- Skip to main content
- Accessibility help

Information
We use cookies to collect anonymous data to help us improve your site browsing experience.
Click 'Accept all cookies' to agree to all cookies that collect anonymous data. To only allow the cookies that make the site work, click 'Use essential cookies only.' Visit 'Set cookie preferences' to control specific cookies.
Your cookie preferences have been saved. You can change your cookie settings at any time.
Literature Review on the Impact of Digital Technology on Learning and Teaching
This literature review was commissioned by the Scottish Government to explore how the use of digital technology for learning and teaching can support teachers, parents, children and young people in improving outcomes and achieving our ambitions for education in Scotland
Digital learning and raising attainment
Key findings
There is conclusive evidence that digital equipment, tools and resources can, where effectively used, raise the speed and depth of learning in science and mathematics for primary and secondary age learners. There is indicative evidence that the same can be said for some aspects of literacy, especially writing and comprehension. Digital technologies appear to be appropriate means to improve basic literacy and numeracy skills, especially in primary settings.
The effect sizes are generally similar to other educational interventions that are effective in raising attainment, though the use of digital learning has other benefits. Also, the extent of the effect may be dampened by the level of capability of teachers to use digital learning tools and resources effectively to achieve learning outcomes. More effective use of digital teaching to raise attainment includes the ability of teachers to identify how digital tools and resources can be used to achieve learning outcomes and adapting their approach, as well as having knowledge and understanding of the technology. This applies in all schools.
Where learners use digital learning at home as well as school for formal and non-formal learning activities these have positive effects on their attainment, because they have extended their learning time. This is particularly important for secondary age learners.
The assessment framework, set out in Annex 2 , identifies a number of educational benefits that digital learning and teaching has the potential to help learners aged 5 to 18 to realise, through the opportunity to learn in different ways, access more sources of information, and be tested and get feedback differently. In terms of raising attainment, these benefits include short term outcomes, such as having a greater feeling of control over learning and more confidence to practise a skill, through to medium term outcomes such as faster acquisition of knowledge and skills, and improved impacts in terms of learners achieving higher exam or test results where digital technology has been used.
In this section, the impact of digital technology on children's attainment in a range of areas is discussed, followed by the impact on aspects of numeracy, literacy and science learning.
Raising children's attainment
There is a substantial body of research that has examined the impact of digital tools and resources on children's attainment in a range of areas.
Higgins et al (2012) provide a summary of research findings from studies with experimental and quasi-experimental designs, which have been combined in meta-analyses to assess the impact of digital learning in schools. Their search identified 48 studies which synthesised empirical research of the impact of digital tools and resources on the attainment of school age learners (5-18 year olds).
They found consistent but small positive associations between digital learning and educational outcomes. For example, Harrison et al (2004) identified statistically significant findings, positively associating higher levels of ICT use with school achievement at each Key Stage in England, and in English, maths, science, modern foreign languages and design technology. Somekh et al (2007) identified a link between high levels of ICT use and improved school performance. They found that the rate of improvement in tests in English at the end of primary education was faster in ICT Test Bed education authorities in England than in equivalent comparator areas. However, Higgins et al note that while these associations show, on average, schools with higher than average levels of ICT provision also have learners who perform slightly higher than average, it may be the case that high performing schools are more likely to be better equipped or more prepared to invest in technology or more motivated to bring about improvement.
Higgins et al report that in general analyses of the impact of digital technology on learning, the typical overall effect size is between 0.3 and 0.4 - just slightly below the overall average for researched interventions in education (Sipe & Curlette, 1997; Hattie, 2008) and no greater than other researched changes to teaching to raise attainment, such as peer tutoring or more focused feedback to learners. The range of effect sizes is also very wide (-0.03 to 1.05),which suggests that it is essential to take into account the differences between technologies and how they are used.
Table 4: Summary of meta-analyses published between 2000 and 2012 (in Higgins et al 2012)
Focus | No of studies | Overall Study Effect ( ) | Impact on |
---|---|---|---|
General | 7 | 0.24-1.05 | Academic success; academic outcomes; learner achievement; school achievement; cognitive outcomes |
Mathematics | 4 | 0.33-0.71 | Mathematics; mathematics performance. |
Mathematics and Science | 1 | 0.01-0.38 | Mathematics; computer tutorials in science; science simulations; live 'labs' |
Science | 3 | 0.19-0.38 | Lower order outcomes; higher order outcomes; retention follow up test; science academic achievements |
Literacy | 12 | -0.03-0.55 | Reading skills and comprehension; writing quantity and quality; accelerated reader; standardised reading tests; spelling; word processing on writing; on spelling; computer texts on reading |
Other Focus | 6 | 0.07-0.46 | Academic achievement; individual achievement; learning outcomes; mathematics achievement; cognitive gains. |
In an earlier meta-analysis, Liao et al (2007), considered the effects of digital tools and resources on elementary school learners' achievement in Taiwan. Synthesizing research comparing the effects of digital learning (equipment, tools and resources) with traditional instruction on elementary school learners' achievement, they considered quantitative and qualitative information from 48 studies including over 5,000 learners. Of the 48 studies, 44 (92%) showed positive effects in favour of a computer assisted intervention, while four (8%) were negative and favoured a traditional instruction method. Nearly 60% of the studies examined the effects of computer aided instruction for teaching mathematics or science. Another 11% of the studies concentrated on the teaching of reading and language. They found an overall positive effect size across all the studies of 0.45 (study-weighted grand mean), which is considered to be a moderate effect, with a wide range of effect sizes (from 0.25 to 2.67).
No significant differences were found between subject areas, and the authors suggest that digital learning has the potential to be implemented in many different subject areas. They found that the two subjects that showed the highest effects were reading and languages, which had a high positive effect size of 0.7. Studies using computer simulations also had higher effects. The authors suggest this may be because simulations can provide learners with the opportunity to engage in a learning activity which could not be replicated in a classroom.
More qualitative studies have identified how improvements in attainment are achieved. From a wide study of primary and secondary schools in England that were early adopters in using digital learning and teaching, Jewitt et al (2011) concluded that:
- Using digital resources provided learners with more time for active learning in the classroom;
- Digital tools and resources provided more opportunity for active learning outside the classroom, as well as providing self-directed spaces, such as blogs and forums, and access to games with a learning benefit;
- Digital resources provided learners with opportunities to choose the learning resources;
- The resources provided safer spaces for formative assessment and feedback.
The sections below focus on specific key areas of attainment: literacy, numeracy, and science learning.
There is a large body of research that has examined the impact of digital equipment, tools and resources on children's literacy. The effects are generally positive, though not as large as the effects found where digital learning is used to improve numeracy, and consistent in finding that ICT helps improve reading and writing skills, as well as developing speaking and listening skills.
Effect of context
Archer and Savage (2014) undertook a meta-analysis to reassess the outcomes presented in three previous meta-analyses considering the impact of digital learning on language and literacy learning: Slavin et al (2008 and 2009) and Torgenson and Zhu (2003). Overall they found a relatively small average positive effect size of 0.18, with a few of the studies having a negative effect and three studies showing moderate to large effect sizes. The authors found that programmes with a small number of participants tended to show larger effect sizes than larger programmes but that not all were statistically significant.
Archer and Savage sought to understand whether the context within which the digital tool or resource was used has an impact on outcomes. In particular, they examined whether training and support given to the teachers or other staff delivering the programme had an impact. The authors found that training and support could be identified in around half of the studies and that it did appear to have a positive impact on the effectiveness of the literacy intervention, with the average effect size rising to 0.57. The authors conclude that this indicates the importance of including implementation factors, such as training and support, when considering the relative effectiveness of digital learning and teaching.
Effect on specific literacy skills
In their meta-analysis, Higgins et al (2012) found that digital learning has a greater impact on writing than on reading or spelling. For example, Torgenson and Zhu (2003) reviewed the impact of using digital technology on the literacy competences of 5-16 year-olds in English and found effect sizes on spelling (0.2) and reading (0.28) much lower than the high effect size for writing (0.89).
In their meta-analysis of studies investigating the effects of digital technology on primary schools in Taiwan, Laio et al (2007) considered studies over a range of curriculum areas; 11 of which addressed the effects of using digital learning in one or more literacy competence. They found no significant differences in effect size between the different subject areas, suggesting the potential for digital technology to raise outcomes is equal across different subjects. However, they did note that the two areas that showed the highest effect sizes (over 0.7) were reading and comprehension.
Effect of specific digital tools and resources
Somekh et al (2007) evaluated the Primary School Whiteboard Expansion ( PSWB ) project in England. They found that the length of time learners were taught with interactive whiteboards ( IWB s) was a major factor in learner attainment at the end of primary schooling, and that there were positive impacts on literacy (and numeracy) once teachers had experienced sustained use and the technology had become embedded in pedagogical practice. This equated to improvements at Key Stage 2 writing (age 11), where boys with low prior attainment made 2.5 months of additional progress.
Hess (2014) investigated the impact of using e-readers and e-books in the classroom, among 9-10 year olds in the USA . The e-books were used in daily teacher-led guided reading groups, replacing traditional print books in these sessions. Teachers also regularly used the e-readers in sessions where the class read aloud, and e-readers were available to learners during the school day for silent reading. The study found a significant difference in reading assessment scores for the group using the e-readers. Scores improved for both male and female learners and the gap between males and females decreased.
The use of digital tools and resources also appears to affect levels of literacy. Lysenko and Abrami (2014) investigated the use of two digital tools on reading comprehension for elementary school children (aged 6-8) in Quebec, Canada. The first was a multimedia tool which linked learning activities to interactive digital stories. The tool included games to engage learners in reading and writing activities, and instructions were provided orally to promote listening comprehension. The second tool was a web-based electronic portfolio in which learners could create a personalised portfolio of their reading and share work with peers, teachers and parents to get feedback. The authors found that in classes where both tools were used together during the whole school year learners performed significantly better both in vocabulary and reading comprehension (with medium-level effect sizes) than learners in classes where the tools were not part of English language instruction.
Rosen and Beck-Hill (2012) reported on a study programme that incorporated an interactive core curriculum and a digital teaching platform. At the time of their report it was available for 9-11 year old learners in English language, arts and mathematics classes in Dallas, Texas. The online platform contained teaching and learning tools. Learners were assessed using standardised tests administered before the programme and after a year's participation. The results of increased achievement scores demonstrated that in each of the two school year groups covered, the experimental learners significantly outperformed the control learners in reading and maths scores. In observations in classrooms that used the programme, the researchers observed higher teacher-learner interaction, a greater number and type of teaching methods per class, more frequent and complex examples of differentiation processes and skills, more frequent opportunities for learner collaboration, and significantly higher learner engagement. The authors report that the teaching pedagogy observed in the classrooms differed significantly from that observed in more traditional classrooms. The teachers following the programme commented that the digital resources made planning and implementing 'differentiation' more feasible. This is differentiation of teaching in terms of content, process, and product, to reflect learners' readiness, interests, and learning profile, through varied instructional and management strategies.
Effect of the amount and quality of digital technology use
The uses of digital technology and access to it appear to be critical factors. Lee et al (2009) analysed how in the US 15-16 year-old learners' school behaviour and standardised test scores in literacy are related to computer use. Learners were asked how many hours a day they typically used a computer for school work and for other activities. The results indicated that the learners who used the computer for one hour a day for both school work and other activities had significantly better reading test scores and more positive teacher evaluations for their classroom behaviours than any other groups [5] . This was found while controlling for socio-economic status, which has been shown to be a predictor of test scores in other research. The analysis used data from a national 2002 longitudinal study, and it is likely that learners' usage of computers has increased and changed since that time.
Biagi and Loi (2013), using data from the 2009 Programme for International Student Assessment ( PISA ) and information on how learners used digital technology at school and at home (both for school work and for entertainment), assessed the relationship between the intensity with which learners used digital tools and resources and literacy scores. They examined uses for: gaming activities (playing individual or collective online games), collaboration and communication activities (such as linking with others in on-line chat or discussion forums), information management and technical operations (such as searching for and downloading information) and creating content, knowledge and problem solving activities (such as using computers to do homework or running simulations at school). These were then compared to country specific test scores in reading. The authors found a positive and significant relationship between gaming activity and language attainment in 11 of the 23 countries studied. For the other measures, where relationships existed and were significant, they tended to be negative.
The more recent PISA data study ( OECD , 2015, using 2012 results) also found a positive relationship between the use of computers and better results in literacy where it is evident that digital technology is being used by learners to increase study time and practice [6] . In addition, it found that the effective use of digital tools is related to proficiency in reading.
There is a large body of research which has examined the impact of digital equipment, tools and resources on children's numeracy skills and mathematical competences throughout schooling. Higgins et al (2012) found from their meta-analysis that effect sizes of tested gains in knowledge and understanding tend to be greater in mathematics and science than in literacy. The key benefits found relate to problem solving skills, practising number skills and exploring patterns and relationships (Condie and Monroe, 2007), in addition to increased learner motivation and interest in mathematics.
Effect on specific numeracy skills
Li and Ma's (2010) meta-analysis of the impact of digital learning on school learners' mathematics learning found a generally positive effect. The authors considered 46 primary studies involving a total of over 36,000 learners in primary and secondary schools. About half of the mathematics achievement outcomes were measured by locally-developed or teacher-made instruments, and the other half by standardized tests. Almost all studies were well controlled, employing random assignment of learners to experimental or control conditions.
Overall, the authors found that, on average, there was a high, significantly positive effect of digital technology on mathematics achievement (mean effect size of 0.71), indicating that, in general, learners learning mathematics with the use of digital technology had higher mathematics achievement than those learning without digital technology. The authors found that:
- Although the difference was small, younger school learners (under 13 years old) had higher attainment gains than older secondary school learners;
- Gains were more positive where teaching was more learner-centred than teacher-centred. In this regard, the authors differentiate between traditional models, where the teacher tends to teach to the whole class, and a learner-centred teaching model which is discovery-based (inquiry-oriented) or problem-based (application-oriented) learning;
- Shorter interventions (six months or less) were found to be more effective in promoting mathematics achievement than longer interventions (between six and 12 months). It is suggested that such gains in mathematics achievement are a result of the novelty effects of technology, as suggested in other research, and as learners get familiar with the technology the novelty effects tend to decrease;
- The authors found no significant effects from different types of computer technology on mathematics achievement. Whether it was used as communication media, a tutorial device, or exploratory environment, learners displayed similar results in their mathematics achievement;
- Equally, the authors found no significant relationship between the effect of using digital technology and the characteristics of learners included in the samples for studies, such as gender, ethnicity, or socio-economic characteristics.
The studies by Lee et al (2009) and Biagi and Loi (2013) found similar results for mathematics as they did for reading and literacy in relation to the use of digital equipment. Learners who used a computer at least one hour a day for both school work and other activities had significantly better mathematics test scores and more positive teacher evaluations for their classroom behaviour in mathematics classes than those who did not use the computer. Biagi and Loi (2013) found a significant positive relationship between intensity of gaming activity and maths test scores in 15 countries out of the 23 studied. As with language, the authors found that learners' total use of digital technologies was positively and significantly associated with PISA test scores for maths in 18 of the 23 countries studied.
Studies have found that using digital equipment for formal learning is also associated with increases in learners' motivation for learning mathematics. House and Telese (2011 and 2012) found that:
- For learners aged 13 and 14 in South Korea, for example, those who expressed high levels of enjoyment at learning mathematics, more frequently used computers in their mathematics homework. However, learners who more frequently played computer games and used the internet outside of school tended to report that they did not enjoy learning mathematics;
- Learners in the USA and Japan aged 13 and 14 who showed higher levels of algebra achievement also used computers more at home and at school for school work. Those who used computers most for other activities had lower test scores. In each of the USA and Japan they found that overall computer usage which included use for school work was significantly related to improvements in test scores.
Somekh et al (2007) found that, once the use of IWB s was embedded, in Key Stage 1 mathematics (age 7) in England, high attaining girls made gains of 4.75 months, enabling them to catch up with high attaining boys. In Key Stage 2 mathematics (age 11), average and high attaining boys and girls who had been taught extensively with the IWB made the equivalent of an extra 2.5 to 5 months' progress over the course of two years.
Digital tools and resources can also increase some learners' confidence in mathematics as well as their engagement in new approaches to learning and their mathematical competences. Overcoming learners' anxieties about mathematics and their competence in specific aspects of the subject are common concerns in teaching mathematics which hampers their ability to learn (reported in Huang et al 2014).
Huang et al (2014) researched the outcomes, in Taiwan, from a computer game simulating the purchase of commodities, from which 7 and 8 year-old primary school learners can learn addition and subtraction, and apply mathematical concepts. The model combined games-based learning with a diagnosis system. When the learner made a mistake, the system could detect the type of mistake and present corresponding instructions to help the learner improve their mathematical comprehension and application. The authors compared two learning groups: both used the game-based model but one without the diagnostic, feedback element. They found that the learning achievement post-test showed a significant difference and also that the mathematics anxiety level of the two learner groups was decreased by about 3.5%.
Passey (2011) found that among over 300 schools in England using Espresso digital resources, those that had been using them over a longer period made significantly greater increases in end of primary school numeracy test results than schools which were recent users.
Science learning
Effects on science knowledge and skills
In their meta-analysis, Laio et al (2007) considered 11 studies looking at the impact of digital technology on science learning. These had a moderate average effect size of 0.38 and generally had positive effects. Condie and Monroe (2007) identified that digital learning made science more interesting, authentic and relevant for learners and provided more time for post-experiment analysis and discussion.
In their study of the PISA data, Biagi and Loi (2013) found a significant positive relationship between learners' total use of digital equipment and science test scores in 21 of the 23 countries they studied. They also found evidence of a significant positive relationship between the intensity of using gaming activity and science scores in 13 of the 23 countries they studied. Somekh et al (2007) found that in primary school science all learners, except high attaining girls, made greater progress when given more exposure to IWB s, with low attaining boys making as much as 7.5 months' additional progress.
Effects of specific digital tools and resources
Digital tools and resources generally have a positive effect on learners' science learning. This can be seen from a number of studies assessing outcomes for learners in different stages of education.
Hung et al (2012) explored the effect of using multi-media tools in science learning in an elementary school's science course in Taiwan. Learners were asked to complete a digital storytelling project by taking pictures with digital cameras, developing the story based on the pictures taken, producing a film based on the pictures by adding subtitles and a background, and presenting the story. From the experimental results, the authors found that this approach improved the learners' motivation to learn science, their attitude, problem-solving capability and learning achievements. In addition, interviews found that the learners in the experimental group enjoyed the project-based learning activity and thought it helpful because of the digital storytelling aspect.
Hsu et al (2012) investigated the effects of incorporating self-explanation principles into a digital tool facilitating learners' conceptual learning about light and shadow with 8-9 year old learners in Taiwan. While they found no difference in the overall test scores of the experimental and control groups, they found a statistically significant difference in retention test scores. Those learners who had paid more attention to the self-explanation prompts tended to outperform those in the control group.
Anderson and Barnett's (2013) study, in the US , examined how a digital game used by learners aged 12-13 increased their understanding of electromagnetic concepts, compared to learners who conducted a more traditional inquiry-based investigation of the same concepts. There was a significant difference between the control and experimental groups in gains in knowledge and understanding of physics concepts. Additionally, learners in the experimental group were able to give more nuanced responses about the descriptions of electric fields and the influence of distance on the forces that change experience because of what they learnt during the game.
Güven and Sülün (2012) considered the effects of computer-enhanced teaching in science and technology courses on the structure and properties of matter, such as the periodical table, chemical bonding, and chemical reactions, for 13-14 year olds in Turkey. Their proposition was that computer-enhanced teaching can instil a greater sense of interest in scientific and technological developments, make abstract concepts concrete through simulation and modelling, and help to carry out some dangerous experiments in the classroom setting. They found a significant difference in achievement tests between the mean scores of the group of learners who were taught with the computer-enhanced teaching method and the control group who were taught with traditional teaching methods.
Belland (2009) investigated the extent to which a digital tool improved US middle school children's ability to form scientific arguments. Taking the premise that being able to construct and test an evidence-based argument is critical to learning science, he studied the impact of using a digital problem based learning tool on 12-14 year olds. Learners worked in small groups and were asked to develop and present proposals for spending a grant to investigate an issue relating to the human genome project. Those in the experimental group used an online system which structured the project into stages of scientific enquiry. The system prompted the learners to structure and organise their thinking in particular ways: by prompting the learners individually, sharing group members' ideas, tasking the group to form a consensus view, and prompting the group to assign specific tasks among themselves.
Using pre- and post- test scores to assess the impact on learners' abilities to evaluate arguments, Belland found a high positive effect size of 0.62 for average-achieving learners compared to their peers in the control group. No significant impacts were found for higher or lower-achieving learners. Belland suggests that for high-achieving learners, this may be because they already have good argument making skills and are already able to successfully structure how they approach an issue and gather evidence. The study also used qualitative information to consider how the learners used the digital tool and compared this to how learners in the control group worked. The author found that in the experimental group they made more progress and were more able to divide tasks up between them, which saved time. They also used the tool more and the teacher less to provide support.
Kucukozer et al (2009) examined the impact of digital tools on teaching basic concepts of astronomy to 11-13 year old school children in Turkey. Learners were asked to make predictions about an astronomical phenomenon such as what causes the seasons or the phases of the moon. A digital tool was used to model the predictions and display their results. The learners were then asked to explain the differences and the similarities between their predictions and their observations. In the prediction and explanation phase the learners worked in groups to discuss their ideas and come to a conclusion. In the observation phase they watched the 3D models presented by their teacher. Thereafter, they were asked to discuss and make conclusions about what they had watched. The authors found that instruction supported by observations and the computer modelling was significantly effective in bringing about better conceptual understanding and learning on the subject.
Ingredients of success
Where studies examine the process that brings about positive results from digital learning and teaching compared to traditional approaches, it is evident that these are more likely to be achieved where digital equipment, tools and resources are used for specific learning outcomes and built into a teaching model from the outset. This broadly supports Higgins et al's (2012) conclusions that:
- Digital technology is best used as a supplement to normal teaching rather than as a replacement for it;
- It is not whether technology is used (or not) which makes the difference, but how well the technology is applied to support teaching and learning by teachers;
- More effective schools and teachers are more likely to use digital technologies effectively than other schools.
Differences in effect sizes and the extent that learners achieve positive gains in attainment are ascribed by most authors of the studies above to:
- The quality of teaching and the ability of teachers to use the digital equipment and tools effectively for lessons;
- The preparation and training teachers are given to use equipment and tools;
- The opportunities teachers have to see how digital resources can be used and pedagogies adapted (Rosen and Beck-Hill, 2012; Belland, 2009).
Teachers have to adapt to learner-centred approaches to learning if they are to use digital tools and resources (Li and Ma, 2010).
As well as ensuring digital tools and resources are supporting learning goals, success appears to also be linked to some other factors:
- The availability of equipment and tools within schools (and at home);
- How learners use digital equipment. Higgins et al (2012) found that collaborative use of technology (in pairs or small groups) is usually more effective than individual use, though some learners - especially younger children - may need guidance in how to collaborate effectively and responsibly;
- The extent that teaching continues to innovate using digital tools and resources (Higgins et al, 2012).
Fullan (2013) suggested four criteria that schools should meet if their use of digital technology to support increased attainment is to be successful. These were that systems should be engaging for learners and teachers; easy to adapt and use; ubiquitous - with access to the technology 24/7; and steeped in real life problem solving.
Fullan and Donnelly (2013) developed these themes further, proposing an evaluation tool to enable educators to systematically evaluate new companies, products and school models, using the context of what they have seen as necessary for success. Questions focus on the three key criteria of pedagogy (clarity and quality of intended outcome, quality of pedagogy and the relationship between teacher and learner, and quality of assessment platform and functioning); system change (implementation support, value for money, and whole system change potential) and technology (quality of user experience/model design, ease of adaptation, and comprehensiveness and integration).
Email: Catriona Rooke
There is a problem
Thanks for your feedback
Your feedback helps us to improve this website. Do not give any personal information because we cannot reply to you directly.

An official website of the United States government
The .gov means it’s official. Federal government websites often end in .gov or .mil. Before sharing sensitive information, make sure you’re on a federal government site.
The site is secure. The https:// ensures that you are connecting to the official website and that any information you provide is encrypted and transmitted securely.
- Publications
- Account settings
Preview improvements coming to the PMC website in October 2024. Learn More or Try it out now .
- Advanced Search
- Journal List
- Springer Nature - PMC COVID-19 Collection

Impacts of digital technologies on education and factors influencing schools' digital capacity and transformation: A literature review
Stella timotheou.
1 CYENS Center of Excellence & Cyprus University of Technology (Cyprus Interaction Lab), Cyprus, CYENS Center of Excellence & Cyprus University of Technology, Nicosia-Limassol, Cyprus
Ourania Miliou
Yiannis dimitriadis.
2 Universidad de Valladolid (UVA), Spain, Valladolid, Spain
Sara Villagrá Sobrino
Nikoleta giannoutsou, romina cachia.
3 JRC - Joint Research Centre of the European Commission, Seville, Spain
Alejandra Martínez Monés
Andri ioannou, associated data.
Data sharing not applicable to this article as no datasets were generated or analysed during the current study.
Digital technologies have brought changes to the nature and scope of education and led education systems worldwide to adopt strategies and policies for ICT integration. The latter brought about issues regarding the quality of teaching and learning with ICTs, especially concerning the understanding, adaptation, and design of the education systems in accordance with current technological trends. These issues were emphasized during the recent COVID-19 pandemic that accelerated the use of digital technologies in education, generating questions regarding digitalization in schools. Specifically, many schools demonstrated a lack of experience and low digital capacity, which resulted in widening gaps, inequalities, and learning losses. Such results have engendered the need for schools to learn and build upon the experience to enhance their digital capacity and preparedness, increase their digitalization levels, and achieve a successful digital transformation. Given that the integration of digital technologies is a complex and continuous process that impacts different actors within the school ecosystem, there is a need to show how these impacts are interconnected and identify the factors that can encourage an effective and efficient change in the school environments. For this purpose, we conducted a non-systematic literature review. The results of the literature review were organized thematically based on the evidence presented about the impact of digital technology on education and the factors that affect the schools’ digital capacity and digital transformation. The findings suggest that ICT integration in schools impacts more than just students’ performance; it affects several other school-related aspects and stakeholders, too. Furthermore, various factors affect the impact of digital technologies on education. These factors are interconnected and play a vital role in the digital transformation process. The study results shed light on how ICTs can positively contribute to the digital transformation of schools and which factors should be considered for schools to achieve effective and efficient change.
Introduction
Digital technologies have brought changes to the nature and scope of education. Versatile and disruptive technological innovations, such as smart devices, the Internet of Things (IoT), artificial intelligence (AI), augmented reality (AR) and virtual reality (VR), blockchain, and software applications have opened up new opportunities for advancing teaching and learning (Gaol & Prasolova-Førland, 2021 ; OECD, 2021 ). Hence, in recent years, education systems worldwide have increased their investment in the integration of information and communication technology (ICT) (Fernández-Gutiérrez et al., 2020 ; Lawrence & Tar, 2018 ) and prioritized their educational agendas to adapt strategies or policies around ICT integration (European Commission, 2019 ). The latter brought about issues regarding the quality of teaching and learning with ICTs (Bates, 2015 ), especially concerning the understanding, adaptation, and design of education systems in accordance with current technological trends (Balyer & Öz, 2018 ). Studies have shown that despite the investment made in the integration of technology in schools, the results have not been promising, and the intended outcomes have not yet been achieved (Delgado et al., 2015 ; Lawrence & Tar, 2018 ). These issues were exacerbated during the COVID-19 pandemic, which forced teaching across education levels to move online (Daniel, 2020 ). Online teaching accelerated the use of digital technologies generating questions regarding the process, the nature, the extent, and the effectiveness of digitalization in schools (Cachia et al., 2021 ; König et al., 2020 ). Specifically, many schools demonstrated a lack of experience and low digital capacity, which resulted in widening gaps, inequalities, and learning losses (Blaskó et al., 2021 ; Di Pietro et al, 2020 ). Such results have engendered the need for schools to learn and build upon the experience in order to enhance their digital capacity (European Commission, 2020 ) and increase their digitalization levels (Costa et al., 2021 ). Digitalization offers possibilities for fundamental improvement in schools (OECD, 2021 ; Rott & Marouane, 2018 ) and touches many aspects of a school’s development (Delcker & Ifenthaler, 2021 ) . However, it is a complex process that requires large-scale transformative changes beyond the technical aspects of technology and infrastructure (Pettersson, 2021 ). Namely, digitalization refers to “ a series of deep and coordinated culture, workforce, and technology shifts and operating models ” (Brooks & McCormack, 2020 , p. 3) that brings cultural, organizational, and operational change through the integration of digital technologies (JISC, 2020 ). A successful digital transformation requires that schools increase their digital capacity levels, establishing the necessary “ culture, policies, infrastructure as well as digital competence of students and staff to support the effective integration of technology in teaching and learning practices ” (Costa et al, 2021 , p.163).
Given that the integration of digital technologies is a complex and continuous process that impacts different actors within the school ecosystem (Eng, 2005 ), there is a need to show how the different elements of the impact are interconnected and to identify the factors that can encourage an effective and efficient change in the school environment. To address the issues outlined above, we formulated the following research questions:
a) What is the impact of digital technologies on education?
b) Which factors might affect a school’s digital capacity and transformation?
In the present investigation, we conducted a non-systematic literature review of publications pertaining to the impact of digital technologies on education and the factors that affect a school’s digital capacity and transformation. The results of the literature review were organized thematically based on the evidence presented about the impact of digital technology on education and the factors which affect the schools’ digital capacity and digital transformation.
Methodology
The non-systematic literature review presented herein covers the main theories and research published over the past 17 years on the topic. It is based on meta-analyses and review papers found in scholarly, peer-reviewed content databases and other key studies and reports related to the concepts studied (e.g., digitalization, digital capacity) from professional and international bodies (e.g., the OECD). We searched the Scopus database, which indexes various online journals in the education sector with an international scope, to collect peer-reviewed academic papers. Furthermore, we used an all-inclusive Google Scholar search to include relevant key terms or to include studies found in the reference list of the peer-reviewed papers, and other key studies and reports related to the concepts studied by professional and international bodies. Lastly, we gathered sources from the Publications Office of the European Union ( https://op.europa.eu/en/home ); namely, documents that refer to policies related to digital transformation in education.
Regarding search terms, we first searched resources on the impact of digital technologies on education by performing the following search queries: “impact” OR “effects” AND “digital technologies” AND “education”, “impact” OR “effects” AND “ICT” AND “education”. We further refined our results by adding the terms “meta-analysis” and “review” or by adjusting the search options based on the features of each database to avoid collecting individual studies that would provide limited contributions to a particular domain. We relied on meta-analyses and review studies as these consider the findings of multiple studies to offer a more comprehensive view of the research in a given area (Schuele & Justice, 2006 ). Specifically, meta-analysis studies provided quantitative evidence based on statistically verifiable results regarding the impact of educational interventions that integrate digital technologies in school classrooms (Higgins et al., 2012 ; Tolani-Brown et al., 2011 ).
However, quantitative data does not offer explanations for the challenges or difficulties experienced during ICT integration in learning and teaching (Tolani-Brown et al., 2011 ). To fill this gap, we analyzed literature reviews and gathered in-depth qualitative evidence of the benefits and implications of technology integration in schools. In the analysis presented herein, we also included policy documents and reports from professional and international bodies and governmental reports, which offered useful explanations of the key concepts of this study and provided recent evidence on digital capacity and transformation in education along with policy recommendations. The inclusion and exclusion criteria that were considered in this study are presented in Table Table1 1 .
Inclusion and exclusion criteria for the selection of resources on the impact of digital technologies on education
Inclusion criteria | Exclusion criteria |
---|---|
• Published in 2005 or later • Review and meta-analysis studies • Formal education K-12 • Peer-reviewed articles • Articles in English • Reports from professional/international bodies • Governmental reports • Book chapters | • Ph.D. dissertations and theses • Conference poster papers • Conference papers without proceedings • Resources on higher education • Resources on pre-school education • Individual studies |
To ensure a reliable extraction of information from each study and assist the research synthesis we selected the study characteristics of interest (impact) and constructed coding forms. First, an overview of the synthesis was provided by the principal investigator who described the processes of coding, data entry, and data management. The coders followed the same set of instructions but worked independently. To ensure a common understanding of the process between coders, a sample of ten studies was tested. The results were compared, and the discrepancies were identified and resolved. Additionally, to ensure an efficient coding process, all coders participated in group meetings to discuss additions, deletions, and modifications (Stock, 1994 ). Due to the methodological diversity of the studied documents we began to synthesize the literature review findings based on similar study designs. Specifically, most of the meta-analysis studies were grouped in one category due to the quantitative nature of the measured impact. These studies tended to refer to student achievement (Hattie et al., 2014 ). Then, we organized the themes of the qualitative studies in several impact categories. Lastly, we synthesized both review and meta-analysis data across the categories. In order to establish a collective understanding of the concept of impact, we referred to a previous impact study by Balanskat ( 2009 ) which investigated the impact of technology in primary schools. In this context, the impact had a more specific ICT-related meaning and was described as “ a significant influence or effect of ICT on the measured or perceived quality of (parts of) education ” (Balanskat, 2009 , p. 9). In the study presented herein, the main impacts are in relation to learning and learners, teaching, and teachers, as well as other key stakeholders who are directly or indirectly connected to the school unit.
The study’s results identified multiple dimensions of the impact of digital technologies on students’ knowledge, skills, and attitudes; on equality, inclusion, and social integration; on teachers’ professional and teaching practices; and on other school-related aspects and stakeholders. The data analysis indicated various factors that might affect the schools’ digital capacity and transformation, such as digital competencies, the teachers’ personal characteristics and professional development, as well as the school’s leadership and management, administration, infrastructure, etc. The impacts and factors found in the literature review are presented below.
Impacts of digital technologies on students’ knowledge, skills, attitudes, and emotions
The impact of ICT use on students’ knowledge, skills, and attitudes has been investigated early in the literature. Eng ( 2005 ) found a small positive effect between ICT use and students' learning. Specifically, the author reported that access to computer-assisted instruction (CAI) programs in simulation or tutorial modes—used to supplement rather than substitute instruction – could enhance student learning. The author reported studies showing that teachers acknowledged the benefits of ICT on pupils with special educational needs; however, the impact of ICT on students' attainment was unclear. Balanskat et al. ( 2006 ) found a statistically significant positive association between ICT use and higher student achievement in primary and secondary education. The authors also reported improvements in the performance of low-achieving pupils. The use of ICT resulted in further positive gains for students, namely increased attention, engagement, motivation, communication and process skills, teamwork, and gains related to their behaviour towards learning. Evidence from qualitative studies showed that teachers, students, and parents recognized the positive impact of ICT on students' learning regardless of their competence level (strong/weak students). Punie et al. ( 2006 ) documented studies that showed positive results of ICT-based learning for supporting low-achieving pupils and young people with complex lives outside the education system. Liao et al. ( 2007 ) reported moderate positive effects of computer application instruction (CAI, computer simulations, and web-based learning) over traditional instruction on primary school student's achievement. Similarly, Tamim et al. ( 2011 ) reported small to moderate positive effects between the use of computer technology (CAI, ICT, simulations, computer-based instruction, digital and hypermedia) and student achievement in formal face-to-face classrooms compared to classrooms that did not use technology. Jewitt et al., ( 2011 ) found that the use of learning platforms (LPs) (virtual learning environments, management information systems, communication technologies, and information- and resource-sharing technologies) in schools allowed primary and secondary students to access a wider variety of quality learning resources, engage in independent and personalized learning, and conduct self- and peer-review; LPs also provide opportunities for teacher assessment and feedback. Similar findings were reported by Fu ( 2013 ), who documented a list of benefits and opportunities of ICT use. According to the author, the use of ICTs helps students access digital information and course content effectively and efficiently, supports student-centered and self-directed learning, as well as the development of a creative learning environment where more opportunities for critical thinking skills are offered, and promotes collaborative learning in a distance-learning environment. Higgins et al. ( 2012 ) found consistent but small positive associations between the use of technology and learning outcomes of school-age learners (5–18-year-olds) in studies linking the provision and use of technology with attainment. Additionally, Chauhan ( 2017 ) reported a medium positive effect of technology on the learning effectiveness of primary school students compared to students who followed traditional learning instruction.
The rise of mobile technologies and hardware devices instigated investigations into their impact on teaching and learning. Sung et al. ( 2016 ) reported a moderate effect on students' performance from the use of mobile devices in the classroom compared to the use of desktop computers or the non-use of mobile devices. Schmid et al. ( 2014 ) reported medium–low to low positive effects of technology integration (e.g., CAI, ICTs) in the classroom on students' achievement and attitude compared to not using technology or using technology to varying degrees. Tamim et al. ( 2015 ) found a low statistically significant effect of the use of tablets and other smart devices in educational contexts on students' achievement outcomes. The authors suggested that tablets offered additional advantages to students; namely, they reported improvements in students’ notetaking, organizational and communication skills, and creativity. Zheng et al. ( 2016 ) reported a small positive effect of one-to-one laptop programs on students’ academic achievement across subject areas. Additional reported benefits included student-centered, individualized, and project-based learning enhanced learner engagement and enthusiasm. Additionally, the authors found that students using one-to-one laptop programs tended to use technology more frequently than in non-laptop classrooms, and as a result, they developed a range of skills (e.g., information skills, media skills, technology skills, organizational skills). Haßler et al. ( 2016 ) found that most interventions that included the use of tablets across the curriculum reported positive learning outcomes. However, from 23 studies, five reported no differences, and two reported a negative effect on students' learning outcomes. Similar results were indicated by Kalati and Kim ( 2022 ) who investigated the effect of touchscreen technologies on young students’ learning. Specifically, from 53 studies, 34 advocated positive effects of touchscreen devices on children’s learning, 17 obtained mixed findings and two studies reported negative effects.
More recently, approaches that refer to the impact of gamification with the use of digital technologies on teaching and learning were also explored. A review by Pan et al. ( 2022 ) that examined the role of learning games in fostering mathematics education in K-12 settings, reported that gameplay improved students’ performance. Integration of digital games in teaching was also found as a promising pedagogical practice in STEM education that could lead to increased learning gains (Martinez et al., 2022 ; Wang et al., 2022 ). However, although Talan et al. ( 2020 ) reported a medium effect of the use of educational games (both digital and non-digital) on academic achievement, the effect of non-digital games was higher.
Over the last two years, the effects of more advanced technologies on teaching and learning were also investigated. Garzón and Acevedo ( 2019 ) found that AR applications had a medium effect on students' learning outcomes compared to traditional lectures. Similarly, Garzón et al. ( 2020 ) showed that AR had a medium impact on students' learning gains. VR applications integrated into various subjects were also found to have a moderate effect on students’ learning compared to control conditions (traditional classes, e.g., lectures, textbooks, and multimedia use, e.g., images, videos, animation, CAI) (Chen et al., 2022b ). Villena-Taranilla et al. ( 2022 ) noted the moderate effect of VR technologies on students’ learning when these were applied in STEM disciplines. In the same meta-analysis, Villena-Taranilla et al. ( 2022 ) highlighted the role of immersive VR, since its effect on students’ learning was greater (at a high level) across educational levels (K-6) compared to semi-immersive and non-immersive integrations. In another meta-analysis study, the effect size of the immersive VR was small and significantly differentiated across educational levels (Coban et al., 2022 ). The impact of AI on education was investigated by Su and Yang ( 2022 ) and Su et al. ( 2022 ), who showed that this technology significantly improved students’ understanding of AI computer science and machine learning concepts.
It is worth noting that the vast majority of studies referred to learning gains in specific subjects. Specifically, several studies examined the impact of digital technologies on students’ literacy skills and reported positive effects on language learning (Balanskat et al., 2006 ; Grgurović et al., 2013 ; Friedel et al., 2013 ; Zheng et al., 2016 ; Chen et al., 2022b ; Savva et al., 2022 ). Also, several studies documented positive effects on specific language learning areas, namely foreign language learning (Kao, 2014 ), writing (Higgins et al., 2012 ; Wen & Walters, 2022 ; Zheng et al., 2016 ), as well as reading and comprehension (Cheung & Slavin, 2011 ; Liao et al., 2007 ; Schwabe et al., 2022 ). ICTs were also found to have a positive impact on students' performance in STEM (science, technology, engineering, and mathematics) disciplines (Arztmann et al., 2022 ; Bado, 2022 ; Villena-Taranilla et al., 2022 ; Wang et al., 2022 ). Specifically, a number of studies reported positive impacts on students’ achievement in mathematics (Balanskat et al., 2006 ; Hillmayr et al., 2020 ; Li & Ma, 2010 ; Pan et al., 2022 ; Ran et al., 2022 ; Verschaffel et al., 2019 ; Zheng et al., 2016 ). Furthermore, studies documented positive effects of ICTs on science learning (Balanskat et al., 2006 ; Liao et al., 2007 ; Zheng et al., 2016 ; Hillmayr et al., 2020 ; Kalemkuş & Kalemkuş, 2022 ; Lei et al., 2022a ). Çelik ( 2022 ) also noted that computer simulations can help students understand learning concepts related to science. Furthermore, some studies documented that the use of ICTs had a positive impact on students’ achievement in other subjects, such as geography, history, music, and arts (Chauhan, 2017 ; Condie & Munro, 2007 ), and design and technology (Balanskat et al., 2006 ).
More specific positive learning gains were reported in a number of skills, e.g., problem-solving skills and pattern exploration skills (Higgins et al., 2012 ), metacognitive learning outcomes (Verschaffel et al., 2019 ), literacy skills, computational thinking skills, emotion control skills, and collaborative inquiry skills (Lu et al., 2022 ; Su & Yang, 2022 ; Su et al., 2022 ). Additionally, several investigations have reported benefits from the use of ICT on students’ creativity (Fielding & Murcia, 2022 ; Liu et al., 2022 ; Quah & Ng, 2022 ). Lastly, digital technologies were also found to be beneficial for enhancing students’ lifelong learning skills (Haleem et al., 2022 ).
Apart from gaining knowledge and skills, studies also reported improvement in motivation and interest in mathematics (Higgins et. al., 2019 ; Fadda et al., 2022 ) and increased positive achievement emotions towards several subjects during interventions using educational games (Lei et al., 2022a ). Chen et al. ( 2022a ) also reported a small but positive effect of digital health approaches in bullying and cyberbullying interventions with K-12 students, demonstrating that technology-based approaches can help reduce bullying and related consequences by providing emotional support, empowerment, and change of attitude. In their meta-review study, Su et al. ( 2022 ) also documented that AI technologies effectively strengthened students’ attitudes towards learning. In another meta-analysis, Arztmann et al. ( 2022 ) reported positive effects of digital games on motivation and behaviour towards STEM subjects.
Impacts of digital technologies on equality, inclusion and social integration
Although most of the reviewed studies focused on the impact of ICTs on students’ knowledge, skills, and attitudes, reports were also made on other aspects in the school context, such as equality, inclusion, and social integration. Condie and Munro ( 2007 ) documented research interventions investigating how ICT can support pupils with additional or special educational needs. While those interventions were relatively small scale and mostly based on qualitative data, their findings indicated that the use of ICTs enabled the development of communication, participation, and self-esteem. A recent meta-analysis (Baragash et al., 2022 ) with 119 participants with different disabilities, reported a significant overall effect size of AR on their functional skills acquisition. Koh’s meta-analysis ( 2022 ) also revealed that students with intellectual and developmental disabilities improved their competence and performance when they used digital games in the lessons.
Istenic Starcic and Bagon ( 2014 ) found that the role of ICT in inclusion and the design of pedagogical and technological interventions was not sufficiently explored in educational interventions with people with special needs; however, some benefits of ICT use were found in students’ social integration. The issue of gender and technology use was mentioned in a small number of studies. Zheng et al. ( 2016 ) reported a statistically significant positive interaction between one-to-one laptop programs and gender. Specifically, the results showed that girls and boys alike benefitted from the laptop program, but the effect on girls’ achievement was smaller than that on boys’. Along the same lines, Arztmann et al. ( 2022 ) reported no difference in the impact of game-based learning between boys and girls, arguing that boys and girls equally benefited from game-based interventions in STEM domains. However, results from a systematic review by Cussó-Calabuig et al. ( 2018 ) found limited and low-quality evidence on the effects of intensive use of computers on gender differences in computer anxiety, self-efficacy, and self-confidence. Based on their view, intensive use of computers can reduce gender differences in some areas and not in others, depending on contextual and implementation factors.
Impacts of digital technologies on teachers’ professional and teaching practices
Various research studies have explored the impact of ICT on teachers’ instructional practices and student assessment. Friedel et al. ( 2013 ) found that the use of mobile devices by students enabled teachers to successfully deliver content (e.g., mobile serious games), provide scaffolding, and facilitate synchronous collaborative learning. The integration of digital games in teaching and learning activities also gave teachers the opportunity to study and apply various pedagogical practices (Bado, 2022 ). Specifically, Bado ( 2022 ) found that teachers who implemented instructional activities in three stages (pre-game, game, and post-game) maximized students’ learning outcomes and engagement. For instance, during the pre-game stage, teachers focused on lectures and gameplay training, at the game stage teachers provided scaffolding on content, addressed technical issues, and managed the classroom activities. During the post-game stage, teachers organized activities for debriefing to ensure that the gameplay had indeed enhanced students’ learning outcomes.
Furthermore, ICT can increase efficiency in lesson planning and preparation by offering possibilities for a more collaborative approach among teachers. The sharing of curriculum plans and the analysis of students’ data led to clearer target settings and improvements in reporting to parents (Balanskat et al., 2006 ).
Additionally, the use and application of digital technologies in teaching and learning were found to enhance teachers’ digital competence. Balanskat et al. ( 2006 ) documented studies that revealed that the use of digital technologies in education had a positive effect on teachers’ basic ICT skills. The greatest impact was found on teachers with enough experience in integrating ICTs in their teaching and/or who had recently participated in development courses for the pedagogical use of technologies in teaching. Punie et al. ( 2006 ) reported that the provision of fully equipped multimedia portable computers and the development of online teacher communities had positive impacts on teachers’ confidence and competence in the use of ICTs.
Moreover, online assessment via ICTs benefits instruction. In particular, online assessments support the digitalization of students’ work and related logistics, allow teachers to gather immediate feedback and readjust to new objectives, and support the improvement of the technical quality of tests by providing more accurate results. Additionally, the capabilities of ICTs (e.g., interactive media, simulations) create new potential methods of testing specific skills, such as problem-solving and problem-processing skills, meta-cognitive skills, creativity and communication skills, and the ability to work productively in groups (Punie et al., 2006 ).
Impacts of digital technologies on other school-related aspects and stakeholders
There is evidence that the effective use of ICTs and the data transmission offered by broadband connections help improve administration (Balanskat et al., 2006 ). Specifically, ICTs have been found to provide better management systems to schools that have data gathering procedures in place. Condie and Munro ( 2007 ) reported impacts from the use of ICTs in schools in the following areas: attendance monitoring, assessment records, reporting to parents, financial management, creation of repositories for learning resources, and sharing of information amongst staff. Such data can be used strategically for self-evaluation and monitoring purposes which in turn can result in school improvements. Additionally, they reported that online access to other people with similar roles helped to reduce headteachers’ isolation by offering them opportunities to share insights into the use of ICT in learning and teaching and how it could be used to support school improvement. Furthermore, ICTs provided more efficient and successful examination management procedures, namely less time-consuming reporting processes compared to paper-based examinations and smooth communications between schools and examination authorities through electronic data exchange (Punie et al., 2006 ).
Zheng et al. ( 2016 ) reported that the use of ICTs improved home-school relationships. Additionally, Escueta et al. ( 2017 ) reported several ICT programs that had improved the flow of information from the school to parents. Particularly, they documented that the use of ICTs (learning management systems, emails, dedicated websites, mobile phones) allowed for personalized and customized information exchange between schools and parents, such as attendance records, upcoming class assignments, school events, and students’ grades, which generated positive results on students’ learning outcomes and attainment. Such information exchange between schools and families prompted parents to encourage their children to put more effort into their schoolwork.
The above findings suggest that the impact of ICT integration in schools goes beyond students’ performance in school subjects. Specifically, it affects a number of school-related aspects, such as equality and social integration, professional and teaching practices, and diverse stakeholders. In Table Table2, 2 , we summarize the different impacts of digital technologies on school stakeholders based on the literature review, while in Table Table3 3 we organized the tools/platforms and practices/policies addressed in the meta-analyses, literature reviews, EU reports, and international bodies included in the manuscript.
The impact of digital technologies on schools’ stakeholders based on the literature review
Impacts | References |
---|---|
Students | |
Knowledge, skills, attitudes, and emotions | |
• Learning gains from the use of ICTs across the curriculum | Eng, ; Balanskat et al., ; Liao et al., ; Tamim et al., ; Higgins et al., ; Chauhan, ; Sung et al., ; Schmid et al., ; Tamim et al., ; Zheng et al., ; Haßler et al., ; Kalati & Kim, ; Martinez et al., ; Talan et al., ; Panet al., ; Garzón & Acevedo, ; Garzón et al., ; Villena-Taranilla, et al., ; Coban et al., |
• Positive learning gains from the use of ICTs in specific school subjects (e.g., mathematics, literacy, language, science) | Arztmann et al., ; Villena-Taranilla, et al., ; Chen et al., ; Balanskat et al., ; Grgurović, et al., ; Friedel et al., ; Zheng et al., ; Savva et al., ; Kao, ; Higgins et al., ; Wen & Walters, ; Liao et al., ; Cheung & Slavin, ; Schwabe et al., ; Li & Ma, ; Verschaffel et al., ; Ran et al., ; Liao et al., ; Hillmayr et al., ; Kalemkuş & Kalemkuş, ; Lei et al., ; Condie & Munro, ; Chauhan, ; Bado, ; Wang et al., ; Pan et al., |
• Positive learning gains for special needs students and low-achieving students | Eng, ; Balanskat et al., ; Punie et al., ; Koh, |
• Oportunities to develop a range of skills (e.g., subject-related skills, communication skills, negotiation skills, emotion control skills, organizational skills, critical thinking skills, creativity, metacognitive skills, life, and career skills) | Balanskat et al., ; Fu, ; Tamim et al., ; Zheng et al., ; Higgins et al., ; Verschaffel et al., ; Su & Yang, ; Su et al., ; Lu et al., ; Liu et al., ; Quah & Ng, ; Fielding & Murcia, ; Tang et al., ; Haleem et al., |
• Oportunities to develop digital skills (e.g., information skills, media skills, ICT skills) | Zheng et al., ; Su & Yang, ; Lu et al., ; Su et al., |
• Positive attitudes and behaviours towards ICTs, positive emotions (e.g., increased interest, motivation, attention, engagement, confidence, reduced anxiety, positive achievement emotions, reduction in bullying and cyberbullying) | Balanskat et al., ; Schmid et al., ; Zheng et al., ; Fadda et al., ; Higgins et al., ; Chen et al., ; Lei et al., ; Arztmann et al., ; Su et al., |
Learning experience | |
• Enhance access to resources | Jewitt et al., ; Fu, |
• Opportunities to experience various learning practices (e.g., active learning, learner-centred learning, independent and personalized learning, collaborative learning, self-directed learning, self- and peer-review) | Jewitt et al., ; Fu, |
• Improved access to teacher assessment and feedback | Jewitt et al., |
Equality, inclusion, and social integration | |
• Improved communication, functional skills, participation, self-esteem, and engagement of special needs students | Condie & Munro, ; Baragash et al., ; Koh, |
• Enhanced social interaction for students in general and for students with learning difficulties | Istenic Starcic & Bagon, |
• Benefits for both girls and boys | Zheng et al., ; Arztmann et al., |
Teachers | |
Professional practice | |
• Development of digital competence | Balanskat et al., |
• Positive attitudes and behaviours towards ICTs (e.g., increased confidence) | Punie et al., , |
• Formalized collaborative planning between teachers | Balanskat et al., |
• Improved reporting to parents | Balanskat et al., |
Teaching practice | |
• Efficiency in lesson planning and preparation | Balanskat et al., |
• Facilitate assessment through the provision of immediate feedback | Punie et al., |
• Improvements in the technical quality of tests | Punie et al., |
• New methods of testing specific skills (e.g., problem-solving skills, meta-cognitive skills) | Punie et al., |
• Successful content delivery and lessons | Friedel et al., |
• Application of different instructional practices (e.g., scaffolding, synchronous collaborative learning, online learning, blended learning, hybrid learning) | Friedel et al., ; Bado, ; Kazu & Yalçin, ; Ulum, |
Administrators | |
Data-based decision-making | |
• Improved data-gathering processes | Balanskat et al., |
• Support monitoring and evaluation processes (e.g., attendance monitoring, financial management, assessment records) | Condie & Munro, |
Organizational processes | |
• Access to learning resources via the creation of repositories | Condie & Munro, |
• Information sharing between school staff | Condie & Munro, |
• Smooth communications with external authorities (e.g., examination results) | Punie et al., |
• Efficient and successful examination management procedures | Punie et al., |
Home-school communication | |
• Support reporting to parents | Condie & Munro, |
• Improved flow of communication between the school and parents (e.g., customized and personalized communications) | Escueta et al., |
School leaders | |
Professional practice | |
• Reduced headteacher isolation | Condie & Munro, |
• Improved access to insights about practices for school improvement | Condie & Munro, |
Parents | |
Home-school relationships | |
• Improved home-school relationships | Zheng et al., |
• Increased parental involvement in children’s school life | Escueta et al., |
Tools/platforms and practices/policies addressed in the meta-analyses, literature reviews, EU reports, and international bodies included in the manuscript
Technologies/tools/practices/policies | References |
---|---|
ICT general – various types of technologies | Eng, (review) Moran et al., (meta-analysis) Balanskat et al., (report) Punie et al., (review) Fu, (review) Higgins et al., (report) Chauhan, (meta-analysis) Schmid et al., (meta-analysis) Grgurović et al., (meta-analysis) Higgins et al., (meta-analysis) Wen & Walters, (meta-analysis) Cheung & Slavin, (meta-analysis) Li & Ma, (meta-analysis) Hillmayr et al., (meta-analysis) Verschaffel et al., (systematic review) Ran et al., (meta-analysis) Fielding & Murcia, (systematic review) Tang et al., (review) Haleem et al., (review) Condie & Munro, (review) Underwood, (review) Istenic Starcic & Bagon, (review) Cussó-Calabuig et al., (systematic review) Escueta et al. ( ) (review) Archer et al., (meta-analysis) Lee et al., (meta-analysis) Delgado et al., (review) Di Pietro et al., (report) |
Practices/policies on schools’ digital transformation | Bingimlas, (review) Hardman, (review) Hattie, (synthesis of multiple meta-analysis) Trucano, (book-Knowledge maps) Ređep, (policy study) Conrads et al, (report) European Commission, (EU report) Elkordy & Lovinelli, (book chapter) Eurydice, (EU report) Vuorikari et al., (JRC paper) Sellar, (review) European Commission, (EU report) OECD, (international paper) |
Computer-assisted instruction, computer simulations, activeboards, and web-based learning | Liao et al., (meta-analysis) Tamim et al., (meta-analysis) Çelik, (review) Moran et al., (meta-analysis) Eng, (review) |
Learning platforms (LPs) (virtual learning environments, management information systems, communication technologies and information and resource sharing technologies) | Jewitt et al., (report) |
Mobile devices—touch screens (smart devices, tablets, laptops) | Sung et al., (meta-analysis and research synthesis) Tamim et al., (meta-analysis) Tamim et al., (systematic review and meta-analysis) Zheng et al., (meta-analysis and research synthesis) Haßler et al., (review) Kalati & Kim, (systematic review) Friedel et al., (meta-analysis and review) Chen et al., (meta-analysis) Schwabe et al., (meta-analysis) Punie et al., (review) |
Digital games (various types e.g., adventure, serious; various domains e.g., history, science) | Wang et al., (meta-analysis) Arztmann et al., (meta-analysis) Martinez et al., (systematic review) Talan et al., (meta-analysis) Pan et al., (systematic review) Chen et al., (meta-analysis) Kao, (meta-analysis) Fadda et al., (meta-analysis) Lu et al., (meta-analysis) Lei et al., (meta-analysis) Koh, (meta-analysis) Bado, (review) |
Augmented reality (AR) | Garzón & Acevedo, (meta-analysis) Garzón et al., (meta-analysis and research synthesis) Kalemkuş & Kalemkuş, (meta-analysis) Baragash et al., (meta-analysis) |
Virtual reality (VR) Immersive virtual reality (IVR) | Villena-Taranilla et al., (meta-analysis) Chen et al., (meta-analysis) Coban et al., (meta-analysis) |
Artificial intelligence (AI) and robotics | Su & Yang, (review) Su et al., (meta review) |
Online learning/elearning | Ulum, (meta-analysis) Cheok & Wong, (review) |
Blended learning | Grgurović et al., (meta-analysis) |
Synchronous parallel participation | Friedel et al., (meta-analysis and review) |
Electronic books/digital storytelling | Savva et al., (meta-analysis) Quah & Ng, (systematic review) |
Multimedia technology | Liu et al., (meta-analysis) |
Hybrid learning | Kazu & Yalçin, (meta-analysis) |
Additionally, based on the results of the literature review, there are many types of digital technologies with different affordances (see, for example, studies on VR vs Immersive VR), which evolve over time (e.g. starting from CAIs in 2005 to Augmented and Virtual reality 2020). Furthermore, these technologies are linked to different pedagogies and policy initiatives, which are critical factors in the study of impact. Table Table3 3 summarizes the different tools and practices that have been used to examine the impact of digital technologies on education since 2005 based on the review results.
Factors that affect the integration of digital technologies
Although the analysis of the literature review demonstrated different impacts of the use of digital technology on education, several authors highlighted the importance of various factors, besides the technology itself, that affect this impact. For example, Liao et al. ( 2007 ) suggested that future studies should carefully investigate which factors contribute to positive outcomes by clarifying the exact relationship between computer applications and learning. Additionally, Haßler et al., ( 2016 ) suggested that the neutral findings regarding the impact of tablets on students learning outcomes in some of the studies included in their review should encourage educators, school leaders, and school officials to further investigate the potential of such devices in teaching and learning. Several other researchers suggested that a number of variables play a significant role in the impact of ICTs on students’ learning that could be attributed to the school context, teaching practices and professional development, the curriculum, and learners’ characteristics (Underwood, 2009 ; Tamim et al., 2011 ; Higgins et al., 2012 ; Archer et al., 2014 ; Sung et al., 2016 ; Haßler et al., 2016 ; Chauhan, 2017 ; Lee et al., 2020 ; Tang et al., 2022 ).
Digital competencies
One of the most common challenges reported in studies that utilized digital tools in the classroom was the lack of students’ skills on how to use them. Fu ( 2013 ) found that students’ lack of technical skills is a barrier to the effective use of ICT in the classroom. Tamim et al. ( 2015 ) reported that students faced challenges when using tablets and smart mobile devices, associated with the technical issues or expertise needed for their use and the distracting nature of the devices and highlighted the need for teachers’ professional development. Higgins et al. ( 2012 ) reported that skills training about the use of digital technologies is essential for learners to fully exploit the benefits of instruction.
Delgado et al. ( 2015 ), meanwhile, reported studies that showed a strong positive association between teachers’ computer skills and students’ use of computers. Teachers’ lack of ICT skills and familiarization with technologies can become a constraint to the effective use of technology in the classroom (Balanskat et al., 2006 ; Delgado et al., 2015 ).
It is worth noting that the way teachers are introduced to ICTs affects the impact of digital technologies on education. Previous studies have shown that teachers may avoid using digital technologies due to limited digital skills (Balanskat, 2006 ), or they prefer applying “safe” technologies, namely technologies that their own teachers used and with which they are familiar (Condie & Munro, 2007 ). In this regard, the provision of digital skills training and exposure to new digital tools might encourage teachers to apply various technologies in their lessons (Condie & Munro, 2007 ). Apart from digital competence, technical support in the school setting has also been shown to affect teachers’ use of technology in their classrooms (Delgado et al., 2015 ). Ferrari et al. ( 2011 ) found that while teachers’ use of ICT is high, 75% stated that they needed more institutional support and a shift in the mindset of educational actors to achieve more innovative teaching practices. The provision of support can reduce time and effort as well as cognitive constraints, which could cause limited ICT integration in the school lessons by teachers (Escueta et al., 2017 ).
Teachers’ personal characteristics, training approaches, and professional development
Teachers’ personal characteristics and professional development affect the impact of digital technologies on education. Specifically, Cheok and Wong ( 2015 ) found that teachers’ personal characteristics (e.g., anxiety, self-efficacy) are associated with their satisfaction and engagement with technology. Bingimlas ( 2009 ) reported that lack of confidence, resistance to change, and negative attitudes in using new technologies in teaching are significant determinants of teachers’ levels of engagement in ICT. The same author reported that the provision of technical support, motivation support (e.g., awards, sufficient time for planning), and training on how technologies can benefit teaching and learning can eliminate the above barriers to ICT integration. Archer et al. ( 2014 ) found that comfort levels in using technology are an important predictor of technology integration and argued that it is essential to provide teachers with appropriate training and ongoing support until they are comfortable with using ICTs in the classroom. Hillmayr et al. ( 2020 ) documented that training teachers on ICT had an important effecton students’ learning.
According to Balanskat et al. ( 2006 ), the impact of ICTs on students’ learning is highly dependent on the teachers’ capacity to efficiently exploit their application for pedagogical purposes. Results obtained from the Teaching and Learning International Survey (TALIS) (OECD, 2021 ) revealed that although schools are open to innovative practices and have the capacity to adopt them, only 39% of teachers in the European Union reported that they are well or very well prepared to use digital technologies for teaching. Li and Ma ( 2010 ) and Hardman ( 2019 ) showed that the positive effect of technology on students’ achievement depends on the pedagogical practices used by teachers. Schmid et al. ( 2014 ) reported that learning was best supported when students were engaged in active, meaningful activities with the use of technological tools that provided cognitive support. Tamim et al. ( 2015 ) compared two different pedagogical uses of tablets and found a significant moderate effect when the devices were used in a student-centered context and approach rather than within teacher-led environments. Similarly, Garzón and Acevedo ( 2019 ) and Garzón et al. ( 2020 ) reported that the positive results from the integration of AR applications could be attributed to the existence of different variables which could influence AR interventions (e.g., pedagogical approach, learning environment, and duration of the intervention). Additionally, Garzón et al. ( 2020 ) suggested that the pedagogical resources that teachers used to complement their lectures and the pedagogical approaches they applied were crucial to the effective integration of AR on students’ learning gains. Garzón and Acevedo ( 2019 ) also emphasized that the success of a technology-enhanced intervention is based on both the technology per se and its characteristics and on the pedagogical strategies teachers choose to implement. For instance, their results indicated that the collaborative learning approach had the highest impact on students’ learning gains among other approaches (e.g., inquiry-based learning, situated learning, or project-based learning). Ran et al. ( 2022 ) also found that the use of technology to design collaborative and communicative environments showed the largest moderator effects among the other approaches.
Hattie ( 2008 ) reported that the effective use of computers is associated with training teachers in using computers as a teaching and learning tool. Zheng et al. ( 2016 ) noted that in addition to the strategies teachers adopt in teaching, ongoing professional development is also vital in ensuring the success of technology implementation programs. Sung et al. ( 2016 ) found that research on the use of mobile devices to support learning tends to report that the insufficient preparation of teachers is a major obstacle in implementing effective mobile learning programs in schools. Friedel et al. ( 2013 ) found that providing training and support to teachers increased the positive impact of the interventions on students’ learning gains. Trucano ( 2005 ) argued that positive impacts occur when digital technologies are used to enhance teachers’ existing pedagogical philosophies. Higgins et al. ( 2012 ) found that the types of technologies used and how they are used could also affect students’ learning. The authors suggested that training and professional development of teachers that focuses on the effective pedagogical use of technology to support teaching and learning is an important component of successful instructional approaches (Higgins et al., 2012 ). Archer et al. ( 2014 ) found that studies that reported ICT interventions during which teachers received training and support had moderate positive effects on students’ learning outcomes, which were significantly higher than studies where little or no detail about training and support was mentioned. Fu ( 2013 ) reported that the lack of teachers’ knowledge and skills on the technical and instructional aspects of ICT use in the classroom, in-service training, pedagogy support, technical and financial support, as well as the lack of teachers’ motivation and encouragement to integrate ICT on their teaching were significant barriers to the integration of ICT in education.
School leadership and management
Management and leadership are important cornerstones in the digital transformation process (Pihir et al., 2018 ). Zheng et al. ( 2016 ) documented leadership among the factors positively affecting the successful implementation of technology integration in schools. Strong leadership, strategic planning, and systematic integration of digital technologies are prerequisites for the digital transformation of education systems (Ređep, 2021 ). Management and leadership play a significant role in formulating policies that are translated into practice and ensure that developments in ICT become embedded into the life of the school and in the experiences of staff and pupils (Condie & Munro, 2007 ). Policy support and leadership must include the provision of an overall vision for the use of digital technologies in education, guidance for students and parents, logistical support, as well as teacher training (Conrads et al., 2017 ). Unless there is a commitment throughout the school, with accountability for progress at key points, it is unlikely for ICT integration to be sustained or become part of the culture (Condie & Munro, 2007 ). To achieve this, principals need to adopt and promote a whole-institution strategy and build a strong mutual support system that enables the school’s technological maturity (European Commission, 2019 ). In this context, school culture plays an essential role in shaping the mindsets and beliefs of school actors towards successful technology integration. Condie and Munro ( 2007 ) emphasized the importance of the principal’s enthusiasm and work as a source of inspiration for the school staff and the students to cultivate a culture of innovation and establish sustainable digital change. Specifically, school leaders need to create conditions in which the school staff is empowered to experiment and take risks with technology (Elkordy & Lovinelli, 2020 ).
In order for leaders to achieve the above, it is important to develop capacities for learning and leading, advocating professional learning, and creating support systems and structures (European Commission, 2019 ). Digital technology integration in education systems can be challenging and leadership needs guidance to achieve it. Such guidance can be introduced through the adoption of new methods and techniques in strategic planning for the integration of digital technologies (Ređep, 2021 ). Even though the role of leaders is vital, the relevant training offered to them has so far been inadequate. Specifically, only a third of the education systems in Europe have put in place national strategies that explicitly refer to the training of school principals (European Commission, 2019 , p. 16).
Connectivity, infrastructure, and government and other support
The effective integration of digital technologies across levels of education presupposes the development of infrastructure, the provision of digital content, and the selection of proper resources (Voogt et al., 2013 ). Particularly, a high-quality broadband connection in the school increases the quality and quantity of educational activities. There is evidence that ICT increases and formalizes cooperative planning between teachers and cooperation with managers, which in turn has a positive impact on teaching practices (Balanskat et al., 2006 ). Additionally, ICT resources, including software and hardware, increase the likelihood of teachers integrating technology into the curriculum to enhance their teaching practices (Delgado et al., 2015 ). For example, Zheng et al. ( 2016 ) found that the use of one-on-one laptop programs resulted in positive changes in teaching and learning, which would not have been accomplished without the infrastructure and technical support provided to teachers. Delgado et al. ( 2015 ) reported that limited access to technology (insufficient computers, peripherals, and software) and lack of technical support are important barriers to ICT integration. Access to infrastructure refers not only to the availability of technology in a school but also to the provision of a proper amount and the right types of technology in locations where teachers and students can use them. Effective technical support is a central element of the whole-school strategy for ICT (Underwood, 2009 ). Bingimlas ( 2009 ) reported that lack of technical support in the classroom and whole-school resources (e.g., failing to connect to the Internet, printers not printing, malfunctioning computers, and working on old computers) are significant barriers that discourage the use of ICT by teachers. Moreover, poor quality and inadequate hardware maintenance, and unsuitable educational software may discourage teachers from using ICTs (Balanskat et al., 2006 ; Bingimlas, 2009 ).
Government support can also impact the integration of ICTs in teaching. Specifically, Balanskat et al. ( 2006 ) reported that government interventions and training programs increased teachers’ enthusiasm and positive attitudes towards ICT and led to the routine use of embedded ICT.
Lastly, another important factor affecting digital transformation is the development and quality assurance of digital learning resources. Such resources can be support textbooks and related materials or resources that focus on specific subjects or parts of the curriculum. Policies on the provision of digital learning resources are essential for schools and can be achieved through various actions. For example, some countries are financing web portals that become repositories, enabling teachers to share resources or create their own. Additionally, they may offer e-learning opportunities or other services linked to digital education. In other cases, specific agencies of projects have also been set up to develop digital resources (Eurydice, 2019 ).
Administration and digital data management
The digital transformation of schools involves organizational improvements at the level of internal workflows, communication between the different stakeholders, and potential for collaboration. Vuorikari et al. ( 2020 ) presented evidence that digital technologies supported the automation of administrative practices in schools and reduced the administration’s workload. There is evidence that digital data affects the production of knowledge about schools and has the power to transform how schooling takes place. Specifically, Sellar ( 2015 ) reported that data infrastructure in education is developing due to the demand for “ information about student outcomes, teacher quality, school performance, and adult skills, associated with policy efforts to increase human capital and productivity practices ” (p. 771). In this regard, practices, such as datafication which refers to the “ translation of information about all kinds of things and processes into quantified formats” have become essential for decision-making based on accountability reports about the school’s quality. The data could be turned into deep insights about education or training incorporating ICTs. For example, measuring students’ online engagement with the learning material and drawing meaningful conclusions can allow teachers to improve their educational interventions (Vuorikari et al., 2020 ).
Students’ socioeconomic background and family support
Research show that the active engagement of parents in the school and their support for the school’s work can make a difference to their children’s attitudes towards learning and, as a result, their achievement (Hattie, 2008 ). In recent years, digital technologies have been used for more effective communication between school and family (Escueta et al., 2017 ). The European Commission ( 2020 ) presented data from a Eurostat survey regarding the use of computers by students during the pandemic. The data showed that younger pupils needed additional support and guidance from parents and the challenges were greater for families in which parents had lower levels of education and little to no digital skills.
In this regard, the socio-economic background of the learners and their socio-cultural environment also affect educational achievements (Punie et al., 2006 ). Trucano documented that the use of computers at home positively influenced students’ confidence and resulted in more frequent use at school, compared to students who had no home access (Trucano, 2005 ). In this sense, the socio-economic background affects the access to computers at home (OECD, 2015 ) which in turn influences the experience of ICT, an important factor for school achievement (Punie et al., 2006 ; Underwood, 2009 ). Furthermore, parents from different socio-economic backgrounds may have different abilities and availability to support their children in their learning process (Di Pietro et al., 2020 ).
Schools’ socioeconomic context and emergency situations
The socio-economic context of the school is closely related to a school’s digital transformation. For example, schools in disadvantaged, rural, or deprived areas are likely to lack the digital capacity and infrastructure required to adapt to the use of digital technologies during emergency periods, such as the COVID-19 pandemic (Di Pietro et al., 2020 ). Data collected from school principals confirmed that in several countries, there is a rural/urban divide in connectivity (OECD, 2015 ).
Emergency periods also affect the digitalization of schools. The COVID-19 pandemic led to the closure of schools and forced them to seek appropriate and connective ways to keep working on the curriculum (Di Pietro et al., 2020 ). The sudden large-scale shift to distance and online teaching and learning also presented challenges around quality and equity in education, such as the risk of increased inequalities in learning, digital, and social, as well as teachers facing difficulties coping with this demanding situation (European Commission, 2020 ).
Looking at the findings of the above studies, we can conclude that the impact of digital technologies on education is influenced by various actors and touches many aspects of the school ecosystem. Figure 1 summarizes the factors affecting the digital technologies’ impact on school stakeholders based on the findings from the literature review.

Factors that affect the impact of ICTs on education
The findings revealed that the use of digital technologies in education affects a variety of actors within a school’s ecosystem. First, we observed that as technologies evolve, so does the interest of the research community to apply them to school settings. Figure 2 summarizes the trends identified in current research around the impact of digital technologies on schools’ digital capacity and transformation as found in the present study. Starting as early as 2005, when computers, simulations, and interactive boards were the most commonly applied tools in school interventions (e.g., Eng, 2005 ; Liao et al., 2007 ; Moran et al., 2008 ; Tamim et al., 2011 ), moving towards the use of learning platforms (Jewitt et al., 2011 ), then to the use of mobile devices and digital games (e.g., Tamim et al., 2015 ; Sung et al., 2016 ; Talan et al., 2020 ), as well as e-books (e.g., Savva et al., 2022 ), to the more recent advanced technologies, such as AR and VR applications (e.g., Garzón & Acevedo, 2019 ; Garzón et al., 2020 ; Kalemkuş & Kalemkuş, 2022 ), or robotics and AI (e.g., Su & Yang, 2022 ; Su et al., 2022 ). As this evolution shows, digital technologies are a concept in flux with different affordances and characteristics. Additionally, from an instructional perspective, there has been a growing interest in different modes and models of content delivery such as online, blended, and hybrid modes (e.g., Cheok & Wong, 2015 ; Kazu & Yalçin, 2022 ; Ulum, 2022 ). This is an indication that the value of technologies to support teaching and learning as well as other school-related practices is increasingly recognized by the research and school community. The impact results from the literature review indicate that ICT integration on students’ learning outcomes has effects that are small (Coban et al., 2022 ; Eng, 2005 ; Higgins et al., 2012 ; Schmid et al., 2014 ; Tamim et al., 2015 ; Zheng et al., 2016 ) to moderate (Garzón & Acevedo, 2019 ; Garzón et al., 2020 ; Liao et al., 2007 ; Sung et al., 2016 ; Talan et al., 2020 ; Wen & Walters, 2022 ). That said, a number of recent studies have reported high effect sizes (e.g., Kazu & Yalçin, 2022 ).

Current work and trends in the study of the impact of digital technologies on schools’ digital capacity
Based on these findings, several authors have suggested that the impact of technology on education depends on several variables and not on the technology per se (Tamim et al., 2011 ; Higgins et al., 2012 ; Archer et al., 2014 ; Sung et al., 2016 ; Haßler et al., 2016 ; Chauhan, 2017 ; Lee et al., 2020 ; Lei et al., 2022a ). While the impact of ICTs on student achievement has been thoroughly investigated by researchers, other aspects related to school life that are also affected by ICTs, such as equality, inclusion, and social integration have received less attention. Further analysis of the literature review has revealed a greater investment in ICT interventions to support learning and teaching in the core subjects of literacy and STEM disciplines, especially mathematics, and science. These were the most common subjects studied in the reviewed papers often drawing on national testing results, while studies that investigated other subject areas, such as social studies, were limited (Chauhan, 2017 ; Condie & Munro, 2007 ). As such, research is still lacking impact studies that focus on the effects of ICTs on a range of curriculum subjects.
The qualitative research provided additional information about the impact of digital technologies on education, documenting positive effects and giving more details about implications, recommendations, and future research directions. Specifically, the findings regarding the role of ICTs in supporting learning highlight the importance of teachers’ instructional practice and the learning context in the use of technologies and consequently their impact on instruction (Çelik, 2022 ; Schmid et al., 2014 ; Tamim et al., 2015 ). The review also provided useful insights regarding the various factors that affect the impact of digital technologies on education. These factors are interconnected and play a vital role in the transformation process. Specifically, these factors include a) digital competencies; b) teachers’ personal characteristics and professional development; c) school leadership and management; d) connectivity, infrastructure, and government support; e) administration and data management practices; f) students’ socio-economic background and family support and g) the socioeconomic context of the school and emergency situations. It is worth noting that we observed factors that affect the integration of ICTs in education but may also be affected by it. For example, the frequent use of ICTs and the use of laptops by students for instructional purposes positively affect the development of digital competencies (Zheng et al., 2016 ) and at the same time, the digital competencies affect the use of ICTs (Fu, 2013 ; Higgins et al., 2012 ). As a result, the impact of digital technologies should be explored more as an enabler of desirable and new practices and not merely as a catalyst that improves the output of the education process i.e. namely student attainment.
Conclusions
Digital technologies offer immense potential for fundamental improvement in schools. However, investment in ICT infrastructure and professional development to improve school education are yet to provide fruitful results. Digital transformation is a complex process that requires large-scale transformative changes that presuppose digital capacity and preparedness. To achieve such changes, all actors within the school’s ecosystem need to share a common vision regarding the integration of ICTs in education and work towards achieving this goal. Our literature review, which synthesized quantitative and qualitative data from a list of meta-analyses and review studies, provided useful insights into the impact of ICTs on different school stakeholders and showed that the impact of digital technologies touches upon many different aspects of school life, which are often overlooked when the focus is on student achievement as the final output of education. Furthermore, the concept of digital technologies is a concept in flux as technologies are not only different among them calling for different uses in the educational practice but they also change through time. Additionally, we opened a forum for discussion regarding the factors that affect a school’s digital capacity and transformation. We hope that our study will inform policy, practice, and research and result in a paradigm shift towards more holistic approaches in impact and assessment studies.
Study limitations and future directions
We presented a review of the study of digital technologies' impact on education and factors influencing schools’ digital capacity and transformation. The study results were based on a non-systematic literature review grounded on the acquisition of documentation in specific databases. Future studies should investigate more databases to corroborate and enhance our results. Moreover, search queries could be enhanced with key terms that could provide additional insights about the integration of ICTs in education, such as “policies and strategies for ICT integration in education”. Also, the study drew information from meta-analyses and literature reviews to acquire evidence about the effects of ICT integration in schools. Such evidence was mostly based on the general conclusions of the studies. It is worth mentioning that, we located individual studies which showed different, such as negative or neutral results. Thus, further insights are needed about the impact of ICTs on education and the factors influencing the impact. Furthermore, the nature of the studies included in meta-analyses and reviews is different as they are based on different research methodologies and data gathering processes. For instance, in a meta-analysis, the impact among the studies investigated is measured in a particular way, depending on policy or research targets (e.g., results from national examinations, pre-/post-tests). Meanwhile, in literature reviews, qualitative studies offer additional insights and detail based on self-reports and research opinions on several different aspects and stakeholders who could affect and be affected by ICT integration. As a result, it was challenging to draw causal relationships between so many interrelating variables.
Despite the challenges mentioned above, this study envisaged examining school units as ecosystems that consist of several actors by bringing together several variables from different research epistemologies to provide an understanding of the integration of ICTs. However, the use of other tools and methodologies and models for evaluation of the impact of digital technologies on education could give more detailed data and more accurate results. For instance, self-reflection tools, like SELFIE—developed on the DigCompOrg framework- (Kampylis et al., 2015 ; Bocconi & Lightfoot, 2021 ) can help capture a school’s digital capacity and better assess the impact of ICTs on education. Furthermore, the development of a theory of change could be a good approach for documenting the impact of digital technologies on education. Specifically, theories of change are models used for the evaluation of interventions and their impact; they are developed to describe how interventions will work and give the desired outcomes (Mayne, 2015 ). Theory of change as a methodological approach has also been used by researchers to develop models for evaluation in the field of education (e.g., Aromatario et al., 2019 ; Chapman & Sammons, 2013 ; De Silva et al., 2014 ).
We also propose that future studies aim at similar investigations by applying more holistic approaches for impact assessment that can provide in-depth data about the impact of digital technologies on education. For instance, future studies could focus on different research questions about the technologies that are used during the interventions or the way the implementation takes place (e.g., What methodologies are used for documenting impact? How are experimental studies implemented? How can teachers be taken into account and trained on the technology and its functions? What are the elements of an appropriate and successful implementation? How is the whole intervention designed? On which learning theories is the technology implementation based?).
Future research could also focus on assessing the impact of digital technologies on various other subjects since there is a scarcity of research related to particular subjects, such as geography, history, arts, music, and design and technology. More research should also be done about the impact of ICTs on skills, emotions, and attitudes, and on equality, inclusion, social interaction, and special needs education. There is also a need for more research about the impact of ICTs on administration, management, digitalization, and home-school relationships. Additionally, although new forms of teaching and learning with the use of ICTs (e.g., blended, hybrid, and online learning) have initiated several investigations in mainstream classrooms, only a few studies have measured their impact on students’ learning. Additionally, our review did not document any study about the impact of flipped classrooms on K-12 education. Regarding teaching and learning approaches, it is worth noting that studies referred to STEM or STEAM did not investigate the impact of STEM/STEAM as an interdisciplinary approach to learning but only investigated the impact of ICTs on learning in each domain as a separate subject (science, technology, engineering, arts, mathematics). Hence, we propose future research to also investigate the impact of the STEM/STEAM approach on education. The impact of emerging technologies on education, such as AR, VR, robotics, and AI has also been investigated recently, but more work needs to be done.
Finally, we propose that future studies could focus on the way in which specific factors, e.g., infrastructure and government support, school leadership and management, students’ and teachers’ digital competencies, approaches teachers utilize in the teaching and learning (e.g., blended, online and hybrid learning, flipped classrooms, STEM/STEAM approach, project-based learning, inquiry-based learning), affect the impact of digital technologies on education. We hope that future studies will give detailed insights into the concept of schools’ digital transformation through further investigation of impacts and factors which influence digital capacity and transformation based on the results and the recommendations of the present study.
Acknowledgements
This project has received funding under Grant Agreement No Ref Ares (2021) 339036 7483039 as well as funding from the European Union’s Horizon 2020 Research and Innovation Program under Grant Agreement No 739578 and the Government of the Republic of Cyprus through the Deputy Ministry of Research, Innovation and Digital Policy. The UVa co-authors would like also to acknowledge funding from the European Regional Development Fund and the National Research Agency of the Spanish Ministry of Science and Innovation, under project grant PID2020-112584RB-C32.
Data availability statement
Declarations.
Publisher's note
Springer Nature remains neutral with regard to jurisdictional claims in published maps and institutional affiliations.
- Archer K, Savage R, Sanghera-Sidhu S, Wood E, Gottardo A, Chen V. Examining the effectiveness of technology use in classrooms: A tertiary meta-analysis. Computers & Education. 2014; 78 :140–149. doi: 10.1016/j.compedu.2014.06.001. [ CrossRef ] [ Google Scholar ]
- Aromatario O, Van Hoye A, Vuillemin A, Foucaut AM, Pommier J, Cambon L. Using theory of change to develop an intervention theory for designing and evaluating behavior change SDApps for healthy eating and physical exercise: The OCAPREV theory. BMC Public Health. 2019; 19 (1):1–12. doi: 10.1186/s12889-019-7828-4. [ PMC free article ] [ PubMed ] [ CrossRef ] [ Google Scholar ]
- Arztmann, M., Hornstra, L., Jeuring, J., & Kester, L. (2022). Effects of games in STEM education: A meta-analysis on the moderating role of student background characteristics. Studies in Science Education , 1-37. 10.1080/03057267.2022.2057732
- Bado N. Game-based learning pedagogy: A review of the literature. Interactive Learning Environments. 2022; 30 (5):936–948. doi: 10.1080/10494820.2019.1683587. [ CrossRef ] [ Google Scholar ]
- Balanskat, A. (2009). Study of the impact of technology in primary schools – Synthesis Report. Empirica and European Schoolnet. Retrieved 30 June 2022 from: https://erte.dge.mec.pt/sites/default/files/Recursos/Estudos/synthesis_report_steps_en.pdf
- Balanskat, A. (2006). The ICT Impact Report: A review of studies of ICT impact on schools in Europe, European Schoolnet. Retrieved 30 June 2022 from: https://en.unesco.org/icted/content/ict-impact-report-review-studies-ict-impact-schools-europe
- Balanskat, A., Blamire, R., & Kefala, S. (2006). The ICT impact report. European Schoolnet . Retrieved from: http://colccti.colfinder.org/sites/default/files/ict_impact_report_0.pdf
- Balyer, A., & Öz, Ö. (2018). Academicians’ views on digital transformation in education. International Online Journal of Education and Teaching (IOJET), 5 (4), 809–830. Retrieved 30 June 2022 from http://iojet.org/index.php/IOJET/article/view/441/295
- Baragash RS, Al-Samarraie H, Moody L, Zaqout F. Augmented reality and functional skills acquisition among individuals with special needs: A meta-analysis of group design studies. Journal of Special Education Technology. 2022; 37 (1):74–81. doi: 10.1177/0162643420910413. [ CrossRef ] [ Google Scholar ]
- Bates, A. W. (2015). Teaching in a digital age: Guidelines for designing teaching and learning . Open Educational Resources Collection . 6. Retrieved 30 June 2022 from: https://irl.umsl.edu/oer/6
- Bingimlas KA. Barriers to the successful integration of ICT in teaching and learning environments: A review of the literature. Eurasia Journal of Mathematics, Science and Technology Education. 2009; 5 (3):235–245. doi: 10.12973/ejmste/75275. [ CrossRef ] [ Google Scholar ]
- Blaskó Z, Costa PD, Schnepf SV. Learning losses and educational inequalities in Europe: Mapping the potential consequences of the COVID-19 crisis. Journal of European Social Policy. 2022; 32 (4):361–375. doi: 10.1177/09589287221091687. [ CrossRef ] [ Google Scholar ]
- Bocconi S, Lightfoot M. Scaling up and integrating the selfie tool for schools' digital capacity in education and training systems: Methodology and lessons learnt. European Training Foundation. 2021 doi: 10.2816/907029,JRC123936. [ CrossRef ] [ Google Scholar ]
- Brooks, D. C., & McCormack, M. (2020). Driving Digital Transformation in Higher Education . Retrieved 30 June 2022 from: https://library.educause.edu/-/media/files/library/2020/6/dx2020.pdf?la=en&hash=28FB8C377B59AFB1855C225BBA8E3CFBB0A271DA
- Cachia, R., Chaudron, S., Di Gioia, R., Velicu, A., & Vuorikari, R. (2021). Emergency remote schooling during COVID-19, a closer look at European families. Retrieved 30 June 2022 from https://publications.jrc.ec.europa.eu/repository/handle/JRC125787
- Çelik B. The effects of computer simulations on students’ science process skills: Literature review. Canadian Journal of Educational and Social Studies. 2022; 2 (1):16–28. doi: 10.53103/cjess.v2i1.17. [ CrossRef ] [ Google Scholar ]
- Chapman, C., & Sammons, P. (2013). School Self-Evaluation for School Improvement: What Works and Why? . CfBT Education Trust. 60 Queens Road, Reading, RG1 4BS, England.
- Chauhan S. A meta-analysis of the impact of technology on learning effectiveness of elementary students. Computers & Education. 2017; 105 :14–30. doi: 10.1016/j.compedu.2016.11.005. [ CrossRef ] [ Google Scholar ]
- Chen, Q., Chan, K. L., Guo, S., Chen, M., Lo, C. K. M., & Ip, P. (2022a). Effectiveness of digital health interventions in reducing bullying and cyberbullying: a meta-analysis. Trauma, Violence, & Abuse , 15248380221082090. 10.1177/15248380221082090 [ PubMed ]
- Chen B, Wang Y, Wang L. The effects of virtual reality-assisted language learning: A meta-analysis. Sustainability. 2022; 14 (6):3147. doi: 10.3390/su14063147. [ CrossRef ] [ Google Scholar ]
- Cheok ML, Wong SL. Predictors of e-learning satisfaction in teaching and learning for school teachers: A literature review. International Journal of Instruction. 2015; 8 (1):75–90. doi: 10.12973/iji.2015.816a. [ CrossRef ] [ Google Scholar ]
- Cheung, A. C., & Slavin, R. E. (2011). The Effectiveness of Education Technology for Enhancing Reading Achievement: A Meta-Analysis. Center for Research and reform in Education .
- Coban, M., Bolat, Y. I., & Goksu, I. (2022). The potential of immersive virtual reality to enhance learning: A meta-analysis. Educational Research Review , 100452. 10.1016/j.edurev.2022.100452
- Condie, R., & Munro, R. K. (2007). The impact of ICT in schools-a landscape review. Retrieved 30 June 2022 from: https://oei.org.ar/ibertic/evaluacion/sites/default/files/biblioteca/33_impact_ict_in_schools.pdf
- Conrads, J., Rasmussen, M., Winters, N., Geniet, A., Langer, L., (2017). Digital Education Policies in Europe and Beyond: Key Design Principles for More Effective Policies. Redecker, C., P. Kampylis, M. Bacigalupo, Y. Punie (ed.), EUR 29000 EN, Publications Office of the European Union, Luxembourg, 10.2760/462941
- Costa P, Castaño-Muñoz J, Kampylis P. Capturing schools’ digital capacity: Psychometric analyses of the SELFIE self-reflection tool. Computers & Education. 2021; 162 :104080. doi: 10.1016/j.compedu.2020.104080. [ CrossRef ] [ Google Scholar ]
- Cussó-Calabuig R, Farran XC, Bosch-Capblanch X. Effects of intensive use of computers in secondary school on gender differences in attitudes towards ICT: A systematic review. Education and Information Technologies. 2018; 23 (5):2111–2139. doi: 10.1007/s10639-018-9706-6. [ CrossRef ] [ Google Scholar ]
- Daniel SJ. Education and the COVID-19 pandemic. Prospects. 2020; 49 (1):91–96. doi: 10.1007/s11125-020-09464-3. [ PMC free article ] [ PubMed ] [ CrossRef ] [ Google Scholar ]
- Delcker J, Ifenthaler D. Teachers’ perspective on school development at German vocational schools during the Covid-19 pandemic. Technology, Pedagogy and Education. 2021; 30 (1):125–139. doi: 10.1080/1475939X.2020.1857826. [ CrossRef ] [ Google Scholar ]
- Delgado, A., Wardlow, L., O’Malley, K., & McKnight, K. (2015). Educational technology: A review of the integration, resources, and effectiveness of technology in K-12 classrooms. Journal of Information Technology Education Research , 14, 397. Retrieved 30 June 2022 from http://www.jite.org/documents/Vol14/JITEv14ResearchP397-416Delgado1829.pdf
- De Silva MJ, Breuer E, Lee L, Asher L, Chowdhary N, Lund C, Patel V. Theory of change: A theory-driven approach to enhance the Medical Research Council's framework for complex interventions. Trials. 2014; 15 (1):1–13. doi: 10.1186/1745-6215-15-267. [ PMC free article ] [ PubMed ] [ CrossRef ] [ Google Scholar ]
- Di Pietro G, Biagi F, Costa P, Karpiński Z, Mazza J. The likely impact of COVID-19 on education: Reflections based on the existing literature and recent international datasets. Publications Office of the European Union; 2020. [ Google Scholar ]
- Elkordy A, Lovinelli J. Competencies, Culture, and Change: A Model for Digital Transformation in K12 Educational Contexts. In: Ifenthaler D, Hofhues S, Egloffstein M, Helbig C, editors. Digital Transformation of Learning Organizations. Springer; 2020. pp. 203–219. [ Google Scholar ]
- Eng TS. The impact of ICT on learning: A review of research. International Education Journal. 2005; 6 (5):635–650. [ Google Scholar ]
- European Commission. (2020). Digital Education Action Plan 2021 – 2027. Resetting education and training for the digital age. Retrieved 30 June 2022 from https://ec.europa.eu/education/sites/default/files/document-library-docs/deap-communication-sept2020_en.pdf
- European Commission. (2019). 2 nd survey of schools: ICT in education. Objective 1: Benchmark progress in ICT in schools . Retrieved 30 June 2022 from: https://data.europa.eu/euodp/data/storage/f/2019-03-19T084831/FinalreportObjective1-BenchmarkprogressinICTinschools.pdf
- Eurydice. (2019). Digital Education at School in Europe , Luxembourg: Publications Office of the European Union. Retrieved 30 June 2022 from: https://eacea.ec.europa.eu/national-policies/eurydice/content/digital-education-school-europe_en
- Escueta, M., Quan, V., Nickow, A. J., & Oreopoulos, P. (2017). Education technology: An evidence-based review. Retrieved 30 June 2022 from https://ssrn.com/abstract=3031695
- Fadda D, Pellegrini M, Vivanet G, Zandonella Callegher C. Effects of digital games on student motivation in mathematics: A meta-analysis in K-12. Journal of Computer Assisted Learning. 2022; 38 (1):304–325. doi: 10.1111/jcal.12618. [ CrossRef ] [ Google Scholar ]
- Fernández-Gutiérrez M, Gimenez G, Calero J. Is the use of ICT in education leading to higher student outcomes? Analysis from the Spanish Autonomous Communities. Computers & Education. 2020; 157 :103969. doi: 10.1016/j.compedu.2020.103969. [ CrossRef ] [ Google Scholar ]
- Ferrari, A., Cachia, R., & Punie, Y. (2011). Educational change through technology: A challenge for obligatory schooling in Europe. Lecture Notes in Computer Science , 6964 , 97–110. Retrieved 30 June 2022 https://link.springer.com/content/pdf/10.1007/978-3-642-23985-4.pdf
- Fielding, K., & Murcia, K. (2022). Research linking digital technologies to young children’s creativity: An interpretive framework and systematic review. Issues in Educational Research , 32 (1), 105–125. Retrieved 30 June 2022 from http://www.iier.org.au/iier32/fielding-abs.html
- Friedel, H., Bos, B., Lee, K., & Smith, S. (2013). The impact of mobile handheld digital devices on student learning: A literature review with meta-analysis. In Society for Information Technology & Teacher Education International Conference (pp. 3708–3717). Association for the Advancement of Computing in Education (AACE).
- Fu JS. ICT in education: A critical literature review and its implications. International Journal of Education and Development Using Information and Communication Technology (IJEDICT) 2013; 9 (1):112–125. [ Google Scholar ]
- Gaol FL, Prasolova-Førland E. Special section editorial: The frontiers of augmented and mixed reality in all levels of education. Education and Information Technologies. 2022; 27 (1):611–623. doi: 10.1007/s10639-021-10746-2. [ PMC free article ] [ PubMed ] [ CrossRef ] [ Google Scholar ]
- Garzón J, Acevedo J. Meta-analysis of the impact of Augmented Reality on students’ learning gains. Educational Research Review. 2019; 27 :244–260. doi: 10.1016/j.edurev.2019.04.001. [ CrossRef ] [ Google Scholar ]
- Garzón, J., Baldiris, S., Gutiérrez, J., & Pavón, J. (2020). How do pedagogical approaches affect the impact of augmented reality on education? A meta-analysis and research synthesis. Educational Research Review , 100334. 10.1016/j.edurev.2020.100334
- Grgurović M, Chapelle CA, Shelley MC. A meta-analysis of effectiveness studies on computer technology-supported language learning. ReCALL. 2013; 25 (2):165–198. doi: 10.1017/S0958344013000013. [ CrossRef ] [ Google Scholar ]
- Haßler B, Major L, Hennessy S. Tablet use in schools: A critical review of the evidence for learning outcomes. Journal of Computer Assisted Learning. 2016; 32 (2):139–156. doi: 10.1111/jcal.12123. [ CrossRef ] [ Google Scholar ]
- Haleem A, Javaid M, Qadri MA, Suman R. Understanding the role of digital technologies in education: A review. Sustainable Operations and Computers. 2022; 3 :275–285. doi: 10.1016/j.susoc.2022.05.004. [ CrossRef ] [ Google Scholar ]
- Hardman J. Towards a pedagogical model of teaching with ICTs for mathematics attainment in primary school: A review of studies 2008–2018. Heliyon. 2019; 5 (5):e01726. doi: 10.1016/j.heliyon.2019.e01726. [ PMC free article ] [ PubMed ] [ CrossRef ] [ Google Scholar ]
- Hattie J, Rogers HJ, Swaminathan H. The role of meta-analysis in educational research. In: Reid AD, Hart P, Peters MA, editors. A companion to research in education. Springer; 2014. pp. 197–207. [ Google Scholar ]
- Hattie J. Visible learning: A synthesis of over 800 meta-analyses relating to achievement. Routledge. 2008 doi: 10.4324/9780203887332. [ CrossRef ] [ Google Scholar ]
- Higgins S, Xiao Z, Katsipataki M. The impact of digital technology on learning: A summary for the education endowment foundation. Education Endowment Foundation and Durham University; 2012. [ Google Scholar ]
- Higgins, K., Huscroft-D’Angelo, J., & Crawford, L. (2019). Effects of technology in mathematics on achievement, motivation, and attitude: A meta-analysis. Journal of Educational Computing Research , 57(2), 283-319.
- Hillmayr D, Ziernwald L, Reinhold F, Hofer SI, Reiss KM. The potential of digital tools to enhance mathematics and science learning in secondary schools: A context-specific meta-analysis. Computers & Education. 2020; 153 (1038):97. doi: 10.1016/j.compedu.2020.103897. [ CrossRef ] [ Google Scholar ]
- Istenic Starcic A, Bagon S. ICT-supported learning for inclusion of people with special needs: Review of seven educational technology journals, 1970–2011. British Journal of Educational Technology. 2014; 45 (2):202–230. doi: 10.1111/bjet.12086. [ CrossRef ] [ Google Scholar ]
- Jewitt C, Clark W, Hadjithoma-Garstka C. The use of learning platforms to organise learning in English primary and secondary schools. Learning, Media and Technology. 2011; 36 (4):335–348. doi: 10.1080/17439884.2011.621955. [ CrossRef ] [ Google Scholar ]
- JISC. (2020). What is digital transformation?. Retrieved 30 June 2022 from: https://www.jisc.ac.uk/guides/digital-strategy-framework-for-university-leaders/what-is-digital-transformation
- Kalati, A. T., & Kim, M. S. (2022). What is the effect of touchscreen technology on young children’s learning?: A systematic review. Education and Information Technologies , 1-19. 10.1007/s10639-021-10816-5
- Kalemkuş, J., & Kalemkuş, F. (2022). Effect of the use of augmented reality applications on academic achievement of student in science education: Meta-analysis review. Interactive Learning Environments , 1-18. 10.1080/10494820.2022.2027458
- Kao C-W. The effects of digital game-based learning task in English as a foreign language contexts: A meta-analysis. Education Journal. 2014; 42 (2):113–141. [ Google Scholar ]
- Kampylis P, Punie Y, Devine J. Promoting effective digital-age learning - a European framework for digitally competent educational organisations. JRC Technical Reports. 2015 doi: 10.2791/54070. [ CrossRef ] [ Google Scholar ]
- Kazu IY, Yalçin CK. Investigation of the effectiveness of hybrid learning on academic achievement: A meta-analysis study. International Journal of Progressive Education. 2022; 18 (1):249–265. doi: 10.29329/ijpe.2022.426.14. [ CrossRef ] [ Google Scholar ]
- Koh C. A qualitative meta-analysis on the use of serious games to support learners with intellectual and developmental disabilities: What we know, what we need to know and what we can do. International Journal of Disability, Development and Education. 2022; 69 (3):919–950. doi: 10.1080/1034912X.2020.1746245. [ CrossRef ] [ Google Scholar ]
- König J, Jäger-Biela DJ, Glutsch N. Adapting to online teaching during COVID-19 school closure: Teacher education and teacher competence effects among early career teachers in Germany. European Journal of Teacher Education. 2020; 43 (4):608–622. doi: 10.1080/02619768.2020.1809650. [ CrossRef ] [ Google Scholar ]
- Lawrence JE, Tar UA. Factors that influence teachers’ adoption and integration of ICT in teaching/learning process. Educational Media International. 2018; 55 (1):79–105. doi: 10.1080/09523987.2018.1439712. [ CrossRef ] [ Google Scholar ]
- Lee, S., Kuo, L. J., Xu, Z., & Hu, X. (2020). The effects of technology-integrated classroom instruction on K-12 English language learners’ literacy development: A meta-analysis. Computer Assisted Language Learning , 1-32. 10.1080/09588221.2020.1774612
- Lei, H., Chiu, M. M., Wang, D., Wang, C., & Xie, T. (2022a). Effects of game-based learning on students’ achievement in science: a meta-analysis. Journal of Educational Computing Research . 10.1177/07356331211064543
- Lei H, Wang C, Chiu MM, Chen S. Do educational games affect students' achievement emotions? Evidence from a meta-analysis. Journal of Computer Assisted Learning. 2022; 38 (4):946–959. doi: 10.1111/jcal.12664. [ CrossRef ] [ Google Scholar ]
- Liao YKC, Chang HW, Chen YW. Effects of computer application on elementary school student's achievement: A meta-analysis of students in Taiwan. Computers in the Schools. 2007; 24 (3–4):43–64. doi: 10.1300/J025v24n03_04. [ CrossRef ] [ Google Scholar ]
- Li Q, Ma X. A meta-analysis of the effects of computer technology on school students’ mathematics learning. Educational Psychology Review. 2010; 22 (3):215–243. doi: 10.1007/s10648-010-9125-8. [ CrossRef ] [ Google Scholar ]
- Liu, M., Pang, W., Guo, J., & Zhang, Y. (2022). A meta-analysis of the effect of multimedia technology on creative performance. Education and Information Technologies , 1-28. 10.1007/s10639-022-10981-1
- Lu Z, Chiu MM, Cui Y, Mao W, Lei H. Effects of game-based learning on students’ computational thinking: A meta-analysis. Journal of Educational Computing Research. 2022 doi: 10.1177/07356331221100740. [ CrossRef ] [ Google Scholar ]
- Martinez L, Gimenes M, Lambert E. Entertainment video games for academic learning: A systematic review. Journal of Educational Computing Research. 2022 doi: 10.1177/07356331211053848. [ CrossRef ] [ Google Scholar ]
- Mayne J. Useful theory of change models. Canadian Journal of Program Evaluation. 2015; 30 (2):119–142. doi: 10.3138/cjpe.230. [ CrossRef ] [ Google Scholar ]
- Moran J, Ferdig RE, Pearson PD, Wardrop J, Blomeyer RL., Jr Technology and reading performance in the middle-school grades: A meta-analysis with recommendations for policy and practice. Journal of Literacy Research. 2008; 40 (1):6–58. doi: 10.1080/10862960802070483. [ CrossRef ] [ Google Scholar ]
- OECD. (2015). Students, Computers and Learning: Making the Connection . PISA, OECD Publishing, Paris. Retrieved from: 10.1787/9789264239555-en
- OECD. (2021). OECD Digital Education Outlook 2021: Pushing the Frontiers with Artificial Intelligence, Blockchain and Robots. Retrieved from: https://www.oecd-ilibrary.org/education/oecd-digital-education-outlook-2021_589b283f-en
- Pan Y, Ke F, Xu X. A systematic review of the role of learning games in fostering mathematics education in K-12 settings. Educational Research Review. 2022; 36 :100448. doi: 10.1016/j.edurev.2022.100448. [ CrossRef ] [ Google Scholar ]
- Pettersson F. Understanding digitalization and educational change in school by means of activity theory and the levels of learning concept. Education and Information Technologies. 2021; 26 (1):187–204. doi: 10.1007/s10639-020-10239-8. [ CrossRef ] [ Google Scholar ]
- Pihir, I., Tomičić-Pupek, K., & Furjan, M. T. (2018). Digital transformation insights and trends. In Central European Conference on Information and Intelligent Systems (pp. 141–149). Faculty of Organization and Informatics Varazdin. Retrieved 30 June 2022 from https://www.proquest.com/conference-papers-proceedings/digital-transformation-insights-trends/docview/2125639934/se-2
- Punie, Y., Zinnbauer, D., & Cabrera, M. (2006). A review of the impact of ICT on learning. Working Paper prepared for DG EAC. Retrieved 30 June 2022 from: http://www.eurosfaire.prd.fr/7pc/doc/1224678677_jrc47246n.pdf
- Quah CY, Ng KH. A systematic literature review on digital storytelling authoring tool in education: January 2010 to January 2020. International Journal of Human-Computer Interaction. 2022; 38 (9):851–867. doi: 10.1080/10447318.2021.1972608. [ CrossRef ] [ Google Scholar ]
- Ran H, Kim NJ, Secada WG. A meta-analysis on the effects of technology's functions and roles on students' mathematics achievement in K-12 classrooms. Journal of computer assisted learning. 2022; 38 (1):258–284. doi: 10.1111/jcal.12611. [ CrossRef ] [ Google Scholar ]
- Ređep, N. B. (2021). Comparative overview of the digital preparedness of education systems in selected CEE countries. Center for Policy Studies. CEU Democracy Institute .
- Rott, B., & Marouane, C. (2018). Digitalization in schools–organization, collaboration and communication. In Digital Marketplaces Unleashed (pp. 113–124). Springer, Berlin, Heidelberg.
- Savva M, Higgins S, Beckmann N. Meta-analysis examining the effects of electronic storybooks on language and literacy outcomes for children in grades Pre-K to grade 2. Journal of Computer Assisted Learning. 2022; 38 (2):526–564. doi: 10.1111/jcal.12623. [ CrossRef ] [ Google Scholar ]
- Schmid RF, Bernard RM, Borokhovski E, Tamim RM, Abrami PC, Surkes MA, Wade CA, Woods J. The effects of technology use in postsecondary education: A meta-analysis of classroom applications. Computers & Education. 2014; 72 :271–291. doi: 10.1016/j.compedu.2013.11.002. [ CrossRef ] [ Google Scholar ]
- Schuele CM, Justice LM. The importance of effect sizes in the interpretation of research: Primer on research: Part 3. The ASHA Leader. 2006; 11 (10):14–27. doi: 10.1044/leader.FTR4.11102006.14. [ CrossRef ] [ Google Scholar ]
- Schwabe, A., Lind, F., Kosch, L., & Boomgaarden, H. G. (2022). No negative effects of reading on screen on comprehension of narrative texts compared to print: A meta-analysis. Media Psychology , 1-18. 10.1080/15213269.2022.2070216
- Sellar S. Data infrastructure: a review of expanding accountability systems and large-scale assessments in education. Discourse: Studies in the Cultural Politics of Education. 2015; 36 (5):765–777. doi: 10.1080/01596306.2014.931117. [ CrossRef ] [ Google Scholar ]
- Stock WA. Systematic coding for research synthesis. In: Cooper H, Hedges LV, editors. The handbook of research synthesis, 236. Russel Sage; 1994. pp. 125–138. [ Google Scholar ]
- Su, J., Zhong, Y., & Ng, D. T. K. (2022). A meta-review of literature on educational approaches for teaching AI at the K-12 levels in the Asia-Pacific region. Computers and Education: Artificial Intelligence , 100065. 10.1016/j.caeai.2022.100065
- Su J, Yang W. Artificial intelligence in early childhood education: A scoping review. Computers and Education: Artificial Intelligence. 2022; 3 :100049. doi: 10.1016/j.caeai.2022.100049. [ CrossRef ] [ Google Scholar ]
- Sung YT, Chang KE, Liu TC. The effects of integrating mobile devices with teaching and learning on students' learning performance: A meta-analysis and research synthesis. Computers & Education. 2016; 94 :252–275. doi: 10.1016/j.compedu.2015.11.008. [ CrossRef ] [ Google Scholar ]
- Talan T, Doğan Y, Batdı V. Efficiency of digital and non-digital educational games: A comparative meta-analysis and a meta-thematic analysis. Journal of Research on Technology in Education. 2020; 52 (4):474–514. doi: 10.1080/15391523.2020.1743798. [ CrossRef ] [ Google Scholar ]
- Tamim, R. M., Bernard, R. M., Borokhovski, E., Abrami, P. C., & Schmid, R. F. (2011). What forty years of research says about the impact of technology on learning: A second-order meta-analysis and validation study. Review of Educational research, 81 (1), 4–28. Retrieved 30 June 2022 from 10.3102/0034654310393361
- Tamim, R. M., Borokhovski, E., Pickup, D., Bernard, R. M., & El Saadi, L. (2015). Tablets for teaching and learning: A systematic review and meta-analysis. Commonwealth of Learning. Retrieved from: http://oasis.col.org/bitstream/handle/11599/1012/2015_Tamim-et-al_Tablets-for-Teaching-and-Learning.pdf
- Tang C, Mao S, Xing Z, Naumann S. Improving student creativity through digital technology products: A literature review. Thinking Skills and Creativity. 2022; 44 :101032. doi: 10.1016/j.tsc.2022.101032. [ CrossRef ] [ Google Scholar ]
- Tolani-Brown, N., McCormac, M., & Zimmermann, R. (2011). An analysis of the research and impact of ICT in education in developing country contexts. In ICTs and sustainable solutions for the digital divide: Theory and perspectives (pp. 218–242). IGI Global.
- Trucano, M. (2005). Knowledge Maps: ICTs in Education. Washington, DC: info Dev / World Bank. Retrieved 30 June 2022 from https://files.eric.ed.gov/fulltext/ED496513.pdf
- Ulum H. The effects of online education on academic success: A meta-analysis study. Education and Information Technologies. 2022; 27 (1):429–450. doi: 10.1007/s10639-021-10740-8. [ PMC free article ] [ PubMed ] [ CrossRef ] [ Google Scholar ]
- Underwood, J. D. (2009). The impact of digital technology: A review of the evidence of the impact of digital technologies on formal education. Retrieved 30 June 2022 from: http://dera.ioe.ac.uk/id/eprint/10491
- Verschaffel, L., Depaepe, F., & Mevarech, Z. (2019). Learning Mathematics in metacognitively oriented ICT-Based learning environments: A systematic review of the literature. Education Research International , 2019 . 10.1155/2019/3402035
- Villena-Taranilla R, Tirado-Olivares S, Cózar-Gutiérrez R, González-Calero JA. Effects of virtual reality on learning outcomes in K-6 education: A meta-analysis. Educational Research Review. 2022; 35 :100434. doi: 10.1016/j.edurev.2022.100434. [ CrossRef ] [ Google Scholar ]
- Voogt J, Knezek G, Cox M, Knezek D, ten Brummelhuis A. Under which conditions does ICT have a positive effect on teaching and learning? A call to action. Journal of Computer Assisted Learning. 2013; 29 (1):4–14. doi: 10.1111/j.1365-2729.2011.00453.x. [ CrossRef ] [ Google Scholar ]
- Vuorikari, R., Punie, Y., & Cabrera, M. (2020). Emerging technologies and the teaching profession: Ethical and pedagogical considerations based on near-future scenarios (No. JRC120183). Joint Research Centre. Retrieved 30 June 2022 from: https://publications.jrc.ec.europa.eu/repository/handle/JRC120183
- Wang LH, Chen B, Hwang GJ, Guan JQ, Wang YQ. Effects of digital game-based STEM education on students’ learning achievement: A meta-analysis. International Journal of STEM Education. 2022; 9 (1):1–13. doi: 10.1186/s40594-022-00344-0. [ CrossRef ] [ Google Scholar ]
- Wen X, Walters SM. The impact of technology on students’ writing performances in elementary classrooms: A meta-analysis. Computers and Education Open. 2022; 3 :100082. doi: 10.1016/j.caeo.2022.100082. [ CrossRef ] [ Google Scholar ]
- Zheng B, Warschauer M, Lin CH, Chang C. Learning in one-to-one laptop environments: A meta-analysis and research synthesis. Review of Educational Research. 2016; 86 (4):1052–1084. doi: 10.3102/0034654316628645. [ CrossRef ] [ Google Scholar ]
- Open access
- Published: 06 February 2023
A critical evaluation, challenges, and future perspectives of using artificial intelligence and emerging technologies in smart classrooms
- Eleni Dimitriadou ORCID: orcid.org/0000-0001-9257-0999 1 , 2 &
- Andreas Lanitis 1 , 2
Smart Learning Environments volume 10 , Article number: 12 ( 2023 ) Cite this article
22k Accesses
41 Citations
4 Altmetric
Metrics details
The term "Smart Classroom" has evolved over time and nowadays reflects the technological advancements incorporated in educational spaces. The rapid advances in technology, and the need to create more efficient and creative classes that support both in-class and remote activities, have led to the integration of Artificial Intelligence and smart technologies in smart classes. In this paper we discuss the concept of Artificial Intelligence in Education and present a literature review related to smart classroom technology, with an emphasis on emerging technologies such as AI-related technologies. As part of this survey key technologies related to smart classes used for effective class management that enhance the convenience of classroom environments, the use of different types of smart teaching aids during the educational process and the use of automated performance assessment technologies are presented. Apart from discussing a variety of technological accomplishments in each of the aforementioned areas, the role of AI is discussed, allowing the readers to comprehend the importance of AI in key technologies related to smart classes. Furthermore, through a SWOT analysis, the Strengths, Weaknesses, Opportunities, and Threats of adopting AI in smart classes are presented, while the future perspectives and challenges in utilizing AI-based techniques in smart classes are discussed. This survey targets educators and AI professionals so that the former get informed about the potential, and limitations of AI in education, while the latter can get inspiration from the challenges and peculiarities of educational AI-based systems.
Introduction
The term “S.M.A.R.T” Classroom stands for Showing, Manageable, Accessible, Real-time Interactive, and Testing (Huang et al., 2019 ), and refers to a setting where the physical space is infused with carefully constructed digital tools and resources to encourage student connection on various social levels, enhance face-to-face interaction in real-time, and record the collective knowledge of the entire class (Lui & Slotta, 2014 ). A smart classroom is defined as a combination of several high-end technologies that aim to assist educators and students in optimising their overall leaning experience (Micrea et al., 2021 ). A Smart classroom combines school education and technology (Li et al., 2019 ) such as mobile technologies, automatic communication and learning tools, video projectors, cameras, sensors, facial recognition software, and other modules that keep track of a variety of environmental factors (Mircea et al., 2021 ). The role of teachers in the smart classroom is to enhance students' performance, creative and thinking skills (Palanisamy et al., 2020 ) while also using new teaching methodologies such as social learning, mobile learning, ubiquitous learning (Chen et al., 2016 ). Although a smart class combines technology with other elements, such as teaching strategies and classroom models, in this paper we focus our attention on the technological dimension of a smart class.
The introduction of Artificial Intelligence (AI) combined with emerging technologies having the form of interactive, remote, and mobile computing in physical and/ or virtual environments constitutes an evident trend in the development of the concept of smart classroom. Most of the technologies employed in a smart class rely on Artificial Intelligence (AI) that empowers the interactive, adaptive, and smart usage of those technologies during the learning process. In the work presented in this paper, a smart classroom is defined as physical or remote space which integrates emerging technologies (Have et al., 2021 ) and AI to provide an enhanced learning experience (see Fig. 1 ).
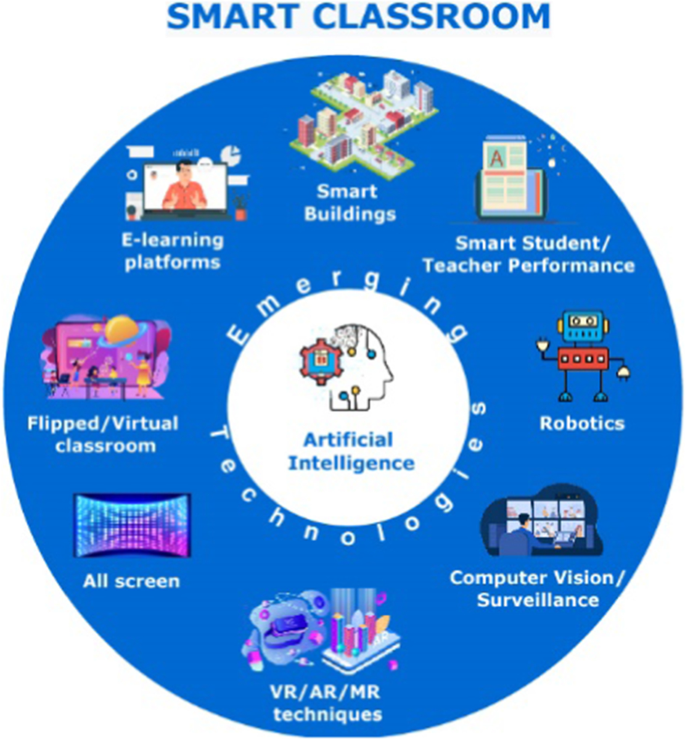
The main technologies encountered in a smart classroom
The term “ Artificial Intelligence ” (AI) was first mentioned by John McCarthyin in 1956 and refers to the ability of computer systems to undertake human tasks (like learning and thinking) that frequently can only be attained through human intelligence (Sadiku et al., 2021 ). Since the 1970s, the specific field of Artificial Intelligence in Education (AIED) has begun to influence the application of technology to instruction and learning, to improve the learning process, and promote student achievements (Southgate et al., 2019 ). The aim of AIED is to establish AI-powered systems such as virtual pedagogical agents, AI robots and intelligent systems which allow flexible, engaging and personalised learning as well as to automate daily tasks of teaching (e.g. feedback and assessment) (AlFarsi et al., 2021 ). The last few years, the topic of AI has been empowered by the groundbreaking technology of deep learning (Sejnowski, 2020 ) that allowed the successful application of AI to several complex machine learning tasks.
Several surveys related to smart classes appear in the literature. Saini and Goel ( 2019 ) focus on technologies related to smart content preparation and distribution, smart student engagement, smart assessment, and smart physical environment. For each pillar Saini and Goel ( 2019 ) provide a review of different technologies and techniques used in a smart classroom and provide recommendations for future research directions. While this survey has some similarities to our approach towards the presentation of concepts related to smart classes, in our case we focus our attention on the use of emerging technologies in conjunction with artificial intelligence in smart classes. Furthermore, when compared to the article by Saini and Goel ( 2019 ), a wider range of smart technologies are presented. Chen et al. ( 2020 ) focus their attention on the use of AI in education. They state that ΑΙ has been extensively used in education in different forms such as computer programs, humanoid robots, web-based chatbots, and online platforms. Despite the wide range of technologies presented by Chen et al. ( 2020 ), this survey also includes an extended range of educational technologies coupled with a comprehensive discussion of the advantages and disadvantages of AI in education, that also includes a SWOT analysis. Chen et al. ( 2022 ) indicate the usefulness of AI in education, which may be used in the form of intelligent tutoring systems for special education, natural language processing, educational robots, performance prediction, discourse analysis, teaching evaluation, learner emotion detection and personalized learning. Their survey is mainly focused on presenting statistical figures related to the use of AI in education, such as the size of research community per subject, and the most frequent AI terms in literature. More recently, Dimitriadou and Lanitis ( 2022a ) presented a short survey on the topic of the use of AI in smart classes. When compared to this work the current paper provides a more extensive coverage of several key technologies related to smart classes, and in addition it provides a comprehensive discussion of advantages and limitations of using AI in smart classes.
In relation to previous surveys reported in the literature, the main contributions of this survey include the review of the latest technologies and discussion of future directions that could support the creation of a next-generation smart classroom, and the understanding of the use of AI in connection to the technologies used in a smart classroom, allowing the readers to get acquainted with the potential of using AI in smart classes, and the main advantages, disadvantages and potential dangers of using this technology. In the remainder of the survey, we present a literature review for studies related to smart classes and AI in education, and present key smart class technologies related to classroom management, teaching aids, and performance assessment. In Sect. " Impact of smart classroom ", we describe the advantages, disadvantages of key technologies related to smart classes. In Sect. " Discussion-future directions ", we elaborate on the role of AI in smart classes, followed by a discussion and possible future research directions.
Key technologies related to smart classes
In this section the key technologies related to smart classes are presented while emphasis is given to the role of AI in the technologies described. The main topics presented are separated in three main categories that refer to class management technologies, teaching aids and performance assessment technologies (see Table 1 ).
Classroom management
The term classroom management refers to the way or approach that a teacher uses to control / manage his / her classroom. Within this scope the management aims to maintain a comfortable and safe teaching environment that contributes to the efficient class delivery. In relation to class management technologies, in this survey we focus on the issues of Computer Vision-based surveillance/security and Smart Environment.
Computer vision-based surveillance
Computer Vision techniques in smart classroom are often used for the tasks of Attendance Recognition or Action (Behaviours) Recognition.
Attendance recognition
In an attempt to reduce the time need for keeping student attendance records, the process of attendance recognition is often automated based on facial identification technologies (Kawaguchi et al. 2005 ). For example Chowdhury et al ( 2020 ), use a suggested a Convolutional Neural networks (CNN’s) based facial identification system to identify students in a real-time video stream captured by a static camera. Several variations to this technique involve systems that can utilize images captured by moving cameras (Mery et al., 2019 ), systems that utilize cameras fixed in the entrance of a class (Chintalapati & Raghunadh, 2013 ), or systems that utilize rotating cameras (Gupta et al., 2018 ).
Action (behaviour) recognition
Human action recognition is a vision-based technique that can identify a complete action performed by a human in a video sequence (Kong & Fu, 2022 ). The ability to recognize human behavior can be extremely important inside a smart classroom (Wang, 2021 ) as it enables the recognition of student behavior and emotions allowing the detection of uncomfortable situations for students, such as high anxiety or reduced concertation levels. Smart classrooms with developed AI-powered surveillance system can detect students who are not paying attention in class and alert the teacher (Parambil et al., 2022 ). In addition, action recognition systems can analyse students’ behaviour during the course and estimate their engagement (Thomas & Jayagopi, 2017 ). Furthermore, automated action recognition also helps students with special needs by monitoring them and warning teachers for potential episodes, for example in case they have an epilepsy episode (Lau et al., 2014 ).
Recently, automated methods for the behaviour analysis of the students and their engagement estimation are widely utilized in a classroom. Ngoc Anh et al. ( 2019 ), presented a system to monitor the behaviour of students in the classroom. Similarly, Thomas and Jayagopi ( 2017 ), used a machine learning algorithm to analyze the students’ engagement in a classroom by analyzing students’ head position, eye gaze direction and facial expressions. Furthermore, Yang and Chen ( 2011 ), presented an automatic smart class system which was focused on eye and face detection to determine if the students were active or not.
Previous studies related to recognizing students’ actions in smart classroom include the work of Li et al. ( 2019 ) who proposed a new spontaneous actions database that show 15 different student actions. The smart classroom, in this study, included round-tables for students and four cameras, which were fixed on the wall (front and back of classroom), to record the students’ actions from various viewpoints. Recently, Dimitriadou and Lanitis ( 2022a , 2022b ) proposed an action recognition system that recognizes seven actions performed by students attending online courses, which are recognized using CNN architectures. In this case both the images captured and the action recognition process is performed on the personal computer of each student, allowing in that way the application of this method to remote teaching activities.
Ashwin and Guddeti ( 2020 ), demonstrated a Hybrid Convolutional Neural Network to analyze students’ body postures, gestures and facial expressions to investigate engagement. Three states of student’s engagement were examined: boredom, engaged and neutral. Rashmi et al. ( 2021 ), proposed an automatic system, that monitors the students’ activities, such as the actions of sleeping, eating, using phone, discussion and being engage. The aim of this study is to localize and recognize multiple actions of the students in an image frame.
Similar technologies can be used for recognizing student actions in school yards. For example, suspicious actions, such as student fights, drug delivery, bullying incidents could be detected automatically allowing the prevention of mental and physical health injuries of students. Ye et al. ( 2018 ) suggested a strategy to identify occurrences of abuse in the environment of school utilizing motion and audio sensors to evaluate activities and verbal expression. Gutierrez and Troyer ( 2014 ) describe a simulator named SimBully to illustrate the impact of public belief and attitudes on abuse occurrences by classmates. Ali et al. ( 2020 ) use the YOLOv3 network to recognize student behaviours such as calling, napping, or reading a book indoors or outdoors with the goal to discover any undesirable behaviours.
- Smart environment
Smart buildings are described as whole structures that use existing technology resources and AI to produce a secure, functioning and friendly setting that utilizes resources wisely and economically (Dryjanski et al., 2020 ). Sensor technology (Abbasy & Quesada, 2017 ), the Internet of things (IoT) (Abdel-Basset et al., 2019 ), external telecommunications and smartphone software technology are commonly used to power advanced technologies in smart classrooms (Wu et al., 2020 ).
AI plays an important role in the creation and implementation of computer applications in order to successfully manage the administrative issues of a school and assist workers in their everyday tasks. Data collected instantly from sensors and cameras can be utilized for the surveillance of students and in collaboration with AI create a safer environment for students. The use of AI in smart environments and smart classes can increase efficiency and result in high performance of students and teachers as well (Augusto et al., 2009 ).
Teaching aids
In a modern smart-classroom, the teaching process is assisted by a plethora of technological means, to maximize the engagement and interaction of students. In this section we provide an overview of these technologies.
Educational Robots can be ‘Real’ devices (Shiomi et al., 2015 ; Weibel et al., 2020 ), or they can be software agents in the form of chatbots (Kollia & Siolas, 2016 ; Pereira, 2016 ).
‘Real’ robots
A real robot is a device that can perform actions usually undertaken by humans. Since the introduction of the first robot back in 1980 (Johal et al., 2018 ) several educational animal-like or human-like robots have been presented to suit different levels of education. Educational robots constitute a subgroup of educational technology, as they are employed to make learning easier, enhance the educational performance of students (Mubin et al., 2013 ) and assist the students in their active participation in the process of problem solving. The main driving force in introducing robots in the learning process is for creating systems that offer more social interaction and support learning (Timms, 2016 ). Examples of educational robots used over the years are summarized in Table 2 .
Initially, robots were constructed to perform repetitive tasks, without any AI. However, the importance of having intelligent machines that may perform advanced tasks eventually led to the use of a series of sensors that provide information about the environment along with the integration of AI for processing and making decisions based on the information received by the sensors (Brady et al., 2012 ; West, 2018 ). Typical sensors used in robotics include microphones, Time-of-flight (ToF) optic sensors and motion detectors (Ben-Ari & Mondada, 2018 ) used in conjunction with AI algorithms for sensing an environment (Poppinga & Laue, 2019 ). In addition, ToF cameras on robots can be enhanced by utilizing CCD cameras, and infrared depth cameras. All of the data received by sensors is usually used to train neural network models and educate robots to execute all of their duties, from comprehending a user to effectively reacting (Vega & Cañas, 2019 ). AI-based functionality incorporated in robots include speech recognition, motion control, computer vision, natural language processing, smart agent technology, movement control, and control for grasping objects.
Students accept and form relationships with robots far more effortlessly because of their interaction, which has been shown to improve psychosocial and physical development (Feil-Seifer & Matarić, 2009 ), as well as their capacity to interact, which improves the process of learning, makes it more exciting and help learners acquire more knowledge (Han et al., 2005 ). Social robots, specifically, have been successful in assisting kids with autism in comprehending concepts such as boundaries between individuals and emotional intimacy and in improving independent learning skills (Woo et al., 2021 ). Robots can become familiar with the personal needs of each student and respond accordingly (Jones & Castellano, 2018 ). Another important feature of robots is their ability to record students’ expressions and mood changes. Robots not only assist students during their courses, but they are also in advance evaluating their behavior and any emotional disturbances that may suggest despair or stress (Werner-Seidler, 2017 ). For example, Researchers at MIT have developed a robot called "Teacher bot" that can detect and respond to student emotions to provide personalized feedback and support (Bourguet et al., 2020 ).
While educational robotics can be extremely useful within a class environment, their use can also extend to teaching activities for students who cannot be physically present be in class supporting in that way remote teaching activities. For example, children that are obliged to stay home or being treated at hospitals may face serious consequences regarding their social development. Thus, missing out long periods of school and social interactions with their peers, due to factors that are beyond the control of children, may result to social isolation and feeling lonely (Helms et al., 2016 ). To combat the above negative situation, robots may ensure that no classes and time with friends are missed, by enabling children have a continuous connection with their teacher and peers (Soares et al., 2017 ).”
The notion chatbot is a combination of two words: “chat” demonstrating conversation and “bot” standing for robot (Chocarro et al., 2021 ). Chatbots simulate conversations with human users via the use of instant messaging services. Chatbots, demonstrate high potential as a learning teaching tool for remote students and can offer personal assistance, educational content support (Colace et al., 2018 ), while they can be used as tutors accompanying the process of learning (Chocarro et al., 2021 ). Examples of educational chatbots used over the years are summarized in Table 3 .
The operation of chatbots is a mix of artificial intelligence and Natural Language Processing (NLP). Natural Language Processing is a branch of artificial intelligence concerned with computers' capacity to grasp written and auditory speech in the same way that humans do (Chowdhury et al., 2003 ). NLP is consisted of three primary elements strongly correlated with AI, speech recognition and speech generation. All three topics are based on AI approaches, like GAN deep neural networks, in order to enhance the quality of the generated speech (Hsu et al., 2019 ) and reasoning, that helps bots make predictions and draw conclusions with the aim to respond appropriately in every interaction with a human. Voice interactive interfaces arose primarily as a result of breakthroughs in computer and speech recognition technology (Guttormsen et al., 2011 ). For example the Amazon Echo is an instance of a voice-interaction-based technology (Teja, 2020 ). It uses DNN to process any given dataset and translate any language for example and Recurrent Neural Networks (RNN) as a controller.
Virtual/augmented/mixed reality
Smart classrooms often incorporate virtual, augmented, and mixed reality as a means of introducing immersive learning experiences. Virtual Reality (VR) concerns the 3D simulation of an imaginary or real environment, that the user can visualize, explore and interact with it (Górski et al., 2016 ). On the other hand, Augmented Reality (AR) offers an interactive experience to users, by adding virtual information to the physical environment of the students and enabling them to use their whole body as a means of interacting with both virtual and real content (Billinghurst et al., 2015 ). Mixed Reality (MR) refers to a blending of real-world and virtual/digital world objects which are visualized together on only one display in a coherent space (Kasapakis et al., 2019 ) (see Fig. 2 ).
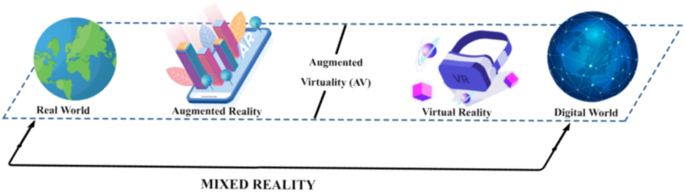
The differences between virtual, augmented and mixed reality
The last few years, the idea of metaverse has risen and is expected to be part of our reality the next few decades. The concept of the metaverse has been around since the 1990s and has gained renewed attention in recent years due to the increasing prevalence of virtual and augmented reality technologies. Metaverse is a parallel digital universe which allows multiple users to emerge into environments that combine both the physical and digital world (Mystakides et al., 2022 ). Metaverse employs technologies like virtual reality, augmented reality and blockchain to achieve the immersion, as these technologies can achieve multisensory interactions. It provides genuine, physical user interaction and complex interrelationships with virtual objects. In education, Metaverse is not a new concept as several researchers and educators have discussed its implications for learning. Metaverse can be the online space where individuals can meet and socially interact for educational and not only, reasons. So, in the field of education, the metaverse has the potential to revolutionize the way that students learn and interact with educational content. By using virtual reality and other immersive technologies, students can experience educational content in a more interactive and engaging way, which can help to improve retention and understanding. Although, there are some challenges concerning the use of Metaverse in education. Some of them that we are addressing here are data privacy, technology implementation, efficiency, cost, lack of standardization and addiction.
Virtual reality in education
VR has been used as an educational tool for numerous subjects (see Table 4 ). Sobota et al. ( 2017 ), states that the two techniques widely used to offer immersive and semi-immersive experience regarding virtual reality in smart classroom are: (a) CAVE (Cave automatic virtual environment) and HMDs (Head-mounted displays) and (b) Interactive school desk. CAVE constitutes a room sized pace including several projection walls where the user is able to move freely in the space and experience their body in immediate interaction with virtual scene and HMDs are suitable devices that offer virtual environment to one user every time. Furthermore, there are different VR accessories which can combine with HMDs and CAVEs, such as gloves, suits or controllers which can offer more exciting experience.
The application of VR in education changed some of the previous teaching ideas, but also some of the already existing teaching models (Chen & Tsai, 2012 ; Gu, 2017 ). Several studies concluded that VR technologies are more likely to influence the motivation and academic performance of students in a positive way (Ibáñez et al., 2014 ; Martín-Gutiérrez et al., 2017 ). Furthermore, Hampel and Dancsházy ( 2014 ) argues that the creation of an environment of virtual learning is quite helpful for students, since students are able to acquire knowledge by themselves. Additionally, there is evidence that VR technologies enhance students’ collaborative and communicative skills along with their cognitive and psychomotor skills (Kaufmann & Schmalstieg, 2002 ; Martín-Gutiérrez et al., 2017 ; Zhou et al., 2008 ) whereas VR technologies can be used for the training of the educators as well (Stavroulia et al., 2016 ).
Augmented reality in education
There are different types of augmented display devices in a smart class that include tablets, smartphones, smartboards and different software which enables the creation of augmented scenarios such as Aurasma, Footnote 1 Layar, Footnote 2 Augment Footnote 3 and Aumentaty Footnote 4 (Chamba-Eras & Aguilar, 2017 ). Oculus Quest, Microsoft HoloLens and Windows Mixed Reality are AR headsets/glasses utilized as augmented display devices. According to Torres et al. ( 2011 ), AR in smart classrooms can be used in the following forms: Enlarged book, Virtual models of specific complicated structures, Educational games for the classroom, Virtual models which produce sounds, Magic eyeglasses, Magic mirrors, Magic doors and windows, Navigation support and Cooperative space. Chamba-Eras and Aguilar ( 2017 ) state that AR is recommended to compensate various deficiencies that might occur in a smart classroom such as difficulties in doing complicated and dangerous experiments, carrying out actual experiments due to equipment costs, and unavailability of appropriate facilities. Furthermore, the study from Stanford University has shown that students who learn with AR technology demonstrate greater knowledge retention and improved problem-solving skills (Queiroz et al., 2022 ). The importance of Augmented Reality technology in education has led to its implementation in various topics as shown in Table 5 :
Mixed reality in education
MR applications in real and smart classroom have many benefits from the perspective of the students in learning and the procedure of obtaining knowledge or skills. According to Dascalu et al. ( 2014 ), some benefits by MR to educational uses are: (a) students remain focused on the task at-hand, (b) it is fostered the affective side of learning, (c) computer-based learning gets more human-oriented, and (d) students’ interest and motivation towards learning is enhanced. Furthermore, MR offers immersive and engaging experiences via creative problem solving. MR worlds achieve high levels of immersion through Head Mounted Displays (HDMs), such as Microsoft HoloLens, HTC Vive, Oculus Rift and Magic Leap One, or AjnaLens. Different educational tools were developed to enhance the efficiency of teaching-process such as Virtual Toolkit (Mateu et al., 2015 ), SMALLable (Tolentino et al., 2009 ), TIWE Linguistico (Fiore et al., 2014 ) and Robostage (Chang et al., 2010 ).
The role of AI for VR, AR and MR
The integration of AI in VR/AR applications has the potential to improve its effectiveness, enabling programmers to develop more engaging and fascinating applications (Kaviyaraj & Uma, 2021 ). The key areas where AI is used in conjunction with VR/AR/MR include the generation of 3D assets, Interaction, Reasoning, Visualization. In the case of AR and MR computer vision capabilities such as pose estimation, object detection, scene labeling and semantic segmentation are used to control content, project an object in the scene, and trigger a spot or occlude objects from the scene (Sahu et al., 2021 ). Furthermore, AI-based techniques are utilized to generate avatars, digital humanoid characters or complementing users that interact and take decisions immediately according to the gamers' choices, resulting in more engaging experiences (El Beheiry et al., 2019 ).
E-learning platforms
Beetham and Sharpe ( 2013 ) state that E-learning platforms (see Table 6 ) are on-line systems that aim to support synchronous, asynchronous or hybrid learning activities. Within this context, synchronous learning is done in real time, asynchronous learning, is done at a convenient time for the student (Potode & Manjare, 2015 ), and hybrid learning constitutes of a combination of synchronous and asynchronous learning activities.
It has been demonstrated that kids who spend a lot of time on e-learning platforms are more engaged and obtain greater grades because they modify their perception of their schoolwork (Benta et al., 2015 ). Students seem to be more activated by the way these platforms work and managed to do the assignments they had and create a sense of responsibility to their submissions, as well as to complete some difficult activities (Benta et al., 2014 ). Students also claimed that this type of environment intrigued them to participate in extra lectures and seminars.
Quite often AI is introduced in E-learning platforms in order to maximize the learning experience of students through the use of adaptive educational systems. The latter can adapt to individual needs and offer support that is tailored to each student, aiming to help students meet their individual goals in the best possible way that fits their personalities and characteristics (Colchester et al., 2017 ). To this end, adaptive educational systems use the learner profile to diagnose individual characteristics and abilities, the taught model to present the learning material and the instructional model to formulate the content in a dynamic and adaptive way. Recently, a new AI-based e-learning platform called "Edu4AI" has been developed to personalize the curriculum for each student based on their learning style, ability and progress (Geramani et al., 2022 ). The efficiency of adaptive educational systems proves the capability of AI to assist leaning in multiple ways (Durlach & Lesgold, 2012 ). Apart from supporting adaptive learning AI is also utilized in other aspects of the learning process. For example, natural language processing algorithms (Chowdhary, 2020 ) are often used to identify plagiarism and avoid transcribing in assignments submitted by students (Chong et al., 2010 ). Furthermore, E-learning platforms are used in different classroom models such as Flipped classroom and Virtual classroom.
Flipped classroom is an innovative educational method where tasks usually performed in the classroom, like presenting the lecture, are conducted at home, while homework is discussed and performed in class (Akçayır et al., 2018 ) whereas a Virtual classroom is defined as a new educational environment that allows students to attend courses online while also facilitating interaction and collaboration by using the artificial intelligence tools and abilities the platform offers (Rufai et al., 2015 ). Lo et al. ( 2017 ) believe that AI has great potential in the flipped classroom approach, because it may enable the personalization and adaptation of the learning process to the students’ needs. Shan and Liu ( 2021 ) suggest a model of Hybrid Teaching of Artificial Intelligence and Flipped Classroom, which combines big data, cloud and online applications to implement comprehensive and individualized learning.
All Screen refers to the ability to project multimedia including audio, photographs, and movies on many screens such as TVs and smartphones. Screen mirroring allows users to access and view the same image or video in two or more screens (Brudy et al., 2019 ). Screen mirroring is valuable since it might improve the connectivity among a cellphone and a different device, such as smart TVs (Ouyang & Zhou, 2019 ). Thus, touch gesture input, with virtual buttons on the screen, is a frequent technique for interacting with screens (Ouyang et al., 2021 ). There are several advantages that all screens may provide to a smart class. To begin with, only wireless screen mirroring makes the connection between instructors' and students' devices straightforward and reliable (Ellern & Buchanan, 2018 ). Furthermore, practical issues related to the use of projectors, such as connectivity issues, requirement for low-light conditions, and projector noise, are mitigated through the adoption of all screen technology (Sahlström et al., 2019 ).
Performance assessment
Student and educator performance assessment and feedback is a highly important education task. Although traditionally performance assessment/prediction has been a quite complex and time-question process, it has been extremely facilitated through automated assessment in a smart classroom (Balfour, 2013 ).
Smart student performance assessment/prediction
Student performance assessment aims on the one hand to inform the teacher about the degree to which students have learnt the content of the lesson and how well they are expected to perform in the future, and on the other, to grade students and provide feedback to them about their performance during the learning process (Saini & Goel, 2019 ). Traditionally, performance assessment was carried out in a paper or oral format. However, the aforementioned method has many disadvantages, since it is a time-consuming and tiring process, while it results to piles of wasted paper and writing material (Vimal & Kumbharana, 2016 ).
In a smart classroom dedicated tools can facilitate the performance assessment/prediction through the automation of the assessment. The easiest tool to assess students in a smart classroom is the employment of multiple-choice questions, which allow automated evaluation and feedback, with the aid of an online web server that compares students’ answers with the configured correct answer (Balfour, 2013 ). An important application of AI in student assessment is plagiarism checking, with Turnitin as a frequently used tool (Ahmed, 2015 ). Bhatia and Kaur ( 2021 ) add an innovative performance assessment/prediction tool based on quantum game theoretic (QGT) decision making. This tool incorporates IoT to gather information and data about students, which are evaluated over a computing platform, aiming to analyze performance and determine the academic enhancement of students. The above methods give the ability to teachers to offer continuous assessment to students, avoiding all the tedious work, while students receive feedback constantly.
Numerous machine learning techniques have been proposed for predicting student performance. Amra and Maghari ( 2017 ) propose a system giving predictions regarding the future performance of secondary students based on several attributes. They compared two distinct machine learning algorithms: K-Nearest Neighbors (KNN) and the Naïve Bayes classifier by feeding them with educational data set of secondary schools, collected from the ministry of education in Gaza Strip. Waheed et al. ( 2020 ), propose a system to predict the students’ academic performance in a virtual learning environment. Their system used artificial neural networks to classify students in two classes: failure and success, receiving as input data of the assessment performance of 32,593 students provided from an open dataset. Authors made a comparison of the results using baseline methods: logistic regression, support vector machines and Artificial Neural Networks (ANN). The ANN method had the best performance out of the tested models. Warschauer and Grimes ( 2008 ), propose the automatic assessment of writing essay assignments with the use of artificial intelligence, using interview data and observations notes as inputs. Students and teachers had positive approach (i.e., student motivation rising, proposing autonomous student activity, constituting a saver of time for teachers).
Educator performance assessment
Traditional assessment methods are usually based on the observation of teachers from experts during course time something that can be expensive, not accurate and usually the feedback provided is infrequent and is related to the performance and not on how teachers can enhance their techniques (Archer et al., 2016 ). To overcome this crucial impediment in teacher development, new technologies are used to produce high quality and meaningful, and continuous automatic feedback for the educators.
Bhatia and Kaur ( 2021 ) use IoT systems are used in classes to collect information regarding students and educators to identify their progress. For this purpose, they utilize a Bayesian model and the collected data are assessed through a fog-cloud computing device over time for both students’ and educators’ performance. Jensen et al. ( 2020 ) devised a method for teachers to effortlessly audiotape the conversations and lectures in a classroom. They utilized voice recognition and Machine Learning (ML) algorithms to provide generalized estimations of essential aspects of educator speech. Therefore, they state that actual instructor conversation can be captured and evaluated for automated feedback. Jensen et al. ( 2021 ) address the issue of designing a framework for automatic educator feedback, that necessitates several considerations about data harvesting processes, automatic assessment and the way feedback is displayed. For this purpose, they employ machine learning techniques, such as Random Forest classifiers, and use transfer learning techniques from BERT algorithm for NLP.
Impact of smart classroom
In this section, based on information derived from the literature, in combination with critical assessment, the impact of smart classroom on the learning process is analyzed while disadvantages of using key technologies related to smart classes and the impact of AI are also discussed.
The integration of AI systems that process data collected by Internet of Things (IoT) and other sensors, can help monitor the circumstances of the classroom, offering a safe and eco-friendly environment while they can also monitor students and inform teachers in the case of a student misconduct or potential accidents. AI algorithms can be used to optimize lighting and temperature in classrooms based on occupancy, ambient temperature, and other factors. All these tools and systems can contribute to the establishment of a better and safer learning environment. Furthermore, the interaction with the learning material offered by key technologies related to smart classes can help boost students’ learning, information may be retained more easily and self-efficiency may increase (León et al., 2016 ). The enhanced interactively offered in smart classes help students have an active role in the class delivery process rather than having a passive role that causes loss of concentration and interest. However, the use of technology for teaching and learning may be related to disconnectedness, which is usually expressed as feelings of separation from learning, the curriculum, the peers and the teachers and the learning devices. Disconnection of students may jeopardize the learning outcomes of students because it results in disengagement, decreased student ownership and absence of student agency. For this reason, teachers in smart classrooms should find ways to remove the barriers to meaningful student involvement and encourage their engagement with the school and the learning process (Blessinger & Wankel, 2013 ).
Lin et al. ( 2019 ) suggest that an adjustable smart system can assist students, improve the learning process and foster a considerable quantity of intellectual learners. Smart classrooms and emerging technologies may overcome the problems related to the provision of timely and individualized support to students, using smart applications that respond to students and provide automated feedback immediately, by comparing current and previous student performance and by motivating students (Vimal & Kumbharana, 2016 ). In addition, Smart classes support the provision of synchronous and asynchronous education while they support both teacher-led and student-centered activities (Beetham & Sharpe, 2013 ). Moreover, the above combination allows to enrich learning with the provision of extra material, improve and retain knowledge through the students’ longer interaction with the learning subject, the teachers and their peers. At the same time, the style of the traditional classroom that is offered on scheduled dates and time is retained, resulting to keeping students aware and alert. Having this in mind, and knowing the fact that many teachers are not familiar with technology, while some of them focus on the use of usual software such as Word, PowerPoint, etc., the teachers may face difficulties, or they may need technical support for the full utilization of the emerging and artificial intelligence tools. These teachers need appropriate education to support the successful implementation of a smart classroom with new technologies.
E-learning involves the use of the internet as a platform for educational activities, that can include three-dimensional environments or real-time virtual interactions between students and teachers. The e-learning environment is seen as a crucial tool for supporting conventional learning formats and is changing how higher education is provided. E-learning platforms have several benefits, including improving student-centered learning, helping students become more independent while educators take on innovative roles, encouraging educators to be more reflective and methodical in creating better e-learning resources, and ultimately giving students the skills to adapt to a constantly changing technology-driven environment. AI-powered personalization and adaptive learning can be used to adjust the pace and difficulty level of content for individual students. E-learning enables students to receive learning activities from educators at a distance, allows for larger classes, and makes it easier to identify and record behaviors and errors to improve learning activities. Even though face-to-face learning is better for daily discussions and contributes to an active environment (Valdez et al., 2015 ), this generation of adolescents dislikes traditional classroom education. Although they like to learn at their own pace, they are curious. Because of their addiction to technology, they rely increasingly on online teaching and learning resources. Through collaborative learning, they enjoy sharing their knowledge with their peers and benefiting from the strengths of their competitors (Agarwal et al., 2019 ).
Virtual spaces in a smart classroom resemble real places allowing students to have an immersive experience and create real memories. Moreover, seeing, ‘touching’ and hearing involve more senses in the learning process and link the learning subjects in multiple ways. Therefore, enriched presentation of the learning material and better visualization, which resembles reality and involves more senses, enhances students’ experience and learning becomes sustainable (Lui & Slotta, 2014 ). Furthermore, the student’s motivation is triggered, situated scaffolding is provided and learning is connected with the student’s everyday life (Bower et al., 2014 ) through an experiential learning process. AI can be used to create more immersive and interactive learning experiences by tracking student movements and adjusting the virtual environment accordingly. Since a smart classroom is equipped with contemporary visualization technologies, which include interactive whiteboards, projectors, all-screen technology, virtual/augmented reality headsets, cameras, and sensors, students can better visualize the content they are taught, enhancing in that way the learning experience. In a smart classroom, students may be immersed in online virtual environments using headsets; as a result, distractions are removed, and the student’s attention is captured. Furthermore, perspective-changing in virtual reality visualizations allows students to become actors rather than just observers, transforming the learning process into a highly experiential experience (Krüger et al., 2019 ).
Computer vision based surveillance
Smart classrooms provide real-time video analysis to educators that want to recognize the behavioural participation and behavioural disaffection of their students (Michalsky, 2021 ). AI-enabled cameras can be used to monitor the classroom for safety and security, as well as to track student attendance and participation . The employment of smart tools and applications, such as cameras, plagiarism checking, and recording, combined with the continuous gathering of data, allows teachers to control student attendance and supervise them both in class and during online assessments (Saini & Goel, 2019 ).
A challenge concerning the use of advanced technological systems is bias in AI. More specifically, there is a concern regarding how fair can AI systems be to every single student despite the personal attributes of a student (i.e., race and gender) (Li et al., 2021 ). Groups that face discrimination in the community of technology, like female students, might face more severe inequalities if the creation of AI systems doesn’t consider how to mitigate such biases. Technologically advanced key technologies related to smart classes incorporating AI capabilities are threatened by security and privacy issues because they store and process data that contain personal and sensitive information that may be exposed to potential invaders (Manca et al., 2016 ). Furthermore, sensors used in classes (i.e. camera sensors or microphones) often used as part of smart-class technological tools, are associated with privacy issues.
One of the greatest challenges in introducing key technologies related to smart classes in schools is the overall cost of equipment required for a comprehensive smart-class deployment is high (Saini & Goel, 2019 ), preventing in that way the widespread use of smart-class technologies. Bearing in mind that the cost does not refer only to the equipment purchase and initial installation, but also to the continuous upgrade and maintenance, the use of smart class technology involves significant running expenses. Also, because all components of a smart class are somehow disconnected from each other, it is not seamless to integrate all technologies under a common framework, and as a result, the task of setting up the equipment can be a lengthy and time-consuming procedure.
Learning about computers, electronics, mechanical engineering, and languages may be interesting thanks to robots. It has been demonstrated that when language acquisition was facilitated by a robot as opposed to audiotapes and books, young children did better on post-learning assessments and displayed greater enthusiasm (Mubin et al., 2013 ). AI can be used to control and program educational robots that can interact with students and enhance their learning experience. The teacher in smart classroom takes on the role of a facilitator if the robot is the focal point of the learning activity (i.e., used as a teaching tool, as in the case of teaching about robotics). If the robot plays a passive role, the teacher must provide fundamental knowledge (e.g., by using the robot in language classes). In such cases, robotics curriculum implementation and teacher training are crucial. Looking ahead, it is evident that more has to be done to secure teacher support before robots can be completely included into our schools. Teachers were rated less favorably than parents and children in a survey regarding school robots. Teachers need to be reassured that the objective is not to replace them with robots but to give them a teaching tool or aid that can enhance the educational process and inspire students.
AI in smart classes: A SWOT analysis
Based on the analysis presented in the previous subsections, the advantages of using AI and emerging technologies may also involve risks that may jeopardise the learning efficiency and experience. In this subsection a SWOT analysis of using AI in smart classes is presented as a means of summarizing the potential of using AI in smart classes, along with possible drawbacks. These observations are presented in a SWOT analysis presented in Table 7 .
Discussion-future directions
In this section we outline the main issues in the dimensions of technology infrastructure, personnel, and data handling that need to be addressed by the research community in order to maximize the impact of AI in enhancing smart classes.
Technology infrastructure
To enhance the capabilities of a smart classroom it is necessary to integrate all technologies, hence a combination of emerging technologies and AI is essential. A central AI system that can manage the use of different technologies, suggest optimum ways of integrating each technology in specific classes, and provide a comprehensive evaluation of students and the educational process will be a highly desirable feature of future smart classes.
Since the teaching process is a highly dynamic process where educators need to adapt to the changes in student attitudes and overall class requirements, it is important to deploy AI-based systems that continuously monitor the student requirements and adjust to respond to all changes. While this can take the form of reinforcement learning (Liu et al., 2018 ) dedicated techniques for AI systems that deal with in-class scenarios, need to be devised.
The integration of special technical equipment is usually an issue due to the expensive cost. As a result, it is critical to adopt new low-cost technical equipment that students may use anywhere, at any time. Experts should develop techniques and technologies that can run on personal equipment rather than dedicated machines, for example using smart phones or low-end personal computers. When it comes to AI-based systems that need to be re-trained continuously, efficient training methods that allow the training process to be completed using ordinary computer systems, need to be employed, so that costs associated with the purchase of dedicated equipment or the purchase of computational time, are decreased.
The need to adopt in practice appropriate teacher training programs regarding the use of technology in education has become urgent. Apart from training for using emerging technologies, educators should also receive adequate training for AI related issues, so that they learn how to harness the power of AI systems for the benefit of the education process. Thus, it is imperative that dedicated AI courses for educators are created, so that smart class teachers are well aware of the potential and risks of using AI empowered emerging technologies. Furthermore, dedicated user-friendly tools that will allow educators to train and use Machine Learning modules should be developed.
Classroom overlays for teachers that incorporate grades, special arrangements, and medical and social information are probably among the educational applications of the future. As technology advances, it will be able to alert teachers to students' learning needs and behavioral issues in real-time and provide solutions. Teachers are sometimes untrained to handle the technical challenges that may occur when a device does not function as planned. As a result, for teachers to succeed, there may be a significant amount of assistance required. To prevent the design of learning from being largely the responsibility of computer scientists who have a limited understanding of successful pedagogy, it is essential that educators learn how to integrate technology into their teaching (Krüger et al., 2019 ). With the recognition that students' abilities can be impacted by their cognitive, motor, and spatial capabilities, technology also makes it easier for teachers to teach content and learning objectives. Students can become more actively involved in the learning process as they develop their motivation and foundational knowledge (Liono et al., 2021 ).
Empathy is the ability to recognize somebody's emotional reactions and motives, care for them and their sentiments (Srinivasan & González, 2022 ). It is vital to develop specialized AI systems that take into consideration the unique characteristics of each student, through an empathetic nature. The topic of producing “empathetic” AI systems can open up several research directions.
Data handling
One of the most crucial issues of the future of smart classes is ethics in the use of data in AI systems (Borenstein & Howard, 2021 ). It is vital to address the way data are collected and used by those systems in order to avoid the violation of privacy. Regulations regarding the collection of data must be established and adopted by the scientific community. Data can also be encrypted and anonymised, so in case a hack occurs it won’t be feasible to find correlations between provided data and individuals. Within this scope, new methods that guarantee data security, but at the same time allows the access to the necessary information by different stakeholders, within a smart-class needs to be developed.
Bias in AI is an issue that needs be addressed while using advanced technological systems. More precisely, there is worry about how fair AI systems can be to all students, regardless the attributes of each student such as ability level, race, religion, appearance, or gender (Li et al., 2021 ). Developers must consider all the biases that may rise due to their personal beliefs and eliminate them. In this way, any form of discrimination towards minorities will be alleviated and students will be able to attend education and receive fair feedback compared to their peers. Furthermore, since supervised AI systems often rely on annotated data, techniques that ensure that any form of bias in the annotation process is eliminated, so that the resulting AI systems are not subjected to any kind of discrimination. AI systems should include machine learning techniques with explainable AI to analyze the educational factors that lead to more fair and effective decision making for students since the ML-based black box model is more understandable to educators (Guleria & Sood, 2022 ).
Using data collection, user profiling, and adaptive learning can be useful in creating a more personalized and effective learning experience, and artificial intelligence can play a role in supporting these efforts. However, it is important to carefully consider the ethical implications of collecting and using data, and to ensure that students' privacy is protected. The use of AI in education can also raise questions about the role of technology in learning and the potential for it to replace human teachers. While AI can certainly be a useful tool for supporting and enhancing education, it is important to consider the limitations of technology and the value of human interaction and guidance in the learning process. Overall, it is necessary to carefully evaluate the potential benefits and risks of using AI and other technology in education, and to strike a balance between the use of technology and more traditional teaching methods.
Conclusions
A range of AI-assisted emerging technologies, that include technologies related to class management, teaching aids and performance assessment have been presented. For each smart class technology presented the role of AI was discussed, allowing the in that way the determination of the role of AI in smart classes. Furthermore, through the analysis of advantages and disadvantages of smart classes, along with a SWOT analysis, the prospects, and trends related to the use of AI on smart classes have been discussed, allowing in that way the definition of several future research directions. The future directions presented can provide motivation to the AI, and educational technology research communities to engage in research activities that aim to deal with the identified challenges. Since the new era of technological advancement and the proliferation of digital devices and applications that are routinely used in everyday life has been integrated in education, there is a continuous need to invest in improving the services offered to students and the further development of AI-based smart classes definitely leads those efforts in the right direction.
Since the concept of smart-classes is continuously enriched through the introduction of requirements and new technologies, in the future we plan to monitor this area and produce updated surveys to reflect future developments and conduct investigations in the area of intelligent learning environment. In addition, in the future we plan to provide specific comparative evaluations of different technologies, so that to quantify the effect of existing technologies and highlight the need for future improvements.
https://www.aurasma.com .
https://www.layar.com/ .
http://www.augment.com/es/ .
http://www.aumentaty.com/ .
Abbreviations
ARTIFICIAL intelligence
Artificial intelligence in education
Artificial neural networks
Augmented reality
Cave automatic virtual environment
Head-mounted displays
Internet of things
Machine learning
Mixed reality
Virtual reality
Abbasy, M. B., & Quesada, E. V. (2017). Predictable influence of IoT in the higher education. International Journal of Information and Education Technology .
Abd-Elaal, E. S., Gamage, S. H., & Mills, J. E. (2019). Artificial intelligence is a tool for cheating academic integrity. In 30th Annual Conference for the Australasian Association for Engineering Education, (pp. 397–403).
Abdel-Basset, M., Manogaran, G., Mohamed, M., & Rushdy, E. (2019). Internet of things in smart education environment: Supportive framework in the decision-making process. Concurrency and Computation: Practice and Experience, 31 (10), e4515.
Article Google Scholar
Adams, J. C., & Hotrop, J. (2008). Building an economical VR system for CS education. ACM SIGCSE Bulletin, 40 (3), 148–152.
Agarwal, A., Naidu, V. R., & Al Mamari, R. (2019). A framework to enhance learning experience in flipped classroom based on student accountability towards active participation. EDULEARN19 Proceedings , 1 , 1569-1577.
Ahmed, R. A. (2015). Overview of different plagiarism detection tools. International Journal of Futuristic Trends in Engineering and Technology, 2 (10), 1–3.
Google Scholar
Akçayır, G., & Akçayır, M. (2018). The flipped classroom: A review of its advantages and challenges. Computers & Education, 126 , 334–345.
Alakärppä, I., Jaakkola, E., Väyrynen, J., & Häkkilä, J. (2017). Using nature elements in mobile AR for education with children. In Proceedings of the 19th International Conference on Human-Computer Interaction with Mobile Devices and Services (pp. 1–13).
Alfalah, S. F., Falah, J. F., Alfalah, T., Elfalah, M., Muhaidat, N., & Falah, O. (2019). A comparative study between a virtual reality heart anatomy system and traditional medical teaching modalities. Virtual Reality, 23 (3), 229–234.
AlFarsi, G., Tawafak, R. M., ElDow, A., Malik, S. I., Jabbar, J., & Al Sideiri, A. (2021). Smart classroom technology in artificial intelligence: A review paper. In International Conference on Culture Heritage, Education, Sustainable Tourism, and Innovation Technologies (pp. 229–235).
Ali, M. Y., Zhang, X. D., & Harun-Ar-Rashid, M. (2020). Student activities detection of SUST using YOLOv3 on deep learning. Indonesian Journal of Electrical Engineering and Informatics, 8 (4), 757–769.
Amra, I. A. A., & Maghari, A. Y. (2017). Students performance prediction using KNN and Naïve Bayesian. In 2017 8th International Conference on Information Technology (pp. 909–913).
Archer, J., Cantrell, S., Holtzman, S. L., Joe, J. N., Tocci, C. M., & Wood, J. (2016). Better feedback for better teaching: A practical guide to improving classroom observations .
Augusto, J. C. (2009). Ambient intelligence: Opportunities and consequences of its use in smart classrooms. Innovation in Teaching and Learning in Information and Computer Sciences, 8 (2), 53–63.
Balfour, S. P. (2013). Assessing writing in MOOCs: automated essay scoring and calibrated peer review™. Research & Practice in Assessment, 8 , 40–48.
Beetham, H., & Sharpe, H. (2013). Rethinking pedagogy for a digital age: Designing for 21st century learning Abingdon . Routledge.
Book Google Scholar
Ben-Ari, M., & Mondada, F. (2018). Robots and their applications. In Elements of Robotics .
Benta, D., Bologa, G., & Dzitac, I. (2014). E-learning platforms in higher education. Case Study. Procedia Computer Science, 31 , 1170–1176.
Benta, D., Bologa, G., Dzitac, S., & Dzitac, I. (2015). University level learning and teaching via e-learning platforms. Procedia Computer Science, 55 , 1366–1373.
Bhatia, M., & Kaur, A. (2021). Quantum computing inspired framework of student performance assessment in smart classroom. Transactions on Emerging Telecommunications Technologies , 32 (9).
Billinghurst, M., Clark, A., & Lee, G. (2015). A survey of augmented reality. Foundations and Trends® in Human–Computer Interaction , 8 (2–3), 73–272.
Blessinger, P., & Wankel, C. (Eds.). (2013). Increasing student engagement and retention using classroom technologies: Classroom response systems and mediated discourse technologies .
Blyth, C. (2018). Immersive technologies and language learning. Foreign Language Annals .
Borenstein, J., & Howard, A. (2021). Emerging challenges in AI and the need for AI ethics education. AI and Ethics, 1 (1), 61–65.
Bourguet, M.-L., Jin, Y., Shi, Y., Chen, Y., Rincon-Ardila, L., & Venture, G. (2020). Social robots that can sense and improve student engagement. In 2020 IEEE International Conference on Teaching, Assessment, and Learning for Engineering (TALE) , 127–134.
Bower, M., Howe, C., McCredie, N., Robinson, A., & Grover, D. (2014). Augmented Reality in education–cases, places and potentials. Educational Media International, 51 (1), 1–15.
Brady, M., Gerhardt, L. A., & Davidson, H. F. (2012). Robotics and artificial intelligence.
Brudy, F., Holz, C., Rädle, R., Wu, C. J., Houben, S., Klokmose, C. N., & Marquardt, N. (2019). Cross-device taxonomy: Survey, opportunities and challenges of interactions spanning across multiple devices. In Proceedings of the 2019 chi conference on human factors in computing systems (pp. 1–28).
Burrack, F., & Thompson, D. (2021). Canvas (LMS) as a means for effective student learning assessment across an institution of higher education. Journal of Assessment in Higher Education, 2 (1), 1–19.
Cai, S., Liu, E., Yang, Y., & Liang, J. C. (2019). Tablet-based AR technology: Impacts on students’ conceptions and approaches to learning mathematics according to their self-efficacy. British Journal of Educational Technology, 50 (1), 248–263.
Causo, A., Vo, G. T., Chen, I., & Yeo, S. H. (2016). Design of robots used as education companion and tutor. In Robotics and mechatronics (pp. 75–84).
Chamba-Eras, L., & Aguilar, J. (2017). Augmented reality in a smart classroom—Case study: SaCI. IEEE Revista Iberoamericana De Tecnologias Del Aprendizaje, 12 (4), 165–172.
Chang, C. W., Lee, J. H., Wang, C. Y., & Chen, G. D. (2010). Improving the authentic learning experience by integrating robots into the mixed-reality environment. Computers & Education, 55 (4), 1572–1578.
Chen, L., Chen, P., & Lin, Z. (2020). Artificial intelligence in education: A review. Ieee Access .
Chen, C. M., & Tsai, Y. N. (2012). Interactive augmented reality system for enhancing library instruction in elementary schools. Computers & Education, 59 (2), 638–652.
Chen, N. S., Cheng, I. L., & Chew, S. W. (2016). Evolution is not enough: Revolutionizing current learning environments to smart learning environments. International Journal of Artificial Intelligence in Education, 26 (2), 561–581.
Chen, X., Zou, D., Xie, H., Cheng, G., & Liu, C. (2022). Two decades of artificial intelligence in education. Educational Technology & Society, 25 (1), 28–47.
Chintalapati, S., & Raghunadh, M. V. (2013). Automated attendance management system based on face recognition algorithms. In 2013 IEEE International conference on computational intelligence and computing research (pp. 1–5).
Chocarro, R., Cortiñas, M., & Marcos-Matás, G. (2021). Teachers’ attitudes towards chatbots in education: a technology acceptance model approach considering the effect of social language, bot proactiveness, and users’ characteristics. Educational Studies (pp. 1–19).
Chong, M., Specia, L., & Mitkov, R. (2010). Using natural language processing for automatic detection of plagiarism. In Proceedings of the 4th International Plagiarism Conference .
Chowdhary, K. (2020). Natural language processing. Fundamentals of artificial intelligence .
Chowdhury, G.G. (2003). Natural Language Processing. Annual Review of Information Science and Technology.
Chowdhury, S., Nath, S., Dey, A., & Das, A. (2020). Development of an automatic class attendance system using cnn-based face recognition. In 2020 Emerging Technology in Computing, Communication and Electronics (pp. 1–5).
Colace, F., De Santo, M., Lombardi, M., Pascale, F., Pietrosanto, A., & Lemma, S. (2018). Chatbot for e-learning: A case of study. International Journal of Mechanical Engineering and Robotics Research, 7 (5), 528–533.
Colchester, K., Hagras, H., Alghazzawi, D., & Aldabbagh, G. (2017). A survey of artificial intelligence techniques employed for adaptive educational systems within e-learning platforms. Journal of Artificial Intelligence and Soft Computing Research, 7 (1), 47–64.
Dascalu, M. I., Moldoveanu, A., & Shudayfat, E. A. (2014). Mixed reality to support new learning paradigms. In 2014 18th International Conferences on System Theory, Control and Computing (pp. 692–697).
Demitriadou, E., Stavroulia, K. E., & Lanitis, A. (2020). Comparative evaluation of virtual and augmented reality for teaching mathematics in primary education. Education and Information Technologies, 25 , 381–401.
Díaz León, C. A., Hincapié Montoya, E. M., Guirales Arredondo, E. A., & Moreno López, G. A. (2016). Design and development of an interaction system in order to be implemented in a smart classroom. Revista EIA, 26 , 95–109.
Dimitriadou, E., & Lanitis, A. (2022b). Using student action recognition to enhance the efficiency of tele-education. In Proceedings of the 17th International Joint Conference on Computer Vision, Imaging and Computer Graphics Theory and Applications (pp. 543–549).
Dimitriadou, E., & Lanitis, A. (2022a). The role of artificial intelligence in smart classes: A survey. In 2022a IEEE 21st Mediterranean Electrotechnical Conference (pp. 642–647).
Dobre, I. (2015). Learning management systems for higher education-an overview of available options for higher education organizations. Procedia-Social and Behavioral Sciences, 180 , 313–320.
Dryjanski, M., Buczkowski, M., Ould-Cheikh-Mouhamedou, Y., & Kliks, A. (2020). Adoption of smart cities with a practical smart building implementation. IEEE Internet of Things Magazine, 3 (1), 58–63.
Dünser, A., & Hornecker, E. (2007). Lessons from an AR book study. In Proceedings of the 1st international conference on Tangible and embedded interaction (pp. 179–182).
Durlach, P. J., & Lesgold, A. M. (2012). Adaptive technologies for training and education .
El Beheiry, M., Doutreligne, S., Caporal, C., Ostertag, C., Dahan, M., & Masson, J. B. (2019). Virtual reality: Beyond visualization. Journal of Molecular Biology, 431 (7), 1315–1321.
Ellern, G. J. D., & Buchanan, H. E. (2018). No strings attached? Challenges and successes in creating a flexible, wire-free active learning classroom. Library Hi Tech .
Feil-Seifer, D., & Matarić, M. J. (2009). Toward socially assistive robotics for augmenting interventions for children with autism spectrum disorders. In Experimental robotics , (pp. 201–210).
Fiore, A., Mainetti, L., & Vergallo, R. (2014). An innovative educational format based on a mixed reality environment: A case study and benefit evaluation. In International Conference on E-Learning, E-Education, and Online Training (pp. 93–100).
Geramani, K., Mazzucato, A., Gaudiello, I., & Ioannidis, G. (2022). Artificial Intelligence in the secondary education: The paradigm of Edu4AI. In E Third Workshop on Technology Enhanced Learning Environments .
Górski, F., Buń, P., Wichniarek, R., Zawadzki, P., & Hamrol, A. (2016). Effective design of educational virtual reality applications for medicine using knowledge-engineering techniques. EURASIA Journal of Mathematics, Science and Technology Education.
GU, W. (2017). The Application of Virtual Reality in Education. DEStech Transactions on Computer Science and Engineering .
Guleria, P., & Sood, M. (2022). Explainable AI and machine learning: performance evaluation and explainability of classifiers on educational data mining inspired career counseling. Education and Information Technologies , 1–36.
Gupta, S. K., Ashwin, T. S., & Guddeti, R. M. R. (2018). Cvucams: Computer vision based unobtrusive classroom attendance management system. In 2018 IEEE 18th International Conference on Advanced Learning Technologies (pp. 101–102).
Gutierrez, E. O. C., & De Troyer, O. (2014). SimBully: A'bullying in schools' simulation.
Guttormsen, M., Bürger, A., Hansen, T. E., & Lietaer, N. (2011). The SiRi particle-telescope system. Nuclear Instruments and Methods in Physics Research Section a: Accelerators, Spectrometers, Detectors and Associated Equipment, 648 (1), 168–173.
Hampel, G., & Dancsházy, K. (2014). Creating a virtual learning environment. Agrárinformatika/journal of Agricultural Informatics, 5 (1), 46–55.
Han, J., Jo, M., Park, S., & Kim, S. (2005). The educational use of home robots for children. In ROMAN 2005. IEEE International Workshop on Robot and Human Interactive Communication (pp. 378–383).
Han, J., & Kim, D. (2009). r-Learning services for elementary school students with a teaching assistant robot. In 4th ACM/IEEE Intl Conf. on Human-Robot Interaction (pp. 255–256).
Hashimoto, T., Kato, N., & Kobayashi, H. (2011). Development of educational system with the android robot SAYA and evaluation. Intl. Journal of Advanced Robotic Systems, 8 (3), 28.
Have, H. & Neves, M. d. C. P. (2021). Emerging technologies. Dictionary of Global Bioethics .
Helms, A. S., Schmiegelow, K., Brok, J., Johansen, C., Thorsteinsson, T., Simovska, V., & Larsen, H. B. (2016). Facilitation of school re-entry and peer acceptance of children with cancer: A review and meta-analysis of intervention studies. European Journal of Cancer Care, 25 (1), 170–179.
Hsu, P. C., Wang, C. H., Liu, A. T., & Lee, H. Y. (2019). Towards robust neural vocoding for speech generation: A survey .
Huang, L. S., Su, J. Y., & Pao, T. L. (2019). A context aware smart classroom architecture for smart campuses. Applied Sciences, 9 (9), 1837.
Ibáñez, M. B., Di Serio, Á., Villarán, D., & Kloos, C. D. (2014). Experimenting with electromagnetism using augmented reality: Impact on flow student experience and educational effectiveness. Computers & Education, 71 , 1–13.
Ip, H. H., Wong, S. W., Chan, D. F., Byrne, J., Li, C., Yuan, V. S., Lau, K. S., & Wong, J. Y. (2018). Enhance emotional and social adaptation skills for children with autism spectrum disorder: A virtual reality enabled approach. Computers & Education, 117 , 1–15.
Ishiguro, H., Ono, T., Imai, M., Maeda, T., Kanda, T., & Nakatsu, R. (2001). Robovie: An interactive humanoid robot. Industrial Robot: An International Journal, 28 (6), 498–504.
Jensen, E., Dale, M., Donnelly, P. J., Stone, C., Kelly, S., Godley, A., & D'Mello, S. K. (2020). Toward automated feedback on teacher discourse to enhance teacher learning. In Proceedings of the 2020 CHI Conference on Human Factors in Computing Systems .
Jensen, E., L. Pugh, S., & K. D'Mello, S. (2021). A deep transfer learning approach to modeling teacher discourse in the classroom. In LAK21: 11th International Learning Analytics and Knowledge Conference (pp. 302–312).
Johal, W., Castellano, G., Tanaka, F., & Okita, S. (2018). Robots for learning. International Journal of Social Robotics, 10 (3), 293–294.
Jones, A., & Castellano, G. (2018). Adaptive robotic tutors that support self-regulated learning: A longer-term investigation with primary school children. International Journal of Social Robotics, 10 (3), 357–370.
Karri, S. P. R., & Kumar, B. S. (2020). Deep learning techniques for implementation of chatbots. In 2020 International Conference on Computer Communication and Informatics (pp. 1–5).
Kasapakis, V., Gavalas, D., & Dzardanova, E. (2019). Mixed Reality .
Kaufmann, H., & Schmalstieg, D. (2002). Mathematics and geometry education with collaborative augmented reality. In ACM SIGGRAPH 2002 conference abstracts and applications.
Kaviyaraj, R., & Uma, M. (2021). A Survey on Future of Augmented Reality with AI in Education. In 2021 International Conference on Artificial Intelligence and Smart Systems (pp. 47–52).
Kawaguchi, Y., Shoji, T., Lin, W., Kakusho, K., & Minoh, M. (2005). Face recognition-based lecture attendance system. In The 3rd AEARU workshop on network education (pp. 70–75).
Kc, D. (2017). Evaluation of moodle features at kajaani university of applied sciences–case study. Procedia Computer Science, 116 , 121–128.
Kennedy, J., Baxter, P., & Belpaeme, T. (2015). Comparing robot embodiments in a guided discovery learning interaction with children. International Journal of Social Robotics, 7 (2), 293–308.
Kerr, J., & Lawson, G. (2020). Augmented reality in design education: Landscape architecture studies as AR experience. International Journal of Art & Design Education, 39 (1), 6–21.
Kim, J., & Shim, J. (2022). Development of an AR-based AI education app for non-majors. IEEE Access, 10 , 14149–14156.
Knudsen, C. J., & Naeve, A. (2002). Presence production in a distributed shared virtual environment for exploring mathematics. In Advanced Computer Systems (pp. 149–159).
Kollia, I., & Siolas, G. (2016). Using the IBM Watson cognitive system in educational contexts. In 2016 IEEE Symposium Series on Computational Intelligence (pp. 1–8).
Kong, Y., & Fu, Y. (2022). Human action recognition and prediction: A survey. International Journal of Computer Vision, 130 (5), 1366–1401.
Krüger, J. M., Buchholz, A., & Bodemer, D. (2019). Augmented reality in education: three unique characteristics from a user’s perspective. In Proc. 27th Int. Conf. on Comput. in Educ .
Lau, T. B., Ong, A. C., & Putra, F. A. (2014). Non-invasive monitoring of people with disabilities via motion detection. International Journal of Signal Processing Systems, 2 (1), 37–41.
Lee, J. H., Yang, H., Shin, D., & Kim, H. (2020). Chatbots .
Li, X., Wang, M., Zeng, W., & Lu, W. (2019). A students’ action recognition database in smart classroom. In 2019 14th International Conference on Computer Science & Education.
Li, C., Xing, W., & Leite, W. (2021). Using fair AI with debiased network embeddings to support help seeking in an online math learning platform. In International Conference on Artificial Intelligence in Education (pp. 245–250).
Lin, H., Xie, S., Xiao, Z., Deng, X., & Cai, K. (2019). Adaptive Recommender System for an Intelligent Classroom Teaching Model. International journal of emerging technologies in learning , 14 (5).
Liono, R. A., Amanda, N., Pratiwi, A., & Gunawan, A. A. (2021). A systematic literature review: Learning with visual by the help of augmented reality helps students learn better. Procedia Computer Science, 179 , 144–152.
Liu, S., Chen, Y., Huang, H., Xiao, L., & Hei, X. (2018). Towards smart educational recommendations with reinforcement learning in classroom. In 2018 IEEE International Conference on Teaching, Assessment, and Learning for Engineering (pp. 1079–1084).
Lo, C. K., Hew, K. F., & Chen, G. (2017). Toward a set of design principles for mathematics flipped classrooms: A synthesis of research in mathematics education. Educational Research Review, 22 , 50–73.
Loos, R. (2015). CoWriter: Children using robots to learn writing. Robotics Today, March , 8 .
Lu, S. J., & Liu, Y. C. (2015). Integrating augmented reality technology to enhance children’s learning in marine education. Environmental Education Research, 21 (4), 525–541.
Lui, M., & Slotta, J. D. (2014). Immersive simulations for smart classrooms: exploring evolutionary concepts in secondary science. Technology, Pedagogy and Education .
Manca, S., Caviglione, L., & Raffaghelli, J. (2016). Big data for social media learning analytics: potentials and challenges. Journal of e-Learning and Knowledge Society , 12 (2).
Martín-Gutiérrez, J., Mora, C. E., Añorbe-Díaz, B., & González-Marrero, A. (2017). Virtual technologies trends in education. Eurasia Journal of Mathematics, Science and Technology Education, 13 (2), 469–486.
Mateu, J., Lasala, M. J., & Alamán, X. (2015). Developing mixed reality educational applications: The virtual touch toolkit. Sensors, 15 (9), 21760–21784.
Mery, D., Mackenney, I., & Villalobos, E. (2019). Student attendance system in crowded classrooms using a smartphone camera. In 2019 IEEE Winter Conference on Applications of Computer Vision (pp. 857–866)
Michalsky, T. (2021). Integrating video analysis of teacher and student behaviors to promote Preservice teachers’ teaching meta-strategic knowledge. Metacognition and Learning, 16 (3), 595–622.
Mintz, R., Litvak, S., & Yair, Y. (2001). 3D-virtual reality in science education: An implication for astronomy teaching. Journal of Computers in Mathematics and Science Teaching, 20 (3), 293–305.
Mircea, M., Stoica, M., & Ghilic-Micu, B. (2021). Investigating the impact of the internet of things in higher education environment. IEEE Access, 9 , 33396–33409.
Morrissey, K., & Kirakowski, J. (2013). ‘Realness’ in chatbots: establishing quantifiable criteria. In International conference on human-computer interaction (pp. 87–96).
Moseley, S., & Ajani, T. (2015). USERS'PERCEPTIONS ON THE BRIGHTSPACE LEARNING MANAGEMENT SYSTEM. Issues in Information Systems , 16 (4).
Mubin, O., Stevens, C. J., Shahid, S., Al Mahmud, A., & Dong, J. J. (2013). A review of the applicability of robots in education. Journal of Technology in Education and Learning, 1 (209–0015), 13.
Mystakidis, S. (2022). Metaverse. Encyclopedia, 2 (1), 486–497.
Ngoc Anh, B., Tung Son, N., Truong Lam, P., Phuong Chi, L., Huu Tuan, N., Cong Dat, N., Huu Trung, N., Umar Aftab, M., & Van Dinh, T. (2019). A computer-vision based application for student behavior monitoring in classroom. Applied Sciences, 9 (22), 4729.
Okita, S. Y., Ng-Thow-Hing, V., & Sarvadevabhatla, R. (2009). Learning together: ASIMO developing an interactive learning partnership with children. In RO-MAN 2009-The 18th IEEE International Symposium on Robot and Human Interactive Communication .
Osada, J., Ohnaka, S., & Sato, M. (2006). The scenario and design process of childcare robot, PaPeRo. In Proceedings of the 2006 ACM SIGCHI international conference on Advances in computer entertainment technology (pp. 80-es).
Ouyang, X., & Zhou, J. (2019). How to help older adults move the focus on a smart TV? Exploring the effects of arrow hints and element size consistency. International Journal of Human-Computer Interaction, 35 (15), 1420–1436.
Ouyang, X., Zhou, J., & Xiang, H. (2021). Screen mirroring is not as easy as it seems: A closer look at older adults’ cross-device experience through touch gestures. International Journal of Human-Computer Interaction, 37 (12), 1173–1189.
Pakanati, D., Thanner, G., & Reddy, R. R. (2020). Design of College Chatbot using Amazon Web Services .
Palanisamy, P., Paavizhi, K., & Saravanakumar, A. R. (2020). Techno pedagogical skills for teaching-learning process in smart class. Talent Development & Excellence, 12 (1), 4984–4994.
Parambil, M. M. A., Ali, L., Alnajjar, F., & Gochoo, M. (2022). Smart classroom: A deep learning approach towards attention assessment through class behavior detection. Advances in Science and Engineering Technology International Conferences (ASET), 2022 , 1–6. https://doi.org/10.1109/ASET53988.2022.9735018
Parmar, D., Isaac, J., Babu, S. V., D'Souza, N., Leonard, A. E., Jörg, S., Gundersen, K. & Daily, S. B. (2016). Programming moves: Design and evaluation of applying embodied interaction in virtual environments to enhance computational thinking in middle school students. In 2016 IEEE Virtual Reality (VR) (pp. 131–140).
Pereira, J. (2016). Leveraging chatbots to improve self-guided learning through conversational quizzes. In Proceedings of the fourth international conference on technological ecosystems for enhancing multiculturality (pp. 911–918).
Pham, T. V., Nguyen, A. T. T., Ngo, T. D., Le, D. H., Le, K. C., Nguyen, T. H., & Le, H. Q. (2020, November). Proposed smart university model as a sustainable living lab for university digital transformation. In 2020 5th International Conference on Green Technology and Sustainable Development (pp. 472–479)
Poppinga, B., & Laue, T. (2019). JET-Net: Real-time object detection for mobile robots. In Robot World Cup (pp. 227–240).
Potode, A., & Manjare, P. (2015). E-learning using artificial intelligence. International Journal of Computer Science and Information Technology Research, 3 (1), 78–82.
Queiroz, A. C. M., Fauville, G., Herrera, F., Da Leme, M. I. S., & Bailenson, J. N. (2022). Do students learn better with immersive virtual reality videos than conventional videos? A comparison of media effects with middle school girls. Technology, Mind, and Behavior . https://doi.org/10.1037/tmb0000082
Rashmi, M., Ashwin, T. S., & Guddeti, R. M. R. (2021). Surveillance video analysis for student action recognition and localization inside computer laboratories of a smart campus. Multimedia Tools and Applications, 80 (2), 2907–2929.
Reeves, L. E., Bolton, E., Bulpitt, M., Scott, A., Tomey, I., Gates, M., & Baldock, R. A. (2021). Use of augmented reality (AR) to aid bioscience education and enrich student experience. Research in Learning Technology, 29 , 2572.
Rufai, M. M., Alebiosu, S. O., & Adeakin, O. A. S. (2015). A conceptual model for virtual classroom management. International Journal of Computer Science, Engineering and Information Technology, 5 (1), 27–32.
Sadiku, M. N., Ashaolu, T. J., Ajayi-Majebi, A., & Musa, S. M. (2021). Artificial intelligence in education. International Journal of Scientific Advances, 2 (1), 5–11.
Sahlström, F., Tanner, M., & Valasmo, V. (2019). Connected youth, connected classrooms. Smartphone use and student and teacher participation during plenary teaching. Learning, Culture and Social Interaction, 21 , 311–331.
Sahu, C. K., Young, C., & Rai, R. (2021). Artificial intelligence (AI) in augmented reality (AR)-assisted manufacturing applications: A review. International Journal of Production Research, 59 (16), 4903–4959.
Saini, M. K., & Goel, N. (2019). How smart are smart classrooms? A review of smart classroom technologies. ACM Computing Surveys, 52 (6), 1–28.
Salichs, M. A., Barber, R., Khamis, A. M., Malfaz, M., Gorostiza, J. F., Pacheco, R., Rivas, R., Corrales, A., Delgado, E., & Garcia, D. (2006). Maggie: A robotic platform for human-robot social interaction. In 2006 IEEE conference on robotics, automation and mechatronics (pp. 1–7)
Sampaio, A. Z., Ferreira, M. M., Rosário, D. P., & Martins, O. P. (2010). 3D and VR models in civil engineering education: Construction, rehabilitation and maintenance. Automation in Construction, 19 (7), 819–828.
Sejnowski, T. J. (2020). The unreasonable effectiveness of deep learning in artificial intelligence. Proceedings of the National Academy of Sciences, 117 (48), 30033–30038.
Shan, S., & Liu, Y. (2021). Blended teaching design of college students’ mental health education course based on artificial intelligence flipped class. Mathematical Problems in Engineering , 2021 .
Shiomi, M., Kanda, T., Howley, I., Hayashi, K., & Hagita, N. (2015). Can a social robot stimulate science curiosity in classrooms? International Journal of Social Robotics, 7 (5), 641–652.
Singh, J., Joesph, M. H., & Jabbar, K. B. A. (2019). Rule-based chatbot for student enquiries. In Journal of Physics: Conference Series, 1228 (1), 012060.
Soares, N., Kay, J. C., & Craven, G. (2017). Mobile robotic telepresence solutions for the education of hospitalized children. Perspectives in Health Information Management , 14 .
Sobota, B., Korečko, Š., Jacho, L., Pastornický, P., Hudák, M., & Sivý, M. (2017). Virtual-reality technologies and smart environments in the process of disabled people education. In 2017 15th International Conference on Emerging eLearning Technologies and Applications.
Southgate, E., Blackmore, K., Pieschl, S., Grimes, S., McGuire, J., & Smithers, K. (2019). Artificial intelligence and emerging technologies in schools .
Srinivasan, R., & González, B. S. M. (2022). The role of empathy for artificial intelligence accountability. Journal of Responsible Technology, 9 , 100021.
Stavroulia, K. E., Ruiz-Harisiou, A., Manouchou, E., Georgiou, K., Sella, F., & Lanitis, A. (2016). A 3D virtual environment for training teachers to identify bullying. In 2016 18th Mediterranean Electrotechnical Conference (pp. 1–6).
Teja, S. V. (2020). Chatbot using deep learning. Academic Leadership-Online Journal .
Thomas, C., & Jayagopi, D. B. (2017). Predicting student engagement in classrooms using facial behavioral cues. In Proceedings of the 1st ACM SIGCHI International Workshop on Multimodal Interaction for education (pp. 33–40).
Tian, X., Risha, Z., Ahmed, I., Lekshmi Narayanan, A. B., & Biehl, J. (2021). Let's talk it out: A chatbot for effective study habit behavioral change. In Proceedings of the ACM on Human-Computer Interaction (pp.1–32).
Timms, M. J. (2016). Letting artificial intelligence in education out of the box: educational cobots and smart classrooms. International Journal of Artificial Intelligence in Education .
Tolentino, L., Birchfield, D., & Kelliher, A. (2009). SMALLab for special needs: Using a mixed-reality platform to explore learning for children with autism. In Proceedings of the NSF Media Arts, Science and Technology Conference, Santa Barbara, CA, USA (pp. 29–30).
Torres, D. R. (2011). Realidad Aumentada, educación y museos. Revista ICONO 14. Revista científica de Comunicación y Tecnologías emergentes , 9 (2), 212–226.
TS, A., & Guddeti, R. M. R. (2020). Automatic detection of students’ affective states in classroom environment using hybrid convolutional neural networks. Education and Information Technologies, 25 (2), 1387–1415.
Valdez, M. T., Ferreira, C. M., Martins, M. J. M., & Barbosa, F. M. (2015). 3D virtual reality experiments to promote electrical engineering education. In 2015 International Conference on Information Technology Based Higher Education and Training (pp. 1–4).
Vega, J., & Cañas, J. M. (2019). Open vision system for low-cost robotics education. Electronics, 8 (11), 1295.
Vimal, P. P., & Kumbharana, C. K. (2016). Analysis of different examination patterns having question answer formulation, evaluation techniques and comparison of MCQ type with one word answer for automated online examination. International Journal of Scientific and Research Publications , 6 (3).
Waheed, H., Hassan, S. U., Aljohani, N. R., Hardman, J., Alelyani, S., & Nawaz, R. (2020). Predicting academic performance of students from VLE big data using deep learning models. Computers in Human Behavior, 104 , 106189.
Wang, R., Zhang, G., Zhang, F., Dong, Z., & Qi, M. (2021). Student Behavior Recognition in Remote Video Classrooms. In Advances in Intelligent Information Hiding and Multimedia Signal Processing (pp. 496–504).
Warschauer, M., & Grimes, D. (2008). Automated writing assessment in the classroom. Pedagogies: an International Journal, 3 (1), 22–36.
Weibel, M., Nielsen, M. K. F., Topperzer, M. K., Hammer, N. M., Møller, S. W., Schmiegelow, K., & Bækgaard Larsen, H. (2020). Back to school with telepresence robot technology: A qualitative pilot study about how telepresence robots help school-aged children and adolescents with cancer to remain socially and academically connected with their school classes during treatment. Nursing Open, 7 (4), 988–997.
Werner-Seidler, A., Perry, Y., Calear, A. L., Newby, J. M., & Christensen, H. (2017). School-based depression and anxiety prevention programs for young people: A systematic review and meta-analysis. Clinical Psychology Review, 51 , 30–47.
West, D. M. (2018). The future of work: Robots, AI, and automation . Brookings Institution Press.
Woo, H., LeTendre, G. K., Pham-Shouse, T., & Xiong, Y. (2021). The use of social robots in classrooms: A review of field-based studies. Educational Research Review, 33 , 100388.
Wu, X., Yang, Y., Yu, X., & Zheng, C. (2020). Intelligent Classroom System Based on Internet of Things Technology. In International Conferences on Big Data Analytics for Cyber-Physical-Systems (pp. 610–616).
Yang, S., & Chen, L. (2011). A face and eye detection based feedback system for smart classroom. In Proceedings of 2011 International Conference on Electronic & Mechanical Engineering and Information Technology (Vol. 2, pp. 571–574).
Ye, L., Wang, P., Wang, L., Ferdinando, H., Seppänen, T., & Alasaarela, E. (2018). A combined motion-audio school bullying detection algorithm. Intlernational Journal of Pattern Recognition and Artificial Intelligence, 32 (12), 1850046.
Yoon, S. A., Elinich, K., Wang, J., Steinmeier, C., & Tucker, S. (2012). Using augmented reality and knowledge-building scaffolds to improve learning in a science museum. International Journal of Computer-Supported Collaborative Learning, 7 (4), 519–541.
Zhou, F., Duh, H. B. L., & Billinghurst, M. (2008). Trends in augmented reality tracking, interaction and display: A review of ten years of ISMAR. In 2008 7th IEEE/ACM Intl. Symposium on Mixed and Augmented Reality (pp. 193–202).
Zulkifli, N. A., & Rozimela, Y. (2021). Online applications to support remote classroom dialogue and assessment. Journal of Physics: Conference Series, 1779 (1), 012039.
Download references
Acknowledgements
This project has received funding from the European Union’s Horizon 2020 Research and Innovation Programme under Grant Agreement No 739578 and the Government of the Republic of Cyprus through the Directorate General for European Programmes, Coordination and Development.
This project has received partial funding from the European Union’s Horizon 2020 Research and Innovation Programme under Grant Agreement No 739578 and the Government of the Republic of Cyprus through the Directorate General for European Programmes, Coordination and Development.
Author information
Authors and affiliations.
Visual Media Computing Lab, Department of Multimedia and Graphic Arts, Cyprus University of Technology, Limassol, Cyprus
Eleni Dimitriadou & Andreas Lanitis
CYENS Centre of Excellence, Nicosia, Cyprus
You can also search for this author in PubMed Google Scholar
Contributions
ED carried out the literature review and authored the paper under the supervision of AL. Both authors read and approved the final manuscript
Corresponding author
Correspondence to Eleni Dimitriadou .
Ethics declarations
Ethics approval and consent to participate.
Not applicable–This is a survey paper that does not require ethics approval.
Consent for publication
All data generated or analysed during this study are included in this published article.
Competing interests
The authors have no financial or proprietary interests in any material discussed in this article.
Additional information
Publisher's note.
Springer Nature remains neutral with regard to jurisdictional claims in published maps and institutional affiliations.
Rights and permissions
Open Access This article is licensed under a Creative Commons Attribution 4.0 International License, which permits use, sharing, adaptation, distribution and reproduction in any medium or format, as long as you give appropriate credit to the original author(s) and the source, provide a link to the Creative Commons licence, and indicate if changes were made. The images or other third party material in this article are included in the article's Creative Commons licence, unless indicated otherwise in a credit line to the material. If material is not included in the article's Creative Commons licence and your intended use is not permitted by statutory regulation or exceeds the permitted use, you will need to obtain permission directly from the copyright holder. To view a copy of this licence, visit http://creativecommons.org/licenses/by/4.0/ .
Reprints and permissions
About this article
Cite this article.
Dimitriadou, E., Lanitis, A. A critical evaluation, challenges, and future perspectives of using artificial intelligence and emerging technologies in smart classrooms. Smart Learn. Environ. 10 , 12 (2023). https://doi.org/10.1186/s40561-023-00231-3
Download citation
Received : 29 August 2022
Accepted : 31 January 2023
Published : 06 February 2023
DOI : https://doi.org/10.1186/s40561-023-00231-3
Share this article
Anyone you share the following link with will be able to read this content:
Sorry, a shareable link is not currently available for this article.
Provided by the Springer Nature SharedIt content-sharing initiative
- Educational technology
- Artificial intelligence
- Emerging technologies
- Smart classroom
Thank you for visiting nature.com. You are using a browser version with limited support for CSS. To obtain the best experience, we recommend you use a more up to date browser (or turn off compatibility mode in Internet Explorer). In the meantime, to ensure continued support, we are displaying the site without styles and JavaScript.
- View all journals
- Explore content
- About the journal
- Publish with us
- Sign up for alerts
- Open access
- Published: 05 June 2024
Classroom communications post pandemic: a case study
- Marwa Abdelmonem 1 &
- Sherin Karawia 1
Humanities and Social Sciences Communications volume 11 , Article number: 728 ( 2024 ) Cite this article
407 Accesses
1 Altmetric
Metrics details
- Environmental studies
This cross-sectional case study ( n = 20) took place in Qatar over a 3-month period spanning from September until the end of November in 2021. It examined the impacts of a post-COVID classroom arrangement that incorporated preventive measures like social distancing and the use of face coverings. Using the case study methodology, we employed two qualitative research methods, namely non-participant observation and semi-structured interviews, to gather primary data. We also conducted an extensive literature review to identify the key themes for investigation in the field. The primary focus of our study was on proxemics (personal space and crowding), technology (Zoom), non-verbal communication, and ergonomics (lighting and acoustics). Our study uncovered new areas for future research, particularly in how proxemics change in socially distanced classrooms, affecting personal space and perceptions of crowding. Additionally, it highlighted themes in post-pandemic classroom design, emphasizing the impact of factors like seating arrangements and available amenities (e.g., pinup walls) on student engagement, especially in design-studio classrooms. The study also revealed unexpected challenges, such as issues with microphone usage due to users’ lack of familiarity with their operation. In summary, the research underscores the ongoing importance of adaptable learning methods and communication strategies, particularly in response to preventive measures like social distancing and face-covering, which have reshaped our concept of personal space and emphasized the crucial role of telecommunication in crisis resilience for communities and organizations.
Similar content being viewed by others
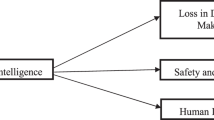
Impact of artificial intelligence on human loss in decision making, laziness and safety in education
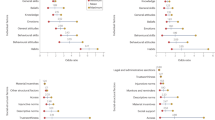
Determinants of behaviour and their efficacy as targets of behavioural change interventions
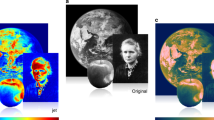
The misuse of colour in science communication
Introduction.
The COVID-19 pandemic, caused by the novel coronavirus (SARS-CoV-2), was declared a global pandemic by the World Health Organization (WHO) due to its rapid spread to multiple countries ( WHO Director-General’s Opening Remarks at the Media Briefing on COVID-19 - 11 March 2020 , 2020 ). It is important to note that COVID-19 is not an isolated event in history, as previous pandemics like the 1918 American Polio epidemic, the 1918 Spanish flu, the 1957 Asian flu, the 1981 AIDS pandemic, the 2009 H1N1 Swine flu, the 2014 West African Ebola, and the 2014 Zika Virus epidemic have occurred (Cennimo, 2020 ; Jarus, 2023 ). COVID-19 can have severe health consequences, particularly for the elderly and those with pre-existing health conditions (World Health Organization, n.d. ) .
Preventing the transmission of COVID-19 involves a combination of physical and medical interventions. The WHO recommends physical measures such as handwashing, social distancing, and wearing face coverings (Centers for Disease Control and Prevention, n.d. ). Medical interventions include vaccines, although their long-term effectiveness is still being studied (Frequently Asked Questions about COVID-19 Vaccination, 2023 ). Vaccines have proven effective in reducing hospitalization rates by 92–96% (Robson, 2021 ), but ongoing research is examining their long-term impacts.
The COVID-19 pandemic led to significant changes in the operations of various institutions, including educational establishments. Measures like remote work and restricted access to buildings were implemented, and as vaccines were developed, some restrictions were lifted. In Qatar, strict COVID-19 measures included travel bans and public place closures, and the use of the Ehteraz app for exposure alerts (Al Khal et al., 2020 ). Mandatory face masks, protective equipment distribution, and clear public communication highlighted preventive measures, linking access to public facilities with a healthy Ehteraz status during the lockdown (Al Khal et al., 2020 ). The gradual reopening and removal of restrictions occurred in four phases, commencing on May 21, 2021 (Government Communications Office, 2020 ). Our study coincided with the second phase, marked by the partial lifting of restrictions, enabling a return to campus within designated daily hours and specific building capacities that varied across different structures.
The transition back to in-person learning has brought challenges in ensuring safety in alignment with WHO guidelines, including social distancing measures within classrooms. Given the mixed sentiments surrounding the reopening, communication within the classroom under setups adhering to WHO guidelines was unforeseen and unfamiliar to everyone. With varied opinions on the reopening, communication within classrooms following WHO guidelines was unexpected. Hence, our research study aimed to explore classroom communication post-online learning during the pandemic, as there is limited existing research on the effects of preventive measures on this aspect.
Student engagement is related to academic performance, and a conducive learning environment plays a crucial role in fostering effective communication and learning (Shernoff et al., 2016 , as cited in Kelly, 2008; Kopec, 2012 ). Therefore, this study aims to investigate the effects of preventive measures like face-covering and social distancing on classroom communication in a post-pandemic, socially-distanced classroom setting. In essence, our study seeks to answer the research question: “What impact do preventive measures have on classroom communication within a post-pandemic classroom environment?” This study was conducted on two interconnected classrooms, which were intentionally furnished to comply with social distancing requirements and create a cohesive socially distanced learning environment, prompting further exploration of this phenomenon.
Literature review
To investigate how preventive measures might impact classroom communication dynamics, our study conducted a review of the literature to identify and extract certain themes from this body of work. These predetermined themes were integral to addressing the aforementioned research question. Out of more than 50 studies examined, only 29 were selected to form the basis of the literature review for this study. Additional sources were incorporated for specific definitions and concepts. The literature selection criteria included original works in environmental design pertaining to proxemics, exploration of blended learning and technology use, considerations in classroom design, and aspects of verbal and non-verbal communication in classrooms.
Personal space
Due to the physical nature of the social distancing measure, literature on personal space was reviewed to better understand the impact of the changing proxemics. The first scholar to introduce the concept of personal space was Edward Hall in 1959 (Beaulieu, 2004 , p. 794). Hall defined personal space as “an invisible three-dimensional zone surrounding a person, which allows that person to regulate his interactions with the outside world” (Beaulieu, 2004 , p. 294). Research shows that “the idea of personal space is actually an interpersonal phenomenon” (Kopec, 2012 ). In other words, it requires the existence of others in order to occur. This was the definition pertaining to the psychological nature of this entity. However, it is also defined based on its physical characteristics as the variable and subjective distance at which one person feels comfortable talking to another ( Dictionary.com | Meanings & Definitions of English Words , 2021 ).
According to Hall ( 1990 ), the hidden boundaries of personal space are not fixed and can vary from one culture to another, as culture plays an important role in defining personal space. Nevertheless, in a socially distanced classroom, personal space that was long referred to as an invisible bubble has become well-identified with its visible physical boundaries after the COVID-19 pandemic. Spacing mechanisms used in design, in general, and in a socially distanced classroom, in particular, serve to maintain one’s perception of their personal space (Kopec, 2012 ).
The importance of the concept of personal space lies in regulating communication and human interaction. Research shows that “the concept of personal space is viewed as variable and permeable in different settings” (Beaulieu, 2004 , p. 297). According to Hall ( 1990 ), interpersonal distance zones, which govern our interactions, can be categorized into four zones. The first category is the intimate, which ranges from 0 to 18”, typically reserved for individuals with close relationships, such as family members or individuals in deeply connected interpersonal bonds (Kopec, 2012 ). The second interpersonal zone is the personal which can range from 18” up to 4’ (Kopec, 2012 , p. 78). This zone is usually used in casual interactions between friends and acquaintances (Kopec, 2012 ). The third level is social , which is usually maintained in business relations between coworkers (Beaulieu, 2004 ) and ranges from 4’ to 12’ (Kopec, 2012 , p. 78). Lastly, the public zone which is usually used by people in public places where they find themselves surrounded by a number of strangers (Kopec, 2012 ). This zone varies from 12’ to 25’ (Kopec, 2012 . p. 78). In a recent study, Mehta ( 2020 ) investigated Hall’s proxemics in urban settings, focusing on changes in street and public space dynamics in residential areas during COVID-19. The research highlighted how residents in mid- to low-density areas repurpose spaces, fostering sociability despite social distancing measures.
In our study, we chose to explore the personal space perceived by participants in an attempt to analyze the various elements that define this concept within the new classroom setup. Our study was mostly exploratory and was not focused on a certain type of interpersonal distance.
Crowding concept
We also explored another concept in environmental psychology that is closely related to personal space, which is Crowding. In normal situations, the occupant load, which is “the number of people that a building code assumes will occupy a given building or a portion of a building”, is based on the building classification (Ballast, 2013 ). The number, placement, and capacity of exits are based on more than just occupant load. The type of occupancy and use, travel distance and remoteness or separation of exits are examples of other factors typically considered when designing a building.
As Kopec ( 2012 , p. 77) indicated, “spacing mechanisms used in design serve to help maintain an individual’s sense of personal space”. To put it differently, the use of physical boundaries such as partitions, furniture and layout allows the individual to identify their own personal space. Crowding, on the other hand, is defined as the “psychological tension produced in environments of high population density, especially when individuals feel that the amount of space available to them is insufficient for their needs” (American Psychological Association, n.d. ). In that sense, it can be argued that implementing social distancing in a classroom can potentially eliminate any sensation of crowding. However, our study examines this concept further, as the perception of crowding can be subjective in nature.
Some researchers such as Evans and Wener ( 2007 ) analyzed the concept of personal space and crowding in trains. According to their review of the literature, incursions into personal space by unfamiliar individuals lead to greater stress compared to those committed by individuals with positive interpersonal relationships. Evans and Wener ( 2007 ) suggested that crowding occurs when the regulation of social interaction fails, leading to a higher level of social interaction than desired. Worchel and Teddie ( 1976 ) also asserted that a feeling of overcrowding is associated with violations of personal space. Crowding can have negative impacts on mental health, causing decreased performance on complex tasks, stress aftermaths, and increased physiological stress (American Psychological Association, n.d. ). The lack of control over social interaction (i.e. privacy) and the decline of socially supportive relationships are considered to be two key mechanisms that contribute to crowding (American Psychological Association, n.d. ).
Upon reviewing the existing literature, specific inquiries emerge concerning the concept of crowding. Our principal emphasis lies in examining how the notion of crowding, closely intertwined with the concept of personal space, has evolved within a socially distanced classroom environment.
Communication
According to Wahyuni ( 2018 ), teachers are required to excel in specific nonverbal communication skills, which encompass body language, the utilization of eye contact, maintaining appropriate distance from students, and the use of touch. Wahyuni ( 2018 ), who further cited Darn (Darn, n.d. ), noted that nearly 75% of classroom management relies on nonverbal communication. In this research, our objective was to investigate the alterations in both verbal and nonverbal communication within the new setup. This change was prompted by the introduction of social distancing measures through the rearrangement of furniture and the adoption of Zoom as an auxiliary tool to facilitate communication between two interconnected classrooms.
Non-verbal communication
It is evident that nonverbal communication plays an important role in classroom interaction (Hall et al., 1977 ). French ( 1977 ) described the non-verbal experience as a multi-dimensional, multi-sensory experience. In his study, French ( 1977 ) emphasized the need for incorporating the non-verbal experience into curriculum development. “The non-verbal experience focused both on self and others”, which requires “an active engagement rather than a passive one” (French, 1977 , p. 176). French’s ( 1977 ) findings resonate well with Astin’s ( 1984 ) regarding the degree of student involvement. Astin ( 1984 ) defines student involvement as the extent of physical and psychological energy invested in the college experience, including academic absorption, extracurricular participation, and interaction with faculty. Education literature distinguishes between student engagement and involvement. Engagement , according to Campus Intelligence (Gay, 2019 ), entails dedicating time and effort to educationally purposeful activities, surpassing mere involvement. Engaged students actively pursue endeavors that contribute to their educational goals, going beyond classroom actions like note-taking and questioning. In their recent research, Walker and Koralesky ( 2021 ) employed the definition of student engagement found in various scholarly reviews, indicating that student engagement is a multi-dimensional construct with three interconnected dimensions: affective, behavioral, and cognitive engagement (Walker and Koralesky, 2021 ; as cited in Chapman, 2002; Fredricks et al., 2004 ; Mandernach, 2015 ; Trowler, 2010 ). Our comprehension of the distinction between these two terms aided us in pinpointing the themes of observation. This is because engagement, in this context, is not the behavior we are focusing on; instead, we are interested in examining classroom dynamics and communication.
Hall et al. ( 1977 ) indicated that non-verbal communication involves a sender and a recipient. Hall et al. ( 1977 ) highlighted that there are some variables that make this sending-receiving type of interaction more effective. Among those variables that Hall et al. ( 1977 ) listed is the “climate”, which refers to how friendly the teacher is in interacting with students. Students are encouraged to actively participate in class discussions if they receive these cues about friendliness and positivity through the sender’s facial expressions and voice tone (Hall et al., 1977 ). This coincides with Zeki’s ( 2009 ) findings that the teacher’s non-verbal communication creates a comfortable and relaxing climate, which consequently enables the students to effectively and confidently engage in class discussions.
In the literature, we discovered another element that affects the student’s engagement in class, which is the locus of control (Astin, 1984 ; Richmond and McCroskey, 2012 , p. 13). According to Astin ( 1984 ), “students’ degree of involvement in learning tasks can be influenced by whether they believe that their behavior is controlled by internal or by external factors.” This is related to belief systems, which are often rooted in one’s locus of control (Kopec, 2012 ). Those who have “a strong external locus of control believe that they are controlled by external forces” (Kopec, 2012 , p. 98). On the other hand, people who have an “internal locus of control believe that their actions, choices, and pursuits control their destiny” (Kopec, 2012 , p. 98). This can be an indicative measure of the student’s level of motivation (Zeki, 2009 ). A number of studies (Zeki, 2009 ; Kopec, 2012 ; Hall, 1959 ) stated that the components of non-verbal communication include, but are not limited to; facial expressions (i.e. mimics), eye contact, angulation, and gestures (i.e. body language). The amount of information one receives from their surroundings comes from all senses but mainly from the eyes and ears (Zeki, 2009 ). This highlights the importance of visual and auditory environmental cues in a classroom.
Verbal communication
Additionally, we sought to comprehend the influence of the new environment on verbal communication. Consequently, we delved into the literature pertaining to physical ergonomics, such as classroom layout, acoustics, and lighting within the field of environmental design.
Classroom layout
Through an examination of early literature centered on the physical ergonomics of classroom configurations, we came across a notable study conducted by Sommer ( 1977 ) that underscored the significance of classroom layout as a means of non-verbal communication. Sommer emphasized that a classroom should not be viewed as a single homogeneous space but rather as a collection of interconnected micro-environments. Elements such as windows, lighting, heating vents, blackboards, and display equipment were identified as factors influencing interactions within the classroom. Additionally, the arrangement of classroom furniture was found to impact body angulation, which in turn affects nonverbal communication (Kopec, 2012 ).
In the realm of classroom design, the establishment of structures known as “affordances” gives rise to opportunities for engagement or limitations in that regard (Metzger and Langley, 2020 ). Affordances pertain to both the perceived and tangible characteristics of objects or surroundings, influencing how they can be employed (Metzger and Langley, 2020 , as cited in Gibson, 1979). In other words, these affordances represent resources within an environment for those who perceive and interact with them. In the context of our study, affordances are represented by movable chairs, movable tables, Zoom technology, and microphones. These affordances are meant to enhance communication while also imposing limitations on social proximity.
Verbal communication in a classroom involves the teacher conveying speech to students through a combination of direct and reflected sound (Berg et al., 1996 ). Effective acoustics are achieved when the reflected sound enhances the quality of the direct sound (Berg et al., 1996 ). Sound within the classroom space exhibits three behaviors: reflection off obstacles, diffraction around obstacles, and transmission into obstacles or new media (Timeline: Sound Wave, n.d. ). Reflected sound can lead to two issues: echoes or reverberation (Timeline: Sound Wave, n.d. ). An echo occurs when a reflected sound wave reaches the ear more than 0.1 s after the original sound wave was heard (Timeline: Sound Wave, n.d. ). On the other hand, reverberation is the prolongation of sound as it repeatedly bounces off hard surfaces (Ballast, 2013 ). Reverberation is generally preferred in classroom acoustics as it enhances speech intelligibility in the interior environment (Ballast, 2013 ). To achieve good acoustics in a classroom, a well-designed space includes an appropriate combination of absorbent, reflective, and diffusive materials (Berg et al., 1996 ).
Classroom acoustics can be affected by sound transmission through barriers, such as HVAC systems and external noise from corridors or streets (Ballast, 2013 ). Research efforts have been directed towards exploring issues such as listening problems among special students, student disengagement, the teacher’s voice, and teacher fatigue (Berg et al., 1996 ). This information is relevant to our study as it pertains to the investigation of a socially distanced classroom setup. We also aimed to investigate acoustics because of its significant impact on both verbal and nonverbal communication, especially when considering the use of face coverings.
Daytime light exposure, particularly from sunlight, full-spectrum LEDs, and digital screens, is significant for learning as it affects both vision and a non-visual system tied to sleep-wake cycles and cognitive performance. Blue light has the most powerful impact on alertness and reduced sleepiness compared to other wavelengths, influencing hormonal secretion in the non-visual system (Uncapher, 2016 ).
In their case study, Tureková et al. ( 2018 ) aimed to show how the school environment significantly affects students’ academic performance. They found that factors like accurate lighting influence visual comfort and fatigue during visual tasks. Assessing the daylight factor in a university classroom revealed insufficient values, prompting the design of a potential software-based alternative to improve visual comfort. They also highlighted that natural light’s impact goes beyond academics, influencing physiological, psychological, and behavioral aspects of both students and workers (Tureková et al., 2018 ).
Al-Sallal ( 2010 ) studied daylighting in UAE classrooms, analyzing visual performance and quality. His research involved data collection from architectural drawings, compliance documents, site visits, and photography. Crucial design issues affecting visual quality, such as space size, depth-to-height ratio, window orientation, lighting direction, and desk position, were investigated through simulation. The study revealed design issues impacting daylighting and visual quality, including depth, glazing area, orientation, and daylighting direction. Visual discomfort was found due to contrasting luminance, high window brightness, and uneven daylight distribution. Mitigation strategies discussed focused on solar shading, glare protection, and daylight redirection, considering UAE climate design requirements. This study holds great relevance to our research because Qatar and the UAE share a similar climate. Our objective was to investigate whether there are any unexpected issues related to lighting in the new setup.
Joia and Lorenzo ( 2021 ) investigated the effectiveness of using Zoom, a popular choice for virtual communication during the pandemic (Joia and Lorenzo, 2021 ). The study’s findings emphasize the importance of a teacher’s digital proficiency on the platform and the availability of metacognitive support within the digital environment as key factors in achieving successful pedagogical objectives. Additionally, the research highlights that disciplines involving hard skills are more prone to not meeting their educational goals when transitioning to technology-mediated environments compared to soft skill disciplines undergoing a similar transition (Joia and Lorenzo, 2021 ).
Another study highlighted the benefits of video communication platforms, including expanding educational reach and enabling multimedia-based pedagogy (Earon, n.d. ). Online learning via Zoom showed satisfactory student levels in a study conducted in Pakistan (Minhas et al., 2021 ), but some students reported dissatisfaction due to technical issues (Kim, 2020 ). While online learning cannot replace face-to-face instruction (Ramadani and Xhaferi, 2020 ; Alawamleh et al., 2020 ), instructors’ digital competence and metacognitive support on Zoom can enhance the online experience (Joia and Lorenzo, 2021 ). The potential of using Zoom in a socially distanced classroom requires further exploration, given the COVID-19 pandemic’s impact and new social distancing measures. In our study, we aim to explore the use of Zoom as a supplementary communication medium in socially distanced classrooms.
To bridge the research gap on socially distanced classrooms that are specific to the context of Qatar, we embraced the philosophy of Epistemic Contextualism, asserting that knowledge attribution varies based on context (Rysiew, 2021 ). Following Morgan et al.’s ( 2016 ) recommendation, we adopted the case study methodology, drawing inspiration from the Case Study Observational Research (CSOR) approach. This approach entails conducting observations before interviews to delve deeper into aspects within the contextual framework. Our choice of a case study methodology aligns with qualitative research principles, suitable for exploring new fields or theorizing important issues (Jamshed, 2014 ). Consequently, we employed two data collection methods: observations followed by semi-structured interviews. Semi-structured interviews, rooted in the belief in socially constructed reality, offer flexibility for cross-verification within and between interviews (Azungah, 2018 ). The main objective was to investigate the impact of socially distanced classrooms on student engagement and classroom communication. This cross-sectional study was conducted within a three-month timeframe. Data were analyzed using thematic analysis (Lawless and Chen, 2018 ) for both the observation and interviews. The study was conducted in a unique setting. Prior to the COVID-19 pandemic, the classroom was designed as a studio for a single group, separated into two interconnected classrooms, illustrated in Fig. 1 as Classroom A and Classroom B. Additionally, two vestibules were designated as break areas for students. Classroom A had a dual function, serving as both a studio space and a location where students assembled their tables in the center for lectures. However, in response to the COVID-19 pandemic, social distancing measures were implemented, mandating that each student remain in their assigned seat even during lectures. In other words, students were assigned to design-studio classrooms A and B. The instructor’s workstation, which contained all the necessary equipment, was located in classroom A. As a result, Classroom A was equipped with a TV screen for presentations, while Classroom B was equipped with a projector. Both the screen and the projector were connected to the audio/speakers’ area that was located next to the instructor’s workstation in Classroom A (see Fig. 2 ).
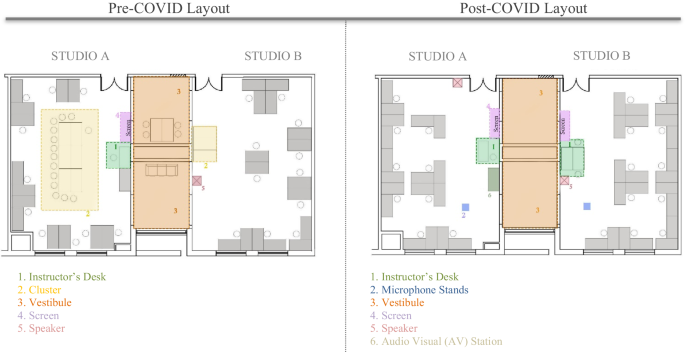
This figure demonstrates Pre- and Post-COVID layouts showcasing a floor plan of the two design-studio classrooms (A and B) interconnected by two vestibules, forming a unified socially distanced classroom. It also illustrates the locations of screens and instructor’s workstation in both classrooms.
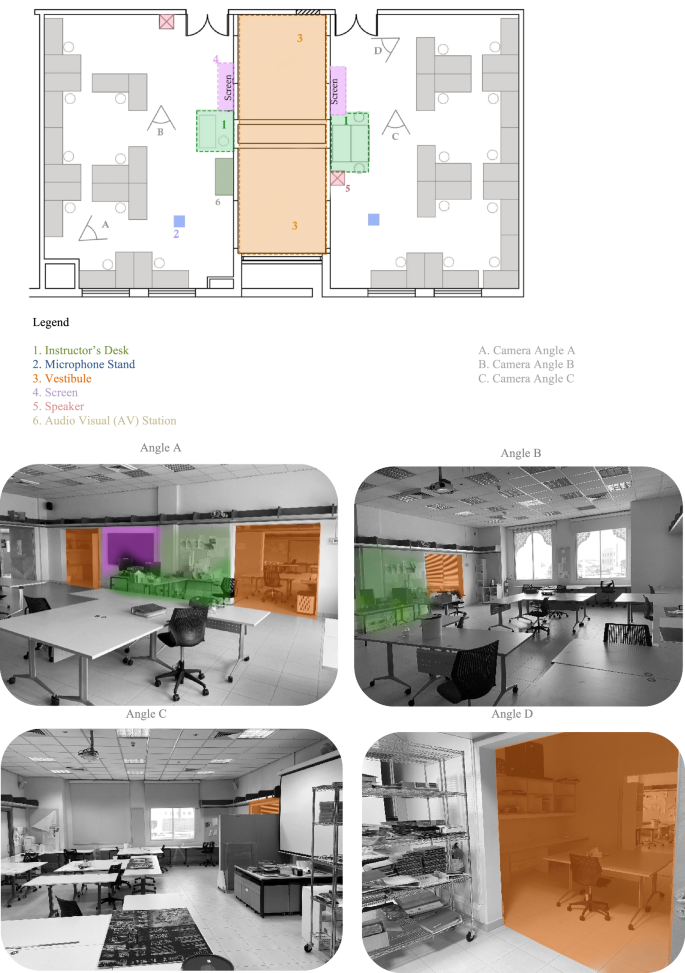
A floor plan depicting the layout of design-studio classrooms A and B , along with four perspectives from both classrooms viewed from various camera angles (angles A , B , C , and D ).
Non-participant observations
Observational documentation spanned three months, from September to November, commencing at the start of the semester before obtaining Institutional Review Board (IRB) approval for interviews, as no direct participant contact was required. Field notes encompassed written observations, hand sketches, and circulation maps, providing visual representations of classroom communications. The observer, the second researcher, attended the bi-weekly class sessions for approximately 60 min, ensuring comprehensive consideration of any potential confounding variables. The observation method was guided by findings from the literature review, shaping the framework for the study.
The observations involved observing students without actively participating, providing an overview of the setting and narrowing focus to specific elements of interest (Non-participant Observation, n.d. ). Students were closely monitored in their natural environments, with observations aligned with several themes derived from the literature review mentioned earlier. Field notes were recorded, specifically focusing on non-verbal communication aspects like angulation, body language, and gestures. Additionally, the observer examined any unanticipated data that had not been emphasized in the literature review. The aim of this method was to examine our predetermined themes extracted from the literature review and explore the emerging themes resulting from the new setup. Data were collected through field notes, sketches, and movement mapping over a period of three months.
Access to the classrooms was granted by both the Interim Chair of the Department and the Research Department. The first author served as the instructor for a lecture-based course during the research period, while the second author conducted the non-participant observations and subsequent semi-structured interviews. To maintain confidentiality, only data that had been de-identified and coded were provided to the first author for the purpose of data analysis.
It’s important to mention that we initially piloted a participant-observation method at the start of the semester, involving observations from both researchers. However, this proved impractical as the first researcher was also the course instructor, heavily engaged in fulfilling teaching obligations. Consequently, we opted for a non-participant observation approach, allowing one researcher to fully immerse in observing and documenting the identified variables from the literature review. Generally, “observational data can be integrated as auxiliary or confirmatory research” (Jamshed, 2014 , p. 88). Essentially, the use of observational data is intended to confirm the applicability of the predetermined themes identified in our literature review and to identify any unforeseen themes.
Semi-structured interviews
The second method utilized involved the conducting of face-to-face semi-structured interviews with college students who were pursuing a major in interior design. This was carried out following the approval granted by a national Institutional Review Board. Data was collected from a group of students ( n = 20) aged between 18 and 20 years old. Ultimately, a total of 17 interviews were conducted, with three participants choosing to withdraw due to scheduling conflicts that arose during the final weeks of the semester. The inclusion and exclusion criteria can be found below in Table 1 .
Interviews were recorded and transcribed verbatim, with each session lasting approximately 30 min (Jamshed, 2014 ). The interview questions, derived from the literature review and observations, were intentionally designed as semi-structured to provide participants with the opportunity to elaborate on their responses. The inquiries primarily consisted of open-ended questions, aiming to delve into participants’ authentic feelings and attitudes regarding the new setup. However, close-ended questions were also incorporated to ensure clarity and consistency in responses.
The interviewing process employed a funnel approach, focusing on building trust and rapport and presenting questions in an unbiased manner to minimize biases from both the interviewer and interviewee (Azungah, 2018 ).
Data analysis
We adopted the inductive/deductive hybrid thematic analysis approach. According to Proudfoot ( 2022 ), thematic analysis that combines elements of both inductive and deductive approaches presents promising prospects for researchers. We initiated the coding process with a deductive approach, employing pre-established themes crafted before engaging with the collected data. These themes were formulated based on the literature review findings, detailed in Tables 2 and 3 . As we analyzed the interview data, we identified new themes that emerged from the study. Consequently, we adopted an inductive coding approach to highlight these additional insights.
Observations
During a 3-month period, the various movements of communication behaviors were captured with sketches. Video or audio recordings were not viable options due to cultural considerations. The outcomes of the non-participant observations align with the previously identified findings from literature review as shown below in Table 2 .
Based on our field observations, the instructor’s position within the room has a significant impact on student engagement. When the instructor is physically present in the room, it positively influences student-instructor interaction. Some students actively participate in class discussions and take notes only when the instructor is within their line of sight. However, there are other students who seem eager to participate regardless of the instructor’s location. While this observation might seem apparent, it proved valuable as it allowed us to connect it to the concept of locus of control. We were particularly interested in exploring whether student engagement stemmed from an internal or external source. Although this observation didn’t offer in-depth information, it provided an insight into how some students might possess an internal locus of control, motivating them to participate actively in class discussions regardless of classroom conditions or arrangements.
Additionally, our observations highlight that establishing eye contact plays a significant role in enhancing verbal communication and increasing student engagement. It is quite normal to see students eagerly joining classroom discussions by raising their hands, especially when they are in a position where the instructor can easily see them. In instances where students cannot recall technical terms for architectural concepts (e.g., forms, shapes, etc.), they resort to using hand gestures to convey their ideas. However, this might pose a challenge for those participating in the class discussion through Zoom from the adjacent room (classroom A or B), as they may not be able to see or interpret these hand gestures effectively. Body language encompasses a broad spectrum of non-verbal cues involving different parts of the body. Emphasizing the significance of hands in particular, they play a pivotal role in enhancing the effectiveness of verbal communication. Our observations also reveal that students commonly adjust their body position, specifically their angulation, to enhance their ability to actively engage in classroom discussions. This adjustment often involves orienting themselves towards key focal points within the classroom, which typically include the instructor’s position or the screen/display, particularly in lecture-style classes. This angulation adjustment may have potential musculoskeletal consequences, which is an aspect we aimed to explore further during the interviews.
This setup is relatively novel and has yet to receive attention in the existing literature. Our field observations underscore the significance of technology utilization in a socially distanced classroom setting. It notably reduces the time spent on communication between two separate rooms. Without the aid of microphones and speakers, instructors are required to repeat discussions for both rooms, effectively duplicating their verbal communication efforts. This duplication of effort can lead to fatigue, occasionally diminishing the instructor’s overall productivity in a socially distanced classroom. Conversely, the positioning of the microphone held significant importance for student engagement. Students seated in closer proximity to the microphone or those with unobstructed access to it demonstrated higher levels of participation in class activities and discussions as illustrated in the mapping diagram (Fig. 3 ).
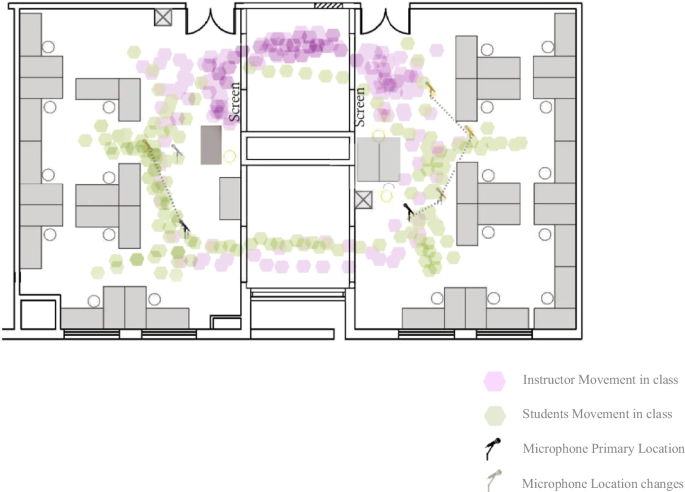
A mapping diagram that layers the instructor’s movement and students’ movement in the classrooms. In this diagram, the instructor’s movement frequency is highlighted with purple hexagons, while student movements are depicted with green hexagons.The dark microphone symbol denotes the primary location of the microphone, while the gray microphone symbol indicates changes in its position based on student movement.
Personal space and crowding
We also aimed to investigate the concept of crowding concerning personal space within the socially distanced setup. Most responses indicated that this feeling was barely experienced within the new arrangement. It appears that some students confused the psychological meaning of crowding with the physical aspects of overcrowding, and a follow-up question might have been helpful to clarify the investigation’s focus. Nonetheless, we suggest that the absence of the feeling of crowding could be attributed to individual personality traits, particularly whether one is a screener or non-screener (Kopec, 2012 ). Screeners are skilled at filtering out irrelevant stimuli and are less easily distracted or upset (“The Oxford Dictionary of Sports Science & Medicine,” 2006 ), while non-screeners have difficulty shifting attention and tend to experience higher levels of anxiety and empathy ( Nonscreener , n.d. ). Participant 4 exhibited a generally calm demeanor and a resilience to the influence of environmental factors. This disposition may shed light on the coping strategy that was observed, which includes either texting or stepping outside the classroom as a response to feeling overwhelmed. This coping strategy was also highlighted by participant 17.
Participant 17: “I actually try to take a break and try to go to the toilet or get a coffee, just a five-minute break, try to look to my phone to distract myself” .
Regarding the concept of personal space, the majority of participants perceived it positively in the socially distanced classroom. They appreciated the spaciousness of the setup and the generous size of their workspace, aligning with existing literature findings related to the concept of personal space as indicated below in Table 3 .
Furniture arrangement was generally perceived positively by 11 out of 20 participants, who appreciated the spacious personal space it provided in studio classes. However, some participants found the layout inconvenient, especially during lecture classes. The availability of a personal pin-up wall was appreciated by some students, but those sitting in the middle of the classroom, such as participant 13, felt disadvantaged due to the lack of this feature. Socially distanced classrooms were better tolerated in studio classes than in lecture classes, with students enjoying the increased personal space regardless of their location in the classroom.
Participant 13: “It is good, but at the same time I feel I don’t like it. I need a corner to sit in, in my area I sit in the middle, so I don’t have a pin up wall. Like in the Junior studio, I feel it is better because they have more corners, their tables are arranged in a U-shape, so most of them have corners and the area in the middle is empty. Having our tables in the middle, ok it is good for me to concentrate during the lecture classes, but in the studio classes it is uncomfortable” .
One drawback of this layout was the limited number of electrical outlets, which could become crowded when students needed to charge their laptops simultaneously. Another concern raised was the L-shaped table setup, which provided more space but required students to frequently turn their bodies and necks to view the screen during lectures, leading to potential musculoskeletal problems as emphasized by participant 14.
Participant 14: “The L-shape table setup is nice, because it gives us more space. However, the layout is not good, because we are not facing the screen, so we have to turn our bodies and neck in order to see the screen, which is very tiring. To open the laptop, take notes, following the lectures on the big screen with a layout that is not comfortable makes me lose focus” .
Instructors moving between the two rooms during class meetings to ensure equal engagement posed a distraction for some participants. They described maintaining two classrooms simultaneously as overwhelming for the instructor. Furthermore, some students found communication with instructors more convenient through Zoom compared to the socially distanced setup.
Nonverbal communication
Body language significantly influences a student’s attentiveness, as highlighted by 16 participants who emphasized that they are more focused when the instructor is physically present in the same classroom. Participant 14 expressed that seeing the instructor’s body language and personality creates a more engaging experience compared to feeling like they are listening to a machine, which helps them concentrate better. The term “focus” was used by those 16 participants to describe their level of engagement in class. Regardless of whether the interactions are one-way or two-way conversations, most students indicated that body language plays a vital role in keeping them attentive during class. However, it is essential to note that in-class participation does not always imply complete engagement with the class content. Rather, it is a term we used to assess the effectiveness of communication within this setting.
Participant 14: “Because focusing makes me understand the class materials, for example, if he or she (referring to the instructor) is here, I will be able to focus and understand, however, if they are in the other room, I try to listen to them but I cannot focus much “ .
Participant 13 emphasized the significance of body language, especially eye contact, in enhancing vocal communication. She mentioned that being able to see the instructor in front of her helps her understand the class materials better compared to when the instructor is in another room, and she struggles to maintain focus. This aligns with the observation that students tend to be more engaged when the instructor is within their line of sight.
Non-verbal communication or body language encompasses various aspects such as eye contact, gestures, voice, facial expressions, touch, space, and body movement/posture (Segal et al., 2023 ). Among these elements, the eyes and ears are the most utilized in any form of vocal communication, whether in one-way or two-way conversations. Hall’s ( 1966 ) findings indicate that the unaided ear’s effective coverage is limited, making visual cues crucial for effective communication.
Participant 14 mentioned relying more on visual cues than auditory ones, suggesting that visual learners may find it challenging to adapt to socially distanced classroom environments, especially when they cannot consistently see the speaker. This lack of human connection, as described by Participant 16, can hinder effective communication between the speaker and the audience.
Participant 10 emphasized the significance of visual access provided by Zoom in a socially distanced classroom. She expressed that when the instructor makes eye contact with the camera, it creates a sense of direct connection and engagement. To her, establishing eye contact is essential for comprehending lessons and establishing a connection with the instructor. She also noted that recalling the instructor’s body language while reviewing PowerPoint slides after class enhances information retention compared to audio-only classes, where relying on notes is necessary.
Additionally, Participant 10 highlighted the importance of eye contact, even in virtual settings, through the Zoom camera. When the instructor looks directly at the camera, it gives the remote audience the impression of maintained eye contact. This effort allows visually inaccessible students to stay involved and potentially engaged with the class content. Furthermore, the participant mentioned that body language helps in mentally noting information for better retention.
Participant 10: “somehow it does work, especially if the instructor is making eye contact with the camera or something. It makes me feel she is directed towards the students and she is looking at us. And for me eye contact is very important in communication, especially when it comes to understanding a lesson. Also, it is like having a relationship with the instructor, it is not just audio, but it is visual as well. Sometimes, when I go over the PowerPoint slides again after class to study, I sort of remember the instructor’s body language and it is like remembering the information that was said during the class. I feel I retain the information better, however, if it was only audio, I feel I will need to go back to my notes to remember and to refresh my memories.”
Participant 1 mentioned the advantage of immediate interaction in studio or graphics classes, where movement and questions are encouraged. However, there was confusion between audience etiquette and lack of participation, as remaining silent during a lecture is expected to avoid distractions. Questions were facilitated through raised hands, achieved by sharing screens via Zoom to connect both rooms. The perception of student engagement may have been skewed because of the room’s dual function (serving as both a studio and a lecture room), potentially causing some misinterpretation.
Three participants mentioned that the socially distanced setup facilitated effective communication with their classmates within the same classroom more than with those in other classrooms. Participant 6 expressed developing stronger relationships and feeling comfortable talking to nearby classmates, whereas communication with students in the other room was less frequent. This sentiment was shared by other participants, highlighting that student-to-student communication was confined within microenvironments, typically within a 1-m radius.
The type of course, whether studio-based or lecture-based, played a significant role in defining student-to-student communication. Studio classes held in those classrooms allowed for more walking around and talking, leading to increased interactions among students. On the other hand, lecture courses tended to limit communication to those sitting nearby or using microphones for questions.
Participant 10 acknowledged the ease of communication within their class but found it challenging to interact with students in the other room. However, efforts were made to maintain personal connections and engage in group discussions, even with students in the adjacent room. Similar experiences were shared by other participants, who expressed greater communication with those sitting closer to them, particularly in lecture classes.
Student location
The quality of a student’s learning experience is correlated with their location in the classroom, as indicated by several responses. Participants mentioned that being closer to the instructor’s position resulted in a better learning experience, allowing for comfortable, conversational distance and improved concentration.
Apart from proximity to the instructor, students also considered other factors when choosing their seats, such as adequate daylighting. Some preferred corner spots for privacy, storage space, and access to pinup walls, while middle spots were perceived as lacking privacy due to the absence of vertical partitions. However, certain desk locations led to musculoskeletal issues for students as they tried to adjust their positions to see the screen or instructor clearly. Participants 5 and 17 pointed out that poor ergonomics could result in disengagement from class activities.
Participant 17: “As a social distance I have no issues. But after the midterms I changed my location because I got neck issues. My table was facing the wall, so I had to twist my whole body to follow the lecture on the screen and that was very tiring”. She added, “…. and after the midterms, I did not like my grades, they were average but I did not like them. So, I felt I had to change, I had to be more comfortable, so I can focus more. Then I found another available place” .
Participant 6 indicated that the instructors’ movement between classrooms was influenced by the layout, with the primary workstation attracting them to stay longer in that room. This affected student engagement and communication with the instructor during class.
Participant 6 said, “Now that I think about it, I mainly talk with people sitting closer to me. However, my location is near the junior partition, which is annoying because I can hear everything through a little crack in the partition—their lectures, jokes, fights, everything.” The external noise from adjacent rooms with similar setups poses additional distractions, especially for students sitting on the wall-side. Despite having absorptive materials (fabric) on the pinup walls, the doors in between the classrooms require further treatment to address noise issues, considering the use of microphones and speakers as part of the socially distanced setup. Acoustics are significantly impacted by the flooring in the classroom, and footsteps and flooring noises can also contribute to distractions.
Circulation
Some participants described the circulation as tight, with Participant 1 stating, “I think the circulation is a bit tight, but overall it is good.” This perception may be attributed to the limited pathways intentionally designed to restrict each student’s space and minimize potential areas of congregation. On the other hand, other participants found the circulation to be convenient for their work and overall smooth. Participant 5 expressed appreciation for the circulation, particularly the provision of two desks for each student, which was beneficial for their work and required ample space. They also found the circulation easy to navigate, with no challenges in moving around the classroom.
Upon analyzing the layout and comparing the seating positions of Participant 1 and Participant 5, we observed that both locations were unobstructed and conveniently accessible from the classroom entrance. Both participants were equidistant from the instructor’s position and the TV screen. However, it is worth mentioning that while Participant 5 was seated near a window, Participant 1 occupied a seat in the middle of classroom A. Despite this difference, they were still relatively close to each other. This variation in seating may have influenced their perceptions of the room’s overall circulation, with the window-side location potentially contributing to a greater sense of spaciousness.
Participant 1 expressed a preference for dimmer environments during lecture courses, stating, “Sometimes when I see it is dark there, I wish I was in the other room. My side has too much light.” This suggests that there is a variation in lighting preferences among students in a classroom setting. The proximity to windows is considered a privilege by some students, providing them with a view and the ability to control daylight, as described by Participant 1. Participant 14, on the other hand, mentioned being situated far from the window and lacking direct sunlight. Additionally, she found the artificial lighting in her class to be either excessively bright or too dim. As someone who enjoys natural light, she expressed a desire for more sunlight in her space. The quality of light, whether natural or artificial, significantly impacts students’ perceptions of the environment. For instance, Participant 14 attributed feelings of lethargy and tiredness during afternoon classes to the absence of sunlight. She believed that more windows would increase her energy and alertness. Participant 5 indicated that having her desk somehow close to the window and receiving sunlight in the morning helps awaken her, suggesting a positive association between productivity and good daylighting. However, Participant 12 pointed out that sitting near windows could be overwhelming in hot weather, expressing a preference for blinds to regulate natural light and maintain a comfortable temperature. Surprisingly, glare was not as problematic as initially thought, as students found ways to adjust their seating to avoid it, utilizing the spacious workspaces provided. In fact, several participants, including Participant 1, stated they had no glare issues due to the L-shaped tables, which allowed them to adjust their laptops accordingly.
While many participants appreciated the adequate amount of daylight in their classroom, they felt that the artificial lighting was inadequate and, to some extent, contributed to feelings of drowsiness. For instance, Participant 16 mentioned that the artificial light in her class (referring to classroom B) was low and dull. Furthermore, Participant 6, who occupies a seat in classroom B, found screen light during presentations or lectures to be bothersome for her eyes despite not receiving direct sunlight due to her window being closed. The room’s darkness and strong light from the screen caused discomfort and eye irritation for her.
The placement of microphones and their limited number discouraged several students from actively participating in class discussions during lecture-based courses. Participant 14 expressed frustration, describing walking to the fixed microphone location as a “nightmare.” A significant issue, pointed out by 12 participants, is the challenging distance between their desks and the microphone, which hinders their ability to engage in class discussions effectively. Participants used terms like “time-consuming” and “waste of time” to describe the inconvenience of walking to the microphone, which can impact the overall communication in the class.
Some students prefer to participate in discussions while remaining seated, especially if the microphone’s location is prominently visible in the room. Shyness was identified as a reason for some students’ hesitance to use the fixed microphone. It is speculated that shyness may stem from cultural factors or personality traits, but further investigation is required to understand the underlying reasons behind this sentiment.
Another theme that emerged from the interviews is that students intentionally refrain from participating due to spatial and technical challenges. For example, participant 4 explained that she holds back from answering questions because she believes others in the second room will reach the microphone faster or the instructor will notice them first. As a result, she remains silent and stays in her place. Participant 10 echoed a similar sentiment, stating that sometimes it takes the instructor time to realize that they want to ask or answer a question, leading to a reluctance to disturb the class or interrupt the instructor. The time required to walk to the microphone makes them hesitant, as by the time they reach there, the instructor might have moved on to the next topic. This situation can be embarrassing for students, as they do not want to interrupt the lecture inadvertently.
Participant 6 shared a similar experience, stating that she refrained from participating altogether during lecture classes due to the effort and stress involved. The process of walking to the microphone, ensuring it works, and repeating herself to those in the other room feels overwhelming. Participant 12 also acknowledged this challenge, indicating that she often weighs whether her question or comment is worth the time and potential disruption to the class.
Participant 3 expressed that she avoids participation due to the lengthy process involved in a socially distanced classroom. She feels that someone else will answer, so she does not need to speak up. Similarly, Participant 4 chooses not to participate in certain situations, believing that others will answer before her.
We propose that this pattern of hesitating to participate may be linked to a concept known as “learned helplessness.” This phenomenon occurs when individuals are repeatedly exposed to uncontrollable stressors, leading them to develop a belief that they lack control over their environment. Thus, this diminished sense of control can reduce their motivation to take action or attempt to change the situation ( APA Dictionary of Psychology , n.d.)
Microphone manners
One emerging sub-theme from the study is the intimidation some users, especially students, experience when using microphones. Instructors are also not effectively trained in microphone usage. Participants reported instances where they could not hear the instructor clearly due to audio volume fluctuations. This was attributed to instructors holding the microphone too far away or pointing it at their chin instead of their mouth, causing acoustic issues for students in the other classroom. Consequently, students tended to zone out when unable to hear clearly, impacting their engagement in class discussions.
Participant 5 mentioned that although instructors were generally audible, there were instances of sound cut-offs or distractions when the microphone was too close to the professor’s mouth, picking up unwanted sounds like breathing. Participant 7 also noted that some students had soft voices even when using the microphone, affecting clarity.
Zoom was deliberately chosen to visually connect people in both rooms for this study, as the transition from full online learning for two years made it a suitable platform. However, interview results revealed two main perspectives on using Zoom in a socially distanced classroom. Some participants found Zoom distracting, perceiving it as a reminder of online classes, while others appreciated its usefulness, especially visual learners who could easily view lecture slides through the sharing feature.
Distraction emerged as a recurring concern among several participants, particularly related to visual and auditory stimuli. These distractions encompassed factors such as noise originating from neighboring classrooms, the movements of the instructor, and technical issues. Notably, some students, like Participant 14, disclosed that they had Attention Deficit Hyperactivity Disorder (ADHD) and experienced heightened sensitivity to various forms of movement, which made both virtual and in-person classes equally challenging in terms of maintaining focus. Conversely, some participants held a positive perspective regarding Zoom’s role in enhancing communication between the two interconnected classrooms. For example, participant 10 expressed gratitude for the opportunity to observe the lecturer’s body language, which contributed to a sense of human interaction, even within a semi-virtual learning environment.
Technical issues occasionally led to student disengagement, but Zoom proved helpful for those in the no-instructor classroom. Students utilized Zoom’s sharing feature to follow lecture slides and virtually raise their hands for participation. However, overall, students preferred face-to-face interaction over Zoom communication, finding the latter tiring and distracting. Observations during the study supported this preference for face-to-face interaction.
Locus of control
Four participants indicated that they had little control over their classroom location and settled for what was available. However, some, like Participant 5, displayed an internal locus of control, stating she could teach herself and adapt to any situation. Participant 5 emphasized that the physical presence of the instructor was not crucial for her learning, indicating a strong internal strength and adaptability.
Conversely, Participant 17 exhibited a blend of external and internal locus of control. Initially, her seat choice was influenced by the location of her friends, reflecting an external factor. However, she later opted to change her seat with the goal of “improving her grades,” indicating a degree of internal control in her decision-making process.
Participant 17: “At the beginning I was just walking with my friends, and they sat so I sat. So, we sat and we were chatting, then I realized oh ok this is my place. But then I felt I cannot continue, and after the midterms, I did not like my grades, they were average but I did not like them. So, I felt I had to change, I had to be more comfortable, so I can focus more. Then I found another available place” .
Participant 15, on the other hand, favored keeping her laptop active during lectures, utilizing Zoom’s sharing feature to manage slides and search for answers when necessary. In doing so, she demonstrated an external locus of control, relying on available resources such as Zoom to address technical and acoustic challenges.
To our knowledge, this study is the first exploration of post-pandemic classroom design and its effects on classroom communication and student engagement under preventive measures such as face covering and social distancing. To investigate the research question in this case study, a thorough examination of both old and recent literature was methodically undertaken. This approach serves as both a limitation and a strength. The absence of pertinent studies prior to the research in 2021 posed a significant challenge. Nevertheless, delving into well-established older literature proved instrumental in overcoming this hurdle and establishing the groundwork for this exploratory research.
With the implementation of social distancing and the redefined proxemics, our study aimed to investigate the variations in the concept of personal space in this context. Hall’s work provided the foundation for our research in this specific area. While reviewing several studies referencing proxemics theory, none closely resembled ours. Thus, we utilized the original work as a starting point for exploration. This approach mirrors Mehta’s study ( 2020 ), which explored the impact of COVID-19 measures in urban settings, noting the transformation of spaces in response to social distancing. Mehta’s findings on sociable spaces resonated with our results regarding furniture arrangement and classroom layout. Our findings on the redefined proxemics in the new setup reveal that the post-pandemic classroom was widely praised for its redefined personal space. A majority of 11 out of 17 participants expressed positive sentiments about the spaciousness and convenience of the newly defined personal space (Table 3 ). Crowding, a psychological concept associated with personal space, was seldom experienced in the new setup. Some participants mentioned that crowding was only felt when there was limited access to charging outlets for their laptops. Additionally, individuals who experienced any form of stress in class identified checking their phones or taking short breaks as coping strategies.
Our findings also show that the furniture arrangement of the post-pandemic classroom is key in facilitating class communication. While being in close proximity to the instructor in the front or middle of the classroom is deemed conducive to student engagement, as it enables close and direct communication that helps students stay on task (Berg et al., 1996 ; as cited by Gullup, 1986), our study reveals that these middle seats do not cater to the spatial requirements of design students in studio classes, mainly due to the absence of pin-up walls. The findings indicate insufficient attention to the needs of students situated in the middle, exposing them to more visibility and diminished privacy compared to their counterparts along the perimeter. This situation is akin to the airplane aisle and window seats’ dilemma. While middle-seat students benefit from being close to the lecturer as a source of information, wall-side students enjoy privacy, access to a pin-up wall, and window advantages. A socio-petal (Mehta, 2020 ) furniture arrangement is recommended as it can provide equal opportunities and amenities for art and design students while facilitating communication in a socially distanced classroom.
The results of interviews and observations highlight the substantial influence of the instructor’s positioning in the classroom on communication with students, encompassing both verbal and non-verbal aspects. The students are more inclined to engage in class discussion if the instructor’s location in the classroom, as well as their body language are visually accessible. This highlights Kopec’s insights about the benefits of a rectangular classroom layout and how it supports the instructor’s capacity to establish eye contact with students since they fall within “the instructor’s primary visual field” (Kopec, 2012 , p. 224). Moreover, our research shows that 80% of the interview responses highlight the positive impact of body language on students’ attentiveness and engagement. This finding is consistent with the established literature, specifically Zeki’s ( 2009 ), emphasizing the significance of body language in non-verbal communication within the classroom. Put differently, the arrangement of the classroom is pivotal for both verbal and non-verbal communication, both in a general classroom setting and specifically in the post-pandemic classroom. Our study contributes to the existing knowledge by examining the utilization of Zoom to enhance body language, including eye contact, in a scenario involving the coordination of two or more classrooms simultaneously, necessitated by insufficient space in the facility. Fifteen percent of our sample expressed positive perceptions of using Zoom as an alternative to face-to-face communication to address spatial challenges in this context (Table 3 ). Based on these findings, we propose that new hidden dimensions arise, shaping communication and interactions within socially distanced classrooms. The noted expansion of personal, social, and public spheres, with the public sphere arguably encompassing the use of Zoom, prompts further exploration in these settings. The distances between students and instructors have the potential to redefine proxemics in post-pandemic classroom arrangements.
Our investigation explored lighting aspects, revealing that they had the least impact on the socially distanced classroom setup. Students’ main concerns revolved around having more control over lighting levels in their designated spaces. Responses showed diverse preferences for lighting conditions, with some favoring dim lighting and others opting for brighter classrooms. The L-shaped workspace effectively addressed glare issues, allowing students to adjust their positions for control if the problem arose. In a prior UAE study (Al-Sallal, 2010 ), it was observed that simulation runs revealed three key issues causing visual discomfort: sharp contrasts in luminance between the task surface (e.g., whiteboard) and nearby surfaces, excessive brightness from windows and uneven distribution of daylight in the space. In his study, the student’s sitting location was identified as a crucial factor influencing visual quality. The most problematic location was reported to be “the rear, opposite side of the windows” (Al-Sallal, 2010 , p. 208) in terms of acceptable luminance. While our study primarily addressed lighting preferences and emerging behaviors impacting post-pandemic classroom communications, certain findings resonate with Al-Sallal’s ( 2010 ), especially those related to student location. Six out of 17 students favored daylighting and found glare issues insignificant, attributing this to their ability to adjust positions within the spacious workspace. Additionally, four out of 17 participants expressed a preference for more control and the ability to adjust lighting levels according to their individual needs to enhance attention and focus (Table 3 ). Some participants positively associated proximity to windows with productivity, as highlighted in the lighting results subsection. To prioritize confidentiality, our study analyzed responses without linking them to specific student locations, resulting in a lack of specificity in our results. This limitation is acknowledged, but the decision was made to safeguard confidentiality, especially since one researcher served as the course instructor, creating a potential challenge in handling information related to student locations. This aspect is worth exploring in future research to understand the correlation between lighting and student location in post-pandemic classrooms.
In addition, we investigated acoustics in this distinctive setup utilizing technology to facilitate the operation of two classrooms simultaneously, aiming to improve verbal communication. Our research focused on exploring the consequences of preventive measures on classroom communication in a post-pandemic setting. Considering earlier research in the field, notably Berg et al.’s ( 1996 ) review article, which synthesized results from diverse studies, emphasizing the influence of noisy classrooms on students’ effective listening, impacting task engagement, discipline, and cooperation. They highlighted that teachers adjust their vocal approach, exhibiting variations in strength (Berg et al., 1996 ; as cited in Gallup, 1986; Ray, 1990). While some effectively project their voices, individuals with weaker voices may encounter stress when elevating their vocal levels (Berg et al., 1996 ; as cited in Berg, 1993). These findings proved valuable as we sought to comprehend the challenges faced by instructors in both general and specific settings. Our findings show that the adoption of microphones eliminated the need for vocal exertion by instructors. One of the acoustic concerns pointed out by some participants was noise. In this setup, noise primarily originated from adjacent classrooms and internal sources, such as speakers’ echoing sound and unwanted noise resulting from improper microphone use (e.g., instructor breathing). Seven out of 17 interviewees expressed more concern about noise related to technical issues, while three out of 17 interviewees identified external noise as more distracting (Table 3 ). For instance, participant 6 mentioned that her location is mainly distracting due to external noise from the neighboring classroom. These findings emphasize emerging challenges in temporary arrangements for socially distanced classrooms, suggesting areas for further exploration, such as the impact of using speakers in acoustically unprepared lecture spaces during emergencies.
This research uncovered novel findings not present in existing literature. One such discovery is the concept of microphone etiquette, an aspect not previously addressed in the specific context of our study. This introduces a new avenue for exploration by researchers and designers. The intentional inclusion of microphones in this setting aimed to improve verbal communication between two interconnected classrooms. Further investigation into blended learning or hybrid approaches utilizing microphones could be valuable, potentially mitigating challenges during local or global outbreaks.
An aspect warranting future exploration in research is students’ perceptions of microphone usage. Approximately 70.6% of participants indicated that microphone location affected their level of engagement in class. Three out of 17 participants explicitly reported abstaining from engagement due to recurrent technical issues, leading to delayed verbal communication. Students refrained from participating due to shyness, as they had to walk to the microphone location and their apprehension that technical problems might arise (Table 3 ). Arguably, this behavior can be attributed to learned helplessness, which is defined as “occurring when an individual continuously faces a negative, uncontrollable situation and stops trying to change their circumstances, even when they have the ability to do so” (Psychology Today, n.d. ). This finding resonates with Polat’s study ( 2022 , p. 95), which suggests that the students’ “individual uncertainties” can lead to learned helplessness, diminishing motivation and resulting in decreased academic performance and passive behaviors. However, given the sample’s size and homogeneity, it remains challenging to definitively associate learned helplessness with the socially-distanced setup or other variables in this case study. Additional data is needed to establish this as a dependent variable specific to this context. The locus of control was also identified within the responses, as outlined in the results section. A more comprehensive understanding of the data regarding locus of control could have been achieved with a larger sample and the incorporation of additional qualitative methods, such as surveys. The four responses collected in this aspect may be influenced by unanticipated variables specific to this context beyond the locus of control.
It is essential to recognize that the findings derived from this case study are highly contextual. If a similar setup is implemented in a different context or country, the results, especially concerning student attitudes toward microphone usage and privacy concerns, may vary. The transferability of our findings may be constrained both geographically and across disciplines. Since the study exclusively targeted interior design students, its relevance to other fields in the hard sciences may be restricted due to the unique nature of their work. It is noteworthy that cultural aspects were not explicitly addressed within the scope of this research.
It is worth mentioning that the non-participant observation method proved valuable in aligning predetermined themes from the literature review with the research question. Given this unprecedented environmental context, we opted for deductive coding to stay within the scope of this research study on classroom communication post-pandemic. However, the semi-structured interviews revealed unexpected themes, providing opportunities for further research, as mentioned earlier. Hence, we utilized a hybrid approach, incorporating both deductive and inductive coding, to identify patterns in the interview responses. This method facilitated the emergence of new themes, including the consideration of microphone etiquette.
In addressing potential biases arising from observations, we took measures to clearly delineate the role of each researcher (Glesne, 2011 ) to ensure transparency and maintain participant data confidentiality. Throughout the study, we consistently practiced reflexivity ( Reflexivity , n.d. ), allowing us to continually scrutinize our own biases and perspectives during data collection and analysis. The decision to employ a single observer was influenced by regulations limiting classroom capacity and other restrictions related to external visitors. Additionally, we incorporated the Devil’s Advocate approach (Trochim, 2020 ) during data analysis, actively challenging our interpretations of the findings.
This research holds relevance for administrators as they strategize for campus emergencies. Future studies could delve into the optimization of furniture arrangement, considering aspects like privacy and body language, particularly in emergency scenarios necessitating preventive measures like face covering and social distancing. The insights gleaned from our findings might aid administrators in effectively organizing designated spaces for concurrent use or incorporating hybrid modes to ensure instructional continuity during emergencies. A deeper understanding of enhancing hybrid learning environments post-pandemic could contribute to user satisfaction and reduce reliance on lockdown measures.
Conclusions
The pandemic has instilled in us the importance of carefully designing successful learning experiences and programs for our students. It forced us to reevaluate our priorities and life choices, leading to significant changes in communication due to preventive measures like face coverings and social distancing. In times of hardship and uncertainty, telecommunication has become indispensable for businesses, governments, and communities to sustain themselves. As a result, interior environments should be adaptable to accommodate such emergencies.
Our study investigated communication dynamics within a classroom environment adapted to mitigate the spread of the coronavirus while ensuring instructional continuity. We conclude that the impact of preventive measures on classroom communication in a post-pandemic setting is multifaceted and context-dependent. While we have delved into specific aspects we believe are linked to communication, there may be more to consider. Among these aspects, we have explored proxemics, which have evolved beyond its cultural definition to incorporate new physical dimensions to accommodate social distancing. Our findings indicated that psychological concepts like crowding in this socially distanced setting require reassessment. The increased spaciousness in classrooms resulting from the setup with social distancing has reduced crowding. Interestingly, the use of cell phones during class, not resulting in disciplinary action, has served as a positive distraction for students experiencing crowding or stress.
Furthermore, post-COVID spacing mechanisms, including occupant load and furniture arrangement with social distancing in mind, have reshaped our perception of personal space. People became less concerned with non-verbal cues that differ across cultures and define their personal space. Instead, the COVID-preventative measures have introduced new hidden dimensions to our understanding of personal space. These new hidden dimensions encompass factors such as proximity to microphones, the instructor’s desk, the pinup walls, and/or windows, all of which regulate communication within a socially distanced classroom. For instance, adopting a socio-petal layout in the classroom could significantly enhance these new hidden dimensions, thereby facilitating communication in a socially distanced setting. Our findings indicate that crowding, often associated with personal space and spatial density, is less prevalent in the post-COVID setup, as each student benefits from a more spacious personal area dictated by COVID protocols. This reduction in crowding contributes to improved performance as individuals regain control over their social interactions.
In times of emergency, like the pandemic, technology becomes essential to facilitate both verbal and non-verbal communication. This exploratory case study highlights the significance of technology in socially distanced setups in design-studio classrooms, particularly in addressing the communication challenges identified. However, further research is needed to investigate the potential for tailored technology usage and its effectiveness within these distinctive educational environments.

Data availability
The data supporting the findings of this study are accessible upon request from the corresponding author (MA). The data cannot be publicly released as they may contain information that could compromise the privacy or consent of research participants.
Alawamleh M, Al-Twait LM, Al-Saht GR (2020) The effect of online learning on communication between instructors and students during Covid-19 pandemic. Asian Educ Dev Stud 11(2):380–400
Article Google Scholar
Al-Sallal KA (2010) Daylighting and visual performance: evaluation of classroom design issues in the UAE. Int J Low-Carbon Technol 5(4):201–209. https://doi.org/10.1093/ijlct/ctq025
American Psychological Association. (n.d.). Crowding. In APA Dictionary of Psychology . Retrieved from https://dictionary.apa.org/crowding
American Psychological Association. (n.d.). Learned helplessness. In APA Dictionary of Psychology . Retrieved from https://dictionary.apa.org/learned-helplessness
Astin AW (1984) Student involvement: a developmental theory for higher education. J Coll Stud Pers 25(4):297–308
Google Scholar
Azungah T (2018) Qualitative research: deductive and inductive approaches to data analysis. Qual Res J 18(4):383–400. https://doi.org/10.1108/QRJ-D-18-00035
Ballast DK (2013) Interior design reference manual: everything you need to know to pass the NCIDQ® Exam. Professional Publications Incorporated
Beaulieu C (2004) Intercultural study of personal space: a case study. J Appl Soc Psychol 34(4):794–805. https://doi.org/10.1111/j.1559-1816.2004.tb02571.x
Berg FS, Blair JC, Benson PV (1996) Classroom acoustics. Lang Speech Hear Serv Sch 27(1):16–20. https://doi.org/10.1044/0161-1461.2701.16
Centers for Disease Control and Prevention. (n.d.). How COVID-19 spreads. Retrieved from https://www.cdc.gov/coronavirus/2019-ncov/easy-to-read/prevent-gettingsick/how-covid-spreads.html
Cennimo DJ (2020) Coronavirus Disease 2019 (COVID-19): practice essentials, background, route of transmission. EMedicine https://emedicine.medscape.com/article/2500114-overview#a1
Darn, S. (n.d.). Nonverbal communication. The Internet TESL Journal . Retrieved from http://iteslj.org/Articles/Darn-Nonverbal/
Dictionary.com Meanings & Definitions of English Words (2021) Dictionary.com. https://www.dictionary.com/browse/personal-space
Earon SA (n.d.) The value of video communications in education. https://explore.zoom.us/docs/doc/The%20Value%20of%20Video%20Communications%20in%20Education.pdf
Evans GW, Wener RE (2007) Crowding and personal space invasion on the train: please don’t make me sit in the middle. J Environ Psychol 27(1):90–94. https://doi.org/10.1016/j.jenvp.2006.10.002
Fredricks JA, Blumenfeld PC, Paris AH (2004) School engagement: potential of the concept, state of the evidence. Rev Educ Res 74(1):59–109. https://doi.org/10.3102/00346543074001059
French RL (1977) Teaching the nonverbal experience. Theory Pract 16(3):176–182. https://doi.org/10.1080/00405847709542695
Frequently Asked Questions about COVID-19 Vaccination (2023) Centers for Disease Control and Prevention. https://www.cdc.gov/coronavirus/2019-ncov/vaccines/faq.html?s_cid=10492:what%20is%20in%20covid%20vaccine:sem.ga:p:RG:GM:gen:PTN:FY21
Gay A (2019) Upgrading from student involvement to engagement. Adobe Blog. https://blog.adobe.com/en/publish/2019/04/12/upgrading-from-student-involvement-to-engagement#:~:text=your%20students%20are.-,Educators%20recognize%20that%20student%20engagement%20goes%20beyond%20attention%20and%20involvement,prepared%20for%20the%20modern%20workplace
Government Communications Office (2020) Statement by the Supreme Committee for Crisis Management on Phase Four of the Gradual Lifting of Coronavirus Restrictions. Supreme Committee for Crisis Management. https://www.gco.gov.qa/en/top-news/phase-4-statement/
Glesne C (2011) Becoming qualitative researchers: an introduction, 4th edn. Pearson, Boston, MA
Hall ET (1966) The hidden dimension. Doubleday, Garden City, NY
Hall ET (1990) The hidden Dimension. Anchor
Hall ET (1959) The silent language . New York, NY: Anchor
Hall JA, Rosenthal R, Archer D, DiMatteo MR, Rogers PL (1977) Nonverbal, skills in the classroom. Theory Pract 16(3):162–166. https://doi.org/10.1080/00405847709542692
Jamshed S (2014) Qualitative research method-Interviewing and observation. J Basic Clin Pharm 5(4):87. https://doi.org/10.4103/0976-0105.141942
Article PubMed PubMed Central Google Scholar
Joia LA, Lorenzo M (2021) Zoom in, zoom out: the impact of the COVID-19 pandemic in the classroom. Sustainability 13(5):2531. https://doi.org/10.3390/su13052531
Jarus O (2023) The worst epidemics and pandemics in history. livescience.com . https://www.livescience.com/worst-epidemics-and-pandemics-in-history.html
Khal AA, Al-Kaabi S, Checketts RJ (2020) Qatar’s response to COVID-19 pandemic. Heart Views 21(3) https://doi.org/10.4103/HEARTVIEWS.HEARTVIEWS_161_20
Kim H (2020) The efficacy of zoom technology as an educational tool for English reading comprehension achievement in EFL classroom. https://doi.org/10.17703/ijact.2020.8.3.198
Kopec D (2012) Environmental psychology for design. Fairchild Books
Lawless B, Chen YW (2018) Developing a method of critical thematic analysis for qualitative communication inquiry. Howard J Commun 30(1):92–106. https://doi.org/10.1080/10646175.2018.1439423
Mandernach BJ, Gonzales RM, Garrett AL (2006) An examination of online instructor presence via threaded discussion participation. J Online Learning Technol 2(4):248–260
Mehta V (2020) The new proxemics: COVID-19, social distancing, and sociable space. J Urban Des 25(6):669–674. https://doi.org/10.1080/13574809.2020.1785283
Minhas S, Hussain T, Sajid K (2021) Exploring Students Online Learning: A Study of Zoom Application. Gazi Univ J Sci 34(2). https://doi.org/10.35378/gujs.691705
Metzger KJ, Langley D (2020) The room itself is not enough: student engagement in active learning classrooms. Coll Teach 68(3):150–160. https://doi.org/10.1080/87567555.2020.1768357
Morgan SJ, Pullon SRH, Macdonald LM, McKinlay EM, Gray BV (2017) Case Study Observational Research: A Framework for Conducting Case Study Research Where Observation Data Are the Focus. Qual Health Res 27(7):1060–1068. https://doi.org/10.1177/1049732316649160
Article PubMed Google Scholar
Non-participant observation (n.d.) Better evaluation. https://www.betterevaluation.org/methods-approaches/methods/non-participant-observation
Nonscreener. (n.d.). Oxford Reference. https://www.oxfordreference.com/display/10.1093/oi/authority.20110803100237930
Polat M (2022) A case study of preservice teachers’ classroom management dilemma in a climate of uncertainty during the COVID-19 pandemic. Int J Educ Lit Stud 10(1):90. https://doi.org/10.7575/aiac.ijels.v.10n.1p.90
Proudfoot K (2022) Inductive/deductive hybrid thematic analysis in mixed methods research. J Mixed Methods Res 17(3):155868982211268
Psychology Today (n.d.) Learned helplessness. https://www.psychologytoday.com/us/basics/learned-helplessness#:~:text=Learned%20helplessness%20occurs%20when%20an,try%20and%20fail%20to%20quit
Ramadani A, Xhaferi B (2020) Teachers’ experiences with online teaching using the zoom platform with EFL teachers in high schools in Kumanova. SEEU Rev 15(1):142–155. https://doi.org/10.2478/seeur-2020-0009
Reflexivity (n.d.) Melbourne Medical School. https://medicine.unimelb.edu.au/school-structure/medical-education/research/qualitative-journey/themes/reflexivity#:~:text=Reflexivity%20is%20about%20acknowledging%20your
Richmond VP, McCroskey JC (2012) Power in the classroom: communication, control, and concern. Routledge
Rysiew P (2021) Epistemic contextualism. In: Zalta EN (ed) Stanford encyclopedia of philosophy. Metaphysics Research Lab, Stanford University
Robson D (2021) Why some people don’t want a Covid-19 vaccine. BBC https://www.bbc.com/future/article/20210720-the-complexities-of-vaccine-hesitancy
Sommer R (1977) Classroom layout. Theory Pract 16(3):174–175. https://doi.org/10.1080/00405847709542694
Shernoff DJ, Kelly S, Tonks SM, Anderson B, Cavanagh RF, Sinha S, Abdi B (2016) Student engagement as a function of environmental complexity in high school classrooms. Learn Instr 43:52–60. https://doi.org/10.1016/j.learninstruc.2015.12.003
Segal J, Smith M, Robinson L, Boose G (2023) Nonverbal communication and body language. HelpGuide. https://www.helpguide.org/articles/relationships-communication/nonverbal-communication.htm
The Oxford Dictionary of Sports Science & Medicine. (2006). In Oxford University Press eBooks. https://doi.org/10.1093/acref/9780198568506.001.0001
Timeline: Sound Wave and Music—Lesson 3 behavior of sound waves: reflection, refraction, and diffraction (n.d.). The Physics Classroom. https://www.physicsclassroom.com/class/sound/Lesson-3/Reflection,-Refraction,-and-Diffraction
Trochim WMK (2020) The research methods knowledge base. Conjointly.com ; Conjoint.ly . https://conjointly.com/kb/qualitative-validity/
Trowler V (2010) Student engagement literature review. The Higher Education Academy
Tureková I, Lukáčová D, Bánesz G (2018) Quality assessment of the university classroom lighting—a case study. TEM J 7(4):829–836. https://doi.org/10.18421/TEM74-21
Uncapher M (2016) The science of effective learning spaces. https://www.edutopia.org/article/science-of-effective-learning-spaces-melina-uncapher . Accessed 3 Oct 2021
Wahyuni A (2018) The power of verbal and nonverbal communication in learning. https://doi.org/10.2991/icigr-17.2018.19
Walker KA, Koralesky KE (2021) Student and instructor perceptions of engagement after the rapid online transition of teaching due to COVID‐19. Nat Sci Educ 50(1):e20038. https://doi.org/10.1002/nse2.20038
WHO Director-General’s opening remarks at the media briefing on COVID-19 - 11 March 2020. (2020) https://www.who.int/director-general/speeches/detail/who-director-general-s-opening-remarks-at-the-media-briefing-on-covid-19---11-march-2020
World Health Organization. (n.d.). COVID-19 vaccines: Advice. Retrieved from https://www.who.int/emergencies/diseases/novel-coronavirus-2019/covid-19-vaccines/advice
Worchel S, Teddie C (1976) The experience of crowding: a two-factor theory. J Personal Soc Psychol 34(1):30–40. https://doi.org/10.1037/0022-3514.34.1.30
Article CAS Google Scholar
Zeki CP (2009) The importance of non-verbal communication in classroom management. Procedia–Soc Behav Sci 1(1):1443–1449. https://doi.org/10.1016/j.sbspro.2009.01.254
Download references
Acknowledgements
We thank all the students who participated in this study for sharing their time and valuable insights. We also appreciate the support and funding provided by Virginia Commonwealth School of the Arts in Qatar for this research.
Author information
Authors and affiliations.
Virginia Commonwealth School of the Arts in Qatar, Doha, Qatar
Marwa Abdelmonem & Sherin Karawia
You can also search for this author in PubMed Google Scholar
Contributions
Conceptualization, MA, methodology, MA; formal analysis, MA; investigation, MA, SK; data curation, MA, SK; writing—original draft preparation, MA, SK; writing—review and editing, MA, SK; visualization, SK; supervision, MA.
Corresponding author
Correspondence to Marwa Abdelmonem .
Ethics declarations
Competing interests.
The authors declare no competing interests.
Ethical approval
This study was performed in line with the principles of the Declaration of Helsinki. Approval was granted by the Qatar Biomedical Research Institute (QBRI) Institutional Review Board at Hamad Bin Khalifa University (Date: 23 November 2021/No. QBRI-IRB-2022-40).
Informed consent
The authors confirm obtaining informed consent from all study participants, ensuring a thorough process. Comprehensive details on the research’s purpose, procedures, risks, and benefits were provided. Importantly, participants willingly joined after understanding the information, highlighting voluntary involvement. The consent process adhered to ethical guidelines, assuring participants’ full awareness and the freedom to withdraw without repercussions. The authors emphasize their commitment to ethical research, prioritizing participant rights and well-being.
Additional information
Publisher’s note Springer Nature remains neutral with regard to jurisdictional claims in published maps and institutional affiliations.
Rights and permissions
Open Access This article is licensed under a Creative Commons Attribution 4.0 International License, which permits use, sharing, adaptation, distribution and reproduction in any medium or format, as long as you give appropriate credit to the original author(s) and the source, provide a link to the Creative Commons licence, and indicate if changes were made. The images or other third party material in this article are included in the article’s Creative Commons licence, unless indicated otherwise in a credit line to the material. If material is not included in the article’s Creative Commons licence and your intended use is not permitted by statutory regulation or exceeds the permitted use, you will need to obtain permission directly from the copyright holder. To view a copy of this licence, visit http://creativecommons.org/licenses/by/4.0/ .
Reprints and permissions
About this article
Cite this article.
Abdelmonem, M., Karawia, S. Classroom communications post pandemic: a case study. Humanit Soc Sci Commun 11 , 728 (2024). https://doi.org/10.1057/s41599-024-03171-6
Download citation
Received : 14 November 2023
Accepted : 13 May 2024
Published : 05 June 2024
DOI : https://doi.org/10.1057/s41599-024-03171-6
Share this article
Anyone you share the following link with will be able to read this content:
Sorry, a shareable link is not currently available for this article.
Provided by the Springer Nature SharedIt content-sharing initiative
Quick links
- Explore articles by subject
- Guide to authors
- Editorial policies

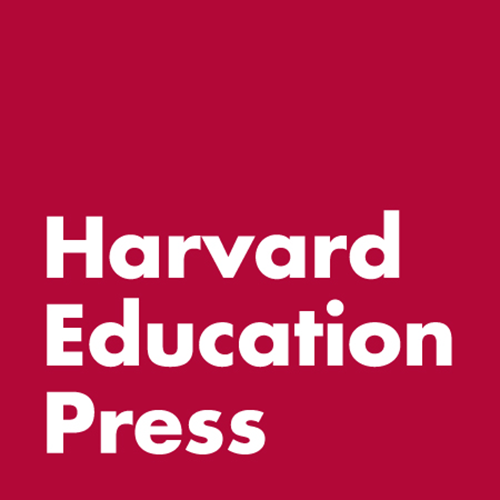
On The Site
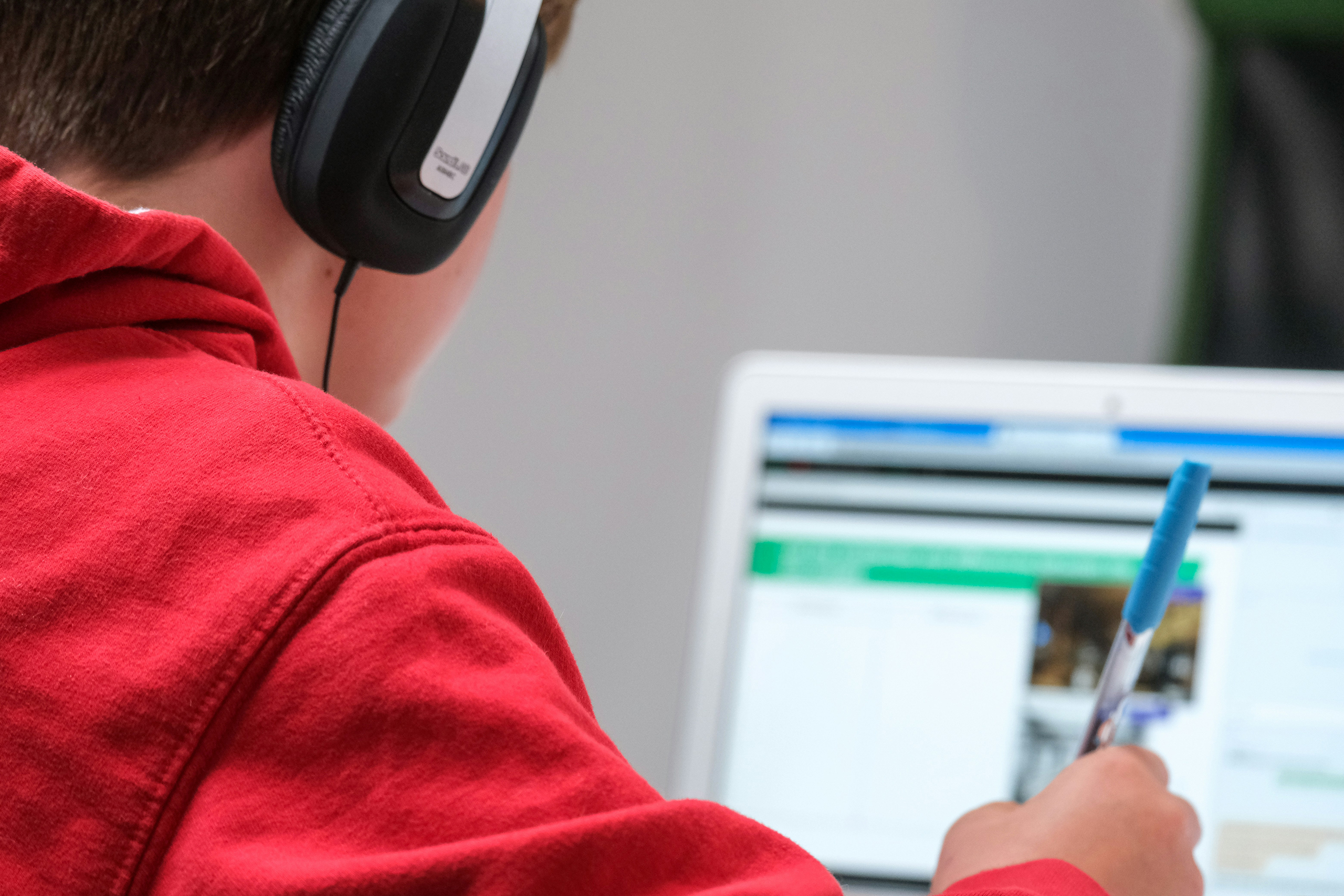
Teaching About Technology in Schools Through Technoskeptical Inquiry
June 3, 2024 | victorialynn | Harvard Educational Review Contributors , Voices in Education
By Jacob Pleasants, Daniel G. Krutka, and T. Philip Nichols
New technologies are rapidly transforming our societies, our relationships, and our schools. Look no further than the intense — and often panicked — discourse around generative AI , the metaverse , and the creep of digital media into all facets of civic and social life . How are schools preparing students to think about and respond to these changes?
In various ways, students are taught how to use technologies in school. Most schools teach basic computing skills and many offer elective vocational-technical classes. But outside of occasional conversations around digital citizenship, students rarely wrestle with deeper questions about the effects of technologies on individuals and society.
Decades ago, Neil Postman (1995) argued for a different form of technology education focused on teaching students to critically examine technologies and their psychological and social effects. While Postman’s ideas have arguably never been more relevant, his suggestion to add technology education as a separate subject to a crowded curriculum gained little traction. Alternatively, we argue that technology education could be an interdisciplinary endeavor that occurs across core subject areas. Technology is already a part of English Language Arts (ELA), Science, and Social Studies instruction. What is missing is a coherent vision and common set of practices and principles that educators can use to align their efforts.
To provide a coherent vision, in our recent HER article , we propose “technoskepticism” as an organizing goal for teaching about technology. We define technoskepticism as a critical disposition and practice of investigating the complex relationships between technologies and societies. A technoskeptical person is not necessarily anti-technology, but rather one who deeply examines technological issues from multiple dimensions and perspectives akin to an art critic.
We created the Technoskepticism Iceberg as a framework to support teachers and students in conducting technological inquiries. The metaphor of an iceberg conveys how many important influences of technology lie beneath our conscious awareness. People often perceive technologies as tools (the “visible” layer of the iceberg), but technoskepticism requires that they be seen as parts of systems (with interactions that produce many unintended effects) and embedded with values about what is good and desirable (and for whom). The framework also identifies three dimensions of technology that students can examine. The technical dimension concerns the design and functions of a technology, including how it may work differently for different people. The psychosocial dimension addresses how technologies change our individual cognition and our larger societies. The political dimension considers who makes decisions concerning the terms, rules, or laws that govern technologies.
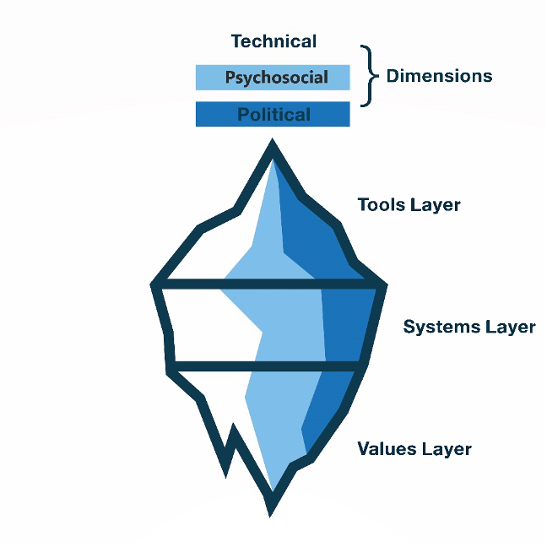
To illustrate these ideas, how might we use the Technoskeptical Iceberg to interrogate generative AI such as ChatGPT in the core subject areas?
A science/STEM classroom might focus on the technical dimension by investigating how generative AI works and demystifying its ostensibly “intelligent” capabilities. Students could then examine the infrastructures involved in AI systems , such as immense computing power and specialized hardware that in turn have profound environmental consequences. A teacher could ask students to use their values to weigh the costs and potential benefits of ChatGPT.
A social studies class could investigate the psychosocial dimension through the longer histories of informational technologies (e.g., the printing press, telegraph, internet, and now AI) to consider how they shifted people’s lives. They could also explore political questions about what rules or regulations governments should impose on informational systems that include people’s data and intellectual property.
In an ELA classroom, students might begin by investigating the psychosocial dimensions of reading and writing, and the values associated with different literacy practices. Students could consider how the concept of “authorship” shifts when one writes by hand, with word processing software, or using ChatGPT. Or how we are to engage with AI-generated essays, stories, and poetry differently than their human-produced counterparts. Such conversations would highlight how literary values are mediated by technological systems .
Students who use technoskepticism to explore generative AI technologies should be better equipped to act as citizens seeking to advance just futures in and out of schools. Our questions are, what might it take to establish technoskepticism as an educational goal in schools? What support will educators need? And what might students teach us through technoskeptical inquiries?
Postman, N. (1995). The End of Education: Redefining the Value of School. Vintage Books.
About the Authors
Jacob Pleasants is an assistant professor of science education at the University of Oklahoma. Through his teaching and research, he works to humanize STEM education by helping students engage with issues at the intersection of STEM and society.
Daniel G. Krutka is a dachshund enthusiast, former high school social studies teacher, and associate professor of social studies education at the University of North Texas. His research concerns technology, democracy, and education, and he is the cofounder of the Civics of Technology project ( www.civicsoftechnology.org ).
T. Philip Nichols is an associate professor in the Department of Curriculum and Instruction at Baylor University. He studies the digitalization of public education and the ways science and technology condition the ways we practice, teach, and talk about literacy.
They are the authors of “ What Relationships Do We Want with Technology? Toward Technoskepticism in Schools ” in the Winter 2023 issue of Harvard Educational Review .
- Gift Guides
- Voices in Education
- Open access
- Published: 15 June 2024
Title and abstract screening for literature reviews using large language models: an exploratory study in the biomedical domain
- Fabio Dennstädt ORCID: orcid.org/0000-0002-5374-8720 1 , 3 ,
- Johannes Zink 2 ,
- Paul Martin Putora 1 , 3 ,
- Janna Hastings 4 , 5 , 6 &
- Nikola Cihoric 3
Systematic Reviews volume 13 , Article number: 158 ( 2024 ) Cite this article
Metrics details
Systematically screening published literature to determine the relevant publications to synthesize in a review is a time-consuming and difficult task. Large language models (LLMs) are an emerging technology with promising capabilities for the automation of language-related tasks that may be useful for such a purpose.
LLMs were used as part of an automated system to evaluate the relevance of publications to a certain topic based on defined criteria and based on the title and abstract of each publication. A Python script was created to generate structured prompts consisting of text strings for instruction, title, abstract, and relevant criteria to be provided to an LLM. The relevance of a publication was evaluated by the LLM on a Likert scale (low relevance to high relevance). By specifying a threshold, different classifiers for inclusion/exclusion of publications could then be defined. The approach was used with four different openly available LLMs on ten published data sets of biomedical literature reviews and on a newly human-created data set for a hypothetical new systematic literature review.
The performance of the classifiers varied depending on the LLM being used and on the data set analyzed. Regarding sensitivity/specificity, the classifiers yielded 94.48%/31.78% for the FlanT5 model, 97.58%/19.12% for the OpenHermes-NeuralChat model, 81.93%/75.19% for the Mixtral model and 97.58%/38.34% for the Platypus 2 model on the ten published data sets. The same classifiers yielded 100% sensitivity at a specificity of 12.58%, 4.54%, 62.47%, and 24.74% on the newly created data set. Changing the standard settings of the approach (minor adaption of instruction prompt and/or changing the range of the Likert scale from 1–5 to 1–10) had a considerable impact on the performance.
Conclusions
LLMs can be used to evaluate the relevance of scientific publications to a certain review topic and classifiers based on such an approach show some promising results. To date, little is known about how well such systems would perform if used prospectively when conducting systematic literature reviews and what further implications this might have. However, it is likely that in the future researchers will increasingly use LLMs for evaluating and classifying scientific publications.
Peer Review reports
Systematic literature reviews (SLRs) summarize knowledge about a specific topic and are an essential ingredient for evidence-based medicine. Performing an SLR involves a lot of effort, as it requires researchers to identify, filter, and analyze substantial quantities of literature. Typically, the most relevant out of thousands of publications need to be identified for the topic and key information needs to be extracted for the synthesis. Some estimates indicate that systematic reviews typically take several months to complete [ 1 , 2 ], which is why the latest evidence may not always be taken into consideration.
Title and abstract screening forms a considerable part of the systematic reviewing workload. In this step, which typically follows defining a search strategy and precedes the full-text screening of a smaller number of search results, researchers determine whether a certain publication is relevant for inclusion in the systematic review based on title and abstract. Automating title and abstract screening has the potential to save time and thereby accelerate the translation of evidence into practice. It may also make the reviewing methodology more consistent and reproducible. Thus, the automation or semi-automation of this part of the reviewing workflow has been of longstanding interest [ 3 , 4 , 5 ].
Several approaches have been developed that use machine learning (ML) to automate or semi-automate screening [ 1 , 6 ]. For example, systematic review software applications such as Covidence [ 7 ] and EPPI-Reviewer [ 8 ] (which use the same algorithm) offer ML-assisted ranking algorithms that aim to show the most relevant publications for the search criteria higher in the reviewing to speed up the manual review process. Elicit [ 9 ] is a standalone literature discovery tool that also offers an ML-assisted literature search facility. Furthermore, several dedicated tools have been developed to specifically automate title and abstract screening [ 1 , 10 ]. Examples include Rayyan [ 11 ], DistillerSR [ 12 ], Abstrackr [ 13 ], RobotAnalyst [ 14 ], and ASReview [ 5 ]. These tools typically work via different technical strategies drawn from ML and topic modeling to enable the system to learn how similar new articles are to a core set of identified ‘good’ results for the topic. These approaches have been found to lead to a considerable reduction in the time taken to complete systematic reviews [ 15 ].
Most of these systems require some sort of pre-selection or specific training for the larger corpus of publications to be analyzed (e.g., identification of some “relevant” publications by a human so that the algorithm can select similar papers) and are thus not fully automated.
Furthermore, dedicated models are required that are built for the specific purpose together with appropriate training data. Fully automated systems that achieve high levels of performance and can be flexibly applied to various topics have not yet been realized.
Large language models (LLMs) are an approach to natural language processing in which very large-scale neural networks are trained on vast amounts of textual data to generate sequences of words in response to input text. These capable models are then subject to different strategies for additional training to improve their performance on a wide range of tasks. Recent technological advancements in model size, architecture, and training strategies have led to general-purpose dialog LLMs achieving and exceeding state-of-the-art performance on many benchmark tasks including medical question answering [ 16 ] and text summarization [ 17 ].
Recent progress in the development of LLMs led to very capable models. While models developed by private companies such as GPT-3/GPT-3.5/GPT-4 from OpenAI [ 18 ] or PaLM and Gemini from Google [ 19 , 20 ] are among the most powerful LLMs currently available, openly available models are actively being developed by different stakeholders and in some cases achieve performances not far from the state of the art [ 21 ].
LLMs have shown remarkable capabilities in a variety of subjects and tasks that would require a profound understanding of text and knowledge for a human to perform. Among others, LLMs can be used for classification [ 22 ], information extraction [ 23 ], and knowledge access [ 24 ]. Furthermore, they can be flexibly adapted via prompt engineering techniques [ 25 ] and parameter settings, to behave in a desired way. At the same time, considerable problems with the usage of LLMs such as “hallucinations” of models [ 26 ], inherent biases [ 27 , 28 ], and weak alignment with human evaluation [ 29 ] have been described. Therefore, even though the text output generated by LLMs is based on objective statistical calculations, the text output itself is not necessarily factual and correct and furthermore incorporates subjectivity based on the training data. This implies, that an LLM-based evaluation system has a priori some fundamental limitations. However, using LLMs for evaluating scientific publications is a novel and interesting approach that may be helpful in creating fully automated and still flexible systems for screening and evaluating scientific literature.
To investigate whether and how well openly available LLMs can be used for evaluating the relevance of publications as part of an automated title and abstract screening system, we conducted a study to evaluate the performance of such an approach in the biomedical domain with modern openly available LLMs.
Using LLMs for title and abstract screening
We designed an approach for evaluating the relevance of publications based on title and abstract using an LLM. This approach is based on the following strategy:
An instruction prompt to evaluate the relevance of a scientific publication for inclusion into an SLR is given to an LLM.
The prompt includes the title and abstract of the publication and the criteria that are considered relevant.
The prompt furthermore includes the request to return just a number as an answer, which corresponds to the relevance of the publication on a Likert scale (“not relevant” to “highly relevant”).
The prompt for each publication is created in a structured and automated way.
A numeric threshold may be defined which separates relevant publications from irrelevant publications (corresponding to the definition of a classifier).
The prompts are created in the following way:
Prompt = [Instruction] + [Title of publication] + [Abstract of publication] + [Relevant Criteria ] .
(“ + ” is not part of the final prompt but indicates the merge of the text strings).
[Instruction] is the text string describing the general instruction for the LLM to evaluate the publication. The LLM is asked to evaluate the relevance of a publication for an SLR on a numeric scale (low relevance to high relevance) based on the title and abstract of the publication and based on defined relevant criteria.
[Title of publication] is the text string “Title:” together with the title of the publication.
[Abstract of publication] is the text string “, Abstract:” together with the abstract of the publication.
[Relevant Criteria] is the text that describes the criteria to evaluate the relevance of a publication. The relevant criteria are defined beforehand by the researchers depending on the topic to determine which publications are relevant. The [Relevant Criteria] text string remains unchanged for all the publications that should be checked for relevance.
The answer to the LLM usually consists just of a digit on a numeric scale (e.g., 1–5). However, variations are acceptable if the answer can unambiguously be assigned to one of the possible scores on the Likert scale (e.g., the answer “The relevance of the publication is 3.” can unambiguously be assigned to the score 3). This assignment of answers to a score can be automated with a string-search command, meaning a simple regular expression command searching for a positive integer number, which will be extracted from the text string.
A request is sent to the LLM for each publication in the corpus. In cases for which an LLM provided an invalid (unprocessable) response for a publication, that response was excluded from the direct downstream analysis. It was determined for how many publications invalid responses were given and how many of these publications would have been relevant.
A schematic illustration of the approach is shown in Fig. 1 . An example of a prompt is provided in Supplementary material 1: Appendix 1.
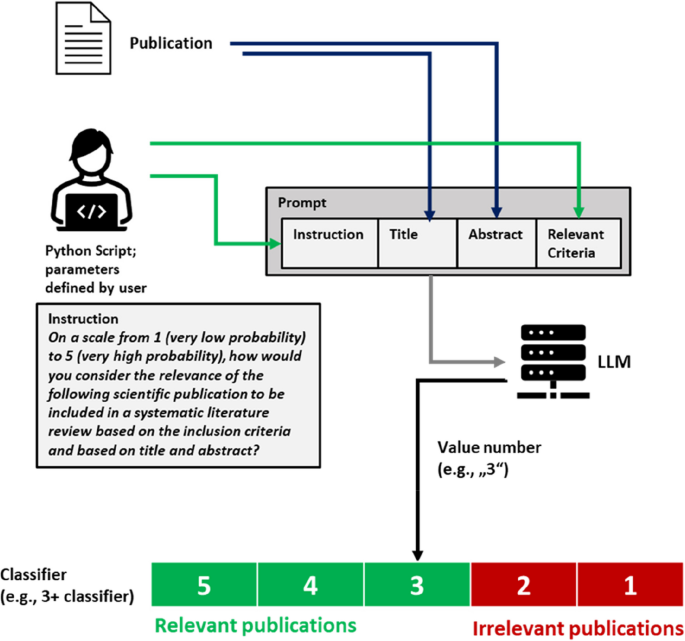
Schematic illustration of the LLM-based approach for evaluating the relevance of a scientific publication. In this example, a 1–5 scale and a 3 + classifier are used
A Python script was created to automate the process and to apply it to a data set with a collection of different publications.
With the publications being sorted into different relevance groups, a threshold can be defined, which is used by a classifier to separate relevant from irrelevant publications. For example, a 3 + classifier would classify publications with a score of ≥ 3 as relevant, and publications with a score < 3 as irrelevant.
The performance of the approach was tested with different LLMs, data sets and settings as described in the following:
Language models
A variety of different models were tested. To investigate the approach with different LLMs (that are also diverse regarding design and training data), the following four models were used in the experiments:
FlanT5-XXL (FlanT5) is an LLM developed by Google Research. It’s a variant of the T5 (text-to-text) model, that utilizes a unified text-to-text framework allowing it to perform a wide range of NLP tasks with the same model architecture, loss function, and hyperparameters. FlanT5 is a variant that was enhanced through fine-tuning over a thousand additional tasks and supporting more languages. It is primarily used for research in various areas of natural language processing, such as reasoning and question-answering [ 30 , 31 ].
OpenHermes-2.5-neural-chat-7b-v3-1-7B (OHNC) [ 32 ] is a powerful open-source LLM, which was merged from the two models OpenHermes 2.5 Mistral 7B [ 33 ] and Neural-Chat (neural-chat-7b-v3-1) [ 34 ]. Despite having only 7 billion parameters it performs better than some larger models on various benchmarks.
Mixtral-8 × 7B-Instruct v0.1 (Mixtral) is a pretrained generative Sparse Mixture of Experts LLM developed by Mistral AI [ 35 , 36 ]. It was reported to outperform powerful models like gpt-3.5-turbo, Claude-2.1, Gemini Pro, and Llama 2 70B-chat on human benchmarks.
Platypus2-70B-Instruct (Platypus 2) is a powerful language model with 70 Billion parameters [ 37 ]. The model itself is a merge of the models Platypus2-70B and SOLAR-0-70b-16bit (previously published as LLaMa-2-70b-instruct-v2) [ 38 ].
Published data sets
A list of several data sets for SLRs is provided to the public by the research group of the ASReview tool [ 39 ]. The list contains data sets on a variety of different biomedical subjects of previously published SLRs. For testing the LLM approach on an individual data set, the [Relevant Criteria] string for each data set was created based on the description in the publication of the corresponding SLR. We tested the approach on a total of ten published data sets covering different biomedical topics (Table 1 , Supplementary material 2: Appendix 2).
Newly created data set on CDSS in radiation oncology
To test the approach also in a prospective setting on a not previously published review, we created a data set for a new, hypothetical SLR, for which title and abstract screening should be performed.
The use case was an SLR on “Clinical Decision Support System (CDSS) tools for physicians in radiation oncology”. A CDSS is an information technology system developed to support clinical decision-making. This general definition may include diagnostic tools, knowledge bases, prognostic models, or patient decision aids [ 50 ]. We decided that the hypothetical SLR should be only about software-based systems to be used by clinicians for decision-making purposes in radiation oncology. We defined the following criteria for the [Relevant Criteria] text of the provided prompt:
Only inclusion of original articles, exclusion of review articles.
Publications examining one or several clinical decision-support systems relevant to radiation therapy.
Decision-support systems are software-based.
Exclusion of systems intended for support of non-clinicians (e.g., patient decision aids).
Publications about models (e.g., prognostic models) should only be included if the model is intended to support clinical decision-making as part of a software application, which may resemble a clinical decision support system.
The following query was used for searching relevant publications on PubMed: “(clinical decision support system) AND (radiotherapy OR radiation therapy)”.
Titles and abstracts of all publications found with the query were collected. A human-based title and abstract screening was performed to obtain the ground truth data set. Two researchers (FD and NC) independently labeled the publications as relevant/not relevant based on the title and abstract and based on the [Relevant criteria] string. The task was to label those publications relevant that may be of interest and should be analyzed as full text, while all other publications should be labeled irrelevant. After labeling all publications, some of the publications were deemed relevant only by one of the two researchers. To obtain a final decision, a third researcher (PMP) independently did the labeling for the undecided cases.
The aim was to create a human-based data set purely representing the process of title and abstract screening without further information or analysis.
A manual title and abstract screening was conducted on 521 publications identified in the search with 36 publications being identified as relevant and labeled accordingly in the data set. This data set was named “CDSS_RO”. It should be noted that this data set is qualitatively different from the 10 published data sets, as not only the publications that may be finally included in an SLR are labeled as relevant, but all publications that should be analyzed in full text based on title and abstract. The file is provided at https://github.com/med-data-tools/title-abstract-screening-ai ).
Parameters and settings of LLM-based title and abstract screening
Standard parameters.
The LLM-based title and abstract screening as described above requires the definition of some parameters. The standard settings for the approach were the following:
[Instruction] string: We used the following standard [Instruction] string:
“On a scale from 1 (very low probability) to X (very high probability), how would you rate the relevance of the following scientific publication to be included in a systematic literature review based on the relevant criteria and based on title and abstract?”
Range of scale: defines the range of the Likert scale mentioned in the [Instruction] string (marked as X in the standard string above). For the standard settings, a value of 5 was used.
Model parameters of the LLMs were defined in the source code. To obtain reproducible results, the model parameters were set accordingly for the model to become deterministic (e.g., the temperature value is a parameter that defines how much variation a response of a model should have. Values greater than 0 add a random element to the output, which should be avoided for the reproducibility of the LLM-based title and abstract screening).
Adaptation of instruction prompt and range
The behavior of an LLM is highly dependent on the provided prompt. Adequate adaptation of the prompt may be used to improve the performance of an LLM for certain tasks [ 25 ]. To investigate what impact a slightly adapted version of the Instruction prompt would have on the results, we added the string “(Note: Give a low score if not all criteria are fulfilled. Give only a high score if all or almost all criteria are fulfilled.)” in the instruction prompt as additional instruction and examined the impact on the performance. Furthermore, the range of the scale was changed from 1–5 to 1–10 in some experiments to investigate what impact this would have on the performance.
Statistical analyses
The performance of the approach, depending on models and threshold, was determined by calculating the sensitivity (= recall), specificity, accuracy, precision, and F1-score of the system, based on the amount of correctly and incorrectly included/excluded publications for each data set.
Comparison with the automated classifier of Natukunda et al.
The LLM-based title and abstract screening was compared to another, recently published approach for fully automated title and abstract screening. This approach, developed by Natukunda et al., uses an unsupervised Latent Dirichlet Allocation-based topic model for screening [ 51 ]. Unlike the LLM-based approach, it does not require an additional [Relevant Criteria] string, but defined search keywords to determine which publications are relevant. The approach was used to do a screening on the ten published data sets as well as on the CDSS_RO data set. To obtain the required keywords we processed the text of the used search terms by splitting combined text into individual words and removing stop words, duplicates, and punctuation (as described in the original publication of Natukunda et al.).
Performance of LLM-based title and abstract screening of different models on published data sets
The LLM-based screening with a Likert scale of 1–5 provided clear results for evaluating the relevance of a publication in the majority of cases. Out of the total of 44,055 publications among the 10 published data sets, valid and unambiguously assignable answers were given for 44,055 publications (100%) by the FlanT5 model, for 44,052 publications (99.993%) by the OHNC model, for 44,026 publications (99.93%) by the Mixtral model and for 44,054 publications (99.998%) by the Platypus 2 model. The few publications for which an invalid answer was given were excluded from further analysis. None of the excluded publications was relevant. The distribution of scores given was different between the different models. For example, the OHNC model ranked the majority of publications with a score of 3 (47.2%) or 4 (34.2%), while the FlanT5 model ranked almost all publications with a score of either 4 (68.1%) or 2 (31.7%). For all models, the group of publications labeled as relevant in the data sets was ranked with higher scores compared to the overall group of publications (mean score of 3.89 compared to 3.38 for FlanT5, 3.86 compared to 3.14 for OHNC, 4.16 compared to 2.12 for Mixtral and 3.80 compared to 2.92 for Platypus 2). An overview is provided in Fig. 2 .
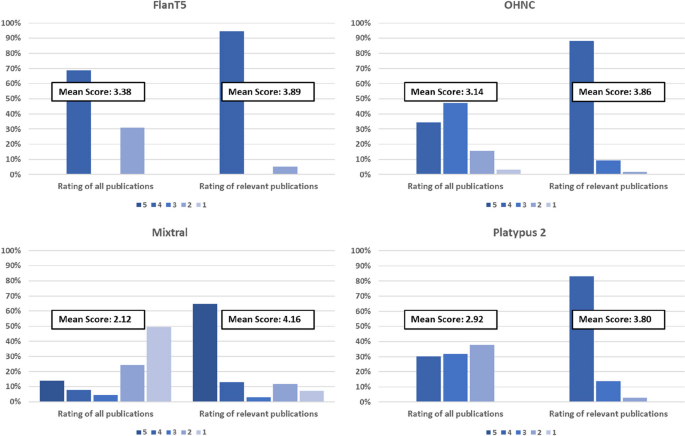
Distribution of scores given by the different models
Based on the scores given, according classifiers that label publications with a score of greater than or equal to “X” as relevant, have higher rates of sensitivity and lower rates of specificity with decreasing threshold (decreasing “X”).
Classifiers with a threshold of ≥ 3 (3 + classifiers) were further analyzed, as these classifiers were considered to correctly identify the vast majority of relevant publications (high sensitivity) without including too many irrelevant publications (sufficient specificity). The 3 + classifiers had a sensitivity/specificity of 94.8%/31.8% for the FlanT5 model, of 97.6%/19.1% for the OHNC model, of 81.9%/75.2% for the Mixtral model, and of 97.2%/38.3% for the Platypus 2 model on all ten published data sets. The performance of the classifiers was quite different depending on the data set used (Fig. 3 ). Detailed results on the individual data sets are presented in Supplementary material 3: Appendix 3.
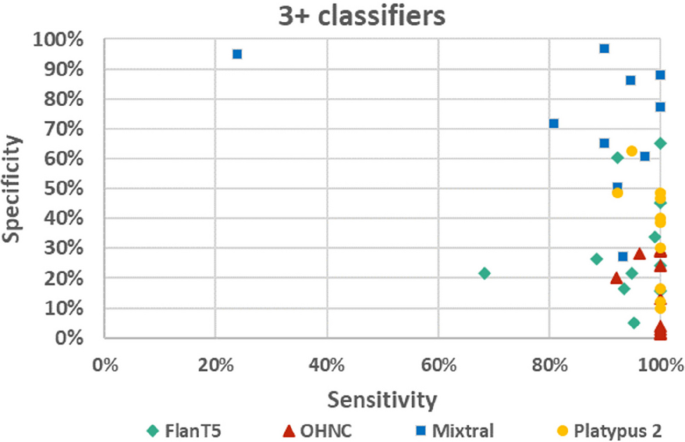
Sensitivity and specificity of the 3 + classifiers on different data sets using different models. Each data point represents the results of one of the data sets
The highest specificity at 100% sensitivity was seen for the Mixtral model on the data set Wolters_2018 with all 19 relevant publications being scored with 3–5, while 4410 of 5019 irrelevant publications were scored with 1 or 2 (specificity of 87.87%). The lowest sensitivity was observed with the Mixtral model on the dataset Jeyaraman_2021 with 23.96% sensitivity at 94.63% specificity.
Using LLM-based title and abstract screening for a new systematic literature review
On the newly created manually labeled data set, the 3 + classifiers had 100% sensitivity for all four models with specificity ranging from 4.54 to 62.47%. The results of the LLM-based title and abstract screening, dependent on the threshold for the classifiers are presented as receiver operating characteristics (ROC) curves in Fig. 4 as well as in Supplementary material 3: Appendix 3.
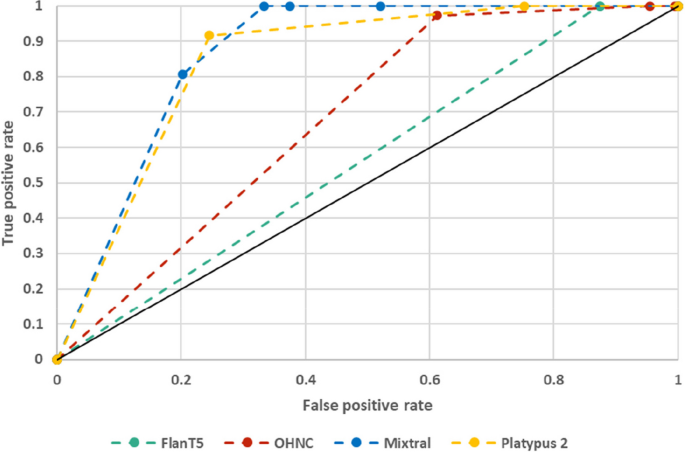
Receiver operating characteristics (ROC) curves of the LLM-based title and abstract screening for the different models on the CDSS_RO data set
Dependence of LLM-based title and abstract screening on Instruction prompt and on a range of scale
Several runs of the Python script with different settings (adapted [Instruction] string and/or range of scale 1–10 instead of 1–5) were performed, which led to different results. Minor adaptation of the Instruction string with an additional demand to focus on the mentioned criteria had a different impact on the performance of the classifiers depending on the LLM used. While the sensitivity of the 3 + classifiers remained at 100% for all four models, the specificity was lower for the OHNC model (2.89% vs. 4.54%), the Mixtral model (56.29% vs. 62.47%) and the Platypus 2 model (15.88% vs. 24.74%), while it was higher for the FlanT5 model (25.15% vs. 12.58%).
Changing the range of scale from 1–5 to 1–10 and using a 6 + classifier instead of a 3 + classifier led to a lower sensitivity for the OHNC model (97.22% vs. 100%), while increasing the specificity (13.49% vs. 4.54%). For the other models, the sensitivity remained at 100% with higher specificity for the Platypus 2 model (51.34% vs. 24.74%) and the FlanT5 model (50.52% vs. 12.58%). The specificity was unchanged for the Mixtral model at 62.47%, which was the highest value among all combinations at 100% sensitivity. No combination of the settings for a range of scales and with/without prompt adaptation was superior among all models. An overview of the results is provided in Fig. 5 .
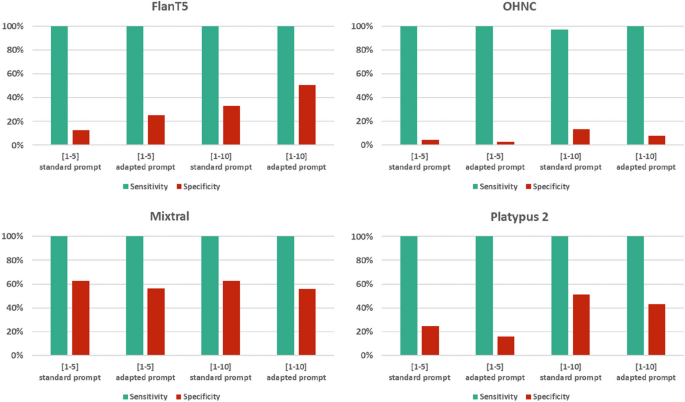
Performance of the classifiers depending on adaptation of the prompt and on the range of scale
Comparison with unsupervised title and abstract screening of Natukunda et al.
The screening approach developed by Natukunda et al. achieved an overall sensitivity of 52.75% at 56.39% specificity on the ten published data sets. As for the LLM-based screening, the performance of this approach was dependent on the data set analyzed. The lowest sensitivity was observed for the Jeyaraman_2021 data set (1.04%), while the highest sensitivity was observed for the Wolters_2018 dataset (100%). Compared to the 3 + classifier with the Mixtral model, the LLM-based approach had higher sensitivity on 9 data sets and equal sensitivity on 1 data set, while it had higher specificity on 6 data sets and lower specificity on 4 data sets.
On the CDSS_RO data set, the approach of Natukunda et al. achieved 94.44% sensitivity (lower than all four LLMs) at 39.59% specificity (lower than the Mixtral model and higher than the FlanT5, OHNC, and Platypus 2 models). Further data on the comparison is provided in Supplementary material 4: Appendix 4.
We developed and elaborated a flexible approach to use LLMs for automated title and abstract screening that has shown some promising results on a variety of biomedical topics. Such an approach could potentially be used to automatically pre-screen the relevance of publications based on title and abstract. While the results are far from perfect, using LLMs for evaluating the relevance of publications could potentially be helpful (e.g., as a pre-processing step) when performing an SLR. Furthermore, the approach is widely applicable without the development of custom tools or training custom models.
Automated and semi-automated screening
A variety of different ML and AI tools have been developed to assist researchers in performing SLRs [ 5 , 10 , 52 , 53 ]. Fully automated systems (like the LLM-based approach presented in our study) still fail to differentiate relevant from irrelevant publications near the level of human evaluation [ 51 , 54 ].
A well-functioning fully automated title and abstract screening system that could be used on different subjects in the biomedical domain and possibly also in other scientific areas would be very valuable. While human-based screening is the current gold standard, it has considerable drawbacks. From a methodological point of view, one major problem of human-based literature evaluation, including title and abstract screening, is the subjectivity of the process [ 55 ]. Evaluating the publications (based on title and abstract) is dependent on the experience and individual judgments of the person doing the screening. To overcome this issue, SLRs of high quality require multiple independent researchers to do the evaluation with specific criteria upon inclusion/exclusion defined beforehand [ 56 ]. Nevertheless, subjectivity remains an unresolved issue, which also limits the reproducibility of results. From a practical point of view, another major problem is the considerable workload needed to be performed by humans, especially if thousands of publications need to be assessed, which is multiplied by the need to have multiple reviewers and to discuss disagreements. The challenge of workload is not just a matter of inconvenience, as SLRs on subjects that require tens of thousands of publications to be searched, may just not be feasible for small research teams to do, or may already be outdated after the time it would take to do the screening and analyze the results.
While fully automated screening approaches may also be affected by subjectivity (since the training data of models is itself generated by processes which are affected by subjectivity), the results would at least be more reproducible, and automation can be applied at scale in order to overcome the problem of practicability.
While current fully automated systems cannot replace humans in title and abstract screening, they may nevertheless be helpful. Such systems are already being used in systematic reviews and most likely their usage will continue to grow [ 57 ].
Ideally, a fully automated system should not miss a single relevant publication (100% sensitivity) while minimizing as far as possible the number of irrelevant publications included. This would allow confident exclusion of some of the retrieved search results which is a big asset to reducing time taken in manual screening.
LLMs for title and abstract screening
By creating structured prompts with clear instructions, an LLM can feasibly be used for evaluating the relevance of a scientific publication. In comparison to some other solutions, the LLM-based screening may have some advantages. On the one hand, the flexible nature of the approach allows adaptation to a specific subject. Depending on the question, different prompts for relevant criteria and instructions can be used to address the individual research question. On the other hand, the approach can create reproducible results, given a fixed model, parameters, prompting strategy, and defined threshold. At the same time, it is scalable to process large numbers of publications. As we have seen, such an approach is feasible with a performance similar to or even better in comparison to other current solutions like the approach of Natukunda et al. However, it should be noted that the performance varied considerably depending on which of the 10 + 1 data sets were used.
Further applications of LLMs in literature analysis
While we investigated LLMs for evaluating the relevance of publications and in particular for title and abstract screening, it is being discussed how these models may be used for a variety of tasks in literature analysis [ 58 , 59 ]. For example, Wang et al. obtained promising results when investigating if ChatGPT may be used for writing Boolean Queries for SLRs [ 60 ]. Aydin et al., also using ChatGPT, employed the LLM to write an entire Literature Review about Digital Twins in Healthcare [ 61 ].
Guo et al. recently performed a study using the OpenAI API with gpt-3.5 and gpt-4 to create a classifier for clinical reviews [ 62 ]. They observed promising results when comparing the performance of the classifier against human-based screening with a sensitivity of 76% at 91% specificity on six different review papers. In contrast to our approach, they used a Boolean classifier instead of a Likert scale. Another approach was developed by Akinseloyin et al., who used ChatGPT to create a method for citation screening by ranking the relevance of publications using a question-answering framework [ 63 ].
The question may arise what the purpose of using a Likert scale instead of a direct binary classifier is (also since some models only rarely use some of the score values; see e.g., FlanT5 in Fig. 2 ). The rationale for using the Likert scale arose out of some preliminary, unsystematic explorations we conducted using different models and ranges of scale (including binary). We realized that using a Likert scale has some advantages as it sorts the publications into several groups depending on the estimated relevance. This also allows flexible adjustment of the threshold (which may potentially also be useful if the user wants to rather focus on sensitivity or rather on specificity).
However, there seem to be several feasible approaches and frameworks to use LLMs for the screening of publications.
It should be noted that an LLM-based approach for evaluating the relevance of publications might just as well be used for a variety of different classification tasks in literature analysis. For example, one may adopt the [Instruction prompt] asking the LLM not to evaluate the relevance of a publication on a Likert scale, but for classification into several groups like “original article”, “trial”, “letter to the editor”, etc. From this point of view, the title and abstract screening is just a special use case of LLM-based classification.
Future developments
The capabilities of LLMs and other AI models will continue to evolve, which will increase the performance of fully automated systems. As we have seen, the results are highly dependent on the LLM used for the approach. In any case, there may still be substantial room for improvement and optimization and it currently is unclear what LLM-based approach with which prompts, models, and settings yields the best results over a large variety of data sets.
Furthermore, LLMs may not only be used for the screening of titles and abstracts but for the analysis of full-text documents. The newest generation of language and multimodal models may process whole articles or potentially also image data from publications [ 64 , 65 ]. Beyond that, LLM-based evaluation of scientific data and publications may only be one of several options for AI assistance in literature analysis. Future systems may combine different ML and AI approaches for optimal automated processing of literature and scientific data.
Limitations of LLM-based title and abstract screening
Even though the LLM-based screening presented in our work shows some promising results, it also has some drawbacks and limitations. While the open framework with adaptable prompts makes the approach flexible, the performance of the approach is highly dependent on the used model, the input parameters/settings, and the data set analyzed. If a slightly different instruction or another scale (1–10 instead of 1–5) is used, this can have a considerable impact on the performance. The classifiers analyzed in our study failed to consistently identify relevant publications at 100% sensitivity without considerably impairing the specificity. In academic research, the bar for automated screening tools needs to be very high, as ideally not a single relevant publication should be missed. The LLM-based title and abstract screening requires the definition of clear criteria for inclusion/exclusion. For research questions with less clear relevance criteria, LLMs may not be that useful for the evaluation. This may potentially be one reason, why the performance of the approach was quite different in our study depending on the data set analyzed. Overall, there are still many open questions, and it is unclear if and how high levels of performance can be consistently guaranteed so that such a system can be relied on. It is interesting that the Mixtral model, even though it seemed to have the highest level of performance on average, performed poorly with low sensitivity on one data set (Fig. 3 ). Further research is needed to investigate the requirements for good performance of the LLMs in evaluating scientific literature.
Another limitation of the approach in its current form is a considerable demand for resources regarding calculation power and hardware equipment. Answering thousands of long text prompts with modern, multi-billion-parameter LLMs requires sufficient IT infrastructure and calculation power to perform. The issue of resource demand is especially relevant if many thousand publications are evaluated and if very complex models are used.
Fundamental issues of using LLMs in literature analysis
On a more fundamental level, there are some general issues regarding the use of LLMs for literature studies. LLMs calculate the probability for a sequence of words based on their training data which derives from past observations and knowledge. They can thereby inherit unwanted features and biases (such as for example ethnic or gender biases) [ 29 , 66 ]. In a recent study by Koo et al., it was shown that the cognitive biases and preferences of LLMs are not the same as the ones of humans as a low correlation between ratings given by LLMs and humans was observed [ 67 ]. The authors therefore stated that LLMs are currently not suitable as fair and reliable automatic evaluators. Considering that using LLMs for evaluating and processing scientific publications may be seen as a problematic and questionable undertaking. However, the biases present in language models affect different tasks differently, and it remains to be seen how they might differentially affect different screening tasks in the literature review [ 28 ].
Nevertheless, it is most likely that LLMs and other AI solutions will be increasingly used in conducting and evaluating scientific research [ 68 ]. While this certainly will provide a lot of chances and opportunities, it is also potentially concerning. The amount and proportion of text being written by AI models is increasing. This includes not only public text on the Internet but also scientific literature and publications [ 69 , 70 ]. The fact that ChatGPT has been chosen as one of the top researchers of the year 2023 by Nature and has frequently been listed as co-author, shows how immediate the impact of the development has already been [ 71 ]. At the same time, most LLMs are trained on large amounts of text provided on the Internet. The idea that in the future LLMs might be used to evaluate publications written with the help of LLMs that may themselves be trained on data created by LLMs may lead to disturbing negative feedback loops which decrease the quality of the results over time [ 72 ]. Such a development could actually undermine academia and evidence-based science [ 73 ], also due to the known fact that LLMs tend to “hallucinate”, meaning that a model may generate text with illusory statements not based on correct data [ 26 ]. It is important to be aware that LLMs are not directly coupled to evidence and that there is no restriction preventing a model from generating incorrect statements. As part of a screening tool assigning just a score value to the relevance of a publication, this may be a mere factor impairing the performance of the system – yet for LLM-based analysis in general this is a major problem.
The majority of studies that so far have been published on using LLMs for publication screening used the currently most powerful models that are operated by private companies—most notably the ChatGPT models GPT-3.5 and GPT-4 developed by OpenAI [ 18 , 74 ]. Using models that are owned and controlled by private companies and that may change over time is associated with additional major problems when using them for publication screening, such as a lack of reproducibility. Therefore, after initial experiments with such models, we decided to use openly available models for our study.
Limitations of the study
Our study has some limitations. While we present a strategy for using LLMs to evaluate the relevance of publications for an SLR, our work does not provide a comprehensive analysis of all possible capabilities and limitations. Even though we achieved promising results on ten published data sets and a newly created one in our study, generalization of the results may be limited as it is not clear how the approach would perform on many other subjects within the biomedical domain more broadly and within other domains. To get a more comprehensive understanding, thorough testing with many more data sets about different topics would be needed, which is beyond the scope of this work. Testing the screening approach on retrospective data sets is also per se problematic. While a good performance on retrospective data should hopefully indicate a good performance if used prospectively on a new topic, this does not have to be the case [ 75 ]. Indeed, naively assuming a classifier that was tested on retrospective data will perform equally on a new research question is clearly problematic, since a new research question in science is by definition new and unfamiliar and therefore will not be represented in previously tested data sets.
Furthermore, models that are trained on vast amounts of scientific literature may even have been trained on some publications or the reviews that are used in the retrospective benchmarking of an LLM-based classifier, which obviously creates a considerable bias. To objectively assess how well an LLM-based solution can evaluate scientific publications for new research questions, large cultivated and independent prospective data sets on many different topics would be needed, which will be very challenging to create. It is interesting that the LLM-based title and abstract screening in our study would have also performed well on our new hypothetical SLR on CDSS in radiation therapy, but of course, this alone is a too limited data basis from which to draw general conclusions. Therefore, it currently cannot be reliably known in which situations such an LLM-based evaluation may succeed or may fail.
Regarding the ten published data sets, the results also need to be interpreted with caution. These data sets may not truly represent the singular task of title and abstract screening. For example, in the Appenzeller-Herzog_2020 data set, only the 26 publications that were finally included (not only after title and abstract screening but also after further analysis) were labeled as relevant [ 40 ]. While these publications ideally should be correctly identified by an AI-classifier, there may be other publications in the data set, that per se cannot be excluded solely based on title and abstract. Furthermore, we had to retrospectively define the [Relevant Criteria] string based on the text in the publication of the SLR. This obviously is a suboptimal way to define inclusion and exclusion criteria, as the defined string may not completely align with the criteria intended by the researchers of the SLR.
We also want to emphasize that the comparison with the approach of Natukunda et al. needs to be interpreted with caution since the two approaches are not based on exactly the same prerequisites: the LLM-based approach requires a [Relevant Criteria] string, while the approach of Natukunda et al. requires defined keywords.
While overall our work shows that LLM-based title and abstract screening is possible and shows some promising results on the analyzed data sets, our study cannot fully answer the question of how well LLMs would perform if they were used for new research. Even more importantly, we cannot answer the question of to what extent LLMs should be used for conducting literature reviews and for doing research.
Large language models can be used for evaluating the relevance of publications for SLRs. We were able to implement a flexible and cross-domain system with promising results on different biomedical subjects. With the continuing progress in the fields of LLMs and AI, fully automated computer systems may assist researchers in performing SLRs and other forms of scientific knowledge synthesis. However, it remains unclear how well such systems will perform when being used in a prospective manner and what implications this will have on the conduction of SLRs.
Availability of data and materials
All data generated and analyzed during this study are either included in this published article (and its supplementary information files) or publicly available on the Internet. The Python script as well as the CDSS_RO data set are available under https://github.com/med-data-tools/title-abstract-screening-ai . The ten published data sets analyzed in our study are available on the GitHub Repository of the research group of the ASReview Tool [ 39 ].
Abbreviations
Artificial intelligence
Application programming interface
Clinical Decision Support System
FlanT5-XXL model
Generative pre-trained transformer
Mixtral-8 × 7B-Instruct v0.1 model
Machine learning
Large language model
OpenHermes-2.5-neural-chat-7b-v3-1-7B model
Platypus2-70B-Instruct model
Receiver operating characteristic
- Systematic literature review
Khalil H, Ameen D, Zarnegar A. Tools to support the automation of systematic reviews: a scoping review. J Clin Epidemiol. 2022;144:22–42.
Article PubMed Google Scholar
Clark J, Scott AM, Glasziou P. Not all systematic reviews can be completed in 2 weeks—But many can be (and should be). J Clin Epidemiol. 2020;126:163.
Clark J, Glasziou P, Del Mar C, Bannach-Brown A, Stehlik P, Scott AM. A full systematic review was completed in 2 weeks using automation tools: a case study. J Clin Epidemiol. 2020;121:81–90.
Pham B, Jovanovic J, Bagheri E, Antony J, Ashoor H, Nguyen TT, et al. Text mining to support abstract screening for knowledge syntheses: a semi-automated workflow. Syst Rev. 2021;10(1):156.
Article PubMed PubMed Central Google Scholar
van de Schoot R, de Bruin J, Schram R, Zahedi P, de Boer J, Weijdema F, et al. An open source machine learning framework for efficient and transparent systematic reviews. Nat Mach Intell. 2021;3(2):125–33.
Article Google Scholar
Hamel C, Hersi M, Kelly SE, Tricco AC, Straus S, Wells G, et al. Guidance for using artificial intelligence for title and abstract screening while conducting knowledge syntheses. BMC Med Res Methodol. 2021;21(1):285.
Covidence [Internet]. [cited 2024 Jan 14]. Available from: www.covidence.org .
Machine learning functionality in EPPI-Reviewer [Internet]. [cited 2024 Jan 14]. Available from: https://eppi.ioe.ac.uk/CMS/Portals/35/machine_learning_in_eppi-reviewer_v_7_web_version.pdf .
Elicit [Internet]. [cited 2024 Jan 14]. Available from: https://elicit.org/ .
Harrison H, Griffin SJ, Kuhn I, Usher-Smith JA. Software tools to support title and abstract screening for systematic reviews in healthcare: an evaluation. BMC Med Res Methodol. 2020;20(1):7.
Rayyan [Internet]. [cited 2024 Jan 14]. Available from: https://www.rayyan.ai/ .
DistillerSR [Internet]. [cited 2024 Jan 14]. Available from: https://www.distillersr.com/products/distillersr-systematic-review-software .
Abstrackr [Internet]. [cited 2024 Jan 14]. Available from: http://abstrackr.cebm.brown.edu/account/login .
RobotAnalyst [Internet]. [cited 2024 Jan 14]. Available from: http://www.nactem.ac.uk/robotanalyst/ .
Clark J, McFarlane C, Cleo G, Ishikawa Ramos C, Marshall S. The impact of systematic review automation tools on methodological quality and time taken to complete systematic review Tasks: Case Study. JMIR Med Educ. 2021;7(2): e24418.
Ayers JW, Poliak A, Dredze M, Leas EC, Zhu Z, Kelley JB, et al. Comparing physician and artificial intelligence chatbot responses to patient questions posted to a public social media forum. JAMA Intern Med. 2023 [cited 2024 Jan 14]; Available from: https://jamanetwork.com/journals/jamainternalmedicine/fullarticle/2804309 .
Tang L, Sun Z, Idnay B, Nestor JG, Soroush A, Elias PA, et al. Evaluating Large Language Models on Medical Evidence Summarization [Internet]. Health Informatics; 2023 Apr [cited 2024 Jan 14]. Available from: http://medrxiv.org/lookup/doi/ https://doi.org/10.1101/2023.04.22.23288967 .
OpenAI: GPT3-apps [Internet]. [cited 2024 Jan 14]. Available from: https://openai.com/blog/gpt-3-apps .
Google: PaLM [Internet]. [cited 2024 Jan 14]. Available from: https://ai.googleblog.com/2022/04/pathways-language-model-palm-scaling-to.html .
Google: Gemini [Internet]. [cited 2024 Jan 14]. Available from: https://deepmind.google/technologies/gemini/#hands-on .
Zhao WX, Zhou K, Li J, Tang T, Wang X, Hou Y, et al. A Survey of Large Language Models. 2023 [cited 2024 Jan 14]; Available from: https://arxiv.org/abs/2303.18223 .
McNichols H, Zhang M, Lan A. Algebra error classification with large language models [Internet]. arXiv; 2023 [cited 2023 May 25]. Available from: http://arxiv.org/abs/2305.06163 .
Wadhwa S, Amir S, Wallace BC. Revisiting relation extraction in the era of large language models [Internet]. arXiv; 2023 [cited 2024 Jan 14]. Available from: http://arxiv.org/abs/2305.05003 .
Trajanoska M, Stojanov R, Trajanov D. Enhancing knowledge graph construction using large language models [Internet]. arXiv; 2023 [cited 2024 Jan 14]. Available from: http://arxiv.org/abs/2305.04676 .
Reynolds L, McDonell K. Prompt programming for large language models: beyond the few-shot paradigm [Internet]. arXiv; 2021 [cited 2024 Jan 14]. Available from: http://arxiv.org/abs/2102.07350 .
Guerreiro NM, Alves D, Waldendorf J, Haddow B, Birch A, Colombo P, et al. Hallucinations in Large Multilingual Translation Models [Internet]. arXiv; 2023 [cited 2024 Jan 14]. Available from: http://arxiv.org/abs/2303.16104 .
Zack T, Lehman E, Suzgun M, Rodriguez JA, Celi LA, Gichoya J, et al. Assessing the potential of GPT-4 to perpetuate racial and gender biases in health care: a model evaluation study. Lancet Digital Health. 2024;6(1):e12-22.
Article CAS PubMed Google Scholar
Hastings J. Preventing harm from non-conscious bias in medical generative AI. Lancet Digital Health. 2024;6(1):e2-3.
Digutsch J, Kosinski M. Overlap in meaning is a stronger predictor of semantic activation in GPT-3 than in humans. Sci Rep. 2023;13(1):5035.
Article CAS PubMed PubMed Central Google Scholar
Huggingface: FlanT5-XXL [Internet]. [cited 2024 Jan 14]. Available from: https://huggingface.co/google/flan-t5-xxl .
Chung HW, Hou L, Longpre S, Zoph B, Tay Y, Fedus W, et al. Scaling Instruction-Finetuned Language Models [Internet]. arXiv; 2022 [cited 2024 Jan 14]. Available from: http://arxiv.org/abs/2210.11416 .
Huggingface: OpenHermes-2.5-neural-chat-7b-v3–1–7B [Internet]. [cited 2024 Jan 14]. Available from: https://huggingface.co/Weyaxi/OpenHermes-2.5-neural-chat-7b-v3-1-7B .
Huggingface: OpenHermes-2.5-Mistral-7B [Internet]. [cited 2024 Jan 14]. Available from: https://huggingface.co/teknium/OpenHermes-2.5-Mistral-7B .
Huggingface: neural-chat-7b-v3–1 [Internet]. [cited 2024 Jan 14]. Available from: https://huggingface.co/Intel/neural-chat-7b-v3-1 .
Huggingface: Mixtral-8x7B-Instruct-v0.1 [Internet]. [cited 2024 Jan 14]. Available from: https://huggingface.co/mistralai/Mixtral-8x7B-Instruct-v0.1 .
Jiang AQ, Sablayrolles A, Roux A, Mensch A, Savary B, Bamford C, et al. Mixtral of Experts [Internet]. [cited 2024 Jan 14]. Available from: http://arxiv.org/abs/2401.04088 .
Huggingface: Platypus2–70B-Instruct [Internet]. [cited 2024 Jan 14]. Available from: https://huggingface.co/garage-bAInd/Platypus2-70B-instruct .
Huggingface: SOLAR-0–70b-16bit [Internet]. [cited 2024 Jan 14]. Available from: https://huggingface.co/upstage/SOLAR-0-70b-16bit#updates .
Systematic Review Datasets: ASReview [Internet]. [cited 2024 Jan 14]. Available from: https://github.com/asreview/systematic-review-datasets .
Appenzeller-Herzog C, Mathes T, Heeres MLS, Weiss KH, Houwen RHJ, Ewald H. Comparative effectiveness of common therapies for Wilson disease: a systematic review and meta-analysis of controlled studies. Liver Int. 2019;39(11):2136–52.
Bos D, Wolters FJ, Darweesh SKL, Vernooij MW, De Wolf F, Ikram MA, et al. Cerebral small vessel disease and the risk of dementia: a systematic review and meta-analysis of population-based evidence. Alzheimer’s & Dementia. 2018;14(11):1482–92.
Donners AAMT, Rademaker CMA, Bevers LAH, Huitema ADR, Schutgens REG, Egberts TCG, et al. Pharmacokinetics and associated efficacy of emicizumab in humans: a systematic review. Clin Pharmacokinet. 2021;60(11):1395–406.
Jeyaraman M, Muthu S, Ganie PA. Does the source of mesenchymal stem cell have an effect in the management of osteoarthritis of the knee? Meta-analysis of randomized controlled trials. CARTILAGE. 2021 Dec;13(1_suppl):1532S-1547S.
Leenaars C, Stafleu F, De Jong D, Van Berlo M, Geurts T, Coenen-de Roo T, et al. A systematic review comparing experimental design of animal and human methotrexate efficacy studies for rheumatoid arthritis: lessons for the translational value of animal studies. Animals. 2020;10(6):1047.
Meijboom RW, Gardarsdottir H, Egberts TCG, Giezen TJ. Patients retransitioning from biosimilar TNFα inhibitor to the corresponding originator after initial transitioning to the biosimilar: a systematic review. BioDrugs. 2022;36(1):27–39.
Muthu S, Ramakrishnan E. Fragility analysis of statistically significant outcomes of randomized control trials in spine surgery: a systematic review. Spine. 2021;46(3):198–208.
Oud M, Arntz A, Hermens ML, Verhoef R, Kendall T. Specialized psychotherapies for adults with borderline personality disorder: a systematic review and meta-analysis. Aust N Z J Psychiatry. 2018;52(10):949–61.
Van De Schoot R, Sijbrandij M, Depaoli S, Winter SD, Olff M, Van Loey NE. Bayesian PTSD-trajectory analysis with informed priors based on a systematic literature search and expert elicitation. Multivar Behav Res. 2018;53(2):267–91.
Wolters FJ, Segufa RA, Darweesh SKL, Bos D, Ikram MA, Sabayan B, et al. Coronary heart disease, heart failure, and the risk of dementia: A systematic review and meta-analysis. Alzheimer’s Dementia. 2018;14(11):1493–504.
Sutton RT, Pincock D, Baumgart DC, Sadowski DC, Fedorak RN, Kroeker KI. An overview of clinical decision support systems: benefits, risks, and strategies for success. npj Digit Med. 2020 Feb 6;3(1):17.
Natukunda A, Muchene LK. Unsupervised title and abstract screening for systematic review: a retrospective case-study using topic modelling methodology. Syst Rev. 2023;12(1):1.
Marshall IJ, Wallace BC. Toward systematic review automation: a practical guide to using machine learning tools in research synthesis. Syst Rev. 2019 Dec;8(1):163, s13643–019–1074–9.
Wallace BC, Trikalinos TA, Lau J, Brodley C, Schmid CH. Semi-automated screening of biomedical citations for systematic reviews. BMC Bioinformatics. 2010;11(1):55.
Li D, Wang Z, Wang L, Sohn S, Shen F, Murad MH, et al. A text-mining framework for supporting systematic reviews. Am J Inf Manag. 2016;1(1):1–9.
PubMed PubMed Central Google Scholar
de Almeida CPB, de Goulart BNG. How to avoid bias in systematic reviews of observational studies. Rev CEFAC. 2017;19(4):551–5.
Siddaway AP, Wood AM, Hedges LV. How to do a systematic review: a best practice guide for conducting and reporting narrative reviews, meta-analyses, and meta-syntheses. Annu Rev Psychol. 2019;70(1):747–70.
Santos ÁOD, Da Silva ES, Couto LM, Reis GVL, Belo VS. The use of artificial intelligence for automating or semi-automating biomedical literature analyses: a scoping review. J Biomed Inform. 2023;142: 104389.
Haman M, Školník M. Using ChatGPT to conduct a literature review. Account Res. 2023;6:1–3.
Liu R, Shah NB. ReviewerGPT? An exploratory study on using large language models for paper reviewing [Internet]. arXiv; 2023 [cited 2024 Jan 14]. Available from: http://arxiv.org/abs/2306.00622
Wang S, Scells H, Koopman B, Zuccon G. Can ChatGPT write a good boolean query for systematic review literature search? [Internet]. arXiv; 2023 [cited 2024 Jan 14]. Available from: http://arxiv.org/abs/2302.03495 .
Aydın Ö, Karaarslan E. OpenAI ChatGPT generated literature review: digital twin in healthcare. SSRN Journal [Internet]. 2022 [cited 2024 Jan 14]; Available from: https://www.ssrn.com/abstract=4308687 .
Guo E, Gupta M, Deng J, Park YJ, Paget M, Naugler C. Automated paper screening for clinical reviews using large language models [Internet]. arXiv; 2023 [cited 2024 Jan 14]. Available from: http://arxiv.org/abs/2305.00844 .
Akinseloyin O, Jiang X, Palade V. A novel question-answering framework for automated citation screening using large language models [Internet]. Health Informatics; 2023 Dec [cited 2024 Jan 14]. Available from: http://medrxiv.org/lookup/doi/ https://doi.org/10.1101/2023.12.17.23300102 .
Koh JY, Salakhutdinov R, Fried D. Grounding language models to images for multimodal inputs and outputs. 2023 [cited 2024 Jan 14]; Available from: https://arxiv.org/abs/2301.13823 .
Wang L, Lyu C, Ji T, Zhang Z, Yu D, Shi S, et al. Document-level machine translation with large language models [Internet]. arXiv; 2023 [cited 2024 Jan 14]. Available from: http://arxiv.org/abs/2304.02210 .
Brown TB, Mann B, Ryder N, Subbiah M, Kaplan J, Dhariwal P, et al. Language Models are Few-Shot Learners [Internet]. arXiv; 2020 [cited 2024 Jan 14]. Available from: http://arxiv.org/abs/2005.14165 .
Koo R, Lee M, Raheja V, Park JI, Kim ZM, Kang D. Benchmarking cognitive biases in large language models as evaluators [Internet]. arXiv; 2023 [cited 2024 Jan 14]. Available from: http://arxiv.org/abs/2309.17012 .
Editorial —Artificial Intelligence language models in scientific writing. EPL. 2023 Jul 1;143(2):20000.
Grimaldi G, Ehrler BAI, et al. Machines Are About to Change Scientific Publishing Forever. ACS Energy Lett. 2023;8(1):878–80.
Article CAS Google Scholar
Grillo R. The rising tide of artificial intelligence in scientific journals: a profound shift in research landscape. Eur J Ther. 2023;29(3):686–8.
nature: ChatGPT and science: the AI system was a force in 2023 — for good and bad [Internet]. [cited 2024 Jan 14]. Available from: https://www.nature.com/articles/d41586-023-03930-6 .
Chiang CH, Lee H yi. Can large language models be an alternative to human evaluations? 2023 [cited 2024 Jan 6]; Available from: https://arxiv.org/abs/2305.01937 .
Erler A. Publish with AUTOGEN or perish? Some pitfalls to avoid in the pursuit of academic enhancement via personalized large language models. Am J Bioeth. 2023;23(10):94–6.
OpenAI: ChatGPT [Internet]. [cited 2024 Jan 14]. Available from: https://openai.com/blog/chatgpt .
Gates A, Gates M, Sebastianski M, Guitard S, Elliott SA, Hartling L. The semi-automation of title and abstract screening: a retrospective exploration of ways to leverage Abstrackr’s relevance predictions in systematic and rapid reviews. BMC Med Res Methodol. 2020;20(1):139.
Download references
Acknowledgements
Not applicable.
Author information
Authors and affiliations.
Department of Radiation Oncology, Cantonal Hospital of St. Gallen, St. Gallen, Switzerland
Fabio Dennstädt & Paul Martin Putora
Institute for Computer Science, University of Würzburg, Würzburg, Germany
Johannes Zink
Department of Radiation Oncology, Inselspital, Bern University Hospital and University of Bern, Bern, Switzerland
Fabio Dennstädt, Paul Martin Putora & Nikola Cihoric
Institute for Implementation Science in Health Care, University of Zurich, Zurich, Switzerland
Janna Hastings
School of Medicine, University of St. Gallen, St. Gallen, Switzerland
Swiss Institute of Bioinformatics, Lausanne, Switzerland
You can also search for this author in PubMed Google Scholar
Contributions
All authors contributed to designing the concept and methodology of the presented approach of LLM-based evaluation of the relevance of a publication to an SLR. The Python script was created by FD and JZ. The experiments were conducted by FD and JH. All authors contributed in writing and revising the manuscript. All authors have read and approved the final version of the manuscript.
Corresponding author
Correspondence to Fabio Dennstädt .
Ethics declarations
Ethics approval and consent to participate, consent for publication, competing interests.
NC is a technical lead for the SmartOncology© project and medical advisor for Wemedoo AG, Steinhausen AG, Switzerland. The authors declare that they have no other competing interests.
Additional information
Publisher’s note.
Springer Nature remains neutral with regard to jurisdictional claims in published maps and institutional affiliations.
Supplementary Information
Supplementary material 1: appendix 1: sample prompt., supplementary material 2: appendix 2: relevant criteria of published datasets., supplementary material 3: appendix 3: performance of models on data sets., supplementary material 4: appendix 4: comparison with other approach., rights and permissions.
Open Access This article is licensed under a Creative Commons Attribution 4.0 International License, which permits use, sharing, adaptation, distribution and reproduction in any medium or format, as long as you give appropriate credit to the original author(s) and the source, provide a link to the Creative Commons licence, and indicate if changes were made. The images or other third party material in this article are included in the article's Creative Commons licence, unless indicated otherwise in a credit line to the material. If material is not included in the article's Creative Commons licence and your intended use is not permitted by statutory regulation or exceeds the permitted use, you will need to obtain permission directly from the copyright holder. To view a copy of this licence, visit http://creativecommons.org/licenses/by/4.0/ . The Creative Commons Public Domain Dedication waiver ( http://creativecommons.org/publicdomain/zero/1.0/ ) applies to the data made available in this article, unless otherwise stated in a credit line to the data.
Reprints and permissions
About this article
Cite this article.
Dennstädt, F., Zink, J., Putora, P.M. et al. Title and abstract screening for literature reviews using large language models: an exploratory study in the biomedical domain. Syst Rev 13 , 158 (2024). https://doi.org/10.1186/s13643-024-02575-4
Download citation
Received : 17 June 2023
Accepted : 30 May 2024
Published : 15 June 2024
DOI : https://doi.org/10.1186/s13643-024-02575-4
Share this article
Anyone you share the following link with will be able to read this content:
Sorry, a shareable link is not currently available for this article.
Provided by the Springer Nature SharedIt content-sharing initiative
- Natural language processing
- Biomedicine
- Title and abstract screening
- Large language models
Systematic Reviews
ISSN: 2046-4053
- Submission enquiries: Access here and click Contact Us
- General enquiries: [email protected]
for Education
- Google Classroom
- Google Workspace Admin
- Google Cloud
Where teaching and learning come together
Google Classroom helps educators create engaging learning experiences they can personalize, manage, and measure. Part of Google Workspace for Education, it empowers educators to enhance their impact and prepare students for the future.
- Contact sales
- Sign in to Classroom
150M people worldwide use Google Classroom
Google Classroom is designed with feedback from the educational community, influencing the development of new features that let educators focus on teaching and students focus on learning.
Enrich and personalize learning
Drive student agency with tools that meet students where they are – and build skills for their future.
Premium features that inspire new ways of teaching and learning
Power student potential.
Create interactive assignments, even from existing PDFs, that provide real-time feedback and individual guidance with prompts and hints with the help of AI.
Help students develop literacy skills
Assign differentiated reading activities using the Classroom integration with Read Along, a fun, speech-based tool from Google that helps students independently build their reading skills, while giving educators insight into their progress.
Reinforce concepts with self-paced learning
Assign interactive questions for YouTube videos, giving students real-time feedback as they move through a lesson, while viewing insights into their performance.
Enhance lessons with popular integrations
Easily find, add, use and grade content with add-ons from popular EdTech tools, right within Classroom.
Make learning more personal and foster student agency
Support differentiated instruction.
Customize classwork for every student and support them with real-time feedback and easy communication tools.
Foster academic integrity
Encourage original thinking and identify potential plagiarism with originality reports that compare student work against billions of web pages and over 40 million books.
Make learning accessible and inclusive
Help students customize their learning environment to reduce barriers to learning.
Prepare students for the future
Encourage organization and time management skills with interactive to-do lists, automatic due dates, and industry-leading productivity tools.
- Explore all features
Amplify instruction with tools that simplify everyday tasks
Boost instructional time with tools purpose-built for teaching, productivity, and collaboration.
Premium features that elevate teaching
Support originality with plagiarism detection.
Help students integrate citations and avoid unintentional plagiarism with unlimited originality reports and a school-owned repository of past work.
Streamline lesson planning
Create a link to your class, then share it with peers in your organization, so they can easily preview, select, and import high-quality classwork into their classes.
Inform instruction with data-driven insights
Classroom analytics provide educators with insights and visibility into how students turn in, perform on, and engage with assignments, so educators can make informed decisions about the best way to provide support.
Simplify and connect grading workflows
Sync gradebooks to seamlessly manage and export grades from Classroom to your school’s SIS – available for PowerSchool (coming soon), Infinite Campus, Skyward SMS, Skyward Qmlativ, and Follett Aspen. Educators can customize grading periods (e.g., quarters, semesters, terms) and grading scales (e.g., letter, numeric) in their class settings to align to their school’s grading structure or system, reduce errors for SIS grade export and allow educators to more easily filter and analyze assignments.
Tools designed for seamless teaching
Save time on everyday tasks.
Assign, grade, and provide feedback across multiple classes, and even on the go with iOS and Android versions of the Classroom app.
Elevate communication, collaboration, and connection
Connect with students and parents instantly with embedded chat and meeting tools while leveraging built-in chat and comment features to leave students feedback as they’re working.
Grade more efficiently
Assess student progress with customizable rubrics that students can see, and save time with efficient feedback and grade export tools.
Get creative with hundreds of apps
Hundreds of EdTech apps integrate with Classroom to spark creativity and enable more opportunities for learning.
Operate with solutions designed to gain visibility, insights, and control
Create learning environments that are easier to manage and support educators and students with connected, safer tools.
Premium features to support your organization and foster stronger learning outcomes
Make data-driven decisions.
Gain visibility into everything from class performance to individual student assignment completion with Classroom analytics (coming soon), or export Classroom logs to BigQuery to analyze adoption, engagement, and more.
Distribute high-quality class templates to educators
Easily share high-quality class templates so educators in your organization can preview and import classwork into their own classes.
Virtually visit classes to support teachers and students
Designated education leaders and staff can temporarily access classes to support educators, manage substitute teachers, see information for guardian conversations, and more.
Manage classes at scale
Create classes automatically and sync class lists from your student information system (SIS) with Clever .
A secure, reliable, and extensible platform for school communities of all sizes
Benefit from industry-leading privacy and security.
Classroom uses the same infrastructure as other Google Workspace products, meeting rigorous privacy standards with regular third-party audits. Access a centralized Admin console with controlled entry and insights into performance and security.
Stay flexible and reliable
Scale your school community with a global network with full-stack security and 99% uptime.
Extend and scale Classroom
Integrate with your student information system (SIS) and customize Classroom to work for your unique needs with APIs.
Support staff and enhance collaboration
Empower educators with instructional resources , professional development programs , and online training courses , available at no cost.
Level up your Classroom with apps
Discover a world of apps that seamlessly integrate with Chromebooks and Google Workspace for Education.
- Explore App Hub
How Classroom can make a difference for you
Education leaders, it administrators.
Classroom can be learned in minutes and serves all types of learners and educators, regardless of their tech savviness. Empower educators, and encourage adoption and proficiency with new tools and techniques, with a broad range of resources.
- Get a quick overview of the benefits of Classroom
- Read customer stories
- Explore trainings and resources for educators
- 40+ ways to use Google Workspace for Education paid editions
- Learn about sustainability in Google for Education products
Teachers can immediately set up classes, easily create coursework, distribute it to the whole class, and grade it efficiently and transparently.
- Find an educator community
- Download the Classroom user guide
- View product guides
Admins have as much control as they need while they access and analyze their data for insights and choose from a range of upgrade options for additional capabilities to fit their specific needs.
- Get started with the paid editions of Workspace for Education
- View product demos
- Explore 40+ ways to use Google Workspace for Education paid editions
- Learn more about Google for Education security and privacy
- Guardian's Guide to Google Classroom
Need more information about Classroom?
- Visit the Help Center
Bring all of your tools together with Google Workspace for Education
Google Workspace for Education empowers your school community with easy-to-use tools that elevate teaching, learning, collaboration, and productivity – all on one secure platform.
- Explore Google Workspace for Education
150 million users
Active around the world, ready to transform your school, you're now viewing content for a different region..
For content more relevant to your region, we suggest:
Sign up here for updates, insights, resources, and more.
Purdue Online Writing Lab Purdue OWL® College of Liberal Arts
Welcome to the Purdue Online Writing Lab
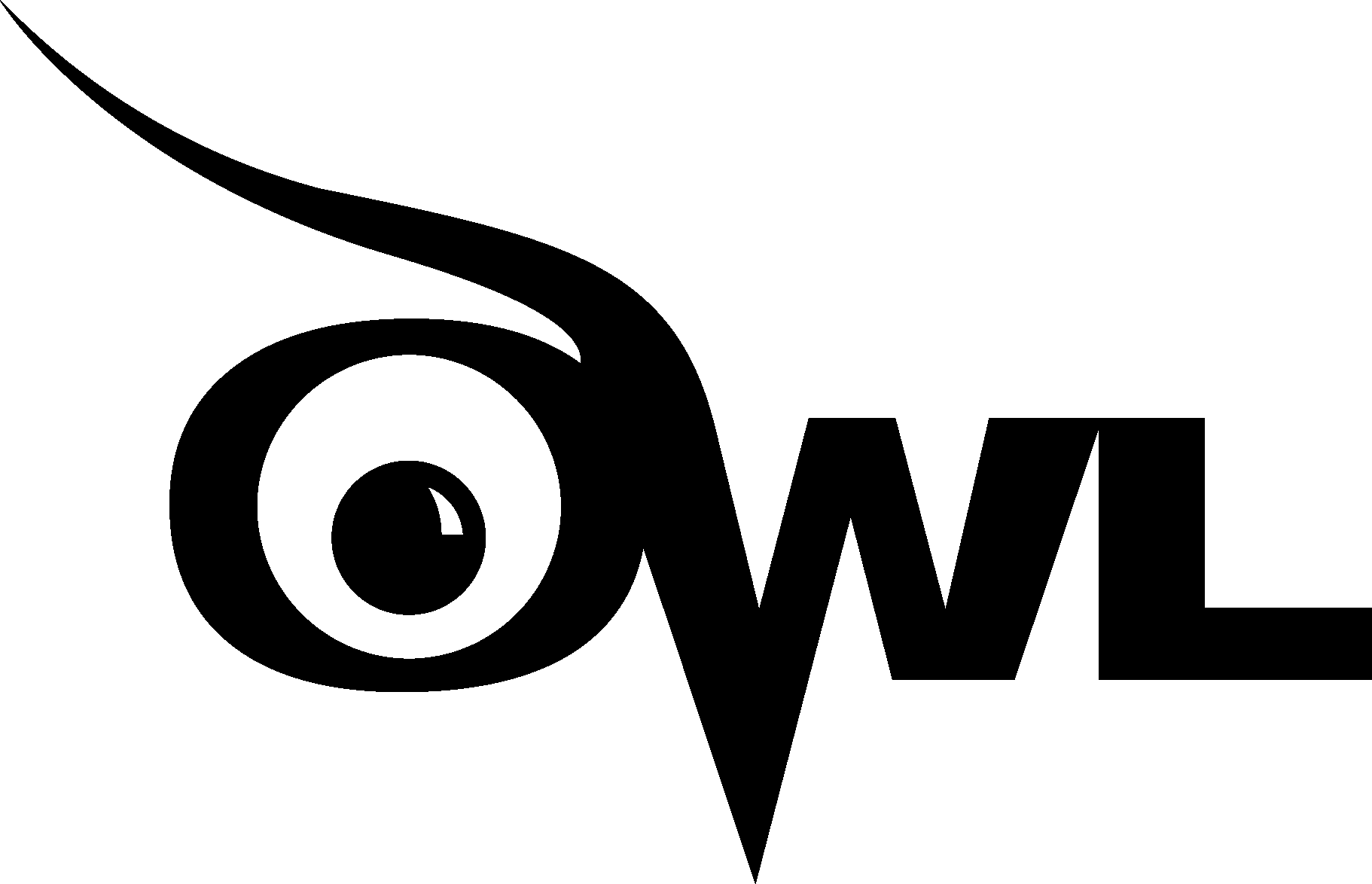
Welcome to the Purdue OWL
This page is brought to you by the OWL at Purdue University. When printing this page, you must include the entire legal notice.
Copyright ©1995-2018 by The Writing Lab & The OWL at Purdue and Purdue University. All rights reserved. This material may not be published, reproduced, broadcast, rewritten, or redistributed without permission. Use of this site constitutes acceptance of our terms and conditions of fair use.
The Online Writing Lab (the Purdue OWL) at Purdue University houses writing resources and instructional material, and we provide these as a free service at Purdue. Students, members of the community, and users worldwide will find information to assist with many writing projects. Teachers and trainers may use this material for in-class and out-of-class instruction.
The On-Campus and Online versions of Purdue OWL assist clients in their development as writers—no matter what their skill level—with on-campus consultations, online participation, and community engagement. The Purdue OWL serves the Purdue West Lafayette and Indianapolis campuses and coordinates with local literacy initiatives. The Purdue OWL offers global support through online reference materials and services.
Social Media
Facebook twitter.

IMAGES
VIDEO
COMMENTS
that technology should play a more integral role in students' education. However, the question of whether the introduction of technology into the classroom has a positive impact on teaching and learning is still under intense debate within the educational community. This Literature Review
Abstract. The following article d iscusses one of the most curren t topics: techn ology in. education, emphasizing in a very crucial aspect as it is technology integration. Consequently, the text ...
This study is a literature review to investigate the effects of implementing technology into a high school mathematics classroom. ... One of these areas of improvement that can be used in the high school mathematics classroom is the use of technology by not only the teacher in the delivery of the content, but also by the students in the ...
the classroom, the benefits and drawbacks of the use of technology in education, and particularly the impact on students' learning. For the purpose of this study, technology included only educational technology, i.e. internet and computer-mediated tools. It is important to understand the impact of technology on student learning because
Overall, the authors found that, on average, there was a high, significantly positive effect of digital technology on mathematics achievement (mean effect size of 0.71), indicating that, in general, learners learning mathematics with the use of digital technology had higher mathematics achievement than those learning without digital technology.
This systematic review aims to explore (1) English teachers' practices of digital technologies integration and (2) the factors that influence this process. In terms of the former, first, digital technologies are mainly used for teacher-centered purposes, mostly to fulfill the instructional purpose of presentation (e.g., Ding et al., 2019; Li ...
5. Conclusion. The complex educational ecosystem involving students, parents, teachers, and school systems is highly interconnected. Digital technology provides positive learning outcomes, inspiring and motivating to students as learners in a digital world beyond the constraints of the classroom.
Articles that were selected for this literature review span from 1986 to 2014. The literature pri-marily focuses on technology use in education; outcomes of technology in the classroom; and online learning environments. The purpose of selecting these key themes was to convey how technology integration has changed across the years; accurately ...
Students' Engagement through Technology and Cooperative Learning: A Systematic Literature Review July 2022 International Journal of Learning and Development 12(3):23
Keywords: educational technology, systematic literature review, English classrooms, teaching English, English skills INTRODUCTION Technology changes education and affects both the acquisition of skills that students require to prepare for university and a career, and the integration of digital instructional strategies by teachers (Delgado et al.,
perception of technology use in the classroom vs how they actually us it. The final section of this literature review will review the challenges identified in the studies related to increasing use of technology in the classroom. This includes studies about educators training on technology, resources needed to complete the task,
Teachers' lack of ICT skills and familiarization with technologies can become a constraint to the effective use of technology in the classroom (Balanskat et al., 2006; Delgado et al., 2015). ... Our literature review, which synthesized quantitative and qualitative data from a list of meta-analyses and review studies, provided useful insights ...
Research has been conducted at all educational levels, and this review will. address studies conducted at the elementary, middle and high, and collegiate levels. Studies seek. to prove whether or not the addition of technology in the classroom does lead to an increase in. student learning and retention of information.
10 Use of technology in. the classroom. Suzanne V assallo and Diana W arren. The use of information and communication technology. (ICT) in schools has increased dramatically in recent. years ...
Barriers to technology integration model. The ways in which teachers use technology in the classroom are influenced by multiple factors. The Barrier to Technology Integration model (Ertmer, Citation 1999; Hew & Brush, Citation 2007) described these factors as 'barriers' that hinder how and how much teachers integrate technology.According to Ertmer's model (1999) these barriers may be ...
The term "Smart Classroom" has evolved over time and nowadays reflects the technological advancements incorporated in educational spaces. The rapid advances in technology, and the need to create more efficient and creative classes that support both in-class and remote activities, have led to the integration of Artificial Intelligence and smart technologies in smart classes. In this paper we ...
Results of this review of literature reveal that technology is used in the classroom for instructional support, information referencing and, communication and ... Using the theme "teachers' use of technology in the classroom", the said search engine reveal 19,500 related studies. However, majority of these focused on the following topics
ABSTRACT. This review summarizes the relevant research on the use of information and communication technology (ICT) in education. Specifically, it reviews studies that have touched upon the merits of ICT integration in schools, barriers or challenges encountered in the use of ICT, factors influencing successful ICT integration, in-service and ...
The literature review will also identify some pitfalls of technology in the classroom. Some of the pitfalls discussed in the literature review include cell phones, lack of school policy and lack of help from technology departments. The review will conclude with where future research is needed.
This study is a literature review to investigate the effects of implementing technology into a high school mathematics classroom. Mathematics has a hierarchical structure in learning and it is essential that students get a firm understanding of mathematics early in education. Some students that miss beginning concepts may continue to struggle with mathematical computation and concepts ...
(Buabbas, A., Al-Mass, Al-Tawari & Buabbas, M., 2020). Technology use needs to be limited within the classroom in order to enhance intrapersonal relationships, social skills, and to avoid the negative health impacts that it is having on our students. Throughout this literature review, one will learn how technology plays an important role
2 TECHNOLOGY INSTRUCTION IN THE CLASSROOM . Abstract. Technology use within the writing classroom for writers who struggle can offer independence and lead to success. This literature review examines the use of technology with struggling writers, the effects of technology use of student engagement, specific strategies used
Literature Review. The idea for conducting this research came from the fact that most teachers in Kosovo mainly use the PowerPoint application of the Microsoft Office suite to create various presentations, through which they organize the lessons. ... Students can learn useful life skills using technology. Using technology in the classroom ...
The literature selection criteria included original works in environmental design pertaining to proxemics, exploration of blended learning and technology use, considerations in classroom design ...
A technoskeptical person is not necessarily anti-technology, but rather one who deeply examines technological issues from multiple dimensions and perspectives akin to an art critic. We created the Technoskepticism Iceberg as a framework to support teachers and students in conducting technological inquiries. The metaphor of an iceberg conveys ...
March 2007 Abstract. Technology has been viewed as a lodestone for improving student academic performance and for increasing the flexibility of public schools. This review provides a synthesis that addresses the effect of technology on teaching and learning and analyzes these effects through the lens of diffusion theory.
2. Background: technology integration in teacher education in Ghana. Ghana's policymakers in education consider technology integration and teacher training in pedagogy as topmost priorities to improve classroom instruction and quality of student learning, as well as to equip learners with the skills needed to thrive in today's changing digital world (Abedi et al., Citation 2024).
Systematically screening published literature to determine the relevant publications to synthesize in a review is a time-consuming and difficult task. Large language models (LLMs) are an emerging technology with promising capabilities for the automation of language-related tasks that may be useful for such a purpose. LLMs were used as part of an automated system to evaluate the relevance of ...
Classroom can be learned in minutes and serves all types of learners and educators, regardless of their tech savviness. Empower educators, and encourage adoption and proficiency with new tools and techniques, with a broad range of resources. Get a quick overview of the benefits of Classroom; Read customer stories
The Online Writing Lab (the Purdue OWL) at Purdue University houses writing resources and instructional material, and we provide these as a free service at Purdue. Students, members of the community, and users worldwide will find information to assist with many writing projects. Teachers and trainers may use this material for in-class and out ...