

FOR EMPLOYERS
Top 10 real-world data science case studies.
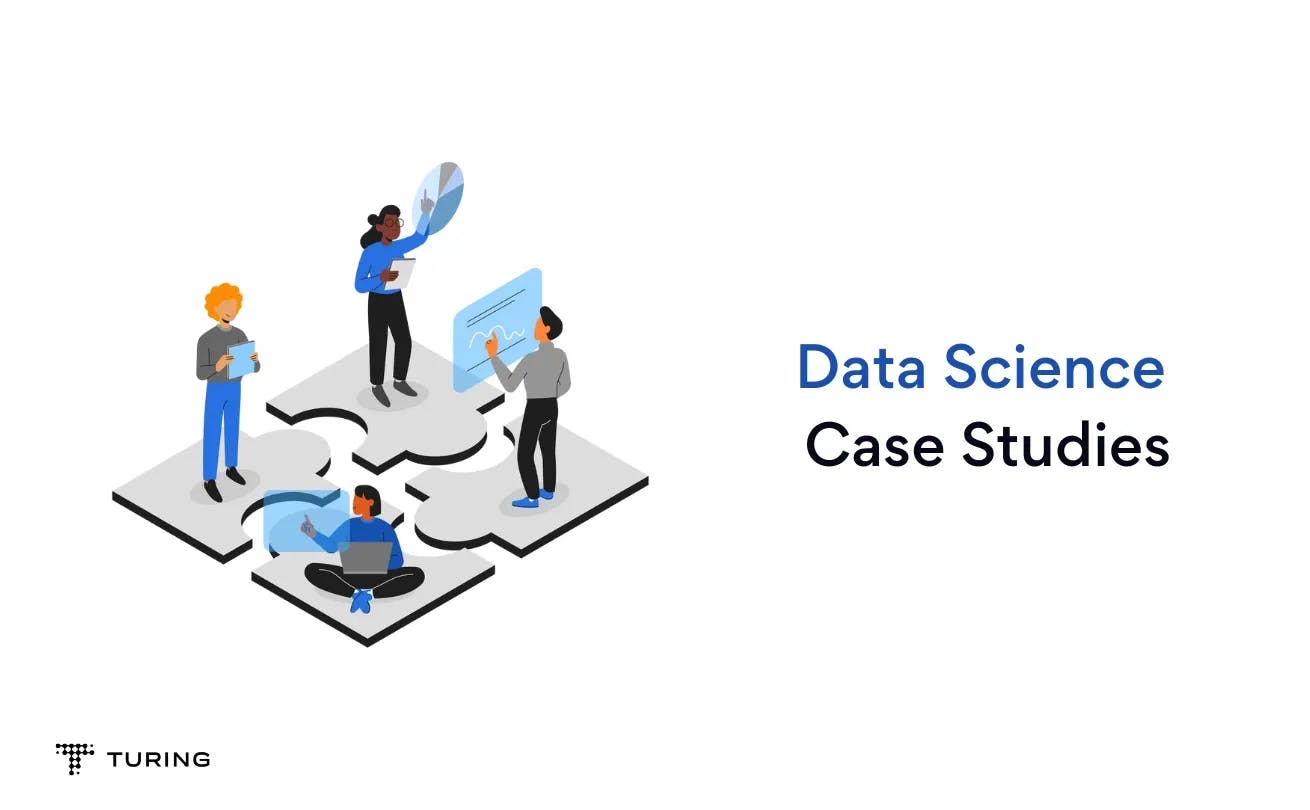
Aditya Sharma
Aditya is a content writer with 5+ years of experience writing for various industries including Marketing, SaaS, B2B, IT, and Edtech among others. You can find him watching anime or playing games when he’s not writing.
Frequently Asked Questions
Real-world data science case studies differ significantly from academic examples. While academic exercises often feature clean, well-structured data and simplified scenarios, real-world projects tackle messy, diverse data sources with practical constraints and genuine business objectives. These case studies reflect the complexities data scientists face when translating data into actionable insights in the corporate world.
Real-world data science projects come with common challenges. Data quality issues, including missing or inaccurate data, can hinder analysis. Domain expertise gaps may result in misinterpretation of results. Resource constraints might limit project scope or access to necessary tools and talent. Ethical considerations, like privacy and bias, demand careful handling.
Lastly, as data and business needs evolve, data science projects must adapt and stay relevant, posing an ongoing challenge.
Real-world data science case studies play a crucial role in helping companies make informed decisions. By analyzing their own data, businesses gain valuable insights into customer behavior, market trends, and operational efficiencies.
These insights empower data-driven strategies, aiding in more effective resource allocation, product development, and marketing efforts. Ultimately, case studies bridge the gap between data science and business decision-making, enhancing a company's ability to thrive in a competitive landscape.
Key takeaways from these case studies for organizations include the importance of cultivating a data-driven culture that values evidence-based decision-making. Investing in robust data infrastructure is essential to support data initiatives. Collaborating closely between data scientists and domain experts ensures that insights align with business goals.
Finally, continuous monitoring and refinement of data solutions are critical for maintaining relevance and effectiveness in a dynamic business environment. Embracing these principles can lead to tangible benefits and sustainable success in real-world data science endeavors.
Data science is a powerful driver of innovation and problem-solving across diverse industries. By harnessing data, organizations can uncover hidden patterns, automate repetitive tasks, optimize operations, and make informed decisions.
In healthcare, for example, data-driven diagnostics and treatment plans improve patient outcomes. In finance, predictive analytics enhances risk management. In transportation, route optimization reduces costs and emissions. Data science empowers industries to innovate and solve complex challenges in ways that were previously unimaginable.
Hire remote developers
Tell us the skills you need and we'll find the best developer for you in days, not weeks.

An official website of the United States government
Here’s how you know
The .gov means it’s official. Federal government websites often end in .gov or .mil. Before sharing sensitive information, make sure you’re on a federal government site.
The site is secure. The https:// ensures that you are connecting to the official website and that any information you provide is encrypted and transmitted securely.
Case studies & examples
Agencies mobilize to improve emergency response in puerto rico through better data.
Federal agencies' response efforts to Hurricanes Irma and Maria in Puerto Rico was hampered by imperfect address data for the island. In the aftermath, emergency responders gathered together to enhance the utility of Puerto Rico address data and share best practices for using what information is currently available.
Federal Data Strategy
BUILDER: A Science-Based Approach to Infrastructure Management
The Department of Energy’s National Nuclear Security Administration (NNSA) adopted a data-driven, risk-informed strategy to better assess risks, prioritize investments, and cost effectively modernize its aging nuclear infrastructure. NNSA’s new strategy, and lessons learned during its implementation, will help inform other federal data practitioners’ efforts to maintain facility-level information while enabling accurate and timely enterprise-wide infrastructure analysis.
Department of Energy
data management , data analysis , process redesign , Federal Data Strategy
Business case for open data
Six reasons why making your agency's data open and accessible is a good business decision.
CDO Council Federal HR Dashboarding Report - 2021
The CDO Council worked with the US Department of Agriculture, the Department of the Treasury, the United States Agency for International Development, and the Department of Transportation to develop a Diversity Profile Dashboard and to explore the value of shared HR decision support across agencies. The pilot was a success, and identified potential impact of a standardized suite of HR dashboards, in addition to demonstrating the value of collaborative analytics between agencies.
Federal Chief Data Officer's Council
data practices , data sharing , data access
CDOC Data Inventory Report
The Chief Data Officers Council Data Inventory Working Group developed this paper to highlight the value proposition for data inventories and describe challenges agencies may face when implementing and managing comprehensive data inventories. It identifies opportunities agencies can take to overcome some of these challenges and includes a set of recommendations directed at Agencies, OMB, and the CDO Council (CDOC).
data practices , metadata , data inventory
DSWG Recommendations and Findings
The Chief Data Officer Council (CDOC) established a Data Sharing Working Group (DSWG) to help the council understand the varied data-sharing needs and challenges of all agencies across the Federal Government. The DSWG reviewed data-sharing across federal agencies and developed a set of recommendations for improving the methods to access and share data within and between agencies. This report presents the findings of the DSWG’s review and provides recommendations to the CDOC Executive Committee.
data practices , data agreements , data sharing , data access
Data Skills Training Program Implementation Toolkit
The Data Skills Training Program Implementation Toolkit is designed to provide both small and large agencies with information to develop their own data skills training programs. The information provided will serve as a roadmap to the design, implementation, and administration of federal data skills training programs as agencies address their Federal Data Strategy’s Agency Action 4 gap-closing strategy training component.
data sharing , Federal Data Strategy
Data Standdown: Interrupting process to fix information
Although not a true pause in operations, ONR’s data standdown made data quality and data consolidation the top priority for the entire organization. It aimed to establish an automated and repeatable solution to enable a more holistic view of ONR investments and activities, and to increase transparency and effectiveness throughout its mission support functions. In addition, it demonstrated that getting top-level buy-in from management to prioritize data can truly advance a more data-driven culture.
Office of Naval Research
data governance , data cleaning , process redesign , Federal Data Strategy
Data.gov Metadata Management Services Product-Preliminary Plan
Status summary and preliminary business plan for a potential metadata management product under development by the Data.gov Program Management Office
data management , Federal Data Strategy , metadata , open data
PDF (7 pages)
Department of Transportation Case Study: Enterprise Data Inventory
In response to the Open Government Directive, DOT developed a strategic action plan to inventory and release high-value information through the Data.gov portal. The Department sustained efforts in building its data inventory, responding to the President’s memorandum on regulatory compliance with a comprehensive plan that was recognized as a model for other agencies to follow.
Department of Transportation
data inventory , open data
Department of Transportation Model Data Inventory Approach
This document from the Department of Transportation provides a model plan for conducting data inventory efforts required under OMB Memorandum M-13-13.
data inventory
PDF (5 pages)
FEMA Case Study: Disaster Assistance Program Coordination
In 2008, the Disaster Assistance Improvement Program (DAIP), an E-Government initiative led by FEMA with support from 16 U.S. Government partners, launched DisasterAssistance.gov to simplify the process for disaster survivors to identify and apply for disaster assistance. DAIP utilized existing partner technologies and implemented a services oriented architecture (SOA) that integrated the content management system and rules engine supporting Department of Labor’s Benefits.gov applications with FEMA’s Individual Assistance Center application. The FEMA SOA serves as the backbone for data sharing interfaces with three of DAIP’s federal partners and transfers application data to reduce duplicate data entry by disaster survivors.
Federal Emergency Management Agency
data sharing
Federal CDO Data Skills Training Program Case Studies
This series was developed by the Chief Data Officer Council’s Data Skills & Workforce Development Working Group to provide support to agencies in implementing the Federal Data Strategy’s Agency Action 4 gap-closing strategy training component in FY21.
FederalRegister.gov API Case Study
This case study describes the tenets behind an API that provides access to all data found on FederalRegister.gov, including all Federal Register documents from 1994 to the present.
National Archives and Records Administration
PDF (3 pages)
Fuels Knowledge Graph Project
The Fuels Knowledge Graph Project (FKGP), funded through the Federal Chief Data Officers (CDO) Council, explored the use of knowledge graphs to achieve more consistent and reliable fuel management performance measures. The team hypothesized that better performance measures and an interoperable semantic framework could enhance the ability to understand wildfires and, ultimately, improve outcomes. To develop a more systematic and robust characterization of program outcomes, the FKGP team compiled, reviewed, and analyzed multiple agency glossaries and data sources. The team examined the relationships between them, while documenting the data management necessary for a successful fuels management program.
metadata , data sharing , data access
Government Data Hubs
A list of Federal agency open data hubs, including USDA, HHS, NASA, and many others.
Helping Baltimore Volunteers Find Where to Help
Bloomberg Government analysts put together a prototype through the Census Bureau’s Opportunity Project to better assess where volunteers should direct litter-clearing efforts. Using Census Bureau and Forest Service information, the team brought a data-driven approach to their work. Their experience reveals how individuals with data expertise can identify a real-world problem that data can help solve, navigate across agencies to find and obtain the most useful data, and work within resource constraints to provide a tool to help address the problem.
Census Bureau
geospatial , data sharing , Federal Data Strategy
How USDA Linked Federal and Commercial Data to Shed Light on the Nutritional Value of Retail Food Sales
Purchase-to-Plate Crosswalk (PPC) links the more than 359,000 food products in a comercial company database to several thousand foods in a series of USDA nutrition databases. By linking existing data resources, USDA was able to enrich and expand the analysis capabilities of both datasets. Since there were no common identifiers between the two data structures, the team used probabilistic and semantic methods to reduce the manual effort required to link the data.
Department of Agriculture
data sharing , process redesign , Federal Data Strategy
How to Blend Your Data: BEA and BLS Harness Big Data to Gain New Insights about Foreign Direct Investment in the U.S.
A recent collaboration between the Bureau of Economic Analysis (BEA) and the Bureau of Labor Statistics (BLS) helps shed light on the segment of the American workforce employed by foreign multinational companies. This case study shows the opportunities of cross-agency data collaboration, as well as some of the challenges of using big data and administrative data in the federal government.
Bureau of Economic Analysis / Bureau of Labor Statistics
data sharing , workforce development , process redesign , Federal Data Strategy
Implementing Federal-Wide Comment Analysis Tools
The CDO Council Comment Analysis pilot has shown that recent advances in Natural Language Processing (NLP) can effectively aid the regulatory comment analysis process. The proof-ofconcept is a standardized toolset intended to support agencies and staff in reviewing and responding to the millions of public comments received each year across government.
Improving Data Access and Data Management: Artificial Intelligence-Generated Metadata Tags at NASA
NASA’s data scientists and research content managers recently built an automated tagging system using machine learning and natural language processing. This system serves as an example of how other agencies can use their own unstructured data to improve information accessibility and promote data reuse.
National Aeronautics and Space Administration
metadata , data management , data sharing , process redesign , Federal Data Strategy
Investing in Learning with the Data Stewardship Tactical Working Group at DHS
The Department of Homeland Security (DHS) experience forming the Data Stewardship Tactical Working Group (DSTWG) provides meaningful insights for those who want to address data-related challenges collaboratively and successfully in their own agencies.
Department of Homeland Security
data governance , data management , Federal Data Strategy
Leveraging AI for Business Process Automation at NIH
The National Institute of General Medical Sciences (NIGMS), one of the twenty-seven institutes and centers at the NIH, recently deployed Natural Language Processing (NLP) and Machine Learning (ML) to automate the process by which it receives and internally refers grant applications. This new approach ensures efficient and consistent grant application referral, and liberates Program Managers from the labor-intensive and monotonous referral process.
National Institutes of Health
standards , data cleaning , process redesign , AI
FDS Proof Point
National Broadband Map: A Case Study on Open Innovation for National Policy
The National Broadband Map is a tool that provide consumers nationwide reliable information on broadband internet connections. This case study describes how crowd-sourcing, open source software, and public engagement informs the development of a tool that promotes government transparency.
Federal Communications Commission
National Renewable Energy Laboratory API Case Study
This case study describes the launch of the National Renewable Energy Laboratory (NREL) Developer Network in October 2011. The main goal was to build an overarching platform to make it easier for the public to use NREL APIs and for NREL to produce APIs.
National Renewable Energy Laboratory
Open Energy Data at DOE
This case study details the development of the renewable energy applications built on the Open Energy Information (OpenEI) platform, sponsored by the Department of Energy (DOE) and implemented by the National Renewable Energy Laboratory (NREL).
open data , data sharing , Federal Data Strategy
Pairing Government Data with Private-Sector Ingenuity to Take on Unwanted Calls
The Federal Trade Commission (FTC) releases data from millions of consumer complaints about unwanted calls to help fuel a myriad of private-sector solutions to tackle the problem. The FTC’s work serves as an example of how agencies can work with the private sector to encourage the innovative use of government data toward solutions that benefit the public.
Federal Trade Commission
data cleaning , Federal Data Strategy , open data , data sharing
Profile in Data Sharing - National Electronic Interstate Compact Enterprise
The Federal CDO Council’s Data Sharing Working Group highlights successful data sharing activities to recognize mature data sharing practices as well as to incentivize and inspire others to take part in similar collaborations. This Profile in Data Sharing focuses on how the federal government and states support children who are being placed for adoption or foster care across state lines. It greatly reduces the work and time required for states to exchange paperwork and information needed to process the placements. Additionally, NEICE allows child welfare workers to communicate and provide timely updates to courts, relevant private service providers, and families.
Profile in Data Sharing - National Health Service Corps Loan Repayment Programs
The Federal CDO Council’s Data Sharing Working Group highlights successful data sharing activities to recognize mature data sharing practices as well as to incentivize and inspire others to take part in similar collaborations. This Profile in Data Sharing focuses on how the Health Resources and Services Administration collaborates with the Department of Education to make it easier to apply to serve medically underserved communities - reducing applicant burden and improving processing efficiency.
Profile in Data Sharing - Roadside Inspection Data
The Federal CDO Council’s Data Sharing Working Group highlights successful data sharing activities to recognize mature data sharing practices as well as to incentivize and inspire others to take part in similar collaborations. This Profile in Data Sharing focuses on how the Department of Transportation collaborates with the Customs and Border Patrol and state partners to prescreen commercial motor vehicles entering the US and to focus inspections on unsafe carriers and drivers.
Profiles in Data Sharing - U.S. Citizenship and Immigration Service
The Federal CDO Council’s Data Sharing Working Group highlights successful data sharing activities to recognize mature data sharing practices as well as to incentivize and inspire others to take part in similar collaborations. This Profile in Data Sharing focuses on how the U.S. Citizenship and Immigration Service (USCIS) collaborated with the Centers for Disease Control to notify state, local, tribal, and territorial public health authorities so they can connect with individuals in their communities about their potential exposure.
SBA’s Approach to Identifying Data, Using a Learning Agenda, and Leveraging Partnerships to Build its Evidence Base
Through its Enterprise Learning Agenda, Small Business Administration’s (SBA) staff identify essential research questions, a plan to answer them, and how data held outside the agency can help provide further insights. Other agencies can learn from the innovative ways SBA identifies data to answer agency strategic questions and adopt those aspects that work for their own needs.
Small Business Administration
process redesign , Federal Data Strategy
Supercharging Data through Validation as a Service
USDA's Food and Nutrition Service restructured its approach to data validation at the state level using an open-source, API-based validation service managed at the federal level.
data cleaning , data validation , API , data sharing , process redesign , Federal Data Strategy
The Census Bureau Uses Its Own Data to Increase Response Rates, Helps Communities and Other Stakeholders Do the Same
The Census Bureau team produced a new interactive mapping tool in early 2018 called the Response Outreach Area Mapper (ROAM), an application that resulted in wider use of authoritative Census Bureau data, not only to improve the Census Bureau’s own operational efficiency, but also for use by tribal, state, and local governments, national and local partners, and other community groups. Other agency data practitioners can learn from the Census Bureau team’s experience communicating technical needs to non-technical executives, building analysis tools with widely-used software, and integrating efforts with stakeholders and users.
open data , data sharing , data management , data analysis , Federal Data Strategy
The Mapping Medicare Disparities Tool
The Centers for Medicare & Medicaid Services’ Office of Minority Health (CMS OMH) Mapping Medicare Disparities Tool harnessed the power of millions of data records while protecting the privacy of individuals, creating an easy-to-use tool to better understand health disparities.
Centers for Medicare & Medicaid Services
geospatial , Federal Data Strategy , open data
The Veterans Legacy Memorial
The Veterans Legacy Memorial (VLM) is a digital platform to help families, survivors, and fellow veterans to take a leading role in honoring their beloved veteran. Built on millions of existing National Cemetery Administration (NCA) records in a 25-year-old database, VLM is a powerful example of an agency harnessing the potential of a legacy system to provide a modernized service that better serves the public.
Veterans Administration
data sharing , data visualization , Federal Data Strategy
Transitioning to a Data Driven Culture at CMS
This case study describes how CMS announced the creation of the Office of Information Products and Data Analytics (OIPDA) to take the lead in making data use and dissemination a core function of the agency.
data management , data sharing , data analysis , data analytics
PDF (10 pages)
U.S. Department of Labor Case Study: Software Development Kits
The U.S. Department of Labor sought to go beyond merely making data available to developers and take ease of use of the data to the next level by giving developers tools that would make using DOL’s data easier. DOL created software development kits (SDKs), which are downloadable code packages that developers can drop into their apps, making access to DOL’s data easy for even the most novice developer. These SDKs have even been published as open source projects with the aim of speeding up their conversion to SDKs that will eventually support all federal APIs.
Department of Labor
open data , API
U.S. Geological Survey and U.S. Census Bureau collaborate on national roads and boundaries data
It is a well-kept secret that the U.S. Geological Survey and the U.S. Census Bureau were the original two federal agencies to build the first national digital database of roads and boundaries in the United States. The agencies joined forces to develop homegrown computer software and state of the art technologies to convert existing USGS topographic maps of the nation to the points, lines, and polygons that fueled early GIS. Today, the USGS and Census Bureau have a longstanding goal to leverage and use roads and authoritative boundary datasets.
U.S. Geological Survey and U.S. Census Bureau
data management , data sharing , data standards , data validation , data visualization , Federal Data Strategy , geospatial , open data , quality
USA.gov Uses Human-Centered Design to Roll Out AI Chatbot
To improve customer service and give better answers to users of the USA.gov website, the Technology Transformation and Services team at General Services Administration (GSA) created a chatbot using artificial intelligence (AI) and automation.
General Services Administration
AI , Federal Data Strategy
resources.data.gov
An official website of the Office of Management and Budget, the General Services Administration, and the Office of Government Information Services.
This section contains explanations of common terms referenced on resources.data.gov.
Data and Analytics Case Study Roll-up Report
Made possible by ey, exclusive global insights case study sponsor.
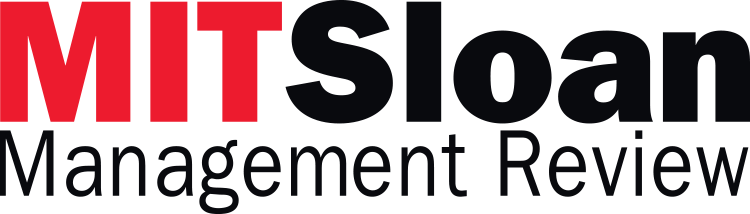
Lessons from Becoming a Data-Driven Organization
October 18, 2016, introduction.
The case studies gathered and presented here tell, in a sense, a single story. It’s the story of a “management revolution,” brought about by the widespread adoption of big data and analytics in both the public and private sectors. 1 In these dispatches from the front lines of that revolution, we see four strikingly dissimilar organizations — a health care system, a bank, a major industrial company, and a municipal government — in the process of becoming data-driven. (The organizations are Intermountain Healthcare , Nedbank , GE , and the city of Amsterdam . Other MIT SMR published case studies cited in this report can be found at sloanreview.mit.edu/case-study/ .) We see them struggle and, to a greater or lesser extent, succeed at using analytics to improve the quality and variety of their products and services, engage in new and deeper ways with patients, customers, and citizens, and transform the way they operate. And crucially, we see them use data and analytics not just to improve productivity and make their operations more efficient, but also to change their fundamental business models.
A leading character in this story is technology. Quite simply, there would be no data and analytics revolution without easily accessible, increasingly inexpensive computing power: the cloud, the Internet, and powerful, versatile software and algorithms. Yet technology is only part of the story. People are equally important. The leaders who mobilize their organizations to embrace and fulfill the promise of analytics, the frontline people who experience how analytics are changing their roles and responsibilities, the data scientists and IT engineers who do the hard work of collecting, classifying, organizing, and deploying staggering amounts of information — they all help us gain a better understanding of the scope and meaning of this revolution.
The technology and the people who deploy it also need a process or system of rules to guide how people create and use information. Rules help transform the noise of disordered information into legible signals with the power to sharpen and deepen the focus on the customer (broadly defined), and in the process improve health outcomes, the customer experience, the realization of business value, and civic life and engagement.
MIT Sloan Management Review has prepared and presented these case studies to show how the analytics revolution is currently transforming organizations as well as the economies and societies in which they operate. This transformation is occurring at every level, from the microeconomic to the macroeconomic, from the way individual stores stock their shelves, to the way manufacturers and their customers connect to realize new forms of value, to the way economies and societies function.
At the same time, these case studies reveal that many organizations struggle mightily just to get a handle on the data they have. And once they’ve located, collected, classified, and organized the data, they must then manage the processes needed to ensure its timeliness, accuracy, completeness, and reliability. Integrating data into decision making can be another fraught step, especially among managers accustomed to acting on gut instinct, intuition, and experience. Organizations and their leaders need considerable fortitude and persistence — not to mention a judicious blend of patience and impatience — to do the grinding, unglamorous work that is the foundation of successful analytics initiatives. That’s a big ask, and it helps explain why so many such initiatives fall short of expectations.
These reports from the front offer a distinctive view of the organizations that are realizing, or aiming to realize, the promise of analytics. As disparate as they are, they share a few key success factors, and they have also faced common hurdles and reaped the benefits that flow from perseverance and dogged adherence to their digital vision. That vision, shaped by each organization’s senior leaders and elevated by those working most closely with the data, focuses not merely on using digital technology to make the existing organization better but using it to transform the organization along three crucial dimensions: improving the customer experience; overhauling operational processes; and designing and executing new, digitally powered business models.
How the Data-Driven Organization Takes Shape
The data-driven organization can be pictured as a pyramid that rests on a base of well-governed data, partnerships, and sustained commitment from leadership and employees alike. With that foundation in place, the organization can move to the next level, where it treats data as a core asset that is an essential element of strategy, fashions its data into commercial offerings, and uses it to deepen the engagement of employees and customers. Then finally, as the organization’s data and analytics capabilities mature, they can underpin innovative new business models — models that alter, sometimes radically, power arrangements within the organization.
Mastering the Basics of Information Management
Many organizations have been slow in compiling, classifying, and organizing the data sitting in siloes and dark corners. It’s “a boring, boring job,” says Ger Baron, Amsterdam’s first-ever chief technology officer. “But very useful!” He ought to know. The Netherlands’ capital has 12,000 different datasets, and even they can’t tell him everything about the city. For example, no one knows exactly how many bridges span Amsterdam’s famous canals, because the city’s individual districts have not centralized their infrastructure data.
That story underscores the challenges organizations face in the realm of data governance, or the methods and rules that organizations use to assure the quality of data, manage it, integrate it into business processes (see the sidebar, “Process Innovation Comes in Different Shapes and Sizes”), and manage its risks.
Mobilizing and motivating an organization to conduct a data inventory can, as Baron implies, be a significant challenge for senior leaders. It’s tough to persuade people to undertake a tedious, laborious job, especially when the payoff seems distant or insubstantial. So leadership needs to recognize and celebrate small wins along the way.
Organizational leaders must decide who will be responsible for data governance. They must determine who will define and disseminate a common vocabulary around data, specify who has access to what data and how and with whom they can share it, and establish how the entire organization can use that data to create value. Some cutting-edge organizations have created data councils, composed of leaders from across the organization, to address such questions. The work of data councils involves mapping workflows, assigning ownership and accountability for key deliverables, defining and enforcing the cultural norms for the use of data in decision making, and measuring what data and analytics contribute to organizational performance. Without such councils to make these and similar high-level decisions, organizations may find it difficult to sustain their analytics initiatives, much less take them to the next level.
The council’s first job is to create a common language for data. The language of data is, like any other language, a social construct, and it’s the job of the data council to define and enforce the social norms around that language, to ensure not just clear communication but organizational cohesion. But even defining data can be a stiff challenge. Although it may seem obvious that data simply means raw information, people involved in analytics can attest that many of their colleagues don’t really understand the term. Does data mean numbers? Social media postings? Location information? Customer activity? Readouts on machine performance? It is all these things and more, but many people have trouble grasping that a single concept can span so many different categories. Without that understanding, it’s all but impossible to sell, price, or even describe data and analytics product offerings.
When that understanding is achieved, though, language can expand listeners’ view of the world. Jeff Liberman, COO of Spanish-language media company Entravision Communications Corp., based in Santa Monica, California, was baffled when he first heard Franklin Rios, the driving force behind the company’s Luminar analytics unit, deliver a board presentation about big data. He simply didn’t see its relevance to the broadcast business where he had spent his professional life. “I was thinking as a broadcaster, saying, ‘How am I going to use this?’” Liberman recalls. “Then I said, ‘Wait a second, I have to take off my broadcaster’s hat and start looking at this as just a flow of information.’ It just really clicked at that point.” The point is that leaders should not underestimate the power of language to make the subject of data “click” in the minds of users.
In addition to finding a common language, those responsible for data governance must also create definitions of success that are consistent with the organization’s data strategy. Consider Intermountain Healthcare Inc. (IMH), a system of 22 hospitals and 185 clinics in Utah and Idaho, headquartered in Salt Lake City. Whether or not he knew it, IMH chief of surgery Mark Ott was socializing a new definition of success when he met with surgeons at IMH’s flagship hospital and showed them data that proved that their postoperative infection rates were in line with national averages. “You think you’re great,” he told them, “but compared to other hospitals in the country, you’re average.” Such blunt language, especially directed at people with as much professional pride as surgeons, can help promote thinking about data as a source of truth, however painful the truth may be. And it can be a powerful force for improving the experience of customers — or in this case, patients.
Data governance also entails determining what data matters in a given context. That’s what Caesars Entertainment Corp. , the Las Vegas-based international gaming company, discovered when it tried to develop a scorecard to promote and advance its initiative to make its hotels, casinos, and other properties more energy efficient and environmentally friendly. Contention quickly arose over the specific information to include in the scorecard.
Some stakeholders thought the scorecard should highlight progress toward cost-reduction and financial goals — which led to debates over whether to track energy consumption or cost savings. Some stakeholders then asked if it was fair to compare older buildings with newer ones built to greener specifications. What about buildings located in hot climates? Was it fair to compare them with buildings in more moderate zones without adjusting for temperature differences?
Other organizations will be sure to confront similar questions as they deliberate what to measure and what purpose the measurements should serve. The answers will vary according to the organization’s strategy and goals, but in any case, it’s up to senior leadership to arrive at a common data language and manage the debate over what to measure — that is, over what data matters most.
In addition to establishing a common language for data, nearly every organization has to close gaps in data quality, as IMH learned when it started to present surgeons with data about their patients’ health outcomes. IMH discovered that data listing a patient as under the care of a particular doctor was sometimes incorrect because the doctor’s partner was seeing that patient. This inconsistency risked undermining physicians’ trust in the data, which in turn posed a risk to the company’s efforts to promote data-driven decision making. In response, IMH encouraged physicians to trust the data by allowing them to see patient data and challenge it.
Categorizing data is another challenge. Amsterdam’s Baron has to pin down the definition of a bridge, which varies from one district of the city to the next. WellPoint (now called Anthem), a leading U.S. provider of health care benefits and insurance, has had to reconcile definitions of emergency-room visits that varied across the 14 regions it serves. And a leading central bank has had to harmonize the widely varying terminology that various bank departments used to refer to the different categories of private-sector institutions under their purview.
Unstructured data can also be a headache. Vince Golla, social media director at Kaiser Foundation Health Plan, one of the largest health care providers in the United States, tells of running an experiment to measure its members’ satisfaction with the parking facilities at the organization’s many locations. For 30 days, he and his team searched Twitter for mentions of Kaiser Permanente . Problem was, most people usually refer to Kaiser Permanente just as Kaiser, and as a result, the Twitter search threw up confounding references to Kaiser rolls, Kaiser beer, and the English rock band Kaiser Chiefs. “It required a little work to sift the wheat from that chaff,” Golla says, with some understatement.
Heavily siloed organizations have their own set of data problems. Wayde Fleener, a data scientist and senior manager in General Mills’ Consumer Insights group , encountered resistance when he set out to discover what his organization knew about its customers since no one had a data diagram that mapped out where data was or how it was being used. “People felt threatened because I was coming into their role and questioning them,” says Fleener. “So there was a lot of resistance, and ‘Are you trying to take over what I’m doing?’ I had to keep saying, ‘No, I’m just trying to help you do things better.’” While sifting through thousands of datasets that referenced the company’s Cheerios cereal, Fleener discovered that some data sources spelled “Cheerios” all the way out, and others spelled it “chrs.” 2 Resolving such discrepancies is a job for a team of “data stewards,” who spend much of their time reviewing reports of such terminological inconsistencies every time they run a data refresh.
Jeanne Ross, research director of the MIT Sloan Center for Information Systems Research, believes that many organizations need a “ data dictator ” to resolve issues such as definitional inconsistencies. As she said in an interview with MIT Sloan Management Review , they need “somebody who says, ‘This is how we will define sales, this is how we will define returns, this is when we will register revenue, and we are all living by this rule. Until we do that, we don’t have data that’s useful for most kinds of analytics. We can still go out and buy demographic data and probably learn something quite useful. But if we want to know how to avoid stock-outs in our stores or what products are of greatest interest to a particular customer segment, we’re going to need the data cleaned up.’” 3 Not every company has the will and resources to make that kind of commitment.
Ultimately, effective information management requires the intervention of people guided by rules, norms, and culture, none of which are static. It’s often tedious, painstaking work and requires a significant investment in human and financial resources.
The New World of Data Partnerships
Anyone involved in a data and analytics initiative soon learns that they can’t go it alone — data and analytics applications inevitably drift across departmental and organizational boundaries, requiring collaboration within and between organizations to reach their full potential. At IMH, for instance, endocrinologists partnered with data scientists and one another to learn how best to manage patients with diabetes.
The most consistently successful physicians shared with their colleagues the methods they used to help patients maintain low blood-sugar levels, ranging from motivational tools to regular calls from the physician’s team. This information-sharing partnership enabled IMH’s physicians to care so well for their diabetic patients that those patients now face no higher risk of heart disease than the general population.
Data partnerships also extend beyond the walls of the organization. Amsterdam relies heavily on the Amsterdam Smart City initiative, a public-private platform, to address many issues of urban life. The initiative encompasses projects across eight categories: smart mobility, smart living, smart society, smart areas, smart economy, big and open data, infrastructure, and living labs. Early wins include replacing most of the city’s parking meters with a pay-by-phone app; an initiative to improve crowd control and traffic flow at large gatherings like the SAIL Amsterdam Festival, which brings more than a million visitors to Amsterdam to view traditional wooden sailing ships; and Rain Sense, which examines where rain falls in the city to help reduce the effect of flooding on traffic flow. (See the sidebar, “How Data Drives Productivity Improvement.”)
At Nedbank Group Ltd., the fourth-largest bank by revenues in South Africa, the company’s card-acquiring business developed a commercial offering, Market Edge, for its merchant business customers that included a suite of analytical tools integrating the customers’ own client data with historical, demographic, weather, and other data to which the bank had access. Each Market Edge offering is tailored to and co-developed with each Nedbank customer to address their particular business needs. (See the sidebar, “Building Better Relationships With Data.”)
Meanwhile, GE’s Industrial Internet offerings are transforming sales agreements into partnerships as the company migrates away from a licensing-based pricing model for its Predix platform to a subscription model. Rather than charging a fixed price for, say, a turbine, GE sells a hardware, software, and service solution whose value is determined in part by whether a customer reaches a certain level of savings in operational costs. If that level is achieved, GE gets a cut. “If we improve, say, [a customer’s] power consumption by X, we get $1; by Y, we get $1.50,” says Ron Holsey, a senior executive at GE Oil & Gas. A crucial aspect of these agreements is that GE and its customer must work together to realize those savings — they become co-creators of value. And that itself changes GE’s relationship with its customer.
Engaged customers can be a company’s most effective sales force. To win new business, GE runs scores of pilot programs with customers in the oil and gas industry to demonstrate how Predix can improve asset performance, increase efficiency, and cut downtime — a crucial issue in an industry where a single idle well can cost millions of dollars a day. Successful programs generate positive word of mouth and convince oil and gas engineers (a conservative group, reluctant to try anything new that isn’t empirically supported and low-risk) to find out what Predix can do for them. “To get anywhere in the oil and gas industry, we need help selling,” says one GE executive. “We need customer voices out in the industry with success stories, or we’re just not going to come to the table with the credibility that we need. So we need to inspire our customers to want to do that.”
Data can also turn businesses and their vendors into partners. When IMH decided to overhaul its electronic health records (EHR) system, it chose to work with Cerner Corp., a leading EHR vendor. IMH’s leadership team liked Cerner’s careful attention to the secondary use of data for back-end analytics as well as its clinical transaction system, which helped clinicians make better patient-care decisions. But IMH wanted to retain its own data management and analytic systems, a condition that called for increased coordination between the organizations. Cerner set up shop in offices next to IMH’s main medical facility and relocated some of its top development talent to Utah.
As a result of their close work together, IMH and Cerner partnered to win a huge contract to provide health care services to the Department of Defense and are brainstorming new products based on IMH’s data management services and data warehouse framework.
In each of these cases, data and analytics fundamentally altered the scope, depth, and quality of the engagements between and among organizations and individuals. Rather than one party buying what the other is selling, they are working together, supported by data, to create value for both sides.
Yet even the closest partnerships have their points of contention and conflict. A handful of forward-looking companies are starting to explore the possibility of gathering, developing, and exploiting IoT data from not only their own physical assets but also those of their customers and vendors. In some cases, customers and vendors do not hesitate to share their data, viewing data sharing as a low-cost, low-risk way to acquire analytics capabilities. But as companies as different as GE and Luminar have learned, customers and vendors can be difficult to sell on the concept, because it suggests a loss of autonomy, a compromise of trade secrets, or the giveaway of a valuable asset. In the public sector, data sharing can raise troubling issues, even when the data concerns something people want to get rid of: garbage. When AEB Amsterdam tried to improve its waste stream by having citizens separate recyclables into different colored bags, it had to confront public concern that the city might be spying on its residents through their trash.
Data partnerships, and data sharing more broadly, are creating important new sources of business value but at the same time generating new and as yet unanswered questions regarding data ownership, data accessibility, and data rights. To the extent that companies rely on data sharing for business value, who will manage the risks that accompany such dependence?
Treating Data as a Core Asset
Organizations can use data to improve virtually anything they do, but a relative handful of standout organizations are using data in highly intentional, systematic ways to address strategic challenges and react to — and in many cases anticipate — sweeping changes in the markets they serve.
What these organizations have in common is that they view data as a core asset. Their leaders are convinced that analytics can improve their ability to innovate and give them a competitive advantage. They support the systematic use of data in decision making and strategy throughout the organizations they lead. These organizations don’t just use analytics to address tactical and operational issues or to support basic reporting and marketing — with a strong push from their leaders, they’ve taken the next step up and are using analytics to allocate resources and create new products and business models. (See the sidebar, “How Data Enhances Sales, Pricing, and Procurement.”)
Today, GE views its competencies around data and analytics, as well as its various Internet of Things offerings, as central elements of its new digital business identity. Data is changing what GE sells, who sells what they sell, and how they sell, in addition to expanding the range of buyers to whom they sell. IMH, a pioneer in the use of data and analytics to improve health outcomes and lower costs, has long believed that data is core to operational decision making and strategic planning. The City of Amsterdam is now using data, its own as well as that of myriad partners, to improve municipal management, and in the process has been named Europe’s most innovative city. Like many large financial institutions, Nedbank is striving to use data to improve its operations and customer focus and achieve strategic goals. All these organizations are, to varying degrees, organizing their activities around the use of data.
For instance, IMH made a data-driven, strategic decision to perform cardiovascular operations (surgeries and catheterizations) at only four of its hospitals. By concentrating expertise at those facilities, IMH has sharply cut response times to treat ST-elevated myocardial infarctions (STEMI) — heart attacks that follow the sudden, complete blockage of coronary arteries. Research has shown that up to 15% of people who suffer STEMI die within 30 days of the event, but survival rates improve if patients receive treatment to unblock the artery within 90 minutes of arriving at the hospital. IMH tracks each STEMI event at its hospitals and shares its measurements with its surgical teams, who use the information to boost their response times. As of 2014, the median response time had been cut to 57 minutes — dramatically lower than the national average of 90 minutes — leading to a marked improvement in health outcomes, with 96% of STEMI patients surviving longer than 30 days after hospitalization.
Commercializing Data and Business Model Innovation
Virtually every power user of data and analytics is discovering the commercial potential of the information they’re collecting, generating, and analyzing (see the sidebar, “Data-Driven Product Innovation”). All the companies covered in this series — GE, IMH, and Nedbank — are beginning to commercialize data, related analytics, or a software platform. GE’s cloud-based Predix software platform is a standout example. Originally developed to provide GE’s own machine operators and maintenance engineers with real-time information to schedule maintenance checks, improve efficiency, and reduce downtime, Predix is now available to GE’s channel and technology partners as well as other customers who can use the platform to build their own set of bespoke analytics.
Like Predix, virtually all efforts to commercialize data and analytics are built from the foundation of a prior internal application. Nedbank’s Market Edge began life as an innovative way for the bank to develop value-added services to differentiate its credit- and debit-card offerings. The products that IMH is brainstorming with Cerner spring from the analytics developed to improve clinical care at IMH’s own facilities.
Bringing those commercial ventures to scale is a pressing question for senior leaders. Do they do it from the inside? That choice inevitably entails deep and difficult changes to functions such as sales and finance, as GE discovered while commercializing the Predix platform. Or do they scale up from the outside? Entravision chose that course when it set up its Luminar analytics unit as a freestanding division, with a separate P&L and its own sales teams. In either case, the scaling effort will blur inter- and intra-organizational boundaries — Luminar’s sales teams, for example, now join Entravision’s teams on sales calls to some advertisers.
Partnerships can serve as a powerful tool for scaling commercial offerings. By joining forces with its small-business clients to improve their performance and fine-tune their strategy, Nedbank builds a following for its consumer analytics offering while enhancing customer satisfaction and engagement. GE, as we have seen, partners with its industrial customers to boost their efficiency, with revenues determined by the savings generated by its analytics.
Organizational constraints, however, can impede efforts to scale commercial analytics offerings. Sometimes other organizational priorities take precedence, while in other cases the organization simply lacks the capabilities needed to bring a product or service to scale.
Nedbank encountered several of these challenges when trying to scale Market Edge, the analytics product for its merchant customers. The internal product team recruited 12 salespeople from its card division to sell Market Edge to merchants but soon learned that despite receiving specialized training, the salespeople were simply not comfortable discussing the product with their clients. Leaders of the scaling campaign have realized that they’ll need to recruit a whole new sales team for Market Edge — the current sales force just isn’t fit for purpose. At the same time, the Market Edge team has run up against resource constraints, because a concurrent program to retool the bank’s IT infrastructure has slowed the drive to move commercialization effort beyond the pilot stage.
GE is using data and analytics to refashion several elements of its business model, including service delivery, its sales force, and the way it prices equipment. It has created a huge software division to support Predix (its new cloud-based platform to host and analyze asset productivity data), develop new machine data applications, and bring together a community of customers and developers (see the sidebar, “Toward Data-Driven Strategic Planning”). The industrial giant is committing $1 billion to install sensors on jet engines, gas turbines, and other machines, connect them to the cloud, and analyze the resulting flow of data to find ways to boost machine reliability and productivity.
The case studies discussed in this report reveal the emerging opportunities with data, along with related challenges: The contours of the “management revolution” are taking shape. Those companies that are winning with data are mastering the data they have access to, co-creating business value from that data, and in some instances, elaborating their business models to take advantage of these new possibilities.
A 2015 MIT Sloan Management Review survey of 2,719 managers in organizations around the world found that the chief barrier to creating business value from analytics was not data management or complex modeling skills. Rather, the main obstacle was translating analytics into business actions — or, as one survey respondent put it, “developing middle management skills at interpretation.”
There are ways to close that gap, notably formal training and experiential learning. But mindsets also have to change. To become effective analytics consumers, managers must learn to distinguish between facts and data. Facts are immutable; data can be spun and massaged to support the preconceptions of the interpreter — a deeply ingrained cognitive tendency known as confirmation bias. A line from Paul Simon’s song “The Boxer” neatly sums up confirmation bias: “A man hears what he wants to hear and disregards the rest.”
In the Nedbank case study, local merchants thought they knew who their best customers were, but data offered a different view. Surgeons at IMH thought they were above average until data showed them otherwise. GE’s Oil & Gas customers believed they knew best how to manage their wells and rigs, until data showed them more productive ways. For managers accustomed to making decisions based entirely on their own experience and intuition, there is a tendency to believe that they are the right people with the right information at the right time. It is noteworthy that managers changed their minds and behavior in each of these cases when counterintuitive data was introduced into a business environment in which they trusted the people presenting the data and were prepared to trust the data itself.
In order to create business value from data, it may not be sufficient for the right information to be received and trusted by the right person at the right time; the right information must also have the right salience to the right person. In an era of information overload, the ability to identify the right information from other information remains a critically important management skill. Blending data and experience in decision making is not a balancing act; neither is a substitute for the other.
Organizational leaders can take a page from the legendary Alfred P. Sloan, who, when faced with unanimous support from his General Motors executive team for one of his decisions, responded: “I propose we postpone further discussion of this matter until our next meeting, to give ourselves time to develop disagreement and perhaps gain understanding of what the decision is all about.”
Data will often underdetermine a course of action, if only because data is subjective in nature; how data is created, weighted, and analyzed is the product of many individual decisions that can introduce personal or political biases. Data-based evidence is often weighed with experience, bolstered with faith, and buffeted by politics. As analytics becomes a more common path to business value, experience, belief, and politics as well as luck will continue to play crucial roles in determining what kind of business value can be derived from data.
1. The term “management revolution” was applied to this data phenomenon by Andrew MacAfee and Erik Brynjolfsson in 2012. See A. MacAfee and E. Brynjolfsson, “Big Data: The Management Revolution,” Harvard Business Review 90, no. 10 (October 2012): 60-68.
2. W. Fleener, M. Fitzgerald, “General Mills Builds Up Big Data to Answer Big Questions,” MIT Sloan Management Review, May 29, 2015. www.sloanreview.mit.edu.
3. J. Ross, D. Kiron, and R.B. Ferguson, “Do You Need a Data Dictator?” MIT Sloan Management Review, August 28, 2012, www.sloanreview.mit.edu.
More Like This
Add a comment cancel reply.
You must sign in to post a comment. First time here? Sign up for a free account : Comment on articles and get access to many more articles.
Comment (1)
Richard ordowich.
- Digital Marketing
- Facebook Marketing
- Instagram Marketing
- Ecommerce Marketing
- Content Marketing
- Data Science Certification
- Machine Learning
- Artificial Intelligence
- Data Analytics
- Graphic Design
- Adobe Illustrator
- Web Designing
- UX UI Design
- Interior Design
- Front End Development
- Back End Development Courses
- Business Analytics
- Entrepreneurship
- Supply Chain
- Financial Modeling
- Corporate Finance
- Project Finance
- Harvard University
- Stanford University
- Yale University
- Princeton University
- Duke University
- UC Berkeley
- Harvard University Executive Programs
- MIT Executive Programs
- Stanford University Executive Programs
- Oxford University Executive Programs
- Cambridge University Executive Programs
- Yale University Executive Programs
- Kellog Executive Programs
- CMU Executive Programs
- 45000+ Free Courses
- Free Certification Courses
- Free DigitalDefynd Certificate
- Free Harvard University Courses
- Free MIT Courses
- Free Excel Courses
- Free Google Courses
- Free Finance Courses
- Free Coding Courses
- Free Digital Marketing Courses
Top 25 Data Science Case Studies [2024]
In an era where data is the new gold, harnessing its power through data science has led to groundbreaking advancements across industries. From personalized marketing to predictive maintenance, the applications of data science are not only diverse but transformative. This compilation of the top 25 data science case studies showcases the profound impact of intelligent data utilization in solving real-world problems. These examples span various sectors, including healthcare, finance, transportation, and manufacturing, illustrating how data-driven decisions shape business operations’ future, enhance efficiency, and optimize user experiences. As we delve into these case studies, we witness the incredible potential of data science to innovate and drive success in today’s data-centric world.
Related: Interesting Data Science Facts
Top 25 Data Science Case Studies [2024]
Case study 1 – personalized marketing (amazon).
Challenge: Amazon aimed to enhance user engagement by tailoring product recommendations to individual preferences, requiring the real-time processing of vast data volumes.
Solution: Amazon implemented a sophisticated machine learning algorithm known as collaborative filtering, which analyzes users’ purchase history, cart contents, product ratings, and browsing history, along with the behavior of similar users. This approach enables Amazon to offer highly personalized product suggestions.
Overall Impact:
- Increased Customer Satisfaction: Tailored recommendations improved the shopping experience.
- Higher Sales Conversions: Relevant product suggestions boosted sales.
Key Takeaways:
- Personalized Marketing Significantly Enhances User Engagement: Demonstrating how tailored interactions can deepen user involvement and satisfaction.
- Effective Use of Big Data and Machine Learning Can Transform Customer Experiences: These technologies redefine the consumer landscape by continuously adapting recommendations to changing user preferences and behaviors.
This strategy has proven pivotal in increasing Amazon’s customer loyalty and sales by making the shopping experience more relevant and engaging.
Case Study 2 – Real-Time Pricing Strategy (Uber)
Challenge: Uber needed to adjust its pricing dynamically to reflect real-time demand and supply variations across different locations and times, aiming to optimize driver incentives and customer satisfaction without manual intervention.
Solution: Uber introduced a dynamic pricing model called “surge pricing.” This system uses data science to automatically calculate fares in real time based on current demand and supply data. The model incorporates traffic conditions, weather forecasts, and local events to adjust prices appropriately.
- Optimized Ride Availability: The model reduced customer wait times by incentivizing more drivers to be available during high-demand periods.
- Increased Driver Earnings: Drivers benefitted from higher earnings during surge periods, aligning their incentives with customer demand.
- Efficient Balance of Supply and Demand: Dynamic pricing matches ride availability with customer needs.
- Importance of Real-Time Data Processing: The real-time processing of data is crucial for responsive and adaptive service delivery.
Uber’s implementation of surge pricing illustrates the power of using real-time data analytics to create a flexible and responsive pricing system that benefits both consumers and service providers, enhancing overall service efficiency and satisfaction.
Case Study 3 – Fraud Detection in Banking (JPMorgan Chase)
Challenge: JPMorgan Chase faced the critical need to enhance its fraud detection capabilities to safeguard the institution and its customers from financial losses. The primary challenge was detecting fraudulent transactions swiftly and accurately in a vast stream of legitimate banking activities.
Solution: The bank implemented advanced machine learning models that analyze real-time transaction patterns and customer behaviors. These models are continuously trained on vast amounts of historical fraud data, enabling them to identify and flag transactions that significantly deviate from established patterns, which may indicate potential fraud.
- Substantial Reduction in Fraudulent Transactions: The advanced detection capabilities led to a marked decrease in fraud occurrences.
- Enhanced Security for Customer Accounts: Customers experienced greater security and trust in their transactions.
- Effectiveness of Machine Learning in Fraud Detection: Machine learning models are greatly effective at identifying fraud activities within large datasets.
- Importance of Ongoing Training and Updates: Continuous training and updating of models are crucial to adapt to evolving fraudulent techniques and maintain detection efficacy.
JPMorgan Chase’s use of machine learning for fraud detection demonstrates how financial institutions can leverage advanced analytics to enhance security measures, protect financial assets, and build customer trust in their banking services.
Case Study 4 – Optimizing Healthcare Outcomes (Mayo Clinic)
Challenge: The Mayo Clinic aimed to enhance patient outcomes by predicting diseases before they reach critical stages. This involved analyzing large volumes of diverse data, including historical patient records and real-time health metrics from various sources like lab results and patient monitors.
Solution: The Mayo Clinic employed predictive analytics to integrate and analyze this data to build models that predict patient risk for diseases such as diabetes and heart disease, enabling earlier and more targeted interventions.
- Improved Patient Outcomes: Early identification of at-risk patients allowed for timely medical intervention.
- Reduction in Healthcare Costs: Preventing disease progression reduces the need for more extensive and costly treatments later.
- Early Identification of Health Risks: Predictive models are essential for identifying at-risk patients early, improving the chances of successful interventions.
- Integration of Multiple Data Sources: Combining historical and real-time data provides a comprehensive view that enhances the accuracy of predictions.
Case Study 5 – Streamlining Operations in Manufacturing (General Electric)
Challenge: General Electric needed to optimize its manufacturing processes to reduce costs and downtime by predicting when machines would likely require maintenance to prevent breakdowns.
Solution: GE leveraged data from sensors embedded in machinery to monitor their condition continuously. Data science algorithms analyze this sensor data to predict when a machine is likely to disappoint, facilitating preemptive maintenance and scheduling.
- Reduction in Unplanned Machine Downtime: Predictive maintenance helped avoid unexpected breakdowns.
- Lower Maintenance Costs and Improved Machine Lifespan: Regular maintenance based on predictive data reduced overall costs and extended the life of machinery.
- Predictive Maintenance Enhances Operational Efficiency: Using data-driven predictions for maintenance can significantly reduce downtime and operational costs.
- Value of Sensor Data: Continuous monitoring and data analysis are crucial for forecasting equipment health and preventing failures.
Related: Data Engineering vs. Data Science
Case Study 6 – Enhancing Supply Chain Management (DHL)
Challenge: DHL sought to optimize its global logistics and supply chain operations to decreases expenses and enhance delivery efficiency. It required handling complex data from various sources for better route planning and inventory management.
Solution: DHL implemented advanced analytics to process and analyze data from its extensive logistics network. This included real-time tracking of shipments, analysis of weather conditions, traffic patterns, and inventory levels to optimize route planning and warehouse operations.
- Enhanced Efficiency in Logistics Operations: More precise route planning and inventory management improved delivery times and reduced resource wastage.
- Reduced Operational Costs: Streamlined operations led to significant cost savings across the supply chain.
- Critical Role of Comprehensive Data Analysis: Effective supply chain management depends on integrating and analyzing data from multiple sources.
- Benefits of Real-Time Data Integration: Real-time data enhances logistical decision-making, leading to more efficient and cost-effective operations.
Case Study 7 – Predictive Maintenance in Aerospace (Airbus)
Challenge: Airbus faced the challenge of predicting potential failures in aircraft components to enhance safety and reduce maintenance costs. The key was to accurately forecast the lifespan of parts under varying conditions and usage patterns, which is critical in the aerospace industry where safety is paramount.
Solution: Airbus tackled this challenge by developing predictive models that utilize data collected from sensors installed on aircraft. These sensors continuously monitor the condition of various components, providing real-time data that the models analyze. The predictive algorithms assess the likelihood of component failure, enabling maintenance teams to schedule repairs or replacements proactively before actual failures occur.
- Increased Safety: The ability to predict and prevent potential in-flight failures has significantly improved the safety of Airbus aircraft.
- Reduced Costs: By optimizing maintenance schedules and minimizing unnecessary checks, Airbus has been able to cut down on maintenance expenses and reduce aircraft downtime.
- Enhanced Safety through Predictive Analytics: The use of predictive analytics in monitoring aircraft components plays a crucial role in preventing failures, thereby enhancing the overall safety of aviation operations.
- Valuable Insights from Sensor Data: Real-time data from operational use is critical for developing effective predictive maintenance strategies. This data provides insights for understanding component behavior under various conditions, allowing for more accurate predictions.
This case study demonstrates how Airbus leverages advanced data science techniques in predictive maintenance to ensure higher safety standards and more efficient operations, setting an industry benchmark in the aerospace sector.
Case Study 8 – Enhancing Film Recommendations (Netflix)
Challenge: Netflix aimed to improve customer retention and engagement by enhancing the accuracy of its recommendation system. This task involved processing and analyzing vast amounts of data to understand diverse user preferences and viewing habits.
Solution: Netflix employed collaborative filtering techniques, analyzing user behaviors (like watching, liking, or disliking content) and similarities between content items. This data-driven approach allows Netflix to refine and personalize recommendations continuously based on real-time user interactions.
- Increased Viewer Engagement: Personalized recommendations led to longer viewing sessions.
- Higher Customer Satisfaction and Retention Rates: Tailored viewing experiences improved overall customer satisfaction, enhancing loyalty.
- Tailoring User Experiences: Machine learning is pivotal in personalizing media content, significantly impacting viewer engagement and satisfaction.
- Importance of Continuous Updates: Regularly updating recommendation algorithms is essential to maintain relevance and effectiveness in user engagement.
Case Study 9 – Traffic Flow Optimization (Google)
Challenge: Google needed to optimize traffic flow within its Google Maps service to reduce congestion and improve routing decisions. This required real-time analysis of extensive traffic data to predict and manage traffic conditions accurately.
Solution: Google Maps integrates data from multiple sources, including satellite imagery, sensor data, and real-time user location data. These data points are used to model traffic patterns and predict future conditions dynamically, which informs updated routing advice.
- Reduced Traffic Congestion: More efficient routing reduced overall traffic buildup.
- Enhanced Accuracy of Traffic Predictions and Routing: Improved predictions led to better user navigation experiences.
- Integration of Multiple Data Sources: Combining various data streams enhances the accuracy of traffic management systems.
- Advanced Modeling Techniques: Sophisticated models are crucial for accurately predicting traffic patterns and optimizing routes.
Case Study 10 – Risk Assessment in Insurance (Allstate)
Challenge: Allstate sought to refine its risk assessment processes to offer more accurately priced insurance products, challenging the limitations of traditional actuarial models through more nuanced data interpretations.
Solution: Allstate enhanced its risk assessment framework by integrating machine learning, allowing for granular risk factor analysis. This approach utilizes individual customer data such as driving records, home location specifics, and historical claim data to tailor insurance offerings more accurately.
- More Precise Risk Assessment: Improved risk evaluation led to more tailored insurance offerings.
- Increased Market Competitiveness: Enhanced pricing accuracy boosted Allstate’s competitive edge in the insurance market.
- Nuanced Understanding of Risk: Machine learning provides a deeper, more nuanced understanding of risk than traditional models, leading to better risk pricing.
- Personalized Pricing Strategies: Leveraging detailed customer data in pricing strategies enhances customer satisfaction and business performance.
Related: Can you move from Cybersecurity to Data Science?
Case Study 11 – Energy Consumption Reduction (Google DeepMind)
Challenge: Google DeepMind aimed to significantly reduce the high energy consumption required for cooling Google’s data centers, which are crucial for maintaining server performance but also represent a major operational cost.
Solution: DeepMind implemented advanced AI algorithms to optimize the data center cooling systems. These algorithms predict temperature fluctuations and adjust cooling processes accordingly, saving energy and reducing equipment wear and tear.
- Reduction in Energy Consumption: Achieved a 40% reduction in energy used for cooling.
- Decrease in Operational Costs and Environmental Impact: Lower energy usage resulted in cost savings and reduced environmental footprint.
- AI-Driven Optimization: AI can significantly decrease energy usage in large-scale infrastructure.
- Operational Efficiency Gains: Efficiency improvements in operational processes lead to cost savings and environmental benefits.
Case Study 12 – Improving Public Safety (New York City Police Department)
Challenge: The NYPD needed to enhance its crime prevention strategies by better predicting where and when crimes were most likely to occur, requiring sophisticated analysis of historical crime data and environmental factors.
Solution: The NYPD implemented a predictive policing system that utilizes data analytics to identify potential crime hotspots based on trends and patterns in past crime data. Officers are preemptively dispatched to these areas to deter criminal activities.
- Reduction in Crime Rates: There is a notable decrease in crime in areas targeted by predictive policing.
- More Efficient Use of Police Resources: Enhanced allocation of resources where needed.
- Effectiveness of Data-Driven Crime Prevention: Targeting resources based on data analytics can significantly reduce crime.
- Proactive Law Enforcement: Predictive analytics enable a shift from reactive to proactive law enforcement strategies.
Case Study 13 – Enhancing Agricultural Yields (John Deere)
Challenge: John Deere aimed to help farmers increase agricultural productivity and sustainability by optimizing various farming operations from planting to harvesting.
Solution: Utilizing data from sensors on equipment and satellite imagery, John Deere developed algorithms that provide actionable insights for farmers on optimal planting times, water usage, and harvest schedules.
- Increased Crop Yields: More efficient farming methods led to higher yields.
- Enhanced Sustainability of Farming Practices: Improved resource management contributed to more sustainable agriculture.
- Precision Agriculture: Significantly improves productivity and resource efficiency.
- Data-Driven Decision-Making: Enables better farming decisions through timely and accurate data.
Case Study 14 – Streamlining Drug Discovery (Pfizer)
Challenge: Pfizer faced the need to accelerate the process of discoverying drug and improve the success rates of clinical trials.
Solution: Pfizer employed data science to simulate and predict outcomes of drug trials using historical data and predictive models, optimizing trial parameters and improving the selection of drug candidates.
- Accelerated Drug Development: Reduced time to market for new drugs.
- Increased Efficiency and Efficacy in Clinical Trials: More targeted trials led to better outcomes.
- Reduction in Drug Development Time and Costs: Data science streamlines the R&D process.
- Improved Clinical Trial Success Rates: Predictive modeling enhances the accuracy of trial outcomes.
Case Study 15 – Media Buying Optimization (Procter & Gamble)
Challenge: Procter & Gamble aimed to maximize the ROI of their extensive advertising budget by optimizing their media buying strategy across various channels.
Solution: P&G analyzed extensive data on consumer behavior and media consumption to identify the most effective times and channels for advertising, allowing for highly targeted ads that reach the intended audience at optimal times.
- Improved Effectiveness of Advertising Campaigns: More effective ads increased campaign impact.
- Increased Sales and Better Budget Allocation: Enhanced ROI from more strategic media spending.
- Enhanced Media Buying Strategies: Data analytics significantly improves media buying effectiveness.
- Insights into Consumer Behavior: Understanding consumer behavior is crucial for optimizing advertising ROI.
Related: Is Data Science Certificate beneficial for your career?
Case Study 16 – Reducing Patient Readmission Rates with Predictive Analytics (Mount Sinai Health System)
Challenge: Mount Sinai Health System sought to reduce patient readmission rates, a significant indicator of healthcare quality and a major cost factor. The challenge involved identifying patients at high risk of being readmitted within 30 days of discharge.
Solution: The health system implemented a predictive analytics platform that analyzes real-time patient data and historical health records. The system detects patterns and risk factors contributing to high readmission rates by utilizing machine learning algorithms. Factors such as past medical history, discharge conditions, and post-discharge care plans were integrated into the predictive model.
- Reduced Readmission Rates: Early identification of at-risk patients allowed for targeted post-discharge interventions, significantly reducing readmission rates.
- Enhanced Patient Outcomes: Patients received better follow-up care tailored to their health risks.
- Predictive Analytics in Healthcare: Effective for managing patient care post-discharge.
- Holistic Patient Data Utilization: Integrating various data points provides a more accurate prediction and better healthcare outcomes.
Case Study 17 – Enhancing E-commerce Customer Experience with AI (Zalando)
Challenge: Zalando aimed to enhance the online shopping experience by improving the accuracy of size recommendations, a common issue that leads to high return rates in online apparel shopping.
Solution: Zalando developed an AI-driven size recommendation engine that analyzes past purchase and return data in combination with customer feedback and preferences. This system utilizes machine learning to predict the best-fit size for customers based on their unique body measurements and purchase history.
- Reduced Return Rates: More accurate size recommendations decreased the returns due to poor fit.
- Improved Customer Satisfaction: Customers experienced a more personalized shopping journey, enhancing overall satisfaction.
- Customization Through AI: Personalizing customer experience can significantly impact satisfaction and business metrics.
- Data-Driven Decision-Making: Utilizing customer data effectively can improve business outcomes by reducing costs and enhancing the user experience.
Case Study 18 – Optimizing Energy Grid Performance with Machine Learning (Enel Group)
Challenge: Enel Group, one of the largest power companies, faced challenges in managing and optimizing the performance of its vast energy grids. The primary goal was to increase the efficiency of energy distribution and reduce operational costs while maintaining reliability in the face of fluctuating supply and demand.
Solution: Enel Group implemented a machine learning-based system that analyzes real-time data from smart meters, weather stations, and IoT devices across the grid. This system is designed to predict peak demand times, potential outages, and equipment failures before they occur. By integrating these predictions with automated grid management tools, Enel can dynamically adjust energy flows, allocate resources more efficiently, and schedule maintenance proactively.
- Enhanced Grid Efficiency: Improved distribution management, reduced energy wastage, and optimized resource allocation.
- Reduced Operational Costs: Predictive maintenance and better grid management decreased the frequency and cost of repairs and outages.
- Predictive Maintenance in Utility Networks: Advanced analytics can preemptively identify issues, saving costs and enhancing service reliability.
- Real-Time Data Integration: Leveraging data from various sources in real-time enables more agile and informed decision-making in energy management.
Case Study 19 – Personalizing Movie Streaming Experience (WarnerMedia)
Challenge: WarnerMedia sought to enhance viewer engagement and subscription retention rates on its streaming platforms by providing more personalized content recommendations.
Solution: WarnerMedia deployed a sophisticated data science strategy, utilizing deep learning algorithms to analyze viewer behaviors, including viewing history, ratings given to shows and movies, search patterns, and demographic data. This analysis helped create highly personalized viewer profiles, which were then used to tailor content recommendations, homepage layouts, and promotional offers specifically to individual preferences.
- Increased Viewer Engagement: Personalized recommendations resulted in extended viewing times and increased interactions with the platform.
- Higher Subscription Retention: Tailored user experiences improved overall satisfaction, leading to lower churn rates.
- Deep Learning Enhances Personalization: Deep learning algorithms allow a more nuanced knowledge of consumer preferences and behavior.
- Data-Driven Customization is Key to User Retention: Providing a customized experience based on data analytics is critical for maintaining and growing a subscriber base in the competitive streaming market.
Case Study 20 – Improving Online Retail Sales through Customer Sentiment Analysis (Zappos)
Challenge: Zappos, an online shoe and clothing retailer, aimed to enhance customer satisfaction and boost sales by better understanding customer sentiments and preferences across various platforms.
Solution: Zappos implemented a comprehensive sentiment analysis program that utilized natural language processing (NLP) techniques to gather and analyze customer feedback from social media, product reviews, and customer support interactions. This data was used to identify emerging trends, customer pain points, and overall sentiment towards products and services. The insights derived from this analysis were subsequently used to customize marketing strategies, enhance product offerings, and improve customer service practices.
- Enhanced Product Selection and Marketing: Insight-driven adjustments to inventory and marketing strategies increased relevancy and customer satisfaction.
- Improved Customer Experience: By addressing customer concerns and preferences identified through sentiment analysis, Zappos enhanced its overall customer service, increasing loyalty and repeat business.
- Power of Sentiment Analysis in Retail: Understanding and reacting to customer emotions and opinions can significantly impact sales and customer satisfaction.
- Strategic Use of Customer Feedback: Leveraging customer feedback to drive business decisions helps align product offerings and services with customer expectations, fostering a positive brand image.
Related: Data Science Industry in the US
Case Study 21 – Streamlining Airline Operations with Predictive Analytics (Delta Airlines)
Challenge: Delta Airlines faced operational challenges, including flight delays, maintenance scheduling inefficiencies, and customer service issues, which impacted passenger satisfaction and operational costs.
Solution: Delta implemented a predictive analytics system that integrates data from flight operations, weather reports, aircraft sensor data, and historical maintenance records. The system predicts potential delays using machine learning models and suggests optimal maintenance scheduling. Additionally, it forecasts passenger load to optimize staffing and resource allocation at airports.
- Reduced Flight Delays: Predictive insights allowed for better planning and reduced unexpected delays.
- Enhanced Maintenance Efficiency: Maintenance could be scheduled proactively, decreasing the time planes spend out of service.
- Improved Passenger Experience: With better resource management, passenger handling became more efficient, enhancing overall customer satisfaction.
- Operational Efficiency Through Predictive Analytics: Leveraging data for predictive purposes significantly improves operational decision-making.
- Data Integration Across Departments: Coordinating data from different sources provides a holistic view crucial for effective airline management.
Case Study 22 – Enhancing Financial Advisory Services with AI (Morgan Stanley)
Challenge: Morgan Stanley sought to offer clients more personalized and effective financial guidance. The challenge was seamlessly integrating vast financial data with individual client profiles to deliver tailored investment recommendations.
Solution: Morgan Stanley developed an AI-powered platform that utilizes natural language processing and ML to analyze financial markets, client portfolios, and historical investment performance. The system identifies patterns and predicts market trends while considering each client’s financial goals, risk tolerance, and investment history. This integrated approach enables financial advisors to offer highly customized advice and proactive investment strategies.
- Improved Client Satisfaction: Clients received more relevant and timely investment recommendations, enhancing their overall satisfaction and trust in the advisory services.
- Increased Efficiency: Advisors were able to manage client portfolios more effectively, using AI-driven insights to make faster and more informed decisions.
- Personalization through AI: Advanced analytics and AI can significantly enhance the personalization of financial services, leading to better client engagement.
- Data-Driven Decision Making: Leveraging diverse data sets provides a comprehensive understanding crucial for tailored financial advising.
Case Study 23 – Optimizing Inventory Management in Retail (Walmart)
Challenge: Walmart sought to improve inventory management across its vast network of stores and warehouses to reduce overstock and stockouts, which affect customer satisfaction and operational efficiency.
Solution: Walmart implemented a robust data analytics system that integrates real-time sales data, supply chain information, and predictive analytics. This system uses machine learning algorithms to forecast demand for thousands of products at a granular level, considering factors such as seasonality, local events, and economic trends. The predictive insights allow Walmart to dynamically adjust inventory levels, optimize restocking schedules, and manage distribution logistics more effectively.
- Reduced Inventory Costs: More accurate demand forecasts helped minimize overstock and reduce waste.
- Enhanced Customer Satisfaction: Improved stock availability led to better in-store experiences and higher customer satisfaction.
- Precision in Demand Forecasting: Advanced data analytics and machine learning significantly enhance demand forecasting accuracy in retail.
- Integrated Data Systems: Combining various data sources provides a comprehensive view of inventory needs, improving overall supply chain efficiency.
Case Study 24: Enhancing Network Security with Predictive Analytics (Cisco)
Challenge: Cisco encountered difficulties protecting its extensive network infrastructure from increasingly complex cyber threats. The objective was to bolster their security protocols by anticipating potential breaches before they happen.
Solution: Cisco developed a predictive analytics solution that leverages ML algorithms to analyze patterns in network traffic and identify anomalies that could suggest a security threat. By integrating this system with their existing security protocols, Cisco can dynamically adjust defenses and alert system administrators about potential vulnerabilities in real-time.
- Improved Security Posture: The predictive system enabled proactive responses to potential threats, significantly reducing the incidence of successful cyber attacks.
- Enhanced Operational Efficiency: Automating threat detection and response processes allowed Cisco to manage network security more efficiently, with fewer resources dedicated to manual monitoring.
- Proactive Security Measures: Employing predictive cybersecurity analytics helps organizations avoid potential threats.
- Integration of Machine Learning: Machine learning is crucial for effectively detecting patterns and anomalies that human analysts might overlook, leading to stronger security measures.
Case Study 25 – Improving Agricultural Efficiency with IoT and AI (Bayer Crop Science)
Challenge: Bayer Crop Science aimed to enhance agricultural efficiency and crop yields for farmers worldwide, facing the challenge of varying climatic conditions and soil types that affect crop growth differently.
Solution: Bayer deployed an integrated platform that merges IoT sensors, satellite imagery, and AI-driven analytics. This platform gathers real-time weather conditions, soil quality, and crop health data. Utilizing machine learning models, the system processes this data to deliver precise agricultural recommendations to farmers, including optimal planting times, watering schedules, and pest management strategies.
- Increased Crop Yields: Tailored agricultural practices led to higher productivity per hectare.
- Reduced Resource Waste: Efficient water use, fertilizers, and pesticides minimized environmental impact and operational costs.
- Precision Agriculture: Leveraging IoT and AI enables more precise and data-driven agricultural practices, enhancing yield and efficiency.
- Sustainability in Farming: Advanced data analytics enhance the sustainability of farming by optimizing resource utilization and minimizing waste.
Related: Is Data Science Overhyped?
The power of data science in transforming industries is undeniable, as demonstrated by these 25 compelling case studies. Through the strategic application of machine learning, predictive analytics, and AI, companies are solving complex challenges and gaining a competitive edge. The insights gleaned from these cases highlight the critical role of data science in enhancing decision-making processes, improving operational efficiency, and elevating customer satisfaction. As we look to the future, the role of data science is set to grow, promising even more innovative solutions and smarter strategies across all sectors. These case studies inspire and serve as a roadmap for harnessing the transformative power of data science in the journey toward digital transformation.
- What is Narrow AI [Pros & Cons] [Deep Analysis] [2024]
- Use of AI in Medicine: 5 Transformative Case Studies [2024]

Team DigitalDefynd
We help you find the best courses, certifications, and tutorials online. Hundreds of experts come together to handpick these recommendations based on decades of collective experience. So far we have served 4 Million+ satisfied learners and counting.
How to Hire the Right Data Engineer? [2024]

15 High Paying Data Engineering Jobs & Career Paths [2024]

Is Data Science Over-Hyped? [2024]

Role of Data Science in Cybersecurity & Threat Detection[2024]

Should You Hire Data Scientist or Data Engineer? [2024]

Is Data Analytics a Safe Career Option? [2024]

- 14 min read
Data-Driven Decision-Making Case Studies: Insights from Real-World Examples
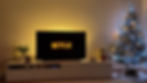
Data has become crucial for making informed decisions in today's fast-paced and ever-changing business environment. Companies use data to gain valuable insights, improve processes, and foster innovation. By studying successful examples of data-driven decision-making, we can gain valuable insights and comprehend the impact of data-driven strategies on business outcomes.
Define Data-Driven Decision Making (DDDM)
Are you tired of making business decisions based on gut instincts and guesswork? It's time to adopt Data-Driven Decision Making (DDDM). DDDM is a strategic approach that leverages collected data to inform and guide your business decisions. You can gain insights by identifying patterns and making informed choices using relevant and accurate data. "This can enhance the precision and efficiency of your decision-making procedure." allowing you to optimize outcomes, mitigate risks, and adapt more dynamically to changing circumstances in today's data-rich environment. Switch to DDDM and give your business the competitive edge it needs!
Importance of DDDM in Modern Businesses
In today's fast-paced and competitive business world, making informed and accurate decisions is more critical than ever. Data-Driven Decision Making (DDDM) is a powerful tool to help modern businesses achieve this goal. By using data and insights to inform business decisions rather than relying on guesswork, companies "Businesses that strategically position themselves to gain a competitive advantage are more likely to achieve success." With DDDM, businesses can make data-backed decisions, leading to better outcomes and tremendous success. So, if you want to stay ahead of your competition and make helpful decisions that drive success, embracing DDDM is the way to go!
Brief Overview of the Success Stories to be Discussed
Discover the success stories showcasing how businesses leverage advanced technologies to drive growth and profitability. Join me for an engaging and thought-provoking session where we will delve into the intricacies of these fascinating case studies. Your active participation will help us uncover valuable insights and unlock new perspectives that can benefit your work. "Make the most of this valuable opportunity to enhance your knowledge and skills!"
1. Netflix's Personalized Recommendations
2. Amazon's Supply Chain Optimization
3. Starbucks Location Analytics
4. American Express Fraud Detection
5. Zara's Fast Fashion Foresight.
You can benefit greatly from this unique opportunity to learn from some of the most innovative companies in the industry. Ensure you take advantage of this chance to expand your knowledge and skills!
Case Study 1: Netflix's Personalized Recommendation
Overview of netflix's challenges in content delivery.
Netflix faced challenges delivering content due to the diverse viewer preferences and vast content library. However, the company has been working hard to address these challenges and ensure users can discover content that aligns with their tastes. By doing so, Netflix aims to improve user satisfaction and retention rates.
How Netflix Used Viewer Data to Tailor Recommendations
By leveraging extensive viewer data, Netflix confidently tackled the challenge of recommending relevant content to its users. The platform thoroughly analyzed user behavior, viewing history, and preferences to create highly sophisticated algorithms. These algorithms were based on machine learning and could personalize content recommendations for each user. This approach significantly increased the likelihood of viewers engaging with content that resonated with their interests.
The Impact on Customer Retention and Satisfaction
The personalized content recommendations profoundly affected customer retention and satisfaction rates. Netflix enhanced the value of its service by providing users with content that closely matched their preferences. This created a stronger bond between users and the platform, leading to longer subscription durations and increased satisfaction.
Lessons Learned and Key Takeaways
Data is a Strategic Asset: Netflix's strategic use of data has wholly revolutionized content delivery. By utilizing excellent viewer data, they have successfully met the needs and preferences of each viewer in an incredibly effective manner.
Personalization Enhances Customer Experience: Personalized recommendations are essential to enhancing the overall customer experience. They can increase engagement, satisfaction, loyalty, and retention. Make no mistake - if you want to take your business to new heights, personalized recommendations are a must!
Continuous Adaptation is Crucial: It is crucial to adapt to achieve success, as Netflix continuously demonstrates. With the ever-evolving preferences of viewers, it is imperative to perform ongoing analysis and make necessary adjustments to algorithms to ensure that recommendations stay consistently relevant.
Balancing Privacy and Personalization: When utilizing viewer data, it is crucial to hit the right balance between personalization and privacy. Netflix has accomplished this by delivering highly personalized recommendations without compromising user privacy.
Netflix's approach to content delivery serves as an inspiration for the transformative power of data-driven decision-making. The personalized recommendations derived from customer data have proven to be a game changer regarding customer retention and satisfaction. The significance of adaptability, strategic use of data, and the balance between personalization and privacy, as highlighted by Netflix's success, can serve as a guide for other businesses looking to impact their customers positively.
Case study 2: amazon's supply chain optimization.
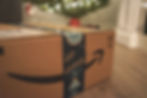
Understanding Amazon's Complex Supply Chain
Amazon has a complex supply chain involves various stages, from sourcing the products to delivering them to customers. The company manages a vast network of fulfillment centers, distribution hubs, and transportation systems. The complexity arises due to the need to manage different types of products, fluctuating demand, and the commitment to fast and efficient delivery.
Implementation of Predictive Analytics for Inventory Management
Using predictive analytics, Amazon has optimized inventory management by accurately forecasting future demand by analyzing historical data, current market trends, and seasonality. This has helped them prevent stockouts and overstock situations, improving their overall business efficiency and customer satisfaction.
Results Achieved in Cost Savings and Delivery Times
Anticipating the future, the implementation of predictive analytics is expected to yield significant results. Amazon will likely achieve cost savings by minimizing excess inventory and improving warehouse efficiency. Additionally, streamlined inventory management contributes to faster order fulfillment, which reduces delivery times and enhances the customer experience. We can expect a boost in efficiency and a better customer experience shortly.
Insights Gained and How Businesses Can Apply Similar Strategies
Data-Driven Decision-Making: In today's business landscape, data-driven decision-making has become the cornerstone of success. If you want your business to thrive, you must leverage advanced analytics to gain actionable insights into your supply chains. This will enable you to make proactive and strategic decisions to enhance your projects. Don't fall behind the competition - take charge and start leveraging the power of data-driven decision-making now.
Dynamic Inventory Optimization: Incorporating a dynamic approach to inventory management that relies on predictive analytics is "Businesses must prioritize their competitive edge and remain ahead of the industry. This is crucial to ensure success and longevity.". It helps them to quickly adjust to changing market conditions and meet the ever-evolving demands of consumers. This not only optimizes the utilization of resources but also reduces wastage, making it a sound strategy crucial for any business that wishes to survive in today's competitive market landscape.
Focus on Customer-Centric Logistics: To improve customer satisfaction, businesses can focus on optimizing logistics and reducing delivery times. Amazon's customer-centric approach demonstrates the importance of fast and reliable delivery. Companies can boost customer loyalty and drive growth by enhancing the customer experience.
Investment in Technology: To stay ahead in supply chain optimization, businesses must adopt cutting-edge technologies like AI and machine learning. Amazon's supply chain success is a testament to the power of continuous investment in technology. So, if you want to thrive in today's competitive market, it's high time you leverage these technologies to your advantage.
Amazon's journey toward optimizing its supply chain through predictive analytics has tremendously impacted cost savings and delivery times. Other businesses can achieve similar results by utilizing data-driven decision-making, implementing dynamic inventory management, prioritizing customer-centric logistics, and investing in advanced technologies.
Case study 3: starbucks location analytics.
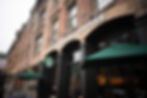
The Problem with Traditional Site Selection
The traditional approach to retail site selection, which relied on broad demographic data and market trends, must be improved in identifying optimal locations. Adopting a more precise approach that considers specific local factors influencing consumer behavior and store performance is imperative to ensure success.
How Starbucks Leveraged Geographic Information Systems (GIS)
Starbucks has transformed its approach to selecting store locations by leveraging Geographic Information Systems (GIS). This innovative technology has enabled Starbucks to systematically evaluate and visualize location-specific data, such as foot traffic patterns, nearby businesses, demographics, and local economic factors. By conducting this comprehensive analysis, Starbucks can gain a more nuanced understanding of potential store locations and make informed decisions.
Outcomes in Terms of New Store Performance and Sales
Starbucks, the renowned coffeehouse chain, has achieved notable success in its site selection strategy by implementing a Geographic Information System (GIS). GIS technology has enabled Starbucks to strategically place its new stores in locations that cater to the preferences and traffic patterns of the local population. As a result, the company has witnessed a significant improvement in the performance of its new stores, surpassing the sales of those selected through traditional methods. The successful implementation of GIS in site selection has contributed to optimizing location decisions, leading to a more efficient and effective expansion strategy for Starbucks.
Broader Implications for Retail Location Decision-Making
Precision in Site Selection: Geographic Information System (GIS) technology has opened new doors for retailers to make informed decisions. By leveraging GIS, businesses can analyze and interpret specific geographic data to optimize their site selection process. This helps them understand local nuances and customer behavior more precisely, allowing them to make data-driven decisions that lead to better business outcomes.
Adaptability to Local Factors: To establish a closer relationship with their customers, retailers must consider various local factors such as competition, demographics, and cultural preferences. By doing so, they can customize their offerings and marketing strategies to fit the local communities' specific needs and preferences. This approach can lead to better customer engagement and loyalty and, ultimately, higher sales for the retailer.
Cost Efficiency: Regarding retail businesses, selecting the right location for a store is crucial for success. Optimal site selection can significantly reduce the risk of underperforming stores and minimize the financial impact of poor location decisions. Retail businesses can enhance their overall cost efficiency and profitability by doing so. This is why it is essential for companies to carefully analyze and consider factors before making any site selection decisions.
Strategic Expansion: Geographic Information System (GIS) provides retailers with a powerful tool to make informed decisions about expanding their business. By leveraging location-based data, retailers can discover new markets and potential locations for growth. This data-driven approach helps retailers create a more sustainable and prosperous expansion plan, resulting in long-term prosperity for the business.
Enhanced Customer Experience: Retailers can improve the shopping experience for their customers by strategically selecting store locations that cater to their preferences and habits. Retailers can attract more foot traffic and enhance customer satisfaction by offering conveniently located stores. In this case, it can increase sales and customer loyalty.
To make it more understandable, Starbucks has been using fantastic GIS technology to help them pick the best locations for their stores. It's like a digital map that allows them to look at much different information, like how many people live nearby, how much traffic there is, and what other businesses are in the area. By using this technology, Starbucks can make better choices about where to put their stores and how they can be successful. Other businesses can also use GIS to make better decisions about where to open new stores and how to compete in the changing world of retail.
Case study 4: american express fraud detection.
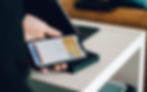
Rise in Credit Card Fraud and the Challenge for Card Issuers
As more and more people turn to digital transactions and online commerce, it's essential to be aware of the increased incidence of credit card fraud. Protect yourself and others from financial crimes by staying informed and proactively safeguarding your financial information. Thus, posing a significant challenge for card issuers. Fraudsters are constantly devising new and innovative tactics to steal sensitive information and exploit vulnerabilities in payment systems. This makes it imperative for financial institutions to stay ahead of the game in detecting and preventing fraudulent activities. Today, with advanced technologies like machine learning and artificial intelligence, card issuers can analyze big data that can identify patterns and anomalies and indicate fraudulent behavior. By adopting a proactive approach to fraud detection and prevention, financial institutions can safeguard their customers' personal information and financial assets, thus building trust and loyalty among their clients.
American Express's Use of Machine Learning for Early Detection
American Express always prioritizes the security of its customers' financial transactions. The company has employed advanced machine-learning algorithms to analyze vast amounts of real-time transaction data to achieve this. These algorithms can identify patterns, anomalies, and behavioral indicators typically associated with fraudulent activities. By continuously learning from new data, the system adapts to evolving fraud tactics and enhances its ability to detect irregularities early on. This advanced technology is a critical component of American Express's fraud prevention strategy, and it helps the company safeguard its customers against potential financial losses.
Effectiveness in Preventing Fraud and Protecting Customers
American Express utilizes an advanced fraud detection system powered by machine learning that has demonstrated exceptional efficacy in preventing fraudulent activities and safeguarding customers. By detecting fraudulent transactions early, the company can promptly take necessary measures, such as notifying customers of suspicious activities or blocking them altogether, thus reinforcing trust in the company's commitment to security and ensuring customer satisfaction.
What Companies Can Learn About Proactive Data Monitoring
Invest in Advanced Analytics: Advanced analytics, such as machine learning, helps companies proactively monitor data for potential fraud indicators and unusual patterns. It identifies issues before they become significant problems, saving the company millions. It also identifies new business opportunities, market trends, and operational inefficiencies, enhancing customer satisfaction and the bottom line.
Real-Time Analysis: Real-time data analysis is a powerful tool for detecting and responding to suspicious activities. By monitoring data in real-time, organizations can quickly "Identify possible threats and take prompt action to reduce their impact." we can overcome any challenges that come our way and pave the path to success. This approach reduces the window of vulnerability and enhances the effectiveness of fraud prevention measures. Therefore, real-time data analysis can help organizations prevent fraudsters from exploiting them. It's important to stay vigilant and proactive in protecting yourself and your finances from potential threats. Please take action now and don't give them the chance to cause any harm. Remember, it's better to be safe than sorry. their interests.
Continuous Learning Systems: Adopting systems that can learn and adapt to new fraud patterns is highly recommended. This approach ensures that the monitoring mechanisms remain up-to-date and effective despite the constantly evolving threats. Embracing such systems can protect businesses. The objective is to safeguard individuals and organizations against financial losses and reputational harm resulting from fraudulent activities.
Customer Communication: Implementing solid and effective communication methods is essential to inform customers of any potential fraud promptly. Through transparent communication, customers can be informed of the situation and take immediate action, building trust between them and the organization.
Collaboration with Industry Partners: Collaborating with industry partners and sharing insights on emerging fraud trends is essential. By working together, we can enhance our ability to combat fraud and protect the entire ecosystem. We can stay informed and better equipped to prevent fraudulent activities through a collective effort.
Balancing Security and User Experience: It's crucial to balance strong security measures with a seamless user experience for online platform security. While taking all necessary steps to prevent fraud and unauthorized access to your system is critical, ensuring that your legitimate customers don't face any inconvenience or dissatisfaction due to stringent security protocols is equally essential. Therefore, adopting a multi-layered approach to security is recommended to shield your system from potential threats without making the user experience cumbersome or frustrating. This may involve utilizing two-factor and risk-based authentication and real-time fraud detection. Furthermore, educating users on secure online practices and equipping them with the necessary tools and resources to protect their personal information and transactions is essential.
American Express has implemented machine learning techniques to detect and prevent fraud at an early stage. This is an excellent example for businesses seeking to improve their proactive data monitoring capabilities. By adopting advanced analytical tools, real-time analysis, continuous learning systems, and effective communication, companies can establish a solid and proactive strategy to combat emerging threats in the digital realm.
Case study 5: zara's fast fashion foresight.
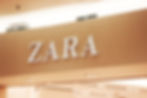
Fast Fashion Industry Challenges in Demand Forecasting
The fast fashion industry, known for producing trendy and affordable clothing rapidly, faces significant challenges in accurately predicting consumer demand. One of the main reasons for this is the constantly changing nature of fashion trends. What is popular today may be out of fashion tomorrow, making it difficult for companies to plan their production processes effectively.
Additionally, fast fashion products have short life cycles, meaning they are only in style for a limited time. As a result, companies need to be able to respond to market shifts and adjust their production accordingly quickly. This can be challenging, as traditional forecasting methods rely on historical data, which may need to be more relevant in a fast-changing market.
To overcome these challenges, the fast fashion industry needs innovative and agile forecasting methods to keep up with the dynamic nature of consumer preferences. This may involve leveraging data analytics and machine learning algorithms to identify emerging trends and predict future demand. Companies can enhance efficiency, reduce waste, and provide excellent customer value.
Zara's Integration of Real-Time Sales Data into Production Decisions
Zara, one of the world's leading fashion retailers, has redefined the fashion industry by leveraging real-time sales data. Zara has integrated real-time sales data into its production decisions, allowing the company to stay ahead of the competition. Zara's vertically integrated supply chain and responsive production model enable it to capture up-to-the-minute sales data from its stores worldwide. This data is then fed back to the design and production teams, who use it to rapidly adjust inventory levels and introduce new designs based on current demand trends. Using real-time sales data, Zara can create a customer-centric approach, ensuring its customers always have access to the latest and most stylish designs.
Benefits Seen in Reduced Waste and Increased Sales
Zara has adopted a real-time analytics approach that has proven to be highly beneficial. The company's production is now closely aligned with actual customer demand, which results in a significant reduction in overstock and markdowns. This approach has minimized the environmental impact of excessive inventory. In addition, the quick response to emerging trends and consumer preferences has led to an increase in full-price sales, boosting revenue and profitability for Zara.
Strategies for Incorporating Real-Time Analytics into Product Development
Connected Supply Chain: Establishing a connected and transparent supply chain that enables seamless real-time data flow from sales channels to production and design teams is imperative. This will ensure that all the teams are on the same page and can make quick, informed decisions based on accurate, up-to-date information. Failure to do so can result in costly delays, inefficiencies, and missed opportunities. So, let's prioritize this and set up a robust supply chain that works for us!
Agile Production Processes: To maintain a competitive edge, it is crucial to adopt constructive and flexible production processes that can quickly respond to changes in demand. This involves embracing shorter production cycles and smaller batch sizes, which makes us more efficient and proactive in meeting customer needs.
Advanced Data Analytics: To optimize your sales strategies, you can use advanced data analytics tools to process and analyze real-time sales data efficiently. You can accurately forecast demand and make data-driven decisions by implementing predictive modeling and machine learning algorithms.
Cross-Functional Collaboration: Promoting collaboration among different organizational departments is crucial to ensure the sales, marketing, design, and production teams have a unified interpretation of real-time data. This way, they can collectively make informed decisions that are in the company's best interest. The organization can improve its efficiency, productivity, and profitability by promoting open communication and collaboration between departments.
Customer Feedback Integration: One way to enhance the accuracy of real-time analytics is to consider customer feedback and preferences. Social media listening and direct customer interactions can provide valuable insights into emerging trends and demands.
Technology Integration: As we look towards the future, it's becoming increasingly clear that investing in technologies that facilitate real-time data collection and processing will be crucial. With the rise of automation and the growing need for instant information, businesses that have point-of-sale systems, inventory management software, and communication tools that streamline information flow will be better equipped to thrive in the fast-paced and ever-changing world of tomorrow. So, it's never too soon to start thinking about how you can integrate these technologies into your business strategy.
Zara's outstanding achievement in the fast fashion industry is a remarkable example of how incorporating real-time sales data into production decisions can lead to immense success. By reducing waste, swiftly responding to market trends, and utilizing advanced analytics, Zara has set a benchmark for other companies in integrating real-time insights into their product development strategies. This approach enhances efficiency and competitiveness in the highly dynamic and ever-evolving fashion industry.
In the constantly shifting business realm, the adage' knowledge is power' has never been more accurate, particularly about tangible, data-derived knowledge. data-driven decision making (dddm) presents an approach where critical business decisions are made not on intuition or experience alone, but on deep dives into data analysis. empirical evidence garnered through this method provides actionable insights leading to strategic, evidence-based decisions..
The stories of Netflix, Amazon, Starbucks, American Express, and Zara demonstrate the immense potential of Data-Driven Decision Making (DDDM). By analyzing vast data points and leveraging advanced analytics, these companies transformed their businesses and achieved unparalleled success.
For instance, Netflix utilized DDDM to create tailor-made recommendations, engaging existing users and attracting new ones. Amazon used data analytics to optimize its supply chain, lowering costs and accelerating shipping times. Starbucks leveraged location analytics to predict the profitability of new store locations with impressive accuracy. American Express used machine learning algorithms to identify frauds faster than ever, saving millions in potential losses. Lastly, Zara demonstrated agility in the competitive fast fashion market by adapting its production and supply chain to meet real-time demand.
As seen in these success stories, data-driven decision-making can powerfully impact business, from customer engagement to trend forecasting. They underscore the importance of meticulous data analysis in navigating the present and forecasting an ever-changing future, paving the way for unparalleled business success. Companies can draw inspiration from these cases and embark on their DDDM journey to achieve similar outcomes.
Recent Posts
Maximizing the potential of WFM in the Gig Economy: Achieving the perfect balance between Flexibility and Stability through Case Studies.
WFM in the Gig Economy: Balancing Flexibility and Stability
Data-Driven Decision Making: Utilizing Analytics in Workforce Management

- SUGGESTED TOPICS
- The Magazine
- Newsletters
- Managing Yourself
- Managing Teams
- Work-life Balance
- The Big Idea
- Data & Visuals
- Reading Lists
- Case Selections
- HBR Learning
- Topic Feeds
- Account Settings
- Email Preferences
Data management
- Technology and analytics
- Analytics and data science
- Performance indicators

10 Steps to Creating a Data-Driven Culture
- David Waller
- February 06, 2020

Why It's So Hard for Users to Control Their Data
- Bhaskar Chakravorti
- January 30, 2020

Do You Care About Privacy as Much as Your Customers Do?
- Thomas C. Redman
- Robert M Waitman
- January 28, 2020

How Large Language Models Reflect Human Judgment
- Ajay Agrawal
- Joshua Gans
- Avi Goldfarb
- June 12, 2023

What Makes the Best Infographics So Convincing
- Andrea Ovans
- April 22, 2014

GenAI Can Help Small Companies Level the Playing Field
- Oguz A Acar
- Andrés Gvirtz
- February 01, 2024

AI Regulation Is Coming
- François Candelon
- Rodolphe Charme di Carlo
- Midas De Bondt
- Theodoros Evgeniou
- From the September–October 2021 Issue

The Real Revolution in Online Education Isn't MOOCs
- Michelle Weise
- October 17, 2014

How Tech Is Transforming Entry-Level, Customer-Facing Jobs
- Emily Potosky
- Melissa Fletcher
- Christopher Sladdin
- May 01, 2023
3 Things Are Holding Back Your Analytics, and Technology Isn't One of Them
- Dan Wiesenfeld
- June 08, 2017

Is Your Company’s Data Ready for Generative AI?
- Thomas H. Davenport
- Priyanka Tiwari
- March 26, 2024

Are You Ready for the Quantum Computing Revolution?
- Shohini Ghose
- September 17, 2020

How to Tell If Machine Learning Can Solve Your Business Problem
- Anastassia Fedyk
- November 25, 2016

Is Your Data Infrastructure Ready for AI?
- Seth Earley
- Josh Bernoff
- April 28, 2020

What the Companies on the Right Side of the Digital Business Divide Have in Common
- Marco Iansiti
- Karim R. Lakhani
- Robert Bock
- January 31, 2017

How to Make Your Company Machine Learning Ready
- James Hodson
- November 07, 2016

AI Adoption Skyrocketed Over the Last 18 Months
- Joe McKendrick
- September 27, 2021

Why We Need to Audit Algorithms
- James Guszcza
- Iyad Rahwan
- Manuel Cebrian
- November 28, 2018

Are You Still Prioritizing Intuition Over Data?
- Tomas Chamorro-Premuzic PhD.
- February 27, 2020

- Leslie K. John
- October 05, 2018

Strategic Analytics: Tools for Preparing Your Team for the Future
- Harvard Business Review
- Eric Siegel
- Edward L. Glaeser
- Cassie Kozyrkov
- May 07, 2020

Smart City Strategy: Amsterdam, Barcelona, and Atlanta
- Alexandra Houghtalin
- March 14, 2018

The Year in Tech, 2024: The Insights You Need from Harvard Business Review
- David De Cremer
- Richard Florida
- Ethan Mollick
- Nita A. Farahany
- October 24, 2023

Blockchain: Tools for Preparing Your Team for the Future
- Catherine Tucker
- Don Tapscott
- October 15, 2019

HBR's Year in Business and Technology: 2021 (2 Books)
- October 20, 2020

Radically Human: How New Technology Is Transforming Business and Shaping Our Future
- Paul Daugherty
- H. James Wilson
- April 26, 2022

Big Data at Work: Dispelling the Myths, Uncovering the Opportunities
- February 25, 2014
Managing with Analytics at Procter & Gamble
- Alain Serels
- April 03, 2013
Tabular Labs 2018
- William Barnett
- Adam Markovitz
- April 28, 2018
Learning the Machine: Anovo Ibérica Introduces AI in Operations
- Javier Zamora
- Josep Valor Sabatier
- Isaac Sastre Boquet
- July 20, 2021

Human + Machine, Updated and Expanded: Reimagining Work in the Age of AI
- September 10, 2024
TradeIX: Blockchain-Enabled Trade Finance in Global Supply Chains
- Erik Hofmann
- Maximilian Enthoven
- Sara Fallegger
- August 11, 2020
TD Bank Group: Building an Effective Enterprise Data Management Policy
- Murat Kristal
- Glenda Crisp
- Connie Bonello
- Katherine Heighington
Oakland A's (A)
- Phillip E. Pfeifer
- John L. Colley
- April 05, 1991
Verisk: Trailblazing in the Big Data Jungle
- Andrew Wasynczuk
- Francesca Gino
- Karim Sameh
- September 05, 2018
From Theme Park To Resort: Customer Information Management At Port Aventura
- Mariano A Hervas
- Marc Planell
- Xavier Sala
- July 05, 2011

The Year in Tech, 2023: The Insights You Need from Harvard Business Review
- Beena Ammanath
- Michael Luca
- Bhaskar Ghosh
- October 25, 2022
Artea: Designing Targeting Strategies
- Eva Ascarza
- Ayelet Israeli
- September 25, 2020
Ubiquitous Surveillance (B)
- Mary Gentile
- David Danks
- Maralee Harrell
- July 07, 2022
Carnival Cruise Lines
- Lynda M. Applegate
- Robert J. Kwortnik
- Gabriele Piccoli
- July 07, 2005

Harvard Business Review, March/April 2024
- March 01, 2024
Popular Topics
Partner center.

IMAGES
VIDEO
COMMENTS
Real-world data science case studies play a crucial role in helping companies make informed decisions. By analyzing their own data, businesses gain valuable insights into customer behavior, market trends, and operational efficiencies.
Case studies & examples. Articles, use cases, and proof points describing projects undertaken by data managers and data practitioners across the federal government.
It’s the story of a “management revolution,” brought about by the widespread adoption of big data and analytics in both the public and private sectors. 1 In these dispatches from the front lines of that revolution, we see four strikingly dissimilar organizations — a health care system, a bank, a major industrial company, and a municipal governme...
Top 25 Data Science Case Studies [2024] Case Study 1 – Personalized Marketing (Amazon) Challenge: Amazon aimed to enhance user engagement by tailoring product recommendations to individual preferences, requiring the real-time processing of vast data volumes.
Data-Driven Decision-Making Case Studies: Insights from Real-World Examples. Netflix. Data has become crucial for making informed decisions in today's fast-paced and ever-changing business environment. Companies use data to gain valuable insights, improve processes, and foster innovation.
Collect data and build trust. With the rise of data science and machine learning, companies are awash in customer data and powerful new ways to gain insight...