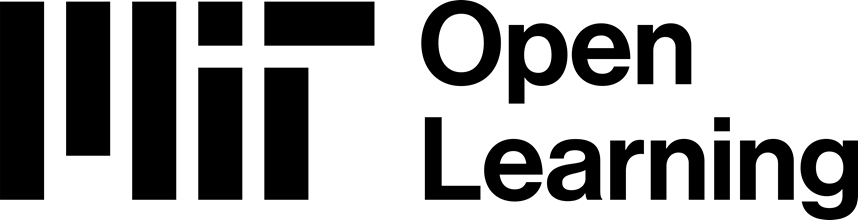
- Data Science
- Engineering
- Entrepreneurship
- Technology Insider
- Manufacturing
- MIT Bootcamps
- MIT Open Learning
- MITx MicroMasters Programs
- Online Education
- Professional Development
- Quantum Computing
View All Posts
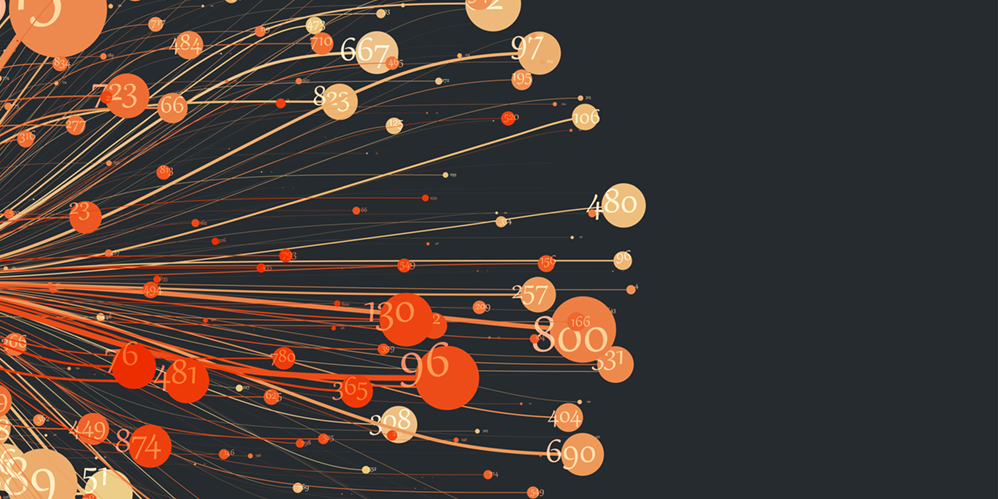
By: MIT xPRO on August 5th, 2020 3 Minute Read
Print/Save as PDF

Here are the Most Common Problems Being Solved by Machine Learning
Machine Learning
Although machine learning offers important new capabilities for solving today’s complex problems, more organizations may be tempted to apply machine learning techniques as a one-size-fits all solution.
To use machine learning effectively, engineers and scientists need a clear understanding of the most common issues that machine learning can solve. In a recent MIT xPRO Machine Learning whitepaper titled " Applications For Machine Learning In Engineering and the Physical Sciences,” Professor Youssef Marzouk and fellow MIT colleagues outlined the potentials and limitations of machine learning in STEM.
Here are some common challenges that can be solved by machine learning:
Accelerate processing and increase efficiency Machine learning can wrap around existing science and engineering models to create fast and accurate surrogates, identify key patterns in model outputs, and help further tune and refine the models. All this helps more quickly and accurately predict outcomes at new inputs and design conditions.
Quantify and manage risk. Machine learning can be used to model the probability of different outcomes in a process that cannot easily be predicted due to randomness or noise. This is especially valuable for situations where reliability and safety are paramount.
Compensate for missing data. Gaps in a data set can severely limit accurate learning, inference, and prediction. Models trained by machine learning improve with more relevant data. When used correctly, machine learning can also help synthesize missing data that round out incomplete datasets.
Make more accurate predictions or conclusions from your data . You can streamline your data-to-prediction pipeline by tuning how your machine learning model’s parameters will be updated and learning during training. Building better models of your data will also improve the accuracy of subsequent predictions.
Solve complex classification and prediction problems. Predicting how an organism’s genome will be expressed or what the climate will be like in fifty years are examples of highly complex problems. Many modern machine learning problems take thousands or even millions of data samples (or far more) across many dimensions to build expressive and powerful predictors, often pushing far beyond traditional statistical methods.
Create new designs. There is often a disconnect between what designers envision and how products are made. It’s costly and time-consuming to simulate every variation of a long list of design variables. Machine learning can identify key variables, automatically generate good options, and help designers identify which best fits their requirements.
Increase yields. Manufacturers aim to overcome inconsistency in equipment performance and predict maintenance by applying machine learning to flag defects and quality issues before products ship to customers, improve efficiency on the production line, and increase yields by optimizing the use of manufacturing resources.
Machine learning is undoubtedly hitting its stride, as engineers and physical scientists leverage the competitive advantage of big data across industries — from aerospace, to construction, to pharmaceuticals, transportation, and energy. But it has never been more important to understand the physics-based models, computational science, and engineering paradigms upon which machine learning solutions are built.
The list above details the most common problems that organizations can solve with machine learning. For more specific applications across engineering and the physical sciences, download MIT xPRO’s free Machine Learning whitepaper .
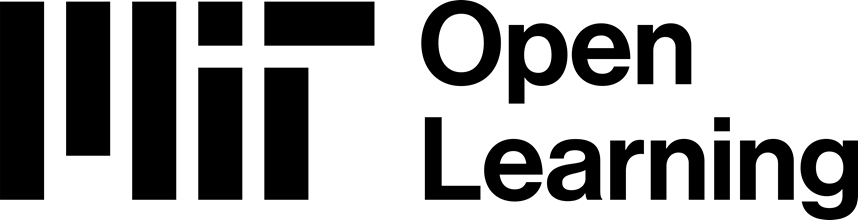
- More about MIT xPRO
- About this Site
- Terms of Service
- Privacy Policy

How to Approach Machine Learning Problems
How do you approach machine learning problems? Are neural networks the answer to nearly every challenge you may encounter?
In this article, Toptal Freelance Python Developer Peter Hussami explains the basic approach to machine learning problems and points out where neural may fall short.
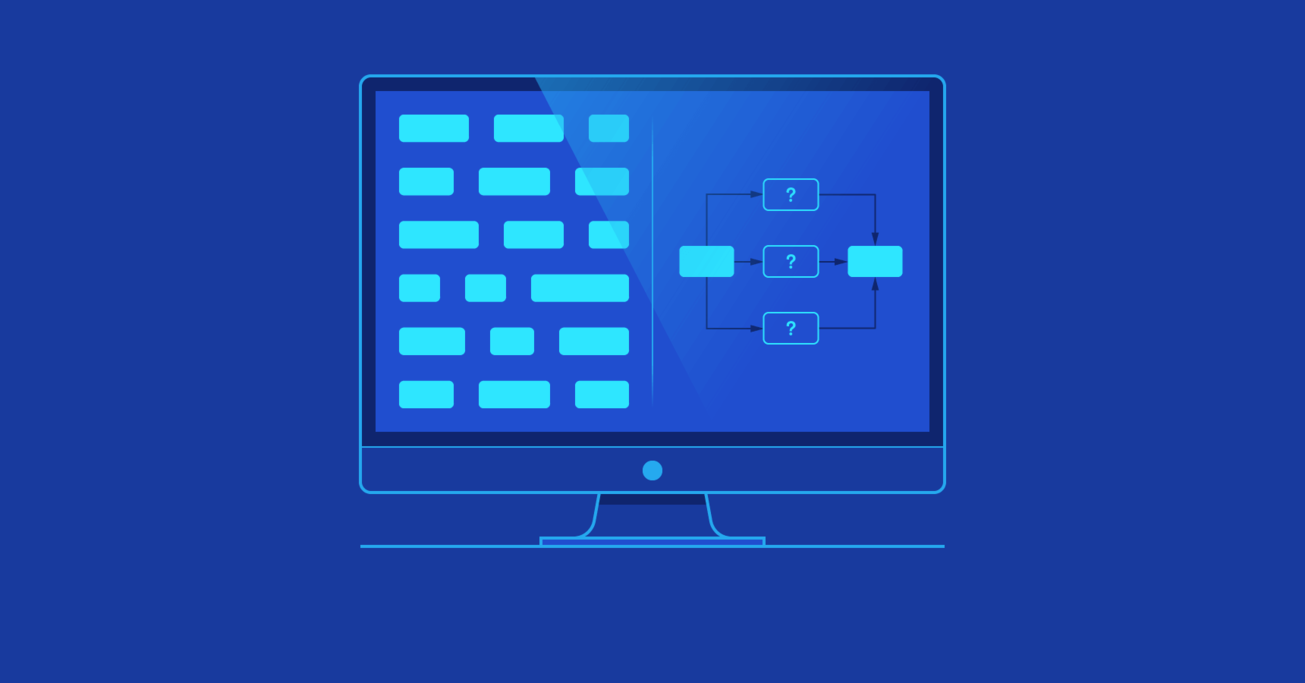
By Peter Hussami
Peter’s rare math-modeling expertise includes audio and sensor analysis, ID verification, NPL, scheduling, routing, and credit scoring.
PREVIOUSLY AT
One of the main tasks of computers is to automate human tasks. Some of these tasks are simple and repetitive, such as “move X from A to B.” It gets far more interesting when the computer has to make decisions about problems that are far more difficult to formalize. That is where we start to encounter basic machine learning problems.
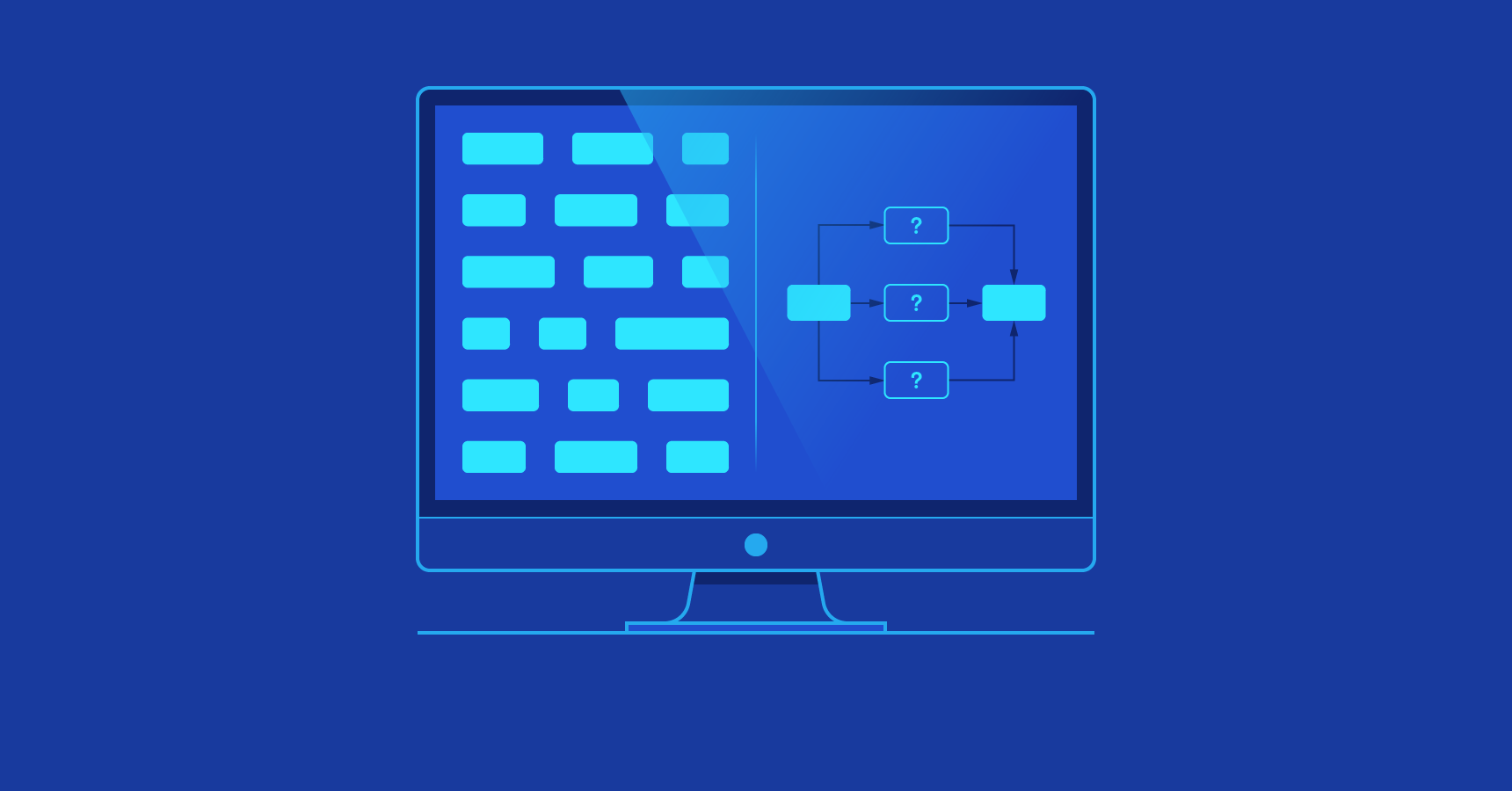
Historically, such algorithms were built by scientists or experts that had intimate knowledge of their field and were largely based on rules. With the explosion of computing power and the availability of large and diverse data sets, the focus has shifted toward a more computational approach.
Most popularized machine learning concepts these days have to do with neural networks, and in my experience, this created the impression in many people that neural networks are some kind of a miracle weapon for all inference problems. Actually, this is quite far from the truth. In the eyes of the statistician, they form one class of inference approaches with their associated strengths and weaknesses, and it completely depends on the problem whether neural networks are going to be the best solution or not.
Quite often, there are better approaches.
In this article, we will outline a structure for attacking machine learning problems. There is no scope for going into too much detail about specific machine learning models , but if this article generates interest, subsequent articles could offer detailed solutions for some interesting machine learning problems.
First, however, let us spend some effort showing why you should be more circumspect than to automatically think “ neural network ” when faced with a machine learning problem.
Pros and Cons of Neural Networks
With neural networks, the inference is done through a weighted “network.” The weights are calibrated during the so-called “learning” process, and then, subsequently, applied to assign outcomes to inputs.
As simple as this may sound, all the weights are parameters to the calibrated network, and usually, that means too many parameters for a human to make sense of.
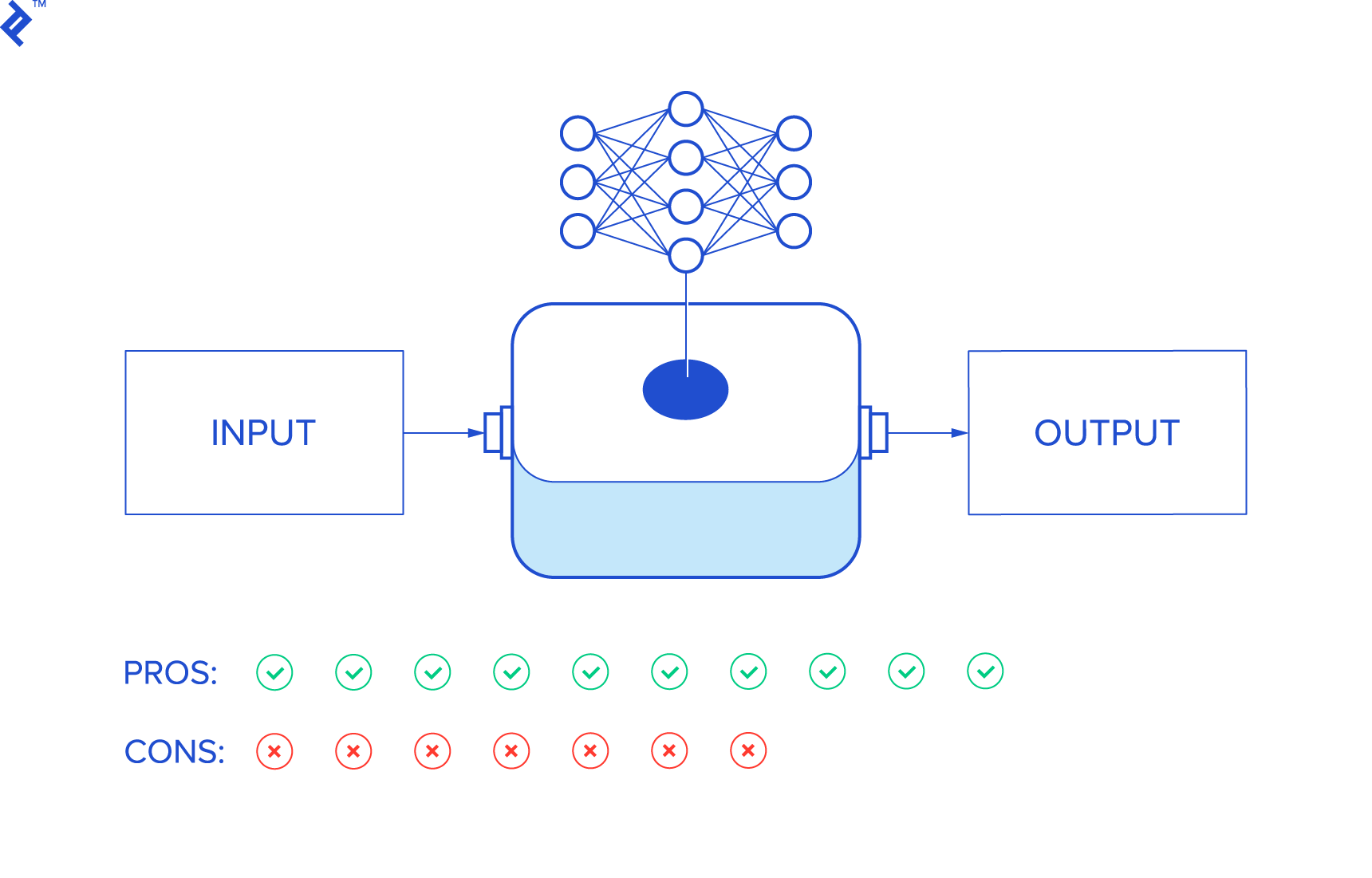
So we might as well just consider neural networks as some kind of an inference black box that connects the input to output, with no specific model in between.
Let us take a closer look at the pros and cons of this approach.
Advantages of Neural Networks
- The input is the data itself. Usable results even with little or no feature engineering.
- Trainable skill. With no feature engineering, there is no need for such hard-to-develop skills as intuition or domain expertise. Standard tools are available for generic inferences.
- Accuracy improves with the quantity of data. The more inputs it sees, the better a neural network performs.
- May outperform classical models when there is not full information about the model. Think of public sentiment, for one.
- Open-ended inference can discover unknown patterns. If you use a model and leave a consideration out of it, it will not detect the corresponding phenomenon. Neural networks might.
Successful neural network example: Google’s AI found a planet orbiting a distant star—where NASA did not—by analyzing accumulated telescope data.
Disadvantages of Neural Networks
- They require a lot of (annotated!) data. First, this amount of data is not always available. Convergence is slow. A solid model (say, in physics) can be calibrated after a few observations—with neural networks, this is out of the question. Annotation is a lot of work, not to mention that it, in itself, is not foolproof.
- No information about the inner structure of the data. Are you interested in what the inference is based on? No luck here. There are situations where manually adjusting the data improves inference by a leap, but a neural network will not be able to help with that.
- Overfitting issues. It happens often that the network has more parameters than the data justifies, which leads to suboptimal inference.
- Performance depends on information. If there is full information about a problem, a solid model tends to outperform a neural network.
- There are sampling problems. Sampling is always a delicate issue, but with a model, one can quickly develop a notion of problematic sampling. Neural networks learn only from the data, so if they get biased data, they will have biased conclusions.
An example of failure: A personal relation told me of a major corporation (that I cannot name) that was working on detecting military vehicles on aerial photos. They had images where there were such vehicles and others that did not. Most images of the former class were taken on a rainy day, while the latter were taken in sunny weather. As a result, the system learned to distinguish light from shadow.
To sum up, neural networks form one class of inference methods that have their pros and cons.
The fact that their popularity outshines all other statistical methods in the eyes of the public has likely more to do with corporate governance than anything else.
Training people to use standard tools and standardized neural network methods is a far more predictable process than hunting for domain experts and artists from various fields. This, however, does not change the fact that using a neural network for a simple, well-defined problem is really just shooting a sparrow with a cannon: It needs a lot of data, requires a lot of annotation work, and in return might just underperform when compared to a solid model. Not the best package.
Still, there is huge power in the fact that they “democratize” statistical knowledge. Once a neural network-based inference solution is viewed as a mere programming tool, it may help even those who don’t feel comfortable with complex algorithms. So, inevitably, a lot of things are now built that would otherwise not exist if we could only operate with sophisticated models.
Approaching Machine Learning Problems
When approaching machine learning problems, these are the steps you will need to go through:
- Setting acceptance criteria
- Cleaning your data and maximizing ist information content
- Choosing the most optimal inference approach
- Train, test, repeat
Let us see these items in detail.
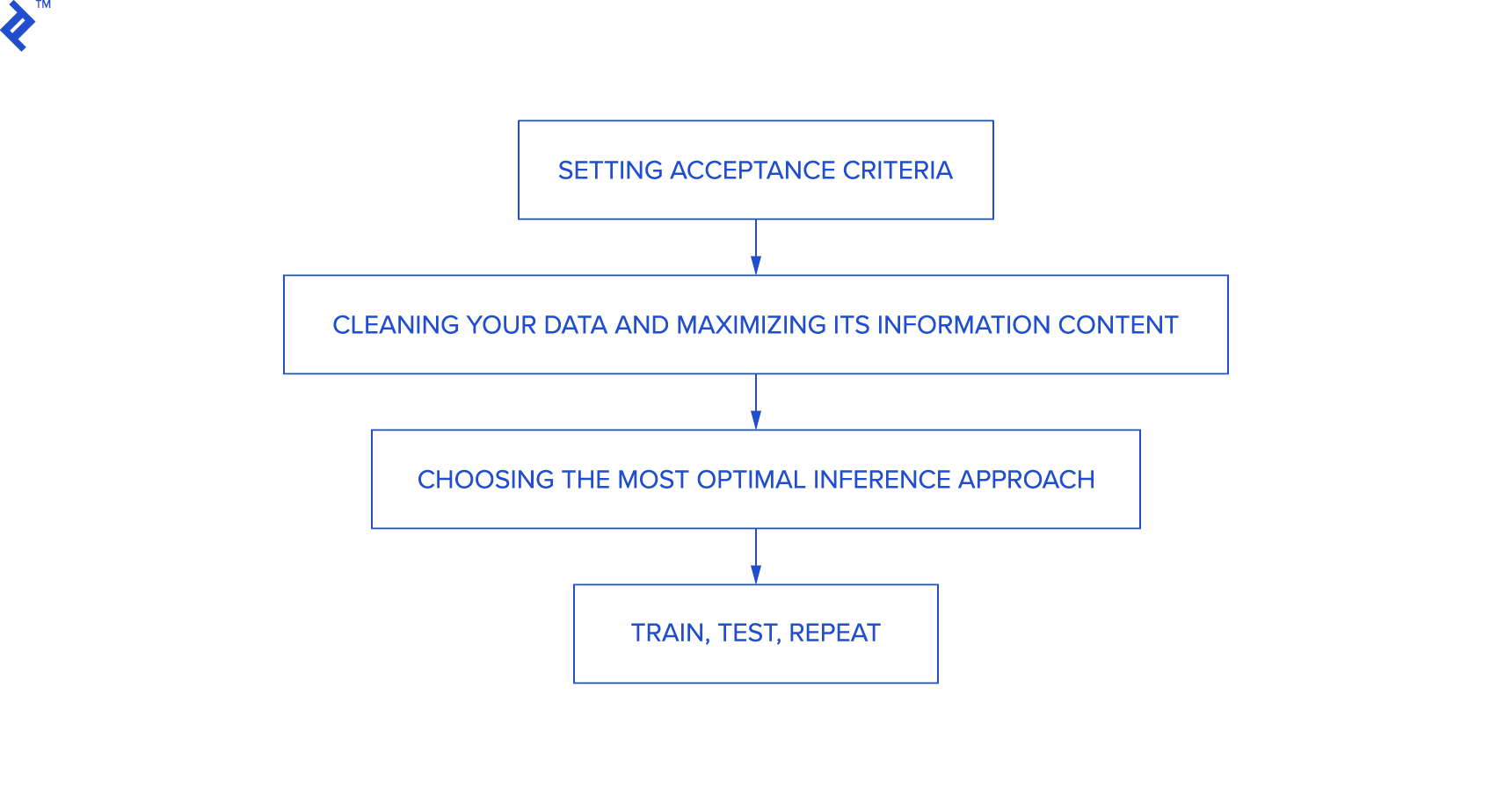
Setting Acceptance Criteria
You should have an idea of your target accuracy as soon as possible, to the extent possible. This is going to be the target you work towards.
Cleansing Your Data and Maximizing Its Information Content
This is the most critical step. First of all, your data should have no (or few) errors. Cleansing it of these is an essential first step. Substitute missing values, try to identify patterns that are obviously bogus, eliminate duplicates and any other anomaly you might notice.
As for information, if your data is very informative (in the linear sense), then practically any inference method will give you good results. If the required information is not in there, then the result will be noise. Maximizing the information means primarily finding any useful non-linear relationships in the data and linearizing them. If that improves the inputs significantly, great. If not, then more variables might need to be added. If all of this does not yield fruit, target accuracy may suffer.
With some luck, there will be single variables that are useful. You can identify useful variables if you—for instance—plot them against the learning target variable(s) and find the plot to be function-like (i.e., narrow range in the input corresponds to narrow range in the output). This variable can then be linearized—for example, if it plots as a parabola, subtract some values and take the square root.
For variables that are noisy—narrow range in input corresponds to a broad range in the output—we may try combining them with other variables.
To have an idea of the accuracy, you may want to measure conditional class probabilities for each of your variables (for classification problems) or to apply some very simple form of regression, such as linear regression (for prediction problems). If the information content of the input improves, then so will your inference, and you simply don’t want to waste too much time at this stage calibrating a model when the data is not yet ready. So keep testing as simple as possible.
Choosing the Most Optimal Inference Approach
Once your data is in decent shape, you can go for the inference method (the data might still be polished later, if necessary).
Should you use a model? Well, if you have good reason to believe that you can build a good model for the task, then you probably should. If you don’t think so, but there is ample data with good annotations, then you may go hands-free with a neural network. In practical machine learning applications, however, there is often not enough data for that.
Playing accuracy vs. cover often pays off tremendously. Hybrid approaches are usually completely fine. Suppose the data is such that you can get near-100% accuracy on 80% of it with a simple model? This means you can demonstrable results quickly, and if your system can identify when it’s operating on the 80% friendly territory, then you’ve basically covered most of the problem. Your client may not yet be fully happy, but this will earn you their trust quickly. And there is nothing to prevent you from doing something similar on the remaining data: with reasonable effort now you cover, say, 92% of the data with 97% accuracy. True, on the rest of the data, it’s a coin flip, but you already produced something useful.
For most practical applications, this is very useful. Say, you’re in the lending business and want to decide whom to give a loan, and all you know is that on 70% of the clients your algorithm is very accurate. Great—true, the other 30% of your applicants will require more processing, but 70% can be fully automated. Or: suppose you’re trying to automate operator work for call centers, you can do a good (quick and dirty) job on the most simple tasks only, but these tasks cover 50% of the calls? Great, the call center saves money if they can automate 50% of their calls reliably.
To sum up: If the data is not informative enough, or the problem is too complex to handle in its entirety, think outside the box. Identify useful and easy-to-solve sub-problems until you have a better idea.
Once you have your system ready, learn, test and loop it until you’re happy with the results.
Train, Test, Repeat
After the previous steps, little of interest is left. You have the data, you have the machine learning method, so it’s time to extract parameters via learning and then test the inference on the test set. Literature suggests 70% of the records should be used for training, and 30% for testing.
If you’re happy with the results, the task is finished. But, more likely, you developed some new ideas during the procedure, and these could help you notch up in accuracy. Perhaps you need more data? Or just more data cleansing? Or another model? Either way, chances are you’ll be busy for quite a while.
So, good luck and enjoy the work ahead!
Further Reading on the Toptal Blog:
- Identifying the Unknown With Clustering Metrics
- Advantages of AI: Using GPT and Diffusion Models for Image Generation
- Stars Realigned: Improving the IMDb Rating System
- Machines and Trust: How to Mitigate AI Bias
Understanding the basics
Machine learning vs. deep learning: what's the difference.
Machine learning includes all inference techniques while deep learning aims at uncovering meaningful non-linear relationships in the data. So deep learning is a subset of machine learning and also a means of automated feature engineering applied to a machine learning problem.
Which language is best for machine learning?
The ideal choice is a language that has both broad programming library support and allows you to focus on the math rather than infrastructure. The most popular language is Python, but algorithmic languages such as Matlab or R or mainstreamers like C++ and Java are all valid choices as well.
Machine learning vs. neural networks: What's the difference?
Neural networks represent just one approach within machine learning with its pros and cons as detailed above.
What is the best way to learn machine learning?
There are some good online courses and summary pages. It all depends on one’s skills and tastes. My personal advice: Think of machine learning as statistical programming. Beef up on your math and avoid all sources that equate machine learning with neural networks.
What are the advantages and disadvantages of artificial neural networks?
Some advantages: no math, feature engineering, or artisan skills required; easy to train; may uncover aspects of the problem not originally considered. Some disadvantages: requires relatively more data; tedious preparation work; leaves no explanation as to why they decide the way they do, overfitting.
- NeuralNetwork
- MachineLearning
Peter Hussami
Budapest, Hungary
Member since July 3, 2017
About the author
World-class articles, delivered weekly.
By entering your email, you are agreeing to our privacy policy .
Toptal Developers
- Algorithm Developers
- Angular Developers
- AWS Developers
- Azure Developers
- Big Data Architects
- Blockchain Developers
- Business Intelligence Developers
- C Developers
- Computer Vision Developers
- Django Developers
- Docker Developers
- Elixir Developers
- Go Engineers
- GraphQL Developers
- Jenkins Developers
- Kotlin Developers
- Kubernetes Experts
- Machine Learning Engineers
- Magento Developers
- .NET Developers
- R Developers
- React Native Developers
- Ruby on Rails Developers
- Salesforce Developers
- SQL Developers
- Tableau Developers
- Unreal Engine Developers
- Xamarin Developers
- View More Freelance Developers
Join the Toptal ® community.
- Machine Learning
- Español – América Latina
- Português – Brasil
- Tiếng Việt
- Foundational courses
- Problem Framing
Introduction to Machine Learning Problem Framing
Introduction to Machine Learning Problem Framing teaches you how to determine if machine learning (ML) is a good approach for a problem and explains how to outline an ML solution.
Except as otherwise noted, the content of this page is licensed under the Creative Commons Attribution 4.0 License , and code samples are licensed under the Apache 2.0 License . For details, see the Google Developers Site Policies . Java is a registered trademark of Oracle and/or its affiliates.
Last updated 2022-07-18 UTC.
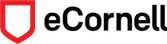
Outside USA: +1‑607‑330‑3200
Problem‑Solving with Machine Learning Cornell Course
Select start date, problem-solving with machine learning, course overview, key course takeaways.
- Define and reframe problems using machine learning (supervised learning) concepts and terminology
- Identify the applicability, assumptions, and limitations of the k-NN algorithm
- Simplify and make Python code efficient with matrix operations using NumPy, a library for the Python programming language
- Build a face recognition system using the k-nearest neighbors algorithm
- Compute the accuracy of an algorithm by implementing loss functions
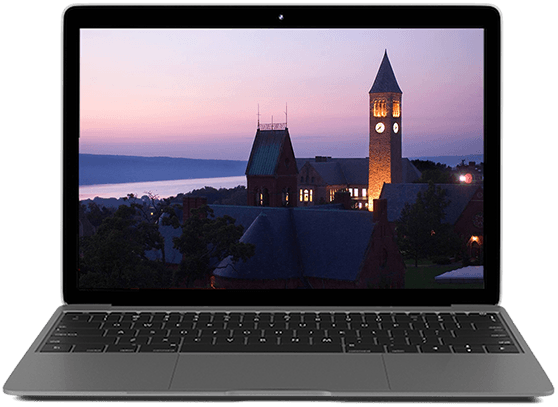
How It Works
Course author.
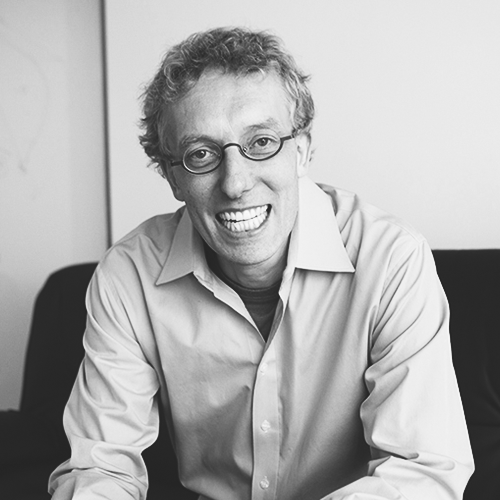
- Certificates Authored
Kilian Weinberger is an Associate Professor in the Department of Computer Science at Cornell University. He received his Ph.D. from the University of Pennsylvania in Machine Learning under the supervision of Lawrence Saul and his undergraduate degree in Mathematics and Computer Science from the University of Oxford. During his career he has won several best paper awards at ICML (2004), CVPR (2004, 2017), AISTATS (2005) and KDD (2014, runner-up award). In 2011 he was awarded the Outstanding AAAI Senior Program Chair Award and in 2012 he received an NSF CAREER award. He was elected co-Program Chair for ICML 2016 and for AAAI 2018. In 2016 he was the recipient of the Daniel M Lazar ’29 Excellence in Teaching Award. Kilian Weinberger’s research focuses on Machine Learning and its applications. In particular, he focuses on learning under resource constraints, metric learning, machine learned web-search ranking, computer vision and deep learning. Before joining Cornell University, he was an Associate Professor at Washington University in St. Louis and before that he worked as a research scientist at Yahoo! Research in Santa Clara.
- Machine Learning
Who Should Enroll
- Programmers
- Data analysts
- Statisticians
- Data scientists
- Software engineers
Stack To A Certificate
Request information now by completing the form below..
Enter your information to get access to a virtual open house with the eCornell team to get your questions answered live.
9 Real-World Problems that can be Solved by Machine Learning
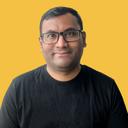
Machine Learning has gained a lot of prominence in the recent years because of its ability to be applied across scores of industries to solve complex problems effectively and quickly. Contrary to what one might expect, Machine Learning use cases are not that difficult to come across. The most common examples of problems solved by machine learning are image tagging by Facebook and spam detection by email providers.
Hey there! This blog is almost about 2000+ words long and may take ~8 mins to go through the whole thing. We understand that you might not have that much time.
This is precisely why we made a short video on the topic. It is less than 2 mins, and summarizes how can Machine Learning be used in everyday life? . We hope this helps you learn more and save your time. Cheers!
AI for business can resolve incredible challenges across industry domains by working with suitable datasets. In this post, we will learn about some typical problems solved by machine learning and how they enable businesses to leverage their data accurately.
What is Machine Learning?
A sub-area of artificial intelligence, machine learning, is an IT system's ability to recognize patterns in large databases to find solutions to problems without human intervention. It is an umbrella term for various techniques and tools to help computers learn and adapt independently.
Unlike traditional programming, a manually created program that uses input data and runs on a computer to produce the output, in Machine Learning or augmented analytics, the input data and output are given to an algorithm to create a program. It leads to powerful insights that can be used to predict future outcomes.
Machine learning algorithms do all that and more, using statistics to find patterns in vast amounts of data that encompass everything from images, numbers, words, etc. If the data can be stored digitally, it can be fed into a machine-learning algorithm to solve specific problems.
Types Of Machine Learning
Today, Machine Learning algorithms are primarily trained using three essential methods. These are categorized as three types of machine learning, as discussed below –
1. Supervised Learning
One of the most elementary types of machine learning, supervised learning, is one where data is labeled to inform the machine about the exact patterns it should look for. Although the data needs to be labeled accurately for this method to work, supervised learning is compelling and provides excellent results when used in the right circumstances.
For instance, when we press play on a Netflix show, we’re informing the Machine Learning algorithm to find similar shows based on our preference.
How it works –
- The Machine Learning algorithm here is provided with a small training dataset to work with, which is a smaller part of the bigger dataset.
- It serves to give the algorithm an idea of the problem, solution, and various data points to be dealt with.
- The training dataset here is also very similar to the final dataset in its characteristics and offers the algorithm with the labeled parameters required for the problem.
- The Machine Learning algorithm then finds relationships between the given parameters, establishing a cause and effect relationship between the variables in the dataset.
2. Unsupervised Learning
Unsupervised learning, as the name suggests, has no data labels. The machine looks for patterns randomly. It means that there is no human labor required to make the dataset machine-readable. It allows much larger datasets to be worked on by the program. Compared to supervised learning, unsupervised Machine Learning services aren’t much popular because of lesser applications in day-to-day life.
How does it work?
- Since unsupervised learning does not have any labels to work off, it creates hidden structures.
- Relationships between data points are then perceived by the algorithm randomly or abstractly, with absolutely no input required from human beings.
- Instead of a specific, defined, and set problem statement, unsupervised learning algorithms can adapt to the data by changing hidden structures dynamically.
3. Reinforcement Learning
Reinforcement learning primarily describes a class of machine learning problems where an agent operates in an environment with no fixed training dataset. The agent must know how to work using feedback.
- Reinforcement learning features a machine learning algorithm that improves upon itself.
- It typically learns by trial and error to achieve a clear objective.
- In this Machine Learning algorithm, favorable outputs are reinforced or encouraged, whereas non-favorable outputs are discouraged.
Top 4 Issues with Implementing Machine Learning
While Machine learning is extensively used across industries to make data-driven decisions, its implementation observes many problems that must be addressed. Here’s a list of organizations' most common machine learning challenges when inculcating ML in their operations.
1. Inadequate Training Data
Data plays a critical role in the training and processing of machine learning algorithms. Many data scientists attest that insufficient, inconsistent, and unclean data can considerably hamper the efficacy of ML algorithms.
2. Underfitting of Training Data
This anomaly occurs when data fails to link the input and output variables explicitly. In simpler terms, it means trying to fit in an undersized t-shirt. It indicates that data isn’t too coherent to forge a precise relationship.
3. Overfitting of Training Data
Overfitting denotes an ML model trained with enormous amounts of data that negatively affects performance. It's similar to trying an oversized jeans.
4. Delayed Implementation
ML models offer efficient results but consume a lot of time due to data overload, slow programs, and excessive requirements. Additionally, they demand timely monitoring and maintenance to deliver the best output.
9 Real-World Problems Solved by Machine Learning
Applications of Machine learning are many, including external (client-centric) applications such as product recommendation , customer service, and demand forecasts, and internally to help businesses improve products or speed up manual and time-consuming processes.
Machine learning algorithms are typically used in areas where the solution requires continuous improvement post-deployment. Adaptable machine learning solutions are incredibly dynamic and are adopted by companies across verticals.

Here we are discussing nine Machine Learning use cases –
1. Identifying Spam
Spam identification is one of the most basic applications of machine learning. Most of our email inboxes also have an unsolicited, bulk, or spam inbox, where our email provider automatically filters unwanted spam emails.
But how do they know that the email is spam?
They use a trained Machine Learning model to identify all the spam emails based on common characteristics such as the email, subject, and sender content.
If you look at your email inbox carefully, you will realize that it is not very hard to pick out spam emails because they look very different from real emails. Machine learning techniques used nowadays can automatically filter these spam emails in a very successful way.
Spam detection is one of the best and most common problems solved by Machine Learning. Neural networks employ content-based filtering to classify unwanted emails as spam. These neural networks are quite similar to the brain, with the ability to identify spam emails and messages.
2. Making Product Recommendations
Recommender systems are one of the most characteristic and ubiquitous machine learning use cases in day-to-day life. These systems are used everywhere by search engines, e-commerce websites (Amazon), entertainment platforms (Google Play, Netflix), and multiple web & mobile apps.
Prominent online retailers like Amazon and eBay often show a list of recommended products individually for each of their consumers. These recommendations are typically based on behavioral data and parameters such as previous purchases, item views, page views, clicks, form fill-ins, purchases, item details (price, category), and contextual data (location, language, device), and browsing history.
These recommender systems allow businesses to drive more traffic, increase customer engagement, reduce churn rate, deliver relevant content and boost profits. All such recommended products are based on a machine learning model’s analysis of customer’s behavioral data. It is an excellent way for online retailers to offer extra value and enjoy various upselling opportunities using machine learning.
3. Customer Segmentation
Customer segmentation, churn prediction and customer lifetime value (LTV) prediction are the main challenges faced by any marketer. Businesses have a huge amount of marketing relevant data from various sources such as email campaigns, website visitors and lead data.
Using data mining and machine learning , an accurate prediction for individual marketing offers and incentives can be achieved. Using ML, savvy marketers can eliminate guesswork involved in data-driven marketing.
For example, given the pattern of behavior by a user during a trial period and the past behaviors of all users, identifying chances of conversion to paid version can be predicted. A model of this decision problem would allow a program to trigger customer interventions to persuade the customer to convert early or better engage in the trial.
4. Image & Video Recognition
Advances in deep learning (a subset of machine learning) have stimulated rapid progress in image & video recognition techniques over the past few years. They are used for multiple areas, including object detection, face recognition, text detection, visual search, logo and landmark detection, and image composition.
Since machines are good at processing images, Machine Learning algorithms can train Deep Learning frameworks to recognize and classify images in the dataset with much more accuracy than humans.
Similar to image recognition , companies such as Shutterstock , eBay , Salesforce , Amazon , and Facebook use Machine Learning for video recognition where videos are broken down frame by frame and classified as individual digital images.

5. Fraudulent Transactions
Fraudulent banking transactions are quite a common occurrence today. However, it is not feasible (in terms of cost involved and efficiency) to investigate every transaction for fraud, translating to a poor customer service experience.
Machine Learning in finance can automatically build super-accurate predictive maintenance models to identify and prioritize all kinds of possible fraudulent activities. Businesses can then create a data-based queue and investigate the high priority incidents.
It allows you to deploy resources in an area where you will see the greatest return on your investigative investment. Further, it also helps you optimize customer satisfaction by protecting their accounts and not challenging valid transactions. Such fraud detection using machine learning can help banks and financial organizations save money on disputes/chargebacks as one can train Machine Learning models to flag transactions that appear fraudulent based on specific characteristics.
6. Demand Forecasting
The concept of demand forecasting is used in multiple industries, from retail and e-commerce to manufacturing and transportation. It feeds historical data to Machine Learning algorithms and models to predict the number of products, services, power, and more.
It allows businesses to efficiently collect and process data from the entire supply chain, reducing overheads and increasing efficiency.
ML-powered demand forecasting is very accurate, rapid, and transparent. Businesses can generate meaningful insights from a constant stream of supply/demand data and adapt to changes accordingly.
7. Virtual Personal Assistant
From Alexa and Google Assistant to Cortana and Siri, we have multiple virtual personal assistants to find accurate information using our voice instruction, such as calling someone, opening an email, scheduling an appointment, and more.
These virtual assistants use Machine Learning algorithms for recording our voice instructions, sending them over the server to a cloud, followed by decoding them using Machine Learning algorithms and acting accordingly.
8. Sentiment Analysis
Sentiment analysis is one of the beneficial and real-time machine learning applications that help determine the emotion or opinion of the speaker or the writer.
For instance, if you’ve written a review, email, or any other form of a document, a sentiment analyzer will be able to assess the actual thought and tone of the text. This sentiment analysis application can be used to analyze decision-making applications, review-based websites, and more.
9. Customer Service Automation
Managing an increasing number of online customer interactions has become a pain point for most businesses. It is because they simply don’t have the customer support staff available to deal with the sheer number of inquiries they receive daily.
Machine learning algorithms have made it possible and super easy for chatbots and other similar automated systems to fill this gap. This application of machine learning enables companies to automate routine and low priority tasks, freeing up their employees to manage more high-level customer service tasks.
Further, Machine Learning technology can access the data, interpret behaviors and recognize the patterns easily. This could also be used for customer support systems that can work identical to a real human being and solve all of the customers’ unique queries. The Machine Learning models behind these voice assistants are trained on human languages and variations in the human voice because it has to efficiently translate the voice to words and then make an on-topic and intelligent response.
If implemented the right way, problems solved by machine learning can streamline the entire process of customer issue resolution and offer much-needed assistance along with enhanced customer satisfaction.
Wrapping Up
As advancements in machine learning evolve, the range of use cases and applications of machine learning too will expand. To effectively navigate the business issues in this new decade, it’s worth keeping an eye on how machine learning applications can be deployed across business domains to reduce costs, improve efficiency and deliver better user experiences.
However, to implement machine learning accurately in your organization, it is imperative to have a trustworthy partner with deep-domain expertise. At Maruti Techlabs, we offer advanced machine learning services that involve understanding the complexity of varied business issues, identifying the existing gaps, and offering efficient and effective tech solutions to manage these challenges.
If you wish to learn more about how machine learning solutions can increase productivity and automate business processes for your business, get in touch with us .
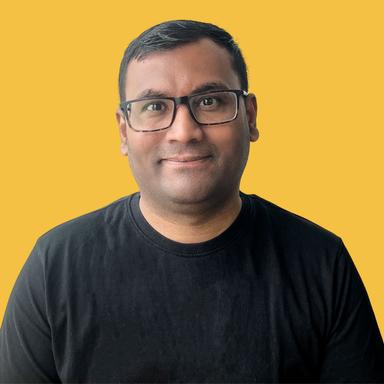
Pinakin is the VP of Data Science and Technology at Maruti Techlabs. With about two decades of experience leading diverse teams and projects, his technological competence is unmatched.
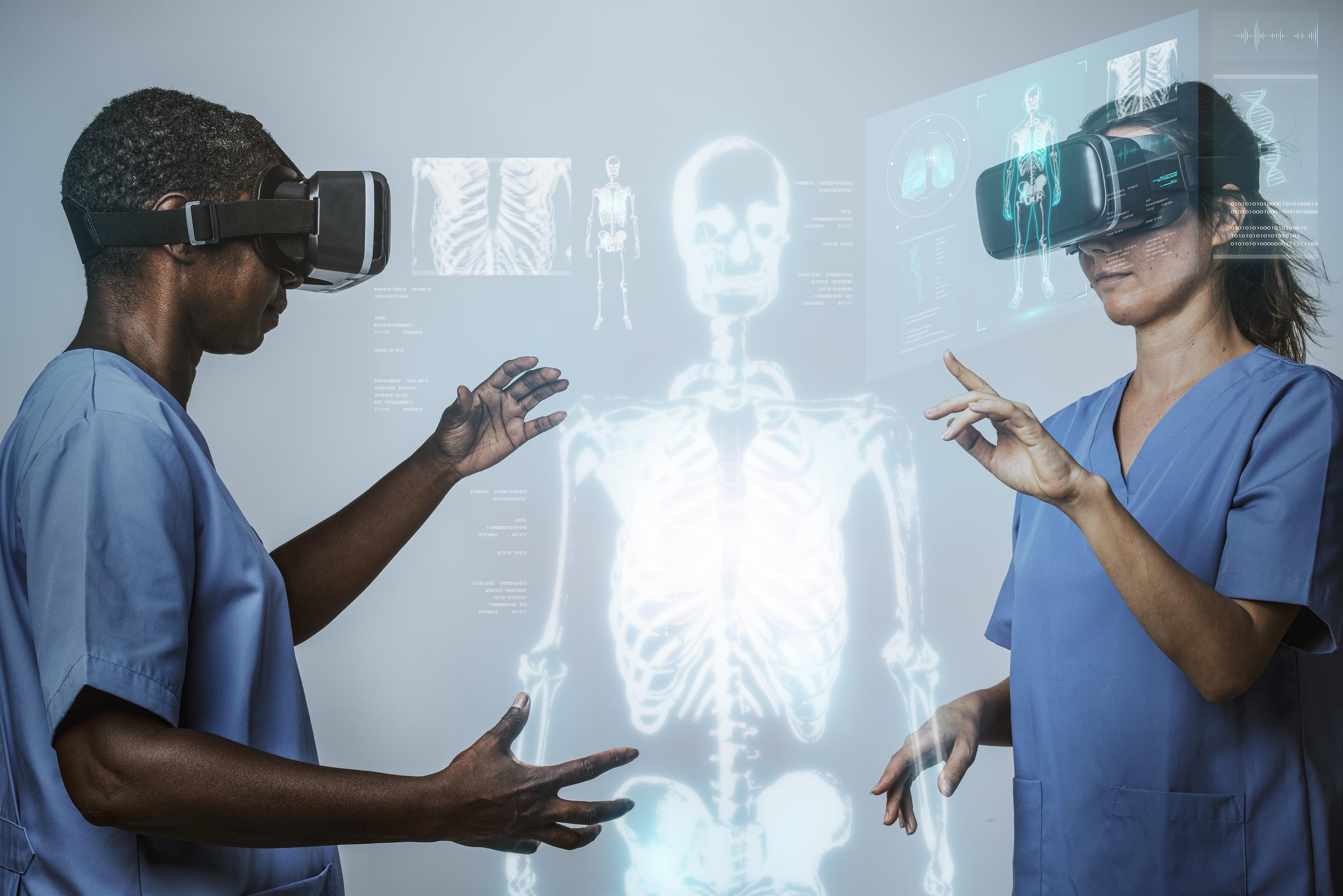
Artificial Intelligence and Machine Learning - 9 MIN READ
Streamlining the Healthcare Space Using Machine Learning and mHealth

Artificial Intelligence and Machine Learning - 7 MIN READ
Machine Learning Algorithms: Obstacles with Implementation
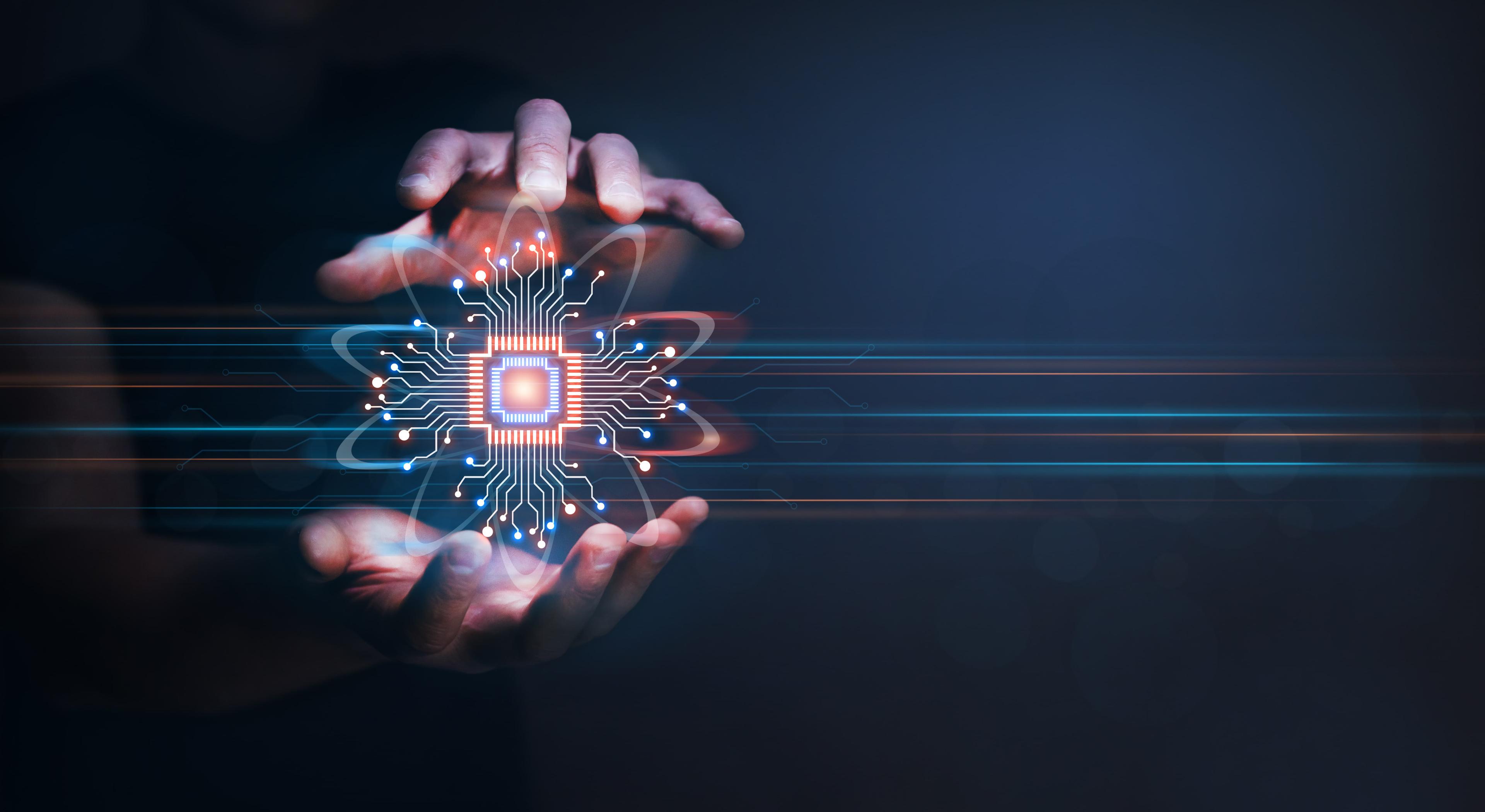
Understanding the Basics of Artificial Intelligence and Machine Learning
- Software Product Development
- Artificial Intelligence
- Data Engineering
- Product Strategy
- DelightfulHomes (Product Development)
- Sage Data (Product Development)
- PhotoStat (Computer Vision)
- UKHealth (Chatbot)
- A20 Motors (Data Analytics)
- Acme Corporation (Product Development)
- Staff Augmentation
- IT Outsourcing
- Privacy Policy
USA 5900 Balcones Dr Suite 100 Austin, TX 78731, USA
India 10th Floor The Ridge Opp. Novotel, Iscon Cross Road Ahmedabad, Gujarat - 380060
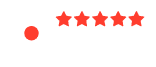
Technologies
Advertisement
Machine Learning: Algorithms, Real-World Applications and Research Directions
- Review Article
- Published: 22 March 2021
- Volume 2 , article number 160 , ( 2021 )
Cite this article
- Iqbal H. Sarker ORCID: orcid.org/0000-0003-1740-5517 1 , 2
511k Accesses
1437 Citations
23 Altmetric
Explore all metrics
In the current age of the Fourth Industrial Revolution (4 IR or Industry 4.0), the digital world has a wealth of data, such as Internet of Things (IoT) data, cybersecurity data, mobile data, business data, social media data, health data, etc. To intelligently analyze these data and develop the corresponding smart and automated applications, the knowledge of artificial intelligence (AI), particularly, machine learning (ML) is the key. Various types of machine learning algorithms such as supervised, unsupervised, semi-supervised, and reinforcement learning exist in the area. Besides, the deep learning , which is part of a broader family of machine learning methods, can intelligently analyze the data on a large scale. In this paper, we present a comprehensive view on these machine learning algorithms that can be applied to enhance the intelligence and the capabilities of an application. Thus, this study’s key contribution is explaining the principles of different machine learning techniques and their applicability in various real-world application domains, such as cybersecurity systems, smart cities, healthcare, e-commerce, agriculture, and many more. We also highlight the challenges and potential research directions based on our study. Overall, this paper aims to serve as a reference point for both academia and industry professionals as well as for decision-makers in various real-world situations and application areas, particularly from the technical point of view.
Similar content being viewed by others
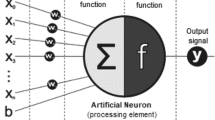
Deep Learning: A Comprehensive Overview on Techniques, Taxonomy, Applications and Research Directions
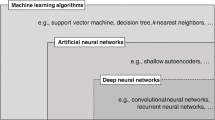
Machine learning and deep learning
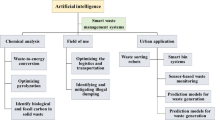
Artificial intelligence for waste management in smart cities: a review
Avoid common mistakes on your manuscript.
Introduction
We live in the age of data, where everything around us is connected to a data source, and everything in our lives is digitally recorded [ 21 , 103 ]. For instance, the current electronic world has a wealth of various kinds of data, such as the Internet of Things (IoT) data, cybersecurity data, smart city data, business data, smartphone data, social media data, health data, COVID-19 data, and many more. The data can be structured, semi-structured, or unstructured, discussed briefly in Sect. “ Types of Real-World Data and Machine Learning Techniques ”, which is increasing day-by-day. Extracting insights from these data can be used to build various intelligent applications in the relevant domains. For instance, to build a data-driven automated and intelligent cybersecurity system, the relevant cybersecurity data can be used [ 105 ]; to build personalized context-aware smart mobile applications, the relevant mobile data can be used [ 103 ], and so on. Thus, the data management tools and techniques having the capability of extracting insights or useful knowledge from the data in a timely and intelligent way is urgently needed, on which the real-world applications are based.
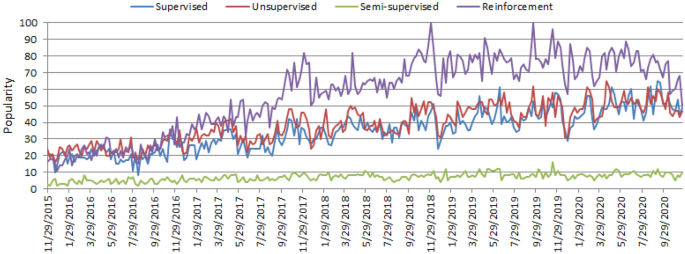
The worldwide popularity score of various types of ML algorithms (supervised, unsupervised, semi-supervised, and reinforcement) in a range of 0 (min) to 100 (max) over time where x-axis represents the timestamp information and y-axis represents the corresponding score
Artificial intelligence (AI), particularly, machine learning (ML) have grown rapidly in recent years in the context of data analysis and computing that typically allows the applications to function in an intelligent manner [ 95 ]. ML usually provides systems with the ability to learn and enhance from experience automatically without being specifically programmed and is generally referred to as the most popular latest technologies in the fourth industrial revolution (4 IR or Industry 4.0) [ 103 , 105 ]. “Industry 4.0” [ 114 ] is typically the ongoing automation of conventional manufacturing and industrial practices, including exploratory data processing, using new smart technologies such as machine learning automation. Thus, to intelligently analyze these data and to develop the corresponding real-world applications, machine learning algorithms is the key. The learning algorithms can be categorized into four major types, such as supervised, unsupervised, semi-supervised, and reinforcement learning in the area [ 75 ], discussed briefly in Sect. “ Types of Real-World Data and Machine Learning Techniques ”. The popularity of these approaches to learning is increasing day-by-day, which is shown in Fig. 1 , based on data collected from Google Trends [ 4 ] over the last five years. The x - axis of the figure indicates the specific dates and the corresponding popularity score within the range of \(0 \; (minimum)\) to \(100 \; (maximum)\) has been shown in y - axis . According to Fig. 1 , the popularity indication values for these learning types are low in 2015 and are increasing day by day. These statistics motivate us to study on machine learning in this paper, which can play an important role in the real-world through Industry 4.0 automation.
In general, the effectiveness and the efficiency of a machine learning solution depend on the nature and characteristics of data and the performance of the learning algorithms . In the area of machine learning algorithms, classification analysis, regression, data clustering, feature engineering and dimensionality reduction, association rule learning, or reinforcement learning techniques exist to effectively build data-driven systems [ 41 , 125 ]. Besides, deep learning originated from the artificial neural network that can be used to intelligently analyze data, which is known as part of a wider family of machine learning approaches [ 96 ]. Thus, selecting a proper learning algorithm that is suitable for the target application in a particular domain is challenging. The reason is that the purpose of different learning algorithms is different, even the outcome of different learning algorithms in a similar category may vary depending on the data characteristics [ 106 ]. Thus, it is important to understand the principles of various machine learning algorithms and their applicability to apply in various real-world application areas, such as IoT systems, cybersecurity services, business and recommendation systems, smart cities, healthcare and COVID-19, context-aware systems, sustainable agriculture, and many more that are explained briefly in Sect. “ Applications of Machine Learning ”.
Based on the importance and potentiality of “Machine Learning” to analyze the data mentioned above, in this paper, we provide a comprehensive view on various types of machine learning algorithms that can be applied to enhance the intelligence and the capabilities of an application. Thus, the key contribution of this study is explaining the principles and potentiality of different machine learning techniques, and their applicability in various real-world application areas mentioned earlier. The purpose of this paper is, therefore, to provide a basic guide for those academia and industry people who want to study, research, and develop data-driven automated and intelligent systems in the relevant areas based on machine learning techniques.
The key contributions of this paper are listed as follows:
To define the scope of our study by taking into account the nature and characteristics of various types of real-world data and the capabilities of various learning techniques.
To provide a comprehensive view on machine learning algorithms that can be applied to enhance the intelligence and capabilities of a data-driven application.
To discuss the applicability of machine learning-based solutions in various real-world application domains.
To highlight and summarize the potential research directions within the scope of our study for intelligent data analysis and services.
The rest of the paper is organized as follows. The next section presents the types of data and machine learning algorithms in a broader sense and defines the scope of our study. We briefly discuss and explain different machine learning algorithms in the subsequent section followed by which various real-world application areas based on machine learning algorithms are discussed and summarized. In the penultimate section, we highlight several research issues and potential future directions, and the final section concludes this paper.
Types of Real-World Data and Machine Learning Techniques
Machine learning algorithms typically consume and process data to learn the related patterns about individuals, business processes, transactions, events, and so on. In the following, we discuss various types of real-world data as well as categories of machine learning algorithms.
Types of Real-World Data
Usually, the availability of data is considered as the key to construct a machine learning model or data-driven real-world systems [ 103 , 105 ]. Data can be of various forms, such as structured, semi-structured, or unstructured [ 41 , 72 ]. Besides, the “metadata” is another type that typically represents data about the data. In the following, we briefly discuss these types of data.
Structured: It has a well-defined structure, conforms to a data model following a standard order, which is highly organized and easily accessed, and used by an entity or a computer program. In well-defined schemes, such as relational databases, structured data are typically stored, i.e., in a tabular format. For instance, names, dates, addresses, credit card numbers, stock information, geolocation, etc. are examples of structured data.
Unstructured: On the other hand, there is no pre-defined format or organization for unstructured data, making it much more difficult to capture, process, and analyze, mostly containing text and multimedia material. For example, sensor data, emails, blog entries, wikis, and word processing documents, PDF files, audio files, videos, images, presentations, web pages, and many other types of business documents can be considered as unstructured data.
Semi-structured: Semi-structured data are not stored in a relational database like the structured data mentioned above, but it does have certain organizational properties that make it easier to analyze. HTML, XML, JSON documents, NoSQL databases, etc., are some examples of semi-structured data.
Metadata: It is not the normal form of data, but “data about data”. The primary difference between “data” and “metadata” is that data are simply the material that can classify, measure, or even document something relative to an organization’s data properties. On the other hand, metadata describes the relevant data information, giving it more significance for data users. A basic example of a document’s metadata might be the author, file size, date generated by the document, keywords to define the document, etc.
In the area of machine learning and data science, researchers use various widely used datasets for different purposes. These are, for example, cybersecurity datasets such as NSL-KDD [ 119 ], UNSW-NB15 [ 76 ], ISCX’12 [ 1 ], CIC-DDoS2019 [ 2 ], Bot-IoT [ 59 ], etc., smartphone datasets such as phone call logs [ 84 , 101 ], SMS Log [ 29 ], mobile application usages logs [ 137 ] [ 117 ], mobile phone notification logs [ 73 ] etc., IoT data [ 16 , 57 , 62 ], agriculture and e-commerce data [ 120 , 138 ], health data such as heart disease [ 92 ], diabetes mellitus [ 83 , 134 ], COVID-19 [ 43 , 74 ], etc., and many more in various application domains. The data can be in different types discussed above, which may vary from application to application in the real world. To analyze such data in a particular problem domain, and to extract the insights or useful knowledge from the data for building the real-world intelligent applications, different types of machine learning techniques can be used according to their learning capabilities, which is discussed in the following.
Types of Machine Learning Techniques
Machine Learning algorithms are mainly divided into four categories: Supervised learning, Unsupervised learning, Semi-supervised learning, and Reinforcement learning [ 75 ], as shown in Fig. 2 . In the following, we briefly discuss each type of learning technique with the scope of their applicability to solve real-world problems.
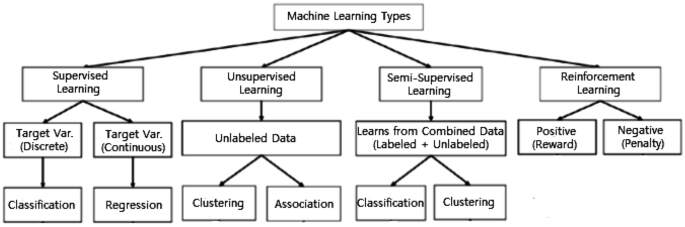
Various types of machine learning techniques
Supervised: Supervised learning is typically the task of machine learning to learn a function that maps an input to an output based on sample input-output pairs [ 41 ]. It uses labeled training data and a collection of training examples to infer a function. Supervised learning is carried out when certain goals are identified to be accomplished from a certain set of inputs [ 105 ], i.e., a task-driven approach . The most common supervised tasks are “classification” that separates the data, and “regression” that fits the data. For instance, predicting the class label or sentiment of a piece of text, like a tweet or a product review, i.e., text classification, is an example of supervised learning.
Unsupervised: Unsupervised learning analyzes unlabeled datasets without the need for human interference, i.e., a data-driven process [ 41 ]. This is widely used for extracting generative features, identifying meaningful trends and structures, groupings in results, and exploratory purposes. The most common unsupervised learning tasks are clustering, density estimation, feature learning, dimensionality reduction, finding association rules, anomaly detection, etc.
Semi-supervised: Semi-supervised learning can be defined as a hybridization of the above-mentioned supervised and unsupervised methods, as it operates on both labeled and unlabeled data [ 41 , 105 ]. Thus, it falls between learning “without supervision” and learning “with supervision”. In the real world, labeled data could be rare in several contexts, and unlabeled data are numerous, where semi-supervised learning is useful [ 75 ]. The ultimate goal of a semi-supervised learning model is to provide a better outcome for prediction than that produced using the labeled data alone from the model. Some application areas where semi-supervised learning is used include machine translation, fraud detection, labeling data and text classification.
Reinforcement: Reinforcement learning is a type of machine learning algorithm that enables software agents and machines to automatically evaluate the optimal behavior in a particular context or environment to improve its efficiency [ 52 ], i.e., an environment-driven approach . This type of learning is based on reward or penalty, and its ultimate goal is to use insights obtained from environmental activists to take action to increase the reward or minimize the risk [ 75 ]. It is a powerful tool for training AI models that can help increase automation or optimize the operational efficiency of sophisticated systems such as robotics, autonomous driving tasks, manufacturing and supply chain logistics, however, not preferable to use it for solving the basic or straightforward problems.
Thus, to build effective models in various application areas different types of machine learning techniques can play a significant role according to their learning capabilities, depending on the nature of the data discussed earlier, and the target outcome. In Table 1 , we summarize various types of machine learning techniques with examples. In the following, we provide a comprehensive view of machine learning algorithms that can be applied to enhance the intelligence and capabilities of a data-driven application.
Machine Learning Tasks and Algorithms
In this section, we discuss various machine learning algorithms that include classification analysis, regression analysis, data clustering, association rule learning, feature engineering for dimensionality reduction, as well as deep learning methods. A general structure of a machine learning-based predictive model has been shown in Fig. 3 , where the model is trained from historical data in phase 1 and the outcome is generated in phase 2 for the new test data.
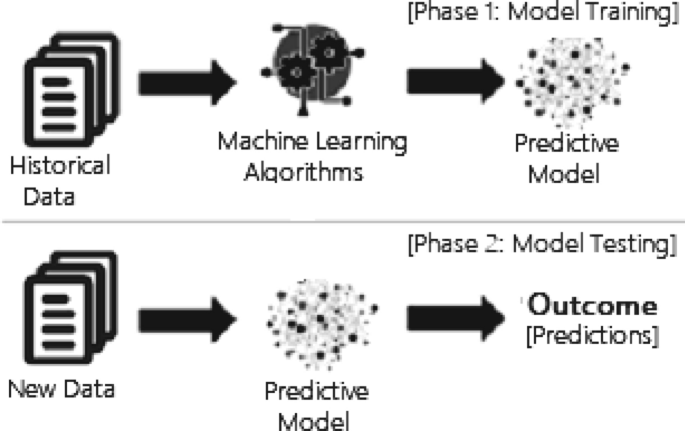
A general structure of a machine learning based predictive model considering both the training and testing phase
Classification Analysis
Classification is regarded as a supervised learning method in machine learning, referring to a problem of predictive modeling as well, where a class label is predicted for a given example [ 41 ]. Mathematically, it maps a function ( f ) from input variables ( X ) to output variables ( Y ) as target, label or categories. To predict the class of given data points, it can be carried out on structured or unstructured data. For example, spam detection such as “spam” and “not spam” in email service providers can be a classification problem. In the following, we summarize the common classification problems.
Binary classification: It refers to the classification tasks having two class labels such as “true and false” or “yes and no” [ 41 ]. In such binary classification tasks, one class could be the normal state, while the abnormal state could be another class. For instance, “cancer not detected” is the normal state of a task that involves a medical test, and “cancer detected” could be considered as the abnormal state. Similarly, “spam” and “not spam” in the above example of email service providers are considered as binary classification.
Multiclass classification: Traditionally, this refers to those classification tasks having more than two class labels [ 41 ]. The multiclass classification does not have the principle of normal and abnormal outcomes, unlike binary classification tasks. Instead, within a range of specified classes, examples are classified as belonging to one. For example, it can be a multiclass classification task to classify various types of network attacks in the NSL-KDD [ 119 ] dataset, where the attack categories are classified into four class labels, such as DoS (Denial of Service Attack), U2R (User to Root Attack), R2L (Root to Local Attack), and Probing Attack.
Multi-label classification: In machine learning, multi-label classification is an important consideration where an example is associated with several classes or labels. Thus, it is a generalization of multiclass classification, where the classes involved in the problem are hierarchically structured, and each example may simultaneously belong to more than one class in each hierarchical level, e.g., multi-level text classification. For instance, Google news can be presented under the categories of a “city name”, “technology”, or “latest news”, etc. Multi-label classification includes advanced machine learning algorithms that support predicting various mutually non-exclusive classes or labels, unlike traditional classification tasks where class labels are mutually exclusive [ 82 ].
Many classification algorithms have been proposed in the machine learning and data science literature [ 41 , 125 ]. In the following, we summarize the most common and popular methods that are used widely in various application areas.
Naive Bayes (NB): The naive Bayes algorithm is based on the Bayes’ theorem with the assumption of independence between each pair of features [ 51 ]. It works well and can be used for both binary and multi-class categories in many real-world situations, such as document or text classification, spam filtering, etc. To effectively classify the noisy instances in the data and to construct a robust prediction model, the NB classifier can be used [ 94 ]. The key benefit is that, compared to more sophisticated approaches, it needs a small amount of training data to estimate the necessary parameters and quickly [ 82 ]. However, its performance may affect due to its strong assumptions on features independence. Gaussian, Multinomial, Complement, Bernoulli, and Categorical are the common variants of NB classifier [ 82 ].
Linear Discriminant Analysis (LDA): Linear Discriminant Analysis (LDA) is a linear decision boundary classifier created by fitting class conditional densities to data and applying Bayes’ rule [ 51 , 82 ]. This method is also known as a generalization of Fisher’s linear discriminant, which projects a given dataset into a lower-dimensional space, i.e., a reduction of dimensionality that minimizes the complexity of the model or reduces the resulting model’s computational costs. The standard LDA model usually suits each class with a Gaussian density, assuming that all classes share the same covariance matrix [ 82 ]. LDA is closely related to ANOVA (analysis of variance) and regression analysis, which seek to express one dependent variable as a linear combination of other features or measurements.
Logistic regression (LR): Another common probabilistic based statistical model used to solve classification issues in machine learning is Logistic Regression (LR) [ 64 ]. Logistic regression typically uses a logistic function to estimate the probabilities, which is also referred to as the mathematically defined sigmoid function in Eq. 1 . It can overfit high-dimensional datasets and works well when the dataset can be separated linearly. The regularization (L1 and L2) techniques [ 82 ] can be used to avoid over-fitting in such scenarios. The assumption of linearity between the dependent and independent variables is considered as a major drawback of Logistic Regression. It can be used for both classification and regression problems, but it is more commonly used for classification.
K-nearest neighbors (KNN): K-Nearest Neighbors (KNN) [ 9 ] is an “instance-based learning” or non-generalizing learning, also known as a “lazy learning” algorithm. It does not focus on constructing a general internal model; instead, it stores all instances corresponding to training data in n -dimensional space. KNN uses data and classifies new data points based on similarity measures (e.g., Euclidean distance function) [ 82 ]. Classification is computed from a simple majority vote of the k nearest neighbors of each point. It is quite robust to noisy training data, and accuracy depends on the data quality. The biggest issue with KNN is to choose the optimal number of neighbors to be considered. KNN can be used both for classification as well as regression.
Support vector machine (SVM): In machine learning, another common technique that can be used for classification, regression, or other tasks is a support vector machine (SVM) [ 56 ]. In high- or infinite-dimensional space, a support vector machine constructs a hyper-plane or set of hyper-planes. Intuitively, the hyper-plane, which has the greatest distance from the nearest training data points in any class, achieves a strong separation since, in general, the greater the margin, the lower the classifier’s generalization error. It is effective in high-dimensional spaces and can behave differently based on different mathematical functions known as the kernel. Linear, polynomial, radial basis function (RBF), sigmoid, etc., are the popular kernel functions used in SVM classifier [ 82 ]. However, when the data set contains more noise, such as overlapping target classes, SVM does not perform well.
Decision tree (DT): Decision tree (DT) [ 88 ] is a well-known non-parametric supervised learning method. DT learning methods are used for both the classification and regression tasks [ 82 ]. ID3 [ 87 ], C4.5 [ 88 ], and CART [ 20 ] are well known for DT algorithms. Moreover, recently proposed BehavDT [ 100 ], and IntrudTree [ 97 ] by Sarker et al. are effective in the relevant application domains, such as user behavior analytics and cybersecurity analytics, respectively. By sorting down the tree from the root to some leaf nodes, as shown in Fig. 4 , DT classifies the instances. Instances are classified by checking the attribute defined by that node, starting at the root node of the tree, and then moving down the tree branch corresponding to the attribute value. For splitting, the most popular criteria are “gini” for the Gini impurity and “entropy” for the information gain that can be expressed mathematically as [ 82 ].
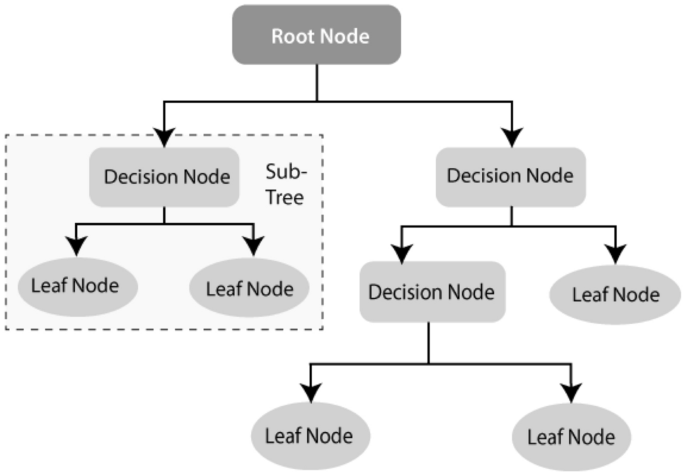
An example of a decision tree structure
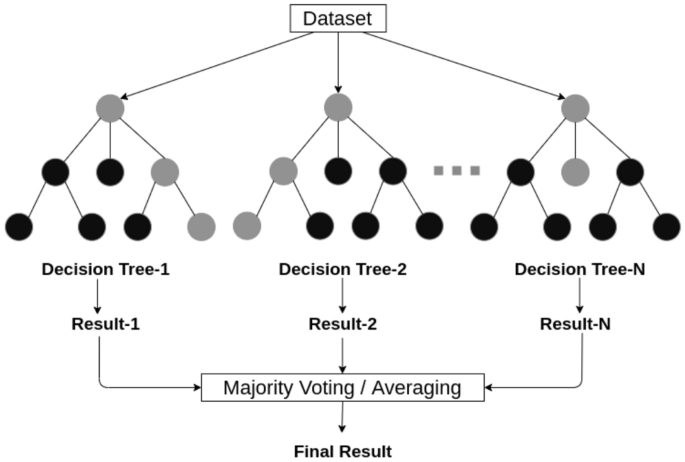
An example of a random forest structure considering multiple decision trees
Random forest (RF): A random forest classifier [ 19 ] is well known as an ensemble classification technique that is used in the field of machine learning and data science in various application areas. This method uses “parallel ensembling” which fits several decision tree classifiers in parallel, as shown in Fig. 5 , on different data set sub-samples and uses majority voting or averages for the outcome or final result. It thus minimizes the over-fitting problem and increases the prediction accuracy and control [ 82 ]. Therefore, the RF learning model with multiple decision trees is typically more accurate than a single decision tree based model [ 106 ]. To build a series of decision trees with controlled variation, it combines bootstrap aggregation (bagging) [ 18 ] and random feature selection [ 11 ]. It is adaptable to both classification and regression problems and fits well for both categorical and continuous values.
Adaptive Boosting (AdaBoost): Adaptive Boosting (AdaBoost) is an ensemble learning process that employs an iterative approach to improve poor classifiers by learning from their errors. This is developed by Yoav Freund et al. [ 35 ] and also known as “meta-learning”. Unlike the random forest that uses parallel ensembling, Adaboost uses “sequential ensembling”. It creates a powerful classifier by combining many poorly performing classifiers to obtain a good classifier of high accuracy. In that sense, AdaBoost is called an adaptive classifier by significantly improving the efficiency of the classifier, but in some instances, it can trigger overfits. AdaBoost is best used to boost the performance of decision trees, base estimator [ 82 ], on binary classification problems, however, is sensitive to noisy data and outliers.
Extreme gradient boosting (XGBoost): Gradient Boosting, like Random Forests [ 19 ] above, is an ensemble learning algorithm that generates a final model based on a series of individual models, typically decision trees. The gradient is used to minimize the loss function, similar to how neural networks [ 41 ] use gradient descent to optimize weights. Extreme Gradient Boosting (XGBoost) is a form of gradient boosting that takes more detailed approximations into account when determining the best model [ 82 ]. It computes second-order gradients of the loss function to minimize loss and advanced regularization (L1 and L2) [ 82 ], which reduces over-fitting, and improves model generalization and performance. XGBoost is fast to interpret and can handle large-sized datasets well.
Stochastic gradient descent (SGD): Stochastic gradient descent (SGD) [ 41 ] is an iterative method for optimizing an objective function with appropriate smoothness properties, where the word ‘stochastic’ refers to random probability. This reduces the computational burden, particularly in high-dimensional optimization problems, allowing for faster iterations in exchange for a lower convergence rate. A gradient is the slope of a function that calculates a variable’s degree of change in response to another variable’s changes. Mathematically, the Gradient Descent is a convex function whose output is a partial derivative of a set of its input parameters. Let, \(\alpha\) is the learning rate, and \(J_i\) is the training example cost of \(i \mathrm{th}\) , then Eq. ( 4 ) represents the stochastic gradient descent weight update method at the \(j^\mathrm{th}\) iteration. In large-scale and sparse machine learning, SGD has been successfully applied to problems often encountered in text classification and natural language processing [ 82 ]. However, SGD is sensitive to feature scaling and needs a range of hyperparameters, such as the regularization parameter and the number of iterations.
Rule-based classification : The term rule-based classification can be used to refer to any classification scheme that makes use of IF-THEN rules for class prediction. Several classification algorithms such as Zero-R [ 125 ], One-R [ 47 ], decision trees [ 87 , 88 ], DTNB [ 110 ], Ripple Down Rule learner (RIDOR) [ 125 ], Repeated Incremental Pruning to Produce Error Reduction (RIPPER) [ 126 ] exist with the ability of rule generation. The decision tree is one of the most common rule-based classification algorithms among these techniques because it has several advantages, such as being easier to interpret; the ability to handle high-dimensional data; simplicity and speed; good accuracy; and the capability to produce rules for human clear and understandable classification [ 127 ] [ 128 ]. The decision tree-based rules also provide significant accuracy in a prediction model for unseen test cases [ 106 ]. Since the rules are easily interpretable, these rule-based classifiers are often used to produce descriptive models that can describe a system including the entities and their relationships.
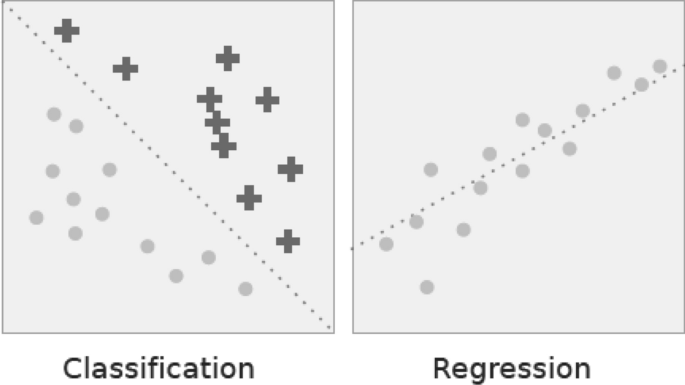
Classification vs. regression. In classification the dotted line represents a linear boundary that separates the two classes; in regression, the dotted line models the linear relationship between the two variables
Regression Analysis
Regression analysis includes several methods of machine learning that allow to predict a continuous ( y ) result variable based on the value of one or more ( x ) predictor variables [ 41 ]. The most significant distinction between classification and regression is that classification predicts distinct class labels, while regression facilitates the prediction of a continuous quantity. Figure 6 shows an example of how classification is different with regression models. Some overlaps are often found between the two types of machine learning algorithms. Regression models are now widely used in a variety of fields, including financial forecasting or prediction, cost estimation, trend analysis, marketing, time series estimation, drug response modeling, and many more. Some of the familiar types of regression algorithms are linear, polynomial, lasso and ridge regression, etc., which are explained briefly in the following.
Simple and multiple linear regression: This is one of the most popular ML modeling techniques as well as a well-known regression technique. In this technique, the dependent variable is continuous, the independent variable(s) can be continuous or discrete, and the form of the regression line is linear. Linear regression creates a relationship between the dependent variable ( Y ) and one or more independent variables ( X ) (also known as regression line) using the best fit straight line [ 41 ]. It is defined by the following equations:
where a is the intercept, b is the slope of the line, and e is the error term. This equation can be used to predict the value of the target variable based on the given predictor variable(s). Multiple linear regression is an extension of simple linear regression that allows two or more predictor variables to model a response variable, y, as a linear function [ 41 ] defined in Eq. 6 , whereas simple linear regression has only 1 independent variable, defined in Eq. 5 .
Polynomial regression: Polynomial regression is a form of regression analysis in which the relationship between the independent variable x and the dependent variable y is not linear, but is the polynomial degree of \(n^\mathrm{th}\) in x [ 82 ]. The equation for polynomial regression is also derived from linear regression (polynomial regression of degree 1) equation, which is defined as below:
Here, y is the predicted/target output, \(b_0, b_1,... b_n\) are the regression coefficients, x is an independent/ input variable. In simple words, we can say that if data are not distributed linearly, instead it is \(n^\mathrm{th}\) degree of polynomial then we use polynomial regression to get desired output.
LASSO and ridge regression: LASSO and Ridge regression are well known as powerful techniques which are typically used for building learning models in presence of a large number of features, due to their capability to preventing over-fitting and reducing the complexity of the model. The LASSO (least absolute shrinkage and selection operator) regression model uses L 1 regularization technique [ 82 ] that uses shrinkage, which penalizes “absolute value of magnitude of coefficients” ( L 1 penalty). As a result, LASSO appears to render coefficients to absolute zero. Thus, LASSO regression aims to find the subset of predictors that minimizes the prediction error for a quantitative response variable. On the other hand, ridge regression uses L 2 regularization [ 82 ], which is the “squared magnitude of coefficients” ( L 2 penalty). Thus, ridge regression forces the weights to be small but never sets the coefficient value to zero, and does a non-sparse solution. Overall, LASSO regression is useful to obtain a subset of predictors by eliminating less important features, and ridge regression is useful when a data set has “multicollinearity” which refers to the predictors that are correlated with other predictors.
Cluster Analysis
Cluster analysis, also known as clustering, is an unsupervised machine learning technique for identifying and grouping related data points in large datasets without concern for the specific outcome. It does grouping a collection of objects in such a way that objects in the same category, called a cluster, are in some sense more similar to each other than objects in other groups [ 41 ]. It is often used as a data analysis technique to discover interesting trends or patterns in data, e.g., groups of consumers based on their behavior. In a broad range of application areas, such as cybersecurity, e-commerce, mobile data processing, health analytics, user modeling and behavioral analytics, clustering can be used. In the following, we briefly discuss and summarize various types of clustering methods.
Partitioning methods: Based on the features and similarities in the data, this clustering approach categorizes the data into multiple groups or clusters. The data scientists or analysts typically determine the number of clusters either dynamically or statically depending on the nature of the target applications, to produce for the methods of clustering. The most common clustering algorithms based on partitioning methods are K-means [ 69 ], K-Mediods [ 80 ], CLARA [ 55 ] etc.
Density-based methods: To identify distinct groups or clusters, it uses the concept that a cluster in the data space is a contiguous region of high point density isolated from other such clusters by contiguous regions of low point density. Points that are not part of a cluster are considered as noise. The typical clustering algorithms based on density are DBSCAN [ 32 ], OPTICS [ 12 ] etc. The density-based methods typically struggle with clusters of similar density and high dimensionality data.
Hierarchical-based methods: Hierarchical clustering typically seeks to construct a hierarchy of clusters, i.e., the tree structure. Strategies for hierarchical clustering generally fall into two types: (i) Agglomerative—a “bottom-up” approach in which each observation begins in its cluster and pairs of clusters are combined as one, moves up the hierarchy, and (ii) Divisive—a “top-down” approach in which all observations begin in one cluster and splits are performed recursively, moves down the hierarchy, as shown in Fig 7 . Our earlier proposed BOTS technique, Sarker et al. [ 102 ] is an example of a hierarchical, particularly, bottom-up clustering algorithm.
Grid-based methods: To deal with massive datasets, grid-based clustering is especially suitable. To obtain clusters, the principle is first to summarize the dataset with a grid representation and then to combine grid cells. STING [ 122 ], CLIQUE [ 6 ], etc. are the standard algorithms of grid-based clustering.
Model-based methods: There are mainly two types of model-based clustering algorithms: one that uses statistical learning, and the other based on a method of neural network learning [ 130 ]. For instance, GMM [ 89 ] is an example of a statistical learning method, and SOM [ 22 ] [ 96 ] is an example of a neural network learning method.
Constraint-based methods: Constrained-based clustering is a semi-supervised approach to data clustering that uses constraints to incorporate domain knowledge. Application or user-oriented constraints are incorporated to perform the clustering. The typical algorithms of this kind of clustering are COP K-means [ 121 ], CMWK-Means [ 27 ], etc.
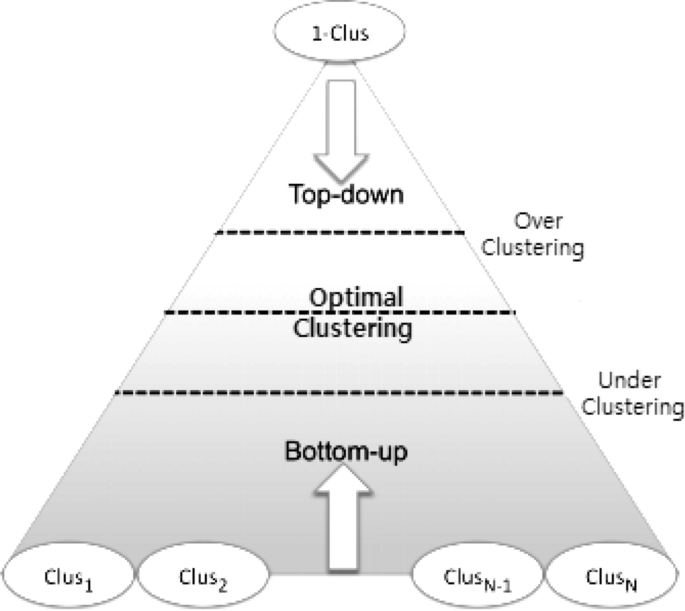
A graphical interpretation of the widely-used hierarchical clustering (Bottom-up and top-down) technique
Many clustering algorithms have been proposed with the ability to grouping data in machine learning and data science literature [ 41 , 125 ]. In the following, we summarize the popular methods that are used widely in various application areas.
K-means clustering: K-means clustering [ 69 ] is a fast, robust, and simple algorithm that provides reliable results when data sets are well-separated from each other. The data points are allocated to a cluster in this algorithm in such a way that the amount of the squared distance between the data points and the centroid is as small as possible. In other words, the K-means algorithm identifies the k number of centroids and then assigns each data point to the nearest cluster while keeping the centroids as small as possible. Since it begins with a random selection of cluster centers, the results can be inconsistent. Since extreme values can easily affect a mean, the K-means clustering algorithm is sensitive to outliers. K-medoids clustering [ 91 ] is a variant of K-means that is more robust to noises and outliers.
Mean-shift clustering: Mean-shift clustering [ 37 ] is a nonparametric clustering technique that does not require prior knowledge of the number of clusters or constraints on cluster shape. Mean-shift clustering aims to discover “blobs” in a smooth distribution or density of samples [ 82 ]. It is a centroid-based algorithm that works by updating centroid candidates to be the mean of the points in a given region. To form the final set of centroids, these candidates are filtered in a post-processing stage to remove near-duplicates. Cluster analysis in computer vision and image processing are examples of application domains. Mean Shift has the disadvantage of being computationally expensive. Moreover, in cases of high dimension, where the number of clusters shifts abruptly, the mean-shift algorithm does not work well.
DBSCAN: Density-based spatial clustering of applications with noise (DBSCAN) [ 32 ] is a base algorithm for density-based clustering which is widely used in data mining and machine learning. This is known as a non-parametric density-based clustering technique for separating high-density clusters from low-density clusters that are used in model building. DBSCAN’s main idea is that a point belongs to a cluster if it is close to many points from that cluster. It can find clusters of various shapes and sizes in a vast volume of data that is noisy and contains outliers. DBSCAN, unlike k-means, does not require a priori specification of the number of clusters in the data and can find arbitrarily shaped clusters. Although k-means is much faster than DBSCAN, it is efficient at finding high-density regions and outliers, i.e., is robust to outliers.
GMM clustering: Gaussian mixture models (GMMs) are often used for data clustering, which is a distribution-based clustering algorithm. A Gaussian mixture model is a probabilistic model in which all the data points are produced by a mixture of a finite number of Gaussian distributions with unknown parameters [ 82 ]. To find the Gaussian parameters for each cluster, an optimization algorithm called expectation-maximization (EM) [ 82 ] can be used. EM is an iterative method that uses a statistical model to estimate the parameters. In contrast to k-means, Gaussian mixture models account for uncertainty and return the likelihood that a data point belongs to one of the k clusters. GMM clustering is more robust than k-means and works well even with non-linear data distributions.
Agglomerative hierarchical clustering: The most common method of hierarchical clustering used to group objects in clusters based on their similarity is agglomerative clustering. This technique uses a bottom-up approach, where each object is first treated as a singleton cluster by the algorithm. Following that, pairs of clusters are merged one by one until all clusters have been merged into a single large cluster containing all objects. The result is a dendrogram, which is a tree-based representation of the elements. Single linkage [ 115 ], Complete linkage [ 116 ], BOTS [ 102 ] etc. are some examples of such techniques. The main advantage of agglomerative hierarchical clustering over k-means is that the tree-structure hierarchy generated by agglomerative clustering is more informative than the unstructured collection of flat clusters returned by k-means, which can help to make better decisions in the relevant application areas.
Dimensionality Reduction and Feature Learning
In machine learning and data science, high-dimensional data processing is a challenging task for both researchers and application developers. Thus, dimensionality reduction which is an unsupervised learning technique, is important because it leads to better human interpretations, lower computational costs, and avoids overfitting and redundancy by simplifying models. Both the process of feature selection and feature extraction can be used for dimensionality reduction. The primary distinction between the selection and extraction of features is that the “feature selection” keeps a subset of the original features [ 97 ], while “feature extraction” creates brand new ones [ 98 ]. In the following, we briefly discuss these techniques.
Feature selection: The selection of features, also known as the selection of variables or attributes in the data, is the process of choosing a subset of unique features (variables, predictors) to use in building machine learning and data science model. It decreases a model’s complexity by eliminating the irrelevant or less important features and allows for faster training of machine learning algorithms. A right and optimal subset of the selected features in a problem domain is capable to minimize the overfitting problem through simplifying and generalizing the model as well as increases the model’s accuracy [ 97 ]. Thus, “feature selection” [ 66 , 99 ] is considered as one of the primary concepts in machine learning that greatly affects the effectiveness and efficiency of the target machine learning model. Chi-squared test, Analysis of variance (ANOVA) test, Pearson’s correlation coefficient, recursive feature elimination, are some popular techniques that can be used for feature selection.
Feature extraction: In a machine learning-based model or system, feature extraction techniques usually provide a better understanding of the data, a way to improve prediction accuracy, and to reduce computational cost or training time. The aim of “feature extraction” [ 66 , 99 ] is to reduce the number of features in a dataset by generating new ones from the existing ones and then discarding the original features. The majority of the information found in the original set of features can then be summarized using this new reduced set of features. For instance, principal components analysis (PCA) is often used as a dimensionality-reduction technique to extract a lower-dimensional space creating new brand components from the existing features in a dataset [ 98 ].
Many algorithms have been proposed to reduce data dimensions in the machine learning and data science literature [ 41 , 125 ]. In the following, we summarize the popular methods that are used widely in various application areas.
Variance threshold: A simple basic approach to feature selection is the variance threshold [ 82 ]. This excludes all features of low variance, i.e., all features whose variance does not exceed the threshold. It eliminates all zero-variance characteristics by default, i.e., characteristics that have the same value in all samples. This feature selection algorithm looks only at the ( X ) features, not the ( y ) outputs needed, and can, therefore, be used for unsupervised learning.
Pearson correlation: Pearson’s correlation is another method to understand a feature’s relation to the response variable and can be used for feature selection [ 99 ]. This method is also used for finding the association between the features in a dataset. The resulting value is \([-1, 1]\) , where \(-1\) means perfect negative correlation, \(+1\) means perfect positive correlation, and 0 means that the two variables do not have a linear correlation. If two random variables represent X and Y , then the correlation coefficient between X and Y is defined as [ 41 ]
ANOVA: Analysis of variance (ANOVA) is a statistical tool used to verify the mean values of two or more groups that differ significantly from each other. ANOVA assumes a linear relationship between the variables and the target and the variables’ normal distribution. To statistically test the equality of means, the ANOVA method utilizes F tests. For feature selection, the results ‘ANOVA F value’ [ 82 ] of this test can be used where certain features independent of the goal variable can be omitted.
Chi square: The chi-square \({\chi }^2\) [ 82 ] statistic is an estimate of the difference between the effects of a series of events or variables observed and expected frequencies. The magnitude of the difference between the real and observed values, the degrees of freedom, and the sample size depends on \({\chi }^2\) . The chi-square \({\chi }^2\) is commonly used for testing relationships between categorical variables. If \(O_i\) represents observed value and \(E_i\) represents expected value, then
Recursive feature elimination (RFE): Recursive Feature Elimination (RFE) is a brute force approach to feature selection. RFE [ 82 ] fits the model and removes the weakest feature before it meets the specified number of features. Features are ranked by the coefficients or feature significance of the model. RFE aims to remove dependencies and collinearity in the model by recursively removing a small number of features per iteration.
Model-based selection: To reduce the dimensionality of the data, linear models penalized with the L 1 regularization can be used. Least absolute shrinkage and selection operator (Lasso) regression is a type of linear regression that has the property of shrinking some of the coefficients to zero [ 82 ]. Therefore, that feature can be removed from the model. Thus, the penalized lasso regression method, often used in machine learning to select the subset of variables. Extra Trees Classifier [ 82 ] is an example of a tree-based estimator that can be used to compute impurity-based function importance, which can then be used to discard irrelevant features.
Principal component analysis (PCA): Principal component analysis (PCA) is a well-known unsupervised learning approach in the field of machine learning and data science. PCA is a mathematical technique that transforms a set of correlated variables into a set of uncorrelated variables known as principal components [ 48 , 81 ]. Figure 8 shows an example of the effect of PCA on various dimensions space, where Fig. 8 a shows the original features in 3D space, and Fig. 8 b shows the created principal components PC1 and PC2 onto a 2D plane, and 1D line with the principal component PC1 respectively. Thus, PCA can be used as a feature extraction technique that reduces the dimensionality of the datasets, and to build an effective machine learning model [ 98 ]. Technically, PCA identifies the completely transformed with the highest eigenvalues of a covariance matrix and then uses those to project the data into a new subspace of equal or fewer dimensions [ 82 ].
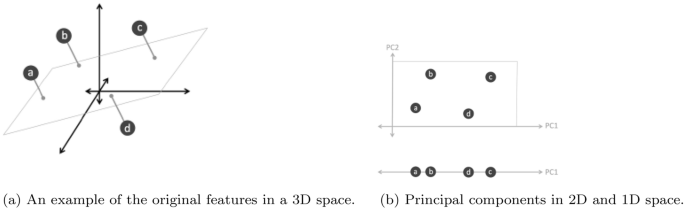
An example of a principal component analysis (PCA) and created principal components PC1 and PC2 in different dimension space
Association Rule Learning
Association rule learning is a rule-based machine learning approach to discover interesting relationships, “IF-THEN” statements, in large datasets between variables [ 7 ]. One example is that “if a customer buys a computer or laptop (an item), s/he is likely to also buy anti-virus software (another item) at the same time”. Association rules are employed today in many application areas, including IoT services, medical diagnosis, usage behavior analytics, web usage mining, smartphone applications, cybersecurity applications, and bioinformatics. In comparison to sequence mining, association rule learning does not usually take into account the order of things within or across transactions. A common way of measuring the usefulness of association rules is to use its parameter, the ‘support’ and ‘confidence’, which is introduced in [ 7 ].
In the data mining literature, many association rule learning methods have been proposed, such as logic dependent [ 34 ], frequent pattern based [ 8 , 49 , 68 ], and tree-based [ 42 ]. The most popular association rule learning algorithms are summarized below.
AIS and SETM: AIS is the first algorithm proposed by Agrawal et al. [ 7 ] for association rule mining. The AIS algorithm’s main downside is that too many candidate itemsets are generated, requiring more space and wasting a lot of effort. This algorithm calls for too many passes over the entire dataset to produce the rules. Another approach SETM [ 49 ] exhibits good performance and stable behavior with execution time; however, it suffers from the same flaw as the AIS algorithm.
Apriori: For generating association rules for a given dataset, Agrawal et al. [ 8 ] proposed the Apriori, Apriori-TID, and Apriori-Hybrid algorithms. These later algorithms outperform the AIS and SETM mentioned above due to the Apriori property of frequent itemset [ 8 ]. The term ‘Apriori’ usually refers to having prior knowledge of frequent itemset properties. Apriori uses a “bottom-up” approach, where it generates the candidate itemsets. To reduce the search space, Apriori uses the property “all subsets of a frequent itemset must be frequent; and if an itemset is infrequent, then all its supersets must also be infrequent”. Another approach predictive Apriori [ 108 ] can also generate rules; however, it receives unexpected results as it combines both the support and confidence. The Apriori [ 8 ] is the widely applicable techniques in mining association rules.
ECLAT: This technique was proposed by Zaki et al. [ 131 ] and stands for Equivalence Class Clustering and bottom-up Lattice Traversal. ECLAT uses a depth-first search to find frequent itemsets. In contrast to the Apriori [ 8 ] algorithm, which represents data in a horizontal pattern, it represents data vertically. Hence, the ECLAT algorithm is more efficient and scalable in the area of association rule learning. This algorithm is better suited for small and medium datasets whereas the Apriori algorithm is used for large datasets.
FP-Growth: Another common association rule learning technique based on the frequent-pattern tree (FP-tree) proposed by Han et al. [ 42 ] is Frequent Pattern Growth, known as FP-Growth. The key difference with Apriori is that while generating rules, the Apriori algorithm [ 8 ] generates frequent candidate itemsets; on the other hand, the FP-growth algorithm [ 42 ] prevents candidate generation and thus produces a tree by the successful strategy of ‘divide and conquer’ approach. Due to its sophistication, however, FP-Tree is challenging to use in an interactive mining environment [ 133 ]. Thus, the FP-Tree would not fit into memory for massive data sets, making it challenging to process big data as well. Another solution is RARM (Rapid Association Rule Mining) proposed by Das et al. [ 26 ] but faces a related FP-tree issue [ 133 ].
ABC-RuleMiner: A rule-based machine learning method, recently proposed in our earlier paper, by Sarker et al. [ 104 ], to discover the interesting non-redundant rules to provide real-world intelligent services. This algorithm effectively identifies the redundancy in associations by taking into account the impact or precedence of the related contextual features and discovers a set of non-redundant association rules. This algorithm first constructs an association generation tree (AGT), a top-down approach, and then extracts the association rules through traversing the tree. Thus, ABC-RuleMiner is more potent than traditional rule-based methods in terms of both non-redundant rule generation and intelligent decision-making, particularly in a context-aware smart computing environment, where human or user preferences are involved.
Among the association rule learning techniques discussed above, Apriori [ 8 ] is the most widely used algorithm for discovering association rules from a given dataset [ 133 ]. The main strength of the association learning technique is its comprehensiveness, as it generates all associations that satisfy the user-specified constraints, such as minimum support and confidence value. The ABC-RuleMiner approach [ 104 ] discussed earlier could give significant results in terms of non-redundant rule generation and intelligent decision-making for the relevant application areas in the real world.
Reinforcement Learning
Reinforcement learning (RL) is a machine learning technique that allows an agent to learn by trial and error in an interactive environment using input from its actions and experiences. Unlike supervised learning, which is based on given sample data or examples, the RL method is based on interacting with the environment. The problem to be solved in reinforcement learning (RL) is defined as a Markov Decision Process (MDP) [ 86 ], i.e., all about sequentially making decisions. An RL problem typically includes four elements such as Agent, Environment, Rewards, and Policy.
RL can be split roughly into Model-based and Model-free techniques. Model-based RL is the process of inferring optimal behavior from a model of the environment by performing actions and observing the results, which include the next state and the immediate reward [ 85 ]. AlphaZero, AlphaGo [ 113 ] are examples of the model-based approaches. On the other hand, a model-free approach does not use the distribution of the transition probability and the reward function associated with MDP. Q-learning, Deep Q Network, Monte Carlo Control, SARSA (State–Action–Reward–State–Action), etc. are some examples of model-free algorithms [ 52 ]. The policy network, which is required for model-based RL but not for model-free, is the key difference between model-free and model-based learning. In the following, we discuss the popular RL algorithms.
Monte Carlo methods: Monte Carlo techniques, or Monte Carlo experiments, are a wide category of computational algorithms that rely on repeated random sampling to obtain numerical results [ 52 ]. The underlying concept is to use randomness to solve problems that are deterministic in principle. Optimization, numerical integration, and making drawings from the probability distribution are the three problem classes where Monte Carlo techniques are most commonly used.
Q-learning: Q-learning is a model-free reinforcement learning algorithm for learning the quality of behaviors that tell an agent what action to take under what conditions [ 52 ]. It does not need a model of the environment (hence the term “model-free”), and it can deal with stochastic transitions and rewards without the need for adaptations. The ‘Q’ in Q-learning usually stands for quality, as the algorithm calculates the maximum expected rewards for a given behavior in a given state.
Deep Q-learning: The basic working step in Deep Q-Learning [ 52 ] is that the initial state is fed into the neural network, which returns the Q-value of all possible actions as an output. Still, when we have a reasonably simple setting to overcome, Q-learning works well. However, when the number of states and actions becomes more complicated, deep learning can be used as a function approximator.
Reinforcement learning, along with supervised and unsupervised learning, is one of the basic machine learning paradigms. RL can be used to solve numerous real-world problems in various fields, such as game theory, control theory, operations analysis, information theory, simulation-based optimization, manufacturing, supply chain logistics, multi-agent systems, swarm intelligence, aircraft control, robot motion control, and many more.
Artificial Neural Network and Deep Learning
Deep learning is part of a wider family of artificial neural networks (ANN)-based machine learning approaches with representation learning. Deep learning provides a computational architecture by combining several processing layers, such as input, hidden, and output layers, to learn from data [ 41 ]. The main advantage of deep learning over traditional machine learning methods is its better performance in several cases, particularly learning from large datasets [ 105 , 129 ]. Figure 9 shows a general performance of deep learning over machine learning considering the increasing amount of data. However, it may vary depending on the data characteristics and experimental set up.
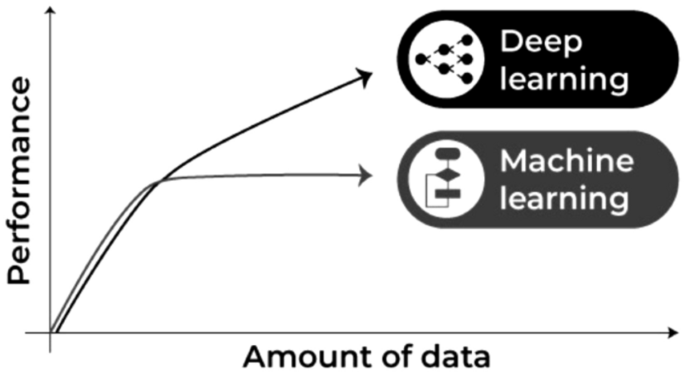
Machine learning and deep learning performance in general with the amount of data
The most common deep learning algorithms are: Multi-layer Perceptron (MLP), Convolutional Neural Network (CNN, or ConvNet), Long Short-Term Memory Recurrent Neural Network (LSTM-RNN) [ 96 ]. In the following, we discuss various types of deep learning methods that can be used to build effective data-driven models for various purposes.
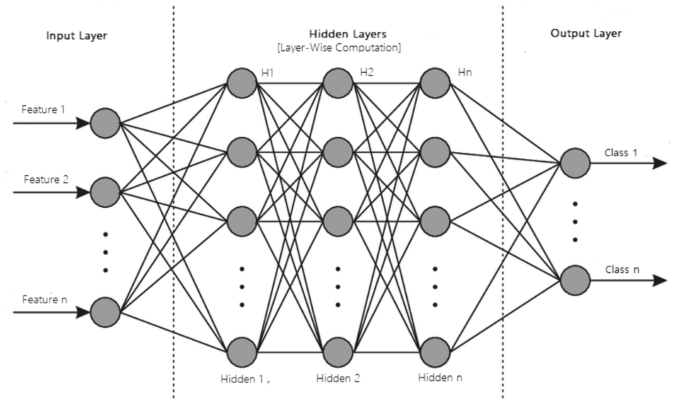
A structure of an artificial neural network modeling with multiple processing layers
MLP: The base architecture of deep learning, which is also known as the feed-forward artificial neural network, is called a multilayer perceptron (MLP) [ 82 ]. A typical MLP is a fully connected network consisting of an input layer, one or more hidden layers, and an output layer, as shown in Fig. 10 . Each node in one layer connects to each node in the following layer at a certain weight. MLP utilizes the “Backpropagation” technique [ 41 ], the most “fundamental building block” in a neural network, to adjust the weight values internally while building the model. MLP is sensitive to scaling features and allows a variety of hyperparameters to be tuned, such as the number of hidden layers, neurons, and iterations, which can result in a computationally costly model.
CNN or ConvNet: The convolution neural network (CNN) [ 65 ] enhances the design of the standard ANN, consisting of convolutional layers, pooling layers, as well as fully connected layers, as shown in Fig. 11 . As it takes the advantage of the two-dimensional (2D) structure of the input data, it is typically broadly used in several areas such as image and video recognition, image processing and classification, medical image analysis, natural language processing, etc. While CNN has a greater computational burden, without any manual intervention, it has the advantage of automatically detecting the important features, and hence CNN is considered to be more powerful than conventional ANN. A number of advanced deep learning models based on CNN can be used in the field, such as AlexNet [ 60 ], Xception [ 24 ], Inception [ 118 ], Visual Geometry Group (VGG) [ 44 ], ResNet [ 45 ], etc.
LSTM-RNN: Long short-term memory (LSTM) is an artificial recurrent neural network (RNN) architecture used in the area of deep learning [ 38 ]. LSTM has feedback links, unlike normal feed-forward neural networks. LSTM networks are well-suited for analyzing and learning sequential data, such as classifying, processing, and predicting data based on time series data, which differentiates it from other conventional networks. Thus, LSTM can be used when the data are in a sequential format, such as time, sentence, etc., and commonly applied in the area of time-series analysis, natural language processing, speech recognition, etc.
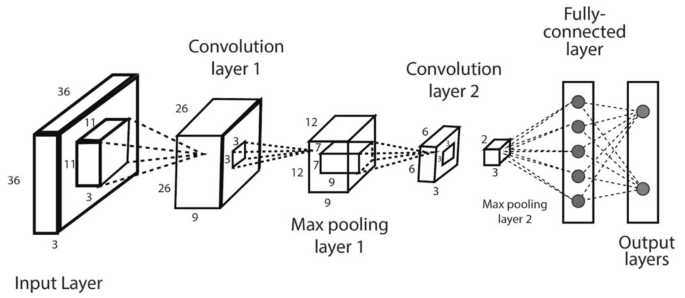
An example of a convolutional neural network (CNN or ConvNet) including multiple convolution and pooling layers
In addition to these most common deep learning methods discussed above, several other deep learning approaches [ 96 ] exist in the area for various purposes. For instance, the self-organizing map (SOM) [ 58 ] uses unsupervised learning to represent the high-dimensional data by a 2D grid map, thus achieving dimensionality reduction. The autoencoder (AE) [ 15 ] is another learning technique that is widely used for dimensionality reduction as well and feature extraction in unsupervised learning tasks. Restricted Boltzmann machines (RBM) [ 46 ] can be used for dimensionality reduction, classification, regression, collaborative filtering, feature learning, and topic modeling. A deep belief network (DBN) is typically composed of simple, unsupervised networks such as restricted Boltzmann machines (RBMs) or autoencoders, and a backpropagation neural network (BPNN) [ 123 ]. A generative adversarial network (GAN) [ 39 ] is a form of the network for deep learning that can generate data with characteristics close to the actual data input. Transfer learning is currently very common because it can train deep neural networks with comparatively low data, which is typically the re-use of a new problem with a pre-trained model [ 124 ]. A brief discussion of these artificial neural networks (ANN) and deep learning (DL) models are summarized in our earlier paper Sarker et al. [ 96 ].
Overall, based on the learning techniques discussed above, we can conclude that various types of machine learning techniques, such as classification analysis, regression, data clustering, feature selection and extraction, and dimensionality reduction, association rule learning, reinforcement learning, or deep learning techniques, can play a significant role for various purposes according to their capabilities. In the following section, we discuss several application areas based on machine learning algorithms.

Applications of Machine Learning
In the current age of the Fourth Industrial Revolution (4IR), machine learning becomes popular in various application areas, because of its learning capabilities from the past and making intelligent decisions. In the following, we summarize and discuss ten popular application areas of machine learning technology.
Predictive analytics and intelligent decision-making: A major application field of machine learning is intelligent decision-making by data-driven predictive analytics [ 21 , 70 ]. The basis of predictive analytics is capturing and exploiting relationships between explanatory variables and predicted variables from previous events to predict the unknown outcome [ 41 ]. For instance, identifying suspects or criminals after a crime has been committed, or detecting credit card fraud as it happens. Another application, where machine learning algorithms can assist retailers in better understanding consumer preferences and behavior, better manage inventory, avoiding out-of-stock situations, and optimizing logistics and warehousing in e-commerce. Various machine learning algorithms such as decision trees, support vector machines, artificial neural networks, etc. [ 106 , 125 ] are commonly used in the area. Since accurate predictions provide insight into the unknown, they can improve the decisions of industries, businesses, and almost any organization, including government agencies, e-commerce, telecommunications, banking and financial services, healthcare, sales and marketing, transportation, social networking, and many others.
Cybersecurity and threat intelligence: Cybersecurity is one of the most essential areas of Industry 4.0. [ 114 ], which is typically the practice of protecting networks, systems, hardware, and data from digital attacks [ 114 ]. Machine learning has become a crucial cybersecurity technology that constantly learns by analyzing data to identify patterns, better detect malware in encrypted traffic, find insider threats, predict where bad neighborhoods are online, keep people safe while browsing, or secure data in the cloud by uncovering suspicious activity. For instance, clustering techniques can be used to identify cyber-anomalies, policy violations, etc. To detect various types of cyber-attacks or intrusions machine learning classification models by taking into account the impact of security features are useful [ 97 ]. Various deep learning-based security models can also be used on the large scale of security datasets [ 96 , 129 ]. Moreover, security policy rules generated by association rule learning techniques can play a significant role to build a rule-based security system [ 105 ]. Thus, we can say that various learning techniques discussed in Sect. Machine Learning Tasks and Algorithms , can enable cybersecurity professionals to be more proactive inefficiently preventing threats and cyber-attacks.
Internet of things (IoT) and smart cities: Internet of Things (IoT) is another essential area of Industry 4.0. [ 114 ], which turns everyday objects into smart objects by allowing them to transmit data and automate tasks without the need for human interaction. IoT is, therefore, considered to be the big frontier that can enhance almost all activities in our lives, such as smart governance, smart home, education, communication, transportation, retail, agriculture, health care, business, and many more [ 70 ]. Smart city is one of IoT’s core fields of application, using technologies to enhance city services and residents’ living experiences [ 132 , 135 ]. As machine learning utilizes experience to recognize trends and create models that help predict future behavior and events, it has become a crucial technology for IoT applications [ 103 ]. For example, to predict traffic in smart cities, parking availability prediction, estimate the total usage of energy of the citizens for a particular period, make context-aware and timely decisions for the people, etc. are some tasks that can be solved using machine learning techniques according to the current needs of the people.
Traffic prediction and transportation: Transportation systems have become a crucial component of every country’s economic development. Nonetheless, several cities around the world are experiencing an excessive rise in traffic volume, resulting in serious issues such as delays, traffic congestion, higher fuel prices, increased CO \(_2\) pollution, accidents, emergencies, and a decline in modern society’s quality of life [ 40 ]. Thus, an intelligent transportation system through predicting future traffic is important, which is an indispensable part of a smart city. Accurate traffic prediction based on machine and deep learning modeling can help to minimize the issues [ 17 , 30 , 31 ]. For example, based on the travel history and trend of traveling through various routes, machine learning can assist transportation companies in predicting possible issues that may occur on specific routes and recommending their customers to take a different path. Ultimately, these learning-based data-driven models help improve traffic flow, increase the usage and efficiency of sustainable modes of transportation, and limit real-world disruption by modeling and visualizing future changes.
Healthcare and COVID-19 pandemic: Machine learning can help to solve diagnostic and prognostic problems in a variety of medical domains, such as disease prediction, medical knowledge extraction, detecting regularities in data, patient management, etc. [ 33 , 77 , 112 ]. Coronavirus disease (COVID-19) is an infectious disease caused by a newly discovered coronavirus, according to the World Health Organization (WHO) [ 3 ]. Recently, the learning techniques have become popular in the battle against COVID-19 [ 61 , 63 ]. For the COVID-19 pandemic, the learning techniques are used to classify patients at high risk, their mortality rate, and other anomalies [ 61 ]. It can also be used to better understand the virus’s origin, COVID-19 outbreak prediction, as well as for disease diagnosis and treatment [ 14 , 50 ]. With the help of machine learning, researchers can forecast where and when, the COVID-19 is likely to spread, and notify those regions to match the required arrangements. Deep learning also provides exciting solutions to the problems of medical image processing and is seen as a crucial technique for potential applications, particularly for COVID-19 pandemic [ 10 , 78 , 111 ]. Overall, machine and deep learning techniques can help to fight the COVID-19 virus and the pandemic as well as intelligent clinical decisions making in the domain of healthcare.
E-commerce and product recommendations: Product recommendation is one of the most well known and widely used applications of machine learning, and it is one of the most prominent features of almost any e-commerce website today. Machine learning technology can assist businesses in analyzing their consumers’ purchasing histories and making customized product suggestions for their next purchase based on their behavior and preferences. E-commerce companies, for example, can easily position product suggestions and offers by analyzing browsing trends and click-through rates of specific items. Using predictive modeling based on machine learning techniques, many online retailers, such as Amazon [ 71 ], can better manage inventory, prevent out-of-stock situations, and optimize logistics and warehousing. The future of sales and marketing is the ability to capture, evaluate, and use consumer data to provide a customized shopping experience. Furthermore, machine learning techniques enable companies to create packages and content that are tailored to the needs of their customers, allowing them to maintain existing customers while attracting new ones.
NLP and sentiment analysis: Natural language processing (NLP) involves the reading and understanding of spoken or written language through the medium of a computer [ 79 , 103 ]. Thus, NLP helps computers, for instance, to read a text, hear speech, interpret it, analyze sentiment, and decide which aspects are significant, where machine learning techniques can be used. Virtual personal assistant, chatbot, speech recognition, document description, language or machine translation, etc. are some examples of NLP-related tasks. Sentiment Analysis [ 90 ] (also referred to as opinion mining or emotion AI) is an NLP sub-field that seeks to identify and extract public mood and views within a given text through blogs, reviews, social media, forums, news, etc. For instance, businesses and brands use sentiment analysis to understand the social sentiment of their brand, product, or service through social media platforms or the web as a whole. Overall, sentiment analysis is considered as a machine learning task that analyzes texts for polarity, such as “positive”, “negative”, or “neutral” along with more intense emotions like very happy, happy, sad, very sad, angry, have interest, or not interested etc.
Image, speech and pattern recognition: Image recognition [ 36 ] is a well-known and widespread example of machine learning in the real world, which can identify an object as a digital image. For instance, to label an x-ray as cancerous or not, character recognition, or face detection in an image, tagging suggestions on social media, e.g., Facebook, are common examples of image recognition. Speech recognition [ 23 ] is also very popular that typically uses sound and linguistic models, e.g., Google Assistant, Cortana, Siri, Alexa, etc. [ 67 ], where machine learning methods are used. Pattern recognition [ 13 ] is defined as the automated recognition of patterns and regularities in data, e.g., image analysis. Several machine learning techniques such as classification, feature selection, clustering, or sequence labeling methods are used in the area.
Sustainable agriculture: Agriculture is essential to the survival of all human activities [ 109 ]. Sustainable agriculture practices help to improve agricultural productivity while also reducing negative impacts on the environment [ 5 , 25 , 109 ]. The sustainable agriculture supply chains are knowledge-intensive and based on information, skills, technologies, etc., where knowledge transfer encourages farmers to enhance their decisions to adopt sustainable agriculture practices utilizing the increasing amount of data captured by emerging technologies, e.g., the Internet of Things (IoT), mobile technologies and devices, etc. [ 5 , 53 , 54 ]. Machine learning can be applied in various phases of sustainable agriculture, such as in the pre-production phase - for the prediction of crop yield, soil properties, irrigation requirements, etc.; in the production phase—for weather prediction, disease detection, weed detection, soil nutrient management, livestock management, etc.; in processing phase—for demand estimation, production planning, etc. and in the distribution phase - the inventory management, consumer analysis, etc.
User behavior analytics and context-aware smartphone applications: Context-awareness is a system’s ability to capture knowledge about its surroundings at any moment and modify behaviors accordingly [ 28 , 93 ]. Context-aware computing uses software and hardware to automatically collect and interpret data for direct responses. The mobile app development environment has been changed greatly with the power of AI, particularly, machine learning techniques through their learning capabilities from contextual data [ 103 , 136 ]. Thus, the developers of mobile apps can rely on machine learning to create smart apps that can understand human behavior, support, and entertain users [ 107 , 137 , 140 ]. To build various personalized data-driven context-aware systems, such as smart interruption management, smart mobile recommendation, context-aware smart searching, decision-making that intelligently assist end mobile phone users in a pervasive computing environment, machine learning techniques are applicable. For example, context-aware association rules can be used to build an intelligent phone call application [ 104 ]. Clustering approaches are useful in capturing users’ diverse behavioral activities by taking into account data in time series [ 102 ]. To predict the future events in various contexts, the classification methods can be used [ 106 , 139 ]. Thus, various learning techniques discussed in Sect. “ Machine Learning Tasks and Algorithms ” can help to build context-aware adaptive and smart applications according to the preferences of the mobile phone users.
In addition to these application areas, machine learning-based models can also apply to several other domains such as bioinformatics, cheminformatics, computer networks, DNA sequence classification, economics and banking, robotics, advanced engineering, and many more.
Challenges and Research Directions
Our study on machine learning algorithms for intelligent data analysis and applications opens several research issues in the area. Thus, in this section, we summarize and discuss the challenges faced and the potential research opportunities and future directions.
In general, the effectiveness and the efficiency of a machine learning-based solution depend on the nature and characteristics of the data, and the performance of the learning algorithms. To collect the data in the relevant domain, such as cybersecurity, IoT, healthcare and agriculture discussed in Sect. “ Applications of Machine Learning ” is not straightforward, although the current cyberspace enables the production of a huge amount of data with very high frequency. Thus, collecting useful data for the target machine learning-based applications, e.g., smart city applications, and their management is important to further analysis. Therefore, a more in-depth investigation of data collection methods is needed while working on the real-world data. Moreover, the historical data may contain many ambiguous values, missing values, outliers, and meaningless data. The machine learning algorithms, discussed in Sect “ Machine Learning Tasks and Algorithms ” highly impact on data quality, and availability for training, and consequently on the resultant model. Thus, to accurately clean and pre-process the diverse data collected from diverse sources is a challenging task. Therefore, effectively modifying or enhance existing pre-processing methods, or proposing new data preparation techniques are required to effectively use the learning algorithms in the associated application domain.
To analyze the data and extract insights, there exist many machine learning algorithms, summarized in Sect. “ Machine Learning Tasks and Algorithms ”. Thus, selecting a proper learning algorithm that is suitable for the target application is challenging. The reason is that the outcome of different learning algorithms may vary depending on the data characteristics [ 106 ]. Selecting a wrong learning algorithm would result in producing unexpected outcomes that may lead to loss of effort, as well as the model’s effectiveness and accuracy. In terms of model building, the techniques discussed in Sect. “ Machine Learning Tasks and Algorithms ” can directly be used to solve many real-world issues in diverse domains, such as cybersecurity, smart cities and healthcare summarized in Sect. “ Applications of Machine Learning ”. However, the hybrid learning model, e.g., the ensemble of methods, modifying or enhancement of the existing learning techniques, or designing new learning methods, could be a potential future work in the area.
Thus, the ultimate success of a machine learning-based solution and corresponding applications mainly depends on both the data and the learning algorithms. If the data are bad to learn, such as non-representative, poor-quality, irrelevant features, or insufficient quantity for training, then the machine learning models may become useless or will produce lower accuracy. Therefore, effectively processing the data and handling the diverse learning algorithms are important, for a machine learning-based solution and eventually building intelligent applications.
In this paper, we have conducted a comprehensive overview of machine learning algorithms for intelligent data analysis and applications. According to our goal, we have briefly discussed how various types of machine learning methods can be used for making solutions to various real-world issues. A successful machine learning model depends on both the data and the performance of the learning algorithms. The sophisticated learning algorithms then need to be trained through the collected real-world data and knowledge related to the target application before the system can assist with intelligent decision-making. We also discussed several popular application areas based on machine learning techniques to highlight their applicability in various real-world issues. Finally, we have summarized and discussed the challenges faced and the potential research opportunities and future directions in the area. Therefore, the challenges that are identified create promising research opportunities in the field which must be addressed with effective solutions in various application areas. Overall, we believe that our study on machine learning-based solutions opens up a promising direction and can be used as a reference guide for potential research and applications for both academia and industry professionals as well as for decision-makers, from a technical point of view.
Canadian institute of cybersecurity, university of new brunswick, iscx dataset, http://www.unb.ca/cic/datasets/index.html/ (Accessed on 20 October 2019).
Cic-ddos2019 [online]. available: https://www.unb.ca/cic/datasets/ddos-2019.html/ (Accessed on 28 March 2020).
World health organization: WHO. http://www.who.int/ .
Google trends. In https://trends.google.com/trends/ , 2019.
Adnan N, Nordin Shahrina Md, Rahman I, Noor A. The effects of knowledge transfer on farmers decision making toward sustainable agriculture practices. World J Sci Technol Sustain Dev. 2018.
Agrawal R, Gehrke J, Gunopulos D, Raghavan P. Automatic subspace clustering of high dimensional data for data mining applications. In: Proceedings of the 1998 ACM SIGMOD international conference on Management of data. 1998; 94–105
Agrawal R, Imieliński T, Swami A. Mining association rules between sets of items in large databases. In: ACM SIGMOD Record. ACM. 1993;22: 207–216
Agrawal R, Gehrke J, Gunopulos D, Raghavan P. Fast algorithms for mining association rules. In: Proceedings of the International Joint Conference on Very Large Data Bases, Santiago Chile. 1994; 1215: 487–499.
Aha DW, Kibler D, Albert M. Instance-based learning algorithms. Mach Learn. 1991;6(1):37–66.
Article Google Scholar
Alakus TB, Turkoglu I. Comparison of deep learning approaches to predict covid-19 infection. Chaos Solit Fract. 2020;140:
Amit Y, Geman D. Shape quantization and recognition with randomized trees. Neural Comput. 1997;9(7):1545–88.
Ankerst M, Breunig MM, Kriegel H-P, Sander J. Optics: ordering points to identify the clustering structure. ACM Sigmod Record. 1999;28(2):49–60.
Anzai Y. Pattern recognition and machine learning. Elsevier; 2012.
MATH Google Scholar
Ardabili SF, Mosavi A, Ghamisi P, Ferdinand F, Varkonyi-Koczy AR, Reuter U, Rabczuk T, Atkinson PM. Covid-19 outbreak prediction with machine learning. Algorithms. 2020;13(10):249.
Article MathSciNet Google Scholar
Baldi P. Autoencoders, unsupervised learning, and deep architectures. In: Proceedings of ICML workshop on unsupervised and transfer learning, 2012; 37–49 .
Balducci F, Impedovo D, Pirlo G. Machine learning applications on agricultural datasets for smart farm enhancement. Machines. 2018;6(3):38.
Boukerche A, Wang J. Machine learning-based traffic prediction models for intelligent transportation systems. Comput Netw. 2020;181
Breiman L. Bagging predictors. Mach Learn. 1996;24(2):123–40.
Article MATH Google Scholar
Breiman L. Random forests. Mach Learn. 2001;45(1):5–32.
Breiman L, Friedman J, Stone CJ, Olshen RA. Classification and regression trees. CRC Press; 1984.
Cao L. Data science: a comprehensive overview. ACM Comput Surv (CSUR). 2017;50(3):43.
Google Scholar
Carpenter GA, Grossberg S. A massively parallel architecture for a self-organizing neural pattern recognition machine. Comput Vis Graph Image Process. 1987;37(1):54–115.
Chiu C-C, Sainath TN, Wu Y, Prabhavalkar R, Nguyen P, Chen Z, Kannan A, Weiss RJ, Rao K, Gonina E, et al. State-of-the-art speech recognition with sequence-to-sequence models. In: 2018 IEEE International Conference on Acoustics, Speech and Signal Processing (ICASSP), 2018 pages 4774–4778. IEEE .
Chollet F. Xception: deep learning with depthwise separable convolutions. In: Proceedings of the IEEE conference on computer vision and pattern recognition, pages 1251–1258, 2017.
Cobuloglu H, Büyüktahtakın IE. A stochastic multi-criteria decision analysis for sustainable biomass crop selection. Expert Syst Appl. 2015;42(15–16):6065–74.
Das A, Ng W-K, Woon Y-K. Rapid association rule mining. In: Proceedings of the tenth international conference on Information and knowledge management, pages 474–481. ACM, 2001.
de Amorim RC. Constrained clustering with minkowski weighted k-means. In: 2012 IEEE 13th International Symposium on Computational Intelligence and Informatics (CINTI), pages 13–17. IEEE, 2012.
Dey AK. Understanding and using context. Person Ubiquit Comput. 2001;5(1):4–7.
Eagle N, Pentland AS. Reality mining: sensing complex social systems. Person Ubiquit Comput. 2006;10(4):255–68.
Essien A, Petrounias I, Sampaio P, Sampaio S. Improving urban traffic speed prediction using data source fusion and deep learning. In: 2019 IEEE International Conference on Big Data and Smart Computing (BigComp). IEEE. 2019: 1–8. .
Essien A, Petrounias I, Sampaio P, Sampaio S. A deep-learning model for urban traffic flow prediction with traffic events mined from twitter. In: World Wide Web, 2020: 1–24 .
Ester M, Kriegel H-P, Sander J, Xiaowei X, et al. A density-based algorithm for discovering clusters in large spatial databases with noise. Kdd. 1996;96:226–31.
Fatima M, Pasha M, et al. Survey of machine learning algorithms for disease diagnostic. J Intell Learn Syst Appl. 2017;9(01):1.
Flach PA, Lachiche N. Confirmation-guided discovery of first-order rules with tertius. Mach Learn. 2001;42(1–2):61–95.
Freund Y, Schapire RE, et al. Experiments with a new boosting algorithm. In: Icml, Citeseer. 1996; 96: 148–156
Fujiyoshi H, Hirakawa T, Yamashita T. Deep learning-based image recognition for autonomous driving. IATSS Res. 2019;43(4):244–52.
Fukunaga K, Hostetler L. The estimation of the gradient of a density function, with applications in pattern recognition. IEEE Trans Inform Theory. 1975;21(1):32–40.
Article MathSciNet MATH Google Scholar
Goodfellow I, Bengio Y, Courville A, Bengio Y. Deep learning. Cambridge: MIT Press; 2016.
Goodfellow I, Pouget-Abadie J, Mirza M, Xu B, Warde-Farley D, Ozair S, Courville A, Bengio Y. Generative adversarial nets. In: Advances in neural information processing systems. 2014: 2672–2680.
Guerrero-Ibáñez J, Zeadally S, Contreras-Castillo J. Sensor technologies for intelligent transportation systems. Sensors. 2018;18(4):1212.
Han J, Pei J, Kamber M. Data mining: concepts and techniques. Amsterdam: Elsevier; 2011.
Han J, Pei J, Yin Y. Mining frequent patterns without candidate generation. In: ACM Sigmod Record, ACM. 2000;29: 1–12.
Harmon SA, Sanford TH, Sheng X, Turkbey EB, Roth H, Ziyue X, Yang D, Myronenko A, Anderson V, Amalou A, et al. Artificial intelligence for the detection of covid-19 pneumonia on chest ct using multinational datasets. Nat Commun. 2020;11(1):1–7.
He K, Zhang X, Ren S, Sun J. Spatial pyramid pooling in deep convolutional networks for visual recognition. IEEE Trans Pattern Anal Mach Intell. 2015;37(9):1904–16.
He K, Zhang X, Ren S, Sun J. Deep residual learning for image recognition. In: Proceedings of the IEEE conference on computer vision and pattern recognition, 2016: 770–778.
Hinton GE. A practical guide to training restricted boltzmann machines. In: Neural networks: Tricks of the trade. Springer. 2012; 599-619
Holte RC. Very simple classification rules perform well on most commonly used datasets. Mach Learn. 1993;11(1):63–90.
Hotelling H. Analysis of a complex of statistical variables into principal components. J Edu Psychol. 1933;24(6):417.
Houtsma M, Swami A. Set-oriented mining for association rules in relational databases. In: Data Engineering, 1995. Proceedings of the Eleventh International Conference on, IEEE.1995:25–33.
Jamshidi M, Lalbakhsh A, Talla J, Peroutka Z, Hadjilooei F, Lalbakhsh P, Jamshidi M, La Spada L, Mirmozafari M, Dehghani M, et al. Artificial intelligence and covid-19: deep learning approaches for diagnosis and treatment. IEEE Access. 2020;8:109581–95.
John GH, Langley P. Estimating continuous distributions in bayesian classifiers. In: Proceedings of the Eleventh conference on Uncertainty in artificial intelligence, Morgan Kaufmann Publishers Inc. 1995; 338–345
Kaelbling LP, Littman ML, Moore AW. Reinforcement learning: a survey. J Artif Intell Res. 1996;4:237–85.
Kamble SS, Gunasekaran A, Gawankar SA. Sustainable industry 4.0 framework: a systematic literature review identifying the current trends and future perspectives. Process Saf Environ Protect. 2018;117:408–25.
Kamble SS, Gunasekaran A, Gawankar SA. Achieving sustainable performance in a data-driven agriculture supply chain: a review for research and applications. Int J Prod Econ. 2020;219:179–94.
Kaufman L, Rousseeuw PJ. Finding groups in data: an introduction to cluster analysis, vol. 344. John Wiley & Sons; 2009.
Keerthi SS, Shevade SK, Bhattacharyya C, Radha Krishna MK. Improvements to platt’s smo algorithm for svm classifier design. Neural Comput. 2001;13(3):637–49.
Khadse V, Mahalle PN, Biraris SV. An empirical comparison of supervised machine learning algorithms for internet of things data. In: 2018 Fourth International Conference on Computing Communication Control and Automation (ICCUBEA), IEEE. 2018; 1–6
Kohonen T. The self-organizing map. Proc IEEE. 1990;78(9):1464–80.
Koroniotis N, Moustafa N, Sitnikova E, Turnbull B. Towards the development of realistic botnet dataset in the internet of things for network forensic analytics: bot-iot dataset. Fut Gen Comput Syst. 2019;100:779–96.
Krizhevsky A, Sutskever I, Hinton GE. Imagenet classification with deep convolutional neural networks. In: Advances in neural information processing systems, 2012: 1097–1105
Kushwaha S, Bahl S, Bagha AK, Parmar KS, Javaid M, Haleem A, Singh RP. Significant applications of machine learning for covid-19 pandemic. J Ind Integr Manag. 2020;5(4).
Lade P, Ghosh R, Srinivasan S. Manufacturing analytics and industrial internet of things. IEEE Intell Syst. 2017;32(3):74–9.
Lalmuanawma S, Hussain J, Chhakchhuak L. Applications of machine learning and artificial intelligence for covid-19 (sars-cov-2) pandemic: a review. Chaos Sol Fract. 2020:110059 .
LeCessie S, Van Houwelingen JC. Ridge estimators in logistic regression. J R Stat Soc Ser C (Appl Stat). 1992;41(1):191–201.
LeCun Y, Bottou L, Bengio Y, Haffner P. Gradient-based learning applied to document recognition. Proc IEEE. 1998;86(11):2278–324.
Liu H, Motoda H. Feature extraction, construction and selection: A data mining perspective, vol. 453. Springer Science & Business Media; 1998.
López G, Quesada L, Guerrero LA. Alexa vs. siri vs. cortana vs. google assistant: a comparison of speech-based natural user interfaces. In: International Conference on Applied Human Factors and Ergonomics, Springer. 2017; 241–250.
Liu B, HsuW, Ma Y. Integrating classification and association rule mining. In: Proceedings of the fourth international conference on knowledge discovery and data mining, 1998.
MacQueen J, et al. Some methods for classification and analysis of multivariate observations. In: Proceedings of the fifth Berkeley symposium on mathematical statistics and probability, 1967;volume 1, pages 281–297. Oakland, CA, USA.
Mahdavinejad MS, Rezvan M, Barekatain M, Adibi P, Barnaghi P, Sheth AP. Machine learning for internet of things data analysis: a survey. Digit Commun Netw. 2018;4(3):161–75.
Marchand A, Marx P. Automated product recommendations with preference-based explanations. J Retail. 2020;96(3):328–43.
McCallum A. Information extraction: distilling structured data from unstructured text. Queue. 2005;3(9):48–57.
Mehrotra A, Hendley R, Musolesi M. Prefminer: mining user’s preferences for intelligent mobile notification management. In: Proceedings of the International Joint Conference on Pervasive and Ubiquitous Computing, Heidelberg, Germany, 12–16 September, 2016; pp. 1223–1234. ACM, New York, USA. .
Mohamadou Y, Halidou A, Kapen PT. A review of mathematical modeling, artificial intelligence and datasets used in the study, prediction and management of covid-19. Appl Intell. 2020;50(11):3913–25.
Mohammed M, Khan MB, Bashier Mohammed BE. Machine learning: algorithms and applications. CRC Press; 2016.
Book Google Scholar
Moustafa N, Slay J. Unsw-nb15: a comprehensive data set for network intrusion detection systems (unsw-nb15 network data set). In: 2015 military communications and information systems conference (MilCIS), 2015;pages 1–6. IEEE .
Nilashi M, Ibrahim OB, Ahmadi H, Shahmoradi L. An analytical method for diseases prediction using machine learning techniques. Comput Chem Eng. 2017;106:212–23.
Yujin O, Park S, Ye JC. Deep learning covid-19 features on cxr using limited training data sets. IEEE Trans Med Imaging. 2020;39(8):2688–700.
Otter DW, Medina JR , Kalita JK. A survey of the usages of deep learning for natural language processing. IEEE Trans Neural Netw Learn Syst. 2020.
Park H-S, Jun C-H. A simple and fast algorithm for k-medoids clustering. Expert Syst Appl. 2009;36(2):3336–41.
Liii Pearson K. on lines and planes of closest fit to systems of points in space. Lond Edinb Dublin Philos Mag J Sci. 1901;2(11):559–72.
Pedregosa F, Varoquaux G, Gramfort A, Michel V, Thirion B, Grisel O, Blondel M, Prettenhofer P, Weiss R, Dubourg V, et al. Scikit-learn: machine learning in python. J Mach Learn Res. 2011;12:2825–30.
MathSciNet MATH Google Scholar
Perveen S, Shahbaz M, Keshavjee K, Guergachi A. Metabolic syndrome and development of diabetes mellitus: predictive modeling based on machine learning techniques. IEEE Access. 2018;7:1365–75.
Santi P, Ram D, Rob C, Nathan E. Behavior-based adaptive call predictor. ACM Trans Auton Adapt Syst. 2011;6(3):21:1–21:28.
Polydoros AS, Nalpantidis L. Survey of model-based reinforcement learning: applications on robotics. J Intell Robot Syst. 2017;86(2):153–73.
Puterman ML. Markov decision processes: discrete stochastic dynamic programming. John Wiley & Sons; 2014.
Quinlan JR. Induction of decision trees. Mach Learn. 1986;1:81–106.
Quinlan JR. C4.5: programs for machine learning. Mach Learn. 1993.
Rasmussen C. The infinite gaussian mixture model. Adv Neural Inform Process Syst. 1999;12:554–60.
Ravi K, Ravi V. A survey on opinion mining and sentiment analysis: tasks, approaches and applications. Knowl Syst. 2015;89:14–46.
Rokach L. A survey of clustering algorithms. In: Data mining and knowledge discovery handbook, pages 269–298. Springer, 2010.
Safdar S, Zafar S, Zafar N, Khan NF. Machine learning based decision support systems (dss) for heart disease diagnosis: a review. Artif Intell Rev. 2018;50(4):597–623.
Sarker IH. Context-aware rule learning from smartphone data: survey, challenges and future directions. J Big Data. 2019;6(1):1–25.
Sarker IH. A machine learning based robust prediction model for real-life mobile phone data. Internet Things. 2019;5:180–93.
Sarker IH. Ai-driven cybersecurity: an overview, security intelligence modeling and research directions. SN Comput Sci. 2021.
Sarker IH. Deep cybersecurity: a comprehensive overview from neural network and deep learning perspective. SN Comput Sci. 2021.
Sarker IH, Abushark YB, Alsolami F, Khan A. Intrudtree: a machine learning based cyber security intrusion detection model. Symmetry. 2020;12(5):754.
Sarker IH, Abushark YB, Khan A. Contextpca: predicting context-aware smartphone apps usage based on machine learning techniques. Symmetry. 2020;12(4):499.
Sarker IH, Alqahtani H, Alsolami F, Khan A, Abushark YB, Siddiqui MK. Context pre-modeling: an empirical analysis for classification based user-centric context-aware predictive modeling. J Big Data. 2020;7(1):1–23.
Sarker IH, Alan C, Jun H, Khan AI, Abushark YB, Khaled S. Behavdt: a behavioral decision tree learning to build user-centric context-aware predictive model. Mob Netw Appl. 2019; 1–11.
Sarker IH, Colman A, Kabir MA, Han J. Phone call log as a context source to modeling individual user behavior. In: Proceedings of the 2016 ACM International Joint Conference on Pervasive and Ubiquitous Computing (Ubicomp): Adjunct, Germany, pages 630–634. ACM, 2016.
Sarker IH, Colman A, Kabir MA, Han J. Individualized time-series segmentation for mining mobile phone user behavior. Comput J Oxf Univ UK. 2018;61(3):349–68.
Sarker IH, Hoque MM, MdK Uddin, Tawfeeq A. Mobile data science and intelligent apps: concepts, ai-based modeling and research directions. Mob Netw Appl, pages 1–19, 2020.
Sarker IH, Kayes ASM. Abc-ruleminer: user behavioral rule-based machine learning method for context-aware intelligent services. J Netw Comput Appl. 2020; page 102762
Sarker IH, Kayes ASM, Badsha S, Alqahtani H, Watters P, Ng A. Cybersecurity data science: an overview from machine learning perspective. J Big Data. 2020;7(1):1–29.
Sarker IH, Watters P, Kayes ASM. Effectiveness analysis of machine learning classification models for predicting personalized context-aware smartphone usage. J Big Data. 2019;6(1):1–28.
Sarker IH, Salah K. Appspred: predicting context-aware smartphone apps using random forest learning. Internet Things. 2019;8:
Scheffer T. Finding association rules that trade support optimally against confidence. Intell Data Anal. 2005;9(4):381–95.
Sharma R, Kamble SS, Gunasekaran A, Kumar V, Kumar A. A systematic literature review on machine learning applications for sustainable agriculture supply chain performance. Comput Oper Res. 2020;119:
Shengli S, Ling CX. Hybrid cost-sensitive decision tree, knowledge discovery in databases. In: PKDD 2005, Proceedings of 9th European Conference on Principles and Practice of Knowledge Discovery in Databases. Lecture Notes in Computer Science, volume 3721, 2005.
Shorten C, Khoshgoftaar TM, Furht B. Deep learning applications for covid-19. J Big Data. 2021;8(1):1–54.
Gökhan S, Nevin Y. Data analysis in health and big data: a machine learning medical diagnosis model based on patients’ complaints. Commun Stat Theory Methods. 2019;1–10
Silver D, Huang A, Maddison CJ, Guez A, Sifre L, Van Den Driessche G, Schrittwieser J, Antonoglou I, Panneershelvam V, Lanctot M, et al. Mastering the game of go with deep neural networks and tree search. nature. 2016;529(7587):484–9.
Ślusarczyk B. Industry 4.0: Are we ready? Polish J Manag Stud. 17, 2018.
Sneath Peter HA. The application of computers to taxonomy. J Gen Microbiol. 1957;17(1).
Sorensen T. Method of establishing groups of equal amplitude in plant sociology based on similarity of species. Biol Skr. 1948; 5.
Srinivasan V, Moghaddam S, Mukherji A. Mobileminer: mining your frequent patterns on your phone. In: Proceedings of the International Joint Conference on Pervasive and Ubiquitous Computing, Seattle, WA, USA, 13-17 September, pp. 389–400. ACM, New York, USA. 2014.
Szegedy C, Liu W, Jia Y, Sermanet P, Reed S, Anguelov D, Erhan D, Vanhoucke V, Rabinovich A. Going deeper with convolutions. In: Proceedings of the IEEE conference on computer vision and pattern recognition. 2015; pages 1–9.
Tavallaee M, Bagheri E, Lu W, Ghorbani AA. A detailed analysis of the kdd cup 99 data set. In. IEEE symposium on computational intelligence for security and defense applications. IEEE. 2009;2009:1–6.
Tsagkias M. Tracy HK, Surya K, Vanessa M, de Rijke M. Challenges and research opportunities in ecommerce search and recommendations. In: ACM SIGIR Forum. volume 54. NY, USA: ACM New York; 2021. p. 1–23.
Wagstaff K, Cardie C, Rogers S, Schrödl S, et al. Constrained k-means clustering with background knowledge. Icml. 2001;1:577–84.
Wang W, Yang J, Muntz R, et al. Sting: a statistical information grid approach to spatial data mining. VLDB. 1997;97:186–95.
Wei P, Li Y, Zhang Z, Tao H, Li Z, Liu D. An optimization method for intrusion detection classification model based on deep belief network. IEEE Access. 2019;7:87593–605.
Weiss K, Khoshgoftaar TM, Wang DD. A survey of transfer learning. J Big data. 2016;3(1):9.
Witten IH, Frank E. Data Mining: Practical machine learning tools and techniques. Morgan Kaufmann; 2005.
Witten IH, Frank E, Trigg LE, Hall MA, Holmes G, Cunningham SJ. Weka: practical machine learning tools and techniques with java implementations. 1999.
Wu C-C, Yen-Liang C, Yi-Hung L, Xiang-Yu Y. Decision tree induction with a constrained number of leaf nodes. Appl Intell. 2016;45(3):673–85.
Wu X, Kumar V, Quinlan JR, Ghosh J, Yang Q, Motoda H, McLachlan GJ, Ng A, Liu B, Philip SY, et al. Top 10 algorithms in data mining. Knowl Inform Syst. 2008;14(1):1–37.
Xin Y, Kong L, Liu Z, Chen Y, Li Y, Zhu H, Gao M, Hou H, Wang C. Machine learning and deep learning methods for cybersecurity. IEEE Access. 2018;6:35365–81.
Xu D, Yingjie T. A comprehensive survey of clustering algorithms. Ann Data Sci. 2015;2(2):165–93.
Zaki MJ. Scalable algorithms for association mining. IEEE Trans Knowl Data Eng. 2000;12(3):372–90.
Zanella A, Bui N, Castellani A, Vangelista L, Zorzi M. Internet of things for smart cities. IEEE Internet Things J. 2014;1(1):22–32.
Zhao Q, Bhowmick SS. Association rule mining: a survey. Singapore: Nanyang Technological University; 2003.
Zheng T, Xie W, Xu L, He X, Zhang Y, You M, Yang G, Chen Y. A machine learning-based framework to identify type 2 diabetes through electronic health records. Int J Med Inform. 2017;97:120–7.
Zheng Y, Rajasegarar S, Leckie C. Parking availability prediction for sensor-enabled car parks in smart cities. In: Intelligent Sensors, Sensor Networks and Information Processing (ISSNIP), 2015 IEEE Tenth International Conference on. IEEE, 2015; pages 1–6.
Zhu H, Cao H, Chen E, Xiong H, Tian J. Exploiting enriched contextual information for mobile app classification. In: Proceedings of the 21st ACM international conference on Information and knowledge management. ACM, 2012; pages 1617–1621
Zhu H, Chen E, Xiong H, Kuifei Y, Cao H, Tian J. Mining mobile user preferences for personalized context-aware recommendation. ACM Trans Intell Syst Technol (TIST). 2014;5(4):58.
Zikang H, Yong Y, Guofeng Y, Xinyu Z. Sentiment analysis of agricultural product ecommerce review data based on deep learning. In: 2020 International Conference on Internet of Things and Intelligent Applications (ITIA), IEEE, 2020; pages 1–7
Zulkernain S, Madiraju P, Ahamed SI. A context aware interruption management system for mobile devices. In: Mobile Wireless Middleware, Operating Systems, and Applications. Springer. 2010; pages 221–234
Zulkernain S, Madiraju P, Ahamed S, Stamm K. A mobile intelligent interruption management system. J UCS. 2010;16(15):2060–80.
Download references
Author information
Authors and affiliations.
Swinburne University of Technology, Melbourne, VIC, 3122, Australia
Iqbal H. Sarker
Department of Computer Science and Engineering, Chittagong University of Engineering & Technology, 4349, Chattogram, Bangladesh
You can also search for this author in PubMed Google Scholar
Corresponding author
Correspondence to Iqbal H. Sarker .
Ethics declarations
Conflict of interest.
The author declares no conflict of interest.
Additional information
Publisher's note.
Springer Nature remains neutral with regard to jurisdictional claims in published maps and institutional affiliations.
This article is part of the topical collection “Advances in Computational Approaches for Artificial Intelligence, Image Processing, IoT and Cloud Applications” guest edited by Bhanu Prakash K N and M. Shivakumar.
Rights and permissions
Reprints and permissions
About this article
Sarker, I.H. Machine Learning: Algorithms, Real-World Applications and Research Directions. SN COMPUT. SCI. 2 , 160 (2021). https://doi.org/10.1007/s42979-021-00592-x
Download citation
Received : 27 January 2021
Accepted : 12 March 2021
Published : 22 March 2021
DOI : https://doi.org/10.1007/s42979-021-00592-x
Share this article
Anyone you share the following link with will be able to read this content:
Sorry, a shareable link is not currently available for this article.
Provided by the Springer Nature SharedIt content-sharing initiative
- Machine learning
- Deep learning
- Artificial intelligence
- Data science
- Data-driven decision-making
- Predictive analytics
- Intelligent applications
- Find a journal
- Publish with us
- Track your research

- SUGGESTED TOPICS
- The Magazine
- Newsletters
- Managing Yourself
- Managing Teams
- Work-life Balance
- The Big Idea
- Data & Visuals
- Reading Lists
- Case Selections
- HBR Learning
- Topic Feeds
- Account Settings
- Email Preferences
A Guide to Solving Social Problems with Machine Learning
- Jon Kleinberg,
- Jens Ludwig,
- Sendhil Mullainathan
Predictive technology can improve public policy — if we use it right.
It’s Sunday night. You’re the deputy mayor of a big city. You sit down to watch a movie and ask Netflix for help. (“Will I like Birdemic? Ishtar? Zoolander 2?”) The Netflix recommendation algorithm predicts what movie you’d like by mining data on millions of previous movie-watchers using sophisticated machine learning tools. And then the next day you go to work and every one of your agencies will make hiring decisions with little idea of which candidates would be good workers; community college students will be largely left to their own devices to decide which courses are too hard or too easy for them; and your social service system will implement a reactive rather than preventive approach to homelessness because they don’t believe it’s possible to forecast which families will wind up on the streets.

- Jon Kleinberg is a professor of computer science at Cornell University and the coauthor of the textbooks Algorithm Design (with Éva Tardos) and Networks, Crowds, and Markets (with David Easley).
- JL Jens Ludwig is the McCormick Foundation Professor of Social Service Administration, Law and Public Policy at the University of Chicago.
- SM Sendhil Mullainathan is a professor of economics at Harvard University and the coauthor (with Eldar Shafir) of Scarcity: Why Having Too Little Means So Much.
Partner Center
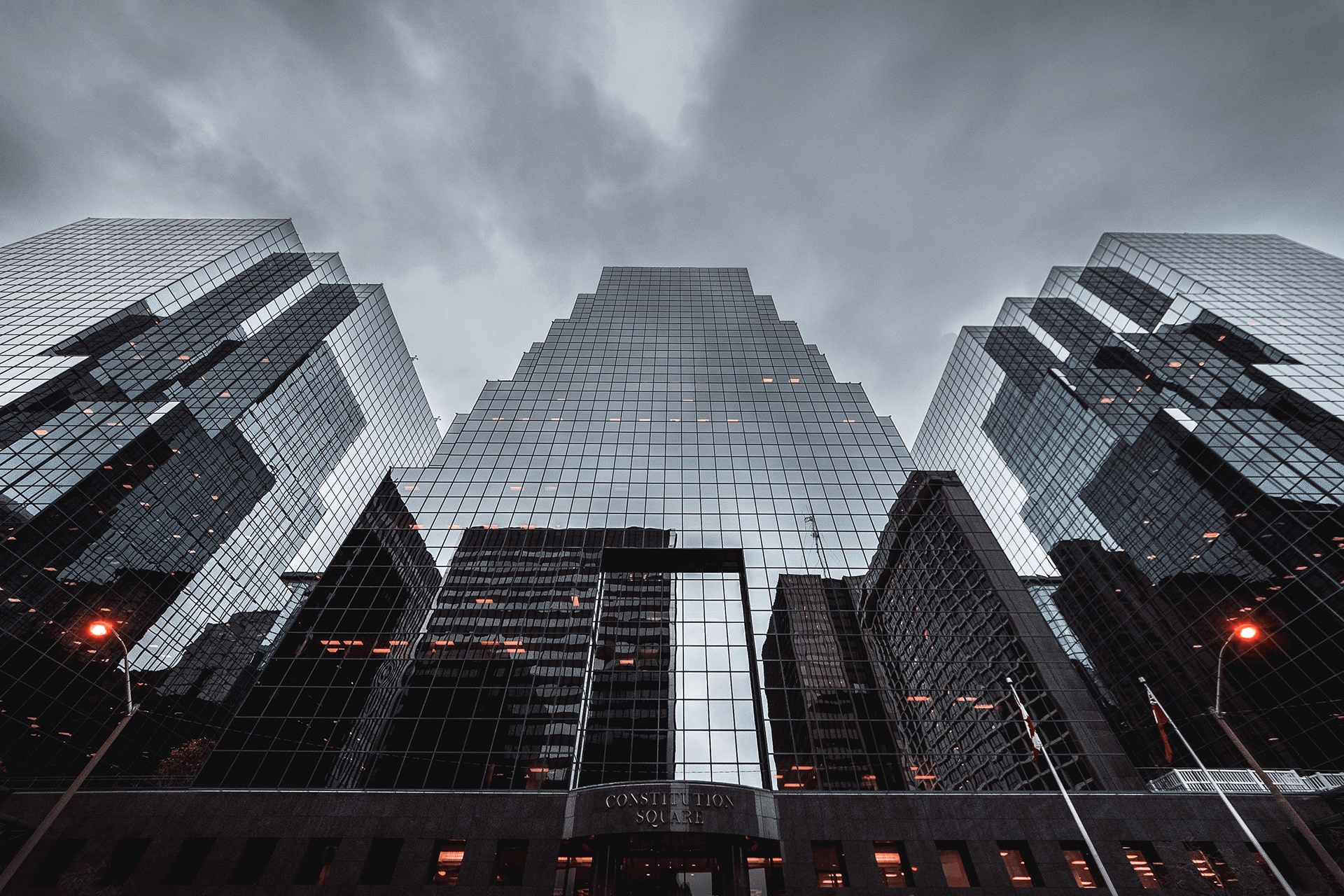
- 10 Real World Problems That Machine Learning Can Solve
Machine Learning is one of the modern-day technologies, which was also just an idea a few years back. None of us ever believed the fact that our vision can be evolved to a point where it starts to feel like science fiction. But we have narrowed the possibilities as we are getting closer to automation by building intelligent machines capable of performing tasks without any human touch or intelligence.
It all started in 1943 when the brilliant mathematician Alan Turing created “The Bombe,” a machine that was cracking a staggering total of 80,000 Enigma messages each month. Not only did it help Allied Forces win World War II, but it also asked a simple question “Can machines figure out ideas on their own?”
We strongly believe that introduction of Artificial Intelligence and its sub-parts like Machine Learning is the answer to that question. Today we are not talking about one of the trending advances in technology which is hot today and forgotten tomorrow; we are discussing Machine Learning which is here to stay and make lives easier on both ends. You might have no idea about “Machine Learning,” but after reading this blog, you will know how it shapes and streamlines the way we work, live, and communicate, in short, “Our Future.”
What is Machine Learning?
Machine Learning is a sub-array of artificial intelligence where computer algorithms independently learn from data and information without any human intervention. It’s a practice of using built-in algorithms to analyze data and further learn from it.
The objective of machine learning is to adapt to new data independently and make decisions and suggestions based on thousands of calculations and analyses. As it is a sub-part of artificial intelligence, the process is accomplished by infusing deep learning applications and AI machines from their fed data.
How Do Problem-Solving And Machine Learning Co-Relates?
The basic definition of problem-solving is to find the most accurate process of finding solutions to complex problems. When we look at the ML, then it is pretty vast and is expanding rapidly. How do problem-solving and machine learning correlate?
In 1977, Tom Mitchell shared a “well-conditioned” definition to the ML, which we believe is the perfect representation of today’s market scenario and how we can use ML to make things easier for businesses from all around the globe. He stated, “An ML is a computer program which is said to learn from experience E to some task T and some performance measure P, if its performance on T, as measured by P, improves with experience E.”
So, suppose you want your program to predict something, for example, identifying a person from a group of individuals using their interaction methods (task T). In that case, you can feed every individual’s way of interaction data (experience E) to a machine learning algorithm. It will successfully learn to PREDICT the individual pattern through their respective way of interaction (performance measure P). It means you are always one step ahead as you already know what they might say or do.
With the inclusion of machine learning in your business, you can use the previously gathered data from your industry to develop better ways than the existing solutions using Machine Learning. The main objective of ML is never to make “perfect” speculations because ML trades in domains. Its main goal is to come up with good enough estimates so these predictions can be helpful in different ways.
Who’s Using It?
The principal element of machine learning is data. All the major industries that work with large amounts of data have already acknowledged the worth of machine learning technology. With endless data, you have endless possibilities. It opens gates to many practical ways that can help scale your business to further domains. By gathering valuable insights from this data, organizations are finding a more efficient way to work and gaining an edge over their competitors.
Some of the top industries in the world that are currently using Machine Learning are:
- Financial Services
Banks and other financial institutions in Singapore are using ML for two key purposes: preventing fraud and identifying essential insights in data.
- Transportation
ML has already become a crucial prospect to delivery companies and other transportation enterprises. They use it to analyze data to identify patterns and even trends, making routes more efficient and even predicting potential problems to increase profitability.
- Health and Care
Health Care is also reaping the benefit of ML as it is helping medical experts analyze data to identify patterns to offer better diagnoses and treatment to the patients.
Ecommerce has already become part of our daily lives. Retail businesses are using ML to recommend items that users might LIKE by analyzing their previous purchases.
- Oil & Gas
Machine learning in the Oil and Gas industry is immense and will expand to various factors very soon. It is currently being used to detect new energy sources, analyze minerals in the ground, and even anticipate any kind of refinery sensor failures.
10 Real-World Problems that Machine Learning can solve
1. recommending products after collecting previous data.
Recommendation systems are one of the most common machine learning use cases in day-to-day life. These systems are used mainly by search engines like Google and Bing and the top eCommerce platforms like Amazon and eBay.
The ML integrated systems show a list of recommended products individually for each of their consumers. These suggestions are based on data like previous purchases, wish lists, searches, clicks, inquiries, and browsing history. This data is fed to a comprehensive ML algorithm to strike the user at the right moment and enhance their customer engagement.
2. Works as the Best Image and Video Recognition Tool
If you have come across features like face recognition, text detection, object detection, and landmark detection, it is because of the integration of deep learning in machine learning. When ML algorithms are trained with innovative deep learning frameworks, they can quickly identify and classify objects and make things easier for a non-native person.
MNL can also be used to determine handwritten text by segmenting a piece of writing into smaller images, each containing a single character.
3. Your Virtual Assistant
A virtual assistant, which is also very common as an AI assistant, is an application program that comprehends natural language voice commands and finishes the tasks for the users like searching the web, booking an appointment, etc. If you have also asked Google Assistant in your android phones to wake you up at 5 AM or asked Siri on your iPhones for directions to the nearest restaurant, then ML has also made your life easier.
Some principal personal assistants or smart assistants available in the market are Siri, Google Assistants, Alexa, Echo, and Google mini. These assistants can help you look for information by voice commands or answer your questions by searching your query on the web.
4. Ingenious Gaming Using ML
With the advancement in technologies, we can improve the graphics of the games and give them a mind at the same time. Lately, if you have been facing difficulties beating the bot in a chess game, then ML might take it over. Today’s games not only simply analyze your moves but are also learning how to play the game better than you by practicing numerous times. Now using your mind against such an intelligent system will surely give you brains and make you smarter at the same time.
5. Devising Superior Health Care Methods
Even hospitals are utilizing machine learning to cure and treat patients. Thanks to our wearable devices, doctors can get accurate data on our health from anywhere in the world and suggest an aid if they find something helpful. The integration of ML in some essential tools can quickly provide real-time insights and combine with the explosion of computing power.
It can help doctors to diagnose critically faster and more accurately. Not only this, AI is assisting in the development of new medications and treatments, predicting harmful reactions at the early stages, and working towards finding a way to lower the costs of healthcare for providers and patients.
6. Protecting Environment in the Most Impactful Way
Aforesaid, possibilities are endless with ML, and it’s just the beginning. Recently IBM’s Green Horizon Project was acknowledged by experts worldwide as it accurately predicted weather and pollution forecasts. We can use it to save and predict natural forecasts with the expertise of the professionals from Singapore by our side. It is helping city planners to run every kind of scenario just by feeding previous data to their ML algorithm to find ways for minimum environmental impact.
7. Real-Time Dynamic Pricing
You might have already encountered this scenario while booking a flight ticket to travel on Christmas or booking a cab at peak hours. You will notice a big gap between the regular pricing and pricing at that particular time. So, in these scenarios, the ML and data analysis techniques are helping businesses to get to know more about their users. It answers two critical questions.
First, how are customers reacting to surge prices? And second, whether they are looking for customers because of surge pricing? The integration of AI and ML helps the businesses and the users as it helps determine when customers are looking for the best promotional and discounted prices.
8. Innovations in the Finance Sector Including Stock Market
The functioning of the finance sector is about to change in the upcoming years completely. Thanks to technologies like mobile app development and machine learning, the stock market is at its all-time high.
Thanks to AI, deep learning, and machine learning, it has become easy for users to predict the market price by feeding it the previous data. It will allow traders to make better and steady decisions which means less financial loss. Not only this, the machine learning-based anomaly detection models can easily monitor your every transaction request and alert you of any kind of suspicious activity.
9. Commute Predictions Using Machine Learning
Almost everyone uses GPS services while driving. A programmed GPS helps us in finding the proper navigation to our destination. But it’s the integration of ML and its features like congestion analysis GPS that helps us by telling us the path to avoid traffic and reach our destination on time. It saves the data like our location and velocities of the vehicles on the same path to determine the traffic and let us know whether it would be the right path to follow.
10. Online Video OTT Streaming Applications
The pioneers of online video streaming services are Netflix and Amazon Prime; both of them combined have killed the traditional way of watching television. But how were they able to keep the customer engaged on their platforms? First by offering impressive content, secondly by getting personalized with the users.
They were able to capture mass audiences using machine learning. At the right time, they integrated ML in their program and fed it the user’s data like day and time they watch content, type of content they like to watch, browsing pattern, whether they like to watch trailers before they watch a movie or a show, etc. They are using practical machine learning frameworks to engage their audience by providing quality streaming service right to their homes.
The possibilities with machine learning are limitless. All you need to do is find a comprehensive way to use it in your particular business domain to improve your services. Sometimes it can be indispensable to understand the problem at hand, as you can’t use any ML algorithm for your business needs.
Every problem is different from the previous one in machine learning. This means that you can’t just feed some data to a machine learning algorithm with a neural network and pray for the results.
Every situation demands a different approach and that is why it is crucial to consider looking for professional Machine Learning experts.
People who must have enough experience in the field and can work with an open mind to first understand your business requirements. And then come up with the incredible machine learning algorithm that benefits your business immensely without wasting anyone’s time.
At ICore Singapore, you get all-inclusive Artificial Intelligence and Machine Learning Development services, redefining the way your business operates.
With the right mix of AI/ML development teams, you can trust us for high-quality solutions that cover all your needs.
Our Writing team believes you had a great time reading this piece. If this helped you in any possible way, a pat on our back would be great!
Share your thoughts via comments or just a thumbs up would do!
Our IT Solutions
- Web Development Services
- PHP Web Development Services
- Laravel Development Services
- CakePHP Development Services
- CodeIgniter Development Services
- Python Development Services
- Full Stack Development Services
- React JS Development Services
- Node JS Development Services
- Angular JS Development Services
- Web Design Services
- eCommerce Development Services
- iOS/iPhone App Development Services
- Android App Development Services
- Hybrid App Development Services
- WooCommerce Development Services
- OpenCart Development Services
- Shopify Development Services
- CRM Software Development Services
- ERP Software Development Services
- Shopify Website Design & Development Services
- WordPress Development Services
- Magento Development Services
- Digital Marketing Services
- Search Engine Optimization (SEO) Services
- Search Engine Marketing (SEM) Services
- Social Media Marketing (SMM) Services
- Content Marketing Services
Recent Blog Posts
- UI vs. UX Design: Definition, Uses & Differences [2024]
- Magento ecommerce Platform: Is It Worth Choosing?
- Top 9 eCommerce Sites in Singapore
- How Much it Costs to build a Website in Singapore?
- Choosing Magento Web Development Service for ecommerce?
- Must Have Tools Required For UI/UX Design? [2024]
- Top Skills You Need to Become UI/UX Designer in Singapore
- How to increase eCommerce sales of your online store?
- Why IoT is the Future of Mobile App Development
- 6 Reasons Developers Should Use Webflow
- List of Key Factors to Consider For Measuring eCommerce Success
- Website SEO Optimization Tips to Follow in 2024
- Guide to Choose the Right eCommerce Platform in Singapore
- Best Flutter Development Tools in 2024
- Social Media Trends You Cannot Ignore in 2024
- Digital Marketing and Small Business: Understanding the bond
- New Possibilities with Python App Development
- React Native for Cross-Platform Mobile App Development
- Website Maintenance Cost in Singapore: Detailed Explanation
- Top Reasons Why Python Development is Widely Used in Enterprise
- Top Python Image-Processing Libraries for Machine Learning
- Integrating Python in Power BI Accomplishing for Better Results
- 7 Best Web Design Software in 2024
- Top OpenCart Development Companies in Singapore 2024
- Is your eCommerce Website Design Search Engine Friendly?
- Top CodeIgniter Web Development Companies 2024
- How to Scale your Web Application
- Why Choose Magento 2 for Your eCommerce Website
- Django Web Framework For App Development
- Comprehensive Guide on Web Scraping Using Python
- Common Web Design Mistakes to Avoid in 2024
- Tips to Hire a Web Development Team
- Top UI/UX Design Agencies in Singapore 2024
- Top Appointment Scheduling Apps to Streamline Your Business
- Social Media Strategy and Budget: 2024
- Django vs Flask: The Best Python Web Framework?
- Top Features to Look for in a Maid App in Singapore
- Top Magento Ecommerce Agency in Singapore 2024
- Top Front End Development Tools for 2024
- AI to automating routine IT tasks and optimizing service delivery!
- Python 2 Vs Python 3 and Why is it the Right Time for Migration
- Top 20 Python Based CMS Of All Time
- Which ERP Software Solution will be best for future?
- Top 7 Python Web Development Libraries to Choose From in 2024
- What is the Cost of UI/UX Design Agency in Singapore?
- Top Automotive Industries Utilizing Mobile Apps
- How Food Ordering App helps to scale up Restaurant Business
- 11 Tips for automotive website design
- Ecommerce SEO: A Need of Online Marketing

An official website of the United States government
The .gov means it’s official. Federal government websites often end in .gov or .mil. Before sharing sensitive information, make sure you’re on a federal government site.
The site is secure. The https:// ensures that you are connecting to the official website and that any information you provide is encrypted and transmitted securely.
- Publications
- Account settings
Preview improvements coming to the PMC website in October 2024. Learn More or Try it out now .
- Advanced Search
- Journal List
- Springer Nature - PMC COVID-19 Collection

Machine Learning: Algorithms, Real-World Applications and Research Directions
Iqbal h. sarker.
1 Swinburne University of Technology, Melbourne, VIC 3122 Australia
2 Department of Computer Science and Engineering, Chittagong University of Engineering & Technology, 4349 Chattogram, Bangladesh
In the current age of the Fourth Industrial Revolution (4 IR or Industry 4.0), the digital world has a wealth of data, such as Internet of Things (IoT) data, cybersecurity data, mobile data, business data, social media data, health data, etc. To intelligently analyze these data and develop the corresponding smart and automated applications, the knowledge of artificial intelligence (AI), particularly, machine learning (ML) is the key. Various types of machine learning algorithms such as supervised, unsupervised, semi-supervised, and reinforcement learning exist in the area. Besides, the deep learning , which is part of a broader family of machine learning methods, can intelligently analyze the data on a large scale. In this paper, we present a comprehensive view on these machine learning algorithms that can be applied to enhance the intelligence and the capabilities of an application. Thus, this study’s key contribution is explaining the principles of different machine learning techniques and their applicability in various real-world application domains, such as cybersecurity systems, smart cities, healthcare, e-commerce, agriculture, and many more. We also highlight the challenges and potential research directions based on our study. Overall, this paper aims to serve as a reference point for both academia and industry professionals as well as for decision-makers in various real-world situations and application areas, particularly from the technical point of view.
Introduction
We live in the age of data, where everything around us is connected to a data source, and everything in our lives is digitally recorded [ 21 , 103 ]. For instance, the current electronic world has a wealth of various kinds of data, such as the Internet of Things (IoT) data, cybersecurity data, smart city data, business data, smartphone data, social media data, health data, COVID-19 data, and many more. The data can be structured, semi-structured, or unstructured, discussed briefly in Sect. “ Types of Real-World Data and Machine Learning Techniques ”, which is increasing day-by-day. Extracting insights from these data can be used to build various intelligent applications in the relevant domains. For instance, to build a data-driven automated and intelligent cybersecurity system, the relevant cybersecurity data can be used [ 105 ]; to build personalized context-aware smart mobile applications, the relevant mobile data can be used [ 103 ], and so on. Thus, the data management tools and techniques having the capability of extracting insights or useful knowledge from the data in a timely and intelligent way is urgently needed, on which the real-world applications are based.
Artificial intelligence (AI), particularly, machine learning (ML) have grown rapidly in recent years in the context of data analysis and computing that typically allows the applications to function in an intelligent manner [ 95 ]. ML usually provides systems with the ability to learn and enhance from experience automatically without being specifically programmed and is generally referred to as the most popular latest technologies in the fourth industrial revolution (4 IR or Industry 4.0) [ 103 , 105 ]. “Industry 4.0” [ 114 ] is typically the ongoing automation of conventional manufacturing and industrial practices, including exploratory data processing, using new smart technologies such as machine learning automation. Thus, to intelligently analyze these data and to develop the corresponding real-world applications, machine learning algorithms is the key. The learning algorithms can be categorized into four major types, such as supervised, unsupervised, semi-supervised, and reinforcement learning in the area [ 75 ], discussed briefly in Sect. “ Types of Real-World Data and Machine Learning Techniques ”. The popularity of these approaches to learning is increasing day-by-day, which is shown in Fig. Fig.1, 1 , based on data collected from Google Trends [ 4 ] over the last five years. The x - axis of the figure indicates the specific dates and the corresponding popularity score within the range of 0 ( m i n i m u m ) to 100 ( m a x i m u m ) has been shown in y - axis . According to Fig. Fig.1, 1 , the popularity indication values for these learning types are low in 2015 and are increasing day by day. These statistics motivate us to study on machine learning in this paper, which can play an important role in the real-world through Industry 4.0 automation.

The worldwide popularity score of various types of ML algorithms (supervised, unsupervised, semi-supervised, and reinforcement) in a range of 0 (min) to 100 (max) over time where x-axis represents the timestamp information and y-axis represents the corresponding score
In general, the effectiveness and the efficiency of a machine learning solution depend on the nature and characteristics of data and the performance of the learning algorithms . In the area of machine learning algorithms, classification analysis, regression, data clustering, feature engineering and dimensionality reduction, association rule learning, or reinforcement learning techniques exist to effectively build data-driven systems [ 41 , 125 ]. Besides, deep learning originated from the artificial neural network that can be used to intelligently analyze data, which is known as part of a wider family of machine learning approaches [ 96 ]. Thus, selecting a proper learning algorithm that is suitable for the target application in a particular domain is challenging. The reason is that the purpose of different learning algorithms is different, even the outcome of different learning algorithms in a similar category may vary depending on the data characteristics [ 106 ]. Thus, it is important to understand the principles of various machine learning algorithms and their applicability to apply in various real-world application areas, such as IoT systems, cybersecurity services, business and recommendation systems, smart cities, healthcare and COVID-19, context-aware systems, sustainable agriculture, and many more that are explained briefly in Sect. “ Applications of Machine Learning ”.
Based on the importance and potentiality of “Machine Learning” to analyze the data mentioned above, in this paper, we provide a comprehensive view on various types of machine learning algorithms that can be applied to enhance the intelligence and the capabilities of an application. Thus, the key contribution of this study is explaining the principles and potentiality of different machine learning techniques, and their applicability in various real-world application areas mentioned earlier. The purpose of this paper is, therefore, to provide a basic guide for those academia and industry people who want to study, research, and develop data-driven automated and intelligent systems in the relevant areas based on machine learning techniques.
The key contributions of this paper are listed as follows:
- To define the scope of our study by taking into account the nature and characteristics of various types of real-world data and the capabilities of various learning techniques.
- To provide a comprehensive view on machine learning algorithms that can be applied to enhance the intelligence and capabilities of a data-driven application.
- To discuss the applicability of machine learning-based solutions in various real-world application domains.
- To highlight and summarize the potential research directions within the scope of our study for intelligent data analysis and services.
The rest of the paper is organized as follows. The next section presents the types of data and machine learning algorithms in a broader sense and defines the scope of our study. We briefly discuss and explain different machine learning algorithms in the subsequent section followed by which various real-world application areas based on machine learning algorithms are discussed and summarized. In the penultimate section, we highlight several research issues and potential future directions, and the final section concludes this paper.
Types of Real-World Data and Machine Learning Techniques
Machine learning algorithms typically consume and process data to learn the related patterns about individuals, business processes, transactions, events, and so on. In the following, we discuss various types of real-world data as well as categories of machine learning algorithms.
Types of Real-World Data
Usually, the availability of data is considered as the key to construct a machine learning model or data-driven real-world systems [ 103 , 105 ]. Data can be of various forms, such as structured, semi-structured, or unstructured [ 41 , 72 ]. Besides, the “metadata” is another type that typically represents data about the data. In the following, we briefly discuss these types of data.
- Structured: It has a well-defined structure, conforms to a data model following a standard order, which is highly organized and easily accessed, and used by an entity or a computer program. In well-defined schemes, such as relational databases, structured data are typically stored, i.e., in a tabular format. For instance, names, dates, addresses, credit card numbers, stock information, geolocation, etc. are examples of structured data.
- Unstructured: On the other hand, there is no pre-defined format or organization for unstructured data, making it much more difficult to capture, process, and analyze, mostly containing text and multimedia material. For example, sensor data, emails, blog entries, wikis, and word processing documents, PDF files, audio files, videos, images, presentations, web pages, and many other types of business documents can be considered as unstructured data.
- Semi-structured: Semi-structured data are not stored in a relational database like the structured data mentioned above, but it does have certain organizational properties that make it easier to analyze. HTML, XML, JSON documents, NoSQL databases, etc., are some examples of semi-structured data.
- Metadata: It is not the normal form of data, but “data about data”. The primary difference between “data” and “metadata” is that data are simply the material that can classify, measure, or even document something relative to an organization’s data properties. On the other hand, metadata describes the relevant data information, giving it more significance for data users. A basic example of a document’s metadata might be the author, file size, date generated by the document, keywords to define the document, etc.
In the area of machine learning and data science, researchers use various widely used datasets for different purposes. These are, for example, cybersecurity datasets such as NSL-KDD [ 119 ], UNSW-NB15 [ 76 ], ISCX’12 [ 1 ], CIC-DDoS2019 [ 2 ], Bot-IoT [ 59 ], etc., smartphone datasets such as phone call logs [ 84 , 101 ], SMS Log [ 29 ], mobile application usages logs [ 137 ] [ 117 ], mobile phone notification logs [ 73 ] etc., IoT data [ 16 , 57 , 62 ], agriculture and e-commerce data [ 120 , 138 ], health data such as heart disease [ 92 ], diabetes mellitus [ 83 , 134 ], COVID-19 [ 43 , 74 ], etc., and many more in various application domains. The data can be in different types discussed above, which may vary from application to application in the real world. To analyze such data in a particular problem domain, and to extract the insights or useful knowledge from the data for building the real-world intelligent applications, different types of machine learning techniques can be used according to their learning capabilities, which is discussed in the following.
Types of Machine Learning Techniques
Machine Learning algorithms are mainly divided into four categories: Supervised learning, Unsupervised learning, Semi-supervised learning, and Reinforcement learning [ 75 ], as shown in Fig. Fig.2. 2 . In the following, we briefly discuss each type of learning technique with the scope of their applicability to solve real-world problems.

Various types of machine learning techniques
- Supervised: Supervised learning is typically the task of machine learning to learn a function that maps an input to an output based on sample input-output pairs [ 41 ]. It uses labeled training data and a collection of training examples to infer a function. Supervised learning is carried out when certain goals are identified to be accomplished from a certain set of inputs [ 105 ], i.e., a task-driven approach . The most common supervised tasks are “classification” that separates the data, and “regression” that fits the data. For instance, predicting the class label or sentiment of a piece of text, like a tweet or a product review, i.e., text classification, is an example of supervised learning.
- Unsupervised: Unsupervised learning analyzes unlabeled datasets without the need for human interference, i.e., a data-driven process [ 41 ]. This is widely used for extracting generative features, identifying meaningful trends and structures, groupings in results, and exploratory purposes. The most common unsupervised learning tasks are clustering, density estimation, feature learning, dimensionality reduction, finding association rules, anomaly detection, etc.
- Semi-supervised: Semi-supervised learning can be defined as a hybridization of the above-mentioned supervised and unsupervised methods, as it operates on both labeled and unlabeled data [ 41 , 105 ]. Thus, it falls between learning “without supervision” and learning “with supervision”. In the real world, labeled data could be rare in several contexts, and unlabeled data are numerous, where semi-supervised learning is useful [ 75 ]. The ultimate goal of a semi-supervised learning model is to provide a better outcome for prediction than that produced using the labeled data alone from the model. Some application areas where semi-supervised learning is used include machine translation, fraud detection, labeling data and text classification.
- Reinforcement: Reinforcement learning is a type of machine learning algorithm that enables software agents and machines to automatically evaluate the optimal behavior in a particular context or environment to improve its efficiency [ 52 ], i.e., an environment-driven approach . This type of learning is based on reward or penalty, and its ultimate goal is to use insights obtained from environmental activists to take action to increase the reward or minimize the risk [ 75 ]. It is a powerful tool for training AI models that can help increase automation or optimize the operational efficiency of sophisticated systems such as robotics, autonomous driving tasks, manufacturing and supply chain logistics, however, not preferable to use it for solving the basic or straightforward problems.
Thus, to build effective models in various application areas different types of machine learning techniques can play a significant role according to their learning capabilities, depending on the nature of the data discussed earlier, and the target outcome. In Table Table1, 1 , we summarize various types of machine learning techniques with examples. In the following, we provide a comprehensive view of machine learning algorithms that can be applied to enhance the intelligence and capabilities of a data-driven application.
Various types of machine learning techniques with examples
Machine Learning Tasks and Algorithms
In this section, we discuss various machine learning algorithms that include classification analysis, regression analysis, data clustering, association rule learning, feature engineering for dimensionality reduction, as well as deep learning methods. A general structure of a machine learning-based predictive model has been shown in Fig. Fig.3, 3 , where the model is trained from historical data in phase 1 and the outcome is generated in phase 2 for the new test data.

A general structure of a machine learning based predictive model considering both the training and testing phase
Classification Analysis
Classification is regarded as a supervised learning method in machine learning, referring to a problem of predictive modeling as well, where a class label is predicted for a given example [ 41 ]. Mathematically, it maps a function ( f ) from input variables ( X ) to output variables ( Y ) as target, label or categories. To predict the class of given data points, it can be carried out on structured or unstructured data. For example, spam detection such as “spam” and “not spam” in email service providers can be a classification problem. In the following, we summarize the common classification problems.
- Binary classification: It refers to the classification tasks having two class labels such as “true and false” or “yes and no” [ 41 ]. In such binary classification tasks, one class could be the normal state, while the abnormal state could be another class. For instance, “cancer not detected” is the normal state of a task that involves a medical test, and “cancer detected” could be considered as the abnormal state. Similarly, “spam” and “not spam” in the above example of email service providers are considered as binary classification.
- Multiclass classification: Traditionally, this refers to those classification tasks having more than two class labels [ 41 ]. The multiclass classification does not have the principle of normal and abnormal outcomes, unlike binary classification tasks. Instead, within a range of specified classes, examples are classified as belonging to one. For example, it can be a multiclass classification task to classify various types of network attacks in the NSL-KDD [ 119 ] dataset, where the attack categories are classified into four class labels, such as DoS (Denial of Service Attack), U2R (User to Root Attack), R2L (Root to Local Attack), and Probing Attack.
- Multi-label classification: In machine learning, multi-label classification is an important consideration where an example is associated with several classes or labels. Thus, it is a generalization of multiclass classification, where the classes involved in the problem are hierarchically structured, and each example may simultaneously belong to more than one class in each hierarchical level, e.g., multi-level text classification. For instance, Google news can be presented under the categories of a “city name”, “technology”, or “latest news”, etc. Multi-label classification includes advanced machine learning algorithms that support predicting various mutually non-exclusive classes or labels, unlike traditional classification tasks where class labels are mutually exclusive [ 82 ].
Many classification algorithms have been proposed in the machine learning and data science literature [ 41 , 125 ]. In the following, we summarize the most common and popular methods that are used widely in various application areas.
- Naive Bayes (NB): The naive Bayes algorithm is based on the Bayes’ theorem with the assumption of independence between each pair of features [ 51 ]. It works well and can be used for both binary and multi-class categories in many real-world situations, such as document or text classification, spam filtering, etc. To effectively classify the noisy instances in the data and to construct a robust prediction model, the NB classifier can be used [ 94 ]. The key benefit is that, compared to more sophisticated approaches, it needs a small amount of training data to estimate the necessary parameters and quickly [ 82 ]. However, its performance may affect due to its strong assumptions on features independence. Gaussian, Multinomial, Complement, Bernoulli, and Categorical are the common variants of NB classifier [ 82 ].
- Linear Discriminant Analysis (LDA): Linear Discriminant Analysis (LDA) is a linear decision boundary classifier created by fitting class conditional densities to data and applying Bayes’ rule [ 51 , 82 ]. This method is also known as a generalization of Fisher’s linear discriminant, which projects a given dataset into a lower-dimensional space, i.e., a reduction of dimensionality that minimizes the complexity of the model or reduces the resulting model’s computational costs. The standard LDA model usually suits each class with a Gaussian density, assuming that all classes share the same covariance matrix [ 82 ]. LDA is closely related to ANOVA (analysis of variance) and regression analysis, which seek to express one dependent variable as a linear combination of other features or measurements.
- Logistic regression (LR): Another common probabilistic based statistical model used to solve classification issues in machine learning is Logistic Regression (LR) [ 64 ]. Logistic regression typically uses a logistic function to estimate the probabilities, which is also referred to as the mathematically defined sigmoid function in Eq. 1 . It can overfit high-dimensional datasets and works well when the dataset can be separated linearly. The regularization (L1 and L2) techniques [ 82 ] can be used to avoid over-fitting in such scenarios. The assumption of linearity between the dependent and independent variables is considered as a major drawback of Logistic Regression. It can be used for both classification and regression problems, but it is more commonly used for classification. g ( z ) = 1 1 + exp ( - z ) . 1
- K-nearest neighbors (KNN): K-Nearest Neighbors (KNN) [ 9 ] is an “instance-based learning” or non-generalizing learning, also known as a “lazy learning” algorithm. It does not focus on constructing a general internal model; instead, it stores all instances corresponding to training data in n -dimensional space. KNN uses data and classifies new data points based on similarity measures (e.g., Euclidean distance function) [ 82 ]. Classification is computed from a simple majority vote of the k nearest neighbors of each point. It is quite robust to noisy training data, and accuracy depends on the data quality. The biggest issue with KNN is to choose the optimal number of neighbors to be considered. KNN can be used both for classification as well as regression.
- Support vector machine (SVM): In machine learning, another common technique that can be used for classification, regression, or other tasks is a support vector machine (SVM) [ 56 ]. In high- or infinite-dimensional space, a support vector machine constructs a hyper-plane or set of hyper-planes. Intuitively, the hyper-plane, which has the greatest distance from the nearest training data points in any class, achieves a strong separation since, in general, the greater the margin, the lower the classifier’s generalization error. It is effective in high-dimensional spaces and can behave differently based on different mathematical functions known as the kernel. Linear, polynomial, radial basis function (RBF), sigmoid, etc., are the popular kernel functions used in SVM classifier [ 82 ]. However, when the data set contains more noise, such as overlapping target classes, SVM does not perform well.

An example of a decision tree structure

An example of a random forest structure considering multiple decision trees
- Adaptive Boosting (AdaBoost): Adaptive Boosting (AdaBoost) is an ensemble learning process that employs an iterative approach to improve poor classifiers by learning from their errors. This is developed by Yoav Freund et al. [ 35 ] and also known as “meta-learning”. Unlike the random forest that uses parallel ensembling, Adaboost uses “sequential ensembling”. It creates a powerful classifier by combining many poorly performing classifiers to obtain a good classifier of high accuracy. In that sense, AdaBoost is called an adaptive classifier by significantly improving the efficiency of the classifier, but in some instances, it can trigger overfits. AdaBoost is best used to boost the performance of decision trees, base estimator [ 82 ], on binary classification problems, however, is sensitive to noisy data and outliers.
- Extreme gradient boosting (XGBoost): Gradient Boosting, like Random Forests [ 19 ] above, is an ensemble learning algorithm that generates a final model based on a series of individual models, typically decision trees. The gradient is used to minimize the loss function, similar to how neural networks [ 41 ] use gradient descent to optimize weights. Extreme Gradient Boosting (XGBoost) is a form of gradient boosting that takes more detailed approximations into account when determining the best model [ 82 ]. It computes second-order gradients of the loss function to minimize loss and advanced regularization (L1 and L2) [ 82 ], which reduces over-fitting, and improves model generalization and performance. XGBoost is fast to interpret and can handle large-sized datasets well.
- Stochastic gradient descent (SGD): Stochastic gradient descent (SGD) [ 41 ] is an iterative method for optimizing an objective function with appropriate smoothness properties, where the word ‘stochastic’ refers to random probability. This reduces the computational burden, particularly in high-dimensional optimization problems, allowing for faster iterations in exchange for a lower convergence rate. A gradient is the slope of a function that calculates a variable’s degree of change in response to another variable’s changes. Mathematically, the Gradient Descent is a convex function whose output is a partial derivative of a set of its input parameters. Let, α is the learning rate, and J i is the training example cost of i th , then Eq. ( 4 ) represents the stochastic gradient descent weight update method at the j th iteration. In large-scale and sparse machine learning, SGD has been successfully applied to problems often encountered in text classification and natural language processing [ 82 ]. However, SGD is sensitive to feature scaling and needs a range of hyperparameters, such as the regularization parameter and the number of iterations. w j : = w j - α ∂ J i ∂ w j . 4
- Rule-based classification : The term rule-based classification can be used to refer to any classification scheme that makes use of IF-THEN rules for class prediction. Several classification algorithms such as Zero-R [ 125 ], One-R [ 47 ], decision trees [ 87 , 88 ], DTNB [ 110 ], Ripple Down Rule learner (RIDOR) [ 125 ], Repeated Incremental Pruning to Produce Error Reduction (RIPPER) [ 126 ] exist with the ability of rule generation. The decision tree is one of the most common rule-based classification algorithms among these techniques because it has several advantages, such as being easier to interpret; the ability to handle high-dimensional data; simplicity and speed; good accuracy; and the capability to produce rules for human clear and understandable classification [ 127 ] [ 128 ]. The decision tree-based rules also provide significant accuracy in a prediction model for unseen test cases [ 106 ]. Since the rules are easily interpretable, these rule-based classifiers are often used to produce descriptive models that can describe a system including the entities and their relationships.
Regression Analysis
Regression analysis includes several methods of machine learning that allow to predict a continuous ( y ) result variable based on the value of one or more ( x ) predictor variables [ 41 ]. The most significant distinction between classification and regression is that classification predicts distinct class labels, while regression facilitates the prediction of a continuous quantity. Figure Figure6 6 shows an example of how classification is different with regression models. Some overlaps are often found between the two types of machine learning algorithms. Regression models are now widely used in a variety of fields, including financial forecasting or prediction, cost estimation, trend analysis, marketing, time series estimation, drug response modeling, and many more. Some of the familiar types of regression algorithms are linear, polynomial, lasso and ridge regression, etc., which are explained briefly in the following.
- Simple and multiple linear regression: This is one of the most popular ML modeling techniques as well as a well-known regression technique. In this technique, the dependent variable is continuous, the independent variable(s) can be continuous or discrete, and the form of the regression line is linear. Linear regression creates a relationship between the dependent variable ( Y ) and one or more independent variables ( X ) (also known as regression line) using the best fit straight line [ 41 ]. It is defined by the following equations: y = a + b x + e 5 y = a + b 1 x 1 + b 2 x 2 + ⋯ + b n x n + e , 6 where a is the intercept, b is the slope of the line, and e is the error term. This equation can be used to predict the value of the target variable based on the given predictor variable(s). Multiple linear regression is an extension of simple linear regression that allows two or more predictor variables to model a response variable, y, as a linear function [ 41 ] defined in Eq. 6 , whereas simple linear regression has only 1 independent variable, defined in Eq. 5 .
- Polynomial regression: Polynomial regression is a form of regression analysis in which the relationship between the independent variable x and the dependent variable y is not linear, but is the polynomial degree of n th in x [ 82 ]. The equation for polynomial regression is also derived from linear regression (polynomial regression of degree 1) equation, which is defined as below: y = b 0 + b 1 x + b 2 x 2 + b 3 x 3 + ⋯ + b n x n + e . 7 Here, y is the predicted/target output, b 0 , b 1 , . . . b n are the regression coefficients, x is an independent/ input variable. In simple words, we can say that if data are not distributed linearly, instead it is n th degree of polynomial then we use polynomial regression to get desired output.
- LASSO and ridge regression: LASSO and Ridge regression are well known as powerful techniques which are typically used for building learning models in presence of a large number of features, due to their capability to preventing over-fitting and reducing the complexity of the model. The LASSO (least absolute shrinkage and selection operator) regression model uses L 1 regularization technique [ 82 ] that uses shrinkage, which penalizes “absolute value of magnitude of coefficients” ( L 1 penalty). As a result, LASSO appears to render coefficients to absolute zero. Thus, LASSO regression aims to find the subset of predictors that minimizes the prediction error for a quantitative response variable. On the other hand, ridge regression uses L 2 regularization [ 82 ], which is the “squared magnitude of coefficients” ( L 2 penalty). Thus, ridge regression forces the weights to be small but never sets the coefficient value to zero, and does a non-sparse solution. Overall, LASSO regression is useful to obtain a subset of predictors by eliminating less important features, and ridge regression is useful when a data set has “multicollinearity” which refers to the predictors that are correlated with other predictors.

Classification vs. regression. In classification the dotted line represents a linear boundary that separates the two classes; in regression, the dotted line models the linear relationship between the two variables
Cluster Analysis
Cluster analysis, also known as clustering, is an unsupervised machine learning technique for identifying and grouping related data points in large datasets without concern for the specific outcome. It does grouping a collection of objects in such a way that objects in the same category, called a cluster, are in some sense more similar to each other than objects in other groups [ 41 ]. It is often used as a data analysis technique to discover interesting trends or patterns in data, e.g., groups of consumers based on their behavior. In a broad range of application areas, such as cybersecurity, e-commerce, mobile data processing, health analytics, user modeling and behavioral analytics, clustering can be used. In the following, we briefly discuss and summarize various types of clustering methods.
- Partitioning methods: Based on the features and similarities in the data, this clustering approach categorizes the data into multiple groups or clusters. The data scientists or analysts typically determine the number of clusters either dynamically or statically depending on the nature of the target applications, to produce for the methods of clustering. The most common clustering algorithms based on partitioning methods are K-means [ 69 ], K-Mediods [ 80 ], CLARA [ 55 ] etc.
- Density-based methods: To identify distinct groups or clusters, it uses the concept that a cluster in the data space is a contiguous region of high point density isolated from other such clusters by contiguous regions of low point density. Points that are not part of a cluster are considered as noise. The typical clustering algorithms based on density are DBSCAN [ 32 ], OPTICS [ 12 ] etc. The density-based methods typically struggle with clusters of similar density and high dimensionality data.

A graphical interpretation of the widely-used hierarchical clustering (Bottom-up and top-down) technique
- Grid-based methods: To deal with massive datasets, grid-based clustering is especially suitable. To obtain clusters, the principle is first to summarize the dataset with a grid representation and then to combine grid cells. STING [ 122 ], CLIQUE [ 6 ], etc. are the standard algorithms of grid-based clustering.
- Model-based methods: There are mainly two types of model-based clustering algorithms: one that uses statistical learning, and the other based on a method of neural network learning [ 130 ]. For instance, GMM [ 89 ] is an example of a statistical learning method, and SOM [ 22 ] [ 96 ] is an example of a neural network learning method.
- Constraint-based methods: Constrained-based clustering is a semi-supervised approach to data clustering that uses constraints to incorporate domain knowledge. Application or user-oriented constraints are incorporated to perform the clustering. The typical algorithms of this kind of clustering are COP K-means [ 121 ], CMWK-Means [ 27 ], etc.
Many clustering algorithms have been proposed with the ability to grouping data in machine learning and data science literature [ 41 , 125 ]. In the following, we summarize the popular methods that are used widely in various application areas.
- K-means clustering: K-means clustering [ 69 ] is a fast, robust, and simple algorithm that provides reliable results when data sets are well-separated from each other. The data points are allocated to a cluster in this algorithm in such a way that the amount of the squared distance between the data points and the centroid is as small as possible. In other words, the K-means algorithm identifies the k number of centroids and then assigns each data point to the nearest cluster while keeping the centroids as small as possible. Since it begins with a random selection of cluster centers, the results can be inconsistent. Since extreme values can easily affect a mean, the K-means clustering algorithm is sensitive to outliers. K-medoids clustering [ 91 ] is a variant of K-means that is more robust to noises and outliers.
- Mean-shift clustering: Mean-shift clustering [ 37 ] is a nonparametric clustering technique that does not require prior knowledge of the number of clusters or constraints on cluster shape. Mean-shift clustering aims to discover “blobs” in a smooth distribution or density of samples [ 82 ]. It is a centroid-based algorithm that works by updating centroid candidates to be the mean of the points in a given region. To form the final set of centroids, these candidates are filtered in a post-processing stage to remove near-duplicates. Cluster analysis in computer vision and image processing are examples of application domains. Mean Shift has the disadvantage of being computationally expensive. Moreover, in cases of high dimension, where the number of clusters shifts abruptly, the mean-shift algorithm does not work well.
- DBSCAN: Density-based spatial clustering of applications with noise (DBSCAN) [ 32 ] is a base algorithm for density-based clustering which is widely used in data mining and machine learning. This is known as a non-parametric density-based clustering technique for separating high-density clusters from low-density clusters that are used in model building. DBSCAN’s main idea is that a point belongs to a cluster if it is close to many points from that cluster. It can find clusters of various shapes and sizes in a vast volume of data that is noisy and contains outliers. DBSCAN, unlike k-means, does not require a priori specification of the number of clusters in the data and can find arbitrarily shaped clusters. Although k-means is much faster than DBSCAN, it is efficient at finding high-density regions and outliers, i.e., is robust to outliers.
- GMM clustering: Gaussian mixture models (GMMs) are often used for data clustering, which is a distribution-based clustering algorithm. A Gaussian mixture model is a probabilistic model in which all the data points are produced by a mixture of a finite number of Gaussian distributions with unknown parameters [ 82 ]. To find the Gaussian parameters for each cluster, an optimization algorithm called expectation-maximization (EM) [ 82 ] can be used. EM is an iterative method that uses a statistical model to estimate the parameters. In contrast to k-means, Gaussian mixture models account for uncertainty and return the likelihood that a data point belongs to one of the k clusters. GMM clustering is more robust than k-means and works well even with non-linear data distributions.
- Agglomerative hierarchical clustering: The most common method of hierarchical clustering used to group objects in clusters based on their similarity is agglomerative clustering. This technique uses a bottom-up approach, where each object is first treated as a singleton cluster by the algorithm. Following that, pairs of clusters are merged one by one until all clusters have been merged into a single large cluster containing all objects. The result is a dendrogram, which is a tree-based representation of the elements. Single linkage [ 115 ], Complete linkage [ 116 ], BOTS [ 102 ] etc. are some examples of such techniques. The main advantage of agglomerative hierarchical clustering over k-means is that the tree-structure hierarchy generated by agglomerative clustering is more informative than the unstructured collection of flat clusters returned by k-means, which can help to make better decisions in the relevant application areas.
Dimensionality Reduction and Feature Learning
In machine learning and data science, high-dimensional data processing is a challenging task for both researchers and application developers. Thus, dimensionality reduction which is an unsupervised learning technique, is important because it leads to better human interpretations, lower computational costs, and avoids overfitting and redundancy by simplifying models. Both the process of feature selection and feature extraction can be used for dimensionality reduction. The primary distinction between the selection and extraction of features is that the “feature selection” keeps a subset of the original features [ 97 ], while “feature extraction” creates brand new ones [ 98 ]. In the following, we briefly discuss these techniques.
- Feature selection: The selection of features, also known as the selection of variables or attributes in the data, is the process of choosing a subset of unique features (variables, predictors) to use in building machine learning and data science model. It decreases a model’s complexity by eliminating the irrelevant or less important features and allows for faster training of machine learning algorithms. A right and optimal subset of the selected features in a problem domain is capable to minimize the overfitting problem through simplifying and generalizing the model as well as increases the model’s accuracy [ 97 ]. Thus, “feature selection” [ 66 , 99 ] is considered as one of the primary concepts in machine learning that greatly affects the effectiveness and efficiency of the target machine learning model. Chi-squared test, Analysis of variance (ANOVA) test, Pearson’s correlation coefficient, recursive feature elimination, are some popular techniques that can be used for feature selection.
- Feature extraction: In a machine learning-based model or system, feature extraction techniques usually provide a better understanding of the data, a way to improve prediction accuracy, and to reduce computational cost or training time. The aim of “feature extraction” [ 66 , 99 ] is to reduce the number of features in a dataset by generating new ones from the existing ones and then discarding the original features. The majority of the information found in the original set of features can then be summarized using this new reduced set of features. For instance, principal components analysis (PCA) is often used as a dimensionality-reduction technique to extract a lower-dimensional space creating new brand components from the existing features in a dataset [ 98 ].
Many algorithms have been proposed to reduce data dimensions in the machine learning and data science literature [ 41 , 125 ]. In the following, we summarize the popular methods that are used widely in various application areas.
- Variance threshold: A simple basic approach to feature selection is the variance threshold [ 82 ]. This excludes all features of low variance, i.e., all features whose variance does not exceed the threshold. It eliminates all zero-variance characteristics by default, i.e., characteristics that have the same value in all samples. This feature selection algorithm looks only at the ( X ) features, not the ( y ) outputs needed, and can, therefore, be used for unsupervised learning.
- Pearson correlation: Pearson’s correlation is another method to understand a feature’s relation to the response variable and can be used for feature selection [ 99 ]. This method is also used for finding the association between the features in a dataset. The resulting value is [ - 1 , 1 ] , where - 1 means perfect negative correlation, + 1 means perfect positive correlation, and 0 means that the two variables do not have a linear correlation. If two random variables represent X and Y , then the correlation coefficient between X and Y is defined as [ 41 ] r ( X , Y ) = ∑ i = 1 n ( X i - X ¯ ) ( Y i - Y ¯ ) ∑ i = 1 n ( X i - X ¯ ) 2 ∑ i = 1 n ( Y i - Y ¯ ) 2 . 8
- ANOVA: Analysis of variance (ANOVA) is a statistical tool used to verify the mean values of two or more groups that differ significantly from each other. ANOVA assumes a linear relationship between the variables and the target and the variables’ normal distribution. To statistically test the equality of means, the ANOVA method utilizes F tests. For feature selection, the results ‘ANOVA F value’ [ 82 ] of this test can be used where certain features independent of the goal variable can be omitted.
- Chi square: The chi-square χ 2 [ 82 ] statistic is an estimate of the difference between the effects of a series of events or variables observed and expected frequencies. The magnitude of the difference between the real and observed values, the degrees of freedom, and the sample size depends on χ 2 . The chi-square χ 2 is commonly used for testing relationships between categorical variables. If O i represents observed value and E i represents expected value, then χ 2 = ∑ i = 1 n ( O i - E i ) 2 E i . 9
- Recursive feature elimination (RFE): Recursive Feature Elimination (RFE) is a brute force approach to feature selection. RFE [ 82 ] fits the model and removes the weakest feature before it meets the specified number of features. Features are ranked by the coefficients or feature significance of the model. RFE aims to remove dependencies and collinearity in the model by recursively removing a small number of features per iteration.
- Model-based selection: To reduce the dimensionality of the data, linear models penalized with the L 1 regularization can be used. Least absolute shrinkage and selection operator (Lasso) regression is a type of linear regression that has the property of shrinking some of the coefficients to zero [ 82 ]. Therefore, that feature can be removed from the model. Thus, the penalized lasso regression method, often used in machine learning to select the subset of variables. Extra Trees Classifier [ 82 ] is an example of a tree-based estimator that can be used to compute impurity-based function importance, which can then be used to discard irrelevant features.

An example of a principal component analysis (PCA) and created principal components PC1 and PC2 in different dimension space
Association Rule Learning
Association rule learning is a rule-based machine learning approach to discover interesting relationships, “IF-THEN” statements, in large datasets between variables [ 7 ]. One example is that “if a customer buys a computer or laptop (an item), s/he is likely to also buy anti-virus software (another item) at the same time”. Association rules are employed today in many application areas, including IoT services, medical diagnosis, usage behavior analytics, web usage mining, smartphone applications, cybersecurity applications, and bioinformatics. In comparison to sequence mining, association rule learning does not usually take into account the order of things within or across transactions. A common way of measuring the usefulness of association rules is to use its parameter, the ‘support’ and ‘confidence’, which is introduced in [ 7 ].
In the data mining literature, many association rule learning methods have been proposed, such as logic dependent [ 34 ], frequent pattern based [ 8 , 49 , 68 ], and tree-based [ 42 ]. The most popular association rule learning algorithms are summarized below.
- AIS and SETM: AIS is the first algorithm proposed by Agrawal et al. [ 7 ] for association rule mining. The AIS algorithm’s main downside is that too many candidate itemsets are generated, requiring more space and wasting a lot of effort. This algorithm calls for too many passes over the entire dataset to produce the rules. Another approach SETM [ 49 ] exhibits good performance and stable behavior with execution time; however, it suffers from the same flaw as the AIS algorithm.
- Apriori: For generating association rules for a given dataset, Agrawal et al. [ 8 ] proposed the Apriori, Apriori-TID, and Apriori-Hybrid algorithms. These later algorithms outperform the AIS and SETM mentioned above due to the Apriori property of frequent itemset [ 8 ]. The term ‘Apriori’ usually refers to having prior knowledge of frequent itemset properties. Apriori uses a “bottom-up” approach, where it generates the candidate itemsets. To reduce the search space, Apriori uses the property “all subsets of a frequent itemset must be frequent; and if an itemset is infrequent, then all its supersets must also be infrequent”. Another approach predictive Apriori [ 108 ] can also generate rules; however, it receives unexpected results as it combines both the support and confidence. The Apriori [ 8 ] is the widely applicable techniques in mining association rules.
- ECLAT: This technique was proposed by Zaki et al. [ 131 ] and stands for Equivalence Class Clustering and bottom-up Lattice Traversal. ECLAT uses a depth-first search to find frequent itemsets. In contrast to the Apriori [ 8 ] algorithm, which represents data in a horizontal pattern, it represents data vertically. Hence, the ECLAT algorithm is more efficient and scalable in the area of association rule learning. This algorithm is better suited for small and medium datasets whereas the Apriori algorithm is used for large datasets.
- FP-Growth: Another common association rule learning technique based on the frequent-pattern tree (FP-tree) proposed by Han et al. [ 42 ] is Frequent Pattern Growth, known as FP-Growth. The key difference with Apriori is that while generating rules, the Apriori algorithm [ 8 ] generates frequent candidate itemsets; on the other hand, the FP-growth algorithm [ 42 ] prevents candidate generation and thus produces a tree by the successful strategy of ‘divide and conquer’ approach. Due to its sophistication, however, FP-Tree is challenging to use in an interactive mining environment [ 133 ]. Thus, the FP-Tree would not fit into memory for massive data sets, making it challenging to process big data as well. Another solution is RARM (Rapid Association Rule Mining) proposed by Das et al. [ 26 ] but faces a related FP-tree issue [ 133 ].
- ABC-RuleMiner: A rule-based machine learning method, recently proposed in our earlier paper, by Sarker et al. [ 104 ], to discover the interesting non-redundant rules to provide real-world intelligent services. This algorithm effectively identifies the redundancy in associations by taking into account the impact or precedence of the related contextual features and discovers a set of non-redundant association rules. This algorithm first constructs an association generation tree (AGT), a top-down approach, and then extracts the association rules through traversing the tree. Thus, ABC-RuleMiner is more potent than traditional rule-based methods in terms of both non-redundant rule generation and intelligent decision-making, particularly in a context-aware smart computing environment, where human or user preferences are involved.
Among the association rule learning techniques discussed above, Apriori [ 8 ] is the most widely used algorithm for discovering association rules from a given dataset [ 133 ]. The main strength of the association learning technique is its comprehensiveness, as it generates all associations that satisfy the user-specified constraints, such as minimum support and confidence value. The ABC-RuleMiner approach [ 104 ] discussed earlier could give significant results in terms of non-redundant rule generation and intelligent decision-making for the relevant application areas in the real world.
Reinforcement Learning
Reinforcement learning (RL) is a machine learning technique that allows an agent to learn by trial and error in an interactive environment using input from its actions and experiences. Unlike supervised learning, which is based on given sample data or examples, the RL method is based on interacting with the environment. The problem to be solved in reinforcement learning (RL) is defined as a Markov Decision Process (MDP) [ 86 ], i.e., all about sequentially making decisions. An RL problem typically includes four elements such as Agent, Environment, Rewards, and Policy.
RL can be split roughly into Model-based and Model-free techniques. Model-based RL is the process of inferring optimal behavior from a model of the environment by performing actions and observing the results, which include the next state and the immediate reward [ 85 ]. AlphaZero, AlphaGo [ 113 ] are examples of the model-based approaches. On the other hand, a model-free approach does not use the distribution of the transition probability and the reward function associated with MDP. Q-learning, Deep Q Network, Monte Carlo Control, SARSA (State–Action–Reward–State–Action), etc. are some examples of model-free algorithms [ 52 ]. The policy network, which is required for model-based RL but not for model-free, is the key difference between model-free and model-based learning. In the following, we discuss the popular RL algorithms.
- Monte Carlo methods: Monte Carlo techniques, or Monte Carlo experiments, are a wide category of computational algorithms that rely on repeated random sampling to obtain numerical results [ 52 ]. The underlying concept is to use randomness to solve problems that are deterministic in principle. Optimization, numerical integration, and making drawings from the probability distribution are the three problem classes where Monte Carlo techniques are most commonly used.
- Q-learning: Q-learning is a model-free reinforcement learning algorithm for learning the quality of behaviors that tell an agent what action to take under what conditions [ 52 ]. It does not need a model of the environment (hence the term “model-free”), and it can deal with stochastic transitions and rewards without the need for adaptations. The ‘Q’ in Q-learning usually stands for quality, as the algorithm calculates the maximum expected rewards for a given behavior in a given state.
- Deep Q-learning: The basic working step in Deep Q-Learning [ 52 ] is that the initial state is fed into the neural network, which returns the Q-value of all possible actions as an output. Still, when we have a reasonably simple setting to overcome, Q-learning works well. However, when the number of states and actions becomes more complicated, deep learning can be used as a function approximator.
Reinforcement learning, along with supervised and unsupervised learning, is one of the basic machine learning paradigms. RL can be used to solve numerous real-world problems in various fields, such as game theory, control theory, operations analysis, information theory, simulation-based optimization, manufacturing, supply chain logistics, multi-agent systems, swarm intelligence, aircraft control, robot motion control, and many more.
Artificial Neural Network and Deep Learning
Deep learning is part of a wider family of artificial neural networks (ANN)-based machine learning approaches with representation learning. Deep learning provides a computational architecture by combining several processing layers, such as input, hidden, and output layers, to learn from data [ 41 ]. The main advantage of deep learning over traditional machine learning methods is its better performance in several cases, particularly learning from large datasets [ 105 , 129 ]. Figure Figure9 9 shows a general performance of deep learning over machine learning considering the increasing amount of data. However, it may vary depending on the data characteristics and experimental set up.

Machine learning and deep learning performance in general with the amount of data
The most common deep learning algorithms are: Multi-layer Perceptron (MLP), Convolutional Neural Network (CNN, or ConvNet), Long Short-Term Memory Recurrent Neural Network (LSTM-RNN) [ 96 ]. In the following, we discuss various types of deep learning methods that can be used to build effective data-driven models for various purposes.

A structure of an artificial neural network modeling with multiple processing layers

An example of a convolutional neural network (CNN or ConvNet) including multiple convolution and pooling layers
- LSTM-RNN: Long short-term memory (LSTM) is an artificial recurrent neural network (RNN) architecture used in the area of deep learning [ 38 ]. LSTM has feedback links, unlike normal feed-forward neural networks. LSTM networks are well-suited for analyzing and learning sequential data, such as classifying, processing, and predicting data based on time series data, which differentiates it from other conventional networks. Thus, LSTM can be used when the data are in a sequential format, such as time, sentence, etc., and commonly applied in the area of time-series analysis, natural language processing, speech recognition, etc.
In addition to these most common deep learning methods discussed above, several other deep learning approaches [ 96 ] exist in the area for various purposes. For instance, the self-organizing map (SOM) [ 58 ] uses unsupervised learning to represent the high-dimensional data by a 2D grid map, thus achieving dimensionality reduction. The autoencoder (AE) [ 15 ] is another learning technique that is widely used for dimensionality reduction as well and feature extraction in unsupervised learning tasks. Restricted Boltzmann machines (RBM) [ 46 ] can be used for dimensionality reduction, classification, regression, collaborative filtering, feature learning, and topic modeling. A deep belief network (DBN) is typically composed of simple, unsupervised networks such as restricted Boltzmann machines (RBMs) or autoencoders, and a backpropagation neural network (BPNN) [ 123 ]. A generative adversarial network (GAN) [ 39 ] is a form of the network for deep learning that can generate data with characteristics close to the actual data input. Transfer learning is currently very common because it can train deep neural networks with comparatively low data, which is typically the re-use of a new problem with a pre-trained model [ 124 ]. A brief discussion of these artificial neural networks (ANN) and deep learning (DL) models are summarized in our earlier paper Sarker et al. [ 96 ].
Overall, based on the learning techniques discussed above, we can conclude that various types of machine learning techniques, such as classification analysis, regression, data clustering, feature selection and extraction, and dimensionality reduction, association rule learning, reinforcement learning, or deep learning techniques, can play a significant role for various purposes according to their capabilities. In the following section, we discuss several application areas based on machine learning algorithms.
Applications of Machine Learning
In the current age of the Fourth Industrial Revolution (4IR), machine learning becomes popular in various application areas, because of its learning capabilities from the past and making intelligent decisions. In the following, we summarize and discuss ten popular application areas of machine learning technology.
- Predictive analytics and intelligent decision-making: A major application field of machine learning is intelligent decision-making by data-driven predictive analytics [ 21 , 70 ]. The basis of predictive analytics is capturing and exploiting relationships between explanatory variables and predicted variables from previous events to predict the unknown outcome [ 41 ]. For instance, identifying suspects or criminals after a crime has been committed, or detecting credit card fraud as it happens. Another application, where machine learning algorithms can assist retailers in better understanding consumer preferences and behavior, better manage inventory, avoiding out-of-stock situations, and optimizing logistics and warehousing in e-commerce. Various machine learning algorithms such as decision trees, support vector machines, artificial neural networks, etc. [ 106 , 125 ] are commonly used in the area. Since accurate predictions provide insight into the unknown, they can improve the decisions of industries, businesses, and almost any organization, including government agencies, e-commerce, telecommunications, banking and financial services, healthcare, sales and marketing, transportation, social networking, and many others.
- Cybersecurity and threat intelligence: Cybersecurity is one of the most essential areas of Industry 4.0. [ 114 ], which is typically the practice of protecting networks, systems, hardware, and data from digital attacks [ 114 ]. Machine learning has become a crucial cybersecurity technology that constantly learns by analyzing data to identify patterns, better detect malware in encrypted traffic, find insider threats, predict where bad neighborhoods are online, keep people safe while browsing, or secure data in the cloud by uncovering suspicious activity. For instance, clustering techniques can be used to identify cyber-anomalies, policy violations, etc. To detect various types of cyber-attacks or intrusions machine learning classification models by taking into account the impact of security features are useful [ 97 ]. Various deep learning-based security models can also be used on the large scale of security datasets [ 96 , 129 ]. Moreover, security policy rules generated by association rule learning techniques can play a significant role to build a rule-based security system [ 105 ]. Thus, we can say that various learning techniques discussed in Sect. Machine Learning Tasks and Algorithms , can enable cybersecurity professionals to be more proactive inefficiently preventing threats and cyber-attacks.
- Internet of things (IoT) and smart cities: Internet of Things (IoT) is another essential area of Industry 4.0. [ 114 ], which turns everyday objects into smart objects by allowing them to transmit data and automate tasks without the need for human interaction. IoT is, therefore, considered to be the big frontier that can enhance almost all activities in our lives, such as smart governance, smart home, education, communication, transportation, retail, agriculture, health care, business, and many more [ 70 ]. Smart city is one of IoT’s core fields of application, using technologies to enhance city services and residents’ living experiences [ 132 , 135 ]. As machine learning utilizes experience to recognize trends and create models that help predict future behavior and events, it has become a crucial technology for IoT applications [ 103 ]. For example, to predict traffic in smart cities, parking availability prediction, estimate the total usage of energy of the citizens for a particular period, make context-aware and timely decisions for the people, etc. are some tasks that can be solved using machine learning techniques according to the current needs of the people.
- Traffic prediction and transportation: Transportation systems have become a crucial component of every country’s economic development. Nonetheless, several cities around the world are experiencing an excessive rise in traffic volume, resulting in serious issues such as delays, traffic congestion, higher fuel prices, increased CO 2 pollution, accidents, emergencies, and a decline in modern society’s quality of life [ 40 ]. Thus, an intelligent transportation system through predicting future traffic is important, which is an indispensable part of a smart city. Accurate traffic prediction based on machine and deep learning modeling can help to minimize the issues [ 17 , 30 , 31 ]. For example, based on the travel history and trend of traveling through various routes, machine learning can assist transportation companies in predicting possible issues that may occur on specific routes and recommending their customers to take a different path. Ultimately, these learning-based data-driven models help improve traffic flow, increase the usage and efficiency of sustainable modes of transportation, and limit real-world disruption by modeling and visualizing future changes.
- Healthcare and COVID-19 pandemic: Machine learning can help to solve diagnostic and prognostic problems in a variety of medical domains, such as disease prediction, medical knowledge extraction, detecting regularities in data, patient management, etc. [ 33 , 77 , 112 ]. Coronavirus disease (COVID-19) is an infectious disease caused by a newly discovered coronavirus, according to the World Health Organization (WHO) [ 3 ]. Recently, the learning techniques have become popular in the battle against COVID-19 [ 61 , 63 ]. For the COVID-19 pandemic, the learning techniques are used to classify patients at high risk, their mortality rate, and other anomalies [ 61 ]. It can also be used to better understand the virus’s origin, COVID-19 outbreak prediction, as well as for disease diagnosis and treatment [ 14 , 50 ]. With the help of machine learning, researchers can forecast where and when, the COVID-19 is likely to spread, and notify those regions to match the required arrangements. Deep learning also provides exciting solutions to the problems of medical image processing and is seen as a crucial technique for potential applications, particularly for COVID-19 pandemic [ 10 , 78 , 111 ]. Overall, machine and deep learning techniques can help to fight the COVID-19 virus and the pandemic as well as intelligent clinical decisions making in the domain of healthcare.
- E-commerce and product recommendations: Product recommendation is one of the most well known and widely used applications of machine learning, and it is one of the most prominent features of almost any e-commerce website today. Machine learning technology can assist businesses in analyzing their consumers’ purchasing histories and making customized product suggestions for their next purchase based on their behavior and preferences. E-commerce companies, for example, can easily position product suggestions and offers by analyzing browsing trends and click-through rates of specific items. Using predictive modeling based on machine learning techniques, many online retailers, such as Amazon [ 71 ], can better manage inventory, prevent out-of-stock situations, and optimize logistics and warehousing. The future of sales and marketing is the ability to capture, evaluate, and use consumer data to provide a customized shopping experience. Furthermore, machine learning techniques enable companies to create packages and content that are tailored to the needs of their customers, allowing them to maintain existing customers while attracting new ones.
- NLP and sentiment analysis: Natural language processing (NLP) involves the reading and understanding of spoken or written language through the medium of a computer [ 79 , 103 ]. Thus, NLP helps computers, for instance, to read a text, hear speech, interpret it, analyze sentiment, and decide which aspects are significant, where machine learning techniques can be used. Virtual personal assistant, chatbot, speech recognition, document description, language or machine translation, etc. are some examples of NLP-related tasks. Sentiment Analysis [ 90 ] (also referred to as opinion mining or emotion AI) is an NLP sub-field that seeks to identify and extract public mood and views within a given text through blogs, reviews, social media, forums, news, etc. For instance, businesses and brands use sentiment analysis to understand the social sentiment of their brand, product, or service through social media platforms or the web as a whole. Overall, sentiment analysis is considered as a machine learning task that analyzes texts for polarity, such as “positive”, “negative”, or “neutral” along with more intense emotions like very happy, happy, sad, very sad, angry, have interest, or not interested etc.
- Image, speech and pattern recognition: Image recognition [ 36 ] is a well-known and widespread example of machine learning in the real world, which can identify an object as a digital image. For instance, to label an x-ray as cancerous or not, character recognition, or face detection in an image, tagging suggestions on social media, e.g., Facebook, are common examples of image recognition. Speech recognition [ 23 ] is also very popular that typically uses sound and linguistic models, e.g., Google Assistant, Cortana, Siri, Alexa, etc. [ 67 ], where machine learning methods are used. Pattern recognition [ 13 ] is defined as the automated recognition of patterns and regularities in data, e.g., image analysis. Several machine learning techniques such as classification, feature selection, clustering, or sequence labeling methods are used in the area.
- Sustainable agriculture: Agriculture is essential to the survival of all human activities [ 109 ]. Sustainable agriculture practices help to improve agricultural productivity while also reducing negative impacts on the environment [ 5 , 25 , 109 ]. The sustainable agriculture supply chains are knowledge-intensive and based on information, skills, technologies, etc., where knowledge transfer encourages farmers to enhance their decisions to adopt sustainable agriculture practices utilizing the increasing amount of data captured by emerging technologies, e.g., the Internet of Things (IoT), mobile technologies and devices, etc. [ 5 , 53 , 54 ]. Machine learning can be applied in various phases of sustainable agriculture, such as in the pre-production phase - for the prediction of crop yield, soil properties, irrigation requirements, etc.; in the production phase—for weather prediction, disease detection, weed detection, soil nutrient management, livestock management, etc.; in processing phase—for demand estimation, production planning, etc. and in the distribution phase - the inventory management, consumer analysis, etc.
- User behavior analytics and context-aware smartphone applications: Context-awareness is a system’s ability to capture knowledge about its surroundings at any moment and modify behaviors accordingly [ 28 , 93 ]. Context-aware computing uses software and hardware to automatically collect and interpret data for direct responses. The mobile app development environment has been changed greatly with the power of AI, particularly, machine learning techniques through their learning capabilities from contextual data [ 103 , 136 ]. Thus, the developers of mobile apps can rely on machine learning to create smart apps that can understand human behavior, support, and entertain users [ 107 , 137 , 140 ]. To build various personalized data-driven context-aware systems, such as smart interruption management, smart mobile recommendation, context-aware smart searching, decision-making that intelligently assist end mobile phone users in a pervasive computing environment, machine learning techniques are applicable. For example, context-aware association rules can be used to build an intelligent phone call application [ 104 ]. Clustering approaches are useful in capturing users’ diverse behavioral activities by taking into account data in time series [ 102 ]. To predict the future events in various contexts, the classification methods can be used [ 106 , 139 ]. Thus, various learning techniques discussed in Sect. “ Machine Learning Tasks and Algorithms ” can help to build context-aware adaptive and smart applications according to the preferences of the mobile phone users.
In addition to these application areas, machine learning-based models can also apply to several other domains such as bioinformatics, cheminformatics, computer networks, DNA sequence classification, economics and banking, robotics, advanced engineering, and many more.
Challenges and Research Directions
Our study on machine learning algorithms for intelligent data analysis and applications opens several research issues in the area. Thus, in this section, we summarize and discuss the challenges faced and the potential research opportunities and future directions.
In general, the effectiveness and the efficiency of a machine learning-based solution depend on the nature and characteristics of the data, and the performance of the learning algorithms. To collect the data in the relevant domain, such as cybersecurity, IoT, healthcare and agriculture discussed in Sect. “ Applications of Machine Learning ” is not straightforward, although the current cyberspace enables the production of a huge amount of data with very high frequency. Thus, collecting useful data for the target machine learning-based applications, e.g., smart city applications, and their management is important to further analysis. Therefore, a more in-depth investigation of data collection methods is needed while working on the real-world data. Moreover, the historical data may contain many ambiguous values, missing values, outliers, and meaningless data. The machine learning algorithms, discussed in Sect “ Machine Learning Tasks and Algorithms ” highly impact on data quality, and availability for training, and consequently on the resultant model. Thus, to accurately clean and pre-process the diverse data collected from diverse sources is a challenging task. Therefore, effectively modifying or enhance existing pre-processing methods, or proposing new data preparation techniques are required to effectively use the learning algorithms in the associated application domain.
To analyze the data and extract insights, there exist many machine learning algorithms, summarized in Sect. “ Machine Learning Tasks and Algorithms ”. Thus, selecting a proper learning algorithm that is suitable for the target application is challenging. The reason is that the outcome of different learning algorithms may vary depending on the data characteristics [ 106 ]. Selecting a wrong learning algorithm would result in producing unexpected outcomes that may lead to loss of effort, as well as the model’s effectiveness and accuracy. In terms of model building, the techniques discussed in Sect. “ Machine Learning Tasks and Algorithms ” can directly be used to solve many real-world issues in diverse domains, such as cybersecurity, smart cities and healthcare summarized in Sect. “ Applications of Machine Learning ”. However, the hybrid learning model, e.g., the ensemble of methods, modifying or enhancement of the existing learning techniques, or designing new learning methods, could be a potential future work in the area.
Thus, the ultimate success of a machine learning-based solution and corresponding applications mainly depends on both the data and the learning algorithms. If the data are bad to learn, such as non-representative, poor-quality, irrelevant features, or insufficient quantity for training, then the machine learning models may become useless or will produce lower accuracy. Therefore, effectively processing the data and handling the diverse learning algorithms are important, for a machine learning-based solution and eventually building intelligent applications.
In this paper, we have conducted a comprehensive overview of machine learning algorithms for intelligent data analysis and applications. According to our goal, we have briefly discussed how various types of machine learning methods can be used for making solutions to various real-world issues. A successful machine learning model depends on both the data and the performance of the learning algorithms. The sophisticated learning algorithms then need to be trained through the collected real-world data and knowledge related to the target application before the system can assist with intelligent decision-making. We also discussed several popular application areas based on machine learning techniques to highlight their applicability in various real-world issues. Finally, we have summarized and discussed the challenges faced and the potential research opportunities and future directions in the area. Therefore, the challenges that are identified create promising research opportunities in the field which must be addressed with effective solutions in various application areas. Overall, we believe that our study on machine learning-based solutions opens up a promising direction and can be used as a reference guide for potential research and applications for both academia and industry professionals as well as for decision-makers, from a technical point of view.
Declaration
The author declares no conflict of interest.
This article is part of the topical collection “Advances in Computational Approaches for Artificial Intelligence, Image Processing, IoT and Cloud Applications” guest edited by Bhanu Prakash K N and M. Shivakumar.
Publisher's Note
Springer Nature remains neutral with regard to jurisdictional claims in published maps and institutional affiliations.
Suggestions or feedback?
MIT News | Massachusetts Institute of Technology
- Machine learning
- Social justice
- Black holes
- Classes and programs
Departments
- Aeronautics and Astronautics
- Brain and Cognitive Sciences
- Architecture
- Political Science
- Mechanical Engineering
Centers, Labs, & Programs
- Abdul Latif Jameel Poverty Action Lab (J-PAL)
- Picower Institute for Learning and Memory
- Lincoln Laboratory
- School of Architecture + Planning
- School of Engineering
- School of Humanities, Arts, and Social Sciences
- Sloan School of Management
- School of Science
- MIT Schwarzman College of Computing
Scientists use generative AI to answer complex questions in physics
Press contact :, media download.
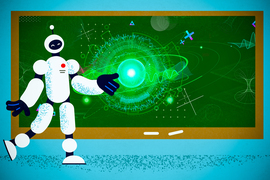
*Terms of Use:
Images for download on the MIT News office website are made available to non-commercial entities, press and the general public under a Creative Commons Attribution Non-Commercial No Derivatives license . You may not alter the images provided, other than to crop them to size. A credit line must be used when reproducing images; if one is not provided below, credit the images to "MIT."
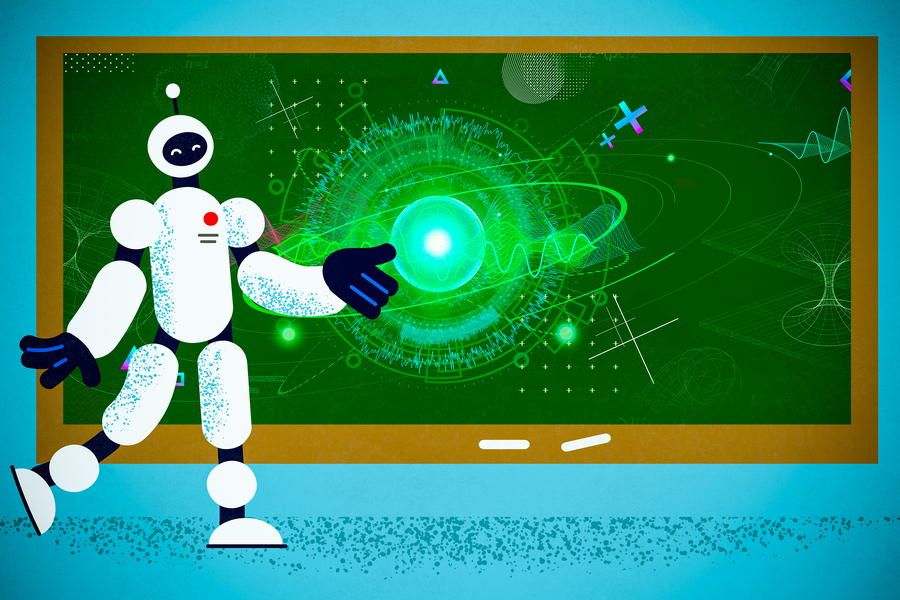
Previous image Next image
When water freezes, it transitions from a liquid phase to a solid phase, resulting in a drastic change in properties like density and volume. Phase transitions in water are so common most of us probably don’t even think about them, but phase transitions in novel materials or complex physical systems are an important area of study.
To fully understand these systems, scientists must be able to recognize phases and detect the transitions between. But how to quantify phase changes in an unknown system is often unclear, especially when data are scarce.
Researchers from MIT and the University of Basel in Switzerland applied generative artificial intelligence models to this problem, developing a new machine-learning framework that can automatically map out phase diagrams for novel physical systems.
Their physics-informed machine-learning approach is more efficient than laborious, manual techniques which rely on theoretical expertise. Importantly, because their approach leverages generative models, it does not require huge, labeled training datasets used in other machine-learning techniques.
Such a framework could help scientists investigate the thermodynamic properties of novel materials or detect entanglement in quantum systems, for instance. Ultimately, this technique could make it possible for scientists to discover unknown phases of matter autonomously.
“If you have a new system with fully unknown properties, how would you choose which observable quantity to study? The hope, at least with data-driven tools, is that you could scan large new systems in an automated way, and it will point you to important changes in the system. This might be a tool in the pipeline of automated scientific discovery of new, exotic properties of phases,” says Frank Schäfer, a postdoc in the Julia Lab in the Computer Science and Artificial Intelligence Laboratory (CSAIL) and co-author of a paper on this approach.
Joining Schäfer on the paper are first author Julian Arnold, a graduate student at the University of Basel; Alan Edelman, applied mathematics professor in the Department of Mathematics and leader of the Julia Lab; and senior author Christoph Bruder, professor in the Department of Physics at the University of Basel. The research is published today in Physical Review Letters.
Detecting phase transitions using AI
While water transitioning to ice might be among the most obvious examples of a phase change, more exotic phase changes, like when a material transitions from being a normal conductor to a superconductor, are of keen interest to scientists.
These transitions can be detected by identifying an “order parameter,” a quantity that is important and expected to change. For instance, water freezes and transitions to a solid phase (ice) when its temperature drops below 0 degrees Celsius. In this case, an appropriate order parameter could be defined in terms of the proportion of water molecules that are part of the crystalline lattice versus those that remain in a disordered state.
In the past, researchers have relied on physics expertise to build phase diagrams manually, drawing on theoretical understanding to know which order parameters are important. Not only is this tedious for complex systems, and perhaps impossible for unknown systems with new behaviors, but it also introduces human bias into the solution.
More recently, researchers have begun using machine learning to build discriminative classifiers that can solve this task by learning to classify a measurement statistic as coming from a particular phase of the physical system, the same way such models classify an image as a cat or dog.
The MIT researchers demonstrated how generative models can be used to solve this classification task much more efficiently, and in a physics-informed manner.
The Julia Programming Language , a popular language for scientific computing that is also used in MIT’s introductory linear algebra classes, offers many tools that make it invaluable for constructing such generative models, Schäfer adds.
Generative models, like those that underlie ChatGPT and Dall-E, typically work by estimating the probability distribution of some data, which they use to generate new data points that fit the distribution (such as new cat images that are similar to existing cat images).
However, when simulations of a physical system using tried-and-true scientific techniques are available, researchers get a model of its probability distribution for free. This distribution describes the measurement statistics of the physical system.
A more knowledgeable model
The MIT team’s insight is that this probability distribution also defines a generative model upon which a classifier can be constructed. They plug the generative model into standard statistical formulas to directly construct a classifier instead of learning it from samples, as was done with discriminative approaches.
“This is a really nice way of incorporating something you know about your physical system deep inside your machine-learning scheme. It goes far beyond just performing feature engineering on your data samples or simple inductive biases,” Schäfer says.
This generative classifier can determine what phase the system is in given some parameter, like temperature or pressure. And because the researchers directly approximate the probability distributions underlying measurements from the physical system, the classifier has system knowledge.
This enables their method to perform better than other machine-learning techniques. And because it can work automatically without the need for extensive training, their approach significantly enhances the computational efficiency of identifying phase transitions.
At the end of the day, similar to how one might ask ChatGPT to solve a math problem, the researchers can ask the generative classifier questions like “does this sample belong to phase I or phase II?” or “was this sample generated at high temperature or low temperature?”
Scientists could also use this approach to solve different binary classification tasks in physical systems, possibly to detect entanglement in quantum systems (Is the state entangled or not?) or determine whether theory A or B is best suited to solve a particular problem. They could also use this approach to better understand and improve large language models like ChatGPT by identifying how certain parameters should be tuned so the chatbot gives the best outputs.
In the future, the researchers also want to study theoretical guarantees regarding how many measurements they would need to effectively detect phase transitions and estimate the amount of computation that would require.
This work was funded, in part, by the Swiss National Science Foundation, the MIT-Switzerland Lockheed Martin Seed Fund, and MIT International Science and Technology Initiatives.
Share this news article on:
Press mentions.
Researchers at MIT and elsewhere have developed a new machine-learning model capable of “predicting a physical system’s phase or state,” report Kyle Wiggers and Devin Coldewey for TechCrunch .
Previous item Next item
Related Links
- Frank Schäfer
- Alan Edelman
- Computer Science and Artificial Intelligence Laboratory
- Department of Mathematics
- Department of Electrical Engineering and Computer Science
Related Topics
- Mathematics
- Computer science and technology
- Artificial intelligence
- Computer modeling
- Computer Science and Artificial Intelligence Laboratory (CSAIL)
- Electrical Engineering & Computer Science (eecs)
Related Articles
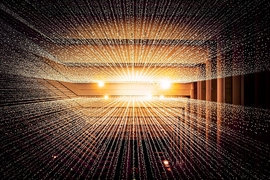
Technique could efficiently solve partial differential equations for numerous applications
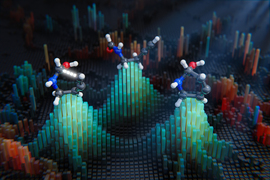
Computational model captures the elusive transition states of chemical reactions
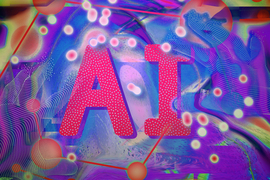
Explained: Generative AI
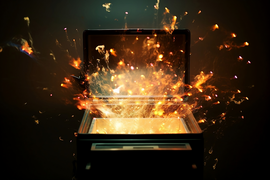
From physics to generative AI: An AI model for advanced pattern generation
More mit news.
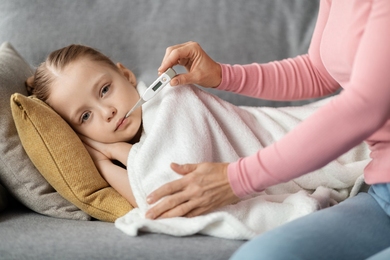
Understanding why autism symptoms sometimes improve amid fever
Read full story →
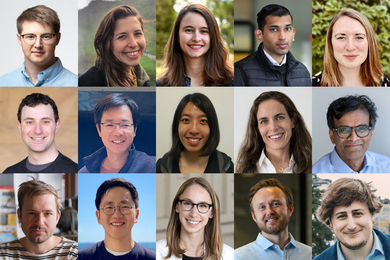
School of Engineering welcomes new faculty
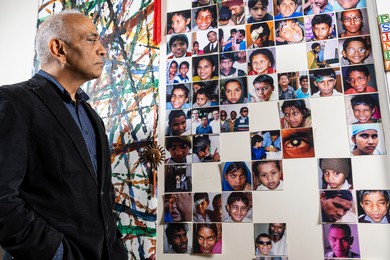
Study explains why the brain can robustly recognize images, even without color
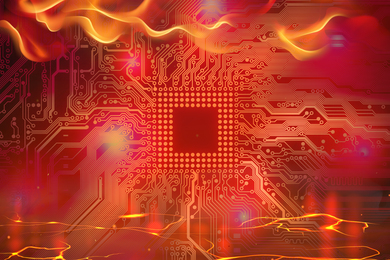
Turning up the heat on next-generation semiconductors
Sarah Millholland receives 2024 Vera Rubin Early Career Award
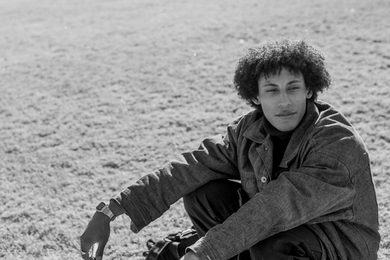
A community collaboration for progress
- More news on MIT News homepage →
Massachusetts Institute of Technology 77 Massachusetts Avenue, Cambridge, MA, USA
- Map (opens in new window)
- Events (opens in new window)
- People (opens in new window)
- Careers (opens in new window)
- Accessibility
- Social Media Hub
- MIT on Facebook
- MIT on YouTube
- MIT on Instagram
Help | Advanced Search
Computer Science > Machine Learning
Title: actively learning combinatorial optimization using a membership oracle.
Abstract: We consider solving a combinatorial optimization problem with an unknown linear constraint using a membership oracle that, given a solution, determines whether it is feasible or infeasible with absolute certainty. The goal of the decision maker is to find the best possible solution subject to a budget on the number of oracle calls. Inspired by active learning based on Support Vector Machines (SVMs), we adapt a classical framework in order to solve the problem by learning and exploiting a surrogate linear constraint. The resulting new framework includes training a linear separator on the labeled points and selecting new points to be labeled, which is achieved by applying a sampling strategy and solving a 0-1 integer linear program. Following the active learning literature, one can consider using SVM as a linear classifier and the information-based sampling strategy known as Simple margin. We improve on both sides: we propose an alternative sampling strategy based on mixed-integer quadratic programming and a linear separation method inspired by an algorithm for convex optimization in the oracle model. We conduct experiments on the pure knapsack problem and on a college study plan problem from the literature to show how different linear separation methods and sampling strategies influence the quality of the results in terms of objective value.
Submission history
Access paper:.
- Other Formats
References & Citations
- Google Scholar
- Semantic Scholar
BibTeX formatted citation

Bibliographic and Citation Tools
Code, data and media associated with this article, recommenders and search tools.
- Institution
arXivLabs: experimental projects with community collaborators
arXivLabs is a framework that allows collaborators to develop and share new arXiv features directly on our website.
Both individuals and organizations that work with arXivLabs have embraced and accepted our values of openness, community, excellence, and user data privacy. arXiv is committed to these values and only works with partners that adhere to them.
Have an idea for a project that will add value for arXiv's community? Learn more about arXivLabs .

IMAGES
VIDEO
COMMENTS
Compensate for missing data. Gaps in a data set can severely limit accurate learning, inference, and prediction. Models trained by machine learning improve with more relevant data. When used correctly, machine learning can also help synthesize missing data that round out incomplete datasets. Make more accurate predictions or conclusions from ...
Master the essential skills needed to recognize and solve complex problems with machine learning and deep learning. Using real-world examples that leverage the popular Python machine learning ecosystem, this book is your perfect companion for learning the art and science of machine learning to become a successful practitioner.
Approaching Machine Learning Problems. When approaching machine learning problems, these are the steps you will need to go through: Setting acceptance criteria. Cleaning your data and maximizing ist information content. Choosing the most optimal inference approach. Train, test, repeat. Let us see these items in detail.
Machine Learning problems are abound. They make up core or difficult parts of the software you use on the web or on your desktop everyday. Think of the "do you want to follow" suggestions on twitter and the speech understanding in Apple's Siri. Below are 10 examples of machine learning that really ground what machine learning is all about.
Introduction to Machine Learning Problem Framing teaches you how to determine if machine learning (ML) is a good approach for a problem and explains how to outline an ML solution. Identify if ML is a good solution for a problem. Learn how to frame an ML problem. Understand how to pick the right model and define success metrics.
But that's OK — In fact, this is is part two of a series of articles in which I'll try and walk you through the main concepts of machine learning. You can find part one here. Once you followed the steps highlighted in the introduction article you should be in a position where you have a very clear and clearly articulated problem and a ...
Machine learning is a powerful problem-solving tool. However, it also has its limitations. Listed below are the main advantages and current challenges of machine learning: Advantages. Scale of data. Machine learning can handle problems that require processing massive volumes of data.
This is how an end-to-end machine learning project is designed and successfully executed. In this article, I wanted to give you a process or a template which you can re-use in your own projects. Applying machine learning to a completely new problem can be a little overwhelming and sometimes straight-up daunting.
Course Overview. This course begins by helping you reframe real-world problems in terms of supervised machine learning. Through understanding the "ingredients" of a machine learning problem, you will investigate how to implement, evaluate, and improve machine learning algorithms. Ultimately, you will implement the k-Nearest Neighbors (k-NN ...
Problem solving. Projects foster innovative problem-solving and critical thinking, enabling a deeper understanding of machine learning functionalities. ... You need to understand the business problem and come up with a rough idea of how you are going to use machine learning to solve it. Look for research papers, open source projects, tutorials ...
Real-world examples make the abstract description of machine learning become concrete. In this post you will go on a tour of real world machine learning problems. You will see how machine learning can actually be used in fields like education, science, technology and medicine. Each machine learning problem listed also includes a link to the publicly available dataset.
Machine learning is a key enabler of automation. By learning from data and improving over time, machine learning algorithms can perform previously manual tasks, freeing humans to focus on more complex and creative tasks. This not only increases efficiency but also opens up new possibilities for innovation.
1.1. Solving Machine Learning Problems This work is the rst to successfully solve Machine Learning problems (or questions) using Machine Learning. Speci cally, our model handles the wide variety of topics covered in MIT's Introduction to Machine Learning course (6.036), except for coding questions and
Spam detection is one of the best and most common problems solved by Machine Learning. Neural networks employ content-based filtering to classify unwanted emails as spam. These neural networks are quite similar to the brain, with the ability to identify spam emails and messages. 2.
Supervised: Supervised learning is typically the task of machine learning to learn a function that maps an input to an output based on sample input-output pairs [].It uses labeled training data and a collection of training examples to infer a function. Supervised learning is carried out when certain goals are identified to be accomplished from a certain set of inputs [], i.e., a task-driven ...
A Guide to Solving Social Problems with Machine Learning. by. Jon Kleinberg, Jens Ludwig, and. Sendhil Mullainathan. December 08, 2016. It's Sunday night. You're the deputy mayor of a big city.
The case study in this article will go over a popular Machine learning concept called classification. Classification. In Machine Learning (ML), classification is a supervised learning concept that groups data into classes. Classification usually refers to any kind of problem where a specific type of class label is the result to be predicted ...
From classification to regression, here are seven algorithms you need to know as you begin your machine learning career: 1. Linear regression. Linear regression is a supervised learning algorithm that predicts and forecasts values within a continuous range, such as sales numbers or prices. Originating from statistics, linear regression performs ...
10 Real-World Problems that Machine Learning can solve. 1. Recommending Products after Collecting Previous Data. Recommendation systems are one of the most common machine learning use cases in day-to-day life. These systems are used mainly by search engines like Google and Bing and the top eCommerce platforms like Amazon and eBay.
The learning algorithms can be categorized into four major types, such as supervised, unsupervised, semi-supervised, and reinforcement learning in the area [ 75 ], discussed briefly in Sect. " Types of Real-World Data and Machine Learning Techniques ". The popularity of these approaches to learning is increasing day-by-day, which is shown ...
Can a machine learn Machine Learning? This work trains a machine learning model to solve machine learning problems from a University undergraduate level course. We generate a new training set of questions and answers consisting of course exercises, homework, and quiz questions from MIT's 6.036 Introduction to Machine Learning course and train a machine learning model to answer these questions ...
This article will define imbalanced data, resampling strategies as solution, appropriate evaluation metrics, kinds of algorithmic approaches, and the utility of synthetic data and data augmentation to address this imbalance. 1. Understanding the Problem. The most important tip really is to understand the problem.
A problem to be solved; can you separate the two sets of points using just a straight line? Source: Author. Left scratching your head? Read on to find an answer to this problem and three practical techniques to solve it and any other problem for that matter! Problem solving strategy 1: Simplify
More recently, researchers have begun using machine learning to build discriminative classifiers that can solve this task by learning to classify a measurement statistic as coming from a particular phase of the physical system, the same way such models classify an image as a cat or dog.
Using the example of AI technology, we illustrate the potential benefits and inherent challenges of integrating new technologies into education. Generative AI is a cogent example, as it presents unique opportunities for personalizing learning and engaging students in creative problem solving around sustainability issues.
We consider solving a combinatorial optimization problem with an unknown linear constraint using a membership oracle that, given a solution, determines whether it is feasible or infeasible with absolute certainty. The goal of the decision maker is to find the best possible solution subject to a budget on the number of oracle calls. Inspired by active learning based on Support Vector Machines ...
DNNs are a popular tool within machine learning due to their ability to model complex patterns and distributions. However, the performance of these networks is highly dependent on the quality of the data used to train the models. ... This paper aims to solve this problem using the approach proposed by Ren et al. (2018) using meta-training and ...