- Original Research Article
- Open access
- Published: 17 August 2020

Wind turbine performance analysis for energy cost minimization
- Yassine Charabi ORCID: orcid.org/0000-0003-2054-688X 1 &
- Sabah Abdul-Wahab 2
Renewables: Wind, Water, and Solar volume 7 , Article number: 5 ( 2020 ) Cite this article
24 Citations
3 Altmetric
Metrics details
A Correction to this article was published on 17 January 2021
This article has been updated
The use of wind energy worldwide has overgrown in recent years to reduce greenhouse gas emissions. Wind power is free, but the installation and maintenance of wind turbines remain very costly. The size of the installation of the wind turbine is not only determined by wind statistics at a given location, but also by turbine infrastructure and maintenance costs. The payback time of the turbine is dependent on turbine energy costs. This study estimates the wind power generation capacity of Northern and Southern Oman and discusses the selection of the most economical, efficient and reliable wind turbines in Oman. HOMER Pro Software was used in this paper to evaluate the wind energy data in the north and south of Oman and to provide well-informed guidance on the most suitable turbines for the power needs of each area. Six different standard wind turbines were measured and compared in terms of the cost of energy and performance. The simulation analysis reveals that the DW54 turbine is the best possible turbine to generate electricity in northern Oman at $0.119/kW. Due to the difference in the wind regime between the north and the south of Oman, the simulation showed that the Hummer H25.0–200 kW turbine is the best option for south Oman with power generation at $0.070/kW. The northern wind turbine plant can efficiently contribute to decarbonization of the energy sector in Oman, with a potential reduction of CO 2 emission approximately 19,000 tons/year in comparison to natural gas and 28,000 tons/year in comparison to diesel. In the Southern Power Plant, carbon emissions are reduced by 18,000 and 12,000 tons/year compared to diesel and natural gas.
Introduction
The rise in global temperature and severe climate change worldwide has increased environmental concerns. Nowadays, more than 90% of the world’s electricity comes from fossil fuels (World-Bank 2015 ), and that energy production plays a vital role in global warming. Any changes in this field can have a significant impact on the environment. Numerous researchers, therefore, have attempted to change or alleviate the negative impacts of global warming, with much of this effort coming from the energy sector (Ghodsi et al. 2019 ; Khare et al. 2016 ; Sahu et al. 2018 ). In comparison to fossil fuels, the impact of renewable energy sources on the environment is negligible. These sources, for example, have no direct CO 2 or NOx emissions. From solar panels to wind turbine generators, a wide range of devices can convert ambient energy into a more useful form, like electricity (Charabi et al. 2019 ). Among these devices, wind turbines are some of the most popular and accessible methods of converting ambient energy to electricity (Yang et al. 2018 ). However, wind energy, like most other sources of renewable energy, has high capital costs, but during the past decade, this trend has changed tremendously. Statics show that the cost of wind production has dropped enormously in recent years, from two million dollars per M.W. to one million in the last decade (Moné 2017 ). This achievement has made it possible to see wind power plants with increasing frequency in both developed and developing countries (Sahu 2018 ).
As a Middle Eastern, oil-dependent country, Oman has started in a new direction on its path of development. The country is trying to change its electricity production industry from one that is entirely oil-based to one that is more reliant on sustainable “greener” energy sources (Abdul-Wahab et al. 2019a ; Al-Suleiman et al. 2019 ). The main two options for this plan are solar and wind energy. Although Oman’s sunny weather provides a unique opportunity for solar energy generation, the country’s wind power potential must not be neglected. As of this article’s writing, Oman has no industrial wind power stations, and the country’s wind turbines are mainly used for research purposes. However, this situation is changing, beginning with developing an understanding of the country’s wind power potential. An incorrect estimation of wind energy needs or the use of low-performance equipment not only reduces the benefits of the project, but also might lead to economic disaster (Dolatabadi et al. 2017 ).
Over the last decade, considerable information on wind resource mapping across Oman has been accumulated to stimulate the deployment of wind power (Al-Yayai and Charabi 2015 ; Al-Yahyai et al. 2012 , 2013 ; Charabi et al. 2011 ; Al Yahyai el al. 2010). Despite the availability of wind mapping information, the deployment of wind energy across Oman is still lagging due to the lack of accurate information on turbine energy cost. Without access to sound information on the cost of wind power technology, it is difficult for decision-makers, if not impossible; to evaluate which wind turbine technologies will most fit their national circumstances. The fast growth and cost reductions in the installed wind energy technologies mean that even data aged one or 2 years will substantially overestimate the cost of power from wind energy technologies. There is also a significant amount of perceived knowledge about the cost and performance of wind power generation technologies that are not accurate or is misleading. Significant knowledge of the cost and performance of wind generation technologies is also viewed that is not right or misleading. This paper fills a significant information gap because there is a lack of precise, comparable, and the latest data on the costs and performance of wind turbines in Oman.
Studies on the viability and economic potential of wind energy have recently spread worldwide.
Kumar and Gaddada ( 2015 ) have explored the outputs of four statistical methods to evaluate Weibull parameters for wind energy applications in four selected sites, located in northern Ethiopia. Gaddada and Kodicherla ( 2016 ) have evaluated wind power capacity and wind energy cost estimates for electricity generation systems in eight selected locations in Tigray (Ethiopia). Kodicherla et al. ( 2017 ) explored the potential of wind energy and developed an economic assessment of the water pumping system in various wind power conversion systems. In three selected Fiji Island stations, Kodicherla et al. ( 2018 ) have investigated the potential of wind power-assisted wind hydrogen production using different types of turbines. The literature also reflects different foci around wind turbines. Many researchers have worked on defining the shape and structure of wind turbines and their effects on aerodynamics (Cai 2019 ; Nema et al. 2009 ; Akpinar and Akpinar 2006 ). Others have tried to improve the performance of current turbines by optimizing placement and hub height (Abdul-Wahab et al. 2019b ; Elkinton et al. 2008 ).
Despite these efforts, the stochastic nature of wind speed makes wind energy generation difficult for some places (Padrón et al. 2019 ). A deep understanding of the specifications of each wind turbine and complete statistical data on wind velocity in any given location can begin to address this problem. These data must be processed and matched to a potential turbine to give a realistic and feasible answer to the suitability of any given piece of wind power equipment. In this paper, HOMER Pro software (HOMER Energy L.L.C., Boulder, Colorado, U.S.A.) was used to analyze wind data for the north and south of Oman and make a well-informed recommendation on the most suitable turbines for each region’s power needs. HOMER Pro software can combine data associated with wind regime, the specifications of wind turbines, and the power demands of consumers to estimate the cost of producing energy using different generators.
In this study, the researchers tried to estimate the potential for wind energy production in Oman’s north and south and suggest the feasibility of using wind turbines in the country. To this end, the performance of six different popular wind turbines was calculated and compared. By considering the performance and cost of energy (C.O.E.), suggestions on the best possible turbines for the north and south of Oman are provided.
Study areas
As has been mentioned previously, two locations were selected for the wind power plants. The northern site was located in Al Batinah North Governorate (24° 42′ 23″ N 56° 28′ 48″ E). The southern site was Mirbat, Dhofar Governorate (16° 58′ 22″ N 54° 42′ 56″ E) (Fig. 1 ). Both plants are located in rural areas with low populations and, therefore, low power demands. Population, power consumption per capita and power consumption patterns change power demands in an area. Demand also changes daily, hourly, and even in the summer and winter. The last reported data from Oman show that each Omani annually consumes around 6550 kWh on average (S.A.O.C 2017 ). Based on this information and the population of the area, the size of the wind power plant is considered at 10 MW. This size can cover current electricity consumption and any possible future growth. Even with a highly accurate prediction, real conditions can have unexpected variations. In order to consider this variation, the monthly 2% day-to-day random variability and 2% time-to-time step of random variability was considered. Figure 2 shows the power consumption patterns in Oman’s households. As can be seen in the figure, April to October is Oman’s summer season and has high electricity demand, while in wintertime, November to March, the power demand decreases significantly. The high demand for energy by cooling systems in the long summer of Oman is the main reason for this trend.
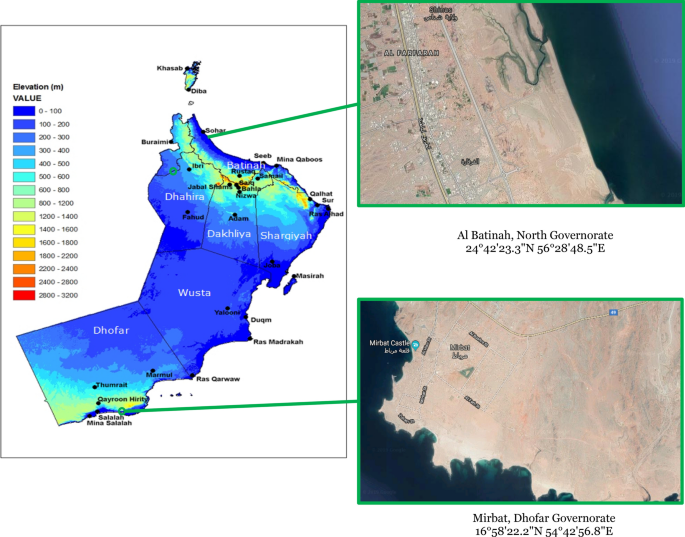
The location of the wind farms in north and south of Oman
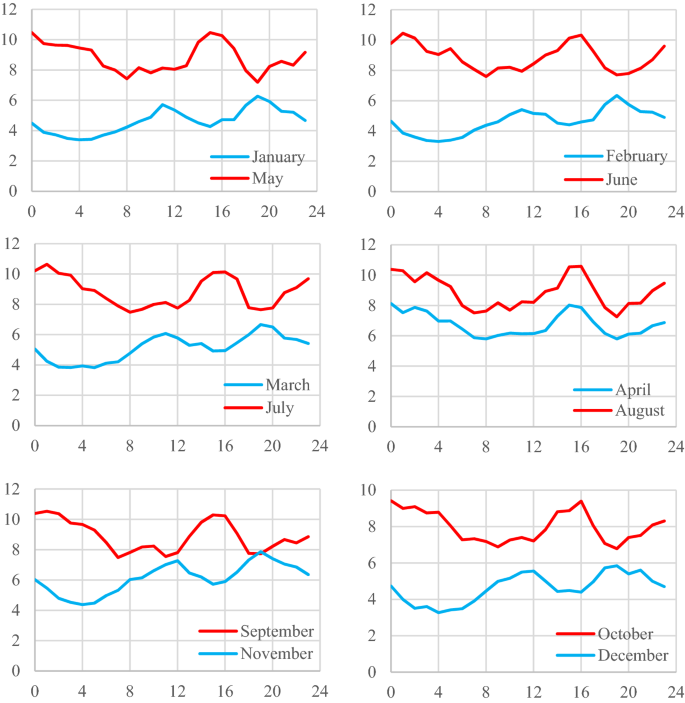
Monthly average of power demand (MW)
HOMER software
Wind turbine performance analysis.
A realistic estimation of power production requires accurate statistical data on wind velocity for an extended period, like a year or more, if possible. The accuracy of the output results entirely depends on the accuracy of this information. Wind velocity is usually measured on an hourly basis. Due to the high number of measurements in a calendar year, however, further processing for such an extended period would be time-consuming and difficult. Therefore, when making calculations based on such large data sets, the average wind velocity is usually used to reduce the processing load. Although using the monthly average seems practical, such a simple average can be misleading. For instance, by using a wind velocity of 0 m/s for 50% of the time and using a velocity of 6 m/s for the rest of the time, the simple average of the wind velocity would be 3 m/s.
Considering a wind turbine with a maximum output power of 3 m/s, the output performance would be wrongly calculated at 100% all day long. Such a system would have 100% output at 50% of the time at best. In order to address such miscalculations, in this research, the two-parameter Weibull distribution was used (Wang et al. 2018 ). In this method, both wind velocity and its probability over time are considered, and the distribution of the wind velocity is used for the following calculations (Moein et al. 2018 ). The probability density (f) and cumulative distribution (F) of the wind based on Weibull distribution are:
where c is the Weibull scale (m/s), and k is the Weibull shape factor.
The different wind turbines on the market have very different specifications. Considering and analyzing all of these turbines in this paper is not possible. Six of the most popular turbines on the market were selected and analyzed in order to make the article descriptive, rational, and practical. In some countries, other brands and models of turbines might be more popular, but the present approach can be used in those countries, too. In making this comparison, the C.O.E. production for each turbine must be calculated and compared carefully. Moreover, the whole system of a wind power plant consisting of one or more turbines must be able to handle the load demand of consumers with no or limited access to the main power line, for such a scenario where there is no access to the power grid, the power generation system has to be equipped with a sufficiently sized battery bank or a fossil fuel generator to cover non-windy hours or days. In order to simplify the problem and eliminate the calculation of fossil fuel generators, the system under consideration was conceptualized as having up to a 10% deficiency in a limited number of days. In real conditions, this amount of energy can be obtained from the main power lines (if accessible) or local generators. However, in this article, further calculations based on these generators were not considered.
Wind speed calculations represent the first phase of the HOMER Pro simulation. The wind velocity was measured and recorded every hour for 1 year. The system measured wind speed at a 10-m height above the sea level, which is the standard height for the measurement. Table 1 shows a sample of the measurements from the northern site for 1 week. For the calculation of the velocity at a different height (based on the height of each wind turbine), the measured values must be modified as in Eq. ( 3 ):
where \(V_{\text{Turbine}}\) and \(V\) show the wind velocity at the turbine and standard anemometer height, \(Z_{\text{Turbine}}\) and \(Z_{\text{anm}}\) are the height of the turbine and the anemometer (m) and \(Z_{0}\) is the surface roughness (m). Surface roughness characterizes the roughness of the field around the turbine. In this project, based on the local properties of the site location, \(Z_{0}\) was considered 0.03 m, which indicates a smooth field with some crops and no trees or buildings in the surrounding area (Homer-Energy 2016 ).
By combining the Weibull equation and Eq. ( 3 ), the average wind velocity can be written as:
And the output power in a wind turbine can be written in the form:
where \(\tau\) is the time, \(C_{p}\) is the turbine’s nominal capacity, and \(f_{v}\) is the wind velocity distribution.
The producers also provide the power curve of each turbine by testing different wind velocities. The power curve shows the real output power of the system in different ranges of wind velocity. Figure 3 shows the power curves of the six selected turbines with data extracted from the producers’ datasheet for the following turbine models:
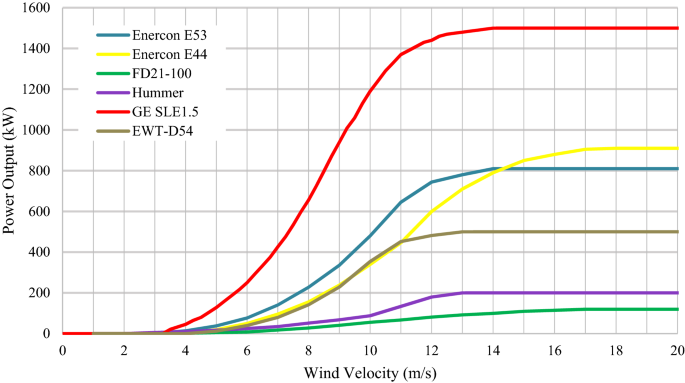
The power curves of the selected turbines
GE 1.5 SLE (GE Power, Schenectady, New York, USA).
Enercon E44 (Enercon, Aurich, Germany).
Enercon E53 (Enercon, Aurich, Germany).
FD21-100 (Enercon, Aurich, Germany).
EWT DW54 (Emergya Wind Turbines Pvt. Ltd., Amersfoort, The Netherlands);
Hummer H25.0–200 kW (Anhui Hummer Dynamo Co., Ltd., Hefei, Anhui, People’s Republic of China).
Economic analysis
In project planning, economic analysis is the most critical factor in decision-making. In this study, an economic analysis was the only indicator considered to show the feasibility of wind projects. Economic feasibility incorporates long-term performance, pointing to the best possible option among the wind turbines. In order to make an accurate estimation of economic feasibility, the total cost of the project must be calculated, including the capital cost (initial cost of the construction and devices), replacement cost as necessary, and maintenance costs. Operation costs should also be considered for the whole project. However, due to the low cost of operation in wind turbines, the operation cost can be considered part of maintenance costs. By accurately estimating these costs, the price of power generation per kW can be estimated. This price is a suitable indicator for choosing the best possible turbine for a wind power plant. In this research, the cost of energy (C.O.E.) per kW was the distinguishing feature considered among the turbines studied. HOMER sensitivity and optimization algorithms were used to select the best wind turbine (Pahlavan et al. 2018 ; Vahdatpour et al. 2017 ). The equations of the method of optimal system measuring, which has a minimum amount of total net present cost (N.P.C.), are as follows:
where C ann,total , C.R.F. i and R proj are the total annual cost, cost recovery factor, real interest rate and lifetime of the project, respectively.
All costs and incomes are evaluated at a constant interest rate over the year. The actual interest rate resulting from inflation is calculated and the effect of the change in interest rate on final N.P.C. is applied to purpose of influencing inflation in calculations. The cost recovery factor (C.R.F.), which indicates the cost recovery over the N years, is calculated as follows:
Software is able to calculate the annual interest rate through the following equation:
Also, the cost of per kW of energy during the lifetime of the project is obtained by software from the following equation:
In the above equation, E Load served is the real electric load in the hybrid system by unit kW/year.
Table 2 shows all costs associated with the selected turbines and which include:
The Capital cost is the initial purchase price,
The Replacement cost is the cost of replacing the generator at the end of its lifetime, the O&M cost is the annual cost of operating and maintaining the generator.
No energy battery storage system storage was taken into consideration for the current simulation focusing on the selection of the best wind turbine, and an annual interest rate of 6% was taken into account.
Results and discussion
Comparison between the proposed wind turbines.
Implementing big data associated with turbine measurements and specifications is difficult. HOMER Pro helps analyze this data and simulate plans for 20 years. The results of the simulation for each turbine are presented in Table 3 .
The main findings from the turbines simulation were as follows:
G.E. Energy 1.5 SLE This turbine is designed and manufactured by G.E. Power, a subsidiary of the General Electric Energy Company, and is a 1500-kW-rated power producer. This model has the highest power output among the selected turbines. It has a three-blade rotor with a 77-m diameter and 85-m hub height. The cut-in wind velocity for this model is 3 m/s, and the cut-off speed is 25 m/s. Cut-in and cut-off velocities can have a significant impact on the performance of the turbine. A turbine with a lower cut-off speed has the advantage of generating power in lower wind speed locations, like the north of Oman. The results of the simulation show that the C.O.E. for this turbine is USD$0.171 for each kW of energy in the north and USD$0.089 in the south. This cost contains the USD$1.75 million dollar maintenance cost for 20 years of operation and a capital cost of USD$3.38 million.
Enercon E44 This turbine, produced in Germany, has the second-highest power output of those considered, with a 900-kW-rated generator, 55-m hub height, and 44-m blade size. This Enercon production has a minimum cut-off wind velocity of 3 m/s, and a 28 m/s maximum cut-off. The HOMER Pro results showed that, by considering the capital cost of USD$2.34m and a maintenance cost of around USD$1 million, the C.O.E. would be USD$0.303 for each kW of energy in the north and USD$0.135/kW in the south.
Enercon E53 This turbine has a 53-m rotor diameter and 800 kW power production potential. Due to the lower power output, this model has lower capital and maintenance costs. Considering all of the costs of the turbine, the system would be able to generate power at USD$0.163/kW and USD$0.088/kW in the north and south, respectively.
FD21 - 100 This Enercon model uses GHREPOWER production with 100-kW output power. The lower output power makes it suitable for smaller wind power plants. FD21-100 has a 3–25 m/s range of working speed, and its highest possible hub height is 42 m. The HOMER Pro software simulation for this turbine showed that the C.O.E. would reach up to USD$0.290 per kW in the north and USD$0.144 kW in the south. In comparison to other turbines, this model has the highest cost of power generation for both locations.
DW54 This turbine is a 500-kW generator designed and produced by Energy Wind Technology (E.W.T.) in Amersfoort, The Netherlands. It has a 54-m rotor diameter and a working velocity between 3 and 10 m/s. With a USD$1.2 million capital cost and USD$750,000 maintenance cost over 20 years, the power generation cost would be USD$0.119/kW. This cost is the lowest possible for generating power in the north of Oman. However, the simulation showed that, due to differences in the wind regime in the north and south, this model is not the best possible option for the south. Each kW of energy produced in the south would cost USD$0.071. However, with its C.O.E., this model is the second best possible turbine for Oman’s north.
Hummer H25.0 – 200 K.W. This model is a 200-kW-rated wind turbine produced by the Anhui Hummer Dynamo Company of Hefei, China. In comparison to other analyzed turbines, this model has a lower cut-in wind velocity by 2.5 m/s and a smaller blade size (12 m). The simulation showed that while the capital cost of the turbine could be as low as USD$300,000, this model’s C.O.E. is not the best for all situations. In the north, power production would cost USD$0.132/kW. While this price is not the best possible option for the north, the results for the south are different. The simulation showed that the turbine would have the best possible results in the south among the selected models, generating power at USD$0.070/kW.
Considering the above-mentioned findings, the DW54 turbine is the best possible turbine for the north of Oman. On the other hand, the Hummer H25.0–200 KW turbine is the best option for Oman’s south. These models can generate electricity at the lowest possible cost. Figure 4 shows the graph of energy production cost for each turbine in the northern and southern sites.
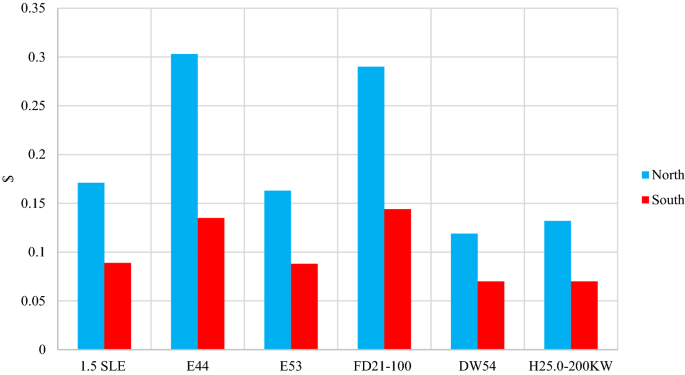
Cost of electricity for different turbines
Advantages of provided wind turbines over natural gas and diesel generators
The current power plants in Oman mostly use natural gas for electricity production. On the other hand, for off-grid consumers (some rural regions), the diesel generators are the primary source of electricity. It is clear that fossil fuel generators emit pollutant gases into the atmosphere and have negative impacts on the environment. In short, the diesel generator’s gas emission is calculated using the same energy production as the best wind turbines. For comparison, the unmet electrical load of wind turbines is considered (Fig. 5 ). Table 4 shows the emitted pollutant gases over one year of use. As it can be seen in Table 3 , the wind turbine power plant in the north can stop the CO 2 emission approximately 19,000 ton/year in comparison to natural gas and 28,000 ton/year in comparison to diesel. In the southern power plant, the reduced gas emission in comparison to diesel and natural gas are 18,000 and 12,000 ton/year, respectively.
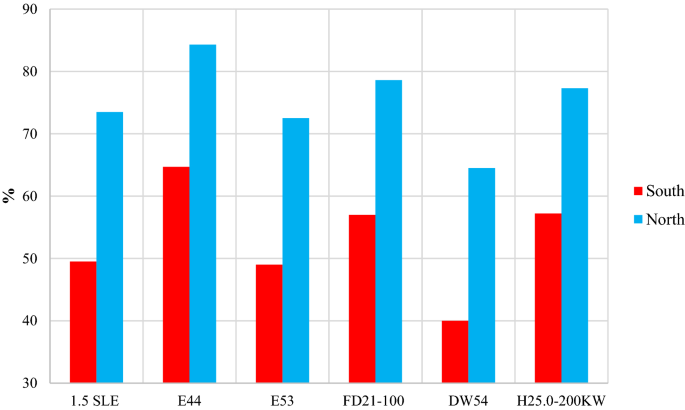
Unmet electrical loads for different turbines
In this study, the feasibility of using wind energy as a source of power production was calculated by collecting and analyzing hourly data on wind regimes over a 1-year period. HOMER Pro software was used to calculate the C.O.E. production of six different wind turbines, in order to select the most suitable wind turbine for two distinct locations in the north and south of Oman. The study’s main findings can be summarized as follows:
DW54 turbine produced by Energy Wind Technology in Amersfoort, The Netherlands, would have the best performance for Oman’s northern regions and can generate the cheapest possible energy from wind at $0.119/kW.
H25.0–200 kW turbine manufactured by the Anhui Hummer Dynamo Company of Hefeit, China, gives the best C.O.E. production for the southern regions of Oman and the lowest possible wind energy can be produced at $0.70/KW.
The difference of the wind regime between the northern and southern parts of Oman and the power curves of the turbines are the main reasons for the selection of two different wind turbines form different manufacturers.
The northern wind turbine plant is estimated to decrease CO 2 emissions by around 19,000 tons per year, compared to natural gas, while diesel emissions by around by 28,000 tons per year.
The southern wind turbines have a potential carbon emission reduction of about 18,000 and 12,000 tons per year compared to diesel and natural gas.
The application of the turbine selection using the HOMER Model described in this paper determined that the H25.0–200 kW turbine selected for the southern parts of Oman has a C.O.E. that is 58.8% lower than the DW54 turbine that was selected for the northern parts of the country. The application of the method followed in this research by developers during the planning stage could significantly improve the financial performance of their investment. Similarly, such techniques could be added to tools such as WAsP to improve decision-making during the initial planning stage.
Availability of data and materials
Data are openly available with HOMER software. HOMER uses the monthly average wind speeds, plus four parameters (Weibull k, 1-h autocorrelation factor, Diurnal pattern strength and Hour of peak wind speed) to synthesize wind data for simulation.
Change history
17 january 2021.
An amendment to this paper has been published and can be accessed via the original article.
Abdul-Wahab, S., Charabi, Y., Al-Mahruqi, A. M., et al. (2019a). Selection of the best solar photovoltaic (P.V.) for Oman. Solar Energy, 188, 1156–1168.
Article Google Scholar
Abdul-Wahab, S., Fessehaye, M., Yetilmezsoy, K., et al. (2019b). Optimization of an inverted multi-stage double slope solar still: An environmentally friendly system for seawater purification. Desalination and water treatment, 141, 42–50.
Akpinar, E. K., & Akpinar, S. (2006). An assessment of wind turbine characteristics and wind energy characteristics for electricity production. Energy Sources, Part A433A: Recovery, Utilization, and Environmental Effects + A465, 28, 941–953.
Al-Suleiman, S., Fessehaye, M., Yetilmezsoy, K., Al-Ghafir, A., Al-Brashdi, A., Al-Hajri, N., & Al-Bulushi, S. (2019). Optimization of an inverted multi-stage double slope solar still: an environmentally friendly system for seawater purification. Desalination and Water Treatment , 141 , 42–50. https://doi.org/10.5004/dwt.2019.23474 .
Al-Yahyai, S., Charabi, Y., Al Badi, A., & Gastli, A. (2013). Wind resource assessment using numerical weather prediction models and multi-criteria decision making technique: Case Study Masirah Island, Oman. International Journal of Renewable Energy Technology. . https://doi.org/10.1504/IJRET.2013.051070 .
Al-Yahyai, S., Charabi, Y., Gastli, A., & Al-Badi, A. (2012). Wind farm land suitability indexing using multi-criteria analysis. Renewable Energy, 44, 80–87. https://doi.org/10.1016/j.renene.2012.01.004 .
Al-Yayai, S., & Charabi, Y. (2015). Assessment of large-scale wind energy potential in the emerging city of Duqm (Oman). Renewable and Sustainable Energy Reviews, 47, 438–447. https://doi.org/10.1016/j.rser.2015.03.024 .
Cai X, Zhang Y, Ding W, et al. (2019) The aerodynamic performance of H-type darrieus V.A.W.T. rotor with and without winglets: C.F.D. simulations. Energy Sources, Part A: Recovery, Utilization, and Environmental Effects: 1–12.
Charabi, Y., Al Hinai, A., Al-Yahyai, S., et al. (2019). Offshore wind potential and wind atlas over the Oman Maritime Zone. Energy, Ecology and Environment, 4, 1–14.
Charabi, Y., Al-Yhyai, S., & Gastli, A. (2011). Evaluation of NWP performance for wind energy resources assessment in Oman. Renewable and Sustainable Energy Reviews, 15 (3), 1545–1555. https://doi.org/10.1016/j.rser.2010.11.055 .
Dolatabadi, A., Mohammadi-Ivatloo, B., Abapour, M., et al. (2017). Optimal stochastic design of wind integrated energy hub. IEEE Transactions on Industrial Informatics, 13, 2379–2388.
Elkinton, C. N., Manwell, J. F., & McGowan, J. G. (2008). Algorithms for offshore wind farm layout optimization. Wind Engineering, 32, 67–84.
Gaddada, S., & Kodicherla, S. P. K. (2016). Wind energy potential and cost estimation of wind energy conversion systems (W.E.C.S.s) for electricity generation in the eight selected locations of Tigray region (Ethiopia). Renewables, 2016 (3), 10. https://doi.org/10.1186/s40807-016-0030-8 .
Ghodsi, M., Ziaiefar, H., Mohammadzaheri, M., et al. (2019). Modeling and characterization of permendur cantilever beam for energy harvesting. Energy, 176, 561–569.
Homer-Energy (2016) HOMER pro version 3.7 user manual. HOMER Energy: Boulder, CO, U.S.A.
Khare, V., Nema, S., & Baredar, P. (2016). Solar–wind hybrid renewable energy system: A review. Renewable and Sustainable Energy Reviews, 58, 23–33.
Kodicherla, S. P. K., Gaddada, S., & Shaik, N. (2017). Wind energy potential and economic evaluation of WPS using WECSs in three selected locations of Northern Ethiopia. African Journal of Science Technology Innovation and Development., 9 (2), 179–187. https://doi.org/10.1080/20421338.2017.1303990 .
Kodicherla, S. P. K., Kan, C., & Nanduri, P. M. B. R. K. (2018). Likelihood of wind energy assisted hydrogen production in three selected stations of Fiji Islands. International Journal of Ambient Energy, 41 (7), 823–832. https://doi.org/10.1080/01430750.2018.1492444 .
Kumar, K. S. P., & Gaddada, S. (2015). Statistical scrutiny of Weibull parameters for wind energy potential appraisal in the area of northern Ethiopia. Renewables, 2, 14. https://doi.org/10.1186/s40807-015-0014-0 .
Moein, M., Pahlavan, S., Jahangiri, M., & Alidadi Shamsabadi, A. (2018). Finding the minimum distance from the national electricity grid for the cost-effective use of diesel generator-based hybrid renewable systems in Iran. Journal of Renewable Energy and Environment, 5 (1), 8–22. https://doi.org/10.30501/jree.2018.88377 .
Moné C, Hand M, Bolinger M, et al. (2017) 2015 cost of wind energy review. National Renewable Energy Lab.(NREL), Golden, CO (United States).
Nema, P., Nema, R., & Rangnekar, S. (2009). A current and future state of art development of hybrid energy system using wind and PV-solar: A review. Renewable and Sustainable Energy Reviews, 13, 2096–2103.
Padrón, I., Avila, D., Marichal, G. N., et al. (2019). Assessment of hybrid renewable energy systems to supplied energy to autonomous desalination systems in two islands of the Canary Archipelago. Renewable and Sustainable Energy Reviews, 101, 221–230.
Pahlavan, S., Jahangiri, M., Sahamsabadi, A. A., & Ariae, A. R. (2018). Assessment of PV-based CHP system: The effect of heat recovery factor and fuel type. Journal of Energy Mangement and Technology., 3 (1), 40–47.
Google Scholar
Sahu, B. K. (2018). Wind energy developments and policies in China: A short review. Renewable and Sustainable Energy Reviews, 81, 1393–1405.
S.A.O.C. (2017) 7 Years Statement of Oman Power & Water Production. Oman Power & Water Procurement Co.
Sahu A, Gupta S, Singh VK, et al. (2018) Design of permanent magnet synchronous generator for wind energy conversion system. Advances in Smart Grid and Renewable Energy. Springer, 23–32.
Vahdatpour, S., Adfar, S. B., Siampour, L., Veisi, E., & Jahangiri, M. (2017). Evaluation of off-grid hybrid renewable systems in the four climate regions of Iran. Journal of Renewable Energy and Environment., 4 (1), 61–70.
Wang, L., Yuan, J., Cholette, M. E., et al. (2018). Comparative study of discretization method and Monte Carlo method for wind farm layout optimization under Weibull distribution. Journal of Wind Engineering and Industrial Aerodynamics, 180, 148–155.
World-Bank. (2015). Renewable energy consumption (% of total final energy consumption) . World Bank: Sustainable Energy for All database.
Yang, B., Yu, T., Shu, H., et al. (2018). Robust sliding-mode control of wind energy conversion systems for optimal power extraction via nonlinear perturbation observers. Applied Energy, 210, 711–723.
Download references
Acknowledgements
The authors would like to thank the anonymous reviewers for their insightful suggestions and careful reading of the manuscript.
The authors received no specific funding for this work.
Author information
Authors and affiliations.
Center for Environmental Studies and Research, Sultan Qaboos University, P.O. Box 32, 123, Muscat, Oman
Yassine Charabi
Department of Mechanical and Industrial Engineering, Sultan Qaboos University, Muscat, Oman
Sabah Abdul-Wahab
You can also search for this author in PubMed Google Scholar
Contributions
The first and the second author contributed to the design and implementation of the research, to the analysis of the results and to the writing of the manuscript. All authors read and approved the final manuscript.
Corresponding author
Correspondence to Yassine Charabi .
Ethics declarations
Competing interests.
The authors declare no competing interests.
Additional information
Publisher's note.
Springer Nature remains neutral with regard to jurisdictional claims in published maps and institutional affiliations.
Rights and permissions
Open Access This article is licensed under a Creative Commons Attribution 4.0 International License, which permits use, sharing, adaptation, distribution and reproduction in any medium or format, as long as you give appropriate credit to the original author(s) and the source, provide a link to the Creative Commons licence, and indicate if changes were made. The images or other third party material in this article are included in the article's Creative Commons licence, unless indicated otherwise in a credit line to the material. If material is not included in the article's Creative Commons licence and your intended use is not permitted by statutory regulation or exceeds the permitted use, you will need to obtain permission directly from the copyright holder. To view a copy of this licence, visit http://creativecommons.org/licenses/by/4.0/ .
Reprints and permissions
About this article
Cite this article.
Charabi, Y., Abdul-Wahab, S. Wind turbine performance analysis for energy cost minimization. Renewables 7 , 5 (2020). https://doi.org/10.1186/s40807-020-00062-7
Download citation
Received : 28 May 2020
Accepted : 10 August 2020
Published : 17 August 2020
DOI : https://doi.org/10.1186/s40807-020-00062-7
Share this article
Anyone you share the following link with will be able to read this content:
Sorry, a shareable link is not currently available for this article.
Provided by the Springer Nature SharedIt content-sharing initiative
- Wind turbine Homer Pro
- Wind energy
- Load demand
- Cost of energy
- Sultanate of Oman
Thank you for visiting nature.com. You are using a browser version with limited support for CSS. To obtain the best experience, we recommend you use a more up to date browser (or turn off compatibility mode in Internet Explorer). In the meantime, to ensure continued support, we are displaying the site without styles and JavaScript.
- View all journals
- My Account Login
- Explore content
- About the journal
- Publish with us
- Sign up for alerts
- Open access
- Published: 22 October 2021
Geophysical constraints on the reliability of solar and wind power worldwide
- Dan Tong ORCID: orcid.org/0000-0003-3787-0707 1 , 2 , 3 ,
- David J. Farnham ORCID: orcid.org/0000-0002-6690-4251 3 ,
- Lei Duan ORCID: orcid.org/0000-0002-6540-1847 3 ,
- Qiang Zhang ORCID: orcid.org/0000-0002-8376-131X 1 ,
- Nathan S. Lewis 3 , 4 ,
- Ken Caldeira ORCID: orcid.org/0000-0002-4591-643X 3 , 5 &
- Steven J. Davis ORCID: orcid.org/0000-0002-9338-0844 2 , 3 , 6
Nature Communications volume 12 , Article number: 6146 ( 2021 ) Cite this article
51k Accesses
88 Citations
556 Altmetric
Metrics details
- Climate change
- Climate-change mitigation
- Renewable energy
If future net-zero emissions energy systems rely heavily on solar and wind resources, spatial and temporal mismatches between resource availability and electricity demand may challenge system reliability. Using 39 years of hourly reanalysis data (1980–2018), we analyze the ability of solar and wind resources to meet electricity demand in 42 countries, varying the hypothetical scale and mix of renewable generation as well as energy storage capacity. Assuming perfect transmission and annual generation equal to annual demand, but no energy storage, we find the most reliable renewable electricity systems are wind-heavy and satisfy countries’ electricity demand in 72–91% of hours (83–94% by adding 12 h of storage). Yet even in systems which meet >90% of demand, hundreds of hours of unmet demand may occur annually. Our analysis helps quantify the power, energy, and utilization rates of additional energy storage, demand management, or curtailment, as well as the benefits of regional aggregation.
Similar content being viewed by others
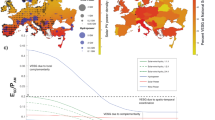
Spatiotemporal management of solar, wind and hydropower across continental Europe
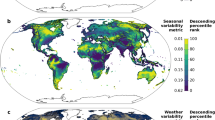
Identification of reliable locations for wind power generation through a global analysis of wind droughts
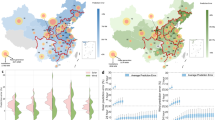
Inherent spatiotemporal uncertainty of renewable power in China
Introduction.
Stabilizing mean global temperatures requires a global transition to energy systems with near-zero (or net-negative) carbon dioxide equivalent emissions 1 , 2 , 3 . In cost-optimized scenarios that accomplish this transition, solar and wind resources often supply a large share (e.g., >60%) of electricity 4 , 5 , 6 , 7 , 8 , 9 , 10 . Designing and operating a highly reliable electricity system that is dependent on such large shares of wind and solar generation can be a challenge, however, due to the variable and uncertain nature of solar and wind resources 11 , 12 . The efficacy of meeting electricity demands with generation from solar and wind resources depends on factors such as location and weather; the area over which generating assets are distributed; the mix and magnitude of solar and wind generation capacities; the availability of energy storage; and firm generation capacity 11 , 12 , 13 , 14 , 15 , 16 . Meanwhile, reliability standards in industrialized countries are typically very high (e.g., targeting <2–3 h of unplanned outages per year, or ~99.97% 17 ). Resource adequacy planning standards for “1-in-10” are also high: in North America (BAL-502-RF-03) 18 , generating resources must be adequate to provide no more than 1 day of unmet electricity demand—or in some cases 1 loss of load event—in 10 years (i.e., 99.97% or 99.99%, respectively) 19 .
Here, we present a systematic analysis of the ability of specified amounts of solar and wind generation to meet electricity demands in 42 major countries across a range of assumptions associated with transmission, energy storage, and generation amounts. In particular, we assess spatial and temporal gaps between electricity demand and the availability of solar and wind resources, which represent gaps that must be filled by other non-emitting generation technologies or operating strategies in reliable electricity systems based on zero-carbon sources. The complementarity of renewable energy sources for this study is defined as a hybridization of solar-wind resources over a given area (here, countries), which we estimate by the Kendall correlation coefficient of these resources across 39-years of resource data 20 . Our goal is to identify the opportunities, complementarity, and challenges of variable renewable resources in greater detail than can be done by integrated assessment models that have multi-year time steps. Our results do not account for realistic power system specifications. Rather, we examine fundamental geophysical constraints on wind- and solar-dominated power systems independent of cost estimates. Note that we do not mean to suggest that the temporal variability of such resources would ever make it physically impossible to meet a given electricity demand (with enough capacity the solar and wind resources would be able to meet demand), but rather the extent to which such variability may determine the economic or socio-political feasibility of reliable systems. Our results will thus continue to be informative even as technological and socio-political feasibility evolves.
Details of our analytical approach are in the “Methods” section. In summary, we use 39 years (1980–2018) of gridded (0.5° × 0.625°) and hourly reanalysis data 21 , 22 and actual/projected hourly electricity demand from a single recent year to evaluate the adequacy of solar and wind resources to meet electricity demand in each of 42 major countries (data sources and countries are listed in Supplementary Data 1 ). First, hourly, area-weighted capacity factors for both solar and wind resources are calculated over each country (or region), assuming perfect transmission within the country or region. Then we exogenously specify (1) the mix of solar and wind generation, (2) the overall level of annual generation from these sources, and (3) the capacity of energy storage, and analyze the ability of the specified technologies to meet hourly demand. We analyze systems ranging from 100% solar (no wind) to 100% wind (no solar), in which total annual generation ranges from equal to annual demand (“1x generation”) to up to three times annual demand (“3x generation”), and in which available energy storage ranges from none (“0 h”) to 12 h of mean demand (“12 h”). In addition, we simulate the impacts of different demands (i.e., demand load profiles) and technologies (i.e., single-axis and dual-axis solar tracking systems) on electricity system reliabilities as sensitivity tests. The number of countries, years of reanalysis data, and different system configurations we analyze require computation and analysis of ~300,000 year-long simulations.
Resources and demand variability
Figure 1 shows the seasonal and daily variability of solar and wind resources and electricity demand in the six countries with the greatest electricity demand on every continents except Antarctica (results from six other major countries and continent-level aggregated regions are shown in Supplementary Figs. 1 and 2 , respectively). Solar and wind consistently peak in summer and winter, respectively, in countries of the Northern Hemisphere (seasons are reversed in countries of the Southern Hemisphere; Fig. 1a–f ). The seasonal cycles of solar and wind thus suggest potential complementarity in many countries (e.g., China, Fig. 1a ; and Germany, Fig. 1b ). However, during the 39-year period, interannual variability of wind is consistently much greater than that of solar in most countries (Fig. 1a–f ), though the magnitude of these resources’ variability differs substantially between two particular countries. For example, Germany’s small area (0.36 million km 2 ) and high latitude (centroid 51.2 °N) result in large interannual variations in both solar (measured by the robust coefficient of variation 23 ; RCoV = 58.8%) and wind resources (RCoV = 47.2%, Fig. 1b ), whereas solar resource variability is very low (RCoV = 6.6%) in the larger and tropical country of Brazil (8.52 million km 2 and centroid 14.2 °S; Fig. 1e ). Wind resources are also more variable than solar resources on the time scale of days to weeks in each country, which acts to limit and undermine the resources’ seasonal complementarity. Electricity demand profiles for each country are determined by factors such as economic conditions, prevailing weather conditions and consumer usage patterns 24 . Therefore, electricity demand for two countries can have unique seasonal shapes and a range of variabilities even if they have similar wind and solar resources. For example, seasonal variability of demand in France (RCoV = 14.4%; Supplementary Fig. 1e ) is greater than that in Germany (RCoV = 7.4%; Fig. 1b ), despite the countries’ similar wind and solar resource profiles.
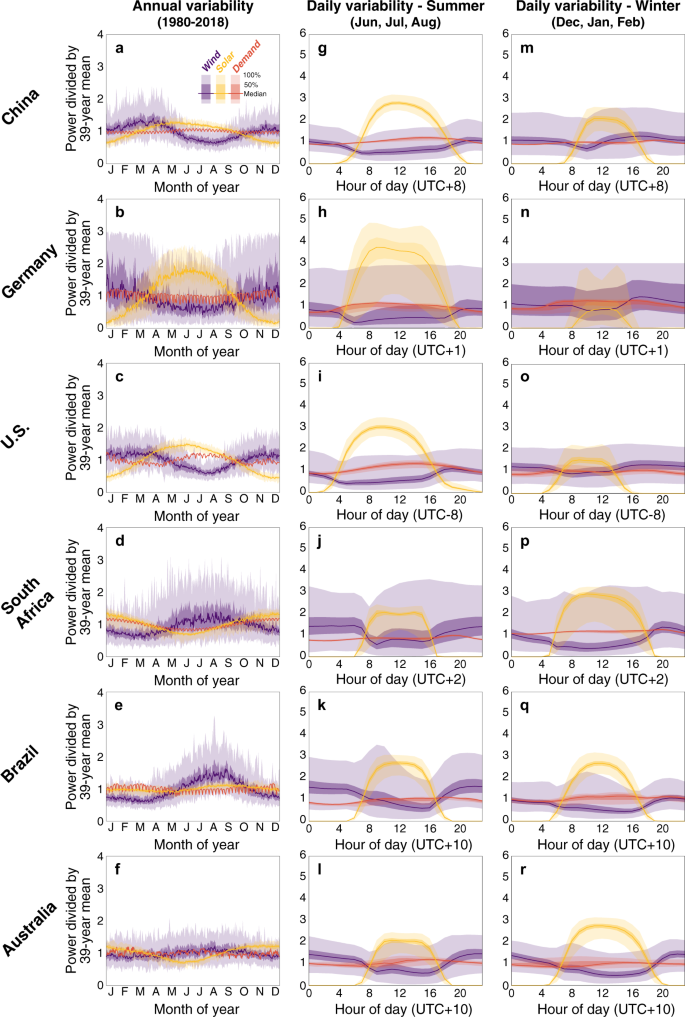
Climatological variability of the area-weighted median power from solar (orange) and wind (blue) resources for the selected country from six continents during the 39-year period 1980–2018. The countries (from the top row to the bottom row) are China ( a , g , m ), Germany ( b , h , n ), contiguous U.S. ( c , i , o ), South Africa ( d , j , p ), Brazil ( e , k , q ), and Australia ( f , l , r ). The left column ( a – f ) depicts the daily and seasonal variability, the middle column ( g – l ) depicts hourly summer (June, July, and August) variability, and the right column ( m – r ) depicts hourly winter (December, January, and February) variability. The lines represent the median, the dark shading represents the inner 50% of observations (25th to 75th percentile) and the light shading represents the outer 50% of observations (0th to 100th percentile) of the daily averaged value for that same day in each of the 39 years of record. Red curves in each panel represent electricity demand for a single, most recent, available year for each country. The time of day shown is the local time of each country and its relation to Coordinated Universal Time (UTC) is shown. Note that the middle of local time zones has been selected for the countries with multiple time zones. The solar, wind, and demand data are each normalized by dividing by their respective 39-year mean value.
Daily cycles of solar and wind resources in each country are also somewhat complementary. Wind power usually peaks at night and rarely falls to zero when resources are aggregated over an entire country. This daily cycle is not substantially different during the summer and winter months (comparing Fig. 1g–l with Fig. 1m–r ). Thirty-four (of the 42) countries have higher average wind power availability during the nighttime than during the daytime. Solar power peaks in the middle of the day and drops off sharply to zero at dusk. The amplitude and duration of the daily cycles for solar power availability is consistently different during the summer and winter months across countries (Fig. 1g–l versus Fig. 1m–r ). The daily cycle of solar resources is a barrier to realizing reliable solar-dominated electricity systems without energy storage and/or complementary wind generation to meet demand during the hours when the solar resource is not available. In addition, given our assumption of single-axis solar tracking, available solar power tends to be flat for several hours around its daytime peak during the daily cycles (Fig. 1g–r ), though in some countries (e.g., Germany, South Africa, Australia) there is a consistent dip near noon, perhaps related to our adjustments of the direct radiation (details in Supplementary Note 1 ). Kendall’s correlation coefficients of solar and wind resources in the 42 main countries range from −0.91 to −0.83 (see Supplementary Data 2 ), another indication of good complementarity (where −1 is the best possible complementarity) 20 .
The most reliable generation systems
The colors in Fig. 2 show the reliability of electricity systems (i.e., the average percentage of electricity demand that is met each year from 1980 to 2018) based only on solar and wind resources for 18 major countries (4 from each of Asia, Europe, Africa, and the Americas, and 2 from Oceania; horizontal axes of each panel), according to: the mix of solar and wind generation (vertical axes), the level of annual generation relative to annual demand (1x in left panels and 1.5x in right panels), and the capacity of energy storage relative to mean electricity demand (0, 3, and 12 h in the first, second, and third rows of panels, respectively). Results for 24 other countries are shown in Supplementary Fig. 3 and Supplementary Data 3 . Figure 2a shows that without any excess annual generation or energy storage (assuming perfect national transmission), the most reliable mixes (white circles) of solar and wind generation could potentially meet 72–91% (average 83%) of electricity demand in these countries. Under these generation and storage assumptions, the most reliable solar-wind generation mixes range from 65 to 85% wind power (73% on average), with countries with substantial desert (like Algeria, Egypt, South Africa) favoring slightly more solar and less wind (65–70% wind) and with higher-latitude countries like Russia and Canada favoring more wind (85% wind; Fig. 2a ).
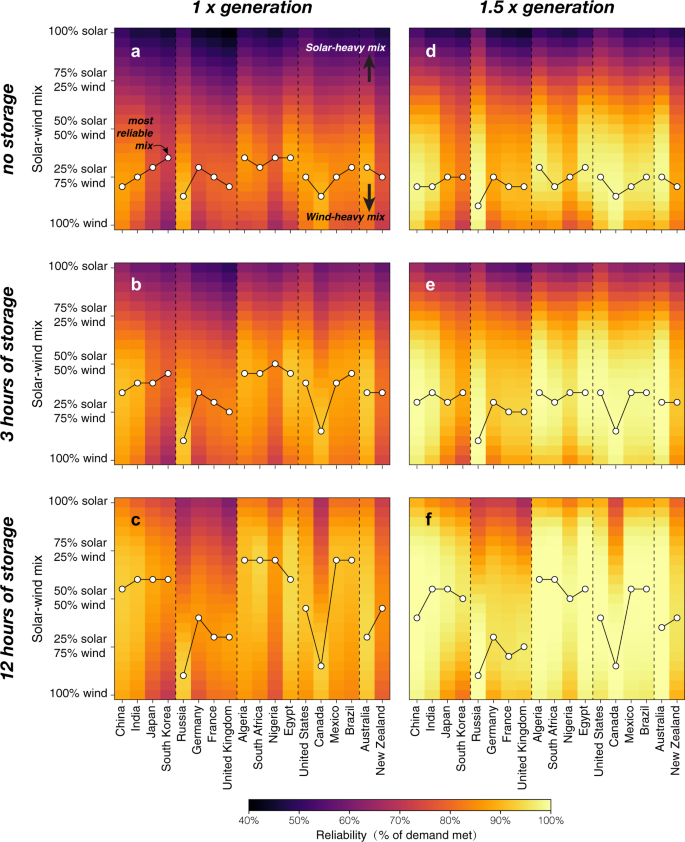
Shading in each panel represents the 39-year average estimated reliability (% of total annual electricity demand met) by a mix of solar and wind resources ranging from 100% solar to 100% wind (every 5% change for solar-wind generation mix). 18 main countries are chosen to show their ability to meet total annual electricity demand, including 16 main countries from four continents (Asia, Europe, Africa, and the Americas) and 2 main countries from Oceania. The white circles represent the highest reliability within each country under 21 sets of solar and wind generation mix (called the most reliable mix). Storage and generation quantities are varied in each panel: a 1x generation without storage; b 1x generation with 3 h of storage; c 1x generation with 12 h of storage; d 1.5x generation without storage; e 1.5x generation with 3 h of storage; and f 1.5x generation with 12 h of storage.
Adding 3 h of energy storage, but still without excess annual generation, increases the reliability so that the most reliable mixes (white circles) meet 78–93% (average 87%) of electricity demand. The share of solar generation in these most reliable mixes increases to 15–50% (36% on average; Fig. 2b ). However, the share of solar generation increases less, or even decreases, in higher-latitude countries like Russia, Canada, and Germany (Fig. 2b ). These trends continue as more storage is added, so that with 12 h of energy storage and no excess annual generation, 83–94% (average 90%) of electricity demand is met with mixes of 10–70% solar power (49% on average; Fig. 2c ).
If generating capacities are instead increased so that annual generation exceeds annual demand in each country by 50% (i.e., 1.5x generation), but without energy storage, the most reliable mixes meet 83–99% (average 94%) of electricity demand. The 1.5x generation most reliable mixes are substantially more reliable than in the 1x generation systems but include more wind power: 70–90% wind power (78% on average; Fig. 2d ). These “overbuilt” systems are more reliable in all of these 18 countries than the systems with 12 h of energy storage but no excess generation (Fig. 2c ). Adding energy storage to systems whose generation is 1.5x annual demand again increases both the system reliability (89–100%, average 98%) and the share of solar generation (most reliable mixes have 10–60% solar power, 36% on average; Fig. 2e, f ).
The unmet demand
The scatter plots in Fig. 3 show the relationships among reliability, energy storage, excess annual generation, and countries’ land area for the most reliable solar-wind mixes of all 42 countries analyzed (see relationships with a log y-axis in Supplementary Fig. 4 ). The linear fits in each panel show that solar-wind systems are generally less reliable in countries with smaller land areas (e.g., Fig. 3a ). Specifically, our results across countries indicate that the reliability of solar-wind systems that lack energy storage increases by 7.2% for every factor of 10 increase in land area; this relationship further suggests the improvement in system reliability that might be expected by expanding transmission systems within large countries. However, excess annual generation tends to alleviate the disadvantage of small country area more than energy storage (this can be seen by comparing the slopes of the linear fits in panels of Fig. 3c and d ). In addition, within each country, to compare the gains in reliability from excess annual generation and energy storage, a nonlinear function was fit to the reliability given the land area, the level of annual generation, and the capacity of energy storage (see Supplementary Information ). Our results indicate that a 10% increase in excess annual generation is equivalent to 3.9 h of storage (Supplementary Note 2 ).
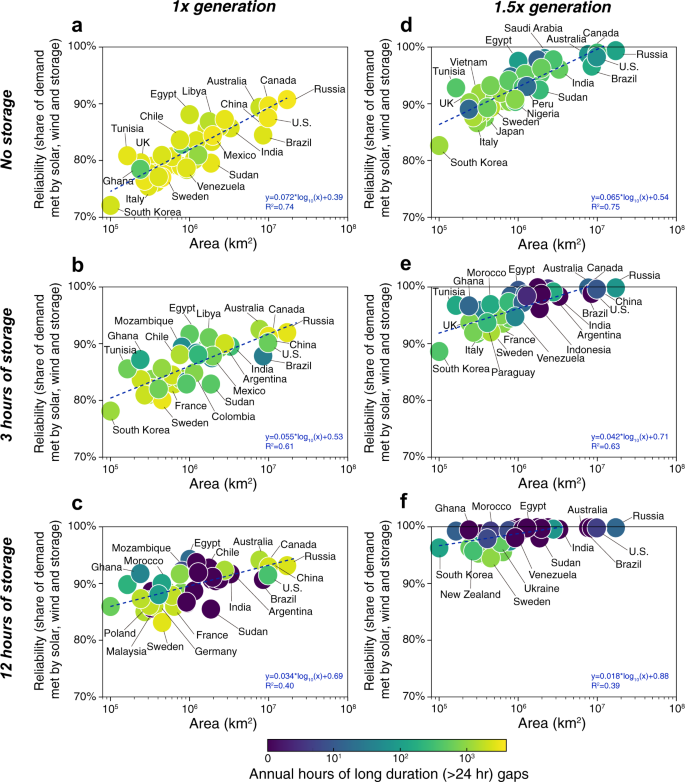
Shading of bubbles represents the annual average hours of long-duration (>24 h) power supply gaps. Storage and generation quantities are varied in each panel: a 1x generation without storage; b 1x generation with 3 h of storage; c 1x generation with 12 h of storage; d 1.5x generation without storage; e 1.5x generation with 3 h of storage; and f 1.5x generation with 12 h of storage.
Figure 3 also points to the nature of systems’ unreliability: the color of bubbles indicates the average number of events in which there would be unmet demand in each of at least 24 contiguous hours (i.e., “long-duration gaps”). In systems that meet >95% of a countrie’s demand, dozens of such long-duration gaps often remain each year (yellow and green circles). In some countries, excess annual generation reduces the number of such long-duration gaps more than adding 12 h of energy storage (e.g., compare Sweden, Australia, Canada, and Russia in Fig. 3c and d ).
Figure 4 further characterizes the magnitude and duration of unmet demand in 16 major countries (removing two African countries from the 18 countries shown in Fig. 1 for figure symmetry; in descending order of their land area), with curves showing gaps of different system configurations sorted by their magnitude and according to the number of hours each year that such a gap occurred (power supply gap represents the fraction of unmet demand to the total demand in that hour averaging over 1980–2018; see relationships with a log y-axis in Supplementary Fig. 5 ). For example, the pale purple curves show that systems with no excess annual generation and 12 h of energy storage consistently have gaps in which >50% of demand is unmet for >1000 h per year (Fig. 4 ). Pale green curves show that systems with 50% excess annual generation and 12 h of energy storage may have much smaller and shorter gaps in some countries (e.g., <10% of demand unmet in fewer than 100 h per year in Russia, China, and Australia), but the gaps may still be >20% of demand for tens of hours or more in countries with relatively large land areas (e.g., Canada, Brazil, India, and Mexico) and >60% of demand for several hundred hours per year in countries with smaller areas (e.g., France, Japan, Germany, New Zealand, the U.K., and South Korea; Fig. 4 ). Indeed, in smaller countries, substantial gaps (>30% of demand for >20 h per year; pale orange curves in Fig. 4 ) remain in systems even with 12 h of energy storage and annual generation that is 3x annual demand.
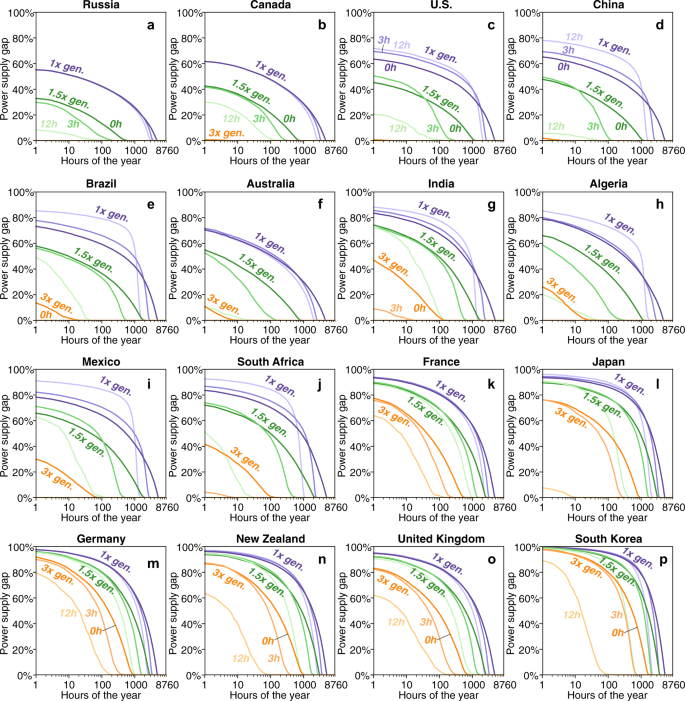
Areas under each curve show the share and hours of unmet electricity demand of the most reliable solar-wind systems in selected countries assuming specified storage and generation quantities: a Russia; b Canada; c contiguous U.S.; d China; e Brazil; f Australia; g India; h Algeria; i Mexico; j South Africa; k France; l Japan; m Germany; n New Zealand; o United Kingdom; p South Korea (see data in Supplementary Data 6 ). Color of lines represents different generation quantities: 1x generation in purple, 1.5x generation in green, and 3x generation in orange. Shading of lines represents different storage quantities: darkest shading represents without storage, medium shading represents 3 h of storage, and lightest shading represents 12 h of storage. Note that the y-axis of power supply gap represents the fraction of unmet demand to the total demand in that hour.
Benefits from sharing resources of multiple nations
We also evaluate the reliability benefits of regional electricity interconnections whereby the solar and wind resources of multiple nations are pooled and shared, again assuming perfect transmission within these regions. The maps in Fig. 5 present the effects of such spatial aggregation, showing the highest reliability of solar-wind generation with no excess annual generation or energy storage at the national level (Supplementary Data 7 ; Fig. 5a ), as well as when a system is aggregated into 19 separate, contiguous multinational regions (Fig. 5b ; categorization in Supplementary Data 4 ) and 6 continents (Supplementary Data 7 ; Fig. 5c ). Each step produces substantial improvements in reliability, with >89.8% of hourly demand met everywhere when resources are aggregated at the continental level (Fig. 5c ). Figure 5c also indicates the additional reliability gains in these systems that would be achieved as a consequence of specific intercontinental connections. Supplementary Fig. 6 shows that the supply gaps in continental-scale solar-wind systems might be entirely eliminated in Africa, Asia, and South America, and limited to <2% of demand and 49, 26, and 13 h in Europe, Oceania, and North America, respectively, given excess annual generation of 50% and 12 h of storage. Substantial supply gaps remain for continental-scale systems when excess annual generation and energy storage are not available (Supplementary Fig. 7 ).
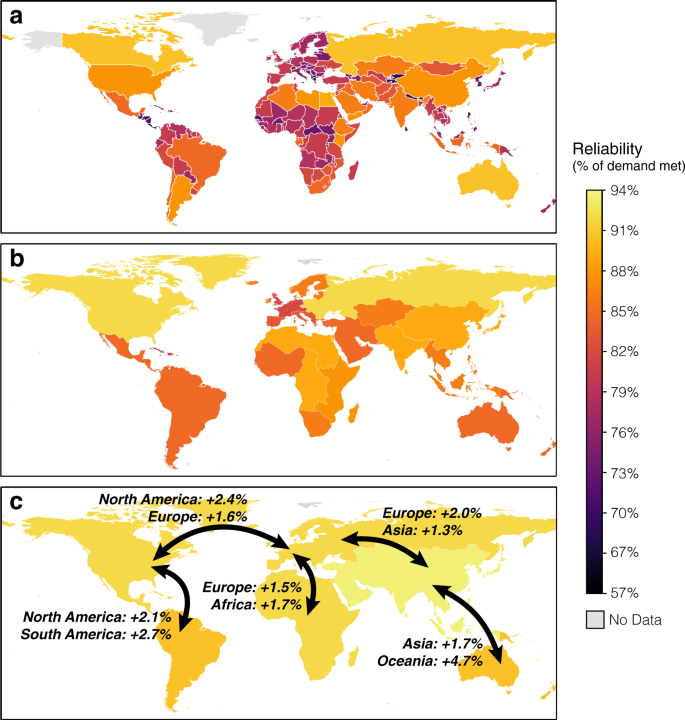
Maps show the reliability (i.e., hourly averaged resource adequacy) at country/region scale ( a ; Supplementary Data 4 ), the subcontinent scale ( b ; 19 multinational regions, and listed in the SI), and at continental scale ( c ; 6 continents: Asia, Europe, Africa, North America, South America, and Oceania). We also evaluated the reliability of the power supply system assuming several intercontinental connections (shown as the arrows: Asia–Oceania, Europe–Asia, Europe–Africa, North America–Europe, and North America–South America). The added reliabilities for each continental power system under various connections are labeled.
Our results suggest that, neglecting transmission constraints, with systems sized to meet time-integrated annual electricity demand, major countries’ solar and wind resources could meet at least 72% of instantaneous electricity demand without excess annual generation or energy storage. For instance, in the contiguous U.S., a solar and wind power system could provide ~85% of total electricity demand, which is consistent with the prior studies and reports 12 , 25 . Solar and wind resources can achieve greater levels of reliability by adding energy storage, increasing deployed capacities (i.e., generating electricity in excess of annual demand), or pooling resources of contiguous, multinational regions 26 . However, the marginal improvements in reliability related to these options differ considerably across countries and regions, according to their land area, location, and geophysical resources (Supplementary Figs. 8 and 9 ).
In small, high-latitude countries, the highest reliability systems are usually wind-heavy (e.g., as high as 95% wind power), with particularly large reliability gains achievable by regional aggregation. In contrast, the most reliable systems in temperate/tropical countries tend to include more solar. Meanwhile, the most reliable systems are not always the same systems that would minimize the frequency of long-duration (≥24 h) power supply gaps (Supplementary Fig. 9 ). In general, more solar in the wind-solar mix reduces the frequency of long-duration gaps. Although reasonably high levels of reliability can be reached by solar-wind resources alone, the defining challenge of such systems are the longer-duration gaps, often associated with extreme weather episodes. For instance, historical solar and wind resources data in Germany reveal that there were nearly 2 weeks in which dispatchable generation had to cover practically all of the demand because of a period with very low solar and wind power availability (called “dark doldrums”) 27 . Although with vast enough wind and solar capacity it might still be possible to meet demand in all hours, the required capacity increases exponentially after a point that depends on the renewable resources of that country, and it is this geophysically-dependent point that will largely determine the cost-effectiveness of highly-reliable, renewables-based electricity systems. Although dispatchable fossil fuel generators with 100% effective carbon capture storage (CCS) could provide system reliability without emissions 2 , such underutilized and capital-intensive backup electricity would require higher investments and variable costs. In contrast, combustion turbines or combined cycle plants burning carbon-neutral biogas, syngas, or hydrogen might have comparatively low capital costs, but would require additional and large capital investments to produce such fuels (e.g., biodigestion, direct air capture, Fischer-Tropsch, and/or electrolysis). Sector-coupling or right-sizing of these net-zero emissions fuel-production facilities could nonetheless make infrequent operation of generators feasible 28 . More firm generation would mean less solar and wind capacity in a given system, which might or might not be cost-effective depending on technology costs. But many jurisdictions and advocates are interested in “maxing out” solar and wind. Our results are especially relevant in that context, highlighting the implications of country-level differences in the variability of solar and wind resources, including how much storage and firm generation might be required to ensure resource adequacy. Although our methods are simple and transparent, our goals and findings are remarkably consistent with much more complex approaches. For example, the recently published Net-Zero America report includes a cost-optimized “all-renewables” scenario which decarbonizes U.S. electricity without nuclear or CCS: by 2050, ~81.6% of primary energy in the E + RE + scenario is from solar and wind 29 .
Our analysis has important limitations and uncertainties. To improve the generality of our results, our analysis focuses exclusively on geophysical constraints and does not consider economic feasibility. As noted throughout, our reliability estimates are a best case given the assumption that electricity can be transmitted losslessly throughout a region of interest. Also, we use area-weighted averages for solar and wind generation potential without regard to existing protections or uses. This use of area-weighted averages affects our estimates in two important ways. First, our estimates may include areas where currently generation cannot be sited. Second, our derivation of solar and wind capacity factors implies uniform distribution of wind and solar generation technology (i.e., a horizontal single-axis tracking system applied in this work), which does not allow us to select locations with particularly high capacity factors or to strategically select a set of locations whose generating potential is mutually negatively correlated. This second point has the effect of making our estimates for the efficacy of solar and wind resources to meet electricity demand more conservative by using the horizontal single-axis tracking system compared to the dual-axis solar tracking systems. For this case, dual-axis solar tracking systems are added to test the impacts on the system reliabilities (see Supplementary Note 3 ), we find that different solar tracking systems have very small impacts on the electricity system reliabilities and the reliability change ratios are within ±5% under the 1x generation system and less sensitive under 3x generation system (Supplementary Fig. 10 ). However, either method to calculate capacity factors of national and regional area-weighted averages may also reduce the resource variability and thereby increase estimates of reliability. Third, hourly variations of solar and wind capacity factors in the reanalysis data MERRA-2 we used may be biased. A new analysis based on a different and independent reanalysis product, ERA5 30 , 31 , has been added and compared to the original results (see Supplementary Note 3 and Supplementary Figs. 11 - 12 ). Our estimates of the system reliabilities by using ERA5 data in the 42 major countries are in good agreement with results of MERRA-2: under 1x generation and the most reliable mixes without storage, reliability under the different loads varies on average from −9.4 to 1.3% (see Supplementary Fig. 9a ). The differences are similar in systems with excess generation (Figs. S11b-c). We also compared the magnitude and duration of unmet demand in 16 major countries like Fig. 4 (see Supplementary Fig. 12 ). The data products of MERRA-2 and ERA5 both can essentially capture the number of hours each year that such a gap occurred. By contrast, the MERRA-2 data has a better performance of meeting hourly demand in larger countries (i.e., Russia and Canada) but a similar performance in small countries (i.e., United Kingdom). The somewhat different patterns of resource variability in the two datasets do not alter our main conclusions.
Our estimates show that the marginal reliability benefit of increased capacity of storage or increased overbuild of wind/solar declines steadily. Under a given capacity of energy storage (e.g., 3 h), our results of 1x, 1.5x, and 3x generation show that the first 10% excess generation increase is larger than the next 10% excess generation increase (i.e., the marginal benefit for system reliability decreases as excess generation increases). As might be expected, the diminishing marginal benefits between excess generation and increased storage apply in both directions. Our fitting model performs well across different nations, overbuild levels, and storage levels. The differences in reliability between the estimates and the model predicted values are between −5.5 and 5.8% and ~80% of the differences are within ±2%, with no systematic bias related to region or the magnitude of overbuild or storage. Nonetheless, our model and conclusions are limited by our experimental design and the discrete levels of excess generation (1x, 1.5x, and 3x) and storage (0, 3, and 12 h) we evaluated.
We compare the reliability improvements obtainable by energy storage, excess capacity, and regional aggregation but not the relative costs of the different options. For example, the energy storage capacities we consider are in some cases quite large: energy storage equal to 12 h of mean electricity demand in the contiguous U.S., Germany, and Japan represents 5.6, 0.7, and 1.4 TWh, respectively (Supplementary Data 5 ). These combined storage capacities represent ~35 times the capacity of Li-ion batteries produced globally to date 32 , and more than 200 times the pumped hydro storage capacities that now exist in those countries 33 . The feasibility of 12 or more hours of energy storage may depend on continued innovation and learning related to the associated materials and technologies 34 , 35 , 36 , 37 . Similarly, the feasibility of pooling solar and wind resources over national or multinational regions may depend on both technological advances that reduce the costs, losses, and risks of power transmission 38 , 39 , 40 as well as shifts in the socio-political support for such infrastructure 41 , 42 . In addition, setting up purely solar and wind supplied electricity systems requires a large number of solar panels and wind turbines to be installed, and we do not incorporate the impacts or interactions (e.g., wakes) from these hypothetical build-outs. Last, in this work, only 1-year of demand data is employed to assess the geophysical constraints of 39-year solar and wind resources. On one hand, we understand that the hourly patterns of countries electricity demand will of course change over time with changes in population, economic activities, power generation structure, and technology. For example, stronger positive correlation between solar/wind availability and demand may be observed as renewable energy gradually dominates the power system. However, our analysis compares resources and demand in different years and at the country-level, which should preclude any bias related to specific subnational weather events. On the other hand, electricity demand profile may also dramatically change with future high electrification. We therefore perform additional analysis using the demand pattern from the future high electrification scenario (i.e., combining the high electrification scenario and rapid technology advancement) 43 and use the results to discuss the sensitivity of our results to such different load profiles (see Supplementary Fig. 13 ), and the results of this test for the U.S. show that reliability is not especially sensitive to the high electrification demand profile: under 1x generation without storage, reliability under the different renewable mixes varies on average from −1 to 2.5%. The differences are even smaller in solar-heavy systems with excess generation. In addition, we test the sensitivity of our results to such changes in demand by simulating the reliability of U.S. resources in meeting current loads from each other region (see Supplementary Note 4 ). These tests show that reliability is not especially sensitive to demand profiles: under 1x generation and the most reliable mixes without storage, reliability under the different loads varies on average from −9 to 2% (see Supplementary Fig. 14a ). The differences become even smaller in systems with excess generation (Supplementary Fig. 14 ).
Despite these simplifying assumptions, our results offer insights from those provided by multi-year time step integrated assessment modes (IAMs) or hourly, cost-optimized energy system models. Specifically, hourly resolution over several decades allows us to evaluate the adequacy of regional solar and wind resources independent of costs. For example, cost-optimizing models which either require renewables sources to meet a very high share of demand or else assume extremely cheap renewable costs generally find substantial increases in system costs related to, e.g., energy storage. Our geophysically-focused results help to explain such results irrespective of cost assumptions. Indeed, we compare the estimates of reliability and capacities in this study with several techno-economic studies that have used independent approaches to model regional solar- and wind-dominated electricity systems in detail 29 , 44 , 45 . In each case, focusing on the U.S., these studies find that the share of non-emitting (or carbon neutral) electricity contributed by solar and wind in cost-optimized systems is typically ~80%, with the residual demand for non-emitting generation met by firmer renewables such as biomass, hydroelectricity, and geothermal 29 , 44 , 45 .
Variable solar and wind energy are projected by many to be the dominant sources of electricity in net-zero emissions energy systems of the future. With solar and wind capacities sized such that total annual generation meets total annual demand, seasonal and daily complementarities of these resources make them capable of meeting three-quarters of hourly electricity demand in larger countries. Increasing the share of demand that can be met by solar and wind generation will require either “overbuilding” (i.e., excess annual generation), the introduction of large-scale energy storage, and/or aggregating resources across multinational regions (Supplementary Data 6 ). We highlight the geophysical considerations related to these options, but economics and geopolitics will also strongly influence which strategies are ultimately adopted and are therefore important topics for further research. Our analysis for the 39-year record of solar and wind resources is in part to obtain a statistically significant analysis of interannual variability and rare events (such as prolonged storms). Establishing estimates for interannual variability and the frequency of rare events that impact solar and wind generation potential is important when considering the lifetime of the capital asset stock in an electricity grid and requires the use of many years of data. Our normalized analysis of the reliability for purely solar and wind supplied electricity system would apply as well to a system with other slowly time-varying generation (e.g., coal, hydro, geothermal, or nuclear) because the variability of solar and wind generation and related long-duration gaps in electricity supply will have to be managed either by ramping backup technologies up and down or by curtailing excess solar and wind generation. Our results reveal national and regional differences in solar and wind resources that may be useful to decision makers and researchers prioritizing their investments in pursuit of reliable and cost-effective electricity systems based predominantly on solar and wind energy.
Hourly solar and wind capacity factors
The capacity factor describes the actual energy output as compared to the systems’ rated energy output (power capacity multiplied by 1 h) 12 . To calculate the wind and solar capacity factors for this study, we first obtained the hourly climatology data from the Modern-Era Retrospective analysis for Research and Application, Version-2 (MERRA-2) reanalysis product, which spans 39 years (1980–2018) and has a horizontal resolution of 0.5° by latitude [−90–90°] and 0.625° by longitude [−180–179.375°] with 361 × 576 grid cells worldwide 21 , 22 . Here we used the surface incoming shortwave flux [W m −2 ] (variable name: SWGDN), top-of-atmosphere incoming shortwave flux [W m −2 ] (variable name: SWTDN), and surface air temperature [K] (variable name: T) for deriving solar capacity factors; and wind speed at 100 m [m s −1 ], estimated based on wind speed at 10 m and 50 m (variable names: U10M, V10M, U50M, and V50M) and a power-law relationship, to derive wind capacity factors. Wind and solar capacity factors were calculated for each grid cell and each hour. Each raw data point (an hourly energy density (solar) or wind speed (wind) value at a specific location and time) was then converted into the corresponding capacity factor based on the following procedures.
For solar capacity factor, we first calculated the solar zenith angle and the solar incidence angle for each grid based on the latitude/longitude location and local time 46 , 47 , and then estimated the in-panel solar radiation 48 . Here we separated the direct and diffuse solar radiation components based on an empirical piecewise model 49 that takes into account both ratios of surface to top-of-atmosphere solar radiation (i.e., the clearness index) and the local zenith angle. We assumed a horizontal single-axis tracking system (north-south direction) with a tilt of the solar panel to be 0° and a maximum tuning angle of 45°. Solar power output from a given panel is calculated using the performance model described by Huld et al. 50 and Pfenninger and Staffell 51 , which considers both the surrounding temperature and the effect of irradiance. It is noted that we assumed the single-axis trackers for calculating solar capacity factors, which may be unsuitable enough for the small countries such as Japan, South Korea, and United Kingdom. These small countries do not have enough uncommitted land area for that and are going very likely to have to favor no tracking with rooftop photovoltaic system. Therefore, we further assessed the impacts of different solar tracking systems (i.e., single-axis and dual-axis for both a horizontal and a vertical axis) on the electricity system reliability. The detailed comparisons are shown in the SI (Supplementary Note 3 and Supplementary Fig. 10 ).
For wind capacity factor, by assuming a wind turbine hub height of 100 m, the raw wind speed data is first interpolated to 100 m by employing a power law, based on wind speed at 10 and 50 m. The 100-m-height wind speed is estimated by employing the following Eqs. ( 1 ) and ( 2 ):
where \(i,\alpha\) represent grid and alpha exponent for wind profile, and \({U}_{10},{U}_{50},\) and \({U}_{100}\) represent wind speed at 10, 50, and 100 m.
The wind capacity factor calculation employed a piecewise function consisting of four parts: (i) below a cut-in speed ( \({u}_{{ci}}\) ) of 3 m s −1 the capacity factor is zero, (ii) between the cut-in speed of 3 m s −1 and rated speed ( \({u}_{r}\) ) of 12 m s −1 the capacity factor is \({{u}_{{ci}}}^{3}/{{u}_{r}}^{3}\) , (iii) between the rated speed of 12 m s −1 and the cut-out speed ( \({u}_{{co}}\) ) of 25 m s −1 the capacity factor is 1.0, and (iv) above the cut-out speed of 25 m s −1 the capacity factor is zero 12 , 52 , 53 . The process yielded the solar and wind capacity factors for each grid cell and each hour.
An area-weighed mean hourly energy generation profile was created for the solar and wind resources individually for each region of interest. In this work, hourly solar and wind capacity factors for 168 countries/regions were produced. Capacity factors derived from reanalysis data were known to differ from real-world systems 12 , 54 , and thus these calculated capacity factors from the reanalysis dataset were rescaled. That is, the reanalysis data were used herein only for reflecting the temporal and spatial characteristics of the resource. For consistency, we normalized capacity factor values using the 25th percentile calculated capacity factor data for a region of interest due to data availability of real-world wind and solar capacity factors from public datasets or reports for all the countries and regions of interest. Our estimates represent real-world wind and solar capacity factors that are in good agreement with available observational data 55 . We then obtained the time-series hourly normalized wind and solar capacity factor dataset at the country/region level.
Country-level hourly electricity demand data
In this work, country-level hourly electricity demand data were estimated in various ways, such as from government and electricity market websites, public power systems datasets, and previous studies (Supplementary Data 1 ). As shown in Supplementary Data 1 , we compiled 168 countries and regions’ demand data, including real-word hourly demand data of 62 countries and regions, and projected hourly demand data of the rest due to data availability 56 . Toktarova et al. developed a multiple linear regression model to project electricity demand in hourly resolution for all countries globally by incorporating 57 real load data profiles of diverse countries to analyze the cyclical pattern of the data. In addition, given the different self-consistent continuous gapless time series of hourly electricity demand among different countries and regions, a single latest year of hourly electricity demand data was used in our following simulations to investigate the impact of diversity of solar and wind resources across years on power system reliability for countries and regions with available real-world electricity data. For the rest of the countries and regions, we chose the hourly electricity demand data of the most recent year of future (i.e., the year of 2020) from the projection model 56 . We herein obtained the country-level demand dataset by joining the 1-year hourly demand data together 39 times to form a 39-year record consistent with the resource data. In addition, for regional demand data, we combined all the demand data of available countries within the according region at hourly scale to represent the temporal characteristics.
Simulation design
A set of forward simulations were performed to track the ability of wind, solar installed capacity, and energy storage, if present, to meet demand in every hour. In this study, we used a Macro Energy Model (MEM), which is developed for optimizing electricity system (or electricity and fuels) without considering any spatial variation, policy, capacity markets 57 . Without considering any power system cost, generation technology, and transmission loss, we modeled the idealized hourly power supply process through dispatching wind and solar energy, as well as charging or discharging of storage, if present. Here we specified the wind and solar installed capacity, and storage capacity under the various capacity mixes of solar and wind fractions (i.e., every 5% change of solar fraction from 0% solar and 100% wind to 100% solar and 0% wind) and different levels of excess annual generation (i.e., 1x, 1.5x, and 3x generation) and energy storage (i.e., maximun 3 and 12 h of charging time) assumptions. The installed capacities for solar, wind, and storage for individual countries/regions are estimated using the Eqs. ( 3 )–( 5 ).
where \(y\) and \(s\) represent the year and size, respectively. \({{{{Capacity}}}}_{{{{solar}}}}\) , \({{{{Capacity}}}}_{{{{wind}}}}\) , and \({{{{Capacity}}}}_{{{{{{{\mathrm{storage}}}}}}}}\) represent the solar, wind, and storage capacities, respectively. \({{{{{\rm{SF}}}}}}\) represents the fraction of energy generated from solar (from 0 to 100% at intervals of 5%); \({{{{{\rm{OB}}}}}}\) represents the overbuilding of capacity, equaling 1, 1.5, or 3; \({{{{{\rm{Pwr}}}}}}\_{{{{{\rm{avg}}}}}}\) represents the mean power demand; \({{{{{\rm{Hrs}}}}}}\) represents the total hours in the year; \({{{{{\rm{CF}}}}}}_{{solar}}\) and \({{{{{{\rm{CF}}}}}}}_{{{{wind}}}}\) represent normalized capacity factors of solar and wind, respectively. And \({{{{{\rm{Bat}}}}}}\) represents battery storage, equaling 0 (i.e., no storage), 3, or 12.
When storage was assumed to be available, we assumed the initial status of storage was the same as the final status for each year, which means the charging and discharging process is balanced. We also assumed a storage charging round-trip efficiency and storage decay rate of, respectively, 90% and 1.14 × 10 −6 per hour (i.e., 1% of stored electricity lost per month) 2 , reflecting the high-end performance of current batteries 58 , 59 . Dispatchable energy used to charge a battery (called the maximum hourly storage charging) was no more than the storage power rating, equaling storage capacity divided by storage charging time.
Given the restriction of computing resources, we chose ten major countries by comprehensively considering the electricity demand and growth domestic product (GDP) from each continent except Oceania (i.e., Asia, Africa, Europe, and the America), within which only two main countries were selected (i.e., Australia and New Zealand). For each main country, 21 sets of the solar and wind mix from 0% solar and 100% wind to 100% solar and 0% wind with 5% change under 3 groups of overbuilt (1x, 1.5x, and 3x generation) and 3 groups of storage (no storage, 3 h, and 12 h of storage) were simulated, totaling 7938 simulations for all the main countries. To investigate the ability to supply power at multinational regions, continental, and intercontinental scales, we further applied the same simulation design for the main countries to multinational regions, continents, and multi-continental regions (Supplementary Data 4 ). In addition, except the abovementioned main countries, 103,194 one-year simulations consisting of 21 sets of the solar and wind mix with no excess generation or energy storage, were added for each of the remaining 126 countries worldwide.
Hourly electricity supply process
For only solar-wind electricity systems without storage, in a given hour, the MEM model estimates the ability of power to be produced by assessing whether dispatchable solar and wind energy is no less than electricity demand. Excess solar and wind energy can be curtailed due to no available storage. 100% reliability results if the solar and wind power supply system can meet all the electricity demand in every hour of the simulation.
When storage is assumed to be available in a given hour, if the solar and wind energy could meet the electricity demand, storage would be charged with excess solar and wind generation, if available, until the storage is full under the constraint of the maximum hourly storage charging, after which solar and wind energy can be curtailed. In contrast, if wind and solar energy cannot meet electricity demand, storage would be discharged to fill the power supply gap until storage is emptied or the power supply gap is filled.
Here, we define reliability assuming electricity systems use only wind/solar/storage resources to meet current demand for electricity services. If one allows for other backup electricity (e.g., using natural gas with or without CCS), then issues of reliability with excess annual generation and/or storage are largely moot.
Data availability
The electricity demand, solar, and wind capacity factors data generated for this study have been deposited in Dantong2021/Geophysical_constraints: Data of electricity demand, solar and wind capacity factors (v1.0). Zenodo. https://doi.org/10.5281/zenodo.5463202 .
Code availability
The Macro Electricity Model (MEM) code is available on GitHub via https://github.com/ClabEnergyProject/MEM .
Rogelj, J. et al. Energy system transformations for limiting end-of-century warming to below 1.5 °C. Nat. Clim. Change 5 , 519–527 (2015).
Article ADS Google Scholar
Davis, S. J. et al. Net-zero emissions energy systems. Science 360 , eaas9793 (2018).
Article PubMed CAS Google Scholar
Tong, D. et al. Committed emissions from existing energy infrastructure jeopardize 1.5 °C climate target. Nature 572 , 373–377 (2019).
Article CAS PubMed PubMed Central Google Scholar
Luderer, G. et al. The role of renewable energy in climate stabilization: results from the EMF27 scenarios. Clim. Change 123 , 427–441 (2014).
Millstein, D., Wiser, R., Bolinger, M. & Barbose, G. The climate and air-quality benefits of wind and solar power in the United States. Nat. Energy 2 , 17134 (2017).
Pehl, M. et al. Understanding future emissions from low-carbon power systems by integration of life-cycle assessment and integrated energy modelling. Nat. Energy 2 , 939–945 (2017).
Article ADS CAS Google Scholar
Grubler, A. et al. A low energy demand scenario for meeting the 1.5 °C target and sustainable development goals without negative emission technologies. Nat. Energy 3 , 515–527 (2018).
Rogelj, J. et al. In Global warming of 1.5 °C. An IPCC Special Report on the Impacts of Global warming of 1.5 °C above Pre-Industrial Levels and Related Global Greenhouse Gas Emission Pathways, in the Context of Strengthening the Global Response to the Threat of Climate Change, Sustainable Development, and Efforts to Eradicate Poverty (eds Masson-Delmotte, V. et al.) https://www.ipcc.ch/site/assets/uploads/sites/2/2019/05/SR15_Chapter2_Low_Res.pdf (2018).
Rogelj, J. et al. Scenarios towards limiting global mean temperature increase below 1.5 °C. Nat. Clim. Change 8 , 325–332 (2018).
Luderer, G. et al. Environmental co-benefits and adverse side-effects of alternative power sector decarbonization strategies. Nat. Commun. 10 , 1–13 (2019).
Article CAS Google Scholar
Drechsler, M. et al. Efficient and equitable spatial allocation of renewable power plants at the country scale. Nat. Energy 2 , 17124 (2017).
Shaner, M. R., Davis, S. J., Lewis, N. S. & Caldeira, K. Geophysical constraints on the reliability of solar and wind power in the United States. Energy Environ. Sci. 11 , 914–925 (2018).
Article Google Scholar
Jacobson, M. Z. et al. 100% clean and renewable wind, water, and sunlight all-sector energy roadmaps for 139 countries of the world. Joule 1 , 108–121 (2017).
Heard, B. P., Brook, B. W., Wigley, T. M. L. & Bradshaw, C. J. A. Burden of proof: a comprehensive review of the feasibility of 100% renewable-electricity systems. Renew. Sust. Energ. Rev. 76 , 1122–1133 (2017).
Arbabzadeh, M., Sioshansi, R., Johnson, J. X. & Keoleian, G. A. The role of energy storage in deep decarbonization of electricity production. Nat. Commun. 10 , 1–11 (2019).
CAS Google Scholar
Sepulveda, N. A., Jenkins, J. D., de Sisternes, F. J. & Lester, R. K. The role of firm low-carbon electricity resources in deep decarbonization of power generation. Joule 2 , 2403–2420 (2018).
Budischak, C. et al. Cost-minimized combinations of wind power, solar power and electrochemical storage, powering the grid up to 99.9% of the time. J. Power Sources 225 , 60–74 (2013).
Planning Resource Adequacy Analysis, Assessment and Documentation (Standard BAL-502-RF-03). https://www.nerc.com/pa/Stand/Reliability%20Standards/BAL-502-RF-03.pdf (2017).
Carden. K., Wintermantel, N. & Pfeifenberger J. The Economics of Resource Adequacy Planning: Why Reserve Margins Are Not Just About Keeping the Lights On (National Regulatory Research Institute, 2011).
Jurasz, J., Canales, F. A., Kies, A., Guezgouz, M. & Beluco, A. A review on the complementarity of renewable energy sources: concept, metrics, application and future research directions. Sol. Energy 195 , 703–724 (2020).
Modern-Era Retrospective analysis for Research and Applications, Version 2 (MERRA-2). https://gmao.gsfc.nasa.gov/reanalysis/MERRA-2/ (2021).
Gelaro, R. et al. The modern-era retrospective analysis for research and applications, version 2 (MERRA-2). J. Clim. 30 , 5419–5454 (2017).
Article ADS PubMed Google Scholar
Lee, J. C. Y., Fields, M. J. & Lundquist, J. K. Assessing variability of wind speed: comparison and validation of 27 methodologies. Wind Energy Sci . 3 , 845–868 (2018).
Hyndman, R. J. & Fan, S. Density forecasting for long-term peak electricity demand. IEEE T. Power Syst. 25 , 1142–1153 (2010).
Mai, T., Sandor, D., Wiser, R. & Schneider, T. Renewable Electricity Futures Study: Executive Summary Vol. 55, https://www.nrel.gov/docs/fy13osti/52409-ES.pdf (2012).
Brinkerink, M., Gallachóir, B. Ó. & Deane, P. A comprehensive review on the benefits and challenges of global power grids and intercontinental interconnectors. Renew. Sust. Energ. Rev. 107 , 274–287 (2019).
Craig, M. Germany’s worse-case scenario in the power sector. https://energytransition.org/2017/07/germanys-worse-case-scenario-in-the-power-sector/#more-15335 (2017).
Dowling, J. A. et al. Role of long-duration energy storage in variable renewable electricity systems. Joule 4 , 1907–1928 (2020).
Larson, E. et al. Net-Zero America: Potential Pathways, Infrastructure, and Impacts . Interim report, Princeton University, Princeton, NJ (2020).
Jourdier, B. Evaluation of ERA5, MERRA-2, COSMO-REA6, NEWA and AROME to simulate wind power production over France. Adv. Sci. Res. 17 , 63 (2020).
Sianturi, Y., Marjuki & Sartika, K. Evaluation of ERA5 and MERRA2 reanalyses to estimate solar irradiance using ground observations over Indonesia region. AIP Conf. Proc. 2223 , 020002 (2020).
Battery Megafactory Forecast: 400% Increase in Capacity to 1 TWh by 2028. https://www.visualcapitalist.com/battery-megafactory-forecast-1-twh-capacity-2028/ (2018).
The International Renewable Energy Agency (IRENA): Renewable Capacity Statistics 2018. https://www.hydroreview.com/2018/04/10/data-source-irena-releases-renewable-capacity-statistics-2018-report/#gref (2018).
Braff, W. A., Mueller, J. M. & Trancik, J. E. Value of storage technologies for wind and solar energy. Nat. Clim. Change 6 , 964–969 (2016).
Kittner, N., Lill, F. & Kammen, D. M. Energy storage deployment and innovation for the clean energy transition. Nat. Energy 2 , 17125 (2017).
Sepulveda, N. A., Jenkins, J. D., Edington, A., Mallapragada, D. S. & Lester, R. K. The design space for long-duration energy storage in decarbonized power systems. Nat. Energy 6 , 506–516 (2021).
Ziegler, M. S. et al. Storage requirements and costs of shaping renewable energy toward grid decarbonization. Joule 3 , 2134–2153 (2019).
Wu, G. C. et al. Strategic siting and regional grid interconnections key to low-carbon futures in African countries. Proc. Natl Acad. Sci. USA 114 , E3004–E3012 (2017).
CAS PubMed PubMed Central Google Scholar
Clack, C. T. M. et al. Evaluation of a proposal for reliable low-cost grid power with 100% wind, water, and solar. Proc. Natl Acad. Sci. USA 114 , 6722–6727 (2017).
Article ADS CAS PubMed PubMed Central Google Scholar
Barasa, M., Bogdanov, D., Oyewo, A. S. & Breyer, C. A cost optimal resolution for Sub-Saharan Africa powered by 100% renewables in 2030. Renew. Sust. Energ. Rev. 92 , 440–457 (2018).
Geels, F. W., Sovacool, B. K., Schwanen, T. & Sorrell, S. The socio-technical dynamics of low-carbon transitions. Joule 1 , 463–479 (2017).
Lauber, V. & Jacobsson, S. The politics and economics of constructing, contesting and restricting socio-political space for renewables—The German Renewable Energy Act. Environ. Innov. Soc. Tr. 18 , 147–163 (2016).
Mai, T. et al. Electrification Futures Study: Scenarios of Electric Technology Adoption and Power Consumption for the United States . National Renewable Energy Laboratory. NREL/TP-6A20-71500. https://doi.org/10.2172/1459351 (2018).
Williams, J. H. et al. Carbon-neutral pathways for the United States. AGU Adv. 2 , e2020AV000284 (2021).
MacDonald, A. E. et al. Future cost-competitive electricity systems and their impact on US CO 2 emissions. Nat. Clim. Change 6 , 526–531 (2016).
Braun, J. E. & Mitchell, J. C. Solar geometry for fixed and tracking surfaces. Sol. Energy 31 , 439–444 (1983).
Meeus, J. H. Astronomical Algorithms (Willmann-Bell, Incorporated, 1991).
Perez, R., Ineichen, P., Seals, R., Michalsky, J. & Stewart, R. Modeling daylight availability and irradiance components from direct and global irradiance. Sol. Energy 44 , 271–289 (1990).
Reindl, D. T., Beckman, W. A. & Duffie, J. A. Diffuse fraction correlations. Sol. Energy 45 , 1–7 (1990).
Huld, T., Gottschalg, R., Beyer, H. G. & Topič, M. Mapping the performance of PV modules, effects of module type and data averaging. Sol. Energy 84 , 324–338 (2010).
Pfenninger, S. & Staffell, I. Long-term patterns of European PV output using 30 years of validated hourly reanalysis and satellite data. Energy 114 , 1251–1265 (2016).
Bett, P. E. & Thornton, H. E. The climatological relationships between wind and solar energy supply in Britain. Renew. Energy 87 , 96–110 (2016).
Rose, S. & Apt, J. Quantifying sources of uncertainty in reanalysis derived wind speed. Renew. Energy 94 , 157–165 (2016).
Staffell, I. & Pfenninger, S. Using bias-corrected reanalysis to simulate current and future wind power output. Energy 114 , 1224–1239 (2016).
Form EIA-860 detailed data with previous form data (EIA-860A/860B). https://www.eia.gov/electricity/data/eia860/ (2019).
Toktarova, A., Gruber, L., Hlusiak, M., Bogdanov, D. & Breyer, C. Long term load projection in high resolution for all countries globally. Int. J. Elec. Power 111 , 160–181 (2019).
Macro Energy Model (MEM). https://github.com/ClabEnergyProject/MEM (2019).
Pellow, M. A., Emmott, C. J., Barnhart, C. J. & Benson, S. M. Hydrogen or batteries for grid storage? A net energy analysis. Energy Environ. Sci. 8 , 1938–1952 (2015).
Wang, H., Jiang, Y. & Manthiram, A. Long cycle life, low self-discharge sodium–selenium batteries with high selenium loading and suppressed polyselenide shuttling. Adv. Energy Mater. 8 , 1701953 (2018).
Download references
Acknowledgements
This work was supported by the National Natural Science Foundation of China (41921005). D.T., D.J.F., L.D., and K.C. were supported by the Carnegie Institution for Science endowment and a gift from Gates Ventures, Inc. S.J.D. was supported by the US National Science Foundation (Innovations at the Nexus of Food, Energy and Water Systems (INFEWS) grant EAR 1639318).
Author information
Authors and affiliations.
Ministry of Education Key Laboratory for Earth System Modeling, Department of Earth System Science, Tsinghua University, Beijing, 100084, China
Dan Tong & Qiang Zhang
Department of Earth System Science, University of California, Irvine, CA, 92697, USA
Dan Tong & Steven J. Davis
Department of Global Ecology, Carnegie Institution for Science, Stanford, CA, 94035, USA
Dan Tong, David J. Farnham, Lei Duan, Nathan S. Lewis, Ken Caldeira & Steven J. Davis
Division of Chemistry and Chemical Engineering, California Institute of Technology, Pasadena, CA, 91125, USA
Nathan S. Lewis
Breakthrough Energy, 4110 Carillon Pt., Kirkland, WA, 98033, USA
Ken Caldeira
Department of Civil and Environmental Engineering, University of California, Irvine, CA, 92697, USA
Steven J. Davis
You can also search for this author in PubMed Google Scholar
Contributions
K.C., N.S.L., S.J.D., and D.T. designed the study. D.T. performed the analyses, with support from D.J.F. on simulations and L.D. on resources estimates, and from S.J.D., Q.Z., N.S.L., and K.C. on analytical approaches. D.T. and S.J.D. led the writing with input from all coauthors.
Corresponding author
Correspondence to Dan Tong .
Ethics declarations
Competing interests.
The authors declare no competing interests.
Additional information
Peer review information Nature Communications thanks Jesse Jenkin and the other, anonymous, reviewer(s) for their contribution to the peer review of this work. Peer reviewer reports are available.
Publisher’s note Springer Nature remains neutral with regard to jurisdictional claims in published maps and institutional affiliations.
Supplementary information
Supplementary information, peer review file, description of additional supplementary files, supplementary data 1, supplementary data 2, supplementary data 3, supplementary data 4, supplementary data 5, supplementary data 6, rights and permissions.
Open Access This article is licensed under a Creative Commons Attribution 4.0 International License, which permits use, sharing, adaptation, distribution and reproduction in any medium or format, as long as you give appropriate credit to the original author(s) and the source, provide a link to the Creative Commons license, and indicate if changes were made. The images or other third party material in this article are included in the article’s Creative Commons license, unless indicated otherwise in a credit line to the material. If material is not included in the article’s Creative Commons license and your intended use is not permitted by statutory regulation or exceeds the permitted use, you will need to obtain permission directly from the copyright holder. To view a copy of this license, visit http://creativecommons.org/licenses/by/4.0/ .
Reprints and permissions
About this article
Cite this article.
Tong, D., Farnham, D.J., Duan, L. et al. Geophysical constraints on the reliability of solar and wind power worldwide. Nat Commun 12 , 6146 (2021). https://doi.org/10.1038/s41467-021-26355-z
Download citation
Received : 24 February 2021
Accepted : 24 September 2021
Published : 22 October 2021
DOI : https://doi.org/10.1038/s41467-021-26355-z
Share this article
Anyone you share the following link with will be able to read this content:
Sorry, a shareable link is not currently available for this article.
Provided by the Springer Nature SharedIt content-sharing initiative
This article is cited by
- Anders Wörman
- Ilias Pechlivanidis
- Cintia Bertacchi Uvo
Communications Engineering (2024)
An assessment of different transition pathways to a green global economy
- Soheil Shayegh
- Severin Reissl
- Matteo Calcaterra
Communications Earth & Environment (2023)
Reliance on fossil fuels increases during extreme temperature events in the continental United States
- Pierre Gentine
Global transcontinental power pools for low-carbon electricity
- Haozhe Yang
- Ranjit Deshmukh
- Sangwon Suh
Nature Communications (2023)
Climate influence on compound solar and wind droughts in Australia
- D. Richardson
- A. J. Pitman
- N. N. Ridder
npj Climate and Atmospheric Science (2023)
By submitting a comment you agree to abide by our Terms and Community Guidelines . If you find something abusive or that does not comply with our terms or guidelines please flag it as inappropriate.
Quick links
- Explore articles by subject
- Guide to authors
- Editorial policies
Sign up for the Nature Briefing newsletter — what matters in science, free to your inbox daily.

Long, short, and medium terms wind speed prediction model based on LSTM optimized by improved moth flame optimization algorithm
- Research Article
- Published: 21 May 2024
Cite this article
- Runze Li 1 ,
- Jianzhou Wang ORCID: orcid.org/0000-0001-6070-9888 1 ,
- Jingrui Li 1 &
- Menggang Kou 1
97 Accesses
Explore all metrics
Time series prediction of wind speed has been widely used in wind power generation. The volatility and instability of wind speed have a large negative impact on wind turbines and power systems, which can lead to grid collapse in severe cases. Therefore, accurate wind speed prediction is crucial for wind power generation. In this paper, considering the influence of different parameters on algorithm training and prediction, an improved moth flame optimization algorithm is constructed to optimize the LSTM wind energy prediction system to obtain better performance. The system consists of three modules: data preprocessing, optimization, and prediction. The data preprocessing module uses fuzzy information granulation to blur the input data. On this basis, the combination of swarm intelligent optimization algorithm and prediction model can effectively predict wind speed time series. Taking the California wind farm as an example, the MAPE of the experiment in the short-term forecast is 3.15%, the MAPE of the medium-term forecast is 4.38%, and the MAPE of the long-term forecast is 18.28%. The experimental results show that the proposed model has obvious advantages over the previous model.
This is a preview of subscription content, log in via an institution to check access.

Access this article
Price includes VAT (Russian Federation)
Instant access to the full article PDF.
Rent this article via DeepDyve
Institutional subscriptions
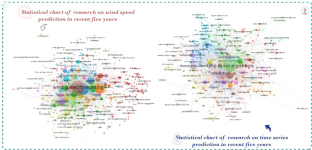
Similar content being viewed by others
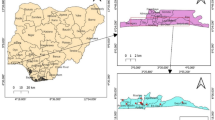
Investigating the influence of clustering techniques and parameters on a hybrid PSO-driven ANFIS model for electricity prediction
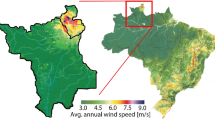
Exploring wind energy for small off-grid power generation in remote areas of Northern Brazil
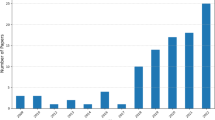
A guided genetic algorithm-based ensemble voting of polynomial regression and LSTM (GGA-PolReg-LSTM) for congestion prediction using IoT and air quality data in sustainable cities
Data availability.
The data sets generated and analyzed during the current study are available from the corresponding author upon reasonable request.
Abou Houran M, Bukhari SMS, Zafar MH, Mansoor M, Chen W (2023) COA-CNN-LSTM: cCoati optimization algorithm-based hybrid deep learning model for PV/wind power forecasting in smart grid applications, Applied Energy, Volume 349. ISSN 121638:0306–2619. https://doi.org/10.1016/j.apenergy.2023.121638
Article Google Scholar
Abualigah L, Hanandeh ES, Zitar RA, Thanh CL, Khatir S, Gandomi AH (2023) Revolutionizing sustainable supply chain management: aA review of metaheuristics, Engineering Applications of Artificial Intelligence, Volume 126, Part A. ISSN 106839:0952–1976. https://doi.org/10.1016/j.engappai.2023.106839
Al-qaness MA, Ewees AA, Fan H, Abualigah L, Abd Elaziz M (2022) Boosted ANFIS model using augmented marine predator algorithm with mutation operators for wind power forecasting. Appl Energy 314:118851
Cai Z, Dai S, Ding Q, Zhang J, Xu D, Li Y (2023) Gray wolf optimization-based wind power load mid-long term forecasting algorithm. Comput Electr Eng 109:108769
Dai Z, Zhang Z, Chen M (2023) The home health care location-routing problem with a mixed fleet and battery swapping stations using a competitive simulated annealing algorithm, Expert Systems with Applications, Volume 228. ISSN 120374:0957–4174. https://doi.org/10.1016/j.eswa.2023.120374
Ehteram M, Ghanbari-Adivi E (2023) Self-attention (SA) temporal convolutional network (SATCN)-long short-term memory neural network (SATCN-LSTM): an advanced python code for predicting groundwater level. Environ Sci Pollut Res 30:92903–92921. https://doi.org/10.1007/s11356-023-28771-8
Gao P, Zhou L, Zhao X, Shao B (2023) Research on ship collision avoidance path planning based on modified potential field ant colony algorithm, Ocean & Coastal Management, Volume 235. ISSN 106482:0964–5691. https://doi.org/10.1016/j.ocecoaman.2023.106482
Gers FA, Schmidhuber J, Cummins F (2000) Learning to forget: continual prediction with LSTM. Neural Computation 12(10):2451–2471. https://doi.org/10.1162/089976600300015015
Article CAS Google Scholar
Graves A, Fernández S, Schmidhuber J (2005) Bidirectional LSTM networks for improved phoneme classification and recognition. In International conference on artificial neural networks. Springer, Berlin Heidelberg, Berlin, Heidelberg
Google Scholar
Han B, Li B, Qin C (2023) A novel hybrid particle swarm optimization with marine predators, Swarm and Evolutionary Computation. ISSN 101375:2210–6502. https://doi.org/10.1016/j.swevo.2023.101375
He P, Fang Q, Jin H, Ji Y, Gong Z, Dong J (2022) Coordinated design of PSS and STATCOM-POD based on the GA-PSO algorithm to improve the stability of wind-PV-thermal-bundled power system, International Journal of Electrical Power & Energy Systems, Volume 141. ISSN 108208:0142–0615. https://doi.org/10.1016/j.ijepes.2022.108208
Hochreiter S, Schmidhuber J (1997) Long short-term memory. Neural Computation 9(8):1735–1780. https://doi.org/10.1162/neco.1997.9.8.1735
Huang Y, Li Y, Zhang Z, Sun Q (2023) A novel path planning approach for AUV based on improved whale optimization algorithm using segment learning and adaptive operator selection, Ocean Engineering, Volume 280. ISSN 114591:0029–8018. https://doi.org/10.1016/j.oceaneng.2023.114591
Khamees AK, Abdelaziz AY, Ali ZM, Alharthi MM, Ghoneim SS, Eskaros MR, Attia MA (2022) Mixture probability distribution functions using novel metaheuristic method in wind speed modeling, Ain Shams Engineering Journal, 13(3). ISSN 101613:2090–4479. https://doi.org/10.1016/j.asej.2021.10.008
Li J, Song Z, Wang X, Wang Y, Jia Y (2022) A novel offshore wind farm typhoon wind speed prediction model based on PSO–Bi-LSTM improved by VMD, Energy, Volume 251. ISSN 123848:0360–5442. https://doi.org/10.1016/j.energy.2022.123848
Li H, You X, Liu S (2023) Multi-ant colony optimization algorithm based on finite history archiving and boxed pigs game, Applied Soft Computing, Volume 138. ISSN 110193:1568–4946. https://doi.org/10.1016/j.asoc.2023.110193
Liu J, Han C (2023) Design and optimization of heat extraction section in energy tunnel using simulated annealing algorithm, Renewable Energy, Volume 213. ISSN 218–232:0960–1481. https://doi.org/10.1016/j.renene.2023.05.135
Liu L, Wang Q, Wang J, Liu M (2014) A rolling grey model optimized by particle swarm optimization in economic prediction. Comput Intell 32(3):391–419. https://doi.org/10.1111/coin.12059
Liu X, Lin Z, Feng Z (2021) Short-term offshore wind speed forecast by seasonal ARIMA - aA comparison against GRU and LSTM, Energy, Volume 227. ISSN 120492:0360–5442. https://doi.org/10.1016/j.energy.2021.120492
Luo J, Gong Y (2023) Air pollutant prediction based on ARIMA-WOA-LSTM model, Atmospheric Pollution Research, 14(6). ISSN 101761:1309–1042. https://doi.org/10.1016/j.apr.2023.101761
May R (1976) Simple mathematical models with very complicated dynamics. Nature 261:459–467. https://doi.org/10.1038/261459a0
Mirjalili S (2015) Moth-flame optimization algorithm: A a novel nature-inspired heuristic paradigm, Knowledge-Based Systems, Volume 89. ISSN 228–249:0950–7051. https://doi.org/10.1016/j.knosys.2015.07.006
Nguyen THT, Phan QB (2022) Hourly day ahead wind speed forecasting based on a hybrid model of EEMD, CNN-Bi-LSTM embedded with GA optimization, Energy Reports, Volume 8, Supplement 10. ISSN 53–60:2352–4847. https://doi.org/10.1016/j.egyr.2022.05.110
Pan S, Yang B, Wang S, Guo Z, Wang L, Liu J, Wu S (2021) Oil well production prediction based on CNN-LSTM model with self-attention mechanism, Energy, Volume 284. ISSN 128701:0360–5442. https://doi.org/10.1016/j.energy.2023.128701
Patidar H, Shende V, Baredar P et al (2023) Comparative evaluation of optimal Weibull parameters for wind power predictions using numerical and metaheuristic optimization methods for different Indian terrains. Environ Sci Pollut Res 30:30874–30891. https://doi.org/10.1007/s11356-022-24395-6
Pedrycz W (1991) Neurocomputations in relational systems. IEEE Trans Pattern Anal Mach Intell 13(3):289–297. https://doi.org/10.1109/34.75517
Qian Y, Wang J, Zhang H et al (2023) Research of a combination system based on fuzzy sets and multi-objective marine predator algorithm for point and interval prediction of wind speed. Environ Sci Pollut Res 30:35781–35807. https://doi.org/10.1007/s11356-022-23773-4
Rahmatian M, Nazarian A, Moeini S (2023) Single-objective optimization design of convergent-divergent ducts of ducted wind turbine using RSM and GA, to increase power coefficient of a small-scale horizontal axis wind turbine, Energy, Volume 269. ISSN 126822:0360–5442. https://doi.org/10.1016/j.energy.2023.126822
Santos VO, Rocha PAC, Scott J, Thé JVG, Gharabaghi B (2023) Spatiotemporal analysis of bidimensional wind speed forecasting: dDevelopment and thorough assessment of LSTM and ensemble graph neural networks on the Dutch database, Energy, Volume 278, Part A. ISSN 127852:0360–5442. https://doi.org/10.1016/j.energy.2023.127852
Singh M, Singh U (2020) An optimized moth-flame optimization algorithm for solving mechanical engineering problems. J Comput Design Eng 7(2):169–175. https://doi.org/10.1016/j.jcde.2019.09.005
Su J, Fu Y, Gao K, Dong H, Mou J (2023) Integrated scheduling problems of open shop and vehicle routing using an ensemble of group teaching optimization and simulated annealing, Swarm and Evolutionary Computation, Volume 83. ISSN 101373:2210–6502. https://doi.org/10.1016/j.swevo.2023.101373
Tang G, Chiclana F, Liu P (2020) A decision-theoretic rough set model with q-rung orthopair fuzzy information and its application in stock investment evaluation, Applied Soft Computing, Volume 91. ISSN 106212:1568–4946. https://doi.org/10.1016/j.asoc.2020.106212
Tang Y, Yu F, Pedrycz W, Yang X, Wang J, Liu S (2022) building trend fuzzy granulation-based LSTM recurrent neural network for long-term time-series forecasting. IEEE Trans Fuzzy Syst 30(6):1599–1613. https://doi.org/10.1109/TFUZZ.2021.3062723
Wang H, Wu M (2018) Global shape optimization of free-form cable-stiffened latticed shell based on local optimal solutions, Engineering Structures, Volume 168. ISSN 576–588:0141–0296. https://doi.org/10.1016/j.engstruct.2018.05.008
Wang JZ, Li YJ, Sun YQ, Li HM, Zhang WG (2019) Multiscale decomposition and fuzzy information granulation LSTM model for wind speed forecasting. Appl Soft Comput 84:105653. https://doi.org/10.1016/j.asoc.2019.105653
Wang J, Li H, Wang Y, Lu H (2021) A hesitant fuzzy wind speed forecasting system with novel defuzzification method and multi-objective optimization algorithm, Expert Systems with Applications, Volume 168. ISSN 114364:0957–4174. https://doi.org/10.1016/j.eswa.2020.114364
Wang J, Wang S, Zeng B, Lu H (2022) A novel ensemble probabilistic forecasting system for uncertainty in wind speed, Applied Energy, Volume 313. ISSN 118796:0306–2619. https://doi.org/10.1016/j.apenergy.2022.118796
Wang J, Zhou Y, Jiang H (2023b) A novel interval system based on multi-objective optimization and hybrid data reconstruct strategy, Expert Systems with Applications, Volume 217. ISSN 119539:0957–4174. https://doi.org/10.1016/j.eswa.2023.119539
Wang K, Hua Y, Huang L, Guo X, Liu X, Ma Z, Ma R, Jiang X (2023c) A novel GA-LSTM-based prediction method of ship energy usage based on the characteristics analysis of operational data, Energy. ISSN 128910:0360–5442. https://doi.org/10.1016/j.energy.2023.128910
Wang W, Wang Q, Zhong R, Chen L, Shi X (2023d) Stacking sequence optimization of arbitrary quadrilateral laminated plates for maximum fundamental frequency by hybrid whale optimization algorithm, Composite Structures, Volume 310. ISSN 116764:0263–8223. https://doi.org/10.1016/j.compstruct.2023.116764
Wang J, Bei J, Song H, Zhang H, Zhang P (2023e) A whale optimization algorithm with combined mutation and removing similarity for global optimization and multilevel thresholding image segmentation. Appl Soft Computing 137:110130. https://doi.org/10.1016/j.asoc.2023.110130
Wang, J, Wang, K, Li, Z, Lu, H, Jiang, H, Xing, Q (2023a) A multitask integrated deep-learning probabilistic prediction for load forecasting, in IEEE Trans Power Syst, https://doi.org/10.1109/TPWRS.2023.3257353
Wei J, Wu X, Yang T, Jiao R (2023) Ultra-short-term forecasting of wind power based on multi-task learning and LSTM, International Journal of Electrical Power & Energy Systems, Volume 149. ISSN 109073:0142–0615. https://doi.org/10.1016/j.ijepes.2023.109073
Wen S, Wang H, Qian J, Men X (2023) A novel combined model based on echo state network optimized by whale optimization algorithm for blast furnace gas prediction. Energy 279:128048. https://doi.org/10.1016/j.energy.2023.128048
Yang B, Wu L, Xiong J, Zhang Y, Chen L (2023) Location and path planning for urban emergency rescue by a hybrid clustering and ant colony algorithm approach, Applied Soft Computing. ISSN 110783:1568–4946. https://doi.org/10.1016/j.asoc.2023.110783
Yuan E, Yang G (2023) SA–EMD–LSTM: a novel hybrid method for long-term prediction of classroom PM25 concentration. Exp Syst Appl 230:120670. https://doi.org/10.1016/j.eswa.2023.120670
Zadeh LA (1965) Fuzzy Sets. Inf Control 8(3):338–353
Zhang Y, Pan G, Chen B, Han J, Zhao Y, Zhang C (2020) Short-term wind speed prediction model based on GA-ANN improved by VMD, Renewable Energy, Volume 156. ISSN 1373–1388:0960–1481. https://doi.org/10.1016/j.renene.2019.12.047
Zhang L, Wang J, Li Z, Zeng B, Huang X (2022) Uncertainty quantification of PM2.5 concentrations using a hybrid model based oncharacteristic decomposition and fuzzy granulation. J Environ Manag 324:116282. https://doi.org/10.1016/j.jenvman.2022.116282
Zhao X, Fang Y, Ma S, Liu Z (2022) Multi-swarm improved moth–flame optimization algorithm with chaotic grouping and Gaussian mutation for solving engineering optimization problems, Expert Systems with Applications, Volume 204. ISSN 117562:0957–4174. https://doi.org/10.1016/j.eswa.2022.117562
Zheng L, Lu W, Zhou Q (2023) Weather image-based short-term dense wind speed forecast with a ConvLSTM-LSTM deep learning model, Building and Environment, Volume 239. ISSN 110446:0360–1323. https://doi.org/10.1016/j.buildenv.2023.110446
Zhou Y, Wang J, Lu H, Zhao W (2022) Short-term wind power prediction optimized by multi-objective dragonfly algorithm based on variational mode decomposition, Chaos, Solitons & Fractals, Volume 157. ISSN 111982:0960–779. https://doi.org/10.1016/j.chaos.2022.111982
Zhu Y (2023) Research on adaptive combined wind speed prediction for each season based on improved gray relational analysis. Environ Sci Pollut Res 30:12317–12347. https://doi.org/10.1007/s11356-022-22957-2
Download references
This work was supported by the General Scientific Research Funding of the Science and Technology Development Fund (FDCT) in Macao under grant 0150/2022/A and the Faculty Research Grants of Macau University of Science and Technology (FRG-22–074-FIE).
Author information
Authors and affiliations.
Institute of Systems Engineering, Macau University of Science and Technology, Macau, 999078, China
Runze Li, Jianzhou Wang, Jingrui Li & Menggang Kou
You can also search for this author in PubMed Google Scholar
Contributions
Conceptualization: Jianzhou Wang. Methodology: Jingrui Li. Formal analysis and investigation: Runze Li. Writing—original draft preparation: Runze Li. Writing—review and editing: Jianzhou Wang. Funding acquisition: Jianzhou Wang. Resources: Menggang Kou. Supervision: Jianzhou Wang
Corresponding author
Correspondence to Jianzhou Wang .
Ethics declarations
Ethics approval.
Not applicable
Consent to participate
Consent for publication, competing interests.
The authors declare no competing interests.
We have not submitted our manuscript to a preprint server before submitting it to Environmental Science and Pollution Research.
Additional information
Responsible Editor: Marcus Schulz
Publisher's Note
Springer Nature remains neutral with regard to jurisdictional claims in published maps and institutional affiliations.
Rights and permissions
Springer Nature or its licensor (e.g. a society or other partner) holds exclusive rights to this article under a publishing agreement with the author(s) or other rightsholder(s); author self-archiving of the accepted manuscript version of this article is solely governed by the terms of such publishing agreement and applicable law.
Reprints and permissions
About this article
Li, R., Wang, J., Li, J. et al. Long, short, and medium terms wind speed prediction model based on LSTM optimized by improved moth flame optimization algorithm. Environ Sci Pollut Res (2024). https://doi.org/10.1007/s11356-024-33580-8
Download citation
Received : 06 January 2024
Accepted : 01 May 2024
Published : 21 May 2024
DOI : https://doi.org/10.1007/s11356-024-33580-8
Share this article
Anyone you share the following link with will be able to read this content:
Sorry, a shareable link is not currently available for this article.
Provided by the Springer Nature SharedIt content-sharing initiative
- Fuzzy information granulation
- Wind speed prediction
- Improved moth flame optimization algorithm
- Long and short terms memory network
- Find a journal
- Publish with us
- Track your research
- Reference Manager
- Simple TEXT file
People also looked at
Review article, wind energy-harvesting technologies and recent research progresses in wind farm control models.
- 1 Bahir Dar Energy Center, Bahir Dar Institute of Technology, Bahir Dar University, Bahir Dar, Ethiopia
- 2 Department of Physics, College of Natural and Computational Science, Wolaita Sodo University, Sodo, Ethiopia
- 3 Department of Physics, Addis Ababa University, Addis Ababa, Ethiopia
In order to sustain the overall competitiveness of the wind power industry, unrelenting focus is required on working toward the advancement of enabling technologies and research studies that are associated with wind farm systems. First, wind farm technologies that include various turbine generator systems coupled with different power transmission configurations have enormous impact in determining the quality of wind power production. In addition, modern wind farms are expected to implement robust power control algorithms to meet more advanced requirements of electricity generation. Accordingly, this study explores the statuses of wind energy harvesting technologies and wind farm control strategies by discussing their recent and future impact on transforming the wind power industry. Doubly fed induction generator (DFIG)-based wind energy harvesting technology is well-matured and has exhibited an excellent track-record in past and recent experiences, but its capability of being further scalable for large-scale power production is limited as it is largely incompatible with high-voltage power transmission networks. On the other hand, permanent magnet synchronous generator (PMSG)-based technology is making significant advancements to attain the maximum possible efficiency level in greatly facilitating larger scale power generation, although the construction of bulky and costly power transmission systems is required. In this regard, future technological advances in the wind farm industry are expected to reasonably optimize the design and cost of high-voltage power transmission systems. Similarly, an increasing number of research studies are introducing a number of power optimization-based control models to create an ideal integration of the aforementioned wind farm technologies so as to ultimately enhance the reliability of electricity production by maintaining the systems’ safety. Yet, additional work is still expected to be undertaken in the future for a more extended evaluation of the performances of many different control models under a similar environment.
1 Introduction
As a part of ensuring successful improvements in global cumulative installations of wind power as shown in Figure 1 , various systems of wind power technologies were proposed, developed, and used by researchers, manufacturers, and wind farm industries as the solutions for enhancing the extraction and transportation of onshore and offshore wind energy. In this regard, generator technologies and wind farm transmission systems have a considerable cumulative effect on onshore and offshore power production. More interestingly, wind generators and power transmission systems provide researchers and engineers with the options required for the significant achievement of wind power generation objectives, including a reduction in energy costs and maximization in wind power production. Consequently, the scale and cost of wind power production largely rely on the efficiencies, reliabilities, and configurations of the generators and power transmission systems ( Cheng and Zhu, 2014 ; Biswas et al., 2021 ). In the case of generator systems, two main technologies are well-proven to be the leading candidates for onshore and offshore wind power applications: a partial-scale converter-based doubly fed induction generator (DFIG) system is widely popular for its better compatibility with onshore power generation ( Mwaniki et al., 2017a ), whereas a full-scale converter-based permanent magnet synchronous generator (PMSG)-based system is regarded as an attractive solution for offshore and multi-mega-scale wind power generation ( Mohan and Vittal, 2018 ). Moreover, compared to the partial-scale converter DFIG-based wind farm, the reactive power capability of a full-scale converter PMSG-based wind farm can be significantly extended, and the grid-side converter in each configuration unit can provide the required reactive power locally. Regardless of its higher cost, full-scale converter-based wind energy conversion technology nowadays receives increasing recognition because of its superior performing efficiency and reliability, particularly in offshore wind farm applications ( Chaithanya et al., 2019 ; Yaramasu and Wu, 2016 ).
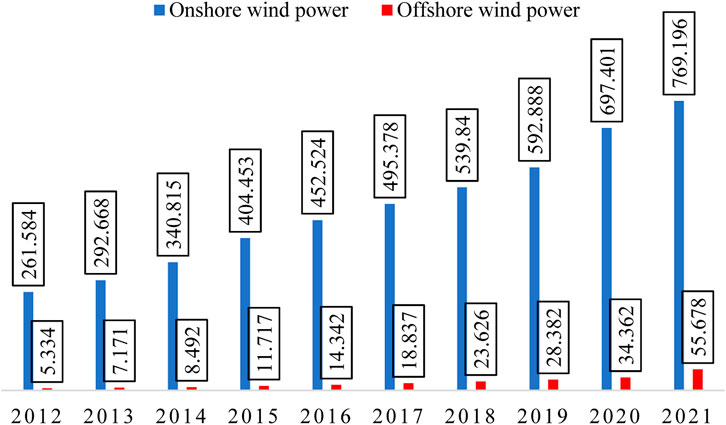
FIGURE 1 . Global onshore and offshore wind power installation trends (in GW) from 2012 to 2021 ( I. Renewable Energy Agency, 2022 ).
Two options of electric power transmission systems can be implemented in interconnecting power generation systems (electric generators and power electronics converters) with wind farm substations, which are high-voltage alternating current (HVAC) and high-voltage direct current (HVDC). The HVAC transmission option is efficient and economical for the wind power industries for which the power substations can be built closer to the power-generating points. More specifically, when the distances between power-generating points and substations can be limited to shorter ranges (usually less than 50 km), the HVAC system shows excellent power transmission capability and economic benefits compared with the HVDC system for both onshore and offshore power applications ( Wei et al., 2017 ). Nevertheless, substations are usually commissioned at points very far away from power-generating sites, particularly in the case of multi-mega-scale offshore wind power generation. Under this situation (for longer transmission lines and multi-scale offshore power generation), the HVAC option is not compatible with the power transmission application. The reason is that HVAC transmission cables are inherently characterized by a large capacitance per length, and thus, in addition to electrical current delivery, power transmission via extended cables of HVAC could also cause generation of capacitive currents. These capacitive currents are regularly rippling, and this significantly affects the transmission capability of electrical currents, and hence, the quality of power production. Consequently, excessive reactive power is required for the HVAC extended power transmission cables. This reactive power requirement can be met by making use of reactive shunt compensation; however, this results in the addition of extra expenses related to capital and operating costs ( Machado et al., 2015 ).
Unlike HVAC-based configuration, the HVDC system is the standard solution in terms of power transmission capability and cost-effectiveness for applications of offshore wind farms that are commissioned far away (usually greater than 140 km) from power load centers. Moreover, the operation of HVDC-based power transmission configurations can be effectively scalable and no reactive power compensators are required at longer ranges. Different configurations of AC and DC power converter-connected HVDC transmission systems can be used for offshore wind farm applications ( Kalair et al., 2016 ; Ryndzionek and Sienkiewicz, 2020 ). Currently, modular multilevel voltage source converter (MM VSC)-connected HVDC in AC parallel configuration, with 864 MW capacity, a voltage of ± 320 k V , and transmission cable length 160 km, is in operation ( Li et al., 2021 ). Additional MM converter models with varying capacities, voltages, and cable lengths are also under construction for applications in the near future. Another AC converter topology that makes a parallel connection with an HVDC system is a cascaded rectifier. Cascaded rectifier-based HVDC configuration was reported to increase energy conversion efficiency by 20% and reduce power system complexity by 65% ( Blaabjerg and Ma, 2017 ).
Furthermore, DC-based converter-HVDC parallel-connected transmission networks including three-level neutral point clamped (3L NPC) HVDC; DC converter-HVDC series connected transmission networks, such as solid-state transformer (SST)-HVDC; and pulse width modulator current source converter (PWM CSC)-HVDC were presented as potential alternatives for high-power transmission applications in recent literature reports. The DC-HVDC/3L NPC-HVDC design in parallel connection was proposed to simplify the complexity of the configuration of offshore power transmission substations by offering the benefit of cost. Series DC-connected designs (SST-HVDC and PWM CSC-HVDC) are also expected to further reduce the costs of bulky offshore wind power transmission substations ( Yaramasu and Wu, 2016 ; Wei et al., 2017 ; Ryndzionek and Sienkiewicz, 2020 ; Peng et al., 2021 ).
On the other hand, research advances have achieved promising milestones in introducing potential strategies that could be practically implemented to enhance the operation of wind farms for maximizing and securing wind power production. These strategies mainly involve the application of optimization-based algorithms for wind farm control. In this context, wind farm control provides a cooperative strategy for the design and operation of the wind power plants, and it is a crucial development to alleviate the losses resulting from the turbine-to-turbine interaction within the plant. Many recent research works have revealed the improvement in wind farm performances, particularly in terms of power production, by using different optimization-based algorithms and models. In this work, the research results from implementing optimization-based wind farm control strategies that use feedforward, model-based closed-loop, and model-free closed-loop algorithms have been presented. For example, under the feedforward control approach, gradient-based optimization algorithms that include sequential quadratic programming, steepest descent, and conjugate gradient and heuristic algorithms that include genetic algorithm, particle swarm optimization (PSO) ( Dursun et al., 2021 ), and artificial bee colony were implemented by using different optimization and evaluation models to increase wind farm power production, and varying results were reported.
With the model-based closed-loop approach, a model predictive optimization strategy was implemented by different researchers including Fontanella et al. (2021) , and the output power of wind farms was claimed to increase with varying levels; the implementation of data-driven optimization methods, such as Bayesian optimization, knowledge-assisted deep deterministic policy gradient algorithm, and support vector machine (SVM) algorithm, also supported the achievability of increments in wind farm power productions. Furthermore, based on the model-free closed-loop approach, different optimization algorithms including simultaneous perturbation stochastic algorithm and nested extremum-seeking controller (NESC) were shown to have capabilities of maximizing wind farms’ output power. The model-based closed-loop approach is generally popular because it would help develop robust wind farm control designs with reduced complexity, cost, etc., in comparison to the other control strategies ( Jain et al., 2021 ).
According to recent research trends, the increase in wind farms’ power production could be generally achieved by the implementation of different optimization-based algorithms along with various design optimization and evaluation models. The amount of increase in power production differs depending on various factors, which include the type of optimization algorithm that could be implemented, wind farm input control parameter that could be optimized, and models that could be used for optimization and evaluation. On the other hand, evaluating the performances of multiple wind farm optimization strategies under a single model environment is challenging; this could affect the acceptance of research reports and their practical implementation on real-world wind farms. In response to this challenge, the benchmark named FarmConners was recently introduced, and a project was also launched, aiming to determine the practical effect of wind farm control on loads of power systems. In its most recent report, the National Renewable Energy Laboratory (NREL) ( Engaging Autopilot, 2021 ) revealed that it tested the practical implementation of the study model analyzed by Martínez-Tossas (2021 ), through the Composites Manufacturing Education and Technology (CoMET) facility, and validated that the ultimate objective of this study (to reduce the turbines’ cost) can be applied to real-world wind operation. Yet, more advanced works are still underway on the practical achievements of further wind farm control objectives that include design optimization, power reliability enhancement, and cost reduction.
Finally, more extended discussions of this work are organized under the sections to follow. Accordingly, Section 2 presents the two most popular wind turbine generator technologies (DFIG and PMSG systems); Section 3 explores technological trends in high-power transmission systems; Section 4 provides summaries of previous studies on different strategies of optimization-based wind farm control models; Section 5 presents brief assessments on the recent and future prospects of wind power systems control; and Section 6 summarizes the impact of relevant technologies and research studies on wind power production.
2 Popular generator technologies for wind energy harvesting
The most common device components used for energy conversion from wind to electrical power in a modern wind energy conversion system comprise a rotor with turbine blades, optionally a gearbox (it can be removed in gearless technologies), an electric generator, a power electronic converter, and a transformer, as shown in Figure 2 . Designs of wind energy conversion systems can be classified into various notions on the basis of the type of generator, speed regulation capability, and strategy by which the aerodynamic power is restricted. In these notions of wind energy conversion systems, the power electronics plays entirely special roles and contributes to power ratings of the system with varying capacities. Two main wind energy harvesting technologies are widely adopted in modern wind energy industries. In the past decade, the DFIG technology designed with partial-scale power electronic converters was a prime choice in wind energy industries; however, the PMSG topology developed with a full-scale power electronic converter is recently receiving prominence due to the fact that it involves full power-regulation capability.
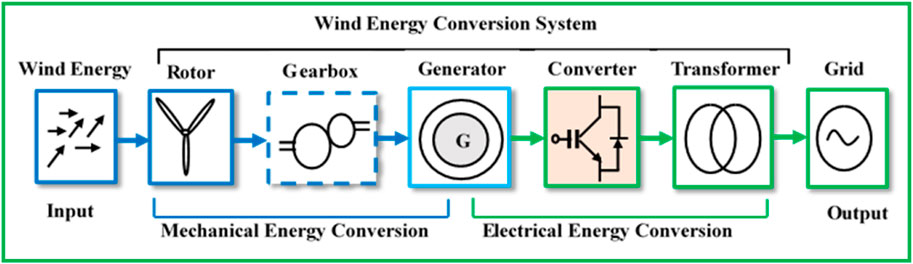
FIGURE 2 . Energy conversion levels in a modern wind energy conversion system.
2.1 Doubly fed induction generator-based turbine technologies
The DFIG (its configuration is shown in Figure 3 ) is the most recognized technology yet, and it has been installed widely since 2000. This wind energy harvesting technology uses both multiple- and single-gearbox systems; but the system with multiple gearboxes had widely gained acceptance until recent years, while single-gearbox technology is currently reported to have outstanding features in several research studies. The stator windings in a DFIG are directly tied to the grid by means of a transformer, and the rotor windings are tied to the power grid via a power electronic converter with approximately 30% power rating of the generator ( Cheng and Zhu, 2014 ; Desalegn et al., 11912 ). In this technology, the frequency and the current in the rotor of the generator can be smoothly regulated by the power electronic converter, and hence, the rotational speed of rotor blades can be adjusted in an acceptable range to increase energy harvesting and minimize the mechanical stress. The comparatively lower rating of the power converter makes this technology preferable in terms of cost. Yet, the major limitations of this technology are the application of slip rings with poor reliability and inadequate power regulation capability with regard to grid or generator power fluctuations. This technology has a globally dominant share in onshore wind power generation, and it is less suitable for offshore wind power application.
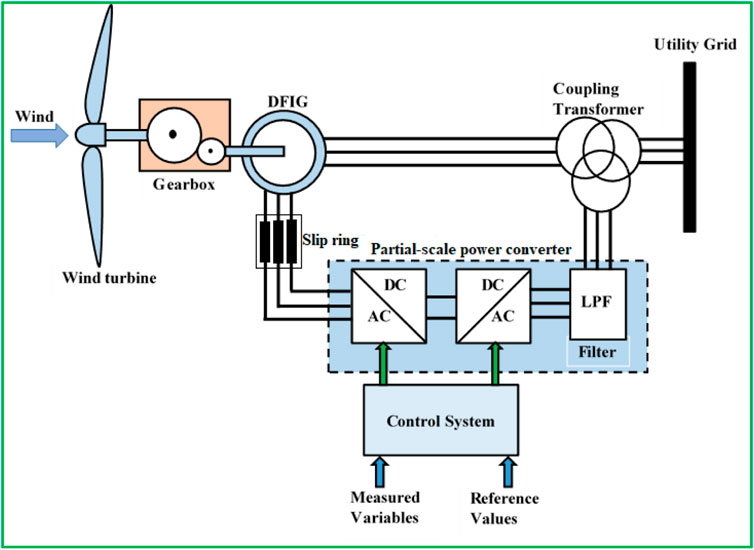
FIGURE 3 . Topology of DFIG-based wind energy conversion technology.
Due to the fact that the power rating capacity for the power electronics converter in DFIG-based wind energy conversion technology is comparatively small, the two-stage voltage source converter (VSC) topology is widely recognized in this technology. Conventionally, two-stage VSCs are developed with a back-to-back design along with a common direct current (DC) link. A special feature of this back-to-back design is that it can help to implement complete power regulation under system operation. Moreover, this design has comparatively low structure complexity with a low component number, and this contributes to excellent efficiency and reduced cost of the DFIG-based system.
2.2 Permanent magnet synchronous generator-based turbine technologies
PMSG-based wind energy conversion technology ( Figure 4 ) is another interesting system, and it is highly recognized in the most recently installed wind farm industries. By developing a full-scale power electronics converter and transformer to couple the power grid and the stator windings of the machine, the energy harvested by this wind energy conversion system can be entirely handled ( Le et al., 12023 ). In comparison to the DFIG-based wind energy conversion design, the most important features that can be recognized are the absence of slip rings, uncomplicated or even unneeded gearbox, enhanced power and speed regulation on the broader scale, and superior grid compensation efficiency. However, increased stress and high cost of power electronics devices and increased power loss in the converter phase are the major disadvantages ( Yaramasu et al., 2017 ). This design is usually not preferable in recently installed onshore wind energy conversion systems. On the other hand, PMSG-based wind power technology has been reported in multiple recent studies as a highly attractive candidate for recent and future offshore wind power applications because its converter device can be scalable to large-scale MW power.
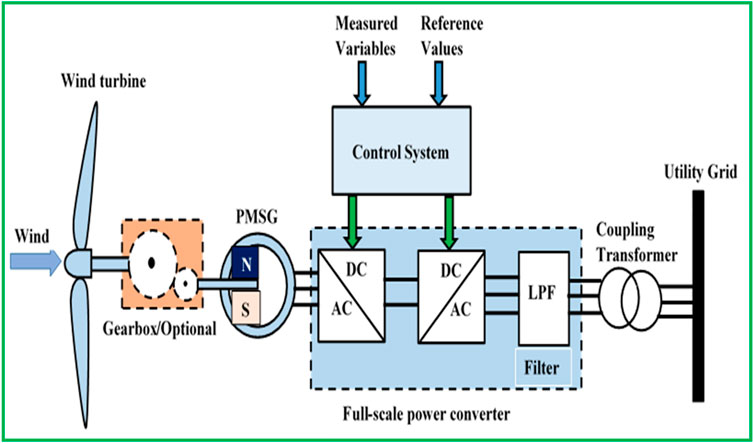
FIGURE 4 . Topology of PMSG-based wind energy conversion technology.
Since the power electronics converter in the PMSG-based wind energy harvesting technology needs to embrace the full power harvested at large-scale megawatts, the two-stage VSC topology may be prone to maximum loss at this power scale. In addition, the cabling in the instance of low-voltage scales below 1 kV with increased current is a design limitation. In order to get along with increasing power rating, various multi-cell converter configurations have been developed for different synchronous generator technology-based systems. The multi-cell converter topology generally has the benefits of standard and robust low-voltage converter technologies. For instance, multi-cell two-stage VSC in parallel connection is the state-of-the-art option for PMSG-based wind energy-harvesting technologies exceeding 3 MW ( Yaramasu and Wu, 2016 ). The advanced configurations of power electronics converters for offshore wind farm applications are presented in Section 3 .
2.3 Comparison of performances of DFIG- and PMSG-based wind energy-harvesting technologies
The performances of DFIG- and PMSG-based wind electric power generation systems are qualitatively summarized and compared in Table 1 . Based on their design types of mechanical and electrical system alignments, different configurations of DFIG- and PMSG-based wind power technologies have been introduced to wind farms for harnessing onshore and offshore wind power. In some cases, the nature of the alignments between mechanical and electrical devices can serve to generally characterize these technologies as geared and direct-drive systems. For example, DFIG-based wind turbines are generally geared technologies as they may depend on either three-stage gearbox or single-stage gearbox design systems, while PMSG-based wind turbines can depend on either single-stage gearbox design or they are direct-drive (gearless) technologies. However, the recent state-of-the-art wind power plants generally rely on gearbox-based technologies, such as three-stage gearbox-based DFIG, single-stage gearbox-based DFIG, and single-stage gearbox-based PMSG. Direct-drive PMSG technology has recently been undergoing research and technological advancements, and reports have claimed its promising development for the application of offshore wind multi-mega scale power generation.
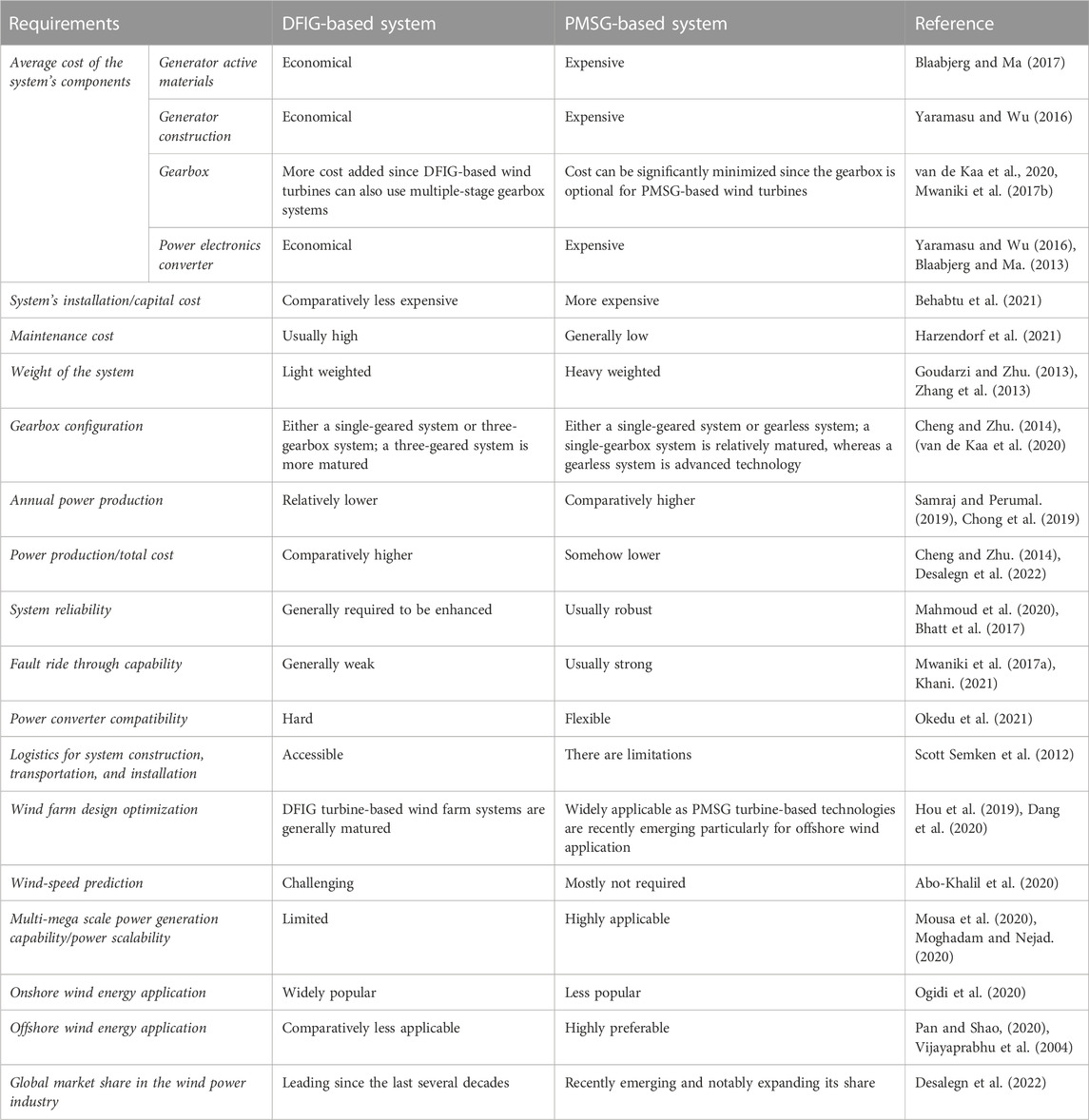
TABLE 1 . Comparison of DFIG- and PMSG-based wind energy harvesting technologies.
Table 1 provides comparative summaries pertinent to the performances and operation characteristics of DFIG- and PMSG-based wind power systems based on multiple requirements. These summaries are designed such that the listed requirements for comparisons generally apply to all configurations of DFIG-based systems (three-stage and single-stage gearbox technologies) and PMSG-based systems (single-stage gearbox and direct-drive technologies). In consideration of the cost of the system as one of the requirements for the comparisons, an average of the costs of two configurations (three-stage and single-stage gearbox) of DFIG technologies is compared with an average of the costs of two configurations (single-stage and direct-drive) of PMSG technologies. As shown in Table 1 , DFIG-based systems are desirable for their lower general cost than PMSG-based systems, while PMSG-based systems are generally attractive solutions due to their suitability for multi-scale offshore wind power application.
The operational characteristics of DFIG- and PMSG-based wind power systems can be quantitatively evaluated based on research findings. Most research works were focused on the studies of DFIG- and PMG-based systems’ reliabilities, active and reactive power performances, etc. Here, the capability of power generation reliability for both generator systems is analyzed and compared based on the reports that were presented in the research works. In addition, active and reactive power performances and energy harvesting ranges of these two generator-based systems are considered for comparative discussion.
The junction temperature of power devices that correspond to DFIG- and PMSG-based systems of a 2-MW rated power capacity is shown in Figures 5A, B comparatively. For these two generator-based wind energy-harvesting technologies, the power devices’ thermal cycling is demonstrated within 0.2 s. According to Figure 5A , the performance of a partial-scale power converter in the DFIG-based system could deteriorate due to its thermal cycle that is characterized by a larger amplitude than the performance of a full-scale power converter in the PMSG-based system, whose thermal cycles are characterized by a smaller amplitude as shown in Figure 5B . This indicates that the reliability performance of the power device in a DFIG-based system could be severely affected due to a larger amplitude, which is associated with the system’s thermal characteristics. Consequently, advanced modeling and testing approaches should be proposed in helping adjust the reliability by establishing the power devices’ thermal behavior more effectively based on the wind power converter’s mission profile ( Blaabjerg and Ma, 2017 ). A robust strategy has been presented by Ma et al. (2015) , which resembles lenses with varying focal lengths used in photography. The wind power converter’s loading analysis and modeling are partitioned subject to some given time constants and various modeling methods and tools.
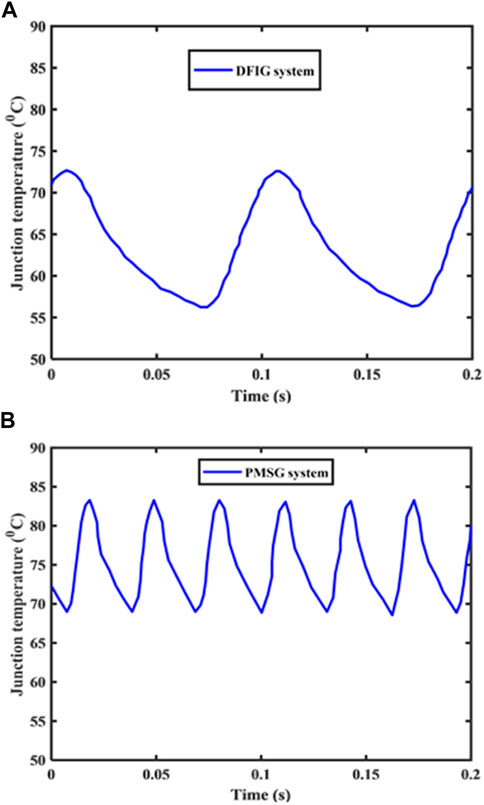
FIGURE 5 . Junction temperature of power devices with generator systems of a 2-MW wind power converter: (A) DFIG system’s rotor side converter and (B) PMSG system’s machine side converter ( Blaabjerg and Ma, 2017 ).
Furthermore, Figures 6A, B show active power control performances for DFIG- and PMSG-based marine current wind energy-harvesting systems under the variable speed control strategy. As shown in Figure 6A , the major advantage of the DFIG-based marine current system is its capability to supply fixed voltage and frequency within the range of ± 30 % speed change with respect to normal synchronous speed. An additional option for the range of speed variation (30%–50%) can also possibly be used. A minimum power rating of the rotor converter is directly associated with the range of 30% speed variation. Similarly, in Figure 6B , the active power control of the PMSG-based marine current system also indicates good power-tracking performance. In both systems, the differences between the estimated and simulated power are negligible. These negligible differences primarily result from the implementation of variable speed control rather than a direct power control.
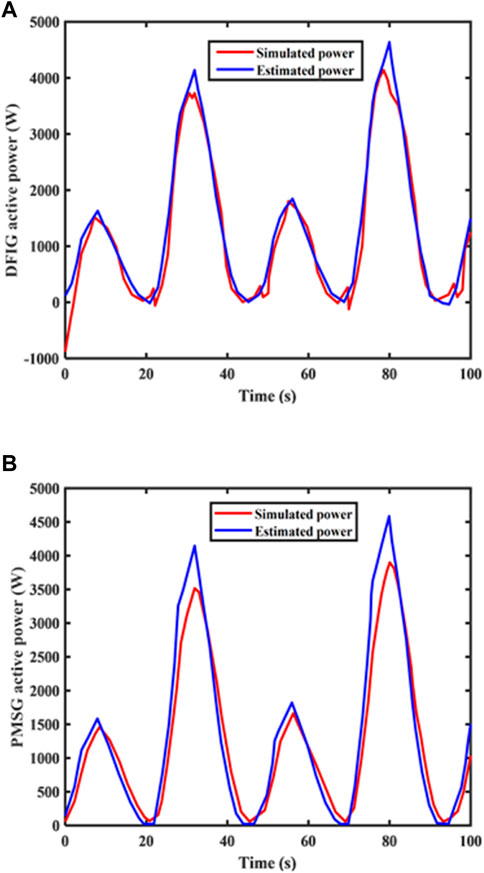
FIGURE 6 . Active power performances of (A) DFIG-based wind energy harvesting technology and (B) PMSG-based wind energy harvesting technology ( Benelghali et al., 2010 ).
As noted, the comparison of DFIG- and PMSG-based wind energy-harvesting systems is qualitatively shown in Table 1 by considering their annual power production capability as one of the criteria. Herein, Figures 7A, B show the annual energy-harvesting performances of DFIG- and PMSG-based marine current turbine technologies for the given ranges of tidal velocities, based on the research findings by Benelghali et al. (2010) . Accordingly, Figure 7A represents the annual energy harvested by a DFIG-based marine current wind power system; the annual harvested energy corresponding to different tidal velocities, with DFIG technology, is calculated to be around 1,530 MWh/year according to the study. On the other hand, by the application of a PMSG-based marine current wind power system ( Figure 7B ), the cumulative generated power under the similar ranges of tidal velocities is reported to be about 1,916 MWh/year by the same study. As noticed, there is nearly a 25% difference in the power produced over a year between these two power systems, and this difference can be further extended when using a larger turbine system. The DFIG-based power system is characterized by its restricted speeds, and this is the reason for the reduction in its annual power production compared to the full-scale PMSG-based system. Meanwhile, according to a study by Fischereit et al. (2015) , the relationship between tidal currents and wind speed was quantified such that the wind speed over a marine was observed to increase or decrease by around 0.2 m/s depending on the direction of the tidal flow with respect to wind direction.
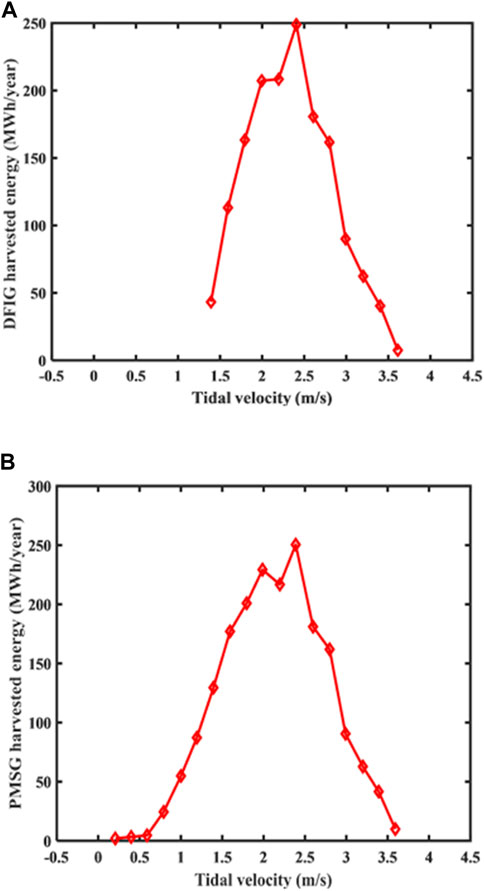
FIGURE 7 . Comparison of energy ranges harvested by (A) DFIG-based system and (B) PMSG-based system ( Benelghali et al., 2010 ).
3 High-power transmission systems for wind farms: Technological advances
Under this section, the general comparison of power transmission systems of wind farms that are based on HVAC and HVDC technologies is shown in Table 2 based on capital expenditure. Parallel AC-connected HVAC, parallel AC-connected HVDC, parallel DC-connected HVDC, and series DC-connected HVDC transmission configurations are also comparatively shown in Table 3 based on main energy criteria, which include the operational condition of the system, cost of the system, and system’s capability for power scalability. Moreover, different HVDC-based state-of-the-art and more advanced technologies for the transmissions of offshore wind farms are shown in Table 4 on the basis of important requirements: the technology’s energy conversion quality, range of applications for recent offshore wind power generation, and the possibility of the development for future offshore wind power application.
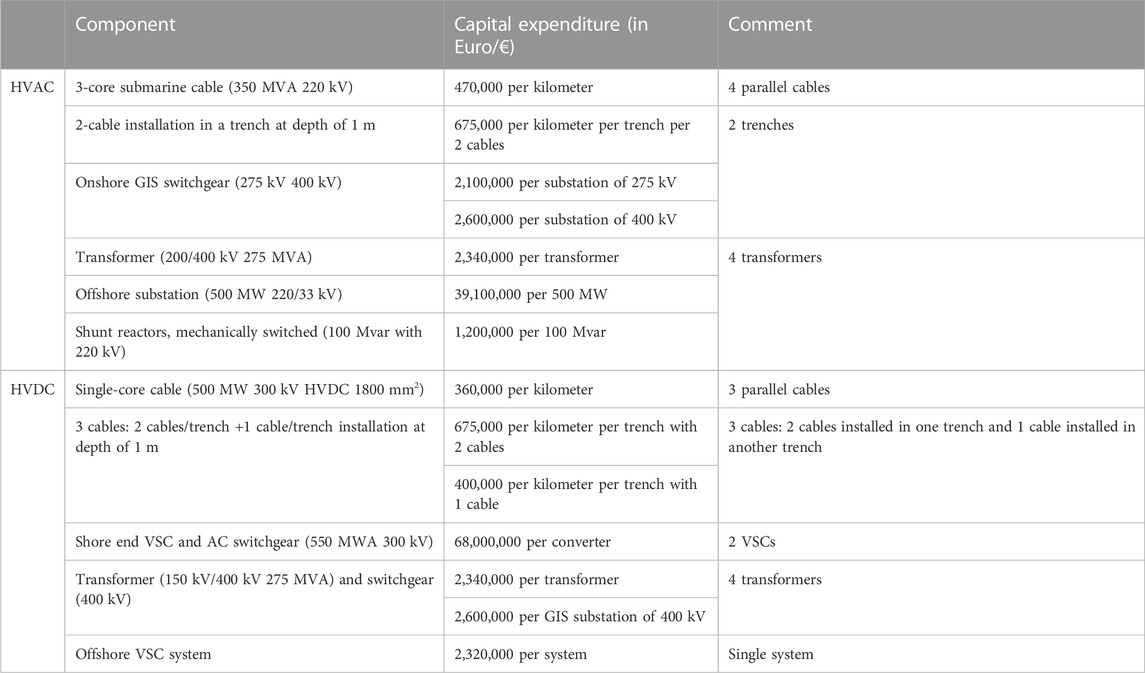
TABLE 2 . Comparison of HVAC and HVDC in terms of their capital costs ( Li et al., 2021 ).
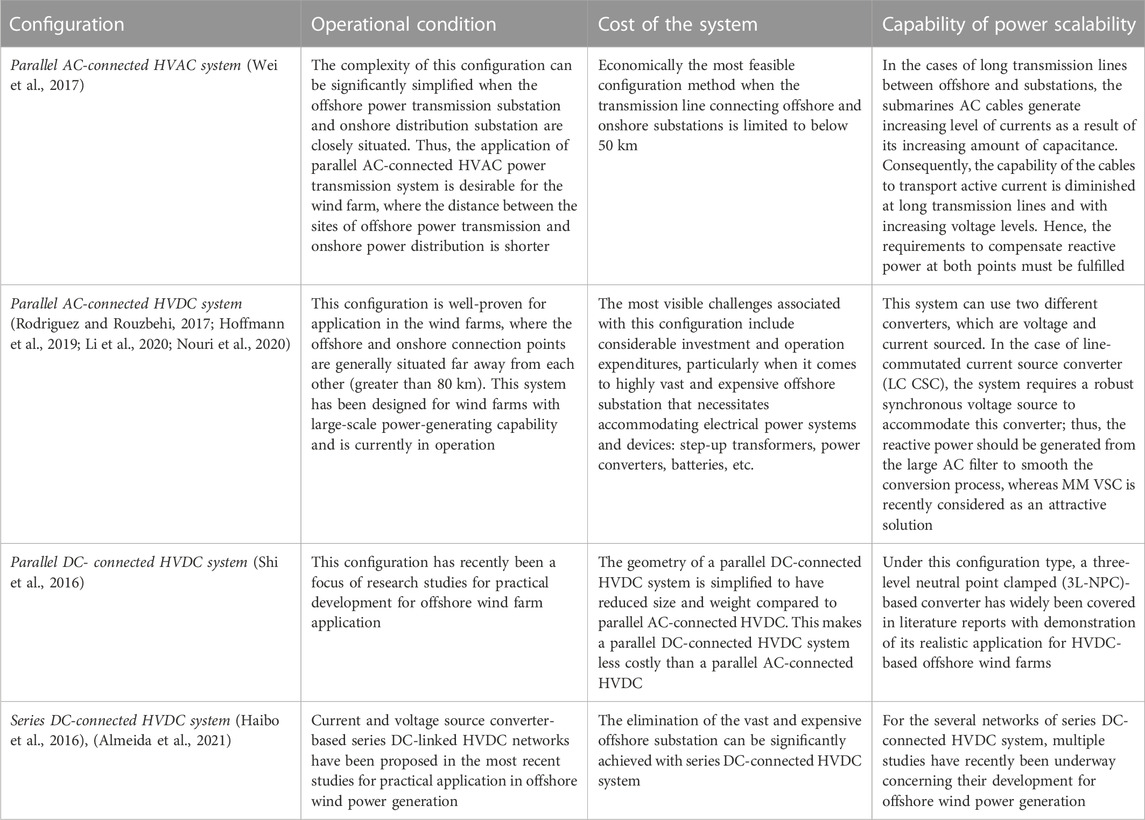
TABLE 3 . Comparison of different configurations of HVAC and HVDC converter systems.
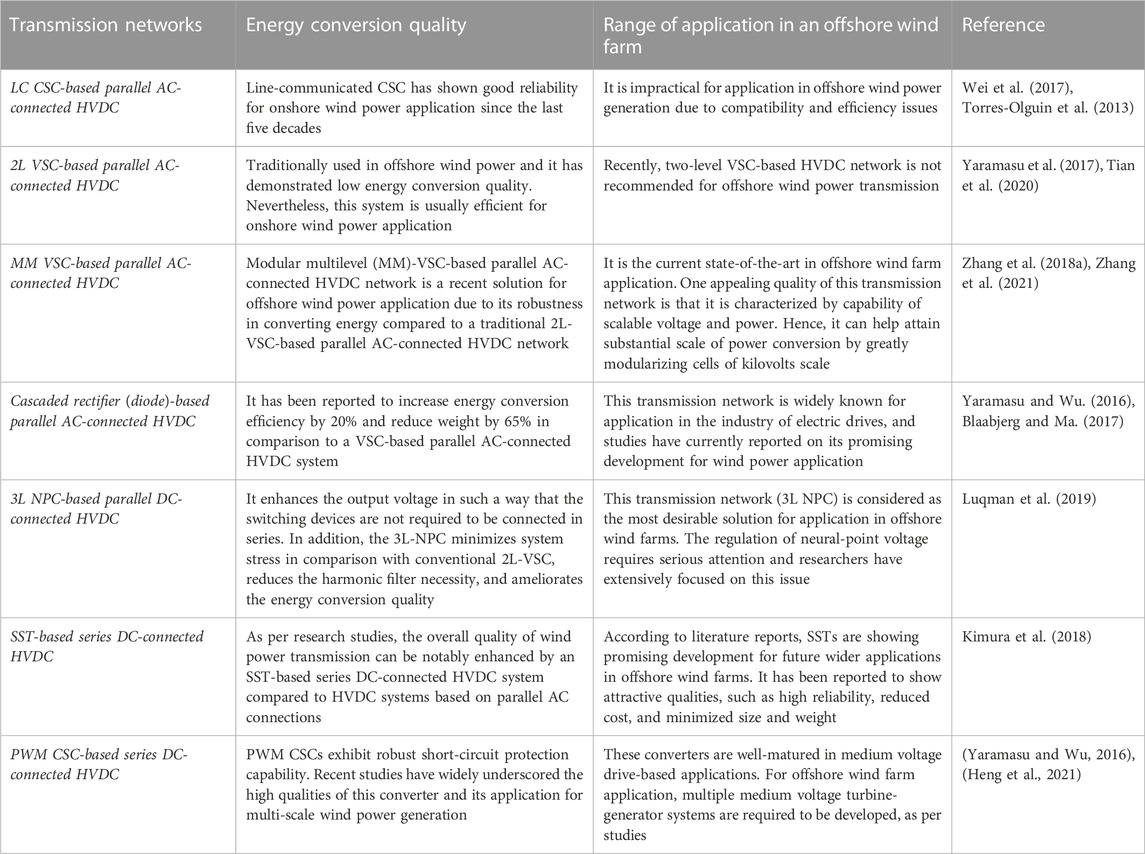
TABLE 4 . Comparison of different converter–HVDC networks for offshore power transmission applications.
3.1 Applications of HVAC and HVDC in wind farms
The general structure of offshore wind farm transmission with the application of HVDC and HVAC systems that include the components, such as power converter, transformer, offshore substation, and onshore substation, is shown in Figure 8 . Here, the main focus is to evaluate the economic efficiencies of HVAC- and HVDC-based transmission systems by comparing the capital expenditures required for their respective components in commissioning offshore wind farms. Accordingly, the outlines of capital costs for the electrical components of HVAC and HVDC systems are shown in Table 2 based on the most recent study by Li et al. (2021) . According to this study, the required capital expenditures are mainly allotted to cover the costs of transformers, onshore GIS switchgear, offshore substations, cable trench, shunt reactors, and submarine cable in the case of the HVAC system. In the case of the HVDC system, the capital expenditures are divided among the costs of cable trench, HVDC cable, onshore inverter, offshore rectifier, transformers, and additional offshore facilities. Based on the outlines of these costs for the components of HVAC and HVDC systems, it can be deduced that at a bigger power capacity and extended transmission line, HVDC presents more attractive cost benefits.
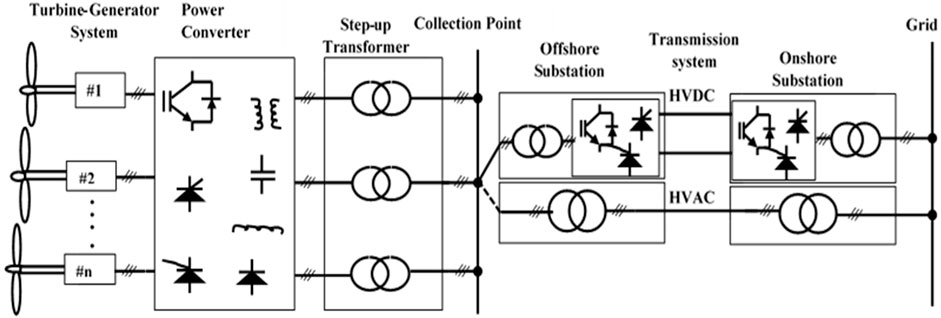
FIGURE 8 . General scheme of an offshore wind farm.
The HVAC system produces a huge amount of capacitive current that corresponds to the length of the transmission line. Here, the real power transmission capability may be determined with specified and reactive currents of a specific HVAC cable. With 50 Hz HVAC, the highest transmission length is around 140 km without compensation for reactive power. The HVDC system is characterized by considerable capability of power transmission compared to the HVAC system, particularly over an extended distance.
Moreover, the HVDC system offers additional advantages without being limited to cost and transmission capability by outperforming the HVAC system over an extended distance; for the vast offshore wind farm power transmission, the HVDC system also demonstrates lower transmission losses and its cables are commercially available (for larger voltages, HVAC cables are not available), and therefore, the HVDC system helps control excessive load as its power can be inherently adjusted. In general, HVDC is a more desirable solution than HVAC in the transmission of multi-mega-scale offshore wind power that is commissioned far away from the shore.
3.2 Advanced configurations of HVAC and HVDC for wind farms
Different configurations of HVAC and HVDC systems are given in Table 3 based on various factors, which include operational condition, overall cost, and capability of the system’s configuration. The configurations include parallel AC-connected HVAC system, parallel AC-connected HVDC system, parallel DC-connected HVDC system, and series DC-connected HVDC system. Parallel AC-connected HVAC system-based configuration is characterized by its low complexity under the condition that the transmission distance between offshore and onshore sites is not long and is economically efficient under the same condition. However, this configuration shows serious disadvantages when the distance between offshore power and onshore substations is extended.
Parallel AC-connected HVDC configuration, which is based on the modular multilevel converter (MMC) topology, is the state-of-the-art solution to offshore wind farms with larger power capacity and longer transmission lines. However, the main disadvantage of this configuration is the high overall cost of power conversion components and the requirement of a bulk offshore substation. On the other hand, this configuration is characterized by its high capability of power scalability, and MMC topology with a power capacity of 864 MW, voltage of ± 320 k V , and cable length 160/45 km is currently in operation. In addition, MMC topology with a power capacity of 900 MW, voltage of ± 320 k V , and cable length of 45/45 km is under construction for offshore wind farm commercial application by 2023; MMC topology with 900 MW, ± 320 k V , and 100/30 km is under development for application by 2024 ( Li et al., 2021 ).
Furthermore, parallel and series DC-connected HVDC configurations are designed to have reduced size and weight, and are recently under development for commercial use in offshore wind farms. The disadvantages that are being posed with the applications of other AC-connected HVDC configurations could be eliminated by DC-connected HVDC configurations. The discussions for different power converter–HVDC networks for offshore wind power transmission are given in Table 4 based on the criteria mainly related to energy conversion quality and range of applications in recent wind farms and possibility for future developments. The power converter–HVDC networks that are considered for discussions here include line-commutated current source converter (LC CSC)-based parallel medium voltage-alternating current (MVAC)-connected HVDC, two-level voltage source converter (2L-VSC)-based parallel MVAC-connected HVDC, multi-modular voltage source converter (MM VSC)-based parallel MVAC-connected HVDC, cascaded rectifier (diode)-based parallel MVAC-connected HVDC, three-level neutral point clamped (3L-NPC)-based parallel MVDC-connected HVDC, solid-state transformer (SST)-based series medium voltage direct current (MVDC)-connected HVDC, and pulse width modulator current source converter (PWM CSC)-based series MVDC-connected HVDC. Each of these HVDC-based power transmission networks is discussed in the following paragraph.
The application of LC CSC-based parallel MVAC connection with HVDC has shown well-proven performance in onshore wind power generation for about the last five decades. Nevertheless, this LC CSC-based network is inapplicable for offshore wind power, because it is incompatible with huge-scale power generation. On the other hand, the application of 2L back-to-back (BTB) VSC topology that is based on parallel MVAC connection with an HVDC system has been dominating over the onshore wind farm, and is less suitable for offshore wind farm due to its compatibility issue with multi-scale wind power generation. Modular multilevel (MM) VSC, based on parallel MVAC connection with an HVDC system, has been applied to offshore wind power transmission with various power capacity, voltage, and cable length levels. Currently, MM VSC is under further development for further improvement so that it would help ensure power maximization from offshore wind farms. At a wind power scale of 200 MW, cascaded rectifier (diode)-based parallel MVAC connection with HVDC was implemented, and it improved power production by 20% and reduced weight by 60% against traditional 2L-VSC-based parallel connection with HVDC according to study assessment by Blaabjerg and Ma (2017) .
Furthermore, various offshore wind power transmission networks that are based on different converter technologies have been proposed in studies to enhance wind power conversion. For example, a three-level (3L) NPC-HVDC parallel MVDC-connected power transmission network has been developed in literature reports in response to the limitations posed while using a power transmission network that is based on the parallel MVAC-connected 2L VSC-HVDC. Series MVDC-connected transmission networks can also be implemented with a (3L) NPC converter ( Peng et al., 2021 ). A series MVDC-connected (3L) NPC-HVDC system can be configured to have a relatively uncomplicated structure, higher power density, and lower cost than a parallel DC-connected system. In addition, SST- and PWM CSC-based series MVDC-connected HVDC configurations are undergoing promising development with attractive features, such as lower costs and weight than parallel MVAC and MVDC-connected networks such as 2L VSC-HVDC, MM VSC-HVDC, and (3L) NPC-HVDC ( Wei et al., 2017 ). Further discussions on this section are given in Tables 3 , 4 .
4 Optimization-based wind farm control models: Research perspectives
Wind farms with larger capacities are required to perform under grid integration in order to ensure more reliable and efficient ways of generating wind power, which include reduced energy losses, maximized power production, lowered energy costs, enhanced power quality, and minimized loads on power systems as the ultimate objectives. Multiple recent studies have indicated that implementing control to individual wind turbines is not an effective strategy to achieve these objectives due to the fact that this strategy is unable to emphasize the complicated aerodynamic interactions across different turbines. Consequently, the strategy for wind farm control design has been aimed at enhancing controllers, which regulate and oversee the performance of a group of wind turbines from a supervisory level as shown in Figure 9 with a hierarchical method. The wind farm controller operates as the supervisory control system and comprises control levels that supervise production of power, system operation and maintenance, and power system services. The supervisory control system uses power grid requirement, energy costs, and turbine condition inputs to deliver the reference inputs to all turbines so that the desired operation can be ultimately met by the wind farm. The hierarchical control structure ( Figure 9 ) enables robust and efficient control of turbines and wind farms by handling the turbines’ power output and affecting the power transfer in the electrical devices so as to achieve the aforementioned objectives.
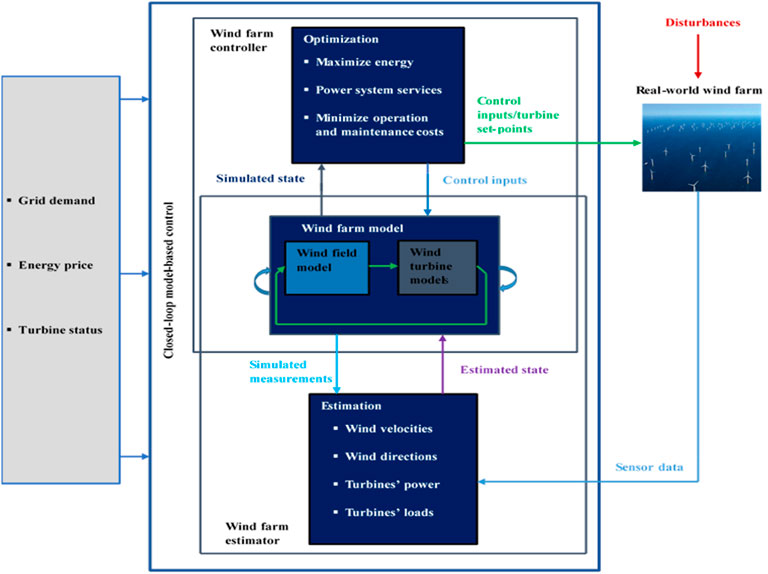
FIGURE 9 . Scheme of the model-based closed-loop wind farm control approach.
The purpose of the discussion under this particular section is to assess the recent research results that have been reported in claiming to achieve the improvements in wind turbines/wind farm performances as per the aforementioned objectives by using different control approaches. In the research practices, two types of universal control approaches are usually proposed for the implementation of the required control objectives. A number of control strategies that rely on non-optimization- and optimization-based approaches are introduced by different researchers in aiming to achieve various wind farm control objectives, such as power maximization, power system load reduction, and grid services provision. In the most recent works, the optimization-based approach, which encompasses standard feedforward, model-based closed-loop, and model-free closed-loop control strategies has been consistently underlined to show outstanding performances compared with the non-optimization-based approach that uses conventional feedback control and feedforward control strategies. Therefore, this study summarizes research findings that have been recently reported in the efforts to realize some wind farm control objectives (primarily power gain/maximization) based on standard feedforward, model-based closed-loop, and model-free closed-loop control algorithms with the implementation of different wind farm optimization strategies and optimization and evaluation models while considering various input parameters, such as yaw angle, blade pitch angle, tip–speed ratio, and axial induction factor. Summaries of the studies are given in Sections 4.1 – 4.3 and Tables 5–7 .
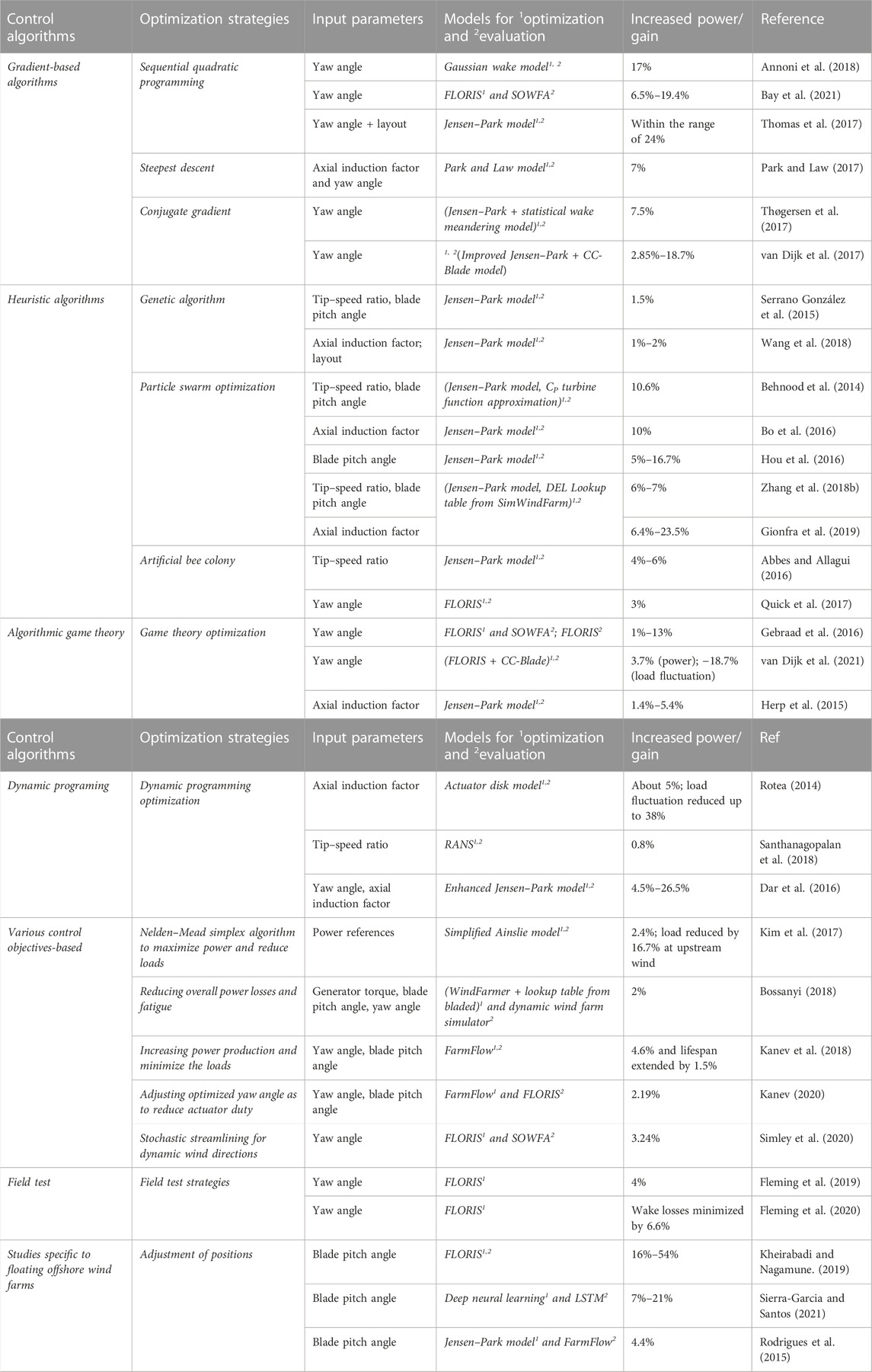
TABLE 5 . Performances of feedforward control strategies with different optimization and evaluation models.
4.1 Standard feedforward control
The research studies on wind farm optimization using the concepts of standard feedforward control are summarized in Table 5 . This approach uses different control algorithms, optimization strategies, input parameters, and wind farm design optimization and evaluation models in enhancing wind farm performances so as to achieve the desired wind farm control objectives. Accordingly, the gradient-based control algorithms with different optimization strategies, such as sequential quadratic programming, steepest descent, and conjugate gradient, were proposed ( Table 5 ) to maximize wind farm power production by adjusting wind turbines’ yaw angles and induction factors. For instance, Annoni et al. (2018) used sequential quadratic programing by implementing the Gaussian wake concept as both the optimization and evaluation model in increasing power production by adjusting the wind turbine’s yaw angle. Under this framework, power production was claimed to increase by 17% compared with the greedy control algorithm. On the other hand, Thomas et al. (2017) , with a similar strategy (sequential quadratic programming), used the Jensen–Park model to optimize the yaw angle and layout of the wind farm, and power production was reported to increase up to 24% compared with the greedy control algorithm. Moreover, steepest descent- and conjugate gradient-based optimizations were proposed by Park and Law (2017 ) and Thøgersen et al. (2017 ) to adjust the axial induction factor and yaw angle (in the case of the steepest descent strategy) and the yaw angle (in the case of the conjugate gradient strategy) of wind farms, and the results indicated that the power production could be maximized by 7% and 7.5%, respectively.
As shown in Table 5 , further standard feedforward optimization strategies that are based on heuristic algorithms (genetic algorithm, particle swarm optimization, and artificial bee colony) and game theory optimization were proposed by different researchers for optimizing various input parameters of wind farms. For instance, a genetic algorithm was proposed by Serrano González et al. (2015) to optimize wind turbines’ tip–speed ratio and blade pitch angle based on the Jensen–Park evaluation model, and it resulted in 1.5% increase in power production. The genetic algorithm was also used by Wang et al. (2018) , aimed at maximizing power production by adjusting the axial induction factor and layout of the wind power system based on the Jensen–Park optimization and evaluation model, and it ended up increasing wind power production by 1%–2%. Another powerful heuristic algorithm-based standard feedforward strategy includes particle swarm optimization, which has been widely proposed in recent research works to achieve various wind farm control objectives. In Table 5 , the results of several studies that implemented the particle swarm algorithm in optimizing wind farm performance are given. Accordingly, in Behnood et al. (2014) , the tip–speed ratio and blade pitch angle were adjusted for a wind farm of 16 turbines based on the Jensen–Park model, and approximation of the power coefficient (as a function of the turbines’ tip–speed ratio and blade pitch angle) and 10.6% increase in power production were reported. The study results that were presented by Bo et al. (2016 ), Hou et al. (2016 ), and Gionfra et al. (2019) also indicated the robustness of particle swarm optimization in helping to maximize wind farm output power. In addition, the heuristic algorithm-based wind farm optimization strategy, artificial bee colony, was proposed to adjust the tip–speed ratio and yaw angle of wind turbines based on the Jensen–Park model and FLORIS for design optimization and evaluation in Abbes and Allagui (2016 ) and Quick et al. (2017 ) and output power increment of 4%–6% and 3% were, respectively, reported to be achieved.
The algorithmic game theory-based wind farm optimization strategy can be implemented to minimize load fluctuations of power systems in addition to maximizing output power by facilitating the adjustments of the yaw angle and axial induction factor. By using this strategy along with different wind farm performance optimization and evaluation models, some research works were introduced by researchers. Accordingly, in Gebraad et al. (2016) , the yaw angle was optimized by using the FLORIS model, whereas output power performance was evaluated by using both SOWFA and FLORIS models, and the wind farm power production was reported to be improved by 1% with FLORIS and SOWFA optimization and evaluation models, and by 13% when the FLORIS platform was used as both the optimization and evaluation model. In addition, in van Dijk et al. (2021) , the FLORIS model was used as both a single platform and in combination with the CC-Blade model for optimizing wind turbines’ yaw angle and evaluating wind farm output performance, where power production was reported to be maximized by 3.7% wind and a single model (FLORIS) and load fluctuation of power systems decreased by 18.7% with combined modes (FLORIS + CC-Blade). More work was also introduced in Herp et al. (2015) , where the axial induction factor was optimized with the Jensen–Park model under different settings and resulted in 1.4%–5.4% increase in wind farm power production.
Additional works that are based on standard feedforward control strategies involving different algorithms and optimization and evaluation models for the design and performance of wind farms are given in Table 5 as extended summary from Table 5 . A dynamic programming strategy was proposed by Rotea (2014) to optimize axial induction of a wind farm by applying the actuator disk model as the design optimization and evaluation standard. The result of this study shows that the power production was maximized by around 5% and wind farm load fluctuation was reduced up to 38% compared to the greedy algorithm strategy. In addition, a dynamic programming optimization strategy was implemented by Santhanagopalan et al. (2018 ) and Dar et al. (2016) by adjusting the tip–speed ratio through the RANS model and the yaw angle and axial induction factor through the enhanced Jensen–Park model to achieve output power increment by 0.8% and 4.5%–26.5%, respectively.
Additional standard feedforward optimization strategies that are based on different wind farm control objectives and with various wind farm design optimization and evaluation models were also presented in many research works, and the summaries of some of their parts are given in Table 5 . Accordingly, the Nelden–Mead simplex algorithm was implemented with the simplified Ainslie model for optimization and evaluation power reference of wind farms in Kim et al. (2017) , and the results showed an increase in the output power by 2.4% and reduction in the load fluctuation at upstream wind by 16.7%. According to a study presented in Bossanyi (2018) , the generator torque, blade pitch angle, and yaw angle were adjusted and evaluated in aiming at minimizing the overall power losses and power system stress, and power production was saved by 2%. In addition, the research works based on field test optimization were discussed by Fleming et al. (2019 ) and Fleming et al. (2020 ) claiming a power gain of 2% and minimization of wake losses by 6.6%, respectively, by using FLORIS as the optimization model in adjusting the yaw angle. In addition, some studies that are specific to floating offshore wind farms were conducted based on blade pitch angle adjustments, and the results of these studies are given in the last row of Table 5 . For instance, in the study by Kheirabadi and Nagamune (2019) , the FLORIS benchmark was used as the optimization and evaluation model for maximizing power production by adjusting the floating offshore wind farm blade pitch angle under different settings, and 16%–54% increase in output power was reported. In addition, the adjustment of floating offshore wind farms under different design optimization and evaluation benchmarks: deep neural learning optimization model and LSTM evaluation model by Sierra-Garcia and Santos (2021) and the Jensen–Park optimization model and FarmFlow evaluation model by Rodrigues et al. (2015) were implemented; power production growth of 7%–21% and 4.4% were, respectively, reported.
4.2 Model-based closed-loop control
Under this control approach, the research works that are based on model predictive optimization and data-driven optimization strategies are summarized in Table 6 . Accordingly, a model predictive optimization algorithm was implemented by Heer et al. (2014) , using the Jensen–Park platform as the optimization model and the SimWindFarm platform as evaluation in aiming to increase wind farm power production with adjustments of the blade pitch angle and tip–speed ratio. Under these two adjustments, the output power showed an increment of 0.4%–1.4%. On the other hand, the axial induction factor was adjusted in the study by Vali et al. (2016) , making use of another wind farm performance optimization and evaluation platform (WFSim) based on the model predictive optimization strategy to achieve a power production growth of 3.8%. Furthermore, with the implementation of the model predictive optimization strategy through the application of the WFSim model for optimizing the axial induction factor and evaluating wind farm output power performance, additional works were presented by Vali et al. (2017 ) and Vali et al. (2019 ), reporting an improvement in power production by 2%–8% and 4%, respectively. On the other hand, larger increments of power production were reported to be achieved while using the SP-wind benchmark as the optimization and evaluation model for adjusting thrust coefficients ( Goit and Meyers, 2015 ; Munters and Meyers, 2016 ; Munters and Meyers, 2017 ), and thrust coefficients and yaw angle rates ( Munters and Meyers, 2018a ; Munters and Meyers, 2018b ) of wind farms under different control settings.
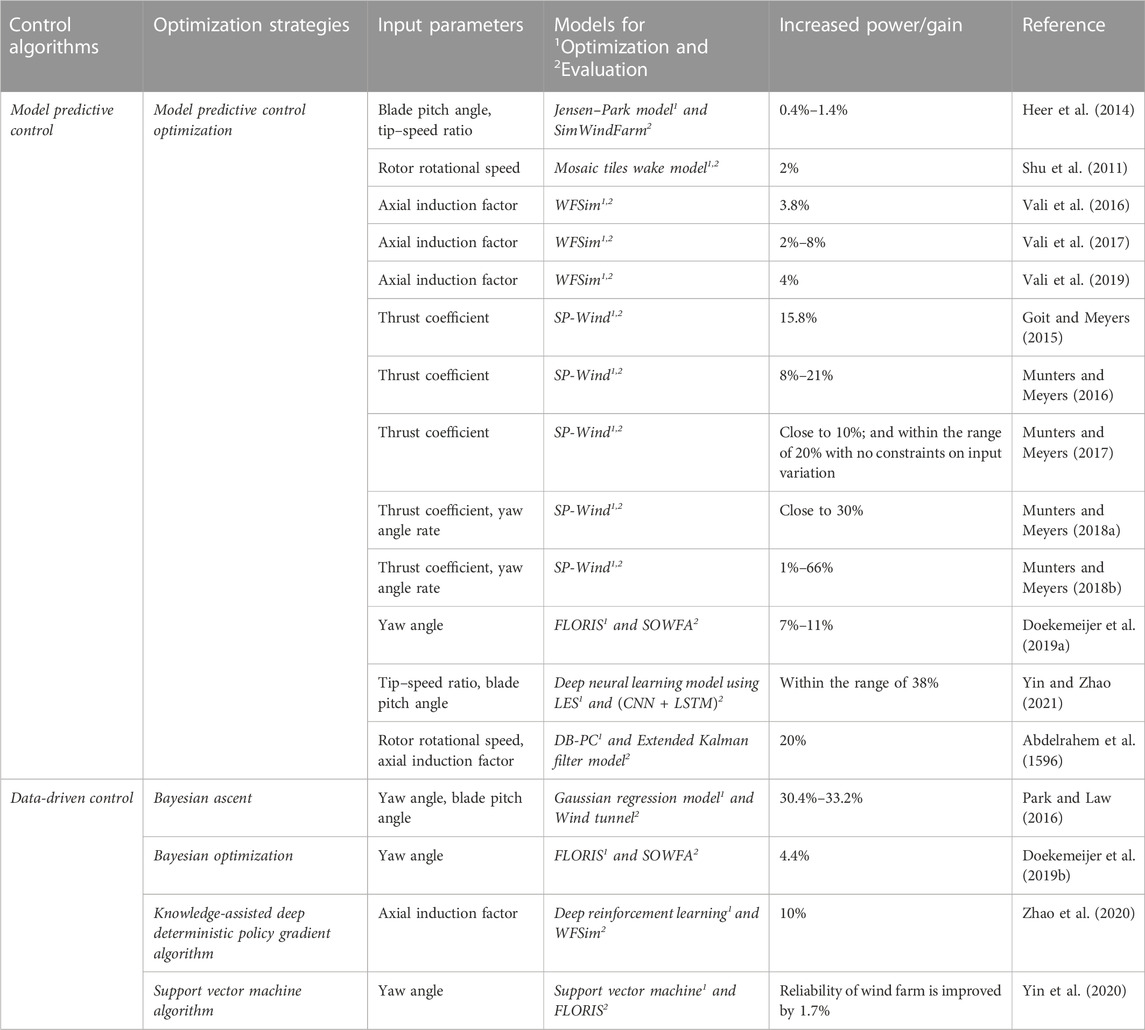
TABLE 6 . Performances of model-based closed-loop control strategies with different optimization and evaluation models.
Different wind farm performance optimization and evaluation models (FLORIS and SOWFA, deep neural learning model using LES and CNN + LSTM, and DB-PC and extended Kalman filter model) were used to adjust the yaw angle ( Doekemeijer et al., 2019a ), tip–speed ratio and blade pitch angle ( Yin and Zhao, 2021 ), and rotor rotational speed and axial induction factor ( Abdelrahem et al., 1596 ); output power growths of 7%–11%, up to 38%, and 20%, respectively, were claimed to be achieved. On the other hand, various data-driven model-based optimization strategies (Bayesian ascent, Bayesian optimization, etc.) were implemented by researchers such as Park and Law (2016 ), Zhao et al. (2020 ), and Yin et al. (2020) by making use of different wind farm optimization models (Gaussian regression model, FLORIS, deep reinforcement learning, and support vector machine, respectively), and evaluation models (wind tunnel, SOWFA, WFSim, and FLORIS, respectively) in adjusting input parameters (yaw angle and blade pitch angle, yaw angle, axial induction factor, and yaw angle, respectively) to determine the levels of increments in the resulted power productions and reliability improvement. Accordingly, output power growths were reported to be achieved by 30.4%–33.2%, 4.4%, and 10%, and reliability was enhanced by 1.7%, respectively.
4.3 Model-free closed-loop control
As shown in Table 7 , model-free closed-loop control algorithm-based wind farm optimization strategies, which include multiple resolution-based simultaneous perturbation stochastic algorithm, game theory, gradient descent, and nested extremum controller, were implemented in optimizing wind turbines’ input parameters, such as axial induction factors and generator torque gains in order to evaluate the possible outcomes in generated power. Axial induction factors were optimized under different settings [simultaneous perturbation stochastic algorithm ( Ahmad et al., 2014) , game theory ( Marden et al., 2013) , and gradient descent ( Gebraad et al., 2013) ], and the outcomes were evaluated with the same model (Jensen–Park), and different levels of increments (32%, up to 25%, and 1%) in the output power were reported to be achieved. On the other hand, generator torque gains and yaw angle were adjusted by implementing the same optimization strategy (nested extremum-seeking controller) and using different evaluation models [SimWindFarm ( Yang et al., 2015) , UTD-WF ( Ciri et al., 2016) ; Ciri et al., 2017) , and wind tunnel ( Campagnolo et al., 2016) ]; varying levels of increase (1.3%, 10%/7.8%, and 15%) in the power production were claimed to be achieved.
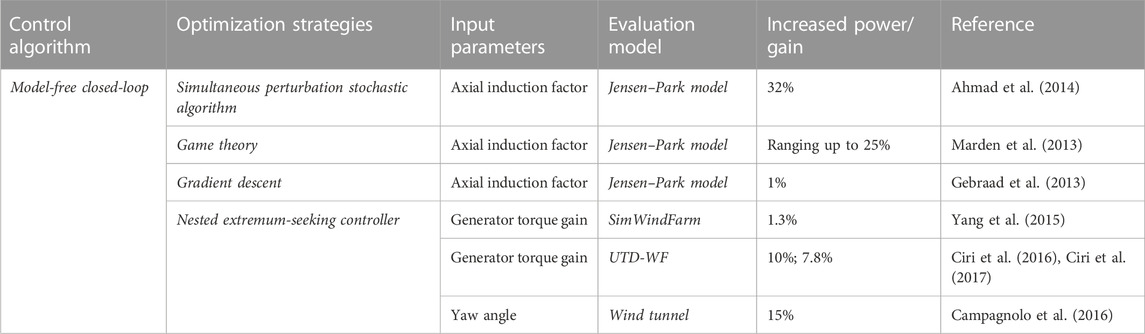
TABLE 7 . Performances of model-free closed-loop control strategies with evaluation models.
5 Recent challenges and future prospects of research studies on wind farm control
Standardizing the effect of wind farm optimization strategies on loads of wind power plants would be highly helpful in applying the results of the research studies for their practical implementation in real-world wind farm industries. This is because the optimization of wind farm performance through research studies is modeled and evaluated by using different strategies (as the summaries of multiple studies in Table 5 – 7 indicate), and this still raises challenges in evaluating the performances of different optimization models under a unified environment. As a result, the technological approach for collaborated wind farm control is presently under advancement in multiple research institutions and industries. In particular, the FarmConners environment was introduced recently for the purpose of producing datasets as the basis for the evaluation of different control models in aiming to alleviate the barriers for wind farm control acceptance ( Göcmen et al., 2020 ). In addition, the project was started by FarmConners to eliminate the challenges associated with the commercial application of wind farm control by collaborating a genuine assessment of the state-of-the-art of wind farm control in the FarmConners environment. Thus, a reliable evaluation of the efficiency of wind farm control models should be conducted so as to accurately determine the performance of wind farm controllers. Correspondingly, in order to maximize the acceptance of the wind farm control models and, ultimately, the FarmConners environment, FarmConners presents an extensive validation framework for wind farm control-oriented flow and load models, at which high-fidelity simulation generates (imitative dataset), wind tunnel experiments, and the field data from a fully operating wind farm in natural environmental conditions are drawn together (as shown in Figure 10 ).

FIGURE 10 . Data texture of the FarmConners environment.
Regardless of this technological progress by FarmConners, and even though recent studies demonstrated through simulations that the power production by wind farms can be maximized with collaborative control strategies, the practical confirmation on a real-world wind farm is still lacking. For instance, it raises the question of whether axial induction control can effectively result in increasing the wind farm’s annual power generation. Even for the more promising approach in simulation, wake-steering wind farm control, its direct practical applicability for a real-world wind farm has not been implemented yet. The considerable measurement noise and unpredictability is a challenge in wake-steering control, making it hard to evaluate the outcomes of measurement campaigns. Because of the unpredictability in models such as wind direction and aerodynamics, collaborative control strategies can become counterproductive over a number of periods, and this may not lead to enhancing the annual power output. However, even if these control concepts are unable to maximize the production of power, they may still be effective in reducing wind farms loads.
In general, wake steering is assumed to be a favorable control strategy for wind farm optimization. Yet, it is not fully convincing whether wake steering can practically be effective in helping to minimize the cost of energy. Turbines are not oriented to being yawed into the wind all the time. These will result in the addition of dynamic fatigue on various components of the turbines, which could result in increased costs for maintenance. Reports by several simulation studies demonstrated that the fatigue load on the blade and drive-train can be significantly improved. However, more advanced studies supported with extended experiments are required to accurately determine the effects of the wake-steering control strategy on the lifespan of the components of the turbines. If this control strategy proves to be effective, it could probably even help redesign various components of wind power systems ( Andersson et al., 2021 ). On the other hand, the most recently published report by the National Renewable Energy Laboratory (NREL) has revealed that it has practically validated that the wake-steering wind farm control, which was based on the study model in Martínez-Tossas (2021) , can be implemented to minimize the costs of wind turbines.
Many related studies were carried out to explore these problems and they came up with various indications. The study by Howland et al. (2019) proposed a wake-steering approach based on yaw misalignment that deflects wakes away from downstream turbines in order to maximize wind farm power production. The evaluation of this approach was conducted with site-specific analytic gradient ascent by using historical operational data; power increases of 7%–13% for wind speeds near the site average and 28%–47% for low wind speeds were observed. This study also reported that the wake steering minimized the power production variability of the wind farm by up to 72%. This work finally indicated that even though the wake-steering results demonstrated the potential to enhance the efficiency and predictability of power production, the resulting gains in annual power production were insignificant at the wind farm. Another study that focused on wind turbines under yawed conditions is a work by Rahimi et al. (2018) , which claimed to present a significantly improved engineering model for the prediction of the loads in yawed flow based on the skewed wake effect. This work put particular emphasis on the contribution of the root vorticity to the azimuthal variation of induced velocity for the prediction of fatigue loads and determining the yawing moment; the new model was derived from computational fluid dynamics of three multi-megawatt wind turbines (each rated 5 MW) and two 10-MW turbines. Simulations were conducted by means of an actuator line model, whereas the proposed model was evaluated based on results from a free vortex wake code and actuator line model simulations for all wind turbines and different yaw angles. The results of this study finally indicated that the proposed model significantly improved the estimation of the azimuthal variation of the axial induction factor, and also considerably improved the prediction of the resulting variation in blade loads.
Furthermore, a dynamic wind farm wake modeling approach that was based on a bilateral convolutional neural network and high-fidelity LES data was proposed by Li et al. (2022) ; another approach that relied on a point vortex transportation model for yawed wind turbine wakes was introduced by Zong and Porté-Agel (2020) . The former ( Li et al., 2022 ) used a novel deep learning method, named the bilateral convolutional neural network (BiCNN), for accurate modeling of dynamic wind farm wakes based on flow field data generated by high-fidelity simulations; its discussion indicated that the developed machine learning-based wake model would capture the spatial variations of the dynamic wakes closely as high-fidelity wake models and would run as fast as low-fidelity static wake models. In addition, this model was shown to outperform high-fidelity numerical models that would be used for the same scenario. On the other hand, the latter ( Zong and Porté-Agel, 2020 ) performed stereo particle imaging velocimetry measurements at multiple stream-wise locations behind a yawed wind turbine in order to study the formation mechanisms of the counter-rotating vortex pair; the results of this study showed that the counter-rotating vortex pair formed behind a yawed wind turbine would originate from the complex interactions between the hub vortex and stream-wise components of the blade tip vortices, which was observed to be essentially different from a yawed drag disk case where the hub vortex would be absent. Furthermore, the model by this study was considered to be the first model that would be capable of accurately simulating the wake deformation behind a yawed wind turbine.
As it has been generally indicated, the popular collaborative wind farm control approaches are based on optimization, which involves model-based closed-loop control, etc. In order to implement a model-based optimizer in a closed-loop, a state estimator needs to be used. However, the utilization of a state estimator will reduce the capability of the optimization approach in some way compared to the non-optimization-based noise-free full state feedback strategy. Recently, a very limited number of studies have been conducted by proposing the enhanced state estimators in optimizing input parameters of wind farms for enabling the provision of grid services.
6 Conclusion
This work presents a comprehensive review on the wind energy engineering approaches by incorporating research and technological issues in order to reflect the recent and future advances, challenges, and opportunities in wind power industry development. Wind power research and technological advancements have greatly contributed to the progress achieved by the wind power industry so far, and the future fate of this industry will also largely rely on the potential of wind power-related studies and technologies. Evidently, the energy-related policies and projections would be directed based on the status of ongoing research studies and technological innovations in the given energy field, which can also be a usual trend in the case of wind power installation. Based on this fundamental premise, advanced research studies and technological progresses that could potentially impact the recent and future onshore and offshore wind power developments have been discussed in this study in line with the directions (zero-emission goals) set by the United Nations Convention on Climate Change (UNCCC).
Numerous wind energy system-related recent research studies are largely inclined toward the technological developments of offshore wind power due to the reason that offshore power conversion technologies can be further scalable for high-power generation applications. Many HVDC-power transmission system-based converters are currently undergoing promising developments with various designs to achieve the weight reduction, cost optimization, and further enhancement of power conversion efficiency of offshore systems in the future. In general, based on the recent advances in the power transmission technologies, the future of the wind power industry seems be more dependent on the offshore wind farms than those onshore.
Moreover, the current advancements in automation-based research projects indicated a very promising future about the objective implementation of some optimization theories on real-world farms. Challenges with wind farm control modeling and evaluation tools were generally projected to be addressed toward the realization of fundamental wind farm optimization objectives. One of the objectives (wind turbines’ cost minimization) has already been reported to be practically validated based on the results of the recently proposed research model. These recent developments and more enhanced works that may be subsequently conducted by researchers and engineers on the wind farm optimal control are of great importance, which could partly contribute to enabling the wind power industry to see grand transition in the not-too-distant future.
Author contributions
All authors listed made a substantial, direct, and intellectual contribution to the manuscript and approved it for publication.
This work was supported by Bahir Dar University Institute of Technology, Bahir Dar Energy Center, and Wolaita Sodo University.
Conflict of interest
The authors declare that the research was conducted in the absence of any commercial or financial relationships that could be construed as a potential conflict of interest.
Publisher’s note
All claims expressed in this article are solely those of the authors and do not necessarily represent those of their affiliated organizations, or those of the publisher, the editors, and the reviewers. Any product that may be evaluated in this article, or claim that may be made by its manufacturer, is not guaranteed or endorsed by the publisher.
Abbreviations
CC, component certification; CoMET, composites manufacturing education and technology; DFIG, doubly fed induction generator; FLORIS, flow redirection and induction in steady state; HVAC, high-voltage alternating current; HVDC, high-voltage direct current; MM VSC, modular multilevel - voltage source converter; NREL, national renewable energy laboratory; PMSG, permanent magnet synchronous generator; PSO, particle swarm optimization; 3L NPC, three-level neural point clamped; SST, solid-state transformer; PWM CSC, pulse width modulator-current source converter; SVM, support vector machine; NESC, nested extremum-seeking controller; SOWFA, simulator for wind farm applications; RANS, reynolds-averaged navier–stokes; LSTM, long short-term memory; WFSim, wind farm simulator.
Abbes, M., and Allagui, M. (2016). Centralized control strategy for energy maximization of large array wind turbines. Sustain Cities Soc. C25, 82–89. doi:10.1016/J.SCS.2015.11.007
CrossRef Full Text | Google Scholar
Abdelrahem, M., Hackl, C., and Kennel, R. (1596). Robust predictive control scheme for permanent-magnet synchronous generators based modern wind turbines. Electron. (Basel) 10 (13), 1596. doi:10.3390/electronics10131596
Abo-Khalil, A. G., Eltamaly, A. M., Praveen, R. P., Alghamdi, A. S., and Tlili, I. (2020). A sensorless wind speed and rotor position control of pmsg in wind power generation systems. Sustain. Switz. 12 (20), 19. doi:10.3390/su12208481
Ahmad, M. A., Azuma, S., and Sugie, T. (2014). A model-free approach for maximizing power production of wind farm using multi-resolution simultaneous perturbation stochastic approximation. Energies 77 (9), 56245624–56465646. doi:10.3390/EN7095624
Almeida, A. O., Lopes, I. F., Almeida, P. M., Tomim, M. A., Passos Filho, J. A., and Barbosa, P. G. (2021). Series-DC connection of Offshore wind generating units - modeling, control and galvanic isolation. Electr. Power Syst. Res. 195, 107149. doi:10.1016/J.EPSR.2021.107149
Andersson, L. E., Anaya-Lara, O., Tande, J. O., Merz, K. O., and Imsland, L. (2021). “Wind farm control - Part I: A review on control system concepts and structures,” in IET renewable power generation ( John Wiley and Sons ), 15, 2085–2108. doi:10.1049/rpg2.12160
Annoni, J., Bay, C., Taylor, T., Pao, L., Fleming, P., and Johnson, K. (2018). Efficient optimization of large wind farms for real-time control. Proc. Am. Control Conf. , 6200–6205. doi:10.23919/ACC.2018.8430751
Bay, C. J., King, J., Fleming, P., Mudafort, R., Martínez-Tossas, L. A., and Bay, C. (2021). Unlocking the full potential of wake steering: Implementation and assessment of a controls-oriented. Model.” . doi:10.5194/wes-2019-19
Behabtu, H. A., Coosemans, T., Berecibar, M., Fante, K. A., Kebede, A. A., Mierlo, J. V., et al. (2021). Performance evaluation of grid-connected wind turbine generators. Energies (Basel) 14 (20), 6807. doi:10.3390/en14206807
Behnood, A., Gharavi, H., Vahidi, B., and Riahy, G. H. (2014). Optimal output power of not properly designed wind farms, considering wake effects. Int. J. Electr. Power Energy Syst. 63, 44–50. doi:10.1016/J.IJEPES.2014.05.052
Benelghali, S., Benbouzid, M. E. H., and Charpentier, J. F. (2010). Comparison of PMSG and DFIG for marine current turbine applications. 19th Int. Conf. Electr. Mach. ICEM . doi:10.1109/ICELMACH.2010.5608118
Bhatt, P., Long, C., Wu, J., and Mehta, B. (2017). Dynamic participation of DFIG for frequency regulation in electrical power systems. Energy Procedia 142, 2183–2188. doi:10.1016/j.egypro.2017.12.586
Biswas, A. K., Ahmed, S. I., Akula, S. K., and Salehfar, H. (20212021). High voltage AC (HVAC) and high voltage DC (HVDC) transmission topologies of offshore wind power and reliability analysis. IEEE Green Technol. Conf. , 271–278. doi:10.1109/GREENTECH48523.2021.00051
Blaabjerg, F., and Ma, K. (2013). Future on power electronics for wind turbine systems. IEEE J. Emerg. Sel. Top. Power Electron 1 (3), 139–152. doi:10.1109/JESTPE.2013.2275978
Blaabjerg, F., and Ma, K. (2017). Wind energy systems. Proc. IEEE 105 (11), 2116–2131, Nov. doi:10.1109/JPROC.2017.2695485
Bo, G., Yongqian, L., Jie, Y., Li, L., and Shun, K. (2016). A wind farm optimal control algorithm based on wake fast-calculation model. J. Sol. Energy Eng. 138 (2). doi:10.1115/1.4032297
Bossanyi, E. (2018). Combining induction control and wake steering for wind farm energy and fatigue loads optimisation. J. Phys. Conf. Ser. 1037 (3), 032011. doi:10.1088/1742-6596/1037/3/032011
Campagnolo, F., Petrovic, V., Schreiber, J., Nanos, E. M., Croce, A., and Bottasso, C. L. (2016). Wind tunnel testing of a closed-loop wake deflection controller for wind farm power maximization. JPhCS 753 (3), 032006. doi:10.1088/1742-6596/753/3/032006
Chaithanya, S., Reddy, V. N. B., and Kiranmayi, R. (2019). Performance evaluation of PMSG-based LFAC system for offshore wind power. Int. J. Ambient Energy 42 (14), 1643–1648. doi:10.1080/01430750.2019.161166110.1080/01430750.2019.1611661
Cheng, M., and Zhu, Y. (2014). The state of the art of wind energy conversion systems and technologies: A review. Energy Convers. Manag. 88, 332–347. doi:10.1016/J.ENCONMAN.2014.08.037
Chong, C. H., Rigit, A. R. H., and Ali, I. (2019). Wind turbine modelling and simulation using Matlab/SIMULINK. IOP Conf. Ser. Mater Sci. Eng. 1101 (1), 012034. doi:10.1088/1757-899x/1101/1/012034
Ciri, U., Rotea, M. A., and Leonardi, S. (2017). Nested extremum seeking control for wind farm power optimization. Proc. Am. Control Conf. , 25–30. doi:10.23919/ACC.2017.7962925
Ciri, U., Rotea, M., Santoni, C., and Leonardi, S. (20162016). Large eddy simulation for an array of turbines with extremum seeking control. Proc. Am. Control Conf. , 531–536. doi:10.1109/ACC.2016.7524968
Dang, L., Samb, S. O., and Bernard, N. (2020). “Design optimization of a direct-drive PMSG considering the torque-speed profile Application for Offshore wind energy,” in Proceedings - 2020 international conference on electrical machines (New York, NY: ICEM ), 1875–1881, Aug. doi:10.1109/ICEM49940.2020.9271034
Dar, Z., Kar, K., Sahni, O., and Chow, J. H. (2016). Optimization of windfarm power by controlling the yaw angle using dynamic programming .
Google Scholar
Desalegn, B., Gebeyehu, D., and Tamirat, B. (2022). Wind energy conversion technologies and engineering approaches to enhancing wind power generation: A review . Amsterdam: Heliyon . doi:10.1016/j.heliyon.2022.e11263
Desalegn, B., Gebeyehu, D., and Tamrat, B. (1191). Evaluating the performances of PI controller (2DOF) under linear and nonlinear operations of DFIG-based wecs: A simulation study. Heliyon 8 (12), e11912. doi:10.1016/J.HELIYON.2022.E11912
Doekemeijer, B. M., Fleming, P. A., and van Wingerden, J. W. (2019). A tutorial on the synthesis and validation of a closed-loop wind farm controller using a steady-state surrogate model. Proc. Am. Control Conf. 2019, 2825–2836. doi:10.23919/ACC.2019.8815126
Doekemeijer, B. M., van der Hoek, D. C., and van Wingerden, J. W. (2019). “Model-based closed-loop wind farm control for power maximization using bayesian optimization: A large eddy simulation study,” in Proceedings of the 3rd IEEE conference on control technology and applications ( CCTA ), New York, NY : 284–289. doi:10.1109/CCTA.2019.8920587
Dursun, E. H., Koyuncu, H., and Kulaksiz, A. A. (2021). A novel unified maximum power extraction framework for PMSG based WECS using chaotic particle swarm optimization derivatives. Eng. Sci. Technol. Int. J. 24 (1), 158–170. doi:10.1016/J.JESTCH.2020.05.005
Engaging Autopilot (2021). NREL explores automation to build better blades, lower costs, and transform wind technology manufacturing | news | NREL . https://www.nrel.gov/news/program/2021/automation-wind-manufacturing.html . (accessed Nov. 20, 2021).
Fischereit, J., et al. (2015). Influence of tides on the sea breeze in the German Bight: How much model complexity is needed? EGUGA 17 (2), 11807. [Online]. Available: https://ui.adsabs.harvard.edu/abs/2015EGUGA.1711807F/abstract (Accessed Jan 08, 2023).
Fleming, P., King, J., Dykes, K., Simley, E., Roadman, J., Scholbrock, A., et al. (2019). Initial results from a field campaign of wake steering applied at a commercial wind farm – Part 1. Wind Energy Sci. 4 (2), 273–285. doi:10.5194/WES-4-273-2019
Fleming, P., King, J., Simley, E., Roadman, J., Scholbrock, A., Murphy, P., et al. (2020). Continued results from a field campaign of wake steering applied at a commercial wind farm - Part 2. Wind Energy Sci. 5 (3), 945–958. doi:10.5194/WES-5-945-2020
Fontanella, A., Al, M., van Wingerden, J. W., and Belloli, M. (2021). Model-based design of a wave-feedforward control strategy in floating wind turbines. Wind Energy Sci. 6 (3), 885–901. doi:10.5194/WES-6-885-2021
Gebraad, P. M. O., Teeuwisse, F. W., van Wingerden, J. W., Fleming, P. A., Ruben, S. D., Marden, J. R., et al. (2016). Wind plant power optimization through yaw control using a parametric model for wake effects - a CFD simulation study. Wind Energy 19 (1), 95–114. doi:10.1002/WE.1822
Gebraad, P. M. O., van Dam, F. C., and van Wingerden, J. W. (2013). A model-free distributed approach for wind plant control. Proc. Am. Control Conf. , 628–633. doi:10.1109/ACC.2013.6579907
Gionfra, N., Sandou, G., Siguerdidjane, H., Faille, D., and Loevenbruck, P. (2019). Wind farm distributed PSO-based control for constrained power generation maximization. Renew. Energy 133, 103–117. doi:10.1016/J.RENENE.2018.09.084
Göcmen, T., Kolle, K., Andersen, S. J., Eguinoa, I., Duc, T., Campagnolo, F., et al. (2020). Launch of the FarmConners wind farm control benchmark for code comparison. J. Phys. Conf. Ser. 1618, 022040. doi:10.1088/1742-6596/1618/2/022040
Goit, J. P., and Meyers, J. (2015). Optimal control of energy extraction in wind-farm boundary layers. J. Fluid Mech. 768, 5–50. doi:10.1017/JFM.2015.70
Goudarzi, N., and Zhu, W. D. (2013). A review on the development of wind turbine generators across the world. Int. J. Dyn. Control 1 (2), 192–202. doi:10.1007/s40435-013-0016-y
Haibo, Z., Florez, D., Saudemont, C., and Gruson, F. (2016). Control strategies of a dc based offshore wind farm with series connected collection grid. In 2016 IEEE International Energy Conference . New York, NY: ENERGYCON 2016 . doi:10.1109/ENERGYCON.2016.7514062
Harzendorf, F., Schelenz, R., and Jacobs, G. (2021). Reducing cost uncertainty in the drivetrain design decision with a focus on the operational phase. Wind Energy Sci. 6 (2), 571–584. doi:10.5194/wes-6-571-2021
Heer, F., Esfahani, P. M., Kamgarpour, M., and Lygeros, J. (2014). “Model based power optimisation of wind farms,” in 2014 European control conference (New York, NY: ECC 2014 ), 1145–1150. doi:10.1109/ECC.2014.6862365
Herp, J., Poulsen, U. v., and Greiner, M. (2015). Wind farm power optimization including flow variability. Renew. Energy 81, 173–181. doi:10.1016/J.RENENE.2015.03.034
Hoffmann, M., Rathke, C., Menze, A., Hemdan, N. G. A., and Kurrat, M. (2019). Parallel operation of HVDC DRU and VSC converters for offshore wind farm connection: Technical and economic feasibility. IET Conf. Publ. 2019, CP751. doi:10.1049/CP.2019.0057
Hou, P., Hu, W., Zhang, B., Soltani, M., Chen, C., and Chen, Z. (2016). Optimised power dispatch strategy for offshore wind farms. IET Renew. Power Gener. 10 (3), 399–409. doi:10.1049/IET-RPG.2015.0176
Hou, P., Zhu, J., Ma, K., Yang, G., Hu, W., and Chen, Z. (2019). A review of offshore wind farm layout optimization and electrical system design methods. J. Mod. Power Syst. Clean Energy 7 (5), 975–986. doi:10.1007/s40565-019-0550-5
Howland, M. F., Lele, S. K., and Dabiri, J. O. (2019). Wind farm power optimization through wake steering. Proc. Natl. Acad. Sci. U. S. A. 116 (29), 14495–14500. doi:10.1073/pnas.1903680116
PubMed Abstract | CrossRef Full Text | Google Scholar
Heng, Z., Jing, C., Huan, Z., Xinmeng, L., Jiachen, L., Hanyu, C., et al. (2021). A multi-functional de-icing equipment using hybrid type parallel current source converters E3S Web of Conferences , 256. doi:10.1051/e3sconf/202125602016
I. Renewable Energy Agency (2022). Renewable capacity statistics 2022 statistiques de capacité renouvelable 2022 estadísticas de capacidad renovable 2022 . [Online]. Available: www.irena.org .
Jain, A., Chan, L., Brown, D. S., and Dragan, A. D. (2021). Optimal cost design for model predictive control. Proc. Mach. Learn Res. 144 (1–13). Accessed: Nov. 25, 2021. [Online]. Available: https://arxiv.org/abs/2104.11353v2 .
Kalair, A., Abas, N., and Khan, N. (2016)., Comparative study of HVAC and HVDC transmission systems Renew. Sustain. Energy Rev. 59. C , 1653–1675, Jun. doi:10.1016/J.RSER.2015.12.288
Kanev, S. (2020). Dynamic wake steering and its impact on wind farm power production and yaw actuator duty. Renew. Energy 146, 9–15. doi:10.1016/J.RENENE.2019.06.122
Kanev, S. K., Savenije, F. J., and Engels, W. P. (2018). Active wake control: An approach to optimize the lifetime operation of wind farms. Wind Energy 21 (7), 488–501. doi:10.1002/WE.2173
Khani, N. G. (2021). Improving fault ride through capability of induction generator-based wind farm using static compensator during asymmetrical faults. Int. Trans. Electr. Energy Syst. 31 (11), 2021. doi:10.1002/2050-7038.13103
Kheirabadi, A. C., and Nagamune, R. (20192019). Modeling and power optimization of floating offshore wind farms with yaw and induction-based turbine repositioning. Proc. Am. Control Conf. , 5458–5463, Jul. doi:10.23919/ACC.2019.8814600
Kim, H., Kim, K., and Paek, I. (2017). Model based open-loop wind farm control using active power for power increase and load reduction. Appl. Sci. 7, 1068. doi:10.3390/APP7101068
Kimura, N., Morizane, T., Iyoda, I., Nakao, K., and Yokoyama, T. (2018). Application of solid-state transformer for HVDC transmission from offshore windfarm. In 7th International IEEE Conference on Renewable Energy Research and Applications . New York, NY: ICRERA 2018 , 902–907. doi:10.1109/ICRERA.2018.8566702
Le, X. C., Duong, M. Q., and Le, K. H. (12023)., Review of the modern maximum power tracking algorithms for permanent magnet synchronous generator of wind power conversion systems 16. Basel, Switzerland: MDPI . doi:10.3390/en16010402 Energies 1
Li, R., Yu, L., Xu, L., and Adam, G. P. (2020). Coordinated control of parallel DR-HVDC and MMC-HVDC systems for offshore wind energy transmission. IEEE J. Emerg. Sel. Top. Power Electron 8 (3), 2572–2582, Sep. doi:10.1109/JESTPE.2019.2931197
Li, R., Zhang, J., and Zhao, X. (2022). Dynamic wind farm wake modeling based on a Bilateral Convolutional Neural Network and high-fidelity LES data. Energy 258, 1248452022. doi:10.1016/J.ENERGY.2022.124845
Li, Z., Song, Q., An, F., Zhao, B., Yu, Z., and Zeng, R. (2021). Review on DC transmission systems for integrating large-scale offshore wind farms. Energy Convers. Econ. 2 (1), 1–14. doi:10.1049/ENC2.12023
Luqman, M., Yao, G., Zhou, L., Zhang, T., and Lamichhane, A. (2019). A novel hybrid converter proposed for Multi-MW wind generator for offshore applications. Energies (Basel) 12, 16. doi:10.3390/en12214167
Ma, K., Liserre, M., Blaabjerg, F., and Kerekes, T. (2015). Thermal loading and lifetime estimation for power device considering mission profiles in wind power converter. IEEE Trans. Power Electron 30 (2), 590–602. doi:10.1109/TPEL.2014.2312335
Machado, J., Neves, M. V., and Santos, P. J. (2015). “Economic limitations of the HVAC transmission system when applied to offshore wind farms,” in Proceedings - 2015 9th international conference on compatibility and power electronics (New York, NY: CPE ), 69–75. doi:10.1109/CPE.2015.7231051
Mahmoud, M. M., Aly, M. M., and Abdel-Rahim, A. M. M. (2020). Enhancing the dynamic performance of a wind-driven PMSG implementing different optimization techniques. SN Appl. Sci. 2 (4), 19. doi:10.1007/s42452-020-2439-3
Marden, J. R., Ruben, S. D., and Pao, L. Y. (2013). A model-free approach to wind farm control using game theoretic methods. IEEE Trans. Control Syst. Technol. 21 (4), 1207–1214. doi:10.1109/TCST.2013.2257780
Martínez-Tossas, L. A. (2021). Numerical investigation of wind turbine wakes under high thrust coefficient . Hoboken, New Jersey: Wind Energy . doi:10.1002/we.2688
Moghadam, F. K., and Nejad, A. R. (2020). Evaluation of PMSG-based drivetrain technologies for 10-MW floating offshore wind turbines: Pros and cons in a life cycle perspective. Wind Energy 23 (7), 1542–1563, Jul. doi:10.1002/WE.2499
Mohan, M., and Vittal, K. P. (2018). “Modeling and simulation of PMSG-based wind power generation system,” in 2018 3rd IEEE international conference on recent trends in electronics, information and communication technology (New York, NY: RTEICT 2018 - Proceedings ), 57–62. doi:10.1109/RTEICT42901.2018.9012620
Mousa, H. H. H., Youssef, A. R., and Mohamed, E. E. M. (2020). Optimal power extraction control schemes for five-phase PMSG based wind generation systems. Eng. Sci. Technol. Int. J. 23 (1), 144–155. doi:10.1016/J.JESTCH.2019.04.004
Munters, W., and Meyers, J. (2017). An optimal control framework for dynamic induction control of wind farms and their interaction with the atmospheric boundary layer. Philosophical Trans. R. Soc. A Math. Phys. Eng. Sci. 375, 20160100. doi:10.1098/RSTA.2016.0100
Munters, W., and Meyers, J. (2018). Dynamic strategies for yaw and induction control of wind farms based on large-eddy simulation and optimization. Energies 11, 177. doi:10.3390/EN11010177
Munters, W., and Meyers, J. (2016). Effect of wind turbine response time on optimal dynamic induction control of wind farms. J. Phys. Conf. Ser. 753 (5), 052007. doi:10.1088/1742-6596/753/5/052007
Munters, W., and Meyers, J. (2018). Optimal dynamic induction and yaw control of wind farms: Effects of turbine spacing and layout. J. Phys. Conf. Ser. 1037 (3), 032015. doi:10.1088/1742-6596/1037/3/032015
Mwaniki, J., Lin, H., and Dai, Z. (20172017). A concise presentation of doubly fed induction generator wind energy conversion systems challenges and solutions. J. Eng. (United Kingdom) 2017, 1–13. doi:10.1155/2017/4015102
Mwaniki, J., Lin, H., and Dai, Z. (2017). A condensed introduction to the doubly fed induction generator wind energy conversion systems J. Eng . (United Kingdom: Hindawi Limited ). doi:10.1155/2017/2918281
Nouri, B., Goksu, O., Gevorgian, V., and Ejnar Sørensen, P. (2020). Generic characterization of electrical test benches for AC- and HVDC-connected wind power plants. Wind Energy Sci. 5 (2), 561–575. doi:10.5194/wes-5-561-2020
Ogidi, O. O., Khan, A., and Dehnavifard, H. (2020). Deployment of onshore wind turbine generator topologies: Opportunities and challenges. Int. Trans. Electr. Energy Syst. 30 (5). doi:10.1002/2050-7038.12308
Okedu, K. E., al Tobi, M., and al Araimi, S. (2021). Comparative study of the effects of machine parameters on DFIG and PMSG variable speed wind turbines during grid fault. Front. Energy Res. 9, 174. doi:10.3389/fenrg.2021.681443
Pan, L., and Shao, C. (2020). Wind energy conversion systems analysis of PMSG on offshore wind turbine using improved SMC and Extended State Observer. Renew. Energy 161, 149–161. doi:10.1016/J.RENENE.2020.06.057
Park, J., and Law, K. H. (2016). A data-driven, cooperative wind farm control to maximize the total power production. Appl. Energy 165, 151–165. doi:10.1016/j.apenergy.2015.11.064
Park, J., and Law, K. H. (2017). Cooperative wind turbine control for maximizing wind farm power 1 using sequential. Convex Program. 2 3.
Peng, X., Liu, Z., and Jiang, D. (2021). A review of multiphase energy conversion in wind power generation. In Renewable and Sustainable Energy Reviews , 147. Elsevier . doi:10.1016/j.rser.2021.111172
Quick, J., Annoni, J., King, R., Dykes, K., Fleming, P., and Ning, A. (2017). Optimization under uncertainty for wake steering strategies. J. Phys. Conf. Ser. 854 (1), 012036. doi:10.1088/1742-6596/854/1/012036
Rahimi, H., Martinez Garcia, A., Stoevesandt, B., Peinke, J., and Schepers, G. (2018). An engineering model for wind turbines under yawed conditions derived from high fidelity models. Wind Energy 21 (8), 618–633. doi:10.1002/we.2182
Rodrigues, S. F., Teixeira Pinto, R., Soleimanzadeh, M., Bosman, P. A. N., and Bauer, P. (2015). Wake losses optimization of offshore wind farms with moveable floating wind turbines. Energy Convers. Manag. 89, 933–941. doi:10.1016/j.enconman.2014.11.005
Rodriguez, P., and Rouzbehi, K. (2017). Multi-terminal DC grids: Challenges and prospects. J. Mod. Power Syst. Clean Energy 5 (4), 515–523. doi:10.1007/s40565-017-0305-0
Rotea, M. A. (2014). Dynamic programming framework for wind power maximization. IFAC Proc. Vol. (IFAC-PapersOnline) 19, 3639–3644. doi:10.3182/20140824-6-ZA-1003.02071
Ryndzionek, R., and Sienkiewicz, Ł. (2020). Evolution of the HVDC link connecting offshore wind farms to onshore power systems. Energies (Basel) 13 (8), 1914. doi:10.3390/en13081914
Samraj, D. B., and Perumal, M. P. (2019). Compatibility of electrical generators for harvesting extended power from wind energy conversion system. Meas. Control 52 (9–10), 1240–1251. doi:10.1177/002029401985818310.1177/0020294019858183
Santhanagopalan, V., Rotea, M. A., and Iungo, G. v. (2018). Performance optimization of a wind turbine column for different incoming wind turbulence. Renew. Energy 116, 232–243. doi:10.1016/j.renene.2017.05.046
Scott Semken, R., Polikarpova, M., Röyttä, P., Alexandrova, J., Pyrhönen, J., Nerg, J., et al. (2012). Direct-drive permanent magnet generators for high-power wind turbines: Benefits and limiting factors. IET Renew. Power Gener. 6 (1), 1–8. doi:10.1049/iet-rpg.2010.0191
Serrano González, J., Burgos Payán, M., Riquelme Santos, J., and González Rodríguez, Á. G. (2015). Maximizing the overall production of wind farms by setting the individual operating point of wind turbines. Renew. Energy 80, 219–229. doi:10.1016/J.RENENE.2015.02.009
Shi, G., Cai, X., Sun, C., Chang, Y., and Yang, R. (2016). All-DC offshore wind farm with parallel connection: An overview , IET Conf. Publ. 2016, CP696. doi:10.1049/CP.2016.0455
CrossRef Full Text
Shu, J., Zhang, B. H., and Bo, Z. Q. (2011). A wind farm coordinated controller for power optimization. IEEE Power Energy Soc. General Meet . doi:10.1109/PES.2011.6039552
Sierra-Garcia, J. E., and Santos, M. (2021). Deep learning and fuzzy logic to implement a hybrid wind turbine pitch control. Neural Comput. Appl. 2021 , 1–15. doi:10.1007/S00521-021-06323-W
Simley, E., Fleming, P., and King, J. (2020). Design and analysis of a wake steering controller with wind direction variability. Wind Energy Sci. 5 (2), 451–468. doi:10.5194/WES-5-451-2020
Thøgersen, E., Tranberg, B., Herp, J., and Greiner, M. (2017). Statistical meandering wake model and its application to yaw-angle optimisation of wind farms. J. Phys. Conf. Ser. 854 (1), 012017. doi:10.1088/1742-6596/854/1/012017
Thomas, J. J., Gebraad, P. M., and Ning, A. (2017). Improving the FLORIS wind plant model for compatibility with gradient-based optimization. Wind Eng. 41 (5), 313–329. doi:10.1177/0309524X1772200010.1177/0309524X17722000
Tian, S., Campos-Gaona, D., Lacerda, V. A., Torres-Olguin, R. E., and Anaya-Lara, O. (2020). Novel control approach for a hybrid grid-forming HVDC offshore transmission system. Energies (Basel) 13 (7), 1681. doi:10.3390/EN13071681
Torres-Olguin, R. E., Garces, A., Molinas, M., and Undeland, T. (2013). Integration of offshore wind farm using a hybrid HVDC transmission composed by the PWM current-source converter and line-commutated converter. IEEE Trans. Energy Convers. 28 (1), 125–134. doi:10.1109/TEC.2012.2230535
Vali, M., Petrović, V., Boersma, S., van Wingerden, J. W., and Kühn, M. (2017). Adjoint-based model predictive control of wind farms: Beyond the quasi steady-state power maximization * *this work has been funded by the ministry for sciences and culture of the federal state of lower saxony, Germany as part of the PhD programme on system integration of renewable energies (SEE) and by the German ministry of economic affairs and energy (BMWi) in the scope of the WIMS-cluster project (FKZ 0324005). IFAC-PapersOnLine 50 (1), 4510–4515. doi:10.1016/J.IFACOL.2017.08.382
Vali, M., Petrović, V., Boersma, S., van Wingerden, J. W., Pao, L. Y., and Kühn, M. (2019). Adjoint-based model predictive control for optimal energy extraction in waked wind farms. Control Eng. Pract. 84, 48–62. doi:10.1016/J.CONENGPRAC.2018.11.005
Vali, M., van Wingerden, J. W., Boersma, S., Petrović, V., and Kühn, M. (2016). A predictive control framework for optimal energy extraction of wind farms. J. Phys. Conf. Ser. 753 (5), 052013. doi:10.1088/1742-6596/753/5/052013
van de Kaa, G., van Ek, M., Kamp, L. M., and Rezaei, J. (2020). Wind turbine technology battles: Gearbox versus direct drive - opening up the black box of technology characteristics. Technol. Forecast Soc. Change 153, 1199332020. doi:10.1016/J.TECHFORE.2020.119933
van Dijk, M. T., van Wingerden, J.-W., Ashuri, T., Li, Y., and Rotea, M. A. (2021). Yaw-misalignment and its impact on wind turbine loads and wind farm power output. J. Phys. Conf. Ser. 753, 062013. doi:10.1088/1742-6596/753/6/062013
van Dijk, M. T., van Wingerden, J. W., Ashuri, T., and Li, Y. (2017). Wind farm multi-objective wake redirection for optimizing power production and loads. Energy 121, 561–569. doi:10.1016/J.ENERGY.2017.01.051
Vijayaprabhu, A., Bhaskar, K. B., Jasmine susila, D., and Dinesh, M. (2004). Review and comparison of various types of generation using WECS topologies. IOP Conf. Ser. Mater Sci. Eng. 1177 (1), 012004. doi:10.1088/1757-899x/1177/1/012004
Wang, L., Cholette, M. E., Zhou, Y., Yuan, J., Tan, A. C. C., and Gu, Y. (2018). Effectiveness of optimized control strategy and different hub height turbines on a real wind farm optimization. Renew. Energy 126, 819–829. doi:10.1016/J.RENENE.2018.04.004
Wei, Q., Wu, B., Xu, D., and Zargari, N. R. (2017). “Overview of offshore wind farm configurations,” in IOP conference series: Earth and environmental science , 93. (1) doi:10.1088/1755-1315/93/1/012009
Yang, Z., Li, Y., and Seem, J. E. (2015). Optimizing energy capture of cascaded wind turbine array with nested-loop extremum seeking control. J. Dyn. Syst. Meas. Control 137 (12). doi:10.1115/1.4031593
Yaramasu, V., Dekka, A., Durán, M. J., Kouro, S., and Wu, B. (2017). PMSG-Based wind energy conversion systems: Survey on power converters and controls. IET Electr. Power Appl. 11 (6), 956–968. doi:10.1049/IET-EPA.2016.0799
Yaramasu, V., and Wu, B. (2016). “Basics of wind energy conversion systems (wecs),” in Model predictive control of wind energy conversion systems , 1–60. doi:10.1002/9781119082989.CH1
Yin, X., Zhang, W., Jiang, Z., and Pan, L. (2020). Data-driven multi-objective predictive control of offshore wind farm based on evolutionary optimization. Renew. Energy 160, 974–986. doi:10.1016/J.RENENE.2020.05.015
Yin, X., and Zhao, X. (2021). Deep neural learning based distributed predictive control for offshore wind farm using high-fidelity les Data. IEEE Trans. Industrial Electron. 68 (4), 3251–3261, Apr. doi:10.1109/TIE.2020.2979560
Zhang, B., Soltani, M., Hu, W., Hou, P., Huang, Q., and Chen, Z. (2018). Optimized power dispatch in wind farms for power maximizing considering fatigue loads. IEEE Trans. Sustain Energy 9 (2), 862–871. doi:10.1109/TSTE.2017.2763939
Zhang, H., Gruson, F., Flórez Rodriguez, D., Saudemont, C., Gruson, F., and Flórez Rodriguez, D. M. (2018). Overvoltage limitation method of an offshore wind farm with DC series-parallel collection grid. IEEE Trans. Sustain. Energy , IEEE 10 (1), 204–213. doi:10.1109/tste.2018.2829929
Zhang, J., Li, K. J., Liu, W., Sun, K., Liu, Z., and di Wang, Z. (2021). Grid side reactive power support strategy for MMC-HVDC connected to the wind farms based on unloading resistor. Electr. Power Syst. Res. 193, 1070102021. doi:10.1016/J.EPSR.2020.107010
Zhang, Z., Chen, A., Matveev, A., Nilssen, R., and Nysveen, A. (2013). High-power generators for offshore wind turbines. Energy Procedia 35, 52–61. doi:10.1016/j.egypro.2013.07.158
Zhao, H., Zhao, J., Qiu, J., Liang, G., and Dong, Z. Y. (2020). Cooperative wind farm control with deep reinforcement learning and knowledge-assisted learning. IEEE Trans. Ind. Inf. 16 (11), 6912–6921, Nov. doi:10.1109/TII.2020.2974037
Zong, H., and Porté-Agel, F. (2020). A point vortex transportation model for yawed wind turbine wakes. J. Fluid Mech. 890, A8. doi:10.1017/JFM.2020.123
Keywords: wind energy harvesting technologies, doubly fed induction generator system, permanent magnet synchronous generator system, high-voltage power transmission systems, optimization-based wind farm control models
Citation: Desalegn B, Gebeyehu D, Tamrat B and Tadiwose T (2023) Wind energy-harvesting technologies and recent research progresses in wind farm control models. Front. Energy Res. 11:1124203. doi: 10.3389/fenrg.2023.1124203
Received: 14 December 2022; Accepted: 26 January 2023; Published: 15 February 2023.
Reviewed by:
Copyright © 2023 Desalegn, Gebeyehu, Tamrat and Tadiwose. This is an open-access article distributed under the terms of the Creative Commons Attribution License (CC BY). The use, distribution or reproduction in other forums is permitted, provided the original author(s) and the copyright owner(s) are credited and that the original publication in this journal is cited, in accordance with accepted academic practice. No use, distribution or reproduction is permitted which does not comply with these terms.
*Correspondence: Belachew Desalegn, [email protected]
This article is part of the Research Topic
Climate Change Challenge: A Wind Energy Perspective
A review on wind energy conversion system and enabling technology
Ieee account.
- Change Username/Password
- Update Address
Purchase Details
- Payment Options
- Order History
- View Purchased Documents
Profile Information
- Communications Preferences
- Profession and Education
- Technical Interests
- US & Canada: +1 800 678 4333
- Worldwide: +1 732 981 0060
- Contact & Support
- About IEEE Xplore
- Accessibility
- Terms of Use
- Nondiscrimination Policy
- Privacy & Opting Out of Cookies
A not-for-profit organization, IEEE is the world's largest technical professional organization dedicated to advancing technology for the benefit of humanity. © Copyright 2024 IEEE - All rights reserved. Use of this web site signifies your agreement to the terms and conditions.

Quick links
- Climate change
- COVID-19 research
- Staff profiles
GenCost: cost of building Australia’s future electricity needs
Each year, CSIRO and the Australian Energy Market Operator (AEMO) collaborate with industry stakeholders to update GenCost. This leading economic report estimates the cost of building new electricity generation, storage, and hydrogen production in Australia out to 2050.
GenCost 2023-24 report released
The latest release was shaped by an unprecedented level of industry participation.
What’s new?
- Renewables (solar and wind + firming) remains the lowest cost new build electricity technology.
- Large-scale nuclear technology costs included for the first time.
- Future wind costs revised upwards.
- An extensive FAQ section addressing common questions from current and past consultations.
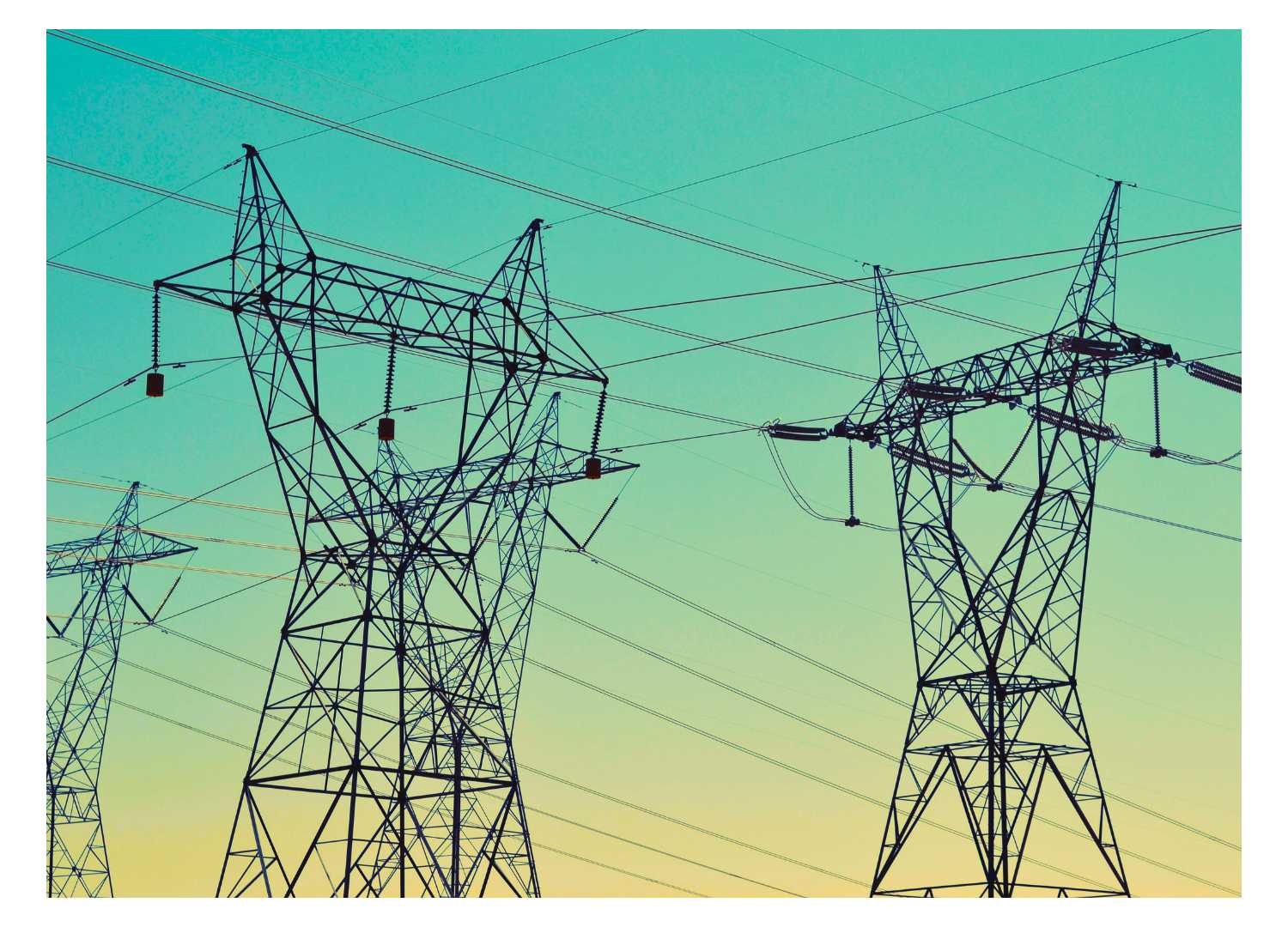
What is GenCost?
GenCost is a leading economic report for business leaders and decision-makers planning reliable and affordable energy solutions to achieve net zero emissions by 2050.
Published annually in collaboration with the Australian Energy Market Operator (AEMO), GenCost offers accurate, policy and technology-neutral cost estimates for new electricity generation, storage, and hydrogen technologies, through to 2050.
GenCost is highly collaborative and transparent, leveraging the expertise of energy industry stakeholders and involving extensive consultation to ensure accuracy prior to publication.
Transcript to be supplied
CSIRO is committed to ensuring its online content is accessible to everyone regardless of ability.
Request accessibility assistance
- View transcript
- Copy embed code
Share & embed this video
https://vimeo.com/896412073?share=copy
<iframe src="//player.vimeo.com/video/896412073?share=copy" width="640" height="360" frameborder="0" allow="autoplay; fullscreen" allowfullscreen></iframe>
GenCost 2023-24 report
Explore key insights from the latest report.
GenCost projects the cost of electricity generation and storage for a wide range of technologies up to the year 2050.
Renewables remain lowest cost
The report highlights wind power’s slower recovery from global inflationary pressures, resulting in upward revisions for both onshore and offshore wind costs over the next decade.
Despite this, updated analysis reaffirms that renewables, including associated storage and transmission costs, remain the lowest cost, new build technology out to 2050.
This competitive position reflects a decade of cost reductions in wind, solar photovoltaics (PV) and batteries before the pandemic. This is in contrast with costs of mature competitors which have remained flat.
Large-scale nuclear costs introduced
The inclusion of large-scale nuclear costs this year was prompted by increased stakeholder interest in nuclear technology following the updated cost estimates for SMRs in the 2023-24 consultation draft.
Applying overseas costs to large-scale nuclear projects in Australia is complex due to the lack of a domestic nuclear industry and significant global differences in labor costs, workforce expertise, governance, and standards. The GenCost 2023-24 report team estimated large-scale nuclear costs using South Korea’s successful nuclear program. They adjusted for differences in Australian and South Korean deployment costs by comparing the cost ratio of new coal generation in each country. GenCost found nuclear power to be more expensive than renewables and estimated a development timeline of at least 15 years, including construction. This reflects the absence of a local development pipeline, additional legal, safety and security requirements, and stakeholder evidence. Achieving the reported nuclear costs depends on Australia committing to a continuous building program like South Korea’s. Initial units are likely to incur higher costs, and a first-of-a-kind premium of up to 100 per cent is possible, although not included in the cost estimates for nuclear or other new electricity technologies in the report.
Explore answers to commonly asked questions about GenCost.
- 2023-24 GenCost report
- 2023-24 GenCost media release
- 2023-24 GenCost infographics
- 2023-24 GenCost report (text version)
- GenCost project data and previous reports
Our in-depth explainers take a closer look at Australia’s evolving electricity sector. What are the challenges and opportunities that lie ahead in Australia's energy transition?
Understanding the cost of Australia's energy transition
Understanding the costs of new-build electricity generation technologies is essential if we are to evolve Australia’s energy system to limit emissions.
The question of nuclear in Australia’s energy sector
In Australia's transition to net zero emissions, the electricity sector has a major role to play. But does nuclear power have a place in our future grid?
Latest news
There are no news releases to show.
Find out how we can help you and your business. Get in touch using the form below and our experts will get in contact soon!
CSIRO will handle your personal information in accordance with the Privacy Act 1988 (Cth) and our Privacy Policy .
Enter a valid email address, for example [email protected]
A Country value must be provided
First name must be filled in
Surname must be filled in
Please choose an option
Organisation must be filled in
Please provide a subject for the enquriy
We'll need to know what you want to contact us about so we can give you an answer
We have received your enquiry and will reply soon.
We're Sorry
The contact form is currently unavailable. Please try again later. If this problem persists, please call us with your enquiry on 1300 363 400 or +61 3 9545 2176. We are available from 9.00 am to 4.00 pm AEST Monday - Friday.

COMMENTS
Wind Energy: A Review Paper. Atul Kumar Muhammad Zafar Ullah Khan Bishwajeet Pandey. M S College, University of Management & Gyancity Rese arch Lab, 1 Motihari, India Technology, Sialkot Campus ...
This paper provides an overview of how the analysis of wind energy has evolved over the last years. ... A high LCS means that a paper is relevant in the research field, whereas a high GCS indicates the paper is considered important by the academic field worldwide. The closeness centrality identifies the papers, in the network, ...
Wind energy's contribution to primary energy supply has increased in the past decade, while technology costs have decreased faster than predicted. New work finds that recent expert estimates of ...
This paper reviews the wind energy technologies used, mainly focusing on the types of turbines used and their future scope. Further, the paper briefly discusses certain future wind generation technologies, namely airborne, offshore, smart rotors, multi-rotors, and other small wind turbine technologies.
Drawing from a recent international workshop, we identify three grand challenges in wind energy research that require further progress from the scientific community: (i) improved understanding of the physics of atmospheric flow in the critical zone of wind power plant operation, (ii) materials and system dynamics of individual wind turbines, and (iii) optimization and control of fleets of wind ...
Wind Energy offers a major forum for the reporting of advances in this rapidly developing technology with the goal of realising the world-wide potential to harness clean energy from land-based and offshore wind. The journal aims to reach all those with an interest in this field from academic research, industrial development through to applications, including individual wind turbines and ...
Wind energy papers that have a thermal energy component or are related to national energy systems are in the scope of Energy, not Wind Energy and Engineering. Finally, research in atmospheric science and fundamental aspects of electrical components (generators, motors, power converters, transmission and distribution grids, etc.) are considered ...
Wind Engineering is the oldest and most authoritative peer-reviewed English language journal devoted entirely to the technology of wind energy. Under the direction of a distinguished editor and editorial board, Wind Engineering appears bimonthly with fully refereed contributions from active figures in the field, book notices, and summaries of the more interesting papers from other sources...
In this editorial paper, the progress and future outlook of wind energy research in two main aspects are discussed. The first aspect is in the area of wind turbine design and computations which covers engineering modeling and high-fidelity approaches. The second part of the paper discusses the usage of data-driven approaches in wind energy ...
Wind, as an open access journal, is dedicated to disseminating rigorously peer-reviewed publications to advance knowledge and technology in wind-energy-related areas.The journal brings many opportunities for actively spreading novel concepts and advancements in multi-disciplinary wind technology and related issues by covering the wind-related scientific and engineering aspects, including but ...
See all (26) Learn more about Research Topics. Part of an innovative journal, this section focuses on the further development of wind energy as one of the essential renewable energy technologies.
The use of wind energy worldwide has overgrown in recent years to reduce greenhouse gas emissions. Wind power is free, but the installation and maintenance of wind turbines remain very costly. The size of the installation of the wind turbine is not only determined by wind statistics at a given location, but also by turbine infrastructure and maintenance costs. The payback time of the turbine ...
In this research paper, wind turbines and solar modules are combined with pumped hydro storage, batteries, and green hydrogen. Energy management strategies are described for five different scenarios of hybrid renewable energy systems, based on single or hybrid storage technologies. ... Wind energy is an important renewable energy source. Rotor ...
Wind power now represents a major and growing source of renewable energy. Large wind turbines (with capacities of up to 6-8 MW) are widely installed in power distribution networks. Increasing numbers of onshore and offshore wind farms, acting as power plants, are connected directly to power transmission networks at the scale of hundreds of megawatts. As its level of grid penetration has ...
Abstract. If future net-zero emissions energy systems rely heavily on solar and wind resources, spatial and temporal mismatches between resource availability and electricity demand may challenge ...
Wind energy is one of the fastest-growing green technologies as it provides clean, safe, and renewable electricity generation. This study provides insights into the available methodologies for sustainable power harnessing using wind resources, scrutinizing the developments in the recent decades and the future potential of global wind power industries. Contrasting and comparing the expected ...
Printed on paper containing at least 50% wastepaper, including 20% postconsumer waste. Wind Energy Technology: Current Status and R&D Future. Robert Threshera, Michael Robinsonaand Paul Veersb. aNational Renewable Energy Laboratory, Golden CO 80401. 1. b. Sandia National Laboratories, Albuquerque, NM 87185. 2.
As a key aspect of wind energy research, wind speed prediction plays an important role in the formulation of future development strategy of power plants. IMFO-FIG-LSTM model provides a technique that meets the requirements of long, medium, and short terms prediction at the same time and can predict wind speed with high accuracy.
Accordingly, this paper explores the statuses of wind energy harvesting tech-nologies, and wind farm control strategies by discussing on their recent and future impacts in transforming wind power industry. ... Wind power research and technological advancements have greatly contributed to the progress achieved by the wind power industry so far ...
The global benchmark levelized cost of electricity has been dropping for onshore wind energy, which was $44 per MWh in 2020, dropping 9 % from 2019 (Eckhouse, 2020).In addition, the global benchmark levelized cost of electricity for offshore wind energy dropped to $78 per MWh in 2020 from $90 per MWh in 2019 (Eckhouse, 2020).One of the drivers of wind energy generation is the energy policies.
The purpose of the paper [15] is to provide a quantitative and qualitative analysis of the growth of wind energy in the world, looking at its development over the past ten years, its distribution ...
As a result of extensive research, wind energy is now being widely used for electrical power generation. Current paper make an effort to exhibit a short review of wind energy conversion system, highlighting its aerodynamics, electrical, mechanical aspects, and different control strategies. The crucial survey findings, ongoing research and ...
Onshore wind energy technologies are already being manufactured and deployed on large scale (Edenhofer et al., Citation 2011). Wind turbines convert the energy of wind into electricity. ... A qualitative research was employed by reviewing papers in the scope of the study. Even though, the complete lifecycle of renewable energy sources have no ...
In order to improve the reliability and life of the wind turbine, this paper takes the rolling bearing in the experimental platform of the wind turbine as the research object. In order to obtain the intrinsic mode function (IMF) of each fault type, the original signals of different fault states of the rolling bearing on the experimental platform are decomposed by using the overall average ...
Wind Research and Development. The U.S. Department of Energy's (DOE) Wind Energy Technologies Office's mission is to fund wind energy research through technology development that will facilitate the decarbonization of our electric grid and achieve a robust U.S. clean energy economy. WETO aims to provide abundant, low-cost, wind power at ...
In the area of marine renewable energy research, the combined development of offshore wind and wave energy has received additional attention in recent years [5]. A number of support schemes and research projects have been successively launched, such as the MARINA [6], H2OCEAN [7], MERMAID [8], TROPOS [9], ORECCA [10], etc.
The question of nuclear in Australia's energy sector. Each year, CSIRO and the Australian Energy Market Operator (AEMO) collaborate with industry stakeholders to update GenCost. This leading economic report estimates the cost of building new electricity generation, storage, and hydrogen production in Australia out to 2050.