- How it works
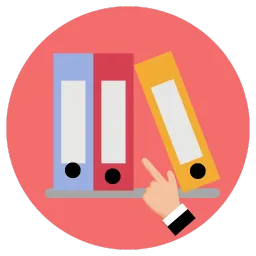

What is Response Bias – Types & Examples
Published by Owen Ingram at September 4th, 2023 , Revised On September 4, 2023
In research, ensuring the accuracy of responses is crucial to obtaining valid and reliable results. When participants’ answers are systematically influenced by external factors or internal beliefs rather than reflecting their genuine opinions or facts, it introduces response bias. This kind of bias can significantly skew the results of a study, making them less trustworthy. Researchers often refer to a scholarly source to understand the nuances of such biases.
Example of Response Bias
Suppose a researcher is conducting a survey about people’s exercise habits. They ask the question:
“How many days a week do you engage in at least 30 minutes of physical exercise?”
The bias here is that participants may not give honest answers due to the perceived social desirability of the behaviour in question, which can be tied to explicit bias . Researchers need to be wary of this and might consider using alternative methods, such as anonymised surveys or indirect questioning, to obtain more accurate responses.
But what exactly is response bias, why does it matter, and how can it manifest? Let’s discuss this in detail.
What is Response Bias?
Response bias refers to the tendency of respondents to answer questions in a way that does not accurately reflect their true beliefs, feelings, or behaviours. This bias can be introduced into survey or research results due to various factors, including the way questions are phrased, the presence of an interviewer, or the respondents’ perceived social desirability of certain answers.
Different Types of Response Bias
The different types of response bias and how to minimise each one are discussed below. These biases can be understood better with the source evaluation method and by referring to a primary source or a secondary source .
Acquiescence Bias (or “Yes-Saying”)
Acquiescence bias occurs when respondents have a tendency to agree with statements or answer ‘yes’ to questions, regardless of their actual opinion. This can particularly distort results in surveys that consist mostly of positive statements, as respondents could be more agreeable or favourable than they truly are.
How to Minimise
Ensure a balance of positive and negative statements in the survey. Also, include a neutral response option where appropriate.
Social Desirability Bias
Social desirability bias comes into play when respondents provide answers they believe will be viewed favourably by others. They might over-report behaviours considered ‘good’ and underreport ‘undesirable’ to present themselves positively.
Assure respondents of the confidentiality of their responses. Employ indirect questioning or third-person techniques to make respondents more comfortable.
Recall Bias
In recall bias , the respondent’s memory of past events is not accurate. They might forget details or remember events differently than they happened. This is particularly common in longitudinal studies or health surveys asking about past behaviours or occurrences.
Use shorter time frames for recall or provide aids (e.g., calendars) to help jog respondents’ memories.
Anchoring Bias
In anchoring bias , respondents rely too heavily on the first piece of information they encounter (the “anchor”) and then adjust their responses based on this anchor. For instance, respondents may anchor around these numbers if a survey asks about the number of books read in the past month and offers an example (“like 10, 20, or 30 books”).
Avoid providing unnecessary examples or information that could act as anchors.
Extreme Responding
Some people are prone to choose the most extreme response options, whether on a scale from 1-5 or 1-10. This might give the impression of very strong opinions, even if the respondent does not feel that strongly.
Use balanced scales with clear delineations between options and provide a neutral midpoint.
Non-Response Bias
Non-response bias occurs when certain groups or individuals choose not to participate in a survey or skip specific questions. If the non-responders are systematically different from those who do respond, this can skew results.
Encourage participation through reminders and incentives. Also, design surveys to be as engaging and concise as possible.
Leading Question Bias
When questions are worded to suggest a particular answer or lead respondents in a certain direction, it can create this bias.
Ensure questions are neutrally worded. Pre-test surveys with a sample group to identify potential leading questions.
Confirmation Bias
While this is more of a cognitive bias than a response bias, it is essential to mention it. When analysing results, researchers might look for data confirming their beliefs or hypotheses and overlook data that contradicts them.
Approach data analysis with an open mind. Use blind analyses when appropriate, and have multiple people review the results.
Order Bias (or Sequence Bias)
The order in which questions or options are presented can influence responses. Respondents might focus more on the first or last items due to primacy and recency effects.
Randomise the order of questions or response options for different respondents.
Halo Effect
If a respondent has a strong positive or negative feeling about one aspect of a subject, that feeling can influence their responses to related questions. For instance, someone who loves a brand might rate it highly across all metrics, even if they have had specific negative experiences.
Design questions to be as specific as possible and separate unrelated concepts.
Causes of Response Bias
Response bias in surveys or questionnaires is a systematic pattern of deviation from the true response due to factors other than the actual subject of the study. Here are some causes of response bias:
Wording of Questions
The way questions are phrased can influence the way respondents answer them. Leading or loaded questions can prompt respondents to answer in a specific way.
Interviewer’s Behaviour or Appearance
How an interviewer behaves, or their personal characteristics can influence respondents’ answers, especially in face-to-face interviews. For example, the respondent might try to please the interviewer or avoid conflict.
Hire an Expert Writer
Proposal and research paper orders completed by our expert writers are
- Formally drafted in academic style
- Plagiarism free
- 100% Confidential
- Never Resold
- Include unlimited free revisions
- Completed to match exact client requirements
Recall Errors
Especially in surveys asking about past events or behaviours, respondents might not remember accurately, and hence, their answers could be biased.
Mood and Context of the Respondent
The environment, the timing, or the respondent’s personal mood or situation can affect their answers. For instance, someone might respond differently to a survey about job satisfaction when they face a lot of work stress compared to a more relaxed day.
Order of Questions
The sequence in which questions are presented can influence how respondents answer subsequent questions. Earlier questions can set a context or frame of mind that affects answers to later questions. This effect can sometimes be due to the ceiling effect or even affinity bias .
Pressure to Conform
In some settings, especially when others are present or watching, respondents might feel pressure to answer in a way that conforms with the majority view or avoids standing out, reflecting a bias for action .
Lack of Anonymity
If respondents believe that their answers will be tied back to them, and they could face negative consequences, they might not answer truthfully.
Fatigue or Boredom
In lengthy surveys or interviews, respondents might get tired or bored and might not answer later questions with the same care and attention as earlier ones.
Misunderstanding of Questions
If respondents do not understand a question fully or interpret it differently than intended, their answers can be biased.
Differential Participation Rates
If certain groups are more or less likely to participate in a survey (e.g., because of its mode or topic), this can introduce bias if the non-participants have answered questions differently than those who did participate.
If respondents believe that their answers will be tied back to them and they could face negative consequences, they might not answer truthfully.
Offering participation incentives might attract respondents with different opinions or characteristics than those who wouldn’t participate without the incentive.
Response Bias Examples
Some response bias examples are:
- When asked about charitable donations, a respondent might exaggerate the amount they have donated because they think it makes them look good, even if they have given little or nothing.
- A participant in a survey agrees with every statement presented to them without really considering the content, such as “I enjoy sunny days” followed by “I prefer rainy days.”
- On a performance review scale of 1 to 7, a manager consistently rates an employee as a ‘4’ for every trait, irrespective of the employee’s true performance.
- A person always uses the ends of a Likert scale (e.g., 1 or 7) regardless of the statement or their true feelings.
- In a feedback survey about a year-long course, a student only recalls and gives feedback on topics from the past few weeks.
- A person is asked to guess the number of candies in a jar after being told that the last guess was 500. They guessed 520, even though their initial thought was 800, because the “500” anchored their response.
- In a survey about a product, the question is phrased as “How much did you love our product?” This can lead respondents to give a more positive answer than if they were asked neutrally.
- A survey about workplace conditions is sent out, but only those with strong opinions (either very positive or very negative) choose to respond. The middle-ground voices are not represented.
- During a face-to-face survey, the interviewer subtly nods when the respondent gives certain answers, unintentionally encouraging the respondent to answer in a particular direction. This can sometimes be a result of the Pygmalion effect , where the expectations of the interviewer influence the responses of the participants.
- On a list of ten movies to rate, respondents give higher ratings to the first and last movies they review, simply because of their positioning on the list.
- Ecological Fallacy
- Pygmalion Effect
- Nonresponse Bias
- Social Desirability Bias
- Ceiling Effect
- Affinity Bias
How to Minimise Response Bias
Minimising response bias is crucial to obtaining valid and reliable data. Here are some strategies to minimise this bias:
- Ensure participants that their responses will remain anonymous and confidential. If they believe their responses can’t be traced back to them, they may be more honest.
- Frame questions in a neutral manner to avoid leading the respondent towards a particular answer.
- Questions that imply a correct answer can skew results. For example, instead of asking, “Don’t you think that X is good?” ask, “What is your opinion on X?”
- For multiple-choice questions, randomising the order of response options can reduce order bias.
- If using Likert scales, offer a neutral middle option and make sure the scale is balanced with an equal number of positive and negative choices.
- Before the main study, conduct a pilot test to identify any potential sources of bias in the questions.
- If the survey or experiment is too long, respondents might rush through it without giving thoughtful answers. Consider breaking up long surveys or providing breaks.
- Assure participants that there are no right or wrong answers and emphasise the importance of honesty.
- If feasible, use multiple measures or methods to assess the same construct. This helps in triangulating the data and checking for consistency.
- If the study involves personal interviews, ensure interviewers are trained to ask questions consistently and without introducing their biases. Publication bias can also have an impact if only studies with certain results are being published and others are not.
- Ensure that participants understand the questions and how to respond. Clarify terms or concepts that might be misunderstood. Checking with a scholarly source can be a good way to ensure the quality of your questions.
- After collecting initial data, consider giving feedback to participants on their responses. This may help clarify or correct misunderstandings.
- After participants have completed the survey or experiment, debrief them to understand their thought processes. This can provide insights into potential sources of bias.
The data collection method you choose (e.g., face-to-face interviews, phone interviews, online surveys) can influence response bias. For example, some people might be more honest in an online survey than in a face-to-face interview.
Frequently Asked Questions
What is response bias.
Response bias refers to the tendency of participants to answer questions untruthfully or misleadingly. This can be due to social desirability, leading questions, or misunderstandings. It affects the validity of survey or questionnaire results, making them not truly representative of the participant’s actual thoughts or feelings.
What is response bias in statistics?
In statistics, response bias occurs when participants’ answers are systematically influenced by external factors rather than reflecting their true feelings, beliefs, or behaviours. This can arise from question-wording, survey method, or the desire to present oneself in a favourable light, potentially skewing the results and reducing data accuracy.
What is voluntary response bias?
Voluntary response bias occurs when individuals choose whether to participate in a survey or study, leading to non-random samples. Those with strong opinions or feelings are more likely to respond, skewing results. This lack of representativeness can lead to inaccurate conclusions that do not reflect the broader population’s true views or characteristics.
How to minimise response bias?
To minimise response bias: use neutral question wording, ensure anonymity, randomise question order, avoid leading or loaded questions, provide a range of response options, pilot test the survey, use trained interviewers, and educate participants about the importance of honesty and accuracy. Choosing appropriate survey methods also helps reduce bias.
How does randomisation in an experiment combat response bias?
Randomisation in an experiment assigns participants to various groups randomly, ensuring that confounding variables are equally distributed. This reduces the likelihood that external factors influence the outcome. By minimising differences between groups, except for the experimental variable, randomisation combats potential biases, including response bias, leading to more valid conclusions.
You May Also Like
Social desirability refers to the tendency of some individuals to respond to questions in a manner that others will view favourably. This can lead to over-reporting “good behaviour” or under-reporting “bad behaviour”.
We all have filled out multiple surveys and questionnaires in our college days. These surveys are conducted to gather data. The common problem is that not everyone can take that survey, meaning individuals select themselves whether they want to respond or not. People have all the authority of this decision.
Have you ever noticed that we often tend to overestimate ourselves? We think we are more skilled than others in performing a certain task, ignoring the complexity of the project.
USEFUL LINKS
LEARNING RESOURCES
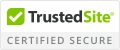
COMPANY DETAILS
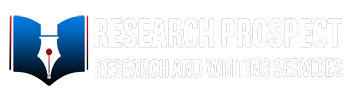
- How It Works
Response Bias: Definition and Examples
Design of Experiments > Response Bias
What is Response Bias?
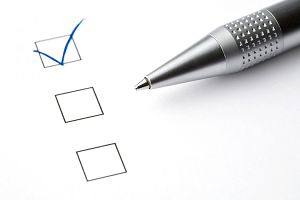
Self-Reporting Issues
People tend to want to portray themselves in the best light, and this can affect survey responses. According to psychology professor Delroy Paulhus , response bias is a common occurrence in the field of psychology, especially when it comes to self-reporting on:
- Personal traits
- Attitudes, like racism or sexism
- Behavior, like alcohol use or unusual sexual behaviors.
Questionnaire Format Issues
Misleading questions can cause response bias; the wording of the question may influence the way a person responds. For example, a person may be asked about their satisfaction for a recent online purchase and may be presented with three options: very satisfied, satisfied, and dissatisfied. By being given only one option for dissatisfaction, the consumer may be less inclined to pick that option. In some cases, the entire questionnaire may result in response bias. For example, this study showed that patients who are more satisfied tend to respond to surveys in higher numbers than patients who were dissatisfied. This leads to the overestimation of satisfaction levels.
Other questionnaire format problems include:
- Unfamiliar content : the person may not have the background knowledge to fully understand the question.
- Fatigue : giving a survey when a person is tired or ill may affect their responses.
- Faulty recall: asking a person about an event that happened in the distant past may result in erroneous responses.
Many of the above issues can be averted by providing an opt-out choice like “undecided” or “not sure.”
Experimenter Bias (Definition + Examples)

In the early 1900s, a German high school teacher named Wilhelm Von Osten thought that the intelligence of animals was underrated. He decided to teach his horse, Hans, some basic arithmetics to prove his point. Clever Hans, as the horse came to be known, was learning quickly. Soon he could add, subtract, multiply, and divide and would give correct answers by tapping his hoof. It took scientists over a year to prove that the horse wasn’t doing the calculations himself. It turned out that Clever Hans was picking up subtle cues from his owner’s facial expressions and gestures.
Influencing the outcome of an experiment in this way is called "experimenter bias" or "observer-expectancy bias."
What is Experimenter Bias?
Experimenter bias occurs when a researcher either intentionally or unintentionally affects data, participants, or results in an experiment.
The phenomenon is also known as observer bias, information bias, research bias, expectancy bias, experimenter effect, observer-expectancy effect, experimenter-expectancy effect, and observer effect.
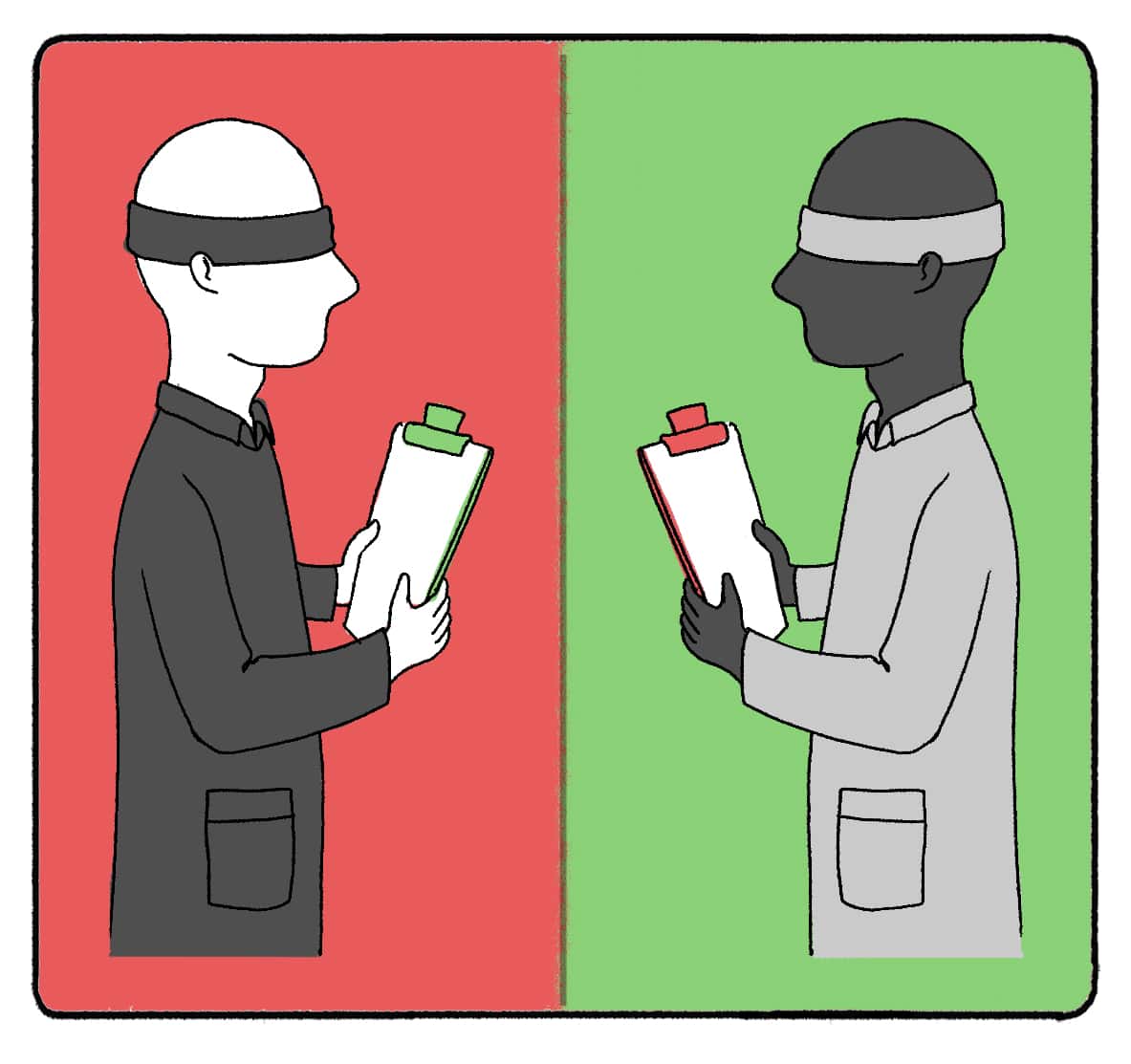
One of the leading causes of experimenter bias is the human inability to remain completely objective. Biases like confirmation bias and hindsight bias affect our judgment every day! In the case of the experimenter bias, people conducting research may lean toward their original expectations about a hypothesis without the experimenter being aware of making an error or treating participants differently. These expectations can influence how studies are structured, conducted, and interpreted. They may negatively affect the results, making them flawed or irrelevant. In a way, this is often a more specific case of confirmation bias .
Rosenthal and Fode Experiment
One of the best-known examples of experimenter bias is the experiment conducted by psychologists Robert Rosenthal and Kermit Fode in 1963.
Rosenthal and Kermit asked two groups of psychology students to assess the ability of rats to navigate a maze. While one group was told their rats were “bright”, the other was convinced they were assigned “dull” rats. The rats were randomly chosen, and no significant difference existed between them.
Interestingly, the students who were told their rats were maze-bright reported faster running times than those who did not expect their rodents to perform well. In other words, the students’ expectations directly influenced the obtained results.
Rosenthal and Fode’s experiment shows how the outcomes of a study can be modified as a consequence of the interaction between the experimenter and the subject.
However, experimenter-subject interaction is not the only source of experimenter bias. (It's not the only time bias may appear as one observes another person's actions. We are influenced by the actor-observer bias daily, whether or not we work in a psychology lab!)
Types of Experimenter Bias
Experimenter bias can occur in all study phases, from the initial background research and survey design to data analysis and the final presentation of results.
Design bias
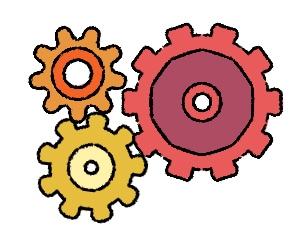
Design bias is one of the most frequent types of experimenter biases. It happens when researchers establish a particular hypothesis and shape their entire methodology to confirm it. Rosenthal showed that 70% of experimenter biases influence outcomes in favor of the researcher‘s hypothesis.
Example of Experimenter Bias (Design Bias)
An experimenter believes separating men and women for long periods eventually makes them restless and hostile. It's a silly hypothesis, but it could be "proven" through design bias. Let's say a psychologist sets this idea as their hypothesis. They measure participants' stress levels before the experiment begins. During the experiment, the participants are separated by gender and isolated from the world. Their diets are off. Routines are shifted. Participants don't have access to their friends or family. Surely, they are going to get restless. The psychologist could argue that these results prove his point. But does it?
Not all examples of design bias are this extreme, but it shows how it can influence outcomes.
Sampling bias
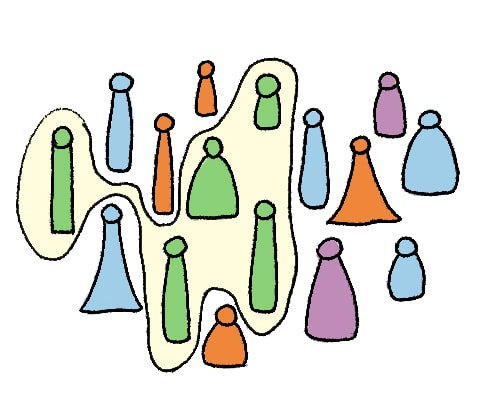
Sampling or selection bias refers to choosing participants so that certain demographics are underrepresented or overrepresented in a study. Studies affected by the sampling bias are not based on a fully representative group.
The omission bias occurs when participants of certain ethnic or age groups are omitted from the sample. In the inclusive bias, on the contrary, samples are selected for convenience, such as all participants fitting a narrow demographic range.
Example of Experimenter Bias (Sampling Bias)
Philip Zimbardo created the Stanford Prison Experiment to answer the question, "What happens when you put good people in an evil place?" The experiment is now one of the most infamous experiments in social psychology. But there is (at least) one problem with Zimbardo's attempt to answer such a vague question. He does not put all types of "good people" in an evil place. All the participants in the Stanford Prison Experiment were young men. Can 24 young men of the same age and background reflect the mindsets of all "good people?" Not really.
Procedural bias
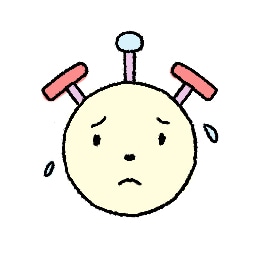
Procedural bias arises when how the experimenter carries out a study affects the results. If participants are given only a short time to answer questions, their responses will be rushed and not correctly show their opinions or knowledge.
Example of Experimenter Bias (Procedural Bias)
Once again, the Stanford Prison Experiment offers a good example of experimenter bias. This example is merely an accusation. Years after the experiment made headlines, Zimbardo was accused of "coaching" the guards. The coaching allegedly encouraged the guards to act aggressively toward the prisoners. If this is true, then the findings regarding the guards' aggression should not reflect the premise of the experiment but the procedure. What happens when you put good people in an evil place and coach them to be evil?
Measurement bias
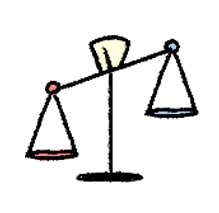
Measurement bias is a systematic error during the data collection phase of research. It can take place when the equipment used is faulty or when it is not being used correctly.
Example of Experimenter Bias (Measurement Bias)
Failing to calibrate scales can drastically change the results of a study! Another example of this is rounding up or down. If an experimenter is not exact with their measurements, they could skew the results. Bias does not have to be nefarious, it can just be neglectful.
Interviewer bias
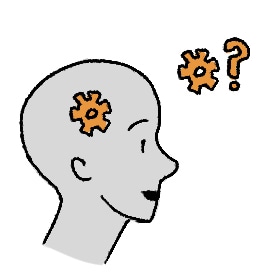
Interviewers can consciously or subconsciously influence responses by providing additional information and subtle clues. As we have seen in the rat-maze experiment, the subject's response will inevitably lean towards the interviewer’s opinions.
Example of Experimenter Bias (Interview Bias)
Think about the difference between the following sets of questions:
- "How often do you bathe?" vs. "I'm sure you're very hygienic, right?"
- "On a scale from 1-10, how much pain did you experience?" vs. "Was the pain mild, moderate, or excruciating?"
- "Who influenced you to become kind?" vs. "Did your mother teach you to use manners?"
The differences between these questions are subtle. In some contexts, researchers may not consider them to be biased! If you are creating questions for an interview, be sure to consult a diverse group of researchers. Interview bias can come from our upbringing, media consumption, and other factors we cannot control!
Response bias
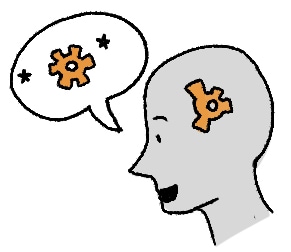
Response bias is a tendency to answer questions inaccurately. Participants may want to provide the answers they think are correct, for instance, or those more socially acceptable than they truly believe. Responders are often subject to the Hawthorne effect , a phenomenon where people make more efforts and perform better in a study because they know they are being observed.
Example of Experimenter Bias (Response Bias)
The Asch Line Study is a great example of this bias. Of course, researchers created this study to show the impact of response bias. In the study, participants sat among several "actors." The researcher asked the room to identify a certain line. Every actor in the room answered incorrectly. To confirm, many participants went along with the wrong answer. This is response bias, and it happens more often than you think.
Reporting bias

Reporting bias, also called selective reporting, arises when the nature of the results influences the dissemination of research findings. This type of bias is usually out of the researcher’s control. Even though studies with negative results can be just as significant as positive ones, the latter are much more likely to be reported, published, and cited by others.
Example of Experimenter Bias (Reporting Bias)
Why do we hear about the Stanford Prison Experiment more than other experiments? Reporting bias! The Stanford Prison Experiment is fascinating. The drama surrounding the results makes great headlines. Stanford is a prestigious school. There is even a movie about it! Yes, some biases went into the study. However, psychologists and content creators will continue discussing this experiment for many years.
How Can You Remove Experimenter Bias From Research?
Unfortunately, experimenter bias cannot be wholly stamped out as long as humans are involved in the experiment process. Our upbringing, education, and experience may always color how we gather and analyze data. However, experimenter bias can be controlled by sharing this phenomenon with people involved in conducting experiments first!
How Can Experimenter Bias Be Controlled?
One way to control experimenter bias is to intentionally put together a diverse team and encourage open communication about how to conduct experiments. The larger the group, the more perspectives will be shared, and biases will be revealed. Biases should be considered at every step of the process.
Strategies to Avoid Experimenter Bias
Most modern experiments are designed to reduce the possibility of bias-distorted results. In general, biases can be kept to a minimum if experimenters are properly trained and clear rules and procedures are implemented.
There are several concrete ways in which researchers can avoid experimenter bias.
Blind analysis
A blind analysis is an optimal way of reducing experimenter bias in many research fields. All the information which may influence the outcome of the experiment is withheld. Researchers are sometimes not informed about the true results until they have completed the analysis. Similarly, when participants are unaware of the hypothesis, they cannot influence the experiment's outcome.
Double-blind study
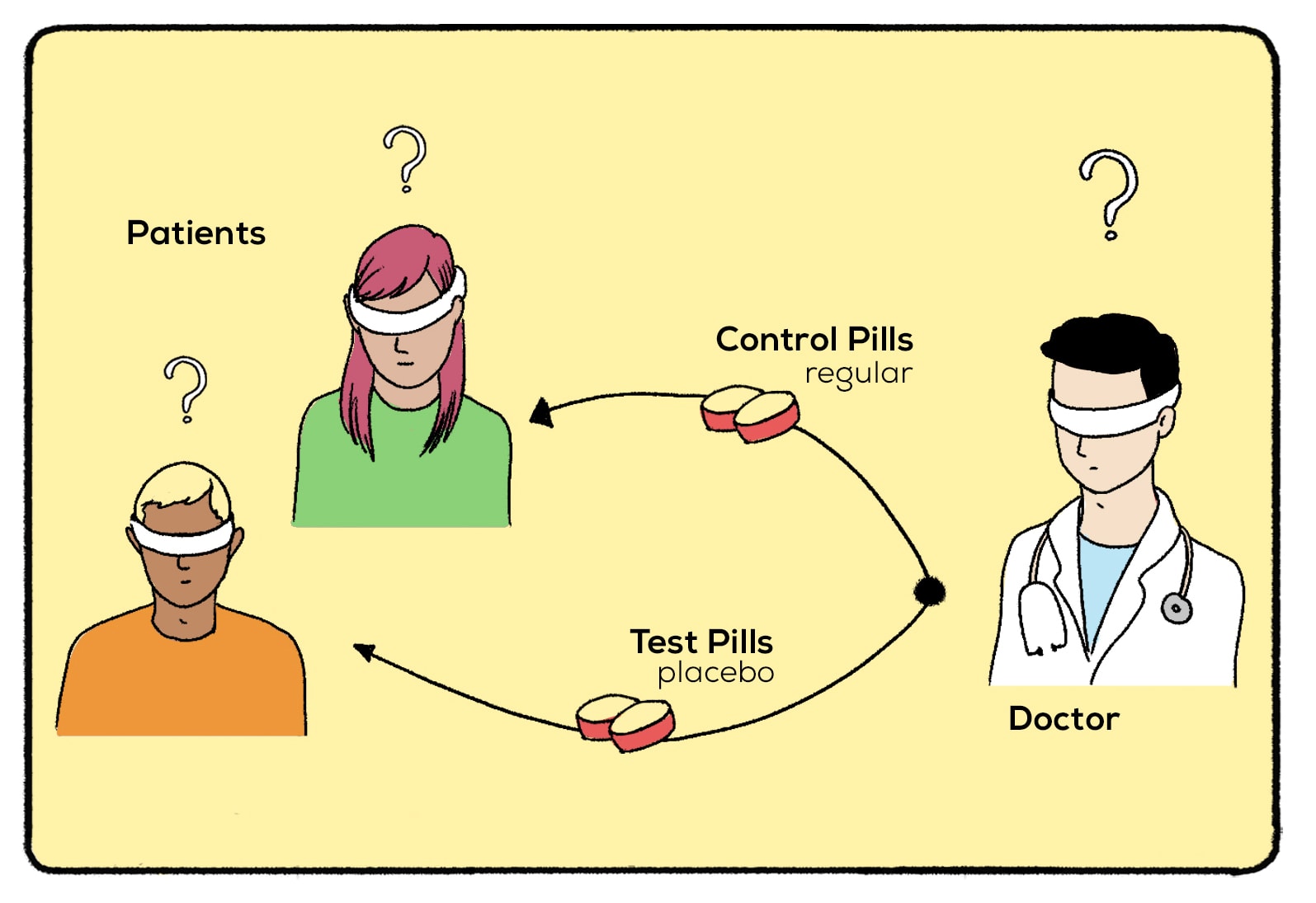
Double-blind techniques are commonly used in clinical research. In contrast to an open trial, a double-blind study is done so that neither the clinician nor the patients know the nature of the treatment. They don’t know who is receiving an actual treatment and who is given a placebo, thus eliminating any design or interview biases from the experiment.
Minimizing exposure
The less exposure respondents have to experimenters, the less likely they will pick up any cues that would impact their answers. One of the common ways to minimize the interaction between participants and experimenters is to pre-record the instructions.
Peer review
Peer review involves assessing work by individuals possessing comparable expertise to the researcher. Their role is to identify potential biases and thus make sure that the study is reliable and worthy of publication.
Understanding and addressing experimenter bias is crucial in psychological research and beyond. It reminds us that human perception and interpretation can significantly shape outcomes, whether it's Clever Hans responding to his owner's cues or students' expectations influencing their rats' performances.
Researchers can strive for more accurate, reliable, and meaningful results by acknowledging and actively working to minimize these biases. This awareness enhances the integrity of scientific research. It deepens our understanding of the complex interplay between observer and subject, ultimately leading to more profound insights into the human mind and behavior.
Related posts:
- 19+ Experimental Design Examples (Methods + Types)
- Backward Design (Lesson Planning + Examples)
- Actor Observer Bias (Definition + Examples)
- Philip Zimbardo (Biography + Experiments)
- Confirmation Bias (Examples + Definition)
Reference this article:
About The Author
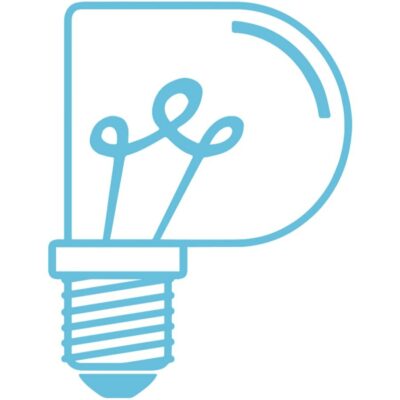
Free Personality Test
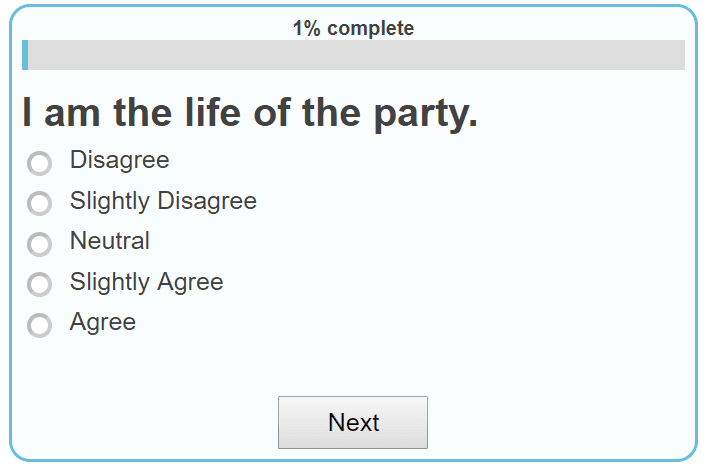
Free Memory Test
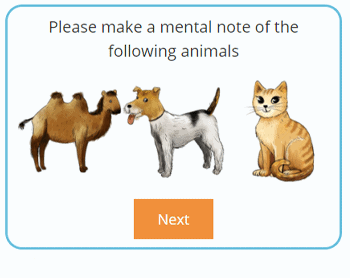
Free IQ Test
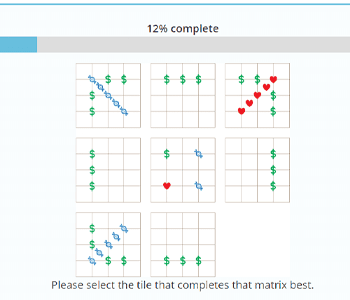
PracticalPie.com is a participant in the Amazon Associates Program. As an Amazon Associate we earn from qualifying purchases.
Follow Us On:
Youtube Facebook Instagram X/Twitter
Psychology Resources
Developmental
Personality
Relationships
Psychologists
Serial Killers
Psychology Tests
Personality Quiz
Memory Test
Depression test
Type A/B Personality Test
© PracticalPsychology. All rights reserved
Privacy Policy | Terms of Use
Root out friction in every digital experience, super-charge conversion rates, and optimize digital self-service
Uncover insights from any interaction, deliver AI-powered agent coaching, and reduce cost to serve
Increase revenue and loyalty with real-time insights and recommendations delivered to teams on the ground
Know how your people feel and empower managers to improve employee engagement, productivity, and retention
Take action in the moments that matter most along the employee journey and drive bottom line growth
Whatever they’re saying, wherever they’re saying it, know exactly what’s going on with your people
Get faster, richer insights with qual and quant tools that make powerful market research available to everyone
Run concept tests, pricing studies, prototyping + more with fast, powerful studies designed by UX research experts
Track your brand performance 24/7 and act quickly to respond to opportunities and challenges in your market
Explore the platform powering Experience Management
- Free Account
- Product Demos
- For Digital
- For Customer Care
- For Human Resources
- For Researchers
- Financial Services
- All Industries
Popular Use Cases
- Customer Experience
- Employee Experience
- Net Promoter Score
- Voice of Customer
- Customer Success Hub
- Product Documentation
- Training & Certification
- XM Institute
- Popular Resources
- Customer Stories
- Artificial Intelligence
- Market Research
- Partnerships
- Marketplace
The annual gathering of the experience leaders at the world’s iconic brands building breakthrough business results, live in Salt Lake City.
- English/AU & NZ
- Español/Europa
- Español/América Latina
- Português Brasileiro
- REQUEST DEMO
- Experience Management
- What is a survey?
- Response Bias
Try Qualtrics for free
What is response bias and how can you avoid it.
13 min read Response bias can hinder the results and success of your survey data. In this ultimate guide, we'll discover how to find it and prevent this bias before it's too late.
For a survey to provide usable data, it’s essential that responses are honest and unbiased.
The reason for this is simple: biased, misleading and dishonest responses lead to inaccurate information that prevents good decision making.
Indeed, if you can’t get an accurate overview of how respondents feel about something — whether it’s your brand, product, service or otherwise — how can you make the right decisions?
Now, when people respond inaccurately or falsely to questions, whether accidentally or deliberately, we call this response bias (also known as survey bias). And it’s a major problem when it comes to getting accurate survey data.
So how do you avoid response bias? In this guide, we’ll introduce the concept of response bias, what it is, why it’s a problem and how to reduce response bias in your future surveys.
Free eBook: The qualitative research design handbook
Response bias definition
As mentioned, response bias is a general term that refers to conditions or factors that influence survey responses.
There are several reasons as to why a respondent might provide inaccurate responses, from a desire to comply with social desirability and answer in a way the respondent thinks they ‘should’ to the nature of the survey and the questions asked.
Typically, response bias arises in surveys that focus on individual behavior or opinions — for example, their political allegiance or drinking habits. As perception plays a huge role in our lives, people tend to respond in a way they think is positive.
Using the drinking example, if a respondent was asked how often they consume alcohol and the options were: ‘frequently, sometimes and infrequently’, they’re more likely to choose sometimes or infrequently so they’re perceived positively.
However, dishonest answers that don’t represent the views of your sample can lead to inaccurate data and information that gradually becomes less useful as you scale your research.
Ultimately, this can have devastating effects on organizations that rely heavily on data-driven research initiatives as it leads to poor decision-making. It can also affect an organization’s reputation if they’re known for publishing highly accurate reports.
Types of Response bias
While ‘response bias’ is the widely understood term for biased or dishonest survey responses, there are actually several different types of response bias. Each one has the potential to skew or even ruin your survey data.
In this section, we’ll look at the different types of response bias and provide examples:
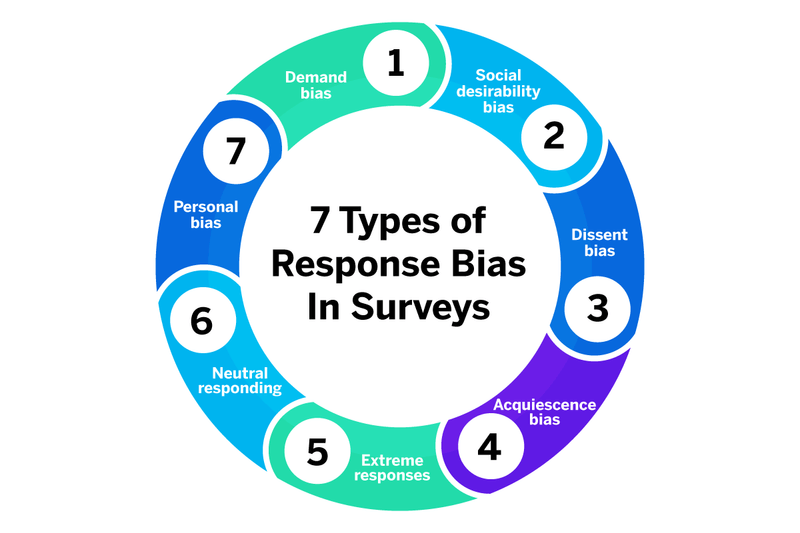
Social desirability bias
Social desirability bias often occurs when respondents are asked sensitive questions and — rather than answer honestly — provide what they believe is the more socially desirable response. The idea behind social desirability bias is that respondents overreport ‘good behavior’ and underreport ‘bad behavior’.
These types of socially desirable answers are often the result of poorly worded questions, leading questions or sensitive topics. For example, alcohol consumption, personal income, intellectual achievements, patriotism, religion, health, indicators of charity and so on.
In surveys that are poorly worded or leading, questions are posed in a way in which encourages respondents to provide a specific answer. One of the most obvious examples of a question that will trigger social desirability bias is: “Do you think it’s appropriate to drink alcohol every day?”
Even if the respondent wants to answer honestly, they’ll respond in a more socially acceptable way.
Social desirability bias also works with affirmative actions. For example, asking someone if they think everyone should donate part of their salary to charity is guaranteed to generate a positive response, even if those in the survey don’t do it.
Non-response bias
Non-response bias — which is sometimes called late response bias — is when people who don’t respond to a survey question differ significantly to those who do respond.
This type of bias is inherent in analytical or experimental research, for example, those assessing health and wellbeing.
Non-response bias is tricky for researchers, precisely because the experiences or outcomes of those who don’t respond could wildly differ to the experiences of those who do respond. As a consequence, the results may then over or underrepresent a particular perspective.
For example, imagine you’re conducting psychiatric research to analyze and diagnose major depression in a population. The behaviors of those who do respond could be vastly different to those who don’t, but you end up overreporting one perspective from your sample.
In this example, there’s no way to make an accurate assumption or fully understand what the survey data is telling you.
Demand bias
Demand bias (demand characteristics or sometimes called survey bias ) occurs when participants change their behaviors or views simply because they assume to know or do know the research agenda.
Demand characteristics are problematic because they can bias your research findings and arise from several sources. Think of these as clues about the research hypotheses:
- The title of the study
- Information about the study or rumors
- How researchers interact with participants
- Study procedure (order of tasks)
- Tools and instruments used (e.g. cameras, apparel)
All of these demand characteristics place hidden demands on participants to respond in a particular way once they perceive them. For example, in one classic experiment published in Psychosomatic Medicine , researchers examined whether demand characteristics and expectations could influence menstrual cycle symptoms reported by study participants.
Some participants were informed of the purpose of the study, while the rest were left unaware. The informed participants were significantly more likely to report negative premenstrual and menstrual symptoms than participants who were aware of the study’s purpose.
Survey research methods can also determine the risks of common demand characteristics, e.g. structured interviews when the responder is physically asked survey questions by another person, or when participants take part in group research.
As a result of the above, rather than present their true feelings, respondents are more likely to give a more socially acceptable answer — or an answer which they believe is what the researchers want them to say.
Extreme response bias
Extreme response bias occurs when the respondents answer a question with an extreme view, even if they don’t have an extreme opinion on the subject.
This bias is most common when conducting research through satisfaction surveys. What usually happens is respondents are asked to rank or rate something (whether it’s an experience, the quality of service or a product) out of 5 points and choose the highest or lowest option, even if it’s not their true stance.
For example, with extreme response bias, if you asked respondents to rate the quality of service they received at a restaurant out of 5, they’re much more likely to say 5 or 1, rather than give a neutral response.
Similarly, a respondent might strongly disagree with a given statement, even if they have no strong feelings towards the topic.
Extreme responding can often occur due to a willingness to please the person asking the question or due to the wording of the question, which triggers a response bias and pushes the respondent to answer in a more extreme way than they otherwise would.
For example: “We have a 5* star customer satisfaction rating, would you agree that we provide a good service to customers?”
Neutral responses
As you can guess, neutral response bias is the opposite of extreme response bias and occurs when a respondent simply provides a neutral response to every question.
Neutral responses typically occur as a result of the participant being uninterested in the survey and/or pressed for time. As a result, they answer the questions as quickly as possible.
For example, if you’re conducting research about the development or HR technology but send it to a sample of the general public — it’s highly unlikely that they’ll be interested and will therefore aim to complete the survey as quickly as possible.
This is why it’s so important that your research methodology takes into consideration the sample and the nature of your survey before you put it live.
Acquiescence bias
Acquiescence bias is like an extreme form of social desirability, but instead of responding in a ‘socially acceptable’ way, respondents simply agree with research statements — regardless of their own opinion.
To put it simply, acquiescence bias is based on respondents’ perceptions of how they think the researcher wants them to respond, leading to potential demand effects. For example, respondents might acquiesce and respond favorably to new ideas or products because they think that’s what a market researcher wants to hear.
Similarly, depending on how a question is asked or how an interviewer reacts, respondents can infer cues as to how they should respond.
You can read more about acquiescence bias here .
Dissent bias
Dissent bias, as its name suggests, is the complete opposite of acquiescence bias in that respondents simply disagree with the statements they’re presented with, rather than give true opinions.
Sometimes, dissent bias is intentional and representative of a respondent’s views or (more likely) their lack of attention and/or desire to get through the survey faster.
Dissent bias can negatively affect your survey results, and you’ll need to consider your survey design or question wording to avoid it.
Voluntary response bias
Voluntary response biases in surveys occur when your sample is made of people who have volunteered to take part in the survey.
While this isn’t inherently detrimental to your survey or data collection, it can result in a study that overreports on one aspect as you’re more likely to have a highly opinionated sample.
For example, call-in radio shows that solicit audience participation in surveys or discussions on controversial topics (e.g. abortion, affirmative action). Similarly, if your sample is composed of people who all feel the same way about a particular issue or topic, you’ll overreport on specific aspects of that issue or topic
This type of voluntary bias can make it particularly difficult to generate accurate results as they tend to overrepresent one particular side.
Cognitive bias
Cognitive bias is a subconscious error in thinking that leads people to misinterpret information from the world around them, affecting their rationality and accuracy of decisions. This includes trying to alter facts to fit a personal view or looking at information differently to align with predetermined thoughts.
For example, a customer who has had negative experiences with products like yours is most likely to respond negatively to questions about your product, even if they’ve never used it.
Cognitive biases can also manifest in several ways, from how we put more emphasis on recent events (recency bias) to irrational escalation — e.g. how we tend to justify increased investment in a decision, especially when it’s something we want. Empathy and social desirability are also considered cognitive biases as they alter how we respond to questions.
Having this response bias in your data collection can lead you to either over or underreport certain samples, influencing how and what decisions are made.
How to reduce, avoid and prevent response bias
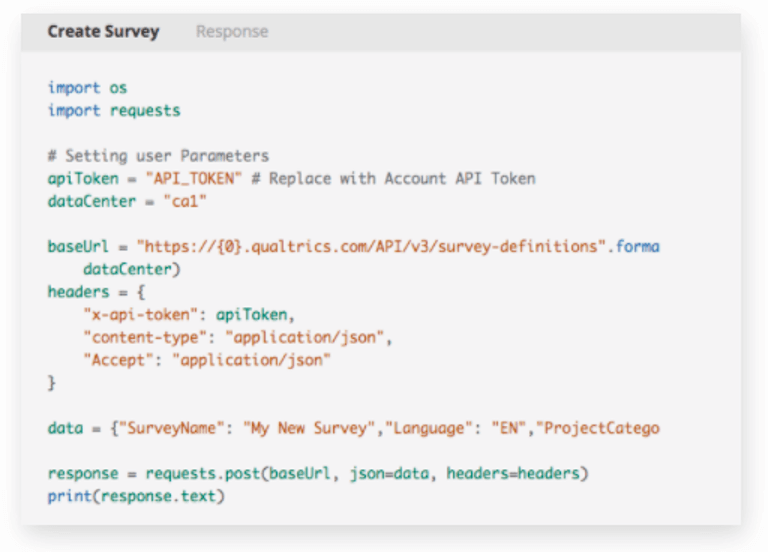
Whether it’s response bias as a result of overrepresenting a certain sample, the way questions are worded or otherwise, it can quickly become a problem that can compromise the validity of your study.
Having said that, it’s easily avoidable, especially if you have the correct survey tools, methodologies, and software in place. And Qualtrics can help.
With Qualtrics CoreXM , you have an all-in-one solution for everything from simple surveys to comprehensive market research . Empower everyone in your organization to carry out research projects, improve your research quality, reduce the risk of response bias and start generating accurate results from any survey type.
Reach the right respondents wherever they are with our survey and panel management tools. Then, leverage our data analytics capabilities to uncover trends and opportunities from your data.
Plus, you can use our free templates that provide hundreds of carefully created questions that further reduce response bias from your survey and ensure you’re analyzing data that will promote better business decisions.
Related resources
Double barreled question 11 min read, likert scales 14 min read, survey research 15 min read, survey bias types 24 min read, post event survey questions 10 min read, best survey software 16 min read, close-ended questions 7 min read, request demo.
Ready to learn more about Qualtrics?
- Media Center
Why do we give false survey responses?
Response bias, what is response bias.
The response bias refers to our tendency to provide inaccurate, or even false, answers to self-report questions, such as those asked on surveys or in structured interviews.
Where this bias occurs
Researchers who rely on participant self-report methods for data collection are faced with the challenge of structuring questionnaires in a way that increases the likelihood of respondents answering honestly. Take, for example, a researcher investigating alcohol consumption on college campuses through a survey administered to the student population. In this case, a major concern would be ensuring that the survey is neutral and non-judgmental in tone. If the survey comes across as disapproving of heavy alcohol consumption, respondents may be more likely to underreport their drinking, leading to biased survey results.
Individual effects
When this bias occurs, we come up with an answer based on external factors, such as societal norms or what we think the researcher wants to hear. This prevents us from taking time to self-reflect and think about how the topic being assessed is actually relevant to us. Not only is this a missed opportunity for critical thinking about oneself and one’s actions, but, in the case of research, it results in the provision of inaccurate data.
Systemic effects
Researchers need to proceed with caution when designing surveys or structured interviews in order to minimize the likelihood of respondents committing response bias. If they fail to do so, this systematic error could be detrimental to the entire study. Instead of progressing knowledge, biased results can lead researchers to draw inaccurate conclusions, which can have wide implications. Research is expensive to conduct and the questions under investigation tend to be of importance. For these reasons, tremendous effort is required in research design to ensure that all findings are as accurate as possible.
Why it happens
Response bias can occur for a variety of reasons. To categorize the possible causes, different forms of response bias have been defined.
Social desirability bias
First is social desirability bias, which refers to when sensitive questions are answered not with the truth, but with a response that conforms to societal norms. While there’s no real “right” answer to the survey question, social expectations may have deemed one viewpoint more acceptable than the other. In order to conform with what we feel is the appropriate stance, we tend to under- or over-report our own position.
Demand characteristics
Second, are demand characteristics. This is when we attempt to predict how the researcher wants us to answer, and adjust our survey responses to align with that. Simply being part of a study can influence the way we respond. Anything from our interactions with the researcher to the extent of our knowledge about the research topic can have an effect on our answers. This is why it’s such a challenge for the principal investigator to design a study that eliminates, or at least minimizes, this bias.
Acquiescence bias
Third, is acquiescence bias, which is the tendency to agree with all “Yes/No” or “Agree/Disagree” questions. This may occur because we are striving to please the researcher, or, as posited by Cronbach, 1 because we are motivated to call to mind information that supports the given statement. He suggests that we selectively focus on information that agrees with the statement, and unconsciously ignore any memories that contradict it.
Extreme responding
A final example of a type of response bias is extreme responding. It’s commonly seen in surveys that use Likert scales - a type of scaled response format with several possible responses ranging from the most negative to the most positive. Responses are biased when respondents select the extremity responses almost exclusively. That is to say, if the Likert scale ranges from 1 to 7, they only ever answer 1 or 7. This can happen when respondents are disinterested and don’t feel like taking the time to actively consider the options. Other times, it happens because demand characteristics have led the participant to believe that the researcher desires a certain response.
Why it is important
In order to conduct well-designed research and obtain the most accurate results possible, academics must have a comprehensive understanding of response bias. However, it’s not just researchers who need to understand this effect. Most of us have, or will go onto, participate in research of some kind, even if it’s as simple as filling out a quick online survey. By being aware of this bias, we can work on being more critical and honest in answering these kinds of questions, instead of responding automatically.
How to avoid it
By knowing about response bias and answering surveys and structured interviews actively, instead of passively, respondents can help researchers by providing more accurate information. However, when it comes to reducing the effects of this bias, the onus is on the creator of the questionnaire.
Wording is of particular importance when it comes to combating response bias. Leading questions can prompt survey-takers to respond in a certain way, even if it’s not how they really feel. For example, in a customer-satisfaction survey a question like “Did you find our customer service satisfactory?” subtly leans towards a more favorable response, whereas asking the respondent to “Rate your customer service experience” is more neutral.
Emphasizing the anonymity of the survey can help reduce social desirability bias, as people feel more comfortable answering sensitive questions honestly when their names aren’t attached to their answers. Utilizing a professional, non-judgemental tone is also important for this.
To avoid bias from demand characteristics, participants should be given as little information about the study as possible. Say, for example, you’re a psychologist at a university, investigating gender differences in shopping habits . A question on this survey might be something like: “How often do you go clothing shopping?”, with the following answer choices: “At least once a week”, “At least once a month”, “At least once a year”, and “Every few years”. If your participants figure out what you’re researching they may answer differently than they otherwise would have.
Many of us resort to response bias, specifically extreme responding and acquiescence bias, when we get bored. This is because it’s easier than putting in the effort to actively consider each statement. For that reason, it’s important to factor in length when designing a survey or structured interview. If it’s too long, participants may zone out and respond less carefully, thereby giving less accurate information.
How it all started
Interestingly, the response bias wasn’t originally considered much of an issue. Gove and Geerken claimed that “response bias variables act largely as random noise," which doesn’t significantly affect the results as long as the sample size is big enough. 2 They weren’t the only researchers to try and quell concerns over this bias but, more recently, it has become increasingly recognized as a genuine source of concern in academia. This is due to the overwhelming amount of research that has come out supporting the presence of an effect, for example, Furnham’s literature review. 3 Knäuper and Wittchen’s 1994 study also demonstrates this bias, specifically, in the context of standardized diagnostic interviews administered to the elderly, who engage in a form of response bias by tending to attribute symptoms of depression to physical conditions. 4
Example 1 - Depression
An emotion-specific response bias has been observed in patients with major depression, as evidenced by a study conducted by Surguladze et al. in 2004. 5 The results of this study showed that patients with major depression had greater difficulty discriminating between happy and sad faces presented for a short duration of time than did the healthy control group. This discrimination impairment wasn’t observed when facial expressions were presented for a longer duration. On these longer trials, patients with major depression exhibited a response bias towards sad faces. It’s important to note that discrimination impairment and response bias did not occur simultaneously, so it’s clear that one can’t be attributed to the other.
Understanding this emotion-specific response bias allows for further insight into the mechanisms of major depression, particularly into the impairments in social functioning associated with the disorder. It’s been suggested that the bias towards sad stimuli may cause people with major depression to interpret situations more negatively. 6
Researchers working outside of mental health should be aware of this bias as well, so that they know to screen for major depression should their survey include questions pertaining to emotion or interpersonal interactions.
Example 2 - Social media
Social media is a useful tool, thanks to both its versatility and its wide reach. However, while most of the surveys used in academic studies have gone through rigorous scrutiny and have been peer-reviewed by experts in the field, this isn’t always the case with social media polls.
Many businesses will administer surveys over social media to gauge their audience’s views on a certain matter. There are many reasons why the results of these kinds of polls should be taken with a grain of salt - for one thing, the sample is most certainly not random. In these situations, response bias is also likely at play.
Take, for example, a poll conducted by a makeup company, where the question is “How much did you love our new mascara?”, with the possible answers: “So much!” and “Not at all.” This is a leading question, which essentially asks for a positive response. Additionally, respondents may be prone to commit acquiescence bias in order to please the company, since there’s no option for a middle-ground response. Even if results of this survey are overwhelmingly positive, you might not want to immediately splurge on the mascara. The positive response could have more to do with the structure of the survey than with the quality of the product.
Response bias describes our tendency to provide inaccurate responses on self-report measures.
Social pressures, disinterest in the survey, and eagerness to please the researcher are all possible causes of response bias. Furthermore, the design of the survey itself can prompt participants to adjust their responses.
Example 1 - Major depression
People with major depression are more likely to identify a given facial expression as sad than people without major depression. This can impact daily interpersonal interactions, in addition to influencing responses on surveys related to emotion-processing.
Example 2 - Interpreting social media surveys
Surveys that aren’t designed to prevent response bias provide misleading results. For this reason, social media surveys, which can be created by anyone, shouldn’t be taken at face value.
When filling out a survey, actively considering each response, instead of answering automatically, can decrease the amount to which we engage in response bias. Anyone conducting research should take care to craft surveys that are anonymous, that are neutral in tone, that provide sufficient answer options, and that don’t give away too much about the research question.
Related Articles
How does society influence one’s behavior.
This article evaluates the ways in which our behaviors are molded by societal influences. The author breaks down the different influences our peers have on our actions, which is pertinent when it comes to exploring social desirability bias.
The Framing Effect
The framing effect describes how the way factors such as wording, setting, and situation influence our choices and opinions. The way survey questions are framed can lead to response bias, by causing respondents to over- or under-report their true viewpoint. This article elaborates on the implications of the framing effect, which are powerful and widespread.
Case studies
From insight to impact: our success stories, is there a problem we can help with.
- Cronbach, L. J. (1942). Studies of acquiescence as a factor in the true-false test. Journal of Educational Psychology, 33 (6), 401–415. doi: 10.1037/h0054677
- Gove, W. R., and Geerken, M. R. (1977). "Response bias in surveys of mental health: An empirical investigation". American Journal of Sociology . 82(6), 1289–1317. doi : 10.1086/226466
- Furnham, Adrian (1986). "Response bias, social desirability and dissimulation". Personality and Individual Differences . 7(3), 385–400. doi: 10.1016/0191-8869(86)90014-0
- Knäuper, Bärbel, and Wittchen, Hans-Ulrich (1994). "Diagnosing major depression in the elderly: Evidence for response bias in standardized diagnostic interviews?". Journal of Psychiatric Research . 28(2), 147–164. doi: 10.1016/0022-3956(94)90026-4
- Surguladze, S. A., Young, A. W., Senior, C., Brébion, G., Travis, M. J., & Phillips, M. L. (2004). Recognition Accuracy and Response Bias to Happy and Sad Facial Expressions in Patients With Major Depression. Neuropsychology, 18 (2), 212–218. doi: 10.1037/0894-4105.18.2.212
About the Authors

Dan is a Co-Founder and Managing Director at The Decision Lab. He is a bestselling author of Intention - a book he wrote with Wiley on the mindful application of behavioral science in organizations. Dan has a background in organizational decision making, with a BComm in Decision & Information Systems from McGill University. He has worked on enterprise-level behavioral architecture at TD Securities and BMO Capital Markets, where he advised management on the implementation of systems processing billions of dollars per week. Driven by an appetite for the latest in technology, Dan created a course on business intelligence and lectured at McGill University, and has applied behavioral science to topics such as augmented and virtual reality.

Dr. Sekoul Krastev
Sekoul is a Co-Founder and Managing Director at The Decision Lab. He is a bestselling author of Intention - a book he wrote with Wiley on the mindful application of behavioral science in organizations. A decision scientist with a PhD in Decision Neuroscience from McGill University, Sekoul's work has been featured in peer-reviewed journals and has been presented at conferences around the world. Sekoul previously advised management on innovation and engagement strategy at The Boston Consulting Group as well as on online media strategy at Google. He has a deep interest in the applications of behavioral science to new technology and has published on these topics in places such as the Huffington Post and Strategy & Business.
We are the leading applied research & innovation consultancy
Our insights are leveraged by the most ambitious organizations.

I was blown away with their application and translation of behavioral science into practice. They took a very complex ecosystem and created a series of interventions using an innovative mix of the latest research and creative client co-creation. I was so impressed at the final product they created, which was hugely comprehensive despite the large scope of the client being of the world's most far-reaching and best known consumer brands. I'm excited to see what we can create together in the future.
Heather McKee
BEHAVIORAL SCIENTIST
GLOBAL COFFEEHOUSE CHAIN PROJECT
OUR CLIENT SUCCESS
Annual revenue increase.
By launching a behavioral science practice at the core of the organization, we helped one of the largest insurers in North America realize $30M increase in annual revenue .
Increase in Monthly Users
By redesigning North America's first national digital platform for mental health, we achieved a 52% lift in monthly users and an 83% improvement on clinical assessment.
Reduction In Design Time
By designing a new process and getting buy-in from the C-Suite team, we helped one of the largest smartphone manufacturers in the world reduce software design time by 75% .
Reduction in Client Drop-Off
By implementing targeted nudges based on proactive interventions, we reduced drop-off rates for 450,000 clients belonging to USA's oldest debt consolidation organizations by 46%
Noble Edge Effect
Why do we tend to favor brands that show care for societal issues, look-elsewhere effect, why do scientists keep looking for a statistically significant result after failing to find one initially, naive allocation, why do we prefer to spread limited resources across our options.
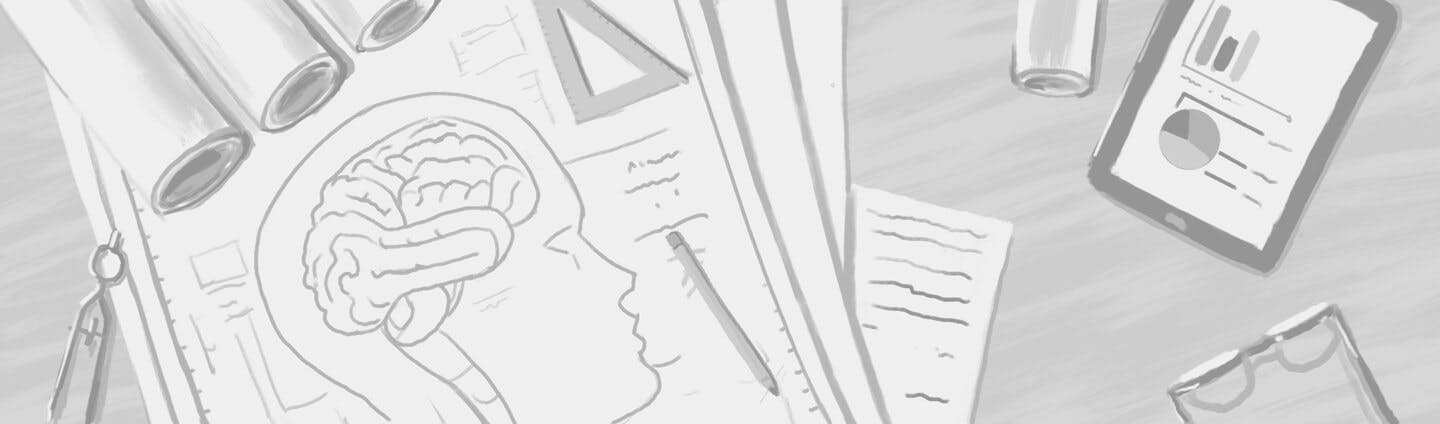
Eager to learn about how behavioral science can help your organization?
Get new behavioral science insights in your inbox every month..
Meet our leadership team
See how we care for the community
Get help, support, or just say hello
Lean how you can become a partner
See our upcoming events
Learn about our culture and jobs
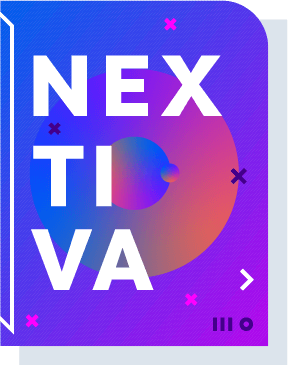
Grow Your Business
Nextiva is shaping the future of growth for all businesses. Start learning how your company can take everything to the next level.
See every product, app, and suite
VoIP phone service
Pipeline and customer management
VoIP, CRM, Live Chat, & Surveys
Worry-free phone service
Advanced business VoIP devices
Learn & Connect
Access our self-help portal
Find phone and product guides
See what people are saying about us
See fees for international calling
One-click VoIP readiness test
Uptime transparency
Nextiva Blog
- Customer Experience
- Business Communication
- Productivity
- Marketing & Sales
- Product Updates
Understanding the 6 Types of Response Bias (With Examples)
June 10, 2019 11 min read

Cameron Johnson
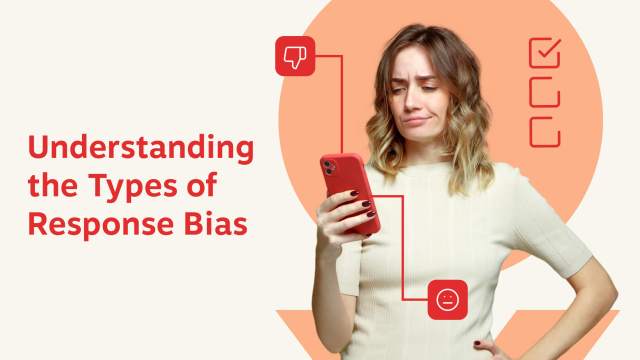
In this guide, we’ll breakdown one of the biggest challenges researchers face when it comes to surveying an audience — Response Bias. Let’s dive in. You need to know exactly what response bias is, what causes it, and crucially, how to avoid response set bias in your surveys. In this article, we cover it all:
- What is response bias?
- Why does response bias matter?
- What types of response bias are there?
- How do you get rid of response bias?
- Which survey tools should your company use?
What Is Response Bias?
This term refers to the various conditions and biases that can influence survey responses. The bias can be intentional or accidental, but with biased responses, survey data becomes less useful as it is inaccurate. This can become a particular issue with self-reporting participant surveys. Bias response is central to any survey, because it dictates the quality of the data, and avoiding bias really is essential if you want meaningful survey responses. Leading bias is one of the more common types. An example would be if your question asks about customer satisfaction , and the options given are Very Satisfied, Satisfied and Dissatisfied. In this instance there is bias that can affect results. To avoid bias here, you could balance the survey questions by including two of each of the positive and negative options.
Why Does Response Bias Matter?
A survey is a powerful tool for businesses because it provides the ability to obtain data and opinions from real members of the target audience, which gives a more accurate assessment of market position and performance than any trial-and-error tests could ever produce. When the goal of the survey is data collection, having the right sample size and survey methodology matter most.
What Types of Response Bias Are There?
One of the key things to avoid response bias is to fully understand how it happens. There are several types of response bias that can affect your surveys, and the ability to recognize each one can help you avoid bias in your surveys as you create them, rather than spotting it later. However, even with this understanding, it is always wise to have several people go through any survey design to check for possible causes of response bias before any survey is sent to respondents. This ensures that the resulting data is as accurate as possible. We will cover the main types of response bias here, and we will provide examples of response bias to show just how easy it is to introduce bias within the survey.
1) Demand Characteristics
One of the more common types of response bias, demand bias, comes from the respondents being influenced simply by being part of the study. This happens as respondents actually change their behavior and opinions as a result of taking part in the study itself. This can happen for several reasons, but it is important to understand each to be able to deal with each form of this response bias problem.
Participants who look to understand the purpose of the survey
For instance, if the survey is looking into a user experience of a website, a participant may see that the aim is to gather data to support making changes to layout or content. The participant may then answer in a way that supports those changes, rather than as they really think, resulting in untruthful or inaccurate responses.
The setting of the survey or study
This is more applicable to surveys carried in person, where researchers conducting the survey can have an influence on the respondents, but it can apply to digital surveys too.
Interaction between researcher and respondent
This can influence how the survey is approached. Note that if it’s a digital survey that researcher-to-respondent interaction is still possible, occurring in the email or message used to invite the respondent to participate.
Wording bias can come into effect here as well
This type of bias influences the entire gamut of responses from individual or multiple participants. For instance, if the researcher knows the participant personally, even greeting them in a friendly manner can have a subconscious effect on the responses. This is as true in an email as it is in person, so by retaining a formal approach to all participants regardless of who they are you can ensure a uniform response from all participants.
Prior knowledge of the survey
Whether the questions themselves, or the general aims of the survey, or how it is being put together, prior knowledge of some aspects of the survey deliver response bias. This is because the participants can become preoccupied with the survey itself, resulting in those participants second-guessing their own answers and providing inaccurate responses as a result.
2) Social Desirability Bias
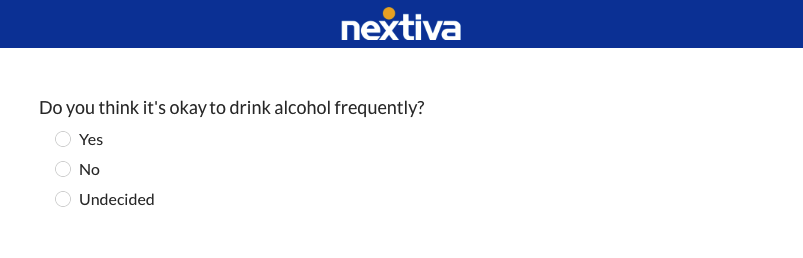

Maintain survey integrity
Participants second guessing research motives or finding out motives before taking the survey both result in response bias. Do this by maintaining the integrity of the survey and ensuring participants do not have additional information. To ascertain if participants have any understanding of the survey motives, a short after-survey questionnaire can be used.
6) Avoid Emotionally Charged Terms
Your questions should be clear, precise, and easily understandable. That means simple, unbiased language that avoids using words that evoke an emotional (rather than through-based) response. This includes words such as:
And so on. You want answers that are thought through, and these so-called ‘lightening’ words instead elicit an emotional response that is not as valuable for your research. In addition, try and avoid using a lot of negative words, as these can have an effect on how the participant perceives the survey and skew their responses. Finally, avoid word tricks. Be transparent with the audience and allow them to develop their answers. All of these are relatively simple steps to deliver improved survey results. Removing response bias will help you acquire accurate, unbiased data, and reflect the real views of your audience.
What Survey Tools Should Your Company Use?
Even if you know the various types of response bias, you still need to monitor the survey for problems and inaccurate data. Nextiva has two tools designed to do this for you, providing performance combined with ease of use for seamless integration into your workflow.
Nextiva Survey Analytics
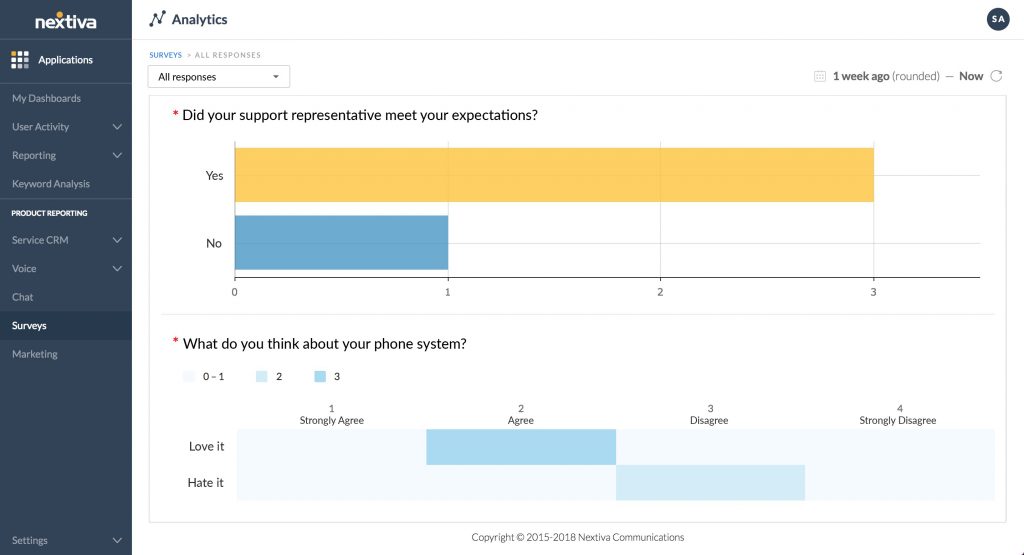
Survey analytics provides business intelligence efficiency with a comprehensive feature set that tracks survey response data throughout your research. This provides simple, clear, visual presentation of the data you need. Through this simple interface you can drill down to get a complete performance picture, analyzing results right down to the individual respondent if necessary. All data is easily accessible, saving time and frustration. With a visual representation of aggregate responses to any question, you can quickly identify trends and anomalies as they are occurring.
Nextiva Surveys
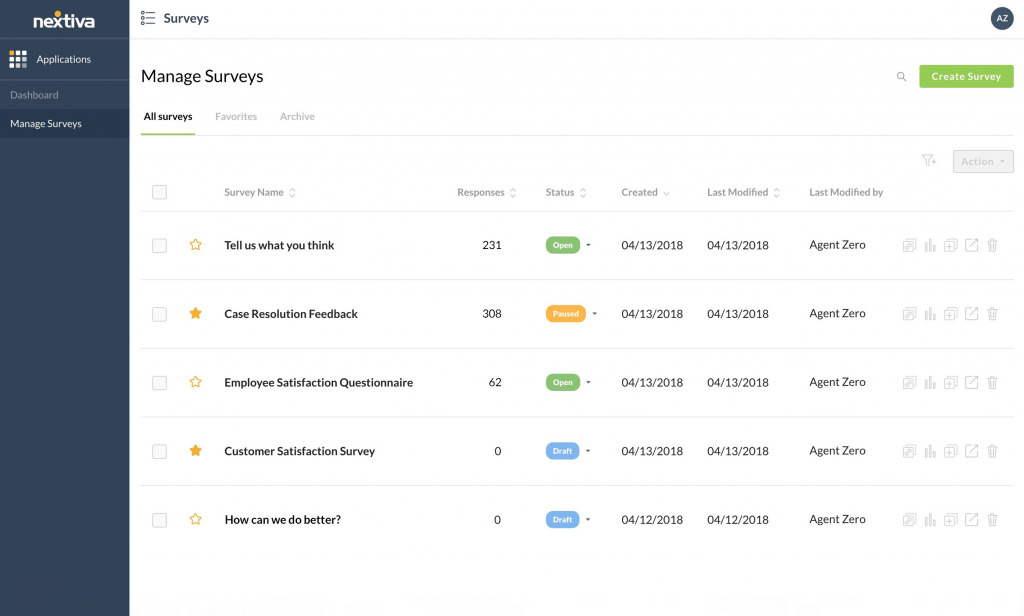
A complete software solution for all your surveys, Nextiva Surveys provides the perfect platform for all your research. With a simple, fast design solution, your surveys will look great. And full customization ensures they always reflect your brand image.
With no coding required, you can get beautiful, rich surveys put together in just a few minutes, saving time and money without sacrificing quality or control. There are templates for all types of questions, complete security, and features including skip logic for a personalized experience. Nextiva Surveys has all the tools you need to create response bias-free, effective surveys that produce exceptional results. Platform free, you can deliver surveys in an email or via the web. The responsive design provides an exceptional user experience even on mobile devices. When it comes to response bias-free surveys, Nextiva has your back. With our easy-to-use survey software creating effective surveys that avoid response bias are just a few clicks away. Try them now and see how easy surveys can be!
ABOUT THE AUTHOR
Cameron Johnson was a market segment leader at Nextiva. Along with his well-researched contributions to the Nextiva Blog, Cameron has written for a variety of publications including Inc. and Business.com. Cameron was recently recognized as Utah's Marketer of the Year.

- Oct 18, 2023
Response Bias Project Makeover

Leigh Nataro teaches elementary statistics, math for business, and math for teaching at Moravian University in Bethlehem, PA. Leigh has been an AP Exam Reader and Table Leader and was on the AP Statistics Instructional Design Team, where she helped to tag items for the AP Classroom question bank. In addition to leading AP Statistics workshops, Leigh leads in-person and virtual Desmos workshops with Amplify. Leigh can be reached on Twitter at @mathteacher24 .
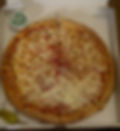
A picture of a pizza is shown to a student and then the student is randomly assigned to answer one of two questions.
Question 1: Would you eat this pizza?
Question 2: Would you eat this vegan pizza?
Does telling a person that a food is vegan impact their response? This was the experiment created by a pair of my students for one of the most engaging projects done in AP Statistics - The Response Bias Project . Students create an experiment to see if they can purposefully bias the results of a question.
Does inserting the word “vegan” into this question while showing the exact same picture bias the results? Here are the results obtained by the students. What do you think?

In my original iteration of this project, students would create various colorful (and often glitter-laden) graphs to compare the results.
But how likely would it be to get these results by chance if no bias was present?
To answer this question, The Response Bias Project needed a makeover.
The Makeover: Include a Simulation
One of the most challenging AP Stats topics for students is estimating p-values from simulations. This is one of the reasons we do a simulation on the first day of class with playing cards called “Hiring Discrimination: It Just Won’t Fly”. (An online version of the scenario and simulation can be found here: Hiring Discrimination .) Several times during the first semester, we use playing cards to perform simulations and then interpret the results to determine if a given claim is convincing. Although we never formally call the results a p-value, examining the dotplots from simulated results sets the stage for tests of significance in the second half of the course. Making over this project with a simulation leads to more robust conclusions and reinforces the idea of a p-value based on simulations.
Originally my students used the free trial of Fathom to create their simulations. However, in recent years Fathom has not been supported on newer Mac operating systems. This led me to investigate using the Common Online Data Analysis Platform or CODAP . This is a free online tool for data analysis and it is specifically designed for use with students in grades 6-14. Students can save their work in google drive, but an account is not required to access CODAP.
To understand what students need to do to create their simulation, I share an instructional video related to one of the projects. Here is the video that goes with the vegan pizza project: CODAP for Response Bias Project and the CODAP file: Vegan Pizza Simulation .

Based on their results from the simulation, the students determined if adding "vegan" created biased results or if it was possible to get results like what they saw in their experiment due to chance alone. Students display their work on posters that are hung around the room. (A sample of posters is included at the end of the blog.) Then, half the class stands by their posters and they present to the few students that are in front of them. This takes about five minutes. Then, the students move on to another poster and pair of presenters. Students get to give their presentation about three or four times to different small groups of their peers. There are no powerpoints, no notecards, less nervousness and stress for students and this gives them practice with more informal presentations they might need to give at some point in the future. Another benefit is students get to see multiple instances of simulated results which helps to lay the foundation of the concept of a p-value for future units in the course.
Why Is This Makeover Helpful?
Although students learn about some common topics from AP Statistics earlier in their math careers, simulation is a topic that is new and often challenging for many students. Creating the simulation in CODAP helps students to understand what each dot in the simulation represents and how the overall distribution shows more likely and less likely outcomes. Students also identify where the value of their statistic (the count of yes answers) falls on the dotplot. They are essentially answering the test of significance question, that is “assuming there was no bias, how many times did the observed outcome or a more extreme outcome occur by chance alone?” Reading about the results on their classmates' posters and hearing about it in multiple presentations solidifies the concept of using probabilities from simulations to draw conclusions.
The Concept of Simulations from the AP Statistics Course and Exam Description
Skill 3.A: Determine relative frequencies, proportions, or probabilities using simulation or calculations.
UNC.2.A.4 Simulation is a way to model random events, such that simulated outcomes closely match real-world outcomes. All possible outcomes are associated with a value to be determined by chance. Record the counts of simulated outcomes and the count total.
UNC.2.A.5 The relative frequency of an outcome or event in simulated or empirical data can be used to estimate the probability of that outcome or event.
Note: On the 2023 AP Statistics Operational Exam, determining a probability based on data from a simulation was part of free-response question 6 .
To understand how students are expected to use simulations on the AP Exam, consider Free Response Question 5 from the 2022 AP exam . Students were asked to use results from a simulation to estimate a p-value from a dotplot. Understanding what each dot represented and that they needed to determine where the sample statistic of 5.66 fell relative to the dots on the plot were the two key concepts needed to calculate the correct p-value. On the dotplot only 3 of the 120 simulated differences in means was 5.66 or higher. Students then needed to also compare the p-value to 0.05 and state their conclusion in context.
2022 #5 estimating a P-value from a dotplot

Examples of Student Posters:

- AP Statistics
Recent Posts
Response Bias Project
What Does This Dot Represent?
The Holy Grail of AP Statistics: The P-value
Instant insights, infinite possibilities
What is response bias and how do you avoid it?
Last updated
18 March 2023
Reviewed by
Response bias can significantly impact the research results, ultimately introducing errors and inaccuracies in the data.
Researchers can use statistical techniques to detect and correct response bias in the data analysis phase. They can also use other techniques, such as asking follow-up questions to clarify responses and ensuring the research instrument is culturally sensitive and appropriate for the target population.
Free template to analyze your survey results
Analyze your survey results in a way that's easy to digest for your clients, colleagues or users.
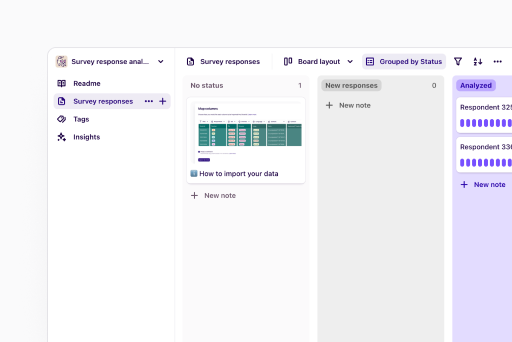
- Response bias definition
Response bias refers to the tendency of survey respondents to answer questions inaccurately or in a particular direction, resulting in biased or distorted data. The bias can arise from various factors, such as:
Social desirability
Acquiescence
Confirmation bias
Response bias can lead to inaccurate or misleading data, making it difficult to draw reliable conclusions. It can also be challenging to detect and correct response bias, especially if it's not recognized or addressed during the study design or data collection process.
If not appropriately addressed, response bias can have significant implications for the validity and reliability of survey results and can lead to erroneous conclusions.
- Types of response bias
The different types of response bias that can occur in survey research include:
1. Social desirability bias
Social desirability bias happens when survey respondents give answers they perceive as socially desirable or acceptable rather than their actual beliefs or experiences.
This can manifest in various ways, such as over-reporting socially desirable behaviors like volunteering or exercising or under-reporting socially undesirable behaviors like smoking or substance abuse.
For example, if a survey asks respondents about their alcohol consumption, those who believe heavy drinking is socially frowned upon may underreport their drinking habits or provide responses that reflect lower consumption levels.
Similarly, respondents may overstate their charitable giving or community involvement activities to appear more socially responsible or altruistic.
2. Non-response bias
Non-response bias occurs when a group of people selected to participate in a survey don't respond, resulting in a sample unrepresentative of the population. This bias can arise due to various reasons, such as:
Lack of interest or motivation to participate
Inability to reach or locate certain individuals
Feeling uncomfortable with the research topic
For example, if you’re using telephone calls to conduct a survey on political preferences, your sample will exclude people who don’t own a telephone. This may lead to a biased estimate of political preferences.
In addition, individuals who participate less in a survey may be less likely to respond, leading to an under-representation of their perspectives.
3. Demand bias
When survey respondents provide answers they think the researcher wants to hear rather than their actual beliefs or experiences, they create demand bias. This occurs because respondents may perceive the researcher has a particular agenda or hypothesis, and they adjust their responses accordingly.
For example, if a company produces a survey about one of its products or services, respondents will likely provide positive feedback to avoid upsetting the sponsor or to improve their chances of receiving future incentives or rewards.
Alternatively, if a survey asks respondents about their political views, respondents may feel pressure to align their responses with the perceived political leanings of the researcher.
4. Extreme response bias
Extreme response bias occurs when survey participants consistently choose the most extreme response options rather than providing moderate responses.
For example, if a survey asks respondents to rate their satisfaction with a product or service on a scale of 1 to 10, some may consistently choose the lowest or highest possible rating rather than providing an accurate response.
Researchers should consider using techniques such as reversing the polarity of some questions or using a response scale with an unprejudiced midpoint.
5. Neutral responses
Neutral responses refer to survey responses where the participant selects the middle option on a response scale or chooses a response indicating a lack of opinion or knowledge on the surveyed topic.
While using neutral responses can help avoid a forced choice and allow respondents to express their ambivalence or lack of knowledge on a particular topic, it can also result in uninformative or meaningless responses. The overuse of neutral responses can skew the distribution of responses and lead to inaccurate conclusions.
To mitigate these biases, researchers can add a "N/A" response or an "I don't have an opinion on this topic" option. They can also create a more forced-choice option with no middle point on the scale, which can help to elicit more decisive responses. These steps can help researchers reduce the impact of response bias and improve the accuracy of their findings.
6. Acquiescence bias
A response bias in which survey respondents tend to agree with statements or questions regardless of their beliefs or attitudes is acquiescence bias. This bias can lead to inaccurate data because respondents may not provide honest or accurate answers.
Acquiescence bias can arise for several reasons. Some people may be more agreeable to avoid conflict, while others may feel pressure to provide socially desirable responses. Additionally, respondents may not fully understand the question or feel it's easier to agree than to think deeply about their response.
For example, if a survey asks respondents to rate their agreement with a series of statements on a scale of 1 to 5, a respondent who exhibits acquiescence bias may tend to select higher ratings even if they disagree with the statements.
This act can lead to overestimating the prevalence of certain attitudes or beliefs in a population. It can also skew the results of factor analysis or other statistical techniques relying on the assumption respondents are answering truthfully.
7. Dissent bias
Dissent bias is a response bias that can arise when respondents feel the survey is biased or they are suspicious of the research intent.
Dissent bias can lead to inaccurate data because the responses provided by respondents don't reflect their true beliefs or attitudes. It can be problematic in surveys aiming to measure public opinion or attitudes toward controversial topics.
Additionally, some respondents may hold opinions that differ from those of the survey administrator or the prevailing social norms.
8. Voluntary response bias
Voluntary response bias happens in survey research when individuals self-select themselves into a sample. This method can lead to inaccurate data because the sample may not represent the studied population.
Voluntary response bias can arise when you conduct a survey using a method that allows individuals to choose whether or not to participate. For example, a television station may ask viewers to call in with their opinions on a particular issue. In this case, the sample comprises only those individuals who choose to call in, which may not represent the broader population
9. Cognitive bias
A cognitive bias is a systematic error of deviating from rational and objective thinking. Individuals can make judgments and decisions based on subjective factors rather than objective facts or evidence. Cognitive biases can occur in various aspects of human cognition, such as memory, perception, attention, and reasoning, leading to inaccurate or illogical judgments and decisions.
There are numerous different types of cognitive biases, including:
Availability bias
Anchoring bias
Framing effect
Hindsight bias
For example, in confirmation bias, people tend to seek out and interpret information to confirm their pre-existing beliefs while ignoring information contradicting those beliefs. The result can lead to overconfidence in the accuracy of their beliefs, preventing them from considering alternative viewpoints.
- How do you get rid of response bias?
Response bias occurs when the responses given by a participant in a survey or study do not accurately reflect their true thoughts, feelings, or behaviors.
Here are some methods researchers can use to reduce response bias:
1. Understand your demographic
Understanding your demographic can help minimize response bias by allowing researchers to design surveys or studies tailored to the target population's specific characteristics and experiences. This approach can increase the questions' relevance and clarity and help ensure participants can provide more accurate and honest responses.
Additionally, understanding the target population's demographic characteristics can allow researchers to identify potential sources of response bias, such as social desirability or acquiescence bias. By anticipating these biases and designing questions that decrease their impact, researchers can increase the accuracy and reliability of the survey results.
2. Avoid question-wording bias
Avoiding question-wording bias is essential in lessening response bias because it helps ensure you phrase questions in a clear, neutral, and unbiased manner. Question-wording bias occurs when the wording of a question is unintentionally or intentionally biased, leading to inaccurate or unreliable responses.
Here are some ways in which avoiding question-wording bias can help decrease response bias:
Use clear and simple language. Clear and simple language is important because it helps ensure respondents understand the questions asked. For example, instead of asking "Do you support a carbon tax to reduce greenhouse gas emissions?", a clearer and simpler way to phrase the question would be "Do you support a tax on carbon pollution?"
Avoid biased or leading language. Biased or leading language can influence respondents' answers by suggesting a particular response or perspective. For example, instead of asking "Don't you agree that our new policy is an improvement?", a more neutral and unbiased way to phrase the question would be "What is your opinion of our new policy?"
Avoid double-barreled questions. Double-barreled questions ask about two different issues or concepts at once. For example, instead of asking "Do you think the government should prioritize healthcare and education?", it would be better to ask "Do you think the government should prioritize healthcare?" and "Do you think the government should prioritize education?" separately.
Use a small sample of participants. Using a small sample of participants may not directly help to decrease response bias. However, it can help to improve the quality and accuracy of data collected by allowing researchers to pilot test their survey questions and identify potential biases or issues before administering the survey to a larger sample.
Shunning question-wording bias can help minimize response bias by ensuring survey questions are clear, unambiguous, and unbiased, increasing the accuracy and reliability of the survey results.
3. Diversify questions
By comprehensively understanding participants' thoughts, feelings, and behaviors, broadening questions can reduce response bias. This approach can help capture a wider range of perspectives and experiences, reducing the risk of bias resulting from relying on a narrow set of questions or perspectives.
Researchers can consider using a mix of questions, such as open-ended questions , multiple choices, or rating scales , vary the specificity question level, and include questions from different perspectives, like exploring an issue's positive and negative aspects.
4. Allow participants to say "no"
Letting participants say "no" can help reduce response bias by allowing them to opt out of questions they feel uncomfortable answering, don't have an opinion about, or don’t understand. This strategy can reduce social desirability bias, where participants may feel pressure to answer in a socially acceptable or desirable way, even if it doesn't reflect their true thoughts or feelings.
Researchers should ensure they present the study materials and questions in a non-coercive and non-judgmental manner. It will help create a safe and comfortable environment for participants, which increases their willingness to participate in the study and provide honest and accurate responses.
5. Effective administration
Effective administration is vital to lessening response bias and maintaining survey integrity. Researchers must always remain neutral and avoid behavior or actions that may influence participants' responses or compromise the survey's integrity.
Ways to ensure the effective administration of a survey can include:
Standardizing the survey procedures
Train survey administrators
Ensure confidentiality in participant's responses
Minimize participant's burden by having clear and unbiased survey questions
Monitor participant's response rate
Effective administration involves a range of practices and strategies designed to create a comfortable and respectful environment for participants. It also ensures you conduct the study in a way that is neutral, unbiased, and respectful of participants' time and privacy.
6. Avoid emotionally charged terms
Using emotionally charged terms can lead to response bias, as it can influence participants to respond in a way that may not reflect their true thoughts or feelings. Emotionally charged terms can evoke strong emotions or personal values, such as "fair," "unfair," "moral," "immoral," "right," and "wrong."
By avoiding emotionally charged terms in a survey or research study, researchers can help reduce response bias and increase the reliability of the data collected. This approach can enhance the overall value of the research and ensure the results reflect participants' thoughts, feelings, and behaviors.
How do you identify a biased question?
A biased question attempts to influence the respondent towards a particular answer or point of view. Look for leading language, emotionally-charged words, false assumptions, limited answer choices, and omitted information.
What is response vs. non-response bias?
Response bias occurs when respondents provide inaccurate or untruthful answers. Non-response bias happens when individuals selected for a study do not participate, leading to a potentially unrepresentative sample.
Can the wording of a question create response bias?
Yes, the wording of a question can create response bias. It's known as question-wording bias. It occurs when you word a question in a way that influences how people interpret and respond to it, leading to inaccurate or misleading data.
Should you be using a customer insights hub?
Do you want to discover previous survey findings faster?
Do you share your survey findings with others?
Do you analyze survey data?
Start for free today, add your research, and get to key insights faster
Editor’s picks
Last updated: 4 March 2023
Last updated: 28 June 2024
Last updated: 16 April 2023
Last updated: 20 March 2024
Last updated: 22 February 2024
Last updated: 23 May 2023
Last updated: 21 December 2023
Last updated: 26 July 2023
Last updated: 14 February 2024
Last updated: 11 March 2023
Last updated: 13 May 2024
Last updated: 30 January 2024
Latest articles
Related topics, .css-je19u9{-webkit-align-items:flex-end;-webkit-box-align:flex-end;-ms-flex-align:flex-end;align-items:flex-end;display:-webkit-box;display:-webkit-flex;display:-ms-flexbox;display:flex;-webkit-flex-direction:row;-ms-flex-direction:row;flex-direction:row;-webkit-box-flex-wrap:wrap;-webkit-flex-wrap:wrap;-ms-flex-wrap:wrap;flex-wrap:wrap;-webkit-box-pack:center;-ms-flex-pack:center;-webkit-justify-content:center;justify-content:center;row-gap:0;text-align:center;max-width:671px;}@media (max-width: 1079px){.css-je19u9{max-width:400px;}.css-je19u9>span{white-space:pre;}}@media (max-width: 799px){.css-je19u9{max-width:400px;}.css-je19u9>span{white-space:pre;}} decide what to .css-1kiodld{max-height:56px;display:-webkit-box;display:-webkit-flex;display:-ms-flexbox;display:flex;-webkit-align-items:center;-webkit-box-align:center;-ms-flex-align:center;align-items:center;}@media (max-width: 1079px){.css-1kiodld{display:none;}} build next, decide what to build next, log in or sign up.
Get started for free
- Skip to primary navigation
- Skip to main content
- Skip to primary sidebar
- Skip to footer
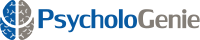
Psychologenie
The Diverse Types of Response Bias Explained With Examples
Response bias is a type of bias which influences a person's response away from facts and reality. This bias is mostly evident in studies interested in collecting participants' self-report, mostly employing a questionnaire format. A survey is a very good example of such a study, and is certainly prone to response biases. PsycholoGenie explains the different types of response biases, and illustrates them with simple examples.

Response bias is a type of bias which influences a person’s response away from facts and reality. This bias is mostly evident in studies interested in collecting participants’ self-report, mostly employing a questionnaire format. A survey is a very good example of such a study, and is certainly prone to response biases. PsycholoGenie explains the different types of response biases, and illustrates them with simple examples.
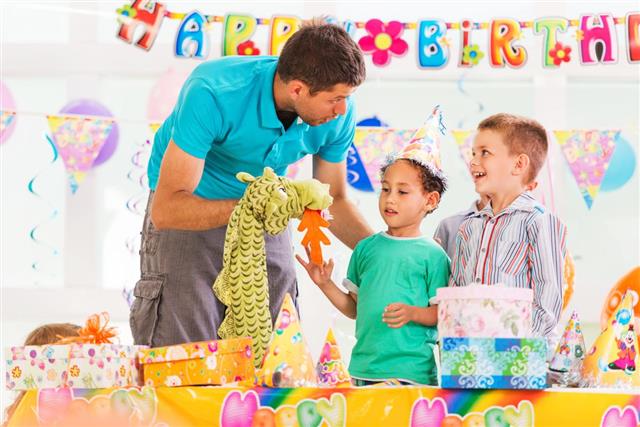
Non-response Bias
This shouldn’t be confused as the opposite of response bias. Non-response bias is not a cognitive bias, and is evident in statistical surveys wherein the responses of participants vary from the potential responses of those who didn’t respond.
The concept of response bias originates from psychology where it is categorized under cognitive biases. Cognitive bias simply means deviation from logical thought and judgment; this type of bias occurs especially when people try to behave in a socially desirable manner. Coming back to response biases, these are as said above, mostly seen during instances where people are asked to report their own behavior by the medium of a questionnaire.
Such biases have a great impact on the validity of the testing instrument (questionnaire), and could lead to inappropriate results and conclusions. Although the reliability (consistency in results) of such tests is comparatively higher, response biases hamper their validity (sole purpose of the test) and hence yield partial results.
Researchers should therefore be aware of these biases beforehand, and should take suitable measures to avoid them.
Types of Response Bias
Acquiescence bias.
As the name suggests, acquiescence bias is the type in which a respondent tends to agree to all the questions in the questionnaire. This type of bias is likely to result in untruthful reporting as the participant might agree with two contradictory responses. For instance, if the questionnaire has a question – “Are you a very bubbly and social person?”, and another one – “Would you prefer reading a novel to attending a party?”. If the participant agrees in both cases, he/she is subject to the acquiescence bias.
This type of bias has been reasoned in two major ways. First – the bias could emerge when a participant tries to be agreeable or compliant in order to avoid displeasure or disapproval of the researcher. However, this view was strongly criticized by the American educational psychologist, Lee Cronbach who proposed a second justification to this bias. According to Cronbach, this bias emerges more from the active cognitive (mental) processes of the participant and less from the motivation to please the researcher. His version suggests that as a participant thinks upon a question, he tries to dig out information from his/her memory to support the endorsement of a statement.
To illustrate Cronbach’s theory, let’s consider the two questions used above. “Are you a very bubbly and social person?”. The participant may agree to this as he is truly an extrovert and enjoys attending social gatherings and chitchatting. The second question however, contradicts the first – “Would you prefer reading a novel to attending a party?”, In this case, the participant thinks of an event wherein he wasn’t in a mood to attend some party and hence resorted to reading a detective novel. This makes him endorse the second statement as well.
The above bias could also occur in terms of disagreeing or denying statements.
Demand Characteristics
In this type of bias, the response of participants is simply influenced because they are a part of a study. To make it even simpler, the participants when in an experiment might adopt behavior they believe is correct in an experimental setting and not be themselves. There are several possibilities which could lead to the emergence of this bias. Firstly, if a participant tries to figure out the hypothesis being tested, he/she might alter his/her behavior in an attempt to support the hypothesis. For example, let’s say a college student is invited to be a participant to a certain study, the participant with the curiosity of why he’d be tested, finds out the hypothesis of the study and goes through the study with this information at the back of his mind.
Another reason for this bias to emerge could be the setting in which the study or the experiment is conducted. For instance the way the researcher greets, or interacts during the experiment could lead to this bias especially if the participant knows the researcher informally; he/she would reciprocate to the researcher in an altered, experiment-centered way instead of being him/herself. Let’s use the previous example of the college student; assuming that the student knows the researcher as a good friend, the researcher instead of saying “Hey what’s up buddy?” would say, “Hello Robert, how are you?”. This would automatically make Robert reply in a formal tone. Same would happen with the study/experiment.
Finally, a third reason for this bias could be the prior experiences of the participant of being in such an experiment or rumors about the experiment lead to a certain level of preoccupation in the participants’ minds, and they take the test while trying to confirm to the things they know about it. For instance, if Robert has attended a similar study before, he might try and relate things from the previous study to the current one. Similarly, if he has heard rumors about the current study, he might try to confirm those when participating
Extreme Responding
Quite clear from the name itself, extreme responding refers to giving extreme answers to questions – extremely negative or extremely positive. This is mostly evident in case the questionnaire offers a scale which expects the user to rate a certain aspect. Most of us do answer such questions wherein we have to rate or give feedback on a scale of 1-5, 5 being the highest; star-ratings are a good example of this type of questionnaires.
Several reasons could contribute to this bias, the first being cultural influences. Researchers have found that certain cultures are more prone than others to be affected by this kind of bias; these include people from Middle East, and Latin America. However, people from East Asia and Western Europe are less likely to be affected by it.
A second reason for this type of a bias could be the intelligence level of the participants, directly related to education. Less educated and less intelligent people are subject to extreme responses as compared to the converse. Also, the wording of the questions could yield an extreme response from the participant; for instance, “Are you of the opinion that any type of addiction is caused by the influence of peers?”. In such question which blame someone of something, the participant might give an extreme response.
A last reason which could lead to this bias could be the motivation to please the researcher. This reason is mostly the case in service or product feedback. People try to please the provider of the service or product by claiming that they like it very much even if they don’t to that extent.
The exact opposite of this bias is neutral responding, wherein a subject may not be very serious about giving the test and would simply check neutral answers to speed up.
Social Desirability Bias
This type results in the participant providing socially desirable, and hence fake answers to sensitive questions. Questions that hint anti-social behavior, for instance “Do you feel it is alright to consume drugs occasionally?” is a question that would surely force the participant to give a negative answer, mostly the one that denies his/her involvement in such an activity.
This bias can affect in both ways; in places where a certain phenomenon or concept is appreciated, participants are likely to over-report, or give extremely positive answer and vice versa. The social desirability bias can prove detrimental to the validity of the test being conducted and can severely hamper the results.
To conduct a just and minimally biased experiment/test, researchers should take certain precautions. Following are several methods to tackle these four response biases.
Tips to Overcome Response Bias
Rephrase questions to create balanced response sets. In simple terms, to recognize the hypothesized phenomenon, half of the questions should require a positive response and vice versa.
Prevent participants from discovering the hypothesis being studied; this can be achieved by the means of deception, wherein the researcher tells the participant/s about one or more aspects of the study which could be associated but isn’t the sole aim.
The researcher or the person conducting the experiment should be trained to stay neutral throughout the experiment, and avoid projecting an experiment-like attitude. A modern approach to introduce complete neutrality is to administer the test via a computer.
Rephrase the questionnaire such that there are forced-choice (either yes or no) questions as well as questions allowing a neutral response. For instance, a forced-choice question could be, “Are you of the opinion that cigarette smoking to a certain extent is okay?”. Whereas a similar question with a neutral response could be, “Even occasional cigarette smoking can prove injurious to health”.
Instead of a random participant, the researcher could approach a person who is close to him/her and knows him/her well. This could help minimizing the demand characteristics bias.
Another way to tackle demand characteristics bias is to give the participant a post-experimental questionnaire which contains items inquiring about how much the subject knows about the hypothesis of the study.
Always remember that minimizing biases would help make the most of the study and yield more valid and reliable results.

Like it? Share it!
Get Updates Right to Your Inbox
Further insights.
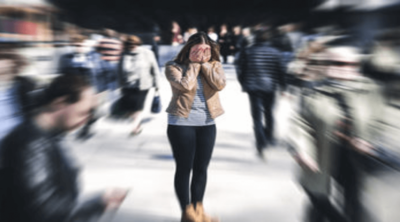
Privacy Overview
Customer Feedback
Turn experience signals into transformative, actionable insights
Reputation Management
Turn social ratings and reviews into revenue
Conversational Intelligence
Find opportunities to elevate customer and agent experiences
Digital Listening
Drive better online experiences and increase user satisfaction
Market Research
Gain a competitive advantage by uncovering customer trends
Advanced Analytics & AI
Let AI take the guesswork out of your CX program
Employee Experience
Turn company culture into your competitive edge
Advisory & Platform Services
Human expertise to complement leading technology
View Plans & Packages
Discover the benefits of investing in the enhancement of experiences
Build an Integrated CX Program
Download our guide to build an integrated CX program in 10 steps.
XI Platform
XI Platform Overview
Get to know the world’s most recommended CX platform
Collect feedback from every perspective, from every channel
Create and share stories that inspire business-wide customer-centricity
Dive into your experience data and uncover meaningful insight
Take informed action to recover at-risk customers and improve results
Mobile Application
Improve customer experiences anywhere, from the palm of your hand
Enterprise Architecture
Text Analytics
Make smarter decisions with industry leading natural language processing
Integrations
Infuse experience intelligence into every enterprise system
Data Management
Conquer your most demanding data management needs
Artificial Intelligence
Revolutionize CX with award-winning AI
Security & Scalability
Built for scalability and best-in-class data security
Learn & Connect
Customer Stories
Learn how leading brands grow their business with InMoment
Blogs & Podcasts
Actionable industry content to gain insight and spark ideas
Resource Library
Proprietary data and strategies from top experience improvement experts
Learn with live webinars, roundtables, and panel discussions, or browse the on-demand catalog of past events
About InMoment
Take a break and learn about our team and what makes us tick
Want to unlock your full potential? Explore our current careers
Learn about leading-edge innovations and employee and company accolades
2024 Online Reputation Benchmark Report
Get insights on review and rating data in key industries and verticals
2024 Consumer Experience Trends
Download the reports to learn what consumers want in 2024
Voluntary Response Bias in Sampling
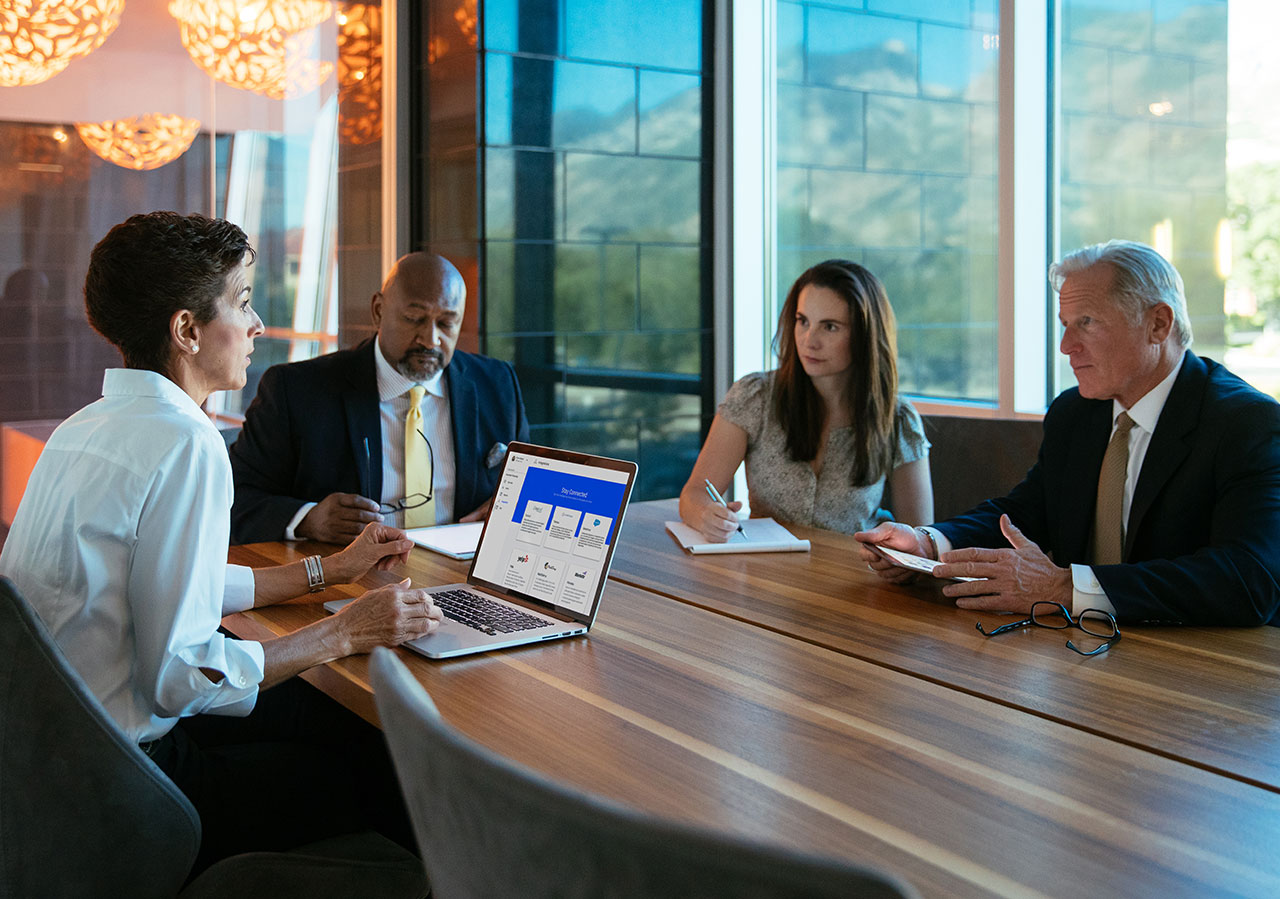
If you received an invitation to take a survey, you would probably be more likely to actually participate if the topic of the survey interested you. That’s the heart of voluntary response sampling. Like all other methods of sampling, voluntary surveys have their pros and cons. It’s one of the easiest ways to sample quickly and get responses, but it can also result in voluntary response bias.
What is Voluntary Response Bias?
A voluntary response is when someone volunteers to be a part of your sample. In doing so, you’re allowing them to skew your data and you don’t get results that are representative of the whole population. Thus, you get biased feedback.
Voluntary response bias refers to how allowing your sample to self-select skews your data, and you don’t actually get results that are representative of your whole population. Voluntary response bias isn’t always inherently bad; it’s not considered the worst of the biases that could arise in your sampling. But it can lead to more extreme results than would actually be true for your population as a whole.
Why Is Voluntary Response Sampling Biased?
When you create a survey, you want to get results that are representative of your population, so you can make the right decisions based on the data. If you’re allowing your sample to self-select, you’re not getting data that shows your entire population. You’re only getting data that reflects your sample. That leaves you with results that aren’t generalizable, and generalizing them anyway is where bias becomes a real problem.
Voluntary response also opens your survey up to the possibility of favoring more extreme results than your population actually experiences. Think about it this way: respondents are more likely to volunteer for a survey if they’re passionate about the topic. The passionate responses can skew your results. You’ll have the customers who loved your product the most (or had a terrible experience) responding instead of your average customer. That could lead to bias problems. You could end up making decisions on products and services that are slightly skewed by voluntary response bias.
What Is an Example of Voluntary Response Bias?
To illustrate voluntary response bias, let’s consider a scenario involving a survey on customer satisfaction with an online retail platform.
Survey Design:
Imagine a company conducts an online survey to gather feedback on customer satisfaction with its e-commerce platform. The survey is distributed through email newsletters and social media, allowing customers to voluntarily respond to questions about their shopping experience.
Voluntary Response Bias in Action:
- Customers who had an exceptionally positive or negative experience with the online retail platform may be more motivated to participate in the survey.
- Customers with neutral or average experiences may be less inclined to take the time to provide feedback, potentially leading to a skewed representation of customer satisfaction.
Resulting Bias:
- The survey results may disproportionately reflect the views of customers who had either highly positive or negative experiences.
- The findings might inaccurately suggest that the majority of customers either love or strongly dislike the platform, creating a potential misrepresentation of overall customer sentiment.
Impact on Generalization:
- If the company relies solely on these biased survey results, they may make decisions based on an exaggerated understanding of customer satisfaction, potentially overlooking the needs and opinions of the more moderate majority.
This example emphasizes how voluntary response bias can manifest in retail surveys when individuals with extreme opinions are more likely to participate, leading to a sample that may not accurately represent the broader spectrum of customer experiences. Recognizing and addressing such biases is crucial for obtaining a more balanced and reliable understanding of customer sentiments in the retail sector.
How to Avoid Voluntary Response Bias
Voluntary response bias can significantly impact the quality of your research results, and potentially lead to skewed and inaccurate conclusions. There are several steps researchers can take to minimize or avoid voluntary response bias and enhance the overall quality of their research.
1. Use Random Sampling Techniques
Employing random sampling methods such as systematic sampling ensures that every member of the population has an equal chance of being selected. This reduces the likelihood of biased participation and contributes to a more representative sample.
2. Ensure Anonymity and Confidentiality
Assure participants of the anonymity and confidentiality of their responses. When individuals feel that their privacy is protected, they may be more willing to participate, reducing concerns about potential repercussions and encouraging a broader range of participants.
3. Implement Post-Stratification Techniques
After data collection, post-stratification techniques can be used to adjust the weights of different groups to match the known population distribution. This helps correct any imbalances that may have occurred during the voluntary response process.
4. Pilot Test Surveys
Before launching a full-scale survey, conduct pilot tests to identify and address any issues with clarity, wording, or potential biases in the questions. Pilot testing helps refine the survey instrument and improve the quality of data collected.
5. Be Transparent About Study Objectives
Clearly communicate the objectives and goals of the research to participants. Providing transparency can attract individuals who are genuinely interested in the topic, rather than those with extreme opinions or biases, leading to a more balanced representation.
By incorporating these strategies into the research design, researchers can minimize the impact of voluntary response bias and enhance the reliability and validity of their study findings. It’s essential to carefully consider the potential biases inherent in voluntary response sampling and take proactive steps to address them throughout the research process.
Advantages of Voluntary Response Sampling
Voluntary response sampling has its own set of advantages in certain situations. Randomly selecting a population and getting those chosen to participate in the survey can be difficult, time-consuming, and expensive. Voluntary response bias can eliminate that. You aren’t spending time tracking down participants and designing your survey since your sample is just those who are already willing to participate in your survey. Here are some key advantages to consider:
1. Cost-Effective
Voluntary response sampling is often a cost-effective method as it involves minimal resources to collect data. Participants voluntarily choose to respond, reducing the need for extensive outreach efforts or financial investment.
2. Quick Data Collection
The process of collecting data through voluntary responses is generally rapid. Since individuals willingly participate, there is no need for lengthy recruitment processes or follow-ups, making it a swift method for gathering information.
3. Ease of Implementation
Implementing voluntary response sampling is relatively simple. Researchers can easily distribute surveys or questionnaires through online platforms, social media, or other accessible channels, allowing for a quick and straightforward data collection process.
4. Wide Geographic Reach
Voluntary response sampling often allows for a broad geographical reach. Through online surveys or other digital means, researchers can attract participants from diverse locations, contributing to a more extensive and varied dataset.
5. Potential for Unbiased Insights
In some cases, voluntary response sampling may provide insights into niche or underrepresented groups. Participants who choose to respond may have a genuine interest in the topic, leading to a diverse set of perspectives that might not be captured through other sampling methods.
Disadvantages of Voluntary Response Sampling
Voluntary response sampling has some very obvious disadvantages. Using voluntary responses can allow bias to creep in on the results and skew data. Voluntary response also can introduce undercoverage bias. Your population could potentially be a complex and diverse group of people. When you use voluntary responses, only those who are inclined to respond are represented in the results. While voluntary response sampling has its advantages, it is crucial to be aware of its limitations and potential drawbacks. Here are some key disadvantages to keep in mind when deciding to participate in voluntary response sampling:
1. Selection Bias
One of the most significant challenges with voluntary response sampling is the potential for selection bias. Individuals who choose to participate may differ systematically from those who do not, leading to a skewed representation of the population and compromising the generalizability of the findings.
2. Lack of Randomization
Voluntary response sampling lacks the randomization inherent in more rigorous sampling methods. This absence of random selection can contribute to a non-representative sample, making it difficult to draw accurate conclusions about the broader population.
3. Limited Control Over Sample Composition
Researchers have limited control over the composition of the sample in voluntary response sampling. Certain demographic groups may be overrepresented or underrepresented, impacting the reliability of the collected data and potentially introducing confounding variables.
4. Potential for Misleading Conclusions
Participants in voluntary response surveys may have strong opinions or experiences related to the subject matter, leading to biased results. This can result in findings that are not reflective of the overall population and may lead to misleading conclusions.
5. Difficulty in Establishing Causation
Establishing causal relationships is challenging with voluntary response sampling, as the self-selected nature of participants makes it difficult to determine whether observed correlations are causal or influenced by other factors.
When utilizing voluntary response sampling, researchers should be aware of these disadvantages and carefully consider the appropriateness of this method for their specific research goals and the nature of the population under study.
Collecting Responses and Feedback
Voluntary response bias is a real risk researchers face when using voluntary response sampling. But considering what voluntary response bias does to a survey also opens up a discussion of the larger challenges with surveying. Choosing methods and creating accurate, simple, and powerful surveys is important, and shouldn’t be taken lightly. With InMoment, you’ll have access to best-in-class survey tools that will help you collect, analyze, and act on customer feedback. Schedule a demo today to see it for yourself!
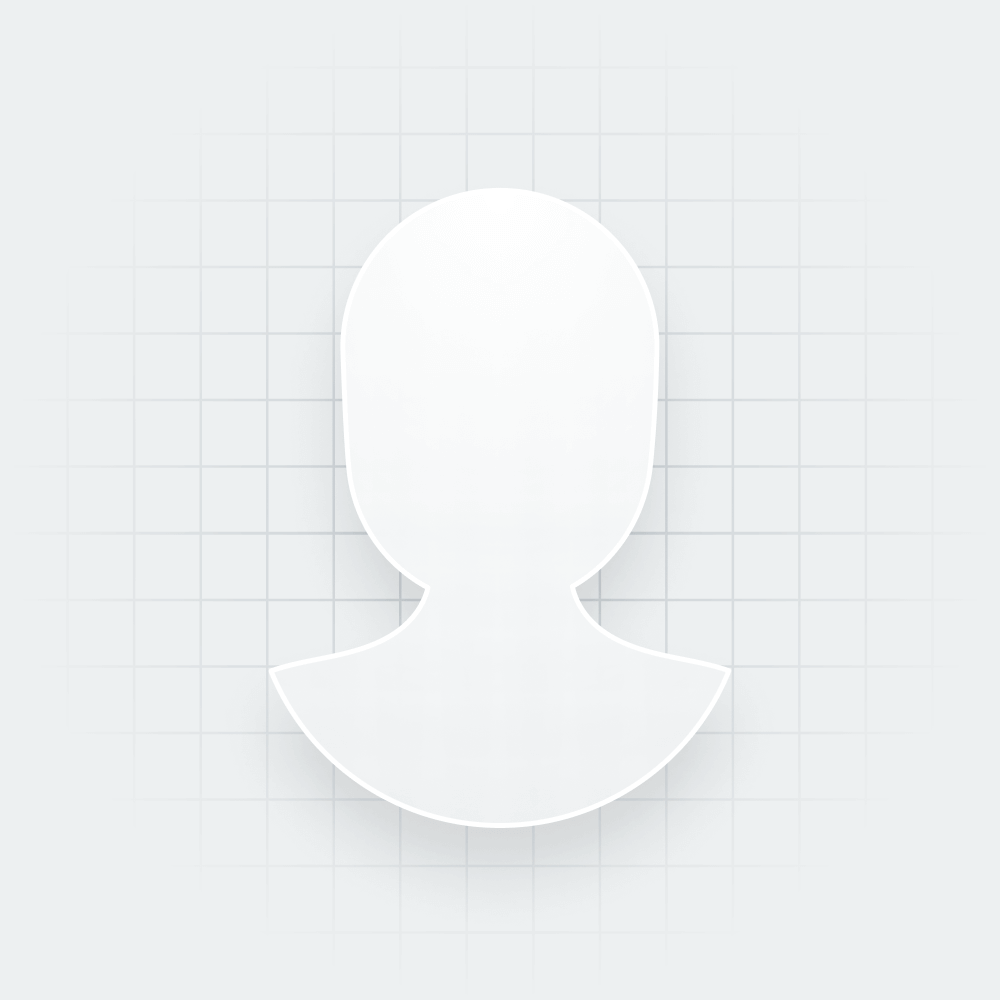
Mike is a passionate professional dedicated to uncovering and reporting on the latest trends and best practices in the Customer Experience (CX) and Reputation Management industries. With a keen eye for innovation and a commitment to excellence, Mike strives to deliver insightful content that empowers CX practitioners to enhance their businesses. His work is driven by a genuine interest in exploring the dynamic landscape of CX and reputation management and providing valuable insights to help businesses thrive in the ever-evolving market.
In this Post
Watch the video – get more out of your cx program.
Watch this demo video to see how InMoment’s award-winning AI tech does the heavy lifting on analysis so you can spend more time lifting CX results.
Get More Out of Your CX Program
The 2024 consumer trends report.
This isn't your average trends report – read on to see how your business can lead the charge into a new era of CX
10 Steps to Easily Build An Integrated Customer Experience Program
A game-changing guide for your team on how to build & implement a competitive, fully-stacked integrated CX program.
Related Articles
- advance-ai-nlp | September 20, 2024
Understand Active Listening and How It’s Revolutionizing Feedback Collection
By: Stephanie Mix
Capturing genuine and actionable feedback from customers and employees can be a challenge, especially when traditional surveys feel long, tedious,…
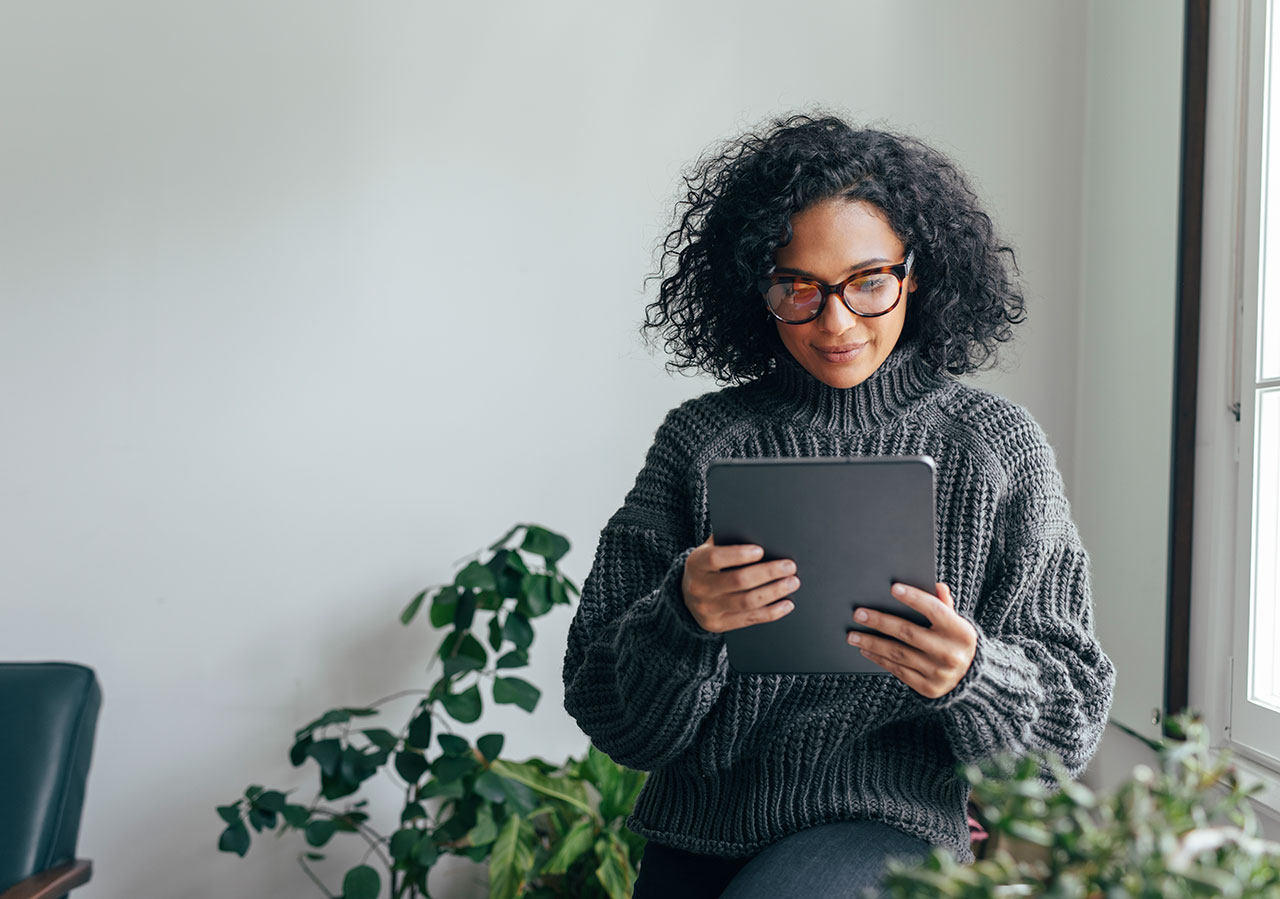
- customer-experience | September 19, 2024
Why the End-to-End Customer Experience Should Be A Priority
By: Mike Henry
When you think of your most recent purchase, you don’t think of the experience in silos. You don’t remember how…
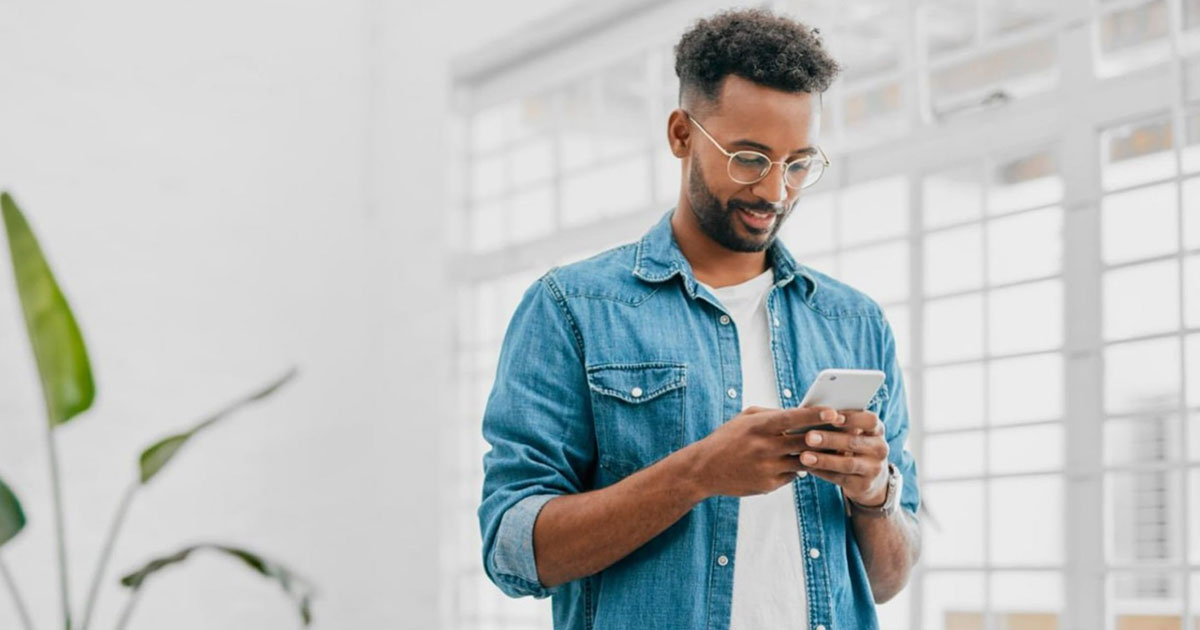
- customer-experience | September 18, 2024
Customer Experience in Healthcare: Transforming Patient Care
By: Cori Lindsey
In today’s healthcare landscape, patient expectations are higher than ever. It’s not just about treating ailments anymore—it’s about delivering care…
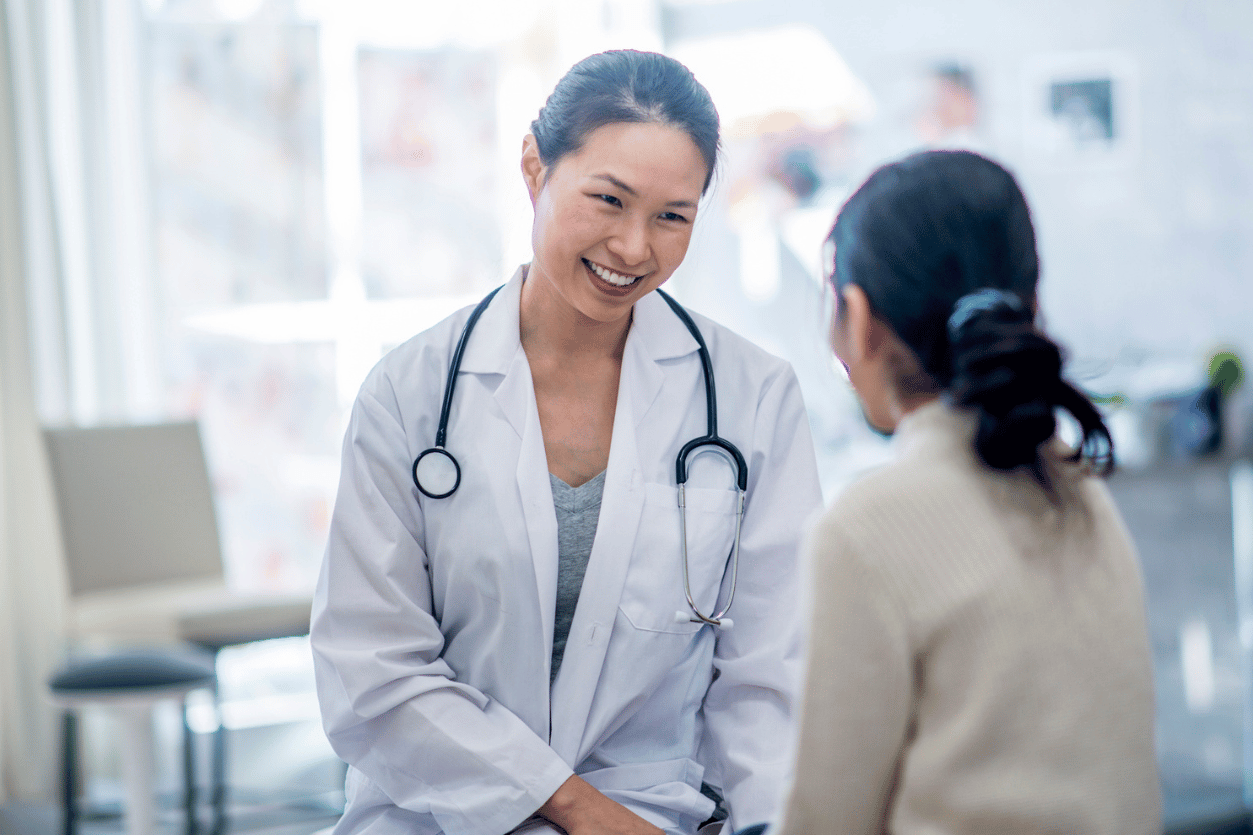
Change Region
Selecting a different region will change the language and content of inmoment.com
North America
Asia pacific.
Have a language expert improve your writing
Run a free plagiarism check in 10 minutes, generate accurate citations for free.
- Knowledge Base
- Research bias
- What Is Nonresponse Bias? | Definition & Example
What Is Nonresponse Bias?| Definition & Example
Published on November 9, 2022 by Kassiani Nikolopoulou . Revised on June 11, 2024.
Nonresponse bias happens when those unwilling or unable to take part in a research study are different from those who do.
In other words, this bias occurs when respondents and nonrespondents categorically differ in ways that impact the research. As a result, the sample is no longer representative of the population as a whole.
Table of contents
What is nonresponse bias, why is nonresponse bias a problem, nonresponse bias example, how to minimize nonresponse bias, other types of research bias, frequently asked questions.
Nonresponse bias can occur when individuals who refuse to take part in a study, or who drop out before the study is completed, are systematically different from those who participate fully. Nonresponse prevents the researcher from collecting data for all units in the sample. It is a common source of error, particularly in survey research .
Causes of nonresponse include:
- Poor survey design or errors in data collection
- Wrong target audience (e.g., asking residents of an elderly home about participation in extreme sports)
- Asking questions likely to be skipped (e.g., sensitive questions about drugs, sexual behavior, or infidelity)
- Inability to contact potential respondents (e.g., when your sample includes individuals who don’t have a steady home address)
- Conducting multiple waves of data collection (e.g., asking the same respondents to fill in the same survey at different points in time)
- Not taking into account linguistic or technical difficulties (e.g., a language barrier)
Types of nonresponse
Usually, a distinction is made between two types of nonresponse:
- Unit nonresponse encompasses instances where all data for a sampled unit is missing—i.e., a number of respondents didn’t complete the survey at all ( missing data ).
- Item nonresponse occurs where only part of the data could not be obtained—i.e., a number of respondents selectively skipped the same survey question.
It is important to keep in mind that nonresponse bias is always associated with a specific variable (like manager workload in the previous example). Respondents and nonrespondents differ with respect to that variable (workload) specifically.
Because managers’ decision to participate or not in the survey relates to their workload, the data is not randomized, leading respondents and nonrespondents to differ in a way that is significant to the research.
Components of nonresponse
Nonresponse bias consists of two components:
- Nonresponse rate
- Differences between respondents and nonrespondents
The extent of bias depends on both the nonresponse rate and the extent to which nonrespondents differ from respondents on the variable(s) of interest. This means that a high level of nonresponse alone does not necessarily lead to research bias , as nonresponse can also be due to random error .
Does this mean that nonresponse bias is present in your research?
It may, but only if:
- The individuals who missed the survey share a common characteristic that differentiates them from those who did receive the survey and filled it in
and
- This common characteristic is directly relevant to your research question
If nonrespondents missed your email due to poor computer skills, then this makes them a distinct group in terms of a unifying characteristic (i.e., poor computer skills). This skill is relevant to your research (information literacy).
Response rate and nonresponse bias
The response rate , or the percentage of sampled units who filled in a survey, can indicate the amount of nonresponse present in your data. For example, a survey with a 70% response rate has a 30% nonresponse rate.
The response rate is often used to estimate the magnitude of nonresponse bias. The assumption is that the higher the response rate , the lower the nonresponse bias .
However, keep in mind that a low response rate (or high nonresponse rate ) is only an indication of the potential for nonresponse bias. Nonresponse bias may be low even when the response rate is low, provided that the nonresponse is random. This occurs when the differences between respondents and nonrespondents on that particular variable are minor.
Nonresponse bias can lead to several issues:
- Because the obtained sample size doesn’t correspond to the intended sample size, nonresponse bias increases sampling error.
- Results are not representative of the target population, as respondents are systematically different from nonrespondents.
- Researchers must devise more elaborate or time-intensive data collection procedures to achieve the requisite response rate and sample size. This, in turn, increases the cost of research.
Nonresponse bias is a common source of bias in research , especially in studies related to health.
Unfortunately, nonresponse is higher among people with heart disease, leading to an underestimation of the association between smoking and heart disease. This is a common problem in health surveys.
Studies generally show that respondents report better health outcomes and more positive health-related behaviors than nonrespondents. They often report lower alcohol consumption, less risky sexual behavior, more physical activity, etc.
It’s possible to minimize nonresponse by designing the survey in a way that obtains the highest possible response rate. There are several steps you can take that will help you in that direction:
During data collection
- During data analysis
To minimize nonresponse bias during data collection, first try to identify individuals in the sample that are less likely to participate in your survey. These could be individuals who are hard to reach or hard to motivate.
It’s a good idea to prepare strategies that may incentivize their cooperation. Some ideas could include:
- Offering incentives , monetary or otherwise (e.g., gifts, donations, raffles). Incentives motivate respondents and make them feel that the survey is worth their time.
- Considering how you contact sample units and what is best suited to your research. Before you launch your survey, think about the total number of contacts you need to have, the timing of the first contact, the interval between contacts, etc. For example, personal contact through face-to-face survey interviews generally increases response rates but may not work for all potential respondents.
- Ensuring respondents’ anonymity and providing ethical considerations . Surveys that require personal or sensitive information should include instructions that make respondents feel at ease, reassuring them that their answers will be kept strictly confidential.
- Keeping your data collection flexible . Consider using multiple modes of data collection, such as online and offline. If data collection is done in person, participants should be able to schedule the appointment whenever convenient for them.
- Sending reminders . Sending a few reminder emails during your data collection period is an effective way to gather more responses. For example, you can send your first reminder halfway through the data collection period and a second near the end.
- Making participation mandatory instead of voluntary whenever possible. For example, asking students to fill in a survey during class time is more effective than inviting them to fill it in via a letter sent to their home address.
During data analysis
During data analysis, the goal is to identify the magnitude of nonresponse bias. Luckily, the nonresponse rate is easy to estimate. However, identifying whether the difference between respondents and nonrespondents is due to a particular characteristic is not so easy.
There are a number of ways you can approach this problem, including:
- Comparing early respondents to late respondents . Later respondents can often resemble nonrespondents in terms of unifying characteristics. In this way, you can infer the characteristics of nonrespondents.
- Using information that is already available for the entire survey sample (respondents and nonrespondents). Relevant information may already be included in the sampling frame itself—for example, sociodemographic characteristics like age or gender, employment data, or information about the duration of membership in the case of a survey of members of a club or sports team. The prerequisite here is that the collected information is related to the survey variables of interest and can be linked to participation behavior.
- Using follow-up surveys to collect at least some key variables, either from all nonrespondents or from a randomly selected sample of them. The drawback here is the additional cost of the survey.
Cognitive bias
- Confirmation bias
- Baader–Meinhof phenomenon
Selection bias
- Sampling bias
- Ascertainment bias
- Attrition bias
- Self-selection bias
- Survivorship bias
- Nonresponse bias
- Undercoverage bias
- Hawthorne effect
- Observer bias
- Omitted variable bias
- Publication bias
- Pygmalion effect
- Recall bias
- Social desirability bias
- Placebo effect
Response bias is a general term used to describe a number of different conditions or factors that cue respondents to provide inaccurate or false answers during surveys or interviews. These factors range from the interviewer’s perceived social position or appearance to the the phrasing of questions in surveys.
Nonresponse bias occurs when the people who complete a survey are different from those who did not, in ways that are relevant to the research topic. Nonresponse can happen because people are either not willing or not able to participate.
Nonresponse bias occurs when those who opt out of a survey are systematically different from those who complete it, in ways that are significant for the research study.
Because of this, the obtained sample is not what the researchers aimed for and is not representative of the population. This is a problem, as it can invalidate the results.
Common types of selection bias are:
- Sampling bias or ascertainment bias
- Volunteer or self-selection bias
Sources in this article
We strongly encourage students to use sources in their work. You can cite our article (APA Style) or take a deep dive into the articles below.
Nikolopoulou, K. (2024, June 11). What Is Nonresponse Bias?| Definition & Example. Scribbr. Retrieved September 23, 2024, from https://www.scribbr.com/research-bias/nonresponse-bias/
Bose, Jonaki. (2001). Nonresponse bias analyses at the national center for education statistics.
Koch, A., & Blohm, M. (2016). Nonresponse Bias. GESIS Survey Guidelines. Mannheim, Germany: GESIS – Leibniz Institute for the Social Sciences. doi: 10.15465/gesis-sg_en_004
Is this article helpful?
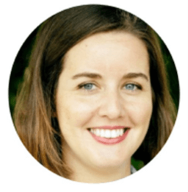
Kassiani Nikolopoulou
Other students also liked, what is response bias | definition & examples, random vs. systematic error | definition & examples, survey research | definition, examples & methods.
Advertisement
Response bias in recognition memory as a cognitive trait
- Published: 08 August 2012
- Volume 40 , pages 1163–1177, ( 2012 )
Cite this article
- Justin Kantner 1 , 2 &
- D. Stephen Lindsay 1
6868 Accesses
70 Citations
3 Altmetric
Explore all metrics
According to signal detection theory, old–new recognition decisions can be affected by response bias, a general proclivity to respond either “old” or “new.” In recognition experiments, response bias is usually analyzed at a group level, but substantial individual differences in bias can underlie group means. These differences suggest that, independent of any experimental manipulation, some people require more memory evidence than do others before they are willing to call an item “old.” In four experiments, we investigated the possibility that recognition response bias is a partial function of a trait-like predisposition. Bias was highly correlated across two recognition study–test cycles separated by 10 min (Experiment 1 ). A nearly identical correlation was observed when the tasks were separated by one week (Experiment 2 ). Bias correlations remained significant even when the stimuli differed sharply between the first and second study–test cycles (Experiment 3 ). No relationship was detected between bias and response strategies in two general knowledge tests (Experiments 2 and 4 ), but bias did weakly predict frequency of false recall in the Deese/Roediger–McDermott (DRM) paradigm (Experiment 4 ). This evidence of trait-like stability suggests an entirely different aspect of response bias than that studied by examining its modulation by task variables, one for which complete theories of recognition memory may need to account.
Similar content being viewed by others
Three regularities of recognition memory: the role of bias.
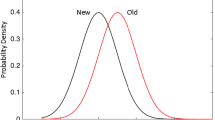
One mirror effect: The regularities of recognition memory
The reliability of criterion shifting in recognition memory is task dependent.
Avoid common mistakes on your manuscript.
Suppose that two participants studied an identical list of commonplace items and each took an identical yes–no recognition test containing equal numbers of items that were and were not on the study list. Suppose further that one participant achieved a hit rate of .90 and a false alarm rate of .40, while the other achieved a hit rate of .60 and a false alarm rate of .10. Although these two participants were equally accurate (75 % correct), their performances were strikingly different. This difference would be characterized as one of response bias: The first participant tended to favor “old” responses and was liberally biased, while the second favored “new” responses and was conservatively biased.
Recent years have seen an accelerating interest in response bias as a measure revealing important strategic influences on recognition memory. It is well established that participants adjust bias according to instructional motivation (e.g., Egan, 1958 ), payoff schedules that encourage “old” or “new” responses (e.g., Healy & Kubovy, 1978 ; Van Zandt, 2000 ), and the proportion of old test items (e.g., Van Zandt, 2000 ). Further work has revealed stimulus-specific properties that affect bias, such as the emotional content of the test items (e.g., Dougal & Rotello, 2007 ) and their subjective memorability (e.g., Brown, Lewis, & Monk, 1977 ). Another class of studies has investigated the extent to which participants can adjust criterion during the course of a test when conditions such as the memory strength of probes or target–distractor similarity are changed midstream (e.g., Benjamin & Bawa, 2004 ; G. E. Cox & Shiffrin, 2012 ; Dobbins & Kroll, 2005 ; Singer, 2009 ; for a review, see Hockley, 2011 ).
The present experiments were designed to examine recognition response bias from a different perspective. The objective was to ask whether bias is strictly a function of the prevailing experimental conditions or inheres to a degree in individual recognizers as a cognitive trait. Often, average response bias on recognition memory tests is neutral, unless manipulations are employed to push bias in a liberal or conservative direction. However, substantial individual differences in bias often underlie group means. Figure 1 illustrates an example of this phenomenon from an experiment reported by Kantner and Lindsay ( 2010 ), which involved a standard item recognition task. In the control condition of that experiment, the mean response bias (bold line) was statistically neutral, but estimates of criteria from the 23 individual participants (gray lines) showed considerable variability. Why would participants in an experiment containing no biasing instructions or incentives differ to such a degree on this measure? While this variability might simply have resulted from measurement error, an intriguing possibility is that such variability reflects bias proclivities within individuals that are independent of the parameters of the recognition task. In the present experiments, we tested the hypothesis that the level of memory evidence participants require before committing to an “old” decision is a cognitive trait, and that the bias they display on a recognition test is a manifestation of that trait.
Spread of individual criterion values in Kantner and Lindsay’s ( 2010 ) Experiment 1 , control condition. The curves representing the new and old strength distributions are purely illustrative, not derived from the data
In referring to recognition response bias as a potential trait, we mean to suggest a characterization of bias as an aspect of cognition that typifies an individual. We use the label cognitive trait to distinguish response bias from a personality trait, although we do not imply that cognitive and personality traits are independent constructs. Response bias might be thought of as a cognitive trait in the same sense as recognition sensitivity: All experimental conditions being equal, one would not expect sensitivity to the difference between old and new items to vary haphazardly within an individual from one test to another. Rather, sensitivity measures on the two tests would be expected to have a predictive relationship. We used this metric to assess the within-individual stability of response bias in the present experiments. The notion of bias as a trait also implies that it holds behavioral consequences that extend beyond the domain of recognition memory; we conducted some initial tests of this possibility in Experiments 2 and 4 .
Although response bias is not generally characterized as representing a trait in the recognition literature, studies have identified a number of populations exhibiting a more liberal response bias than appropriate comparison groups, such as the elderly (e.g., Huh, Kramer, Gazzaley, & Delis, 2006 ), patients with Alzheimer’s disease (e.g., Beth, Budson, Waring, & Ally, 2009 ), schizophrenia patients (e.g., Moritz, Woodward, Jelinek, & Klinge, 2008 ), dementia patients (e.g., Woodard, Axelrod, Mordecai, & Shannon, 2004 ), individuals with mental retardation (Carlin et al., 2008 ), and panic disorder patients (Windmann & Krüger, 1998 ). The association of liberal response bias and particular populations is consistent with the idea that groups of individuals may be differentiated from one another on the basis of response bias without a specific experimental intervention, which is, in turn, consistent with the notion of response bias as a cognitive trait. Relatedly, a small literature on the “yea-saying bias” suggests that some individuals are predisposed to respond affirmatively to questions, a phenomenon demonstrated by young children and individuals with impaired cognitive development (e.g., Couch & Keniston, 1960 ).
At least two studies have examined the relationship of response bias to cognitive or personality traits within individuals; correlations between recognition bias and established traits suggest that bias has trait-like qualities. Because frontal brain regions are often implicated in criterion setting (see, e.g., Kramer et al., 2005 ), Huh et al. ( 2006 ) correlated response bias on a recognition test with performance on four measures of executive function in 293 adults ranging from 35 to 89 years old. Among these measures, inhibition (indexed via a Stroop task) was the only significant predictor of response bias ( r = .31, estimated from the reported beta values); Huh et al. declared the analysis inconclusive. In a study of 28 undergraduates, Gillespie and Eysenck ( 1980 ) found that introverts used a more conservative recognition criterion than extraverts, and described introverts as exercising greater “response cautiousness.” This finding is consistent with the possibility that response bias may arise from a trait corresponding to a required level of evidence before action is taken—a trait that, like introversion/extraversion, is stable within an individual.
A number of studies have investigated individual differences in false memory proneness via the Deese/Roediger–McDermott (DRM) paradigm (Roediger & McDermott, 1995 ). Given that a liberal recognition bias is associated with increased endorsement of test probes that were not studied, evidence that DRM false recognition has trait-like qualities could suggest the same characterization of response bias. DRM performance has been correlated with a number of individual-difference measures (e.g., age, working memory, and frequency of dissociative experiences; for a review, see Gallo, 2010 ), and some experiments have identified populations with particularly high rates of DRM errors (e.g., Geraerts et al., 2009 ). These findings suggest that some individuals are inherently more prone than others to accept memories as true even when memory evidence is weak, making them especially vulnerable to false memories.
Two studies have assessed the within-individual stability of DRM false recognition. Salthouse and Siedlecki ( 2007 ) found reliable stability within a single test but not across separate tests differing by stimulus type, and false recognition of critical lures was uncorrelated with a host of cognitive and personality measures in two experiments. However, Blair, Lenton, and Hastie ( 2002 ) found high levels of reliability in tests of the same DRM lists given two weeks apart, indicating that false recognition does not vary unpredictably within an individual.
Two further findings from the DRM literature are suggestive with respect to trait response bias. Blair et al. ( 2002 ) reported a significant correlation between critical and noncritical false alarms on the first test, a result that hints at a relationship between general recognition bias and DRM false memories (although that correlation was not significant in the second test). Relatedly, Qin, Ogle, and Goodman ( 2008 ) found that response bias calculated from the noncritical DRM trials was significantly (albeit weakly) predictive of susceptibility to adopting fictitious childhood events as autobiographical. These results are consistent with the possibility that response bias is a trait that generalizes to tasks outside of recognition memory.
Measurement of response bias
The measurement of response bias raises complex theoretical and statistical questions relevant to any recognition memory experiment, and the optimal method for estimating bias has been a matter of extensive debate. There are many options for calculating bias, and each is tied to model-based assumptions that may or may not hold for a given data set (see Rotello & Macmillan, 2008 ). In testing the trait-like stability of bias, then, it is important to establish that any evidence of within-individual consistency is not tied to a particular index. Therefore, in addition to our primary bias measure ( c ), we calculated consistency using four other well-known estimates of bias ( c a , ln[ β ], B'' , and FA) in each of the present experiments. A brief description of these measures follows.
We describe and illustrate our results in terms of the bias estimate c (Macmillan, 1993 ), a simple and widely used measure given as the opposite of half the sum of the z -converted hit and false alarm rates. Positive values of c indicate a criterion to the right of the intersection of the old- and new-item distributions and a bias to respond “new”; negative values indicate a criterion to the left of the intersection of the distributions and a bias to respond “old.” One criticism of c is the assumption inherent in its calculation that the two distributions have equal variance; evidence from receiver operating characteristic (ROC) curves suggests that the variance is often greater for the old than for the new distribution (e.g., Ratcliff, Sheu, & Gronlund, 1992 ). An alternative to c that addresses this shortcoming is c a , which produces estimates of response criterion at multiple levels of confidence that take into account the relative variances of the old- and new-item distributions (Macmillan & Creelman, 2005 ). When the two variances truly are equal, c will be equivalent to c a at the middle (neutral) confidence level; to the extent that the variances differ, c will deviate from the middle c a value. We calculated c a from confidence ratings collected at test and report the middle- c a values obtained in each of the present experiments.
The additional bias measures computed in the present experiments were chosen to represent a range of approaches to estimating bias. The ln( β ) measure is the natural log of the ratio of the target and lure likelihoods at a given (criterial) point on the strength-of-evidence axis. B'' is a prominent nonparametric bias statistic, although its status as a “model-free” estimate has been questioned (Macmillan & Creelman, 1996 ). Calculations and discussion of ln( β ) and B'' can be found in Stanislaw and Todorov ( 1999 ). Finally, the false alarm rate simply estimates bias with no reference to the hit rate.
An additional consideration in the measurement of response bias is the estimation of recognition sensitivity; changes in bias can be difficult to disentangle from changes in sensitivity, particularly when both are indexed by hit and false alarm rates. For example, if a participant completes two recognition tests and achieves the same false alarm rate on each but a higher hit rate on the second test, the resulting increase in d' and decrease in c across tests leaves unclear whether sensitivity has increased, bias has become more liberal, or both. To help minimize the statistical confounding of bias and recognition sensitivity, we used the sensitivity estimate A z , the area under the ROC curve (Verde, Macmillan, & Rotello, 2006 ). Both A z and c a were computed using Lewis O. Harvey’s ( 2005 ) RSCORE program.
Experiment 1
If response bias represents a cognitive trait, it should remain consistent within an individual across time. Therefore, an important first step in establishing response bias as trait-like is to determine whether a given participant will show the same level of bias on two different recognition tests. Experiment 1 tested this possibility in a straightforward manner: Each participant took two recognition tests (each preceded by its own study list) that were separated by a filled 10-min interval. The measure of interest was the correlation between the bias on Test 1 and bias on Test 2.
Participants
In each of the present experiments, University of Victoria students participated for optional bonus credit in an undergraduate psychology course. A total of 41 participants took part in Experiment 1 .
The stimuli were 192 four- to eight-letter medium- to high-frequency English nouns drawn from the MRC psycholinguistic database ( www.psy.uwa.edu.au/mrcdatabase/uwa_mrc.htm ; Coltheart, 1981 ). Study and test lists were created via random selection from the 192-word pool for each participant. Forty-eight randomly selected words composed Study List 1. Test List 1 consisted of the 48 words from Study List 1 and 48 nonstudied words. Study and Test Lists 2 were populated in the same way, with no words repeated from the first study–test cycle. Each study list included three primacy and three recency buffers. The study and test lists were each presented in a randomized order. All of the present experiments were conducted with E-Prime software (Psychology Software Tools, Inc., Sharpsburg, PA).
Study items were presented for 1 s each, with a blank 1-s interstimulus interval (ISI). Participants were instructed to remember each word for a subsequent memory test. Upon completion of the study list, participants received test instructions informing them that they would see another list of words, that some of these words had appeared in the preceding study list and some had not, and that their task was to indicate whether or not each item had been studied. Recognition judgments were made on a 6-point, confidence-graded scale (1 = definitely not studied , 2 = probably not studied , 3 = maybe not studied , 4 = maybe studied , 5 = probably studied , and 6 = definitely studied ). Responses were nonspeeded. A 1-s intertrial interval (ITI) separated test trials.
After the first study–test cycle, participants spent 8 min writing down the names of as many countries as they could. The procedure for the second study–test cycle was identical to that of the first, with the exception of a minor instructional modification to inform participants that no words would be repeated from the first cycle.
Results and discussion
In this and each subsequent experiment, recognition rating data were converted to hits (H) and false alarms (FA) by scoring responses of 4, 5, and 6 as hits on target trials and as false alarms on lure trials. Occasional false alarm rates of 0 and hit rates of 1 were replaced according to Macmillan and Kaplan ( 1985 ). Across experiments, such replacements were made for 0.65 % of scores.
The means of interest are displayed in Table 1 . Bias was roughly neutral and did not differ significantly between Test 1 and Test 2, t (40) = 1.59, p = .12. Recognition sensitivity was statistically equivalent across tests, t < 1.
Bias varied greatly at the level of the individual, ranging from extremely conservative to extremely liberal. The highest value of c in a single test was 1.10 (H = .44, FA = .02); the lowest value was –1.01 (H = .92, FA = .73). The question was whether these values were predictive of bias across the two recognition tests.
Five bias statistics ( c , c a , ln[ β ], B'' , and FA) were used to calculate Test 1–Test 2 bias correlations in Experiments 1 – 3 . To minimize the influence of outliers on the observed correlations, any bias scores more than 3 SD s from the mean for a given measure in a given experiment were removed prior to correlational analysis; across all measures and experiments, this cutoff resulted in the removal of 0.73 % of bias scores, or 1.47 % of data points in the correlational analyses. Most of the bias scores removed were ln( β ) (53 %) or FA (29 %) values. All correlations were calculated with Pearson’s r statistic and controlled for sensitivity ( A z ) on Tests 1 and 2.
Test 1 c is plotted against Test 2 c for each participant in Fig. 2 . As is clear from inspection of the figure, the direction and magnitude of individuals’ biases tended to be consistent from Test 1 to Test 2. Overall, a strong positive correlation between bias on the first and second tests was observed, r (37) = .67, p < .001. Correlations based on the supplementary bias measures are displayed in Table 2 . These analyses indicated positive, highly significant bias relationships across tests regardless of the measure used, suggesting that the within-individual stability of bias in Experiment 1 was not an artifact of the properties of any particular estimate.
Correlation of recognition bias at Test 1 and Test 2 in Experiment 1
To establish a benchmark against which to compare the intertest bias correlations, the split-half reliability of c within a single test was measured. The within-test correlations were .69 and .78 on Tests 1 and 2, respectively, for a mean within-test correlation of .73. Thus, the level of stability in bias across tests in Experiment 1 was similar to that observed within a single test, an indication that a delay of 10 min and a separate study–test cycle had very little effect on participants’ response biases.
Experiment 2
The finding of bias consistency when 10 min separated two recognition study–test cycles shows that variability in bias across participants is not solely the result of measurement error. Experiment 2 was designed to provide a stronger test of lasting consistency in bias by separating two study–test cycles by one week.
A second goal of Experiment 2 was to investigate a second dimension of trait-like stability in response bias: its transfer to nonrecognition memory tasks. The idea is that if response bias is the manifestation of an “evidence requirement” trait, it should correlate with performance on other tasks in which an evidence requirement might influence responses.
This possibility was tested with two such tasks in Experiment 2 . The first was a DRM recall task. Given the decreased caution exercised by liberal recognizers in accepting words as having been encountered previously, the prediction was that such participants would be more likely than those exhibiting a conservative recognition bias to commit false recall of critical DRM lures. The second nonrecognition measure was grain size in estimating answers to general knowledge questions (Goldsmith, Koriat, & Weinberg-Eliezer, 2002 ). Participants were asked questions (e.g., “What year did Elvis Presley die?”) and responded with numerical ranges that they believed were likely to contain the exact answer. Fine-grained answers (e.g., “1973–1978”) are less likely to be accurate but are more informative than coarse-grained answers (e.g., “1950–1990”). The grain size with which one answers a question is understood to reflect a preference for accuracy versus informativeness in responding (Ackerman & Goldsmith, 2008 ). We predicted that participants exhibiting a more conservative recognition bias would tend to use wider ranges than would liberal recognizers, on the basis that recognition response bias is a reflection of a “required evidence” trait: Conservative recognizers were hypothesized to require more evidence of their knowledge of a topic before committing to a narrow-range answer.
A total of 46 participants took part in Experiment 2 .
The stimuli used in the recognition portions of the experiment were identical to those used in Experiment 1 . The stimuli used in the DRM task were the doctor, window, rough, bread, anger, sweet, couch, and smell lists from Stadler, Roediger, and McDermott ( 1999 ). Each list contained 15 words in decreasing order of semantic relatedness to the category prototype (Roediger & McDermott, 1995 ).
The general knowledge task included 50 trivia-style questions, each with an exact numerical answer, written by the first author and a research assistant. All questions selected for use in Experiment 2 called for answers in the form of specific years. Each question began with the words “In what year” and referred to a historical, political, scientific, or pop cultural event from the last 200 years.
Participants took part in two sessions at the same time of day exactly one week apart. Session 1 consisted of a recognition study–test cycle and either the general knowledge or DRM task. Session 2 consisted of a second recognition study–test cycle (with different words from those used in Session 1) and whichever of the general knowledge or DRM tasks was not included in Session 1. The assignment of the nonrecognition tasks to Sessions 1 and 2 was random for each participant, as was the order of the two tasks within each session.
The procedure for the recognition phases was identical to that of Experiment 1 . The procedure for the DRM and general knowledge tasks was as follows:
Participants were informed that on each trial they would see a list of words presented one at a time on the computer screen, that they were to read each word aloud, and that they would subsequently be asked to write down as many words from the list as they could recall within a 2-min time limit. Words were presented for 2 s each with a 1-s ISI. The ordering of the eight lists was random for each participant.
General knowledge task
Instructions stated that participants were to respond to each of a series of questions with a range of years within which they were “reasonably certain the event in question occurred, such that you would be comfortable giving this information to a friend if asked.” Responses were nonspeeded. A 1-s ITI separated each trial. A single practice trial preceded the 50 test trials.
The general knowledge task data from five participants were removed from the analyses reported below. Three of these participants were new to North America, one had lived during an inordinate number of the events in question, and one gave several ranges beginning much earlier than 200 years ago, despite instructions to the contrary. These participants’ DRM and recognition data were analyzed.
Recognition test means are displayed in Table 3 . Performance on the two tests was very similar; sensitivity and bias were both statistically equivalent (both t s < 1). Mean bias across all participants was again approximately neutral.
As in Experiment 1 , variability in bias was substantial across participants, with single test values ranging from 1.15 (H = .40, FA = .02) to –0.78 (H = .92, FA = .56). Test 1 c is plotted against Test 2 c in Fig. 3 . The correlation of bias across the two tests was again highly significant, r (42) = .73, p < .001, and directionally greater than in Experiment 1 , when the two tests were only 10 min apart. Variability in the coefficients calculated from the supplementary bias measures was greater than in Experiment 1 (see Table 2 ), but each correlation was again indicative of a strong positive relationship, with three of the five correlations directionally higher than their counterparts in Experiment 1 .
Correlation of recognition bias at Test 1 and Test 2 in Experiment 2
On the DRM test, participants falsely recalled an average of 2.86 critical lures out of eight possible ( SD = 1.73, range = 0 to 7). The correlation of the number of critical lures recalled and the mean of Test 1 c and Test 2 c for each participant (correcting for mean A z ) was negative but did not approach significance, r (40) = –.12, p = .45 (see Fig. 4 , top panel). The supplementary bias measures also yielded negative but very weak correlations (strongest r = –.17, lowest p = .28).
Correlation of recognition bias and performance on nonrecognition tasks in Experiment 2 : Frequency of DRM false recall (top panel) and mean range width in the general knowledge task (bottom panel)
Across participants, the mean range width in the general knowledge task was 25.4 years ( SD = 17.9, range = 7.3 to 86.2). The split-half reliability of the range width measure was .82. Mean range width was not significantly correlated with c , r (38) = .12, p = .47; see Fig. 4 , bottom panel), nor with the supplementary measures (strongest r = –.19 [using FA], lowest p = .24).
While bias was highly correlated across a one-week interval, evidence of a relationship between bias and performance on the DRM and general knowledge tasks was not obtained. Unfortunately, the null relationships are difficult to interpret. Despite the tendency for participants to overestimate their own knowledge levels in the general knowledge task, prior knowledge may have driven variability in range sizes to a far greater degree than did response bias. Even more problematic was the timing of Experiment 2 , which coincided with lectures on the DRM paradigm in several of the psychology courses taken by our participants. Interviews conducted during the debriefing revealed that more than half of the participants came to the experiment with fresh insight that they should avoid recall of critical lures. We addressed these potential impediments to identifying a relationship between recognition bias and the DRM and grain size tasks in Experiment 4 .
Experiment 3
In Experiments 1 and 2 , the correlated bias measures were derived from two tests of word recognition, leaving open the possibility that bias is consistent for words (or, more generally, that it is consistent within the same stimulus domain) but differs unpredictably when the to-be-recognized stimuli change. To address this possibility, Experiment 3 included conditions in which two recognition study–test cycles varied in the class of materials used.
The stimulus domains chosen for the experiment were words and digital images of masterwork paintings. These materials are well suited to an examination of bias consistency across stimuli in two respects. First, words and paintings share few features beyond their visual presentation modality and contrast sharply along several dimensions: Paintings are richly detailed, complex in subject matter, and thematically (and sometimes emotionally) evocative, whereas the common word stimuli used in the present experiments possess none of these attributes. The use of such qualitatively distinct stimulus sets provides a strong test of the within-individual consistency of bias across materials.
A second advantage of words and paintings in providing a rigorous test of bias consistency is their tendency to elicit very different magnitudes of bias on recognition tests: Whereas common words tend to produce roughly neutral responding on average, paintings are associated with dramatic conservatism (Lindsay & Kantner, 2011 ). Note that bias consistency does not require that the obtained measure of bias be the same, or even similar, for a given participant across tests if the two tests use different stimuli. Rather, it requires that bias on Test 1 predict bias on Test 2, such that a participant with a more liberal than average word recognition bias should show a more liberal than average painting recognition bias. A finding that bias remained correlated across words and paintings despite sharp cross-stimulus differences would provide substantial evidence of trait-like stability across materials.
A total of 143 undergraduates participated, each randomly assigned to one of four conditions: the word–word (WW) condition (i.e., words in the first study–test cycle and words in the second study–test cycle), the painting–painting (PP) condition, the word–painting (WP) condition, and the painting–word (PW) condition. The WW, PP, WP, and PW conditions included 40, 37, 35, and 31 participants, respectively.
Word stimuli were identical to those used in Experiments 1 and 2 . Several hundred images of masterwork paintings were obtained from a computer-based memory training game called Art Dealer by permission of its creator, Jeffrey P. Toth. This set contains large, full-color, high-definition images of works by well-known artists from the 17th to the early 20th centuries (e.g., Rembrandt, Matisse, Modigliani). In all, 204 of these images, representing a wide array of artists, styles, and themes, were selected for use in Experiment 3 . Very famous works (e.g., Van Gogh’s self-portraits) were avoided. Paintings and words were assigned to study and test phases by the same method as the words in Experiments 1 and 2 .
The procedure was essentially identical to that of Experiment 1 , with the exceptions of the materials manipulation and a slight modification to the filler task.
Recognition means are displayed in Table 4 . Test 1 bias is plotted against Test 2 bias for each condition in Fig. 5 . Individuals’ bias values were again marked by considerable variability. Values of c ranged from 1.27 (H = .31, FA = .02) to –0.96 (H = .88, FA = .77) in the WW condition, 1.10 (H = .33, FA = .04) to –0.68 (H = 1 [uncorrected], FA = .17) in the PP condition, 0.90 (H = .60, FA = .02) to –1.21 (H = .96, FA = .75) in the PW condition, and 0.75 (H = .46, FA = .08) to –0.65 (H = .92, FA = .46) in the WP condition.
Correlations of recognition bias at Test 1 and Test 2 in the four conditions of Experiment 3
WW condition
Neither A z nor c differed significantly across tests, both p s > .28. Values of c were significantly correlated across the tests, r (36) = .81, p < .001, replicating the findings of Experiments 1 and 2. The strength of the relationship was consistent across all bias measures (see Table 2 ).
PP condition
Sensitivity rose significantly from Test 1 to Test 2, t (36) = –3.45, p < .01, while bias was unchanged ( t < 1). c was positively correlated across tests, but the relationship did not survive the partialing out of A z , r (33) = .31, p = .07. The supplementary measures yielded similar results: In all but one case (FA), the magnitude of the correlation was reduced below significance when controlling for sensitivity. The average p value for the bias correlation across all five measures was .056.
PW condition
There was no significant difference in sensitivity on the painting and word tests ( t < 1). As expected, painting bias was much more conservative than word bias, t (30) = 3.837, p < .001. The bias correlation across tests was significant, r (27) = .64, p < .001. The supplementary measures yielded concordant results.
WP condition
Group differences in sensitivity and bias followed the opposite pattern from the PW condition: Sensitivity differed significantly, t (34) = 3.246, p < .01, while bias was statistically equivalent ( t < 1). The correlation of c was again significant, r (31) = .45, p < .01. The spread of correlation coefficients across bias measures was unusually wide (range = .31 to .75), partially due to differences across measures in the presence or absence of score removals via the 3- SD cutoff. Correlations were significant in four of five cases, however, and were substantially positive in all cases.
Thus, Test 1 bias remained strongly predictive of Test 2 bias when different materials were used in the two tests. Stability was not equivalent across all four conditions, however. Fisher’s tests on bias correlations averaged across the five measures confirmed that the magnitude of the WW correlation ( M = .76) was significantly greater than that of the PP condition ( M = .33), z = 2.59, p < .01. Correlations in the PW ( M = .60) and WP ( M = .52) conditions did not differ significantly from those of any other condition. The directionally lower stability in the PW and WP conditions than in the WW condition is not surprising and suggests that consistency in stimuli contributes to consistency in bias across tests. The relatively weak bias relationship observed in the PP condition, however, was an unexpected result, given that the materials did not differ in this condition. As suggested by the increase in sensitivity across tests, it may be that some participants in the PP condition used the experience gained in the first study–test cycle to alter their approach to the second, producing a change in response bias across the tests and thereby reducing the correlation. For the present purposes, the important point is that even when the two tests used different materials, individuals who were conservative (or liberal) on one test tended to be conservative (or liberal) on the other.
Experiment 4
While Experiments 1 – 3 provided support for the characterization of recognition bias as a trait, evidence in the form of generalization beyond recognition memory has been absent. In Experiment 2 , bias was uncorrelated with performance on a DRM free recall test and a general knowledge task tapping strategic adjustments of grain size, two tasks hypothesized to involve the same evidence criterion at work in producing recognition bias. Experiment 4 returned to the DRM and grain size paradigms under conditions expected to increase the likelihood of detecting a relationship with recognition bias if one exists.
The DRM task in Experiment 4 was unchanged from that in Experiment 2 , but the timing of the experiment within the course of the academic term was believed to better suit the DRM paradigm. Experiment 2 took place mid-semester, and, as noted above, overlapped with lectures on the DRM paradigm in some psychology courses, giving many participants recent preexperimental insight regarding the nature of the test lists. In experimental settings, warnings about the critical lure decrease DRM false alarm rates (see Starns, Lane, Alonzo, & Roussel, 2007 , for a review). Variability in this foreknowledge across Experiment 2 participants may have driven differences in false recall of critical lures, undermining the detection of other mediators (e.g., inherent response bias). Experiment 4 was conducted in the first half of the fall semester, at which time very few of the introductory psychology students who constitute the majority of research participants had been familiarized with the DRM paradigm. As in Experiment 2 , liberal recognizers were predicted to recall a higher proportion of critical lures than conservative recognizers.
The general knowledge task was revised for Experiment 4 on the suspicion that the null result in Experiment 2 arose from the use of range size as the dependent measure. Given that participants were free to choose whatever range sizes they felt were appropriate, this measure might have been driven by variability in knowledge of the subject matter (either actual or assumed) to the extent that any potential relationship with response bias was obscured. Moreover, the incentive for using particular interval sizes in Experiment 2 (accurate yet informative answers) was merely implied; any response bias associated with the general knowledge task might be brought out more effectively with more explicit consequences for responses. Therefore, a new version of the task was created in which each question was accompanied by two response options, one of which was correct (e.g., “What did year did CBC make its first television broadcast? a. 1953 b. 1963”). Participants were informed that they would gain 10 cents for every correct answer and lose 10 cents for every incorrect answer. They were also given the right to “pass” on any question to which they did not feel confident giving an answer (called report option by Koriat & Goldsmith, 1994 ), in which case they neither gained nor lost money on that trial. In this scenario, any question to which the participant does not have prior knowledge of the answer is a small gamble in which giving a response incurs risk that can be avoided with the exercise of report option.
The dependent measure of interest was the proportion of trials on which liberal versus conservative recognizers would use the pass option. Conservative recognizers, who were assumed to require more memory evidence than liberal recognizers before committing to an “old” judgment, were hypothesized to require more confidence in their knowledge of the answer to a given question before committing to the gamble. Thus, conservative recognizers should exercise report option significantly more often. This task is particularly appealing, given the goals of this experiment, in that risk-taking behaviors have been associated with extraversion (Patterson & Newman, 1993 ), and extraversion, in turn, has been associated with a liberal recognition bias (Gillespie & Eysenck, 1980 ).
A total of 50 participants took part in Experiment 4 .
The same words used in Experiments 1 – 3 served as recognition task materials. DRM task materials were identical to those of Experiment 2 . The general knowledge task included 50 questions, each with two response alternatives. Approximately half of the questions were retained from Experiment 2 ; in order to increase variety within the task, the remainder of the set comprised questions requiring numerical responses other than the names of years. These items were drawn from the original pool of 208 questions (see the Exp. 2 Method).
Two response alternatives were prepared for each question. One alternative was always the correct answer. The second option was chosen by the first author and was designed to pose as a plausible alternative that would generate uncertainty without making the task overly difficult. Generally, the incorrect alternative was a value of moderate numerical distance from the correct answer.
Experiment 4 consisted of three stages: a recognition study–test cycle, the DRM task, and the general knowledge task with report option. Participants were tested in groups of one to three, a measure taken to increase the efficiency of data collection, given the unusual length of the experiment. In sessions of more than one participant, a second experimenter was present and aided in the transition between phases. The order of the recognition, DRM, and general knowledge tasks was counterbalanced across groups; within groups, each participant completed the tasks in the same order.
The recognition task followed a procedure identical to that of Experiments 1 – 3 (note, however, that only one study–test cycle was included in Exp. 4 ). The procedure for the DRM task was identical to that of Experiment 2 , with two exceptions required by the group testing format. First, participants were asked to read list words silently. Second, a black-and-white flashing screen (rather than an auditory tone) alerted participants to the end of each 2-min recall period.
The general knowledge task was similar to the one used in Experiment 2 , with differences reflecting the change to a two-alternative forced choice (2AFC) response format with report option. Task instructions were analogous to those in Experiment 2 , with an additional component informing participants that they would gain 10 cents for each correct response, lose 10 cents for each incorrect response, and gain or lose nothing by choosing to “pass” on answering a given question. The instructions emphasized that a negative balance at the end of the task would not result in any loss of money.
Questions were again presented near the top of the screen, with two boxes positioned underneath; these boxes contained response options A and B. Near the bottom of the screen appeared the words “Press spacebar to pass.” Participants chose an answer by entering “a” or “b,” or passed by hitting the spacebar. Passing initiated the next trial. Selection of one of the response alternatives prompted the appearance of a confidence scale ranging from 50 % to 100 % near the top of the screen. Participants indicated their confidence in the selected answer via keypress, initiating the next trial.
The data of two participants who were near chance in recognition accuracy were removed from subsequent analyses. The general knowledge test data of one additional participant were deleted due to a failure to follow task instructions. Therefore, the following analyses included 48 participants in the DRM and recognition tasks and 47 participants in the general knowledge task.
Group recognition measures are displayed in Table 5 . Values of c ranged from 1.40 (H = .31, FA = 0 [uncorrected]) to –0.53 (H = .81, FA = .58). As in Experiments 1 – 3 , all bias scores greater than 3 SD from the mean for a given parameter were removed prior to correlational analysis (0.83 % of all scores), and all bias correlations controlled for A z . In the DRM task, participants falsely recalled an average of 2.98 critical lures out of eight possible ( SD = 1.92, range = 0 to 7). Unlike in Experiment 2 , values of c were significantly correlated with frequency of false recall, r (44) = –.32, p < .05, with a negative relationship indicating that increasing liberality of recognition bias was associated with increasing frequency of false recall (see Fig. 6 ). The results using the supplementary bias measures were consistent with those using c (see Table 6 ); only ln( β ) yielded a nonsignificant relationship. The correlation of A z and DRM false recall (controlling for c ) was nearly reliable, r (44) = –.29, p = .051. These analyses suggest that both bias and sensitivity are related to recall of critical lures in the DRM paradigm.
Correlations of recognition bias and frequency of DRM false recall (top panel) and correlations of bias and number of passes in the general knowledge task (bottom panel) in Experiment 4
Participants chose to pass on an average of 12.53 ( SD = 8.69) out of 50 general knowledge questions (25.1 %). Individual participants’ use of the pass option ranged from 0 to 31 times. When questions were answered with one of the two response alternatives, mean accuracy was 68.9 % ( SD = 10.5 %) and mean confidence was 58.2 % ( SD = 12.5 %). The split-half reliability of the pass measure was high (.81). No relationship was detected between c and frequency of passing ( r = .06), accuracy ( r < .001), or confidence ( r = –.08). The strongest relationship yielded by the supplementary bias estimates was r = –.14, p = .35. Recognition sensitivity was also unrelated to these measures (strongest r = –.06).
One further analysis concerned individuals’ frequencies of passing versus giving responses at a 50 % confidence level. Since both types of responses signify an expectation of chance-level ability in answering a question, it was expected that this comparison would discriminate liberal and conservative responders: The former should be more likely to risk an incorrect response, while the latter should be more likely to pass. However, preference for the pass option (the number of passes minus the number of 50 % confidence responses) was uncorrelated with c ( r = .09) and with the supplementary measures (strongest r = .08)
Experiment 4 provided the first indication of a relationship between recognition response bias and performance on a nonrecognition task: Individuals using a more lax criterion for calling items “old” in a recognition test also used a more lax standard for recalling related but nonpresented list items in a DRM procedure. Though replication of this relationship is warranted, its presence in Experiment 4 is consistent with the suspicion that the lack of relationship in Experiment 2 was due to the noise added by widespread foreknowledge of the task (although we note that, contrary to that speculation, the absolute rate of DRM intrusions was not higher in Exp. 4 than in Exp. 2 ; it may simply be that the bias–DRM relationship is weak and that there was insufficient power to detect it in Exp. 2 ).
No relationship was observed between bias and performance in the general knowledge task, consistent with the results of Experiment 2 . It might be that conservatism in a recognition task and conservatism in a general knowledge test have independent cognitive substrates, and that trait-like stability in recognition bias is not relevant to the class of decisions exemplified in the general knowledge task. Alternatively, the general knowledge task might not have elicited systematic biases relevant to a required evidence criterion. We consider this possibility in the General Discussion.
General discussion
The construct of response bias provides a basis for understanding how recognition decisions are reached under conditions of uncertainty and how they are affected by factors independent of memory. In the present experiments, we examined whether measured response bias might also provide a basis for understanding individual recognizers. The perspective taken was a departure from that of most previous research on bias: Instead of asking what factors influence bias from without (e.g., task and stimulus manipulations), the present work asked to what extent bias is founded within an individual as a stable cognitive trait.
The present experiments provided evidence of substantial within-individual consistency. Experiment 1 established that one’s response bias does not vary freely from one recognition test to the next; rather, bias on an initial test is highly predictive of bias on a subsequent test. Experiment 2 extended this finding by demonstrating a similar correlation of bias on two tests one week apart. While this comparison spans separate experiments and groups of participants, it is nonetheless worth emphasizing that the differences between the 10-min and one-week intervals transcend duration. With a 10-min interval, participants remain within the context of the experiment between tests, changing only the task with which they are engaged; when one week separates the tests, by contrast, participants return to the laboratory for Test 2 having accumulated a week of life experiences since Test 1. The fact that these two intervals were associated with similar correlations of bias is strongly suggestive of trait-like stability.
In Experiment 3 , significant bias correlations were observed even with substantial differences in the stimuli across two tests (the PW and WP conditions). The presence of large, significant correlations across stimulus classes sharing few common features suggests that participant predisposition accounts for a good deal of the variance in bias. The similarity of the average correlations observed in the PW and WP conditions is sensible, given the identical content of the two conditions. It is informative, however, in light of the divergent trends distinguishing the two conditions. The PW condition showed a sizable shift in bias from paintings to words, but no change in sensitivity; the WP condition showed no shift in bias across stimuli (contrary to expectation), but significantly greater sensitivity to paintings than to words. Bias stability, then, is apparently not reliant on a match in general discrimination or response bias across tests. The former finding is consistent with the signal detection theory assumption that discrimination and bias are independent properties, while the latter confirms the hypothesis that bias need not be similar across tests to have a predictive relationship across tests.
Is the bias observed in recognition tests a special case of a general proclivity to act on the basis of a certain level of evidence? Experiments 2 and 4 explored this question by allowing recognition bias to be correlated with performance on nonrecognition tasks. These experiments produced mixed results. Bias did not predict DRM false recall in Experiment 2 , but the interpretation of this result was clouded by the fact that many participants had very recently learned of the DRM paradigm in classroom lectures. Experiment 4 was timed to resolve this concern and did show a significant tendency for participants with a more liberal recognition bias to recall more critical lures in the DRM task. These findings are noteworthy in two respects: First, they represent a correspondence of recognition bias with performance on a task outside of recognition memory (i.e., free recall), suggesting a common cognitive substrate. Second, they suggest that liberality in response bias is related to general false memory proneness, which has itself been discussed as a possible trait (e.g., Geraerts et al., 2009 ).
Recognition bias was not, however, associated with degree of conservatism in answering general knowledge questions. Whether conservatism was operationalized as the use of wide ranges in numerical estimates (Exp. 2 ) or the frequent use of a “pass” option to avoid the risk of an incorrect response (Exps. 4 ), we found no apparent relationship with recognition bias. As noted earlier, these null findings may result from a true lack of relationship between recognition bias and risk tolerance in estimation. On the basis of the present findings, it might be the case that bias correlates with performance on memory tasks that are episodic in nature (e.g., DRM), but not with other forms of decision making, such as estimation. However, it might be that the general knowledge task did not motivate patterns of responding driven by a liberal or conservative bias. The payoffs and losses associated with individual responses were small (10 cents), and participants were aware that they could not lose money on balance. A design fostering loss aversion (Kahneman & Tversky, 1984 ) or including trial-by-trial feedback might increase investment in responses and bring out a decisional bias related to recognition bias.
We view the present evidence for within-individual bias stability as broadly consistent with past research (cited in the introduction) indicating that certain groups of participants defined by inherent characteristics (e.g., Alzheimer’s patients, panic disorder patients) can be characterized as liberally biased recognizers. We believe the present results are also consistent with the Blair et al. ( 2002 ) finding of substantial within-individual consistency in false recognition of critical lures from the same DRM lists tested two weeks apart, although Blair et al. argued against response bias consistency as an account of their results (p. 595). Our conclusions seem at odds, however, with those of Salthouse and Siedlecki ( 2007 ), who did not find predictive relationships of false DRM recognition across tests differing in materials, nor reliable correlations between false recognition and established individual-difference variables. The Salthouse and Siedlecki results suggest that recognition of critical lures is governed largely by task-specific factors, while our results point to an element of response bias that is inherent to an individual and is consistent across time and task variations. These findings are not irreconcilable, however: While response bias and the tendency to commit DRM errors are likely related to one another (Miller, Guerin, & Wolford, 2011 ), the relationship is imperfect. In the present Experiment 4 , for example, bias on a recognition test predicted the number of critical lures recalled by participants in a DRM free recall task, but the modest strength of the relationship ( r = –.32) suggests independent properties as well. It might be that individual differences in recognition response bias are more stable than are individual differences in DRM false recognition. Future research should determine whether bias is related to the individual-difference measures tested by Salthouse and Siedlecki.
The possibility that response bias is a trait carries at least three general implications. First, it suggests that bias proclivities at the level of the individual represent a source of variance in recognition memory experiments, one that can be identified in models of recognition. Models of individual participant data can be constrained if an individual’s predisposition to bias is identified beforehand. If not, trait bias suggests a psychological interpretation of model parameters that index response bias.
Second, the concept of trait response bias suggests that an individual’s bias on a recognition test is a product both of inherent tendencies and of any experimental manipulations that affect bias. The relative influences of predisposition and of a given experimental manipulation in determining a participant’s overall level of bias is an open question, but there are clearly scenarios in which the two factors could come into conflict. For example, a growing literature has demonstrated that participants tend not to make appropriate criterion shifts in response to changing classes of items (e.g., strongly vs. weakly encoded) when the shifts must occur within a single test list (Stretch & Wixted, 1998 ; Verde & Rotello, 2007 ). Generally, within-list criterion shifts are not observed unless the two item classes are strikingly different (Bruno, Higham, & Perfect, 2009 ; Singer, 2009 ; Singer & Wixted, 2006 ) or corrective feedback is administered (Rhodes & Jacoby, 2007 ; Verde & Rotello, 2007 ). Participants’ resistance to within-list criterion shifting might be a partial result of inherent bias tendencies that anchor shifting behavior. Similarly, the remarkable unwillingness of participants to exercise appropriate levels of bias even when they are aware that test lists are composed only of targets or lures (J. C. Cox & Dobbins, 2011 ) might be explained in part by such an anchoring effect. Even when manipulations such as feedback, instructional motivation, and payoffs are successful in moving response bias, such shifts are usually suboptimal (e.g., Aminoff et al., 2012 ), another potential influence of trait bias.
The third and most significant implication of the results presented here is that individuals can be placed along a continuum of recognition bias from more conservative to more liberal, and, critically, that this placement characterizes the individual , not just his or her performance on a given test. An important question is whether the trait-like qualities of response bias are limited to recognition memory measures or extend to multiple classes of decisions. Preliminary evidence for the generalization of bias beyond recognition memory was obtained with the DRM free recall task used in Experiment 4 , but not with the general knowledge task. Further work with such tasks and with personality measures relevant to criterial levels of decision evidence (e.g., maximizing tendencies; Simon, 1956 ) will help to determine the extent to which recognition bias is a special case of a more general, intraindividually stable decision-making heuristic.
The idea that people are inherently disposed to some tendency or other is commonplace in the domain of personality psychology; indeed, the very notion of a personality trait implies that persons possess enduring attributes that guide their thinking and behavior across different situations. The notion of a memory trait is less explored. The present experiments suggest that response bias observed in a recognition test is tethered to a predisposition internal to the recognizer, and that a complete theory of recognition memory performance should account for this factor.
Ackerman, R., & Goldsmith, M. (2008). Control over grain size in memory reporting—With and without satisficing knowledge. Journal of Experimental Psychology: Learning, Memory, and Cognition, 34, 1224–1245. doi: 10.1037/a0012938
Article PubMed Google Scholar
Aminoff, E. M., Clewett, D., Freeman, S., Frithsen, A., Tipper, C., Johnson, A., . . . Miller, M. B. (2012). Individual differences in shifting decision criterion: A recognition memory study. Memory & Cognition . doi: 10.3758/s13421-012-0204-6
Benjamin, A. S., & Bawa, S. (2004). Distractor plausibility and criterion placement in recognition. Journal of Memory and Language, 51, 159–172. doi: 10.1016/j.jml.2004.04.001
Article Google Scholar
Beth, E. H., Budson, A. E., Waring, J. D., & Ally, B. A. (2009). Response bias for picture recognition in patients with Alzheimer disease. Cognitive and Behavioral Neurology, 22, 229–235. doi: 10.1097/WNN.0b013e3181b7f3b1
Blair, I. V., Lenton, A. P., & Hastie, R. (2002). The reliability of the DRM paradigm as a measure of individual differences in false memories. Psychonomic Bulletin & Review, 9, 590–596. doi: 10.3758/BF03196317
Brown, J., Lewis, V. J., & Monk, A. F. (1977). Memorability, word frequency and negative recognition. Quarterly Journal of Experimental Psychology, 29, 461–473. doi: 10.1080/14640747708400622
Bruno, D., Higham, P. A., & Perfect, T. J. (2009). Global subjective memorability and the strength-based mirror effect in recognition memory. Memory & Cognition, 37, 807–818. doi: 10.3758/MC.37.6.807
Carlin, M. T., Toglia, M. P., Wakeford, Y., Jakway, A., Sullivan, K., & Hasel, L. (2008). Veridical and false pictorial memory in individuals with and without mental retardation. American Journal on Mental Retardation, 113, 201–213.
Coltheart, M. (1981). The MRC psycholinguistic database. Quarterly Journal of Experimental Psychology, 33A, 497–505. doi: 10.1080/14640748108400805
Google Scholar
Couch, A., & Keniston, K. (1960). Yeasayers and naysayers: Agreeing response set as a personality variable. Journal of Abnormal and Social Psychology, 60, 151–174.
Cox, J. C., & Dobbins, I. G. (2011). The striking similarities between standard, distractor-free, and target-free recognition. Memory & Cognition, 39, 925–940. doi: 10.3758/s13421-011-0090-3
Cox, G. E., & Shiffrin, R. M. (2012). Criterion setting and the dynamics of recognition memory. Topics in Cognitive Science, 4, 135–150. doi: 10.1111/j.1756-8765.2011.01177.x
Dobbins, I. G., & Kroll, N. E. A. (2005). Distinctiveness and the recognition mirror effect: Evidence for an item-based criterion placement heuristic. Journal of Experimental Psychology: Learning, Memory, and Cognition, 31, 1186–1198. doi: 10.1037/0278-7393.31.6.1186
Dougal, S., & Rotello, C. M. (2007). “Remembering” emotional words is based on response bias, not recollection. Psychonomic Bulletin & Review, 14, 423–429. doi: 10.3758/BF03194083
Egan, J. P. (1958). Recognition memory and the operating characteristic. USAF Operational Applications Laboratory Technical Note , 58–51.
Gallo, D. A. (2010). False memories and fantastic beliefs: 15 years of the DRM illusion. Memory & Cognition, 38, 833–848. doi: 10.3758/MC.38.7.833
Geraerts, E., Lindsay, D. S., Merckelbach, H., Jelicic, M., Raymaekers, L., Arnold, M. M., & Schooler, J. W. (2009). Cognitive mechanisms underlying recovered-memory experiences of childhood sexual abuse. Psychological Science, 20, 92–98. doi: 10.1111/j.1467-9280.2008.02247.x
Gillespie, C. R., & Eysenck, M. W. (1980). Effects of introversion–extraversion on continuous recognition memory. Bulletin of the Psychonomic Society, 15, 233–235.
Goldsmith, M., Koriat, A., & Weinberg-Eliezer, A. (2002). Strategic regulation of grain size memory reporting. Journal of Experimental Psychology: General, 131, 73–95. doi: 10.1037/0096-3445.131.1.73
Harvey, L. O., Jr. (2005). Parameter estimation of signal detection models: RscorePlus (Version 5.6.0). [Computer software and manual]. Retrieved from http://psych.colorado.edu/~lharvey/html/software.html
Healy, A. F., & Kubovy, M. (1978). The effects of payoffs and prior probabilities on indices of performance and cutoff location in recognition memory. Memory & Cognition, 6, 544–553. doi: 10.3758/BF03198243
Hockley, W. E. (2011). Criterion changes: How flexible are recognition decision processes? In P. A. Higham & J. P. Leboe (Eds.), Constructions of remembering and metacognition: Essays in honour of Bruce Whittlesea . Palgrave Macmillan: Basingstoke, U.K.
Huh, T. J., Kramer, J. H., Gazzaley, A., & Delis, D. C. (2006). Response bias and aging on a recognition memory task. Journal of the International Neuropsychological Society, 12, 1–7. doi: 10.1017/S1355617706060024
Kahneman, D., & Tversky, A. (1984). Choices, values, and frames. American Psychologist, 39, 341–350. doi: 10.1037/0003-066X.39.4.341
Kantner, J., & Lindsay, D. S. (2010). Can corrective feedback improve recognition memory? Memory & Cognition, 38, 389–406. doi: 10.3758/MC.38.4.389
Koriat, A., & Goldsmith, M. (1994). Memory in naturalistic and laboratory contexts: Distinguishing the accuracy-oriented and quantity-oriented approaches to memory assessment. Journal of Experimental Psychology: General, 123, 297–315. doi: 10.1037/0096-3445.123.3.297
Kramer, J. H., Rosen, H. J., Du, A. T., Schuff, N., Hollnagel, C., Weiner, M. W., & Delis, D. C. (2005). Dissociations in hippocampal and frontal contributions to episodic memory performance. Neuropsychology, 19, 799–805. doi: 10.1037/0894-4105.19.6.7999
Lindsay, D. S., & Kantner, J. (2011). A search for influences of feedback on recognition of music, poetry, and art. In P. A. Higham & J. P. Leboe (Eds.), Constructions of remembering and metacognition: Essays in honour of Bruce Whittlesea . Palgrave Macmillan: Basingstoke, U.K.
Macmillan, N. A. (1993). Signal detection theory as data analysis method and psychological decision model. In G. Keren & C. Lewis (Eds.), A handbook for data analysis in the behavioral sciences: Methodological issues (pp. 21–57). Hillsdale, NJ: Erlbaum.
Macmillan, N. A., & Creelman, C. D. (1996). Triangles in ROC space: History and theory of “nonparametric” measures of sensitivity and response bias. Psychonomic Bulletin & Review, 3, 164–170. doi: 10.3758/BF03212415
Macmillan, N. A., & Creelman, C. D. (2005). Detection theory: A user’s guide (2nd ed.). Mahwah, NJ: Erlbaum.
Macmillan, N. A., & Kaplan, H. L. (1985). Detection theory analysis of group data: Estimating sensitivity from average hit and false-alarm rates. Psychological Bulletin, 98, 185–199. doi: 10.1037/0033-2909.98.1.185
Miller, M. B., Guerin, S. A., & Wolford, G. L. (2011). The strategic nature of false recognition in the DRM paradigm. Journal of Experimental Psychology: Learning, Memory, and Cognition, 37, 1228–1235. doi: 10.1037/a0024539
Moritz, S., Woodward, T. S., Jelinek, L., & Klinge, R. (2008). Memory and metamemory in schizophrenia: A liberal acceptance account of psychosis. Psychological Medicine, 38, 825–832. doi: 10.1017/S0033291707002553
Patterson, C. M., & Newman, J. P. (1993). Reflectivity and learning from aversive events: Toward a psychological mechanism for the syndromes of disinhibition. Psychological Review, 100, 716–736. doi: 10.1037/0033-295X.100.4.716
Qin, J., Ogle, C. M., & Goodman, G. S. (2008). Adults’ memories of childhood: True and false reports. Journal of Experimental Psychology: Applied, 14, 373–391. doi: 10.1037/a0014309
Ratcliff, R., Sheu, C., & Gronlund, S. D. (1992). Testing global memory models using ROC curves. Psychological Review, 99, 518–535. doi: 10.1037/0033-295X.99.3.518
Rhodes, M. G., & Jacoby, L. L. (2007). On the dynamic nature of response criterion in recognition memory: Effects of base rate, awareness, and feedback. Journal of Experimental Psychology: Learning, Memory, and Cognition, 33, 305–320. doi: 10.1037/0278-7393.33.2.305
Roediger, H. L., III, & McDermott, K. B. (1995). Creating false memories: Remembering words not presented in lists. Journal of Experimental Psychology: Learning, Memory, and Cognition, 21, 803–814. doi: 10.1037/0278-7393.21.4.803
Rotello, C. M., & Macmillan, N. A. (2008). Response bias in recognition memory. In A. S. Benjamin & B. H. Ross (Eds.), Skill and strategy in memory use (The Psychology of Learning and Motivation) (Vol. 48, pp. 61–94). San Diego, CA: Elsevier/Academic Press.
Chapter Google Scholar
Salthouse, T. A., & Siedlecki, K. L. (2007). An individual difference analysis of false recognition. American Journal of Psychology, 120, 429–458.
PubMed Google Scholar
Simon, H. A. (1956). Rational choice and the structure of the environment. Psychological Review, 63, 129–138. doi: 10.1037/h0042769
Singer, M. (2009). Strength-based criterion shifts in recognition memory. Memory & Cognition, 37, 976–984. doi: 10.3758/MC.37.7.976
Singer, M., & Wixted, J. T. (2006). Effect of delay on recognition decisions: Evidence for a criterion shift. Memory & Cognition, 34, 125–137. doi: 10.3758/BF03193392
Stadler, M. A., Roediger, H. L., III, & McDermott, K. B. (1999). Norms for word lists that create false memories. Memory & Cognition, 27, 494–500. doi: 10.3758/BF03211543
Stanislaw, H., & Todorov, N. (1999). Calculation of signal detection theory measures. Behavior Research Methods, Instruments, & Computers, 31, 137–149. doi: 10.3758/BF03207704
Starns, J. J., Lane, S. M., Alonzo, J. D., & Roussel, C. C. (2007). Metamnemonic control over the discriminability of memory evidence: A signal detection analysis of warning effects in the associative list paradigm. Journal of Memory and Language, 56, 592–607. doi: 10.1016/j.jml.2006.08.013
Stretch, V., & Wixted, J. T. (1998). On the difference between strength-based and frequency-based mirror effects in recognition memory. Journal of Experimental Psychology: Learning, Memory, and Cognition, 24, 1379–1396. doi: 10.1037/0278-7393.24.6.1379
Van Zandt, T. (2000). ROC curves and confidence judgments in recognition memory. Journal of Experimental Psychology: Learning, Memory, and Cognition, 26, 582–600. doi: 10.1037/0278-7393.26.3.582
Verde, M. F., Macmillan, N. A., & Rotello, C. M. (2006). Measures of sensitivity based on a single hit rate and false alarm rate: The accuracy, precision, and robustness of d' , A z , and A' . Perception & Psychophysics, 68, 643–654. doi: 10.3758/BF03208765
Verde, M. F., & Rotello, C. M. (2007). Memory strength and the decision process in recognition memory. Memory & Cognition, 35, 254–262. doi: 10.3758/BF03193446
Windmann, S., & Krüger, T. (1998). Subconscious detection of threat as reflected by an enhanced response bias. Consciousness and Cognition, 7, 603–633. doi: 10.1006/ccog.1998.0337
Woodard, J. L., Axelrod, B. N., Mordecai, K. L., & Shannon, K. D. (2004). Value of signal detection theory indexes for Wechsler Memory Scale–III recognition measures. Journal of Clinical and Experimental Neuropsychology, 26, 577–586. doi: 10.1080/13803390490496614
Download references
Author Note
Justin Kantner and D. Stephen Lindsay, Department of Psychology, University of Victoria, Victoria, British Columbia, Canada.
This research was supported by a Discovery Grant from the Natural Sciences and Engineering Research Council of Canada to D.S.L. We thank Mayumi Okamoto, Caitlin Malli, Graeme Austin, Emily Cameron, Jordy Freeman, and Priya Rosenberg for their assistance with data collection, and Jordy Freeman for suggesting one of the Experiment 4 analyses. J.K. is now at the University of California, Santa Barbara.
Correspondence concerning this article should be addressed to Justin Kantner, Department of Psychological and Brain Sciences, University of California, Santa Barbara, Santa Barbara, California, 93106. Email: [email protected]. Phone: (805) 845-1906.
Author information
Authors and affiliations.
Department of Psychology, University of Victoria, Victoria, BC, Canada
Justin Kantner & D. Stephen Lindsay
Department of Psychological and Brain Sciences, University of California, Santa Barbara, Santa Barbara, CA, 93106, USA
Justin Kantner
You can also search for this author in PubMed Google Scholar
Corresponding author
Correspondence to Justin Kantner .
Rights and permissions
Reprints and permissions
About this article
Kantner, J., Lindsay, D.S. Response bias in recognition memory as a cognitive trait. Mem Cogn 40 , 1163–1177 (2012). https://doi.org/10.3758/s13421-012-0226-0
Download citation
Published : 08 August 2012
Issue Date : November 2012
DOI : https://doi.org/10.3758/s13421-012-0226-0
Share this article
Anyone you share the following link with will be able to read this content:
Sorry, a shareable link is not currently available for this article.
Provided by the Springer Nature SharedIt content-sharing initiative
- Recognition memory
- Response bias
- Individual differences
- Find a journal
- Publish with us
- Track your research
Observer Bias: Definition, Examples & Prevention
Julia Simkus
Editor at Simply Psychology
BA (Hons) Psychology, Princeton University
Julia Simkus is a graduate of Princeton University with a Bachelor of Arts in Psychology. She is currently studying for a Master's Degree in Counseling for Mental Health and Wellness in September 2023. Julia's research has been published in peer reviewed journals.
Learn about our Editorial Process
Saul McLeod, PhD
Editor-in-Chief for Simply Psychology
BSc (Hons) Psychology, MRes, PhD, University of Manchester
Saul McLeod, PhD., is a qualified psychology teacher with over 18 years of experience in further and higher education. He has been published in peer-reviewed journals, including the Journal of Clinical Psychology.
Olivia Guy-Evans, MSc
Associate Editor for Simply Psychology
BSc (Hons) Psychology, MSc Psychology of Education
Olivia Guy-Evans is a writer and associate editor for Simply Psychology. She has previously worked in healthcare and educational sectors.
Observer bias is a type of experimenter bias that occurs when a researcher’s expectations, perspectives, opinions, or prejudices impact the results of an experiment. This type of research bias is also called detection bias or ascertainment bias.
This typically occurs when a researcher is aware of the purpose and hypotheses of a study and holds expectations about what will happen.
If a researcher is trying to find a particular result to support the hypothesis of the study or has a predetermined idea of what the results should be, they will have the incentive to twist the data to make them more in line with their predictions.
This bias occurs most often in observational studies or any type of research where measurements are taken and recorded manually. In observational studies, a researcher records behaviors or takes measurements from participants without trying to influence the outcome of the experiment.
Observational studies are used in a number of different research fields, most specifically medicine, psychology, behavioral science, and ethnography.
For Example
You are performing an observational study to investigate the effects of a new medication to treat nausea. Group A receives the actual treatment with the new medication, while group B receives a placebo.
The participants do not know which group they are a part of, but you – the researcher – do.
Unconsciously, you treat the two groups differently, framing questions more negatively towards Group B and commenting that those in Group A seem more energized and upbeat.
Impact of Observer Bias
Observer bias can result in misleading and unreliable results. A researcher’s biases and prejudices can affect data collection and observer interpretation, leading to results that fail to represent accurately what exists in reality.
It might also result in inaccurate data sets, misleading information, or biased treatment from researchers.
Observer bias can damage scientific research and policy decisions and lead to negative outcomes for people involved in the research studies.
Why Observer Bias Can Happen
Subjective methods.
Subjective research methods are those that involve some type of interpretation before you record the observations. Subjectivity refers to the way research is influenced by the perspectives, values, emotions, social experiences, and viewpoints of the researcher.
This could lead a researcher to record some observations as relevant while ignoring other equally important observations.
Even if a researcher is subconsciously primed to see only what they expect to observe, subjective research methods could lead to skewed conclusions.
Objective Methods
Although objective research tends to be impartial and fact-based, observer bias might still influence studies that use more objective methods.
This is because researchers tend to interpret or record readings differently, skewing the results to be more in line with their predictions.
For example, when measuring blood pressure using a blood pressure monitor, a researcher might round up the blood pressure to the nearest whole number.
Or, due to familiarity with the procedures of measuring blood pressure, a researcher might be less careful when taking the measurements and thus record inaccurate results.
How to Minimize Observer Bias
Blinding, or masking, ensures that the participants and researchers are all unsure of the goals of the study.
This will help eliminate some of the research expectations that come from knowing the study’s purpose so observers are less likely to be biased.
Additionally, in double-blind studies , neither the researchers nor the subjects know which treatments are being used or which group they belong to.
Random Assignment
Randomly assigning subjects to groups instead of choosing the subjects themselves will help minimize observer bias.
Multiple Observers
Having multiple researchers involved in the research study will ensure that your data is consistent and make it less likely that one researcher’s biases will significantly affect the project’s outcome.
It can also be beneficial to use multiple data collection methods for the same observations to corroborate your findings and check that they line up with each other.
Train Observers
Before beginning a study, it is beneficial to train all observers in the procedures to ensure everyone collects and records data exactly the same way.
This will eliminate any variation in how different observers report the same observation, keeping interrater reliability high and minimizing observer bias.
Standardized Procedures
It is important to create standardized procedures or protocols that are easy for all observers to follow.
You can record these procedures so that the researchers can refer back to them at any point in the research process.
Related Biases
Observer bias is closely related to several other types of research bias.
Observer-Expectancy Effect
The observer-expectancy effect occurs when a researcher’s cognitive bias causes them to subconsciously influence the results of their own study through interactions with participants.
Researchers might unconsciously or deliberately treat certain subjects differently, leading to unequal results between groups.
For example, a researcher might ask different questions or give different directions to one group and not another, or influence certain participants’ behavior by changing their body language, posture, tone of voice, or appearance in certain ways.
Actor-Observer Bias
Actor-observer bias is an attributional bias where a researcher attributes their own actions to external factors while attributing other people’s behaviors to internal causes.
This bias can help explain why we are inclined to blame others for things that happen, even when we would not blame ourselves for acting in the same way.
For example, if you perform poorly on a test, you might blame the result on external factors such as teacher bias or the questions being harder than usual.
However, if a classmate fails the same test, you might attribute their failure to a lack of intelligence or preparation.
Hawthorne Effect
The Hawthorne effect refers to some participants’ tendency to work harder and perform better when they know they are being observed.
This effect also suggests that individuals may change their behavior due to the attention they are receiving from researchers rather than because of any manipulation of independent variables.
Experimenter Bias
Experimenter bias is any type of cognitive bias that occurs when experimenters allow their expectations to affect their interpretation of observations.
Experimenter bias typically refers to all types of biases from researchers that might influence a study, including observer bias, the observer-expectancy effect, actor-observer bias, and the Hawthorne effect.
When a researcher has a predetermined idea of the results of their study, they might conduct the study or record results in a way that confirms their theory.
What is the difference between observer bias and confirmation bias?
Observer bias is a type of experimenter bias where a researcher’s predetermined expectations, perspectives, opinions, or prejudices can impact the results of an experiment.
Confirmation bias is a type of cognitive bias that occurs when a researcher favors information or interprets findings to favor their existing beliefs.
Unlike observer bias which can be intentional in some instances, confirmation bias happens due to the natural way our brains work, so it cannot be eliminated.
What is the difference between observer bias and the observer effect?
The observer effect in psychology is also known as the Hawthorne effect. It refers to how people change their behavior when they know they are being observed in a study.
Observer bias is a related term in the social sciences that refers to the error that results from an observer’s cognitive biases, particularly when observers overemphasize behavior they expect to find and fail to notice behavior they do not expect.
The observer effect is not to be confused with the observer-expectancy effect or the actor-observer bias, discussed above.
Further Reading
Burghardt, G. M., Bartmess‐LeVasseur, J. N., Browning, S. A., Morrison, K. E., Stec, C. L., Zachau, C. E., & Freeberg, T. M. (2012). Perspectives–minimizing observer bias in behavioral studies: a review and recommendations . Ethology , 118 (6), 511-517.
Hróbjartsson, A., Thomsen, A. S. S., Emanuelsson, F., Tendal, B., Hilden, J., Boutron, I., … & Brorson, S. (2013). Observer bias in randomized clinical trials with measurement scale outcomes: a systematic review of trials with both blinded and nonblinded assessors . Cmaj , 185 (4), E201-E211.
Salvia, J. A., & Meisel, C. J. (1980). Observer bias: A methodological consideration in special education research. The Journal of Special Education , 14 (2), 261-270.


An official website of the United States government
The .gov means it’s official. Federal government websites often end in .gov or .mil. Before sharing sensitive information, make sure you’re on a federal government site.
The site is secure. The https:// ensures that you are connecting to the official website and that any information you provide is encrypted and transmitted securely.
- Publications
- Account settings
The PMC website is updating on October 15, 2024. Learn More or Try it out now .
- Advanced Search
- Journal List
- Health Serv Res
- v.37(5); 2002 Oct
A Demonstration of the Impact of Response Bias on the Results of Patient Satisfaction Surveys
The purposes of the present study were to examine patient satisfaction survey data for evidence of response bias, and to demonstrate, using simulated data, how response bias may impact interpretation of results.
Data Sources
Patient satisfaction ratings of primary care providers (family practitioners and general internists) practicing in the context of a group-model health maintenance organization and simulated data generated to be comparable to the actual data.
Study Design
Correlational analysis of actual patient satisfaction data, followed by a simulation study where response bias was modeled, with comparison of results from biased and unbiased samples.
Principal Findings
A positive correlation was found between mean patient satisfaction rating and response rate in the actual patient satisfaction data. Simulation results suggest response bias could lead to overestimation of patient satisfaction overall, with this effect greatest for physicians with the lowest satisfaction scores.
Conclusions
Findings suggest that response bias may significantly impact the results of patient satisfaction surveys, leading to overestimation of the level of satisfaction in the patient population overall. Estimates of satisfaction may be most inflated for providers with the least satisfied patients, thereby threatening the validity of provider-level comparisons.
In recent years, health care organizations, policymakers, advocacy groups, and individual consumers have become increasingly concerned about the quality of health care. One result of this concern is the widespread use of patient satisfaction measures as indicators of health care quality ( Carlson et al. 2000 ; Ford, Bach, and Fottler 1997 ; Rosenthal and Shannon 1997 ; Young, Meterko, and Desai 2000 ). In some organizations, patient satisfaction survey results are used in determining provider compensation ( Gold et al. 1995 ).
As in any measurement procedure, biased results pose a severe threat to validity. Random selection is often used to ensure that patients who receive a questionnaire are representative, but random selection does not ensure that those who respond are also representative. Researchers have become increasingly aware that systematic differences between respondents and nonrespondents are a greater cause for concern than low response rates alone ( Asch, Jedrziewski, and Christakis 1997 ; Krosnick 1999 ; Williams and Macdonald 1986 ).
Numerous studies have assessed the differences between responders and nonresponders (or initial responders and initial nonresponders) on demographic variables, respondent characteristics, health status, and health-related behaviors; most have found differences on at least some variables ( van den Akker et al. 1998 ; Band et al. 1999 ; Benfante et al. 1989 ; Diehr et al. 1992 ; Etter and Perneger 1997 ; Goodfellow et al. 1988 ; Heilbrun et al. 1991 ; Hill et al. 1997 ; Hoeymans et al. 1998 ; Jay et al. 1993 ; Lasek et al. 1997 ; Launer, Wind, and Deeg 1994 ; Livingston et al. 1997 ; Macera et al. 1990 ; Norton et al. 1994 ; O'Neill, Marsden, and Silman 1995 ; Panser et al. 1994 ; Prendergast, Beal, and Williams 1993 ; Rockwood et al. 1989 ; Smith and Nutbeam 1990 ; Templeton et al. 1997 ; Tennant and Badley 1991 ; Vestbo and Rasmussen 1992 ). However, differences between responders and nonresponders are difficult to identify when the difference is on a variable for which prior information is not available for the full sample. For instance, it is relatively straightforward to assess whether older patients respond at a higher rate than younger patients by comparing age distributions of the survey sample and the respondent sample. In contrast, for a variable such as satisfaction, the underlying distribution in the full sample is not known, and so there is no straightforward means of determining whether the distribution of this variable for respondents differs from the distribution for the full sample. However, in spite of the difficulties inherent in this sort of investigation, there are a number of studies that have attempted to assess differences in satisfaction between respondents and nonrespondents (or early and late respondents). Findings from these studies suggest that nonrespondents or late respondents may evaluate care differently, and perhaps less favorably, than respondents or early respondents, respec-tively ( Barkley and Furse 1996 ; Etter, Perneger, and Rougemont 1996 ; Lasek et al. 1997 ; Pearson and Maier 1995 ; Woolliscroft et al. 1994 ).
The first objective of the present study was to examine actual patient satisfaction data for evidence of response bias. The second objective was to examine how response bias (in this case, differential likelihood of response as a function of level of satisfaction) might impact patient satisfaction survey results using simulated data.
The setting for the patient satisfaction survey was a group-model health maintenance organization, in central and eastern Massachusetts, with a total enrollment of more than 150,000 members. Members are covered for most outpatient medical costs with a nominal copayment for outpatient services and are served by a large multispecialty group practice of more than two hundred physicians.
The present study was limited to family practitioners and general internists. Patient satisfaction questionnaires were mailed to a random sample of patients who visited their primary care provider during the previous three months. For each provider, up to 150 patients per quarter were selected to receive a questionnaire. Patients who had been sent a survey during the previous two years were not eligible. While patient satisfaction with all providers in the medical group is periodically assessed, the organization places a special focus on satisfaction with primary care providers because each health plan member is assigned to a specific primary care provider to manage their health care needs.
Data collection occurred during the spring of 1997 and the spring of 1998. All responses were anonymous; administration consisted of a single mailing, without follow-up. Data from these two periods (chosen to minimize the impact of secular trends) were combined after determining that the average difference in mean satisfaction rating (for the same provider for the two quarters) was approximately .08, and the corresponding correlation (for pairs of satisfaction ratings for the two time periods) was .91. In addition, the average difference in response rate for providers was 8 percent, and the corresponding correlation (for matched pairs of response rates) was .58.
The items on the questionnaire were standard patient satisfaction questions from an operational survey implemented as part of ongoing organizational quality assurance efforts. Items addressed areas that the health care organization identified as important. Eleven questions related to satis-faction with the provider were selected for this analysis (see Appendix ). Ratings were on a 5-point scale, with 1 the lowest possible rating, and 5 the highest possible rating.
For the second phase of this study, data were generated to simulate one hundred patient satisfaction ratings (on a 1 to 5 scale) for each of one hundred physicians. The goal of the simulation was to produce realistic data, com-parable to the observed data, but with a known underlying distribution.
We constructed a stochastic model using the assumption of a linear relationship between the characteristics we were modeling. Preliminary analysis of the real dataset provided evidence of the appropriateness of a linear model in this case (see Figure 1 ). The first step in the simulation was to generate satisfaction scores for one hundred simulated physicians. These scores were normally distributed. With these scores as a starting point, one hundred patient ratings were generated for each physician, allowing differences between patients of the same provider. This procedure simulated differences between patients by allowing “easier” and “harder” raters, but did not explicitly constrain simulated patients to vary in a specified way. The simulated ratings were put on a 1 to 5 metric, comparable to the actual patient satisfaction scores. The mean was near the high end of the scale, as is typical of patient satisfaction ratings in general, and of the actual dataset analyzed here in particular.

Mean Satisfaction Rating by Response Rate by Provider. A circle represents one provider. If there is more than one provider at a single point, the number of lines extending out from the center of the circle equals the total number of providers at that point
From this simulated population of one hundred ratings per physician, two different samples were selected: a random sample, and a biased sample. In order to select a sample comparable to the observed sample, and simulate the bias posited to underlie the observed data, simulated patients were selected to be “respondents” for the biased sample in such a way that the more satisfied a patient was, the more likely that patient was to be included. The probabilities used to differentially select simulated patients as respondents were determined using an iterative process, working backwards from the real dataset until the biased sample matched the actual data in terms of response rate, satisfaction mean, and standard deviation, and the correlation between the two. It was considered desirable that the simulated data be comparable to the observed data in order that the simulation produce realistic data.
This data generation model and sampling procedure resulted in data that closely approximated the real patient satisfaction data. Once this model was determined, one hundred replications were conducted resulting in a total of simulated ratings for ten thousand physicians, each rated by one hundred patients.
For the actual (observed) patient satisfaction data, the response rate for each provider was calculated from the number of returned questionnaires divided by the total number of questionnaires sent. Satisfaction scores were calculated for all physicians with more than one completed patient satisfaction questionnaire, if the patient had responded to at least 10 of the 11 items identified for this study (thus the number of usable surveys was less than the number of returned surveys).
A generalizability study was conducted to estimate the generalizability of the satisfaction ratings at the provider level. In this case, a generalizability analysis was considered more appropriate than classical test theory-based estimates of reliability such as Cronbach's alpha, as the former allows assessment of multiple sources of error simultaneously, for example, both items and patients (raters). Generalizability analyses produce estimates of the variance components associated with each source of error, and also allow computation of a generalizability coefficient (g), which is a reliability coefficient comparable to alpha. Finally, the variance components from the generalizability analysis can be used to estimate reliability for different numbers of patients and items, in a manner similar to using the Spearman-Brown formula with classical reliability estimates.
The correlation between mean patient satisfaction scores and response rate was calculated. For the simulated data, three satisfaction ratings were calculated for each physician. The first, referred to as the “Full Mean,” was the mean of all one hundred simulated patient ratings, without sampling. The second, referred to as the “Random Mean,” was calculated based on a random sample of the patient ratings. The third, referred to as the “Biased Mean,” was the mean of the sample of patient ratings that resulted from differentially sampling patients as described above, where the most satisfied patients had a greater probability of being included. For each physician, the difference between these three mean ratings was calculated.
The dataset of actual patient satisfaction ratings contained ratings of 82 physicians by 6,681 patients, with an average of 81 patients rating each physician (the range was 14 to 158). Descriptive statistics are reported in Table 1 . The overall response rate was 32 percent; response rates for individual providers ranged from 11 to 55 percent.
Descriptive Statistics for Real Observed and Simulated Data
Data Source | Mean | SD | Correlation between Response Rate and Satisfaction Rating | Response Rate |
---|---|---|---|---|
Observed data | 4.54 | .14 | .52 | 32% |
Simulated full sample | 4.41 | .14 | NA | 100% |
Simulated random sample | 4.41 | .15 | −.01 | 31% |
Simulated biased sample | 4.53 | .13 | .55 | 32% |
Variance components estimates from the generalizability study revealed the following percentages of variance associated with each facet: provider 3 percent; patient (nested within provider) 65 percent; item 1 percent; patient by item interaction .4 percent; error 31 percent. These results make clear that varying the number of items is likely to have minimal impact on the generalizability estimate—that is, changing the number of items would have almost no impact on the reliability of the scores. Therefore, estimates of the g-coefficient were calculated holding the number of items constant at 11, but varying the number of patients providing ratings. It was found that a g-coefficient of .80 or greater would require approximately one hundred patient raters per provider. If only 10 patient raters were available per provider, the g-coefficient would be .31; with 50 patients the reliability would increase to .69; and with one hundred patients it would increase to .81. The average number of respondents per provider in this data set was 81; with 81 respondents and 11 items the g-coefficient is estimated to be .78.
The correlation between response rate and mean satisfaction rating was .52, which is statistically significant ( p <.01). Figure 1 displays response rate by mean satisfaction rating for each provider.
Means and standard deviations of the simulated satisfaction data are also presented in Table 1 . The simulated data generation and biased sampling procedure produced data that closely resembled the real dataset (see Table 1 ), as was our intent. Considering the simulated data only, the mean for the random sample was identical to the mean for the full sample, but the difference between the biased sample mean and the full mean was .12, or just less than a full standard deviation.
Table 2 contains the primary results of the simulation. As expected, differences were found between the mean ratings based on the entire sample and the mean ratings after sampling. Differences were not uniform across all satisfaction levels; discrepancies were greater for those physicians with lower true satisfaction ratings. Figure 2 provides a graphic summary of typical results and highlights that differences between the biased mean and the full mean varied across physicians. Differences were greatest when true satisfaction scores were lowest.

Differences between Full Sample Mean Ratings and Biased Mean Ratings by Provider
Average Difference between Biased Sample Mean and Full Mean
Physician Standing Based on Full Mean | Difference |
---|---|
Bottom quartile | .16 |
Second quartile | .13 |
Third quartile | .11 |
Top quartile | .09 |
Overall | .12 |
Note : In all cases the mean ratings after sampling were higher than the mean ratings based on the full sample.
The results of the generalizability study demonstrate that for the observed satisfaction data, a high percentage of the variation in scores between providers is associated with differences in patients' ratings, and a very small percentage is associated with different items. This means that while a given patient is likely to provide similar ratings across a number of items referring to a given provider, different patients rating the same provider are likely to give different ratings. This finding highlights that the score for any physician will depend to a large extent on how many patients, and which patients, provide ratings.
The relatively high correlation between response rate and mean patient satisfaction rating in the real dataset analyzed here suggests that in this instance more satisfied patients were more likely to respond than those who were less satisfied. This finding is consistent with the findings of other studies of patient satisfaction ( Barkley and Furse 1996 ; Etter, Perneger, and Rougemont 1996 ; Lasek et al. 1997 ; Pearson and Maier 1995 ; Woolliscroft et al. 1994 ).
The results of our simulation study demonstrated that if response bias is present, it will have a meaningful impact on the results of patient satisfaction surveys. If less-satisfied patients are less likely to respond, patient satisfaction will be overestimated overall and the magnitude of the error will be greatest for physicians with the lowest patient satisfaction. For physicians who are “better” at satisfying patients, a high percentage of patients may be likely to respond and provide high ratings; for physicians with less-satisfied patients, a smaller percentage of patients will be likely to respond, and further, these respondents may be the most satisfied of the low-satisfaction physician's patients. This results in a bias in satisfaction scores for low-satisfaction physicians, inflating their scores relative to those of high-satisfaction physicians, thereby minimizing differences between the two. Thus, for both high- and low-satisfaction physicians, the most satisfied patients will be most likely to respond, but the difference between respondents and nonrespondents (and therefore the difference between true scores and observed scores) is likely to be greater for low satisfaction physicians than for high satisfaction physicians.
While the magnitude of the difference between the full-sample satisfaction ratings and the biased-sample satisfaction ratings may seem small (.12 across all simulations), in fact this difference is close to a full standard deviation. In addition, the difference in mean ratings for the full sample compared to the biased sample was almost twice as large for those physicians in the lowest satisfaction quartile (.16), as compared to those in the highest quartile (.09).
It is important to be clear on the impact of bias compared to random error. Both random error and bias may result in changes in relative rankings. Random error may add to or subtract from a provider's true score, and if the effect is in one direction (increase) for one provider, and in the opposite direction (decrease) for another provider, and if the true scores for these two providers are relatively close, then the rank of their observed scores may reverse as a result. The extent of the effect, and the resultant impact on relative rank, depend on the magnitude of the error relative to the variance in true scores of the providers. Even if there is no bias in the scores, if only a small number of patients respond, the magnitude of the error may be relatively large.
While random error introduces “noise” in the manner described above, bias could mask positive changes in scores, as the magnitude of the bias is a function of satisfaction. An example may help illustrate this. Imagine that provider A has relatively low true satisfaction ratings at time one. Now imagine that provider A changes, so that at time two his patients are in fact more satisfied. More patients will be likely to respond to the survey, and his “observed” score will be higher. However, at the same time, his observed score will be likely to be more accurate, and therefore the difference between his observed score and his true score will be less. Thus, provider A's observed score will increase less than his true score.
Our results provide an illustration of the impact of response bias under one set of realistic conditions. However, it is important to note that while we believe our simulation was realistic, other circumstances are likely to be encountered in practice. For instance, the actual survey studied here had a relatively low response rate overall, which was likely due at least in part to the absence of follow-up procedures. Surveys that do include follow-up procedures are likely to yield higher response rates, and increases in response rates are likely to reduce the impact of bias. In addition, the magnitude of the bias may also vary depending on circumstances, and we do not know at present whether the magnitude of the bias investigated here could be considered typical.
There is another important issue to consider with respect to the number of respondents. Other things being equal, fewer respondents will result in larger standard errors for satisfaction estimates for providers. In the case of patient satisfaction data analyzed at the level of the individual provider, it is possible to construct confidence intervals for each provider based on the number of patients providing ratings for that provider, so that the width of the intervals for low response-rate providers would be greater than that for high response rate providers. Used appropriately, such confidence intervals would discourage unwarranted conclusions about differences between providers, or between an individual provider and a set standard. However, it is important to note that response bias serves to change the mean of a distribution of a set of scores, rather than simply reduce the precision of measurement. Thus, while it is advisable take standard errors into account when making comparisons, they will not correct for biased scores.
It is important to note the limitations of this study. First, evidence of a positive relationship between response rate and satisfaction ratings in the real data analysis was based on data on primary care providers working in the context of a single health care organization. Clearly, additional research is needed to determine whether this relationship is typical of data from other organizations, other parts of the country, and other types of providers. A second limitation is the fact that the patient satisfaction questionnaire used in this study was anonymous, and therefore we were unable to link patient responses with patient characteristics.
It is also important to highlight that the simulation results are limited to the extent that the models used are reflective of what is likely to occur with real data. The model used to generate the simulated patient satisfaction ratings produced variability between providers and between patients, but did not explicitly model other factors (beyond provider and patient facets) that might produce this variability. The simulated dataset therefore is consistent with the assumption that some providers are “better” at satisfying patients than others, and that different patients are likely to give differing ratings of the same provider. We did not attempt to model the complex relationships between satisfaction and the numerous factors that may influence satisfaction, such as differences in experience with the provider, the medical issue involved, patient expectations, differences in interpretations of the items and the scale, and differences in provider characteristics including race/ethnicity, language, gender, and age. With respect to selecting respondents to simulate a biased sample, level of satisfaction was the only variable considered in determining likelihood of responding. There are almost certainly nonrandom factors that contribute to likelihood of response, and to the extent that these mitigate the relationship between satisfaction and response rate, our model is an oversimplification. While the simplicity of our model may be a limitation, our results highlight a simple but important point—if likelihood of responding is related to satisfaction, then results will be biased, regardless of what factors influence satisfaction. For example, if certain providers tend to receive lower ratings, and they have sicker patients, this does not invalidate our argument or our findings. In fact, the impact of health status may be underestimated if the least satisfied of the sick may be least likely to respond.
In light of the results of this study, and the limitations discussed above, it is clear that further research in this area is needed. We have provided a demonstration of how response bias attributable to differences in satisfaction could impact validity. However, in actual datasets, numerous factors may influence both satisfaction and response likelihood. Further research is needed to generate and test more complex models. Our research highlights the importance of considering not only the factors that influence satisfaction, but also response biases that serve as filters and thereby influence obtained satisfaction ratings. Additional research is needed to determine whether evidence of response bias due to differences in satisfaction exists in other datasets, and to examine possible interactions between satisfaction, response likelihood, and patient and provider characteristics.
In spite of the exploratory nature of this study, our findings do have practical implications. First, health care organization administrators and others who use patient satisfaction surveys should be aware that response biases may impact the results of such surveys, giving the impression that patients are more satisfied than they in fact are. If results are used to evaluate and compare individual providers, providers who are better at satisfying patients may be disadvantaged relative to less-satisfying peers. Not only may the relative rankings of providers change, as may happen even with random sampling error, but estimates of the magnitude of differences between providers will also be influenced. This bias could systematically deflate estimates of positive change and mask signs of improvement. Researchers working in applied settings can look for evidence of response bias by instituting follow-up procedures, and comparing early responders to more reluctant responders, although even lack of differences between these two groups will not be definitive evidence of lack of bias. Organizations should continue to strive to maximize response rates, as the impact of such biases will be minimized at higher response rates.
In summary, our findings raise concerns about the possibility of response bias in patient satisfaction surveys. Our analysis of actual patient satisfaction data suggests that the most satisfied patients may be the most likely to respond, and our simulation demonstrated how such a response bias might jeopardize the validity of interpretations based on a biased sample. Further research is needed to determine whether the effect demonstrated here is more than a theoretical possibility.
Questionnaire Items with Item Means and Standard Deviations
Item | Mean (SD) | Minimum Maximum |
My provider is concerned for me as a person. | 4.57 (.16) | 4.17 |
4.92 | ||
My provider spends enough time with me. | 4.51 (.15) | 4.23 |
4.89 | ||
My provider listens carefully to what I am saying. | 4.58 (.14) | 4.28 |
4.89 | ||
My provider explains my condition to my satisfaction. | 4.53 (.14) | 4.19 |
4.82 | ||
My provider explains medication to my satisfaction. | 4.50 (.15) | 4.08 |
4.81 | ||
My provider supplies me with the results of my tests | 4.46 (.19) | 3.76 |
in a timely fashion. | 4.78 | |
My provider supplies information so I can make decisions | 4.46 (.16) | 4.06 |
regarding my own care. | 4.79 | |
My provider refers me to specialists as needed. | 4.53 (.15) | 4.00 |
4.77 | ||
My provider treats me with respect and courtesy. | 4.70 (.11) | 4.44 |
4.89 | ||
My provider returns my telephone calls within a reasonable | 4.49 (.18) | 3.75 |
period of time. | 4.77 | |
I would recommend my provider to family and friends. | 4.61 (.15) | 4.23 |
4.86 |
Number of providers=82.
All responses were on a 5-point scale with 1=Strongly Disagree and 5=Strongly Agree.
- Asch D, Jedrziewski M, Christakis N. “Response Rates to Mail Surveys Published in Medical Journals.” Clinical Epidemiology. 1997; 50 (10):1129–36. [ PubMed ] [ Google Scholar ]
- Band P, Spinelli J, Threlfall W, Fang R, Le N, Gallagher R. “Identification of Occupational Cancer Risks in British Columbia. Part 1: Methodology Descriptive Results and Analysis of Cancer Risks by Cigarette Smoking Categories of 15,463 Incident Cancer Cases.” Journal of Occupational and Environmental Medicine. 1999; 41 (4):224–32. [ PubMed ] [ Google Scholar ]
- Barkley WM, Furse DH. “Changing Priorities for Improvement: The Impact of Low Response Rates in Patient Satisfaction.” Joint Commission Journal on Quality Improvement. 1996; 22 (6):427–33. [ PubMed ] [ Google Scholar ]
- Benfante R, Reed D, MacLean C, Kagan A. “Response Bias in the Honolulu Heart Program.” American Journal of Epidemiology. 1989; 130 (6):1088–100. [ PubMed ] [ Google Scholar ]
- Carlson MJ, Blustein J, Fiorentino N, Prestianni F. “Socioeconomic Status and Dissatisfaction Among HMO Enrollees.” Medical Care. 2000; 38 (5):508–16. [ PubMed ] [ Google Scholar ]
- Diehr P, Koepsell T, Cheadle A, Psaty B. “Assessing Response Bias in Random-Digit Dialing Surveys: The Telephone-Prefix Method.” Statistics in Medicine. 1992; 11 (8):1009–21. [ PubMed ] [ Google Scholar ]
- Etter J, Perneger T. “Analysis of Non-Response Bias in a Mailed Health Survey.” Journal of Clinical Epidemiology. 1997; 50 (10):1123–8. [ PubMed ] [ Google Scholar ]
- Etter JF, Perneger TV, Rougemont A. “Does Sponsorship Matter in Patient Satisfaction Surveys? A Randomized Trial.” Medical Care. 1996; 34 (4):327–35. [ PubMed ] [ Google Scholar ]
- Ford RC, Bach SA, Fottler MD. “Methods of Measuring Patient Satisfaction in Health Care Organizations.” Health Care Management Review. 1997; 22 (2):74–89. [ PubMed ] [ Google Scholar ]
- Gold M, Hurley R, Lake T, Ensor T, Berenson R. “A National Survey of the Arrangements Managed-Care Plans Make with Physicians.” New England Journal of Medicine. 1995; 333 (25):1678–83. [ PubMed ] [ Google Scholar ]
- Goodfellow M, Kiernan NE, Ahern F, Smyer MA. “Response Bias Using Two-Stage Data Collection: A Study of Elderly Participants in a Program.” Evaluation Review. 1988; 12 (6):638–54. [ Google Scholar ]
- Heilbrun LK, Ross PD, Wasnich RD, Yano K, Vogel JM. “Characteristics of Respondents and Nonrespondents in a Prospective Study of Osteoporosis.” Journal of Clinical Epidemiology. 1991; 44 (3):233–9. [ PubMed ] [ Google Scholar ]
- Hill A, Roberts J, Ewings P, Gunnell D. “Non-Response Bias in a Lifestyle Survey.” Journal of Public Health Medicine. 1997; 19 (2):203–7. [ PubMed ] [ Google Scholar ]
- Hoeymans N, Feskens E, Bos GVD, Kromhout D. “Non-Response Bias in a Study of Cardiovascular Diseases Functional Status and Self-Rated Health Among Elderly Men.” Age and Ageing. 1998; 27 (1):35–40. [ PubMed ] [ Google Scholar ]
- Jay GM, Liang J, Liu X, Sugisawa H. “Patterns of Nonresponse in a National Survey of Elderly Japanese.” Journal of Gerontology. 1993; 48 (3):S143–52. [ PubMed ] [ Google Scholar ]
- Krosnick JA. “Survey Research.” Annual Review of Psychology. 1999; 50 :537–67. [ PubMed ] [ Google Scholar ]
- Lasek RJ, Barkley W, Harper DL, Rosenthal GE. “An Evaluation of the Impact of Nonresponse Bias on Patient Satisfaction Surveys.” Medical Care. 1997; 35 (6):646–52. [ PubMed ] [ Google Scholar ]
- Launer LJ, Wind AW, Deeg DJH. “Nonresponse Pattern and Bias in a Community-Based Cross-Sectional Study of Cognitive Functioning among the Elderly.” American Journal of Epidemiology. 1994; 139 (8):803–12. [ PubMed ] [ Google Scholar ]
- Livingston P, Lee S, McCarty C, Taylor H. “A Comparison of Participants with Non-Participants in a Population-Based Epidemiologic Study: The Melbourne Visual Impairment Project.” Ophthalmic Epidemiology. 1997; 4 (2):73–81. [ PubMed ] [ Google Scholar ]
- Macera C, Jackson K, Davis D, Kronenfeld J, Blair S. “Patterns of Non-Response to a Mail Survey.” Journal of Clinical Epidemiology. 1990; 43 (12):1427–30. [ PubMed ] [ Google Scholar ]
- Norton MC, Breitner JCS, Welsh KA, Wyse BW. “Characteristics of Nonresponders in a Community Survey of the Elderly.” Journal of the American Geriatrics Society. 1994; 42 :1252–6. [ PubMed ] [ Google Scholar ]
- O'Neill T, Marsden D, Silman A. “Differences in the Characteristics of Responders and Non-Responders in a Prevalence Survey of Vertebral Osteoporosis.” Osteoporosis International. 1995; 5 (5):327–34. The European Vertebral Osteoporosis Study Group. [ PubMed ] [ Google Scholar ]
- Panser L, Chute C, Guess H, Larsonkeller J, Girman C, Oesterling J, Lieber M, Jacobsen S. “The Natural History of Prostatism: The Effects of Non-Response Bias.” International Journal of Epidemiology. 1994; 23 (6):1198–205. [ PubMed ] [ Google Scholar ]
- Pearson D, Maier ML. “Assessing Satisfaction and Non-Response Bias in an HMO-Sponsored Employee Assistance Program.” Employee Assistance Quarterly. 1995; 10 (3):21–34. [ Google Scholar ]
- Prendergast M, Beal J, Williams S. “An Investigation of Non-Response Bias by Comparison of Dental Health in 5-Year-Old Children According to Parental Response to a Questionnaire.” Community Dental Health. 1993; 10 (3):225–34. [ PubMed ] [ Google Scholar ]
- Rockwood K, Stolee P, Robertson D, Shillington ER. “Response Bias in a Health Status Survey of Elderly People.” Age and Ageing. 1989; 18 :177–82. [ PubMed ] [ Google Scholar ]
- Rosenthal GE, Shannon SE. “The Use of Patient Perceptions in the Evaluation of Health-Care Delivery Systems.” Medical Care. 1997; 35 (11, supplement):NS58–68. [ PubMed ] [ Google Scholar ]
- Smith C, Nutbeam D. “Assessing Non-Response Bias: A Case Study from the 1985 Welsh Heart Health Survey.” Health Education Research. 1990; 5 (3):381–6. [ Google Scholar ]
- Templeton L, Deehan A, Taylor C, Drummond C, Strang J. “Surveying General Practitioners: Does a Low Response Rate Matter?” British Journal of General Practice. 1997; 47 (415):91–4. [ PMC free article ] [ PubMed ] [ Google Scholar ]
- Tennant A, Badley E. “Investigating Non-Response Bias in a Survey of Disablement in the Community: Implications for Survey Methodology.” Journal of Epidemiology and Community Health. 1991; 45 (3):247–50. [ PMC free article ] [ PubMed ] [ Google Scholar ]
- van den Akker M, Buntinx F, Metsemakers J, Knottnerus J. “Morbidity in Responders and Non-Responders in a Register-Based Population Survey.” Family Practice. 1998; 15 (3):261–3. [ PubMed ] [ Google Scholar ]
- Vestbo J, Rasmussen F. “Baseline Characteristics Are Not Sufficient Indicators of Non-Response Bias Follow-Up Studies.” Journal of Epidemiology and Community Health. 1992; 46 (6):617–9. [ PMC free article ] [ PubMed ] [ Google Scholar ]
- Williams P, Macdonald A. “The Effect of Non-Response Bias on the Results of Two-Stage Screening Surveys of Psychiatric Disorder.” Social Psychiatry. 1986; 21 (4):182–6. [ PubMed ] [ Google Scholar ]
- Woolliscroft JO, Howell JD, Patel BP, Swanson DE. “Resident–Patient Interactions: The Humanistic Qualities of Internal Medicine Residents Assessed by Patients Attending Physicians Program Supervisors and Nurses.” Academic Medicine. 1994; 69 (3):216–24. [ PubMed ] [ Google Scholar ]
- Young GJ, Meterko M, Desai KR. “Patient Satisfaction with Hospital Care: Effects of Demographic and Institutional Characteristics.” Medical Care. 2000; 38 (3):325–34. [ PubMed ] [ Google Scholar ]

IMAGES
VIDEO
COMMENTS
Revised on March 17, 2023. Response bias refers to several factors that can lead someone to respond falsely or inaccurately to a question. Self-report questions, such as those asked on surveys or in structured interviews, are particularly prone to this type of bias. Example: Response bias. A job applicant is asked to take a personality test ...
What is Response Bias - Types & Examples. Published by Owen Ingram at September 4th, 2023 , Revised On September 4, 2023. In research, ensuring the accuracy of responses is crucial to obtaining valid and reliable results. When participants' answers are systematically influenced by external factors or internal beliefs rather than reflecting ...
Response bias (also called survey bias) is the tendency of a person to answer questions on a survey untruthfully or misleadingly. For example, they may feel pressure to give answers that are socially acceptable. The respondent may not be aware that they aren't answering the questions in the way the researcher intended: the format of the ...
Example of Experimenter Bias (Response Bias) The Asch Line Study is a great example of this bias. Of course, researchers created this study to show the impact of response bias. In the study, participants sat among several "actors." The researcher asked the room to identify a certain line. Every actor in the room answered incorrectly.
Information bias occurs during the data collection step and is common in research studies that involve self-reporting and retrospective data collection. It can also result from poor interviewing techniques or differing levels of recall from participants. The main types of information bias are: Recall bias. Observer bias.
Non-response bias — which is sometimes called late response bias — is when people who don't respond to a survey question differ significantly to those who do respond. This type of bias is inherent in analytical or experimental research, for example, those assessing health and wellbeing. Non-response bias is tricky for researchers ...
Non-response bias is a type of bias that arises when people who refuse to participate or drop out of a study systematically differ from those who take part. For example, if conducting a study on the prevalence of depression in a community, your results may be an underestimation if those with depression are less likely to participate than those ...
A final example of a type of response bias is extreme responding. It's commonly seen in surveys that use Likert scales - a type of scaled response format with several possible responses ranging from the most negative to the most positive. Responses are biased when respondents select the extremity responses almost exclusively.
2) Social Desirability Bias. This type of response bias results from participants answering sensitive questions with socially desirable, rather than truthful answers. The key here is how response bias questions are worded. To better illustrate this, here is an example:
A survey using a Likert style response set. This is one example of a type of survey that can be highly vulnerable to the effects of response bias. Response bias is a general term for a wide range of tendencies for participants to respond inaccurately or falsely to questions. These biases are prevalent in research involving participant self-report, such as structured interviews or surveys. [1]
The Makeover: Include a Simulation. One of the most challenging AP Stats topics for students is estimating p-values from simulations. This is one of the reasons we do a simulation on the first day of class with playing cards called "Hiring Discrimination: It Just Won't Fly". (An online version of the scenario and simulation can be found ...
Response bias can significantly impact the research results, ultimately introducing errors and inaccuracies in the data. If a survey or research is affected by response bias, the results may fail to accurately reflect the target population's views, opinions, or behaviors. As a result, the outcome can have serious implications for decision-making, policy development, and other applications.
Channeling bias is a type of selection bias noted in observational studies. It occurs most frequently when patient characteristics, such as age or severity of illness, affect cohort assignment. This can occur, for example, in surgical studies where different interventions carry different levels of risk.
A survey is a very good example of such a study, and is certainly prone to response biases. PsycholoGenie explains the different types of response biases, and illustrates them with simple examples. Response bias is a type of bias which influences a person's response away from facts and reality. This bias is mostly evident in studies ...
A voluntary response is when someone volunteers to be a part of your sample. In doing so, you're allowing them to skew your data and you don't get results that are representative of the whole population. Thus, you get biased feedback. Voluntary response bias refers to how allowing your sample to self-select skews your data, and you don't ...
For example, their baseline preference for or against a particular response may undulate throughout the course of the experiment, and this too may be attenuated through practice. The values reported in the present work should therefore be considered only lower bounds, and may increase once other forms of bias are accounted for.
response bias in perceptual experiments has a substan-tial sensory component. However, previous studies provide limited insight into perhaps the most confounding aspect of response bias: the fact that different people can exhibit opposite biases for the exact same task. For example, people have been shown to have idiosyncratic perceptual
Something went wrong. Please try again. | Khan Academy. If you're seeing this message, it means we're having trouble loading external resources on our website. If you're behind a web filter, please make sure that the domains *.kastatic.org and *.kasandbox.org are unblocked. Explore. Search. AI for Teachers Donate Log in Sign up.
Response rate and nonresponse bias. The response rate, or the percentage of sampled units who filled in a survey, can indicate the amount of nonresponse present in your data. For example, a survey with a 70% response rate has a 30% nonresponse rate. The response rate is often used to estimate the magnitude of nonresponse bias.
Experiment 1. If response bias represents a cognitive trait, it should remain consistent within an individual across time. Therefore, an important first step in establishing response bias as trait-like is to determine whether a given participant will show the same level of bias on two different recognition tests.
Observer bias is a type of experimenter bias that occurs when a researcher's expectations, perspectives, opinions, or prejudices impact the results of an experiment. This type of research bias is also called detection bias or ascertainment bias. This typically occurs when a researcher is aware of the purpose and hypotheses of a study and ...
A positive correlation was found between mean patient satisfaction rating and response rate in the actual patient satisfaction data. Simulation results suggest response bias could lead to overestimation of patient satisfaction overall, with this effect greatest for physicians with the lowest satisfaction scores.