Society Homepage About Public Health Policy Contact
Data-driven hypothesis generation in clinical research: what we learned from a human subject study, article sidebar.
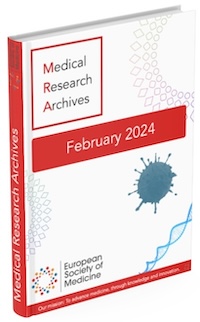

Submit your own article
Register as an author to reserve your spot in the next issue of the Medical Research Archives.
Join the Society
The European Society of Medicine is more than a professional association. We are a community. Our members work in countries across the globe, yet are united by a common goal: to promote health and health equity, around the world.
Join Europe’s leading medical society and discover the many advantages of membership, including free article publication.
Main Article Content
Hypothesis generation is an early and critical step in any hypothesis-driven clinical research project. Because it is not yet a well-understood cognitive process, the need to improve the process goes unrecognized. Without an impactful hypothesis, the significance of any research project can be questionable, regardless of the rigor or diligence applied in other steps of the study, e.g., study design, data collection, and result analysis. In this perspective article, the authors provide a literature review on the following topics first: scientific thinking, reasoning, medical reasoning, literature-based discovery, and a field study to explore scientific thinking and discovery. Over the years, scientific thinking has shown excellent progress in cognitive science and its applied areas: education, medicine, and biomedical research. However, a review of the literature reveals the lack of original studies on hypothesis generation in clinical research. The authors then summarize their first human participant study exploring data-driven hypothesis generation by clinical researchers in a simulated setting. The results indicate that a secondary data analytical tool, VIADS—a visual interactive analytic tool for filtering, summarizing, and visualizing large health data sets coded with hierarchical terminologies, can shorten the time participants need, on average, to generate a hypothesis and also requires fewer cognitive events to generate each hypothesis. As a counterpoint, this exploration also indicates that the quality ratings of the hypotheses thus generated carry significantly lower ratings for feasibility when applying VIADS. Despite its small scale, the study confirmed the feasibility of conducting a human participant study directly to explore the hypothesis generation process in clinical research. This study provides supporting evidence to conduct a larger-scale study with a specifically designed tool to facilitate the hypothesis-generation process among inexperienced clinical researchers. A larger study could provide generalizable evidence, which in turn can potentially improve clinical research productivity and overall clinical research enterprise.
Article Details
The Medical Research Archives grants authors the right to publish and reproduce the unrevised contribution in whole or in part at any time and in any form for any scholarly non-commercial purpose with the condition that all publications of the contribution include a full citation to the journal as published by the Medical Research Archives .
Warning: The NCBI web site requires JavaScript to function. more...

An official website of the United States government
The .gov means it's official. Federal government websites often end in .gov or .mil. Before sharing sensitive information, make sure you're on a federal government site.
The site is secure. The https:// ensures that you are connecting to the official website and that any information you provide is encrypted and transmitted securely.
- Publications
- Account settings
- Browse Titles
NCBI Bookshelf. A service of the National Library of Medicine, National Institutes of Health.
Walker HK, Hall WD, Hurst JW, editors. Clinical Methods: The History, Physical, and Laboratory Examinations. 3rd edition. Boston: Butterworths; 1990.

Clinical Methods: The History, Physical, and Laboratory Examinations. 3rd edition.
Chapter 2 collecting and analyzing data: doing and thinking.
David A. Nardone .
Clinicians embrace problem solving as one of their primary goals in patient care and value this skill as the major determinant of clinical competence. Despite these tenets, there is little conscious utilization of diagnostic reasoning strategies in clinical medicine. The focus is usually placed on pathophysiological knowledge base and not on collecting and analyzing data. When the latter are discussed, it is almost always in reference to algorithms, decision analysis, Bayes's theorem, and the clinicopathological conference exercise.
The physician, as decision maker, must possess a propensity for taking risks, a willingness to be dogmatic at times, and a dogged determination to make adequate decisions based on inadequate information. It is necessary to recognize patterns and to conceptualize, correlate, and compare data analytically. Even the experienced problem solver, however, is limited by cognitive strain. Only a few bits and chunks of data can be processed consciously through operative channels simultaneously. The clinician is limited also by the natural history of the disease. For instance, a symptom or sign may not have been manifested as yet; or certain manifestations may occur only in a small percentage of patients, that is, the sensitivity is low. Finally, the success or failure in the diagnostic process is dependent upon the quality of the patient–physician relationship. The physician must be caring and command sufficient competence in the psychosocial aspects of clinical medicine to facilitate the development of a trusting bond and structure an environment that is conducive to interchange. On the other hand, the patient must be cooperative and capable of relating problems, priorities, and expectations.
Reduction of cognitive strain is dependent primarily on implementing certain strategies of doing and thinking. Figure 2.1 and Table 2.1 represent a compilation of these general concepts. Whereas it might be assumed that problem solving begins after the pertinent manifestations from the history and physical examination have been gleaned and collated, it actually begins at the moment the patient and physician make initial contact. At this point, the diagnostic possibilities are infinite. The strategies then enable the clinician to sense the existence of a symptom or a sign (problem identification); formulate potential causes (hypothesis generation); collect data methodically (hypothesis evaluation and hypothesis analysis); and finally, to organize, synthesize, and prioritize the significant clinical findings (hypothesis assembling) for subsequent steps in diagnostic reasoning.
Collecting and analyzing data (Adapted from Feinstein, 1973.).
Collecting and Analyzing Data.
This series of methods directs the evaluation and interpretation of disease manifestations and the handling of rival hypotheses and discordant data. They determine the content and sequence of questions posed to the patient, of maneuvers performed during the physical examination, and of laboratory procedures utilized. The physician obviously does not proceed rigidly in the manner outlined. There is constant movement back and forth from one modality to another. The positive outcome of such a process is that the clinician can effectively proceed from infinity, the diagnostic unknown so to speak, to a point quite proximal to the diagnosis utilizing the doing and thinking strategies in the history and physical alone. The diagnosis is reached ultimately, in most circumstances, by implementing the same techniques as they pertain to the laboratory.
It is important to note the differences in doing and thinking when considering the issue of collecting and analyzing data. Doing refers to asking questions during the history, performing both general and specific maneuvers in the physical examination, and performing appropriate laboratory procedures. Thinking strategies reflect the intellectual tasks required throughout the encounter. The clinician continually generates and reformulates hypotheses, grapples with concepts of choosing appropriate labels or manifestations, and assembles each symptom and sign elicited in the history, physical, and laboratory into problem lists and diagnostic impressions. Thus, thinking forms the basis for all the action-oriented (doing) strategies.
- Problem Identification
One cannot solve a problem without first determining that it exists. In the earliest stages of the history, the clinician elicits the chief complaint and other health concerns. This technique of symptom and problem listing provides the interviewer with diagnostic leads to generate hypotheses and assists in prioritizing the patient's concerns so the problem solver can grasp the big picture and appreciate any potential interrelationship between the various symptoms and problems identified. Another advantage of symptom listing is that it may avoid the dilemma posed by the patient with a positive review of systems.
As symptoms are evaluated and analyzed, other problems are frequently uncovered. Consider a patient who presents with joint pains. During review of this problem, the physician learns that salicylate therapy alleviated the symptoms but was discontinued. Querying the patient discloses that there was an episode of black stools. Thus, the additional problem of melena is identified.
Obviously, there is no correlate of symptom listing in the physical and laboratory areas. However, problems are identified in a fashion similar to the patient with melena above. As an example, a clinician has interviewed a patient with chest pain and has a strong suspicion that it represents angina pectoris. During routine cardiac examination, the patient is found to have a systolic crescendo–decrescendo murmur heard best at the second right intercostal space and radiating to the carotid vessels. Heretofore, aortic stenosis had not been a problem. As for the laboratory, consider a woman who presents with peripheral edema. Neither the history nor the physical examination supports a cardiac, hepatic, venous, or renal cause. Preliminary laboratory investigation includes obtaining a serum albumin level to test the hypothesis of decreased colloid oncotic pressure from liver disease and a urinalysis to confirm or eliminate the possibility of nephrotic syndrome. Both are negative, but the urine does contain moderate amounts of glucose. The possibility of diabetes mellitus now enters this patient's medical profile.
- Hypothesis Generation
Formulation and revision of hypotheses are constant features of diagnostic reasoning and pervade the entire encounter ( Fig. 2.1 ). This is somewhat contrary to what is commonly believed, since hypothesis generation is usually ascribed to the history. Hypotheses may be general and refer to topographic parts of the anatomy such as domains (organ, system, region, channel) and foci (a subset of domain). When domains are diseased, certain symptoms and signs emanate. Hypotheses may be specific as well and refer to certain explicatory sets ( Table 2.1 ). These sets may be further categorized into disorders (congestive heart failure), derangements (myocardial infarction), pathoanatomic entities (coronary thrombosis), and pathophysiologic entities (hyperlipoproteinemia). The more specific the symptom or sign elicited, the better chance of activating specific hypotheses. For instance, nausea, inspiratory rales, and an elevated sedimentation rate are nonspecific, whereas syncope, S 3 gallop, and heavy proteinuria are specific. The latter three examples evoke a more narrow differential diagnosis. Some clinical manifestations may even be pathognomonic, that is, only one hypothesis fits. Consider the significance of paroxysmal nocturnal dyspnea, Cheyne-Stokes respirations, an arterial plaque in the fundus, etc. The seasoned practitioner more reliably generates specific hypotheses.
Hypothesis generation is predicated on informed intuition. It is imaginative and to a great extent subconscious. Frequently, armed with the mere knowledge of age, sex, and chief complaint, the clinician can entertain general and specific hypotheses that implicate common, reversible, and even exotic disease states. In fact, early hypothesis generation is the rule. Nevertheless, this is more readily achievable if the case is familiar. Conversely, with an unfamiliar case, effective hypothesis generation is often delayed until a higher percentage of the complete data base is collected. In this latter situation, it is best to concentrate on topographic and not explicatory sets.
Typically only a few hypotheses can be entertained at any time. Clinical findings elicited during the medical interview and physical examination generates the most hypotheses, but positive laboratory data contribute very little to generation of new hypotheses. Usually laboratory procedures are utilized to confirm or reject hypotheses. The number of hypotheses generated depends on the experience of the clinician.
- Hypothesis Evaluation
This is the strategy in which the clinician obtains the patient's story and performs the core physical and laboratory examinations in order to clarify and refine hypotheses generated to date. The major elements of hypothesis evaluation are characterization (doing) and choosing manifestations (thinking). Ultimately, these contribute in a meaningful way to the reformulation of hypotheses for appropriate analysis later. To this point, there has been identification of problems and generation of hypotheses. In order to resolve a problem of unknown cause, as is often the case at the bedside, the physician is confronted with the decision either to search in a nonbiased manner for information through hypothesis evaluation or proceed directly with hypothesis analysis. The option of evaluation grants the potential to convert an open-ended problem into one that is more defined, and in the history dramatically increases the probability of eliciting affirmative responses that are of significantly greater value than when directly testing hypotheses. If one resorts to hypothesis analysis immediately, there is a certain risk to assume. On the one hand, the problem in question may be solved promptly. If the result is negative or not particularly helpful, however, then premature closure is likely and very little has been accomplished.
The following two cases are illustrative: A 72-year-old man presents with progressive dyspnea. No doubt the topographic hypotheses of cardiac and pulmonary causes of dyspnea come to mind immediately. Perhaps such explicatory set hypotheses as chronic obstructive pulmonary disease and congestive heart failure are entertained. Hypothesis evaluation dictates that the physician obtain a clearer picture of dyspnea by determining the circumstances and characteristics of dyspnea (What? How? When? Where?), whereas hypothesis analysis would cause the clinician to query the patient immediately about tobacco usage and a prior history of myocardial infarction, etc.
In the second case, a 47-year-old man consults his physician for anorexia, weight gain, and increased abdominal girth. There is a strong suspicion of heavy alcohol intake. The examining physician may choose to evaluate the patient thoroughly by a careful and methodical examination of all core systems or resort to an hypothesis-driven examination where only the supraclavicular fossae are palpated (neoplastic nodes), the abdomen is inspected for distention (ascites), palpated for masses (hepatosplenomegaly) and fluid wave (ascites), and the skin checked for spider angiomas, the breasts examined for gynecomastia, and the testicles palpated for atrophy. The latter three represent direct testing for potential complications from alcoholism.
Characterization
The hallmarks of characterization are chronology, severity, influential factors, and expert witness. Chronology is applicable to the interview only and is the crux of any present illness. Just as virtually every pathophysiologic process has a beginning, an intermediate stage, and current status, so does each clinical manifestation of disease. Frequently just determining the chronology of a symptom carries clinical significance for diagnostic purposes. Consider the implications of the 45-year-old woman with intermittent disabling headaches for 22 years versus the patient who has suffered similar headaches but only for the last 2 weeks.
Severity is an index of the magnitude, progression, and impact of the disease on the patient's lifestyle. This technique assists the clarification process immeasurably. Reflect on the significance of the following: (1) a patient with extertional and nonexertional chest pain who consumes 5 to 10 sublingual trinitroglycerin tablets per day and is unable to work; (2) a patient with longstanding peptic ulcer disease who presents with an exacerbation and on physical examination is found to have marked epigastric involuntary guarding; and (3) the patient with progressive dyspnea whose pulmonary function testing reveals marked airflow obstruction on all parameters.
Precipitating events, alleviating elements, exacerbating stimuli, and associated symptoms or signs form the components of influential factors. These are well-known aspects of symptom characterization in the present illness but perhaps not appreciated when performing physical examination maneuvers and laboratory procedures. For instance, when examining an elderly woman who injured her hip in a fall, palpation and observation reveal that the pain is partially alleviated with hip flexion, exacerbated with other movements, and that there are associated signs of adductor muscle spasm and external rotation of the hip. As for the laboratory, a 41 -year-old man presents with substernal pressure-like chest pain occurring more commonly at rest than after exertion. Both the physical examination and resting electrocardiogram are normal. During a treadmill electrocardiogram, 2 mm of ST depression developed in the inferior leads (precipitated), one episode of six-beat atrial tachycardia was observed (associated), and all changes reverted to baseline 6 minutes after completion of the procedure (alleviated).
The expert witness technique is a method to validate, to collect additional information of diagnostic importance, and to assist in the determination of severity. The physician implements this strategy during the interview with the patient, when communicating with family, friends, ambulance technicians, nurses, etc., and during the physical and laboratory examinations by requesting informal and formal second-opinion consultations from colleagues. There are distinct advantages in the diagnostic and therapeutic framework when a critical finding is confirmed by a trusted associate. Is there asymmetry of the supraclavicular fossae? Is this nevus suspicious for neoplasm? Do you see an infiltrate in the lingula? Are these atypical lymphocytes? As a result of such exchange, clinical certainty is increased and the probability of premature closure is lessened.
Choosing Manifestations
There is a constant interplay between characterization and choosing manifestations. The latter is an intermediate step of hypothesis evaluation in which symptoms, signs, and problems are translated into medically meaningful terms with appropriate pathophysiological significance. Two categories exist under choosing manifestations: labeling and deviance.
Labeling permits the matching of symptoms and signs with accepted medical terminology. Accuracy depends on the clinician's and patient's ability to perceive, interact, and respond to the other individual's verbal comments. When examining the patient, the physician's psychomotor skills, perception, and interpretation of findings are necessary to label correctly. Frequently, when describing or inscribing physical findings, they are not expressed literally and only the interpretative statement is made. The term "spider angiomas" quite adequately and completely accounts for the description: "there are multiple erythematous dot-like lesions with serpiginous processes radiating in several different directions; they blanch on pressure and refill centrally when the pressure is released." The exception is the situation when findings cannot be labeled because the clinician is not knowledgeable enough to do so. In a laboratory study, the process is the same as in the physical examination despite the fact that labeling may be the province of a consultant. As an example, a radiologic procedure would probably be interpreted more expertly by a radiologist. Obviously, there are many pitfalls and errors in labeling because the process is complex, subjective, and dynamic.
The technique of deviance applies to understanding the ranges of normalcy and abnormality in assessing the significance of symptoms and signs being evaluated. Discussion of laboratory normal ranges are contained elsewhere in this book. There is considerable difficulty in assigning normalcy and abnormality to clinical manifestations collected during the history and physical. The physician's challenge in the history depends not only on his or her clinical skills, zeal, and biases but also on the patient's level of cooperation and memory. Thus, without attention to details, misperceptions and verbal misstatements occur. Likewise, signs elicited during the physical examination are at best semiquantitative. The danger, of course, is either overinterpretation or underinterpretation of manifestations. The consequences of the former are needless worry, unnecessary investigations, operations, treatments, and excessive costs. In the opposite situation, an underlying disease is not detected.
There is rarely difficulty in determining the abnormal state when marked deviations from normal exist. It is when the manifestation is less than severe that the clinician has a dilemma. It is no wonder that items in the history and physical are reviewed and repeated, that the same laboratory procedure is reordered, and that an advanced level test of a more invasive and costly nature is requested. Furthermore, these situations typically result in soliciting second opinions from other experts.
- Hypothesis Analysis
Whether it be soliciting a response during the history, performing a physical examination maneuver, or utilizing a laboratory test, the physician proceeds from an open-ended data collection mode in characterization to direct and specific ones in hypothesis analysis. Implicit in this definition is either a yes–no response in the history or a positive–negative result in the physical and laboratory examinations. Although clinical experience and knowledge of pathophysiology are central to any aspect of the patient–physician encounter, they are infinitely more essential when testing hypotheses. Frequently clinicians may characterize with only topographic-based hypotheses in mind, but it is impossible to analyze without explanatory sets relating to explicit entities, etiologies, and complications at hand. The intellectual preparatory mechanisms embodied in analyzing result in questions, maneuvers, and procedures that reflect more synthesis, development, and creativity. While employing this strategy, the clinician focuses on solidifying or refuting hypotheses entertained. The reward for the yes–no response and the positive–negative result justifies any inherent risk assumed by thwarting spontaneous symptom-related statements from the patient and by sacrificing detailed evaluation of every aspect of the physical and laboratory examinations. The danger of premature closure is no longer a factor.
Pertinent Systems Review
The presence of a symptom, problem, or physical sign, already localized to a domain and focus, requires the search for other symptoms and signs that, if present, may be manifestations of disease in the same domain. This is explorative direct questioning and examining in a nondirective manner. The clinician assumes that pursuing symptoms and signs within the same system is more likely to yield positive results than embarking on a questioning and examining process in an unrelated system area. It also compensates for the physician's fallibility in remembering and recognizing all disease patterns, provides additional thinking time, and permits one to rule out more remote, or even more common, possibilities.
Pathophysiology
This strategy depends on that basic fund of knowledge related to etiologies and complications of disease processes. In this context, as one applies the principles of the history, physical, and laboratory medicine to resolve a patient's problems, the pervasive and primary concern is to affix causality. This is the essence of the physician's expectations as a problem solver. Inherent in utilizing the technique of pathophysiology is that no question, maneuver, or procedure can be effected without a specific hypothetical explicatory set in mind ( Figure 2.1 , Table 2.1 ). Searching for etiological clues assists the clinician in isolating the problem according to the pathoanatomic and pathophysiologic entities, whereas seeking complications facilitates focusing on derangements and disorders. Note that the latter two, as explicatory sets, do explain symptoms and signs, but rarely, if ever, account for ultimate causation. For instance, when determining that a patient has paroxysmal nocturnal dyspnea, the theory is that congestive heart failure is responsible for the symptom. As a disorder, however, congestive heart failure is a complication of a more basic disease mechanism, perhaps myocardial infarction secondary to atherosclerosis, which in itself may be caused by acquired hyperlipidemia, etc.
Clinician Priority
This particular subsection of hypothesis analysis addresses techniques that transcend purity in diagnostic reasoning. In all preceding sections the goal was strictly the pursuit of a diagnosis. The concept of clinician priority attempts to focus the diagnostic process into the practical perspective of clinical medicine. These techniques are utilized subconsciously throughout the encounter, but are rarely appreciated as such by the clinician. They represent the "art of medicine" and "picking up the game" strategies that one assimilates, perhaps by osmosis, during clinical training apprenticeships and through continuing professional experience. There are five such categories: urgency, uncertainty, threshold, reversibility, and commonness.
When a clinician acts out of urgency , it is because the presence of a particular symptom or sign implies that immediate diagnostic or therapeutic intervention is indicated. It is action oriented. Attention is directed toward the acutely ill and potentially acutely ill, who may have serious life-threatening or fatal diseases. Accordingly, uncommon entities may be ranked higher than those with greater frequency of occurrence. With this in mind, it is easy to comprehend why the physician chooses to elicit the presence of rigors in a patient with fever, dysuria, and flank pain ("Is the patient bacteremic?") and dedicates more than a few moments to observe the same patient carefully for pilo-erection and decreased skin perfusion. There are even occasions in which the search for a particular complication (hypovolemia from diarrhea) provides a much stronger stimulus for the clinician than the cause of the diarrhea itself.
Coping with uncertainty at the bedside is universal. It plagues the physician, but protects the patient. Basically this strategy forces one to collect more historical, physical examination, and laboratory data than may be necessary because the consequences of diagnostic error without doing so are too great. A decision must be made at what level uncertainty is tolerable. The challenge is to be conservative and to avoid errors of omission. It is as if the fear of clinical consequences and its attendant penalties enable clinicians to be more effective. Unfortunately, there is excessive reliance on laboratory medicine. It is infinitely more acceptable to implement the strategy of uncertainty to its fullest during history taking and the physical examination, when patient risk is virtually nonexistent, but it is not necessarily more judicious when utilizing laboratory procedures.
Threshold is the converse of uncertainty. It is that point at which further data could be collected but neither a positive nor a negative response would contribute to the analytic process or change the predictive value significantly. Uncertainty prevails until that critical point when the remaining doubt can be tolerated. The dilemma is whether to continue being driven by uncertainty or to invoke threshold. If the threshold is set too high, then redundant, and often needless, information is sought. When set too low, the physician may negate an opportunity to make a diagnosis or institute therapy.
The emphasis on reversibility or treatability embodies the essence of medicine, that is, cure the patient. Relevant data must be collected to aid therapeutic decision making. If presented with two competing hypotheses, the one with the greatest potential for treatment will be ranked higher. The payoff for doing so is greater. The following case exemplifies this: A 59-year-old man has had progressively worsening dyspnea for 2½ years without associated wheezing. Physical examination reveals findings compatible with chronic obstructive pulmonary disease. Pulmonary function spirometry with and without bronchodilators is ordered despite the fact the patient has clinical evidence of irreversible disease. It is hoped that a reactive obstructive component, responsive to bronchodilator therapy, will be uncovered.
The adage "common things are common" aptly describes the technique of commonness. Of those techniques discussed previously, it is most likely to be in the clinician's awareness. The issue is one of good sense. It is not helpful to entertain an uncommon hypothesis unless there is good reason, as when invoking urgency and uncertainty. Thus, in the patient with abdominal pain in whom pancreatitis is under consideration, why collect data about symptoms relevant to renal disease, emboli, vasculitis, etc., when it is more appropriate to investigate the presence of symptoms of cholelithiasis and alcohol intake, both of which account for 95% of the cases of acute pancreatitis? Similarly, in a patient with an enlarging abdomen and swollen ankles, it will be a much higher priority to check for signs of cardiac and hepatic diseases as opposed to those implicating inferior vena cava obstruction.
Case Building
This process involves both consolidation of clinical data and refinement and modification of diagnostic possibilities to assist in solidifying hypotheses, refuting them, and distinguishing between two likely candidates. Elimination enables one to disprove a hypothesis in a convincing manner by seeking negative responses and results to questions and maneuvers of high sensitivity (true positive rate) for a given hypothesis. Thus it is difficult to entertain seriously the diagnosis of infectious mononucleosis without sore throat, reactive airway disease without prolonged expiration, and nephrotic syndrome in the patient without proteinuria. Discriminating between two closely related hypotheses is a frequent challenge. In the patient with several episodes of hematochezia, determining whether the blood is on the outside of the stool or mixed in with the stool helps to distinguish between anal disease and luminal pathology more proximal to the anus. A comparable example in the physical examination is attempting to transilluminate a scrotal mass, and in the laboratory arena when ordering a serum gamma-glutamyltransferase in a patient with elevated alkaline phosphatase. Finally, with confirmation one attempts to clinch a diagnosis by seeking clinical manifestations of high specificity despite the fact that one or more bits of data already support such. Thus, discerning that a patient has low back pain that radiates to the thigh and lateral calf is suggestive of radiculopathy. But determining that this pain is associated with numbness and that it is exacerbated by coughing, sneezing, and straining is even more convincing. Similarly in the physical examination of a patient with dyspnea on exertion, the findings of peripheral edema, hepatomegaly, inspiratory rales, and distended neck veins support the contention that left-sided heart failure is the cause, but the finding of an S 3 , gallop is definitive and confirms the suspicion.
- Hypothesis Assembling
This element in the sequential strategic process of diagnostic reasoning encompasses the synthesis and integration of multiple clinical clues from the vast amount of data collected. Assembling is governed by the principle that a hierarchical organizational structure of facts exists in the scheme of diagnosis. The stimuli are to reduce the scope of the problem and sort out the complexities encountered to date. Ultimately, a working problem list will be developed to guide any further investigative pursuits and therapeutic management. To be functional, the problem list must be both coherent and adequate in the context of the patient being evaluated. In history taking, hypothesis assembling encompasses the formulation of a narrowed set of hypotheses to permit further characterization and analysis during the physical examination and laboratory testing. At the conclusion of the physical examination, all clues and elicited manifestations from the history and physical undergo the same process to direct laboratory data collection. Finally, after all appropriate laboratory tests are completed, the problem list is transformed into the refined product of impressions and diagnoses.
Many positive and negative items elicited at the bedside are not relevant and must be filtered out. Pertinence determines which normal and abnormal manifestations will be retained or disregarded and which will require further attention. In general, findings with pathophysiological significance, especially those with a high clinician priority, will be kept. Likewise, symptoms and signs will be retained if there is a potential for inclusion in a particular explicatory set. For example, an 85-year-old man has a 7-day history of nausea, vomiting (once daily), diarrhea (five to ten times daily), headache, nasal stuffiness, and dizziness. He was treated with antibiotics for a carbuncle 4 weeks ago and has a past history of left inguinal herniorrhaphy and cholecystectomy. On physical examination, he had a 25 mm Hg orthostatic drop in systolic blood pressure, poor skin turgor, scarred tympanic membranes, anisocoria, S 4 gallop, right upper quadrant and left inguinal scars, active bowel sounds, diffuse abdominal tenderness, perianal erythema, perianal skin tag, and a mildly enlarged prostate. Are headache, nasal stuffiness, left inguinal herniorrhaphy, scarred tympanic membranes, anisocoria, etc., pertinent? Are they truly clinical problems worthy of note at this time?
Clustering or lumping is the aggregation of several symptoms and signs into recognizable patterns that fit under the sets of disorders, derangements, pathoanatomic entities, and pathophysiologic entities. They may be related to one another by cause and effect (dependent clustering) or by virtue of their clinical significance (independent clustering). In the former category polyuria, polydipsia, and polyphagia are classic symptoms for diabetes mellitus. The osmotic effect of glucose is responsible for polyuria and calorie loss, which cause both polydipsia and polyphagia. In the independent category, one can cluster orthopnea, paroxysmal nocturnal dyspnea, distended neck veins, positive hepatojugular reflux, S 3 gallop, and peripheral edema under the umbrella of congestive heart failure. Lumping then is consistent with the law of parsimony, which dictates that clinicians should make as few diagnoses as possible. This is monopathic reasoning.
Frequently patients have many positive symptoms and signs. Whereas clustering supports economy in diagnosis, splitting promotes polypathic reasoning with the retention of certain clinical manifestations as separate problem entities because inappropriate aggregation may jeopardize the diagnostic and therapeutic processes. The dilemma posed to physicians by splitting is similar to uncertainty in some respects. There is a fear of missing a diagnosis. In the 85-year-old patient described above under pertinence, orthostatic hypotension was found on physical examination. There is a high probability that this objective sign is caused by vomiting and diarrhea. Thus, they could be lumped together as cause and effect. In so doing, however, the clinician is at risk of excluding a completely separate and important problem, namely, extracellular volume depletion. Splitting prevents this from happening.
Problem listing is the identification of a formalized working set of symptoms and signs, aggregated symptoms and signs, as well as hypothesized derangements and disorders. Either by lumping or splitting, the clinician must account for all positive findings elicited in the history, physical, and laboratory sections of the patient's evaluation. In order to qualify for listing, each must have an importance diagnostically, therapeutically, or both.
Referring once again to the patient described earlier, original laboratory work-up revealed azotemia (blood urea nitrogen, 38 mg/dl; creatinine, 2.4 mg/dl), hypoalbuminemia (albumin, 3.1 g/dl), elevated alkaline phosphatase (alkaline phosphatase, 212 U/L), hyperuricemia (uric acid, 9.2 mg/dl), and moderate stool leukocytes. Thus an appropriate problem list for this patient at the end of the history might include (1) diarrhea, (2) headache, (3) dizziness, and (4) prior surgeries. After the physical examination, the list might be revised to state (1) diarrhea, (2) dizziness, (3) prior surgeries, (4) extracellular volume depletion, (5) perianal erythema, and (6) mildly enlarged prostate. With the knowledge of the laboratory data the refined version might be listed accordingly: (1) inflammatory diarrhea, (2) extracellular volume depletion, (3) dizziness, (4) perianal erythema, (5) mildly enlarged prostate, (6) azotemia, (7) hypoalbuminemia, (8) hyperuricemia, and (9) elevated alkaline phosphatase. Obviously, the patient's problems are not totally resolved, but they are certainly narrowed to the point that focused supportive therapy can be administered and second-line hypothesis-driven laboratory testing can be planned ultimately to confirm definitive diagnoses.
Collecting and analyzing data involve problem identification, generation of general and specific hypotheses, methodical information gathering during evaluation and analysis, and assembling of pertinent clinical clues as problems to direct further investigation and treatment. This process is a continuum and quite dynamic. It never seems to end because the "patient" host and disease are changing variables. The clinician must proceed in a flexible manner throughout the entire framework, based on judgment, learned behavior, and knowledge of pathophysiologic principles.
- Bashook PG. A conceptual framework for measuring clinical problem-solving. J Med Educ. 1976; 51 :109–14. [ PubMed : 1249820 ]
- Benbassat J, Bachar-Bassan E. A comparison of initial diagnostic hypotheses of medical students and internists. J Med Educ. 1984; 59 :951–56. [ PubMed : 6502664 ]
- Blois MS. Clinical judgment and computers. N Engl J Med. 1980; 303 :192–97. [ PubMed : 7383090 ]
- Bollet AJ. Analyzing the diagnostic process. Res Staff Phys Dec 1978; 41–42.
- Christensen-Szalanski JJJ, Bushyhead JB. Physicians" misunderstanding of normal findings. Med Decis Making. 1983; 3 :169–75. [ PubMed : 6633186 ]
- Connelly DP, Johnson PE. The medical problem-solving process. Hum Pathol. 1980; 11 :412–18. [ PubMed : 7429488 ]
- Cox KR. How do you decide what it is and what to do? Med J Aust. 1975; 2 :57–59. [ PubMed : 1160724 ]
- de Dombal FT, Horrorcks JC, Staniland JR, Guillou PJ. Production of artificial "case histories" by using a small computer. Br Med J. 1971; 2 :578–81. [ PMC free article : PMC1795846 ] [ PubMed : 5579198 ]
- Dudley HAF. The clinical task. Lancet. 1970; 2 :1352–54. [ PubMed : 4098919 ]
- *Eddy DM, Clanton CH. The art of diagnosis: solving the clinicopathological exercise. N Engl J Med. 1982; 306 :1263–67. [ PubMed : 7070446 ]
- Ekwo EE. An analysis of the problem-solving process of third year medical students. Annu Conf Res Med Educ. 1977; 16 :317–22. [ PubMed : 75707 ]
- *Elstein AS, Shulman LS, Sprafka SA. Medical problem solving: an analysis of clinical reasoning. Cambridge: Harvard University Press, 1978.
- *Feinstein AR. An analysis of diagnostic reasoning: I. The domains and disorders of clinical macrobiology. Yale J Biol Med. 1973; 46 :212–32. [ PMC free article : PMC2591978 ] [ PubMed : 4803623 ]
- *Feinstein AR. An analysis of diagnostic reasoning: II. The strategy of intermediate decisions. Yale J Biol Med. 1973; 46 :264–83. [ PMC free article : PMC2591913 ] [ PubMed : 4775683 ]
- Illingworth RS. The importance of knowing what is normal. Publ Health Lond. 1981; 95 :66–68. [ PubMed : 7244078 ]
- Johnson PE, Duran AS, Hassebrock F, Moller J, Prietula M, Fel-tovich PJ, Swanson DB. Expertise and error in diagnostic reasoning. Cog Sci. 1981; 5 :235–83.
- *Kassirer JP, Gorry GA. Clinical problem solving: a behavioral analysis. Ann Intern Med. 1978; 89 :245–55. [ PubMed : 677593 ]
- Komaroff AL. The variability and inaccuracy of medical data. IEEE Proc. 1979; 67 :1196–1207.
- Lipkin M. Diagnosis, the assessment of the patient and his problems. In: The care of patients: concepts and tactics. New York: Oxford University Press 1974:103–56.
- Miller GA. The magical number seven, plus or minus two: some limits on our capacity for processing information. Psychol Rev. 1956; 63 :81–97. [ PubMed : 13310704 ]
- *Miller PB. Strategy selection in medical diagnosis. MAC-TR-153. Massachusetts Institute of Technology, Project MAC, Cambridge, 1975.
- Nardone DA, Reuler JB, Girard DE. Teaching history-taking: where are we? Yale J Biol Med. 1980; 53 :233–50. [ PMC free article : PMC2595873 ] [ PubMed : 7405275 ]
- Pauker SG, Kassirer JP. The threshold approach to clinical decision making. N Engl J Med. 1980; 302 :1109–17. [ PubMed : 7366635 ]
- Pople HE Jr., Myers JD, Miller RA. DIALOG: A model of diagnostic logic for internal medicine. In: Proceedings of the Fourth International Conference on Artificial Intelligence, 1975:848–55.
- *Rubin AD. Hypothesis formation and evaluation in medical diagnosis. AI-TR-316, Artificial Intelligence Laboratory, Massachusetts Institute of Technology, Cambridge, 1975.
- Sox HC; Jr., Blatt MA, Higgins MC, Marlon, KI. Medical decision making. Boston: Bulterworths, 1988.
- *Sprosty PJ. The use of questions in the diagnostic problem solving process. In: The diagnostic process, Jacquez JA, (ed). Ann Arbor: University of Michigan Press, 1964:281–310.
- Style A. Intuition and problem solving. J Roy Coll Gen Pract. 1979; 29 :71–74. [ PMC free article : PMC2159128 ] [ PubMed : 480297 ]
- Vovtovich AF, Rippey RM. Knowledge, realism, and diagnostic reasoning in a physical diagnosis course. J Med Educ. 1982; 57 :461–67. [ PubMed : 7077636 ]
- Cite this Page Nardone DA. Collecting and Analyzing Data: Doing and Thinking. In: Walker HK, Hall WD, Hurst JW, editors. Clinical Methods: The History, Physical, and Laboratory Examinations. 3rd edition. Boston: Butterworths; 1990. Chapter 2.
- PDF version of this page (2.3M)
In this Page
Related items in bookshelf.
- All Textbooks
Related information
- PMC PubMed Central citations
- PubMed Links to PubMed
Similar articles in PubMed
- [Issues of research in medicine]. [Acta Med Croatica. 2006] [Issues of research in medicine]. Topić E. Acta Med Croatica. 2006; 60 Suppl 1:5-16.
- Guilty? Not guilty? Of "doing" without "thinking" "thinking" without "doing" or 'thinking with doing--doing with thinking'. [Iowa Dent J. 1977] Guilty? Not guilty? Of "doing" without "thinking" "thinking" without "doing" or 'thinking with doing--doing with thinking'. Richey DW. Iowa Dent J. 1977 Jan; 63(1):11.
- Perspective: Whither the problem list? Organ-based documentation and deficient synthesis by medical trainees. [Acad Med. 2010] Perspective: Whither the problem list? Organ-based documentation and deficient synthesis by medical trainees. Kaplan DM. Acad Med. 2010 Oct; 85(10):1578-82.
- Review A taxonomy of prospection: introducing an organizational framework for future-oriented cognition. [Proc Natl Acad Sci U S A. 2014] Review A taxonomy of prospection: introducing an organizational framework for future-oriented cognition. Szpunar KK, Spreng RN, Schacter DL. Proc Natl Acad Sci U S A. 2014 Dec 30; 111(52):18414-21. Epub 2014 Nov 21.
- Review Milestones of critical thinking: a developmental model for medicine and nursing. [Acad Med. 2014] Review Milestones of critical thinking: a developmental model for medicine and nursing. Papp KK, Huang GC, Lauzon Clabo LM, Delva D, Fischer M, Konopasek L, Schwartzstein RM, Gusic M. Acad Med. 2014 May; 89(5):715-20.
Recent Activity
- Collecting and Analyzing Data: Doing and Thinking - Clinical Methods Collecting and Analyzing Data: Doing and Thinking - Clinical Methods
Your browsing activity is empty.
Activity recording is turned off.
Turn recording back on
Connect with NLM
National Library of Medicine 8600 Rockville Pike Bethesda, MD 20894
Web Policies FOIA HHS Vulnerability Disclosure
Help Accessibility Careers

Scientific hypothesis generation process in clinical research: a secondary data analytic tool versus experience study protocol
- Find this author on Google Scholar
- Find this author on PubMed
- Search for this author on this site
- ORCID record for Xia Jing
- For correspondence: [email protected]
- Info/History
- Supplementary material
- Preview PDF
Background Scientific hypothesis generation is a critical step in scientific research that determines the direction and impact of any investigation. Despite its vital role, we have limited knowledge of the process itself, hindering our ability to address some critical questions.
Objective To what extent can secondary data analytic tools facilitate scientific hypothesis generation during clinical research? Are the processes similar in developing clinical diagnoses during clinical practice and developing scientific hypotheses for clinical research projects? We explore the process of scientific hypothesis generation in the context of clinical research. The study is designed to compare the role of VIADS, our web-based interactive secondary data analysis tool, and the experience levels of study participants during their scientific hypothesis generation processes.
Methods Inexperienced and experienced clinical researchers are recruited. In this 2×2 study design, all participants use the same data sets during scientific hypothesis-generation sessions, following pre-determined scripts. The inexperienced and experienced clinical researchers are randomly assigned into groups with and without using VIADS. The study sessions, screen activities, and audio recordings of participants are captured. Participants use the think-aloud protocol during the study sessions. After each study session, every participant is given a follow-up survey, with participants using VIADS completing an additional modified System Usability Scale (SUS) survey. A panel of clinical research experts will assess the scientific hypotheses generated based on pre-developed metrics. All data will be anonymized, transcribed, aggregated, and analyzed.
Results This study is currently underway. Recruitment is ongoing via a brief online survey 1 . The preliminary results show that study participants can generate a few to over a dozen scientific hypotheses during a 2-hour study session, regardless of whether they use VIADS or other analytic tools. A metric to assess scientific hypotheses within a clinical research context more accurately, comprehensively, and consistently has also been developed.
Conclusion The scientific hypothesis-generation process is an advanced cognitive activity and a complex process. Clinical researchers can quickly generate initial scientific hypotheses based on data sets and prior experience based on our current results. However, refining these scientific hypotheses is much more time-consuming. To uncover the fundamental mechanisms of generating scientific hypotheses, we need breakthroughs that capture thinking processes more precisely.
Competing Interest Statement
The authors have declared no competing interest.
Clinical Trial
This study is not a clinical trial per NIH definition.
Funding Statement
The project is supported by a grant from the National Library of Medicine of the United States National Institutes of Health (R15LM012941) and partially supported by the National Institute of General Medical Sciences of the National Institutes of Health (P20 GM121342). The content is solely the author's responsibility and does not necessarily represent the official views of the National Institutes of Health.
Author Declarations
I confirm all relevant ethical guidelines have been followed, and any necessary IRB and/or ethics committee approvals have been obtained.
The details of the IRB/oversight body that provided approval or exemption for the research described are given below:
The study has been approved by the Institutional Review Board (IRB) at Clemson University (IRB2020-056).
I confirm that all necessary patient/participant consent has been obtained and the appropriate institutional forms have been archived, and that any patient/participant/sample identifiers included were not known to anyone (e.g., hospital staff, patients or participants themselves) outside the research group so cannot be used to identify individuals.
I understand that all clinical trials and any other prospective interventional studies must be registered with an ICMJE-approved registry, such as ClinicalTrials.gov. I confirm that any such study reported in the manuscript has been registered and the trial registration ID is provided (note: if posting a prospective study registered retrospectively, please provide a statement in the trial ID field explaining why the study was not registered in advance).
I have followed all appropriate research reporting guidelines and uploaded the relevant EQUATOR Network research reporting checklist(s) and other pertinent material as supplementary files, if applicable.
Data Availability
This manuscript is the study protocol. After we analyze and publish the results, transcribed, aggregated, de-identified data can be requested from the authors.
Abbreviations
View the discussion thread.
Supplementary Material
Thank you for your interest in spreading the word about medRxiv.
NOTE: Your email address is requested solely to identify you as the sender of this article.

Citation Manager Formats
- EndNote (tagged)
- EndNote 8 (xml)
- RefWorks Tagged
- Ref Manager
- Tweet Widget
- Facebook Like
- Google Plus One
Subject Area
- Health Informatics
- Addiction Medicine (336)
- Allergy and Immunology (664)
- Anesthesia (178)
- Cardiovascular Medicine (2600)
- Dentistry and Oral Medicine (314)
- Dermatology (218)
- Emergency Medicine (391)
- Endocrinology (including Diabetes Mellitus and Metabolic Disease) (920)
- Epidemiology (12135)
- Forensic Medicine (10)
- Gastroenterology (752)
- Genetic and Genomic Medicine (4031)
- Geriatric Medicine (380)
- Health Economics (670)
- Health Informatics (2605)
- Health Policy (994)
- Health Systems and Quality Improvement (966)
- Hematology (359)
- HIV/AIDS (832)
- Infectious Diseases (except HIV/AIDS) (13616)
- Intensive Care and Critical Care Medicine (785)
- Medical Education (397)
- Medical Ethics (109)
- Nephrology (426)
- Neurology (3797)
- Nursing (208)
- Nutrition (564)
- Obstetrics and Gynecology (728)
- Occupational and Environmental Health (689)
- Oncology (1989)
- Ophthalmology (576)
- Orthopedics (235)
- Otolaryngology (303)
- Pain Medicine (249)
- Palliative Medicine (72)
- Pathology (470)
- Pediatrics (1098)
- Pharmacology and Therapeutics (456)
- Primary Care Research (443)
- Psychiatry and Clinical Psychology (3379)
- Public and Global Health (6470)
- Radiology and Imaging (1379)
- Rehabilitation Medicine and Physical Therapy (801)
- Respiratory Medicine (866)
- Rheumatology (395)
- Sexual and Reproductive Health (403)
- Sports Medicine (337)
- Surgery (438)
- Toxicology (51)
- Transplantation (185)
- Urology (165)
- Comprehensive Learning Paths
- 150+ Hours of Videos
- Complete Access to Jupyter notebooks, Datasets, References.

Hypothesis Testing – A Deep Dive into Hypothesis Testing, The Backbone of Statistical Inference
- September 21, 2023
Explore the intricacies of hypothesis testing, a cornerstone of statistical analysis. Dive into methods, interpretations, and applications for making data-driven decisions.

In this Blog post we will learn:
- What is Hypothesis Testing?
- Steps in Hypothesis Testing 2.1. Set up Hypotheses: Null and Alternative 2.2. Choose a Significance Level (α) 2.3. Calculate a test statistic and P-Value 2.4. Make a Decision
- Example : Testing a new drug.
- Example in python
1. What is Hypothesis Testing?
In simple terms, hypothesis testing is a method used to make decisions or inferences about population parameters based on sample data. Imagine being handed a dice and asked if it’s biased. By rolling it a few times and analyzing the outcomes, you’d be engaging in the essence of hypothesis testing.
Think of hypothesis testing as the scientific method of the statistics world. Suppose you hear claims like “This new drug works wonders!” or “Our new website design boosts sales.” How do you know if these statements hold water? Enter hypothesis testing.
2. Steps in Hypothesis Testing
- Set up Hypotheses : Begin with a null hypothesis (H0) and an alternative hypothesis (Ha).
- Choose a Significance Level (α) : Typically 0.05, this is the probability of rejecting the null hypothesis when it’s actually true. Think of it as the chance of accusing an innocent person.
- Calculate Test statistic and P-Value : Gather evidence (data) and calculate a test statistic.
- p-value : This is the probability of observing the data, given that the null hypothesis is true. A small p-value (typically ≤ 0.05) suggests the data is inconsistent with the null hypothesis.
- Decision Rule : If the p-value is less than or equal to α, you reject the null hypothesis in favor of the alternative.
2.1. Set up Hypotheses: Null and Alternative
Before diving into testing, we must formulate hypotheses. The null hypothesis (H0) represents the default assumption, while the alternative hypothesis (H1) challenges it.
For instance, in drug testing, H0 : “The new drug is no better than the existing one,” H1 : “The new drug is superior .”
2.2. Choose a Significance Level (α)
When You collect and analyze data to test H0 and H1 hypotheses. Based on your analysis, you decide whether to reject the null hypothesis in favor of the alternative, or fail to reject / Accept the null hypothesis.
The significance level, often denoted by $α$, represents the probability of rejecting the null hypothesis when it is actually true.
In other words, it’s the risk you’re willing to take of making a Type I error (false positive).
Type I Error (False Positive) :
- Symbolized by the Greek letter alpha (α).
- Occurs when you incorrectly reject a true null hypothesis . In other words, you conclude that there is an effect or difference when, in reality, there isn’t.
- The probability of making a Type I error is denoted by the significance level of a test. Commonly, tests are conducted at the 0.05 significance level , which means there’s a 5% chance of making a Type I error .
- Commonly used significance levels are 0.01, 0.05, and 0.10, but the choice depends on the context of the study and the level of risk one is willing to accept.
Example : If a drug is not effective (truth), but a clinical trial incorrectly concludes that it is effective (based on the sample data), then a Type I error has occurred.
Type II Error (False Negative) :
- Symbolized by the Greek letter beta (β).
- Occurs when you accept a false null hypothesis . This means you conclude there is no effect or difference when, in reality, there is.
- The probability of making a Type II error is denoted by β. The power of a test (1 – β) represents the probability of correctly rejecting a false null hypothesis.
Example : If a drug is effective (truth), but a clinical trial incorrectly concludes that it is not effective (based on the sample data), then a Type II error has occurred.
Balancing the Errors :
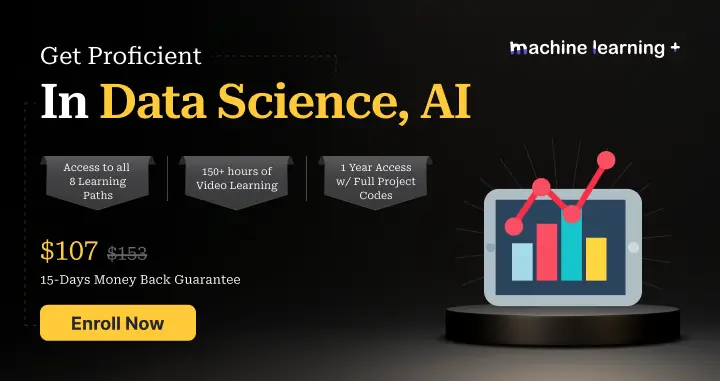
In practice, there’s a trade-off between Type I and Type II errors. Reducing the risk of one typically increases the risk of the other. For example, if you want to decrease the probability of a Type I error (by setting a lower significance level), you might increase the probability of a Type II error unless you compensate by collecting more data or making other adjustments.
It’s essential to understand the consequences of both types of errors in any given context. In some situations, a Type I error might be more severe, while in others, a Type II error might be of greater concern. This understanding guides researchers in designing their experiments and choosing appropriate significance levels.
2.3. Calculate a test statistic and P-Value
Test statistic : A test statistic is a single number that helps us understand how far our sample data is from what we’d expect under a null hypothesis (a basic assumption we’re trying to test against). Generally, the larger the test statistic, the more evidence we have against our null hypothesis. It helps us decide whether the differences we observe in our data are due to random chance or if there’s an actual effect.
P-value : The P-value tells us how likely we would get our observed results (or something more extreme) if the null hypothesis were true. It’s a value between 0 and 1. – A smaller P-value (typically below 0.05) means that the observation is rare under the null hypothesis, so we might reject the null hypothesis. – A larger P-value suggests that what we observed could easily happen by random chance, so we might not reject the null hypothesis.
2.4. Make a Decision
Relationship between $α$ and P-Value
When conducting a hypothesis test:
We then calculate the p-value from our sample data and the test statistic.
Finally, we compare the p-value to our chosen $α$:
- If $p−value≤α$: We reject the null hypothesis in favor of the alternative hypothesis. The result is said to be statistically significant.
- If $p−value>α$: We fail to reject the null hypothesis. There isn’t enough statistical evidence to support the alternative hypothesis.
3. Example : Testing a new drug.
Imagine we are investigating whether a new drug is effective at treating headaches faster than drug B.
Setting Up the Experiment : You gather 100 people who suffer from headaches. Half of them (50 people) are given the new drug (let’s call this the ‘Drug Group’), and the other half are given a sugar pill, which doesn’t contain any medication.
- Set up Hypotheses : Before starting, you make a prediction:
- Null Hypothesis (H0): The new drug has no effect. Any difference in healing time between the two groups is just due to random chance.
- Alternative Hypothesis (H1): The new drug does have an effect. The difference in healing time between the two groups is significant and not just by chance.
Calculate Test statistic and P-Value : After the experiment, you analyze the data. The “test statistic” is a number that helps you understand the difference between the two groups in terms of standard units.
For instance, let’s say:
- The average healing time in the Drug Group is 2 hours.
- The average healing time in the Placebo Group is 3 hours.
The test statistic helps you understand how significant this 1-hour difference is. If the groups are large and the spread of healing times in each group is small, then this difference might be significant. But if there’s a huge variation in healing times, the 1-hour difference might not be so special.
Imagine the P-value as answering this question: “If the new drug had NO real effect, what’s the probability that I’d see a difference as extreme (or more extreme) as the one I found, just by random chance?”
For instance:
- P-value of 0.01 means there’s a 1% chance that the observed difference (or a more extreme difference) would occur if the drug had no effect. That’s pretty rare, so we might consider the drug effective.
- P-value of 0.5 means there’s a 50% chance you’d see this difference just by chance. That’s pretty high, so we might not be convinced the drug is doing much.
- If the P-value is less than ($α$) 0.05: the results are “statistically significant,” and they might reject the null hypothesis , believing the new drug has an effect.
- If the P-value is greater than ($α$) 0.05: the results are not statistically significant, and they don’t reject the null hypothesis , remaining unsure if the drug has a genuine effect.
4. Example in python
For simplicity, let’s say we’re using a t-test (common for comparing means). Let’s dive into Python:
Making a Decision : “The results are statistically significant! p-value < 0.05 , The drug seems to have an effect!” If not, we’d say, “Looks like the drug isn’t as miraculous as we thought.”
5. Conclusion
Hypothesis testing is an indispensable tool in data science, allowing us to make data-driven decisions with confidence. By understanding its principles, conducting tests properly, and considering real-world applications, you can harness the power of hypothesis testing to unlock valuable insights from your data.
More Articles
F statistic formula – explained, correlation – connecting the dots, the role of correlation in data analysis, sampling and sampling distributions – a comprehensive guide on sampling and sampling distributions, law of large numbers – a deep dive into the world of statistics, central limit theorem – a deep dive into central limit theorem and its significance in statistics, similar articles, complete introduction to linear regression in r, how to implement common statistical significance tests and find the p value, logistic regression – a complete tutorial with examples in r.
Subscribe to Machine Learning Plus for high value data science content
© Machinelearningplus. All rights reserved.

Machine Learning A-Z™: Hands-On Python & R In Data Science
Free sample videos:.

Thank you for visiting nature.com. You are using a browser version with limited support for CSS. To obtain the best experience, we recommend you use a more up to date browser (or turn off compatibility mode in Internet Explorer). In the meantime, to ensure continued support, we are displaying the site without styles and JavaScript.
- View all journals
- Explore content
- About the journal
- Publish with us
- Sign up for alerts
- Open access
- Published: 09 July 2024
Automating psychological hypothesis generation with AI: when large language models meet causal graph
- Song Tong ORCID: orcid.org/0000-0002-4183-8454 1 , 2 , 3 , 4 na1 ,
- Kai Mao 5 na1 ,
- Zhen Huang 2 ,
- Yukun Zhao 2 &
- Kaiping Peng 1 , 2 , 3 , 4
Humanities and Social Sciences Communications volume 11 , Article number: 896 ( 2024 ) Cite this article
1512 Accesses
4 Altmetric
Metrics details
- Science, technology and society
Leveraging the synergy between causal knowledge graphs and a large language model (LLM), our study introduces a groundbreaking approach for computational hypothesis generation in psychology. We analyzed 43,312 psychology articles using a LLM to extract causal relation pairs. This analysis produced a specialized causal graph for psychology. Applying link prediction algorithms, we generated 130 potential psychological hypotheses focusing on “well-being”, then compared them against research ideas conceived by doctoral scholars and those produced solely by the LLM. Interestingly, our combined approach of a LLM and causal graphs mirrored the expert-level insights in terms of novelty, clearly surpassing the LLM-only hypotheses ( t (59) = 3.34, p = 0.007 and t (59) = 4.32, p < 0.001, respectively). This alignment was further corroborated using deep semantic analysis. Our results show that combining LLM with machine learning techniques such as causal knowledge graphs can revolutionize automated discovery in psychology, extracting novel insights from the extensive literature. This work stands at the crossroads of psychology and artificial intelligence, championing a new enriched paradigm for data-driven hypothesis generation in psychological research.
Similar content being viewed by others
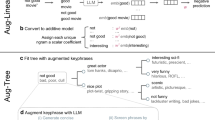
Augmenting interpretable models with large language models during training
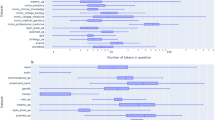
ThoughtSource: A central hub for large language model reasoning data
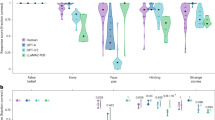
Testing theory of mind in large language models and humans
Introduction.
In an age in which the confluence of artificial intelligence (AI) with various subjects profoundly shapes sectors ranging from academic research to commercial enterprises, dissecting the interplay of these disciplines becomes paramount (Williams et al., 2023 ). In particular, psychology, which serves as a nexus between the humanities and natural sciences, consistently endeavors to demystify the complex web of human behaviors and cognition (Hergenhahn and Henley, 2013 ). Its profound insights have significantly enriched academia, inspiring innovative applications in AI design. For example, AI models have been molded on hierarchical brain structures (Cichy et al., 2016 ) and human attention systems (Vaswani et al., 2017 ). Additionally, these AI models reciprocally offer a rejuvenated perspective, deepening our understanding from the foundational cognitive taxonomy to nuanced esthetic perceptions (Battleday et al., 2020 ; Tong et al., 2021 ). Nevertheless, the multifaceted domain of psychology, particularly social psychology, has exhibited a measured evolution compared to its tech-centric counterparts. This can be attributed to its enduring reliance on conventional theory-driven methodologies (Henrich et al., 2010 ; Shah et al., 2015 ), a characteristic that stands in stark contrast to the burgeoning paradigms of AI and data-centric research (Bechmann and Bowker, 2019 ; Wang et al., 2023 ).
In the journey of psychological research, each exploration originates from a spark of innovative thought. These research trajectories may arise from established theoretical frameworks, daily event insights, anomalies within data, or intersections of interdisciplinary discoveries (Jaccard and Jacoby, 2019 ). Hypothesis generation is pivotal in psychology (Koehler, 1994 ; McGuire, 1973 ), as it facilitates the exploration of multifaceted influencers of human attitudes, actions, and beliefs. The HyGene model (Thomas et al., 2008 ) elucidated the intricacies of hypothesis generation, encompassing the constraints of working memory and the interplay between ambient and semantic memories. Recently, causal graphs have provided psychology with a systematic framework that enables researchers to construct and simulate intricate systems for a holistic view of “bio-psycho-social” interactions (Borsboom et al., 2021 ; Crielaard et al., 2022 ). Yet, the labor-intensive nature of the methodology poses challenges, which requires multidisciplinary expertise in algorithmic development, exacerbating the complexities (Crielaard et al., 2022 ). Meanwhile, advancements in AI, exemplified by models such as the generative pretrained transformer (GPT), present new avenues for creativity and hypothesis generation (Wang et al., 2023 ).
Building on this, notably large language models (LLMs) such as GPT-3, GPT-4, and Claude-2, which demonstrate profound capabilities to comprehend and infer causality from natural language texts, a promising path has emerged to extract causal knowledge from vast textual data (Binz and Schulz, 2023 ; Gu et al., 2023 ). Exciting possibilities are seen in specific scenarios in which LLMs and causal graphs manifest complementary strengths (Pan et al., 2023 ). Their synergistic combination converges human analytical and systemic thinking, echoing the holistic versus analytic cognition delineated in social psychology (Nisbett et al., 2001 ). This amalgamation enables fine-grained semantic analysis and conceptual understanding via LLMs, while causal graphs offer a global perspective on causality, alleviating the interpretability challenges of AI (Pan et al., 2023 ). This integrated methodology efficiently counters the inherent limitations of working and semantic memories in hypothesis generation and, as previous academic endeavors indicate, has proven efficacious across disciplines. For example, a groundbreaking study in physics synthesized 750,000 physics publications, utilizing cutting-edge natural language processing to extract 6368 pivotal quantum physics concepts, culminating in a semantic network forecasting research trajectories (Krenn and Zeilinger, 2020 ). Additionally, by integrating knowledge-based causal graphs into the foundation of the LLM, the LLM’s capability for causative inference significantly improves (Kıcıman et al., 2023 ).
To this end, our study seeks to build a pioneering analytical framework, combining the semantic and conceptual extraction proficiency of LLMs with the systemic thinking of the causal graph, with the aim of crafting a comprehensive causal network of semantic concepts within psychology. We meticulously analyzed 43,312 psychological articles, devising an automated method to construct a causal graph, and systematically mining causative concepts and their interconnections. Specifically, the initial sifting and preparation of the data ensures a high-quality corpus, and is followed by employing advanced extraction techniques to identify standardized causal concepts. This results in a graph database that serves as a reservoir of causal knowledge. In conclusion, using node embedding and similarity-based link prediction, we unearthed potential causal relationships, and thus generated the corresponding hypotheses.
To gauge the pragmatic value of our network, we selected 130 hypotheses on “well-being” generated by our framework, comparing them with hypotheses crafted by novice experts (doctoral students in psychology) and the LLM models. The results are encouraging: Our algorithm matches the caliber of novice experts, outshining the hypotheses generated solely by the LLM models in novelty. Additionally, through deep semantic analysis, we demonstrated that our algorithm contains more profound conceptual incorporations and a broader semantic spectrum.
Our study advances the field of psychology in two significant ways. Firstly, it extracts invaluable causal knowledge from the literature and converts it to visual graphics. These aids can feed algorithms to help deduce more latent causal relations and guide models in generating a plethora of novel causal hypotheses. Secondly, our study furnishes novel tools and methodologies for causal analysis and scientific knowledge discovery, representing the seamless fusion of modern AI with traditional research methodologies. This integration serves as a bridge between conventional theory-driven methodologies in psychology and the emerging paradigms of data-centric research, thereby enriching our understanding of the factors influencing psychology, especially within the realm of social psychology.
Methodological framework for hypothesis generation
The proposed LLM-based causal graph (LLMCG) framework encompasses three steps: literature retrieval, causal pair extraction, and hypothesis generation, as illustrated in Fig. 1 . In the literature gathering phase, ~140k psychology-related articles were downloaded from public databases. In step two, GPT-4 were used to distil causal relationships from these articles, culminating in the creation of a causal relationship network based on 43,312 selected articles. In the third step, an in-depth examination of these data was executed, adopting link prediction algorithms to forecast the dynamics within the causal relationship network for searching the highly potential causality concept pairs.
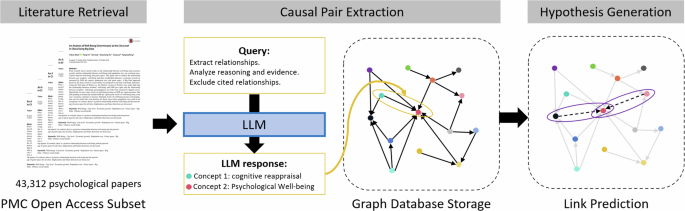
Note: LLM stands for large language model; LLMCG algorithm stands for LLM-based causal graph algorithm, which includes the processes of literature retrieval, causal pair extraction, and hypothesis generation.
Step 1: Literature retrieval
The primary data source for this study was a public repository of scientific articles, the PMC Open Access Subset. Our decision to utilize this repository was informed by several key attributes that it possesses. The PMC Open Access Subset boasts an expansive collection of over 2 million full-text XML science and medical articles, providing a substantial and diverse base from which to derive insights for our research. Furthermore, the open-access nature of the articles not only enhances the transparency and reproducibility of our methodology, but also ensures that the results and processes can be independently accessed and verified by other researchers. Notably, the content within this subset originates from recognized journals, all of which have undergone rigorous peer review, lending credence to the quality and reliability of the data we leveraged. Finally, an added advantage was the rich metadata accompanying each article. These metadata were instrumental in refining our article selection process, ensuring coherent thematic alignment with our research objectives in the domains of psychology.
To identify articles relevant to our study, we applied a series of filtering criteria. First, the presence of certain keywords within article titles or abstracts was mandatory. Some examples of these keywords include “psychol”, “clin psychol”, and “biol psychol”. Second, we exploited the metadata accompanying each article. The classification of articles based on these metadata ensured alignment with recognized thematic standards in the domains of psychology and neuroscience. Upon the application of these criteria, we managed to curate a subset of approximately 140K articles that most likely discuss causal concepts in both psychology and neuroscience.
Step 2: Causal pair extraction
The process of extracting causal knowledge from vast troves of scientific literature is intricate and multifaceted. Our methodology distils this complex process into four coherent steps, each serving a distinct purpose. (1) Article selection and cost analysis: Determines the feasibility of processing a specific volume of articles, ensuring optimal resource allocation. (2) Text extraction and analysis: Ensures the purity of the data that enter our causal extraction phase by filtering out nonrelevant content. (3) Causal knowledge extraction: Uses advanced language models to detect, classify, and standardize causal factors relationships present in texts. (4) Graph database storage: Facilitates structured storage, easy retrieval, and the possibility of advanced relational analyses for future research. This streamlined approach ensures accuracy, consistency, and scalability in our endeavor to understand the interplay of causal concepts in psychology and neuroscience.
Text extraction and cleaning
After a meticulous cost analysis detailed in Appendix A , our selection process identified 43,312 articles. This selection was strategically based on the criterion that the journal titles must incorporate the term “Psychol”, signifying their direct relevance to the field of psychology. The distributions of publication sources and years can be found in Table 1 . Extracting the full texts of the articles from their PDF sources was an essential initial step, and, for this purpose, the PyPDF2 Python library was used. This library allowed us to seamlessly extract and concatenate titles, abstracts, and main content from each PDF article. However, a challenge arose with the presence of extraneous sections such as references or tables, in the extracted texts. The implemented procedure, employing regular expressions in Python, was not only adept at identifying variations of the term “references” but also ascertained whether this section appeared as an isolated segment. This check was critical to ensure that the identified that the “references” section was indeed distinct, marking the start of a reference list without continuation into other text. Once identified as a standalone entity, the next step in the method was to efficiently remove the reference section and its subsequent content.
Causal knowledge extraction method
In our effort to extract causal knowledge, the choice of GPT-4 was not arbitrary. While several models were available for such tasks, GPT-4 emerged as a frontrunner due to its advanced capabilities (Wu et al., 2023 ), extensive training on diverse data, with its proven proficiency in understanding context, especially in complex scientific texts (Cheng et al., 2023 ; Sanderson, 2023 ). Other models were indeed considered; however, the capacity of GPT-4 to generate coherent, contextually relevant responses gave our project an edge in its specific requirements.
The extraction process commenced with the segmentation of the articles. Due to the token constraints inherent to GPT-4, it was imperative to break down the articles into manageable chunks, specifically those of 4000 tokens or fewer. This approach ensured a comprehensive interpretation of the content without omitting any potential causal relationships. The next phase was prompt engineering. To effectively guide the extraction capabilities of GPT-4, we crafted explicit prompts. A testament to this meticulous engineering is demonstrated in a directive in which we asked the model to elucidate causal pairs in a predetermined JSON format. For a clearer understanding, readers are referred to Table 2 , which elucidates the example prompt and the subsequent model response. After extraction, the outputs were not immediately cataloged. A filtering process was initiated to ascertain the standardization of the concept pairs. This process weeded out suboptimal outputs. Aiding in this quality control, GPT-4 played a pivotal role in the verification of causal pairs, determining their relevance, causality, and ensuring correct directionality. Finally, while extracting knowledge, we were aware of the constraints imposed by the GPT-4 API. There was a conscious effort to ensure that we operated within the bounds of 60 requests and 150k tokens per minute. This interplay of prompt engineering and stringent filtering was productive.
In addition, we conducted an exploratory study to assess GPT-4’s discernment between “causality” and “correlation” involved four graduate students (mean age 31 ± 10.23), each evaluating relationship pairs extracted from their familiar psychology articles. The experimental details and results can be found in Appendix A and Table A1. The results showed that out of 289 relationships identified by GPT-4, 87.54% were validated. Notably, when GPT-4 classified relationships as causal, only 13.02% (31/238) were recognized as non-relationship, while 65.55% (156/238) agreed upon as causality. This shows that GPT-4 can accurately extract relationships (causality or correlation) in psychological texts, underscoring the potential as a tool for the construction of causal graphs.
To enhance the robustness of the extracted causal relationships and minimize biases, we adopted a multifaceted approach. Recognizing the indispensable role of human judgment, we periodically subjected random samples of extracted causal relationships to the scrutiny of domain experts. Their valuable feedback was instrumental in the real-time fine-tuning the extraction process. Instead of heavily relying on referenced hypotheses, our focus was on extracting causal pairs, primarily from the findings mentioned in the main texts. This systematic methodology ultimately resulted in a refined text corpus distilled from 43,312 articles, which contained many conceptual insights and were primed for rigorous causal extraction.
Graph database storage
Our decision to employ Neo4j as the database system was strategic. Neo4j, as a graph database (Thomer and Wickett, 2020 ), is inherently designed to capture and represent complex relationships between data points, an attribute that is essential for understanding intricate causal relationships. Beyond its technical prowess, Neo4j provides advantages such as scalability, resilience, and efficient querying capabilities (Webber, 2012 ). It is particularly adept at traversing interconnected data points, making it an excellent fit for our causal relationship analysis. The mined causal knowledge finds its abode in the Neo4j graph database. Each pair of causal concepts is represented as a node, with its directionality and interpretations stored as attributes. Relationships provide related concepts together. Storing the knowledge graph in Neo4j allows for the execution of the graph algorithms to analyze concept interconnectivity and reveal potential relationships.
The graph database contains 197k concepts and 235k connections. Table 3 encapsulates the core concepts and provides a vivid snapshot of the most recurring themes; helping us to understand the central topics that dominate the current psychological discourse. A comprehensive examination of the core concepts extracted from 43,312 psychological papers, several distinct patterns and focal areas emerged. In particular, there is a clear balance between health and illness in psychological research. The prominence of terms such as “depression”, “anxiety”, and “symptoms of depression magnifies the commitment in the discipline to understanding and addressing mental illnesses. However, juxtaposed against these are positive terms such as “life satisfaction” and “sense of happiness”, suggesting that psychology not only fixates on challenges but also delves deeply into the nuances of positivity and well-being. Furthermore, the significance given to concepts such as “life satisfaction”, “sense of happiness”, and “job satisfaction” underscores an increasing recognition of emotional well-being and job satisfaction as integral to overall mental health. Intertwining the realms of psychology and neuroscience, terms such as “microglial cell activation”, “cognitive impairment”, and “neurodegenerative changes” signal a growing interest in understanding the neural underpinnings of cognitive and psychological phenomena. In addition, the emphasis on “self-efficacy”, “positive emotions”, and “self-esteem” reflect the profound interest in understanding how self-perception and emotions influence human behavior and well-being. Concepts such as “age”, “resilience”, and “creativity” further expand the canvas, showcasing the eclectic and comprehensive nature of inquiries in the field of psychology.
Overall, this analysis paints a vivid picture of modern psychological research, illuminating its multidimensional approach. It demonstrates a discipline that is deeply engaged with both the challenges and triumphs of human existence, offering holistic insight into the human mind and its myriad complexities.
Step 3: Hypothesis generation using link prediction
In the quest to uncover novel causal relationships beyond direct extraction from texts, the technique of link prediction emerges as a pivotal methodology. It hinges on the premise of proposing potential causal ties between concepts that our knowledge graph does not explicitly connect. The process intricately weaves together vector embedding, similarity analysis, and probability-based ranking. Initially, concepts are transposed into a vector space using node2vec, which is valued for its ability to capture topological nuances. Here, every pair of unconnected concepts is assigned a similarity score, and pairs that do not meet a set benchmark are quickly discarded. As we dive deeper into the higher echelons of these scored pairs, the likelihood of their linkage is assessed using the Jaccard similarity of their neighboring concepts. Subsequently, these potential causal relationships are organized in descending order of their derived probabilities, and the elite pairs are selected.
An illustration of this approach is provided in the case highlighted in Figure A1. For instance, the behavioral inhibition system (BIS) exhibits ties to both the behavioral activation system (BAS) and the subsequent behavioral response of the BAS when encountering reward stimuli, termed the BAS reward response. Simultaneously, another concept, interference, finds itself bound to both the BAS and the BAS Reward Response. This configuration hints at a plausible link between the BIS and interference. Such highly probable causal pairs are not mere intellectual curiosity. They act as springboards, catalyzing the genesis of new experimental designs or research hypotheses ripe for empirical probing. In essence, this capability equips researchers with a cutting-edge instrument, empowering them to navigate the unexplored waters of the psychological and neurological domains.
Using pairs of highly probable causal concepts, we pushed GPT-4 to conjure novel causal hypotheses that bridge concepts. To further elucidate the process of this method, Table 4 provides some examples of hypotheses generated from the process. Such hypotheses, as exemplified in the last row, underscore the potential and power of our method for generating innovative causal propositions.
Hypotheses evaluation and results
In this section, we present an analysis focusing on quality in terms of novelty and usefulness of the hypotheses generated. According to existing literature, these dimensions are instrumental in encapsulating the essence of inventive ideas (Boden, 2009 ; McCarthy et al., 2018 ; Miron-Spektor and Beenen, 2015 ). These parameters have not only been quintessential for gauging creative concepts, but they have also been adopted to evaluate the caliber of research hypotheses (Dowling and Lucey, 2023 ; Krenn and Zeilinger, 2020 ; Oleinik, 2019 ). Specifically, we evaluate the quality of the hypotheses generated by the proposed LLMCG algorithm in relation to those generated by PhD students from an elite university who represent human junior experts, the LLM model, which represents advanced AI systems, and the research ideas refined by psychological researchers which represents cooperation between AI and humans.
The evaluation comprises three main stages. In the first stage, the hypotheses are generated by all contributors, including steps taken to ensure fairness and relevance for comparative analysis. In the second stage, the hypotheses from the first stage are independently and blindly reviewed by experts who represent the human academic community. These experts are asked to provide hypothesis ratings using a specially designed questionnaire to ensure statistical validity. The third stage delves deeper by transforming each research idea into the semantic space of a bidirectional encoder representation from transformers (BERT) (Lee et al., 2023 ), allowing us to intricately analyze the intrinsic reasons behind the rating disparities among the groups. This semantic mapping not only pinpoints the nuanced differences, but also provides potential insights into the cognitive constructs of each hypothesis.
Evaluation procedure
Selection of the focus area for hypothesis generation.
Selecting an appropriate focus area for hypothesis generation is crucial to ensure a balanced and insightful comparison of the hypothesis generation capacities between various contributors. In this study, our goal is to gauge the quality of hypotheses derived from four distinct contributors, with measures in place to mitigate potential confounding variables that might skew the results among groups (Rubin, 2005 ). Our choice of domain is informed by two pivotal criteria: the intricacy and subtlety of the subject matter and familiarity with the domain. It is essential that our chosen domain boasts sufficient complexity to prompt meaningful hypothesis generation and offer a robust assessment of both AI and human contributors” depth of understanding and creativity. Furthermore, while human contributors should be well-acquainted with the domain, their expertise need not match the vast corpus knowledge of the AI.
In terms of overarching human pursuits such as the search for happiness, positive psychology distinguishes itself by avoiding narrowly defined, individual-centric challenges (Seligman and Csikszentmihalyi, 2000 ). This alignment with our selection criteria is epitomized by well-being, a salient concept within positive psychology, as shown in Table 3 . Well-being, with its multidimensional essence that encompass emotional, psychological, and social facets, and its central stature in both research and practical applications of positive psychology (Diener et al., 2010 ; Fredrickson, 2001 ; Seligman and Csikszentmihalyi, 2000 ), becomes the linchpin of our evaluation. The growing importance of well-being in the current global context offers myriad novel avenues for hypothesis generation and theoretical advancement (Forgeard et al., 2011 ; Madill et al., 2022 ; Otu et al., 2020 ). Adding to our rationale, the Positive Psychology Research Center at Tsinghua University is a globally renowned hub for cutting-edge research in this domain. Leveraging this stature, we secured participation from specialized Ph.D. students, reinforcing positive psychology as the most fitting domain for our inquiry.
Hypotheses comparison
In our study, the generated psychological hypotheses were categorized into four distinct groups, consisting of two experimental groups and two control groups. The experimental groups encapsulate hypotheses generated by our algorithm, either through random selection or handpicking by experts from a pool of generated hypotheses. On the other hand, control groups comprise research ideas that were meticulously crafted by doctoral students with substantial academic expertise in the domains and hypotheses generated by representative LLMs. In the following, we elucidate the methodology and underlying rationale for each group:
LLMCG algorithm output (Random-selected LLMCG)
Following the requirement of generating hypotheses centred on well-being, the LLMCG algorithm crafted 130 unique hypotheses. These hypotheses were derived by LLMCG’s evaluation of the most likely causal relationships related to well-being that had not been previously documented in research literature datasets. From this refined pool, 30 research ideas were chosen at random for this experimental group. These hypotheses represent the algorithm’s ability to identify causal relationships and formulate pertinent hypotheses.
LLMCG expert-vetted hypotheses (Expert-selected LLMCG)
For this group, two seasoned psychological researchers, one male aged 47 and one female aged 46, in-depth expertise in the realm of Positive Psychology, conscientiously handpicked 30 of the most promising hypotheses from the refined pool, excluding those from the Random-selected LLMCG category. The selection criteria centered on a holistic understanding of both the novelty and practical relevance of each hypothesis. With an illustrious postdoctoral journey and a robust portfolio of publications in positive psychology to their names, they rigorously sifted through the hypotheses, pinpointing those that showcased a perfect confluence of originality and actionable insight. These hypotheses were meticulously appraised for their relevance, structural coherence, and potential academic value, representing the nexus of machine intelligence and seasoned human discernment.
PhD students’ output (Control-Human)
We enlisted the expertise of 16 doctoral students from the Positive Psychology Research Center at Tsinghua University. Under the guidance of their supervisor, each student was provided with a questionnaire geared toward research on well-being. The participants were given a period of four working days to complete and return the questionnaire, which was distributed during vacation to ensure minimal external disruptions and commitments. The specific instructions provided in the questionnaire is detailed in Table B1 , and each participant was asked to complete 3–4 research hypotheses. By the stipulated deadline, we received responses from 13 doctoral students, with a mean age of 31.92 years (SD = 7.75 years), cumulatively presenting 41 hypotheses related to well-being. To maintain uniformity with the other groups, a random selection was made to shortlist 30 hypotheses for further analysis. These hypotheses reflect the integration of core theoretical concepts with the latest insights into the domain, presenting an academic interpretation rooted in their rigorous training and education. Including this group in our study not only provides a natural benchmark for human ingenuity and expertise but also underscores the invaluable contribution of human cognition in research ideation, serving as a pivotal contrast to AI-generated hypotheses. This juxtaposition illuminates the nuanced differences between human intellectual depth and AI’s analytical progress, enriching the comparative dimensions of our study.
Claude model output (Control-Claude)
This group exemplifies the pinnacle of current LLM technology in generating research hypotheses. Since LLMCG is a nascent technology, its assessment requires a comparative study with well-established counterparts, creating a key paradigm in comparative research. Currently, Claude-2 and GPT-4 represent the apex of AI technology. For example, Claude-2, with an accuracy rate of 54. 4% excels in reasoning and answering questions, substantially outperforming other models such as Falcon, Koala and Vicuna, which have accuracy rates of 17.1–25.5% (Wu et al., 2023 ). To facilitate a more comprehensive evaluation of the new model by researchers and to increase the diversity and breadth of comparison, we chose Claude-2 as the control model. Using the detailed instructions provided in Table B2, Claude-2 was iteratively prompted to generate research hypotheses, generating ten hypotheses per prompt, culminating in a total of 50 hypotheses. Although the sheer number and range of these hypotheses accentuate the capabilities of Claude-2, to ensure compatibility in terms of complexity and depth between all groups, a subsequent refinement was considered essential. With minimal human intervention, GPT-4 was used to evaluate these 50 hypotheses and select the top 30 that exhibited the most innovative, relevant, and academically valuable insights. This process ensured the infusion of both the LLM”s analytical prowess and a layer of qualitative rigor, thus giving rise to a set of hypotheses that not only align with the overarching theme of well-being but also resonate with current academic discourse.
Hypotheses assessment
The assessment of the hypotheses encompasses two key components: the evaluation conducted by eminent psychology professors emphasizing novelty and utility, and the deep semantic analysis involving BERT and t -distributed stochastic neighbor embedding ( t -SNE) visualization to discern semantic structures and disparities among hypotheses.
Human academic community
The review task was entrusted to three eminent psychology professors (all male, mean age = 42.33), who have a decade-long legacy in guiding doctoral and master”s students in positive psychology and editorial stints in renowned journals; their task was to conduct a meticulous evaluation of the 120 hypotheses. Importantly, to ensure unbiased evaluation, the hypotheses were presented to them in a completely randomized order in the questionnaire.
Our emphasis was undeniably anchored to two primary tenets: novelty and utility (Cohen, 2017 ; Shardlow et al., 2018 ; Thompson and Skau, 2023 ; Yu et al., 2016 ), as shown in Table B3 . Utility in hypothesis crafting demands that our propositions extend beyond mere factual accuracy; they must resonate deeply with academic investigations, ensuring substantial practical implications. Given the inherent challenges of research, marked by constraints in time, manpower, and funding, it is essential to design hypotheses that optimize the utilization of these resources. On the novelty front, we strive to introduce innovative perspectives that have the power to challenge and expand upon existing academic theories. This not only propels the discipline forward but also ensures that we do not inadvertently tread on ground already covered by our contemporaries.
Deep semantic analysis
While human evaluations provide invaluable insight into the novelty and utility of hypotheses, to objectively discern and visualize semantic structures and the disparities among them, we turn to the realm of deep learning. Specifically, we employ the power of BERT (Devlin et al., 2018 ). BERT, as highlighted by Lee et al. ( 2023 ), had a remarkable potential to assess the innovation of ideas. By translating each hypothesis into a high-dimensional vector in the BERT domain, we obtain the profound semantic core of each statement. However, such granularity in dimensions presents challenges when aiming for visualization.
To alleviate this and to intuitively understand the clustering and dispersion of these hypotheses in semantic space, we deploy the t -SNE ( t -distributed Stochastic Neighbor Embedding) technique (Van der Maaten and Hinton, 2008 ), which is adept at reducing the dimensionality of the data while preserving the relative pairwise distances between the items. Thus, when we map our BERT-encoded hypotheses onto a 2D t -SNE plane, an immediate visual grasp on how closely or distantly related our hypotheses are in terms of their semantic content. Our intent is twofold: to understand the semantic terrains carved out by the different groups and to infer the potential reasons for some of the hypotheses garnered heightened novelty or utility ratings from experts. The convergence of human evaluations and semantic layouts, as delineated by Algorithm 1 in Appendix B , reveal the interplay between human intuition and the inherent semantic structure of the hypotheses.
Qualitative analysis by topic analysis
To better understand the underlying thought processes and the topical emphasis of both PhD students and the LLMCG model, qualitative analyses were performed using visual tools such as word clouds and connection graphs, as detailed in Appendix B . The word cloud, as a graphical representation, effectively captures the frequency and importance of terms, providing direct visualization of the dominant themes. Connection graphs, on the other hand, elucidate the relationships and interplay between various themes and concepts. Using these visual tools, we aimed to achieve a more intuitive and clear representation of the data, allowing for easy comparison and interpretation.
Observations drawn from both the word clouds and the connection graphs in Figures B1 and B2 provide us with a rich tapestry of insights into the thought processes and priorities of Ph.D. students and the LLMCG model. For instance, the emphasis in the Control-Human word cloud on terms such as “robot” and “AI” indicates a strong interest among Ph.D. students in the nexus between technology and psychology. It is particularly fascinating to see a group of academically trained individuals focusing on the real world implications and intersections of their studies, as shown by their apparent draw toward trending topics. This not only underscores their adaptability but also emphasizes the importance of contextual relevance. Conversely, the LLMCG groups, particularly the Expert-selected LLMCG group, emphasize the community, collective experiences, and the nuances of social interconnectedness. This denotes a deep-rooted understanding and application of higher-order social psychological concepts, reflecting the model”s ability to dive deep into the intricate layers of human social behavior.
Furthermore, the connection graphs support these observations. The Control-Human graph, with its exploration of themes such as “Robot Companionship” and its relation to factors such as “heart rate variability (HRV)”, demonstrates a confluence of technology and human well-being. The other groups, especially the Random-selected LLMCG group, yield themes that are more societal and structural, hinting at broader determinants of individual well-being.
Analysis of human evaluations
To quantify the agreement among the raters, we employed Spearman correlation coefficients. The results, as shown in Table B5, reveal a spectrum of agreement levels between the reviewer pairs, showcasing the subjective dimension intrinsic to the evaluation of novelty and usefulness. In particular, the correlation between reviewer 1 and reviewer 2 in novelty (Spearman r = 0.387, p < 0.0001) and between reviewer 2 and reviewer 3 in usefulness (Spearman r = 0.376, p < 0.0001) suggests a meaningful level of consensus, particularly highlighting their capacity to identify valuable insights when evaluating hypotheses.
The variations in correlation values, such as between reviewer 2 and reviewer 3 ( r = 0.069, p = 0.453), can be attributed to the diverse research orientations and backgrounds of each reviewer. Reviewer 1 focuses on social ecology, reviewer 3 specializes in neuroscientific methodologies, and reviewer 2 integrates various views using technologies like virtual reality, and computational methods. In our evaluation, we present specific hypotheses cases to illustrate the differing perspectives between reviewers, as detailed in Table B4 and Figure B3. For example, C5 introduces the novel concept of “Virtual Resilience”. Reviewers 1 and 3 highlighted its originality and utility, while reviewer 2 rated it lower in both categories. Meanwhile, C6, which focuses on social neuroscience, resonated with reviewer 3, while reviewers 1 and 2 only partially affirmed it. These differences underscore the complexity of evaluating scientific contributions and highlight the importance of considering a range of expert opinions for a comprehensive evaluation.
This assessment is divided into two main sections: Novelty analysis and usefulness analysis.
Novelty analysis
In the dynamic realm of scientific research, measuring and analyzing novelty is gaining paramount importance (Shin et al., 2022 ). ANOVA was used to analyze the novelty scores represented in Fig. 2 a, and we identified a significant influence of the group factor on the mean novelty score between different reviewers. Initially, z-scores were calculated for each reviewer”s ratings to standardize the scoring scale, which were then averaged. The distinct differences between the groups, as visualized in the boxplots, are statistically underpinned by the results in Table 5 . The ANOVA results revealed a pronounced effect of the grouping factor ( F (3116) = 6.92, p = 0.0002), with variance explained by the grouping factor (R-squared) of 15.19%.
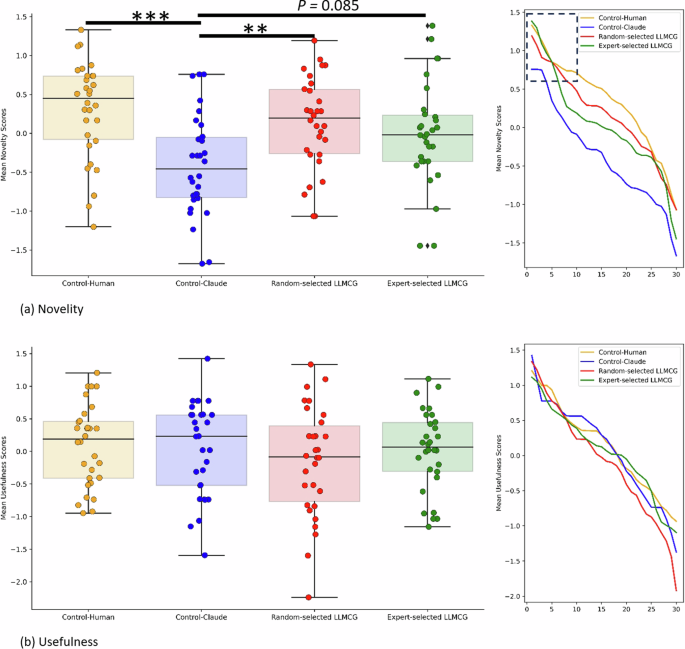
Box plots on the left ( a ) and ( b ) depict distributions of novelty and usefulness scores, respectively, while smoothed line plots on the right demonstrate the descending order of novelty and usefulness scores and subjected to a moving average with a window size of 2. * denotes p < 0.05, ** denotes p <0.01.
Further pairwise comparisons using the Bonferroni method, as delineated in Table 5 and visually corroborated by Fig. 2 a; significant disparities were discerned between Random-selected LLMCG and Control-Claude ( t (59) = 3.34, p = 0.007) and between Control-Human and Control-Claude ( t (59) = 4.32, p < 0.001). The Cohen’s d values of 0.8809 and 1.1192 respectively indicate that the novelty scores for the Random-selected LLMCG and Control-Human groups are significantly higher than those for the Control-Claude group. Additionally, when considering the cumulative distribution plots to the right of Fig. 2 a, we observe the distributional characteristics of the novel scores. For example, it can be observed that the Expert-selected LLMCG curve portrays a greater concentration in the middle score range when compared to the Control-Claude , curve but dominates in the high novelty scores (highlighted in dashed rectangle). Moreover, comparisons involving Control-Human with both Random-selected LLMCG and Expert-selected LLMCG did not manifest statistically significant variances, indicating aligned novelty perceptions among these groups. Finally, the comparisons between Expert-selected LLMCG and Control-Claude ( t (59) = 2.49, p = 0.085) suggest a trend toward significance, with a Cohen’s d value of 0.6226 indicating generally higher novelty scores for Expert-selected LLMCG compared to Control-Claude .
To mitigate potential biases due to individual reviewer inclinations, we expanded our evaluation to include both median and maximum z-scores from the three reviewers for each hypothesis. These multifaceted analyses enhance the robustness of our results by minimizing the influence of extreme values and potential outliers. First, when analyzing the median novelty scores, the ANOVA test demonstrated a notable association with the grouping factor ( F (3,116) = 6.54, p = 0.0004), which explained 14.41% of the variance. As illustrated in Table 5 , pairwise evaluations revealed significant disparities between Control-Human and Control-Claude ( t (59) = 4.01, p = 0.001), with Control-Human performing significantly higher than Control-Claude (Cohen’s d = 1.1031). Similarly, there were significant differences between Random-selected LLMCG and Control-Claude ( t (59) = 3.40, p = 0.006), where Random-selected LLMCG also significantly outperformed Control-Claude (Cohen’s d = 0.8875). Interestingly, the comparison of Expert-selected LLMCG with Control-Claude ( t (59) = 1.70, p = 0.550) and other group pairings did not include statistically significant differences.
Subsequently, turning our attention to maximum novelty scores provided crucial insights, especially where outlier scores may carry significant weight. The influence of the grouping factor was evident ( F (3,116) = 7.20, p = 0.0002), indicating an explained variance of 15.70%. In particular, clear differences emerged between Control-Human and Control-Claude ( t (59) = 4.36, p < 0.001), and between Random-selected LLMCG and Control-Claude ( t (59) = 3.47, p = 0.004). A particularly intriguing observation was the significant difference between Expert-selected LLMCG and Control-Claude ( t (59) = 3.12, p = 0.014). The Cohen’s d values of 1.1637, 1.0457, and 0.6987 respectively indicate that the novelty scores for the Control-Human , Random-selected LLMCG , and Expert-selected LLMCG groups are significantly higher than those for the Control-Claude group. Together, these analyses offer a multifaceted perspective on novelty evaluations. Specifically, the results of the median analysis echo and support those of the mean, reinforcing the reliability of our assessments. The discerned significance between Control-Claude and Expert-selected LLMCG in the median data emphasizes the intricate differences, while also pointing to broader congruence in novelty perceptions.
Usefulness analysis
Evaluating the practical impact of hypotheses is crucial in scientific research assessments. In the mean useful spectrum, the grouping factor did not exert a significant influence ( F (3,116) = 5.25, p = 0.553). Figure 2 b presents the utility score distributions between groups. The narrow interquartile range of Control-Human suggests a relatively consistent assessment among reviewers. On the other hand, the spread and outliers in the Control-Claude distribution hint at varied utility perceptions. Both LLMCG groups cover a broad score range, demonstrating a mixture of high and low utility scores, while the Expert-selected LLMCG gravitates more toward higher usefulness scores. The smoothed line plots accompanying Fig. 2 b further detail the score densities. For instance, Random-selected LLMCG boasts several high utility scores, counterbalanced by a smattering of low scores. Interestingly, the distributions for Control-Human and Expert-selected LLMCG appear to be closely aligned. While mean utility scores provide an overarching view, the nuances within the boxplots and smoothed plots offer deeper insights. This comprehensive understanding can guide future endeavors in content generation and evaluation, spotlighting key areas of focus and potential improvements.
Comparison between the LLMCG and GPT-4
To evaluate the impact of integrating a causal graph with GPT-4, we performed an ablation study comparing the hypotheses generated by GPT-4 alone and those of the proposed LLMCG framework. For this experiment, 60 hypotheses were created using GPT-4, following the detailed instructions in Table B2 . Furthermore, 60 hypotheses for the LLMCG group were randomly selected from the remaining pool of 70 hypotheses. Subsequently, both sets of hypotheses were assessed by three independent reviewers for novelty and usefulness, as previously described.
Table 6 shows a comparison between the GPT-4 and LLMCG groups, highlighting a significant difference in novelty scores (mean value: t (119) = 6.60, p < 0.0001) but not in usefulness scores (mean value: t (119) = 1.31, p = 0.1937). This indicates that the LLMCG framework significantly enhances hypothesis novelty (all Cohen’s d > 1.1) without affecting usefulness compared to the GPT-4 group. Figure B6 visually contrasts these findings, underlining the causal graph’s unique role in fostering novel hypothesis generation when integrated with GPT-4.
The t -SNE visualizations (Fig. 3 ) illustrate the semantic relationships between different groups, capturing the patterns of novelty and usefulness. Notably, a distinct clustering among PhD students suggests shared academic influences, while the LLMCG groups display broader topic dispersion, hinting at a wider semantic understanding. The size of the bubbles reflects the novelty and usefulness scores, emphasizing the diverse perceptions of what is considered innovative versus beneficial. Additionally, the numbers near the yellow dots represent the participant IDs, which demonstrated that the semantics of the same participant, such as H05 or H06, are closely aligned. In Fig. B4 , a distinct clustering of examples is observed, particularly highlighting the close proximity of hypotheses C3, C4, and C8 within the semantic space. This observation is further elucidated in Appendix B , enhancing the comprehension of BERT’s semantic representation. Instead of solely depending on superficial textual descriptions, this analysis penetrates into the underlying understanding of concepts within the semantic space, a topic also explored in recent research (Johnson et al., 2023 ).
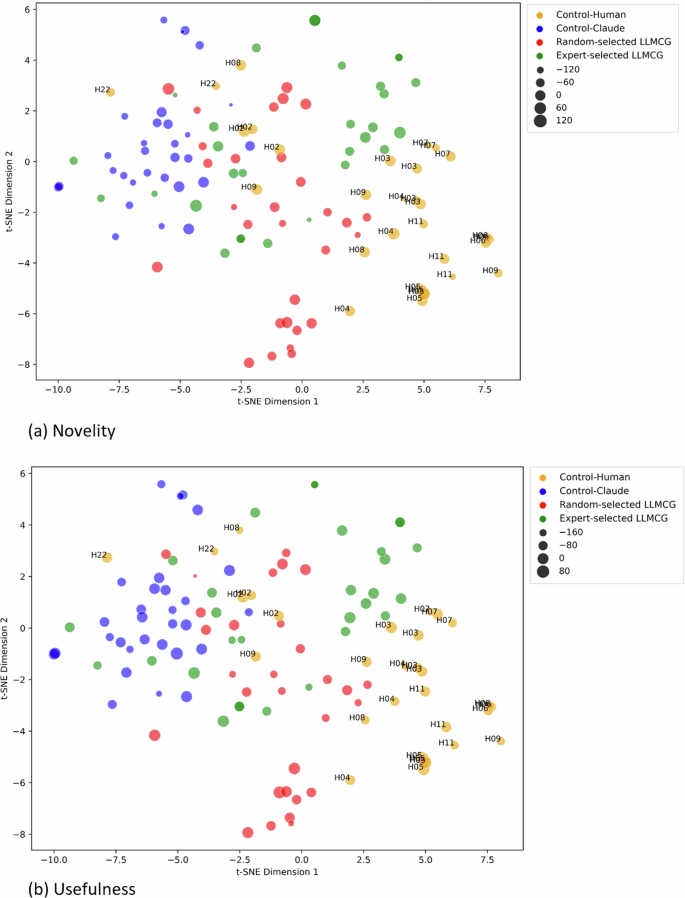
Comparison of ( a ) novelty and ( b ) usefulness scores (bubble size scaled by 100) among the different groups.
In the distribution of semantic distances (Fig. 4 ), we observed that the Control-Human group exhibits a distinctively greater semantic distance in comparison to the other groups, emphasizing their unique semantic orientations. The statistical support for this observation is derived from the ANOVA results, with a significant F-statistic ( F (3,1652) = 84.1611, p < 0.00001), underscoring the impact of the grouping factor. This factor explains a remarkable 86.96% of the variance, as indicated by the R -squared value. Multiple comparisons, as shown in Table 7 , further elucidate the subtleties of these group differences. Control-Human and Control-Claude exhibit a significant contrast in their semantic distances, as highlighted by the t value of 16.41 and the adjusted p value ( < 0.0001). This difference indicates distinct thought patterns or emphasis in the two groups. Notably, Control-Human demonstrates a greater semantic distance (Cohen’s d = 1.1630). Similarly, a comparison of the Control-Claude and LLMCG models reveals pronounced differences (Cohen’s d > 0.9), more so with the Expert-selected LLMCG ( p < 0.0001). A comparison of Control-Human with the LLMCG models shows divergent semantic orientations, with statistically significant larger distances than Random-selected LLMCG ( p = 0.0036) and a trend toward difference with Expert-selected LLMCG ( p = 0.0687). Intriguingly, the two LLMCG groups—Random-selected and Expert-selected—exhibit similar semantic distances, as evidenced by a nonsignificant p value of 0.4362. Furthermore, the significant distinctions we observed, particularly between the Control-Human and other groups, align with human evaluations of novelty. This coherence indicates that the BERT space representation coupled with statistical analyses could effectively mimic human judgment. Such results underscore the potential of this approach for automated hypothesis testing, paving the way for more efficient and streamlined semantic evaluations in the future.
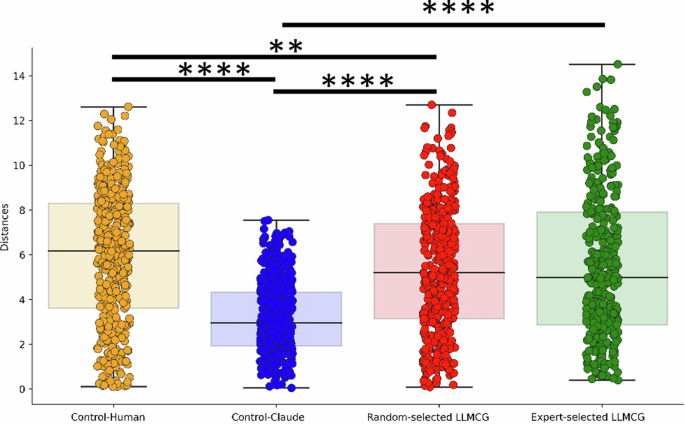
Note: ** denotes p < 0.01, **** denotes p < 0.0001.
In general, visual and statistical analyses reveal the nuanced semantic landscapes of each group. While the Ph.D. students’ shared background influences their clustering, the machine models exhibit a comprehensive grasp of topics, emphasizing the intricate interplay of individual experiences, academic influences, and algorithmic understanding in shaping semantic representations.
This investigation carried out a detailed evaluation of the various hypothesis contributors, blending both quantitative and qualitative analyses. In terms of topic analysis, distinct variations were observed between Control-Human and LLMCG, the latter presenting more expansive thematic coverage. For human evaluation, hypotheses from Ph.D. students paralleled the LLMCG in novelty, reinforcing AI’s growing competence in mirroring human innovative thinking. Furthermore, when juxtaposed with AI models such as Control-Claude , the LLMCG exhibited increased novelty. Deep semantic analysis via t -SNE and BERT representations allowed us to intuitively grasp semantic essence of hypotheses, signaling the possibility of future automated hypothesis assessments. Interestingly, LLMCG appeared to encompass broader complementary domains compared to human input. Taken together, these findings highlight the emerging role of AI in hypothesis generation and provide key insights into hypothesis evaluation across diverse origins.
General discussion
This research delves into the synergistic relationship between LLM and causal graphs in the hypothesis generation process. Our findings underscore the ability of LLM, when integrated with causal graph techniques, to produce meaningful hypotheses with increased efficiency and quality. By centering our investigation on “well-being” we emphasize its pivotal role in psychological studies and highlight the potential convergence of technology and society. A multifaceted assessment approach to evaluate quality by topic analysis, human evaluation and deep semantic analysis demonstrates that AI-augmented methods not only outshine LLM-only techniques in generating hypotheses with superior novelty and show quality on par with human expertise but also boast the capability for more profound conceptual incorporations and a broader semantic spectrum. Such a multifaceted lens of assessment introduces a novel perspective for the scholarly realm, equipping researchers with an enriched understanding and an innovative toolset for hypothesis generation. At its core, the melding of LLM and causal graphs signals a promising frontier, especially in regard to dissecting cornerstone psychological constructs such as “well-being”. This marriage of methodologies, enriched by the comprehensive assessment angle, deepens our comprehension of both the immediate and broader ramifications of our research endeavors.
The prominence of causal graphs in psychology is profound, they offer researchers a unified platform for synthesizing and hypothesizing diverse psychological realms (Borsboom et al., 2021 ; Uleman et al., 2021 ). Our study echoes this, producing groundbreaking hypotheses comparable in depth to early expert propositions. Deep semantic analysis bolstered these findings, emphasizing that our hypotheses have distinct cross-disciplinary merits, particularly when compared to those of individual doctoral scholars. However, the traditional use of causal graphs in psychology presents challenges due to its demanding nature, often requiring insights from multiple experts (Crielaard et al., 2022 ). Our research, however, harnesses LLM’s causal extraction, automating causal pair derivation and, in turn, minimizing the need for extensive expert input. The union of the causal graphs’ systematic approach with AI-driven creativity, as seen with LLMs, paves the way for the future of psychological inquiry. Thanks to advancements in AI, barriers once created by causal graphs’ intricate procedures are being dismantled. Furthermore, as the era of big data dawns, the integration of AI and causal graphs in psychology augments research capabilities, but also brings into focus the broader implications for society. This fusion provides a nuanced understanding of the intricate sociopsychological dynamics, emphasizing the importance of adapting research methodologies in tandem with technological progress.
In the realm of research, LLMs serve a unique purpose, often by acting as the foundation or baseline against which newer methods and approaches are assessed. The demonstrated productivity enhancements by generative AI tools, as evidenced by Noy and Zhang ( 2023 ), indicate the potential of such LLMs. In our investigation, we pit the hypotheses generated by such substantial models against our integrated LLMCG approach. Intriguingly, while these LLMs showcased admirable practicality in their hypotheses, they substantially lagged behind in terms of innovation when juxtaposed with the doctoral student and LLMCG group. This divergence in results can be attributed to the causal network curated from 43k research papers, funneling the vast knowledge reservoir of the LLM squarely into the realm of scientific psychology. The increased precision in hypothesis generation by these models fits well within the framework of generative networks. Tong et al. ( 2021 ) highlighted that, by integrating structured constraints, conventional neural networks can accurately generate semantically relevant content. One of the salient merits of the causal graph, in this context, is its ability to alleviate inherent ambiguity or interpretability challenges posed by LLMs. By providing a systematic and structured framework, the causal graph aids in unearthing the underlying logic and rationale of the outputs generated by LLMs. Notably, this finding echoes the perspective of Pan et al. ( 2023 ), where the integration of structured knowledge from knowledge graphs was shown to provide an invaluable layer of clarity and interpretability to LLMs, especially in complex reasoning tasks. Such structured approaches not only boost the confidence of researchers in the hypotheses derived but also augment the transparency and understandability of LLM outputs. In essence, leveraging causal graphs may very well herald a new era in model interpretability, serving as a conduit to unlock the black box that large models often represent in contemporary research.
In the ever-evolving tapestry of research, every advancement invariably comes with its unique set of constraints, and our study was no exception. On the technical front, a pivotal challenge stemmed from the opaque inner workings of the GPT. Determining the exact machinations within the GPT that lead to the formation of specific causal pairs remains elusive, thereby reintroducing the age-old issue of AI’s inherent lack of transparency (Buruk, 2023 ; Cao and Yousefzadeh, 2023 ). This opacity is magnified in our sparse causal graph, which, while expansive, is occasionally riddled with concepts that, while semantically distinct, converge in meaning. In tangible applications, a careful and meticulous algorithmic evaluation would be imperative to construct an accurate psychological conceptual landscape. Delving into psychology, which bridges humanities and natural sciences, it continuously aims to unravel human cognition and behavior (Hergenhahn and Henley, 2013 ). Despite the dominance of traditional methodologies (Henrich et al., 2010 ; Shah et al., 2015 ), the present data-centric era amplifies the synergy of technology and humanities, resonating with Hasok Chang’s vision of enriched science (Chang, 2007 ). This symbiosis is evident when assessing structural holes in social networks (Burt, 2004 ) and viewing novelty as a bridge across these divides (Foster et al., 2021 ). Such perspectives emphasize the importance of thorough algorithmic assessments, highlighting potential avenues in humanities research, especially when incorporating large language models for innovative hypothesis crafting and verification.
However, there are some limitations to this research. Firstly, we acknowledge that constructing causal relationship graphs has potential inaccuracies, with ~13% relationship pairs not aligning with human expert estimations. Enhancing the estimation of relationship extraction could be a pathway to improve the accuracy of the causal graph, potentially leading to more robust hypotheses. Secondly, our validation process was limited to 130 hypotheses, however, the vastness of our conceptual landscape suggests countless possibilities. As an exemplar, the twenty pivotal psychological concepts highlighted in Table 3 alone could spawn an extensive array of hypotheses. However, the validation of these surrounding hypotheses would unquestionably lead to a multitude of speculations. A striking observation during our validation was the inconsistency in the evaluations of the senior expert panels (as shown in Table B5 ). This shift underscores a pivotal insight: our integration of AI has transitioned the dependency on scarce expert resources from hypothesis generation to evaluation. In the future, rigorous evaluations ensuring both novelty and utility could become a focal point of exploration. The promising path forward necessitates a thoughtful integration of technological innovation and human expertise to fully realize the potential suggested by our study.
In conclusion, our research provides pioneering insight into the symbiotic fusion of LLMs, which are epitomized by GPT, and causal graphs from the realm of psychological hypothesis generation, especially emphasizing “well-being”. Importantly, as highlighted by (Cao and Yousefzadeh, 2023 ), ensuring a synergistic alignment between domain knowledge and AI extrapolation is crucial. This synergy serves as the foundation for maintaining AI models within their conceptual limits, thus bolstering the validity and reliability of the hypotheses generated. Our approach intricately interweaves the advanced capabilities of LLMs with the methodological prowess of causal graphs, thereby optimizing while also refining the depth and precision of hypothesis generation. The causal graph, of paramount importance in psychology due to its cross-disciplinary potential, often demands vast amounts of expert involvement. Our innovative approach addresses this by utilizing LLM’s exceptional causal extraction abilities, effectively facilitating the transition of intense expert engagement from hypothesis creation to evaluation. Therefore, our methodology combined LLM with causal graphs, propelling psychological research forward by improving hypothesis generation and offering tools to blend theoretical and data-centric approaches. This synergy particularly enriches our understanding of social psychology’s complex dynamics, such as happiness research, demonstrating the profound impact of integrating AI with traditional research frameworks.
Data availability
The data generated and analyzed in this study are partially available within the Supplementary materials . For additional data supporting the findings of this research, interested parties may contact the corresponding author, who will provide the information upon receiving a reasonable request.
Battleday RM, Peterson JC, Griffiths TL (2020) Capturing human categorization of natural images by combining deep networks and cognitive models. Nat Commun 11(1):5418
Article ADS PubMed PubMed Central Google Scholar
Bechmann A, Bowker GC (2019) Unsupervised by any other name: hidden layers of knowledge production in artificial intelligence on social media. Big Data Soc 6(1):2053951718819569
Article Google Scholar
Binz M, Schulz E (2023) Using cognitive psychology to understand GPT-3. Proc Natl Acad Sci 120(6):e2218523120
Article CAS PubMed PubMed Central Google Scholar
Boden MA (2009) Computer models of creativity. AI Mag 30(3):23–23
Google Scholar
Borsboom D, Deserno MK, Rhemtulla M, Epskamp S, Fried EI, McNally RJ (2021) Network analysis of multivariate data in psychological science. Nat Rev Methods Prim 1(1):58
Article CAS Google Scholar
Burt RS (2004) Structural holes and good ideas. Am J Sociol 110(2):349–399
Buruk O (2023) Academic writing with GPT-3.5: reflections on practices, efficacy and transparency. arXiv preprint arXiv:2304.11079
Cao X, Yousefzadeh R (2023) Extrapolation and AI transparency: why machine learning models should reveal when they make decisions beyond their training. Big Data Soc 10(1):20539517231169731
Chang H (2007) Scientific progress: beyond foundationalism and coherentism1. R Inst Philos Suppl 61:1–20
Cheng K, Guo Q, He Y, Lu Y, Gu S, Wu H (2023) Exploring the potential of GPT-4 in biomedical engineering: the dawn of a new era. Ann Biomed Eng 51:1645–1653
Article ADS PubMed Google Scholar
Cichy RM, Khosla A, Pantazis D, Torralba A, Oliva A (2016) Comparison of deep neural networks to spatio-temporal cortical dynamics of human visual object recognition reveals hierarchical correspondence. Sci Rep 6(1):27755
Article ADS CAS PubMed PubMed Central Google Scholar
Cohen BA (2017) How should novelty be valued in science? Elife 6:e28699
Article PubMed PubMed Central Google Scholar
Crielaard L, Uleman JF, Châtel BD, Epskamp S, Sloot P, Quax R (2022) Refining the causal loop diagram: a tutorial for maximizing the contribution of domain expertise in computational system dynamics modeling. Psychol Methods 29(1):169–201
Article PubMed Google Scholar
Devlin J, Chang M W, Lee K & Toutanova (2019) Bert: pre-training of deep bidirectional transformers for language understanding. In Proceedings of the 2019 Conference of the North American Chapter of the Association for Computational Linguistics: Human Language Technologies, Volume 1 (Long and Short Papers) (pp. 4171–4186)
Diener E, Wirtz D, Tov W, Kim-Prieto C, Choi D-W, Oishi S, Biswas-Diener R (2010) New well-being measures: short scales to assess flourishing and positive and negative feelings. Soc Indic Res 97:143–156
Dowling M, Lucey B (2023) ChatGPT for (finance) research: the Bananarama conjecture. Financ Res Lett 53:103662
Forgeard MJ, Jayawickreme E, Kern ML, Seligman ME (2011) Doing the right thing: measuring wellbeing for public policy. Int J Wellbeing 1(1):79–106
Foster J G, Shi F & Evans J (2021) Surprise! Measuring novelty as expectation violation. SocArXiv
Fredrickson BL (2001) The role of positive emotions in positive psychology: The broaden-and-build theory of positive emotions. Am Psychol 56(3):218
Gu Q, Kuwajerwala A, Morin S, Jatavallabhula K M, Sen B, Agarwal, A et al. (2024) ConceptGraphs: open-vocabulary 3D scene graphs for perception and planning. In 2nd Workshop on Language and Robot Learning: Language as Grounding
Henrich J, Heine SJ, Norenzayan A (2010) Most people are not WEIRD. Nature 466(7302):29–29
Article ADS CAS PubMed Google Scholar
Hergenhahn B R, Henley T (2013) An introduction to the history of psychology . Cengage Learning
Jaccard J, Jacoby J (2019) Theory construction and model-building skills: a practical guide for social scientists . Guilford publications
Johnson DR, Kaufman JC, Baker BS, Patterson JD, Barbot B, Green AE (2023) Divergent semantic integration (DSI): Extracting creativity from narratives with distributional semantic modeling. Behav Res Methods 55(7):3726–3759
Kıcıman E, Ness R, Sharma A & Tan C (2023) Causal reasoning and large language models: opening a new frontier for causality. arXiv preprint arXiv:2305.00050
Koehler DJ (1994) Hypothesis generation and confidence in judgment. J Exp Psychol Learn Mem Cogn 20(2):461–469
Krenn M, Zeilinger A (2020) Predicting research trends with semantic and neural networks with an application in quantum physics. Proc Natl Acad Sci 117(4):1910–1916
Lee H, Zhou W, Bai H, Meng W, Zeng T, Peng K & Kumada T (2023) Natural language processing algorithms for divergent thinking assessment. In: Proc IEEE 6th Eurasian Conference on Educational Innovation (ECEI) p 198–202
Madill A, Shloim N, Brown B, Hugh-Jones S, Plastow J, Setiyawati D (2022) Mainstreaming global mental health: Is there potential to embed psychosocial well-being impact in all global challenges research? Appl Psychol Health Well-Being 14(4):1291–1313
McCarthy M, Chen CC, McNamee RC (2018) Novelty and usefulness trade-off: cultural cognitive differences and creative idea evaluation. J Cross-Cult Psychol 49(2):171–198
McGuire WJ (1973) The yin and yang of progress in social psychology: seven koan. J Personal Soc Psychol 26(3):446–456
Miron-Spektor E, Beenen G (2015) Motivating creativity: The effects of sequential and simultaneous learning and performance achievement goals on product novelty and usefulness. Organ Behav Hum Decis Process 127:53–65
Nisbett RE, Peng K, Choi I, Norenzayan A (2001) Culture and systems of thought: holistic versus analytic cognition. Psychol Rev 108(2):291–310
Article CAS PubMed Google Scholar
Noy S, Zhang W (2023) Experimental evidence on the productivity effects of generative artificial intelligence. Science 381:187–192
Oleinik A (2019) What are neural networks not good at? On artificial creativity. Big Data Soc 6(1):2053951719839433
Otu A, Charles CH, Yaya S (2020) Mental health and psychosocial well-being during the COVID-19 pandemic: the invisible elephant in the room. Int J Ment Health Syst 14:1–5
Pan S, Luo L, Wang Y, Chen C, Wang J & Wu X (2024) Unifying large language models and knowledge graphs: a roadmap. IEEE Transactions on Knowledge and Data Engineering 36(7):3580–3599
Rubin DB (2005) Causal inference using potential outcomes: design, modeling, decisions. J Am Stat Assoc 100(469):322–331
Article MathSciNet CAS Google Scholar
Sanderson K (2023) GPT-4 is here: what scientists think. Nature 615(7954):773
Seligman ME, Csikszentmihalyi M (2000) Positive psychology: an introduction. Am Psychol 55(1):5–14
Shah DV, Cappella JN, Neuman WR (2015) Big data, digital media, and computational social science: possibilities and perils. Ann Am Acad Political Soc Sci 659(1):6–13
Shardlow M, Batista-Navarro R, Thompson P, Nawaz R, McNaught J, Ananiadou S (2018) Identification of research hypotheses and new knowledge from scientific literature. BMC Med Inform Decis Mak 18(1):1–13
Shin H, Kim K, Kogler DF (2022) Scientific collaboration, research funding, and novelty in scientific knowledge. PLoS ONE 17(7):e0271678
Thomas RP, Dougherty MR, Sprenger AM, Harbison J (2008) Diagnostic hypothesis generation and human judgment. Psychol Rev 115(1):155–185
Thomer AK, Wickett KM (2020) Relational data paradigms: what do we learn by taking the materiality of databases seriously? Big Data Soc 7(1):2053951720934838
Thompson WH, Skau S (2023) On the scope of scientific hypotheses. R Soc Open Sci 10(8):230607
Tong S, Liang X, Kumada T, Iwaki S (2021) Putative ratios of facial attractiveness in a deep neural network. Vis Res 178:86–99
Uleman JF, Melis RJ, Quax R, van der Zee EA, Thijssen D, Dresler M (2021) Mapping the multicausality of Alzheimer’s disease through group model building. GeroScience 43:829–843
Van der Maaten L, Hinton G (2008) Visualizing data using t-SNE. J Mach Learn Res 9(11):2579–2605
Vaswani A, Shazeer N, Parmar N, Uszkoreit J, Jones L, Gomez A N & Polosukhin I (2017) Attention is all you need. In Advances in Neural Information Processing Systems
Wang H, Fu T, Du Y, Gao W, Huang K, Liu Z (2023) Scientific discovery in the age of artificial intelligence. Nature 620(7972):47–60
Webber J (2012) A programmatic introduction to neo4j. In Proceedings of the 3rd annual conference on systems, programming, and applications: software for humanity p 217–218
Williams K, Berman G, Michalska S (2023) Investigating hybridity in artificial intelligence research. Big Data Soc 10(2):20539517231180577
Wu S, Koo M, Blum L, Black A, Kao L, Scalzo F & Kurtz I (2023) A comparative study of open-source large language models, GPT-4 and Claude 2: multiple-choice test taking in nephrology. arXiv preprint arXiv:2308.04709
Yu F, Peng T, Peng K, Zheng SX, Liu Z (2016) The Semantic Network Model of creativity: analysis of online social media data. Creat Res J 28(3):268–274
Download references
Acknowledgements
The authors thank Dr. Honghong Bai (Radboud University), Dr. ChienTe Wu (The University of Tokyo), Dr. Peng Cheng (Tsinghua University), and Yusong Guo (Tsinghua University) for their great comments on the earlier version of this manuscript. This research has been generously funded by personal contributions, with special acknowledgment to K. Mao. Additionally, he conceived and developed the causality graph and AI hypothesis generation technology presented in this paper from scratch, and generated all AI hypotheses and paid for its costs. The authors sincerely thank K. Mao for his support, which enabled this research. In addition, K. Peng and S. Tong were partly supported by the Tsinghua University lnitiative Scientific Research Program (No. 20213080008), Self-Funded Project of Institute for Global Industry, Tsinghua University (202-296-001), Shuimu Scholars program of Tsinghua University (No. 2021SM157), and the China Postdoctoral International Exchange Program (No. YJ20210266).
Author information
These authors contributed equally: Song Tong, Kai Mao.
Authors and Affiliations
Department of Psychological and Cognitive Sciences, Tsinghua University, Beijing, China
Song Tong & Kaiping Peng
Positive Psychology Research Center, School of Social Sciences, Tsinghua University, Beijing, China
Song Tong, Zhen Huang, Yukun Zhao & Kaiping Peng
AI for Wellbeing Lab, Tsinghua University, Beijing, China
Institute for Global Industry, Tsinghua University, Beijing, China
Kindom KK, Tokyo, Japan
You can also search for this author in PubMed Google Scholar
Contributions
Song Tong: Data analysis, Experiments, Writing—original draft & review. Kai Mao: Designed the causality graph methodology, Generated AI hypotheses, Developed hypothesis generation techniques, Writing—review & editing. Zhen Huang: Statistical Analysis, Experiments, Writing—review & editing. Yukun Zhao: Conceptualization, Project administration, Supervision, Writing—review & editing. Kaiping Peng: Conceptualization, Writing—review & editing.
Corresponding authors
Correspondence to Yukun Zhao or Kaiping Peng .
Ethics declarations
Competing interests.
The author(s) declared no potential conflicts of interest with respect to the research, authorship, and/or publication of this article.
Ethical approval
In this study, ethical approval was granted by the Institutional Review Board (IRB) of the Department of Psychology at Tsinghua University, China. The Research Ethics Committee documented this approval under the number IRB202306, following an extensive review that concluded on March 12, 2023. This approval indicates the research’s strict compliance with the IRB’s guidelines and regulations, ensuring ethical integrity and adherence throughout the study.
Informed consent
Before participating, all study participants gave their informed consent. They received comprehensive details about the study’s goals, methods, potential risks and benefits, confidentiality safeguards, and their rights as participants. This process guaranteed that participants were fully informed about the study’s nature and voluntarily agreed to participate, free from coercion or undue influence.
Additional information
Publisher’s note Springer Nature remains neutral with regard to jurisdictional claims in published maps and institutional affiliations.
Supplementary information
Supplemental material, rights and permissions.
Open Access This article is licensed under a Creative Commons Attribution 4.0 International License, which permits use, sharing, adaptation, distribution and reproduction in any medium or format, as long as you give appropriate credit to the original author(s) and the source, provide a link to the Creative Commons licence, and indicate if changes were made. The images or other third party material in this article are included in the article’s Creative Commons licence, unless indicated otherwise in a credit line to the material. If material is not included in the article’s Creative Commons licence and your intended use is not permitted by statutory regulation or exceeds the permitted use, you will need to obtain permission directly from the copyright holder. To view a copy of this licence, visit http://creativecommons.org/licenses/by/4.0/ .
Reprints and permissions
About this article
Cite this article.
Tong, S., Mao, K., Huang, Z. et al. Automating psychological hypothesis generation with AI: when large language models meet causal graph. Humanit Soc Sci Commun 11 , 896 (2024). https://doi.org/10.1057/s41599-024-03407-5
Download citation
Received : 08 November 2023
Accepted : 25 June 2024
Published : 09 July 2024
DOI : https://doi.org/10.1057/s41599-024-03407-5
Share this article
Anyone you share the following link with will be able to read this content:
Sorry, a shareable link is not currently available for this article.
Provided by the Springer Nature SharedIt content-sharing initiative
Quick links
- Explore articles by subject
- Guide to authors
- Editorial policies

Have a language expert improve your writing
Run a free plagiarism check in 10 minutes, generate accurate citations for free.
- Knowledge Base
Hypothesis Testing | A Step-by-Step Guide with Easy Examples
Published on November 8, 2019 by Rebecca Bevans . Revised on June 22, 2023.
Hypothesis testing is a formal procedure for investigating our ideas about the world using statistics . It is most often used by scientists to test specific predictions, called hypotheses, that arise from theories.
There are 5 main steps in hypothesis testing:
- State your research hypothesis as a null hypothesis and alternate hypothesis (H o ) and (H a or H 1 ).
- Collect data in a way designed to test the hypothesis.
- Perform an appropriate statistical test .
- Decide whether to reject or fail to reject your null hypothesis.
- Present the findings in your results and discussion section.
Though the specific details might vary, the procedure you will use when testing a hypothesis will always follow some version of these steps.
Table of contents
Step 1: state your null and alternate hypothesis, step 2: collect data, step 3: perform a statistical test, step 4: decide whether to reject or fail to reject your null hypothesis, step 5: present your findings, other interesting articles, frequently asked questions about hypothesis testing.
After developing your initial research hypothesis (the prediction that you want to investigate), it is important to restate it as a null (H o ) and alternate (H a ) hypothesis so that you can test it mathematically.
The alternate hypothesis is usually your initial hypothesis that predicts a relationship between variables. The null hypothesis is a prediction of no relationship between the variables you are interested in.
- H 0 : Men are, on average, not taller than women. H a : Men are, on average, taller than women.
Receive feedback on language, structure, and formatting
Professional editors proofread and edit your paper by focusing on:
- Academic style
- Vague sentences
- Style consistency
See an example
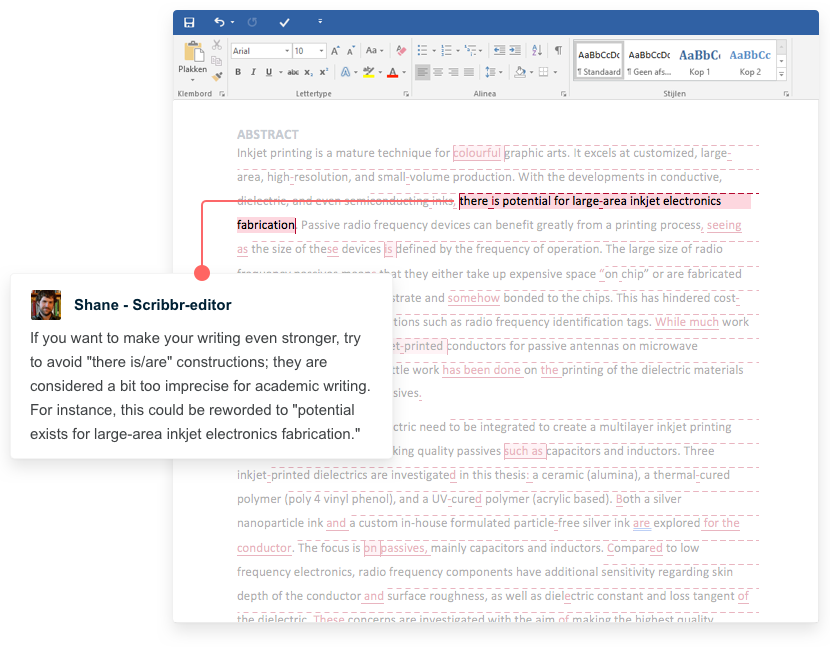
For a statistical test to be valid , it is important to perform sampling and collect data in a way that is designed to test your hypothesis. If your data are not representative, then you cannot make statistical inferences about the population you are interested in.
There are a variety of statistical tests available, but they are all based on the comparison of within-group variance (how spread out the data is within a category) versus between-group variance (how different the categories are from one another).
If the between-group variance is large enough that there is little or no overlap between groups, then your statistical test will reflect that by showing a low p -value . This means it is unlikely that the differences between these groups came about by chance.
Alternatively, if there is high within-group variance and low between-group variance, then your statistical test will reflect that with a high p -value. This means it is likely that any difference you measure between groups is due to chance.
Your choice of statistical test will be based on the type of variables and the level of measurement of your collected data .
- an estimate of the difference in average height between the two groups.
- a p -value showing how likely you are to see this difference if the null hypothesis of no difference is true.
Based on the outcome of your statistical test, you will have to decide whether to reject or fail to reject your null hypothesis.
In most cases you will use the p -value generated by your statistical test to guide your decision. And in most cases, your predetermined level of significance for rejecting the null hypothesis will be 0.05 – that is, when there is a less than 5% chance that you would see these results if the null hypothesis were true.
In some cases, researchers choose a more conservative level of significance, such as 0.01 (1%). This minimizes the risk of incorrectly rejecting the null hypothesis ( Type I error ).
The results of hypothesis testing will be presented in the results and discussion sections of your research paper , dissertation or thesis .
In the results section you should give a brief summary of the data and a summary of the results of your statistical test (for example, the estimated difference between group means and associated p -value). In the discussion , you can discuss whether your initial hypothesis was supported by your results or not.
In the formal language of hypothesis testing, we talk about rejecting or failing to reject the null hypothesis. You will probably be asked to do this in your statistics assignments.
However, when presenting research results in academic papers we rarely talk this way. Instead, we go back to our alternate hypothesis (in this case, the hypothesis that men are on average taller than women) and state whether the result of our test did or did not support the alternate hypothesis.
If your null hypothesis was rejected, this result is interpreted as “supported the alternate hypothesis.”
These are superficial differences; you can see that they mean the same thing.
You might notice that we don’t say that we reject or fail to reject the alternate hypothesis . This is because hypothesis testing is not designed to prove or disprove anything. It is only designed to test whether a pattern we measure could have arisen spuriously, or by chance.
If we reject the null hypothesis based on our research (i.e., we find that it is unlikely that the pattern arose by chance), then we can say our test lends support to our hypothesis . But if the pattern does not pass our decision rule, meaning that it could have arisen by chance, then we say the test is inconsistent with our hypothesis .
If you want to know more about statistics , methodology , or research bias , make sure to check out some of our other articles with explanations and examples.
- Normal distribution
- Descriptive statistics
- Measures of central tendency
- Correlation coefficient
Methodology
- Cluster sampling
- Stratified sampling
- Types of interviews
- Cohort study
- Thematic analysis
Research bias
- Implicit bias
- Cognitive bias
- Survivorship bias
- Availability heuristic
- Nonresponse bias
- Regression to the mean
Hypothesis testing is a formal procedure for investigating our ideas about the world using statistics. It is used by scientists to test specific predictions, called hypotheses , by calculating how likely it is that a pattern or relationship between variables could have arisen by chance.
A hypothesis states your predictions about what your research will find. It is a tentative answer to your research question that has not yet been tested. For some research projects, you might have to write several hypotheses that address different aspects of your research question.
A hypothesis is not just a guess — it should be based on existing theories and knowledge. It also has to be testable, which means you can support or refute it through scientific research methods (such as experiments, observations and statistical analysis of data).
Null and alternative hypotheses are used in statistical hypothesis testing . The null hypothesis of a test always predicts no effect or no relationship between variables, while the alternative hypothesis states your research prediction of an effect or relationship.
Cite this Scribbr article
If you want to cite this source, you can copy and paste the citation or click the “Cite this Scribbr article” button to automatically add the citation to our free Citation Generator.
Bevans, R. (2023, June 22). Hypothesis Testing | A Step-by-Step Guide with Easy Examples. Scribbr. Retrieved August 13, 2024, from https://www.scribbr.com/statistics/hypothesis-testing/
Is this article helpful?
Rebecca Bevans
Other students also liked, choosing the right statistical test | types & examples, understanding p values | definition and examples, what is your plagiarism score.
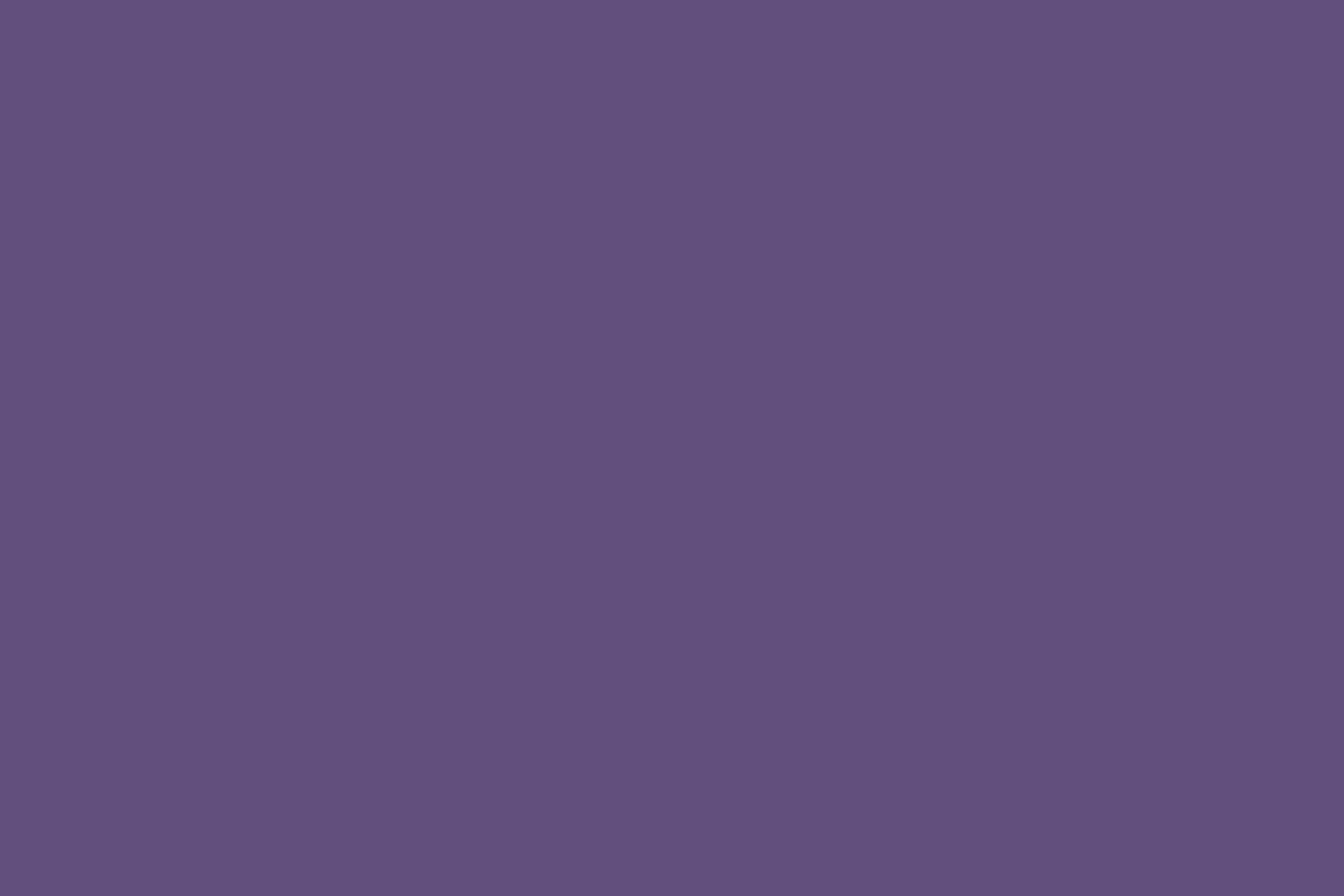
How to Implement Hypothesis-Driven Development
Remember back to the time when we were in high school science class. Our teachers had a framework for helping us learn – an experimental approach based on the best available evidence at hand. We were asked to make observations about the world around us, then attempt to form an explanation or hypothesis to explain what we had observed. We then tested this hypothesis by predicting an outcome based on our theory that would be achieved in a controlled experiment – if the outcome was achieved, we had proven our theory to be correct.
We could then apply this learning to inform and test other hypotheses by constructing more sophisticated experiments, and tuning, evolving or abandoning any hypothesis as we made further observations from the results we achieved.
Experimentation is the foundation of the scientific method, which is a systematic means of exploring the world around us. Although some experiments take place in laboratories, it is possible to perform an experiment anywhere, at any time, even in software development.
Practicing Hypothesis-Driven Development is thinking about the development of new ideas, products and services – even organizational change – as a series of experiments to determine whether an expected outcome will be achieved. The process is iterated upon until a desirable outcome is obtained or the idea is determined to be not viable.
We need to change our mindset to view our proposed solution to a problem statement as a hypothesis, especially in new product or service development – the market we are targeting, how a business model will work, how code will execute and even how the customer will use it.
We do not do projects anymore, only experiments. Customer discovery and Lean Startup strategies are designed to test assumptions about customers. Quality Assurance is testing system behavior against defined specifications. The experimental principle also applies in Test-Driven Development – we write the test first, then use the test to validate that our code is correct, and succeed if the code passes the test. Ultimately, product or service development is a process to test a hypothesis about system behaviour in the environment or market it is developed for.
The key outcome of an experimental approach is measurable evidence and learning.
Learning is the information we have gained from conducting the experiment. Did what we expect to occur actually happen? If not, what did and how does that inform what we should do next?
In order to learn we need use the scientific method for investigating phenomena, acquiring new knowledge, and correcting and integrating previous knowledge back into our thinking.
As the software development industry continues to mature, we now have an opportunity to leverage improved capabilities such as Continuous Design and Delivery to maximize our potential to learn quickly what works and what does not. By taking an experimental approach to information discovery, we can more rapidly test our solutions against the problems we have identified in the products or services we are attempting to build. With the goal to optimize our effectiveness of solving the right problems, over simply becoming a feature factory by continually building solutions.
The steps of the scientific method are to:
- Make observations
- Formulate a hypothesis
- Design an experiment to test the hypothesis
- State the indicators to evaluate if the experiment has succeeded
- Conduct the experiment
- Evaluate the results of the experiment
- Accept or reject the hypothesis
- If necessary, make and test a new hypothesis
Using an experimentation approach to software development
We need to challenge the concept of having fixed requirements for a product or service. Requirements are valuable when teams execute a well known or understood phase of an initiative, and can leverage well understood practices to achieve the outcome. However, when you are in an exploratory, complex and uncertain phase you need hypotheses.
Handing teams a set of business requirements reinforces an order-taking approach and mindset that is flawed.
Business does the thinking and ‘knows’ what is right. The purpose of the development team is to implement what they are told. But when operating in an area of uncertainty and complexity, all the members of the development team should be encouraged to think and share insights on the problem and potential solutions. A team simply taking orders from a business owner is not utilizing the full potential, experience and competency that a cross-functional multi-disciplined team offers.
Framing hypotheses
The traditional user story framework is focused on capturing requirements for what we want to build and for whom, to enable the user to receive a specific benefit from the system.
As A…. <role>
I Want… <goal/desire>
So That… <receive benefit>
Behaviour Driven Development (BDD) and Feature Injection aims to improve the original framework by supporting communication and collaboration between developers, tester and non-technical participants in a software project.
In Order To… <receive benefit>
As A… <role>
When viewing work as an experiment, the traditional story framework is insufficient. As in our high school science experiment, we need to define the steps we will take to achieve the desired outcome. We then need to state the specific indicators (or signals) we expect to observe that provide evidence that our hypothesis is valid. These need to be stated before conducting the test to reduce biased interpretations of the results.
If we observe signals that indicate our hypothesis is correct, we can be more confident that we are on the right path and can alter the user story framework to reflect this.
Therefore, a user story structure to support Hypothesis-Driven Development would be;
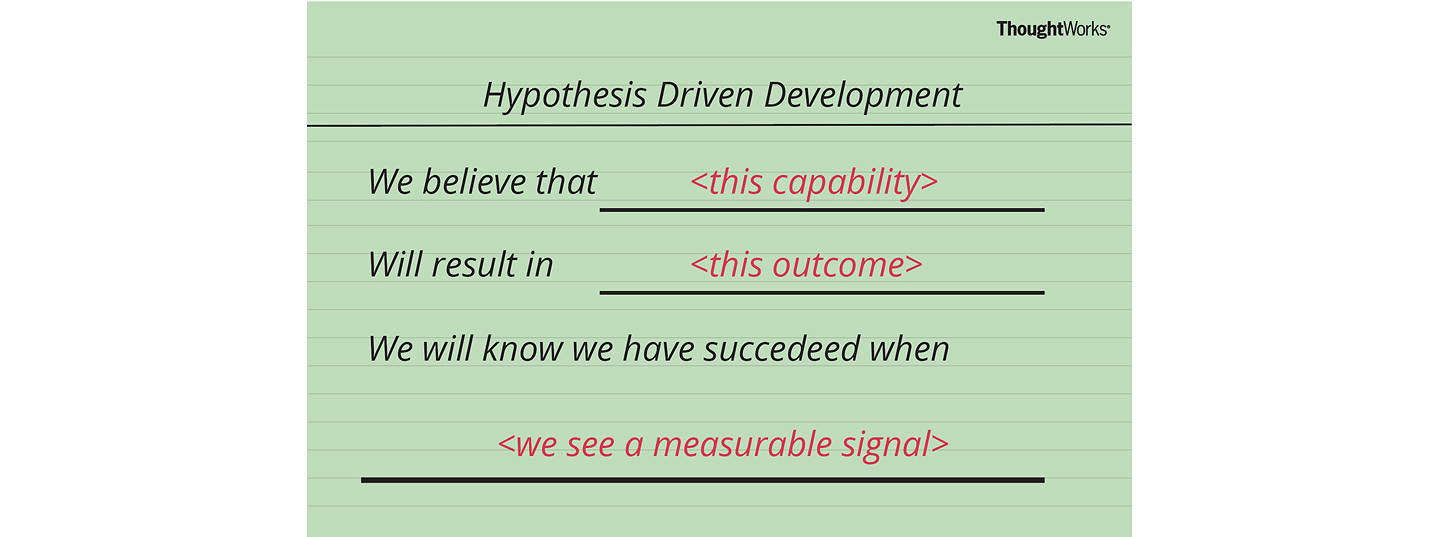
We believe < this capability >
What functionality we will develop to test our hypothesis? By defining a ‘test’ capability of the product or service that we are attempting to build, we identify the functionality and hypothesis we want to test.
Will result in < this outcome >
What is the expected outcome of our experiment? What is the specific result we expect to achieve by building the ‘test’ capability?
We will know we have succeeded when < we see a measurable signal >
What signals will indicate that the capability we have built is effective? What key metrics (qualitative or quantitative) we will measure to provide evidence that our experiment has succeeded and give us enough confidence to move to the next stage.
The threshold you use for statistically significance will depend on your understanding of the business and context you are operating within. Not every company has the user sample size of Amazon or Google to run statistically significant experiments in a short period of time. Limits and controls need to be defined by your organization to determine acceptable evidence thresholds that will allow the team to advance to the next step.
For example if you are building a rocket ship you may want your experiments to have a high threshold for statistical significance. If you are deciding between two different flows intended to help increase user sign up you may be happy to tolerate a lower significance threshold.
The final step is to clearly and visibly state any assumptions made about our hypothesis, to create a feedback loop for the team to provide further input, debate and understanding of the circumstance under which we are performing the test. Are they valid and make sense from a technical and business perspective?
Hypotheses when aligned to your MVP can provide a testing mechanism for your product or service vision. They can test the most uncertain areas of your product or service, in order to gain information and improve confidence.
Examples of Hypothesis-Driven Development user stories are;
Business story
We Believe That increasing the size of hotel images on the booking page
Will Result In improved customer engagement and conversion
We Will Know We Have Succeeded When we see a 5% increase in customers who review hotel images who then proceed to book in 48 hours.
It is imperative to have effective monitoring and evaluation tools in place when using an experimental approach to software development in order to measure the impact of our efforts and provide a feedback loop to the team. Otherwise we are essentially blind to the outcomes of our efforts.
In agile software development we define working software as the primary measure of progress.
By combining Continuous Delivery and Hypothesis-Driven Development we can now define working software and validated learning as the primary measures of progress.
Ideally we should not say we are done until we have measured the value of what is being delivered – in other words, gathered data to validate our hypothesis.
Examples of how to gather data is performing A/B Testing to test a hypothesis and measure to change in customer behaviour. Alternative testings options can be customer surveys, paper prototypes, user and/or guerrilla testing.
One example of a company we have worked with that uses Hypothesis-Driven Development is lastminute.com . The team formulated a hypothesis that customers are only willing to pay a max price for a hotel based on the time of day they book. Tom Klein, CEO and President of Sabre Holdings shared the story of how they improved conversion by 400% within a week.
Combining practices such as Hypothesis-Driven Development and Continuous Delivery accelerates experimentation and amplifies validated learning. This gives us the opportunity to accelerate the rate at which we innovate while relentlessly reducing cost, leaving our competitors in the dust. Ideally we can achieve the ideal of one piece flow: atomic changes that enable us to identify causal relationships between the changes we make to our products and services, and their impact on key metrics.
As Kent Beck said, “Test-Driven Development is a great excuse to think about the problem before you think about the solution”. Hypothesis-Driven Development is a great opportunity to test what you think the problem is, before you work on the solution.
How can you achieve faster growth?
January 13, 2024
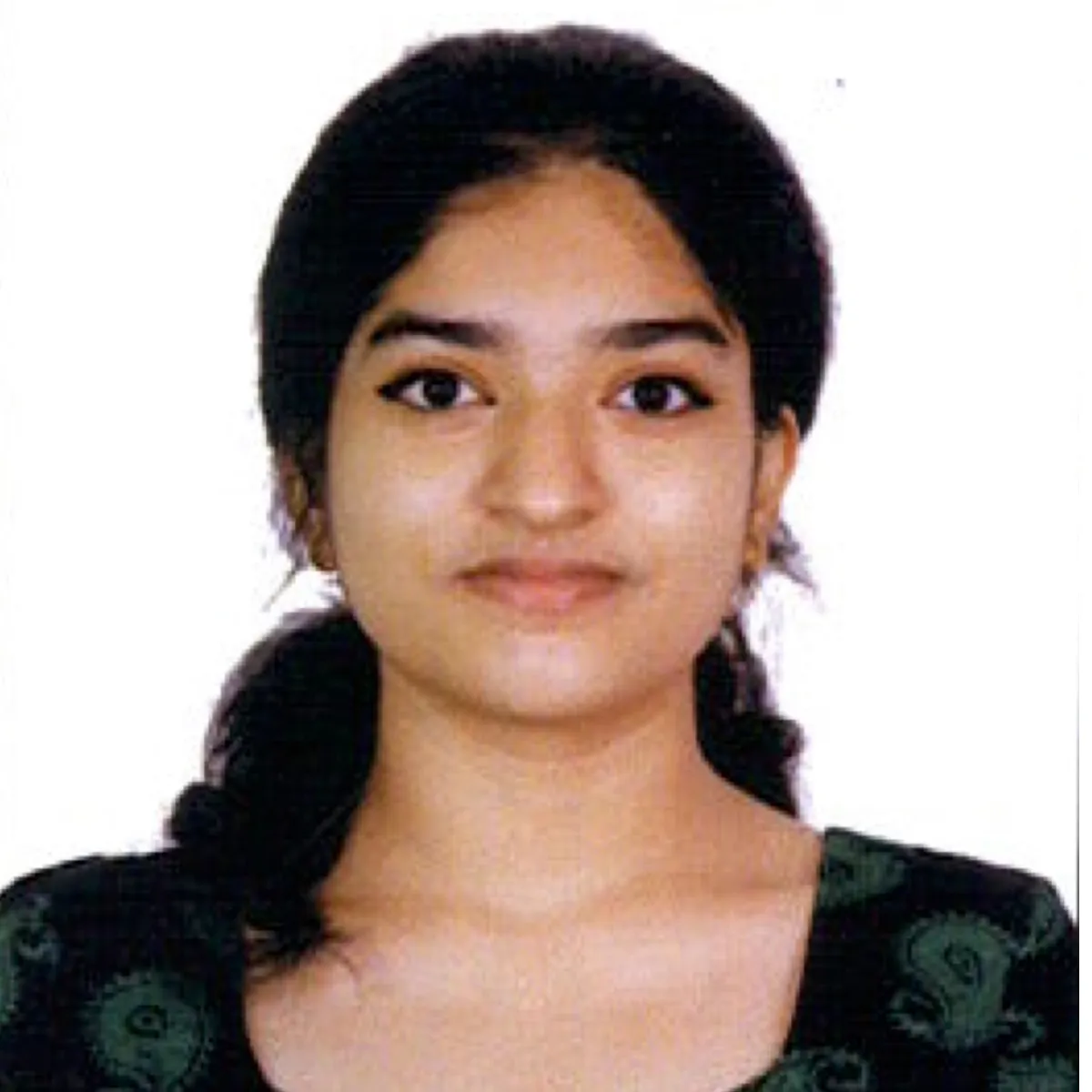
Demystifying Hypothesis Generation: A Guide to AI-Driven Insights
Hypothesis generation involves making informed guesses about various aspects of a business, market, or problem that need further exploration and testing. This article discusses the process you need to follow while generating hypothesis and how an AI tool, like Akaike's BYOB can help you achieve the process quicker and better.

What is Hypothesis Generation?
Hypothesis generation involves making informed guesses about various aspects of a business, market, or problem that need further exploration and testing. It's a crucial step while applying the scientific method to business analysis and decision-making.
Here is an example from a popular B-school marketing case study:
A bicycle manufacturer noticed that their sales had dropped significantly in 2002 compared to the previous year. The team investigating the reasons for this had many hypotheses. One of them was: “many cycling enthusiasts have switched to walking with their iPods plugged in.” The Apple iPod was launched in late 2001 and was an immediate hit among young consumers. Data collected manually by the team seemed to show that the geographies around Apple stores had indeed shown a sales decline.
Traditionally, hypothesis generation is time-consuming and labour-intensive. However, the advent of Large Language Models (LLMs) and Generative AI (GenAI) tools has transformed the practice altogether. These AI tools can rapidly process extensive datasets, quickly identifying patterns, correlations, and insights that might have even slipped human eyes, thus streamlining the stages of hypothesis generation.
These tools have also revolutionised experimentation by optimising test designs, reducing resource-intensive processes, and delivering faster results. LLMs' role in hypothesis generation goes beyond mere assistance, bringing innovation and easy, data-driven decision-making to businesses.
Hypotheses come in various types, such as simple, complex, null, alternative, logical, statistical, or empirical. These categories are defined based on the relationships between the variables involved and the type of evidence required for testing them. In this article, we aim to demystify hypothesis generation. We will explore the role of LLMs in this process and outline the general steps involved, highlighting why it is a valuable tool in your arsenal.
Understanding Hypothesis Generation
A hypothesis is born from a set of underlying assumptions and a prediction of how those assumptions are anticipated to unfold in a given context. Essentially, it's an educated, articulated guess that forms the basis for action and outcome assessment.
A hypothesis is a declarative statement that has not yet been proven true. Based on past scholarship , we could sum it up as the following:
- A definite statement, not a question
- Based on observations and knowledge
- Testable and can be proven wrong
- Predicts the anticipated results clearly
- Contains a dependent and an independent variable where the dependent variable is the phenomenon being explained and the independent variable does the explaining
In a business setting, hypothesis generation becomes essential when people are made to explain their assumptions. This clarity from hypothesis to expected outcome is crucial, as it allows people to acknowledge a failed hypothesis if it does not provide the intended result. Promoting such a culture of effective hypothesising can lead to more thoughtful actions and a deeper understanding of outcomes. Failures become just another step on the way to success, and success brings more success.
Hypothesis generation is a continuous process where you start with an educated guess and refine it as you gather more information. You form a hypothesis based on what you know or observe.
Say you're a pen maker whose sales are down. You look at what you know:
- I can see that pen sales for my brand are down in May and June.
- I also know that schools are closed in May and June and that schoolchildren use a lot of pens.
- I hypothesise that my sales are down because school children are not using pens in May and June, and thus not buying newer ones.
The next step is to collect and analyse data to test this hypothesis, like tracking sales before and after school vacations. As you gather more data and insights, your hypothesis may evolve. You might discover that your hypothesis only holds in certain markets but not others, leading to a more refined hypothesis.
Once your hypothesis is proven correct, there are many actions you may take - (a) reduce supply in these months (b) reduce the price so that sales pick up (c) release a limited supply of novelty pens, and so on.
Once you decide on your action, you will further monitor the data to see if your actions are working. This iterative cycle of formulating, testing, and refining hypotheses - and using insights in decision-making - is vital in making impactful decisions and solving complex problems in various fields, from business to scientific research.
How do Analysts generate Hypotheses? Why is it iterative?
A typical human working towards a hypothesis would start with:
1. Picking the Default Action
2. Determining the Alternative Action
3. Figuring out the Null Hypothesis (H0)
4. Inverting the Null Hypothesis to get the Alternate Hypothesis (H1)
5. Hypothesis Testing
The default action is what you would naturally do, regardless of any hypothesis or in a case where you get no further information. The alternative action is the opposite of your default action.
The null hypothesis, or H0, is what brings about your default action. The alternative hypothesis (H1) is essentially the negation of H0.
For example, suppose you are tasked with analysing a highway tollgate data (timestamp, vehicle number, toll amount) to see if a raise in tollgate rates will increase revenue or cause a volume drop. Following the above steps, we can determine:
Default Action | “I want to increase toll rates by 10%.” |
Alternative Action | “I will keep my rates constant.” |
H | “A 10% increase in the toll rate will not cause a significant dip in traffic (say 3%).” |
H | “A 10% increase in the toll rate will cause a dip in traffic of greater than 3%.” |
Now, we can start looking at past data of tollgate traffic in and around rate increases for different tollgates. Some data might be irrelevant. For example, some tollgates might be much cheaper so customers might not have cared about an increase. Or, some tollgates are next to a large city, and customers have no choice but to pay.
Ultimately, you are looking for the level of significance between traffic and rates for comparable tollgates. Significance is often noted as its P-value or probability value . P-value is a way to measure how surprising your test results are, assuming that your H0 holds true.
The lower the p-value, the more convincing your data is to change your default action.
Usually, a p-value that is less than 0.05 is considered to be statistically significant, meaning there is a need to change your null hypothesis and reject your default action. In our example, a low p-value would suggest that a 10% increase in the toll rate causes a significant dip in traffic (>3%). Thus, it is better if we keep our rates as is if we want to maintain revenue.
In other examples, where one has to explore the significance of different variables, we might find that some variables are not correlated at all. In general, hypothesis generation is an iterative process - you keep looking for data and keep considering whether that data convinces you to change your default action.

Internal and External Data
Hypothesis generation feeds on data. Data can be internal or external. In businesses, internal data is produced by company owned systems (areas such as operations, maintenance, personnel, finance, etc). External data comes from outside the company (customer data, competitor data, and so on).
Let’s consider a real-life hypothesis generated from internal data:
Multinational company Johnson & Johnson was looking to enhance employee performance and retention. Initially, they favoured experienced industry candidates for recruitment, assuming they'd stay longer and contribute faster. However, HR and the people analytics team at J&J hypothesised that recent college graduates outlast experienced hires and perform equally well. They compiled data on 47,000 employees to test the hypothesis and, based on it, Johnson & Johnson increased hires of new graduates by 20% , leading to reduced turnover with consistent performance.
For an analyst (or an AI assistant), external data is often hard to source - it may not be available as organised datasets (or reports), or it may be expensive to acquire. Teams might have to collect new data from surveys, questionnaires, customer feedback and more.
Further, there is the problem of context. Suppose an analyst is looking at the dynamic pricing of hotels offered on his company’s platform in a particular geography. Suppose further that the analyst has no context of the geography, the reasons people visit the locality, or of local alternatives; then the analyst will have to learn additional context to start making hypotheses to test.
Internal data, of course, is internal, meaning access is already guaranteed. However, this probably adds up to staggering volumes of data.
Looking Back, and Looking Forward
Data analysts often have to generate hypotheses retrospectively, where they formulate and evaluate H0 and H1 based on past data. For the sake of this article, let's call it retrospective hypothesis generation.
Alternatively, a prospective approach to hypothesis generation could be one where hypotheses are formulated before data collection or before a particular event or change is implemented.
For example:
A pen seller has a hypothesis that during the lean periods of summer, when schools are closed, a Buy One Get One (BOGO) campaign will lead to a 100% sales recovery because customers will buy pens in advance. He then collects feedback from customers in the form of a survey and also implements a BOGO campaign in a single territory to see whether his hypothesis is correct, or not.
The HR head of a multi-office employer realises that some of the company’s offices have been providing snacks at 4:30 PM in the common area, and the rest have not. He has a hunch that these offices have higher productivity. The leader asks the company’s data science team to look at employee productivity data and the employee location data. “Am I correct, and to what extent?”, he asks.
These examples also reflect another nuance, in which the data is collected differently:
- Observational: Observational testing happens when researchers observe a sample population and collect data as it occurs without intervention. The data for the snacks vs productivity hypothesis was observational.
- Experimental: In experimental testing, the sample is divided into multiple groups, with one control group. The test for the non-control groups will be varied to determine how the data collected differs from that of the control group. The data collected by the pen seller in the single territory experiment was experimental.
Such data-backed insights are a valuable resource for businesses because they allow for more informed decision-making, leading to the company's overall growth. Taking a data-driven decision, from forming a hypothesis to updating and validating it across iterations, to taking action based on your insights reduces guesswork, minimises risks, and guides businesses towards strategies that are more likely to succeed.
How can GenAI help in Hypothesis Generation?
Of course, hypothesis generation is not always straightforward. Understanding the earlier examples is easy for us because we're already inundated with context. But, in a situation where an analyst has no domain knowledge, suddenly, hypothesis generation becomes a tedious and challenging process.
AI, particularly high-capacity, robust tools such as LLMs, have radically changed how we process and analyse large volumes of data. With its help, we can sift through massive datasets with precision and speed, regardless of context, whether it's customer behaviour, financial trends, medical records, or more. Generative AI, including LLMs, are trained on diverse text data, enabling them to comprehend and process various topics.
Now, imagine an AI assistant helping you with hypothesis generation. LLMs are not born with context. Instead, they are trained upon vast amounts of data, enabling them to develop context in a completely unfamiliar environment. This skill is instrumental when adopting a more exploratory approach to hypothesis generation. For example, the HR leader from earlier could simply ask an LLM tool: “Can you look at this employee productivity data and find cohorts of high-productivity and see if they correlate to any other employee data like location, pedigree, years of service, marital status, etc?”
For an LLM-based tool to be useful, it requires a few things:
- Domain Knowledge: A human could take months to years to acclimatise to a particular field fully, but LLMs, when fed extensive information and utilising Natural Language Processing (NLP), can familiarise themselves in a very short time.
- Explainability: Explainability is its ability to explain its thought process and output to cease being a "black box".
- Customisation: For consistent improvement, contextual AI must allow tweaks, allowing users to change its behaviour to meet their expectations. Human intervention and validation is a necessary step in adoptingAI tools. NLP allows these tools to discern context within textual data, meaning it can read, categorise, and analyse data with unimaginable speed. LLMs, thus, can quickly develop contextual understanding and generate human-like text while processing vast amounts of unstructured data, making it easier for businesses and researchers to organise and utilise data effectively.LLMs have the potential to become indispensable tools for businesses. The future rests on AI tools that harness the powers of LLMs and NLP to deliver actionable insights, mitigate risks, inform decision-making, predict future trends, and drive business transformation across various sectors.
Together, these technologies empower data analysts to unravel hidden insights within their data. For our pen maker, for example, an AI tool could aid data analytics. It can look through historical data to track when sales peaked or go through sales data to identify the pens that sold the most. It can refine a hypothesis across iterations, just as a human analyst would. It can even be used to brainstorm other hypotheses. Consider the situation where you ask the LLM, " Where do I sell the most pens? ". It will go through all of the data you have made available - places where you sell pens, the number of pens you sold - to return the answer. Now, if we were to do this on our own, even if we were particularly meticulous about keeping records, it would take us at least five to ten minutes, that too, IF we know how to query a database and extract the needed information. If we don't, there's the added effort required to find and train such a person. An AI assistant, on the other hand, could share the answer with us in mere seconds. Its finely-honed talents in sorting through data, identifying patterns, refining hypotheses iteratively, and generating data-backed insights enhance problem-solving and decision-making, supercharging our business model.
Top-Down and Bottom-Up Hypothesis Generation
As we discussed earlier, every hypothesis begins with a default action that determines your initial hypotheses and all your subsequent data collection. You look at data and a LOT of data. The significance of your data is dependent on the effect and the relevance it has to your default action. This would be a top-down approach to hypothesis generation.
There is also the bottom-up method , where you start by going through your data and figuring out if there are any interesting correlations that you could leverage better. This method is usually not as focused as the earlier approach and, as a result, involves even more data collection, processing, and analysis. AI is a stellar tool for Exploratory Data Analysis (EDA). Wading through swathes of data to highlight trends, patterns, gaps, opportunities, errors, and concerns is hardly a challenge for an AI tool equipped with NLP and powered by LLMs.
EDA can help with:
- Cleaning your data
- Understanding your variables
- Analysing relationships between variables
An AI assistant performing EDA can help you review your data, remove redundant data points, identify errors, note relationships, and more. All of this ensures ease, efficiency, and, best of all, speed for your data analysts.
Good hypotheses are extremely difficult to generate. They are nuanced and, without necessary context, almost impossible to ascertain in a top-down approach. On the other hand, an AI tool adopting an exploratory approach is swift, easily running through available data - internal and external.
If you want to rearrange how your LLM looks at your data, you can also do that. Changing the weight you assign to the various events and categories in your data is a simple process. That’s why LLMs are a great tool in hypothesis generation - analysts can tailor them to their specific use cases.
Ethical Considerations and Challenges
There are numerous reasons why you should adopt AI tools into your hypothesis generation process. But why are they still not as popular as they should be?
Some worry that AI tools can inadvertently pick up human biases through the data it is fed. Others fear AI and raise privacy and trust concerns. Data quality and ability are also often questioned. Since LLMs and Generative AI are developing technologies, such issues are bound to be, but these are all obstacles researchers are earnestly tackling.
One oft-raised complaint against LLM tools (like OpenAI's ChatGPT) is that they 'fill in' gaps in knowledge, providing information where there is none, thus giving inaccurate, embellished, or outright wrong answers; this tendency to "hallucinate" was a major cause for concern. But, to combat this phenomenon, newer AI tools have started providing citations with the insights they offer so that their answers become verifiable. Human validation is an essential step in interpreting AI-generated hypotheses and queries in general. This is why we need a collaboration between the intelligent and artificially intelligent mind to ensure optimised performance.
Clearly, hypothesis generation is an immensely time-consuming activity. But AI can take care of all these steps for you. From helping you figure out your default action, determining all the major research questions, initial hypotheses and alternative actions, and exhaustively weeding through your data to collect all relevant points, AI can help make your analysts' jobs easier. It can take any approach - prospective, retrospective, exploratory, top-down, bottom-up, etc. Furthermore, with LLMs, your structured and unstructured data are taken care of, meaning no more worries about messy data! With the wonders of human intuition and the ease and reliability of Generative AI and Large Language Models, you can speed up and refine your process of hypothesis generation based on feedback and new data to provide the best assistance to your business.
Related Posts
The latest industry news, interviews, technologies, and resources.
.jpg)
Analyst 2.0: How is AI Changing the Role of Data Analysts
The future belongs to those who forge a symbiotic relationship between Human Ingenuity and Machine Intelligence

From Development to Deployment: Exploring the LLMOps Life Cycle
Discover how Large Language Models (LLMs) are revolutionizing enterprise AI with capabilities like text generation, sentiment analysis, and language translation. Learn about LLMOps, the specialized practices for deploying, monitoring, and maintaining LLMs in production, ensuring reliability, performance, and security in business operations.
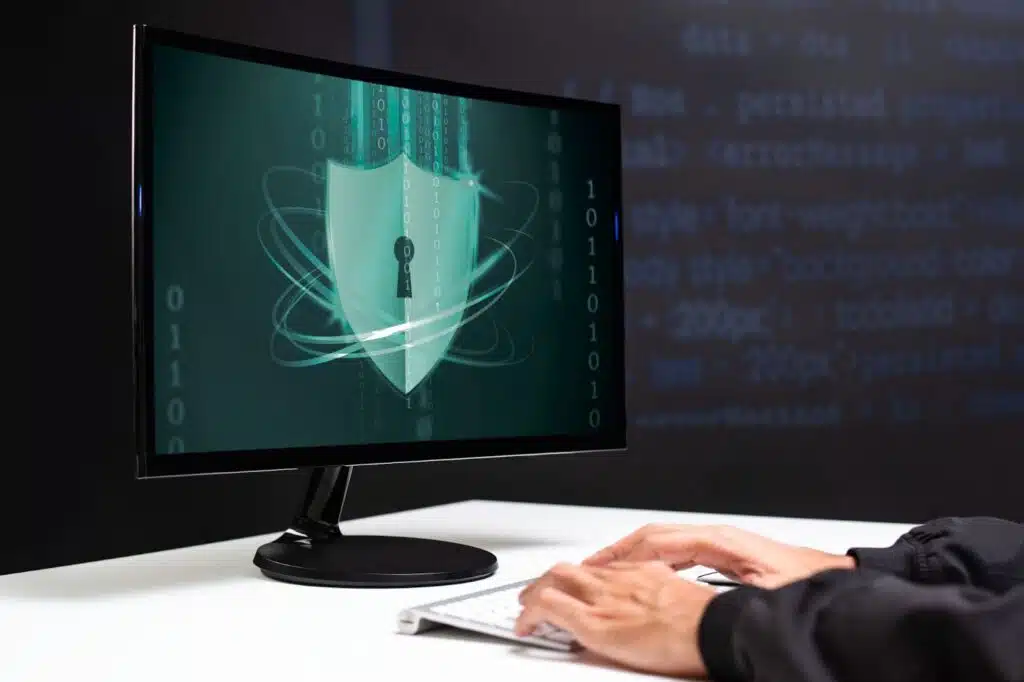
8 Ways By Which AI Fraud Detection Helps Financial Firms
In the era of the Digital revolution, financial systems and AI fraud detection go hand-in-hand as they share a common characteristic.
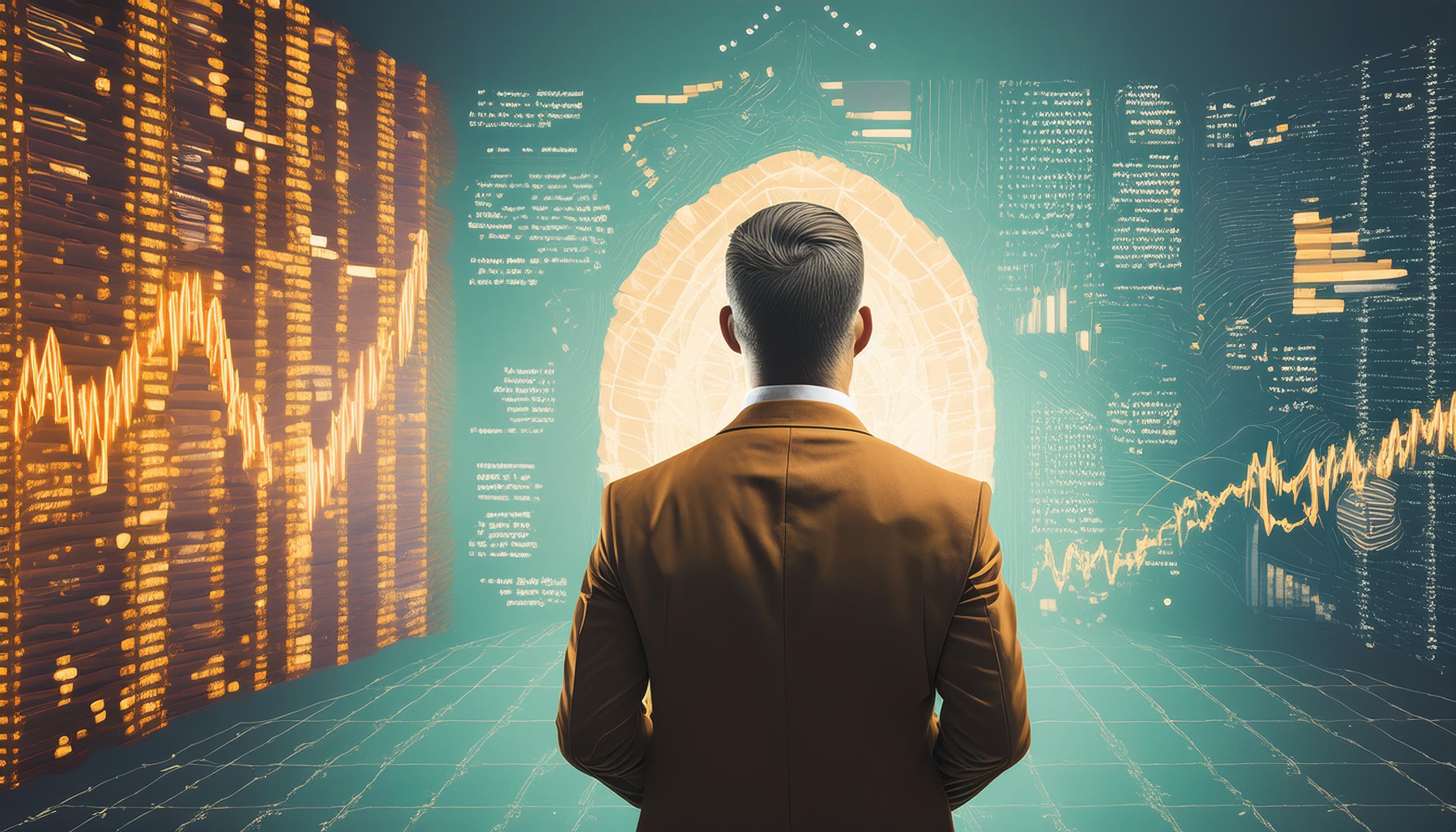
Akaike's Top 5 Game-Changing Generative AI Solutions in Banking, Finance, and Insurance
Knowledge Center
Case Studies
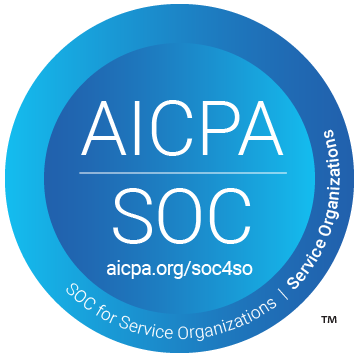
© 2023 Akaike Technologies Pvt. Ltd. and/or its associates and partners
Terms of Use
Privacy Policy
Terms of Service
© Akaike Technologies Pvt. Ltd. and/or its associates and partners
Link prediction for hypothesis generation: an active curriculum learning infused temporal graph-based approach
- Open access
- Published: 12 August 2024
- Volume 57 , article number 244 , ( 2024 )
Cite this article
You have full access to this open access article
- Uchenna Akujuobi 1 na1 ,
- Priyadarshini Kumari 2 na1 ,
- Jihun Choi 3 ,
- Samy Badreddine 1 ,
- Kana Maruyama 3 ,
- Sucheendra K. Palaniappan 4 &
- Tarek R. Besold 1
218 Accesses
Explore all metrics
Over the last few years Literature-based Discovery (LBD) has regained popularity as a means to enhance the scientific research process. The resurgent interest has spurred the development of supervised and semi-supervised machine learning models aimed at making previously implicit connections between scientific concepts/entities within often extensive bodies of literature explicit—i.e., suggesting novel scientific hypotheses. In doing so, understanding the temporally evolving interactions between these entities can provide valuable information for predicting the future development of entity relationships. However, existing methods often underutilize the latent information embedded in the temporal aspects of the interaction data. Motivated by applications in the food domain—where we aim to connect nutritional information with health-related benefits—we address the hypothesis-generation problem using a temporal graph-based approach. Given that hypothesis generation involves predicting future (i.e., still to be discovered) entity connections, in our view the ability to capture the dynamic evolution of connections over time is pivotal for a robust model. To address this, we introduce THiGER , a novel batch contrastive temporal node-pair embedding method. THiGER excels in providing a more expressive node-pair encoding by effectively harnessing node-pair relationships. Furthermore, we present THiGER-A , an incremental training approach that incorporates an active curriculum learning strategy to mitigate label bias arising from unobserved connections. By progressively training on increasingly challenging and high-utility samples, our approach significantly enhances the performance of the embedding model. Empirical validation of our proposed method demonstrates its effectiveness on established temporal-graph benchmark datasets, as well as on real-world datasets within the food domain.
Similar content being viewed by others
Connecting the Dots: Hypotheses Generation by Leveraging Semantic Shifts
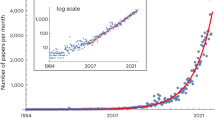
Forecasting the future of artificial intelligence with machine learning-based link prediction in an exponentially growing knowledge network
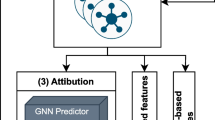
Dyport: dynamic importance-based biomedical hypothesis generation benchmarking technique
Explore related subjects.
- Artificial Intelligence
Avoid common mistakes on your manuscript.
1 Introduction
The saddest aspect of life right now is that science gathers knowledge faster than society gathers wisdom. — Isaac Asimov. Science is advancing at an increasingly quick pace, as evidenced, for instance, by the exponential growth in the number of published research articles per year (White 2021 ). Effectively navigating this ever-growing body of knowledge is tedious and time-consuming in the best of cases, and more often than not becomes infeasible for individual scientists (Brainard 2020 ). In order to augment the efforts of human scientists in the research process, computational approaches have been introduced to automatically extract hypotheses from the knowledge contained in published resources. Swanson ( 1986 ) systematically used a scientific literature database to find potential connections between previously disjoint bodies of research, as a result hypothesizing a (later confirmed) curative relationship between dietary fish oils and Raynaud’s syndrome . Swanson and Smalheiser then automatized the search and linking process in the ARROWSMITH system (Swanson and Smalheiser 1997 ). Their work and other more recent examples (Fan and Lussier 2017 ; Trautman 2022 ) clearly demonstrate the usefulness of computational methods in extracting latent information from the vast body of scientific publications.
Over time, various methodologies have been proposed to address the Hypothesis Generation (HG) problem. Swanson and Smalheiser (Smalheiser and Swanson 1998 ; Swanson and Smalheiser 1997 ) pioneered the use of a basic ABC model grounded in a stringent interpretation of structural balance theory (Cartwright and Harary 1956 ). In essence, if entities A and B, as well as entities A and C, share connections, then entities B and C should be associated. Subsequent years have seen the exploration of more sophisticated machine learning-based approaches for improved inference. These encompass techniques such as text mining (Spangler et al. 2014 ; Spangler 2015 ), topic modeling (Sybrandt et al. 2017 ; Srihari et al. 2007 ; Baek et al. 2017 ), association rules (Hristovski et al. 2006 ; Gopalakrishnan et al. 2016 ; Weissenborn et al. 2015 ), and others (Jha et al. 2019 ; Xun et al. 2017 ; Shi et al. 2015 ; Sybrandt et al. 2020 )
In the context of HG, where the goal is to predict novel relationships between entities extracted from scientific publications, comprehending prior relationships is of paramount importance. For instance, in the domain of social networks, the principles of social theory come into play when assessing the dynamics of connections between individuals. When there is a gradual reduction in the social distance between two distinct individuals, as evidenced by factors such as the establishment of new connections with shared acquaintances and increased geographic proximity, there emerges a heightened likelihood of a subsequent connection between these two individuals (Zhang and Pang 2015 ; Gitmez and Zárate 2022 ). This concept extends beyond social networks and finds relevance in predicting scientific relationships or events through the utilization of temporal information (Crichton et al. 2018 ; Krenn et al. 2023 ; Zhang et al. 2022 ). In both contexts, the principles of proximity and evolving relationships serve as valuable indicators, enabling a deeper understanding of the intricate dynamics governing these complex systems.
Modeling these relationships’ temporal evolution assumes a critical role in constructing an effective and resilient hypothesis generation model. To harness the temporal dynamics, Akujuobi et al. ( 2020b , 2020a ) and Zhou et al. ( 2022 ) conceptualize the HG task as a temporal graph problem. More precisely, given a sequence of graphs \(G = \{G_{0}, G_{2},\ldots , G_{T} \}\) , the objective is to deduce which previously unlinked nodes in \(G_{T}\) ought to be connected. In this framework, nodes denote biomedical entities, and the graphs \(G_{\tau }\) represent temporal graphlets (see Fig. 1 ).
Definition 1
Temporal graphlet : A temporal graphlet \(G_{\tau } = \{V^{\tau },E^{\tau }\}\) is a temporal subgraph at time point \(\tau\) , where \(V^{\tau } \subset V\) and \(E^{\tau } \subset E\) are the temporal set of nodes and edges of the subgraph.
Their approach tackles the HG problem by introducing a temporal perspective. Instead of relying solely on the final state \(E_{T}\) on a static graph, it considers how node pairs evolve over discrete time steps \(E^{\tau }: \tau = 0 \dots T\) . To model this sequential evolution effectively, Akujuobi et al. and Zhou et al. leverage the power of recurrent neural networks (RNNs) (see Fig. 2 a). However, it is essential to note that while RNNs have traditionally been the preferred choice for HG, their sequential nature may hinder capturing long-range dependencies, impacting performance for lengthy sequences.
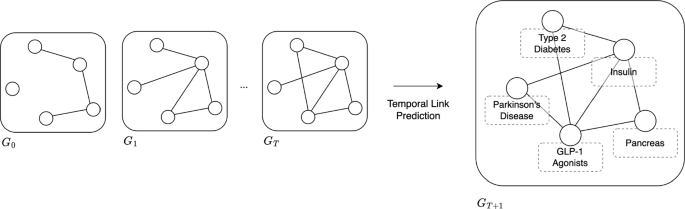
Modeling hypothesis generation as a temporal link prediction problem
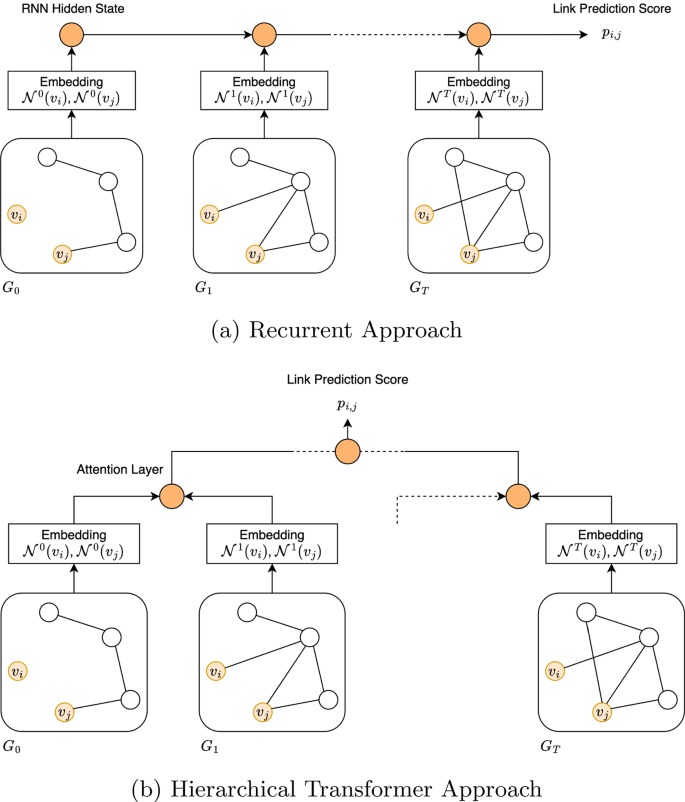
Predicting the link probability \(p_{i,j}\) for a node pair \(v_i\) and \(v_j\) using a a Recurrent Neural Network approach (Akujuobi et al. 2020b ; Zhou et al. 2022 ), b THiGER, our approach. The recurrent approach aggregates the neighborhood information \({{\mathcal {N}}}^t(v_i)\) and \({{\mathcal {N}}}^t(v_j)\) sequentially while THiGER aggregates the neighborhood information hierarchically in parallel
To shed these limitations, we propose THiGER ( T emporal Hi erarchical G raph-based E ncoder R epresentation), a robust transformer-based model designed to capture the evolving relationships between node pairs. THiGER overcomes the constraints of previous methods by representing temporal relationships hierarchically (see Fig. 2 b). The proposed hierarchical layer-wise framework presents an incremental approach to comprehensively model the temporal dynamics among given concepts. It achieves this by progressively extracting the temporal interactions between consecutive time steps, thus enabling the model to prioritize attention to the informative regions of temporal evolution during the process. Our method effectively addresses issues arising from imbalanced temporal information (see Sect. 5.2 ). Moreover, it employs a contrastive learning strategy to improve the quality of task-specific node embeddings for node-pair representations and relationship inference tasks.
An equally significant challenge in HG is the lack of negative-class samples for training. Our dataset provides positive-class samples, which represent established connections between entities, but it lacks negative-class samples denoting non-existent connections (as opposed to undiscovered connections, which could potentially lead to scientific breakthroughs). This situation aligns with the positive-unlabeled (PU) learning problem. Prior approaches have typically either discarded unobserved connections as uninformative or wrongly treated them as negative-class samples. The former approach leads to the loss of valuable information, while the latter introduces label bias during training.
In response to these challenges, we furthermore introduce THiGER-A, an active curriculum learning strategy designed to train the model incrementally. THiGER-A utilizes progressively complex positive samples and highly informative, diverse unobserved connections as negative-class samples. Our experimental results demonstrate that by employing incremental training with THiGER-A, we achieve enhanced convergence and performance for hypothesis-generation models compared to training on the entire dataset in one go. Remarkably, our approach demonstrates strong generalization capabilities, especially in challenging inductive test scenarios where the entities were not part of the seen training dataset.
Inspired by Swanson’s pioneering work, we chose the food domain as a promising application area for THiGER. This choice is motivated by the increasing prevalence of diet-related health conditions, such as obesity and type-2 diabetes, alongside the growing recognition and utilization of the health benefits associated with specific food products in wellness and medical contexts.
In summary, our contributions are as follows:
Methodology: We propose a novel temporal hierarchical transformer-based architecture for node pair encoding. In utilizing the temporal batch-contrastive strategy, our architecture differs from existing approaches that learn in conventional static or temporal graphs. In addition, we present a novel incremental training strategy for temporal graph node pair embedding and future relation prediction. This strategy effectively mitigates negative-label bias through active learning and improves generalization by training the model progressively on increasingly complex positive samples using curriculum learning.
Evaluation: We test the model’s efficacy on several real-world graphs of different sizes to give evidence for the model’s strength for temporal graph problems and hypothesis generation. The model is trained end-to-end and shows superior performance on HG tasks.
Application: To the best of our knowledge, this is the first application of temporal hypothesis generation in the health-related food domain. Through case studies, we validate the practical relevance of our findings.
The remaining sections of this paper include a discussion of related work in Sect. 2 , a detailed introduction of the proposed THiGER model and the THiGER-A active curriculum learning strategy in Sect. 3 , an overview of the datasets, the model setup and parameter tuning, and our evaluation approach in Sect. 4 , the results of our experimental evaluations in Sect. 5 , and finally, our conclusions and a discussion of future work in Sect. 6 .
2 Related works
2.1 hypothesis generation.
The development of effective methods for machine-assisted discovery is crucial in pushing scientific research into the next stage (Kitano 2021 ). In recent years, several approaches have been proposed in a bid to augment human abilities relevant to the scientific research process including tools for research design and analysis (Tabachnick and Fidell 2000 ), process modelling and simulation (Klein et al. 2002 ), or scientific hypothesis generation (King et al. 2004 , 2009 ).
The early pioneers of the hypothesis generation domain proposed the so called ABC model for generating novel scientific hypothesis based on existing knowledge (Swanson 1986 ; Swanson and Smalheiser 1997 ). ABC-based models are simple and efficient, and have been implemented in classical hypothesis generation systems such as ARROWSMITH (Swanson and Smalheiser 1997 ). However, several drawbacks remain, including the need for similarity metrics defined on heuristically determined term lists and significant costs in terms of computational complexity with respect to the size of common entities.
More recent approaches, thus, have aimed to curtain the limitation of the ABC model. Spangler et al. ( 2014 ); Spangler ( 2015 ) proposed text mining techniques to identify entity relationships from unstructured medical texts. AGATHA (Sybrandt et al. 2020 ) used a transformer encoder architecture to learn the ranking criteria between regions of a given semantic graph and the plausibility of new research connections. Srihari et al. ( 2007 ); Baek et al. ( 2017 ) proposed several text mining approaches to detect how concepts are linked within and across multiple text documents. Sybrandt et al. ( 2017 ) proposed incorporating machine learning techniques such as clustering and topical phrase mining. Shi et al. ( 2015 ) modeled the probability that concepts will be linked based on a given time window using random walks.
The previously mentioned methods do not consider temporal attributes of the data. More recent works (Jha et al. 2019 ; Akujuobi et al. 2020a ; Zhou et al. 2022 ; Xun et al. 2017 ) argue that capturing the temporal information available in scholarly data can lead to better predictive performance. Jha et al. ( 2019 ) explored the co-evolution of concepts across knowledge bases using a temporal matrix factorization framework. Xun et al. ( 2017 ) modeled concepts’ co-occurrence probability using their temporal embedding. Akujuobi et al. ( 2020a , 2020b ) and Zhou et al. ( 2022 ) captured the temporal information in the scholarly data using RNN techniques.
Our approach captures the dynamic relationship information using a temporal hierarchical transformer encoder model. This strategy alleviates the limitations of the RNN-based models. Furthermore, with the incorporation of active curriculum learning strategies, our model can incrementally learn from the data.
2.2 Temporal graph learning
Learning on temporal graphs has received considerable attention from the research community in recent years. Some works (Hisano 2018 ; Ahmed et al. 2016 ; Milani Fard et al. 2019 ) apply static methods on aggregated graph snapshots. Others, including (Zhou et al. 2018 ; Singer et al. 2019 ), utilize time as a regularizer over consecutive snap-shots of the graph to impose a smoothness constraint on the node embeddings. A popular category of approaches for dynamic graphs is to introduce point processes that are continuous in time. DyRep (Trivedi et al. 2019 ) models the occurrence of an edge as a point process using graph attention on the destination node neighbors. Dynamic-Triad (Zhou et al. 2018 ) models the evolution patterns in a graph by imposing a triadic closure-where a triad with three nodes is developed from an open triad (i.e., with two nodes not connected).
Some recent works on temporal graphs apply several combinations of GNNs and recurrent architectures (e.g., GRU). EvolveGCN (Pareja et al. 2020 ) adapts the graph convolutional network (GCN) model along the temporal dimension by using an RNN to evolve the GCN parameters. T-PAIR (Akujuobi et al. 2020b , a ) recurrently learns a node pair embedding by updating GraphSAGE parameters using gated neural networks (GRU). TGN (Rossi et al. 2020 ) introduces a memory module framework for learning on dynamic graphs. TDE (Zhou et al. 2022 ) captures the local and global changes in the graph structure using hierarchical RNN structures. TNodeEmbed (Singer et al. 2019 ) proposes the use of orthogonal procrustes on consecutive time-step node embeddings along the time dimension.
However, the limitation of RNN remains due to their sequential nature and robustness especially when working on a long timeline. Since the introduction of transformers, there has been interest in their application on temporal graph data. More related to this work, Zhong and Huang ( 2023 ) and Wang et al. ( 2022 ) both propose the use of a transformer architecture to aggregate the node neighborhood information while updating the memory of the nodes using GRU. TLC (Wang et al. 2021a ) design a two-stream encoder that independently processes temporal neighborhoods associated with the two target interaction nodes using a graph-topology-aware Transformer and then integrates them at a semantic level through a co-attentional Transformer.
Our approach utilizes a single hierarchical encoder model to better capture the temporal information in the network while simultaneously updating the node embedding on the task. The model training and node embedding learning is performed end-to-end.
2.3 Active curriculum learning
Active learning (AL) has been well-explored for vision and learning tasks (Settles 2012 ). However, most of the classical techniques rely on single-instance-oracle strategies, wherein, during each training round, a single instance with the highest utility is selected using measures such as uncertainty sampling (Kumari et al. 2020 ), expected gradient length (Ash et al. 2020 ), or query by committee (Gilad-Bachrach et al. 2006 ). The single-instance-oracle approach becomes computationally infeasible with large training datasets such as ours. To address these challenges, several batch-mode active learning methods have been proposed (Priyadarshini et al. 2021 ; Kirsch et al. 2019 ; Pinsler et al. 2019 ). Priyadarshini et al. ( 2021 ) propose a method for batch active metric learning, which enables sampling of informative and diverse triplet data for relative similarity ordering tasks. In order to prevent the selection of correlated samples in a batch, Kirsch et al. ( 2019 ); Pinsler et al. ( 2019 ) develop distinct methods that integrate mutual information into the utility function. All three approaches demonstrate effectiveness in sampling diverse batches of informative samples for metric learning and classification tasks. However, none of these approaches can be readily extended to our specific task of hypothesis prediction on an entity-relationship graph.
Inspired by human learning, Bengio et al. ( 2009 ) introduced the concept of progressive training, wherein the model is trained on increasingly difficult training samples. Various prior works have proposed different measures to quantify the difficulty of training examples. Hacohen and Weinshall ( 2019 ) introduced curriculum learning by transfer, where they developed a score function based on the prediction confidence of a pre-trained model. Wang et al. ( 2021b ) proposed a curriculum learning approach specifically for graph classification tasks. Another interesting work is relational curriculum learning (RCL) (Zhang et al. 2023 ) suggests training the model progressively on complex samples. Unlike most prior work, which typically consider data to be independent, RCL quantifies the difficulty level of an edge by aggregating the embeddings of the neighboring nodes. While their approach utilizes similar relational data to ours, their method does not specifically tackle the challenges inherent to the PU learning setting, which involves sampling both edges and unobserved relationships from the training data. In contrast, our proposed method introduces an incremental training strategy that progressively trains the model by focusing on positive edges of increasing difficulty, as well as incorporating highly informative and diverse negative edges.
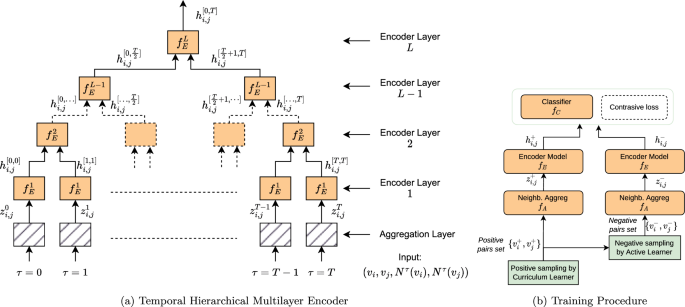
Schematic representation of the proposed model for temporal node-pair link prediction. In a , the hierarchical graph transformer model takes as input the aggregated node pair embeddings obtained at each time step \(\tau\) , these temporal node pair embeddings are further encoded and aggregated at each encoder layer. The final output is the generalized node pair embedding across all time steps. In b , a general overview of the model is given, highlighting the incorporation of the Active Curriculum Learning strategy
3 Methodology
3.1 notation.
\(G = \{G_0, \dots , G_T\}\) is a temporal graph such that \(G_\tau = \{V^\tau , E^\tau \}\) evolves over time \(\tau =0\dots T\) ,
\(e(v_i, v_j)\) or \(e_{ij}\) is used to denote the edge between nodes \(v_i\) and \(v_j\) , and \((v_i, v_j)\) is used to denote the node pair corresponding to the edge,
\(y_{i,j}\) is the label associated with the edge \(e(v_i,v_j)\) ,
\({{\mathcal {N}}}^{{\tau }}(v_{})\) gives the neighborhood of a node v in \(V^\tau\) ,
\(x_{v_{}}\) is the embedding of a node v and is static across time steps,
\(z_{i,j}^{\tau }\) is the embedding of a node pair \(\langle v_i, v_j \rangle\) . It depends on the neighborhood of the nodes at a time step \(\tau\) ,
\(h_{i,j}^{[\tau _0,\tau _f]}\) is the embedding of a node pair over a time step window \(\tau _0, \dots , \tau _f\) where \(0 \le \tau _0 \le \tau _f \le T\) ,
\(f(.; \theta )\) is a neural network depending on a set of parameters \(\theta\) . For brevity, \(\theta\) can be omitted if it is clear from the context.
\(E^+\) and \(E^-\) are the subsets of positive and negative edges, denoting observed and non-observed connections between biomedical concepts, respectively.
L is the number of encoder layers in the proposed model.
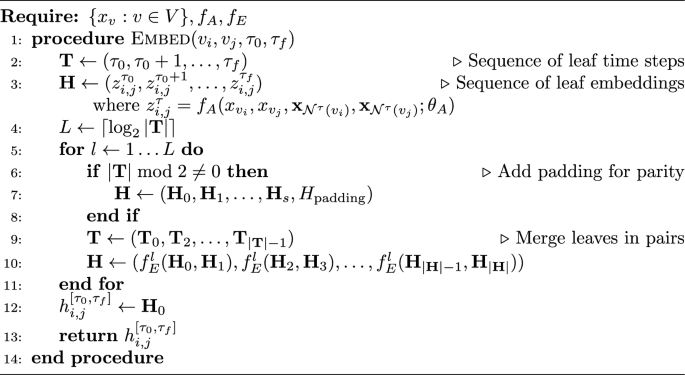
Hierarchical Node-Pair Embedding \(h_{i,j}^{[\tau _0,\tau _f]}\)
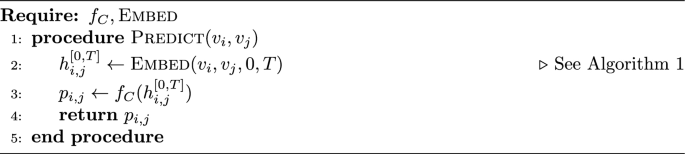
Link Prediction
3.2 Model overview
The whole THiGER(-A) model is shown in Fig. 3 b. Let \(v_i, v_j \in V_T\) be nodes denoting two concepts. The pair is assigned a positive label \(y_{i,j} = 1\) if a corresponding edge (i.e., a link) is observed in \(G_T\) . That is, \(y_{i,j} = 1\) iff \(e(v_i, v_j) \in E^{T}\) , otherwise 0. The model predicts a score \(p_{i,j}\) that reflects \(y_{i,j}\) . The prediction procedure is presented in Algorithm 2.
The link prediction score is given by a neural classifier \(p_{i,j} = f_C(h_{i,j}^{[0,T]}; \theta _C)\) , where \(h_{i,j}^{[0,T]}\) is an embedding vector for the node pair. This embedding is calculated in Algorithm 1 using a hierarchical transformer encoder and illustrated in Fig. 3 a.
The input to the hierarchical encoder layer is the independent local node pair embedding aggregation at each time step shown in line 3 of algorithm 1 as
where \({\textbf{x}}_{{{\mathcal {N}}}^{{\tau }}(v_{i})} = \{x_{v'}: v' \in {{\mathcal {N}}}^{{\tau }}(v_{i})\}\) and \({\textbf{x}}_{{{\mathcal {N}}}^{{\tau }}(v_{j})} = \{x_{v'}: v' \in {{\mathcal {N}}}^{{\tau }}(v_{j})\}\) are the embeddings of the neighbors of \(x_{v_{i}}\) and \(x_{v_{j}}\) at the given time step.
Subsequently, the local node pair embeddings aggregation is processed by the aggregation layer illustrated in Fig. 3 a and shown in line 10 of Algorithm 1. At each hierarchical layer, temporal node pair embeddings are calculated for a sub-window using
where n represents the sub-window size. When necessary, we ensure an even number of leaves to aggregate by adding zero padding values \(H_\textrm{padding} = {\textbf{0}}_d\) , where d is the dimension of the leaf embeddings. The entire encoder architecture is denoted as \(f_E = { f^l_E: l=1\dots L}\) .
In this work, the classifier \(f_C(.; \theta _C)\) is modeled using a multilayer perceptron network (MLP), \(f_A(.; \theta _A)\) is elaborated in Sect. 3.3 , and \(f_E(.;\theta _E)\) is modeled by a multilayer transformer encoder network, which is detailed in Sect. 3.4 .
3.3 Neighborhood aggregation
The neighborhood aggregation is modeled using GraphSAGE (Hamilton et al. 2017 ). GraphSAGE uses K layers to iteratively aggregate a node embedding \(x_{v_{}}\) and its neighbor embeddings \({\textbf{x}}_{{{\mathcal {N}}}^{{\tau }}(v_{})} = \{x_{v'}, v' \in {{\mathcal {N}}}^{{\tau }}(v_{})\}\) . \(f_A\) uses the GraphSAGE block to aggregate \((x_{v_{i}}, {\textbf{x}}_{{{\mathcal {N}}}^{{\tau }}(v_{i})})\) and \((x_{v_{j}}, {\textbf{x}}_{{{\mathcal {N}}}^{{\tau }}(v_{j})})\) in parallel, then merges the two aggregated representations using a MLP layer. In this paper, we explore three models based on the aggregation technique used at each iterative step of GraphSAGE.
Mean Aggregation: This straightforward technique amalgamates neighborhood representations by computing element-wise means of each node’s neighbors and subsequently propagating this information iteratively. For all nodes within the specified set:
Here, \(\beta _{v}^{k}\) denotes the aggregated vector at iteration k , and \(\beta ^{k-1}_{v}\) at iteration \(k-1\) . \(W^S\) and \(W^N\) represent trainable weights, and \(\sigma\) constitutes a sigmoid activation, collectively forming a conventional MLP layer.
GIN (Graph Isomorphism Networks): Arguing that traditional graph aggregation methods, like mean aggregation, possess limited expressive power, GIN introduces the concept of aggregating neighborhood representations as follows:

In this formulation, \(\epsilon ^{k}\) governs the relative importance of the node compared to its neighbors at layer k and can be a learnable parameter or a fixed scalar.
Multi-head Attention: We introduce a multi-head attention-based aggregation technique. This method aggregates neighborhood representations by applying multi-head attention to the node and its neighbors at each iteration:
Here, \(\phi\) represents a multi-head attention function, as detailed in Vaswani et al. ( 2017 ).
3.3.1 Neighborhood definition
To balance performance and scalability considerations, we adopt the neighborhood sampling approach utilized in GraphSAGE to maintain a consistent computational footprint for each batch of neighbors. In this context, we employ a uniform sampling method to select a neighborhood node set of fixed size, denoted as \({{\mathcal {N}}}^{'}(v_{}) \subset {{\mathcal {N}}}^{{\tau }}(v_{})\) , from the original neighbor set at each step. This sampling procedure is essential as, without it, the memory and runtime complexity of a single batch becomes unpredictable and, in the worst-case scenario, reaches a prohibitive \({{\mathcal {O}}}(|V|)\) , making it impractical for handling large graphs.
3.4 Temporal hierarchical multilayer encoder layer
The temporal hierarchical multilayer encoder is the fundamental component of our proposed model, responsible for processing neighborhood representations collected over multiple time steps, specifically \((z_{i,j}^{0}, z_{i,j}^{1}, \dots , z_{i,j}^{T})\) . These neighborhood representations are utilized to construct a hierarchical tree.
At the initial hierarchical layer, we employ an encoder, denoted as \(f_E^1\) , to distill adjacent sequential local node-pair embeddings, represented as \((z_{i,j}^{\tau }, z_{i,j}^{\tau + 1})\) , combining them into a unified embedding, denoted as \(h_{i,j}^{[\tau ,\tau +1]}\) . In cases where the number of time steps is not an even multiple of 2, a zero-vector dummy input is appended.
This process repeats at each hierarchical level l within the tree, with \(h_{i,j}^{[\tau -n,\tau ]} = f^l_E(h_{i,j}^{[\tau -n,\tau -\frac{n}{2}]}, h_{i,j}^{[(\tau -\frac{n}{2}) + 1,\tau ]};\theta ^l_E)\) . Each layer \(f_E^l\) consists of a transformer encoder block and may contain \(N - 1\) encoder sublayers, where \(N \ge 1\) . This mechanism can be viewed as an iterative knowledge aggregation process, wherein the model progressively summarizes the information from pairs of local node pair embeddings.
The output of each encoder layer, denoted as \(h_{i,j}^{[\tau _0,\tau _f]}\) , offers a comprehensive summary of temporal node pair information from time step \(\tau _0\) to \(\tau _f\) . Finally, the output of the last layer, \(h_{i,j}^{[0,T]}\) , is utilized for inferring node pair relationships.
3.5 Parameter learning
The trainable parts of the architecture are the weights and parameters of the neighborhood aggregator \(f_A\) , the transformer network \(f_E\) , the classifier \(f_C\) and the embedding representations \(\{x_{v_{}}: v \in V\}\) .
To obtain suitable representations, we employ a combination of supervised and contrastive loss functions on the output of the hierarchical encoder layer \(h_{i,j}^{[0,T]}\) . The contrastive loss function encourages the embeddings of positive (i.e. a link exists in \(E^T\) ) node pairs to be closer while ensuring that the embeddings of negative node pairs are distinct.
We adopt a contrastive learning framework (Chen et al. 2020 ) to distinguish between positive and negative classes. For brevity, we temporarily denote \(h_{i,j}^{[0,T]}\) as \(h_{i,j}\) . Given two positive node pairs with corresponding embeddings \(e(v_i, v_j) \rightarrow h_{i,j}\) and \(e(v_o, v_n) \rightarrow h_{o,n}\) , the loss function is defined as follows:
where \(\alpha\) represents a temperature parameter, B is a set of node pairs in a given batch, and \(\mathbbm {1}_{(k,w) \ne (i,j)}\) indicates that the labels of node pair ( k , w ) and ( i , j ) are different. We employ the angular similarity function \(\textrm{sim}(x)=1 - \arccos (x)/\pi\) . We do not explicitly sample negative examples, following the methodology outlined in Chen et al. ( 2020 ).
The contrastive loss is summed over the positive training data \(E^+\) :
To further improve the discriminative power of the learned features, we also minimize the center loss:
where E is the data of positive and negative edges, \(y_{i,j}\) is the class of the pair (0 or 1), \(c_{y_{{i,j}}} \in R^d\) denotes the corresponding class center. The class centers are updated after each mini-batch step following the method proposed in Wen et al. ( 2016 ).
Finally, a good node pair vector \(h_{i,j}^{[0,T]}\) should minimize the binary cross entropy loss of the node pair prediction task:
We adopt the joint supervision of the prediction loss, contrastive loss, and center loss to jointly train the model for discriminative feature learning and relationship inference:
As is usual, the losses are applied over subsets of the entire dataset. In this case, we have an additional requirement for pairs of nodes in \(E^-\) : at least one of the two nodes needs to appear in \(E^+\) . An elaborate batch sampling strategy is proposed in the following section. The model parameters are trained end to end.
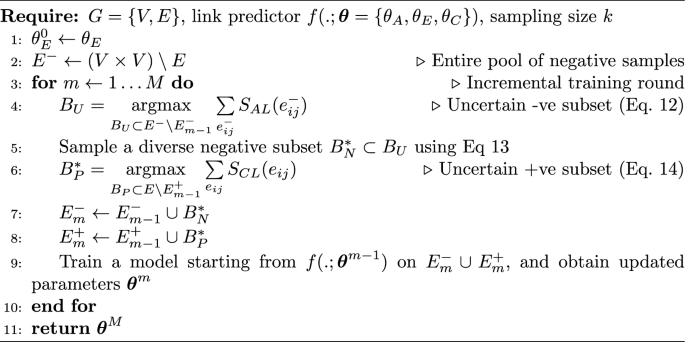
Training Procedure in THiGER-A
3.6 Incremental training strategy
This section introduces the incremental training strategy THiGER-A , which extends our base THiGER model. The pseudo-code for THiGER-A is presented in Algorithm 3. We represent the parameters used in the entire architecture as \(\varvec{\theta }= (\theta _A, \theta _E, \theta _C)\) . Let \(P(y \mid e_{i,j}; \varvec{\theta })\) , where \(y\in \{0,1\}\) , denote the link predictor for the nodes \((v_i, v_j)\) . Specifically, in shorthand, we denote \(P(y=1 \mid e_{i,j};\varvec{\theta })\) by \(p_{i,j}\) as in line 3 of Algorithm 2, likewise \(P(y=0\mid e_{i,j}; \varvec{\theta }) = 1 - p_{i,j}\) .
We define \(E^- = (V \times V) \setminus E\) as the set of negative edges representing non-observed connections in the graph. The size of the negative set grows quadratically with the number of nodes, resulting in a computational complexity of \({{\mathcal {O}}}(|V|^2)\) . For large, sparse graphs like ours, the vast number of negative edges makes it impractical to use all of them for model training.
Randomly sampling negative examples may introduce noise and hinder training convergence. To address this challenge, we propose an approach to sample a smaller subset of “informative” negative edges that effectively capture the entity relationships within the graph. Leveraging active learning, a technique for selecting high-utility datasets, we aim to choose a subset \(B^*_N \subset E^-\) that leads to improved model learning.
3.6.1 Negative Edge Sampling using Active Learning
Active learning (AL) is an iterative process centered around acquiring a high-utility subset of samples and subsequently retraining the model. The initial step involves selecting a subset of samples with high utility, determined by a specified informativeness measure. Once this subset is identified, it is incorporated into the training data, and the model is subsequently retrained. This iterative cycle, involving sample acquisition and model retraining, aims to improve the model’s performance and generalizability through the learning process.
In this context, we evaluate the informativeness of edges using a score function denoted as \(S_{AL}: (v_{i}^{-}, v_{j}^{-}) \rightarrow {\mathbb {R}}\) . An edge \((v_{i}^{-}, v_{j}^{-})\) is considered more informative than \((v_{k}^{-}, v_{l}^{-})\) if \(S_{AL}(v_{i}^{-}, v_{j}^{-}) > S_{AL}(v_{k}^{-}, v_{l}^{-})\) . The key challenge in AL lies in defining \(S_{AL}\) , which encodes the learning of the model \(P(.;\varvec{\theta })\) trained in the previous iteration.
We gauge the informativeness of an edge based on model uncertainty. An edge is deemed informative when the current model \(P(.;\varvec{\theta })\) exhibits high uncertainty in predicting its label. Uncertainty sampling is one of the most popular choices for the quantification of informativeness due to its simplicity and high effectiveness in selecting samples for which the model lacks sufficient knowledge. Similar to various previous techniques, we use Shannon entropy to approximate informativeness (Priyadarshini et al. 2021 ; Kirsch et al. 2019 ). It is important to emphasize that ground truth labels are unavailable for negative edges, which represent unobserved entity connections. Therefore, to estimate the informativeness of negative edges, we calculate the expected Shannon entropy across all possible labels. Consequently, the expected entropy for a negative edge \((v_{i}^{-}, v_{j}^{-})\) at the \(m^{th}\) training round is defined as:
Here, \(\varvec{\theta }^{m-1}\) is the base hypothesis predictor model trained at the \((m-1)^{th}\) training round and \(m = 0, 1, \cdots , M\) denotes the AL training round. Selecting a subset of uncertain edges, \(B_{U}\) using Eq. 12 unfortunately does not ensure diversity among the selected subset. The diversity metric is crucial in subset selection as it encourages the selection of diverse samples within the embedding space. This, in turn, results in a higher cumulative informativeness for the selected subset, particularly when the edges exhibit overlapping features. The presence of a highly-correlated edges in the selected subset can lead to a sub-optimal batch with high redundancy. The importance of diversity in selecting informative edges has been emphasized in several prior works (Kirsch et al. 2019 ; Priyadarshini et al. 2021 ). To obtain a diverse subset, both approaches aim to maximize the joint entropy (and consequently, minimize mutual information) among the samples in the selected batch. However, maximizing joint entropy is an expensive combinatorial optimization problem and does not scale well for larger datasets, as in our case.
We adopt a similar approach as (Kumari et al. 2020 ) and utilize the k-means++ algorithm (Arthur and Vassilvitskii 2006 ) to cluster the selected batch \(B_U\) into diverse landmark points. While (Kumari et al. 2020 ) is tailored for metric learning tasks with the triplet samples as inputs, our adaptation of the k-means++ algorithm is designed for graph datasets, leading to the selection of diverse edges within the gradient space. Although diversity in the gradient space is effective for gradient-based optimizer, a challenge arises due to the high dimensionality of the gradient space, particularly when the model is large. To overcome this challenge, we compute the expected gradient of the loss function with respect to only the penultimate layer of the network, \(\nabla _{\theta _{out}}{{\mathcal {L}}}_{e_{ij}^{-}}\) , assuming it captures task-specific features. We begin to construct an optimal subset \(B_{N}^{*} \in B_{U}\) by initially (say, at \(k=0\) ) selecting the two edges with the most distinct gradients. Subsequently, we iteratively select the most dissimilar gradient edge from the selected subset using the maxmin optimization objective defined in Eq. 13 .
Here \(d_{E}\) represents the Euclidean distance between two vectors in the gradient space, consisting of \(\nabla _{\theta _{out}}{{\mathcal {L}}}_{e_{ij}^{-1}}\) , which denotes the gradient of the loss function \({{\mathcal {L}}}\) with respect to the penultimate layer of the network \(\theta _{out}\) . The process continues until we reach the allocated incremental training budget, \(|B_{N}^{*}| = K\) . The resulting optimal subset of negative edges, \(B_{N}^{*}\) , comprises negative edges that are both diverse and informative.
3.6.2 Positive Edge Sampling
Inspired by Curriculum Learning (CL), a technique mimicking certain aspects of human learning, we investigate its potential to enhance the performance and generalization of the node pair predictor model. Curriculum Learning involves presenting training data to the model in a purposeful order, starting with easier examples and gradually progressing to more challenging ones. We hypothesize that applying CL principles can benefit our node pair predictor model. By initially emphasizing the learning of simpler connections and leveraging prior knowledge, the model can effectively generalize to more complex connections during later stages of training. Although Active Learning (AL) and CL both involve estimating the utility of training samples, they differ in their approach to label availability. AL operates in scenarios where labels are unknown and estimates sample utility based on expected scores. In contrast, CL uses known labels to assess sample difficulty. For our model, we use one of the common approaches to define a difficulty score \(S_{CL}\) based on the model’s prediction confidence. The model’s higher prediction confidence indicates easier samples.
Here, \(S_{CL}(v_{i}, v_{j})\) indicates predictive uncertainty of an edge \(e_{ij}\) to be positive by an existing trained model \(\theta ^{m-1}\) at \((m-1)^{th}\) iteration. In summary, for hypothesis prediction using a large training dataset, Active Curriculum Learning provides a natural approach to sample an informative and diverse subset of high-quality samples, helping to alleviate the challenges associated with label bias.
4 Experimental setup
In this section, we present the experimental setup for our evaluation. We compare our proposed model, THiGER(-A), against several state-of-the-art (SOTA) methods to provide context for the empirical results on benchmark datasets. To ensure fair comparisons, we utilize publicly available baseline implementations and modify those as needed to align with our model’s configuration and input requirements. All experiments were conducted using Python. For the evaluation of the interaction datasets, we train all models on a single NVIDIA A10G GPU. In the case of the food-related biomedical dataset, we employ 4 NVIDIA V100 GPUs for model training. Notably, all models are trained on single machines. In our experiments, we consider graphs as undirected. The node attribute embedding dimension is set to \(d=128\) for all models evaluated. For baseline methods, we performed a parameter search on the learning rate and training steps, and we report the best results achieved. Our model is implemented in TensorFlow.
4.1 Datasets and model setup
Table 1 shows the statistics of the datasets used in this study. Unless explicitly mentioned, all methods, including our model, share the same initial node attributes provided by pretrained Node2Vec (Grover and Leskovec 2016 ). The pre-trained Node2vec embedding effectively captures the structural information of nodes in the training graph. In our proposed framework, the choice of a fixed node embedding is to enable the model capture the temporal evolution of network relations, given that the node embeddings are in the same vector space. While employing a dynamic node embedding framework may enhance results, it introduces complexities associated with aligning vector spaces across different timestamps. This aspect is deferred to future research. It is important to note that the Node2vec embeddings serve solely as initializations for the embedding layer, and the embedding vectors undergo fine-tuning during the learning process to further capture the dynamic evolution of node relationships. For models that solely learn embedding vectors for individual nodes, we represent the \(h_{i,j}\) of a given node pair as the concatenation of the embedding vectors for nodes \(\langle x_i, x_j \rangle\) .
4.1.1 Interaction datasets
We have restructured the datasets to align with our specific use case. We partition the edges in the temporal graphs into five distinct groups based on their temporal labels. For example, if a dataset is labeled up to 500 time units, we reorganize them as follows: \(\{0, \dots , 100\} \rightarrow 0\) , \(\{101, \dots , 200\} \rightarrow 1\) , \(\{201, \dots , 300\} \rightarrow 2\) , \(\{301, \dots , 400\} \rightarrow 3\) , and \(\{401, \dots , 500\} \rightarrow 4\) . These User-Item based datasets create bipartite graphs. For all inductive evaluations, we assume knowledge of three nearest node neighbors for each of the unseen nodes. Neighborhood information is updated after model training to incorporate this knowledge, with zero vectors assigned to new nodes.
4.1.2 Food-related biomedical temporal datasets
To construct the relationship graph, we extract sentences containing predefined entities (Genes, Diseases, Chemical Compounds, Nutrition, and Food Ingredients). We establish connections between two concepts that appear in the same sentence within any publication in the dataset. The time step for each relationship between concept pairs corresponds to the publication year when the first mention was identified (i.e., the oldest publication year among all the publications where the concepts are associated). We generate three datasets for evaluation based on concept pair domains: Ingredient, disease pairs, Ingredient, Chemical compound pairs, and all pairs (unfiltered). Graph statistics are provided in Table 1 . For training and testing sets, we divide the graph into 10-year intervals starting from 1940 (i.e., { \(\le 1940\) }, {1941–1950}, \(\dots\) , {2011–2020}). The splits \(\le\) 2020 are used for training, and the split {2021–2022} is used for testing. In accordance with the problem configuration in the interaction dataset, we update the neighborhood information and also assume knowledge of three nearest node neighbors pertaining to each of the unseen nodes for inductive evaluations.
4.1.3 Model setup & parameter tuning
Model Configuration: We employ a hierarchical encoder with \(N\lceil \log _{2} T \rceil\) layers, where N is a multiple of each hierarchical layer (i.e., with \(N-1\) encoder sublayers), and T represents the number of time steps input to each hierarchical encoder layer. In our experiments, we set the number of encoder layer multiples to \(N=2\) . We use 8 attention heads with 128 dimensional states. For the position-wise feed-forward networks, we use 512 dimensional inner states. For the activation function, we applied the Gaussian Error Linear Unit (GELU, Hendrycks and Gimpel 2016 ). We apply a dropout (Srivastava et al. 2014 ) to the output of each sub-layer with a rate of \(P_{drop} = 0.1\) .
Optimizer: Our models are trained using the AdamW optimizer (Loshchilov and Hutter 2017 ), with the following hyper-parameters: \(\beta _1 = 0.9\) , \(\beta _2 = 0.99\) , and \(\epsilon = 10^{-7}\) . We use a linear decay of the learning rate. We set the number of warmup steps to \(10\%\) of the number of train steps. We vary the learning rate with the size of the training data.
Time Embedding: We use Time2Vec (T2V, Kazemi et al. 2019 ) to generate time-step embeddings which encode the temporal sequence of the time steps. The T2V model is learned and updated during the model training.
Active learning: The size of subset \(B_U\) is twice the size of the optimal subset \(B^{*}\) . The model undergoes seven training rounds for the Wikipedia, Reddit, and Last FM datasets, while it is trained for three rounds for the food-related biomedical dataset (All, Ingredient-Disease, Ingredient-Chemical). Due to the large size of biomedical dataset, we limit the model training to only three rounds. However, we anticipate that increasing the number of training rounds will lead to further improvements in performance.
4.2 Evaluation metrics
In this study, we assess the efficacy of the models by employing the binary F1 score and average precision score (AP) as the performance metrics. The binary F1 score is defined as the harmonic mean of precision and recall, represented by the formula:
Here, precision denotes the ratio of true positive predictions to the total predicted positives, while recall signifies the ratio of true positive predictions to the total actual positives.
The average precision score is the weighted mean of precisions achieved using different thresholds, using the incremental change in recall from the previous threshold as weight:
where N is the total number of thresholds, \(P_{k}\) is the precision at cut-off k , and \(\Delta R_{k} = R_{k} - R_{k - 1}\) is a sequential change in the recall value. Our emphasis on positive predictions in the evaluations is driven by our preference for models that efficiently forecast future connections between pairs of nodes.
4.3 Method categories
We categorize the methods into two main groups based on their handling of temporal information:
Static Methods: These methods treat the graph as static data and do not consider the temporal aspect. The static methods under consideration include the Logistic regression model, GraphSAGE (Hamilton et al. 2017 ), and AGATHA (Sybrandt et al. 2020 ).
Temporal Methods: These state-of-the-art methods leverage temporal information to create more informative node representations. We evaluate the performance of our base model, THiGER, and the final model, THiGER-A, against the following temporal methods: CTDNE (Nguyen et al. 2018 ), TGN (Rossi et al. 2020 ), JODIE (Kumar et al. 2019 ), TNodeEmbed (Singer et al. 2019 ), DyRep (Trivedi et al. 2019 ), T-PAIR (Akujuobi et al. 2020b ), and TDE (Zhou et al. 2022 ).
5 Experiments
The performance of THiGER-A is rigorously assessed across multiple benchmark datasets, as presented in Tables 2 and 3 . The experimental evaluations are primarily geared toward two distinct objectives:
Assessing the model’s effectiveness in handling interaction datasets pertinent to temporal graph problems.
Evaluating the model’s proficiency in dealing with food-related biomedical datasets, specifically for predicting relationships between food-related concepts and other biomedical terms.
In Sects. 4.1.1 and 4.1.2 , a comprehensive overview of the used datasets is provided. Our evaluations encompass two fundamental settings:
Transductive setup: This scenario involves utilizing data from all nodes during model training.
Inductive setup: In this configuration, at least one node in each evaluated node pair has not been encountered during the model’s training phase.
These experiments are designed to rigorously assess THiGER-A’s performance across diverse datasets, offering insights into its capabilities under varying conditions and problem domains.
5.1 Quantitative evaluation: interaction temporal datasets
We assess the performance of our proposed model in the context of future interaction prediction (Rossi et al. 2020 ; Kumar et al. 2019 ). The datasets record interactions between users and items.
We evaluate the performance on three distinct datasets: (i) Reddit, (ii) LastFM, and (iii) Wikipedia, considering both transductive and inductive settings. In the transductive setting, THiGER-A outperforms other models across all datasets, except Wikipedia, where AGATHA exhibits significant superiority. Our analysis reveals that AGATHA’s advantage lies in its utilization of the entire graph for neighborhood and negative sampling, which gives it an edge over models using a subset of the graph due to computational constraints. This advantage is more evident in the transductive setup since AGATHA’s training strategy leans towards seen nodes. Nevertheless, THiGER-A consistently achieves comparable or superior performance even in the presence of AGATHA’s implicit bias. It is imperative to clarify that AGATHA was originally designed for purposes other than node-pair predictions. Nonetheless, we have adapted the algorithm to align with the node-pair configuration specifically for our research evaluations.
In the inductive setup, our method excels in the Wikipedia and Reddit datasets but lags behind some baselines in the LastFM dataset. Striking a balance between inductive and transductive performance, THiGER-A’s significant performance gain over THiGER underscores the effectiveness of the proposed incremental learning strategy. This advantage is particularly pronounced in the challenging inductive test setting.
5.2 Quantitative evaluation: food-related biomedical temporal datasets
This section presents the quantitative evaluation of our proposed model on temporal node pair (or “link”) prediction, explicitly focusing on food-related concept relationships extracted from scientific publications in the PMC dataset. The evaluation encompasses concept pairs from different domains, including Ingredient, Disease pairs (referred to as F-ID), Ingredient, Chemical Compound pairs (F-IC), and all food-related pairs (F-A). The statistical characteristics of the dataset are summarized in Table 1 .
Table 3 demonstrates that our model outperforms the baseline models in both inductive and transductive setups. The second-best performing model is AGATHA, which, as discussed in the previous section, exhibits certain advantages over alternative methods. It is noteworthy that the CTDNE method exhibits scalability issues with larger datasets.
An intriguing observation from this evaluation is that, aside from our proposed model, static methods outperform temporal methods on this dataset. Further investigation revealed that the data is more densely distributed toward the later time steps. Notably, a substantial increase in information occurs during the last time steps. Up to the year 2000, the average number of edges per time step is approximately 100, 000. However, this number surges to about 1 million in the time window from 2001 to 2010, followed by another leap to around 4 million in the 2011–2020 time step. This surge indicates a significant influx of knowledge in food-related research in recent years.
We hypothesize that while this influx is advantageous for static methods, it might adversely affect some temporal methods due to limited temporal information. To test this hypothesis, we conduct an incremental evaluation, illustrated in Fig. 4 , using two comparable link prediction methods (Logistic Regression and GraphSAGE) and the two best temporal methods (tNodeEmbed and THiGER). In this evaluation, we incrementally assess the transductive performance on testing pairs up to the year 2000. Specifically, we evaluate the model performance on the food dataset (F-A) in the time intervals 1961–1970 by using all available training data up to 1960, and similarly for subsequent time intervals.
From Fig. 4 , it is evident that temporal methods outperform static methods when the temporal data is more evenly distributed, i.e., when there is an incremental increase in temporal data. The sudden exponential increase in data during the later years biases the dataset towards the last time steps. However, THiGER consistently outperforms the baseline methods in the incremental evaluation, underscoring its robustness and flexibility.
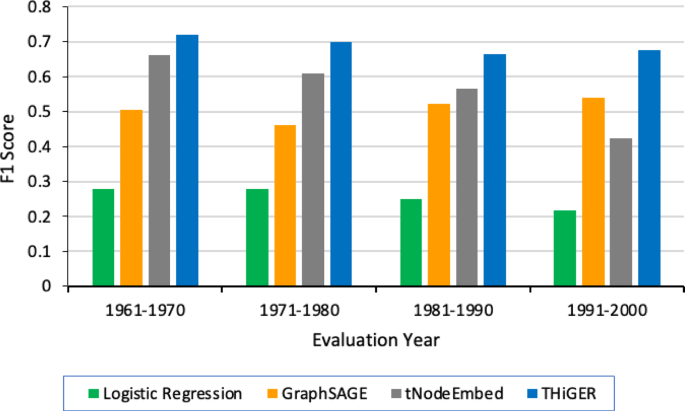
Transductive F1 score of incremental prediction (per year) made by THiGER and three other baselines. The models are incrementally trained with data before the displayed evaluation time window
5.3 Ablation study
In this section, we conduct an ablation study to assess the impact of various sampling strategies on the base model’s performance. The results are presented in Table 4 , demonstrating the performance improvements achieved by the different versions of the THiGER model (-mean, -gin and -attn) for each dataset. Due to the much larger size of the food-related biomedical dataset, we conduct the ablation study only for the baseline datasets.
First, we investigate the influence of the active learning (AL)-based negative sampler on the base THiGER model. A comparison of the model’s performance with and without the AL-based negative sampler reveals significant improvements across all datasets. Notably, the performance gains are more pronounced in the challenging inductive test cases where at least one node of an edge is unseen in the training data. This underscores the effectiveness and generalizability of the AL-based learner for the hypothesis prediction model in the positive-unlabeled (PU) learning setup.
Next, we integrate curriculum learning (CL) as a positive data sampler, resulting in further enhancements to the base model. Similar to the AL-based sampling, the performance gains are more pronounced in the inductive test setting. The relatively minor performance improvement in the transductive case may be attributed to the limited room for enhancement in that specific context. Nevertheless, both AL alone and AL combined with CL enhance the base model’s performance and generalizability, particularly in the inductive test scenario.
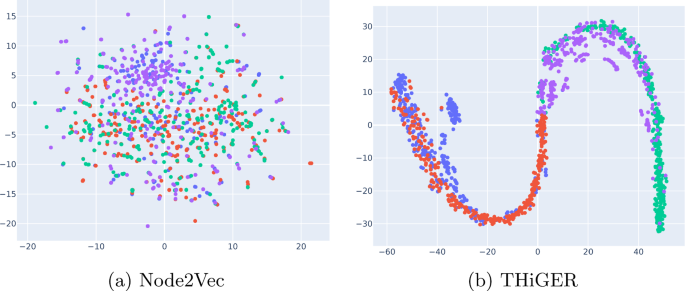
Pair embedding visualization. The blue color denotes the true negative samples, the red points are false negative, the green points are true positive, and the purple points are false positive
5.4 Pair embedding visualization
In this section, we conduct a detailed analysis of the node pair embeddings generated by THiGER using the F-ID dataset. To facilitate visualization, we randomly select 900 pairs and employ t-SNE (Van der Maaten and Hinton 2008 ) to compare these embeddings with those generated by Node2Vec, as shown in Fig. 5 . We employ color-coding to distinguish between the observed labels and the predicted labels. Notably, we observe distinct differences in the learned embeddings. THiGER effectively separates positive and negative node pairs in the embedding space. True positives (denoted in green) and true negatives (denoted in blue) are further apart in the embedding space, while false positives (indicated in red) and false negatives (shown in purple) occupy an intermediate region. This observation aligns with the idea that unknown connections are not unequivocal in our application domain, possibly due to missing data or discoveries yet to be made.
5.5 Case study
To assess the predictive accuracy of our model, we conducted a detailed analysis using the entire available food-related biomedical temporal dataset. We collaborated with biologists to evaluate the correctness of the generated hypotheses. Unlike providing binary predictions (1 or 0), we take a probabilistic approach by assigning a probability score within the range of 0 to 1. This score reflects the likelihood of a connection existing between the predicted node pairs. Consequently, the process of ranking a set of relation predictions associated with a specific node is tantamount to ranking the corresponding predicted probabilities.
Using this methodology, we selected 402 node pairs and presented them to biomedical researchers for evaluation. The researchers sought hypotheses related to specific oils. Subsequently, we generated hypotheses representing potential future connections between the oil nodes and other nodes, resulting in a substantial list. Given the anticipated extensive list, we implemented a filtering process based on the associated probability scores. This enabled us to selectively identify predictions with high probabilities, which were then communicated to the biomedical researchers for evaluation. The evaluation encompassed two distinct approaches.
First, they conducted manual searches for references to the predicted positive node pairs in various biology texts, excluding our dataset. Their findings revealed relationships in 70 percent of the node pairs through literature searches and reviews.
Secondly, to explore cases where no direct relationship was apparent in existing literature, they randomly selected and analyzed three intriguing node pairs: (i) Flaxseed oil and Root caries , (ii) Benzoxazinoid and Gingelly oil , and (iii) Senile osteoporosis and Soybean oil .
5.5.1 Flaxseed oil and root caries
Root caries refers to a dental condition characterized by the decay and demineralization of tooth root surfaces. This occurs when tooth roots become exposed due to gum recession, allowing bacterial invasion and tooth structure erosion. While the scientific literature does not explicitly mention the use of flaxseed oil for root caries, it is well-established that flaxseed oil possesses antibacterial properties (Liu et al. 2022 ). These properties may inhibit bacterial species responsible for root caries. Furthermore, flaxseed oil is a rich source of omega-3 fatty acids and lignans, factors potentially relevant to this context. Interestingly, observational studies are investigating the oil’s effects on gingivitis (Deepika 2018 ).
5.5.2 Benzoxazinoid and gingelly oil
Benzoxazinoids are plant secondary metabolites synthesized in many monocotyledonous species and some dicotyledonous plants (Schullehner et al. 2008 ). Gingelly oil, derived from sesame seeds, originates from a dicotyledonous plant. In the biologists’ opinion, this concurrence suggests a valid basis for the hypothesized connection.
5.5.3 Senile osteoporosis and soybean oil
Senile osteoporosis is a subtype of osteoporosis occurring in older individuals due to age-related bone loss. Soybean oil, a common vegetable oil derived from soybeans, contains phytic acid (Anderson and Wolf 1995 ). Phytic acid is known to inhibit the absorption of certain minerals, including calcium, which is essential for bone strength (Lönnerdal et al. 1989 ). Again, in the experts’ opinion, this suggests a valid basis for a (unfortunately detrimental) connection between the oil and the health condition.
6 Conclusions
We introduce an innovative approach to tackle the hypothesis generation problem within the context of temporal graphs. We present THiGER, a novel transformer-based model designed for node pair prediction in temporal graphs. THiGER leverages a hierarchical framework to effectively capture and learn from temporal information inherent in such graphs. This framework enables efficient parallel temporal information aggregation. We also introduce THiGER-A, an incremental training strategy that enhances the model’s performance and generalization by training it on high-utility samples selected through active curriculum learning, particularly benefiting the challenging inductive test setting. Quantitative experiments and analyses demonstrate the efficiency and robustness of our proposed method when compared to various state-of-the-art approaches. Qualitative analyses illustrate its practical utility.
For future work, an enticing avenue involves incorporating additional node-pair relationship information from established biomedical and/or food-related knowledge graphs. In scientific research, specific topics often experience sudden exponential growth, leading to temporal data distribution imbalances. Another intriguing research direction, thus, is the study of the relationship between temporal data distribution and the performance of temporal graph neural network models. We plan to analyze the performance of several temporal GNN models across diverse temporal data distributions and propose model enhancement methods tailored to such scenarios.
Due to the vast scale of the publication graph, training the hypothesis predictor with all positive and negative edges is impractical and limits the model’s ability to generalize, especially when the input data is noisy. Thus, it is crucial to train the model selectively on a high-quality subset of the training data. Our work presents active curriculum learning as a promising approach for feasible and robust training for hypothesis predictors. However, a static strategy struggles to generalize well across different scenarios. An exciting direction for future research could be to develop dynamic policies for data sampling that automatically adapt to diverse applications. Furthermore, improving time complexity is a critical challenge, particularly for applications involving large datasets and models.
Ahmed NM, Chen L, Wang Y et al. (2016) Sampling-based algorithm for link prediction in temporal networks. Inform Sci 374:1–14
Article MathSciNet Google Scholar
Akujuobi U, Chen J, Elhoseiny M et al. (2020) Temporal positive-unlabeled learning for biomedical hypothesis generation via risk estimation. Adv Neural Inform Proc Syst 33:4597–4609
Google Scholar
Akujuobi U, Spranger M, Palaniappan SK et al. (2020) T-pair: Temporal node-pair embedding for automatic biomedical hypothesis generation. IEEE Trans Knowledge Data Eng 34(6):2988–3001
Anderson RL, Wolf WJ (1995) Compositional changes in trypsin inhibitors, phytic acid, saponins and isoflavones related to soybean processing. J Nutr 125(suppl–3):581S-588S
Arthur D, Vassilvitskii S (2006) \(k\) -means++: The advantages of careful seeding. Stanford University, Tech. rep
Ash JT, Zhang C, Krishnamurthy A et al. (2020) Deep batch active learning by diverse, uncertain gradient lower bounds. ICLR, Vienna
Baek SH, Lee D, Kim M et al. (2017) Enriching plausible new hypothesis generation in pubmed. PloS One 12(7):e0180539
Article Google Scholar
Bengio Y, Louradour J, Collobert R, et al. (2009) Curriculum learning. In: Proceedings of the 26th Annual International Conference on Machine Learning, 41–48
Brainard J (2020) Scientists are drowning in COVID-19 papers. Can new tools keep them afloat? — science.org. https://www.science.org/content/article/scientists-are-drowning-covid-19-papers-can-new-tools-keep-them-afloat , [Accessed 25-May-2023]
Cartwright D, Harary F (1956) Structural balance: a generalization of Heider’s theory. Psychol Rev 63(5):277
Chen T, Kornblith S, Norouzi M, et al. (2020) A simple framework for contrastive learning of visual representations. In: International Conference on Machine Learning, PMLR, 1597–1607
Crichton G, Guo Y, Pyysalo S et al. (2018) Neural networks for link prediction in realistic biomedical graphs: a multi-dimensional evaluation of graph embedding-based approaches. BMC Bioinform 19(1):1–11
Deepika A (2018) Effect of flaxseed oil in plaque induced gingivitis-a randomized control double-blind study. J Evid Based Med Healthc 5(10):882–5
Fan Jw, Lussier YA (2017) Word-of-mouth innovation: hypothesis generation for supplement repurposing based on consumer reviews. In: AMIA Annual Symposium Proceedings, American Medical Informatics Association, p 689
Gilad-Bachrach R, Navot A, Tishby N (2006) Query by committee made real. NeurIPS, Denver
Gitmez AA, Zárate RA (2022) Proximity, similarity, and friendship formation: Theory and evidence. arXiv preprint arXiv:2210.06611
Gopalakrishnan V, Jha K, Zhang A, et al. (2016) Generating hypothesis: Using global and local features in graph to discover new knowledge from medical literature. In: Proceedings of the 8th International Conference on Bioinformatics and Computational Biology, BICOB, 23–30
Grover A, Leskovec J (2016) node2vec: Scalable feature learning for networks. In: Proceedings of the 22nd ACM SIGKDD International Conference on Knowledge Discovery and Data Mining, 855–864
Hacohen G, Weinshall D (2019) On the power of curriculum learning in training deep networks. In: International Conference on Machine Learning, PMLR, 2535–2544
Hamilton W, Ying Z, Leskovec J (2017) Inductive representation learning on large graphs. Adv Neural Inform Proc Syst. https://doi.org/10.48550/arXiv.1706.02216
Hendrycks D, Gimpel K (2016) Bridging nonlinearities and stochastic regularizers with gaussian error linear units. CoRR, abs/160608415 3
Hisano R (2018) Semi-supervised graph embedding approach to dynamic link prediction. In: Complex Networks IX: Proceedings of the 9th Conference on Complex Networks CompleNet 2018 9, Springer, 109–121
Hristovski D, Friedman C, Rindflesch TC, et al. (2006) Exploiting semantic relations for literature-based discovery. In: AMIA Annual Symposium Proceedings, 349
Jha K, Xun G, Wang Y, et al. (2019) Hypothesis generation from text based on co-evolution of biomedical concepts. In: Proceedings of the 25th ACM SIGKDD International Conference on Knowledge Discovery & Data Mining, ACM, 843–851
Kazemi SM, Goel R, Eghbali S, et al. (2019) Time2vec: Learning a vector representation of time. arXiv preprint arXiv:1907.05321
King RD, Whelan KE, Jones FM et al. (2004) Functional genomic hypothesis generation and experimentation by a robot scientist. Nature 427(6971):247–252
King RD, Rowland J, Oliver SG et al. (2009) The automation of science. Science 324(5923):85–89
Kirsch A, van Amersfoort J, Gal Y (2019) BatchBALD: efficient and diverse batch acquisition for deep Bayesian active learning. NeurIPS, Denver
Kitano H (2021) Nobel turing challenge: creating the engine for scientific discovery. npj Syst Biol Appl 7(1):29
Klein MT, Hou G, Quann RJ et al. (2002) Biomol: a computer-assisted biological modeling tool for complex chemical mixtures and biological processes at the molecular level. Environ Health Perspect 110(suppl 6):1025–1029
Krenn M, Buffoni L, Coutinho B et al. (2023) Forecasting the future of artificial intelligence with machine learning-based link prediction in an exponentially growing knowledge network. Nat Machine Intell 5(11):1326–1335
Kumari P, Goru R, Chaudhuri S et al. (2020) Batch decorrelation for active metric learning. IJCAI-PRICAI, Jeju Island
Book Google Scholar
Kumar S, Zhang X, Leskovec J (2019) Predicting dynamic embedding trajectory in temporal interaction networks. In: Proceedings of the 25th ACM SIGKDD International Conference on Knowledge Discovery & Data Mining, 1269–1278
Liu Y, Liu Y, Li P et al. (2022) Antibacterial properties of cyclolinopeptides from flaxseed oil and their application on beef. Food Chem 385:132715
Lönnerdal B, Sandberg AS, Sandström B et al. (1989) Inhibitory effects of phytic acid and other inositol phosphates on zinc and calcium absorption in suckling rats. J Nutr 119(2):211–214
Loshchilov I, Hutter F (2017) Decoupled weight decay regularization. arXiv preprint arXiv:1711.05101
Milani Fard A, Bagheri E, Wang K (2019) Relationship prediction in dynamic heterogeneous information networks. In: Advances in Information Retrieval: 41st European Conference on IR Research, ECIR 2019, Cologne, Germany, April 14–18, 2019, Proceedings, Part I 41, Springer, 19–34
Nguyen GH, Lee JB, Rossi RA et al. (2018) Continuous-time dynamic network embeddings. Companion Proc Web Conf 2018:969–976
Pareja A, Domeniconi G, Chen J, et al. (2020) Evolvegcn: Evolving graph convolutional networks for dynamic graphs. In: Proceedings of the AAAI conference on artificial intelligence, 5363–5370
Pinsler R, Gordon J, Nalisnick E et al. (2019) Bayesian batch active learning as sparse subset approximation. NeurIPS, Denver
Priyadarshini K, Chaudhuri S, Borkar V, et al. (2021) A unified batch selection policy for active metric learning. In: Machine Learning and Knowledge Discovery in Databases. Research Track: European Conference, ECML PKDD 2021, Bilbao, Spain, September 13–17, 2021, Proceedings, Part II 21, Springer, 599–616
Rossi E, Chamberlain B, Frasca F, et al. (2020) Temporal graph networks for deep learning on dynamic graphs. arXiv preprint arXiv:2006.10637
Schullehner K, Dick R, Vitzthum F et al. (2008) Benzoxazinoid biosynthesis in dicot plants. Phytochemistry 69(15):2668–2677
Settles B (2012) Active learning. SLAIML, Shimla
Shi F, Foster JG, Evans JA (2015) Weaving the fabric of science: dynamic network models of science’s unfolding structure. Soc Networks 43:73–85
Singer U, Guy I, Radinsky K (2019) Node embedding over temporal graphs. arXiv preprint arXiv:1903.08889
Smalheiser NR, Swanson DR (1998) Using Arrowsmith: a computer-assisted approach to formulating and assessing scientific hypotheses. Comput Methods Prog Biomed 57(3):149–153
Spangler S (2015) Accelerating discovery: mining unstructured information for hypothesis generation. Chapman and Hall/CRC, Boca Raton
Spangler S, Wilkins AD, Bachman BJ, et al. (2014) Automated hypothesis generation based on mining scientific literature. In: Proceedings of the 20th ACM SIGKDD International Conference on Knowledge Discovery and Data Mining, ACM, 1877–1886
Srihari RK, Xu L, Saxena T (2007) Use of ranked cross document evidence trails for hypothesis generation. In: Proceedings of the 13th ACM SIGKDD International Conference on Knowledge Discovery and Data Mining, ACM, 677–686
Srivastava N, Hinton G, Krizhevsky A et al. (2014) Dropout: a simple way to prevent neural networks from overfitting. J Machine Learn Res 15(1):1929–1958
MathSciNet Google Scholar
Swanson DR (1986) Fish oil, Raynaud’s syndrome, and undiscovered public knowledge. Perspect Biol Med 30(1):7–18
Swanson DR, Smalheiser NR (1997) An interactive system for finding complementary literatures: a stimulus to scientific discovery. Artif Intell 91(2):183–203
Sybrandt J, Shtutman M, Safro I (2017) Moliere: Automatic biomedical hypothesis generation system. In: Proceedings of the 23rd ACM SIGKDD International Conference on Knowledge Discovery and Data Mining, 1633–1642
Sybrandt J, Tyagin I, Shtutman M, et al. (2020) Agatha: automatic graph mining and transformer based hypothesis generation approach. In: Proceedings of the 29th ACM International Conference on Information & Knowledge Management, 2757–2764
Tabachnick BG, Fidell LS (2000) Computer-assisted research design and analysis. Allyn & Bacon Inc, Boston
Trautman A (2022) Nutritive knowledge based discovery: Enhancing precision nutrition hypothesis generation. PhD thesis, The University of North Carolina at Charlotte
Trivedi R, Farajtabar M, Biswal P, et al. (2019) Dyrep: Learning representations over dynamic graphs. In: International Conference on Learning Representations
Van der Maaten L, Hinton G (2008) Visualizing data using t-sne. J Machine Learn Res 9(11):2579–2605
Vaswani A, Shazeer N, Parmar N et al. (2017) Attention is all you need. Adv Neural Inform Proc Syst. https://doi.org/10.48550/arXiv.1706.03762
Wang Y, Wang W, Liang Y et al. (2021) Curgraph: curriculum learning for graph classification. Proc Web Conf 2021:1238–1248
Wang Z, Li Q, Yu D et al. (2022) Temporal graph transformer for dynamic network. In: Part II (ed) Artificial Neural Networks and Machine Learning-ICANN 2022: 31st International Conference on Artificial Neural Networks, Bristol, UK, September 6–9, 2022, Proceedings. Springer, Cham, pp 694–705
Chapter Google Scholar
Wang L, Chang X, Li S, et al. (2021a) Tcl: Transformer-based dynamic graph modelling via contrastive learning. arXiv preprint arXiv:2105.07944
Weissenborn D, Schroeder M, Tsatsaronis G (2015) Discovering relations between indirectly connected biomedical concepts. J Biomed Semant 6(1):28
Wen Y, Zhang K, Li Z, et al. (2016) A discriminative feature learning approach for deep face recognition. In: Computer Vision–ECCV 2016: 14th European Conference, Amsterdam, The Netherlands, October 11–14, 2016, Proceedings, Part VII 14, Springer, 499–515
White K (2021) Publications Output: U.S. Trends and International Comparisons | NSF - National Science Foundation — ncses.nsf.gov. https://ncses.nsf.gov/pubs/nsb20214 , [Accessed 25-May-2023]
Xun G, Jha K, Gopalakrishnan V, et al. (2017) Generating medical hypotheses based on evolutionary medical concepts. In: 2017 IEEE International Conference on Data Mining (ICDM), IEEE, 535–544
Zhang R, Wang Q, Yang Q et al. (2022) Temporal link prediction via adjusted sigmoid function and 2-simplex structure. Sci Rep 12(1):16585
Zhang Y, Pang J (2015) Distance and friendship: A distance-based model for link prediction in social networks. In: Asia-Pacific Web Conference, Springer, 55–66
Zhang Z, Wang J, Zhao L (2023) Relational curriculum learning for graph neural networks. https://openreview.net/forum?id=1bLT3dGNS0
Zhong Y, Huang C (2023) A dynamic graph representation learning based on temporal graph transformer. Alexandria Eng J 63:359–369
Zhou H, Jiang H, Yao W et al. (2022) Learning temporal difference embeddings for biomedical hypothesis generation. Bioinformatics 38(23):5253–5261
Zhou L, Yang Y, Ren X, et al. (2018) Dynamic network embedding by modeling triadic closure process. In: Proceedings of the AAAI Conference on Artificial Intelligence
Download references
Author information
Uchenna Akujuobi and Priyadarshini Kumari have contributed equally to this work.
Authors and Affiliations
Sony AI, Barcelona, Spain
Uchenna Akujuobi, Samy Badreddine & Tarek R. Besold
Sony AI, Cupertino, USA
Priyadarshini Kumari
Sony AI, Tokyo, Japan
Jihun Choi & Kana Maruyama
The Systems Biology Institute, Tokyo, Japan
Sucheendra K. Palaniappan
You can also search for this author in PubMed Google Scholar
Contributions
U.A. and P.K. co-lead the reported work and the writing of the manuscript, J.C., S.B., K.M., and S.P. supported the work and the writing of the manuscript. T.B. supervised the work overall. All authors reviewed the manuscript and contributed to the revisions based on the reviewers’ feedback.
Corresponding authors
Correspondence to Uchenna Akujuobi or Priyadarshini Kumari .
Ethics declarations
Conflict of interest.
The authors have no Conflict of interest to declare that are relevant to the content of this article.
Additional information
Publisher's note.
Springer Nature remains neutral with regard to jurisdictional claims in published maps and institutional affiliations.
Rights and permissions
Open Access This article is licensed under a Creative Commons Attribution-NonCommercial-NoDerivatives 4.0 International License, which permits any non-commercial use, sharing, distribution and reproduction in any medium or format, as long as you give appropriate credit to the original author(s) and the source, provide a link to the Creative Commons licence, and indicate if you modified the licensed material. You do not have permission under this licence to share adapted material derived from this article or parts of it. The images or other third party material in this article are included in the article’s Creative Commons licence, unless indicated otherwise in a credit line to the material. If material is not included in the article’s Creative Commons licence and your intended use is not permitted by statutory regulation or exceeds the permitted use, you will need to obtain permission directly from the copyright holder. To view a copy of this licence, visit http://creativecommons.org/licenses/by-nc-nd/4.0/ .
Reprints and permissions
About this article
Akujuobi, U., Kumari, P., Choi, J. et al. Link prediction for hypothesis generation: an active curriculum learning infused temporal graph-based approach. Artif Intell Rev 57 , 244 (2024). https://doi.org/10.1007/s10462-024-10885-1
Download citation
Accepted : 25 July 2024
Published : 12 August 2024
DOI : https://doi.org/10.1007/s10462-024-10885-1
Share this article
Anyone you share the following link with will be able to read this content:
Sorry, a shareable link is not currently available for this article.
Provided by the Springer Nature SharedIt content-sharing initiative
- Temporal graph neural network
- Active learning
- Hierarchical transformer
- Curriculum learning
- Literature based discovery
- Edge prediction
- Find a journal
- Publish with us
- Track your research
- Data Science
- Data Analysis
- Data Visualization
- Machine Learning
- Deep Learning
- Computer Vision
- Artificial Intelligence
- AI ML DS Interview Series
- AI ML DS Projects series
- Data Engineering
- Web Scrapping
Understanding Hypothesis Testing
Hypothesis testing involves formulating assumptions about population parameters based on sample statistics and rigorously evaluating these assumptions against empirical evidence. This article sheds light on the significance of hypothesis testing and the critical steps involved in the process.
What is Hypothesis Testing?
A hypothesis is an assumption or idea, specifically a statistical claim about an unknown population parameter. For example, a judge assumes a person is innocent and verifies this by reviewing evidence and hearing testimony before reaching a verdict.
Hypothesis testing is a statistical method that is used to make a statistical decision using experimental data. Hypothesis testing is basically an assumption that we make about a population parameter. It evaluates two mutually exclusive statements about a population to determine which statement is best supported by the sample data.
To test the validity of the claim or assumption about the population parameter:
- A sample is drawn from the population and analyzed.
- The results of the analysis are used to decide whether the claim is true or not.
Example: You say an average height in the class is 30 or a boy is taller than a girl. All of these is an assumption that we are assuming, and we need some statistical way to prove these. We need some mathematical conclusion whatever we are assuming is true.
Defining Hypotheses
- Null hypothesis (H 0 ): In statistics, the null hypothesis is a general statement or default position that there is no relationship between two measured cases or no relationship among groups. In other words, it is a basic assumption or made based on the problem knowledge. Example : A company’s mean production is 50 units/per da H 0 : [Tex]\mu [/Tex] = 50.
- Alternative hypothesis (H 1 ): The alternative hypothesis is the hypothesis used in hypothesis testing that is contrary to the null hypothesis. Example: A company’s production is not equal to 50 units/per day i.e. H 1 : [Tex]\mu [/Tex] [Tex]\ne [/Tex] 50.
Key Terms of Hypothesis Testing
- Level of significance : It refers to the degree of significance in which we accept or reject the null hypothesis. 100% accuracy is not possible for accepting a hypothesis, so we, therefore, select a level of significance that is usually 5%. This is normally denoted with [Tex]\alpha[/Tex] and generally, it is 0.05 or 5%, which means your output should be 95% confident to give a similar kind of result in each sample.
- P-value: The P value , or calculated probability, is the probability of finding the observed/extreme results when the null hypothesis(H0) of a study-given problem is true. If your P-value is less than the chosen significance level then you reject the null hypothesis i.e. accept that your sample claims to support the alternative hypothesis.
- Test Statistic: The test statistic is a numerical value calculated from sample data during a hypothesis test, used to determine whether to reject the null hypothesis. It is compared to a critical value or p-value to make decisions about the statistical significance of the observed results.
- Critical value : The critical value in statistics is a threshold or cutoff point used to determine whether to reject the null hypothesis in a hypothesis test.
- Degrees of freedom: Degrees of freedom are associated with the variability or freedom one has in estimating a parameter. The degrees of freedom are related to the sample size and determine the shape.
Why do we use Hypothesis Testing?
Hypothesis testing is an important procedure in statistics. Hypothesis testing evaluates two mutually exclusive population statements to determine which statement is most supported by sample data. When we say that the findings are statistically significant, thanks to hypothesis testing.
One-Tailed and Two-Tailed Test
One tailed test focuses on one direction, either greater than or less than a specified value. We use a one-tailed test when there is a clear directional expectation based on prior knowledge or theory. The critical region is located on only one side of the distribution curve. If the sample falls into this critical region, the null hypothesis is rejected in favor of the alternative hypothesis.
One-Tailed Test
There are two types of one-tailed test:
- Left-Tailed (Left-Sided) Test: The alternative hypothesis asserts that the true parameter value is less than the null hypothesis. Example: H 0 : [Tex]\mu \geq 50 [/Tex] and H 1 : [Tex]\mu < 50 [/Tex]
- Right-Tailed (Right-Sided) Test : The alternative hypothesis asserts that the true parameter value is greater than the null hypothesis. Example: H 0 : [Tex]\mu \leq50 [/Tex] and H 1 : [Tex]\mu > 50 [/Tex]
Two-Tailed Test
A two-tailed test considers both directions, greater than and less than a specified value.We use a two-tailed test when there is no specific directional expectation, and want to detect any significant difference.
Example: H 0 : [Tex]\mu = [/Tex] 50 and H 1 : [Tex]\mu \neq 50 [/Tex]
To delve deeper into differences into both types of test: Refer to link
What are Type 1 and Type 2 errors in Hypothesis Testing?
In hypothesis testing, Type I and Type II errors are two possible errors that researchers can make when drawing conclusions about a population based on a sample of data. These errors are associated with the decisions made regarding the null hypothesis and the alternative hypothesis.
- Type I error: When we reject the null hypothesis, although that hypothesis was true. Type I error is denoted by alpha( [Tex]\alpha [/Tex] ).
- Type II errors : When we accept the null hypothesis, but it is false. Type II errors are denoted by beta( [Tex]\beta [/Tex] ).
Null Hypothesis is True | Null Hypothesis is False | |
---|---|---|
Null Hypothesis is True (Accept) | Correct Decision | Type II Error (False Negative) |
Alternative Hypothesis is True (Reject) | Type I Error (False Positive) | Correct Decision |
How does Hypothesis Testing work?
Step 1: define null and alternative hypothesis.
State the null hypothesis ( [Tex]H_0 [/Tex] ), representing no effect, and the alternative hypothesis ( [Tex]H_1 [/Tex] ), suggesting an effect or difference.
We first identify the problem about which we want to make an assumption keeping in mind that our assumption should be contradictory to one another, assuming Normally distributed data.
Step 2 – Choose significance level
Select a significance level ( [Tex]\alpha [/Tex] ), typically 0.05, to determine the threshold for rejecting the null hypothesis. It provides validity to our hypothesis test, ensuring that we have sufficient data to back up our claims. Usually, we determine our significance level beforehand of the test. The p-value is the criterion used to calculate our significance value.
Step 3 – Collect and Analyze data.
Gather relevant data through observation or experimentation. Analyze the data using appropriate statistical methods to obtain a test statistic.
Step 4-Calculate Test Statistic
The data for the tests are evaluated in this step we look for various scores based on the characteristics of data. The choice of the test statistic depends on the type of hypothesis test being conducted.
There are various hypothesis tests, each appropriate for various goal to calculate our test. This could be a Z-test , Chi-square , T-test , and so on.
- Z-test : If population means and standard deviations are known. Z-statistic is commonly used.
- t-test : If population standard deviations are unknown. and sample size is small than t-test statistic is more appropriate.
- Chi-square test : Chi-square test is used for categorical data or for testing independence in contingency tables
- F-test : F-test is often used in analysis of variance (ANOVA) to compare variances or test the equality of means across multiple groups.
We have a smaller dataset, So, T-test is more appropriate to test our hypothesis.
T-statistic is a measure of the difference between the means of two groups relative to the variability within each group. It is calculated as the difference between the sample means divided by the standard error of the difference. It is also known as the t-value or t-score.
Step 5 – Comparing Test Statistic:
In this stage, we decide where we should accept the null hypothesis or reject the null hypothesis. There are two ways to decide where we should accept or reject the null hypothesis.
Method A: Using Crtical values
Comparing the test statistic and tabulated critical value we have,
- If Test Statistic>Critical Value: Reject the null hypothesis.
- If Test Statistic≤Critical Value: Fail to reject the null hypothesis.
Note: Critical values are predetermined threshold values that are used to make a decision in hypothesis testing. To determine critical values for hypothesis testing, we typically refer to a statistical distribution table , such as the normal distribution or t-distribution tables based on.
Method B: Using P-values
We can also come to an conclusion using the p-value,
- If the p-value is less than or equal to the significance level i.e. ( [Tex]p\leq\alpha [/Tex] ), you reject the null hypothesis. This indicates that the observed results are unlikely to have occurred by chance alone, providing evidence in favor of the alternative hypothesis.
- If the p-value is greater than the significance level i.e. ( [Tex]p\geq \alpha[/Tex] ), you fail to reject the null hypothesis. This suggests that the observed results are consistent with what would be expected under the null hypothesis.
Note : The p-value is the probability of obtaining a test statistic as extreme as, or more extreme than, the one observed in the sample, assuming the null hypothesis is true. To determine p-value for hypothesis testing, we typically refer to a statistical distribution table , such as the normal distribution or t-distribution tables based on.
Step 7- Interpret the Results
At last, we can conclude our experiment using method A or B.
Calculating test statistic
To validate our hypothesis about a population parameter we use statistical functions . We use the z-score, p-value, and level of significance(alpha) to make evidence for our hypothesis for normally distributed data .
1. Z-statistics:
When population means and standard deviations are known.
[Tex]z = \frac{\bar{x} – \mu}{\frac{\sigma}{\sqrt{n}}}[/Tex]
- [Tex]\bar{x} [/Tex] is the sample mean,
- μ represents the population mean,
- σ is the standard deviation
- and n is the size of the sample.
2. T-Statistics
T test is used when n<30,
t-statistic calculation is given by:
[Tex]t=\frac{x̄-μ}{s/\sqrt{n}} [/Tex]
- t = t-score,
- x̄ = sample mean
- μ = population mean,
- s = standard deviation of the sample,
- n = sample size
3. Chi-Square Test
Chi-Square Test for Independence categorical Data (Non-normally distributed) using:
[Tex]\chi^2 = \sum \frac{(O_{ij} – E_{ij})^2}{E_{ij}}[/Tex]
- [Tex]O_{ij}[/Tex] is the observed frequency in cell [Tex]{ij} [/Tex]
- i,j are the rows and columns index respectively.
- [Tex]E_{ij}[/Tex] is the expected frequency in cell [Tex]{ij}[/Tex] , calculated as : [Tex]\frac{{\text{{Row total}} \times \text{{Column total}}}}{{\text{{Total observations}}}}[/Tex]
Real life Examples of Hypothesis Testing
Let’s examine hypothesis testing using two real life situations,
Case A: D oes a New Drug Affect Blood Pressure?
Imagine a pharmaceutical company has developed a new drug that they believe can effectively lower blood pressure in patients with hypertension. Before bringing the drug to market, they need to conduct a study to assess its impact on blood pressure.
- Before Treatment: 120, 122, 118, 130, 125, 128, 115, 121, 123, 119
- After Treatment: 115, 120, 112, 128, 122, 125, 110, 117, 119, 114
Step 1 : Define the Hypothesis
- Null Hypothesis : (H 0 )The new drug has no effect on blood pressure.
- Alternate Hypothesis : (H 1 )The new drug has an effect on blood pressure.
Step 2: Define the Significance level
Let’s consider the Significance level at 0.05, indicating rejection of the null hypothesis.
If the evidence suggests less than a 5% chance of observing the results due to random variation.
Step 3 : Compute the test statistic
Using paired T-test analyze the data to obtain a test statistic and a p-value.
The test statistic (e.g., T-statistic) is calculated based on the differences between blood pressure measurements before and after treatment.
t = m/(s/√n)
- m = mean of the difference i.e X after, X before
- s = standard deviation of the difference (d) i.e d i = X after, i − X before,
- n = sample size,
then, m= -3.9, s= 1.8 and n= 10
we, calculate the , T-statistic = -9 based on the formula for paired t test
Step 4: Find the p-value
The calculated t-statistic is -9 and degrees of freedom df = 9, you can find the p-value using statistical software or a t-distribution table.
thus, p-value = 8.538051223166285e-06
Step 5: Result
- If the p-value is less than or equal to 0.05, the researchers reject the null hypothesis.
- If the p-value is greater than 0.05, they fail to reject the null hypothesis.
Conclusion: Since the p-value (8.538051223166285e-06) is less than the significance level (0.05), the researchers reject the null hypothesis. There is statistically significant evidence that the average blood pressure before and after treatment with the new drug is different.
Python Implementation of Case A
Let’s create hypothesis testing with python, where we are testing whether a new drug affects blood pressure. For this example, we will use a paired T-test. We’ll use the scipy.stats library for the T-test.
Scipy is a mathematical library in Python that is mostly used for mathematical equations and computations.
We will implement our first real life problem via python,
import numpy as np from scipy import stats # Data before_treatment = np . array ([ 120 , 122 , 118 , 130 , 125 , 128 , 115 , 121 , 123 , 119 ]) after_treatment = np . array ([ 115 , 120 , 112 , 128 , 122 , 125 , 110 , 117 , 119 , 114 ]) # Step 1: Null and Alternate Hypotheses # Null Hypothesis: The new drug has no effect on blood pressure. # Alternate Hypothesis: The new drug has an effect on blood pressure. null_hypothesis = "The new drug has no effect on blood pressure." alternate_hypothesis = "The new drug has an effect on blood pressure." # Step 2: Significance Level alpha = 0.05 # Step 3: Paired T-test t_statistic , p_value = stats . ttest_rel ( after_treatment , before_treatment ) # Step 4: Calculate T-statistic manually m = np . mean ( after_treatment - before_treatment ) s = np . std ( after_treatment - before_treatment , ddof = 1 ) # using ddof=1 for sample standard deviation n = len ( before_treatment ) t_statistic_manual = m / ( s / np . sqrt ( n )) # Step 5: Decision if p_value <= alpha : decision = "Reject" else : decision = "Fail to reject" # Conclusion if decision == "Reject" : conclusion = "There is statistically significant evidence that the average blood pressure before and after treatment with the new drug is different." else : conclusion = "There is insufficient evidence to claim a significant difference in average blood pressure before and after treatment with the new drug." # Display results print ( "T-statistic (from scipy):" , t_statistic ) print ( "P-value (from scipy):" , p_value ) print ( "T-statistic (calculated manually):" , t_statistic_manual ) print ( f "Decision: { decision } the null hypothesis at alpha= { alpha } ." ) print ( "Conclusion:" , conclusion )
T-statistic (from scipy): -9.0 P-value (from scipy): 8.538051223166285e-06 T-statistic (calculated manually): -9.0 Decision: Reject the null hypothesis at alpha=0.05. Conclusion: There is statistically significant evidence that the average blood pressure before and after treatment with the new drug is different.
In the above example, given the T-statistic of approximately -9 and an extremely small p-value, the results indicate a strong case to reject the null hypothesis at a significance level of 0.05.
- The results suggest that the new drug, treatment, or intervention has a significant effect on lowering blood pressure.
- The negative T-statistic indicates that the mean blood pressure after treatment is significantly lower than the assumed population mean before treatment.
Case B : Cholesterol level in a population
Data: A sample of 25 individuals is taken, and their cholesterol levels are measured.
Cholesterol Levels (mg/dL): 205, 198, 210, 190, 215, 205, 200, 192, 198, 205, 198, 202, 208, 200, 205, 198, 205, 210, 192, 205, 198, 205, 210, 192, 205.
Populations Mean = 200
Population Standard Deviation (σ): 5 mg/dL(given for this problem)
Step 1: Define the Hypothesis
- Null Hypothesis (H 0 ): The average cholesterol level in a population is 200 mg/dL.
- Alternate Hypothesis (H 1 ): The average cholesterol level in a population is different from 200 mg/dL.
As the direction of deviation is not given , we assume a two-tailed test, and based on a normal distribution table, the critical values for a significance level of 0.05 (two-tailed) can be calculated through the z-table and are approximately -1.96 and 1.96.
The test statistic is calculated by using the z formula Z = [Tex](203.8 – 200) / (5 \div \sqrt{25}) [/Tex] and we get accordingly , Z =2.039999999999992.
Step 4: Result
Since the absolute value of the test statistic (2.04) is greater than the critical value (1.96), we reject the null hypothesis. And conclude that, there is statistically significant evidence that the average cholesterol level in the population is different from 200 mg/dL
Python Implementation of Case B
import scipy.stats as stats import math import numpy as np # Given data sample_data = np . array ( [ 205 , 198 , 210 , 190 , 215 , 205 , 200 , 192 , 198 , 205 , 198 , 202 , 208 , 200 , 205 , 198 , 205 , 210 , 192 , 205 , 198 , 205 , 210 , 192 , 205 ]) population_std_dev = 5 population_mean = 200 sample_size = len ( sample_data ) # Step 1: Define the Hypotheses # Null Hypothesis (H0): The average cholesterol level in a population is 200 mg/dL. # Alternate Hypothesis (H1): The average cholesterol level in a population is different from 200 mg/dL. # Step 2: Define the Significance Level alpha = 0.05 # Two-tailed test # Critical values for a significance level of 0.05 (two-tailed) critical_value_left = stats . norm . ppf ( alpha / 2 ) critical_value_right = - critical_value_left # Step 3: Compute the test statistic sample_mean = sample_data . mean () z_score = ( sample_mean - population_mean ) / \ ( population_std_dev / math . sqrt ( sample_size )) # Step 4: Result # Check if the absolute value of the test statistic is greater than the critical values if abs ( z_score ) > max ( abs ( critical_value_left ), abs ( critical_value_right )): print ( "Reject the null hypothesis." ) print ( "There is statistically significant evidence that the average cholesterol level in the population is different from 200 mg/dL." ) else : print ( "Fail to reject the null hypothesis." ) print ( "There is not enough evidence to conclude that the average cholesterol level in the population is different from 200 mg/dL." )
Reject the null hypothesis. There is statistically significant evidence that the average cholesterol level in the population is different from 200 mg/dL.
Limitations of Hypothesis Testing
- Although a useful technique, hypothesis testing does not offer a comprehensive grasp of the topic being studied. Without fully reflecting the intricacy or whole context of the phenomena, it concentrates on certain hypotheses and statistical significance.
- The accuracy of hypothesis testing results is contingent on the quality of available data and the appropriateness of statistical methods used. Inaccurate data or poorly formulated hypotheses can lead to incorrect conclusions.
- Relying solely on hypothesis testing may cause analysts to overlook significant patterns or relationships in the data that are not captured by the specific hypotheses being tested. This limitation underscores the importance of complimenting hypothesis testing with other analytical approaches.
Hypothesis testing stands as a cornerstone in statistical analysis, enabling data scientists to navigate uncertainties and draw credible inferences from sample data. By systematically defining null and alternative hypotheses, choosing significance levels, and leveraging statistical tests, researchers can assess the validity of their assumptions. The article also elucidates the critical distinction between Type I and Type II errors, providing a comprehensive understanding of the nuanced decision-making process inherent in hypothesis testing. The real-life example of testing a new drug’s effect on blood pressure using a paired T-test showcases the practical application of these principles, underscoring the importance of statistical rigor in data-driven decision-making.
Frequently Asked Questions (FAQs)
1. what are the 3 types of hypothesis test.
There are three types of hypothesis tests: right-tailed, left-tailed, and two-tailed. Right-tailed tests assess if a parameter is greater, left-tailed if lesser. Two-tailed tests check for non-directional differences, greater or lesser.
2.What are the 4 components of hypothesis testing?
Null Hypothesis ( [Tex]H_o [/Tex] ): No effect or difference exists. Alternative Hypothesis ( [Tex]H_1 [/Tex] ): An effect or difference exists. Significance Level ( [Tex]\alpha [/Tex] ): Risk of rejecting null hypothesis when it’s true (Type I error). Test Statistic: Numerical value representing observed evidence against null hypothesis.
3.What is hypothesis testing in ML?
Statistical method to evaluate the performance and validity of machine learning models. Tests specific hypotheses about model behavior, like whether features influence predictions or if a model generalizes well to unseen data.
4.What is the difference between Pytest and hypothesis in Python?
Pytest purposes general testing framework for Python code while Hypothesis is a Property-based testing framework for Python, focusing on generating test cases based on specified properties of the code.
Please Login to comment...
Similar reads.
- data-science
Improve your Coding Skills with Practice
What kind of Experience do you want to share?

Outbreak Toolkit
Hypothesis generation, on this page, questionnaires and interviewing, exposure analysis.
Generating hypotheses is an important, but often challenging, step in an outbreak investigation . When generating hypotheses, it is best to keep an open mind and to cast a wide net. A good starting place would be to identify exposures that have been previously been associated with the pathogen under investigation. This can be done by:
- searching an outbreak database such as Outbreak Summaries , the Marler-Clark database, and the CDC Foodborne Outbreak Online database (see Tools for links to these databases)
- reviewing the published literature using a search engine such as PubMed or Google Scholar.
If the case definition for the illnesses under investigation includes laboratory information in the form of Whole Genome Sequencing (WGS) results, consider investigating where and when the sequence has been seen before. Provincial and federal public health laboratories maintain WGS databases that can contain valuable information for outbreak investigation purposes. PulseNet Canada can provide information about how common or rare the serotype or sequence is nationally, where and when it was last seen, and if it has been detected in any food samples in the past. PulseNet Canada will also be able to check the United States’ PulseNet WGS databases for matches. FoodNet Canada can provide information about whether the sequence has previously been seen in farm or retail samples from its sentinel sites.
While it is important to gather such historical information, the most effective way to generate a high-quality hypothesis is to identify common exposures amongst cases. This can be achieved by interviewing cases using a hypothesis generating questionnaire and analysing exposures.
Back to top
Hypothesis generating questionnaires
Hypothesis generating questionnaires (or shotgun questionnaires) are intended to obtain detailed information on what a person’s exposures were in the days leading up to their illness. They are typically quite long and ask about many exposures such as travel history, contact with animals, restaurants, events attended, and a comprehensive food history. The time period of interest varies between pathogens, as the exposure period is equal to the maximum incubation period of the pathogen.
When designing a questionnaire, it is important to ensure that the questions are gathering the intended information. Questions should be concise, informal, and specific. Before interviewing cases, questionnaires should be tested to ensure clarity and identify any potential errors.
Read more – Questionnaire Design
Case interviewing
Once the questionnaire is developed and piloted, it should be administered to cases in a consistent and unbiased manner. Case interviews can be conducted by one or multiple interviewers. A centralized approach allows a single interviewer to standardize interviews, detect patterns, and probe for items of interest. However, a multiple- interviewer approach is more time-efficient and allows for multiple perspectives when it comes time to identify the source.
Although case interviewing is an important outbreak investigation tool, it is not without its challenges. By the time the outbreak team is ready to conduct the interview, it could be weeks to months after the onset of symptoms. It is difficult for people to recall what they ate over a month ago. Sometimes cases might need to be interviewed multiple times as the hypothesis is developed and refined.
Read more- Case interviews
Once the interviews are complete, the data can be entered into a database or line list . The frequency of exposures for the cases is then obtained (e.g., % of cases that consumed each food item).
It is tempting to conclude that the most commonly consumed food items are the most likely suspects, but it is possible that these foods are commonly consumed amongst the general population as well. What is needed is a baseline proportion to compare the exposure frequencies to. Reference population studies, such as the CDC Food Atlas, the Nesbitt Waterloo study and Foodbook (see Tools ), can be used for this purpose. These studies provide investigators with the expected food frequencies based on 7-day food histories from thousands of respondents. These data can be used as a point of comparison for questionnaire data to identify exposures such as food items with higher than expected frequencies. Statistical tests (e.g., binomial probability tests) can then be used to test whether the differences between the proportion of cases exposed is significantly different from the proportion of “controls” (i.e., people included in the population studies) (see Tools ).
There are many limitations to using expected food frequencies, such as some studies not accounting for:
- Seasonality (e.g., consumption of cherries is higher in the summer, however the expected levels are the same year-round)
- Differences in consumption between sexes, adults and children
- Geographic location
- Various ethnic/religious/cultural groups
Further, since specific questions differ among surveys, it is often difficult to find the most appropriate comparison group. For example, the CDC Atlas of Exposures differentiates between hamburgers eaten at home or outside the home, while questionnaires used in investigations typically do not. Such differences in food definitions can make it challenging to determine which reference variable is the most appropriate to use as an “expected” level.
It is important to keep in mind that some foods with high expected consumption levels (e.g., chicken) may not flag statistically, but could still be potential sources. Further, there are other common exposures amongst cases that can carry important clues about the source of the outbreak. Cases that report common restaurants, events, or grocery stores can be considered sub-clusters. These sub-clusters should be investigated thoroughly by obtaining menus, receipts, or shopper card information if possible.
- Case study, Module 2: Hypothesis generation
- Case study, Module 2 – Exercise 3: Food frequency analysis in Excel
- Case study, Module 2: Interpreting hypothesis generation results
- Hypothesis generation through case exposures in multiple restaurant clusters example: Barton Behravesh C, et al . 2012. Multistate outbreak of Salmonella serotype Typhimurium infections associated with consumption of restaurant tomatoes, USA, 2006: hypothesis generation through case exposures in multiple restaurant clusters. Epidemiol Infect . 140 (11): 2053-2061 .
- Case-case study example: Galanis, E., et al . 2014. The association between campylobacteriosis, agriculture and drinking water: a case-case study in a region of British Columbia, Canada, 2005–2009. Epidemiol Infect . 142 (10): 2075-2084 .
- Exact Probability Calculation and Case-Case Study example: Gaulin, C., et al. 2012. Escherichia coli O157:H7 Outbreak Linked to Raw Milk Cheese in Quebec, Canada: Use of Exact Probability Calculation and Case-Case Study Approaches to Foodborne Outbreak Investigation. J Food Prot . 5: 812-818 .
Toolkit binomial probability calculation tool for food exposures
- This Microsoft Excel document allows users to enter outbreak case food exposure numbers for 300 food items and automatically calculates binomial probabilities using two reference populations and flags exposures of interest for follow-up (Reference populations: CDC Population Survey Atlas of Exposures, 2006-2007 and Waterloo Region, Ontario Food Consumption Survey, November 2005 to March 2006).
Toolkit Outbreak Summaries overview
- This PDF document provides an overview of the Outbreak Summaries application, its key features and benefits, and an example of how it can be used during an outbreak investigation.
CDC National Outbreak Reporting System (NORS) Dashboard
- The NORS dashboard allows users to view and download data on disease outbreaks reported to CDC.Data can be filtered by type of outbreak, year, state, etiology (genus only), setting, food/ingredient, water exposure, and water type.
Food Consumption Patterns in the Waterloo Region
- This food frequency study by Nesbitt et. al. was conducted in Waterloo, Ontario in 2005-2006. The study collected 7-day food consumption data from 2,332 Canadians.
CDC Food Atlas 2006-2007
- This study by CDC was conducted in 10 U.S. states in 2006-2007. The study asked 17,000 respondents about their exposure to a comprehensive list of foods as well as animal exposure.
FoodNet Canada Reports and Publications
- FoodNet Canada reports and publications provide information on the areas of greatest risk to human health to help direct food safety actions and programming as well as public health interventions, and to evaluate their effectiveness.
CDC FoodNet Reports
- The Foodborne Diseases Active Surveillance Network (FoodNet) Annual Reports are summaries of information collected through active surveillance of nine pathogens.
Marler Clark Foodborne Illness Outbreak Database
- This database provides summaries of food and water related outbreaks caused by various enteric pathogens dating back to 1984.
FDA Foodborne Illness-Causing Organisms Cheat Sheet
- A quick summary chart on foodborne illnesses, organisms involved, symptom onset times, signs and symptoms to expect, and food sources.
CFIA: Canada’s 10 Least Wanted Foodborne Pathogens
- This infographic prepared by the CFIA includes information on symptoms, onset time, transmission, potential sources, and preventative measures for ten foodborne pathogens.
Foodbook: Canadian Food Exposure Study to Strengthen Outbreak Response
- Foodbook is a population-based telephone survey that was conducted in all Canadian provinces and territories. It provides essential data on food, animal and water exposure which is used by the Agency, as well as other federal, provincial, and territorial (F/P/T) partners to understand, respond to, control and prevent enteric illness in Canada.
Toolkit outbreak response database*
*Due to the Government of Canada’s Standard on Web Accessibility, this tool cannot be posted, but it is available upon request. Please contact us at [email protected] to request a copy. Please let us know if you need support or an accessible format.

IMAGES
COMMENTS
Hypothesis generation is an early and critical step in any hypothesis-driven clinical research project. Because it is not yet a well-understood cognitive process, the need to improve the process goes unrecognized. Without an impactful hypothesis, the significance of any research project can be questionable, regardless of the rigor or diligence applied in other steps of the study, e.g., study ...
Thus, hypothesis generation is an important initial step in the research workflow, reflecting accumulating evidence and experts' stance. In this article, we overview the genesis and importance of scientific hypotheses and their relevance in the era of the coronavirus disease 2019 (COVID-19) pandemic.
From there, data necessary for hypothesis generation and verification are selected. Furthermore, data generally provide a basis for hypotheses and theories. In other words, data support hypotheses as evidences. Therefore, getting to know obtained data in depth is one of the essential steps leading to better hypothesis generation.
The first few steps of selecting a scientific problem and developing a hypothesis are similar, with the additional step of rigorously defining a phenotype and then carefully selecting research participants with and without that trait. As in the basic science paradigm, the hypothesis is tested by the application of a specific assay to the cases ...
Hypothesis generation is a crucial step in any data science project. If you skip this or skim through this, the likelihood of the project failing increases exponentially. Hypothesis Generation vs. Hypothesis Testing. This is a very common mistake data science beginners make.
Developing a hypothesis (with example) Step 1. Ask a question. Writing a hypothesis begins with a research question that you want to answer. The question should be focused, specific, and researchable within the constraints of your project. Example: Research question.
A hypothesis is an educated guess or statement about the relationship between 2 or more variables 2,3. Scientific hypothesis generation is a critical step in scientific research that determines the direction and impact of research investigations. However, despite its vital role, we do not know the answers to some basic questions about the process.
Hypothesis generation is predicated on informed intuition. It is imaginative and to a great extent subconscious. Frequently, armed with the mere knowledge of age, sex, and chief complaint, the clinician can entertain general and specific hypotheses that implicate common, reversible, and even exotic disease states.
Academic investigators and practitioners working on the further development and application of hypothesis generation and interpretation in big data computing, with backgrounds in data science and engineering, or the study of problem solving and scientific methods or who employ those ideas in fields like machine learning will find this book of ...
Background Scientific hypothesis generation is a critical step in scientific research that determines the direction and impact of any investigation. Despite its vital role, we have limited knowledge of the process itself, hindering our ability to address some critical questions. Objective To what extent can secondary data analytic tools facilitate scientific hypothesis generation during ...
Explore the intricacies of hypothesis testing, a cornerstone of statistical analysis. Dive into methods, interpretations, and applications for making data-driven decisions. In this Blog post we will learn: What is Hypothesis Testing? Steps in Hypothesis Testing 2.1. Set up Hypotheses: Null and Alternative 2.2. Choose a Significance Level (α) 2.3.
Step 3: Hypothesis generation using link prediction. In the quest to uncover novel causal relationships beyond direct extraction from texts, the technique of link prediction emerges as a pivotal ...
itute the two major drivers. These are the steps for Qu. drant Hypothesis Generation: Identify the two main drivers by using techniques such as Structured Brainstorming or by surv. ying subject-matter experts. A discussion to identify the two main drivers can be. a useful exercise in itself. Construct a 2 × 2.
Step 5: Present your findings. The results of hypothesis testing will be presented in the results and discussion sections of your research paper, dissertation or thesis.. In the results section you should give a brief summary of the data and a summary of the results of your statistical test (for example, the estimated difference between group means and associated p-value).
Hypothesis generation is an early and critical step in any hypothesis-driven clinical research project. Because it is not yet a well-understood cognitive process, the need to improve the process ...
The steps of the scientific method are to: Make observations. Formulate a hypothesis. Design an experiment to test the hypothesis. State the indicators to evaluate if the experiment has succeeded. Conduct the experiment. Evaluate the results of the experiment. Accept or reject the hypothesis.
The generated hypothesis are a subset of a tremendously large space of possibilities. Our goal is to understand how humans generate that subset. In general, probabilistic inference is comprised of two steps: hypothesis generation and hypothesis evaluation, with feedback between these two processes.
What is Hypothesis Generation? Hypothesis generation involves making informed guesses about various aspects of a business, market, or problem that need further exploration and testing. It's a crucial step while applying the scientific method to business analysis and decision-making. Here is an example from a popular B-school marketing case study:
Overview of hypothesis generation. When an outbreak has been identi-fied, demographic, clinical and/or laboratory data are usually ob-tained from the health department, clinicians, or laboratories, and these data are organized in a line listing (see FOCUS Issue 4 for more information about line listings). The next step in the investigation in ...
2.1 Hypothesis generation. The development of effective methods for machine-assisted discovery is crucial in pushing scientific research into the next stage (Kitano 2021).In recent years, several approaches have been proposed in a bid to augment human abilities relevant to the scientific research process including tools for research design and analysis (Tabachnick and Fidell 2000), process ...
Hypothesis Generation (HG) is a task that aims to uncover hidden associations between disjoint scientific terms, which influences innovations in prevention, treatment, and overall public health. ... to establish temporal dependencies of node-pair (term-pair) embeddings between any two time-steps for smoothing spatiotemporal node-pair embeddings ...
Hypothesis generation is the formation of guesses as to what the segment of code does; this step can also guide a re- segmentation of the code. Finally, verification is the process of examining the code and associated documentation to determine the consistency of the code with the current hypotheses.
Step 1: Define the Hypothesis. Null Hypothesis (H 0): The average cholesterol level in a population is 200 mg/dL. ... Analysis required in Natural Language Generation (NLG) and Understanding (NLU) Language is the method to share and communicate our understanding and knowledge with one another. Language plays an essential factor when it comes to ...
Generating hypotheses is an important, but often challenging, step in an outbreak investigation. When generating hypotheses, it is best to keep an open mind and to cast a wide net. A good starting place would be to identify exposures that have been previously been associated with the pathogen under investigation. ... 2006: hypothesis generation ...
Retrieval augmented generation (RAG) is a type of information retrieval process. It modifies interactions with a large language model (LLM) so that it responds to queries with reference to a specified set of documents, using it in preference to information drawn from its own vast, static training data.This allows LLMs to use domain-specific and/or updated information. [1]