Artificial Intelligence in Interventional Radiology: A Literature Review and Future Perspectives
Affiliations.
- 1 Fondazione Policlinico Universitario A. Gemelli IRCCS, UOC di Radiologia, Dipartimento di Diagnostica per Immagini, Radioterapia Oncologica ed Ematologia, Roma, Italy.
- 2 Università Cattolica del Sacro Cuore, Istituto di Radiologia, Roma, Italy.
- 3 Department of Radiology, Hadassah Hebrew University Medical Center, Jerusalem, Israel.
- 4 Department of Radiology, AFaR-IRCCS Fatebenefratelli Hospital Foundation for Health Research and Education, via di Ponte Quattro Capi 39, 00186 Roma, Italy.
- 5 Fondazione Policlinico Universitario A. Gemelli IRCCS, UOC di Radioterapia Oncologica, Dipartimento di Diagnostica per Immagini, Radioterapia Oncologica ed Ematologia, Roma, Italy.
- 6 Università Cattolica del Sacro Cuore, Istituto di Radioterapia Oncologica, Roma, Italy.
- PMID: 31781215
- PMCID: PMC6874978
- DOI: 10.1155/2019/6153041
The term "artificial intelligence" (AI) includes computational algorithms that can perform tasks considered typical of human intelligence, with partial to complete autonomy, to produce new beneficial outputs from specific inputs. The development of AI is largely based on the introduction of artificial neural networks (ANN) that allowed the introduction of the concepts of "computational learning models," machine learning (ML) and deep learning (DL). AI applications appear promising for radiology scenarios potentially improving lesion detection, segmentation, and interpretation with a recent application also for interventional radiology (IR) practice, including the ability of AI to offer prognostic information to both patients and physicians about interventional oncology procedures. This article integrates evidence-reported literature and experience-based perceptions to assist not only residents and fellows who are training in interventional radiology but also practicing colleagues who are approaching to locoregional mini-invasive treatments.
Copyright © 2019 Roberto Iezzi et al.

Publication types

An official website of the United States government
The .gov means it’s official. Federal government websites often end in .gov or .mil. Before sharing sensitive information, make sure you’re on a federal government site.
The site is secure. The https:// ensures that you are connecting to the official website and that any information you provide is encrypted and transmitted securely.
- Publications
- Account settings
Preview improvements coming to the PMC website in October 2024. Learn More or Try it out now .
- Advanced Search
- Journal List
- v.2019; 2019

Artificial Intelligence in Interventional Radiology: A Literature Review and Future Perspectives
Roberto iezzi.
1 Fondazione Policlinico Universitario A. Gemelli IRCCS, UOC di Radiologia, Dipartimento di Diagnostica per Immagini, Radioterapia Oncologica ed Ematologia, Roma, Italy
2 Università Cattolica del Sacro Cuore, Istituto di Radiologia, Roma, Italy
S. N. Goldberg
3 Department of Radiology, Hadassah Hebrew University Medical Center, Jerusalem, Israel
4 Department of Radiology, AFaR-IRCCS Fatebenefratelli Hospital Foundation for Health Research and Education, via di Ponte Quattro Capi 39, 00186 Roma, Italy
V. Valentini
5 Fondazione Policlinico Universitario A. Gemelli IRCCS, UOC di Radioterapia Oncologica, Dipartimento di Diagnostica per Immagini, Radioterapia Oncologica ed Ematologia, Roma, Italy
6 Università Cattolica del Sacro Cuore, Istituto di Radioterapia Oncologica, Roma, Italy
R. Manfredi
The term “artificial intelligence” (AI) includes computational algorithms that can perform tasks considered typical of human intelligence, with partial to complete autonomy, to produce new beneficial outputs from specific inputs. The development of AI is largely based on the introduction of artificial neural networks (ANN) that allowed the introduction of the concepts of “computational learning models,” machine learning (ML) and deep learning (DL). AI applications appear promising for radiology scenarios potentially improving lesion detection, segmentation, and interpretation with a recent application also for interventional radiology (IR) practice, including the ability of AI to offer prognostic information to both patients and physicians about interventional oncology procedures. This article integrates evidence-reported literature and experience-based perceptions to assist not only residents and fellows who are training in interventional radiology but also practicing colleagues who are approaching to locoregional mini-invasive treatments.
1. Introduction
The term “artificial intelligence” (AI) includes computational algorithms that can perform tasks considered typical of human intelligence, with partial to complete autonomy, to produce new beneficial outputs from specific inputs [ 1 ]. Although premises to the development of AI were achieved in the early era of computers, it has only been with the introduction of new powerful computational hardware, in association with the capability of collecting and storing huge amounts of data, that it has become feasible to explore its potential in tasks most relevant to the field of radiology such as pattern recognition, pattern identification, planning, language comprehension, object and sound recognition, problem solving, prognosticating diseases, and deciding when and whether therapy is not needed or of limited use or in offering patients and physicians prognostic data on treatment outcomes. Indeed, although healthcare represents a challenging field for AI application, medical imaging is currently one of the most promising areas to apply this technology [ 2 ].
From the beginning, it has been quite clear that computers could be potentially useful in assisting the radiologist in the routine tasks of detection and diagnosis. The idea fostering the use of the so-called computer-aided detection/diagnosis (CAD) systems, precursors of modern AI, was to provide radiologists with the assistance in the detection and interpretations of potential lesions (especially in mammography and chest or musculoskeletal radiography) in order to discriminate between benign and malignant lesions, reduce false negatives, and boost radiologists' productivity, especially in terms of discovery and identification of significant findings requiring a prompt human validation [ 3 ]. Main limitations of CAD systems were their task-specific orientation which is suited to only one particular given task in a corresponding specific imaging modality and, moreover, their reliability and the risk of false positive results implied mandatory validation by a trained radiologist [ 3 ]. Since then, ever-increasing attempts have been made to improve upon the diagnostic performance of AI and facilitate the help it could provide in daily clinical practice.
The development of AI is largely based on the introduction of artificial neural networks (ANN) in the early 1950s [ 4 ] and their subsequent further evolution (from single to multilayer ANN), introducing the concepts of “computational learning models,” machine learning (ML) and deep learning (DL).
ML is based upon the so-called “reverse training” method, in which computer systems focus on specific pathological features identified during a training period [ 5 ]. Thus, ML applications require a set of data on a specific pathology on which the computer can train itself, and those data must necessarily contain the desired outcome that needs to be predicted (e.g., nodules or emphysema on chest X-rays, focal liver lesions, hemorrhage in head CT, and so on). Big data is the type of data that may be supplied into the analytical system so that an ML model could learn, improving the accuracy of its predictions. Once trained, the computer can apply this information even to new cases never seen before [ 6 , 7 ]. ML can be supervised or unsupervised, depending, respectively, on the “labeled” input previously selected by human experts, or directly extracted by the machine using several computational methods [ 6 , 8 ]. Among the evaluated features, the ideal ML model should include those most relevant to the outcome and the most generic ones which can be applied to the general population, even though it may not be possible to identify these features beforehand. Typical ML tasks in radiology are the identification of specific patterns/conditions or image segmentation , which can be defined as the representation through partitioning of the digital image into meaningful parts (i.e., pixels or segments) for interpretation. Both have been successfully applied over a wide range of clinical settings including for the detection of fatty liver using ultrasound [ 9 ], CT carotid plaque characterization [ 10 ], and prediction of lesion-specific ischaemia from quantitative coronary CT angiography [ 11 ].
A significant step forward is represented by deep learning (DL), which is based on the implementation of a large number of ANN layers, allowing determination of more complex relationships (similar to neuronal networks) and a more sophisticated performance, attributes particularly suited for imaging. More important, DL is able to perform higher level classification tasks and to automatically extract and learn features, which is valuable when managing the information content of digital images that are only partially detectable and usable by a human reader. This concept unveils the extraordinary potential of DL in comparison with conventional imaging management.
The presence of numerous neural layers between input and output and the use of several techniques (most commonly called convolutional neural networks—CNN) contribute to the plasticity of DL and offer the potential to mimic human brain mechanisms in the training process. Crucial to success of the method is the exposure of CNN to data, in particular images, which can be processed during “training” (supervised or unsupervised). If data are unlabeled, the learning process is based on the automatic clustering of image findings according to their natural variability. Hybrid learning models that include some human guidance are most often used, due to the difficulty of successfully achieving truly unsupervised training. DL represents a hot topic in research, literally exploding in the last years.
Matching ML/DL image processing with clinical and when available pathological/histological data, to correlate intrinsic diagnostic patterns and features of a CT or MRI scan to a specific pathology and histological subtype, has opened a new window in research establishing so-called radiomics [ 12 – 14 ]. In this setting, CAD can also be taken to a higher performance level. ML-based CAD can be taught on the intrinsic differences of a population and then detect and/or diagnose the variations of a single lesion, allowing the identification of common as well as uncommon cases [ 15 ].
Supervised and unsupervised learning are largely based on statistical algorithms [ 16 ], with important differences between them. Supervised learning deals primarily with classification (i.e., identification of categories for new observations using the same collected on labelled training data sets) and regression (i.e., predictions on continued variables for new observations inferred on training sets). Unsupervised learning cannot take advantage on the labelling process and manages unclassified data; therefore, recognition of latent patterns is performed by applying clustering (aimed to define groups within data) and dimensionality reduction [ 16 ]. The sense of such a classification needs a subsequent validation to assess its utility.
Whichever the ML technique used, each approach presents advantages and disadvantages. General pros have to be considered for ML ability to process large volumes of data, to identify trends and patterns only partly detectable by humans, to face with complexity (multidimensionality of data), and to perform high computational tasks.
These advantages are not without cons. First, huge data sets are necessary to train ML machines, whose collection has been limited for a long time in healthcare (although the development of large databases in the era of the so-called “big data” is going to be more widespread). But even when available, the “quality” of data is a major challenge both for the supervised training (due to the large amount of effort needed for labelling data) and the unsupervised training (process of selection and validation).
Moreover, ML assessment represents a critical aspect in terms of statistical power definition (sensitivity, specificity, error susceptibility, and so on) of ML within the task (especially in clinical settings), often in the absence of “disclosure” about “how and why” machines elaborate their tasks, which raises problems when ML applications are introduced in routine medical activity [ 1 , 2 , 6 , 8 , 16 ].
The aim of this article is to integrate evidence-reported literature and experience-based perceptions, while attempting to make the information easy to access, assisting not only residents and fellows who are training in interventional radiology, but also practicing colleagues who are attempting to gain further expertise with these locoregional mini-invasive treatments.
2. AI and Interventional Radiology
2.1. treatment response.
AI applications appear promising for radiology scenarios, as they naturally affect and potentially improve upon lesion detection, segmentation, and interpretation of imaging—prerequisites for good interventional radiology (IR) practice [ 17 ]. Moreover, advantages are foreseen even in areas previously not addressed.
One of the biggest challenges of interventional radiology is to estimate/forecast the outcomes and/or the benefits of a treatment before actually performing it [ 18 ]. The identification of an accurate method to predict the success rate of a specific treatment in a specific patient could reduce unnecessary and useless procedures and interventions, reducing healthcare costs and dramatically decreasing the risk for the patient. It should also be useful to investigate how a patient's demographic and pathologic characteristics before the treatment can influence treatment efficacy, which can then be measured with posttreatment evaluations.
This type of challenge can be readily taken up using AI and DL, using a computer which autoimproves itself by learning from given inputted data. A patient's baseline diagnostic images, clinical data, and characteristics and outcomes of the planned intervention can be retrospectively applied to a cohort of patients to teach the computer to construct and work on a model that can correlate and “learn” the relationship between those model variables and procedural results. The resultant refined model would then allow the prediction of the procedural outcome in future new patients even before performing the procedure, assuming the characteristics of the intervention are specified. Classification of patients as a responder (complete or partial) or nonresponder could potentially be used in daily clinical practice as an indicator to decide whether or not a specific intervention should be performed [ 19 ]. DL-based prediction models can assist interventional radiologists in making decisions as to what procedure will offer the best outcome for each patient. Obviously, these prediction models would require a continuous evaluation and validation to limit or even eliminate possible errors and improve performance in both terms of diagnostic and therapeutic efficiencies.
The field of interventional oncology could greatly benefit from AI, given the great variety of data on which the prediction for daily clinical practice can be made, even though there is the need for more data to help implement ML in the best way [ 18 ]. A robust and trustworthy perspective on procedural outcomes could give interventional radiologist more and more solid data upon which to recommend a particular and specific treatment to each patient. In particular, Abajan et al. evaluated the capacity of artificial intelligence to predict chemoembolization outcomes in patients with hepatocellular carcinoma, based on baseline magnetic resonance imaging, dividing patients into responders and nonresponders. They obtained a very good negative predictive value (88.5%) based upon the ML models that relied upon the two features of tumour signal intensity and the presence or absence of cirrhosis [ 19 ]. In another anatomic site, the brain, Asadi et al. performed studies on prediction of procedural outcome in stroke and brain arteriovenous malformations patients and successfully individualized treatment based on predicting features [ 20 , 21 ]. Nonetheless, even if AI can provide information on disease and treatment correlation, it does not necessarily provide an insight on causality and pathophysiology; this information can be, however, obtained from randomized controlled trials, making these two approaches complementary to each other, to design the best treatment strategy.
2.2. Procedural Guidance and Support
Owing to the evolution of ML/DL, we are currently surrounded by technology to such an extent that it can assist us, among other tasks, to overcome distances and grant access to extensive knowledge. Touch and touchless devices are everywhere, simplifying our life in many ways, from phone and home assistants to intelligent lights or thermostats, to smart-locks and navigators, and with the introduction of sharing platforms and networks, streaming channels, and live-chat channels as well, our world can be seen as a great, unique web of people.
In an operating room setting, and more specifically in the interventional radiology suite, one of the most important things in procedural planning is the assessment of the patient's anatomy and its pathophysiologic changes. There is also much other valuable information archived in online databases or literature, ranging from (1) individual patient characteristics such as those on tumour characteristics and behaviour which are useful in the specific field of oncological interventions; (2) evidence to support or overcome a particular and unforeseen problem or finding; and (3) local hospital information on angio suite supplies, on the availability of specific devices such as a microcatheter, guidewire, or metallic coils. Currently, however, in large part but not exclusively due to sterility issues, procedural information must be collected beforehand, in the preprocedural planning, whereas, during the procedure, the interaction between the operator and the great amount of patient, literature, and supply data can only be achieved through sterile covers, or indirectly made by other team members, which implies a certain amount of distraction, errors, and time consumption. Nevertheless, these obstacles could be overcome with the implementation, in medical clinical practice, and particularly in operatory theaters and angio suites, of touchless interaction devices, ranging from eye-tracking systems to inertial sensors, to cameras or webcams, to voice-driven smart assistants [ 22 ].
Gesture-capture camera systems, with or without utilization of inertial sensors, have been experimented with defining and associating specific actions to a variety of gestures to control medical image viewers while in surgical scrub [ 23 – 25 ]. Indeed, voice recognition interfaces have been demonstrated to enable significant time sparing when dealing with switching on and off operating room components [ 26 ]. Navigation systems constructed using inertial sensors worn under sterile gloves have been tried for needle insertion path planning, with a claimed gesture-recognition rate of 100% for 3/4 gestures [ 27 ]. Augmented reality devices, such as glasses, which interactively display to the operator the whole variety of relevant information or diagnostic images have also been tested [ 28 , 29 ].
A group of researchers from the University of California, San Francisco, tested the possibility to question a smart assistant—previously instructed with a large database of information on sheath sizes and compatibility—to obtain suggestions as to which sheath is likely to be most appropriate for the deployment of a particular endovascular stent, during a specific interventional procedure, without removing the sterile surgical scrub, with good results both in terms of time sparing and accuracy [ 30 ].
As in the above-mentioned case, questions regarding the correct size of a device or on the time-consuming task of assess for the availability of a particular device or instrument according to the hospital stocks could be directly and instantaneously answered by the smart computer. Questions to the smart assistant could also imply a cost analysis, allowing the operator to choose between two devices not only assessing their dimensions but also their expensiveness in relation to outcome data, providing to all angio-suite staff the perception of the real global cost of a procedure, which must not be taken lightly, minimizing the waste and the inappropriate utilization of guidewires, catheters, coils, and other devices [ 18 ].
3. Future Perspectives
Most researchers agree that the future of AI lies in enhancing and assisting interventional radiology, not taking over from interventionalists.
Augmented reality , in which additional information about the patient can be provided to the operator in real time during the operation, is another technology already being put into practice. When this is combined with machine learning, the algorithm could help the radiologist to make more rapid proper and accurate decisions in terms of diagnosis, treatment management, and planning. Earlier diagnosis through quicker, more accurate reading of scans might enable cancer to be detected earlier, enabling treatment at an earlier stage, with less need for invasive standard surgical approaches. Collaboration between computer algorithms—with their ability to synthesize and spot patterns in vast data sets—and skilled operators—who are able to make sense of the “messiness” of the human body by arriving at correct conclusions despite the multiplicity and complexity of the situation—could raise the standard of IR across the board. Yet, there are significant challenges to overcome before these technologies can be considered mainstream. Regardless, currently, there is intense enthusiasm on the part of clinicians who are calling for increased collaboration between computer scientists, biomedical engineers, and interventional radiologists as machine learning is posited to play a more prominent role in interventional radiology procedures, from informing the initial diagnosis to patient selection and intraprocedural guidance.
4. Conclusions
The emerging role of AI may offer the opportunity to better tailor treatment to patients according to “big data” that can be rapidly analyzed, uncovering new insights that may otherwise have required decades of prospective trials. Thus, this new approach could most likely result in a paradigm shift in the near future, definitively changing the current conventional treatment algorithms of tumour therapy, providing superior really personalized care to patients.
Conflicts of Interest
The authors declare that they have no conflicts of interest.
- Narrative review
- Open access
- Published: 02 May 2024
Artificial intelligence in interventional radiology: state of the art
- Pierluigi Glielmo ORCID: orcid.org/0009-0009-1692-2986 1 ,
- Stefano Fusco 1 ,
- Salvatore Gitto 1 , 2 ,
- Giulia Zantonelli 1 ,
- Domenico Albano 2 , 3 ,
- Carmelo Messina 1 , 2 ,
- Luca Maria Sconfienza 1 , 2 &
- Giovanni Mauri 4
European Radiology Experimental volume 8 , Article number: 62 ( 2024 ) Cite this article
622 Accesses
2 Altmetric
Metrics details
Artificial intelligence (AI) has demonstrated great potential in a wide variety of applications in interventional radiology (IR). Support for decision-making and outcome prediction, new functions and improvements in fluoroscopy, ultrasound, computed tomography, and magnetic resonance imaging, specifically in the field of IR, have all been investigated. Furthermore, AI represents a significant boost for fusion imaging and simulated reality, robotics, touchless software interactions, and virtual biopsy. The procedural nature, heterogeneity, and lack of standardisation slow down the process of adoption of AI in IR. Research in AI is in its early stages as current literature is based on pilot or proof of concept studies. The full range of possibilities is yet to be explored.
Relevance statement Exploring AI’s transformative potential, this article assesses its current applications and challenges in IR, offering insights into decision support and outcome prediction, imaging enhancements, robotics, and touchless interactions, shaping the future of patient care.
• AI adoption in IR is more complex compared to diagnostic radiology.
• Current literature about AI in IR is in its early stages.
• AI has the potential to revolutionise every aspect of IR.
Graphical Abstract
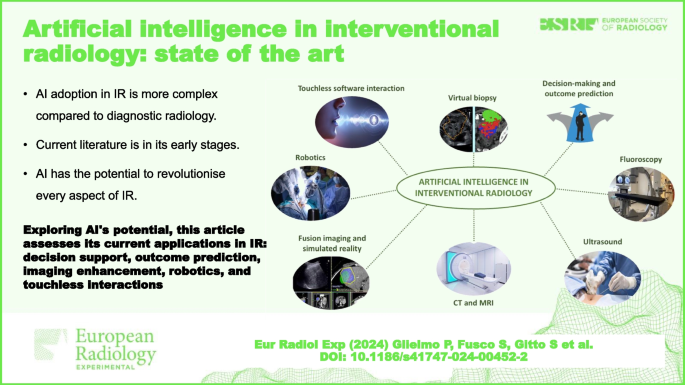
Perspectives in artificial intelligence (AI) differ and are more complex for interventional radiology (IR) than for diagnostic radiology because IR encompasses diagnostic imaging, imaging guidance, and early imaging evaluation as well as therapeutic tools [ 1 ].
Whilst diagnostic radiology is largely based on data acquired in a standardised format, IR, due to its procedural nature, relies on mostly unstructured data. Nevertheless, preprocedural, procedural, and postprocedural imaging constitutes a sizable dataset when compared to other specialties in medicine. In addition, machine learning (ML) and data augmentation techniques reduce the dataset size required for effective training. ML techniques exploit expedients such as in supervised learning (where models learn from labelled examples), few-shot learning (capable of generalising effectively from minimal examples per category), and transfer learning (leveraging knowledge from one task to improve performance on another). Data augmentation techniques enable the creation of synthetic training examples through the transformation of original data ( e.g., elastic transformations, affine image transformations, pixel-level transformations) or from the generation of artificial data. Researchers have developed generative AI algorithms that generate artificial radiological images for training. Similar techniques could be adapted for IR obtaining more representative and extensive training data [ 1 , 2 ].
Another factor that contributes to slowing down the process of adoption of AI in IR is the heterogeneous nature of this subspecialty. IR provides mini-invasive solutions for many different pathologies across multiple organ systems. Intraprocedural imaging, be it ultrasound (US), fluoroscopy, or even computed tomography (CT) or magnetic resonance imaging, can be heavily operator dependent as is the choice of the preferred percutaneous/intravascular approach, guidance, and different devices. This lack of standardisation poses challenges in creating adequate datasets for training and implies the need for flexibility of AI to a number of different situations and options for the same task. Furthermore, being a relatively young technology-based subspecialty, IR is constantly evolving which exacerbates issues related to its inner heterogeneity.
AI in IR is still in its early stages. Much of the literature relies on preliminary and hypothetical use cases. That being said, AI has the potential to improve and transform every aspect of IR. Acknowledging that every improvement of AI in diagnostic radiology affects IR more or less directly, in the following paragraphs, we cover the most promising AI applications present in literature specifically regarding the field of IR. These applications can be divided into the following areas of improvement: decision-making and outcome prediction, fluoroscopy, US, CT, MRI, fusion imaging and simulated reality, robotics, touchless software interaction, and virtual biopsy, as synthesised in Table 1 .
Decision-making and outcome prediction
AI support in decision-making concerns a great variety of fields other than IR and other specialties in medicine. Interventional radiologists use clinical information and image interpretation for diagnosis and treatment often relying on multidisciplinary boards to improve patient care due to the interdisciplinarity of IR. Traditionally, clinical risk calculators have been developed using scoring systems or linear models validated on a limited patient sample. ML offers the potential to uncover nonlinear associations amongst the input variables missed by these older models. It could incorporate all available data, along with radiomic information, to perform descriptive analysis, assess risks, and make predictions to help tailor the management of a specific patient [ 3 ].
In interventional oncology, many AI applications focus on predicting the response of hepatocellular carcinoma to transarterial chemoembolisation (TACE) [4−6]. Up to 60% of patients with hepatocellular carcinoma who undergo TACE do not benefit from it despite multiple sessions. Patient selection guidelines for TACE are based on the Barcelona Clinic liver cancer—BCLC staging system [ 7 ]. Higher arterial enhancement and grey-level co-occurrence matrix, lower homogeneity, and smaller tumour size at pretherapeutic dynamic CT texture analysis were shown to be significant predictors of complete response after TACE [ 8 ]. However, the accuracy of this method is limited based on traditional statistics. Morshid et al. [ 4 ] developed a predictive model by extracting image texture features from neural network-based segmentation of hepatocellular carcinoma lesions and the background liver in 105 patients. The accuracy rate for distinguishing TACE-susceptible versus TACE-refractory cases was 74.2%, surpassing the predictive capability of the Barcelona Clinic liver cancer staging system alone (62.9%). Another study predicted TACE treatment response by combining clinical patient data and baseline MRI [ 9 ].
Sinha et al. [ 3 ] built and evaluated their AI models on large national datasets and achieved excellent predictions regarding two different outcomes in two different clinical settings: iatrogenic pneumothorax after CT-guided transthoracic biopsy and occurrence of length of stay > 3 days after uterine artery embolisation. Area under the receiver operating characteristic curve was 0.913 for the transthoracic biopsy model and 0.879 for the uterine artery embolisation model. All model input features were available before hospital admission.
In another study, a ML algorithm, the model for end-stage liver disease—MELD score, and Child–Pugh score were compared for predicting 30-day mortality following transjugular intrahepatic portosystemic shunt—TIPS. Model for end-stage liver disease and Child–Pugh are popular tools to predict outcomes in patients with cirrhosis, but they are not specifically designed for patients with transjugular intrahepatic portosystemic shunt. However, they performed better than AI that was still able to make predictions out of mere demographic factors and medical comorbidities, data that are absent in these scores [ 10 ].
In a pilot retrospective study, Daye et al. [ 11 ] used AI to predict local tumour progression and overall survival in 21 patients with adrenal metastases treated with percutaneous thermal ablation. The AI software had an accuracy of 0.93 in predicting local tumour response and overall survival when clinical data were combined with features extracted from pretreatment contrast-enhanced CT.
In another study [ 12 ], AI outperformed traditional radiological biomarkers from CT angiography for good reperfusion and functional outcome prediction after endovascular treatment in acute ischemic stroke patients on a registry dataset with 1,301 patients. The predictive value was overall relatively low. Similarly, Hofmeister et al. [ 13 ] obtained information on the success of different endovascular treatments based on non-contrast CT in a prospective validation cohort of 47 patients. A small subset of radiomic features was predictive of first-attempt recanalisation with thromboaspiration (area under the receiver operating characteristic curve = 0.88). The same subset also predicted the overall number of passages required for successful recanalisation.
Mechanical thrombectomy success in acute ischemic stroke is commonly assessed by the thrombolysis in cerebral infarction (TICI) score, assigned by visual inspection of digital subtraction angiography during the intervention. Digital subtraction angiography interpretation and subsequent TICI scoring is highly observer dependent. Application of AI in this setting has been investigated. Digital subtraction angiography image data are rarely used in AI due to the complex nature of angiographic runs. Nielsen et al. [ 14 ] evaluated the general suitability of a deep learning (DL) model at producing an objective TICI score in case of occlusion of the M1 segment of the middle cerebral artery.
Fluoroscopy
AI has proved its utility in enhancing performance and diagnostic power and in facilitating the interpretation of fluoroscopic imaging.
Although major vessels have standard views for angiographic acquisition, the angiographic characteristics are influenced by clinical settings, such as view angle, magnification ratio, use of contrast media, and imaging system [ 44 ]. Most of the presented models based on angiographic images have the advantage that image preprocessing steps were minimised or cancelled because they are seamlessly integrated into the DL model.
Yang et al. [ 15 ] proposed a robust method for major vessels segmentation on coronary angiography using four DL models constructed on the basis of U-Net architecture. This could be a valuable tool for target vessel identification and for easily understanding the tree structure of regional vasculature.
Segmentation and extraction of catheter and guidewire from fluoroscopic images will aid in virtual road mapping of the vasculature from pre-operative imaging. Segmentation methods for electrophysiology electrodes and catheter have been proposed [ 16 , 17 ]. Electrodes are clearly visible in two-dimensional x-ray images and this specific feature facilitates their segmentation. Ambrosini et al. [ 18 ] introduced a fully automatic approach based on the U-Net model that can be run in real time for segmentation of catheter with no specific features.
Due to the spatial inconsistency between mask image (no contrast agent) and live image (with contrast agent) caused by inevitable and complex patient motion, subtraction angiography usually contains motion artefacts and the vessels are blurred, a phenomenon known as inter-scan motion [ 44 ]. Numerous image coregistration algorithms have been proposed to reduce motion artefacts, but they are computationally intensive and have not had widespread adoption [ 45 ]. AI demonstrated better performances than the compared registration algorithms. In particular, Gao et al. [ 19 ] trained a residual dense block on single live images fed into the generator and satisfactorily subtracted images as output. This resulted in subtraction images generated without the preliminary non-contrast acquisition, avoiding the issue of translational motion entirely and reducing the radiation dose [ 19 ].
Radiation exposure to the operator remains a relevant issue in IR. Whilst its relevance has diminished in diagnostic radiology with the emergence of radiation-free imaging modalities and the widespread use of CT, which allows a safe distance from the radiation source, interventional radiologists continue to rely on nearby x-rays.
Radiation exposure to both the operator and the patient has been significantly reduced using an AI-equipped fluoroscopy unit with ultrafast collimation during endoscopy [ 20 ]. It is easy to imagine its adaptability to IR. During an endoscopic procedure requiring fluoroscopy, the endoscopist is usually focused only on a small region of the displayed field of view that correlates with procedural activities such as the movement of a guidewire or a catheter. The larger area around the region of interest (ROI) receives much less attention but is needed for reference and orientation purposes. With the present technology, the larger area outside the ROI is exposed to the same radiation dosage as the small ROI. The AI-equipped fluoroscopy system can minimise radiation exposure via a secondary collimator by constantly adjusting the shutter’s lead blade orientation to block radiation to the area outside of the ROI for a majority of image frames and overlying the real-time ROI images over a full field of view image acquired a few frames before. Image outside of the ROI aids only in the orientation, and this effect is not perceptible to the operator. Although the ROI is automatically targeted using AI, there is also an optional provision for manual control by the operator [ 20 ].
Accurate needle placement is crucial in IR procedures aiming at tissue sampling. Needle localisation during US-guided manoeuvres is not always optimal because of lower detection with steep needle-probe angles, deep insertions, reflective signal losses, hyperechoic surrounding tissues, and intrinsic needle visibility [ 46 ]. Furthermore, current US systems are not specifically designed for IR and are limited to the diagnostic aspects. Hardware-based approaches for improving needle shaft and tip localisation, for example, external trackers and specialised needles/probes, exist [ 47 , 48 ]. However, image processing-based methods that do not require additional hardware are easier to adapt in the standard clinical workflow.
Mwikirize et al. [ 21 ] used a faster region-based convolutional neural network (Faster R-CNN) to improve two-dimensional US-guided needle insertion. A Faster R-CNN is translational invariant, allowing needles of various sizes to be inserted at different depths and insertion angles, and the detector will perform accurately regardless of the needle’s geometrical transformation. The system allows automatic detection of needle insertion side, estimation of the needle insertion trajectory, and facilitating automatic localisation of the tip. It achieved a precision of 99.6%, recall of 99.8%, and an F1 score of 0.99 on scans collected over a bovine/porcine lumbosacral spine phantom. Accurate tip localisation is obtained even in cases where, due to needle discontinuity, various regions of the needle may be detected separately but this applies only to non-bending needles.
The shortage of high-quality training data from US-guided interventions is particularly pronounced when compared to other imaging modalities. US is inherently operator dependent and susceptible to artefact. Furthermore, the manual annotation of images is more challenging and time-consuming. To address these problems, Arapi et al. [ 22 ] employed synthetic US data generated from CT and MRI to train a DL detection algorithm. They validated their model for the localisation of needle tip and target anatomy on real in vitro US images, showing promising results for this data generation approach.
The efficacy of thermal ablation in treating tumours is linked to achieving complete tumour coverage with minimal ablative margin, ideally at least 5 mm, enhancing local tumour control. Manual segmentation and registration of tumour and ablation zones invariably introduce operator bias in ablative margin analysis and are time-consuming. The registration in particular is challenging due to errors induced by breathing motion and heating-related tissue deformation. Current methodologies lack intra-procedural accuracy, posing limitations in assessing ablative margin and tissue contraction. Several retrospective studies have employed DL to address these difficulties, demonstrating its utility in achieving deformable image registration and auto-segmentation [ 23 , 24 , 25 ]. The COVER-ALL randomised controlled trial investigated a novel AI-based intra-procedural approach to optimise tumour coverage and minimise non-target tissue ablation, potentially elevating liver ablation efficacy [ 26 ]. Similarly, a separate study [ 27 ] demonstrates the effectiveness of DL in segmenting Lipiodol on cone-beam CT during TACE, outperforming conventional methods. This would allow physicians to feel comfortable relying heavily on cone-beam CT imaging and using obtained cone-beam CT data to make predictive inferences about treatment success and even patient outcome.
Regarding cone-beam CT, DL techniques have been successfully used to generate a synthetic CT image from cone-beam CT imaging [ 28 , 29 ] overcoming the limitations in image contrast compared to multi-detector CT and enhancing a frequently used image guidance system in the IR suite.
Creating synthetic contrast-enhanced CT images has been proposed in diagnostic radiology [ 30 , 31 ] to reduce usage of iodinated contrast agents. Pinnock et al. proposed a first study on synthetic contrast-enhanced CT in IR, which poses challenges such as organ displacement and needle insertion [ 32 ].
MRI-guided interventions are not widespread performed in IR, and most of the time, MRI use is limited to bioptic procedures or fusion imaging [ 49 ]. Needle placement is crucial even in these cases. A group of researchers applied three-dimensional CNNs to create a more sophisticated and automatic needle localisation system for MRI-guided transperineal prostate biopsies. Although some of their results were not statistically significant, this group demonstrated a potential for ML applications to improve needle segmentation and localisation with MRI assistance in a clinical setting [ 33 ].
Fusion imaging and simulated reality
Multimodality image fusion is increasingly used in IR and in a variety of clinical situations [ 50 , 51 , 52 , 53 , 54 , 55 , 56 ]. It allows the generation of a composite image from multiple input images containing complementary information of the same anatomical site for vascular and non-vascular procedures. Pixel level image fusion algorithms are at the base of this technology. By integrating the information contained in multiple images of the same scene into one composite image, pixel level image fusion is recognised as having high significance in a variety of fields. DL-based image fusion is currently in its early stages; however, DL-based image fusion methods have been proposed for other fields such as digital photography and multimodality imaging too, showing advantages over conventional methods and huge potential for future improvement [ 34 ].
Simulated reality, along with AI and robotics, represents some of the most exciting technology advancements in the future of medicine and particularly in radiology. Virtual reality and augmented reality (AR) provide stereoscopic and three-dimensional immersion of a simulated object. Virtual reality simulates a virtual environment whilst AR overlays simulated objects into the real-world background [ 57 ]. This technology can be used to display volumetric medical images, such as CT and MRI allowing for a more accurate representation of the three-dimensional nature of anatomical structures, thereby being beneficial in diagnosis, education, and interventional procedures.
Interacting with volumetric images in a virtual space with a stereoscopic view has several advantages over the conventional monoscopic two-dimensional slices on a flat panel as perception of depth and distance. Virtual three-dimensional anatomy/trajectory is overlaid onto visual surface anatomy using a variety of technologies to create a fused real-time AR image. The technique permits accurate visual navigation, theoretically without need for fluoroscopy.
Many studies have already utilised simulated reality in IR procedures [ 58 , 59 , 60 , 61 ]. Fritz et al. [ 62 , 63 ] employed AR for a variety of IR procedures performed on cadavers, including spinal injection and MRI-guided vertebroplasty. Solbiati et al. [ 64 ] reported the first in vivo study of an AR system for the guidance of percutaneous interventional oncology procedures. Recently, Albano et al. [ 65 ] performed bone biopsies guided by AR in eight patients with 100% technical success.
The primary advantages secured by utilising AI in this setting include automated landmark recognition, compensation for motion artefact, and generation/validation of a safe needle trajectory.
Auloge et al. [ 35 ] conducted a 20-patient randomised clinical study to test the efficacy of percutaneous vertebroplasty for patients with vertebral compression fractures. Patients were randomised to two groups: procedures performed with standard fluoroscopy and procedures augmented with AI guidance. Following cone beam CT acquisition of the target volume, the AI software automatically recognises osseous landmarks, numerically identifies each vertebral level, and displays two/three-dimensional planning images on the user interface. The target vertebra is manually selected and the software suggests an optimal transpedicular approach which may be adjusted in multiple planes ( e.g., intercostovertebral access for thoracic levels). Once the trajectory is validated, the C-arm automatically rotates to the ‘bulls-eye view’ along the planned insertional axis. The ‘virtual’ trajectory is then superimposed over the ‘real-world’ images from cameras integrated in the flat-panel detector of a standard C-arm fluoroscopy machine, and the monitor displays live video output from the four cameras (including ‘bulls-eye’ and ‘sagittal’/perpendicular views) with overlaid, motion-compensated needle trajectories. The metrics studied included trocar placement accuracy, complications, trocar deployment time, and fluoroscopy time. All procedures in both groups were successful with no complications observed in either group. No statistically significant differences in accuracy were observed between the groups. Fluoroscopy time was lower in the AI-guided group, whilst deployment time was lower in the standard-fluoroscopy group.
Robotic assistance is becoming essential in surgery, increasing precision and accuracy as well as the operator’s degrees of freedom compared to human ability alone. Its increased use is inevitable in IR where robotic assistance with remote control also allows for radiation protection during interventional procedures.
The majority of robotic systems currently employed in clinical practice are primarily teleoperators or assistants for tasks involving holding and precise aiming. The advancement of systems capable of operating at higher autonomy levels, especially in challenging conditions, presents considerable research hurdles. A critical aspect for such systems is their ability to consistently track surgical and IR tools and relevant anatomical structures throughout interventional procedures, accounting for organ movement and breathing. The application of deep artificial neural networks to robotic systems helps in handling multimodal data generated in robotic sensing applications [ 36 ].
Fagogenis et al. [ 37 ] demonstrated autonomous catheter navigation in the cardiovascular system using endoscopic sensors located at the catheter tip to perform paravalvular leak closure. Beating-heart navigation is particularly challenging because the blood is opaque and the cardiac tissue is moving. The endovascular endoscope acts as a combined contact and imaging sensor. ML processes camera input data from the sensor providing clear images of whatever the catheter tip is touching whilst also inferring what it is touching ( e.g., the blood, tissue, and valve) and how hard it is pressing.
In an article published in Nature Machine Intelligence, Chen et al. [ 38 ] described a DL driven robotic guidance system for obtaining vascular access. AI based on a recurrent fully convolutional network—Rec-FCN takes bimodal near-infrared and duplex US imaging sequences as its inputs and performs a series of complex vision tasks, including vessel segmentation, classification, and depth estimation. A three-dimensional map of the arm surface and vasculature is derived, from which the operator may select a target vessel that is subsequently tracked in real time in the presence of arm motion.
Touchless software interaction
IR is highly technology dependent and IR suites rank amongst the most technologically advanced operating rooms in medicine. The interventionalist must interact with various hardware during procedures within the confines of a sterile environment. Furthermore, in some cases, this necessitates verbal communication with the circulating nurse or technician for the manipulation of computers in the room. Touchless software interaction could simplify and speed up direct interaction with computers, eliminating the need for an intermediary and thereby enhancing efficiency. For instance, one study utilised a voice recognition interface to adjust various parameters during laparoscopic surgery such as the initial setup of the light sources and the camera, as well as procedural steps such as the activation of the insufflator [ 39 ].
AI has emerged as an important approach to streamline these touchless software-assisted interactions using voice and gesture commands. ML frameworks can be trained to classify voice commands and gestures that physicians may employ during a procedure. These methods can contribute to improved recognition rates of these actions by cameras, speakers, and other touchless devices [ 40 , 41 ].
In a study by Schwarz et al. [ 42 ], body gestures were learned by a ML software using inertial sensors worn on the head and body of the operator with a recognition rate of 90%. Body sensors eliminate issues associated with cameras such as ensuring adequate illumination or the need to perform gestures in the camera’s line of sight.
Virtual biopsy
Virtual biopsy refers to the application of radiomics for the extraction of quantitative information not accessible through visual inspection from radiological images for tissue characterisation [ 66 , 67 ].
Features from radiological images can be fed into AI models in order to derive lesions’ pathological characteristics and molecular status. Barros et al. [ 43 ] developed an AI model for digital mammography that achieved an area under the receiver operating characteristic curve of 0.76 (95% confidence interval 0.72–0.83), 0.85 (0.82–0.89), and 0.82 (0.77–0.87) for the pathologic classification of ductal carcinoma in situ , invasive carcinomas, and benign lesions, respectively.
In the future, virtual biopsy could partially substitute traditional biopsy, avoiding biopsy complications or providing additional information to that obtained by biopsy, especially in core biopsies where only a small amount of tissue is taken from lesions that may be very heterogeneous. However, virtual biopsy has the disadvantage of having low spatial and contrast resolution, with respect to tissue biopsy that is able to explore processes at a subcellular level.
Conclusions
AI opens the door to a multitude of major improvements in every step of the interventional radiologist’s workflow and to completely new possibilities in the field. ML is flexible, as it learns to work for virtually any kind of application.
The evolution of AI in IR is anticipated to drive precision medicine to new heights. Tailoring treatment plans to individual patient profiles by leveraging AI-based predictive analytics could lead to more accurate diagnoses and optimised procedural strategies. The prospect of dynamic adaptation to procedural variations is on the horizon, potentially revolutionising treatment customisation in real-time.
AI-driven enhanced imaging capabilities, coupled with advanced navigational aids, are set to provide interventional radiologists with unprecedented accuracy and real-time guidance during complex procedures. Furthermore, the integration of AI with robotics is a compelling avenue, potentially steering IR towards more autonomous procedures.
The applications currently explored in medical literature just give a clue of what will be the real scenario in the future of IR. Nevertheless, AI research in IR is nascent and will encounter many technical and ethical problems, similar to those faced in diagnostic radiology. Collaborative initiatives amongst healthcare institutions to pool standardised anonymised data and promote the sharing of diverse datasets must be encouraged. Federated learning is a ML approach where models are trained collaboratively across decentralised devices without sharing raw data. It enables collaborative model development whilst preserving patient privacy [ 68 ].
Expectations are high, probably beyond the capabilities of current AI tools. A lot of work has to be done to see AI in the IR suite, with patient care improvement always as the primary goal.
Abbreviations
- Artificial intelligence
Augmented reality
Convolutional neural network
Computed tomography
- Deep learning
Interventional radiology
- Machine learning
Magnetic resonance imaging
Region of interest
Transarterial chemoembolisation
Thrombolysis in cerebral infarction
von Ende E, Ryan S, Crain MA, Makary MS (2023) Artificial intelligence, augmented reality, and virtual reality advances and applications in interventional radiology. Diagnostics 13:892. https://doi.org/10.3390/diagnostics13050892
Article Google Scholar
Nalepa J, Marcinkiewicz M, Kawulok M (2019) Data augmentation for brain-tumor segmentation: a review. Front Comput Neurosci 3:83. https://doi.org/10.3389/fncom.2019.00083
Sinha I, Aluthge DP, Chen ES, Sarkar IN, Ahn SH (2020) Machine learning offers exciting potential for predicting postprocedural outcomes: a framework for developing random forest models in IR. J Vasc Interv Radiol 31:1018-1024.e4. https://doi.org/10.1016/j.jvir.2019.11.030
Article PubMed PubMed Central Google Scholar
Morshid A, Elsayes KM, Khalaf AM et al (2019) A machine learning model to predict hepatocellular carcinoma response to transcatheter arterial chemoembolization. Radiol Artif Intell 1:e180021. https://doi.org/10.1148/ryai.2019180021
Peng J, Kang S, Ning Z et al (2020) Residual convolutional neural network for predicting response of transarterial chemoembolization in hepatocellular carcinoma from CT imaging. Eur Radiol 30:413–424. https://doi.org/10.1007/s00330-019-06318-1
Article PubMed Google Scholar
Kim J, Choi SJ, Lee SH, Lee HY, Park H (2018) Predicting survival using pretreatment CT for patients with hepatocellular carcinoma treated with transarterial chemoembolization: comparison of models using radiomics. AJR Am J Roentgenol 211:1026–1034. https://doi.org/10.2214/AJR.18.19507
Reig M, Forner A, Rimola J et al (2022) BCLC strategy for prognosis prediction and treatment recommendation: the 2022 update. J Hepatol 76:681–693. https://doi.org/10.1016/j.jhep.2021.11.018
Park HJ, Kim JH, Choi SY et al (2017) Prediction of therapeutic response of hepatocellular carcinoma to transcatheter arterial chemoembolization based on pretherapeutic dynamic CT and textural findings. AJR Am J Roentgenol 209:W211–W220. https://doi.org/10.2214/AJR.16.17398
Abajian A, Murali N, Savic LJ et al (2018) Predicting treatment response to intra-arterial therapies for hepatocellular carcinoma with the use of supervised machine learning - an artificial intelligence concept. J Vasc Interv Radiol 29:850-857.e1. https://doi.org/10.1016/j.jvir.2018.01.769
Gaba RC, Couture PM, Bui JT, et al (2013) Prognostic capability of different liver disease scoring systems for prediction of early mortality after transjugular intrahepatic portosystemic shunt creation. J Vasc Interv Radiol 24:411–420, 420.e1–4; quiz 421. https://doi.org/10.1016/j.jvir.2012.10.026
Daye D, Staziaki PV, Furtado VF et al (2019) CT texture analysis and machine learning improve post-ablation prognostication in patients with adrenal metastases: a proof of concept. Cardiovasc Intervent Radiol 42:1771–1776. https://doi.org/10.1007/s00270-019-02336-0
Hilbert A, Ramos LA, van Os HJA et al (2019) Data-efficient deep learning of radiological image data for outcome prediction after endovascular treatment of patients with acute ischemic stroke. Comput Biol Med 115:103516. https://doi.org/10.1016/j.compbiomed.2019.103516
Article CAS PubMed Google Scholar
Hofmeister J, Bernava G, Rosi A et al (2020) Clot-based radiomics predict a mechanical thrombectomy strategy for successful recanalization in acute ischemic stroke. Stroke 51:2488–94. https://doi.org/10.1161/STROKEAHA.120.030334
Nielsen M, Waldmann M, Fro¨lich AM, et al. Deep learning-based automated thrombolysis in cerebral infarction scoring: a timely proof-of-principle study. Stroke 2021;52:3497–504. https://doi.org/10.1161/STROKEAHA.120.033807
Yang S, Kweon J, Roh JH et al (2019) Deep learning segmentation of major vessels in x-ray coronary angiography. Sci Rep 9:1–11. https://doi.org/10.1038/s41598-019-53254-7
Article CAS Google Scholar
Baur C, Albarqouni S, Demirci S, Navab N, Fallavollita P. Cathnets: detection and single-view depth prediction of catheter electrodes. International Conference on Medical Imaging and Virtual Reality. pp. 38–49. Springer (2016). https://doi.org/10.1007/978-3-319-43775-0_4
Wu X, Housden J, Ma Y, Razavi B, Rhode K, Rueckert D (2015) Fast catheter segmentation from echocardiographic sequences based on segmentation from corresponding x-ray fluoroscopy for cardiac catheterization interventions. IEEE Trans Med Imag 34:861–876. https://doi.org/10.1109/TMI.2014.2360988
Ambrosini P, Ruijters D, Niessen WJ, et al. Fully automatic and real-time catheter segmentation in x-ray fluoroscopy. Lect Notes Comput Sci. 2017; 10434:577-85. https://doi.org/10.48550/arXiv.1707.05137
Gao Y, Song Y, Yin X et al (2019) Deep learning-based digital subtraction angiography image generation. Int J Comput Assist Radiol Surg 14:1775–84. https://doi.org/10.1007/s11548-019-02040-x
Bang JY, Hough M, Hawes RH, Varadarajulu S. Use of artificial intelligence to reduce radiation exposure at fluoroscopy-guided endoscopic procedures. Am J Gastroenterol 2020;115:555-61. https://doi.org/10.14309/ajg.0000000000000565
Mwikirize C, Nosher JL, Hacihaliloglu I (2018) Convolution neural networks for real-time needle detection and localization in 2D ultrasound. Int J Comput Assist Radiol Surg 13:647–57. https://doi.org/10.1007/s11548-018-1721-y
Arapi V, Hardt-Stremayr A, Weiss S, Steinbrener J (2023) Bridging the simulation-to-real gap for AI-based needle and target detection in robot-assisted ultrasound-guided interventions. Eur Radiol Exp 7:30. https://doi.org/10.1186/s41747-023-00344-x
Laimer G, Jaschke N, Schullian et al (2021) Volumetric assessment of the periablational safety margin after thermal ablation of colorectal liver metastases. Eur Radiol 31:6489–6499. https://doi.org/10.1007/s00330-020-07579-x
Lin YM, Paolucci I, O’Connor CS et al (2023) Ablative margins of colorectal liver metastases using deformable CT image registration and autosegmentation. Radiology 307:e221373. https://doi.org/10.1148/radiol.221373
An C, Jiang Y, Huang Z et al (2020) Assessment of ablative margin after microwave ablation for hepatocellular carcinoma using deep learning-based deformable image registration. Front Oncol 10:573316. https://doi.org/10.3389/fonc.2020.573316
Lin YM, Paolucci I, Anderson BM et al (2022) Study protocol COVER-ALL: clinical impact of a volumetric image method for confirming tumour coverage with ablation on patients with malignant liver lesions. Cardiovasc Interv Radiol 45:1860–1867. https://doi.org/10.1007/s00270-022-03255-3
Malpani R, Petty CW, Yang J et al (2022) Quantitative automated segmentation of lipiodol deposits on cone-beam CT imaging acquired during transarterial chemoembolization for liver tumors: deep learning approach. JVIR 33:324-332.e2. https://doi.org/10.1016/j.jvir.2021.12.017
Chen L, Liang X, Shen C, Jiang S, Wang J (2020) Synthetic CT generation from CBCT images via deep learning. Med Phys 47:1115–1125. https://doi.org/10.1002/mp.13978
Xue X, Ding Y, Shi J et al (2021) Cone beam CT (CBCT) based synthetic CT generation using deep learning methods for dose calculation of nasopharyngeal carcinoma radiotherapy. Technol Cancer ResTreat 20:15330338211062416. https://doi.org/10.1177/15330338211062415
Choi JW, Cho YJ, Ha JY et al (2021) Generating synthetic contrast enhancement from non-contrast chest computed tomography using a generative adversarial network. Sci Rep 11:20403. https://doi.org/10.1038/s41598-021-00058-3
Article CAS PubMed PubMed Central Google Scholar
Kim SW, KimKwak JH et al (2021) The feasibility of deep learning-based synthetic contrast-enhanced CT from nonenhanced CT in emergency department patients with acute abdominal pain. Sci Rep 11:20390. https://doi.org/10.1038/s41598-021-99896-4
Pinnock MA, Hu Y, Bandula S, Barratt DC (2023) Multi-phase synthetic contrast enhancement in interventional computed tomography for guiding renal cryotherapy. Int J Comput Assist Radiol Surg 18:1437–1449. https://doi.org/10.1007/s11548-023-02843-z
Mehrtash A, Ghafoorian M, Pernelle G et al (2019) Automatic needle segmentation and localization in MRI with 3-D convolutional neural networks: application to MRI-targeted prostate biopsy. IEEE Trans Med Imaging 38:1026–36. https://doi.org/10.1109/TMI.2018.2876796
Liu Y, Chen X, Wang Z, Jane Wang Z, Ward RK, Wang X (2018) Deep learning for pixel-level image fusion: recent advances and future prospects. Inf Fusion 42:158–73. https://doi.org/10.1016/j.inffus.2017.10.007
Auloge P, Cazzato RL, Ramamurthy N et al (2020) Augmented reality and artificial intelligence-based navigation during percutaneous vertebroplasty: a pilot randomised clinical trial. Eur Spine J 29:1580–1589. https://doi.org/10.1007/s00586-019-06054-6
Malpani R, Petty CW, Bhatt N, Staib LH, Chapiro J (2021) Use of artificial intelligence in non-oncologic interventional radiology: current state and future directions. Dig Dis Interv 5:331–337. https://doi.org/10.1055/s-0041-1726300
Fagogenis G, Mencattelli M, Machaidze Z et al (2019) Autonomous robotic intracardiac catheter navigation using haptic vision. Sci Robot 4:eaaw1977. https://doi.org/10.1126/scirobotics.aaw1977
Chen AI, Balter ML, Maguire TJ et al (2020) Deep learning robotic guidance for autonomous vascular access. Nat Mach Intell 2:104–115. https://doi.org/10.1038/s42256-020-0148-7
El-Shallaly GEH, Mohammed B, Muhtaseb MS, Hamouda AH, Nassar AHM (2005) Voice recognition interfaces (VRI) optimize the utilization of theatre staff and time during laparoscopic cholecystectomy. Minim Invasive Ther Allied Technol 14:369–371. https://doi.org/10.1080/13645700500381685
Mewes A, Hensen B, Wacker F et al (2017) Touchless interaction with software in interventional radiology and surgery: a systematic literature review. Int J Comput Assist Radiol Surg 12:291–305. https://doi.org/10.1007/s11548-016-1480-6
Seals K, Al-Hakim R, Mulligan P. et al. The development of a machine learning smart speaker application for device sizing in interventional radiology. J Vasc Interv Radiol. 30:S20. https://doi.org/10.1016/j.jvir.2018.12.077
Schwarz LA, Bigdelou A, Navab N (2011) Learning gestures for customizable human-computer interaction in the operating room. Med Image Comput Comput Assist Interv 14:129–36. https://doi.org/10.1007/978-3-642-23623-5_17
Barros V, Tlusty T, Barkan E et al (2023) Virtual biopsy by using artificial intelligence-based multimodal modeling of binational mammography data. Radiology 306:e220027. https://doi.org/10.1148/radiol.220027
Baum S, Pentecost MJ (2006) Abrams’ angiography interventional radiology. Lippincott Williams & Wilkins, Philadelphia
Google Scholar
Seah J, Boeken T, Sapoval M et al (2022) Prime time for artificial intelligence in interventional radiology. Cardiovasc Intervent Radiol 45:283–289. https://doi.org/10.1007/s00270-021-03044-4
Cohen M, Jacob D (2007) Ultrasound guided interventional radiology. J Radiol 88:1223–1229. https://doi.org/10.1016/s0221-0363(07)91330-x
Moore J, Clarke C, Bainbridge D et al (2009) Image guidance for spinal facet injections using tracked ultrasound. Med Image Comput Comput Assist Interv 12:516–523. https://doi.org/10.1007/978-3-642-04268-3_64
Xia W, West S, Finlay M et al (2017) Looking beyond the imaging plane: 3D needle tracking with a linear array ultrasound probe. Sci Rep 7:3674. https://doi.org/10.1038/s41598-017-03886-4
Mauri G, Cova L, De Beni S et al (2015) Real-time US-CT/MRI image fusion for guidance of thermal ablation of liver tumors undetectable with US: results in 295 cases. Cardiovasc Intervent Radiol. 38(1):143–51. https://doi.org/10.1007/s00270-014-0897-y
Carriero S, Della Pepa G, Monfardini L et al (2021) Role of fusion imaging in image-guided thermal ablations. Diagnostics (Basel) 11:549. https://doi.org/10.3390/diagnostics11030549
Orlandi D, Viglino U, Dedone G et al (2022) US-CT fusion-guided percutaneous radiofrequency ablation of large substernal benign thyroid nodules. Int J Hyperthermia 39:847–854. https://doi.org/10.1080/02656736.2022.2091167
Monfardini L, Orsi F, Caserta R et al (2018) Ultrasound and cone beam CT fusion for liver ablation: technical note. Int J Hyperthermia 35:500–504. https://doi.org/10.1080/02656736.2018.1509237
Mauri G, Mistretta FA, Bonomo G et al (2020) Long-term follow-up outcomes after percutaneous US/CT-guided radiofrequency ablation for cT1a-b renal masses: experience from single high-volume referral center. Cancers (Basel) 12:1183. https://doi.org/10.3390/cancers12051183
Mauri G, Monfardini L, Della Vigna P et al (2021) Real-time US-CT fusion imaging for guidance of thermal ablation in of renal tumors invisible or poorly visible with US: results in 97 cases. Int J Hyperthermia 38(1):771–776. https://doi.org/10.1080/02656736.2021.1923837
Monfardini L, Gennaro N, Orsi F et al (2021) Real-time US/cone-beam CT fusion imaging for percutaneous ablation of small renal tumours: a technical note. Eur Radiol 31(10):7523–7528. https://doi.org/10.1007/s00330-021-07930-w
Mauri G, Gennaro N, De Beni S et al (2019) Real-Time US-18FDG-PET/CT image fusion for guidance of thermal ablation of 18FDG-PET-positive liver metastases: the added value of contrast enhancement. Cardiovasc Intervent Radiol 42:60–68. https://doi.org/10.1007/s00270-018-2082-1
Uppot RN, Laguna B, McCarthy CJ et al (2019) Implementing virtual and augmented reality tools for radiology education and training, communication, and clinical care. Radiology 291:570–580. https://doi.org/10.1148/radiol.2019182210
Mauri G (2015) Expanding role of virtual navigation and fusion imaging in percutaneous biopsies and ablation. Abdom Imaging 40:3238–9. https://doi.org/10.1007/s00261-015-0495-8
Mauri G, Gitto S, Pescatori LC, Albano D, Messina C, Sconfienza LM (2022) Technical feasibility of electromagnetic US/CT fusion imaging and virtual navigation in the guidance of spine biopsies. Ultraschall Med 43:387–392. https://doi.org/10.1055/a-1194-4225
Mauri G, Solbiati L (2015) Virtual navigation and fusion imaging in percutaneous ablations in the neck. Ultrasound Med Biol 41(3):898. https://doi.org/10.1016/j.ultrasmedbio.2014.10.022
Calandri M, Mauri G, Yevich S et al (2019) Fusion imaging and virtual navigation to guide percutaneous thermal ablation of hepatocellular carcinoma: a review of the literature. Cardiovasc Intervent Radiol 42(5):639–647. https://doi.org/10.1007/s00270-019-02167-z
Fritz J, P UT, Ungi T et al Augmented reality visualisation using an image overlay system for MR-guided interventions: technical performance of spine injection procedures in human cadavers at 1.5 Tesla. Eur Radiol. 2013;23:235–245. https://doi.org/10.1007/s00330-012-2569-0
Fritz J, P UT, Ungi T, et al. MR-guided vertebroplasty with augmented reality image overlay navigation. Cardiovasc Intervent Radiol 2014;37:1589–1596. https://doi.org/10.1007/s00270-014-0885-2
Solbiati M, Ierace T, Muglia R et al (2022) Thermal ablation of liver tumors guided by augmented reality: an initial clinical experience. Cancers 14:1312. https://doi.org/10.3390/cancers14051312
Albano D, Messina C, Gitto S, Chianca V, Sconfienza LM (2023) Bone biopsies guided by augmented reality: a pilot study. Eur Radiol Exp 7:40. https://doi.org/10.1186/s41747-023-00353-w
Defeudis A, Panic J, Nicoletti G, Mazzetti S, Giannini V, Regge D (2023) Virtual biopsy in abdominal pathology: where do we stand? BJR Open 5(1):20220055. https://doi.org/10.1259/bjro.20220055
Arthur A, Johnston EW, Winfield JM, et al (2022) Virtual biopsy in soft tissue sarcoma. How close are we?. Front Oncol. 12, 892620. https://doi.org/10.3389/fonc.2022.892620
Xu J, Glicksberg BS, Su C, Walker P, Bian J, Wang F (2021) Federated learning for healthcare informatics. J Healthc Inform Res 5:1–19. https://doi.org/10.1007/s41666-020-00082-4
Download references
Acknowledgements
Large language models were not used for this paper.
No funding was received to assist with the preparation of this manuscript.
Author information
Authors and affiliations.
Dipartimento di Scienze Biomediche per la Salute, Università degli Studi di Milano, Via Mangiagalli, 31, 20133, Milan, Italy
Pierluigi Glielmo, Stefano Fusco, Salvatore Gitto, Giulia Zantonelli, Carmelo Messina & Luca Maria Sconfienza
IRCCS Istituto Ortopedico Galeazzi, Via Cristina Belgioioso, 173, 20157, Milan, Italy
Salvatore Gitto, Domenico Albano, Carmelo Messina & Luca Maria Sconfienza
Dipartimento di Scienze Biomediche, Chirurgiche ed Odontoiatriche, Università degli Studi di Milano, Via della Commenda, 10, 20122, Milan, Italy
Domenico Albano
Divisione di Radiologia Interventistica, IEO, IRCCS Istituto Europeo di Oncologia, Milan, Italy
Giovanni Mauri
You can also search for this author in PubMed Google Scholar
Contributions
PG, SF, SG, and GZ evaluated the literature and drafted the manuscript. DA and CM contributed to evaluate the literature for writing the paper. GM and LMS provided the final approval, expertise, and final revision.
Corresponding author
Correspondence to Pierluigi Glielmo .
Ethics declarations
Ethics approval and consent to participate.
Not applicable.
Consent for publication
Competing interests.
LMS is a member of the Advisory Editorial Board for European Radiology Experimental (Musculoskeletal Radiology). They have not taken part in the selection or review process for this article. The remaining authors declare that they have no competing interests.
Additional information
Publisher’s note.
Springer Nature remains neutral with regard to jurisdictional claims in published maps and institutional affiliations.
Rights and permissions
Open Access This article is licensed under a Creative Commons Attribution 4.0 International License, which permits use, sharing, adaptation, distribution and reproduction in any medium or format, as long as you give appropriate credit to the original author(s) and the source, provide a link to the Creative Commons licence, and indicate if changes were made. The images or other third party material in this article are included in the article's Creative Commons licence, unless indicated otherwise in a credit line to the material. If material is not included in the article's Creative Commons licence and your intended use is not permitted by statutory regulation or exceeds the permitted use, you will need to obtain permission directly from the copyright holder. To view a copy of this licence, visit http://creativecommons.org/licenses/by/4.0/ .
Reprints and permissions
About this article
Cite this article.
Glielmo, P., Fusco, S., Gitto, S. et al. Artificial intelligence in interventional radiology: state of the art. Eur Radiol Exp 8 , 62 (2024). https://doi.org/10.1186/s41747-024-00452-2
Download citation
Received : 28 September 2023
Accepted : 26 February 2024
Published : 02 May 2024
DOI : https://doi.org/10.1186/s41747-024-00452-2
Share this article
Anyone you share the following link with will be able to read this content:
Sorry, a shareable link is not currently available for this article.
Provided by the Springer Nature SharedIt content-sharing initiative
- Neural networks (computer)
- Radiology (interventional)
Europe PMC requires Javascript to function effectively.
Either your web browser doesn't support Javascript or it is currently turned off. In the latter case, please turn on Javascript support in your web browser and reload this page.
Search life-sciences literature (44,147,901 articles, preprints and more)
- Free full text
- Citations & impact
- Similar Articles
Artificial Intelligence in Interventional Radiology: A Literature Review and Future Perspectives.
Author information, affiliations.
- Merlino B 1
- Manfredi R 1
- Goldberg SN 2
- Valentini V 4
ORCIDs linked to this article
- Iezzi R | 0000-0002-2791-481X
Journal of Oncology , 03 Nov 2019 , 2019: 6153041 https://doi.org/10.1155/2019/6153041 PMID: 31781215 PMCID: PMC6874978
Abstract
Free full text .

Artificial Intelligence in Interventional Radiology: A Literature Review and Future Perspectives
Roberto iezzi.
1 Fondazione Policlinico Universitario A. Gemelli IRCCS, UOC di Radiologia, Dipartimento di Diagnostica per Immagini, Radioterapia Oncologica ed Ematologia, Roma, Italy
2 Università Cattolica del Sacro Cuore, Istituto di Radiologia, Roma, Italy

S. N. Goldberg
3 Department of Radiology, Hadassah Hebrew University Medical Center, Jerusalem, Israel
4 Department of Radiology, AFaR-IRCCS Fatebenefratelli Hospital Foundation for Health Research and Education, via di Ponte Quattro Capi 39, 00186 Roma, Italy
V. Valentini
5 Fondazione Policlinico Universitario A. Gemelli IRCCS, UOC di Radioterapia Oncologica, Dipartimento di Diagnostica per Immagini, Radioterapia Oncologica ed Ematologia, Roma, Italy
6 Università Cattolica del Sacro Cuore, Istituto di Radioterapia Oncologica, Roma, Italy
R. Manfredi
The term “artificial intelligence” (AI) includes computational algorithms that can perform tasks considered typical of human intelligence, with partial to complete autonomy, to produce new beneficial outputs from specific inputs. The development of AI is largely based on the introduction of artificial neural networks (ANN) that allowed the introduction of the concepts of “computational learning models,” machine learning (ML) and deep learning (DL). AI applications appear promising for radiology scenarios potentially improving lesion detection, segmentation, and interpretation with a recent application also for interventional radiology (IR) practice, including the ability of AI to offer prognostic information to both patients and physicians about interventional oncology procedures. This article integrates evidence-reported literature and experience-based perceptions to assist not only residents and fellows who are training in interventional radiology but also practicing colleagues who are approaching to locoregional mini-invasive treatments.
- 1. Introduction
The term “artificial intelligence” (AI) includes computational algorithms that can perform tasks considered typical of human intelligence, with partial to complete autonomy, to produce new beneficial outputs from specific inputs [ 1 ]. Although premises to the development of AI were achieved in the early era of computers, it has only been with the introduction of new powerful computational hardware, in association with the capability of collecting and storing huge amounts of data, that it has become feasible to explore its potential in tasks most relevant to the field of radiology such as pattern recognition, pattern identification, planning, language comprehension, object and sound recognition, problem solving, prognosticating diseases, and deciding when and whether therapy is not needed or of limited use or in offering patients and physicians prognostic data on treatment outcomes. Indeed, although healthcare represents a challenging field for AI application, medical imaging is currently one of the most promising areas to apply this technology [ 2 ].
From the beginning, it has been quite clear that computers could be potentially useful in assisting the radiologist in the routine tasks of detection and diagnosis. The idea fostering the use of the so-called computer-aided detection/diagnosis (CAD) systems, precursors of modern AI, was to provide radiologists with the assistance in the detection and interpretations of potential lesions (especially in mammography and chest or musculoskeletal radiography) in order to discriminate between benign and malignant lesions, reduce false negatives, and boost radiologists' productivity, especially in terms of discovery and identification of significant findings requiring a prompt human validation [ 3 ]. Main limitations of CAD systems were their task-specific orientation which is suited to only one particular given task in a corresponding specific imaging modality and, moreover, their reliability and the risk of false positive results implied mandatory validation by a trained radiologist [ 3 ]. Since then, ever-increasing attempts have been made to improve upon the diagnostic performance of AI and facilitate the help it could provide in daily clinical practice.
The development of AI is largely based on the introduction of artificial neural networks (ANN) in the early 1950s [ 4 ] and their subsequent further evolution (from single to multilayer ANN), introducing the concepts of “computational learning models,” machine learning (ML) and deep learning (DL).
ML is based upon the so-called “reverse training” method, in which computer systems focus on specific pathological features identified during a training period [ 5 ]. Thus, ML applications require a set of data on a specific pathology on which the computer can train itself, and those data must necessarily contain the desired outcome that needs to be predicted (e.g., nodules or emphysema on chest X-rays, focal liver lesions, hemorrhage in head CT, and so on). Big data is the type of data that may be supplied into the analytical system so that an ML model could learn, improving the accuracy of its predictions. Once trained, the computer can apply this information even to new cases never seen before [ 6 , 7 ]. ML can be supervised or unsupervised, depending, respectively, on the “labeled” input previously selected by human experts, or directly extracted by the machine using several computational methods [ 6 , 8 ]. Among the evaluated features, the ideal ML model should include those most relevant to the outcome and the most generic ones which can be applied to the general population, even though it may not be possible to identify these features beforehand. Typical ML tasks in radiology are the identification of specific patterns/conditions or image segmentation , which can be defined as the representation through partitioning of the digital image into meaningful parts (i.e., pixels or segments) for interpretation. Both have been successfully applied over a wide range of clinical settings including for the detection of fatty liver using ultrasound [ 9 ], CT carotid plaque characterization [ 10 ], and prediction of lesion-specific ischaemia from quantitative coronary CT angiography [ 11 ].
A significant step forward is represented by deep learning (DL), which is based on the implementation of a large number of ANN layers, allowing determination of more complex relationships (similar to neuronal networks) and a more sophisticated performance, attributes particularly suited for imaging. More important, DL is able to perform higher level classification tasks and to automatically extract and learn features, which is valuable when managing the information content of digital images that are only partially detectable and usable by a human reader. This concept unveils the extraordinary potential of DL in comparison with conventional imaging management.
The presence of numerous neural layers between input and output and the use of several techniques (most commonly called convolutional neural networks—CNN) contribute to the plasticity of DL and offer the potential to mimic human brain mechanisms in the training process. Crucial to success of the method is the exposure of CNN to data, in particular images, which can be processed during “training” (supervised or unsupervised). If data are unlabeled, the learning process is based on the automatic clustering of image findings according to their natural variability. Hybrid learning models that include some human guidance are most often used, due to the difficulty of successfully achieving truly unsupervised training. DL represents a hot topic in research, literally exploding in the last years.
Matching ML/DL image processing with clinical and when available pathological/histological data, to correlate intrinsic diagnostic patterns and features of a CT or MRI scan to a specific pathology and histological subtype, has opened a new window in research establishing so-called radiomics [ 12 – 14 ]. In this setting, CAD can also be taken to a higher performance level. ML-based CAD can be taught on the intrinsic differences of a population and then detect and/or diagnose the variations of a single lesion, allowing the identification of common as well as uncommon cases [ 15 ].
Supervised and unsupervised learning are largely based on statistical algorithms [ 16 ], with important differences between them. Supervised learning deals primarily with classification (i.e., identification of categories for new observations using the same collected on labelled training data sets) and regression (i.e., predictions on continued variables for new observations inferred on training sets). Unsupervised learning cannot take advantage on the labelling process and manages unclassified data; therefore, recognition of latent patterns is performed by applying clustering (aimed to define groups within data) and dimensionality reduction [ 16 ]. The sense of such a classification needs a subsequent validation to assess its utility.
Whichever the ML technique used, each approach presents advantages and disadvantages. General pros have to be considered for ML ability to process large volumes of data, to identify trends and patterns only partly detectable by humans, to face with complexity (multidimensionality of data), and to perform high computational tasks.
These advantages are not without cons. First, huge data sets are necessary to train ML machines, whose collection has been limited for a long time in healthcare (although the development of large databases in the era of the so-called “big data” is going to be more widespread). But even when available, the “quality” of data is a major challenge both for the supervised training (due to the large amount of effort needed for labelling data) and the unsupervised training (process of selection and validation).
Moreover, ML assessment represents a critical aspect in terms of statistical power definition (sensitivity, specificity, error susceptibility, and so on) of ML within the task (especially in clinical settings), often in the absence of “disclosure” about “how and why” machines elaborate their tasks, which raises problems when ML applications are introduced in routine medical activity [ 1 , 2 , 6 , 8 , 16 ].
The aim of this article is to integrate evidence-reported literature and experience-based perceptions, while attempting to make the information easy to access, assisting not only residents and fellows who are training in interventional radiology, but also practicing colleagues who are attempting to gain further expertise with these locoregional mini-invasive treatments.
- 2. AI and Interventional Radiology
2.1. Treatment Response
AI applications appear promising for radiology scenarios, as they naturally affect and potentially improve upon lesion detection, segmentation, and interpretation of imaging—prerequisites for good interventional radiology (IR) practice [ 17 ]. Moreover, advantages are foreseen even in areas previously not addressed.
One of the biggest challenges of interventional radiology is to estimate/forecast the outcomes and/or the benefits of a treatment before actually performing it [ 18 ]. The identification of an accurate method to predict the success rate of a specific treatment in a specific patient could reduce unnecessary and useless procedures and interventions, reducing healthcare costs and dramatically decreasing the risk for the patient. It should also be useful to investigate how a patient's demographic and pathologic characteristics before the treatment can influence treatment efficacy, which can then be measured with posttreatment evaluations.
This type of challenge can be readily taken up using AI and DL, using a computer which autoimproves itself by learning from given inputted data. A patient's baseline diagnostic images, clinical data, and characteristics and outcomes of the planned intervention can be retrospectively applied to a cohort of patients to teach the computer to construct and work on a model that can correlate and “learn” the relationship between those model variables and procedural results. The resultant refined model would then allow the prediction of the procedural outcome in future new patients even before performing the procedure, assuming the characteristics of the intervention are specified. Classification of patients as a responder (complete or partial) or nonresponder could potentially be used in daily clinical practice as an indicator to decide whether or not a specific intervention should be performed [ 19 ]. DL-based prediction models can assist interventional radiologists in making decisions as to what procedure will offer the best outcome for each patient. Obviously, these prediction models would require a continuous evaluation and validation to limit or even eliminate possible errors and improve performance in both terms of diagnostic and therapeutic efficiencies.
The field of interventional oncology could greatly benefit from AI, given the great variety of data on which the prediction for daily clinical practice can be made, even though there is the need for more data to help implement ML in the best way [ 18 ]. A robust and trustworthy perspective on procedural outcomes could give interventional radiologist more and more solid data upon which to recommend a particular and specific treatment to each patient. In particular, Abajan et al. evaluated the capacity of artificial intelligence to predict chemoembolization outcomes in patients with hepatocellular carcinoma, based on baseline magnetic resonance imaging, dividing patients into responders and nonresponders. They obtained a very good negative predictive value (88.5%) based upon the ML models that relied upon the two features of tumour signal intensity and the presence or absence of cirrhosis [ 19 ]. In another anatomic site, the brain, Asadi et al. performed studies on prediction of procedural outcome in stroke and brain arteriovenous malformations patients and successfully individualized treatment based on predicting features [ 20 , 21 ]. Nonetheless, even if AI can provide information on disease and treatment correlation, it does not necessarily provide an insight on causality and pathophysiology; this information can be, however, obtained from randomized controlled trials, making these two approaches complementary to each other, to design the best treatment strategy.
2.2. Procedural Guidance and Support
Owing to the evolution of ML/DL, we are currently surrounded by technology to such an extent that it can assist us, among other tasks, to overcome distances and grant access to extensive knowledge. Touch and touchless devices are everywhere, simplifying our life in many ways, from phone and home assistants to intelligent lights or thermostats, to smart-locks and navigators, and with the introduction of sharing platforms and networks, streaming channels, and live-chat channels as well, our world can be seen as a great, unique web of people.
In an operating room setting, and more specifically in the interventional radiology suite, one of the most important things in procedural planning is the assessment of the patient's anatomy and its pathophysiologic changes. There is also much other valuable information archived in online databases or literature, ranging from (1) individual patient characteristics such as those on tumour characteristics and behaviour which are useful in the specific field of oncological interventions; (2) evidence to support or overcome a particular and unforeseen problem or finding; and (3) local hospital information on angio suite supplies, on the availability of specific devices such as a microcatheter, guidewire, or metallic coils. Currently, however, in large part but not exclusively due to sterility issues, procedural information must be collected beforehand, in the preprocedural planning, whereas, during the procedure, the interaction between the operator and the great amount of patient, literature, and supply data can only be achieved through sterile covers, or indirectly made by other team members, which implies a certain amount of distraction, errors, and time consumption. Nevertheless, these obstacles could be overcome with the implementation, in medical clinical practice, and particularly in operatory theaters and angio suites, of touchless interaction devices, ranging from eye-tracking systems to inertial sensors, to cameras or webcams, to voice-driven smart assistants [ 22 ].
Gesture-capture camera systems, with or without utilization of inertial sensors, have been experimented with defining and associating specific actions to a variety of gestures to control medical image viewers while in surgical scrub [ 23 – 25 ]. Indeed, voice recognition interfaces have been demonstrated to enable significant time sparing when dealing with switching on and off operating room components [ 26 ]. Navigation systems constructed using inertial sensors worn under sterile gloves have been tried for needle insertion path planning, with a claimed gesture-recognition rate of 100% for 3/4 gestures [ 27 ]. Augmented reality devices, such as glasses, which interactively display to the operator the whole variety of relevant information or diagnostic images have also been tested [ 28 , 29 ].
A group of researchers from the University of California, San Francisco, tested the possibility to question a smart assistant—previously instructed with a large database of information on sheath sizes and compatibility—to obtain suggestions as to which sheath is likely to be most appropriate for the deployment of a particular endovascular stent, during a specific interventional procedure, without removing the sterile surgical scrub, with good results both in terms of time sparing and accuracy [ 30 ].
As in the above-mentioned case, questions regarding the correct size of a device or on the time-consuming task of assess for the availability of a particular device or instrument according to the hospital stocks could be directly and instantaneously answered by the smart computer. Questions to the smart assistant could also imply a cost analysis, allowing the operator to choose between two devices not only assessing their dimensions but also their expensiveness in relation to outcome data, providing to all angio-suite staff the perception of the real global cost of a procedure, which must not be taken lightly, minimizing the waste and the inappropriate utilization of guidewires, catheters, coils, and other devices [ 18 ].
- 3. Future Perspectives
Most researchers agree that the future of AI lies in enhancing and assisting interventional radiology, not taking over from interventionalists.
Augmented reality , in which additional information about the patient can be provided to the operator in real time during the operation, is another technology already being put into practice. When this is combined with machine learning, the algorithm could help the radiologist to make more rapid proper and accurate decisions in terms of diagnosis, treatment management, and planning. Earlier diagnosis through quicker, more accurate reading of scans might enable cancer to be detected earlier, enabling treatment at an earlier stage, with less need for invasive standard surgical approaches. Collaboration between computer algorithms—with their ability to synthesize and spot patterns in vast data sets—and skilled operators—who are able to make sense of the “messiness” of the human body by arriving at correct conclusions despite the multiplicity and complexity of the situation—could raise the standard of IR across the board. Yet, there are significant challenges to overcome before these technologies can be considered mainstream. Regardless, currently, there is intense enthusiasm on the part of clinicians who are calling for increased collaboration between computer scientists, biomedical engineers, and interventional radiologists as machine learning is posited to play a more prominent role in interventional radiology procedures, from informing the initial diagnosis to patient selection and intraprocedural guidance.
- 4. Conclusions
The emerging role of AI may offer the opportunity to better tailor treatment to patients according to “big data” that can be rapidly analyzed, uncovering new insights that may otherwise have required decades of prospective trials. Thus, this new approach could most likely result in a paradigm shift in the near future, definitively changing the current conventional treatment algorithms of tumour therapy, providing superior really personalized care to patients.
- Conflicts of Interest
The authors declare that they have no conflicts of interest.
Full text links
Read article at publisher's site: https://doi.org/10.1155/2019/6153041
Citations & impact
Impact metrics, citations of article over time, alternative metrics.

Article citations
Artificial intelligence, augmented reality, and virtual reality advances and applications in interventional radiology..
von Ende E , Ryan S , Crain MA , Makary MS
Diagnostics (Basel) , 13(5):892, 27 Feb 2023
Cited by: 8 articles | PMID: 36900036 | PMCID: PMC10000832
Technological Advancements in Interventional Oncology.
Posa A , Barbieri P , Mazza G , Tanzilli A , Natale L , Sala E , Iezzi R
Diagnostics (Basel) , 13(2):228, 07 Jan 2023
Cited by: 1 article | PMID: 36673038 | PMCID: PMC9857620
Artificial Intelligence in Interventional Radiology.
Kallini JR , Moriarty JM
Semin Intervent Radiol , 39(3):341-347, 01 Jun 2022
Cited by: 0 articles | PMID: 36062217 | PMCID: PMC9433147
Review Free full text in Europe PMC
Applications and challenges of artificial intelligence in diagnostic and interventional radiology.
Waller J , O'Connor A , Rafaat E , Amireh A , Dempsey J , Martin C , Umair M
Pol J Radiol , 87:e113-e117, 25 Feb 2022
Cited by: 9 articles | PMID: 35280945 | PMCID: PMC8906183
Challenges of Implementing Artificial Intelligence in Interventional Radiology.
Mazaheri S , Loya MF , Newsome J , Lungren M , Gichoya JW
Semin Intervent Radiol , 38(5):554-559, 24 Nov 2021
Cited by: 2 articles | PMID: 34853501 | PMCID: PMC8612837
Similar Articles
To arrive at the top five similar articles we use a word-weighted algorithm to compare words from the Title and Abstract of each citation.
Interventional Radiology ex-machina: impact of Artificial Intelligence on practice.
Gurgitano M , Angileri SA , Rodà GM , Liguori A , Pandolfi M , Ierardi AM , Wood BJ , Carrafiello G
Radiol Med , 126(7):998-1006, 16 Apr 2021
Cited by: 51 articles | PMID: 33861421 | PMCID: PMC8050998
AI musculoskeletal clinical applications: how can AI increase my day-to-day efficiency?
Shin Y , Kim S , Lee YH
Skeletal Radiol , 51(2):293-304, 03 Aug 2021
Cited by: 9 articles | PMID: 34341865
Prime Time for Artificial Intelligence in Interventional Radiology.
Seah J , Boeken T , Sapoval M , Goh GS
Cardiovasc Intervent Radiol , 45(3):283-289, 14 Jan 2022
Cited by: 7 articles | PMID: 35031822 | PMCID: PMC8921296
Artificial Intelligence and the Trainee Experience in Radiology.
Simpson SA , Cook TS
J Am Coll Radiol , 17(11):1388-1393, 01 Oct 2020
Cited by: 9 articles | PMID: 33010211
Europe PMC is part of the ELIXIR infrastructure
- Search Menu
- Sign in through your institution
- Advance articles
- Themed Content
- AI and Machine Learning
- Gastrointestinal
- Genitourinary
- Head and Neck
- Interventional
- Medical Physics: Diagnostic
- Medical Physics: Radiotherapy
- Musculoskeletal and Soft Tissue
- Nuclear Medicine and Molecular Imaging
- Obstetrics and Gynaecology
- Paediatrics
- Radiation Protection
- Radiobiology
- Radiotherapy and Oncology
- Respiratory and Chest
- Why publish in BJR
- Author Guidelines
- Submission Site
- Reviewer Guidelines
- Open Access
- Read and Publish
- Self-Archiving Policy
- Author Testimonials
- About British Institute Radiology
- Editorial Board
- Advertising & Corporate Services
- BIR Journals
- Journals on Oxford Academic
- Books on Oxford Academic

- < Previous
Imaging of calcific tendinopathy: natural history, migration patterns, pitfalls, and management: a review

- Article contents
- Figures & tables
- Supplementary Data
Sonal Saran, Joban Ashish Babhulkar, Harun Gupta, Basavaraj Chari, Imaging of calcific tendinopathy: natural history, migration patterns, pitfalls, and management: a review, British Journal of Radiology , Volume 97, Issue 1158, June 2024, Pages 1099–1111, https://doi.org/10.1093/bjr/tqae039
- Permissions Icon Permissions
Calcific tendinopathy is characterized by the deposition of calcium hydroxyapatite crystals in various tendons of the body. Terms like calcium tendinitis, tendinosis, and tendinopathy are used interchangeably. Calcific tendinopathy is a common and well-documented ailment in the literature. Although common, the natural history, aetiology, and progression of calcific tendinitis are poorly understood. The treatment options include conservative and interventional measures. However, these measures cannot be applied as a blanket and are often tailored depending on the stage/phase of the disease. Out of the recognized stages of the disease, the resorptive stage causes the utmost symptoms when the calcium is rather soft and unstable. During this stage, the calcium may migrate beyond expected resorption and get deposited in the adjacent tissues contiguous with the calcium focus. The common destinations include bursal migration, intraosseous migration, muscular migration, and other less common migration sites. Such atypical presentations can lead to dilemmas in the diagnosis, prolongation of the diagnostic pathway, unwarranted apprehension, and treatment delay. Radiologists’ role in this situation is to correctly recognize the imaging findings of atypical presentations of calcific tendinopathy and prevent unnecessary diagnostic and interventional studies. In this review article, we describe the pathogenic pathway and natural history of calcific tendinopathy from a radiologist’s perspective and discuss different migratory patterns of calcium in calcific tendinopathy not only around the shoulder but also in other areas of the body on different imaging modalities. We also show a few examples of mimics and pitfalls on imaging. Finally, we discuss the appropriate management option of this condition.
Email alerts
Related articles in.
- Google Scholar
Citing articles via
- Recommend to your Library
- Advertising & Corporate Services
- Journals Career Network
Affiliations
- Online ISSN 1748-880X
- Print ISSN 0007-1285
- Copyright © 2024 British Institute of Radiology
- About Oxford Academic
- Publish journals with us
- University press partners
- What we publish
- New features
- Open access
- Institutional account management
- Rights and permissions
- Get help with access
- Accessibility
- Advertising
- Media enquiries
- Oxford University Press
- Oxford Languages
- University of Oxford
Oxford University Press is a department of the University of Oxford. It furthers the University's objective of excellence in research, scholarship, and education by publishing worldwide
- Copyright © 2024 Oxford University Press
- Cookie settings
- Cookie policy
- Privacy policy
- Legal notice
This Feature Is Available To Subscribers Only
Sign In or Create an Account
This PDF is available to Subscribers Only
For full access to this pdf, sign in to an existing account, or purchase an annual subscription.
Announcing the launch of the Journal of Medical Imaging and Interventional Radiology
- Open access
- Published: 29 May 2024
- Volume 11 , article number 1 , ( 2024 )
Cite this article
You have full access to this open access article
- Antonio Pinto ORCID: orcid.org/0000-0002-5949-2631 1
12 Accesses
Explore all metrics
Avoid common mistakes on your manuscript.
I’m honored to announce the launch of the Journal of Medical Imaging and Interventional Radiology (previously named Journal of Radiological Review ), the second official publication of the Italian Society of Medical and Interventional Radiology (SIRM). This is a peer-reviewed, full open access journal publishing topics within radiology, diagnostic imaging and interventional radiology, including but not limited to the following areas: Radiography, Ultrasound, Computed Tomography (CT), Magnetic Resonance Imaging (MRI), Hybrid Imaging (such as PET-CT, PET-MRI, and SPECT-CT), and their clinical utility.
The Journal of Medical Imaging and Interventional Radiology (JMIIR) will publish a wide spectrum of papers, including original researches, review articles, pictorial essays, technical notes, case reports, editorials, commentaries, and letters to the editor. The JMIIR follows a double-blind peer review process. This means that the author’s identity will be concealed from the reviewers throughout peer review: it is the author’s responsibility to anonymize the manuscript and any associated materials. Two experts reviewers will assess submitted articles, evaluating the manuscript’s ethical and scientific soundness, coherence, potential duplication of previously published work, and suitability for publication. The Editors will then make a decision based on these assessments, and if required, they may consult with members of the Editorial Board. Particolar attention is given to the editorial and advisory board of the journal concerning gender bias in the peer-review and publication process: a significant issue lies in the limited representation of women in the realm of scientific publications [ 1 ]. Taking into account the first official Journal of SIRM, “La Radiologia Medica”, during the years 2017–2021, the percentages of women as first authors, corresponding authors and last authors were 33–44%, 20–36%, and 15–21%, respectively [ 2 ].
I’m excited to redefine our mission and vision for serving our great radiological community: new initiatives will be introduced to reflect changes in radiology literature and to more effectively promote evidence-based, patient-centric, artificial intelligence-conscious, and radiologist-friendly journalism.
JMIIR is published by Springer: over the last few years, the publishing industry has faced the challenge of unethical individuals or organisations attempting to publish fabricated manuscripts. Springer Nature is committed to detecting such manuscripts upon submission, ensuring they are not published. This dedication aims to safeguard the integrity of the scientific record and prevent Editors and reviewers from investing valuable time and energy in fraudulent papers. As such, the journal may use software to screen for plagiarism ( Geppetto and PySciDetect , two tools that can detect different types of generated text). These will support the actual policies and practices in order to prevent publishing misconduct.
JMIIR encourages authors worldwide to submit original research manuscripts on the topics described above, offering the opportunity to share findings and contributions within the community interested in the areas addressed.
Finally, I would like to express my gratitude to the Board of Directors of the Italian Society of Medical and Interventional Radiology, the SIRM President Prof. Andrea Giovagnoni, and Prof. Antonio Barile for granting me the opportunity to serve as JMIIR Editor-in-Chief. I would also express my gratitude to our publisher Springer, and their team of experts, for their outstanding work, guidance and encouragement.
Antonio Pinto,
Editor-in-Chief,
Journal of Medical Imaging and Interventional Radiology
Data availability
I do not analyze or generate any datasets, because this work is an Editorial.
Pinto A, Fornasa F (2022) Equity, diversity and inclusion in radiology: a great opportunity. J Radiol Rev 9:169–170. https://doi.org/10.23736/S2723-9284.22.00218-1
Article Google Scholar
Giovagnoni A, Gandolfo N, Miele V (2022) Women in radiology: the SIRM believes in equal opportunity. Radiol Med 127:225–227. https://doi.org/10.1007/s11547-022-01474-9
Article PubMed PubMed Central Google Scholar
Download references
No funds, grants, or other support was received.
Author information
Authors and affiliations.
Department of Radiology, CTO Hospital, Azienda Ospedaliera dei Colli, Viale Colli Aminei 21, 80141, Naples, Italy
Antonio Pinto
You can also search for this author in PubMed Google Scholar
Contributions
The author approved the final version of the manuscript.
Corresponding author
Correspondence to Antonio Pinto .
Ethics declarations
Conflict of interest.
The author has no financial or proprietary interests in any material discussed in this article.
Rights and permissions
Open Access This article is licensed under a Creative Commons Attribution 4.0 International License, which permits use, sharing, adaptation, distribution and reproduction in any medium or format, as long as you give appropriate credit to the original author(s) and the source, provide a link to the Creative Commons licence, and indicate if changes were made. The images or other third party material in this article are included in the article's Creative Commons licence, unless indicated otherwise in a credit line to the material. If material is not included in the article's Creative Commons licence and your intended use is not permitted by statutory regulation or exceeds the permitted use, you will need to obtain permission directly from the copyright holder. To view a copy of this licence, visit http://creativecommons.org/licenses/by/4.0/ .
Reprints and permissions
About this article
Pinto, A. Announcing the launch of the Journal of Medical Imaging and Interventional Radiology . J Med Imaging Intervent Radiol 11 , 1 (2024). https://doi.org/10.1007/s44326-024-00007-0
Download citation
Received : 13 February 2024
Accepted : 01 May 2024
Published : 29 May 2024
DOI : https://doi.org/10.1007/s44326-024-00007-0
Share this article
Anyone you share the following link with will be able to read this content:
Sorry, a shareable link is not currently available for this article.
Provided by the Springer Nature SharedIt content-sharing initiative
- New journal
- Journal policy statement
- Open-access
- Editorial procedure
- Find a journal
- Publish with us
- Track your research
- Download PDF
- Share X Facebook Email LinkedIn
- Permissions
Early vs Late Anticoagulation in Minor, Moderate, and Major Ischemic Stroke With Atrial Fibrillation : Post Hoc Analysis of the ELAN Randomized Clinical Trial
- 1 Department of Neurology, Inselspital, Bern University Hospital, University of Bern, Bern, Switzerland
- 2 University Institute of Diagnostic and Interventional Neuroradiology, Inselspital, Bern University Hospital, University of Bern, Bern, Switzerland
- 3 Clinical Trials Unit, University of Bern, Bern, Switzerland
- 4 Department of Neurology, University of Basel and University Hospital Basel, Basel, Switzerland
- 5 Department of Neurology, Medical University of Graz, Graz, Austria
- 6 Division of Neuroradiology, Vascular and Interventional Radiology, Department of Radiology, Medical University of Graz, Graz, Austria
- 7 Support Center for Advanced Neuroimaging, Inselspital, Bern University Hospital and University of Bern, Bern, Switzerland
- 8 Department of Neurology, Helsinki University Hospital, University of Helsinki, Helsinki, Finland
- 9 Internal, Vascular, and Emergency Medicine, Stroke Unit, Santa Maria della Misericordia Hospital, University of Perugia, Perugia, Italy
- 10 Department of Neurology, University Medical Center Hamburg–Eppendorf, Hamburg, Germany
- 11 Department of Neurology, University Hospital Lausanne, University of Lausanne, Lausanne, Switzerland
- 12 Department of Neurology, Cantonal Hospital Aarau, Aarau, Switzerland
- 13 Department of Neurology, Oslo University Hospital, Oslo, Norway
- 14 Research Department, Reha Rheinfelden, Rheinfelden, Switzerland
- 15 Stroke Center, Lisbon Central University Hospital, Lisbon, Portugal
- 16 Faculty of Medicine, Institute of Anatomy, University of Lisbon, Lisbon, Portugal
- 17 Department of Neurology, Sree Chitra Tirunal Institute for Medical Sciences and Technology, Thiruvananthapuram, India
- 18 Department of Internal Medicine, Faculty of Medicine, School of Health Sciences, University of Thessaly, Larissa, Greece
- 19 Department of Cerebrovascular Medicine, National Cerebral and Cardiovascular Center, Osaka, Japan
- 20 Department of Neurology, Pavol Jozef Šafárik University, Košice, Slovakia
- 21 Faculty of Medicine, Louis Pasteur University Hospital, Košice, Slovakia
- 22 Department of Neurosciences, Experimental Neurology, KU Leuven, Leuven, Belgium
- 23 Department of Neurology, University Hospitals Leuven, Leuven, Belgium
- 24 Department of Neurology, Shaare-Zedek Medical Center, Jerusalem, Israel
- 25 Stroke Clinical Trials Network Ireland, School of Medicine, University College Dublin and Department of Neurology, Mater University Hospital, Dublin, Ireland
- 26 School of Cardiovascular and Metabolic Health, Queen Elizabeth University Hospital, University of Glasgow, Glasgow, United Kingdom
Question Does infarct size modify the safety and efficacy of early vs late initiation of direct oral anticoagulation (DOAC) after ischemic stroke in people with atrial fibrillation?
Findings In this post hoc analysis of 1962 participants from the Early Versus Later Anticoagulation for Stroke With Atrial Fibrillation (ELAN) randomized clinical trial, the odds of the primary outcome (recurrent ischemic stroke, symptomatic intracranial hemorrhage, extracranial bleeding, systemic embolism, or vascular death within 30 days) were similar for early vs late DOAC initiation among participants with minor, moderate, and major stroke.
Meaning Early DOAC treatment was not associated with a higher rate of bleeding complications, especially symptomatic intracranial hemorrhage, or any adverse events regardless of infarct size, including major stroke.
Importance Whether infarct size modifies the treatment effect of early vs late direct oral anticoagulant (DOAC) initiation in people with ischemic stroke and atrial fibrillation is unknown.
Objective To assess whether infarct size modifies the safety and efficacy of early vs late DOAC initiation.
Design, Setting, and Participants Post hoc analysis of participants from the multinational (>100 sites in 15 countries) randomized clinical Early Versus Later Anticoagulation for Stroke With Atrial Fibrillation (ELAN) trial who had (1) acute ischemic stroke, (2) atrial fibrillation, and (3) brain imaging available before randomization. The ELAN trial was conducted between October 2017 and December 2022. Data were analyzed from October to December 2023 for this post hoc analysis.
Intervention Early vs late DOAC initiation after ischemic stroke. Early DOAC initiation was within 48 hours for minor or moderate stroke or on days 6 to 7 for major stroke; late DOAC initiation was on days 3 to 4 for minor stroke, days 6 to 7 for moderate stroke, and days 12 to 14 for major stroke.
Main Outcomes and Measures The primary outcome was a composite of recurrent ischemic stroke, symptomatic intracranial hemorrhage, extracranial bleeding, systemic embolism, or vascular death within 30 days. The outcome was assessed according to infarct size (minor, moderate, or major) using odds ratios and risk differences between treatment arms. Interrater reliability for infarct size between the core laboratory and local raters was assessed, and whether this modified the estimated treatment effects was also examined.
Results A total of 1962 of the original 2013 participants (909 [46.3%] female; median [IQR] age, 77 [70-84] years) were included. The primary outcome occurred in 10 of 371 participants (2.7%) with early DOAC initiation vs 11 of 364 (3.0%) with late DOAC initiation among those with minor stroke (odds ratio [OR], 0.89; 95% CI, 0.38-2.10); in 11 of 388 (2.8%) with early DOAC initiation vs 14 of 392 (3.6%) with late DOAC initiation among those with moderate stroke (OR, 0.80; 95% CI, 0.35-1.74); and in 8 of 219 (3.7%) with early DOAC initiation vs 16 of 228 (7.0%) with late DOAC initiation among those with major stroke (OR, 0.52; 95% CI, 0.21-1.18). The 95% CI for the estimated risk difference of the primary outcome in early anticoagulation was −2.78% to 2.12% for minor stroke, −3.23% to 1.76% for moderate stroke, and −7.49% to 0.81% for major stroke. There was no significant treatment interaction for the primary outcome. For infarct size, interrater reliability was moderate (κ = 0.675; 95% CI, 0.647-0.702) for local vs core laboratory raters and strong (κ = 0.875; 95% CI, 0.855-0.894) between core laboratory raters.
Conclusions and Relevance The treatment effect of early DOAC initiation did not differ in people with minor, moderate, or major stroke assessed by brain imaging. Early treatment was not associated with a higher rate of adverse events, especially symptomatic intracranial hemorrhage, for any infarct size, including major stroke.
Trial Registration ClinicalTrials.gov Identifier: NCT03148457
Read More About
Goeldlin MB , Hakim A , Branca M, et al. Early vs Late Anticoagulation in Minor, Moderate, and Major Ischemic Stroke With Atrial Fibrillation : Post Hoc Analysis of the ELAN Randomized Clinical Trial . JAMA Neurol. Published online May 28, 2024. doi:10.1001/jamaneurol.2024.1450
Manage citations:
© 2024
Artificial Intelligence Resource Center
Neurology in JAMA : Read the Latest
Browse and subscribe to JAMA Network podcasts!
Others Also Liked
Select your interests.
Customize your JAMA Network experience by selecting one or more topics from the list below.
- Academic Medicine
- Acid Base, Electrolytes, Fluids
- Allergy and Clinical Immunology
- American Indian or Alaska Natives
- Anesthesiology
- Anticoagulation
- Art and Images in Psychiatry
- Artificial Intelligence
- Assisted Reproduction
- Bleeding and Transfusion
- Caring for the Critically Ill Patient
- Challenges in Clinical Electrocardiography
- Climate and Health
- Climate Change
- Clinical Challenge
- Clinical Decision Support
- Clinical Implications of Basic Neuroscience
- Clinical Pharmacy and Pharmacology
- Complementary and Alternative Medicine
- Consensus Statements
- Coronavirus (COVID-19)
- Critical Care Medicine
- Cultural Competency
- Dental Medicine
- Dermatology
- Diabetes and Endocrinology
- Diagnostic Test Interpretation
- Drug Development
- Electronic Health Records
- Emergency Medicine
- End of Life, Hospice, Palliative Care
- Environmental Health
- Equity, Diversity, and Inclusion
- Facial Plastic Surgery
- Gastroenterology and Hepatology
- Genetics and Genomics
- Genomics and Precision Health
- Global Health
- Guide to Statistics and Methods
- Hair Disorders
- Health Care Delivery Models
- Health Care Economics, Insurance, Payment
- Health Care Quality
- Health Care Reform
- Health Care Safety
- Health Care Workforce
- Health Disparities
- Health Inequities
- Health Policy
- Health Systems Science
- History of Medicine
- Hypertension
- Images in Neurology
- Implementation Science
- Infectious Diseases
- Innovations in Health Care Delivery
- JAMA Infographic
- Law and Medicine
- Leading Change
- Less is More
- LGBTQIA Medicine
- Lifestyle Behaviors
- Medical Coding
- Medical Devices and Equipment
- Medical Education
- Medical Education and Training
- Medical Journals and Publishing
- Mobile Health and Telemedicine
- Narrative Medicine
- Neuroscience and Psychiatry
- Notable Notes
- Nutrition, Obesity, Exercise
- Obstetrics and Gynecology
- Occupational Health
- Ophthalmology
- Orthopedics
- Otolaryngology
- Pain Medicine
- Palliative Care
- Pathology and Laboratory Medicine
- Patient Care
- Patient Information
- Performance Improvement
- Performance Measures
- Perioperative Care and Consultation
- Pharmacoeconomics
- Pharmacoepidemiology
- Pharmacogenetics
- Pharmacy and Clinical Pharmacology
- Physical Medicine and Rehabilitation
- Physical Therapy
- Physician Leadership
- Population Health
- Primary Care
- Professional Well-being
- Professionalism
- Psychiatry and Behavioral Health
- Public Health
- Pulmonary Medicine
- Regulatory Agencies
- Reproductive Health
- Research, Methods, Statistics
- Resuscitation
- Rheumatology
- Risk Management
- Scientific Discovery and the Future of Medicine
- Shared Decision Making and Communication
- Sleep Medicine
- Sports Medicine
- Stem Cell Transplantation
- Substance Use and Addiction Medicine
- Surgical Innovation
- Surgical Pearls
- Teachable Moment
- Technology and Finance
- The Art of JAMA
- The Arts and Medicine
- The Rational Clinical Examination
- Tobacco and e-Cigarettes
- Translational Medicine
- Trauma and Injury
- Treatment Adherence
- Ultrasonography
- Users' Guide to the Medical Literature
- Vaccination
- Venous Thromboembolism
- Veterans Health
- Women's Health
- Workflow and Process
- Wound Care, Infection, Healing
- Register for email alerts with links to free full-text articles
- Access PDFs of free articles
- Manage your interests
- Save searches and receive search alerts

IMAGES
VIDEO
COMMENTS
Artificial Intelligence in Interventional Radiology: A Literature Review and Future Perspectives J Oncol. 2019 Nov 3:2019:6153041. doi: 10.1155/2019/6153041. ... and interpretation with a recent application also for interventional radiology (IR) practice, including the ability of AI to offer prognostic information to both patients and ...
Since its inception in the early 20th century, interventional radiology (IR) has evolved tremendously and is now a distinct clinical discipline with its own training pathway. The arsenal of modalities at work in IR includes x-ray radiography and fluoroscopy, CT, MRI, US, and molecular and multimodality imaging within hybrid interventional environments. This article briefly reviews the major ...
Mewes A., Hensen B., Wacker F., Hansen C. Touchless interaction with software in interventional radiology and surgery: a systematic literature review. International Journal of Computer Assisted Radiology and Surgery. 2017; 12 (2):291-305. doi: 10.1007/s11548-016-1480-6. [Google Scholar]
Artificial intelligence (AI) has demonstrated great potential in a wide variety of applications in interventional radiology (IR). Support for decision-making and outcome prediction, new functions and improvements in fluoroscopy, ultrasound, computed tomography, and magnetic resonance imaging, specifically in the field of IR, have all been investigated. Furthermore, AI represents a significant ...
Current applications of algorithmic artificial intelligence in interventional radiology: A review of the literature. Andrew J O'Brien, Corresponding Author. Andrew J O'Brien ... This article reviews the literature to contextualise how AI is currently being implemented in interventional radiology. This review considers the literature from a ...
This article reviews the literature to contextualise how AI is currently being implemented in interventional radiology. This review considers the literature from a preoperative, intraoperative and ...
The term "artificial intelligence" (AI) includes computational algorithms that can perform tasks considered typical of human intelligence, with partial to complete autonomy, to produce new beneficial outputs from specific inputs. The development of AI is largely based on the introduction of artificial neural networks (ANN) that allowed the introduction of the concepts of " ...
DOI: 10.1155/2019/6153041 Corpus ID: 208333956; Artificial Intelligence in Interventional Radiology: A Literature Review and Future Perspectives @article{Iezzi2019ArtificialII, title={Artificial Intelligence in Interventional Radiology: A Literature Review and Future Perspectives}, author={Roberto Iezzi and Shraga Nahum Goldberg and Biagio Merlino and Alessandro Posa and Vincenzo Valentini and ...
ReviewArticle Artificial Intelligence in Interventional Radiology: A Literature Review and Future Perspectives Roberto Iezzi ,1,2 S. N. Goldberg,3 B. Merlino,1,2 A ...
This review is a brief overview of the current status and the potential role of robotics in interventional radiology. Literature published in the last decades, with an emphasis on the last 5 years ...
Purpose The objective of this comprehensive review is to investigate the studies assessing the interventional radiology knowledge among medical students worldwide and inspect the feasible solutions for improving their perspective on this specialty. Methods A comprehensive literature search was accomplished on PubMed, Scopus, Web of science, and Embase databases. The quality of eligible ...
Interventional radiology, and particularly interventional oncology, represents one of the medical subspecialties in which technological advancements and innovations play an utterly fundamental role. Artificial intelligence, consisting of big data analysis and feature extrapolation through computational algorithms for disease diagnosis and treatment response evaluation, is nowadays playing an ...
Interventional radiology (IR) nurses are responsible for providing conscious sedation and nursing care for patients undergoing invasive procedures. ... In this literature review, the frequency of interruptions has been studied in a variety of clinical settings and during various aspects of care. Most articles focused on learning how to prevent ...
This systematic review evaluates the ways in which PROs have been used in the interventional radiology literature. Methods Systematic review was conducted in accordance with the Preferred Reporting Items for Systematic Reviews and Meta-Analyses guidelines and was designed and conducted by a medical librarian.
Rajat Chand, Adam E. M. Eltorai, Terrance Healey, Sun Ahn. Offers a rapid-fire review of core interventional radiology concepts through a question and answer format. Sections are organized by general information and body area. Includes examples of classic and foundational cases and relevant images. 84k Accesses.
The typical review lists 10-20 comments. Aim at improving the manuscript quality and making it understandable to the reader. It is essential that these comments (a) are numbered, (b) follow in order according the sections of the manuscript, and (c) are prefaced by a subject heading such as "Results" or "Methods.".
Purpose In this article, we systematically examine the current state of research of systems that focus on touchless human-computer interaction in operating rooms and interventional radiology suites. We further discuss the drawbacks of current solutions and underline promising technologies for future development. Methods A systematic literature search of scientific papers that deal with ...
Search worldwide, life-sciences literature Search. Advanced Search
Terms like calcium tendinitis, tendinosis, and tendinopathy are used interchangeably. Calcific tendinopathy is a common and well-documented ailment in the literature. Although common, the natural history, aetiology, and progression of calcific tendinitis are poorly understood. The treatment options include conservative and interventional measures.
Interventional Radiology Specialty Board Examinations Tatsushi Oura 1, Hiroyuki Tatekawa, Daisuke Horiuchi , ... they referred to textbooks and the literature to determine the correct answers. If the answers were in agreement, they were considered correct. In case of disagreement, a ... A systematic review and meta-analysis [Internet]. bioRxiv ...
The Journal of Medical Imaging and Interventional Radiology (JMIIR) will publish a wide spectrum of papers, including original researches, review articles, pictorial essays, technical notes, case reports, editorials, commentaries, and letters to the editor. The JMIIR follows a double-blind peer review process. This means that the author's identity will be concealed from the reviewers ...
Key Points. Question Does infarct size modify the safety and efficacy of early vs late initiation of direct oral anticoagulation (DOAC) after ischemic stroke in people with atrial fibrillation?. Findings In this post hoc analysis of 1962 participants from the Early Versus Later Anticoagulation for Stroke With Atrial Fibrillation (ELAN) randomized clinical trial, the odds of the primary outcome ...