Click through the PLOS taxonomy to find articles in your field.
For more information about PLOS Subject Areas, click here .
Loading metrics
Open Access
Peer-reviewed
Research Article

Clustering algorithms: A comparative approach
Roles Data curation, Formal analysis, Investigation, Methodology, Software, Validation, Visualization, Writing – original draft, Writing – review & editing
Affiliation Institute of Mathematics and Computer Science, University of São Paulo, São Carlos, São Paulo, Brazil
Roles Conceptualization, Data curation, Formal analysis, Investigation, Methodology, Validation, Writing – original draft, Writing – review & editing
* E-mail: [email protected]
Affiliation Department of Computer Science, Federal University of São Carlos, São Carlos, São Paulo, Brazil

Roles Validation, Writing – original draft, Writing – review & editing
Affiliation Federal University of Technology, Paraná, Paraná, Brazil
Roles Funding acquisition, Project administration, Supervision, Validation, Writing – review & editing
Affiliation São Carlos Institute of Physics, University of São Paulo, São Carlos, São Paulo, Brazil
Roles Funding acquisition, Project administration, Supervision, Validation, Writing – original draft, Writing – review & editing
Roles Conceptualization, Formal analysis, Funding acquisition, Methodology, Project administration, Resources, Supervision, Validation, Writing – original draft, Writing – review & editing
- Mayra Z. Rodriguez,
- Cesar H. Comin,
- Dalcimar Casanova,
- Odemir M. Bruno,
- Diego R. Amancio,
- Luciano da F. Costa,
- Francisco A. Rodrigues
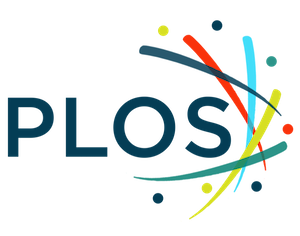
- Published: January 15, 2019
- https://doi.org/10.1371/journal.pone.0210236
- Reader Comments
Many real-world systems can be studied in terms of pattern recognition tasks, so that proper use (and understanding) of machine learning methods in practical applications becomes essential. While many classification methods have been proposed, there is no consensus on which methods are more suitable for a given dataset. As a consequence, it is important to comprehensively compare methods in many possible scenarios. In this context, we performed a systematic comparison of 9 well-known clustering methods available in the R language assuming normally distributed data. In order to account for the many possible variations of data, we considered artificial datasets with several tunable properties (number of classes, separation between classes, etc). In addition, we also evaluated the sensitivity of the clustering methods with regard to their parameters configuration. The results revealed that, when considering the default configurations of the adopted methods, the spectral approach tended to present particularly good performance. We also found that the default configuration of the adopted implementations was not always accurate. In these cases, a simple approach based on random selection of parameters values proved to be a good alternative to improve the performance. All in all, the reported approach provides subsidies guiding the choice of clustering algorithms.
Citation: Rodriguez MZ, Comin CH, Casanova D, Bruno OM, Amancio DR, Costa LdF, et al. (2019) Clustering algorithms: A comparative approach. PLoS ONE 14(1): e0210236. https://doi.org/10.1371/journal.pone.0210236
Editor: Hans A. Kestler, University of Ulm, GERMANY
Received: December 26, 2016; Accepted: December 19, 2018; Published: January 15, 2019
Copyright: © 2019 Rodriguez et al. This is an open access article distributed under the terms of the Creative Commons Attribution License , which permits unrestricted use, distribution, and reproduction in any medium, provided the original author and source are credited.
Data Availability: All datasets used for evaluating the algorithms can be obtained from Figshare: https://figshare.com/s/29005b491a418a667b22 .
Funding: This work has been supported by FAPESP - Fundação de Amparo à Pesquisa do Estado de São Paulo (grant nos. 15/18942-8 and 18/09125-4 for CHC, 14/20830-0 and 16/19069-9 for DRA, 14/08026-1 for OMB and 11/50761-2 and 15/22308-2 for LdFC), CNPq - Conselho Nacional de Desenvolvimento Científico e Tecnológico (grant nos. 307797/2014-7 for OMB and 307333/2013-2 for LdFC), Núcleo de Apoio à Pesquisa (LdFC) and CAPES - Coordenação de Aperfeiçoamento de Pessoal de Nível Superior (Finance Code 001).
Competing interests: The authors have declared that no competing interests exist.
Introduction
In recent years, the automation of data collection and recording implied a deluge of information about many different kinds of systems [ 1 – 8 ]. As a consequence, many methodologies aimed at organizing and modeling data have been developed [ 9 ]. Such methodologies are motivated by their widespread application in diagnosis [ 10 ], education [ 11 ], forecasting [ 12 ], and many other domains [ 13 ]. The definition, evaluation and application of these methodologies are all part of the machine learning field [ 14 ], which became a major subarea of computer science and statistics due to their crucial role in the modern world.
Machine learning encompasses different topics such as regression analysis [ 15 ], feature selection methods [ 16 ], and classification [ 14 ]. The latter involves assigning classes to the objects in a dataset. Three main approaches can be considered for classification: supervised, semi-supervised and unsupervised classification. In the former case, the classes, or labels, of some objects are known beforehand, defining the training set, and an algorithm is used to obtain the classification criteria. Semi-supervised classification deals with training the algorithm using both labeled and unlabeled data. They are commonly used when manually labeling a dataset becomes costly. Lastly, unsupervised classification, henceforth referred as clustering , deals with defining classes from the data without knowledge of the class labels. The purpose of clustering algorithms is to identify groups of objects, or clusters, that are more similar to each other than to other clusters. Such an approach to data analysis is closely related to the task of creating a model of the data, that is, defining a simplified set of properties that can provide intuitive explanation about relevant aspects of a dataset. Clustering methods are generally more demanding than supervised approaches, but provide more insights about complex data. This type of classifiers constitute the main object of the current work.
Because clustering algorithms involve several parameters, often operate in high dimensional spaces, and have to cope with noisy, incomplete and sampled data, their performance can vary substantially for different applications and types of data. For such reasons, several different approaches to clustering have been proposed in the literature (e.g. [ 17 – 19 ]). In practice, it becomes a difficult endeavor, given a dataset or problem, to choose a suitable clustering approach. Nevertheless, much can be learned by comparing different clustering methods. Several previous efforts for comparing clustering algorithms have been reported in the literature [ 20 – 29 ]. Here, we focus on generating a diversified and comprehensive set of artificial, normally distributed data containing not only distinct number of classes, features, number of objects and separation between classes, but also a varied structure of the involved groups (e.g. possessing predefined correlation distributions between features). The purpose of using artificial data is the possibility to obtain an unlimited number of samples and to systematically change any of the aforementioned properties of a dataset. Such features allow the clustering algorithms to be comprehensive and strictly evaluated in a vast number of circumstances, and also grants the possibility of quantifying the sensitivity of the performance with respect to small changes in the data. It should be observed, nevertheless, that the performance results reported in this work are therefore respective and limited to normally distributed data, and other results could be expected for other types of data following other statistical behavior. Here we associate performance with the similarity between the known labels of the objects and those found by the algorithm. Many measurements have been defined for quantifying such similarity [ 30 ], we compare the Jaccard index [ 31 ], Adjusted Rand index [ 32 ], Fowlkes-Mallows index [ 33 ] and Normalized mutual information [ 34 ]. A modified version of the procedure developed by [ 35 ] was used to create 400 distinct datasets, which were used in order to quantify the performance of the clustering algorithms. We describe the adopted procedure and the respective parameters used for data generation. Related approaches include [ 36 ].
Each clustering algorithm relies on a set of parameters that needs to be adjusted in order to achieve viable performance, which corresponds to an important point to be addressed while comparing clustering algorithms. A long standing problem in machine learning is the definition of a proper procedure for setting the parameter values [ 37 ]. In principle, one can apply an optimization procedure (e.g., simulated annealing [ 38 ] or genetic algorithms [ 39 ]) to find the parameter configuration providing the best performance of a given algorithm. Nevertheless, there are two major problems with such an approach. First, adjusting parameters to a given dataset may lead to overfitting [ 40 ]. That is, the specific values found to provide good performance may lead to lower performance when new data is considered. Second, parameter optimization can be unfeasible in some cases, given the time complexity of many algorithms, combined with their typically large number of parameters. Ultimately, many researchers resort to applying classifier or clustering algorithms using the default parameters provided by the software. Therefore, efforts are required for evaluating and comparing the performance of clustering algorithms in the optimization and default situations. In the following, we consider some representative examples of algorithms applied in the literature [ 37 , 41 ].
Clustering algorithms have been implemented in several programming languages and packages. During the development and implementation of such codes, it is common to implement changes or optimizations, leading to new versions of the original methods. The current work focuses on the comparative analysis of several clustering algorithm found in popular packages available in the R programming language [ 42 ]. This choice was motivated by the popularity of the R language in the data mining field, and by virtue of the well-established clustering packages it contains. This study is intended to assist researchers who have programming skills in R language, but with little experience in clustering of data.
The algorithms are evaluated on three distinct situations. First, we consider their performance when using the default parameters provided by the packages. Then, we consider the performance variation when single parameters of the algorithms are changed, while the rest are kept at their default values. Finally, we consider the simultaneous variation of all parameters by means of a random sampling procedure. We compare the results obtained for the latter two situations with those achieved by the default parameters, in such a way as to investigate the possible improvements in performance which could be achieved by modifying the algorithms.
The algorithms were evaluated on 400 artificial, normally distributed, datasets generated by a robust methodology previously described in [ 36 ]. The number of features, number of classes, number of objects for each class and average distance between classes can be systematically changed among the datasets.
The text is divided as follows. We start by revising some of the main approaches to clustering algorithms comparison. Next, we describe the clustering methods considered in the analysis, we also present the R packages implementing such methods. The data generation method and the performance measurements used to compare the algorithms are presented, followed by the presentation of the performance results obtained for the default parameters, for single parameter variation and for random parameter sampling.
Related works
Previous approaches for comparing the performance of clustering algorithms can be divided according to the nature of used datasets. While some studies use either real-world or artificial data, others employ both types of datasets to compare the performance of several clustering methods.
A comparative analysis using real world dataset is presented in several works [ 20 , 21 , 24 , 25 , 43 , 44 ]. Some of these works are reviewed briefly in the following. In [ 43 ], the authors propose an evaluation approach based in a multiple criteria decision making in the domain of financial risk analysis over three real world credit risk and bankruptcy risk datasets. More specifically, clustering algorithms are evaluated in terms of a combination of clustering measurements, which includes a collection of external and internal validity indexes. Their results show that no algorithm can achieve the best performance on all measurements for any dataset and, for this reason, it is mandatory to use more than one performance measure to evaluate clustering algorithms.
In [ 21 ], a comparative analysis of clustering methods was performed in the context of text-independent speaker verification task, using three dataset of documents. Two approaches were considered: clustering algorithms focused in minimizing a distance based objective function and a Gaussian models-based approach. The following algorithms were compared: k-means, random swap, expectation-maximization, hierarchical clustering, self-organized maps (SOM) and fuzzy c-means. The authors found that the most important factor for the success of the algorithms is the model order, which represents the number of centroid or Gaussian components (for Gaussian models-based approaches) considered. Overall, the recognition accuracy was similar for clustering algorithms focused in minimizing a distance based objective function. When the number of clusters was small, SOM and hierarchical methods provided lower accuracy than the other methods. Finally, a comparison of the computational efficiency of the methods revealed that the split hierarchical method is the fastest clustering algorithm in the considered dataset.
In [ 25 ], five clustering methods were studied: k-means, multivariate Gaussian mixture, hierarchical clustering, spectral and nearest neighbor methods. Four proximity measures were used in the experiments: Pearson and Spearman correlation coefficient, cosine similarity and the euclidean distance. The algorithms were evaluated in the context of 35 gene expression data from either Affymetrix or cDNA chip platforms, using the adjusted rand index for performance evaluation. The multivariate Gaussian mixture method provided the best performance in recovering the actual number of clusters of the datasets. The k-means method displayed similar performance. In this same analysis, the hierarchical method led to limited performance, while the spectral method showed to be particularly sensitive to the proximity measure employed.
In [ 24 ], experiments were performed to compare five different types of clustering algorithms: CLICK, self organized mapping-based method (SOM), k-means, hierarchical and dynamical clustering. Data sets of gene expression time series of the Saccharomyces cerevisiae yeast were used. A k-fold cross-validation procedure was considered to compare different algorithms. The authors found that k-means, dynamical clustering and SOM tended to yield high accuracy in all experiments. On the other hand, hierarchical clustering presented a more limited performance in clustering larger datasets, yielding low accuracy in some experiments.
A comparative analysis using artificial data is presented in [ 45 – 47 ]. In [ 47 ], two subspace clustering methods were compared: MAFIA (Adaptive Grids for Clustering Massive Data Sets) [ 48 ] and FINDIT (A Fast and Intelligent Subspace Clustering Algorithm Using Dimension Voting) [ 49 ]. The artificial data, modeled according to a normal distribution, allowed the control of the number of dimensions and instances. The methods were evaluated in terms of both scalability and accuracy. In the former, the running time of both algorithms were compared for different number of instances and features. In addition, the authors assessed the ability of the methods in finding adequate subspaces for each cluster. They found that MAFIA discovered all relevant clusters, but one significant dimension was left out in most cases. Conversely, the FINDIT method performed better in the task of identifying the most relevant dimensions. Both algorithms were found to scale linearly with the number of instances, however MAFIA outperformed FINDIT in most of the tests.
Another common approach for comparing clustering algorithms considers using a mixture of real world and artificial data (e.g. [ 23 , 26 – 28 , 50 ]). In [ 28 ], the performance of k-means, single linkage and simulated annealing (SA) was evaluated, considering different partitions obtained by validation indexes. The authors used two real world datasets obtained from [ 51 ] and three artificial datasets (having two dimensions and 10 clusters). The authors proposed a new validation index called I index that measures the separation based on the maximum distance between clusters and compactness based on the sum of distances between objects and their respective centroids. They found that such an index was the most reliable among other considered indices, reaching its maximum value when the number of clusters is properly chosen.
A systematic quantitative evaluation of four graph-based clustering methods was performed in [ 27 ]. The compared methods were: markov clustering (MCL), restricted neighborhood search clustering (RNSC), super paramagnetic clustering (SPC), and molecular complex detection (MCODE). Six datasets modeling protein interactions in the Saccharomyces cerevisiae and 84 random graphs were used for the comparison. For each algorithm, the robustness of the methods was measured in a twofold fashion: the variation of performance was quantified in terms of changes in the (i) methods parameters and (ii) dataset properties. In the latter, connections were included and removed to reflect uncertainties in the relationship between proteins. The restricted neighborhood search clustering method turned out to be particularly robust to variations in the choice of method parameters, whereas the other algorithms were found to be more robust to dataset alterations. In [ 52 ] the authors report a brief comparison of clustering algorithms using the Fundamental clustering problem suite (FPC) as dataset. The FPC contains artificial and real datasets for testing clustering algorithms. Each dataset represents a particular challenge that the clustering algorithm has to handle, for example, in the Hepta and LSum datasets the clusters can be separated by a linear decision boundary, but have different densities and variances. On the other hand, the ChainLink and Atom datasets cannot be separated by linear decision boundaries. Likewise, the Target dataset contains outliers. Lower performance was obtained by the single linkage clustering algorithm for the Tetra, EngyTime, Twodiamonds and Wingnut datasets. Although the datasets are quite versatile, it is not possible to control and evaluate how some of its characteristics, such as dimensions or number of features, affect the clustering accuracy.
Clustering methods
Many different types of clustering methods have been proposed in the literature [ 53 – 56 ]. Despite such a diversity, some methods are more frequently used [ 57 ]. Also, many of the commonly employed methods are defined in terms of similar assumptions about the data (e.g., k-means and k-medoids) or consider analogous mathematical concepts (e.g, similarity matrices for spectral or graph clustering) and, consequently, should provide similar performance in typical usage scenarios. Therefore, in the following we consider a choice of clustering algorithms from different families of methods. Several taxonomies have been proposed to organize the many different types of clustering algorithms into families [ 29 , 58 ]. While some taxonomies categorize the algorithms based on their objective functions [ 58 ], others aim at the specific structures desired for the obtained clusters (e.g. hierarchical) [ 29 ]. Here we consider the algorithms indicated in Table 1 as examples of the categories indicated in the same table. The algorithms represent some of the main types of methods in the literature. Note that some algorithms are from the same family, but in these cases they posses notable differences in their applications (e.g., treating very large datasets using clara). A short description about the parameters of each considered algorithm is provided in S1 File of the supplementary material.
- PPT PowerPoint slide
- PNG larger image
- TIFF original image
The first column shows the name of the algorithms used throughout the text. The second column indicates the category of the algorithms. The third and fourth columns contain, respectively, the function name and R library of each algorithm.
https://doi.org/10.1371/journal.pone.0210236.t001
Regarding partitional approaches, the k-means [ 68 ] algorithm has been widely used by researchers [ 57 ]. This method requires as input parameters the number of groups ( k ) and a distance metric. Initially, each data point is associated with one of the k clusters according to its distance to the centroids (clusters centers) of each cluster. An example is shown in Fig 1(a) , where black points correspond to centroids and the remaining points have the same color if the centroid that is closest to them is the same. Then, new centroids are calculated, and the classification of the data points is repeated for the new centroids, as indicated in Fig 1(b) , where gray points indicate the position of the centroids in the previous iteration. The process is repeated until no significant changes of the centroids positions is observed at each new step, as shown in Fig 1(c) and 1(d) .
Each plot shows the partition obtained after specific iterations of the algorithm. The centroids of the clusters are shown as a black marker. Points are colored according to their assigned clusters. Gray markers indicate the position of the centroids in the previous iteration. The dataset contains 2 clusters, but k = 4 seeds were used in the algorithm.
https://doi.org/10.1371/journal.pone.0210236.g001
The a priori setting of the number of clusters is the main limitation of the k-means algorithm. This is so because the final classification can strongly depend on the choice of the number of centroids [ 68 ]. In addition, the k-means is not particularly recommended in cases where the clusters do not show convex distribution or have very different sizes [ 59 , 60 ]. Moreover, the k-means algorithm is sensitive to the initial seed selection [ 41 ]. Given these limitations, many modifications of this algorithm have been proposed [ 61 – 63 ], such as the k-medoid [ 64 ] and k-means++ [ 65 ]. Nevertheless, this algorithm, besides having low computational cost, can provide good results in many practical situations such as in anomaly detection [ 66 ] and data segmentation [ 67 ]. The R routine used for k-means clustering was the k-means from the stats package, which contains the implementation of the algorithms proposed by Macqueen [ 68 ], Hartigan and Wong [ 69 ]. The algorithm of Hartigan and Wong is employed by the stats package when setting the parameters to their default values, while the algorithm proposed by Macqueen is used for all other cases. Another interesting example of partitional clustering algorithms is the clustering for large applications (clara) [ 70 ]. This method takes into account multiple fixed samples of the dataset to minimize sampling bias and, subsequently, select the best medoids among the chosen samples, where a medoid is defined as the object i for which the average dissimilarity to all other objects in its cluster is minimal. This method tends to be efficient for large amounts of data because it does not explore the whole neighborhood of the data points [ 71 ], although the quality of the results have been found to strongly depend on the number of objects in the sample data [ 62 ]. The clara algorithm employed in our analysis was provided by the clara function contained in the cluster package. This function implements the method developed by Kaufman and Rousseeuw [ 70 ].
The Ordering Points To Identify the Clustering Structure (OPTICS) [ 72 , 73 ] is a density-based cluster ordering based on the concept of maximal density-reachability [ 72 ]. The algorithm starts with a data point and expands its neighborhood using a similar procedure as in the dbscan algorithm [ 74 ], with the difference that the neighborhood is first expanded to points with low core-distance. The core distance of an object p is defined as the m -th smallest distance between p and the objects in its ϵ -neighborhood (i.e., objects having distance less than or equal to ϵ from p ), where m is a parameter of the algorithm indicating the smallest number of points that can form a cluster. The optics algorithm can detect clusters having large density variations and irregular shapes. The R routine used for optics clustering was the optics from the dbscan package. This function considers the original algorithm developed by Ankerst et al. [ 72 ]. An hierarchical clustering structure from the output of the optics algorithm can be constructed using the function extractXi from the dbscan package. We note that the function extractDBSCAN , from the same package, provides a clustering from an optics ordering that is similar to what the dbscan algorithm would generate.
Clustering methods that take into account the linkage between data points, traditionally known as hierarchical methods, can be subdivided into two groups: agglomerative and divisive [ 59 ]. In an agglomerative hierarchical clustering algorithm, initially, each object belongs to a respective individual cluster. Then, after successive iterations, groups are merged until stop conditions are reached. On the other hand, a divisive hierarchical clustering method starts with all objects in a single cluster and, after successive iterations, objects are separated into clusters. There are two main packages in the R language that provide routines for performing hierarchical clustering, they are the stats and cluster . Here we consider the agnes routine from the cluster package which implements the algorithm proposed by Kaufman and Rousseeuw [ 70 ]. Four well-known linkage criteria are available in agnes , namely single linkage, complete linkage, Ward’s method, and weighted average linkage [ 75 ].
Model-based methods can be regarded as a general framework for estimating the maximum likelihood of the parameters of an underlying distribution to a given dataset. A well-known instance of model-based methods is the expectation-maximization (EM) algorithm. Most commonly, one considers that the data from each class can be modeled by multivariate normal distributions, and, therefore, the distribution observed for the whole data can be seen as a mixture of such normal distributions. A maximum likelihood approach is then applied for finding the most probable parameters of the normal distributions of each class. The EM approach for clustering is particularly suitable when the dataset is incomplete [ 76 , 77 ]. On the other hand, the clusters obtained from the method may strongly depend on the initial conditions [ 54 ]. In addition, the algorithm may fail to find very small clusters [ 29 , 78 ]. In the R language, the package mclust [ 79 , 80 ]. provides iterative EM (Expectation-Maximization) methods for maximum likelihood estimation using parameterized Gaussian mixture models. Functions estep and mstep implement the individual steps of an EM iteration. A related algorithm that is also analyzed in the current study is the hcmodel, which can be found in the hc function of the mclust package. The hcmodel algorithm, which is also based on Gaussian-mixtures, was proposed by Fraley [ 81 ]. The algorithm contains many additional steps compared to traditional EM methods, such as an agglomerative procedure and the adjustment of model parameters through a Bayes factor selection using the BIC aproximation [ 82 ].
In recent years, the efficient handling of high dimensional data has become of paramount importance and, for this reason, this feature has been desired when choosing the most appropriate method for obtaining accurate partitions. To tackle high dimensional data, subspace clustering was proposed [ 49 ]. This method works by considering the similarity between objects with respect to distinct subsets of the attributes [ 88 ]. The motivation for doing so is that different subsets of the attributes might define distinct separations between the data. Therefore, the algorithm can identify clusters that exist in multiple, possibly overlapping, subspaces [ 49 ]. Subspace algorithms can be categorized into four main families [ 89 ], namely: lattice, statistical, approximation and hybrid. The hddc function from package HDclassif implements the subspace clustering method of Bouveyron [ 90 ] in the R language. The algorithm is based on statistical models, with the assumption that all attributes may be relevant for clustering [ 91 ]. Some parameters of the algorithm, such as the number of clusters or model to be used, are estimated using an EM procedure.
So far, we have discussed the application of clustering algorithms on static data. Nevertheless, when analyzing data, it is important to take into account whether the data are dynamic or static. Dynamic data, unlike static data, undergo changes over time. Some kinds of data, like the network packets received by a router and credit card transaction streams, are transient in nature and they are known as data stream . Another example of dynamic data are time series because its values change over time [ 92 ]. Dynamic data usually include a large number of features and the amount of objects is potentially unbounded [ 59 ]. This requires the application of novel approaches to quickly process the entire volume of continuously incoming data [ 93 ], the detection of new clusters that are formed and the identification of outliers [ 94 ].
Materials and methods
Artificial datasets.
The proper comparison of clustering algorithms requires a robust artificial data generation method to produce a variety of datasets. For such a task, we apply a methodology based on a previous work by Hirschberger et al. [ 35 ]. The procedure can be used to generate normally distributed samples characterized by F features and separated into C classes. In addition, the method can control both the variance and correlation distributions among the features for each class. The artificial dataset can also be generated by varying the number of objects per class, N e , and the expected separation, α , between the classes.
For each class i in the dataset, a covariance matrix R i of size F × F is created, and this matrix is used for generating N e objects for the classes. This means that pairs of features can have distinct correlation for each generated class. Then, the generated class values are divided by α and translated by s i , where s i is a random variable described by a uniform random distribution defined in the interval [−1, 1]. Parameter α is associated with the expected distances between classes. Such distances can have different impacts on clusterization depending on the number of objects and features used in the dataset. The features in the generated data have a multivariate normal distribution. In addition, the covariance among the features also have a normal distribution. Notice that such a procedure for the generation of artificial datasets was previously used in [ 36 ].
In Fig 2 , we show some examples of artificially generated data. For visualization purposes, all considered cases contain F = 2 features. The parameters used for each case are described in the caption of the figure. Note that the methodology can generate a variety of dataset configurations, including variations in features correlation for each class.
The parameters used for each case are (a) C = 2, Ne = 100 and α = 3.3. (b) C = 2, Ne = 100 and α = 2.3. (c) C = 10, Ne = 50 and α = 4.3. (d) C = 10, Ne = 50 and α = 6.3. Note that each class can present highly distinct properties due to differences in correlation between their features.
https://doi.org/10.1371/journal.pone.0210236.g002
- Number of classes ( C ): The generated datasets are divided into C = {2, 10, 50} classes.
- Number of features ( F ): The number of features to characterize the objects is F = {2, 5, 10, 50, 200}.
- Number of object per class ( N e ): we considered Ne = {5, 50, 100, 500, 5000} objects per class. In our experiments, in a given generated dataset, the number of instances for each class is constant.
- Mixing parameter ( α ): This parameter has a non-trivial dependence on the number of classes and features. Therefore, for each dataset, the value of this parameter was tuned so that no algorithm would achieve an accuracy of 0% or 100%.
We refer to datasets containing 2, 10, 50 and 200 features as DB2F, DB10F, DB50F, DB200F respectively. Such datasets are composed of all considered number of classes, C = {2, 10, 50}, and 50 elements for each class (i.e., Ne = 50). In some cases, we also indicate the number of classes considered for the dataset. For example, dataset DB2C10F contains 2 classes, 10 features and 50 elements per class.
For each case, we consider 10 realizations of the dataset. Therefore, 400 datasets were generated in total.
Evaluating the performance of clustering algorithms
The evaluation of the quality of the generated partitions is one of the most important issues in cluster analysis [ 30 ]. Indices used for measuring the quality of a partition can be categorized into two classes, internal and external indices. Internal validation indices are based on information intrinsic to the data, and evaluates the goodness of a clustering structure without external information. When the correct partition is not available it is possible to estimate the quality of a partition measuring how closely each instance is related to the cluster and how well-separated a cluster is from other clusters. They are mainly used for choosing an optimal clustering algorithm to be applied on a specific dataset [ 96 ]. On the other hand, external validation indices measure the similarity between the output of the clustering algorithm and the correct partitioning of the dataset. The Jaccard, Fowlkes-Mallows and adjusted rand index belong to the same pair counting category, making them closely related. Some differences include the fact that they can exhibit biasing with respect to the number of clusters or the distribution of class sizes in a partition. Normalization helps prevent this unwanted effect. In [ 97 ] the authors discuss several types of bias that may affect external cluster validity indices. A total of 26 pair-counting based external cluster validity indices were used to identify the bias generated by the number of clusters. It was shown that the Fowlkes Mallows and Jaccard index monotonically decrease as the number of clusters increases, favoring partitions with smaller number of clusters, while the Adjusted Rand Index tends to be indifferent to the number of clusters.
Note that when the two sets of labels have a perfect one-to-one correspondence, the quality measures are all equal to unity.
Previous works have shown that there is no single internal cluster validation index that outperforms the other indices [ 100 , 101 ]. In [ 101 ] the authors compare a set of internal cluster validation indices in many distinct scenarios, indicating that the Silhouette index yielded the best results in most cases.
Results and discussion
The accuracy of each considered clustering algorithm was evaluated using three methodologies. In the first methodology, we consider the default parameters of the algorithms provided by the R package. The reason for measuring performance using the default parameters is to consider the case where a researcher applies the classifier to a dataset without any parameter adjustment. This is a common scenario when the researcher is not a machine learning expert. In the second methodology, we quantify the influence of the algorithms parameters on the accuracy. This is done by varying a single parameter of an algorithm while keeping the others at their default values. The third methodology consists in analyzing the performance by randomly varying all parameters of a classifier. This procedure allows the quantification of certain properties such as the maximum accuracy attained and the sensibility of the algorithm to parameter variation.
Performance when using default parameters
In this experiment, we evaluated the performance of the classifiers for all datasets described in Section Artificial datasets . All unsupervised algorithms were set with their default configuration of parameters. For each algorithm, we divide the results according to the number of features contained in the dataset. In other words, for a given number of features, F , we used datasets with C = {2, 10, 50, 200} classes, and N e = {5, 50, 100} objects for each class. Thus, the performance results obtained for each F corresponds to the performance averaged over distinct number of classes and objects per class. We note that the algorithm based on subspaces cannot be applied to datasets containing 2 features, and therefore its accuracy was not quantified for such datasets.
In Fig 3 , we show the obtained values for the four considered performance metrics. The results indicate that all performance metrics provide similar results. Also, the hierarchical method seems to be strongly affected by the number of features in the dataset. In fact, when using 50 and 200 features the hierarchical method provided lower accuracy. The k-means, spectral, optics and dbscan methods benefit from an increment in the number of features. Interestingly, the hcmodel has a better performance in the datasets containing 10 features than in those containing 2, 50 and 200 features, which suggests an optimum performance for this algorithm for datasets containing around 10 features. It is also clear that for 2 features the performance of the algorithms tend to be similar, with the exception of the optics and dbscan methods. On the other hand a larger number of features induce marked differences in performance. In particular, for 200 features, the spectral algorithm provides the best results among all classifiers.
All artificial datasets were used for evaluation. The averages were calculated separately for datasets containing 2, 10 and 50 features. The considered performance indexes are (a) adjusted Rand, (b) Jaccard, (c) normalized mutual information and (d) Fowlkes Mallows.
https://doi.org/10.1371/journal.pone.0210236.g003
We use the Kruskal-Wallis test [ 102 ], a nonparametric test, to explore the statistical differences in performance when considering distinct number of features in clustering methods. First, we test if the difference in performance is significant for 2 features. For this case, the Kruskal-Wallis test returns a p-value of p = 6.48 × 10 −7 , with a chi-squared distance of χ 2 = 41.50. Therefore, the difference in performance is statistically significant when considering all algorithms. For datasets containing 10 features, a p-value of p = 1.53 × 10 −8 is returned by the Kruskal-Wallis test, with a chi-squared distance of χ 2 = 52.20). For 50 features, the test returns a p-value of p = 1.56 × 10 −6 , with a chi-squared distance of χ 2 = 41.67). For 200 features, the test returns a p-value of p = 2.49 × 10 −6 , with a chi-squared distance of χ 2 = 40.58). Therefore, the null hypothesis of the Kruskal–Wallis test is rejected. This means that the algorithms indeed have significant differences in performance for 2, 10, 50 and 200 features, as indicated in Fig 3 .
In order to verify the influence of the number of objects used for classification, we also calculated the average accuracy for datasets separated according to the number of objects N e . The result is shown in Fig 4 . We observe that the impact that changing N e has on the accuracy depends on the algorithm. Surprisingly, the hierarchical, k-means and clara methods attain lower accuracy when more data is used. The result indicates that these algorithms tend to be less robust with respect to the larger overlap between the clusters due to an increase in the number of objects. We also observe that a larger N e enhances the performance of the hcmodel, optics and dbscan algorithms. This results is in agreement with [ 90 ].
All artificial datasets were used for evaluation. The averages were calculated separately for datasets containing 5, 50 and 100 objects per class. The considered performance indexes are (a) adjusted Rand, (b) Jaccard, (c) normalized mutual information and (d) Fowlkes Mallows.
https://doi.org/10.1371/journal.pone.0210236.g004
In most clustering algorithms, the size of the data has an effect on the clustering quality. In order to quantify this effect, we considered a scenario where the data has a high number of instances. Datasets with F = 5, C = 10 and Ne = {5, 50, 500, 5000} instances per class were created. This dataset will be referenced as DB10C5F. In Fig 5 we can observe that the subspace and spectral methods lead to improved accuracy when the number of instances increases. On the other hand, the size of the dataset does not seem to influence the accuracy of the kmeans, clara, hcmodel and EM algorithms. For the spectral, hierarchical and hcmodel algorithms, the accuracy could not be calculated when 5000 instances per class was used due to the amount of memory used by these methods. For example, in the case of the spectral algorithm method, a lot of processing power is required to compute and store the kernel matrix when the algorithm is executed. When the size of the dataset is too small, we see that the subspace algorithm results in low accuracy.
The plots correspond to the ARI, Jaccard and FM indexes averaged for all datasets containing 10 classes and 5 features (DB10C5F).
https://doi.org/10.1371/journal.pone.0210236.g005
It is also interesting to verify the performance of the clustering algorithms when setting distinct values for the expected number of classes K in the dataset. Such a value is usually not known beforehand in real datasets. For instance, one might expect the data to contain 10 classes, and, as a consequence, set K = 10 in the algorithm, but the objects may actually be better accommodated into 12 classes. An accurate algorithm should still provide reasonable results even when a wrong number of classes is assumed. Thus, we varied K for each algorithm and verified the resulting variation in accuracy. Observe that the optics and dbscan methods were not considered in this analysis as they do not have a parameter for setting the number of classes. In order to simplify the analysis, we only considered datasets comprising objects described by 10 features and divided into 10 classes (DB10C10F). The results are shown in Fig 6 . The top figures correspond to the average ARI and Jaccard indexes calculated for DB10C10F, while the Silhoute and Dunn indexes are shown at the bottom of the figure. The results indicate that setting K < 10 leads to a worse performance than obtained for the cases where K > 10, which suggests that a slight overestimation of the number of classes has smaller effect on the performance. Therefore, a good strategy for choosing K seems to be setting it to values that are slightly larger than the number of expected classes. An interesting behavior is observed for hierarchical clustering. The accuracy improves as the number of expected classes increases. This behavior is due to the default value of the method parameter, which is set as “average”. The “average” value means that the unweighted pair group method with arithmetic mean (UPGMA) is used to agglomerate the points. UPGMA is the average of the dissimilarities between the points in one cluster and the points in the other cluster. The moderate performance of UPGMA in recovering the original groups, even with high subgroup differentiation, is probably a consequence of the fact that UPGMA tends to result in more unbalanced clusters, that is, the majority of the objects are assigned to a few clusters while many other clusters contain only one or two objects.
The upper plots correspond to the ARI and Jaccard indices averaged for all datasets containing 10 classes and 10 features (DB10C10F). The lower plots correspond to the Silhouette and Dunn indices for the same dataset. The red line indicates the actual number of clusters in the dataset.
https://doi.org/10.1371/journal.pone.0210236.g006
The external validation indices show that most of the clustering algorithms correctly identify the 10 main clusters in the dataset. Naturally, this knowledge would not be available in a real life cluster analysis. For this reason, we also consider internal validation indices, which provides feedback on the partition quality. Two internal validation indices were considered, the Silhouette index (defined in the range [−1,1]) and the Dunn index (defined in the range [0, ∞]). These indices were applied to the DB10C10F and DB10C2F dataset while varying the expected number of clusters K . The results are presented in Figs 6 and 7 . In Fig 6 we can see that the results obtained for the different algorithms are mostly similar. The results for the Silhouette index indicate high accuracy around k = 10. The Dunn index displays a slightly lower performance, misestimating the correct number of clusters for the hierarchical algorithm. In Fig 7 Silhouette and Dunn show similar behavior.
The upper plots correspond to the ARI and Jaccard indices averaged for all datasets containing 10 classes and 2 features (DB10C2F). The lower plots correspond to the Silhouette and Dunn indices for the same dataset. The red line indicates the actual number of clusters in the dataset.
https://doi.org/10.1371/journal.pone.0210236.g007
The results obtained for the default parameters are summarized in Table 2 . The table is divided into four parts, each part corresponds to a performance metric. For each performance metric, the value in row i and column j of the table represents the average performance of the method in row i minus the average performance of the method in column j . The last column of the table indicates the average performance of each algorithm. We note that the averages were taken over all generated datasets.
In general, the spectral algorithm provides the highest accuracy rate among all evaluated methods.
https://doi.org/10.1371/journal.pone.0210236.t002
The results shown in Table 2 indicate that the spectral algorithm tends to outperform the other algorithms by at least 10%. On the other hand, the hierarchical method attained lower performance in most of the considered cases. Another interesting result is that the k-means and clara provided equivalent performance when considering all datasets. In the light of the results, the spectral method could be preferred when no optimitization of parameters values is performed.
One-dimensional analysis
In addition to the aforementioned quantities, we also measured, for each dataset, the maximum accuracy obtained when varying each single parameter of the algorithm. We then calculate the average of maximum accuracies, 〈max Acc〉, obtained over all considered datasets. In Table 3 , we show the values of 〈 S 〉, max S , Δ S and 〈max Acc〉 for datasets containing two features. When considering a two-class problem (DB2C2F), a significant improvement in performance (〈 S 〉 = 10.75% and 〈 S 〉 = 13.35%) was observed when varying parameter modelName , minPts and kpar of, respectively, the EM, optics and spectral methods. For all other cases, only minor average gain in performance was observed. For the 10-class problem, we notice that an inadequate value for parameter method of the hierarchical algorithm can lead to substantial loss of accuracy (16.15% on average). In most cases, however, the average variation in performance was small.
This analysis is based on the performance (measured through the ARI index) obtained when varying a single parameter of the clustering algorithm, while maintaining the others in their default configuration. 〈 S 〉, max S , Δ S are associated with the average, standard deviation and maximum difference between the performance obtained when varying a single parameter and the performance obtained for the default parameter values. We also measure 〈max Acc〉, the average of best ARI values obtained when varying each parameter, where the average is calculated over all considered datasets.
https://doi.org/10.1371/journal.pone.0210236.t003
In Table 4 , we show the values of 〈 S 〉, max S , Δ S and 〈max Acc〉 for datasets described by 10 features. For the the two-class clustering problem, a moderate improvement can be observed for the k-means, hierarchical and optics algorithm through the variation of, respectively, parameter nstart , method and minPts . A large increase in accuracy was observed when varying parameter modelName of the EM method. Changing the modelName used by the algorithm led to, on average, an improvement of 18.8%. A similar behavior was obtained when the number of classes was set to C = 10. For 10 classes, the variation of method in the hierarchical algorithm provided an average improvement of 6.72%. A high improvement was also observed when varying parameter modelName of the EM algorithm, with an average improvement of 13.63%.
This analysis is based on the performance obtained when varying a single parameter, while maintaining the others in their default configuration. 〈 S 〉, max S , Δ S are associated with the average, standard deviation and maximum difference between the performance obtained when varying a single parameter and the performance obtained for the default parameter values. We also measure 〈max Acc〉, the average of best ARI values obtained when varying each parameter, where the average is calculated over all considered datasets.
https://doi.org/10.1371/journal.pone.0210236.t004
Differently from the parameters discussed so far, the variation of some parameters plays a minor role in the discriminative power of the clustering algorithms. This is the case, for instance, of parameters kernel and iter of the spectral clustering algorithm and parameter iter.max of the kmeans clustering. In some cases, the effect of a unidimensional variation of parameter resulted in reduction of performance. For instance, the variation of min.individuals and models of the subspace algorithm provided an average loss of accuracy on the order of 〈 S 〉 = 20%, depending on the dataset. Similar behavior is observed for the dbscan method, for which the variation of minPts causes and average loss of accuracy of 20.32%. Parameters metric and rngR of the clara algorithm also led to marked decrease in performance.
In Table 5 , we show the values of 〈 S 〉, max S , Δ S and 〈max Acc〉 for datasets described by 200 features. For the two-class clustering problem, a significant improvement in performance was observed when varying nstart in the k-means method, method in the hierarchical algorithm, modelName in the hcmodel method and modelName in the EM method. On the other hand, when varying metric , min.individuals and use in, respectively, the clara, subspace, and hcmodel methods an average loss of accuracy larger than 10% was verified. The largest loss of accuracy happens with parameter minPts (49.47%) of the dbscan method. For the 10-class problem, similar results were observed, with the exception of the clara method, for which any parameter change resulted in a large loss of accuracy.
This analysis is based on the performance obtained when varying a single parameter, while maintaining the others in their default configuration. 〈 S 〉, max S , Δ S are associated with the average, standard deviation and maximum difference between the performance obtained when varying a single parameter and the performance obtained for the default parameter values. We also measure 〈max Acc〉, the average of best ARI values obtained when varying each parameter.
https://doi.org/10.1371/journal.pone.0210236.t005
Multi-dimensional analysis
A complete analysis of the performance of a clustering algorithm requires the simultaneous variation of all of its parameters. Nevertheless, such a task is difficult to do in practice, given the large number of parameter combinations that need to be taken into account. Therefore, here we consider a random variation of parameters aimed at obtaining a sampling of each algorithm performance for its complete multi-dimensional parameter space.
The performance of the algorithms for the different sets of parameters was evaluated according to the following procedure. Consider the histogram of ARI values obtained for the random sampling of parameters for the k-means algorithm, shown in Fig 8 . The red dashed line indicates the ARI value obtained for the default parameters of the algorithm. The light blue shaded region indicates the parameters configurations where the performance of the algorithm improved. From this result we calculated four main measures. The first, which we call p-value, is given by the area of the blue region divided by the total histogram area, multiplied by 100 in order to result in a percentage value. The p-value represents the percentage of parameter configurations where the algorithm performance improved when compared to the default parameters configuration. The second, third and fourth measures are given by the mean, 〈 R 〉, standard deviation, Δ R , and maximum value, max R , of the relative performance for all cases where the performance is improved (e.g. the blue shaded region in Fig 8 ). The relative performance is calculated as the difference in performance between a given realization of parameter values and the default parameters. The mean indicates the expected improvement of the algorithm for the random variation of parameters. The standard deviation represents the stability of such improvement, that is, how certain one is that the performance will be improved when doing such random variation. The maximum value indicates the largest improvement obtained when random parameters are considered. We also measured the average of the maximum accuracies 〈max ARI〉 obtained for each dataset when randomly selecting the parameters. In the S2 File of the supplementary material we show the distribution of ARI values obtained for the random sampling of parameters for all clustering algorithms considered in our analysis.
The algorithm was applied to dataset DB10C10F, and 500 sets of parameters were drawn.
https://doi.org/10.1371/journal.pone.0210236.g008
In Table 6 we show the performance (ARI) of the algorithms for dataset DB2C2F when applying the aforementioned random selection of parameters. The optics and EM methods are the only algorithms with a p-value larger than 50%. Also, a high average gain in performance was observed for the EM (22.1%) and hierarchical (30.6%) methods. Moderate improvement was observed for the hcmodel, kmeans, spectral, optics and dbscan algorithms.
The p-value represents the probability that the classifier set with a random configuration of parameters outperform the same classifier set with its default parameters. 〈 R 〉, Δ R and max R represent the average, standard deviation and maximum value of the improvement obtained when random parameters are considered. Column 〈max ARI〉 indicates the average of the best accuracies obtained for each dataset.
https://doi.org/10.1371/journal.pone.0210236.t006
The performance of the algorithms for dataset DB10C2F is presented in Table 7 . A high p-value was obtained for the optics (96.6%), EM (76.5%) and k-means (77.7%). Nevertheless, the average improvement in performance was relatively low for most algorithms, with the exception of the optics method, which led to an average improvement of 15.9%.
https://doi.org/10.1371/journal.pone.0210236.t007
A more marked variation in performance was observed for dataset DB2C10F, with results shown in Table 8 . The EM, kmeans, hierarchical and optics clustering algorithms resulted in a p-value larger than 50%. In such cases, when the performance was improved, the average gain in performance was, respectively, 30.1%, 18.0%, 25.9% and 15.5%. This means that the random variation of parameters might represent a valid approach for improving these algorithms. Actually, with the exception of clara and dbscan, all methods display significant average improvement in performance for this dataset. The results also show that a maximum accuracy of 100% can be achieved for the EM and subspace algorithms.
https://doi.org/10.1371/journal.pone.0210236.t008
In Table 9 we show the performance of the algorithms for dataset DB10C10F. The p-values for the EM, clara, k-means and optics indicate that the random selection of parameters usually improves the performance of these algorithms. The hierarchical algorithm can be significantly improved by the considered random selection of parameters. This is a consequence of the default value of parameter method , which, as discussed in the previous section, is not appropriate for this dataset.
https://doi.org/10.1371/journal.pone.0210236.t009
The performance of the algorithms for the dataset DB2C200F is presented in Table 10 . A high p-value was obtained for the EM (65.1%) and k-means (65.6%) algorithms. The average gain in performance in such cases was 39.1% and 35.4%, respectively. On the other hand, only in approximately 16% of the cases the Spectral and Subspace methods resulted in an improved ARI. Interestingly, the random variation of parameters led to, on average, large performance improvements for all algorithms.
https://doi.org/10.1371/journal.pone.0210236.t010
In Table 11 we show the performance of the algorithms for dataset DB10C200F. A high p-value was obtained for all methods. On the other hand, the average improvement in accuracy tended to be lower than in the case of the dataset DB2C200F.
https://doi.org/10.1371/journal.pone.0210236.t011
Conclusions
Clustering data is a complex task involving the choice between many different methods, parameters and performance metrics, with implications in many real-world problems [ 63 , 103 – 108 ]. Consequently, the analysis of the advantages and pitfalls of clustering algorithms is also a difficult task that has been received much attention. Here, we approached this task focusing on a comprehensive methodology for generating a large diversity of heterogeneous datasets with precisely defined properties such as the distances between classes and correlations between features. Using packages in the R language, we developed a comparison of the performance of nine popular clustering methods applied to 400 artificial datasets. Three situations were considered: default parameters, single parameter variation and random variation of parameters. It should be nevertheless be borne in mind that all results reported in this work are respective to specific configurations of normally distributed data and algorithmic implementations, so that different performance can be obtained in other situations. Besides serving as a practical guidance to the application of clustering methods when the researcher is not an expert in data mining techniques, a number of interesting results regarding the considered clustering methods were obtained.
Regarding the default parameters, the difference in performance of clustering methods was not significant for low-dimensional datasets. Specifically, the Kruskal-Wallis test on the differences in performance when 2 features were considered resulted in a p-value of p = 6.48 × 10 −7 (with a chi-squared distance of χ 2 = 41.50). For 10 features, a p-value of p = 1.53 × 10 −8 ( χ 2 = 52.20) was obtained. Considering 50 features resulted in a p-value of p = 1.56 × 10 −6 for the Kruskal-Wallis test ( χ 2 = 41.67). For 200 features, the obtained p-value was p = 2.49 × 10 −6 ( χ 2 = 40.58).
The Spectral method provided the best performance when using default parameters, with an Adjusted Rand Index (ARI) of 68.16%, as indicated in Table 2 . In contrast, the hierarchical method yielded an ARI of 21.34%. It is also interesting that underestimating the number of classes in the dataset led to worse performance than in overestimation situations. This was observed for all algorithms and is in accordance with previous results [ 44 ].
Regarding single parameter variations, for datasets containing 2 features, the hierarchical, optics and EM methods showed significant performance variation. On the other hand, for datasets containing 10 or more features, most methods could be readily improved through changes on selected parameters.
With respect to the multidimensional analysis for datasets containing ten classes and two features, the performance of the algorithms for the multidimensional selection of parameters was similar to that using the default parameters. This suggests that the algorithms are not sensitive to parameter variations for this dataset. For datasets containing two classes and ten features, the EM, hcmodel, subspace and hierarchical algorithm showed significant gain in performance. The EM algorithm also resulted in a high p-value (70.8%), which indicates that many parameter values for this algorithm can provide better results than the default configuration. For datasets containing ten classes and ten features, the improvement was significantly lower for almost all the algorithms, with the exception of the hierarchical clustering. When a large number of features was considered, such as in the case of the datasets containing 200 features, large gains in performance were observed for all methods.
In Tables 12 , 13 and 14 we show a summary of the best accuracies obtained during our analysis. The tables contain the best performance, measured as the ARI of the resulting partitions, achieved by each algorithm in the three considered situations (default, one- and multi-dimensional adjustment of parameters). The results are respective to datasets DB2C2F, DB10C2F, DB2C10F, DB10C10F, DB2C200F and DB10C200F. We observe that, for datasets containing 2 features, the algorithms tend to show similar performance, specially when the number of classes is increased. For datasets containing 10 features or more, the spectral algorithm seems to consistently provide the best performance, although the EM, hierarchical, k-means and subspace algorithms can also achieve similar performance with some parameter tuning. It should be observed that several clustering algorithms, such as optics and dbscan, aim at other data distributions such as elongated or S-shaped [ 72 , 74 ]. Therefore, different results could be obtained for non-normally distributed data.
https://doi.org/10.1371/journal.pone.0210236.t012
https://doi.org/10.1371/journal.pone.0210236.t013
https://doi.org/10.1371/journal.pone.0210236.t014
Other algorithms could be compared in future extensions of this work. An important aspect that could also be explored is to consider other statistical distributions for modeling the data. In addition, an analogous approach could be applied to semi-supervised classification.
Supporting information
S1 file. description of the clustering algorithms’ parameters..
We provide a brief description about the parameters of the clustering algorithms considered in the main text.
https://doi.org/10.1371/journal.pone.0210236.s001
S2 File. Clustering performance obtained for the random selection of parameters.
The file contains figures showing the histograms of ARI values obtained for identifying the clusters of, respectively, datasets DB10C10F and DB2C10F using a random selection of parameters. Each plot corresponds to a clustering method considered in the main text.
https://doi.org/10.1371/journal.pone.0210236.s002
- View Article
- PubMed/NCBI
- Google Scholar
- 7. Aggarwal CC, Zhai C. In: Aggarwal CC, Zhai C, editors. A Survey of Text Clustering Algorithms. Boston, MA: Springer US; 2012. p. 77–128.
- 13. Joachims T. Text categorization with support vector machines: Learning with many relevant features. In: European conference on machine learning. Springer; 1998. p. 137–142.
- 14. Witten IH, Frank E. Data Mining: Practical Machine Learning Tools and Techniques. 2nd ed. San Francisco: Morgan Kaufmann; 2005.
- 37. Berkhin P. In: Kogan J, Nicholas C, Teboulle M, editors. A Survey of Clustering Data Mining Techniques. Berlin, Heidelberg: Springer Berlin Heidelberg; 2006. p. 25–71.
- 42. R Development Core Team. R: A Language and Environment for Statistical Computing; 2006. Available from: http://www.R-project.org .
- 44. Erman J, Arlitt M, Mahanti A. Traffic classification using clustering algorithms. In: Proceedings of the 2006 SIGCOMM workshop on mining network data. ACM; 2006. p. 281–286.
- 47. Parsons L, Haque E, Liu H. Evaluating subspace clustering algorithms. In: Workshop on Clustering High Dimensional Data and its Applications, SIAM Int. Conf. on Data Mining. Citeseer; 2004. p. 48–56.
- 48. Burdick D, Calimlim M, Gehrke J. MAFIA: A Maximal Frequent Itemset Algorithm for Transactional Databases. In: Proceedings of the 17th International Conference on Data Engineering. Washington, DC, USA: IEEE Computer Society; 2001. p. 443–452.
- 51. UCI. breast-cancer-wisconsin;. Available from: https://http://archive.ics.uci.edu/ml/machine-learning-databases/breast-cancer-wisconsin/ .
- 52. Ultsch A. Clustering wih som: U* c. In: Proceedings of the 5th Workshop on Self-Organizing Maps. vol. 2; 2005. p. 75–82.
- 54. Aggarwal CC, Reddy CK. Data Clustering: Algorithms and Applications. vol. 2. 1st ed. Chapman & Hall/CRC; 2013.
- 58. Jain AK, Topchy A, Law MH, Buhmann JM. Landscape of clustering algorithms. In: Pattern Recognition, 2004. ICPR 2004. Proceedings of the 17th International Conference on. vol. 1. IEEE; 2004. p. 260–263.
- 64. Kaufman L, Rousseeuw PJ. Finding groups in data: an introduction to cluster analysis. Series in Probability& Mathematical Statistics. 2009;.
- 65. Arthur D, Vassilvitskii S. k-means++: The advantages of careful seeding. In: Proceedings of the eighteenth annual ACM-SIAM symposium on Discrete algorithms. Society for Industrial and Applied Mathematics; 2007. p. 1027–1035.
- 66. Sequeira K, Zaki M. ADMIT: anomaly-based data mining for intrusions. In: Proceedings of the eighth ACM SIGKDD international conference on Knowledge discovery and data mining. ACM; 2002. p. 386–395.
- 67. Williams GJ, Huang Z. Mining the knowledge mine. In: Australian Joint Conference on Artificial Intelligence. Springer; 1997. p. 340–348.
- 68. MacQueen J. Some methods for classification and analysis of multivariate observations. In: Proceedings of the Fifth Berkeley Symposium on Mathematical Statistics and Probability, Volume 1: Statistics. Berkeley, Calif: University of California Press; 1967. p. 281–297.
- 70. Kaufman L, Rousseeuw PJ. Finding Groups in Data: an introduction to cluster analysis. John Wiley & Sons; 1990.
- 71. Han J, Kamber M. Data Mining. Concepts and Techniques. vol. 2. 2nd ed. -: Morgan Kaufmann; 2006.
- 73. Ankerst M, Breunig MM, Kriegel HP, Sander J. OPTICS: Ordering Points To Identify the Clustering Structure. ACM Press; 1999. p. 49–60.
- 74. Ester M, Kriegel HP, Sander J, Xu X. A Density-based Algorithm for Discovering Clusters a Density-based Algorithm for Discovering Clusters in Large Spatial Databases with Noise. In: Proceedings of the Second International Conference on Knowledge Discovery and Data Mining. KDD’96. AAAI Press; 1996. p. 226–231.
- 86. Ng AY, Jordan MI, Weiss Y. On spectral clustering: Analysis and an algorithm. In: Advances in Neural Information Processing Systems 14. MIT Press; 2001. p. 849–856.
- 95. Horn RA, Johnson CR. Matrix Analysis. 2nd ed. New York, NY, USA: Cambridge University Press; 2012.
- 96. Liu Y, Li Z, Xiong H, Gao X, Wu J. Understanding of internal clustering validation measures. In: Data Mining (ICDM), 2010 IEEE 10th International Conference on. IEEE; 2010. p. 911–916.
- 98. Cover TM, Thomas JA. Elements of Information Theory. vol. 2. Wiley; 2012.
- 102. McKight PE, Najab J. Kruskal-Wallis Test. Corsini Encyclopedia of Psychology. 2010;.
Data clustering: a review

New Citation Alert added!
This alert has been successfully added and will be sent to:
You will be notified whenever a record that you have chosen has been cited.
To manage your alert preferences, click on the button below.
New Citation Alert!
Please log in to your account
Information & Contributors
Bibliometrics & citations, view options.
- Wani A (2024) Comprehensive analysis of clustering algorithms: exploring limitations and innovative solutions PeerJ Computer Science 10.7717/peerj-cs.2286 10 (e2286) Online publication date: 29-Aug-2024 https://doi.org/10.7717/peerj-cs.2286
- Duan G Zou C (2024) A clustering effectiveness measurement model based on merging similar clusters PeerJ Computer Science 10.7717/peerj-cs.1863 10 (e1863) Online publication date: 29-Feb-2024 https://doi.org/10.7717/peerj-cs.1863
- Khodadad N Khalili A Mirza Kochch Khoshnevis A (2024) Bibliometric Analysis and Systematic Review of Infill Development Based on Mapping the Scientific Fields Structure Journal of Researches in Islamic Architecture 10.61186/jria.12.1.7 12 :1 (0-0) Online publication date: 1-Mar-2024 https://doi.org/10.61186/jria.12.1.7
- Show More Cited By
Recommendations
Survey of clustering: algorithms and applications.
This article is a survey into clustering applications and algorithms. A number of important well-known clustering methods are discussed. The authors present a brief history of the development of the field of clustering, discuss various types of ...
A new approach to clustering data with arbitrary shapes
In this paper we propose a clustering algorithm to cluster data with arbitrary shapes without knowing the number of clusters in advance. The proposed algorithm is a two-stage algorithm. In the first stage, a neural network incorporated with an ART-like ...
A novel optimization approach towards improving separability of clusters
The objective functions in optimization models of the sum-of-squares clustering problem reflect intra-cluster similarity and inter-cluster dissimilarities and in general, optimal values of these functions can be considered as ...
- New optimization model is formulated for hard partitional clustering problem.
Reviewer: Jose M. Ramirez
Data clustering is not defined the same way in each of the disciplines that use it to deal with problems that involve the extraction of information or structure from data. The authors have produced a good survey of this slippery topic. They devote a considerable amount of space to presenting clustering techniques from the perspective of several disciplines, including fuzzy systems, neural networks, and searching. The section of definitions and notations is weak: it is just a glossary of terms, with no context provided. It would have been better to define the terms when they were needed for each technique described. References are numerous, as expected in a survey, but are not annotated sufficiently to enable readers to define a research plan on a given aspect. In some places the paper does not reflect the state of the art in the use of clustering, as, for examples in neural networks and fuzzy systems. One cause of this weakness is the problem of dealing with a multidisciplinary subject whose advances are reported in a wide range of journals and proceedings. The other cause, namely the extremely long review process, is completely out of the authors' control: the paper was received in March 1997, but accepted in January 1999.

Access critical reviews of Computing literature here
Become a reviewer for Computing Reviews.
Information
Published in.

Association for Computing Machinery
New York, NY, United States
Publication History
Permissions, check for updates, author tags.
- cluster analysis
- clustering applications
- exploratory data analysis
- incremental clustering
- similarity indices
- unsupervised learning
Contributors
Other metrics, bibliometrics, article metrics.
- 9,579 Total Citations View Citations
- 76,760 Total Downloads
- Downloads (Last 12 months) 5,616
- Downloads (Last 6 weeks) 596
- Figueiredo I Frediani J Araujo M Prado S Costa K (2024) MODELO DE AUTOENCODER COM ENSEMBLE LEARNING E CLUSTERIZAÇÃO PARA DETECÇÃO DE INTRUSÃO EM REDES Revista Contemporânea 10.56083/RCV4N6-223 4 :6 (e4910) Online publication date: 28-Jun-2024 https://doi.org/10.56083/RCV4N6-223
- ALASALI T ORTAKCI Y (2024) Clustering Techniques in Data Mining: A Survey of Methods, Challenges, and ApplicationsVeri Madenciliğinde Kümeleme Teknikleri: Yöntemler, Zorluklar ve Uygulamalar Üzerine Bir Araştırma Computer Science 10.53070/bbd.1421527 Online publication date: 5-Mar-2024 https://doi.org/10.53070/bbd.1421527
- Sharma V Srivastava D Kumar L Payal M (2024) A Novel Study on IoT and Machine Learning-Based Transportation Machine Learning Techniques and Industry Applications 10.4018/979-8-3693-5271-7.ch001 (1-28) Online publication date: 3-May-2024 https://doi.org/10.4018/979-8-3693-5271-7.ch001
- Hajamydeen A Yassin W (2024) Beyond Supervision Recent Trends and Future Direction for Data Analytics 10.4018/979-8-3693-3609-0.ch007 (170-196) Online publication date: 12-Jul-2024 https://doi.org/10.4018/979-8-3693-3609-0.ch007
- Poly A Banu P (2024) Discovering the Micro-Clusters From a Group of DHH Learners Transforming Education for Personalized Learning 10.4018/979-8-3693-0868-4.ch010 (159-175) Online publication date: 26-Apr-2024 https://doi.org/10.4018/979-8-3693-0868-4.ch010
- Jevsejev R Bereiša M (2024) IMPROVEMENT OF INCIDENT MANAGEMENT MODEL USING MACHINE LEARNING METHODS Mokslas - Lietuvos ateitis 10.3846/mla.2024.21633 16 (1-6) Online publication date: 5-Jan-2024 https://doi.org/10.3846/mla.2024.21633
- Liu Z Zhou J Yang X Zhao Z Lv Y (2024) Research on Water Resource Modeling Based on Machine Learning Technologies Water 10.3390/w16030472 16 :3 (472) Online publication date: 31-Jan-2024 https://doi.org/10.3390/w16030472
View options
View or Download as a PDF file.
View online with eReader .
Login options
Check if you have access through your login credentials or your institution to get full access on this article.
Full Access
Share this publication link.
Copying failed.
Share on social media
Affiliations, export citations.
- Please download or close your previous search result export first before starting a new bulk export. Preview is not available. By clicking download, a status dialog will open to start the export process. The process may take a few minutes but once it finishes a file will be downloadable from your browser. You may continue to browse the DL while the export process is in progress. Download
- Download citation
- Copy citation
We are preparing your search results for download ...
We will inform you here when the file is ready.
Your file of search results citations is now ready.
Your search export query has expired. Please try again.
IEEE Account
- Change Username/Password
- Update Address
Purchase Details
- Payment Options
- Order History
- View Purchased Documents
Profile Information
- Communications Preferences
- Profession and Education
- Technical Interests
- US & Canada: +1 800 678 4333
- Worldwide: +1 732 981 0060
- Contact & Support
- About IEEE Xplore
- Accessibility
- Terms of Use
- Nondiscrimination Policy
- Privacy & Opting Out of Cookies
A not-for-profit organization, IEEE is the world's largest technical professional organization dedicated to advancing technology for the benefit of humanity. © Copyright 2024 IEEE - All rights reserved. Use of this web site signifies your agreement to the terms and conditions.
Help | Advanced Search
Statistics > Methodology
Title: a model-based approach for clustering binned data.
Abstract: Binned data often appears in different fields of research, and it is generated after summarizing the original data in a sequence of pairs of bins (or their midpoints) and frequencies. There may exist different reasons to only provide this summary, but more importantly, it is necessary being able to perform statistical analyses based only on it. We present a Bayesian nonparametric model for clustering applicable for binned data. Clusters are modeled via random partitions, and within them a model-based approach is assumed. Inferences are performed by a Markov chain Monte Carlo method and the complete proposal is tested using simulated and real data. Having particular interest in studying marine populations, we analyze samples of Lobatus (Strobus) gigas' lengths and found the presence of up to three cohorts along the year.
Subjects: | Methodology (stat.ME); Statistics Theory (math.ST) |
Cite as: | [stat.ME] |
(or [stat.ME] for this version) | |
Focus to learn more arXiv-issued DOI via DataCite |
Submission history
Access paper:.
- HTML (experimental)
- Other Formats

References & Citations
- Google Scholar
- Semantic Scholar
BibTeX formatted citation

Bibliographic and Citation Tools
Code, data and media associated with this article, recommenders and search tools.
- Institution
arXivLabs: experimental projects with community collaborators
arXivLabs is a framework that allows collaborators to develop and share new arXiv features directly on our website.
Both individuals and organizations that work with arXivLabs have embraced and accepted our values of openness, community, excellence, and user data privacy. arXiv is committed to these values and only works with partners that adhere to them.
Have an idea for a project that will add value for arXiv's community? Learn more about arXivLabs .

An official website of the United States government
The .gov means it’s official. Federal government websites often end in .gov or .mil. Before sharing sensitive information, make sure you’re on a federal government site.
The site is secure. The https:// ensures that you are connecting to the official website and that any information you provide is encrypted and transmitted securely.
- Publications
- Account settings
Preview improvements coming to the PMC website in October 2024. Learn More or Try it out now .
- Advanced Search
- Journal List
- Springer Nature - PMC COVID-19 Collection

An Improved K-means Clustering Algorithm Towards an Efficient Data-Driven Modeling
1 Department of Computer Science and Engineering, Chittagong University of Engineering & Technology, Chittagong, 4349 Bangladesh
MD. Asif Iqbal
Avijeet shil, m. j. m. chowdhury.
2 Department of Computer Science and Information Technology, La Trobe University, Victoria, 3086 Australia
Mohammad Ali Moni
3 School of Health and Rehabilitation Sciences, Faculty of Health and Behavioural Sciences, The University of Queensland, St Lucia, QLD 4072 Australia
Iqbal H. Sarker
Associated data.
Data and codes used in this work can be made available upon reasonable request
K-means algorithm is one of the well-known unsupervised machine learning algorithms. The algorithm typically finds out distinct non-overlapping clusters in which each point is assigned to a group. The minimum squared distance technique distributes each point to the nearest clusters or subgroups. One of the K-means algorithm’s main concerns is to find out the initial optimal centroids of clusters. It is the most challenging task to determine the optimum position of the initial clusters’ centroids at the very first iteration. This paper proposes an approach to find the optimal initial centroids efficiently to reduce the number of iterations and execution time . To analyze the effectiveness of our proposed method, we have utilized different real-world datasets to conduct experiments. We have first analyzed COVID-19 and patient datasets to show our proposed method’s efficiency. A synthetic dataset of 10M instances with 8 dimensions is also used to estimate the performance of the proposed algorithm. Experimental results show that our proposed method outperforms traditional kmeans++ and random centroids initialization methods regarding the computation time and the number of iterations.
Introduction
Machine learning is a subset of Artificial Intelligence that makes applications capable of learning and improves the result through the experience, not being programmed explicitly through computation [ 1 ]. Supervised and unsupervised are the basic approaches of the machine learning algorithm. The unsupervised algorithm identifies hidden data structures from unlabelled data contained in the dataset [ 2 ]. According to the hidden structures of the datasets, clustering algorithm is typically used to find the similar data groups which also can be considered as a core part of data science as mentioned in Sarker et al. [ 3 ].
In the context of data science and machine learning, K-Means clustering is known as one of the powerful unsupervised techniques to identify the structure of a given dataset. The clustering algorithm is the best choice for separating the data into groups and is extensively exercised for its simplicity [ 4 ]. Its applications have been seen in different important real-world scenarios, for example, recommendation systems, various smart city services as well as cybersecurity and many more to cluster the data. Beyond this, clustering is one of the most useful techniques for business data analysis [ 5 ]. Also, the K-means algorithm has been used to analyze the users’ behavior and context-aware services [ 6 ]. Moreover, the K-means algorithm plays a vital role in complicated feature extraction.
In terms of problem type, K-means algorithm is considered an NP-Hard problem [ 7 ]. It is widely used to find the number of clusters so that it is possible to divide the unlabelled dataset into clusters to solve real-world problems in various application domains, mentioned above. It is done by calculating the distances from a centroid of a cluster. We need to fix the initial centroids’ coordinates to find the number of clusters at initialization. Thus, this step has a crucial role in the K-means algorithm. Generally, we randomly select the initial centroids. If we can determine the initial centroids efficiently, it will take fewer steps to converge. According to D. T. Pham et al. [ 8 ], the overall complexity of the K-means algorithm is
Where t is the number of iterations, k is the number of clusters and n is the number of data points.
Optimization plays an important role both for supervised and unsupervised learning algorithms [ 9 ]. So, it will be a great advantage if we can save some computational costs by optimization. The paper will give an overview to find the initial centroids more efficiently with the help of principal component analysis (PCA) and percentile concept for estimating the initial centroids. We need to have fewer iterations and execution times than the conventional method.
In this paper, recent datasets of COVID-19, a healthcare dataset and a synthetic dataset size of 10 Million instances are used to analyze our proposed method. In the COVID-19 dataset, K-means clustering is used to divide the countries into different clusters based on the health care quality. A patient dataset with relatively high instances and low dimensions is used for clustering the patient and inspecting the performance of the proposed algorithm. And finally, a high instance of 10M synthetic dataset is used to evaluate the performance. We have also compared our method with the kmeans++ and random centroids selection methods.
The key contributions of our work are as follows-
- We propose an improved K-means clustering algorithm that can be used to build an efficient data-driven model.
- Our approach finds the optimal initial centroids efficiently to reduce the number of iterations and execution time.
- To show the efficiency of our model comparing with the existing approaches, we conduct experimental analysis utilizing a COVID-19 real-world dataset. A 10M synthetic dataset as well as a health care dataset have also been analyzed to determine our proposed method’s efficiency compared to the benchmark models.
This paper provides an algorithmic overview of our proposed method to develop an efficient k-means clustering algorithm. It is an extended and refined version of the paper [ 10 ]. Elaborations from the previous paper are (i) analysis of the proposed algorithm with two additional datasets along with the COVID-19 dataset [ 10 ], (ii) the comparison with random centroid selection and kmeans++ centroid selection methods, (iii) analysis of our proposed method more generalized way in different fields, and (iv) including more recent related works and summarizing several real-world applications.
In Sect. 2 , we’ll discuss the works of similar concepts. In Sect. 3 , we will further discuss our proposed methodology with a proper example. In Sect. 4 , we will show some experimental results, description of the datasets and a comparative analysis. In Sects. 5 and 6 , discussion and conclusion have been included.
Related Work
Several approaches have been made to find the initial cluster centroids more efficiently. In this section, we will bring some of these works. M. S. Rahman et al. [ 11 ], provided a centroids selection method based on radial and angular coordinates. The authors showed experimental evaluations for his proposed work for small(10k-20k) and large(1M-2M) datasets. However, the number of iterations of his proposed method isn’t constant for all the test cases. Thus, the runtime of his proposed method increases drastically with the increment of the cluster number. A. Kumar et al. also proposed to find initial centroids based on the dissimilarity tree [ 12 ]. This method improves k-means clustering slightly, but the execution time isn’t significantly enhanced. In [ 13 ], M.S. Mahmud et al. proposed a novel weighted average approach to finding the initial centroids by calculating the mean of every data point’s distance. It only describes the execution time of 3 clusters with 3 datasets. Improvement of execution time is also trivial. In [ 14 ], authors M. Goyal et al. also tried to find the centroids by dividing the sorted distances with k, the number of equal partitions. This method’s execution time has not been demonstrated. M. A. Lakshmi et al. [ 15 ] proposed a method to find initial centroids with the help of the nearest neighbour method. They compared their method using SSE(Sum of the Squared Differences)with random and kmeans++ initial centroids selection methods. SSE of their method was roughly similar to random and kmeans++ initial centroids selection methods. Moreover, they didn’t provide any comparison regarding execution time as well. K. B. Sawant [ 16 ] has proposed a method to find the initial cluster with the distance of the neighbourhood. The proposed method calculates and sorts all the distances from the first point. Then, the entire dataset was divided into equal portions. But the author didn’t mention any comparative analysis to prove his proposed method better than the existing one. In [ 17 ] , the authors proposed to save the distance to the nearest cluster of the previous iteration and used the distance to compare in the next iteration. But still, initial centroids are selected randomly. In [ 18 ], M. Motwani et al. proposed a method with the farthest distributed centroids clustering (FDCC) algorithm. The authors failed to include information on how this approach performs with a distributed dataset and a comparison of execution times. M. Yedla et al. [ 19 ] proposed a method where the algorithm sorted the data point by the distance from the origin and subdivided it into k (number of clusters needed) sets.
COVID-19 has been the subject of several major studies lately. The K-means algorithm has a significant influence on these studies. S. R. Vadyala et al. proposed a combined algorithm with k-means and LSTM to predict the number of confirmed cases of COVID-19 [ 20 ]. In [ 21 ], author A. Poompaavai et al. attempted to identify the affected areas by COVID-19 in India by using the k-means clustering algorithm. Many approaches have been attempted to solve the COVID-19 problem using k-means clustering. In [ 22 ], S.K. Sonbhadra et al. proposed a novel bottom-up approach for COVID-19 articles using k-means clustering along with DBSCAN and HAC. S. Chinchorkar used the K-means algorithm for defining Covid-19 containment zones. In this paper [ 23 ], the size and locations of such zones (affected by Coronapositive patients) are considered dynamic. K-means algorithm is proposed to handle the zones dynamically. But if the number of Corona-positive patient outbreaks, K-means may not be effective as it will take huge computational power and resources to handle the dataset. N. Aydin et al. used K-means in accessing countries’ performance against COVID-19. In this paper [ 24 ], K-means and hierarchical clustering methods are used for cluster analysis to find out the optimum number of classes in order to categorize the countries for further performance analysis. In this paper [ 25 ], a comparison is made based on the GDP declines and deaths in China and OECD countries. K-means is used for clustering analysis to find out the current impact of GDP growth rate, deaths, and account balances.T. Zhang used a generalized K-means algorithm in GLMs to group the state-level time series patterns for the analysis of the outbreak of COVID-19 in the United States [ 26 ]
K-means clustering algorithm has a huge impact on patient and medically related work. Many researchers use the k-means algorithm for their research purpose. Ldl Fuente-Tomas et al. [ 27 ] used the k-means algorithm to classify patients with bipolar disorder. P. Sili-tonga et al. [ 28 ] used a k-means clustering algorithm for clustering patient disease data. N. Das et al. [ 29 ] used the k-means algorithm to find the nearest blood & plasma donor. MS Alam et al. [ 30 ] used the k-means algorithm m for detecting human brain tumors in a magnetic resonance imaging (MRI) image. Optimized data mining and clustering models can provide an insightful information about the transmission pattern of COVID-19 outbreak [ 31 ].
Among all the improved and efficient k-means clustering algorithms proposed previously, they take the initial center by randomization [ 15 , 17 ] or the k-means++ algorithm [ 15 , 32 ]. Those processes of selecting the initial cluster take more time. In contrast, our proposed k-means algorithm chooses initial centroids using principal component analysis(PCA) & percentile.
Proposed Methodology
The K-means clustering is the NP-Hard optimization problem [ 33 ]. The efficiency of the k-means clustering algorithm depends on the selection or assignment of initial clusters’ centroids [ 34 ]. So, it is important to select the centroids more systematically to improve the K-means clustering algorithm’s performance and execution time. This section introduces our proposed method of assignment of initial centroids by using Principal Component Analysis (PCA) and dividing the values into percentiles to get the efficient initial coordinate of centroids. The flowchart in Fig. Fig.1 1 depicts the overall process of our proposed method.

Flowchart of the proposed method
In the next subsection, We will describe our proposed method.
Input Dataset and Pre-processing
In Sect. 4.1 , a vivid description has been given about the dataset of our model. Every dataset has properties that requires manual pre-processing to make it competent for feeding the K-means clustering algorithm. K-means clustering algorithm can only perform its operation on numerical data. So any value which is not numerical or categorical must need to be transformed. Again, handling missing values needs to be performed during manual pre-processing.
As we have tried to solve real-world problems with our proposed method, all attributes are not equally important. So, we have selected some of the attributes for the K-means clustering algorithm’s implementation. So, choosing the right attributes must be specified before applying Principal Component Analysis (PCA)and percentile.
Principal Component Analysis (PCA)
PCA is a method, mostly discussed in mathematics, that utilizes an orthogonal transformation to translate a set of observations of potentially correlated variables into a set of values of linearly uncorrelated variables, called principal components. PCA is widely used in data analysis and making a predictive model [ 35 ]. PCA reduces the dimension of datasets by increasing interpretability but minimizing the loss of information simultaneously. For this purpose, the orthogonal transformation is used. Thus, the PCA algorithm helps to quantify the relationship between the large related dataset [ 36 ], and it helps to reduce computational complexity. A pseudo-code of PCA algorithm is provided below 1 .

PCA tries to fit as much information as possible into the first component, then the second component, and so on.
We convert the multi-dimensional dataset into two dimensions for our proposed method using PCA. Because with these two dimensions, we can easily split the data into horizontal and vertical planes.
The percentile model is a well-known method used in statistics. It divides the whole dataset into 100 different parts. Each part contains 1 percent data of the total dataset. For example, the 25th percentile means this part contains 25 percent of data of the total dataset. That implies, using the percentile method, we can split our dataset into different distributions according to our given values [ 37 ].
The percentile formula is given below:
Here, P = The Percentile to find, n = Total Number of values, R = Percentile at P
After reducing the dataset into two dimensions by applying PCA, the percentile method is used on the dataset. The first component of PCA holds the majority of information, and Percentiles can only be applied to one-dimensional data. As we have found most of the information in the first component of PCA, we have considered only the first component.
Finally, dataset is partitioned according to the desired number of clusters with the help of the percentile method. It splits the two-dimensional data into equal parts of the desired number of clusters.
Dataset Split and Mean Calculation
After splitting the reduced dimensional dataset through percentile, we extract the split data from the primary dataset by indexing for every percentile. In this process, we can get back the original data. After retrieving the original data for each percentile, we have calculated the mean of each attribute. These means from the split dataset are the initial centroids of clusters of our proposed method.
Centroids Determination
After splitting the dataset and calculating the mean according to Subsect. 3.4 , we select each split dataset’s mean as a centroid. These centroids are considered as our proposed initial centroids for the efficient k-means clustering algorithm. K-means is an iterative method that attempts to make partitions in an unsupervised dataset. The sub-groups formed after the division are non-overlapping subgroups. This algorithm tries to make a group as identical as possible for the inter-cluster data points and aims to remain separate from the other cluster. In Algorithm 2, a pseudo-code is provided of the k-means algorithm:

Cluster Generation
At the last step, we have executed our modified k-means algorithm until the centroids converge. Passing our proposed centroids instead of random or kmeans++ centroids through the k-means algorithm we have generated the final clusters [ 32 ]. The proposed method always considers the same centroids for each test. The pseudocode of our whole proposed methodology is given in the algorithm 3. In the next section, evaluation and experimental results of our proposed model are discussed.

Evaluation and Experimental Result
We have gone through a couple of experiments to measure the effectiveness and validate our proposed model for selecting the optimum initial centroids for the k-means clustering algorithm. The proposed model is tested with the high dimension, relatively high instances, and very high instances datasets. We have used a few COVID-19 datasets and merged them to have a handful of features for clustering the countries according to their health quality during COVID-19. We have tested the model for clustering the health care patients [ 38 ]. The mode is also tested with 10 million data created with the scikit-learn library [ 39 ]. A detailed explanation of the datasets is given in the following subsection.
Dataset Exploration
We experimented with many different datasets to descry our model’s efficiency. Properties of our used datasets are
- Low instances with a high dimensional dataset
- Relatively high instances with a low dimensional dataset
- Very high instances dataset
In the next Subsect. of 4.1.1 , 4.1.2 and 4.1.3 , a brief explanation of those datasets is given.

COVID-19 Dataset
Many machine learning algorithms, including supervised and unsupervised methods, were applied to the covid-19 dataset. For creating our model, we used a few datasets for selecting the features required for analyzing the health care quality of the countries. The selected datasets are owid-covid-data [ 40 ], covid-19-testing-policy [ 41 ], public-events-covid [ 41 ], covid-containment-and-health-index [ 41 ], inform-covid-indicators [ 42 ]. It is worth mentioning that we used the data up to 11 th August 2020.
For instance, some of the attributes of the owid-covid-data [ 40 ] are shown in Table 1 . Covid-19-testing-policy [ 41 ] dataset contains the categorical values of the testing policy of the countries shown in Table Table2 2 .
Sample data of COVID-19 dataset
Country | Total cases per million | New cases per million | Total deaths per million | New deaths per million | Cardiovasc death rate | Hospital beds per thousand | life expectancy |
---|---|---|---|---|---|---|---|
Australia | 839.102 | 12.275 | 12.275 | 0.706 | 107.791 | 3.84 | 83.44 |
Bangladesh | 1581.808 | 17.651 | 20.876 | 0.237 | 298.003 | 0.8 | 72.59 |
China | 61.769 | 0.079 | 3.258 | 0.003 | 261.899 | 4.34 | 76.91 |
Sample data of Covid-19-testing-policy
Entity | Code | Date | Testing policy |
---|---|---|---|
Australia | AUS | Aug 11, 2020 | 3 |
Bangladesh | BGD | Aug 11, 2020 | 2 |
China | CHN | Aug 11, 2020 | 3 |
Other datasets also contained such features required to ensure the health care quality of a country. These real-world datasets helped us to analyze our proposed method for real-world scenarios.
We merged the datasets according to the country name with the regular expression pre-processing. Some pre-processing and data cleaning had been conducted in case of merging the data, and we had also handled some missing data consciously. There are so many attributes regarding COVID-19; among them, 25 attributes were finally selected, as these attributes closely signify the health care quality of a country. The attributes represent categorical and numerical values. These are country name, cancellation of public events (due to public health awareness), stringency index 1 , testing policy ( category of testing facility available to the mass people), total positive case per million, new cases per million, total death per million, new deaths per million, cardiovascular death rate, hospital beds available per thousand, life expectancy, inform the COVID-19 risk (rate), hazard and exposure dimension rate, people using at least basic sanitation services (rate), inform vulnerability(rate), inform health conditions (rate), inform epidemic vulnerability (rate), mortality rate, prevalence of undernourishment, lack of coping capacity, access to healthcare, physicians density, current health expenditure per capita, maternal mortality ratio. We have consciously selected the features before feeding the model. A two-dimensional plot of the dataset is shown in Fig. Fig.2 2 .

2D distribution plot of COVID-19 dataset
It is a dataset of low instances with high dimensions.
Medical Dataset
Our second dataset is Medical related dataset with 100k instances. It is an open-source dataset for research purposes. It has a random sample of 6 million patient records from our Medical Quality Improvement Consortium (MQIC) database [ 38 ]. Any personal information has been excluded. The final attributes of the dataset are: gender, age, diabetes, hypertension, stroke, heart disease, smoking history, and BMI.
A glimpse of the Medical dataset is given in Table Table3. 3 . It is a dataset of relatively high instances with low dimensional data. Figure Figure3 3 provides a graphical representation of the dataset, which gives meaningful insights into the distribution of the data.
Sample data of Medical Dataset
Gender | Age | Diabetes | Hypertension | Stroke | Heart disease | Moking history | BMI |
---|---|---|---|---|---|---|---|
Female | 80.0 | 0 | 0 | 0 | 1 | Never | 25.19 |
Female | 36.0 | 0 | 0 | 0 | 0 | Current | 23.45 |
Female | 44.0 | 1 | 0 | 0 | 0 | Never | 19.31 |
Male | 42.0 | 0 | 0 | 0 | 0 | Never | 33.64 |
Male | 18.0 | 0 | 0 | 0 | 0 | Never | 21.78 |

2D distribution plot of medical dataset
Synthetic Dataset
A final syntactic dataset has been made to cover the very high instances dataset category. This synthetic dataset is created with the scikit-learn library [ 39 ]. The dataset has 8 dimensions with 10M (Ten Million) instances. The two-dimensional distribution is shown in Fig. Fig.4 4 .

2D distribution plot of scikit-learn library dataset
Experimental Setup
We have selected the following questions to evaluate our proposed model.
- Is the proposed method for selecting efficient clusters of centroids working well both for the high and low dimensional dataset?
- Does the method reduce the iteration for finding the final clusters with the k-means algorithm compared to the existing methods?
- Does the method reduce the execution time to some extent compared to the existing methods for the k-means clustering algorithm?
To answer these questions, we have used real-time COVID-19 healthcare quality data of different countries, patient data, and a scikit-learn library dataset with 10M instances. In the following sub-sections, we have briefly discussed experimental results and comparison with its’ effectiveness.
Evaluation Process
In machine learning and data science, computational power is one of the main issues. Because at the same time, the computer needs to process a large amount of data. So, reducing computational costs is a big deal. K-means clustering is a popular unsupervised machine learning algorithm. It is widely used in different clustering processes. The algorithm randomly selects the initial clusters’ centroids, which sometimes causes many iterations and high computational power. As discussed in the methodology section, we have implemented our proposed method. However, many researchers proposed many ideas discussed in the related work Sect. 2 . We have compared our method with the best existing k-means++ and random method [ 32 ]. We have measured the effectiveness of the model with
- Number of iterations needed for finding the final clusters
- Execution time for reaching out to the final clusters
These two things will be measured in the upcoming subsections.
Experimental Result
Analysis with covid-19 dataset.
Firstly, we are starting our experiment with the COVID-19 dataset. Details explanation of the dataset is provided in Subsect. 4.1.1 . As we are making clusters for the countries with similar types of health care quality, we have defined the optimum number of clusters with the elbow method [ 44 ]. For the COVID-19dataset, the optimum number of clusters is 4. So, we are looking forward to the results with 4 clusters. Here, each type of cluster contains the same types of healthcare quality.
In Fig. Fig.5, 5 , we have shown the experimental result in terms of iteration number for the COVID-19 dataset of 50 tests. Here, we have compared the results of our proposed method to the traditional random centroid selection method and existing the best centroid selection method kmeans++. The yellow, red, and blue lines in Fig. Fig.5 5 represent the random, kmeans++, and our proposed method consecutively. The graphical representation clearly shows that the number of iterations of our model is constant and outperforms most of the cases. On the other hand, the random and kmeans++ method propagates randomly, and in most cases, the number of iterations is higher than our proposed method. So, we can claim that our model outperforms in terms of iteration number and execution time.

Iteration for 4 clusters with COVID-19 dataset
The graph in Fig. Fig.6 6 represents the experimental result for execution time with the COVID-19 data. Execution time is closely related to the number of iterations. In Fig. Fig.6, 6 , the yellow line represents the results for the random method, the red line for the existing kmeans++method and the blue line for our proposed method. As the centroids of our proposed method are constant for each iteration, the execution time is always nearly constant. Figure Figure6 6 depicts that in the case of the random and kmeans++ method, the execution time varies randomly while our proposed methods outperform in every test case.

Execution time for 4 clusters with COVID-19 dataset
Analysis with Medical Dataset
K-means clustering algorithm is widely used in medical sectors as well. Patient clustering is also one of the important tasks where clustering is usually used. We have analyzed our model with the patient data mentioned in Subsect. 4.1.2 . As it is a real-world implementation, we have used the elbow method to find out the clusters’ optimum number. For the dataset, the optimum number of clusters is 3
We have conducted 50 tests over the dataset. The final outcome in terms of iteration is shown in Fig. Fig.7. 7 . The blue line represents the execution time for 3 clusters with the dataset, and it is nearly constant. Compared to the other two methods represented with the yellow line for random and red line for kmeans++, our model notably outperforms.

Iteration for 3 clusters with Medical Dataset
In Fig. Fig.8, 8 , we have shown the experimental result for execution time. The blue line represents the execution time for 3 clusters with the dataset. It is nearly constant. Compared to the other two methods represented with the yellow line for random and red line for kmeans++, our model notably outperforms.

Execution time for 3 clusters with Medical Dataset
Analysis with Synthetic Dataset
In practical scenarios, clustering data may be massive. For that reason, we have created a synthetic dataset described in Subsect. 4.1.3 for getting insight into our model, whether it works for a very large dataset or not. This synthetic dataset contains about 10 million instances, and the dataset is only created for demonstration purposes. We have also created the clusters randomly for testing our model. We have run the model with random, kmeans++ and our proposed model 50 times simultaneously with the dataset. The model is tested with 3, 4 and 5 clusters. Figure Figure9 9 graphically represents the experimental results with the synthetic dataset. The blue, yellow and red lines represent the results for the proposed, random and kmeans++ methods consecutively. The left side graphs show the experimental results in terms of iteration, and the right side graphs show the experimental results for the execution time of 3, 4, and 5 clusters.

Total iteration and execution time for different number of clusters with Synthetic Dataset. (a) 3 clusters (b) 4 clusters (c) 5 clusters
For the clusters, the number of iterations is constant for our proposed method, and it also outperforms most of the test cases. Other models are random in nature. The kmeans++ and random models have not reduced the iteration significantly. It is a remarkable contribution.
Execution time is a vital issue for improving an algorithm’s performance. The right side graphs of Fig. Fig.9 9 show the results in terms of execution time for 3, 4, and 5 clusters. The execution time needed for our proposed method is nearly constant and outperforms compared to the kmeans++ and random methods.
Effectiveness Comparison Based on Number of Iterations and Execution Time
Computation cost is one of the fundamental issues in data science. If we can reduce the number of iterations to some extent, the performance will be improved. A Constant iteration of the algorithm helps us to have the same performance over time.
In Fig. Fig.5, 5 , we find the experimental result for covid-19 dataset. When our proposed method is applied, we have found a constant number of iterations in each test. But the existing kmeans++ and random methods’ iteration are random. It varies for different test cases. Figure Figure6 6 provides the execution time comparison for existing kmeans++, random and our proposed method. Our model executed the k-means clustering algorithm for each test case in the shortest time.
The medical dataset contains a relatively high instance of data with real-world hospital patient data. Figures Figures7 7 and and8 8 represent the experimental results for random, kmeans++ and our proposed methods. We have found that our model converged to the final clusters with a reduced number of constant iterations and improved execution time.
In Fig. Fig.9, 9 , we have shown the experimental results of the K-means clustering algorithm for 3,4 and 5 clusters consecutively for the number of iterations along with execution time. This experiment has been done on a synthetic dataset described in Subsect. 4.1.3 . Constant optimum iteration compared to the existing model kmeans++, random and shortest execution time signify that our model outperforms for large datasets.
Based on the experimental results, we claim that our model outperforms in real-world applications and reduces the computational power of the K-means clustering algorithm. It also outperforms for a huge instance of datasets.
The above discussion answers the last two questions discussed in the experimental setup Subsect. 4.2 .
K-means clustering is one of the most popular unsupervised clustering algorithms. By default k-means clustering algorithm randomly selects the initial centroids. Sometimes, it consumes a huge computational power and time. Over the years, many researchers tried to select the initial centroids more systematically. Some of the works have been mentioned in Sect. 2 , and most of the previous works are not detailed enough and well recognized. From equation 1 , we presume that the execution time will be reduced significantly if we can reduce the number of iteration. And our proposed method focuses on minimizing the iteration. Two statistical models, PCA(principal component analysis) [ 35 ] and percentile [ 37 ] are used to deploy the proposed method. It is also mentionable that the proposed method provides the optimal number of iterations for applying the K-means clustering algorithm. However, the most popular and standard method is kmeans++ [ 32 ]. So, we have compared our model with the kmeans++ method and the default random method to analyze the efficiency. We haven’t modified the main K-means clustering algorithm instead developed an efficient centroid selection process that provides an optimum number of constant iterations. As the time complexity is directly related to the iteration shown in equation 1 and our model gives less number of iterations, the overall execution time is reduced.
We have made clusters of the countries with similar types of health care quality for solving the real-world problem with our proposed method. It is high-dimensional data. The medical-related dataset is also used for making patient clusters. We have also used a synthetic dataset created with scikit-learn consisting of 10M instances to ensure that our model is also outperforming for a large number of instances. This model performs well for both low and high-dimensional datasets. Thus, this technique could be applied to solve many unsupervised learning problems in various real-world application areas ranging from personalized services to today’s various smart city services and security, i.e., to detect cyber-anomalies [ 6 , 45 ]. Internet of Things (IoT) is another cutting edge technology where clustering is widely used [ 46 ].
Our proposed method reduces the computational power. So, the proposed model will work faster in case of a clustering problem where the data volume is too large. This proposed method is easy to implement, and no extra setup is needed.
In this article, we have proposed an improved K-means clustering method that increases the performance of the traditional one. We have significantly reduced the number of iterations by systematically selecting the initial centroids for generating the clusters. PCA and Percentile techniques have been used to reduce the dimension of data and segregate the dataset according to the number of clusters. Finally, these segregated data have been used to select our initials centroids. Thus, we have successfully minimized the number of iterations. As the complexity of the traditional K-means clustering algorithm is directly related to the number of iterations, our proposed approach outperformed compared to the existing methods. We believe this method could play a significant role for data-driven solutions in various real-world application domains.
Author Contributions
All authors equally contributed to preparing and revising the manuscript.
Not Applicable
Data Availability Statement
Declarations.
The authors declare no conflict of interest.
The authors follow all the relevant ethical rules.
1 It is one of the matrices used by Oxford COVID-19 Government Response Tracker [ 43 ]. It delivers a picture of the country’s enforced strongest measures.
Publisher's Note
Springer Nature remains neutral with regard to jurisdictional claims in published maps and institutional affiliations.
Data Clustering for Classification of Vegetable Biomass from Compositional Data: A Tool for Biomass Valorization
59 Pages Posted: 13 Sep 2024
Daniel David Durán-Aranguren
Universidad de los Andes, Colombia
Juan Toro-Delgado
Universidad de los Andes
Valentina Núñez-Barrero
Valentina florez-bulla, rocío sierra, john a. posada.
Delft University of Technology
Solange I. Mussatto
Technical University of Denmark
Compositional data on vegetable biomass is widely available from research papers and online databases. However, the high diversity of biomass characteristics and composition represents a challenge for researchers and companies willing to produce novel substances from residues, and that should decide on the best and most feasible options for their use as feedstocks. The present study constructed a database with information gathered from the proximate, ultimate, and chemical composition of different biomass residues that can be used for data analysis and classification to elucidate better how they can be valorized. Different data clustering techniques were implemented to determine how compositional data can be segmented. The identified groups, that contained residues with similar characteristics, allowed to have an insight into the valorization of these biomasses, which can be used as an initial tool for biorefinery design. The use of data clustering facilitated the identification of different types of biomasses in a systematic way, which until now has not been reported in the literature.
Keywords: biomass composition, biomass classification, biorefinery design, machine learning, Principal Component Analysis, data clustering
Suggested Citation: Suggested Citation
Daniel David Durán-Aranguren (Contact Author)
Universidad de los andes, colombia ( email ).
Carrera Primera # 18A-12 Bogota, DC D.C. 110311 Colombia
Universidad de los Andes ( email )
Av. Plaza 1905 Santiago Chile
Delft University of Technology ( email )
Stevinweg 1 Stevinweg 1 Delft, 2628 CN Netherlands
Technical University of Denmark ( email )
Anker Engelunds Vej 1 Building 101A Lyngby, 2800 Denmark
Do you have a job opening that you would like to promote on SSRN?
Paper statistics, related ejournals, horticulture & forestry ejournal.
Subscribe to this fee journal for more curated articles on this topic
Agronomy & Soil Science eJournal
Agriculture products ejournal, environmental data analysis ejournal, environmental chemistry ejournal.
- Skip to Guides Search
- Skip to breadcrumb
- Skip to main content
- Skip to footer
- Skip to chat link
- Report accessibility issues and get help
- Go to Penn Libraries Home
- Go to Franklin catalog
CWP: What Data Can Tell Us — Fall 2024: Researching the White Paper
- Getting started
- News and Opinion Sites
- Academic Sources
- Grey Literature
- Substantive News Sources
- What to Do When You Are Stuck
- Understanding a citation
- Examples of Quotation
- Examples of Paraphrase
- Chicago Manual of Style: Citing Images
- Researching the Op-Ed
- Researching Prospective Employers
- Resume Resources
- Cover Letter Resources
Research the White Paper
Researching the white paper:.
The process of researching and composing a white paper shares some similarities with the kind of research and writing one does for a high school or college research paper. What’s important for writers of white papers to grasp, however, is how much this genre differs from a research paper. First, the author of a white paper already recognizes that there is a problem to be solved, a decision to be made, and the job of the author is to provide readers with substantive information to help them make some kind of decision--which may include a decision to do more research because major gaps remain.
Thus, a white paper author would not “brainstorm” a topic. Instead, the white paper author would get busy figuring out how the problem is defined by those who are experiencing it as a problem. Typically that research begins in popular culture--social media, surveys, interviews, newspapers. Once the author has a handle on how the problem is being defined and experienced, its history and its impact, what people in the trenches believe might be the best or worst ways of addressing it, the author then will turn to academic scholarship as well as “grey” literature (more about that later). Unlike a school research paper, the author does not set out to argue for or against a particular position, and then devote the majority of effort to finding sources to support the selected position. Instead, the author sets out in good faith to do as much fact-finding as possible, and thus research is likely to present multiple, conflicting, and overlapping perspectives. When people research out of a genuine desire to understand and solve a problem, they listen to every source that may offer helpful information. They will thus have to do much more analysis, synthesis, and sorting of that information, which will often not fall neatly into a “pro” or “con” camp: Solution A may, for example, solve one part of the problem but exacerbate another part of the problem. Solution C may sound like what everyone wants, but what if it’s built on a set of data that have been criticized by another reliable source? And so it goes.
For example, if you are trying to write a white paper on the opioid crisis, you may focus on the value of providing free, sterilized needles--which do indeed reduce disease, and also provide an opportunity for the health care provider distributing them to offer addiction treatment to the user. However, the free needles are sometimes discarded on the ground, posing a danger to others; or they may be shared; or they may encourage more drug usage. All of those things can be true at once; a reader will want to know about all of these considerations in order to make an informed decision. That is the challenging job of the white paper author. The research you do for your white paper will require that you identify a specific problem, seek popular culture sources to help define the problem, its history, its significance and impact for people affected by it. You will then delve into academic and grey literature to learn about the way scholars and others with professional expertise answer these same questions. In this way, you will create creating a layered, complex portrait that provides readers with a substantive exploration useful for deliberating and decision-making. You will also likely need to find or create images, including tables, figures, illustrations or photographs, and you will document all of your sources.
South Asian Studies Librarian

Connect to a Librarian Live Chat or "Ask a Question"
- Librarians staff live chat from 9-5 Monday through Friday . You can also text to chat: 215-543-7674
- You can submit a question 24 hours a day and we aim to respond within 24 hours
- You can click the "Schedule Appointment" button above in librarian's profile box (to the left), to schedule a consultation with her in person or by video conference.
- You can also make an appointment with a Librarian by subject specialization .
- Connect by email with a subject librarian
Find more easy contacts at our Quick Start Guide
- Next: Getting started >>
- Last Updated: Sep 12, 2024 3:12 PM
- URL: https://guides.library.upenn.edu/c.php?g=1424358
- Biomedical Signal Processing
- Machine Learning
- Cluster Analysis
RESEARCH PAPER ON CLUSTER TECHNIQUES OF DATA VARIATIONS
- This person is not on ResearchGate, or hasn't claimed this research yet.

- VIT University
Abstract and Figures

Discover the world's research
- 25+ million members
- 160+ million publication pages
- 2.3+ billion citations
- Raquel Ausejo-Marcos
- María Teresa Tejedor
- Sara Miguel-Jiménez
- María Victoria Falceto

- Chantelle Y van Staden

- Tejaswini Katale
- Aishwarya Katale

- Nur Ulfah Maisurah Mohd Faiz

- Mohd Najib Mohd Salleh
- Tan Kang Leng

- Borra Sivaiah
- Riyam Patel
- P. Sandhiyadevi
- G.K. Mohanapriya

- Dongshik Kang

- J TERRAMECHANICS

- Cecilia M. Procopiuc

- Jong Soo Park
- Alexander Hinneburg
- Daniel A. Keim

- Patrick J. Flynn
- J OPER RES SOC
- Thomas H. Cormen

- Clifford Stein

- Johannes Gehrke

- Prabhakar Raghavan
- INFORM SYST
- Sudipto Guha
- Rajeev Rastogi
- Kyuseok Shim

- Richard C. Dubes
- R.A. Jarvis
- Edward A. Patrick
- Recruit researchers
- Join for free
- Login Email Tip: Most researchers use their institutional email address as their ResearchGate login Password Forgot password? Keep me logged in Log in or Continue with Google Welcome back! Please log in. Email · Hint Tip: Most researchers use their institutional email address as their ResearchGate login Password Forgot password? Keep me logged in Log in or Continue with Google No account? Sign up
- Environment
- Science & Technology
- Business & Industry
- Health & Public Welfare
- Topics (CFR Indexing Terms)
- Public Inspection
- Presidential Documents
- Document Search
- Advanced Document Search
- Public Inspection Search
- Reader Aids Home
- Office of the Federal Register Announcements
- Using FederalRegister.Gov
- Understanding the Federal Register
- Recent Site Updates
- Federal Register & CFR Statistics
- Videos & Tutorials
- Developer Resources
- Government Policy and OFR Procedures
- Congressional Review
- My Clipboard
- My Comments
- My Subscriptions
- Sign In / Sign Up
- Site Feedback
- Search the Federal Register
This site displays a prototype of a “Web 2.0” version of the daily Federal Register. It is not an official legal edition of the Federal Register, and does not replace the official print version or the official electronic version on GPO’s govinfo.gov.
The documents posted on this site are XML renditions of published Federal Register documents. Each document posted on the site includes a link to the corresponding official PDF file on govinfo.gov. This prototype edition of the daily Federal Register on FederalRegister.gov will remain an unofficial informational resource until the Administrative Committee of the Federal Register (ACFR) issues a regulation granting it official legal status. For complete information about, and access to, our official publications and services, go to About the Federal Register on NARA's archives.gov.
The OFR/GPO partnership is committed to presenting accurate and reliable regulatory information on FederalRegister.gov with the objective of establishing the XML-based Federal Register as an ACFR-sanctioned publication in the future. While every effort has been made to ensure that the material on FederalRegister.gov is accurately displayed, consistent with the official SGML-based PDF version on govinfo.gov, those relying on it for legal research should verify their results against an official edition of the Federal Register. Until the ACFR grants it official status, the XML rendition of the daily Federal Register on FederalRegister.gov does not provide legal notice to the public or judicial notice to the courts.
Design Updates: As part of our ongoing effort to make FederalRegister.gov more accessible and easier to use we've enlarged the space available to the document content and moved all document related data into the utility bar on the left of the document. Read more in our feature announcement .
Electronic Common Technical Document; Data Standards; Center for Drug Evaluation and Research and Center for Biologics Evaluation and Research Supporting Electronic Common Technical Document Version 4.0
A Notice by the Food and Drug Administration on 09/16/2024
This document has been published in the Federal Register . Use the PDF linked in the document sidebar for the official electronic format.
- Document Details Published Content - Document Details Agencies Department of Health and Human Services Food and Drug Administration Agency/Docket Number Docket No. FDA-2018-D-1216 Document Citation 89 FR 75545 Document Number 2024-20897 Document Type Notice Pages 75545-75546 (2 pages) Publication Date 09/16/2024 Published Content - Document Details
- View printed version (PDF)
- Document Dates Published Content - Document Dates Dates Text Support for eCTDv4.0 electronic submissions begins September 16, 2024. FDA will also continue to support eCTDv3.2.2 electronic submissions. Submit either electronic or written comments at any time. Published Content - Document Dates
This table of contents is a navigational tool, processed from the headings within the legal text of Federal Register documents. This repetition of headings to form internal navigation links has no substantive legal effect.
Electronic Submissions
Written/paper submissions, for further information contact:, supplementary information:.
This feature is not available for this document.
Additional information is not currently available for this document.
- Sharing Enhanced Content - Sharing Shorter Document URL https://www.federalregister.gov/d/2024-20897 Email Email this document to a friend Enhanced Content - Sharing
- Print this document
Document page views are updated periodically throughout the day and are cumulative counts for this document. Counts are subject to sampling, reprocessing and revision (up or down) throughout the day.
This document is also available in the following formats:
More information and documentation can be found in our developer tools pages .
This PDF is the current document as it appeared on Public Inspection on 09/13/2024 at 8:45 am.
It was viewed 30 times while on Public Inspection.
If you are using public inspection listings for legal research, you should verify the contents of the documents against a final, official edition of the Federal Register. Only official editions of the Federal Register provide legal notice of publication to the public and judicial notice to the courts under 44 U.S.C. 1503 & 1507 . Learn more here .
Document headings vary by document type but may contain the following:
- the agency or agencies that issued and signed a document
- the number of the CFR title and the number of each part the document amends, proposes to amend, or is directly related to
- the agency docket number / agency internal file number
- the RIN which identifies each regulatory action listed in the Unified Agenda of Federal Regulatory and Deregulatory Actions
See the Document Drafting Handbook for more details.
Department of Health and Human Services
Food and drug administration.
- [Docket No. FDA-2018-D-1216]
Food and Drug Administration, HHS.
The Food and Drug Administration's (FDA or Agency) Center for Drug Evaluation and Research and Center for Biologics Evaluation and Research are announcing support for Electronic Common Technical Document (eCTD) Version 4.0 (v4.0)-based electronic submissions.
Support for eCTDv4.0 electronic submissions begins September 16, 2024. FDA will also continue to support eCTDv3.2.2 electronic submissions. Submit either electronic or written comments at any time.
You may submit comments as follows:
Submit electronic comments in the following way:
- Federal eRulemaking Portal: https://www.regulations.gov . Follow the instructions for submitting comments. Comments submitted electronically, including attachments, to https://www.regulations.gov will be posted to the docket unchanged. Because your comment will be made public, you are solely responsible for ensuring that your comment does not include any confidential information that you or a third party may not wish to be posted, such as medical information, your or anyone else's Social Security number, or confidential business information, such as a manufacturing process. Please note that if you include your name, contact information, or other information that identifies you in the body of your comments, that information will be posted on https://www.regulations.gov .
- If you want to submit a comment with confidential information that you do not wish to be made available to the public, submit the comment as a written/paper submission and in the manner detailed (see “Written/Paper Submissions” and “Instructions”).
Submit written/paper submissions as follows:
- Mail/Hand Delivery/Courier (for written/paper submissions): Dockets Management Staff (HFA-305), Food and Drug Administration, 5630 Fishers Lane, Rm. 1061, Rockville, MD 20852.
- For written/paper comments submitted to the Dockets Management Staff, FDA will post your comment, as well as any attachments, except for information submitted, marked and identified, as confidential, if submitted as detailed in “Instruction.”
Instructions: All submissions received must include the Docket No. FDA-2018-D-1216 for “Electronic Common Technical Document; Data Standards; Center for Drug Evaluation and Research and Center for Biologics Evaluation and Research Supporting Electronic Common Technical Document Version 4.0.” Received comments will be placed in the docket and, except for those submitted as “Confidential Submissions,” publicly viewable at https://www.regulations.gov or at the Dockets Management Staff between 9 a.m. and 4 p.m., Monday through Friday, 240-402-7500.
- Confidential Submissions—To submit a comment with confidential information that you do not wish to be ( print page 75546) made publicly available, submit your comments only as a written/paper submission. You should submit two copies total. One copy will include the information you claim to be confidential with a heading or cover note that states “THIS DOCUMENT CONTAINS CONFIDENTIAL INFORMATION.” The Agency will review this copy, including the claimed confidential information, in its consideration of comments. The second copy, which will have the claimed confidential information redacted/blacked out, will be available for public viewing and posted on https://www.regulations.gov . Submit both copies to the Dockets Management Staff. If you do not wish your name and contact information to be made publicly available, you can provide this information on the cover sheet and not in the body of your comments and you must identify this information as “confidential.” Any information marked as “confidential” will not be disclosed except in accordance with 21 CFR 10.20 and other applicable disclosure law. For more information about FDA's posting of comments to public dockets, see 80 FR 56469 , September 18, 2015, or access the information at: https://www.govinfo.gov/content/pkg/FR-2015-09-18/pdf/2015-23389.pdf .
Docket: For access to the docket to read background documents or the electronic and written/paper comments received, go to https://www.regulations.gov and insert the docket number, found in brackets in the heading of this document, into the “Search” box and follow the prompts and/or go to the Dockets Management Staff, 5630 Fishers Lane, Rm. 1061, Rockville, MD 20852, 240-402-7500.
Jonathan Resnick, Center for Drug Evaluation and Research, Food and Drug Administration, [email protected] ; or James Myers, Center for Biologics Evaluation and Research, Food and Drug Administration, Bldg. 71, Rm. 7301, Silver Spring, MD 20993-0002, 240-402-7911.
According to the guidance for industry entitled “Providing Regulatory Submissions in Electronic Format—Certain Human Pharmaceutical Product Applications and Related Submissions Using the eCTD Specifications” (available at https://www.fda.gov/regulatory-information/search-fda-guidance-documents/providing-regulatory-submissions-electronic-format-certain-human-pharmaceutical-product-applications ), submissions subject to section 745A(a) of the Federal Food, Drug, and Cosmetic Act ( 21 U.S.C. 379k-1(a) ) must be submitted in eCTD format using the version of eCTD currently supported by FDA unless such submission is exempt from the electronic submission requirements or if FDA has granted a waiver. The version of eCTD currently supported by FDA is specified in the FDA Data Standards Catalog (available at https://www.fda.gov/regulatory-information/search-fda-guidance-documents/data-standards-catalog ). FDA plans to update the FDA Data Standards Catalog to add eCTDv4.0 upon publication of this notice. FDA will support both eCTDv3.2.2 and eCTDv4.0 submissions before eventually only supporting eCTDv4.0 submissions. FDA will provide advance notice of when the Agency will begin supporting electronic submission only in eCTDv4.0. Specifications for eCTDv3.2.2 and v4.0 are available on FDA's eCTD web page (available at: https://www.fda.gov/drugs/electronic-regulatory-submission-and-review/electronic-common-technical-document-ectd ).
Dated: September 10, 2024.
Lauren K. Roth,
Associate Commissioner for Policy.
[ FR Doc. 2024-20897 Filed 9-13-24; 8:45 am]
BILLING CODE 4164-01-P
- Executive Orders
Reader Aids
Information.
- About This Site
- Legal Status
- Accessibility
- No Fear Act
- Continuity Information
Adaptive structural enhanced representation learning for deep document clustering
- Published: 12 September 2024
Cite this article
- Jingjing Xue ORCID: orcid.org/0000-0001-5797-9713 1 ,
- Ruizhang Huang 1 ,
- Ruina Bai 1 ,
- Yanping Chen 1 ,
- Yongbin Qin 1 &
- Chuan Lin 1
Structural deep document clustering methods, which leverage both structural information and inherent data properties to learn document representations using deep neural networks for clustering, have recently garnered increased research interest. However, the structural information used in these methods is usually static and remains unchanged during the clustering process. This can negatively impact the clustering results if the initial structural information is inaccurate or noisy. In this paper, we present an adaptive structural enhanced representation learning network for document clustering. This network can adjust the structural information with the help of clustering partitions and consists of two components: an adaptive structure learner, which automatically evaluates and adjusts structural information at both the document and term levels to facilitate the learning of more effective structural information, and a structural enhanced representation learning network. The latter incorporates integrates this adjusted structural information to enhance text document representations while reducing noise, thereby improving the clustering results. The iterative process between clustering results and the adaptive structural enhanced representation learning network promotes mutual optimization, progressively enhancing model performance. Extensive experiments on various text document datasets demonstrate that the proposed method outperforms several state-of-the-art methods.
Graphical abstract
The overall framework of adaptive structural enhanced representation learning network
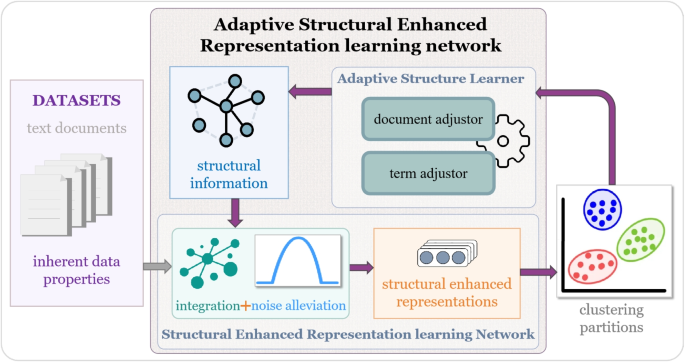
This is a preview of subscription content, log in via an institution to check access.
Access this article
Subscribe and save.
- Get 10 units per month
- Download Article/Chapter or eBook
- 1 Unit = 1 Article or 1 Chapter
- Cancel anytime
Price includes VAT (Russian Federation)
Instant access to the full article PDF.
Rent this article via DeepDyve
Institutional subscriptions
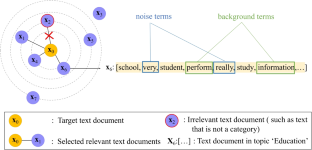
Similar content being viewed by others
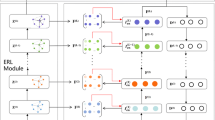
Deep structural enhanced network for document clustering
Representation Learning for Short Text Clustering
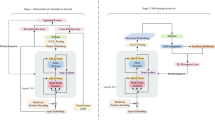
DEC-transformer: deep embedded clustering with transformer on Chinese long text
Explore related subjects.
- Artificial Intelligence
Data Availability
Data will be made available on request.
http://mlg.ucd.ie/datasets/bbc.html
https://www.aminer.cn/data
https://msnews.github.io/
http://mlg.ucd.ie/files/datasets/bbcsport.zip
The experiments were conducted on an NVIDIA Tesla P40 GPU with 24 GB of memory.
Xie J, Girshick R, Farhadi A (2016) Unsupervised deep embedding for clustering analysis. In: International Conference on Machine Learning. PMLR, pp 478–487
Guo X, Gao L, Liu X, Yin J (2017) Improved deep embedded clustering with local structure preservation. In: Proceedings of the twenty-sixth international joint conference on artificial intelligence. International Joint Conferences on Artificial Intelligence Organization
Ren L, Qin Y, Chen Y, Bai R, Xue J, Huang R (2023) Deep structural enhanced network for document clustering. Appl Intell 53(10):12163–12178
Article Google Scholar
Bai R, Huang R, Zheng L, Chen Y, Qin Y (2022) Structure enhanced deep clustering network via a weighted neighbourhood auto-encoder. Neural Netw 155:144–154
Bo D, Wang X, Shi C, Zhu M, Lu E, Cui P (2020) Structural deep clustering network. Proc Web Conf 2020:1400–1410
Google Scholar
Ng A et al (2011) Sparse autoencoder. CS294A Lecture Notes 72(2011):1–19
Lopez R, Boyeau P, Yosef N, Jordan M, Regier J (2020) Decision-making with auto-encoding variational bayes. Adv Neural Inf Process Syst 33:5081–5092
Scarselli F, Gori M, Tsoi AC, Hagenbuchner M, Monfardini G (2008) The graph neural network model. IEEE Trans Neural Netw 20(1):61–80
Guo X, Liu X, Zhu E, Yin J (2017) Deep clustering with convolutional autoencoders. In: International conference on neural information processing. Springer, pp 373–382
Ahmed U, Srivastava G, Yun U, Lin JC-W (2022) Eandc: An explainable attention network based deep adaptive clustering model for mental health treatment. Futur Gener Comput Syst 130:106–113
Pitchandi P, Balakrishnan M (2023) Document clustering analysis with aid of adaptive jaro winkler with jellyfish search clustering algorithm. Adv Eng Softw 175:103322
Hazratgholizadeh R, Balafar M, Derakhshi M (2023) Active constrained deep embedded clustering with dual source. Appl Intell 53(5):5337–5367
Sadok S, Leglaive S, Girin L, Alameda-Pineda X, Séguier R (2024) A multimodal dynamical variational autoencoder for audiovisual speech representation learning. Neural Netw 172:106120
Rocha MB, Krohling RA (2024) Vae-gna: a variational autoencoder with gaussian neurons in the latent space and attention mechanisms. Knowl Inf Sys 1–23
Ji Q, Sun Y, Gao J, Hu Y, Yin B (2021) A decoder-free variational deep embedding for unsupervised clustering. IEEE Trans Neural Netw Learning Sys 33(10):5681–5693
Article MathSciNet Google Scholar
Stauffer C, Grimson WEL (1999) Adaptive background mixture models for real-time tracking. In: Proceedings. 1999 IEEE computer society conference on computer vision and pattern recognition (Cat. No PR00149), vol 2, pp 246–252. IEEE
Miao Y, Yu L, Blunsom P (2016) Neural variational inference for text processing. In: International conference on machine learning. PMLR, pp 1727–1736
Bakhouya M, Ramchoun H, Hadda M, Masrour T (2024) Implicitly adaptive optimal proposal in variational inference for bayesian learning. Int J Data Sci Anal 1–16
Bai R, Huang R, Qin Y, Chen Y, Lin C (2023) Hvae: A deep generative model via hierarchical variational auto-encoder for multi-view document modeling. Inf Sci 623:40–55
Liu Y, Liu Z, Li S, Yu Z, Guo Y, Liu Q, Wang G (2023) Cloud-vae: Variational autoencoder with concepts embedded. Pattern Recogn 140:109530
Zhang H, Lu G, Zhan M, Zhang B (2022) Semi-supervised classification of graph convolutional networks with laplacian rank constraints. Neural Process Lett 1–12
Peng Z, Liu H, Jia Y, Hou J (2021) Attention-driven graph clustering network. In: Proceedings of the 29th ACM international conference on multimedia. pp 935–943
Schlichtkrull M, Kipf TN, Bloem P, Van Den Berg R, Titov I, Welling M (2018) Modeling relational data with graph convolutional networks. In: European semantic web conference. Springer, pp 593–607
Guo L, Dai Q (2022) Graph clustering via variational graph embedding. Pattern Recognition 122:L108334
Tsitsulin A, Palowitch J, Perozzi B, Müller E (2023) Graph clustering with graph neural networks. J Mach Learn Res 24(127):1–21
MathSciNet Google Scholar
Tu W, Guan R, Zhou S, Ma C, Peng X, Cai Z, Liu Z, Cheng J, Liu X (2024) Attribute-missing graph clustering network. Proc AAAI Conf Artif Intell 38:15392–15401
Peng Z, Liu H, Jia Y, Hou J (2022) Deep attention-guided graph clustering with dual self-supervision. IEEE Trans Circ Sys Video Technol
Xu J, Li T, Zhang D, Wu J (2024) Ensemble clustering via fusing global and local structure information. Expert Syst Appl 237:121557
Müller E (2023) Graph clustering with graph neural networks. J Mach Learn Res 24:1–21
Liu Y, Yang X, Zhou S, Liu X, Wang S, Liang K, Tu W, Li L (2023) Simple contrastive graph clustering. IEEE Trans Neural Netw Learn Sys
Joachims T (1996) A probabilistic analysis of the rocchio algorithm with tfidf for text categorization. Technical report, Carnegie-mellon univ pittsburgh pa dept of computer science
Greene D, Cunningham P (2006) Practical solutions to the problem of diagonal dominance in kernel document clustering. In: Proceedings of the 23rd international conference on machine learning. pp 377–384
Lewis DD, Yang Y, Russell-Rose T, Li F (2004) Rcv1: A new benchmark collection for text categorization research. J Mach Learn Res 5(Apr):361–397
Wu F, Qiao Y, Chen J-H, Wu C, Qi T, Lian J, Liu D, Xie X, Gao J, Wu W,et al (2020) Mind: A large-scale dataset for news recommendation. In: Proceedings of the 58th annual meeting of the association for computational linguistics. pp 3597–3606
MacQueen J, et al (1967) Some methods for classification and analysis of multivariate observations. In: Proceedings of the fifth berkeley symposium on mathematical statistics and probability, vol 1, pp 281–297. Oakland, CA, USA
Blei DM, Ng AY, Jordan MI (2003) Latent dirichlet allocation. J Mach Learn Res 3:993–1022
Peterson LE (2009) K-nearest neighbor. Scholarpedia 4(2):1883
Gan G, Ma C, Wu J (2007) Data clustering: theory, algorithms, and applications. Soc Industr Appl Math 20
Download references
Acknowledgements
This work is supported by the National Natural Science Foundation of China under Grant No. 62066007, the Key Technology R&D Program of Guizhou Province No. [2023]300 and No. [2022]277.
Author information
Authors and affiliations.
Text Computing & Cognitive Intelligence Engineering Research Center of National Education Ministry, State Key Laboratory of Public Big Data, College of Computer Science and Technology, Guizhou University, Guiyang, 550000, Guizhou, China
Jingjing Xue, Ruizhang Huang, Ruina Bai, Yanping Chen, Yongbin Qin & Chuan Lin
You can also search for this author in PubMed Google Scholar
Contributions
Jingjing Xue: Writing - original draft, visualization, validation, methodology, investigation, formal analysis, data curation, conceptualization. Ruizhang Huang: Writing - review & editing, supervision, resources, project administration, methodology, funding acquisition, conceptualization. Ruina Bai: Writing - review, writing - review, data verification Yanping Chen: Supervision, resources, project administration, funding acquisition. Yongbin Qin: Supervision, resources, project administration, funding acquisition. Chuan Lin: Supervision, resources, project administration.
Corresponding author
Correspondence to Ruizhang Huang .
Ethics declarations
Competing of interest.
The authors declare that they have no known competing financial interests or personal relationships that could have appeared to influence the work reported in this paper.
Ethical and Informed Consent for Data Used
This study strictly adheres to ethical guidelines. Participants provided informed consent before data collection, ensuring privacy and voluntary participation. For inquiries, contact the principal investigator.
Additional information
Publisher's note.
Springer Nature remains neutral with regard to jurisdictional claims in published maps and institutional affiliations.
Rights and permissions
Springer Nature or its licensor (e.g. a society or other partner) holds exclusive rights to this article under a publishing agreement with the author(s) or other rightsholder(s); author self-archiving of the accepted manuscript version of this article is solely governed by the terms of such publishing agreement and applicable law.
Reprints and permissions
About this article
Xue, J., Huang, R., Bai, R. et al. Adaptive structural enhanced representation learning for deep document clustering. Appl Intell (2024). https://doi.org/10.1007/s10489-024-05791-6
Download citation
Accepted : 18 August 2024
Published : 12 September 2024
DOI : https://doi.org/10.1007/s10489-024-05791-6
Share this article
Anyone you share the following link with will be able to read this content:
Sorry, a shareable link is not currently available for this article.
Provided by the Springer Nature SharedIt content-sharing initiative
- Adaptive learning
- Document clustering
- Representation learning
- Find a journal
- Publish with us
- Track your research

IMAGES
VIDEO
COMMENTS
1. Introduction. Clustering (an aspect of data mining) is considered an active method of grouping data into many collections or clusters according to the similarities of data points features and characteristics (Jain, 2010, Abualigah, 2019).Over the past years, dozens of data clustering techniques have been proposed and implemented to solve data clustering problems (Zhou et al., 2019 ...
Clustering has primarily been used as an analytical technique to group unlabeled data for extracting meaningful information. The fact that no clustering algorithm can solve all clustering problems has resulted in the development of several clustering algorithms with diverse applications. We review data clustering, intending to underscore recent applications in selected industrial sectors and ...
Despite these limitations, the K-means clustering algorithm is credited with flexibility, efficiency, and ease of implementation. It is also among the top ten clustering algorithms in data mining [59], [217], [105], [94].The simplicity and low computational complexity have given the K-means clustering algorithm a wide acceptance in many domains for solving clustering problems.
Data analysis is used as a common method in modern science research, which is across communication science, computer science and biology science. Clustering, as the basic composition of data analysis, plays a significant role. On one hand, many tools for cluster analysis have been created, along with the information increase and subject intersection. On the other hand, each clustering ...
Introduction. In recent years, the automation of data collection and recording implied a deluge of information about many different kinds of systems [1-8].As a consequence, many methodologies aimed at organizing and modeling data have been developed [].Such methodologies are motivated by their widespread application in diagnosis [], education [], forecasting [], and many other domains [].
Abstract. Clustering is a common technique for statistical data analysis, which is used in many fields, including machine learning, data mining, pattern recognition, image analysis and ...
A Rapid Review of Clustering Algorithms. Clustering algorithms aim to organize data into groups or clusters based on the inherent patterns and similarities within the data. They play an important role in today's life, such as in marketing and e-commerce, healthcare, data organization and analysis, and social media.
The goal is to group unlabeled data so that the data objects whose characteristics and attributes are similar are together in a cluster such that the similarities of data objects within the same clusters are higher when compared with other clusters' data objects. In other words, data clustering analysis classifies unlabeled data to ensure ...
Deep Clustering: A Comprehensive Survey. Yazhou Ren, Jingyu Pu, Zhimeng Yang, Jie Xu, Guofeng Li, Xiaorong Pu, Philip S. Yu, Lifang He. View a PDF of the paper titled Deep Clustering: A Comprehensive Survey, by Yazhou Ren and 7 other authors. Cluster analysis plays an indispensable role in machine learning and data mining.
Clustering of Large Data Sets. Research Studies Press Ltd., Taunton, UK. Google Scholar. Cited By View all. ... In this paper we propose a clustering algorithm to cluster data with arbitrary shapes without knowing the number of clusters in advance. The proposed algorithm is a two-stage algorithm. In the first stage, a neural network ...
Data is useless if information or knowledge that can be used for further reasoning cannot be inferred from it. Cluster analysis, based on some criteria, shares data into important, practical or both categories (clusters) based on shared common characteristics. In research, clustering and classification have been used to analyze data, in the field of machine learning, bioinformatics, statistics ...
Then, for each subject, we therefore provide an up-to-date review of research papers. Discover the world's research. 25+ million members; ... In real-world data clustering analysis problems, the ...
This paper deals with a research area-based bibliometric study on automatic clustering algorithms (ACA), covering the background of clustering algorithms. ... This paper is "Data clustering: A review" by Jain et al. in the ACM computing surveys, which has received a total of 6154 citations since September 1999. There are three other papers ...
Components and classifications for data clustering. Our article selection in this work follows a similar literature search approach of Govender and Sivakumar where google scholar (which provides indirect links to databases such as science direct) was indicated as the main search engine.In addition to key reference word combinations, they used such as "clustering", "clustering analysis", we ...
Various clustering algorithms have been developed under different paradigms for grouping scattered data points and forming efficient cluster shapes with minimal outliers. This paper attempts to address the problem of creating evenly shaped clusters in detail and aims to study, review and analyze few clustering algorithms falling under different ...
A Survey of Data Clustering Methods. Saima Bano and M. N. A. Khan. Shaheed Zulfikar Ali Bhutto Institute of Science and Technology, Islamabad, Pakistan. [email protected], [email protected] ...
In hierarchical clustering methods, clusters are formed by iteratively dividing the patterns using top-down or bottom up approach. There are two forms of hierarchical method namely agglomerative and divisive hierarchical clustering [32].The agglomerative follows the bottom-up approach, which builds up clusters starting with single object and then merging these atomic clusters into larger and ...
Clustering analysis method is one of the main analytical methods in data mining, the method of clustering algorithm will influence the clustering results directly. This paper discusses the standard k-means clustering algorithm and analyzes the shortcomings of standard k-means algorithm, such as the k-means clustering algorithm has to calculate the distance between each data object and all ...
View a PDF of the paper titled A model-based approach for clustering binned data, by Asael Fabian Mart\'inez and Carlos D\'iaz-Avalos View PDF HTML (experimental) Abstract: Binned data often appears in different fields of research, and it is generated after summarizing the original data in a sequence of pairs of bins (or their midpoints) and ...
Graph data models enable efficient storage, visualization, and analysis of highly interlinked data, by providing the benefits of horizontal scalability and high query performance. Clustering techniques, such as K-means, hierarchical clustering, are highly beneficial tools in data mining and machine learning to find meaningful similarities and differences between data points. Recent ...
K-means is an iterative method that attempts to make partitions in an unsupervised dataset. The sub-groups formed after the division are non-overlapping subgroups. This algorithm tries to make a group as identical as possible for the inter-cluster data points and aims to remain separate from the other cluster.
This paper focuses on document clustering algorithms that build such hierarchical so- lutions and (i) presents a comprehensive study of partitional and agglomerative algorithms that use different ...
Abstract. Compositional data on vegetable biomass is widely available from research papers and online databases. However, the high diversity of biomass characteristics and composition represents a challenge for researchers and companies willing to produce novel substances from residues, and that should decide on the best and most feasible options for their use as feedstocks.
The divisive hierarchical clustering method first sets all data points into one initial cluster, then divides the initial cluster into several sub-clusters, and iteratively partitions these sub-clusters into smaller ones until each cluster contains only one data point or data points within each cluster are similar enough (Turi 2001).The left branch in Fig. 1 demonstrates the procedure in detail.
Unlike a school research paper, the author does not set out to argue for or against a particular position, and then devote the majority of effort to finding sources to support the selected position. Instead, the author sets out in good faith to do as much fact-finding as possible, and thus research is likely to present multiple, conflicting ...
This paper proposes the role of data governance for non-technical aspects of the migration process. This paper presents a case study of the high-level data migration process and how data governance comes into play. ... Research Center for Data and Information Sciences, National Research and Innovation Agency (BRIN), Central Jakarta and Bandung ...
Introduction. Cluster analys is divides data into meaningful or useful. groups (clusters). If meaningful clusters are our ob jective, then the res ulting clusters should capture the "natural ...
The Food and Drug Administration's (FDA or Agency) Center for Drug Evaluation and Research and Center for Biologics Evaluation and Research are announcing support for Electronic Common Technical Document (eCTD) Version 4.0 (v4.0)-based electronic submissions.
Abstract Structural deep document clustering methods, which leverage both structural information and inherent data properties to learn document representations using deep neural networks for clustering, have recently garnered increased research interest. However, the structural information used in these methods is usually static and remains unchanged during the clustering process. This can ...