- Skip to main content
- Skip to primary sidebar
- Skip to footer
- QuestionPro

- Solutions Industries Gaming Automotive Sports and events Education Government Travel & Hospitality Financial Services Healthcare Cannabis Technology Use Case NPS+ Communities Audience Contactless surveys Mobile LivePolls Member Experience GDPR Positive People Science 360 Feedback Surveys
- Resources Blog eBooks Survey Templates Case Studies Training Help center

Home Audience

Sample Size Determination: Definition, Formula, and Example
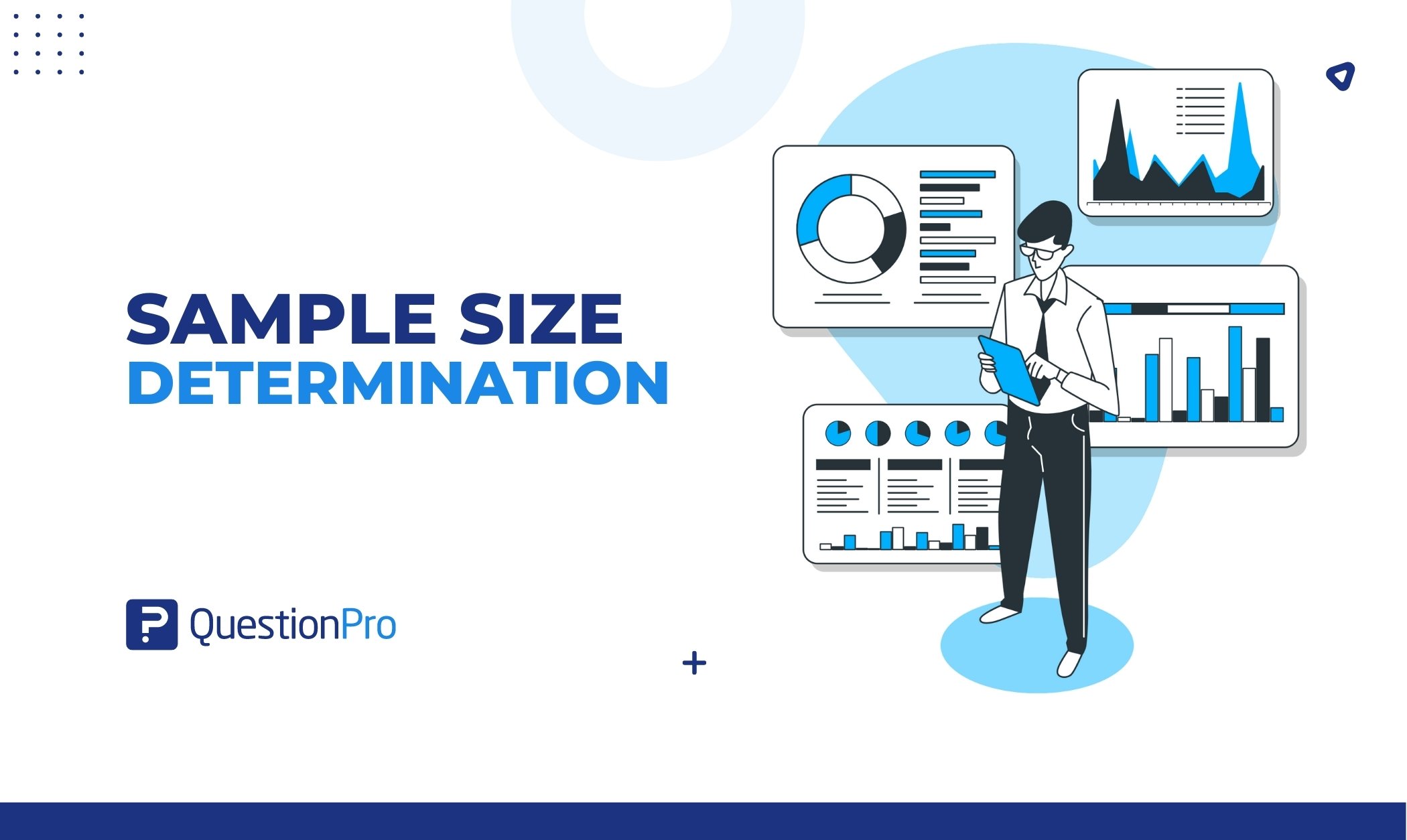
Are you ready to survey your research target? Research surveys help you gain insights from your target audience. The data you collect gives you insights to meet customer needs, leading to increased sales and customer loyalty. Sample size calculation and determination are imperative to the researcher to determine the right number of respondents, keeping in mind the research study’s quality.
So, how should you do the sample size determination? How do you know who should get your survey? How do you decide on the number of the target audience?
Sending out too many surveys can be expensive without giving you a definitive advantage over a smaller sample. But if you send out too few, you won’t have enough data to draw accurate conclusions.
Knowing how to calculate and determine the appropriate sample size accurately can give you an edge over your competitors. Let’s take a look at what a good sample includes. Also, let’s look at the sample size calculation formula so you can determine the perfect sample size for your next survey.
What is Sample Size?
‘Sample size’ is a market research term used for defining the number of individuals included in conducting research. Researchers choose their sample based on demographics, such as age, gender questions , or physical location. It can be vague or specific.
For example, you may want to know what people within the 18-25 age range think of your product. Or, you may only require your sample to live in the United States, giving you a wide population range. The total number of individuals in a particular sample is the sample size.
What is sample size determination?
Sample size determination is the process of choosing the right number of observations or people from a larger group to use in a sample. The goal of figuring out the sample size is to ensure that the sample is big enough to give statistically valid results and accurate estimates of population parameters but small enough to be manageable and cost-effective.
In many research studies, getting information from every member of the population of interest is not possible or useful. Instead, researchers choose a sample of people or events that is representative of the whole to study. How accurate and precise the results are can depend a lot on the size of the sample.
Choosing the statistically significant sample size depends on a number of things, such as the size of the population, how precise you want your estimates to be, how confident you want to be in the results, how different the population is likely to be, and how much money and time you have for the study. Statistics are often used to figure out how big a sample should be for a certain type of study and research question.
Figuring out the sample size is important in ensuring that research findings and conclusions are valid and reliable.
Why do you need to determine the sample size?
Let’s say you are a market researcher in the US and want to send out a survey or questionnaire . The survey aims to understand your audience’s feelings toward a new cell phone you are about to launch. You want to know what people in the US think about the new product to predict the phone’s success or failure before launch.
Hypothetically, you choose the population of New York, which is 8.49 million. You use a sample size determination formula to select a sample of 500 individuals that fit into the consumer panel requirement. You can use the responses to help you determine how your audience will react to the new product.
However, determining a sample size requires more than just throwing your survey at as many people as possible. If your estimated sample sizes are too big, it could waste resources, time, and money. A sample size that’s too small doesn’t allow you to gain maximum insights, leading to inconclusive results.
LEARN ABOUT: Survey Sample Sizes
What are the terms used around the sample size?
Before we jump into sample size determination, let’s take a look at the terms you should know:
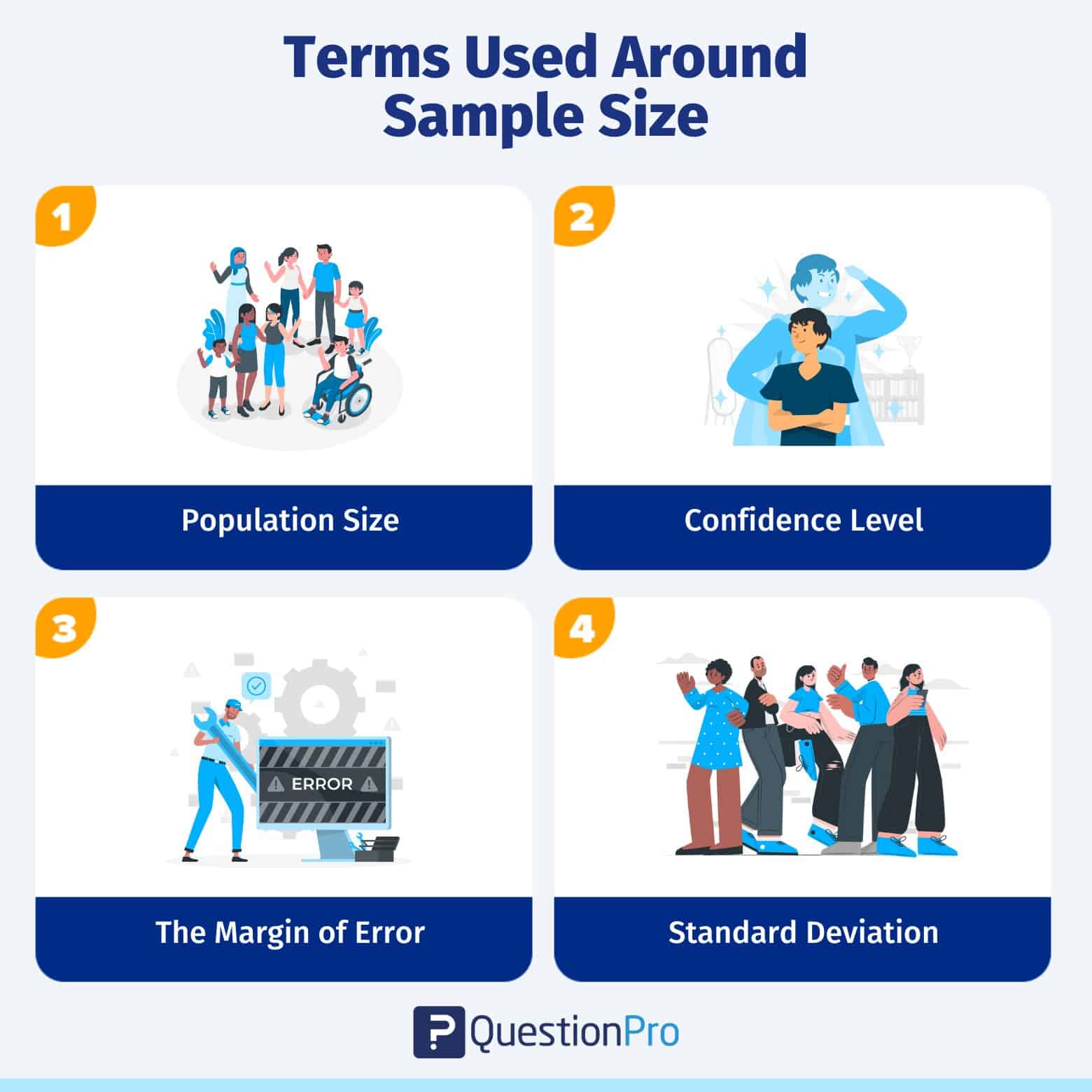
1. Population size:
Population size is how many people fit your demographic. For example, you want to get information on doctors residing in North America. Your population size is the total number of doctors in North America.
Don’t worry! Your population size doesn’t always have to be that big. Smaller population sizes can still give you accurate results as long as you know who you’re trying to represent.
2. Confidence level:
The confidence level tells you how sure you can be that your data is accurate. It is expressed as a percentage and aligned to the confidence interval. For example, if your confidence level is 90%, your results will most likely be 90% accurate.
3. The margin of error (confidence interval):
There’s no way to be 100% accurate when it comes to surveys. Confidence intervals tell you how far off from the population means you’re willing to allow your data to fall.
A margin of error describes how close you can reasonably expect a survey result to fall relative to the real population value. Remember, if you need help with this information, use our margin of error calculator .
4. Standard deviation:
Standard deviation is the measure of the dispersion of a data set from its mean. It measures the absolute variability of a distribution. The higher the dispersion or variability, the greater the standard deviation and the greater the magnitude of the deviation.
For example, you have already sent out your survey. How much variance do you expect in your responses? That variation in response is the standard deviation.
Sample size calculation formula – sample size determination
With all the necessary terms defined, it’s time to learn how to determine sample size using a sample calculation formula.
Your confidence level corresponds to a Z-score. This is a constant value needed for this equation. Here are the z-scores for the most common confidence levels:
90% – Z Score = 1.645
95% – Z Score = 1.96
99% – Z Score = 2.576
If you choose a different confidence level, various online tools can help you find your score.
Necessary Sample Size = (Z-score)2 * StdDev*(1-StdDev) / (margin of error)2
Here is an example of how the math works, assuming you chose a 90% confidence level, .6 standard deviation, and a margin of error (confidence interval) of +/- 4%.
((1.64)2 x .6(.6)) / (.04)2
( 2.68x .0.36) / .0016
.9648 / .0016
603 respondents are needed, and that becomes your sample size.
Free Sample Size Calculator
How is a sample size determined?
Determining the right sample size for your survey is one of the most common questions researchers ask when they begin a market research study. Luckily, sample size determination isn’t as hard to calculate as you might remember from an old high school statistics class.
Before calculating your sample size, ensure you have these things in place:
Goals and objectives:
What do you hope to do with the survey? Are you planning on projecting the results onto a whole demographic or population? Do you want to see what a specific group thinks? Are you trying to make a big decision or just setting a direction?
Calculating sample size is critical if you’re projecting your survey results on a larger population. You’ll want to make sure that it’s balanced and reflects the community as a whole. The sample size isn’t as critical if you’re trying to get a feel for preferences.
For example, you’re surveying homeowners across the US on the cost of cooling their homes in the summer. A homeowner in the South probably spends much more money cooling their home in the humid heat than someone in Denver, where the climate is dry and cool.
For the most accurate results, you’ll need to get responses from people in all US areas and environments. If you only collect responses from one extreme, such as the warm South, your results will be skewed.
Precision level:
How close do you want the survey results to mimic the true value if everyone responded? Again, if this survey determines how you’re going to spend millions of dollars, then your sample size determination should be exact.
The more accurate you need to be, the larger the sample you want to have, and the more your sample will have to represent the overall population. If your population is small, say, 200 people, you may want to survey the entire population rather than cut it down with a sample.
Confidence level:
Think of confidence from the perspective of risk. How much risk are you willing to take on? This is where your Confidence Interval numbers become important. How confident do you want to be — 98% confident, 95% confident?
Understand that the confidence percentage you choose greatly impacts the number of completions you’ll need for accuracy. This can increase the survey’s length and how many responses you need, which means increased costs for your survey.
Knowing the actual numbers and amounts behind percentages can help make more sense of your correct sample size needs vs. survey costs.
For example, you want to be 99% confident. After using the sample size determination formula, you find you need to collect an additional 1000 respondents.
This, in turn, means you’ll be paying for samples or keeping your survey running for an extra week or two. You have to determine if the increased accuracy is more important than the cost.
Population variability:
What variability exists in your population? In other words, how similar or different is the population?
If you are surveying consumers on a broad topic, you may have lots of variations. You’ll need a larger sample size to get the most accurate picture of the population.
However, if you’re surveying a population with similar characteristics, your variability will be less, and you can sample fewer people. More variability equals more samples, and less variability equals fewer samples. If you’re not sure, you can start with 50% variability.
Response rate:
You want everyone to respond to your survey. Unfortunately, every survey comes with targeted respondents who either never open the study or drop out halfway. Your response rate will depend on your population’s engagement with your product, service organization, or brand.
The higher the response rate, the higher your population’s engagement level. Your base sample size is the number of responses you must get for a successful survey.
Consider your audience:
Besides the variability within your population, you need to ensure your sample doesn’t include people who won’t benefit from the results. One of the biggest mistakes you can make in sample size determination is forgetting to consider your actual audience.
For example, you don’t want to send a survey asking about the quality of local apartment amenities to a group of homeowners.
Select your respondents
Focus on your survey’s objectives:
You may start with general demographics and characteristics, but can you narrow those characteristics down even more? Narrowing down your audience makes getting a more accurate result from a small sample size easier.
For example, you want to know how people will react to new automobile technology. Your current population includes anyone who owns a car in a particular market.
However, you know your target audience is people who drive cars that are less than five years old. You can remove anyone with an older vehicle from your sample because they’re unlikely to purchase your product.
Once you know what you hope to gain from your survey and what variables exist within your population, you can decide how to calculate sample size. Using the formula for determining sample size is a great starting point to get accurate results.
After calculating the sample size, you’ll want to find reliable customer survey software to help you accurately collect survey responses and turn them into analyzed reports.
LEARN MORE: Population vs Sample
In sample size determination, statistical analysis plan needs careful consideration of the level of significance, effect size, and sample size.
Researchers must reconcile statistical significance with practical and ethical factors like practicality and cost. A well-designed study with a sufficient sample size can improve the odds of obtaining statistically significant results.
To meet the goal of your survey, you may have to try a few methods to increase the response rate, such as:
- Increase the list of people who receive the survey.
- To reach a wider audience, use multiple distribution channels, such as SMS, website, and email surveys.
- Send reminders to survey participants to complete the survey.
- Offer incentives for completing the survey, such as an entry into a prize drawing or a discount on the respondent’s next order.
- Consider your survey structure and find ways to simplify your questions. The less work someone has to do to complete the survey, the more likely they will finish it.
- Longer surveys tend to have lower response rates due to the length of time it takes to complete the survey. In this case, you can reduce the number of questions in your survey to increase responses.
QuestionPro’s sample size calculator makes it easy to find the right sample size for your research based on your desired level of confidence, your margin of error, and the size of the population.
FREE TRIAL LEARN MORE
Frequently Asked Questions (FAQ)
The four ways to determine sample size are: 1. Power analysis 2. Convenience sampling, 3. Random sampling , 4. Stratified sampling
The three factors that determine sample size are: 1. Effect size, 2. Level of significance 3. Power
Using statistical techniques like power analysis, the minimal detectable effect size, or the sample size formula while taking into account the study’s goals and practical limitations is the best way to calculate the sample size.
The sample size is important because it affects how precise and accurate the results of a study are and how well researchers can spot real effects or relationships between variables.
The sample size is the number of observations or study participants chosen to be representative of a larger group
MORE LIKE THIS
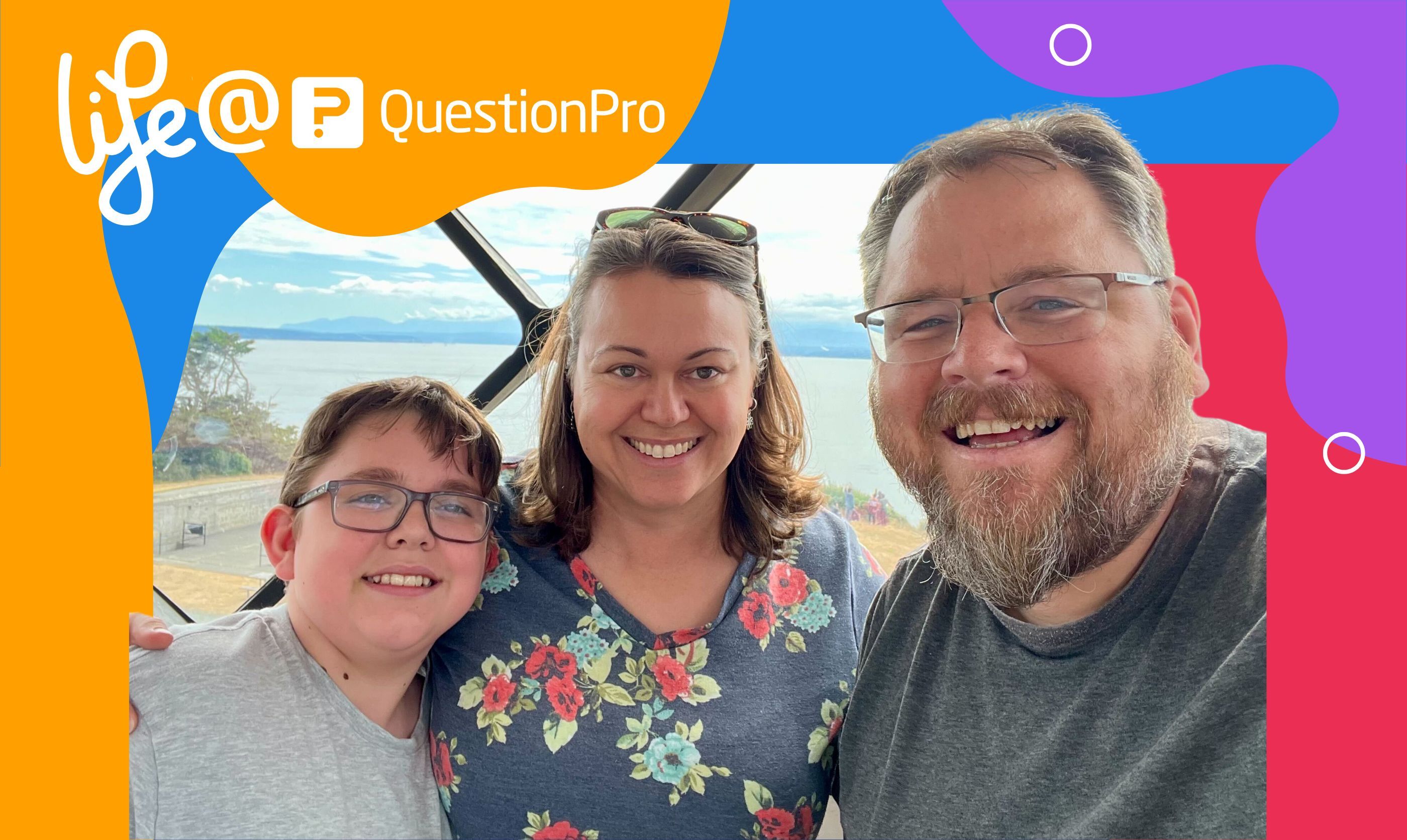
Life@QuestionPro: The Journey of Kristie Lawrence
Jun 7, 2024
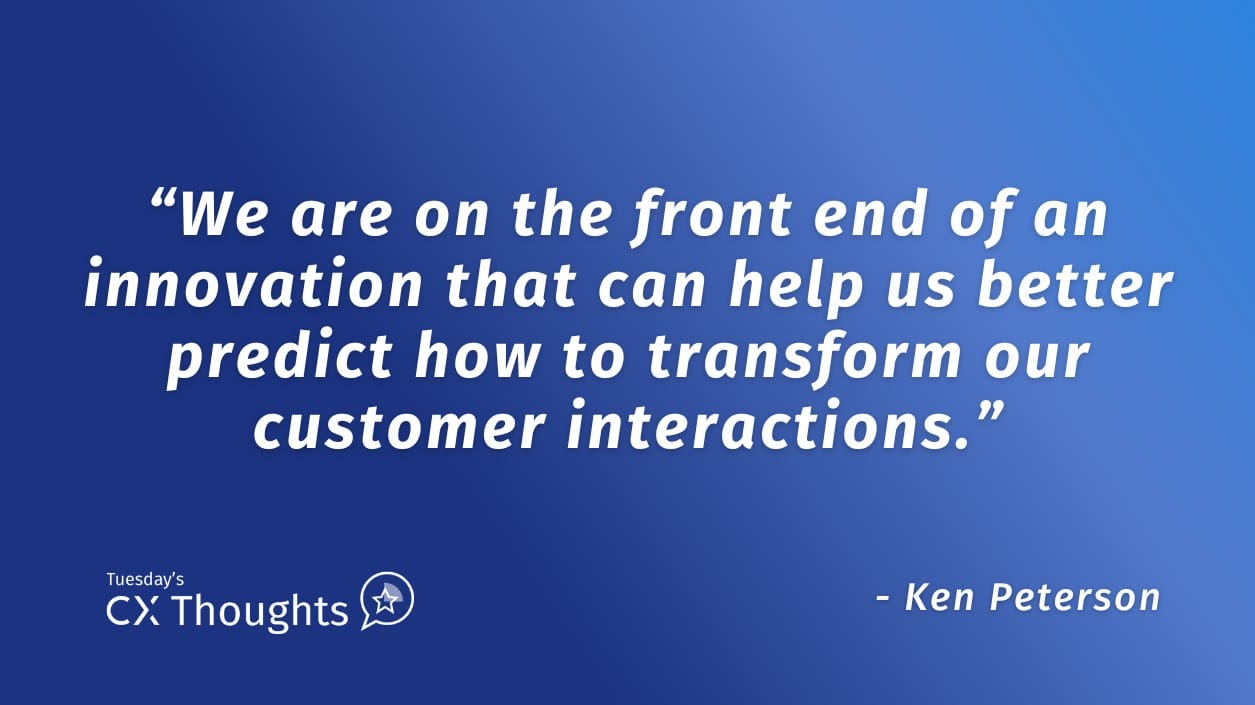
How Can I Help You? — Tuesday CX Thoughts
Jun 5, 2024
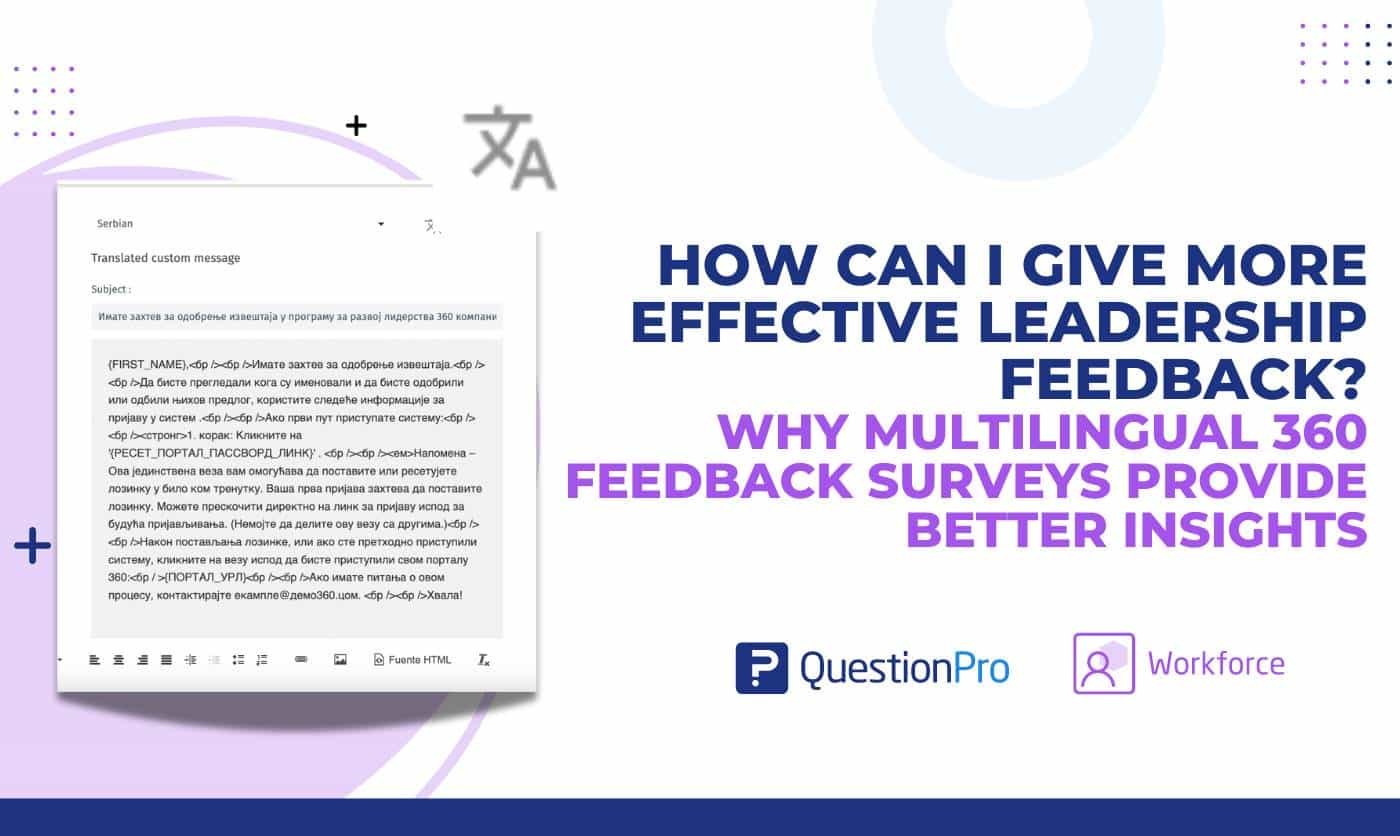
Why Multilingual 360 Feedback Surveys Provide Better Insights
Jun 3, 2024
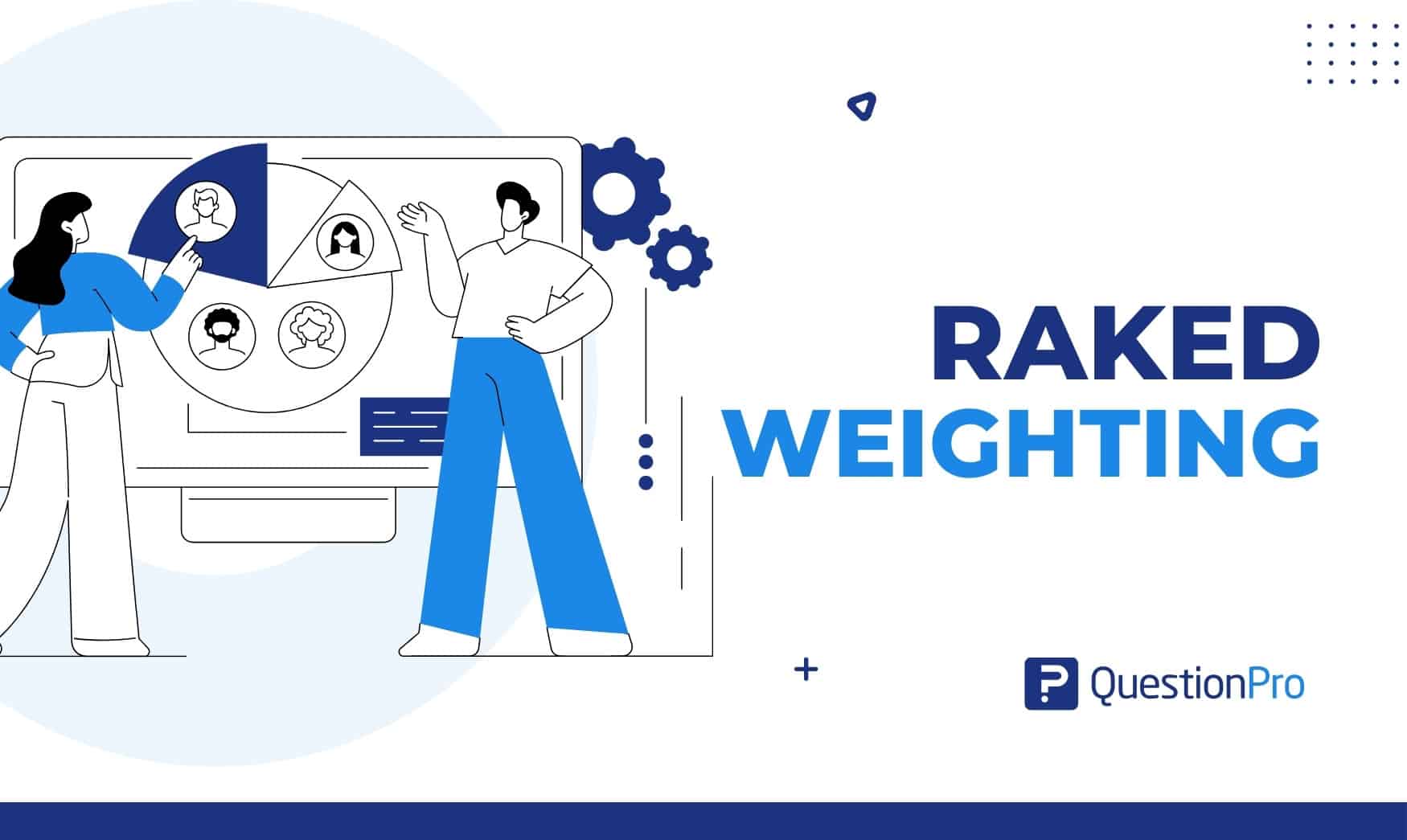
Raked Weighting: A Key Tool for Accurate Survey Results
May 31, 2024
Other categories
- Academic Research
- Artificial Intelligence
- Assessments
- Brand Awareness
- Case Studies
- Communities
- Consumer Insights
- Customer effort score
- Customer Engagement
- Customer Experience
- Customer Loyalty
- Customer Research
- Customer Satisfaction
- Employee Benefits
- Employee Engagement
- Employee Retention
- Friday Five
- General Data Protection Regulation
- Insights Hub
- Life@QuestionPro
- Market Research
- Mobile diaries
- Mobile Surveys
- New Features
- Online Communities
- Question Types
- Questionnaire
- QuestionPro Products
- Release Notes
- Research Tools and Apps
- Revenue at Risk
- Survey Templates
- Training Tips
- Uncategorized
- Video Learning Series
- What’s Coming Up
- Workforce Intelligence
- Technical Support
- Technical Papers
- Knowledge Base
- Question Library
Call our friendly, no-pressure support team.
Figuring Out (Determining) Sample Size for Survey Research
Table of Contents
Figuring Out Sample Size (Sample Size Determination)
Folks wanting to learn how to determine the right sample size for their research studies are badly underserved: nearly every article you can find on the internet tells, at best, just half the story. An inadequate sample size could lead to results that are far from the truth, costing your company millions in misguided investments.
The most common advice you’ll find on the internet often leads straight to those inadequate sample sizes. There are different samples size calculations for different purposes – for means (single or multiple, independent or dependent), for proportions (single, paired, independent), for multivariate statistics (factor analysis, regression, logit, etc.) and for experiments (e.g., conjoint, MaxDiff). For brevity’s sake we’ll focus on figuring out sample size for single proportions, leaving the reader to generalize for cases of two proportions, and for single, paired and independent means.
We’ll cover some rules of thumb about multivariate statistics and experiments. We’ll also differentiate between sample size for confidence intervals (the topic of almost every other article about sample size that you’ll find) and sample size for statistical testing (a topic that is almost uniformly neglected).
In this comprehensive guide, we'll dive deep into:
- The definition of sample size and its significance in research
- Factors influencing the determination of sample size
- Step-by-step calculation methods for figuring out both sample size needs, confidence intervals and hypotheses testing.
- Sample size advice for studies with complex analyses
Sample Size Definition
When we talk about sample size we just mean the number of respondents (people) that you include in your study . This number depends on whether you want to ensure that the results will (a) reflect the overall population's characteristics or (b) support managerially valuable hypothesis tests, or both.
Significance of Sample Size in Market Research?
Sample size is the currency with which you buy accuracy in survey research , both by generating quantifiable margins of error around any statistics we generate and by delivering credible hypothesis testing results.
Figuring out a properly defined sample size balances cost-efficiency with statistical rigor . It gives your study credibility and it offers a clearer lens through which you can understand your research findings.
To Summarize:
- Sample Size Definition : The number of observations or respondents in a study.
- Significance of Sample Size in Market Research : It directly impacts the credibility and value of the research.
Need Sample for Your Research?
Let us connect you with your ideal audience! Reach out to us to request sample for your survey research.
Request Sample
Factors Influencing Sample Size Determination
How to find the appropriate sample size depends on a few factors. Each requires careful consideration. Let's delve into these key factors.
Confidence versus power
This factor depends on whether you want your sample size scaled for precision (your margin of error or your confidence interval) or for power (i.e., for supporting hypothesis testing). Just for purposes of a sneak preview, the two formulas are slightly different (the formula for statistical power of a hypothesis test has one extra variable in it).
Population Size
Population sizes only matter in the rare case when your sample size will exceed 5% of the total population size. This happens so infrequently that we can refer anyone interested to Google “finite population correction factor,” which you can then add straightforwardly to your sample size formula.
More information about population vs sample
Margin of Error (Confidence Interval)
The margin of error is the range within which the population parameter is expected to fall. Smaller margins require larger sample sizes. Simply put, the more precise you want to be, the larger your sample size needs to be.
Confidence Level
Confidence level refers to the probability that the sample results will represent the population within the margin of error. Common levels are 90%, 95%, and 99%. Higher confidence levels require larger sample sizes.
Standard Deviation
Standard deviation measures how spread out the values in your data set are. When you expect a high variation, you'll need a larger sample size to capture it accurately.
Quick Reference Table:
Sample Size Formulas
Sample size formula for margin of error (confidence interval, precision).
You may recall when learning statistics that your professor showed a formula for a confidence interval, then did some algebra to use it to solve for sample size (n). That’s where this formula comes from, from the confidence interval around a single proportion:
- n = Sample Size
- Z a/2 = Z-value that corresponds to desired confidence level (1.96 corresponds with the typical 95% confidence level)
- p = Proportion of the population (since this is often not known, we usually use a worst case estimate of 0.5)
- d = Margin of error (the radius of the confidence interval, or the precision)
Sample size formula for hypothesis testing
What your professor didn’t show you is that there’s a different formula when you want your sample size to support statistical testing. That’s where this formula comes from:
- n, Z a/2 , p and d are as above and
- Zb =the Z-value that corresponds to the desired level of statistical power (0.84 corresponds to the commonly used 80% power)
Figuring Out Sample Size: The Process
The sample size calculation process looks harder than it is. Just break it down into systematic steps. Here's how you can approach it, complete with real-world examples.
Step 1: Determine Confidence Level—Choose Wisely
The confidence level you select specifies how confident you can be that your sample results will reflect the true population parameter (a de facto standard is to shoot for 95% confidence). A higher confidence level, such as 99%, will provide greater assurance but will demand a larger sample size. A level like 99% might be appropriate for projects that carry high stakes, such as healthcare studies or regulatory compliance assessments.
On the flip side, a lower confidence level, like 90%, may suffice for quick market assessments or pilot studies. While it reduces the sample size needed, it does come at the cost of confidence in your findings. Here you accept a slightly higher risk that your sample results may not perfectly represent the broader population.
Rule of Thumb : For most business or academic research, a confidence level of 95% is considered a good starting point. For high-stakes, mission-critical projects, aim for 99%. For more exploratory or pilot projects where you can tolerate a bit more risk, 90% might be acceptable.
Z a/2 -the Z score for Confidence Level
In the context of confidence levels, this Z-score gives us the confidence level we want to have that the population score (mean, proportion, whatever you’re measuring) is within the margin of error, or contained within the confidence interval.
To calculate the Z-score, you can look it up in the standard normal distribution table, or use statistical software. The Z-score table below shows the Z-scores for the most commonly used confidence levels in market research (90%, 95%, and 99%) .
Z-score Table for Common Confidence Levels
Remember, the choice of confidence level dictates how much risk you're willing to accept, and in turn, influences the sample size and potentially, the viability of your project.
Example : Let's say you're researching consumer preferences for a new type of organic snack bar. You decide to go with a 95% confidence level, that is a 95% chance that your margin of error will include the population’s preference for the new snack bar. This equates to a Z-score of 1.96.
Step 2: Choose the Margin of Error/Precision
The margin of error measures the precision of your survey results. Simply put, a smaller margin of error (e.g., 2%) provides more accurate insights but requires a larger sample size. This can be particularly valuable when you're working on high-stakes projects or research where even minor errors could have significant business or policy implications.
Conversely, a larger margin of error (e.g., 5% or 10%) may suffice for exploratory studies or when resource constraints are a significant concern. In these cases, the benefit of a larger sample size may not outweigh the additional time and costs involved.
Rule of Thumb: Always weigh the trade-off between precision and resources to arrive at an optimal margin of error for your study. Larger samples give you more precision but they also cost more. Your margin of error directly influences both the quality and feasibility of your market research. This selection is not merely a statistical decision; it’s a strategic one that can have a meaningful impact on your project's success.
Example : Continuing with the organic snack bar study, you decide a 5% (0.05) margin of error is acceptable: you want your estimate to be accurate to with +/- 5% of the population percentage.
Step 3: Estimate Standard Deviation
The standard deviation is a measure of the dispersion or spread of your data points around their average value. A high standard deviation implies more variability, whereas a low standard deviation indicates that the values are more bunched around the mean.
Why Standard Deviation Matters : A high standard deviation, means that there's a larger spread in the opinions, attitudes, or behaviors of your target population. This level of variability could require a larger sample size to capture the differences adequately. In contrast, a low standard deviation simplifies things; the closer your data points are to the mean, the less sample you may need for precise results.
Rule of Thumb : If you don't have prior data to calculate the actual standard deviation, a typical approach for proportions is to assume a 50:50 split or a proportion (p) of 0.05. This conservative estimate maximizes your sample size and thereby reduces the chance of underestimating it. However, if you have historical data or pilot studies to draw from, use the observed standard deviation as it will provide a more accurate sample size tailored to your research.
Example : Given the lack of preliminary data on consumer preferences for organic snack bars, you choose p = 0.5 to maximize your sample size.
Step 4: Determine Your Level of Power (for Hypothesis Testing Only)
Power is your ability to identify a difference of a particular size in hypothesis testing. If being able to detect a difference of 5% is really important to you, then you want to have a lot of power to detect that size of difference.
Why Power Matters: In a statistical test we have to worry about both confidence and power, because we seek to avoid both false positives (through the confidence level) and false negatives (via the power level). If you calculate sample size and ignore power, your sample sill be too small to detect the things that matter to you and you increase your risk of experiencing a false negative. False negatives can be very costly in practice. Let’s say a new ad campaign will be so successful that it will increase sales by 10%. If your product has $500 million in sales, that 10% increase is $50 million. If you cut costs on sample size and get a false negative result, however, you could conclude that the new ad isn’t a success, and cost your company $50 million in lost sales.
Rule of Thumb : We usually want at least 70% or 80% power to detect differences when they are real. In truth, however, when setting both the confidence level and power, we should consider how costly are false negatives (concluding the advertising doesn’t work when in fact it does) and false positives (concluding a new ad is successful when it is not) and then tailor our confidence and power to reflect those costs.
Step 5: Apply the Appropriate Sample Size Formula
This is where determining the correct sample size formula comes into play. Let’s say we want to make sure our study can identify the percentage of respondents who want our new product. We want 95% confidence the proportion we measure will be within 10 percentage points of the population proportion, but we don’t really have a clue what that might be.
Example : Plug in the Z-score (1.96), estimated proportion (0.5), and margin of error (0.05) into the sample size formula for margin of error:
Note that we rounded our answer up to 385 because we can’t interview 0.16 of a respondent.
Actually, it turns out management wants to know the results of a statistical test. The current advertising scored 50% while it was in the testing phase, so we want to know if our new ad can beat the old one by 5%. Moreover, because we stand to lose sales if we get a false negative here, we want to have 80% power to detect a significant difference. Now we use the sample size formula for power:
Note that when we took power into account because we wanted to avoid a false negative) our sample size requirement more than doubled, from 385 to 784. Had the company gone out with a sample of 385, it would have had only a 50% chance of identifying a successful ad campaign! That’s research money very poorly spent, but it’s exactly what happens if you don’t take power into account.
Summary Checklist: Sample Size Determination Steps
- Determine Confidence Level : Usually 95%, but sometimes 90% or 99%.
- Choose Margin of Error : A small percentage (2-5%) is common.
- Estimate Proportion of Population : Often 0.5 to maximize sample size.
- Choose a level of power (hypothesis testing only) : 80% is common, 70% is usually a minimum recommendation
- Apply the Appropriate Sample Size Formula : Use the formula to find the ideal sample size.
By following these steps, you're well on your way to figuring out sample size correctly for your study. This is a cornerstone of robust and credible market research, one that balances the risks of false positives and false negatives so as to maximize the value of your findings.
Using Sample Size Calculators
Though the sample size formula is a reliable tool for manual calculations, let's face it—math can be tedious. Sample size calculators can offer a more convenient route , often giving you the same level of accuracy with just a few clicks. However, most online sample size calculators use only the sample size for precision formula and thus do not take into account power. To remedy this, you may want just to double the sample size from an online calculator (because when we chose 80% power in the example above, the sample size, 784, was about double the one that came from considering only the confidence interval.
Key Takeaway: Sample size calculators are your go-to tools for quick, accurate, and convenient calculations. Most sample size calculators neglect statistical power, however, so use them with caution.
Troubleshooting Sample Size Issues
Sometimes your calculated sample size may be impractical (unaffordable). However, there are some strategies you can employ to come up with a more affordable sample size (hopefully without compromising your research too much).
Lowering the Confidence Level
If your sample size is turning out too large for your resources, one option is to lower the confidence level . A move from a 99% to a 95% confidence level can noticeably reduce the needed sample size. Remember though, this makes your results less robust.
Lowering the Power
While this comes with risks, lowering your power to 70% from 80%, say, can reduce your sample size.
Increasing the Margin of Error
Similarly, widening the margin of error will also decrease your required sample size. While this increases the range within which your population parameter is expected to fall, it's a trade-off that can sometimes make the research process more feasible.
Key Takeaway: Tweaking your confidence level, power or margin of error can reduce sample size needs, but always weigh the pros and cons.
Troubleshooting Options
Remember, these are options to help make your study feasible, but they do come with trade-offs. Always consider the impact of these adjustments on the reliability and credibility of your findings.
Real-Life Sample Size Applications
Understanding the mechanics of how to figure out sample size is great, but what does this mean in real-world settings? How has accurate sample size determination influenced the outcomes of actual market research projects?
Success Story
Let's consider a tech company that recently launched a new feature and wanted to gauge user satisfaction. By carefully calculating a sample size that took into account a 95% confidence level and a 4% margin of error, the company was able to reliably conclude that the feature was well-received, leading to its continued investment and improvement.
Consequences of Poor Sample Size
On the flip side, another business failed to adequately figure out sample size for a similar user-satisfaction survey. They concluded there was no change in user satisfaction, but there was and they missed it leading to misguided business decisions.
Key Takeaway: Accurate sample size determination isn't just academic; it has tangible implications for your business decisions and overall strategy.
Real-Life Implications
- Success Scenarios : Precise sample size -> Reliable data -> Informed Decisions
- Failure Scenarios : Inaccurate sample size -> Unreliable Data -> Misguided Decisions
Figuring out sample size is more than a statistical necessity; it's a vital business tool that can guide a company toward success or contribute to its failure.
Get Started with Your Survey Research Today!
Ready for your next research study? Get access to our free survey research tool. In just a few minutes, you can create powerful surveys with our easy-to-use interface.
Start Survey Research for Free or Request a Product Tour
Sample Sizes for Different Research Methods
The calculations above work for a single proportion. Similar equations exist for confidence intervals and statistical tests involving differences in proportions and differences in means. Complex statistical models have their own sample size requirements.
Regression analysis/driver analysis
The old rule of thumb of 10 observations per variable in the model is useful and works for data of average condition. When using particularly clean data we may get by with as few as 5 observations per variable. More common will be data with higher than average levels of multicollinearity and this will require larger sample sizes. So if our regression model has 12 variables, the basic recommendation would be n = 10k = 10(12) = 120.
Because it estimates the shape of an S-curve rather than a straight line, logit is more sample size intensive than regression. The rule of thumb is 10 times the number of variables in the model divided by the smaller of the two percentages of the binary response: n = 10k/p. So if our model has 2 predictors and we expect the response will be about 60/40 we’d go with n = 10(12)/(0.40) = 300.
Segmentation
Previous advice was a bit all over the board, but the most recent paper on the topic suggested a sample size of 100 for every basis variable included in the segmentation analysis. So if we have 20 basis variables, that suggests n=2,000.
Factor analysis
One source suggests that samples of less than a hundred are held to be “poor,” 200 to be “fair” and 300 “good.” Others suggest that when the number of factors is small and correlations are large and reliable, samples of as few as 50 may be workable. Given the messiness of most survey research data, erring on the side of larger sample size seems prudent.
Tree-Based Segmentation
In classification or regression trees, sample is split and then split again, repeatedly. After three levels of pairwise splits, a tree model could have eight groups. For this reason, we usually recommend having at least 1000 respondents.
Conjoint Analysis/MaxDiff
Our usual recommendation about multivariate statistics (like conjoint analysis and MaxDiff analysis ) is to have at least 300 respondents, or at least 200 per separately reportable subgroup. Another way to think about conjoint analysis is to work backward from the simulator: what size differences in shares would be worth capturing, and what size of sample do you need to capture them (using a sample size formula for the difference in two proportions).
Key Takeaway : The methodology you choose can significantly impact your sample size needs, so choose wisely and calculate accordingly. Tailoring your sample size to the specific demands of your chosen methodology isn't just best practice; it's crucial for obtaining valid, actionable insights.
FAQ: Frequently Asked Questions about Figuring Out Sample Size
You've journeyed through the intricate maze of sample size determination, but you may still have lingering questions. Let's tackle some of those.
How do you define sample size?
Sample size refers to the number of individual data points or subjects that are included in a study. It's a crucial aspect of market research that impacts the reliability and credibility of your findings.
What is a good sample size?
A "good" sample size is one that allows for a high confidence level and a low margin of error (and for statistical testing, a high level of power), all while remaining manageable and cost-effective. Figuring out the ideal sample size can vary based on the research methodology.
How do I calculate sample size?
To calculate the ideal sample size, you typically use a sample size formula that takes into account the statistic you want to study, your desired levels of confidence (and power), and the acceptable margin of error. Some online calculators can also do this for you.
And there you have it—a detailed guide on Understanding and Figuring Out Sample Size for Surveys .
Sawtooth Software
3210 N Canyon Rd Ste 202
Provo UT 84604-6508
United States of America
Support: [email protected]
Consulting: [email protected]
Sales: [email protected]
Products & Services
Support & Resources
- Sign Up Now
- -- Navigate To -- CR Dashboard Connect for Researchers Connect for Participants
- Log In Log Out Log In
- Recent Press
- Papers Citing Connect
- Connect for Participants
- Connect for Researchers
- Connect AI Training
- Managed Research
- Prime Panels
- MTurk Toolkit
- Health & Medicine
- Conferences
- Knowledge Base
- A Researcher’s Guide To Statistical Significance And Sample Size Calculations
Determining Sample Size: How Many Survey Participants Do You Need?
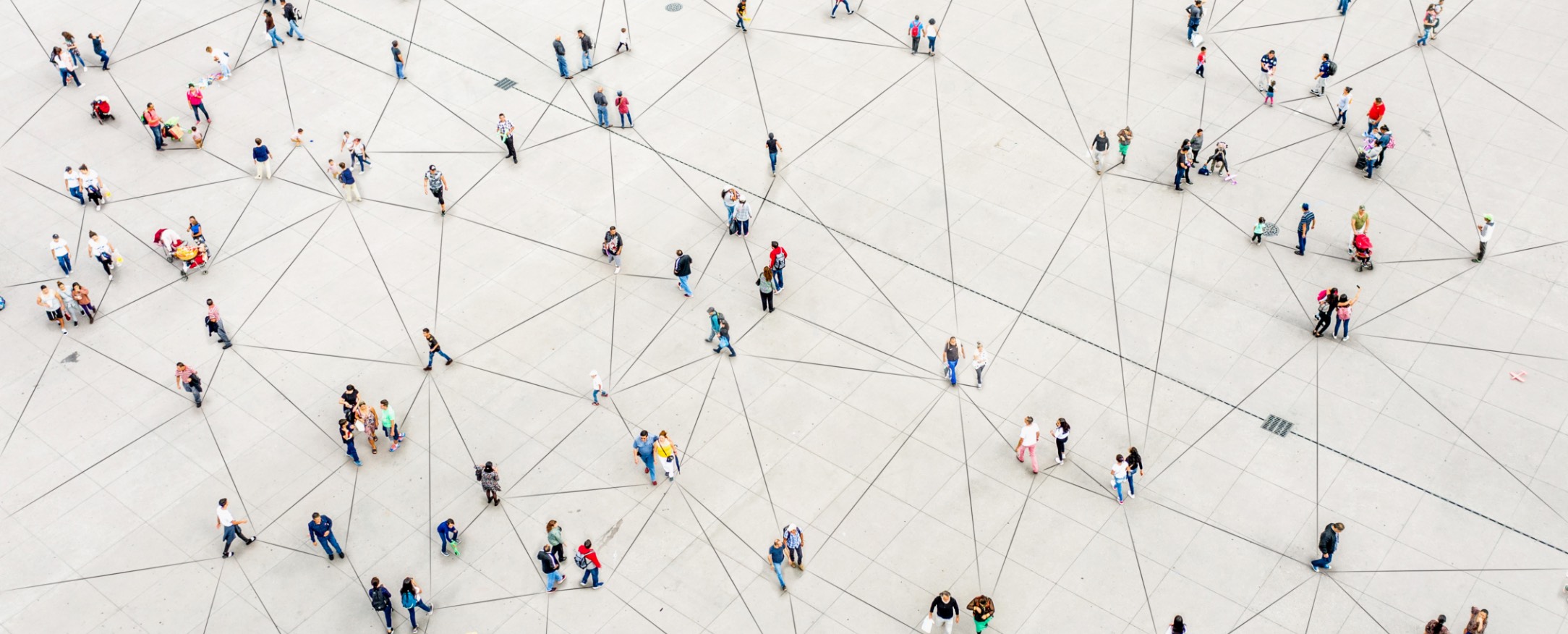
Quick Navigation:
How to calculate a statistically significant sample size in research, determining sample size for probability-based surveys and polling studies, determining sample size for controlled surveys, determining sample size for experiments, how to calculate sample size for simple experiments, an example sample size calculation for an a/b test, what if i don’t know what size difference to expect, part iii: sample size: how many participants do i need for a survey to be valid.
In the U.S., there is a Presidential election every four years. In election years, there is a steady stream of polls in the months leading up to the election announcing which candidates are up and which are down in the horse race of popular opinion.
If you have ever wondered what makes these polls accurate and how each poll decides how many voters to talk to, then you have thought like a researcher who seeks to know how many participants they need in order to obtain statistically significant survey results.
Statistically significant results are those in which the researchers have confidence their findings are not due to chance . Obtaining statistically significant results depends on the researchers’ sample size (how many people they gather data from) and the overall size of the population they wish to understand (voters in the U.S., for example).
Calculating sample sizes can be difficult even for expert researchers. Here, we show you how to calculate sample size for a variety of different research designs.
Before jumping into the details, it is worth noting that formal sample size calculations are often based on the premise that researchers are conducting a representative survey with probability-based sampling techniques. Probability-based sampling ensures that every member of the population being studied has an equal chance of participating in the study and respondents are selected at random.
For a variety of reasons, probability sampling is not feasible for most behavioral studies conducted in industry and academia . As a result, we outline the steps required to calculate sample sizes for probability-based surveys and then extend our discussion to calculating sample sizes for non-probability surveys (i.e., controlled samples) and experiments.
Determining how many people you need to sample in a survey study can be difficult. How difficult? Look at this formula for sample size.
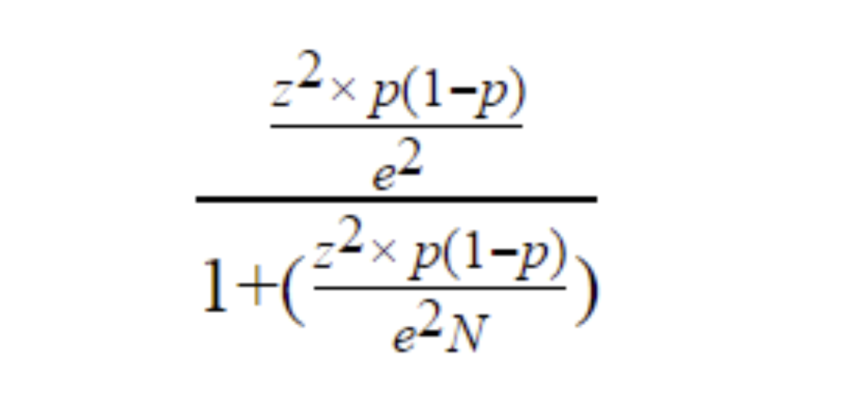
No one wants to work through something like that just to know how many people they should sample. Fortunately, there are several sample size calculators online that simplify knowing how many people to collect data from.
Even if you use a sample size calculator, however, you still need to know some important details about your study. Specifically, you need to know:
- What is the population size in my research?
Population size is the total number of people in the group you are trying to study. If, for example, you were conducting a poll asking U.S. voters about Presidential candidates, then your population of interest would be everyone living in the U.S.—about 330 million people.
Determining the size of the population you’re interested in will often require some background research. For instance, if your company sells digital marketing services and you’re interested in surveying potential customers, it isn’t easy to determine the size of your population. Everyone who is currently engaged in digital marketing may be a potential customer. In situations like these, you can often use industry data or other information to arrive at a reasonable estimate for your population size.
- What margin of error should you use?
Margin of error is a percentage that tells you how much the results from your sample may deviate from the views of the overall population. The smaller your margin of error, the closer your data reflect the opinion of the population at a given confidence level.
Generally speaking, the more people you gather data from the smaller your margin of error. However, because it is almost never feasible to collect data from everyone in the population, some margin of error is necessary in most studies.
- What is your survey’s significance level?
The significance level is a percentage that tells you how confident you can be that the true population value lies within your margin of error. So, for example, if you are asking people whether they support a candidate for President, the significance level tells you how likely it is that the level of support for the candidate in the population (i.e., people not in your sample) falls within the margin of error found in your sample.
Common significance levels in survey research are 90%, 95%, and 99%.
Once you know the values above, you can plug them into a sample size formula or more conveniently an online calculator to determine your sample size.
The table below displays the necessary sample size for different sized populations and margin of errors. As you can see, even when a population is large, researchers can often understand the entire group with about 1,000 respondents.
- How Many People Should I Invite to My Study?
Sample size calculations tell you how many people you need to complete your survey. What they do not tell you, however, is how many people you need to invite to your survey. To find that number, you need to consider the response rate.
For example, if you are conducting a study of customer satisfaction and you know from previous experience that only about 30% of the people you contact will actually respond to your survey, then you can determine how many people you should invite to the survey to wind up with your desired sample size.
All you have to do is take the number of respondents you need, divide by your expected response rate, and multiple by 100. For example, if you need 500 customers to respond to your survey and you know the response rate is 30%, you should invite about 1,666 people to your study (500/30*100 = 1,666).
Sample size formulas are based on probability sampling techniques—methods that randomly select people from the population to participate in a survey. For most market surveys and academic studies, however, researchers do not use probability sampling methods. Instead they use a mix of convenience and purposive sampling methods that we refer to as controlled sampling .
When surveys and descriptive studies are based on controlled sampling methods, how should researchers calculate sample size?
When the study’s aim is to measure the frequency of something or to describe people’s behavior, we recommend following the calculations made for probability sampling. This often translates to a sample of about 1,000 to 2,000 people. When a study’s aim is to investigate a correlational relationship, however, we recommend sampling between 500 and 1,000 people. More participants in a study will always be better, but these numbers are a useful rule of thumb for researchers seeking to find out how many participants they need to sample.
If you look online, you will find many sources with information for calculating sample size when conducting a survey, but fewer resources for calculating sample size when conducting an experiment. Experiments involve randomly assigning people to different conditions and manipulating variables in order to determine a cause-and-effect relationship. The reason why sample size calculators for experiments are hard to find is simple: experiments are complex and sample size calculations depend on several factors.
The guidance we offer here is to help researchers calculate sample size for some of the simplest and most common experimental designs: t -tests, A/B tests, and chi square tests.
Many businesses today rely on A/B tests. Especially in the digital environment, A/B tests provide an efficient way to learn what kinds of features, messages, and displays cause people to spend more time or money on a website or an app.
For example, one common use of A/B testing is marketing emails. A marketing manager might create two versions of an email, randomly send one to half the company’s customers and randomly send the second to the other half of customers and then measure which email generates more sales.
In many cases , researchers may know they want to conduct an A/B test but be unsure how many people they need in their sample to obtain statistically significant results. In order to begin a sample size calculation, you need to know three things.
1. The significance level .
The significance level represents how sure you want to be that your results are not due to chance. A significance level of .05 is a good starting point, but you may adjust this number up or down depending on the aim of your study.
2. Your desired power.
Statistical tests are only useful when they have enough power to detect an effect if one actually exists. Most researchers aim for 80% power—meaning their tests are sensitive enough to detect an effect 8 out of 10 times if one exists.
3. The minimum effect size you are interested in.
The final piece of information you need is the minimum effect size, or difference between groups, you are interested in. Sometimes there may be a difference between groups, but if the difference is so small that it makes little practical difference to your business, it probably isn’t worth investigating. Determining the minimum effect size you are interested in requires some thought about your goals and the potential impact on your business.
Once you have decided on the factors above, you can use a sample size calculator to determine how many people you need in each of your study’s conditions.
Let’s say a marketing team wants to test two different email campaigns. They set their significance level at .05 and their power at 80%. In addition, the team determines that the minimum response rate difference between groups that they are interested in is 7.5%. Plugging these numbers into an effect size calculator reveals that the team needs 693 people in each condition of their study, for a total of 1,386.
Sending an email out to 1,386 people who are already on your contact list doesn’t cost too much. But for many other studies, each respondent you recruit will cost money. For this reason, it is important to strongly consider what the minimum effect size of interest is when planning a study.
When you don’t know what size difference to expect among groups, you can default to one of a few rules of thumb. First, use the effect size of minimum practical significance. By deciding what the minimum difference is between groups that would be meaningful, you can avoid spending resources investigating things that are likely to have little consequences for your business.
A second rule of thumb that is particularly relevant for researchers in academia is to assume an effect size of d = .4. A d = .4 is considered by some to be the smallest effect size that begins to have practical relevance . And fortunately, with this effect size and just two conditions, researchers need about 100 people per condition.
After you know how many people to recruit for your study, the next step is finding your participants. By using CloudResearch’s Prime Panels or MTurk Toolkit, you can gain access to more than 50 million people worldwide in addition to user-friendly tools designed to make running your study easy. We can help you find your sample regardless of what your study entails. Need people from a narrow demographic group? Looking to collect data from thousands of people? Do you need people who are willing to engage in a long or complicated study? Our team has the knowledge and expertise to match you with the right group of participants for your study. Get in touch with us today and learn what we can do for you.
Continue Reading: A Researcher’s Guide to Statistical Significance and Sample Size Calculations
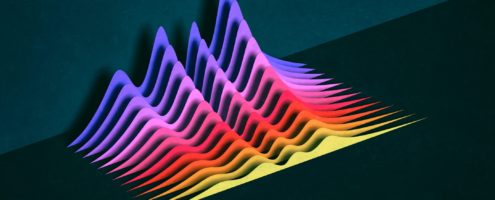
Part 1: What Does It Mean for Research to Be Statistically Significant?
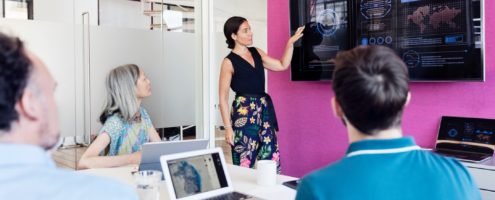
Part 2: How to Calculate Statistical Significance
Related articles, what is data quality and why is it important.
If you were a researcher studying human behavior 30 years ago, your options for identifying participants for your studies were limited. If you worked at a university, you might be...
How to Identify and Handle Invalid Responses to Online Surveys
As a researcher, you are aware that planning studies, designing materials and collecting data each take a lot of work. So when you get your hands on a new dataset,...
SUBSCRIBE TO RECEIVE UPDATES
2024 grant application form, personal and institutional information.
- Full Name * First Last
- Position/Title *
- Affiliated Academic Institution or Research Organization *
Detailed Research Proposal Questions
- Project Title *
- Research Category * - Antisemitism Islamophobia Both
- Objectives *
- Methodology (including who the targeted participants are) *
- Expected Outcomes *
- Significance of the Study *
Budget and Grant Tier Request
- Requested Grant Tier * - $200 $500 $1000 Applicants requesting larger grants may still be eligible for smaller awards if the full amount requested is not granted.
- Budget Justification *
Research Timeline
- Projected Start Date * MM slash DD slash YYYY Preference will be given to projects that can commence soon, preferably before September 2024.
- Estimated Completion Date * MM slash DD slash YYYY Preference will be given to projects that aim to complete within a year.
- Project Timeline *
- Email This field is for validation purposes and should be left unchanged.
- Name * First Name Last Name
- I would like to request a demo of the Sentry platform
- Name This field is for validation purposes and should be left unchanged.
- Name * First name Last name
- Name * First Last
- Comments This field is for validation purposes and should be left unchanged.
- Name * First and Last
- Please select the best time to discuss your project goals/details to claim your free Sentry pilot for the next 60 days or to receive 10% off your first Managed Research study with Sentry.
- Email * Enter Email Confirm Email
- Organization
- Job Title *
.webp)
How To Determine Survey Sample Size: A Short Guide

Imagine you want to know how many people in the US drink coffee every morning. If you ask 10 people, that likely isn’t representative of such a large group.
But if you want to know how many of your 50 coworkers do so, 10 respondents may be enough to get the right idea.
That is what a survey sample size is in a nutshell. It’s not usually possible to collect feedback from an entire group you are trying to survey. So you have to decide how many people you need answers from to get reliable results.
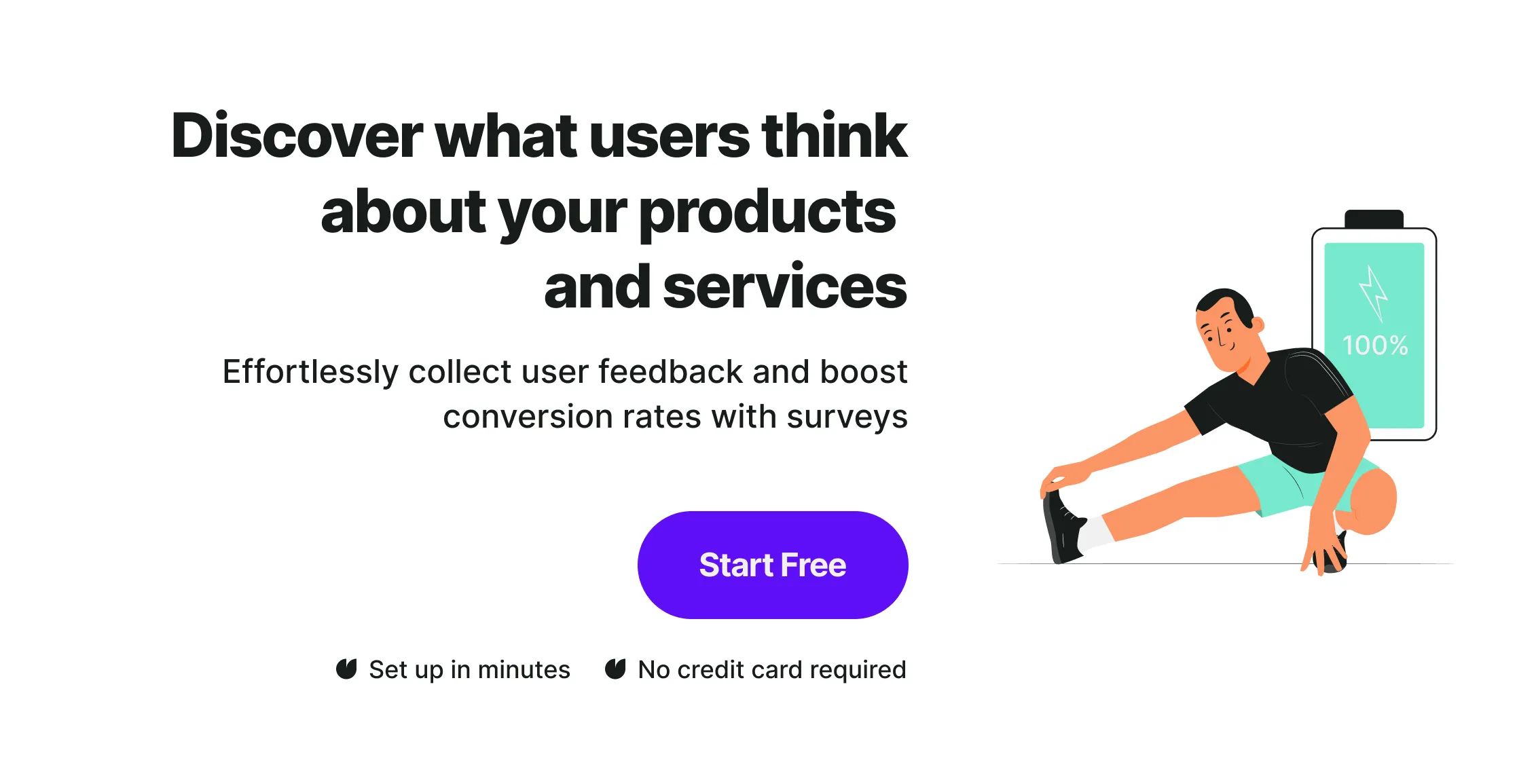
What is Survey Sample Size?
A survey sample size is the number of participants in a study. In other words, the number of people who responded to your survey.
When conducting any survey, one of your main goals is to get results that are statistically significant. This means enough responses to infer conclusions with confidence.
Sample size usually refers to surveying large populations. So, if all you want to do is find out your 500 customers’ satisfaction, you likely won’t have trouble getting a representative sample.
On the other hand, if you want to see how many marketers in the US are potential customers for you, you’ll have to make do with a statistically significant portion of them.
PS: Check out this persona survey template to figure out where to look for potential customers!
What is a Good Sample Size for a Survey?
When the sample size is too small, you may get a disproportionately small or large number of outliers. This can skew results significantly.
On the other hand, while surveying more people will always get you more accurate results, it is often unfeasible, complex, and expensive. Oftentimes, surveying the entire population is not worth the time and effort.
So, what’s the golden mean?
Many statisticians concur that a sample size of 100 is the minimum you need for meaningful results. If your population is smaller than that, you should aim to survey all of the members.
The same source states that the maximum number of respondents should be 10% of your population, but it should not exceed 1000.
For instance, if you have a population of 250,000, 10% would be 25,000. Since this is higher than 1,000, a sample size of 1,000 should be enough to get you statistically significant results.
How to Determine Sample Size for a Survey
Calculating sample size can be made easier with software such as a calculator. Still, let’s have a look at what the process is like behind the scenes.
Sample Size Formula
The survey sample size is usually determined with a take on Slovin’s formula .
.png)
Here is what you’ll need.
“P”, which is your standard deviation
This is how confident you can be that a population will select an answer within a given range. In plain English, this would be how extreme you can expect the responses to be.
A low standard deviation means that you don’t expect your respondents to be extreme. A higher standard deviation will mean that you expect respondents to choose answers from the ends of the spectrum.
Standard deviation is given as a number between 0 and 1. If you are unsure, go with 0.5, which is the exact midway point. Bear in mind, though, that this might make your results more estimated.
“Z”, which represents your “z score”
Your z score is the number of standard deviations a given group is away from the mean.
Thankfully, you can use a cheat sheet to determine your “z score”, depending on how confident you want to be about your results.
For example
- 99% desired confidence level would equal a z score of 2.58
- 95% would equal 1.96
- 90% is 1.65
- 85% is 1.44
According to Lisa Sullivan, Professor of Biostatistics
“a 95% confidence interval means that if we were to take 100 different samples and compute a 95% confidence interval for each sample, then approximately 95 of the 100 confidence intervals will contain the true mean value”.
In layman’s terms, if you calculate your sample size with a z score of 1.96, then if you were to repeat your survey 100 times, you would get the same mean result about 95 times.
“E”, which is your margin of error
The margin of error is basically how sure you need to be that the results are accurate for the entire population. The bigger the margin of error, the less reliable your results are.
You usually want the margin of error at around 5% or less. This means you can be 95% or more sure that your results are accurate.
For example, let’s say you discover that 85% of your respondents find your customer service effective. If you assumed a 5% margin of error, you must add 5% to either side of the score.
In essence, you can assume that 80-90% of your customer base is satisfied with your customer service.
The margin of error should be provided in decimal form. Note that the smaller margin of error you want, the larger your sample size will be.
And finally, “N”, which is your population size
The population is all the people whose attitudes or stances you are trying to learn about.
The population could be anything from all of your customers to all people on Earth. The bigger your population, the bigger your sample will need to be in order to get reliable results.
When you know what your population is, you need to figure out how many people are in that category.
For instance, if you wanted to get accurate survey results for the entire US, your population size would be 329.5 million (source: World Bank, 2020 ).
If you wanted to run an NPS campaign , you’d consider all of your existing customers.
Calculation: What Sample Size is Needed for a Survey?
Determining the sample size begins with considering the population in a study.
So, let’s say I wanted to survey a population of 500, and I want to be 5% confident that I will get accurate results 95% of the time. I would need to survey 218 people.
This formula may seem confusing. You can use this spreadsheet I prepared to skip the manual labor. Make sure to copy the spreadsheet and edit only the green fields.
Let’s work with another example. We’ll assume the following values:
- 95% confidence level
- 0.5 standard deviation
- 5% margin of error
- Population of 10,000
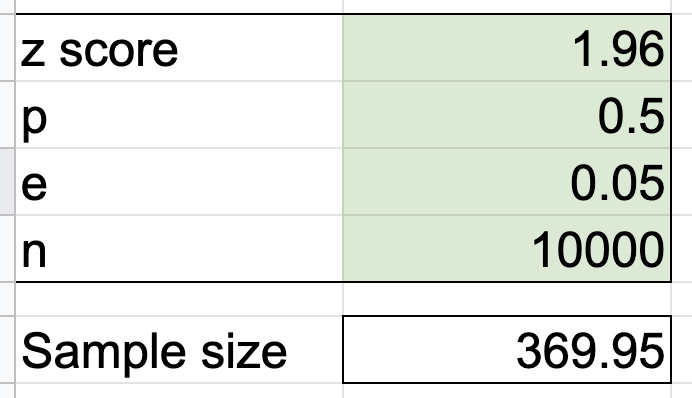
Using our spreadsheet, you can see that you should survey at least 370 people. And Survicate is currently working on a sample size calculator, so stay tuned!
Once you have your sample size, you have to account for your response rate . You can expect it to be more or less 20-30%. If you expect to get fewer responses, send the survey to a larger number of people to reach your sample size milestone.
How to Decide Who to Include in Your Sample?
For small to medium-size businesses, the method of getting a sample is usually to send the survey to the entire customer base and hope to get enough responses.
But you may want to target your survey as well. For example, if your previous survey showed you have relatively few NPS detractors , you may want to target them specifically to find out whether their score had changed or to get more details.
Nonetheless, choosing a random sample will usually get you the most reliable results for your population.
What if You Can’t Get Enough Responses?
Sometimes, you just can’t get the number of responses to match that magical sample size you desire. Using best survey tools may help boost your response rate, but what if you still can’t get close to 100?
Calculating sample size in survey research is still important. You can benchmark how many more responses you need and thus, how you can treat incomplete results.
While your results may not be statistically significant, you can still use the feedback to fuel your business decisions. The answers will still be valuable, especially if you make good use of open-ended questions for context.

Send surveys through any channel with Survicate
Generally speaking, most surveyors want the results to be as comprehensive as possible. The more responses you get, the better. The only limiting factors are time and money.
With survey software like Survicate, you can target your audience directly through your website, email, or app, without having to manually reach out or ask bystanders. This cuts down on the required time and effort considerably.
Simply use one of our ready-to-send templates and start gathering feedback . Now, you can take advantage of our 10-day free trial to test all Business plan features with up to 25 survey responses.
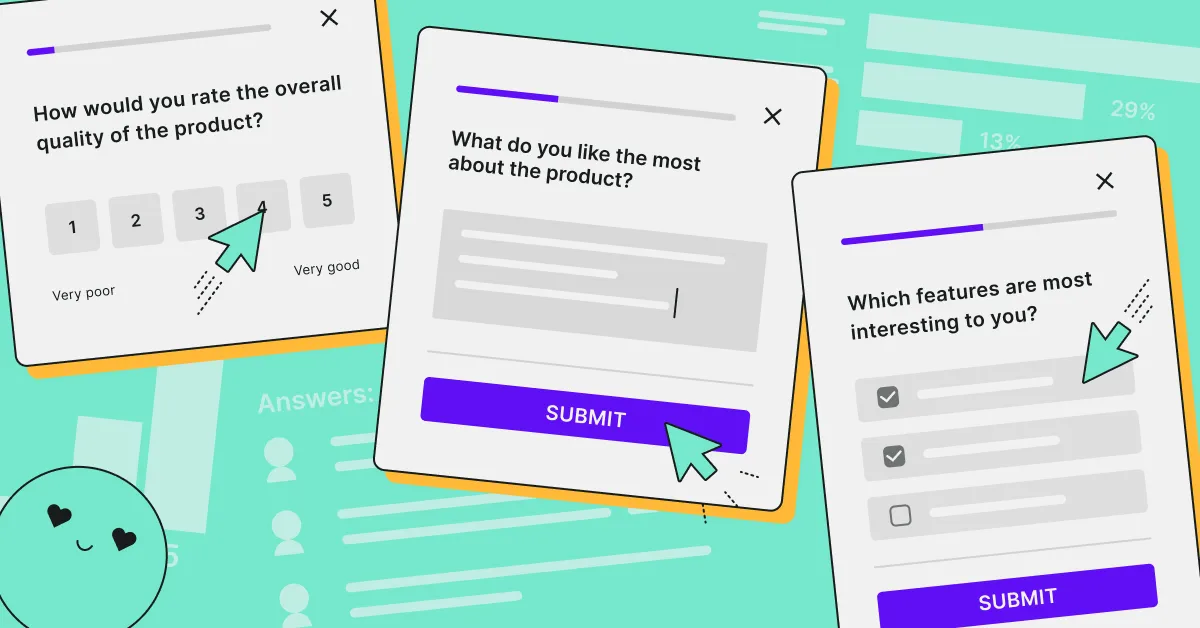
We’re also there

Sample Size Calculator
Find out the sample size.
This calculator computes the minimum number of necessary samples to meet the desired statistical constraints.
Find Out the Margin of Error
This calculator gives out the margin of error or confidence interval of observation or survey.
Related Standard Deviation Calculator | Probability Calculator
In statistics, information is often inferred about a population by studying a finite number of individuals from that population, i.e. the population is sampled, and it is assumed that characteristics of the sample are representative of the overall population. For the following, it is assumed that there is a population of individuals where some proportion, p , of the population is distinguishable from the other 1-p in some way; e.g., p may be the proportion of individuals who have brown hair, while the remaining 1-p have black, blond, red, etc. Thus, to estimate p in the population, a sample of n individuals could be taken from the population, and the sample proportion, p̂ , calculated for sampled individuals who have brown hair. Unfortunately, unless the full population is sampled, the estimate p̂ most likely won't equal the true value p , since p̂ suffers from sampling noise, i.e. it depends on the particular individuals that were sampled. However, sampling statistics can be used to calculate what are called confidence intervals, which are an indication of how close the estimate p̂ is to the true value p .
Statistics of a Random Sample
The uncertainty in a given random sample (namely that is expected that the proportion estimate, p̂ , is a good, but not perfect, approximation for the true proportion p ) can be summarized by saying that the estimate p̂ is normally distributed with mean p and variance p(1-p)/n . For an explanation of why the sample estimate is normally distributed, study the Central Limit Theorem . As defined below, confidence level, confidence intervals, and sample sizes are all calculated with respect to this sampling distribution. In short, the confidence interval gives an interval around p in which an estimate p̂ is "likely" to be. The confidence level gives just how "likely" this is – e.g., a 95% confidence level indicates that it is expected that an estimate p̂ lies in the confidence interval for 95% of the random samples that could be taken. The confidence interval depends on the sample size, n (the variance of the sample distribution is inversely proportional to n , meaning that the estimate gets closer to the true proportion as n increases); thus, an acceptable error rate in the estimate can also be set, called the margin of error, ε , and solved for the sample size required for the chosen confidence interval to be smaller than e ; a calculation known as "sample size calculation."
Confidence Level
The confidence level is a measure of certainty regarding how accurately a sample reflects the population being studied within a chosen confidence interval. The most commonly used confidence levels are 90%, 95%, and 99%, which each have their own corresponding z-scores (which can be found using an equation or widely available tables like the one provided below) based on the chosen confidence level. Note that using z-scores assumes that the sampling distribution is normally distributed, as described above in "Statistics of a Random Sample." Given that an experiment or survey is repeated many times, the confidence level essentially indicates the percentage of the time that the resulting interval found from repeated tests will contain the true result.
Confidence Interval
In statistics, a confidence interval is an estimated range of likely values for a population parameter, for example, 40 ± 2 or 40 ± 5%. Taking the commonly used 95% confidence level as an example, if the same population were sampled multiple times, and interval estimates made on each occasion, in approximately 95% of the cases, the true population parameter would be contained within the interval. Note that the 95% probability refers to the reliability of the estimation procedure and not to a specific interval. Once an interval is calculated, it either contains or does not contain the population parameter of interest. Some factors that affect the width of a confidence interval include: size of the sample, confidence level, and variability within the sample.
There are different equations that can be used to calculate confidence intervals depending on factors such as whether the standard deviation is known or smaller samples (n<30) are involved, among others. The calculator provided on this page calculates the confidence interval for a proportion and uses the following equations:
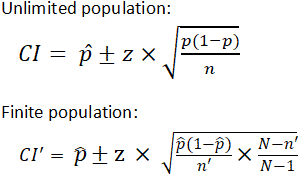
Within statistics, a population is a set of events or elements that have some relevance regarding a given question or experiment. It can refer to an existing group of objects, systems, or even a hypothetical group of objects. Most commonly, however, population is used to refer to a group of people, whether they are the number of employees in a company, number of people within a certain age group of some geographic area, or number of students in a university's library at any given time.
It is important to note that the equation needs to be adjusted when considering a finite population, as shown above. The (N-n)/(N-1) term in the finite population equation is referred to as the finite population correction factor, and is necessary because it cannot be assumed that all individuals in a sample are independent. For example, if the study population involves 10 people in a room with ages ranging from 1 to 100, and one of those chosen has an age of 100, the next person chosen is more likely to have a lower age. The finite population correction factor accounts for factors such as these. Refer below for an example of calculating a confidence interval with an unlimited population.
EX: Given that 120 people work at Company Q, 85 of which drink coffee daily, find the 99% confidence interval of the true proportion of people who drink coffee at Company Q on a daily basis.
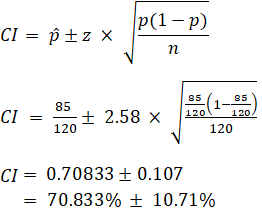

Sample Size Calculation
Sample size is a statistical concept that involves determining the number of observations or replicates (the repetition of an experimental condition used to estimate the variability of a phenomenon) that should be included in a statistical sample. It is an important aspect of any empirical study requiring that inferences be made about a population based on a sample. Essentially, sample sizes are used to represent parts of a population chosen for any given survey or experiment. To carry out this calculation, set the margin of error, ε , or the maximum distance desired for the sample estimate to deviate from the true value. To do this, use the confidence interval equation above, but set the term to the right of the ± sign equal to the margin of error, and solve for the resulting equation for sample size, n . The equation for calculating sample size is shown below.
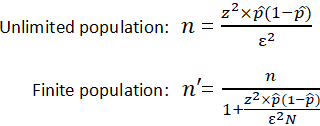
EX: Determine the sample size necessary to estimate the proportion of people shopping at a supermarket in the U.S. that identify as vegan with 95% confidence, and a margin of error of 5%. Assume a population proportion of 0.5, and unlimited population size. Remember that z for a 95% confidence level is 1.96. Refer to the table provided in the confidence level section for z scores of a range of confidence levels.
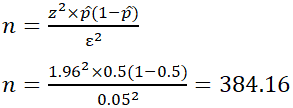
Thus, for the case above, a sample size of at least 385 people would be necessary. In the above example, some studies estimate that approximately 6% of the U.S. population identify as vegan, so rather than assuming 0.5 for p̂ , 0.06 would be used. If it was known that 40 out of 500 people that entered a particular supermarket on a given day were vegan, p̂ would then be 0.08.
2024 Theses Doctoral
Statistically Efficient Methods for Computation-Aware Uncertainty Quantification and Rare-Event Optimization
He, Shengyi
The thesis covers two fundamental topics that are important across the disciplines of operations research, statistics and even more broadly, namely stochastic optimization and uncertainty quantification, with the common theme to address both statistical accuracy and computational constraints. Here, statistical accuracy encompasses the precision of estimated solutions in stochastic optimization, as well as the tightness or reliability of confidence intervals. Computational concerns arise from rare events or expensive models, necessitating efficient sampling methods or computation procedures. In the first half of this thesis, we study stochastic optimization that involves rare events, which arises in various contexts including risk-averse decision-making and training of machine learning models. Because of the presence of rare events, crude Monte Carlo methods can be prohibitively inefficient, as it takes a sample size reciprocal to the rare-event probability to obtain valid statistical information about the rare-event. To address this issue, we investigate the use of importance sampling (IS) to reduce the required sample size. IS is commonly used to handle rare events, and the idea is to sample from an alternative distribution that hits the rare event more frequently and adjusts the estimator with a likelihood ratio to retain unbiasedness. While IS has been long studied, most of its literature focuses on estimation problems and methodologies to obtain good IS in these contexts. Contrary to these studies, the first half of this thesis provides a systematic study on the efficient use of IS in stochastic optimization. In Chapter 2, we propose an adaptive procedure that converts an efficient IS for gradient estimation to an efficient IS procedure for stochastic optimization. Then, in Chapter 3, we provide an efficient IS for gradient estimation, which serves as the input for the procedure in Chapter 2. In the second half of this thesis, we study uncertainty quantification in the sense of constructing a confidence interval (CI) for target model quantities or prediction. We are interested in the setting of expensive black-box models, which means that we are confined to using a low number of model runs, and we also lack the ability to obtain auxiliary model information such as gradients. In this case, a classical method is batching, which divides data into a few batches and then constructs a CI based on the batched estimates. Another method is the recently proposed cheap bootstrap that is constructed on a few resamples in a similar manner as batching. These methods could save computation since they do not need an accurate variability estimator which requires sufficient model evaluations to obtain. Instead, they cancel out the variability when constructing pivotal statistics, and thus obtain asymptotically valid t-distribution-based CIs with only few batches or resamples. The second half of this thesis studies several theoretical aspects of these computation-aware CI construction methods. In Chapter 4, we study the statistical optimality on CI tightness among various computation-aware CIs. Then, in Chapter 5, we study the higher-order coverage errors of batching methods. Finally, Chapter 6 is a related investigation on the higher-order coverage and correction of distributionally robust optimization (DRO) as another CI construction tool, which assumes an amount of analytical information on the model but bears similarity to Chapter 5 in terms of analysis techniques.
- Operations research
- Stochastic processes--Mathematical models
- Mathematical optimization
- Bootstrap (Statistics)
- Sampling (Statistics)
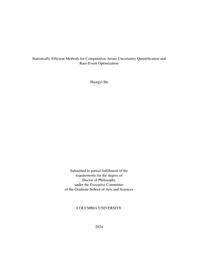
More About This Work
- DOI Copy DOI to clipboard
Root out friction in every digital experience, super-charge conversion rates, and optimize digital self-service
Uncover insights from any interaction, deliver AI-powered agent coaching, and reduce cost to serve
Increase revenue and loyalty with real-time insights and recommendations delivered to teams on the ground
Know how your people feel and empower managers to improve employee engagement, productivity, and retention
Take action in the moments that matter most along the employee journey and drive bottom line growth
Whatever they’re are saying, wherever they’re saying it, know exactly what’s going on with your people
Get faster, richer insights with qual and quant tools that make powerful market research available to everyone
Run concept tests, pricing studies, prototyping + more with fast, powerful studies designed by UX research experts
Track your brand performance 24/7 and act quickly to respond to opportunities and challenges in your market
Explore the platform powering Experience Management
- Free Account
- For Digital
- For Customer Care
- For Human Resources
- For Researchers
- Financial Services
- All Industries
Popular Use Cases
- Customer Experience
- Employee Experience
- Employee Exit Interviews
- Net Promoter Score
- Voice of Customer
- Customer Success Hub
- Product Documentation
- Training & Certification
- XM Institute
- Popular Resources
- Customer Stories
- Artificial Intelligence
- Market Research
- Partnerships
- Marketplace
The annual gathering of the experience leaders at the world’s iconic brands building breakthrough business results, live in Sydney.
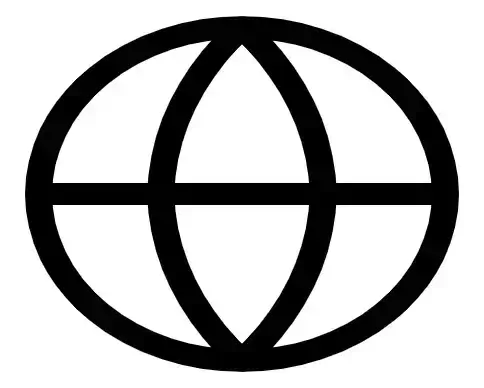
- English/AU & NZ
- Español/Europa
- Español/América Latina
- Português Brasileiro
- REQUEST DEMO
- Experience Management
- Determining Sample Size
Try Qualtrics for free
How to determine sample size.
12 min read Sample size can make or break your research project. Here’s how to master the delicate art of choosing the right sample size.
Sample size is the beating heart of any research project. It’s the invisible force that gives life to your data, making your findings robust, reliable and believable.
Sample size is what determines if you see a broad view or a focus on minute details; the art and science of correctly determining it involves a careful balancing act. Finding an appropriate sample size demands a clear understanding of the level of detail you wish to see in your data and the constraints you might encounter along the way.
Remember, whether you’re studying a small group or an entire population, your findings are only ever as good as the sample you choose.
Free eBook: The ultimate guide to conducting market research
“How much sample do we need?” is one of the most commonly-asked questions and stumbling points in the early stages of research design . Finding the right answer to it requires first understanding and answering two other questions:
How important is statistical significance to you and your stakeholders?
What are your real-world constraints.
At the heart of this question is the goal to confidently differentiate between groups, by describing meaningful differences as statistically significant. Statistical significance isn’t a difficult concept, but it needs to be considered within the unique context of your research and your measures.
First, you should consider when you deem a difference to be meaningful in your area of research. While the standards for statistical significance are universal, the standards for “meaningful difference” are highly contextual.
For example, a 10% difference between groups might not be enough to merit a change in a marketing campaign for a breakfast cereal, but a 10% difference in efficacy of breast cancer treatments might quite literally be the difference between life and death for hundreds of patients. The exact same magnitude of difference has very little meaning in one context, but has extraordinary meaning in another. You ultimately need to determine the level of precision that will help you make your decision.
Within sampling, the lowest amount of magnification – or smallest sample size – could make the most sense, given the level of precision needed, as well as timeline and budgetary constraints.
If you’re able to detect statistical significance at a difference of 10%, and 10% is a meaningful difference, there is no need for a larger sample size, or higher magnification. However, if the study will only be useful if a significant difference is detected for smaller differences – say, a difference of 5% — the sample size must be larger to accommodate this needed precision. Similarly, if 5% is enough, and 3% is unnecessary, there is no need for a larger statistically significant sample size.
You should also consider how much you expect your responses to vary. When there isn’t a lot of variability in response, it takes a lot more sample to be confident that there are statistically significant differences between groups.
For instance, it will take a lot more sample to find statistically significant differences between groups if you are asking, “What month do you think Christmas is in?” than if you are asking, “How many miles are there between the Earth and the moon?”. In the former, nearly everybody is going to give the exact same answer, while the latter will give a lot of variation in responses. Simply put, when your variables do not have a lot of variance, larger sample sizes make sense.
Statistical significance
The likelihood that the results of a study or experiment did not occur randomly or by chance, but are meaningful and indicate a genuine effect or relationship between variables.
Magnitude of difference
The size or extent of the difference between two or more groups or variables, providing a measure of the effect size or practical significance of the results.
Actionable insights
Valuable findings or conclusions drawn from data analysis that can be directly applied or implemented in decision-making processes or strategies to achieve a particular goal or outcome.
It’s crucial to understand the differences between the concepts of “statistical significance”, “magnitude of difference” and “actionable insights” – and how they can influence each other:
- Even if there is a statistically significant difference, it doesn’t mean the magnitude of the difference is large: with a large enough sample, a 3% difference could be statistically significant
- Even if the magnitude of the difference is large, it doesn’t guarantee that this difference is statistically significant: with a small enough sample, an 18% difference might not be statistically significant
- Even if there is a large, statistically significant difference, it doesn’t mean there is a story, or that there are actionable insights
There is no way to guarantee statistically significant differences at the outset of a study – and that is a good thing.
Even with a sample size of a million, there simply may not be any differences – at least, any that could be described as statistically significant. And there are times when a lack of significance is positive.
Imagine if your main competitor ran a multi-million dollar ad campaign in a major city and a huge pre-post study to detect campaign effects, only to discover that there were no statistically significant differences in brand awareness . This may be terrible news for your competitor, but it would be great news for you.
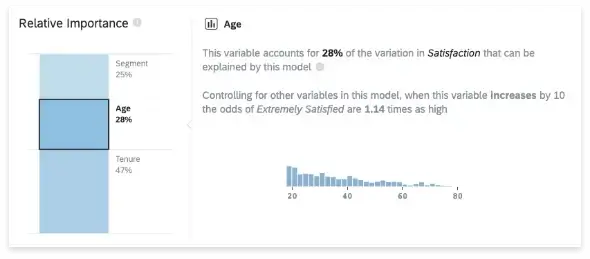
With Stats iQ™ you can analyze your research results and conduct significance testing
As you determine your sample size, you should consider the real-world constraints to your research.
Factors revolving around timings, budget and target population are among the most common constraints, impacting virtually every study. But by understanding and acknowledging them, you can definitely navigate the practical constraints of your research when pulling together your sample.
Timeline constraints
Gathering a larger sample size naturally requires more time. This is particularly true for elusive audiences, those hard-to-reach groups that require special effort to engage. Your timeline could become an obstacle if it is particularly tight, causing you to rethink your sample size to meet your deadline.
Budgetary constraints
Every sample, whether large or small, inexpensive or costly, signifies a portion of your budget. Samples could be like an open market; some are inexpensive, others are pricey, but all have a price tag attached to them.
Population constraints
Sometimes the individuals or groups you’re interested in are difficult to reach; other times, they’re a part of an extremely small population. These factors can limit your sample size even further.
What’s a good sample size?
A good sample size really depends on the context and goals of the research. In general, a good sample size is one that accurately represents the population and allows for reliable statistical analysis.
Larger sample sizes are typically better because they reduce the likelihood of sampling errors and provide a more accurate representation of the population. However, larger sample sizes often increase the impact of practical considerations, like time, budget and the availability of your audience. Ultimately, you should be aiming for a sample size that provides a balance between statistical validity and practical feasibility.
4 tips for choosing the right sample size
Choosing the right sample size is an intricate balancing act, but following these four tips can take away a lot of the complexity.
1) Start with your goal
The foundation of your research is a clearly defined goal. You need to determine what you’re trying to understand or discover, and use your goal to guide your research methods – including your sample size.
If your aim is to get a broad overview of a topic, a larger, more diverse sample may be appropriate. However, if your goal is to explore a niche aspect of your subject, a smaller, more targeted sample might serve you better. You should always align your sample size with the objectives of your research.
2) Know that you can’t predict everything
Research is a journey into the unknown. While you may have hypotheses and predictions, it’s important to remember that you can’t foresee every outcome – and this uncertainty should be considered when choosing your sample size.
A larger sample size can help to mitigate some of the risks of unpredictability, providing a more diverse range of data and potentially more accurate results. However, you shouldn’t let the fear of the unknown push you into choosing an impractically large sample size.
3) Plan for a sample that meets your needs and considers your real-life constraints
Every research project operates within certain boundaries – commonly budget, timeline and the nature of the sample itself. When deciding on your sample size, these factors need to be taken into consideration.
Be realistic about what you can achieve with your available resources and time, and always tailor your sample size to fit your constraints – not the other way around.
4) Use best practice guidelines to calculate sample size
There are many established guidelines and formulas that can help you in determining the right sample size.
The easiest way to define your sample size is using a sample size calculator , or you can use a manual sample size calculation if you want to test your math skills. Cochran’s formula is perhaps the most well known equation for calculating sample size, and widely used when the population is large or unknown.
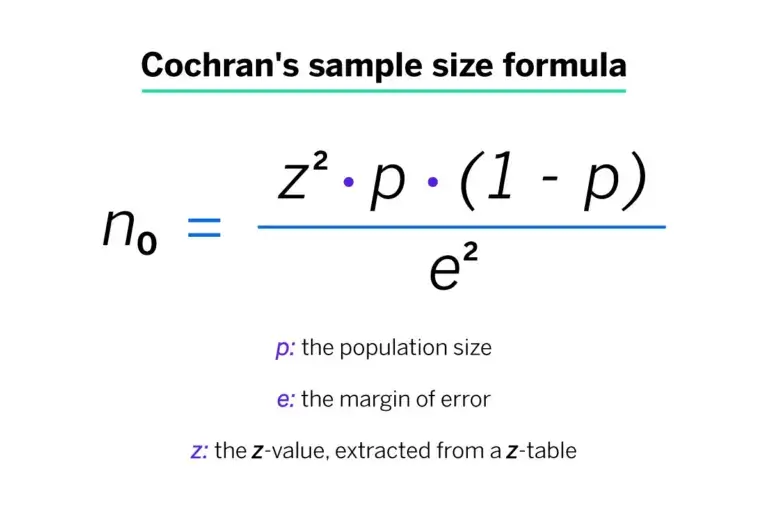
Beyond the formula, it’s vital to consider the confidence interval, which plays a significant role in determining the appropriate sample size – especially when working with a random sample – and the sample proportion. This represents the expected ratio of the target population that has the characteristic or response you’re interested in, and therefore has a big impact on your correct sample size.
If your population is small, or its variance is unknown, there are steps you can still take to determine the right sample size. Common approaches here include conducting a small pilot study to gain initial estimates of the population variance, and taking a conservative approach by assuming a larger variance to ensure a more representative sample size.
Empower your market research
Conducting meaningful research and extracting actionable intelligence are priceless skills in today’s ultra competitive business landscape. It’s never been more crucial to stay ahead of the curve by leveraging the power of market research to identify opportunities, mitigate risks and make informed decisions.
Equip yourself with the tools for success with our essential eBook, “The ultimate guide to conducting market research” .
With this front-to-back guide, you’ll discover the latest strategies and best practices that are defining effective market research. Learn about practical insights and real-world applications that are demonstrating the value of research in driving business growth and innovation.
Related resources
Selection bias: how to avoid errors in research 11 min read, systematic random sampling 12 min read, convenience sampling 18 min read, non-probability sampling 17 min read, simple random sampling 9 min read, sampling methods 15 min read, sampling and non-sampling errors 10 min read, request demo.
Ready to learn more about Qualtrics?
Advertisement
Population-level norm values by EQ-5D-3L in Hungary - a comparison of survey results from 2022 with those from 2000
- Open access
- Published: 05 June 2024
Cite this article
You have full access to this open access article
- András Inotai ORCID: orcid.org/0000-0002-0663-2733 1 ,
- Dávid Nagy 1 , 2 ,
- Zoltán Kaló 1 , 2 &
- Zoltán Vokó 1 , 2
Explore all metrics
Although population norms of the EQ-5D-3L instrument had been available in Hungary since 2000, their evaluation was based on a United Kingdom (UK) value set. Our objective was to estimate the population norms for EQ-5D-3L by using the new Hungarian value set available since 2020, to extend the scope to adolescents, and to compare with norms from 2000.
A cross sectional EQ-5D-3L survey representative of the Hungarian population was conducted in 2022. The EQ-5D-3L dimensional responses were analyzed by age and sex and compared with the survey from 2000, by estimating population frequencies with their 95% confidence intervals; index values were evaluated by both value sets.
Altogether, 11,910 respondents, aged 12 or more (578 between 12 and 17), completed the EQ-5D-3L. There was a notable improvement in reporting problems for both sexes (age 35–64) regarding the pain/discomfort and anxiety/depression compared to 2000. Below the age 44, both sexes had an EQ-5D-3L index plateau of 0.98, while above the age 55, men tended to have numerically higher index values compared to women, with the difference increasing with older age. Improvement in dimensional responses were also translated to numerically higher index values for both sexes between ages 18 and 74 compared to 2000. Multivariate regression analysis showed that higher educational attainment, lower age, larger household size, and active occupational status were associated with higher index values.
Over the past 22 years, there was a large improvement in HRQoL of the middle-aged to elderly men and women in Hungary.
Plain English Summary
Health states can be described by a combination of statements of health-related quality of life measures. ‘Value sets’ are numerical expressions of how preferred a health state is. The provision of population-level health-related quality of life estimates (also known as ‘population norms’) are expected to improve the precision of patient-level clinical decision making, and health economic and public health studies. However, preference towards these health states is influenced by culture, resulting in differences across populations. While responses for the EQ-5D-3L instrument for adults have been available in Hungary since 2000, the evaluation of these responses was based on a ‘value set’ from the United Kingdom, rather than a Hungarian one.
This research, utilizing the newly introduced Hungarian ‘value set’ (available since 2020) for the EQ-5D-3L instrument, offers a larger sample size, inclusion of adolescents and potentially improved sampling compared to the prior research conducted in 2000. Comparison of the two surveys allows us to estimate changes in both dimensional responses and overall health-related quality of life of the population over a 20-year time horizon, while we also compare the impact of different ‘value sets’ on health-related quality of life assessment. A large EQ-5D-3L improvement was observed in middle-aged-to-elderly people.
Avoid common mistakes on your manuscript.
Introduction
Health-related quality of life (HRQoL) is a subjective multi-dimensional concept that includes dimensions related to physical, mental, emotional, and social functioning and that goes beyond clinical measures of health status [ 1 ]. Standardized and validated generic and disease-specific measures are used to estimate the HRQoL of individuals [ 2 ]. Generic measures are universally applicable in a wide range of diseases.
One of the most widely used generic HRQoL instrument is the EQ-5D developed by the EuroQoL Group. All EQ-5D questionnaires include a descriptive system focusing on five dimensions: mobility, self-care, usual activities, pain/discomfort and anxiety/depression, and a vertical visual analogue scale for self-assessment of health status (EQ VAS). EQ-5D-3L and −5L are popular generic measures among adults, and EQ-5D-Y-3L and −5L are targeted for the younger population with a child-friendly wording [ 3 ]. In the EQ-5D-3L index, each dimension has three levels (3L), with level 1 (L1) denoting no problems and level 3 (L3) denoting ‘extreme problems/unable to/confined to bed’. (In the 5L version, each dimension has five levels). The EQ VAS (also known as EQ-5D Thermometer) is ranged from 0 to 100 (‘the best and worst health you can imagine’) [ 4 , 5 ].
EQ-5D instruments’ patient-reported values (profile scores) can be converted to an index score using a selected algorithm. Such algorithms are based on surveying the general public’s preferences for different combinations of health states, resulting in ‘value sets’ that are numerical expressions of how preferred a health state is. Therefore, measures such as EQ-5D are also referred to as a ‘preference-based’ or ‘preference-accompanied measure’. The measurement interval of the value set developed originally for EQ-5D-3L by Dolan (using the time trade-off (TTO) method, based on a United Kingdom (UK) population sample) is between -0.594 (health state 33333) to 1 (health state 11111), where the value of 0.0 refers to being dead, 1.0 refers to full health (‘UK value set’) [ 6 , 7 , 8 , 9 ].
However, as individuals in different cultures or countries may assign different values to certain health states, many countries other than the UK have also developed their own value sets since the introduction of the EQ-5D-3L [ 10 ]. Since 2020, a Hungarian value set has also been available for both the EQ-5D-3L and the 5L, based on a non-probability quota sample of 1000 respondents in the Hungarian general population, by using a composite TTO method [ 11 ] (‘Hungarian (HU) value set’). Quotas are set by age and sex. Index values in the Hungarian value set range from -0.865 to 1 for the EQ-5D-3L health states. EQ-5D is also among the preferred instruments for health technology assessment (HTA) in Hungary [ 12 ].
Population norm values are used as a reference to estimate the HRQoL decrement of a patient population with different diseases. They are applied both in micro-level clinical decision-making (estimating HRQoL) and in health economic models, and macro-level public health decisions (estimating health loss/burden of disease [ 13 ]). In Hungary, the poor general health status of the population has led to several population-level health surveys by using the EQ-5D-3L instrument. Most importantly, in 2000, a national health survey was conducted on a representative sample of 5503 individuals representing the whole population, age and sex, by using a paper-based self-administered EQ-5D-3L [ 14 , 15 ]. This was followed by the European Value of a Quality Adjusted Life Year (EuroVaQ) project in 2010, in which a population sample of 2281 individuals completed a web-based EQ-5D-3L (although it was intended to be representative, the authors reported that the sample overrepresented women and underrepresented the elderly population) [ 16 ]. Both surveys applied the UK value set and used an adult sample. The publication of the Hungarian value set in 2020 provided a solid platform for re-estimating the index values of the EQ-5D-3L for the Hungarian adult population. In parallel, the increasing number of HTA submission dossiers with pediatric/adolescent indication also necessitated defining population norm values for the under-18 population to have a more accurate estimate of their cost effectiveness in the Hungarian setting. This led to the need for population health surveys covering an extended age range, also including the 12–17 age band.
This paper aims to present an overview of the HRQoL of the Hungarian population aged 12 years and older based on a representative random sample using the EQ-5D-3L instrument, and to compare the results with the prior survey from 2000.
This survey was part of a larger cross-sectional national survey on travelling habits of Hungarian people, conducted by the Hungarian Central Statistical Office on a quarterly basis. EQ-5D-3L was an add-on to this survey using standard demographic questions, but without any other health care-related questions.
Selection of instrument and age
Although the EQ-5D-5L was also considered for the study, the EQ-5D-3L was ultimately selected. This allows for a comparison with prior population surveys [ 14 , 15 , 16 ], with several existing disease-specific HRQoL studies using EQ-5D-3L (conducted both at Semmelweis University [e.g. 17 – 22 ] and at other research centers in Hungary [e.g. 23 – 28 ]), and also with high quality validation studies [ 29 , 30 , 31 , 32 ]. As the EuroQoL Group recommends the EQ-5D-3L adult version for adolescents aged 16 and above, and considers both the EQ-5D-3L adult version and the EQ-5D-Y-3L to be acceptable for adolescents aged 12–15 years, for logistical simplicity (i.e., using only one instrument) and in concordance with EQ-5D-Y-3L user guide, the minimum eligible age was 12 years in this study, while the EQ-5D-3L version was applied also for adolescents aged 12–17 years. As health economic models benefit from more precise index value data and also to minimize residual confounding by age, index values are reported per a 5-year age band. However, to ensure comparability with the national health survey from 2000, also a 10-year age band was applied in this study.
Research ethics
The study protocol was approved by the Medical Research Council – Scientific and Ethical Committee in Hungary (number of ethical approval: IV/2292-1 2022/EKU), and research was performed in accordance with the ethical standards of the 1964 Declaration of Helsinki [ 33 ].
Population survey sampling
Primary sampling units (PSUs) were settlements, and the secondary sampling units were dwellings in Hungary. The settlements were stratified by county and size. Larger settlements were selected with certainty. No general national threshold was applied to define certainty PSUs, it varied from county to county. The probability of selecting smaller PSUs was proportional to their size in terms of dwellings. Dwellings were randomly selected within a settlement. All household members aged 12 years or older were included in the survey.
Population survey weighting
Design weights were calculated based on the sampling design. After the data collection, these were calibrated to correct for nonresponse by geographical region to population size, sex and age distribution, development category of the settlement, and household size, so that we could provide unbiased estimates on the level of the population. These analytical weights were used in the statistical analysis. They had a range of 140–2500, reflecting the number of people a study participant represented. To include 11,910 respondents aged 12 or more, 15,058 individuals were contacted in 7578 households, resulting in a response rate of 79%. Of all the EQ-5D-3L questionnaires, 2.81% were self-administered online, 46.64% by telephone interview, and 50.55% by personal interview, between 1st April and 2nd May 2022. The questionnaire was designed in such a way that it did not allow item nonresponse, ‘I do not know/I do not respond’ answers. As the questionnaire was short, withdrawing the participation in the meantime did not happen. No deletion of responders due to lack of data or imputation was necessary. The participant (unit)-level non-response was corrected for in the weighting, the description of which was provided earlier.
Statistical analysis methods
We used the survey module of the statistical software STATA 16.1 [ 34 ]. Dimensional distribution analysis was performed by estimating population frequencies with their 95% confidence intervals, by using the same age bands and reporting structure as applied in the 2000 national health survey. Mean EQ-5D-3L index values with their 95% confidence intervals were estimated for the target population by age and sex using “svy: mean” procedure with an analytically derived variance estimator associated with the sample mean. Weighted multiple linear regression analyses were applied by using sex, age (adults only), education, occupation, and household size as explanatory variables applying “svy: regress” procedure. Additionally, we fitted a regression model with the interaction terms between sex and age bands adjusted for education, occupation, and household size. Design-based standard errors were estimated taking into account the stratified cluster sampling.
Sample characteristics
Table 1 describes the baseline characteristics of the sample including age, sex, geographical region, education, occupation and household size, also by the mode of administration. In the unweighted sample 54.3% of participants were women, 33.8% were students and 11.5% participants were from Budapest. Online responders tend to be younger and living in Budapest with a higher educational attainment.
EQ-5D-3L dimensional, index (using HU value set), and VAS norms by age and sex
Supplementary Table 1 reports weighted EQ-5D-3L questionnaire responses by mode of administration. Problems (L2 + L3) in anxiety/depression were reported slightly more frequently in the case of online self-administration, compared to the telephone and online interviews. Supplementary Table 2 reports the weighted EQ-5D-3L dimensional responses for 5- and 10-year age bands. Among the five dimensions, anxiety/depression was the one where both sexes reported problems (L2 + L3) even in the younger age bands. Towards higher age bands, mobility, pain/discomfort and usual activities were increasingly associated with problems. Similarly, L3 impairments were reported mainly in the dimensions of usual activities and pain/discomfort by older adults. Comparing the two sexes, younger men tended to report slightly more problems in all dimensions; on contrary, above 75 women tended to report more problems. Along with older ages, women tended to report more L3 impairments in the dimension of pain/discomfort, while men tended to report more L3 impairments in self-care, compared to the other sex.
Table 2 reports the weighted EQ-5D-3L index values from the 2022 population survey (evaluated by using the Hungarian value set) of the 12-year-old and older by 5- and 10-year age bands. Using the Hungarian value set, index values showed a plateau of 0.98 under age of 45. Men older than 54 years generally had numerically higher, although statistically not significantly different index values compared to women, with the difference slightly increasing with older ages. In contrast to adults, the index values for girls were minimally higher than for boys among participants under 18 years of age.
Table 2 also reports EQ VAS data in a similar age structure. Above the age of 34, men tended to have minimally higher EQ VAS compared to women in every 10-year age bands. Above age 39, EQ VAS tended to decrease in every consecutive 5-year age band in both sexes.
Comparison of the dimensional responses between 2000 and 2022
Supplementary Table 3 reports comparison of problems by dimension between 2000 and 2022, using the data structure of the 2000 national survey. There was a notable improvement in reporting problems (L2 + L3) for both sexes in the age band of 35–64 in all dimensions except for self-care, with a major improvement in both pain/discomfort and anxiety/depression, especially for women. Some improvement was also seen in pain/discomfort (for age 18–34) and anxiety/depression (for both age 18–34 and 65+) from 2000 to 2022 for both sexes, but a numerically larger one for women.
Comparison of index values (using the UK value set) between 2022 and 2000
Figure 1 shows a comparison of weighted EQ-5D-3L index values of the 2022 population survey with the 2000 national health survey for 10-year age bands, both using the UK value set to ensure consistency. Supplementary Table 4 reports the weighted EQ-5D-3L index values for the same comparison for 10-year age bands. The 2022 population survey resulted in higher index values than the 2000 national health survey between age 18–74, especially between the ages 35 and 64, where better dimensional responses of the 2022 population survey were also translated into higher weighted index values for both sexes compared to the 2000 national health survey. On contrary, for the 85+ age band, the 2022 population survey showed markedly lower weighted index values compared to the 2000 national health survey. In that study, the difference by sex was numerically even larger in all relevant age bands.
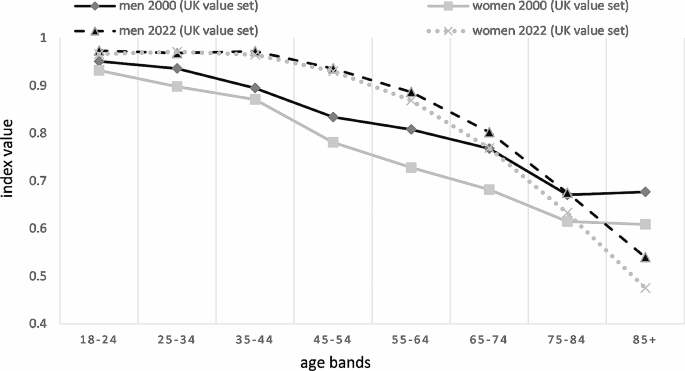
Comparison of EQ-5D-3L index values (using the UK value set) in both the 2022 population survey and the 2000 national health survey
Comparison of index values derived by the HU and UK value sets for the 2022 population survey
As an overview, Fig. 2 presents the weighted EQ-5D-3L index data for both sexes from the 2022 population survey by using both the Hungarian (default) and the UK value sets for 10-year age bands. Index values derived by the UK value set from the 2022 population survey (see Supplementary Table 4 ) showed a similar trend to those indices derived by the HU value set from 2022 (see Table 2 ), but with lower weighted index values above age of 45 and a larger, statistically not significant difference between men and women, especially in older age groups.
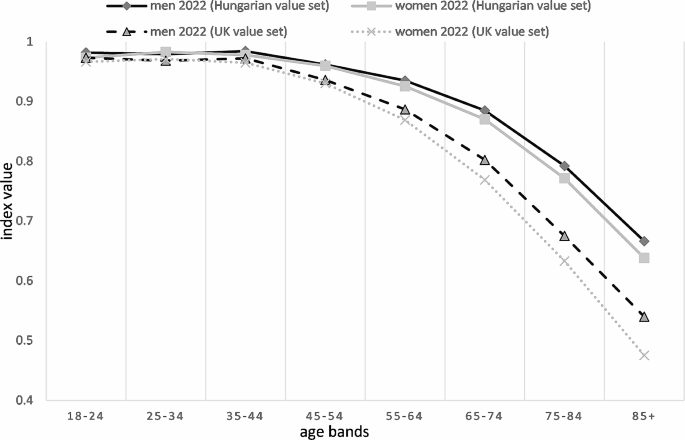
EQ-5D-3L index values (using the UK and Hungarian value set) per 10-year age bands and sex in 2022 population survey
Comparison of VAS results
Supplementary Table 5 reports the weighted EQ VAS numbers for the same comparison for 10-year age bands. EQ VAS numbers were consistently higher in both sexes in 2022 compared to 2000 for with the smallest difference for those aged 85+.
Regression results
Finally, Table 3 presents the results of the weighted multiple linear regression analysis estimating the EQ-5D-3L index values for adults. Multivariate analysis showed that, after controlling for other variables, sex had no significant impact on the index values, but there was a significant interaction between sex and age (adjusted Wald-test p -value: 0.014). In terms of age, the index values significantly differ from the index value of the reference age band (18–24 years) above the age of 49 years. Higher educational attainment was associated with higher index values. Economic activity was used as a nominal variable in the model and showed a significant impact on the index values: employed respondents and students had significantly higher index values than those who were unemployed, retired or inactive for other reasons, the latter having the lowest index values. Finally, a larger household size (a larger number of people living in a household) was also associated with higher index values.
Our results showed that in 2022 among the five dimensions, anxiety/depression was the one where both sexes reported problems in younger age bands; towards higher age bands, mobility, pain/discomfort and usual activities were increasingly associated with problems. Both EQ-5D-3L index values and EQ VAS showed reduction along with age above the age of 44 in both sexes, with men having somewhat higher values compared to women. EQ VAS numbers were consistently higher in 2022 compared to 2000 for both sexes, and there was a large improvement in EQ-5D-3L index values between age 35–64. According to the multivariable analysis, younger age, higher education, being active, and larger household size are associated with better HRQoL.
Comparison with the national health survey 2000
Over the past 22 years the HRQoL of women aged 25–74 and men aged 35–64 improved considerably. Interestingly, our study could not replicate the relatively high index values observed in the 2000 national health survey for the age 85+ (for both men and women). However, in that survey, only 1% of respondents had this age which meant that some of the outliers may have had a potentially larger impact. Since 2000, the health status of elderly people has improved (life expectancy at age 65 improved from 17.3 to 18.7 for women and 13.4 to 14.6 for men between 2004 and 2016) [ 35 ], which (if better HRQoL is also assumed) may contradict our results showing lower index values. It seems that the elderly population with a better HRQoL may have been overrepresented in the 2000 national health survey, and the small number of participants of this age provided less robust estimates for this age band.
Overall, beyond using a more relevant value set for EQ-5D-3L, our study also offers larger sample size, thus enhanced statistical power, narrow age bands for more precise economic evaluations, inclusion of adolescents and potentially improved sampling, compared to the 2000 national health survey. We strongly believe that these factors contribute to more credible population norm estimates.
Impact of UK and HU value sets on index values from the 2022 population survey
Higher index values derived using the Hungarian value set compared to the UK value set (shown in Fig. 2 ) can be explained by two key factors. Firstly, the Hungarian value set uses a 0.020 constant (decrement, to be used for health states other than 11111) instead of 0.081 used by UK value set. Secondly and more importantly, the new Hungarian value set does not apply the constant N3 (an additional -0.269 decrement for L3 responses in any dimension used by UK value set, beyond the respective dimension-specific L3 decrement), as its impact has been considered in larger L3 decrements in the Hungarian value set compared to the UK one. On the other hand, for L2 responses which were reported much more frequently (Supplementary Table 2 ), the new Hungarian value set tend use smaller decrements compared to the UK one.
Comparison with other population norms in the region
Nikl et al. published population norms for Hungarian population on a sample of 2000 adults, reported to be broadly representative in terms of sex, age groups, highest level of education, geographical region, and settlement type, using the 15D instrument [ 36 ]. The mean 15D index value was 0.810 using the Norwegian 15D value set. In that study, with advancing age categories, the 15D index values showed an inverse U-shaped curve with highest index values of 0.82 for both age bands of 25–34 and 45–54; and numerical results could be considered somewhat consistent with index values (derived by the UK value set) of this research. However, different HRQoL instruments, sample sizes, value sets, recruitment (i.e., voluntary registration from online panel) make the more detailed comparison of the two studies difficult. In Poland, Golicki and Niewada published population norms on a sample of 3963 adults, representative of the Polish population in terms of age, sex, geographical region, education, and socio-professional group, by using a self-administered EQ-5D-5L instrument [ 37 ]. To calculate index values, an interim EQ-5D-5L value set for Poland was used based on a crosswalk methodology. Index values (0.96 for those aged 18–24, 0.94 for 35–44, 0.9 for 45–54 and 0.81 for 65–74, respectively) were broadly consistent yet somewhat lower than the Hungarian ones (derived by the Hungarian value set). Again, differences in the applied instruments, sample sizes and administration make further direct comparison of the norms between the two countries difficult. In both studies, similarly to our results, men tend to have higher values in almost all age bands, especially above 35 years. Finally, Zrubka et al. compared EQ-5D-3L population studies from Hungary, Slovenia and Poland and reported issues in terms of comparability due different national characteristics, different data collection methodologies and times [ 38 ]. Importantly, data gaps for age 65+ were reported to be a general concern, confirming our findings in the oldest age category in the 2000 national health survey.
Regression analyses: younger age, higher education, being active and having a larger household size were associated with better HRQoL
To minimize the impact of adult value set applied also for adolescents, our regression analysis included adults only. The lower EQ-5D-3L index in elderly individuals may be explained by the fact that elderly people tend to suffer from more diseases, including multi-morbid conditions [ 39 ], which potentially have a major impact on HRQoL. Better education is shown to be associated with healthier lifestyles [ 40 , 41 , 42 ], higher participation in prevention programs, and appreciation of being healthy in general. Active occupation (i.e., employee, student) may lead to more physical activities and/or social contact, which may contribute to higher index values. On the contrary, inactive people may lack these, while those involved in childcare may feel isolated, sleepless or experience maternal depression, potentially associated with poorer HRQoL. Finally, interpersonal relationships are likely to be stronger in households with two or more people. Moreover, in larger families, the average household index value seems to be increasing significantly with household size even after controlling for the other factors listed in Table 3 . Our findings on the association of age and education with HRQoL were also confirmed by similar conclusions from the 2000 national health survey [ 14 ] and the EuroVaQ study [ 16 ]. Interestingly, these prior studies also found that sex had a significant impact on the EQ-5D-3L index, as did household income (however, this latter variable was not included in the 2022 population survey). This is in line with our observation that there was an interaction between sex and age in our study.
Implications and future research
This research has significant policy implications. The new population norm values for those under 18 introduced by this research will have a significant impact both on the accuracy of health burden estimates and the economic evaluation of health technologies for adolescents. Similarly, the application of the new Hungarian value set that truly reflects the preferences of Hungarian people for different health states are expected to improve the accuracy of health burden estimates and economic evaluations for adults. Moreover, as a Hungarian value set for the EQ-5D-Y-3L has also been available since 2022 [ 43 ], future research could compare the impact of using the EQ-3D-3L (with the adult value set) and the future use of the EQ-5D-Y-3L (with the new value set for adolescents) to conclude on the applicability of the adult EQ-5D-3L for adolescents aged 12–15 years, as considered to be acceptable by the EuroQoL Group [ 44 ]. Finally, this study together with the research conducted in 2000, with some limitations (difference in sampling methodology, mixing EQ-5D-3L administration methods, various factors influencing population change over 20 years etc.), allows researchers to estimate changes in HRQoL of the population over a 20-year time horizon.
Strengths and limitations
The large representative random sample and the wide age range are the main strengths of this study. Compared to some other large-scale surveys, our study had more robust outreach for those above 65, a cohort especially relevant from public health and health economic point-of-view. However, it has some limitations, too. First, as the intention with the population-level health survey was to establish norm values for previous and ongoing disease-specific research in Hungary, and also to ensure comparability with the 2000 national health survey, the EQ-5D-5L was not considered for this research. Second, different administration methods of the questionnaire may have introduced bias even in a homogeneous sample. Third, the analytical weights had a relatively large range and, as noted by Potter and Zeng [ 45 ], ‘extreme variation in the sampling weights can result in excessively large sampling variances when the data and the selection probabilities are not positively correlated’. Finally, using the adult EQ-5D-3L also for 12-15-year-old adolescents may also have had an impact on the results.
Over the past 22 years, there was a large improvement in reporting problems for both sexes (especially for women) in age 35–64 in EQ-5D-3L dimensions of pain/discomfort and anxiety/depression, compared to 2000. This was also translated to considerably higher index values for middle-aged women and men. Younger age, higher education, being active, and larger household size are associated with better HRQoL. The study, using the new national value set and extended age, is expected to improve the accuracy of economic evaluations and disease burden studies in Hungary.
Data availability
All data generated or analyzed during this study are available from the authors upon reasonable request and with permission of the Hungarian Central Statistical Office.
Office of Disease Prevention and Health Promotion (2020). Health-Related Quality of Life and Well-Being. Health-Related Quality of Life and Well-Being | Healthy People 2020. Retrieved October 13, 2022, from: https://www.healthypeople.gov/2020/about/foundation-health-measures/Health-Related-Quality-of-Life-and-Well-Being
Guyatt, G. H., Feeny, D. H., & Patrick, D. L. (1993). Measuring health-related quality of life. Annals of Internal Medicine , 118 (8), 622–629. https://doi.org/10.7326/0003-4819-118-8-199304150-00009
Article CAS PubMed Google Scholar
EuroQoL Group (2022). EuroQoL instruments. Retrieved October 13, 2022, from: https://euroqol.org/
Brooks, R. (1996). EuroQoL: The current state of play. Health Policy , 37 (1), 53–72. https://doi.org/10.1016/0168-8510(96)00822-6
EuroQoL Group. (1990). EuroQoL–a new facility for the measurement of health-related quality of life. Health Policy , 16 (3), 199–208. https://doi.org/10.1016/0168-8510(90)90421-9
Article Google Scholar
Dolan, P. (1997). Modeling valuations for EuroQoL health states. Medical Care , 35 , 1095–1108.
Lugnér, A. K., & Krabbe, P. F. M. (2020). An overview of the time trade-off method: Concept, foundation, and the evaluation of distorting factors in putting a value on health. Expert Rev Pharmacoecon Outcomes Res , 20 (4), 331–342. https://doi.org/10.1080/14737167.2020.1779062
Article PubMed Google Scholar
Drummond, M. F., O’Brein, B., Stoddart, G. L., & Torrance, G. W. (1997). Methods for economic evaluations of healthcare programmes . Oxford University Press.
Berger, M. L., Bingefors, K., Hedblom, E. C., Pashos, C. L., & Torrance, G. W. (2003). Health care cost, quality and outcomes – ISPOR book of terms . ISPOR.
Roudijk, B., Donders, A. R. T., Stalmeier, P. F. M., Cultural, & Values Group. (2019). Cultural values: Can they explain differences in Health utilities between countries? Medical Decision Making , 39 (5), 605–616. https://doi.org/10.1177/0272989X19841587
Article PubMed PubMed Central Google Scholar
Rencz, F., Brodszky, V., Gulácsi, L., Golicki, D., Ruzsa, G., Pickard, A. S., et al. (2020). Parallel valuation of the EQ-5D-3L and EQ-5D-5L by Time Trade-Off in Hungary. Value In Health : The Journal of the International Society for Pharmacoeconomics and Outcomes Research , 23 (9), 1235–1245. https://doi.org/10.1016/j.jval.2020.03.019
Rencz, F., Gulácsi, L., Drummond, M., Golicki, D., Rupel, P., Simon, V., J., et al. (2016). EQ-5D in Central and Eastern Europe: 2000–2015. Quality of Life Research , 25 (11), 2693–2710. https://doi.org/10.1007/s11136-016-1375-6
Inotai, A., Ágh, T., & Mészáros, Á. (2012). Quality of life, utility and health burden in asthma, chronic obstructive pulmonary disease and rheumatoid arthritis. International Journal of Person Centered Medicine , 2 , 505–510.
Google Scholar
Szende, A., & Nemeth, R. (2003). Health-related quality of life of the Hungarian population. Orvosi Hetilap , 144 (34), 1667–1674.
PubMed Google Scholar
Boros, J., Németh, R., & Vitrai, J. (2002). National Health Survey 2000. [In Hungarian] Retrieved October 13, 2022, from: https://www.nnk.gov.hu/attachments/article/846/Kutatasi_jelentes2000.pdf
Baji, P., Brodszky, V., Rencz, F., Boncz, I., Gulácsi, L., & Péntek, M. (2015). Health state of the Hungarian population between 2000–2010. Orv Hetil , 156 (50), 2035–2044. [In Hungarian.].
Szekeres, G., Rozsa, S., Dome, P., Barsony, G., & Gonda, X. (2021). A Real-World, prospective, Multicenter, single-arm observational study of Duloxetine in patients with major depressive disorder or generalized anxiety disorder. Frontiers in Psychiatry , 12 , 689143. https://doi.org/10.3389/fpsyt.2021.689143
Rieckmann, N., Neumann, K., Feger, S., Ibes, P., Napp, A., Preuß, D., et al. (2020). Health-related qualify of life, angina type and coronary artery disease in patients with stable chest pain. Health and Quality of Life Outcomes , 18 (1), 140. https://doi.org/10.1186/s12955-020-01312-4 . Erratum in: Health Qual Life Outcomes, 18(1), 205.
Rencz, F., Gulácsi, L., Péntek, M., Poór, A. K., Sárdy, M., Holló, P., et al. (2018). Proposal of a new scoring formula for the Dermatology Life Quality Index in psoriasis. British Journal of Dermatology , 179 (5), 1102–1108. https://doi.org/10.1111/bjd.16927
Hankó, B., Kázmér, M., Kumli, P., Hrágyel, Z., Samu, A., Vincze, Z., et al. (2007). Self-reported medication and lifestyle adherence in Hungarian patients with type 2 diabetes. Pharmacy World & Science , 29 (2), 58–66. https://doi.org/10.1007/s11096-006-9070-2
Inotai, A., Rojkovich, B., Fülöp, A., Jászay, E., Agh, T., & Mészáros, A. (2012). Health-related quality of life and utility in patients receiving biological and non-biological treatments in rheumatoid arthritis. Rheumatology International , 32 (4), 963–969. https://doi.org/10.1007/s00296-010-1721-x
Ágh, T., Inotai, A., & Mészáros, Á. (2011). Factors associated with medication adherence in patients with chronic obstructive pulmonary disease. Respiration , 82 (4), 328–334. https://doi.org/10.1159/000324453
Fekete, H., Guillemin, F., Pallagi, E., Fekete, R., Lippai, Z., Luterán, F., et al. (2020). Evaluation of osteoarthritis knee and hip quality of life (OAKHQoL): Adaptation and validation of the questionnaire in the Hungarian population. Ther Adv Musculoskelet Dis , 12 , 1759720X20959570. https://doi.org/10.1177/1759720X20959570
Péntek, M., Gulácsi, L., Herszényi, L., Banai, J., Palatka, K., Lakatos, P. L., et al. (2021). Subjective expectations regarding longevity and future health: A cross-sectional survey among patients with Crohn’s disease. Colorectal Disease , 23 (1), 105–113. https://doi.org/10.1111/codi.15357
Farkas, K., Kolossváry, E., & Járai, Z. (2020). Simple assessment of quality of life and lower limb functional capacity during cilostazol treatment – results of the Short-tERm cIlostazol eFFicacy and quality of life (SHERIFF) study. Vasa , 49 (3), 235–242. https://doi.org/10.1024/0301-1526/a000845
Brodszky, V., Péntek, M., Bálint, P. V., Géher, P., Hajdu, O., Hodinka, L., et al. (2010). Comparison of the psoriatic arthritis quality of life (PsAQoL) questionnaire, the functional status (HAQ) and utility (EQ-5D) measures in psoriatic arthritis: Results from a cross-sectional survey. Scandinavian Journal of Rheumatology , 39 (4), 303–309. https://doi.org/10.3109/03009740903468982
Vokó, Z., Németh, R., Nagyjánosi, L., Jermendy, G., Winkler, G., Hídvégi, T., et al. (2014). Mapping the Nottingham Health Profile onto the preference-based EuroQoL-5D instrument for patients with diabetes. Value Health Reg Issues , 4 , 31–36. https://doi.org/10.1016/j.vhri.2014.06.002
López-Bastida, J., Linertová, R., Oliva-Moreno, J., Serrano-Aguilar, P., Posada-de-la-Paz, M., Kanavos, P., et al. (2016). Social/economic costs and health-related quality of life in patients with scleroderma in Europe. The European Journal of Health Economics , 17 (Suppl 1), 109–117. https://doi.org/10.1007/s10198-016-0789-y
Koszorú, K., Hajdu, K., Brodszky, V., Bató, A., Gergely, L. H., Kovács, A., et al. (2023). Comparing the psychometric properties of the EQ-5D-3L and EQ-5D-5L descriptive systems and utilities in atopic dermatitis. The European Journal of Health Economics , 24 (1), 139–152. https://doi.org/10.1007/s10198-022-01460-y
Bató, A., Brodszky, V., Gergely, L. H., Gáspár, K., Wikonkál, N., Kinyó, Á., et al. (2021). The measurement performance of the EQ-5D-5L versus EQ-5D-3L in patients with hidradenitis suppurativa. Quality of Life Research , 30 (5), 1477–1490. https://doi.org/10.1007/s11136-020-02732-x
Poór, A. K., Rencz, F., Brodszky, V., Gulácsi, L., Beretzky, Z., Hidvégi, B., et al. (2017). Measurement properties of the EQ-5D-5L compared to the EQ-5D-3L in psoriasis patients. Quality of Life Research , 26 (12), 3409–3419. https://doi.org/10.1007/s11136-017-1699-x
Rencz, F., Lakatos, P. L., Gulácsi, L., Brodszky, V., Kürti, Z., Lovas, S., et al. (2019). Validity of the EQ-5D-5L and EQ-5D-3L in patients with Crohn’s disease. Quality of Life Research , 28 (1), 141–152. https://doi.org/10.1007/s11136-018-2003-4
World Medical Association (2013). Declaration Of Helsinki – Ethical Principles For Medical Research Involving Human Subjects. Retrieved November 30, 2022, from: https://www.wma.net/policies-post/wma-declaration-of-helsinki-ethical-principles-for-medical-research-involving-human-subjects/
StataCorp (2019). Stata Statistical Software: Release 16. College Station . StataCorp LLC.
Hungarian Central Statistical Office (2016). Healthy life expectancy at age of 65, by gender (2004-16). [in Hungarian] Retrieved, November 30, 2022, from: https://www.ksh.hu/docs/hun/eurostat_tablak/tabl/tsdph220.html
Nikl, A., Janssen, M. F., Brodszky, V., & Rencz, F. (2023). Hungarian population norms for the 15D generic preference-accompanied health status measure. Quality of Life Research , 14 . https://doi.org/10.1007/s11136-023-03514-x
Golicki, D., & Niewada, M. (2017). EQ-5D-5L Polish population norms. Arch Med Sci, 1;13(1):191–200. https://doi.org/10.5114/aoms.2015.52126
Zrubka, Z., Golicki, D., Prevolnik-Rupel, V., Baji, P., Rencz, F., Brodszky, V., et al. (2019). Towards a central-eastern European EQ-5D-3L population norm: Comparing data from Hungarian, Polish and Slovenian population studies. The European Journal of Health Economics , 20 (Suppl 1), 141–154. https://doi.org/10.1007/s10198-019-01071-0
Holzer, B. M., Siebenhuener, K., Bopp, M., & Minder, C. E. (2017). Evidence-based design recommendations for prevalence studies on multimorbidity: Improving comparability of estimates. Popul Health Metr , 15 (1), 9. https://doi.org/10.1186/s12963-017-0126-4
Hungarian Central Statistical Office (2019). Physical activities. [in Hungarian] Retrieved November 30, 2022, from: https://www.ksh.hu/docs/hun/xftp/idoszaki/elef/testmozgas_2019/testmozgas_2019.pdf
Hungarian Central Statistical Office (2019). Smoking habits. [in Hungarian] Retrieved November 30, 2022, from: https://www.ksh.hu/docs/hun/xftp/idoszaki/elef/dohanyzas_2019/dohanyzas_2019.pdf
Hungarian Central Statistical Office (2019). Health overview. [in Hungarian]. Retrieved November 30, 2022, from: https://www.ksh.hu/docs/hun/xftp/idoszaki/pdf/egeszsegugyi_helyzetkep_2019.pdf
Rencz, F., Ruzsa, G., Bató, A., Yang, Z., Finch, A. P., & Brodszky, V. (2022). Value Set for the EQ-5D-Y-3L in Hungary. Pharmacoeconomics , 20 , 1–11. https://doi.org/10.1007/s40273-022-01190-2
EuroQoL Group (2020). EQ-5D-Y User Guide. Recommended age range of users of the EQ-5D-Y version. Retrieved October 13, 2022, from: https://euroqol.org/publications/user-guides/
Potter, F., & Zeng, Y. (2015). Methods and Issues in Trimming Extreme Weights in Sample Surveys. In: Proceedings of the Joint Statistical Meetings 2015 Survey Research Methods Section. Retrieved March 8, 2024, from: http://www.asasrms.org/Proceedings/y2015/files/234115.pdf
Download references
Acknowledgements
Authors would like to acknowledge the work of employees of Hungarian Central Statistical Office, who designed and supervised the sampling and the data collection.
Project no. 2020 − 1.1.6-JÖVŐ-2021-00013 has been implemented with the support provided by the Ministry of Culture and Innovation of Hungary from the National Research, Development and Innovation Fund, financed under the 2020 − 1.1.6-JÖVŐ funding scheme. The funding source was not involved in study design; in the collection, analysis and interpretation of data; in the writing of the report; and in the decision to submit the article for publication.
Open access funding provided by Semmelweis University.
Author information
Authors and affiliations.
Center for Health Technology Assessment, Semmelweis University, Üllői út 25, Budapest, 1091, Hungary
András Inotai, Dávid Nagy, Zoltán Kaló & Zoltán Vokó
Syreon Research Institute, Mexikói út 65, Budapest, 1142, Hungary
Dávid Nagy, Zoltán Kaló & Zoltán Vokó
You can also search for this author in PubMed Google Scholar
Contributions
Conceptualization: ZV, AI. Funding acquisition: ZV. Formal analyses, methodology: ZV, DN. Visualization: AI. Validation: ZV, DN. Project administration: AI. Investigation: ZK, ZV, AI. Writing, original draft: AI. Writing, review and editing: ZK, ZV and DN. All authors read and approved the final manuscript.
Corresponding author
Correspondence to András Inotai .
Ethics declarations
Ethics approval and consent to participate.
The study protocol was approved by the Medical Research Council – Scientific and Ethical Committee in Hungary (number of ethical approval: IV/2292-1 2022/EKU), and the research was performed in accordance with the ethical standards of the 1964 Declaration of Helsinki. Written consent was obtained from each participant.
Consent for publication
Not applicable as only aggregated health data has been published.
Competing interests
Authors state that they have no conflict of interest.
Additional information
Publisher’s note.
Springer Nature remains neutral with regard to jurisdictional claims in published maps and institutional affiliations.
Electronic supplementary material
Below is the link to the electronic supplementary material.
Supplementary Material
Rights and permissions.
Open Access This article is licensed under a Creative Commons Attribution 4.0 International License, which permits use, sharing, adaptation, distribution and reproduction in any medium or format, as long as you give appropriate credit to the original author(s) and the source, provide a link to the Creative Commons licence, and indicate if changes were made. The images or other third party material in this article are included in the article’s Creative Commons licence, unless indicated otherwise in a credit line to the material. If material is not included in the article’s Creative Commons licence and your intended use is not permitted by statutory regulation or exceeds the permitted use, you will need to obtain permission directly from the copyright holder. To view a copy of this licence, visit http://creativecommons.org/licenses/by/4.0/ .
Reprints and permissions
About this article
Inotai, A., Nagy, D., Kaló, Z. et al. Population-level norm values by EQ-5D-3L in Hungary - a comparison of survey results from 2022 with those from 2000. Qual Life Res (2024). https://doi.org/10.1007/s11136-024-03699-9
Download citation
Accepted : 24 May 2024
Published : 05 June 2024
DOI : https://doi.org/10.1007/s11136-024-03699-9
Share this article
Anyone you share the following link with will be able to read this content:
Sorry, a shareable link is not currently available for this article.
Provided by the Springer Nature SharedIt content-sharing initiative
- Health-related quality of life
- Population survey
- Find a journal
- Publish with us
- Track your research

Federal Acquisition Regulation
Full far download in various formats, browse far part/subpart and download in various formats.
- Data Initiatives
- Regulations
- Smart Matrix
- Regulations Search
- Acquisition Regulation Comparator (ARC)
- Large Agencies
- Small Agencies
- CAOC History
- CAOC Charter
- Civilian Agency Acquisition Council (CAAC)
- Federal Acquisition Regulatory Council
- Interagency Suspension and Debarment Committee (ISDC)
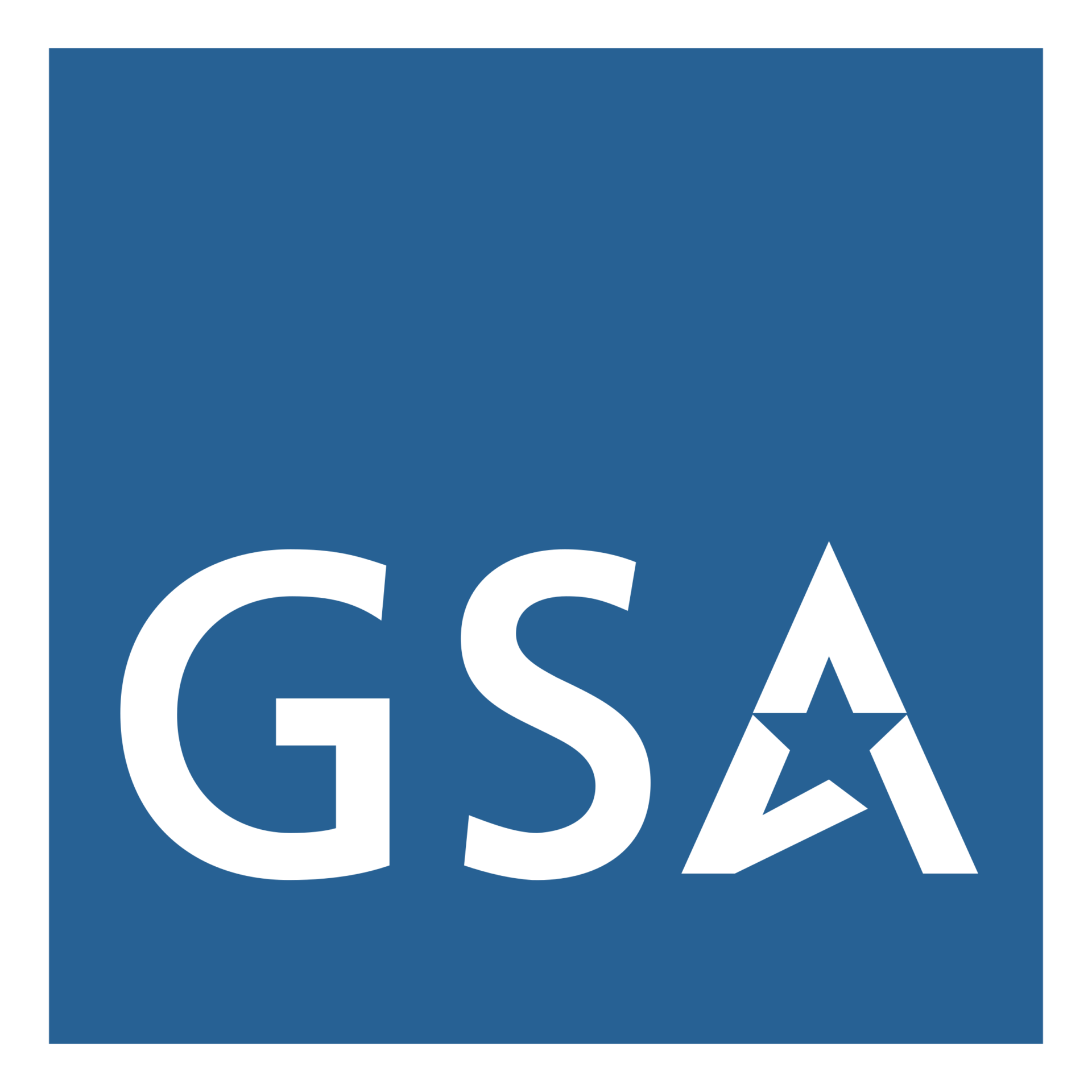
ACQUISITION.GOV
An official website of the General Services Administration
- Open access
- Published: 01 June 2024
Comprehensive knowledge of mother-to-child HIV/AIDS transmission, prevention, and associated factors among reproductive-age women in East Africa: insights from recent demographic and national health surveys
- Bewuketu Terefe 1 ,
- Mahlet Moges Jembere 2 &
- Bikis Liyew 2
BMC Women's Health volume 24 , Article number: 318 ( 2024 ) Cite this article
191 Accesses
1 Altmetric
Metrics details
More than 90% of babies acquire HIV/AIDS through vertical transmission, primarily due to low maternal comprehensive knowledge about Mother-To-Child Transmission (MTCT) of HIV/AIDS and its prevention, which is a cornerstone for eliminating MTCT of HIV/AIDS. However, there are limitations in terms of population data and literature evidence based on recent Demographic and Health Surveys (DHS) reports in East Africa. Therefore, this study aims to assess the comprehensive knowledge and PMTCT of HIV/AIDS among women, as well as the associated factors in East Africa.
Our data was obtained from the most recent DHS conducted in East African countries between 2011 and 2022. For our research, we included DHS data from ten nations, resulting in a total weighted sample of 133,724 women for our investigation. A generalized linear model (GLM) with a log link and binomial family to directly estimate prevalence ratios (PR) and 95% confidence intervals (CI) for the association between the independent variables, and the outcome variable. Finally, we reported the adjusted prevalence ratios along with their corresponding 95% CIs. Factors with p-values ≤ 0.2 for univariate logistic regression and < 0.05 were considered statistically significant factors of HIV/AIDS knowledge and prevention in the final model.
In this study, 59.41% (95% CI: 59.15–59.67) of respondents had a comprehensive knowledge about MTCT of HIV/AIDS and its prevention among reproductive-age women in East Africa. Being in the older age group, better education level, being from a rich household, employment status, having ANC follow up, institutional delivery, and modern contraception usage were associated with higher prevalence ratios of comprehensive knowledge about MTCT of HIV/AIDS and its prevention. However, being single in marital status, rural women, and traditional contraception utilization were associated with lower ratios of comprehensive knowledge about MTCT of HIV/AIDS and its prevention.
Our findings indicate a significant deficiency in comprehensive knowledge and prevention of HIV/AIDS MTCT among women in East Africa. These results emphasize the need for significant improvements in maternal-related health services. It is crucial to effectively target high-risk populations during interventions, raise awareness about this critical public health issue, and address the catastrophic consequences associated with MTCT. By implementing these measures, we can make substantial progress in reducing the transmission of HIV/AIDS from mother to child and ensuring better health outcomes for both mothers and their children.
Peer Review reports
Introduction
Vertical transmission of Human Immunodeficiency Virus (HIV) from mother to child during pregnancy, birth, and breast feeding remains a serious public health concern and is the leading source of HIV infection in children under the age of 15 worldwide [ 1 , 2 ]. Morbidity and mortality from HIV infection have declined globally over the last decade as a result of preventive measures such as greater coverage of Antiretroviral Therapy (ART) and prevention of HIV/AIDS transmission from mother to child (PMTCT) [ 3 , 4 ]. However, over 90% of new infections of HIV in babies and young children are transmitted from mother to child still [ 5 ]. In 2022, there was around 39 million HIV-positive people worldwide [ 6 ]. Among these, about 37.5 million, and 1.5 million were adults and children (15 and under), 53% were women and girls [ 6 , 7 ]. Similarly, the USAIDS, in 2023 estimated, more than 39 million individuals were infected with HIV, and lived with the virus [ 8 ]. Additionally, AIDS-related illnesses claimed the lives of almost 630 thousand people this year [ 8 ]. However, Eastern and Southern Africa making over half of that number [ 9 , 10 ]. Using the above references, it is obvious that the number of people getting infected with HIV is increasing over time, and rigorous research related to it is expected from various individuals and organizations [ 6 ].
According to the UNAIDS 2023 report, in terms of women and girls in 2022, women and girls of all ages accounted for 46% of all new HIV infections worldwide [ 11 ]. Women and girls (of all ages) accounted for 63% of all new HIV infections in Sub-Saharan Africa (SSA) [ 6 , 11 ]. In all other geographical regions, men and boys accounted for more than 70% of new HIV infections in 2022. In 2022, 4000 adolescent girls and young women aged 15–24 years would be infected with HIV per week over the world. SSA was responsible for 3100 of these illnesses [ 12 ].
In 2017, approximately 50% of the 180,000 new pediatric HIV infections occurred during breastfeeding, and it is estimated that in the absence of any intervention to prevent MTCT, the risk of transmission ranges from 15 to 45% (5–10% during pregnancy, 10–20% during childbirth, and 10–20% via mixed infant feeding) [ 13 ]. This rate, however, can be reduced to less than 5% with appropriate interventions [ 13 ]. The recent 2023 UNAIDS reports indicated that, each day, HIV infection affects 4,000 individuals, including 1,100 young people aged 15 to 24. If present patterns persist, it is projected that 1.2 million individuals will acquire HIV in 2025, which is three times higher than the targeted number of 370,000 new infections for that year [ 14 ].
Knowledge of MTCT and PMTCT for HIV/AIDS is associated with characteristics such as maternal age, maternal education, wealth level, occupation, marital status, media exposure, and domicile [ 15 , 16 , 17 , 18 , 19 , 20 ]. Maternal awareness of HIV/AIDS MTCT and prevention is essential for HIV MTCT elimination. Despite the fact that the majority of the population in SSA lives in rural areas with limited availability and accessibility of health facilities, the majority of studies on HIV/AIDS knowledge and prevention were conducted among available women, such as those who came to the health facility for their antenatal care follow up [ 17 , 21 , 22 , 23 , 24 , 25 ]. Since East Africa is the second most affected region by HIV/AIDS, women are the primary vulnerable group among the population in the region, and no current study has revealed the situation utilizing nationally representative data from recent DHS surveys that this study aims to investigate. Hence, studying women’s comprehensive knowledge about HIV/AIDS will help reduce stigma and discrimination, improve health outcomes for mothers and children, and decrease MTCT [ 26 ]. Furthermore, by understanding the factors involved, the findings of this study can provide valuable insights for policymakers, healthcare providers, and public health practitioners in East Africa. Therefore, using the recent national demographic health survey data, this study aimed to assess the comprehensive knowledge and PMTCT of HIV/AIDS among women, as well as its associated factors in East Africa.
Data sources and study population
Our data was obtained from the most recent Demographic and Health Surveys (DHS) conducted in East African countries between 2011 and 2022. This study included DHS data from 10 countries as shown in Table 1 . To conduct our research, we incorporated DHS data from these 10 nations using the corresponding Stata command. The survey utilized stratified, two-stage cluster sampling. In the first step, enumeration areas (EAs) were selected with a probability proportional to their size within each sampling stratum. Subsequently, households were sampled in the second step. The source population consisted of mothers of reproductive age. Consequently, classical logistic regression was deemed more appropriate. Ultimately, our study utilized a weighted sample of 133,724 women of reproductive age.
Data management and statistical analysis
Stata version 17 is used to extract, recode, and analyze data. Weighting was used throughout the study to ensure representativeness and non-response rate, as well as to obtain a suitable statistical estimate (robust standard error) [ 27 ]. In the univariate analysis, variables with a p-value of ≤ 0.2 were considered for the multivariable analysis. The multivariable logistic model provided the adjusted prevalence ratio (APR) with a 95% confidence interval to identify the associated factors of knowledge of PMTCT use. We used generalized linear models (GLM) with a log link and binomial family to directly estimate prevalence ratios (PR) and 95% confidence intervals (CI) for the association between the independent variables and the binary outcome of comprehensive knowledge of PMTCT. This approach allows for the estimation of PRs without the common issue of overestimation that can occur when using logistic regression to estimate odds ratios for common outcomes. We specified robust standard errors to account for potential heteroscedasticity in the model. The log-binomial GLM allowed us to directly estimate prevalence ratios, which are more readily interpretable than odds ratios for this cross-sectional study with a relatively common outcome. The use of robust standard errors ensures valid statistical inferences in the presence of any violation of model assumptions.
Since the data had a potential hierarchical structure, we assessed it to determine if multilevel model analysis could be conducted by calculating the intra-class correlation (ICC) coefficient. However, the ICC coefficient was found to be only approximately 1.7%, which did not meet the minimum criterion for conducting multilevel analysis. Descriptive data were summarized using measures such as frequency count and proportion for categorical variables. To examine multicollinearity among the independent variables, a logistic regression was fitted using the variance inflation factor. The Hosmer and Lemeshow test were also used to evaluate the overall fitness of the final regression model. The statistical significance for the final model was set at p < 0.05.
Variables of the study
The outcome variable.
The outcome variable of this study was the comprehensive knowledge of PMTCT among women of reproductive age. This outcome was measured using two percentages: the percentage of women who were aware that HIV can be transmitted from mother to child during pregnancy, delivery, and breastfeeding, and in all three ways; and the percentage of women who knew that the risk of mother-to-child transmission can be reduced by the mother taking special drugs. Women who responded “Yes” to both questions were considered knowledgeable about PMTCT, whereas those who missed either of them were classified as not knowledgeable. The study population included all women of reproductive age, specifically those aged 15–49 years old, as determined by the IR file, and the time period was defined by the current status at the time of the survey interview. The outcome variable was subsequently recategorized as “Yes = 1” if the women knew the correct answers to both questions, and “No = 0” if they missed either of them. All classifications and analyses were conducted following the guidelines provided in the DHS statistics book [ 28 ].
The independent variables
Independent variables: Various maternal-related factors were included, such as maternal age, educational status, types of places of residence, marital status, household wealth index, current employment status, mass media exposure, ANC follow-up, place of delivery, number of health visits in the past 12 months, under-five children, contraceptive utilization, distance to the health facility, knowledge of HIV/AIDS, sex of the household head, country, and breastfeeding status.
Sociodemographic characteristics of the study participant
In this study, a total weighted sample of 133,724 women of reproductive age were enrolled in East African countries. Nearly half of them, 53,712 (40.17%), fell within the 15–24 years age group. In terms of marital status, approximately half of the mothers, 66,037 (49.38%), were married. Regarding place of residence, educational status, wealth index, place of delivery, and ANC follow-up, the majority of mothers, 97,636 (73.01%), 97,637 (46.81%), 34,309 (25.66%), 120,494 (90.11%), and 129,855 (97.11%), respectively, were from rural areas, had primary educational status, belonged to the richest households, opted for institutional delivery, and had at least one ANC follow-up during their pregnancies. Similarly, approximately 67,551 (50.52%) and 79,879 (59.73%) of women did not have access to any form of mass media exposure (such as radio, television, or magazines/newspapers) and were unemployed, respectively. However, more than half of the mothers, 88,376 (66.09%), and 51,509 (38.52%), did not utilize any contraceptive methods and reported facing challenges related to the distance to the health facility. Furthermore, around 107,992 (80.76%) participants had only one health facility visit per year, and 93,094 (69.62%) reported having male household heads (Table 2 ).
Knowledge of women about PMTCT of HIV/AIDS
The overall comprehensive knowledge of PMTCT of HIV/AIDS was about 79,447(59.41%). The transmission of HIV/AIDS during pregnancy 110,349(82.52%), during delivery 120,735(90.29%), during breastfeeding 119,955(89.70%), and about a special drug to avoid HIV during pregnancy 108,782(81.35%) was replied correctly (Table 3 ).
Factors associated with comprehensive knowledge of PMTCT of HIV/AIDS among women in East Africa
The adjusted prevalence ratio (APR) of having comprehensive knowledge about PMTCT of HIV increased by 1.09 times (APR = 1.09, 95% CI: 1.07, 1.11) and 1.05 times (APR = 1.05, 95% CI: 1.03, 1.08) among women aged 25–34 years and 35–49 years, respectively, compared to women aged 15–24 years. Similarly, compared to participants with no education, mothers who had completed primary education and secondary/higher education had higher prevalence ratios of being knowledgeable about PMTCT of HIV, with prevalence ratios of 1.08 (APR = 1.08, 95% CI: 1.05, 1.10) and 1.06 (APR = 1.06, 95% CI: 1.03, 1.13) respectively. Regarding the household wealth index, mothers from middle, richer, and richest households showed higher ratios of having comprehensive knowledge of PMTCT of HIV compared to mothers from the poorest households, with prevalence ratios of 1.06 (APR = 1.06, 95% CI: 1.02, 1.11), (APR = 1.09, 95% CI: 1.04, 1.13), and (APR = 1.08, 95% CI: 1.05, 1.11) respectively. The prevalence ratio of comprehensive knowledge about HIV were 1.04 times higher among employed mothers (APR = 1.04, 95% CI: 1.03, 1.06) compared to unemployed mothers. The ratios of knowledge about HIV among married and divorced/widowed women were (APR = 1.19, 95% CI: 1.15, 1.26) and (APR = 1.16, 95% CI: 1.14, 1.19) times higher, respectively, when compared to never married women. Women who gave birth at health institutions had 1.25 times higher ratios of (APR = 1.25, 95% CI: 1.23, 1.28) of being knowledgeable about PMTCT of HIV compared to those who gave birth at home. Moreover, women who had at least one ANC visit showed more comprehensive knowledge about PMTCT, with a prevalence ratio of 1.22 (95% CI: 1.17, 1.27) compared to those who did not have an ANC visit. On the other hand, regarding contraceptive method types, mothers who utilized traditional methods had 0.13 times lower ratios (APR = 0.87, 95% CI: 0.84, 0.91), while those who used modern methods had 1.09 times higher ratios (APR = 1.09, 95% CI: 1.07, 1.10), of being knowledgeable about PMTCT of HIV compared to mothers who did not use any type of contraceptives. Finally, women from rural areas showed less comprehensive knowledge about PMTCT, with a prevalence ratio of 0.98 (95% CI: 0.97, 0.99) compared to urban residential women (Table 4 ).
The purpose of this study was to examine comprehensive knowledge regarding HIV/AIDS transmission from mother to child, as well as its prevention and associated factors, among reproductive-age women in East Africa using recent DHS data. In this survey, about 59.41% of respondents were comprehensively knowledgeable with HIV/AIDS MTCT and its prevention. This result is lower than in previous studies conducted in Zimbabwe [ 16 ], Tanzania [ 29 ], and Nigeria [ 30 ]. However, our study’s findings are slightly higher than those of research conducted in SSA [ 19 ], Ethiopia [ 17 ], and Uganda [ 31 ]. Firstly, the disparity may be due to the fact that the study conducted a pooled analysis that included data from multiple East African countries. Since each country may have different contexts, healthcare systems, and population characteristics, the combined analysis might have introduced variations in the results. Secondly, differences in the study time, sample size, outcome ascertainment criteria, approach of analysis, and the study population could contribute to the observed disparity. These methodological variations can influence the findings and interpretations. For example, if the studies were conducted at different time points, there could have been changes in healthcare policies, interventions, or awareness campaigns that could impact the knowledge levels about the specific topic being studied. Additionally, differences in sample sizes, criteria for determining the outcome, analytical approaches, and characteristics of the study population (e.g., age groups, socioeconomic status) can all introduce variations in the results. Overall, the observed disparity in the findings may be due to a combination of factors related to the diverse nature of the pooled analysis, as well as differences in study methodology and population characteristics. These factors need to be considered when interpreting and comparing the results of studies conducted in different settings or at different time points. In the multiple logistic regression analysis, older age, attendance at primary and secondary school, coming from a wealthy family, marital status, at least one ANC follow-up, institutional delivery, and contraception use were associated with a higher likelihood of knowing about HIV/AIDS MTCT and prevention.
The study found that older age groups had higher ratios of knowing about MTCT of HIV/AIDS and its prophylaxis than younger age groups (women aged 15–24 years). This is consistent with research conducted in SSA, Ethiopia, and Zimbabwe [ 16 , 19 , 20 ]. This could be linked to older women’s proximity to various maternal health services during each consecutive pregnancy. Furthermore, this could imply that initiatives to support younger women (adolescents) in raising HIV awareness, reducing MTCT, and promoting ART adherence and viral suppression are insufficient [ 13 ]. As a result, more attention should be placed on HIV/AIDS and MTCT ideas for those young moms in order to prevent HIV transmission from mother to child. The study’s findings regarding the association between age groups and knowledge about MTCT of HIV/AIDS align with the Social Cognitive Theory (SCT) proposed by Bandura (1986) [ 32 ]. According to SCT, individuals acquire knowledge and behavior through observational learning and social interactions. In this context, older women’s higher ratios of knowing about MTCT and its prophylaxis could be attributed to their increased exposure to maternal health services, which provide opportunities for information exchange and learning from healthcare professionals. This finding supports the notion that access to healthcare services and exposure to educational interventions play a crucial role in knowledge acquisition and behavior change. Furthermore, the paragraph suggests that the lack of sufficient initiatives targeting younger women, particularly adolescents, raises questions about the effectiveness of current interventions based on the Theory of Planned Behavior (TPB). According to TPB, individuals’ attitudes, subjective norms, and perceived behavioral control influence their intentions and subsequent behaviors [ 33 ].
Similarly, when compared to uneducated participants, women with primary and secondary/higher educational attainment had significantly higher likelihood of being knowledgeable about HIV PMTCT. This is consistent with prior research done elsewhere SSA [ 19 ], and Ethiopia [ 15 , 20 , 34 ]. This could be because educated women have better access to health-related information and can grasp HIV/AIDS and associated MTCT. The findings regarding the association between educational attainment and knowledge about HIV PMTCT align with several theoretical perspectives. One such framework is the Health Belief Model (HBM), which suggests that individuals’ health-related beliefs and perceptions influence their adoption of preventive behaviors. In this context, educated women may have a higher level of perceived susceptibility to HIV/AIDS and recognize the significance of PMTCT knowledge in protecting their own health and that of their children [ 35 , 36 , 37 ]. Education can also enhance their perceived benefits of adopting preventive measures, such as adhering to antiretroviral therapy and practicing safe delivery methods, leading to a higher likelihood of being knowledgeable about PMTCT [ 35 ]. Furthermore, the findings resonate with the Diffusion of Innovations theory, which posits that knowledge and new ideas are more readily adopted by individuals with higher education levels [ 36 , 38 ].
In terms of the household wealth index, and employment status, the current study discovered that mothers from the middle, richer, and richest households were more likely to have comprehensive knowledge of HIV PMTCT than mothers from the worst household wealth index, and unemployed mothers respectively. This is consistent with research undertaken in SSA [ 19 ], Ethiopia [ 15 ], and Tanzania [ 39 ]. The higher degree of awareness among women from well-off households could be attributed to their easy access to maternal health services such as PMTCT programs and mass media exposure. Employed mothers may have more social interaction and independence than unemployed mothers.
In terms of marital status, married and divorced/widowed women were more educated about HIV PMTCT than never married women. Women who were married or divorced were more likely to have comprehensive understanding about MTCT and its eradication. This conclusion is similar with findings from Rwanda [ 40 ], Nigeria [ 41 , 42 ], and Ethiopia [ 15 , 43 ]. The most obvious explanation is that married and divorced women obtain health information at health care centers during ANC visits and related family planning services [ 15 ]. Women who gave birth in health facilities, those who used modern contraception, and those who had ANC follow-up during their pregnancy periods had a higher likelihood of understanding HIV PMTCT than their counterparts. This could be because women who have a history of ANC follow-up may have the opportunity to learn from health experts, and this information may improve women’s knowledge of PMTCT. Similarly, women with a history of institutional delivery and contemporary contraception use may be eligible for PMTCT services from health experts at a health facility. This finding is similar to the findings of an Ethiopian investigation [ 18 , 44 ].
Women from rural areas in developing countries and Sub-Saharan Africa tend to exhibit lower comprehensive knowledge about Prevention of Mother-to-Child Transmission (PMTCT) of HIV compared to urban residential women. Research indicates that various factors influence this disparity in PMTCT knowledge among women in different settings. Studies have shown that women with access to mass media, formal education, and occupation are more likely to have correct knowledge of MTCT and PMTCT [ 15 , 45 ]. Urban areas often provide better access to health information and education through media and workplaces, contributing to higher knowledge levels among urban women. Women’s decision-making power, wealth index, and occupation type play a significant role in their PMTCT knowledge [ 46 , 47 ]. Women with decision-making power, manual occupations, and higher wealth status are more likely to have better PMTCT knowledge.
Factors like ANC follow-up and utilization of maternal health services are associated with higher PMTCT knowledge among women [ 45 , 48 ]. Women who engage in ANC services have increased opportunities to learn about PMTCT from health professionals. Rural residents face challenges in accessing PMTCT services due to limited infrastructure and media coverage, contributing to lower knowledge levels compared to urban areas [ 45 , 48 ]. Efforts are needed to intensify health education and PMTCT services in rural and emerging regions.
This study relied on nationally representative data, as well as adequate statistical analysis and a large number of factors. As a result, it can assist policymakers, as well as governmental and non-governmental groups, in making appropriate actions. However, the study had certain shortcomings. First, because it was based on survey data, some characteristics that may be related with the outcome variable, such as the quality and availability of health care and knowledge about HIV/AIDS, were not addressed. Second, because it is based on survey data, we are unable to demonstrate the temporal relationship between the result variable and the independent variables that were included. Furthermore, we used DHS from the preceding ten years, and there may have been changes in MTCT and ART regimen awareness, as well as ART uptake before to and during pregnancy (Option B+) over time. As a result, due to time constraints, caution is advised when interpreting study findings.
Conclusions, and implications
The study findings reveal that HIV/AIDS MTCT and preventive knowledge among reproductive-age women in East Africa is rated as low. However, certain factors were identified to be associated with a higher likelihood of knowledge about MTCT of HIV/AIDS and its prevention. These factors include older age, attending primary and secondary school, coming from a wealthy family and rural areas, being married, having at least one antenatal care (ANC) follow-up, opting for institutional delivery, and using contraception.
These findings have important implications for addressing the knowledge gap and improving the prevention of HIV/AIDS MTCT among reproductive-age women in East Africa. The study highlights the need for targeted interventions and educational programs that focus on improving knowledge and awareness of HIV/AIDS transmission and prevention methods. Specifically, efforts should be directed towards younger women, those with limited education, and those from lower socioeconomic backgrounds, as they are more likely to have lower levels of knowledge.
Furthermore, the study underscores the importance of ANC utilization and institutional delivery, as these factors were associated with higher knowledge levels. Strengthening and expanding ANC services, particularly in terms of HIV/AIDS education and counseling, can enhance women’s understanding of MTCT and its prevention. Similarly, promoting contraception use among reproductive-age women can serve as an additional avenue to disseminate information on MTCT prevention.
Policy makers, healthcare providers, and public health practitioners in East Africa should consider incorporating these findings into their strategies and interventions. By addressing the identified factors and tailoring interventions to the specific needs of different subgroups, it is possible to improve knowledge levels, reduce stigma and discrimination, enhance health outcomes for mothers and children, and ultimately reduce the incidence of HIV/AIDS MTCT in the region. As a result, it is preferable to prioritize high-risk populations during the intervention in order to raise awareness about this critical public health issue and address its catastrophic consequences. Improving maternal-related services such as ANC, institutional delivery, and family planning are examples of good possibilities for women to have a more thorough understanding of HIV/AIDS vertical transmission.
Data availability
All data concerning this study are accommodated and presented in this document. The detailed data set can be freely accessible from the www.dhsprogram.com website.
HIV/AIDS JUNPo. UNAIDS Report on the Global AIDS Epidemic. Geneva. World Health Organization; 2012.
Piot P, Quinn TC. Response to the AIDS pandemic—a global health model. N Engl J Med. 2013;368(23):2210–8.
Article CAS PubMed PubMed Central Google Scholar
Granich R, Gupta S, Hersh B, Williams B, Montaner J, Young B, Zuniga JM. Trends in AIDS deaths, new infections and ART coverage in the top 30 countries with the highest AIDS mortality burden; 1990–2013. PLoS ONE. 2015;10(7):e0131353.
Article PubMed PubMed Central Google Scholar
Organization WH. United Nations Programe on HIV/AIDS. Initiating second generation HIV surveillance systems: practical guidelines Ginebra. WHOS/UNAIDS; 2006.
Schouten EJ, Jahn A, Midiani D, Makombe SD, Mnthambala A, Chirwa Z, et al. Prevention of mother-to-child transmission of HIV and the health-related Millennium Development Goals: time for a public health approach. Lancet. 2011;378(9787):282–4.
Article PubMed Google Scholar
HIV JUNPo. World AIDS Day Report 2022: dangerous inequalities. UN; 2022.
Services UDHH. HIV and AIDS global statistics:The Global HIV and AIDS Epidemic: https://www.hiv.gov/hiv-basics/overview/data-and-trends/global-statistics/ . 2023.
USAIDS, THE PATH THAT ENDS. AIDS 2023 USAIDS Global AIDS Update: https://www.unaids.org/en . 2023.
Gelaw YA, Magalhães RJS, Assefa Y, Williams G. Spatial clustering and socio-demographic determinants of HIV infection in Ethiopia, 2015–2017. Int J Infect Dis. 2019;82:33–9.
Safer WHODMP, Organization WH. Counselling for maternal and newborn health care: a handbook for building skills. World Health Organization; 2010.
UNAIDS, Global HIV. & AIDS statistics — Fact sheet: https://www.unaids.org/en/resources/fact-sheet . 2023.
UNAIDS, Global HIV. & AIDS statistics — Fact sheet 2023: https://www.unaids.org/sites/default/files/media_asset/UNAIDS_FactSheet_en.pdf . 2023.
UNAIDS. Start Free stay free AIDS Free—2017 progress report. Switzerland: UNAIDS Geneva; 2017.
Google Scholar
UNAIDS G. IN DANGER: Global AIDS Update 2022: Joint United Nations Programme on HIV/ AIDS. 2022: https://www.unaids.org/en/resources/documents/2022/in-danger-global-aids-update . 2022.
Luba TR, Feng Z, Gebremedhin SA, Erena AN, Nasser AM, Bishwajit G, Tang S. Knowledge about mother–to–child transmission of HIV, its prevention and associated factors among Ethiopian women. J Global Health. 2017;7(2).
Masaka A, Dikeleko P, Moleta K, David M, Kaisara T, Rampheletswe F, Rwegerera GM. Determinants of comprehensive knowledge of mother to child transmission (MTCT) of HIV and its prevention among Zimbabwean women: analysis of 2015 Zimbabwe demographic and Health Survey. Alexandria J Med. 2019;55(1):68–75.
Article Google Scholar
Alemu YM, Habtewold TD, Alemu SM. Mother’s knowledge on prevention of mother-to-child transmission of HIV, Ethiopia: a cross sectional study. PLoS ONE. 2018;13(9):e0203043.
Liyeh TM, Cherkose EA, Limenih MA, Yimer TS, Tebeje HD. Knowledge of prevention of mother to child transmission of HIV among women of reproductive age group and associated factors at Mecha district, Northwest Ethiopia. BMC Res Notes. 2020;13(1):1–6.
Teshale AB, Tessema ZT, Alem AZ, Yeshaw Y, Liyew AM, Alamneh TS, et al. Knowledge about mother to child transmission of HIV/AIDS, its prevention and associated factors among reproductive-age women in sub-saharan Africa: evidence from 33 countries recent demographic and health surveys. PLoS ONE. 2021;16(6):e0253164.
Terefe B, Techane MA, Assimamaw NT. Comprehensive knowledge, attitudes, behaviors, and associated factors of HIV/AIDS in Gondar City Public Health Facilities Among HIV Testing and Counselling Service Users, Northwest Ethiopia, 2022; an ordinal logistic regression analysis. HIV/AIDS-Research and Palliative Care. 2023:713 – 26.
Nyarko V, Pencille L, Akoku DA, Tarkang EE. Knowledge, attitudes and practices regarding the prevention of mother-to-child transmission of HIV among pregnant women in the Bosome Freho District in the Ashanti region of Ghana: a descriptive cross-sectional design. PAMJ-Clinical Med. 2019;1(69).
Mukhtar M, Quansar R, Bhat SN, Khan S. Knowledge, attitude and practice regarding mother-to-child transmission of HIV, its prevention, and associated factors among antenatal women attending a health care facility in district Srinagar, North India: a cross sectional study. Int J Community Med Public Health. 2020;7(7):2622.
Abebe AM, Kassaw MW, Shewangashaw NE. Level of knowledge about prevention of mother-to-child transmission of HIV option B + and associated factors among ANC clients in Kombolcha Town, South Wollo Amhara Regional State, Ethiopia, 2017. HIV/AIDS-Research and Palliative Care. 2020:79–86.
Abiodun MO, Munir’deen AI, Aboyeji PA. Awareness and knowledge of mother-to-child transmission of HIV among pregnant women. J Natl Med Assoc. 2007;99(7):758.
PubMed PubMed Central Google Scholar
Terefe B, Mekonnen BA, Tamir TT, Assimamaw NT, Limenih MA. Evaluation of Quality of Prevention of Mother to Child Transmission of HIV Service Provision and its determinants: the case of Health Facility readiness and mothers’ perspectives. J Multidisciplinary Healthc. 2024:93–110.
Terefe B, Jembere MM. Discrimination against HIV/AIDS patients and associated factors among women in east African countries: using the most recent DHS data (2015–2022). J Health Popul Nutr. 2024;43(1):3.
Elkasabi M. Sampling and Weighting with DHS Data: https://blog.dhsprogram.com/sampling-weighting-at-dhs/ . 2015.
Croft T, Marshall AM, Allen CK, Arnold F, Assaf S, Balian S, et al. Guide to DHS statistics: DHS-7 (version 2). Rockville, MD: ICF; 2020.
Wangwe P, Nyasinde M, Charles D. Counselling at primary health facilities and level of knowledge of antenatal attendees and their attitude on prevention of mother to child transmission of HIV in Dar-Es Salaam, Tanzania. Afr Health Sci. 2013;13(4):914–9.
Ashimi A, Omole-Ohonsi A, Amole T, Ugwa E. Pregnant women’s knowledge and attitude to mother to child transmission of human immuno-deficiency virus in a rural community in Northwest Nigeria. West Afr J Med. 2014;33(1):68–73.
CAS PubMed Google Scholar
Byamugisha R, Tumwine JK, Ndeezi G, Karamagi CA, Tylleskär T. Attitudes to routine HIV counselling and testing, and knowledge about prevention of mother to child transmission of HIV in eastern Uganda: a cross-sectional survey among antenatal attendees. J Int AIDS Soc. 2010;13(1):1–11.
Bandura A. Social foundations of thought and action. Englewood Cliffs NJ. 1986;1986:23–8.
Conner M, Armitage CJ. Extending the theory of planned behavior: a review and avenues for further research. J Appl Soc Psychol. 1998;28(15):1429–64.
Malaju MT, Alene GD. Determinant factors of pregnant mothers’ knowledge on mother to child transmission of HIV and its prevention in Gondar town, North West Ethiopia. BMC Pregnancy Childbirth. 2012;12(1):1–7.
Strecher VJ, Champion VL, Rosenstock IM. The health belief model and health behavior. 1997.
Jeihooni AK, Arameshfard S, Hatami M, Mansourian M, Kashfi SH, Rastegarimehr B, et al. The effect of educational program based on health belief model about HIV/AIDS among high school students. Int J Pediatrics-Mashhad. 2018;6(3):7285–96.
Tarkang EE, Zotor FB. Application of the health belief model (HBM) in HIV prevention: a literature review. Cent Afr J Public Health. 2015;1(1):1–8.
Bertrand JT. Diffusion of innovations and HIV/AIDS. J Health Communication. 2004;9(S1):113–21.
Haile ZT, Teweldeberhan AK, Chertok IR. Correlates of women’s knowledge of mother-to-child transmission of HIV and its prevention in Tanzania: a population-based study. AIDS Care. 2016;28(1):70–8.
Deynu M, Nutor JJ. Determinants of comprehensive knowledge on mother-to-child transmission of HIV and its prevention among childbearing women in Rwanda: insights from the 2020 Rwandan demographic and Health Survey. BMC Public Health. 2023;23(1):1–14.
Olopha PO, Fasoranbaku AO, Gayawan E. Spatial pattern and determinants of sufficient knowledge of mother to child transmission of HIV and its prevention among Nigerian women. PLoS ONE. 2021;16(6):e0253705.
Olugbenga-Bello A, Adebimpe W, Osundina F, Abdulsalam S. Perception on prevention of mother-to-child-transmission (PMTCT) of HIV among women of reproductive age group in Osogbo, Southwestern Nigeria. Int J Women’s Health. 2013:399–405.
Abtew S, Awoke W, Asrat A. Knowledge of pregnant women on mother-to-child transmission of HIV, its prevention, and associated factors in Assosa town, Northwest Ethiopia. HIV/AIDS-Research and Palliative Care. 2016:101-7.
Abajobir AA, Zeleke AB. Knowledge, attitude, practice and factors associated with prevention of mother-to-child transmission of HIV/AIDS among pregnant mothers attending antenatal clinic in Hawassa referral hospital, South Ethiopia. J Aids Clin Res. 2013;4(6):2–7.
Liyeh TM, Cherkose EA, Limenih MA, Yimer TS, Tebeje HD. Knowledge of prevention of mother to child transmission of HIV among women of reproductive age group and associated factors at Mecha district, Northwest Ethiopia. BMC Res Notes. 2020;13:1–6.
Zegeye B, Ahinkorah BO, Ameyaw EK, Seidu A-A, Olorunsaiye CZ, Yaya S. Women’s decision-making power and knowledge of prevention of mother to child transmission of HIV in sub-saharan Africa. BMC Womens Health. 2022;22(1):115.
Sama C-B, Feteh VF, Tindong M, Tanyi JT, Bihle NM, Angwafo FF III. Prevalence of maternal HIV infection and knowledge on mother–to–child transmission of HIV and its prevention among antenatal care attendees in a rural area in northwest Cameroon. PLoS ONE. 2017;12(2):e0172102.
Deynu M, Nutor JJ. Determinants of comprehensive knowledge on mother-to-child transmission of HIV and its prevention among childbearing women in Rwanda: insights from the 2020 Rwandan demographic and Health Survey. BMC Public Health. 2023;23(1):5.
Download references
Acknowledgements
We would like to acknowledge the DHS program for providing permission for this study following research ethics.
This study was not supported financially by anyone.
Author information
Authors and affiliations.
Department of Community Health Nursing, School of Nursing, College of Medicine and Health Sciences, University of Gondar, Gondar, Ethiopia
Bewuketu Terefe
Department of Emergency and Critical Care Nursing, School of Nursing, College of Medicine and Health Sciences, University of Gondar, Gondar, Ethiopia
Mahlet Moges Jembere & Bikis Liyew
You can also search for this author in PubMed Google Scholar
Contributions
BT was involved in conceptualization, design, data extraction, statistical analysis, language editing, and original manuscript writing. MMJ reviewed the study’s design and the draft manuscript, checked the analysis, and made a significant contribution. BL data interpretation, data curation, article review, and validation, critical revision for intellectual substance, and article review. The authors approved the final version of the manuscript.
Corresponding author
Correspondence to Bewuketu Terefe .
Ethics declarations
Ethics approval and consent to participate.
The study was conducted after obtaining a permission letter from www.dhsprogram.com on an online request to access East African DHS data after reviewing the submitted brief descriptions of the survey to the DHS program. The datasets were treated with the utmost confidence. This study was done based on secondary data from East Africa DHS. Issues related to informed consent, confidentiality, anonymity, and privacy of the study participants are already done ethically by the DHS office. We did not manipulate and apply the microdata other than in this study. There was no patient or public involvement in this study.
Consent for publication
Not applicable.
Competing interests
The authors declare no competing interests.
Additional information
Publisher’s note.
Springer Nature remains neutral with regard to jurisdictional claims in published maps and institutional affiliations.
Rights and permissions
Open Access This article is licensed under a Creative Commons Attribution 4.0 International License, which permits use, sharing, adaptation, distribution and reproduction in any medium or format, as long as you give appropriate credit to the original author(s) and the source, provide a link to the Creative Commons licence, and indicate if changes were made. The images or other third party material in this article are included in the article’s Creative Commons licence, unless indicated otherwise in a credit line to the material. If material is not included in the article’s Creative Commons licence and your intended use is not permitted by statutory regulation or exceeds the permitted use, you will need to obtain permission directly from the copyright holder. To view a copy of this licence, visit http://creativecommons.org/licenses/by/4.0/ . The Creative Commons Public Domain Dedication waiver ( http://creativecommons.org/publicdomain/zero/1.0/ ) applies to the data made available in this article, unless otherwise stated in a credit line to the data.
Reprints and permissions
About this article
Cite this article.
Terefe, B., Jembere, M.M. & Liyew, B. Comprehensive knowledge of mother-to-child HIV/AIDS transmission, prevention, and associated factors among reproductive-age women in East Africa: insights from recent demographic and national health surveys. BMC Women's Health 24 , 318 (2024). https://doi.org/10.1186/s12905-024-03173-1
Download citation
Received : 26 August 2023
Accepted : 29 May 2024
Published : 01 June 2024
DOI : https://doi.org/10.1186/s12905-024-03173-1
Share this article
Anyone you share the following link with will be able to read this content:
Sorry, a shareable link is not currently available for this article.
Provided by the Springer Nature SharedIt content-sharing initiative
- Comprehensive knowledge
- Mother-to-child transmission
- HIV/AIDS prevention
- Reproductive-age women
- East Africa
- Associated factors
BMC Women's Health
ISSN: 1472-6874
- Submission enquiries: [email protected]
- General enquiries: [email protected]
The state of AI in early 2024: Gen AI adoption spikes and starts to generate value
If 2023 was the year the world discovered generative AI (gen AI) , 2024 is the year organizations truly began using—and deriving business value from—this new technology. In the latest McKinsey Global Survey on AI, 65 percent of respondents report that their organizations are regularly using gen AI, nearly double the percentage from our previous survey just ten months ago. Respondents’ expectations for gen AI’s impact remain as high as they were last year , with three-quarters predicting that gen AI will lead to significant or disruptive change in their industries in the years ahead.
About the authors
This article is a collaborative effort by Alex Singla , Alexander Sukharevsky , Lareina Yee , and Michael Chui , with Bryce Hall , representing views from QuantumBlack, AI by McKinsey, and McKinsey Digital.
Organizations are already seeing material benefits from gen AI use, reporting both cost decreases and revenue jumps in the business units deploying the technology. The survey also provides insights into the kinds of risks presented by gen AI—most notably, inaccuracy—as well as the emerging practices of top performers to mitigate those challenges and capture value.
AI adoption surges
Interest in generative AI has also brightened the spotlight on a broader set of AI capabilities. For the past six years, AI adoption by respondents’ organizations has hovered at about 50 percent. This year, the survey finds that adoption has jumped to 72 percent (Exhibit 1). And the interest is truly global in scope. Our 2023 survey found that AI adoption did not reach 66 percent in any region; however, this year more than two-thirds of respondents in nearly every region say their organizations are using AI. 1 Organizations based in Central and South America are the exception, with 58 percent of respondents working for organizations based in Central and South America reporting AI adoption. Looking by industry, the biggest increase in adoption can be found in professional services. 2 Includes respondents working for organizations focused on human resources, legal services, management consulting, market research, R&D, tax preparation, and training.
Also, responses suggest that companies are now using AI in more parts of the business. Half of respondents say their organizations have adopted AI in two or more business functions, up from less than a third of respondents in 2023 (Exhibit 2).
Gen AI adoption is most common in the functions where it can create the most value
Most respondents now report that their organizations—and they as individuals—are using gen AI. Sixty-five percent of respondents say their organizations are regularly using gen AI in at least one business function, up from one-third last year. The average organization using gen AI is doing so in two functions, most often in marketing and sales and in product and service development—two functions in which previous research determined that gen AI adoption could generate the most value 3 “ The economic potential of generative AI: The next productivity frontier ,” McKinsey, June 14, 2023. —as well as in IT (Exhibit 3). The biggest increase from 2023 is found in marketing and sales, where reported adoption has more than doubled. Yet across functions, only two use cases, both within marketing and sales, are reported by 15 percent or more of respondents.
Gen AI also is weaving its way into respondents’ personal lives. Compared with 2023, respondents are much more likely to be using gen AI at work and even more likely to be using gen AI both at work and in their personal lives (Exhibit 4). The survey finds upticks in gen AI use across all regions, with the largest increases in Asia–Pacific and Greater China. Respondents at the highest seniority levels, meanwhile, show larger jumps in the use of gen Al tools for work and outside of work compared with their midlevel-management peers. Looking at specific industries, respondents working in energy and materials and in professional services report the largest increase in gen AI use.
Investments in gen AI and analytical AI are beginning to create value
The latest survey also shows how different industries are budgeting for gen AI. Responses suggest that, in many industries, organizations are about equally as likely to be investing more than 5 percent of their digital budgets in gen AI as they are in nongenerative, analytical-AI solutions (Exhibit 5). Yet in most industries, larger shares of respondents report that their organizations spend more than 20 percent on analytical AI than on gen AI. Looking ahead, most respondents—67 percent—expect their organizations to invest more in AI over the next three years.
Where are those investments paying off? For the first time, our latest survey explored the value created by gen AI use by business function. The function in which the largest share of respondents report seeing cost decreases is human resources. Respondents most commonly report meaningful revenue increases (of more than 5 percent) in supply chain and inventory management (Exhibit 6). For analytical AI, respondents most often report seeing cost benefits in service operations—in line with what we found last year —as well as meaningful revenue increases from AI use in marketing and sales.
Inaccuracy: The most recognized and experienced risk of gen AI use
As businesses begin to see the benefits of gen AI, they’re also recognizing the diverse risks associated with the technology. These can range from data management risks such as data privacy, bias, or intellectual property (IP) infringement to model management risks, which tend to focus on inaccurate output or lack of explainability. A third big risk category is security and incorrect use.
Respondents to the latest survey are more likely than they were last year to say their organizations consider inaccuracy and IP infringement to be relevant to their use of gen AI, and about half continue to view cybersecurity as a risk (Exhibit 7).
Conversely, respondents are less likely than they were last year to say their organizations consider workforce and labor displacement to be relevant risks and are not increasing efforts to mitigate them.
In fact, inaccuracy— which can affect use cases across the gen AI value chain , ranging from customer journeys and summarization to coding and creative content—is the only risk that respondents are significantly more likely than last year to say their organizations are actively working to mitigate.
Some organizations have already experienced negative consequences from the use of gen AI, with 44 percent of respondents saying their organizations have experienced at least one consequence (Exhibit 8). Respondents most often report inaccuracy as a risk that has affected their organizations, followed by cybersecurity and explainability.
Our previous research has found that there are several elements of governance that can help in scaling gen AI use responsibly, yet few respondents report having these risk-related practices in place. 4 “ Implementing generative AI with speed and safety ,” McKinsey Quarterly , March 13, 2024. For example, just 18 percent say their organizations have an enterprise-wide council or board with the authority to make decisions involving responsible AI governance, and only one-third say gen AI risk awareness and risk mitigation controls are required skill sets for technical talent.
Bringing gen AI capabilities to bear
The latest survey also sought to understand how, and how quickly, organizations are deploying these new gen AI tools. We have found three archetypes for implementing gen AI solutions : takers use off-the-shelf, publicly available solutions; shapers customize those tools with proprietary data and systems; and makers develop their own foundation models from scratch. 5 “ Technology’s generational moment with generative AI: A CIO and CTO guide ,” McKinsey, July 11, 2023. Across most industries, the survey results suggest that organizations are finding off-the-shelf offerings applicable to their business needs—though many are pursuing opportunities to customize models or even develop their own (Exhibit 9). About half of reported gen AI uses within respondents’ business functions are utilizing off-the-shelf, publicly available models or tools, with little or no customization. Respondents in energy and materials, technology, and media and telecommunications are more likely to report significant customization or tuning of publicly available models or developing their own proprietary models to address specific business needs.
Respondents most often report that their organizations required one to four months from the start of a project to put gen AI into production, though the time it takes varies by business function (Exhibit 10). It also depends upon the approach for acquiring those capabilities. Not surprisingly, reported uses of highly customized or proprietary models are 1.5 times more likely than off-the-shelf, publicly available models to take five months or more to implement.
Gen AI high performers are excelling despite facing challenges
Gen AI is a new technology, and organizations are still early in the journey of pursuing its opportunities and scaling it across functions. So it’s little surprise that only a small subset of respondents (46 out of 876) report that a meaningful share of their organizations’ EBIT can be attributed to their deployment of gen AI. Still, these gen AI leaders are worth examining closely. These, after all, are the early movers, who already attribute more than 10 percent of their organizations’ EBIT to their use of gen AI. Forty-two percent of these high performers say more than 20 percent of their EBIT is attributable to their use of nongenerative, analytical AI, and they span industries and regions—though most are at organizations with less than $1 billion in annual revenue. The AI-related practices at these organizations can offer guidance to those looking to create value from gen AI adoption at their own organizations.
To start, gen AI high performers are using gen AI in more business functions—an average of three functions, while others average two. They, like other organizations, are most likely to use gen AI in marketing and sales and product or service development, but they’re much more likely than others to use gen AI solutions in risk, legal, and compliance; in strategy and corporate finance; and in supply chain and inventory management. They’re more than three times as likely as others to be using gen AI in activities ranging from processing of accounting documents and risk assessment to R&D testing and pricing and promotions. While, overall, about half of reported gen AI applications within business functions are utilizing publicly available models or tools, gen AI high performers are less likely to use those off-the-shelf options than to either implement significantly customized versions of those tools or to develop their own proprietary foundation models.
What else are these high performers doing differently? For one thing, they are paying more attention to gen-AI-related risks. Perhaps because they are further along on their journeys, they are more likely than others to say their organizations have experienced every negative consequence from gen AI we asked about, from cybersecurity and personal privacy to explainability and IP infringement. Given that, they are more likely than others to report that their organizations consider those risks, as well as regulatory compliance, environmental impacts, and political stability, to be relevant to their gen AI use, and they say they take steps to mitigate more risks than others do.
Gen AI high performers are also much more likely to say their organizations follow a set of risk-related best practices (Exhibit 11). For example, they are nearly twice as likely as others to involve the legal function and embed risk reviews early on in the development of gen AI solutions—that is, to “ shift left .” They’re also much more likely than others to employ a wide range of other best practices, from strategy-related practices to those related to scaling.
In addition to experiencing the risks of gen AI adoption, high performers have encountered other challenges that can serve as warnings to others (Exhibit 12). Seventy percent say they have experienced difficulties with data, including defining processes for data governance, developing the ability to quickly integrate data into AI models, and an insufficient amount of training data, highlighting the essential role that data play in capturing value. High performers are also more likely than others to report experiencing challenges with their operating models, such as implementing agile ways of working and effective sprint performance management.
About the research
The online survey was in the field from February 22 to March 5, 2024, and garnered responses from 1,363 participants representing the full range of regions, industries, company sizes, functional specialties, and tenures. Of those respondents, 981 said their organizations had adopted AI in at least one business function, and 878 said their organizations were regularly using gen AI in at least one function. To adjust for differences in response rates, the data are weighted by the contribution of each respondent’s nation to global GDP.
Alex Singla and Alexander Sukharevsky are global coleaders of QuantumBlack, AI by McKinsey, and senior partners in McKinsey’s Chicago and London offices, respectively; Lareina Yee is a senior partner in the Bay Area office, where Michael Chui , a McKinsey Global Institute partner, is a partner; and Bryce Hall is an associate partner in the Washington, DC, office.
They wish to thank Kaitlin Noe, Larry Kanter, Mallika Jhamb, and Shinjini Srivastava for their contributions to this work.
This article was edited by Heather Hanselman, a senior editor in McKinsey’s Atlanta office.
Explore a career with us
Related articles.

Moving past gen AI’s honeymoon phase: Seven hard truths for CIOs to get from pilot to scale
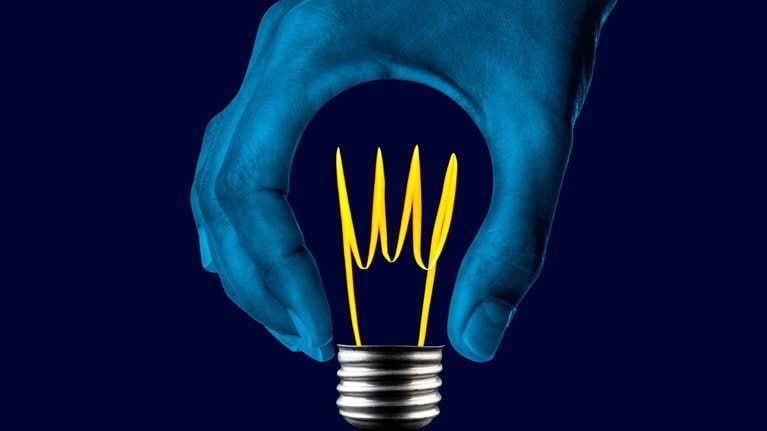
A generative AI reset: Rewiring to turn potential into value in 2024

Implementing generative AI with speed and safety

An official website of the United States government
The .gov means it’s official. Federal government websites often end in .gov or .mil. Before sharing sensitive information, make sure you’re on a federal government site.
The site is secure. The https:// ensures that you are connecting to the official website and that any information you provide is encrypted and transmitted securely.
- Publications
- Account settings
Preview improvements coming to the PMC website in October 2024. Learn More or Try it out now .
- Advanced Search
- Journal List
- Dental Press J Orthod
- v.19(4); Jul-Aug 2014
Language: English | Portuguese
How sample size influences research outcomes
Jorge faber.
1 Adjunct professor, Department of Orthodontics, University of Brasília.
Lilian Martins Fonseca
2 Invited Professor, Department of Orthodontics, University of Brasília.
Sample size calculation is part of the early stages of conducting an epidemiological, clinical or lab study. In preparing a scientific paper, there are ethical and methodological indications for its use. Two investigations conducted with the same methodology and achieving equivalent results, but different only in terms of sample size, may point the researcher in different directions when it comes to making clinical decisions. Therefore, ideally, samples should not be small and, contrary to what one might think, should not be excessive. The aim of this paper is to discuss in clinical language the main implications of the sample size when interpreting a study.
O cálculo amostral faz parte dos estágios iniciais de realização de um estudo epidemiológico, clínico ou laboratorial. Há indicações éticas e metodológicas para o seu emprego na elaboração de um trabalho científico. Duas pesquisas, realizadas com a mesma metodologia obtendo resultados equivalentes, e que diferem apenas no tamanho da amostra, podem apontar para diferentes direções no processo de tomada de decisão clínica. Portanto, as amostras estudadas idealmente não devem ser pequenas e, ao contrário do que pode-se pensar, não devem ser excessivas. O objetivo desse artigo é discutir, numa linguagem clínica, as principais implicações do tamanho das amostras na interpretação de um estudo.
In recent years a growing concern has overwhelmed the scientific community in the healthcare area: Sample size calculation. Although at first blush it may seem like an overriding concern over methodological issues, notably to clinicians, such concern is utterly justifiable. This issue is of paramount importance.
Samples should not be either too big or too small since both have limitations that can compromise the conclusions drawn from the studies. Too small a sample may prevent the findings from being extrapolated, whereas too large a sample may amplify the detection of differences, emphasizing statistical differences that are not clinically relevant. 1 We will discuss in this article the major impacts of sample size on orthodontic studies.
FACTORS THAT AFFECT SAMPLE SIZE
The purpose of estimating the appropriate sample size is to produce studies capable of detecting clinically relevant differences. Bearing this point in mind, there are different formulas to calculate sample size. 2 , 3 These formulas comprise several aspects which are listed below. Most sample size calculators available on the web have limited validity because they use a single formula - which is usually not divulged - to generate sample sizes for the studies.
The first aspect is the type of variable being studied. For example, it should be determined if the variable is categorical like the Angle classification (Class I, II or III), or continuous like the length of the dental arch (usually measured in millimeters).
It is then necessary to determine the relationship between the groups that will be evaluated and the statistical analysis that will be employed. Are we going to evaluate groups that are independent, i.e., the measurements of one group do not influence the other? Are they dependent groups like the measurements taken before and after treatment? Are we going to use a split-mouth design, whereby treatment is performed on one quadrant and a different therapy on another quadrant? Will we be using t-test or chi-square test? All these questions lead to different sample size calculation formulas.
Subsequently, we have to answer the question concerning which results we envisage if a standard treatment is performed. What is the mean value or the expected ratio? The answer to this question is usually obtained from the literature or by means of pilot studies.
It is also important to determine what is the smallest magnitude of the effect and the extent to which it is clinically relevant. For example, how many degrees of difference in the ANB angle can be considered relevant? It is vital that we address this issue. The smaller the difference that we wish to identify, the greater the number of cases in a study. If researchers wish to detect a difference as small as 0.1° in an ANB angle, they will probably need thousands of patients in their study. If this value rises to 1°, the number of cases required falls drastically.
Finally, it is essential that the researcher determine the level of significance and the type II error, which is the probability of not rejecting the null hypothesis, although the hypothesis is actually false, which the study will accept as reasonable.
With this information in hand, we will apply the appropriate formula according to the study design in question, and determine the sample size. Today, this calculation is typically carried out with the aid of a computer program. For example, Pocock's formula 2 for continuous variables is frequently used in our specialty. It is used in studies where one wishes to examine the difference between data means with normal distribution and equal-size, independent groups.
PROBLEMS WITH VERY SMALL SAMPLES
Try to envision the following scenario. A researcher conducts a study on patients who are being treated with a new device which although very uncomfortable has the potential to improve treatment of Class II malocclusions. The researcher wishes to compare the new functional device with the Herbst appliance. Patients will be randomly assigned to each group. The researcher is not aware, but we are, that s/he needs 60 subjects (30 patients in each group) to ensure sufficient power to be able to extrapolate the statistical analysis results to the overall population. In other words, so that we can feel confident that these results will serve as a parameter on which to base the proposed treatment. Furthermore, we also know, although the researcher does not, that this new therapy is less effective than the traditional method.
However, the researcher used only 15 patients in each group. The results of the study showed that the new device is inferior to conventional treatment. What are the implications?
The first is that using a sample smaller than the ideal increases the chance of assuming as true a false premise. Thus, chances are that the proposed device has no disadvantage compared to traditional therapy. Furthermore, it is assumed that people were subjected to a study, and had to undergo in vain all additional suffering associated with the therapy, given that the goals of the study were not achieved. In addition, financial and time resources were squandered since ultimately it will contribute absolutely nothing to improve clinical practice or quality of life. The situation becomes even worse if the research involves public funding: A total waste of taxpayer money.
PROBLEMS WITH VERY LARGE SAMPLES
There is a widespread belief that large samples are ideal for research or statistical analysis. However, this is not always true. Using the above example as a case study, very large samples that exceed the value estimated by sample size calculation present different hurdles.
The first is ethical. Should a study be performed with more patients than necessary? This means that more people than needed are exposed to the new therapy. Potentially, this implies increased hassle and risk. Obviously the problem is compounded if the new protocol is inferior to the traditional method: More patients are involved in a new, uncomfortable therapy that yields inferior results.
The second obstacle is that the use of a larger number of cases can also involve more financial and human resources than necessary to obtain the desired response.
In addition to these factors, there is another noteworthy issue that has to do with statistics. Statistical tests were developed to handle samples, not populations. When numerous cases are included in the statistics, analysis power is substantially increased. This implies an exaggerated tendency to reject null hypotheses with clinically negligible differences. What is insignificant becomes significant. Thus, a potential statistically significant difference in the ANB angle of 0.1° between the groups cited in the previous example would obviously produce no clinical difference in the effects of wearing an appliance.
When very large samples are available in a retrospective study, the researcher needs first to collect subsamples randomly, and only then perform the statistical test. If it is a prospective study, the researcher should collect only what is necessary, and include a few more individuals to compensate for subjects that leave the study.
CONCLUSIONS
In designing a study, sample size calculation is important for methodological and ethical reasons, as well as for reasons of human and financial resources. When reading an article, the reader should be on the alert to ascertain that the study they are reading was subjected to sample size calculation. In the absence of this calculation, the findings of the study should be interpreted with caution.
An appropriate sample renders the research more efficient: Data generated are reliable, resource investment is as limited as possible, while conforming to ethical principles. The use of sample size calculation directly influences research findings. Very small samples undermine the internal and external validity of a study. Very large samples tend to transform small differences into statistically significant differences - even when they are clinically insignificant. As a result, both researchers and clinicians are misguided, which may lead to failure in treatment decisions.
How to cite this article: Faber J, Fonseca LM. How sample size influences research outcomes. Dental Press J Orthod. 2014 July-Aug;19(4):27-9. DOI: http://dx.doi.org/10.1590/2176-9451.19.4.027-029.ebo

IMAGES
VIDEO
COMMENTS
As a standard parameter, a reasonable sample size falls between 200 and 400 (Hair et al., 2014). A desired sample-to-variable ratio of 15:1 or 20:1 is suitable for establishing sample size (Hair ...
For education surveys, we recommend getting a statistically significant sample size that represents the population.If you're planning on making changes in your school based on feedback from students about the institution, instructors, teachers, etc., a statistically significant sample size will help you get results to lead your school to success.
3) Plan for a sample that meets your needs and considers your real-life constraints. Every research project operates within certain boundaries - commonly budget, timeline and the nature of the sample itself. When deciding on your sample size, these factors need to be taken into consideration.
Sample size is a term used in market research to define the number of subjects included in a survey, study, or experiment. In surveys with large populations, sample size is incredibly important. The reason for this is because it's unrealistic to get answers or results from everyone - instead, you can take a random sample of individuals that ...
Determining the right sample size for your survey is one of the most common questions researchers ask when they begin a market research study. Luckily, sample size determination isn't as hard to calculate as you might remember from an old high school statistics class. Before calculating your sample size, ensure you have these things in place:
Unlock the secrets of figuring out sample size. Learn why finding the correct sample size is critical for accurate research outcomes. Explore step-by-step methods for calculating sample size for confidence intervals and hypothesis testing. Dive deep into factors influencing sample size determination and gain insights into real-life applications ...
Stage 2: Calculate sample size. Now that you've got answers for steps 1 - 4, you're ready to calculate the sample size you need. This can be done using an online sample size calculator or with paper and pencil. 1. Find your Z-score. Next, you need to turn your confidence level into a Z-score.
This part's easy! Just divide the number you got from Step #3 by the number you got from Step #4. That's your magic number. So, for example, if you need 100 women who use shampoo to fill out your survey and you think about 10% of these shampoo-using women that you send the survey to will actually fill it out, then you need to send it to 100 ...
Determining Sample Size for Controlled Surveys. Sample size formulas are based on probability sampling techniques—methods that randomly select people from the population to participate in a survey. For most market surveys and academic studies, however, researchers do not use probability sampling methods.
The same source states that the maximum number of respondents should be 10% of your population, but it should not exceed 1000. For instance, if you have a population of 250,000, 10% would be 25,000. Since this is higher than 1,000, a sample size of 1,000 should be enough to get you statistically significant results.
Predominantly, the sample size should be determined based on statistical analysis. 2, 21, 22 The type of analysis should be closely related to the study design, study objective, research question(s), or primary research outcome. Most sample size calculation software packages include the option to select the required statistical test related to ...
This free sample size calculator determines the sample size required to meet a given set of constraints. Also, learn more about population standard deviation. ... It is an important aspect of any empirical study requiring that inferences be made about a population based on a sample. Essentially, sample sizes are used to represent parts of a ...
In the initial stage of planning a research study, sample size calcu-lation—or power calculation—answers the question, "How many participants or observations need to be included in this study?" If the sample size is low, the research outcome might not be reproduc - ible.1 Informal guidelines for sample size based on the experience
The sample size for a study needs to be estimated at the time the study is proposed; too large a sample is unnecessary and unethical, and too small a sample is unscientific and also unethical. The necessary sample size can be calculated, using statistical software, based on certain assumptions. If no assumptions can be made, then an arbitrary ...
It is the ability of the test to detect a difference in the sample, when it exists in the target population. Calculated as 1-Beta. The greater the power, the larger the required sample size will be. A value between 80%-90% is usually used. Relationship between non-exposed/exposed groups in the sample.
So there was no uniform answer to the question and the ranges varied according to methodology. In fact, Shaw and Holland (2014) claim, sample size will largely depend on the method. (p. 87), "In truth," they write, "many decisions about sample size are made on the basis of resources, purpose of the research" among other factors. (p. 87).
Sample size estimations for the Passing-Bablok and Deming method comparison studies are exemplified in Table 7 and Table 8 respectively. As seen in these tables, sample size estimations are based on slope, analytical precision (% CV), and range ratio (c) value (66, 67). These tables might seem quite complicated for some researchers that are not ...
The bottom line is, you need to survey a lot of people before you can start having any confidence in your results. Bibliography. This webpage calculates the sample size required for a desired confidence interval, or the confidence interval for a given sample size: Creative Research Systems, 2003.
Based on Statistical Power: This parameter is the power that is need from the s tudy. As power ... Appropriate Sample Size in Survey Research. Learning and Performance Journal, 19, 43-50.
Survey research means collecting information about a group of people by asking them questions and analyzing the results. To conduct an effective survey, follow these six steps: ... Based on this question, you need to determine exactly who you will target to participate in the survey. ... The sample size will be smaller, ...
In order to make up for a rough estimate of 20.0% of non-response rate, the minimum sample size requirement is calculated to be 254 patients (i.e. 203/0.8) by estimating the sample size based on the EPV 50, and is calculated to be 375 patients (i.e. 300/0.8) by estimating the sample size based on the formula n = 100 + 50i.
Abstract. An underlying assumption of most church -based research is that the. sample from which the data is collected is representative of a wider. collection of church members, church leaders ...
The thesis covers two fundamental topics that are important across the disciplines of operations research, statistics and even more broadly, namely stochastic optimization and uncertainty quantification, with the common theme to address both statistical accuracy and computational constraints. Here, statistical accuracy encompasses the precision of estimated solutions in stochastic optimization ...
Stage 2: Calculate sample size. Now that you've got answers for steps 1 - 4, you're ready to calculate the sample size you need. This can be done using the online sample size calculator above or with paper and pencil. 5. Find your Z-score. Next, you need to turn your confidence level into a Z-score.
Purpose Although population norms of the EQ-5D-3L instrument had been available in Hungary since 2000, their evaluation was based on a United Kingdom (UK) value set. Our objective was to estimate the population norms for EQ-5D-3L by using the new Hungarian value set available since 2020, to extend the scope to adolescents, and to compare with norms from 2000. Methods A cross sectional EQ-5D-3L ...
FAC Number Effective Date HTML DITA PDF Word EPub Apple Books Kindle; 2024-05: 05/22/2024
To conduct our research, we incorporated DHS data from these 10 nations using the corresponding Stata command. The survey utilized stratified, two-stage cluster sampling. In the first step, enumeration areas (EAs) were selected with a probability proportional to their size within each sampling stratum.
If 2023 was the year the world discovered generative AI (gen AI), 2024 is the year organizations truly began using—and deriving business value from—this new technology. In the latest McKinsey Global Survey on AI, 65 percent of respondents report that their organizations are regularly using gen AI, nearly double the percentage from our ...
An appropriate sample renders the research more efficient: Data generated are reliable, resource investment is as limited as possible, while conforming to ethical principles. The use of sample size calculation directly influences research findings. Very small samples undermine the internal and external validity of a study.