
- Previous Article
- Next Article

Promises and Pitfalls of Technology
Politics and privacy, private-sector influence and big tech, state competition and conflict, author biography, how is technology changing the world, and how should the world change technology.
[email protected]
- Split-Screen
- Article contents
- Figures & tables
- Supplementary Data
- Peer Review
- Open the PDF for in another window
- Guest Access
- Get Permissions
- Cite Icon Cite
- Search Site
Josephine Wolff; How Is Technology Changing the World, and How Should the World Change Technology?. Global Perspectives 1 February 2021; 2 (1): 27353. doi: https://doi.org/10.1525/gp.2021.27353
Download citation file:
- Ris (Zotero)
- Reference Manager
Technologies are becoming increasingly complicated and increasingly interconnected. Cars, airplanes, medical devices, financial transactions, and electricity systems all rely on more computer software than they ever have before, making them seem both harder to understand and, in some cases, harder to control. Government and corporate surveillance of individuals and information processing relies largely on digital technologies and artificial intelligence, and therefore involves less human-to-human contact than ever before and more opportunities for biases to be embedded and codified in our technological systems in ways we may not even be able to identify or recognize. Bioengineering advances are opening up new terrain for challenging philosophical, political, and economic questions regarding human-natural relations. Additionally, the management of these large and small devices and systems is increasingly done through the cloud, so that control over them is both very remote and removed from direct human or social control. The study of how to make technologies like artificial intelligence or the Internet of Things “explainable” has become its own area of research because it is so difficult to understand how they work or what is at fault when something goes wrong (Gunning and Aha 2019) .
This growing complexity makes it more difficult than ever—and more imperative than ever—for scholars to probe how technological advancements are altering life around the world in both positive and negative ways and what social, political, and legal tools are needed to help shape the development and design of technology in beneficial directions. This can seem like an impossible task in light of the rapid pace of technological change and the sense that its continued advancement is inevitable, but many countries around the world are only just beginning to take significant steps toward regulating computer technologies and are still in the process of radically rethinking the rules governing global data flows and exchange of technology across borders.
These are exciting times not just for technological development but also for technology policy—our technologies may be more advanced and complicated than ever but so, too, are our understandings of how they can best be leveraged, protected, and even constrained. The structures of technological systems as determined largely by government and institutional policies and those structures have tremendous implications for social organization and agency, ranging from open source, open systems that are highly distributed and decentralized, to those that are tightly controlled and closed, structured according to stricter and more hierarchical models. And just as our understanding of the governance of technology is developing in new and interesting ways, so, too, is our understanding of the social, cultural, environmental, and political dimensions of emerging technologies. We are realizing both the challenges and the importance of mapping out the full range of ways that technology is changing our society, what we want those changes to look like, and what tools we have to try to influence and guide those shifts.
Technology can be a source of tremendous optimism. It can help overcome some of the greatest challenges our society faces, including climate change, famine, and disease. For those who believe in the power of innovation and the promise of creative destruction to advance economic development and lead to better quality of life, technology is a vital economic driver (Schumpeter 1942) . But it can also be a tool of tremendous fear and oppression, embedding biases in automated decision-making processes and information-processing algorithms, exacerbating economic and social inequalities within and between countries to a staggering degree, or creating new weapons and avenues for attack unlike any we have had to face in the past. Scholars have even contended that the emergence of the term technology in the nineteenth and twentieth centuries marked a shift from viewing individual pieces of machinery as a means to achieving political and social progress to the more dangerous, or hazardous, view that larger-scale, more complex technological systems were a semiautonomous form of progress in and of themselves (Marx 2010) . More recently, technologists have sharply criticized what they view as a wave of new Luddites, people intent on slowing the development of technology and turning back the clock on innovation as a means of mitigating the societal impacts of technological change (Marlowe 1970) .
At the heart of fights over new technologies and their resulting global changes are often two conflicting visions of technology: a fundamentally optimistic one that believes humans use it as a tool to achieve greater goals, and a fundamentally pessimistic one that holds that technological systems have reached a point beyond our control. Technology philosophers have argued that neither of these views is wholly accurate and that a purely optimistic or pessimistic view of technology is insufficient to capture the nuances and complexity of our relationship to technology (Oberdiek and Tiles 1995) . Understanding technology and how we can make better decisions about designing, deploying, and refining it requires capturing that nuance and complexity through in-depth analysis of the impacts of different technological advancements and the ways they have played out in all their complicated and controversial messiness across the world.
These impacts are often unpredictable as technologies are adopted in new contexts and come to be used in ways that sometimes diverge significantly from the use cases envisioned by their designers. The internet, designed to help transmit information between computer networks, became a crucial vehicle for commerce, introducing unexpected avenues for crime and financial fraud. Social media platforms like Facebook and Twitter, designed to connect friends and families through sharing photographs and life updates, became focal points of election controversies and political influence. Cryptocurrencies, originally intended as a means of decentralized digital cash, have become a significant environmental hazard as more and more computing resources are devoted to mining these forms of virtual money. One of the crucial challenges in this area is therefore recognizing, documenting, and even anticipating some of these unexpected consequences and providing mechanisms to technologists for how to think through the impacts of their work, as well as possible other paths to different outcomes (Verbeek 2006) . And just as technological innovations can cause unexpected harm, they can also bring about extraordinary benefits—new vaccines and medicines to address global pandemics and save thousands of lives, new sources of energy that can drastically reduce emissions and help combat climate change, new modes of education that can reach people who would otherwise have no access to schooling. Regulating technology therefore requires a careful balance of mitigating risks without overly restricting potentially beneficial innovations.
Nations around the world have taken very different approaches to governing emerging technologies and have adopted a range of different technologies themselves in pursuit of more modern governance structures and processes (Braman 2009) . In Europe, the precautionary principle has guided much more anticipatory regulation aimed at addressing the risks presented by technologies even before they are fully realized. For instance, the European Union’s General Data Protection Regulation focuses on the responsibilities of data controllers and processors to provide individuals with access to their data and information about how that data is being used not just as a means of addressing existing security and privacy threats, such as data breaches, but also to protect against future developments and uses of that data for artificial intelligence and automated decision-making purposes. In Germany, Technische Überwachungsvereine, or TÜVs, perform regular tests and inspections of technological systems to assess and minimize risks over time, as the tech landscape evolves. In the United States, by contrast, there is much greater reliance on litigation and liability regimes to address safety and security failings after-the-fact. These different approaches reflect not just the different legal and regulatory mechanisms and philosophies of different nations but also the different ways those nations prioritize rapid development of the technology industry versus safety, security, and individual control. Typically, governance innovations move much more slowly than technological innovations, and regulations can lag years, or even decades, behind the technologies they aim to govern.
In addition to this varied set of national regulatory approaches, a variety of international and nongovernmental organizations also contribute to the process of developing standards, rules, and norms for new technologies, including the International Organization for Standardization and the International Telecommunication Union. These multilateral and NGO actors play an especially important role in trying to define appropriate boundaries for the use of new technologies by governments as instruments of control for the state.
At the same time that policymakers are under scrutiny both for their decisions about how to regulate technology as well as their decisions about how and when to adopt technologies like facial recognition themselves, technology firms and designers have also come under increasing criticism. Growing recognition that the design of technologies can have far-reaching social and political implications means that there is more pressure on technologists to take into consideration the consequences of their decisions early on in the design process (Vincenti 1993; Winner 1980) . The question of how technologists should incorporate these social dimensions into their design and development processes is an old one, and debate on these issues dates back to the 1970s, but it remains an urgent and often overlooked part of the puzzle because so many of the supposedly systematic mechanisms for assessing the impacts of new technologies in both the private and public sectors are primarily bureaucratic, symbolic processes rather than carrying any real weight or influence.
Technologists are often ill-equipped or unwilling to respond to the sorts of social problems that their creations have—often unwittingly—exacerbated, and instead point to governments and lawmakers to address those problems (Zuckerberg 2019) . But governments often have few incentives to engage in this area. This is because setting clear standards and rules for an ever-evolving technological landscape can be extremely challenging, because enforcement of those rules can be a significant undertaking requiring considerable expertise, and because the tech sector is a major source of jobs and revenue for many countries that may fear losing those benefits if they constrain companies too much. This indicates not just a need for clearer incentives and better policies for both private- and public-sector entities but also a need for new mechanisms whereby the technology development and design process can be influenced and assessed by people with a wider range of experiences and expertise. If we want technologies to be designed with an eye to their impacts, who is responsible for predicting, measuring, and mitigating those impacts throughout the design process? Involving policymakers in that process in a more meaningful way will also require training them to have the analytic and technical capacity to more fully engage with technologists and understand more fully the implications of their decisions.
At the same time that tech companies seem unwilling or unable to rein in their creations, many also fear they wield too much power, in some cases all but replacing governments and international organizations in their ability to make decisions that affect millions of people worldwide and control access to information, platforms, and audiences (Kilovaty 2020) . Regulators around the world have begun considering whether some of these companies have become so powerful that they violate the tenets of antitrust laws, but it can be difficult for governments to identify exactly what those violations are, especially in the context of an industry where the largest players often provide their customers with free services. And the platforms and services developed by tech companies are often wielded most powerfully and dangerously not directly by their private-sector creators and operators but instead by states themselves for widespread misinformation campaigns that serve political purposes (Nye 2018) .
Since the largest private entities in the tech sector operate in many countries, they are often better poised to implement global changes to the technological ecosystem than individual states or regulatory bodies, creating new challenges to existing governance structures and hierarchies. Just as it can be challenging to provide oversight for government use of technologies, so, too, oversight of the biggest tech companies, which have more resources, reach, and power than many nations, can prove to be a daunting task. The rise of network forms of organization and the growing gig economy have added to these challenges, making it even harder for regulators to fully address the breadth of these companies’ operations (Powell 1990) . The private-public partnerships that have emerged around energy, transportation, medical, and cyber technologies further complicate this picture, blurring the line between the public and private sectors and raising critical questions about the role of each in providing critical infrastructure, health care, and security. How can and should private tech companies operating in these different sectors be governed, and what types of influence do they exert over regulators? How feasible are different policy proposals aimed at technological innovation, and what potential unintended consequences might they have?
Conflict between countries has also spilled over significantly into the private sector in recent years, most notably in the case of tensions between the United States and China over which technologies developed in each country will be permitted by the other and which will be purchased by other customers, outside those two countries. Countries competing to develop the best technology is not a new phenomenon, but the current conflicts have major international ramifications and will influence the infrastructure that is installed and used around the world for years to come. Untangling the different factors that feed into these tussles as well as whom they benefit and whom they leave at a disadvantage is crucial for understanding how governments can most effectively foster technological innovation and invention domestically as well as the global consequences of those efforts. As much of the world is forced to choose between buying technology from the United States or from China, how should we understand the long-term impacts of those choices and the options available to people in countries without robust domestic tech industries? Does the global spread of technologies help fuel further innovation in countries with smaller tech markets, or does it reinforce the dominance of the states that are already most prominent in this sector? How can research universities maintain global collaborations and research communities in light of these national competitions, and what role does government research and development spending play in fostering innovation within its own borders and worldwide? How should intellectual property protections evolve to meet the demands of the technology industry, and how can those protections be enforced globally?
These conflicts between countries sometimes appear to challenge the feasibility of truly global technologies and networks that operate across all countries through standardized protocols and design features. Organizations like the International Organization for Standardization, the World Intellectual Property Organization, the United Nations Industrial Development Organization, and many others have tried to harmonize these policies and protocols across different countries for years, but have met with limited success when it comes to resolving the issues of greatest tension and disagreement among nations. For technology to operate in a global environment, there is a need for a much greater degree of coordination among countries and the development of common standards and norms, but governments continue to struggle to agree not just on those norms themselves but even the appropriate venue and processes for developing them. Without greater global cooperation, is it possible to maintain a global network like the internet or to promote the spread of new technologies around the world to address challenges of sustainability? What might help incentivize that cooperation moving forward, and what could new structures and process for governance of global technologies look like? Why has the tech industry’s self-regulation culture persisted? Do the same traditional drivers for public policy, such as politics of harmonization and path dependency in policy-making, still sufficiently explain policy outcomes in this space? As new technologies and their applications spread across the globe in uneven ways, how and when do they create forces of change from unexpected places?
These are some of the questions that we hope to address in the Technology and Global Change section through articles that tackle new dimensions of the global landscape of designing, developing, deploying, and assessing new technologies to address major challenges the world faces. Understanding these processes requires synthesizing knowledge from a range of different fields, including sociology, political science, economics, and history, as well as technical fields such as engineering, climate science, and computer science. A crucial part of understanding how technology has created global change and, in turn, how global changes have influenced the development of new technologies is understanding the technologies themselves in all their richness and complexity—how they work, the limits of what they can do, what they were designed to do, how they are actually used. Just as technologies themselves are becoming more complicated, so are their embeddings and relationships to the larger social, political, and legal contexts in which they exist. Scholars across all disciplines are encouraged to join us in untangling those complexities.
Josephine Wolff is an associate professor of cybersecurity policy at the Fletcher School of Law and Diplomacy at Tufts University. Her book You’ll See This Message When It Is Too Late: The Legal and Economic Aftermath of Cybersecurity Breaches was published by MIT Press in 2018.
Recipient(s) will receive an email with a link to 'How Is Technology Changing the World, and How Should the World Change Technology?' and will not need an account to access the content.
Subject: How Is Technology Changing the World, and How Should the World Change Technology?
(Optional message may have a maximum of 1000 characters.)
Citing articles via
Email alerts, affiliations.
- Special Collections
- Review Symposia
- Info for Authors
- Info for Librarians
- Editorial Team
- Emerging Scholars Forum
- Open Access
- Online ISSN 2575-7350
- Copyright © 2024 The Regents of the University of California. All Rights Reserved.
Stay Informed
Disciplines.
- Ancient World
- Anthropology
- Communication
- Criminology & Criminal Justice
- Film & Media Studies
- Food & Wine
- Browse All Disciplines
- Browse All Courses
- Book Authors
- Booksellers
- Instructions
- Journal Authors
- Journal Editors
- Media & Journalists
- Planned Giving
About UC Press
- Press Releases
- Seasonal Catalog
- Acquisitions Editors
- Customer Service
- Exam/Desk Requests
- Media Inquiries
- Print-Disability
- Rights & Permissions
- UC Press Foundation
- © Copyright 2024 by the Regents of the University of California. All rights reserved. Privacy policy Accessibility
This Feature Is Available To Subscribers Only
Sign In or Create an Account
Feb 13, 2023
200-500 Word Example Essays about Technology
Got an essay assignment about technology check out these examples to inspire you.
Technology is a rapidly evolving field that has completely changed the way we live, work, and interact with one another. Technology has profoundly impacted our daily lives, from how we communicate with friends and family to how we access information and complete tasks. As a result, it's no surprise that technology is a popular topic for students writing essays.
But writing a technology essay can be challenging, especially for those needing more time or help with writer's block. This is where Jenni.ai comes in. Jenni.ai is an innovative AI tool explicitly designed for students who need help writing essays. With Jenni.ai, students can quickly and easily generate essays on various topics, including technology.
This blog post aims to provide readers with various example essays on technology, all generated by Jenni.ai. These essays will be a valuable resource for students looking for inspiration or guidance as they work on their essays. By reading through these example essays, students can better understand how technology can be approached and discussed in an essay.
Moreover, by signing up for a free trial with Jenni.ai, students can take advantage of this innovative tool and receive even more support as they work on their essays. Jenni.ai is designed to help students write essays faster and more efficiently, so they can focus on what truly matters – learning and growing as a student. Whether you're a student who is struggling with writer's block or simply looking for a convenient way to generate essays on a wide range of topics, Jenni.ai is the perfect solution.
The Impact of Technology on Society and Culture
Introduction:.
Technology has become an integral part of our daily lives and has dramatically impacted how we interact, communicate, and carry out various activities. Technological advancements have brought positive and negative changes to society and culture. In this article, we will explore the impact of technology on society and culture and how it has influenced different aspects of our lives.
Positive impact on communication:
Technology has dramatically improved communication and made it easier for people to connect from anywhere in the world. Social media platforms, instant messaging, and video conferencing have brought people closer, bridging geographical distances and cultural differences. This has made it easier for people to share information, exchange ideas, and collaborate on projects.
Positive impact on education:
Students and instructors now have access to a multitude of knowledge and resources because of the effect of technology on education . Students may now study at their speed and from any location thanks to online learning platforms, educational applications, and digital textbooks.
Negative impact on critical thinking and creativity:
Technological advancements have resulted in a reduction in critical thinking and creativity. With so much information at our fingertips, individuals have become more passive in their learning, relying on the internet for solutions rather than logic and inventiveness. As a result, independent thinking and problem-solving abilities have declined.
Positive impact on entertainment:
Technology has transformed how we access and consume entertainment. People may now access a wide range of entertainment alternatives from the comfort of their own homes thanks to streaming services, gaming platforms, and online content makers. The entertainment business has entered a new age of creativity and invention as a result of this.
Negative impact on attention span:
However, the continual bombardment of information and technological stimulation has also reduced attention span and the capacity to focus. People are easily distracted and need help focusing on a single activity for a long time. This has hampered productivity and the ability to accomplish duties.
The Ethics of Artificial Intelligence And Machine Learning
The development of artificial intelligence (AI) and machine learning (ML) technologies has been one of the most significant technological developments of the past several decades. These cutting-edge technologies have the potential to alter several sectors of society, including commerce, industry, healthcare, and entertainment.
As with any new and quickly advancing technology, AI and ML ethics must be carefully studied. The usage of these technologies presents significant concerns around privacy, accountability, and command. As the use of AI and ML grows more ubiquitous, we must assess their possible influence on society and investigate the ethical issues that must be taken into account as these technologies continue to develop.
What are Artificial Intelligence and Machine Learning?
Artificial Intelligence is the simulation of human intelligence in machines designed to think and act like humans. Machine learning is a subfield of AI that enables computers to learn from data and improve their performance over time without being explicitly programmed.
The impact of AI and ML on Society
The use of AI and ML in various industries, such as healthcare, finance, and retail, has brought many benefits. For example, AI-powered medical diagnosis systems can identify diseases faster and more accurately than human doctors. However, there are also concerns about job displacement and the potential for AI to perpetuate societal biases.
The Ethical Considerations of AI and ML
A. Bias in AI algorithms
One of the critical ethical concerns about AI and ML is the potential for algorithms to perpetuate existing biases. This can occur if the data used to train these algorithms reflects the preferences of the people who created it. As a result, AI systems can perpetuate these biases and discriminate against certain groups of people.
B. Responsibility for AI-generated decisions
Another ethical concern is the responsibility for decisions made by AI systems. For example, who is responsible for the damage if a self-driving car causes an accident? The manufacturer of the vehicle, the software developer, or the AI algorithm itself?
C. The potential for misuse of AI and ML
AI and ML can also be used for malicious purposes, such as cyberattacks and misinformation. The need for more regulation and oversight in developing and using these technologies makes it difficult to prevent misuse.
The developments in AI and ML have given numerous benefits to humanity, but they also present significant ethical concerns that must be addressed. We must assess the repercussions of new technologies on society, implement methods to limit the associated dangers, and guarantee that they are utilized for the greater good. As AI and ML continue to play an ever-increasing role in our daily lives, we must engage in an open and frank discussion regarding their ethics.
The Future of Work And Automation
Rapid technological breakthroughs in recent years have brought about considerable changes in our way of life and work. Concerns regarding the influence of artificial intelligence and machine learning on the future of work and employment have increased alongside the development of these technologies. This article will examine the possible advantages and disadvantages of automation and its influence on the labor market, employees, and the economy.
The Advantages of Automation
Automation in the workplace offers various benefits, including higher efficiency and production, fewer mistakes, and enhanced precision. Automated processes may accomplish repetitive jobs quickly and precisely, allowing employees to concentrate on more complex and creative activities. Additionally, automation may save organizations money since it removes the need to pay for labor and minimizes the danger of workplace accidents.
The Potential Disadvantages of Automation
However, automation has significant disadvantages, including job loss and income stagnation. As robots and computers replace human labor in particular industries, there is a danger that many workers may lose their jobs, resulting in higher unemployment and more significant economic disparity. Moreover, if automation is not adequately regulated and managed, it might lead to stagnant wages and a deterioration in employees' standard of life.
The Future of Work and Automation
Despite these difficulties, automation will likely influence how labor is done. As a result, firms, employees, and governments must take early measures to solve possible issues and reap the rewards of automation. This might entail funding worker retraining programs, enhancing education and skill development, and implementing regulations that support equality and justice at work.
IV. The Need for Ethical Considerations
We must consider the ethical ramifications of automation and its effects on society as technology develops. The impact on employees and their rights, possible hazards to privacy and security, and the duty of corporations and governments to ensure that automation is utilized responsibly and ethically are all factors to be taken into account.
Conclusion:
To summarise, the future of employment and automation will most certainly be defined by a complex interaction of technological advances, economic trends, and cultural ideals. All stakeholders must work together to handle the problems and possibilities presented by automation and ensure that technology is employed to benefit society as a whole.
The Role of Technology in Education
Introduction.
Nearly every part of our lives has been transformed by technology, and education is no different. Today's students have greater access to knowledge, opportunities, and resources than ever before, and technology is becoming a more significant part of their educational experience. Technology is transforming how we think about education and creating new opportunities for learners of all ages, from online courses and virtual classrooms to instructional applications and augmented reality.
Technology's Benefits for Education
The capacity to tailor learning is one of technology's most significant benefits in education. Students may customize their education to meet their unique needs and interests since they can access online information and tools.
For instance, people can enroll in online classes on topics they are interested in, get tailored feedback on their work, and engage in virtual discussions with peers and subject matter experts worldwide. As a result, pupils are better able to acquire and develop the abilities and information necessary for success.
Challenges and Concerns
Despite the numerous advantages of technology in education, there are also obstacles and considerations to consider. One issue is the growing reliance on technology and the possibility that pupils would become overly dependent on it. This might result in a lack of critical thinking and problem-solving abilities, as students may become passive learners who only follow instructions and rely on technology to complete their assignments.
Another obstacle is the digital divide between those who have access to technology and those who do not. This division can exacerbate the achievement gap between pupils and produce uneven educational and professional growth chances. To reduce these consequences, all students must have access to the technology and resources necessary for success.
In conclusion, technology is rapidly becoming an integral part of the classroom experience and has the potential to alter the way we learn radically.
Technology can help students flourish and realize their full potential by giving them access to individualized instruction, tools, and opportunities. While the benefits of technology in the classroom are undeniable, it's crucial to be mindful of the risks and take precautions to guarantee that all kids have access to the tools they need to thrive.
The Influence of Technology On Personal Relationships And Communication
Technological advancements have profoundly altered how individuals connect and exchange information. It has changed the world in many ways in only a few decades. Because of the rise of the internet and various social media sites, maintaining relationships with people from all walks of life is now simpler than ever.
However, concerns about how these developments may affect interpersonal connections and dialogue are inevitable in an era of rapid technological growth. In this piece, we'll discuss how the prevalence of digital media has altered our interpersonal connections and the language we use to express ourselves.
Direct Effect on Direct Interaction:
The disruption of face-to-face communication is a particularly stark example of how technology has impacted human connections. The quality of interpersonal connections has suffered due to people's growing preference for digital over human communication. Technology has been demonstrated to reduce the usage of nonverbal signs such as facial expressions, tone of voice, and other indicators of emotional investment in the connection.
Positive Impact on Long-Distance Relationships:
Yet there are positives to be found as well. Long-distance relationships have also benefited from technological advancements. The development of technologies such as video conferencing, instant messaging, and social media has made it possible for individuals to keep in touch with distant loved ones. It has become simpler for individuals to stay in touch and feel connected despite geographical distance.
The Effects of Social Media on Personal Connections:
The widespread use of social media has had far-reaching consequences, especially on the quality of interpersonal interactions. Social media has positive and harmful effects on relationships since it allows people to keep in touch and share life's milestones.
Unfortunately, social media has made it all too easy to compare oneself to others, which may lead to emotions of jealousy and a general decline in confidence. Furthermore, social media might cause people to have inflated expectations of themselves and their relationships.
A Personal Perspective on the Intersection of Technology and Romance
Technological advancements have also altered physical touch and closeness. Virtual reality and other technologies have allowed people to feel physical contact and familiarity in a digital setting. This might be a promising breakthrough, but it has some potential downsides.
Experts are concerned that people's growing dependence on technology for intimacy may lead to less time spent communicating face-to-face and less emphasis on physical contact, both of which are important for maintaining good relationships.
In conclusion, technological advancements have significantly affected the quality of interpersonal connections and the exchange of information. Even though technology has made it simpler to maintain personal relationships, it has chilled interpersonal interactions between people.
Keeping tabs on how technology is changing our lives and making adjustments as necessary is essential as we move forward. Boundaries and prioritizing in-person conversation and physical touch in close relationships may help reduce the harm it causes.
The Security and Privacy Implications of Increased Technology Use and Data Collection
The fast development of technology over the past few decades has made its way into every aspect of our life. Technology has improved many facets of our life, from communication to commerce. However, significant privacy and security problems have emerged due to the broad adoption of technology. In this essay, we'll look at how the widespread use of technological solutions and the subsequent explosion in collected data affects our right to privacy and security.
Data Mining and Privacy Concerns
Risk of Cyber Attacks and Data Loss
The Widespread Use of Encryption and Other Safety Mechanisms
The Privacy and Security of the Future in a Globalized Information Age
Obtaining and Using Individual Information
The acquisition and use of private information is a significant cause for privacy alarm in the digital age. Data about their customers' online habits, interests, and personal information is a valuable commodity for many internet firms. Besides tailored advertising, this information may be used for other, less desirable things like identity theft or cyber assaults.
Moreover, many individuals need to be made aware of what data is being gathered from them or how it is being utilized because of the lack of transparency around gathering personal information. Privacy and data security have become increasingly contentious as a result.
Data breaches and other forms of cyber-attack pose a severe risk.
The risk of cyber assaults and data breaches is another big issue of worry. More people are using more devices, which means more opportunities for cybercriminals to steal private information like credit card numbers and other identifying data. This may cause monetary damages and harm one's reputation or identity.
Many high-profile data breaches have occurred in recent years, exposing the personal information of millions of individuals and raising serious concerns about the safety of this information. Companies and governments have responded to this problem by adopting new security methods like encryption and multi-factor authentication.
Many businesses now use encryption and other security measures to protect themselves from cybercriminals and data thieves. Encryption keeps sensitive information hidden by encoding it so that only those possessing the corresponding key can decipher it. This prevents private information like bank account numbers or social security numbers from falling into the wrong hands.
Firewalls, virus scanners, and two-factor authentication are all additional security precautions that may be used with encryption. While these safeguards do much to stave against cyber assaults, they are not entirely impregnable, and data breaches are still possible.
The Future of Privacy and Security in a Technologically Advanced World
There's little doubt that concerns about privacy and security will persist even as technology improves. There must be strict safeguards to secure people's private information as more and more of it is transferred and kept digitally. To achieve this goal, it may be necessary to implement novel technologies and heightened levels of protection and to revise the rules and regulations regulating the collection and storage of private information.
Individuals and businesses are understandably concerned about the security and privacy consequences of widespread technological use and data collecting. There are numerous obstacles to overcome in a society where technology plays an increasingly important role, from acquiring and using personal data to the risk of cyber-attacks and data breaches. Companies and governments must keep spending money on security measures and working to educate people about the significance of privacy and security if personal data is to remain safe.
In conclusion, technology has profoundly impacted virtually every aspect of our lives, including society and culture, ethics, work, education, personal relationships, and security and privacy. The rise of artificial intelligence and machine learning has presented new ethical considerations, while automation is transforming the future of work.
In education, technology has revolutionized the way we learn and access information. At the same time, our dependence on technology has brought new challenges in terms of personal relationships, communication, security, and privacy.
Jenni.ai is an AI tool that can help students write essays easily and quickly. Whether you're looking, for example, for essays on any of these topics or are seeking assistance in writing your essay, Jenni.ai offers a convenient solution. Sign up for a free trial today and experience the benefits of AI-powered writing assistance for yourself.
Start Writing With Jenni Today
Sign up for a free Jenni AI account today. Unlock your research potential and experience the difference for yourself. Your journey to academic excellence starts here.
Suggestions or feedback?
MIT News | Massachusetts Institute of Technology
- Machine learning
- Sustainability
- Black holes
- Classes and programs
Departments
- Aeronautics and Astronautics
- Brain and Cognitive Sciences
- Architecture
- Political Science
- Mechanical Engineering
Centers, Labs, & Programs
- Abdul Latif Jameel Poverty Action Lab (J-PAL)
- Picower Institute for Learning and Memory
- Lincoln Laboratory
- School of Architecture + Planning
- School of Engineering
- School of Humanities, Arts, and Social Sciences
- Sloan School of Management
- School of Science
- MIT Schwarzman College of Computing
A comprehensive study of technological change
Press contact :.
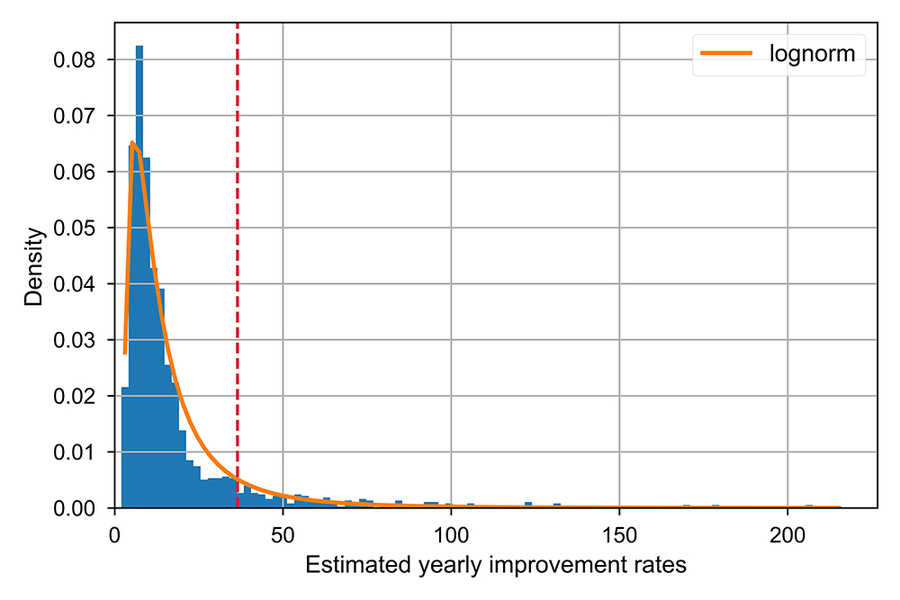
Previous image Next image
The societal impacts of technological change can be seen in many domains, from messenger RNA vaccines and automation to drones and climate change. The pace of that technological change can affect its impact, and how quickly a technology improves in performance can be an indicator of its future importance. For decision-makers like investors, entrepreneurs, and policymakers, predicting which technologies are fast improving (and which are overhyped) can mean the difference between success and failure.
New research from MIT aims to assist in the prediction of technology performance improvement using U.S. patents as a dataset. The study describes 97 percent of the U.S. patent system as a set of 1,757 discrete technology domains, and quantitatively assesses each domain for its improvement potential.
“The rate of improvement can only be empirically estimated when substantial performance measurements are made over long time periods,” says Anuraag Singh SM ’20, lead author of the paper. “In some large technological fields, including software and clinical medicine, such measures have rarely, if ever, been made.”
A previous MIT study provided empirical measures for 30 technological domains, but the patent sets identified for those technologies cover less than 15 percent of the patents in the U.S. patent system. The major purpose of this new study is to provide predictions of the performance improvement rates for the thousands of domains not accessed by empirical measurement. To accomplish this, the researchers developed a method using a new probability-based algorithm, machine learning, natural language processing, and patent network analytics.
Overlap and centrality
A technology domain, as the researchers define it, consists of sets of artifacts fulfilling a specific function using a specific branch of scientific knowledge. To find the patents that best represent a domain, the team built on previous research conducted by co-author Chris Magee, a professor of the practice of engineering systems within the Institute for Data, Systems, and Society (IDSS). Magee and his colleagues found that by looking for patent overlap between the U.S. and international patent-classification systems, they could quickly identify patents that best represent a technology. The researchers ultimately created a correspondence of all patents within the U.S. patent system to a set of 1,757 technology domains.
To estimate performance improvement, Singh employed a method refined by co-authors Magee and Giorgio Triulzi, a researcher with the Sociotechnical Systems Research Center (SSRC) within IDSS and an assistant professor at Universidad de los Andes in Colombia. Their method is based on the average “centrality” of patents in the patent citation network. Centrality refers to multiple criteria for determining the ranking or importance of nodes within a network.
“Our method provides predictions of performance improvement rates for nearly all definable technologies for the first time,” says Singh.
Those rates vary — from a low of 2 percent per year for the “Mechanical skin treatment — Hair removal and wrinkles” domain to a high of 216 percent per year for the “Dynamic information exchange and support systems integrating multiple channels” domain. The researchers found that most technologies improve slowly; more than 80 percent of technologies improve at less than 25 percent per year. Notably, the number of patents in a technological area was not a strong indicator of a higher improvement rate.
“Fast-improving domains are concentrated in a few technological areas,” says Magee. “The domains that show improvement rates greater than the predicted rate for integrated chips — 42 percent, from Moore’s law — are predominantly based upon software and algorithms.”
TechNext Inc.
The researchers built an online interactive system where domains corresponding to technology-related keywords can be found along with their improvement rates. Users can input a keyword describing a technology and the system returns a prediction of improvement for the technological domain, an automated measure of the quality of the match between the keyword and the domain, and patent sets so that the reader can judge the semantic quality of the match.
Moving forward, the researchers have founded a new MIT spinoff called TechNext Inc. to further refine this technology and use it to help leaders make better decisions, from budgets to investment priorities to technology policy. Like any inventors, Magee and his colleagues want to protect their intellectual property rights. To that end, they have applied for a patent for their novel system and its unique methodology.
“Technologies that improve faster win the market,” says Singh. “Our search system enables technology managers, investors, policymakers, and entrepreneurs to quickly look up predictions of improvement rates for specific technologies.”
Adds Magee: “Our goal is to bring greater accuracy, precision, and repeatability to the as-yet fuzzy art of technology forecasting.”
Share this news article on:
Related links.
- Sociotechnical Systems Research Center
- Institute for Data, Systems, and Society (IDSS)
Related Topics
- Technology and society
- Innovation and Entrepreneurship (I&E)
Related Articles
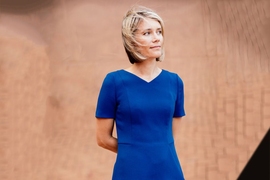
Shaping technology’s future
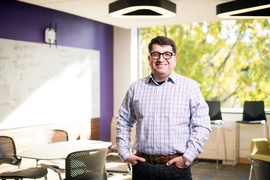
Tackling society’s big problems with systems theory
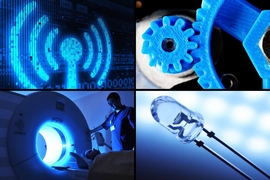
Patents forecast technological change
Previous item Next item
More MIT News
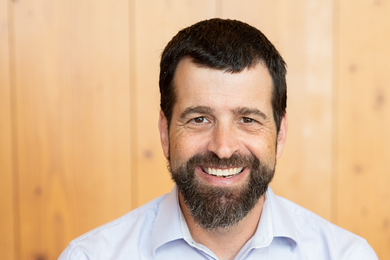
Sam Madden named faculty head of computer science in EECS
Read full story →
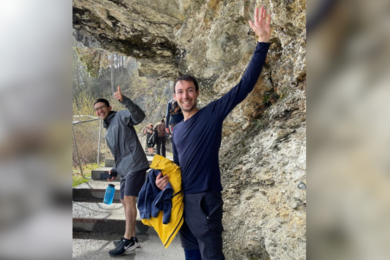
Remembering Mathieu Le Provost: AeroAstro researcher, adventurer, and friend
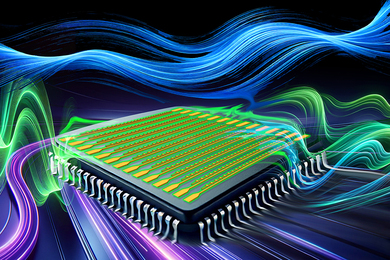
Nanostructures enable on-chip lightwave-electronic frequency mixer
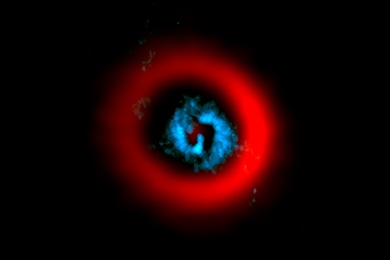
3 Questions: Evidence for planetary formation through gravitational instability

Enabled by a significant gift, MIT’s Security Studies Program launches the Center for Nuclear Security Policy
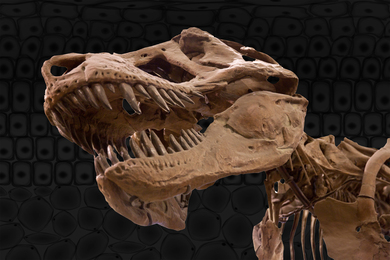
MIT chemists explain why dinosaur collagen may have survived for millions of years
- More news on MIT News homepage →
Massachusetts Institute of Technology 77 Massachusetts Avenue, Cambridge, MA, USA
- Map (opens in new window)
- Events (opens in new window)
- People (opens in new window)
- Careers (opens in new window)
- Accessibility
- Social Media Hub
- MIT on Facebook
- MIT on YouTube
- MIT on Instagram
You are using an outdated browser. Upgrade your browser today or install Google Chrome Frame to better experience this site.
Technology pros and cons: is technology good or bad for society?

Find out what are the main the pros and cons of technology. We discuss the history, importance and role of technology, as well as its impact on society. Is technological progress good or is it becoming a threat to us? Vote in our poll and debate (see below)
Is technology good for society? A brief history
The impact of technology on society is undeniable. Technology and science have played a central role in human history and help shape entire civilizations. Technological progress was key for the emergence and downfall of empires. The development of hunting and farming tools allowed our ancestors to dominate other hominid species. The invention of the wheel and writing, as well as the introduction of metal tools and weapons were other landmarks in the history of technology . Many successive civilizations have contributed to the world's advancement. Often the development of technology also helped these societies to dominate militarily , politically, and economically their neighbors, as well as increase the welfare of their citizens.
The Egyptians invented many farming, medical and construction technologies. The Mesopotamians are credited for introducing irrigation and drainage systems, as well as sophisticated mud-brick and stone architecture techniques. Greeks were responsible for many inventions, such as the watermill, and the improvement on many existing technologies. Still today Greek mathematicians, engeneers and philosophers are recognised as fundamental to the history of human thought and technology. The Romans brought technology to a new level, and their monumental amphitheatres, aqueducts, bridges, harbours, dams and public baths help them dominate the Western world for centuries. Ancient Indian civilizations are credited for developing good understanding of seafaring, sanitation and hydrological technologies. Chinese discoveries include paper, matches, the cross-bow, seismological detectors, the wheelbarrow, the suspension bridge and the compass, among others.
During the Middle Ages architecture, navigation, papermaking and military technologies were developed. The Arab conquest of the Iberian Peninsula helped to introduce Europe to many technological advances developed in the east. The Renaissance and the Age of Explorations also demonstrated how innovation shaped societies. Research and inventions were put into practice. The use of artillery, new cranes and medical techniques marked a beginning of a scientific revolution. The Portuguese and Spanish discoveries, were enabled by technological progress but also help connecting different civilization which accelerated the spread of innovation. The industrial revolution brought the steam engine and developments in mining, metalurgy, manufacturing and transport. Since the 19th century, science and technology have evolved even faster. The 20th century brought the expansion of electrification and communication technologies, mass industrial production, electronic computing, nuclear technology and space exploration among others. It also demonstrated the devastating power of some of the technologies developed by humans. The weapons developed during that century, including the weapons of mass destruction, caused the deaths of millions.
The 21st century seems to have accelerated even faster these processes and intensified the impact of technology on society. Technology colleges such as MIT and Stanford have help accelerate scientific discovery. Genetic engineering, nanotechnology, 3D printing, wireless powered devices, augmented reality, articifical intelligence, drones , quantum computers and superconductivity are among the many new technologies we are witnessing today. But what come next? Can technology continue to help our lives or is it becoming a real threat to us? Can we keep scientific progress under control or will technology control our lives? Let's discuss the advantages and disadvantages or technology.
Pros and cons of technology
Pros of technological progress
- Thanks to technological progress humans live longer and much more comfortable lives. The medical advancements have helped us develop vaccines and treatment for diseases which were previously lethal. Giving birth is not as dangerous as it used to be. Technological progress has allowed develop new techniques for diagnosis and mitigation of diseases and other conditions. Scientific research has improved our understanding of nutrition and contributed to healthier lifestyles.
- Technology has allowed humans to travel faster and trade goods all over the world. Crossing the Atlantic was historically an adventure that would take weeks. Now in just a few hours you can travel from New York to London or Paris . Thanks to technology we can have holidays in remote locations and capture these moments through pictures. We can now buy and consume goods produced far away. In the same supermarket you can find French cheeses, South African wines, Spanish olive oils, Brazilian coffees, and Japanese fish.
- Without technology we would still be nomads hunting and gathering fruits and vegetables. Industrial societies heavily rely on technological progress. We can feed a fast growing world population thanks to the continuous innovation in production techniques. New inventions help foster the production, storage, treatment and transportation of goods. Societies which invest in research and development have a competitive edge and thrive. The people in technologically advanced societies live more comfortable lives.
- Genetically modified foods (GMO) may help fight hunger and ensure that world population continues to be fed. Genetic modification techniques contribute to produce more food and to maintain agricultural production at affordable prices.
- The Internet, computers and mobile phones illustrate the role of technology in improving society. Efficiency has skyrocketed thanks to these inventions. Our work and social lives have been transformed. People can now work from home and collaborate with teams located in other towns, countries or even continents. We can keep a fluid communication and relationship with friends and family living abroad. News of events cross the globe in seconds. Social netwoks such as facebook and twitter are extremely useful. Thanks to technology grandparents get to see and chat with their grandchildren much more often. People today get to meet others sharing similar hobbies or interests.
- Thanks to new technologies, alternative forms of entertainment and art have developed during the last century. Photography, radio, movies, television shows, music and video games occupy a central spot in people's lives. There are new forms of entertainment at our doorstep, such as virtual reality . Additionally, IT is facilitating the work of creators and help increase the quality of entertainment.
- The importance of technology in the delopment of renewable energies is evident. Without technological progress it would be difficult to envisage a green future in which the problem of climate change could be kept under control. Scientific advancements are making electric cars more affordable and enhancing the effectiveness and efficiency of solar and eolic energy, as well as that hydropower .
Cons and risks of technology
- Technology is altering our lifestyle and will alter the cognitive and social development of current and future generations. There are many different ways in which the evolution of technology and society are connected. Technological innovation has changed our lives. Computers , smartphones and the internet have strongly affected how we interact with other people. Many claim that they are dehumanizing our lives and making us more solitary people. Technology may be also facilitating cultural colonialism and reducing diversity. Today, children play less with other children and spend much of their time watching videos in their tablets and playing video games. People are doing less physical exercise than their ancestors. We are becoming increasingly detached from nature and attached to technological gadgets.
- Human cloning technology is a reality and in addition to some obvious advantages, human cloning brings some risks. For instance it could create worrisome divides in society between those genetically divided to be smarter or physically more attractive and the rest. Human cloning will be difficult to regulate and will bring concerns regarding its interference with nature and religious beliefs.
- Weaponization of viruses . For instance, viruses such as Ebola or AIDS could be transformed into a virus that could be transmitted through the air. This could endanger or even cause the extinction of the the human race . Lifeforms can be created through genetic manipulation. With techonological progress the techniques to create or manipulate lifeforms will be increasingly accessible to us. Potentially even high school children will be able to create life in science experiments. Genomes of infectious diseases will be available to download from the internet. Terrorists, psychopaths and other criminals will have the capacity to use genetic manipulation to harm or threaten others.
- Similarly, scientific experimentation might create enormous dangers for society. Risky experiments may go wrong . Researchers are currently mutating microorganism in order to find cures to diseases. By accident these diseases could escape the laboratory and spread. Experiments with particle accelerators, such as the Large Hadron Collider , entail some serious risks. Some scientists even claimed that humans could create a black hole that could destroy Earth.
- Enrichment of uranium is becoming an increasingly cheaper process. Traditionally the infrastructure required to produce nuclear power and build nuclear bombs was extremely expensive. Thanks to technological progress and the use of laser beams to separate U-235 and U-238, in the not so distant future, people might be able to enrich uranium home. Nuclear weapons in the hands of the wrong organizations or individuals could create enormous unrest in the world population and a serious security threat.
- Technological progress is to be blamed for the negative effects of global warming and climate change . The role of technology fostering economic progress is difficult to deny. However, at the same time the generation of enegy necessary to the increased production and transportation of goods, for instance through combustion engines and thermoelectric generators, has produced an increased in the emission and concentration of greenhouse gases in the atmosphere.
- Technology can be also used as an undesirable tool of control . For instance, scientists are working to develop brain scanning machines which could allow read a person's thoughts. This would have great benefits as could allow people with disabilities or people having suffered brain damage to communicate. Moreover if through a magnetic resoncance we could enquire criminals and terrorist we could also prevent harm for society. Similarly, thanks to advanced IT, it is possible to analyse the communications of millions of people and identify potential crimes and wrongdoers. Unfortunately, there is a very real possibility that technology will end up used by some governments in a draconian way. A dystopian future where people are constantly inquisitorially surveilled by a Big Brother as in Orwell's science fiction classic 1984.
What do you think, is technology good or bad? Is technological progress out of control? Will the rest of the 21st century see the importance of technology reduced?
Vote to see result and collect 1 XP. Your vote is anonymous. If you change your mind, you can change your vote simply by clicking on another option.
Voting results
New to netivist?
Join with confidence, netivist is completely advertisement free. You will not receive any promotional materials from third parties.
Or sign in with your favourite Social Network:
Join the debate
In order to join the debate you must be logged in.
Already have an account on netivist? Just login . New to netivist? Create your account for free .
Report Abuse and Offensive language
Was there any kind of offensive or inappropriate language used in this comment.
If you feel this user's conduct is unappropriate, please report this comment and our moderaters will review its content and deal with this matter as soon as possible.
NOTE: Your account might be penalized should we not find any wrongdoing by this user. Only use this feature if you are certain this user has infringed netivist's Terms of Service .
Our moderators will now review this comment and act accordingly. If it contains abusive or inappropriate language its author will be penalized.
Posting Comment
Your comment is being posted. This might take a few seconds, please wait.
Error Posting Comment
error.
We are having trouble saving your comment. Please try again .
Most Voted Debates
Rank | |
---|---|
Start a Debate
Would you like to create a debate and share it with the netivist community? We will help you do it!
Found a technical issue?
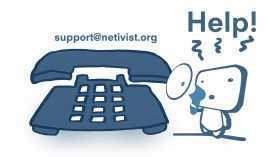
Are you experiencing any technical problem with netivist? Please let us know!
Help netivist
Help netivist continue running free!
Please consider making a small donation today. This will allow us to keep netivist alive and available to a wide audience and to keep on introducing new debates and features to improve your experience.
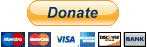
- What is netivist?
- Entertainment
- Top Debates
- Top Campaigns
- Provide Feedback
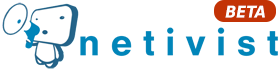
Follow us on social media:

Share by Email
There was an error...
Email successfully sent to:

Join with confidence, netivist is completely advertisement free You will not recive any promotional materials from third parties
Join netivist
Already have a netivist account?
If you already created your netivist account, please log in using the button below.
If you are new to netivist, please create your account for free and start collecting your netivist points!
You just leveled up!
Congrats you just reached a new level on Netivist. Keep up the good work.

Together we can make a difference
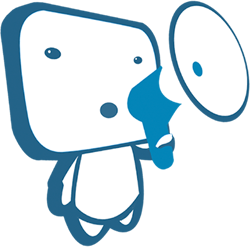
Follow us and don't miss out on the latest debates!
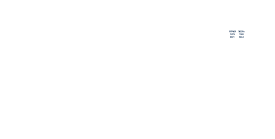
Technological Change
Technological change underpins many of the developments we've seen in health, agriculture, energy, and global development.
By: Max Roser , Hannah Ritchie and Edouard Mathieu
Almost every development we cover on Our World in Data is underpinned by technological change.
Medical innovations contributed to the decline in child mortality and the improvement in life expectancy . Thanks to the advances in agricultural technologies, higher crop yields and less undernourishment became possible. The long-term decline of global poverty was primarily driven by increased productivity from technological change. Access to energy , electricity, sanitation , and clean water has transformed the lives of billions. Transport, telephones, and the Internet have allowed us to collaborate on problems at a global level.
Emerging technologies are often expensive and, therefore, initially limited to society's richest. A key part of technological progress is making these life-changing and often life-saving innovations affordable for everyone.
Technology has improved our lives in many ways, but these developments are not always positive. Many of humanity’s largest threats — such as nuclear weapons and potentially artificial intelligence — result from technological advances. To mitigate these risks, good governance can be as important as the technology itself.
On this page, you can find our data, visualizations, and writing on the most fundamental technological changes that have shaped our world.
Research & Writing
Key articles on technological change.
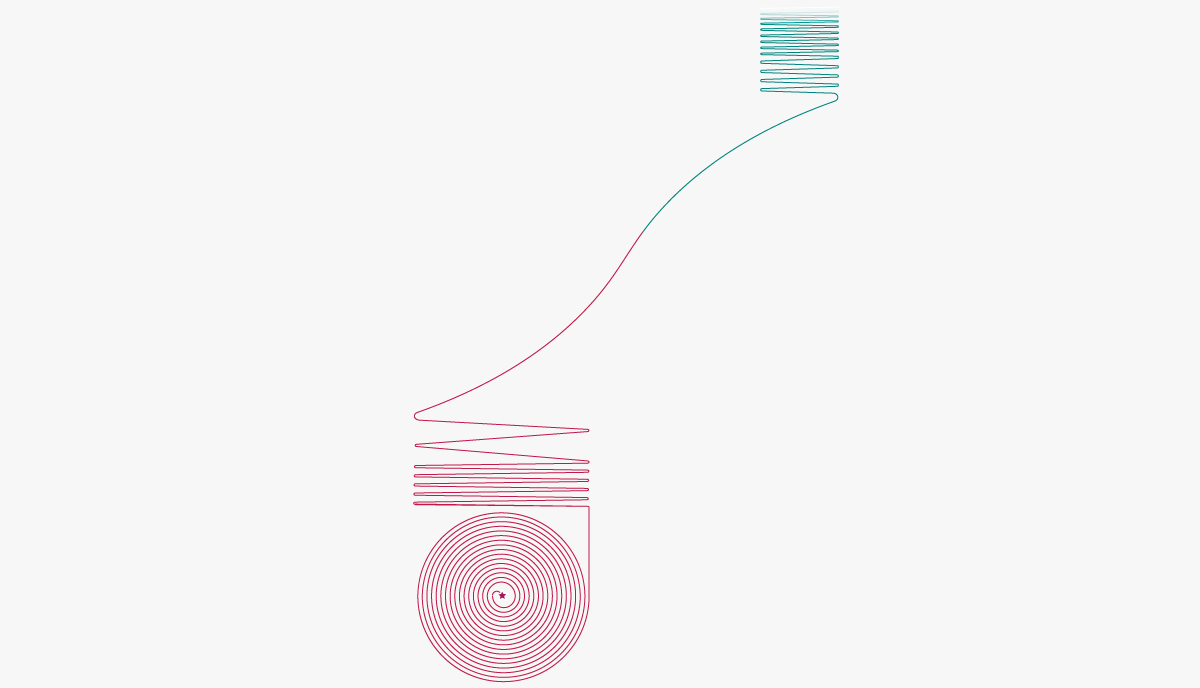
Technology over the long run: zoom out to see how dramatically the world can change within a lifetime
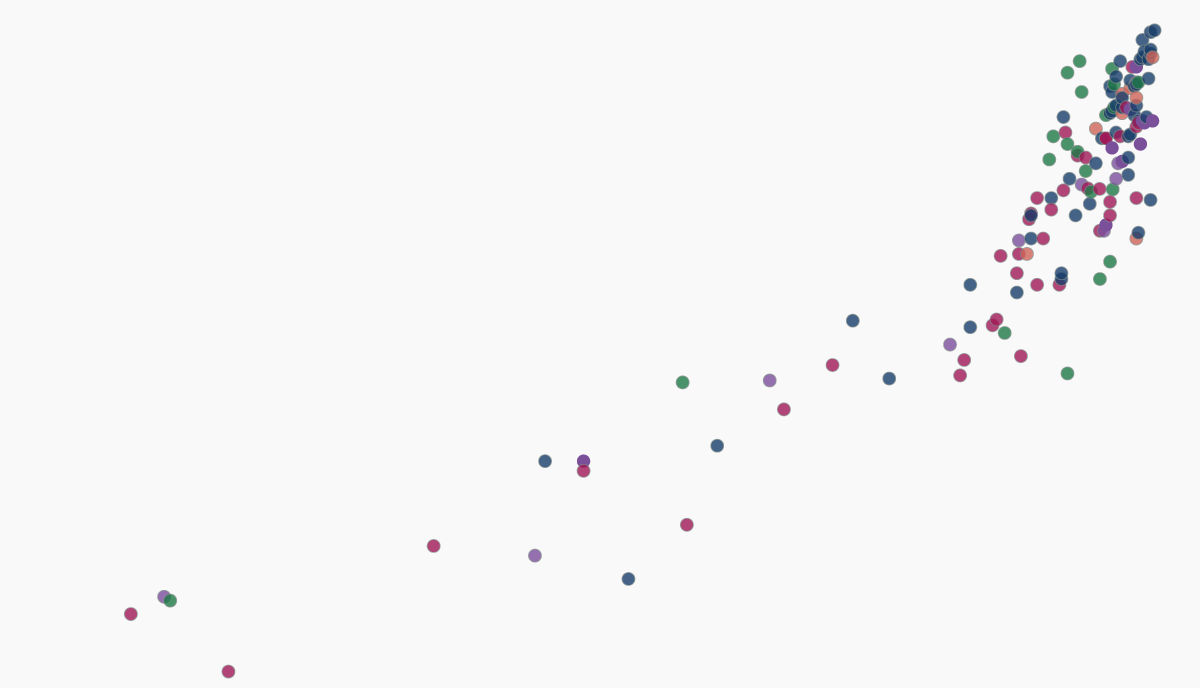
The brief history of artificial intelligence: the world has changed fast — what might be next?
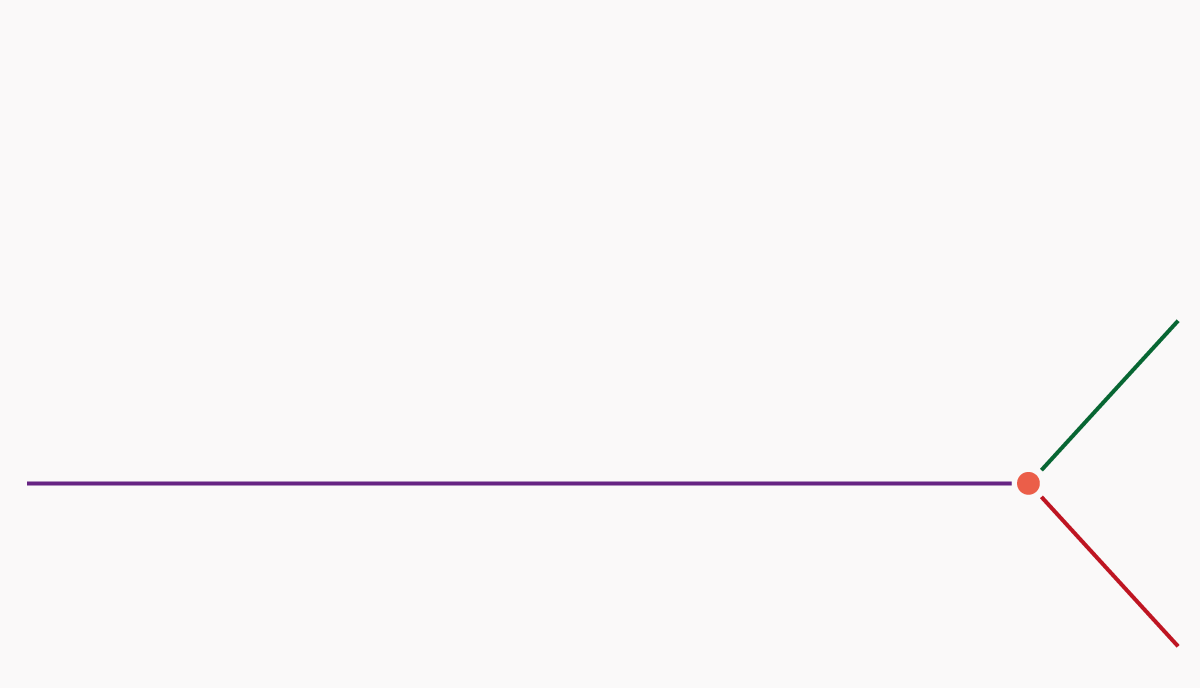
Artificial intelligence is transforming our world — it is on all of us to make sure that it goes well
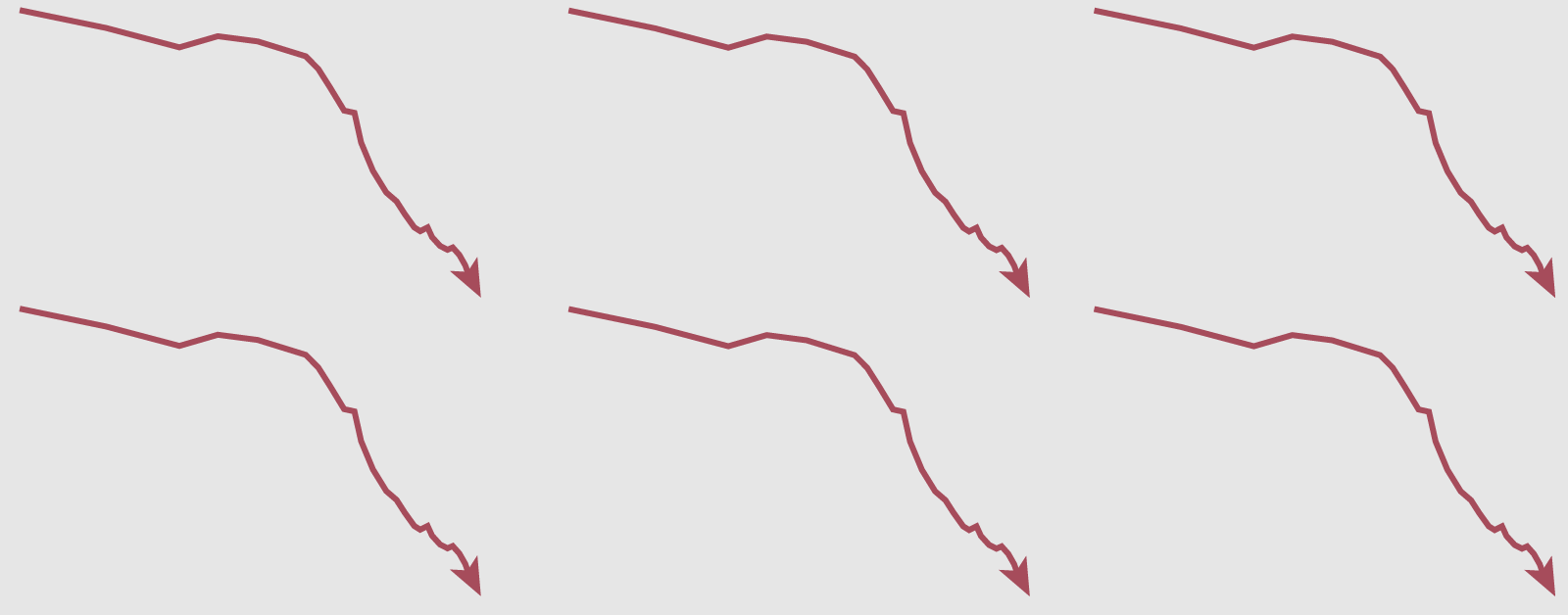
The price of batteries has declined by 97% in the last three decades
Hannah Ritchie
Artificial Intelligence (AI) and Communication Technologies
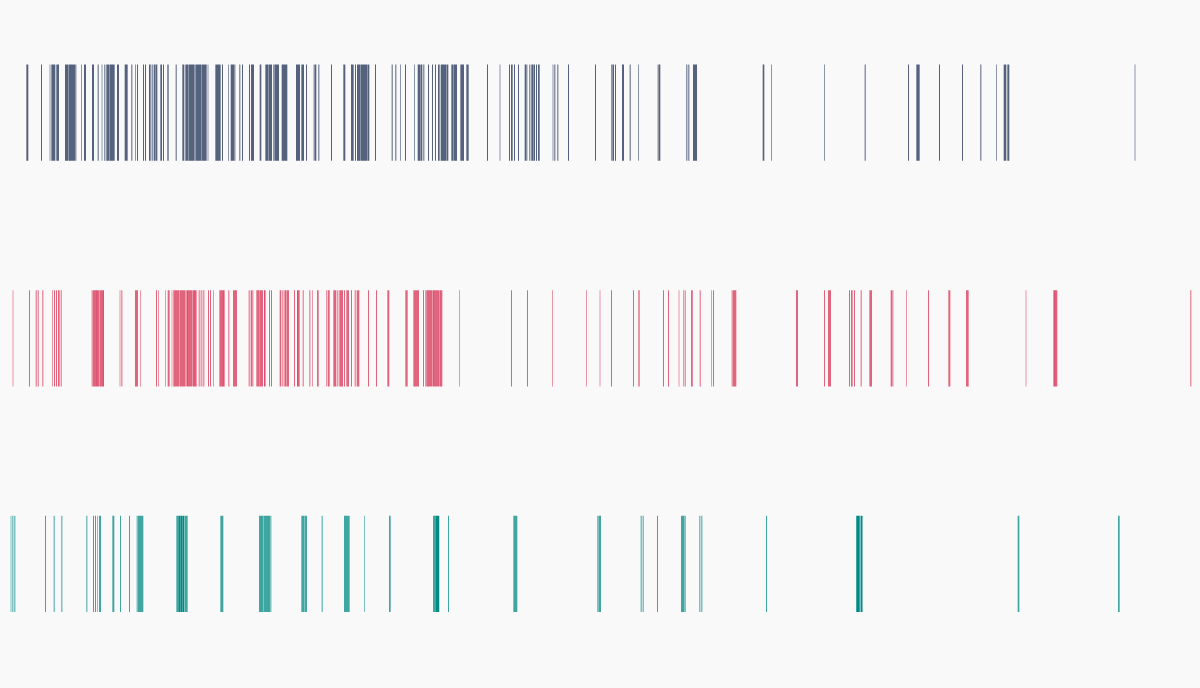
AI timelines: What do experts in artificial intelligence expect for the future?
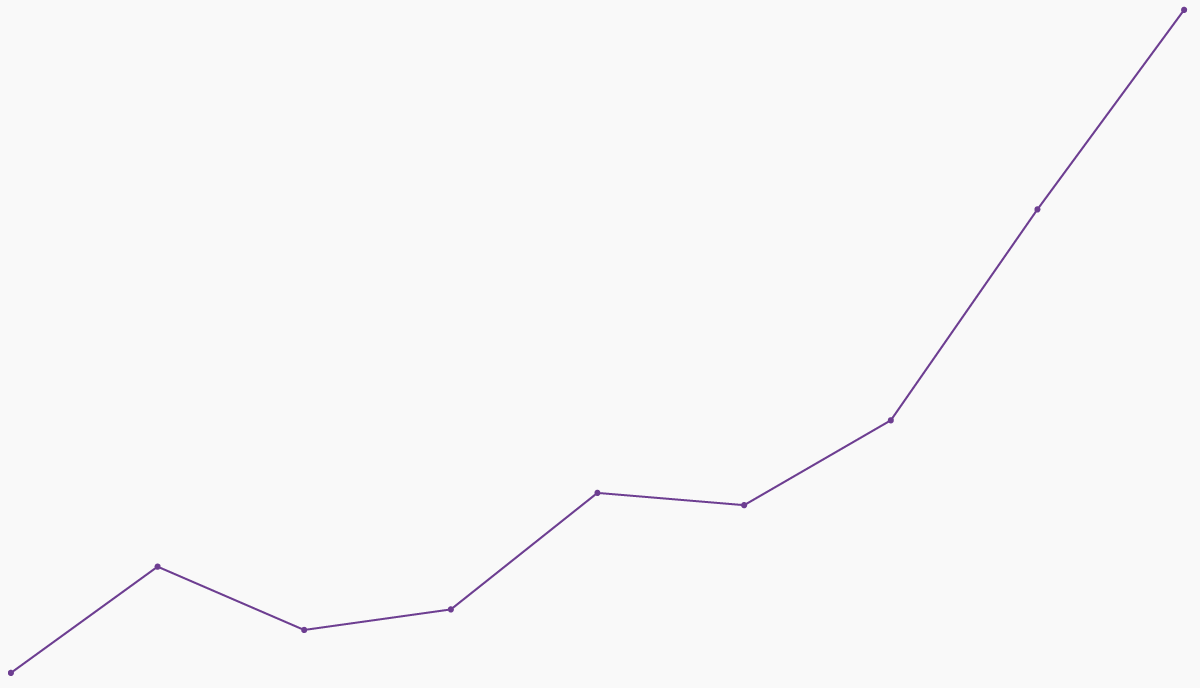
Artificial intelligence has advanced despite having few resources dedicated to its development – now investments have increased substantially
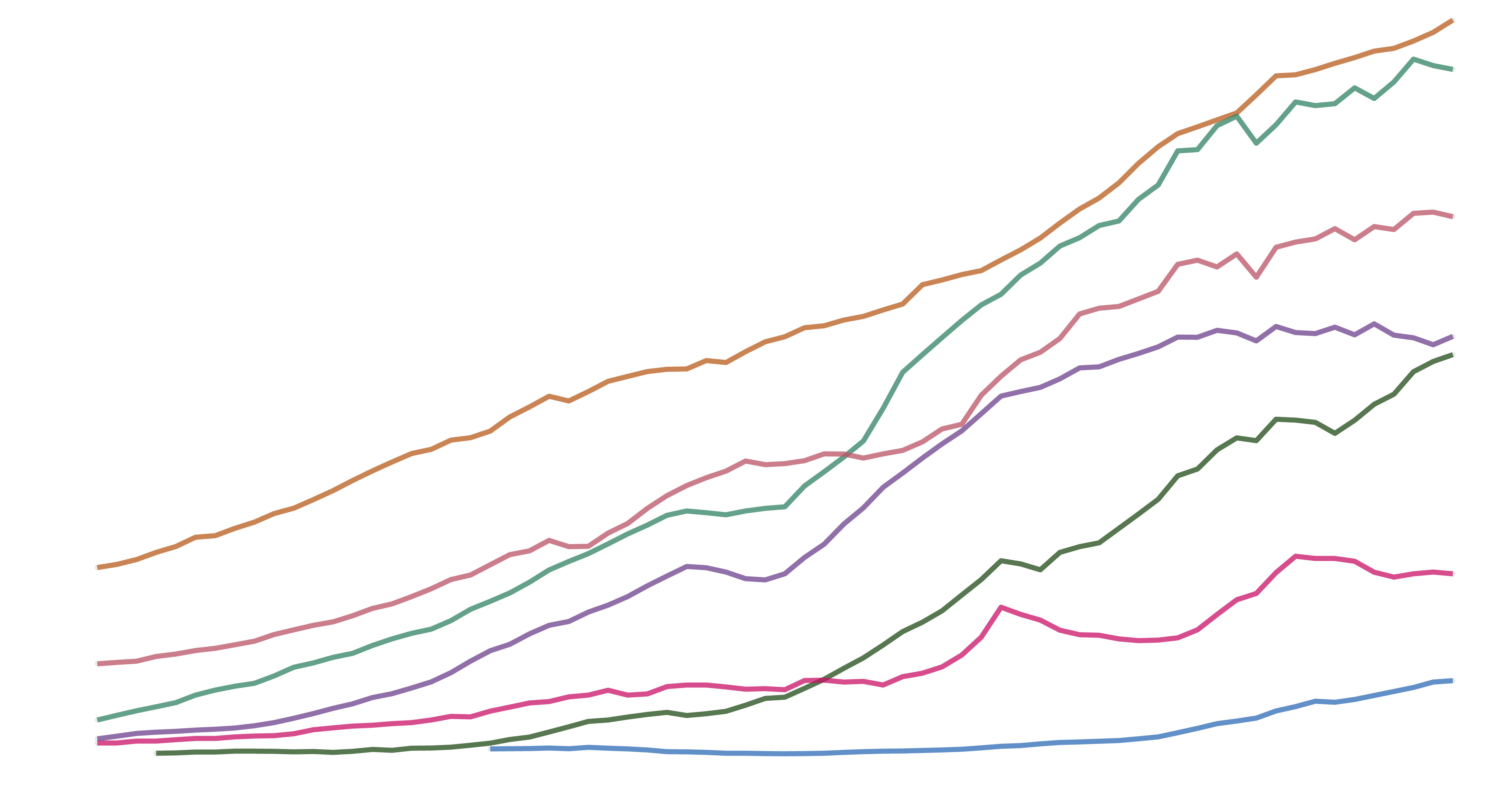
The importance of social networks for innovation and productivity
Esteban Ortiz-Ospina
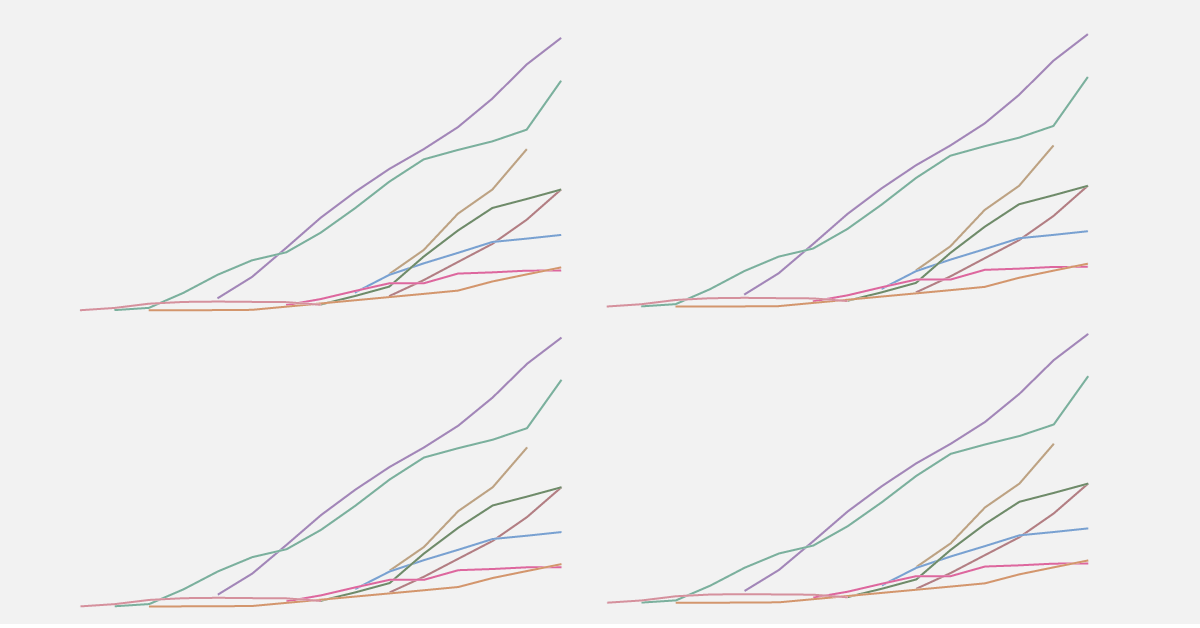
The rise of social media
Energy and agricultural technologies.
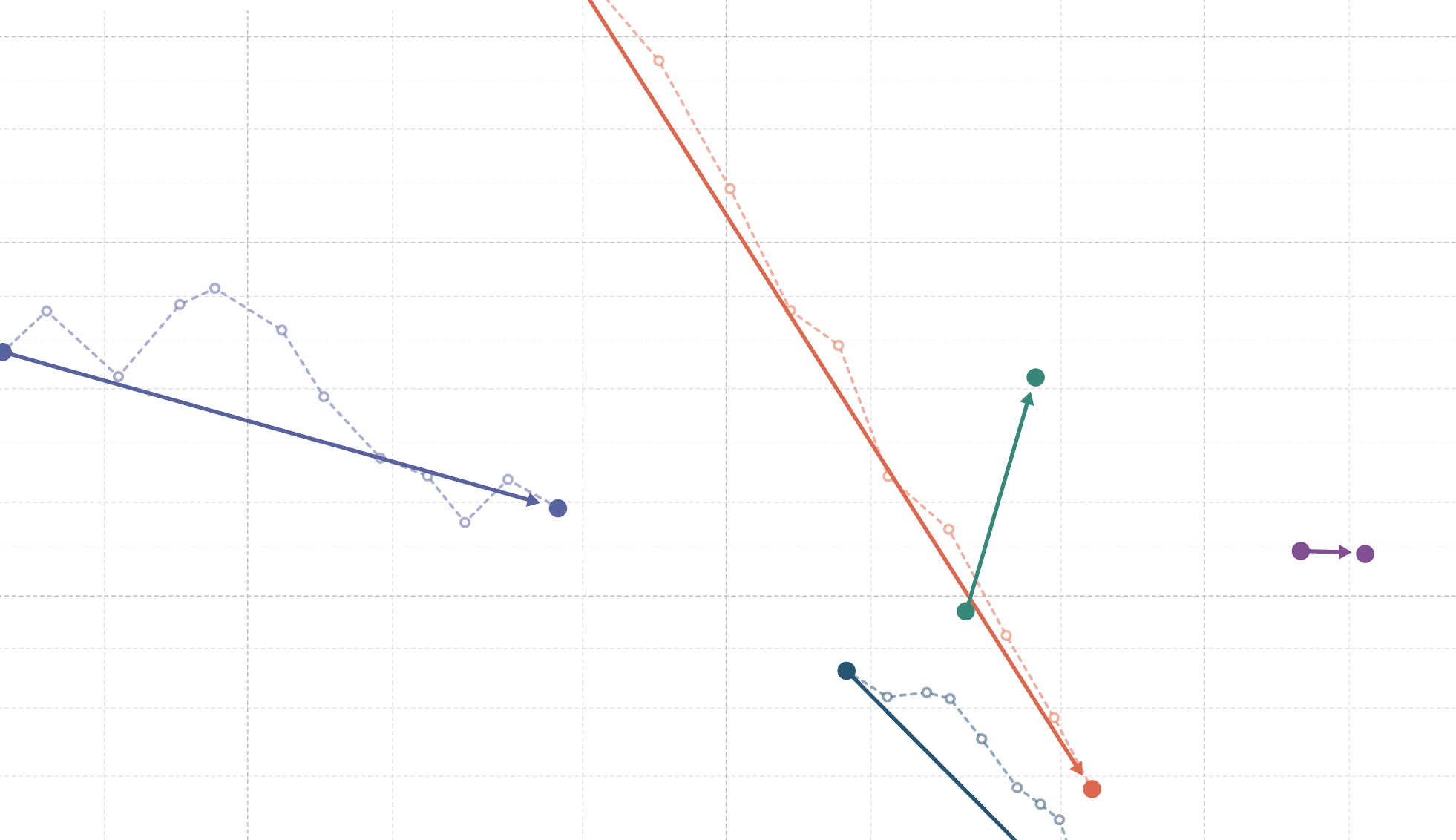
Why did renewables become so cheap so fast?
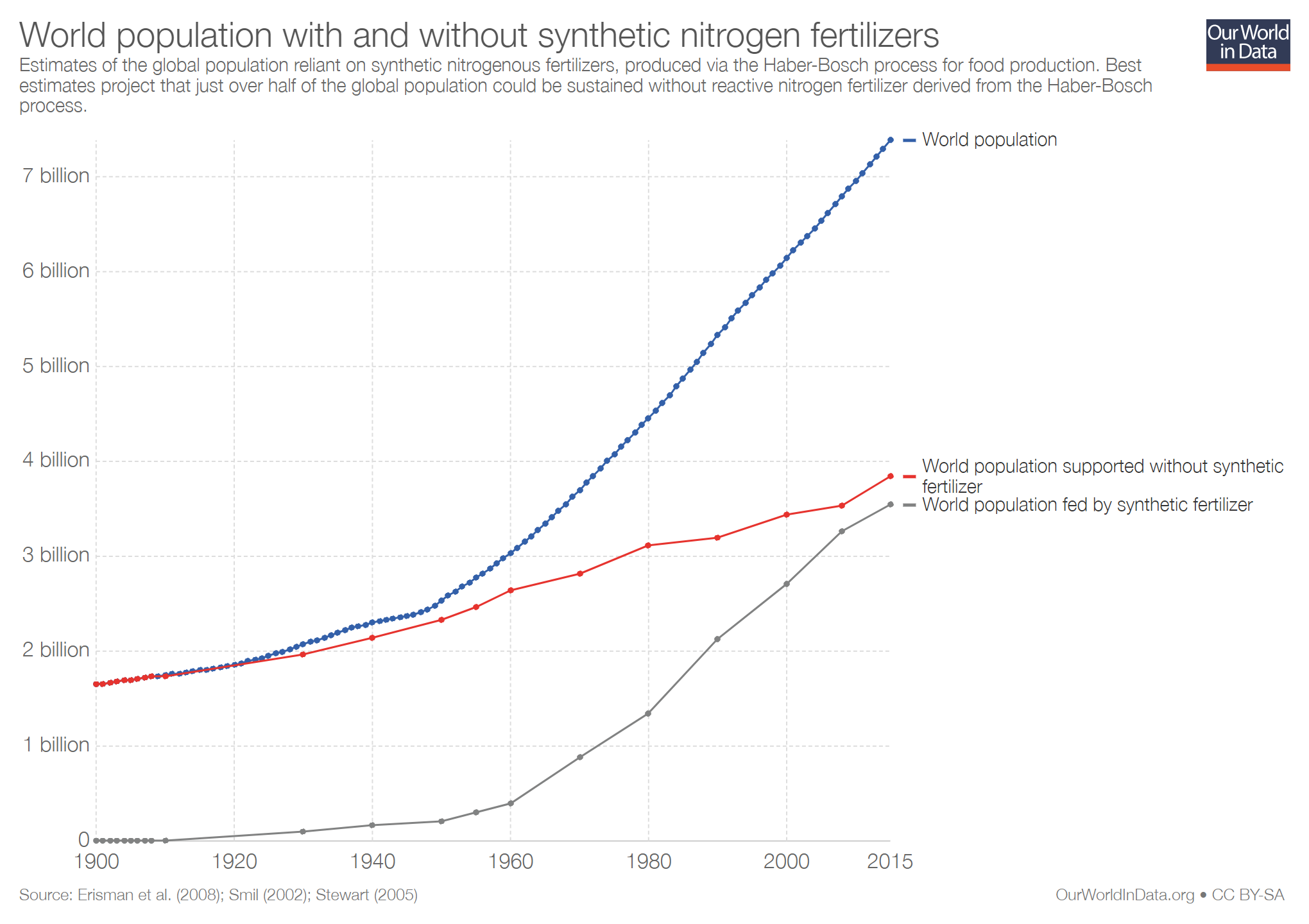
How many people does synthetic fertilizer feed?
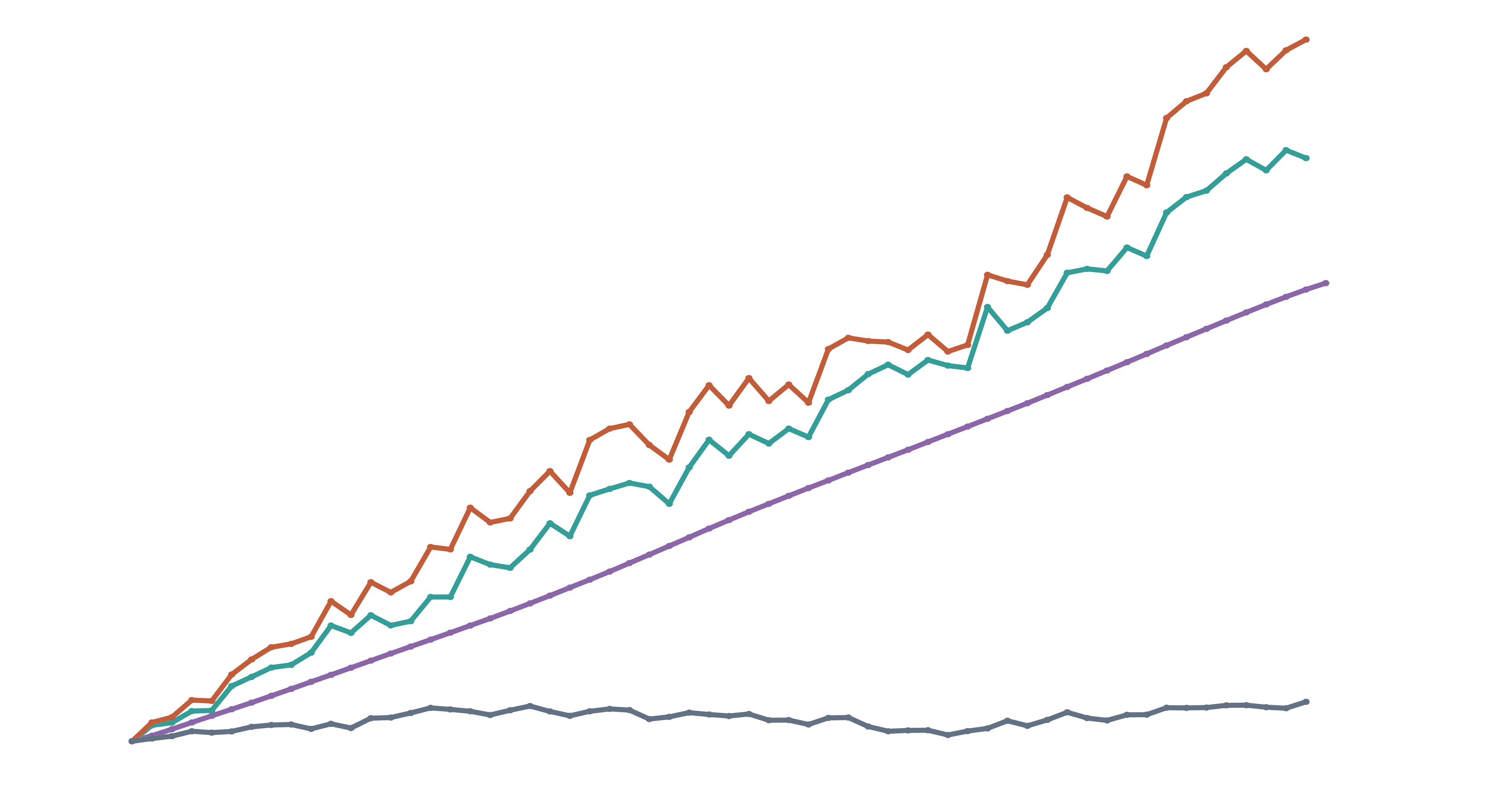
Yields vs. land use: how the Green Revolution enabled us to feed a growing population
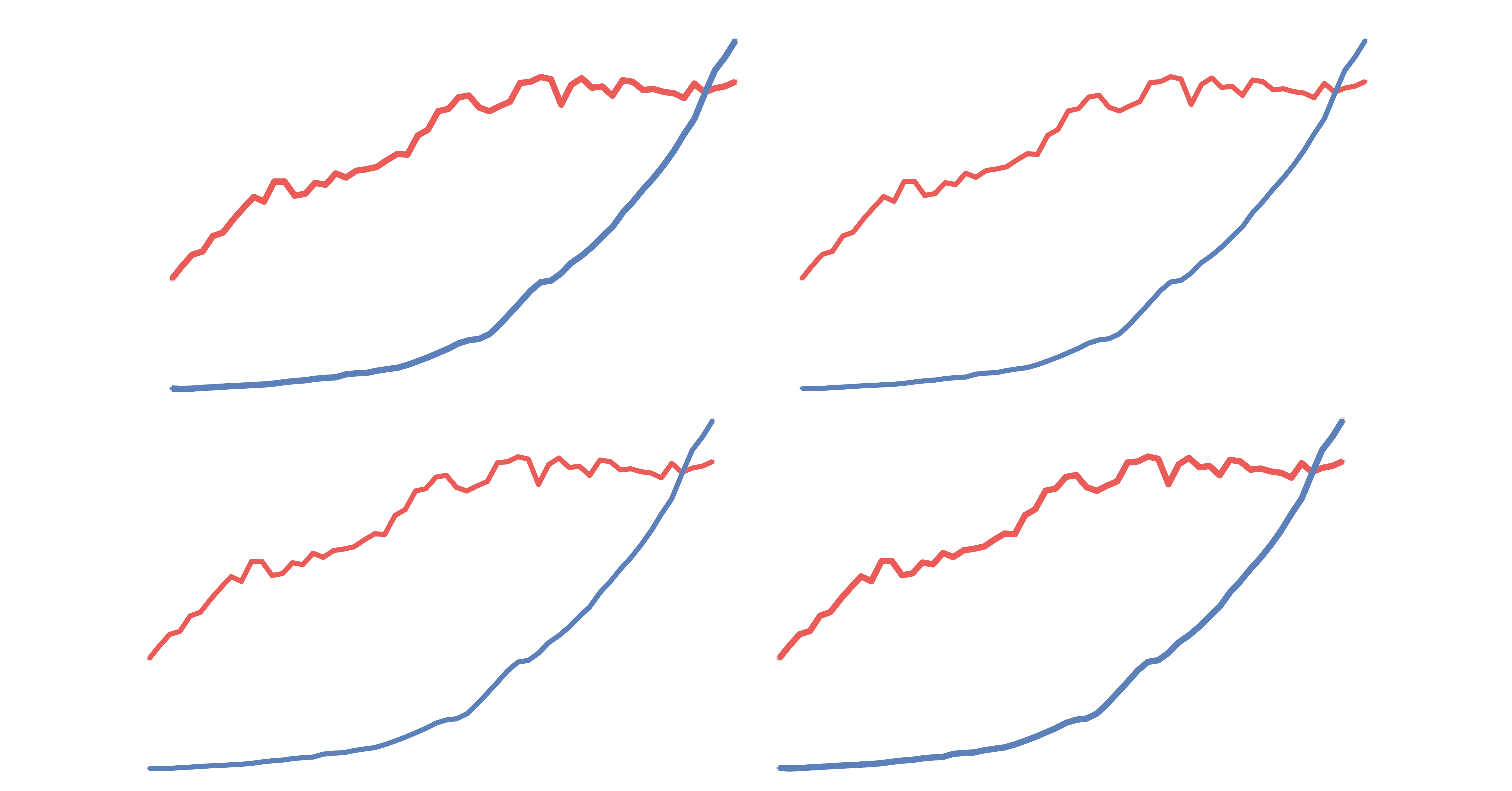
The world now produces more seafood from fish farms than wild catch
Theory of technological change.
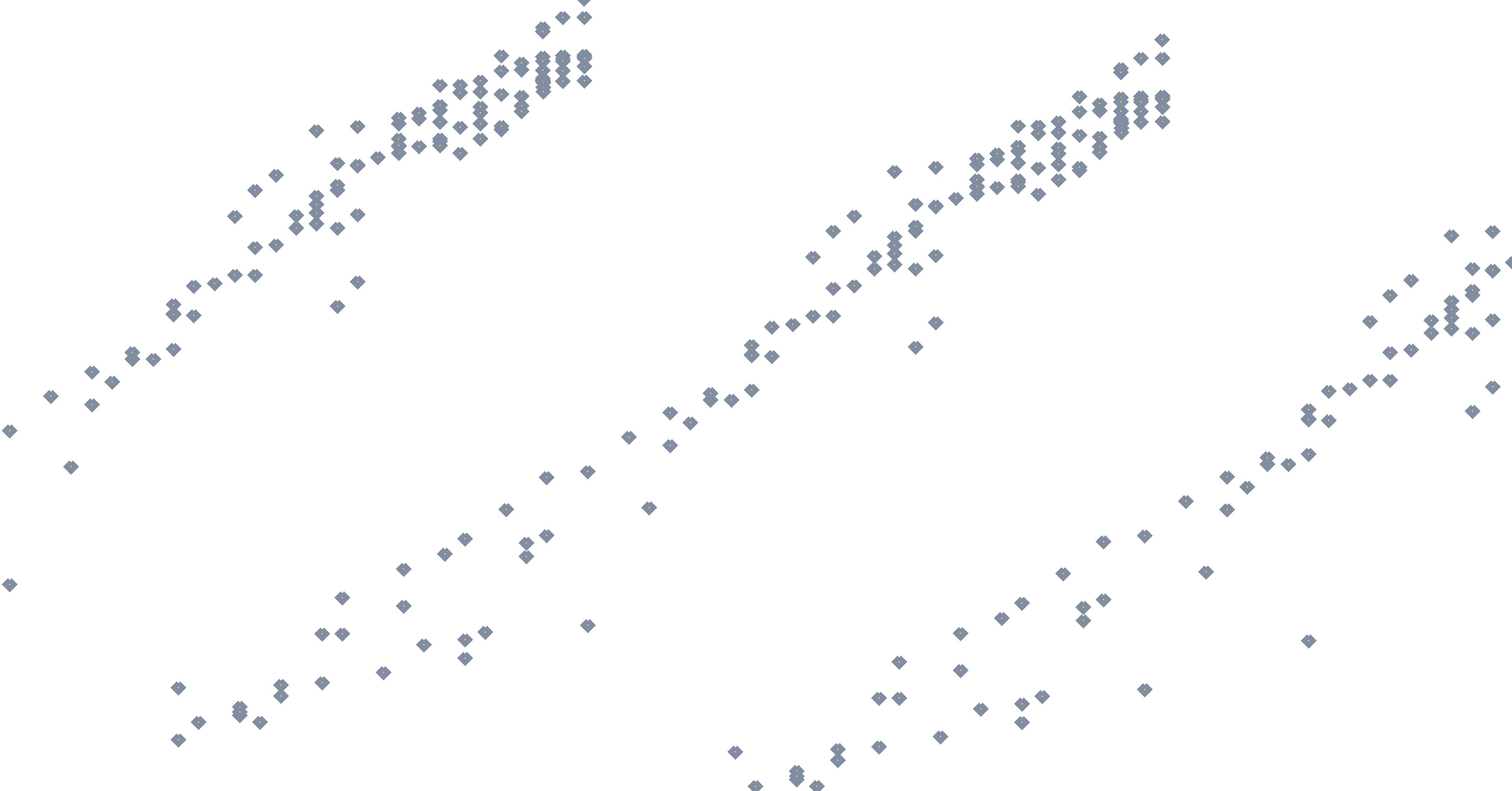
What is Moore's Law?
Max Roser, Hannah Ritchie and Edouard Mathieu
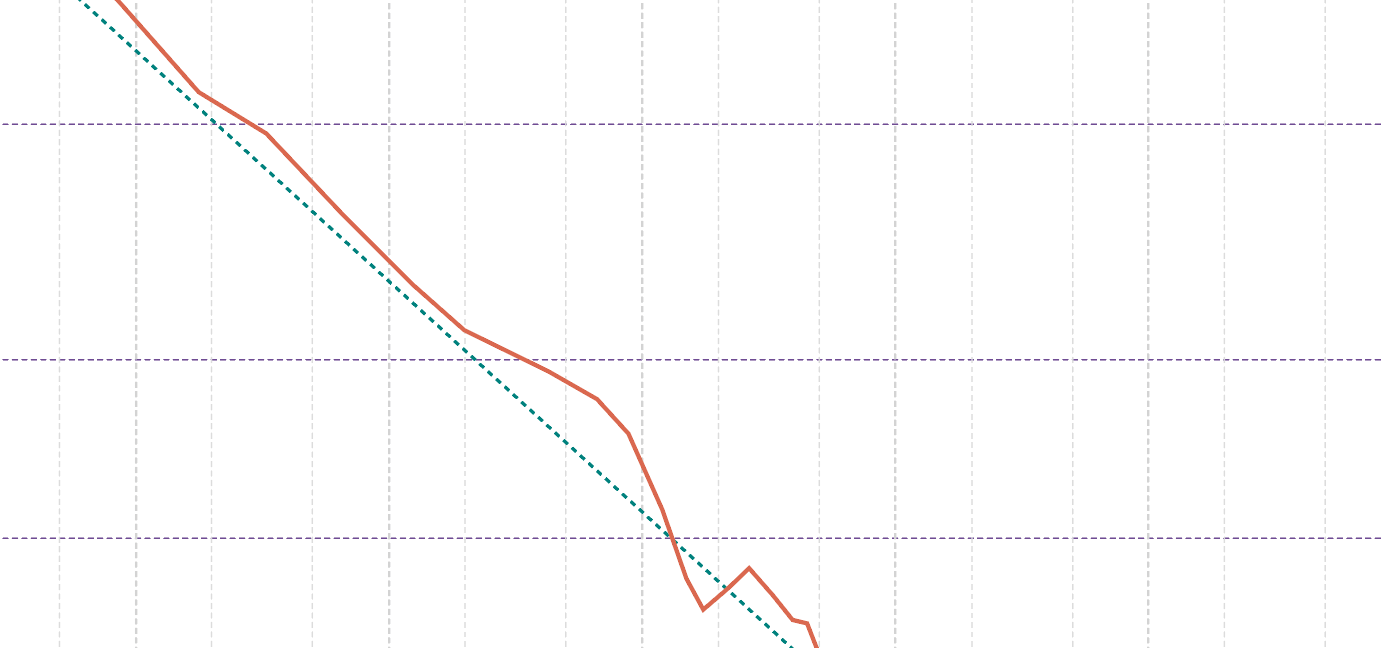
Learning curves: What does it mean for a technology to follow Wright’s Law?
Interactive charts on technological change, cite this work.
Our articles and data visualizations rely on work from many different people and organizations. When citing this topic page, please also cite the underlying data sources. This topic page can be cited as:
BibTeX citation
Reuse this work freely
All visualizations, data, and code produced by Our World in Data are completely open access under the Creative Commons BY license . You have the permission to use, distribute, and reproduce these in any medium, provided the source and authors are credited.
The data produced by third parties and made available by Our World in Data is subject to the license terms from the original third-party authors. We will always indicate the original source of the data in our documentation, so you should always check the license of any such third-party data before use and redistribution.
All of our charts can be embedded in any site.
Our World in Data is free and accessible for everyone.
Help us do this work by making a donation.
Thank you for visiting nature.com. You are using a browser version with limited support for CSS. To obtain the best experience, we recommend you use a more up to date browser (or turn off compatibility mode in Internet Explorer). In the meantime, to ensure continued support, we are displaying the site without styles and JavaScript.
- View all journals
- Explore content
- About the journal
- Publish with us
- Sign up for alerts
- Published: 04 January 2023
Papers and patents are becoming less disruptive over time
- Michael Park ORCID: orcid.org/0000-0001-8373-5480 1 ,
- Erin Leahey 2 &
- Russell J. Funk ORCID: orcid.org/0000-0001-6670-4981 1
Nature volume 613 , pages 138–144 ( 2023 ) Cite this article
377k Accesses
257 Citations
4583 Altmetric
Metrics details
- Scientific community
Theories of scientific and technological change view discovery and invention as endogenous processes 1 , 2 , wherein previous accumulated knowledge enables future progress by allowing researchers to, in Newton’s words, ‘stand on the shoulders of giants’ 3 , 4 , 5 , 6 , 7 . Recent decades have witnessed exponential growth in the volume of new scientific and technological knowledge, thereby creating conditions that should be ripe for major advances 8 , 9 . Yet contrary to this view, studies suggest that progress is slowing in several major fields 10 , 11 . Here, we analyse these claims at scale across six decades, using data on 45 million papers and 3.9 million patents from six large-scale datasets, together with a new quantitative metric—the CD index 12 —that characterizes how papers and patents change networks of citations in science and technology. We find that papers and patents are increasingly less likely to break with the past in ways that push science and technology in new directions. This pattern holds universally across fields and is robust across multiple different citation- and text-based metrics 1 , 13 , 14 , 15 , 16 , 17 . Subsequently, we link this decline in disruptiveness to a narrowing in the use of previous knowledge, allowing us to reconcile the patterns we observe with the ‘shoulders of giants’ view. We find that the observed declines are unlikely to be driven by changes in the quality of published science, citation practices or field-specific factors. Overall, our results suggest that slowing rates of disruption may reflect a fundamental shift in the nature of science and technology.
Similar content being viewed by others
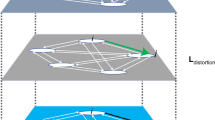
Leading countries in global science increasingly receive more citations than other countries doing similar research
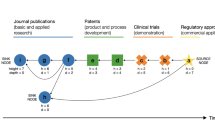
Dynamics of technology emergence in innovation networks
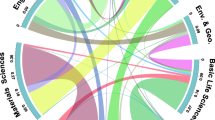
Interdisciplinarity revisited: evidence for research impact and dynamism
Although the past century witnessed an unprecedented expansion of scientific and technological knowledge, there are concerns that innovative activity is slowing 18 , 19 , 20 . Studies document declining research productivity in semiconductors, pharmaceuticals and other fields 10 , 11 . Papers, patents and even grant applications have become less novel relative to prior work and less likely to connect disparate areas of knowledge, both of which are precursors of innovation 21 , 22 . The gap between the year of discovery and the awarding of a Nobel Prize has also increased 23 , 24 , suggesting that today’s contributions do not measure up to the past. These trends have attracted increasing attention from policymakers, as they pose substantial threats to economic growth, human health and wellbeing, and national security, along with global efforts to combat grand challenges such as climate change 25 , 26 .
Numerous explanations for this slowdown have been proposed. Some point to a dearth of ‘low-hanging fruit’ as the readily available productivity-enhancing innovations have already been made 19 , 27 . Others emphasize the increasing burden of knowledge; scientists and inventors require ever more training to reach the frontiers of their fields, leaving less time to push those frontiers forward 18 , 28 . Yet much remains unknown, not merely about the causes of slowing innovative activity, but also the depth and breadth of the phenomenon. The decline is difficult to reconcile with centuries of observation by philosophers of science, who characterize the growth of knowledge as an endogenous process, wherein previous knowledge enables future discovery, a view captured famously in Newton’s observation that if he had seen further, it was by ‘standing on the shoulders of giants’ 3 . Moreover, to date, the evidence pointing to a slowdown is based on studies of particular fields, using disparate and domain-specific metrics 10 , 11 , making it difficult to know whether the changes are happening at similar rates across areas of science and technology. Little is also known about whether the patterns seen in aggregate indicators mask differences in the degree to which individual works push the frontier.
We address these gaps in understanding by analysing 25 million papers (1945–2010) in the Web of Science (WoS) ( Methods ) and 3.9 million patents (1976–2010) in the United States Patent and Trademark Office’s (USPTO) Patents View database ( Methods ). The WoS data include 390 million citations, 25 million paper titles and 13 million abstracts. The Patents View data include 35 million citations, 3.9 million patent titles and 3.9 million abstracts. Subsequently, we replicate our core findings on four additional datasets—JSTOR, the American Physical Society corpus, Microsoft Academic Graph and PubMed—encompassing 20 million papers. Using these data, we join a new citation-based measure 12 with textual analyses of titles and abstracts to understand whether papers and patents forge new directions over time and across fields.
Measurement of disruptiveness
To characterize the nature of innovation, we draw on foundational theories of scientific and technological change 2 , 29 , 30 , which distinguish between two types of breakthroughs. First, some contributions improve existing streams of knowledge, and therefore consolidate the status quo. Kohn and Sham (1965) 31 , a Nobel-winning paper used established theorems to develop a method for calculating the structure of electrons, which cemented the value of previous research. Second, some contributions disrupt existing knowledge, rendering it obsolete, and propelling science and technology in new directions. Watson and Crick (1953) 32 , also a Nobel winner, introduced a model of the structure of DNA that superseded previous approaches (for example, Pauling’s triple helix). Kohn and Sham and Watson and Crick were both important, but their implications for scientific and technological change were different.
We quantify this distinction using a measure—the CD index 12 —that characterizes the consolidating or disruptive nature of science and technology (Fig. 1 ). The intuition is that if a paper or patent is disruptive, the subsequent work that cites it is less likely to also cite its predecessors; for future researchers, the ideas that went into its production are less relevant (for example, Pauling’s triple helix). If a paper or patent is consolidating, subsequent work that cites it is also more likely to cite its predecessors; for future researchers, the knowledge upon which the work builds is still (and perhaps more) relevant (for example, the theorems Kohn and Sham used). The CD index ranges from −1 (consolidating) to 1 (disruptive). We measure the CD index five years after the year of each paper’s publication (indicated by CD 5 , see Extended Data Fig. 1 for the distribution of CD 5 among papers and patents and Extended Data Fig. 2 for analyses using alternative windows) 33 . For example, Watson and Crick and Kohn and Sham both received over a hundred citations within five years of being published. However, the Kohn and Sham paper has a CD 5 of −0.22 (indicating consolidation), whereas the Watson and Crick paper has a CD 5 of 0.62 (indicating disruption). The CD index has been validated extensively in previous research, including through correlation with expert assessments 12 , 34 .
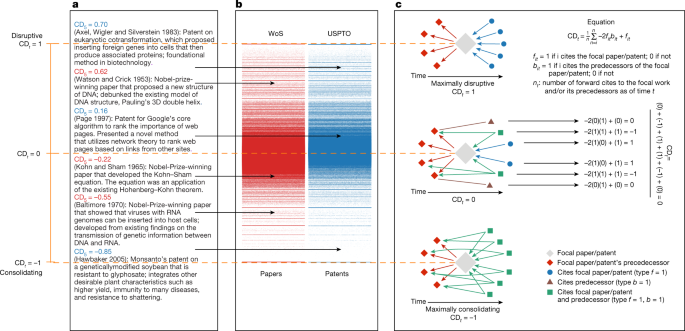
This figure shows a schematic visualization of the CD index. a , CD index value of three Nobel Prize-winning papers 31 , 32 , 58 and three notable patents 59 , 60 , 61 in our sample, measured as of five years post-publication (indicated by CD5). b , Distribution of CD 5 for papers from WoS ( n = 24,659,076) between 1945 and 2010 and patents from Patents View ( n = 3,912,353) between 1976 and 2010, where a single dot represents a paper or patent. The vertical (up–down) dimension of each ‘strip’ corresponds to values of the CD index (with axis values shown in orange on the left). The horizontal (left–right) dimension of each strip helps to minimize overlapping points. Darker areas on each strip plot indicate denser regions of the distribution (that is, more commonly observed CD 5 values). Additional details on the distribution of the CD index are given in Extended Data Fig. 1 . c , Three hypothetical citation networks, where the CD index is at the maximally disruptive value (CD t = 1), midpoint value (CD t = 0), and maximally consolidating value (CD t = −1). The panel also provides the equation for the CD index and an illustrative calculation.
Declining disruptiveness
Across fields, we find that science and technology are becoming less disruptive. Figure 2 plots the average CD 5 over time for papers (Fig. 2a ) and patents (Fig. 2b ). For papers, the decrease between 1945 and 2010 ranges from 91.9% (where the average CD 5 dropped from 0.52 in 1945 to 0.04 in 2010 for ‘social sciences’) to 100% (where the average CD 5 decreased from 0.36 in 1945 to 0 in 2010 for ‘physical sciences’); for patents, the decrease between 1980 and 2010 ranges from 78.7% (where the average CD 5 decreased from 0.30 in 1980 to 0.06 in 2010 for ‘computers and communications’) to 91.5% (where the average CD 5 decreased from 0.38 in 1980 to 0.03 in 2010 for ‘drugs and medical’). For both papers and patents, the rates of decline are greatest in the earlier parts of the time series, and for patents, they appear to begin stabilizing between the years 2000 and 2005. For papers, since about 1980, the rate of decline has been more modest in ‘life sciences and biomedicine’ and physical sciences, and most marked and persistent in social sciences and ‘technology’. Overall, however, relative to earlier eras, recent papers and patents do less to push science and technology in new directions. The general similarity in trends we observe across fields is noteworthy in light of ‘low-hanging fruit’ theories 19 , 27 , which would probably predict greater heterogeneity in the decline, as it seems unlikely fields would ‘consume’ their low-hanging fruit at similar rates or times.
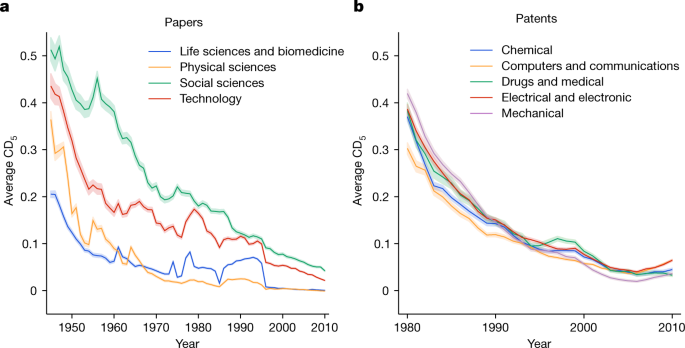
a , b , Decline in CD 5 over time, separately for papers ( a , n = 24,659,076) and patents ( b , n = 3,912,353). For papers, lines correspond to WoS research areas; from 1945 to 2010 the magnitude of decline ranges from 91.9% (social sciences) to 100% (physical sciences). For patents, lines correspond to National Bureau of Economic Research (NBER) technology categories; from 1980 to 2010 the magnitude of decline ranges from 93.5% (computers and communications) to 96.4% (drugs and medical). Shaded bands correspond to 95% confidence intervals. As we elaborate in the Methods , this pattern of decline is robust to adjustment for confounding from changes in publication, citation and authorship practices over time.
Source data
Linguistic change.
The decline in disruptive science and technology is also observable using alternative indicators. Because they create departures from the status quo, disruptive papers and patents are likely to introduce new words (for example, words used to create a new paradigm might differ from those that are used to develop an existing paradigm) 35 , 36 . Therefore, if disruptiveness is declining, we would expect a decline in the diversity of words used in science and technology. To evaluate this, Fig. 3a , d documents the type-token ratio (that is, unique/total words) of paper and patent titles over time (Supplementary Information section 1 ). We observe substantial declines, especially in the earlier periods, before 1970 for papers and 1990 for patents. For paper titles (Fig. 3a ), the decrease (1945–2010) ranges from 76.5% (social sciences) to 88% (technology); for patent titles (Fig. 3d ), the decrease (1980–2010) ranges from 32.5% (chemical) to 81% (computers and communications). For paper abstracts (Extended Data Fig. 3a ), the decrease (1992–2010) ranges from 23.1% (life sciences and biomedicine) to 38.9% (social sciences); for patent abstracts (Extended Data Fig. 3b ), the decrease (1980–2010) ranges from 21.5% (mechanical) to 73.2% (computers and communications). In Fig. 3b , e , we demonstrate that these declines in word diversity are accompanied by similar declines in combinatorial novelty; over time, the particular words that scientists and inventors use in the titles of their papers and patents are increasingly likely to have been used together in the titles of previous work. Consistent with these trends in language, we also observe declining novelty in the combinations of previous work cited by papers and patents, based on a previously established measure of ‘atypical combinations’ 14 (Extended Data Fig. 4 ).
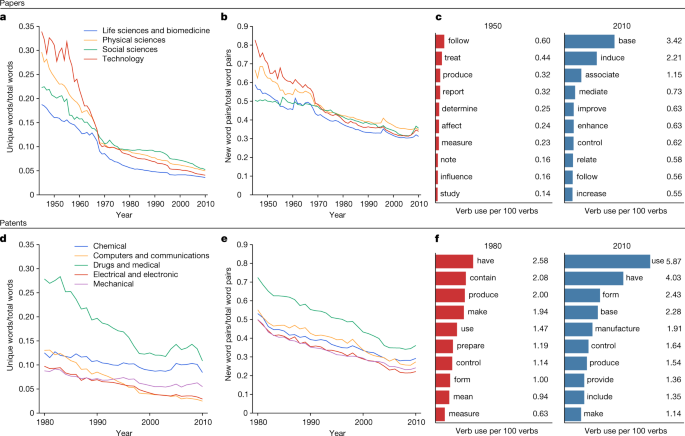
a , d , Figures showing a decline in the diversity of language used in science and technology based on the unique/total words of paper titles from 1945 to 2010 ( a , n = 24,659,076) and of patent titles from 1980 to 2010 ( d , n = 3,912,353). b , e , Figures showing a decline in the novelty of language used in science and technology based on the number of new word pairs/total word pairs introduced each year in WoS paper titles from 1945 to 2010 ( b ) and in Patents View patent titles from 1980 to 2010 (refs. 1 , 17 ) ( e ). For papers in both a and b , lines correspond to WoS research areas ( n = 264 WoS research area × year observations). For patents in both d and e , lines correspond to NBER technology categories ( n = 229 NBER technology category × year observations). c , f , Figures showing the frequency of the most commonly used verbs in paper titles for the first (red) and last (blue) decades of the observation period in paper ( c , n = 24,659,076) and patent ( f , n = 3,912,353) titles.
The decline in disruptive activity is also apparent in the specific words used by scientists and inventors. If disruptiveness is declining, we reasoned that verbs alluding to the creation, discovery or perception of new things should be used less frequently over time, whereas verbs alluding to the improvement, application or assessment of existing things may be used more often 35 , 36 . Figure 3 shows the most common verbs in paper (Fig. 3c ) and patent titles (Fig. 3f ) in the first and last decade of each sample (Supplementary Information section 2 ). Although precisely and quantitatively characterizing words as ‘consolidating’ or ‘disruptive’ is challenging in the absence of context, the figure highlights a clear and qualitative shift in language. In the earlier decades, verbs evoking creation (for example, ‘produce’, ‘form’, ‘prepare’ and ‘make’), discovery (for example, ‘determine’ and ‘report’) and perception (for example, ‘measure’) are prevalent in both paper and patent titles. In the later decades, however, these verbs are almost completely displaced by those tending to be more evocative of the improvement (for example, ‘improve’, ‘enhance’ and ‘increase’), application (for example, ‘use’ and ‘include’) or assessment (for example, ‘associate’, ‘mediate’ and ‘relate’) of existing scientific and technological knowledge and artefacts. Taken together, these patterns suggest a substantive shift in science and technology over time, with discovery and invention becoming less disruptive in nature, consistent with our results using the CD index.
Conservation of highly disruptive work
The aggregate trends we document mask considerable heterogeneity in the disruptiveness of individual papers and patents and remarkable stability in the absolute number of highly disruptive works ( Methods and Fig. 4 ). Specifically, despite large increases in scientific productivity, the number of papers and patents with CD 5 values in the far right tail of the distribution remains nearly constant over time. This ‘conservation’ of the absolute number of highly disruptive papers and patents holds despite considerable churn in the underlying fields responsible for producing those works (Extended Data Fig. 5 , inset). These results suggest that the persistence of major breakthroughs—for example, measurement of gravity waves and COVID-19 vaccines—is not inconsistent with slowing innovative activity. In short, declining aggregate disruptiveness does not preclude individual highly disruptive works.
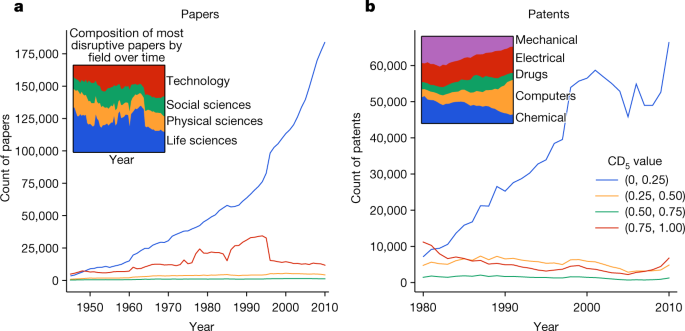
This figure shows the number of disruptive papers ( a , n = 5,030,179) and patents ( b , n = 1,476,004) across four different ranges of CD 5 (papers and patents with CD 5 values in the range [−1.0, 0) are not represented in the figure). Lines correspond to different levels of disruptiveness as measured by CD 5 . Despite substantial increases in the numbers of papers and patents published each year, there is little change in the number of highly disruptive papers and patents, as evidenced by the relatively flat red, green and orange lines. This pattern helps to account for simultaneous observations of both aggregate evidence of slowing innovative activity and seemingly major breakthroughs in many fields of science and technology. The inset plots show the composition of the most disruptive papers and patents (defined as those with CD 5 values >0.25) by field over time. The observed stability in the absolute number of highly disruptive papers and patents holds despite considerable churn in the underlying fields of science and technology responsible for producing those works. ‘Life sciences’ denotes the life sciences and biomedicine research area; ‘electrical’ denotes the electrical and electronic technology category; ‘drugs’ denotes the drugs and medical technology category; and ‘computers’ denotes the computers and communications technology category.
Alternative explanations
What is driving the decline in disruptiveness? Earlier, we suggested our results are not consistent with explanations that link slowing innovative activity to diminishing ‘low-hanging fruit’. Extended Data Fig. 5 shows that the decline in disruptiveness is unlikely to be due to other field-specific factors by decomposing variation in CD 5 attributable to field, author and year effects ( Methods ).
Declining rates of disruptive activity are unlikely to be caused by the diminishing quality of science and technology 22 , 37 . If they were, then the patterns seen in Fig. 2 should be less visible in high-quality work. However, when we restrict our sample to articles published in premier publication venues such as Nature , Proceedings of the National Academy of Sciences and Science or to Nobel-winning discoveries 38 (Fig. 5 ), the downward trend persists.
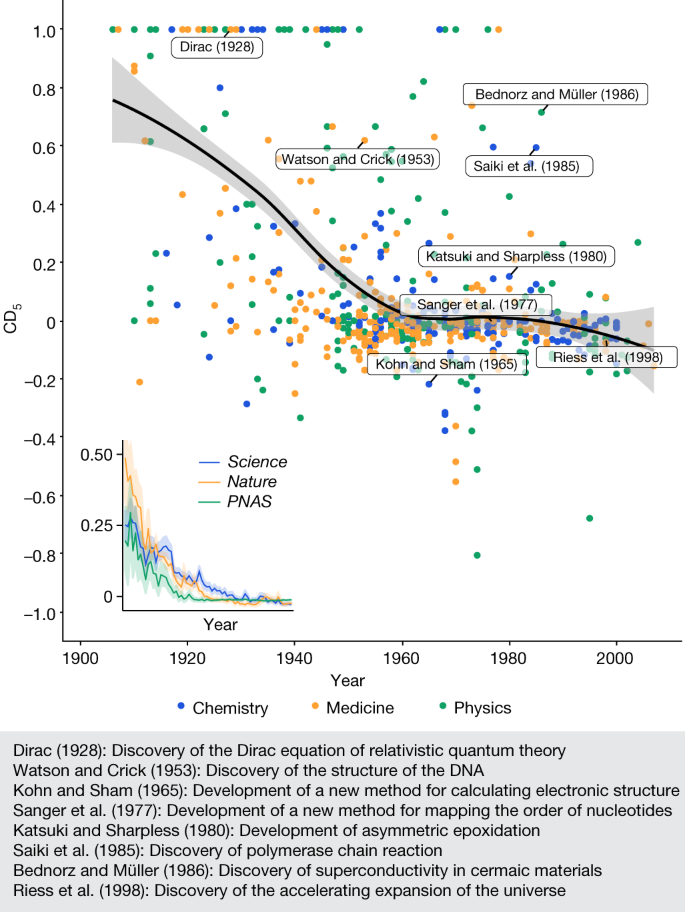
This figure shows changes in CD 5 over time for papers published in Nature , Proceedings of the National Academy of Sciences ( PNAS ) and Science (inset plot, n = 223,745) and Nobel Prize-winning papers (main plot, n = 635), with several notable examples 31 , 32 , 58 , 62 , 63 , 64 , 65 , 66 highlighted. Colours indicate the three different journals in the inset plot; colours indicate the three different fields in which the Nobel Prize is awarded in the main plot. Shaded bands correspond to 95% confidence intervals. For historical completeness, we plot CD index scores for all Nobel papers back to 1900 (the first year in which the prize was awarded); however, our main analyses begin in the post-1945 era, when the WoS data are generally more reliable. The figure indicates that changes in the quality of published science over time is unlikely to be responsible for the decline in disruption.
Furthermore, the trend is not driven by characteristics of the WoS and UPSTO data or our particular derivation of the CD index; we observe similar declines in disruptiveness when we compute CD 5 on papers in JSTOR, the American Physical Society corpus, Microsoft Academic Graph and PubMed ( Methods ), the results of which are shown in Extended Data Fig. 6 . We further show that the decline is not an artefact of the CD index by reporting similar patterns using alternative derivations 13 , 15 ( Methods and Extended Data Fig. 7 ).
Declines in disruptiveness are also not attributable to changing publication, citation or authorship practices ( Methods ). First, using approaches from the bibliometrics literature 39 , 40 , 41 , 42 , 43 , we computed several normalized versions of the CD index that adjusted for the increasing tendency for papers and patents to cite previous work 44 , 45 . Results using these alternative indicators (Extended Data Fig. 8a , d ) were similar to those we reported in Fig. 2 . Second, using regression, we estimated models of CD 5 as a function of indicator variables for each paper or patent’s publication year, along with specific controls for field × year level—number of new papers/patents, mean number of papers/patents cited, mean number of authors or inventors per paper—and paper or patent-level—number of papers or patents cited—factors. Predictions from these models indicated a decline in disruptive papers and patents (Extended Data Fig. 8b , e and Supplementary Table 1 ) that was consistent with our main results. Finally, using Monte Carlo simulations, we randomly rewired the observed citation networks while preserving key characteristics of scientists’ and inventors’ citation behaviour, including the number of citations made and received by individual papers and patents and the age gap between citing and cited works. We find that observed CD 5 values are lower than those from the simulated networks (Extended Data Fig. 8 c, f ), and the gap is widening: over time, papers and patents are increasingly less disruptive than would be expected by chance. Taken together, these additional analyses indicate that the decline in CD 5 is unlikely to be driven by changing publication, citation or authorship practices.
Growth of knowledge and disruptiveness
We also considered how declining disruptiveness relates to the growth of knowledge (Extended Data Fig. 9 ). On the one hand, scientists and inventors face an increasing knowledge burden, which may inhibit discoveries and inventions that disrupt the status quo. On the other hand, as previously noted, philosophers of science suggest that existing knowledge fosters discovery and invention 3 , 6 , 7 . Using regression models, we evaluated the relationship between the stock of papers and patents (a proxy for knowledge) within fields and their CD 5 (Supplementary Information section 3 and Supplementary Table 2 ). We find a positive effect of the growth of knowledge on disruptiveness for papers, consistent with previous work 20 ; however, we find a negative effect for patents.
Given these conflicting results, we considered the possibility that the availability of knowledge may differ from its use. In particular, the growth in publishing and patenting may lead scientists and inventors to focus on narrower slices of previous work 18 , 46 , thereby limiting the ‘effective’ stock of knowledge. Using three proxies, we document a decline in the use of previous knowledge among scientists and inventors (Fig. 6 ). First, we see a decline in the diversity of work cited (Fig. 6a,d ), indicating that contemporary science and technology are engaging with narrower slices of existing knowledge. Moreover, this decline in diversity is accompanied by an increase in the share of citations to the 1% most highly cited papers and patents (Fig. 6a (i) , d(i) ), which are also decreasing in semantic diversity (Fig. 6a (ii) , d (ii) ). Over time, scientists and inventors are increasingly citing the same previous work, and that previous work is becoming more topically similar. Second, we see an increase in self-citation (Fig. 6b,e ), a common proxy for the continuation of one’s pre-existing research stream 47 , 48 , 49 , which is consistent with scientists and inventors relying more on highly familiar knowledge. Third, the mean age of work cited, a common measure for the use of dated knowledge 50 , 51 , 52 , is increasing (Fig. 6c,f ), suggesting that scientists and inventors may be struggling to keep up with the pace of knowledge expansion and instead relying on older, familiar work. All three indicators point to a consistent story: a narrower scope of existing knowledge is informing contemporary discovery and invention.
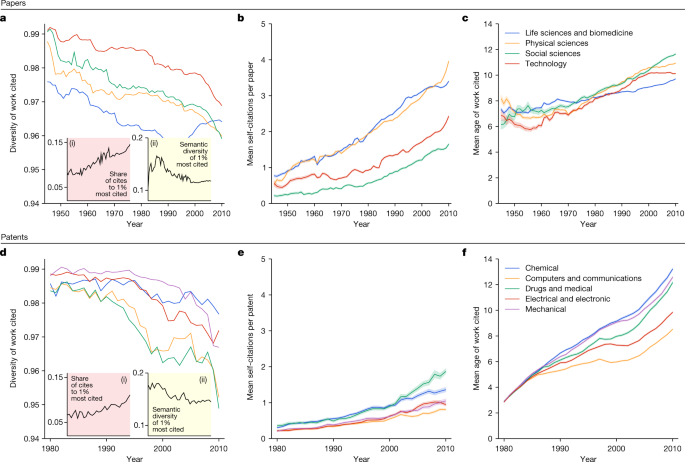
a – f , Changes in the level of diversity of existing scientific and technological knowledge use among papers ( a , n = 264 WoS research area × year observations; b and c , n = 24,659,076 papers) and patents ( d , 229 NBER technology category × year observations; e and f , n = 3,912,353 patents) based on following measures: diversity of work cited ( a and d ), mean number of self-citations ( b and e ) and mean age of cited work ( c and f ). Shaded bands ( b , c , e and f ) correspond to 95% confidence intervals. The inset plots of a and d show changes in the share of citations to the top 1% most highly cited papers ( a (i) and d (i)) and in the semantic diversity of the top 1% most cited over time ( a (ii) and d (ii)). Values of both measures are computed within field and year, and are subsequently averaged across fields for plotting. Semantic diversity is based on paper and patent titles; values correspond to the ratio of the standard deviation to the mean pairwise cosine similarity (that is, the coefficient of variation) among the titles of the 1% most cited papers and patents by field and year. To enable semantic comparisons, titles were vectorized using pretrained word embeddings. For papers, lines are shown for each WoS research area; for patents, lines are shown for each NBER technology category. In subsequent regression analyses using these measures, we find that using less diverse work, more of one’s own work and older work is associated with less disruptive papers and patents ( Methods and Extended Data Table 1 ).
Results from a subsequent series of regression models suggest that use of less diverse work, more of one’s own work and older work are all negatively associated with disruption ( Methods , Extended Data Table 1 and Supplementary Table 3 ), a pattern that holds even after accounting for the average age and number of previous works produced by team members. When the range of work used by scientists and inventors narrows, disruptive activity declines.
In summary, we report a marked decline in disruptive science and technology over time. Our analyses show that this trend is unlikely to be driven by changes in citation practices or the quality of published work. Rather, the decline represents a substantive shift in science and technology, one that reinforces concerns about slowing innovative activity. We attribute this trend in part to scientists’ and inventors’ reliance on a narrower set of existing knowledge. Even though philosophers of science may be correct that the growth of knowledge is an endogenous process—wherein accumulated understanding promotes future discovery and invention—engagement with a broad range of extant knowledge is necessary for that process to play out, a requirement that appears more difficult with time. Relying on narrower slices of knowledge benefits individual careers 53 , but not scientific progress more generally.
Moreover, even though the prevalence of disruptive works has declined, we find that the sheer number has remained stable. On the one hand, this result may suggest that there is a fixed ‘carrying capacity’ for highly disruptive science and technology, in which case, policy interventions aimed at increasing such work may prove challenging. On the other hand, our observation of considerable churn in the underlying fields responsible for producing disruptive science and technology suggests the potential importance of factors such as the shifting interests of funders and scientists and the ‘ripeness’ of scientific and technologicalknowledge for breakthroughs, in which case the production of disruptive work may be responsive to policy levers. In either case, the stability we observe in the sheer number of disruptive papers and patents suggests that science and technology do not appear to have reached the end of the ‘endless frontier’. Room remains for the regular rerouting that disruptive works contribute to scientific and technological progress.
Our study is not without limitations. Notably, even though research to date supports the validity of the CD index 12 , 34 , it is a relatively new indicator of innovative activity and will benefit from future work on its behaviour and properties, especially across data sources and contexts. Studies that systematically examine the effect of different citation practices 54 , 55 , which vary across fields, would be particularly informative.
Overall, our results deepen understanding of the evolution of knowledge and may guide career planning and science policy. To promote disruptive science and technology, scholars may be encouraged to read widely and given time to keep up with the rapidly expanding knowledge frontier. Universities may forgo the focus on quantity, and more strongly reward research quality 56 , and perhaps more fully subsidize year-long sabbaticals. Federal agencies may invest in the riskier and longer-term individual awards that support careers and not simply specific projects 57 , giving scholars the gift of time needed to step outside the fray, inoculate themselves from the publish or perish culture, and produce truly consequential work. Understanding the decline in disruptive science and technology more fully permits a much-needed rethinking of strategies for organizing the production of science and technology in the future.
We limit our focus to research papers published between 1945 and 2010. Although the WoS data begin in the year 1900, the scale and social organization of science shifted markedly in the post-war era, thereby making comparisons with the present difficult and potentially misleading 67 , 68 , 69 . We end our analyses of papers in 2010 because some of our measures require several subsequent years of data following paper publication. The WoS data archive 65 million documents published in 28,968 journals between 1900 and 2017 and 735 million citations among them. In addition, the WoS data include the titles and the full text of abstracts for 65 and 29 million records, respectively, published between 1913 and 2017. After eliminating non-research documents (for example, book reviews and commentaries) and subsetting the data to the 1945–2010 window, the analytical sample consists of n = 24,659,076 papers.
Patents View data
We limit our focus to patents granted from 1976, which is the earliest year for which machine-readable records are available in the Patents View data. As we did with papers, we end our analyses in 2010 because some measures require data from subsequent years for calculation. The Patents View data are the most exhaustive source of historical data on inventions, with information on 6.5 million patents granted between 1976 and 2017 and their corresponding 92 million citations. The Patents View data include the titles and abstracts for 6.5 million patents granted between 1976 and 2017. Following previous work 12 , we focused our attention on utility patents, which cover the vast majority (91% in our data) of patented inventions. After eliminating non-utility patents and subsetting the data to the 1976–2010 window, the analytical sample consists of n = 3,912,353 patents.
Highly disruptive papers and patents
Observations (and claims) of slowing progress in science and technology are increasingly common, supported not only by the evidence we report, but also by previous research from diverse methodological and disciplinary perspectives 10 , 11 , 18 , 19 , 20 , 21 , 22 , 23 , 24 . Yet as noted in the main text, there is a tension between observations of slowing progress from aggregate data on the one hand, and continuing reports of seemingly major breakthroughs in many fields of science and technology—spanning everything from the measurement of gravity waves to the sequencing of the human genome—on the other. In an effort to reconcile this tension, we considered the possibility that whereas overall, discovery and invention may be less disruptive over time, the high-level view taken in previous work may mask considerable heterogeneity. Put differently, aggregate evidence of slowing progress does not preclude the possibility that some subset of discoveries and inventions is highly disruptive.
To evaluate this possibility, we plot the number of disruptive papers (Fig. 4a ) and patents (Fig. 4b ) over time, where disruptive papers and patents are defined as those with CD 5 values >0. Within each panel, we plot four lines, corresponding to four evenly spaced intervals—(0, 0.25], (0.25, 0.5], (0.5, 0.75], (0.75, 1.00]—over the positive values of CD 5 . The first two intervals therefore correspond to papers and patents that are relatively weakly disruptive, whereas the latter two correspond to those that are more strongly so (for example, where we may expect to see major breakthroughs such as some of those mentioned above). Despite major increases in the numbers of papers and patents published each year, we see little change in the number of highly disruptive papers and patents, as evidenced by the relatively flat red, green and orange lines. Notably, this ‘conservation’ of disruptive work holds even despite fluctuations over time in the composition of the scientific and technological fields responsible for producing the most disruptive work (Fig. 4 , inset plots). Overall, these results help to account for simultaneous observations of both major breakthroughs in many fields of science and technology and aggregate evidence of slowing progress.
Relative contribution of field, year and author or inventor effects
Our results show a steady decline in the disruptiveness of science and technology over time. Moreover, the patterns we observe are generally similar across broad fields of study, which suggests that the factors driving the decline are not unique to specific domains of science and technology. The decline could be driven by other factors, such as the conditions of science and technology at a point in time or the particular individuals who produce science and technology. For example, exogenous factors such as economic conditions may encourage research or invention practices that are less disruptive. Similarly, scientists and inventors of different generations may have different approaches, which may result in greater or lesser tendencies for producing disruptive work. We therefore sought to understand the relative contribution of field, year and author (or inventor) factors to the decline of disruptive science and technology.
To do so, we decomposed the relative contribution of field, year and author fixed effects to the predictive power of regression models of the CD index. The unit of observation in these regressions is the author (or inventor) × year. We enter field fixed effects using granular subfield indicators (that is, 150 WoS subject areas for papers, 138 NBER subcategories for patents). For simplicity, we did not include additional covariates beyond the fixed effects in our models. Field fixed effects capture all field-specific factors that do not vary by author or year (for example, the basic subject matter); year fixed effects capture all year-specific factors that do not vary by field or author (for example, the state of communication technology); author (or inventor) fixed effects capture all author-specific factors that do not vary by field or year (for example, the year of PhD awarding). After specifying our model, we determine the relative contribution of field, year and author fixed effects to the overall model adjusted R 2 using Shapley–Owen decomposition. Specifically, given our n = 3 groups of fixed effects (field, year and author) we evaluate the relative contribution of each set of fixed effects by estimating the adjusted R 2 separately for the 2 n models using subsets of the predictors. The relative contribution of each set of fixed effects is then computed using the Shapley value from game theory 70 .
Results of this analysis are shown in Extended Data Fig. 5 , for both papers (top bar) and patents (bottom bar). Total bar size corresponds to the value of the adjusted R 2 for the fully specified model (that is, with all three groups of fixed effects). Consistent with our observations from plots of the CD index over time, we observe that for both papers and patents, field-specific factors make the lowest relative contribution to the adjusted R 2 (0.02 and 0.01 for papers and patents, respectively). Author fixed effects, by contrast, appear to contribute much more to the predictive power of the model, for both papers (0.20) and patents (0.17). Researchers and inventors who entered the field in more recent years may face a higher burden of knowledge and thus resort to building on narrower slices of existing work (for example, because of more specialized doctoral training), which would generally lead to less disruptive science and technology being produced in later years, consistent with our findings. The pattern is more complex for year fixed effects; although year-specific factors that do not vary by field or author hold more explanatory power than field for both papers (0.02) and patents (0.16), they appear to be substantially more important for the latter than the former. Taken together, these findings suggest that relatively stable factors that vary across individual scientists and inventors may be particularly important for understanding changes in disruptiveness over time. The results also confirm that domain-specific factors across fields of science and technology play a very small role in explaining the decline in disruptiveness of papers and patents.
Alternative samples
We also considered whether the patterns we document may be artefacts of our choice of data sources. Although we observe consistent trends in both the WoS and Patents View data, and both databases are widely used by the Science of Science community, our results may conceivably be driven by factors such as changes in coverage (for example, journals added or excluded from WoS over time) or even data errors rather than fundamental changes in science and technology. To evaluate this possibility, we therefore calculated CD 5 for papers in four additional databases—JSTOR, the American Physical Society corpus, Microsoft Academic Graph and PubMed. We included all records from 1930 to 2010 from PubMed (16,774,282 papers), JSTOR (1,703,353 papers) and American Physical Society (478,373 papers). The JSTOR data were obtained via a special request from ITHAKA, the data maintainer ( http://www.ithaka.org ), as were the American Physical Society data ( https://journals.aps.org/datasets ). We downloaded the Microsoft Academic Graph data from CADRE at Indiana University ( https://cadre.iu.edu/ ). The PubMed data were downloaded from the National Library of Medicine FTP server ( ftp://ftp.ncbi.nlm.nih.gov/pubmed/baseline ). Owing to the exceptionally large scale of Microsoft Academic Graph and the associated computational burden, we randomly extracted 1 million papers. As shown in Extended Data Fig. 6 , the downward trend in disruptiveness is evident across all samples.
Alternative bibliometric measures
Several recent papers have introduced alternative specifications of the CD index 12 . We evaluated whether the declines in disruptiveness we observe are corroborated using two alternative variations. One criticism of the CD index has been that the number of papers that cite only the focal paper’s references dominates the measure 13 . Bornmann et al. 13 proposes \({{\rm{DI}}}_{l}^{{\rm{nok}}}\) as a variant that is less susceptible to this issue. Another potential weakness of the CD index is that it could be very sensitive to small changes in the forward citation patterns of papers that make no backward citations 15 . Leydesdorff et al. 15 suggests DI* as an alternate indicator of disruption that addresses this issue. Therefore, we calculated \({{\rm{DI}}}_{l}^{{\rm{nok}}}\) where l = 5 and DI* for 100,000 randomly drawn papers and patents each from our analytic sample. Results are presented in Extended Data Fig. 7a (papers) and b (patents). The blue lines indicate disruption based on Bornmann et al. 13 and the orange lines indicate disruption based on Leydesdorff et al. 15 . Across science and technology, the two alternative measures both show declines in disruption over time, similar to the patterns observed with the CD index. Taken together, these results suggest that the declines in disruption we document are not an artefact of our particular operationalization.
Robustness to changes in publication, citation and authorship practices
We also considered whether our results may be attributable to changes in publication, citation or authorship practices, rather than by substantive shifts in discovery and invention. Perhaps most critically, as noted in the main text, there has been a marked expansion in publishing and patenting over the period of our study. This expansion has naturally increased the amount of previous work that is relevant to current science and technology and therefore at risk of being cited, a pattern reflected in the marked increase in the average number of citations made by papers and patents (that is, papers and patents are citing more previous work than in previous eras) 44 , 45 . Recall that the CD index quantifies the degree to which future work cites a focal work together with its predecessors (that is, the references in the bibliography of the focal work). Greater citation of a focal work independently of its predecessors is taken to be evidence of a social process of disruption. As papers and patents cite more previous work, however, the probability of a focal work being cited independently of its predecessors may decline mechanically; the more citations a focal work makes, the more likely future work is to cite it together with one of its predecessors, even by chance. Consequently, increases in the number of papers and patents available for citing and in the average number of citations made by scientists and inventors may contribute to the declining values of the CD index. In short, given the marked changes in science and technology over our long study window, the CD index of papers and patents published in earlier periods may not be directly comparable to those of more recent vintage, which could in turn render our conclusions about the decline in disruptive science and technology suspect. We addressed these concerns using three distinctive but complementary approaches—normalization, regression adjustment and simulation.
Verification using normalization
First, following common practice in bibliometric research 39 , 40 , 41 , 42 , 43 , we developed two normalized versions of the CD index, with the goal of facilitating comparisons across time. Among the various components of the CD index, we focused our attention on the count of papers or patents that only cite the focal work’s references ( N k ), as this term would seem most likely to scale with the increases in publishing and patenting and in the average number of citations made by papers and patents to previous work 13 . Larger values of N k lead to smaller values of the CD index. Consequently, marked increases in N k over time, particularly relative to other components of the measure, may lead to a downward bias, thereby inhibiting our ability to accurately compare disruptive science and technology in later years with earlier periods.
Our two normalized versions of the CD index aim to address this potential bias by attenuating the effect of increases in N k . In the first version, which we call ‘Paper normalized’, we subtract from N k the number of citations made by the focal paper or patent to previous work ( N b ). The intuition behind this adjustment is that when a focal paper or patent cites more previous work, N k is likely to be larger because there are more opportunities for future work to cite the focal paper or patent’s predecessors. This increase in N k would result in lower values of the CD index, although not necessarily as a result of the focal paper or patent being less disruptive. In the second version, which we call ‘field × year normalized’, we subtract N k by the average number of backward citations made by papers or patents in the focal paper or patent’s WoS research area or NBER technology category, respectively, during its year of publication (we label this quantity \({N}_{{\rm{b}}}^{{\rm{m}}{\rm{e}}{\rm{a}}{\rm{n}}}\) ). The intuition behind this adjustment is that in fields and time periods in which there is a greater tendency for scientists and inventors to cite previous work, N k is also likely to be larger, thereby leading to lower values of the CD index, although again not necessarily as a result of the focal paper or patent being less disruptive. In cases in which either N b or \({N}_{{\rm{b}}}^{{\rm{m}}{\rm{e}}{\rm{a}}{\rm{n}}}\) exceed the value of N k , we set N k to 0 (that is, N k is never negative in the normalized measures). Both adaptations of the CD index are inspired by established approaches in the scientometrics literature, and may be understood as a form of ‘citing side normalization’ (that is, normalization by correcting for the effect of differences in lengths of references lists) 40 .
In Extended Data Fig. 8 , we plot the average values of both normalized versions of the CD index over time, separately for papers (Extended Data Fig. 8a ) and patents (Extended Data Fig. 8d ). Consistent with our findings reported in the main text, we continue to observe a decline in the CD index over time, suggesting that the patterns we observe in disruptive science and technology are unlikely to be driven by changes in citation practices.
Verification using regression adjustment
Second, we adjusted for potential confounding using a regression-based approach. This approach complements the bibliometric normalizations just described by allowing us to account for a broader array of changes in publication, citation and authorship practices in general (the latter of which is not directly accounted for in either the normalization approach or the simulation approach described next), and increases the amount of previous work that is relevant to current science and technology in particular. In Supplementary Table 1 , we report the results of regression models predicting CD 5 for papers (Models 1–4) and patents (Models 5–8), with indicator variables included for each year of our study window (the reference categories are 1945 and 1980 for papers and patents, respectively). Models 1 and 4 are the baseline models, and include no other adjustments beyond the year indicators. In Models 2 and 5, we add subfield fixed effects (WoS subject areas for papers and NBER technology subcategories for patents). Finally, in Models 3–4 and 7–8, we add control variables for several field × year level—number of new papers orpatents, mean number of papers or patents cited, mean number of authors or inventors per paper—and paper- or patent-level—number of papers or patents cited—characteristics, thereby enabling more robust comparisons in patterns of disruptive science and technology over the long time period spanned by our study. For the paper models, we also include a paper-level control for the number of unlinked references (that is, the number of citations to works that are not indexed in WoS). We find that the inclusion of these controls improves model fit, as indicated by statistically significant Wald tests presented below the relevant models.
Across all eight models shown in Supplementary Table 1 , we find that the coefficients on the year indicators are statistically significant and negative, and growing in magnitude over time, which is consistent with the patterns we reported based on unadjusted CD 5 values index in the main text (Fig. 2 ). In Extended Data Fig. 8 , we visualize the results of our regression-based approach by plotting the predicted CD 5 values separately for each of the year indicators included in Models 4 (papers) and 8 (patents). To enable comparisons with raw CD 5 values shown in the main text, we present the separate predictions made for each year as a line graph. As shown in the figure, we continue to observe declining values of the CD index across papers and patents, even when accounting for changes in publication, citation and authorship practices.
Verification using simulation
Third, following related work in the Science of Science 14 , 71 , 72 , 73 , we considered whether our results may be an artefact of changing patterns in publishing and citation practices by using a simulation approach. In essence, the CD index measures disruption by characterizing the network of citations around a focal paper or patent. However, many complex networks, even those resulting from random processes, exhibit structures that yield non-trivial values on common network measures (for example, clustering) 74 , 75 , 76 . During the period spanned by our study, the citation networks of science and technology experienced significant change, with marked increases in both the numbers of nodes (that is, papers or patents) and edges (that is, citations). Thus, rather than reflecting a meaningful social process, the observed declines in disruption may result from these structural changes in the underlying citation networks.
To evaluate this possibility, we followed standard techniques from network science 75 , 77 and conducted an analysis in which we recomputed the CD index on randomly rewired citation networks. If the patterns we observe in the CD index are the result of structural changes in the citation networks of science and technology (for example, growth in the number of nodes or edges) rather than a meaningful social process, then these patterns should also be visible in comparable random networks that experience similar structural changes. Therefore, finding that the patterns we see in the CD index differ for the observed and random citation networks would serve as evidence that the decline in disruption is not an artefact of the data.
We began by creating copies of the underlying citation network on which the values of the CD index used in all analyses reported in the main text were based, separately for papers and patents. For each citation network (one for papers, one for patents), we then rewired citations using a degree-preserving randomization algorithm. In each iteration of the algorithm, two edges (for example, A–B and C–D) are selected from the underlying citation network, after which the algorithm attempts to swap the two endpoints of the edges (for example, A–B becomes A–D, and C–D becomes C–B). If the degree centrality of A, B, C and D remains the same after the swap, the swap is retained; otherwise, the algorithm discards the swap and moves on to the next iteration. When evaluating degree centrality, we consider ‘in-degree’ (that is, citations from other papers or patents to the focal paper or patent) and ‘out-degree’ (that is, citations from the focal paper or patent to other papers or patents) separately. Furthermore, we also required that the age distribution of citing and cited papers or patents was identical in the original and rewired networks. Specifically, swaps were only retained when the publication year of the original and candidate citations was the same. In light of these design choices, our rewiring algorithm should be seen as fairly conservative, as it preserves substantial structure from the original network. There is no scholarly consensus on the number of swaps necessary to ensure the original and rewired networks are sufficiently different from one another; the rule we adopt here is 100 × m , where m is the number of edges in the network being rewired.
Following previous work 14 , we created ten rewired copies of the observed citation networks for both papers and patents. After creating these rewired citation networks, we then recomputed CD 5 . Owing to the large scale of the WoS data, we base our analyses on a random subsample of ten million papers; CD 5 was computed on the rewired network for all patents. For each paper and patent, we then compute a z score that compares the observed CD 5 value to those of the same paper or patent in the ten rewired citation networks. Positive z scores indicate that the observed CD 5 value is greater (that is, more disruptive) than would be expected by chance; negative z scores indicate that the observed values are lesser (that is, more consolidating).
The results of these analyses are shown in Extended Data Fig. 8 , separately for papers (Extended Data Fig. 8c ) and patents (Extended Data Fig. 8f ). Lines correspond to the average z score among papers or patents published in the focal year. The plots reveal a pattern of change in the CD index over and beyond that ‘baked in’ to the changing structure of the network. We find that on average, papers and patents tend to be less disruptive than would be expected by chance, and moreover, the gap between the observed CD index values and those from the randomly rewired networks is increasing over time, which is consistent with our findings of a decline in disruptive science and technology.
Taken together, the results of the foregoing analyses suggest that although there have been marked changes in science and technology over the course of our long study window, particularly with respect to publication, citation and authorship practices, the decline in disruptive science and technology that we document using the CD index is unlikely to be an artefact of these changes, and instead represents a substantive shift in the nature of discovery and invention.
Regression analysis
We evaluate the relationship between disruptiveness and the use of previous knowledge using regression models, predicting CD 5 for individual papers and patents, based on three indicators of previous knowledge use—the diversity of work cited, mean number of self-citations and mean age of work cited. Our measure of the diversity of work cited is measured at the field × year level; all other variables included in the regressions are defined at the level of the paper or patent. To account for potential confounding factors, our models included year and field fixed effects. Year fixed effects account for time variant factors that affect all observations (papers or patents) equally (for example, global economic trends). Field fixed effects account for field-specific factors that do not change over time (for example, some fields may intrinsically value disruptive work over consolidating ones). In contrast to our descriptive plots, for our regression models, we adjust for field effects using the more granular 150 WoS ‘extended subjects’ (for example, ‘biochemistry and molecular biology’, ‘biophysics’, ‘biotechnology and applied microbiology’, ‘cell biology’, ‘developmental biology’, ‘evolutionary biology’ and ‘microbiology’ are extended subjects within the life sciences and biomedicine research area) and 38 NBER technology subcategories (for example, ‘agriculture’, ‘food’, ‘textile’; ‘coating’; ‘gas’; ‘organic’; and ‘resins’ are subcategories within the chemistry technology category).
In addition, we also include controls for the ‘mean age of team members’ (that is, ‘career age’, defined as the difference between the publication year of the focal paper or patent and the first year in which each author or inventor published a paper or patent) and the ‘mean number of previous works produced by team members’. Although increases in rates of self-citations may indicate that scientists and inventors are becoming more narrowly focused on their own work, these rates may also be driven in part by the amount of previous work available for self-citing. Similarly, although increases in the age of work cited in papers and patents may indicate that scientists and inventors are struggling to keep up, they may also be driven by the rapidly aging workforce in science and technology 78 , 79 . For example, older scientists and inventors may be more familiar with or more attentive to older work, or may actively resist change 80 . These control variables help to account for these alternative explanations.
Supplementary Table 3 shows summary statistics for variables used in the ordinary-least-squares regression models. The diversity of work cited is measured by normalized entropy, which ranges from 0 to 1. Greater values on this measure indicate a more uniform distribution of citations to a wider range of existing work; lower values indicate a more concentrated distribution of citations to a smaller range of existing work. The tables show that the normalized entropy in a given field and year has a nearly maximal average entropy of 0.98 for both science and technology. About 16% of papers cited in a paper are by an author of the focal paper; the corresponding number for patents is about 7%. Papers tend to rely on older work and work that varies more greatly in age (measured by standard deviation) than patents. In addition, the average CD 5 of a paper is 0.04 whereas the average CD 5 of a patent is 0.12, meaning that the average paper tends to be less disruptive than the average patent.
We find that using more diverse work, less of one’s own work and older work tends to be associated with the production of more disruptive science and technology, even after accounting for the average age and number of previous works produced by team members. These findings are based on our regression results, shown in Extended Data Table 1 . Models 6 and 12 present the full regression models. The models indicate a consistent pattern for both science and technology, wherein the coefficients for diversity of work cited are positive and significant for papers (0.159, P < 0.01) and patents (0.069, P < 0.01), indicating that in fields in which there is more use of diverse work, there is greater disruption. Holding all other variables at their means, the predicted CD 5 of papers and patents increases by 303.5% and 1.3%, respectively, when the diversity of work cited increases by 1 s.d. The coefficients of the ratio of self-citations to total work cited is negative and significant for papers (−0.011, P < 0.01) and patents (−0.060, P < 0.01), showing that when researchers or inventors rely more on their own work, discovery and invention tends to be less disruptive. Again holding all other variables at their means, the predicted CD 5 of papers and patents decreases by 622.9% and 18.5%, respectively, with a 1 s.d. increase in the ratio. The coefficients of the interaction between mean age of work cited and dispersion in age of work cited is positive and significant for papers (0.000, P < 0.01) and patents (0.001, P < 0.01), suggesting that—holding the dispersion of the age of work cited constant—papers and patents that engage with older work are more likely to be disruptive. The predicted CD 5 of papers and patents increases by a striking 2,072.4% and 58.4%, respectively, when the mean age of work cited increases by 1 s.d. (about nine and eight years for papers and patents, respectively), again holding all other variables at their means. In summary, the regression results suggest that changes in the use of previous knowledge may contribute to the production of less disruptive science and technology.
Reporting summary
Further information on research design is available in the Nature Portfolio Reporting Summary linked to this article.
Data availability
Data associated with this study are freely available in a public repository at https://doi.org/10.5281/zenodo.7258379 . Our study draws on data from six sources: the American Physical Society, JSTOR, Microsoft Academic Graph, Patents View, PubMed and WoS. Data from Microsoft Academic Graph, Patents View and PubMed are publicly available, and our repository includes complete data for analyses from these sources. Data from the American Physical Society, JSTOR and WoS are not publicly available, and were used under licence from their respective publishers. To facilitate replication, our repository includes limited versions of the data from these sources, which will enable calculation of basic descriptive statistics. The authors will make full versions of these data available upon request and with permission from their respective publishers. Source data are provided with this paper.
Code availability
Open-source code related to this study is available at https://doi.org/10.5281/zenodo.7258379 and http://www.cdindex.info . We used Python v.3.10.6 (pandas v.1.4.3, numpy v.1.23.1, matplotlib v.3.5.2, seaborn v.0.11.2, spacy v.2.2, jupyterlab v.3.4.4) to wrangle, analyse and visualize data and to conduct statistical analyses. We used MariaDB v.10.6.4 to wrangle data. We used R v.4.2.1 (ggplot2 v.3.36, ggrepel v.0.9.0) to visualize data. We used StataMP v.17.0 (reghdfe v.5.7.3) to conduct statistical analyses.
Fleming, L. Recombinant uncertainty in technological search. Manage. Sci. 47 , 117–132 (2001).
Article Google Scholar
Schumpeter, J. Capitalism, Socialism and Democracy (Perennial, 1942).
Koyré, A. An unpublished letter of Robert Hooke to Isaac Newton. ISIS 43 , 312–337 (1952).
Article MathSciNet MATH Google Scholar
Popper, K. Conjectures and Refutations: The Growth of Scientific Knowledge (Routledge, 2014).
Fleck, L. Genesis and Development of a Scientific Fact (Univ. Chicago Press, 2012).
Acemoglu, D., Akcigit, U. & Kerr, W. R. Innovation network. Proc. Natl Acad. Sci. USA 113 , 11483–11488 (2016).
Article ADS CAS Google Scholar
Weitzman, M. L. Recombinant growth. Q. J. Econ. 113 , 331–360 (1998).
Tria, F., Loreto, V., Servedio, V. D. P. & Strogatz, S. H. The dynamics of correlated novelties. Sci. Rep. 4 , 1–8 (2014).
Fink, T. M. A., Reeves, M., Palma, R. & Farr, R. S. Serendipity and strategy in rapid innovation. Nat. Commun. 8 , 1–9 (2017).
Article CAS Google Scholar
Pammolli, F., Magazzini, L. & Riccaboni, M. The productivity crisis in pharmaceutical R&D. Nat. Rev. Drug Discov. 10 , 428–438 (2011).
Bloom, N., Jones, C. I., Van Reenen, J. & Webb, M. Are ideas getting harder to find? Am. Econ. Rev. 110 , 1104–1144 (2020).
Funk, R. J. & Owen-Smith, J. A dynamic network measure of technological change. Manage. Sci. 63 , 791–817 (2017).
Bornmann, L., Devarakonda, S., Tekles, A. & Chacko, G. Are disruption index indicators convergently valid? The comparison of several indicator variants with assessments by peers. Quant. Sci. Stud. 1 , 1242–1259 (2020).
Uzzi, B., Mukherjee, S., Stringer, M. & Jones, B. Atypical combinations and scientific impact. Science 342 , 468–472 (2013).
Leydesdorff, L., Tekles, A. & Bornmann, L. A proposal to revise the disruption index. Prof. Inf. 30 , e300121 (2021).
Lu, C. et al. Analyzing linguistic complexity and scientific impact. J. Informetr. 13 , 817–829 (2019).
Hofstra, B. et al. The diversity–innovation paradox in science. Proc. Natl Acad. Sci. USA 117 , 9284–9291 (2020).
Jones, B. F. The burden of knowledge and the ‘death of the renaissance man’: is innovation getting harder? Rev. Econ. Stud. 76 , 283–317 (2009).
Article MATH Google Scholar
Gordon, R. J. The Rise and Fall of American Growth (Princeton Univ. Press, 2016).
Chu, J. S. G. & Evans, J. A. Slowed canonical progress in large fields of science. Proc. Natl Acad. Sci. USA 118 , e2021636118 (2021).
Packalen, M. & Bhattacharya, J. NIH funding and the pursuit of edge science. Proc. Natl Acad. Sci. USA 117 , 12011–12016 (2020).
Jaffe, A. B. & Lerner, J. Innovation and its Discontents: How Our Broken Patent System Is Endangering Innovation and Progress, and What To Do About It (Princeton Univ. Press, 2011).
Horgan, J. The End of Science: Facing the Limits of Knowledge in the Twilight of the Scientific Age (Basic Books, 2015).
Collison, P. & Nielsen, M. Science Is Getting Less Bang for its Buck (Atlantic, 2018).
Nolan, A. Artificial intelligence and the future of science. oecd.ai , https://oecd.ai/en/wonk/ai-future-of-science (25 October 2021).
Effective Policies to Foster High-risk/High-reward Research. OECD Science, Technology, and Industry Policy Papers (OECD, 2021).
Cowen, T. The Great Stagnation: How America Ate All the Low-Hanging Fruit of Modern History, Got Sick, and Will (Eventually) Feel Better (Penguin, 2011).
Einstein, A. The World As I See It (Citadel Press, 1949).
Arthur, W. B. The structure of invention. Res. Policy 36 , 274–287 (2007).
Tushman, M. L. & Anderson, P. Technological discontinuities and organizational environments. Adm. Sci. Q. 31 , 439–465 (1986).
Kohn, W. & Sham, L. J. Self-consistent equations including exchange and correlation effects. Phys. Rev. 140 , A1133 (1965).
Article ADS MathSciNet Google Scholar
Watson, J. D. & Crick, F. H. C. Molecular structure of nucleic acids: a structure for deoxyribose nucleic acid. Nature 171 , 737–738 (1953).
Bornmann, L. & Tekles, A. Disruption index depends on length of citation window. Prof. Inf. 28 , e280207 (2019).
Wu, L., Wang, D. & Evans, J. A. Large teams develop and small teams disrupt science and technology. Nature 566 , 378–382 (2019).
Kuhn, T. S. The Structure of Scientific Revolutions (Univ. Chicago Press, 1962).
Brad Wray, K. Kuhn and the discovery of paradigms. Philos. Soc. Sci. 41 , 380–397 (2011).
Ioannidis, J. P. A. Why most published research findings are false. PLoS Med. 2 , e124 (2005).
Li, J., Yin, Y., Fortunato, S. & Wang, D. A dataset of publication records for Nobel laureates. Sci. Data 6 , 1–10 (2019).
Bornmann, L. & Marx, W. Methods for the generation of normalized citation impact scores in bibliometrics: which method best reflects the judgements of experts? J. Informetr. 9 , 408–418 (2015).
Waltman, L. A review of the literature on citation impact indicators. J. Informetr. 10 , 365–391 (2016).
Waltman, L. & van Eck, N. J. in Springer Handbook of Science and Technology Indicators (eds. Glänzel, W. et al.) 281–300 (Springer, 2019).
Bornmann, L. How can citation impact in bibliometrics be normalized? A new approach combining citing-side normalization and citation percentiles. Quant. Sci. Stud. 1 , 1553–1569 (2020).
Petersen, A. M., Pan, R. K., Pammolli, F. & Fortunato, S. Methods to account for citation inflation in research evaluation. Res. Policy 48 , 1855–1865 (2019).
Bornmann, L. & Mutz, R. Growth rates of modern science: a bibliometric analysis based on the number of publications and cited references. J. Assoc. Inf. Sci. Technol. 66 , 2215–2222 (2015).
Bornmann, L., Haunschild, R. & Mutz, R. Growth rates of modern science: a latent piecewise growth curve approach to model publication numbers from established and new literature databases. Humanit. Soc. Sci. Commun. 8 , 1–15 (2021).
Jones, B. F. & Weinberg, B. A. Age dynamics in scientific creativity. Proc. Natl Acad. Sci. USA 108 , 18910–18914 (2011).
Bonzi, S. & Snyder, H. Motivations for citation: a comparison of self citation and citation to others. Scientometrics 21 , 245–254 (1991).
Fowler, J. & Aksnes, D. Does self-citation pay? Scientometrics 72 , 427–437 (2007).
King, M. M., Bergstrom, C. T., Correll, S. J., Jacquet, J. & West, J. D. Men set their own cites high: gender and self-citation across fields and over time. Socius 3 , 2378023117738903 (2017).
Mukherjee, S., Romero, D. M., Jones, B. & Uzzi, B. The nearly universal link between the age of past knowledge and tomorrow’s breakthroughs in science and technology: the hotspot. Sci. Adv. 3 , e1601315 (2017).
Article ADS Google Scholar
Merton, R. K. Singletons and multiples in scientific discovery: a chapter in the sociology of science. Proc. Am. Philos. Soc. 105 , 470–486 (1961).
Google Scholar
Wang, D., Song, C. & Barabási, A.-L. Quantifying long-term scientific impact. Science 342 , 127–132 (2013).
Leahey, E. Not by productivity alone: how visibility and specialization contribute to academic earnings. Am. Sociol. Rev. 72 , 533–561 (2007).
Tahamtan, I. & Bornmann, L. Core elements in the process of citing publications: conceptual overview of the literature. J. Informetr. 12 , 203–216 (2018).
Tahamtan, I. & Bornmann, L. What do citation counts measure? An updated review of studies on citations in scientific documents published between 2006 and 2018. Scientometrics 121 , 1635–1684 (2019).
Bhattacharya, J. & Packalen, M. Stagnation and Scientific Incentives (Working Paper 26752) , https://www.nber.org/papers/w26752 (2020).
Azoulay, P., Graff Zivin, J. S. & Manso, G. Incentives and creativity: evidence from the academic life sciences. RAND J. Econ. 42 , 527–554 (2011).
Baltimore, D. Viral RNA-dependent DNA polymerase: RNA-dependent DNA polymerase in virions of RNA tumour viruses. Nature 226 , 1209–1211 (1970).
Page, L. Method for node ranking in a linked database. US patent 6,285,999 (2001).
Axel, R., Wigler, M. H. & Silverstein, S. J. Processes for inserting DNA into eucaryotic cells and for producing proteinaceous materials. US patent 4,634,665 (1983).
Hawbaker, M. S. Soybean variety SE90346. US patent 6,958,436 (2005).
Katsuki, T. & Sharpless, K. B. The first practical method for asymmetric epoxidation. J. Am. Chem. Soc. 102 , 5974–5976 (1980).
Riess, A. G., et al. Observational evidence from supernovae for an accelerating universe and a cosmological constant. Astron. J. 116 , 1009 (1998).
Dirac, P. A. M. The quantum theory of the electron. Proc. R. Soc. Lond. A Math. Phys. Sci. 117 , 610–624 (1928).
ADS MATH Google Scholar
Sanger, F., Nicklen, S. & Coulson, A. R. DNA sequencing with chain-terminating inhibitors. Proc. Natl Acad. Sci. USA 74 , 5463–5467 (1977).
Bednorz, J. G. & Müller, K. A. Possible high T c superconductivity in the Ba-La-Cu-O system. Z. Phys. B Condens. Matter 64 , 189–193 (1986).
Wuchty, S., Jones, B. F. & Uzzi, B. The increasing dominance of teams in production of knowledge. Science 316 , 1036–1039 (2007).
Guimera, R., Uzzi, B., Spiro, J. & Amaral, L. A. N. Team assembly mechanisms determine collaboration network structure and team performance. Science 308 , 697–702 (2005).
Jones, B. F., Wuchty, S. & Uzzi, B. Multi-university research teams: shifting impact, geography, and stratification in science. Science 322 , 1259–1262 (2008).
Grömping, U. Estimators of relative importance in linear regression based on variance decomposition. Am. Stat. 61 , 139–147 (2007).
Article MathSciNet Google Scholar
Mukherjee, S., Uzzi, B., Jones, B. & Stringer, M. A new method for identifying recombinations of existing knowledge associated with high-impact innovation. J. Prod. Innov. Manage. 33 , 224–236 (2016).
Christianson, N. H., Sizemore Blevins, A. & Bassett, D. S. Architecture and evolution of semantic networks in mathematics texts. Proc. R. Soc. A 476 , 20190741 (2020).
Article ADS MathSciNet MATH Google Scholar
Newman, M. E. J. The structure of scientific collaboration networks. Proc. Natl Acad. Sci. USA 98 , 404–409 (2001).
Article ADS MathSciNet CAS MATH Google Scholar
Newman, M. E. J. Scientific collaboration networks. I. Network construction and fundamental results. Phys. Rev. E 64 , 016131 (2001).
Uzzi, B. & Spiro, J. Collaboration and creativity: the small world problem. Am. J. Sociol. 111 , 447–504 (2005).
Funk, R. J. Making the most of where you are: geography, networks, and innovation in organizations. Acad. Manage. J. 57 , 193–222 (2014).
Barabási, A.-L. Network Science (Cambridge Univ. Press, 2016).
Blau, D. M. & Weinberg, B. A. Why the US science and engineering workforce is aging rapidly. Proc. Natl Acad. Sci. USA 114 , 3879–3884 (2017).
Cui, H., Wu, L. & Evans, J. A. Aging scientists and slowed advance. Preprint at https://doi.org/10.48550/arXiv.2202.04044 (2022).
Azoulay, P., Fons-Rosen, C. & Graff Zivin, J. S. Does science advance one funeral at a time? Am. Econ. Rev. 109 , 2889–2920 (2019).
Download references

Acknowledgements
This study was supported by the National Science Foundation (grant Nos. 1829168, 1932596 and 1829302).
Author information
Authors and affiliations.
Carlson School of Management, University of Minnesota, Minneapolis, MN, USA
Michael Park & Russell J. Funk
School of Sociology, University of Arizona, Tucson, AZ, USA
Erin Leahey
You can also search for this author in PubMed Google Scholar
Contributions
R.J.F. and E.L. collaboratively contributed to the conception and design of the study. R.J.F. and M.P. collaboratively contributed to the acquisition, analysis and interpretation of the data. R.J.F. created software used in the study. R.J.F., E.L. and M.P. collaboratively drafted and revised the manuscript.
Corresponding author
Correspondence to Russell J. Funk .
Ethics declarations
Competing interests.
The authors declare no competing interests.
Peer review
Peer review information.
Nature thanks Diana Hicks and the other, anonymous, reviewer(s) for their contribution to the peer review of this work. Peer reviewer reports are available.
Additional information
Publisher’s note Springer Nature remains neutral with regard to jurisdictional claims in published maps and institutional affiliations.
Extended data figures and tables
Extended data fig. 1 distribution of cd 5 ..
This figure gives an overview of the distribution of CD 5 for papers (n = 24,659,076) and patents (n = 3,912,353). Panels a and c show counts of papers and patents over discrete intervals of CD 5 . Panels b and d show the distribution of CD 5 over time, within 10 (papers) and 5 (patents) year intervals, using letter-value plots. These plots are similar to boxplots, but generally provide more reliable summaries for large datasets. They are drawn by identifying the median of the underlying distribution and then recursively drawing boxes outward from there in either direction that encompass half of the remaining data.
Extended Data Fig. 2 CD index measured using alternative forward citation windows.
This figure evaluates the sensitivity of our results to the use of different forward citation windows when computing the CD index for papers (n = 24,659,076) and patents (n = 3,912,353). In the main text, the index is computed based on citations made to papers and patents and their backward references as of 5 years after the year of publication. a and c plot the CD index using a longer, 10 year forward window, for papers and patents, respectively. b and d plot the CD index using all forward citations made to sample papers and patents as of the year 2017. Shaded bands correspond to 95% confidence intervals. Overall, the results mirror those reported in the main text, although the decline is somewhat steeper using longer forward citation windows, suggesting our primary results may represent a more conservative estimate.
Extended Data Fig. 3 Diversity of language use in science and technology over time.
This figure shows changes in the ratio of unique to total words (also known as the type-token ratio) over time based on data from the abstracts of papers ( a , n = 76 WoS research area × year observations) and patents ( b , n = 229 NBER technology category × year observations). For papers, lines correspond to WoS research areas; for patents, lines correspond to NBER technology categories. For paper abstracts, lines begin in 1992 because WoS does not reliably record abstracts for papers published prior to the early 1990s. The ratio of unique to total words is computed separately by field (i.e., the uniqueness of words and total word counts are determined within WoS research areas and NBER technology categories). If disruption is decreasing, we may plausibly expect to see a decrease in the diversity of words used by scientists and inventors, as discoveries and inventions will be less likely to create departures from the status quo, and will therefore be less likely to need to introduce new terminology. For both papers and patents, we observe declining diversity in word use over time, which is consistent with this expectation and corroborates our findings using the CD index.
Extended Data Fig. 4 Declining combinatorial novelty.
This figure shows changing patterns in the combinatorial novelty/conventionality of papers ( a , n = 24,659,076) and patents ( b , n = 3,912,353), using a previously proposed measure of “atypical combinations” 14 . The measure quantifies the degree to which the prior work cited by a paper or patent would be expected by chance. For papers, we follow prior work 14 and consider combinations of cited journals. If a paper made three citations to prior work, and that work was published in three different journals— Nature , Cell , and Science —then there are three combinations— Nature × Cell , Nature × Science , and Science × Cell . To determine the degree to which each combination would be expected by chance, the frequency of observed pairings is compared to those in 10 “rewired” copies of the overall citation network, using a z-score. For patents, there is no natural analogue to journals, and therefore we consider pairings of primary United States Patent Classification (USPC) system codes. We present the results of this analysis following the approach of prior work 14 , which plots the cumulative distribution function of the measure. In general, there is a rightward shift in the cumulative distributions over time, suggesting that for both papers and patents, combinations are more conventional than would be expected by chance, consistent with what we would anticipate based on our results using the CD index. For patents, there is also a smaller shift in the opposite direction on the left side of the distribution, suggesting that novel patents in recent decades are somewhat more novel than novel patents in earlier decades. Overall, however, the bulk of the distribution is moving rightward, indicating greater conventionality.
Extended Data Fig. 5 Contribution of field, year, and author effects.
This figure shows the relative contribution of field, year, and author fixed effects to the adjusted R 2 in regression models predicting CD 5 . The top bar shows the results for papers (n = 80,607,091 paper × author observations); the bottom bar shows the results for patents (n = 8,319,826 patent × inventor observations). The results suggest that for both papers and patents, stable characteristics of authors contribute significantly to patterns of disruptiveness. Moreover, relatively little of the variation is accounted for by field-specific factors.
Extended Data Fig. 6 CD index over time across data sources.
This figure shows changes in CD 5 over time across four additional data sources (the WoS [n = 24,659,076] and Patents View [n = 3,912,353] lines are included for reference): JSTOR (n = 1,703,353), the American Physical Society corpus (n = 478,373), Microsoft Academic Graph (n = 1,000,000), and PubMed (n = 16,774,282). Colours indicate the six different data sources. Shaded bands correspond to 95% confidence intervals. The figure indicates that the decline in disruption is unlikely to be driven by our sample choice of WoS papers and Patents View patents.
Extended Data Fig. 7 Alternative measures of disruption.
This figure shows the decline in the disruption of papers ( a , n = 100,000) and patents ( b , n = 100,000) based on two alternative measures of disruption. The blue lines calculate disruption using a measure proposed in Bornmann et al. 13 , \({{DI}}_{l}^{{nok}}\) where l = 5, which makes the measure more resilient to marginal changes in the number of papers or patents that only cite the focal work’s references. The orange lines calculate disruption using a measure proposed in Leydesdorff et al. 15 , DI*, which makes the measure less sensitive to small changes in the forward citation patterns of papers or patents that make no backward citations. Shaded bands correspond to 95% confidence intervals. With both alternative measures, we observe decreases in disruption for papers and patents, suggesting that the decline is not an artefact of our operationalization of disruption.
Extended Data Fig. 8 Robustness to changes in publication, citation, and authorship practices.
This figure evaluates whether declines in disruptiveness may be attributable to changes in publication, citation, and authorship practices for papers (n = 24,659,076) and patents (n = 3,912,353). Panels a and d adjust for these changes using a normalization approach. We present two alternative versions of the CD index, both of which account for the tendency for papers and patents to cite more prior work over time. Blue lines indicate normalization at the paper level (accounting for the number of citations made by the focal paper/patent). Orange lines indicate normalization at the field and year level (accounting for the mean number of citations made by papers/patents in the focal field and year). Panels b (papers) and e (patents) adjust for changes in publication, citation, and authorship practices using a regression approach. The panels show predicted values of CD 5 based on regressions reported in Models 4 (papers) and 8 (patents) of Supplementary Table 1 , which adjust for field × year— Number of new papers/patents , Mean number of papers/patents cited , Mean number of authors/inventors per paper/patent —and paper/patent-level— Number of papers/patents cited , Number of unlinked references —characteristics. Predictions are made separately for each year indicator included in the models; we then connect these separate predictions with lines to aid interpretation. Finally, Panels c (papers) and f (patents) adjust for changes in publication, citation, and authorship practices using a simulation approach. The panels plot z-scores that compare values of CD 5 obtained from the observed citation networks to those obtained from randomly rewired copies of the observed networks. Across all six panels, shaded bands correspond to 95% confidence intervals.
Extended Data Fig. 9 Growth of scientific and technological knowledge.
This figure shows the number of papers (n = 24,659,076) published ( a ) and patents (n = 3,912,353) granted ( b ) over time. For papers, lines correspond to WoS research areas; for patents, lines correspond to NBER technology categories.
Supplementary information
Supplementary information.
Supplementary Sections 1–3, Tables 1–3 and References.
Peer Review File
Source data fig. 2., source data fig. 3., source data fig. 4., source data fig. 5., source data fig. 6., source data extended data fig. 1., source data extended data fig. 2., source data extended data fig. 3., source data extended data fig. 5., source data extended data fig. 6., source data extended data fig. 7., source data extended data fig. 8., source data extended data fig. 9., rights and permissions.
Springer Nature or its licensor (e.g. a society or other partner) holds exclusive rights to this article under a publishing agreement with the author(s) or other rightsholder(s); author self-archiving of the accepted manuscript version of this article is solely governed by the terms of such publishing agreement and applicable law.
Reprints and permissions
About this article
Cite this article.
Park, M., Leahey, E. & Funk, R.J. Papers and patents are becoming less disruptive over time. Nature 613 , 138–144 (2023). https://doi.org/10.1038/s41586-022-05543-x
Download citation
Received : 14 February 2022
Accepted : 08 November 2022
Published : 04 January 2023
Issue Date : 05 January 2023
DOI : https://doi.org/10.1038/s41586-022-05543-x
Share this article
Anyone you share the following link with will be able to read this content:
Sorry, a shareable link is not currently available for this article.
Provided by the Springer Nature SharedIt content-sharing initiative
This article is cited by
Recsoi: recommending research directions using statements of ignorance.
- Adrien Bibal
- Nourah M. Salem
- Lawrence E. Hunter
Journal of Biomedical Semantics (2024)
Mapping the scientific output of organoids for animal and human modeling infectious diseases: a bibliometric assessment
- Jean Monlong
- Agnès Wiedemann
Veterinary Research (2024)
Redefining cancer research for therapeutic breakthroughs
- Arseniy E. Yuzhalin
British Journal of Cancer (2024)
In search of innovative potential
- Lutz Bornmann
- Christoph Ettl
- Christian Leibel
EMBO Reports (2024)
BrainSwarming, blockchain, and bioethics: applying Innovation Enhancing Techniques to healthcare and research
- Anuraag A. Vazirani
- Tony McCaffrey
- Sebastian Porsdam Mann
Scientific Reports (2024)
By submitting a comment you agree to abide by our Terms and Community Guidelines . If you find something abusive or that does not comply with our terms or guidelines please flag it as inappropriate.
Quick links
- Explore articles by subject
- Guide to authors
- Editorial policies
Sign up for the Nature Briefing newsletter — what matters in science, free to your inbox daily.


- Previous Article
Advances in artificial intelligence and automation have the potential to be labor-saving and to increase inequality and poverty around the globe. They also give rise to winner-takes-all dynamics that advantage highly skilled individuals and countries that are at the forefront of technological progress. We analyze the economic forces behind these developments and delineate domestic economic policies to mitigate the adverse effects while leveraging the potential gains from technological advances. We also propose reforms to the global system of governance that make the benefits of advances in artificial intelligence more inclusive.
- I. Introduction
Advances in artificial intelligence (AI) and related forms of automation technologies have led to growing fears about job losses and increasing inequality. This concern is widespread in high-income countries. Developing countries and emerging market economies should be even more concerned than high-income countries, as their comparative advantage in the world economy relies on abundant labor and natural resources. Declining returns to labor and natural resources as well as the winner-takes-all dynamics brought on by new information technologies could lead to further immiseration in the developing world. This could undermine the rapid gains that have been the hallmark of success in development over the past fifty years and threaten the progress made in reducing poverty and inequality.
For many decades, there was a presumption that advances in technology would benefit all— embodied by the trickle-down dogma that characterized neoliberalism. And for some time, this presumption was in fact justified. For example, for the three decades following World War II, the US economy and many other high-income and developing countries experienced broadly shared increases in living standards. However, over the past half-century, output growth and median worker incomes started to decouple.
Moreover, economic theory cautions that technological progress is likely to create both winners and losers (see Korinek and Stiglitz, 2019 , for a review). As long as the winners and losers from technological progress are located within the same country, there is at least the possibility that domestic policy measures can compensate the losers. However, when technological progress deteriorates the terms of trade and thus undermines the comparative advantage of entire countries, then entire nations may be worse off except if the winners within one country compensate the losers in other countries, which seems politically very difficult.
This paper argues that concerns about whether technological progress leads to inclusive growth are indeed justified – and that especially developing countries may face a stark new set of challenges going forward. However, we propose policies that can mitigate the adverse effects so that advances in technology lead to a world with greater shared prosperity. This will require new domestic polices and development strategies as well as strong international cooperation and a rewriting of the global rules governing the information economy.
We start by laying out the key properties of AI and related automation technologies that underlie the concerns about recent technological progress. 2 AI is likely to be labor-saving and resource-saving, devaluing the sources of comparative advantage of many developing countries and deteriorating their terms of trade. Being an information technology, AI also tends to give rise to natural monopolies, creating a small set of so-called superstar firms that are located in a few powerful countries but serve the entire world economy. Moreover, under reasonable assumptions, the rate and direction of technological progress chosen by the market are generally suboptimal ( Korinek and Stiglitz, 2019 ). This creates the possibility of steering innovation in AI and other technologies in directions that are more beneficial to humanity at large, for example, preserving the planet or creating satisfying employment opportunities, rather than substituting for labor and creating more unemployment and inequality.
Taking a step back, we evaluate to what extent the discussed concerns about technological progress are justified, given what we know at present. There is vast uncertainty about the impact of AI, even among experts in the field. Some argue that AI is less important than the big innovations of the 20 th century and will have rather limited impact on the economy, whereas others go as far as predicting that AI will lead to more rapid technological progress than mankind has ever seen before.
In this context, we discuss how to reconcile the buzz among technologists over the past decade with economic data that suggests rather modest productivity increases over the period – encapsulated by the so-called productivity puzzle. We also analyze how the forces generated by progress in AI interact with other recent developments, in particular with the recovery from COVID-19, with secular population dynamics, and with the need for a Green Transition.
Despite the uncertainties surrounding AI, its potentially dramatic consequences suggest that we should steer our own research in directions where the expected social value added of economic analysis is greatest: we need to think particularly hard about potential events that would be highly disruptive to our society.
To grasp the historical nature of what is going on, we look at the broader history of technological progress. Humanity spent much of its history at a Malthusian stage in which the vast majority of the population lived at subsistence levels. The Industrial Revolution that lifted living standards started a bit over two centuries ago, making it a mere blip in the history of human civilization. For developing countries, the era of manufacturing-based export-led growth that enabled the East Asian Miracle stretched over the past half-century – only one quarter of the history of the Industrial Revolution. It is conceivable that we are now going into another era. There is even a risk that the terms-of-trade losses generated by progress in AI may erase much of the gains that the developing countries have made in recent decades.
However, the Industrial Revolution also offers ample lessons on how to manage innovation in a positive way: technological revolutions are very disruptive, but collective action can mitigate the adverse effects and generate an environment in which the gains are shared broadly. The labor-using nature of the Industrial Revolution ushered in an Age of Labor in which the economic gains of workers also shifted political dynamics in their favor, but there is a risk that future labor-saving progress may do the opposite. The decline of manufacturing will require a new development model that follows a more multi-pronged strategy to replace the manufacturing-based export-led growth model.
The key policy question is how countries can improve the likelihood of benign outcomes from technological progress. This is especially pertinent for developing countries, but it is also a challenge for advanced economies to develop policies that ensure that technological advances lead to broadly shared prosperity and that their adverse effects are mitigated. We delineate here a number of such policies. Taxation and redistribution are a first line of defense to compensate the losers of progress, although the scope for redistribution may be limited in developing countries.
Targeted expenditure policies can serve double duty by providing both income to workers and a valuable social return – for example, investments in education or infrastructure are labor intensive and enhance human capital and the physical infrastructure of countries, both of which are important in bridging the digital divide and ensuring that all citizens can participate in the opportunities afforded by digital technologies.
To replace the manufacturing-based export-led growth model, developing countries will need to steer technological progress and technology adoption in new directions, in part by leveraging the opportunities that AI and other digital technologies afford in agriculture and services.
Finally, we describe a set of policies at the supra-national level to reform our global system of governance in a way that developing countries can benefit from advances in AI and other information technologies while addressing the downsides of these new technologies. We need to design a global tax regime for the digital age that enables countries to raise taxes on transactions that occur within their borders. Competition policy is also increasingly a question that transcends national borders as the footprint of the digital giants is global and authorities in their countries of origin do not face the correct incentives to ensure a competitive marketplace. Intellectual property regimes need to be adapted so they are attuned to the needs and circumstances of developing countries. Moreover, information policy including the regulation of data needs to be discussed at the supra-national level to provide a voice to developing countries that could otherwise not influence the design of such policies.
The remainder of this paper is organized as follows. In the second section, we provide an overview of the downside risks of technological progress, with special emphasis on potential AI-induced economic disruptions; in the third, we discuss the uncertainties surrounding the nature and level of the impacts as well as the broader context. The fourth section reviews what we can learn from the bigger historical picture of technological progress. The fifth section distills the critical role of government policy in managing the effects of technological progress and enabling the benefits of innovation to be widely shared. The sixth section analyzes how our global system of governance needs to be updated to allow developing countries to maximize the benefits and minimize the costs of advances in AI and related technologies.
II. Downside Risks of Technological Progress
Many technology optimists suggest that productivity gains go hand in hand with real wage gains. This presumption that technological progress would benefit all was also embodied by the trickle-down dogma that has characterized neoliberalism. However, the presumption was supported neither by theory nor evidence; indeed, economic theory has always held that advances in technology do not necessarily benefit all and may create winners and losers. The data ( Figure 1 ) show that in recent decades, many countries have experienced episodes during which wages lagged productivity growth. Moreover, as we argue below, even where average wages did keep up with productivity, median wages may not have, and there is a risk that any positive gains seen in the past may not continue.
Productivity and Earnings Growth
Citation: IMF Working Papers 2021, 166; 10.5089/9781513583280.001.A001
- Download Figure
- Download figure as PowerPoint slide
Figure 2 illustrates that the income gains associated with technological progress have been highly unevenly distributed. In the US and other high-income countries, most of the benefits of growth have gone to those at the top, resulting in widening income inequality in most advanced economies since the early 1980s, reversing an earlier downward trend in many countries.
Income Inequality over Time, 1960–2015
How can we reconcile this with economic theory? In the context of a competitive economy, we can think of technological progress as moving out the production possibility frontier: one can get more of any output for a given amount of inputs. But this increase in production possibilities does not tell us how the gains from progress will be distributed. In our simplest economic models, for example, if we assume a competitive economy with a Cobb-Douglas production function, relative shares are fixed.
However, in the more general case, technical change may change the distribution of income, so that, for instance, labor gets a smaller share of a larger pie. If its share decreases enough, workers could even be worse off. Whether wages increase or decrease depends on what happens to the demand for labor at existing wages. Using the terminology first introduced by Hicks, technical change that leads to a decrease in the relative share of labor is called capital-biased; if it leads to a decrease in the share of unskilled labor, it is called skill-biased; if it leads to an outright reduction in wages, it is called labor-saving. The US, for example, has experienced routine-biased technological change that has replaced workers engaged in both manual and cognitive routine activities since the 1980s and that has contributed to the hollowing out of the middle class ( Autor et al., 2003 ).
Korinek and Stiglitz (2019) show that the distributive effects of innovations can be seen as generating quasi-rents -aside from delivering direct gains to innovators, innovations lead to changes in factor demands, for example lowering demand for unskilled labor and raising demand for skilled labor, and the affected workers experience gains or losses. The winners of progress (e.g. the skilled workers in our example) experience these gains without having contributed to the innovation, obtaining quasi-rents, whereas the losers experience losses without any fault of their own. That, in turn, has an important implication: governments can capture some of the quasi-rents by taxing the winners and redistributing it; and given the nature of the gains, governments may even be able to raise taxes in ways that have no or limited distortionary effects, for example if the winners include owners of fixed factors such as land. Thus, “managed” technological progress could allow for Pareto-improving outcomes.
However, there is a big difference between looking at the impacts of AI within a single country and from a global perspective. When the benefits are experienced in one country and the cost is borne in another, a Pareto improvement would require that the winners compensate the losers across national boundaries. Today, such cross-border transfers are voluntary and limited.
As a result, the fruits of technological progress will be unequally shared; but more troublesome is that while some countries may gain a great deal, others will lose. These differences will be reflected, respectively, in improvements and deteriorations of countries’ terms of trade. In the following, we will analyze several of the specific forms of progress that the AI revolution and related automation technologies are likely to induce, with particular focus on how they may hurt developing countries.
- A. Labor-Saving Technological Progress
Many observers are concerned that AI may be labor-saving, that is, cause a decline in the demand for labor at existing factor prices. If this occurs, equilibrium wages will decrease and workers will be worse off.
As we have noted, over the past half-century, the US and many other countries seem to have experienced technological progress that was biased against workers with lower levels of education performing routine tasks, sufficiently biased that it may even have been labor-saving in that segment, reducing such workers’ real incomes. For example, Autor et al. (2003) observe that from the 1970s to the 1990s, while computerization was a substitute for an increasing number of routine tasks, technological change increased the productivity of workers in non-routine jobs that involved problem-solving and complex communications tasks. These changes in technology may have explained nearly 2/3 of the relative demand shift toward college-educated labor over that period. Similarly, more recently, Acemoglu and Restrepo (2020) estimated significant adverse employment and wage effects from the introduction of industrial robots in the US, concentrated in manufacturing and among routine manual, blue-collar, assembly, and related occupations, helping to explain the dramatic increase in wage dispersion across skill groups over the past five decades ( Figure 3 ).
Rising Wage-Skill Premia in the US
(Real Wages of Full Time U.S. Male Workers, 1963 = 100)
This job polarization in terms of wages has also been reflected in relative employment dynamics. Employment in nonroutine jobs has continued to grow steadily in the US, while that in routine jobs has stagnated, or in some periods declined, since around 1990, contributing, as we have noted, to a “hollowing out of the middle” ( Figure 4 ). 3 OECD (2019) note that middle-skilled jobs may be the most prone to both automation and offshoring, as they most encompass routine tasks that are relatively easy to automate (or offshore). 4
Employment in Routine vs. Non-Routine Jobs
(Persons, millions)
Standard models of aggregate production functions with skilled and unskilled labor-augmenting progress and capital-augmenting progress can generate the observed patterns of movements in factor prices and shares, depending on patterns of progress as well as elasticities and cross-elasticities of substitution. Acemoglu and Restrepo (2019a) formulate a particular model in which the displacement of workers by robots will reduce the labor share of income and may be labor-saving if the productivity gains from the robots are modest. Berg et al. (2018) focus on the differential effects of technological progress across worker groups and shows that technological progress may be unskilled-labor-saving because that type of labor is easily substituted for by robots; by contrast, high-skilled labor is likely complementary to robots and will benefit from technological progress; as a result, technological advances risk bringing about large increases in inequality. Automation may also worsen inequality along other dimensions—for example, in sectors where women occupy more routine jobs ( Brussevich et al., 2018 ).
Even if technological progress is labor-saving in the short run, it may also trigger additional accumulation of capital that is complementary to labor, benefiting labor in the long run. For example, Stiglitz (2015) and Caselli and Manning (2019) show that in an economy with capital and labor only, in which long-run capital accumulation is determined by an exogenous interest rate, labor will always gain. 5 Ultimately, however, impacts on inequality depend on whether there are other scarce limiting factors in the economy, for example, natural resources or land, which would benefit from technological progress and ultimately become more scarce as the factors “capital” and “machine-replacing labor” become more abundant and cheaper. Indeed, Korinek and Stiglitz (2021a) show that if this is the case, then, without government intervention, labor may lose out from technological progress even in the long run.
At a global level, similar dynamics may play out. Although labor-saving technological progress would make the world as a whole richer, it would hit developing countries that have a comparative advantage in cheap labor particularly hard. If worldwide demand for labor, or for unskilled labor, declines, such countries would experience a significant deterioration in their terms of trade and lose a substantial fraction of their export income. Labor-saving progress may not only create winners and losers within the affected developing countries, but it may make entire countries on net worse off. Alonso et al. (2020) find that improvements in the productivity of “robots” could drive divergence, as advanced countries benefit from computerization more given their higher initial capital stock.
However, it is also conceivable that other forms of advances in technology could benefit workers: intelligence-assisting devices and algorithms (IA) may be complementary to labor rather than substituting for it, thus enhancing the prospects of labor. Innovations that fall into this category may include augmented reality (AR), machine learning (ML) algorithms that help analyze complex data, and other forms of integration of AI with humans. 6 Automation technologies frequently affect particular tasks but not (entire) jobs, which consist of multiple tasks (see, e.g., Acemoglu and Autor, 2011 )—IA innovations may help workers be more productive in their jobs by taking over, or improving, certain tasks. For example, a doctor is engaged in diagnosis but also in explaining the diagnosis to the patient. AI may do a better job in diagnosis – for example, in radiology – but it may not quite replace the doctor in communicating with the patient, at least not yet.
Driverless trucks provide another example: truck driving provides significant employment opportunities for men with only a high school education so there is understandably concern for the disruption that self-driving trucks might bring about. But truck drivers also perform a number of related tasks – they fill orders, load and unload, monitor the truck, and more – not all of which may be easily automated. More generally, most jobs have multiple dimensions and consist of multiple tasks. With some tasks automated, workers will be able to devote more attention to, and perform better at, those tasks that are not. Importantly, both AI and IA imply extensive restructuring of the economy.
The central concern of this paper remains: there may be a reduction in the demand for labor, especially for unskilled labor. We will further evaluate whether or not these fears are justified below in Section 3. If, however, it turns out that AI is labor saving, and especially if it is unskilled labor saving, the consequences for developing countries would be severe. This is the “resource” which constitutes their comparative advantage and in which they are relatively rich. The convergence in standards of living between developing countries and developed that has marked the past half century would be arrested, even reversed. It would also present great challenges to domestic policy within developing countries. In many parts of the world, inequalities within developing countries are greater than in developed. AI would exacerbate those inequalities – and developing countries often lack the institutional capacities to counteract them.
- B. Resource-Saving Technological Progress
Another type of progress that is of great concern to some developing countries is resource-saving technological progress. This has gotten less attention than labor-saving progress so far (e.g., Solow, 2009 ), but AI and other digital technologies have often been praised for their potential to produce more output with fewer natural resources. For instance, they may help reduce the demand for depletable natural resources and lower carbon emissions. Examples include algorithms that optimize efficiency in data centers or that make transportation networks more efficient. Technologies that enable telework may also reduce the carbon footprint of workers. 7 Thus, such resource-saving innovations may have adverse distributional effects on developing countries that have a comparative advantage in natural resources, and that have specialized in exporting them. The impact on exporters of different types of natural resources may be quite different – for example, exporters of carbon-based energy will fare differently from exporters of rare earth metals.
Consider oil-exporting countries, which have already experienced many developmental challenges while being resource-rich. Resource-saving AI, while saving the planet, would make them resource-poor countries that still experience the same developmental challenges. The challenges of addressing global inequality under such a scenario would be an order of magnitude larger than they are even today, posing a test for the global community. A number of oil-exporting countries rely on their export revenue to buy food and other basic essentials—if they lose their ability to export oil, the consequences would be dire. Thus, as in the case of labor-saving technological progress, the world as a whole may be better off—in this case by undoing resource scarcity and reducing climate change—but not all countries would benefit. 8
- C. Information, Digital Monopolies and Superstars
So far we have considered the effects of technological change in a competitive environment. However, the rise of AI and other information technologies may also lead to greater concentrations of market power. As a result, the economy may move to an equilibrium that is less competitive and more distorted by market power, with greater rents for dominant firms. Actors with market power will use that power to advantage themselves. The resulting distortions may offset part of the benefits of innovation, exacerbating the adverse distributive effects of labor-saving or resource-saving innovation. With any inequality-averse social welfare function, societal welfare could decrease.
While the assumption of competitive markets often provides a useful benchmark, that model becomes less appropriate as one considers an economy that is dominated by AI. It is hard to conceive of an AI economy being competitive, or at least well-described by the standard competitive equilibrium model.
There are several reasons why advances in AI may intensify market power. First, AI is an information good, and information goods are different from other goods in that they are non-rivalrous – they can be used at close-to-zero marginal cost, implying that a single firm can serve a very large market. Moreover, the creation of AI codes or ML algorithms typically involves high sunk costs and/or fixed costs – in a private market, firms need to earn monopoly rents to recoup these costs. Moreover, even small sunk costs may result in markets not being contestable, i.e., there could be sustained rents and profits. In addition, AI applications and platforms typically involve significant network externalities. Some of these arise because firms accumulate vast amounts of data that allow them to train their algorithms better than those of the competition. All of these effects create large barriers to entry and a tendency towards creating large monopolies, sometimes also called “superstar” effects (see, e.g., Korinek and Ng, 2019 , and Stiglitz and Greenwald, 2014a ).
Some authors have identified a growing number of “superstar firms” in the economy that are “super profitable” (see, e.g., Autor et al., 2020 ). However, rather than reflecting “super-productive” technology, much of these profits may arise from the exercise of monopoly power that is derived from the nature of these information technologies. For example, in the US, a large fraction of the gains in the stock market over the past decade have been concentrated in digital giants, to an important extent driven by their market power. Moreover, algorithmic advances have also enabled digital firms to extract more consumer surplus through discriminatory pricing.
Such superstar and monopoly effects are likely to play out not only at a company level but also at a country level, and they are likely to be particularly severe in the context of AI. They may be exacerbated by agglomeration economies associated with R&D in AI. There is a risk that those countries that lead in the advancement in AI may reap all the benefits, becoming “superstar countries” and reaping all the rents associated with the development of AI. The rest of the world, and in particular most developing and emerging economies, may be left behind, with the notable exception of China – one of the leaders in AI. Moreover, to the extent that firms or countries can protect their knowledge, the resulting monopolization of knowledge may also impede the catching-up process. Importantly, even if competitors could “steal” a superstar’s knowledge, this may not necessarily be sufficient as the superstars can continuously improve their algorithms based on their users’ data, thus remaining, perhaps permanently, ahead. In the past, advances in technology were driven to an important extent by basic research that was financed by governments in high-income countries and that was freely available to all—including to developing countries. This too may change with AI.
Some observers suggest as a silver lining for developing countries in that ML technologies are reliant on data and that more diverse data contain more information. Thus, selling data might generate some income for developing countries. However, this is unlikely to make up for their lost income as the marginal return to more diverse data may be limited. Moreover, future advances in ML algorithms may make them less reliant on large quantities of data and instead require more specific, tailored data.
- D. Misguided Technological Progress
Economic theory has illuminated why the nature of innovation (e.g., the factor bias) may not be welfare maximizing. Much of economics takes the factor bias of technological change as exogenously given, and the standard economic welfare theorems assert the efficiency of competitive market economies for a given level of technology. However, the direction and rate of technological progress are themselves economic decisions, as emphasized by the literature on induced innovation (e.g. Kennedy, 1964 ; von Weizsäcker, 1966 ; Samuelson, 1965 ; Atkinson and Stiglitz, 1969 ; Acemoglu, 1998 , 2002 ; Stiglitz, 2006 ). There is no analogue of the welfare theorems for innovation: markets on their own will not in general be efficient either in the level or direction (nature) of innovative activity and technological change. The market may even provide incentives for innovations that reduce efficiency by absorbing more resources than they create for society, as may be the case, for example, for high-frequency trading. This calls for policy to actively steer technological progress, as we will discuss further below.
The fundamental problem is that knowledge is a public good, in the Samuelsonian sense. If it is to be privately financed and produced, there must be inefficient restraints on the use of knowledge, and those restraints typically also give rise to market power. If there are no restraints on the use of knowledge, then innovators cannot appropriate the returns to their production of knowledge, and so they will have little incentive to innovate. 9 When knowledge is produced as a by-product of learning or investing, the inability to fully appropriate all the learning benefits will lead to under production or underinvestment in sectors of the economy associated with high learning and learning spillovers. As Greenwald and Stiglitz (2006) and Stiglitz and Greenwald (2014a) point out, this has important implications for developmental policy, providing a rationale for industrial and trade policies. 10
More recent literature has drawn attention not only to biases in the level and pace of innovation but also to the direction. In economies with incomplete risk markets and imperfect and/or asymmetric information (i.e., in all real-world economies), the equilibrium is not constrained Pareto efficient, and prices do not necessarily give the “correct” signal to innovators on the direction of innovation. There are pecuniary externalities that matter. 11 For instance, in the Shapiro-Stiglitz (1984) efficiency wage model, where unemployment acts as a disciplining device to discourage shirking in the context of a labor market with imperfect and costly monitoring, there will be too much labor-augmenting technological progress, resulting in too high a level of unemployment ( Stiglitz, 2006 ). There are multiple other biases, for example, towards innovative activities in which intellectual property rights are more easily secured, and in drugs, to me-too innovations, where private returns can markedly exceed social returns.
Markets do not care about income distribution. Market forces may drive economic decisions towards efficiency—in the narrow, microeconomic sense— but will not give any consideration to the distributive consequences. Recent contributions, however, have emphasized that overall economic performance can be affected by inequality ( Ostry et al., 2019 ; Stiglitz, 2013 ); obviously, individual entrepreneurs will not take into account this macroeconomic externality, and accordingly the market will be biased towards producing too much labor-saving innovation, creating a role for redistributive policies. In addition, Korinek and Stiglitz (2020) show that in the presence of constraints on redistribution, policy can improve welfare by steering innovation to take into account its distributive implications.
There are some self-correcting forces: for example, if labor is getting cheaper, innovators face smaller incentives to save on labor, providing a corrective mechanism within the market economy to an ever-decreasing share of labor, but this mechanism no longer works when wages are set by efficiency wage considerations or reach subsistence levels. 12
What is most relevant for developing countries is that these distributive implications extend across borders, and so decisions made in one country have effects on other countries that the innovating country and the innovators within that country have no incentive to consider. Even if markets were efficient in the choice of technology for the conditions of the country in which the innovation occurs, those conditions are markedly different from the conditions in other countries. In developing countries, a key question is about adopting appropriate technologies rather than innovating, but the same kind of analysis that argues for the need for government intervention in steering technological innovation also provides arguments for intervention in steering technology adoption. This is especially so if, after the initial adoption of technology from abroad, there is further adaptation to local circumstances, and the benefits and costs of the technological evolution are not fully appropriated, for example, in the process of learning by doing. These concerns have long been at the center of concern of industrial policy.
- E. Broader Harms Associated with AI
There are also a number of broader harms associated with AI that have recently received a lot of attention—the ways in which new technology can affect security (including cybersecurity), privacy, incitement to “bad” behavior, including through hate speech, political manipulation, and, in the economic arena, price discrimination, sometimes exacerbating pre-existing societal divides.
While these matters affect both high-income and developing economies, an important concern is that the international community may address them in a way that does not reflect the priorities and needs of developing countries. Policymakers in many countries are beginning to discuss appropriate regulatory regimes and a set of rules to address these potential harms. It is unclear whether developing countries and emerging markets will be sufficiently represented at the table when these discussions take place. In fact, many of the standards, rules and regulations are likely to be set by high-income countries and China (e.g., Ding et al., 2018 ; Sacks, 2018 ), even though the impacts may be larger, and potentially different, on developing countries and emerging markets.
Moreover, the institutional capacity of developing countries to counter these harms may be more limited—especially when facing off against the technology giants. Weaker institutional foundations may make some countries more prone to abuses of autocratic and totalitarian leaders using mis-/disinformation and surveillance technologies. Less educated populations may suffer more from the consequences of mis-/disinformation, such as those associated with the anti-vaccine movement.
III. Evaluating the Uncertainties and Opportunities
- A. Uncertainty about the Pace and Scale of Progress
The impact of technological change depends heavily on its pace and scale. If it occurs slowly, there is time to adjust. If automation is limited to a few tasks or sectors at a time, the impacts will be limited. However, there is a great degree of uncertainty about the pace of change and the magnitude of the coming disruption, even among experts in this area. Some economists (e.g., Gordon, 2016 ) assert that we are not in an era of unprecedented innovation, and that economic growth will be less rapid in the future than it has been over the past century. In fact, Gordon (2016) argues that indoor toilets and electricity had far bigger consequences on people’s standards of living than more recent innovations. Another view is that AI is a truly transformative technology—a General-Purpose Technology (GPT)—that has the potential to revolutionize every sector of the economy (e.g., Trajtenberg, 2019 ). Like steam engines or electricity in previous technological revolutions, this view predicts that AI will lead to significant productivity gains and structural changes across the entire economy.
An even more radical perspective that goes back to John von Neumann is that AI may eventually advance to a point where AI systems reach human levels of general intelligence. This may imply that they can also do research, design better versions of themselves and thereby recursively self-improve, giving rise to accelerating technological progress and, in the words of von Neumann, “the appearance of approaching some essential singularity in the history of the race beyond which human affairs, as we know them, could not continue” (see Ulam, 1958 ). 13 The concept of such a singularity has been popularized by Good (1965) , Vinge (1993) and Kurzweil (2005) , and is being increasingly discussed among economists (e.g. Nordhaus, 2015 ; Aghion et al., 2017 ). Predictions of when such a chain of events might occur, however, continue to be perpetually revised— Armstrong et al. (2014) note that over the past six decades or so analysts have continued to expect “the development of [general] AI [to occur] within 15–25 years from whenever the prediction is made.” 14
This last perspective emphasizes that AI-driven machines may not only be physically stronger than humans and better and faster at processing information, but in an increasing number of domains, they may also learn better and faster than humans. 15 Thus, AI may be much more disruptive than a “mere” GPT; AI programs are increasingly replacing tasks previously performed by humans. If machines can engage in all tasks that have traditionally been performed by labor, and if they can do so at ever lower cost, then traditional labor would eventually become redundant, with the marginal product of human labor possibly falling so low that it no longer covers the subsistence cost necessary to keep a human alive ( Korinek and Stiglitz, 2019 ). This would represent the extreme case of labor-saving innovation: it is in fact labor-replacing innovation – employing labor would become a strictly dominated technology. 16
We discussed earlier some studies examining which jobs may be replaced by automation and AI in coming decades, typically based on job- or task-level data. The predictions in these studies vary widely, ranging from a relatively small percentage of 14% of all jobs ( OECD, 2019 ) to an estimate of 20–25% (Bain and Company, 2018) and almost 50% by Frey and Osborne (2017) and McKinsey Global Institute (2017) . Even the lower numbers suggest a significant effect, especially because the impact may be concentrated in certain industries and among certain groups of workers, specifically among unskilled and routine jobs. Knowing what fraction of all jobs will be lost to AI therefore does not necessarily provide a good metric of the impact on income distribution, and especially so in the short run.
Applying our earlier insights on steering innovation to economic research, economists should steer their research in directions where the expected social value added of economic analysis is greatest, that is, where it has the highest welfare impact.
Even if some of the described scenarios have a relatively low probability, it is important to think particularly hard about events that will be highly disruptive to society, to think through the consequences, and to prepare for how we might ameliorate some of the more adverse effects. Extensive labor replacing innovation would be such an event. Even if one places a relatively low probability on such an event—and one may argue that it is not actually a low-probability event—the associated social repercussions would be sufficiently large that it makes sense to focus attention on such an event. Studying scenarios that pose the most adverse social impacts would better prepare economies to deal with them when they occur— and they also provide valuable lessons for scenarios in which the impact is less stark.
- B. The Productivity Puzzle: Are We Really in an Era of Unprecedented Innovation?
In relating the debate about the economic significance of AI-based innovation to recent economic data, we encounter a well-known puzzle: if we are really living in an era of significant technological disruption, why are the increases in innovation not showing up in GDP data? This is analogous to the puzzle of missing productivity growth from computerization that Bob Solow described in the 1980s when the GPT of the time – computers – spread throughout the economy ( Solow, 1987 ). It took until the following decade for US national accounts to show a pickup in productivity growth. Part of the explanation for the productivity puzzle is that there are long lags, as was the case for computerization. At present, AI is influential in a limited number of sectors, like inventing better ways of advertising. Even if AI is transforming advertising, this will not transform our overall standard of living. (In this particular case, it may actually lower overall efficiency, as it may undermine the price system by enabling pervasive discriminatory pricing.) Going forward, many sectors of the economy will require complementary investments and changes in processes and organization as well as new skills among their workers to take full advantage of AI (see e.g. Brynjolfsson et al., 2019 ).
Another part of the explanation of the productivity puzzle is that there are difficult measurement problems. Many recent technologies may have led to increases in societal welfare that are not captured by GDP (see e.g. Brynjolfsson, 2020 ). For example, when online services are exchanged against “eyeballs,” i.e., when users are exposed to advertisements instead of paying for services, the benefits to consumers are not included. 17
- C. Putting AI in the Broader Context of Development
There are several other important factors that are relevant when it comes to managing the potential adverse effects of AI on developing countries in coming decades.
The COVID-19 pandemic has imposed an extra shadow cost on physical interaction with humans, which is likely to accelerate the automation of jobs that require physical interaction (see e.g. Korinek and Stiglitz, 2021b ). The resulting changes will have long-lasting effects on the economy, even after the pandemic is overcome. The new technologies that are introduced now will reduce the demand for labor worldwide for some time to come. 18
Population Dynamics
Population dynamics will interact in important ways with labor-saving or -replacing technologies (see e.g. Varian, 2020 ). In countries with rapidly growing working-age populations, such as in many African countries, lots of new jobs will have to be created to maintain a given employment rate. Advances in automation that are developed in high-income countries and easily deployed around the world will make this more difficult. However, the large supply of labor may slow down the development and adoption of automation technologies within such countries (although the evidence in several countries suggests that at least in large export-oriented manufacturing, the technologies employed are remarkably similar to those in advanced countries; see Rodrik, 2011 ). 19 Moreover, young populations also generate significant demand for education, which in turn creates jobs. Overall, even countries like India face difficulties in creating enough formal sector jobs to keep pace with the growing working age population. The faster growth of population makes capital deepening more difficult, slowing the pace of growth in income per capita.
Conversely, in countries in which the working-age population is declining, such as China, the impact of job automation on the workforce is mitigated as workers that are replaced by technological progress can simply retire. Moreover, aging populations create large service sector needs, particularly in healthcare. Many of these service sector jobs are unlikely to be replaced by automation or AI in the near future. Overall, the evidence suggests that aging societies adopt new technologies and automate ( Acemoglu and Restrepo, 2019b and Figure 5 ). 20
Population Aging and Automation
The Green Transition
A third important force affecting developing countries in coming decades is the threat of global warming, which calls for significant public policy interventions to facilitate the Green Transition, i.e., the transition away from an economy that is dependent on fossil fuels to one that is more environmentally sustainable and relies more on renewable energy. Without global policies to save our planet, developing countries will experience some of the largest losses from global warming.
There are many similarities between the effects of AI and the Green Transition. Both involve large changes in relative prices and generate significant redistributions, and many developing countries will be strongly affected. The Green Transition is similar to resource-saving innovation and risks undermining the standard of living of oil-exporting countries, among which there are a number of low-income countries.
There is also an important complementarity between the Green Transition and AI: the Green Transition is likely to increase the demand for labor which could offset some of the negative effects on labor demand of automation and AI. Indeed, given the labor needed for the Green Transition, the labor replacement due to automation and AI in many activities, including manufacturing, could be considered a fortunate development enabling countries to better address the challenges of climate change. There is thus an inherent tension in frequent claims that on the one hand economies cannot afford to mitigate climate change (i.e., that there are insufficient resources), and on the other hand concerns over a potential crisis with a surplus of labor arising from labor-saving AI. 21, 22
However, we do face challenges in how to channel surplus resources into what is required for the Green Transition. Some of the skill sets of those labor resources freed up by technological progress will differ from those needed in the Green Transition, although Louie and Pearce (2016) argue that the retraining costs would be moderate , and many of the investments (such as installing solar panels) require only limited skills.
There may be institutional constraints that make it difficult to reallocate capital towards green investment. While many sources of savings are long term (pension funds and sovereign wealth funds) and the investments needed for the Green Transition are long term, standing in between are short-term financial markets. Local, national, and multilateral Green Development Banks may be helpful in financing the private green transition. Better disclosure to investors of risks associated with “brown” investments (i.e., ones that contribute to pollution) and changes in fiduciary standards for asset managers towards their investors, would help move resources into green investments. Of course, without strong incentives, provided by price signals and environmental regulatory constraints, incentives for green investments and innovation will be greatly attenuated.
IV. Lessons from Past Technological Transformations
To grasp the historical nature of what is going on, it is necessary to put the advent of AI and related technologies in the context of the broader history of technological progress. Humanity spent much of its history at a Malthusian stage. The Industrial Revolution started a little over two centuries ago, and was but a blip in the history of mankind. The era of manufacturing-based export-led growth that enabled the East Asian Miracle stretched over the past half-century – one quarter of the history of the Industrial Revolution. It is easily conceivable that we are now going into another era.
Many are far more sanguine than we are about the disruptive potential of AI. They point to the automobile and other innovations at the end of the nineteenth century. Jobs were lost, making buggy whips and horse carriages obsolete, but overall, labor demand increased, and more jobs were created. Our analytical discussion made clear that there is no inherent reason that innovation has these effects. This time could well be different. Looking at the time before the Industrial Revolution and the early decades of the revolution itself serves as a reminder.
- A. Pre-Industrial Revolution
Before the Industrial Revolution, innovation proceeded at a far slower pace than today. There were still many innovations, but the actual living standard of the vast majority of people was stagnant ( Maddison, 2003 ). The interpretation of Malthus (1798) was that every time an innovation took place, the population started to grow and absorbed the surplus that was generated.
This pre-industrial state of affairs may be still relevant in the least developed countries and is particularly problematic in some African countries, where the death rate has been greatly reduced by medical innovations, but reproductive rates have continued to be very high. The affected countries have been slow to go through the demographic transition that marked the rise of living standards in Asia. As a result, several countries are facing a difficult-to-manage explosion in population combined with stagnant living standards.
There is a risk that poor countries may see a return to Malthusian dynamics if technological progress undermines the source of their comparative advantage. Consider a country that exports manufacturing goods produced using cheap labor but is not very productive in agriculture, for example because of a shortage of land and a high population density. The country uses its export revenues to import food for workers in the manufacturing sector, granting them a living standard that is above subsistence levels. If a new technology produces the manufacturing goods more cheaply, the wages of the manufacturing workers will fall, and they may well fall below the subsistence cost of workers. If that is the case, the country may return to a Malthusian state of affairs in which part of the population suffers from hunger and deprivation. Increasing agricultural productivity may mitigate this dire state of affairs but the question is, would they be sufficient to support a population that was previously supported by imported food? Thus, populations may decline not as a result of choice, as in many developed countries, but from Malthusian dynamics. In today’s globally connected world, that presents ugly alternatives: Will the rich countries simply look away, as they see this suffering and near-starvation in poorer countries? Will they create ever-increasing barriers to stave off the inevitable pressures of migration?
- B. Industrial Revolution
The Industrial Revolution marked the beginning of rapid growth in high-income countries. After centuries in which standards of living had been stagnant, growth started to increase markedly. It transformed the world. The Industrial Revolution thus provides us with a number of lessons that are very relevant today:
Innovation Can Be Very Disruptive
Even when an innovation ultimately proves to be beneficial for society at large, not everyone benefits. It can give rise to very large disruptions during the transition. In the short run, there was significant social upheaval from the industrial revolution—Charles Dickens’ novels make it clear that not everyone prospered. In the UK, some people were living under much worse conditions in the cities of the mid-19 th century than they had been in the rural areas prior to that. Even indicators such as life expectancy initially went down. Looking at those who suffered, the Industrial Revolution was clearly not a Pareto improvement.
Collective Action Can Mitigate the Adverse Effects
The onset of the industrial revolution posed many challenges that required collective action. However, it took time for societies to put in place the collective mechanisms to respond to these challenges. This is why the industrial revolution had significant negative effects on the masses for some time. Eventually, governments played an important role in mitigating the adverse effects, including the problems posed by urbanization, such as challenges in sanitation, environmental degradation, public health, infrastructure, and congestion.
Government took a strong role too in advancing the positive effects of the new economy. Education was an important element in creating a productive workforce – it was therefore also in the interests of capitalists, and public education received broad public support.
In high-income countries, institutions related to labor legislation, unionization, and social safety nets were not created until the end of the 19 th century and beginning of the 20 th century. In the United States, the ready availability of land implied that labor was relatively scarce, limiting the extent to which labor could be exploited. Nonetheless, in the early years of the 20 th century, labor was not doing very well. It was only dramatic events like the 1911 Triangle Shirtwaist Factory fire in New York City that led to labor legislation that really protected workers. In most high-income countries, labor legislation today is taken for granted, but in 1900, it was not obvious if meaningful labor legislation would ever be enacted. Strikingly, some of the tough political battles that made the adoption of such legislation problematic a century ago are playing out once again in the United States, where there has been an erosion of protections, for example, those associated with minimum wages, health and safety standards, or overtime pay, among others.
These labor market reforms helped support the structural transformation that occurred with the rise of manufacturing, and they showed that equality and growth are complementary (e.g., Ostry et al., 2019 ). At a basic level, they were necessary to sustain social peace and democracy. And they ushered in what might be called an “Age of Labor.” Most developing countries have not gone through this process yet.
This Age of Labor may not last forever. In the US, minimum wages have declined in real terms in recent decades, below the level of fifty years ago ( Figure 6 ), and many protections on hours and working conditions have been eviscerated. Advances in AI may further contribute to undermining labor’s bargaining position and thus these social protections. And in developing countries, they may do so before workers have ever acquired similar levels of rights and protections as they have in high-income countries.
Federal Minimum Wage (adjusted for inflation) in the US, 1938–2020
Politics and Political Economy
The Age of Labor conferred not only unprecedented economic returns upon workers in the form of rising wages, but also, in parallel, unprecedented political power. However, this power has been eroded more recently (see e.g. Boix, 2019 ). In simple models of democracy, the median voter (or more broadly, the “majority”) determines political outcomes. But the evidence is that that model provides a poor description of the outcomes of the political process. For instance, the majority of voters want a more egalitarian society (see, e.g., chapter 1 in Cerra et al., forthcoming ). But in recent decades, in many countries, the political and economic rules have evolved in the opposite direction, giving more influence to the power of “money”. 23 , 24
- C. Manufacturing-Based Export-Led Growth
In developing countries, there has been a single model of development that has proved enormously successful over the past fifty years: manufacturing-based export-led growth (see Stiglitz, 2018a ). It enabled many East Asian countries to close the gap between themselves and high-income countries, increasing per capita incomes in these countries multifold.
One big change inherent in this development strategy was moving from discussions of static comparative advantage to more dynamic comparative advantage. This was central to the East Asia “Miracle.” Half a century ago, South Korea was seen by many to have a comparative advantage in agriculture. It instead pursued a strategy of creating its own dynamic comparative advantage via an industrial policy that led it towards industrialization. That model served most of East Asia remarkably well, in a way few had anticipated (e.g., Myrdal (1968) who predicted that Asia would never develop). See also Aghion et al. (2021) .
The path to development in East Asia has been via exports of cheap labor-intensive manufactured goods. This development strategy combined learning, the provision of employment opportunities, foreign exchange, tax revenue—everything that was needed for a quick developmental transition.
While their development trajectory began with taking advantage of their static comparative advantage in cheap labor, and especially cheap unskilled labor, over time, many East Asian countries moved up the “value” chain, producing higher value added and more complex products and developing their dynamic comparative advantage.
Earlier advances in technology have already reduced the importance of cheap labor; but now advances in AI may erode it further still. Going forward, growth led solely by exports of labor-intensive manufacturing goods will no longer be available as a strategy of development. Indeed, the share of manufacturing employment is decreasing globally. Moreover, the jobs that can be outsourced may be more easily automated. There may be reshoring of production that had previously been outsourced, using highly automated production processes, and the process may have been accelerated by the Covid pandemic.
The forces that facilitated the development in East Asia may thus be going in reverse, making it difficult for other developing countries to follow the strategy.
One of the critical reasons for the success of the export-led growth model based on manufacturing goods was that it enabled developing countries to catch up in multiple domains. 25 Developing countries are poorer than developed countries not only because there is a gap in material resources but also because of a gap in knowledge ( World Bank, 1998 ). A quarter century ago, the World Bank began thinking of itself as a knowledge bank, not only helping countries to catch up in resources but also to catch up in knowledge.
AI may have characteristics that will increase the gap in knowledge and make it more difficult to catch up. While technology adoption lags have declined over the past centuries ( Comin and Hobijn, 2010 and Figure 7 ), the specific nature of AI may reverse that. Cutting-edge AI technology is highly specialized, and improvements are driven to a large degree by learning from large datasets, creating a winner-takes-all dynamic, as we noted earlier. In addition, a disproportionate share of the people working in AI are in private companies, and a significant share of the knowledge is not in the public domain and therefore not easily accessible to developing and emerging economies. (This contrasts with many past technologies, when publicly financed knowledge production was more central, so access to knowledge was more easily available to developing and emerging economies.) Moreover, an important resource input to AI is data, and access to data is concentrated and not globally public. The implication is that the nature of AI technology and how these advances are generated will make it more difficult to catch up than in the past. In fact, the exponential nature of growth in AI technology may imply that laggards not only cannot catch up, but that the gap between them and the front runners may grow, compounding the potential adverse effects that developing countries may suffer from labor-saving or resource-saving technological progress. 26
Technology Adoption Lags
- D. What is Different This Time
Not only may the AI revolution make it more difficult for developing countries to catch up, the AI revolution may also be more difficult to manage for economic policymakers than earlier technological transitions. The structural transformation from an agrarian rural economy to an industrial urban economy eventually led to a more egalitarian society. As we have noted, the reasons included that innovation associated with that transition overall was unskilled-biased, i.e., it increased the relative productivity of unskilled labor. Moreover, industrial production provided a strong force towards mass education. Furthermore, industrial production typically involved large establishments that could be unionized relatively easily, and the unions advocated for wage compression. All these forces led to greater equality. In the current transition, what risks becoming our “destination”—a service sector economy, marked by greater inequality, with less support for public education and more concentrations of market power—may be less attractive in many ways than the current situation, and the process of getting there may be more disruptive; that is, unless countervailing policy interventions are made.
AI may be labor-saving and resource-saving, and it is likely more biased towards ever-higher skills so that general education becomes less important. 27 This may reduce support for equality-enhancing public education, which has been one of the strong forces for more equalitarian outcomes in the past. Moreover, the service sector which is becoming an increasingly important part of the economy is marked by smaller establishments. In addition, worker tenure has declined, making it harder to unionize the workforce ( Choi and Spletzer, 2012 ). Digital technologies are likely to create more barriers to entry and give rise to more monopoly power and winner-takes-all dynamics, with rents going to a small number of extremely wealthy individuals and enterprises, disproportionately located in high-income countries.
Although for many developing countries, average income per capita may increase, large fractions of society may be left behind. Moreover, some developing countries may experience declines in income per capita as innovation erodes their comparative advantage. Unskilled workers in these countries may suffer the most.
Although greater inequality would increase the need for social protection, it may result in a less egalitarian politico-economic equilibrium, as the new concentrations of economic and political power may reduce support for the critical role of government in mitigating the adverse distributional consequences of technological change. (See, e.g., Gilens, 2005.)
V. Domestic Policy Responses
We have seen how economic policy played a critical role in shaping economic outcomes in previous eras of innovation; the same will be true in the case of AI. In this section, we discuss what policy levers can be employed to address the effects of technological disruption, both in developing countries and to protect vulnerable segments in advanced economies. Some of these are similar to what worked in earlier periods of technological change; some are attuned to the special problems posed by AI and labor-replacing innovation. In section 6, we will discuss changes in global policies, norms and rules that would assist developing countries in their response to technological change. In this short paper, we can only touch on a few of the more salient policies.
- A. Taxation, Redistribution, and Government Expenditures
Among the critical policies to combat rising inequality are those of taxation and redistribution, with a particularly important role for progressive taxation. However, in recent years, a number of countries have actually made their tax systems more and more regressive. For example, many countries tax the returns to capital and rents (such as land rents, monopoly rents, and other forms of exploitation rents) at lower rates than workers. In the US, the rich pay a lower fraction of their income in taxes than the majority of the population ( Saez and Zucman, 2019 ).
Raising taxes is a particular challenge for developing countries, in which the informal sector is typically much larger than in high-income economies. However, this also means that there is significant scope for developing economies to enhance their tax structures and expenditure systems (e.g., scrapping harmful subsidies and tax exemptions) to build fiscal space for public spending, and improvements in tax capacity. Also, new digital tools and new data may actually give governments new policy tools to increase tax compliance. For example, when an activity becomes intermediated via centralized digital platforms, it becomes easier for governments to access business transactions and levy taxes on them. For example, governments have long found it difficult to monitor and tax the earnings of taxi drivers. But if driving is intermediated via digital platforms, all their earnings – including most tips – are recorded. 28
One of the dilemmas when it comes to taxation and redistribution is that labor-saving technological progress reduces tax revenue from labor – traditionally the most highly taxed factor in the economy – precisely at the time when the need for redistribution rises (see e.g. Korinek, 2020 ). This necessitates that taxation increasingly shifts towards other factors and rents. From the perspective of efficiency, the taxation of rents is particularly desirable ( George, 1879 ). Imposing taxes on fixed factors, such as land, acts like a lump sum tax, and taxing rents generated by market power and political activity may discourage such rent-seeking, enhancing efficiency.
We have argued earlier that technological progress creates winners and losers, and the gains of the winners are quasi-rents that governments may be able to tax without introducing distortions. In particular, some of the monopoly rents of digital giants can be taxed without introducing major distortions into the economy.
In designing tax systems, an important concern is about incidence: the possibility that general equilibrium effects imply that taxes are ultimately borne by other factors and agents than those on whom they are levied, undermining the desired redistributive objectives. For example, a common result in simple models is that capital taxation discourages capital accumulation by capitalists. However, the adverse effects may be more than offset by public investments in human and physical capital (see e.g. Stiglitz, 2018b ). High on the list of what is desirable to tax are “bads” rather than goods, i.e., Pigouvian taxes on activities and goods that create negative externalities, for example, polluting or carbon-emitting goods. This would contribute to the Green Transition in a dual way, not only by providing tax revenue for public investments but also by correcting market prices to reflect the negative externalities. 29
Social Protection
If individuals could obtain insurance against the adverse effects of disruptive innovations, then it would be more likely that these innovations would be Pareto improvements ( Korinek and Stiglitz, 2019 ). But such insurance is not available. One of the functions of social insurance is to socialize these risks that otherwise would have been borne by individuals. But in developing countries, systems of social protection are typically less developed, making it even more likely that there be significant groups that are worse off.
Universal Basic Income
Many commentators have responded to concerns about the impact of technological progress on employment by advocating a universal basic income (UBI). While proposals differ in their detail, they typically entail that all individuals are paid a UBI independent of their employment or wealth status, and with a level of UBI payments geared above the poverty line. While such programs would imply formidable fiscal costs, and with it, possibly large distortionary taxes, those could be contained if a UBI replaced other social safety programs (such as social security, welfare, or unemployment insurance systems). By doing so, it would also reduce the overall administration costs.
From a global welfare perspective, a global UBI that was truly “universal” as the name suggests, i.e., that covers all citizens of the world equally, would be most desirable, given the potentially large global implications of AI. Currently, access to prototypes of a UBI is exclusive to people who were lucky to be born in specific locations that have the fiscal capacity to afford such programs (e.g., in Alaska where oil revenue is collected in the Alaska Permanent Fund and distributed to the residents of the state). But given the limitations on cross-border transfers that have been the center of attention of this paper, a global UBI is clearly still in the realm of fantasy. 30 , 31
However, in the short- to medium-run, the focus should be on creating jobs for everyone who is able and willing to work, especially in light of the earlier discussion of how much labor will be needed for the Green Transition, to provide services to the young, the sick, and the elderly, and to invest in infrastructure. Governments may have a role to play in helping match the need for work and people willing and able to work. However, while a clear need for a UBI may be in a more distant future, there are other policies that may achieve similar objectives to a UBI. For example, one approach to ensuring a modicum of income for all over the long run, with co-benefits of perhaps increasing social cohesion and solidarity, is shared capital ownership (e.g., Solow, 2009 ): as part of government assistance programs (such as those enacted in the wake of COVID-19 in 2020), firms receiving government help should contribute shares to a sovereign wealth fund—owned by everyone within the nation. Similarly, firms that build on or employ innovations that are based in part on government-funded research should be required to do the same. 32
Starting with Keynes (1931), economists have argued that technological progress and automation would in principle enable people to work less and spend more time on more meaningful activities rather than tedious and repetitive tasks – a point also emphasized, for example, by Varian (2020) . However, this requires either that wages go up in tandem with productivity growth, unlike in recent decades, or that the fruits of progress are shared more widely using transfers. If these questions of distribution can be solved satisfactorily, then individuals could indeed respond to productivity growth by working less without experiencing material losses. There is considerable evidence that many workers would prefer to work less and with more flexible work sessions. The Dutch model, which provides all workers with a right to part-time work (at pro-rated wages) could serve as an example, assuming that wages are sufficiently high. 33 , 34
Expenditure and Infrastructure Policy
Expenditure policy can be as important in offsetting the adverse effects of AI as taxation and direct redistribution, and it carries several benefits over transfers that are particularly relevant in developing countries: government expenditures may be easier to target based on need, and for whom the social returns of those expenditures may be high. For instance, expenditures on human well-being, such as on education and health, are naturally targeted to those who need education and healthcare, rather than being spent on those who already are educated or on those who are healthy. Expenditures to protect the environment help those who bear the brunt of environmental degradation, including climate change, which disproportionately affect the poor. 35
Expenditure policies that increase the demand for unskilled labor may serve double duty: they raise demand for unskilled labor, increasing the equality of market income (what is often now called pre-distribution), and sometimes they can be targeted so that the benefits of the expenditure go disproportionately to the less well-off. One important example is infrastructure investments in poorer neighborhoods, which are a labor-intensive expenditure that can be designed to be pro-egalitarian.
Of particular importance are investments in digital infrastructure that reduce the “digital divide” and allow citizens to access the vast services provided by the Internet. Recent advances in network technology allow developing countries to leapfrog older technologies in which high-income countries have invested fortunes, for example by using wireless 5G technologies instead of laying vast networks of cables.
Other infrastructure investments include public transportation systems that connect especially lower income workers with jobs and enhance the opportunities available to them. Another example of labor-demand increasing public expenditures is creating service sector jobs, for example in healthcare, caring for the elderly, and some aspects of education, which can again be designed to serve double duty – disproportionately benefiting the poor and needy as they increase wages by increasing the demand for labor.
- B. Pre-Distribution
Our concern here is the distribution of consumption (or more broadly, of well-being) among the citizens of a country. That is affected by inequalities in market incomes and the extent of redistribution. The previous subsection discussed redistribution through tax and expenditure policies. But a society with a more equalitarian market distribution needs to place less burden on redistribution. Good policy entails an optimal mix of “pre-distribution”—actions to increase the equality of market income—and redistribution. This is especially so because some of the actions to increase the equality of market distribution are actually efficiency-enhancing, i.e., have a negative cost. For instance, actions which reduce market power, the ability of firms to exploit information asymmetries, or to engage in a variety of other exploitive practices.
There are two categories of policies which affect the distribution of market incomes: (1) Policies that affect individuals endowments of assets—human capital (education) and financial assets. These are affected by the public provision of education and more broadly, policies which affect the intergenerational transmission of advantage and disadvantage (such as inheritance taxes.) And (2) policies that affect the returns on factors, which include the laws and regulations that determine the “rules of the game.” These include competition laws, labor legislation, and rules governing globalization, the financial sector, and corporate governance. These rules affect simultaneously efficiency and distribution. 36
Education Regarding the first set of such policies, the fact that more educated workers receive higher incomes than less educated ones may invite the conclusion that education is the solution to inequality. While providing more equal access to high-quality education especially for the poor may reduce inequality—and is absolutely essential to avoid an education-based digital divide whereby some simply do not know how to access and benefit from the resources and opportunities offered by the Internet and related digital technologies—education is far from a panacea. Indeed, if there are large innate differences in ability, education can identify and amplify these differences, actually increasing inequalities in market income. ( Stiglitz, 1975b ). Moreover, education cannot address the problems arising from the declining share of labor income overall.
Steering Innovation in AI in High-income Countries
The overall direction of innovation in AI will be set to a large extent by high-income countries plus China. This implies that the direction of technological progress in those countries – how labor-saving it is – also matters for developing countries that will be exposed to the new technologies.
Korinek and Stiglitz (2020) make the case for actively steering technological progress so that it is more labor-using. They show that whenever lump-sum transfers are not available, it is desirable to encourage technological progress that leads to higher demand for those types of workers with the lowest incomes. This can be done by nudging entrepreneurs, by considering the labor market implications of government-sponsored research, or by explicit incentives provided to the private sector. Klinova and Korinek (2021) and Partnership on AI (2021) describe how to develop and how to operationalize frameworks for steering advances in AI towards greater shared prosperity.
Many governmental policies have indirect effects on incentives for innovation. For example, at least in the short run, the cost of capital is influenced by monetary policy, with the goal of stabilizing aggregate demand. In recent years, monetary authorities in many countries have set interest rates such that real returns on safe assets have been very low or even negative, likely below the social shadow price of capital. Stiglitz (2014) shows that this encourages excessive automation in high-income countries. Acemoglu et al (2020) observe that tax policies that favor capital over labor also distort the direction of progress towards saving labor.
And there are immediate implications for developing countries: Once the cost of developing a labor-saving innovation has been incurred in high-income countries, it can frequently be rolled out globally at comparatively low cost, potentially imposing significant welfare costs on workers in developing countries. Examples include self-checkout kiosks that harm workers, whatever their benefits or costs may be for consumers and global corporates.
Pritchett (2019) observes that migration policies in high-income countries restrict labor supply and lead to comparatively high wages that do not reflect the abundance of labor, and in particular of unskilled labor, at the global level. The high wages then provide innovators in high-income countries with excessive incentives to invest in the automation of tasks that are performed by unskilled labor compared to what is desirable from the perspective of developing countries (or from the perspective of global efficiency). 37
Economists are also becoming increasingly aware of the importance of regional heterogeneity. Unlike in stylized models in which only national borders exist, labor does not move seamlessly across regions within countries. Even in high-income countries, large disparities between regions or between rural and urban areas persist, as illustrated, for example, by the case of northern and southern Italy or by the rural/urban differential in the United States and many other countries. Looking ahead, the “good” jobs of the future (e.g., those hard to automate and/or complementary to AI) might be located in major urban centers (such as non-routine manual occupations and personal services). Such geographical disparities call for location-based policies in fostering development, although the details of such policies can be complex. For example, they may entail a trade-off between income growth (which benefits from geographical concentration) and geographical inequality (which does not).
New Development Strategies
Developing countries will need a new multi-pronged development strategy to replace the manufacturing-led export-based growth model. Industrial policies have traditionally been among the most important aspects of countries’ development strategies—interventions that shape the direction in which the economy is moving, with particular emphasis on the secondary sector. However, in an age of increasing automation in manufacturing, development strategies have to broaden their focus beyond manufacturing and the secondary sector to other sectors of the economy, including agriculture and services. 38
Greenwald and Stiglitz (2014b) point out that every country has, in effect, a sectoral development policy—shaped by infrastructure and education investments and tax and regulatory policy. It is only that some countries do not know (or admit) that they have such policies. The danger then is that such policies can be more easily captured by special interests. 39 In developing countries development policies are much more at the center of economic policy. They need to be designed to manage innovations and mitigate the effects of and adapt to the disruptions that innovations may engender, to ensure that the net societal benefits, broadly defined, are maximized.
A lot of innovation in developing countries focuses on technology adoption and adaptation rather than developing entirely novel technologies. Whereas high-income countries focus on “steering innovation,” developing countries need to pay attention to “steering the adoption of technologies.” Their development strategy should intentionally focus on steering the adoption of labor-using technologies that have already been developed in high-income countries, adapting them to their own circumstances and needs, redesigning them, and building on them. Decisions on what type of inward FDI to encourage should also be informed by these objectives.
In designing the new development strategies, developing countries will need to think carefully about the rationale for public interventions: how can government improve upon the decisions made by decentralized agents? Of particular importance is that the direction of technological progress and technology adoption is endogenous, and there is no presumption that market decisions in this area are socially desirable. Decisions made at one date have effects in later periods, with firms making the decisions appropriating only a fraction of the benefits and bearing only part of the costs of their decisions. For example, this is clearly manifest when there are knowledge spillovers to other firms and when technology evolves over time, e.g., through learning by doing. Firms acting on their own will not fully consider the dynamic implications of their decisions today on others.
There are also market failures beyond the ability to appropriate the returns from current choices—for instance, imperfections of risk and capital markets. The capital market imperfections that impede the reallocation of labor in high-income countries in response to innovation—and that can result in innovations which decrease welfare —are even more important in developing countries, making it imperative to combine industrial policies with active labor market policies (see, e.g., delli Gati et al., 2012a, 2012b).
Relatedly, part of the problem is that market prices do not adequately reflect social shadow values. A well-known example is that, in the absence of appropriate regulation, the price of carbon in the market is zero, but this does not reflect the social cost of carbon. Similarly, market prices do not reflect the social value of an equitable distribution of resources and do not guide innovation in that direction. Given the constraints on redistribution, this leaves an important role for the government to steer innovation and foster economic development in a socially desirable direction ( Korinek and Stiglitz, 2020 ). For example, much could be gained from encouraging innovators to shift their focus from labor-saving towards more labor-using technologies.
Fortunately, while the new technologies necessitate a change away from the old and highly successful development strategies of the past half century, they also open up new opportunities. In agriculture, AI offers the potential for large productivity increases based on algorithms that help farmers fine-tune and optimize a range of decisions that increase their yield. Such algorithms depend on crops, soil and weather conditions and need to be customized to local conditions. Just as agricultural extension services, which extended general knowledge about agriculture to local farmers, played a critical role in the development of the US, there is an important role for government agricultural extension services today in developing countries. 40 Digital platforms can also enhance the ability of small farmers to trade their products at fair market prices, reducing the market power of middle men that frequently absorb a significant fraction of the surplus generated in agriculture.
Developing the service sector is crucial for economic development as the role of the primary and secondary sectors is declining. Many developing countries may carve out new areas of comparative advantage in services that will, however, depend on good internet connections and a certain degree of education of the workforce. For example, call centers and similar business and consumer services rely on requisite language skills. There is also a growing market for simple human services that can be broken down into small components and fed into AI systems (e.g., labeling images). However, as we noted earlier, services that can be outsourced are often also more easily automated. Other services such as tourism have proven a more automation-resistant (although not pandemic-resistant) source of export revenue for countries that have managed to fashion themselves into desirable tourist destinations. Exporting services offers many of the potential growth benefits of the manufacturing-based export-led growth model.
Services that are aimed at a domestic audience, for example, healthcare, caring for the elderly, as well as education, may not deliver much export revenue but are important for economic development and welfare. There is much scope for employing AI to improve the delivery and efficiency of these services, and it requires government policy to do so since private service providers are frequently small in size and cannot afford the necessary investments. And even in these areas, there may be significant opportunities for cross border trade, for example, via medical tourism and via retirees from advanced countries relocating to warmer climates, if adequate health care is available.
VI. Global Governance
In a globally integrated economy—from which developing countries and emerging markets have benefited enormously in many ways—global rules matter. The global rules have always been set to favor high-income countries; they are, to a large extent, set by the large powerful countries, and frequently by powerful special interests within them, whereas developing countries do not have a seat at the table, or are at least underrepresented.
The global rules have large effects on the ability of these countries to levy taxes in the digital era, on high-income countries’ ability to extract rents from the developing countries (say through market power and intellectual property rights), and more broadly on the global terms of trade and distribution of income. 41 While developing countries may realize these inequities—and the inefficiencies—of our global economic system, it often seems that there is little they can do.
AI has provided a new arena in which rules need to be set, at the same time that it may exacerbate the imbalances in economic power, as our earlier discussion emphasized. However, there are reasons for cautious hope when it comes to the rules governing information and AI. First, the rules in this area are still in the process of being set so there is hope that international institutions and civil society may have a positive impact on the shape of these rules. Still, the fact that recent trade agreements between the US and other countries have contained provisions reflecting the interests of big-tech companies—with limited open debate and limiting the scope for these trading partners to design regimes that reflect a broader public interest—is of concern.
Secondly, it should be in the self-interest of high-income countries to avoid the possibility of a strong backlash to globalization in developing countries. The possibility of such a backlash is considerable: The United States and a number of other high-income countries, which have been big beneficiaries of globalization, have experienced such a backlash – in part because they have not ensured that the losers of globalization were compensated. In the past, there was at least some sense that globalization created mutual gains for high-income and developing countries. The backlash in developing countries would be even greater if they come to see globalization as a mechanism of rent extraction from their economies (even if the truth may be that technological change is making them lose some of the earlier gains from globalization).
Moreover, international institutions, some of which are less and less dominated by high-income countries, may play a role in ensuring that the rules are set in a way that more adequately reflects the interests and concerns of all countries, including developing countries. As the rules for new technologies are being written, there are several areas of particular concern in which reforms in global governance would help developing countries better adapt to advances in AI.
- A. A Global Tax Regime for the Digital Age
The inadequacies in the global tax regime make it difficult for developing countries to capture much of the rents that the global digital giants earn within their borders, even as their activities take away business from domestic firms and thereby reduce the domestic tax base. Indeed, even high-income countries have had difficulty with adequately taxing global tech giants. Some of the issues are now being discussed at the OECD in an attempt to establish a global tax regime.
The current global tax regime allows multinational firms to avoid much taxation—often paying taxes at rates markedly lower than local small businesses. It also impairs the ability of developing countries and emerging markets to tax the economic activity which occurs within their territories. This system is both inefficient and inequitable.
The controversy over digital taxation has exposed the deeper problems of multinational corporate taxation based on transfer prices, which are easily manipulated. The issue could be addressed by moving to a formulary apportionment system, whereby the worldwide profits of a corporation are apportioned to different countries according to a formula (see, e.g., Clausing and Avi-Yonah, 2007 ). The exact formula could have large distributive effects across countries. For instance, a simple formula based just on sales, while less manipulatable than other formulae, may disadvantage developing countries. A particular controversy associated with the digital economy is the value assigned to the data that are collected in the process of economic transactions and how and whether that value should be taxed.
The broader debate over international taxation has also led to renewed attention on closing down fiscal paradises, on international initiatives for transparency in capital ownership, which would help developing countries to increase their tax base, and on creating a global minimum multinational corporate tax rate, to prevent a race to the bottom.
- B. Global Competition Policy
The tendency of digital technologies to give rise to natural monopolies makes competition policy especially important. One challenge is that the countries in which tech giants are based have incentives to protect their own tech firms since they share in the rents that these firms earn globally. For example, when the European Union investigated Google for anticompetitive practices or when Germany investigated the privacy practices of Facebook, the US treated it as a political question rather than a matter of economic policy and responded by accusing Europe of being anti-American. While the policy remedies suggested by the Europeans may have reduced the rents the companies could earn in Europe, their purported aim was to ascertain that these firms’ practices did not violate the norms on competition and privacy established in Europe. The tendency for matters of competition policy to turn into arguments over rents may get worse, given the global concentration of market power in AI in two countries, China and the United States.
Individual developing countries and emerging market economies stand little chance in reining in the behaviors of powerful global corporations on their own – in many instances, the corporations have a higher market capitalization than the GDP of the countries in question. This makes it important for developing countries to coordinate and develop competition policy together, for example, via a common competition authority for developing and emerging economies that can exert sufficient power over large global corporations, just as the countries of Europe would not be able to police the competitive behavior of American corporations on their own but are able to do so through the European Union.
Given the breadth and reach of the new digital giants, there is a need for stronger rules preventing conflicts of interest for companies that simultaneously own a marketplace and participate in it, and stronger rules preventing pre-emptive mergers, i.e., mergers and acquisitions designed to stifle the threat of a competitive marketplace in the future. There will also be a need for more ex-post remediation: breaking up mergers when they prove to be anti-competitive. 42 As the experiences cited above have shown, the countries in which digital giants are based may not have the correct incentives to police these companies’ competitive practices, given the large global rents that are at stake.
- C. Intellectual Property Rights
The current system of intellectual property (IP) rights is designed to give (temporary) monopoly rents to innovators to compensate and reward them for their innovative activities. There has been much concern in recent years that the prevailing IP system gives excessive protection to innovators, with particularly adverse effects on developing countries. As the World Commission on the Social Dimensions of Globalization (2004) emphasized, there is a need to rebalance the international IP regime to ensure an equitable distribution of the gains from technological progress. Korinek and Stiglitz (2019) demonstrated that reducing the length of patent protection can ensure that the gains from AI-based innovations are better shared among society and can thus lead to a welfare improvement.
The most efficient way of distributing technological advances is to keep them in the public domain, financed via governments, international organizations, donors or charities. This avoids restrictions in access to new technologies and the creation of monopolies that concentrate rents and power. There is much scope for publicly financed research and development to benefit developing countries, for example, in the areas of agriculture where new technologies increase the productivity of crops, or in healthcare where developing countries face unique challenges that do not attract sufficient research by private corporations in high-income countries.
When research and development is financed privately, there is a strong case for granting different patent protection in developing countries than in high-income economies. The length of patent protection trades off how much surplus to allocate to innovators to compensate them for their efforts versus how much to let the broader public benefit from an innovation. Most patents are developed in high-income countries and are financed by the surplus that innovators extract from the patent protection there; innovators would not incur significant losses if developing countries could use their technology for free before their patents expire in high-income countries. Indeed, in many sectors, including pharmaceuticals, there is extensive cross-border price discrimination; drug companies could offer life-saving drugs to some of the poorest countries at steeply discounted prices. Compulsory licenses (part of TRIPS and other international agreements) give the right to access such life-saving IP at appropriate royalties, but many developing countries do not have the capacity to exercise those rights; and those that do have the capacity are intimidated from doing so by threats from developed countries. Trade agreements have done everything they can to impede access to generic medicines, forcing developing countries to pay high prices for drugs.
Before the advent of AI, it was clear that there was a need for a developmentally oriented IP regime—in some ways markedly different from that currently prevailing ( Cimoli et al 2014 ). But AI has made the challenge of access to knowledge even greater. Part of the nature of AI is that it may not even need much protection by the patent system. Algorithms can be kept proprietary, and they are always evolving. Requiring disclosure of certain key algorithms is imperative to ascertain whether algorithms are discriminatory, for example, by engaging in price discrimination. 43
- D. Data and Information Policy
Data is a critical input underlying the new AI economy. That is why information policy – the rules governing the control over and use of data – has moved to the top of the policy agenda. Global tech firms are setting the data regulatory agenda in their interest without sufficient public oversight. This has already happened in recent trade agreements. For instance, while the new trade agreement between Canada, Mexico and the US had stronger provisions protecting labor and access to healthcare as well as better investor-state dispute settlement provisions, rules on the digital economy moved in the opposite direction, providing better protection for the tech giants. Being part of an international agreement, it may be difficult to change the data regulation regime in the future. This is particularly important for developing countries: the rules are currently being set with little concern for the views of citizens in the high-income countries, let alone those in the rest of the world.
Moreover, the monopolization of data by global AI firms also makes it more difficult for developing countries to catch up and develop their own AI-based companies. Global firms can use their access to vast troves of data from across the world to refine their products and offerings to consumers ever further. This makes it more and more difficult for newcomers in developing countries to close the gap between themselves and the leading firms.
Europe has actively worked on rules to ensure that the benefits of new digital technologies are shared and the harms are minimized. For instance, the EU has put forward proposals to require data sharing, with the goal of preventing accretion of monopoly power by monopolizing data. But giving control rights over data to individuals will not suffice; without proper regulation, individuals turn their data over to the digital giants and internet providers, receiving but a pittance: asymmetries in information and power are just too great to ensure an equitable outcome.
New transparency regulations, for example, regarding the algorithms and targeting of advertising, are necessary, but again not sufficient. Policymakers must be able to address the discriminatory impacts of pricing and advertising.
There is also a need for stronger rules protecting privacy and the rapid spread of misinformation and messages that promote violence and hate as well as other harmful messaging, even when conducted as part of a political campaign. In the US, the Section 230 provision which reduces the accountability of internet companies—unlike other publishers— is an example of a regulation that should be reconsidered.
As in the case of competition policy, the countries in which tech giants are based may not face the correct incentives to police the worldwide behavior of their companies since they share in the rents that these companies earn around the world. Developing countries need to cooperate and band together to have sufficient clout to impose regulation on global giants that reflects their developmental interests.
- VII. Conclusion
Advances in AI and related technologies may, like the Industrial Revolution, represent a critical turning point in history. Increasing automation in manufacturing may lead to increases in wage inequality, declining labor demand, and increased skill premia in most countries; as well as to the demise of the manufacturing-export-led developmental model, which has historically had profound positive effects on many emerging market economies. The worst-case scenario is the unravelling of much of the gains in development and poverty reduction that could be observed over the last half century.
While earlier technological advances were associated with more shared prosperity and increasing equality between and within countries, the new advances may result in increasing inequality along both dimensions unless policies are designed to counterbalance them.
The new era will be governed by different rules and will require a different kind of economic analysis. Just as the production functions that Ricardo used to analyze agrarian and rural economies are very different from those in the models of a manufacturing economy that dominated the mid-20 th century, current economic frameworks must be adjusted and updated to think about the models that will describe the next 50 years. For instance, the competitive equilibrium model may be even less relevant to the 21 st century AI economy than it was to the 20 th century manufacturing economy.
There is a particular high degree of uncertainty across the possible scenarios of technological development and their impact, but what we do know is that there are large potential downside risks that should not be ignored. Economic analysis, based on models appropriate to this new era, has the potential to help in the development of policies—both at the global and national level—that can mitigate these adverse effects, to ensure that this new era of innovation will lead to increased standards of living for all, including the billions living in developing countries.
Acemoglu , Daron ( 1998 ), “ Why Do New Technologies Complement Skills? Directed Technical Change and Wage Inequality ,” Quarterly Journal of Economics , 113 ( 4 ), pp. 1055 – 1089 .
- Search Google Scholar
- Export Citation
Acemoglu , Daron ( 2002 ), “ Directed Technical Change ,” Review of Economic Studies , 69 ( 4 ), pp. 781 – 809 .
Acemoglu , Daron and David Autor ( 2011 ), “ Skills, Tasks and Technologies: Implications for Employment and Earnings ,” Handbook of Labor Economics 4b , pp. 1043 – 1171 .
Acemoglu , Daron , Andrea Manera , and Pascual Restrepo ( 2020 ), “ Does the US tax code favor automation? ” Brookings Papers on Economic Activity 2020 ( 1 ).
Acemoglu , Daron , and Pascual Restrepo ( 2019a ), “ Automation and New Tasks: How Technology Displaces and Reinstates Labor ,” Journal of Economic Perspectives 33 ( 2 ), pp. 3 – 30 .
Acemoglu , Daron , and Pascual Restrepo ( 2019b ), “ Demographics and Automation ,” Unpublished Working Paper, MIT . https://economics.mit.edu/files/16788
Acemoglu , Daron , and Pascual Restrepo ( 2020 ), “ Robots and Jobs: Evidence from US Labor Markets ,” Journal of Political Economy 128 ( 6 ), pp. 2188 – 2244 .
Aghion , Philippe , Ufuk Akcigit , Antonin Bergeaud , Richard Blundell , and David Hemous ( 2019 ), “ Innovation and Top Income Inequality ,” Review of Economic Studies 86 ( 1 ), pp. 1 – 45 .
Aghion , Philippe , Reda Cherif , and Fuad Hasanov ( 2021 ), “ Competition, Innovation, and Inclusive Growth ,” IMF Working Paper No. 2021/080. Also forthcoming as Chapter 6 in Cerra et al. (forthcoming) .
Aghion , Philippe , Benjamin F. Jones & Charles I. Jones ( 2017 ), “ Artificial Intelligence and Economic Growth ,” NBER WP 23928 .
Akcigit , Ufuk , Wenjie Chen , Federico J. Díez , Romain Duval , Philipp Engler , Jiayue Fan , Chiara Maggi , Marina M. Tavares , Daniel Schwarz , Ippei Shibata , and Carolina Villegas-Sánchez ( 2021 ), “ Rising Corporate Market Power: Emerging Policy Issues ,” IMF Staff Discussion Note SDN/21/01 .
Alonso , Cristian , Andrew Berg , Siddharth Kothari , Chris Papageorgiou , and Sidra Rehman ( 2020 ), “ Will the AI Revolution Cause a Great Divergence? ,” IMF Working Paper 20/184 .
Armstrong , Stuart , Kaj Sotala and Seán S. ÓhÉigeartaigh ( 2014 ), “ The errors, insights and lessons of famous AI predictions – and what they mean for the future ,” Journal of Experimental & Theoretical Artificial Intelligence , 26 ( 3 ), pp. 317 – 342 . http://www.fhi.ox.ac.uk/wp-content/uploads/FAIC.pdf
Arrow , Kenneth ( 1962a ), “ Economic Welfare and the Allocation of Resources for Invention ,” in: R. Nelson , ed., The Rate and Direction of Inventive Activity: Economic and Social Factors , Princeton University Press .
Arrow , Kenneth ( 1962b ), “ The Economic Implications of Learning by Doing ,” The Review of Economic Studies 29 ( 3 ), pp. 155 – 173 .
Atkinson , Anthony , and Joseph Stiglitz ( 1969 ), “ A New View of Technological Change ,” Economic Journal 79 ( 315 ), pp. 573 – 578
Autor , David H. , ( 2014 ), “ Skills, Education, and the Rise of Earnings Inequality Among the ’Other 99 Percent’ ,” Science , 23 May 2014 : 344 ( 6186 ), pp. 843 – 851 .
Autor , David ( 2015 ), “ Why Are There Still So Many Jobs? The History and Future of Workplace Automation ,” Journal of Economic Perspectives 29 ( 3 ), pp. 3 – 30 .
Autor , David H. ( 2019 ), “ Work of the Past, Work of the Future ,” AEA Papers and Proceedings 109 , pp. 1 – 32 .
Autor , David , David Dorn , Lawrence F Katz , Christina Patterson , and John Van Reenen ( 2020 ), “ The Fall of the Labor Share and the Rise of Superstar Firms ,” Quarterly Journal of Economics 135 ( 2 ), pp. 645 – 709 .
Autor , David , Frank Levy , and Richard J. Murnane , ( 2003 ), “ The Skill Content of Recent Technological Change: An Empirical Exploration ,” Quarterly Journal of Economics 118 ( 4 ), pp. 1279 – 1333 .
Berg , Andrew , Edward F. Buffie , and Luis-Felipe Zanna ( 2018 ), “ Should we fear the robot revolution? (The correct answer is yes) ,” Journal of Monetary Economics 97 , pp. 117 – 148 .
Boix , Carles ( 2019 ), Democratic Capitalism at the Crossroads: Technological Change and the Future of Politics , Princeton University Press .
Brussevich , Mariya , Era Dabla-Norris , Christine Kamunge , Pooja Karnane , Salma Khalid , and Kalpana Kochhar ( 2018 ), “ Gender, Technology, and the Future of Work ,” IMF Staff Discussion Note, SDN/18/07, October 2018 .
Brynjolfsson , Erik , Avinash Collis , Erwin Diewert , Felix Eggers , and Kevin Fox ( 2020 ), “ Measuring the Impact of Free Goods on Real Household Consumption ,” AEA Papers and Proceedings 110 , pp. 25 – 30 .
Brynjolfsson , Erik , Daniel Rock , and Chad Syverson ( 2019 ), “ Artificial Intelligence and the Modern Productivity Paradox: A Clash of Expectations and Statistics ,” The Economics of Artificial Intelligence: An Agenda, Agrawal, Gans, and Goldfarb .
Caselli , Francesco , and Alan Manning ( 2019 ), “ Robot Arithmetic: New Technology and Wages ,” American Economic Review: Insights 2019 1 ( 1 ), pp. 1 – 12 .
Cerra , Valerie , Barry Eichengreen , Asmaa El-Ganainy , and Martin Schindler (Eds.) (forthcoming), How to Achieve Inclusive Growth , Oxford University Press and IMF .
Charlton , Andrew , and Joseph E. Stiglitz ( 2005 ), Fair Trade for All , Oxford University Press .
Choi , Eleanor J. , and James R. Spletzer ( 2012 ), “ The declining average size of establishments: evidence and explanations ,” BLS Monthly Labor Review, March 2012 . https://www.bls.gov/opub/mlr/2012/03/art4full.pdf
Cimoli , Mario , Giovanni Dosi , Keith E. Maskus , Ruth L. Okediji , Jerome H. Reichman , and Joseph Stiglitz (eds.) ( 2014 ), Intellectual Property Rights: Legal and Economic Challenges for Development , Oxford University Press
Clausing , Kimberley and Reuben Avi-Yonah ( 2007 ), “ Reforming Corporate Taxation in a Global Economy: A Proposal to Adopt Formulary Apportionment ,” The Hamilton Project, Brookings Institution .
Colmer , Jonathan , Ian Hardman , Jay Shimshack , and John Voorheis ( 2020 ), “ Disparities in PM2.5 air pollution in the United States ,” Science , Vol. 369 , Issue 6503 , pp. 575 – 578 .
Comin , Diego , and Bart Hobijn ( 2010 ), “ An Exploration of Technology Diffusion ,” American Economic Review 100 ( 5 ), pp. 2031 – 59 .
Delli Gatti , Domenico , Mauro Gallegati , Bruce Greenwald , Alberto Russo , and Joseph E. Stiglitz ( 2012a ), “ Sectoral Imbalances and Long Run Crises ,”The Global Macro Economy and Finance , Franklin Allen, Masahiko Aoki, Jean-Paul Fitoussi, Nobuhiro Kiyotaki, Roger Gordon, and Joseph Stiglitz, eds., IEA Conference Volume No. 150-III, Palgrave, pp. 61 – 97 .
Delli Gatti , Domenico , Mauro Gallegati , Bruce Greenwald , Alberto Russo , and Joseph E. Stiglitz ( 2012b ), “ Mobility Constraints, Productivity Trends, and Extended Crises ,” Journal of Economic Behavior & Organization 83 ( 3 ), pp. 375 – 393 .
Ding , Jeffrey , Paul Triolo , and Samm Sacks ( 2018 ), “ Chinese Interests Take a Big Seat at the AI Governance Table ,” New America blog, June 20, 2018 . https://www.newamerica.org/cybersecurity-initiative/digichina/blog/chinese-interests-take-big-seat-ai-governance-table/
El-Ganainy , Asmaa , Ekkehard Ernst , Rossana Merola , Richard Rogerson , and Martin Schindler ( 2021 ), “ Inclusivity in the Labor Market ,” IMF Working Paper No. 2021/141. Also forthcoming as Chapter 3 in Cerra et al. (forthcoming) .
Frey , Carl Benedikt and Michael A. Osborne ( 2017 ), “ The future of employment: How susceptible are jobs to computerisation? ” Technological Forecasting and Social Change 114 , pp. 254 – 280 .
George , Henry ( 1879 ), Progress and poverty: An inquiry into the cause of industrial depressions, and of increase of want with increase of wealth, the remedy , D Appleton and Company .
Good , Irving John ( 1965 ), “ Speculations Concerning the First Ultraintelligent Machine ,” Advances in Computers , Vol. 6 .
Gordon , Robert ( 2016 ), The Rise and Fall of American Growth: The U.S. Standard of Living since the Civil War , Princeton University Press .
Grace , Katja , John Salvatier , Allan Dafoe , Baobao Zhang , and Owain Evans ( 2018 ), “ When Will AI Exceed Human Performance? Evidence from AI Experts ,” Journal of Artificial Intelligence Research , https://arxiv.org/abs/1705.08807v3 .
Greenwald , Bruce and Joseph Stiglitz ( 1986 ) “ Externalities in Economies with Imperfect Information and Incomplete Markets ,” Quarterly Journal of Economics , Vol. 101 , No. 2 , May, pp. 229 – 264 .
Greenwald , Bruce and Joseph Stiglitz ( 2006 ) “ Helping Infant Economies Grow: Foundations of Trade Policies for Developing Countries ,” American Economic Review: AEA Papers and Proceedings 96 ( 2 ), pp. 141 – 146 .
Greenwald , Bruce and Joseph Stiglitz ( 2014a ), Creating a Learning Society: A New Approach to Growth, Development, and Social Progress , Columbia University Press .
Greenwald , Bruce and Joseph E. Stiglitz ( 2014b ), “ Industrial Policies, the Creation of a Learning Society, and Economic Development ,” The Industrial Policy Revolution I: The Role of Government Beyond Ideology , Joseph E. Stiglitz and Justin Yifu Lin (eds.), Palgrave Macmillan , pp. 43 – 71 .
Harari , Yuval Noah ( 2017 ), Homo Deus: A Brief History of Tomorrow , Harper .
Harris , Karen , Austin Kimson , and Andrew Schwedel ( 2018 ), “ Labor 2030: The Collision of Demographics, Automation and Inequality ,” Bain and Company Reports .
Kennedy , Charles ( 1964 ), “ Induced Bias in Innovation and the Theory of Distribution ,” Economic Journal LXXIV , pp. 541 – 47 .
Klinova , Katya and Anton Korinek ( 2021 ), “ AI and Shared Prosperity ,” Proceedings of the 2021 AAAI/ACM Conference on AI, Ethics, and Society (AIES ’21) .
Korinek , Anton ( 2019 ), “ The Rise of Artificially Intelligent Agents ,” working paper , University of Virginia .
Korinek , Anton ( 2020 ), “ Taxation and the Vanishing Labor Market in the Age of AI ,” Ohio State Technology Law Journal 16 ( 1 ), pp. 244 – 257 .
Korinek , Anton and Megan Juelfs ( 2021 ), “ Preparing for the (Non-Existent?) Future of Work ,” in preparation for Justin Bullock et al. (Eds.), Oxford Handbook of AI Governance , Oxford University Press .
Korinek , Anton and Ding Xuan Ng ( 2019 ), “ Digitization and the Macro-Economics of Superstars ,” working paper , University of Virginia .
Korinek , Anton and Joseph E. Stiglitz ( 2019 ), “ Artificial Intelligence and Its Implications for Income Distribution and Unemployment ,” in Agrawal et al.: The Economics of Artificial Intelligence , NBER and University of Chicago Press .
Korinek , Anton and Joseph E. Stiglitz ( 2020 ), “ Steering Technological Progress ,” working paper .
Korinek , Anton and Joseph E. Stiglitz ( 2021a ), “ Artificial Intelligence, Globalization, and Strategies for Economic Development ,” NBER Working Paper w28453 .
Korinek , Anton and Joseph E. Stiglitz ( 2021b ), “ Will COVID-19 Drive Advances in Automation and AI that Exacerbate Economic Inequality?” BMJ 372:n367 .
Kurzweil , Ray ( 2005 ) The Singularity is Near , Penguin Group , pp. 135 – 136 .
Louie , Edward P. , and Joshua M. Pearce ( 2016 ), “ Retraining investment for U.S. transition from coal to solar photovoltaic employment ,” Energy Economics 57 , pp. 295 – 302 .
Maddison , Angus ( 2003 ), Contours of the World Economy 1–2030 AD: Essays in Macro-Economic History , Oxford University Press .
Malthus , Thomas R. ( 1798 ), An Essay on the Principle of Population , J. Johnson .
McKinsey Global Institute ( 2017 ), “ Jobs lost, jobs gained: What the future of work will mean for jobs, skills, and wages ,” McKinsey Reports .
McMillan , Margaret S. , and Dani Rodrik ( 2011 ), “ Globalization, Structural Change and Productivity Growth ,” NBER Working Paper 17143 .
Myrdal , Gunnar ( 1968 ), Asian Drama: An Inquiry into the Poverty of Nations , Pantheon Books .
Nordhaus , William ( 2015 ), “ Are We Approaching an Economic Singularity? Information Technology and the Future of Economic Growth ,” NBER Working Paper No. 21547 .
OECD ( 2019 ), The Future of Work , OECD Employment Outlook 2019 .
Ostry , Jonathan , Prakash Loungani , and Andrew Berg ( 2019 ), Confronting Inequality: How Societies Can Choose Inclusive Growth , Columbia University Press .
Partnership on AI ( 2021 ), Redesigning AI for Shared Prosperity: An Agenda . Technical Report . Available at: partnershiponai.org/shared-prosperity
Peralta-Alva , Adrian , and Agustin Roitman ( 2018 ), “ Technology and the Future of Work ,” IMF Working Paper WP/18/207. Also published as IMF Staff Note to Group of Twenty: April 2018 .
Peszko , Grzegorz; van der Mensbrugghe , Dominique; Golub , Alexander; Ward , John; Zenghelis , Dimitri; Marijs , Cor; Schopp , Anne; Rogers , John A. l Midgley , Amelia ( 2020 ). Diversification and Cooperation in a Decarbonizing World: Climate Strategies for Fossil Fuel-Dependent Countries . Washington, DC : World Bank .
Peterson Institute for International Economics (PIIE) ( 2020 ), “ How to Fix Economic Inequality? ” https://www.piie.com/sites/default/files/documents/how-to-fix-economic-inequality.pdf .
Pouokam , Nathalie ( 2021 ), “ Sharing Resource Wealth and Addressing Fragility ,” IMF Working Paper No. 2021/097. Also forthcoming as Chapter 6 in Cerra et al. (forthcoming) .
Pritchett , Lant ( 2019 ), “ The Future of Jobs is Facing One, Maybe Two, of the Biggest Price Distortions Ever ,” Economic Research Forum Working Paper 1370 . https://erf.org.eg/app/uploads/2019/12/1370.pdf
Rodrik , Dani ( 2011 ), “ Unconditional Convergence ,” NBER Working Paper w17546 .
Romer , Paul M. ( 1986 ), “ Increasing Returns and Long-Run Growth ,” Journal of Political Economy , Vol. 94 , No. 5 ( October ), pp. 1002 – 1037 .
Rubin , Richard ( 2020 ), “ The ’Robot Tax’ Debate Heats Up ,” Wall Street Journal , January 20, 2020 . https://www.wsj.com/articles/the-robot-tax-debate-heats-up-11578495608.
Sacks , Samms ( 2018 ), “ Beijing Wants to Rewrite the Rules of the Internet ,” The Atlantic , June 18, 2018 . https://amp.theatlantic.com/amp/article/563033/
Saez , Emmanuel and Gabriel Zucman ( 2019 ), The Triumph of Injustice: How the Rich Dodge Taxes and How to Make Them Pay , Norton .
Samuelson , Paul ( 1965 ), “ A Theory of Induced Innovations along Kennedy-Weisäcker Lines ,” Review of Economics and Statistics XLVII: 444 – 64 .
Shapiro , Carl and J. E. Stiglitz ( 1984 ) “ Equilibrium Unemployment as a Worker Discipline Device ,” American Economic Review , 74 ( 3 ), June 1984 , pp. 433 – 444 .
Solow , Robert ( 1987 ), “ We’d Better Watch Out .” New York Times Book Review , July 12 , pp. 36 .
Solow , Robert ( 2009 ), “ Does Growth Have a Future? Does Growth Theory Have a Future? Are These Questions Related? ,” History of Political Economy 41 , pp. 27 – 34
Stiglitz , Joseph E. ( 1975a ) “ Information and Economic Analysis ,” Current Economic Problems , J.M. Parkin and A.R. Nobay (eds.), Cambridge : Cambridge University Press , pp. 27 – 52 . ( Proceedings of the Association of University Teachers of Economics, Manchester, England, April 1974 .)
Stiglitz , Joseph ( 1975b ) “ The Theory of Screening, Education and the Distribution of Income ,” American Economic Review , 65 ( 3 ), June 1975, pp. 283 – 300
Stiglitz , Joseph E. ( 1987a ) “ On the Microeconomics of Technical Progress ,” Technology Generation in Latin American Manufacturing Industries , Jorge M. Katz (ed.), New York : St. Martin’s Press , pp. 56 – 77 . ( Presented to IDB-CEPAL Meetings, Buenos Aires, November 1978 .)
Stiglitz , Joseph E. ( 1987b ) “ Learning to Learn, Localized Learning and Technological Progress ,” Economic Policy and Technological Performance , P. Dasgupta and Stoneman (eds.), Cambridge University Press , 1987 , pp. 125 – 153 .
Stiglitz , Joseph E. ( 2006 ), “ Samuelson and the Factor Bias of Technological Change ,” Samuelsonian Economics and the Twenty-First Century , M. Szenberg et al, eds., Oxford University Press : New York , pp. 235 – 251
Stiglitz , Joseph E. ( 2013 ) The Price of Inequality: How Today’s Divided Society Endangers Our Future , New York : W.W. Norton & Company .
Stiglitz , Joseph E. ( 2014 ), “ Unemployment and Innovation ,” NBER Working Paper no. 20670 .
Stiglitz , Joseph E. ( 2015 ), “ Leaders and followers: Perspectives on the Nordic model and the economics of innovation ,” Journal of Public Economics, Elsevier , 127 ( C ), pp 3 – 16 .
Stiglitz , Joseph E. ( 2018a ), “ From Manufacturing-Led Export Growth To A Twenty-First-Century Inclusive Growth Strategy: Explaining The Demise Of A Successful Growth Model And What To Do About It ,” WIDER Working Paper 2018/176 Helsinki: UNU-WIDER , accessible at https://www.wider.unu.edu/publication/manufacturing-led-export-growth-twenty-first-century-inclusive-growth-strategy
Stiglitz , Joseph E. ( 2018b ), “ Pareto Efficient Taxation and Expenditures: Pre- and Redistribution ,” Journal of Public Economics Special Issue in Honor of Sir Tony Atkinson (1944-2017) , 162 , pp. 101 – 119 .
Stiglitz , Joseph E. ( 2019 ), People, Power, and Profits: Progressive Capitalism for an Age of Discontent , Norton .
Stiglitz , Joseph E. , Nell Abernathy , Adam Hersh , Susan Holmberg and Mike Konczal ( 2015 ), Rewriting the Rules of the American Economy: An Agenda for Growth and Shared Prosperity , A Roosevelt Institute Book , Norton .
Stiglitz , Joseph E. , Carter Daugherty , and the Foundation for European Progressive Studies ( 2019 ), Rewriting the Rules of the European Economy: An Agenda for Growth and Shared Prosperity , Norton .
Summers , Lawrence ( 2017 ), “ Picking on robots won’t deal with job destruction ,” The Washington Post Opinion , March 5, 2017 .
Trajtenberg , Manuel ( 2019 ) “ Artificial Intelligence as the Next GPT: A Political-Economy Perspective ,” pp. 175 – 186 , in Agrawal , Ajay , Joshua Gans and Avi Goldfarb ( 2019 ), The Economics of Artificial Intelligence: An Agenda , National Bureau of Economic Research and University of Chicago Press .
Ulam , Stanislaw ( 1958 ), “ Tribute to John von Neumann ,” Bulletin of the American Mathematical Society 64 , # 3 , part 2, pp. 5 .
Varian , Hal ( 2020 ), “ Automation versus procreation (aka bots versus tots) ,” VoxEU , https://voxeu.org/article/automation-versus-procreation-aka-bots-versus-tots
Von Weizsäcker , Carl ( 1966 ), “ Tentative Notes on a Two-Sector Model with Induced Technical Progress ,” Review of Economic Studies 33 , pp. 245 – 51 .
Vinge , Vernor ( 1993 ), “ The Coming Technological Singularity: How to Survive in the Post-Human Era ,” Vision-21: Interdisciplinary Science and Engineering in the Era of Cyberspace , Geoffrey Landis , ed., NASA Publication CP-10129 , pp. 11 – 22 . https://edoras.sdsu.edu/~vinge/misc/singularity.html
Walsh , Toby ( 2016 ), “ The Singularity May Never Be Near ,” https://arxiv.org/abs/1602.06462v1 .
World Bank ( 1998 ), World Development Report 1998/1999: Knowledge for Development , Oxford University Press . https://openknowledge.worldbank.org/handle/10986/5981
World Commission on the Social Dimension of Globalization ( 2004 ), A Fair Globalization: Creating Opportunities for All , https://www.ilo.org/public/english/wcsdg/docs/report.pdf
World Economic Outlook (WEO) ( 2018 ), “ Manufacturing Jobs: Implications for Productivity,” Chapter 3, April 2018 .
Wu , Tim ( 2018 ), The Curse of Bigness: Antitrust in the New Gilded Age , Columbia Global Reports .
This paper is an extended version of a chapter that has been accepted for publication by Oxford University Press in the forthcoming book on “How to Achieve Inclusive Growth,” edited by V. Cerra, B. Eichengreen, A. El-Ganainy, and M. Schindler due for publication in 2021. Parts of this paper subsume ideas presented in previous work by Korinek and Stiglitz (2021a) under the title “Artificial Intelligence, Globalization, and Strategies for Economic Development.” We thank Avital Balwit, Andy Berg, Valerie Cerra, Sharmini Coorey, Romain Duval, Barry Eichengreen, Katya Klinova, Nikola Spatafora, Jeromin Zettelmeyer, participants in the IMF IG seminar series, and numerous IMF colleagues for insightful comments and suggestions; David Autor, Adrian Peralta-Alva and Agustin Roitman for helpful data and charts; and Jaime Sarmiento for excellent research assistance. Financial support from the Institute for New Economic Thinking is gratefully acknowledged.
AI is frequently defined as the ability of machines to perform tasks that previously required human intelligence. This implies that AI carries by its nature the potential to replace human labor. A number of arguments put forward in this paper are applicable not only to AI but also to other forms of technological progress.
As can be seen in the figure, the COVID shock in 2020 has clearly accelerated the trend, at least temporarily, giving rise to a large decline in employment in routine manual jobs but only a modest dip in nonroutine cognitive jobs.
See also WEO (2018) for a broader review of employment and earnings dynamics across countries and sectors.
The result is intuitive: the dual to the production function is the factor price frontier. Technological change shifts out the factor price frontier, implying that if the interest rate is unchanged, wages must increase.
One extreme example is Elon Musk’s Neuralink which aims to achieve a symbiosis of humans and AI by surgically implanting technology into the brain.
As always, calculating the full consequences of a new technology on the demand for any natural resource, or carbon emissions, is complex. It must be done on a full life-cycle basis, incorporating initial investment, maintenance, as well as day-to-day operations. That said, for instance, data centers running cutting-edge AI applications are typically energy-intensive and may lead to increases in demand for electricity and depletable natural resources. Still, on net, it is likely that the demand for carbon-based energy sources will decrease. Some natural-resource-rich economies may benefit, such as those rich in rare earths or other metals that are inputs in the production of batteries, microchips, solar panels, wind turbines etc.
In addition, many fossil-fuel-dependent countries have not yet diversified their export base, and may face limited options to diversify into job-rich manufacturing growth given this sector’s vulnerability to automation. See, e.g., Peszko et al. (2020) .
There is a large literature on the welfare economics of innovation, dating back to Arrow (1962a) . Stiglitz (1975a , 1987 ) drew attention explicitly to the public good aspects of knowledge, and the similarity between the economics of information and the economics of knowledge. See also Romer (1986) .
The inefficiencies in economies with learning by doing was first noted by Arrow (1962b) .
See Greenwald and Stiglitz (1986) . These, in turn, give rise to macroeconomic externalities, the consequences in the context of innovation have been studied by Korinek and Stiglitz (2019) .
More generally, the direction of innovation is affected by the share of the factor. If the elasticity of substitution is high, a lower factor price will be associated with an increased factor share, and this can induce greater efforts at increasing the productivity of that factor. In that case, the equilibrating force just described does not arise, and the opposite occurs ( Stiglitz, 2014 ).
As Vinge (1993) noted: “Within thirty years, we will have the technological means to create superhuman intelligence. Shortly after, the human era will be ended.” However, it should be noted that general AI does not in itself imply the singularity (e.g., Walsh, 2016 ).
Responding to Kurzweil’s (2005) thesis that “The Singularity is Near,” Walsh (2016) provides arguments for why “The Singularity May Never Be Near.”
There is even a perspective that holds that AI-powered machines could become agents of their own ( Korinek, 2019 ).
Note that this is in contrast to a long tradition in the traditional economics literature that viewed labor as an essential input for any production process.
The measurement problems are still more complicated: advertising is an “intermediate” product and does not directly enter into the value of the final goods and service that constitutes GDP. If advertising were a normal input, and markets were competitive, an increase in the efficiency of production of an intermediate good would be reflected in a lowering of the final goods price, and that in turn would be associated with an increase in GDP. Better advertising engines may, as we noted earlier, actually increase market power and decrease overall economic efficiency. Moreover, they may induce an adverse redistribution, lowering welfare still more.
Any innovations to deal with Covid-19 will still be available in the post-Covid-19 world. Moreover, the development of research strategies in response to Covid-19 may set in motion a process of “learning to learn,” learning better how to innovate in human-replacing dimensions. See Atkinson and Stiglitz (1969) and Stiglitz (1987b ).
This would not, of course, be true if the factor price equalization theorem held. More generally, differences in domestic factor ratios do not necessarily align well with differences in factor prices.
There are countervailing forces to the scarcity of labor associated with a declining working age population. A younger population may be more tech savvy, better able to pick up, adopt and adapt to new technologies. The figure suggests that the scarcity effect dominates. There are other factors too that play a role in robotization.
There is a similar dissonance between those who argue that the economy faces secular stagnation and those who say there are not the resources required for a rapid green transition.
Over the long run, the effect of the green transition on the demand for labor is more problematic. While many of the green technologies have higher upfront costs, maintenance costs are markedly lower, and not only are life-cycle carbon emissions lower, but so is labor usage.
For example, based on data for 1981–2002, Gilens (2005) finds that in the US actual policy outcomes strongly reflected the preferences of higher-income groups, with little relationship to the preferences of the poor or middle-income citizens. For a broader discussion of the interplay of economic and political inequality, see Stiglitz (2013 , 2019 ).
Harari (2017) also explores the implications of super-human artificial intelligence on society and politics.
The emphasis here is on (traded) goods rather than (non-traded) services—while learning by doing could occur in both, it is the former that drives export-led development. See, e.g., McMillan and Rodrik (2011) who note that non-traded service sector development on its own typically has not had a substantial impact on overall productivity.
Stiglitz (2015) models the relationship between technological leaders and followers.
We emphasize that the focus here is on the more adverse scenarios, to help prepare policies; should they not materialize, so much the better. We noted countervailing forces—the need for labor for the green transition, that even within advanced economies, people may still be needed for service jobs requiring physical proximity and/or the “human touch” (such as elderly care, housekeeping, etc.). Most important, these outcomes are not inevitable: we can steer innovation in a different direction and, as the discussion below will hopefully make clear, there are multiple actions that can be taken to mitigate some of the adverse effects.
Some are justifiably concerned that digital platforms are in fact very efficient at exploiting workers. But platforms can also provide information on whether workers are exploited and, with proper regulation, make it easier to address such exploitation than it used to be before the digital age.
See also Pouokam (2021) .
Some countries have started to experiment with schemes that have some characteristics of a UBI. E.g., Spain introduced in early 2020 a “minimum vital income” to ensure a guaranteed minimum income for the poorest. However, it is not unconditional, but instead tops up incomes below the minimum income, which may create disincentive effects to continue work in jobs that pay below that threshold. Several other countries have run pilot programs, often on a small scale and/or for a limited time. Overall, these programs appear to indicate that such schemes tend to have little impact on labor supply (see, e.g., https://www.vox.com/future-perfect/2020/2/19/21112570/universal-basic-income-ubi-map ). Earlier research on a negative income tax in the US suggested that by enabling individuals to search more for a better matching job may actually enhance productivity.
UBI programs may turn out to be important policies in a future in which labor truly becomes redundant ( Korinek and Juelfs, 2021 ). There is uncertainty over when that future may arise, as the earlier discussions indicated—but given the complexities of transitioning to such a new regime, there may be a rationale for countries to start experimenting with UBI systems.
Notably, some have discussed a “robot tax” that could help finance redistributive fiscal measures (e.g., Rubin, 2020 ). However, such a robot tax may be difficult to implement (e.g., what distinguishes a “robot” from traditional capital?) and may discourage innovation (e.g., Summers, 2017 ). Conceptually, government ownership of capital is equivalent to taxes on capital with exemptions on new investment that avoid any negative incentive effects of capital taxation, although it may be insufficient to provide funding for large-scale redistributive programs that may be needed in a long-term equilibrium with low employment levels. See also Korinek (2020) .
The reduced labor supply may itself help sustain higher wages.
For a broader view of how to achieve inclusivity in the labor market beyond the challenges posed by technological advances, see El-Ganainy et al. (2021) .
For example, Colmer et al. (2020) find that while fine-particle air pollution has decreased overall in the US over the past four decades, whiter and richer neighborhoods have become relatively less polluted, while poor and minority communities are (still) the most polluted.
For an extensive discussion of some of the critical “rules,” see Stiglitz et al (2015 , 2019 ). Later, we discuss a particularly important set of policies that can affect the returns to factors—those associated with steering the development and adoption of technologies.
The alternative – to allow for greater migration – would of course also put downward pressure on wages in advanced countries and might increase inequality within those countries.
Curiously, such policies have continued to be referred to as “industrial policies” even when they move the economy away from the industrial sector. We use the more generic term sectoral policies , but they are broader: they can also be used to change technology within a sector (e.g., towards green or more labor-intensive technologies).
For example, US bankruptcy provisions favoring derivatives can be thought of a sectoral policy encouraging the growth of derivatives; but until the 2008 financial crisis, few outside of that sector were even aware of the favorable treatment that derivatives have received.
In 1914, the U.S. Department of Agriculture created a system of “extension” services, with the aim of providing farmers with expert advice on agriculture and farming. See, e.g., https://www.almanac.com/cooperative-extension-services .
For a discussion of how this plays out in trade rules, see, e.g., Charlton and Stiglitz (2005) .
There is by now a large literature describing the new competition policies that may be required. See Stiglitz (2019) and Wu (2018) , as well as Akcigit et al. (2021) for an overview of emerging issues and complexities in competition policy.
It is sometimes argued that such disclosure is not possible because algorithms are always evolving. While they are always changing, they could still be disclosed as of a particular moment in time. There are other (often costly) ways of monitoring the behavior of algorithms at any point in time.
- Share on facebook Share on linkedin Share on twitter
Table of Contents
- View raw image
- Download Powerpoint Slide
International Monetary Fund Copyright © 2010-2021. All Rights Reserved.
- [185.80.151.9]
- 185.80.151.9
Character limit 500 /500
Technological Innovation and Economic Growth: A Brief Report on the Evidence
Mercatus Research Paper
29 Pages Posted: 5 Mar 2019
James Broughel
Competitive Enterprise Institute (CEI)
Adam D. Thierer
R Street Institute
Date Written: March 4, 2019
Technological innovation is a fundamental driver of economic growth and human progress. Yet some critics want to deny the vast benefits that innovation has bestowed and continues to bestow on mankind. To inform policy discussions and address the technology critics’ concerns, this paper summarizes relevant literature documenting the impact of technological innovation on economic growth and, more broadly, on living standards and human well-being. The historical record is unambiguous regarding how ongoing innovation has improved the way we live; however, the short-term disruptive aspects of technological change are real and deserve attention as well. The paper concludes with an extended discussion about the relevance of these findings for shaping cultural attitudes toward technology and the role that public policy can play in fostering innovation, growth, and ongoing improvements in the quality of life of citizens.
Keywords: technological innovation, economic growth, permissionless innovation, regulation, risk-taking, entrepreneurship, total factor productivity
JEL Classification: K2, O3, O4
Suggested Citation: Suggested Citation
James Broughel (Contact Author)
Competitive enterprise institute (cei) ( email ), r street institute ( email ).
1050 17th Street Northwest #1150 Washington, DC 20036 United States
Do you have a job opening that you would like to promote on SSRN?
Paper statistics, related ejournals, io: productivity, innovation & technology ejournal.
Subscribe to this fee journal for more curated articles on this topic
Sustainability & Economics eJournal
Mercatus center research paper series.
Subscribe to this free journal for more curated articles on this topic
Sustainable Technology eJournal
International political economy: globalization ejournal, political economy - development: domestic development strategies ejournal.
Interdisciplinary Teams and Innovation: Questioning, Networking, Observing and Experimenting
Technological triumphs and failures of 2018, openmind books, scientific anniversaries, the geologist who discovered parkinson’s disease, featured author, latest book, technological progress and potential future risks.
The list of new technologies grows every day. Robots, Augmented Reality, algorithms, and machine-to-machine communications help people with a range of different tasks.(1) These technologies are broad-based in their scope and significant in their ability to transform existing businesses and personal lives. They have the potential to ease people’s lives and improve their personal and business dealings.(2) Technology is becoming much more sophisticated and this is having a substantial impact on the workforce.(3)

In this paper, I explore the impact of robots, artificial intelligence, and machine learning on the workforce and public policy. If society needs fewer workers due to automation and robotics, and many social benefits are delivered through jobs, how are people outside the workforce for a lengthy period of time going to get health care and pensions? These are profound questions for public policy and we need to figure out how to deliver social benefits in the new digital economy.
Emerging Technologies
Industrial robots are expanding in magnitude around the developed world. In 2013, for example, there were an estimated 1.2 million robots in use. This total rose to around 1.5 million in 2014 and is projected to increase to about 1.9 million in 2017.(4) Japan has the largest number with 306,700, followed by North America (237,400), China (182,300), South Korea (175,600), and Germany (175,200). Overall, robotics is expected to rise from a $15-billion sector now to $67 billion by 2025.(5)
According to an RBC Global Asset Management study, the costs of robots and automation have fallen substantially. It used to be that the “high costs of industrial robots restricted their use to few high-wage industries like the auto industry. However, in recent years, the average costs of robots have fallen, and in a number of key industries in Asia, the cost of robots and the unit costs of low-wage labor are converging… Robots now represent a viable alternative to labor.”(6)
In the contemporary world, there are many robots that perform complex functions. According to a presentation on robots:
The early 21st century saw the first wave of companionable social robots. They were small cute pets like AIBO, Pleo, and Paro. As robotics become more sophisticated, thanks largely to the smart phone, a new wave of social robots has started, with humanoids Pepper and Jimmy and the mirror-like Jibo, as well as Geppetto Avatars’ software robot, Sophie. A key factor in a robot’s ability to be social is their ability to correctly understand and respond to people’s speech and the underlying context or emotion.(7)
These machines are capable of creative actions. Anthropologist Eitan Wilf of Hebrew University of Jerusalem says that sociable robots represent “a cultural resource for negotiating problems of intentionality.”(8) He describes a “jazz-improvising humanoid robot marimba player” that can interpret music context and respond creatively to improvisations on the part of other performers. Designers can put it with a jazz band, and the robot will ad lib seamlessly with the rest of the group. If someone were listening to the music, that person could not discern the human from the robot performer.
Amazon has organized a “picking challenge” designed to see if robots can “autonomously grab items from a shelf and place them in a tub.” The firm has around 50,000 people working in its warehouses and it wants to see if robots can perform the tasks of selecting items and moving them around the warehouse. During the competition, a Berlin robot successfully completed ten of the twelve tasks. To move goods around the facility, the company already uses 15,000 robots and it expects to purchase additional ones in the future.(9)
In the restaurant industry, firms are using technology to remove humans from parts of food delivery. Some places, for example, are using tablets that allow customers to order directly from the kitchen with no requirement of talking to a waiter or waitress. Others enable people to pay directly, obviating the need for cashiers. Still others tell chefs how much of an ingredient to add to a dish, which cuts down on food expenses.(10) Other experimentalists are using a robot known as Nao to help people deal with stress. In a pilot project called “Stress Game,” Thi-Hai-Ha Dang and Adriana Tapus subject people to a board game where they have to collect as many hand objects as they can. During the test, stress is altered through game difficulty and noises when errors are made. The individuals are wired to a heart monitor so that Nao can help people deal with stress. When the robot feels human stress levels increasing, it provides coaching designed to decrease the tension. Depending on the situation, it can respond in empathetic, encouraging, or challenging ways. In this way, the “robot with personality” is able to provide dynamic feedback to the experimental subjects and help them deal with tense activities.(11)
Computerized Algorithms
There are computerized algorithms that have taken the place of human transactions. We see this in the stock exchanges, where high-frequency trading by machines has replaced human decision making. People submit buy and sell orders, and computers match them in the blink of an eye without human intervention. Machines can spot trading inefficiencies or market differentials at a very small scale and execute trades that make money for people.(12)
Some individuals specialize in arbitrage trading, whereby the algorithms see the same stocks having different market values. Humans are not very efficient at spotting price differentials but computers can use complex mathematical formulas to determine where there are trading opportunities. Fortunes have been made by mathematicians who excel in this type of analysis.(13)
Artificial Intelligence
Artificial intelligence refers to “machines that respond to stimulation consistent with traditional responses from humans, given the human capacity for contemplation, judgment and intention.”(14) It incorporates critical reasoning and judgment into response decisions. Long considered a visionary advance, AI is now here and being incorporated in a variety of different areas. It is being used in finance, transportation, aviation, and telecommunications. Expert systems “make decisions which normally require a human level of expertise.”(15) These systems help humans anticipate problems or deal with difficulties as they come up.
There is growing applicability of artificial intelligence in many industries.(16) It is being used to take the place of humans in a variety of areas. For example, it is being used in space exploration, advanced manufacturing, transportation, energy development, and health care. By tapping into the extraordinary processing power of computers, humans can supplement their own skills and improve productivity through artificial intelligence.
Impact on the Workforce
The rapid increase in emerging technologies suggests that they are having a substantial impact on the workforce. Many of the large tech firms have achieved broad economic scale without a large number of employees. For example, Derek Thompson writes: “Google is worth $370 billion but has only about 55,000 employees—less than a tenth the size of AT&T’s workforce in its heyday [in the 1960s].”(17) According to economist Andrew McAfee: “We are facing a time when machines will replace people for most of the jobs in the current economy, and I believe it will come not in the crazy distant future.”(18)
In a number of fields, technology is substituting for labor, and this has dramatic consequences for middle-class jobs and incomes. Cornell University engineer Hod Lipson argues that “for a long time the common understanding was that technology was destroying jobs but also creating new and better ones. Now the evidence is that technology is destroying jobs and indeed creating new and better ones but also fewer ones.”(19)
Martin Ford issues an equally strong warning. In his book, The Lights in the Tunnel, he argues that “as technology accelerates, machine automation may ultimately penetrate the economy to the extent that wages no longer provide the bulk of consumers with adequate discretionary income and confidence in the future. If this issue is not addressed, the result will be a downward economic spiral.”(20) Continuing, he warns that “at some point in the future—it might be many years or decades from now—machines will be able to do the jobs of a large percentage of the ‘average’ people in our population, and these people will not be able to find new jobs.”
Firms have discovered that robotics, machine learning, and artificial intelligence can replace humans and improve accuracy, productivity, and efficiency of operations. During the Great Recession of 2008–09, many businesses were forced to downsize their workforce for budgetary reasons. They had to find ways to maintain operations through leaner workforces. One business leader I know had five hundred workers for his $100 million business and now has the same size workforce even though the company has grown to $250 million in revenues. He did this by automating certain functions and using robots and advanced manufacturing techniques to operate the firm.
The US Bureau of Labor Statistics (BLS) compiles future employment projections. In its most recent analysis, the agency predicts that 15.6 million new positions will be created between 2012 and 2022. This amounts to growth of about 0.5 percent per year in the labor force.
The health-care and social assistance sector is expected to grow the most with an annual rate of 2.6 percent. This will add around five million new jobs over that decade. That is about one-third of all the new jobs expected to be created.(21) Other areas that are likely to experience growth include professional services (3.5 million), construction (1.6 million), leisure and hospitality (1.3 million), state and local government (929,000), finance (751,000), and education (675,000).
Interestingly, in light of technology advances, the information sector is one of the areas expected to shrink in jobs. BLS projections anticipate that about 65,000 jobs will be lost there over the coming decade. Even though technology is revolutionizing many businesses, it is doing this by transforming operations, not increasing the number of jobs. Technology can boost productivity and improve efficiency, but does so by reducing the number of employees needed to generate the same or even higher levels of production.
Manufacturing is another area thought to lose jobs. The BLS expects the United States to lose 550,000 jobs, while the federal government will shed 407,000 positions, and agriculture, forestry, fishing, and hunting will drop 223,000 jobs.(22) These sectors are the ones thought to be least likely to generate new positions in the coming decade.
Since BLS projections make few assumptions about emerging technologies, it is likely that their numbers underestimate the disruptive impact of these developments. It is hard to quantify the way that robots, artificial intelligence, and sensors will affect the workforce because we are in the early stages of the technology revolution. It is hard to be definitive about emerging trends because it is not clear how new technologies will affect various jobs.
But there are estimates of the likely impact of computerization on many occupations. Oxford University researchers Carl Frey and Michael Osborn claim that technology will transform many sectors of life. They studied 702 occupational groupings and found that “forty-seven percent of US workers have a high probability of seeing their jobs automated over the next twenty years.”(23) According to their analysis, telemarketers, title examiners, hand sewers, mathematical technicians, insurance underwriters, watch repairers, cargo agents, tax preparers, photographic process workers, new accounts clerks, library technicians, and data entry keyers have a ninety-nine percent of having their jobs computerized. At the other end of the spectrum, recreational therapists, mechanic supervisors, emergency management directors, mental health social workers, audiologists, occupational therapists, health-care social workers, oral surgeons, supervisors of fire fighters, and dieticians have less than a one percent chance of having their tasks computerized. They base their analysis of improving levels of computerization, wage levels, and education required in different fields.(24) In addition, we know that fields such as health care and education have been slow to embrace the technology revolution, but are starting to embrace new models. Innovations in personalized learning and mobile health mean that many schools and hospitals are shifting from traditional to computerized service delivery. Educators are using massive, open, online courses (MOOCs) and tablet-based instruction, while health-care providers are relying on medical sensors, electronic medical records, and machine learning to diagnose and evaluate health treatments.
Hospitals used to be staffed with people who personally delivered the bulk of medical treatment. But health providers now are storing information in electronic medical records and data-sharing networks are connecting lab tests, clinical data, and administration information in order to promote greater efficiency. Patients surf the web for medical information and supplement professional advice with online resources. Both education and health-care sectors are seeing the disruption that previously has transformed other fields.
Given the uncertainties surrounding job projections, it is not surprising that experts disagree over the impact of emerging technologies. For example, in their highly acclaimed book, The Second Machine Age: Work, Progress, and Prosperity in a Time of Brilliant Technologies , economists Erik Brynjolfsson and Andrew McAfee argue that technology is producing major changes in the workforce. According to them:
Technological progress is going to leave behind some people, perhaps even a lot of people, as it races ahead. As we will demonstrate, there has never been a better time to be a worker with special skills or the right education because these people can use technology to create and capture value. However, there has never been a worse time to be a worker with only “ordinary” skills and abilities to offer, because computers, robots, and other digital technologies are acquiring these skills and abilities at an extraordinary rate.(25)
Former US Treasury Secretary Lawrence Summers is equally pessimistic about the employment impact. He argues that “if current trends continue, it could well be that a generation from now a quarter of middle-aged men will be out of work at any given moment.” From his standpoint, “providing enough work” will be the major economic challenge facing the world. (26)
However, some economists dispute these claims. They recognize that many jobs will be lost through technology improvements, but say that new ones will be created. There may be fewer people sorting items in a warehouse because machines can do that better than humans. But jobs analyzing big data, mining information, and managing data-sharing networks will be created. According to those individuals, the job gains and losses will even out over the long run. In future decades, work will be transformed but humans will still be needed to manage the digital world.
For example, MIT economist David Autor has analyzed data on jobs and technology but “doubts that technology could account for such an abrupt change in total employment […] The sudden slowdown in job creation is a big puzzle, but there is not a lot of evidence it is linked to computers.”(27) In the same vein, Harvard economist Richard Freeman is “skeptical that technology would change a wide range of business sectors fast enough to explain recent job numbers.”(28)
Northwestern economist Robert Gordon takes an even stronger stance. He argues that:
Recent progress in computing and automation is less transformative than electrification, cars, and wireless communication, and perhaps even indoor plumbing. Previous advances that enabled people to communicate and travel rapidly over long distances may end up being more significant to society’s advancement than anything to come in the twenty-first century.(29)
Based on this reasoning, he does not anticipate dramatic workforce effects from emerging technologies, even though many other experts already see the substitution of technology for labor.
A Pew Research Center study asked 1,896 experts about the impact of emerging technologies. Its researchers found that:
Half of these experts (48%) envision a future in which robots and digital agents have displaced significant numbers of both blue- and white-collar workers—with many expressing concern that this will lead to vast increases in income inequality, masses of people who are effectively unemployable, and breakdowns in the social order.(30)
Implications for Public Policy
In the classic Edward Bellamy book, Looking Backwards, protagonist Julian West wakes up from a 113-year slumber and finds that the United States in 2000 is completely different from 1887. People stop working at age forty-five and devote their lives to mentoring other people and contributing to the overall sense of community.(31) There are shorter work weeks for ordinary people and everyone receives full benefits, food, and housing.
Similar to our time period, new technologies at that time enabled people to be very productive in a short period of time. Society did not need a large number of workers so people could devote much of their lives to education, volunteerism, and community development. In conjunction with these employment trends, public policy shifted to encourage new lifestyles and ways of living.
In flash-forwarding to the current era, we may be on the verge of a similar technology transition. Robotics and machine learning have improved productivity and enhanced the overall economy of developed nations. Countries that have invested in innovation have seen tremendous growth in overall economic performance. In the future, it may be possible that society will not need as many workers as seen today.
Yet unlike Bellamy’s utopia, there has been little public discussion regarding the economic or policy impact of emerging technologies. Observers worry that knowledge societies are destroying industrial and manufacturing jobs, and exacerbating social and economic divisions. In its most pointed form, skeptics fear that technology will eliminate jobs, reduce incomes, and create a permanent underclass of unemployable people. As argued by Nicolas Colin and Bruno Palier: “Employment is becoming less routine, less steady, and generally less well remunerated. Social policy will therefore have to cover the needs of not just outside the labor market but even many inside it.”(32)
If technology innovation allows businesses to provide goods and services with far fewer employees, what will that mean for workers? A significant increase in the number of people without full-time jobs would exacerbate divisions within society and complicate the distribution of benefits such as pensions, health care, and insurance. Most benefits are tied to employment so if the economy requires fewer workers due to technological advancement, we need to consider how this will affect social benefit delivery.
In this section, I review short- and long-term steps we should consider to deal with emerging technologies. This includes thinking about how to deliver benefits outside of jobs, considering a basic income guarantee, revamping the earned income tax credit, providing activity accounts for lifetime learning and job retraining, encouraging corporate profit-sharing, providing benefit credits for volunteerism, making curricular reforms to assure that students have the skills they need for a twenty-first-century economy, encouraging adult education and continuing learning, expanding arts and culture for leisure time, and avoiding a permanent underclass suffering the ill effects of income inequality.
Benefits Outside of Jobs
If we end up in a situation with many people unemployed or underemployed for significant periods of time, we need a way to provide health care, disability, and pension benefits outside of employment. Called “flexicurity” or flexible security, this idea “separate(s) the provision of benefits from jobs.”(33) It offers health care, education, and housing assistance on a universal basis.
Currently, people must work sixty percent of the time (around twenty-four hours a week) in order to qualify for full-time benefits. When they are fully employed, they are eligible for company-sponsored health-care plans and pensions. During the period since World War II, jobs have been a primary distribution system for social benefits. Except for the poor and elderly, this keeps benefits outside of the public sector and places the onus on private companies.
That approach worked well in an era when most of the people who wanted jobs were able to get them. People with limited skills were able to get well-paying jobs with benefits in factories, warehouses, and production facilities. They could educate their children, achieve a reasonable standard of living, and guard against disabling illnesses.
The complication came when the economy shifted, wages stagnated, and technology made it possible for companies to get by with fewer workers. The advent of robotics, machine learning, artificial intelligence, and machine-to-machine communications eliminated a number of jobs and put a number of people outside the typical workforce.
For health care, people need access to quality care through plans outside of employment. It is possible through commercial insurers to purchase catastrophic insurance for extraordinary health claims. Or if people are poor or elderly, there are government programs that guarantee access to medical care. The recent expansion of health insurance through the Affordable Care Act has extended insurance to millions of people who previously lacked coverage.
In regard to retirement planning, many employers have moved to 401-style pension plans. Employees contribute to their own funds and sometimes get a match from the employer. But this does not help those outside the workforce who need retirement assistance. Even Social Security is tied to employment. People who have not worked are not eligible for retirement benefits so we need to figure out ways to take care of those people in the newly emerging economy.
Provide Activity Accounts for Lifetime Learning and Job Retraining
We should consider the establishment of activity accounts for lifetime learning and job retraining. In an era of fast technology innovation and job displacement, there needs to be a means for people to gain new skills throughout their adulthood. When people are employed, their companies could contribute a set amount to an individual’s fund. This account could be augmented by contributions from the person him or herself as well as the government. Similar to a retirement account, money in the fund could be invested tax-free in investment options including cash reserves, stocks, and bonds. The owner of the account could draw on it to finance lifetime learning and job retraining expenses. It would be portable, meaning that if the person moved or switched jobs, the account would migrate with that individual.
The goal of this account is to provide incentives for continuing education. Under virtually any scenario, people are going to have to continue their education beyond the first twenty years of their lives. Emerging jobs are going to require different skills than what people gain in school. There will be new jobs created that may not exist today. As pointed out by Brookings Institution scholar Kemal Dervis, it will be crucial as technology innovation continues in the future to provide people with a means to upgrade their skills and knowledge levels.(34) He notes that France has established “individual activity accounts” that provide social benefits.
With the expected increase in leisure time, adults need time and financial support for continued learning. We should not envision education merely as a time for young people to learn new skills or pursue areas of interest. Instead, we need to think about education as a continuing activity that broadens people’s horizons over the course of their entire lives. Education is an enrichment activity and we need to view it as a general benefit for the individual as well as the society as a whole.
Incentives for Volunteerism
The trends cited in this analysis suggest that we need to consider income supplements or benefit eligibility through vehicles other than full-time jobs. The workforce ramifications of emerging technologies mean that many people in the future may not be able to provide for their families through regular employment.
One possibility comes through volunteer activities. Even if people have limited employment options, many participate in a wide range of public-minded organizations. They help other people, train the next generation, or provide assistance for the less fortunate in society.
A variety of survey evidence demonstrates that young people are particularly interested in volunteerism. In general, they have different attitudes toward work and leisure time, and many say they want time to pursue outside activities. For example, a survey of American students found that they want “a job that focuses on helping others and improving society.” In addition, they value quality of life considerations, not just financial well-being.(35)
A number of them value volunteer activities outside of their work experience. They have varied interests and want extra-curricular activities that fulfill them. This may involve tutoring in after-school programs, helping English as a Second Language pupils, stopping domestic violence, protecting the environment, or encouraging entrepreneurship. According to a Deloitte study, “63 percent of Millennials donate to charities and 43 percent actively volunteer or are a member of a community organization.”(36)
In a digital world where there may be less work and more leisure time, it makes sense to think about incentives and work credits for volunteerism. This could include credits toward social benefits or public rewards that acknowledge community contributions. In the United Kingdom, for example, volunteers get reimbursed for expenses or earn credits for job training programs through participation in worthy causes. In addition, volunteering counts as “looking for work” so people can use those activities to qualify for national insurance credits.(37)
Going forward, the United States should consider those types of incentives. In the future, people are likely to have more time outside of employment so it makes sense to encourage them toward community engagement and give them incentives to volunteer for nonprofit organizations or charitable causes. This will benefit the overall community and give people purposeful activities in which to engage.
Expanding Arts and Culture for Leisure Time
The so-called “end of work” may create a new kind of economy. According to Harvard economist Lawrence Katz: “It is possible that information technology and robots [will] eliminate traditional jobs and make possible a new artisanal economy […] an economy geared around self-expression, where people would do artistic things with their time.”(38) From his standpoint, this transition would move the world from one of consumption to creativity.
People will use their leisure time to pursue interests in arts and culture, or special areas that they follow. This could include reading, poetry, music, or woodworking. Depending on their background, they could have more time for family and friends. A study of family time found that macroeconomic conditions affect how much time people spend together. When employment problems rise, “fathers spend more time engaging in enriching childcare activities” and “mothers are less likely to work standard hours.”(39) As long as there are opportunities for people to pursue broader interests, reduction in work does not have to eliminate chances for cultural pursuits.
To summarize, advanced societies are at a major turning point in terms of how we think about work, leisure, and social benefit delivery. If these economies need fewer workers to complete needed tasks and benefits are delivered mainly through full-time jobs, there is a danger that many people will have difficulties getting health care, pensions, and the income maintenance they need to sustain their lives. This is of particular concern at a time of large income inequality and highly skewed economic distributions.(40)
The contrast between the age of scarcity in which we have lived and the upcoming age of abundance through new technologies means that we need to pay attention to the social contract. We need to rewrite it in keeping with the dramatic shifts in employment and leisure time that are taking place. People have to understand we are witnessing a fundamental interruption of the current cycle where people are paid for their work and spend their money on goods and services. When a considerable portion of human labor is no longer necessary to run the economy, we have to rethink income generation, employment, and public policy. Our emerging economic system means we will not need all the workers that we have. New technologies will make these individuals obsolete and unemployable.
In this situation, it is important to address the policy and leisure time issues raised by persistent unemployment or underemployment. There is a danger of disruptions and unrest from large groups of people who are not working. That creates poverty and social dissatisfaction and runs the risk of instability for the society as a whole. Stability cannot be enforced through a police presence or having wealthy individuals live in gated communities.
There needs to be ways for people to live fulfilling lives even if society needs relatively few workers. We need to think about ways to address these issues before we have a permanent underclass of unemployed individuals. This includes a series of next steps for society. There needs to be continuous learning avenues, opportunities for arts and culture, and mechanisms to supplement incomes and benefits other than through full-time jobs. Policies that encourage volunteerism and reward those who contribute to worthy causes make sense from the standpoint of society as a whole. Adoption of these steps will help people adapt to the new economic realities.
Acknowledgments
I wish to thank Hillary Schaub for outstanding research assistance on this project.
1. James Manyika, Michael Chui, Jacques Bughin, Richard Dobbs, Peter Bisson, and Alex Marrs, Disruptive Technologies: Advances That Will Transform Life, Business, and the Global Economy (McKinsey Global Institute, May, 2013).
2. Daniela Rus, “How technological breakthroughs will transform everyday life,” Foreign Affairs, July/August, 2015.
3. A more extended discussion of these issues can be found in Darrell M. West, What Happens If Robots Take the Jobs? (Brookings Institution Policy Report, October, 2015).
4. James Hagerty, “Meet the new generation of robots for manufacturing,” Wall Street Journal, June 2, 2015.
5. Alison Sander and Meldon Wolfgang, “The rise of robotics,” The Boston Consulting Group, August 27, 2014. https://www.bcgperspectives.com/content/articles/business_unit_strategy_innovation_rise_of_robotics.
6. RBC Global Asset Management, Global Megatrends: Automation in Emerging Markets (2014).
7. Cynthia Breazeal, “The personal side of robots,” South by Southwest, March 13, 2015.
8. Eitan Wilf. “Sociable robots, jazz music, and divination: contingency as a cultural resource for negotiating problems of intentionality,” American Ethnologist: Journal of the American Ethnological Society, November 6, 2013: 605. http://onlinelibrary.wiley.com/doi/10.1111/amet.12041/abstract.
9. Mike Murphy, “Amazon tests out robots that might one day replace warehouse workers,” Quartz, June 1, 2015.
10. Lydia DePillis, “Minimum-wage offensive could speed arrival of robot-powered restaurants,” Washington Post, August 16, 2015.
11. Thi-Hai-Ha Dang and Adriana Tapus, “Stress game: the role of motivational robotic assistance in reducing user’s task stress,” International Journal of Social Robotics, April, 2015.
12. Michael Lewis, Flash Boys: A Wall Street Revolt (New York: Norton, 2015).
13. Andrei A. Kirilenko and Andrew W. Lo, “Moore’s Law versus Murphy’s Law: algorithmic trading and its discontents,” Journal of Economic Perspectives, 2013. http://www.jstor.org/stable/pdf/23391690.pdf?acceptTC=true.
14. Shukla Shubhendu and Jaiswal Vijay, “Applicability of artificial intelligence in different fields of life,” International Journal of Scientific Engineering and Research, September, 2013.
17. Derek Thompson, “A world without work,” The Atlantic, July/August, 2015.
18. Dawn Nakagawa, “The second machine age is approaching,” Huffington Post, February 24, 2015.
19. MIT Technology Review, “Who will own the robots,” September, 2015.
20. Martin Ford, The Lights in the Tunnel: Automation, Accelerating Technology, and the Economy of the Future (CreateSpace Independent Publishing Platform, 2009). Also see his more recent book, Rise of the Robots: Technology and the Threat of a Jobless Future (New York: Basic Books, 2015).
21. US Bureau of Labor Statistics, “Employment projections: 2012–2022 summary,” December 19, 2013. http://www.bls.gov/news.release/ecopro.nr0.htm
23. Quoted in Harold Meyerson, “Technology and trade policy is pointing America toward a job apocalypse,” Washington Post, March 26, 2014. The original paper is by Carl Benedikt Frey and Michael Osborne, “The future of employment: how susceptible are jobs to computerisation,” Oxford University Programme on the Impacts of Future Technology, September 17, 2013.
24. Frey and Osborne, “The future of employment,” op. cit.: 57–72.
25. Erik Brynjolfsson and Andrew McAfee, The Second Machine Age: Work, Progress, and Prosperity in a Time of Brilliant Technologies (New York: W. W. Norton, 2014), 11.
26. Lawrence Summers, “The economic challenge of the future: jobs,” Wall Street Journal, July 7, 2014.
27. Quoted in David Rotman, “How technology is destroying jobs,” MIT Technology Review, June 12, 2013. http://www.technologyreview.com/featuredstory/515926/how-technology-is-destroying-jobs/
29. Quoted in Melissa Kearney, Brad Hershbein, and David Boddy, “The future of work in the age of the machine,” Brookings Institution Hamilton Project, February, 2015.
30. Aaron Smith and Janna Anderson, “AI, robotics, and the future of jobs,” Pew Research Center, August 6, 2014.
31. Edward Bellamy, Looking Backward 2000–1887 (Boston: Ticknor & Co., 1888).
32. Nicolas Colin and Bruno Palier, “Social policy for a digital age,” Foreign Affairs, July/August, 2015.
34. Kemal Dervis, “A new birth for social democracy,” Brookings Institution Project Syndicate, June 10, 2015.
35. The Griffith Insurance Education Foundation, “Millennial generation attitudes about work and the insurance industry,” February 6, 2012.
36. Lindsey Pollack, “Attitudes and attributes of millennials in the workplace,” September 12, 2014.
37. Job Centre Plus, “Volunteering while getting benefits,” UK Department for Work and Pensions, October, 2010. https://www.gov.uk/government/uploads/system/uploads/attachment_data/file/264508/dwp1023.pdf
38. Quoted in Thompson, “A world without work,” op. cit.
39. Melinda Sandler Morill and Sabrina Wulff Pabilonia, “What effects do macroeconomic conditions have on families’ time together?” Leibniz Information Centre for Economics, 2012. http://hdl.handle.net/10419/58561
40. Darrell M. West, Billionaires: Reflections on the Upper Crust (Washington DC: Brookings Institution Press, 2014).
Related publications
- Augmented Environments and New Media Forms
- How Is the Internet Changing the Way We Work?
- Possible Futures and the Future We Want
Download Kindle
Download epub, download pdf, more publications related to this article, more about economy, geopolitics, global economy, comments on this publication.
Morbi facilisis elit non mi lacinia lacinia. Nunc eleifend aliquet ipsum, nec blandit augue tincidunt nec. Donec scelerisque feugiat lectus nec congue. Quisque tristique tortor vitae turpis euismod, vitae aliquam dolor pretium. Donec luctus posuere ex sit amet scelerisque. Etiam sed neque magna. Mauris non scelerisque lectus. Ut rutrum ex porta, tristique mi vitae, volutpat urna.
Sed in semper tellus, eu efficitur ante. Quisque felis orci, fermentum quis arcu nec, elementum malesuada magna. Nulla vitae finibus ipsum. Aenean vel sapien a magna faucibus tristique ac et ligula. Sed auctor orci metus, vitae egestas libero lacinia quis. Nulla lacus sapien, efficitur mollis nisi tempor, gravida tincidunt sapien. In massa dui, varius vitae iaculis a, dignissim non felis. Ut sagittis pulvinar nisi, at tincidunt metus venenatis a. Ut aliquam scelerisque interdum. Mauris iaculis purus in nulla consequat, sed fermentum sapien condimentum. Aliquam rutrum erat lectus, nec placerat nisl mollis id. Lorem ipsum dolor sit amet, consectetur adipiscing elit.
Nam nisl nisi, efficitur et sem in, molestie vulputate libero. Quisque quis mattis lorem. Nunc quis convallis diam, id tincidunt risus. Donec nisl odio, convallis vel porttitor sit amet, lobortis a ante. Cras dapibus porta nulla, at laoreet quam euismod vitae. Fusce sollicitudin massa magna, eu dignissim magna cursus id. Quisque vel nisl tempus, lobortis nisl a, ornare lacus. Donec ac interdum massa. Curabitur id diam luctus, mollis augue vel, interdum risus. Nam vitae tortor erat. Proin quis tincidunt lorem.
Human Legacies When Robots Rule the Earth
Do you want to stay up to date with our new publications.
Receive the OpenMind newsletter with all the latest contents published on our website
OpenMind Books
- The Search for Alternatives to Fossil Fuels
- View all books
About OpenMind
Connect with us.
- Keep up to date with our newsletter
Quote this content
How to Accelerate Technological Progress
What do we know about what works?
This essay is a map of the content on New Things Under the Sun that relate to accelerating technological progress. For this essay I’ll basically take it as a given that technological progress is desirable. While I think that’s true, I don’t necessarily think it is obvious (see the article “ What are the returns to R&D? ” for one theoretical argument for why we should be spending more on R&D and “ When technology goes bad ” for some reasons progress could be bad). The main message is that there are lots of factors that collectively set the rate of technological progress - it’s not down to any one thing.
Unless otherwise noted, all of the below is founded on a base of evidence derived from academic studies. That doesn’t mean it’s correct: academic knowledge isn’t received from on high; it is always contested and always evolving. But it does represent a real effort to think through these issues with data and careful thought by a community of experts. So I think it’s worth hearing.
The raw inputs of innovation: human beings
Innovation comes from human beings (at least for now). So one of the most important inputs to innovation is, simply, more people. And indeed, we do have some evidence that larger populations innovate at a faster rate, though most of this evidence relates to innovation that predates the modern world. The article “ More people leads to more ideas ” reviews some evidence consistent with this view from isolated islands, lab experiments, and the history of the human race.
So anything that increases our population could increase the rate of technological progress .
Of course, not all people become innovators. One reason may be that they lack the resources to innovate (see below, on incentives). But another might be that they lack the very idea of trying new things . People take their cues about what range of options they should consider from the people around them. And if the people around you simply accept problems as unsolvable, you might do the same and not try to innovate yourself. That’s one way that innovation can become self-perpetuating: if lots of people are trying to innovate, that can inspire others to view problems as things to be solved rather than mitigated.
The best evidence we have that this notion is true comes from a variety of studies about entrepreneurship. As discussed in “ Entrepreneurship is contagious ”, we tend to find entrepreneurs in social clusters, and when we expose people who are not themselves predisposed to be entrepreneurs to entrepreneurship, it seems to increase the probability they go on to found businesses. “ The ‘idea’ of being an entrepreneur ” provides further related evidence: the impact of entrepreneurial peers is highest when they occupy a similar social position and lowest when someone probably already has the “idea” of being an entrepreneur. Moreover, entrepreneurs self-report that family/friend role models are important reasons for them starting a business. Finally, we have a bit of evidence you can transmit these ideas even through media. That all suggests people might obtain the “idea” of trying new things from seeing people like themselves try new things.
That implies one way to accelerate technological progress is to expose people to examples of people like themselves innovating.
Now let’s return to an assumption we made at the outset of this section, that humans are necessary inputs to innovation. We’re living in an era of rapidly advancing artificial intelligence; what if we drop that assumption? There’s not much in the way of empirical data on what happens when machines take over the task of innovating because it hasn’t happened yet, but the article “ What if we could automate invention? ” looks at what one particular class of economic models say on this question. These models imply that if you really could replace all the human inventors with equally capable robots, then growth accelerates ever faster into something wild and unparalleled in human history.
But that singularity-style result requires fully replacing humans with robots. A more nuanced model thinks of innovation as consisting of a large number of different tasks, some of which are automated, some of which can only be done by humans, but all of which are necessary for innovation to occur. 1 When humans are still the only ones capable of doing some of the necessary innovation work, then the accelerating dynamic described doesn’t work.
Instead, these models predict the rate of progress depends not on how much of the innovation pipeline is automated, but the rate at which we automate more and more of it.
Innovation with and without a map
So now you’ve got some people who are open to new and better ways of doing things. How successful are they likely to be?
People can actually get pretty far innovating without deep causal knowledge of how things “really” work. This occurs via a process analogous to biological evolution; tinker and retain any improvements. The article “ Progress in programming as evolution ” provides the most direct evidence of innovation as a sort of evolutionary process, at least in the context of coding. The article “ Learning curves are tough to use ” looks into another example of this phenomenon, wherein experience in a particular production process drives progress (though note that a lot of the literature on this kind of innovation greatly overstates how predictable and useful it is - see “ Standard evidence for learning curves is not good enough ”).
So, to encourage innovation (at least to some extent), just encourage people to get started using new production processes.
But to move really quickly and to make the kinds of big jumps that you simply can’t arrive at via a process of blind tinkering, it helps to have a map of the terrain. Knowledge can be that map. The article “ Science as a map of unfamiliar terrain ” reviews evidence from a few papers that patents which use science are more valuable, especially when the patented invention occupies relatively unfamiliar knowledge terrain.
More generally, while modern science has its share of problems, on the whole it generates knowledge that helps us better understand the world. A series of natural experiments where scientific progress was rapidly and unexpectedly sped up or slowed down, discussed in “ More science leads to more innovation ,” goes on to show that basic scientific discoveries are, indeed, spun out into new and valuable technologies. The article “ Science is good at making useful knowledge ” provides some additional, more correlational, evidence: patents that cite science seem to outperform those that don’t. And even technologies that do not directly rely on new scientific discoveries frequently rely indirectly on them. Evidence for this, suggested by the predictive power of patent citations, is discussed in “ Ripples in the river of knowledge .”
Lastly, the articles “ How common is independent discovery? ” and “ Contingency and science ” imply there is plenty of unexplored scientific terrain out there, waiting to be discovered. “How common is independent discovery?” argues it is, in fact, not particularly common for multiple groups to independently discover the same idea. That suggests we have not reached a saturation point wherein additional researchers would mostly end up repeating the work done by others. The article “Contingency and science” complements this line of evidence by reviewing evidence that the direction taken by science has a lot of contingency; that suggests the existence of paths not taken, which might be fruitfully explored by additional scientists.
That means another obvious way to accelerate technological progress is to increase our knowledge of how the world works by expanding basic scientific research.
Steering Science
More science is better, but assuming there are limits, how should we choose which scientific topics get more support and which get less?
In general, targeting the science most likely to be useful for technological progress is actually quite challenging. The article “ Knowledge spillovers are a big deal ” looks at several examples where the intended beneficiary of a specific research project accounted for only a small part of the total benefits. In other words, the R&D had many unintended benefits, implying that precise targeting is challenging. Relatedly, there tends to be quite a long time lag between when scientific discoveries are made and when technological applications become available. The article “ How long does it take to go from science to technology ” looks at citations from patented inventions to academic work, as well as statistical correlations between scientific research and industry productivity, to estimate that the time between a scientific discovery and a technological implementation is often on the order of 20 years.
All told, given a long enough time horizon, scientific funding should not strive to be too targeted to specific problems since spillovers may provide unexpected solutions.
One of the most important ways we actually allocate funds to different scientific priorities at present is via academia. The academic ecosystem has created a system of incentives that pushes researchers to do novel work within a framework of methods and background knowledge sufficiently well understood by a community of experts that its merit can be evaluated. This system works ok - as noted above, science does seem to lead to technological advance - but it’s far from perfect.
To begin, who are the scientists choosing what to work on? The articles “ Gender and what gets researched ” and “ Geography and what gets researched ” provide some evidence that who is able to do research has a measurable impact on what gets researched. Specifically, having more women inventors and researchers in a field helps a field avoid gender-related blindspots, both because women tend to be more likely to work on different things then men and because men are more likely to be responsive to the priorities of women when there are more female researchers in a field. A similar dynamic plays out on a geographical axis: researchers seem to be disproportionately drawn to local problems (though this affinity can be overcome).
In general, if we want research to cater to the priorities of society at large, then it helps to have scientist at large represented in the research community.
Beyond the identity of the scientific community, the processes are also not perfect. Consider anonymous peer review, which forms an important input into how most science funding is allocated. The article “ What does peer review know? ” presents some evidence that peer review does generate useful (albeit pretty noisy) information about the likely success of research projects. However, “ Biases against risky research ” presents some reasons to believe this system might also be biased against certain kinds of risky (but ultimately more valuable) research streams. “ Can taste beat peer review? ” argues that some problems with peer review might be overcome by relying on well incentivized and carefully selected individual decision-makers, but the evidence base here is thin.
The article “ Conservatism in science ” looks at other evidence that science has a bias against very novel research questions, preferring work that is more closely tied to existing paradigms. That article suggests this might, in part, be due to budget constraints; if it’s hard for very unusual proposals to score top marks, and the budget is so tight that only proposals with top marks get funded, then novel research might be underfunded.
Peer review does provide (limited) information on how well research proposals will fare, but may be subject to biases against some kinds of risky or novel research.
Setting aside the questions of what research projects get supported, there are a different set of issues related to the conduct of research and dissemination of results. The articles “ One question many answers ” and “ Publication bias is real ” for example, document serious issues with the research and publication process. The articles “ Publication bias without editors? The case of preprint servers ” and “ Publish-or-perish and the quality of science ” look at some evidence that these problems are at least partially due to factors related to the publication system (and the associated prestige of publishing). Both articles argue these effects are real, but probably not that large in their magnitude. The article “ Why is publication bias worse in some disciplines than in others ” points instead to issues related to the quality of empirical methods as an important cause of publication bias. Essentially, to the extent that empirical work is “more art than science” in a field, the easier it is to justify not publishing work that goes against the prevailing wisdom.
All in all, it seems almost certain that we can improve our processes for selecting which research projects to fund, and how we disseminate and communicate findings. But the evidence base is thin enough that I’m nervous recommending any particular course of action.
To close, let’s assume we have identified a domain where the traditional scientific incentive system is not working well; specifically, we’ll assume it is underinvesting in research on an important topic. Now what?
The article “ Building a new research field ” looks at two specific challenges that come with trying to encourage development of an understudied topic. First, because it is hard to do great science in isolation, there is a coordination challenge with getting a critical mass of scientists to begin work in a new field. One tool for solving this challenge is scientific prizes, discussed at length in the article “ Steering science with prizes .”
It seems scientific prizes can sometimes galvanize research in overlooked areas by creating credible, public, signals of areas of promising research.
A second challenge to building a new research field is that it is challenging to do great work in a new field, at least on average. That creates a strong incentive for scientists to “stay in their lane” rather than branch out and try new things. If we want to encourage scientists to try new things regardless, one approach may be to offer some insulation from the usual demands of academia to continuously produce high profile publications. Alas, as discussed in “Building a new research field” the evidence on the efficacy of this strategy is mixed.
Humans + knowledge = faster innovation
Now, we’ve got people looking to solve problems, and knowledge to help them find solutions. That knowledge isn’t much good if it’s not in the head of the people who need it. For that reason, it’s also important to circulate knowledge. You can do this in a variety of ways.
The article “ Free knowledge and innovation ” looks at several experimental and quasi-experimental studies on different forms of free knowledge depositories (think libraries and wikipedia) to show making knowledge freely accessible has a measurable positive impact on the rate of innovation.
One way to accelerate innovation is to create free knowledge resources.
Not all knowledge is codified in text though; some of it lives primarily in people’s heads. One way to get at this knowledge is just to pick knowledgeable people up and drop them down in a community that lacks the requisite knowledge. The article “ Importing knowledge ” looks at this in the context of immigration, examining a variety of cases where an influx of immigrants with a specific knowledge advantage led to that knowledge advantage being picked up in the host country.
Policies that encourages high skilled immigration, especially from people with different knowledge advantages, can also accelerate innovation.
Lastly, the article “ Urban social infrastructure and innovation ” looks at some evidence that creating ways for people to mix locally - in bars, cafes, and in dense neighborhoods - helps circulate local knowledge. The article “ Innovation at the Office ” surveys similar evidence for colleagues working within the same building and the article “ Why proximity matters: who you know ” complements both articles, highlighting the ways that proximity seems to be important especially for encouraging meetings between people who might not normally interact. The article “ Local Learning ” provides some additional evidence that local interactions facilitate the exchange of novel ideas among academics working together at the same university. Lastly, “ Academic conferences and collaboration ” indicates that social mixing doesn’t only mean where you live and work - it looks at how conferences encourage the formation of new collaborative relationships.
Another way to accelerate innovation is to encourage social mixing of people with different sets of knowledge.
Incentivizing Innovation
Once you’ve got people who are open to trying to solve problems, and who have the knowledge to help them innovate, innovation is now in their choice set. But for them to choose to innovate (instead of doing some other thing in their choice set), you’ve got to make it an attractive option, either by lowering the costs of innovating or raising the benefits of it.
One simple way to lower the costs of innovation is simply to provide the funding for innovation activities, e.g., from the government.
That can be risky. The government might not know who to fund, and recipients might not use the money as intended. But we do have pretty good evidence that this is not an insurmountable problem for governments. “ An example of high returns to publicly funded R&D ” looks at some quasi-experimental evaluations of programs like the US government’s small business innovation and research grants. These studies show the funds make a difference, and probably have quite good returns on investment.
With some caveats, we also have decent evidence that private sector R&D is pretty responsive to market signals. “ When extreme necessity is the mother of invention ” presents some straight-forward evidence that innovation is highly responsive to demand, in this case the kind of very extreme demand that arises in the wake of global crises (think global pandemics, world wars, and energy crises). For some evidence from more “normal” times, “ Medicine and the limits of market-driven innovation ” looks at a number of studies in medicine that show the R&D of pharma and other private sector health companies is quite responsive to market signals. When there’s more money in curing a disease, there’s more R&D. But at the same time, that R&D tends to be limited to stuff that’s already close to the market. More fundamental research doesn’t seem very responsive to market signals. Similarly, “ Pulling more fuel efficient cars into existence ” reviews studies that use patents and direct measures of technological progress in fuel efficiency to argue carmakers are responsive to market signals as well. Specifically, higher fuel prices and explicit emissions standards (enforced by fines for failing to meet them) seem to work quite reliably at inducing incremental innovation, though it is less clear they work for more radical kinds of innovation.
Still, to rapidly pull technologies close to viability onto the market, making it more profitable to do so seems to work.
Competitive markets are good at efficiently allocating resources in an economy, by aggregating information and equating the value people derive from a product with the marginal cost of producing it. But not all products are well served by the market system, especially public goods (of which knowledge is just one example). It can be hard to efficiently allocate resources to public goods without market signals. One alternative that has been proposed is Buterin, Hitzig, and Weyl’s proposal for a mechanism to fund public goods in a decentralized fashion, discussed in the article “ Optimal Kickstarter .”
As a general principle, the way we incentivize innovation is itself a (social) technology, and we should try to make progress on this technology as well, by trying new things.
Organizing Innovators
Now you’ve got knowledgeable people open to solving problems and incentivized to do so. Can we say anything about the way you should approach solving a problem?
One common finding in studies of innovation is that “ The best new ideas combine disparate old ideas .” In other words, creating an environment where inventors are exposed to disparate ideas can sometimes lead to unexpected breakthroughs. As noted earlier, this can be one rationale for innovators to physically locate in a space with many other knowledge workers whether that be a city or building. But there may be other ways to encourage workers to become exposed to unexpected ideas, such as by shuffling workers between project types, allocating some portion of their time to undirected exploration/personal projects, or creating a culture of open communication between different divisions (though note these suggestions are speculations on my part, not derived from studies reviewed on this site).
Important, but perhaps unexpected, innovations may be more likely when inventors are exposed to many different kinds of idea.
Another basic factor to consider is the size of the team that works on a problem. As described in the article “ Are ideas getting harder to find because of the burden of knowledge? ” there has been a trend towards greater use of teams in the innovation process, possibly because the amount of knowledge that must be directed at a problem tends to grow over time. The article “ Highly cited innovation takes a team ” surveys some work on the efficacy of innovative teams in different domains, where impact is mostly measured by creating highly cited patents or projects. It tends to be the case that bigger teams are associated with more impact, with some evidence that avoiding “weak links” in a team is more important than including a super-productive “star.” But a big caveat here is that all this evidence is correlational - we don’t have much in the way of experiments (natural or artificial) to draw on here.
Many problems seem to be best solved with a team.
We also have some work (just one study, so be cautious putting too much weight on it) that while teams are good at producing highly cited work. Coincidentally, you see the same dynamic at the level of the organization. The article “ The size of firms and the nature of innovation ” documents that larger firms seem to develop more incremental innovations than smaller ones, on average (even as they seem to spend on R&D at the same proportional rate as small firms).
A variety of reasons have been proposed for this correlation between the size of an innovating group and its incremental nature. The article “ Big firms have different incentives ” argues, at least for large organizations, part of the problem may be simply be the differing incentives faced by large firms. Since they sell more units, incremental cost reductions are more valuable for large firms than for small ones. On the sales side, large incumbent firms partially cannibalize their own sales when they invent new and superior products, which further disincentivizes this kind of innovation.
Large teams and large organizations appear to produce more incremental innovations, at least on average.
A final question to consider is whether a team of inventors (or scientists) should work together in the same physical space, or remotely. In some cases, there is no question, because work requires physical proximity to research instruments. But in other cases, it is entirely possible for a team to collaborate at a distance most of the time, though likely gathering in one place on at least a few occasions. As discussed in the article “ Remote breakthroughs ” the conventional wisdom that colocation is ideal for generating breakthrough ideas seems to have been true for most of the twentieth century, but is probably less applicable today in the era of digital collaboration. Indeed, some evidence suggests remote teams today are more capable of generating remote breakthroughs, possibly because they can draw on a more varied set of local knowledge environments or possibly because remote communication disrupts some of the social dynamics that normally hinder productive collaboration.
Where it is feasible, remote collaboration among team members should no longer be assumed to be inferior to working together in the same physical space.
To summarize. Innovation happens when people apply knowledge to problems. There are lots of points where we can nudge that process along faster. More people (or more robots to take on some of their tasks). More people considering innovation. More knowledge. Better organization of science. More communication of knowledge. More resources to innovation. More benefits from innovating. Better management of innovators. Each of those might only have a small impact individually; but they stack up. Moreover, they compound, year on year.
There is so much more to say about all this; the academic literature is vast. So this essay will grow, bit by bit, as New Things Under the Sun gets more and more new articles added. But hopefully this has given us some places to start.
Note: this essay is continuously updated as relevant articles are added to New Things Under the Sun. To keep abreast of updates, subscribe to the site newsletter.
Introduction
What are the returns to R&D?
When technology goes bad
More people leads to more ideas
Entrepreneurship is contagious
The ‘idea’ of being an entrepreneur
What if we could automate invention?
Progress in programming as evolution
Learning curves are tough to use
Standard evidence for learning curves is not good enough
Science as a map of unfamiliar terrain
More science leads to more innovation
Science is good at making useful knowledge
Ripples in the river of knowledge
Knowledge spillovers are a big deal
How long does it take to go from science to technology
Gender and what gets researched
Geography and what gets researched
What does peer review know?
Biases against risky research
Can taste beat peer review?
Conservatism in science
One question many answers
Publication bias is real
Publication bias without editors? The case of preprint servers
Publish-or-perish and the quality of science
Why is publication bias worse in some disciplines than in others
Building a new research field
Steering science with prizes
Human + Knowledge = faster innovation
Free knowledge and innovation
Importing knowledge
Urban social infrastructure and innovation
Innovation at the Office
Why proximity matters: who you know
Local Learning
Academic conferences and collaboration
An example of high returns to publicly funded R&D
When extreme necessity is the mother of invention
Medicine and the limits of market-driven innovation
Pulling more fuel efficient cars into existence
Optimal Kickstarter
The best new ideas combine disparate old ideas
Are ideas getting harder to find because of the burden of knowledge?
Highly cited innovation takes a team
The size of firms and the nature of innovation
Big firms have different incentives
Remote breakthroughs

Talk to our experts
1800-120-456-456
- Technology Essay
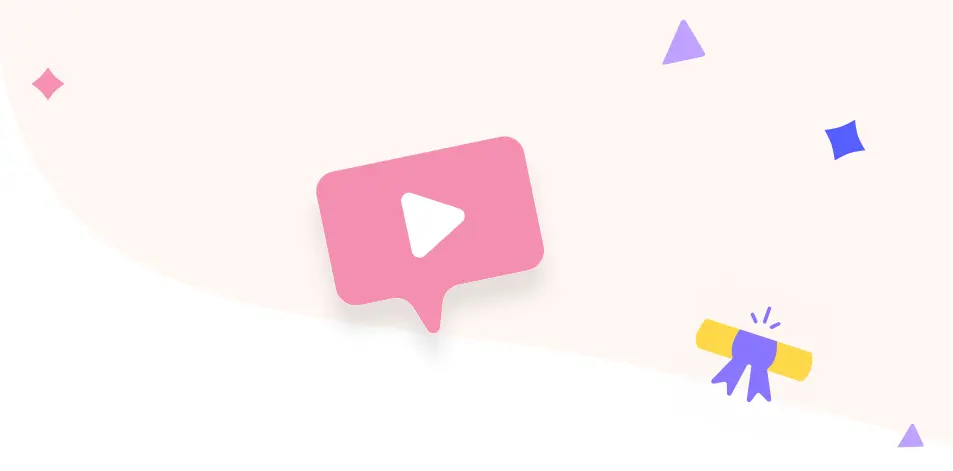
Essay on Technology
The word "technology" and its uses have immensely changed since the 20th century, and with time, it has continued to evolve ever since. We are living in a world driven by technology. The advancement of technology has played an important role in the development of human civilization, along with cultural changes. Technology provides innovative ways of doing work through various smart and innovative means.
Electronic appliances, gadgets, faster modes of communication, and transport have added to the comfort factor in our lives. It has helped in improving the productivity of individuals and different business enterprises. Technology has brought a revolution in many operational fields. It has undoubtedly made a very important contribution to the progress that mankind has made over the years.
The Advancement of Technology:
Technology has reduced the effort and time and increased the efficiency of the production requirements in every field. It has made our lives easy, comfortable, healthy, and enjoyable. It has brought a revolution in transport and communication. The advancement of technology, along with science, has helped us to become self-reliant in all spheres of life. With the innovation of a particular technology, it becomes part of society and integral to human lives after a point in time.
Technology is Our Part of Life:
Technology has changed our day-to-day lives. Technology has brought the world closer and better connected. Those days have passed when only the rich could afford such luxuries. Because of the rise of globalisation and liberalisation, all luxuries are now within the reach of the average person. Today, an average middle-class family can afford a mobile phone, a television, a washing machine, a refrigerator, a computer, the Internet, etc. At the touch of a switch, a man can witness any event that is happening in far-off places.
Benefits of Technology in All Fields:
We cannot escape technology; it has improved the quality of life and brought about revolutions in various fields of modern-day society, be it communication, transportation, education, healthcare, and many more. Let us learn about it.
Technology in Communication:
With the advent of technology in communication, which includes telephones, fax machines, cellular phones, the Internet, multimedia, and email, communication has become much faster and easier. It has transformed and influenced relationships in many ways. We no longer need to rely on sending physical letters and waiting for several days for a response. Technology has made communication so simple that you can connect with anyone from anywhere by calling them via mobile phone or messaging them using different messaging apps that are easy to download.
Innovation in communication technology has had an immense influence on social life. Human socialising has become easier by using social networking sites, dating, and even matrimonial services available on mobile applications and websites.
Today, the Internet is used for shopping, paying utility bills, credit card bills, admission fees, e-commerce, and online banking. In the world of marketing, many companies are marketing and selling their products and creating brands over the internet.
In the field of travel, cities, towns, states, and countries are using the web to post detailed tourist and event information. Travellers across the globe can easily find information on tourism, sightseeing, places to stay, weather, maps, timings for events, transportation schedules, and buy tickets to various tourist spots and destinations.
Technology in the Office or Workplace:
Technology has increased efficiency and flexibility in the workspace. Technology has made it easy to work remotely, which has increased the productivity of the employees. External and internal communication has become faster through emails and apps. Automation has saved time, and there is also a reduction in redundancy in tasks. Robots are now being used to manufacture products that consistently deliver the same product without defect until the robot itself fails. Artificial Intelligence and Machine Learning technology are innovations that are being deployed across industries to reap benefits.
Technology has wiped out the manual way of storing files. Now files are stored in the cloud, which can be accessed at any time and from anywhere. With technology, companies can make quick decisions, act faster towards solutions, and remain adaptable. Technology has optimised the usage of resources and connected businesses worldwide. For example, if the customer is based in America, he can have the services delivered from India. They can communicate with each other in an instant. Every company uses business technology like virtual meeting tools, corporate social networks, tablets, and smart customer relationship management applications that accelerate the fast movement of data and information.
Technology in Education:
Technology is making the education industry improve over time. With technology, students and parents have a variety of learning tools at their fingertips. Teachers can coordinate with classrooms across the world and share their ideas and resources online. Students can get immediate access to an abundance of good information on the Internet. Teachers and students can access plenty of resources available on the web and utilise them for their project work, research, etc. Online learning has changed our perception of education.
The COVID-19 pandemic brought a paradigm shift using technology where school-going kids continued their studies from home and schools facilitated imparting education by their teachers online from home. Students have learned and used 21st-century skills and tools, like virtual classrooms, AR (Augmented Reality), robots, etc. All these have increased communication and collaboration significantly.
Technology in Banking:
Technology and banking are now inseparable. Technology has boosted digital transformation in how the banking industry works and has vastly improved banking services for their customers across the globe.
Technology has made banking operations very sophisticated and has reduced errors to almost nil, which were somewhat prevalent with manual human activities. Banks are adopting Artificial Intelligence (AI) to increase their efficiency and profits. With the emergence of Internet banking, self-service tools have replaced the traditional methods of banking.
You can now access your money, handle transactions like paying bills, money transfers, and online purchases from merchants, and monitor your bank statements anytime and from anywhere in the world. Technology has made banking more secure and safe. You do not need to carry cash in your pocket or wallet; the payments can be made digitally using e-wallets. Mobile banking, banking apps, and cybersecurity are changing the face of the banking industry.
Manufacturing and Production Industry Automation:
At present, manufacturing industries are using all the latest technologies, ranging from big data analytics to artificial intelligence. Big data, ARVR (Augmented Reality and Virtual Reality), and IoT (Internet of Things) are the biggest manufacturing industry players. Automation has increased the level of productivity in various fields. It has reduced labour costs, increased efficiency, and reduced the cost of production.
For example, 3D printing is used to design and develop prototypes in the automobile industry. Repetitive work is being done easily with the help of robots without any waste of time. This has also reduced the cost of the products.
Technology in the Healthcare Industry:
Technological advancements in the healthcare industry have not only improved our personal quality of life and longevity; they have also improved the lives of many medical professionals and students who are training to become medical experts. It has allowed much faster access to the medical records of each patient.
The Internet has drastically transformed patients' and doctors’ relationships. Everyone can stay up to date on the latest medical discoveries, share treatment information, and offer one another support when dealing with medical issues. Modern technology has allowed us to contact doctors from the comfort of our homes. There are many sites and apps through which we can contact doctors and get medical help.
Breakthrough innovations in surgery, artificial organs, brain implants, and networked sensors are examples of transformative developments in the healthcare industry. Hospitals use different tools and applications to perform their administrative tasks, using digital marketing to promote their services.
Technology in Agriculture:
Today, farmers work very differently than they would have decades ago. Data analytics and robotics have built a productive food system. Digital innovations are being used for plant breeding and harvesting equipment. Software and mobile devices are helping farmers harvest better. With various data and information available to farmers, they can make better-informed decisions, for example, tracking the amount of carbon stored in soil and helping with climate change.
Disadvantages of Technology:
People have become dependent on various gadgets and machines, resulting in a lack of physical activity and tempting people to lead an increasingly sedentary lifestyle. Even though technology has increased the productivity of individuals, organisations, and the nation, it has not increased the efficiency of machines. Machines cannot plan and think beyond the instructions that are fed into their system. Technology alone is not enough for progress and prosperity. Management is required, and management is a human act. Technology is largely dependent on human intervention.
Computers and smartphones have led to an increase in social isolation. Young children are spending more time surfing the internet, playing games, and ignoring their real lives. Usage of technology is also resulting in job losses and distracting students from learning. Technology has been a reason for the production of weapons of destruction.
Dependency on technology is also increasing privacy concerns and cyber crimes, giving way to hackers.
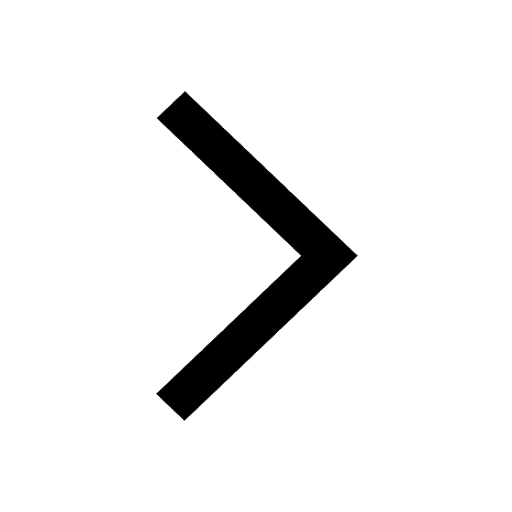
FAQs on Technology Essay
1. What is technology?
Technology refers to innovative ways of doing work through various smart means. The advancement of technology has played an important role in the development of human civilization. It has helped in improving the productivity of individuals and businesses.
2. How has technology changed the face of banking?
Technology has made banking operations very sophisticated. With the emergence of Internet banking, self-service tools have replaced the traditional methods of banking. You can now access your money, handle transactions, and monitor your bank statements anytime and from anywhere in the world. Technology has made banking more secure and safe.
3. How has technology brought a revolution in the medical field?
Patients and doctors keep each other up to date on the most recent medical discoveries, share treatment information, and offer each other support when dealing with medical issues. It has allowed much faster access to the medical records of each patient. Modern technology has allowed us to contact doctors from the comfort of our homes. There are many websites and mobile apps through which we can contact doctors and get medical help.
4. Are we dependent on technology?
Yes, today, we are becoming increasingly dependent on technology. Computers, smartphones, and modern technology have helped humanity achieve success and progress. However, in hindsight, people need to continuously build a healthy lifestyle, sorting out personal problems that arise due to technological advancements in different aspects of human life.
The Measure technological Progress Essay
- To find inspiration for your paper and overcome writer’s block
- As a source of information (ensure proper referencing)
- As a template for you assignment
Introduction
Whether technology can be meaningfully measured.
Progress as understood by many enlightenment thinkers is defined as the idea that humanity or civilizations will change their social existence for the better. In other words, progress is the belief that human beings are generally heading in a desirable direction. Throughout society, man has undergone definite social change and this can be attributed to several reasons; central to this argument is the role that technology (especially communication and transport-related technologies) has played in making this possible. The paper postulates that there is a definite link between social progress and these technologies and a tangible way of measuring that progress will be proposed thereafter.
Enlightenment thinkers put forward the notion that human beings possess an infinite potential to progress. They further argue that the unity of knowledge provides a great degree of reward and minimal suffering. Human beings respond very positively to new things and knowledge because it provides them with an opportunity to know more about their lives. While such notions may seem traditional, it is critical to acknowledge the fact that such assertions have been supported by a lot of events and incidences across history. (Blumenberg, 256) The major problem would be whether there are tangible methods for measuring these technological developments.
To effectively measure technological progress and hence render support to its relation to social progress, it is critical to understand that technology is inspired by the historical, political, social, and economic forces at play within a certain period. Anthropologists and sociologists have studied the concept of cultural and social evolution and a substantial number of them believe that the central propellant of development amongst human civilization is technology especially communication and transport technologies. For instance, Lewis Morgan argued that there are three major stages in social evolution and these include civilization, barbarism, and savagery. One can utilize this model to establish a model for measuring technological progress. This is largely because there is a visible transformation that such advancements caused about the way people lived their lives over the ages. Technological milestones strongly stand out in history and these can be associated with how social evolution has occurred with time. For example, during the savagery era the discovery of fire, the use of the bow, and the development of pottery can be seen as some of the indicators of advancement and these inventions can then be associated with humanity’s improvement. At that time, communication developments were associated with the discovery of fire and life become much easier or progressed because of these inventions. In the barbarian era, the domestication of animals, the development of agriculture, and the commencement of metalworking were critical in changing people’s lives for the better. (Wilston & Kingston, 52)
Through these inventions, transport and communication technologies emanated. For example, animal domestication led to hose riding and easy movement of people from point to point. Additionally, metalwork led to the invention of the wheel and hence efficiency in transport through steam turbines, trains, and the like. (Hellemans & Bunch, 103) In the age of civilization, learning the alphabet and learning to read and write were the most important inventions of that time. They paved the way for fast and convenient communication between various groups and this eventually translated to a closer-knit world. There are various inventions today (or in the recent past) that markedly affect the way people relate to one another through communication and transportation. For instance, when motor vehicles were invented, there was less time spent moving from place to place. When the internet was developed, the entire globe was literarily reduced to an interconnected network of people. This continually makes people grow and develop. (Pacey, 76) In other words, whenever a significant invention is made in human society, then this has usually translated into positive changes in human economic, social and political spheres. Ignoring the positive changes brought by these inventions based on the fatalities or problems that have arisen out of them would be like throwing out the baby with its bathwater. At the heart of every invention is the need to solve a particular problem. (Sampson, 35) This means that every time a technological milestone arises, then a major problem is solved and this makes people’s lives relatively easy. One can therefore measure technological progress effectively through such inventions as these milestones have eliminated rather than created more problems for humanity.
It should be noted that the inventions do not necessarily have to be tangible as they can be service-based (such as banking or the internet) but still lead to further development. The challenge with such a model of measuring technological progress is that it may be difficult trying to find out which inventions should be chosen and which ones should be ignored. The best way of handling this dilemma is by first understanding what development entails. After many arguments, disagreements, and compromises, several philosophers have agreed that there is more to development than just material wealth. Some other indicators are necessary as these other elements can contribute to people’s satisfaction with their lives. Given this stance and for purposes of this paper, it is necessary to think of social progress as a process by which actors and principals promote their spiritual, physical, and social well-being. (Wilson, 59) As a result, good measures of technological progress should deal with all these three aspects. Physical well-being is often denoted by people’s state of wealth and health. If people have lower infant mortalities, fewer health complications, and longer lives, then this is a step in the right direction and it can be treated as social progress. Therefore one can measure such economic perimeters through Lewis Morgan’s model which gives precedence to inventions. About the most important inventions, priorities should go to those that directly contributed to society’s state of the economy.
Social and spiritual well-being can be linked to technological developments as well but through different parameters. (Pursell & Kranzberg, 18)Here, stakeholders often look at society’s ability to include its people in decision-making processes, whether society’s talents are being used and whether people are living lives filled with optimism, dignity, and commitment to one another. To measure such technologies, it would be essential to study how they have contributed towards certain community principles. (Roberti, 3) For instance, equity, justice, diversity, and trustworthiness can be considered as reasonable measures as these are all intangible elements of economic and social progress. An example in the field of education is the development of internet filtering; this has gone a long way in promoting morality because it has reduced the harms associated with too much content accessibility for minors. In the field of science, precedence should be given to those technologies that promote greater cooperation and inclusion of the global community. A case in point was Russia’s launching of an outer space satellite. (World Bank, 13) The latter country first sought consensus from other stakeholders in the field of astronomy such that all humanity could realize some of the benefits that come with utilizing such a piece of technology. Other technology milestones such as weather-related predictions can also be considered monumental. This is because they have significantly contributed to humanity’s ability to deal with natural disasters such as earthquakes. (Pollard, 56)
The paper has shown that it may not be easy to measure technological development about social progress because a definition of social progress takes on physical, social, and spiritual facets; nonetheless, this can still be done. It is possible to achieve some economic and social goals of development while at the same time meeting the social and spiritual needs of humanity as well. In instances where there are several pathways to achieving certain goals through technology, precedence ought to be given to those technologies that can improve people’s economic concerns as well as their social and moral needs as well. This also means that the same approach should be used in measuring how technology has led to the development and progress of man throughout the ages. The indices that will be quite useful here include: technologies that have contributed to substantial economic advancement, technologies that have promoted greater equality and justice, and technologies that have enhanced the state of cooperation and interdependence between various individuals. It may be difficult to merge these different aspects but an effective link between technology and human progress can only be effectively ascertained by using both quantitative (economic measures) and qualitative measures of development.
Polard, Sidney. “The concept of progress.” History of Ideas Journal 28(2004): 56.
Blumenberg, Hanis. Legitimacy of the modern age. Cambridge: Cambridge University press, 1997.
Hellemans, Alexander & Bunch, Bryan. Timetables of technology. NY: Schuster and Simons, 1993.
Pursell, Caroll & Kranzberg, Melvin. Technology in western civilizations. Oxford: oxford University Press, 2004.
Sampson, Richard. Progress in the age of reason. Harvard: Harvard University Press, 2004.
Roberti, Mark. “Civil society declarations amount to abandonment of human progress.” Branford Magazine (2009):3.
Wilson, E. Consilience. Oxford: OUP, 2002.
The World Bank. “Technological development and progress.” Global economic prospects report 2008.
Pacey, Arnold. The maze of ingenuity. Cambridge: MIT press, 1994.
Williams, Trevor & Kingston, Thomas. A short history of technology. NY: Dover Press, 2006.
- Video Distribution: MPEG-4, Multicasting, Encoding
- Multi-Touch Touch Screen Controls in Military Aircraft
- Visiting the Henry Ford Museum
- Tangible Property of the Restaurant Business
- Historical Milestones of AFL.
- Industrial Communication Protocol
- Macau Institute of Electrical and Electronics Engineers
- Fiber Optics Laboratory Experiments
- Analogue to Digital Sound Effect Processor
- Digital Meter of Electricity and Server Communication
- Chicago (A-D)
- Chicago (N-B)
IvyPanda. (2022, March 9). The Measure technological Progress. https://ivypanda.com/essays/the-measure-technological-progress/
"The Measure technological Progress." IvyPanda , 9 Mar. 2022, ivypanda.com/essays/the-measure-technological-progress/.
IvyPanda . (2022) 'The Measure technological Progress'. 9 March.
IvyPanda . 2022. "The Measure technological Progress." March 9, 2022. https://ivypanda.com/essays/the-measure-technological-progress/.
1. IvyPanda . "The Measure technological Progress." March 9, 2022. https://ivypanda.com/essays/the-measure-technological-progress/.
Bibliography
IvyPanda . "The Measure technological Progress." March 9, 2022. https://ivypanda.com/essays/the-measure-technological-progress/.
This website uses cookies to ensure you get the best experience on our website. Without cookies your experience may not be seamless.

- Unpopular Essays on Technological Progress
In this Book

- Nicholas Rescher
- Published by: University of Pittsburgh Press
- View Citation
Table of Contents

- Title, Copyright, Dedication
- pp. vii-viii
- 1. Technological Progress and Human Happiness
- 2. The Enviromental Crisis and the Quality of Life
- 3. The Allocation of Exotic Medical Lifesaving Therapy
- 4. Ethical Issues Regarding the Delivery of Health Care
- 5. Ideals, Justice, and Crime
- 6. Economics Versus Moral Philosophy: Te Pareto Principle as a Case Study
- 7. Why Save Endangered Species?
- 8. Scientific Progress and the "Limits of Growth"
- pp. 107-118
- pp. 119-120
- Subject Index
- pp. 121-122
Additional Information
Project muse mission.
Project MUSE promotes the creation and dissemination of essential humanities and social science resources through collaboration with libraries, publishers, and scholars worldwide. Forged from a partnership between a university press and a library, Project MUSE is a trusted part of the academic and scholarly community it serves.

2715 North Charles Street Baltimore, Maryland, USA 21218
+1 (410) 516-6989 [email protected]
©2024 Project MUSE. Produced by Johns Hopkins University Press in collaboration with The Sheridan Libraries.
Now and Always, The Trusted Content Your Research Requires

Built on the Johns Hopkins University Campus
Technological Progress and Rent Seeking
We model firms’ allocation of resources across surplus-creating (i.e., productive) and surplus-appropriating (i.e., rent-seeking) activities. Our model predicts that industry-wide technological advancements, such as recent progress in data collection and processing, induce a disproportionate and socially inefficient reallocation of resources toward surplus-appropriating activities. As technology improves, firms rely more on appropriation to obtain their profits, endogenously reducing the impact of technological progress on economic progress and inflating the price of the resources used for both types of activities. We apply our theoretical insights to shed light on the rise of high-frequency trading
The authors thank the editor Tarun Ramadorai, two anonymous referees, Salomé Baslandze, Philip Bond, Assa Cohen, Yao Deng, Winston Dou, Maryam Farboodi, Deeksha Gupta, Urban Jermann, Leonid Kogan, Ben Lockwood, Greg Nini, Christian Opp, Tom Sargent, Mathieu Taschereau-Dumouchel, Stephen Terry, Boris Vallée, Ed Van Wesep, Laura Veldkamp, Colin Ward, Ryan Zalla, Yao Zeng, and seminar participants at the American Finance Association meetings, the NBER Corporate Finance meeting, the Northern Finance Association meetings, the SFS Cavalcade, the Wash-U Corporate Finance conference, the University College London, and the Wharton School for their helpful comments. The views expressed herein are those of the authors and do not necessarily reflect the views of the National Bureau of Economic Research.
MARC RIS BibTeΧ
Download Citation Data
Conferences
More from nber.
In addition to working papers , the NBER disseminates affiliates’ latest findings through a range of free periodicals — the NBER Reporter , the NBER Digest , the Bulletin on Retirement and Disability , the Bulletin on Health , and the Bulletin on Entrepreneurship — as well as online conference reports , video lectures , and interviews .
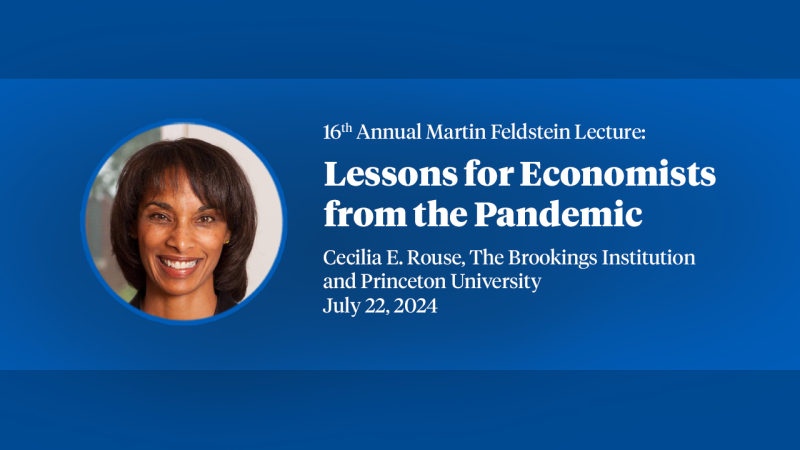
- Are We too Dependent on Technology? Words: 1688
- Negative Effects of Technology on Society Words: 1456
- Does Technology Mean Progress or Not Words: 336
- Technology: Is It Neutral? Words: 573
- How Technology Has Affected Education Words: 559
- The Relationships Between Technology Progress and Communication Words: 567
- Technology and Society. Relative Influence. Words: 1636
- Concepts of How Science and Technology has Shaped the Modern World Words: 1790
- How Do Science and Technology Help Us Define the Modern World? Words: 1635
- Technology in Human Interpretation Words: 1097
Technological Progress and Technologies’ Use Nowadays
Technologies are everywhere in the contemporary world. It is so due to the progress in science and all applied disciplines which provide people with more facilities in life. The world is changing due to the development of the scientific thought. That is why with the flow of time technologies became an inexplicable part of my life. In this respect I try to do my best by means of technological approach trying to implement all possible measures or devices in education, work, and entertainment. Such idea goes beyond any understanding when it touches upon the ad absurdum way of reasoning about the issue of technologies’ use for mankind.
When making a survey on my daily activities it is necessary to admit the beginning of it up to the end. The Internet resources and Web 2.0 technologies help me to communicate with friends and different communities whenever and wherever I want or need. It also considers them. Hence, after getting up I check on Facebook and MySpace status and look at possible messages from my friends living all over the world. It helps me also to communicate at once with more than one user. Then I check e-mail and comments on my blogs posted on different social networks. It helps me to share my thoughts and opinions throughout the communities of young people. The creativeness of youngster, as I see, is fully described and provided or informed via Internet resources. This is why virtual reality becomes more real.
When at college, I keep my I-Phone switched on with active silent profile in order to have SMS from fellows in neighboring classes, or for the purpose to inform somebody about a gathering. In this very device I save my photos and videos with friends and mates just to have fun and to be engaged in the process of global facilitation of nations with technological decisions. Also my I-Phone supports Wi-Fi, and it helps me to stay in touch with global community via supported services as MSN, ICQ, and Blogger. For youth it is too vital to upload some material out of the Internet and post some files in it as well. Thus, I try during a day to gather some informative materials, such as photos from parties, lunch at the refectory and kisses of couples, so that to represent these materials in the net.
Some people say that Internet and computer games do harm to the development of children and youth on the whole. Opposing to such assertion I use internet libraries and different sources for successful readings in education. It helps me to make parallels between different sayings or problems raised in classes, so that to provide personal view. Such opinion may be supposed with the relation to the Google services. Indeed, they are helpful for my educational development. For example, Google Books provides me with one of the biggest electronic libraries in the world. Moreover, there are also some materials being antique and, say, lost for centuries. Those materials which were under “Top Secret” mark are available today. Moreover, this technology saves my time because there is an appropriate fast search. So I can find any citation in a definite book.
To sum up, the world of technological progress is increasing every day. It is vital for a contemporary individual to be familiar with new technologies, because they can take more benefits then one can suppose.
Cite this paper
- Chicago (N-B)
- Chicago (A-D)
StudyCorgi. (2021, November 5). Technological Progress and Technologies’ Use Nowadays. https://studycorgi.com/technological-progress-nowadays/
"Technological Progress and Technologies’ Use Nowadays." StudyCorgi , 5 Nov. 2021, studycorgi.com/technological-progress-nowadays/.
StudyCorgi . (2021) 'Technological Progress and Technologies’ Use Nowadays'. 5 November.
1. StudyCorgi . "Technological Progress and Technologies’ Use Nowadays." November 5, 2021. https://studycorgi.com/technological-progress-nowadays/.
Bibliography
StudyCorgi . "Technological Progress and Technologies’ Use Nowadays." November 5, 2021. https://studycorgi.com/technological-progress-nowadays/.
StudyCorgi . 2021. "Technological Progress and Technologies’ Use Nowadays." November 5, 2021. https://studycorgi.com/technological-progress-nowadays/.
This paper, “Technological Progress and Technologies’ Use Nowadays”, was written and voluntary submitted to our free essay database by a straight-A student. Please ensure you properly reference the paper if you're using it to write your assignment.
Before publication, the StudyCorgi editorial team proofread and checked the paper to make sure it meets the highest standards in terms of grammar, punctuation, style, fact accuracy, copyright issues, and inclusive language. Last updated: March 24, 2022 .
If you are the author of this paper and no longer wish to have it published on StudyCorgi, request the removal . Please use the “ Donate your paper ” form to submit an essay.

Login | Register
- Current Issues
- Previous Issues
- Editorial Team
- Managerial Board
- Become a Reviewer
- Submission Guidelines
- Harvard Citation Style
- Vancouver Citation Style
- APA Citation Style
- Download RIS
- Download BibTeX
Book Review
- Tianhao Zhi (United International College)
This article reviews C. Aspalter’s latest book Super Inequality . The book consists of a collection of Aspalter’s essays in (i) public choice theory, (ii) a theory of social efficiency, and (iii) a theory of super inequality. Not only had it provided us with a timely and systematic explanation regarding the causes of super inequality, but also a myriad of other social issues such as persistent social divide, inflation, a lack of education, and Medicare for many people. The book starts from a foundation of behavioral economics, which attempts to explain why humans make inferior choices when better options are available, with an emphasis on the context of public choices. Aspalter further elaborates on a General Theory of Z-Efficiency. Mainstream economists often focus merely on economic and allocative inefficiency, while ignoring other forms of social inefficiency arising from non-economic factors such as environmental, managerial, and personal and social barriers. The realization of higher general efficiency requires sound social policy design beyond the conventional laissez-faire, neoliberal economic approach. The book continues to build a general theory of super inequality from the lens of the Z-inefficiency measure. In our current world of fast-changing technological progress, thanks to the development of AI, big data, and blockchain technology, it is never a better time to contemplate the ramifications of a technological paradigm shift and how it will affect social efficiency overall. To this end, Aspalter’s General Theory of Z-Efficiency and Super Inequality laid a promising path for future research in this direction.
Keywords: public choice theory, a general theory of Z-efficiency, super inequality
Zhi, T., (2024) “Book Review”, Social Development Issues 46(3): 12. doi: https://doi.org/10.3998/sdi.6776
Downloads: Download XML Download PDF
6 Downloads
Published on 04 sep 2024, creative commons attribution 4.0.
Christian Aspalter (2023) , Super Inequality: Theoretical Essays in Economics and Social Policy. Springer Nature, 158 p. ISBN-13 978-9-81995-168-0 (Hardcover). $ 109.99.
The Laisse-Faire Economics Fairytale Coming to Another End
Once upon a time, all students of economics were imparted with a profound fairytale, and when those economic students became economist adults, they were very often led to believe that the fairytale is how the world actually works. As the fairytale goes: somewhere on an island where people live and trade and prosper; there exists a platonic market that functions to its maximum economic efficiency only when it is not intervened by the authority: in such an economy, every player, consumers, and producers alike, behaves rationally and selfishly. They gather in a marketplace to fully realize their happiness and material wealth: consumers are the utility maximizers while producers are the profit squeezers. Competition ensures the best of goods and services being produced at the most efficient market price where the producers earn zero economic profit in the long-run equilibrium and maximum economic surplus is realized for both consumers and producers. The division of labor will not only allow the individuals to focus on what they are comparatively advantageous, but also give rise to international trade that enables nations to focus on their comparative advantage and benefit from higher economic well-being through the exchange of goods and services produced by other nations with their own distinct comparative advantages. Economic growth will be sustained by innovation that ensures the breaking of old equilibrium to the new and everyone will live happily ever after.
This well-told fairytale, as history had documented, had temporarily, or perhaps illusively aligned with the economic reality for a limited period until a full-blown financial or social crisis that caught people unprepared and devastated when their life savings were wiped out and billions of dollars evaporated in the frightening market stampede: from the 1929–1939 the Great Depression to the 1980 Japanese economic crisis, to the 1996 Asian financial crisis, up to the more recent 2007 Global Financial Crisis. Every crisis reminds us of an old French proverb: “plus ça change, plus c’est la même chose (the more things change, the more they stay the same)” , and every crisis serves as a timely reminder that the economic reality is far more complex than what the economic fairytale depicts.
We often associate the financial and social crises with a simple explanation of market failure , and we often solve the economic issues merely from economic toolkits: environmental pollution arises from negative externality, information asymmetry misled the market-clearing prices, people sometimes behave irrationally, etc. Amid the radical fear and uncertainty, we often apply sub-optimal economic remedies that may alleviate the issue in the short-run and sometimes worsen the situation in the long-run: be it an astronomical fiscal stimulus or a radical monetary expansion, or a combination of both. However, the reoccurring economic crises and social issues, combined with the inability of economic policy to eradicate the long-term issues, have left us to wonder: is it all but a simple ramification of market failures? Are there deeper causes of social imbalances that perpetuate and aggravate the pendulum of economic and social cycles from hundreds of years ago until now? Will it be an ultimate solution that would eternally end the crises and human sufferings, and ultimately project the economy in particular and the society in general on a long-term path of prosperity, equality, and sustainability?
Super Inequality: Going Beyond Laisse-Faire Economics to a Better Understanding of Economic and Social Crises
C. Aspalter’s new book: Super Inequality: Theoretical Essays in Economics and Social Policy provides us with a timely reminder that the malfunctioning of laisse-faire market alone, neglects a deeper root cause of the social-economic crises aforementioned. Super inequality arises not merely as a result of market failures, but more importantly from an inherent imperfection of decision-making in radical uncertainty, combined with systemic institutional construct by political and social elitists.
Distorted Choices: A Perspective from Behavioural Economics
Aspalter starts his analysis with a general theory of distorted choices . The key question is: why do people make inferior choices when better options are available? It may sound a little cliché to claim that people are not always rational when making important decisions: there is already a plethora of literature in behavioral economics regarding bounded rationality of economic agents due to psychological factors, cognitive limits, emotions, greed, and fear, or may simply due to laziness ( Akerlof & Shiller, 2009 ; Kahneman & Tversky, 1979 ). Yet it is less discussed about public choices, which differs from private choices of market behaviors. Some might argue that public choices are much less urgent for individuals (people have ample time to make choices and more information is available compared to private decisions, such as investment decisions when decisions have to be made in a small time frame with limited information), and public officials are often portraited in laissez-faire economics as possessing an ideal-typical, Mahatma Gandhi element of altruism, as Aspalter describes. Hence, the general law of rationality applies, implying that decision can be understood from the decision of a rational, representative individual, in other words, the macro phenomena is micro-founded. Yet there is plenty of evidence that defies this convenient thinking, particularly in the area of the social welfare system, as Aspalter observes:
“This really explains why the vast majority of welfare state systems around the world still stick to horrible social policy choices, such as asset- and means-tests (AMTs), even though already a long-time ago economists and now also social policy scientists have come to see the poverty-exacerbating, poverty-spreading and poverty-cementing effects of any social program that is based on or uses asset- and means-tests, including proxy asset- and means-tests.” (C. Aspalter, 2023 , p. 29)
The distorted choice is not merely an outcome of bounded rationality of the masses but is often exacerbated by the systemic coercion and manipulation by political and corporate elites. More often than not, public officials, like private individuals, are self-interested too. Rent-seeking behavior arises when the self-interested public authorities intertwine with corporate interests ( Holcombe, 2018 ).
With a foundation of behavioral economics and sociology of Holcombe and Foucault, Aspalter identifies six types of determining factors: (i) systems of cultural biases and cultural variance; (ii) aggregate historical factors; (iii) geographical factors; (iv) effects of cultural, social, societal, governmental, judicial, or economic discrimination and exclusion, (v) government interference in terms of availability of resources and opportunities, and (vi) judicial interference in terms of punishments. This serves as a foundation in the discussion of public policy efficiency that stems not only from an allocative factor of Leibenstein sense (X-efficiency) but also from managerial and environmental barriers, as well as from inferior choices of the masses, as is further elaborated in following sections.
Beyond Economic Efficiency: The Theory of Z-efficiency and Super Inequality
When we teach ECON101 class, the concept of economic efficiency is usually introduced right after the first lecture on supply and demand and market-clearing equilibrium. The shaded area of consumer and producer surplus becomes the golden rule to measure economic efficiency. The market functions at its optimum only when it is free from external distortion, such as the benevolent act of the government to set price ceilings and floors, or in a market where monopoly dominates the markets. The lack of competition or the presence of external policy intervention will result in a deadweight loss, thus lowering overall economic efficiency. Furthermore, Leibenstein (1966) expounds on the notion of X-inefficiency due to a lack of competition, which disables the firms to produce on its full production possibility frontier and raises the cost curve. Only later the concept of market failure was introduced in the form of information asymmetry and externality, combined with people’s inability to make rational decisions that often lead to sub-optimal situations. The way we address these types of market inefficiency is usually by resorting back to the market force itself, such as pricing and marketizing externalities and information. The ultimate principle remains unchanged: let the invisible hand to fix the problem of laissez-faire economy itself . This principle is often easily, yet forcefully adopted for other non-economic social issues.
It is true that the economic system is a crucially important subset of a social system, yet they are not equivalent. It is misleading to gauge other types of social efficiency in the same way as we measure economic efficiency, such as the provision of public goods, the removal of social, cultural, and managerial barriers, and so on. Built from the theoretical foundation of the predecessors ( Foucault, 1954 ; Holcombe, 2018 ; Luhmann, 1984 ), Aspalter proposed a general theory of efficiency taxonomized in four broad categories, namely the M-, N-, Y-, Z-efficiency, in addition to Leibenstein’s X-efficiency aforementioned. M-inefficiency arises due to managerial barriers, N-efficiency due to environmental barriers, and Y- and Z-inefficiency from personal-level influences.
Sound policy ought to be judged by whether it brings forth the maximum social efficiency. From the renewed perspective in measuring efficiency from the four additional dimensions, it is tempting to do a thought experiment of a hypothetical society with the highest possible general efficiency, just like how classical economists describe the way an ideal laissez-faire economy works. We envision that in such a society with the highest general efficiency, not only does it possess a competitive market economy that yields the highest allocative efficiency and the Leibenstein X-efficiency, but also a full managerial/governance efficiency in the public sector, and a complete removal of natural, physical, social, cultural, and environmental barriers, thanks to the advancement of technology and effective governance (the maximum M- and N-efficiency). In such a society, every individual is fully capable of making the best decisions for themselves free of knowledge and physical constraints, thanks to viable social programs that make education and healthcare affordable and in line with the long-term interests of every member of the society (the maximum Y-efficiency and Z-efficiency). This ideal is perhaps still far-fetched, yet it can serve as an ultimate goal of setting optimal social policies.
“Every problem, every mental formation, every social problem can be overcome. The only downsides are time and efforts needed, i.e. the longer a problem lasted, or the more severe the repercussions (suffering and harm caused) were, the longer it may in general take to heal them, i.e. to soften them and in the end to dissolve them.” (C. Aspalter, 2023 , p. 76)
Looking Ahead for a Better Future
“Modern methods of production have given us the possibility of ease and security for all; we have chosen instead to have overwork for some and starvation for others.” — Bertrand Russel, In Praise of Idleness
Toward the end of the book, we are well-equipped with an alternative lens to look at many prevailing social issues in the Western world today that laissez-faire economic fails to explain: high inflation, persistent social divide, myopic corporatism, lack of access to education, and healthcare resources for many due to financial barriers and suboptimal insurance scheme, and above all, the rise of super inequality.
Capitalism can be immensely creative: we constantly see new products emerging: from Ford’s T-model nearly 100 years ago, to the modern-day smartphone and AI technology, and undoubtedly to a promising future of everincreasing standard of living, thanks to the brave entrepreneurial pursuits of the creative, industrious individuals and social economic entities ( Schumpeter, 1939 ). Capitalism can also be frighteningly destructive: the adoption of new technology often outpaces people’s complacency. While new jobs were created, more traditional jobs were lost, leading to anger, frustration, fear, and riots. We benefit tremendously from the creative side of capitalism, yet we often downplay the dark side: myopic, selfish, speculative, unequal, predatory, and eventually self-destructive, as vindicated by countless financial and social crises in history.
Looking back, the profound lack of social efficiency consideration in policymaking in the heyday of neoliberalist optimism had led to a systemic neglect of many social issues, which planted the seeds for many subsequent social and economic crises later on. Not long ago, we had an illusion of “The Great Moderation” , and we were passionately teaching this illusion in macroeconomic lectures. With blind faith in the invisible hand, higher education, Medicare, along with many important public goods were privatized. Since then, education and healthcare have become increasingly unaffordable, pushing more people into lifelong debt and despair. Financial markets and financial institutions that ought to be under tight regulation and public scrutiny, thanks to the bitter experience of the Great Depression of 1929–1939, were once again deregulated and liberated. In the aftermath of the 2007 GFC, radical and unconventional QE , 1 which was meant to provide emergent liquidity to the troubled financial institutions in the hope of eventually alleviating long-term economic issues, had instead worsened the gap between the 1 percent of the financial elitists and the 99 percent of everyone else, aggravating the problem of super inequality.
The pendulum of the business cycle keeps swinging in its own course, and we are currently moving forward from the debris of one historical crisis to a brave new world of unprecedented challenges and opportunities, thanks to the development of AI, big data, and blockchain technology, along with many other emerging technologies. It is never a better time to contemplate the relationship between technological progress and super inequality from the lens of Aspalter’s General Theory of Z-Efficiency, in the hope of preventing future crises. While we cannot retreat to the stinky and ignorant era of the Medieval old days, we need a proper understanding of the complex and intertwining relationship between technological progress and Z-efficiency to adapt to a better new world. Does technological progress benefit everyone, or just a small handful of a few elitists? Would automation and AI reshape our workforce and render it more productive, creative, and innovative, or will it lead to a mass unemployment issue and even a deeper social crisis? What kind of financial market and financial institutions would foster sustainable growth from technological innovation, while reducing Z-inefficiency and delivering higher social well-being? We do not have answers to these questions at the moment, but I am convinced that Aspalter’s general theory of Z-efficiency and super inequality has laid a strong foundation and a promising theoretical framework to tackle these issues in future research endeavors.
- Quantitative Easing. ⮭
Akerlof, George A., & Shiller, Robert J. (2009). Animal spirits: How human psychology drives the economy, and why it matters for global capitalism . New Jersey: Princeton University Press.
Aspalter, Christian. (2023). Super inequality: Theoretical essays in economics and social policy . Springer: Singapore, ISBN: 978-981-99-5168-0.
Foucault, Michel. (1954). Maladie Mentale et personnalité . Paris: Presses Universitaires de France.
Holcombe, Randall G. (2018). Political capitalism: How political influence is made and maintained . Cambridge: Cambridge University Press.
Kahneman, Daniel, & Tversky, Amos. (1979). Prospect theory: An analysis of decision under risk . Econometrica , 47(2), 263–291. https://doi.org/10.2307/1914185 https://doi.org/10.2307/1914185
Leibenstein, Harvey. (1966). Allocative efficiency vs. X-efficiency. The American Economic Review , 56(3), 392–415. https://doi.org/10.2307/2551810 https://doi.org/10.2307/2551810
Luhmann, Niklas. (1984). Soziale Systeme . Frankfurt A.M.: Suhrkamp.
Russel, Bertrand. In praise of idleness. Retrived from https://files.libcom.org/files/Bertrand%20Russell%20-%20In%20Praise%20of%20Idleness.pdf https://files.libcom.org/files/Bertrand%20Russell%20-%20In%20Praise%20of%20Idleness.pdf
Schumpeter, Joseph A. (1939). Business cycles: A theoretical, historical, and statistical analysis of the capitalist process . New York: McGraw Hill.
Tianhao Zhi, Faculty of Science and Technology, BNU-HKBU United International College, China. He can be contacted at [email protected].
Harvard-Style Citation
Zhi, T. (2024) 'Book Review', Social Development Issues . 46(3) doi: 10.3998/sdi.6776
Show: Vancouver Citation Style | APA Citation Style
Vancouver-Style Citation
Zhi, T. Book Review. Social Development Issues. 2024 9; 46(3) doi: 10.3998/sdi.6776
Show: Harvard Citation Style | APA Citation Style
APA-Style Citation
Zhi, T. (2024, 9 4). Book Review. Social Development Issues 46(3) doi: 10.3998/sdi.6776
Show: Harvard Citation Style | {% trans 'Vancouver Citation Style' %}
Non Specialist Summary
This article has no summary

COMMENTS
Understanding technology and how we can make better decisions about designing, deploying, and refining it requires capturing that nuance and complexity through in-depth analysis of the impacts of different technological advancements and the ways they have played out in all their complicated and controversial messiness across the world.
The long-run perspective on technological change The big visualization offers a long-term perspective on the history of technology.1 The timeline begins at the center of the spiral. The first use of stone tools, 3.4 million years ago, marks the beginning of this history of technology.2 Each turn of the spiral represents 200,000 years of history.
Embark on a technological journey with jenni.ai's curated essays. From bite-sized 200-word insights to in-depth 500-word analyses, immerse yourself in discussions on the innovations and implications of today's tech landscape.
This has since changed partly due to the technological advancements over the years that have led to the replacement of human and animal labor with more efficient energy sources as wind power, hydroelectric and steam energies that ultimately led to a significant increase in productivity. Thus, the thesis statement for this essay is to analyze the impact of technological advancement on people ...
Since the dotcom bubble burst back in 2000, technology has radically transformed our societies and our daily lives. From smartphones to social media and healthcare, here's a brief history of the 21st century's technological revolution. Just over 20 years ago, the dotcom bubble burst, causing the stocks of many tech firms to tumble.
The societal impacts of technological change can be seen in many domains, from messenger RNA vaccines and automation to drones and climate change. The pace of that technological change can affect its impact, and how quickly a technology improves in performance can be an indicator of its future importance. For decision-makers like investors, entrepreneurs, and policymakers, predicting which ...
The impact of technology on society is undeniable. Technology and science have played a central role in human history and help shape entire civilizations. Technological progress was key for the emergence and downfall of empires. The development of hunting and farming tools allowed our ancestors to dominate other hominid species.
Technological change underpins many of the developments we've seen in health, agriculture, energy, and global development.
Theories of scientific and technological change view discovery and invention as endogenous processes1,2, wherein previous accumulated knowledge enables future progress by allowing researchers to ...
In recent years, many economists have questioned the ability of technological progress to keep propelling the economy forward despite declining population growth and rising dependency ratios (Gordon 2016). According to those in this camp, the low-hanging fruit have mostly been picked, and further advances will become increasingly difficult (Bloom and others 2017).
The question about the interconnection between technology development and progress has been a matter of discussion since the Enlightenment era. The idea about technological improvement as a base for social development and the level of human well-being and personal happiness has been prevailing in the United States since the late eighteenth century.
This paper argues that concerns about whether technological progress leads to inclusive growth are indeed justified - and that especially developing countries may face a stark new set of challenges going forward.
It is the cloak of mystery that shrouds progress and its popular and uncritical acceptance in our society that inspired my research into technological progress, a study which was funded by a Summer Undergraduate Research Fellowship from the University of New Hampshire.
Technological innovation is a fundamental driver of economic growth and human progress. Yet some critics want to deny the vast benefits that innovation has bestowed and continues to bestow on mankind.
Technological Progress and Potential Future Risks. Emerging technologies, such as industrial robots, artificial intelligence, and machine learning, are advancing at a rapid pace. These developments can improve the speed, quality, and cost of goods and services, but they also displace large numbers of workers.
This essay is a map of the content on New Things Under the Sun that relate to accelerating technological progress. For this essay I'll basically take it as a given that technological progress is desirable.
Essay on Technology. The word "technology" and its uses have immensely changed since the 20th century, and with time, it has continued to evolve ever since. We are living in a world driven by technology. The advancement of technology has played an important role in the development of human civilization, along with cultural changes.
To effectively measure technological progress and hence render support to its relation to social progress, it is critical to understand that technology is inspired by the historical, political, social, and economic forces at play within a certain period. Anthropologists and sociologists have studied the concept of cultural and social evolution ...
Technological Progress Essay | IT in the Last Decade. Some technological inventions have affected our life greatly for the past decades, especially computers. It's true that computers really provide us more convenient life. With computers, we can deal with many documents even faster, we can use computer to control machines to work, and we can ...
Unpopular Essays on Technological Progress. Nicholas Rescher examines a number of controversial social issues using the intellectual tools of the philosopher, in an attempt to clarify some of the complexities of modern society, technology, and economics. He elucidates his thoughts on topics such as: whether technological progress leads to ...
Our model predicts that industry-wide technological advancements, such as recent progress in data collection and processing, induce a disproportionate and socially inefficient reallocation of resources toward surplus-appropriating activities.
This paper, "Technological Progress and Technologies' Use Nowadays", was written and voluntary submitted to our free essay database by a straight-A student.
Technological progress Critique Essay. When we hail to the amazing development that mankind has made in science and technology, there is a widespread concern about its negative influence on our life. In my opinion, technology is a double-edged sword, bringing about substantial benefits as well as some drawbacks.
In our current world of fast-changing technological progress, thanks to the development of AI, big data, and blockchain technology, it is never a better time to contemplate the ramifications of a technological paradigm shift and how it will affect social efficiency overall. ... The book consists of a collection of Aspalter's essays in (i ...
Search calls for papers; Journal Suggester; Open access publishing; ... The rapid development of digital technology today has allowed gamification to be incorporated into various learning environments, ... It also allows you to set goals and has a statistics function that shows the user's progress in completing tasks.