Field experiments, explained
Editor’s note: This is part of a series called “The Day Tomorrow Began,” which explores the history of breakthroughs at UChicago. Learn more here.
A field experiment is a research method that uses some controlled elements of traditional lab experiments, but takes place in natural, real-world settings. This type of experiment can help scientists explore questions like: Why do people vote the way they do? Why do schools fail? Why are certain people hired less often or paid less money?
University of Chicago economists were early pioneers in the modern use of field experiments and conducted innovative research that impacts our everyday lives—from policymaking to marketing to farming and agriculture.

Jump to a section:
What is a field experiment, why do a field experiment, what are examples of field experiments, when did field experiments become popular in modern economics, what are criticisms of field experiments.
Field experiments bridge the highly controlled lab environment and the messy real world. Social scientists have taken inspiration from traditional medical or physical science lab experiments. In a typical drug trial, for instance, participants are randomly assigned into two groups. The control group gets the placebo—a pill that has no effect. The treatment group will receive the new pill. The scientist can then compare the outcomes for each group.
A field experiment works similarly, just in the setting of real life.
It can be difficult to understand why a person chooses to buy one product over another or how effective a policy is when dozens of variables affect the choices we make each day. “That type of thinking, for centuries, caused economists to believe you can't do field experimentation in economics because the market is really messy,” said Prof. John List, a UChicago economist who has used field experiments to study everything from how people use Uber and Lyft to how to close the achievement gap in Chicago-area schools . “There are a lot of things that are simultaneously moving.”
The key to cleaning up the mess is randomization —or assigning participants randomly to either the control group or the treatment group. “The beauty of randomization is that each group has the same amount of bad stuff, or noise or dirt,” List said. “That gets differenced out if you have large enough samples.”
Though lab experiments are still common in the social sciences, field experiments are now often used by psychologists, sociologists and political scientists. They’ve also become an essential tool in the economist’s toolbox.
Some issues are too big and too complex to study in a lab or on paper—that’s where field experiments come in.
In a laboratory setting, a researcher wants to control as many variables as possible. These experiments are excellent for testing new medications or measuring brain functions, but they aren’t always great for answering complex questions about attitudes or behavior.
Labs are highly artificial with relatively small sample sizes—it’s difficult to know if results will still apply in the real world. Also, people are aware they are being observed in a lab, which can alter their behavior. This phenomenon, sometimes called the Hawthorne effect, can affect results.
Traditional economics often uses theories or existing data to analyze problems. But, when a researcher wants to study if a policy will be effective or not, field experiments are a useful way to look at how results may play out in real life.
In 2019, UChicago economist Michael Kremer (then at Harvard) was awarded the Nobel Prize alongside Abhijit Banerjee and Esther Duflo of MIT for their groundbreaking work using field experiments to help reduce poverty . In the 1990s and 2000s, Kremer conducted several randomized controlled trials in Kenyan schools testing potential interventions to improve student performance.
In the 1990s, Kremer worked alongside an NGO to figure out if buying students new textbooks made a difference in academic performance. Half the schools got new textbooks; the other half didn’t. The results were unexpected—textbooks had no impact.
“Things we think are common sense, sometimes they turn out to be right, sometimes they turn out to be wrong,” said Kremer on an episode of the Big Brains podcast. “And things that we thought would have minimal impact or no impact turn out to have a big impact.”
In the early 2000s, Kremer returned to Kenya to study a school-based deworming program. He and a colleague found that providing deworming pills to all students reduced absenteeism by more than 25%. After the study, the program was scaled nationwide by the Kenyan government. From there it was picked up by multiple Indian states—and then by the Indian national government.
“Experiments are a way to get at causal impact, but they’re also much more than that,” Kremer said in his Nobel Prize lecture . “They give the researcher a richer sense of context, promote broader collaboration and address specific practical problems.”
Among many other things, field experiments can be used to:
Study bias and discrimination
A 2004 study published by UChicago economists Marianne Bertrand and Sendhil Mullainathan (then at MIT) examined racial discrimination in the labor market. They sent over 5,000 resumes to real job ads in Chicago and Boston. The resumes were exactly the same in all ways but one—the name at the top. Half the resumes bore white-sounding names like Emily Walsh or Greg Baker. The other half sported African American names like Lakisha Washington or Jamal Jones. The study found that applications with white-sounding names were 50% more likely to receive a callback.
Examine voting behavior
Political scientist Harold Gosnell , PhD 1922, pioneered the use of field experiments to examine voting behavior while at UChicago in the 1920s and ‘30s. In his study “Getting out the vote,” Gosnell sorted 6,000 Chicagoans across 12 districts into groups. One group received voter registration info for the 1924 presidential election and the control group did not. Voter registration jumped substantially among those who received the informational notices. Not only did the study prove that get-out-the-vote mailings could have a substantial effect on voter turnout, but also that field experiments were an effective tool in political science.
Test ways to reduce crime and shape public policy
Researchers at UChicago’s Crime Lab use field experiments to gather data on crime as well as policies and programs meant to reduce it. For example, Crime Lab director and economist Jens Ludwig co-authored a 2015 study on the effectiveness of the school mentoring program Becoming a Man . Developed by the non-profit Youth Guidance, Becoming a Man focuses on guiding male students between 7th and 12th grade to help boost school engagement and reduce arrests. In two field experiments, the Crime Lab found that while students participated in the program, total arrests were reduced by 28–35%, violent-crime arrests went down by 45–50% and graduation rates increased by 12–19%.
The earliest field experiments took place—literally—in fields. Starting in the 1800s, European farmers began experimenting with fertilizers to see how they affected crop yields. In the 1920s, two statisticians, Jerzy Neyman and Ronald Fisher, were tasked with assisting with these agricultural experiments. They are credited with identifying randomization as a key element of the method—making sure each plot had the same chance of being treated as the next.
The earliest large-scale field experiments in the U.S. took place in the late 1960s to help evaluate various government programs. Typically, these experiments were used to test minor changes to things like electricity pricing or unemployment programs.
Though field experiments were used in some capacity throughout the 20th century, this method didn’t truly gain popularity in economics until the 2000s. Kremer and List were early pioneers and first began experimenting with the method in the 1990s.
In 2004, List co-authored a seminal paper defining field experiments and arguing for the importance of the method. In 2008, he and UChicago economist Steven Levitt published another study tracing the history of field experiments and their impact on economics.
In the past few decades, the use of field experiments has exploded. Today, economists often work alongside NGOs or nonprofit organizations to study the efficacy of programs or policies. They also partner with companies to test products and understand how people use services.
There are several ethical discussions happening among scholars as field experiments grow in popularity. Chief among them is the issue of informed consent. All studies that involve human test subjects must be approved by an institutional review board (IRB) to ensure that people are protected.
However, participants in field experiments often don’t know they are in an experiment. While an experiment may be given the stamp of approval in the research community, some argue that taking away peoples’ ability to opt out is inherently unethical. Others advocate for stricter review processes as field experiments continue to evolve.
According to List, another major issue in field experiments is the issue of scale . Many experiments only test small groups—say, dozens to hundreds of people. This may mean the results are not applicable to broader situations. For example, if a scientist runs an experiment at one school and finds their method works there, does that mean it will also work for an entire city? Or an entire country?
List believes that in addition to testing option A and option B, researchers need a third option that accounts for the limitations that come with a larger scale. “Option C is what I call critical scale features. I want you to bring in all of the warts, all of the constraints, whether they're regulatory constraints, or constraints by law,” List said. “Option C is like your reality test, or what I call policy-based evidence.”
This problem isn’t unique to field experiments, but List believes tackling the issue of scale is the next major frontier for a new generation of economists.
Hero photo copyright Shutterstock.com
More Explainers
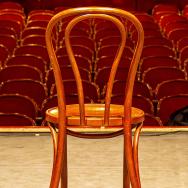
Improv, Explained
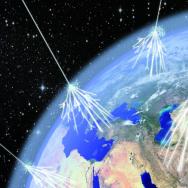
Cosmic rays, explained
Get more with UChicago News delivered to your inbox.
Recommended Stories
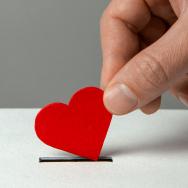
An economist illuminates our giving habits—during the pandemic and…
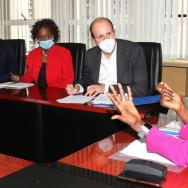
Collaborating with Kenyan government on development innovations is…
Related Topics
Latest news, "an ‘unparalleled experience’: uchicago students attend national conventions.
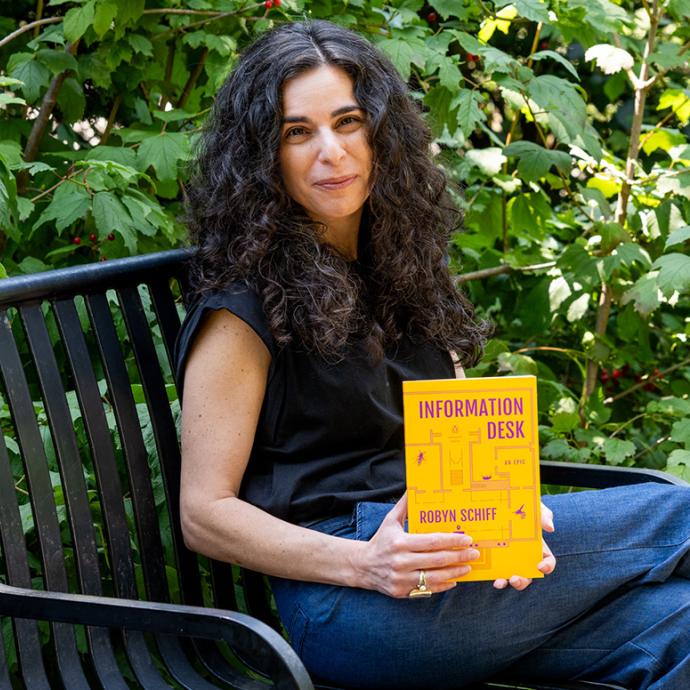
Robyn Schiff’s epic poem ‘Information Desk’ draws critical acclaim
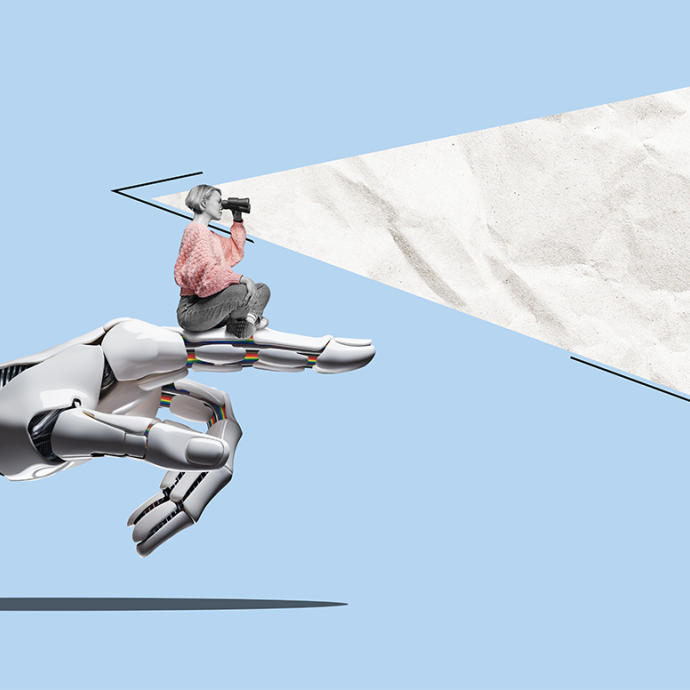
NSF awards $20 million to build AI models that predict scientific discoveries and technological advancements
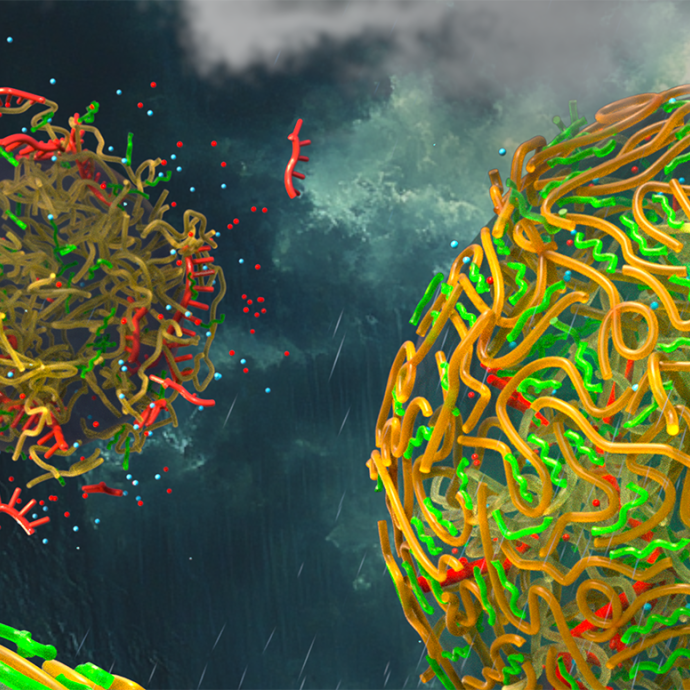
Biochemistry
New research suggests rainwater could have helped form the first protocell walls
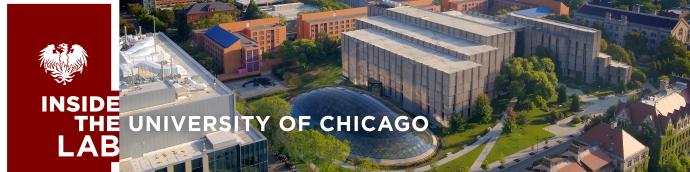
Go 'Inside the Lab' at UChicago
Explore labs through videos and Q&As with UChicago faculty, staff and students
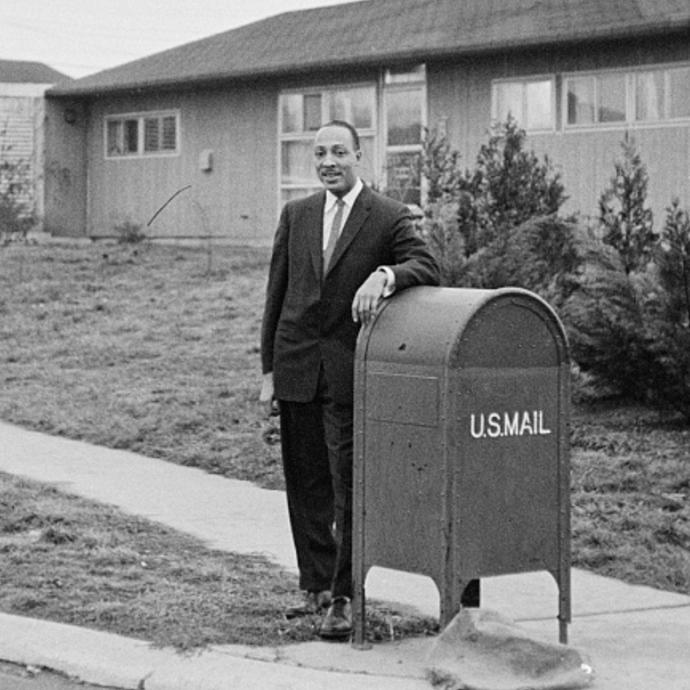
Big Brains podcast
Big Brains podcast: How homeownership shaped race in America
Around uchicago.
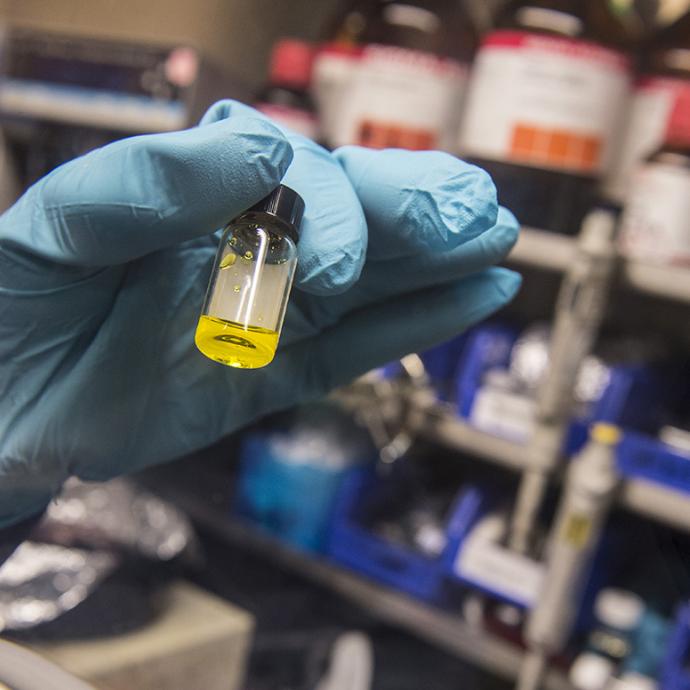
Breakthrough by UChicago scientists could ease notoriously difficult chemical reaction
Quantrell and PhD Teaching Awards
UChicago announces 2024 winners of Quantrell and PhD Teaching Awards
Campus News
Project to improve accessibility, sustainability of Main Quadrangles
National Academy of Sciences
Five UChicago faculty elected to National Academy of Sciences in 2024
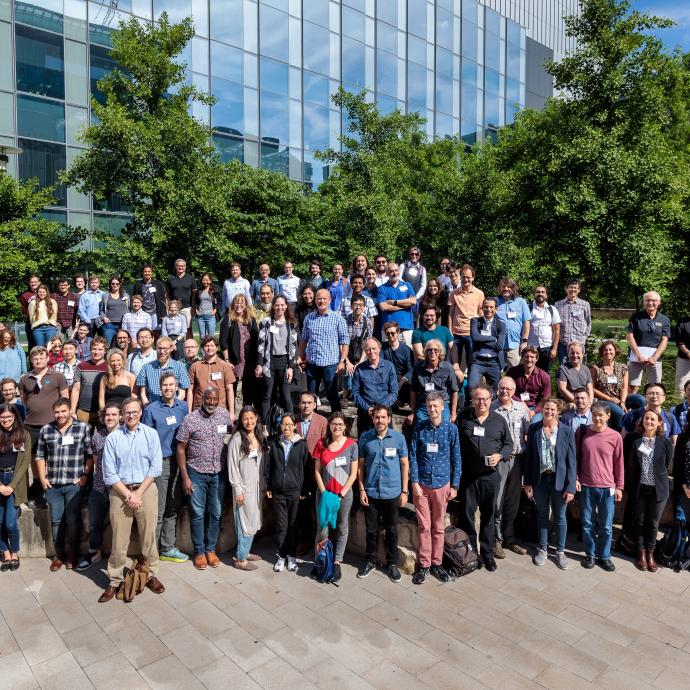
UChicago’s Kavli Institute for Cosmological Physics celebrates 20 years of discovery
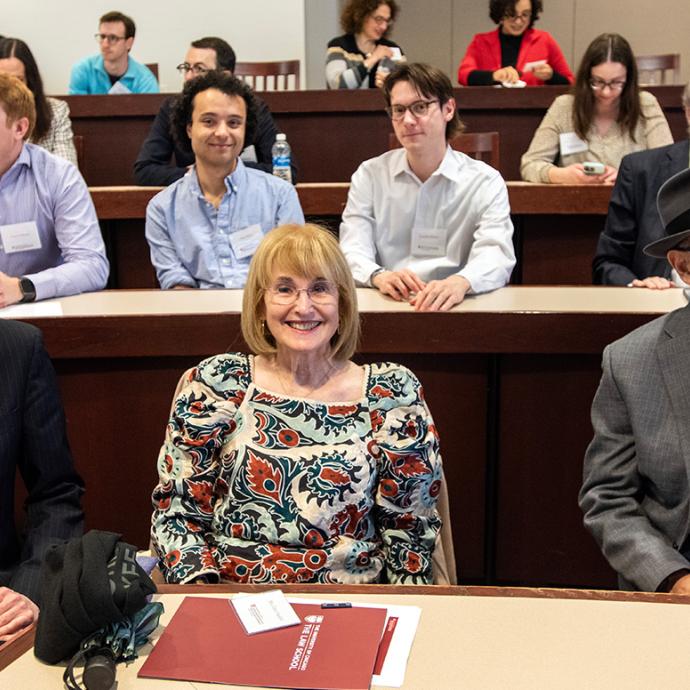
University of Chicago Law School
Coase-Sandor Institute for Law and Economics celebrates decade of impact
Biological Sciences Division
“You have to be open minded, planning to reinvent yourself every five to seven years.”
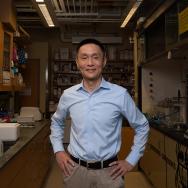
Meet A UChicagoan
Organist pulls out all the stops to bring Bach to UChicago
Introduction to Field Experiments and Randomized Controlled Trials

Have you ever been curious about the methods researchers employ to determine causal relationships among various factors, ultimately leading to significant breakthroughs and progress in numerous fields? In this article, we offer an overview of field experimentation and its importance in discerning cause and effect relationships. We outline how randomized experiments represent an unbiased method for determining what works. Furthermore, we discuss key aspects of experiments, such as intervention, excludability, and non-interference. To illustrate these concepts, we present a hypothetical example of a randomized controlled trial evaluating the efficacy of an experimental drug called Covi-Mapp.
Why experiments?
Every day, we find ourselves faced with questions of cause and effect. Understanding the driving forces behind outcomes is crucial, ranging from personal decisions like parenting strategies to organizational challenges such as effective advertising. This blog aims to provide a systematic introduction to experimentation, igniting enthusiasm for primary research and highlighting the myriad of experimental applications and opportunities available.
The challenge for those who seek to answer causal questions convincingly is to develop a research methodology that doesn't require identifying or measuring all potential confounders. Since no planned design can eliminate every possible systematic difference between treatment and control groups, random assignment emerges as a powerful tool for minimizing bias. In the contentious world of causal claims, randomized experiments represent an unbiased method for determining what works. Random assignment means participants are assigned to different groups or conditions in a study purely by chance. Basically, each participant has an equal chance to be assigned to a control group or a treatment group.
Field experiments, or randomized studies conducted in real-world settings, can take many forms. While experiments on college campuses are often considered lab studies, certain experiments on campus – such as those examining club participation – may be regarded as field experiments, depending on the experimental design. Ultimately, whether a study is considered a field experiment hinges on the definition of "the field."
Researchers may employ two main scenarios for randomization. The first involves gathering study participants and randomizing them at the time of the experiment. The second capitalizes on naturally occurring randomizations, such as the Vietnam draft lottery.
Intervention, Excludability, and Non-Interference
Three essential features of any experiment are intervention, excludability, and non-interference. In a general sense, the intervention refers to the treatment or action being tested in an experiment. The excludability principle is satisfied when the only difference between the experimental and control groups is the presence or absence of the intervention. The non-interference principle holds when the outcome of one participant in the study does not influence the outcomes of other participants. Together, these principles ensure that the experiment is designed to provide unbiased and reliable results, isolating the causal effect of the intervention under study.
Omitted Variables and Non-Compliance
To ensure unbiased results, researchers must randomize as much as possible to minimize omitted variable bias. Omitted variables are factors that influence the outcome but are not measured or are difficult to measure. These unmeasured attributes, sometimes called confounding variables or unobserved heterogeneity, must be accounted for to guarantee accurate findings.
Non-compliance can also complicate experiments. One-sided non-compliance occurs when individuals assigned to a treatment group don't receive the treatment (failure to treat), while two-sided non-compliance occurs when some subjects assigned to the treatment group go untreated or individuals assigned to the control group receive the treatment. Addressing these issues at the design level by implementing a blind or double-blind study can help mitigate potential biases.
Achieving Precision through Covariate Balance
To ensure the control and treatment groups are comparatively similar in all relevant aspects, particularly when the sample size (n) is small, it is essential to achieve covariate balance. Covariance measures the association between two variables, while a covariate is a factor that influences the outcome variable. By balancing covariates, we can more accurately isolate the effects of the treatment, leading to improved precision in our findings.
Fictional Example of Randomized Controlled Trial of Covi-Mapp for COVID-19 Management
Let's explore a fictional example to better understand experiments: a one-week randomized controlled trial of the experimental drug Covi-Mapp for managing Covid. In this case, the control group receives the standard care for Covid patients, while the treatment group receives the standard care plus Covi-Mapp. The outcome of interest is whether patients have cough symptoms on day 7, as subsidizing cough symptoms is an encouraging sign in Covid recovery. We'll measure the presence of cough on day 0 and day 7, as well as temperature on day 0 and day 7. Gender is also tracked. The control represents the standard care for COVID-19 patients, while the treatment includes standard care plus the experimental drug.
In this Covi-Mapp example, the intervention is the Covi-Mapp drug, the excludability principle is satisfied if the only difference in patient care between the groups is the drug administration, and the non-interference principle holds if one patient's outcome doesn't affect another's.
First, let's assume we have a dataset containing the relevant information for each patient, including cough status on day 0 and day 7, temperature on day 0 and day 7, treatment assignment, and gender. We'll read the data and explore the dataset:
library(data.table) d <- fread("../data/COVID_rct.csv") names(d) "temperature_day0" "cough_day0" "treat_zmapp" "temperature_day14" "cough_day14" "male" |
Simple treatment effect of the experimental drug
Without any covariates, let's first look at the estimated effect of the treatment on the presence of cough on day 7. The estimated proportion of patients with a cough on day 7 for the control group (not receiving the experimental drug) is 0.847458. In other words, about 84.7% of patients in the control group are expected to have a cough on day 7, all else being equal. The estimated effect of the experimental drug on the presence of cough on day 7 is -0.23. This means that, on average, receiving the experimental drug reduces the proportion of patients with a cough on day 7 by 23.8% compared to the control group.
covid_1 <- d[ , lm(cough_day7 ~ treat_drug)] coeftest(covid_1, vcovHC) Estimate Std. Error t value Pr(>|t|) (Intercept) 0.847458 0.047616 17.798 < 2e-16 *** treat_covid_mapp -0.237702 0.091459 -2.599 0.01079 * Signif. codes: 0 ‘***’ 0.001 ‘**’ 0.01 ‘*’ 0.05 ‘.’ 0.1 ‘ ’ 1 |
We know that a patient's initial condition would affect the final outcome. If the patient has a cough and a fever on day 0, they might not fare well with the treatment. To better understand the treatment's effect, let's add these covariates:
covid_2 <- d[ , lm(cough_day7 ~ treat_drug + cough_day0 + temperature_day0)] coeftest(covid_2, vcovHC) Estimate Std. Error t value Pr(>|t|) (Intercept) -19.469655 7.607812 -2.5592 0.012054 * treat_covid_mapp -0.165537 0.081976 -2.0193 0.046242 * cough_day0 0.064557 0.178032 0.3626 0.717689 temperature_day0 0.205548 0.078060 2.6332 0.009859 ** Signif. codes: 0 ‘***’ 0.001 ‘**’ 0.01 ‘*’ 0.05 ‘.’ 0.1 ‘ ’ 1 |
The output shows the results of a linear regression model, estimating the effect of the experimental drug (treat_covid_mapp) on the presence of cough on day 7, adjusting for cough on day 0 and temperature on day 0. The experimental drug significantly reduces the presence of cough on day 7 by approximately 16.6% compared to the control group (p-value = 0.046242). The presence of cough on day 0 does not significantly predict the presence of cough on day 7 (p-value = 0.717689). A one-unit increase in temperature on day 0 is associated with a 20.6% increase in the presence of cough on day 7, and this effect is statistically significant (p-value = 0.009859).
Should we add day 7 temperature as a covariate? By including it, we might find that the treatment is no longer statistically significant since the temperature on day 7 could be affected by the treatment itself. It is a post-treatment variable, and by including it, the experiment loses value as we used something that was affected by intervention as our covariate.
However, we'd like to investigate if the treatment affects men or women differently. Since we collected gender as part of the study, we could check for Heterogeneous Treatment Effect (HTE) for male vs. female. The experimental drug has a marginally significant effect on the outcome variable for females, reducing it by approximately 23.1% (p-value = 0.05391).
covid_4 <- d[ , lm(cough_day7 ~ treat_drug + treat_drug * male + cough_day0 + temperature_day0)] coeftest(covid_4, vcovHC) t test of coefficients: Estimate Std. Error t value Pr(>|t|) (Intercept) 48.712690 10.194000 4.7786 6.499e-06 *** treat_zmapp -0.230866 0.118272 -1.9520 0.05391 . male 3.085486 0.121773 25.3379 < 2.2e-16 *** dehydrated_day0 0.041131 0.194539 0.2114 0.83301 temperature_day0 0.504797 0.104511 4.8301 5.287e-06 *** treat_zmapp:male -2.076686 0.198386 -10.4679 < 2.2e-16 *** Signif. codes: 0 ‘***’ 0.001 ‘**’ 0.01 ‘*’ 0.05 ‘.’ 0.1 ‘ ’ 1 |
Which group, those coded as male == 0 or male == 1, have better health outcomes (cough) in control? What about in treatment? How does this help to contextualize any heterogeneous treatment effect that might have been estimated?
Stargazer is a popular R package that enables users to create well-formatted tables and reports for statistical analysis results.
covid_males <- d[male == 1, lm(temperature_day14 ~ treat_drug)] covid_females <- d[male == 0, lm(temperature_day14 ~ treat_drug)] stargazer(covid_males, covid_females, title = "", type = 'text', dep.var.caption = 'Outcome Variable:', dep.var.labels = c('Cough on Day 7'), se = list( sqrt(diag(vcov(covid_males))), sqrt(diag(vcovHC(covid_females)))) ) =============================================================== Outcome Variable: Temperature on Day 14 (1) (2) treat_covid_mapp -2.591*** -0.323* (0.220) (0.174) Constant 101.692*** 98.487*** (0.153) (0.102) Observations 37 63 R2 0.798 0.057 Adjusted R2 0.793 0.041 Residual Std. Error 0.669 (df = 35) 0.646 (df = 61) F Statistic 138.636*** (df = 1; 35) 3.660* (df = 1; 61) =============================================================== Note: *p<0.1; **p<0.05; ***p<0.01 |
Looking at this regression report, we see that males in control have a temperature of 102; females in control have a temperature of 98.6 (which is very nearly a normal temperature). So, in control, males are worse off. In treatment, males have a temperature of 102 - 2.59 = 99.41. While this is closer to a normal temperature, this is still elevated. Females in treatment have a temperature of 98.5 - .32 = 98.18, which is slightly lower than a normal temperature, and is better than an elevated temperature. It appears that the treatment is able to have a stronger effect among male participants than females because males are *more sick* at baseline.
In conclusion, experimentation offers a fascinating and valuable avenue for primary research, allowing us to address causal questions and enhance our understanding of the world around us. Covariate control helps to isolate the causal effect of the treatment on the outcome variable, ensuring that the observed effect is not driven by confounding factors. Proper control of covariates enhances the internal validity of the study and ensures that the estimated treatment effect is an accurate representation of the true causal relationship. By exploring and accounting for sub groups in data, researchers can identify whether the treatment has different effects on different groups, such as men and women or younger and older individuals. This information can be critical for making informed policy decisions and developing targeted interventions that maximize the benefits for specific groups. The ongoing investigation of experimental methodologies and their potential applications represents a compelling and significant area of inquiry.
Gerber, A. S., & Green, D. P. (2012). Field Experiments: Design, Analysis, and Interpretation . W. W. Norton.
“DALL·E 2.” OpenAI , https://openai.com/product/dall-e-2
“Data Science 241. Experiments and Causal Inference.” UC Berkeley School of Information , https://www.ischool.berkeley.edu/courses/datasci/241
- A-Z Publications
Annual Review of Sociology
Volume 43, 2017, review article, field experiments across the social sciences.
- Delia Baldassarri 1 , and Maria Abascal 2
- View Affiliations Hide Affiliations Affiliations: 1 Department of Sociology, New York University, New York, New York 10012; email: [email protected] 2 Department of Sociology, Columbia University, New York, New York 10027; email: [email protected]
- Vol. 43:41-73 (Volume publication date July 2017) https://doi.org/10.1146/annurev-soc-073014-112445
- First published as a Review in Advance on May 22, 2017
- © Annual Reviews
Using field experiments, scholars can identify causal effects via randomization while studying people and groups in their naturally occurring contexts. In light of renewed interest in field experimental methods, this review covers a wide range of field experiments from across the social sciences, with an eye to those that adopt virtuous practices, including unobtrusive measurement, naturalistic interventions, attention to realistic outcomes and consequential behaviors, and application to diverse samples and settings. The review covers four broad research areas of substantive and policy interest: first, randomized controlled trials, with a focus on policy interventions in economic development, poverty reduction, and education; second, experiments on the role that norms, motivations, and incentives play in shaping behavior; third, experiments on political mobilization, social influence, and institutional effects; and fourth, experiments on prejudice and discrimination. We discuss methodological issues concerning generalizability and scalability as well as ethical issues related to field experimental methods. We conclude by arguing that field experiments are well equipped to advance the kind of middle-range theorizing that sociologists value.
Article metrics loading...
Full text loading...
Literature Cited
- Abascal M . 2015 . Us and them: black–white relations in the wake of Hispanic population growth. Am. Sociol. Rev. 80 : 789– 813 [Google Scholar]
- Adida CL , Laitin DD , Valfort MA . 2016 . Why Muslim Integration Fails in Christian-Heritage Societies Cambridge, MA: Harvard Univ. Press [Google Scholar]
- Ahmed AM , Hammarstedt M . 2008 . Discrimination in the rental housing market: a field experiment on the Internet. J. Urban Econ. 64 : 362– 72 [Google Scholar]
- Ahmed AM , Hammarstedt M . 2009 . Detecting discrimination against homosexuals: evidence from a field experiment on the Internet. Economica 76 : 599– 97 [Google Scholar]
- Arceneaux K , Nickerson DW . 2009 . Who is mobilized to vote? A re-analysis of 11 field experiments. Am. J. Political Sci. 53 : 1– 16 [Google Scholar]
- Attanasio O , Augsburg B , De Haas R , Fitzsimons E , Harmgart H . 2012 . Group lending or individual lending? Evidence from a randomised field experiment in Mongolia. Work. Pap. No. 136, Eur. Bank Reconstr. Dev. [Google Scholar]
- Attanasio O , Pellerano L , Reyes SP . 2009 . Building trust? Conditional cash transfer programmes and social capital. Fiscal Stud. 30 : 139– 77 [Google Scholar]
- Avdeenko A , Gilligan MG . 2015 . International interventions to build social capital: evidence from a field experiment in Sudan. Am. Political Sci. Rev. 109 : 427– 49 [Google Scholar]
- Ayres I , Siegelman P . 1995 . Race and gender discrimination in bargaining for a new car. Am. Econ. Rev. 85 : 304– 21 [Google Scholar]
- Baldassarri D . 2015 . Cooperative networks: altruism, group solidarity, and reciprocity in Ugandan farmer organizations. Am. J. Sociol. 121 : 355– 95 [Google Scholar]
- Baldassarri D . 2016 . Prosocial behavior across communities: evidence from a nationwide lost-letter experiment Presented at Advances with Field Experiments Conf., Sept. 16, Univ Chicago: [Google Scholar]
- Banerjee A , Bertrand M , Datta S , Mullainathan S . 2009 . Labor market discrimination in Delhi: evidence from a field experiment. J. Comp. Econ. 37 : 14– 27 [Google Scholar]
- Banerjee A , Duflo E . 2009 . The experimental approach to development economics. Annu. Rev. Econ. 1 : 151– 78 [Google Scholar]
- Banerjee A , Duflo E . 2011 . Poor Economics: A Radical Rethinking of the Way to Fight Global Poverty. New York: Public Affairs [Google Scholar]
- Banerjee A , Duflo E , Glennerster R , Kothari D . 2010a . Improving immunization coverage in rural India: Clustered randomized controlled immunisation campaigns with and without incentives. Br. Med. J. 340:c2220 [Google Scholar]
- Banerjee A , Duflo E , Glennerster R , Kinnan C . 2010b . The miracle of microfinance? Evidence from a randomized evaluation. Work. Pap. No. 13-09, Dep. Econ., MIT [Google Scholar]
- Barr A . 2003 . Trust and expected trustworthiness: experimental evidence from Zimbabwean villages. Econ. J. 113 : 614– 30 [Google Scholar]
- Bauchet J , Marshall C , Starita L , Thomas J , Yalouris A . 2011 . Latest findings from randomized evaluations of microfinance. Access Finance Forum Rep. 2 : 1– 27 [Google Scholar]
- Beath A , Christia F , Enikolopov R . 2013 . Empowering women: evidence from a field experiment in Afghanistan. Am. Political Sci. Rev. 107 : 540– 57 [Google Scholar]
- Benson PL , Karabenick SA , Lerner RM . 1976 . Pretty pleases: the effects of physical attractiveness, race, and sex on receiving help. J. Exp. Soc. Psychol. 12 : 409– 15 [Google Scholar]
- Benz M , Meier S . 2008 . Do people behave in experiments as in the field? Evidence from donations. Exp. Econ. 11 : 278– 81 [Google Scholar]
- Bertrand M , Karlan D , Mullainathan S , Shafir E , Zinman J . 2010 . What's advertising content worth? Evidence from a consumer credit marketing field experiment. Q. J. Econ. 125 : 263– 306 [Google Scholar]
- Bertrand M , Mullainathan S . 2004 . Are Emily and Greg more employable than Lakisha and Jamal? A field experiment on labor market discrimination. Am. Econ. Rev. 94 : 991– 1013 [Google Scholar]
- Besbris M , Faber JW , Rich P , Sharkey P . 2015 . Effect of neighborhood stigma on economic transitions. PNAS 112 : 4994– 98 [Google Scholar]
- Bettinger EP . 2012 . Paying to learn: the effect of financial incentives on elementary school test scores. Rev. Econ. Stat. 94 : 686– 98 [Google Scholar]
- Bigoni M , Bortolotti S , Casari M , Gambetta D , Pancotto F . 2016 . Amoral familism, social capital, or trust? The behavioural foundations of the Italian north–south divide. Econ. J. 126 : 1318– 41 [Google Scholar]
- Blommaert L , Coenders M , van Tubergen F . 2014 . Discrimination of Arabic-named applicants in the Netherlands: an Internet-based field experiment examining different phases in online recruitment procedures. Soc. Forces 92 : 957– 82 [Google Scholar]
- Bond RM , Fariss CJ , Jones JJ , Kramer AD , Marlow C . et al. 2012 . A 61-million-person experiment in social influence and political mobilization. Nature 489 : 295– 98 [Google Scholar]
- Bosch M , Carnero MA , Farré L . 2010 . Information and discrimination in the rental housing market: evidence from a field experiment. Reg. Sci. Urban Econ. 40 : 11– 19 [Google Scholar]
- Brearley HC . 1931 . Experimental sociology in the United States. Soc. Forces 10 : 196– 99 [Google Scholar]
- Butler DM , Broockman DE . 2011 . Do politicians racially discriminate against constituents? A field experiment on state legislators. Am. J. Political Sci. 55 : 463– 77 [Google Scholar]
- Butler DM , Nickerson DW . 2011 . Can learning constituency opinion affect how legislators vote? Results from a field experiment. Q. J. Political Sci. 6 : 55– 83 [Google Scholar]
- Camerer C . 2003 . Behavioral Game Theory: Experiments in Strategic Interaction New York, NY: Russell Sage Found. [Google Scholar]
- Cardenas J , Carpenter J . 2008 . Behavioural development economics: lessons from field labs in the developing world. J. Dev. Stud. 44 : 337– 64 [Google Scholar]
- Casey K , Glennerster R , Miguel E . 2012 . Reshaping institutions: evidence on external aid and local collective action. Q. J. Econ. 127 : 1755– 812 [Google Scholar]
- Castilla EJ , Benard S . 2010 . The paradox of meritocracy in organizations. Adm. Sci. Q. 55 : 543– 676 [Google Scholar]
- Centola D . 2010 . The spread of behavior in an online social network experiment. Science 329 : 1194– 97 [Google Scholar]
- Charness G , Gneezy U . 2009 . Incentives to exercise. Econometrica 77 : 909– 31 [Google Scholar]
- Chetty R , Hendren N , Katz LF . 2015 . The effects of exposure to better neighborhoods on children: new evidence from the moving to opportunity experiment. Work. Pap. 21156, NBER, Cambridge, MA [Google Scholar]
- Chong D , Junn J . 2011 . Politics from the perspective of minority populations. Cambridge Handbook of Experimental Political Science JN Druckman, DP Green, JH Kuklinski, A Lupia, 602– 33 Cambridge, UK: Cambridge Univ. Press [Google Scholar]
- Cialdini RB , Ascani K . 1976 . Test of a concession procedure for inducing verbal, behavioral, and further compliance with a request to give blood. J. Pers. Soc. Psychol. 61 : 295– 300 [Google Scholar]
- Cialdini RB , Vincent JE , Lewis SK , Catalan J , Wheeler D , Darby BL . 1975 . Reciprocal concessions procedure for inducing compliance: the door-in-the-face technique. J. Pers. Soc. Psychol. 31 : 206– 15 [Google Scholar]
- Clampet-Lundquist S , Massey DS . 2008 . Neighborhood effects on economic self-sufficiency: a reconsideration of the Moving to Opportunity experiment. Am. J. Sociol. 114 : 107– 43 [Google Scholar]
- Cohen J , Dupas P . 2010 . Free distribution or cost-sharing? Evidence from a randomized malaria prevention experiment. Q. J. Econ. 125 : 1– 40 [Google Scholar]
- Cole S , Giné X , Tobacman J , Topalova P , Townsend R , Vickery J . 2013 . Barriers to household risk management: evidence from India. Am. Econ. J. Appl. Econ. 5 : 104– 35 [Google Scholar]
- Cook TD , Shadish WR . 1994 . Social experiments: some developments over the past fifteen years. Annu. Rev. Psychol. 45 : 545– 80 [Google Scholar]
- Correll SJ , Benard S , Paik I . 2007 . Getting a job: is there a motherhood penalty?. Am. J. Sociol. 112 : 1297– 339 [Google Scholar]
- Cox D . 1958 . Planning of Experiments New York: Wiley [Google Scholar]
- Crépon B , Devoto F , Duflo E , Parienté W . 2011 . Impact of microcredit in rural areas of Morocco: evidence from a randomized evaluation. Work. Pap., Dep. Econ., MIT [Google Scholar]
- Cross H , Kenney GM , Mell J , Zimmerman W . 1990 . Employer hiring practices: differential treatment of Hispanic and Anglo job seekers. Tech. rep., Urban Inst., Washington, DC [Google Scholar]
- Deaton A . 2010 . Instruments, randomization, and learning about development. J. Econ. Lit. 48 : 424– 55 [Google Scholar]
- Dehejia R , Pop-Eleches C , Samii C . 2015 . From local to global: external validity in a fertility natural experiment. Work. Pap. 21459, NBER, Cambridge, MA [Google Scholar]
- Doob AN , Gross AE . 1968 . Status as an inhibitor of horn-honking responses. J. Soc. Psychol. 76 : 213– 18 [Google Scholar]
- Druckman JN , Green DP , Kuklinski JH , Lupia A . 2011 . Cambridge Handbook of Experimental Political Science Cambridge, UK: Cambridge Univ. Press [Google Scholar]
- Duflo E , Kremer M , Robinson J . 2008 . How high are rates of return to fertilizer? Evidence from field experiments in Kenya. Am. Econ. Rev. 98 : 482– 88 [Google Scholar]
- Duflo E , Kremer M , Robinson J . 2011 . Nudging farmers to use fertilizer: theory and experimental evidence from Kenya. Am. Econ. Rev. 101 : 2350– 90 [Google Scholar]
- Dunn EW , Aknin LB , Norton MI . 2008 . Spending money on others promotes happiness. Science 319 : 1687– 88 [Google Scholar]
- Dunning T . 2012 . Natural Experiments in the Social Sciences: A Design-Based Approach Cambridge, UK: Cambridge Univ. Press [Google Scholar]
- Dupas P . 2009 . What matters (and what does not) in households’ decision to invest in malaria prevention?. Am. Econ. Rev. 99 : 224– 30 [Google Scholar]
- Dupas P . 2011 . Do teenagers respond to HIV risk information? Evidence from a field experiment in Kenya. Am. Econ. J. Appl. Econ. 3 : 1– 34 [Google Scholar]
- Dupas P . 2014 . Short-run subsidies and long-run adoption of new health products: evidence from a field experiment. Econometrica 82 : 197– 228 [Google Scholar]
- Dupas P , Robinson J . 2011 . Savings constraints and microenterprise development: evidence from a field experiment in Kenya. Work. Pap. 14693, NBER, Cambridge, MA [Google Scholar]
- Emswiller T , Deaux K , Willits JE . 1971 . Similarity, sex, and requests for small favors. J. Appl. Soc. Psychol. 1 : 284– 91 [Google Scholar]
- Enos RD . 2014 . Causal effect of intergroup contact on exclusionary attitudes. PNAS 111 : 3699– 704 [Google Scholar]
- Enos RD , Fowler A , Vavreck L . 2014 . Increasing inequality: the effect of GOTV mobilization on the composition of the electorate. J. Polit. 76 : 273– 88 [Google Scholar]
- Fearon JD , Humphreys M , Weinstein JM . 2009 . Can development aid contribute to social cohesion after civil war? Evidence from a field experiment in post-conflict Liberia. Am. Econ. Rev. 99 : 287– 91 [Google Scholar]
- Fearon JD , Humphreys M , Weinstein JM . 2015 . How does development assistance affect collective action capacity? Results from a field experiment in post-conflict Liberia. Am. J. Political Sci. 109 : 450– 69 [Google Scholar]
- Fershtman C , Gneezy U . 2001 . Discrimination in a segmented society: an experimental approach. Q. J. Econ. 116 : 351– 77 [Google Scholar]
- Fisher RA . 1935 . The Design of Experiments New York: Hafner [Google Scholar]
- Fiszbein A , Schady N . 2009 . Conditional cash transfers: reducing present and future poverty. World Bank Policy Res. Rep., World Bank Washington, DC: [Google Scholar]
- Forbes GB , Gromoll HF . 1971 . The lost letter technique as a measure of social variables: some exploratory findings. Soc. Forces 50 : 113– 15 [Google Scholar]
- Freedman JL , Fraser SC . 1966 . Compliance without pressure: the foot-in-the-door technique. J. Pers. Soc. Psychol. 4 : 195– 202 [Google Scholar]
- Freese J , Peterson D . 2017 . Replication in social science. Annu. Rev. Sociol. 43. In press [Google Scholar]
- Fryer R . 2011 . Financial incentives and student achievement: evidence from randomized trials. Q. J. Econ. 126 : 1755– 98 [Google Scholar]
- Gaddis SM . 2015 . Discrimination in the credential society: an audit study of race and college selectivity in the labor market. Soc. Forces 93 : 1451– 79 [Google Scholar]
- Gaddis SM , Ghoshal R . 2015 . Arab American housing discrimination, ethnic competition, and the contact hypothesis. Ann. Am. Acad. Political Soc. Sci. 660 : 282– 99 [Google Scholar]
- Galster G , Constantine P . 1991 . Discrimination against female-headed households in rental housing: theory and exploratory evidence. Rev. Soc. Econ. 49 : 76– 100 [Google Scholar]
- Gantner L . 2007 . PROGRESA: An integrated approach to poverty alleviation in Mexico. Case Studies in Food Policy for Developing Countries: Policies for Health, Nutrition, Food Consumption, and Poverty P Pinstrup-Andersen, F Cheng, Vol 1 211– 20 Ithaca, NY: Cornell Univ. Press [Google Scholar]
- Garfinkel H . 1967 . Studies in Ethnomethodology Englewood Cliffs, NJ: Prentice-Hall [Google Scholar]
- Gelman A . 2014 . Experimental reasoning in social science. Field Experiments and Their Critics: Essays on the Uses and Abuses of Experimentation in the Social Sciences DL Teele 185– 95 New Haven, CT: Yale Univ. Press [Google Scholar]
- Gerber AS . 2011 . Field experiments in political science. Cambridge Handbook of Experimental Political Science JN Druckman, DP Green, JH Kuklinski, A Lupia 115– 38 Cambridge, UK: Cambridge Univ. Press [Google Scholar]
- Gerber AS , Green DP . 2000 . The effects of canvassing, telephone calls, and direct mail on voter turnout: a field experiment. Am. Political Sci. Rev. 94 : 653– 63 [Google Scholar]
- Gerber AS , Green DP . 2012 . Field Experiments New York: Norton [Google Scholar]
- Gerber AS , Green DP , Larimer CW . 2008 . Social pressure and voter turnout: evidence from a large scale field experiment. Am. Political Sci. Rev. 102 : 33– 48 [Google Scholar]
- Gerber AS , Green DP , Shachar R . 2003 . Voting may be habit-forming: evidence from a randomized field experiment. Am. J. Political Sci. 47 : 540– 50 [Google Scholar]
- Gil-White F . 2004 . Ultimatum game with an ethnicity manipulation: results from Kohvdiin Bulgan Sum, Mongolia. Foundations of Human Sociality: Economic Experiments and Ethnographic Evidence from Fifteen Small-Scale Societies J Henrich, R Boyd, S Bowles, C Camerer, E Fehr, H Gintis, 260– 304 Oxford, UK: Oxford Univ. Press [Google Scholar]
- Gilligan MJ , Pasquale BJ , Samii C . 2014 . Civil war and social cohesion: lab-in-the-field evidence from Nepal. Am. J. Political Sci. 58 : 604– 19 [Google Scholar]
- Giné X , Karlan D . 2014 . Group versus individual liability: short and long term evidence from Philippine-microcredit lending groups. J. Dev. Econ. 107 : 65– 83 [Google Scholar]
- Giné X , Karlan D , Zinman J . 2010 . Put your money where your butt is: a commitment contract for smoking cessation. Am. Econ. J. Appl. Econ. 213– 35 [Google Scholar]
- Gneezy U , List J , Price MK . 2012 . Toward an understanding of why people discriminate: evidence from a series of natural field experiments. Work. Pap. 17855, NBER, Cambridge, MA [Google Scholar]
- Gneezy U , Meier S , Rey-Biel P . 2011 . When and why incentives (don't) work to modify behavior. J. Econ. Perspect. 25 : 191– 210 [Google Scholar]
- Gneezy U , Rey-Biel P . 2014 . On the relative efficiency of performance pay and noncontingent incentives. J. Eur. Econ. Assoc. 12 : 62– 72 [Google Scholar]
- Gneezy U , Rustichini A . 2000 . A fine is a price. J. Legal Stud. 29 : 1– 17 [Google Scholar]
- Goel V . 2014 . Facebook tinkers with users’ emotions in news feed experiment, stirring outcry. New York Times , June 30 B1
- Gosnell HF . 1927 . Getting Out the Vote: An Experiment in the Stimulation of Voting Chicago: Chicago Univ. Press [Google Scholar]
- Green DP , Gerber A . 2008 . Get Out the Vote: How to Increase Voter Turnout Washington, DC: Brookings Inst. Press. 2nd ed. [Google Scholar]
- Green DP , Wong J . 2009 . Tolerance and the contact hypothesis: a field experiment. The Political Psychology of Democratic Citizenship 228– 46 Oxford, UK: Oxford Univ. Press [Google Scholar]
- Greenberg D , Shroder M . 2004 . The Digest of Social Experiments. Washington, DC: Urban Inst. Press [Google Scholar]
- Grose CR . 2014 . Field experimental work on political institutions. Annu. Rev. Political Sci. 17 : 355– 70 [Google Scholar]
- Grossman G , Baldassarri D . 2012 . The impact of elections on cooperation: evidence from a lab in the field experiment in Uganda. Am. J. Political Sci. 56 : 964– 85 [Google Scholar]
- Grossman G , Paler L . 2015 . Using experiments to study political institutions. Handbook of Comparative Political Institutions J Gandhi, R Ruiz-Rufino 84– 97 London: Routledge [Google Scholar]
- Habyarimana J , Humphreys M , Posner DN , Weinstein JM . 2009 . Coethnicity: Diversity and the Dilemmas of Collective Action New York: Russell Sage Found. [Google Scholar]
- Harrison GW . 2013 . Field experiments and methodological intolerance. J. Econ. Methodol. 20 : 103– 17 [Google Scholar]
- Harrison GW , List JA . 2004 . Field experiments. J. Econ. Lit. 42 : 1009– 55 [Google Scholar]
- Hausman JA , Wise DA . 1985 . Social Experimentation Chicago: Chicago Univ. Press [Google Scholar]
- Heckman JJ . 1992 . Randomization and social policy evaluation. Evaluating Welfare and Training Programs CF Manski, I Garfinkel 201– 30 Cambridge, MA: Harvard Univ. Press [Google Scholar]
- Heckman JJ . 1998 . Detecting discrimination. J. Econ. Perspect. 12 : 101– 16 [Google Scholar]
- Heckman JJ , Siegelman P . 1993 . The Urban Institute audit studies: their methods and findings. Clear and Convincing Evidence: Measurement of Discrimination in America M Fix, RJ Struyk 187– 258 Washington, DC: Urban Inst. Press [Google Scholar]
- Henrich J , Boyd R , Bowles S , Camerer C , Fehr E . et al. 2001 . In search of homo economicus: behavioral experiments in 15 small-scale societies. Am. Econ. Rev. 91 : 73– 78 [Google Scholar]
- Henrich J , Ensminger J , McElreath R , Barr A , Barrett C . et al. 2010 . Markets, religion, community size, and the evolution of fairness and punishment. Science 327 : 1480– 84 [Google Scholar]
- Henrich J , McElreath R , Barr A , Ensminger J , Barrett C . et al. 2006 . Costly punishment across human societies. Science 312 : 1767– 70 [Google Scholar]
- Henry PJ . 2008 . College sophomores in the laboratory redux: influences of a narrow data base on social psychology's view of the nature of prejudice. Psychol. Inq. 19 : 49– 71 [Google Scholar]
- Herberich DH , List JA , Price MK . 2011 . How many economists does it take to change a light bulb? A natural field experiment on technology adoption Work. Pap., Univ. Chicago [Google Scholar]
- Heyman J , Ariely D . 2004 . Effort for payment: a tale of two markets. Psychol. Sci. 15 : 787– 93 [Google Scholar]
- Holland J , Silva AS , Mace R . 2012 . Lost letter measure of variation in altruistic behaviour in 20 neighbourhoods. PLOS ONE 7 : e43294 [Google Scholar]
- Houlette MA , Gaertner SL , Johnson KM , Banker BS , Riek BM , Dovidio JF . 2004 . Developing a more inclusive social identity: an elementary school intervention. J. Soc. Issues 60 : 35– 55 [Google Scholar]
- Humphreys M , Sanchez de la Sierra R , van der Windt P . 2013 . Fishing, commitment, and communication: a proposal for comprehensive nonbinding research registration. Polit. Anal. 21 : 1– 20 [Google Scholar]
- Imbens G , Wooldridge J . 2009 . Recent developments in the econometrics of program evaluation. J. Econ. Lit. 47 : 5– 86 [Google Scholar]
- Isen AM , Levin PF . 1972 . Effect of feeling good on helping: cookies and kindness. J. Pers. Soc. Psychol. 21 : 384– 88 [Google Scholar]
- Jackson M , Cox DR . 2013 . The principles of experimental design and their application in sociology. Annu. Rev. Sociol. 39 : 27– 49 [Google Scholar]
- Jensen R , Miller N . 2008 . Giffen behavior and subsistence consumption. Am. Econ. Rev. 98 : 1553– 77 [Google Scholar]
- Kamenica E . 2012 . Behavioral economics and psychology of incentives. Annu. Rev. Econ. 4 : 427– 52 [Google Scholar]
- Karlan D . 2005 . Using experimental economics to measure social capital and predict financial decisions. Am. Econ. Rev. 95 : 1688– 99 [Google Scholar]
- Karlan D , Appel J . 2011 . More Than Good Intentions: Improving the Ways the World's Poor Borrow, Save, Farm, Learn, and Stay Healthy New York: Penguin [Google Scholar]
- Karlan D , Goldberg N . 2011 . Microfinance evaluation strategies: notes on methodology and findings. The Handbook of Microfinance B Armendáriz, M Labie 17– 58 London: World Scientific [Google Scholar]
- Karlan D , McConnell M , Mullainathan S , Zinman J . 2014 . Getting to the top of mind: how reminders increase saving. Manag. Sci. 62 : 3393– 3411 [Google Scholar]
- Karlan D , Osei-Akoto I , Osei R , Udry C . 2010 . Examining underinvestment in agriculture: measuring returns to capital and insurance. Work. Pap., Abdul Latif Jameel Poverty Action Lab. https://www.poverty-action.org/sites/default/files/Panel3-3-Farmers-Returns-Capital.pdf [Google Scholar]
- Karlan D , Zinman J . 2011 . Microcredit in theory and practice: using randomized credit scoring for impact. Science 332 : 1278– 84 [Google Scholar]
- Keizer K , Lindenberg S , Steg L . 2008 . The spreading of disorder. Science 322 : 1681– 85 [Google Scholar]
- Kelly E , Moena P , Oakes J , Fan W , Okechukwu C . et al. 2014 . Changing work and work-family conflict: evidence from the work, family, and health network. Am. Sociol. Rev. 79 : 485– 516 [Google Scholar]
- Kling JR , Liebman JB , Katz LF . 2007 . Experimental analysis of neighborhood effects. Econometrica 75 : 83– 119 [Google Scholar]
- Kotran A . 2015 . Opower and utility partners save over eight terawatt-hours of energy power and utility partners save over eight terawatt-hours of energy. News release, May 21
- Kramer ADI , Guillory JE , Hancock JT . 2014 . Experimental evidence of massive-scale emotional contagion through social networks. PNAS 111 : 8788– 90 [Google Scholar]
- Kremer M . 2003 . Randomized evaluations of educational programs in developing countries: some lessons. Am. Econ. Rev. 93 : 102– 6 [Google Scholar]
- Kremer M , Brannen C , Glennerster R . 2013 . The challenge of education and learning in the developing world. Science 340 : 297– 300 [Google Scholar]
- Kremer M , Leino J , Miguel E , Zwane AP . 2011 . Spring cleaning: rural water impacts, valuation, and property rights institutions. Q. J. Econ. 126 : 145– 205 [Google Scholar]
- Kugelmass H . 2016 . “Sorry, I'm not accepting new patients”: an audit study of access to mental health care. J. Health Soc. Behav. 57 : 168– 83 [Google Scholar]
- Lacetera N , Macis M . 2010 . Do all material incentives for pro-social activities backfire? The response to cash and non-cash incentives for blood donations. J. Econ. Psychol. 31 : 738– 48 [Google Scholar]
- Lacetera N , Macis M , Slonim R . 2013 . Economic rewards to motivate blood donations. Science 340 : 927– 28 [Google Scholar]
- Landry CE , Lange A , List JA , Price MK , Rupp NG . 2010 . Is a donor in hand better than two in the bush? Evidence from a natural field experiment. Am. Econ. Rev. 100 : 958– 83 [Google Scholar]
- Langer EJ , Rodin J . 1976 . The effects of choice and enhanced responsibility for the aged: a field experiment in an institutional setting. J. Pers. Soc. Psychol. 34 : 191– 98 [Google Scholar]
- Lauster N , Easterbrook A . 2011 . No room for new families? A field experiment measuring rental discrimination against same-sex couples and single parents. Soc. Probl. 58 : 389– 409 [Google Scholar]
- Leuven E , Oosterbeek H , van der Klaauw B . 2010 . The effect of financial rewards on students’ achievement: evidence from a randomized experiment. J. Eur. Econ. Assoc. 8 : 1243– 65 [Google Scholar]
- Levine M , Prosser A , Evans D , Reicher S . 2005 . Identity and emergency intervention: how social group membership and inclusiveness of group boundaries shape helping behavior. Pers. Soc. Psychol. Bull. 31 : 443– 53 [Google Scholar]
- Levitt SD , List JA . 2009 . Field experiments in economics: the past, the present, and the future. Eur. Econ. Rev. 53 : 1– 18 [Google Scholar]
- Levitt SD , List JA , Neckerman S , Sadoff S . 2012 . The behavioralist goes to school: leveraging behavioral economics to improve educational performance. Work. Pap. 18165, NBER Cambridge, MA: [Google Scholar]
- List JA . 2007 . Field experiments: a bridge between lab and naturally occurring data. B.E. J. Econ. Anal. Policy 5 : 2 [Google Scholar]
- Lucas JW . 2003 . Theory-testing, generalization, and the problem of external validity. Sociol. Theory 21 : 236– 53 [Google Scholar]
- Ludwig J , Duncan GJ , Gennetian LA , Katz LF , Kessler RC . et al. 2013 . Long-term neighborhood effects on low-income families: evidence from moving to opportunity. Am. Econ. Rev. 103 : 226– 31 [Google Scholar]
- Ludwig J , Liebman JB , Kling JR , Duncan GJ , Katz LF . et al. 2008 . What can we learn about neighborhood effects from the moving to opportunity experiment?. Am. J. Sociol. 114 : 144– 88 [Google Scholar]
- Marwell G , Ames RE . 1979 . Experiments on the provision of public goods: resources, interest, group size, and the free-rider problem. Am. J. Sociol. 84 : 1335– 60 [Google Scholar]
- Massey DS , Lundy G . 2001 . Use of Black English and racial discrimination in urban housing markets: new methods and findings. Urban Aff. Rev. 36 : 452– 69 [Google Scholar]
- McDermott R . 2011 . Internal and external validity. Cambridge Handbook of Experimental Political Science JN Druckman, DP Green, JH Kuklinski, A Lupia, 27– 40 Cambridge, UK: Cambridge Univ. Press [Google Scholar]
- McEwan PJ . 2015 . Improving learning in primary schools of developing countries: a meta-analysis of randomized experiments. Rev. Educ. Res. 85 : 353– 94 [Google Scholar]
- McNutt M . 2015 . Editorial retraction of Lacour & Green. Science 346 : 1366– 69 Science 348 : 1100 [Google Scholar]
- Merton RK . 1945 . Sociological theory. Am. J. Sociol. 50 : 462– 73 [Google Scholar]
- Michelson M , Nickerson DW . 2011 . Voter Mobilization Cambridge, UK: Cambridge Univ. Press [Google Scholar]
- Miguel E , Kremer M . 2004 . Worms: identifying impacts on education and health in the presence of treatment externalities. Econometrica 72 : 159– 217 [Google Scholar]
- Milgram S , Liberty HJ , Toledo R , Wackenhut J . 1986 . Response to intrusion into waiting lines. J. Pers. Soc. Psychol. 51 : 683– 89 [Google Scholar]
- Milgram S , Mann L , Hartner S . 1965 . The lost letter technique: a tool of social research. Public Opin. Q. 29 : 437– 38 [Google Scholar]
- Milkman KL , Akinola M , Chugh D . 2015 . What happens before? A field experiment exploring how pay and representation differentially shape bias on the pathway into organizations. J. Appl. Psychol. 100 : 1678– 712 [Google Scholar]
- Milkman KL , Beshears J , Choi JJ , Laibson D , Madrian BC . 2011 . Using implementation intentions prompts to enhance influenza vaccination rates. PNAS 108 : 10415– 20 [Google Scholar]
- Morgan S , Winship C . 2007 . Counterfactuals and Causal Inference Cambridge, UK: Cambridge Univ. Press [Google Scholar]
- Morton R , Williams K . 2010 . Experimental Political Science and the Study of Causality Cambridge, UK: Cambridge Univ. Press [Google Scholar]
- Moss-Racusin CA , Dovidio JF , Brescoll V , Graham MJ , Handelsman J . 2012 . Science faculty's subtle gender biases favor male students. PNAS 109 : 16474– 79 [Google Scholar]
- Munnell AH . 1986 . Lessons from the Income Maintenance Experiments Boston: Fed. Res. Bank of Boston [Google Scholar]
- Mutz DC . 2011 . Population-Based Survey Experiments Princeton, NJ: Princeton Univ. Press [Google Scholar]
- Nagda BRA , Tropp LR , Paluck EL . 2006 . Looking back as we look ahead: integrating research, theory, and practice on intergroup relations. J. Soc. Issues 62 : 439– 51 [Google Scholar]
- Neumark D , Bank RJ , Nort KDV . 1996 . Sex discrimination in restaurant hiring: an audit study. Q. J. Econ. 111 : 915– 41 [Google Scholar]
- Nickerson DW . 2008 . Is voting contagious? Evidence from two field experiments. Am. Political Sci. Rev. 102 : 49– 57 [Google Scholar]
- Nolan JM , Kenefick J , Schultz PW . 2011 . Normative messages promoting energy conservation will be underestimated by experts unless you show them the data. Soc. Influence 6 : 169– 80 [Google Scholar]
- Nolan JM , Schultz PW , Cialdini RB , Goldstein NJ , Griskevicius V . 2008 . Normative social influence is underdetected. Pers. Soc. Psychol. Bull. 34 : 913– 23 [Google Scholar]
- Nosek B , Aarts A , Anderson J , Anderson C , Attridge P . et al. 2015a . Estimating the reproducibility of psychological science. Science 349 : 943– 51 [Google Scholar]
- Nosek B , Alter G , Banks G , Borsboom D , Bowman S . et al. 2015b . Promoting an open research culture. Science 348 : 1422– 25 [Google Scholar]
- Olken B . 2007 . Monitoring corruption: evidence from a field experiment in Indonesia. J. Political Econ. 115 : 200– 49 [Google Scholar]
- Olken B . 2010 . Direct democracy and local public goods: evidence from a field experiment in Indonesia. Am. Political Sci. Rev. 104 : 243– 67 [Google Scholar]
- Pager D . 2003 . The mark of a criminal record. Am. J. Sociol. 108 : 937– 75 [Google Scholar]
- Pager D . 2007 . The use of field experiments for studies of employment discrimination: contributions, critiques, and directions for the future. Ann. Am. Acad. Political Soc. Sci. 609 : 104– 33 [Google Scholar]
- Pager D , Quillian L . 2005 . Walking the talk: what employers say versus what they do. Am. Sociol. Rev. 70 : 355– 80 [Google Scholar]
- Pager D , Western B , Bonikowski B . 2009 . Discrimination in a low-wage labor market: a field experiment. Am. Sociol. Rev. 74 : 777– 99 [Google Scholar]
- Paluck EL . 2009 . Reducing intergroup prejudice and conflict using the media: a field experiment in Rwanda. Interpers. Relat. Group Process. 96 : 574– 87 [Google Scholar]
- Paluck EL , Cialdini RB . 2014 . Field research methods. Handbook of Research Methods in Social and Personality Psychology HT Reis, CM Judd 81– 97 New York: Cambridge Univ. Press, 2nd ed.. [Google Scholar]
- Paluck EL , Green DP . 2009 . Prejudice reduction: what works? A review and assessment of research and practice. Annu. Rev. Psychol. 60 : 339– 67 [Google Scholar]
- Paluck EL , Shepherd H . 2012 . The salience of social referents: a field experiment on collective norms and harassment behavior in a school social network. J. Pers. Soc. Psychol. 103 : 899– 915 [Google Scholar]
- Paluck EL , Shepherd H , Aronow PM . 2016 . Changing climates of conflict: a social network driven experiment in 56 schools. PNAS 113 : 566– 71 [Google Scholar]
- Pedulla DS . 2016 . Penalized or protected? Gender and the consequences of non-standard and mismatched employment histories. Am. Sociol. Rev. 81 : 262– 89 [Google Scholar]
- Pettigrew TF . 1998 . Intergroup contact theory. Annu. Rev. Psychol. 49 : 65– 85 [Google Scholar]
- Riach PA , Rich J . 2002 . Field experiments of discrimination in the market place. Econ. J. 112 : 480– 518 [Google Scholar]
- Rodríguez-Planas N . 2012 . Longer-term impacts of mentoring, educational services, and learning incentives: evidence from a randomized trial in the United States. Am. Econ. J. Appl. Econ. 4 : 121– 39 [Google Scholar]
- Rondeau D , List JA . 2008 . Matching and challenge gifts to charity: evidence from laboratory and natural field experiments. Exp. Econ. 11 : 253– 67 [Google Scholar]
- Ross SL , Turner MA . 2005 . Housing discrimination in metropolitan America: explaining changes between 1989 and 2000. Soc. Probl. 52 : 152– 80 [Google Scholar]
- Rossi PH , Berk RA , Lenihan KJ . 1980 . Money, Work, and Crime: Experimental Evidence New York: Academic Press [Google Scholar]
- Rossi PH , Berk RA , Lenihan KJ . 1982 . Saying it wrong with figures: a comment on Zeisel. Am. J. Sociol. 88 : 390– 93 [Google Scholar]
- Rossi PH , Lyall KC . 1978 . An overview evaluation of the NIT experiment. Eval. Stud. Rev. 3 : 412– 28 [Google Scholar]
- Sabin N . 2015 . Modern microfinance: a field in flux. Social Finance Nicholls A, Paton R, Emerson J Oxford, UK: Oxford Univ. Press [Google Scholar]
- Salganik MJ , Dodds PS , Watts DJ . 2006 . Experimental study of inequality and unpredictability in an artificial cultural market. Science 311 : 854– 56 [Google Scholar]
- Sampson RJ . 2008 . Moving to inequality: neighborhood effects and experiments meet social structure. Am. J. Sociol. 114 : 189– 231 [Google Scholar]
- Sampson RJ . 2012 . Great American City: Chicago and the Enduring Neighborhood Effect Chicago, IL: Chicago Univ. Press [Google Scholar]
- Schuler SR , Hashemi SM , Badal SH . 1998 . Men's violence against women in rural Bangladesh: undermined or exacerbated by microcredit programmes?. Dev. Pract. 8 : 148– 57 [Google Scholar]
- Schultz P . 2004 . School subsidies for the poor: evaluating the Mexican Progresa poverty program. J. Dev. Econ. 74 : 199– 250 [Google Scholar]
- Shadish WR , Cook TD . 2009 . The renaissance of field experimentation in evaluating interventions. Annu. Rev. Psychol. 607– 29 [Google Scholar]
- Shadish WR , Cook TD , Campbell DT . 2002 . Experimental and Quasi-experimental Designs for Generalized Causal Inference. New York: Houghton, Mifflin and Company [Google Scholar]
- Simpson BT , McGrimmon T , Irwin K . 2007 . Are blacks really less trusting than whites? Revisiting the race and trust question. Soc. Forces 86 : 525– 52 [Google Scholar]
- Sniderman PM , Grob DB . 1996 . Innovations in experimental design in attitude surveys. Annu. Rev. Sociol. 22 : 377– 99 [Google Scholar]
- Steinpreis RE , Anders KA , Ritzke D . 1999 . The impact of gender on the review of the curricula vitae of job applicants and tenure candidates: a national empirical study. Sex Roles 41 : 509– 28 [Google Scholar]
- Stutzer A , Goette L , Zehnder M . 2011 . Active decisions and prosocial behaviour: a field experiment on blood donations. Econ. J. 121 : 476– 93 [Google Scholar]
- Teele DL . 2014 . Reflections on the ethics of field experiments. Field Experiments and Their Critics: Essays on the Uses and Abuses of Experimentation in the Social Sciences DL Teele 115– 40 New Haven, CT: Yale Univ. Press [Google Scholar]
- Thornton RL . 2008 . The demand for, and impact of, learning HIV status. Am. Econ. Rev. 98 : 1829– 63 [Google Scholar]
- Tilcsik A . 2011 . Pride and prejudice: employment discrimination against openly gay men in the United States. Am. J. Sociol. 117 : 586– 626 [Google Scholar]
- Travers J , Milgram S . 1969 . An experimental study of the small world problem. Sociometry 32 : 425– 43 [Google Scholar]
- Turner MA , Bednarz BA , Herbig C , Lee SJ . 2003 . Discrimination in metropolitan housing markets phase 2: Asians and Pacific Islanders Tech. rep., Urban Inst., Washington, DC [Google Scholar]
- Turner MA , Fix M , Struyk RJ . 1991 . Opportunities Denied, Opportunities Diminished: Racial Discrimination in Hiring Washington, DC: Urban Inst. Press [Google Scholar]
- Turner MA , Ross SL , Galster GC , Yinger J . 2002 . Discrimination in metropolitan housing markets: national results from phase 1 of the Housing Discrimination Study (HDS) Tech. rep., Urban Inst Washington, DC: [Google Scholar]
- Van Bavel JJ , Mende-Siedlecki P , Brady WJ , Reinero DA . 2016 . Contextual sensitivity in scientific reproducibility. PNAS 113 : 6454– 59 [Google Scholar]
- Van de Rijt A , Kang SM , Restivo M , Patil A . 2014 . Field experiments of success-breeds-success dynamics. PNAS 111 : 6934– 39 [Google Scholar]
- Van Der Merwe WG , Burns J . 2008 . What's in a name? Racial identity and altruism in post-apartheid South Africa. South Afr. J. Econ. 76 : 266– 75 [Google Scholar]
- Vermeersch C , Kremer M . 2005 . School Meals, Educational Achievement, and School Competition: Evidence from a Randomized Evaluation. New York: World Bank [Google Scholar]
- Volpp KG , Troxel AB , Pauly MV , Glick HA , Puig A . et al. 2009 . A randomized, controlled trial of financial incentives for smoking cessation. N. Engl. J. Med. 360 : 699– 709 [Google Scholar]
- Whitt S , Wilson RK . 2007 . The dictator game, fairness and ethnicity in postwar Bosnia. Am. J. Political Sci. 51 : 655– 68 [Google Scholar]
- Wienk RE , Reid CE , Simonson JC , Eggers FJ . 1979 . Measuring racial discrimination in American housing markets: the housing market practices survey. Tech. Rep. HUD-PDR-444(2), Dep. Hous. Urban Dev Washington, DC: [Google Scholar]
- Williams WM , Ceci SJ . 2015 . National hiring experiments reveal 2:1 faculty preference for women on STEM tenure track. PNAS 112 : 5360– 65 [Google Scholar]
- Yamagishi T . 2011 . Trust: The Evolutionary Game of Mind and Society New York: Springer [Google Scholar]
- Yamagishi T , Cook KS , Watabe M . 1998 . Uncertainty, trust, and commitment formation in the United States and Japan. Am. J. Sociol. 104 : 165– 94 [Google Scholar]
- Zeisel H . 1982 . Disagreement over the evaluation of a controlled experiment. Am. J. Sociol. 88 : 378– 89 [Google Scholar]
Data & Media loading...
- Article Type: Review Article
Most Read This Month
Most cited most cited rss feed, birds of a feather: homophily in social networks, social capital: its origins and applications in modern sociology, conceptualizing stigma, framing processes and social movements: an overview and assessment, organizational learning, the study of boundaries in the social sciences, assessing “neighborhood effects”: social processes and new directions in research, social exchange theory, culture and cognition, focus groups.

I want to publish
To find out how to publish or submit your book proposal:
To find a journal or submit your article to a journal:
- Field Experiment
- Medicine and Healthcare
- Medical Statistics & Computing
Experiments
Louis Cohen, Lawrence Manion, Keith Morrison in Research Methods in Education , 2017
The design experiment can be considered as a special case of a field experiment; it has its roots in experimental research, both in ‘true’ and quasi-experiments, and is intended to provide formative feedback on, for example, practical problems in, say, teaching and learning, and to bridge the potential gap between research and practice (Brown, 1992, p. 143; Reinking and Bradley, 2008; Bradley and Reinking, 2011; Engeström, 2011; Seel, 2011, p. 925; Anderson and Shattuck, 2012; Laurillard, 2012), in other words, to enhance the external validity of an experiment. The design experiment strives to avoid the artificial world of the laboratory and the lack of applicability to ‘real-world problems’ that follows from this artificial condition (Bradley and Reinking, 2011; Reinking and Bradley, 2008; Seel, 2011; Laurillard, 2012), and to have direct practical relevance to the complex world of teaching, learning and classrooms. Given their intended direct relevance to classrooms and the field nature – the diverse, complex, ‘real world’ of an actual classroom – design experiments may not be able to fulfil the requirements of a true experiment, for example, in randomization or in the application of controls. In these respects, design experiments are similar to action research (cf. Anderson and Shattuck, 2012).
External validity and public health
Sridhar Venkatapuram, Alex Broadbent in The Routledge Handbook of Philosophy of Public Health , 2023
The other reason they give for doubting the trade-off is because the notion of the “artificiality” of experimental settings, used to justify its existence, is vague and ambiguous (Jimenez-Buedo and Miller 2010: 307). The difficulty in blaming the artificiality of experimental environments for external validity failure is that none of the proponents of this view explain in exactly which respects the environments are meant to differ. In any case, it is clear that there is a lot more to the external validity problem than just a concern about the artificiality of experimental settings. This can be seen by considering the case of so-called field experiments or observational studies, where causes and effects are observed in real-world settings. In these studies, there is nothing artificial about the experimental context; yet, the external validity question is still a legitimate concern. This is because we can have cases where a field experiment exposes a clear causal relation evident in one context, but this causal relation does not hold in a new context because of different confounding factors, for example.
Randomization Tests or Permutation Tests? A Historical and Terminological Clarification
Vance W. Berger in Randomization, Masking, and Allocation Concealment , 2017
Interestingly, another statistical heavyweight, Jerzy Neyman, did something very similar with respect to giving credit to Fisher for the randomization design principle as Edward Pitman did with respect to giving credit to Fisher for developing the test. In his notorious paper, read before the Industrial and Agricultural Research Section of the Royal Statistical Society, Neyman (1935, p. 109) stated:Owing to the work of R. A. Fisher, “Student” and their followers, it is hardly possible to add anything essential to the present knowledge concerning local experiments…. One of the most important achievements of the English School is their method of planning field experiments known as the method of Randomized Blocks and Latin Squares.
Does an economic incentive affect provider behavior? Evidence from a field experiment on different payment mechanisms
Published in Journal of Medical Economics , 2019
Xiaoyu Xi, Ennan Wang, Qianni Lu, Piaopiao Chen, Tian Wo, Kammy Tang
We used a field experiment study design to examine the behaviors of physicians. If a laboratory experiment were performed instead of a field experiment, the conclusions might not be valid due to hyper-abstraction and simplification26. However, the participants in a field study were not restricted to college students, instead, they were adults in society. Moreover, the experimental environment was not confined to a laboratory. A field experiment, as defined by Harrison and List27, was an experiment conducted in multiple locations, including laboratories and actual environments. Its participants included both students and non-college adults. Therefore, under the real social conditional, the experiment subjects could make realistic choices. Above all, because of the differences between the experimental environment and subjects, the field experiment could represent actual conditions in a real environment, and subjects might act instinctively as they do in daily life, increasing the external validity of results28.
Employees’ Improvisational Behavior: Exploring the Role of Leader Grit and Humility
Published in Human Performance , 2022
Arménio Rego, Andreia Vitória, Miguel Pina e Cunha, Bradley P. Owens, Ana Ventura, Susana Leal, Camilo Valverde, Rui Lourenço-Gil
While providing overall support for the proposed causal direction of our model, our research is not without limitations. First, other causalities are possible. For example, employees may develop higher self-efficacy, hope, and optimism after making improvisations that are revealed to be successful. It is also possible that leaders adopt more perseverant efforts in pursuing challenging goals as a consequence of their higher employees’ PsyCap. Although the experiment was designed to enhance realism, which enhances confidence in hypothesized causality, it also suffers from modest external validity and other limitations (Lonati et al., 2018). Future studies should include covariates to rule out confounding and endogeneity effects, should adopt other experimental designs, and should be carried out in real organizational settings (Antonakis, Bendahan, Jacquart, & Lalive, 2014). A field experiment would represent a very important step forward in that endeavor, although abundant obstacles (methodological and practical) may make the endeavor unfeasible. Second, future studies may explore boundary conditions of the PsyCap-improvisation relationship. For example, is the relationship more positive when employees experience psychological safety?
Impact of safety training and interventions on training-transfer: targeting migrant construction workers
Published in International Journal of Occupational Safety and Ergonomics , 2020
Rahat Hussain, Akeem Pedro, Do Yeop Lee, Hai Chien Pham, Chan Sik Park
Notwithstanding, even though the culturally diverse nature of the work crews is a valuable aspect to evaluate migrant worker safety performance, it is hard to engage labourers for such practice. Similarly, the extent of interventions being implemented during and after training sessions is difficult to control. To address these challenges, the experiment design phase of this research has focused more on the reasons for training failures from both the literature and current migrant worker issues in industry. The approach presented in this study measures the combined impact of all interventions in a field experiment; however, in order to improve the reliability and validity in measurement, the individual effects of interventions should also be considered in further studies.
Related Knowledge Centers
- External Validity
- Randomization
- Statistical Inference
- Random Assignment
- Rubin Causal Model
- Standard Deviation
- Accuracy & Precision
- Sample Size Determination
- Stepped-Wedge Trial
Current Research
- Clinical Trials (United States)
- Clinical Trials (Europe)
- Clinical Trials (Australia/New Zealand)
- Clinical Trials (India)
Knowledge is an evolving asset. Help us improve this page for a future release.
- Affiliated Professors
- Invited Researchers
- J-PAL Scholars
- Diversity, Equity, and Inclusion
- Code of Conduct
- Initiatives
- Latin America and the Caribbean
- Middle East and North Africa
- North America
- Southeast Asia
- Agriculture
- Crime, Violence, and Conflict
- Environment, Energy, and Climate Change
- Labor Markets
- Political Economy and Governance
- Social Protection
- Evaluations
- Research Resources
- Policy Insights
- Evidence to Policy
- For Affiliates
- Support J-PAL
The Abdul Latif Jameel Poverty Action Lab (J-PAL) is a global research center working to reduce poverty by ensuring that policy is informed by scientific evidence. Anchored by a network of more than 1,000 researchers at universities around the world, J-PAL conducts randomized impact evaluations to answer critical questions in the fight against poverty.
- Affiliated Professors Our affiliated professors are based at 97 universities and conduct randomized evaluations around the world to design, evaluate, and improve programs and policies aimed at reducing poverty. They set their own research agendas, raise funds to support their evaluations, and work with J-PAL staff on research, policy outreach, and training.
- Board Our Board of Directors, which is composed of J-PAL affiliated professors and senior management, provides overall strategic guidance to J-PAL, our sector programs, and regional offices.
- Diversity, Equity, and Inclusion J-PAL recognizes that there is a lack of diversity, equity, and inclusion in the field of economics and in our field of work. Read about what actions we are taking to address this.
- Initiatives J-PAL initiatives concentrate funding and other resources around priority topics for which rigorous policy-relevant research is urgently needed.
- Events We host events around the world and online to share results and policy lessons from randomized evaluations, to build new partnerships between researchers and practitioners, and to train organizations on how to design and conduct randomized evaluations, and use evidence from impact evaluations.
- Blog News, ideas, and analysis from J-PAL staff and affiliated professors.
- News Browse news articles about J-PAL and our affiliated professors, read our press releases and monthly global and research newsletters, and connect with us for media inquiries.
- Press Room Based at leading universities around the world, our experts are economists who use randomized evaluations to answer critical questions in the fight against poverty. Connect with us for all media inquiries and we'll help you find the right person to shed insight on your story.
- Overview J-PAL is based at MIT in Cambridge, MA and has seven regional offices at leading universities in Africa, Europe, Latin America and the Caribbean, Middle East and North Africa, North America, South Asia, and Southeast Asia.
- Global Our global office is based at the Department of Economics at the Massachusetts Institute of Technology. It serves as the head office for our network of seven independent regional offices.
- Africa J-PAL Africa is based at the Southern Africa Labour & Development Research Unit (SALDRU) at the University of Cape Town in South Africa.
- Europe J-PAL Europe is based at the Paris School of Economics in France.
- Latin America and the Caribbean J-PAL Latin America and the Caribbean is based at the Pontificia Universidad Católica de Chile.
- Middle East and North Africa J-PAL MENA is based at the American University in Cairo, Egypt.
- North America J-PAL North America is based at the Massachusetts Institute of Technology in the United States.
- South Asia J-PAL South Asia is based at the Institute for Financial Management and Research (IFMR) in India.
- Southeast Asia J-PAL Southeast Asia is based at the Faculty of Economics and Business at the University of Indonesia (FEB UI).
- Overview Led by affiliated professors, J-PAL sectors guide our research and policy work by conducting literature reviews; by managing research initiatives that promote the rigorous evaluation of innovative interventions by affiliates; and by summarizing findings and lessons from randomized evaluations and producing cost-effectiveness analyses to help inform relevant policy debates.
- Agriculture How can we encourage small farmers to adopt proven agricultural practices and improve their yields and profitability?
- Crime, Violence, and Conflict What are the causes and consequences of crime, violence, and conflict and how can policy responses improve outcomes for those affected?
- Education How can students receive high-quality schooling that will help them, their families, and their communities truly realize the promise of education?
- Environment, Energy, and Climate Change How can we increase access to energy, reduce pollution, and mitigate and build resilience to climate change?
- Finance How can financial products and services be more affordable, appropriate, and accessible to underserved households and businesses?
- Firms How do policies affecting private sector firms impact productivity gaps between higher-income and lower-income countries? How do firms’ own policies impact economic growth and worker welfare?
- Gender How can we reduce gender inequality and ensure that social programs are sensitive to existing gender dynamics?
- Health How can we increase access to and delivery of quality health care services and effectively promote healthy behaviors?
- Labor Markets How can we help people find and keep work, particularly young people entering the workforce?
- Political Economy and Governance What are the causes and consequences of poor governance and how can policy improve public service delivery?
- Social Protection How can we identify effective policies and programs in low- and middle-income countries that provide financial assistance to low-income families, insuring against shocks and breaking poverty traps?
Handbook of Field Experiments
The last 15 years have seen an explosion in the number, scope, quality, and creativity of field experiments. To take stock of this remarkable progress, we were invited to edit a Handbook of Field Experiments , published at Elsevier. We were fortunate to assemble a volume made of wonderful papers by the best experts in the field. Some chapters are more methodological, while others are focused on results. All of them provide thoughtful reflections on the advances and issues in the field, useful research tips and insights into what the next steps need to be, all of which should be very useful for graduate students. Taken together, these papers offer an incredibly rich overview of the state of literature. This page collects together all the working paper versions of the chapters, and will also link to the final versions as they become available. We hope you enjoy it.
—Abhijit Banerjee and Esther Duflo
Introduction
An Introduction to the "Handbook of Field Experiments" Abhijit Banerjee and Esther Duflo
Many (though by no means all) of the questions that economists and policymakers ask themselves are causal in nature: What would be the impact of adding computers in classrooms? What is the price elasticity of demand for preventive health products? Would increasing interest rates lead to an increase in default rates? Decades ago, the statistician Fisher (Fisher, 1925) proposed a method to answer such causal questions: Randomized Controlled Trials (RCTs) . In an RCT, the assignment of different units to different treatment groups is chosen randomly. This ensures that no unobservable characteristics of the units are reflected in the assignment, and hence that any difference between treatment and control units reflects the impact of the treatment. While the idea is simple, the implementation in the field can be more involved, and it took some time before randomization was considered to be a practical tool for answering questions in economics.
Some Historical Background
The Politics and Practice of Social Experiments: Seeds of a Revolution Judy Gueron
Between 1970 and the early 2000s, there was a revolution in support for the use of randomized experiments to evaluate social programs. Focusing on the welfare reform studies that helped to speed that transformation in the United States, this chapter describes the major challenges to randomized controlled trials (RCTs), how they emerged and were overcome, and how initial conclusions about conditions necessary to success — strong financial incentives, tight operational control, and small scale — proved to be wrong. The final section discusses lessons from this experience for other fields.
Methodology and Practice of RCTs
The Econometrics of Randomized Experiments Susan Athey and Guido Imbens
Randomized experiments have a long tradition in agricultural and biomedical settings. In economics they have a much shorter history. Although there have been notable experiments over the years, such as the RAND health care experiment (Manning, Newhouse, Duan, Keeler and Leibowitz, 1987, see the general discussion in Rothstein and von Wachter, 2016) and the Negative Income Tax experiments (e.g., Robins, 1985), it is only recently that there has been a large number of randomized experiments in economics, and development economics in particular. See Duflo, Glennerster, and Kremer (2006) for a survey. In this chapter we discuss some of the statistical methods that are important for the analysis and design of randomized experiments. A major theme of the chapter is the focus on statistical methods directly justified by randomization, in the spirit of Freedman who wrote “Experiments should be analyzed as experiments, not as observational studies. A simple comparison of rates might be just the right tool, with little value added by ‘sophisticated’ models,” (Freedman, 2006, p. 691) We draw from a variety of literatures. This includes the statistical literature on the analysis and design of experiments, e.g., Wu and Hamada (2009), Cox and Reid (2000), Altman (1991), Cook and DeMets (2008), Kempthorne (1952, 1955), Cochran and Cox (1957), Davies (1954), and Hinkelman and Kempthorne (2005, 2008). We also draw on the literature on causal inference, both in experimental and observational settings, Rosenbaum (1995, 2002, 2009), Rubin (2006), Cox (1992), Morgan and Winship (2007), Morton Williams (2010) and Lee (2005), and Imbens and Rubin (2015). In the economics literature we build on recent guides to practice in randomized experiments in development economics, e.g., Duflo, Glennerster, and Kremer (2006), Glennerster (2016), and Glennerster and Takavarasha (2013) as well as the general empirical micro literature (Angrist and Pischke, 2008).
Decision Theoretic Approaches to Experiment Design and External Validity Abhijit Banerjee, Sylvain Chassang, and Erik Snowberg
A modern, decision-theoretic framework can help clarify important practical questions of experimental design. Building on our recent work, this chapter begins by summarizing our framework for understanding the goals of experimenters, and applying this to re-randomization. We then use this framework to shed light on questions related to experimental registries, pre-analysis plans, and most importantly, external validity. Our framework implies that even when large samples can be collected, external decisionmaking remains inherently subjective. We embrace this conclusion, and argue that in order to improve external validity, experimental research needs to create a space for structured speculation.
The Practicalities of Running Randomized Evaluations: Partnerships, Measurement, Ethics, and Transparency Rachel Glennerster
Economists have known for a long time that randomization could help identify causal connections by solving the problem of selection bias. Chapter 1 in this book and Gueron and Rolston (2013) describe the effort in the US to move experiments out of the laboratory into the policy world in the 1960s and 1970s. This experience was critical in proving the feasibility of field experiments, working through some of the important ethical questions involved, showing how researchers and practitioners could work together, and demonstrating that the results of field experiments were often very different from those generated by observational studies. Interestingly, there was relatively limited academic support for this first wave of field experiments (Gueron and Rolston 2013), most of which were carried out by research groups such as MDRC, Abt, and Mathematica, to evaluate US government programs, and they primarily used individual-level randomization. In contrast, a more recent wave of field experiments starting in the mid-1990s was driven by academics, initially was focused on developing countries, often worked with nongovernmental organizations, and frequently used clustered designs.
The Psychology of Construal in the Design of Field Experiments Elizabeth Levy Paluck and Eldar Shafir
Why might you be interested in this chapter? A fair assumption is that you are reading because you care about good experimental design. To create strong experimental designs that test people’s responses to an intervention, researchers typically consider the classically recognized motivations presumed to drive human behavior. It does not take extensive psychological training to recognize that several types of motivations could affect an individual’s engagement with and honesty during your experimental paradigm. Such motivations include strategic self-presentation, suspicion, lack of trust, level of education or mastery, and simple utilitarian motives such as least effort and optimization. For example, minimizing the extent to which your findings are attributable to high levels of suspicion among participants, or to their decision to do the least amount possible, is important for increasing the generalizability and reliability of your results.
Understanding Preferences and Preference Change
Field Experiments in Markets Omar Al-Ubaydli and John List
This is a review of the literature of field experimental studies of markets. The main results covered by the review are as follows: (1) Generally speaking, markets organize the efficient exchange of commodities; (2) There are some behavioral anomalies that impede efficient exchange; (3) Many behavioral anomalies disappear when traders are experienced.
Field Experiments on Discrimination Marianne Bertrand and Esther Duflo
This article reviews the existing field experimentation literature on the prevalence of discrimination, the consequences of such discrimination, and possible approaches to undermine it. We highlight key gaps in the literature and ripe opportunities for future field work. Section 1 reviews the various experimental methods that have been employed to measure the prevalence of discrimination, most notably audit and correspondence studies; it also describes several other measurement tools commonly used in lab-based work that deserve greater consideration in field research. Section 2 provides an overview of the literature on the costs of being stereotyped or discriminated against, with a focus on self-expectancy effects and self-fulfilling prophecies; section 2 also discusses the thin field-based literature on the consequences of limited diversity in organizations and groups. The final section of the paper, Section 3, reviews the evidence for policies and interventions aimed at weakening discrimination, covering role model and intergroup contact effects, as well as socio-cognitive and technological de-biasing strategies.
Field Experiments on Voter Mobilization: An Overview of a Burgeoning Literature Alan Gerber and Donald Green
In recent years the focus of empirical work in political science has begun to shift from description to an increasing emphasis on the credible estimation of causal effects. A key feature of this change has been the increasing prominence of experimental methods, and especially field experiments. In this chapter we review the use of field experiments to study political participation. Although several important experiments address political phenomena other than voter participation (Bergan 2009; Butler and Broockman 2015; Butler and Nickerson 2011; Broockman 2013, 2014; Grose 2014), the literature measuring the effect of various interventions on voter turnout is the largest and most fully developed, and it provides a good illustration of how the use of field experiments in political science has proceeded. From an initial focus on the relative effects of different modes of communication, scholars began to explore how theoretical insights from social psychology and behavioral economics might be used to craft messages and how voter mobilization experiments could be employed to test the real world effects of theoretical claims. The existence of a large number of experimental turnout studies was essential, because it provided the background against which unusual and important results could be easily discerned.
Lab in the Field: Measuring Preferences in the Wild Uri Gneezy and Alex Imas
In this chapter, we discuss the “lab-in-the-field” methodology, which combines elements of both lab and field experiments in using standardized, validated paradigms from the lab in targeting relevant populations in naturalistic settings. We begin by examining how the methodology has been used to test economic models with populations of theoretical interest. Next, we outline how lab-in-the-field studies can be used to complement traditional Randomized Control Trials in collecting covariates to test theoretical predictions and explore behavioral mechanisms. We proceed to discuss how the methodology can be utilized to compare behavior across cultures and contexts, and test for the external validity of results obtained in the lab. The chapter concludes with an overview of lessons on how to use the methodology effectively.
Field Experiments in Marketing Duncan Simester
Marketing is a diverse field that draws from a rich array of disciplines and a broad assortment of empirical and theoretical methods. One of those disciplines is economics and one of the methods used to investigate economic questions is field experiments. The history of field experiments in the marketing literature is surprisingly long. Early examples include Curhan (1974) and Eskin and Baron (1977), who vary prices, newspaper advertising, and display variables in grocery stores. This chapter reviews the recent history of field experiments in marketing by identifying papers published in the last 20 years (between 1995 and 2014). We report how the number of papers published has increased during this period, and evaluate different explanations for this increase. We then group the papers into five topics and review the papers by topic. The chapter concludes by reflecting on the design of field experiments used in marketing, and proposing topics for future research.
The Challenge of Improving Human Capital
Impacts and Determinants of Health Levels in Low-Income Countries Pascaline Dupas and Ted Miguel
Improved health in low-income countries could considerably improve wellbeing and possibly promote economic growth. The last decade has seen a surge in field experiments designed to understand the barriers that households and governments face in investing in health and how these barriers can be overcome, and to assess the impacts of subsequent health gains. This chapter first discusses the methodological pitfalls that field experiments in the health sector are particularly susceptible to, then reviews the evidence that rigorous field experiments have generated so far. While the link from in utero and child health to later outcomes has increasingly been established, few experiments have estimated the impacts of health on contemporaneous productivity among adults, and few experiments have explored the potential for infrastructural programs to impact health outcomes. Many more studies have examined the determinants of individual health behavior, on the side of consumers as well as among providers of health products and services.
The Production of Human Capital in Developed Countries: Evidence from 196 Randomized Field Experiments Roland Fryer
Randomized field experiments designed to better understand the production of human capital have increased exponentially over the past several decades. This chapter summarizes what we have learned about various partial derivatives of the human capital production function, what important partial derivatives are left to be estimated, and what – together – our collective efforts have taught us about how to produce human capital in developed countries. The chapter concludes with a back of the envelope simulation of how much of the racial wage gap in America might be accounted for if human capital policy focused on best practices gleaned from randomized field experiments.
Field Experiments in Education in Developing Countries Karthik Muralidharan Perhaps no field in development economics in the past decade has benefited as much from the use of experimental methods as the economics of education. The rapid growth in high‐quality studies on education in developing countries (many of which use randomized experiments) is perhaps best highlighted by noting that there have been several systematic reviews of this evidence aiming to synthesize findings for research and policy in just the past three years . These include Muralidharan 2013 (focused on India), Glewwe et al. 2014 (focused on school inputs), Kremer et al. 2013, Krishnaratne et al. 2013, Conn 2014 (focused on sub‐Saharan Africa), McEwan 2014, Ganimian and Murnane (2016), Evans and Popova (2015), and Glewwe and Muralidharan (2016). While these are not all restricted to experimental studies, they typically provide greater weight to evidence from randomized controlled trials (RCT's).
Designing Effective Social Programs
Social Policy: Mechanism Experiments and Policy Evaluations Bill Congdon, Jeffrey Kling, Jens Ludwig, and Sendhil Mullainathan
Policymakers and researchers are increasingly interested in using experimental methods to inform the design of social policy. The most common approach, at least in developed countries, is to carry out large-scale randomized trials of the policies of interest, or what we call here policy evaluations. In this chapter we argue that in some circumstances the best way to generate information about the policy of interest may be to test an intervention that is different from the policy being considered, but which can shed light on one or more key mechanisms through which that policy may operate. What we call mechanism experiments can help address the key external validity challenge that confronts all policy-oriented work in two ways. First, mechanism experiments sometimes generate more policy-relevant information per dollar of research funding than can policy evaluations, which in turn makes it more feasible to test how interventions work in different contexts. Second, mechanism experiments can also help improve our ability to forecast effects by learning more about the way in which local context moderates policy effects, or expand the set of policies for which we can forecast effects. We discuss how mechanism experiments and policy evaluations can complement one another, and provide examples from a range of social policy areas including health insurance, education, labor market policy, savings and retirement, housing, criminal justice, redistribution, and tax policy. Examples focus on the U.S. context.
Field Experiments in Developing Country Agriculture Alain de Janvry, Elisabeth Sadoulet, and Tavneet Suri
This chapter provides a review of the role of field experiments in answering research questions in agriculture that ultimately let us better understand how policy can improve productivity and farmer welfare in developing economies. We first review recent field experiments in this area, highlighting the contributions experiments have already made to this area of research. We then outline areas where experiments can further fill existing gaps in our knowledge on agriculture and how future experiments can address the specific complexities in agriculture.
The Personnel Economics of the State Frederico Finan, Ben Olken, and Rohini Pande
Governments play a central role in facilitating economic development. Yet while economists have long emphasized the importance of government quality, historically they have paid less attention to the internal workings of the state and the individuals who provide the public services. This chapter reviews a nascent but growing body of field experiments that explores the personnel economics of the state. To place the experimental findings in context, we begin by documenting some stylized facts about how public sector employment differs from that in the private sector. In particular, we show that in most countries throughout the world, public sector employees enjoy a significant wage premium over their private sector counterparts. Moreover, this wage gap is largest among low-income countries, which tends to be precisely where governance issues are most severe. These differences in pay, together with significant information asymmetries within government organizations in low-income countries, provide a prima facie rationale for the emphasis of the recent field experiments on three aspects of the state–employee relationship: selection, incentive structures, and monitoring. We review the findings on all three dimensions and then conclude this survey with directions for future research.
Designing Social Protection Programs: Using Theory and Experimentation to Understand how to Help Combat Poverty Rema Hanna and Dean Karlan
“Anti-poverty” programs come in many varieties, ranging from multi-faceted, complex programs to more simple cash transfers. Articulating and understanding the root problem motivating government and nongovernmental organization intervention is critical for choosing amongst many anti-poverty policies, or combinations thereof. Policies should differ depending on whether the underlying problem is about uninsured shocks, liquidity constraints, information failures, or some combination of all of the above. Experimental designs and thoughtful data collection can help diagnose the root problems better, thus providing better predictions for what anti-poverty programs to employ in specific conditions and contexts. However, the more complex theories are likewise more challenging to test, requiring larger samples, and often more nuanced experimental designs, as well as detailed data on many aspects of household and community behavior and outcomes. We provide guidance on these design and testing issues for social protection programs, from how to target programs, to who should implement the program, to whether and what conditions to require for program participation. In short, careful experimentation designed testing can help provide a stronger conceptual understanding of why programs do or not work, thereby allowing one to ultimately make stronger policy prescriptions that further the goal of poverty reduction.
Social Experiments in the Labor Market Jesse Rothstein and Till von Wachter
Large-scale social experiments were pioneered in labor economics, and are the basis for much of what we know about topics ranging from the effect of job training to incentives for job search to labor supply responses to taxation. Random assignment has provided a powerful solution to selection problems that bedevil non- experimental research. Nevertheless, many important questions about these topics require going beyond random assignment. This applies to questions pertaining to both internal and external validity, and includes effects on endogenously observed outcomes, such as wages and hours; spillover effects; site effects; heterogeneity in treatment effects; multiple and hidden treatments; and the mechanisms producing treatment effects. In this Chapter, we review the value and limitations of randomized social experiments in the labor market, with an emphasis on these design issues and approaches to addressing them. These approaches expand the range of questions that can be answered using experiments by combining experimental variation with econometric or theoretical assumptions. We also discuss efforts to build the means of answering these types of questions into the ex ante design of experiments. Our discussion yields an overview of the expanding toolkit available to experimental researchers.
Experimental Method In Psychology
Saul McLeod, PhD
Editor-in-Chief for Simply Psychology
BSc (Hons) Psychology, MRes, PhD, University of Manchester
Saul McLeod, PhD., is a qualified psychology teacher with over 18 years of experience in further and higher education. He has been published in peer-reviewed journals, including the Journal of Clinical Psychology.
Learn about our Editorial Process
Olivia Guy-Evans, MSc
Associate Editor for Simply Psychology
BSc (Hons) Psychology, MSc Psychology of Education
Olivia Guy-Evans is a writer and associate editor for Simply Psychology. She has previously worked in healthcare and educational sectors.
On This Page:
The experimental method involves the manipulation of variables to establish cause-and-effect relationships. The key features are controlled methods and the random allocation of participants into controlled and experimental groups .
What is an Experiment?
An experiment is an investigation in which a hypothesis is scientifically tested. An independent variable (the cause) is manipulated in an experiment, and the dependent variable (the effect) is measured; any extraneous variables are controlled.
An advantage is that experiments should be objective. The researcher’s views and opinions should not affect a study’s results. This is good as it makes the data more valid and less biased.
There are three types of experiments you need to know:
1. Lab Experiment
A laboratory experiment in psychology is a research method in which the experimenter manipulates one or more independent variables and measures the effects on the dependent variable under controlled conditions.
A laboratory experiment is conducted under highly controlled conditions (not necessarily a laboratory) where accurate measurements are possible.
The researcher uses a standardized procedure to determine where the experiment will take place, at what time, with which participants, and in what circumstances.
Participants are randomly allocated to each independent variable group.
Examples are Milgram’s experiment on obedience and Loftus and Palmer’s car crash study .
- Strength : It is easier to replicate (i.e., copy) a laboratory experiment. This is because a standardized procedure is used.
- Strength : They allow for precise control of extraneous and independent variables. This allows a cause-and-effect relationship to be established.
- Limitation : The artificiality of the setting may produce unnatural behavior that does not reflect real life, i.e., low ecological validity. This means it would not be possible to generalize the findings to a real-life setting.
- Limitation : Demand characteristics or experimenter effects may bias the results and become confounding variables .
2. Field Experiment
A field experiment is a research method in psychology that takes place in a natural, real-world setting. It is similar to a laboratory experiment in that the experimenter manipulates one or more independent variables and measures the effects on the dependent variable.
However, in a field experiment, the participants are unaware they are being studied, and the experimenter has less control over the extraneous variables .
Field experiments are often used to study social phenomena, such as altruism, obedience, and persuasion. They are also used to test the effectiveness of interventions in real-world settings, such as educational programs and public health campaigns.
An example is Holfing’s hospital study on obedience .
- Strength : behavior in a field experiment is more likely to reflect real life because of its natural setting, i.e., higher ecological validity than a lab experiment.
- Strength : Demand characteristics are less likely to affect the results, as participants may not know they are being studied. This occurs when the study is covert.
- Limitation : There is less control over extraneous variables that might bias the results. This makes it difficult for another researcher to replicate the study in exactly the same way.
3. Natural Experiment
A natural experiment in psychology is a research method in which the experimenter observes the effects of a naturally occurring event or situation on the dependent variable without manipulating any variables.
Natural experiments are conducted in the day (i.e., real life) environment of the participants, but here, the experimenter has no control over the independent variable as it occurs naturally in real life.
Natural experiments are often used to study psychological phenomena that would be difficult or unethical to study in a laboratory setting, such as the effects of natural disasters, policy changes, or social movements.
For example, Hodges and Tizard’s attachment research (1989) compared the long-term development of children who have been adopted, fostered, or returned to their mothers with a control group of children who had spent all their lives in their biological families.
Here is a fictional example of a natural experiment in psychology:
Researchers might compare academic achievement rates among students born before and after a major policy change that increased funding for education.
In this case, the independent variable is the timing of the policy change, and the dependent variable is academic achievement. The researchers would not be able to manipulate the independent variable, but they could observe its effects on the dependent variable.
- Strength : behavior in a natural experiment is more likely to reflect real life because of its natural setting, i.e., very high ecological validity.
- Strength : Demand characteristics are less likely to affect the results, as participants may not know they are being studied.
- Strength : It can be used in situations in which it would be ethically unacceptable to manipulate the independent variable, e.g., researching stress .
- Limitation : They may be more expensive and time-consuming than lab experiments.
- Limitation : There is no control over extraneous variables that might bias the results. This makes it difficult for another researcher to replicate the study in exactly the same way.
Key Terminology
Ecological validity.
The degree to which an investigation represents real-life experiences.
Experimenter effects
These are the ways that the experimenter can accidentally influence the participant through their appearance or behavior.
Demand characteristics
The clues in an experiment lead the participants to think they know what the researcher is looking for (e.g., the experimenter’s body language).
Independent variable (IV)
The variable the experimenter manipulates (i.e., changes) is assumed to have a direct effect on the dependent variable.
Dependent variable (DV)
Variable the experimenter measures. This is the outcome (i.e., the result) of a study.
Extraneous variables (EV)
All variables which are not independent variables but could affect the results (DV) of the experiment. EVs should be controlled where possible.
Confounding variables
Variable(s) that have affected the results (DV), apart from the IV. A confounding variable could be an extraneous variable that has not been controlled.
Random Allocation
Randomly allocating participants to independent variable conditions means that all participants should have an equal chance of participating in each condition.
The principle of random allocation is to avoid bias in how the experiment is carried out and limit the effects of participant variables.
Order effects
Changes in participants’ performance due to their repeating the same or similar test more than once. Examples of order effects include:
(i) practice effect: an improvement in performance on a task due to repetition, for example, because of familiarity with the task;
(ii) fatigue effect: a decrease in performance of a task due to repetition, for example, because of boredom or tiredness.

Generative AI and labour productivity: a field experiment on coding
Generative artificial intelligence (Gen AI) tools hold significant promise for enhancing worker productivity across various fields. These AI models have demonstrated capabilities comparable to humans in areas like clinical care, education, language modelling, art, music and design. A growing body of literature explores commercial and non-commercial applications, ethical considerations, regulatory frameworks, and implications for security and education. However, empirical research on AI's impact on productivity in tasks requiring cognitive abilities remains scarce.
Contribution
We investigate the impact of Gen AI on labour productivity through a field experiment in the coding industry. In September 2023, Ant Group launched CodeFuse, a large language model (LLM) designed to assist programming teams. In our experiment, one group of programmers had access to CodeFuse (the treatment group), while another group did not (the control group). By comparing similar employees from these two groups, we assessed how AI affected their productivity.
Our findings indicate that LLMs can significantly boost productivity among programmers. Productivity (measured by the number of lines of code produced) increased by 55% for the group using the LLM. Approximately one third of this increase was directly attributable to code generated by the LLM. The remaining productivity gains were likely due to improved efficiency in other coding tasks, as programmers had more time available. However, the productivity gains were statistically significant primarily among junior staff, with a less pronounced effect on senior employees. This difference appears to stem from lower engagement with the LLM by senior programmers, rather than the tool being less useful to them. The rate at which programmers accepted the LLM's suggestions did not vary with experience level, suggesting that the lower impact on senior programmers' productivity was due to less frequent use of the tool.
In this paper we examine the effects of generative artificial intelligence (gen AI) on labour productivity. In September 2023, Ant Group introduced CodeFuse, a large language model (LLM) designed to assist programmer teams with coding. While one group of programmers used it, other programmer teams were not informed about this LLM. Leveraging this event, we conducted a field experiment on these two groups of programmers. We identified employees who used CodeFuse as the treatment group and paired them with comparable employees in the control group, to assess the impact of AI on their productivity. Our findings indicate that the use of gen AI increased code output by more than 50%. However, productivity gains are statistically significant only among entry-level or junior staff, while the impact on more senior employees is less pronounced.
JEL Classification: D22, G31, R30
Keywords: artificial intelligence, productivity, field experiment, big tech
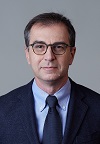
- Share this page
- Sign up to receive email alerts
- Translations
- Legal information
- Terms and conditions
- Copyright and permissions
- Privacy notice
- Cookies notice
- Email scam warning
Incorporation of mechanistic model outputs as features for data-driven models for yield prediction: a case study on wheat and chickpea
- Open access
- Published: 04 September 2024
Cite this article
You have full access to this open access article
- Dhahi Al-Shammari ORCID: orcid.org/0000-0001-6608-8322 1 ,
- Yang Chen 2 ,
- Niranjan S. Wimalathunge 1 ,
- Chen Wang 3 ,
- Si Yang Han 1 &
- Thomas F. A. Bishop 1
Introduction
Context Data-driven models (DDMs) are increasingly used for crop yield prediction due to their ability to capture complex patterns and relationships. DDMs rely heavily on data inputs to provide predictions. Despite their effectiveness, DDMs can be complemented by inputs derived from mechanistic models (MMs).
This study investigated enhancing the predictive quality of DDMs by using as features a combination of MMs outputs, specifically biomass and soil moisture, with conventional data sources like satellite imagery, weather, and soil information. Four experiments were performed with different datasets being used for prediction: Experiment 1 combined MM outputs with conventional data; Experiment 2 excluded MM outputs; Experiment 3 was the same as Experiment 1 but all conventional temporal data were omitted; Experiment 4 utilised solely MM outputs. The research encompassed ten field-years of wheat and chickpea yield data, applying the eXtreme Gradient Boosting (XGBOOST) algorithm for model fitting. Performance was evaluated using root mean square error (RMSE) and the concordance correlation coefficient (CCC).
Results and conclusions
The validation results showed that the XGBOOST model had similar predictive power for both crops in Experiments 1, 2, and 3. For chickpeas, the CCC ranged from 0.89 to 0.91 and the RMSE from 0.23 to 0.25 t ha −1 . For wheat, the CCC ranged from 0.87 to 0.92 and the RMSE from 0.29 to 0.35 t ha −1 . However, Experiment 4 significantly reduced the model's accuracy, with CCCs dropping to 0.47 for chickpeas and 0.36 for wheat, and RMSEs increasing to 0.46 and 0.65 t ha −1 , respectively. Ultimately, Experiments 1, 2, and 3 demonstrated comparable effectiveness, but Experiment 3 is recommended for achieving similar predictive quality with a simpler, more interpretable model using biomass and soil moisture alongside non-temporal conventional features.
Explore related subjects
- Artificial Intelligence
Avoid common mistakes on your manuscript.
Data-driven models (DDMs) have emerged as powerful tools for agricultural applications, including crop yield prediction. These models utilise advanced statistical and machine learning techniques to analyse large amounts of data collected from multiple sources, such as weather stations, satellite imagery, soil sensors, and historical crop yield records. DDMs can capture the complex interactions between environmental factors and crop growth patterns by incorporating these diverse datasets, enabling accurate yield predictions. DDMs, such as Random Forest (Breiman, 2001 ) and Neural Networks (Abdi et al., 1999 ), are commonly used in these models to analyse large datasets and identify patterns that can help predict crop yields. The XGBOOST (eXtreme Gradient Boosting) is another one of these DDMs algorithms that has been shown to be successful in crop yield prediction (Al-Shammari, 2022 ; Huber et al., 2022 ; Jones et al., 2022 ; Kang et al., 2020 ). It excels at handling multicollinearity, which refers to strong correlations between features used for modelling. In agricultural datasets, features, such as remote sensing (RS), soil, and weather factors can exhibit interdependencies or collinearity. XGBOOST addresses this issue by using regularisation techniques, effectively reducing the impact of collinear of features in the final model.
Although DDMs require a minimum knowledge of the processes (Cao & Zhang, 2007 ), DDMs require representative features, which are assumed to help reveal hidden information about a specific phenomenon. One of the biggest challenges is to select features that allow a DDM to reveal hidden information or patterns to estimate the response variable more accurately.
In contrast to traditional DDMs, mechanistic models (MMs) require domain knowledge in a system to produce meaningful relationships between the inputs (features) and the outputs (response) (Fan et al., 2015 ). Unlike DDMs, MMs are governed by some processes defined by the experts who create the proper formulas to solve a specific issue. MMs can explain part of but not all processes in dynamic and complex systems, such as cropping systems. Consequently, many studies have employed DDMs to find empirical relationships rather than using mathematical formulas to describe a specific phenomenon, taking advantage of DDMs' flexibility in accepting a large number and variety of features and less of a need to understand complex mechanisms to provide predictions.
Many studies have used MMs to model processes in agricultural systems. Of relevance to this study is a processed-based model (C-Crop) that has been introduced based on simulating plant growth to predict crop yield (Donohue et al., 2018 ). The authors of C-Crop stated that this model is simple and effective for predicting wheat and canola yield. This model is based on calculating biomass from environmental variables (weather, remote sensing) to describe the processes using mathematical equations. In another study, a processed-based model for soil moisture prediction was developed by Wimalathunge and Bishop ( 2019 ). This model uses rainfall, evapotranspiration (ET), and some soil attributes, including clay, sand, and bulk density, to calculate soil moisture. The advantage of this model is that it can predict soil moisture for the whole profile, which is very important in dryland cropping systems since water availability is a yield-limiting factor in these systems. The advantage of these two models is that they can be easily constructed using readily and freely available geospatial data, capturing the within-field variability and benefiting precision agriculture operations.
Since DDMs are very flexible in dealing with various features, there is a need to select the best representative features, allowing these models to maximise the prediction quality. One way to do that is by combining MMs with DDMs to form what is known as domain-driven models (Cao & Zhang, 2007 ). Several studies have examined the advantages of combining DDMs and MMs (Džeroski & Todorovski, 2003 ; Fan et al., 2015 ; Todorovski & Džeroski, 2006 ). Fan et al. ( 2015 ) incorporated two sub-models derived from MMs and DDMs to build knowledge-and-data-driven modelling (KDDM) for tomato plant growth modelling. They found that the proposed KDDM has several advantages over the MM and the DDM approaches in predicting tomato yield.
Therefore, this study aimed to investigate the potential of incorporating MMs into DDMs for wheat and chickpeas yield prediction at the within-field scale for precision agriculture. Two outputs from MMs, namely biomass and soil moisture, are investigated in this study for use as features. The XGBOOST algorithm was selected, and four experiments are performed to explore scenarios involving different combinations of MM-based features and more general ones such as remote sensing. The results of the experiments are considered in terms of the prediction quality and the feature importance of the features in the models.
Methodology
The study area comprises a single site with 10 field-years of crop yield data near Moree, New South Wales, Australia. The total area of these fields is ~ 1 096 ha. In any season all 4 fields are sown to the same crop. Wheat yield maps were available for the four fields (Fields 1, 2, 3, and 4), whereas chickpea yield maps were unavailable for Field 2 (Fig. 1 and Table 1 ).
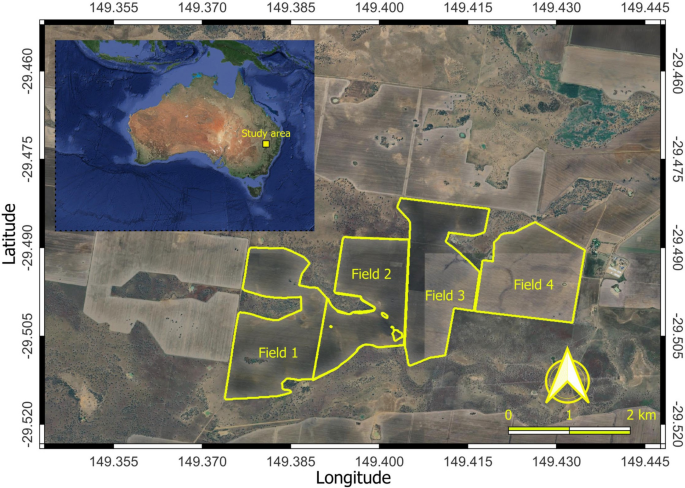
Map of Australia showing the location of the study area. Fields 1, 2, 3, and 4 are shown in yellow colours (Color figure online)
The area receives an average of 450 mm of rainfall annually, mostly occurring during summer. However, the area is also prone to drought and heat waves. The soils in the Moree region are predominantly heavy clays (Hunter & Earl, 1999 ), and clay loams, with some areas of sandy soils (Young & Schwenke, 2013 ). The soils are generally fertile and well-suited for crop production. Soil constraints, such as sodicity can be an issue in some areas (Filippi et al., 2018 ). The climate, topography, and soil characteristics of Moree make it a challenging yet productive agricultural region.
Yield monitor data of wheat and chickpeas was acquired from a private AgTech company and processed to remove anomalies by excluding data points outside the established range of 0.1 to 10 t ha −1 (Taylor et al., 2007 ). Subsequently, values exceeding 2.5 standard deviations from the field mean were also removed from the remaining data. Yield data was then kriged using block kriging (a spatial interpolation method) at a 10 m spatial support onto a 10 m grid. The total size of the yield dataset after interpolation was 259 318 observations.
Derived and processed features
To examine the potential of incorporating the MMs with the DDMs for wheat and chickpeas yield prediction, various sets of features were prepared from Tables 2 , 3 and shown in Fig. 2 . This study used the terms derived and processed to differentiate between the types of features. The difference between derived and processed features lies in the information extraction and refinement level applied to the raw data. Derived features refer to the conventional ones obtained from RS or other platforms that are used as is or after simple calculations. On the other hand, processed refers to the features that are the outputs of mechanistic models (MM).
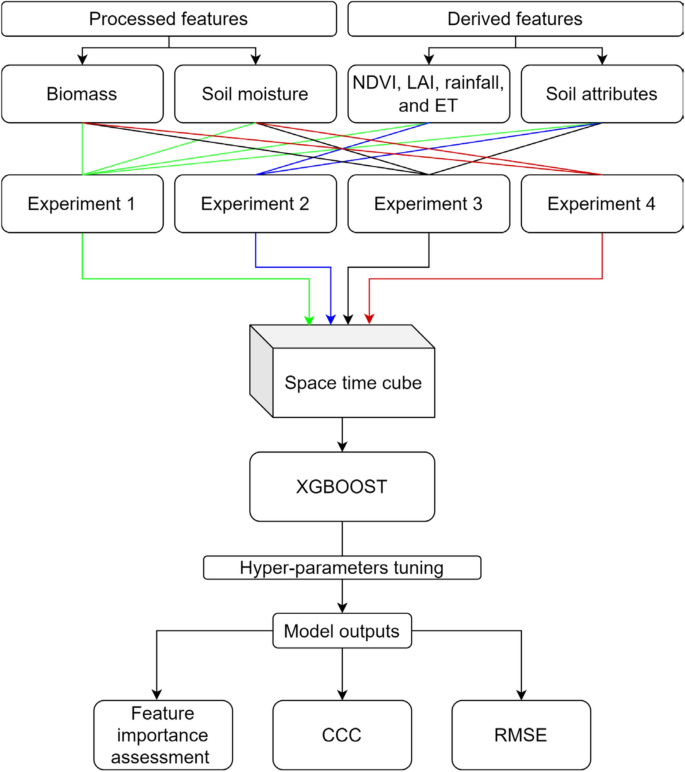
Flowchart illustrating the process of creating the four datasets, models, and the evaluation processes for each experiment. Each coloured line (green, blue, black, and red) refers to a dataset and the features included in it (Color figure online)

Derived features
A set of temporal, elevation and soil-derived features were prepared as a space–time data cube (Tables 2 , 3 ). Google Earth Engine (GEE) (Gorelick et al., 2017 ) was used to obtain all the derived features (except rainfall). The time series of normalized difference vegetation index (NDVI) images (from 1st of May to 1st of September) were acquired from Sentinel-2 at 10 m resolution for each growing season and used to calculate the average of the seasonal NDVI for each pixel. The enhanced vegetation index (EVI) was used to calculate the leaf area index (LAI) for the 1st September (peak LAI) for each growing season, using an equation (Eq. 1 ) developed by (Boegh et al., 2002 ). The peak LAI was used in this study as it was reported to be highly correlated to the final yield (Cai et al., 2019 ).
The ET was derived from a dataset that provides accurate actual evapotranspiration (AET) for Australia using a model that has been developed by the Commonwealth Scientific and Industrial Research Organisation (CSIRO) known as the CSIRO MODIS Reflectance-based Scaling EvapoTranspiration (CMRSET) model (Guerschman et al., 2022 ). The accumulated ET was calculated as the sum of ET from 1st of May to the 1st of September at 30 m resolution. Elevation is a spatially static data, which was accessed a digital elevation model (DEM) at 30 m resolution (Australia, 2015 ). Soil attributes were derived from the Soil and Landscape Grid of Australia (SLGA) at 90 m resolution (Grundy et al., 2015 ). These soil attributes were available as five layers from different soil depths (0–5 cm, 5–15 cm, 15–30 cm, 30–60 cm, and 60–100 cm) for each attribute. Then, the weighted average of each attribute was calculated to extract the attribute as the root zone average (0–100 cm). Daily rainfall grids (~ 5 km) for each growing season have been downloaded from the Australian climate database known as (SILO) (Jeffrey et al., 2001 ). The rainfall was calculated as the accumulated rainfall from the start of the growing season 1st of May to the 1st of September.
In our study, spatial resolution harmonisation involved resampling the original 30 m, 90 m, and 5 km resolution layers to 10 m to ensure consistency across all features used in the final modelling. For data layers with spatial resolutions of 30 m and 90 m, bilinear interpolation was employed to resample the data, chosen for its effectiveness in preserving continuous spatial information without introducing significant artifacts. The rainfall data, was resampled using the nearest neighbour method as the difference from the target grid size of 10 m was too far from 5 km.
Biomass (processed feature)
The C-Crop model is an MM, which was developed to simulate some of the processes in the ecosystem using mathematical equations. This model can predict biomass using the fraction of photosynthetically active radiation ( f par ), which is derived from NDVI, and a carbon mass accumulation and turn-over model (Donohue et al., 2018 ). The Donahue et al. ( 2018 ) model used the 16-day NDVI product with 250 m resolution, the Moderate Resolution Imaging Spectroradiometer (MODIS) (MOD13Q1: collection 5) (Justice et al., 1998 ). However, for this study, Landsat 8 data was used to derive the NDVI at 30 m resolution as the authors stated that this model is unrestricted to a specific source of data where the C-crop can use NDVI time series from any source (e.g., satellite) at any spatial resolution as long as the source provides 16 days or higher temporal resolution. Therefore, Landsat images at 16-day intervals were acquired to preserve the C-Crop structure unchanged. Moreover, the C-Crop model requires the NDVI time series to be prepared according to a nominal start and end of the growing season to calculate biomass. According to the crop calendar for wheat and chickpeas in the study area, wheat sowing begins in April and ends in July, while chickpeas sow in May and end in July, with the growing season for both crops ending in October to December. The nominal start and end of the growing season were determined as the 1st of May and the 1st of October, respectively. Besides the NDVI time series, C-Crop also uses the air temperature, which is a 5 km resolution and 1-day temporal resolution, which was readily available from (Jeffrey et al., 2001 ). Donohue et al. ( 2018 ) study provides more details about C-Crop. After preparing the inputs for the C-Crop model, biomass maps, which represent the seasonal biomass were generated for each field at 10 m resolution. The maximum biomass during the season was calculated from the time series and added to the space time data cube. Maximum biomass is valuable for assessing overall productivity and its impact on final yield.
Soil moisture (processed feature)
The water balance (WB) model, which has been developed by Wimalathunge and Bishop ( 2019 ), has been used to estimate the soil moisture to 100 cm depth (root zone). This WB is a multi-layer, knowledge-based model that better represents the vertical soil moisture variation. It is also an unsaturated model where water infiltrates through layers freely and continuously according to the soil properties. It requires rainfall, evapotranspiration and an estimate of the soil water bucket size. In this study the SLGA was used to represent the soil water bucket size. SLGA soil depth intervals are the WB model’s layer thickness. The corresponding clay, sand and bulk density values were used to calculate the bucket size which is represented by field capacity ( θ FC ) using a pedotransfer function (PTF) (Padarian et al., 2018 ).
For this study, the model used ET from MODIS (MOD16; Mu et al., 2011 ) which is a different source to ET used in the machine learning and shown in Tables 2 , 3 . This is because CMRSET ET provides the daily averages for each month and MODIS ET provides 8 day totals which is closer to the daily time step needed for the modelling. The MODIS ET was resampled using nearest neighbour to downscale the 8 day total to a daily total. The rainfall used was the same as presented in Tables 2 , 3 . The modelling depth was 100 cm, and the spatial resolution of the predictions was 90 m, as determined by the SLGA data. The model was run on each SLGA grid cell with the corresponding value for rainfall and ET. For example, the model uses the same ET value for each 90 m grid cell within the 500 m ET grid cell. The model was run on a daily time step from the 1st of January 2015 to the 31st of September 2023, and the output at 0–100 cm (root zone) was used as an input of the data-driven model. For this study, the sum of soil moisture from the 1st of May to the 1st of September for each growing season was calculated and used as a predictor. The hypothesis is that the soil moisture output can replace the rainfall because it provides a better representation of the available water for crops than the rainfall. Moreover, this output has a much higher resolution (~ 90 m) than the rainfall data, provided at a 5 km resolution.
Description of models
Figure 2 illustrates the main steps followed to evaluate each model with different sets of features. Four experiments were performed based on different datasets and tested individually in the XGBOOST (see next section). The base DDM model was built using all features, both derived and processed (Fig. 2 and Table 1 , Experiment 1). The biomass and soil moisture (processed) features were removed from the model (Experiment 2) to test the impact of removing these on the model's predictive power. Then, the derived temporal features were removed for Experiment 3 to test the potential of using soil moisture and biomass as features instead of their temporal inputs, e.g., rainfall, NDVI, ET. The aim was to create simpler and more interpretable models. The soil features were kept in the model even though some of these are used in the water balance model as inputs. The reason being that also relate to soil fertility which can impact on crop yield. Experiment 4 only uses features produced by the MMs (biomass and soil moisture) to test the possibility of the MMs replacing all the other derived features completely.
Wheat and chickpeas were modelled separately. The reason for this is that different crop types require specific models due to a variety of factors that are unique to each crop type. The factors include biological characteristics, environmental responses, and management practices. For each model, datasets were split randomly into 80 percent for training and 20 percent for validation. Therefore, other strategies such as leave-on-field-out (LOFO) and leave-one-season-out (LOSO) cross-validation were not employed. The models were evaluated individually using the concordance correlation coefficient (CCC), the root mean square error (RMSE) and feature importance to understand the most important features for the model.
These three metrics allow a fair evaluation of the benefits of the addition of processed features to the DDM model. The CCC is a measure that combines measures of both precision and accuracy to determine how well a pair of observations conform to a 1:1 correspondence with each other. The RMSE captures the amount of error (t ha −1 ) in the DDMs. The feature importance highlights the contribution of individual features to model prediction quality.
The XGBOOST is a highly efficient and powerful ML algorithm that has gained immense popularity (Chen & Guestrin, 2016 ). It is a decision-tree-based ensemble algorithm that applies boosting on weak learners (Fauzan & Murfi, 2018 ), such that the weak learners learn sequentially from the residual of the previous weak learner. The idea of learning from the trees sequentially can reduce the bias (Nielsen, 2016 ). L1 regularisation is used to handle the multicollinearity, as L1 adds a penalty term to the objective function during training, which helps control the complexity of the model and discourages large coefficients for correlated features.
The XGBOOST was used for all experiments. Predictions were repeated 100 times, and the mean of CCC, RMSE and feature importance were calculated. The feature importance was calculated for each experiment to evaluate the importance of derived and processed features and their contribution to the models. The feature importance in XGBOOST models is calculated based on the concept of gain, which measures the improvement in model performance resulting from splitting a particular feature. The gain is computed by considering the average loss reduction achieved by using that feature to split data across all trees in the ensemble. The higher the gain value, the more important the feature is considered. This calculation allows XGBOOST to identify features that contribute most significantly to predicting the response variable. According to Chen and Guestrin ( 2016 ), calculating feature importance in XGBOOST provides a robust measure of relevance for each feature. They explain that gain-based methods have advantages over other techniques, such as permutation-based or drop-column-importance approaches because they consider interactions between features and their contributions. Additionally, this method can handle missing values effectively without requiring imputation.
Tuning an XGBOOST model is important for maximising its predictive performance and generalisation capabilities. By fine-tuning hyperparameters, the model's predictive quality can be enhanced significantly, prevent overfitting, and improve the handling of diverse datasets. This study used a combination of grid search and internal cross-validation to tune hyperparameters for an XGBOOST model, aiming to optimise model performance by finding the best hyperparameter values for each model. For each combination of hyperparameters, each model for each experiment underwent a rigorous evaluation through fivefold cross-validation, implemented via the xgb.cv function. This function trains the model on different subsets of the data, ensuring that the evaluation is robust and not biased toward a specific portion of the data. The RMSEs were recorded for both the training and validation phases at the most effective iteration determined by early stopping (a technique used here to prevent overfitting by halting training if there is no improvement in validation error for three consecutive rounds). This systematic approach allowed for careful monitoring and adjusting the model based on its performance across the different data folds. Finally, the hyperparameter settings that yielded the lowest RMSE on the validation data were identified and selected for each model.
Exploratory data analysis
According to the coefficient of variation (CV) values (Tables 2 , 3 ), it is clear that there are notable differences in variability between wheat yield in 2017 and its features, such as biomass, SM, and LAI. Biomass, with a CV of 31.55%, shows greater variability compared to wheat yield, which has a CV of 25.30%. This suggests that biomass may better represent variability for predicting yield. In contrast, the SM showed significantly less variability (CV of 0.39%) compared to yield. This low variability might mean it is less useful for predicting yield than other features. LAI, with a CV close to that of yield (20.25%), might be better aligned as a feature, reflecting similar variability patterns without extreme fluctuations.
Furthermore, the variability of chickpea yield over the two seasons (2019 and 2022) was much higher (as reflected by the CV , where a noticeable trend can be noticed with a significant increase in yield variability from 2019 to 2022. The CV for chickpea yield in 2019 was around 99.95%, indicating considerable fluctuations in yield compared to wheat ( CV = 25.30%). However, in 2022, this variability increased to 162.04%. This increase highlights a greater inconsistency in chickpea production, potentially due to more extreme environmental conditions or other factors affecting agricultural outputs.
When comparing the variability of other features across these two seasons, such as biomass, SM, LAI, and rainfall, distinct patterns are observed. Biomass, while still variable, shows a decrease in CV from 85.30% in 2019 to 31.89% in 2022, suggesting that the fluctuations in biomass production have become less pronounced, possibly due to more consistent growth conditions and better management practices. In contrast, SM and rainfall maintain consistently low variability across both seasons (with CVs under 1% for rainfall and around 1% for SM). LAI showed a reduction in variability, from a CV of 42.97% in 2019 to 18.72% in 2022, hinting at more consistent growth conditions between the seasons. The overall reduction in variability among these features, except for yield, raises important considerations for predictive modelling. It suggests that while environmental and growth conditions measured by SM, rainfall, and LAI have become more stable, the factors specifically affecting yield have diverged, becoming more volatile.
While many soil properties such as AWC, clay content, ECEC, PTO, and SOC are influenced by long-term processes like weathering, organic matter accumulation, and gradual shifts in mineral content and thus remain relatively stable over time, certain properties like BDW can exhibit more immediate changes. For example, BDW can be significantly impacted by soil compaction due to agricultural practices or the use of heavy machinery. These characteristics make soil a relatively static factor in short to medium term, especially when compared to more dynamic, temporal. Therefore, Tables 2 , 3 showed that the CV was much less compared to wheat and chickpeas yield.
The AWC showed little variability, with an average value of about 19% across the study area. The BDW remained relatively constant, averaging around 1.67 g/cm 3 . Clay content in the soil varied significantly between locations, ranging from 46 to 72%, with an average clay content of about 64%. The ECEC varies widely, ranging from 23.95 to 59.65 meq/100 g, indicating the soil's capacity to retain essential nutrients through cation exchange.
Figure 3 shows that the strongest positive correlation in all growing seasons was between ET and yield, and this was expected as ET reflects the amount of water used by a crop for growth and maintaining its physiological processes, and the spatial resolution of ET (30 m) is close to the yield’s resolution (10 m) as compared to other features. There was also strong positive correlation between NDVI and LAI, which is expected as the relationship between NDVI and LAI is generally positive (Smith et al., 2008 ). Similarly, there was a strong relationship between NDVI and ET and LAI and ET in all growing seasons. Yield-biomass and yield-SM correlation had very weak correlations. The yield-ET correlation was stronger in the 2017 growing season (wheat) than in the 2019 and 2022 growing seasons (chickpeas). The multicollinearity was also observed between other features, which can be an issue for the DDMs; however, using the XGBOOST with L1 regularisation can mitigate the multicollinearity.
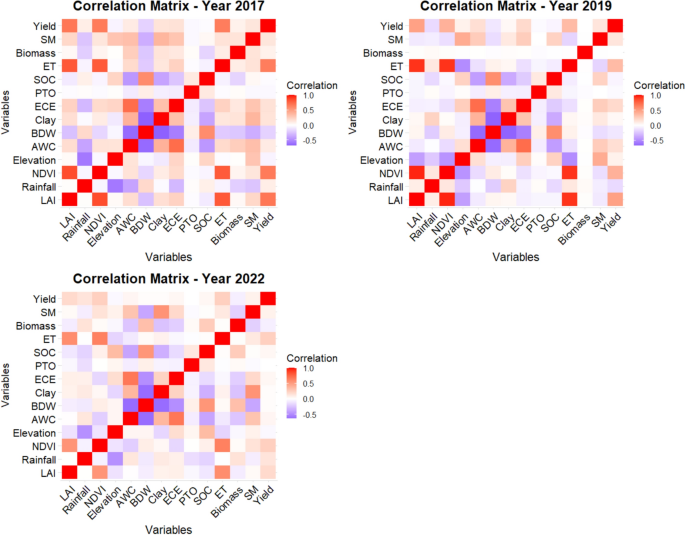
Correlation matrix showing the Peason’s correlation between yield, processed, and derived features
The learning curves of XGBOOST are crucial in understanding the effectiveness and performance of the model, offering insight into how well XGBOOST generalises to new, unseen data. Typically, XGBOOST learning curves demonstrate rapid improvement in training performance with increasing data size, followed by a plateau as the model approaches its optimal capacity.
This study explored and reported the learning curves to examine the ability of the models to learn from the derived and the processed features (Figs. 4 and 5 ). Figure 4 shows the RMSE of training and validation sets over different iterations for the four wheat experiments (1, 2, 3, and 4). All experiments showed a similar initial high RMSE of around 2.3 t ha −1 , rapidly decreasing and stabilising around 0.32 t ha −1 , indicating effective model learning and minimal overfitting. Experiment 4 (Fig. 4 D) stands out with the fastest convergence, stabilising within 50 iterations, compared to the other datasets, which stabilise at around 200 iterations. However, the final RMSE values for Experiment 4 were higher, suggesting lower model performance on this dataset. The close alignment of training and validation RMSE values across all experiments indicates good model generalisation. However, this is not unexpected, as in each field, we have training and validation samples.
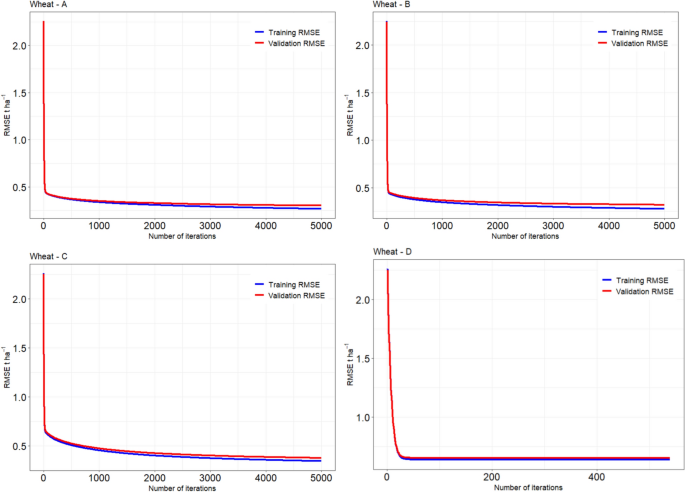
Learning curves for wheat models using XGBOOST. Panels A , B , C , and D represent models built for Experiments 1, 2, 3, and 4, respectively
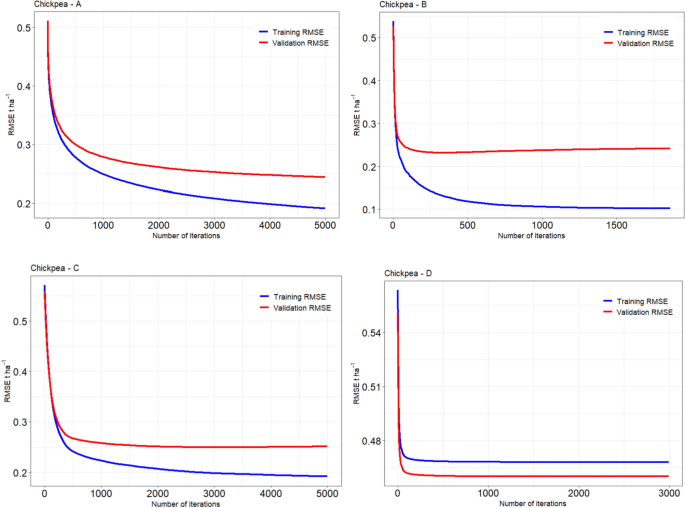
Learning curves for chickpea models using XGBOOST. Panels A , B , C , and D represent models built for Experiments 1, 2, 3, and 4, respectively
The RMSE plots (Fig. 5 ) for the chickpea experiments (1, 2, 3, and 4) reveal distinct patterns in model performance and generalisation. Experiment 1 (Fig. 5 A) shows a gradual decrease in RMSE, stabilising around 0.3 t ha −1 for training and slightly higher for validation after about 2000 iterations, indicating slight overfitting. The learning curve for the model built using Experiment 2 converged quickly, with training RMSE dropping below 0.2 t ha −1 within 500 iterations, but the validation RMSE remains around 0.3 t ha −1 , suggesting significant overfitting. The model built using Experiment 3 followed a similar trend to that built using Experiment 1, with RMSE stabilising at 0.2 t ha −1 for training and above 0.3 t ha −1 for validation, showing moderate overfitting. The model built using Experiment 4 exhibited the best performance, with both training and validation RMSE stabilising around 0.48 t ha −1 within 500 iterations, indicating minimal overfitting and good generalisation. These results suggest that while the models developed in Experiments 1, 2, and 3 exhibited varying degrees of overfitting, the model that used processed data only achieved a balanced and robust fit, highlighting the need for potential regularisation and feature optimisation in the other features in the other experiments.
The results obtained from the validation set show that the predictive power of the XGBOOST for both crops was almost similar, where the CCC ranged from 0.89 to 0.91 and an RMSE ranged from 0.23 to 0.25 t ha −1 for chickpeas, and CCC ranged from 0.87 to 0.92 and an RMSE ranged from 0.29 to 0.35 t ha −1 for wheat, for Experiment 1, Experiment 2, and Experiment 3, respectively (Figs. 6 and 7 ). In the case of Experiment 4 there was a sharp degradation in the XGBOOST model's predictive power, where the CCCs declined to 0.47 and 0.36, with increased RMSEs to 0.46 and 0.65 for chickpeas and wheat, respectively.
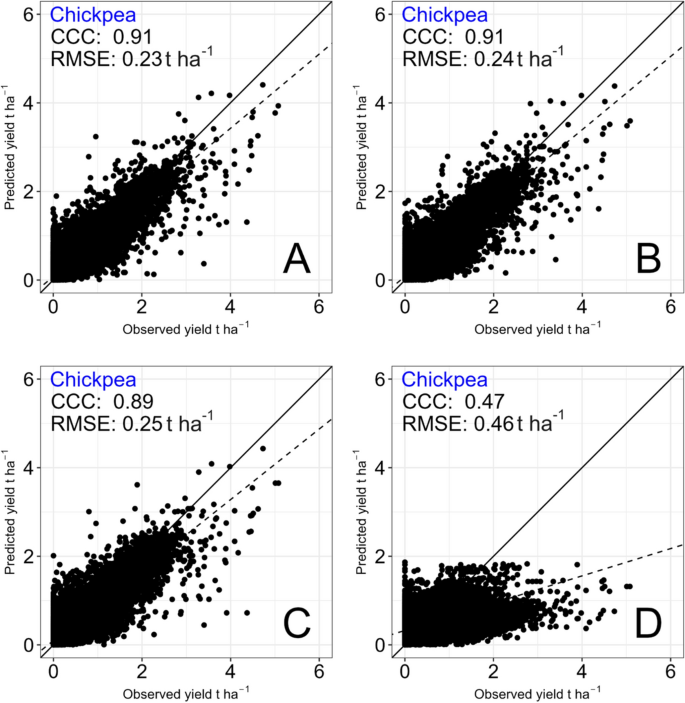
Observed vs predicted yield of chickpeas obtained from XGBOOST model for Experiments 1, 2, 3, and 4, shown in Figures A , B , C , and D , respectively
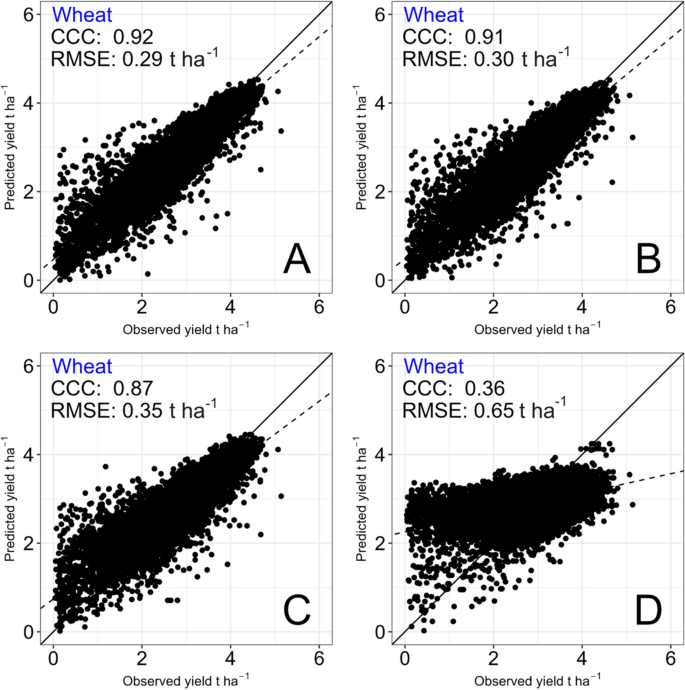
Observed vs predicted yield of wheat obtained from XGBOOST model for Experiments 1, 2, 3, and 4, shown in Figures A , B , C , and D , respectively
Feature importance from XGBOOST was calculated (Figs. 8 and 9 ) to identify the features that have more impact on the model. In general, the temporal (processed and derived) features were more important than soil attributes for both crops, indicating that temporal features provide valuable insights into the temporal dynamics of a system and can significantly affect the outcome of predictive models. The importance of rainfall was evident for chickpeas but not for wheat, whereas the LAI was one of the most important features in Experiments 1 and 2 for both crops. The importance of biomass was not evident in Experiment 1 for both crops as the models seemed to learn from the derived features more than biomass; however, biomass importance was evident in Experiment 3 for both crops, which revealed the impact of biomass on the outcomes with the absence of other temporal features. The NDVI was at the top of the feature importance for wheat and the third most important feature for chickpeas, which is likely due to the correlation with yield (Fig. 3 ). The other soil properties were not important for both models (except ECEC and clay, which appeared to be more important for wheat in dataset 3). Soil moisture appeared to be more important than biomass in Experiment 4 for both crops, indicating the importance of soil moisture for predicting crop yield.
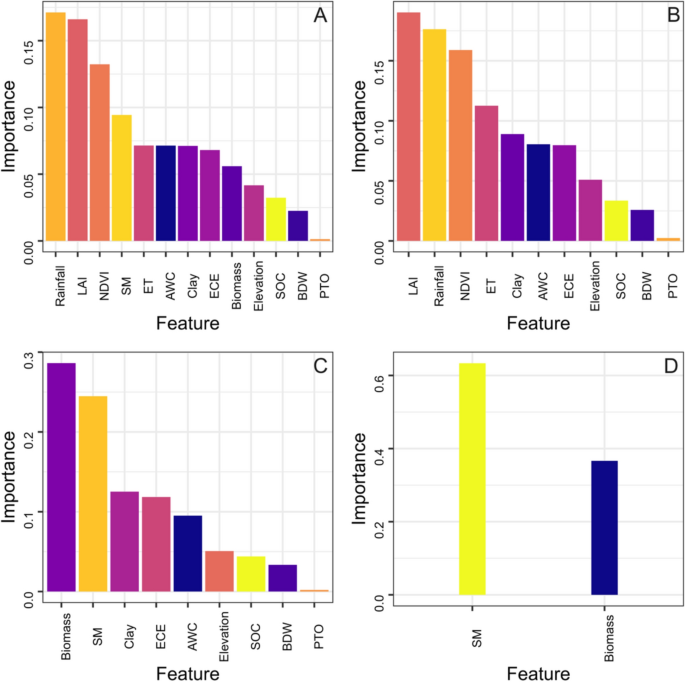
Feature importance obtained from XGBOOST for chickpeas. A , B , C and D correspond to Experiments 1, 2, 3, and 4, respectively
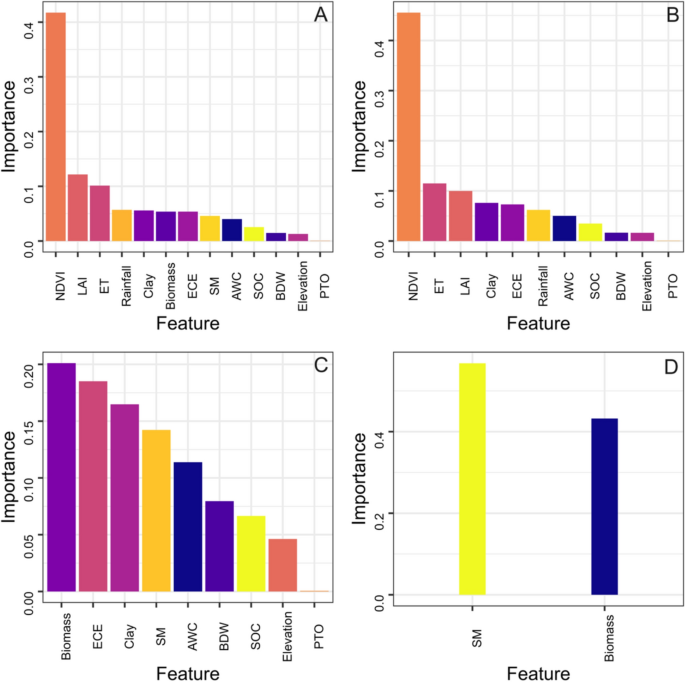
Feature importance obtained from XGBOOST for wheat. A , B , C and D correspond to Experiments 1, 2, 3, and 4, respectively
Although the model built for Experiment 4 converged better, its prediction performance was still lower than the other models. Figure 10 illustrates the difference between observed (top panel) and predicted yield of wheat for Experiment 3 (middle panel) and Experiment 4 (bottom panel). The model developed for Experiment 3 captured the within-field variability and could predict low and high yield values. However, the model that was built for Experiment 4 overpredicted yield and could not capture the within-field variability. This is likely due to the low variability in the soil moisture features.
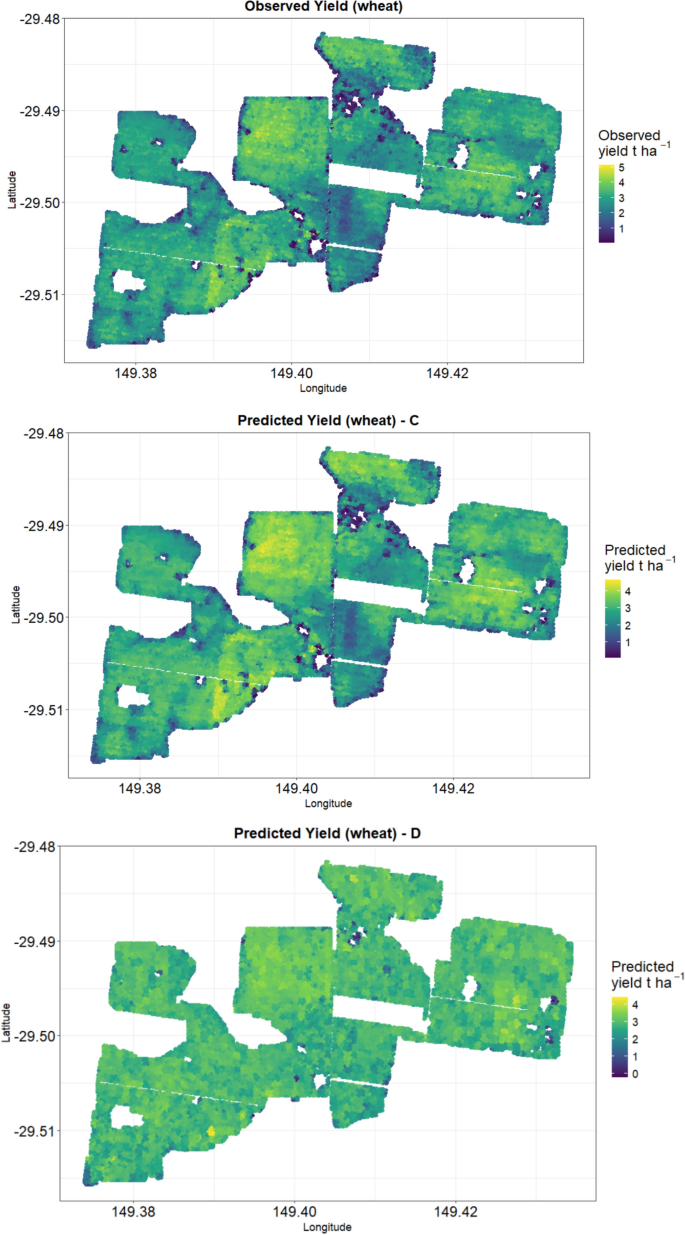
The difference between observed (top panel) and predicted yield of wheat using Experiment 3 (middle panel) and Experiment 4 (bottom panel)
This study investigated the potential of using MMs outputs (biomass and soil moisture) as features in ML models. Four experiments (Experiments 1, 2, 3, and 4) were performed with different combinations of features and tested using the XGBOOST model in this study. The results obtained from all experiments showed the potential for using biomass and soil moisture as features in DDMs. However, several points must be considered when using MMs outputs as features in DDMs. For example, the MMs are usually restricted by expert knowledge, and the quality of the outputs from an MM depends on how these models are designed. Therefore, using these outputs in DDMs might increase/decrease in prediction quality depending on the MMs predictive quality. In this study, the XGBOOST could learn from the derived features;however, the need for a more real representation of the temporal processes or higher-resolution inputs might require using prior knowledge to model one or more inputs (Reichstein et al., 2019 ). For example, in the case of the WB model (Wimalathunge & Bishop, 2019 ), the soil moisture is represented by the root zone depth at a 90 m resolution. This can be considered an advantage for use in the DDMs as the rainfall (5 km) (Jeffrey et al., 2001 ) does not provide such representation. C-Crop also assumes that biomass can be predicted by empirically using the greenness of the vegetation cover as the response to light and temperature. Donohue et al. ( 2018 ) suggest biomass is directly related to yield through the harvest index. As shown in the results, using biomass and soil moisture along with the soil attributes and removing the other temporal features, XGBOOST could preserve the predictive power while reducing the number of features by removing the derived features. However, biomass and soil moisture could only provide reasonable predictions with soil features, which means that soil attributes play an important role in predicting crop yield. Instead, biomass and soil moisture could use multiple features because they provide a more stable and less noisy representation of the temporal features.
The feature importance plots helped us understand different features contribution towards predicting the target variable. It allowed us to gain insights into which features significantly impact the model's performance, aiding in feature selection, understanding relationships between features, and improving interpretability. According to the results in Fig. 8 A, rainfall was the most important feature for chickpeas but not for wheat. This is because chickpeas data were obtained for two seasons, and the rainfall variation in these two seasons was significantly different (Tables 2 , 3 ), which explains the impact of the rainfall on the outcomes. The LAI was also on the top of the feature importance list for both crops, and this is because LAI is a good indicator of final crop yield (Ziliani et al., 2022 ). Several studies have demonstrated the usefulness of LAI for predicting crop yield in various agricultural systems (Cao et al., 2021 ; Ma et al., 2022 ; Mokhtari et al., 2018 ; Tewes et al., 2020 ). The peak LAI contributed significantly to the model, which could be due to the high correlation with final yield that was found here (Report value) and has been reported in the past, e.g., Cai et al. ( 2019 ). The NDVI was also one of the most important features for both crops (Experiments 1 and 2). Research studies showed that NDVI can be a very important feature in DDM models of yield (Al-Shammari et al., 2021 ; Filippi et al., 2017 ; Johnson et al., 2016 ). The accumulated ET was also an important feature to the model for both crops (Figs. 8 .A and 8B and 9A and 8B). ET is a parameter that reflects the impact of various factors on crop growth and is highly correlated with crop growth and yield (Khan et al., 2019 ), and the ET obtained from the CMRSET model was a significant feature for crop yield.
Elevation did not have an impact on the outcomes of the models. Because the elevation in the study area did not vary significantly, the models (Figs. 8 and 9 A, B and C) could not identify the relationship between elevation and other features to discern its impact on the crop yield. This is because the variation will make a difference if it varies within a field. A significant change in elevation leads to variation in temperature frost incidence, and water flow, which leads to variation in yield (Dixit & Chen, 2011 ; Kelleher et al., 2001 ; Thornton et al., 2009 ). However, this was not the case in our study area, therefore, the elevation was not important to the model.
The low importance of soil properties in the XGBOOST model in all datasets was attributed to the uncertainty of these properties at the fine scale (10 m in this study). The SLGA is a valuable resource for understanding soil properties at a national scale. However, it is important to acknowledge some limitations associated with this dataset. Firstly, SLGA relies on predictive modelling techniques that use various environmental covariates to estimate soil properties. This can introduce uncertainty and potential errors in the predictions. These models depend on the availability and quality of input data, which may vary across different regions of Australia. Additionally, SLGA provides point estimates of soil properties at a spatial resolution of 90 m, which may not capture local-scale variability or fine-scale features, such as soil texture gradients within landscapes (Han et al., 2022 ; Kidd et al., 2020 ). Therefore, caution should be exercised when using SLGA for site-specific applications or detailed studies.
The learning curves (Figs. 4 and 5 ) revealed that the models would learn better from the processed data. The learning curves also showed that using data from different years would lead to overfitting the model using the derived features (Fig. 5 A–C). However, using the processed feature (Fig. 5 D) led to minimising the overfit, indicating the potential for modelling the other features (e.g., soil data). This would lead to improving the temporal extrapolation of predictions through time.
Conclusions
The potential of incorporating MMs and DDMs has been investigated. Two outputs from two mechanistic models (C-Crop and WB models) were used with the commonly used LAI, NDVI, weather and soil data. The results of this study indicated that these two outputs could be helpful to replace the other temporal features while preserving the model’s predictive power, reducing noise caused by using many temporal features in the model, predictions at higher resolution, and more interpretable models. Biomass and soil moisture cannot completely replace the soil features used in this study. This is especially important when the scale of a study is small. This study also highlighted the need for improving MMs to improve ML models that use MMs outputs as inputs, as shown in the Experiment 4 results.
Data availability
Not applicable.
Abdi, H., Valentin, D., & Edelman, B. (1999). Neural networks . Sage.
Book Google Scholar
Al-Shammari, D. (2022). A comparison between machine learning and simple mechanistic-type models for yield prediction in site-specific crop yield predictions.
Al-Shammari, D., Whelan, B. M., Wang, C., Bramley, R. G. V., Fajardo, M., & Bishop, T. F. A. (2021). Impact of spatial resolution on the quality of crop yield predictions for site-specific crop management. Agricultural and Forest Meteorology, 310 , 108622. https://doi.org/10.1016/j.agrformet.2021.108622
Article Google Scholar
Australia, G. (2015). Digital elevation model (DEM) of Australia derived from LiDAR 5 Metre grid . Commonwealth of Australia and Geoscience Australia.
Google Scholar
Boegh, E., Soegaard, H., Broge, N., Hasager, C., Jensen, N., Schelde, K., & Thomsen, A. (2002). Airborne multispectral data for quantifying leaf area index, nitrogen concentration, and photosynthetic efficiency in agriculture. Remote Sensing of Environment, 81 (2–3), 179–193. https://doi.org/10.1016/S0034-4257(01)00342-X
Breiman, L. (2001). Random Forests. Machine Learning, 45 , 5–32.
Cai, Y., Guan, K., Lobell, D., Potgieter, A. B., Wang, S., Peng, J., Xu, T., Asseng, S., Zhang, Y., & You, L. (2019). Integrating satellite and climate data to predict wheat yield in Australia using machine learning approaches. Agricultural and Forest Meteorology, 274 , 144–159. https://doi.org/10.1016/j.agrformet.2019.03.010
Cao, J., Zhang, Z., Tao, F., Zhang, L., Luo, Y., Zhang, J., Han, J., & Xie, J. (2021). Integrating multi-source data for rice yield prediction across China using machine learning and deep learning approaches. Agricultural and Forest Meteorology, 297 , 108275. https://doi.org/10.1016/j.agrformet.2019.03.010
Cao, L., & Zhang, C. (2007). The evolution of KDD: Towards domain-driven data mining. International Journal of Pattern Recognition and Artificial Intelligence, 21 (04), 677–692. https://doi.org/10.1142/S0218001407005612
Chen, T., & Guestrin, C. (2016). Xgboost: A scalable tree boosting system. Proceedings of the 22nd Acm Sigkdd International Conference On Knowledge Discovery And Data Mining
Dixit, P. N., & Chen, D. (2011). Effect of topography on farm-scale spatial variation in extreme temperatures in the Southern Mallee of Victoria, Australia. Theoretical and Applied Climatology, 103 , 533–542. https://doi.org/10.1007/s00704-010-0327-2
Donohue, R. J., Lawes, R. A., Mata, G., Gobbett, D., & Ouzman, J. (2018). Towards a national, remote-sensing-based model for predicting field-scale crop yield. Field Crops Research, 227 , 79–90. https://doi.org/10.1016/j.fcr.2018.08.005
Džeroski, S., & Todorovski, L. (2003). Learning population dynamics models from data and domain knowledge. Ecological Modelling, 170 (2–3), 129–140. https://doi.org/10.1016/S0304-3800(03)00221-7
Fan, X.-R., Kang, M.-Z., Heuvelink, E., de Reffye, P., & Hu, B.-G. (2015). A knowledge-and-data-driven modeling approach for simulating plant growth: A case study on tomato growth. Ecological Modelling, 312 , 363–373. https://doi.org/10.1016/j.ecolmodel.2015.06.006
Fauzan, M. A., & Murfi, H. (2018). The accuracy of XGBoost for insurance claim prediction. International Journal Advance in Soft Computing and Its Application, 10 (2), 159–171.
Filippi, P., Cattle, S. R., Bishop, T. F., Odeh, I. O., & Pringle, M. J. (2018). Digital soil monitoring of top-and sub-soil pH with bivariate linear mixed models. Geoderma, 322 , 149–162. https://doi.org/10.1016/j.geoderma.2018.02.033
Article CAS Google Scholar
Filippi, P., Jones, E., Bishop, T., Acharige, N., Dewage, S., Johnson, L., Ugbaje, S., Jephcott, T., Paterson, S., Whelan, B. (2017). A big data approach to predicting crop yield Proceedings of the 7th Asian Australasian Conference on Precision Agriculture, Retrieved from https://core.ac.uk/download/pdf/144867423.pdf
Gorelick, N., Hancher, M., Dixon, M., Ilyushchenko, S., Thau, D., & Moore, R. (2017). Google earth engine: Planetary-scale geospatial analysis for everyone. Remote Sensing of Environment, 202 , 18–27. https://doi.org/10.1016/j.rse.2017.06.031
Grundy, M., Rossel, R. V., Searle, R., Wilson, P., Chen, C., & Gregory, L. (2015). Soil and landscape grid of Australia. Soil Research, 53 (8), 835–844. https://doi.org/10.1071/SR15191
Guerschman, J. P., McVicar, T. R., Vleeshower, J., Van Niel, T. G., Peña-Arancibia, J. L., & Chen, Y. (2022). Estimating actual evapotranspiration at field-to-continent scales by calibrating the CMRSET algorithm with MODIS, VIIRS, Landsat and Sentinel-2 data. Journal of Hydrology, 605 , 127318. https://doi.org/10.1016/j.jhydrol.2021.127318
Han, S. Y., Filippi, P., Singh, K., Whelan, B. M., & Bishop, T. F. (2022). Assessment of global, national and regional-level digital soil mapping products at different spatial supports. European Journal of Soil Science, 73 (5), e13300. https://doi.org/10.1111/ejss.13300
Huber, F., Yushchenko, A., Stratmann, B., & Steinhage, V. (2022). Extreme gradient boosting for yield estimation compared with deep learning approaches. Computers and Electronics in Agriculture, 202 , 107346. https://doi.org/10.1016/j.compag.2022.107346
Hunter, J. T., & Earl, J. (1999). Floristic descriptions of grassland areas on the Moree Plains . NSW Department of Land and Water Conservation and the NSW National Parks and Wildlife Service.
Jeffrey, S. J., Carter, J. O., Moodie, K. B., & Beswick, A. R. (2001). Using spatial interpolation to construct a comprehensive archive of Australian climate data. Environmental Modelling & Software, 16 (4), 309–330. https://doi.org/10.1016/S1364-8152(01)00008-1
Johnson, M. D., Hsieh, W. W., Cannon, A. J., Davidson, A., & Bédard, F. (2016). Crop yield forecasting on the Canadian Prairies by remotely sensed vegetation indices and machine learning methods. Agricultural and Forest Meteorology, 218 , 74–84. https://doi.org/10.1016/j.agrformet.2015.11.003
Jones, E. J., Bishop, T. F., Malone, B. P., Hulme, P. J., Whelan, B. M., Filippi, P. J. C., & Agriculture, E. I. (2022). Identifying causes of crop yield variability with interpretive machine learning. Computers and Electronics in Agriculture, 192 , 106632. https://doi.org/10.1016/j.compag.2021.106632
Justice, C. O., Vermote, E., Townshend, J. R., Defries, R., Roy, D. P., Hall, D. K., Salomonson, V. V., Privette, J. L., Riggs, G., & Strahler, A. (1998). The moderate resolution imaging spectroradiometer (MODIS): Land remote sensing for global change research. IEEE Transactions on Geoscience and Remote Sensing, 36 (4), 1228–1249.
Kang, Y., Ozdogan, M., Zhu, X., Ye, Z., Hain, C., & Anderson, M. (2020). Comparative assessment of environmental variables and machine learning algorithms for maize yield prediction in the US Midwest. Environmental Research Letters, 15 (6), 064005. https://doi.org/10.1088/1748-9326/ab7df9
Kelleher, F., Rollings, N., Poulton, D., & Cornish, P. (2001). Temperature variation and frost risk in undulating cropland. Proceedings of the 10th Australian Agronomy Conference
Khan, A., Stöckle, C. O., Nelson, R. L., Peters, T., Adam, J. C., Lamb, B., Chi, J., & Waldo, S. (2019). Estimating biomass and yield using metric evapotranspiration and simple growth algorithms. Agronomy Journal, 111 (2), 536–544. https://doi.org/10.2134/agronj2018.04.0248
Kidd, D., Searle, R., Grundy, M., McBratney, A., Robinson, N., O’Brien, L., Zund, P., Arrouays, D., Thomas, M., & Padarian, J. (2020). Operationalising digital soil mapping–Lessons from Australia. Geoderma Regional, 23 , e00335. https://doi.org/10.1016/j.geodrs.2020.e00335
Ma, C., Liu, M., Ding, F., Li, C., Cui, Y., Chen, W., & Wang, Y. (2022). Wheat growth monitoring and yield estimation based on remote sensing data assimilation into the SAFY crop growth model. Scientific Reports, 12 (1), 5473. https://doi.org/10.1038/s41598-022-09535-9
Article CAS PubMed PubMed Central Google Scholar
Mokhtari, A., Noory, H., & Vazifedoust, M. (2018). Improving crop yield estimation by assimilating LAI and inputting satellite-based surface incoming solar radiation into SWAP model. Agricultural and Forest Meteorology, 250 , 159–170. https://doi.org/10.1016/j.agrformet.2017.12.250
Mu, Q., Zhao, M., & Running, S. W. (2011). Improvements to a MODIS global terrestrial evapotranspiration algorithm. Remote Sensing of Environment, 115 (8), 1781–1800. https://doi.org/10.1016/j.rse.2011.02.019
Nielsen, D. (2016). Tree boosting with xgboost-why does xgboost win" every" machine learning competition? NTNU.
Padarian, J., Morris, J., Minasny, B., & McBratney, A. B. (2018). Pedotransfer functions and soil inference systems. In Pedometrics (pp. 195-220). Springer.
Reichstein, M., Camps-Valls, G., Stevens, B., Jung, M., Denzler, J., Carvalhais, N., & Prabhat, F. (2019). Deep learning and process understanding for data-driven earth system science. Nature, 566 (7743), 195–204. https://doi.org/10.1038/s41586-019-0912-1
Article CAS PubMed Google Scholar
Smith, A. M., Bourgeois, G., Teillet, P. M., Freemantle, J., & Nadeau, C. (2008). A comparison of NDVI and MTVI2 for estimating LAI using CHRIS imagery: A case study in wheat. Canadian Journal of Remote Sensing, 34 (6), 539–548. https://doi.org/10.5589/m08-071
Taylor, J., McBratney, A., & Whelan, B. (2007). Establishing management classes for broadacre agricultural production. Agronomy Journal, 99 (5), 1366–1376. https://doi.org/10.2134/agronj2007.0070
Tewes, A., Hoffmann, H., Krauss, G., Schäfer, F., Kerkhoff, C., & Gaiser, T. (2020). New approaches for the assimilation of LAI measurements into a crop model ensemble to improve wheat biomass estimations. Agronomy, 10 (3), 446. https://doi.org/10.3390/agronomy10030446
Thornton, P. K., Jones, P. G., Alagarswamy, G., & Andresen, J. (2009). Spatial variation of crop yield response to climate change in East Africa. Global Environmental Change, 19 (1), 54–65. https://doi.org/10.1016/j.gloenvcha.2008.08.005
Todorovski, L., & Džeroski, S. (2006). Integrating knowledge-driven and data-driven approaches to modeling. Ecological Modelling, 194 (1–3), 3–13. https://doi.org/10.1016/j.ecolmodel.2005.10.001
Wimalathunge, N., & Bishop, T. (2019). A space-time observation system for soil moisture in agricultural landscapes. Geoderma, 344 , 1–13. https://doi.org/10.1016/j.geoderma.2019.03.002
Young, R., & Schwenke, T. (2013). Transition to Zero Tillage: A Survey of Farming Practices up until 2003 on the North West Slopes and Plains of NSW. 56 pp. Addendum to Final Report to the GRDC for project DAN 00027 ‘By how much can water use efficiency be increased and deep drainage reduced by optimal cropping system management on Vertosols in North Western NSW’. NSW Department of Primary Industries Tamworth Agricultural Institute Tamworth NSW Australia. Farming Practices in North Western NSW, 3 , 3.
Ziliani, M. G., Altaf, M. U., Aragon, B., Houborg, R., Franz, T. E., Lu, Y., Sheffield, J., Hoteit, I., & McCabe, M. F. (2022). Early season prediction of within-field crop yield variability by assimilating CubeSat data into a crop model. Agricultural and Forest Meteorology, 313 , 108736. https://doi.org/10.1016/j.agrformet.2021.108736
Download references
Acknowledgements
The corresponding author would like to gratefully acknowledge the financial support (CSIRO/Data61 Postgraduate Research Stipend and Supplementary Scholarship in Digital Agriculture) from the Commonwealth Scientific and Industrial Research Organisation (CSIRO). This research was also funded by the University of Sydney, and partly funded by the Cotton Research and Development Corporation (CRDC).
Open Access funding enabled and organized by CAUL and its Member Institutions. The work was supported by CSIRO/Data61.
Author information
Authors and affiliations.
Precision Agriculture Laboratory, School of Life and Environmental Sciences, Sydney Institute of Agriculture, The University of Sydney, Sydney, NSW, Australia
Dhahi Al-Shammari, Niranjan S. Wimalathunge, Si Yang Han & Thomas F. A. Bishop
Department of Transport and Planning, Victoria, Australia
CSIRO Data61, Eveleigh, NSW, 2015, Australia
You can also search for this author in PubMed Google Scholar
Contributions
Conceptualization & methodology: Dhahi Al-Shammari, Thomas F.A. Bishop; Material preparation & data collection: Dhahi Al-Shammari, Si Yang Han, Niranjan S. Wimalathunge; Statistical analysis: Dhahi Al-Shammari; Writing—original draft preparation: Dhahi Al-Shammari, Niranjan S. Wimalathunge, Si Yang Han; Review & editing: Dhahi Al-Shammari, Thomas F.A. Bishop, Si Yang Han; Supervision: Thomas F.A. Bishop, Chen Wang.
Corresponding author
Correspondence to Dhahi Al-Shammari .
Ethics declarations
Conflict of interest.
The authors have no competing interests to declare that are relevant to the content of this article.
Additional information
Publisher's note.
Springer Nature remains neutral with regard to jurisdictional claims in published maps and institutional affiliations.
Rights and permissions
Open Access This article is licensed under a Creative Commons Attribution 4.0 International License, which permits use, sharing, adaptation, distribution and reproduction in any medium or format, as long as you give appropriate credit to the original author(s) and the source, provide a link to the Creative Commons licence, and indicate if changes were made. The images or other third party material in this article are included in the article's Creative Commons licence, unless indicated otherwise in a credit line to the material. If material is not included in the article's Creative Commons licence and your intended use is not permitted by statutory regulation or exceeds the permitted use, you will need to obtain permission directly from the copyright holder. To view a copy of this licence, visit http://creativecommons.org/licenses/by/4.0/ .
Reprints and permissions
About this article
Al-Shammari, D., Chen, Y., Wimalathunge, N.S. et al. Incorporation of mechanistic model outputs as features for data-driven models for yield prediction: a case study on wheat and chickpea. Precision Agric (2024). https://doi.org/10.1007/s11119-024-10184-3
Download citation
Accepted : 23 August 2024
Published : 04 September 2024
DOI : https://doi.org/10.1007/s11119-024-10184-3
Share this article
Anyone you share the following link with will be able to read this content:
Sorry, a shareable link is not currently available for this article.
Provided by the Springer Nature SharedIt content-sharing initiative
- Within-field variation
- Soil moisture
- Precision agriculture
- Find a journal
- Publish with us
- Track your research
Information
- Author Services
Initiatives
You are accessing a machine-readable page. In order to be human-readable, please install an RSS reader.
All articles published by MDPI are made immediately available worldwide under an open access license. No special permission is required to reuse all or part of the article published by MDPI, including figures and tables. For articles published under an open access Creative Common CC BY license, any part of the article may be reused without permission provided that the original article is clearly cited. For more information, please refer to https://www.mdpi.com/openaccess .
Feature papers represent the most advanced research with significant potential for high impact in the field. A Feature Paper should be a substantial original Article that involves several techniques or approaches, provides an outlook for future research directions and describes possible research applications.
Feature papers are submitted upon individual invitation or recommendation by the scientific editors and must receive positive feedback from the reviewers.
Editor’s Choice articles are based on recommendations by the scientific editors of MDPI journals from around the world. Editors select a small number of articles recently published in the journal that they believe will be particularly interesting to readers, or important in the respective research area. The aim is to provide a snapshot of some of the most exciting work published in the various research areas of the journal.
Original Submission Date Received: .
- Active Journals
- Find a Journal
- Proceedings Series
- For Authors
- For Reviewers
- For Editors
- For Librarians
- For Publishers
- For Societies
- For Conference Organizers
- Open Access Policy
- Institutional Open Access Program
- Special Issues Guidelines
- Editorial Process
- Research and Publication Ethics
- Article Processing Charges
- Testimonials
- Preprints.org
- SciProfiles
- Encyclopedia
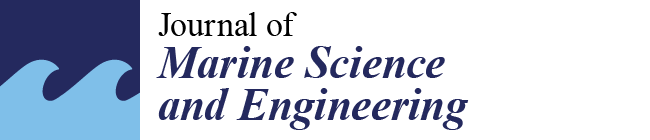
Article Menu
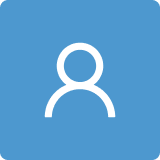
- Subscribe SciFeed
- Recommended Articles
- Google Scholar
- on Google Scholar
- Table of Contents
Find support for a specific problem in the support section of our website.
Please let us know what you think of our products and services.
Visit our dedicated information section to learn more about MDPI.
JSmol Viewer
Hydroelasto-plastic response of a ship model in freak waves: an experimental and numerical investigation.
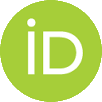
1. Introduction
1.1. background, 1.2. recent studies on hydroelasto-plasticity generated by freak waves, 1.2.1. generation of freak wave, 1.2.2. fsi of freak wave and ship structure, 1.2.3. hydroelasto-plasticity, 1.3. challenges of studying hydroelasto-plastic responses caused by freak waves, 1.4. objectives of this paper, 2. hydroelasto-plastic model experiment of a ship structure in freak waves, 2.1. model description, 2.2. experimental facilities, 2.3. experimental cases, 3. numerical methodology, 3.1. hydroelasto-plastic numerical framework, 3.2. peregrine breather solution theory solved from nonlinear schrödinger’s equation, 3.3. nonlinear fem, 3.4. two-way hydroelasto-plastic coupling cfd and nonlinear fem, 4. numerical modelling, 4.1. generation of numerical freak wave, 4.2. cfd model, 4.3. numerical nonlinear fem model, 5. results analysis, 5.1. wave elevation analysis, 5.2. rotational deformation analysis, 6. discussion, 7. conclusions, author contributions, data availability statement, conflicts of interest.
- Haver, S.; Vestbostad, T.M.; Andersen, O.J.; Jakobsen, J.B. Freak waves and their conditional probability problem. In Proceedings of the Fourteenth International Ship and Polar Engineering Conference, Toulon, France, 23–28 May 2004. [ Google Scholar ]
- Haver, S. Freak Waves ; Ifremer: Brest, France, 2001; pp. 129–140. [ Google Scholar ]
- Haver, S. Freak Wave Event at Draupner Jacket January 1 1995 ; Statoil Technical: Stavanger, Norway, 2003. [ Google Scholar ]
- Gunson, J.; Lehner, S.; Bitner-Gregersen, E. Extreme Wave Conditions from Wave Model Hindcasts and from Synthetic Aperture Radar Images. In Proceedings of the International Conference on Design and Operation for Abnormal Conditions II, London, UK, 6–7 November 2001. [ Google Scholar ]
- Müller, P.; Garrett, C.; Osborne, A. Freak waves. Oceanography 2005 , 18 , 66. [ Google Scholar ] [ CrossRef ]
- Anders, M. Update on incident involving the containership MOL Confort. Am. J. Transp. 2013 , 558 , 19. [ Google Scholar ]
- Vikram, M. Ukrainian Cargo Ship Arvin Sinks off Black Sea. Available online: https://maritime.direct/en/2021/01/18/ukrainian-cargo-ship-arvin-sinks-off-black-sea/ (accessed on 18 January 2021).
- Kriebel, D.L.; Alsina, M.V. Simulation of freak waves in a background random sea. In Proceedings of the Tenth International Ship and Polar Engineering Conference, Seattle, WA, USA, 27 May–2 June 2000. [ Google Scholar ]
- Kim, N.; Kim, C.H. Investigation of a dynamic property of Draupner freak wave. Int. J. Ship Polar Eng. 2003 , 13 , 1–12. [ Google Scholar ]
- Sheng, Y. Numerical Simulation of Freak Wave and the Interactions Between Freak Wave and Ship Structure. Master’s Thesis, Shanghai Jiaotong University, Shanghai, China, 2013. [ Google Scholar ]
- Waseda, T.; Rheem, C.K.; Sawamura, J.; Yuhara, T.; Kinoshita, T.; Tanizawa, K.; Tomita, H. Freak wave generation in Laboratory Wave Tank. In Proceedings of the 15th International Ship and Polar Engineering Conference, Seoul, Republic of Korea, 19–24 June 2005. [ Google Scholar ]
- Hu, Z.; Tang, W.; Xue, H.; Zhang, X. Numerical study of Freak waves as nonlinear Schrödinger breather solutions under finite water depth. Wave Motion 2015 , 52 , 81–90. [ Google Scholar ] [ CrossRef ]
- Chabchoub, A.; Hoffmann, N.; Akhmediev, N. Freak wave observation in a water wave tank. Phys. Rev. Lett. 2011 , 106 , 204502. [ Google Scholar ] [ CrossRef ] [ PubMed ]
- Zakharov, V.E.; Dyachenko, A.I.; Prokofiev, A.O. Freak waves as nonlinear stage of Stokes wave modulation instability. Eur. J. Mech. B Fluids 2006 , 25 , 677–692. [ Google Scholar ] [ CrossRef ]
- Didenkulova, I.I.; Nikolkina, I.F.; Pelinovsky, E.N. Freak waves in the basin of intermediate depth and the possibility of their formation due to the modulational instability. JETP Lett. 2013 , 97 , 194–198. [ Google Scholar ] [ CrossRef ]
- Clauss, G.F.; Kauffeldt, A.; Klein, M. Systematic investigation of loads and motions of a bulk carrier in extreme seas. In Proceedings of the 28th International Conference on Ship Mechanics and Arctic Engineering, Honolulu, HI, USA, 31 May–5 June 2009; Volume 43444, pp. 277–287. [ Google Scholar ]
- Shi, J.S.; Takuji, W.; Katsuyuki, S.; Takeshi, K.; Tetsuo, Y. Wave Loads on Container Ship in Freak Waves ; The Japan Society of Naval Architects and Ocean Engineers: Tokyo, Japan, 2006. [ Google Scholar ]
- Kinoshita, T. Longitudinal Loads on a Container Ship in Extreme Regular Sea and Freak Wave. In Proceedings of the 4th International Conference on Hydroelasticity in Marine Technology, Wuxi, China, 10–14 September 2006. [ Google Scholar ]
- Holst, A.; Gunnar, D.; Celine, F. CFD Analysis of Wave-Induced Loads on Tidal Turbine Blades. IEEE J. Ocean. Eng. 2015 , 40 , 506–521. [ Google Scholar ] [ CrossRef ]
- Wang, J.; Hao, Q.; Zhe, H.; Lin, M. Three-dimensional study on the interaction between a container ship and freak waves in beam sea. Int. J. Nav. Archit. Ocean. Eng. 2022 , 15 , 100509. [ Google Scholar ] [ CrossRef ]
- Liu, Y. Hydroelastic Response of Large Floating Structure in Nonlinear Waves. Master’s Thesis, Jiangsu University of Technology, Zhenjiang, China, 2020. [ Google Scholar ]
- Masaoka, K.; Okada, H. A numerical approach for ship hull girder collapse behavior in waves. In Proceedings of the Thirteenth International Ship and Polar Engineering Conference, Honolulu, HI, USA, 25–30 May 2003. [ Google Scholar ]
- Iijima, K.; Kimura, K.; Xu, W.; Fujikubo, M. Hydroelasto-plasticity approach to predicting the post- ultimate strength behavior of a ship’s hull girder in waves. J. Mar. Sci. Technol. 2011 , 16 , 379–389. [ Google Scholar ] [ CrossRef ]
- Lee, S.; You, J.; Lee, H.; Lim, T.; Park, S.; Seo, J.; Rhee, S.; Rhee, K. Experimental Study on the Six Degree-of-Freedom Motions of a Damaged Ship Floating in Regular Waves. IEEE J. Ocean. Eng. 2016 , 41 , 40–49. [ Google Scholar ]
- Liu, W.; Song, X.; Wu, W.; Suzuki, K. Strength of a Container Ship in Freak waves Obtained by Nonlinear Hydroelasto-plasticity Dynamic Analysis and Finite Element Modeling. J. Ship Mech. Arct. Eng. 2016 , 138 , 031602. [ Google Scholar ]
- Liu, W.; Huang, Y.; Li, Y.; Song, X.; Wei, F.; Wu, X. Numerical and experimental investigation on nonlinear cyclic collapse response of ship model in regular waves. J. Ship Mech. Arct. Eng. 2021 , 143 , 041702. [ Google Scholar ] [ CrossRef ]
- Liu, W.; Luo, W.; Yang, M.; Xia, T.; Huang, Y.; Wang, S.; Li, Y. Development of a fully coupled numerical hydroelasto-plastic approach for ship structure. Ocean Eng. 2022 , 258 , 111713. [ Google Scholar ] [ CrossRef ]
- Liu, W.; Song, X.; Pei, Z.; Li, Y. A Hydroelasto-buckling Experiment Study of Ship Model in Single Wave. Ocean Eng. 2017 , 142 , 102–114. [ Google Scholar ] [ CrossRef ]
- Mei, C.C. The Applied Dynamics of Ocean Surface Waves ; World Scientific: Singapore, 1989; Volume 1. [ Google Scholar ]
Click here to enlarge figure
Case | Wave Height (m) | Wavelength/Model Length | Wavelength (m) | Period (s) |
---|---|---|---|---|
H1 | 0.05 | 1 | 1.6 | 1.0123 |
H2 | 0.07 | 1 | 1.6 | 1.0123 |
H3 | 0.09 | 1 | 1.6 | 1.0123 |
H4 | 0.11 | 1 | 1.6 | 1.0123 |
L2 | 0.11 | 1.5 | 2.4 | 1.2398 |
L3 | 0.11 | 2 | 3.2 | 1.4316 |
L4 | 0.11 | 3 | 4.8 | 1.7534 |
Ultimate Sagging BM (N·mm) | Critical Rotational Angle (°) | Ultimate Hogging BM (N·mm) | Critical Rotational Angle (°) | |
---|---|---|---|---|
Experiment | 1850 | 0.121 | −10269 | −0.3048 |
Simulation | 1792 | 0.101 | −10631 | −0.3811 |
The statements, opinions and data contained in all publications are solely those of the individual author(s) and contributor(s) and not of MDPI and/or the editor(s). MDPI and/or the editor(s) disclaim responsibility for any injury to people or property resulting from any ideas, methods, instructions or products referred to in the content. |
Share and Cite
Liu, W.; Mo, Y.; Xiong, L.; Xu, H.; Song, X.; Li, Y. Hydroelasto-Plastic Response of a Ship Model in Freak Waves: An Experimental and Numerical Investigation. J. Mar. Sci. Eng. 2024 , 12 , 1555. https://doi.org/10.3390/jmse12091555
Liu W, Mo Y, Xiong L, Xu H, Song X, Li Y. Hydroelasto-Plastic Response of a Ship Model in Freak Waves: An Experimental and Numerical Investigation. Journal of Marine Science and Engineering . 2024; 12(9):1555. https://doi.org/10.3390/jmse12091555
Liu, Weiqin, Yining Mo, Luonan Xiong, Haodong Xu, Xuemin Song, and Ye Li. 2024. "Hydroelasto-Plastic Response of a Ship Model in Freak Waves: An Experimental and Numerical Investigation" Journal of Marine Science and Engineering 12, no. 9: 1555. https://doi.org/10.3390/jmse12091555
Article Metrics
Further information, mdpi initiatives, follow mdpi.
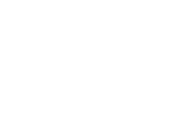
Subscribe to receive issue release notifications and newsletters from MDPI journals
Thank you for visiting nature.com. You are using a browser version with limited support for CSS. To obtain the best experience, we recommend you use a more up to date browser (or turn off compatibility mode in Internet Explorer). In the meantime, to ensure continued support, we are displaying the site without styles and JavaScript.
- View all journals
- Explore content
- About the journal
- Publish with us
- Sign up for alerts
- Open access
- Published: 30 August 2024
Machine learning surrogate for 3D phase-field modeling of ferroelectric tip-induced electrical switching
- Kévin Alhada–Lahbabi ORCID: orcid.org/0009-0006-5514-0088 1 ,
- Damien Deleruyelle 1 &
- Brice Gautier 1
npj Computational Materials volume 10 , Article number: 197 ( 2024 ) Cite this article
Metrics details
- Computational methods
- Electronic devices
Phase-field modeling offers a powerful tool for investigating the electrical control of the domain structure in ferroelectrics. However, its broad application is constrained by demanding computational requirements, limiting its utility in inverse design scenarios. Here, we introduce a machine-learning surrogate to accelerate 3D phase-field modeling of tip-induced electrical switching. By dynamically handling the boundary conditions, the surrogate achieves accurate reproduction of switching trajectories under various tip locations and applied voltages. With stable predictions throughout entire morphological evolution pathways and a relative error inferior to 10% compared to direct solvers, the model efficiently emulates intricate switching sequences. By successfully replicating the boundary conditions, the presented framework strides towards a holistic surrogate for the ferroelectric phase field. With up to 2500-fold speed-ups over classical methods, our approach opens the path for the tractable design of the domain structure and the resolution of realistic inverse problems.
Similar content being viewed by others
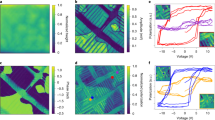
Experimental discovery of structure–property relationships in ferroelectric materials via active learning
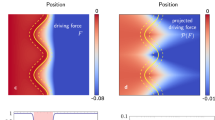
Phase field modeling with large driving forces
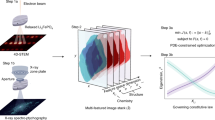
Correlative image learning of chemo-mechanics in phase-transforming solids
Introduction.
Ferroelectric thin films hold promise for the future of modern nanoelectronic devices 1 . Given their potential applications in nonvolatile memories 2 , 3 , extensive research efforts have been directed towards manipulating the domain structure, employing either electrical 4 , 5 or mechanical stresses to accomplish domain switching 6 , 7 .
In recent years, the manipulation of ferroelectric domain walls (DWs) has garnered substantial attention, revealing topological entities with distinct properties compared to traditional ferroelectric domains 4 , 8 , 9 , 10 . Specifically, the observed electrical conductivity near DWs has prompted the emergence of DW nanoelectronics, enabling information storage in these regions rather than within the domains themselves. However, DW memory devices hinge on strategic wall placement, thereby requiring precise control of domain states. Currently, DW engineering often employs electrode setups and metallic scanning probe tips, strategically triggering electrical switching to design domain structures 4 , 8 . Yet, polarization reversal typically exhibits intricate dynamics 2 , 3 , underscoring the necessity for a comprehensive understanding of ferroelectric switching mechanisms.
Phase-field modeling stands out as a prominent mesoscale computational technique, offering valuable physical insights into ferroelectric materials 11 , 12 , 13 . Based on energetic considerations, it is commonly employed to elucidate the domain dynamics encountered in experimental scenarios 10 , 14 , 15 , 16 , 17 . However, its broader adoption is impeded by the substantial computational cost associated with solving complex partial differential equations (PDEs), underscoring the need for faster alternative methods.
Nowadays, machine-learning surrogate models have garnered significant attention for expediting phase-field simulation, due to their capacity to swiftly infer solutions for complex systems of PDEs 18 , 19 , 20 , 21 , 22 , 23 , 24 , 25 , 26 , 27 , 28 , 29 , 30 , 31 , 32 , 33 . These surrogate models, often designed as explicit time-steppers, forecast the subsequent microstructural state based on information from the current input state.
A common approach involves employing dimensionality reduction techniques, such as principal component analysis (PCA) or autoencoders (AE), thus facilitating more efficient learning of trajectory dynamics 19 , 24 , 27 , 29 . For instance, Montes de Oca Zapiain et al. introduced a framework utilizing PCA and recurrent neural networks, demonstrating high accuracy and remarkable speedups in emulating the two-phase mixture problem 19 .
Alternatively, some research groups have opted for convolutional neural networks (CNNs) as surrogate models 18 , 27 , 29 , 30 . Leveraging the inherent image-based structure of phase-field microstructures, CNNs utilize morphology grid representations directly as input. Recent investigations highlight their potential in successfully inferring ferroelectric microstructural evolutionary pathways 18 .
A distinct strategy for developing machine-learning emulators involves physics-informed neural networks (PINNs) 22 , 34 . PINNs incorporate system-specific physical knowledge during training, constructing a physically constrained loss function, and have shown remarkable efficacy in addressing PDE-based problems 35 , 36 . A recent milestone by Lu et al. unveiled Deep Operator Networks (DeepONet), an innovative framework adept at learning the intrinsic nonlinear operator directly from the data 21 . DeepONet-based approaches have successfully been applied to general phase-field problems, where they exploit the free energy as a physics-informed loss function 20 , 37 .
The recent synergy of phase-field modeling and reinforcement learning (RL) has yielded breakthrough results in material inverse design 38 , 39 , 40 . In this context, the specified microstructure serves as the target state, while an RL agent, able to manipulate boundary conditions, learns and implements an optimal strategy to achieve this configuration. In a recent study, Vasudevan et al. explored the application of RL for microstructure optimization, aiming to uncover the physical mechanisms behind enhanced material properties 40 . Utilizing a 2D phase-field model, RL agents were assigned the task of reaching energetically unfavorable configurations, leading to the development of non-intuitive strategies for material design optimization.
In a notable advancement, Smith et al. employed RL to electrically design domain structures using a piezoresponse force microscopy (PFM) tip in an automated manner 41 . By constructing a physical surrogate of domain dynamics based on extensive PFM experiments, they trained an RL agent to optimize tip trajectories to replicate target DW structures. While their experimental surrogate yielded impressive results, employing phase-field modeling as the physical environment for trajectory optimization could extend exploration to more diverse situations and complex phenomena. Unfortunately, traditional phase-field methods are considered prohibitively expensive for such scenarios, given RL’s requirement for thousands to millions of state transitions for meaningful policy learning, as highlighted by the authors. The development of fast surrogate models is therefore crucial to fully leverage RL’s potential and expedite material inverse design tasks.
In a prior study, we introduced a novel CNN-based surrogate to the ferroelectric phase field to efficiently infer the temporal evolution of domain formation in PbZr x Ti 1− x O 3 (PZT) in 2D 18 . By incorporating physical biases, our model achieves accurate long-term forecasts of morphological trajectories, offering over 600× speedup compared to high-fidelity solvers. Unfortunately, the framework was limited to 2D domain formation with static boundary conditions. To address scenarios involving the electrical design of the domain structure, a 3D surrogate capable of replicating time-evolving boundary conditions becomes necessary.
In this work, we introduce a machine learning approach to significantly accelerate 3D phase-field modeling of tip-induced electrical switching. Our framework incorporates dynamic boundary conditions to accurately capture domain dynamics across diverse morphological evolution pathways under complex electrical switching trajectories. Notably, the model successfully emulates tip-induced switching for various tip locations, applied voltages, and application times. Demonstrating high accuracy, with a relative error below 10% compared to traditional phase-field methods, and achieving an acceleration factor of up to 2500, our model serves as a computationally efficient surrogate for investigating the electrical control of polarization in both direct and inverse problems.
Learning tip-induced electrical switching with machine-learning
In this study, our primary goal is to develop a surrogate capable of accurately reproducing the electrical reversal of polarization induced by an atomic force microscopy (AFM) tip. Moreover, for flexible application across diverse situations, the model must handle arbitrary tip placements on the film surface and a broad spectrum of specified voltages. In this section, we focus on introducing the methodology used to forecast electrical domain switching trajectories using machine learning.
Surrogate model operation
In ferroelectric phase-field modeling, the temporal evolution of the microstructure is governed by the time-dependent Ginzburg–Landau (TDGL) equation 12
where \({\mathcal{P}}({\boldsymbol{r}},t)\) is the spontaneous polarization, L is the kinetic coefficient, and ψ signifies the total free energy. For a detailed description of the phase-field methodology and incorporation of the tip-induced electrical boundary conditions, please refer to the dedicated in the “Methods” section.
This study presents a surrogate model designed as an explicit time-stepper to replace the TDGL equation. Based on the current state \({X}^{{t}_{k}}\) at time t k , the model forecasts the subsequent microstructural state \({X}^{{t}_{k+1}}\) at time t k +1 through the operation:
in which \({\mathcal{S}}\) is an operation representing the neural network’s forward pass.
The microstructural morphology can be effectively characterized at any time t k by the polarization components [ \({{\mathcal{P}}}_{x}^{{t}_{k}}\) , \({{\mathcal{P}}}_{y}^{{t}_{k}}\) , \({{\mathcal{P}}}_{z}^{{t}_{k}}\) ] and the electrostatic potential \({{\mathcal{V}}}^{{t}_{k}}\) 18 . To accommodate changes in boundary conditions along the domain-switching trajectory, the machine-learning framework also receives the tip-related boundary conditions as inputs at time t k . Specifically, the tip location \([{y}_{{\rm {tip}}}^{{t}_{k}},{z}_{{\rm {tip}}}^{{t}_{k}}]\) , and prescribed voltage \({u}_{{\rm {T}}}^{{t}_{k}}\) , are incorporated to succinctly characterize the tip’s action. Thus, the microstructural state representation at time t k can be expressed as
The surrogate must then adeptly learn to predict the microstructure one time-step Δ t ahead:
By iteratively using its predictions, the network can generate rollout predictions from the initial state at t 0 to the final time t N across times t = { t 0 , …, t N }, formally expressed as
To mimic the incremental update of the polarization field governed by the TDGL equation, the polarization output [ \({{\mathcal{P}}}_{x}^{{t}_{k+1}}\) , \({{\mathcal{P}}}_{y}^{{t}_{k+1}}\) , \({{\mathcal{P}}}_{z}^{{t}_{k+1}}\) ] was calculated using the residual learning approach, consistent with a previous study 18 .
Surrogate model architecture
The surrogate model employed a 3D CNN based on an encoder–decoder architecture, similar to an anterior work 18 . Specifically, we adopted a 3D U-Net with skip connections, a well-established architecture in computer vision 42 .
At each time step, the model receives the current microstructural state as input, denoted \({X}^{{t}_{k}}\) . It is important to note that the boundary conditions, represented by \({[{u}_{{\rm {T}}},{y}_{{\rm {tip}}},{z}_{{\rm {tip}}}]}^{{t}_{k}}\) are scalar values, whereas the concatenation \({[{{\mathcal{P}}}_{x},{{\mathcal{P}}}_{y},{{\mathcal{P}}}_{z},{\mathcal{V}}]}^{{t}_{k}}\) follows the grid shape [ N x , N y , N z , 4]. During prediction, these scalar boundary conditions are directly integrated into the encoder’s latent space.
Initially, the \({[{{\mathcal{P}}}_{x},{{\mathcal{P}}}_{y},{{\mathcal{P}}}_{z},{\mathcal{V}}]}^{{t}_{k}}\) inputs are fed into the encoder, extracting essential features, and encoding them into a 1D latent vector. At this stage, the scalar boundary conditions are concatenated with the latent encoding. This combined representation is then fed into a multi-layer perceptron (MLP) for further information processing within the latent space. Subsequently, the decoder progressively upsamples the latent information back to the original input shape, ultimately predicting the subsequent state \({X}^{{t}_{k+1}}={[{{\mathcal{P}}}_{x},{{\mathcal{P}}}_{y},{{\mathcal{P}}}_{z},{\mathcal{V}}]}^{{t}_{k+1}}\) at the next time step. Detailed information about the network architecture, including a comprehensive report of the hyperparameters used in different model layers, is provided in Supplementary Note 1 .
Training loss error
In this work, the model was trained in a supervised fashion, employing the \({{\mathcal{L}}}_{2}\) error loss function as detailed in the “Methods” section. The training loss is expressed as
Here, \({Y}^{{t}_{k+1}}\) represents the microstructure labels obtained from high-fidelity phase-field simulations and \({\mathcal{S}}({X}^{t})\) denotes the model outputs. Specifically, the loss formulation involves a contribution of the output components:
where \({{\mathcal{L}}}_{2}^{{{\mathcal{P}}}_{x}}\) , \({{\mathcal{L}}}_{2}^{{{\mathcal{P}}}_{y}}\) , \({{\mathcal{L}}}_{2}^{{{\mathcal{P}}}_{z}}\) and \({{\mathcal{L}}}_{2}^{{\mathcal{V}}}\) denote the polarization and electrostatic potential components of the total loss, and the subscripts distinguish between the variable components.
Electrical switching prediction of c + /c − domains
In this section, we begin by first demonstrating our approach with a PZT thin film vertically oriented along the (001) direction as a representative model, a common system for studying domain switching dynamics 43 , 44 , 45 . In these structures, the significant lattice compressive mismatch (see the “Methods” section) promotes a domain structure comprised solely of vertical c + /c − domains. Consequently, solely the out-of-plane component of the polarization \({{\mathcal{P}}}_{x}\) and the electrostatic potential \({\mathcal{V}}\) are considered as microstructural inputs within this section.
This section details the construction of a diverse and representative dataset of tip-induced switching trajectories, crucial to guarantee comprehensive learning of electrical switching dynamics. Aiming to construct a surrogate model for designing electrical domain structures, each trajectory was initiated with a single vertically oriented monodomain. At every grid point, the polarization was uniformly set either to— P c 0 or P c 0 , with a randomly assigned direction (Upward or Downward) for each simulation. Importantly, applying uniform electrical poling for a sufficient duration readily achieves these desired states, offering a realistic starting point for designing the domain state.
Each trajectory consisted of 10 distinct switching events, each programmed to last 200Δ t , where Δ t denotes the time-step employed in the phase-field simulations. Consequently, the total simulation duration spanned 2000Δ t . For each event, the tip location ( y tip , z tip ) was randomly chosen on the sample surface, as depicted in Fig. 1 a, b. The prescribed voltage u T was randomly selected from the distribution shown in Fig. 1 c. This distribution encompassed a range of voltages leading to electric fields approaching and exceeding the film’s coercive field, thereby ensuring frequent occurrences of electrical switching. Notably, voltages corresponding to electric fields below the coercive field, incapable of inducing domain reversal, were also included to train the model on the nuanced relationship between applied voltage and domain dynamics.
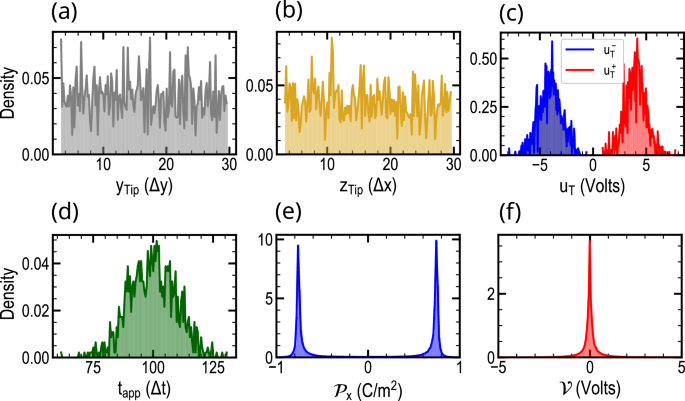
a and b tip locations ( y tip − z tip ), c prescribed voltage of the AFM tip ( u T ), d tip application time ( t app ), e ferroelectric polarization ( \({{\mathcal{P}}}_{x}\) ), and f electrostatic potential ( \({\mathcal{V}}\) ) distributions.
Additionally, within each switching event spanning 200Δ t , the tip application time t app is randomly selected from the distribution shown in Fig. 1 d. This distribution covers a range of approximately 50Δ t –150Δ t , representing diverse tip interaction durations. Throughout the remaining timesteps of each event, the domain undergoes relaxation without any applied voltage. This methodology ensures the model learns not only the temporal aspects of electrical switching but also the subsequent domain dynamics, including potential outcomes such as domain nucleation on the black electrode or back-switching to the initial state.
The microstructure state was then recorded at uniformly spaced time intervals of 20Δ t , resulting in trajectories comprising 100 frames per simulation ({ t 0 , …, t 100 }). Here, we conducted 1400 phase-field simulations to model ferroelectric domain switching on a system size of N x × N y × N z = 16 × 32 × 32, producing \([{{\mathcal{P}}}_{x}^{{t}_{k}},{{\mathcal{V}}}^{{t}_{k}}]\) sequences with a shape of (100, 16, 32, 32, 2). The tip-related electrical boundary conditions [ y tip , z tip , u T ] were recorded at the same intervals along the trajectory as scalar values, resulting in a tensor of shape (100, 3). The dataset was subsequently divided into a training dataset (1000 simulations), a validation dataset (200 simulations), and a test dataset (200 simulations).
Figure 1 e, f illustrates the distributions of polarization \({{\mathcal{P}}}_{x}\) and electrostatic potential \({\mathcal{V}}\) within the training dataset. An overview of a typical electrical domain switching trajectory from the training dataset is presented in Fig. 2 a, depicting the evolution of the polarization and electrostatic potential variables, through a sequence of 10 tip-induced switching events.
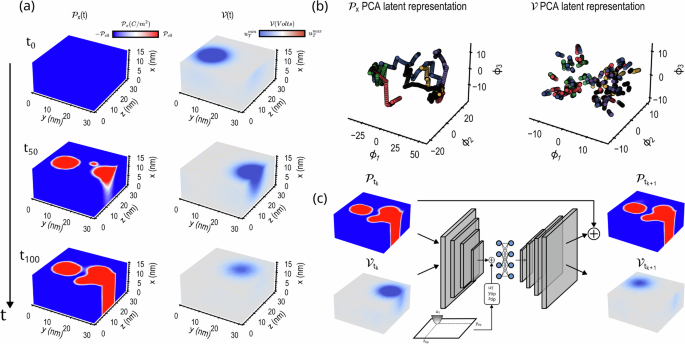
a Evolution of the polarization \({{\mathcal{P}}}_{x}\) and electrostatic potential \({\mathcal{V}}\) fields throughout a trajectory comprising 10 tip-induced switching events. b Visualization of selected trajectory examples for the \({{\mathcal{P}}}_{x}\) and \({\mathcal{V}}\) variables in the PCA lower-dimensional space. c The surrogate model takes the microstructure ( \({[{{\mathcal{P}}}_{x},{\mathcal{V}}]}^{{t}_{k}}\) ) and tip-related boundary conditions (prescribed voltage u T and tip locations [ y tip , z tip ]) at time t k as input to predict the next microstructural state at t k +1 .
The inherently complex nature of phase-field simulations generates highly intricate and nonlinear trajectories. In this study, we employed principal component analysis (PCA) to facilitate a clear and concise visualization of the switching trajectories 18 , 19 (Details on PCA are given in Supplementary Note 2 ). Figure 2 b illustrates five arbitrarily chosen training dataset trajectories in the low-dimensional space delineated by the initial three principal components, for the polarization and electrostratic potential. In this representation, each switching event within a trajectory is characterized by abrupt directional changes, facilitating the observation of alterations in the boundary conditions across the simulation. Furthermore, this visualization approach effectively underscores the extensive diversity of scenarios encompassed within the generated datasets. Finally, the surrogate model architecture specifically tailored for the prediction of electrical switching in c + /c − domains is presented in Fig. 2 c.
The model was trained on the 1200 structures comprising the training/validation dataset over 100 epochs (refer to the “Methods” section for Training details). The training history, illustrated in Supplementary Fig. 1 , depicts the evolution of the total \({{\mathcal{L}}}_{2}\) loss, as well as its two components ( \({{\mathcal{L}}}_{2}^{{{\mathcal{P}}}_{x}}\) and \({{\mathcal{L}}}_{2}^{{\mathcal{V}}}\) ), during the training process. Following training, the model’s performance was evaluated on the 200 test simulations, assessing the model’s ability to accurately forecast the microstructure using both direct one-step and long-timestep rollout strategies.
Evaluation of one-step prediction
The performance of one-step predictions is quantified using the \({{\mathcal{L}}}_{2}\) mean squared error (MSE) and \({{\mathcal{L}}}_{1}\) mean absolute error (MAE) metrics in Table 1 for the two output fields. Detailed metric computation procedures are described in the “Methods” section.
The model demonstrates remarkable accuracy in forecasting subsequent microstructural states during electrical switching, achieving a quantitative MAE of 2.86 × 10 −3 C/m 2 for the polarization field and 0.11 mV for the electrostatic potential. MSE values are also notably low, at 1.10 × 10 −5 and 7.79 × 10 −5 for \({{\mathcal{P}}}_{x}\) and \({\mathcal{V}}\) , respectively, highlighting the model’s ability to capture the influence of boundary condition modifications and anticipate domain state dynamics.
While a model demonstrates accuracy for one-step predictions, it may not necessarily effectively replace high-fidelity methods, especially for longer time frames requiring full simulation unfolding. This can lead to a consistent accumulation of errors over the trajectories, highlighting the crucial need for robust models. Therefore, a thorough assessment is essential to evaluate the model’s ability to sustain error accumulation and ensure stable predictions over long time intervals.
Evaluation of unrolled prediction
For the rollout trajectories, simulations were initiated from diverse initial frames to evaluate the model’s robustness in scenarios with potential error accumulation. Our primary objective is to develop a surrogate that can effectively replace the high-fidelity phase field for a maximized number of frames while forecasting the switching process, leading to significant computational acceleration. As such, the model’s performance was analyzed across a spectrum of initial frames ranging from time t 0 to t 80 with the goal of predicting the complete morphological evolutionary pathway up to time t 100 . Therefore, the surrogate unfolds the simulation from 20 (starting from t 80 ) to 100 (starting from t 20 ) timesteps.
For each starting frame in the test dataset, the mean, 25th, and 75th quartiles of the MSE and the macro average relative error (MARE) were calculated over the 200 unrolled test trajectories for the \({{\mathcal{P}}}_{x}\) ferroelectric morphology (Fig. 3 ). The results highlight that simulations initiated at earlier frames tend to show higher prediction errors. In fact, data-driven surrogates naturally accumulate errors over long-time step inferences, as each prediction builds upon the previous one 26 .
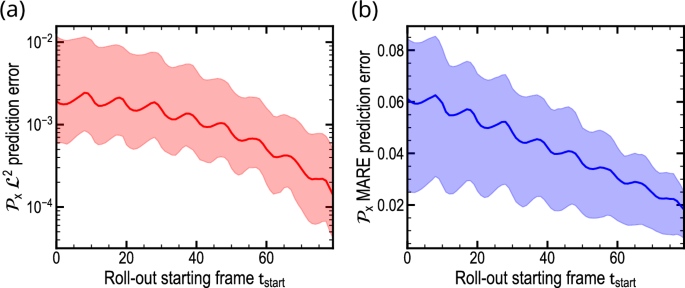
a Evolution of the \({{\mathcal{L}}}_{2}\) error and b MARE during the unrolled trajectory. For each starting frame, complete domain switching trajectories are performed until the final frame at time t 100 . Results are averaged over 200 test dataset simulations, with the solid line indicating the mean and the shaded region representing the interquartile range between the 25th and 75th percentiles.
Despite the observed sensitivity to initial conditions, the model displayed noteworthy robustness and stability. Even when starting from early frames and predicting all 10 switching events, no significant error accumulation was observed. The MARE stayed consistently below 10% across test samples, even for full simulation unrolling. In particular, the mean MARE hovered around 6% when starting from the t 0 state. Even better accuracy was achieved for predictions starting from slightly later frames, between t 20 and t 30 (corresponding roughly to 7 switching events). In these cases, the MARE dropped below 5%. Further reduction of the forecasted timesteps significantly enhances accuracy, ultimately yielding a 2% MARE.
Following these guidelines, it becomes feasible to define an error threshold for the surrogate, aligning with the accuracy requirements imposed by the application. This threshold would determine the acceptable number of predictable timesteps by the surrogate. Additionally, a hybrid solver approach could be envisaged, periodically incorporating high-fidelity phase-field iterations to restore microstructure state accuracy. This restored state could then be used for a new surrogate prediction sequence, as demonstrated in comparable literature 26 .
Interestingly, both the mean and quartile error curves demonstrate consistent oscillations that coincide with individual switching events. This suggests that the model’s performance is sensitive to the specific initial state within a switching event. Notably, the error increases as the initial state approaches the end of a tip application period. This finding implies that the model performs best when starting its prediction at the very beginning of the tip application.
Illustration of forecasted trajectories
Figure 4 depicts the model’s ability to predict domain switching in a complete test simulation, covering the trajectory from t 0 to t 100 and including 10 switching events. The final outputs of \({{\mathcal{P}}}_{x}\) (Fig. 4 a) and \({\mathcal{V}}\) (Fig. 4 b) at t 100 closely match ground truth values. With high accuracy with minimal error accumulation during microstructure evolution, the surrogate proves its efficacy in anticipating domain dynamics during tip-induced electrical switching events. To provide a concise representation of the entire trajectory, both the ground truth and model predictions are depicted in the PCA space in Fig. 4 at each discrete timestep ( t 0 , …, t 100 ). Crucially, the dynamics of the reference solver are faithfully reproduced, capturing the overarching trends in the \({{\mathcal{P}}}_{x}\) and \({\mathcal{V}}\) sequences, respectively. Additional insights into the internal structure of the surrogate predictions are given from the 2D cross-sectional views presented in Supplementary Fig. 2 .
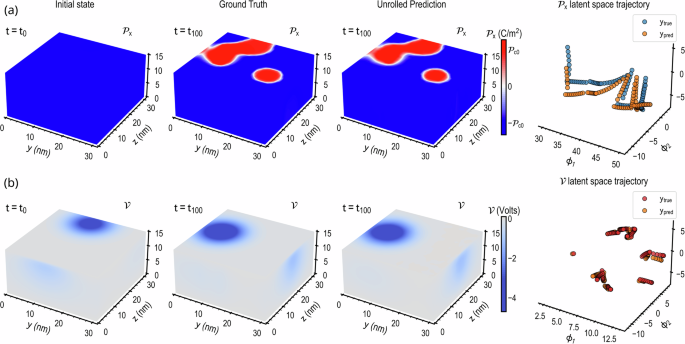
The microstructural states of a polarization ( \({{\mathcal{P}}}_{x}\) ) and b electrostatic potential ( \({\mathcal{V}}\) ) are depicted at the initial ( t 0 ) and final ( t 100 ) times. The prediction and high-fidelity trajectories for both variables are visualized in the low-dimensional space, utilizing the first three principal components.
A detailed overview of the domain state evolution during switching forecasting is presented in Fig. 5 . Here, the model serves as a complete replacement for the reference phase-field, unfolding a test simulation from t 0 to t 100 . The corresponding domain state evolution is depicted at various time steps across the simulation ( t 5 , t 25 , t 50 , t 75 , and t 100 ) for both the prediction and ground truth. Remarkably, the model closely mimics the true domain dynamics with impressive consistency throughout the simulation. While the model closely tracks the overall domain evolution, minor timing-related discrepancies exist. These mainly appear as slight overestimation of domain shrinkage (e.g., at t 25 ), ultimately having minimal impact on the final state. Conversely, the model occasionally diverges more significantly towards the trajectory’s end, missing a small domain formation (e.g., at t 25 ).
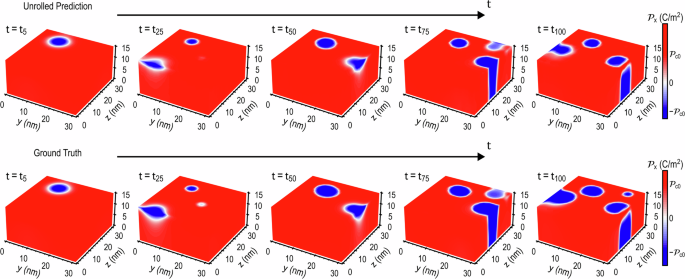
Illustration of an unrolled model prediction versus the high-fidelity reference solution initialized at time t 0 for a test trajectory. Both microstructural states are represented at timesteps t 5 , t 25 , t 50 , t 75 and t 100 .
These findings highlight the model’s overall effectiveness in capturing domain dynamics but also point to areas for further improvement. By successfully replicating entire tip-induced switching sequences with a relative error below 10%, the model demonstrates a remarkable ability to capture the fundamental physical trend governing electrical domain switching. This achievement underscores the model’s potential to serve as a viable alternative to computationally demanding direct numerical solvers, offering a valuable compromise between computational efficiency and accuracy.
Unveiling generalization with unseen domain structure
While the model succeeds at predicting domain switching starting from single-domain states, a critical step for practical use involves its ability to handle unfamiliar initial domain structures. While pooling prior to electrical domain design might be an option in some cases, real-world applications may require operation on randomly configured domains. Therefore, a key question is whether the model can generalize its predictions to arbitrary conditions.
To assess the model’s ability to handle unseen domains, a new test set of 200 simulations (each with 10 switching events) was created. Here, these simulations did not start from single-domain states. Instead, they began with arbitrary domain structures resulting from natural domain formation. For each simulation, prior to tip-induced switching, the polarization was randomly initialized at each grid point, following a uniform distribution between \({{\mathcal{P}}}_{c0}\) and \(-{{\mathcal{P}}}_{c0}\) . Then, a classical domain formation process was simulated until equilibrium was reached (see the “Methods” section). The final domain state then became the starting point ( t 0 ) for the tip-induced electrical trajectory. Supplementary Fig. 3 showcases representative switching trajectories initiated from diverse, realistic domain configurations. These complex starting states reflect real-world scenarios and lead to more intricate dynamics during tip applications, as seen in the figure.
The model’s performance on unseen initial states was directly assessed for unrolled trajectories. The results, reported in Fig. 6 , demonstrate accuracy levels comparable to the single-domain cases, with consistently low MARE even for long-term predictions (100 frames), averaging below 6%. These findings highlight the model’s ability to generalize to unseen domain structures, accurately predicting 10 switching events without compromising accuracy.
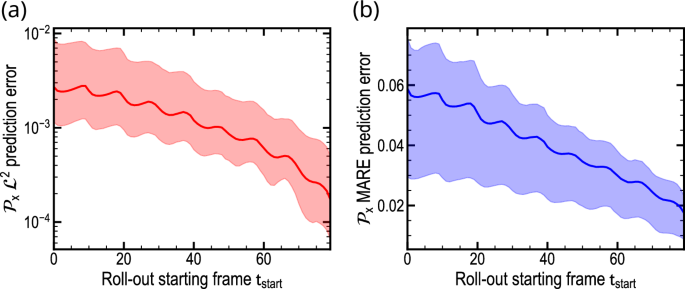
a Evolution of the \({{\mathcal{L}}}_{2}\) error and b MARE during the unrolled prediction. For each starting frame, complete domain switching trajectories are performed until the final time t 100 . Results are averaged over 200 test dataset simulations, with the solid line indicating the mean and the shaded region representing the interquartile range.
Finally, Fig. 7 illustrates the model’s predictions initiated from arbitrary domain configurations throughout an entire simulation. The \({{\mathcal{P}}}_{x}\) microstructure inferred by the surrogate is compared with the corresponding ground truth for the final states, along with its representation in the PCA space. Remarkably, even when starting from unseen initial configurations, the dynamical pathways produced by the surrogate exhibit significant agreement with the high-fidelity trajectories. These observations underscore the surrogate’s comprehension of the underlying evolution equation governing domain switching dynamics, thereby exhibiting remarkable generalization to unseen scenarios and enabling exploration of real-world applications.
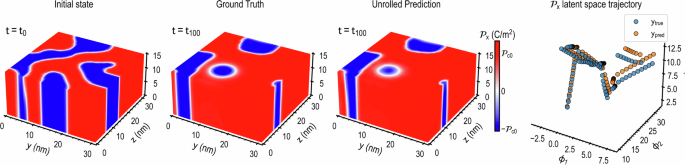
The polarization ( \({{\mathcal{P}}}_{x}\) ) microstructures are depicted at the initial ( t 0 ) and final ( t 100 ) times. The prediction and high-fidelity trajectories for both variables are visualized in the low-dimensional space, utilizing the first three principal components.
Electrical switching prediction of a/c domains
In this section, we address the case of electrical switching in a/c ferroelectric domain states, which are commonly examined in the field of domain and DW control engineering 46 , 47 , 48 . These structures are characterized by mechanical boundary conditions that allow for both in-plane and out-of-plane polarization orientations. When subjected to a tip-induced electric field, such systems have the potential to exhibit both out-of-plane and in-plane domain switching. Thus, all components of the polarization vector ( \({{\mathcal{P}}}_{x}\) , \({{\mathcal{P}}}_{y}\) , \({{\mathcal{P}}}_{z}\) ) were taken into account during the training of the machine learning surrogate specifically developed for predicting a/c domain switching dynamics in this section.
In the context of a/c domains, and more broadly, in analogous ferroelectric domain configurations featuring non-180° DWs, most research efforts have primarily focused on achieving precise control over the DWs displacement using tip scanning technique 41 , 46 , 47 , 48 , 49 , 50 . In consideration of this, we tailored the dataset generation and model training process with the specific aim of developing a surrogate capable of precisely manipulating 90 ∘ DWs through electrical tip scanning as illustrated in Fig. 8 a.
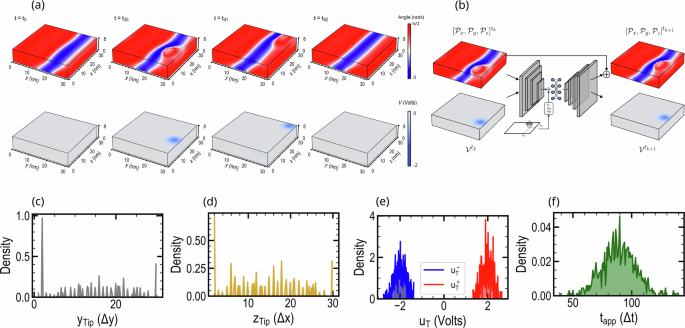
a Example depicting the evolution of the a/c domain structure and electrostatic potential throughout a training trajectory. Domain states are depicted at time t 0 , t 20 , t 41 , and the final time t 62 , highlighting various voltage applications during tip scanning trajectory. b Illustration demonstrating the surrogate model operations over the \({{\mathcal{P}}}_{x}\) , \({{\mathcal{P}}}_{y}\) , and \({{\mathcal{P}}}_{z}\) polarization components, along with the \({\mathcal{V}}\) electrostatic potential, specifically in the scenario of a/c ferroelectric domain switching. Distribution in the training dataset of c and d tip locations ( y tip − z tip ), e prescribed voltages of the AFM tip ( u T ), and f tip application times ( t app ).
To do this, a dataset comprising 1400 phase-field simulations was generated on a grid size of N x × N y × N z = 8 × 32 × 32, with the microstructural state \([{{\mathcal{P}}}_{x},{{\mathcal{P}}}_{y},{{\mathcal{P}}}_{z},{\mathcal{V}}]\) stored at intervals of 20Δ t . An illustration of the surrogate operation in the context of the a/c structure is provided in Fig. 8 b, emphasizing the consideration and prediction of both the in-plane and out-of-plane polarization components. Adaptations made to the network architecture to accommodate this scenario are detailed in Supplementary Note 1 .
Each trajectory began with the domain structure initialized with an in-plane a domain surrounded by out-of-plane c domains (Fig. 8 a). This initialization was achieved through phase-field of a/c domain formation (see the “Methods” section), progressing from a random initial polarization noise to attain domain equilibrium before the commencement of the switching trajectory. It is worth noting that the location and orientation of the in-plane domain differed across various initial states within the dataset.
Subsequently, a random voltage was selected, and tip-induced switching was conducted by scanning the tip along with the 90° DW, emulating real-life tip scanning experiments. The decision to apply the tip on either the left or right side of the a/c domain was made randomly, resulting in occurrences of DW motion in both directions. The number of tip applications along the domain wall (DW) was randomly selected from a uniform distribution ranging between 3 and 8 applications. The tip locations ( y tip , z tip ) along a trajectory were determined based on the number of application steps, ensuring the tip scanned the entire film width along the DW (Fig. 8 c, d). This approach aimed to cover a representative range of DW-tip interactions and DW motions. The prescribed voltage u T was randomly selected from the distribution shown in Fig. 8 e, encompassing both sub and low-coercive electric fields. This voltage selection ensured potential in-plane electric domain switching, enabling the surrogate to generalize across a broad spectrum of domain dynamics. Similarly to the previous case, each switching event lasted 200Δ t , with tip application times randomly ranging from 50Δ t to 150Δ t , and the remaining timesteps were utilized for domain relaxation (Fig. 8 f). Subsequently, the structure underwent relaxation for an additional 100Δ t after the completion of tip scanning. A typical a/c domain switching training trajectory following this methodology is illustrated in Fig. 8 a. Finally, the dataset was divided according to a 1000:200:200 training/validation/test ratio.
Following model training, we directly evaluated the surrogate in the scenario of unrolled a/c tip scanning domain switching prediction on the test dataset. The model was provided with an initial frame close to the start of the simulation ( t 0 – t 30 ) and was assessed by unrolling the entire simulation until the conclusion of tip scanning and final domain state relaxation. The \({{\mathcal{L}}}_{2}\) and MARE prediction errors for the in-plane and out-of-plane polarization components depending on the initial frame are reported in Fig. 9 .
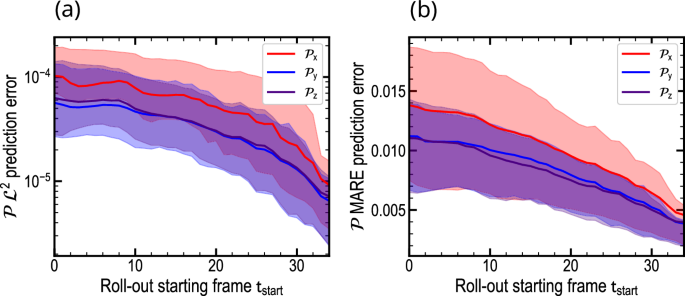
a Evolution of the \({{\mathcal{L}}}_{2}\) error and b MARE during the unrolled prediction for the \({{\mathcal{P}}}_{x}\) , \({{\mathcal{P}}}_{y}\) and \({{\mathcal{P}}}_{z}\) polarization components. For each starting frame, complete domain switching trajectories are performed until the final frame. Results are averaged over 200 test dataset simulations, with the solid line indicating the mean and the shaded region representing the interquartile range.
Our analysis reveals that the surrogate accurately predicts the domain state for all components, exhibiting a relative error below 2%, even when starting from the simulation onset. This underscores the surrogate’s capability to faithfully forecast the domain state throughout the entirety of a tip-scanning trajectory. Notably, errors in the out-of-plane polarization ( \({{\mathcal{P}}}_{x}\) ) are marginally higher than those observed in the in-plane counterparts, likely attributable to the prevalence of out-of-plane domains in a/c ferroelectric structures.
Interestingly, the overall errors are slightly lower than in the previous case of c + /c − domains. This disparity can be attributed to the fact that the typical switching trajectory induced by tip scanning in the case of 90° DW control yields less variation in the global domain state relative to the multiple nucleation events of c + /c − domains arising from the tip trajectory in the preceding section.
Figure 10 presents an illustration of the model performance over an entire tip-scanning trajectory using a simulation from the test dataset. In this simulation, the tip was biased with a voltage of −1.93 V and scanned along the DW by applying the voltage over five application steps. Figure 10 demonstrates the evolution of the a/c domain structure throughout the simulation, from the initial scanning to relaxation completion at the final time, t 67 . It can be observed that the surrogate model adeptly reproduces the domain dynamics during the entire tip-scanning process, progressively moving the domain wall through tip-induced in-plane ferroelectric switching. Additional examples of switching trajectory predictions from test simulations are provided in Supplementary Figs. 4 – 6 . Notably, the surrogate model accurately forecasts not only the final position of the domain wall after scanning but also the underlying domain state transitions. Hence, the proposed framework proves to operate as an effective alternative to traditional phase-field modeling for the entire trajectory length in applications related to 90° DW motion and control.
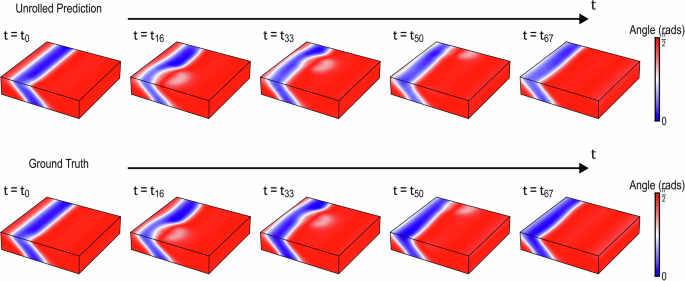
Both predicted and ground truth microstructural states are represented at timesteps t 0 , t 16 , t 33 , t 50 and at the final time t 67 .
Computation efficiency
In this section, we analyze the acceleration provided by the machine learning surrogate model when compared to traditional approaches. The primary advantage of using a neural network surrogate lies in its significantly cheaper computational cost during inference, as opposed to direct numerical solvers. However, quantifying the speed-up achieved by a surrogate model presents a nuanced challenge due to hidden computational costs, such as dataset generation and model training.
To navigate this complexity, we initiate our analysis by focusing on the inference times of both approaches. Assuming the surrogate entirely substitutes the direct solver from time t 0 , we report acceleration factors computed over the 200 test simulations in the case of unrolled simulations Table 2 . This evaluation was conducted on both CPU and GPU material, enabling a fair comparison with simulations using traditional solvers. The analysis reveals significant performance gains with the surrogate, achieving speed-ups of 1390 on CPU and 2550 on GPU, confirming the surrogate’s potential to unlock demanding phase-field problems.
While our approach yields rapid inferences, acknowledging the initial computational investment in surrogate creation is crucial. We present dataset generation and model training times in Table 2 for a comprehensive cost overview. It is essential to emphasize that these results are contingent upon our specific computational material and methodology. Alternative computational configurations or numerical approaches for the phase field, such as employing finite-element methods instead of spectral methods to solve the PDEs set, may yield divergent execution times.
This study presents a machine-learning surrogate for tip-induced electrical switching. Handling time-evolving electrical boundary conditions, the surrogate faithfully predicts polarization and electrostatic potential evolution across multiple switching scenarios. Its versatility spans diverse voltage, tip location, and application times, enabling exploration of vast parameter spaces in realistic settings. Remarkably, it maintains relative errors below 10% even over long timesteps inference. This fast time-stepper offers a 2500× speedup in morphology inference, paving the way for real-time simulations.
Generating training data for data-driven surrogates incurs substantial upfront costs, primarily due to dataset creation. Data augmentation leveraging physically plausible transformations offers a potential solution to mitigate this bottleneck. Additionally, transfer learning across diverse material parameters and system scales expands framework applicability, requiring minimal additional training data. Importantly, surrogate development constitutes a one-time investment. Subsequent use incurs negligible computational expense, unlocking the ability to solve previously intractable optimization problems requiring massive iterations.
In this article, we addressed vertical c + /c − and a/c domain structures, showcasing the surrogate model’s ability to manage complex domain states with both in-plane and out-of-plane polarization components. This framework could be expanded to handle additional domain structures in 3D ferroelectrics, such as the 71°, 109°, and 180° domain walls in BiFeO 3 ferroelectrics 49 , 50 , or the domain states found in (110) oriented PZT thin films 40 , 51 .
Despite incorporating electrical boundary conditions, further development is necessary to create a comprehensive surrogate model for ferroelectric phase-field. Building on the current approach, integrating mechanical conditions like tip location, load, and misfit strain could emulate tip-induced switching and explore domain states in realistic mechanical scenarios 17 . Furthermore, in real-life contexts, parameters related to the experimental setup typically require calibration for accurate modeling. Therefore, an extension of the presented framework to accommodate additional tip parameters can be envisaged. For example, while the current framework operates with a fixed tip radius, we present a potential extension that includes varying tip diameters in Supplementary Note 5 . This example illustrates possible adaptations of the existing framework to effectively address the constraints of real-life experimental setups.
With this work, we aim to provide a promising approach for utilizing phase-field modeling in addressing costly inverse problems through RL 40 , 41 . Our framework lays the foundation for an AI agent that designs domain structures via electrical phase-field simulations. The AI, tasked with achieving a target state, could explore diverse tip locations, voltages, and durations to learn an optimal switching strategy through repeated attempts. Leveraging our efficient surrogate instead of the full phase-field model enables significantly faster learning while accurately capturing switching dynamics. We envision this framework significantly helping the design and comprehension of domain structures in modern DW nanoelectronics.
In conclusion, we presented a machine learning approach to accurately replicate tip-induced electrical switching in 3D ferroelectric phase-field simulations. The surrogate demonstrates remarkable accuracy over extended timescales, providing an efficient alternative to computationally expensive high-fidelity methods. Its ability to rapidly simulate electrical switching trajectories with dynamic boundary conditions creates new opportunities for the electrical design of ferroelectric materials at an unprecedented pace.
Phase-field modeling
In the context of phase-field simulations, the dynamic evolution of ferroelectric polarization is described by the TDGL equation 11 , 12 , 14 :
where P i ( r , t ) is the spontaneous polarization, L is a kinetic coefficient, ψ is the total free energy and r = ( x , y , z ) denotes the spatial vector in 3D. The total free energy includes the bulk, gradient, electric, and elastic free energy density
The polarization was updated at each time step following the explicit scheme:
where Δ t is the time step for integration.
The bulk energy is described by
where α i , α i j , and α i j k are the second-, fourth- and sixth-order PZT Landau coefficients, which are taken from the literature 52 , and \({\alpha }_{1}=\frac{T-{T}_{0}}{2\epsilon C}\) refers to the dielectric permittivity ϵ , the Curie temperature T 0 and the Curie constant C .
The energy caused by the DWs is described by the gradient energy, which in a cubic system is calculated by
where G represents the gradient energy coefficient tensor.
The electric energy is given by
where E i = − ∇ i V is the electric field obtained by solving the electrostatic equilibrium:
Here, ρ represents the electric charge, and − ∇ ⋅ P denotes the depolarization charges induced by the polarization. Electrostatic equilibrium is solved using the fast Fourier transform method (details in refs. 18 , 53 ), with periodic boundary conditions along the y and z in-plane directions.
Tip-induced switching was emulated by adjusting the electrostatic potential under the tip in the Dirichlet boundary conditions at the top electrode ( x = x top ). Consistent with many phase-field studies, the surface electrostatic potential was approximated using a Lorentz-like distribution of the applied bias u T 14 , 54 :
where γ is the half-width of the tip. The distance from the tip center, r , is calculated as \(r=\sqrt{{({y}_{{\rm{tip}}}-y)}^{2}+{({z}_{{\rm{tip}}}-z)}^{2}}\) , accounting for the varying tip location r tip = x top , y tip , z tip ) across switching trajectories.
In microstructure evolution without tip influence, we assume complete charge screening at both the bottom electrode and the top surface. This configuration is employed during relaxation phases post-switching and for the initial domain formation prior to tip application sequence in unforeseen domain structure scenarios. Hence, short-circuit electrostatic conditions were applied:
The elastic energy density is described by
where C is the elastic stiffness tensor, ϵ is the total strain and ϵ 0 is the electrostrictive strain caused by the polarization as
where Q is the electrostrictive tensor. The total strain contains the homogeneous and heterogeneous strains:
which is linked to the mechanical displacement u i by
The mechanical equilibrium equation σ i j , j = 0, solved for the displacements, is given by (using Einstein notation) :
The simulations were conducted on a 3D grid of N x × N y × N z points with uniform spacing Δ x / l 0 = Δ y / l 0 = Δ z / l 0 = 1, where \({l}_{0}=\sqrt{{G}_{110}/{\alpha }_{0}}\approx 1\,{\rm{nm}}\) ( \({\alpha }_{0}=| {\alpha }_{1}{| }_{T = 2{5}\,^{\circ }{\rm {C}}}\) 11 ). Gradient energy coefficients followed ref. 11 : G 11 / G 110 = 0.6, G 12 / G 110 = 0, G 44 / G 110 = 0.3. The time step was Δ t = 0.02 t 0 , where t 0 = 1/( α 0 L 0 ). For the c + /c − domain state scenario, the PZT films were constrained by a −1% in-plane mismatch, aligning with a typical setting in PZT simulations 43 . For the case addressing a/c domain structures, no lattice mismatch was applied. The other PZT parameters utilize values established in literature 11 , 43 , 55 . A listing of these parameters and the associated normalization procedure is provided in Supplementary Note 6 .
Training details
To enhance the stability of rollout predictions, we implemented a progressive noise augmentation strategy on the input training features. Inspired by error accumulation in real-world data (refs. 18 , 56 ), Gaussian noise was incrementally increased along simulation trajectories. Notably, the target labels remained noise-free. The noise magnitudes for polarization and electrostatic fields were set at σ P = 10 −3 and σ V = 10 −5 , respectively, conforming to the methodology established in ref. 18 .
The model parameters were optimized during training using the Adam optimizer with a batch size of 32. The initial learning rate was set at 10 −3 and gradually reduced to 10 −6 , following an exponential decay over during 100 epochs.
Error metrics
Mean squared error (mse).
The mean squared error loss function \({{\mathcal{L}}}_{2}\) can be computed over the \({\{({Y}_{i},{X}_{i})\}}_{i = 1}^{N}\) training samples as
where \({Y}^{{t}_{k+1}}\) is the microstructure labels obtained by real phase-field simulations and \({\mathcal{S}}({X}^{t})\) are the model outputs.
To assess rollout simulations, the score is averaged across each trajectory, such as
where M denotes the number of trajectories in the validation dataset and N is the number of frames per simulation.
Macro average relative error (MARE)
The macro average relative error (MARE) can be computed in the context of rollout evaluations by
Mean absolute error (MAE)
The mean absolute error (MAE) \({{\mathcal{L}}}_{1}\) can be determined by
Computational material
The machine learning framework utilized in this study was implemented using TensorFlow2. The training procedure and assessments of GPU computational efficiency were conducted on an NVIDIA GeForce RTX 3080 with 10 GB of RAM. Dataset generation using the direct numerical solver and assessments of CPU computational efficiency were performed using an INTEL i9 CPU clocked at 5.1 GHz.
Data availability
The data that support the results of this study are available upon reasonable request.
Code availability
The machine learning codes that support the findings of this study are available upon reasonable request.
Scott, J. F. & Paz de Araujo, C. A. Ferroelectric memories. Science 246 , 1400–1405 (1989).
Article CAS PubMed Google Scholar
Crassous, A., Sluka, T., Tagantsev, A. K. & Setter, N. Polarization charge as a reconfigurable quasi-dopant in ferroelectric thin films. Nat. Nanotechnol. 10 , 614–618 (2015).
Sharma, P. et al. Conformational domain wall switch. Adv. Funct. Mater. 29 , 1807523 (2019).
Article Google Scholar
McGilly, L. J., Yudin, P., Feigl, L., Tagantsev, A. K. & Setter, N. Controlling domain wall motion in ferroelectric thin films. Nat. Nanotechnol. 10 , 145–150 (2015).
Setter, N. et al. Ferroelectric thin films: review of materials, properties, and applications. J. Appl. Phys. 100 , 051606 (2006).
Gonzalez Casal, S. et al. Mechanical switching of ferroelectric domains in 33–200 nm-thick sol–gel-grown PbZr 0.2 Ti 0.8 O 3 films assisted by nanocavities. Adv. Electron. Mater. 8 , 1–9 (2022).
Guo, E. J., Roth, R., Das, S. & Dörr, K. Strain induced low mechanical switching force in ultrathin PbZr 0.2 Ti 0.8 O 3 films. Appl. Phys. Lett. 105 , 012903 (2014).
Sharma, P. et al. Nonvolatile ferroelectric domain wall memory. Sci. Adv. 3 , e1700512 (2017).
Article PubMed PubMed Central Google Scholar
Sharma, P., Moise, T. S., Colombo, L. & Seidel, J. Roadmap for ferroelectric domain wall nanoelectronics. Adv. Funct. Mater. 32 , 2110263 (2022).
Article CAS Google Scholar
Wang, J. et al. Ferroelectric domain-wall logic units. Nat. Commun. 13 , 3255 (2022).
Article CAS PubMed PubMed Central Google Scholar
Li, Y. L., Hu, S. Y., Liu, Z. K. & Chen, L. Q. Effect of substrate constraint on the stability and evolution of ferroelectric domain structures in thin films. Acta Mater. 50 , 395–411 (2002).
Chen, L.-Q. Phase-field models for microstructure evolution. Annu. Rev. Mater. Res. 32 , 113–140 (2002).
Zhao, Y. Understanding and design of metallic alloys guided by phase-field simulations. npj Comput. Mater. 9 , 94 (2023).
Wang, J. J., Wang, B. & Chen, L. Q. Understanding, predicting, and designing ferroelectric domain structures and switching guided by the phase-field method. Annu. Rev. Mater. Res. 49 , 127–152 (2019).
Bortis, A., Trassin, M., Fiebig, M. & Lottermoser, T. Manipulation of charged domain walls in geometric improper ferroelectric thin films: a phase-field study. Phys. Rev. Mater. 6 , 064403 (2022).
Vasudevan, R. K. et al. Domain wall geometry controls conduction in ferroelectrics. Nano Lett. 12 , 5524–5531 (2012).
Alhada-Lahbabi, K., Deleruyelle, D. & Gautier, B. Phase-field study of nanocavity-assisted mechanical switching in PbTiO 3 thin films. Adv. Electron. Mater. 10 , 2300744 (2023).
Alhada-Lahbabi, K., Deleruyelle, D. & Gautier, B. Machine learning surrogate model for acceleration of ferroelectric phase-field modeling. ACS Appl. Electron. Mater. 5 , 3894–3907 (2023).
Montes de Oca Zapiain, D., Stewart, J. A. & Dingreville, R. Accelerating phase-field-based microstructure evolution predictions via surrogate models trained by machine learning methods. npj Comput. Mater. 7 , 1–11 (2021).
Li, W., Bazant, M. Z. & Zhu, J. Phase-field DeepONet: physics-informed deep operator neural network for fast simulations of pattern formation governed by gradient flows of free-energy functionals. Comput. Methods Appl. Mech. Eng. 416 , 116299 (2023).
Lu, L., Jin, P., Pang, G., Zhang, Z. & Karniadakis, G. E. Learning nonlinear operators via DeepONet based on the universal approximation theorem of operators. Nat. Mach. Intell. 3 , 218–229 (2021).
Raissi, M., Perdikaris, P. & Karniadakis, G. E. Physics-informed neural networks: a deep learning framework for solving forward and inverse problems involving nonlinear partial differential equations. J. Comput. Phys. 378 , 686–707 (2019).
Hemmasian, A. et al. Surrogate modeling of melt pool temperature field using deep learning. Addit. Manuf. Lett. 5 , 100123 (2023).
Fetni, S. et al. Capabilities of auto-encoders and principal component analysis of the reduction of microstructural images; application on the acceleration of phase-field simulations. Comput. Mater. Sci. 216 , 111820 (2023).
Choi, J. Y., Xue, T., Liao, S. & Cao, J. Accelerating phase-field simulation of three-dimensional microstructure evolution in laser powder bed fusion with composable machine learning predictions. Addit. Manuf. 79 , 103938 (2024).
Google Scholar
Oommen, V., Shukla, K., Desai, S., Dingreville, R. & Karniadakis, G. E. Rethinking materials simulations: blending direct numerical simulations with neural operators. npj Comput. Mater 10 , 145 (2024).
Peivaste, I. et al. Machine-learning-based surrogate modeling of microstructure evolution using phase-field. Comput. Mater. Sci. 214 , 111750 (2022).
Xue, T., Gan, Z., Liao, S. & Cao, J. Physics-embedded graph network for accelerating phase-field simulation of microstructure evolution in additive manufacturing. npj Comput. Mater. 8 , 201 (2022).
Oommen, V., Shukla, K., Goswami, S., Dingreville, R. & Em Karniadakis, G. Learning two-phase microstructure evolution using neural operators and autoencoder architectures. npj Comput. Mater. 8 , 190 (2022).
Yang, K. & Cao, Y. Self-supervised learning and prediction of microstructure evolution with convolutional recurrent neural networks. Patterns 2 , 100243 (2021).
Wu, P., Iquebal, A. S. & Kumar, A. Emulating microstructural evolution during spinodal decomposition using a tensor decomposed convolutional and recurrent neural network. Comput. Mater. Sci. 224 , 112187 (2023).
Kemeth, F. P. et al. Black and gray box learning of amplitude equations: application to phase field systems. Phys. Rev. E 107 , 025305 (2023).
Alhada-Lahbabi, K., Deleruyelle, D. & Gautier, B. Ultrafast and accurate prediction of polycrystalline hafnium oxide phase-field ferroelectric hysteresis using graph neural networks. Nanoscale Adv. 6 , 2350–2362 (2024).
Karniadakis, G. E. et al. Physics-informed machine learning. Nat. Rev. Phys. 3 , 422–440 (2021).
Samaniego, E. et al. An energy approach to the solution of partial differential equations in computational mechanics via machine learning: Concepts, implementation and applications. Comput. Methods Appl. Mech. Eng. 362 , 112790 (2020).
Teichert, G. H., Natarajan, A. R., Van der Ven, A. & Garikipati, K. Machine learning materials physics: integrable deep neural networks enable scale bridging by learning free energy functions. Comput. Methods Appl. Mech. Eng. 353 , 201–216 (2019).
Goswami, S., Yin, M., Yu, Y. & Karniadakis, G. E. A physics-informed variational DeepONet for predicting crack path in quasi-brittle materials. Comput. Methods Appl. Mech. Eng. 391 , 114587 (2022).
Yang, H. & Demkowicz, M. J. Reinforcement learning strategy for control of microstructure evolution in phase field models. Comput. Mater. Sci. 231 , 112577 (2024).
Mianroodi, J. R., Siboni, N. H. & Raabe, D. Computational discovery of energy-efficient heat treatment for microstructure design using deep reinforcement learning. arXiv:abs/2209.11259 (2022).
Vasudevan, R. K., Orozco, E. & Kalinin, S. V. Discovering mechanisms for materials microstructure optimization via reinforcement learning of a generative model. Mach. Learn.: Sci. Technol. 3 , 04LT03 (2022).
Smith, B. et al. Physics-informed models of domain wall dynamics as a route for autonomous domain wall design via reinforcement learning. Digit. Discov. 3 , 456–466 (2024).
Ronneberger, O., Fischer, P. & Brox, T. U-net: convolutional networks for biomedical image segmentation. In Proc. 18th International Conference on Medical Image Computing and Computer-Assisted Intervention—MICCAI 2015, Part III, Munich, Germany, October 5–9, 2015 Vol. 18 234–241 (Springer, 2015).
Cao, Y., Morozovska, A. & Kalinin, S. V. Pressure-induced switching in ferroelectrics: phase-field modeling, electrochemistry, flexoelectric effect, and bulk vacancy dynamics. Phys. Rev. B 96 , 184109 (2017).
Wang, Y.-J., Li, J., Zhu, Y.-L. & Ma, X.-L. Phase-field modeling and electronic structural analysis of flexoelectric effect at 180° domain walls in ferroelectric PbTiO 3 . J. Appl. Phys. 122 , 224101 (2017).
Cao, Y. & Kalinin, S. V. Phase-field modeling of chemical control of polarization stability and switching dynamics in ferroelectric thin films. Phys. Rev. B 94 , 235444 (2016).
Khan, A. I., Marti, X., Serrao, C., Ramesh, R. & Salahuddin, S. Voltage-controlled ferroelastic switching in Pb(Zr 0.2 Ti 0.8 )O 3 thin films. Nano Lett. 15 , 2229–2234 (2015).
Nagarajan, V. et al. Dynamics of ferroelastic domains in ferroelectric thin films. Nat. Mater. 2 , 43–47 (2003).
Gao, P. et al. Atomic-scale mechanisms of ferroelastic domain-wall-mediated ferroelectric switching. Nat. Commun. 4 , 2791 (2013).
Wu, M. et al. Complete selective switching of ferroelastic domain stripes in multiferroic thin films by tip scanning. Adv. Electron. Mater. 10 , 2300640 (2024).
Wu, M. et al. Facile control of ferroelastic domain patterns in multiferroic thin films by a scanning tip bias. ACS Appl. Mater. Interfaces 15 , 11983 (2023).
Liu, D., Wang, J., Wang, J.-S. & Huang, H.-B. Phase field simulation of misfit strain manipulating domain structure and ferroelectric properties in PbZr (1– x ) Ti x O 3 thin films. Acta Phys. Sin. 69 , 127801 (2020).
Hu, H.-L. & Chen, L.-Q. Three-dimensional computer simulation of ferroelectric domain formation. J. Am. Ceram. Soc. 81 , 492–500 (1998).
Wang, J. J., Ma, X. Q., Li, Q., Britson, J. & Chen, L. Q. Phase transitions and domain structures of ferroelectric nanoparticles: phase field model incorporating strong elastic and dielectric inhomogeneity. Acta Mater. 61 , 7591–7603 (2013).
Cao, Y., Li, Q., Chen, L.-Q. & Kalinin, S. V. Coupling of electrical and mechanical switching in nanoscale ferroelectrics. Appl. Phys. Lett. 107 , 202905 (2015).
Wang, J., Shi, S. Q., Chen, L. Q., Li, Y. & Zhang, T. Y. Phase-field simulations of ferroelectric/ferroelastic polarization switching. Acta Mater. 52 , 749–764 (2004).
Sanchez-Gonzalez, A. et al. Learning to simulate complex physics with graph networks. arXiv:abs/2002.09405 (2020).
Download references
Acknowledgements
This work has received the financial support of IPCEI France 2030 Programs POI and eFerroNVM together with ANR-23-CE24-0015-01 ECHOES project.
Author information
Authors and affiliations.
INSA Lyon, CNRS, Ecole Centrale de Lyon, Université Claude Bernard Lyon 1, CPE Lyon, INL, UMR5270, 69621, Villeurbanne, France
Kévin Alhada–Lahbabi, Damien Deleruyelle & Brice Gautier
You can also search for this author in PubMed Google Scholar
Contributions
K.A.L. got the original idea, ran the phase-field simulations, conceived the Machine Learning framework, performed the analysis, and wrote the manuscript. K.A.L., D.D., and B.G. worked on the development of the phase-field modeling code. All authors reviewed and discussed the manuscript.
Corresponding author
Correspondence to Kévin Alhada–Lahbabi .
Ethics declarations
Competing interests.
The authors declare no competing interests.
Additional information
Publisher’s note Springer Nature remains neutral with regard to jurisdictional claims in published maps and institutional affiliations.
Supplementary information
Rights and permissions.
Open Access This article is licensed under a Creative Commons Attribution-NonCommercial-NoDerivatives 4.0 International License, which permits any non-commercial use, sharing, distribution and reproduction in any medium or format, as long as you give appropriate credit to the original author(s) and the source, provide a link to the Creative Commons licence, and indicate if you modified the licensed material. You do not have permission under this licence to share adapted material derived from this article or parts of it. The images or other third party material in this article are included in the article’s Creative Commons licence, unless indicated otherwise in a credit line to the material. If material is not included in the article’s Creative Commons licence and your intended use is not permitted by statutory regulation or exceeds the permitted use, you will need to obtain permission directly from the copyright holder. To view a copy of this licence, visit http://creativecommons.org/licenses/by-nc-nd/4.0/ .
Reprints and permissions
About this article
Cite this article.
Alhada–Lahbabi, K., Deleruyelle, D. & Gautier, B. Machine learning surrogate for 3D phase-field modeling of ferroelectric tip-induced electrical switching. npj Comput Mater 10 , 197 (2024). https://doi.org/10.1038/s41524-024-01375-7
Download citation
Received : 26 February 2024
Accepted : 04 August 2024
Published : 30 August 2024
DOI : https://doi.org/10.1038/s41524-024-01375-7
Share this article
Anyone you share the following link with will be able to read this content:
Sorry, a shareable link is not currently available for this article.
Provided by the Springer Nature SharedIt content-sharing initiative
Quick links
- Explore articles by subject
- Guide to authors
- Editorial policies
Sign up for the Nature Briefing newsletter — what matters in science, free to your inbox daily.

Physical Review E
Covering statistical, nonlinear, biological, and soft matter physics.
- Collections
- Editorial Team
From hydrodynamics to dipolar colloids: Modeling complex interactions and self-organization with generalized potentials
T. j. j. m. van overveld, w. g. ellenbroek, j. m. meijer, h. j. h. clercx, and m. duran-matute, phys. rev. e 110 , 035103 – published 3 september 2024.
- No Citing Articles
- INTRODUCTION
- INTERACTIONS IN THE HYDRODYNAMIC SYSTEM
- MODEL POTENTIAL FOR THE HYDRODYNAMIC…
- ONE-DIMENSIONAL MONTE CARLO SIMULATIONS
- FROM INDIVIDUAL PARTICLES TO PATTERNS
- CONCLUSIONS
The self-organization of clusters of particles is a fundamental phenomenon across various physical systems, including hydrodynamic and colloidal systems. One example is that of dense spherical particles submerged in a viscous fluid and subjected to horizontal oscillations. The interaction of the particles with the oscillating flow leads to the formation of one-particle-thick chains or multiple-particle-wide bands, both oriented perpendicular to the oscillation direction. In this study, we model the hydrodynamic interactions between such particles and parallel chains using simplified potentials. We first focus on the hydrodynamic interactions between chains, which we characterize using data from fully resolved numerical simulations. Based on these interactions, we propose a simplified model potential, called the Siren potential, which combines the representative hydrodynamic interactions: short-range attraction, mid-range repulsion, and long-range attraction. Through one-dimensional Monte Carlo simulations, we successfully replicate the characteristic patterns observed in hydrodynamic experiments and draw the phase diagram for the model potential. We further extend our analysis to two-dimensional systems, introducing a dipole-capillary model potential that accounts for both chain formation and Siren -like chain interactions. This potential is based on a system with colloidal particles at an interface, where chain formation is driven by an external electric field that induces a dipole moment parallel to the interface in each particle. The capillary force contributes the long-range attraction. Starting with parallel chains, the patterns in the two-dimensional Monte Carlo simulations of this colloidal system are similar to those observed in the hydrodynamic experiments. However, we identify that nonlinear interactions are important for some distinct steps in the chain formation. Still, the model potentials help clarify the dynamic behavior of the particles and chains due to the complex interactions encountered in both hydrodynamic and colloidal systems, drawing parallels between them.
- Received 19 February 2024
- Accepted 2 August 2024
DOI: https://doi.org/10.1103/PhysRevE.110.035103
©2024 American Physical Society
Physics Subject Headings (PhySH)
- Research Areas
- Physical Systems
Authors & Affiliations
- Fluids and Flows Group and J.M. Burgers Center for Fluid Mechanics, Department of Applied Physics and Science Education, Eindhoven University of Technology , P. O. Box 513, 5600 MB Eindhoven, The Netherlands
- Soft Matter and Biological Physics Group, Department of Applied Physics and Science Education, Eindhoven University of Technology , P. O. Box 513, 5600 MB Eindhoven, The Netherlands
- * Contact author: [email protected]
Article Text (Subscription Required)
References (subscription required).
Vol. 110, Iss. 3 — September 2024
Access Options
- Buy Article »
- Log in with individual APS Journal Account »
- Log in with a username/password provided by your institution »
- Get access through a U.S. public or high school library »
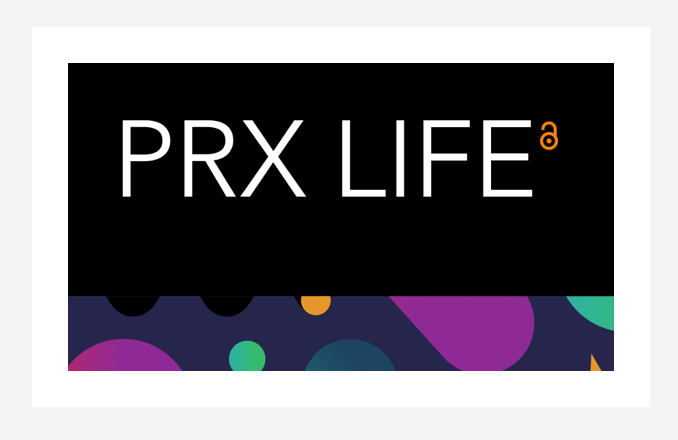
Authorization Required
Other options.
- Buy Article »
- Find an Institution with the Article »
Download & Share
Examples of the patterns observed in the experiments by van Overveld et al. [ 19 ], as a function of the relative particle-fluid excursion length normalized by particle diameter, A r / D , and particle coverage fraction ϕ . The patterns range from one-particle-thick chains to multiple-particle-wide bands, all with an intrinsic spacing between them that varies with A r / D . The colors indicate different regions in the parameter space, with only chains and irregular clusters (blue), chains and bands (green), and more disordered structures (orange).
(a) The dimensionless, average streamwise force per particle F x as a function of the normalized chain spacing λ / D , where positive values of F x correspond to repulsive interactions (see insert). The chains are aligned (as in the insert), except for the cases with open symbols, which correspond to touching chains in a staggered configuration. (b) Collapse of the data displayed in (a). The triangles represent forces from simulations of two touching chains in staggered ( λ / D = 3 / 2 , upward triangle) or straight ( λ / D = 1 , downward triangle) configuration (as shown in the upper left corner), for A r / D = [ 0.5 , 0.6 , ⋯ , 1 ] . Around the first zero crossing, the data roughly follows the black dashed line, given by Eq. ( 1 ).
(a) The parameter space in terms of A / B and C / B that determines the characteristics of the potential in Eq. ( 2 ). A net short-range attraction only occurs for sufficiently large C / B (above the lower black line). The interaction is attractive at every distance if the magnitudes of the two attractive terms are too large (above the upper black line). Above the dotted line, the potential is similar to those commonly used in DLVO theory, with the primary minimum (related to irreversible clustering) below the secondary minimum. (b) Examples of the potentials as indicated by the colored symbols in (a). The Siren potential (solid green curve), characterized by [ A , B , C ] = [ 1 , 3 , 2 ] , is used for the rest of this section. The vertical black line at r / D = 1 represents the shortest possible center-to-center distance between two hard spheres.
The lattice sums are calculated for two distinct one-dimensional configurations: (a) a uniform particle distribution and (b) groups of M particles with an equal spacing Δ . In the example configuration shown here, M = 3 . The particle diameter is D and the unit cell length L .
The average energy per particle u , given by Eq. ( 5 ), as a function of ϕ and Δ / L for the Siren potential, for (a) M = 2 and (b) M = 4 . The red and blue curves indicate local minima and maxima, respectively. The white dashed lines indicate the position of the secondary minimum in the Siren potential ( Δ / D = r min / D ≈ 2.18 ), as shown in Fig. 3 . The Roman numerals (I-V) correspond to the five distinct configurations illustrated for the case with M = 4 in Fig. 6 .
Schematic overview of the five distinct configurations corresponding to the extrema in Fig. 5 , here with M = 4 and ϕ = 0.5 . The dotted lines indicate the edges of the unit cell.
Particle positions over time obtained from one-dimensional Monte Carlo simulations using the Siren potential for N = 6 , ϕ = 0.2 , and k B T = 0 . The simulations are initiated from either a uniform distribution of (a) single particles or (b) pairs of particles. The vertical dashed lines indicate the periodic boundary, and the horizontal dashed line indicates the time at which the system has reached an equilibrium state (after which the particle positions relative to each other remain constant).
Resulting particle distributions from the one-dimensional MC simulations after 10 5 steps for ϕ = [ 0.2 , 0.5 , 0.8 ] . The colors indicate the total potential energy of each particle in the shown configuration. A comparison of the particle distributions in different potentials (Well, Mermaid, and Siren) is given in Fig. 15 in the Appendix.
Normalized histogram of the distances between the particles for the Siren potential simulations in Fig. 8 , averaged over the final 10 4 MC steps, with bin size Δ r / D = 0.45 . The dashed lines indicate the median distances at r / D ≈ [ 1.92 , 2.00 , 1.44 ] for ϕ = [ 0.2 , 0.5 , 0.8 ] , respectively. The insert shows the relative distribution at short distances, with bin size Δ r / D = 0.025 .
Phase diagram illustrating the different states in the one-dimensional system governed by the Siren potential. The control parameters are the particle coverage fraction ϕ and the normalized thermal energy k B T / U max . The different states are identified based on the criterion that at least 50% of the interparticle distances satisfy the corresponding condition. The black dots indicate simulations where no dominant state is found. The black vertical bars at ϕ = 0.35 and 0.77 correspond to the bifurcation points in Fig. 5 for two particles per unit cell ( M = 2 ).
Example of the dipole-capillary potential described by Eq. ( 9 ), incorporating dipolar and capillary interactions, with A c = A d = 1 . The colors correspond to the values of U d c and are separated by black contour lines with steps of 0.25. The arrow represents the direction of the dipole moment.
Examples of the interaction between two parallel particle chains, each consisting of five particles, at various normalized spacings λ / D , for A d = 1 and A c = 0.05 . The colors represent the values of the potential given by Eq. ( 9 ). The black arrows indicate the direction of the forces, with the larger arrows being the forces at the centers of the particles.
The average of the force F x on each particle (in the right chain) for a configuration with two parallel chains in straight (solid) and staggered (dashed) configurations, with A d = 1 . (a) Variation of A c while keeping K = 4 fixed. (b) Variation of K while keeping A c = 0.05 fixed. Positive values of F ¯ x correspond to repulsion between the chains.
Particle positions after 10 5 MC steps, starting from parallel chains, with A d = 1 and A c = 0.01 both constant. We either increase N at constant k B T (left column) or increase k B T at constant N (right column). The colors indicate the energy of each particle within the potential landscape.
The particle distributions from the one-dimensional MC simulations for the Well, Mermaid, and Siren potentials, as given in Fig. 3 . Results are shown after 10 5 steps for ϕ = [ 0.2 , 0.5 , 0.8 ] . The colors indicate the total potential energy of each particle in the shown configuration.
Sign up to receive regular email alerts from Physical Review E
- Forgot your username/password?
- Create an account
Article Lookup
Paste a citation or doi, enter a citation.

IMAGES
VIDEO
COMMENTS
Field experiments, explained. Editor's note: This is part of a series called "The Day Tomorrow Began," which explores the history of breakthroughs at UChicago. Learn more here. A field experiment is a research method that uses some controlled elements of traditional lab experiments, but takes place in natural, real-world settings.
Field experiments are experiments carried out outside of laboratory settings.. They randomly assign subjects (or other sampling units) to either treatment or control groups to test claims of causal relationships. Random assignment helps establish the comparability of the treatment and control group so that any differences between them that emerge after the treatment has been administered ...
In this article, we offer an overview of field experimentation and its importance in discerning cause and effect relationships. We outline how randomized experiments represent an unbiased method for determining what works. Furthermore, we discuss key aspects of experiments, such as intervention, excludability, and non-interference.
First, field experiments are not behavioural economics. The former is a research method, the latter is a field or a body of research insights, some of which have been unearthed using field experimental methods. Second, field experiments are not pilot studies. A field experiment can be done on the national rollout of a policy,
Field experiments have grown significantly in prominence since the 1990s. In this article, we provide a summary of the major types of field experiments, explore their uses, and describe a few examples. ... and examine whether subjects behave as predicted by the model. In a field experiment, one accepts the actual preferences and institutions ...
Quantifying the Role of Theory in Field Experiments. The use of "experimental" (i.e., random-assignment) designs came relatively late to economics.1Over the last 15 years, however, randomized experiments in field settings have proliferated, and in 2010 field experiments represented about 3 percent of the arti-.
Using field experiments, scholars can identify causal effects via randomization while studying people and groups in their naturally occurring contexts. In light of renewed interest in field experimental methods, this review covers a wide range of field experiments from across the social sciences, with an eye to those that adopt virtuous practices, including unobtrusive measurement ...
This brief historical review shows how different the role of theory is in laboratory and fi eld experiments. Models have always played a key role in laboratory experi- ments, with an increasing trend. Field experiments have been largely Descriptive, with only a recent increase in the role for models.
Field Experiment. A field experiment is a type of research study where certain variables are intentionally manipulated and controlled in a natural setting or environment. Unlike a laboratory experiment, field experiments have less control over experimental conditions and extraneous variables, making it more difficult to infer causality.
Sixth, we describe two methodological challenges that field experiments frequently confront, noncompliance and attrition, showing the statistical and design implications of each. Seventh, we discuss the study of natural experiments and discontinuities as alternatives to both randomized interventions and conventional nonexperimental research.
We investigate the practical value of eld experiments by studying the number of ex-periments required. Other studies have investigated the required size of eld experiments. For example, Lewis and Rao (2012) conducted a set of 25 eld experiments involving large display advertising campaigns, each one including over 500,000 unique users and totaling
The history of field experiments in the marketing literature is surprisingly long. Early examples include Curhan (1974) and Eskin and Baron (1977), who vary prices, newspaper advertising, and display variables in grocery stores. This chapter reviews the recent history of field experiments in marketing by identifying papers published in the last ...
Figure 1. The Strawman. The strawman (illustrated in Figure 1) views the researcher as running a small, well-designed, and tightly controlled experiment with 100 treatment schools, 100 control schools), implemented by excellent (say, partners. She uncovers some results. If they are negative, she shelves the paper.
Therefore, to the best of our knowledge, the closest that economists have come to testing the models using field experiments is to find evidence in support of subcomponents of the model 49,50 ...
Field experiments document a positive influence of diverse crop rotations on microbial biomass nitrogen, total carbon and total nitrogen (Lori et al., 2017) and appear to correlate linearly with the volume of 0.2-3.0 μm diameter pores ("protective" pore space), the multifunctional pore system and a decrease in soil bulk density.
Field Experiments in Marketing. Anja Lambrecht and Catherine E. Tucker September 10, 2015. Abstract In a digitally enabled world, experimentation is easier. Here, we explore what this means for marketing researchers, and the subtleties of designing eld experiments for research. It gives guidelines for interpretation and describe the potential ...
Differences between model outcomes and field results are evident. For example, our previous T-FACE experiment demonstrated that spikelet number per unit area was a crucial factor, not percent grain fill, which down-regulated rice yield under future climate (Wang et al., 2018b, Wang et al., 2020).
6.10. Field experiments: Examples. "two randomized experiments conducted in schools in urban India. A remedial education program hired young women to teach students lagging behind in basic literacy and numeracy skills. It increased average test scores of all children in treatment schools by 0.28 standard deviation, mostly due to large gains ...
The simulation results support the field experiments to quantify the effects of suction strength on the stability of homogeneous loess slope. The initial suction strength of loess soil in the experiment site is about 5 kPa, which increases with the increase of the internal friction angle and initial matric suction.
An example of a framed field experiment is Chin, Bond, and Geva's (2000) study of the way in which sixty‐nine congressional staffers made simulated scheduling decisions, an experiment designed to detect whether scheduling preference is given to groups associated with a political action committee. "Natural" field experiments unobtrusively ...
There are three types of experiments you need to know: 1. Lab Experiment. A laboratory experiment in psychology is a research method in which the experimenter manipulates one or more independent variables and measures the effects on the dependent variable under controlled conditions. A laboratory experiment is conducted under highly controlled ...
5.1: Experiments Required elements of an experiment, and how they differ from the elements of an observational study. Basic example of an experimental design. 5.2: Experimental units and sampling units Introduction to sampling units, experimental units, and the concept of level at which units are independent within an experiment.
In September 2023, Ant Group introduced CodeFuse, a large language model (LLM) designed to assist programmer teams with coding. While one group of programmers used it, other programmer teams were not informed about this LLM. Leveraging this event, we conducted a field experiment on these two groups of programmers.
Experiments and surveys show that the art management model development system built by the newly introduced visual image technology, big data technology, and IP algorithm can increase user satisfaction by 24%. ... providing strong technical support for the field of art management, while also providing designers with a more accurate tool for ...
Model and field experiment was applied to evaluate effect of plant density on yield. • Raising plant density in plastic-mulched (PM) fields would not always increase yield. • OPD need derive from combination of field experiment with model simulation. • 45,000 plants ha −1 is the OPD for the area under PM with 300 mm annual precipitation.
The model developed for Experiment 3 captured the within-field variability and could predict low and high yield values. However, the model that was built for Experiment 4 overpredicted yield and could not capture the within-field variability. This is likely due to the low variability in the soil moisture features.
A similar hydroelasto-plastic model is designed, and a hydroelasto-plastic experiment is conducted to observe experimental freak waves and large rotational deformations. The theoretical velocity field from the Peregrine breather solution theory, based on the NLS equation, is defined in a CFD platform to generate 3D numerical freak waves.
Utilizing a 2D phase-field model, RL agents were assigned the task of reaching energetically unfavorable configurations, leading to the development of non-intuitive strategies for material design ...
The self-organization of clusters of particles is a fundamental phenomenon across various physical systems, including hydrodynamic and colloidal systems. One example is that of dense spherical particles submerged in a viscous fluid and subjected to horizontal oscillations. The interaction of the particles with the oscillating flow leads to the formation of one-particle-thick chains or multiple ...