Attention Span
- Reference work entry
- Cite this reference work entry
- Elizabeth Levin 3 &
- Jennifer Bernier 3
1701 Accesses
1 Citations
Sustained attention ; Vigilance
Attention span refers to an individual’s ability to attend to a stimulus or object over a period of time. This ability is also known as sustained attention or vigilance.

Description
Attention includes a number of components, one of which, attention span, is the ability to maintain focus and alertness over a period of time. Sustained attention requires persistence and motivation [ 2 ]. Thus, individuals with short attention spans may appear to give up or not put sufficient effort into tasks. Attention span increases with age, and is related to, and plays a role in other aspects of functioning including learning, memory, academic performance, and the understanding and processing of large quantities of information [ 1 , 3 ].
Research has shown that a child’s sustained attention develops in a linear fashion until the age of four, but then undergoes a dramatic increase between the ages of 4 and 6 years [ 1 ]. Between the ages of 7 and 8 years...
This is a preview of subscription content, log in via an institution to check access.
Access this chapter
- Available as PDF
- Read on any device
- Instant download
- Own it forever
- Available as EPUB and PDF
- Durable hardcover edition
- Dispatched in 3 to 5 business days
- Free shipping worldwide - see info
Tax calculation will be finalised at checkout
Purchases are for personal use only
Institutional subscriptions
Barkley, R. A. (1997). Behavioral inhibition, sustained attention, and executive function: Constructing a unified theory of ADHD. Psychological Bulletin, 121 (1), 65–94.
PubMed Google Scholar
Bennett Murphy, L., Murphy, E. C., & Rose, C. L. (2001). Sustained attention and unintentional injury among preschool-aged children. Child Neuropsychology, 7 (2), 72–83.
Betts, J., Mckay, J., Maruff, P., & Anderson, V. (2006). The Development of sustained attention in children: The effect of age and task load. Child Neuropsychology, 12 , 205–221.
Christakis, D. A., Zimmerman, F. J., DiGiuseppe, D. L., & McCarty, C. A. (2004). Early television exposure and subsequent attention problems in children. Pediatrics, 113 , 708–713.
DeShazo Barry, T., Grofer Klinger, L., Lyman, R. D., Bush, D., & Hawkins, L. (2001). Visual selective attention versus sustained attention in boys with Attention-Deficit/Hyperactivity Disorder. Journal of Attention Disorders, 4 (4), 193–202.
Google Scholar
Rose, C. L., Murphy, L. B., Schickedantz, B., & Tucci, J. (2001). The effects of event rate and signal probability on children’s vigilance. Journal of Clinical and Experimental Neuropsychology, 23 (2), 215–224.
Ruff, H. A., & Capozzoli, M. C. (2003). Development of attention and distractibility in the first 4 years of life. Developmental Psychology, 39 (5), 877–890.
Download references
Author information
Authors and affiliations.
Department of Psychology, Laurentian University, Ramsey Lake Road, Sudbury, ON, P3E2S9, Canada
Elizabeth Levin & Jennifer Bernier
You can also search for this author in PubMed Google Scholar
Editor information
Editors and affiliations.
Neurology, Learning and Behavior Center, 230 South 500 East, Suite 100, Salt Lake City, Utah, 84102, USA
Sam Goldstein Ph.D.
Department of Psychology MS 2C6, George Mason University, Fairfax, VA, 22030, USA
Jack A. Naglieri Ph.D. ( Professor of Psychology ) ( Professor of Psychology )
Rights and permissions
Reprints and permissions
Copyright information
© 2011 Springer Science+Business Media, LLC
About this entry
Cite this entry.
Levin, E., Bernier, J. (2011). Attention Span. In: Goldstein, S., Naglieri, J.A. (eds) Encyclopedia of Child Behavior and Development. Springer, Boston, MA. https://doi.org/10.1007/978-0-387-79061-9_226
Download citation
DOI : https://doi.org/10.1007/978-0-387-79061-9_226
Publisher Name : Springer, Boston, MA
Print ISBN : 978-0-387-77579-1
Online ISBN : 978-0-387-79061-9
eBook Packages : Behavioral Science
Share this entry
Anyone you share the following link with will be able to read this content:
Sorry, a shareable link is not currently available for this article.
Provided by the Springer Nature SharedIt content-sharing initiative
- Publish with us
Policies and ethics
- Find a journal
- Track your research
Thank you for visiting nature.com. You are using a browser version with limited support for CSS. To obtain the best experience, we recommend you use a more up to date browser (or turn off compatibility mode in Internet Explorer). In the meantime, to ensure continued support, we are displaying the site without styles and JavaScript.
- View all journals
- Explore content
- About the journal
- Publish with us
- Sign up for alerts
- Published: 03 March 2022
A brain-based general measure of attention
- Kwangsun Yoo ORCID: orcid.org/0000-0002-5213-4575 1 ,
- Monica D. Rosenberg ORCID: orcid.org/0000-0001-6179-4025 1 , 2 ,
- Young Hye Kwon ORCID: orcid.org/0000-0001-7754-4223 1 ,
- Qi Lin ORCID: orcid.org/0000-0001-9702-8584 1 ,
- Emily W. Avery ORCID: orcid.org/0000-0002-8481-3978 1 ,
- Dustin Sheinost ORCID: orcid.org/0000-0002-6301-1167 3 ,
- R. Todd Constable ORCID: orcid.org/0000-0001-5661-9521 3 , 4 , 5 &
- Marvin M. Chun ORCID: orcid.org/0000-0003-1070-7993 1 , 4 , 6 , 7
Nature Human Behaviour volume 6 , pages 782–795 ( 2022 ) Cite this article
4689 Accesses
11 Citations
75 Altmetric
Metrics details
- Network models
Attention is central to many aspects of cognition, but there is no singular neural measure of a person’s overall attentional functioning across tasks. Here, using original data from 92 participants performing three different attention-demanding tasks during functional magnetic resonance imaging, we constructed a suite of whole-brain models that can predict a profile of multiple attentional components (sustained attention, divided attention and tracking, and working memory capacity) for novel individuals. Multiple brain regions across the salience, subcortical and frontoparietal networks drove accurate predictions, supporting a common (general) attention factor across tasks, distinguished from task-specific ones. Furthermore, connectome-to-connectome transformation modelling generated an individual’s task-related connectomes from rest functional magnetic resonance imaging, substantially improving predictive power. Finally, combining the connectome transformation and general attention factor, we built a standardized measure that shows superior generalization across four independent datasets (total N = 495) of various attentional measures, suggesting broad utility for research and clinical applications.
This is a preview of subscription content, access via your institution
Access options
Access Nature and 54 other Nature Portfolio journals
Get Nature+, our best-value online-access subscription
24,99 € / 30 days
cancel any time
Subscribe to this journal
Receive 12 digital issues and online access to articles
111,21 € per year
only 9,27 € per issue
Buy this article
- Purchase on Springer Link
- Instant access to full article PDF
Prices may be subject to local taxes which are calculated during checkout
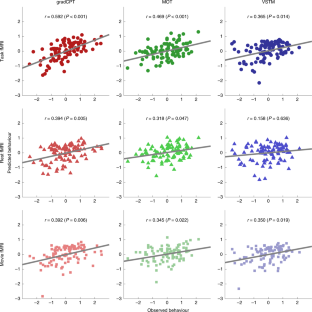
Similar content being viewed by others
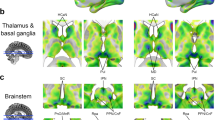
The subcortical and neurochemical organization of the ventral and dorsal attention networks
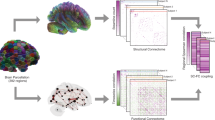
Heritability and interindividual variability of regional structure-function coupling
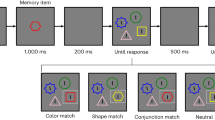
The dynamic process of hyperfocusing and hyperfiltering in schizophrenia
Data availability.
Raw task and rest fMRI data used in the primary analyses ( n = 92) are available at https://doi.org/10.15154/1520622 .
Code availability
Scripts for the predictive model (the general attention model, C2C model and CPM) construction are available for download at https://github.com/rayksyoo/General_Attention . Scripts for the other (statistical) analyses are available from the corresponding author upon request.
Chun, M. M., Golomb, J. D. & Turk-Browne, N. B. A taxonomy of external and internal attention. Annu. Rev. Psychol. 62 , 73–101 (2011).
Article PubMed Google Scholar
Weissman, D. H., Roberts, K. C., Visscher, K. M. & Woldorff, M. G. The neural bases of momentary lapses in attention. Nat. Neurosci. 9 , 971–978 (2006).
Article CAS PubMed Google Scholar
Heinrichs, R. W. & Zakzanis, K. K. Neurocognitive deficit in schizophrenia: a quantitative review of the evidence. Neuropsychology 12 , 426–445 (1998).
Biederman, J., Newcorn, J. & Sprich, S. Comorbidity of attention deficit hyperactivity disorder with conduct, depressive, anxiety, and other disorders. Am. J. Psychiatry 148 , 564–577 (1991).
Levin, H. S. et al. Neurobehavioral outcome following minor head injury: a three-center study. J. Neurosurg. 66 , 234–243 (1987).
Rosenberg, M. D. et al. Functional connectivity predicts changes in attention observed across minutes, days, and months. Proc. Natl Acad. Sci. U. S. A. 117 , 3797–3807 (2020).
Article CAS PubMed PubMed Central Google Scholar
Kucyi, A. et al. Prediction of stimulus-independent and task-unrelated thought from functional brain networks. Nat. Commun. 12 , 1793 (2021).
Deary, I. J., Penke, L. & Johnson, W. The neuroscience of human intelligence differences. Nat. Rev. Neurosci. 11 , 201–211 (2010).
Miyake, A. et al. The unity and diversity of executive functions and their contributions to complex ‘frontal lobe’ tasks: a latent variable analysis. Cogn. Psychol. 41 , 49–100 (2000).
Huang, L., Mo, L. & Li, Y. Measuring the interrelations among multiple paradigms of visual attention: an individual differences approach. J. Exp. Psychol. Hum. Percept. Perform. 38 , 414–428 (2012).
Corbetta, M. & Shulman, G. L. Control of goal-directed and stimulus-driven attention in the brain. Nat. Rev. Neurosci. 3 , 215–229 (2002).
Article CAS Google Scholar
Kanwisher, N. & Wojciulik, E. Visual attention: insights from brain imaging. Nat. Rev. Neurosci. 1 , 91–100 (2000).
Rosenberg, M. D., Finn, E. S., Scheinost, D., Constable, R. T. & Chun, M. M. Characterizing attention with predictive network models. Trends Cogn. Sci. 21 , 290–302 (2017).
Rosenberg, M. D. et al. A neuromarker of sustained attention from whole-brain functional connectivity. Nat. Neurosci. 19 , 165–171 (2016).
Wu, E. X. W. et al. Overlapping attentional networks yield divergent behavioral predictions across tasks: neuromarkers for diffuse and focused attention? Neuroimage 209 , 116535 (2020).
Kucyi, A., Hove, M. J., Esterman, M., Hutchison, R. M. & Valera, E. M. Dynamic brain network correlates of spontaneous fluctuations in attention. Cereb. Cortex 27 , 1831–1840 (2017).
PubMed Google Scholar
Shen, X. et al. Using connectome-based predictive modeling to predict individual behavior from brain connectivity. Nat. Protoc. 12 , 506–518 (2017).
Finn, E. S. et al. Functional connectome fingerprinting: identifying individuals using patterns of brain connectivity. Nat. Neurosci. 18 , 1664–1671 (2015).
Woo, C. W., Chang, L. J., Lindquist, M. A. & Wager, T. D. Building better biomarkers: brain models in translational neuroimaging. Nat. Neurosci. 20 , 365–377 (2017).
Gratton, C. et al. Defining individual-specific functional neuroanatomy for precision psychiatry. Biol. Psychiatry 88 , 28–39 (2020).
Cohen, J. R. & D’Esposito, M. The segregation and integration of distinct brain networks and their relationship to cognition. J. Neurosci. 36 , 12083–12094 (2016).
Yoo, K. et al. Multivariate approaches improve the reliability and validity of functional connectivity and prediction of individual behaviors. Neuroimage 197 , 212–223 (2019).
Rosenberg, M. D. et al. Methylphenidate modulates functional network connectivity to enhance attention. J. Neurosci. 36 , 9547–9557 (2016).
Rosenberg, M. D., Hsu, W.-T., Scheinost, D., Todd Constable, R. & Chun, M. M. Connectome-based models predict separable components of attention in novel individuals. J. Cogn. Neurosci. 30 , 160–173 (2018).
Yoo, K. et al. Connectome-based predictive modeling of attention: comparing different functional connectivity features and prediction methods across datasets. Neuroimage 167 , 11–22 (2018).
Lin, Q. et al. Resting-state functional connectivity predicts cognitive impairment related to Alzheimer’s disease. Front. Aging Neurosci. 10 , 94 (2018).
Article PubMed PubMed Central Google Scholar
Avery, E. W. et al. Distributed patterns of functional connectivity predict working memory performance in novel healthy and memory-impaired individuals. J. Cogn. Neurosci. 32 , 241–255 (2019).
Zhang, H. et al . Do intrinsic brain functional networks predict working memory from childhood to adulthood? Hum. Brain Mapp . https://doi.org/10.1002/hbm.25143 (2020).
Tomasi, D. & Volkow, N. D. Network connectivity predicts language processing in healthy adults. Hum. Brain Mapp. 41 , 3696–3708 (2020).
Beaty, R. E. et al. Robust prediction of individual creative ability from brain functional connectivity. Proc. Natl Acad. Sci. U. S. A. 115 , 1087–1092 (2018).
Hsu, W.-T., Rosenberg, M. D., Scheinost, D., Constable, R. T. & Chun, M. M. Resting-state functional connectivity predicts neuroticism and extraversion in novel individuals. Soc. Cogn. Affect. Neurosci. 13 , 224–232 (2018).
Jiang, R. et al. Connectome-based individualized prediction of temperament trait scores. Neuroimage 183 , 366–374 (2018).
Cai, H., Chen, J., Liu, S., Zhu, J. & Yu, Y. Brain functional connectome-based prediction of individual decision impulsivity. Cortex 125 , 288–298 (2020).
Esterman, M., Noonan, S. K., Rosenberg, M. & Degutis, J. In the zone or zoning out? Tracking behavioral and neural fluctuations during sustained attention. Cereb. Cortex 23 , 2712–2723 (2013).
Fan, J., McCandliss, B. D., Fossella, J., Flombaum, J. I. & Posner, M. I. The activation of attentional networks. Neuroimage 26 , 471–479 (2005).
Kardan, O. et al . Adult neuromarkers of sustained attention and working memory predict inter- and intra-individual differences in these processes in youth. Preprint at bioRxiv https://doi.org/10.1101/2021.08.01.454530 (2021).
Engle, R. W. Working memory capacity as executive attention. Curr. Dir. Psychol. Sci. 11 , 19–23 (2002).
Article Google Scholar
Yoo, K. et al . A cognitive state transformation model for task-general and task-specific subsystems of the brain connectome. Preprint at bioRxiv https://doi.org/10.1101/2020.12.23.424176 (2020).
Noble, S. et al. Influences on the test-retest reliability of functional connectivity MRI and its relationship with behavioral utility. Cereb. Cortex 27 , 5415–5429 (2017).
Varoquaux, G. Cross-validation failure: small sample sizes lead to large error bars. NeuroImage 180 , 68–77 (2018).
Jangraw, D. C. et al. A functional connectivity-based neuromarker of sustained attention generalizes to predict recall in a reading task. Neuroimage 166 , 99–109 (2018).
Fountain-Zaragoza, S., Samimy, S., Rosenberg, M. D. & Prakash, R. S. Connectome-based models predict attentional control in aging adults. Neuroimage 186 , 1–13 (2019).
Van Essen, D. C. et al. The WU-Minn Human Connectome Project: an overview. Neuroimage 80 , 62–79 (2013).
DuPaul, G. J., Power, T. J., Anastopoulos, A. D. & Reid, R. ADHD Rating Scale—IV: Checklists, Norms, and Clinical Interpretation (Guilford, 1998).
Consortium, T. A.-200. The ADHD-200 Consortium: a model to advance the translational potential of neuroimaging in clinical neuroscience. Front. Syst. Neurosci. 6 , 62 (2012).
Google Scholar
Satterthwaite, T. D. et al. Neuroimaging of the Philadelphia neurodevelopmental cohort. NeuroImage 86 , 544–553 (2014).
Casey, B. J. et al. The adolescent brain cognitive development (ABCD) study: imaging acquisition across 21 sites. Dev. Cogn. Neurosci. 32 , 43–54 (2018).
Wojciulik, E. & Kanwisher, N. The generality of parietal involvement in visual attention. Neuron 23 , 747–764 (1999).
Duncan, J. & Owen, A. M. Common regions of the human frontal lobe recruited by diverse cognitive demands. Trends Neurosci. 23 , 475–483 (2000).
Ramnani, N. & Owen, A. M. Anterior prefrontal cortex: insights into function from anatomy and neuroimaging. Nat. Rev. Neurosci. 5 , 184–194 (2004).
Miller, E. K. & Cohen, J. D. An integrative theory of prefrontal cortex function. Annu. Rev. Neurosci. 24 , 167–202 (2001).
Pardo, J. V., Fox, P. T. & Raichle, M. E. Localization of a human system for sustained attention by positron emission tomography. Nature 349 , 61–64 (1991).
Corbetta, M., Shulman, G. L., Miezin, F. M. & Petersen, S. E. Superior parietal cortex activation during spatial attention shifts and visual feature conjunction. Sci. (80-.) 270 , 802–805 (1995).
Hopfinger, J. B., Buonocore, M. H. & Mangun, G. R. The neural mechanisms of top-down attentional control. Nat. Neurosci. 3 , 284–291 (2000).
Sprague, T. C. & Serences, J. T. Attention modulates spatial priority maps in the human occipital, parietal and frontal cortices. Nat. Neurosci. 16 , 1879–1887 (2013).
Wimmer, R. D. et al. Thalamic control of sensory selection in divided attention. Nature 526 , 705–709 (2015).
Heinze, H. J. et al. Combined spatial and temporal imaging of brain activity during visual selective attention in humans. Nature 372 , 543–546 (1994).
Coull, J. T., Vidal, F., Nazarian, B. & Macar, F. Functional anatomy of the attentional modulation of time estimation. Sci. (80-.) 303 , 1506–1508 (2004).
Gao, J. H. et al. Cerebellum implicated in sensory acquisition and discrimination rather than motor control. Sci. (80-.). 272 , 545–547 (1996).
Leiner, H. C., Leiner, A. L. & Dow, R. S. Does the cerebellum contribute to mental skills? Behav. Neurosci. 100 , 443–454 (1986).
Petersen, S. E., Fox, P. T., Posner, M. I., Mintun, M. & Raichle, M. E. Positron emission tomographic studies of the processing of single words. J. Cogn. Neurosci. 1 , 153–170 (1989).
Stoodley, C. J. The cerebellum and cognition: evidence from functional imaging studies. Cerebellum 11 , 352–365 (2012).
Strick, P. L., Dum, R. P. & Fiez, J. A. Cerebellum and nonmotor function. Annu. Rev. Neurosci. 32 , 413–434 (2009).
Buckner, R. L. The cerebellum and cognitive function: 25 years of insight from anatomy and neuroimaging. Neuron 80 , 807–815 (2013).
Allen, G., Buxton, R. B., Wong, E. C. & Courchesne, E. Attentional activation of the cerebellum independent of motor involvement. Sci. (80-.) 275 , 1940–1943 (1997).
Rees, G., Frackowiak, R. & Frith, C. Two modulatory effects of attention that mediate object categorization in human cortex. Sci. (80-.) 275 , 835–838 (1997).
Gottwald, B., Mihajlovic, Z., Wilde, B. & Mehdorn, H. M. Does the cerebellum contribute to specific aspects of attention? Neuropsychologia 41 , 1452–1460 (2003).
Greene, A. S., Gao, S., Scheinost, D. & Constable, R. T. Task-induced brain state manipulation improves prediction of individual traits. Nat. Commun. 9 , 2807 (2018).
Article PubMed PubMed Central CAS Google Scholar
Jiang, R. et al. Task-induced brain connectivity promotes the detection of individual differences in brain–behavior relationships. Neuroimage 207 , 116370 (2020).
Sui, J., Jiang, R., Bustillo, J. & Calhoun, V. Neuroimaging-based individualized prediction of cognition and behavior for mental disorders and health: methods and promises. Biol. Psychiatry 88 , 818–828 (2020).
Gao, S., Greene, A. S., Constable, R. T. & Scheinost, D. Combining multiple connectomes improves predictive modeling of phenotypic measures. Neuroimage 201 , 116038 (2019).
Pujol, J. et al. Clinical application of functional magnetic resonance imaging in presurgical identification of the central sulcus. J. Neurosurg. 88 , 863–869 (1998).
Bullmore, E. The future of functional MRI in clinical medicine. Neuroimage 62 , 1267–1271 (2012).
Vanderwal, T., Kelly, C., Eilbott, J., Mayes, L. C. & Castellanos, F. X. Inscapes: a movie paradigm to improve compliance in functional magnetic resonance imaging. Neuroimage 122 , 222–232 (2015).
Rosenberg, M., Noonan, S., DeGutis, J. & Esterman, M. Sustaining visual attention in the face of distraction: a novel gradual-onset continuous performance task. Atten. Percept. Psychophys. 75 , 426–439 (2013).
Pylyshyn, Z. W. & Storm, R. W. Tracking multiple independent targets: evidence for a parallel tracking mechanism. Spat. Vis. 3 , 179–197 (1988).
Luck, S. J. & Vogel, E. K. The capacity of visual working memory for features and conjunctions. Nature 390 , 279–284 (1997).
Pashler, H. Familiarity and visual change detection. Percept. Psychophys. 44 , 369–378 (1988).
Rouder, J. N., Morey, R. D., Morey, C. C. & Cowan, N. How to measure working memory capacity in the change detection paradigm. Psychon. Bull. Rev. 18 , 324–330 (2011).
Cox, R. W. AFNI: software for analysis and visualization of functional magnetic resonance neuroimages. Comput. Biomed. Res. 29 , 162–173 (1996).
Shen, X., Tokoglu, F., Papademetris, X. & Constable, R. T. Groupwise whole-brain parcellation from resting-state fMRI data for network node identification. Neuroimage 82 , 403–415 (2013).
Scheinost, D. et al. Ten simple rules for predictive modeling of individual differences in neuroimaging. Neuroimage 193 , 35–45 (2019).
Nichols, T. E. & Holmes, A. P. Nonparametric permutation tests for functional neuroimaging: a primer with examples. Hum. Brain Mapp. 15 , 1–25 (2002).
Abbas, K. et al. GEFF: graph embedding for functional fingerprinting. Neuroimage 221 , 117181 (2020).
Amico, E. & Goñi, J. The quest for identifiability in human functional connectomes. Sci. Rep. 8 , 8254 (2018).
Barch, D. M. et al. NeuroImage function in the human connectome: task-fMRI and individual differences in behavior. Neuroimage 80 , 169–189 (2013).
Smith, S. M. et al. Resting-state fMRI in the Human Connectome Project. Neuroimage 80 , 144–168 (2013).
Download references
Acknowledgements
This project was supported by National Institutes of Health grant MH108591 to M.M.C. and by National Science Foundation grant BCS1558497 to M.M.C.
Author information
Authors and affiliations.
Department of Psychology, Yale University, New Haven, CT, USA
Kwangsun Yoo, Monica D. Rosenberg, Young Hye Kwon, Qi Lin, Emily W. Avery & Marvin M. Chun
Department of Psychology, University of Chicago, Chicago, IL, USA
Monica D. Rosenberg
Department of Radiology and Biomedical Imaging, Yale School of Medicine, New Haven, CT, USA
Dustin Sheinost & R. Todd Constable
Interdepartmental Neuroscience Program, Yale University, New Haven, CT, USA
R. Todd Constable & Marvin M. Chun
Department of Neurosurgery, Yale School of Medicine, New Haven, CT, USA
R. Todd Constable
Department of Neuroscience, Yale School of Medicine, New Haven, CT, USA
Marvin M. Chun
Wu Tsai Institute, Yale University, New Haven, CT, USA
You can also search for this author in PubMed Google Scholar
Contributions
K.Y., M.D.R. and M.M.C. designed the study. Y.H.K. and E.W.A. performed fMRI experiments. K.Y. and M.D.R. analysed behavioural data. K.Y. and Y.H.K. analysed fMRI data. K.Y. conducted modelling and visualization. K.Y., M.M.C., M.D.R., Q.L., D.S. and R.T.C. discussed the results and implications. M.M.C. and R.T.C. supervised the project. K.Y., Y.H.K. and M.M.C. wrote the original draft; K.Y., M.M.C., M.D.R., Q.L., E.W.A., D.S. and R.T.C. reviewed the original draft and contributed to the final version of the paper.
Corresponding authors
Correspondence to Kwangsun Yoo or Marvin M. Chun .
Ethics declarations
Competing interests.
The authors declare no competing interests.
Peer review
Peer review information.
Nature Human Behaviour thanks Jing Sui, Francisco Castellanos and the other, anonymous, reviewer(s) for their contribution to the peer review of this work. Peer reviewer reports are available.
Additional information
Publisher’s note Springer Nature remains neutral with regard to jurisdictional claims in published maps and institutional affiliations.
Extended data
Extended data fig. 1 predictive anatomy of three task-based cpms..
a . The scale bar in gradCPT, MOT and VSTM represents the relative ratio of predictive functional connections to all possible number of functional connections between networks with a sign representing whether the connection is in a positive or negative network. The scale bar in overlap represents the actual number of predictive functional connections with a sign representing whether the connection is in a positive or negative network. GradCPT: gradual-onset continuous performance task, MOT: multiple object tracking, and VSTM: visual short-term memory. MF: medial-frontal network, FP: frontoparietal network, DM: default mode network, VI: visual I, VII: visual II, VAs: visual association, SA: salience network, Subc: subcortex, Cbl: cerebellum. b . The number of predictive connections of three task-based CPMs in positive and negative networks.
Extended Data Fig. 2 Cross-prediction results of five common attention factor CPMs.
a . Cross-prediction results when models were applied to predict the common attention factor from different fMRI data. Models’ prediction accuracies were assessed by prediction q 2 and correlation r between observed and predicted common factor measures. P values of significance were obtained using 1,000 permutations and corrected for all 5×5 tests (***: p < 0.001, **: p < 0.01, *: p < 0.05, and ~: p < 0.1). Rows represent different fMRI data used to predict a common attention factor used in model construction, and columns represent the same but in model validation. b . Cross-prediction results, taking into account shared variance (the common factor) between task behaviors. Models’ prediction accuracies were assessed by partial correlation between observed and predicted behavior scores while controlling for the shared variance. P values of significance were obtained using 1,000 permutations and corrected for all 5×9 tests (***: p < 0.001, **: p < 0.01, *: p < 0.05, and ~: p < 0.1). Rows represent different fMRI data used to predict a common attention factor used in model construction, and columns represent combinations of fMRI data and behavior scores used in model validation. GradCPT: gradual-onset continuous performance task, MOT: multiple object tracking, and VSTM: visual short-term memory.
Extended Data Fig. 3 A similarity of individual behaviours between different tasks.
The similarity was assessed by Pearson’s correlation of individual performances between attention tasks. Individual behaviors were significantly correlated between every pair of tasks. GradCPT: gradual-onset continuous performance task, MOT: multiple object tracking, and VSTM: visual short-term memory.
Extended Data Fig. 4 Cross-prediction results of task-specific CPMs.
a . Cross-prediction results, taking into account shared variance between task behaviors. Models’ prediction accuracies were assessed by partial correlation between observed and predicted behavior scores while controlling for the shared variance. P value was obtained using 1,000 permutations and corrected for multiple tests (***: p < 0.001, **: p < 0.01, *: p < 0.05, and ~: p < 0.1). Rows represent combinations of fMRI data and behavior scores used in model construction, and columns represent combinations of fMRI data and behavior scores used in model validation. GradCPT: gradual-onset continuous performance task, MOT: multiple object tracking, and VSTM: visual short-term memory. b . Cross-prediction results when models were applied to predict the common attention factor from different fMRI data. Models’ prediction accuracies were assessed by correlation between observed and predicted common factor. P value was obtained using 1,000 permutations and corrected for all 9×5 tests (***: p < 0.001, **: p < 0.01, *: p < 0.05, and ~: p < 0.1). Rows represent combinations of fMRI data and behavior scores used in model construction, and columns represent different fMRI data used to predict a common attention factor used in model validation.
Extended Data Fig. 5 Cross-prediction using connectivity between the frontoparietal (FP, 2), visual II (VII, 6), salience (SA, 8), subcortical (Subc, 9), cerebellar (Cbl, 10) networks.
Prediction of a model using connectivity between the medial-frontal (1), default mode (3), motor (4), visual I (5), visual association (7) networks was also obtained as a control. A. Rows represent combinations of networks (indicated by numbers) used in each model. Models’ prediction accuracies were assessed by correlating model-predicted and observed behavioral scores. B. Prediction performance of each network obtained by averaging all models that used the network in A. C. The same result as A, but model accuracies were assessed by q2. D. Prediction performance of each network obtained by averaging all models that used the network in C. GradCPT: gradual-onset continuous performance task, MOT: multiple object tracking, and VSTM: visual short-term memory.
Extended Data Fig. 6 Similarity between C2C model-generated task connectomes and empirical task connectomes.
Error bar represents standard deviation from 1,000 iterations. A and C represent a spatial similarity between two connectomes assessed by Pearson’s correlation. Darker bars represent the similarity between empirical task and generated task connectomes, and lighter bars represent the similarity between empirical task and empirical rest connectomes. The higher similarity of the generated connectome indicates that the C2C model accurately generates the target task connectome from the rest connectome. B and D represent root mean square (RMS) difference between two connectomes. The smaller difference of the generated connectome indicates that the C2C model accurately generates the target task connectome from the rest connectome. In a box-whisker plot, a box covers the first to third quartile ( q 1 and q 3, respectively) of the data, and a center line represents the median. A red dot represents the mean. Whisker covers approximately 99.3% of data (±2.7* standrad deviation ), extended to the most extreme point that is not an outlier. A data point is considered an outlier if it is greater than q 3+1.5*( q 3- q 1) or less than q 1-1.5*( q 3- q 1). GradCPT: gradual-onset continuous performance task, MOT: multiple object tracking, and VSTM: visual short-term memory. *: p < 0.001 from 1,000 permutations.
Extended Data Fig. 7 The general attention connectome lookup table.
Out of a total 30,135 edges, 10,885 (36.1%) edges were pulled from gradCPT, 12,542 (41.6%) edges were from MOT, and 6,708 (22.3%) were from VSTM. The Ratio map was obtained based on All map. In each within- or between-network element in Ratio, the number of edges in the element for each task was counted and normalized by the total number of edges of each task. A task with the highest normalized value was assigned.
Extended Data Fig. 8 Scatter plots of predicted and observed attention scores in four external datasets.
Three models, the general attention model and two single task models (model 1 and 4 in Table 1) were trained within the internal dataset and then applied to rest connectomes in the four datasets. If a fitted line closely passes the origin (0,0) with a positive slope (staying within white quadrants), the model could be considered successfully predicting actual attentional abilities. There was no constraint on intercepts in fitting a line. The general model best generalized to predict various attentional measures in four independent external datasets.
Extended Data Fig. 9 Prediction error, assessed by mean square error (MSE), of the general attention model in four independent datasets.
The general model significantly reduced prediction error (assessed by MSE) compared to null models in four datasets. In all datasets, the general attention model produced the lowest prediction error among all models tested. ***: p < 0.001, **: p < 0.01, *: p < 0.05, and ~: p < 0.1 from 1,000 permutations.
Supplementary information
Supplementary methods, results, discussion, references, Tables 1–7 and Figs. 1–11.
Reporting summary
Rights and permissions.
Reprints and permissions
About this article
Cite this article.
Yoo, K., Rosenberg, M.D., Kwon, Y.H. et al. A brain-based general measure of attention. Nat Hum Behav 6 , 782–795 (2022). https://doi.org/10.1038/s41562-022-01301-1
Download citation
Received : 17 June 2021
Accepted : 14 January 2022
Published : 03 March 2022
Issue Date : June 2022
DOI : https://doi.org/10.1038/s41562-022-01301-1
Share this article
Anyone you share the following link with will be able to read this content:
Sorry, a shareable link is not currently available for this article.
Provided by the Springer Nature SharedIt content-sharing initiative
This article is cited by
Gaze-based attention refocusing training in virtual reality for adult attention-deficit/hyperactivity disorder.
- Benjamin Selaskowski
- Laura Marie Asché
- Niclas Braun
BMC Psychiatry (2023)
Quick links
- Explore articles by subject
- Guide to authors
- Editorial policies
Sign up for the Nature Briefing newsletter — what matters in science, free to your inbox daily.

- Reference Manager
- Simple TEXT file
People also looked at
Review article, attention in psychology, neuroscience, and machine learning.
- Gatsby Computational Neuroscience Unit, Sainsbury Wellcome Centre, University College London, London, United Kingdom
Attention is the important ability to flexibly control limited computational resources. It has been studied in conjunction with many other topics in neuroscience and psychology including awareness, vigilance, saliency, executive control, and learning. It has also recently been applied in several domains in machine learning. The relationship between the study of biological attention and its use as a tool to enhance artificial neural networks is not always clear. This review starts by providing an overview of how attention is conceptualized in the neuroscience and psychology literature. It then covers several use cases of attention in machine learning, indicating their biological counterparts where they exist. Finally, the ways in which artificial attention can be further inspired by biology for the production of complex and integrative systems is explored.
1. Introduction
Attention is a topic widely discussed publicly and widely studied scientifically. It has many definitions within and across multiple fields including psychology, neuroscience, and, most recently, machine learning ( Chun et al., 2011 ; Cho et al., 2015 ). As William James wrote at the dawn of experimental psychology, “Everyone knows what attention is. It is the taking possession by the mind, in clear, and vivid form, of one out of what seems several simultaneously possible objects or trains of thought.” Since James wrote this, many attempts have been made to more precisely define and quantify this process while also identifying the underlying mental and neural architectures that give rise to it. The glut of different experimental approaches and conceptualizations to study what is spoken of as a single concept, however, has led to something of a backlash amongst researchers. As was claimed in the title of a recent article arguing for a more evolution-informed approach to the concept, “No one knows what attention is” ( Hommel et al., 2019 ).
Attention is certainly far from a clear or unified concept. Yet despite its many, vague, and sometimes conflicting definitions, there is a core quality of attention that is demonstrably of high importance to information processing in the brain and, increasingly, artificial systems. Attention is the flexible control of limited computational resources. Why those resources are limited and how they can best be controlled will vary across use cases, but the ability to dynamically alter and route the flow of information has clear benefits for the adaptiveness of any system.
The realization that attention plays many roles in the brain makes its addition to artificial neural networks unsurprising. Artificial neural networks are parallel processing systems comprised of individual units designed to mimic the basic input-output function of neurons. These models are currently dominating the machine learning and artificial intelligence (AI) literature. Initially constructed without attention, various mechanisms for dynamically re-configuring the representations or structures of these networks have now been added.
The following section, section 2, will cover broadly the different uses of the word attention in neuroscience and psychology, along with its connection to other common neuroscientific topics. Throughout, the conceptualization of attention as a way to control limited resources will be highlighted. Behavioral studies will be used to demonstrate the abilities and limits of attention while neural mechanisms point to the physical means through which these behavioral effects are manifested. In section 3, the state of attention research in machine learning will be summarized and relationships between artificial and biological attention will be indicated where they exist. And in section 4 additional ways in which findings from biological attention can influence its artificial counterpart will be presented.
The primary aim of this review is to give researchers in the field of AI or machine learning an understanding of how attention is conceptualized and studied in neuroscience and psychology in order to facilitate further inspiration where fruitful. A secondary aim is to inform those who study biological attention how these processes are being operationalized in artificial systems as it may influence thinking about the functional implications of biological findings.
2. Attention in Neuroscience and Psychology
The scientific study of attention began in psychology, where careful behavioral experimentation can give rise to precise demonstrations of the tendencies and abilities of attention in different circumstances. Cognitive science and cognitive psychology aim to turn these observations into models of how mental processes could create such behavioral patterns. Many word models and computational models have been created that posit different underlying mechanisms ( Driver, 2001 ; Borji and Itti, 2012 ).
The influence of single-cell neurophysiology in non-human primates along with non-invasive means of monitoring human brain activity such as EEG, fMRI, and MEG have made direct observation of the underlying neural processes possible. From this, computational models of neural circuits have been built that can replicate certain features of the neural responses that relate to attention ( Shipp, 2004 ).
In the following sub-sections, the behavioral and neural findings of several different broad classes of attention will be discussed.
2.1. Attention as Arousal, Alertness, or Vigilance
In its most generic form, attention could be described as merely an overall level of alertness or ability to engage with surroundings. In this way it interacts with arousal and the sleep-wake spectrum. Vigilance in psychology refers to the ability to sustain attention and is therefore related as well. Note, while the use of these words clusters around the same meaning, they are sometimes used more specifically in different niche literature ( Oken et al., 2006 ).
Studying subjects in different phases of the sleep-wake cycle, under sleep deprivation, or while on sedatives offers a view of how this form of attention can vary and what the behavioral consequences are. By giving subjects repetitive tasks that require a level of sustained attention—such as keeping a ball within a certain region on a screen—researchers have observed extended periods of poor performance in drowsy patients that correlate with changes in EEG signals ( Makeig et al., 2000 ). Yet, there are ways in which tasks can be made more engaging that can lead to higher performance even in drowsy or sedated states. This includes increasing the promise of reward for performing the task, adding novelty or irregularity, or introducing stress ( Oken et al., 2006 ). Therefore, general attention appears to have limited reserves that won't be deployed in the case of a mundane or insufficiently rewarding task but can be called upon for more promising or interesting work.
Interestingly, more arousal is not always beneficial. The Yerkes-Dodson curve ( Figure 1B ) is an inverted-U that represents performance as a function of alertness on sufficiently challenging tasks: at low levels of alertness performance is poor, at medium levels it is good, and at high levels it becomes poor again. The original study used electric shocks in mice to vary the level of alertness, but the finding has been repeated with other measures ( Diamond, 2005 ). It may explain why psychostimulants such as Adderall or caffeine can work to increase focus in some people at some doses but become detrimental for others ( Wood et al., 2014 ).
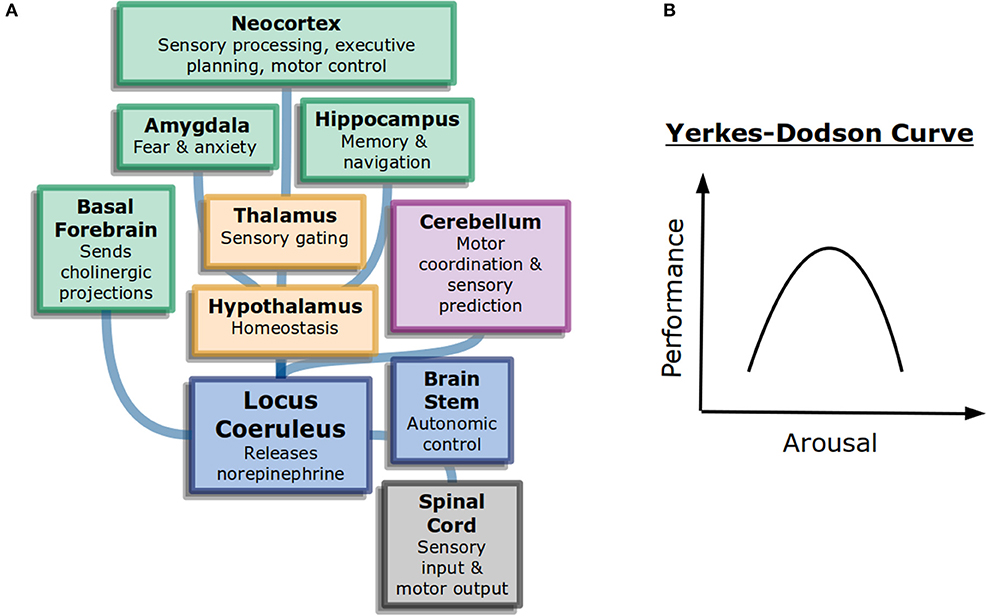
Figure 1 . General attention and alertness (A) Cells in the locus coeruleus release norepinephrine (also known as noradrenaline) onto many parts of the brain with different functions, including onto other neuromodulatory systems. This contributes to overall arousal ( Samuels and Szabadi, 2008 ). Colors here represent different divisions of the brain: forebrain (green), diencephalon (yellow), and brainstem (blue). (B) The Yerkes-Dodson curve describes the nonlinear relationship between arousal and performance on challenging tasks.
The neural circuits underlying the sleep-wake cycle are primarily in the brain stem ( Coenen, 1998 ). These circuits control the flow of information into the thalamus and then onto cortex. Additionally, neuromodulatory systems play a large role in the control of generalized attention. Norepinephrine, acetylcholine, and dopamine are believed to influence alertnesss, orienting to important information, and executive control of attention, respectively ( Posner, 2008 ). The anatomy of neuromodulators matches their function as well. Neurons that release norepinephrine, for example, have their cell bodies in the brain stem but project very broadly across the brain, allowing them to control information processing broadly ( Figure 1A ).
2.2. Sensory Attention
In addition to overall levels of arousal and alertness, attention can also be selectively deployed by an awake subject to specific sensory inputs. Studying attention within the context of a specific sensory system allows for tight control over both stimuli and the locus of attention. Generally, to look for this type of attention the task used needs to be quite challenging. For example, in a change detection task, the to-be-detected difference between two stimuli may be very slight. More generally, task difficulty can be achieved by presenting the stimulus for only a very short period of time or only very weakly.
A large portion of the study of attention in systems neuroscience and psychology centers on visual attention in particular ( Kanwisher and Wojciulik, 2000 ). This may reflect the general trend in these fields to emphasis the study of visual processing over other sensory systems ( Hutmacher, 2019 ), along with the dominant role vision plays in the primate brain. Furthermore, visual stimuli are frequently used in studies meant to address more general, cognitive aspects of attention as well.
Visual attention can be broken down broadly into spatial and feature-based attention.
2.2.1. Visual Spatial Attention
Saccades are small and rapid eye movements made several times each second. As the fovea offers the highest visual resolution on the retina, choosing where to place it is essentially a choice about where to deploy limited computational resources. In this way, eye movements indicate the locus of attention. As this shift of attention is outwardly visible it is known as overt visual attention.
By tracking eye movements as subjects are presented with different images, researchers have identified image patterns that automatically attract attention. Such patterns are defined by oriented edges, spatial frequency, color contrast, intensity, or motion ( Itti and Koch, 2001 ). Image regions that attract attention are considered “salient” and are computed in a “bottom-up” fashion. That is, they don't require conscious or effortful processing to identify and are likely the result of built-in feature detectors in the visual system. As such, saliency can be computed very quickly. Furthermore, different subjects tend to agree on which regions are salient, especially those identified in the first few saccades ( Tatler et al., 2005 ).
Salient regions can be studied in “free-viewing” situations, that is, when the subject is not given any specific instructions about how to view the image. When a particular task is assigned, the interplay between bottom-up and “top-down” attention becomes clear. For example, when instructed to saccade to a specific visual target out of an array, subjects may incorrectly saccade to a particularly salient distractor instead ( van Zoest and Donk, 2005 ). More generally, task instructions can have a significant effect on the pattern of saccades generated when subjects are viewing a complex natural image and given high-level tasks (e.g., asked to assess the age of a person or guess their socio-economic status). Furthermore, the natural pattern of eye movements when subjects perform real world tasks, like sandwich making, can provide insights to underlying cognitive processes ( Hayhoe and Ballard, 2005 ).
When subjects need to make multiple saccades in a row they tend not to return to locations they have recently attended and may be slow to respond if something relevant occurs there. This phenomenon is known as inhibition of return ( Itti and Koch, 2001 ). Such behavior pushes the visual system to not just exploit image regions originally deemed most salient but to explore other areas as well. It also means the saccade generating system needs to have a form of memory; this is believed to be implemented by short-term inhibition of the representation of recently-attended locations.
While eye movements are an effective means of controlling visual attention, they are not the only option. “Covert” spatial attention is a way of emphasizing processing of different spatial locations without an overt shift in fovea location. Generally, in the study of covert spatial attention, subjects must fixate on a central point throughout the task. They are cued to covertly attend to a location in their peripheral vision where stimuli relevant for their visual task will likely appear. For example, in an orientation discrimination task, after the spatial cue is provided an oriented grating will flash in the cued location and the subject will need to indicate its orientation. On invalidly-cued trials (when the stimulus appears in an uncued location), subjects perform worse than on validly-cued (or uncued) trials ( Anton-Erxleben and Carrasco, 2013 ). This indicates that covert spatial attention is a limited resource that can be flexibly deployed and aids in the processing of visual information.
Covert spatial attention is selective in the sense that certain regions are selected for further processing at the expense of others. This has been referred to as the “spotlight” of attention. Importantly, for covert—as opposed to overt—attention the input to the visual system can be identical while the processing of that input is flexibly selective.
Covert spatial attention can be impacted by bottom-up saliency as well. If an irrelevant but salient object is flashed at a location that then goes on to have a task relevant stimulus, the exogenous spatial attention drawn by the irrelevant stimulus can get applied to the task relevant stimulus, possibly providing a performance benefit. If it is flashed at an irrelevant location, however, it will not help, and can harm performance ( Berger et al., 2005 ). Bottom-up/exogenous attention has a quick time course, impacting covert attention for 80–130 ms after the distractor appears ( Anton-Erxleben and Carrasco, 2013 ).
In some theories of attention, covert spatial attention exists to help guide overt attention. Particularly, the pre-motor theory of attention posits that the same neural circuits plan saccades and control covert spatial attention ( Rizzolatti et al., 1987 ). The frontal eye field (FEF) is known to be involved in the control of eye movements. Stimulating the neurons in FEF at levels too low to evoke eye movements has been shown to create effects similar to covert attention ( Moore et al., 2003 ). In this way, covert attention may be a means of deciding where to overtly look. The ability to covertly attend may additionally be helpful in social species, as eye movements convey information about knowledge and intent that may best be kept secret ( Klein et al., 2009 ).
To study the neural correlates of covert spatial attention, researchers identify which aspects of neural activity differ based only on differences in the attentional cue (and not on differences in bottom-up features of the stimuli). On trials where attention is cued toward the receptive field of a recorded neuron, many changes in the neural activity have been observed ( Noudoost et al., 2010 ; Maunsell, 2015 ). A commonly reported finding is an increase in firing rates, typically of 20–30% ( Mitchell et al., 2007 ). However, the exact magnitude of the change depends on the cortical area studied, with later areas showing stronger changes ( Luck et al., 1997 ; Noudoost et al., 2010 ). Attention is also known to impact the variability of neural firing. In particular, it decreases trial-to-trial variability as measured via the Fano Factor and decreases noise correlations between pairs of neurons. Attention has even been found to impact the electrophysiological properties of neurons in a way that reduces their likelihood of firing in bursts and also decreases the height of individual action potentials ( Anderson et al., 2013 ).
In general, the changes associated with attention are believed to increase the signal-to-noise ratio of the neurons that represent the attended stimulus, however they can also impact communication between brain areas. To this end, attention's effect on neural synchrony is important. Within a visual area, attention has been shown to increase spiking coherence in the gamma band—that is at frequencies between 30 and 70 Hz ( Fries et al., 2008 ). When a group of neurons fires synchronously, their ability to influence shared downstream areas is enhanced. Furthermore, attention may also be working to directly coordinate communication across areas. Synchronous activity between two visual areas can be a sign of increased communication and attention has been shown to increase synchrony between the neurons that represent the attended stimulus in areas V1 and V4, for example ( Bosman et al., 2012 ). Control of this cross-area synchronization appears to be carried out by the pulvinar ( Saalmann et al., 2012 ).
In addition to investigating how attention impacts neurons in the visual pathways, studies have also searched for the source of top-down attention ( Noudoost et al., 2010 ; Miller and Buschman, 2014 ). The processing of bottom-up attention appears to culminate with a saliency map produced in the lateral intraparietal area (LIP). The cells here respond when salient stimuli are in their receptive field, including task-irrelevant but salient distractors. Prefrontal areas such as FEF, on the other hand, appear to house the signals needed for top-down control of spatial attention and are less responsive to distractors.
While much of the work on the neural correlates of sensory attention focuses on the cortex, subcortical areas appear to play a strong role in the control and performance benefits of attention as well. In particular, the superior colliculus assists in both covert and overt spatial attention and inactivation of this region can impair attention ( Krauzlis et al., 2013 ). And, as mentioned above, the pulvinar plays a role in attention, particularly with respect to gating effects on cortex ( Zhou et al., 2016 ).
2.2.2. Visual Feature Attention
Feature attention is another form of covert selective attention. In the study of feature attention, instead of being cued to attend to a particular location, subjects are cued on each trial to attend to a particular visual feature such as a specific color, a particular shape, or a certain orientation. The goal of the task may be to detect if the cued feature is present on the screen or readout another one of its qualities (e.g., to answer “what color is the square?” should result in attention first deployed to squares). Valid cueing about the attended feature enhances performance. For example, when attention was directed toward a particular orientation, subjects were better able to detect faint gratings of that orientation than of any other orientation ( Rossi and Paradiso, 1995 ). While the overall task (e.g., detection of an oriented grating) remains the same, the specific instructions (detection of 90° grating vs. 60° vs. 30°) will be cued on each individual trial, or possibly blockwise. Successful trial-wise cueing indicates that this form of attention can be flexibly deployed on fast timescales.
Visual search tasks are also believed to activate feature-based attention ( Figure 2 ). In these tasks, an array of stimuli appears on a screen and subjects need to indicate—frequently with an eye movement—the location of the cued stimulus. As subjects are usually allowed to make saccades throughout the task as they search for the cued stimulus, this task combines covert feature-based attention with overt attention. In fact, signals of top-down feature-based attention have been found in FEF, the area involved in saccade choice ( Zhou and Desimone, 2011 ). Because certain features can create a pop-out effect—for example, a single red shape amongst several black ones will immediately draw attention—visual search tasks also engage bottom-up attention which, depending on the task, may need to be suppressed ( Wolfe and Horowitz, 2004 ).
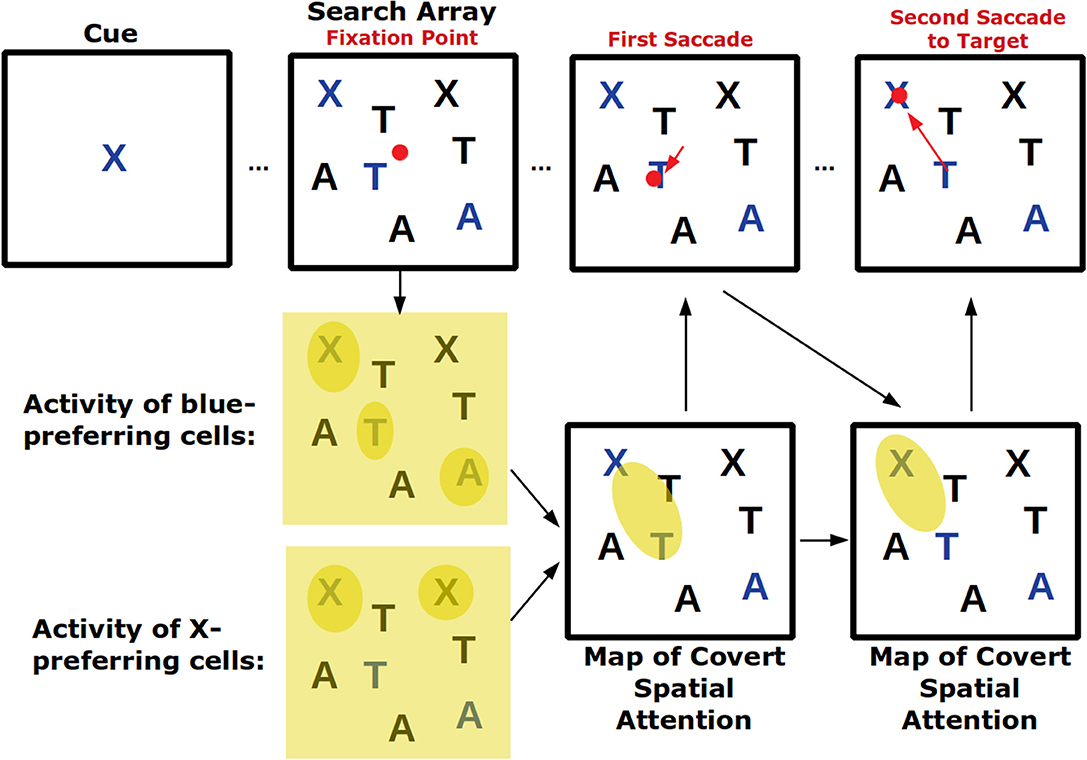
Figure 2 . Visual search tasks engage many forms of visual attention. Across the top row the progression of a visual search task is shown. First, a cue indicates the target of the visual search, in this case a blue X. Then a search array appears with many non-targets. Top-down feature attention to cells that represent the color blue and the shape X will increase their firing throughout the visual field but firing will be strongest where blue or Xs actually occur. These neural response will play a role in generating a map of covert spatial attention which can be used to explore visual space before saccading. After the shift in overt attention with the first saccade, the covert attention map is remade. Finally, the target is located and successfully saccaded to. If the visual array contained a pop-out stimulus (for example a green O) it may have captured covert spatial attention in a bottom-up way and led to an additional incorrect saccade.
Neural effects of feature-based attention in the visual system are generally similar to those of spatial attention. Neurons that represent the attended feature, for example, have increased firing rates, and those that represent very different features have suppressed rates ( Treue and Trujillo, 1999 ). As opposed to spatial attention, however, feature-based attention is spatially-global. This means that when deploying attention to a particular feature the activity of the neurons that represent that feature anywhere in visual space are modulated ( Saenz et al., 2002 ). Another difference between spatial and feature attention is the question of how sources of top-down attention target the correct neurons in the visual system. The retinotopic map, wherein nearby cells represent nearby spatial locations, makes spatial targeting straightforward, but cells are not as neatly organized according to preferred visual features.
The effects of spatial and feature attention appear to be additive ( Hayden and Gallant, 2009 ). Furthermore, both feature and spatial attention are believed to create their effects by acting on the local neural circuits that implement divisive normalization in visual cortex ( Reynolds and Heeger, 2009 ). Modeling work has shown that many of the neural effects of selective attention can be captured by assuming that top-down connections provide targeted synaptic inputs to cells in these circuits ( Lindsay et al., 2019 ). However, models that rely on effects of the neuromodulator acetylcholine can also replicate neural correlates of attention ( Sajedin et al., 2019 ).
Potential sources of top-down feature-based attention have been found in prefrontal cortex where sustained activity encodes the attended feature ( Bichot et al., 2015 ; Paneri and Gregoriou, 2017 ). Inactivating the ventral prearcuate area impairs performance on search tasks. From prefrontal areas, attention signals are believed to travel in a reverse hierarchical way wherein higher visual areas send inputs to those below them ( Ahissar and Hochstein, 2000 ).
A closely related topic to feature attention is object attention. Here, attention is not deployed to an abstract feature in advance of a visual stimulus, but rather it is applied to a particular object in the visual scene ( Chen, 2012 ). The initial feedforward pass of activity through the visual hierarchy is able to pre-attentively segregate objects from their backgrounds in parallel across the visual field, provided these objects have stark and salient differences from the background. In more crowded or complex visual scenes, recurrent and serial processing is needed in order to identify different objects ( Lamme and Roelfsema, 2000 ). Serial processing involves moving limited attentional resources from one location in the image to another; it can take the form of shifts in either covert or overt spatial attention ( Buschman and Miller, 2009 ). Recurrent connections in the visual system—that is, both horizontal connections from nearby neurons in the same visual area and feedback connections from those in higher visual areas—aid in figure-ground segregation and object identification. The question of how the brain performs perceptual grouping of low-level features into a coherent object identity has been studied for nearly a century. It is believed that attention may be required for grouping, particularly for novel or complex objects ( Roelfsema and Houtkamp, 2011 ). This may be especially important in visual search tasks that require locating an object that is defined by a conjunction of several features.
Neurally, the effects of object-based attention can spread slowly through space as parts of an object are mentally traced ( Roelfsema et al., 1998 ). Switching attention to a location outside an object appears to incur a greater cost than switching to the same distance away but within the object ( Brown and Denney, 2007 ). In addition, once attention is applied to a visual object, it is believed to activate feature-based attention for the different features of that object across the visual field ( O'Craven et al., 1999 ).
Another form of attention sometimes referred to as feature attention involves attending to an entire feature dimension. An example of this is the Stroop test, wherein the names of colors are written in different colored ink and subjects either need to read the word itself or say the color of the ink. Here attention cannot be deployed to a specific feature in advance, only to the dimensions word or color. Neurally, the switch between dimensions appears to impact sensory coding in the visual stream and is controlled by frontal areas ( Liu et al., 2003 ).
2.2.3. Computational Models of Visual Attention
Visual attention, being one of the most heavily-studied topics in the neuroscience of attention, has inspired many computational models of how attention works. In general, these models synthesize various neurophysiological findings in order to help explain how the behavioral impacts of attention arise ( Heinke and Humphreys, 2005 ).
Several computational models meant to calculate saliency have been devised ( Itti and Koch, 2001 ). These models use low-level visual feature detectors—usually designed to match those in the visual system—to create an image-specific saliency map that can predict the saccade patterns of humans in response to the same image. Another approach to calculating saliency based on information theoretic first principles has also been explored and was able to account for certain visual search behaviors ( Bruce and Tsotsos, 2009 ).
Some of the behavioral and neural correlates of attention are similar whether the attention is bottom-up or top-down. In the Biased Competition Model of attention, stimuli compete against each other to dominate the neural response ( Desimone, 1998 ). Attention (bottom-up or top-down) can thus work by biasing this competition toward the stimulus that is the target of attention. While the Biased Competition Model is sometimes used simply as a “word model” to guide intuition, explicit computational instantiations of it have also been built. A hierarchical model of the visual pathway that included top-down biasing as well as local competition mediated through horizontal connections was able to replicate multiple neural effects of attention ( Deco and Rolls, 2004 ). A model embodying similar principles but using spiking neurons was also implemented ( Deco and Rolls, 2005 ).
Similar models have been constructed explicitly to deal with attribute naming tasks such as the Stroop test described above. The Selective Attention Model (SLAM), for example, has local competition in both the sensory encoding and motor output modules and can mimic known properties of response times in easier and more challenging Stroop-like tests ( Phaf et al., 1990 ).
Visual perception has been framed and modeled as a problem of Bayesian inference ( Lee and Mumford, 2003 ). Within this context, attention can help resolve uncertainty under settings where inference is more challenging, typically by modulating priors ( Rao, 2005 ). For example, in Chikkerur et al. (2010) spatial attention functions to reduce uncertainty about object identity and feature attention reduces spatial uncertainty. These principles can capture both behavioral and neural features of attention and can be implemented in a biologically-inspired neural model.
The feature similarity gain model of attention (FSGM) is a description of the neural effects of top-down attention that can be applied in both the feature and spatial domain ( Treue and Trujillo, 1999 ). It says that the way in which a neuron's response is modulated by attention depends on that neuron's tuning. Tuning is a description of how a neuron responds to different stimuli, so according to the FSGM a neuron that prefers (that is, responds strongly to), e.g., the color blue, will have its activity enhanced by top-down attention to blue. The FSGM also says attention to non-preferred stimuli will cause a decrease in firing and that, whether increased or decreased, activity is scaled multiplicatively by attention. Though not initially defined as a computational model, this form of neural modulation has since been shown through modeling to be effective at enhancing performance on challenging visual tasks ( Lindsay and Miller, 2018 ).
Other models conceptualize attention as a dynamic routing of information through a network. An implementation of this form of attention can be found in the Selective Attention for Identification Model (SAIM) ( Heinke and Humphreys, 2003 ). Here, attention routes information from the retina to a representation deemed the “focus of attention”; depending on the current task, different parts of the retinal representation will be mapped to the focus of attention.
2.2.4. Attention in Other Sensory Modalities
A famous example of the need for selective attention in audition is the “cocktail party problem”: the difficulty of focusing on the speech from one speaker in a crowded room of multiple speakers and other noises ( Bronkhorst, 2015 ). Solving the problem is believed to involve “early” selection wherein low level features of a voice such as pitch are used to determine which auditory information is passed on for further linguistic processing. Interestingly, selective auditory attention has the ability to control neural activity at even the earliest level of auditory processing, the cochlea ( Fritz et al., 2007 ).
Spatial and feature attention have also been explored in the somatosensory system. Subjects cued to expect a tap at different parts on their body are better able to detect the sensation when that cue is valid. However, these effects seem weaker than they are in the visual system ( Johansen-Berg and Lloyd, 2000 ). Reaction times are faster in a detection task when subjects are cued about the orientation of a stimulus on their finger ( Schweisfurth et al., 2014 ).
In a study that tested subjects' ability to detect a taste they had been cued for it was shown that validly-cued tastes can be detected at lower concentrations than invalidly-cued ones ( Marks and Wheeler, 1998 ). This mimics the behavioral effects found with feature-based visual attention. Attention to olfactory features has not been thoroughly explored, though visually-induced expectations about a scent can aid its detection ( Gottfried and Dolan, 2003 ; Keller, 2011 ).
Attention can also be spread across modalities to perform tasks that require integration of multiple sensory signals. In general, the use of multiple congruent sensory signals aids detection of objects when compared to relying only on a single modality. Interestingly, some studies suggest that humans may have a bias for the visual domain, even when the signal from another domain is equally valid ( Spence, 2009 ). Specifically, the visual domain appears to dominate most in tasks that require identifying the spatial location of a cue ( Bertelson and Aschersleben, 1998 ). This can be seen most readily in ventriloquism, where the visual cue of the dummy's mouth moving overrides auditory evidence about the true location of the vocal source. Visual evidence can also override tactile evidence, for example, in the context of the rubber arm illusion ( Botvinick and Cohen, 1998 ).
Another effect of the cross-modal nature of sensory processing is that an attentional cue in one modality can cause an orienting of attention in another modality ( Spence and Driver, 2004 ). Generally, the attention effects in the non-cued modality are weaker. This cross-modal interaction can occur in the context of both endogenous (“top-down”) and exogenous (“bottom-up”) attention.
2.3. Attention and Executive Control
With multiple simultaneous competing tasks, a central controller is needed to decide which to engage in and when. What's more, how to best execute tasks can depend on history and context. Combining sensory inputs with past knowledge in order to coordinate multiple systems for the job of efficient task selection and execution is the role of executive control, and this control is usually associated with the prefrontal cortex ( Miller and Buschman, 2014 ). As mentioned above, sources of top-down visual attention have also been located in prefrontal regions. Attention can reasonably be thought of as the output of executive control. The executive control system must thus select the targets of attention and communicate that to the systems responsible for implementing it. According to the reverse hierarchy theory described above, higher areas signal to those from which they get input which send the signal on to those below them and so on ( Ahissar and Hochstein, 2000 ). This means that, at each point, the instructions for attention must be transformed into a representation that makes sense for the targeted region. Through this process, the high level goals of the executive control region can lead to very specific changes, for example, in early sensory processing.
Executive control and working memory are also intertwined, as the ability to make use of past information as well as to keep a current goal in mind requires working memory. Furthermore, working memory is frequently identified as sustained activity in prefrontal areas. A consequence of the three-way relationship between executive control, working memory, and attention is that the contents of working memory can impact attention, even when not desirable for the task ( Soto et al., 2008 ). For example, if a subject has to keep an object in working memory while simultaneously performing a visual search for a separate object, the presence of the stored object in the search array can negatively interfere with the search ( Soto et al., 2005 ). This suggests that working memory can interfere with the executive control of attention. However, there still appears to be additional elements of that control that working memory alone does not disrupt. This can be seen in studies wherein visual search performance is even worse when subjects believe they will need to report the memorized item but are shown a search array for the attended item instead ( Olivers and Eimer, 2011 ). This suggests that, while all objects in working memory may have some influence over attention, the executive controller can choose which will have the most.
Beyond the flexible control of attention within a sensory modality, attention can also be shifted between modalities. Behavioral experiments indicate that switching attention either between two different tasks within a sensory modality (for example, going from locating a visual object to identifying it) or between sensory modalities (switching from an auditory task to a visual one) incurs a computational cost ( Pashler, 2000 ). This cost is usually measured as the extent to which performance is worse on trials just after the task has been switched vs. those where the same task is being repeated. Interestingly, task switching within a modality seems to incur a larger cost than switching between modalities ( Murray et al., 2009 ). A similar result is found when switching between or across modes of response (for example, pressing a bottom vs. verbal report), suggesting this is not specific to sensory processing ( Arrington et al., 2003 ). Such findings are believed to stem from the fact that switching within a modality requires a reconfiguration of the same neural circuits, which is more difficult than merely engaging the circuitry of a different sensory system. An efficient executive controller would need to be aware of these costs when deciding to shift attention and ideally try to minimize them; it has been shown that switch costs can be reduced with training ( Gopher, 1996 ).
The final question regarding the executive control of attention is how it evolves with learning. Eye movement studies indicate that searched-for items can be detected more rapidly in familiar settings rather than novel ones, suggesting that previously-learned associations guide overt attention ( Chun and Jiang, 1998 ). Such benefits are believed to rely on the hippocampus ( Aly and Turk-Browne, 2017 ). In general, however, learning how to direct attention is not as studied as other aspects of the attention process. Some studies have shown that subjects can enhance their ability to suppress irrelevant task information, and the generality of that suppression depends on the training procedure ( Kelley and Yantis, 2009 ). Looking at the neural correlates of attention learning, imaging results suggest that the neural changes associated with learning do not occur in the sensory pathways themselves but rather in areas more associated with attentional control ( Kelley and Yantis, 2010 ). Though not always easy to study, the development of attentional systems in infancy and childhood may provide further clues as to how attention can be learned ( Reynolds and Romano, 2016 ).
2.4. Attention and Memory
Attention and memory have many possible forms of interaction. If memory has a limited capacity, for example, it makes sense for the brain to be selective about what is allowed to enter it. In this way, the ability of attention to dynamically select a subset of total information is well-matched to the needs of the memory system. In the other direction, deciding to recall a specific memory is a choice about how to deploy limited resources. Therefore, both memory encoding and retrieval can rely on attention.
The role of attention in memory encoding appears quite strong ( Aly and Turk-Browne, 2017 ). For information to be properly encoded into memory, it is best for it be the target of attention. When subjects are asked to memorize a list of words while simultaneously engaging in a secondary task that divides their attention, their ability to consciously recall those words later is impaired (though their ability to recognize the words as familiar is not so affected) ( Gardiner and Parkin, 1990 ). Imaging studies have shown that increasing the difficulty of the secondary task weakens the pattern of activity related to memory encoding in the left ventral inferior frontal gyrus and anterior hippocampus and increases the representation of secondary task information in dorsolateral prefrontal and superior parietal regions ( Uncapher and Rugg, 2005 ). Therefore, without the limited neural processing power placed on the task of encoding, memory suffers. Attention has also been implicated in the encoding of spatially-defined memories and appears to stabilize the representations of place cells ( Muzzio et al., 2009 ).
Implicit statistical learning can also be biased by attention. For example, in Turk-Browne et al. (2005) subjects watched a stream of stimuli comprised of red and green shapes. The task was to detect when a shape of the attended color appeared twice in a row. Unbeknownst to the subjects, certain statistical regularities existed in the stream such that there were triplets of shapes likely to occur close together. When shown two sets of three shapes—one an actual co-occurring triplet and another a random selection of shapes of the same color—subjects recognized the real triplet as more familiar, but only if the triplets were from the attended color. The statistical regularities of the unattended shapes were not learned.
Yet some learning can occur even without conscious attention. For example, in Watanabe (2003) patients engaged in a letter detection task located centrally in their visual field while random dot motion was shown in the background at sub-threshold contrast. The motion had 10% coherence in a direction that was correlated with the currently-presented letter. Before and after learning this task, subjects performed an above-threshold direction classification task. After learning the task, direction classification improved only for the direction associated with the targeted letters. This suggests a reward-related signal activated by the target led to learning about a non-attended component of the stimulus.
Many behavioral studies have explored the extent to which attention is needed for memory retrieval. For example, by asking subjects to simultaneously recall a list of previously-memorized words and engage in a secondary task like card sorting, researchers can determine if memory retrieval pulls from the same limited pool of attentional resources as the task. Some such studies have found that retrieval is impaired by the co-occurrence of an attention-demanding task, suggesting it is an attention-dependent process. The exact findings, however, depend on the details of the memory and non-memory tasks used ( Lozito and Mulligan, 2006 ).
Even if memory retrieval does not pull from shared attentional resources, it is still clear that some memories are selected for more vivid retrieval at any given moment than others. Therefore, a selection process must occur. An examination of neuroimaging results suggests that the same parietal brain regions responsible for the top-down allocation and bottom-up capture of attention may play analogous roles during memory retrieval ( Wagner et al., 2005 ; Ciaramelli et al., 2008 ).
Studies of memory retrieval usually look at medium to long-term memory but a mechanism for attention to items in working memory has also been proposed ( Manohar et al., 2019 ). It relies on two different mechanisms of working memory: synaptic traces for non-attended items and sustained activity for the attended one.
Some forms of memory occur automatically and within the sensory processing stream itself. Priming is a well-known phenomenon in psychology wherein the presence of a stimulus at one point in time impacts how later stimuli are processed or interpreted. For example, the word “doctor” may be recognized more quickly following the word “hospital” than the word “school.” In this way, priming requires a form of implicit memory to allow previous stimuli to impact current ones. Several studies on conceptual or semantic priming indicate that attention to the first stimulus is required for priming effects to occur ( Ballesteros and Mayas, 2015 ); this mirrors findings that attention is required for memory encoding more generally.
Most priming is positive, meaning that the presence of a stimulus at one time makes the detection and processing of it or a related stimulus more likely at a later time. In this way, priming can be thought of as biasing bottom-up attention. However, top-down attention can also create negative priming. In negative priming, when stimuli that functioned as a distractor on the previous trial serve as the target of attention on the current trial, performance suffers ( Frings et al., 2015 ). This may stem from a holdover effect wherein the mechanisms of distractor suppression are still activated for the now-target stimulus.
Adaptation can also be considered a form of implicit memory. Here, neural responses decrease after repeated exposure to the same stimulus. By reducing the response to repetition, changes in the stimulus become more salient. Attention—by increasing the neural response to attended stimuli—counters the effects of adaptation ( Pestilli et al., 2007 ; Anton-Erxleben et al., 2013 ). Thus, both with priming and adaptation, top-down attention can overcome automatic processes that occur at lower levels which may be guiding bottom-up attention.
3. Attention in Machine Learning
While the concept of artificial attention has come up prior to the current resurgence of artificial neural networks, many of its popular uses today center on ANNs ( Mancas et al., 2016 ). The use of attention mechanisms in artificial neural networks came about—much like the apparent need for attention in the brain—as a means of making neural systems more flexible. Attention mechanisms in machine learning allow a single trained artificial neural network to perform well on multiple tasks or tasks with inputs of variable length, size, or structure. While the spirit of attention in machine learning is certainly inspired by psychology, its implementations do not always track with what is known about biological attention, as will be noted below.
In the form of attention originally developed for ANNs, attention mechanisms worked within an encoder-decoder framework and in the context of sequence models ( Cho et al., 2015 ; Chaudhari et al., 2019 ). Specifically, an input sequence will be passed through an encoder (likely a recurrent neural network) and the job of the decoder (also likely a recurrent neural network) will be to output another sequence. Connecting the encoder and decoder is an attention mechanism.
Commonly, the output of the encoder is a set of a vectors, one for each element in the input sequence. Attention helps determine which of these vectors should be used to generate the output. Because the output sequence is dynamically generated one element at a time, attention can dynamically highlight different encoded vectors at each time point. This allows the decoder to flexibly utilize the most relevant parts of the input sequence.
The specific job of the attention mechanism is to produce a set of scalar weightings, α t i , one for each of the encoded vectors ( v i ). At each step t , the attention mechanism (ϕ) will take in information about the decoder's previous hidden state ( h t −1 ) and the encoded vectors to produce unnormalized weightings:
Because attention is a limited resource, these weightings need to represent relative importance. To ensure that the α values sum to one, the unnormalized weightings are passed through a softmax:
These attention values scale the encoded vectors to create a single context vector on which the decoder can be conditioned:
This form of attention can be made entirely differentiable and so the whole network can be trained end-to-end with simple gradient descent.
This type of artificial attention is thus a form of iterative re-weighting. Specifically, it dynamically highlights different components of a pre-processed input as they are needed for output generation. This makes it flexible and context dependent, like biological attention. As such it is also inherently dynamic. While sequence modeling already has an implied temporal component, this form of attention can also be applied to static inputs and outputs (as will be discussed below in the context of image processing) and will thus introduce dynamics into the model.
In the traditional encoder-decoder framework without attention, the encoder produced a fixed-length vector that was independent of the length or features of the input and static during the course of decoding. This forced long sequences or sequences with complex structure to be represented with the same dimensionality as shorter or simpler ones and didn't allow the decoder to interrogate different parts of the input during the decoding process. But encoding the input as a set of vectors equal in length to the input sequence makes it possible for the decoder to selectively attend to the portion of the input sequence relevant at each time point of the decoding. Again, as in interpretations of attention in the brain, attention in artificial systems is helpful as a way to flexibly wield limited resources. The decoder can't reasonably be conditioned on the entirety of the input so at some point a bottleneck must be introduced. In the system without attention, the fixed-length encoding vector was a bottleneck. When an attention mechanism is added, the encoding can be larger because the bottleneck (in the form of the context vector) will be produced dynamically as the decoder determines which part of the input to attend to.
The motivation for adding such attention mechanisms to artificial systems is of course to improve their performance. But another claimed benefit of attention is interpretability. By identifying on which portions of the input attention is placed (that is, which α i values are high) during the decoding process, it may be possible to gain an understanding of why the decoder produced the output that it did. However, caution should be applied when interpreting the outputs of attention as they may not always explain the behavior of the model as expected ( Jain and Wallace, 2019 ; Wiegreffe and Pinter, 2019 ).
In the following subsections, specific applications of this general attention concept will be discussed, along with some that don't fit neatly into this framework. Further analogies to the biology will also be highlighted.
3.1. Attention for Natural Language Processing
As described above, attention mechanisms have frequently been added to models charged with processing sequences. Natural language processing (NLP) is one of the most common areas of application for sequence modeling. And, though it was not the original domain of attention in machine learning—nor does it have the most in common with biology—NLP is also one of the most common areas of application for attention ( Galassi et al., 2019 ).
An early application of the this form of attention in artificial neural networks was to the task of translation ( Bahdanau et al., 2014 ) ( Figure 3 ). In this work, a recurrent neural network encodes the input sentence as a set of “annotation” vectors, one for each word in the sentence. The output, a sentence in the target language, is generated one word at a time by a recurrent neural network. The probability of each generated word is a function of the previously generated word, the hidden state of the recurrent neural network and a context vector generated by the attention mechanism. Here, the attention mechanism is a small feedforward neural network that takes in the hidden state of the output network as well as the current annotation vector to create the weighting over all annotation vectors.
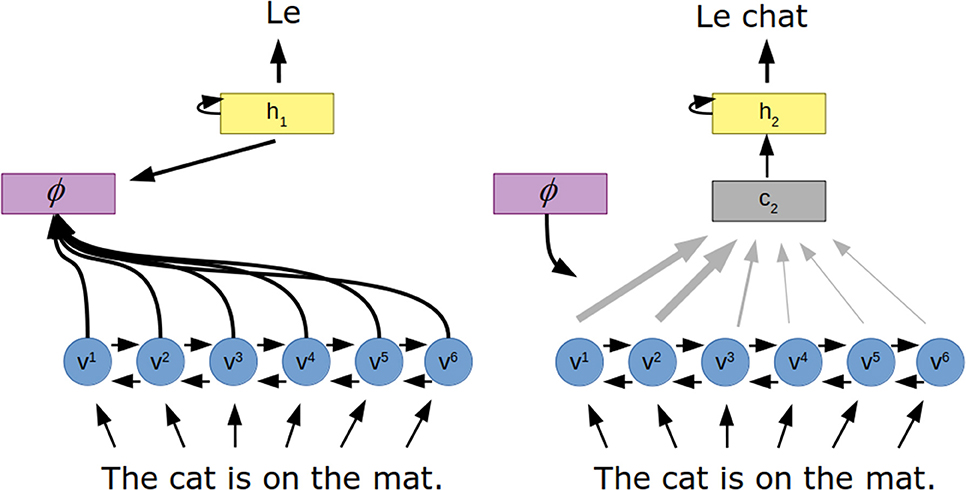
Figure 3 . Attention for neural machine translation. The to-be-translated sentence is encoded to a series of vectors ( v ) via a recurrent neural network. The attention mechanism (ϕ) uses the hidden state of the decoder ( h ) and these vectors to determine how the encoded vectors should be combined to produce a context vector ( c ), which influences the next hidden state of the decoder and thus the next word in the translated sentence.
Blending information from all the words in the sentence this way allows the network to pull from earlier or later parts when generating an output word. This can be especially useful for translating between languages with different standard word orders. By visualizing the locations in the input sentence to which attention was applied the authors observed attention helping with this problem.
Since this initial application, many variants of attention networks for language translation have been developed. In Firat et al. (2016) , the attention mechanism was adapted so it could be used to translate between multiple pairs of languages rather than just one. In Luong et al. (2015) , the authors explore different structures of attention to determine if the ability to access all input words at once is necessary. And in Cheng et al. (2016) , attention mechanisms were added to the recurrent neural networks that perform the sentence encoding and decoding in order to more flexibly create sentence representations.
In 2017, the influential “Attention is All You Need” paper utilized a very different style of architecture for machine translation ( Vaswani et al., 2017 ). This model doesn't have any recurrence, making it simpler to train. Instead, words in the sentence are encoded in parallel and these encodings generate key and query representations that are combined to create attention weightings. These weightings scale the word encodings themselves to create the next layer in the model, a process known as “self-attention.” This process repeats, and eventually interacts with the autoregressive decoder which also has attention mechanisms that allow it to flexibly focus on the encoded input (as in the standard form of attention) and on the previously generated output. The Transformer—the name given to this new attention architecture—outperformed many previous models and quickly became the standard for machine translation as well as other tasks ( Devlin et al., 2018 ).
Interestingly, self-attention has less in common with biological attention than the recurrent attention models originally used for machine translation. First, it reduces the role of recurrence and dynamics, whereas the brain necessarily relies on recurrence in sequential processing tasks, including language processing and attentional selection. Second, self-attention provides a form of horizontal interaction between words—which allows for words in the encoded sentence to be processed in the context of those around them—but this mechanism does not include an obvious top-down component driven by the needs of the decoder. In fact, self-attention has been shown under certain circumstances to simply implement a convolution, a standard feedforward computation frequently used in image processing ( Andreoli, 2019 ; Cordonnier et al., 2019 ). In this way, self-attention is more about creating a good encoding than performing a task-specific attention-like selection based on limited resources. In the context of a temporal task, its closest analogue in psychology may be priming because priming alters the encoding of subsequent stimuli based on those that came before. It is of course not the direct goal of machine learning engineers to replicate the brain, but rather to create networks that can be easily trained to perform well on tasks. These different constraints mean that even large advances in machine learning do not necessarily create more brain-like models.
While the study of attention in human language processing is not as large as other areas of neuroscience research, some work has been done to track eye movements while reading ( Myachykov and Posner, 2005 ). They find that people will look back at previous sections of text in order to clarify what they are currently reading, particularly in the context of finding the antecedent of a pronoun. Such shifts in overt attention indicate what previous information is most relevant for the current processing demands.
3.2. Attention for Visual Tasks
As in neuroscience and psychology, a large portion of studies in machine learning are done on visual tasks. One of the original attention-inspired tools of computer vision is the saliency map, which identifies which regions in an image are most salient based on a set of low-level visual features such as edges, color, or depth and how they differ from their surround ( Itti and Koch, 2001 ). In this way, saliency maps indicate which regions would be captured by “bottom-up” attention in humans and animals. Computer scientists have used saliency maps as part of their image processing pipeline to identify regions for further processing.
In more recent years, computer vision models have been dominated by deep learning. And since their success in the 2012 ImageNet Challenge ( Russakovsky et al., 2015 ), convolutional neural networks have become the default architecture for visual tasks in machine learning.
The architecture of convolutional neural networks is loosely based on the mammalian visual system ( Lindsay, 2020 ). At each layer, a bank of filters is applied to the activity of the layer below (in the first layer this is the image). This creates a H × W × C tensor of neural activity with the number of channels, C equal to the number of filters applied and H and W representing the height and width of the 2-D feature maps that result from the application of a filter.
Attention in convolutional neural networks has been used to enhance performance on a variety of tasks including classification, segmentation, and image-inspired natural language processing. Also, as in the neuroscience literature, these attentional processes can be divided into spatial and feature-based attention.
3.2.1. Spatial Attention
Building off of the structures used for attention in NLP tasks, visual attention has been applied to image captioning. In Xu et al. (2015) , the encoding model is a convolutional neural network. The attention mechanism works over the activity at the fourth convolutional layer. As each word of the caption is generated, a different pattern of weighting across spatial locations of the image representation is created. In this way, attention for caption generation replaces the set of encoded word vectors in a translation task with a set of encoded image locations. Visualizing the locations with high weights, the model appears to attend to the object most relevant to the current word being generated for the caption.
This style of attention is referred to as “soft” because it produces a weighted combination of the visual features over spatial locations ( Figure 4B ). “Hard” attention is an alternative form that chooses a single spatial location to be passed into the decoder at the expense of all others ( Figure 4A ). In Xu et al. (2015) , to decide which location should receive this hard attention, the attention weights generated for each spatial location were treated as probabilities. One location is chosen according to these probabilities. Adding this stochastic element to the network makes training more difficult, yet it was found to perform somewhat better than soft attention.
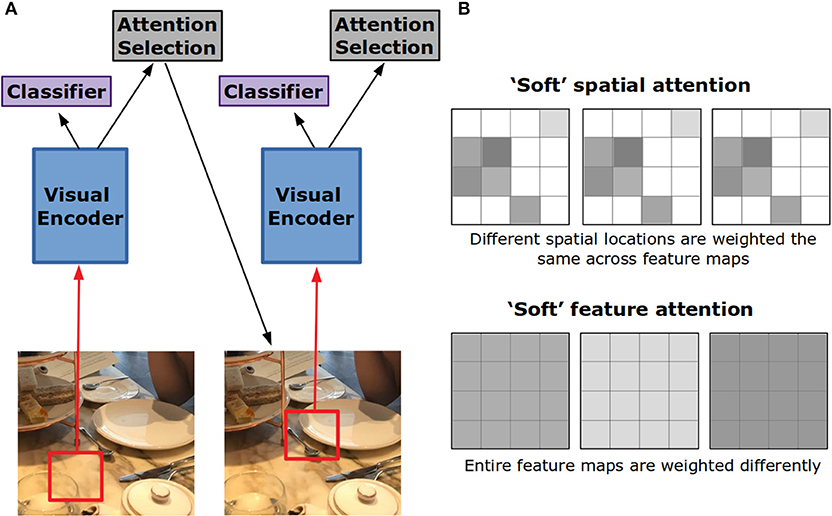
Figure 4 . Hard vs. soft visual attention in artificial neural networks. (A) In hard attention, the network only gets input from a small portion of the whole image. This portion is iteratively chosen by the network through an attention selection mechanism. If the input is foveated, the network can use the lower resolution periphery to guide this selection. (B) Feature maps in convolutional neural networks are 2-D grids of activation created by the application of a filter to the layer below. In soft spatial attention, different locations on these grids are weighted differently. In soft feature attention, different feature maps are weighted differently.
A 2014 study used reinforcement learning to train a hard attention network to perform object recognition in challenging conditions ( Mnih et al., 2014 ). The core of this model is a recurrent neural network that both keeps track of information taken in over multiple “glimpses” made by the network and outputs the location of the next glimpse. For each glimpse, the network receives a fovea-like input (central areas are represented with high resolution and peripheral with lower) from a small patch of the image. The network has to integrate the information gained from these glimpses to find and classify the object in the image. This is similar to the hard attention described above, except the selection of a location here determines which part of the image is sampled next (whereas in the case above it determined which of the already-processed image locations would be passed to the decoder). With the use of these glimpses, the network is not required to process all of the image, saving computational resources. It can also help when multiple objects are present in the image and the network must classify each ( Ba et al., 2014 ). Recent work has shown that adding a pre-training step enhances the performance of hard attention applied to complex images ( Elsayed et al., 2019 ).
In many ways, the correspondence between biological and artificial attention is strongest when it comes to visual spatial attention. For example, this form of hard attention—where different locations of the image are sequentially-sampled for further processing—replicates the process of saccading and is therefore akin to overt visual attention in the neuroscience and psychology literature. Insofar as soft attention dynamically re-weights different regions of the network's representation of the image without any change in the input to the network, it is akin to covert spatial attention. Also, as the mode of application for soft attention involves multiplicative scaling of the activity of all units at a specific location, it replicates neural findings about covert spatial attention.
Soft spatial attention has been used for other tasks, including visual question and answering ( Chen et al., 2015 ; Xu and Saenko, 2016 ; Yang et al., 2016 ) and action recognition in videos ( Sharma et al., 2015 ). Hard attention has also been used for instance segmentation ( Ren and Zemel, 2017 ) and for fine-grained classification when applied using different levels of image resolution ( Fu et al., 2017 ).
3.2.2. Feature Attention
In the case of soft spatial attention, weights are different in different spatial locations of the image representation yet they are the same across all feature channels at that location. That is, the activity of units in the network representing different visual features will all be modified the same way if they represent the same location in image space. Feature attention makes it possible to dynamically re-weight individual feature maps, creating a spatially global change in feature processing.
In Stollenga et al. (2014) , a convolutional neural network is equipped with a feature-based attention mechanism. After an image is passed through the standard feedforward architecture, the activity of the network is passed into a policy that determines how the different feature maps at different layers should be weighted. This re-weighting leads to different network activity which leads to different re-weightings. After the network has run for several timesteps the activity at the final layer is used to classify the object in the image. The policy that determines the weighting values is learned through reinforcement learning, and can be added to any pre-trained convolutional neural network.
The model in Chen et al. (2017) combines feature and spatial attention to aid in image captioning. The activity of the feedforward pass of the convolutional network is passed into the attention mechanism along with the previously generated word to create attention weightings for different channels at each layer in the CNN. These weights are used to scale activity and then a separate attention mechanism does the same procedure for generating spatial weightings. Both spatial and feature attention weights are generated and applied to the network at each time point.
In the model in De Vries et al. (2017) , the content of a question is used to control how a CNN processes an image for the task of visual question and answering. Specifically, the activity of a language embedding network is passed through a multi-layer perceptron to produce the additive and multiplicative parameters for batch normalization of each channel in the CNN. This procedure, termed conditional batch normalization, functions as a form of question-dependent feature attention.
A different form of dynamic feature re-weighting appears in “squeeze-and-excitation” networks ( Hu et al., 2018 ). In this architecture, the weightings applied to different channels are a nonlinear function of the activity of the other channels at the same layer. As with “self-attention” described above, this differs in spirit from more “top-down” approaches where weightings are a function of activity later in the network and/or biased by the needs of the output generator. Biologically speaking, this form of interaction is most similar to horizontal connections within a visual area, which are known to carry out computations such as divisive normalization ( Carandini and Heeger, 2012 ).
In the study of the biology of feature-based attention, subjects are usually cued to attend to or search for specific visual features. In this way, the to-be-attended features are known in advance and relate to the specific sub-task at hand (e.g., detection of a specific shape on a given trial of a general shape detection task). This differs from the above instances of artificial feature attention, wherein no external cue biases the network processing before knowledge about the specific image is available. Rather, the feature re-weighting is a function of the image itself and meant to enhance the performance of the network on a constant task (note this was also the case for the forms of artificial spatial attention described).
The reason for using a cueing paradigm in studies of biological attention is that it allows the experimenter to control (and thus know) where attention is placed. Yet, it is clear that even without explicit cueing, our brains make decisions about where to place attention constantly; these are likely mediated by local and long-range feedback connections to the visual system ( Wyatte et al., 2014 ). Therefore, while the task structure differs between the study of biological feature attention and its use in artificial systems, this difference may only be superficial. Essentially, the artificial systems are using feedforward image information to internally generate top-down attentional signals rather than being given the top-down information in the form of a cue.
That being said, some artificial systems do allow for externally-cued feature attention. For example setting a prior over categories in the network in Cao et al. (2015) makes it better at localizing the specific category. The network in Wang et al. (2014) , though not convolutional, has a means of biasing the detection of specific object categories as well. And in Lindsay and Miller (2018) , several performance and neural aspects of biological feature attention during a cued object detection task were replicated using a CNN. In Luo et al. (2020) , the costs and benefits of using a form of cued attention in CNNs were explored.
As mentioned above, the use of multiplicative scaling of activity is in line with certain findings from biological visual attention. Furthermore, modulating entire feature maps by the same scalar value is aligned with the finding mentioned above that feature attention acts in a spatially global way in the visual system.
3.3. Multi-Task Attention
Multi-task learning is a challenging topic in machine learning. When one network is asked to perform several different tasks—for example, a CNN that must classify objects, detect edges, and identify salient regions—training can be difficult as the weights needed to do each individual task may contradict each other. One option is have a set of task-specific parameters that modulate the activity of the shared network differently for each task. While not always called it, this can reasonably be considered a form of attention, as it flexibly alters the functioning of the network.
In Maninis et al. (2019) , a shared feedforward network is trained on all of multiple tasks, while task specific skip connections and squeeze-and-excitation blocks are trained to modulate this activity only on their specific task. This lets the network benefit from sharing processing that is common to all tasks while still specializing somewhat to each.
A similar procedure was used in Rebuffi et al. (2017) to create a network that performs classification on multiple different image domains. There, the domain could be identified from the input image making it possible to select the set of task-specific parameters automatically at run-time.
In Zhao et al. (2018) , the same image can be passed into the network and be classified along different dimensions (e.g. whether the person in the picture is smiling or not, young or old). Task-specific re-weighting of feature channels is used to execute these different classifications.
The model in Strezoski et al. (2019) uses what could be interpreted as a form of hard feature attention to route information differently in different tasks. Binary masks over feature channels are chosen randomly for each task. These masks are applied in a task-specific way during training on all tasks and at run-time. Note that in this network no task-specific attentional parameters are learned, as these masks are pre-determined and fixed during training. Instead, the network learns to use the different resulting information pathways to perform different tasks.
In a recent work, the notion of task-specific parameters was done away with entirely ( Levi and Ullman, 2020 ). Instead, the activations of a feedforward CNN are combined with a task input and passed through a second CNN to generate a full set of modulatory weights. These weights then scale the activity of the original network in a unit-specific way (thus implementing both spatial and feature attention). The result is a single set of feedforward weights capable of flexibly engaging in multiple visual tasks.
When the same input is processed differently according to many different tasks, these networks are essentially implementing a form of within-modality task switching that relies on feature attention. In this way, it is perhaps most similar to the Stroop test described previously.
3.4. Attention to Memory
Deep neural networks tend not to have explicit memory, and therefore attention to memory is not studied. Neural Turing Machines, however, are a hybrid neural architecture that includes external memory stores ( Graves et al., 2014 ). The network, through training, learns how to effectively interact with these stores to perform tasks such as sorting and repetition of stored sequences. Facilitating this interaction is a form of attention. Memories are stored as a set of vectors. To retrieve information from this store, the network generates a weight for each vector and calculates a weighted sum of the memories. To determine these weights, a recurrent neural network (which receives external and task-relevant input) outputs a vector and memories are weighted in accordance to their similarity to this vector. Thus, at each point in time, the network is able to access context-relevant memories.
As described previously, how the brain chooses what memories to attend to and then attends to them is not entirely clear. The use of a similarity metric in this model means that memories are retrieved based on their overlap with a produced activity vector, similar to associative memory models in the neuroscience literature. This offers a mechanism for the latter question—that is, how attention to memory could be implemented in the brain. The activity vector that the model produces controls what memories get attended and the relationship with biology is less clear here.
4. Ideas for Future Interaction Between Artificial and Biological Attention
As has been shown, some amount of inspiration from biology has already led to several instances of attention in artificial neural networks (summarized in Figure 5 ). While the addition of such attention mechanisms has led to appreciable increases in performance in these systems, there are clearly still many ways in which they fall short and additional opportunities for further inspiration exist. In the near term, this inspiration will likely be in the form of incremental improvements to specialized artificial systems as exist now. However, the true promise of brain-inspired AI should deliver a more integrated, multiple-purpose agent that can engage flexibly in many tasks.
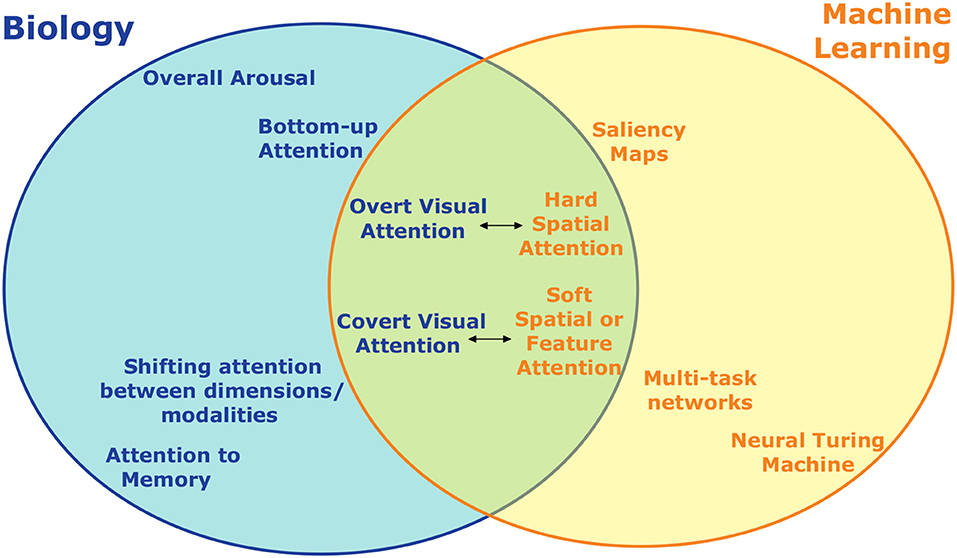
Figure 5 . An incomplete summary of the different types of attention studied in neuroscience/psychology and machine learning and how they relate. On the left are divisions of attention studied biologically, on the right are those developed for artificial intelligence and machine learning. Topics at the same horizontal location are to some extent analogous, with the distance between them indicating how close the analogy is. Forms of visual attention, for example, have the most overlap and are the most directly comparable across biology and machine learning. Some forms of attention, such as overall arousal, don't have an obvious artificial analogue.
4.1. How to Enhance Performance
There are two components to the study of how attention works in the brain that can be considered flip sides of the same coin. The first is the question of how attention enhances performance in the way that it does—that is, how do the neural changes associated with attention make the brain better at performing tasks. The second is how and why attention is deployed in the way that it is—what factors lead to the selection of certain items or tasks for attention and not others.
Neuroscientists have spent a lot of time investigating the former question. In large part, the applicability of these findings to artificial neural systems, however, may not be straightforward. Multiplicative scaling of activity appears in both biological and artificial systems and is an effective means of implementing attention. However, many of the observed effects of attention in the brain make sense mainly as a means of increasing the signal carried by noisy, spiking neurons. This includes increased synchronization across neurons and decreased firing variability. Without analogs for these changes in deep neural networks, it is hard to take inspiration from them. What's more, the training procedures for neural networks can automatically determine the changes in activity needed to enhance performance on a well-defined task and so lessons from biological changes may not be as relevant.
On the other hand, the observation that attention can impact spiking-specific features such as action potential height, burstiness, and precise spike times may indicate the usefulness of spiking networks. Specifically, spiking models offer more degrees of freedom for attention to control and thus allow attention to possibly have larger and/or more nuanced impacts.
Looking at the anatomy of attention may provide usable insights to people designing architectures for artificial systems. For example, visual attention appears to modulate activity more strongly in later visual areas like V4 ( Noudoost et al., 2010 ), whereas auditory attention can modulate activity much earlier in the processing stream. The level at which attention should act could thus be a relevant architectural variable. In this vein, recent work has shown that removing self-attention from the early layers of a Transformer model enhances its performance on certain natural language processing tasks and also makes the model a better predictor of human fMRI signals during language processing ( Toneva and Wehbe, 2019 ).
The existence of cross-modal cueing—wherein attention cued in one sensory modality can cause attention to be deployed to the same object or location in another modality—indicates some amount of direct interaction between different sensory systems. Whereas many multi-modal models in machine learning use entirely separate processing streams that are only combined at the end, allowing some horizontal connections between different input streams may help coordinate their processing.
Attention also interacts with the kind of adaptation that normally occurs in sensory processing. Generally, neural network models do not have mechanisms for adaptation—that is, neurons have no means of reducing their activity if given the same input for multiple time steps. Given that adaptation helps make changes and anomalies stand out, it may be useful to include. In a model with adaption, attention mechanisms should work to reactivate adapted neurons if the repeated stimulus is deemed important.
Finally, some forms of attention appear to act in multiple ways on the same system. For example, visual attention is believed to both: (1) enhance the sensitivity of visual neurons in the cortex by modulating their activity and (2) change subcortical activity such that sensory information is readout differently ( Birman and Gardner, 2019 ; Sreenivasan and Sridharan, 2019 ). In this way, attention uses two different mechanisms, in different parts of the brain, to create its effect. Allowing attention to modulate multiple components of a model architecture in complementary ways may allow it to have more robust and effective impacts.
4.2. How to Deploy Attention
The question of how to deploy attention is likely the more relevant challenge for producing complex and integrated artificial intelligence. Choosing the relevant information in a stream of incoming stimuli, picking the best task to engage in, or deciding whether to engage in anything at all requires that an agent have an integrative understanding of its state, environment, and needs.
The most direct way to take influence from biological attention is to mimic it directly. Scanpath models, for example, have existed in the study of saliency for many years. They attempt to predict the series of fixations that humans make while viewing images ( Borji and Itti, 2019 ). A more direct approach to training attention was used in Linsley et al. (2018) . Here, a large dataset of human top-down attention was collected by having subjects label the regions of images most relevant for object classification. The task-specific saliency maps created through this method were used to train attention in a deep convolutional neural network whose main task was object recognition. They found that influencing the activity of intermediate layers with this method could increase performance. Another way of learning a teacher's saliency map was given in Zagoruyko and Komodakis (2016) .
Combined training on tasks and neural data collected from human visual areas has also helped the performance of CNNs ( Fong et al., 2018 ). Using neural data collected during attention tasks in particular could help train attention models. Such transfer could also be done for other tasks. For example, tracking eye movements during reading could inform NLP models; thus far, eye movements have been used to help train a part-of-speech tagging model ( Barrett et al., 2016 ). Interestingly, infants may learn from attending to what adults around them attend to and the coordination of attention more broadly across agents may be very helpful in a social species. Therefore, the attention of others should influence how attention is guided. Attempts to coordinate joint attention will need to be integrated into attention systems ( Kaplan and Hafner, 2006 ; Klein et al., 2009 ).
Activities would likely need to flexibly decide which of several possible goals should be achieved at any time and therefore where attention should be placed. This problem clearly interacts closely with issues around reinforcement learning—particularly hierarchical reinforcement learning which involves the choosing of subtasks—as such decisions must be based on expected positive or negative outcomes. Indeed, there is a close relationship between attention and reward as previously rewarded stimuli attract attention even in contexts where they no longer provide reward ( Camara et al., 2013 ). A better understanding of how humans choose which tasks to engage in and when should allow human behavior to inform the design of a multi-task AI.
To this end, the theory put forth in Shenhav et al. (2013) , which says that allocation of the brain's limited ability to control different processes is based on the expected value of that control, may be of use. In this framework, the dorsal anterior cingulate cortex is responsible for integrating diverse information—including the cognitive costs of control—in order to calculate the expected value of control and thus direct processes like attention. Another approach for understanding human executive control in complex tasks is inverse reinforcement learning. This method was recently applied to a dataset of eye movements during visual search in order to determine the reward functions and policies used by humans ( Zelinsky et al., 2020 ).
An additional factor that drives biological attention but is perhaps underrepresented in artificial attention systems is curiosity ( Gottlieb et al., 2013 ). In biology, novel, confusing, and surprising stimuli can grab attention, and inferotemporal and perirhinal cortex are believed to signal novel visual situations via an adaptation mechanism that reduces responses to familiar inputs. Reinforcement learning algorithms that include novelty as part of the estimate of the value of a state can encourage this kind of exploration ( Jaegle et al., 2019 ). How exactly to calculate surprise or novelty in different circumstances is not always clear, however. Previous work on biological attention has understood attention selection in Bayesian terms of surprise or information gathering and these framings may be useful for artificial systems ( Itti and Baldi, 2006 ; Mirza et al., 2019 ).
A final issue in the selection of attention is how conflicts are resolved. Given the brain's multiple forms of attention—arousal, bottom-up, top-down, etc.—how do conflicts regarding the appropriate locus of attention get settled? Looking at the visual system, it seems that the local circuits that these multiple systems target are burdened with this task. These circuits receive neuromodulatory input along with top-down signals which they must integrate with the bottom-up input driving their activity. Horizontal connections mediate this competition, potentially using winner-take-all mechanisms. This can be mimicked in the architecture of artificial systems.
4.3. Attention and Learning
Attention, through its role in determining what enters memory, guides learning. Most artificial systems with attention include the attention mechanism throughout training. In this way, the attention mechanism is trained along with the base architecture; however, with the exception of the Neural Turing Machine, the model does not continue learning once the functioning attention system is in place. Therefore, the ability of attention to control learning and memory is still not explicitly considered in these systems.
Attention could help make efficient use of data by directing learning to the relevant components and relationships in the input. For example, saliency maps have been used as part of the pre-processing for various computer vision tasks ( Lee et al., 2004 ; Wolf et al., 2007 ; Bai and Wang, 2014 ). Focusing subsequent processing only on regions that are intrinsically salient can prevent wasteful processing on irrelevant regions and, in the context of network training, could also prevent overfitting to these regions. Using saliency maps in this way, however, requires a definition of saliency that works for the problem at hand. Using the features of images that capture bottom-up attention in humans has worked for some computer vision problems; looking at human data in other modalities may be useful as well.
In a related vein, studies on infants suggest that they have priors that guide their attention to relevant stimuli such as faces. Using such priors could bootstrap learning both of how to process important stimuli and how to better attend to their relevant features ( Johnson, 2001 ).
In addition to deciding which portions of the data to process, top-down attention can also be thought of as selecting which elements of the network should be most engaged during processing. Insofar as learning will occur most strongly in the parts of the network that are most engaged, this is another means by which attention guides learning. Constraining the number of parameters that will be updated in response to any given input is an effective form of regularization, as can be seen in the use of dropout and batch normalization. Attention—rather than randomly choosing which units to engage and disengage—is constrained to choose units that will also help performance on this task. It is therefore a more task-specific form of regularization.
In this way, attention may be particularly helpful for continual learning where the aim is to update a network to perform better on a specific task while not disrupting performance on the other tasks the network has already learned to do. A related concept, conditional computation, has recently been applied to the problem of continual learning ( Lin et al., 2019 ). In conditional computation, the parameters of a network are a function of the current input (it can thus be thought of as an extreme form of the type of modulation done by attention); optimizing the network for efficient continual learning involves controlling the amount of interference between different inputs. More generically, it may be helpful to think of attention, in part, as a means of guarding against undesirable synaptic changes.
Attention and learning also work in a loop. Specifically, attention guides what is learned about the world and internal world models are used to guide attention. This inter-dependency has recently been formalized in terms of a reinforcement learning framework that also incorporates cognitive Bayesian inference models that have succeeded in explaining human learning and decision making ( Radulescu et al., 2019 ). Interconnections between basal ganglia and prefrontal cortex are believed to support the interplay between reinforcement learning and attention selection.
At a more abstract level, the mere presence of attention in the brain's architecture can influence representation learning. The global workspace theory of consciousness says that at any moment a limited amount of information selected from the brain's activity can enter working memory and be available for further joint processing ( Baars, 2005 ). Inspired by this, the ‘consciousness prior' in machine learning emphasizes a neural network architecture with a low-dimensional representation that arises from attention applied to an underlying high-dimensional state representation ( Bengio, 2017 ). This low-D representation should efficiently represent the world at an abstract level such that it can be used to summarize and make predictions about future states. The presence of this attention-mediated bottleneck has a trickle-down effect that encourages disentangled representations at all levels such that they can be flexibly combined to guide actions and make predictions.
Conscious attention is required for the learning of many complex skills such as playing a musical instrument. However once fully learned, these processes can become automatic, possibly freeing attention up to focus on other things ( Treisman et al., 1992 ). The mechanisms of this transformation are not entirely clear but insofar as they seem to rely on moving the burden of the task to different, possibly lower/more reflexive brain areas, it may benefit artificial systems to have multiple redundant pathways that can be engaged differently by attention ( Poldrack et al., 2005 ).
4.4. Limitations of Attention: Bugs or Features?
Biological attention does not work perfectly. As mentioned above, performance can suffer when switching between different kinds of attention, arousal levels need be just right in order to reach peak performance, and top-down attention can be interrupted by irrelevant but salient stimuli. A question when transferring attention to artificial systems is are these limitations bugs to be avoided or features to be incorporated?
Distractability, in general, seems like a feature of attention rather than a bug. Even when attempting to focus on a task it is beneficial to still be aware of—and distractable by—potentially life-threatening changes in the environment. The problem comes only when an agent is overly distractable to inputs that do not pose a threat or provide relevant information. Thus, artificial systems should balance the strength of top down attention such that it still allows for the processing of unexpected but informative stimuli. For example, attentional blink refers to the phenomenon wherein a subject misses a second target in a stream of targets and distractors if it occurs quickly after a first target ( Shapiro et al., 1997 ). While this makes performance worse, it may be necessary to give the brain time to process and act on the first target. In this way, it prevents distractability to ensure follow through.
Any agent, artificial or biological, will have some limitations on its energy resources. Therefore, prudent decisions about when to engage in the world versus enter an energy-saving state such as sleep will always be of relevance. For many animals sleep occurs according to a schedule but, as was discussed, it can also be delayed or interrupted by attention-demanding situations. The decision about when to enter a sleep state must thus be made based on a cost-benefit analysis of what can be gained by staying awake. Because sleep is also known to consolidate memories and perform other vital tasks beyond just energy conservation, this decision may be a complex one. Artificial systems will need to have an integrative understanding of their current state and future demands to make this decision.
5. Conclusions
Attention is a large and complex topic that sprawls across psychology, neuroscience, and artificial intelligence. While many of the topics studied under this name are non-overlapping in their mechanisms, they do share a core theme of the flexible control of limited resources. General findings about flexibility and wise uses of resources can help guide the development of AI, as can specific findings about the best means of deploying attention to specific sensory modalities or tasks.
Author Contributions
GL conceived and wrote the article and generated the figures.
This work was supported by a Marie Skłodowska-Curie Individual Fellowship (No. 844003) and a Sainsbury Wellcome Centre/Gatsby Computational Unit Fellowship.
Conflict of Interest
The author declares that the research was conducted in the absence of any commercial or financial relationships that could be construed as a potential conflict of interest.
The reviewer MR declared a past co-authorship with the author GL to the handling Editor.
Acknowledgments
The author would like to thank Jacqueline Gottlieb and the three reviewers for their insights and pointers to references.
Ahissar, M., and Hochstein, S. (2000). The spread of attention and learning in feature search: effects of target distribution and task difficulty. Vis. Res. 40, 1349–1364. doi: 10.1016/S0042-6989(00)00002-X
PubMed Abstract | CrossRef Full Text | Google Scholar
Aly, M., and Turk-Browne, N. B. (2017). “How hippocampal memory shapes, and is shaped by, attention,” in The Hippocampus From Cells to Systems , eds D. E. Hannula and M. C. Duff (Cham: Springer), 369–403.
Google Scholar
Anderson, E. B., Mitchell, J. F., and Reynolds, J. H. (2013). Attention-dependent reductions in burstiness and action-potential height in macaque area V4. Nat. Neurosci. 16, 1125–1131. doi: 10.1038/nn.3463
Andreoli, J.-M. (2019). Convolution, attention and structure embedding. arXiv [preprint] . arXiv: 1905.01289.
Anton-Erxleben, K., and Carrasco, M. (2013). Attentional enhancement of spatial resolution: linking behavioural and neurophysiological evidence. Nat. Rev. Neurosci. 14, 188–200. doi: 10.1038/nrn3443
Anton-Erxleben, K., Herrmann, K., and Carrasco, M. (2013). Independent effects of adaptation and attention on perceived speed. Psychol. Sci. 24, 150–159. doi: 10.1177/0956797612449178
Arrington, C. M., Altmann, E. M., and Carr, T. H. (2003). Tasks of a feather flock together: Similarity effects in task switching. Mem. Cogn. 31, 781–789. doi: 10.3758/BF03196116
Ba, J., Mnih, V., and Kavukcuoglu, K. (2014). Multiple object recognition with visual attention. arXiv [preprint] . arXiv:1412.7755.
Baars, B. J. (2005). Global workspace theory of consciousness: toward a cognitive neuroscience of human experience. Prog. Brain Res. 150, 45–53. doi: 10.1016/S0079-6123(05)50004-9
Bahdanau, D., Cho, K., and Bengio, Y. (2014). Neural machine translation by jointly learning to align and translate. arXiv [preprint] . arXiv:1409.0473.
Bai, X., and Wang, W. (2014). Saliency-SVM: an automatic approach for image segmentation. Neurocomputing 136, 243–255. doi: 10.1016/j.neucom.2014.01.008
CrossRef Full Text | Google Scholar
Ballesteros, S., and Mayas, J. (2015). Selective attention affects conceptual object priming and recognition: a study with young and older adults. Front. Psychol. 5:1567. doi: 10.3389/fpsyg.2014.01567
Barrett, M., Bingel, J., Keller, F., and Søgaard, A. (2016). “Weakly supervised part-of-speech tagging using eye-tracking data,” in Proceedings of the 54th Annual Meeting of the Association for Computational Linguistics (Volume 2: Short Papers) (Berlin), 579–584.
Bengio, Y. (2017). The consciousness prior. arXiv [preprint] . arXiv:1709.08568.
Berger, A., Henik, A., and Rafal, R. (2005). Competition between endogenous and exogenous orienting of visual attention. J. Exp. Psychol. 134, 207–221. doi: 10.1037/0096-3445.134.2.207
Bertelson, P., and Aschersleben, G. (1998). Automatic visual bias of perceived auditory location. Psychon. Bull. Rev. 5, 482–489. doi: 10.3758/BF03208826
Bichot, N. P., Heard, M. T., DeGennaro, E. M., and Desimone, R. (2015). A source for feature-based attention in the prefrontal cortex. Neuron 88, 832–844. doi: 10.1016/j.neuron.2015.10.001
Birman, D., and Gardner, J. L. (2019). A flexible readout mechanism of human sensory representations. Nat. Commun. 10, 1–13. doi: 10.1038/s41467-019-11448-7
Borji, A., and Itti, L. (2012). State-of-the-art in visual attention modeling. IEEE Trans. Pattern Anal. Mach. Intell. 35, 185–207. doi: 10.1109/TPAMI.2012.89
Borji, A., and Itti, L. (2019). Cat2000: a large scale fixation dataset for boosting saliency research. arXiv [preprint] . arXiv:1505.03581.
Bosman, C. A., Schoffelen, J.-M., Brunet, N., Oostenveld, R., Bastos, A. M., Womelsdorf, T., et al. (2012). Attentional stimulus selection through selective synchronization between monkey visual areas. Neuron 75, 875–888. doi: 10.1016/j.neuron.2012.06.037
Botvinick, M., and Cohen, J. (1998). Rubber hands ‘feel' touch that eyes see. Nature 391, 756–756. doi: 10.1038/35784
Bronkhorst, A. W. (2015). The cocktail-party problem revisited: early processing and selection of multi-talker speech. Attent. Percept. Psychophys. 77, 1465–1487. doi: 10.3758/s13414-015-0882-9
Brown, J. M., and Denney, H. I. (2007). Shifting attention into and out of objects: evaluating the processes underlying the object advantage. Percept. Psychophys. 69, 606–618. doi: 10.3758/BF03193918
Bruce, N. D., and Tsotsos, J. K. (2009). Saliency, attention, and visual search: an information theoretic approach. J. Vis. 9:5. doi: 10.1167/9.3.5
Buschman, T. J., and Miller, E. K. (2009). Serial, covert shifts of attention during visual search are reflected by the frontal eye fields and correlated with population oscillations. Neuron 63, 386–396. doi: 10.1016/j.neuron.2009.06.020
Camara, E., Manohar, S., and Husain, M. (2013). Past rewards capture spatial attention and action choices. Exp. Brain Res. 230, 291–300. doi: 10.1007/s00221-013-3654-6
Cao, C., Liu, X., Yang, Y., Yu, Y., Wang, J., Wang, Z., et al. (2015). “Look and think twice: Capturing top-down visual attention with feedback convolutional neural networks,” in Proceedings of the IEEE International Conference on Computer Vision (Santiago, CA), 2956–2964.
Carandini, M., and Heeger, D. J. (2012). Normalization as a canonical neural computation. Nat. Rev. Neurosci. 13, 51–62. doi: 10.1038/nrn3136
Chaudhari, S., Polatkan, G., Ramanath, R., and Mithal, V. (2019). An attentive survey of attention models. arXiv [preprint] . arXiv:1904.02874.
Chen, K., Wang, J., Chen, L.-C., Gao, H., Xu, W., and Nevatia, R. (2015). ABC-CNN: an attention based convolutional neural network for visual question answering. arXiv [preprint] . arXiv:1511.05960.
Chen, L., Zhang, H., Xiao, J., Nie, L., Shao, J., Liu, W., et al. (2017). “SCA-CNN: Spatial and channel-wise attention in convolutional networks for image captioning,” in Proceedings of the IEEE Conference on Computer Vision and Pattern Recognition (Honolulu, HI), 5659–5667. doi: 10.1109/CVPR.2017.667
Chen, Z. (2012). Object-based attention: a tutorial review. Attent. Percept. Psychophys. 74, 784–802. doi: 10.3758/s13414-012-0322-z
Cheng, J., Dong, L., and Lapata, M. (2016). Long short-term memory-networks for machine reading. arXiv preprint arXiv:1601.06733 . doi: 10.18653/v1/D16-1053
Chikkerur, S., Serre, T., Tan, C., and Poggio, T. (2010). What and where: a bayesian inference theory of attention. Vis. Res. 50, 2233–2247. doi: 10.1016/j.visres.2010.05.013
Cho, K., Courville, A., and Bengio, Y. (2015). Describing multimedia content using attention-based encoder-decoder networks. IEEE Trans. Multimed. 17, 1875–1886. doi: 10.1109/TMM.2015.2477044
Chun, M. M., Golomb, J. D., and Turk-Browne, N. B. (2011). A taxonomy of external and internal attention. Annu. Rev. Psychol. 62, 73–101. doi: 10.1146/annurev.psych.093008.100427
Chun, M. M., and Jiang, Y. (1998). Contextual cueing: implicit learning and memory of visual context guides spatial attention. Cogn. Psychol. 36, 28–71. doi: 10.1006/cogp.1998.0681
Ciaramelli, E., Grady, C. L., and Moscovitch, M. (2008). Top-down and bottom-up attention to memory: a hypothesis (atom) on the role of the posterior parietal cortex in memory retrieval. Neuropsychologia 46, 1828–1851. doi: 10.1016/j.neuropsychologia.2008.03.022
Coenen, A. M. (1998). Neuronal phenomena associated with vigilance and consciousness: from cellular mechanisms to electroencephalographic patterns. Conscious. Cogn. 7, 42–53. doi: 10.1006/ccog.1997.0324
Cordonnier, J.-B., Loukas, A., and Jaggi, M. (2019). On the relationship between self-attention and convolutional layers. arXiv [preprint] . arXiv:1911.03584.
De Vries, H., Strub, F., Mary, J., Larochelle, H., Pietquin, O., and Courville, A. C. (2017). “Modulating early visual processing by language,” in Advances in Neural Information Processing Systems (Long Beach, CA), 6594–6604.
Deco, G., and Rolls, E. T. (2004). A neurodynamical cortical model of visual attention and invariant object recognition. Vis. Res. 44, 621–642. doi: 10.1016/j.visres.2003.09.037
Deco, G., and Rolls, E. T. (2005). Neurodynamics of biased competition and cooperation for attention: a model with spiking neurons. J. Neurophysiol. 94, 295–313. doi: 10.1152/jn.01095.2004
Desimone, R. (1998). Visual attention mediated by biased competition in extrastriate visual cortex. Philos. Trans. R. Soc. Lond. Ser. B Biol. Sci. 353, 1245–1255. doi: 10.1098/rstb.1998.0280
Devlin, J., Chang, M.-W., Lee, K., and Toutanova, K. (2018). BERT: pre-training of deep bidirectional transformers for language understanding. arXiv [preprint] arXiv:1810.04805.
Diamond, D. M. (2005). Cognitive, endocrine and mechanistic perspectives on non-linear relationships between arousal and brain function. Nonlinearity Biolo Toxicol Med. 3, 1–7. doi: 10.2201/nonlin.003.01.001
Driver, J. (2001). A selective review of selective attention research from the past century. Br. J. Psychol. 92, 53–78. doi: 10.1348/000712601162103
Elsayed, G., Kornblith, S., and Le, Q. V. (2019). “Saccader: improving accuracy of hard attention models for vision,” in Advances in Neural Information Processing Systems (Vancouver, BC), 700–712.
Firat, O., Cho, K., and Bengio, Y. (2016). Multi-way, multilingual neural machine translation with a shared attention mechanism. arXiv preprint arXiv:1601.01073 . doi: 10.18653/v1/N16-1101
Fong, R. C., Scheirer, W. J., and Cox, D. D. (2018). Using human brain activity to guide machine learning. Sci. Rep. 8:5397. doi: 10.1038/s41598-018-23618-6
Fries, P., Womelsdorf, T., Oostenveld, R., and Desimone, R. (2008). The effects of visual stimulation and selective visual attention on rhythmic neuronal synchronization in macaque area v4. J. Neurosci. 28, 4823–4835. doi: 10.1523/JNEUROSCI.4499-07.2008
Frings, C., Schneider, K. K., and Fox, E. (2015). The negative priming paradigm: an update and implications for selective attention. Psychon. Bull. Rev. 22, 1577–1597. doi: 10.3758/s13423-015-0841-4
Fritz, J. B., Elhilali, M., David, S. V., and Shamma, S. A. (2007). Auditory attention–focusing the searchlight on sound. Curr. Opin. Neurobiol. 17, 437–455. doi: 10.1016/j.conb.2007.07.011
Fu, J., Zheng, H., and Mei, T. (2017). “Look closer to see better: recurrent attention convolutional neural network for fine-grained image recognition,” in Proceedings of the IEEE Conference on Computer Vision and Pattern Recognition (Honolulu, HI), 4438–4446.
Galassi, A., Lippi, M., and Torroni, P. (2019). Attention, please! a critical review of neural attention models in natural language processing. arXiv [preprint] . arXiv:1902.02181.
Gardiner, J. M., and Parkin, A. J. (1990). Attention and recollective experience in recognition memory. Mem. Cogn. 18, 579–583.
PubMed Abstract | Google Scholar
Gopher, D. (1996). Attention control: explorations of the work of an executive controller. Cogn. Brain Res. 5, 23–38.
Gottfried, J. A., and Dolan, R. J. (2003). The nose smells what the eye sees: crossmodal visual facilitation of human olfactory perception. Neuron 39, 375–386. doi: 10.1016/S0896-6273(03)00392-1
Gottlieb, J., Oudeyer, P.-Y., Lopes, M., and Baranes, A. (2013). Information-seeking, curiosity, and attention: computational and neural mechanisms. Trends Cogn. Sci. 17, 585–593. doi: 10.1016/j.tics.2013.09.001
Graves, A., Wayne, G., and Danihelka, I. (2014). Neural turing machines. arXiv [preprint] . arXiv:1410.5401.
Hayden, B. Y., and Gallant, J. L. (2009). Combined effects of spatial and feature-based attention on responses of v4 neurons. Vis. Res. 49, 1182–1187. doi: 10.1016/j.visres.2008.06.011
Hayhoe, M., and Ballard, D. (2005). Eye movements in natural behavior. Trends Cogn. Sci. 9, 188–194. doi: 10.1016/j.tics.2005.02.009
Heinke, D., and Humphreys, G. W. (2003). Attention, spatial representation, and visual neglect: simulating emergent attention and spatial memory in the selective attention for identification model (SAIM). Psychol. Rev. 110, 29–87. doi: 10.1037/0033-295X.110.1.29
Heinke, D., and Humphreys, G. W. (2005). Computational models of visual selective attention: a review. Connect. Models Cogn. Psychol. 1, 273–312. doi: 10.4324/9780203647110
Hommel, B., Chapman, C. S., Cisek, P., Neyedli, H. F., Song, J.-H., and Welsh, T. N. (2019). No one knows what attention is. Attent. Percept. Psychophys. 81, 2288–2303. doi: 10.3758/s13414-019-01846-w
Hu, J., Shen, L., and Sun, G. (2018). “Squeeze-and-excitation networks,” in Proceedings of the IEEE Conference on Computer Vision and Pattern Recognition (Salt Lake City, UT), 7132–7141.
Hutmacher, F. (2019). Why is there so much more research on vision than on any other sensory modality? Front. Psychol. 10:2246. doi: 10.3389/fpsyg.2019.02246
Itti, L., and Baldi, P. F. (2006). “Bayesian surprise attracts human attention,” in Advances in Neural Information Processing Systems (Vancouver, BC), 547–554.
Itti, L., and Koch, C. (2001). Computational modelling of visual attention. Nat. Rev. Neurosci. 2, 194–203. doi: 10.1038/35058500
Jaegle, A., Mehrpour, V., and Rust, N. (2019). Visual novelty, curiosity, and intrinsic reward in machine learning and the brain. Curr. Opin. Neurobiol. 58, 167–174. doi: 10.1016/j.conb.2019.08.004
Jain, S., and Wallace, B. C. (2019). Attention is not explanation. arXiv [preprint] . arXiv:1902.10186.
Johansen-Berg, H., and Lloyd, D. M. (2000). The physiology and psychology of selective attention to touch. Front. Biosci. 5, D894–D904. doi: 10.2741/A558
Johnson, M. H. (2001). Functional brain development in humans. Nat. Rev. Neurosci. 2, 475–483. doi: 10.1038/35081509
Kanwisher, N., and Wojciulik, E. (2000). Visual attention: insights from brain imaging. Nat. Rev. Neurosci. 1, 91–100. doi: 10.1038/35039043
Kaplan, F., and Hafner, V. V. (2006). The challenges of joint attention. Interact. Stud. 7, 135–169. doi: 10.1075/is.7.2.04kap
Keller, A. (2011). Attention and olfactory consciousness. Front. Psychol. 2:380. doi: 10.3389/fpsyg.2011.00380
Kelley, T. A., and Yantis, S. (2009). Learning to attend: effects of practice on information selection. J. Vis. 9:16. doi: 10.1167/9.7.16
Kelley, T. A., and Yantis, S. (2010). Neural correlates of learning to attend. Front. Hum. Neurosci. 4:216. doi: 10.3389/fnhum.2010.00216
Klein, J. T., Shepherd, S. V., and Platt, M. L. (2009). Social attention and the brain. Curr. Biol. 19, R958–R962. doi: 10.1016/j.cub.2009.08.010
Krauzlis, R. J., Lovejoy, L. P., and Zénon, A. (2013). Superior colliculus and visual spatial attention. Annu. Rev. Neurosci. 36, 165–182. doi: 10.1146/annurev-neuro-062012-170249
Lamme, V. A., and Roelfsema, P. R. (2000). The distinct modes of vision offered by feedforward and recurrent processing. Trends Neurosci. 23, 571–579. doi: 10.1016/S0166-2236(00)01657-X
Lee, S.-H., Shin, J.-K., and Lee, M. (2004). “Non-uniform image compression using biologically motivated saliency map model,” in Proceedings of the 2004 Intelligent Sensors, Sensor Networks and Information Processing Conference, 2004 (Melbourne, VIC), 525–530.
Lee, T. S., and Mumford, D. (2003). Hierarchical bayesian inference in the visual cortex. JOSA A 20, 1434–1448. doi: 10.1364/JOSAA.20.001434
Levi, H., and Ullman, S. (2020). Multi-task learning by a top-down control network. arXiv [Preprint] . arXiv:2002.03335.
Lin, M., Fu, J., and Bengio, Y. (2019). Conditional computation for continual learning. arXiv [preprint] . arXiv:1906.06635.
Lindsay, G. (2020). Convolutional neural networks as a model of the visual system: past, present, and future. J. Cogn. Neurosci. doi: 10.1162/jocn_a_01544. [Epub ahead of print].
Lindsay, G. W., and Miller, K. D. (2018). How biological attention mechanisms improve task performance in a large-scale visual system model. eLife 7:e38105. doi: 10.7554/eLife.38105
Lindsay, G. W., Rubin, D. B., and Miller, K. D. (2019). A simple circuit model of visual cortex explains neural and behavioral aspects of attention. bioRxiv. [preprint]. doi: 10.1101/2019.12.13.875534
Linsley, D., Shiebler, D., Eberhardt, S., and Serre, T. (2018). Learning what and where to attend. arXiv [preprint] . arXiv:1805.08819.
Liu, T., Slotnick, S. D., Serences, J. T., and Yantis, S. (2003). Cortical mechanisms of feature-based attentional control. Cereb. Cortex 13, 1334–1343. doi: 10.1093/cercor/bhg080
Lozito, J. P., and Mulligan, N. W. (2006). Exploring the role of attention during memory retrieval: effects of semantic encoding and divided attention. Mem. Cogn. 34, 986–998. doi: 10.3758/BF03193246
Luck, S. J., Chelazzi, L., Hillyard, S. A., and Desimone, R. (1997). Neural mechanisms of spatial selective attention in areas V1, V2, and V4 of macaque visual cortex. J. Neurophysiol. 77, 24–42. doi: 10.1152/jn.1997.77.1.24
Luo, X., Roads, B. D., and Love, B. C. (2020). The costs and benefits of goal-directed attention in deep convolutional neural networks. arXiv [preprint] . arXiv:2002.02342.
Luong, M.-T., Pham, H., and Manning, C. D. (2015). Effective approaches to attention-based neural machine translation. arXiv preprint] . arXiv:1508.04025.
Makeig, S., Jung, T.-P., and Sejnowski, T. J. (2000). Awareness during drowsiness: dynamics and electrophysiological correlates. Can. J. Exp. Psychol. 54, 266–273. doi: 10.1037/h0087346
Mancas, M., Ferrera, V. P., Riche, N., and Taylor, J. G. (2016). From Human Attention to Computational Attention, Vol. 2 . New York, NY: Springer.
Maninis, K.-K., Radosavovic, I., and Kokkinos, I. (2019). “Attentive single-tasking of multiple tasks,” in Proceedings of the IEEE Conference on Computer Vision and Pattern Recognition (Long Beach, CA), 1851–1860. doi: 10.1109/CVPR.2019.00195
Manohar, S. G., Zokaei, N., Fallon, S. J., Vogels, T., and Husain, M. (2019). Neural mechanisms of attending to items in working memory. Neurosci. Biobehav. Rev . 101, 1–12. doi: 10.1016/j.neubiorev.2019.03.017
Marks, L. E., and Wheeler, M. E. (1998). Attention and the detectability of weak taste stimuli. Chem. Senses 23, 19–29. doi: 10.1093/chemse/23.1.19
Maunsell, J. H. (2015). Neuronal mechanisms of visual attention. Annu. Rev. Vis. Sci. 1, 373–391. doi: 10.1146/annurev-vision-082114-035431
Miller, E. K., and Buschman, T. J. (2014). “Neural mechanisms for the executive control of attention,” in The Oxford Handbook of Attention , eds A. C. Nobre and S. Kastner (Oxford, UK: Oxford University Press).
Mirza, M. B., Adams, R. A., Friston, K., and Parr, T. (2019). Introducing a bayesian model of selective attention based on active inference. Sci. Rep. 9:13915. doi: 10.1038/s41598-019-50138-8
Mitchell, J. F., Sundberg, K. A., and Reynolds, J. H. (2007). Differential attention-dependent response modulation across cell classes in macaque visual area v4. Neuron 55, 131–141. doi: 10.1016/j.neuron.2007.06.018
Mnih, V., Heess, N., Graves, A., et al. (2014). “Recurrent models of visual attention,” in Advances in Neural Information Processing Systems (Montreal, QC), 2204–2212.
Moore, T., Armstrong, K. M., and Fallah, M. (2003). Visuomotor origins of covert spatial attention. Neuron 40, 671–683. doi: 10.1016/S0896-6273(03)00716-5
Murray, M. M., De Santis, L., Thut, G., and Wylie, G. R. (2009). The costs of crossing paths and switching tasks between audition and vision. Brain Cogn. 69, 47–55. doi: 10.1016/j.bandc.2008.05.004
Muzzio, I. A., Kentros, C., and Kandel, E. (2009). What is remembered? Role of attention on the encoding and retrieval of hippocampal representations. J. Physiol. 587, 2837–2854. doi: 10.1113/jphysiol.2009.172445
Myachykov, A., and Posner, M. I. (2005). “Attention in language,” in Neurobiology of Attention , eds L. Itti, G. Rees, and J. K. Tsotsos (Burlington, MA: Elsevier), 324–329.
Noudoost, B., Chang, M. H., Steinmetz, N. A., and Moore, T. (2010). Top-down control of visual attention. Curr. Opin. Neurobiol. 20, 183–190. doi: 10.1016/j.conb.2010.02.003
O'Craven, K. M., Downing, P. E., and Kanwisher, N. (1999). fMRI evidence for objects as the units of attentional selection. Nature 401, 584–587. doi: 10.1038/44134
Oken, B. S., Salinsky, M. C., and Elsas, S. (2006). Vigilance, alertness, or sustained attention: physiological basis and measurement. Clin. Neurophysiol. 117, 1885–1901. doi: 10.1016/j.clinph.2006.01.017
Olivers, C. N., and Eimer, M. (2011). On the difference between working memory and attentional set. Neuropsychologia 49, 1553–1558. doi: 10.1016/j.neuropsychologia.2010.11.033
Paneri, S., and Gregoriou, G. G. (2017). Top-down control of visual attention by the prefrontal cortex. Functional specialization and long-range interactions. Front. Neurosci. 11:545. doi: 10.3389/fnins.2017.00545
Pashler, H. (2000). “Task switching and multitask performance,” in Control of Cognitive Processes: Attention and Performance XVIII , eds S. Monsell and J. Driver (MIT Press), 277. doi: 10.1002/acp.849
Pestilli, F., Viera, G., and Carrasco, M. (2007). How do attention and adaptation affect contrast sensitivity? J. Vis. 7, 9.1–9.12. doi: 10.1167/7.7.9
Phaf, R. H., Van der Heijden, A., and Hudson, P. T. (1990). SLAM: a connectionist model for attention in visual selection tasks. Cogn. Psychol. 22, 273–341. doi: 10.1016/0010-0285(90)90006-P
Poldrack, R. A., Sabb, F. W., Foerde, K., Tom, S. M., Asarnow, R. F., Bookheimer, S. Y., et al. (2005). The neural correlates of motor skill automaticity. J. Neurosci. 25, 5356–5364. doi: 10.1523/JNEUROSCI.3880-04.2005
Posner, M. I. (2008). Measuring alertness. Ann. N. Y. Acad. Sci. 1129, 193–199. doi: 10.1196/annals.1417.011
Radulescu, A., Niv, Y., and Ballard, I. (2019). Holistic reinforcement learning: the role of structure and attention. Trends Cogn. Sci . 23, 278–292. doi: 10.1016/j.tics.2019.01.010
Rao, R. P. (2005). Bayesian inference and attentional modulation in the visual cortex. Neuroreport 16, 1843–1848. doi: 10.1097/01.wnr.0000183900.92901.fc
Rebuffi, S.-A., Bilen, H., and Vedaldi, A. (2017). “Learning multiple visual domains with residual adapters,” in Advances in Neural Information Processing Systems (Long Beach, CA), 506–516.
Ren, M., and Zemel, R. S. (2017). “End-to-end instance segmentation with recurrent attention,” in Proceedings of the IEEE Conference on Computer Vision and Pattern Recognition (Long Beach, CA), 6656–6664.
Reynolds, G. D., and Romano, A. C. (2016). The development of attention systems and working memory in infancy. Front. Syst. Neurosci. 10:15. doi: 10.3389/fnsys.2016.00015
Reynolds, J. H., and Heeger, D. J. (2009). The normalization model of attention. Neuron 61, 168–185. doi: 10.1016/j.neuron.2009.01.002
Rizzolatti, G., Riggio, L., Dascola, I., and Umiltá, C. (1987). Reorienting attention across the horizontal and vertical meridians: evidence in favor of a premotor theory of attention. Neuropsychologia 25, 31–40. doi: 10.1016/0028-3932(87)90041-8
Roelfsema, P. R., and Houtkamp, R. (2011). Incremental grouping of image elements in vision. Attent. Percept. Psychophys. 73, 2542–2572. doi: 10.3758/s13414-011-0200-0
Roelfsema, P. R., Lamme, V. A., and Spekreijse, H. (1998). Object-based attention in the primary visual cortex of the macaque monkey. Nature 395, 376–381. doi: 10.1038/26475
Rossi, A. F., and Paradiso, M. A. (1995). Feature-specific effects of selective visual attention. Vis. Res. 35, 621–634. doi: 10.1016/0042-6989(94)00156-G
Russakovsky, O., Deng, J., Su, H., Krause, J., Satheesh, S., Ma, S., et al. (2015). ImageNet large scale visual recognition challenge. Int. J. Comput. Vis. 115, 211–252. doi: 10.1007/s11263-015-0816-y
Saalmann, Y. B., Pinsk, M. A., Wang, L., Li, X., and Kastner, S. (2012). The pulvinar regulates information transmission between cortical areas based on attention demands. Science 337, 753–756. doi: 10.1126/science.1223082
Saenz, M., Buracas, G. T., and Boynton, G. M. (2002). Global effects of feature-based attention in human visual cortex. Nat. Neurosci. 5, 631–632. doi: 10.1038/nn876
Sajedin, A., Menhaj, M. B., Vahabie, A.-H., Panzeri, S., and Esteky, H. (2019). Cholinergic modulation promotes attentional modulation in primary visual cortex-a modeling study. Sci. Rep. 9:20186. doi: 10.1038/s41598-019-56608-3
Samuels, E. R., and Szabadi, E. (2008). Functional neuroanatomy of the noradrenergic locus coeruleus: its roles in the regulation of arousal and autonomic function part i: principles of functional organisation. Curr. Neuropharmacol. 6, 235–253. doi: 10.2174/157015908785777229
Schweisfurth, M. A., Schweizer, R., and Treue, S. (2014). Feature-based attentional modulation of orientation perception in somatosensation. Front. Hum. Neurosci. 8:519. doi: 10.3389/fnhum.2014.00519
Shapiro, K. L., Raymond, J., and Arnell, K. (1997). The attentional blink. Trends Cogn. Sci. 1, 291–296. doi: 10.1016/S1364-6613(97)01094-2
Sharma, S., Kiros, R., and Salakhutdinov, R. (2015). Action recognition using visual attention. arXiv [preprint] . arXiv:1511.04119.
Shenhav, A., Botvinick, M. M., and Cohen, J. D. (2013). The expected value of control: an integrative theory of anterior cingulate cortex function. Neuron 79, 217–240. doi: 10.1016/j.neuron.2013.07.007
Shipp, S. (2004). The brain circuitry of attention. Trends Cogn. Sci. 8, 223–230. doi: 10.1016/j.tics.2004.03.004
Soto, D., Heinke, D., Humphreys, G. W., and Blanco, M. J. (2005). Early, involuntary top-down guidance of attention from working memory. J. Exp. Psychol. Hum. Percept. Perform. 31, 248–261. doi: 10.1037/0096-1523.31.2.248
Soto, D., Hodsoll, J., Rotshtein, P., and Humphreys, G. W. (2008). Automatic guidance of attention from working memory. Trends Cogn. Sci. 12, 342–348. doi: 10.1016/j.tics.2008.05.007
Spence, C. (2009). Explaining the colavita visual dominance effect. Prog. Brain Res. 176, 245–258. doi: 10.1016/S0079-6123(09)17615-X
Spence, C., and Driver, J. (2004). Crossmodal Space and Crossmodal Attention . Oxford, UK: Oxford University Press.
Sreenivasan, V., and Sridharan, D. (2019). Subcortical connectivity correlates selectively with attention's effects on spatial choice bias. Proc. Natl. Acad. Sci. U.S.A. 116, 19711–19716. doi: 10.1073/pnas.1902704116
Stollenga, M. F., Masci, J., Gomez, F., and Schmidhuber, J. (2014). “Deep networks with internal selective attention through feedback connections,” in Advances in Neural Information Processing Systems (Montreal, QC), 3545–3553.
Strezoski, G., van Noord, N., and Worring, M. (2019). Many task learning with task routing. arXiv preprint arXiv:1903.12117 . doi: 10.1109/ICCV.2019.00146
Tatler, B. W., Baddeley, R. J., and Gilchrist, I. D. (2005). Visual correlates of fixation selection: effects of scale and time. Vis. Res. 45, 643–659. doi: 10.1016/j.visres.2004.09.017
Toneva, M., and Wehbe, L. (2019). “Interpreting and improving natural-language processing (in machines) with natural language-processing (in the brain),” in Advances in Neural Information Processing Systems , 14928–14938.
Treisman, A., Vieira, A., and Hayes, A. (1992). Automaticity and preattentive processing. Am. J. Psychol. 105, 341–362. doi: 10.2307/1423032
Treue, S., and Trujillo, J. C. M. (1999). Feature-based attention influences motion processing gain in macaque visual cortex. Nature 399:575. doi: 10.1038/21176
Turk-Browne, N. B., Jungé, J. A., and Scholl, B. J. (2005). The automaticity of visual statistical learning. J. Exp. Psychol. 134, 552–564. doi: 10.1037/0096-3445.134.4.552
Uncapher, M. R., and Rugg, M. D. (2005). Effects of divided attention on fmri correlates of memory encoding. J. Cogn. Neurosci. 17, 1923–1935. doi: 10.1162/089892905775008616
van Zoest, W., and Donk, M. (2005). The effects of salience on saccadic target selection. Vis. Cogn. 12, 353–375. doi: 10.1080/13506280444000229
Vaswani, A., Shazeer, N., Parmar, N., Uszkoreit, J., Jones, L., Gomez, A. N., et al. (2017). “Attention is all you need,” in Advances in Neural Information Processing Systems (Long Beach, CA), 5998–6008.
Wagner, A. D., Shannon, B. J., Kahn, I., and Buckner, R. L. (2005). Parietal lobe contributions to episodic memory retrieval. Trends Cogn. Sci. 9, 445–453. doi: 10.1016/j.tics.2005.07.001
Wang, Q., Zhang, J., Song, S., and Zhang, Z. (2014). “Attentional neural network: Feature selection using cognitive feedback,” in Advances in Neural Information Processing Systems (Montreal, QC), 2033–2041.
Watanabe, W. (2003). Is subliminal learning really passive? Nature 422:36. doi: 10.1038/422036a
Wiegreffe, S., and Pinter, Y. (2019). Attention is not not explanation. arXiv [preprint] . arXiv:1908.04626.
Wolf, L., Guttmann, M., and Cohen-Or, D. (2007). “Non-homogeneous content-driven video-retargeting,” in 2007 IEEE 11th International Conference on Computer Vision (Rio de Janeiro), 1–6.
Wolfe, J. M., and Horowitz, T. S. (2004). What attributes guide the deployment of visual attention and how do they do it? Nat. Rev. Neurosci. 5, 495–501. doi: 10.1038/nrn1411
Wood, S., Sage, J. R., Shuman, T., and Anagnostaras, S. G. (2014). Psychostimulants and cognition: a continuum of behavioral and cognitive activation. Pharmacol. Rev. 66, 193–221. doi: 10.1124/pr.112.007054
Wyatte, D., Jilk, D. J., and O'Reilly, R. C. (2014). Early recurrent feedback facilitates visual object recognition under challenging conditions. Front. Psychol. 5:674. doi: 10.3389/fpsyg.2014.00674
Xu, H., and Saenko, K. (2016). “Ask, attend and answer: exploring question-guided spatial attention for visual question answering,” in European Conference on Computer Vision (Amsterdam: Springer), 451–466.
Xu, K., Ba, J., Kiros, R., Cho, K., Courville, A., Salakhudinov, R., et al. (2015). “Show, attend and tell: Neural image caption generation with visual attention,” in International Conference on Machine Learning (Lille), 2048–2057.
Yang, Z., He, X., Gao, J., Deng, L., and Smola, A. (2016). “Stacked attention networks for image question answering,” in Proceedings of the IEEE Conference on Computer Vision and Pattern Recognition (Las Vegas, NV), 21–29.
Zagoruyko, S., and Komodakis, N. (2016). Paying more attention to attention: improving the performance of convolutional neural networks via attention transfer. arXiv [preprint] . arXiv:1612.03928.
Zelinsky, G. J., Chen, Y., Ahn, S., Adeli, H., Yang, Z., Huang, L., et al. (2020). Predicting goal-directed attention control using inverse-reinforcement learning. arXiv [preprint] . arXiv:2001.11921.
Zhao, X., Li, H., Shen, X., Liang, X., and Wu, Y. (2018). “A modulation module for multi-task learning with applications in image retrieval,” in Proceedings of the European Conference on Computer Vision (ECCV) (Munich), 401–416.
Zhou, H., and Desimone, R. (2011). Feature-based attention in the frontal eye field and area V4 during visual search. Neuron 70, 1205–1217. doi: 10.1016/j.neuron.2011.04.032
Zhou, H., Schafer, R. J., and Desimone, R. (2016). Pulvinar-cortex interactions in vision and attention. Neuron 89, 209–220. doi: 10.1016/j.neuron.2015.11.034
Keywords: attention, artificial neural networks, machine learning, vision, memory, awareness
Citation: Lindsay GW (2020) Attention in Psychology, Neuroscience, and Machine Learning. Front. Comput. Neurosci. 14:29. doi: 10.3389/fncom.2020.00029
Received: 02 December 2019; Accepted: 23 April 2020; Published: 16 April 2020.
Reviewed by:
Copyright © 2020 Lindsay. This is an open-access article distributed under the terms of the Creative Commons Attribution License (CC BY) . The use, distribution or reproduction in other forums is permitted, provided the original author(s) and the copyright owner(s) are credited and that the original publication in this journal is cited, in accordance with accepted academic practice. No use, distribution or reproduction is permitted which does not comply with these terms.
*Correspondence: Grace W. Lindsay, gracewlindsay@gmail.com
This article is part of the Research Topic
How Can Neuroscience Contribute to the Development of General Artificial Intelligence?
- Open access
- Published: 17 February 2023
A comprehensive review of attention tests: can we assess what we exactly do not understand?
- Ehsan Nasiri ORCID: orcid.org/0000-0002-9679-5623 1 , 2 ,
- Mohammad Khalilzad 1 ,
- Zahra Hakimzadeh ORCID: orcid.org/0000-0002-3046-9753 1 ,
- Ashkan Isari ORCID: orcid.org/0000-0002-4873-8384 1 ,
- Sajjad Faryabi-Yousefabad ORCID: orcid.org/0000-0001-8034-4177 3 ,
- Saeed Sadigh-Eteghad ORCID: orcid.org/0000-0003-2872-1072 2 &
- Amirreza Naseri ORCID: orcid.org/0000-0001-9723-0109 1 , 4
The Egyptian Journal of Neurology, Psychiatry and Neurosurgery volume 59 , Article number: 26 ( 2023 ) Cite this article
20k Accesses
1 Citations
Metrics details
Attention, as it is now defined as a process matching data from the environment to the needs of the organism, is one of the main aspects of human cognitive processes. There are several aspects to attention including tonic alertness (a process of intrinsic arousal that varies by minutes to hours), phasic alertness (a process that causes a quick change in attention as a result of a brief stimulus), selective attention (a process differentiating multiple stimuli), and sustained attention (a process maintaining persistence of response and continuous effort over an extended period). Attention dysfunction is associated with multiple disorders; therefore, there has been much effort in assessing attention and its domains, resulting in a battery of tests evaluating one or several attentional domains; instances of which are the Stroop color-word test, Test of Everyday Attention, Wisconsin Card Sorting Test, and Cambridge Neuropsychological Test Automated Battery. These tests vary in terms of utilities, range of age, and domains. The role of attention in human life and the importance of assessing it merits an inclusive review of the efforts made to assess attention and the resulting tests; Here we highlight all the necessary data regarding neurophysiological tests which assess human attentive function and investigates the evolution of attention tests over time. Also, the ways of assessing the attention in untestable patients who have difficulty in reading or using a computer, along with the lack of ability to comprehend verbal instructions and executive tasks, are discussed. This review can be of help as a platform for designing new studies to researchers who are interested in working on attention and conditions causing deficits in this aspect of body function, by collecting and organizing information on its assessment.
Introduction
Cognition is the general term used to refer to the process of gaining knowledge [ 1 ]. Cognition encompasses several domains, including attention, language, learning, memory, perception, and higher reasoning [ 2 ]. These domains are highly interconnected, for instance, attention and memory cannot function with another lacking operation; as memory capacity is limited comes attention to filter what gets to be encoded [ 3 ]. As a domain of cognition, attention can be defined as a crucial process for keeping thoughts organized, but psychologists and philosophers have always tried to define attention.
By the 1950s several models for attention were proposed [ 4 ]. Now an acceptable definition for Attention is a cognitive process matching data from the environment to the needs of the organism [ 5 ]. Being able to focus on one thing and ignore other stimuli is another definition provided by some [ 6 ]. Despite these definitions Bernhard Hommel and colleagues, after a discussion about the concept of attention, the difference between attention and intention, and the synthetic approach to attention found that “ no one knows, or can ever know, exactly what attention is ” [ 7 ]. This was in contrast with the prior belief about attention which was defined by William James who mentioned “Everyone knows what attention is.”
There are several aspects to attention including tonic alertness (defined as a process of intrinsic arousal that varies by minutes to hours), phasic alertness (defined as a process that causes a quick change in attention as a result of a brief stimulus), selective attention (defined as a process differentiating multiple stimuli), and sustained attention (defined as a process maintaining persistence of response and continuous effort over an extended period) [ 8 , 9 , 10 , 11 ]. Attention is also divided based on where it functions: "bottom-up" which refers to guidance that stems from external stimuli and "top-down" which refers to guidance that comes from intrinsic factors rooted in previously acquired knowledge [ 12 ].
It is generally agreed that attention can be focused on voluntarily using “top-down” signals derived from knowledge of the current task (finding your lost keys), and automatically using “bottom-up” signals captured by salient sensory events in the sensorium (a flashing fire alarm)[ 13 , 14 ]. Studies in neuroimaging and neurophysiology have discovered that a large network, involving the posterior parietal cortex, temporoparietal junction (TPJ), superior temporal sulcus, and dorsal areas of the frontal cortex, supports the orienting of attention [ 14 , 15 , 16 ]. Although there are neural correlations between both types of attention in the frontal and posterior parietal cortex, and both can modulate processing in sensory processing regions; prefrontal neurons reflected the target location first during top-down attention, whereas parietal neurons signaled it earlier during bottom-up attention [ 13 , 17 ]. According to studies, involuntary attention is highly related to ventral regions (temporoparietal junction) and can be engaged more rapidly, and is more resistant to interference than voluntary attention which is related to more dorsal segments of the frontal and parietal lobes [ 14 ].
Regardless of definition, a need for assessing attention, as one of the components of human cognition, leads to designing different attention tests. Testing attention is included in assessing the neuropsychological function of a subject [ 18 ]. Besides a few exceptions, such as the Test of Everyday Attention for Children (TEA-Ch), most attention tests evaluate attention in a wide range of ages (as young as a 4-year-old to as old as one can get) [ 19 ]. Traditionally most of the tests were taken on paper. With the invention and widespread use of computers, many tests are now conducted via the computer, although some remain only on paper or in the form of cards. Using audio tapes are also used as a way of evaluating attention and specifically to assess the auditory domain of attention [ 20 , 21 , 22 , 23 ].
Attention dysfunction is a symptom that is associated with multiple disorders [ 24 , 25 ]. In addition to attention deficit hyperactivity disorders (ADHD), Schizophrenia [ 26 ], anxiety disorder [ 27 ], depression [ 28 ], posttraumatic stress disorder [ 29 ], epilepsy [ 30 ], dementia [ 31 ], hearing loss [ 32 ], even heart disease [ 33 ] and anemia [ 34 ], can cause impairment in attentive function. Studies suggested that episodic memory loss, which is the characteristic symptom of Alzheimer's disease (AD) type dementia, may be secondary to failures of attentional processes [ 35 ]. Also, a recent study found overlapping cognitive profiles between mild cognitive impairment (MCI) and ADHD [ 36 ]. Attention has a vital role in determining one’s ability in dealing with problems, planning, organizing thoughts and behavior and choosing targets and acting towards them. Hence deficits in this domain of cognition can herald difficulties in many everyday activities in subjects suffering aforementioned conditions. Therefore, assessing attention seems necessary in evaluation of these conditions [ 37 ].
Studies on patients with AD revealed that with progressive dysfunction of prefrontal cortex (PFC) and medial temporal lobe, signs of cognitive impairment and inattention appear. Thus, a part of attention circuit consists of PFC and medial temporal lobe [ 38 ]. It is also believed that information related to bottom-up attention comes from visual cortex and is directed toward PFC. It has also been seen that when differentiating stimuli with different color and shape, PFC is activated. PFC and posterior parietal cortex (PPC) are origins for signals responsible for top-down attention. Role of PFC and PPC in maintaining attention was further established by studies stimulating these two areas during an attention-requiring activity which resulted in shorter latency of detecting and responding. PFC is activated earlier in top-down attention than PPC. Deactivation of PFC also impaired performance in all attentive tasks while PPC disruption was noticeable only in more difficult tasks. Timing and difficulty differences give the idea of a more important role for PFC in this aspect of attention [ 39 ].
Previous research has demonstrated a connection between attention dysfunction and several mental and neurological conditions. This association may be explained by the role of immune dysregulation, inflammation, anxiety, and stress in the development of ADHD, which can lead to a wide range of chronic illnesses, including cardiovascular, and metabolic diseases (due to inflammation), as well as psychiatric (such as schizophrenia and neurodegenerative diseases (due to immune dysregulation and increase in kynurenic acid (KYNA) as a result of the activation the tryptophan (TRP)-kynurenine (KYN) metabolic pathway) [ 38 , 40 , 41 ]. Stressors can induce inflammation and inflammation in turn can affect multiple brain sites resulting in impairment of cognitive function. Studies support stress role in inattention by showing a meaningful connection between childhood trauma and severity of ADHD. It has been also shown that in a stressful condition the levels of the proinflammatory cytokines rise in the brain [ 40 ]. Therefore, it is crucial to diagnose and treat attention deficit disorders early on. This makes the assessment of attention function even more important. The interaction between cognition and emotion is also considerable. Biases in attention are identified as central characteristics of depression and anxiety [ 42 ]. Emotions also impact cognition and their effect on attention has been illustrated by studies assessing reaction times under different emotions such as fear [ 43 , 44 ].
In this review, our main objective to pursue is to summarize the different neurophysiological tests which assess human attentive function and investigate the evolution of attention tests over time. We have searched PubMed and Google Scholar databases for articles and studies that evaluated attention assessment methods and tests using the "attention tests" keywords. We have considered those which were relevant to the objectives in hand and reviewed the included.
In this review, we have summarized the available cognitive tests for assessing attentive function. Table 1 briefly presents the results of this review. The table is sorted by the date each test was established and contains brief information on the setting each test is undertaken. The duration of each test, the domain(s) assessed, and the age of each test targeted are also noted. The details of the tests are mentioned afterward.
Digit Span Test (DGS)
The Digit Span Test along with the reaction time test is thought to be among the very first tests assessing cognition and attention used by psychologists. The first time DGS has been introduced and put into work was in 1716 by Gottfried Leibniz [ 45 ]. The most current version which has been used since 2008 is embedded in Wechsler Adult Intelligence Scales IV [ 46 , 47 , 48 ]. This test is also a part of the Wechsler Intelligence Scale for Children and the Working Memory Index [ 47 , 49 , 50 ]. DGS is at large a measure of working memory but is also described to be of importance in evaluating everyday simple attention [ 51 , 52 , 53 ]. DGS in its latest version includes 3 subtasks: Digit Span Forward, Digit Span Backward, and Digit Span Sequencing [ 47 ]. In this task the subject is presented with a series of random digits, then the subject is required to repeat the digits in the same sequence or backward [ 54 ].
Simple and choice reaction time (RT)
This test has been used to measure an individual’s reaction time to an illustrated stimulus since the nineteenth century. This test has two major parts, one in which the participant is timed responding to a predictable single stimulus (simple RT) and another in which the participant is expected to respond appropriately to one of several stimuli (choice RT) [ 55 , 56 ]. RT task is a common test evaluating a subject’s central processing speed which correlates with cognitive efficiency, namely sustained attention and alertness [ 57 , 58 ]. This test is both a simple single-choice and a multiple-choice RT task which consists of five steps, each a more complex chain of reactions than the previous one. In each scenario, the participants have to respond whenever a yellow dot shows up. The dot may show up in one of five designated places, and the subjects have to respond either by pressing a pad, touching the screen, or sometimes doing both. There are 4 pieces of results to the RTI which are categorized into RT and movement time, each including a simple and a five-choice task [ 56 ].
Stroop Color and Word Test
The Stroop Color and Word Test is originally a test that was used to measure the ability to inhibit cognitive interference [ 21 ]; but studies suggested the Stroop test as a measure of attentive function, especially selective attention, too [ 59 , 60 , 61 , 62 , 63 , 64 ]. The standard Stroop Test includes words typed in different colors. Participants are initially asked to read the colored names and they are timed doing so. Following this step, they are asked to identify the printed color of each word. Normally, the required time for naming the color is more than that of reading the color’s name.
The emotional Stroop test
The emotional Stroop, known as the E-Stroop test, employs target and control lists that incorporate variably colored words [ 65 ]. E-Stroop is the most commonly utilized test for measuring attentional biases [ 66 ]. Attentional bias refers to hyper-attention to threatening material [ 67 ]. The sensitivity of the e-Stroop sufficiently differentiates between neutral and emotionally charged words. In the E-Stroop test, participants are required to name the ink color of words with emotional or neutral valence, which is the main difference between the traditional Stroop and E-Stroop. Specifically, the words do not represent names of colors but contain words varying in accordance with their emotional valence [ 68 ]. In the other words in the E-Stroop test, the emotional meaning of the word tends to capture and hold the participant's attention and cause a slowdown in reaction time, regardless of the ink color in the traditional test [ 69 ].
The Wisconsin Card Sorting Test (WCST)
This neuropsychological test was first devised in 1948 and has been used to assess cognitive processes of higher levels since 1963 [ 54 , 70 ]. These processes include attention, perseverance, working memory, abstract thinking, cognitive flexibility, and set-shifting. The test is made up of two card packs; each containing four stimulus cards and 64 response cards. The dimensions of each card are 7 × 7 cm and on them are various shapes of different colors and numbers. The subjects are required to sort the response cards with the stimulus cards using the feedback given to them by pre-established instructions. This study uses the Heaton technique with 128 cards. Each subject took the test separately and 12 sets of points were applied [ 71 ]. Due to the character of the test, carrying out a reliability study was not possible. However, validity studies, done on a Turkish sample, confirmed the credibility of this test [ 54 ]. This test has been used to assess cognitive function in subjects suffering from various disorders; including TBI, schizophrenia, chronic alcoholism, anorexia nervosa, autism spectrum, obsessive–compulsive disorder, and ADHD [ 70 , 72 , 73 , 74 , 75 ].
The Mackworth Clock Test
This test was first devised in 1948 to assess vigilance in radar technicians of the British Air Force [ 76 , 77 , 78 , 79 , 80 ]. This test assesses sustained attention and was evaluated in two different time frames, one from 1962 to 1964 and another from 1980 to 1984 [ 77 , 81 , 82 , 83 ]. Effects of alcohol hangovers, fatigue, and specific serotonin reuptake inhibition on the test results have been studied [ 84 , 85 , 86 , 87 ]. A metal box with a white face of 30.5 cm in diameter and on it a 15.25 cm long pointer, black in color, was used in this test. The face was divided into 100 3.6-degree steps and the pointer jumped one step each second. The target stimuli in this test were called double jumps, where the pointer traveled 7.2 degrees in one second. These stimuli occurred randomly in varying time intervals and each trial took 62 min to carry out. The box, or 'the clock' as it was referred to, was placed at a distance of 1.22 m but could be reduced to a minimum of 0.31 m if the subject had difficulty seeing the pointer sharply [ 76 ]. A computerized version of the test has been available since 2000 [ 76 , 88 ].
Match to sample visual search task
This task is a benchmark measuring speed/accuracy trade. The task assesses how well the subject is in matching visual samples. A complex visual pattern appears in the center of the screen then after a short pause, a number of similar patterns appear at the edge of the screen. Of all these patterns only, one matches that of the center. Efficient performance is defined as the ability to reject all unintended patterns and to recognize and point out the correct ones. Percent correct is the variable of interest in this task. A subject with a better performance gets a better score[ 89 , 90 ].
The rapid visual information processing (RVP)
The RVP task is a 4-min-long visual continuous performance task (CPT). This test was devised by modifying and simplifying Wesnes and Warburton task and is designed to analyze sustained attention [ 56 ]. The test has been utilized in schizophrenic patients and subjects having ADHD and social anxiety disorder [ 56 , 91 , 92 , 93 ]. In the test process numbers from 2 to 9 appear in the center of a screen without any specific arrangement at a rate of 100 numbers/min. Subjects are required to press a specific button whenever the pattern of the numbers is 2–4-6, 3–5-7, or 4–6-8. Seven factors were quantified: (1) number of misses (times in which there is no response despite the pattern being shown); (2) probability of hits (h, times when the subject responds accurately), calculated by dividing the number of hits by the number of hits and misses; (3) total correct rejections (times when subject correctly ignores other patterns); (4) probability of false alarms (f, times 0 when the subject responds inaccurately), calculated by dividing false alarms by total false alarms and correct rejections; (5) A’ (calculated as \(0.5+[(h-f)+(h-f)2]/[4\times h\times (1-f)]\) ), a signal detection measure of sensitivity to the target, regardless of response tendency; (6) B” (calculated as \([(h-h2)-(f-f2)]/[\left(h-h2\right)+\left(f-f2\right)]\) ), a signal detection measure of the strength of trace needed to cause a response (Sahgal, 1987); and (7) mean latency (mean time needed to react in correct responses) [ 56 ].
Dichotic listening test
This test was first designed to assess auditory function and measure hemispheric asymmetry [ 94 ]. It is also used as the standard basis for all the tests studying selective auditory attention. In this test, subjects are provided with two sound sequences. Sequences are played in the same ear in one instance while in the other instance, each sequence is played in a different ear. The subject is then required to only focus on one of the formerly played sequences while rejecting the other. In the instance of dichotic listening, a male voice is presented to one ear and a female voice to the other. On the other hand, in the biotic instance, both ears are presented with both sequences [ 95 ]. This test has been used to evaluate the effect of the attentional deficit on the elderly affected by AD [ 96 ].
The continuous performance test
The continuous performance test that was first introduced in 1956 is administered to assess sustained attention and selective visual attention [ 97 , 98 , 99 ]. In the first round of testing, three tests were undertaken; the Continuous Performance Test, Identical Pairs version (CPT-IP), the Attention Span Task, and the Information Overload Task. The entire round of testing took 1.5 h to carry out. In the initial home visit the Quick Test a demographic questionnaire, and an inventory of perceived attentional styles were also administered. In the second run which is executed for confirming the reliability of the first run over time, only information-processing aspects are re-evaluated [ 98 ]. This test has been used on subjects with schizophrenia, ADHD, mania, and alcoholism [ 100 , 101 , 102 , 103 , 104 ].
Motor screening (MOT)
This task screens for difficulties in the visual, movement, and comprehension zone. Results are stated in the form of two indices, the standard score of mean latency (MOT ML) and mean error (MOT ME) [ 105 ].
The Cambridge Neuropsychological Test Automated Battery (CANTAB)
Cambridge Neuropsychological Test Automated Battery (CANTAB), is a highly sensitive and objective measures series of cognitive tests developed at the University of Cambridge. Culturally neutral and requiring no technical knowledge or prior familiarity with computers make CANTAB suitable for usage in different studies. CANTAB also offered Web-based testing. Reaction Time (CANTAB-RTI), Rapid Visual Information Processing (CANTAB-RVP), Motor Screening Task (CANTAB-MOT), and Match to Sample Visual Search (CANTAB-MTS) are subtests of CANTAB which can assess the attention and psychomotor speed [ 106 , 107 ].
The Ruff 2 and 7 test
This test was designed to assess sustained attention and visual selective attention [ 108 , 109 ]. Using a pen and paper, sustained attention and its voluntary and intentional sides are evaluated using varying distractors. Assessments were done on four groups of patients suffering from a single lesion limited to the right or left anterior or right or left posterior region of the cerebrum. A greater fall in processing rate, independent of the processing mode (serial or parallel), was noticed in patients suffering from a right-hemisphere lesion than in subjects with a left-hemisphere lesion. Moreover, patients with anterior lesions showed a more noticeable contrast between serial and parallel processing, as was forecasted. This difference was the most prominent in subjects with a right frontal lesion [ 110 ]. This test has also been utilized on patients suffering from post-concussion syndrome [ 109 ]. Changes in the indices of this test by aging and depression have been studied [ 111 , 112 ]. The validity of the test has been evaluated for outpatient schizophrenic subjects [ 113 ].
The Test of Variables of Attention (TOVA)
Test of Variables of Attention (TOVA) is derivative of CPTs and commonly administered to assess sustained attention [ 114 , 115 , 116 , 117 ]. Subjects in the TOVA are presented with a screen on which two signals are shown, a square in the upper half or a square in the lower half. The subjects are expected to react based on the location of the square, respond if the square is presented in the upper half ('go' signal), and ignore if the square is presented in the lower half ('no-go' signal). Each task includes two constellations of 'go' and 'no-go' trials, differing only in respect of the ratio of the two kinds. In the first constellation, the majority of the trials are 'go’ trials assessing whether the subject is capable of denying responses when presenting with an occasional ‘no-go’ signal. A higher ratio of ‘no-go’ trials is shown in the later constellation and the subject is assessed whether he or she is capable of a swift response to the infrequent ‘go’ signals [ 114 , 118 , 119 , 120 ]. TOVA has been used to diagnose ADHD and TBI [ 121 , 122 , 123 ].
Test of everyday attention (TEA)
The test of everyday attention (TEA) includes eight subtests, which are standardized to have an age-adjusted mean of 10 with a standard deviation of 3 for ages between 18 and 80, and by this, it is comparable to that of the Third Edition of Wechsler Adult Intelligence Scale and Wechsler Memory Scale–III [ 124 , 125 ]. This test is designed to quantify sustained, selective, divided attention and attentional switching, both[ 20 , 126 , 127 ]. The subtest of the TEA is as follows:
TEA—map search
This subtest is devised to quantify visual selective attention, the subject looks at a tourist map of a city and looks for symbols representing different services. For example, a knife and a fork represent eating facilities.
TEA—elevator counting
This subtest is devised to quantify the sustained aspect of attention, asks the subjects to pretend they are in an elevator whose floor indication is not functioning, and by hearing a set of pre-recorded tapes they should guess which floor they have arrived.
TEA—elevator counting with distraction
A subtest of Elevator counting requires the subject to differentiate between high-tone and low-tone sounds as a means to evaluate auditory selective attention.
Visual elevator: subjects are required to count presented doors as a means to evaluate visual attentional switching.
TEA—auditory elevator with reversal
The same as the visual elevator subtest, evaluating auditory attentional switching.
TEA—telephone search
Subjects are required to look for symbols in a phone directory. This subtest assesses visual selective attention.
TEA—telephone search dual task
Subjects are required to do the same as a telephone search subtest but this time counting presented tones at the same time. This subtest assesses divided attention.
TEA—lottery task
Subjects are required to listen to the numbers announced on an audiotape, then write down the two letters preceding a specified number [ 124 , 128 , 129 , 130 , 131 , 132 , 133 ].
The psychomotor vigilance task (PVT)
The psychomotor vigilance task (PVT), undertaken by a computer, is a test timing widely used to measure reduced fatigue-related changes in alertness due to sleep loss [ 134 ]. PVT is considered a validated task for measuring sustained attention [ 135 , 136 , 137 ], which is one of the primary components of processes of attention [ 138 ]. During the PVT test, the participant reacts to a particular minimal change in the labile environment [ 139 ]. In detail, participants monitor a fixed point on a display for the appearance of a millisecond counter and are taught to press a key on the computer when they want to react to a digital signal. Any error concerning missing a signal or pressing the key without a signal will be registered. Significant results which are called PVT performance lapses are those in which the subject reacts in more than 500 ms or does not respond at all [ 140 ]. The standard 10-min PVT is often considered impractical in applied contexts, so a modified brief 3-min version of the PVT (PVT-B) is suggested as a useful alternative to this test [ 141 ].
Test of everyday attention for children (TEA-Ch)
The Test of Everyday Attention for Children (TEA-Ch), designed for ages 6–16, measures different aspects of attention in children. This test compares a subject's performance to the average score of his age group[ 128 ]. This test has been utilized to assess children with ADHD and traumatic brain injury [ 19 , 142 , 143 , 144 ]. The test provides a means for evaluating selective attention, sustained attention, and executive control [ 145 ]. TEA-Ch tests oriented toward sustained attention are the following:
TEA-Ch—Score!
Subject children are asked to mutely count the tones they hear on an audiotape of 15 min. 10 trials are undertaken and the number of tones in each trial ranges between 9 and 15. Intervals between tones are different, and each successfully undertaken trial is counted as one point.
TEA-Ch—Sky Search dual task
A 'dual-task' in which children are required to do Sky Search and Score! subtests at the same time. Therefore, the scores obtained in this subtest measure both sustained and selective attention.
TEA-Ch—Score dual task
A 'dual-task' in which the child is required to do the Score! subtest combining it with another auditory task where the child needs to listen to a news report on an audiotape and look for an animal's name. By the completion of the 10 trials, the child has to give the number of tones heard and the animal's name.
TEA-Ch—Walk, Do Not Walk
Children are required to note down the path on a piece of paper with a pen every time a tone is heard on the audiotape but do not make a mark if a second tone is immediately heard after the first. There are 20 trials in this subtest and the rate of the tones is increasing as the child is going through them. Each point represents one successfully completed trial.
TEA-Ch—code transmission
An audiotape is played for the children and they are required to listen for two ‘5 s’ in a row. After the two ‘5 s’ are heard the child must tell the number just before the two ‘5 s’.
TEA-Ch tests oriented toward selective attention are the following:
TEA-Ch—Sky Search
Children are tutored to look for specific spaceships on a large piece of paper that is filled with analogous ploy spaceships. In the second part, there is no ploy spaceship, and rules out motor dysfunction.
TEA-Ch—map mission
Children have 1 min to look for target symbols on a map full of distractors.
TEA-Ch tests oriented towards Attentional Control/Switching Focused are the following.
TEA-Ch—Creature Counting
Children start counting numbers until a visual stimulus, which is a creature in a tunnel, is shown. By seeing that stimulus, the child should start counting backward. This process is repeated and both speed and accuracy are considered.
TEA-Ch—Opposite Worlds
The 'Same Worlds' subtest requires the children to say 'one' and 'two' when they see their respective characters. In the later 'Opposite Worlds' subtest children are required to say 'one' when the character for 'two' is visualized and vice versa [ 128 ].
AX-Continuous Performance Test (AX-CPT)
Like TOVA, AX-Continuous Performance Test (AX-CPT) is also a derivative of CPTs and is commonly administered to assess sustained attention [ 114 , 115 , 116 , 117 ]. The AX-CPT is a comparable task in which subjects are required to respond as swiftly as possible to a stimulus or ignore one considering the prior stimulus [ 114 , 146 ].
The Conners Continuous Performance Test—2
Attention problems, most notably in the sustained domain of attention, are assessed using The Conners Continuous Performance Test — 2, utilizing a computer [ 147 , 148 , 149 ]. The Conners' CPT is a helpful measure in diagnosing ADHD and has been studied on other disorders such as schizophrenia, TBI, and idiopathic epilepsy [ 147 , 150 , 151 , 152 , 153 , 154 , 155 ]. 360 stimuli trials are performed on the screen, in letters presented 1, 2, or 4 s apart (ISI: Inter-Stimulus Interval). 18 blocks of 20, is the established division method for the trials. The ISIs are evened out across these blocks. The participation instructions require pressing the spacebar or the appropriate key on the mouse for any letter that appears, excluding the letter "x". Aside from the suggested practice test, it takes fourteen min for the CPT to be put into practice. Non-X stimuli appear 324 times, and the letter "X" (nontarget) appears 36 times during the test. One of the prominent advantages of this paradigm is putting a high quantity of targets to the test; ensuring a larger response database for producing the statistical output [ 148 ].
The Madrid Card Sorting Test
This test is the simplified version of the WCST and is done on the computer [ 156 ]. This test also features the possibility to undertake an ERP study. The test stimulus battery utilizes 24 response cards of the original 64 in the WCST and the subject is required to match these cards with the 4 stimulus cards based only on one criterion. This can be either the color, shape, or number on the card. A sensitive measure of sorting errors and set-shifting ability was possible only by using unequivocal cards. These sets of cards were used in 137 trials and put into 18 series in a semi-random manner. The intended sorting rule for each series differed and was initially unrecognized by the subjects. The stimuli were designed on the STIM package developed by NeuroScan Inc.; however, can be done in any format that supports at least 256 colors and 40 pixels/cm of resolution. Trials started with a compound stimulus displaying 4 key cards above one choice card showing up on an NEC monitor containing 10,243,768 pixels. The cards were fixed at a horizontal angle of 48 and a vertical angle of 3.58 and 1.5 m apart from the monitor. It was not proven that using smaller stimuli would make a meaningful change in EEG considering eye movement artifacts. Moreover, it is thought to be a deteriorating factor in the acuity of vision in the elderly or subjects suffering from neurological issues. The ideal contrast was achieved by using a black outline for shapes on a completely white background, while card stimuli were illustrated upon a dark one. The brightness of both the cards and the background was fixed at all times. Sequence control of the stimuli was provided by the STIM package but was tested successfully with other similar software such as MEL v1.0 and Presentation v0.50. An IBM computer was used for carrying out different parts of the trials [ 157 ].
The Cognitive Assessment Battery (CAB)
In recent years, online mobile-based cognitive assessments developed. One of them is CogniFit General Cognitive Assessment Battery (CAB). Although CAB does not identify the presence or absence of clinical diagnoses, studies found CAB a useful tool for distinguishing between controls and MCI as well as MCI and dementia [ 158 ]. The Concentration Cognitive Assessment (CAB-AT) is a tool for assessing attention. Resolution Test REST-SPER, Processing Test REST-INH, Equivalencies Test INH-REST, and Simultaneity Test DIAT-SHIF are subtests of CAB-AT which are development were inspired by CPT, classic Stroop test, TOVA, and Hooper Visual Organization Task, which was originally developed as a screening instrument for neurological dysfunction but subsequently has been used as a test of visuospatial skills [ 159 ].
Table 1 is a summary of the characteristics of cognitive tests concerning the attentive function in humans. All the mentioned tests are developed for assessing attention in patients with stable conditions and without significant disabilities. The conventional tests, which typically require the ability to read or use a computer, along with the ability to comprehend verbal instructions and executive tasks, are incompatible with some populations due to significant intelligence deficits or low cognitive and social functioning, which have been considered “untestable”[ 160 ]. Such characteristics are common in people with Intellectual Disability or Autism Spectrum Disorder (ASD), who are identified with limited abilities to understand instructions and perform tasks [ 161 ]. Cooperation and communication impairment are two significant factors in the difficulty of testing people with intellectual disability or ASD who have trouble understanding and responding to instructions [ 162 , 163 ]. Ho has trouble understanding and responding to instructions. Hence, in some previous studies, examiners invited examinees to engage in a game to improve their motivation by creating a playful test environment [ 164 , 165 , 166 , 167 ].
Evaluating unstable patients
It is noteworthy that only a few tests are compatible with the needs of individuals who exhibit such characteristics. According to a systematic review study, three tests and three batteries are appropriate for assessing people with intellectual disability or ASD. The tests mentioned were the Test of Auditory Discrimination, the Integrated and Visual and Auditory Continuous Performance Test (IVA-CPT), and Posner's Paradigm, while the batteries identified were the Cognitive Assessment System (CAS), TEA, and the Leiter international performance scale–revised (Leiter-R)[ 161 ].
Integrated and Visual and Auditory Continuous Performance Test (IVACPT)
The Integrated Visual and Auditory (IVA) CPT is made up of 22 subscales that provide information on inattention, inhibition, response consistency, attention diversity, and overall reaction time discrimination. It can assess inattention and impulsivity in the visual and auditory domains on the same task. When a computer screen displays a target stimulus, the individual must hit a button. However, when non-target stimuli flash, the user must not press the button. The IVA can be administered to individuals aged 5 and above. The IVA-CPT was created to aid in the measurement of ADHD symptoms; however, it has been used to assess attention and impulsivity in several neurodevelopmental disorders. The test evaluates sustained attention, processing speed, and attention consistency over time [ 168 , 169 , 170 , 171 ].
Test of auditory discrimination
This test assesses auditory distractibility, attention, and discrimination by asking the participant to detect speech sounds in both quiet and distracting (background noise) listening environments. It can be used on people aged 44 months to 70 years and older [ 170 , 172 ].
Posner's paradigm
The Posner paradigm is a computer task that takes roughly 45 min to complete. This entails identifying a target (for example, a star, letters, or other symbols). The target is preceded by a cue that is either valid (indicates the target location correctly), invalid (indicates the target location incorrectly), or neutral (no indication of target location). Invalid trials necessitate the participant to disengage from the incorrectly cued location and shift to the proper one [ 173 , 174 ].
Cognitive Assessment System (CAS)
CAS consists of three attention subtests, including expressive attention (The task for children aged 5 to 7 years includes pictures of ordinary animals; they must determine whether the animal depicted in real life is large or small, regardless of the relative size of the screen image; and those aged 8 to 18 are asked to name the color of the ink used to print the word rather than read that word), number detection (The aim is to identify specific numbers on a page (for ages 5 to 7 years) or specific numbers in a particular font (for ages 8 to 18 years) by underlying them), as well as receptive attention (The task is to underline pairs of objects or letters that either are identical in appearance or are the same from a lexical perspective.) [ 175 , 176 ].
As previously stated, it was used to evaluate the capacity for selective or focused attention. This test involves quickly scanning a telephone directory page for specific symbols. The summary score is calculated by dividing the amount of time it took to search the page for symbols by the number of symbols correctly identified [ 177 , 178 ].
Leiter International Performance Scale–Revised (Leiter-R)
The Leiter International Performance Scale–Revised (Leiter-R), includes 20 subtests in the visual function and reasoning battery (measuring nonverbal intelligence in the form of visual functioning, reasoning, and visuospatial skills) and the memory and attention battery (evaluating performance in memory and attention domains). Leiter-R is approved for people aged 2 months and 0 months to 20 years and 11 months [ 179 ].
In addition, there are procedures and adaptations in the test set that make it more compatible with this population. Including the training sessions that necessitate more direct interaction between examiner and examinee, extra testing time, and the development of a device that allows the gradual discrimination of each test element. How instructions are communicated is also a significant factor in comprehending the instructions. Many authors point to the value of using short, simple as well as regularly repeating sentences, and speaking enthusiastically and encouragingly [ 161 ].
From another point of view, since the performance of conventional neuropsychological tests is multifaceted and often requires intact upper limb function, their administration is limited in patients with upper limb disability and compromised motor function who are referred to rehabilitation settings and outpatient follow-up clinics due to interpretation difficulty. Upper limb dysfunction resulting in a stroke can range from complete immobility, as in limb amputation and hemiparesis to more subtle motor impairment in neurological disorders such as multiple sclerosis (MS) and Parkinson's disease [ 180 ]. Therefore, two modified tests in this population group have been developed and widely used, which include:
The Oral Trail Making Test (OTMT)
In oral TMT, the subject counts as swiftly as they can from 1 to 25 (OTMT-A) and switches between numbers and letters (OTMT-B; 1-A-2-B-3-c, and so on). At number 13, the timing was stopped. The time to completion is the outcome measure for the OTMT-A and OTMT-B[ 181 ]. An oral TMT paradigm has the potential to be used in a clinical setting as an alternate measure of cognitive flexibility.
The Symbol Digit Modalities Test-oral (SDMT-oral)
Participants are given a written key of symbol-digit pairs with a number ranging from 1 to 9 in the oral format of SDMT. A list of 110 symbols is presented to the participant, who must provide verbal answers to the relevant number for as many of the symbols as possible within a time limit of 90 s[ 182 ]. Since its inception, the SDMT-oral has been utilized with varying degrees of regularity, most notably as part of neuropsychological assessment batteries in MS. The SDMT-oral is more recommended for clinical application[ 180 ].
It should also be mentioned that, due to the wide variety of available psychological tests, selecting the appropriate test for particular clinical groups is not challenging. For example, WCST or CANTAB, which we described before, appear to be appropriate in children with developmental language disorder who are classified as having language functional abnormalities in the absence of a definite etiology [ 183 , 184 ].
Discussion and conclusion
There are multiple batteries for evaluating different parts of attention including, but not limited to, the Stroop test, continuous performance test, test of everyday attention for children, psychomotor vigilance test, and Wisconsin Card Sorting Test. Although the scientific study of attention, began in psychology, at this point, a debate about the different underlying mechanisms of these behavioral patterns is still ongoing. This review can be used for designing future studies regarding this domain of human cognition.
Beyond the historical definition of attention as a selection of wanted messages from unwanted ones (filter mechanism), another proposed mechanism for attention is to take up the level of the most important arriving signal [ 185 , 186 , 187 ]. Attention is vital for the proper functioning of the rest of our cognitive capacities. The application of attention in machine learning has added to its importance in recent years [ 188 ]. Improving the input–output models as well as adopting new primitives in deep learning methods is similar to what happens in the brain during the attention process; therefore, modeling complex systems with attention mechanisms, has multiple benefits such as focusing on a subset of elements and temporal dimensions [ 189 ].
Limitations and future directions
This study's limitations should be taken into consideration when interpreting its results. First, the strategy used to identify additional journals with the scope of untestable patients may have excluded studies published in scientific journals in which these terms do not appear as part of the title/abstract or scoping interests; which suggested getting focused in future studies. Second, we consulted one specialist database for locating papers and search reference lists of the included papers as well as reference lists of literature reviews that were discovered during the screening process. Additional web searches may yield more thorough descriptions of patients who have difficulty applying traditional tests. By properly establishing the search protocol and utilizing numerous pertinent datasets, such bias can be reduced.
Availability of data and materials
Not applicable.
Abbreviations
Deficit hyperactivity disorders
Alzheimer's disease
Mild cognitive impairment
Digit Span Test
Reaction time
Wisconsin Card Sorting Test
Rapid Visual Information Processing
Motor screening
Cambridge Neuropsychological Test Automated Battery
Test of Variables of Attention
Test of everyday attention
Psychomotor vigilance task
AX-Continuous Performance Test
Cognitive Assessment Battery
Autism spectrum disorder
Integrated Visual and Auditory
Cognitive Assessment System
Multiple sclerosis
Oral Trail Making Test
Symbol Digit Modalities Test-oral
Brewer JB, Gabrieli JDE, Preston AR, Vaidya CJ, Rosen AC. Chapter 5—memory. In: Goetz CG, editor. Textbook of clinical neurology. 3rd ed. Philadelphia: W.B. Saunders; 2007. p. 63–78.
Chapter Google Scholar
Bushnell PJ, Tilson HA. 13.21—Cognitive function. In: McQueen CA, editor. Comprehensive toxicology. 2nd ed. Oxford: Elsevier; 2010. p. 367–81.
Chun MM, Turk-Browne NB. Interactions between attention and memory. Curr Opin Neurobiol. 2007;17(2):177–84.
Article CAS PubMed Google Scholar
Burk JA, Blumenthal SA, Maness EB. Neuropharmacology of attention. Eur J Pharmacol. 2018;835:162–8.
Article CAS PubMed PubMed Central Google Scholar
Ben Azouz O, Dellagi L, Kebir O, Tabbane K. The concept of attention. Tunis Med. 2009;87(10):680–4.
PubMed Google Scholar
Wilterdink J. The mental status examination in adults. UpToDate. 2019. https://www.uptodate.com/contents/the-mental-status-examination-in-adults .
Hommel B, Chapman CS, Cisek P, Neyedli HF, Song J-H, Welsh TN. No one knows what attention is. Atten Percept Psychophys. 2019;81(7):2288–303.
Article PubMed PubMed Central Google Scholar
Degutis JM, Van Vleet TM. Tonic and phasic alertness training: a novel behavioral therapy to improve spatial and non-spatial attention in patients with hemispatial neglect. Front Hum Neurosci. 2010. https://doi.org/10.3389/fnhum.2010.00060 .
Valdez P, Ramírez C, García A, Talamantes J, Armijo P, Borrani J. Circadian rhythms in components of attention. Biol Rhythm Res. 2005;36(1–2):57–65.
Article Google Scholar
Ko L-W, Komarov O, Hairston WD, Jung T-P, Lin C-T. Sustained attention in real classroom settings: an EEG study. Front Hum Neurosci. 2017;11:388.
Johnston WA, Dark VJ. Selective attention. Ann Rev Psychol. 1986;37(1):43–75.
Katsuki F, Constantinidis C. Bottom-up and top-down attention. Neuroscientisty. 2013. https://doi.org/10.3389/fnhum.2017.00388 .
Buschman TJ, Miller EK. Top-down versus bottom-up control of attention in the prefrontal and posterior parietal cortices. Science. 2007;315(5820):1860–2.
Bowling JT, Friston KJ, Hopfinger JB. Top-down versus bottom-up attention differentially modulate frontal-parietal connectivity. Hum Brain Mapp. 2020;41(4):928–42.
Article PubMed Google Scholar
Hopfinger JB, Slotnick SD. Attentional control and executive function. Cogn Neurosci. 2020;11(1–2):1–4.
Koziol LF, Joyce AW, Wurglitz G. The neuropsychology of attention: revisiting the “Mirsky model.” Appl Neuropsychol Child. 2014;3(4):297–307.
Long N, Kuhl B. Bottom-up and top-down factors differentially influence stimulus representations across large-scale attentional networks. J Neurosci. 2018;38:2724–817.
Finney GR, Minagar A, Heilman KM. Assessment of mental status. Neurol Clin. 2016. https://doi.org/10.1016/j.ncl.2015.08.001 .
Heaton SC, Reader SK, Preston AS, Fennell EB, Puyana OE, Gill N, et al. The Test of Everyday Attention for Children (TEA-Ch): patterns of performance in children with ADHD and clinical controls. Child Neuropsychol. 2001;7(4):251–64.
Robertson I, Ward T, Ridgeway V, Nimmo-Smith I, Anespie C. The test of everyday attention. Manual. 1994. https://www.researchgate.net/profile/Tony-Ward-11/publication/267552527_The_Test_of_Everyday_Attention_Manual/links/5469b4a80cf2f5eb180500f3/The-Test-of-Everyday-Attention-Manual.pdf .
Stroop JR. Studies of interference in serial verbal reactions. J Exp Psychol. 1935;18(6):643.
Heaton RK, Staff P. Wisconsin card sorting test: computer version 2, vol. 4. Odessa: Psychological Assessment Resources; 1993. p. 1–4.
Google Scholar
Chelune GJ, Baer RA. Developmental norms for the Wisconsin Card Sorting test. J Clin Exp Neuropsychol. 1986;8(3):219–28.
Halperin JM. The clinical assessment of attention. Int J Neurosci. 1991;58(3–4):171–82.
Talebi M, Sadigh-Eteghad S, Talebi M, Naseri A, Zafarani F. Predominant domains and associated demographic and clinical characteristics in multiple sclerosis-related cognitive impairment in mildly disabled patients. Egypt J Neurol Psychiatr Neurosurg. 2022;58(1):48.
Carter JD, Bizzell J, Kim C, Bellion C, Carpenter KLH, Dichter G, et al. Attention deficits in schizophrenia–preliminary evidence of dissociable transient and sustained deficits. Schizophr Res. 2010;122(1–3):104–12.
Najmi S, Kuckertz JM, Amir N. Attentional impairment in anxiety: inefficiency in expanding the scope of attention. Depress Anxiety. 2012;29(3):243–9.
Keller AS, Leikauf JE, Holt-Gosselin B, Staveland BR, Williams LM. Paying attention to attention in depression. Transl Psychiatry. 2019;9(1):279.
Vasterling JJ, Brailey K, Constans JI, Sutker PB. Attention and memory dysfunction in posttraumatic stress disorder. Neuropsychology. 1998;12(1):125–33.
Dunn DW, Kronenberger WG. Childhood epilepsy, attention problems, and ADHD: review and practical considerations. Semin Pediatr Neurol. 2005;12(4):222–8.
McGuinness B, Barrett SL, Craig D, Lawson J, Passmore AP. Attention deficits in Alzheimer’s disease and vascular dementia. J Neurol Neurosurg Psychiatry. 2010;81(2):157–9.
Tharpe AM, Ashmead D, Sladen DP, Ryan HA, Rothpletz AM. Visual attention and hearing loss: past and current perspectives. J Am Acad Audiol. 2008;19(10):741–7.
Gazzellini S, Dettori M, Amadori F, Paoli B, Napolitano A, Mancini F, et al. Association between attention and heart rate fluctuations in pathological worriers. Front Hum Neurosci. 2016;10:648.
Islam K, Seth S, Saha S, Roy A, Das R, Datta AK. A study on association of iron deficiency with attention deficit hyperactivity disorder in a tertiary care center. Indian J Psychiatry. 2018;60(1):131–4.
Malhotra PA. Impairments of attention in Alzheimer’s disease. Curr Opin Psychol. 2019;29:41–8.
Mendonca F, Sudo FK, Santiago-Bravo G, Oliveira N, Assuncao N, Rodrigues F, et al. Mild cognitive impairment or attention-deficit/hyperactivity disorder in older adults? A cross sectional study. Front Psychiatry. 2021;12: 737357.
Lyon RE, Rizeq J, Flora DB, Martinussen R, Andrade BF, Toplak ME. Age-related variance in performance versus ratings of attention and impulse regulation in children: implications for the assessment of ADHD. Brain Sci. 2022;12(8):1033.
Martos D, Tuka B, Tanaka M, Vécsei L, Telegdy G. Memory enhancement with kynurenic acid and its mechanisms in neurotransmission. Biomedicines. 2022;10(4):849.
Katsuki F, Constantinidis C. Bottom-up and top-down attention: different processes and overlapping neural systems. Neuroscientist. 2014;20(5):509–21.
Saccaro LF, Schilliger Z, Perroud N, Piguet C. Inflammation, anxiety, and stress in attention-deficit/hyperactivity disorder. Biomedicines. 2021. https://doi.org/10.3390/biomedicines9101313 .
Ahmed GK, Darwish AM, Khalifa H, Haridy NA. Relationship between Attention Deficit Hyperactivity Disorder and epilepsy: a literature review. Egypt J Neurol Psychiatr Neurosurg. 2022;58(1):52.
Joormann J, Arditte KA. The relational theory of attention: Implications for the processing of emotional stimuli in psychological disorders. Aust Psychol. 2013;48(6):399–401.
Battaglia S, Cardellicchio P, Di Fazio C, Nazzi C, Fracasso A, Borgomaneri S. Stopping in (e)motion: reactive action inhibition when facing valence-independent emotional stimuli. Front Behav Neurosci. 2022;16: 998714.
Battaglia S, Cardellicchio P, Di Fazio C, Nazzi C, Fracasso A, Borgomaneri S. The influence of vicarious fear-learning in “infecting” reactive action inhibition. Front Behav Neurosci. 2022;16: 946263.
Wambach D, Lamar M, Swenson R, Penney DL, Kaplan E, Libon DJ. Digit span. In: Kreutzer JS, DeLuca J, Caplan B, editors. Encyclopedia of clinical neuropsychology. New York: Springer, New York; 2011. p. 844–9.
Bilder R, Sabb F, Parker D, Kalar D, Chu W, Fox J, et al. Cognitive ontologies for neuropsychiatric phenomics research. Cogn Neuropsychiatry. 2009;14:419–50.
Holdnack JA. 4—The development, expansion, and future of the WAIS-IV as a cornerstone in comprehensive cognitive assessments. In: Goldstein G, Allen DN, DeLuca J, editors. Handbook of psychological assessment. 4th ed. San Diego: Academic Press; 2019. p. 103–39.
Raiford SE, Coalson DL, Saklofske DH, Weiss LG. CHAPTER 2—practical issues in WAIS-IV administration and scoring. In: Weiss LG, Saklofske DH, Coalson DL, Raiford SE, editors. WAIS-IV clinical use and interpretation. San Diego: Academic Press; 2010. p. 25–59.
Weiss LG, Saklofske DH, Holdnack JA, Prifitera A. Chapter 1 - WISC-V: Advances in the assessment of intelligence. In: Weiss LG, Saklofske DH, Holdnack JA, Prifitera A, editors. WISC-V. 2nd ed. San Diego: Academic Press; 2019. p. 1–21.
Wahlstrom D, Weiss LG, Saklofske DH. Chapter 2—practical issues in WISC-V administration and scoring. In: Weiss LG, Saklofske DH, Holdnack JA, Prifitera A, editors. WISC-V Assessment and Interpretation. San Diego: Academic Press; 2016. p. 25–62.
Groth-Marnat G, Baker S. Digit Span as a measure of everyday attention: a study of ecological validity. Percept Mot Skills. 2003;97(3 Pt 2):1209–18.
Cullum CM. 4.11—neuropsychological assessment of adults. In: Bellack AS, Hersen M, editors. Comprehensive clinical psychology. Oxford: Pergamon; 1998. p. 303–47.
Cullum CM, Larrabee GJ. CHAPTER 6—WAIS-IV use in neuropsychological assessment. In: Weiss LG, Saklofske DH, Coalson DL, Raiford SE, editors. WAIS-IV clinical use and interpretation. San Diego: Academic Press; 2010. p. 167–87.
Coulacoglou C, Saklofske DH. Chapter 5—executive function, theory of mind, and adaptive behavior. In: Coulacoglou C, Saklofske DH, editors. Psychometrics and psychological assessment. San Diego: Academic Press; 2017. p. 91–130.
Deary IJ, Liewald D, Nissan J. A free, easy-to-use, computer-based simple and four-choice reaction time programme: the Deary-Liewald reaction time task. Behav Res Methods. 2011;43(1):258–68.
Gau SSF, Huang WL. Rapid visual information processing as a cognitive endophenotype of attention deficit hyperactivity disorder. Psychol Med. 2014;44(2):435–46.
Zeinalzadeh A, Nazary-Moghadam S, Sayyed Hoseinian SH, Ebrahimzadeh MH, Imani E, Karimpour S. Intra- and inter-session reliability of methods for measuring reaction time in participants with and without patellofemoral pain syndrome. Arch Bone Jt Surg. 2021;9(1):102–9.
PubMed PubMed Central Google Scholar
Rigoli M, Facchin A, Cardile D, Beschin N, Luzzatti C. Open-source open-access reaction time test (OORTT): an easy tool to assess reaction times. Neurol Sci. 2021;42(6):2461–9.
Jensen AR, Rohwer WD. The stroop color-word test: a review. Acta Physiol (Oxf). 1966;25:36–93.
CAS Google Scholar
Barbarotto R, Laiacona M, Frosio R, Vecchio M, Farinato A, Capitani E. A normative study on visual reaction times and two Stroop colour-word tests. Ital J Neurol Sci. 1998;19(3):161–70.
Valgimigli S, Padovani R, Budriesi C, Leone M, Lugli D, Nichelli P. The Stroop test: a normative Italian study on a paper version for clinical use. G Ital Psicol. 2010;37:945–56.
Scarpina F, Tagini S. The stroop color and word test. Front Psychol. 2017;8:557.
Assef EC, Capovilla AG, Capovilla FC. Computerized stroop test to assess selective attention in children with attention deficit hyperactivity disorder. Span J Psychol. 2007;10(1):33–40.
Wright BC. What Stroop tasks can tell us about selective attention from childhood to adulthood. Br J Psychol. 2017;108(3):583–607.
Williams JMG, Mathews A, MacLeod C. The emotional Stroop task and psychopathology. Psychol Bull. 1996;120(1):3.
Peach N, Jovev M, Foster A, Jackson H. Testing the Stroop effect in a nonclinical sample: hypervigilance or difficulty to disengage? J Exp Psychopathol. 2012;3(3):496–510.
Muris P, Merckelbach H. 620—Specific phobias. In: Bellack AS, Hersen M, editors. Comprehensive clinical psychology. Oxford: Pergamon; 1998. p. 461–74.
De Angelis J, Ricciardelli P. Emotional Stroop task. In: Zeigler-Hill V, Shackelford TK, editors. Encyclopedia of personality and individual differences. Cham: Springer International Publishing; 2017. p. 1–4.
McKenna FP, Sharma D. Reversing the emotional Stroop effect reveals that it is not what it seems: the role of fast and slow components. J Exp Psychol Learn Mem Cogn. 2004;30(2):382.
Gómez-de-Regil L. Assessment of executive function in patients with traumatic brain injury with the Wisconsin card-sorting test. Brain Sci. 2020;10(10):699.
Kafadar H, Orhan İB. The relationship between wisconsin card sorting test and raven standard progressive matrices: a latent variable analysis. Int Online J Educ Sci. 2016;8(1). https://doi.org/10.15345/iojes.2016.01.005 .
Sullivan EV, Mathalon DH, Zipursky RB, Kersteen-Tucker Z, Knight RT, Pfefferbaum A. Factors of the Wisconsin Card Sorting Test as measures of frontal-lobe function in schizophrenia and in chronic alcoholism. Psychiatry Res. 1993;46(2):175–99.
Westwood H, Stahl D, Mandy W, Tchanturia K. The set-shifting profiles of anorexia nervosa and autism spectrum disorder using the Wisconsin Card Sorting Test: a systematic review and meta-analysis. Psychol Med. 2016;46(9):1809–27.
Henry JD. A meta-analytic review of Wisconsin Card Sorting Test and verbal fluency performance in obsessive-compulsive disorder. Cogn Neuropsychiatry. 2006;11(2):156–76.
Arán Filippetti V, Krumm GL, Raimondi W. Computerized versus manual versions of the Wisconsin Card Sorting Test: implications with typically developing and ADHD children. Appl Neuropsychol Child. 2020;9(3):230–45.
Lichstein K, Riedel B, Richman S. The Mackworth clock test: a computerized version. J Psychol. 2000;134:153–61.
Veksler B, Gunzelmann G. Functional equivalence of sleep loss and time on task effects in sustained attention. Cogn Sci. 2018;42(2):600–32. https://doi.org/10.1111/cogs.12489 .
Somma A, Becker S, Leitner C, Fossati A. Reliability, factor validity, and neuropsychological correlates of the child concentration inventory–2 in a community sample of Italian adolescents. Assessment. 2021. https://doi.org/10.1177/10731911211033349 .
Westwood S, Criaud M, Lam S-L, Lukito S, Wallace-Hanlon S, Kowalczyk O, et al. Transcranial direct current stimulation (tDCS) combined with cognitive training in adolescent boys with ADHD: a double-blind, randomised, sham-controlled trial. Psychol Med. 2021. https://doi.org/10.1017/S0033291721001859 .
Jerison HJ. Performance on a simple vigilance task in noise and quiet. J Acoustical Soc Am. 1957;29(11):1163–5.
Giambra L, Quilter R. Sustained attention in adulthood: a unique, large-sample, longitudinal and multicohort analysis using the Mackworth clock-test. Psychol Aging. 1988;3:75–83.
Giambra LM, Quilter RE, Phillips PB. The relationship of age and extraversion to arousal and performance on a sustained attention task: a cross-sectional investigation using the Mackworth clock-test. Personality Individ Differ. 1988;9(2):225–30.
Whittenburg JA, Ross S, Andrews TG. Sustained perceptual efficiency as measured by the Mackworth “Clock” Test. Percept Mot Skills. 1956;6(3):109–16.
Verster JC, van Duin D, Volkerts ER, Schreuder AH, Verbaten MN. Alcohol hangover effects on memory functioning and vigilance performance after an evening of binge drinking. Neuropsychopharmacology. 2003;28(4):740–6.
Williamson AM, Feyer A-M, Mattick RP, Friswell R, Finlay-Brown S. Developing measures of fatigue using an alcohol comparison to validate the effects of fatigue on performance. Accid Anal Prev. 2001;33(3):313–26.
Riedel WJ, Eikmans K, Heldens A, Schmitt JAJ. Specific serotonergic reuptake inhibition impairs vigilance performance acutely and after subchronic treatment. J Psychopharmacol. 2005;19(1):12–20.
Schmitt JAJ, Ramaekers JG, Kruizinga MJ, van Boxtel MPJ, Vuurman EFPM, Riedel WJ. Additional dopamine reuptake inhibition attenuates vigilance impairment induced by serotonin reuptake inhibition in man. J Psychopharmacol. 2002;16(3):207–14.
Johns M. The assessment of sleepiness in human drug trials: a new perspective. Curr Psychopharmacol. 2019;8(1):5–26.
Green R, Till C, Al-Hakeem H, Cribbie R, Téllez-Rojo MM, Osorio E, et al. Assessment of neuropsychological performance in Mexico City youth using the Cambridge Neuropsychological Test Automated Battery (CANTAB). J Clin Exp Neuropsychol. 2019;41(3):246–56.
Levaux MN, Potvin S, Sepehry AA, Sablier J, Mendrek A, Stip E. Computerized assessment of cognition in schizophrenia: promises and pitfalls of CANTAB. Eur Psychiatry. 2007;22(2):104–15.
Cattapan-Ludewig K, Hilti CC, Ludewig S, Vollenweider FX, Feldon J. Rapid visual information processing in schizophrenic patients: the impact of cognitive load and duration of stimulus presentation. A pilot study. Neuropsychobiology. 2005;52(3):130–4.
Hilti CC, Hilti LM, Heinemann D, Robbins T, Seifritz E, Cattapan-Ludewig K. Impaired performance on the Rapid Visual Information Processing task (RVIP) could be an endophenotype of schizophrenia. Psychiatry Res. 2010;177(1–2):60–4.
Tetik D, Gica S, Bestepe EE, Buyukavsar A, Gulec H. Emotional information processing and assessment of cognitive functions in social anxiety disorder: an event-related potential study. Clin EEG Neurosci. 2020. https://doi.org/10.1177/1550059420981506 .
Eskicioglu E, Taslica S, Narin B, Guducu C, Oniz A, Ozgoren M. Brain asymmetry in directing attention during dichotic listening test: an fNIRS study. Laterality. 2019;24(4):377–92.
Werner LA, et al. 350—Human auditory development. In: Masland RH, Albright TD, Albright TD, Masland RH, Dallos P, Oertel D, et al., editors. The senses: a comprehensive reference. New York: Academic Press; 2008. p. 871–93.
Bouma A, Gootjes L. Effects of attention on dichotic listening in elderly and patients with dementia of the Alzheimer type. Brain Cogn. 2011;76(2):286–93.
Kropotov JD. Chapter 12—Psychometrics and neuropsychological assessment. In: Kropotov JD, editor. Functional neuromarkers for psychiatry. San Diego: Academic Press; 2016. p. 9–16.
Cornblatt B, Risch N, Faris G, Friedman D, Erlenmeye’Kimling L. The continuous performance test, identical pairs version (CPT-IP): I. new findings about sustained attention in normal families. Psychiatry Res. 1988;26:223–38.
Gervais NJ, Brake WG, Lacreuse A. 1.16—ovarian hormones and prefrontal cortex-related cognition. In: Pfaff DW, Joëls M, editors. Hormones, Brain and Behavior. 3rd ed. Oxford: Academic Press; 2017. p. 439–51.
Kurtz MM, Ragland JD, Bilker W, Gur RC, Gur RE. Comparison of the continuous performance test with and without working memory demands in healthy controls and patients with schizophrenia. Schizophr Res. 2001;48(2):307–16.
Losier BJ, McGrath PJ, Klein RM. Error patterns on the continuous performance test in non-medicated and medicated samples of children with and without ADHD: a meta-analytic review. J Child Psychol Psychiatry. 1996;37(8):971–87.
Epstein JN, Erkanli A, Conners CK, Klaric J, Costello JE, Angold A. Relations between Continuous Performance Test performance measures and ADHD behaviors. J Abnorm Child Psychol. 2003;31(5):543–54.
Sax KW, Strakowski SM, Zimmerman ME, DelBello MP, Keck PE, Hawkins JM. Frontosubcortical neuroanatomy and the Continuous Performance Test in Mania. Am J Psychiatry. 1999;156(1):139–41.
Dougherty DM, Moeller FG, Steinberg JL, Marsh DM, Hines SE, Bjork JM. Alcohol increases commission error rates for a Continuous Performance Test. Alcoholism. 1999;23(8):1342–51.
Bogaczewicz A, Sobow T, Kowalski J, Ząbek J, Woźniacka A, Bogaczewicz J. Cambridge Neuropsychological Test Automated Battery in assessment of cognitive parameters in patients with systemic lupus erythematosus in relation to autoantibody profile. Reumatologia. 2015;53(3):131–8.
Robbins T, editor Cambridge neuropsychological test automated battery (CANTAB): utility and validation. IEE Colloquium on Computer-Aided Tests of Drug Effectiveness; IET. 1994.
Sabahi Z, Farhoudi M, Naseri A, Talebi M. Working memory assessment using Cambridge neuropsychological test automated battery can help in the diagnosis of mild cognitive impairment: a systematic review and meta-analysis. Dement Neuropsychol. 2022;16(4):444–56.
Messinis L, Kosmidis MH, Tsakona I, Georgiou V, Aretouli E, Papathanasopoulos P. Ruff 2 and 7 Selective Attention Test: normative data, discriminant validity and test–retest reliability in Greek adults. Arch Clin Neuropsychol. 2007;22(6):773–85.
Cicerone KD, Azulay J. Diagnostic utility of attention measures in postconcussion syndrome. Clin Neuropsychol. 2002;16(3):280–9.
Ruff RM, Niemann H, Allen CC, Farrow CE, Wylie T. The ruff 2 and 7 selective attention test: a neuropsychological application. Percept Mot Skills. 1992;75(3 suppl):1311–9.
Knight RG, McMahon J, Skeaff CM, Green TJ. Reliable change indices for the ruff 2 and 7 selective attention test in older adults. Appl Neuropsychol. 2010;17(4):239–45.
Ruff RM. What role does depression play on the performance of the ruff 2 and 7 selective attention test? Percept Mot Skills. 1994;78(1):63–6.
Lee P, Li P-C, Liu C-H, Lin H-Y, Huang C-Y, Hsieh C-L. Practice effects, test-retest reliability, and minimal detectable change of the ruff 2 and 7 selective attention test in patients with schizophrenia. Int J Environ Res Public Health. 2021;18(18):9440.
Gorman TE, Green CS. Chapter 6—young minds on video games. In: Blumberg FC, Brooks PJ, editors. Cognitive development in digital contexts. San Diego: Academic Press; 2017. p. 121–43.
Lewandowski L, Wood W, Miller LA. Chapter 3—technological applications for individuals with learning disabilities and ADHD. In: Luiselli JK, Fischer AJ, editors. Computer-assisted and web-based innovations in psychology, special education, and health. San Diego: Academic Press; 2016. p. 61–93.
Leark RA, Greenberg LM, Kindschi C, Dupuy T, Hughes SJ. TOVA professional manual. Los Alamitos: TOVA Company; 2008.
Mitko A, Rothlein D, Poole V, Robinson M, McGlinchey R, DeGutis J, et al. Individual differences in sustained attention are associated with cortical thickness. Hum Brain Mapp. 2019. https://doi.org/10.1002/hbm.24594 .
Crow A. Associations between neuroticism and executive function outcomes: response inhibition and sustained attention on a continuous performance test. Percept Mot Skills. 2019;126:623–38.
Greenberg L, Waldman I. Developmental normative data on the test of variables of attention (T.O.V.A.?). J Child Psychol Psychiatry. 1993;34:1019–30.
Selander H, Strand N, Almberg M, Lidestam B. Ready for a learner’s permit? Clinical Neuropsychological off-road tests and driving behaviors in a simulator among adolescents with ADHD and ASD. Dev Neurorehabil. 2021;24(4):256–65. https://doi.org/10.1080/17518423.2020.1869339 .
Forbes GB. Clinical utility of the test of variables of attention (TOVA) in the diagnosis of attention-deficit/hyperactivity disorder. J Clin Psychol. 1998;54(4):461–76.
Lambez B, Harwood-Gross A, Zion-Golumbic E, Rassovsky Y. Non-pharmacological interventions for cognitive difficulties in ADHD: a systematic review and meta-analysis. J Psychiatr Res. 2019. https://doi.org/10.1016/j.jpsychires.2018.09.009 .
Welsh MB. May I have your attention? Testing a subjective attention scale. In: Denison S, Mack M, Xu Y, Armstrong BC (eds.) Proceedings of the 42nd Annual Conference of the Cognitive Science Society. Cognitive Science Society. pp. 2535–41.
Hall J, O’Carroll RE, Frith CD. 7—Neuropsychology. In: Johnstone EC, Owens DC, Lawrie SM, McIntosh AM, Sharpe M, editors. Companion to Psychiatric studies. 8th ed. St. Louis: Churchill Livingstone; 2010. p. 121–40.
Gourlay C, Collin P, D’Auteuil C, Jacques M, Caron P-O, Scherzer P. Age differences in social-cognitive abilities across the stages of adulthood and path model investigation of adult social cognition. Neuropsychol Dev Cogn B Aging Neuropsychol Cogn. 2022;29(6):1033–67.
Robertson IH, Ward T, Ridgeway V, Nimmo-Smith I. The structure of normal human attention: The Test of Everyday Attention. J Int Neuropsychol Soc. 1996;2(6):525–34.
Chan RC, Lai MK, Robertson IH. Latent structure of the Test of Everyday Attention in a non-clinical Chinese sample. Arch Clin Neuropsychol. 2006;21(5):477–85.
Gillen G. Chapter 8—managing attention deficits to optimize function. In: Gillen G, editor. Cognitive and perceptual rehabilitation. Saint Louis: Mosby; 2009. p. 184–209.
Jones S, Davies-Thompson J, Tree J. Can machines find the bilingual advantage? Machine learning algorithms find no evidence to differentiate between lifelong bilingual and monolingual cognitive profiles. Front Hum Neurosci. 2021;15:621772.
Pinto J, Dores A, Peixoto B, Geraldo A, Barbosa F. Systematic review of sensory stimulation programs in the rehabilitation of acquired brain injury. Eur Psychol. 2020. https://doi.org/10.1027/1016-9040/a000421 .
Kourtesis P, Collina S, Doumas L, MacPherson S. Validation of the Virtual Reality Everyday Assessment Lab (VR-EAL): an immersive virtual reality neuropsychological battery with enhanced ecological validity. J Int Neuropsychol Soc. 2021;27(2):181–96. https://doi.org/10.1017/S1355617720000764 .
Moran C, McGovern D, Warren G, Grálaigh R, Kenney J, Smeaton A, et al. Young and restless, old and focused: age-differences in mind-wandering frequency and phenomenology. Psychol Aging. 2020. https://doi.org/10.1037/pag0000526 .
David D, Fleminger S, Kopelman M, Lovestone S, Mellers J. Lishman’s organic psychiatry: a textbook of neuropsychiatry. New York: Wiley; 2012.
Grant DA, Honn KA, Layton ME, Riedy SM, Van Dongen HPA. 3-minute smartphone-based and tablet-based psychomotor vigilance tests for the assessment of reduced alertness due to sleep deprivation. Behav Res Methods. 2017;49(3):1020–9.
D’Rozario AL, Field CJ, Hoyos CM, Naismith SL, Dungan GC, Wong KKH, et al. Impaired neurobehavioural performance in untreated obstructive sleep apnea patients using a novel standardised test battery. Front Surg. 2018;5:35.
Wong KF, Teng J, Chee MWL, Doshi K, Lim J. Positive effects of mindfulness-based training on energy maintenance and the EEG correlates of sustained attention in a cohort of nurses. Front Hum Neurosci. 2018;12:80.
Di Muzio M, Diella G, Di Simone E, Novelli L, Alfonsi V, Scarpelli S, et al. Nurses and night shifts: poor sleep quality exacerbates psychomotor performance. Front Neurosci. 2020;14:1050.
Cohen RA. Sustained attention. In: Kreutzer JS, DeLuca J, Caplan B, editors. Encyclopedia of clinical neuropsychology. New York: Springer, New York; 2011. p. 2440–3.
Reifman J, Kumar K, Khitrov MY, Liu J, Ramakrishnan S. PC-PVT 2.0: an updated platform for psychomotor vigilance task testing, analysis, prediction, and visualization. J Neurosci Methods. 2018;304:39–45.
Lee I-S, Bardwell WA, Ancoli-Israel S, Dimsdale JE. Number of lapses during the psychomotor vigilance task as an objective measure of fatigue. J Clin Sleep Med. 2010;6(2):163–8.
Basner M, Mollicone D, Dinges DF. Validity and sensitivity of a Brief Psychomotor Vigilance Test (PVT-B) to total and partial sleep deprivation. Acta Astronaut. 2011;69(11–12):949–59.
Araujo G, Antonini T, Anderson V, Vannatta K, Salley C, Bigler E, et al. Profiles of executive function across children with distinct brain disorders: traumatic brain injury, stroke, and brain tumor. J Int Neuropsychol Soc. 2017;23:1–10.
Manly T, Anderson V, Nimmo-Smith I, Turner A, Watson P, Robertson IH. The differential assessment of children’s attention: the Test of Everyday Attention for Children (TEA-Ch), normative sample and ADHD performance. J Child Psychol Psychiatry. 2001;42(8):1065–81.
Malegiannaki AC, Aretouli E, Metallidou P, Messinis L, Zafeiriou D, Kosmidis MH. Test of Everyday Attention for Children (TEA-Ch): Greek normative data and discriminative validity for children with combined type of attention deficit-hyperactivity disorder. Dev Neuropsychol. 2019;44(2):189–202.
Chan RC, Wang L, Ye J, Leung WW, Mok MY. A psychometric study of the test of everyday attention for Children in the Chinese setting. Arch Clin Neuropsychol. 2008;23(4):455–66.
Gonthier C, Macnamara BN, Chow M, Conway ARA, Braver TS. Inducing proactive control shifts in the AX-CPT. Front Psychol. 2016;7:1822.
Conners CK, Staff M, Connelly V, Campbell S, MacLean M, Barnes J. Conners’ Continuous Performance Test II (CPT II v5). Multi-Health Syst Inc. 2000;29:175–96.
Conners CK, Sitarenios G. Conners’ Continuous Performance Test (CPT). In: Kreutzer JS, DeLuca J, Caplan B, editors. Encyclopedia of Clinical Neuropsychology. New York: Springer, New York; 2011. p. 681–3.
Sanchez L, Chronis-Tuscano A, Hunter S. Improving compliance with diabetes management in young adolescents with attention-deficit/hyperactivity disorder using behavior therapy pediatric diabetes and ADHD. Cogn Behav Pract. 2006;13:134–56.
McGee RA, Clark SE, Symons DK. Does the Conners’ Continuous Performance Test aid in ADHD diagnosis? J Abnorm Child Psychol. 2000;28(5):415–24.
Solanto MV, Etefia K, Marks DJ. The utility of self-report measures and the Continuous Performance Test in the diagnosis of ADHD in adults. CNS Spectr. 2004;9(9):649–59.
Sanz JC, Gómez V, Vargas ML, Marín JJ. Dimensions of attention impairment and negative symptoms in schizophrenia: a multidimensional approach using the Conners Continuous Performance Test in a Spanish population. Cogn Behav Neurol. 2012;25(2):63–71.
Zane KL, Gfeller JD, Roskos PT, Bucholz RD. The clinical utility of the conners’ Continuous Performance Test-II in traumatic brain injury. Arch Clin Neuropsychol. 2016;31(8):996–1005.
Ord JS, Boettcher AC, Greve KW, Bianchini KJ. Detection of malingering in mild traumatic brain injury with the Conners’ Continuous Performance Test–II. J Clin Exp Neuropsychol. 2010;32(4):380–7.
Borgatti R, Piccinelli P, Montirosso R, Donati G, Rampani A, Molteni L, et al. Study of attentional processes in children with idiopathic epilepsy by Conners’ Continuous Performance Test. J Child Neurol. 2004;19(7):509–15.
Periáñez JA, Barceló F. Madrid adaptation of the Wisconsin card sorting test: a comparative study of internal consistency. Rev Neurol. 2001;33(7):611–8.
Barceló F. The Madrid card sorting test (MCST): a task switching paradigm to study executive attention with event-related potentials. Brain Res Brain Res Protoc. 2003;11(1):27–37.
Nordlund A, Påhlsson L, Holmberg C, Lind K, Wallin A. The Cognitive Assessment Battery (CAB): a rapid test of cognitive domains. Int Psychogeriatr. 2011;23(7):1144–51.
Hooper HE. Hooper visual organization test manual. Los Angeles, CA, Western Psychological Services; 1983. https://www.scirp.org/(S(351jmbntvnsjt1aadkposzje))/reference/referencespapers.aspx?referenceid=1420250 .
Alpern GD. Measurement of “untestable” autistic children. J Abnorm Psychol. 1967;72(6):478.
Rosário V, Gomes C, Loureiro C. Systematic review of attention testing in allegedly “Untestable” populations. Int J Psychol Res Rev. 2019. https://escipub.com/ijprr-2019-07-1905/ .
Wolf-Schein EG. Considerations in assessment of children with severe disabilities including deaf-blindness and autism. Int J Disabil Dev Educ. 1998;45(1):35–55. https://doi.org/10.1080/1034912980450104 .
Bathurst K, Gottfried AW. Untestable subjects in child development research: developmental implications. Child Dev. 1987;58(4):1135–44.
Areces D, Dockrell J, García T, González-Castro P, Rodríguez C. Analysis of cognitive and attentional profiles in children with and without ADHD using an innovative virtual reality tool. PLoS ONE. 2018;13(8): e0201039.
Díaz-Orueta U, Garcia-López C, Crespo-Eguílaz N, Sánchez-Carpintero R, Climent G, Narbona J. AULA virtual reality test as an attention measure: convergent validity with Conners’ Continuous Performance Test. Child Neuropsychol. 2014;20(3):328–42.
Oliveira J, Gamito P, Alghazzawi DM, Fardoun HM, Rosa PJ, Sousa T, et al. Performance on naturalistic virtual reality tasks depends on global cognitive functioning as assessed via traditional neurocognitive tests. Appl Neuropsychol Adult. 2018;25(6):555–61.
McPherson J, Burns NR. Assessing the validity of computer-game-like tests of processing speed and working memory. Behav Res Methods. 2008;40(4):969–81.
Corbett BA, Constantine LJ. Autism and attention deficit hyperactivity disorder: assessing attention and response control with the integrated visual and auditory continuous performance test. Child Neuropsychol. 2006;12(4–5):335–48.
Thompson L, Thompson M, Reid A. Neurofeedback outcomes in clients with Asperger’s syndrome. Appl Psychophysiol Biofeedback. 2010;35(1):63–81.
Matson JL, Rieske RD, Williams LW. The relationship between autism spectrum disorders and attention-deficit/hyperactivity disorder: an overview. Res Dev Disabil. 2013;34(9):2475–84.
Sandford JATA. Integrated visual and auditory continuous performance test manual. Richmond: VA Braintrain Inc; 1995.
Reynolds CR, Fletcher-Janzen E (eds). Goldman-Fristoe-Woodcock Test of Auditory Discrimination. In: Encyclopedia of Special Education. 2008. https://doi.org/10.1002/9780470373699.speced0937 .
Klinger LG, Renner P. Performance-based measures in autism: implications for diagnosis, early detection, and identification of cognitive profiles. J Clin Child Psychol. 2000;29(4):479–92.
Posner M. Orienting of attention. Q J Exp Psychol. 1980;32:3–25.
Naglieri JA, Otero TM. The cognitive assessment system—second edition: From theory to practice Contemporary intellectual assessment: Theories, tests, and issues, 4th edn. New York: The Guilford Press; 2018. p. 452–85.
Taddei S, Contena B. Brief report: cognitive performance in autism and Asperger’s syndrome: what are the differences? J Autism Dev Disord. 2013;43(12):2977–83.
de Vries PJ, Watson P. Attention deficits in tuberous sclerosis complex (TSC): rethinking the pathways to the endstate. J Intellect Disabil Res. 2008;52(Pt 4):348–57.
Rose E, Bramham J, Young S, Paliokostas E, Xenitidis K. Neuropsychological characteristics of adults with comorbid ADHD and borderline/mild intellectual disability. Res Dev Disabil. 2009;30(3):496–502.
Borgwardt L, Thuesen AM, Olsen KJ, Fogh J, Dali CI, Lund AM. Cognitive profile and activities of daily living: 35 patients with alpha-mannosidosis. J Inherit Metab Dis. 2015;38(6):1119–27.
Jaywant A, Barredo J, Ahern DC, Resnik L. Neuropsychological assessment without upper limb involvement: a systematic review of oral versions of the Trail Making Test and Symbol-Digit Modalities Test. Neuropsychol Rehabil. 2018;28(7):1055–77.
Ricker JH, Axelrod BN. Analysis of an oral paradigm for the trail making test. Assessment. 1994;1(1):47–52.
Smith A. Symbol Digit Modalities Test (SDMT). Manual (Revised). Los Angeles: Western Psychological Services; 1982.
Aljahlan Y, Spaulding TJ. Attentional shifting in children with developmental language disorder: a meta-analysis. J Commun Disord. 2021;91: 106105.
Bishop DV. What causes specific language impairment in children? Curr Dir Psychol Sci. 2006;15(5):217–21.
Deutsch JA, Deutsch D. Attention: some theoretical considerations. Psychol Rev. 1963;70(1):80.
Kaldas A. Attention: a descriptive taxonomy. Hist Philos Life Sci. 2022;44(4):63.
Wickens C. Attention: theory, principles, models and applications. Int J Hum Comput Interact. 2021;37(5):403–17.
Lindsay GW. Attention in psychology, neuroscience, and machine learning. Front Comput Neurosci. 2020;14:29.
Hernández A, Amigó JM. Attention mechanisms and their applications to complex systems. Entropy (Basel). 2021. https://doi.org/10.3390/e23030283 .
Download references
Acknowledgements
The research protocol was reviewed and approved by the Student Research Committee of Tabriz University of Medical Sciences (grant number: 68480).
This research was supported by a grant from the Student Research Committee of Tabriz University of Medical Sciences (grant number: 68480) to AN.
Author information
Authors and affiliations.
Student Research Committee, Tabriz University of Medical Sciences, Golgasht Street, Tabriz, 5166/15731, East Azerbaijan, Iran
Ehsan Nasiri, Mohammad Khalilzad, Zahra Hakimzadeh, Ashkan Isari & Amirreza Naseri
Neurosciences Research Center (NSRC), Tabriz University of Medical Sciences, Golgasht Street, Tabriz, 5166/15731, East Azerbaijan, Iran
Ehsan Nasiri & Saeed Sadigh-Eteghad
Yuksek Ihtisas University, Ankara, Turkey
Sajjad Faryabi-Yousefabad
Research Center for Evidence-Based Medicine, Iranian EBM Centre: A Joanna Briggs Institute (JBI) Center of Excellence, Tabriz University of Medical Sciences, Golgasht Street, Tabriz, 5166/15731, East Azerbaijan, Iran
Amirreza Naseri
You can also search for this author in PubMed Google Scholar
Contributions
EN, MK, ZH, SFY, AI: investigation; methodology; writing—original draft. SS-E: investigation; supervision; validation; writing—review and editing. AN*: conceptualization; methodology; project administration; funding acquisition; writing—original draft. All authors read and approved the final manuscript.
Corresponding authors
Correspondence to Saeed Sadigh-Eteghad or Amirreza Naseri .
Ethics declarations
Ethics approval and consent to participate.
Ethical approval code: IR.TBZMED.VCR.REC.1400.577.
Consent for publication
Competing interests.
The authors declare that they have no conflicting interests to disclose.
Additional information
Publisher's note.
Springer Nature remains neutral with regard to jurisdictional claims in published maps and institutional affiliations.
Rights and permissions
Open Access This article is licensed under a Creative Commons Attribution 4.0 International License, which permits use, sharing, adaptation, distribution and reproduction in any medium or format, as long as you give appropriate credit to the original author(s) and the source, provide a link to the Creative Commons licence, and indicate if changes were made. The images or other third party material in this article are included in the article's Creative Commons licence, unless indicated otherwise in a credit line to the material. If material is not included in the article's Creative Commons licence and your intended use is not permitted by statutory regulation or exceeds the permitted use, you will need to obtain permission directly from the copyright holder. To view a copy of this licence, visit http://creativecommons.org/licenses/by/4.0/ .
Reprints and permissions
About this article
Cite this article.
Nasiri, E., Khalilzad, M., Hakimzadeh, Z. et al. A comprehensive review of attention tests: can we assess what we exactly do not understand?. Egypt J Neurol Psychiatry Neurosurg 59 , 26 (2023). https://doi.org/10.1186/s41983-023-00628-4
Download citation
Received : 21 October 2022
Accepted : 03 February 2023
Published : 17 February 2023
DOI : https://doi.org/10.1186/s41983-023-00628-4
Share this article
Anyone you share the following link with will be able to read this content:
Sorry, a shareable link is not currently available for this article.
Provided by the Springer Nature SharedIt content-sharing initiative
- Attention tests
- Cognitive processes
- Sustained attention
- Selective attention
- Neuropsychological tests
- Original article
- Open access
- Published: 22 July 2021
How do we measure attention? Using factor analysis to establish construct validity of neuropsychological tests
- Melissa Treviño ORCID: orcid.org/0000-0002-7713-8193 1 ,
- Xiaoshu Zhu 2 ,
- Yi Yi Lu 3 , 4 ,
- Luke S. Scheuer 3 , 4 ,
- Eliza Passell 3 , 4 ,
- Grace C. Huang 2 ,
- Laura T. Germine 3 , 4 &
- Todd S. Horowitz 1
Cognitive Research: Principles and Implications volume 6 , Article number: 51 ( 2021 ) Cite this article
25k Accesses
22 Citations
14 Altmetric
Metrics details
We investigated whether standardized neuropsychological tests and experimental cognitive paradigms measure the same cognitive faculties. Specifically, do neuropsychological tests commonly used to assess attention measure the same construct as attention paradigms used in cognitive psychology and neuroscience? We built on the “general attention factor”, comprising several widely used experimental paradigms (Huang et al., 2012). Participants ( n = 636) completed an on-line battery (TestMyBrain.org) of six experimental tests [Multiple Object Tracking, Flanker Interference, Visual Working Memory, Approximate Number Sense, Spatial Configuration Visual Search, and Gradual Onset Continuous Performance Task (Grad CPT)] and eight neuropsychological tests [Trail Making Test versions A & B (TMT-A, TMT-B), Digit Symbol Coding, Forward and Backward Digit Span, Letter Cancellation, Spatial Span, and Arithmetic]. Exploratory factor analysis in a subset of 357 participants identified a five-factor structure: (1) attentional capacity (Multiple Object Tracking, Visual Working Memory, Digit Symbol Coding, Spatial Span), (2) search (Visual Search, TMT-A, TMT-B, Letter Cancellation); (3) Digit Span; (4) Arithmetic; and (5) Sustained Attention (GradCPT). Confirmatory analysis in 279 held-out participants showed that this model fit better than competing models. A hierarchical model where a general cognitive factor was imposed above the five specific factors fit as well as the model without the general factor. We conclude that Digit Span and Arithmetic tests should not be classified as attention tests. Digit Symbol Coding and Spatial Span tap attentional capacity, while TMT-A, TMT-B, and Letter Cancellation tap search (or attention-shifting) ability. These five tests can be classified as attention tests.
Significance statement
Assessment of cognitive function in clinical populations, for both clinical and research purposes, is primarily based on standardized neuropsychological testing. However, this approach is limited as a clinical research tool due to two major issues: sensitivity and construct validity. Deriving new measures based on contemporary work in cognitive psychology and cognitive neuroscience could help to solve these problems. However, we do not understand the relationship between existing neuropsychological tests and widely used cognitive paradigms. The goal of this paper is to begin to address this problem, using factor analysis tools to map the relationships, specifically in the domain of attention. Our results should provide guidance for which neuropsychological tests should be classified as attention tests, and hopefully provide inspiration for the development of new clinical assessments based on experimental attention paradigms. Furthermore, we hope we have provided a template for other researchers to explore the connections between cognitive paradigms and neuropsychological tests in domains beyond attention. By bringing these fields closer together, we can improve our scientific understanding of cognition, and ultimately improve the welfare of people who suffer from cognitive disorders and deficits.
Introduction
Assessing cognitive functioning across the gamut of health and mental health conditions has traditionally relied on standardized neuropsychological test batteries (Foti et al., 2017 ; Helmstaedter et al., 2003 ; Meade et al., 2018 ; Vives et al., 2015 ). However, this approach may be reaching its limits as a clinical research tool in many fields, due to two major issues: sensitivity and construct validity (Bilder & Reise, 2019 ; Horowitz et al., 2018 ; Howieson, 2019 ; Kessels, 2019 ; Marcopulos & Łojek, 2019 ; Parsons & Duffield, 2019 ). We and others have proposed that deriving new measures based on contemporary work in cognitive psychology and cognitive neuroscience could help to solve these problems (Carter & Barch, 2007 ; Horowitz et al., 2018 ). However, we currently do not understand the relationship between existing neuropsychological tests and widely used cognitive paradigms. The goal of this paper is to begin to address this problem, using factor analysis tools to map the relationships. Specifically, we will address the attention domain, which was the most frequently assessed cognitive domain in our survey of cancer-related cognitive impairment studies (Horowitz et al., 2019 ).
Many neuropsychological tests were originally designed to diagnose severe cognitive difficulties (e.g., resulting from stroke). As a result, they tend to lack sensitivity to the less severe, and often more diffuse cognitive difficulties encountered by many clinical populations (Nelson & Suls, 2013 ). This insensitivity may contribute to the widely observed lack of correlation between objective neuropsychological tests and patients’ subjective reports of their own cognitive problems (Jenkins et al., 2006 ; Srisurapanont et al., 2017 ).
Neuropsychological tests tend to be developed from a practical rather than a theoretical standpoint, and often tap multiple cognitive abilities in a single test (Sohlberg & Mateer, 1989 ). This means that it is often difficult to know exactly what cognitive faculties are being measured by a given test (Kessels, 2019 ; Schmidt et al., 1994 ). The Digit Symbol Coding test, for example, is a widely used neuropsychological test that is variously held to measure attention, psychomotor speed, working memory, processing speed, and executive function (Horowitz et al., 2019 ). In the clinical setting, this lack of specificity can be an advantage. If a patient has cognitive problems, they are likely to show up on the Digit Symbol Coding test. However, the downside is that it is very difficult to pin down which cognitive faculties are affected (Jaeger, 2018 ). For research purposes, this construct validity problem is a major limitation (McFall, 2005 ; McFall & Townsend, 1998 ), and poses a general challenge to integrating neuropsychological research with cognitive neuroscience (Horowitz et al., 2019 ).
In contrast to the neuropsychological tradition, experimental paradigms (“paradigms” rather than “tests”, because there is no standard version; Kessels, 2019 ) in basic cognitive psychology and cognitive neuroscience are explicitly created to test theoretical models of specific cognitive functions and operations. Experimental paradigms often have internal manipulations that allow for separations of subcomponent processes. Consider the visual search paradigm, in which observers search through N items to find a target (e.g., search for the T among Ls). Instead of looking at the overall response time, the experimenter computes the slope of the regression line for response time as a function of N to yield a pure search rate, independent of perceptual, response, and motor stages (Sternberg, 1966 ). Similarly, in the Eriksen flanker paradigm (Eriksen & Eriksen, 1974 ) participants see three letters, and are asked to give one response if the central letter belongs to one category (e.g., X or T) and another response if it belongs to another (e.g., O or C). If the two flanking letters come from the same category as the target (e.g., X T X, compatible trial), responses are typically faster than when they come from different categories (e.g., X C X, incompatible). The primary dependent measure is again not overall response time, but the incompatible-compatible difference score, which provides a measure of the strength of interference from incompatible responses. This sort of logic is rare in neuropsychological tests, perhaps in part because they have been largely administered, even now, as paper-and-pencil tests. Consider the Trail Making Test (Partington & Leiter, 1949 ), in which participants are asked to connect a sequence of digits in order (1, 2, 3, etc., version A) or alternate between digits and letters (1, A, 2, B, etc., version B). The score for each version is overall completion time. This score conflates search ability, motor function, and executive function into a single score. As with the Digit Symbol Coding test, this makes the Trail Making Test sensitive to a wide range of deficits, which contributes to its popularity (along with the fact that it is not proprietary), while making the results difficult to interpret (Kessels, 2019 ) though several groups have attempted to decompose Trail Making Test performance (Crowe, 1998 ; Misdraji & Gass, 2010 ; Salthouse, 2011 ). Interestingly, the Trail Making Test does produce a difference score: the difference between versions A and B is held to measure target-switching or executive function (Crowe, 1998 ; Sánchez-Cubillo, 2009 ). However, this difference score is rarely reported in the scientific literature (e.g., in the cancer-related cognitive impairment field, see Horowitz et al., 2019 ).
One way to bridge this gap is to take experimental paradigms and adapt them to the demands of clinical research and practice. Several recent ventures in this direction have been based on Posner and Petersen’s ( 1990 ) influential Attentional Network theory, which proposed that attention is divided into three separate functional brain networks. The alerting network is defined as maintaining a vigilant and alert state, the orienting network involves directing attention in space, and the executive control is responsible for resolving conflict between responses (MacLeod et al., 2010 ). Based on this conceptualization of attention, Fan et al. ( 2002 ) developed the Attentional Network Test, which combines a flanker task (with incongruent, congruent, and neutral trials) and a cued reaction time task (with central, double, spatial, and no cue trials). The difference between trials with congruent and incongruent flankers measures executive control, the difference between central and spatial cues measures orienting, and the difference between double and no-cue trials measures orienting. The Dalhousie Computerized Attention Battery (Jones et al., 2016 ) was also designed to measure the alerting, orienting, and executive control networks, using a battery of eight tasks adapted from the experimental literature. Simple and choice reaction time tasks measure alerting, visual search measures orienting, while a go/no go task, a dual task, a flanker task, and item and location working memory tasks measure executive control. The NIH Toolbox ( https://www.healthmeasures.net/explore-measurement-systems/nih-toolbox ) is a comprehensive set of computerized tests designed to measure cognitive, emotional, sensory, and motor functions. The NIH Toolbox Cognition Battery, part of the NIH Toolbox initiative, uses a version of the flanker test derived from the ANT to measure inhibitory control (Zelazo et al., 2013 ).
The ANT has been adopted fairly widely. A review by Arora, Lawrence, and Klein (Arora et al., 2020 ) found 889 studies using the ANT through 2019. Similarly, the paper describing the NIH Toolbox Cognition Battery Executive Function and Attention components has been cited in 244 papers as of early 2021. The Dalhousie battery has not had much time to gain traction; we could only find two non-methodological papers that had cited it (Cunningham et al., 2018 ; Sardiwalla et al., 2019 ). However, none of the three batteries showed up in our survey of meta-analyses of cancer-related cognitive impairment (Horowitz et al., 2019 ), or in a survey of practicing clinical neuropsychologists (Rabin et al., 2016 ).
We propose that factor analysis can serve as a useful tool to help establish construct validity. By mapping the relationships between neuropsychological tests and experimental paradigms, we can gain a better understanding of how neuropsychological tests relate to contemporary theories in cognitive psychology and cognitive neuroscience. Our approach is to run a set of participants on a battery composed of experimental cognitive paradigms from the attention literature and neuropsychological tests commonly used to measure attention in clinical populations, and use factor analysis to see whether the neuropsychological tests load on the same factor as the experimental paradigms.
Factor analysis is widely used in the development and validation of neuropsychological test batteries, but typically this is only done to understand the factor structure within a battery (e.g., Jones et al., 2015 ; Price et al., 2002 ). Two studies have used factor analysis to understand the relationships among neuropsychological tests commonly used to assess attention. Mirsky et al. ( 1991 ) found that many tests loaded onto a “perceptual-motor speed” factor (including Stroop, the Trail Making Test, Digit Symbol Coding, and Digit Cancellation), a “numerical-mnemonic” factor (Digit Span and Arithmetic) a “vigilance” factor (Continuous Performance Test) and a “flexibility” factor (Wisconsin Card Sorting Test). Schmidt et al. ( 1994 ) found separate factors for “scanning” (Stroop, TMT-A & -B, Digit Symbol Coding) and “spanning” (Visual Span, Digit Span Forwards, Digit Span Backwards).
In studies of intelligence, the Cattell–Horn–Carroll taxonomy (McGrew, 2009 ) is an influential factor-analytic scheme for organizing tests into domains (termed “broad abilities). While the taxonomy was developed on intelligence batteries, there is a certain amount of overlap with neuropsychological testing, where batteries (or tests from batteries) such as the Wechsler Adult Intelligence Scale often serve clinical purposes. Recent meta-factor-analytic work from Jewsbury et al. ( 2017 ) and Agelink van Rentergem et al. ( 2020 ) show that the Cattell–Horn–Carroll framework also fits well to data from neuropsychological batteries.
Studies that have tried to map clinical neuropsychological tests to laboratory or experimental paradigms are rare. In the working memory domain, Shelton et al. ( 2009 ) showed that clinical tests from the Wechsler batteries (Wechsler Adult Intelligence Scale III and Wechsler Memory Scale) correlated poorly with a factor defined by laboratory paradigms such as operation span (OSPAN, Turner & Engle, 1989 ), while the laboratory paradigms better predicted fluid intelligence. To our knowledge, no one has used factor analysis to study the relationship between neuropsychological attention tests and experimental attention paradigms.
As an object of study, attention lacks the sort of strong criterion construct that fluid intelligence presents for working memory (Shelton et al., 2009 ). However, a study from Huang et al. ( 2012 ) provides a useful starting point. Huang et al. tested 257 participants on 17 different experimental paradigms drawn from the attention literature, including nine “primary” and eight “secondary” paradigms, selected for their theoretical relevance as attention measures (Bundesen, 1990 ; Desimone & Duncan, 1995 ; Huang & Pashler, 2007 ; Posner & Petersen, 1990 ; Treisman & Gelade, 1980 ). In a principal components analysis, all nine primary paradigms loaded strongly on a single general attention factor, which Huang et al. termed a , with analogy to g , the general intelligence factor. The a factor accounted for 35% of total task variance, while 65% of the variance was explained by task-specific mechanisms. This result suggests that there is a single underlying attention mechanism that can be measured with one of the nine primary paradigms. To be precise, a should be regarded as a selective attention factor, as there were no sustained attention paradigms in Huang et al.’s battery.
In contrast, a study from Skogsberg et al. ( 2015 ), who tested 222 participants on 11 tasks, concluded that attention measures could be meaningfully divided into four clusters: spatiotemporal attention, global attention, transient attention, and sustained attention. Unfortunately, the set of attention tests analyzed by Skogsberg et al. has very little overlap with the Huang et al. ( 2012 ) set; only Multiple Object Tracking was included in both analyses. It is possible that Huang et al.’s a is a subset of the four clusters identified by Skogsberg et al. We return to this topic in the discussion.
The plan for the current study was to determine whether neuropsychological tasks used to measure attention would load on to the same factor(s) as tests derived from experimental cognitive paradigms. We chose to design our study around Huang et al. ( 2012 )’s a factor. By using a single factor that would correlate with multiple tests, we thought to achieve more power while simplifying the interpretation of the data.
We designed a battery composed of six experimental tests, and eight neuropsychological tests. The experimental tests included five selective attention paradigms, and one sustained attention test. The five selective attention tests (multiple object tracking, spatial configuration visual search, visual working memory, approximate number sense, and flanker interference) were selected to represent the paradigms that had the strongest correlations (i.e., 0.56–0.67) to the a factor in Huang et al.’s ( 2012 ) study, were widely used in experimental, cognitive psychology, and had strong theoretical justifications as attention measures. It is important to note that our tests were not identical to those used by Huang et al. This project was not an attempt to replicate Huang et al., but to build on the concept of the general attention factor. In some cases (multiple object tracking, visual working memory, approximate number sense, and flanker interference), we opted to use tasks that were already available on the TestMyBrain platform.
The multiple object tracking (MOT) task corresponds to the Tracking paradigm in Huang et al.’s ( 2012 ) battery. In MOT, participants need to remember and track a set of targets among a larger set of identical distractors. This requires selective attention to track targets while excluding distractors. Selective attention is central to successful performance in these tasks (Holcombe et al., 2014 ; Vul et al., 2009 ), and the paradigm has been a useful proving ground for models of attention (Cavanagh & Alvarez, 2005 ; Pylyshyn & Storm, 1988 ; Scholl, 2009 ). A version of the MOT was included in the Skogsberg et al. ( 2015 ) battery. Our MOT task asked participants to track 3–5 out of 10 disks, while Huang et al.’s Tracking paradigm had participants track 4 out of 8 disks.
Visual search has played a central role in attentional theory for decades (Schneider & Shiffrin, 1977 ; Treisman & Gelade, 1980 ). Spatial configuration search, where targets are distinguished from distractors only by the internal arrangement of components, is widely held to index serial shifts of covert attention (Bricolo et al., 2002 ; Wolfe, 2021 ; Woodman & Luck, 1999 ). Here we employed the widely used spatial configuration search for T-shaped targets among L-shaped distractors. This corresponds to Huang et al.’s ( 2012 ) Configuration Search task for squares that were white-above-black among black-above-white.
Visual working memory (VWM) may seem like an odd choice to measure attention, especially when we are trying to distinguish between attention and working memory functions. However, VWM is a distinct, modality-specific memory store (Fougnie & Marois, 2011 ), and is tightly linked to selective attention, in that both encoding (Emrich et al., 2017 ; Praß & de Haan, 2019 ) and maintenance in VWM require visual attention (Makovski et al., 2008 ; Roper & Vecera, 2014 ; Sandry & Ricker, 2020 ). Huang et al. ( 2012 ) used a Visual Short-Term Memory task requiring participants to memorize an array of six colors and then recreate this array from memory after it offset (i.e., full report paradigm). However, it is much more common to use a change-detection paradigm to measure visual short-term or working memory capacity (Luck & Vogel, 1997 ; Pashler, 1988 ). In a change detection paradigm, the whole array is presented at recall, and the participant has to indicate whether or not any element has changed. This approach is more time-efficient, and also avoids the complication of changes in the state of the memory during an extended report process (Peters et al., 2018 ). Accordingly, we measured Visual Working Memory (VWM) in a change-detection paradigm where participants had to memorize four shapes and report whether one of them changed after a brief delay.
Enumeration and numerosity perception are also tightly linked to selective attention. Specifically, enumeration can be described as an attentional individuation mechanism (Mazza & Caramazza, 2015 ). Whether we perceive precise counts or estimates depends on whether attention is focused or distributed (Chong & Evans, 2011 ). To measure numerosity perception, Huang et al. ( 2012 ) employed a Counting task that required participants to report whether the number of dots on the screen was even or odd. We opted for the Approximate Number Sense (ANS, Halberda et al., 2012 ) task that required participants to indicate whether there were more blue dots than yellow dots or vice versa. The ANS task is more strongly linked to attention, since it is a selective enumeration task, requiring participants to filter out irrelevant items.
The final paradigm was response selection, a form of internal attention (Chun et al., 2011 ) involving selection between competing actions. Huang et al. ( 2012 )’s Response Selection task was a 4-alternative forced-choice response to the color of a ball. We chose the Flanker Interference task (Eriksen & Eriksen, 1974 ), which requires participants to respond to a central target in the presence of irrelevant flanking stimuli that could be congruent or incongruent with the correct response. This choice was made partly for theoretical reasons, in that the requirement to filter out distraction makes the Flanker Interference task more of a selective attention task than a forced-choice response time task. Additionally, the Flanker Interference task is more widely used, both in experimental cognitive psychology and in neuropsychology. The Attentional Network Task (Fan et al., 2002 ), the Dalhousie Computerized Attention Battery, and the NIH Toolbox Cognition Battery executive function and attention sub-battery (Zelazo et al., 2013 ) all include a Flanker Interference component.
Finally, we also included a sustained attention test, the Gradual Onset Continuous Performance Test (gradCPT). The gradCPT is similar to continuous performance tasks that require frequent responses, such as the Sustained Attention to Response Task (Robertson et al., 1997 ), except that the gradCPT uses gradual transitions between stimuli, rather than abrupt onsets that may capture attention (Yantis & Jonides, 1990 ) and thus reduce sensitivity to vigilance decrements (Rosenberg et al., 2013 ). The gradCPT has been demonstrated to be sensitive to individual differences (Fortenbaugh et al., 2015 ; Rosenberg et al., 2013 ).
In contrast, the eight neuropsychological tests (Trail Making Test versions A & B (TMT-A, TMT-B), Digit Symbol Coding, Forward and Backward Digit Span, Letter Cancellation, Spatial Span, and Arithmetic) were not chosen for their theoretical or empirical links to attention. We selected the tests most frequently used to measure attention in our review of the literature on cancer-related cognitive impairment in cancer survivors (Horowitz et al., 2019 ). Digit span, arithmetic, letter cancellation, and the Trail Making Test were also among the most frequently used tests for “attention, concentration, and working memory” in a survey of the membership lists of the National Academy of Neuropsychology and the International Neuropsychological Society (Rabin et al., 2016 ), so we believe that this usage is typical of neuropsychological practice.
Historically, the neuropsychological tests used to measure attention have not been grounded in attentional theory (Mirsky et al., 1991 ; Schmidt et al., 1994 ). Tests such as Digit Span (measuring the number of digits participants can recall) and Arithmetic (ability to solve mathematical word problems) would seem to have little relationship to attention, since they do not require any sort of selection or filtering. Indeed, in our database of cancer-related cognitive impairment studies (Horowitz et al., 2019 ), these tests are also frequently classified under working memory, rather than attention. Then again, given that visual working memory and numerosity perception tests do seem to be linked to attention, we should not rule out these tests as attention measures out of hand. The Trail Making and Letter Cancellation tests closely resemble the visual search paradigm. However, as noted above, it is difficult to parse out the search component from motor factors or ability to alternate sequences (in the TMT-B). The Digit Symbol Coding test, in which participants are given a symbol-number key and asked to match a presented symbol to its corresponding number within an allowed time, similarly seems to tap into a number of cognitive domains, including visual search. Spatial Span, a visuospatial analog of the Digit Span tests, may be related to multitarget spatial attention tasks such as MOT (Trick et al., 2012 ). Notably, the Spatial Span test is alone among the neuropsychology tests we used in that it is never classified as anything other than an attention test in our dataset (Horowitz et al., 2019 ).
We hypothesized that the five selective attention paradigms would load on a common factor, a . We included the sustained attention paradigm on a hunch that some of the neuropsychological tests were better at predicting vigilance than selection. The key research question was which of the neuropsychological tests, if any, would also load on the a factor.
Participants
Sample size.
Recommendations for determining the minimal sample size for factor analysis have been diverse and often contradictory (MacCallum et al., 1999 ; Preacher & MacCallum, 2002 ), though Thompson ( 2004 ) suggested that a sample size of 300 is generally considered sufficient. That said, there are some basic guiding principles to take into account when determining sample size, including the strength of the relationship between variables and factors (measured by level of communality), the number of factors, and the number of variables per factor. Smaller sample sizes are required when the communality is higher and the variable-to-factor ratio is higher (Mundfrom et al., 2005 ).
The current study assumes two general factors underlying fourteen measures, a variable to factor ratio of 7:1. According to the Monte Carlo study conducted by MacCallum et al. ( 1999 ), a sample size of 200 can achieve an excellent recovery when the communality is low, if the variable-to-factor ratio is 20:3 or greater. Inter-correlations between measures that are assumed to load on to one factor are expected to be significant. We were able to estimate pairwise correlations among six of our fourteen measures from previous studies in the TestMyBrain database. Some of these correlations were lower than 0.15. To detect a correlation of 0.15 with power of 0.80, the required sample size is 350. We therefore aimed to obtain 350 participants.
Recruitment
We recruited participants from three sources: Visitors to TestMyBrain.org, an online cognitive testing platform, who clicked on a link titled “Cancer and Cognition”; Constant Contact (secure email marketing service) email blasts to participants signed up for the Citizen Science 4 Brain Health community; advertisements shared with cancer-focused mailing lists including the National Cancer Institute’s email list, Cancer Carepoint, Gryt Health, Cancer Survivorship, and American Cancer Society’s network. Recruitment invitations included a brief study description and informed participants that they would get feedback on their scores after completing the study.
Inclusion/exclusion criteria
We included only participants between the ages of 18 and 89 at the time of recruitment. We excluded participants who had a disability that substantially interfered with their ability to complete neurocognitive tests and/or were a current resident of the European Union or European Economic Area. To satisfy the General Data Protection Regulation, the consent form stated that residents from the European Union or European Economic Area were not allowed to participate. Additionally, since we are interested in determining whether our results will generalize to a population of cancer survivors, the exclusion criteria for the current study included a current or past diagnosis of cancer. Data from participants with a current or past diagnosis of cancer will be reported in a separate paper.
Participants began the study by clicking on a link that took them to a study information/online consent form. Once they had read the information and agreed to the consent form, they were then directed to the study and given a link with a coded ID that they could use to access the study at a future time, if needed. Participants were not required to complete the study in a single session. Coded IDs were not linked with email addresses or other personal identifying information.
When participants first clicked on the link to the study, they were taken to a page that asked for their age and the type of device they were using. Participants who reported an age younger than 18 or older than 89 were not allowed to continue the study. Next, participants were informed that they would receive the results of their assessments once they completed the study. They were then asked a series of questions to ascertain their demographics (age, gender, race, ethnicity, educational background) and cancer history (diagnosis and treatment), if any.
Once they had answered these questions, they began the cognitive testing battery. The battery took around 90 min to complete. There were four possible testing orders, counterbalanced across participants. Time of completion of each test was captured in the data to allow for any performance differences that arose from participation across multiple sessions to be modeled in our data analysis.
After participants completed all measures, they were given a debriefing questionnaire which asked about any challenges or technical difficulties they may have experienced during testing. Finally, participants were presented with results of their assessment, based on comparing their scores to the scores of participants from the TestMyBrain normative database, along with links to resources to address any concerns they might have about their cognitive health, or any questions about cancer and cognition.
Measures were divided into those that were adapted from traditional tests of neuropsychological functioning and paradigms adapted from the experimental literature.
Neuropsychological tests
The Arithmetic test required participants to solve a series of 20 arithmetic word problems of increasing difficulty (e.g., “How many hours will it take to walk 24 miles at a rate of 3 miles per hour?”). For each trial, regardless of difficulty, the participant earned 1 point for a correct answer and 2 points for a correct answer given within 10 s. The primary outcome variable was total points earned across the test. The first 5 questions had a 15 s time limit, which increased to 30 s for questions 6–10, 60 s for questions 11–19, and 120 s for the final question. This test was modeled after the arithmetic test of the Wechsler Adult Intelligence Scale, 3rd Edition (Wechsler, 1997 ).
Trail making test, parts A and B
Participants were presented with a display of 25 circled alphanumeric characters. The task was to draw lines, on the device screen, connecting the circles in the proper order. In Part A, the circles contained digits that had to be connected in ascending numerical order (i.e., “1”, “2”, “3”… “25”) starting with “1”. In Part B, the circles contained both digits and letters that had to be connected in ascending numerical and alphabetical order, switching between digits and letters (i.e., “1”, “A”, “2”, “B”, “3”, “C”… “13”). Depending on the device (i.e., laptop/desktop or tablet/smartphone), participants could use either their mouse or their finger to connect the circles. As these two response types have different motor demands, we also corrected for device/input type in all analyses (Germine et al., 2019 ). The primary outcome variable for each part was the total time to connect all circles. This test was modeled after the classic Trail Making Test (Partington & Leiter, 1949 ; Reitan, 1971 ), adapted for digital administration.
Digit span, forward and backward
These tests required participants to recall sequences of digits of increasing length. Sequences were presented serially, one digit at a time. Each digit was presented at the center of the screen for 1 s. Then, the final digit was removed and the participant had to type in the sequence. For the Forward Digit Span, the sequence had to be typed in as it was originally presented. For the Backward Digit Span, the sequence had to be typed in reverse order. They had 4 s to respond. The test began with 2 digit sequences. There were two trials for each sequence length. If the participant was correct on at least one of the two trials, the sequence length was increased for the next trial, up to 11 digits. The test ended when the participant missed two trials of the same length. The primary outcome measure for both tests was the length of the longest sequence where participants got at least one of two trials right. These tests were modeled after the Digit Span Forward and Digit Span Backward tests from the Wechsler Adult Intelligence Scale, 3rd Edition (Wechsler, 1997 ). For details, see Hartshorne and Germine ( 2015 ).
Digit symbol coding
In this test, participants had to match a target symbol with its corresponding digit. On each trial, participants were shown a novel symbol at the top of the screen. Underneath the symbol was a key mapping each of 9 novel symbols to one of the digits 1–3. The participant had to type in the digit that corresponded to the target symbol. Participants had 90 s to complete as many trials as possible. The primary outcome measure was the number of correct trials. This test was modeled after the Digit Symbol Coding test from the Wechsler Adult Intelligence Scale, 3rd Edition (Wechsler, 1997 ). For details, see Hartshorne and Germine ( 2015 ).
Letter cancellation
The Letter Cancellation test was a search test where the target was a conjunction of the letter “d” and two line segments, and the distractors were the letter “d” with one, three, or four line segments, and the letter “p” with one to four line segments. The line segments could be above the letter, below the letter, or both. There were 57 letter + line segment items, arranged in a 6 × 10 grid with the rightmost three spaces on the bottom row blank. The task was to click on all instances of the target. Whenever the participant correctly clicked on a target, it turned red. There were 14 trials. Trials timed out after 20 s. The outcome variable was the total number of targets correctly detected. This test was modeled after the D2 Test (Brickenkamp & Zillmer, 1998 ).
Spatial span
In this test, participants saw an array of 16 circles, arranged in concentric groups of four around a central point. Circles were progressively larger moving out from the center. At the start of a trial, all of the circles flashed briefly. Then, a sequence of circles was flashed, one by one, followed by all of the circles flashing again. At this point, the participant had to click on the previously flashed circles in the proper sequence. They had 12 s to respond. The test began with sequences of length 4. There were two trials for each sequence length. If the participant was correct on at least one of the two trials, the sequence length was increased for the next trial, up to length 7. The test ended when the participant missed two trials of the same length. The primary outcome measure was the length of sequence the participant could accurately recall before making two consecutive mistakes. This test was modeled after the Corsi Spatial Span test (Corsi, P, 1972 ; Della Sala et al., 1999 ).
Experimental paradigms
Approximate number sense dots test.
On each trial, participants were shown an array of blue and yellow dots for 200 ms. There were 5–20 dots of each color, and dot size varied. The participant’s task was to report whether there were more blue dots or more yellow dots. Participants had 10 s to respond. There were 100 trials. The primary outcome measure was accuracy, defined as the proportion of correct responses. For details, see Halberda et al. ( 2008 , 2012 ).
Flanker interference
The Flanker paradigm required participants to indicate the direction of a central arrow flanked by arrows facing either the same direction (congruent) or the opposite direction (incongruent). The flanker arrows were displayed for 100 ms before target onset. The target and flankers were presented together for 50 ms, and then, all arrows disappeared and were replaced by a fixation cross for 70 ms. Participants were instructed to press the “x” key on their keyboard to report a left-pointing target or the “m” key to report a right-pointing target. Participants had three seconds to respond to each trial, and were instructed to respond as quickly as possible. On trials where a participant’s response time exceeded the 85th percentile of their incongruent trial response time distribution, they were warned to go faster. There were 96 trials. The primary outcome measure was the accuracy (proportion of correct trials) difference between congruent trials and incongruent trials. For details, see Passell et al. ( 2019 ).
Gradual onset continuous performance task (GradCPT)
In this task, the participant sees a series of 300 outdoor scenes, comprised of 90% street scenes and 10% mountain scenes, presented within a circular window. Images were presented for roughly 800 ms, and the transition between images was gradual. Participants were instructed to press a button when they saw a street scene, and to withhold response when they saw a mountain scene (10% of images). The primary outcome variable was d’ . To compute d’ , we used the commission error (CE) rate and omission error (OE) rates. The hit rate ( H ) was defined as 1-CE rate, or the proportion of target mountain scenes participants correctly withheld a response to. The false alarm rate ( F ) was the OE rate, or the number of non-target street scenes participants withheld responses to. We used the equation d’ = z ( H ) − z ( F ). In the cases where no CEs ( H = 1.0) or OEs ( F = 0.0) were made, we used the standard procedure (Macmillan & Creelman, 2005 ) of deducting or adding one-half error to each measure to prevent d’ from being undefined. For further methodological details, see Fortenbaugh et al. ( 2015 ).
Multiple object tracking (MOT)
This paradigm presented participants with an array of 10 identical black disks. At the beginning of each trial, a subset of disks would blink, alternating between a black and a green smiley face for 1000 ms to identify them as targets. All disks would then move randomly around the screen for 5 s. The participant’s task was to track the target disks. At the end of the trial, all disks stopped moving and the participant had 15 s to click on all of the target disks. Correct responses were indicated by green smiley faces, incorrect responses by red frowning faces. There were 3 sets of 6 trials, for a total of 18 trials. The number of targets increased from 3 in the first set to 4 in the second set to 5 in the third set. The primary outcome measure was accuracy, computed as the total number of correct responses divided by the total number of targets tracked. For details, see Passell et al. ( 2019 ) or Wilmer et al. ( 2016 ).
Spatial configuration visual search
This paradigm presented participants with an array of line segments arranged into “T” or “L” configurations. The participant’s task was to search for the target letter “T”, and report whether it was rotated 90° to the left or to the right. The letter “L” served as the distractor, and could appear in any of the four 90° rotations. There were two blocks of trials. In the first block, the total number of items (set size) was 4, and in the second block the set size was 12. Each trial had one target; the remaining items were distractors. Participants had 5 s to respond. There were 100 trials. The primary outcome measure was the slope of the reaction time (for correct trials only) by set size function.
Visual working memory (VWM)
In this test, participants were shown a memory array of four novel objects for 1 s. The objects then disappeared. After a 1000-ms retention interval, a single probe object was presented at one of the four locations, and participants were asked to make an unspeeded judgment as to whether or not the probe was the same object that was presented at that location in the original array. There were a total of six novel objects that were randomly sampled throughout the test. There were 4 practice trials and 42 test trials. The primary outcome measure was the number of correct responses (max = 42).
Data analysis
The data were cleaned and formatted for a series of factor analyses to understand the latent characteristics across the 14 measures. Scores were considered outliers if they were three times the interquartile range below the 25 th percentile or above the 75 th percentile of the outcome distribution. Participants with outliers on more than one measure were identified and excluded from the factor analysis. We also log-transformed those measures with highly skewed distributions.
While we had a priori expectations about how the experimental tests would relate to one another (all except perhaps GradCPT would load onto an a factor), the relationships among the neuropsychological tests and between the two classes of tests were left open. We therefore conducted an initial exploratory factor analysis, followed by a set of confirmatory factor analyses. Since our power analysis indicated at least 350 participants for the exploratory factor analysis, we randomly selected 55% of the participants (training group, n = 357) to investigate the underlying factor structure. We held the remaining 45% of the participants (testing group, n = 279) for the confirmatory factor analyses. The two groups showed no statistical difference on the performance of the 14 measures and on the demographic characteristics.
Exploratory factor analysis
We used several converging methods to determine how many factors to retain, beginning with a parallel analysis. Developed by (Horn, 1965 ), parallel analysis compares eigenvalues extracted from the analysis data against eigenvalues calculated from randomly generated correlation matrices using the same number of observations and variables. The number of factors to retain is determined by the number of eigenvalues from the analysis data that are larger than those that were randomly generated. The models with various number of factors were then compared in terms of the degree of fit assessed by three goodness-of-fit indices: Tucker–Lewis Index (TLI), root mean square of residuals (RMSR), and root mean square error of approximation (RMSEA), as well as the RMSEA 90% confidence interval (CI). A good fit is defined by TLI > 0.95, RMSR < 0.05, and RMSEA < 0.06 with lower value of CI close to 0 and upper value no more than 0.08 (Browne & Cudeck, 2016 ; Hu & Bentler, 1999 ). RMSR or RMSEA < 0.08 indicates an acceptable model fit.
Since all the measures are testing some aspects of cognitive ability, it would not be realistic to assume that any extracted latent structure is truly independent from the others. Therefore, we used maximum likelihood for eigenvalue calculation, factor extraction, and oblimin rotation when extracting more than one factor, to allow the factors to correlate.
Confirmatory factor analysis
Confirmatory factor analysis was performed using the held-out testing sample to assess and compare goodness-of-fit between the extracted factor structures and the other candidate structures. Based on prior literature, we hypothesized two factors, the general attention factor ( a ), and a sustained attention factor. We tested whether this hypothesis was supported by the observed data in terms of model fit indices such as comparative fit index (CFI, > 0.90 for acceptable fit, and > 0.95 for good fit) and standardized root mean square residual (SRMR, < 0.05 for good fit, and < 0.08 for acceptable fit, Hu & Bentler, 1999 ), in addition to TLI and RMSEA. Since the competing factor structures were not nested in nature, we followed the non-nested model comparison sequence as recommended by Merkle et al. ( 2016 ). We employed Vuong’s ( 1989 ) test to first determine whether the candidate models had equal fit to the same data, or whether the models were distinguishable. If they were distinguishable, we further tested whether one model fit significantly better than another using the non-nested likelihood ratio test (LRT). A final factor structure was distinguishable from the other candidate models and had acceptable model fit in both the exploratory factor analysis and confirmatory factor analysis.
Given the diverse sample and multiple ways to respond, we further assessed measurement invariance in the entire sample ( n = 636) across demographic groups and response device groups (see Supplemental material in Thornton & Horowitz, 2020 ) using multigroup confirmatory factor analysis. The measurement invariance testing involves comparing models with increasing constraints. This begins with configural invariance, in which the same factorial structure is fitted to subgroups separately and factor loadings are allowed to vary freely (i.e., unconstrained model). Then, metric invariance (also called weak invariance) is tested by assessing the difference on goodness-of-fit indices of models imposing equality in factor loading across subgroups and the unconstrained models. If metric invariance holds, the next step is to test scalar invariance by further constraining intercepts to be equivalent across subgroups. The measurement invariance is determined by the insignificant changes (Δ) in model fit indices such as ΔCFI (≤ 0.01) and ΔRMSEA (≤ 0.015) (Cheung & Rensvold, 2002 ), especially ΔCFI which is more robust to sample size than chi-square (Δ χ 2 ).
Data manipulation and analyses were all conducted using R 3.6.0 (R Core Team, 2020 ). Exploratory factor analyses were obtained using the psych package (v1.8.12, Revelle, 2018 ), the lavaan package (v0.6-5, Rosseel, 2012 ) for the confirmatory factor analyses, nonnest2 (0.5-4, Merkle & You, 2020 ) for the Vuong tests, and semTools (v0.5-2, Jorgenson et al., 2019 ) to test for measurement invariance.
Recruitment and retention
As depicted in Fig. 1 , 4125 people completed the consent form and the demographic questionnaire (see below). Of those, 957 ended up completing the test battery, including 643 who reported not having a diagnosis of cancer. The latter group comprised the sample for this study. Seven people were found to have outliers on more than one measure and thus excluded from analysis. As a result, the analysis group contains 636 participants.
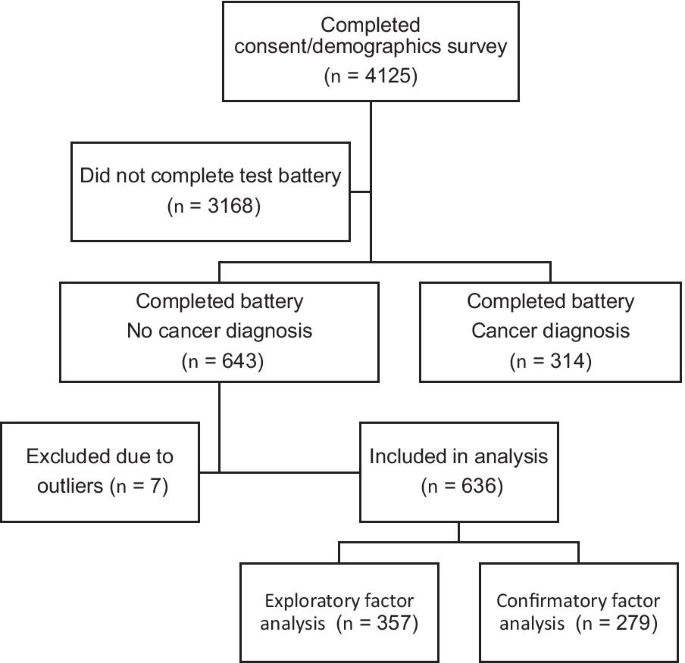
Study participation flow chart
Demographics
The basic demographic characteristics of the participants are shown in Table 1 . Participants were relatively young, but the majority were older than 25. A majority reported female gender. More than half had not finished college. Since the sample was web-based, we do not know participants’ geographic locations. Therefore, we cannot judge whether the racial/ethnic breakdown reflects larger populations (i.e., USA, the continent of North America, etc.). However, the proportion reporting an Asian race was larger than one would expect from a purely US sample.
Measure performance and correlations
Table 2 summarizes the outcomes used for each measure and their descriptive statistics. The two Trail Making Test tests (TMT-A, TMT-B) showed highly skewed distributions. After log-transformation, the distribution of these two tests was close to normal.
Figure 2 presents the correlation matrix of the 14 measures. Since scores for the two Trail Making Test tests and Visual Search were based on time, higher scores indicate poorer performance. In contrast, scores for the remaining measures were accuracy-based, and higher score indicates better performance. Therefore, we flipped the sign of the correlation coefficients for the time-based measures. While the most measures showed positive associations, the Flanker test, when measured by conflict accuracy, was weakly or even negatively correlated with the other tests.
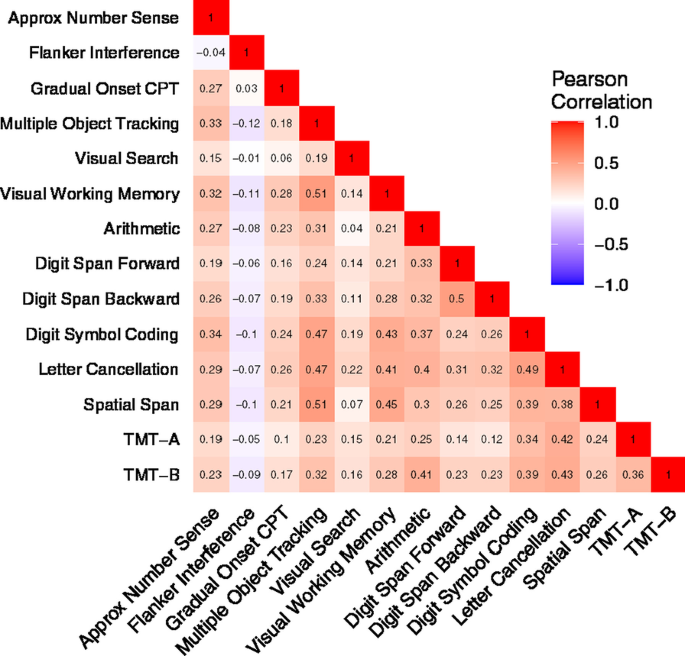
Correlation matrix. Note that TMT-A and TMT-B are log-transformed, and the sign of correlation coefficients was flipped for TMT-A, TMT-B, and Visual Search
The exploratory factor analysis was conducted using the training group of 357 participants randomly selected from the full sample of 636. The parallel analysis shown in Fig. 3 suggested three factors. The first factor accounted for 34.5% of the total variance, and the first three factors together account for over 50% of the variance.
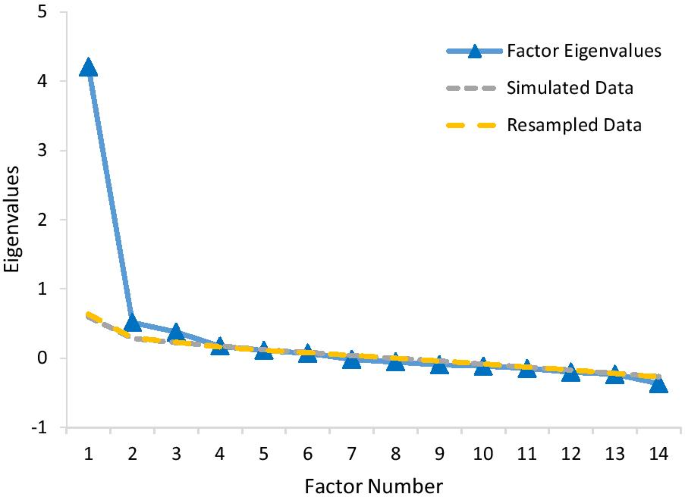
Parallel analysis scree plot
Next, we extracted one- to five-factor structures to compare goodness of fit. In addition to the three factor structure suggested by parallel analysis, the four- and five-factor structures also achieved good model fits (see Table 3 ).
Figure 4 shows the factor loadings of the five-factor structure, also depicted graphically in Fig. 5 . We named the five factors (1) Attentional Capacity, (2) Search, (3) Digit Span, (4) Arithmetic, and (5) Sustained Attention. All but one measure loaded highly on at least one of the five factors, using a minimum factor loading of 0.3. Flanker Inference had low loading on all five factors, which was not surprising given the low correlations observed in the correlation matrix. Although Digit Symbol Coding had a loading over 0.3 on the first (Capacity) factor, its loading onto the second (Search) factor was close to 0.3. Three factors had only one or two measures with high loadings, including the Digit Span factor, the Arithmetic factor, and the Sustained Attention factor (GradCPT only). The factor loadings for the one- to four-factor structures are available in “ Appendix B ”. In the three-factor structure, GradCPT loaded onto the first factor and Arithmetic loaded onto the second factor, while Visual Search did not load onto any factor. (Its loading on the first factor was 0.246, not meeting out 0.300 criterion.) In the four-factor structure, Arithmetic moved onto its own factor, while Visual Search loaded onto the second factor. Finally, the five-factor structure moved GradCPT onto the Sustained Attention factor. Table 4 shows the factor correlation matrix from the five-factor solution. Next, we used confirmatory factor analysis to compare model fit of the three exploratory factor structures and two other candidate structures.

Factor loadings from the five-factor exploratory factor analysis. Blue indicates positive loadings, red negative. The vertical line on each panel denotes the .30 threshold for inclusion of a measure in a given factor
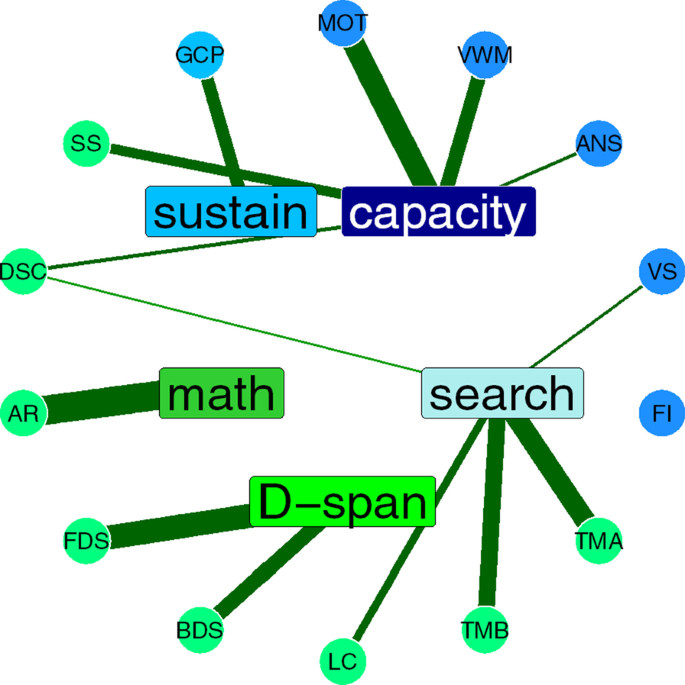
Graphic depiction of five-factor exploratory factor analysis structure. Tests are disks on the outer circle, factors rectangles on the inner circle. Thickness of lines indicates factor loadings, green for positive, red for negative. Darker blue tests were cognitive paradigms predicted to load onto the a factor. The light blue test (GCP) was predicted to load onto sustained attention factor. Neuropsychological tests are depicted in green
Due to consistently low factor loadings on all the extracted factors, Flanker was excluded from the confirmatory factor analysis. We assessed the model fit for all factor solutions using the held out testing group of 279 participants. These included a one-factor solution in which all tests would load onto a general attention factor, and a two-factor solution where experimental and neuropsychological paradigms and tests clustered on independent factors, as well as the three, four, and five factor structures derived from the exploratory factor analysis.
Because the model comparisons require all models to be specified on the same set of measures, we kept Visual Search in the three-factor structure even though its highest factor loading was only 0.246. As shown in Table 5 , the three structures extracted from the exploratory factor analysis had much better model fit than the two a priori structures. The four-factor structure and the five-factor structure had slightly better model fit than the three-factor structure. The Vuong’s test indicated that the three-factor structure and the four-factor structure were distinguishable ( p = 0.018), but they fitted the Testing group equally well based on the non-nested LRT ( p = 0.060). The four-factor structure and the five-factor structure were indistinguishable ( p = 0.375). The five-factor structure, however, is more theoretically plausible, since sustained and selective attention measures should be independent (Parasuraman et al., 1998 ).
Given the positive correlations between the five factors derived from the exploratory factor analysis (see Table 4 ), we further tested a hierarchical factor model (see Fig. 6 ) where a general factor was imposed above the five factors. The model fit was similar to that of the five-factor solution without the general factor. The Chi-square test also showed no significant difference ( p = 0.076 for Δ χ 2 (Δ df = 5) = 9.972) in terms of model fit between the two models. The model comparison result supported the existence of a general cognitive factor. However, the poor model fit of the single-factor structure in both exploratory and confirmatory factor analyses suggested that the five more-specific factors measure unique aspects of cognitive ability. Therefore, the five-factor structure was selected as the final model.
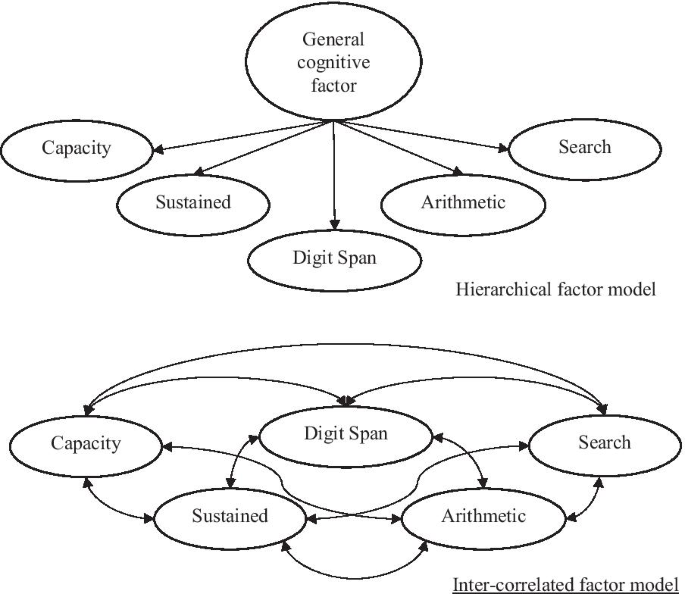
Graphic depiction of hierarchical factor model with five factors and the model with five inter-correlated factors
Measurement invariance
We collected information about the type of device participants used to respond for each measure. There were two general types of response mode, keyboard/mouse click and touchscreen. Participants were allowed to switch response devices between measures. To analyze the possible effect of response mode on the final factor structure, we included only participants who used one device consistently for all measures. There were 535 participants who used one device for all measures. Of these, 418 participants used either keyboard or mouse to respond, while 117 participants used touchscreen only.
The results showed that the metric invariance did not hold between the two mode groups (ΔCFI = 0.01, ΔRMSEA = 0.003, and p < 0.01for Δ χ 2 (Δ df = 8) = 25.694), indicating that individual factor loadings differed as a function of response mode.
Assessments of measurement invariance for demographic factors are reported in “ Appendix C ”—Measurement invariance analysis by demographic characteristics.
Both cognitive neuroscience and neuropsychology purport to measure cognitive functions, using a largely overlapping terminology (“attention”, executive function”, working memory”, etc.). However, the two fields are largely separate, with different goals and different institutional bases, and we know very little about how well concepts and measures from the two fields overlap. Our goal in this paper is to start making connections between the two fields in order to improve both neuropsychological assessment and our broader scientific understanding of cognition and the brain.
We hypothesized that the five selective experimental attention paradigms (ANS, MOT, VWM, Visual Search, and Flanker Interference) would load onto the a general attention factor reported by Huang et al. ( 2012 ). We could then use the degree to which the neuropsychological tests loaded onto a as an index of how well they function as (selective) attention measures. Neuropsychological tests that did not load on to a might load on to a common factor with the GradCPT, suggesting that they measure sustained attention. Or the neuropsychological tests might not be related at all to the experimental paradigms. The results that we actually observed were more complex than the scenarios we envisioned a priori. Our results are more consistent with a five-factor structure that can explain the observed correlations.
The five-factor structure
We settled on a five-factor structure, based on converging evidence from the scree plot, goodness-of-fit metrics, and theoretical considerations. These comprise: (1) an attentional capacity factor, (2) a search factor; (3) a digit span factor; (4) an arithmetic factor, and (5) a sustained attention factor. Flanker Interference did not load on to any factor.
The first factor comprised three experimental paradigms (MOT, VWM, and ANS), and two neuropsychological tests (Digit Symbol Coding and Spatial Span). Based on the nature of the three experimental paradigms, we tentatively label this the attentional capacity factor.
A second factor comprised the experimental Visual Search paradigm and three neuropsychological tests: Letter Cancellation, TMT-A, and TMT-B. All of the neuropsychological tests have a visual search component: the Letter Cancellation test requires the participant to look for the letter “d” with 2 lines among “d” with one or three lines and the letter “p” with one to three lines. It thus closely resembles a conjunction foraging search task (Jóhannesson et al., 2017 ; Kristjánsson et al., 2020 ). Both versions of the Trail Making Test require sequential search for alphanumeric characters. Therefore, we think of this factor as picking up variance related to search or attentional shifting. An important caveat here is that the configural Visual Search paradigm itself loaded less strongly onto this factor than the neuropsychological tests.
One interesting finding is that Digit Symbol Coding loaded almost equally on the first two factors. This is not entirely surprising given that there is a clear search component to the test. Participants need to find the target symbol in the key to find the correct response. Over the course of the test session, the key mappings will become automated and the search component will decrease in importance. If the mappings were to shift from trial to trial, this test would probably load more strongly on the search factor.
The third factor is fairly easy to characterize, as it included both the Forward Digit and Backward Digit Span tests, and nothing else. The Arithmetic test formed the fourth factor. These findings are in line with previous factor analytic studies of neuropsychological tests (Mirsky et al., 1991 ; Schmidt et al., 1994 ).
The fifth factor included just the GradCPT. We had predicted that this paradigm would not load onto the same factor as the other experimental cognitive paradigms, since it should measure sustained attention, rather than selective attention, and these faculties are known to be independent (Parasuraman et al., 1998 ). However, we did expect to see some of the neuropsychological tests to load onto this factor as well, which they did not.
A possible compromise between the single-factor and five-factor structures is the stratified model, where the five specific factors are nested under a general factor. The fit of this model was not statistically distinguishable from the five-factor model in our analyses, so it provides another way to look at the data. We assume that this general factor corresponds to something like the general intelligence g factor of the Cattell–Horn–Carroll model, rather than the general attention factor a proposed by Huang et al. ( 2012 ). In this context, it is worth noting that there was disagreement among the namesakes of the Cattell–Horn–Carroll model as to whether the general stratum was necessary (McGrew, 2009 ), and the recent work fitting the model to neuropsychological data eschew the general factor in favor of intercorrelated lower-order factors (Agelink van Rentergem et al., 2020 ; Jewsbury et al., 2017 ).
Relationship to other factor analyses of attention tasks
We assumed that our five selective attention paradigms would load on to a single factor, corresponding to Huang et al.’s (Huang et al., 2012 ) a . This was not what we observed. Only three paradigms MOT and VWM (and, if we’re generous, ANS) loaded into the first factor. Visual Search loaded onto the second factor with several of the neuropsychological tests, and Flanker Interference did not load onto any factor. This difference from Huang et al.’s analysis is not due to the fact that we found five factors, while Huang et al. found only one. If we limit ourselves to a single factor, Visual Search and Flanker Interference still do not load onto this factor (though all of the other tests do, see Table 6 ).
It is important to note that our study was not intended as a replication of Huang et al. (Huang et al., 2012 ). As we noted in the Introduction, there is no single definitive version of an experimental paradigm. The tests that we employed to instantiate the five paradigms we selected to represent the a factor differed in ways large and small from those used in the original Huang et al. study. In the MOT test, whereas our participants tracked 3–5 out of 10 items, Huang et al.’s tracked 4 of 8. Huang et al. measured VWM using a full-report technique, whereas we used a single-item probe technique. Our Visual Search paradigm was a search for a rotated T target among rotated Ls. Huang et al.’s Configuration Search paradigm was a search for a square composed of a white rectangle above a black rectangle among squares with the opposite spatial configuration. Instead of the even/odd judgment used in Huang et al.’s Counting test, our ANS test requires participants to judge which of two sets visible on the screen is larger. The dependent measure for Counting was a reaction time slope, as opposed to accuracy for the ANS. Furthermore, Huang et al.’s Counting task spanned the subitizing (3–4) and estimation ranges (13–14) ranges, while the ANS samples only the estimation range. As we noted in the Introduction, the Flanker Interference task is substantially different from Huang et al.’s Response Selection test.
Furthermore, factor analysis is sensitive to the context of the battery. Our battery included only four of the nine “primary” paradigms and none of the nine “secondary” paradigms used in Huang et al.’s battery. We also included the GradCPT and eight neuropsychological tests that were not in Huang et al.’s battery. This certainly affected the factor structure.
In contrast to Huang et al. ( 2012 )’s single factor solution, Skogsberg et al. ( 2015 ) obtained a four-factor structure for their battery: Global Attention, Sustained Attention, Transient Attention, and Spatiotemporal attention. Unfortunately, there are only two tasks in common between their battery and ours. Their Central Focusing Task corresponds to Flanker Interference, and both batteries included MOT. Furthermore, the reliability of the Central Focusing task was too low for it to be included in the analysis.
In the Skogsberg et al. ( 2015 ) data, MOT forms part of the Spatiotemporal Attention factor, so it is tempting to identify that with our Attentional Capacity factor. However, while MOT and the Spatial Span task fit that description, it is more difficult to see how VWM and Digit Symbol Coding can be thought of as spatiotemporal tasks. Furthermore, the ANS, which weakly loads onto our Capacity factor, would seem to correspond more closely to Skogsberg et al.’s Global Attention factor, since it requires the observer to segregate by color across the visual field. Meanwhile, Skogsberg et al.’s Spatiotemporal Attention factor includes the Spatial Shifting task, which we would predict should load onto with our Attentional Shifting Factor. Thus, our factor structure does not neatly align with Skogsberg et al.’s, although both analyses agree on the existence of a Sustained Attention Factor.
Similarly, it is difficult to map Huang et al.’s ( 2012 ) general factor to one of the four factors in Skogsberg et al. ( 2015 ). Again, the only paradigm in common between the two datasets is MOT, which would identify Huang et al.’s a with Skogsberg’s Spatiotemporal Attention factor, yet many of the paradigms in a do not fit that description (e.g., Visual Short-Term Memory, Response Selection, Counting). Perhaps it is our verbal descriptions of the factors that are misleading us here. It would be an interesting project, beyond the scope of this paper, to take the correlation matrices from these three studies (our study; Huang et al., 2012 ; and Skogsberg et al., 2015 ), subject them to the same factoring or clustering rules, and attempt some sort of synthesis. Ultimately, however, we are going to need more such studies, ideally using overlapping batteries. The existence of only three factor analytic studies of attention, with only one paradigm in common, points to the severe neglect of individual difference work in this field.
We also think it important to consider the demographic and cultural differences between the three samples. Both of the previous studies used convenience samples of undergraduate students. Huang et al. ( 2012 ) studied 257 students aged 17–22 at South China Normal University in Guangzhou, Guangdong, China. Skogsberg et al. ( 2015 ) studied 222 students, aged 18–26, from Northwestern University in Evanston, Illinois, USA. No demographic data were provided for participants in either study, though by definition they all possessed some college education.
Our study, in contrast, recruited a global internet sample of 636 people. Our participants ranged in age from 18 to 81, with a mean age of 31. More than half of our sample was older than the participants in the undergraduate studies. We also had much more variation in educational level, with 28% of our sample reporting a high school education or less, and 42% reported having completed a college degree. Finally, while we do not know which countries our participants lived in, only 21.1% reported Asian ethnic background, while 61.1% reported white or European ethnic background. Overall, we have good reasons to believe that there was a lot more demographic heterogeneity in our sample than in the two undergraduate samples.
Demographic characteristics seem likely to influence not only performance but also the observed factor structure. Our measurement invariance analysis (see “ Appendix C ”—Measurement invariance analysis by demographic characteristics) showed that metric invariance held for age, gender, and education, indicating that factor loadings did not significantly vary as a function of these characteristics. Nevertheless, we suggest that the greater diversity of our sample contributed to differences between the factor structure we observed and those obtained by previous studies, possibly via other characteristics that we did not consider here. Cultural variables may also influence the observed factor structures. Cross-cultural studies have indicated cultural differences in attention and perception between participants of East Asian and Western descent (Amer et al., 2017 ; Masuda & Nisbett, 2001 ). All of these issues need to be taken into account when comparing across studies or attempting theoretical generalizations. Now that remote testing platforms have become more widespread, future factor analytic studies should aim to cast a wider net, in order to increase both generalizability and variability.
Relationship to the Cattell–Horn–Carroll model of cognitive abilities
As we have mentioned, the Cattell–Horn–Carroll model (McGrew, 2009 ) is an influential model of human cognitive abilities, based in factor analysis. It arose out the field of intelligence measurement, and has recently been shown to fit well to neuropsychological batteries (Agelink van Rentergem et al., 2020 ; Jewsbury et al., 2017 ). The Cattell–Horn–Carroll model therefore provides a theoretically and empirically sound approach to classifying neuropsychological tests.
The relationship between Cattell–Horn–Carroll and the way cognition is thought of in cognitive psychology and cognitive science is not clear. Consider attention, the focus of this paper. Cattell–Horn–Carroll is a hierarchy of abilities, with narrow abilities (e.g., “quantitative reasoning”) organized under broad abilities (e.g., “fluid reasoning), with a general cognitive ability stratum at the top (McGrew & Schneider, 2018 ). There is no “broad ability” corresponding to attention in the Cattell–Horn–Carroll model. Attention is mentioned in many places in the hierarchy under fluid reasoning, working memory capacity, and processing speed ability. In this view, attention is not a single function or ability, but a property of many different subsystems. Jewsbury et al. ( 2017 ) proposed a similar view of executive function. Reconciling this approach with the view of attention as an independent factor or factor on its own will require cross-disciplinary collaboration.

Implications for theories of attention
Our analysis suggests three subcomponents of attention (i.e., attention capacity, search, and sustained attention). For the attention capacity factor, it is not surprising the experimental paradigms of MOT, VWM, and ANS paradigms comprised this factor. The relationship between visual working memory and multiple object tracking has been explored in some depth. It is important to keep in mind that spatial memory and visual working memory are distinct constructs (Klauer & Zhao, 2004 ; Oh & Kim, 2004 ; Vicari et al., 2003 ; Woodman & Luck, 2004 ). While the most intuitive model of multiple object tracking would involve storing the locations of targets in spatial memory, then moving attention in turn as quickly as possible to each target to update its location, this class of model is unable to account for MOT performance (Pylyshyn & Storm, 1988 ; Vul et al., 2009 ), leading theorists to propose additional cognitive structures or operations such as visual indexes (or “FINSTS” – “FINgers of INTsantiation”, Pylyshyn, 1989 , 2001 ) or multifocal attention (Cavanagh & Alvarez, 2005 ). There is also some dispute as to whether spatial working memory is involved. Allen, et al. ( 2006 ) argued that MOT performance was closely linked to Baddeley and Hitch’s spatial working memory store, aka the visuo-spatial sketchpad (Baddeley & Hitch, 1974 ). However, several studies have shown dissociations between MOT and spatial working memory (Bettencourt et al., 2011 ; Carter et al., 2005 ; O’Hearn et al., 2010 ). Furthermore, Trick et al. ( 2012 ) showed that visuospatial ability (including Corsi Blocks, a spatial span variant) but not working memory, predicts MOT.
The most striking link between the two paradigms is that they seem to have a similar capacity limit of around four items (Cowan, 2001 ). Vul et al. ( 2009 ) used an ideal observer model to show that the limit on the number of objects that can be tracked is not a property of the information available in the paradigm and therefore must derive from a limitation in either memory or attention. This analysis does not conclusively link MOT and VWM, but it does raise the possibility that their common variance might derive from reliance on a common attentional resource. Fougnie and Marois ( 2006 ) explicitly posited that the common capacity limit in the two paradigms (as well as rapid serial visual presentation paradigms) derived from a common reliance on visuospatial attention. However, Souza and Oberauer ( 2017 ) argued that VWM and MOT use different attentional resources.
Electrophysiological studies also demonstrate close links between VWM and MOT. The contralateral delay activity, a sustained voltage decrease during the retention interval of a short-term memory test, indexes the number of items held in visual working memory (Luck & Vogel, 1997 ; Luria et al., 2016 ). The amplitude of this activity can also be used to measure the number of targets being tracked in an MOT experiment (Drew & Vogel, 2008 ; Drew et al., 2011 , 2012 ). This suggests an overlap in the neural circuitry involved in the two paradigms.
While the relationship between ANS, on the one hand, and VWM and MOT, on the other, is not well studied, it is worth considering the theoretical relationship between enumeration and attention. Numerosity is just one of a set of summary or ensemble statistics that can be extracted by the visual system (Alvarez, 2011 ). There is some evidence these representations are derived independently from one another (Khvostov & Utochkin, 2019 ; Utochkin & Vostrikov, 2017 ). However, there may be some core faculty for computing statistics that is held in common, and is also useful for tracking and remembering objects. Alternatively, it may be that there is something unique about numerosity or magnitude perception that makes it a probe for attention.
Meanwhile, what does it mean that Visual Search did not load onto the first factor? Visual search has long been identified as a core paradigm in the modern study of attention, dating back to Treisman and Gelade’s seminal work ( 1980 ), yet here it does not load with other commonly used attentional paradigms. These results may be telling us something about the fractionation of attention. Performing a difficult spatial-configuration search with little guiding information will rely on directing focal attention to each item in turn (Moran et al., 2016 ; Woodman & Luck, 1999 ), and therefore much of the variance may be due primarily to variations in the speed with which participants can shift attention. A paradigm like the ANS, on the other hand, requires a global distribution of attention across the display, with no shifting. Similarly, most accounts of MOT assume that attention is continuously distributed to all items in parallel, rather than shifting from one target to another in turn (Howe et al., 2010 ; Pylyshyn & Storm, 1988 ), unless target identities also must be tracked (Oksama & Hyönä, 2008 ). Attention also seems to be required for maintenance in visual working memory (Balestrieri et al., 2019 ; Heuer & Schubö, 2016 ). In some cases, this involves discrete shifts of spatial attention (Williams et al., 2013 ); it is not clear if it is also possible to maintain attention to multiple items in parallel, as in MOT.
Implications for interpreting data from clinical research studies
The major impetus behind this project was our survey of meta-analyses of the neuropsychological research on cancer-related cognitive impairment (Horowitz et al., 2019 ). One of our findings was that there was a great deal of variability in how tests were assigned to domains. For example, the Digit Symbol Coding was classified as a test of processing speed 43.1% of the time, as an attention test 32.3% of the time, and as an executive function test 24.6% of the time. Furthermore, many tests classified as attention tests, such as Digit Span, seemed to us to have little face validity. This project was conceived as a way to provide some empirical guidance for what should be classified as an attention test and what should not, an approach that we hope will be adopted for other domains as well.
One conclusion from this study is that, in line with our initial impressions, Digit Span and Arithmetic tests should not be classified as attention tests. This is not a novel finding. Mirsky et al. ( 1991 ) conducted a factor analysis of putative neuropsychological attention tests, and found that Digit Span and Arithmetic tests did not load onto the same factor as Trail Making, Digit Symbol Coding, Letter Cancellation, Stroop and Continuous Performance tests. Digit Span and Arithmetic are probably best thought of as Working Memory tests, as specified in the Wechsler Adult Intelligence Scale standard model (Reynolds et al., 2013 ). Agelink ven Rentergen et al.’s ( 2020 ) factor analysis of neuropsychological tests also found that in the best-fitting model the Digit Span tests formed their own “working memory” factor.
On the positive side, we found evidence Digit Symbol Coding and Spatial Span do seem to be tapping attentional capacity, while Trail Making and Letter Cancellation measure attentional shifting. These five tests could continue to be classified as attention tests, on the basis of these results, though reports should distinguish between Capacity and Search ability.
Implications for future clinical research studies
The connection between a subset of the neuropsychological tests and the cognitive attention paradigms is a two-way street. Not only does this finding validate that the neuropsychological tests have some connection to the construct of attention, it also suggests that certain experimental paradigms might be usefully adapted to serve clinical purposes.
The standard armamentarium of clinical neuropsychology has a number of limitations (Marcopulos & Łojek, 2019 ), including lack of sensitivity, lack of process-purity (Kessels, 2019 ), and lack of repeatability (Bilder & Reise, 2019 ). Developing tests from cognitive neuroscience paradigms, which tend to be theoretically derived to be more process-pure, and designed for repeatability, is a potential solution (Kessels, 2019 ). Whether such tests would be more sensitive is an empirical question.
Experimental cognitive paradigms do have drawbacks as potential clinical tests (Kessels, 2019 ). Their psychometric properties, including sensitivity and reliability, are generally not known. Most paradigms have been tested primarily on college undergraduates, meaning not only is their generalizability in question, but also that without extensive norming studies, there is no way to adjust an individual’s score for factors like age, sex, and education. Determining clinical utility will require normative data with clinical and nonclinical samples. Many paradigms rely on response time, and may become unreliable when different populations adopt different speed-accuracy tradeoffs. Since each study adapts the paradigm to answer a specific question, there are innumerable variants of each paradigm, so there is no standard to adopt. And while they are typically not proprietary, by the same token they cannot simply be used off the shelf; some investment is necessary to produce a useful version.
We do not mean to minimize these problems. However, we do think that they can be overcome. The Cognitive Neuroscience Treatment Research to Improve Cognition in Schizophrenia initiative, for example, has been leveraging cognitive psychology and cognitive neuroscience paradigms to develop tests to improve our understanding of schizophrenia (Barch et al., 2009 ; Carter & Barch, 2007 ), and a similar initiative is underway for cognitive deficits associated with obesity and diabetes (d’Ardenne et al., 2019 ).
Our findings suggest that if a condition leads to deficits on the Digit Symbol Coding or Spatial Span tests, then a test based on MOT, for example, might be useful. Tullo et al. ( 2018 ) have begun developing MOT as a clinical test of attentional capacity. Similarly, if deficits are observed using the Trail Making Test, it might be worth using a visual search paradigm (Gold et al., 2007 ; Horowitz et al., 2006 ) to determine whether the problem stems specifically from a problem in shifting attention, or whether it might be attributable to the other faculties tapped by the Trail Making Test.
Limitations and caveats
There are a number of important limitations to this study. First, we are sampling from the population of people who are online and self-selected to participate in cognitive studies for free. As noted above, we think that our sample is probably more representative than, say, undergraduate university psychology students. However, it is better-educated, younger, and probably more affluent than the population as a whole. The majority of subjects were recruited through TestMyBrain.org. In past studies using TestMyBrain.org, the top sources for the site have been www.google.com , www.stumbleupon.com , and www.i-am-bored.com , and frequently used search terms leading to TestMyBrain.org were “brain test” and “brain tests”, suggesting that many visitors arrive at the website because they are curious about their cognitive abilities (Germine et al., 2012 ). Furthermore, we do not have a good idea of which countries our participants live in, and our demographic information does not line up with standard US racial/ethnic categories. Critically, we do not know how the factor structure might change for specific clinical populations. We are currently studying a population of cancer survivors, but interested researchers might want to carry out replications in their fields of interest.
Second, our analysis noted significant effects of response mode on the factor structure; whether participants used a keyboard and mouse or a touchscreen made a noticeable difference. We did not have enough participants who used only a touchscreen to fully characterize this effect, but since computing as a whole, and computerized neuropsychological testing in particular, is moving toward touchscreen interfaces, this issue will become increasingly important.
Perhaps the most important limitation of our study is that our neuropsychological tests were not necessarily identical to the tests currently being administered by clinical neuropsychologists. A challenge of the present study was converting traditional paper-and-pencil tests to an online format while keeping the differences between the two to a minimum. Instructions for TestMyBrain measures were given visually, and practices were completed in order to ensure comprehension of instructions. In contrast, a neuropsychologist administers the pencil-and-paper versions and instructions are given orally. The traditional Arithmetic and Digit Span tests require participants to verbally answer, the Trail Making Test, Digit Symbol Coding, and Letter Cancellation necessitate the use of a pen or pencil, and Spatial Span requires finger pointing. Our online measures were modified so that participants could respond using either a keyboard or touchscreen. In “ Appendix A ”—Comparison of online and traditional, we detail the traditional pencil-paper tests and their modified online counterparts. In addition to administration and formatting differences, digitizing pencil-and-pencil tests may alter the perceptional, cognitive and motor performances of tests and introduce measurement bias due to device variations (Germine et al., 2019 ).
Conclusions
The goal of this project is to provide a bridge between theory-driven cognitive research and clinically relevant neuropsychological research. We believe it is important to align neuropsychology with cognitive psychology and cognitive neuroscience to improve the precision and interpretability of cognitive assessments. Our results should provide guidance for which neuropsychological tests should be classified as attention tests, and hopefully provide inspiration for the development of new clinical assessments based on experimental attention paradigms. Furthermore, we hope we have provided a template for other researchers to explore the connections between cognitive paradigms and neuropsychological tests in domains beyond attention. By bringing these fields closer together, we can improve our scientific understanding of cognition, and ultimately improve the welfare of people who suffer from cognitive disorders and deficits.
Availability of data and materials
The datasets generated and/or analyzed during the current study are available in the Open Science Foundation repository, https://osf.io/py83d/ .
Abbreviations
Approximate number sense
Gradual onset continuous performance task
Multiple object tracking
Trail making test, version A
Trail making test, version B
Visual working memory
Comparative fit index
Likelihood ratio test
Root mean square of residuals
Root mean square error of approximation
Tucker–Lewis Index
Agelink van Rentergem, J. A., de Vent, N. R., Schmand, B. A., Murre, J. M. J., Staaks, J. P. C., ANDI Consortium, & Huizenga, H. M. (2020). The factor structure of cognitive functioning in cognitively healthy participants: A meta-analysis and meta-analysis of individual participant data. Neuropsychology Review , 30 (1), 51–96. https://doi.org/10.1007/s11065-019-09423-6
Agelinkvan Rentergem, J. A., de Vent, N. R., Schmand, B. A., et al. (2020). The factor structure of cognitive functioning in cognitively healthy participants: A meta-analysis and meta-analysis of individual participant data. Neuropsychology Review, 30 , 51–96. https://doi.org/10.1007/s11065-019-09423-6
Article Google Scholar
Allen, R., McGeorge, P., Pearson, D. G., & Milne, A. (2006). Multiple-target tracking: A role for working memory? Quarterly Journal of Experimental Psychology, 59 (6), 1101–1116. https://doi.org/10.1080/02724980543000097
Alvarez, G. A. (2011). Representing multiple objects as an ensemble enhances visual cognition. Trends in Cognitive Sciences, 15 (3), 122–131. https://doi.org/10.1016/j.tics.2011.01.003
Article PubMed Google Scholar
Amer, T., Ngo, K. W. J., & Hasher, L. (2017). Cultural differences in visual attention: Implications for distraction processing. British Journal of Psychology, 108 (2), 244–258. https://doi.org/10.1111/bjop.12194
Arora, S., Lawrence, M. A., & Klein, R. M. (2020). The attention network test database: ADHD and cross-cultural applications. Frontiers in Psychology . https://doi.org/10.3389/fpsyg.2020.00388
Article PubMed PubMed Central Google Scholar
Baddeley, A. D., & Hitch, G. (1974). Working memory. In G. H. Bower (Ed.), Psychology of learning and motivation (Vol. 8, pp. 47–89). Academic Press. https://doi.org/10.1016/S0079-7421(08)60452-1
Chapter Google Scholar
Balestrieri, E., Ronconi, L., & Melcher, D. (2019). Shared resources between visual attention and visual working memory are allocated through rhythmic sampling. BioRxiv . https://doi.org/10.1101/567602
Barch, D. M., Carter, C. S., Arnsten, A., Buchanan, R. W., Cohen, J. D., Geyer, M., Green, M. F., Krystal, J. H., Nuechterlein, K., Robbins, T., Silverstein, S., Smith, E. E., Strauss, M., Wykes, T., & Heinssen, R. (2009). Selecting paradigms from cognitive neuroscience for translation into use in clinical trials: Proceedings of the third CNTRICS meeting. Schizophrenia Bulletin, 35 (1), 109–114. https://doi.org/10.1093/schbul/sbn163
Bates, M. E., & Lemay, E. P. (2004). The d2 test of attention: Construct validity and extensions in scoring techniques. Journal of the International Neuropsychological Society, 10 (3), 392–400. https://doi.org/10.1017/S135561770410307X
Bettencourt, K. C., Michalka, S. W., & Somers, D. C. (2011). Shared filtering processes link attentional and visual short-term memory capacity limits. Journal of Vision . https://doi.org/10.1167/11.10.22
Bilder, R. M., & Reise, S. P. (2019). Neuropsychological tests of the future: How do we get there from here? The Clinical Neuropsychologist, 33 (2), 220–245. https://doi.org/10.1080/13854046.2018.1521993
Brickenkamp, R., & Zillmer, E. (1998). The d2 test of attention . Hogrefe & Huber Publishers.
Google Scholar
Bricolo, E., Gianesini, T., Fanini, A., Bundesen, C., & Chelazzi, L. (2002). Serial attention mechanisms in visual search: A direct behavioral demonstration. Journal of Cognitive Neuroscience, 14 (7), 980–993. https://doi.org/10.1162/089892902320474454
Brown, L. A. (2016). Spatial-sequential working memory in younger and older adults: Age predicts backward recall performance within both age groups. Frontiers in Psychology, 7 , 1514–1514. https://doi.org/10.3389/fpsyg.2016.01514
Browne, M. W., & Cudeck, R. (2016). Alternative ways of assessing model fit. Sociological Methods & Research . https://doi.org/10.1177/0049124192021002005
Bundesen, C. (1990). A theory of visual attention. Psychological Review, 97 (4), 523.
Carter, C. S., & Barch, D. M. (2007). Cognitive neuroscience-based approaches to measuring and improving treatment effects on cognition in schizophrenia: The CNTRICS initiative. Schizophrenia Bulletin, 33 (5), 1131–1137. https://doi.org/10.1093/schbul/sbm081
Carter, O. L., Burr, D. C., Pettigrew, J. D., Wallis, G. M., Hasler, F., & Vollenweider, F. X. (2005). Using psilocybin to investigate the relationship between attention, working memory, and the serotonin 1A and 2A receptors. Journal of Cognitive Neuroscience, 17 (10), 1497–1508. https://doi.org/10.1162/089892905774597191
Cavanagh, P., & Alvarez, G. A. (2005). Tracking multiple targets with multifocal attention. Trends in Cognitive Sciences, 9 (7), 349–354. https://doi.org/10.1016/j.tics.2005.05.009
Cheung, G. W., & Rensvold, R. B. (2002). Evaluating goodness-of-fit indexes for testing measurement invariance. Structural Equation Modeling: A Multidisciplinary Journal, 9 (2), 233–255. https://doi.org/10.1207/S15328007SEM0902_5
Chong, S. C., & Evans, K. K. (2011). Distributed versus focused attention (count vs estimate): Distributed versus focused attention. Wiley Interdisciplinary Reviews: Cognitive Science, 2 (6), 634–638. https://doi.org/10.1002/wcs.136
Chun, M. M., Golomb, J. D., & Turk-Browne, N. B. (2011). A Taxonomy of external and internal attention. Annual Review of Psychology, 62 (1), 73–101. https://doi.org/10.1146/annurev.psych.093008.100427
Corsi, P. (1972). Memory and the medial temporal region of the brain. Unpublished doctoral dissertation.
Cowan, N. (2001). The magical number 4 in short-term memory: A reconsideration of mental storage capacity. The Behavioral and Brain Sciences , 24 (1), 87–114; discussion 114–85.
Crowe, S. F. (1998). The differential contribution of mental tracking, cognitive flexibility, visual search, and motor speed to performance on parts A and B of the trail making test. Journal of Clinical Psychology, 54 (5), 585–591. https://doi.org/10.1002/(SICI)1097-4679(199808)54:5%3c585::AID-JCLP4%3e3.0.CO;2-K
Cunningham, J. E. A., Jones, S. A. H., Eskes, G. A., & Rusak, B. (2018). Acute sleep restriction has differential effects on components of attention. Frontiers in Psychiatry . https://doi.org/10.3389/fpsyt.2018.00499
d’Ardenne, K., Savage, C., Small, D., Vainik, U., & Luke E. Stoeckel, P. (2019). Core neuropsychological measures for obesity and diabetes trials project workshop report (Version 2) . https://doi.org/10.31232/osf.io/7jygx
Della Sala, S., Gray, C., Baddeley, A., Allamano, N., & Wilson, L. (1999). Pattern span: A tool for unwelding visuo–spatial memory. Neuropsychologia, 37 (10), 1189–1199. https://doi.org/10.1016/S0028-3932(98)00159-6
Desimone, R., & Duncan, J. (1995). Neural mechanisms of selective visual attention. Annual Review of Neuroscience, 18 (1), 193–222.
Drew, T., Horowitz, T. S., Wolfe, J. M., & Vogel, E. K. (2011). Delineating the neural signatures of tracking spatial position and working memory during attentive tracking. The Journal of Neuroscience, 31 (2), 659–668. https://doi.org/10.1523/JNEUROSCI.1339-10.2011
Drew, T., Horowitz, T. S., Wolfe, J. M., & Vogel, E. K. (2012). Neural measures of dynamic changes in attentive tracking load. Journal of Cognitive Neuroscience, 24 (2), 440–450. https://doi.org/10.1162/jocn_a_00107
Drew, T., & Vogel, E. K. (2008). Neural measures of individual differences in selecting and tracking multiple moving objects. The Journal of Neuroscience: the Official Journal of the Society for Neuroscience, 28 (16), 4183–4191. https://doi.org/10.1523/JNEUROSCI.0556-08.2008
Emrich, S. M., Lockhart, H. A., & Al-Aidroos, N. (2017). Attention mediates the flexible allocation of visual working memory resources. Journal of Experimental Psychology: Human Perception and Performance, 43 (7), 1454–1465. https://doi.org/10.1037/xhp0000398
Eriksen, B. A., & Eriksen, C. W. (1974). Effects of noise letters upon the identification of a target letter in a nonsearch task. Perception and Psychophysics, 16 (1), 143–149. https://doi.org/10.3758/BF03203267
Fan, J., McCandliss, B. D., Sommer, T., Raz, A., & Posner, M. I. (2002). Testing the efficiency and independence of attentional networks. Journal of Cognitive Neuroscience, 14 (3), 340–347. https://doi.org/10.1162/089892902317361886
Fortenbaugh, F. C., DeGutis, J., Germine, L., Wilmer, J. B., Grosso, M., Russo, K., & Esterman, M. (2015). Sustained attention across the life span in a sample of 10,000 dissociating ability and strategy. Psychological Science, 26 (9), 1497–1510. https://doi.org/10.1177/0956797615594896
Foti, M., Lo Buono, V., Corallo, F., Palmeri, R., Bramanti, P., & Marino, S. (2017). Neuropsychological assessment in migraine patients: A descriptive review on cognitive implications. Neurological Sciences, 38 (4), 553–562. https://doi.org/10.1007/s10072-017-2814-z
Fougnie, D., & Marois, R. (2006). Distinct capacity limits for attention and working memory: Evidence from attentive tracking and visual working memory paradigms. Psychological Science: A Journal of the American Psychological Society/APS, 17 (6), 526–534. https://doi.org/10.1111/j.1467-9280.2006.01739.x
Fougnie, D., & Marois, R. (2011). What limits working memory capacity? Evidence for modality-specific sources to the simultaneous storage of visual and auditory arrays. Journal of Experimental Psychology. Learning. Memory. and Cognition . https://doi.org/10.1037/a0024834
Germine, L., Nakayama, K., Duchaine, B. C., Chabris, C. F., Chatterjee, G., & Wilmer, J. B. (2012). Is the Web as good as the lab? Comparable performance from Web and lab in cognitive/perceptual experiments. Psychonomic Bulletin & Review, 19 (5), 847–857. https://doi.org/10.3758/s13423-012-0296-9
Germine, L., Reinecke, K., & Chaytor, N. S. (2019). Digital neuropsychology: Challenges and opportunities at the intersection of science and software. The Clinical Neuropsychologist, 33 (2), 271–286. https://doi.org/10.1080/13854046.2018.1535662
Gold, J. M., Fuller, R. L., Robinson, B. M., Braun, E. L., & Luck, S. J. (2007). Impaired top–down control of visual search in schizophrenia. Schizophrenia Research, 94 (1–3), 148–155. https://doi.org/10.1016/j.schres.2007.04.023
Halberda, J., Ly, R., Wilmer, J. B., Naiman, D. Q., & Germine, L. (2012). Number sense across the lifespan as revealed by a massive Internet-based sample. Proceedings of the National Academy of Sciences, 109 (28), 11116. https://doi.org/10.1073/pnas.1200196109
Halberda, J., Mazzocco, M. M. M., & Feigenson, L. (2008). Individual differences in non-verbal number acuity correlate with maths achievement. Nature, 455 (7213), 665–668. https://doi.org/10.1038/nature07246
Hartshorne, J. K., & Germine, L. T. (2015). When does cognitive functioning peak? The asynchronous rise and fall of different cognitive abilities across the life span. Psychological Science, 26 (4), 433–443. https://doi.org/10.1177/0956797614567339
Helmstaedter, C., Kurthen, M., Lux, S., Reuber, M., & Elger, C. E. (2003). Chronic epilepsy and cognition: A longitudinal study in temporal lobe epilepsy. Annals of Neurology, 54 (4), 425–432. https://doi.org/10.1002/ana.10692
Heuer, A., & Schubö, A. (2016). The focus of attention in visual working memory: protection of focused representations and its individual variation. PLoS ONE, 11 (4), e0154228. https://doi.org/10.1371/journal.pone.0154228
Holcombe, A. O., Chen, W.-Y., & Howe, P. D. L. (2014). Object tracking: Absence of long-range spatial interference supports resource theories. Journal of Vision, 14 (6), 1–1. https://doi.org/10.1167/14.6.1
Horn, J. L. (1965). A rationale and test for the number of factors in factor analysis. Psychometrika, 30 (2), 179–185. https://doi.org/10.1007/BF02289447
Horowitz, T. S., Choi, W. Y., Horvitz, J. C., Côté, L. J., & Mangels, J. A. (2006). Visual search deficits in Parkinson’s disease are attenuated by bottom-up target salience and top-down information. Neuropsychologia, 44 (10), 1962–1977. https://doi.org/10.1016/j.neuropsychologia.2006.01.037
Horowitz, T. S., Suls, J., & Treviño, M. (2018). A call for a neuroscience approach to cancer-related cognitive impairment. Trends in Neurosciences, 41 (8), 493–496. https://doi.org/10.1016/j.tins.2018.05.001
Horowitz, T. S., Treviño, M., Gooch, I. M., & Duffy, K. A. (2019). Understanding the profile of cancer-related cognitive impairments: A critique of meta-analyses. JNCI: Journal of the National Cancer Institute . https://doi.org/10.1093/jnci/djz100
Howe, P. D. L., Cohen, M. A., Pinto, Y., & Horowitz, T. S. (2010). Distinguishing between parallel and serial accounts of multiple object tracking. Journal of Vision, 10 (8), 11. https://doi.org/10.1167/10.8.11
Howieson, D. (2019). Current limitations of neuropsychological tests and assessment procedures. The Clinical Neuropsychologist, 33 (2), 200–208. https://doi.org/10.1080/13854046.2018.1552762
Hu, L., & Bentler, P. M. (1999). Cutoff criteria for fit indexes in covariance structure analysis: Conventional criteria versus new alternatives. Structural Equation Modeling: A Multidisciplinary Journal, 6 (1), 1–55. https://doi.org/10.1080/10705519909540118
Huang, L., Mo, L., & Li, Y. (2012). Measuring the interrelations among multiple paradigms of visual attention: An individual differences approach. Journal of Experimental Psychology: Human Perception and Performance, 38 (2), 414–428. https://doi.org/10.1037/a0026314
Huang, L., & Pashler, H. (2007). A Boolean map theory of visual attention. Psychological Review, 114 (3), 599.
Jaeger, J. (2018). Digit symbol substitution test. Journal of Clinical Psychopharmacology, 38 (5):513–519.
Jenkins, V., Shilling, V., Deutsch, G., Bloomfield, D., Morris, R., Allan, S., Bishop, H., Hodson, N., Mitra, S., & Sadler, G. (2006). A 3-year prospective study of the effects of adjuvant treatments on cognition in women with early stage breast cancer. British Journal of Cancer, 94 (6), 828.
Jewsbury, P. A., Bowden, S. C., & Duff, K. (2017). The Cattell–Horn–Carroll model of cognition for clinical assessment. Journal of Psychoeducational Assessment, 35 (6), 547–567. https://doi.org/10.1177/0734282916651360
Jóhannesson, O. I., Kristjánsson, Á., & Thornton, I. M. (2017). Are foraging patterns in humans related to working memory and inhibitory control? Japanese Psychological Research, 59 (2), 152–166. https://doi.org/10.1111/jpr.12152
Jones, S. A. H., Butler, B. C., Kintzel, F., Johnson, A., Klein, R. M., & Eskes, G. A. (2016). Measuring the performance of attention networks with the dalhousie computerized attention battery (DalCAB): Methodology and reliability in healthy adults. Frontiers in Psychology, 7 , 823. https://doi.org/10.3389/fpsyg.2016.00823
Jones, S. A. H., Butler, B., Kintzel, F., Salmon, J. P., Klein, R. M., & Eskes, G. A. (2015). Measuring the components of attention using the Dalhousie computerized attention battery (DalCAB). Psychological Assessment, 27 (4), 1286–1300. https://doi.org/10.1037/pas0000148
Jorgenson, T. D., Pornprasertmanit, S., Schoemann, A. M., & Rosseel, Y. (2019). semTools: Useful tools for structural equation modeling (0.5–2) [R]. https://CRAN.R-project.org/package=semTools
Kessels, R. P. C. (2019). Improving precision in neuropsychological assessment: Bridging the gap between classic paper-and-pencil tests and paradigms from cognitive neuroscience. The Clinical Neuropsychologist, 33 (2), 357–368. https://doi.org/10.1080/13854046.2018.1518489
Khvostov, V. A., & Utochkin, I. S. (2019). Independent and parallel visual processing of ensemble statistics: Evidence from dual tasks. Journal of Vision, 19 (9), 3–3. https://doi.org/10.1167/19.9.3
Klauer, K. C., & Zhao, Z. (2004). Double dissociations in visual and spatial short-term memory. Journal of Experimental Psychology General, 133 (3), 355–381. https://doi.org/10.1037/0096-3445.133.3.355
Kristjánsson, T., Thornton, I. M., Chetverikov, A., & Kristjánsson, Á. (2020). Dynamics of visual attention revealed in foraging tasks. Cognition, 194 , 104032. https://doi.org/10.1016/j.cognition.2019.104032
Luck, S. J., & Vogel, E. K. (1997). The capacity of visual working memory for features and conjunctions. Nature, 390 (6657), 279–281. https://doi.org/10.1038/36846
Luria, R., Balaban, H., Awh, E., & Vogel, E. K. (2016). The contralateral delay activity as a neural measure of visual working memory. Neuroscience & Biobehavioral Reviews, 62 , 100–108. https://doi.org/10.1016/j.neubiorev.2016.01.003
MacCallum, R. C., Widaman, K. F., Zhang, S., & Hong, S. (1999). Sample size in factor analysis. Psychological Methods, 4 (1), 84.
MacLeod, J. W., Lawrence, M. A., McConnell, M. M., Eskes, G. A., Klein, R. M., & Shore, D. I. (2010). Appraising the ANT: Psychometric and theoretical considerations of the attention network test. Neuropsychology, 24 (5), 637–651. https://doi.org/10.1037/a0019803
Macmillan, N. A., & Creelman, C. D. (2005). Detection theory: A user’s guide . Lawrence Erlbaum Associates.
Makovski, T., Sussman, R., & Jiang, Y. V. (2008). Orienting attention in visual working memory reduces interference from memory probes. Journal of Experimental Psychology. Learning, Memory, and Cognition, 34 (2), 369–380.
Marcopulos, B., & Łojek, E. (2019). Introduction to the special issue: Are modern neuropsychological assessment methods really “modern”? Reflections on the current neuropsychological test armamentarium. The Clinical Neuropsychologist, 33 (2), 187–199. https://doi.org/10.1080/13854046.2018.1560502
Masuda, T., & Nisbett, R. E. (2001). Attending holistically versus analytically: Comparing the context sensitivity of Japanese and Americans. Journal of Personality and Social Psychology, 81 (5), 922–934. https://doi.org/10.1037/0022-3514.81.5.922
Mazza, V., & Caramazza, A. (2015). Multiple object individuation and subitizing in enumeration: A view from electrophysiology. Frontiers in Human Neuroscience . https://doi.org/10.3389/fnhum.2015.00162
McFall, R. M. (2005). Theory and utility-Key themes in evidence-based assessment: Comment on the special section. Psychological Assessment, 17 (3):312–323.
McFall, R. M., & Townsend, J. T. (1998). Foundations of psychological assessment: Implications for cognitive assessment in clinical science. Psychological Assessment, 10 (4), 316.
McGrew, K. S., & Schneider, W. J. (2018). CHC theory revised: A visual graphic summary of Schneider and McGrew’s 2018 CHC Update Chapter . 45.
McGrew, K. S. (2009). CHC theory and the human cognitive abilities project: Standing on the shoulders of the giants of psychometric intelligence research. Intelligence, 37 (1), 1–10. https://doi.org/10.1016/j.intell.2008.08.004
Meade, T., Manolios, N., Cumming, S. R., Conaghan, P. G., & Katz, P. (2018). Cognitive impairment in rheumatoid arthritis: A systematic review. Arthritis Care & Research, 70 (1), 39–52. https://doi.org/10.1002/acr.23243
Merkle, E. C., & You, D. (2020). nonnest2: Tests of non-nested models (0.5–4) [R]. https://CRAN.R-project.org/package=nonnest2
Merkle, E. C., You, D., & Preacher, K. J. (2016). Testing nonnested structural equation models. Psychological Methods, 21 (2), 151–163. https://doi.org/10.1037/met0000038
Mirsky, A. F., Anthony, B. J., Duncan, C. C., Ahearn, M. B., & Kellam, S. G. (1991). Analysis of the elements of attention: A neuropsychological approach. Neuropsychology Review, 2 (2), 109–145. https://doi.org/10.1007/BF01109051
Misdraji, E. L., & Gass, C. S. (2010). The trail making test and its neurobehavioral components. Journal of Clinical and Experimental Neuropsychology, 32 (2), 159–163. https://doi.org/10.1080/13803390902881942
Moran, R., Zehetleitner, M., Liesefeld, H. R., Müller, H. J., & Usher, M. (2016). Serial vs. parallel models of attention in visual search: Accounting for benchmark RT-distributions. Psychonomic Bulletin & Review, 23 (5), 1300–1315. https://doi.org/10.3758/s13423-015-0978-1
Mundfrom, D. J., Shaw, D. G., & Ke, T. L. (2005). Minimum sample size recommendations for conducting factor analyses. International Journal of Testing, 5 (2), 159–168. https://doi.org/10.1207/s15327574ijt0502_4
Nelson, W. L., & Suls, J. (2013). New approaches to understand cognitive changes associated with chemotherapy for non-central nervous system tumors. Journal of Pain and Symptom Management, 46 (5), 707–721. https://doi.org/10.1016/j.jpainsymman.2012.11.005
O’Hearn, K., Hoffman, J. E., & Landau, B. (2010). Developmental profiles for multiple object tracking and spatial memory: Typically developing preschoolers and people with Williams syndrome. Developmental Science, 13 (3), 430–440. https://doi.org/10.1111/j.1467-7687.2009.00893.x
Oh, S.-H., & Kim, M.-S. (2004). The role of spatial working memory in visual search efficiency. Psychonomic Bulletin & Review, 11 (2), 275–281. https://doi.org/10.3758/BF03196570
Oksama, L., & Hyönä, J. (2008). Dynamic binding of identity and location information: A serial model of multiple identity tracking. Cognitive Psychology, 56 (4), 237–283. https://doi.org/10.1016/j.cogpsych.2007.03.001
Parasuraman, R., Warm, J. S., & See, J. E. (1998). Brain systems of vigilance. In The attentive brain (pp. 221–256). The MIT Press.
Parsons, T. D., & Duffield, T. (2019). National Institutes of Health initiatives for advancing scientific developments in clinical neuropsychology. The Clinical Neuropsychologist, 33 (2), 246–270. https://doi.org/10.1080/13854046.2018.1523465
Partington, J. E., & Leiter, R. G. (1949). Partington’s pathways test. The Psychological Service Center Bulletin, 1 (2), 9–20.
Pashler, H. (1988). Familiarity and visual change detection. Perception & Psychophysics, 44 (4), 369–378. https://doi.org/10.3758/BF03210419
Passell, E., Dillon, D. G., Baker, J. T., Vogel, S. C., Scheuer, L. S., Mirin, N. L., & Germine, L. (2019). Digital cognitive assessment: Results from the TestMyBrain NIMH research domain criteria (RDoC) Field Test Battery Report.
Peters, B., Rahm, B., Czoschke, S., Barnes, C., Kaiser, J., & Bledowski, C. (2018). Sequential whole report accesses different states in visual working memory. Journal of Experimental Psychology: Learning, Memory, and Cognition, 44 (4), 588–603. https://doi.org/10.1037/xlm0000466
Posner, M. I., & Petersen, S. E. (1990). The attention system of the human brain. Annual Review of Neuroscience, 13 (1), 25–42.
Praß, M., & de Haan, B. (2019). Multi-target attention and visual short-term memory capacity are closely linked in the intraparietal sulcus. Human Brain Mapping . https://doi.org/10.1002/hbm.24618
Preacher, K. J., & MacCallum, R. C. (2002). Exploratory factor analysis in behavior genetics research: Factor recovery with small sample sizes. Behavior Genetics, 32 (2), 153–161. https://doi.org/10.1023/A:1015210025234
Price, L. R., Tulsky, D., Millis, S., & Weiss, L. (2002). Redefining the factor structure of the wechsler memory scale-III: Confirmatory factor analysis with cross-validation. Journal of Clinical and Experimental Neuropsychology, 24 (5), 574–585. https://doi.org/10.1076/jcen.24.5.574.1013
Pylyshyn, Z. W. (1989). The role of location indexes in spatial perception: A sketch of the FINST spatial-index model. Cognition, 32 , 65–97.
Pylyshyn, Z. W. (2001). Visual indexes, preconceptual objects, and situated vision. Cognition, 80 (1–2), 127–158.
Pylyshyn, Z. W., & Storm, R. W. (1988). Tracking multiple independent targets: Evidence for a parallel tracking mechanism. Spatial Vision, 3 (3), 179–197.
R Core Team. (2020). R: A language and environment for statistical computing . http://www.R-project.org/
Rabin, L. A., Paolillo, E., & Barr, W. B. (2016). Stability in test-usage practices of clinical neuropsychologists in the United States and Canada over a 10-year period: A follow-up survey of INS and NAN members. Archives of Clinical Neuropsychology, 31 (3), 206–230. https://doi.org/10.1093/arclin/acw007
Reitan, R. M. (1971). Trail making test. Manual for administration and scoring . Reitan Neuropsychology Laboratory.
Revelle, W. (2018). psych: Procedures for personality and psychological research (1.8.12) [R]. Northwestern University. https://CRAN.R-project.org/package=psych
Reynolds, M. R., Ingram, P. B., Seeley, J. S., & Newby, K. D. (2013). Investigating the structure and invariance of the Wechsler Adult Intelligence Scales, Fourth edition in a sample of adults with intellectual disabilities. Research in Developmental Disabilities, 34 (10), 3235–3245. https://doi.org/10.1016/j.ridd.2013.06.029
Robertson, I. H., Manly, T., Andrade, J., Baddeley, B. T., & Yiend, J. (1997). `Oops!’: Performance correlates of everyday attentional failures in traumatic brain injured and normal subjects. Neuropsychologia, 35 (6), 747–758. https://doi.org/10.1016/S0028-3932(97)00015-8
Roper, Z. J. J., & Vecera, S. P. (2014). Visual short-term memory load strengthens selective attention. Psychonomic Bulletin & Review, 21 (2), 549–556. https://doi.org/10.3758/s13423-013-0503-3
Rosenberg, M., Noonan, S., DeGutis, J., & Esterman, M. (2013). Sustaining visual attention in the face of distraction: A novel gradual-onset continuous performance task. Attention, Perception, & Psychophysics, 75 (3), 426–439. https://doi.org/10.3758/s13414-012-0413-x
Rosseel, Y. (2012). lavaan: An R package for structural equation modeling. Journal of Statistical Software, 48 (1), 1–36. https://doi.org/10.18637/jss.v048.i02
Salthouse, T. A. (2011). What cognitive abilities are involved in trail-making performance? Intelligence, 39 (4), 222–232. https://doi.org/10.1016/j.intell.2011.03.001
Sánchez-Cubillo, I., Perianez, J., Adrover-Roig, D., Rodriguez-Sanchez, J., Rios-Lago, M., Tirapu, J., & Barcelo, F. (2009). Construct validity of the trail making test: Role of task-switching, working memory, inhibition/interference control, and visuomotor abilities. Journal of the International Neuropsychological Society , 15 (3), 438–450.
Sandry, J., & Ricker, T. J. (2020). Prioritization within visual working memory reflects a flexible focus of attention. Attention, Perception, & Psychophysics, 82 (6), 2985–3004. https://doi.org/10.3758/s13414-020-02049-4
Sardiwalla, Y., Eskes, G., Bernard, A., George, R. B., & Schmidt, M. (2019). Assessing the feasibility of using the Dalhousie computerized attention battery to measure postoperative cognitive dysfunction in older patients. Journal of Perioperative Practice, 29 (10), 328–336. https://doi.org/10.1177/1750458918808163
Schmidt, M., Trueblood, W., Merwin, M., & Durham, R. L. (1994). How much do ‘attention’ tests tell us? Archives of Clinical Neuropsychology, 9 (5), 383–394. https://doi.org/10.1093/arclin/9.5.383
Schneider, W., & Shiffrin, R. M. (1977). Controlled and automatic human information processing: I. Detection, search, and attention. Psychological Review, 84 (1), 1–66.
Scholl, B. J. (2009). What have we learned about attention from multiple object tracking (and vice versa). Computation, Cognition, and Pylyshyn , 49–78.
Shelton, J. T., Elliott, E. M., Hill, B. D., Calamia, M. R., & Gouvier, W. D. (2009). A comparison of laboratory and clinical working memory tests and their prediction of fluid intelligence. Intelligence, 37 (3), 283. https://doi.org/10.1016/j.intell.2008.11.005
Sohlberg, M. M., & Mateer, C. A. (1989). Introduction to cognitive rehabilitation: Theory and practice . Guilford Press.
Skogsberg, K., Grabowecky, M., Wilt, J., Revelle, W., Iordanescu, L., & Suzuki, S. (2015). A relational structure of voluntary visual-attention abilities. Journal of Experimental Psychology: Human Perception and Performance, 41 (3), 761–789. https://doi.org/10.1037/a0039000
Souza, A. S., & Oberauer, K. (2017). The contributions of visual and central attention to visual working memory. Attention, Perception, & Psychophysics, 79 (7), 1897–1916. https://doi.org/10.3758/s13414-017-1357-y
Srisurapanont, M., Suttajit, S., Eurviriyanukul, K., & Varnado, P. (2017). Discrepancy between objective and subjective cognition in adults with major depressive disorder. Scientific Reports, 7 (1), 3901.
Sternberg, S. (1966). High-speed scanning in human memory. Science (new York, NY), 153 (736), 652–654.
Thompson, B. (2004). Exploratory and confirmatory factor analysis: Understanding concepts and applications (pp. x, 195). American Psychological Association. https://doi.org/10.1037/10694-000
Thornton, I. M., & Horowitz, T. S. (2020). MILO mobile: An iPad App to measure search performance in multi-target sequences. I-Perception, 11 (3), 2041669520932587. https://doi.org/10.1177/2041669520932587
Treisman, A. M., & Gelade, G. (1980). A feature-integration theory of attention. Cognitive Psychology, 12 (1), 97–136.
Trick, L. M., Mutreja, R., & Hunt, K. (2012). Spatial and visuospatial working memory tests predict performance in classic multiple-object tracking in young adults, but nonspatial measures of the executive do not. Attention, Perception, & Psychophysics, 74 (2), 300–311. https://doi.org/10.3758/s13414-011-0235-2
Tullo, D., Faubert, J., & Bertone, A. (2018). The characterization of attention resource capacity and its relationship with fluid reasoning intelligence: A multiple object tracking study. Intelligence, 69 , 158–168. https://doi.org/10.1016/j.intell.2018.06.001
Turner, M. L., & Engle, R. W. (1989). Is working memory capacity task dependent? Journal of Memory and Language, 28 (2), 127–154. https://doi.org/10.1016/0749-596X(89)90040-5
Utochkin, I. S., & Vostrikov, K. O. (2017). The numerosity and mean size of multiple objects are perceived independently and in parallel. PLoS ONE, 12 (9), e0185452. https://doi.org/10.1371/journal.pone.0185452
Vicari, S., Bellucci, S., & Carlesimo, G. A. (2003). Visual and spatial working memory dissociation: Evidence from Williams syndrome. Developmental Medicine and Child Neurology, 45 (4), 269–273.
Vives, M., López-Navarro, E., García-Campayo, J., & Gili, M. (2015). Cognitive impairments and depression: A critical review. Actas Españolas De Psiquiatría, 43 (5), 187–193.
PubMed Google Scholar
Vul, E., Frank, M., Tenenbaum, J., & Alvarez, G. A. (2009). Explaining human multiple object tracking as resource-constrained approximate inference in a dynamic probabilistic model. Advances in Neural Information Processing Systems , 22 . http://books.nips.cc/papers/files/nips22/NIPS2009_0980.pdf
Vuong, Q. H. (1989). Likelihood ratio tests for model selection and non-nested hypotheses. Econometrica, 57 (2), 307–333. https://doi.org/10.2307/1912557
Wechsler, D. (1997). Wechsler Adult Intelligence Scale—Third edition (WAIS-III) . Psychological Corporation.
Williams, M., Pouget, P., Boucher, L., & Woodman, G. F. (2013). Visual-spatial attention aids the maintenance of object representations in visual working memory. Memory & Cognition, 41 (5), 698–715. https://doi.org/10.3758/s13421-013-0296-7
Wilmer, J., Martini, P., Germine, L., & Nakayama, K. (2016). Multiple object tracking predicts math potential. Journal of Vision, 16 (12), 421–421. https://doi.org/10.1167/16.12.421
Wolfe, J. M. (2021). Guided search 6.0: An updated model of visual search. Psychonomic Bulletin & Review . https://doi.org/10.3758/s13423-020-01859-9
Woodman, G. F., & Luck, S. J. (1999). Electrophysiological measurement of rapid shifts of attention during visual search. Nature . http://schneider.lrdc.pitt.edu/P2465/Readings/Woodman_1999.PDF
Woodman, G. F., & Luck, S. J. (2004). Visual search is slowed when visuospatial working memory is occupied. Psychonomic Bulletin & Review, 11 (2), 269–274. https://doi.org/10.3758/BF03196569
Yantis, S., & Jonides, J. (1990). Abrupt visual onsets and selective attention: Voluntary versus automatic allocation. Journal of Experimental Psychology. Human Perception and Performance, 16 (1), 121–134.
Zelazo, P. D., Anderson, J. E., Richler, J., Wallner-Allen, K., Beaumont, J. L., & Weintraub, S. (2013). II. NIH toolbox cognition battery (CB): Measuring executive function and attention: nih toolbox cognition battery (CB). Monographs of the Society for Research in Child Development, 78 (4), 16–33. https://doi.org/10.1111/mono.12032
Download references
Acknowledgements
We would like to thank all of the participants for volunteering their time to complete the batter. We are grateful to the American Cancer Society, Cancer Carepoint, Gryt Health, and the National Coalition for Cancer Survivorship for helping to publicize this study.
Open Access funding provided by the National Institutes of Health (NIH). This project has been funded in part from the National Cancer Institute, under contract number # HHSN261201800002B to Westat. The opinions expressed by the authors are their own, and this material should not be interpreted as representing the official viewpoint of the U.S. Department of Health and Human Services, the National Institutes of Health or the National Cancer Institute.
Author information
Authors and affiliations.
Basic Biobehavioral and Psychological Sciences Branch, National Cancer Institute, 9609 Medical Center Drive, Rockville, MD, 20850, USA
Melissa Treviño & Todd S. Horowitz
Westat, 1600 Research Boulevard, Rockville, USA
Xiaoshu Zhu & Grace C. Huang
Institute for Technology in Psychiatry, McLean Hospital, Belmont, USA
Yi Yi Lu, Luke S. Scheuer, Eliza Passell & Laura T. Germine
Department of Psychiatry, Harvard Medical School, Cambridge, USA
You can also search for this author in PubMed Google Scholar
Contributions
MT and TSH contributed to conceptualization. XZ, YYL, LSS and EP helped in data curation. XZ contributed to formal analysis. GCH and TSH contributed to funding acquisition. YYL, LSS and LG helped in investigation. MT, XZ, LG and TSH contributed to methodology. YYL and GCH helped in project administration. LG contributed to resources. LSS, EP and LG contributed to software. GCH, LG and TSH helped in supervision. XZ and TSH contributed to visualization. MT contributed to writing—original draft preparation. MT, XZ, LG and TSH helped in writing—review and editing. All authors read and approved the final manuscript.
Corresponding author
Correspondence to Melissa Treviño .
Ethics declarations
Ethics approval and consent to participate.
All participants gave informed consent. Consent procedures were approved by the Partners Health Care IRB.
Consent for publication
Competing interests.
The authors declare that they have no competing interests.
Additional information
Publisher's note.
Springer Nature remains neutral with regard to jurisdictional claims in published maps and institutional affiliations.
Appendix A: Comparison of online and traditional tests
TMT-A & TMT-B were similar between the online and paper and pencil version except for the implement used for connecting circles. In our online TMT-A & TMT-B, participants had to connect a series of circles on their device screen, in ascending order. For Form A, 25 circles contained numbers and had to be connected in ascending numerical order (e.g., 1-2-3) by drawing a line between circles. For Form B, 25 circles contained letters and numbers, and had to be connected in ascending numerical and alphabetical order, alternating between numbers and letters (e.g., 1-A-2-B-3-C). Depending on the device (i.e., laptop/desktop or tablet/smartphone), participants could use either their mouse or their finger to connect the circles. In the traditional Trail Making Test, participants are given a pencil and are asked to connect the circles. The primary outcome variable for both versions is the time it takes to connect all the circles, calculated separately for Parts A and B.
In the online Digit Symbol Coding test, participants had to choose which number matched a target symbol shown on screen, using a given symbol-number key. Participants could use either a keyboard or touchscreen. Participants in the paper and pencil version are given a pencil and a sheet of paper with the key located at the top of the page and rows of numbers. Participant are tasked to copy the symbol below each number. The primary measure for both online and original versions was how many matches the participant correctly makes in 90 s.
The online and paper and pencil Letter Cancellation tests are comparable in asking participants to search an array of “d” and “p” letters for instances of the lowercase letter “d” flanked by various arrangements of 2 lines. Anytime the participant saw the target letter, they were then asked to cross the letter out (by clicking or touching the letter for the online test or striking through the target letter with a pencil for the original version) until all instances of the letter were found or 20 s passed. Participants’ online score was the total number of correctly identified letters. Various calculations are derived from the traditional letter cancellation test but most notably, the sum of the number of target letters (Bates & Lemay, 2004 ).
The Arithmetic test required participants to solve a series of arithmetic computation and word problems of increasing difficulty. The online version was modeled after the arithmetic test of the Wechsler Adult Intelligence Scales III and was presented visually. For each online question, the participant could earn 1 point for a correct answer and 2 points for a correct answer given within 10 s, regardless of the time allowed or difficulty of the trial. The primary outcome variable was total points earned across the test. There was a total of 20 possible questions. Time limits were given for each question, 15 s for the first 5 questions, then 30 s for the next 5 questions, 60 s for the following 9 questions, and 120 s for the final question. For the traditional test, participants are orally presented with a series of word problems and are not supplied with a pencil or paper. Participants are timed beginning after each problem is read and participants must respond orally within a time limit. Time limits are 15 s for the first 6 questions, then 30 s for 7–11 questions, 60 s for 12 -19 questions, and 120 s for question 20. If the participant gets 4 consecutive wrong answers, then the test is stopped. For questions 1–18, 1 point is obtained for a correct answer given within the time limit; and for questions 9- 20, 2 points are obtained if a correct answer was given within 10 s or 1 point if answer was given within the time limit.
Both online and traditional digit span tests required participants to recall strings of digits of increasing length. The forward digit span test required participants to recall digits in the order they were presented. The backward digit span test required them to recall the digits in reverse order. The online tests were adapted from the Digit Span tests of the Wechsler Adult Intelligence Scales. For the online version, digit sequences are presented visually, and participants are asked to memorize the numbers and then either keyboard or finger press the digits. There are two trials for each sequence length presented and the test ends when the participant misses two trials of the same length. The longest possible sequence length is 11 digits. Participants have 4 s to respond before they are warned to keep responding with the remaining number of digits. Score for the digit span test is calculated as the highest number of digits participants were able to successfully recall at least once—in other words, the length of the longest sequence where participants got at least one of two trials right. For the traditional version, participants are orally presented the digit sequences and asked to verbally recite the sequence. The test ends when the two trials for the same sequence length is incorrect or when the maximal sequence length is reached (9 digits forward, 8 backward). Each correct response is worth one point.
In the Spatial Span test, participants had to learn and recall sequences of visually presented spatial locations. For the online test, sequences were indicated by a shape that changed color. When clicking on each dot in a sequence, participants have 12 s to click on the next dot. If at any point they take more than 12 s to click the next dot, they timeout and the next trial begins. The sequences increased in length from 4 to 7. The primary outcome measure was the length of sequence the participant could accurately recall before making two consecutive mistakes. For the traditional Spatial Span test, the administrator taps the spatial sequence on a board that contains an array of 10 blocks. Participants are then asked to reproduce the sequence by tapping the blocks in the same order they were presented. The test comprises eight sequence lengths, from 2 to 9, with two trials for each sequence length (Brown, 2016 ).
Appendix B: Factor loadings from the exploratory factor analysis
See Tables 6 and 7 .
Appendix C: Measurement invariance analysis by demographic characteristics
We assessed measurement invariance on three demographic characteristics. In addition to age group and gender, we further collapsed education into two subgroups, “Less than college” and “College and above” so each group had over 250 participants.
As shown in Table 8 , the results supported metric invariance for all three characteristics where the changes in fit indices were smaller than the recommended cutoff values for CFI and RMSEA as defined in the Confirmatory Factor Analysis subsection of the Data Analysis section. The scalar invariance held for education only in terms of ΔCFI and ΔRMSEA.
Rights and permissions
Open Access This article is licensed under a Creative Commons Attribution 4.0 International License, which permits use, sharing, adaptation, distribution and reproduction in any medium or format, as long as you give appropriate credit to the original author(s) and the source, provide a link to the Creative Commons licence, and indicate if changes were made. The images or other third party material in this article are included in the article's Creative Commons licence, unless indicated otherwise in a credit line to the material. If material is not included in the article's Creative Commons licence and your intended use is not permitted by statutory regulation or exceeds the permitted use, you will need to obtain permission directly from the copyright holder. To view a copy of this licence, visit http://creativecommons.org/licenses/by/4.0/ .
Reprints and permissions
About this article
Cite this article.
Treviño, M., Zhu, X., Lu, Y.Y. et al. How do we measure attention? Using factor analysis to establish construct validity of neuropsychological tests. Cogn. Research 6 , 51 (2021). https://doi.org/10.1186/s41235-021-00313-1
Download citation
Received : 08 October 2020
Accepted : 22 June 2021
Published : 22 July 2021
DOI : https://doi.org/10.1186/s41235-021-00313-1
Share this article
Anyone you share the following link with will be able to read this content:
Sorry, a shareable link is not currently available for this article.
Provided by the Springer Nature SharedIt content-sharing initiative
- Neuroscience
You Now Have a Shorter Attention Span Than a Goldfish
T he average attention span for the notoriously ill-focused goldfish is nine seconds, but according to a new study from Microsoft Corp., people now generally lose concentration after eight seconds, highlighting the affects of an increasingly digitalized lifestyle on the brain.
Researchers in Canada surveyed 2,000 participants and studied the brain activity of 112 others using electroencephalograms (EEGs). Microsoft found that since the year 2000 (or about when the mobile revolution began) the average attention span dropped from 12 seconds to eight seconds.
“Heavy multi-screeners find it difficult to filter out irrelevant stimuli — they’re more easily distracted by multiple streams of media,” the report read.
On the positive side, the report says our ability to multitask has drastically improved in the mobile age.
Microsoft theorized that the changes were a result of the brain’s ability to adapt and change itself over time and a weaker attention span may be a side effect of evolving to a mobile Internet.
The survey also confirmed generational differences for mobile use; for example, 77% of people aged 18 to 24 responded “yes” when asked, “When nothing is occupying my attention, the first thing I do is reach for my phone,” compared with only 10% of those over the age of 65.
And now congratulate yourself for concentrating long enough to make it through this article.
5 Horrible Habits You Need to Stop Right Now

More Must-Reads from TIME
- How Selena Gomez Is Revolutionizing the Celebrity Beauty Business
- TIME100 Most Influential Companies 2024
- Javier Milei’s Radical Plan to Transform Argentina
- How Private Donors Shape Birth-Control Choices
- The Deadly Digital Frontiers at the Border
- What's the Best Measure of Fitness?
- The 31 Most Anticipated Movies of Summer 2024
- Want Weekly Recs on What to Watch, Read, and More? Sign Up for Worth Your Time
Contact us at [email protected]
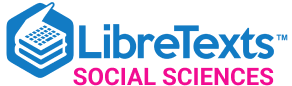
- school Campus Bookshelves
- menu_book Bookshelves
- perm_media Learning Objects
- login Login
- how_to_reg Request Instructor Account
- hub Instructor Commons
Margin Size
- Download Page (PDF)
- Download Full Book (PDF)
- Periodic Table
- Physics Constants
- Scientific Calculator
- Reference & Cite
- Tools expand_more
- Readability
selected template will load here
This action is not available.
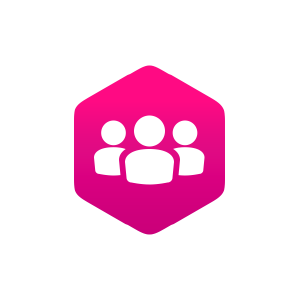
11.2: History of Attention
- Last updated
- Save as PDF
- Page ID 54125
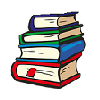
- Mehgan Andrade and Neil Walker
- College of the Canyons
\( \newcommand{\vecs}[1]{\overset { \scriptstyle \rightharpoonup} {\mathbf{#1}} } \)
\( \newcommand{\vecd}[1]{\overset{-\!-\!\rightharpoonup}{\vphantom{a}\smash {#1}}} \)
\( \newcommand{\id}{\mathrm{id}}\) \( \newcommand{\Span}{\mathrm{span}}\)
( \newcommand{\kernel}{\mathrm{null}\,}\) \( \newcommand{\range}{\mathrm{range}\,}\)
\( \newcommand{\RealPart}{\mathrm{Re}}\) \( \newcommand{\ImaginaryPart}{\mathrm{Im}}\)
\( \newcommand{\Argument}{\mathrm{Arg}}\) \( \newcommand{\norm}[1]{\| #1 \|}\)
\( \newcommand{\inner}[2]{\langle #1, #2 \rangle}\)
\( \newcommand{\Span}{\mathrm{span}}\)
\( \newcommand{\id}{\mathrm{id}}\)
\( \newcommand{\kernel}{\mathrm{null}\,}\)
\( \newcommand{\range}{\mathrm{range}\,}\)
\( \newcommand{\RealPart}{\mathrm{Re}}\)
\( \newcommand{\ImaginaryPart}{\mathrm{Im}}\)
\( \newcommand{\Argument}{\mathrm{Arg}}\)
\( \newcommand{\norm}[1]{\| #1 \|}\)
\( \newcommand{\Span}{\mathrm{span}}\) \( \newcommand{\AA}{\unicode[.8,0]{x212B}}\)
\( \newcommand{\vectorA}[1]{\vec{#1}} % arrow\)
\( \newcommand{\vectorAt}[1]{\vec{\text{#1}}} % arrow\)
\( \newcommand{\vectorB}[1]{\overset { \scriptstyle \rightharpoonup} {\mathbf{#1}} } \)
\( \newcommand{\vectorC}[1]{\textbf{#1}} \)
\( \newcommand{\vectorD}[1]{\overrightarrow{#1}} \)
\( \newcommand{\vectorDt}[1]{\overrightarrow{\text{#1}}} \)
\( \newcommand{\vectE}[1]{\overset{-\!-\!\rightharpoonup}{\vphantom{a}\smash{\mathbf {#1}}}} \)
There has been a large increase in research activity in the area of attention since the 1950s. This research has focused not only on attention, but also how attention is related to memory and executive functioning. Human learning and behaviour are dependent on our ability to pay attention to our environment, retain and retrieve information, and use cognitive strategies. An understanding of the development of attention is also critical when we consider that deficits in attention often lead to difficulties in school and in the work force. Thus, attention is an important topic in the study of psychology, specifically in the areas of development (see Part II of this book), learning (Part III), and psychological disorders (see the section on ADHD in Part IV). There is no doubt that an understanding of attention and related concepts is critical to our understanding of human cognition and learning.
Introduction to the History of Research on Attention
The study of attention is a major part of contemporary cognitive psychology and cognitive neuroscience. Attention plays a critical role in essentially all aspects of perception, cognition, and action, influencing the choices we make. The study of attention has been of interest to the field of psychology since its earliest days. However, many ideas about attention can be traced to philosophers in the 18th and 19th centuries, preceding the foundation of the field of psychology. The topic of attention was originally discussed by philosophers. Among the issues considered were the role of attention on conscious awareness and thought, and whether attention was directed voluntarily or involuntarily toward objects or events. The characterization of attention provided by each philosopher reflected that individual's larger metaphysical views of the nature of things and how we come to know the world. For instance, Joan Luis Vives (1492-1540) recognized the role of attention in forming memories. Gottfried Leibniz (1646-1716) introduced the concept of apperception, which refers to an act that is necessary for an individual to become conscious of a perceptual event. He noted that without apperception, information does not enter conscious awareness. Leibniz said, "Attention is a determination of the soul to know something in preference to other things". In summary, many philosophers gave attention a central role in perception and thinking. They introduced several important issues, such as the extent to which attention is directed automatically or intentionally. These topics continue to be examined and evaluated in contemporary research. Although they conducted little experimental research themselves, their conceptual analysis of attention laid the foundation for the scientific study of attention in ensuing years. The philosophical analyses of attention led to some predictions that could be tested experimentally. In addition, in the mid-1800s psychophysical methods were being developed that allowed the relation between physical stimulus properties and their corresponding psychological perceptions to be measured. Wilhelm Wundt, who established the first laboratory devoted to psychological research in 1879, was responsible for introducing the study of attention to the field. In addition, the relation between attention and perception was one of the first topics to be studied in experimental psychology. Wundt held that attention was an inner activity that caused ideas to be present to differing degrees in consciousness. He distinguished between perception, which was the entry into the field of attention, and apperception, which was responsible for entry into the inner focus. He assumed that the focus of attention could narrow or widen. This view that has also enjoyed popularity in recent years. At the end of the 19th century, Hermann von Helmholtz (1821-1894) argued that attention is essential for visual perception. Using himself as a subject and pages of briefly visible printed letters as stimuli, he found that attention could be directed in advance of the stimulus presentation to a particular region of the page, even though the eyes were kept fixed at a central point. He also found that attention was limited: The letters in by far the largest part of the visual field, even in the vicinity of the fixation point, were not automatically perceived.
William James's [1] (1890/1950) views on attention are probably the most well known of the early psychologists. In his famous Principles of Psychology (1980), James asserted that "the faculty of voluntarily bringing back a wandering attention, over and over again, is the very root of judgment, character, and will." His definition of attention is also widely quoted. According to James (1890), “It is taking possession by the mind, in clear and vivid form, of one of what seem several simultaneously possible objects or trains of thought. Focalization, concentration, of consciousness are of its essence. It implies withdrawal from some things in order to deal effectively with others, and is a condition which has a real opposite in the confused, dazed, scatterbrained state." Moreover, according to James, the immediate effects of attention are to make us perceive, conceive, distinguish and remember, better than we otherwise could –both more successive things and each thing more clearly. It also shortens “reaction time”. James’s definition also mentions clearness, which Titchener (1908/1973) viewed as the central aspect of attention. Pillsbury (1908/1973) agreed with Titchener, indicating, “the essence of attention as a conscious process is an increase in the clearness on one idea or a group of ideas at the expense of others”. Researchers at the beginning of the 20th century debated how this increased clearness is obtained. In summary, around 1860, the philosophical approach dominated the study of psychology in general and attention especially. During the period from 1980 to 1909, the study of attention was transformed, as was the field of psychology as a whole, to one of scientific inquiry with emphasis on experimental investigations. However, given that behaviourism came to dominate psychology in the next period, at least in the United States, the study of attentional mechanisms was largely delayed until the middle of the 20th century.
Although one often reads that research on attention essentially ceased during the period of 1910-1949, attention research never disappeared completely. However, there was an increase in interest in the topic with the advent of contemporary cognitive psychology. Lovie (1983) compiled tables showing the numbers of papers on attention listed in Psychological Abstracts and its predecessor, Psychological Index, in five-year intervals from 1910 to 1960, showing that studies on the topic were conducted during these time periods. Among the important works on attention was that of Jersild (1927) who published a classic monograph, “Mental Set and Shift”.
Another significant contribution during this era was the discovery of the psychological refractory period effect by Telford (1931). He noted that numerous studies showed that stimulation of neurons was followed by a refractory phase during which the neurons were less sensitive to stimulation. Stroop (1935/1992) also published what is certainly one of the most widely cited studies in the field of psychology, in which he demonstrated that stimulus information that is irrelevant to the task can have a major impact on performance (see below for John Ridley Stroop and the impact of the Stroop Color-Word Task on research on attention). Paschal (1941), Gibson (1940) and Mowrer, Rayman and Bliss (1940) also conducted research on attention such as that on preparatory set or mental set. In sum, although the proportion of psychological research devoted to the topic of attention was much less during this time period than during preceding decades, many important discoveries were made, which have influenced contemporary research on the topic.
The period from 1950 to 1974 saw a revival of interest in the characterization of human information processing. Research on attention during this period was characterized by an interplay between technical applications and theory. Mackworth (1950) reported experiments on the maintenance of vigilance that exemplified this interaction and set the stage for extensive research on the topic over the remainder of the 20th century. This research originated from concerns about the performance of radar operators in World War II detecting infrequently occurring signals. Cherry (1953) conducted one of the seminal works in attention during this period, studying the problem of selective attention, or, as he called it, “the cocktail party phenomenon”. He used a procedure called dichotic listening in which he presented different messages to each ear through headphones. Broadbent (1958) developed the first complete model of attention, called Filter Theory (see below). Treisman (1960) reformulated Broadbent's Filter Theory into what is now called the Filter-Attenuation Theory (see below). In the early 1970s, there was a shift from studying attention mainly with auditory tasks to studying it mainly with visual tasks. A view that regards attention as a limited-capacity resource that can be directed toward various processes became popular. Kahneman’s (1973) model is the most well known of these unitary capacity or resource theories.
According to this model, attention is a single resource that can be divided among different tasks in different amounts. The basic idea behind these models is that multiple tasks should produce interference when they compete for the limited capacity resources. Also, in this time period, the first controlled experiments that used psychophysiological techniques to study attention were conducted on humans. These experiments used methods that allow brain activity relating to the processing of a stimulus, called event related potentials, to be measured using electrodes placed on the scalp. In sum, the research during this period yielded considerable information about the mechanisms of attention. The most important development was the introduction of detailed information processing models of attention. Research on attention blossomed during the last quarter of the 20th century. Multiple resources models have emerged from many studies showing that it is easier to perform two tasks together when the tasks use different stimulus or response modalities than when they use the same modalities. Treisman and Gelade (1980) also developed a highly influential variant of the Spotlight Theory called the Feature Integration Theory to explain the results from visual search studies, in which subjects are to detect whether a target is present among distracters. Priming studies have also been popular during the most recent period of attention research. In such studies, a prime stimulus precedes the imperative stimulus to which the subject is to respond; the prime can be the same as or different from some aspect of the imperative stimulus. In addition, a major focus has been on gathering neuropsychological evidence pertaining to the brain mechanisms that underlie attention. Cognitive neuroscience, of which studies of attention are a major part, has made great strides due to the continued development of neuroimaging technologies. The converging evidence provided by neuropsychological and behavioral data promises to advance the study of attention significantly in the first half of the 21st century.
Finally, significant advances have also been made toward expanding the theories and methods of attention to address a range of applied problems. Two major areas can be identified. The first one concerns ergonomics in its broadest sense, ranging from human-machine interactions to improvement of work environments such as mental workload and situation awareness. The second major area of application is clinical neuropsychology, which has benefited substantially from adopting cognitive models and experimental methods to describe and investigate cognitive deficits in neurological patients. There is also work being done on the clinical application of attentional strategies (e.g., mindfulness training) in the treatment of a wide range of psychological disorders (see section on mindfulness).
John Ridley Stroop and The Stroop Effect
For over half a century, the Stroop effect has been one of the most well known standard demonstrations in undergraduate psychology courses and laboratories. In this cognitive task, participants asked to name the color of the ink in which an incompatible color word is printed (e.g., to say “red” aloud in response to the stimulus word GREEN printed in red ink) take longer than when asked to name the color in a control condition (e.g., to say "red" to the stimulus XXXXX printed in red ink). This effect, now known as the Stroop effect, was first reported in the classic article “Studies of Interference in Serial Verbal Reactions” published in the Journal of Experimental Psychology in 1935. Since then, this phenomena has become one of the most well known in the history of psychology.
Stroop’s article has become one of the most cited articles in the history of experimental psychology. It has more than 700 studies seeking to explain some nuance of the Stroop effect along with thousands of others directly or indirectly influenced by this article (MacLeod, 1992). However, at the time of its publication, it had relatively little impact because it was published at the height of Behaviourism in America (MacLeod, 1991). For the next thirty years after its publication, almost no experimental investigations of the Stroop effect occurred. For instance, between 1935 and 1964, only 16 articles are cited that directly examined the Stroop effect. In 1960s, with the advent of information processing as the dominant perspective in cognitive psychology, Stroop's work was rediscovered. Since then, the annual number of studies rose quickly, until by 1969 the number of articles settled in at just over 20 annually, where it appears to have remained (MacLeod, 1992).
Donald Broadbent and Dichotic Listening
Donald E. Broadbent has been praised for his outstanding contributions to the field of psychology since the 1950s, most notably in the area of attention. In fact, despite the undeniable role that attention plays in almost all psychological processes, research in this area was neglected by psychologists for the first half of the twentieth century (Massaro, 1996). During that time, behaviourists ignored the role of attention in human behaviour. Behaviourism was characterized by a stimulus-response approach, emphasizing the association between a stimulus and a response, but without identifying the cognitive operations that lead to that response (Reed, 2000). Subsequently, in the mid-1950s, a growing number of psychologists became interested in the information-processing approach as opposed to the stimulus response approach. It was Broadbent’s elaboration of the idea of the human organism as an information-processing system that lead to a systematic study of attention, and more generally, to the interrelation of scientific theory and practical application in the study of psychology.
Dichotic Listening Experiments
In 1952, Broadbent published his first report in a series of experiments that involved a dichotic listening paradigm. In that report, he was concerned with a person’s ability to answer one of two messages that were delivered at the same time, but one of which was irrelevant.
The participants were required to answer a series of Yes-No questions about a visual display over a radio-telephone. For example, the participant would be asked “S-1 from G.D.O. Is there a heart on Position 1?” Over,” to which the participant should answer “G.D.O. from S-1. Yes, over.” Participants in groups I, II, III, and IV heard two successive series of messages, in which two voices (G.D.O and Turret) spoke simultaneously during some of the messages. Only one of the voices was addressing S-1, and the other addressed S-2, S-3, S-4, S-5, or S-6. Participants were assigned to the following five groups:
- Group I: instructed to answer the message for S-1 and ignore the other on both runs
- Group II: instructed on one run to only answer the message from G.D.O. andon the second run was provided with a visual cue before the pairs of messages began for the name of the voice to be answered
- Group III: were given the same directions as Group I on one run, and on the other run had the experimenter indicate the correct voice verbally after the two messages had reached the “over” stage
- Group IV: had the correct voice indicated in all cases, but in one run it was before the messages began (like in Group II) and in the other run it was after the messages had finished (like in Group III)
- Group V: under the same conditions as Group I, heard the same recordings as Groups I, II, III and IV, but then also heard a two new recordings. One recording had a voice that addressed S-1 and a voice that addressed T-2, T-3, T-4, T-5, orT6 (thus the simultaneous messages were more distinct than for the other groups). The other recording had this same differentiation of messages, but also had both voices repeat the call-sign portion of the message (i.e., “S-1 from G.D.O., S-1 from G.D.O.)
For groups I and II, it is important to note that the overall proportion of failures to answer the correct message correctly was 52%. Results from Groups III and IV indicated that delaying knowledge of the correct voice until the message is completed makes that knowledge almost useless. More specifically, Broadbent (1952) stated:
“The present case is an instance of selection in perception (attention). Since the visual cue to the correct voice is useless when it arrives towards the ends of the message, it is clear that process of discarding part of the information contained in the mixed voices has already taken place…It seems possible that one of the two voices is selected for response without reference to its correctness, and that the other is ignored…If one of the two voices is selected (attended to) in the resulting mixture there is no guarantee that it will be the correct one, and both call signs cannot be perceived at once any more than both messages can be received and stored till a visual cue indicates the one to be answered”. (p. 55)
In 1954, Broadbent used the same procedure as discussed above with slight modifications. In that case, he found information that indicated the positive impact that spatial separation of the messages has on paying attention to and understanding the correct message. The dichotic listening paradigm has been utilized in numerous other publications, both by Broadbent and by other psychologists working in the field of cognition. For example, Cherry (1953) investigated how we can recognize what one person is saying when others are speaking at the same time, which be described as the “cocktail party problem” (p. 976). In his experiment, subjects listened to simultaneous messages and were instructed to repeat one of the messages word by word or phrase by phrase.
Information-Processing and the Filter Model of Attention
Cognitive psychology is often called human information processing, which reflects the approach taken by many cognitive psychologists in studying cognition. The stage approach, with the acquisition, storage, retrieval, and use of information in a number of separate stages, was influenced by the computer metaphor and the way people enter, store, and retrieve data from a computer (Reed, 2000). The stages in an information-processing model are:
- Sensory Store: brief storage for information in its original sensory form
- Filter: part of attention in which some perceptual information is blocked out and not recognized, while other information is attended to and recognized
- Pattern Recognition: stage in which a stimulus is recognized
- Selection: stage that determines what information a person will try to remember
- Short-Term Memory: memory with limited capacity, that lasts for about 20-30 seconds without attending to its content
- Long-Term Memory: memory that has no capacity limit and lasts from minutes to a lifetime
Using an information-processing approach, Broadbent collected data on attention (Reed, 2000). He used a dichotic listening paradigm (see above section), asking participants to listen simultaneously to messages played in each ear, and based on the difficulty that participants had in listening to the simultaneous messages, proposed that a listener can attend to only one message at a time (Broadbent, 1952; Broadbent, 1954). More specifically, he asked enlisted men in England's Royal Army to listen to three pairs of digits. One digit from each pair was presented to one ear at the same time that the other digit from the pair was presented to the other ear. The subjects were asked to recall the digits in whatever order they chose, and almost all of the correct reports involved recalling all of the digits presented to one ear, followed by all the digits presented to the other ear. A second group of participants were asked to recall the digits in the order they were presented (i.e., as pairs). Performance was worse than when they were able to recall all digits from one ear and then the other.
To account for these findings, Broadbent hypothesized that the mechanism of attention was controlled by two components: a selective device or filter located early in the nervous system, and a temporary buffer store that precedes the filter (Broadbent, 1958). He proposed that the filter was tuned to one channel or the other, in an all-or-nothing manner. Broadbent’s filter model, described in his book Perception and Communication (1958), was one of the first information-processing models to be examined by psychologists.
Shortly after, it was discovered that if the unattended message became highly meaningful (for example, hearing one’s name as in Cherry's Cocktail Party Effect, as mentioned above), then attention would switch automatically to the new message. This result led to the paradox that the content of the message is understood before it is selected, indicating that Broadbent needed to revise his theory (Craik & Baddeley, 1995). Broadbent did not shy away from this task. In fact, he saw all scientific theories as temporary statements, a method of integrating current evidence in a coherent manner. According to Craik and Baddeley, (1995), although Broadbent always presented his current theories firmly and persuasively, he never took the position of obstinately defending an outmoded theory. When he published his second book on the topic, Decision and Stress (1971), he used his filter model as the starting point, to which he applied modifications and added concepts “to accommodate new findings that the model itself had stimulated” (Massaro, 1996, pp. 141). Despite its inconsistencies with emerging findings, the filter model remains the first and most influential information-processing model of human cognition.
Anne Treisman and Feature Integration Theory
Anne Treisman is one of the most influential cognitive psychologists in the world today. For over four decades, she has been has using innovative research methods to define fundamental issues in the area of attention and perception. Best known for her Feature Integration Theory (1980, 1986), Treisman’s hypotheses about the mechanisms involved in information processing have formed a starting point for many theorists in this area of research.
In 1967, while Treisman worked as a visiting scientist in the psychology department at Bell Telephone Laboratories, she published an influential paper in Psychological Review that was central to the development of selective attention as a scientific field of study. This paper articulated many of the fundamental issues that continue to guide studies of attention to this day. While at Bell, Treisman’s research interests began to expand (Anon, 1991). Although she remained intrigued by the role of attention on auditory perception, she was now also fascinated by the way this construct modulates perception in the visual modality.
In the following years, Treisman returned to Oxford, where she accepted a position as University lecturer in the Psychology Department and was appointed a Fellow of St. Anne’s College (Treisman, 2006). Here, she began to explore the notion that attention is involved in integrating separate features to form visual perceptual representations of objects. Using a stopwatch and her children as research participants, she found that the search for a red ‘X’ among red ‘Os’ and blue ‘Xs’ was slow and laborious compared to the search for either shape or colour alone (Gazzaniga et al., 2002). These findings were corroborated by results from testing adult participants in the laboratory and provided the basis of a new research program, where Treisman conducted experiments exploring the relationships between feature integration, attention and object perception (Triesman & Gelade, 1980).
In 1976, Treisman’s marriage to Michel Treisman ended. She remarried in 1978, to Daniel Kahneman, a fellow psychologist who would go on to win the Nobel Prize for Economics in 2002. Shortly thereafter, Treisman and Kahneman accepted positions at the University of British Columbia, Canada. In 1980, Treisman and Gelade published a seminal paper proposing her enormously influential Feature Integration Theory (FIT). Treisman’s research demonstrated that during the early stages of object perception, early vision encodes features such as color, form, and orientation as separate entities (in "feature maps") (Treisman, 1986). Focused attention to these features recombines the separate features resulting in correct object perception. In the absence of focused attention, these features can bind randomly to form illusory conjunctions (Treisman & Schmidt, 1982; Treisman, 1986). Feature integration theory has had an overarching impact both within and outside the area of psychology.
Feature Integration Theory Experiments
According to Treisman’s Feature Integration Theory perception of objects is divided into two stages:
- Pre-Attentive Stage : The first stage in perception is so named because it happens automatically, without effort or attention by the perceiver. In this stage, an object is analyzed into its features (i.e., color, texture, shapes etc.). Treisman suggests that the reason we are unaware of the breakdown of an object into its elementary features is that this analysis occurs early in the perceptual processes, before we have become conscious of the object. Evidence: Treisman created a display of four objects flanked by two black numbers. This display was flashed on a screen for one-fifth of a second and followed by a random dot masking field in order to eliminate residual perception of the stimuli. Participants were asked to report the numbers first, followed by what they saw at each of the four locations where the shapes had been. In 18 percent of trials, participants reported seeing objects that consisted of a combination of features from two different stimuli (i.e., color and shape). The combinations of features from different stimuli are called illusory conjunctions (Treisman and Schmidt, 1982). The experiment also showed that these illusory conjunctions could occur even if the stimuli differ greatly in shape and size. According to Treisman, illusory conjunctions occur because early in the perceptual process, features may exist independently of one another, and can therefore be incorrectly combined in laboratory settings when briefly flashed stimuli are followed by a masking field (Treisman, 1986).
- Focused Attention Stage : During this second stage of perception features are recombined to form whole objects. Evidence: Treisman repeated the illusory conjunction experiment, but this time, participants were instructed to ignore the flanking numbers, and to focus their attention on the four target objects. Results demonstrated that this focused attention eliminated illusory conjunctions, so that all shapes were paired with their correct colours (Treisman and Schmidt, 1982). The experiment demonstrates the role of attention in the correct perception of objects.
How top students are studying for longer despite shrinking attention spans
Amidst the widely-observed decline in average attention spans, new data from the study app Brainscape reveals the opposite to be true.
The prevailing wisdom is pretty set: according to many experts, attention spans have dropped off a cliff over the past two decades. But new data from a study app called Brainscape —an adaptive flashcard platform—reveals that over the past 10 years, its 6 million users have been putting in longer and longer study sessions.
This begs the following questions: how is it possible that students’ attention spans are improving when the widely-observed trend is declining? Is it even possible to improve your attention span or is it a given trait for your particular brain anatomy? And if it is, how? Supported by the results of our attention span analysis, we’ll be answering all of these questions in this guide.
How have attention spans changed over time?
The purported decline in average attention span is often attributed to advances in technology, social media, and unprecedented access to information thanks to the mobile devices everyone of every socioeconomic class owns.
Short video clips, like those found on TikTok or Instagram Reels, have become the most widely consumed content for younger generations. Even average movie shots are getting shorter, dropping from 12 seconds in 1930 to an average of just 2.5 seconds . Newspaper articles have become significantly shorter too, with the estimated reading length often provided at the top of the page.
“Our attention span averages 47 seconds on a screen, and it is shrinking.” - Gloria Mark, PhD
A study by expert Gloria Mark, who received her PhD in psychology from Columbia University, found that in 2004 the average span of attention was 150 seconds long . By 2012, the average attention span declined to half that at 75 seconds, and by 2018, they decreased even further to a very short 47 seconds.
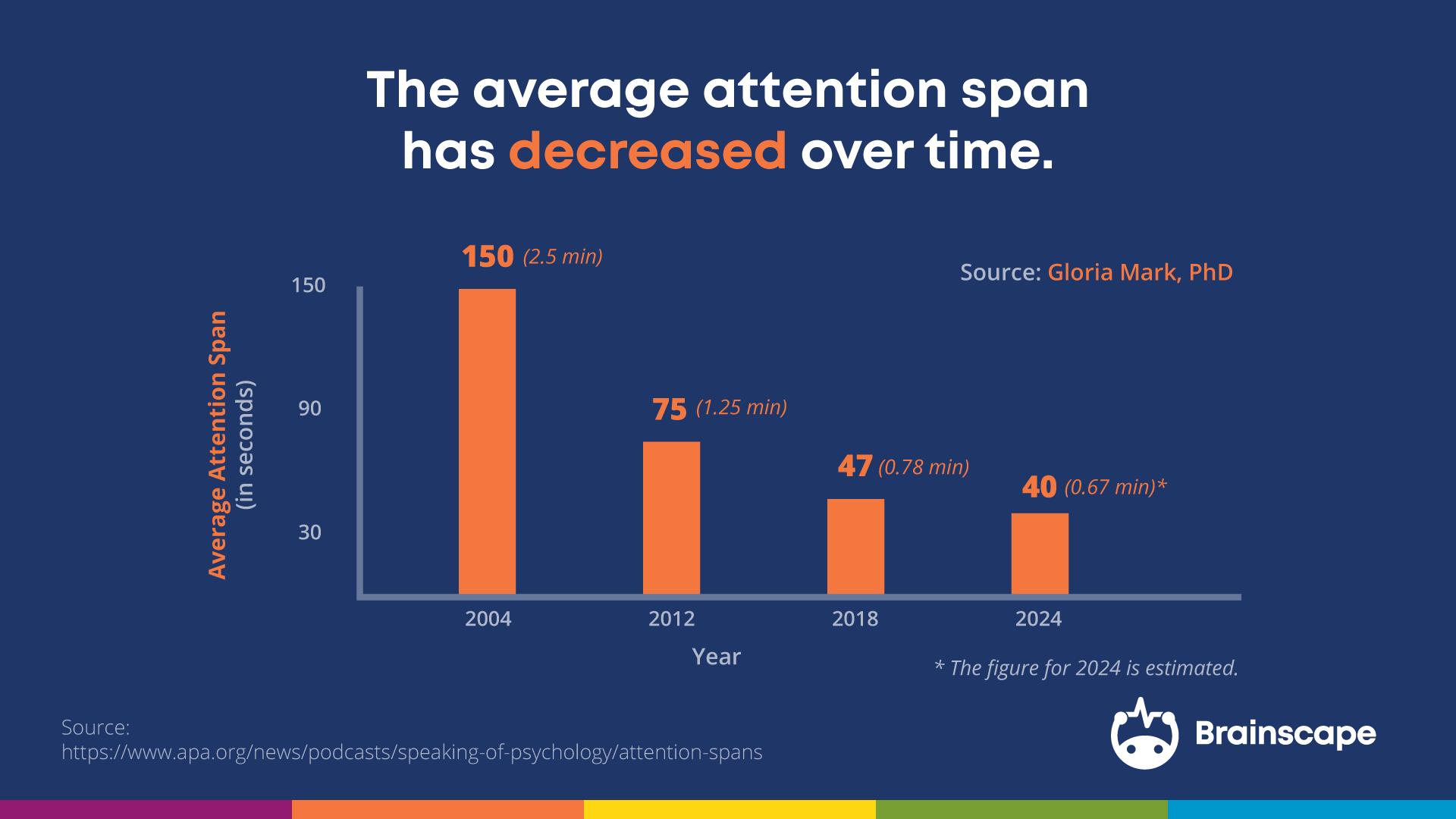
Over the past several years, this low attention span average has been replicated by other researchers to within a few seconds.
You’d think these trends towards a state of constant distraction would be catastrophic for students’ academic performance. Instead, a comprehensive analysis of over 6 million students has revealed that the opposite trend is true for study habits . The attention spans of top students have actually been improving over the past 10 years.
Attention spans while studying are increasing
While already short attention spans seem to be worsening worldwide, Brainscape’s analysis of over 6 million people revealed that this wasn’t the case when it came to the attention span of students using the platform to study. Using our raw data on the length of study sessions, we found that the average study session has increased by 53% over the past ten years. In 2015, the average study session for a Brainscape user was 9.4 minutes, and by 2024 the average session had increased to 14.4 minutes.
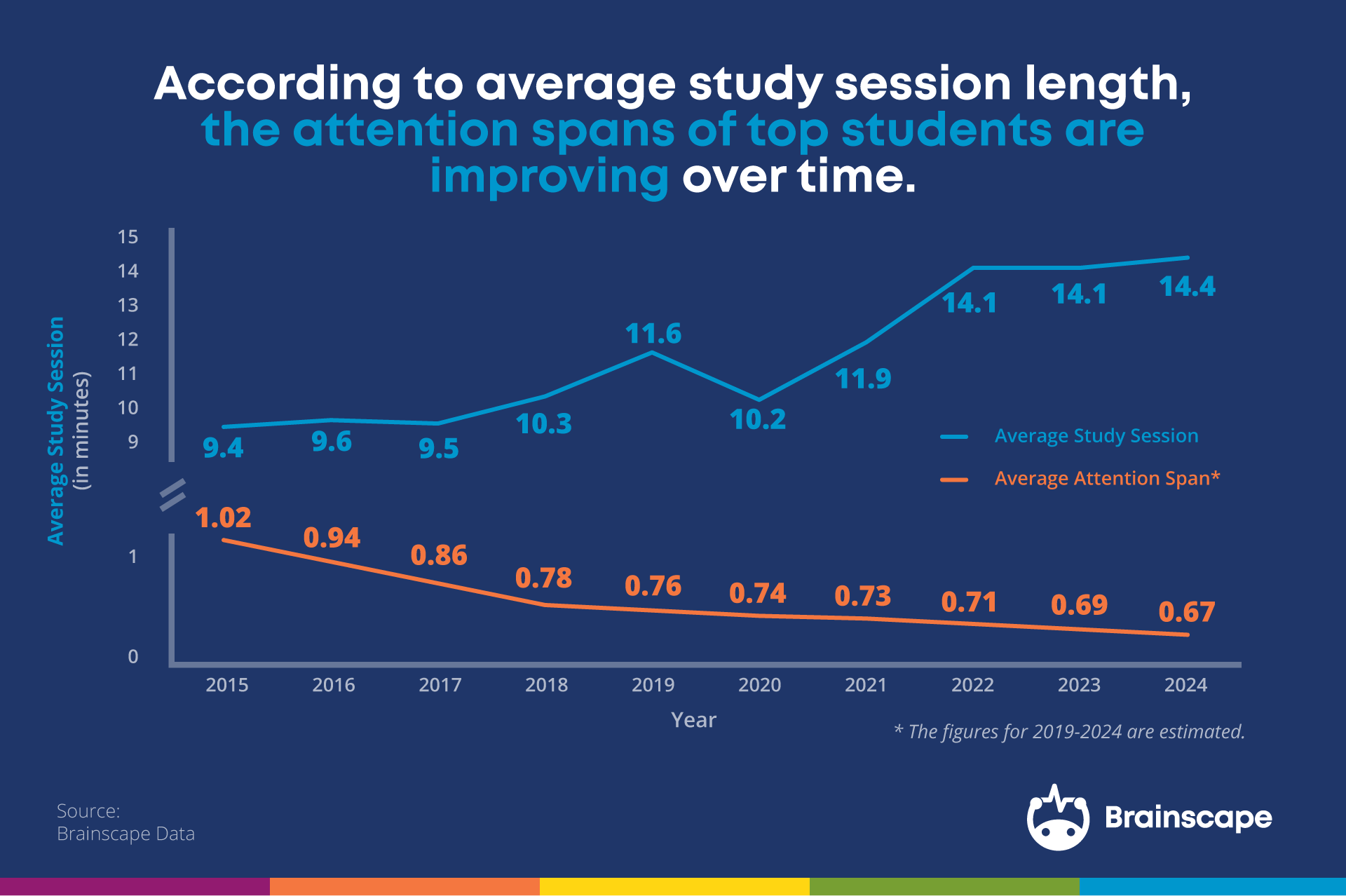
While a 5-minute increase may not seem like much, it needs to be considered in the context of a single study session, defined as opening the app to work through 10, 20, 30, or more flashcards in a single sitting. A student could complete multiple study sessions in a single day, which would then translate into 10 minutes (2 sessions), 15 minutes (3 sessions), or 20 minutes (4 sessions) of additional studying per day, compared with 10 years ago.
Add this up over the standard work week of 5 days and you’ve got as much as 1 hour 40 minutes more time devoted to learning on top of what they're already doing . In one month, that’s 6.67 hours of additional learning. In one year, 80 hours , which is enough time to learn to swim, become proficient in cooking, and get a first aid certification.
In other words, those extra minutes of studying compound tremendously over time. Even if a student studies for just 5 extra minutes each day, that’s 30.5 hours of additional time spent studying each year. That is a huge difference, especially when taking the benefits of spaced repetition into account.
And women are doing even better than men...
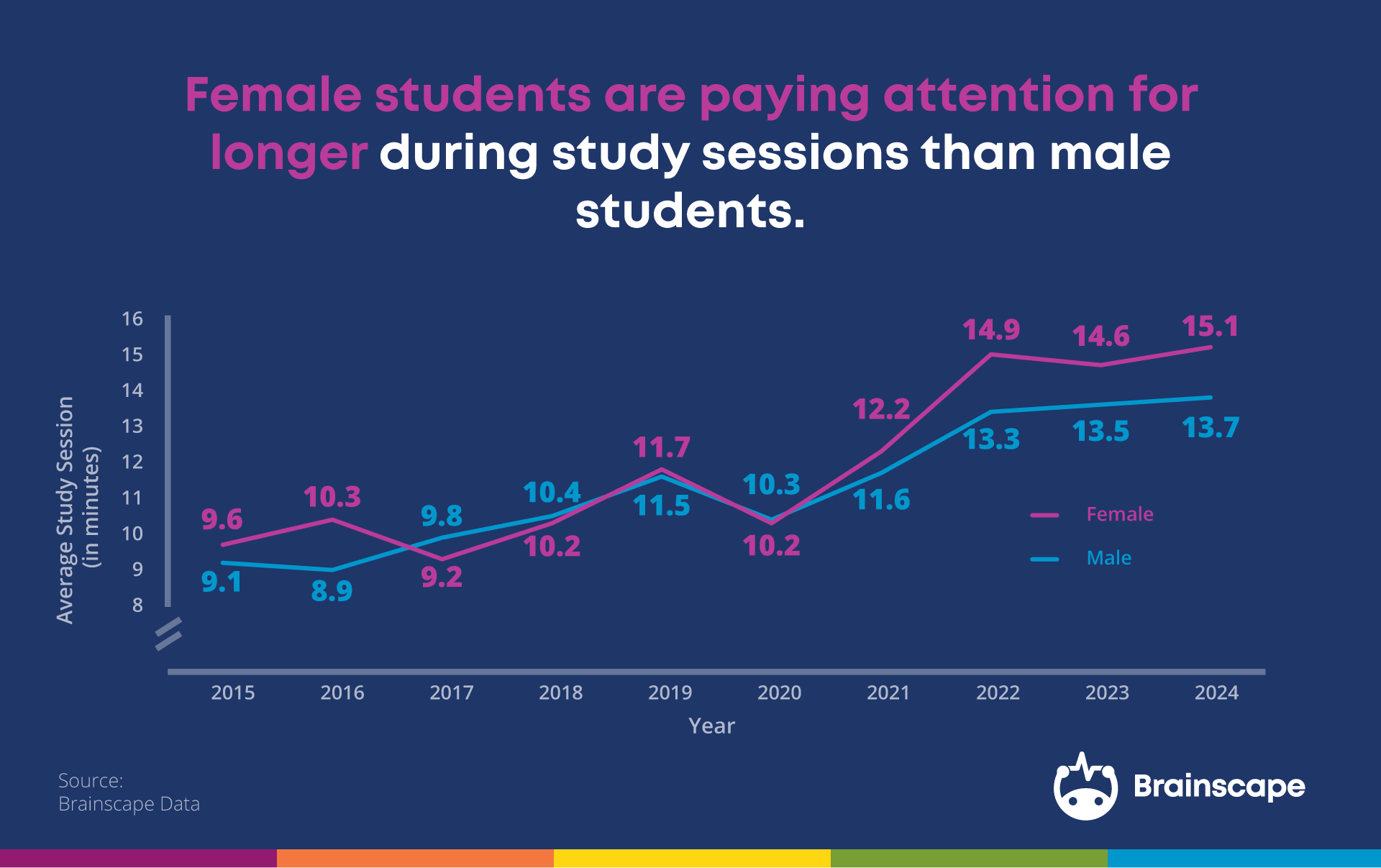
Another surprising finding—although perhaps not all that surprising to women—is that the average attention span of female-identified students is not only longer than their male-identified counterparts but has increased at a faster rate too!
Between 2015 and 2024, female users extended their average study session times to 15.1 minutes, while the average study session for male users increased to 13.7 minutes. In other words, on average, women study for 1.4 minutes longer than men do every time they sit down to study.
Assuming three short study sessions per day, that additional study time equates to 4.2 minutes per day, 21 minutes per week, and 1 hour 20 minutes per month: the standard length of a feature film!
How are students improving their attention spans while studying?
The 53% increase in study session length flies in the face of the prevailing wisdom that attention spans are decreasing, as supported by Mark’s and others’ research. But perhaps more importantly, it illustrates that attention spans are not static things . Like any muscle in the body, you can build and lengthen your attention span , even for cognitively taxing tasks like studying.
If six million students could increase their attention span by 5 minutes per study session, so can you. The question is how.
The answer is that if you tap into the way the human brain was biologically designed to learn, then learning is going to feel a lot less painful and taxing , allowing you to learn for longer before becoming fatigued.
Brainscape does exactly this through a study algorithm that is based upon the learning principles underpinning the cognitive science of how brains learn:
Spaced Repetition
Spaced repetition involves your repeated exposure to concepts based upon how well you understand them. This requires breaking a subject down into its atomic parts, framing them as question-and-answer pairs (as flashcards do), and then repeating each concept based on your confidence in how well you understand them.
In Brainscape, students are required to rate how well they understood a flashcard on a scale of 1 to 5 with 1 being “not at all” and 5 being “perfectly”. Flashcards rated 1’s and 2’s get repeated very often, while those rated 4’s and 5’s much less often, which promotes faster, more efficient learning.
Active Recall
Active recall involves the retrieval of information from memory, rather than recognizing it in your study notes or in a selection of multiple-choice answers. Flashcards are the perfect tool for active recall because they frame every concept as a question, which your brain is then compelled to answer by retrieving the information from memory. This strengthens the brain’s neural pathways to that information, building memory much faster.
Moreover, feeling the burn of the knowledge gap —for the questions you don’t know the answer to—really motivates the brain to hold on to that information for longer.
Metacognition
Metacognition is the self-reflection you do on how well you understand a concept. In other words, it’s “thinking about your thinking”. After flipping over a flashcard, Brainscape asks you to rate how well you knew that concept, which compels you to reflect on your understanding. This establishes additional memory hooks to that concept, allowing it to leave a deeper impression, thereby enhancing memory.
Accessibility, anytime, anywhere
Brainscape is instantly accessible as an app on any device, synchronous across multiple devices, and even allows students to download flashcards for offline study. In other words, no matter where you are—college-bound on a bus or Cambodia-bound on an Airbus A380—you’re never more than a tap or two away from engaging in impactful, efficient studying for any subject or exam.
Study metrics making progress visible
The ability to see your study progress over time is a great motivator for students to keep up with their study habits. Thanks to technology, it’s easier than ever to measure and view your progress, and to hold yourself accountable. Seeing the progress in real-time also motivates students to keep up their study pace, or even surpass it to earn a better score on the next exam.
A checklist for increasing your attention span
Spaced repetition, active recall, metacognition, accessibility, and study metrics are all ways that Brainscape has managed to increase the average attention span of its learners over the past 10 years. Through constant improvements to its flashcard algorithm and study experience, students have found learning increasingly more efficient and less painful, motivating them to spend more and more time studying with the app.
But outside of this study tool, there are many immediately actionable ways you can optimize your attention span. In this article— 12 Focus exercises to build your attention span like a muscle —you’ll find a checklist of 12 exercises you can use to eliminate distractions and improve your intrinsic ability to focus better, no matter what’s going on around you.
In conclusion…
Armed with a learning technology that optimizes study efficiency and minimizes inertia, top students have managed to increase their attention spans dramatically against a backdrop of dramatically decreasing attention spans. If you would like to join this counter-narrative and supercharge your study productivity, you can browse Brainscape’s library, featuring millions of flashcards made by top students, professors, publishers, and experts, spanning hundreds of subjects; or create your own completely free.
Flashcards for serious learners .
- Skip to main content
- Keyboard shortcuts for audio player

Your Health
- Treatments & Tests
- Health Inc.
- Public Health
ADHD diagnoses are rising. 1 in 9 U.S. kids have gotten one, new study finds

Maria Godoy
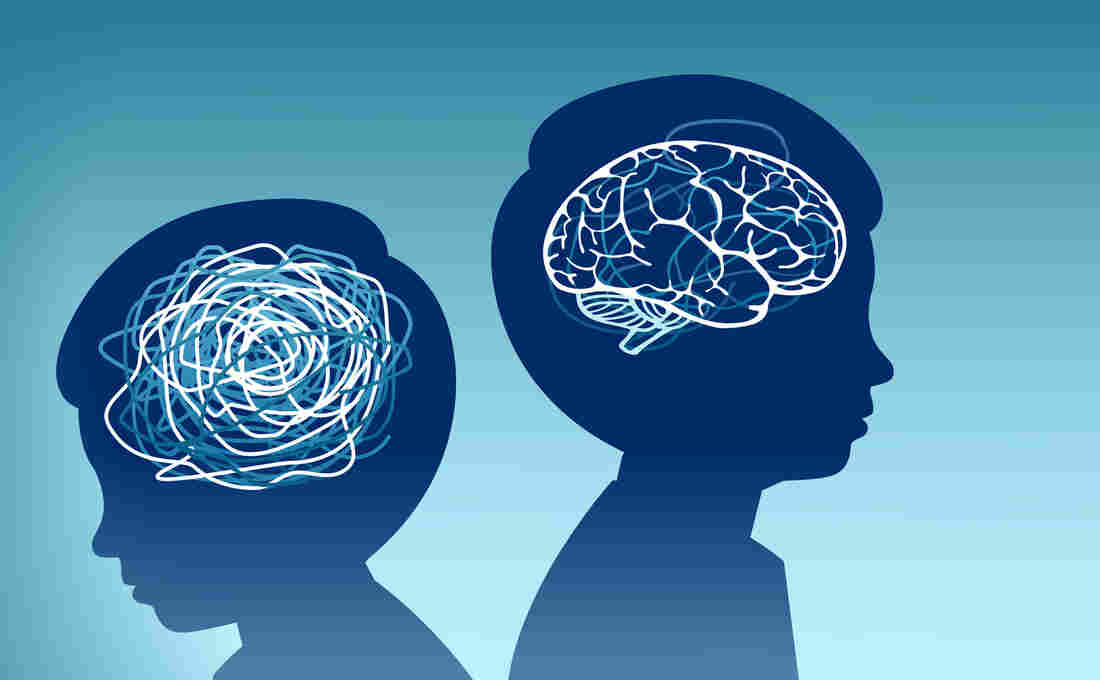
Attention-deficit/hyperactivity disorder (ADHD) is one of the most common neurodevelopment disorders among children. SIphotography/Getty Images hide caption
Attention-deficit/hyperactivity disorder (ADHD) is one of the most common neurodevelopment disorders among children.
About 1 in 9 children in the U.S., between the ages of 3 and 17, have been diagnosed with ADHD. That's according to a new report from the Centers for Disease Control and Prevention that calls attention-deficit/hyperactivity disorder an "expanding public health concern."
Researchers found that in 2022, 7.1 million kids and adolescents in the U.S. had received an ADHD diagnosis – a million more children than in 2016. That jump in diagnoses was not surprising, given that the data was collected during the pandemic, says Melissa Danielson, a statistician with the CDC's National Center on Birth Defects and Developmental Disabilities and the study's lead author.
She notes that other studies have found that many children experienced heightened stress, depression and anxiety during the pandemic. "A lot of those diagnoses... might have been the result of a child being assessed for a different diagnosis, something like anxiety or depression, and their clinician identifying that the child also had ADHD," Danielson says.
The increase in diagnoses also comes amid growing awareness of ADHD — and the different ways that it can manifest in children. Danielson says that may help explain why girls are becoming more commonly diagnosed with ADHD compared to boys than they had been in the past. She says boys have long been diagnosed with ADHD at around two and half times the rate of girls, but the new reports finds that difference is narrowing.

Shots - Health News
Bike riding in middle school may boost mental health, study finds.
Decades ago, ADHD was thought of as a disorder of hyperactivity among boys, Danielson says. "Boys will often have hyperactive or impulsive ADHD, where they'll run into the street or jump off things or do things that might make them more likely to be injured," she says.
"Girls tend to manifest their ADHD in a more inattentive way. They'll be daydreaming or have a lack of focus or be hyper focused on a particular task that maybe is not the task that they need to be focused on," says Danielson.
The study, which appears in the Journal of Clinical Child & Adolescent Psychology, was based on data from the National Survey of Children's Health , which gathers detailed information from parents.
While the report found that the number of kids diagnosed with ADHD had risen since 2016, only about half of them were taking medication to treat the condition – compared with two-thirds of children back in 2016. The data didn't look into reasons why this might be, but Danielson notes that reports of shortages of ADHD medications began around the time the data was collected.
Dr. Max Wiznitzer, a professor of pediatric neurology at Case Western Reserve University, says he suspects some parents may be reluctant to put their kids on ADHD medication out of misguided concerns. "There's the myth that it's addictive, which it's not." He says studies have shown people treated with ADHD have no increased risk of drug abuse.

Want to protect your kids' eyes from myopia? Get them to play outside
Wiznitzer says medication is important because it can help kids focus by controlling symptoms of impulsivity, overactivity and inattention. But ADHD treatment also requires therapy that can teach children — and their parents — behavioral and educational strategies to manage their condition. "It's always a two-pronged approach," he says. He finds it troubling that the report found less than half of kids and adolescents diagnosed with ADHD were getting any behavioral therapy.
The report also found that nearly 78% percent of children diagnosed with ADHD had at least one other diagnosed disorder. The most common were behavioral or conduct problems, anxiety and developmental delays. Autism and depression were also frequently observed, Danielson says.
Kids with ADHD are at increased risk for other conditions including depression, anxiety and substance abuse and if left untreated, ADHD can raise the risk of serious health concerns in adulthood. This includes a higher risk of diabetes, heart disease and shortened life span, Wiznitzer says – which is why increased awareness and diagnosis is important.
Danielson says parents can also find information on treatment and services at CHADD — Children And Adults with ADHD , a non-profit resources organization where Wiznitzer serves on the advisory board.
He says parents seeking treatment for their kids should start with a conversation with their pediatrician.
This story was edited by Jane Greenhalgh.
- attention-deficit/hyperactivity disorder (ADHD)
- kids health
- Latest Latest
- The West The West
- Sports Sports
- Opinion Opinion
- Magazine Magazine
CDC study says 1 in 9 children have ADHD
The 7.1 million is an increase, but could mean diagnosis is getting better.

By Lois M. Collins
By 2022, 7.1 million children — about 1 in 9 — had been diagnosed with attention deficit/hyperactivity disorder, according to a new Centers for Disease Control and Prevention study . About 1 million of them got their diagnosis since 2016, which was when the previous most recent national estimate was done.
The numbers are based on 2022 National Survey of Children’s Health data on 45,000 children ages 3-17, and the study was published in the Journal of Clinical Child & Adolescent Psychology. The researchers also looked at severity, presence of co-occurring disorders and treatments, including medication and behavioral therapy.
The updated numbers come at a time when the U.S. Food and Drug Administration says Adderall, one of the most-prescribed treatments for ADHD, is in short supply nationwide and the situation isn’t likely to get better before the end of the year. Per the FDA statement , “FDA is in frequent communication with all manufacturers of amphetamine mixed salts, and one of those companies, Teva, is experiencing ongoing intermittent manufacturing delays. Other manufacturers continue to produce amphetamine mixed salts, but there is not sufficient supply to continue to meet U.S. market demand through those producers.”
According to the CDC prevalence report, while 7.1 million children have ever been diagnosed with ADHD, 6.5 million of them currently have the disorder. Of those with existing ADHD, the report said nearly 42% had mild ADHD, 45% were classified as moderate and not quite 13% had severe ADHD. Just under 78% had “at least one co-occurring disorder.” About half of the children with current ADHD take medication for the condition and just over 44% have received behavioral treatment in the past year. The report also notes that 3 in 10 children who have ADHD are not being treated for it.
The CDC study authors write that the data “can be used by policymakers, government agencies, healthcare systems, public health practitioners and other partners to plan for needs of children with ADHD.”
The CDC says ADHD is one of the most common neurodevelopmental disorders of children and that symptoms often last into adulthood. They present most often as inattentive or as hyperactive-impulsive. Some people have both.
The study notes that those with complex ADHD may also have mental, behavioral and developmental disorders, in addition to the ADHD. And they add that those with an ADHD diagnosis “are more likely to experience poor health outcomes like obesity, chronic illness, and accidental injury, as well as have increased healthcare utilization compared to peers without ADHD.”
According to Medical News Today , “Attention deficit hyperactivity disorder (ADHD), also referred to as Attention Deficit Disorder (ADD), is one of the most common childhood conditions that involve behavioral symptoms such as inattentiveness, hyperactivity and impulsiveness. Although the condition may begin during childhood, it can continue through adolescence and adulthood. Signs of ADHD include a short attention span or being easily distracted, restlessness, constant fidgeting or overactivity and being impulsive. ADHD can be hard to diagnose and even harder to understand.”
Cambridge scientists believe that children who have trouble regulating their emotions — sometimes seen as depression, anxiety and outbursts — could be exhibiting a core symptom of ADHD. Their study, just published in Nature Mental Health , found that about half of children with ADHD have some emotional dysregulation, but that Ritalin, which is commonly prescribed for ADHD, doesn’t treat that symptom well, they reported in a news release .
Treating ADHD
Treatment for ADHD is very specific, according to the CDC study, which said medication is the most common. Training parents in behavior therapy is the preferred first-line treatment in children younger than 6, while for those 6-11, medication and behavior therapy together is recommended. Medication is recommended for adolescents 12-17, along with behavioral interventions if they are available. Additionally, “behavioral classroom management, behavioral peer interventions, and organizational training have demonstrated well-established effectiveness.”
It’s not clear whether cases are rising or experts are getting better at diagnosing the disorder. But the study’s lead author, Melissa Danielson, a CDC statistician, believes more doctors, parents, teachers and even kids are familiar with the symptoms. “There’s more providers that are comfortable with making those diagnoses and treating ADHD, which can allow for children to be helped by different medications or behavior therapy or school services,” she told NBC News . “So since there are more opportunities for these kids to be helped, I think there’s more incentive to get that kind of diagnosis.”
Log in using your username and password
- Search More Search for this keyword Advanced search
- Latest content
- Current issue
- Topic Collection
- BMJ Journals More You are viewing from: Google Indexer
You are here
- Online First
- Span of control and well-being outcomes among hospital frontline managers: too much to handle?
- Article Text
- Article info
- Citation Tools
- Rapid Responses
- Article metrics

- http://orcid.org/0009-0000-4998-2074 Ane-Kathrine Lundberg Hansen 1 ,
- Christian Bøtcher Jacobsen 1 ,
- Vita Ligaya Dalgaard 1 , 2
- 1 King Frederik Center for Public Leadership, Department of Political Science , Aarhus Universitet , Aarhus , Denmark
- 2 Department of Psychology and Behavioural Sciences , Aarhus Universitet , Aarhus , Denmark
- Correspondence to Ane-Kathrine Lundberg Hansen, King Frederik Center for Public Leadership, Department of Political Science, Aarhus Universitet, Aarhus 8000, Denmark; aklh{at}ps.au.dk
Purpose To examine the consequences of broader spans of control for well-being outcomes among frontline managers.
Method Healthcare managers were surveyed in collaboration with the Central Denmark Region. The response rate was 74.5%. Using regression analysis, we investigate how span of control is associated with outcomes related to well-being understood as perceived stress, burnout, job satisfaction, satisfaction with the work environment, intention to quit their current job and work–life balance.
Findings Span of control may be an important factor in establishing well-being among frontline managers in the Danish hospital sector on several parameters. Span of control is associated the strongest with work–life balance and intention to quit, least but significantly with perceived stress and not significantly with burnout.
Practical implications We recommend that healthcare organisations consider whether it could be more optimal to reduce the span of control for some managers. Furthermore, we recommend that future studies pay attention to span of control and provide stronger causal evidence about its impact on healthcare workers.
- health system
- mental health
Data availability statement
All data relevant to the study are included in the article or uploaded as supplementary information.
https://doi.org/10.1136/leader-2024-000978
Statistics from Altmetric.com
Request permissions.
If you wish to reuse any or all of this article please use the link below which will take you to the Copyright Clearance Center’s RightsLink service. You will be able to get a quick price and instant permission to reuse the content in many different ways.
WHAT IS ALREADY KNOWN ON THIS TOPIC
We know that broad spans of control can impede effective leadership and come with costs for employees and patients. However, there is little knowledge about the association with manager well-being.
WHAT THIS STUDY ADDS
This study directly investigates the associations between span of control and important aspects of the managers’ own well-being understood as job satisfaction, satisfaction with the work environment, work–life balance, burnout, perceived stress and intention to quit.
HOW THIS STUDY MIGHT AFFECT RESEARCH, PRACTICE OR POLICY
We suggest that healthcare organisations consider the managers’ own well-being and its relationship with span of control. Furthermore, we urge future research to provide stronger causal evidence about its impact on healthcare workers and managers.
Introduction
Healthcare managers can make important differences for the well-being and motivation of their staff as well as for patient safety and health. 1 However, many healthcare managers are also challenged by broad spans of control, that is, direct leadership responsibility for a high number of employees. There is scant evidence for the actual spans of control in healthcare sectors, but recent examples from Denmark show average spans of control of around 37 employees for both nursing managers 2 and clinical directors, 3 and in Canada, a study found an average of as high as 77 employees. 4 Broad spans of control can impede effective leadership, 2 and studies have shown how broad spans of control can have downstream costs for employees and patients. 5 However, there is little understanding of the potential costs of broader spans of control for the well-being of frontline managers in terms of, for example, stress, burnout and satisfaction. The work environment of managers is important, since low manager well-being can have negative spillover for employees 6 and higher manager turnover, which can be associated with less effective leadership. 7 Thus, the well-being of managers can be an important mechanism between span of control and outcomes for employees and patients, which we still need to understand in more detail. In addition, ongoing stress and burnout may carry individual negative consequences to the managers themselves such as risk of sickness absence and other negative health consequences. 8 9
Broad spans of control can be demanding for managers. Because they need to oversee a high number of employees, their time for relation building is stretched, and their managerial responsibilities become more complex. 10 From research on job demands and resources, it is well-known that higher job demands can be associated with lower job satisfaction, and higher levels of stress, burnout and turnover. 11 Studies have also shown that demands such as a high level of perceived conflict are associated with stress outcomes among nursing managers. 12 However, in this line of study, there are few studies with attention to objective demand factors such as span of control.
Principles for span of control were developed by early organisation scholars, for example, Gulick, 13 and developed into a theory about span of control by Meier and Bohte. 10 14 The theory states that broader spans of control can be associated with costs incurred by more distant management, while more narrow spans of control may also be associated with high costs from hiring more managers. These studies primarily focus on the staff-related consequences and tend to neglect potential negative consequences of broad spans of control for the managers themselves.
Studies suggest that span of control can have negative implications for satisfaction among managers. Wong et al 15 find that nursing managers have a medium level of satisfaction with their span of control, and that broad spans of control are associated with higher perceived system demands. In a review article, Lee and Cummings 16 found that reducing the span of control can be associated with increased job satisfaction among frontline managers. Thus, there have been suggestions that a broad span of control can come at the cost of lower manager well-being and higher workload. 17 However, to the best of our knowledge, apart from job satisfaction, outcomes related to well-being such as perceived stress and burnout among managers have only to a lesser extent been examined as a consequence of broad spans of control. One recent conceptual article on nurse managers found a few studies that reported role overload, burnout and lower organisational commitment as potential negative consequences of broad spans of control. 18
Span of control is a relatively simple aspect of a manager’s responsibilities, and theory also suggests that it is important to take the organisational context into account. Studies suggest that managers can deal better with broader spans of control, when (1) they need less frequent interactions with staff, (2) work is less complex, and (3) managers and staff have strong capabilities. 19 Thus, it is important to control for these factors in empirical studies.
Departing in these observations, we believe this study is among the first of its kind to directly investigate the associations between frontline managers’ span of control and their own well-being broadly understood as their job satisfaction, work–life balance, burnout, perceived stress and intention to quit their current job. The purpose of the article is twofold: (1) to provide an empirical description of stress and well-being outcomes among frontline managers in hospitals in Denmark and (2) to investigate whether a manager’s span of control is associated with different aspects of manager well-being.
The design is a cross-sectional study design. We have used the Strengthening the Reporting of Observational Studies in Epidemiology cross-sectional reporting guidelines. A reporting checklist can be found online supplemental appendix B . In the following, we first introduce the population and the data collection process, then we operationalise the measures included in the analysis, and finally, we describe our analytical approach.
Supplemental material
Population and data collection.
The Danish healthcare sector is divided into five regions each responsible for hospitals and specialised healthcare within a geographically defined area. This paper is based on an online survey addressed to all functional managers (N=686) with staff responsibility working within the hospital sector of one of these regions, namely the Central Denmark Region (hereafter ‘The Region’). The Human Resources Departments within The Region provided contact information on the managers. The data collection was conducted using Qualtrics and took place in June 2022. The survey was distributed via email and it was optional to fill in the survey. The survey was used as part of the preparation for a field experiment evaluating a leadership training programme among frontline managers on leading the well-being and the psychosocial work environment in Danish hospital settings. 20 In total, 511 managers answered the survey, corresponding to a high response rate of 74.5% while 67.3% were full responses. The partial responses are also included in this study, which explains the varying number of respondents included in each analysis.
Independent variables
The independent variable of the study is span of control. Span of control was measured by asking the functional managers: ‘Towards how many people do you have direct staff responsibility?’. Direct staff responsibility was defined as the number of employees for whom the manager was responsible for having mandatory annual staff development interviews with.
Dependent variables
In this study, we investigate six different dependent variables central to well-being outcomes among managers. Perceived stress was measured with the Danish consensus version of the 10-item Perceived Stress Scale (PSS-10), ranging from 0 to 40. 21 Burnout was measured using a subscale from the Copenhagen Burnout Inventory measuring personal burnout, ranging from 0 to 24. 22 For both scales, missing values were replaced with imputed numbers if respondents had provided answers for more than half the items in the scale. Additionally, both scales were rescaled to 0–100 to increase comparability between results.
The other four dependent variables were measured using single-item approaches. To measure job satisfaction , working environment satisfaction and work–life balance , we used items from the Danish Psychosocial Work Environment Questionnaire. 23 For job satisfaction and work environment satisfaction measures, we asked respondents to indicate their satisfaction with the ‘overall job’ and the ‘working environment’ on 11-point scales ranging from 0 to 10, with 10 being the highest level of satisfaction. Work–life balance was measured on a 5-point Likert scale ranging from 1: ‘to a very low degree’ to 5: ‘to a very high degree’. The question was phrased ‘Does your work take up so much of your time that it affects your personal life?’. To make high values reflect a good work–life balance, this item was reversed. Lastly, to measure intention to quit , we used the question ‘How often do you think about leaving your current job?’. This question has been used in a range of other studies, see for example Frye et al . 24 Answers were provided on a scale from 1: ‘never’ to 5: ‘always’. All four items were rescaled to 0–100 to increase comparability of results.
Sociodemographic and organisational covariates
We included several sociodemographic, career and organisational-level covariates expected to influence the relationship between span of control and manager well-being. As sociodemographic variables, we included age and gender. As career covariates, we included one variable indicating educational background and three variables measuring leadership experience (one variable indicating the number of years as manager and two dummy variables indicating whether the manager had finalised a master or a diploma education, respectively). Finally, as an organisational variable indicating complexity of the management task, we included the number of different types of professions (i.e. nurses, physiotherapists, etc.) for which the healthcare managers had direct staff responsibility.
Analytical approach
First, we perform descriptive statistics. We then use multiple ordinary least squares (OLS) regression models to describe the relationships between span of control and the outlined outcomes. We present our results in four models, where model I contains no control variables, model II contains sociodemographic control variables, model III contains sociodemographic control variables and career variables, and model IV contains sociodemographic control variables, career variables and task complexity. All analyses are conducted in Stata 18.
Table 1 presents descriptive statistics on all included variables. Most of the respondents are women (83%), the mean age is 51 years, and a large share of the respondents are nurses (48%). The mean level of experience among the middle managers is short of 11 years and many have completed leadership training (a bit over 40% has, for example, finalised a diploma education within leadership). Lastly, the managers have responsibility for employees with 2.6 different educational backgrounds on average.
- View inline
Demographic and descriptive statistics
The span of control varies between 2 and 120, with a mean of 34. On scales from 0 to 100, we find the following average scores for the six dependent variables: the average perceived stress score is 36.3 (14.5 on the original scale from 0 to 40); the average level of personal burnout is 40.2 (9.6 on the original scale from 0 to 24); the average level of job satisfaction is 71.8; the average level of satisfaction with the working environment is 66.7; the average intention to quit is 43.5 and finally, the average work–life balance is 47.6 (with 100 indicating high balance).
The table in online supplemental appendix C displays bivariate correlations between all included variables. The included well-being outcomes are highly correlated. The strongest correlations are found between stress and burnout, and between job satisfaction and working environment satisfaction. For the covariates, none of them are consistently associated with all six dependent variables. However, two of the included career variables, namely experience and diploma education, are correlated with several of the well-being outcomes. Both are negatively correlated with perceived stress and burnout, while experience is also positively correlated with working environment satisfaction, and having a diploma education is positively correlated with job satisfaction. Also, task complexity (the number of educational backgrounds among a manager’s group of employees) is positively correlated with perceived stress. Lastly, gender is positively correlated with burnout, while age is negatively associated with perceived stress.
Differences across educational groups in span of control
Table 2 presents the mean of the primary independent variable (span of control) for different educational groups. There are large differences in the mean span of control across educational backgrounds. Midwives (mean span of control=51.8) and radiographers (mean span of control=46.6) have a markedly broader span of control than all other groups on average. The span of control is also broader among nurses than in the full group of managers. Of the studied groups, the laboratory assistant managers have the narrowest mean span of control of 22.
Mean span of control across educational background
The association between span of control and mental well-being
Table 3 shows the results of the OLS regressions on the association between span of control and mental well-being parameters. We find that managers with broader spans of control have a higher level of perceived stress, are less satisfied with their job and their work environment, have a higher intention to quit and less work–life balance. However, we do not find that span of control is significantly related to burnout. The coefficient is largest for work–life balance and intention to quit, and smallest for perceived stress. Figure 1 summarises this by displaying the marginal effects of span of control on the six included aspects of manager well-being.
OLS regression models: span of control and aspects of mental well-being
- Download figure
- Open in new tab
- Download powerpoint
Marginal effects of span of control on aspects of mental well-being. The dotted line for burnout indicates an insignificant result.
It can be difficult to interpret the strength of the associations. For example, perceived stress was rescaled to ensure comparability. If we instead look at the original PSS-10 ranging from 0 to 40, our results in Model IV convert to an average increase in the PSS score of approximately 0.36 for each 10 employees added to the span of control. For example, in an organisation with 30 employees, the mean would be 14.42, while the mean would be 15.51 in an organisation with 60 employees (on average).
In this study, we aimed to provide a description of stress and well-being outcomes among frontline managers in hospitals in Denmark, and to investigate the relationship between span of control and measures of well-being such as stress and satisfaction outcomes, intention to quit and work–life balance in frontline managers in a large Danish hospital setting. Regarding the descriptive results on stress, burnout and job satisfaction among the managers, we can compare our study with studies on the full Danish population. Across three out of four of these aspects, the managers in the hospital setting score worse than the general population. 23 25 26 This is, however, not the case for job satisfaction, which is on average equivalent to the general population. 23 This could suggest that frontline managers generally face high job demands, complexity and relationships that require their attention.
We found no evidence for any relationships between span of control and burnout, but broader spans of control were associated with lower job satisfaction, lower overall satisfaction with the psychosocial work environment, higher perceived stress, higher quit intentions and lower degree of work–life balance. The strongest associations were observed in relation to work–life balance, intention to quit and the satisfaction outcomes. These results suggest that broad spans of control involve hidden costs to managers’ well-being. The results are somewhat in line with a recent conceptual review of span of control on nurse managers which in a few studies found that broader spans of control were associated with role overload, burnout and lower organisational commitment. 18 In this study, we did not find a significant association between span of control and burnout but with related outcomes such as stress and intention to quit. This could be due to the detrimental aspects of burnout on work functioning which may cause those with the highest symptom levels to be on sick leave and thus less represented in the study. However, although the consequences of large spans of control in the current study are clearly significant except for burnout, associations are relatively small, which suggests that many other individual and organisational factors contribute to mental health and work-related well-being outcomes. This is in accordance with other studies that have investigated the relation between work factors and mental health outcomes. 27
In this study, we adjusted for profession, manager experience and education to account for different work settings and some individual differences. However, taken together, the study calls for a better general understanding of the relationship between work organisation and manager well-being and perhaps a more nuanced understanding of the concept of span of control. Span of control measured simply as the number of employees is useful for comparisons, but probably also too crude to understand how challenging a given managerial position is. Others have argued that the concept of span of control needs to be considered in relation to, for example, staff characteristics, work complexity and manager abilities. 18
While span of control has well-known implications on various employee aspects, this study is among the first to investigate the relationship between span of control and several mental health and work-related well-being outcomes among a large group of frontline managers and with a very high response rate. Yet, several limitations should also be mentioned. Caution is advised regarding drawing conclusions on the causal relationships between span of control and the related outcomes given the cross-sectional study design. Since all variables included in our analysis all originate from the same survey (see online supplemental appendix A for a full list of survey items), we acknowledge that there is a risk of common source bias. 28 However, we believe that this risk is to some extent alleviated by our primary independent variable being an objective measure. Furthermore, all results of this article build on a large sample of frontline managers within public hospitals in a geographically defined area. We believe that these results to a great extent can be inferred to other Danish healthcare settings. However, to provide a more nuanced understanding of how span of control is associated with well-being, more research is needed within other parts of the public sector, in the private sector, at different managerial levels and in different countries to disclose whether the finding that a narrower span of control may be more optimal for manager well-being is also relevant to other settings.
Conclusions
Many healthcare managers oversee large numbers of employees, and also much larger than is typically advised in the literature on span of control. We have here demonstrated how broad spans of control are associated with several negative outcomes for frontline managers in hospitals. Frontline managers with broader spans of control experience higher stress, worse work–life balance, lower job satisfaction and satisfaction with their work environment, and higher intention to quit their job. Generally, the coefficients for the associations between span of control and these outcomes are small, which suggests that many other factors are decisive for manager well-being. Still, we recommend that healthcare organisations consider whether it would be more optimal to reduce the span of control for some managers. Furthermore, we recommend that future studies pay attention to span of control and provide stronger causal evidence about its impact on healthcare workers.
Ethics statements
Patient consent for publication.
Not applicable.
Ethics approval
This study involves human participants and was approved by the Institutional Review Board at Aarhus BSS, Aarhus University (BSS-2022-030). Participants gave informed consent to participate in the study before taking part.
- Jacobsen CB ,
- Hansen AKL ,
- Pedersen LD
- Cecchini M ,
- Pedersen LD ,
- Bech M , et al
- Laschinger HKS ,
- Holm-Petersen C ,
- Østergaard S ,
- Andersen PBN
- Dalgaard VL ,
- Aschbacher K ,
- Andersen JH , et al
- Willert MV ,
- Vestergaard JM , et al
- Bakker AB ,
- de Vries JD
- Adriaenssens J ,
- Hamelink A ,
- Elliott-Miller P ,
- Laschinger H , et al
- Cummings GG
- Boned-Galán A ,
- López-Ibort N ,
- Gascón-Catalán A
- Shirey MR ,
- Dick T , et al
- Cathcart D ,
- Karnas J , et al
- Hansen AKL , et al
- Eskildsen A ,
- Nielsen KJ , et al
- Kristensen TS ,
- Borritz M ,
- Villadsen E , et al
- Huh C , et al
- Jensen MM ,
- Pedersen MH , et al
- Kristensen TS
- Rugulies R ,
- Greiner BA , et al
- Jakobsen M ,
Supplementary materials
Supplementary data.
This web only file has been produced by the BMJ Publishing Group from an electronic file supplied by the author(s) and has not been edited for content.
- Data supplement 1
- Data supplement 2
- Data supplement 3
Contributors All authors developed the idea for this study in collaboration. AKLH drafted the manuscript together with VLD and CBJ. AKLH was in charge of the data collection and data analysis. All authors approved the final version of the manuscript. CBJ is the guarantor of this study.
Funding This study was funded by the Novo Nordic Foundation (grant number 28278).
Disclaimer Novo Nordic has not had any role in the study.
Competing interests None declared.
Provenance and peer review Not commissioned; externally peer reviewed.
Supplemental material This content has been supplied by the author(s). It has not been vetted by BMJ Publishing Group Limited (BMJ) and may not have been peer-reviewed. Any opinions or recommendations discussed are solely those of the author(s) and are not endorsed by BMJ. BMJ disclaims all liability and responsibility arising from any reliance placed on the content. Where the content includes any translated material, BMJ does not warrant the accuracy and reliability of the translations (including but not limited to local regulations, clinical guidelines, terminology, drug names and drug dosages), and is not responsible for any error and/or omissions arising from translation and adaptation or otherwise.
Read the full text or download the PDF:
- Open access
- Published: 22 May 2024
Enhancing clinical skills in pediatric trainees: a comparative study of ChatGPT-assisted and traditional teaching methods
- Hongjun Ba 1 , 2 ,
- Lili zhang 1 &
- Zizheng Yi 1
BMC Medical Education volume 24 , Article number: 558 ( 2024 ) Cite this article
301 Accesses
Metrics details
As artificial intelligence (AI) increasingly integrates into medical education, its specific impact on the development of clinical skills among pediatric trainees needs detailed investigation. Pediatric training presents unique challenges which AI tools like ChatGPT may be well-suited to address.
This study evaluates the effectiveness of ChatGPT-assisted instruction versus traditional teaching methods on pediatric trainees’ clinical skills performance.
A cohort of pediatric trainees ( n = 77) was randomly assigned to two groups; one underwent ChatGPT-assisted training, while the other received conventional instruction over a period of two weeks. Performance was assessed using theoretical knowledge exams and Mini-Clinical Evaluation Exercises (Mini-CEX), with particular attention to professional conduct, clinical judgment, patient communication, and overall clinical skills. Trainees’ acceptance and satisfaction with the AI-assisted method were evaluated through a structured survey.
Both groups performed similarly in theoretical exams, indicating no significant difference ( p > 0.05). However, the ChatGPT-assisted group showed a statistically significant improvement in Mini-CEX scores ( p < 0.05), particularly in patient communication and clinical judgment. The AI-teaching approach received positive feedback from the majority of trainees, highlighting the perceived benefits in interactive learning and skill acquisition.
ChatGPT-assisted instruction did not affect theoretical knowledge acquisition but did enhance practical clinical skills among pediatric trainees. The positive reception of the AI-based method suggests that it has the potential to complement and augment traditional training approaches in pediatric education. These promising results warrant further exploration into the broader applications of AI in medical education scenarios.
Peer Review reports
Introduction
The introduction of ChatGPT by OpenAI in November 2022 marked a watershed moment in educational technology, heralded as the third major innovation following Web 2.0’s emergence over a decade earlier [ 1 ] and the rapid expansion of e-learning driven by the COVID-19 pandemic [ 2 ]. In medical education, the integration of state-of-the-art Artificial Intelligence (AI) has been particularly transformative for pediatric clinical skills training—a field where AI is now at the forefront.
Pediatric training, with its intricate blend of extensive medical knowledge and soft skills like empathetic patient interaction, is pivotal for effective child healthcare. The need for swift decision-making, especially in emergency care settings, underscores the specialty’s complexity. Traditional teaching methods often fall short, hindered by logistical challenges and difficulties in providing a standardized training experience. AI tools such as ChatGPT offer a promising solution, with their ability to simulate complex patient interactions and thus improve pediatric trainees’ communication, clinical reasoning, and decision-making skills across diverse scenarios [ 3 , 4 ].
ChatGPT’s consistent, repeatable, and scalable learning experiences represent a significant advancement over traditional constraints, such as resource limitations and standardization challenges [ 5 ], offering a new paradigm for medical training. Its proficiency in providing immediate, personalized feedback could revolutionize the educational journey of pediatric interns. Our study seeks to investigate the full extent of this potential revolution, employing a mixed-methods approach to quantitatively and qualitatively measure the impact of ChatGPT on pediatric trainees’ clinical competencies.
Despite AI’s recognized potential within the academic community, empirical evidence detailing its influence on clinical skills development is limited [ 6 ]. Addressing this gap, our research aims to contribute substantive insights into the efficacy of ChatGPT in enhancing the clinical capabilities of pediatric trainees, establishing a new benchmark for the intersection of AI and medical education.
Participants and methods
Participants.
Our study evaluated the impact of ChatGPT-assisted instruction on the clinical skills of 77 medical interns enrolled in Sun Yat-sen University’s five-year program in 2023. The cohort, consisting of 42 males and 35 females, was randomly allocated into four groups based on practicum rotation, using a computer-generated randomization list. Each group, composed of 3–4 students, was assigned to either the ChatGPT-assisted or traditional teaching group for a two-week pediatric internship rotation. Randomization was stratified by baseline clinical examination scores to ensure group comparability.
Study design
A controlled experimental design was implemented with blind assessment. The interns were randomly assigned to the ChatGPT-assisted group (39 students) or the traditional group (38 students), with no significant differences in gender, age, or baseline clinical examination scores ( p > 0.05). The ChatGPT-assisted group received instruction supplemented with ChatGPT version 4.0, while the traditional group received standard bedside teaching (as depicted in Fig. 1 ). Both groups encountered identical clinical case scenarios involving common pediatric conditions: Kawasaki disease, gastroenteritis, congenital heart disease, nephrotic syndrome, bronchopneumonia, and febrile convulsion. All interns had equal access to the same teaching materials, instructors, and intensity of courses. The core textbook was the 9th edition of “Pediatrics” published by the People’s Medical Publishing House. Ethical approval was obtained from the institutional review board, and informed consent was secured, with special attention to privacy concerns due to the involvement of pediatric patient data.
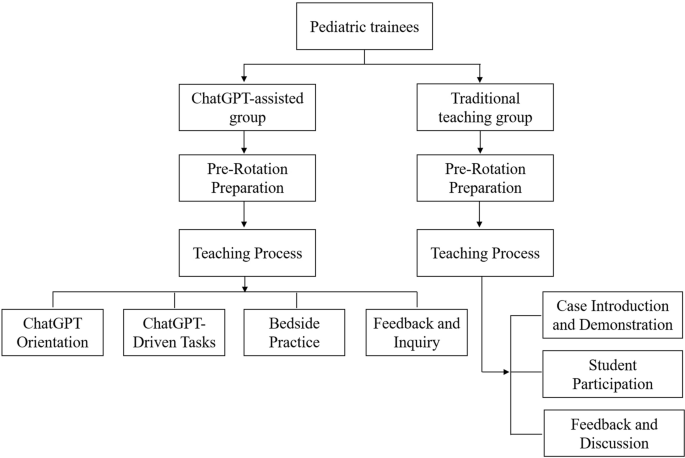
Study design and flow chart
Instructional implementation
Traditional teaching group, pre-rotation preparation.
Instructors designed typical cases representing common pediatric diseases and updated knowledge on the latest diagnostic and therapeutic advancements. They developed multimedia presentations detailing the presentation, diagnostic criteria, and treatment plans for each condition.
Teaching process
The teaching method during the rotation was divided into three stages:
Case introduction and demonstration
Instructors began with a detailed introduction of clinical cases, explaining diagnostic reasoning and emphasizing key aspects of medical history-taking and physical examination techniques.
Student participation
Students then conducted patient interviews and physical assessments independently, with the instructor observing. For pediatric patients, particularly infants, history was provided by the guardians.
Feedback and discussion
At the end of each session, instructors provided personalized feedback on student performance and answered questions, fostering an interactive learning environment.
ChatGPT-assisted teaching group
Educators prepared structured teaching plans focusing on common pediatric diseases and representative cases. The preparation phase involved configuring ChatGPT (version 4.0) settings to align with the educational objectives of the rotation.
The rotation was executed in four consecutive steps:
ChatGPT orientation
Students were familiarized with the functionalities and potential educational applications of ChatGPT version 4.0.
ChatGPT-driven tasks
In our study, ChatGPT version 4.0 was used as a supplementary educational tool within the curriculum. Students engaged with the AI to interactively explore dynamically generated clinical case vignettes based on pediatric medicine. These vignettes encompassed clinical presentations, history taking, physical examinations, diagnostic strategies, differential diagnoses, and treatment protocols, allowing students to query the AI to enhance their understanding of various clinical scenarios.
Students accessed clinical vignettes in both text and video formats, with video particularly effective in demonstrating physical examination techniques and communication strategies with guardians, thereby facilitating a more interactive learning experience.
ChatGPT initially guided students in forming assessments, while educators critically reviewed their work, providing immediate, personalized feedback to ensure proper development of clinical reasoning and decision-making skills. This blend of AI and direct educator involvement aimed to improve learning outcomes by leveraging AI’s scalability alongside expert educators’ insights.
Bedside clinical practice
Students practiced history-taking and physical examinations at the patient’s bedside, with information about infants provided by their guardians.
Feedback and inquiry
Instructors offered feedback on performance and addressed student queries to reinforce learning outcomes.
Assessment methods
The methods used to evaluate the interns’ post-rotation performance included three assessment tools:
Theoretical knowledge exam
Both groups completed the same closed-book exam to test their pediatric theoretical knowledge, ensuring consistency in cognitive understanding assessment.
Mini-CEX assessment
The Mini-CEX has been widely recognized as an effective and reliable method for assessing clinical skills [ 7 , 8 ]. Practical skills were evaluated using the Mini-CEX, which involved students taking histories from parents of pediatric patients and conducting physical examinations on infants, supervised by an instructor. Mini-CEX scoring utilized a nine-point scale with seven criteria, assessing history-taking, physical examination, professionalism, clinical judgment, doctor-patient communication, organizational skills, and overall competence.
History taking
This assessment measures students’ ability to accurately collect patient histories, utilize effective questioning techniques, respond to non-verbal cues, and exhibit respect, empathy, and trust, while addressing patient comfort, dignity, and confidentiality.
Physical examination
This evaluates students on informing patients about examination procedures, conducting examinations in an orderly sequence, adjusting examinations based on patient condition, attending to patient discomfort, and ensuring privacy.
Professionalism
This assesses students’ demonstration of respect, compassion, and empathy, establishment of trust, attention to patient comfort, maintenance of confidentiality, adherence to ethical standards, understanding of legal aspects, and recognition of their professional limits.
Clinical judgment
This includes evaluating students’ selection and execution of appropriate diagnostic tests and their consideration of the risks and benefits of various treatment options.
Doctor-patient communication
This involves explaining test and treatment rationales, obtaining patient consent, educating on disease management, and discussing issues effectively and timely based on disease severity.
Organizational efficiency
This measures how students prioritize based on urgency, handle patient issues efficiently, demonstrate integrative skills, understand the healthcare system, and effectively use resources for optimal service.
Overall competence
This assesses students on judgment, integration, and effectiveness in patient care, evaluating their overall capabilities in caring and efficiency.
The scale ranged from below expectations (1–3 points), meeting expectations (4–6 points), to exceeding expectations (7–9 points). To maintain assessment consistency, all Mini-CEX evaluations were conducted by a single assessor.
ChatGPT method feedback survey
Only for the ChatGPT-assisted group, the educational impact of the ChatGPT teaching method was evaluated post-rotation through a questionnaire. This survey used a self-assessment scale with a Cronbach’s Alpha coefficient of 0.812, confirming its internal consistency and reliability. Assessment items involved active learning engagement, communication skills, empathy, retention of clinical knowledge, and improvement in diagnostic reasoning. Participant satisfaction was categorized as (1) very satisfied, (2) satisfied, (3) neutral, or (4) dissatisfied.
Statistical analysis
Data were analyzed using R software (version 4.2.2) and SPSS (version 26.0). Descriptive statistics were presented as mean ± standard deviation (x ± s), and independent t-tests were performed to compare groups. Categorical data were presented as frequency and percentage (n[%]), with chi-square tests applied where appropriate. A P -value of < 0.05 was considered statistically significant. All assessors of the Mini-CEX were blinded to the group assignments to minimize bias.
Theoretical knowledge exam scores for both groups of trainees
The theoretical knowledge exam revealed comparable results between the two groups, with the ChatGPT-assisted group achieving a mean score of 92.21 ± 2.37, and the traditional teaching group scoring slightly higher at 92.38 ± 2.68. Statistical analysis using an independent t-test showed no significant difference in the exam scores (t = 0.295, p = 0.768), suggesting that both teaching methods similarly supported the trainees’ theoretical learning.
Mini-CEX evaluation results for both groups of trainees
All trainees completed the Mini-CEX evaluation in 38 ± 0.5 min on average, with immediate post-evaluation feedback averaging 5.8 ± 0.6 min per student. The ChatGPT group demonstrated statistically significant improvement in professional conduct, clinical judgment, patient communication, and overall clinical skills compared to the traditional group. A detailed comparison of the CEX scoring for both student groups is presented in Table 1 ; Fig. 2 .
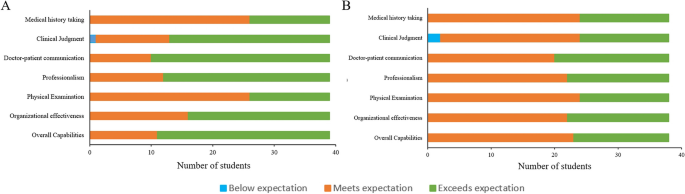
The scale scores of Mini-CEX assessment between the two groups. A : ChatGPT-assisted group; B : Traditional teaching group
Satisfaction survey results of trainees in the ChatGPT-assisted teaching
Feedback from the trainees regarding the ChatGPT-assisted teaching method was overwhelmingly positive. High levels of satisfaction and interest were reported, with no instances of dissatisfaction noted. The summary of these findings, including specific aspects of the teaching method that were rated highly by the students, is detailed in Table 2 .
The integration of ChatGPT into pediatric medical education represents a significant stride in leveraging artificial intelligence (AI) to enhance the learning process. Our findings suggest that while AI does not substantially alter outcomes in theoretical knowledge assessments, it plays a pivotal role in the advancement of clinical competencies.
The parity in theoretical examination scores between the ChatGPT-assisted and traditionally taught groups indicates that foundational medical knowledge can still be effectively acquired through existing educational frameworks. This underscores the potential of ChatGPT as a complementary, rather than a substitutive, educational instrument [ 9 , 10 ].
Mini-CEX evaluations paint a different picture, revealing the ChatGPT group’s superior performance in clinical realms. These competencies are crucial for the comprehensive development of a pediatrician and highlight the value of an interactive learning environment in bridging the gap between theory and practice [ 11 , 12 ].
The unanimous satisfaction with ChatGPT-assisted learning points to AI’s capacity to enhance student engagement. This positive response could be attributed to the personalized and interactive nature of the AI experience, catering to diverse learning styles [ 13 , 14 ]. However, it is critical to consider the potential for overreliance on technology and the need for maintaining an appropriate balance between AI and human interaction in medical training.
The ChatGPT group’s ascendency in clinical skillfulness could be a testament to the repetitive, adaptive learning scenarios proffered by AI technology. ChatGPT’s proficiency in tailoring educational content to individual performance metrics propels a more incisive and efficacious learning journey. Furthermore, the on-site, real-time feedback from evaluators is likely instrumental in consolidating clinical skillsets, echoing findings on the potency of immediate feedback in clinical education [ 15 , 16 ].
The study’s strength lies in its pioneering exploration of ChatGPT in pediatric education and the structured use of Mini-CEX for appraising clinical competencies, but it is not without limitations. The ceiling effect may have masked subtle differences in theoretical knowledge, and our small, single-center cohort limits the generalizability of our findings. The transitory nature of the study precludes assessment of long-term retention, a factor that future research should aim to elucidate [ 17 , 18 ].
Moreover, the ongoing evolution of AI and medical curricula necessitates continuous reevaluation of ChatGPT’s role in education. Future studies should explore multicenter trials, long-term outcomes, and integration strategies within existing curricula to provide deeper insights into AI’s role in medical education. Ethical and practical considerations, including data privacy, resource allocation, and cost, must also be carefully navigated to ensure that AI tools like ChatGPT are implemented responsibly and sustainably.
In conclusion, ChatGPT’s incorporation into pediatric training did not significantly affect the acquisition of theoretical knowledge but did enhance clinical skill development. The high levels of trainee satisfaction suggest that ChatGPT is a valuable adjunct to traditional educational methods, warranting further investigation and thoughtful integration into medical curricula.
Availability of data and materials
All data sets generated for this study were included in the manuscript.
Hollinderbäumer A, Hartz T, Uckert F. Education 2.0 — how has social media and web 2.0 been integrated into medical education? A systematical literature review. GMS Z Med Ausbild. 2013;30(1):Doc14. https://doi.org/10.3205/zma000857 .
Article Google Scholar
Turnbull D, Chugh R, Luck J. Transitioning to E-Learning during the COVID-19 pandemic: how have higher education institutions responded to the challenge? Educ Inf Technol (Dordr). 2021;26(5):6401–19. https://doi.org/10.1007/s10639-021-10633-w .
Bright TJ, Wong A, Dhurjati R, Bristow E, Bastian L, Coeytaux RR, et al. Effect of clinical decision-support systems: a systematic review. Ann Intern Med. 2012;157(1):29–43. https://doi.org/10.7326/0003-4819-157-1-201207030-00450 .
Chan KS, Zary N. Applications and challenges of implementing artificial intelligence in medical education: integrative review. JMIR Med Educ. 2019;5(1):e13930. https://doi.org/10.2196/13930 .
Geoffrey Currie. A conversation with ChatGPT. J Nucl Med Technol. 2023;51(3):255–60. https://doi.org/10.2967/jnmt.123.265864 .
Hossain E, Rana R, Higgins N, Soar J, Barua PD, Pisani AR, et al. Natural language processing in electronic health records in relation to healthcare decision-making: a systematic review. Comput Biol Med. 2023;155:106649. https://doi.org/10.1016/j.compbiomed.2023.106649 .
Mortaz Hejri S, Jalili M, Masoomi R, Shirazi M, Nedjat S, Norcini J. The utility of mini-clinical evaluation Exercise in undergraduate and postgraduate medical education: a BEME review: BEME guide 59. Med Teach. 2020;42(2):125–42. https://doi.org/10.1080/0142159X.2019.1652732 .
Motefakker S, Shirinabadi Farahani A, Nourian M, Nasiri M, Heydari F. The impact of the evaluations made by Mini-CEX on the clinical competency of nursing students. BMC Med Educ. 2022;22(1):634. https://doi.org/10.1186/s12909-022-03667-2 .
Eysenbach G. The role of ChatGPT, generative language models, and artificial intelligence in medical education: a conversation with ChatGPT and a call for papers. JMIR Med Educ. 2023;9:e46885. https://doi.org/10.2196/46885 .
Guo J, Bai L, Yu Z, Zhao Z, Wan B. An AI-application-oriented In-Class teaching evaluation model by using statistical modeling and ensemble learning. Sens (Basel). 2021;21(1):241. https://doi.org/10.3390/s21010241 .
Vishwanathaiah S, Fageeh HN, Khanagar SB, Maganur PC. Artificial Intelligence its uses and application in pediatric dentistry: a review. Biomedicines. 2023;11(3):788. https://doi.org/10.3390/biomedicines11030788 .
Cooper A, Rodman A. AI and Medical Education - A 21st-Century Pandora’s Box. N Engl J Med. 2023;389(5):385–7. https://doi.org/10.1056/NEJMp2304993 .
Arif TB, Munaf U, Ul-Haque I. The future of medical education and research: is ChatGPT a blessing or blight in disguise? Med Educ Online. 2023;28(1):2181052. https://doi.org/10.1080/10872981.2023.2181052 .
Nagi F, Salih R, Alzubaidi M, Shah H, Alam T, Shah Z, et al. Applications of Artificial Intelligence (AI) in medical education: a scoping review. Stud Health Technol Inf. 2023;305:648–51. https://doi.org/10.3233/SHTI230581 .
van de Ridder JM, Stokking KM, McGaghie WC, ten Cate OT. What is feedback in clinical education? Med Educ. 2008;42(2):189–97. https://doi.org/10.1111/j.1365-2923.2007.02973.x .
Wilbur K, BenSmail N, Ahkter S. Student feedback experiences in a cross-border medical education curriculum. Int J Med Educ. 2019;10:98–105. https://doi.org/10.5116/ijme.5ce1.149f .
Morrow E, Zidaru T, Ross F, Mason C, Patel KD, Ream M, et al. Artificial intelligence technologies and compassion in healthcare: a systematic scoping review. Front Psychol. 2023;13:971044. https://doi.org/10.3389/fpsyg.2022.971044 .
Soong TK, Ho CM. Artificial intelligence in medical OSCEs: reflections and future developments. Adv Med Educ Pract. 2021;12:167–73. https://doi.org/10.2147/AMEP.S287926 .
Download references
Acknowledgements
Author information, authors and affiliations.
Department of Pediatric Cardiology, Heart Center, First Affiliated Hospital of Sun Yat-sen University, 58# Zhongshan Road 2, Guangzhou, 510080, China
Hongjun Ba, Lili zhang & Zizheng Yi
Key Laboratory on Assisted Circulation, Ministry of Health, 58# Zhongshan Road 2, Guangzhou, 510080, China
You can also search for this author in PubMed Google Scholar
Contributions
H-JB conceived and designed the ideas for the manuscript. H-JB, L-LZ, and Z-ZY participated in all data collection and processing. H-JB was the major contributors in organizing records and drafting the manuscript. All authors proofread and approved the manuscript.
Corresponding author
Correspondence to Hongjun Ba .
Ethics declarations
Ethics approval and consent to participate.
Ethical approval was obtained from the the Ethics Committee of The First Affiliated Hospital, Sun Yat-sen University, and informed consent was secured, with special attention to privacy concerns due to the involvement of pediatric patient data.
Consent for publication
Not applicable.
Competing interests
The authors declare no competing interests.
Additional information
Publisher’s note.
Springer Nature remains neutral with regard to jurisdictional claims in published maps and institutional affiliations.
Rights and permissions
Open Access This article is licensed under a Creative Commons Attribution 4.0 International License, which permits use, sharing, adaptation, distribution and reproduction in any medium or format, as long as you give appropriate credit to the original author(s) and the source, provide a link to the Creative Commons licence, and indicate if changes were made. The images or other third party material in this article are included in the article's Creative Commons licence, unless indicated otherwise in a credit line to the material. If material is not included in the article's Creative Commons licence and your intended use is not permitted by statutory regulation or exceeds the permitted use, you will need to obtain permission directly from the copyright holder. To view a copy of this licence, visit http://creativecommons.org/licenses/by/4.0/ . The Creative Commons Public Domain Dedication waiver ( http://creativecommons.org/publicdomain/zero/1.0/ ) applies to the data made available in this article, unless otherwise stated in a credit line to the data.
Reprints and permissions
About this article
Cite this article.
Ba, H., zhang, L. & Yi, Z. Enhancing clinical skills in pediatric trainees: a comparative study of ChatGPT-assisted and traditional teaching methods. BMC Med Educ 24 , 558 (2024). https://doi.org/10.1186/s12909-024-05565-1
Download citation
Received : 01 March 2024
Accepted : 16 May 2024
Published : 22 May 2024
DOI : https://doi.org/10.1186/s12909-024-05565-1
Share this article
Anyone you share the following link with will be able to read this content:
Sorry, a shareable link is not currently available for this article.
Provided by the Springer Nature SharedIt content-sharing initiative
- Pediatric training
- Clinical skills
- Medical education
- AI in healthcare
- Mini-clinical evaluation exercise
- Interactive learning
BMC Medical Education
ISSN: 1472-6920
- Submission enquiries: [email protected]
- General enquiries: [email protected]

An official website of the United States government
The .gov means it’s official. Federal government websites often end in .gov or .mil. Before sharing sensitive information, make sure you’re on a federal government site.
The site is secure. The https:// ensures that you are connecting to the official website and that any information you provide is encrypted and transmitted securely.
- Publications
- Account settings
Preview improvements coming to the PMC website in October 2024. Learn More or Try it out now .
- Advanced Search
- Journal List
- HHS Author Manuscripts

Assessment of Attention in Preschoolers
E.m. mahone.
1 Kennedy Krieger Institute
2 Johns Hopkins University School of Medicine
H.E. Schneider
In the past two decades, there has been an increased interest in the assessment and treatment of preschool children presenting with concerns about attention problems. This article reviews the research and clinical literature involving assessment of attention and related skills in the preschool years. While inattention among preschoolers is common, symptoms alone do not necessarily indicate a disorder, and most often represent a normal variation in typical preschool child development. Thus, accurate identification of “disordered” attention in preschoolers can be challenging, and development of appropriate, norm-referenced tests of attention for preschoolers is also difficult. The current review suggests that comprehensive assessment of attention and related functions in the preschool child should include thorough review of the child’s history, planned observations, and formal psychometric testing. The three primary methods of psychometric assessment that have been used to characterize attentional functioning in preschool children include performance-based tests, structured caregiver interviews, and rating scales (parent, teacher, and clinician). Among performance-based methods for measurement of attention in the preschool years, tests have been developed to assess sustained attention, selective (focused) attention, span of attention (encoding/manipulation), and (top-down) controlled attention—including freedom from distractibility and set shifting. Many of these tests remain experimental in nature, and review of published methods yields relatively few commercially available, nationally normed tests of attention for preschoolers, and an overall dearth of reliability and validity studies on the available measures.
Attention Problems in Preschoolers
Attention problems are common among preschool children. By the age of 4 years, as many as 40% of children have sufficient problems with attention to be of concern to parents and preschool teachers ( Palfrey, Levine, Walker, & Sullivan, 1985 ). Symptoms of inattention, even those falling short of formal diagnosis of Attention-deficit/Hyperactivity Disorder (ADHD) are observed to be as high as 3 to 15% in community samples and 50% or higher among clinical referrals ( Christophersen & Mortweet, 2003 ). For example, the number of preschoolers described as “always on the go/driven by a motor” is as high as 72.7% ( Pavuluri, Luk, & McGee, 1999 ). Inattention among preschoolers is not always indicative of ADHD, and may represent a variety of alternative or co-existing conditions, including language disorders, hearing loss, low intellectual functioning, or other forms of psychopathology. Most often, though, inattention is a normal variation observed in typical preschool child development, making identification of “disordered” attention more problematic ( Mahone, 2005 ), especially given the tremendous variability in caregiver ratings of attention and endorsed symptoms of ADHD in this age group ( Stefanatos & Baron, 2007 ). In the past two decades, there has been an increased interest in the assessment and treatment of preschool children presenting with attention problems, especially those associated with symptoms of ADHD, i.e., distractibility and hyperactivity ( Shelton & Barkley, 1993 ). It has been asserted that earlier identification and treatment of attention problems may minimize the harmful impact of childhood disorders, and facilitate appropriate diagnosis, or just as importantly, non-diagnosis ( Wilens et al., 2002b ).
In the preschool years, ADHD is now the most commonly diagnosed form of psychopathology ( Armstrong & Nettleton, 2004 ), and the prevalence estimates appear to be increasing. For example, DeBar and colleagues ( DeBar, Lynch, Powell, & Gale, 2003 ) reported the occurrence of ADHD to be 2% in a sample of 38,664 general pediatric patients under the age of 5 years, while Connor (2000) reported that the incidence might be as high as 59% in child psychiatry clinics. In another sample of 200 children age 6 years and younger referred to an outpatient psychiatric clinic, 86% met diagnostic criteria for ADHD ( Wilens et al., 2002a ). The preponderance of research findings suggest that preschool children presenting with disrupted attentional skills are at significant risk for social ( Rapport, Scanlan, & Denney, 1999 ), developmental ( Newborg, Stock, & Wnek, 1988 ), and academic difficulties ( Stefanatos & Baron, 2007 ), relative to typically developing children ( American Academy of Pediatrics, 2000 ), including multiple developmental deficits ( Yochman, Ornoy, & Parush, 2006 ) as well as diagnosis of ADHD and/or other psychopathology in the school-age years ( Lahey et al., 2004 , 2006 ; Massetti, et al., 2007 ; McGee, Partridge, Williams, & Silva, 1991 ; Wilens et al., 2002b ). Attention problems in preschool are also associated with poor social adjustment and frequent emergency medical visits during adolescence ( Greenhill, Posner, Vaughan, & Kratochvil, 2008 ). Even sub-threshold ADHD in early childhood has been shown to be associated with later academic failure and grade retention ( Bussing, Mason, Bell, Porter, & Garvan, 2010 ). Preschool children who actually meet formal diagnostic criteria for ADHD are at even higher risk for behavior problems 6 years later ( Lahey et al., 2006 ), as well as depression and suicidal behavior by age 18 ( Chronis-Tuscano, et al., 2010 ). The Centers for Disease Control estimated that between 1998 and 2009, nearly 9% of children in the US (1 in 11 children between the ages of 5 and 17) have ADHD, with boys receiving the diagnosis approximately twice as often as girls ( Akinbami, Liu, Pastor, & Reuben, 2011 ). Nevertheless, the proportion of girls exhibiting attention problems has also increased rapidly over the past two decades. A recent large epidemiological study of 3907 children found that of the 8.7% who met DSM-IV-TR criteria for ADHD, 51% were boys and 49% were girls (Froelich et al., 2007).
While early identification and treatment of attention problems in preschoolers may minimize the adverse impact ( Sonuga-Barke, Koerting, Smith, McCann, & Thompson, 2011 ), prior to age 4 years, it is difficult to characterize the behavioral features specifically associated with ADHD (or other co-existing disorders), and to distinguish them from attentional difficulties that occur in typically developing children ( Smidts & Oosterlaan, 2007 ). Some researchers have noted that preschool children who meet criteria for the Inattentive ADHD subtype are actually more likely to go on to have reading, spelling, and mathematics problems compared with those presenting with primarily hyperactive/impulsive behaviors, who are more likely to go on to develop ADHD ( Massetti et al., 2007 ). Other investigators have questioned whether the Inattentive subtype of ADHD is meaningful at all in the preschool years; Egger and colleagues noted that the Inattentive subtype of ADHD occurs in fewer than 1 in 1000 preschoolers in the general population ( Egger, Kondo, & Angold, 2006 ). Given these considerations, development of valid objective methods for assessing attention in preschool children is particularly important. Since diagnostic thresholds tend to be viewed though the expectations of those applying the standards (i.e., parents, teachers), what is considered a “disorder” can change over time ( Sonuga-Barke et al., 2011 ), and relying on parent or teacher reports of child symptoms in isolation can lead to over-identification of disorders ( Gimpel & Kuhn, 2000 ). Further, when impairment criteria from the Diagnostic and Statistical Manual for Mental Disorders, Fourth Edition, Text Revision (DSM-IV-TR; American Psychiatric Association--APA, 2000 ) are used carefully in diagnosing preschoolers, fewer children actually meet formal diagnostic criteria for ADHD (Healy, Miller, Castelli, Marks, & Halperin, 2008). Additionally, while ADHD is presently treated as a categorical phenomenon for the purposes of DSM-IV-TR, the syndrome more likely represents dimensional variations rather than a categorical entity ( Sonuga-Barke et al., 2011 ), and the DSM-V is likely to reflect these changes ( APA, 2012 ; Stefanatos & Baron, 2007 ). Thus, psychometrically sound assessment methods can help quantify these dimensions more accurately, and can facilitate diagnostic accuracy in young children.
Risk Factors for Attention Problems in Preschoolers
Early developmental differences involving attentional control in preschoolers are considered to arise from a variety of influences, including genetic ( Biederman & Faraone, 2002 ), temperamental ( Nigg, Goldsmith, & Sachek, 2004 ), and environmental ( Pauli-Pott & Becker, 2011 ) factors. A recent longitudinal study of over 2000 children from Canada, who were followed from age 5 months to 8 years, showed that the strongest early predictors of attention problems included premature birth, low birth weight, prenatal tobacco exposure, non-intact family, young maternal age, paternal history of antisocial behavior, and maternal depression ( Galera et al., 2011 ). Similarly, a recent study conducted in Israel found that several parameters observed during infancy (birth-1 month) were significantly associated with later development of ADHD: advanced maternal age, lower maternal education, family history of ADHD, and family history of social problems. Between 3–18 months, decrease in head circumference, delay in motor and language milestones, and difficult temperament were all significantly associated with later onset of ADHD, suggesting that temperament, family environment, and early developmental delays are significant risk factors in the preschool years ( Gurevitz, Geva, Varon, & Leitner, 2012 ). As part of the Family Life Project, researchers evaluated behavior and associated risk factors for attention problems among preschoolers ages 3–5 years. They observed that symptoms of inattention and hyperactivity occurred along a single latent factor that did not vary over the preschool years. Further, they observed that low caregiver educational level was the single best predictor of symptom severity ( Willoughby et al., 2012 ).
In the past decade, there has also been increasing concern regarding the amount of screen media used by young children and its relationship with early attention problems. Using retrospective data from the National Longitudinal Survey of Youth, researchers found that number of hours of television watched per day at age 1 and 3 years was associated with attention problems at age 7 years ( Christakis, Zimmerman, DiGiuseppe, & McCarty, 2004 ). There may even be immediate cognitive effects of short-term television viewing in young children. In a recently published study, children who watched a fast-paced television show (specifically a popular fantastical cartoon about an animated sponge that lives under the sea), performed more poorly on a Tower of Hanoi test immediately after viewing than those who had not watched the show ( Lillard & Peterson, 2011 ). These associations remain controversial, however, and there is still a question of whether inattention, per se, represents a cause or an effect of increased television viewing. Nevertheless, the American Academy of Pediatrics (AAP) has taken a conservative approach to television viewing by young children, and has recommended no screen time for children under age 2 years and no more than 1–2 hours a day of quality television and media for older children ( AAP, 2011 ).
Neuropsychological Models of Attention
Attention encompasses several important neuropsychological processes that develop rapidly during the preschool years, including the ability to focus on and attend to stimuli over a period of time and the capacity to take in and report back stimuli immediately after presentation ( White, Campbell, Echeverria, Knox, & Janulwicz, 2009 ). Attention can be considered the foundation on which most other cognitive and neuropsychological functions develop ( Cooley & Morris, 1990 ). Multiple models of attention in the adult brain have been articulated; however, fewer have been translated to apply clinically to the assessment of children ( Mahone, 2005 ). When attentional models are applied to younger children, there exists greater overlap with other developing skills, e.g., executive function, language, visuospatial skills ( White et al., 2009 ), and tests designed to measure attention are affected by (and dependent on) skill development in these other neurobehavioral domains. The prominent neuropsychological models of attention, and their relationship to behavior among preschoolers, are outlined below.
Selective Attention
As humans, our senses are constantly bombarded by stimuli. Because there are limits to the capacity of human information processing, development of goal-directed behavior requires a high degree of selectivity within the attentional system ( Lachter, Forster, & Ruthruff, 2004 ). To this end, Broadbent (1958) proposed a selective filter model of attention in which stimuli are selected via top-down control to be processed further, while non-selected (filtered) information is not processed beyond the extraction of its basic physical features. This filtering mechanism becomes more efficient over the course of early development as children use a larger proportion of their attentional capacities to focus on relevant tasks or stimuli ( Lane & Pearson, 1982 ). Broadbent’s theory provided the basis for other contemporary attentional theories (described below), especially those involving selective attention . The selective attention system includes two competing processes: bottom-up and top-down. Bottom-up attention occurs when the brain automatically orients or attends to sensory features in the environment that stand out in some way. Conversely, top-down attention involves conscious (effortful) control of attention toward some target (including shifting of attention). Bottom-up attention has long been considered to be dependent on the posterior parietal cortex, whereas top-down attention is considered to be dependent on the prefrontal cortex and its connections. However, more recent evidence from studies in primates suggests that the dorsolateral prefrontal cortex is also involved in visually based bottom-up selective attention ( Katsuki & Constantinidis, 2012 ).
Pribram and McGuinness (1975) proposed a related theory in which arousal , activation , and effort act as essential elements in controlled attention. In their model, arousal is defined by the orienting response to sensory input (bottom-up), and is considered dependent on the brainstem, reticular system, and hypothalamus. As children mature beyond the preschool years toward adolescence, forebrain control over this system (top-down) is exerted by the amygdala and frontal cortex—which regulate arousal (phasic, short term attention), and by the basal ganglia—which regulate activation (tonic, longer lasting attention). Pribram and McGuinness highlighted the importance of automatic and effortful cognitive processes in understanding attentional control. In their model, automatic processing is performed without intention. It is necessarily brief, not limited by short-term memory, and preferentially processed by the left cerebral hemisphere. Conversely, effortful (conscious, top-down, frontally-mediated) processing, which invokes limitations on one’s capacity, is necessarily slower, uses serial processing, and is used for novel information ( Goldberg & Costa, 1981 ).
Posner and Peterson ( Peterson & Posner, 2012 ; Posner & Peterson, 1990 ) have asserted that attention is not carried out in a single section of the brain, but rather in a network of regions that complete different, complementary functions. This attentional network is considered separate from the systems of the brain that process data. In the Posner and Peterson model, three primary functions of the attention network are sensory orientation , detection and conscious processing of signals, and maintaining a state of alertness. Visual orienting, which is probably the most relevant to performance-based tests of attention in children, is considered a primary function of the posterior parietal cortex, as well as the lateral pulvinar nucleus of the postereolateral thalamus, and the superior colliculus, with each part carrying out a specific function important in disengaging from a previous focus, shifting, and encoding information from the new stimuli. Posner has asserted that when a target is detected, the brain disengages from a general state of alertness, orients in order to process specific information from that target, and prepares to respond to those specific signals. The brain then maintains a more specific pattern of alertness in order to continue processing (or orienting to) signals with a higher priority. This activation appears to occur primarily in the right hemisphere and involves the noradrenergic system, which is considered to increase the rate of selection of high priority visual information.
Selective or focused attention as described in the models above is often measured using paradigms in which an individual is given two or more concurrent stimuli (or dimensions of stimuli) and must attend to one and ignore the others ( Cooley & Morris, 1990 ). Examples of selective attention measures include: perceptual matching tasks, in which individuals are shown pictures or objects and asked to indicate if a picture is same or different than another; central-incidental learning tasks, in which individuals are given stimuli representing two or more categories, with one designated as central (or important), and then asked to recall both central and non-central (incidental) details; and, visual search or cancellation tasks (described below) in which individuals are asked to search a visual display for a predetermined target.
Divided Attention
A criticism of the Broadbent (1958) and other theories of selective attention is that they do not adequately account for simultaneous or parallel processing, requiring divided attention ( Lachter et al., 2004 ). Divided attention is required when one is given two concurrent tasks or stimuli and instructed to respond to both components. Tasks requiring divided attention differ from selective attention tasks by virtue of the additional demand for number of items attended to, thus requiring additional top-down control ( Cooley & Morris, 1990 ). A common method for assessing divided attention is the dual-task interference paradigm, in which (for example) performance on a task is carried out alone, and then contrasted with performance on the same task performed at the same time as a competing task. NEPSY Statue (described below) is an example of a divided attention task that can be used in preschoolers.
Sustained Attention
Sustained attention or vigilance involves the maintenance of attention over time, and is often based on paradigms that require individuals to detect changes in stimuli over longer periods of time, such as continuous performance tests (Cooley & Morris, 1991). In a model based primarily on factor analytic methods applied to neuropsychological measures in adults and children, Mirsky and colleagues ( Mirsky, Anthony, Duncan, Ahern, & Kellam, 1991 ) were among the first to use continuous performance tests to measure sustained attention. In their studies, Mirsky and colleagues suggested that there are at least four distinct attention functions. These four functions included: focus-execute (i.e., target selection and response—similar to selective attention), sustain (i.e., vigilance, persistence), shift (i.e., flexibility of attentional focus), and encode (i.e., registration, mental manipulation, and recall of information). Similar factors were observed in adults and in children. The authors suggested that while each factor was localized to a different part of the brain, the regions work together as an organized system. “Sustained” attention was dependent on midbrain and brainstem; shifting with the prefrontal cortex; focused attention with the superior temporal cortex, inferior parietal cortex, and corpus striatum; and, encoding with the hippocampus and amygdala. Since Mirsky’s model was based on performance-based tests of attention, it translates easily to the clinical setting, and several computerized continuous performance tests for preschoolers have been developed and normed (see descriptions of tests below).
Attention and Executive Control Functions
There has been a concurrent interest in characterizing problems with attention and executive control functions in preschool children, driven in part by the increase in early identification of psychiatric and neurodevelopmental conditions ( Espy, 2004 ). In the preschool years, these executive function skills have significant overlap with the developmental constructs measured in assessment of attention ( Mahone, 2005 ; Mahone, Pillion, & Hiemenz, 2001 ; Mahone, Pillion, Hoffman, Hiemenz, & Denckla, 2005 ). Like their school-age counterparts, preschool children with attention problems may also present with significant executive dysfunction, although the pattern and trajectory of these behaviors in preschoolers may be different than that observed in school-age children ( Arons, Katz-Leavy, Wittig, & Wayne Holden, 2002 ; Byrne, DeWolfe, & Bawden, 1998 ), with hyperactivity and impulse control (more than inattention and organization) observed as the primary problems ( Byrne, Bawden, Beattie, & DeWolfe, 2000 ; Mahone et al., 2005 ; Mahone & Hoffman, 2007 ). This profile, however, may simply reflect what is demanded or expected of a preschool child. Like attention, individual characteristics of executive dysfunction change and are exhibited differently throughout development, from preschool through adult life ( Root II & Resnick, 2003 ), and are elicited by environmental demands. Among preschoolers, however, both attention and executive function skills can be directly facilitated by provision of externally guided, attention-directing behaviors, usually initiated by the parent ( Conway & Stifter, 2012 ).
A number of theories have attempted to capture the developmental overlap between attention and executive function. For example, Knudsen’s model of attention ( Knudsen, 2007 ) emphasized the role of top-down control of both attention and behavior. The model suggests that there are four essential components of attention: working memory , competitive selection , top-down sensitivity control , and salience filters . Sensory information is filtered for what are either behaviorally important, instinctive stimuli, or infrequent stimuli in a bottom-up approach. The stimuli are encoded and go through competitive selection based on relative signal strength in order to determine what gets access to working memory. Working memory (a core executive function) uses the information to direct subsequent behaviors, as well as direct attention using a frontally mediated, top-down approach. The sensitivity of the information is regulated to improve signal-to-noise and attend only to the information relative to stimuli involved in necessary actions or judgments, and to selectively direct the necessary stimuli to be further encoded. Knudson argued that these tasks are dependent on the development of the prefrontal cortex.
Barkley’s model of executive function ( Barkley, 2011a ) also highlights the dynamic overlap between executive control and elements of attention. His theory emphasized the developmental unfolding of self-regulation and top-down control of behavior as a marker of prefrontal cortex development, and cites at least six key executive functions: self-restraint (executive inhibition), self-directed sensory-motor action (nonverbal working memory), self-directed private speech (verbal working memory), self-directed emotion/motivation (inhibition of strong emotions), self-directed play (planning, innovation, generativity), and self-directed attention (self-awareness). As a theory with a more direct link to top-down control, Barkley’s model has been linked more directly to ADHD than to disorders in which bottom-up or posterior attention systems are more affected. While performance-based tests of the model have been developed, their ecological validity has been criticized, and Barkley has emphasized the importance of rating scales for measuring elements of the model ( Barkley, 2011b ).
Recently, Dennis and colleagues ( Dennis, Sinopoli, Fletcher, & Schachar, 2008 ) highlighted the importance of situational factors in determining the relationship between attention and executive function. Their model considered the integrated nature of stimulus orienting and response control, by considering the elements of each that are more related to attention networks (i.e., bottom-up), or to executive control networks (i.e., top-down). In their model, orientation to stimuli can occur as a result of a bottom-up (passive, external stimulus driven) or top-down (task driven, internally directed) approach. Response to stimuli can occur in a preprogramed, model-driven, performance and reward-based approach (bottom-up), or in a more top-down approach using instructions and attention priorities. In both stimulus orientating and response control, the individual is involved in three (potentially simultaneous) cognitive processes: activation (directing specific attention), inhibition (voluntary or automatic avoidance or cessation of response), and adjustment (performance monitoring and contingency adjustment), all of which interact dynamically to produce goal-directed behaviors.
Attention and Brain Development in Preschoolers
Development of the human nervous system begins 2–3 weeks after conception ( Black, DiCicco-Bloom, & Dreyfus, 1990 ). Brain systems underlying attentional control are among the earliest to develop, with continued maturation into at least early adulthood ( Gogtay et al., 2004 ). The trajectory of functional development, however, is nonlinear, and progresses in a region-specific manner that coincides with maturation of different brain systems ( Halperin & Schulz, 2006 ). By age 2 years, the brain is approximately 80% of its adult size ( Giedd et al., 1999 ). Subsequently, synapse formation ( Huttenlocher & Dabholkar, 1997 ) and myelination ( Kinney, Brody, Kloman, & Gilles, 1988 ) proceed rapidly up to age 2 years, followed by a plateau phase, during which neurons begin to form complex dendritic trees ( Mrzljak, Uylings, Van Eden, & Judas, 1990 ). Maximum synaptic density (i.e., synaptic overproduction) is observed at age 3 months in the primary auditory cortex, but not until age 15 months in the prefrontal cortex ( Huttenlocher & Dabholkar, 1997 ). After age 5 years, brain development is marked by continued neuronal growth, experience-dependent pruning of inefficient synapses, and cortical organization ( Gogtay et al., 2004 ), with eventual reduction of synaptic density to 60% of its earlier maximum ( Huttenlocher & Dabholkar, 1997 ).
Functional development of attention in the preschool years occurs via a process of active neural development—largely genetically determined—but also through a dynamic process of shaping and constraining abilities through experience, potentially modifying genetic expression ( Blair & Raver, 2012 ). The idea of experiential canalization , described by Gottleib (1991), notes that functional development is shaped concurrently by biology as well as experience (or opportunity). In normal development, this “shaping” occurs via progressive myelination and associated cortical thinning, and is associated with improvements in attentional control. Conversely, pathological pruning (affected in part by experience) may contribute to the disrupted development of attentional skills ( Kotrla & Weinberger, 2000 ; Marsh, Gerber, & Peterson, 2008 ), including ADHD ( Sowell, Thompson, Welcome, Henkenius, Toga, & Peterson, 2003 ; Shaw et al., 2007 ).
The prefrontal cortex, which has a prominent role in the development of controlled (top-down) attention, has a protracted period of development, with rapid changes beginning in the first year of life ( Diamond, 2002 ), that continue into young adulthood ( Luna et al., 2001 ). There is evidence that maturation of the prefrontal cortex and its connections during infancy sets the stage for emergence of many “higher” cognitive processes, including top-down control of attention ( Colombo & Cheatham, 2006 ; Kraybill & Bell, 2012 ). Prefrontal development in infancy and toddlerhood has been most commonly measured via electrophysiology (EEG; Fox, Schmidt, Henderson, & Marshall, 2007 ), such that frontal EEG power (considered a measure of neuronal excitability) is observed to be associated with controlled attention in toddlers ( Morasch & Bell, 2011 ).
Over the first year of life, the development of attention is most commonly assessed behaviorally using visual attention paradigms ( Columbo, 2001 ). In the first three months of life, infants are visually attracted to objects with contours and/or edges (e.g., faces, checkerboards), and exhibit long visual fixations. During this period, infants have difficulty disengaging visual attention from objects. This difficulty is likely because the pathways from the basal ganglia to the superior colliculus have begun to develop at that time, whereas the parietal lobes have yet to mature ( Hood & Atkinson, 1993 ). Experimental paradigms used in infancy often include habituation and paired comparison, variants of which have been incorporated into infant development scales (e.g., Bayley Scales of Infant and Toddler Development, Third Edition; Bayley, 2006 ). Object novelty preference typically begins to emerge in infants around age 4–6 months, and is thought to coincide with the maturation of the cortical visual system and the parietal cortex. During this period, infants’ visual gaze time decreases and visual disengagement occurs. By 7–12 months, gaze time begins to increase again, and is accompanied by a shift from involuntary to more voluntary control, which continues to develop throughout toddlerhood and the preschool years ( Columbo, 2001 ).
The development of neural systems supporting attentional control occurs at different rates in boys and girls (De Bellis, 2001). At birth, girls are already considered to be 3 weeks ahead of boys in terms of physical maturation; 1 year ahead by school entry (Eme, 1992). Although there are few reported sex differences in problem behavior in infancy and early childhood; sex differences appear to emerge by age 4 years, with boys showing more aggressive and impulsive behaviors. The change in behavior among girls may be a function of more rapid neurobiological, cognitive, motor, and social development, which, in preschool, may be protective against the manifestation of some ADHD symptoms in early childhood (Biederman et al., 2008). Sexual dimorphism in cerebral development may also contribute to the observed differences in attention problems. Giedd and colleagues compiled normative growth curves, demonstrating the sexual dimorphism in brain development (Lenroot et al., 2007). From age 4 to 20 years, linear increases in white matter volume with age were observed, whereas age-related changes in gray matter were nonlinear, regionally specific, and differed for boys and girls. Total cerebral gray matter volume was 10% larger in boys, but peaked much earlier in girls than boys (10.5 years vs. 14 years). The trajectory was identical for the caudate, which also showed peak at age 10.5 for girls and 14 for boys. Frontal lobe gray matter volume peaked around 10.5 years for boys (9.5 years for girls), and declined during post-adolescence resulting in an overall net decrease across the age span. Results were similar for parietal lobe volumes and differed only in that the slope of the curve was steeper and volumes peaked 1.5 years earlier for each sex (9.0 years for boys, 7.5 years for girls). Similarly, temporal lobe volumes peaked age 11 years for boys and 10 years for girls.
Interventions for Attention Problems in Preschoolers
Behavioral interventions.
A variety of programs have been developed to target improvements in behaviors associated with ADHD, including inattention and self-control. Two such programs, Triple P ( Bor, Sanders, & Markie-Dads, 2002 ) and The Incredible Years ( Jones, Daley, Hutchings, Bywater, & Eames, 2008 ), which emphasize parent training, have been shown to be effective in reducing disruptive behaviors, more so than behaviors associated with attentional control ( Halperin, Bedard, & Curchack-Lichtin, 2012 ). Similarly, in a randomized control trial of a treatment program for preschoolers focusing on self-regulation and mother-child interactions (i.e., the Revised New Forest Parent Programme ), the authors reported a reduction in symptoms associated with ADHD up to 9 weeks after conclusion of treatment ( Thompson et al., 2009 ). More recently, Halperin and colleagues ( Halperin et al., 2012 ) recently developed a novel method for training attention and other cognitive skills in preschoolers that has shown initial promise. Their Training Executive, Attention, and Motor Skills program utilized parent-directed small groups (3–4 parent-child dyads), that employed “games” emphasizing attention, inhibitory control, working memory, planning, visuospatial skills, and motor skills. Groups occurred daily for 30–45 minutes, and treatments lasted approximately 5–8 weeks. Preliminary evidence suggested that parent and teacher ratings of attention improved (relative to baseline) after completion of treatment, with gains maintained at three-month follow up ( Halperin et al., 2012 ).
Pharmacotherapy
The practice of prescribing psychotropic medications for very young children has increased in both the US and Europe ( Connor, 2002 ). For example, Rappley, Eneli, & Mullan (2002) reported on 223 children age 3 years and younger who were receiving treatment for attention problems. More than half of their sample received treatment in an idiosyncratic manner, and had monitoring less than once every three months. In an attempt to improve knowledge about assessment and treatment of attention problems in young children, the NIMH began a clinical trial in 2000 to study the effects of methylphenidate in preschoolers (ages 3–6 years) with ADHD. This study, known as the Preschool ADHD Treatment Study (PATS) has begun to shed light on diagnostic methods and treatments for ADHD and other attentional disorders in the preschool years ( Vitiello et al., 2007 ), including safety of stimulants ( Greenhill et al., 2006 ), short-term ( Abikoff et al., 2007 ) and long-term effectiveness ( Vitiello et al., 2007 ), and associated side effects ( Ghuman et al., 2001 ), including reduced growth rates among preschoolers treated with stimulants ( Swanson et al., 2006 ). In one sample of preschoolers with ADHD treated with stimulant medication, more than 82% of the sample saw improvement in behavior ratings by at least one standard deviation ( Short, Manos, Findling, & Schubel, 2004 ). The presumed mechanism of action of stimulants, which has been established via studies of older children and adults (but not among preschoolers), is by increasing the availability of catecholamines (especially dopamine and norepinephrine) in the synaptic cleft (Plizska, 2007). Given the preponderance of these neurotransmitters in the fronto-striatal circuitry, stimulants have primary action on attentional functions affecting top down control, and improvement in these skills has been observed in preschool children ( Abikoff et al., 2007 ). From these studies, the American Academy of Pediatrics has recently addressed the use of stimulant medication in preschool children (ages 4–5 years), and has recommended using stimulant medications only in those preschool-aged children with symptoms of ADHD who have moderate-to-severe symptoms that have persisted for at least 9 months, with dysfunction manifested in both the home and other settings such as preschool or child care, and who have not responded adequately to behavior therapy ( AAP, 2011 ).
To date, there have been only two published studies of pharmacological treatment of preschool children with non-stimulant medication—both with atomoxetine, a selective norepinephrine reuptake inhibitor. An open-label study of atomoxetine (titrated to 1.8 mg/kg/day) with 12 preschool children ages 3–5 years showed adequate tolerability and reduction of parent reported hyperactivity and impulsivity from baseline ( Ghuman et al., 2009 ). More recently, a larger, 8-week, double blind, placebo-controlled, randomized clinical trial of atomoxetine (titrated to 1.8 mg/kg/day) in 101 children with ADHD (ages 5–6 years) led to significant reductions in core symptoms of inattention (observed by parents and teachers) and hyperactivity (observed by parents), although functional impairment was not significantly affected ( Kratochvil et al., 2011 ). Given the findings from pharmacological studies of preschoolers, it is clear that behavioral treatments are necessary to augment any medication therapy treatment in this age range.
Methods for Assessing Attention in Preschoolers
Comprehensive assessment of attention and related functions in children should include thorough review of the child’s history, planned observations, and formal psychometric testing ( Mahone & Slomine, 2008 ). The three primary methods of psychometric assessment that have been used to characterize attentional functioning in preschool children include performance-based tests, rating scales (parent, teacher, clinician), and structured interviews. Performance-based methods for measurement of attention in the preschool years have been developed to address most of the salient components of attention described by Mirsky, Dennis, and Posner, and include sustained attention, selective (focused) attention, span of attention (encoding/maintaining), and top-down controlled attention, including freedom from distractibility and set-shifting. A review of published methods is provided below, and includes measures that are commercially available, and those that have been published only in the research literature.
Performance-based Tests
Continuous performance tests, kiddie continuous performance test (k-cpt).
The K-CPT ( Conners, 2001 ) is a computerized test of attention for children ages 4 and 5 years. The task lasts 7.5 minutes and uses 11 black and white pictures of common objects (10 targets, 1 non-targets). The child must respond by clicking a mouse or pressing space bar as quickly as possible following all pictures except for the non-target (a soccer ball). Data regarding omissions, commissions, perseverations, hit reaction time, and standard error are collected. Response time data are also used to determine response consistency as the task progresses, as well as in response to the two interstimulus intervals. The normative sample included 454 four- and five-year-old children, of whom 314 were classified as nonclinical, 100 as having ADHD, and 40 as clinical cases without ADHD. The nonclinical sample was relatively even in regard to sex distribution (48% boys, 52% girls); however, the majority of the ADHD sample was boys (75%). Split-half reliability ranged from .72 to .83 ( Conners, 2001 ).
Despite its publication over a decade ago, there have been relatively few validity studies using the K-CPT. In one study combining the K-CPT (ages 3–5) and the CPT-II ( Conners, 2000 ; ages 6–7), omission errors were significantly correlated with the Conners’ Parent Rating Scale-Revised (CPRS-R) Cognitive Problems and Inattention scales; although, commission errors were not found to be associated with the Hyperactivity scale ( Rezazadeh, Wilding, & Cornish, 2011 ). Preschool children without behavioral difficulties are observed to have fewer omissions, lower reaction time standard error, less variability and less deterioration in performance over time than children with hyperactive and oppositional behaviors ( Youngwirth, Harvey, Gates, Hashin, & Friedman-Weieneth, 2007 ). The K-CPT has also been used as an attention measure with healthy preschoolers in research comparing effects of DHA supplements on cognitive functioning ( Ryan & Nelson, 2008 ).
Auditory Continuous Performance Test for Preschoolers (ACPT-P) ( Mahone et al., 2001 )
The ACPT-P is a computerized go/no-go test developed for use in children ages 36 to 78 months. Omissions, commissions, response latency, and variability of response time are the primary performance indices. This is a true go/no-go test, with only two stimuli—one target (dog bark) and one non-target (bell). Auditory stimuli are presented for 690 msec, with a fixed ISI of 5000 msec. During this five-minute test, 15 target and 15 non-target stimuli are presented. The child presses the space bar immediately following the target stimulus, and ignores the non-target. Initial studies showed no significant differences between typically developing boys and girls on any of the indices. Performance (omissions, response latency, and variability) improved with age from age 3–4 years, leveling off by age 5 years and was correlated with parent ratings on the CPRS-R ( Mahone et al., 2001 ). Preschool children with ADHD demonstrate significantly poorer performance on the ACPT-P with regard to omissions, mean response time, and variability ( Mahone et al., 2005 ). Among preschoolers with ADHD, the ACPT-P omissions, response time, and variability were significantly associated with performance on the NEPSY Statue test ( Mahone et al., 2005 ). Among typically developing preschool children, amount of nighttime sleep was significantly (inversely) associated with number of commission errors on the ACPT-P ( Lam, Mahone, Mason, & Scharf, 2011 ).
Continuous Performance Task for Preschoolers (CPTP) ( Corkum, Byrne, & Ellsworth, 1995 )
The CPTP is a computerized test designed for children ages 3–5 years. The CPTP uses pictures for stimuli, includes targets and distractors, a sequential presentation of stimuli in a quasi-random fashion, a slowed rate of presentation to allow for longer viewing time, a longer response window, fewer trials to reduce total task duration, and a training phase ( Corkum et al., 1995 ). All six stimuli (1 target, 5 non-targets) are black and white, hand drawn (and scanned) figures with the same basic circular shape. Non-targets include pictures of a girl’s face, the sun, an ice cream cone, a lollipop, and a flower; the target is a pig face. The test presents 240 stimuli (40 targets) in pseudorandom order in which the target and each non-target occur once every six trials. For evaluation purposes, the session is divided into four 60-trial blocks, and the total test is 9 minutes long. Omissions, commissions and response latency are recorded.
In a sample of 60 typically developing preschoolers, an increase in omissions was observed over the 9-minute course of the CPTP. In all blocks, 3-year-olds showed more omissions than 4- and 5-year-olds; however, only in the last three blocks did 4-year-olds display more omissions than 5-year-olds. A greater number of commissions were displayed by 3-year-olds than by the other two groups, and boys displayed more commissions than girls. The sensitivity to target discrimination improved with age as well. When compared with other measures of preschool attention, commissions and omissions on the CPTP were significantly correlated with performance on a preschool picture cancellation test ( Corkum et al., 1995 ).
Hagelthorn and colleagues ( Hagelthorn, Hiemenz, Pillion, & Mahone, 2003 ) adapted the CPTP by shortening the length to three minutes and reducing the number of non-targets to one to facilitate more direct comparison with a preschool auditory CPT (i.e., the ACPT-P described above). In this adaptation, stimulus duration and interstimulus intervals remained identical to those described by Corkum et al. (1995) . In this sample, typically developing children displayed a significantly higher rate of omissions and commissions on the visual task than on the auditory task. Mean reaction time and omissions errors decreased with age. Of note, the findings from the Hagelthorn study suggested that 3-year olds likely require interstimulus intervals as long as 5000 msec in order to respond within the allotted interval (and not to the preceding stimulus).
The CPTP has also been used in preschool children with ADHD. In a sample of 50 children ages 3–6 years, children with ADHD made more errors of omission and commission than age-matched typically developing peers ( DeWolfe, Byrne, & Bawden, 1999 ). Preschoolers with ADHD who had not been treated with stimulant medications had significantly more omissions and commissions on the CPTP than matched controls; however, once treated, group differences were eliminated ( Byrne et al., 1998 ). Noland and colleagues also used the CPTP to investigate attention in 4-year-olds who had experienced prenatal substance exposure ( Noland et al., 2005 ). The authors reported that children with prenatal cocaine exposure “passed” the pretest portion of the CPTP at a significantly lower rate than non-exposed children. Additionally, cocaine-exposed and cigarette-exposed children display significantly more commission errors than non-exposed children, and increased exposure to cigarettes was associated with a higher rate of commission errors. Severity of marijuana exposure showed a positive, but non-significant correlation with omission errors.
Zoo Runner was developed by Prather, Saramento, and Alexander (1995) to assess sustained attention in children ages 3 to 6 years. It has two components: an auditory attention task and a visual task. On the auditory test, animal sounds are presented to the child for 1000 msec, and with an ISI of 1000 msec. There are a total of 215 trials, and the task takes 7.2 minutes. There is 1 target and 11 non-target stimuli. Children are asked to press a button when they hear the word “tiger.” Mean reaction time, omissions, and commission errors were recorded. The auditory version of Zoo Runner has been used in both clinical and nonclinical samples. In a sample of 50 children ages 3–6, children with ADHD had similar rates of omissions and commissions on this task compared with typically developing peers ( DeWolfe et al., 1999 ). Similar to the CPTP, preschoolers with ADHD who had not been pharmacologically treated displayed significantly more omissions on the auditory Zoo Runner task than matched controls; however, once treated, this difference was eliminated ( Byrne et al., 1998 ).
The visual version of Zoo Runner requires the child to respond after seeing a picture of a cat. Stimulus presentation time, interstimulus intervals, total number of trials, number of targets and non-targets, and total task duration are the same as in the auditory version. In both tasks, typically developing children were noted to improve with age on omissions, commissions, and reaction times. Children at age 3 years demonstrated a higher number of omissions on both tasks, suggesting that the tasks may be too difficult for typical children of that age. After age 5.5 years, little further improvement is observed ( Prather, Sarmento, & Alexander, 1995 ).
Children’s Continuous Performance Test (C-CPT) ( Kerns & Rondeau, 1998 )
The C-CPT is a computerized CPT that uses a combined visual/auditory format. Stimuli are presented at 1500 msec intervals—one target for every six non-targets, for a total duration of 5 minutes. Familiar animal pictures are paired with the common noise associated with those animals. Familiarization to the pictures and sounds are completed prior to test administration, and a practice trial is administered until 100% accuracy is obtained. Correct hits, omission errors, commission errors, and reaction times are recorded. Three subtests can be administered to each participant, for a total of 200 stimuli, including 29 randomly presented targets. Task 1 presents the visual and auditory pairing, during which the child is asked to respond to the sheep. Task 2 presents only the audio stimuli (with an X on the screen), and the child is again asked to respond to the sheep sound. Task 3 pairs the visual and auditory stimuli incorrectly, and the child is asked to respond to the picture of the sheep, regardless of the accompanying animal sound.
When administered to typically developing children ages 40 through 64 months, a significant age effect was found for correct hits on all three tasks, and for commission errors on Task 1. Correct hits increased as age increased, while commission errors decreased with age. As a group, children displayed fewer overall hits on Task 2 (auditory only) than on Tasks 1 and 3, suggesting that the task may have been more difficult. More commission errors were made on Task 3 (incorrect pairings) than on Tasks 1 or 2. Reaction times across all three tasks were faster for commission errors than for correct hits. No significant effects of sex were identified ( Kerns & Rondeau, 1998 ). Among clinically-referred preschoolers with attention problems, only 39% had successful completion of all three C-CPT trials (compared with 98.9% control completion), despite all clinical participants being able to complete the practice trial successfully, suggesting that only Task 1 is appropriate for use in clinical groups ( Kerns & Rondeau, 1998 ).
The Preschool-Continuous Performance Test (P-CPT) is a modification of the C-CPT, and includes only Task 1. The child is told that he or she is responsible for feeding the sheep, and must do so by touching the sheep when it appears on the computer screen. The child is not supposed to touch any of the other animals. The task is 5 minutes in duration, includes 200 stimulus presentations, of which 29 are sheep. Omission errors, commission errors, and reaction times are recorded. In a sample of typically developing children ages 3–5 years from private preschools in a socio-economically disadvantaged area of Canada, correct hits (.65), omissions (.63), and reaction time (.77) were all found to display modest test-retest reliability while commission errors had less stability (.44) ( Muller, Kerns, & Konkin, 2012 ). Preschool children with both early and late pre-term birth made significantly more errors of omission and commission than age-matched term birth children ( Baron, Kerns, Muller, Ahronovich, & Litman, 2011 ).
Gordon Diagnostic System (GDS) ( Gordon, McClure, & Post, 1986 )
The GDS is a self-contained computerized CPT that is most often used with children of school age or adults; however, it can also be used with 4- and 5-year-old children. Normative data for the GDS were collected during the mid-1980s., with data based on more than 1250 children aged 4 to 16 years old ( Gordon & Mettelman, 1987 ). Preschool children (4–5 years-old) are asked to complete two tasks— Delay and Vigilance . On the Delay task, the child is instructed to earn maximum points by pushing a button; however, a delay between presses is required in order to earn points. The child must determine how long to wait, as well as be able to wait that amount of time between presses in order to earn points. Typically, a 6-second delay is required; however, this is decreased to 4 seconds for preschool children. If the child responds sooner than 4 seconds, a buzzer sounds and the timer is reset; however if they wait the 4 seconds before responding, a light flashes and a point is earned. The delay task is 6 minutes in duration. The percentage of correct responses (efficiency ratio) is used to determine the child’s level of impulsivity. The Vigilance task is also modified for children ages 4 and 5 years. Rather than the standard 1/9 combination required for response with older children, the preschool task assesses sustained attention by flashing numbers quickly on the screen and asking the child to respond when a 1 appears. The presentation interval is 2 seconds, and the entire task lasts 6 minutes. Performance is evaluated using the total correct and the total number of commissions.
Mariani and Barkley (1997) used a version of this task with 4- and 5-year old boys in which they extended the standard duration time from 6 minutes to 9 minutes and used the single digit target of 7. Boys with ADHD performed significantly worse on the total correct responses, but not on commission errors. Similarly, Pulsifer and colleagues used the GDS to assess attention in 5-year-old children living in an urban environment, with and without prenatal drug exposure. The authors reported that the drug exposed group had significantly fewer commission errors, although both groups performed below average in regard to sustained attention ( Pulsifer, Butz, O'Reilley, Belcher, 2008 ).
Preschool Vigilance Task (PVT) ( Harper & Ottinger, 1992 )
The PVT is a computerized task that presents a picture of a tree with an extended right-side branch. A bird appears on the branch for 0.5 seconds 25 times at intermittent intervals ranging from 10 to 60 seconds, for total task duration of 14.5 minutes. The ISIs are chosen randomly, but each participant receives the same sequence. The child is instructed to press the response button only when the bird appears on the tree. The computer records errors of commission, errors of omission, and reaction time. This task was evaluated on a sample of 20 hyperactive 4–6 year olds, predominantly boys, and 20 controls matched on gender and age. Errors of omission demonstrated high test-retest reliability (.80); however, commission errors and reaction time did not. Hyperactive children tended to make more errors of omission, but not commission, on the PVT. The PVT was able to correctly classify hyperactive versus typically developing with 82.5% accuracy ( Harper & Ottinger, 1992 ).
Additional Issues with Preschool CPT Tests
Other continuous performance tasks have been developed that assess sustained attention in preschoolers with and without ADHD, as well as with and without other childhood disorders. Some researchers have adapted tasks designed for older children to be more appropriate for preschool groups, for example, by decreasing the total task duration and using more basic stimuli ( Finneran, Francis, & Leonard, 2009 ). Even with these adaptations, other factors, such as ISI can impact performance ( Finneran et al., 2009 ; Hagelthorn et al., 2003 ). In a study of preschool children described as low or high risk for ADHD Berwid and colleagues manipulated the ratio of targets to non-targets in a computerized test. Across groups, rates of omission and commission errors decreased with age and the youngest group displayed significantly more errors than the middle and oldest age groups (which did not differ). An interaction effect was observed, such that group differences in commission errors diminished with age. High-risk children also displayed longer and more variable reaction times, though again, mean response time and response time standard deviation decreased with age when comparing the youngest group (ages 3.44 to 4.54) to the older two groups (ages 4.54–5.57, and 5.58–6.95). The greatest response time discrepancies between risk groups were found to be present in the youngest age groups ( Berwid, Curko, Marks, Santra, Bender, & Halperin, 2005 ). Another common problem is that some CPT tasks for preschoolers use letters or numbers as stimuli ( Harper & Ottinger, 1992 ), which presumes the children taking the test have automaticity of recognition of their letters and numbers.
A summary of the CPT tests reviewed is provided in Table 1 .
Preschool Continuous Performance Tests
Note: All continuous performance measures listed are designed to assess sustained attention, vigilance, and inhibitory control. K-CPT = Conners’ Kiddie Continuous Performance Test; ACPT-P = Auditory Continuous Performance Test for Preschoolers; CPTP = Continuous Performance Test for Preschoolers; C-CPT = Children’s Continuous Performance Test; GDS = Gordon Diagnostic System; PVT = Preschool Vigilance Test; ISI = interstimulus interval;
Visual Cancellation Tasks
Picture deletion test for preschoolers (pdtp) ( corkum et al., 1995 ).
The PDTP presents a quasi-randomized matrix of picture targets and non-targets, and reduces fine motor demands (compared with traditional paper and pencil tasks) via use of a self-inking “bingo” stamper ( Corkum et al., 1995 ). Normative data were collected on 60 Caucasian children ages 3–5 years. Sex distribution in each age group was relatively equal. The PDTP requires the child to search a visual array of targets and non-targets to locate and mark the targets as quickly as possible. The training phase involves teaching the child to identify and mark the appropriate target. The test phase involves four tasks: Geometric Shape Deletion, Cat Deletion, Fish Deletion, and Motor Deletion. The total test phase lasts approximately 10 minutes. The first three tasks include one Discrimination and two Deletion phases. The Discrimination phase is used to determine that the child is able to identify an example of each distractor and the target. In the Deletion phase, the child is presented with two adjacent 10-inch × 6-inch arrays of 15 target and 45 non-target pictures, with an example of the target at the top, and asked to mark the targets as quickly as possible. Performance is measured in time to completion (speed), errors of omission, and errors of commission (accuracy). The Motor Deletion Task assessed the speed of marker use by presenting a single 10-inch × 6-inch array of circles and asking the child to mark all of the circles as quickly as possible.
Across the three age groups, more commissions were observed on the Fish trial than the Cat trial. Three-year olds made more omissions on the Cat trial than on the Shape trial. No significant differences between age groups were observed in regard for omissions and commissions on the Shape trial; however, 3-year olds made more omissions than 4-year-olds on the Cat trial, and the number of omissions decreased on the Fish trial as children got older. Similarly, commissions decreased with age on the Fish trial as well. Age effects were observed in regard to commissions on the Cat trial in the younger two groups as well, with 4-year-olds performing better than 3-year-olds. Performance on the Cat and Fish trials of the PDTP were significantly associated with commission and omission error rates on the CPTP ( Corkum et al., 1995 ).
A revised version of the PDTP has been published, with removal of the Fish trial, an increase in the number of Cat trial arrays, and change in the printed layout of the task ( Byrne et al., 1998 ). Using the revised task in a clinical sample of 50 children with ADHD, ages 3–6 years, there were no group differences (compared with age-matched controls) on the PDTP for omission errors; however, the ADHD group made significantly more commission errors and displayed a longer latency to task completion ( DeWolfe et al., 1999 ). Additionally, preschoolers with ADHD who had not been treated with stimulant medications displayed significantly more commission errors on the PDTP than matched controls; however, once treated, there were no longer group differences ( Byrne et al., 1998 ). The PDTP has more recently been used to assess attention in 4-year-old children with prenatal substance exposure. The children with prenatal cocaine exposure “passed” the pretest portion of this task at a significantly lower rate than non-exposed children. Additionally, preschool children with prenatal tobacco exposure produced a higher rate of commission errors than non-exposed children ( Noland et al., 2005 ).
NEPSY Visual Attention ( Korkman, Kirk, & Kemp, 2007 )
The original version of the NEPSY was designed to assess neuropsychological development of children ages 3 to 12 years. NEPSY subtests were divided into five content Cores—one of which one was Attention/Executive Functions. The Visual Attention subtest was included in this Core. Only two of the subtests on the NEPSY Attention/Executive Function Core were designed for use with preschool children—Visual Attention and Statue. Both of these measures have been used in studies of preschoolers with symptoms of ADHD ( Mahone et al., 2005 ; Youngwirth et al., 2007 ), and in evaluating attention in other clinical groups, such as those with exposure to gestational diabetes mellitus ( Nomura et al., 2012 ). The NEPSY Visual Attention subtest is a visual cancellation task that assesses the child’s ability to use selective focus and attention, along with speed and accuracy. The task requires the child to identify and mark the target stimulus among an array of distractors as quickly as possible. For preschoolers, there are two trials: bunnies (arranged in rows) and cats (arranged in random order). The child is required to search the pages and mark each target (which is represented at the top of the page) with a red crayon. Each trial is 120 seconds. Published reliability coefficients for children ages 3–5 years ranged from .68 to .76. In a sample of 184 typically developing children ages 3½ to 6 years, a moderate association was observed between the NEPSY Visual Attention and Statue subtests in children with a verbal span of ≤ 3 digits, but no relation in children with digit span of ≥ 5 ( Espy, 2004 ). Unfortunately, the Visual Attention subtest was not included in the second edition of the NEPSY due to concerns about insufficient clinical sensitivity ( Korkman, Kirk, & Kemp, 1998 ).
Visual Search Task (Visearch; Wilding, Munir, & Cornish, 2001 )
This is a visual selection task designed for children ages 3–7 years. The task has two conditions, the single-target search condition and the dual-target search condition. In this task, participants were seated in front of a screen showing a display of black and brown color “holes” of different shapes on a green background with a river and some trees. In the single-target search condition, participants were instructed to touch a hole of prior specified shape and color (e.g., black vertical ellipse or a brown horizontal ellipse) in order to uncover the “king of monsters.” Participants are instructed that small monsters (which are blue for black vertical ellipses and yellow for brown horizontal ellipses) will pop from the target holes, but they have to continue looking until the king of monsters was found. The king always appears on the twentieth target touched. Performance is assessed using the number of false alarms to non-target holes and mean response time per hit. A modified version of the original task was used by Wilding et al. in which the “holes” were larger than in the original version, measuring 8 mm or 6 mm in diameter, with the same measurements for the longer and shorter axes of the ellipses. There were 20 targets in the single-target searches and 15 for each target in the dual-target search. Non-targets were large or small circles and ellipses in both colors; there were 50 non-targets in the single search conditions and 40 non-targets in the dual search condition, making 70 objects in all ( Wilding et al., 2001 ).
Additional Performance-based Tests of Attention
Statue ( korkman et al., 1998 ; korkman et al., 2007 ).
The NEPSY Statue subtest assesses motor persistence and inhibition by asking the child to close his or her eyes, remain in a specified body position, and refrain from speaking or laughing for 75 seconds while the examiner performs potential distractions (e.g., dropping a pencil). The published reliability coefficient for the original NEPSY among children ages 3 to 4 years was only .50; nevertheless, the Statue test has been found to be highly sensitive in clinical groups. Preschoolers (ages 3–5 years) with ADHD were observed to have lower Statue scores than age- and sex-matched controls ( Mahone et al., 2005 ), while preschoolers with behavior problems had lower Statue performance than those without behavior problems ( Youngwirth et al., 2007 ). In the revised NEPSY (NEPSY-II; Korkman et al., 2007 ), normative data have been updated and the Statue subtest is administered in exactly the same manner as in the first edition. In the NEPSY-II, the reliability coefficient for children ages 3–4 years on the Statue subtest is considerably improved (.82) ( Korkman et al., 2007 ).
Recognition of Pictures (Differential Ability Scales-Second Edition—DAS-II; Elliott, 2007)
The DAS-II was standardized on a sample of 3,480 children, ages 2:6 through 17:11, stratified by six-month age groups for ages 2, 3, and 4, and one-year age groups for ages 5 through 17. A total of 880 children between the ages of 2:6 and 4:11 were included. On the Recognition of Pictures subtest, the child is presented with pictures of common objects for 5 seconds. This is followed by presentation of a different page, on which the target object(s) is presented with similar distractor items. The task requires the child only to point to the target object(s) to identify the target. The number of target items gradually increases, as does the similarity of the targets to the distractor items. Reliability coefficients for the Recognition of Pictures task for children ages 2:6–5:11 range from .77 to .85 ( Elliot, 2007 ). The task assesses short-term recognition memory, which is highly dependent on focused (selective) attention and visual attention span. School-age children with ADHD have been shown to have poorer performance on the Recognition of Pictures test, compared to controls ( Weisen, 2008 ); however, there is little literature supporting its validity among preschoolers.
Digit Span Measures
There are two similar digit span measures that have been published and normed for preschool aged children. Both assess auditory verbal attention span (i.e., the “encode” component of attention described by Mirsky and colleagues). The first is Number Recall (Kaufman Assessment Battery for Children—KABC-II; Kaufman & Kaufman, 2004 ). The KABC-II was standardized on a sample of 3,025 children, stratified by six-month age groups in ages 3 and 4, and one-year age groups for ages 5 through 18. The Number Recall task requires the child to repeat series of single syllable numbers of increasing length. The numbers in the series are presented orally at a rate of one per second. The series of numbers on the original KABC ranged from two to eight, but was expanded to include nine-digit sequences on the KABC-II. Reliability coefficients for the Number Recall task of the KABC-II for children ages four and five are .87 and .79, respectively ( Kaufman & Kaufman, 2004 ). There is significant improvement in performance among typically developing controls between ages 3–4 years; with slower rate of improvement between 4–5 years ( Lam et al., 2011 ). Mariani and Barkley (1997) reported that preschool boys with ADHD (ages 4–5 years) had significantly lower scores on the Number Recall subtest compared to controls. Among typically developing preschool children in fulltime daycare, the amount of daytime napping was significantly associated with performance on Number Recall ( Lam et al., 2011 ).
The second digit span test for preschoolers is the Recall of Digits Forward (Differential Ability Scales - Second Edition- DAS-II; Elliott, 2007). The DAS-II was standardized on a sample of 3,480 children, ages 2:6 through 17:11, stratified by six-month age groups for ages 2, 3, and 4, and one-year age groups for ages 5 through 17. A total of 880 children between the ages of 2:6 and 4:11 were included. Similar to the KABC-II Number Recall task, the Recall of Digits Forward task requires the child to repeat a series of digits of increasing length. The numbers in the series are presented orally at a rate of two per second in order to prevent rehearsal. The series length begins with 2 digits and gradually increases to 10 digits. There are five trials of each series length. Reliability coefficients for the Recall of Digits Forward task for children ages 2:6–5:11 range from .88 to .91. ( Elliot, 2007 ). Differences have been observed on this task, such that children who were born premature at an extremely low birth weight perform more poorly on this task at age 3 than children who were born at term ( Baron, Kerns, Muller, Ahronovich, & Litman, 2011 ).
Other Verbal Span Tasks
Adaptations to verbal span tasks that have been used in school-age children ( Brocki & Bohlin, 2004 ; Brocki, Randall, Bohlin, & Kerns, 2008 ; Thorell, 2007 ) have been used for preschool children. In these adaptations, the child is typically presented with two words or digits and asked to repeat verbatim for two trials. The span is then increased by a single digit/word every other trial until the child responds incorrectly to at least one of two trials at that span length ( Wahlstedt et al., 2008 ), or failure on both items ( Muller et al., 2012 ). Among preschool children, these tasks typically show moderate test-retest reliability, e.g., forward digit span (.70) and forward word span (.57) ( Muller et al., 2012 ). Muller and colleagues also identified significant practice effects (over a 21-day interval) for forward digit span but not the forward word span task. Wahlstedt and colleagues ( Wahlstedt, Thorell, & Bohlin, 2008 ) used forward and backward verbal span tasks consisting of familiar nouns (e.g., cat, rabbit, clown) in which the child was initially presented with two words, which sequence length increased by a single word every other trial until the child responded incorrectly to at least one of the two trials at that span length. Children with high rates of ADHD symptoms showed significantly lower performance on this verbal span test only if they also had poor performance on other executive function tests ( Muller et al., 2012 ).
Hand Movements (KABC-II) ( Kaufman & Kaufman, 2004 )
The Hand Movements subtest of the KABC and KABC-II requires that the child replicate an exact series of palm, fist, and side hand taps on the table. The task has not changed between the first and second version of the KABC. This task is short in duration (approximately 5 minutes or less), and normative data are available for children ages 4 and 5 years. Split-half reliability coefficients for the Hand Movements subtest of the KABC-II for children ages 4 and 5 years was .57 and .75, respectively, while test-retest reliability in this age group is 0.58. Barkley and colleagues also found significant group differences between preschool boys with and without ADHD on the Hand Movement subtest ( Mariani & Barkley, 1997 ).
Xylophone Task ( Muller et al., 2012 )
Another example of a visual span task is the Xylophone Task, in which an onscreen xylophone displays a sequence of lit bars, and the child is asked to replicate the series on the touch screen monitor. The trials start with sequences of two notes and progress to series of five notes, with the task discontinuing after errors on both trials at a given length. In the backward condition, the child is asked to repeat the sequence in reverse. Forward xylophone was shown to have poor test-retest reliability (.26); however, test-retest reliability of the backward condition was slightly better, although still low (.34).
Spatial Span
An adaptation of the Spatial Span task has also been developed for preschoolers ( Wahlstedt et al., 2008 ). In this version, a 4 × 4 matrix appears on a computer screen and is described as “windows” on a house. Pigs appear in the windows for 1000 msec with a 75 msec ISI. The child is asked to remember the sequence of windows in which the pigs appeared. Two trials are administered at each span length until the child responds incorrectly to at least one of the trials at that length. Preschool children with high levels of ADHD symptoms were shown to have poorer performance on this version of the Spatial Span task, compared to age-matched controls ( Wahlstedt et al., 2008 ).
“Simple” Reaction Time Tasks
Simple reaction time tasks require that the examinee respond as quickly as possible when a stimulus appears. In this most basic format, only one stimulus is included, and the child must always respond. In a sample of typically developing, Russian children (ages 4–6 years), response accuracy for all age groups was found to be high, with no significant effect of sex. Young children required greater response latency (compared to adults), and response times decrease steadily with age through childhood ( Kiselev, Espy, & Sheffield, 2009 ). This type of task may be useful as a control task in behavioral experiments with preschool children (contrasted with go/no-go or choice reaction time tasks), since median “basic” response time does not appear to differ between typically developing preschoolers (ages 3–4), preschoolers with ADHD, and preschoolers with ADHD and ODD ( Gopin, Berwid, Mark, Mlondnicka, & Halperin, 2012 ).
Rating Scales
Child behavior checklist for ages 1.5 to 5 (cbcl 1.5–5) ( achenbach & rescorla, 2000 ).
The CBCL for ages 1.5 to 5 assesses a wide range of internalizing and externalizing problems in young children, including a scale pertaining specifically to attention problems. There are 100 behavioral items that are rated on a scale from 0 (not true) to 2 (very/often true). There is also a version available for completion by a child’s teacher or caregiver (Caregiver-Teacher Report Form; C-TRF) that includes items that pertain to group situations and removes items most specifically related to the home or family environment. Scales included on both the CBCL and the C-TRF include Emotionally Reactive, Somatic Complaints, Aggressive Behavior, Anxious/Depressed, Withdrawn, and Attention Problems, while a Sleep Problems scale is included only on the CBCL. Internalizing, Externalizing, and Total Problems scales are also included. Test retest reliability is high for the majority of scales on both the CBCL and the C-TRF. Mean reliability was 0.85 and 0.81 respectively. Reliability for the Attention Problems scale is 0.78 for the CBCL and .84 for the C-TRF ( Achenbach & Rescorla, 2000 ).
The CBCL has been used in preschoolers for general behavioral screening ( Corkum et al., 1995 ; DeWolfe et al., 1999 ; Egger et al., 2006 ; Posner et al., 2007 ) and to determine correlations with other measures ( Kadesjo, Hagglof, Kadesjo, & Gillberg, 2003 ). When used to specifically evaluate the behavior of children with and without ADHD, parent ratings on the Attention Problems scale were significantly higher than parent ratings of matched controls for an untreated ADHD group; however, once the ADHD group was treated, parent reports on the CBCL Attention Problems scale for the ADHD group were not different than parent ratings of controls ( Byrne et al., 1998 ).
Behavior Assessment Scale for Children-2 (BASC-2) ( Reynolds & Kamphaus, 2004 )
The BASC-2 is a broad band caregiver rating scale for children that was designed to assess a wide range of child behavior problems and psychopathology, including attention problems, hyperactivity, and aggression. The BASC-2 is often used as a general screener of behavioral, emotional, and some adaptive function, as well as to specifically identify hyperactivity and inattention ( Gopin, Healey, Castelli, Marks, & Halperin, 2010 ; Gopin et al., 2012 ; Youngwirth et al., 2007 ) The Preschool version of the BASC-2 consists of a parent and teacher report. Behaviors such as hyperactivity, aggression, anxiety, depression, somatization, withdrawal, attention problems, ability to adapt, social and communication skills, and daily living skills are included on these scales.
Conners-Early Childhood Scales ( Conners, 2000 )
In the current revision of the Conners Rating Scales, a new measure, the Conners Early Childhood (Conners EC; Conners, 2009 ) was developed for use in children ages 2 to 6 years. As with the previous Conners’ scales, parent and teacher forms are available. The Conners EC behavioral scales include Inattention/Hyperactivity, Defiant/Aggressive Behaviors, Anxiety, Mood and Affect, and Physical Symptoms. Developmental Milestone scales include Adaptive Skills, Communication, Motor Skills, Play, and Pre-Academic/Cognitive. The Conners EC can be completed at its full length (behavioral and developmental scales), or these areas can be assessed separately. Validity scales are only included on the full length and Behavior forms. The Conners-EC as standardized on a group of 1600 cases randomly selected (with consideration of racial/ethnic distribution) from a total of 3281 assessments collected. Reliability for the Conners-EC Parent Behavior Scales was as follows: internal consistency 0.86, test-retest 0.87, and inter-rater 0.72. For the Conners EC-Teacher Behavior Scales, reliability was as follows: internal consistency 0.89 and test-retest 0.93. The Conners-EC Behavior subscales are highly correlated with similar subscales on the BASC-2 and the CBCL 1.5–5 ( Conners, 2000 ).
ADHD Rating Scale-IV—Preschool Version ( DuPaul, Power, Anastopoulos, & Reid, 1998 ; McGoey, DuPaul, Haley, & Shleton, 2007 )
The ADHD-RS-IV includes the 18 behavioral descriptors of ADHD as determined by the Diagnostic and Statistical Manual of Mental Disorders, Fourth Edition (DSM-IV;APA, 1994). Raters can consist of parents and teachers who respond to each question on a 4-point scale. Use of this questionnaire tends to consider ratings as 2 (pretty much) or 3 (very much) to reflect symptom presence ( DuPaul et al., 1998 ; Kadesjo et al., 2001; Marks et al., 2005 ).
McGoey and colleagues ( McGoey et al., 2007 ) adapted the instrument and established clinical utility in preschool children. Parent ratings were collected on 902 children ages 3: to 5:11 (53% boys, 47% girls) and teacher data were collected on 977 children (51% boys, 49% girls). Test-retest reliability and concurrent validity were determined by having a subsample of 42 parents and 41 teachers complete the ADHD-RS-IV Preschool Version and the Conners’ Teacher or Parent Rating Scale-Revised four weeks after initial completion. Symptom statements were modified to be more appropriate to the developmental level of preschoolers. Statements are rated on a Likert scale of 0 (not at all) to 3 (very often) to reflect the child’s behavior. Scores can be obtained for Inattention, Hyperactive/Impulsive, and Total Scales. Internal consistency for the three scales ranged from 0.92 to 0.95 on the School Version and from 0.85 to 0.92 on the Home Version. Test-retest reliability correlations for the three scales ranged from 0.93 to 0.96 for the School Version and between 0.80 and 0.87 for the Home Version. Correlations between the ADHD-RS-IV Preschool Version-teacher report and the CTRS-R Oppositional, Cognitive Problems/Inattention, Hyperactivity, and ADHD Index scales were all significant. Correlations between the same scales on the CPRS-R and the parent report were also significant.
The ADHD-RS-IV rating scale has been used as a screener for entry for preschool ADHD studies ( Gopin et al., 2012 ; Nomura et al., 2012 ), to classify preschoolers as “high risk” or “low risk” of ADHD based on parent and teacher ratings (Berwind et al., 2005), and to assess parent perception of ADHD-related symptoms in the context of other problem behaviors ( Kadesjo et al., 2003 ). Other researchers have adapted an 18-item list of symptoms to be more appropriate for the preschool population (e.g., loss of interest during story time or rolling on the floor during circle time) so that care providers can rate the behaviors on a Likert scale (e.g., PATS Diagnostic Interview). Internal consistency of this rating scale is reported at 0.84 ( Posner et al., 2007 ).
Behavior Rating Inventory for Children (BRIC) ( Gopin et al., 2010 )
The BRIC was designed to be a brief clinician rating scale that assesses core ADHD symptoms and associated behaviors in preschool children (ages 3–4 years). The BRIC is a 5-item scale that takes only 1–2 minutes to complete. Items are rated on a 5-point Likert Scale that provides behavioral examples at points 1, 3, and 5 in order to assist with clinician reliability. There are two scales: the ADHD Symptom Triad and the Mood/Sociability Factor. The ADHD Symptom Triad is comprised of three items, each one assessing one of the three core ADHD symptoms-inattention, hyperactivity, and impulsivity. The two items on the Mood/Sociability Factor assess social and mood impairment associated with ADHD. Lower scores reflect more positive behaviors. When used with preschool children, the ADHD Symptom Triad demonstrated adequate test-retest reliability when rated by the same examiner on both occasions (0.78), although reliability declined when rated by different clinicians (0.59). The Mood/Sociability Factor demonstrated only moderate results when rated by the same clinician (0.55) and when rated by different clinicians (0.50). Scores on the ADHD Symptom Triad reflected significant differences between hyperactive/inattentive children and a control group; however, differences on the Mood/Sociability Factor were not observed. There is a moderate association between the ADHD Symptom Triad and the BASC-2 PRS-P and the BASC-2 TRS-P inattention and hyperactivity scales. BRIC clinician ratings were correlated as well with parent and teacher reports (i.e., ADHD-RS-IV) as the parent and teacher reports were with one another. Significant correlations were also observed between the ADHD Symptom Triad and actigraph scores. Overall, the BRIC demonstrated high specificity, but limited sensitivity ( Gopin et al., 2010 ).
Early Child Inventory-4 (ECI-4) ( Sprafkin & Gadow, 1996 )
The ECI-4 is a DSM-IV-based rating scale designed for use with children ages 3–6 years to assess behaviors and emotions. Completion time is approximately 10–15 minutes. The parent version contains 108 items, 77 of which are also included on the teacher version. Behaviors unlikely to occur in the school setting are not included on the teacher version. On both versions, items are rated on a 4-point scale, “never” through “very often.” Scoring is completed in two ways, count (categorical) and severity (dimensional). Symptom count is a categorical score that is obtained from the number of symptoms of particular disorder that are rated as “often” or “very often.” This is compared to the symptom criterion score, which is the minimum symptom count necessary to meet DSM-IV diagnostic criteria. If the symptom count is equal or greater, the screening cutoff score is received for that disorder. Symptom severity is a dimensional score obtained by adding the values (0–4) of each response related to a particular disorder. ADHD Inattentive subtype, ADHD Hyperactive-Impulsive subtype, and ADHD Combined subtype are included on this measure. Other diagnoses include ODD, Conduct Disorder, PDD, Dysthymic Disorder, Major Depressive Disorder, Generalized Anxiety Disorder, and Social Phobia, as well as questions regarding tics, sleeping problems, feeding problems, posttraumatic stress disorder, selective mutism, and other problematic behaviors. The parent form also includes items symptomatic of Separation Anxiety Disorder.
The ECI-4 also includes a Developmental Deficits Index that includes 12 items about language motor, self-help, play behavior, and responsiveness to adults. Items are summed to a total. The parent version contains 12 items rated on a 4-point scale, while the teacher version contains 8 items on a 5 point scale. Internal consistency was high in regard to ADHD (ADHD-I = 0.91, ADHD-HI = 0.90). Sensitivity for ADHD was similar between parent (.66) and teacher (.68) forms, but increased when these forms were considered together (.90). Specificity also improved when the measures were combined (0.41). The ECI-4 demonstrates internal consistency, criterion validity, and convergent/divergent validity with the CBCL and TRF ( Sprafkin, Volpe, Gadow, Nolan, & Kelly, 2002 Nolan,& Kelly, 2002). The ECI-4 has also been used to establish the discriminant validity of other measures, such as the K-SADS ( Birmaher et al., 2009 ).
The ECI-4 has been used to identify prevalence rates of psychiatric disorders in a community sample of preschoolers ( Gadow, Sprafkin, & Nolan, 2001 ). ADHD was one of the most prevalent disorders, and all three subtypes of ADHD were rated as experiencing higher levels of developmental deficits, according to parents. Teacher ratings suggest that only Combined-Type ADHD experiences a significantly lower level of developmental progress. In another study of prevalence rates using the ECI-4, Nolan et al. ( Nolan, Gadow, & Sprafkin, 2001 ) reported that although Combined type ADHD saw a significant difference in prevalence between genders (boys > girls), this was not the case for ADHD-I or ADHD-HI within the preschool population, although boys tend to display more severe symptoms in all categories. Preschoolers with Combined type also displayed more severe social problems than children with just Inattentive or Hyperactive-Impulsive alone. In general, preschoolers with ADHD tended to display higher severity symptoms than older children ( Nolan et al., 2001 ).
A strength of the ECI-4 is that it has been used internationally. In a prevalence study of children in Spain that compared parent and teacher ratings, ADHD Inattentive subtype was determined to be the highest prevalence disorder as rated by teachers; however, the ADHD Hyperactive/Impulsive (HI) subtype was the most prevalent according to parents. Significant differences between urban and rural teacher reports did not occur for ADHD; however, parent ratings reflected significantly more ADHD-HI in urban settings than in rural ( Jane et al., 2006 ). In a small pilot study of 10 Mexican children with a mean age of 51 months, parent and teacher Symptom Severity scores were significantly correlated; however, in a small subsection of that group who had been diagnosed as having ADHD, no correlation was found between the ECI-4 and the Conners’ Rating Scale-Revised. When compared with diagnosis based on psychiatric interview, the ECI-4 failed to demonstrate strong sensitivity and specificity ( Poblano & Romero, 2006 ).
A summary of the reviewed preschool rating scales is listed in Table 3 .
Rating Scales for Attention in Preschoolers
Note: CBCL = Child Behavior Checklist; BASC = Behavior Assessment System for Children; BRIC = Behavior Rating Inventory for Children; ECI-4 = Early Child Inventory-4;
Structured Interviews
Kiddie-schedule for affective disorders and schizophrenia-present and lifetime version (k-sads-pl) ( kaufman et al., 1997 ).
The KSADS-PL is a semi-structured clinical interview developed for children ages 6 to 18 years that can be used to determine the presence of psychiatric disorders in children. Administration to parents of typically developing children takes approximately 45 minutes; however, increased psychopathology can result in a more extended interview, averaging approximately 75 minutes. ADHD was not a part of previous versions of the K-SADS, but was added when the K-SADS-PL was developed. Inter-rater reliability was above 98%, test-retest reliability for ADHD was 0.59 to .63, and concurrent validity was established with the Conners’ Parent Rating Scale in children screened positive for ADHD.
The K-SADS was not initially designed for use with very young children; however, Birmaher and colleagues suggested that the measure can be useful in the preschool population (ages 2 to 5; Birmaher et al., 2009 ). In establishing psychometric properties, comparisons were made between the K-SADS-PL and the Child Behavior Checklist (CBCL), Early Childhood Inventory-4 (ECI-4) and Preschool Age Psychiatric Assessment (PAPA). Convergent validity and discriminant validity were established to be strong for diagnosis of ADHD. Inter-rater reliability reached significance for all diagnoses (ranging from .80 to .90). Few diagnostic differences were observed when compared to the results of a subsequently administered PAPA interview. Thus, while not specifically designed for preschoolers, the K-SADS has been used to assist in establishing appropriate study inclusion and participant groupings, in conjunction with other measures ( Gopin et al., 2012 ; Nomura et al., 2012 ).
Preschool Age Psychiatric Assessment (PAPA) ( Egger & Angold, 2004 )
The PAPA is a semi-structured interview that is completed by trained interviewers with the parents of children ages 2 to 5 years. The questions focus on the three months immediately preceding interview. There are 25 modules that generate symptom, scaled scores, and diagnoses. Clinicians can choose to administer only some of the modules. The PAPA includes developmentally appropriate questions about behaviors that are not a part of diagnostic criteria, such as temper tantrums and toilet training. The measure has been determined to be reliable and valid for preschool age children ( Egger et al., 2006 ). The reported test-retest reliability for the ADHD module is 0.74. The PAPA has been used to determine diagnosis and/or group placement of participants. When the PAPA is used as the diagnostic instrument, significant stability of ADHD diagnosis has been observed between the preschool and school age years ( Tandon, Xuemei, & Luby, 2011 ).
Diagnostic Interview Schedule, Young Children (DISC-YC) ( Lucas, Fisher, & Luby, 1998 )
The current NIMH Diagnostic Interview Schedule for Children-IV (NIMH-DISC-IV) is designed to be a highly structured diagnostic instrument that can be used by non-clinicians to assess children ages 6 to 17 years ( Shaffer, Fisher, Lucas, Dulcan, & Schwab-Stone, 2000 ). There are two versions, the parent (DISC-P) and the youth (DISC-Y, ages 9–17), both of which ask the same questions but differ in the pronouns and structure of the question dependent on respondent. The NIMH DISC-IV–P has been determined to be a reliable and valid measure of psychiatric disorders ( McGrath, 2004 ; Shaffer et al., 2000 ). The DISC-YC ( Lucas et al., 1998 ) is an adaptation of the DISC-P. It is a structured parent interview that asks a series of questions about the child’s behavior in yes/no format. Additional questions are posed (or not) within each module depending on initial parent responses. Follow-up questions about age of onset, level of impairment, parental concern, and treatment are asked in cases when parents have responded to the presence of clinically significant levels of symptomatic behaviors. Because the DISC-YC is a computerized, fully structured interview, it can be conducted by trained individuals who have limited clinical experience, as it does not require the same level of clinical judgment of some other parent interviews (e.g., K-SADS, PAPA).
Lavigne and colleagues ( Lavigne, Lebailly, Hopkins, Gouze, & Binns, 2009 ) compared the prevalence rates of ADHD in 4-year-olds, as determined by the DISC-YC and the Child Symptom Inventory (CSI), and found the DISC-YC resulted in a similar prevalence of ADHD-Combined Subtype, but not of Inattentive or Hyperactive-Impulsive subtypes. Overall presence of any type of ADHD was similar between the two measures. When considering only severe impairment levels (i.e., those that met minimum diagnostic criteria, with at least one symptom meeting severe impairment criteria), the rates of ADHD obtained using the DISC-YC were similar to that of the K-SADS, but higher than those obtained when using the PAPA.
A summary of the reviewed structured psychiatric interviews is listed in Table 4 .
Structured Diagnostic Interviews for Preschoolers
Note: KSADS-PL = Kiddie Schedule for Affective Disorders and Schizophrenia, Present and Lifetime; PAPA = Preschool Age Psychiatric Assessment; DISC-YC = Diagnostic Interview Schedule for Children-Young Children
Summary and Conclusions
Despite the increased interest in assessment of preschool age children, the development of formal measures of attention that are appropriate and valid for use in this age range has been slow. The majority of published assessment methods remain experimental and not commercially available, making access to appropriate tests more difficult for the practicing clinician. Review of the published literature highlights three primary methods for assessing attention in preschool age children: performance-based tests, structured parent interviews, and rating scales. Performance-based tests include a variety of continuous performance tests, aimed at assessing sustained attention , inhibitory control , and consistency of response. Among the CPTs, only the Conners Kiddie CPT (K-CPT) is commercially available in this age range; however, there are remarkably few published validity studies using the K-CPT. Among published CPT tests for preschoolers, those employing a fixed ISI of greater than 2000 msec, and those whose overall length is 5–7 minutes or less, have shown greatest utility in preschool age children. Conversely, preschool children have much more difficulty completing tests with ISI of 1500 msec or less, those whose length is 9 minutes or more, and those in which the pairing of auditory and visual stimuli are not congruent. Additional performance based tests are available to assess other components of attention, including visual selective attention (e.g., cancellation tests), motor persistence (i.e., NEPSY-II Statue), and encoding, working memory, and auditory/visual attention span (e.g., digit and spatial span tests). Compared to the preschool CPTs, these measures have more published validity studies, and also have the advantage of ease of administration, time efficiency, and (in many tests) nationally-representative standardization samples.
Rating scales for preschool children remain a viable and highly practical assessment method. There are a variety of commercially published instruments (e.g., Conners Early Childhood; BASC-2; CBCL) and methods that can be used by parents, teachers, and clinicians. The published rating scales more consistently have nationally-representative standardization samples, and most have scales specific to attention problems in preschool age children. The benefit of the rating scales is that the severity of inattention symptoms can be directly compared to “typical” attention problems observed in this age range, thus helping the clinician distinguish disordered attention in this age range, and to differentiate it from other cognitive and behavioral problems. The rating scale methods also provide data that complement the information obtained via performance-based methods.
Among the three structured psychiatric interviews reviewed, none are commercially available, making them largely impractical for clinical use outside of research settings. Further, all three (K-SADS, PAPA, DISC-YC) require considerable clinician training, which in some cases (e.g., PAPA) is available only through the author. Nevertheless, the PAPA and the DISC-YC, which were developed specifically for preschool children, show considerable promise in characterizing problematic behaviors in preschool age children, and linking them specifically to DSM-IV diagnostic categories.
In conclusion, while there are now more methods available for assessing attention in preschool age children, the research characterizing the validity and utility of these measures remains limited. Future studies are encouraged to broaden the range of skills assessed, and to clarify which methods work best with which children.
Additional Performance-based Tests of Attention for Preschoolers
Note: PDTP = Picture Deletion Test for Preschoolers; Visual Attention = NEPSY Visual Attention subtest; Statue = NEPSY-2 Statue subtest; Number Recall = Kaufman Assessment Battery for Children-2 (KABC-2) Number Recall; Hand Movements = KABC-2 Hand Movements; Recognition of Pictures = Differential Ability Scales-Second Edition (DAS-II); Recall of Digits Forward = DAS-II;
Acknowledgements
This work was supported by the National Institutes of Health Grants R01 HD068425, P30 HD 24061 (Intellectual and Developmental Disabilities Research Center), the Johns Hopkins Brain Sciences Institute, and the Johns Hopkins University School of Medicine Institute for Clinical and Translational Research, an NIH/NCRR CTSA Program, UL1-RR025005.
- Abikoff HB, Vitiello B, Riddle MA, Cunningham C, Greenhill LL, Swanson JM, Wigal T. Methylphenidate effects on functional outcomes in the Preschoolers with Attention-Deficit/Hyperactivity Disorder Treatment Study (PATS) Journal of Child and Adolescent Psychopharmacology. 2007; 17 :581–592. [ PubMed ] [ Google Scholar ]
- Achenbach TM, Rescorla LA. Manual for the ASEBA preschool forms and profiles. Burlington, VT: University of Vermont, Research Center for Children, Youth, & Families; 2000. [ Google Scholar ]
- Akinbami LJ, Liu X, Pastor PN, Reuben CA. NCHS Data Brief. Vol. 70. Hyattsville, MD: National Center for Health Statistics; 2011. Attention deficit hyperactivity disorder among children aged 5–17 years in the United States, 1998–2009. [ PubMed ] [ Google Scholar ]
- American Academy of Pediatrics. Clinical practice guideline: Diagnosis and evaluation of the child with attention-deficit/hyperactivity disorder. Pediatrics. 2000; 105 :1158–1170. [ PubMed ] [ Google Scholar ]
- American Academy of Pediatrics. Policy statement: Media use by children younger than 2 years. Pediatrics. 2011; 128 [ Google Scholar ]
- American Psychiatric Association. Diagnostic and statistical manual of mental disorders, Fourth Edition. Text Revision. Washington, DC: Author; 2000. [ Google Scholar ]
- American Psychiatric Association. Attention Deficit/Hyperactivity Disorder. Washington, DC: Author; 2012. May 1, DSM-5 Development Website ( www.dsm5.org/ProposedRevisions/Pages/proposedrevision.aspx?rid=383 ). [ Google Scholar ]
- Armstrong MB, Nettleton SK. Attention deficit hyperactivity disorder and preschool children. Seminars in Speech Language. 2004; 25 :225–232. [ PubMed ] [ Google Scholar ]
- Arons BS, Katz-Leavy J, Wittig AC, Wayne Holden E. Too young for ADHD: The potential role of systems of care. Journal of Developmental and Behavioral Pediatrics. 2002; 23 (1 Suppl):S57–S63. [ PubMed ] [ Google Scholar ]
- Barkley RA. Executive functioning and self-regulation: integration, extended phenotype, and clinical implications. New York: Guilford Press; 2011a. [ Google Scholar ]
- Barkley RA. The Barkley deficits in executive functioning scale: rating scales, norms, and interpretive guide. New York: Guilford Press; 2011b. [ Google Scholar ]
- Baron IS, Kerns KA, Muller U, Ahronovich MD, Litman F. Executive functions in extremely low birth weight and late preterm preschoolers: Effects on working memory and response inhibition. Neuropsychology. 2011 [ PubMed ] [ Google Scholar ]
- Bayley N. Bayley scales of infant and toddler development-third edition. San Antonio, TX: Harcourt Assessment; 2006. [ Google Scholar ]
- Berwid O, Curko Kera EA, Marks DJ, Santra A, Bender HA, Halperin JM. Sustained attention and response inhibition in young children at risk for attention deficit/hyperactivity disorder. Journal of Child Psychology and Psychiatry. 2005; 46 :1219–1229. [ PubMed ] [ Google Scholar ]
- Biederman J, Faraone SV. Current concepts on the neurobiology of attention-deficit/hyperactivity disorder. Journal of Attention Disorders. 2002; 6 (Suppl. 1):S7–S16. [ PubMed ] [ Google Scholar ]
- Birmaher B, Ehman M, Axelson DA, Goldstein BI, Monk K, Kalas C, Brent DA. Schedule for affective disorders and schizophrenia for school-age children (K-SADS-PL) for the assessment for preschool children -A preliminary psychometric study. Journal of Psychiatric Research. 2009; 43 :680–686. [ PMC free article ] [ PubMed ] [ Google Scholar ]
- Black IB, DiCicco-Bloom E, Dreyfus CF. Nerve growth factor and the issue of mitosis in the nervous system. Current Topics in Developmental Biology. 1990; 24 :161–192. [ PubMed ] [ Google Scholar ]
- Blair C, Raver CC. Child development in the context of adversity: Experiential canalization of brain and behavior. American Psychologist. 2012; 67 :309–318. [ PMC free article ] [ PubMed ] [ Google Scholar ]
- Bor W, Sanders MR, Markie-Dadds C. The effects of the Triple P Positive Parenting Program on preschool children with co-occurring disruptive behavior and attentional/hyperactive difficulties. Journal of Abnormal Child Psychology. 2002; 30 :571–587. [ PubMed ] [ Google Scholar ]
- Broadbent DE. Perception and communication. New York: Pergammon; 1958. [ Google Scholar ]
- Brocki KC, Bohlin G. Executive functions in chidlren aged 6 to 13: A dimensional and developmental study. Deveopmental Neuropsychology. 2004; 26 :571–593. [ PubMed ] [ Google Scholar ]
- Brocki KC, Randall KD, Bohlin G, Kerns KA. Working memory in school-aged children with attention-deficit/hyperactivity disorder combined type: Are deficits modality specific and are they independent of impaired inhibitory control? Journal of Clinical and Experimental Neuropsychology. 2008; 30 :749–759. [ PubMed ] [ Google Scholar ]
- Bussing R, Mason DM, Bell L, Porter P, Garvan C. Adolescent outcomes of childhood attention-deficit/hyperactivity disorder in a diverse community sample. Journal of the American Academy of Child & Adolescent Psychiatry. 2010; 49 :595–605. [ PMC free article ] [ PubMed ] [ Google Scholar ]
- Byrne JM, DeWolfe NA, Bawden HN. Assessment of attention-deficit hyperactivity disorder in preschoolers. Child Neuropsychology. 1998; 4 :49–66. [ PubMed ] [ Google Scholar ]
- Byrne JM, Bawden HN, Beattie TL, DeWolfe NA. Preschoolers classified as having attention-deficit hyperactivity disorder (ADHD): DSM-IV symptom endorsement pattern. Journal of Child Neurology. 2000; 15 :533–538. [ PubMed ] [ Google Scholar ]
- Chatterjee A, Coslett HB. Neglect: Cognitive Neuropsychological Aspects. In: Feinberg T, Farah MJ, editors. Behavioral Neurology and Neuropsychology. Oxford University Press; 2003. [ Google Scholar ]
- Christakis DA, Zimmerman FJ, DiGiuseppe DL, McCarty CA. Early television exposure and subsequent attentional problems in children. Pediatrics. 2004; 113 :708–713. [ PubMed ] [ Google Scholar ]
- Christophersen ER, Mortweet SL. Treatments that work with children: Empirically supported strategies for managing childhood problems. Washinton, DC: American Psychological Association; 2003. [ Google Scholar ]
- Chronis-Tuscano A, Molina BS, Pelham WE, Applegate B, Dahlke A, Overmyer M, Lahey BB. Very early predictors of adolescent depression and suicide attempts in children with attention-deficit/hyperactivity disorder. Archives of General Psychiatry. 2010; 67 :1044–1051. [ PMC free article ] [ PubMed ] [ Google Scholar ]
- Columbo J. The development of visual attention in infancy. Annual Review of Psychology. 2001; 52 :337–367. [ PubMed ] [ Google Scholar ]
- Colombo J, Cheatham CL. The emergence and basis of endogenous attention in infancy and early childhood. In: Kail RV, editor. Advances in child development and behavior. Vol. 34. Amsterdam: Elsevier; 2006. pp. 283–322. [ PubMed ] [ Google Scholar ]
- Conners CK. Conners' Kiddie Continuous Performance Test (K-CPT) North Tonawanda, NY: Multi-Health Systems, Inc.; 2001. [ Google Scholar ]
- Conners CK. Conners Early Childhood. North Tonawanda. New York: Multi-Health Systems, Inc.; 2009. [ Google Scholar ]
- Conners CK MHS Staff. Conners' Continuous Performance Test-II (CPT-II) North Tonawanda, NY: Multi-Health Systems, Inc.; 2000. [ Google Scholar ]
- Connor DF. Preschool attention deficit hyperactivity disorder: a review of prevalence, diagnosis, neurobiology, and stimulant treatment. Journal of Developmental & Behavorial Pediatrics. 2002; 23 :S1–S9. [ PubMed ] [ Google Scholar ]
- Conway A, Stifter CA. Longitudinal antecedents of executive function in preschoolers. Child Development. 2012; 83 :1022–1036. [ PMC free article ] [ PubMed ] [ Google Scholar ]
- Cooley EL, Morris RD. Attention in children: A neuropsychologically based model for assessment. Developmental Neuropsychology. 1990; 6 :239–274. [ Google Scholar ]
- Corkum V, Byrne JM, Ellsworth C. Clinical assessment of sustained attention in preschoolers. Child Neuropsychology. 1995; 1 :3–18. [ Google Scholar ]
- DeBar LL, Lynch F, Powell J, Gale J. Use of psychotropic agents in preschool children: associated symptoms, diagnoses, and health care services in a health maintenance organization. Archives of Pediatrics and Adolescent Medicine. 2003; 157 :150–157. [ PubMed ] [ Google Scholar ]
- Dennis M, Sinopoli KJ, Fletcher JM, Schachar R. Puppets, robots, critics, and actors within a taxonomy of attention in developmental disorders. Journal of the International Neuropsychological Society. 2008; 14 :673–690. [ PMC free article ] [ PubMed ] [ Google Scholar ]
- DeWolfe NA, Byrne JM, Bawden HN. Early clinical assessment of attention. The Clinical Neuropsychologist. 1999; 13 :458–473. [ PubMed ] [ Google Scholar ]
- Diamond A. Normal development of prefrontal cortex from birth to young adulthood: Cognitive functions, anatomy, and biochemistry. In: Stuss DT, Knight RT, editors. Principles of frontal lobe function. New York: Oxford University Press; 2002. pp. 466–503. [ Google Scholar ]
- DuPaul GJ, Power TJ, Anastopoulos AD, Reid R. ADHD Rating Scale-IV. New York: Guilford Press; 1998. [ Google Scholar ]
- Egger H, Angold A. The preschool age psychiatric assessment (PAPA): A structured parent interview for diagnosing psychiatric disorders in preschool children. In: DelCarmen-Wiggins R, Carter A, editors. Handbook of Infant, Toddler, and Preschool Mental Health Assessment. New York: Oxford University Press; 2004. [ Google Scholar ]
- Egger HL, Erkanli A, Keeler G, Potts E, Walter BK, Angold A. Test-retest reliability of the preschool age psychiatric assessment (PAPS) Journal of the American Academy of Child & Adolescent Psychiatry. 2006; 45 :538–549. [ PubMed ] [ Google Scholar ]
- Egger HL, Kondo D, Angold A. The epidemiology and diagnostic issues in preschool attention-deficit/hyperactivity disorder: A review. Infants and Young Children. 2006; 19 :109–122. [ Google Scholar ]
- Elliot CD. Differential Ability Scales-Second Edition. San Antonio, Texas: Harcourt Assessment, Inc.; 2007. [ Google Scholar ]
- Espy KA. Using developmental, cognitive, and neuroscience approaches to understand executive control in young children. Developmental Neuropsychology. 2004; 26 :379–384. [ PubMed ] [ Google Scholar ]
- Espy KA, Bull R. Inhibitory processes in young children and individual variation in short term memory. Development Neuropsychology. 2005; 28 :669–688. [ PMC free article ] [ PubMed ] [ Google Scholar ]
- Finneran D, Francis A, Leonard L. Sustained attention in children with specific language impairment (SLI) Journal of Speech, Lanuage, and Hearing Research. 2009; 52 :915–929. [ PMC free article ] [ PubMed ] [ Google Scholar ]
- Fox NA, Schmidt LA, Henderson HA, Marshall P. Developmental psychophysiology: Conceptual and methodological issues. In: Cacioppo JT, Tassinary LG, Berntson GG, editors. Handbook of psychophysiology. 3rd ed. Cambridge, UK: Cambridge University Press; 2007. pp. 453–481. [ Google Scholar ]
- Froehlich TE, Lanphear BP, Epstein JN, Barbaresi WJ, Katusic SK, Kahn RS. Prevalence, recognition, and treatment of attention-deficit/hyperactivity disorder in a national sample of US children. Archives of Pediatric and Adolescent Medicine. 2007; 161 :857–864. [ PubMed ] [ Google Scholar ]
- Gadow KD, Sprafkin J, Nolan EE. DSM-IV Symptoms in community and clinic preschool children. Journal of the American Academy of Child and Adolescent Psychiatry. 2001; 40 :1383–1392. [ PubMed ] [ Google Scholar ]
- Galera C, Cote SM, Bouvard MP, Pingault J, Melchoir M, Michel G, Boivin M, Tremblay RE. Early risk factors for hyperactivity-impulsivity and inattention from 17 months to 18 years. Archives of General Psychiatry. 2011; 68 :1267–1275. [ PubMed ] [ Google Scholar ]
- Ghuman JK, Aman MG, Ghuman HS, Reichenbacher T, Gelenberg A, Wright R, Rice S, Fort C. Prospective, naturalistic, pilot study of open-label atomoxetine treatment in preschool children with attention-deficit/hyperactivity disorder. Journal of Child and Adolescent Psychopharmacology. 2009; 19 :155–166. [ PMC free article ] [ PubMed ] [ Google Scholar ]
- Ghuman JK, Ginsburg GS, Subramaniam G, Ghuman HS, Kau AS, Riddle MA. Psychostimulants in preschool children with attention-deficit/hyperactivity disorder: Clinical evidence from a developmental disorders institution. The Journal of the American Academy of Child and Adolescent Psychiatry. 2001; 40 :516–524. [ PubMed ] [ Google Scholar ]
- Giedd JN, Blumenthal J, Jeffries NO, Rajapakse JC, Vaituzis AC, Liu H, Castellanos FX. Development of the human corpus callosum during childhood and adolescence: a longitudinal MRI study. Progress in Neuropsychopharmacology & Biological Psychiatry. 1999; 23 :571–588. [ PubMed ] [ Google Scholar ]
- Gimpel GA, Kuhn BR. Maternal report of attention deficit hyperactivity disorder symptoms in preschool children. Child Care Health Development. 2000; 26 :163–176. discussion 176-169. [ PubMed ] [ Google Scholar ]
- Gogtay N, Giedd JN, Lusk L, Hayashi KM, Greenstein D, Vaituzis AC, Thompson PM. Dynamic mapping of human cortical development during childhood through early adulthood. Proceedings of the National Academy of Sciences of the United States of America. 2004; 101 :8174–8179. [ PMC free article ] [ PubMed ] [ Google Scholar ]
- Goldberg E, Costa LD. Hemispheric differences in the acquisition and use of descriptive systems. Brain and Language. 1981; 14 :144–173. [ PubMed ] [ Google Scholar ]
- Gopin C, Healey D, Castelli K, Marks D, Halperin JM. Usefulness of a clinician rating scale in identifying preschool children with ADHD. Journal of Attention Disorders. 2010; 13 :479–488. [ PubMed ] [ Google Scholar ]
- Gopin CB, Berwid O, Mark DJ, Mlodnicka A, Halperin JM. ADHD Preschoolers With and Without ODD: Do They Act Differently Depending On Degree of Task Engagement/Reward? Journal of Attention Disorders. 2012 (published online Feb 8 prior to publication). [ PubMed ] [ Google Scholar ]
- Gordon M, McClure FD, Post EM. Interpretive Guide to the Gordon Diagnostic System. Syracuse, NY: Gordon Systems; 1986. [ Google Scholar ]
- Gordon M, Mettelman BB. Technical Guide to the Gordon Diagnostic System. Syracuse, NY: Gordon Systems, Inc.; 1987. [ Google Scholar ]
- Gottlieb G. Experiential canalization of behavioral development: Theory. Developmental Psychology. 1991; 27 :4–13. [ Google Scholar ]
- Greenhill LL, Muniz R, Ball RR, Levine A, Pestreich L, Jiang H. Efficacy and safety if dexmethylphenidate extended-release capsules in children with attention-deficit/hyperactivity disorder. Journal of the American Academy of Child and Adolescent Psychiatry. 2006; 45 :817–823. [ PubMed ] [ Google Scholar ]
- Greenhill LL, Posner K, Vaughan BS, Kratochvil CJ. Attention deficit hyperactivity disorder in preschool children. Child and Adolescent Psychiatry Clinics of North America. 2008; 17 :347–366. ix. [ PubMed ] [ Google Scholar ]
- Gurevitz M, Geva R, Varon M, Leitner Y. Early markers in infants and toddlers for development of ADHD. Journal of Attention Disorders. 2012 [ PubMed ] [ Google Scholar ]
- Hagelthorn KM, Hiemenz JR, Pillion JP, Mahone EM. Age and performance parameters in continuous performance tests for preschoolers. Perceptual & Motor Skills. 2003; 96 (3 Pt 1):975–989. [ PubMed ] [ Google Scholar ]
- Halperin JM, Bedard AV, Curchack-Lichtin JT. Preventive interventions for ADHD: A neurodevelopmental perspective. Neurotherapeutics. 2012 [ PMC free article ] [ PubMed ] [ Google Scholar ]
- Halperin JM, Marks DJ, Bedard AV, Chacko A, Curchack JT, Yoon CA, Healy DM. Training executive, attention, and motor skills: A proof-of-concept study in preschool children with ADHD. Journal of Attention Disorders. 2012 [ PubMed ] [ Google Scholar ]
- Halperin JM, Schulz KP. Revisiting the role of the prefrontal cortex in the pathophysiology of attention-deficit/hyperactivity disorder. Psychological Bulletin. 2006; 132 :560–581. [ PubMed ] [ Google Scholar ]
- Harper GW, Ottinger DR. The performance of hyperactive and control preschoolers on a new computerized measure of visual vigilance: The preschool Vigilance Task. Journal of Child Psychology & Psychiatry. 1992; 33 :1365–1372. [ PubMed ] [ Google Scholar ]
- Healey D, Miller C, Castelli K, Marks D, Halperin J. The impact of impairment criteria on rates of ADHD diagnoses in preschoolers. Journal of Abnormal Child Psychology. 2008; 36 :771–778. [ PubMed ] [ Google Scholar ]
- Hood BM, Atkinson J. Disengaging visual attention in the infant and adult. Infant Behavior and Development. 1993; 16 :405–422. [ Google Scholar ]
- Huttenlocher PR, Dabholkar AS. Regional differences in synaptogenesis in human cerebral cortex. Journal of Comparative Neurology. 1997; 387 :167–178. [ PubMed ] [ Google Scholar ]
- Jané MC, Canals J, Ballesp S, Viñas F, Esparó G, Domènech E. Parents and teachers reports of DSM-IV psychopathological symptoms in preschool children: differences between urban-rural Spanish areas. Social Psychiatry Psychiatric Epidemiol. 2006; 41 :386–393. [ PubMed ] [ Google Scholar ]
- Jones K, Daley D, Hutchings J, Bywater T, Eames C. Efficacy of the Incredible Years Programme as an early intervention for children with conduct problems and ADHD: Long-term follow-up. Child Care Health and Development. 2008; 34 :380–390. [ PubMed ] [ Google Scholar ]
- Kadesjo C, Hagglof B, Kadesjo B, Gillberg C. Attention-deficit-hyperactivity disorder with and without oppositional defiant disorder in 3-to 7-year-old children. Developmental Medicine & Child Neurology. 2003; 45 :693–699. [ PubMed ] [ Google Scholar ]
- Katsuki F, Constantinidis C. Early involvement of prefrontal cortex in visual bottom-up attention. Nature Neuroscience. 2012; 15 :1160–1166. [ PMC free article ] [ PubMed ] [ Google Scholar ]
- Kaufman J, Birmaher B, Brent D, Rao U, Flynn C, Moreci P, Ryan N. Schedule for Affective Disorders and Schizophrenia for School-Age Children-Present and Lifetime Version (K-SADS-PL): Initial Reliability and Validity Data. Journal of the American Academy of Child & Adolescent Psychiatry. 1997; 36 :980–988. [ PubMed ] [ Google Scholar ]
- Kaufman AS, Kaufman NL. Kaufman assessment battery for children-II. Circle Pines, MN: American Guidance Service; 2004. [ Google Scholar ]
- Kerns K, Rondeau L. Development of a continuous performance test for preschool children. Journal of Attention Disorders. 1998; 2 :229–238. [ Google Scholar ]
- Kinney HC, Brody BA, Kloman AS, Gilles FH. Sequence of central nervous system myelination in human infancy II: Patterns of myelination in autopsied infants. Journal of Neuropathology and Experimental Neurology. 1988; 47 :217–234. [ PubMed ] [ Google Scholar ]
- Kiselev S, Espy KA, Sheffield T. Age-related differences in reaction time task performance in young children. Journal of Experimental Child Psychology. 2009; 102 :150–166. [ PubMed ] [ Google Scholar ]
- Knudsen EI. Fundamental components of attention. Annual Review of Neuroscience. 2007; 30 :57–78. [ PubMed ] [ Google Scholar ]
- Korkman M, Kirk U, Kemp S. NEPSY Clinical and Intrepretative Manual. San Antonio, TX: Harcourt Assessment, Inc.; 1998. [ Google Scholar ]
- Korkman M, Kirk U, Kemp S. NEPSY: Second Edition. San Antonio, TX: Pearson; 2007. [ Google Scholar ]
- Kotrla KJ, Weinberger DR. Developmental neurobiology. In: Sadock BJ, Sadock VA, editors. Comprehensive Textbook of Psychiatry 7th ed. Philadelphia, PA: Lippincott Williams & Wilkins; 2000. pp. 32–40. [ Google Scholar ]
- Kratochvil CJ, Vaughan BS, Stoner JA, Daughton JM, Lubberstedt BD, March JS. A double-blind, placeob-controlled study of atomoxetine in young children with ADHD. Pediatrics. 2011; 127 :e862–e868. [ PMC free article ] [ PubMed ] [ Google Scholar ]
- Kraybill JH, Bell MA. Infant predictors of preschool and post-kindergarten executive function. Developmental Psychobiology. 2012 [ PMC free article ] [ PubMed ] [ Google Scholar ]
- Lachter J, Forster KI, Ruthruff E. Forty-five years after Broadbent (1958): Still no identification without attention. Psychological Review. 2004; 111 :880–913. [ PubMed ] [ Google Scholar ]
- Lahey BB, Pelham WE, Chronis A, Massetti G, Kipp H, Ehrhardt A, Lee SS. Predictive validity of ICD-10 hyperkenetic disorder relative to DSM-IV attention-deficit/hyperactivity disorder among younger children. Journal of Child Psychology & Psychiatry. 2006; 47 :472–479. [ PubMed ] [ Google Scholar ]
- Lahey BB, Pelham WE, Loney J, Kipp H, Ehrhardt A, Lee SS, Massetti G. Three-year predictive validity of DSM-IV attention deficit hyperactivity disorder in children diagnosed at 4–6 years. American Journal of Psychiatry. 2004; 161 :2014–2020. [ PubMed ] [ Google Scholar ]
- Lam JC, Mahone EM, Mason EBA, Schraf SM. The effects of napping on cognitive function in preschoolers. Journal of Developmental & Behavorial Pediatrics. 2011; 32 :90–97. [ PMC free article ] [ PubMed ] [ Google Scholar ]
- Lane DM, Pearson DA. The development of selective attention. Merrill-Palmer Quarterly. 1982; 28 :317–337. [ Google Scholar ]
- Lavigne JV, LeBailly SA, Hopkins J, Gouze KR, Binns HJ. The prevalence of ADHD, ODD, depression, and anxiety in a community sample of 4-Year-olds. Journal of Clinical Child & Adolescent Psychology. 2009; 38 :315–328. [ PubMed ] [ Google Scholar ]
- Lillard AS, Peterson J. The immedicate impact of different types of television on young children's executive function. Pediatrics. 2011; 128 :644–649. [ PMC free article ] [ PubMed ] [ Google Scholar ]
- Lucas CP, Fisher P, Luby JL. Young Child DISC-IV Research Draft: Diagnostic Interview Schedule for Children. New York, NY: Columbia University, Division of Children Psychiatry, Joy and William Ruane Center to Identify and Treat Mood Disorders; 1998. [ Google Scholar ]
- Luna B, Thulborn KR, Munoz DP, Merriam EP, Carver KE, Minshew NJ, Sweeney JA. Maturation of widely distributed brain function subserves cognitive development. NeuroImage. 2001; 13 :786–793. [ PubMed ] [ Google Scholar ]
- Mahone EM. Measurement of attention and related functions in the preschool child. Mental Retardation Developmental Disabilities Research Review. 2005; 11 :216–225. [ PubMed ] [ Google Scholar ]
- Mahone EM, Pillion JP, Hiemenz JR. Initial development of an auditory continuous performance test for preschoolers. Journal of Attention Disorders. 2001; 5 :93–106. [ Google Scholar ]
- Mahone EM, Pillion JP, Hoffman J, Hiemenz JR, Denckla MB. Construct validity of the auditory continuous performance test for preschoolers. Developmental Neuropsychology. 2005; 27 :11–33. [ PubMed ] [ Google Scholar ]
- Mahone EM, Hoffman J. Behavior ratings of executive function among preschoolers with ADHD. Clinical Neuropsychology. 2007; 21 :569–586. [ PubMed ] [ Google Scholar ]
- Mahone EM, Slomine BS. Neurodevelopmental disorders. In: Morgan J, Ricker J, editors. Textbook of Clinical Neuropsychology. New York: Taylor and Francis; 2008. pp. 105–127. [ Google Scholar ]
- Mariani MA, Barkley RA. Neuropsychological and academic functioning in preschool boys with attention deficit hyperactivity disorder. Developmental Neuropsychology. 1997; 13 :111–129. [ Google Scholar ]
- Marks DJ, Berwid OG, Santra A, Kera EC, Cyrulnik SE, Halperin JM. Neuropsychological correlates of ADHD symptoms in preschoolers. Neuropsychology. 2005; 19 :446–455. [ PubMed ] [ Google Scholar ]
- Marsh R, Gerber AJ, Peterson BS. Neuroimaging Studies of Normal Brain Development and Their Relevance for Understanding Childhood Neuropsychiatric Disorders. Journal of the American Academy of Child and Adolescent Psychiatry. 2008; 47 :1233–1251. [ PMC free article ] [ PubMed ] [ Google Scholar ]
- Massetti GM, Lahey BB, Pelham WE, Loney J, Ehrhardt A, Lee SS, Kipp H. Academic achievement over 8 years among children who met modified criteria for Attention-deficit/Hyperactivity Disorder at 4–6 years of age. Journal of Abnormal Child Psychology. 2007; 36 :399–410. [ PMC free article ] [ PubMed ] [ Google Scholar ]
- McGee R, Partridge F, Williams S, Silva PA. A twelve-year follow-up of preschool hyperactive children. Journal of the American Academy of Child & Adolescent Psychiatry. 1991; 30 :224–232. [ PubMed ] [ Google Scholar ]
- McGoey KE, DuPaul GJ, Haley E, Shleton TL. Parent and teacher ratings of attention deficit/hyperactivity disorder in preschool: The ADHD Rating Scale-IV Preschool Version. Journal of Psychopathology Behavioral Assessment. 2007; 29 :269–276. [ Google Scholar ]
- McGrath AM, Handwerk ML, Armstrong KJ, Lucas CP, Friman PC. The validity of the ADHD section of the Diagnostic Interview for Children. Behavioral Modification. 2004; 28 :349–374. [ PubMed ] [ Google Scholar ]
- Mirsky AF, Anthony BJ, Duncan CC, Ahern MB, Kellam SG. Analysis of the elements of attention: A neuropsychological approach. Neuropsychology Review. 1991; 2 :109–145. [ PubMed ] [ Google Scholar ]
- Morasch KC, Bell MA. The role of inhibitory control in behavioral and physiological expressions of toddler executive function. Journal of Experimental Child Psychology. 2011; 108 :593–606. [ PMC free article ] [ PubMed ] [ Google Scholar ]
- Mrzljak L, Uylings HB, Van Eden CG, Judas M. Neuronal development in human prefrontal cortex in prenatal and postnatal stages. Progress in Brain Research. 1990; 85 :185–222. [ PubMed ] [ Google Scholar ]
- Muller U, Kerns KA, Konkin K. Test-retest reliability and practice effects of executive function tasks in preschool children. The Clinical Neuropsychologist. 2012; 26 :271–287. [ PubMed ] [ Google Scholar ]
- Newborg J, Stock JR, Wnek L. The manual for the Battell Developmental Inventory. Boston: Houghton Mifflin; 1988. [ Google Scholar ]
- Nigg JT, Goldsmith HH, Sachek J. Temperament and attention deficit hyperactivity disorder: the development of a multiple pathway model. Journal of Clinical Child and Adolescent Psychology. 2004; 33 :42–53. [ PubMed ] [ Google Scholar ]
- Nolan EE, Gadow KD, Sprafkin J. Teacher reports of DSM-IV ADHD, ODD, and CD symptoms in schoolchildren. Journal of the American Academy of Child and Adolescent Psychiatry. 2001; 40 :241–249. [ PubMed ] [ Google Scholar ]
- Noland JS, Singer LT, Short EJ, Minnes S, Arendt RE, Kirchner HL, Bearer C. Prenatal drug exposure and selective attention in preschoolers. Neurotoxicology and Teratology. 2005; 27 :429–438. [ PubMed ] [ Google Scholar ]
- Nomura Y, Marks DJ, Grossman B, Yoon M, Loudon H, Stone J, Halperin JM. Exposure to gestational diabetes mellitus and low socioeconomic status: Effects on neurocognitive development and risk of attention-deficit/hyperactivity disorder in offspring. Archives of Pediatric and Adolescent Medicine. 2012; 166 :337–343. [ PMC free article ] [ PubMed ] [ Google Scholar ]
- Palfrey JS, Levine MD, Walker DK, Sullivan M. The emergence of attention deficits in early childhood: a prospective study. Journal of Developmental and Behavioral Pediatrics. 1985; 6 :339–348. [ PubMed ] [ Google Scholar ]
- Pavuluri MN, Luk SL, McGee R. Parent reported preschool attention deficit hyperactivity: Measurement and validity. European Child and Adolescent Psychiatry. 1999; 8 :126–133. [ PubMed ] [ Google Scholar ]
- Pauli-Pott U, Becker K. Neuropsychological basic deficits in preschoolers at risk for ADHD: A meta-analysis. Clinical Psychology Review. 2011; 31 :626–637. [ PubMed ] [ Google Scholar ]
- Peterson SE, Posner MI. The attention system of the human brain: 20 years after. Annual Review of Neuroscience. 2012; 35 :73–89. [ PMC free article ] [ PubMed ] [ Google Scholar ]
- Pliszka SR. Pharmacologic treatment of attention-deficit/hyperactivity disorder: Efficacy, safety, and mechanisms of action. Neuropsychology Review. 2007; 17 :61–72. [ PubMed ] [ Google Scholar ]
- Poblano A, Romero E. ECI-4 Screening of attention deficit-hyperactivity disorder and co-morbidity in Mexican preschool children. Arquivos de Neuro-psiquiatria. 2006; 64 :932–936. [ PubMed ] [ Google Scholar ]
- Posner K, Melvin GA, Murray DW, Gugga SS, Fisher P, Skrobala A, Greenhill LL. Clinical presentation of attention-deficit/hyperactivity disorder in preschool children: The Preschoolers with Attention-Deficit/Hyperactivity Disorder Treatment Study (PATS) Journal of Child and Adolescent Psychopharmacology. 2007; 17 :547–562. [ PubMed ] [ Google Scholar ]
- Posner MI, Peterson SE. The attention system of the human brain. Annual Review of Neuroscience. 1990; 13 :25–42. [ PubMed ] [ Google Scholar ]
- Prather PA, Sarmento N, Alexander A. Development of vigilance in preschoolers [abstract] Journal of the International Neuropsychological Society. 1995; 1 :153. [ Google Scholar ]
- Pribram KH, McGuinness D. Arousal, activation, and effort in the control of attention. Psychological Review. 1975; 2 :116–149. [ PubMed ] [ Google Scholar ]
- Pulsifer MB, Butz AM, O'Reilley Foran M, Belcher HME. Prenatal drug exposure: Effects on cognitive functioning at 5 years of age. Clinical Pediatrics. 2008; 47 :58–65. [ PMC free article ] [ PubMed ] [ Google Scholar ]
- Rappley M, Eneli I, Mullan P. Patterns of psychotropic medication use in very young children with attention-deficit hyperactivity disorder. Journal of Developmental and Behavioral Pediatrics. 2002; 23 :23–30. [ PubMed ] [ Google Scholar ]
- Rapport MD, Scanlan SW, Denney CB. Attentiondeficit/hyperactivity disorder and scholastic achievement:A model of dual developmental pathways. Journal of Child Psychology and Psychiatry. 1999; 40 :1169–1183. [ PubMed ] [ Google Scholar ]
- Reynolds C, Kamphaus R. Behavior assessment system for children-2. Circle Pines, MN: American Guidance Systems, Inc.; 2004. [ Google Scholar ]
- Rezazadeh SM, Wilding J, Cornish K. The relationship between measures of cognitive attention and behavioral ratings of attention in typically developing children. Child Neuropsychology. 2011; 17 :197–208. [ PubMed ] [ Google Scholar ]
- Riley C, DuPaul GJ, Pipan M, Kern L, Van Brakle J, Blum NJ. Combined type versus ADHD predominatly hyperactive-impulsive type: Is there a difference in functional impairment? Journal of Developmental & Behavorial Pediatrics. 2008; 29 :270–275. [ PubMed ] [ Google Scholar ]
- Root RW, II, Resnick RJ. An update on the diagnosis and treatment of attention-deficit/hyperactivity disorder in children. Professional Psychology: Research and Practice. 2003; 34 :34–41. [ Google Scholar ]
- Ryan AS, Nelson EB. Assessing the effect of docosahexaenoic acid on cognitive functions in healthy, preschool children: a randomized, placebo-controlled, double-blind study. Clinical Pediatrics. 2008; 47 :355–362. [ PubMed ] [ Google Scholar ]
- Shaffer D, Fisher P, Lucas CP, Dulcan MK, Schwab-Stone ME. NIMH diagnostic interview schedule for children version IV (NIMH DISC-IV): Description, differences from previous versions, and reliability of some common diagnoses. Journal of the American Academy of Child and Adolescent Psychiatry. 2000; 39 :28–38. [ PubMed ] [ Google Scholar ]
- Shaw P, Eckstrand K, Sharp W, Blumenthal J, Lerch JP, Greenstein D, Rapoport JL. Attention-deficit/hyperactivity disorder is characterized by a delay in cortical maturation. Proceedings of the National Academy of Sciences of the United States of America. 2007; 104 :19649–19654. [ PMC free article ] [ PubMed ] [ Google Scholar ]
- Shelton T, Barkley R. Assessment of attention deficit hyperactivity disorder in young children. In: J JC, Willis D, editors. Testing young children. Austin, TX: Pro-Ed.; 1993. pp. 290–318. [ Google Scholar ]
- Short EJ, Manos MJ, Findling RL, Schubel EA. A prospective study of stimulant response in preschool children: Insights from ROC analyses. Journal of the American Academy of Child and Adolescent Psychiatry. 2004; 43 :251–259. [ PubMed ] [ Google Scholar ]
- Smidts DP, Oosterlaan J. How common are symptoms of ADHD in typically developing preschoolers? A study on prevalence rates and prenatal/demographic risk factors. Cortex. 2007; 43 :710–717. [ PubMed ] [ Google Scholar ]
- Sonuga-Barke EJS, Koerting J, Smith E, McCann DC, Thompson M. Early detection and intervention for attention-deficit/hyperactivity disorder. Expert Reviews Neurotheapeutics. 2011; 11 :557–563. [ PubMed ] [ Google Scholar ]
- Sowell ER, Thompson PM, Welcome SE, Henkenius AL, Toga AW, Peterson BS. Cortical abnormalities in children and adolescents with attention-deficit hyperactivity disorder. Lancet. 2003; 362 :1699–1707. [ PubMed ] [ Google Scholar ]
- Sprafkin J, Gadow KD. Early Childhood Symptom Inventories. Stony Brook, NY: Checkmate Plus; 1996. [ Google Scholar ]
- Sprafkin J, Volpe RJ, Gadow KD, Nolan EE, Kelly K. A DSM-IV-Referenced screening instrument for preschool children: The early childhood inventory-4. Journal of the American Academy of Child & Adolescent Psychiatry. 2002; 41 :604–612. [ PubMed ] [ Google Scholar ]
- Stefanatos GA, Baron IS. Attention deficit/hyperactivity disorder: A neuropsychological perspective toward DSM-V. Neuropsychology Review. 2007; 17 :5–38. [ PubMed ] [ Google Scholar ]
- Stevens T, Mulsow M. There is no meaningful relationship between television exposure and symptoms of attention-deficit/hyperactivity disorder. Pediatrics. 2006; 117 :665–672. [ PubMed ] [ Google Scholar ]
- Swanson J, Greenhill L, Wigal T, Kollins S, Stehli A, Davies M, Wigal S. Stimulant-related reductions of growth rates in the PATS. Journal of the American Academy of Child & Adolescent Psychiatry. 2006; 45 :1304–1313. [ PubMed ] [ Google Scholar ]
- Tandon M, Si X, Belden A, Luby J. Attention-deficit/hyperactivity disorder in preschool children: An investigation of validation based on visual attention performance. Journal of Child and Adolescent Psychopharmacology. 2009; 19 :137–146. [ PMC free article ] [ PubMed ] [ Google Scholar ]
- Tandon M, Xuemei S, Luby J. Preschool onset ADHD: Course and predictors of stability over 24m. Journal of Child and Adolescent Psychopharmacology. 2011; 21 :321–330. [ PMC free article ] [ PubMed ] [ Google Scholar ]
- Thompson MJ, Laver-Bradbury C, Ayres M, Sonuga-Barke E. A small scale randomized controlled trial of the revised new forest parenting programme for preschoolers with attention deficit hyperactivity disorder. European Journal of Child and Adolescent Psychiatry. 2009; 18 :605–616. [ PubMed ] [ Google Scholar ]
- Thorell LB. Do delay aversion and executive function deficits make distinct contributions to the functional impact of ADHD symptoms? A study of early academic skill deficits. Journal of Child Psychological Psychiatry. 2007; 48 :1061–1070. [ PubMed ] [ Google Scholar ]
- Vitiello B, Abikoff HB, Chuang SZ, Kollins SH, McCracken JT, Riddle MA, Greenhill LL. Effectiveness of methylphenidate in the 10-month continuation phase of the Preschoolers with Attention-Deficit/Hyperactivity Disorder Treatment Study (PATS) Journal of Child & Adolescent Psychopharmacology. 2007; 17 :593–604. [ PubMed ] [ Google Scholar ]
- Wahlstedt C, Thorell LB, Bohlin G. ADHD symptoms and executive function impairment: Early predictors of later behavior problems. Developmental Psychology. 2008; 33 :160–178. [ PubMed ] [ Google Scholar ]
- Weisen S. Clinical use of the Differential Abilities Scales-Second Edition (DAS-II) for children with attention deficit/hyperactivity disorder [Abstract] Dissertation Abstracts International, Section B: Sciences and Engineering. 2008; 69 (6-B):3863. [ Google Scholar ]
- White RF, Campbell R, Echeverria D, Knox SS, Janulewicz P. Assessment of neuropsychological trajectories in longitudinal population-based studies of children. Journal of Epidemiology and Community Health. 2009; 63 (suppl 1):i15–i26. [ PMC free article ] [ PubMed ] [ Google Scholar ]
- Wilding J, Munir F, Cornish K. The nature of attentional differences between groups of children differentiated by teacher ratings of attention and hyperactivity. British Journal of Psychology Bulletin. 2001; 92 :357–371. [ PubMed ] [ Google Scholar ]
- Wilens TE, Biederman J, Brown S, Monuteaux M, Prince J, Spencer TJ. Patterns of psychopathology and dysfunction in clinically referred preschoolers. Journal of Developmental and Behavioral Pediatrics. 2002a; 23 (1 Suppl):S31–S36. [ PubMed ] [ Google Scholar ]
- Wilens TE, Biederman J, Brown S, Tanguay S, Monuteaux MC, Blake C, Spencer TJ. Psychiatric comorbidity and functioning in clinically referred preschool children and school-age youths with ADHD. Journal of the American Academy of Child and Adolescent Psychiatry. 2002b; 41 :262–268. [ PubMed ] [ Google Scholar ]
- Willoughby MT, Pek J, Greenberg MG, et al. Journal of Abnormal Child Psychology. 2012 [ Google Scholar ]
- Yochman A, Ornoy A, Parush S. Co-occurrence of developmental delays among preschool children with attention-deficit-hyperactivity disorder. Developmental Medicine & Child Neurology. 2006; 48 :483–488. [ PubMed ] [ Google Scholar ]
- Youngwirth SD, Harvey EA, Gates EC, Hashim RL, Friedman-Weieneth JL. Neuropsychological abilities of preschool-aged children who display hyperactivity and/or oppositional defiant behavior problems. Child Neuropsychology. 2007; 13 :422–443. [ PubMed ] [ Google Scholar ]
The Future of Mental Health
Dispatches on the latest efforts in psychological and psychiatric treatment
Can Virtual Coworking Platforms Make Us More Productive?
Membership services like Flow Club, Flown and Caveday offer online study halls complete with proctors and goal setting
/https://tf-cmsv2-smithsonianmag-media.s3.amazonaws.com/accounts/headshot/Frederick_Reimers-edit.png)
Frederick Reimers
Freelance writer
:focal(2048x1375:2049x1376)/https://tf-cmsv2-smithsonianmag-media.s3.amazonaws.com/filer_public/2d/97/2d970291-2df5-4f27-85aa-851bca5869b4/flowclubonlaptop.jpg)
In June 2022, Susan Lieu’s dream of publishing a book was turning into a nightmare. She’d signed a contract with a major publishing house to deliver a memoir about finding her way in the world after her mother, a Vietnamese immigrant who’d established two successful nail salons, died at the age of 38 from a botched tummy tuck procedure. More than a year later, though, Lieu was far behind the word count she’d need in order to publish the book before her own 38th birthday. She was procrastinating, feeling isolated writing in her basement, and juggling the responsibilities of raising a toddler.
Then a friend told her about Flow Club , a membership service that hosts dozens of online coworking sessions a day. Participants in each one-to three-hour live study hall are encouraged by a proctor to set goals and report on progress at the end, but the majority of the time it’s just a group of people working simultaneously in front of their computer cameras.
Lieu quickly ramped up to spending five to ten hours a day in the virtual shared workspaces. Seven months later, she turned in the manuscript to what would become The Manicurist’s Daughter , published this March to significant acclaim. “I don’t think I would have finished my memoir without Flow Club,” says Lieu, who even thanked the company in her acknowledgments. “It was a godsend.”
/https://tf-cmsv2-smithsonianmag-media.s3.amazonaws.com/filer_public/e4/ad/e4ad6dde-afb3-43e8-8199-447bb26cd8e7/flowclubscheduledesktop.jpg)
Why did it work for her? For one thing, says Lieu, Flow Club taps into accountability, the positive social pressure we feel when our actions are answerable to others. “If you end up scrolling the internet for the whole hour you have to own up to that,” she says. Lieu points to studies like the American Society for Training and Development’s in 2010 that found a 65 percent increase in the likelihood of completing a goal if a person shares it with someone else. Even more significantly, it found that the probability rose to 95 percent if that goal was shared during an appointment specifically made for accountability.
Accountability is just one of a basket of psychological mechanisms that makes Flow Club work. Another is minimizing the number of times your brain switches tasks, what some experts call context switching, and yet another is called body doubling, a boost of focus people get while performing tasks alongside a peer. It’s also why Flow Club is one of a burgeoning number of such companies now offering coworking sessions for those looking to get more done, or just feel better while working.
“I’m an extrovert,” says Lieu, “I can feed off the energy of others.” Even, apparently, if that energy is derived from a video gallery of remote workers, though Lieu says the five-minute goal sharing and debrief periods that open and close each session are enough for a morale boost. “There’s also virtual bursts of confetti we can throw at each other to celebrate a win,” she says. “It’s a little hit of dopamine.” A 2019 study published in the journal Trends in Neuroscience showed that even small social interactions can activate our dopamine reward circuitry, another piece of evidence that these coworking platforms are underpinned by scientific efficacy.
I’m not an extrovert, though, so when I logged onto Flow Club, as well as two other coworking platforms, Flown and Caveday , for week-long trials, the social aspects of the experience weren’t as appealing to me. Flown’s sessions can have as many as 40 participants—lawyers, academics, writers, all logging on from locales as far-flung as England, Australia and Uruguay. Even in the fly-eye multitude of video screens, I often felt shy to speak my goals out loud, and gravitated to sessions where participants shared mainly through the group text chat function, an option in most of the platforms. (“I hate those text-only sessions,” says Lieu, an actor by trade.) On Caveday, I felt even more squeamish when hosts broke participants into groups of two, three or four for introductions and goal sharing.
/https://tf-cmsv2-smithsonianmag-media.s3.amazonaws.com/filer_public/53/e1/53e1f62d-8f5f-4217-8209-1d6815f74c3b/flown20lets20do20deep20work20nate.jpg)
Get through that short discomfort, however, and I found the services tremendously helpful. I was first diagnosed with ADHD in 1979, and prescribed Ritalin. Prior to that, I recall grade school as a blur of frustration. I took myself off of the medication in high school, afraid of the stigma, and bashed my way to graduation thanks only to a writing ability that had fortuitously installed during middle school, when I was still on the meds. When I got a new ADHD prescription years later in adulthood, all I could think was, “why did I ever get off this stuff?” I no longer lost my wallet and keys weekly, gained far more domestic tranquility as I followed through on tasks, and became far less stressed about making work deadlines because I simply started them earlier. For a freelance writer competing in a world of diminishing opportunity, however, the drugs are not enough. As someone who works at home and has a toddler himself, I was able to get far more done working on each of the platforms than I normally do left to my own devices at home.
I appreciated the peppy welcome from the session hosts and goal setting, and loved checking tasks off my list, but what was most intriguing was the effect of working alongside others virtually. With my camera on, I felt myself sitting up straighter, and though no one could see what I was working on, I often was able to stop myself from unthinkingly logging onto social media sites. In the corner of my computer screen, the gallery of other participants worked steadily away. It was strangely calming, and most every time I glanced at it, I felt myself relax and settle into the work.
Some experts refer to this phenomenon as body doubling , a term coined in 1996 by Linda Anderson, an executive productivity coach, and now popular in the ADHD community. The basic idea is that the presence of another person allows someone to feel more focused and motivated, says Candan Ertubey, a psychologist at the University of Hertfordshire in England. She likens the effect to that of training with a partner. “We know that jogging with a partner is more effective,” she says. “It’s bringing that coaching model to the work environment.”
In 2023, Ertubey led an unpublished study of the effects of virtual coworking on 101 Flown members. She found that 95 percent of participants reported an above average improvement in focus, that 94 percent reported an above average improvement in productivity, and 85 percent rated their quality of work as higher with the program. Ertubey also found that “work flow and well-being were highly coordinated,” with 87 percent reporting an above average improvement in stress level.
In Ertubey’s estimation, three psychological mechanisms make programs like Flown work. The first is the declaration of intent that triggers accountability. The second is body doubling, and the third is the strict schedule of work time punctuated by interactive breaks. Flown, Caveday and Flow Club all include short interactive breaks within their sessions. Hosts urge participants to take their hands off their keyboards and stretch their shoulders, or share one word about how they are feeling, or high five the screen en-masse. Those small breaks are significant, says Ertubey. “The nature and quality of attention is a very complicated matter, but we know that interspersing short periods of distraction during focused work enhances creativity.”
That last process, alternating stints of focused work with short breaks, is one of the strategies for obtaining better focus prescribed by productivity guru Cal Newport . His book Deep Work was a big inspiration for Flown’s founder Alicia Navarro. Newport describes deep work as, “activities performed in a state of distraction-free concentration that push your cognitive capabilities to their limit.” The company’s name, like Flow Club’s, is a nod to the concept of the “flow state,” that Newport says accompanies successful deep work.
/https://tf-cmsv2-smithsonianmag-media.s3.amazonaws.com/filer_public/d8/f1/d8f1c77f-1459-4947-b4f7-d767af8a5d7a/hremployee20success20optional20employee20benefit202.png)
“There are systematic attacks on our attention by modern technology,” says Navarro. She points to a 2008 study from University of California, Irvine researchers that found office workers were distracted from their work every three minutes and 5 seconds. The authors also found that it took those workers an average of 23 minutes and 15 seconds to return their focus to the original task. Sophie Leroy, a business professor at the University of Washington, has subsequently dubbed the phenomenon “attention residue ,” which she describes as “the brain struggling to move on from interruption.” That struggle, according to Leroy, impedes cognitive performance. As Newport likes to say, there’s a cost to task switching.
“I wanted to build a platform for people to be able to do deep work,” says Navarro. That meant helping people set aside dedicated blocks of time for distraction-free work, and then using positive social pressure like accountability and body doubling to keep them on task.
In my own time on the coworking sites, I found those pressures to be beneficial. Time and again, I course-corrected back to work in the sessions, and while my smartphone called to me like a phantom limb, I could more easily leave it untouched in the desk drawer.
Launched during the Covid-19 pandemic, Flown’s users have tripled every year, says Navarro. She also reports that the average number of focus sessions per user per week has risen from 4.3 to 6 over the last year. “This means that people are using Flown more heavily, on average, as we grow,” she says. Flow Club reports similar growth, quadrupling users since the beginning of 2023 and now offering close to 2,000 sessions a week for nearly round-the-clock coverage.
Interestingly, on all three of the platforms, users don’t limit their productivity to typing on keyboards. A frequent goal I heard on Flow Club was to “relax my shoulders,” which I quickly adopted as one of my own. On Flown, I saw people list tasks like, “make a breakfast smoothie and then clean for 30 minutes,” and “bake a birthday cake for my son.” Navarro heard a report that one Flown participant used the time to practice parallel parking.
Lieu, who still logs three to five Flow Club sessions a week as she markets The Manicurist’s Daughter and works on a new television screenplay, reports all manner of unexpected behavior. She sees artists sketching, or cleaning up their studios. In her afternoon sessions, she often sees Australian users logging on to perform their morning routine: take vitamins, drink water, meditate. “I always think of a morning routine as something very private,” she says, “but if you are living alone and need that Flow Club appointment to make getting your day started more easily, I can understand that.”
Lieu references what many call the “loneliness epidemic,” and cites a 2024 American Psychiatric Association poll finding that 30 percent of adults feel loneliness once a week and that 10 percent feel lonely every day. For her, Flow Club has been an antidote to that. “I really miss coworkers,” she says. People she has met on the platform have attended readings on her book tour, and the effects of coworking provide a sort of existential comfort. “I can observe other people struggling with their work and can relate to that,” she says.
Lieu adds: “It’s comforting, and it helps me get out of my head. I can remind myself everyone isn’t perfect. I’m not alone in this world.”
Get the latest stories in your inbox every weekday.
/https://tf-cmsv2-smithsonianmag-media.s3.amazonaws.com/accounts/headshot/Frederick_Reimers-edit.png)
Frederick Reimers | READ MORE
Frederick Reimers is a Jackson, Wyoming-based writer whose work has appeared in The New York Times , Bloomberg , Outside and Ski magazines.

IMAGES
VIDEO
COMMENTS
Thus many authors would make the case that a lecture session should last no more than 10-15 min to accommodate the biological set point of a student's attention span. In 2015, a study commissioned by Microsoft and discussed in Time magazine found that the average attention span was in fact only 8 s. If indeed this is the case, then even ...
These days, most of us live our lives tethered to our computers and smartphones, which are unending sources of distraction. Research has shown that over the past couple of decades people's attention spans have shrunk in measurable ways. Gloria Mark, PhD, of the University of California Irvine, talks about how the internet and digital devices ...
In order to provide an overview of the impact of technology on learning, Tamim, Bernard, Borokhovski, Abrami, and Schmid [ 14] conducted a second-order meta-analysis on 40 years of research on the role of technology in learning in educational settings. The analysis revealed that the overall effect size of technology use on learning is Cohen's ...
The problem is illustrated by a study that, according to the authors, ... A resource shared between "storage" and "processing" spans both sides of the distinction between attention to things and events (i.e., the information to be stored), and attention to goals and actions (i.e., to the task sets guiding the processing operations ...
IntroductionStudies examining sustained attention abilities typically utilize metrics that quantify performance on vigilance tasks, such as response time and response time variability. However, approaches that assess the duration that an individual can maintain their attention over time are lacking.MethodsHere we developed an objective attention span metric that quantified the maximum amount ...
studies of student attention have focussed on the impacts of active listening, flipped classrooms and ... assessed their attention span before and after a DMS was employed within their semester-long unit. ... good teaching is insufficient in and of itself to control students' interest and attention. In addition to research on teaching ...
Attention includes a number of components, one of which, attention span, is the ability to maintain focus and alertness over a period of time. Sustained attention requires persistence and motivation [ 2 ]. Thus, individuals with short attention spans may appear to give up or not put sufficient effort into tasks.
Attention span is the amount of time spent concentrating on a task before becoming distracted. ... A research study that consisted of 10,430 males and females ages 10 to 70 observed sustained attention time across a lifespan. The study required participants to use a cognitive testing website where data was gathered for seven months. The data ...
Finally, by combining connectomes from the multiple attention tasks in our study, a common attention factor, the C2C framework and CPM behaviour prediction, we were able to derive a general ...
Attention is the important ability to flexibly control limited computational resources. It has been studied in conjunction with many other topics in neuroscience and psychology including awareness, vigilance, saliency, executive control, and learning. It has also recently been applied in several domains in machine learning. The relationship between the study of biological attention and its use ...
Memory span/sustained attention/working memory: Digit Span (WISC-III; 3 measures) ... Two other studies reported improvements in other aspects of attention. Study 13 reported improvements in divided attention and ... 22). These findings suggest that mindfulness training may be particularly helpful for improving selective attention. Five studies ...
Using a qualitative content analysis approach, this study reviewed 45 published studies, research on student's Class attention since 2008, primarily focusing on reasons for students' attention and ...
Abstract: The present research work intends to compare in the form of the study on the span of attention with ... presentation. Sir William Hamilton (1959) was the first to carry experimental study in this field. Later on serial studies were carried on revealing significant facts. Dallerback (1929) studied the span of attention for dots,
Fishy citations. In his 2014 book "Brief: Make a Bigger Impact by Saying Less," Joseph McCormack wrote "Prevailing research says that the average attention span is down to 8 seconds from 12 ...
Attention, as it is now defined as a process matching data from the environment to the needs of the organism, is one of the main aspects of human cognitive processes. There are several aspects to attention including tonic alertness (a process of intrinsic arousal that varies by minutes to hours), phasic alertness (a process that causes a quick change in attention as a result of a brief ...
were used in preliminary studies, 75 in a control study, and 426 in the final studies with six toys. The means of the highest attention spans found in this study on the final testing of 426 children are shown in Table 1. In determining the attention spans, an arbitrary time limit of forty-five minutes was set for the termina-
We investigated whether standardized neuropsychological tests and experimental cognitive paradigms measure the same cognitive faculties. Specifically, do neuropsychological tests commonly used to assess attention measure the same construct as attention paradigms used in cognitive psychology and neuroscience? We built on the "general attention factor", comprising several widely used ...
Thus many authors would make the case that a lecture session should last no more than 10-15 min to accommodate the biological set point of a student's attention span. In 2015, a study commissioned by Microsoft and discussed in Time magazine found that the average attention span was in fact only 8 s.
May 14, 2015 5:09 AM EDT. T he average attention span for the notoriously ill-focused goldfish is nine seconds, but according to a new study from Microsoft Corp., people now generally lose ...
performance task. Results: In a population of 262 individuals aged 7-85, we showed that attention. span was longer in young adults than in children and older adults. Furthermore, declines in ...
In summary, around 1860, the philosophical approach dominated the study of psychology in general and attention especially. During the period from 1980 to 1909, the study of attention was transformed, as was the field of psychology as a whole, to one of scientific inquiry with emphasis on experimental investigations.
2.2. Paradigm and stimuli. Participants from all age groups completed the same visual CPT in the same research lab at the UCSF Neuroscape Center (Figure 1A), except for 16 children who completed the same CPT using identical equipment at Cortica Healthcare's labs in Marin County.The CPT was modeled after the Test of Variables of Attention (TOVA) (Leark et al., 2007) and has been used in ...
A study by expert Gloria Mark, who received her PhD in psychology from Columbia University, found that in 2004 the average span of attention was 150 seconds long. By 2012, the average attention span declined to half that at 75 seconds, and by 2018, they decreased even further to a very short 47 seconds. Research by Gloria Mark, PhD, shows that ...
Emotional processing. Early visual experience plays a critical role in the development of Theory of Mind (ToM; Minter et al., Citation 1998), which involves identifying and understanding other people's emotional states and interpreting emotional cues conveyed through speech.Some studies found individuals with CB to experience delays in the development of ToM as compared to individuals with LB ...
ADHD is an ongoing and expanding public health concern, according to researchers studying the disorder. One million more U.S. children were diagnosed in 2022 compared to 2016, a new study shows.
By 2022, 7.1 million children — about 1 in 9 — had been diagnosed with attention deficit/hyperactivity disorder, according to a new Centers for Disease Control and Prevention study. About 1 million of them got their diagnosis since 2016, which was when the previous most recent national estimate was done. The numbers are based on 2022 ...
Purpose To examine the consequences of broader spans of control for well-being outcomes among frontline managers. Method Healthcare managers were surveyed in collaboration with the Central Denmark Region. The response rate was 74.5%. Using regression analysis, we investigate how span of control is associated with outcomes related to well-being understood as perceived stress, burnout, job ...
Study design. A controlled experimental design was implemented with blind assessment. The interns were randomly assigned to the ChatGPT-assisted group (39 students) or the traditional group (38 students), with no significant differences in gender, age, or baseline clinical examination scores (p > 0.05).The ChatGPT-assisted group received instruction supplemented with ChatGPT version 4.0, while ...
Risk Factors for Attention Problems in Preschoolers. Early developmental differences involving attentional control in preschoolers are considered to arise from a variety of influences, including genetic (Biederman & Faraone, 2002), temperamental (Nigg, Goldsmith, & Sachek, 2004), and environmental (Pauli-Pott & Becker, 2011) factors.A recent longitudinal study of over 2000 children from Canada ...
In 2023, Ertubey led an unpublished study of the effects of virtual coworking on 101 Flown members. She found that 95 percent of participants reported an above average improvement in focus, that ...