's reactions, and be more certain that this move will deliver value.
However, this only makes sense if the validation of hypothesis statements is backed by relevant data that'll allow you to determine whether the hypothesis is valid or not. By doing so, you can be certain that you're developing and testing hypotheses to accelerate your product management and avoiding decisions based on guesswork.
Certainly, a failed experiment may bring you just as much knowledge and findings as one that succeeds. Teams have to learn from their mistakes, boost their hypothesis generation and testing knowledge , and make improvements according to the results of their experiments. This is an ongoing process, of course, as no product can grow if it isn't iterated and improved.
If you're only planning to or are currently building a product, Upsilon can lend you a helping hand. Our team has years of experience providing product development services for growth-stage startups and building MVPs for early-stage businesses , so you can use our expertise and knowledge to dodge many mistakes. Don't be shy to contact us to discuss your needs!

20+ Major SaaS Challenges and How to Solve Them
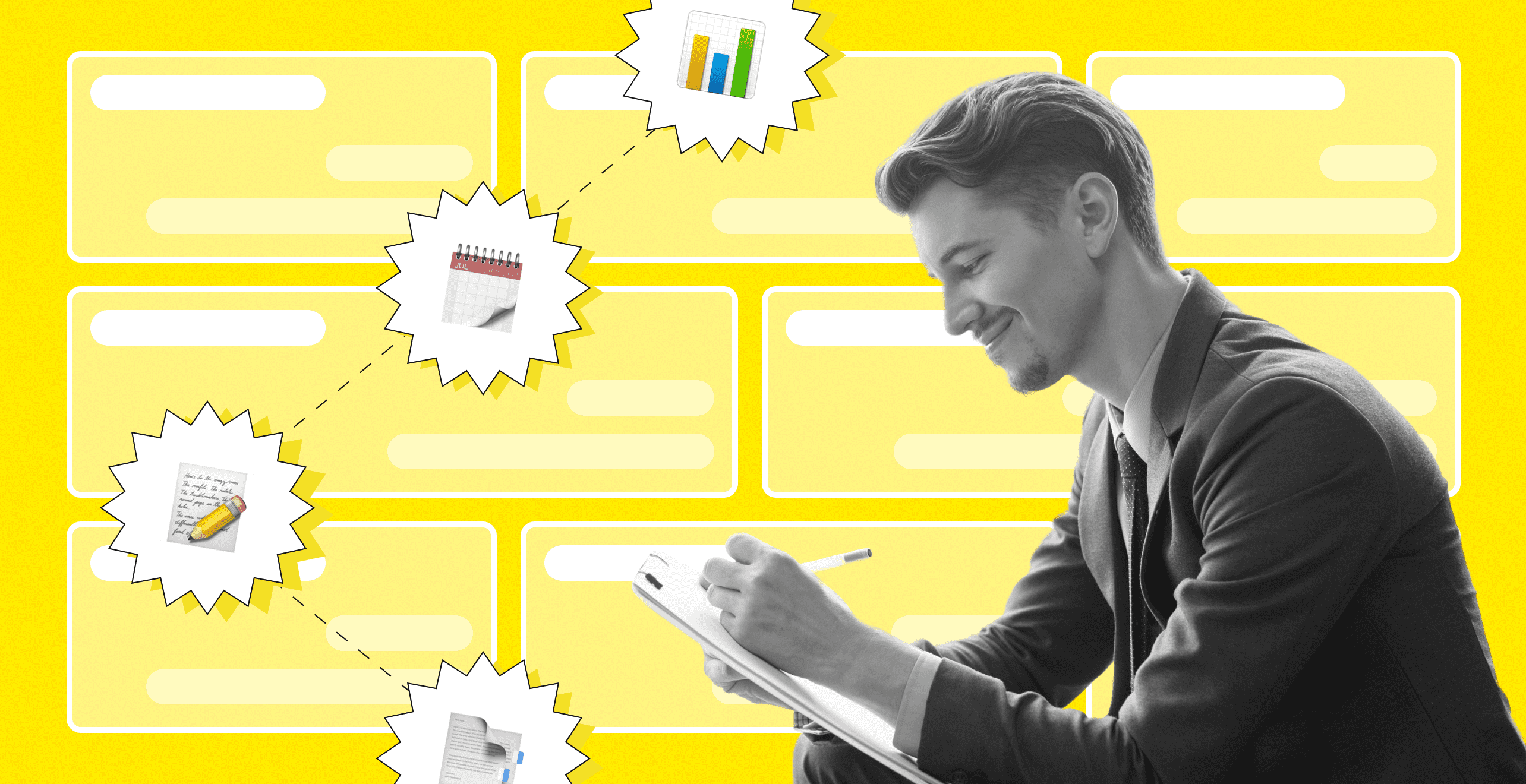
Product Charter: Purpose, Writing Tips, and Examples
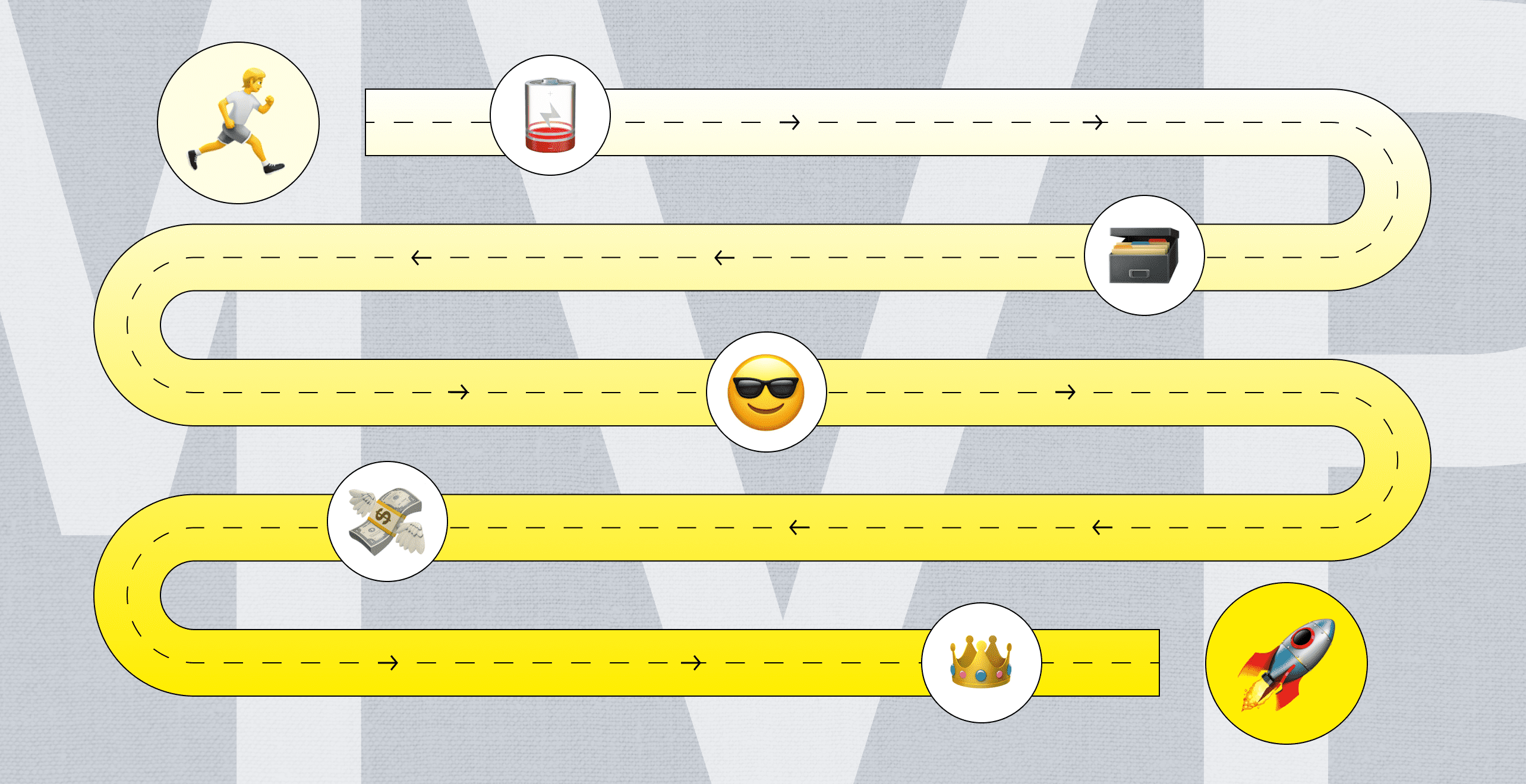
How to Make an MVP Roadmap
Never miss an update.

- Resources Home 🏠
- Try SciSpace Copilot
- Search research papers
- Add Copilot Extension
- Try AI Detector
- Try Paraphraser
- Try Citation Generator
- April Papers
- June Papers
- July Papers

The Craft of Writing a Strong Hypothesis

Table of Contents
Writing a hypothesis is one of the essential elements of a scientific research paper. It needs to be to the point, clearly communicating what your research is trying to accomplish. A blurry, drawn-out, or complexly-structured hypothesis can confuse your readers. Or worse, the editor and peer reviewers.
A captivating hypothesis is not too intricate. This blog will take you through the process so that, by the end of it, you have a better idea of how to convey your research paper's intent in just one sentence.
What is a Hypothesis?
The first step in your scientific endeavor, a hypothesis, is a strong, concise statement that forms the basis of your research. It is not the same as a thesis statement , which is a brief summary of your research paper .
The sole purpose of a hypothesis is to predict your paper's findings, data, and conclusion. It comes from a place of curiosity and intuition . When you write a hypothesis, you're essentially making an educated guess based on scientific prejudices and evidence, which is further proven or disproven through the scientific method.
The reason for undertaking research is to observe a specific phenomenon. A hypothesis, therefore, lays out what the said phenomenon is. And it does so through two variables, an independent and dependent variable.
The independent variable is the cause behind the observation, while the dependent variable is the effect of the cause. A good example of this is “mixing red and blue forms purple.” In this hypothesis, mixing red and blue is the independent variable as you're combining the two colors at your own will. The formation of purple is the dependent variable as, in this case, it is conditional to the independent variable.
Different Types of Hypotheses

Types of hypotheses
Some would stand by the notion that there are only two types of hypotheses: a Null hypothesis and an Alternative hypothesis. While that may have some truth to it, it would be better to fully distinguish the most common forms as these terms come up so often, which might leave you out of context.
Apart from Null and Alternative, there are Complex, Simple, Directional, Non-Directional, Statistical, and Associative and casual hypotheses. They don't necessarily have to be exclusive, as one hypothesis can tick many boxes, but knowing the distinctions between them will make it easier for you to construct your own.
1. Null hypothesis
A null hypothesis proposes no relationship between two variables. Denoted by H 0 , it is a negative statement like “Attending physiotherapy sessions does not affect athletes' on-field performance.” Here, the author claims physiotherapy sessions have no effect on on-field performances. Even if there is, it's only a coincidence.
2. Alternative hypothesis
Considered to be the opposite of a null hypothesis, an alternative hypothesis is donated as H1 or Ha. It explicitly states that the dependent variable affects the independent variable. A good alternative hypothesis example is “Attending physiotherapy sessions improves athletes' on-field performance.” or “Water evaporates at 100 °C. ” The alternative hypothesis further branches into directional and non-directional.
- Directional hypothesis: A hypothesis that states the result would be either positive or negative is called directional hypothesis. It accompanies H1 with either the ‘<' or ‘>' sign.
- Non-directional hypothesis: A non-directional hypothesis only claims an effect on the dependent variable. It does not clarify whether the result would be positive or negative. The sign for a non-directional hypothesis is ‘≠.'
3. Simple hypothesis
A simple hypothesis is a statement made to reflect the relation between exactly two variables. One independent and one dependent. Consider the example, “Smoking is a prominent cause of lung cancer." The dependent variable, lung cancer, is dependent on the independent variable, smoking.
4. Complex hypothesis
In contrast to a simple hypothesis, a complex hypothesis implies the relationship between multiple independent and dependent variables. For instance, “Individuals who eat more fruits tend to have higher immunity, lesser cholesterol, and high metabolism.” The independent variable is eating more fruits, while the dependent variables are higher immunity, lesser cholesterol, and high metabolism.
5. Associative and casual hypothesis
Associative and casual hypotheses don't exhibit how many variables there will be. They define the relationship between the variables. In an associative hypothesis, changing any one variable, dependent or independent, affects others. In a casual hypothesis, the independent variable directly affects the dependent.
6. Empirical hypothesis
Also referred to as the working hypothesis, an empirical hypothesis claims a theory's validation via experiments and observation. This way, the statement appears justifiable and different from a wild guess.
Say, the hypothesis is “Women who take iron tablets face a lesser risk of anemia than those who take vitamin B12.” This is an example of an empirical hypothesis where the researcher the statement after assessing a group of women who take iron tablets and charting the findings.
7. Statistical hypothesis
The point of a statistical hypothesis is to test an already existing hypothesis by studying a population sample. Hypothesis like “44% of the Indian population belong in the age group of 22-27.” leverage evidence to prove or disprove a particular statement.
Characteristics of a Good Hypothesis
Writing a hypothesis is essential as it can make or break your research for you. That includes your chances of getting published in a journal. So when you're designing one, keep an eye out for these pointers:
- A research hypothesis has to be simple yet clear to look justifiable enough.
- It has to be testable — your research would be rendered pointless if too far-fetched into reality or limited by technology.
- It has to be precise about the results —what you are trying to do and achieve through it should come out in your hypothesis.
- A research hypothesis should be self-explanatory, leaving no doubt in the reader's mind.
- If you are developing a relational hypothesis, you need to include the variables and establish an appropriate relationship among them.
- A hypothesis must keep and reflect the scope for further investigations and experiments.
Separating a Hypothesis from a Prediction
Outside of academia, hypothesis and prediction are often used interchangeably. In research writing, this is not only confusing but also incorrect. And although a hypothesis and prediction are guesses at their core, there are many differences between them.
A hypothesis is an educated guess or even a testable prediction validated through research. It aims to analyze the gathered evidence and facts to define a relationship between variables and put forth a logical explanation behind the nature of events.
Predictions are assumptions or expected outcomes made without any backing evidence. They are more fictionally inclined regardless of where they originate from.
For this reason, a hypothesis holds much more weight than a prediction. It sticks to the scientific method rather than pure guesswork. "Planets revolve around the Sun." is an example of a hypothesis as it is previous knowledge and observed trends. Additionally, we can test it through the scientific method.
Whereas "COVID-19 will be eradicated by 2030." is a prediction. Even though it results from past trends, we can't prove or disprove it. So, the only way this gets validated is to wait and watch if COVID-19 cases end by 2030.
Finally, How to Write a Hypothesis
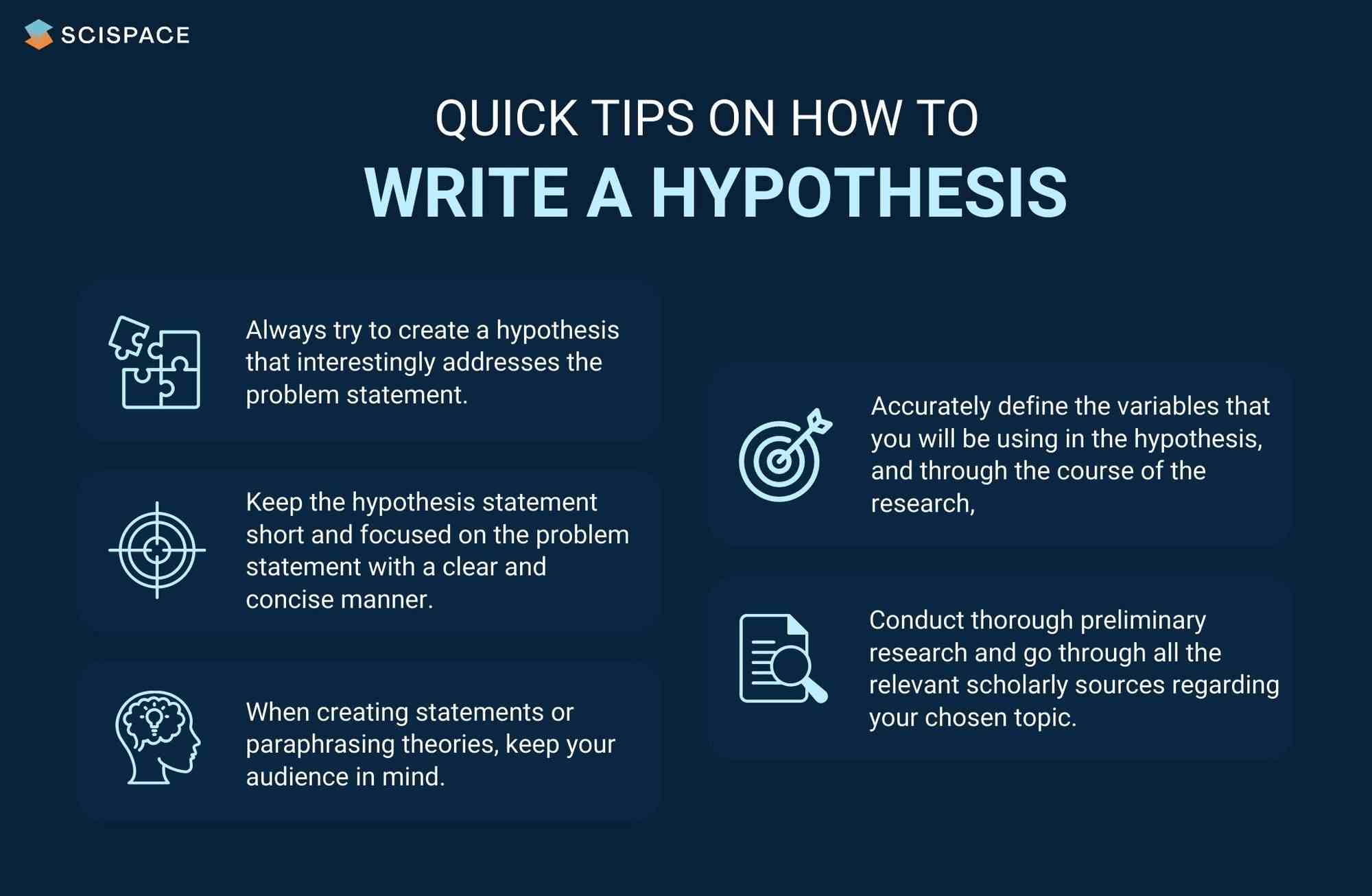
Quick tips on writing a hypothesis
1. Be clear about your research question
A hypothesis should instantly address the research question or the problem statement. To do so, you need to ask a question. Understand the constraints of your undertaken research topic and then formulate a simple and topic-centric problem. Only after that can you develop a hypothesis and further test for evidence.
2. Carry out a recce
Once you have your research's foundation laid out, it would be best to conduct preliminary research. Go through previous theories, academic papers, data, and experiments before you start curating your research hypothesis. It will give you an idea of your hypothesis's viability or originality.
Making use of references from relevant research papers helps draft a good research hypothesis. SciSpace Discover offers a repository of over 270 million research papers to browse through and gain a deeper understanding of related studies on a particular topic. Additionally, you can use SciSpace Copilot , your AI research assistant, for reading any lengthy research paper and getting a more summarized context of it. A hypothesis can be formed after evaluating many such summarized research papers. Copilot also offers explanations for theories and equations, explains paper in simplified version, allows you to highlight any text in the paper or clip math equations and tables and provides a deeper, clear understanding of what is being said. This can improve the hypothesis by helping you identify potential research gaps.
3. Create a 3-dimensional hypothesis
Variables are an essential part of any reasonable hypothesis. So, identify your independent and dependent variable(s) and form a correlation between them. The ideal way to do this is to write the hypothetical assumption in the ‘if-then' form. If you use this form, make sure that you state the predefined relationship between the variables.
In another way, you can choose to present your hypothesis as a comparison between two variables. Here, you must specify the difference you expect to observe in the results.
4. Write the first draft
Now that everything is in place, it's time to write your hypothesis. For starters, create the first draft. In this version, write what you expect to find from your research.
Clearly separate your independent and dependent variables and the link between them. Don't fixate on syntax at this stage. The goal is to ensure your hypothesis addresses the issue.
5. Proof your hypothesis
After preparing the first draft of your hypothesis, you need to inspect it thoroughly. It should tick all the boxes, like being concise, straightforward, relevant, and accurate. Your final hypothesis has to be well-structured as well.
Research projects are an exciting and crucial part of being a scholar. And once you have your research question, you need a great hypothesis to begin conducting research. Thus, knowing how to write a hypothesis is very important.
Now that you have a firmer grasp on what a good hypothesis constitutes, the different kinds there are, and what process to follow, you will find it much easier to write your hypothesis, which ultimately helps your research.
Now it's easier than ever to streamline your research workflow with SciSpace Discover . Its integrated, comprehensive end-to-end platform for research allows scholars to easily discover, write and publish their research and fosters collaboration.
It includes everything you need, including a repository of over 270 million research papers across disciplines, SEO-optimized summaries and public profiles to show your expertise and experience.
If you found these tips on writing a research hypothesis useful, head over to our blog on Statistical Hypothesis Testing to learn about the top researchers, papers, and institutions in this domain.
Frequently Asked Questions (FAQs)
1. what is the definition of hypothesis.
According to the Oxford dictionary, a hypothesis is defined as “An idea or explanation of something that is based on a few known facts, but that has not yet been proved to be true or correct”.
2. What is an example of hypothesis?
The hypothesis is a statement that proposes a relationship between two or more variables. An example: "If we increase the number of new users who join our platform by 25%, then we will see an increase in revenue."
3. What is an example of null hypothesis?
A null hypothesis is a statement that there is no relationship between two variables. The null hypothesis is written as H0. The null hypothesis states that there is no effect. For example, if you're studying whether or not a particular type of exercise increases strength, your null hypothesis will be "there is no difference in strength between people who exercise and people who don't."
4. What are the types of research?
• Fundamental research
• Applied research
• Qualitative research
• Quantitative research
• Mixed research
• Exploratory research
• Longitudinal research
• Cross-sectional research
• Field research
• Laboratory research
• Fixed research
• Flexible research
• Action research
• Policy research
• Classification research
• Comparative research
• Causal research
• Inductive research
• Deductive research
5. How to write a hypothesis?
• Your hypothesis should be able to predict the relationship and outcome.
• Avoid wordiness by keeping it simple and brief.
• Your hypothesis should contain observable and testable outcomes.
• Your hypothesis should be relevant to the research question.
6. What are the 2 types of hypothesis?
• Null hypotheses are used to test the claim that "there is no difference between two groups of data".
• Alternative hypotheses test the claim that "there is a difference between two data groups".
7. Difference between research question and research hypothesis?
A research question is a broad, open-ended question you will try to answer through your research. A hypothesis is a statement based on prior research or theory that you expect to be true due to your study. Example - Research question: What are the factors that influence the adoption of the new technology? Research hypothesis: There is a positive relationship between age, education and income level with the adoption of the new technology.
8. What is plural for hypothesis?
The plural of hypothesis is hypotheses. Here's an example of how it would be used in a statement, "Numerous well-considered hypotheses are presented in this part, and they are supported by tables and figures that are well-illustrated."
9. What is the red queen hypothesis?
The red queen hypothesis in evolutionary biology states that species must constantly evolve to avoid extinction because if they don't, they will be outcompeted by other species that are evolving. Leigh Van Valen first proposed it in 1973; since then, it has been tested and substantiated many times.
10. Who is known as the father of null hypothesis?
The father of the null hypothesis is Sir Ronald Fisher. He published a paper in 1925 that introduced the concept of null hypothesis testing, and he was also the first to use the term itself.
11. When to reject null hypothesis?
You need to find a significant difference between your two populations to reject the null hypothesis. You can determine that by running statistical tests such as an independent sample t-test or a dependent sample t-test. You should reject the null hypothesis if the p-value is less than 0.05.

You might also like

Consensus GPT vs. SciSpace GPT: Choose the Best GPT for Research
Literature Review and Theoretical Framework: Understanding the Differences

Types of Essays in Academic Writing - Quick Guide (2024)
Have a language expert improve your writing
Run a free plagiarism check in 10 minutes, generate accurate citations for free.
- Knowledge Base
Methodology
- How to Write a Strong Hypothesis | Steps & Examples
How to Write a Strong Hypothesis | Steps & Examples
Published on May 6, 2022 by Shona McCombes . Revised on November 20, 2023.
A hypothesis is a statement that can be tested by scientific research. If you want to test a relationship between two or more variables, you need to write hypotheses before you start your experiment or data collection .
Example: Hypothesis
Daily apple consumption leads to fewer doctor’s visits.
Table of contents
What is a hypothesis, developing a hypothesis (with example), hypothesis examples, other interesting articles, frequently asked questions about writing hypotheses.
A hypothesis states your predictions about what your research will find. It is a tentative answer to your research question that has not yet been tested. For some research projects, you might have to write several hypotheses that address different aspects of your research question.
A hypothesis is not just a guess – it should be based on existing theories and knowledge. It also has to be testable, which means you can support or refute it through scientific research methods (such as experiments, observations and statistical analysis of data).
Variables in hypotheses
Hypotheses propose a relationship between two or more types of variables .
- An independent variable is something the researcher changes or controls.
- A dependent variable is something the researcher observes and measures.
If there are any control variables , extraneous variables , or confounding variables , be sure to jot those down as you go to minimize the chances that research bias will affect your results.
In this example, the independent variable is exposure to the sun – the assumed cause . The dependent variable is the level of happiness – the assumed effect .
Receive feedback on language, structure, and formatting
Professional editors proofread and edit your paper by focusing on:
- Academic style
- Vague sentences
- Style consistency
See an example
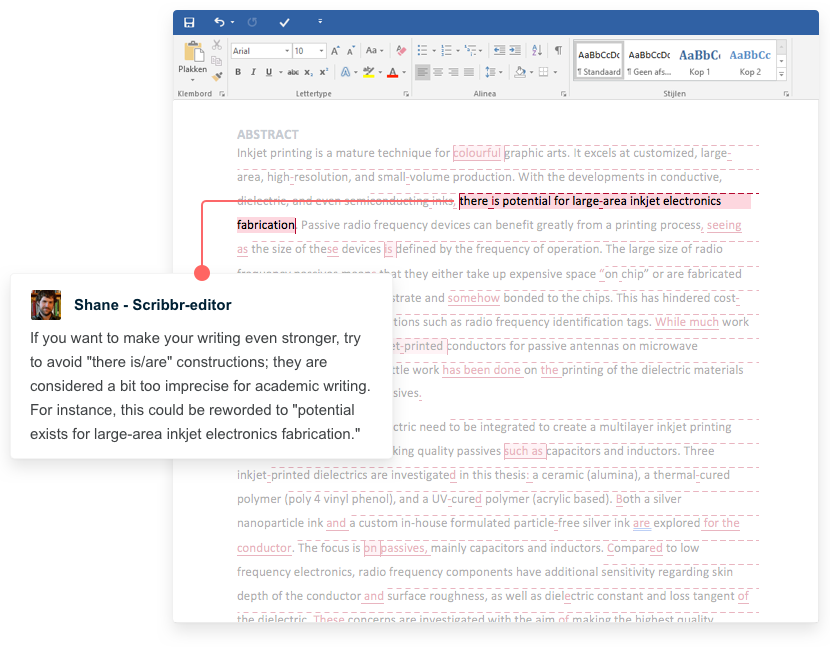
Step 1. Ask a question
Writing a hypothesis begins with a research question that you want to answer. The question should be focused, specific, and researchable within the constraints of your project.
Step 2. Do some preliminary research
Your initial answer to the question should be based on what is already known about the topic. Look for theories and previous studies to help you form educated assumptions about what your research will find.
At this stage, you might construct a conceptual framework to ensure that you’re embarking on a relevant topic . This can also help you identify which variables you will study and what you think the relationships are between them. Sometimes, you’ll have to operationalize more complex constructs.
Step 3. Formulate your hypothesis
Now you should have some idea of what you expect to find. Write your initial answer to the question in a clear, concise sentence.
4. Refine your hypothesis
You need to make sure your hypothesis is specific and testable. There are various ways of phrasing a hypothesis, but all the terms you use should have clear definitions, and the hypothesis should contain:
- The relevant variables
- The specific group being studied
- The predicted outcome of the experiment or analysis
5. Phrase your hypothesis in three ways
To identify the variables, you can write a simple prediction in if…then form. The first part of the sentence states the independent variable and the second part states the dependent variable.
In academic research, hypotheses are more commonly phrased in terms of correlations or effects, where you directly state the predicted relationship between variables.
If you are comparing two groups, the hypothesis can state what difference you expect to find between them.
6. Write a null hypothesis
If your research involves statistical hypothesis testing , you will also have to write a null hypothesis . The null hypothesis is the default position that there is no association between the variables. The null hypothesis is written as H 0 , while the alternative hypothesis is H 1 or H a .
- H 0 : The number of lectures attended by first-year students has no effect on their final exam scores.
- H 1 : The number of lectures attended by first-year students has a positive effect on their final exam scores.
Research question | Hypothesis | Null hypothesis |
---|---|---|
What are the health benefits of eating an apple a day? | Increasing apple consumption in over-60s will result in decreasing frequency of doctor’s visits. | Increasing apple consumption in over-60s will have no effect on frequency of doctor’s visits. |
Which airlines have the most delays? | Low-cost airlines are more likely to have delays than premium airlines. | Low-cost and premium airlines are equally likely to have delays. |
Can flexible work arrangements improve job satisfaction? | Employees who have flexible working hours will report greater job satisfaction than employees who work fixed hours. | There is no relationship between working hour flexibility and job satisfaction. |
How effective is high school sex education at reducing teen pregnancies? | Teenagers who received sex education lessons throughout high school will have lower rates of unplanned pregnancy teenagers who did not receive any sex education. | High school sex education has no effect on teen pregnancy rates. |
What effect does daily use of social media have on the attention span of under-16s? | There is a negative between time spent on social media and attention span in under-16s. | There is no relationship between social media use and attention span in under-16s. |
If you want to know more about the research process , methodology , research bias , or statistics , make sure to check out some of our other articles with explanations and examples.
- Sampling methods
- Simple random sampling
- Stratified sampling
- Cluster sampling
- Likert scales
- Reproducibility
Statistics
- Null hypothesis
- Statistical power
- Probability distribution
- Effect size
- Poisson distribution
Research bias
- Optimism bias
- Cognitive bias
- Implicit bias
- Hawthorne effect
- Anchoring bias
- Explicit bias
Here's why students love Scribbr's proofreading services
Discover proofreading & editing
A hypothesis is not just a guess — it should be based on existing theories and knowledge. It also has to be testable, which means you can support or refute it through scientific research methods (such as experiments, observations and statistical analysis of data).
Null and alternative hypotheses are used in statistical hypothesis testing . The null hypothesis of a test always predicts no effect or no relationship between variables, while the alternative hypothesis states your research prediction of an effect or relationship.
Hypothesis testing is a formal procedure for investigating our ideas about the world using statistics. It is used by scientists to test specific predictions, called hypotheses , by calculating how likely it is that a pattern or relationship between variables could have arisen by chance.
Cite this Scribbr article
If you want to cite this source, you can copy and paste the citation or click the “Cite this Scribbr article” button to automatically add the citation to our free Citation Generator.
McCombes, S. (2023, November 20). How to Write a Strong Hypothesis | Steps & Examples. Scribbr. Retrieved September 23, 2024, from https://www.scribbr.com/methodology/hypothesis/
Is this article helpful?
Shona McCombes
Other students also liked, construct validity | definition, types, & examples, what is a conceptual framework | tips & examples, operationalization | a guide with examples, pros & cons, what is your plagiarism score.
Marketing91
What is a Research Hypothesis And How to Write it?
June 12, 2023 | By Hitesh Bhasin | Filed Under: Marketing
A research hypothesis can be defined as a clear, specific and predictive statement that states the possible outcome of a scientific study. The result of the research study is based on previous research studies and can be tested by scientific research.
The research hypothesis is written before the beginning of any scientific research or data collection .
Table of Contents
What is Research Hypothesis?
The research hypothesis is the first step and basis of all research endeavours. The research hypothesis shows what you want to prove with your research study. Therefore, the research hypothesis should be written first before you begin the study, no matter what kind of research study you are conducting.
The research hypothesis shows the direction to the researcher conducting the research. It states what the researcher expects to find from the study. It is a tentative answer that guides the entire research study.
Writing a research hypothesis is not an easy task. It requires skills to write a testable research hypothesis. The researcher is required to study the research done by other researchers on the same subject and find out the loopholes in those researches to make it the basis for their research.
Make sure to consider the general research question posed in the study before jumping directly to write a research hypothesis. Pointing out the exact question can be very difficult for researchers as most researchers are usually not aware of what they are trying to find from their research study. Moreover, the added excitement to conduct the study makes it even more difficult for the researchers to pin down the exact research hypothesis.
There are two primary criteria to develop a reasonable research hypothesis. First, the research hypothesis should be researchable and second; it must be interesting. By researchable, we mean that the question in the research hypothesis statement should be able to be answered with the help of science and the answer to the question should be answerable within a reasonable period.
The research hypothesis being interesting means that the research question should be valuable in the context of the ongoing scientific research of the topic.
Let us learn about the research hypothesis in quantitative and qualitative studies:
Research hypothesis in Quantitative studies
The research hypothesis in a quantitative study consists of one independent variable and one dependent variable, and the research hypothesis mentions the expected relationship between both of the variables.
The independent variable is mentioned first in the research hypothesis followed by explanations and results, etc. and then the dependent variable is specified. Make sure that the variables are referred to in the same order as they are mentioned in the research hypothesis; otherwise, there are chances that your readers get confused while reading your research proposal .
When both variables are used in continuous nature, then it is easy to describe negative or positive relationships between both of them. In the case of categorical variables, the hypothesis statement about which category of independent variables is associated with which group of dependent variables.
It is good to represent the research hypothesis in directional format. That means, the statement is made about the expected relationship between the variables based on past research, the study of existing research, on an educational guess , or only by observation.
Additionally, the null hypothesis can also be used between two variables which state that there is no relationship between the variables. The null hypothesis is the basis of all types of statistical research.
Lastly, a simple research hypothesis for quantitative research should provide a direction for the study of the relationship between two variables. Still, it should also use phrases like “tend to” or “in general” to soften the tone of the hypothesis.
Research hypothesis in qualitative research
The role of the research hypothesis in qualitative research is different as compared to its role in quantitative research. The research hypothesis is not developed at the beginning of the research because of the inductive nature of the qualitative studies.
The research hypothesis is introduced during the iterative process of data collection and the Interpretation of the data. The research hypothesis helps the researchers ask more questions and look for answers for disconfirming evidence.
The qualitative study is dependent on the questions and subquestions asked by the researchers at the beginning of the qualitative research. Generally, in qualitative studies one or two central questions are developed and based on these central questions a series of five to ten subquestions is built and these sub-questions are further used to develop central questions for the research purpose.
In qualitative studies, these questions are directly asked the participant of the research study usually through focus groups or in-depth interviews. This is done to develop an understanding between participants of the study and the researchers. This helps in creating a collaborative experience between the two.
Variables in hypothesis
In research studies like correlational research and experimental studies, a hypothesis shows a relationship between two or more variables. There is an independent variable and a dependent variable.
An independent variable is a variable that a researcher can control and change, whereas, a dependent variable is a variable that the researcher measures and observes.
For example, regular exercise lowers the chances of a heart attack. In this example, the regular exercise is an independent variable and probabilities of occurrence of heart attack is a dependent variable that researchers can measure by observation.
How to develop a reasonable research hypothesis?
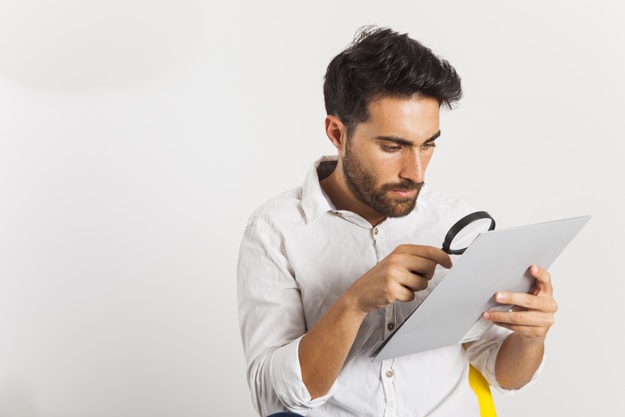
A research hypothesis plays an essential role in the research study. Therefore, it is necessary to develop an accurate and precise research hypothesis. In this section, you will learn how to develop a reasonable research hypothesis. The following are the steps involved in developing a research hypothesis.
Step 1. Have a question?
The first step involved in writing a research hypothesis is having a question that you want to answer. This question should be specific and within the scope of your research area. Make sure that the question that you ask is researchable within the time duration of your research study. The examples of research hypothesis questions can be
- Do students who attend classes regularly score more in exams?
- Do people prefer to buy products that have a high price as compared to the other similar products available in the market ?
Step 2. Do some preliminary research:
Preliminary research is conducted before a researcher decides his research hypothesis. In the preliminary research, all the knowledge available about the question is collected by studying the theories and previous studies.
Having this knowledge helps the researchers to form educational assumptions about the outcomes of the research. At this stage, the researcher might prepare a conceptual framework to determine which variable should be studied and what you think is the relationship between the different variables.
The preliminary study also helps the researcher to change the topic if he feels the problem doesn’t have much scope for research.
Step 3. Formulation of hypothesis:
At this stage, the final research hypothesis is formulated. At this stage, the researcher has some idea of what he should expect from the research study. Write the answer to the question of research hypothesis in concise and clear sentences.
The clearer the research hypothesis, the easier will be for researchers to conduct the research.
Step 4. Refine the final hypothesis:
It is essential to make sure that your research hypothesis is testable and specific. You can define a hypothesis in different ways, but you should make sure that all the words that you use in your research hypothesis have precise definitions.
Besides, your hypothesis should contain a set of variables, the relationship between the variables, specific group being studied, and already predicted the outcome of the research.
Step 5. Use three methods to phrase your hypothesis:
They establish a clear relationship between variables, write the hypothesis in if.. then form. The first part of the sentence should be an independent variable, and the second part of the variable should state the dependent variable.
For example, if a student attends 100% classes in a semester, then he will score more than 90% in the exams.
In academic research, the research hypotheses are formed in terms of correlations or effects. In such hypotheses, the relationship between the variables is directly stated in the research hypothesis.
For example, the high numbers of lectures attended by students have a positive impact on their results.
When you are writing a research hypothesis to compare two groups, the hypothesis should state what the differences you are expecting to find in both the groups are.
For example, the students who have more than 70% attendance will score better in exams than the students who have lower than 50% attendance.
Step 6. Write the Null hypothesis:
A null hypothesis is written when research involves statistical hypothesis testing. A null hypothesis when there is no specific relationship between the variables.
It is a default position that shows that two variables used in the hypothesis are not related to each other. A null hypothesis is usually written as H0, and alternative hypotheses are written as H1 or Ha.
Importance of Research Hypothesis
Research plays an essential role in every field. To experiment, a researcher needs to make sure that the research he wants to conduct is testable. A research hypothesis is developed after conducting a preliminary study.
A preliminary study is the study of previous studies done by researchers and the study of research papers written on the same concept. With the help of the research hypothesis, a researcher makes sure that he is not hidden towards a dead end, and it works as a direction map for the researcher.
Liked this post? Check out the complete series on Market research
Related posts:
- How to Write Research Proposal? Research Proposal Format
- What are the Research Objectives? Types, Examples & How to Write Them
- How to write a Research Question? Types and Tips
- What is a Research Statement and How to Write it
- What is Research Design? Type of Research Designs
- 7 Key Differences between Research Method and Research Methodology
- Qualitative Research: Meaning, and Features of Qualitative Research
- Research Ethics – Importance and Principles of Ethics in Research
- What Are Concept Statements? How To Write A Concept Statement?
- Sales Copy: What it is and How to Write Effective Sales Copy
About Hitesh Bhasin
Hitesh Bhasin is the CEO of Marketing91 and has over a decade of experience in the marketing field. He is an accomplished author of thousands of insightful articles, including in-depth analyses of brands and companies. Holding an MBA in Marketing, Hitesh manages several offline ventures, where he applies all the concepts of Marketing that he writes about.
All Knowledge Banks (Hub Pages)
- Marketing Hub
- Management Hub
- Marketing Strategy
- Advertising Hub
- Branding Hub
- Market Research
- Small Business Marketing
- Sales and Selling
- Marketing Careers
- Internet Marketing
- Business Model of Brands
- Marketing Mix of Brands
- Brand Competitors
- Strategy of Brands
- SWOT of Brands
- Customer Management
- Top 10 Lists
Leave a Reply Cancel reply
Your email address will not be published. Required fields are marked *
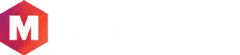
- About Marketing91
- Marketing91 Team
- Privacy Policy
- Cookie Policy
- Terms of Use
- Editorial Policy
WE WRITE ON
- Digital Marketing
- Human Resources
- Operations Management
- Marketing News
- Marketing mix's
- Competitors
BUS602: Marketing Management
Case Study: Role of Marketing Mix
This research article looks at tourism of Lake Samosir in North Sumatra, Indonesia. The underlying question is whether the implementation of the marketing mix influenced tourism in the area.
4.3. Hypothesis Testing
As explained in the previous chapter, there are 5 hypotheses in this study. Hypothesis testing analysis is carried out with a significance level of 5%, resulting in a critical t-value of ± 1.96. The hypothesis is accepted if the t-value obtained ≥ 1.96, while hypothesis is not supported if the t-value obtained < 1.96. The following is a table of hypothesis testing to answer the overall questions of the study:
|
|
|
| ||
H | Marketing Mix Satisfactions on Tourist | 3.78 | Data Supporting the Hypothesis | ||
H | Quality Service on Tourist Satisfactions | 5.94 | Data Supporting the Hypothesis | ||
H | Marketing Mix on Tourists Loyalty | 4.19 | Data Supporting the Hypothesis | ||
H | Quality Service on Tourists Loyalty | 3.23 | Data Supporting the Hypothesis | ||
H | Tourist Satisfactions on Tourists Loyalty | 3.16 | Data Supporting the Hypothesis |
Table 2: Hypothesis Testing of Research Model Based on table 2 above which contains the conclusion of the hypothesis model results, it can be concluded as follows:
a) Marketing Mix has a positive effect on Tourist Satisfactions
Based on data processing results of the structural model, the output of t-value is 3.78. The result of t-value shown is greater than 1.96, so it can be concluded that the variable of marketing mix has a significant positive effect on tourist satisfactions. Thus, hypothesis 1 can be accepted and it can be concluded that the higher marketing mix perceived, the higher tourist satisfactions will be.
b) Service Quality has a positive effect on Tourist Satisfactions
Based on data processing results of the structural model, the output of t-value is 5.94. The result of t-value shown is greater than 1.96, so it can be concluded that the variable of service quality has a significant positive effect on tourist satisfactions. Thus, it can be concluded that the higher service quality perceived, the higher tourist satisfactions will be.
c) Marketing Mix has a positive effect on Tourists Loyalty
Based on data processing results of the structural model, the output of t-value is 4.19. The result of t-value shown is greater than 1.96, so it can be concluded that the variable of marketing mix has a significant positive effect on tourists loyalty. Thus, it can be concluded that the higher marketing mix perceived, the higher tourist loyalty will be.
d) Service Quality has a positive effect on Tourists Loyalty
Based on data processing results of the structural model, the output of t-value is 3.23. The result of t-value shown is greater than 1.96, so it can be concluded that the variable of service quality has a significant positive effect on tourists loyalty. Thus, it can be concluded that the higher the perceived service quality, the higher tourist loyalty will be.
e) Satisfactions has a positive effect on Tourists Loyalty
Based on data processing results of the structural model, the output of t-value is 3.16. The result of t-value shown is greater than 1.96, so it can be concluded that the variable of satisfactions has a significant positive effect on tourists loyalty. Thus, it can be concluded that with higher perceived satisfaction comes higher tourist loyalty.
Hypothesis Testing Of Mediation (Indirect Effects)
As explained in the previous chapter, in this study there are two moderation hypotheses by Tourist Satisfaction variables. Hypothesis testing analysis is carried out with a significance level of 5%, resulting in a critical t-value of ± 1.96. The hypothesis is accepted if the t-value obtained ≥ 1.96, while hypothesis is not supported if the t-value obtained < 1.96.
The following is a table of testing hypotheses to answer indirect influences.
Table 3. Testing of Indirect Influence Hypotheses
Hypothesis | Through |
Indirect Effects (t ) | Tourist Satisfaction |
Effect of Marketing Mix on Loyalty | 2.50 |
Effect of Service Quality on Loyalty | 3.00 |
Based on the results of the LISREL output above, the data from the structural model, obtained the output of t-value (line 3), in the result showed that the variables of tourists satisfaction can mediate the effect between the variable of marketing mix and service quality that has an indirect effect on tourists loyalty. This can be seen from t-count value is greater than 1.96 i.e. 2.50 and 3.00.

IMAGES
VIDEO
COMMENTS
A well-founded marketing hypothesis should also provide you with new, testable clues about your users regardless of whether or not the test wins, loses or yields inconclusive results. These new insights should inform future testing: a solid hypothesis can help you quickly separate worthwhile ideas from the rest when planning follow-up tests.
3. One-Sided vs. Two-Sided Testing. When it's time to test your hypothesis, it's important to leverage the correct testing method. The two most common hypothesis testing methods are one-sided and two-sided tests, or one-tailed and two-tailed tests, respectively. Typically, you'd leverage a one-sided test when you have a strong conviction ...
In essence, hypothesis testing involves making an educated guess about a population parameter and then using data to determine if the hypothesis is supported or rejected. In the context of marketing, hypotheses can be formulated about consumer behavior, product preferences, advertising effectiveness, and many other aspects of the marketing mix.
The Basics: Marketing Experimentation Hypothesis. A hypothesis is a research-based statement that aims to explain an observed trend and create a solution that will improve the result. This statement is an educated, testable prediction about what will happen. It has to be stated in declarative form and not as a question.
Developing a hypothesis is an essential part of marketing experimentation. Qualitative-based research should inform hypotheses that you test with real-world behavior. The hypotheses help you discover how accurate those insights from qualitative research are. If you engage in hypothesis-driven testing, then you ensure your tests are strategic ...
By transforming marketing ideas into hypotheses, we orient our test to learn about our customer rather than merely trying out an idea. Here is a 4-step framework for creating a hypothesis, along with good and bad examples. ... we transform our ideas for solving them into a hypothesis containing four key parts: If [we achieve this in the mind of ...
We examined three approaches to research in marketing: exploratory hypotheses, dominant hypothesis, and competing hypotheses. Our review of empirical studies on scientific methodology suggests that the use of a single dominant hypothesis lacks objectivity relative to the use of exploratory and competing hypotheses approaches. We then conducted a publication audit of over 1,700 empirical papers ...
We then conducted a publication audit of over 1,700 empirical papers in six leading marketing journals during 1984-1999. Of these, 74% used the dominant hypothesis approach, while 13 % used ...
Make a hypothesis. Collect research. Select your metrics. Execute the experiment. Analyze the results. Performing a marketing experiment involves doing research, structuring the experiment, and analyzing the results. Let's go through the seven steps necessary to conduct a marketing experiment. 1.
Hypothesis is a much-underused concept in marketing analytics that can yield significant results.It is a method of testing a marketing theory or proposition before investing significant resources into implementation. Using this approach can ensure that resources are prioritized to achieve better outcomes. So, let's dive into the hypothesis and how it can be used in marketing analytics.
And, being hypothesis-driven was required to have any success at McKinsey. A hypothesis is an idea or theory, often based on limited data, which is typically the beginning of a thread of further investigation to prove, disprove or improve the hypothesis through facts and empirical data. The first step in being hypothesis-driven is to focus on ...
You may want to come up with both a problem statement and a hypothesis. For example: Problem Statement: "The lead generation form is too long, causing unnecessary friction.". Hypothesis: "By changing the amount of form fields from 20 to 10, we will increase number of leads.". Proposed solution.
Hypothesis-driven approach. A marketing experiment should be based on a well-formulated hypothesis that predicts the expected outcome. A reasonable hypothesis is specific, testable, and grounded in existing knowledge or data. It serves as the foundation for experimental design and helps businesses focus on the most relevant variables and outcomes.
For example, a hypothesis might be formulated to test the effects of a new marketing campaign on consumer buying behavior. Engineering: In engineering, hypotheses are used to test the effectiveness of new technologies or designs. For example, a hypothesis might be formulated to test the efficiency of a new solar panel design.
A hypothesis is the next step, when an idea gets wrapped with specifics to become an assumption that may be tested. As such, you can refine the idea by adding details to it. The previously mentioned idea can be worded into a product hypothesis statement like: "The cart abandonment rate is high, and many users flee at checkout.
Simple hypothesis. A simple hypothesis is a statement made to reflect the relation between exactly two variables. One independent and one dependent. Consider the example, "Smoking is a prominent cause of lung cancer." The dependent variable, lung cancer, is dependent on the independent variable, smoking. 4.
Developing a hypothesis (with example) Step 1. Ask a question. Writing a hypothesis begins with a research question that you want to answer. The question should be focused, specific, and researchable within the constraints of your project. Example: Research question.
A research hypothesis can be defined as a clear, specific and predictive statement that states the possible outcome of a scientific study. The result of the research study is based on previous research studies and can be tested by scientific research. The research hypothesis is written before the beginning of any scientific research or data ...
4.3. Hypothesis Testing. As explained in the previous chapter, there are 5 hypotheses in this study. Hypothesis testing analysis is carried out with a significance level of 5%, resulting in a critical t-value of ± 1.96. The hypothesis is accepted if the t-value obtained ≥ 1.96, while hypothesis is not supported if the t-value obtained < 1.96.