- Open access
- Published: 27 July 2018

A systematic literature review of simulation models for non-technical skill training in healthcare logistics
- Chen Zhang ORCID: orcid.org/0000-0003-4057-4124 1 ,
- Thomas Grandits 2 ,
- Karin Pukk Härenstam 3 , 4 ,
- Jannicke Baalsrud Hauge 5 &
- Sebastiaan Meijer 2
Advances in Simulation volume 3 , Article number: 15 ( 2018 ) Cite this article
15k Accesses
35 Citations
2 Altmetric
Metrics details
A Correction to this article was published on 24 April 2019
This article has been updated
Resource allocation in patient care relies heavily on individual judgements of healthcare professionals. Such professionals perform coordinating functions by managing the timing and execution of a multitude of care processes for multiple patients. Based on advances in simulation, new technologies that could be used for establishing realistic representations have been developed. These simulations can be used to facilitate understanding of various situations, coordination training and education in logistics, decision-making processes, and design aspects of the healthcare system. However, no study in the literature has synthesized the types of simulations models available for non-technical skills training and coordination of care.
A systematic literature review, following the PRISMA guidelines, was performed to identify simulation models that could be used for training individuals in operative logistical coordination that occurs on a daily basis. This article reviewed papers of simulation in healthcare logistics presented in the Web of Science Core Collections, ACM digital library, and JSTOR databases. We conducted a screening process to gather relevant papers as the knowledge foundation of our literature study. The screening process involved a query-based identification of papers and an assessment of relevance and quality.
Two hundred ninety-four papers met the inclusion criteria. The review showed that different types of simulation models can be used for constructing scenarios for addressing different types of problems, primarily for training and education sessions. The papers identified were classified according to their utilized paradigm and focus areas. (1) Discrete-event simulation in single-category and single-unit scenarios formed the most dominant approach to developing healthcare simulations and dominated all other categories by a large margin. (2) As we approached a systems perspective (cross-departmental and cross-institutional), discrete-event simulation became less popular and is complemented by system dynamics or hybrid modeling. (3) Agent-based simulations and participatory simulations have increased in absolute terms, but the share of these modeling techniques among all simulations in this field remains low.
Conclusions
An extensive study analyzing the literature on simulation in healthcare logistics indicates a growth in the number of examples demonstrating how simulation can be used in healthcare settings. Results show that the majority of studies create situations in which non-technical skills of managers, coordinators, and decision makers can be trained. However, more system-level and complex system-based approaches are limited and use methods other than discrete-event simulation.
Quality and safety in healthcare depend on the successful interaction between multiple teams, individuals, and support processes aimed at making the right resources, such as medications, medical equipment, information, and people, available at the right time [ 1 , 2 ]. Furthermore, in many healthcare settings, resource utilization must be prioritized such that the person most in need of a resource from a medical perspective will receive it. The cost of failure is high, both in terms of personal tragedies as well as the socio-economic burden of increased costs due to prolonged treatments or hospital stay [ 3 ].
Many of the everyday decisions regarding how resources will be used for patient care are made by individuals and networks of people performing coordinating functions, in the sense that they manage the timing and execution of many care processes of multiple patients. Their decisions often depend on judgements combining perspectives on the relevant medical conditions, the resources at hand, and the urgency of the situation; their decisions also depend on receiving information to help make sense of the situation as well as managing high stakes and competing goals [ 4 ].
Little is known about how these prioritizing and coordination skills are learned, how people performing them build their mental system models, what information and strategies they use, and which work practices are most successful. Most of the individuals performing coordination tasks are trained on the job in an unsystematic manner, and the knowledge remains, for the most part, tacit.
Simulation in healthcare is well known as a method for training individuals and teams in escalating situations surrounding individual patients [ 5 ]. To create meaningful simulations for training the non-technical skills used in coordination [ 6 ], there is a need to develop simulations of logistical challenges in a systematic manner as well as to describe and develop learning outcomes for the non-technical skills used in coordination. To support this development, it is important to know what types of logistical problems can be addressed by what types of simulations.
Logistics is one of many growing fields in healthcare management. This trend is driven by various societal impacts; population growth and an aging society have already put pressure on the operation of healthcare systems [ 7 , 8 ]. While healthcare logistics has been defined in various ways by researchers, in this paper, we define it as “operational handlings for the delivery of care, including its supportive services, from origination to recipient.” Focusing on the recipient of services, healthcare logistics could be patient-centric or material-centric. Patient-centric logistics relate to patient flows through the healthcare system. In this context, quality, safety, and efficiency of services for patients are keywords. Material-centric logistics address the positioning, storage, and circulation of goods and materials, such as blood and pharmaceutical products, within the hospital or the healthcare system.
Computer-based simulation plays an important role in the operational support of healthcare logistics. Generally, simulation can be useful in the design of complex social-technical systems [ 9 ]. As an innovative technology for adding analytical capacity, simulation can be used as an intermediate test in the (re)design of organizational rules and structures, workflow process management, performance, and avoidance of human errors [ 10 , 11 ]. More specifically, according to Jun et al. [ 12 ], simulation could provide benefits such as more effective redesign or innovation, deeper insights into barriers and incentives to adoption, and provision of an environment to “bench-test” final products prior to formal release. A change or improvement in real systems, however, might be expensive or dangerous, and balancing resource allocations is a central non-technical skill for healthcare professionals. Simulation adds value by providing a solution for training individuals to solve customized problems in a virtual, persuasive environment.
The application of discrete-event simulation in healthcare began to grow considerably at the end of the 1990s [ 12 ]; however, it remains unknown what type of simulations could be used to train, develop, and test non-technical aspects of coordination. Many types of simulation paradigms exist today. Discrete-event simulation, system dynamics, and agent-based simulation are the most utilized tools for modeling and analyzing systems according to the user’s interests and the specific task addressed. Discrete-event simulation is a tool for assessing the efficiency of delivery structures, forecasting changes in patient flow and examining resource efficiency in staffing [ 12 ]. System dynamics focus on the effect of structure on behavior [ 13 ]. Instead of addressing individual transactions, system dynamics is commonly used for higher level problems, such as strategic decision making, management controls, or policy changes [ 14 ]. Agent-based simulation is based on a “bottom-up” construction for the provision of emergent phenomena based on individual interactions of resource units [ 15 ].
Literature reviews have been conducted with explicit focus on the application of simulation in patient flow or material flow. However, previous literature reviews have been limited in at least one of the following aspects: (1) Reviews usually address simulation of healthcare logistics in a very narrow manner, analyzing a single key aspect such as low stakeholder engagement [ 16 ], a single simulation technique [ 17 ], or a single department; (2) most reviews have examined papers published before 2012.
This study is a continuation of the work by Dieckmann et al. [ 6 ], with a focus on the identification of available simulation models to provide meaningful training of non-technical skills in healthcare logistics. This is the perspective through which the literature was reviewed and understood. Given the large number of training simulations published, it is of interest to explore the diversity in this genre. The objective of this study is to provide a systematic literature review to answer the following research question:
What types of simulation models are currently available for training non-technical skills in handling logistical issues?
Search strategy
To answer the research question, the Web of Science Core Collection, the ACM Digital Library, and JSTOR were searched to retrieve articles focusing on simulation in healthcare logistics between 1998 and 2017. We utilized papers from these three databases because all of them rigorously select core journals and the keynote proceedings of conferences. The search terms were divided into the following two categories: patient-centric queries and material-centric queries. The papers were screened following the Preferred Reporting Items for Systematic Reviews and Meta-Analysis (PRISMA) guidelines.
The keywords were formulated by the individual reviewers to identify papers on relevant simulation techniques and investigated systems, as summarized in Table 1 . Keywords such as “healthcare,” “patient flow,” “pharma*,” “blood,” and “drug” specify the issues addressed. Keywords such as “simulation,” “system dynamics,” “simulator,” and “game” specify the research methods implemented.
Paper inclusion criteria
The criteria for inclusion in this review were that studies addressed the research question and strive to improve the performance of healthcare logistics. As the focus was logistical issues in healthcare management, publications regarding epidemiology, nutrition process improvement, and statistical analysis of health programs were not included. Abstracts, book reviews of limited length, and papers not granting access to full texts were also discarded. In addition to these general requirements, criteria for classification were implemented:
Application-oriented paper. The paper employs at least one simulation technique and presents a detailed scenario, or experiment, of a real-world healthcare system.
Subjective and methodological paper. The paper focuses on subjective and methodological perspectives on simulation techniques but might not report a use case (Fig. 1 ).
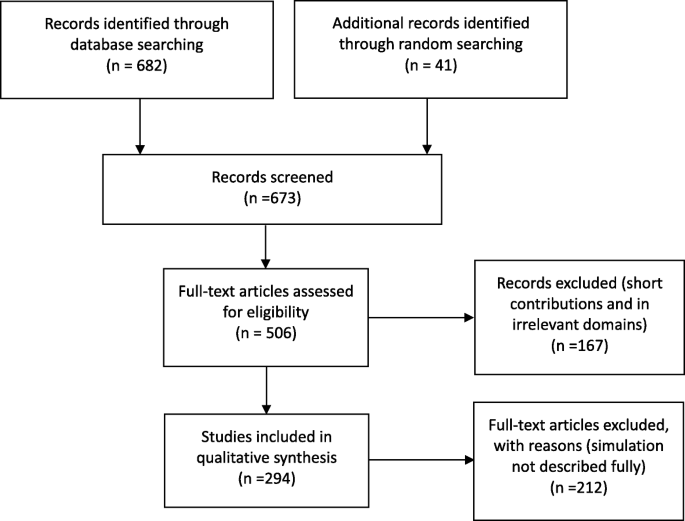
PRISMA flow diagram of assessment procedure and results: number of records included and excluded and reasons
The scope of the research and focus area were decided after the screening process. Simulation paradigms were classified based on statements from the authors; if no simulation technique was stated, the conceptualization framework was checked to determine its relevant category.
Following the retrieval of papers, discarding of duplicates, and review by the authors, the total number of essential publications was 294. The search identified 248 patient-centric and 46 material-centric papers. The patient-centric spectrum included 214 problem-solving papers, among which 114 utilized discrete-event simulation. For material-centric papers, discrete-event simulation was the dominant simulation paradigm as well. The number of publications for the past 5 years remained high, reinforcing our supposition that there is much knowledge to be gained from recent publications. The repository is available in the declaration.
For qualitative analysis, representative papers, listed in Table 2 , were identified. The papers featured statements of the relevant research questions or a description of the investigated system. We considered the number of publications utilizing different simulation techniques, scopes of research, and tools.
Following the screening process, we identified the question levels and the categories of addressed issues. The following question levels were derived: single department/unit, cross-department/unit, and cross-institutional. The category single department/unit included studies that model operation within a single department in an organization. The category cross-department/unit included studies that simulate multiple departments/units within the same organization. The category cross-institutional included the simulation modeling of interactions and flows between healthcare service providers in a large-scale network with widespread distribution regions. We identified the following categories of addressed issues: care pathway and appointment, staffing decision making, work procedures, specialized transport, facility design, healthcare systems, supply chain, inventory management, network distribution and dispatching, network configuration, procurement logistics, methodological contributions, and miscellaneous. The facility design was considered because architectural planning is a strategic decision that has a durable and profound effect on healthcare operation. The miscellaneous category included all research publications that we were not able to clearly classify into at least one of the abovementioned categories.
Logistical simulations—review
Discrete-event simulation
Discrete-event simulation has been applied to model and analyze all aspects of logistics management in healthcare. In particular, patient flow management and planning of staffing requirement are effective applications of this simulation technology. Our profiling of the literature is mostly in line with the findings of previous literature reviews; that is, discrete-event simulation is a useful tool with respect to improving patient flow, managing bed capacity, and scheduling and utilizing of resources [ 16 , 17 ]. DeRienzo et al. addressed the effect of nursing capacity by comparing different nursing sizes and demonstrated the applicability of supporting healthcare managers in handling operative tasks [ 18 ]. Devapriya et al. also developed a decision-supporting tool based on discrete-event simulation for the strategic planning of hospital bed capacity [ 19 ]. Bhattacharjee et al. analyzed appointment scheduling policies for patients to be treated by a medical scanning machine [ 20 ]. Vasilakis et al. developed a discrete-event simulation to study how long it took for patients to obtain their appointments from their referral [ 21 ]. Jørgensen et al. investigated internal blood logistics in hospitals and evaluated the effects of various management controls on the waiting times for accessing blood samples [ 22 ]. This simulation paradigm is most suitable for the realistic representation of processes in health services for analyzing “what-if” scenarios and assessing the performance of a logistical system.
System dynamics
System dynamics is used for organizational simulations. The paradigm is a mechanism-driven one for making decisions strategically for health services and resources from a global perspective. For instance, Rashwan et al. developed a system dynamics simulation to study bed blocking in Irish hospitals [ 23 ]. The focus was twofold: testing the policies for solving delayed discharges and envisaging the counterproductive and unintended consequences of these new policies [ 24 ]. Brailsford et al. simulated patient flow perspectives to identify system-wide bottlenecks [ 25 ]. Through the simulation, Lane et al. showed that the daily variation of used hospital bed capacity could not be balanced in the long run by simply increasing capacity; instead, optimal design of flows should be the core of the operation technology [ 26 ]. One paper investigated logistical outsourcing [ 27 ] and deployed system dynamics simulation with a sensitivity analysis for the evaluation and analysis of sustainability and economic performance. Content holders can use system dynamics simulation to envisage the complexity and identify opportunities and risks of the policies and management controls proposed.
Agent-based simulation
Agent-based simulation could be considered a means of soft computing in healthcare logistics. Agent-based simulation provides a gateway for understanding the behavior of distributed and connected service providers. The associated modeling and analysis are able to handle engineering system problems in complex networks. As an example, this paradigm was introduced to solve the coordination and collaboration difficulty of caregivers in a mental healthcare system [ 28 ]. The positive effect of coordination technology was confirmed by such modeling considerations. The local decision rules of caregivers were relevant for operative decision making with respect to successful provision of home help, a conclusion drawn from Marcon et al.’s work [ 29 ]. Bidding decisions made by distributers and suppliers in the pharmaceutical industry were studied in Jetly et al.’s work [ 30 ]. The performance of a multi-site network was simulated with pre-selected indicators, including the number of released products, degree of consolidation, and the return on assets. Multi-agent systems are not only effective for modeling flows between providers; they could also be applied in hospital environments. In Marin et al.’s work, patients, nurses, doctors, and the department as the manager are specified as agents with simple behavior rules [ 31 ]. With the support of multi-agent languages, the properties and relationships of actors could be simulated and validated for a specific social-technical environment.
Game and participatory simulation
Games and participatory simulation are life-like media that facilitate experimental learning. The use of such media enables the development of non-technical teamwork skills. For instance, Mustafee and Katsaliaki developed a pedagogical business game that simulated the blood supply chain [ 32 , 33 ]. The players were encouraged to propose different solutions, taking costs, time-efficiency, and stock levels of products into account. Focusing on quality of service in healthcare, a web-based organizational simulation was built and deployed for training referral and diagnostic skills [ 34 ]. The results showed that the usefulness of information on symptoms, diseases, and severity levels is associated with the perception of information sources. A board game provided valuable insight into the adoption of future technology in hospital logistical work [ 15 ]. Regarding the practical settings of two hospitals, the adoption of wearable technologies was reflected through role play. Such role playing could also be used to analyze the working environment in wards [ 35 ].
Hybrid modeling
Hybrid modeling is the efficient combination of various simulation modeling techniques described. Most hybrid modeling involves coupling discrete-event simulation and system dynamics. Two case studies, concerning infection control and regional social care system engineering, were simulated using hybrid models [ 36 ]. Zulkepli modeled an integrated ICU by combining system dynamics and discrete-event simulation [ 37 ]. The greatest advantage of hybrid modeling is the ability to integrate different simulation approaches and empirical data from different sources [ 38 ].
Analysis of trends
Discrete-event simulation has been the most prominent paradigm for modeling patient-centric logistics over the past decade. Between 2008 and 2017, as presented in Fig. 2 , more than half of the included papers used discrete-event simulation. However, the distribution of simulation techniques among different periods showed growing methodological diversity in recent years. The presence of system dynamics is observed in all periods, although the number of publications remained small. Agent-based simulation, games, and hybrid modeling were utilized only in the last decade. The specific simulation paradigm used was not stated in some studies, especially between 1998 and 2007, during which the majority of the methods used were classified as miscellaneous. Game-based methods were used in a few studies. Thus, interactive simulations are still quite new and rarely used.
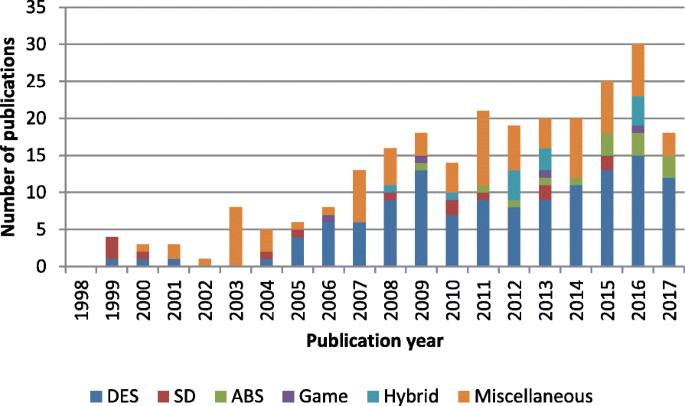
Simulation paradigms for patient-centric logistics
The single category was the predominant level addressed in all periods, as shown in Fig. 3 . Between 2008 and 2012, the majority of studies addressed logistical issues at the single-unit level. The systems perspective was introduced between 2013 and 2017. Work addressing logistics issues at the cross-departmental and cross-institutional levels formed half of all research efforts. However, cross-institutional issues remained largely underexplored in the literature compared with other problems studied.
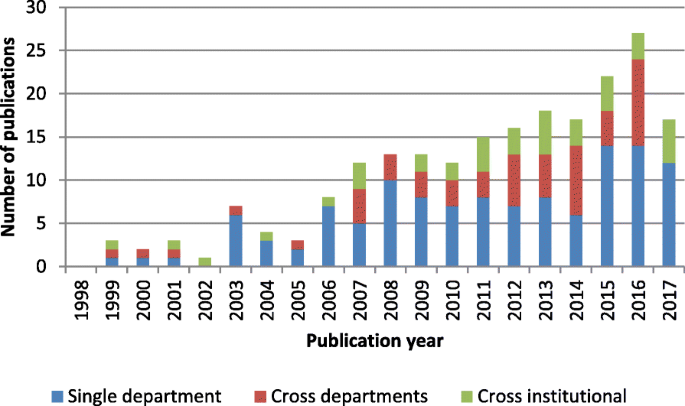
Research scope for patient-centric logistics
Discrete-event simulation was the most prominent simulation paradigm in material-centric approaches as well, as shown in Fig. 4 . The research theme started to develop in 2006, after which the number of publications and the diversity of the utilized paradigms increased. Despite the growth, six of 16 papers utilized discrete-event simulation, and only three papers utilized system dynamics, agent-based simulation, and/or hybrid modeling.
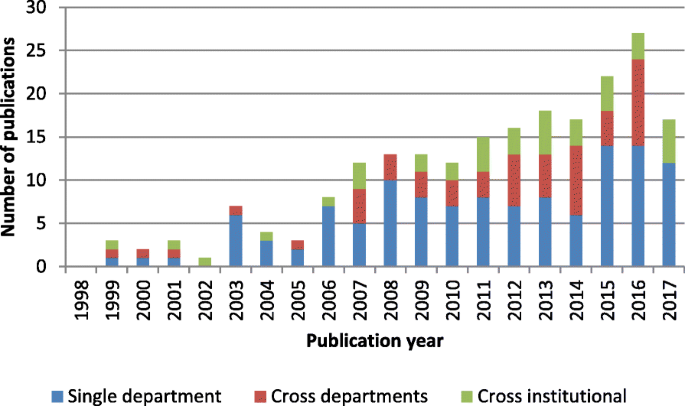
Simulation paradigms for material-centric logistics
Compared with patient-centric logistics, material-centric logistics was covered by a limited number of articles. Shah et al. had already stressed the underexplored potential of this area in 2004 [ 39 ]. We identified few publications on this subject during this period. The period 2013–2017 showed the largest output, but the volume was still not able to catch up with that of papers related to patient-centric logistics.
As shown in Fig. 5 , a growing number of papers have analyzed material handling between multiple units. However, simulation design for cross- and single-department logistics was lacking over the last 5 years, despite studies reporting on the need for improving hospital internal supply chains to reduce costs [ 40 ].
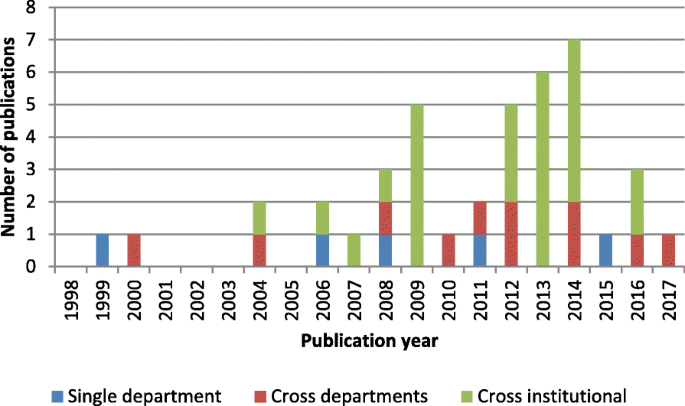
Research scope for material-centric logistics
Discussions
The literature review demonstrated that different simulation techniques could be utilized for different educational purposes, as summarized in Table 3 . Discrete-event simulation is suitable for operational problems, whereas strategic issues are better explored by system dynamics. Agent-based simulation stands out as a versatile tool because that agent method is object-oriented and flexible for describing the anatomy of complex systems formed by multiple actors. Healthcare logistics is a complex socio-technical system characterized by interconnected components and non-rational operation management. Agent-based simulation can explicitly model the interaction between system components, facilitating the understanding of overall performance under uncertainty and dynamics.
Games and participatory simulation are particularly useful for training at the tactical level because games help identify productive or counterproductive human actions. The strength of the agent-based method is the modeling and analysis of human behavior [ 33 , 41 ]. Healthcare logistics are largely characterized by non-rational operative decision making by medical personnel regarding needs of their patients. By modeling decisions at the agent level, it is possible to obtain insight into the reasoning process of decisions being made [ 42 ]. By involving these operational experts in participatory simulations, we can assess their perception of processes and healthcare system operations [ 43 ]. This effort delivers insight at another level of abstraction than technical, often discrete-event-based, simulations can provide.
Regarding training purposes, agent-based simulation and games are suitable for training negotiation and coordination in logistics, whereas discrete-event simulation and system dynamics can be utilized for reducing the uncertainty of decision-making processes by adding details to the model.
Participatory simulation is valuable for validating various simulators that model complex systems. The advantage of participatory simulation corresponds to the delivery structure of the investigated system, as healthcare logistics is carried out by collaborative efforts in which different professionals, knowledge, and skills work together.
Discrete-event simulation has the lowest requirement of technological preparation and is found to support all areas [ 26 , 44 , 45 , 46 ]. System dynamics and agent-based simulation might require formal methods and mathematics pertaining to system design, such as differential equations [ 47 ], decision theories [ 48 ], and game-based approaches [ 33 ].
Complex socio-technical systems, such as air traffic controls [ 49 ], routinely apply flow and logistical simulations. The studies examined in this review indicate a growing practice of implementing simulation in healthcare settings to create situations in which the non-technical skills of managers, coordinators, and decision makers can be trained and developed. Building on existing concepts from other industries [ 50 ], future applications might be hands-on training of teams using gaming and participatory simulation alongside empirical data to create situations for training tricky decision making, for strategic planning, and for exploring the effect of decisions on other parts of the system [ 51 ].
Our review yielded many examples of applications in healthcare, indicating that the issues of training of strategic or operational coordination and decision making in healthcare can all be addressed by simulation. The orthogonal simulation techniques are discrete-event simulation, system dynamics, agent-based simulation, game, and participatory simulation. For patient-centric logistics, discrete-event simulation in single-department/unit scenarios is the most dominant form of simulation, the maturity of which takes the lead over other categories by a large margin. As a systems perspective was applied, discrete-event simulation became less popular and was compensated for by system dynamics or hybrid modeling. The literature review showed that tools for logistical simulations vary in this field, with tools such as AnyLogic, Arena, NetLogo, and board games implemented most frequently. This is an extensive study analyzing the growth in the use of simulation in healthcare settings.
Lack of standardization
The number of miscellaneous simulations was significant, although discrete-event simulation, system dynamics, and agent-based simulation were well-established and well-standardized simulation techniques in many software packages. Most of the miscellaneous simulations were custom-made solutions. A focal point of these papers was implementing the modular design of protocols, revealing a lack of standards. Compared with processes in many other industries, healthcare processes are less standardized, and thus, composition of services varies. We believe that much effort could be saved by employing standardization in both healthcare processes and simulation formulism.
Lack of identification for material-centric logistics
In the domain of material-centric logistics, the focus is on inventory management and network distribution. A general lack of articles indicated limited research effort. One reason is that material-centric logistics is not an independent research stream yet—in many cases, the analysis of material-centric logistics is attached to a larger research project pertaining to physical distribution and logistical management.
Lack of complex system modeling and simulation
System dynamics, agent-based simulation, and hybrid modeling were underdeveloped for handling the complexity of social-technical systems. Digital transformation would change many aspects of the human-technology interaction in the provision of health services. A knowledge gap exists between the promise of future delivery of care that abolishes institutional boundaries and the current methods for testing and demonstrating functionalities. To bridge this gap, we require a better understanding of interconnected relationships between care providers and extensions to model individual-level requirements.
Limitations
The review has limitations. The search terms were formulated by the authors. As a result, the data search might not have been comprehensive. To eliminate the risk of omitting important contributions, the search terms combined keywords related to content and scientific methods, respectively. Second, although both journal and conference contributions were considered, the exclusion of abstracts and posters might lead to publication bias according to the Assessment of Multiple Systematic Reviews (AMSTAR) checklist for assessing the quality of systematic reviews. Because the aim of the review was to identify logistical simulations for training and education purposes, the exclusion is understandable for the short contributions that are not able to document the simulation models in a detailed manner. Therefore, publication bias is not prevalent in our literature review. The review only analyzed papers published since 1998. This approach was taken because the growth in the use of healthcare simulations started as Jun et al. surveyed the practical application of discrete-event simulation in healthcare [ 12 ], which was noted by Persson and Persson [ 52 ]. Therefore, a synthesis of the literature after 1998 should not distort the analysis.
General conclusion
The overview demonstrates that the simulation models available are mainly event-based, which is understandable. The strict regulations and rules associated with the medical field make process simulation particularly suited to handling issues in this area. These perceptions, together with the lack of literature on using agent-based simulation and participatory simulation, suggest a research direction involving the development of ontologies, architectures, and terminologies for their better acceptance in training and education of non-technical skills, with more problem-solving studies performed to demonstrate the corresponding benefits.
It is worth noting that the growth of digitalized healthcare occurs in parallel with the demographic change into an aging society. Currently, digital transformation, provision of homecare, and de-institutionalization are transferring practical applications into the decentralized paradigm. This effort requires coordination between caregivers and stakeholders. Agent-based simulation and participatory simulation can support comprehensive engineering to achieve quality and safety improvements. Therefore, agent-based simulation and participatory simulation are promising approaches for better handling healthcare logistics given current societal trends.
Change history
24 april 2019.
The original article [1] contains a previous iteration of author, Chen Zhang’s name.
Abbreviations
Miscellaneous
Hybrid simulation
Systems dynamics
Klein G, Feltovich PJ, Bradshaw JM, Woods DD. Common ground and coordination in joint activity. In: Rouse WB, Boff KR, editors. Organizational simulation. New Jersey: Wiley; 2005. p. 139–84.
Vincent C, Amalberti R. Safety strategies in hospitals. In: Safer healthcare. Cham: Springer International Publishing. p. 73–91.
Nemeth CP, Nunnally M, O’Connor MF, Brandwijk M, Kowalsky J, Cook RI. Regularly irregular: how groups reconcile cross-cutting agendas and demand in healthcare. Cogn Tech Work. 2007;9:139–48.
Article Google Scholar
Macrae C, Draycott T. Delivering high reliability in maternity care: in situ simulation as a source of organisational resilience. Saf Sci. 2016. https://doi.org/10.1016/j.ssci.2016.10.019 .
Crichton M, O’Connor P, Flin R. Safety at the sharp end: a guide to non-technical skills. Hampshire: Ashgate Publishing, Ltd; 2013.
Dieckmann P, Zeltner LG, Helsø A-M. “Hand-it-on”: an innovative simulation on the relation of non-technical skills to healthcare. Adv Simul. 2016;1:30.
Ulmanen P, Szebehely M. From the state to the family or to the market? Consequences of reduced residential eldercare in Sweden: from the state to the family. Int J Soc Welf. 2015;24:81–92.
Hagihara A, Hasegawa M, Hinohara Y, Abe T, Motoi M. The aging population and future demand for emergency ambulances in Japan. Intern Emerg Med. 2013;8:431–7.
Article PubMed Google Scholar
Kriz WC. Creating effective learning environments and learning organizations through gaming simulation design. Simul Gaming. 2003;34:495–511.
Kriz WC. Types of gaming simulation applications. Simul Gaming. 2017;48:3–7.
Meijer S. The power of sponges: comparing high-tech and low-tech gaming for innovation. Simul Gaming. 2015;46:512–35.
Jun JB, Jacobson SH, Swisher JR. Application of discrete-event simulation in health care clinics: a survey. J Oper Res Soc. 1999;50:109–23.
Brailsford SC. System dynamics: what’s in it for healthcare simulation modelers. In: Proceedings of the winter simulation conference; 2008. p. 1478–83.
Google Scholar
Koelling P, Schwandt MJ. Health systems: a dynamic system-benefits from system dynamics. In: Proceedings of the winter simulation conference; 2005. p. 1321–7.
Mustafee N, Katsaliaki K, Taylor SJE. Profiling literature in healthcare simulation. Simulation. 2010;86:543–8.
Schaefer JJ, Vanderbilt AA, Cason CL, Bauman EB, Glavin RJ, Lee FW, et al. Literature review: instructional design and pedagogy science in healthcare simulation. Simul Healthc. 2011;6:30–41.
Nestel D, Groom J, Eikeland-Husebø S, OʼDonnell JM. Simulation for learning and teaching procedural skills: the state of the science. Simul Healthc. 2011;6:10–3.
DeRienzo CM, Shaw RJ, Meanor P, Lada E, Ferranti J, Tanaka D. A discrete event simulation tool to support and predict hospital and clinic staffing. Health Informatics J. 2016;23:124–33.
Devapriya P, Strömblad CTB, Bailey MD, Frazier S, Bulger J, Kemberling ST, et al. StratBAM: a discrete-event simulation model to support strategic hospital bed capacity decisions. J Med Syst. 2015;39:130.
Bhattacharjee P, Ray PK. Simulation modelling and analysis of appointment system performance for multiple classes of patients in a hospital: a case study. Oper Res Health Care. 2016;8:71–84.
Vasilakis C, Sobolev BG, Kuramoto L, Levy AR. A simulation study of scheduling clinic appointments in surgical care: individual surgeon versus pooled lists. J Oper Res Soc. 2007;58:202–11.
Jørgensen P, Jacobsen P, Poulsen JH. Identifying the potential of changes to blood sample logistics using simulation. Scand J Clin Lab Invest. 2013;73:279–85.
Rashwan W, Ragab MA, Abo-Hamad W, Arisha A. Bed blockage in Irish hospitals: system dynamics methodology. In: Proceedings of the winter simulation conference; 2013. p. 3984–5.
Rashwan W, Abo-Hamad W, Arisha A. A system dynamics view of the acute bed blockage problem in the Irish healthcare system. Eur J Oper Res. 2015;247:276–93.
Brailsford SC, Lattimer VA, Tarnaras P, Turnbull JC. Emergency and on-demand health care: modelling a large complex system. J Oper Res Soc. 2004;55:34–42.
Lane DC, Monefeldt C, Rosenhead JV. Looking in the wrong place for healthcare improvements: a system dynamics study of an accident and emergency department. J Oper Res Soc. 2000;51:518–31.
Azzi A, Persona A, Sgarbossa F, Bonin M. Drug inventory management and distribution: outsourcing logistics to third-party providers. Strateg Outsourcing Int J. 2013;6:48–64.
Kalton A, Falconer E, Docherty J, Alevras D, Brann D, Johnson K. Multi-agent-based simulation of a complex ecosystem of mental health care. J Med Syst. 2016;40:39.
Marcon E, Chaabane S, Sallez Y, Bonte T, Trentesaux D. A multi-agent system based on reactive decision rules for solving the caregiver routing problem in home health care. Simul Model Pract Theory. 2017;74:134–51.
Jetly G, Rossetti CL, Handfield R. A multi-agent simulation of the pharmaceutical supply chain. J Simul. 2012;6:215–26.
Escudero-Marin P, Pidd M. Using ABMS to simulate emergency departments. In: Proceedings of the winter simulation conference; 2011. p. 1239–50.
Mustafee N, Katsaliaki K. The blood supply game. In: Proceedings of the winter simulation conference; 2010. p. 327–38.
Katsaliaki K, Mustafee N, Kumar S. A game-based approach towards facilitating decision making for perishable products: an example of blood supply chain. Expert Syst Appl. 2014;41:4043–59.
Basole RC, Bodner DA, Rouse WB. Healthcare management through organizational simulation. Decis Support Syst. 2013;55:552–63.
Mattarelli E, Fadel KJ, Weisband SP. Design of a role-playing game to study the trajectories of health care workers in an operating room. In: Proceedings of conference on human factors in computing systems; 2006. p. 1091–6.
Brailsford SC, Desai SM, Viana J. Towards the holy grail: combining system dynamics and discrete-event simulation in healthcare. In: Proceedings of the winter simulation conference; 2010. p. 2293–303.
Zulkepli J, Eldabi T, Mustafee N. Hybrid simulation for modelling large systems: an example of integrated care model. In: Proceedings of the winter simulation conference; 2012.
zen MB, Zabawa J. Modeling healthcare demand using a hybrid simulation approach. In: Proceedings of the winter simulation conference; 2016. p. 1535–46.
Shah N. Pharmaceutical supply chains: key issues and strategies for optimisation. Comput Chem Eng. 2004;28:929–41.
Article CAS Google Scholar
Gaba DM. Adapting space science methods for describing and planning research in simulation in healthcare: science traceability and decadal surveys. Simul Healthc. 2012;7:27–31.
Liu Z, Rexachs D, Epelde F, Luque E. An agent-based model for quantitatively analyzing and predicting the complex behavior of emergency departments. J Comput Sci. 2017;21(Suppl C):11–23.
Kolb EMW, Schoening S, Peck J, Lee T. Reducing emergency department overcrowding: five patient buffer concepts in comparison. In: Proceedings of the winter simulation conference; 2008. p. 1516–25.
Kotiadis K, Tako AA, Vasilakis C. A participative and facilitative conceptual modelling framework for discrete event simulation studies in healthcare. J Oper Res Soc. 2014;65:197–213.
Demir E, Gunal MM, Southern D. Demand and capacity modelling for acute services using discrete event simulation. Health Syst. 2017;6:33–40.
Reynolds M, Vasilakis C, McLeod M, Barber N, Mounsey A, Newton S, et al. Using discrete event simulation to design a more efficient hospital pharmacy for outpatients. Health Care Manag Sci. 2011;14:223–36.
Pehlivan C, Augusto V, Xie X. Admission control in a pure loss healthcare network: MDP and DES approach. In: Proceedings of the winter simulation conference; 2013. p. 54–65.
Medeiros DJ, Swenson E, DeFlitch C. Improving patient flow in a hospital emergency department. In: Proceedings of the winter simulation conference; 2008. p. 1526–31.
Ferrin DM, Miller MJ, McBroom DL. Maximizing hospital finanacial impact and emergency department throughput with simulation. In: Proceedings of the winter simulation conference; 2007. p. 1566–73.
Dehlendorff C, Kulahci M, Andersen KK. Designing simulation experiments with controllable and uncontrollable factors for applications in healthcare. Appl Stat. 2011;60:31–49.
Duguay C, Chetouane F. Modeling and improving emergency department systems using discrete event simulation. Simulation. 2007;83:311–20.
Kittipittayakorn C, Ying K-C. Using the integration of discrete event and agent-based simulation to enhance outpatient service quality in an orthopedic department. J Healthc Eng. 2016;2016.
Persson MJ, Persson JA. Analysing management policies for operating room planning using simulation. Health Care Manag Sci. 2010;13:182–91.
Guo M, Wagner M, West C. Outpatient clinic scheduling—a simulation approach. In: Proceedings of the winter simulation conference; 2004. p. 1981–7.
Wijewickrama A, Takakuwa S. Simulation analysis of appointment scheduling in an outpatient department of internal medicine. In: Proceedings of the winter simulation conference; 2005. p. 2264–73.
Johansson B, Jain S, Montoya-Torres J, Hngan J. Integrating balanced scorecard and simulation modeling to improve emergency department performance in Irish hospitals. In: Proceedings of the winter simulation conference; 2010. p. 2340–51.
Lin Y, Zhang J. An ant colony optimization approach for efficient admission scheduling of elective inpatients. In: Proceedings of the annual conference on genetic and evolutionary computation; 2011. p. 15–6.
Helm JE, AhmadBeygi S, Van Oyen MP. Design and analysis of hospital admission control for operational effectiveness. Prod Oper Manag. 2011;20:359–74.
Yokouchi M, Aoki S, Sang H, Zhao R, Takakuwa S. Operations analysis and appointment scheduling for an outpatient chemotherapy department. In: Proceedings of the winter simulation conference; 2012.
Khurma N, Salamati F, Pasek ZJ. Simulation of patient discharge process and its improvement. In: Proceedings of the winter simulation conference; 2013. p. 2452–62.
Kooij R, Mes MRK, Hans EW. Simulation framework to analyze operating room release mechanisms. In: Proceedings of the winter simulation conference; 2014. p. 1144–55.
Huggins A, Claudio D, Waliullah M. A detailed simulation model of an infusion treatment center. In: Proceedings of the winter simulation conference; 2014. p. 1198–209.
Prodel M, Augusto V, Xie X. Hospitalization admission control of emergency patients using markovian decision processes and discrete event simulation. In: Proceedings of the winter simulation conference; 2014. p. 1433–44.
Giesen E, Ketter W, Zuidwijk R. Dynamic agent-based scheduling of treatments: evidence from the Dutch youth health care sector. In: Müller JP, Ketter W, Kaminka G, Wagner G, Bulling N, editors. Multiagent system technologies. Cham: Springer International Publishing; 2015. p. 173–99.
Chapter Google Scholar
Chen Y, Kuo YH, Balasubramanian H, Wen C. Using simulation to examine appointment overbooking schemes for a medical imaging center. In: Proceedings of the winter simulation conference; 2015. p. 1307–18.
Crowe S, Vasilakis C, Gallivan S, Bull C, Fenton M. Informing the management of pediatric heart transplant waiting lists: complementary use of simulation and analytical modeling. In: Proceedings of the winter simulation conference; 2015. p. 1654–65.
Chen P-S, Robielos RAC, Palaña PKVC, Valencia PLL, GY-H C. Scheduling patients’ appointments: allocation of healthcare service using simulation optimization. J Healthc Eng. 2015;6:259–80.
Kim S-H, Chan CW, Olivares M, Escobar G. ICU admission control: an empirical study of capacity allocation and its implication for patient outcomes. Manag Sci. 2015;61:19–38.
Khanna S, Boyle J, Good N, Bell A, Lind J. Analysing the emergency department patient journey: discovery of bottlenecks to emergency department patient flow. Emerg Med Australas. 2017;29:18–23.
Kim BBJ, Delbridge TR, Kendrick DB. Adjusting patients streaming initiated by a wait time threshold in emergency department for minimizing opportunity cost. Int J Health Care Qual Assur. 2017;30:516–27.
Ozcan YA, Tànfani E, Testi A. Improving the performance of surgery-based clinical pathways: a simulation-optimization approach. Health Care Manag Sci. 2017;20:1–15.
Bozzetto M, Rota S, Vigo V, Casucci F, Lomonte C, Morale W, et al. Clinical use of computational modeling for surgical planning of arteriovenous fistula for hemodialysis. BMC Med Inform Decis Mak. 2017;17:26.
Article PubMed PubMed Central Google Scholar
Vile JL, Allkins E, Frankish J, Garland S, Mizen P, Williams JE. Modelling patient flow in an emergency department to better understand demand management strategies. J Simul. 2017;11:115–27.
Demir E, Southern D. Enabling better management of patients: discrete event simulation combined with the STAR approach. J Oper Res Soc. 2017;68:577–90.
Chepenik L, Pinker E. The impact of increasing staff resources on patient flow in a psychiatric emergency service. Psychiatr Serv. 2017;68:470–5.
Saltzman R, Roeder T, Lambton J, Param L, Frost B, Fernandes R. The impact of a discharge holding area on the throughput of a pediatric unit. Serv Sci. 2017;9:121–35.
Toth DJA, Khader K, Slayton RB, Kallen AJ, Gundlapalli AV, O’Hagan JJ, et al. The potential for interventions in a long-term acute care hospital to reduce transmission of carbapenem-resistant enterobacteriaceae in affiliated healthcare facilities. Clin Infect Dis. 2017;65:581–7.
Steward D, Glass TF, Ferrand YB. Simulation-based design of ed operations with care streams to optimize care delivery and reduce length of stay in the emergency department. J Med Syst. 2017;41:162.
Tako AA, Kotiadis K. PartiSim: a multi-methodology framework to support facilitated simulation modelling in healthcare. Eur J Oper Res. 2015;244:555–64.
Monks T, Pearn K, Allen M. Simulation of stroke care systems. In: Proceedings of the winter simulation conference; 2015. p. 1391–402.
Djanatliev A, Meier F. Hospital processes within an integrated system view: a hybrid simulation approach. In: Proceedings of the winter simulation conference; 2016. p. 1364–75.
Alahäivälä T, Oinas-Kukkonen H. Understanding persuasion contexts in health gamification: a systematic analysis of gamified health behavior change support systems literature. Int J Med Inform. 2016;96:62–70.
Zhong X, Lee HK, Li J. From production systems to health care delivery systems: a retrospective look on similarities, difficulties and opportunities. Int J Prod Res. 2017;55:4212–27.
Lim ME, Worster A, Goeree R, Tarride J-É. Simulating an emergency department: the importance of modeling the interactions between physicians and delegates in a discrete event simulation. BMC Med Inform Decis Mak. 2013;13:59.
Vanderby S, Carter MW. An evaluation of the applicability of system dynamics to patient flow modelling. J Oper Res Soc. 2010;61:1572–81.
Chockalingam A, Jayakumar K, Lawley MA. A stochastic control approach to avoiding emergency department overcrowding. In: Proceedings of the winter simulation conference; 2010. p. 2399–411.
Djanatliev A, German R. Prospective healthcare decision-making by combined system dynamics, discrete-event and agent-based simulation. In: Proceedings of the winter simulation conference; 2013. p. 270–81.
Wolstenholme E. A patient flow perspective of U.K. health services: exploring the case for new “intermediate care” initiatives. Syst Dyn Rev. 1999;15:253–71.
Jansen FJA, Etman LFP, Rooda JE, Adan IJBF. Aggregate simulation modeling of an MRI department using effective process times. In: Proceedings of the winter simulation conference; 2012.
Zambrano F, Concha P, Ramis F, Neriz L, Bull M, Veloz P, et al. Improving patient access to a public hospital complex using agent simulation. In: Proceedings of the winter simulation conference; 2016. p. 1277–88.
Lamé G, Jouini O, Cardinal JS-L, Carvalho M, Tournigand C, Wolkenstein P. Patient-hospital communication: a platform to improve outpatient chemotherapy. In: Proceedings of the winter simulation conference; 2016. p. 2099–110.
April J, Better M, Glover F, Kelly J, Laguna M. Enhancing business process management with simulation optimization. In: Proceedings of the winter simulation conference; 2006. p. 642–9.
Bowers J, Ghattas M, Mould G. Exploring alternative routes to realising the benefits of simulation in healthcare. J Oper Res Soc. 2012;63:1457–66.
Chavis J, Cochran AL, Kocher KE, Washington VN, Zayas-Cabán G. A simulation model of patient flow through the emergency department to determine the impact of a short stay unit on hospital congestion. In: Proceedings of the winter simulation conference; 2016. p. 1982–93.
Patvivatsiri L. A simulation model for bioterrorism preparedness in an emergency room. In: Proceedings of the winter simulation conference; 2006. p. 501–8.
Comas M, Castells X, Hoffmeister L, Román R, Cots F, Mar J, et al. Discrete-event simulation applied to analysis of waiting lists: evaluation of a prioritization system for cataract surgery. Value Health. 2008;11:1203–13.
Kuhl ME. A simulation study of patient flow for day of surgery admission. In: Proceedings of the winter simulation conference; 2012.
Miller M, Ferrin D, Shahi N. Estimating patient surge impact on boarding time in several regional emergency departments. In: Proceedings of the winter simulation conference; 2009. p. 1906–15.
Roure M, Halley Q, Augusto V. Modelling and simulation of an outpatient surgery unit. In: Proceedings of the winter simulation conference; 2015. p. 1525–36.
Doğan NÖ, Unutulmaz O. Lean production in healthcare: a simulation-based value stream mapping in the physical therapy and rehabilitation department of a public hospital. Total Qual Manag Bus Excell. 2016;27:64–80.
Batarseh OG, Goldlust EJ, Day TE. SysML for conceptual modeling and simulation for analysis: a case example of a highly granular model of an emergency department. In: Proceedings of the winter simulations conference; 2013. p. 2398–409.
Schonherr O, Rose O. A general model description for discrete processes. In: Proceedings of the winter simulation conference; 2011. p. 2201–13.
Southard PB, Chandra C, Kumar S. RFID in healthcare: a six sigma DMAIC and simulation case study. Int J Health Care Qual Assur. 2012;25:291–321.
Santibáñez P, Chow VS, French J, Puterman ML, Tyldesley S. Reducing patient wait times and improving resource utilization at British Columbia Cancer Agency’s ambulatory care unit through simulation. Health Care Manag Sci. 2009;12:392–407.
Konrad RA, Lawley MA. Input modeling for hospital simulation models using electronic messages. In: Proceedings of the winter simulation conference; 2009. p. 134–47.
Hagtvedt R, Ferguson M, Griffin P, Jones GT, Keskinocak P. Cooperative strategies to reduce ambulance diversion. In: Proceedings of the winter simulation conference; 2009. p. 1861–74.
Maull RS, Smart PA, Harris A, AA-F K. An evaluation of ‘fast track’ in A&E: a discrete event simulation approach. Serv Ind J. 2009;29:923–41.
Roberts SD. Tutorial on the simulation of healthcare systems. In: Proceedings of the winter simulation conference; 2011. p. 1408–19.
Hosseini S, Jannat S. Discrete event simulation technique for evaluating performance of oncology department: a case study. In: Proceedings of the winter simulation conference; 2015. p. 1343–54.
Levin S, Garifullin M. Simulating wait time in healthcare: accounting for transition process variability using survival analyses. In: Proceedings of the winter simulation conference. 2015. p. 1252–60.
Levin S, Dittus R, Aronsky D, Weinger M, France D. Evaluating the effects of increasing surgical volume on emergency department patient access. Qual Saf Health Care. 2011;20:146–52.
CAS Google Scholar
Mahapatra S, Koelling CP, Patvivatsiri L, Fraticelli B, Eitel D, Grove L. Pairing emergency severity index5-level triage data with computer aided system design to improve emergency department access and throughput. In: Proceedings of the winter simulation conference; 2003. p. 1917–25.
McClean S, Barton M, Garg L, Fullerton K. A modeling framework that combines Markov models and discrete-event simulation for stroke patient care. ACM Trans Model Comput Simul. 2011;21:25.
Reindl S, Mönch L, Mönch M, Scheider A. Modeling and simulation of cataract surgery processes. In: Proceedings of the winter simulation conference; 2009. p. 1937–45.
Takakuwa S, Wijewickrama A. Optimizing staffing schedule in light of patient satisfaction for the whole outpatient hospital ward. In: Proceedings of the winter simulation conference; 2008. p. 1500–8.
Takakuwa S, Katagiri D. Modeling of patient flows in a large-scale outpatient hospital ward by making use of electronic medical records. In: Proceedings of the winter simulation conference; 2007. p. 1523–31.
Takakuwa S, Shiozaki H. Functional analysis for operating emergency department of a general hospital. In: Proceedings of the winter simulation conference; 2004. p. 2003–11.
Coelli FC, Ferreira RB, Almeida RMVR, Pereira WCA. Computer simulation and discrete-event models in the analysis of a mammography clinic patient flow. Comput Methods Prog Biomed. 2007;87:201–7.
Rohleder TR, Lewkonia P, Bischak DP, Duffy P, Hendijani R. Using simulation modeling to improve patient flow at an outpatient orthopedic clinic. Health Care Manag Sci. 2011;14:135–45.
Wang T, Guinet A, Belaidi A, Besombes B. Modelling and simulation of emergency services with ARIS and Arena. Case study: the emergency department of Saint Joseph and Saint Luc Hospital. Prod Plan Control. 2009;20:484–95.
Abo-Hamad W, Arisha A. Multi-criteria framework for emergency department in Irish hospital. In: Proceedings of the winter simulation conference; 2012.
Abo-Hamad W, Arisha A. Simulation-based framework to improve patient experience in an emergency department. Eur J Oper Res. 2013;224:154–66.
Wang Y, Lee LH, Chew EP, Lam SSW, Low SK, Ong MEH, et al. Multi-objective optimization for a hospital inpatient flow process via discrete event simulation. In: Proceedings of the winter simulation conference; 2015. p. 3622–31.
Zhao Y, Peng Q, Strome T, Weldon E, Zhang M, Chochinov A. Bottleneck detection for improvement of emergency department efficiency. Bus Process Manag J. 2015;21:564–85.
Rashwan W, Habib H, Arisha A, Courtney G, Kennelly S. An integrated approach of multi-objective optimization model for evaluating new supporting program in Irish hospitals. In: Proceedings of the winter simulation conference; 2016. p. 1904–15.
Rashwan W, Arisha A. Modeling behavior of nurses in clinical medical unit in university hospital: burnout implications. In: Proceedings of the winter simulation conference; 2015. p. 3880–91.
Bair AE, Song WT, Chen Y, Morris BA. The impact of inpatient boarding on emergency department crowding: a discrete-event simulation study. In: Proceedings of the spring computer simulation conference; 2009.
Bountourelis T, Eckman D, Luangkesorn L, Schaefer A, Nabors SG, Clermont G. Sensitivity analysis of an ICU simulation model. In: Proceedings of the winter simulation conference; 2012.
Hamrock E, Paige K, Parks J, Scheulen J, Levin S. Discrete event simulation for healthcare organizations: a tool for decision making. J Healthc Manag. 2013;58:110–24.
Centeno MA, Albacete C, Terzano DO, Carrillo M, Ogazon T. Project and process improvements in healthcare organizations: a simulation study of the radiology department at JMH. In: Proceedings of the winter simulation conference; 2000. p. 1978–84.
Ramis FJ, Palma JL, Baesler FF. The use of simulation for process improvement at an ambulatory surgery center. In: Proceedings of the winter simulation conference; 2001. p. 1401–4.
Pasin F, Jobin MH, Cordeau JF. An application of simulation to analyse resource sharing organisations among health-care organisations. Int J Oper Prod Manag. 2002;22:381–93.
Wiinamaki A, Dronzek R. Using simulation in the architectural concept phase of an emergency department design. In: Proceedings of the winter simulation conference; 2003. p. 1912–6.
Samaha S, Armel WS, Starks DW. The use of simulation to reduce the length of stay in an emergency department. In: Proceedings of the winter simulation conference; 2003. p. 1907–11.
Blasak RE, Starks DW, Armel WS, Hayduk MC. Healthcare process analysis: the use of simulation to evaluate hospital operations between the emergency department and a medical telemetry unit. In: Proceedings of the winter simulation conference; 2003. p. 1887–93.
Baesler FF, Jahnsen HE, DaCosta M. The use of simulation and design of experiments for estimating maximum capacity in an emergency room. In: Proceedings of the winter simulation conference; 2003. p. 1903–6.
Centeno MA, Giachetti R, Linn R, Ismail AM. A simulation-ilp based tool for scheduling ER staff. In: Proceedings of the winter simulation conference; 2003. p. 1930–8.
Schenk JR, Huang D, Zheng N, Allen TT. Multiple fidelity simulation optimization of hospital performance under high consequence event scenarios. In: Proceedings of the winter simulation conference; 2005. p. 936–42.
Spry CW, Lawley MA. Evaluating hospital pharmacy staffing and work scheduling using simulation. In: Proceedings of the winter simulation conference; 2005. p. 2256–63.
Hay AM, Valentin EC, Bijlsma RA. Modeling emergency care in hospitals: a paradox-the patient should not drive the process. In: Proceedings of the winter simulation conference; 2006. p. 439–45.
Wijewickrama AKA, Takakuwa S. Simulation analysis of an outpatient department of internal medicine in a university hospital. In: Proceedings of the winter simulation conference; 2006. p. 425–32.
Ballard SM, Kuhl ME. The use of simulation to determine maximum capacity in the surgical suite operating room. In: Proceedings of the winter simulation conference; 2006. p. 433–8.
Taaffe K, Johnson M, Steinmann D. Improving hospital evacuation planning using simulation. In: Proceedings of the winter simulation conference; 2006. p. 509–15.
VanBerkel PT, Blake JT. A comprehensive simulation for wait time reduction and capacity planning applied in general surgery. Health Care Manag Sci. 2007;10:373–85.
Rico F, Salari E, Centeno G. Emergency departments nurse allocation to face a pandemic influenza outbreak. In: Proceedings of the winter simulation conference; 2007. p. 1292–8.
Miller M, Ferrin D, Ashby M, Flynn T, Shahi N. Merging six emergency departments into one: a simulation approach. In: Proceedings of the winter simulation conference; 2007. p. 1574–8.
Patvivatsiri L, Montes EJ Jr, Xi O. Modeling bioterrorism preparedness with simulation in rural healthcare system. In: Proceedings of the winter simulation conference; 2007. p. 1155–60.
Paul JA, Hariharan G. Hospital capacity planning for efficient disaster mitigation during a bioterrorist attack. In: Proceedings of the winter simulation conference; 2007. p. 1139–47.
Song WT, Bair AE, Chih M. A simulation study on the impact of physician starting time in a physical examination service. In: Proceedings of the winter simulation conference; 2008. p. 1553–62.
Protil RM, Stroparo JR, Bichinho GL. Applying computer simulation to increase the surgical center occupation rate at a university hospital in Curitiba-Brazil. In: Proceedings of the winter simulation conference; 2008. p. 1609–16.
Nielsen AL, Hilwig H, Kissoon N, Teelucksingh S. Discrete event simulation as a tool in optimization of a professional complex adaptive system. Stud Health Technol Inform. 2008;136:247.
PubMed Google Scholar
Huschka TR, Denton BT, Narr BJ, Thompson AC. Using simulation in the implementation of an outpatient procedure center. In: Proceedings of the winter simulation conference; 2008. p. 1547–52.
Oddoye JP, Jones DF, Tamiz M, Schmidt P. Combining simulation and goal programming for healthcare planning in a medical assessment unit. Eur J Oper Res. 2009;193:250–61.
Raunak M, Osterweil L, Wise A, Clarke L, Henneman P. Simulating patient flow through an emergency department using process-driven discrete event simulation. In: Proceedings of the international conference on software engineering; 2009. p. 73–83.
Efe K, Raghavan V, Choubey S. Simulation modeling movable hospital assets managed with RFID sensors. In: Proceedings of the winter simulation conference; 2009. p. 2054–64.
Ferrand Y, Magazine M, Rao U. Comparing two operating-room-allocation policies for elective and emergency surgeries. In: Proceedings of the winter simulation conference; 2010. p. 2364–74.
Zeltyn S, Marmor YN, Mandelbaum A, Carmeli B, Greenshpan O, Mesika Y, et al. Simulation-based models of emergency departments: operational, tactical, and strategic staffing. ACM Trans Model Comput Simul. 2011;21:24.
Weng S-J, Tsai B-S, Wang L-M, Chang C-Y, Gotcher D. Using simulation and data envelopment analysis in optimal healthcare efficiency allocations. In: Proceedings of the winter simulation conference; 2011. p. 1295–305.
Geis GL, Pio B, Pendergrass TL, Moyer MR, Patterson MD. Simulation to assess the safety of new healthcare teams and new facilities. Simul Healthc. 2011;6:125.
Cabrera E, Luque E, Taboada M, Epelde F, Iglesias ML. ABMS optimization for emergency departments. In: Proceedings of the winter simulation conference; 2012.
Kuo Y-H, Leung JM, Graham CA. Simulation with data scarcity: developing a simulation model of a hospital emergency department. In: Proceedings of the winter simulation conference; 2012.
Mustafee N, Lyons T, Rees P, Davies L, Ramsey M, Williams MD. Planning of bed capacities in specialized and integrated care units: incorporating bed blockers in a simulation of surgical throughput. In: Proceedings of the winter simulation conference; 2012.
Rashwan W, Ragab M, Abo-Hamad W, Arisha A. Evaluating policy interventions for delayed discharge: a system dynamics approach. In: Proceedings of the winter simulation conference; 2013. p. 2463–74.
Shin SY, Balasubramanian H, Brun Y, Henneman PL, Osterweil LJ. Resource scheduling through resource-aware simulation of emergency departments. In: Proceedings of the international workshop on software engineering in health care; 2013. p. 64–70.
Amyot D. Real-time simulations to support operational decision making in healthcare. In: Proceedings of the summer computer simulation conference; 2013. p. 64–70.
Verma S, Gupta A. Improving services in outdoor patient departments by focusing on process parameters: a simulation approach. In: Proceedings of the winter simulation conference; 2013. p. 2250–61.
Yaylali E, Simmons J, Taheri J. Systems engineering methods for enhancing the value stream in public health preparedness: the role of Markov models, simulation, and optimization. Public Health Rep. 2014;129:145–53.
Pinto LR, Perpétuo IHO, de Campos FCC, Ribeiro YCNMB. Analysis of hospital bed capacity via queuing theory and simulation. In: Proceedings of the winter simulation conference; 2014. p. 1281–92.
Ozen A, Balasubramanian H, Samra P, Ehresman M, Li H, Fairman T, et al. The impact of hourly discharge rates and prioritization on timely access to inpatient beds. In: Proceedings of the winter simulation conference; 2014. p. 1210–20.
Kalbasi A, Krishnamurthy D, Rolia J, Singhal S. Simulation by example for complex systems. In: Proceedings of the winter simulation conference; 2014. p. 974–85.
Wurzer G, Lorenz WE. Causality in hospital simulation based on utilization chains. In: Proceedings of the symposium on simulation for architecture and urban design; 2014.
Aboueljinane L, Sahin E, Jemai Z, Marty J. A simulation study to improve the performance of an emergency medical service: application to the French Val-de-Marne department. Simul Model Pract Theory. 2014;47:46–59.
Ghanes K, Jouini O, Jemai Z, Wargon M, Hellmann R, Thomas V, et al. A comprehensive simulation modeling of an emergency department: a case study for simulation optimization of staffing levels. In: Proceedings of the winter simulation conference; 2014. p. 1421–32.
Zhou Z, Wang Y, Li L. Process mining based modeling and analysis of workflows in clinical care—a case study in a Chicago outpatient clinic. In: Proceedings of the IEEE international conference on networking, sensing and control; 2014. p. 590–5.
van Buuren M, Kommer GJ, van der Mei R, Bhulai S. A simulation model for emergency medical services call centers. In: Proceedings of the winter simulation conference; 2015. p. 844–55.
Franck T, Augusto V, Xie X, Gonthier R, Achour E. Performance evaluation of an integrated care for geriatric departments using discrete-event simulation. In: Proceedings of the winter simulation conference; 2015. p. 1331–42.
Ghanes K, Wargon M, Jouini O, Jemai Z, Diakogiannis A, Hellmann R, et al. Simulation-based optimization of staffing levels in an emergency department. Simulation. 2015;91:942–53.
Carmen R, Defraeye M, Van Nieuwenhuyse I. A decision support system for capacity planning in emergency departments. Int J Simul Model. 2015;14:299–312.
dos Santos M, Quintal RS, da PAC, Gomes CFS. Simulation of operation of an integrated information for emergency pre-hospital care in Rio de Janeiro municipality. Procedia Comput Sci. 2015;55:931–8.
Agor J, McKenzie K, Ozaltin O, Mayorga M, Parikh RS, Huddleston J. Simulation of triaging patients into an internal medicine department to validate the use of an optimization based workload score. In: Proceedings of the winter simulation conference; 2016. p. 3708–9.
Lee W, Shin K, Lee H-R, Shin H, Lee T. A structured approach for constructing high fidelity ED simulation. In: Proceedings of the winter simulation conference; 2016. p. 1950–60.
Pujowidianto NA, Lee LH, Pedrielli G, Chen C-H, Li H. Constrained optimizaton for hospital bed allocation via discrete event simulation with nested partitions. In: Proceedings of the winter simulation conference; 2016. p. 1916–25.
Augusto V, Xie X, Prodel M, Jouaneton B, Lamarsalle L. Evaluation of discovered clinical pathways using process mining and joint agent-based discrete-event simulation. In: Proceedings of the winter simulation conference; 2016. p. 2135–46.
Tiwari V, Sandberg WS. Perioperative bed capacity planning guided by theory of constraints. In: Proceedings of the winter simulation conference; 2016. p. 1894–903.
Thorwarth M, Rashwan W, Arisha A. An analytical representation of flexible resource allocation in hospitals. Flex Serv Manuf J. 2016;28:148–65.
Kuo Y-H, Rado O, Lupia B, Leung JMY, Graham CA. Improving the efficiency of a hospital emergency department: a simulation study with indirectly imputed service-time distributions. Flex Serv Manuf J. 2016;28:120–47.
Kadı D, Kuvvetli Y, Çolak S. Performance analysis of a university hospital blood laboratory via discrete event simulation. Simulation. 2016;92:473–84.
Cimellaro GP, Piqué M. Resilience of a hospital emergency department under seismic event. Adv Struct Eng. 2016;19:825–36.
Zhong X, Lee HK, Williams M, Kraft S, Sleeth J, Welnick R, et al. Staffing ratio analysis in primary care redesign: a simulation approach. In: Matta A, Sahin E, Li J, Guinet A, Vandaele NJ, editors. Health care systems engineering for scientists and practitioners. New York: Springer; 2016. p. 133–44.
Lambton J, Roeder T, Saltzman R, Param L, Fernandes R. Using simulation to model improvements in pediatric bed placement in an acute care hospital. Jona J Nurs Adm. 2017;47:88–93.
Bakker M, Tsui K-L. Dynamic resource allocation for efficient patient scheduling: a data-driven approach. J Syst Sci Syst Eng. 2017;26:448–62.
Weng S-J, Xu Y-Y, Gotcher D, Wang L-M. A pilot study of available bed forecasting system (ABFS) in the emergency healthcare network. In: Proceedings of the summer computer simulation conference; 2017.
Tànfani E, Testi A. Improving surgery department performance via simulation and optimization. In: Proceedings of the IEEE workshop on health care management; 2010.
Friemann F, Schönsleben P. Reducing global supply chain risk exposure of pharmaceutical companies by further incorporating warehouse capacity planning into the strategic supply chain planning process. J Pharm Innov. 2016;11:162–76.
Ramis FJ, Baesler F, Berho E, Neriz L, Sepulveda JA. A simulator to improve waiting times at a medical imaging center. In: Proceedings of the winter simulation conference; 2008. p. 1572–7.
Zeinali F, Mahootchi M, Sepehri MM. Resource planning in the emergency departments: a simulation-based metamodeling approach. Simul Model Pract Theory. 2015;53:123–38.
Wang J, Zhong X, Li J, Howard PK. Modeling and analysis of care delivery services within patient rooms: a system-theoretic approach. IEEE Trans Autom Sci Eng. 2014;11:379–93.
Luangkesorn KL, Bountourelis T, Schaefer A, Nabors S, Clermont G. The case against utilization: deceptive performance measures in inpatient care capacity models. In: Proceedings of the winter simulation conference; 2012.
Holm LB, Dahl FA. Simulating the influence of a 45% increase in patient volume on the emergency department of Akershus University Hospital. In: Proceedings of the winter simulation conference; 2010. p. 2455–61.
Miller MJ, Ferrin DM, Szymanski JM. Simulating six sigma improvement ideas for a hospital emergency department. In: Proceedings of the winter simulation conference; 2003. p. 1926–9.
Mackay M, Qin S, Clissold A, Hakendorf P, Ben-Tovim D, McDonnell G. Patient flow simulation modelling-an approach conducive to multi-disciplinary collaboration towards hospital capacity management. In: Proceedings of the international congress on modelling and simulation; 2013. p. 50–6.
Mes M, Bruens M. A generalized simulation model of an integrated emergency post. In: Proceedings of the winter simulation conference; 2012.
Khurma N, Bacioiu GM, Pasek ZJ. Simulation-based verification of lean improvement for emergency room process. In: Proceedings of the winter simulation conference; 2008. p. 1490–9.
Centeno MA, Lee MA, Lopez E, Fernandez HR, Carrillo M, Ogazon T. A simulation study of the labor and delivery rooms at JMH. In: Proceeding of the winter simulation conference; 2001. p. 1392–400.
Ramakrishnan S, Nagarkar K, DeGennaro M, Srihari K, Courtney AK, Emick F. A study of the CT scan area of a healthcare provider. In: Proceedings of the winter simulation conference; 2004. p. 2025–31.
Ruohonen T, Neittaanmäki P, Teittinen J. Simulation model for improving the operation of the emergency department of special health care. In: Proceedings of the winter simulation conference; 2006. p. 453–8.
Bountourelis T, Luangkesorn L, Schaefer A, Maillart L, Nabors SG, Clermont G. Development and validation of a large scale ICU simulation model with blocking. In: Proceedings of the winter simulation conference; 2011. p. 1143–53.
Al-Araidah O, Boran A, Wahsheh A. Reducing delay in healthcare delivery at outpatients clinics using discrete event simulation. Int J Simul Model. 2012;11:185–95.
Pasupathy R. Performance evaluation in a laboratory medicine unit. In: Proceedings of the winter simulation conference; 2013. p. 3972–3.
Taylor SJE, Abbott P, Young T, Grocott-Mason R. Student modeling & simulation projects in healthcare: experiences with Hillingdon Hospital. In: Proceedings of the winter simulation conference; 2014. p. 3650–61.
Oh C, Novotny AM, Carter PL, Ready RK, Campbell DD, Leckie MC. Use of a simulation-based decision support tool to improve emergency department throughput. Oper Res Health Care. 2016;9:29–39.
Eskandari H, Riyahifard M, Khosravi S, Geiger CD. Improving the emergency department performance using simulation and MCDM methods. In: Proceedings of the winter simulation conference; 2011. p. 1211–22.
Mielczarek B, Uziałko J. Using simulation to forecast the demand for hospital emergency services at the regional level. In: Proceedings of the winter simulation conference; 2012.
Perimal-Lewis L. Health intelligence: discovering the process model using process mining by constructing start-to-end patient journeys. In: Proceedings of the Australasian workshop on health informatics and knowledge management; 2014. p. 59–67.
Hosseini N, Taaffe K. Evaluation of optimal scheduling policy for accommodating elective and non-elective surgery via simulation. In: Proceedings of the winter simulation conference; 2014. p. 1377–86.
Esengul Tayfur TK. Allocation of resources for hospital evacuations via simulation. In: Proceedings of the winter simulation conference; 2007. p. 1148–54.
Ashby M, Miller M, Ferrin D, Flynn T. Simulating the patient move: transitioning to a replacement hospital. In: Proceedings of the winter simulation conference; 2007. p. 1562–5.
Pérez ES, Yepez LA, de la Mota IF. Simulation and optimization of the pre-hospital care system of the National University of Mexico using travelling salesman problem algorithms. In: Proceedings of the summer computer simulation conference; 2010. p. 364–70.
Güttinger D, Godehardt E, Zinnen A. Optimizing emergency supply for mass events. In: Proceedings of the 4th international ICST (Institute for Computer Sciences, social-informatics and telecommunications engineering) conference on simulation tools and techniques; 2011. p. 125–33.
Noreña D, Yamín L, Akhavan-Tabatabaei R, Ospina W. Using discrete event simulation to evaluate the logistics of medical attention during the relief operations in an earthquake in Bogota. In: Proceedings of the winter simulation conference; 2011. p. 2666–78.
Ullrich C, Van Utterbeeck F, Dejardin E, Debacker M, Dhondt E. Pre-hospital simulation model for medical disaster management. In: Proceedings of the winter simulation conference; 2013. p. 2432–3.
Noei S, Santana H, Sargolzaei A, Noei M. Reducing traffic congestion using geo-fence technology: application for emergency car. In: Proceedings of the international workshop on emerging multimedia applications and services for smart cities; 2014. p. 15–20.
Moon I-C, Bae JW, Lee J, Kim D, Lee H, Lee T, et al. EMSSIM: emergency medical service simulator with geographic and medical details. In: Proceedings of the winter simulation conference; 2015. p. 1272–84.
Gibson IW. An approach to hospital planning and design using discrete event simulation. In: Proceedings of the winter simulation conference; 2007. p. 1501–9.
Miller MJ, Ferrin DM, Shahi N, LaVecchia R. Allocating outpatient clinic services using simulation and linear programming. In: Proceedings of the winter simulation conference; 2008. p. 1637–44.
Ashby M, Ferrin D, Miller M, Shahi N. Discrete event simulation: optimizing patient flow and redesign in a replacement facility. In: Proceedings of the winter simulation conference; 2008. p. 1632–6.
Boucherie RJ, Hans EW, Hartmann T. Health care logistics and space: accounting for the physical build environment. In: Proceedings of the winter simulation conference; 2012.
Wurzer G. In-process agent simulation for early stages of hospital planning. Math Comput Model Dyn Syst. 2013;19:331–43.
Wurzer G, Lorenz WE, Rössler M, Hafner I, Popper N, Glock BMODYPLAN. Early-stage hospital simulation with emphasis on cross-clinical treatment chains. In: Proceedings of the symposium on simulation for architecture and urban design; 2015. p. 160–3.
Schaumann D, Pilosof NP, Date K, Kalay YE. A study of human behavior simulation in architectural design for healthcare facilities. Ann Dell Ist Super Sanita. 2016;52:24–32.
Cimellaro GP, Malavisi M, Mahin S. Using discrete event simulation models to evaluate resilience of an emergency department. J Earthq Eng. 2017;21:203–26.
Pulat PS, Kasap S, Splinter GL. Simulation study of an ideal primary care delivery system. Simulation. 2001;76:78–86.
Moeke D, van de Geer R, Koole G, Bekker R. On the performance of small-scale living facilities in nursing homes: a simulation approach. Oper Res Health Care. 2016;11:20–34.
Maroufkhani A, Lanzarone E, Castelnovo C, Di Mascolo M. A discrete event simulation model for the admission of patients to a home care rehabilitation service. In: Matta A, Sahin E, Li J, Guinet A, Vandaele NJ, editors. Health care systems engineering for scientists and practitioners. New York: Springer; 2016. p. 91–100.
Bhattacharjee P, Ray PK. Patient flow modelling and performance analysis of healthcare delivery processes in hospitals: a review and reflections. Comput Ind Eng. 2014;78:299–312.
Hu S, Heim JA. Developing domain-specific simulation objects for modeling clinical laboratory operations. In: Proceedings of the winter simulation conference; 2014. p. 1341–52.
Workman RW. Simulation of the drug development process: a case study from the pharmaceutical industry. In: Proceedings of the winter simulation conference; 2000. p. 1995–8.
Blau GE, Pekny JF, Varma VA, Bunch PR. Managing a portfolio of interdependent new product candidates in the pharmaceutical industry. J Prod Innov Manag. 2004;21:227–45.
Chen Y, Mockus L, Orcun S, Reklaitis GV. Simulation-optimization approach to clinical trial supply chain management with demand scenario forecast. Comput Chem Eng. 2012;40:82–96.
Perez-Escobedo JL, Azzaro-Pantel C, Pibouleau L. Multiobjective strategies for New Product Development in the pharmaceutical industry. Comput Chem Eng. 2012;37:278–96.
Chen Y, Pekny JF, Reklaitis GV. Integrated planning and optimization of clinical trial supply chain system with risk pooling. Ind Eng Chem Res. 2013;52:152–65.
El Afia A, Mezouar H. A global mapping of the Moroccan supply chain of hospital drugs, and a simulation of the dispensation process. In: Proceedings of the international conference on big data, cloud and applications; 2017.
Huyghe J, Nouwen M, Vanattenhoven J. Involving end-users in game based ideation: a case study in hospital logistics. In: Proceedings of the Nordic conference on human-computer interaction; 2016.
Best AM, Dixon CA, Kelton WD, Lindsell CJ, Ward MJ. Using discrete event computer simulation to improve patient flow in a Ghanaian acute care hospital. Am J Emerg Med. 2014;32:917–22.
Caputo AC, Pelagagge PM. Management criteria of automated order picking systems in high-rotation high-volume distribution centers. Ind Manag Data Syst. 2006;106:1359–83.
Katsaliaki K, Brailsford SC. Using simulation to improve the blood supply chain. J Oper Res Soc. 2007;58:219–27.
Vila-Parrish AR, Ivy JS, King RE. A simulation-based approach for inventory modeling of perishable pharmaceuticals. In: Proceedings of the winter simulation conference; 2008. p. 1532–8.
Jung JY, Blau G, Pekny JF, Reklaitis GV, Eversdyk D. Integrated safety stock management for multi-stage supply chains under production capacity constraints. Comput Chem Eng. 2008;32:2570–81.
Mustafee N, Taylor SJE, Katsaliaki K, Brailsford S. Facilitating the analysis of a UK national blood service supply chain using distributed simulation. Simulation. 2009;85:113–28.
Rossetti MD, Liu Y. Simulating SKU proliferation in a health care supply chain. In: Proceedings of the winter simulation conference; 2009. p. 2365–74.
Ren C, Wang W, He M, Shao B, Wang Q, Dong J. The use of simulation for Global Supply Network rationalization. In: Proceedings of IEEE international conference on service operations and logistics, and informatics. 2010. p. 276–81.
Babaï MZ, Syntetos AA, Dallery Y, Nikolopoulos K. Dynamic re-order point inventory control with lead-time uncertainty: analysis and empirical investigation. Int J Prod Res. 2009;47:2461–83.
Baesler F, Bastías A, Nemeth M, Martínez C. Blood centre inventory analysis using discrete simulation. In: Proceedings of the winter simulation conference; 2012.
Onggo BS. Elements of a hybrid simulation model: a case study of the blood supply chain in low-and middle-income countries. In: Proceedings of the winter simulation conference; 2014. p. 1597–607.
Gebicki M, Mooney E, Chen S-J, Mazur LM. Evaluation of hospital medication inventory policies. Health Care Manag Sci. 2014;17:215–29.
Duan Q, Liao TW. Optimization of blood supply chain with shortened shelf lives and ABO compatibility. Int J Prod Econ. 2014;153:113–29.
Baesler F, Nemeth M, Martínez C, Bastías A. Analysis of inventory strategies for blood components in a regional blood center using process simulation. Transfusion. 2014;54:323–30.
Wang K-M, Ma Z-J. Age-based policy for blood transshipment during blood shortage. Transp Res Part E Logist Transp Rev. 2015;80:166–83.
Leung N-HZ, Chen A, Yadav P, Gallien J. The impact of inventory management on stock-outs of essential drugs in Sub-Saharan Africa: secondary analysis of a field experiment in Zambia. PLoS One. 2016;11:e0156026.
Article CAS PubMed PubMed Central Google Scholar
Yurtkuran A, Emel E. Simulation based decision-making for hospital pharmacy management. In: Proceedings of the winter simulation conference; 2008. p. 1539–46.
Lee YM. Analyzing dispensing plan for emergency medical supplies in the event of bioterrorism. In: Proceedings of the winter simulation conference; 2008. p. 2600–8.
Lee YM, Ghosh S, Ettl M. Simulating distribution of emergency relief supplies for disaster response operations. In: Proceedings of the winter simulation conference; 2009. p. 2797–808.
Ozdamar L. Planning helicopter logistics in disaster relief. Spectrum. 2011;33:655–72.
Kulkarni NS, Niranjan S. Multi-echelon network optimization of pharmaceutical cold chains: a simulation study. In: Proceedings of the winter simulations conference; 2013. p. 3486–98.
Postacchini L, Ciarapica FE, Bevilacqua M, Mazzuto G, Paciarotti C. A way for reducing drug supply chain cost for a hospital district: a case study. J Ind Eng Manag. 2016;9:207–30.
Abdelkafi C, Beck BHL, David B, Druck C, Horoho M. Balancing risk and costs to optimize the clinical supply chain-a step beyond simulation. J Pharm Innov. 2009;4:96–106.
Alfonso E, Xie X, Augusto V, Garraud O. Modelling and simulation of blood collection systems: improvement of human resources allocation for better cost-effectiveness and reduction of candidate donor abandonment. Vox Sang. 2013;104:225–33.
Article CAS PubMed Google Scholar
Cho S-H, Jang H, Lee T, Turner J. Simultaneous location of trauma centers and helicopters for emergency medical service planning. Oper Res. 2014;62:751–71.
Liao H-C, Chang H-H. The optimal approach for parameter settings based on adjustable contracting capacity for the hospital supply chain logistics system. Expert Syst Appl. 2011;38:4790–7.
Akcay A, Martagan T. Stochastic simulation under input uncertainty for contract-manufacturer selection in pharmaceutical industry. In: Proceedings of the winter simulation conference; 2016. p. 2292–303.
Jacobs EA, Bickel WK. Modeling drug consumption in the clinic using simulation procedures: demand for heroin and cigarettes in opioid-dependent outpatients. Exp Clin Psychopharmacol. 1999;7:412–26.
Royston G, Dost A, Townshend J, Turner H. Using system dynamics to help develop and implement policies and programmes in health care in England. Syst Dyn Rev. 1999;15:293–313.
Mustafee N, Taylor SJE, Katsaliaki K, Brailsford S. Distributed simulation with COTS simulation packages: a case study in health care supply chain simulation. In: Proceedings of the winter simulation conference; 2006. p. 1136–42.
Muller J, Popke C, Urbat M, Zeier A, Plattner H. A simulation of the pharmaceutical supply chain to provide realistic test data. In: Proceedings of the international conference on advances in system simulation; 2009. p. 44–9.
Devi SP, Rao KS, Krishnaswamy S, Wang S. System dynamics model for simulation of the dynamics of corneal transplants. OPSEARCH. 2010;47:284–92.
Ng Adam TS, Sy C, Li J. A system dynamics model of Singapore healthcare affordability. In: Proceedings of the winter simulation conference; 2011. p. 1306–18.
Djanatliev A, German R, Kolominsky-Rabas P, Hofmann BM. Hybrid simulation with loosely coupled system dynamics and agent-based models for prospective health technology assessments. In: Proceedings of the winter simulation conference; 2012.
zen MB. Estimating future demand for hospital emergency services at the regional level. In: Proceedings of the winter simulation conference; 2013. p. 2386–97.
Elleuch H, Hachicha W, Chabchoub H. A combined approach for supply chain risk management: description and application to a real hospital pharmaceutical case study. J Risk Res. 2014;17:641–63.
Padilla JJ, Diallo SY, Kavak H, Sahin O, Sokolowski JA, Gore RJ. Semi-automated initialization of simulations: an application to healthcare. J Def Model Simul. 2015;13:171–82.
Taaffe K, Zinouri N, Kamath AG. Integrating simulation modeling and mobile technology to improve day-of-surgery patient care. In: Proceedings of the winter simulation conference; 2016. p. 2111–2.
Pitt M, Monks T, Crowe S, Vasilakis C. Systems modelling and simulation in health service design, delivery and decision making. BMJ Qual Saf. 2016;25:38–45.
Jahangirian M, Borsci S, Shah SGS, Taylor SJE. Causal factors of low stakeholder engagement: a survey of expert opinions in the context of healthcare simulation projects. Simulation. 2015;91:511–26.
Dangerfield BC. System dynamics applications to European health care issues. J Oper Res Soc. 1999;50:45–353.
Harrell CR, Price RN. Healthcare simulation modeling and optimization using MedModel. In: Proceedings of the winter simulation conference; 2000. p. 203–7.
Joustra P, van der Sluis E, van Dijk NM. To pool or not to pool in hospitals: a theoretical and practical comparison for a radiotherapy outpatient department. Ann Oper Res. 2010;178:77–89.
Morrison BP, Bird BC. Healthcare process analysis: a methodology for modeling front office and patient care processes in ambulatory health care. In: Proceedings of the winter simulation conference; 2003. p. 1882–6.
Baldwin LP, Eldabi T, Paul RJ. Simulation in healthcare management: a soft approach (MAPIU). Simul Model Pract Theory. 2004;12:541–57.
White KP. A survey of data resources for simulating patient flows in healthcare delivery systems. In: Proceedings of the winter simulation conference; 2005. p. 926–35.
Brailsford S. Overcoming the barriers to implementation of operations research simulation models in healthcare. Clin Investig Med Med Clin Exp. 2005;28:312–5.
Eldabi T, Paul RJ, Young T. Simulation modelling in healthcare: reviewing legacies and investigating futures. J Oper Res Soc. 2007;58:262–70.
Chahal K, Eldabi T. Applicability of hybrid simulation to different modes of governance in UK healthcare. In: Proceedings of the winter simulation conference; 2008. p. 1469–77.
Gupta D, Denton B. Appointment scheduling in health care: challenges and opportunities. IIE Trans. 2008;40:800–19.
Brailsford SC, Harper PR, Patel B, Pitt M. An analysis of the academic literature on simulation and modelling in health care. J Simul. 2009;3:130–40.
Katsaliaki K, Mustafee N, Taylor SJE, Brailsford S. Comparing conventional and distributed approaches to simulation in a complex supply-chain health system. J Oper Res Soc. 2009;60:43–51.
Forsythe L. Action research, simulation, team communication, and bringing the tacit into voice society for simulation in healthcare. Simul Healthc. 2009;4:143–8.
Seropian M, Lavey R. Design considerations for healthcare simulation facilities. Simul Healthc. 2010;5:338–45.
Katsaliaki K, Mustafee N. Applications of simulation within the healthcare context. J Oper Res Soc. 2011;62:1431–51.
LeBlanc VR, Manser T, Weinger MB, Musson D, Kutzin J, Howard SK. The study of factors affecting human and systems performance in healthcare using simulation. Simul Healthc. 2011;6:24–9.
Beliën J, Forcé H. Supply chain management of blood products: a literature review. Eur J Oper Res. 2012;217:1–16.
Jahangirian M, Naseer A, Stergioulas L, Young T, Eldabi T, Brailsford S, et al. Simulation in health-care: lessons from other sectors. Oper Res. 2012;12:45–55.
Hong TS, Shang PP, Arumugam M, Yusuff RM. Use of simulation to solve outpatient clinic problems: a review of the literature. South Afr J Ind Eng. 2013;24:27–42.
Holm LB, Dahl FA. Simulating the effect of physician triage in the emergency department of Akershus University Hospital. In: Proceedings of the winter simulation conference; 2009. p. 1896–905.
Günal MM, Pidd M. DGHPSim: supporting smart thinking to improve hospital performance. In: Proceedings of the winter simulation conference; 2008. p. 1484–9.
Yeon N, Lee T, Jang H. Outpatients appointment scheduling with multi-doctor sharing resources. In: Proceedings of the winter simulation conference; 2010. p. 3318–29.
Sugiyama T, Goryoda S, Inoue K, Sugiyama-Ihana N, Nishi N. Construction of a simulation model and evaluation of the effect of potential interventions on the incidence of diabetes and initiation of dialysis due to diabetic nephropathy in Japan. BMC Health Serv Res. 2017;17:833.
Download references
This work is supported by the Stockholm County Council, in the design of the study and collection, analysis, interpretation of data, and writing the manuscript.
Availability of data and materials
The datasets used and/or analyzed during the current study are available from the corresponding author on reasonable request.
Author information
Authors and affiliations.
School of Engineering Sciences in Chemistry, Biotechnology and Health, Royal Institute of Technology, 2010, Röntgenvägen 1, 14152, Huddinge, Sweden
School of Engineering Sciences in Chemistry, Biotechnology and Health, Royal Institute of Technology, Hälsovägen 11, 14152, Huddinge, Sweden
Thomas Grandits & Sebastiaan Meijer
Pediatric Emergency Department, Karolinska University Hospital, Tomtebodavägen 18a, 17177, Stockholm, Sweden
Karin Pukk Härenstam
Department of Learning, Informatics, Management and Ethics, Karolinska Institute, Tomtebodavägen 18a, 17177, Stockholm, Sweden
School of Industrial Engineering and Management, Royal Institute of Technology, Mariekällgatan 3, 15144, Södertälje, Sweden
Jannicke Baalsrud Hauge
You can also search for this author in PubMed Google Scholar
Contributions
CZ, TG, and SM designed the study and located the articles from the databases. CZ and TG performed the screening process. CZ, TG, SM, KH, and JH analyzed and interpreted the systematic review results. CZ and TG were responsible for writing the paper. KH, JH, and SM were the major contributors for revising the manuscript. All authors read and approved the final manuscript.
Corresponding author
Correspondence to Chen Zhang .
Ethics declarations
Ethics approval and consent to participate.
Not applicable
Consent for publication
Competing interests.
The authors declare that they have no competing interests.
Publisher’s Note
Springer Nature remains neutral with regard to jurisdictional claims in published maps and institutional affiliations.
Rights and permissions
Open Access This article is distributed under the terms of the Creative Commons Attribution 4.0 International License ( http://creativecommons.org/licenses/by/4.0/ ), which permits unrestricted use, distribution, and reproduction in any medium, provided you give appropriate credit to the original author(s) and the source, provide a link to the Creative Commons license, and indicate if changes were made. The Creative Commons Public Domain Dedication waiver ( http://creativecommons.org/publicdomain/zero/1.0/ ) applies to the data made available in this article, unless otherwise stated.
Reprints and permissions
About this article
Cite this article.
Zhang, C., Grandits, T., Härenstam, K.P. et al. A systematic literature review of simulation models for non-technical skill training in healthcare logistics. Adv Simul 3 , 15 (2018). https://doi.org/10.1186/s41077-018-0072-7
Download citation
Received : 06 March 2018
Accepted : 25 June 2018
Published : 27 July 2018
DOI : https://doi.org/10.1186/s41077-018-0072-7
Share this article
Anyone you share the following link with will be able to read this content:
Sorry, a shareable link is not currently available for this article.
Provided by the Springer Nature SharedIt content-sharing initiative
- Logistical simulations
- Non-technical skills
Advances in Simulation
ISSN: 2059-0628
- General enquiries: [email protected]
An official website of the United States government
Official websites use .gov A .gov website belongs to an official government organization in the United States.
Secure .gov websites use HTTPS A lock ( Lock Locked padlock icon ) or https:// means you've safely connected to the .gov website. Share sensitive information only on official, secure websites.
- Publications
- Account settings
- Advanced Search
- Journal List

Simulation modeling to assess performance of integrated healthcare systems: Literature review to characterize the field and visual aid to guide model selection
Nicolas larrain, oliver groene.
- Author information
- Article notes
- Copyright and License information
Competing Interests: The authors declare no conflict of interest. Both authors are employed at OptiMedis AG, a commercial company. This does not alter our adherence to PLOS ONE policies on sharing data and materials.
* E-mail: [email protected]
Received 2020 Apr 15; Accepted 2021 Jun 28; Collection date 2021.
This is an open access article distributed under the terms of the Creative Commons Attribution License , which permits unrestricted use, distribution, and reproduction in any medium, provided the original author and source are credited.
The guiding principle of many health care reforms is to overcome fragmentation of service delivery and work towards integrated healthcare systems. Even though the value of integration is well recognized, capturing its drivers and its impact as part of health system performance assessment is challenging. The main reason is that current assessment tools only insufficiently capture the complexity of integrated systems, resulting in poor impact estimations of the actions taken towards the ‘Triple Aim’. We describe the unique nature of simulation modeling to consider key health reform aspects: system complexity, optimization of actions, and long-term assessments.
Research question
How can the use and uptake of simulation models be characterized in the field of performance assessment of integrated healthcare systems?
A systematic search was conducted between 2000 and 2018, in 5 academic databases (ACM D. Library, CINAHL, IEEE Xplore, PubMed, Web of Science) complemented with grey literature from Google Scholar. Studies using simulation models with system thinking to assess system performance in topics relevant to integrated healthcare were selected for revision.
After screening 2274 articles, 30 were selected for analysis. Five modeling techniques were characterized, across four application areas in healthcare. Complexity was defined in nine aspects, embedded distinctively in each modeling technique. ‘What if?’ & ‘How to?’ scenarios were identified as methods for system optimization. The mean time frame for performance assessments was 18 years.
Conclusions
Simulation models can evaluate system performance emphasizing the complex relations between components, understanding the system’s adaptability to change in short or long-term assessments. These advantages position them as a useful tool for complementing performance assessment of integrated healthcare systems in their pursuit of the ‘Triple Aim’. Besides literacy in modeling techniques, accurate model selection is facilitated after identification and prioritization of the complexities that rule system performance. For this purpose, a tool for selecting the most appropriate simulation modeling techniques was developed.
1. Introduction
The guiding principle of many health care reforms is to overcome fragmentation of service delivery and work towards integrated healthcare systems (IHS) [ 1 , 2 ]. Integrated healthcare comes in the form of linkage and coordination of providers along the continuum of care [ 3 ]. By focusing on the nature and strength of the links between the system components, IHS rely on and enhance the complexity of the health system [ 4 ] to achieve a threefold objective; improve health of the population, improve patient (and carer) experience while reducing healthcare costs (‘Triple Aim’) [ 5 ]. To reach the Triple Aim, IHS introduce solutions to ensure the sustainability of health care provision through investment in preventive care and constant improvements in clinical practice [ 3 ].
IHS success has been evidenced in numerous publications. However, healthcare managers encounter problems when assessing the drivers of this success [ 6 – 8 ]. These challenges arise because assessment tools are not specific to integrated care and don’t consider the unique nature of the approach [ 9 , 10 ]. Acknowledging a lack of specific assessment tools, the Expert Group on Health System Performance Assessment of the EU created a standard framework for performance assessment of integrated care [ 3 , 6 , 7 ]. The framework consists of a series of key performance indicators in topics relevant to IHS. However, even though specific to IHS, the indicators compiled by the expert group are insufficient to capture the full value of integrated care. The problem is not in the completeness of the indicator list, but in monitoring indicators as a performance assessment approach. Indicators are developed based on assumptions about the interrelation between a measure and the system objectives [ 11 ], but the causal pathways are not described and are known to be multiple, non-linear, with changing causal effects and affected by several individuals and contextual factors [ 12 , 13 ]. In other words, the traditional approach can’t capture the complexity of the health system [ 4 ]. Because IHS enhance system complexity and strive for efficiency and accountability in every component of the system, indicators alone are insuficient to guide improvement [ 9 , 12 ].
Complex health policy issues can be better assessed with methods that enable research synthesis and utilize a complex systems perspective [ 14 , 15 ]. As defined by Petticrew et al. (2018) [ 15 ]; a complex system perspective (or just ‘systems thinking’) is defined by acknowledging the value arising from the relationships between the system components and their dynamic properties. When used for evaluating system performance, system thinking answers an essential concern for IHS ‘how did the intervention reshape the system in favorable ways?’.
Simulation modeling (SM) is a discipline with the features necessary to implement systems thinking when assessing performance of a health system [ 14 , 16 , 17 ]. A simulation is a virtual recreation of a real system. It is used to test situations and understand the effect of interventions on the performance of the system over time. Combining expert opinion with observational and experimental results, SM provides a relatively inexpensive way to estimate individual and population-level effects of changes in the system’s determinants of performance.
There is extensive literature reviewing simulation models in the healthcare sector. Salleh et al. [ 18 ] published an umbrella review including 37 reviews, that together cover articles from 1950 to 2016 and explore the wide range of applications in healthcare, software tools and data sources used in the field of healthcare simulation. Meanwhile, the paper by Günal & Pidd [ 19 ] starts by narrating the historic progression of simulation modeling an its applications in healthcare, giving some idea of the long history of the field. Most recently (2021), Roy et al. [ 17 ] analyzed healthcare simulation literature of the past decade, addressing issues in various healthcare service delivery levels and categorizing the literature accordingly. Altogether, literature in the field provides a comprehensive characterization of simulation models in healthcare, including; the areas and types of application where the discipline has been used, the techniques available, data sources, simulation software [ 18 , 20 , 21 ], type of outputs and level of insight, inputs and resources required [ 22 ], relative frequency of use and level of implementation [ 23 ] and specific aspects of a care facility operations where techniques are most common [ 17 , 24 , 25 ]. These topics are most commonly analyzed following a structure similar to the one best represented by Mielczarek et al. [ 25 ], who creates a system of classification of health care topic areas assessed with simulation methods. The objective is to investigate the usefulness of modeling techniques and their correlation with a corresponding health care application. While authors add innovations to this common structure, such as the identification of research gaps influencing the limited uptake of the discipline [ 20 , 23 , 24 , 26 ] or exploring the link between interventions and key performance indicators (KPI) [ 26 ], complexities in the relationships of system components have been heavily underassessed. Roy [ 17 ] recognizes the complexity of the health system and the ability of simulation modeling to address this complexity, but his review focuses on capturing specific health issues addressed, operations management concepts applied, simulation methods used, and identifying major research gaps—a framework similar to the one by Mielczarek et al. [ 25 ]. Vanbrabant et al. [ 26 ] also acknowledges simulations as the technique most suitable to capture the randomness and complexity of patient flow through the emergency department. But the analysis is limited to providing insights into which interventions influence which KPI. In the same line, Laker [ 27 ] also recognizes the usefulness of simulation models to integrate complexity, and provides an excellent summary of the properties of four simulation techniques. However, it fails on providing a common framework to characterize and contrast the complexities that can be represented in each technique. Complexity is also indirectly mentioned in identified research gaps, when both Vanbrand et al. and Yousefi et al. [ 20 ] state the underuse of simulation models in multi-objective evaluations and Brailsford et al. and Roy et al. [ 17 , 24 ] suggests that healthcare is an area of application for hybrid simulation due in part to increasing system complexity.
By overlooking complexity, the advantages of simulation modeling and the challenges of IHS performance assessment remain unmatched. Furthermore, simulation time frames and optimization capabilities, standard knowledge for simulation experts but not for healthcare managers [ 28 ], are also overlooked in reviews summarizing the use of SM in healthcare. The gap results in simulation models not been systematically picked up by integrated healthcare managers to assess performance of IHS. The issue was partially addressed in 2015 by the ISOPOR task force [ 14 , 29 ], who published a series of papers describing how three of the most common simulation modeling techniques can be used to evaluate complex health systems and provide descriptions and tools to implement them accurately. However, at the time there was no common understanding of the drivers affecting IHS performance hence a clear explanation and exemplification of how these particularly complex health systems could make use of simulation models to assess performance was not possible.
This literature review is intended to bring together the field of performance assessment of integrated healthcare systems and the discipline of simulation modeling. We contribute to the vast literature characterizing the use of simulation modeling in health system performance assessment by focusing specifically on the discipline’s ability to implement a complex system perspective in topics relevant to IHS. Our research is directed to readers that seek to expand performance assessment tools while considering the enhanced complexity embedded in the integrated care approach. We conclude our analysis with the creation of a practical tool for selecting the most appropriate simulation modeling technique depending on the characteristics of the system to be modeled.
2.1 Search strategy
A comprehensive search strategy was performed directed to find articles that allowed us to understand how simulation modeling techniques implemented a complex system perspective in topics relevant to IHS. The systematic search was conducted in 5 academic databases (ACM Digital Library, CINAHL, IEEE Xplore, PubMed & Web of Science). Grey literature was searched for in Google Scholar and only considered if articles complied with all the criteria in the AACODS checklist for critically appraising grey literature [ 30 ]. Finally, papers were also added through snowballing. The search was conducted for the period 01/01/2000–31/12/2018 as an increased interest in SM has been documented after this starting date, supported by technology advances [ 18 ]. The review was registered in PROSPERO (Registration number: CRD42020149658).
A Boolean search code was developed with three scopes of terms. The first scope, “ Technique ”, filters for simulation modeling techniques and combines 17 systematic search strategies extracted from the umbrella review by Salleh et al. [ 18 ] added to the list of simulation modeling techniques described in Jun et al. [ 22 ]. The second scope, “ Integrated healthcare systems topics of interest ” is defined by 76 search terms, extracted from the indicator types and domains stated in the framework for performance assessment of IHS developed by the Expert Group of the European Commission [ 3 , 6 , 7 ], the systematic review of methods for IHS performance assessment by Strandberg-Larsen et al. [ 31 ] and the “Care Coordination Measures Atlas” by McDonald et al. [ 32 ]. Finally, the third scope refers to the healthcare sector. Terms in the first scope (“ Techniques ”) were restricted to the title, and terms in the other scopes were restricted to title/abstract. The complete list of terms can be found in S1 Table .
2.2 Selection criteria
Inclusion criteria.
Only health system evaluations taking a complex system perspective were considered. Furthermore, we only included articles that used a simulation model in the list of techniques described in Salleh et al. [ 18 ] or in Jun et al. [ 22 ] as SM techniques or self-identified as such. Finally, articles further had to address the performance assessment of an IHS topic-of-interest. The lists of SM techniques and IHS topics of interest can be found in S2 Table .
Exclusion criteria
We excluded studies that described non-computer-based simulation models. Also, we excluded studies that were not calibrated and validated against data from a real situation. Finally, we excluded from the data extraction and analysis studies whose reporting standards were insufficient to replicate the assessment or did not fully enable reviewers the complete understanding of the implementation of systems thinking. To comply with the latter criteria, only studies graded ‘A’ in quality assessment were selected.

2.3 Quality assessment
Two independent reviewers (SW & NL) assessed the quality of papers during the screening process. Using the quality assessment tool developed by Fone et al. [ 33 ] to appraise simulation modeling studies, reviewers gave a score of 0, 1, or 2 in ten criteria and created four quality groups (A to D). The quality assessment was followed with an assessment of the credibility and relevance of the articles for the purpose of this review and aided reviewers to select articles for revision. Given the focus of the review, an assessment of the risk of bias in the study’s results was not considered.
2.4 Data extraction and analysis
Data extraction was made by the main author (NL), based on the template used by Brailsford et al. in their analysis of simulation and modeling techniques for healthcare [ 23 ]. The final extraction sheet was modified focusing on two main topics. First, to characterize the different modeling techniques, their area of application, key features for implementation, together with data requirements and outcomes. Second, to characterize the complex aspects of the health system that each technique can represent. The detailed data extraction sheet can be found in S3 Table .
The analysis was conducted in two phases. First, using a ‘Deductive a priori template approach’ [ 34 ] articles were classified and characterized according to previous assessments of SM made by Jun et al. [ 22 ], Salleh et al. [ 18 ], and Rueckel et al. [ 21 ]. Subsequently, in a ‘Data-driven inductive approach’ [ 35 ], simulation modeling techniques were re-characterized in five items following the objectives of this review. Item (1.) presents the IHS topics-of-interest where SM has been successfully applied. The item aims to inform and exemplify in what situation of interest to IHS can the discipline be useful, in a similar structure of the analysis of previous literature. Even though the selection of articles is primarly intended to understand simulation models’ ability to integrate the shortcomings of IHS performance assessment, and not to identify the link between simulation technique and helathcare area, a similar analysis to that of previous literature will allow us to validate our findinds when compared to conclusions of other authors. In item (2.) we supported the analysis of the reviewed papers with further literature and present an introductory description of the identified simulation techniques, explaining how they are applied in the topics of interest to IHS. The last three items were selected to explore how simulation models deal with challenges that are particularly harmful to integrated care and are not yet mirrored in traditional assessment tools [ 8 ]. Item (3.) presents the modeled complexities in relationships between system components, summarized per modeling technique. The item allows us to understand the capacities of each technique to correctly model causality paths and co-existing effects, essential concerns for several integrated care interest areas [ 8 , 10 ]. Item (4.) presents the identified optimization capabilities, essential function of any tool guiding healthcare to the Triple Aim [ 3 , 10 , 11 , 36 ]. Preventive medicine and overall population health improvements are known to show effects only after several years after intervention [ 7 ] and as they comprise an essential part of IHS, Item (5.) presents the time frame of the selected papers to understand the capacity for long-term assessments.
The search resulted in 2271 unique articles. Screenings at title/abstract and full text were made by two separate reviewers (SW & NL) and resulted in seventy-six articles selected for quality assessment. Out of these, thirty studies were included for data extraction and analysis because of their reporting quality and detailed description of system thinking. Fig 1 presents the PRISMA diagram of the selection process. Selected papers are described in Table 1 .
Fig 1. PRISMA diagram.
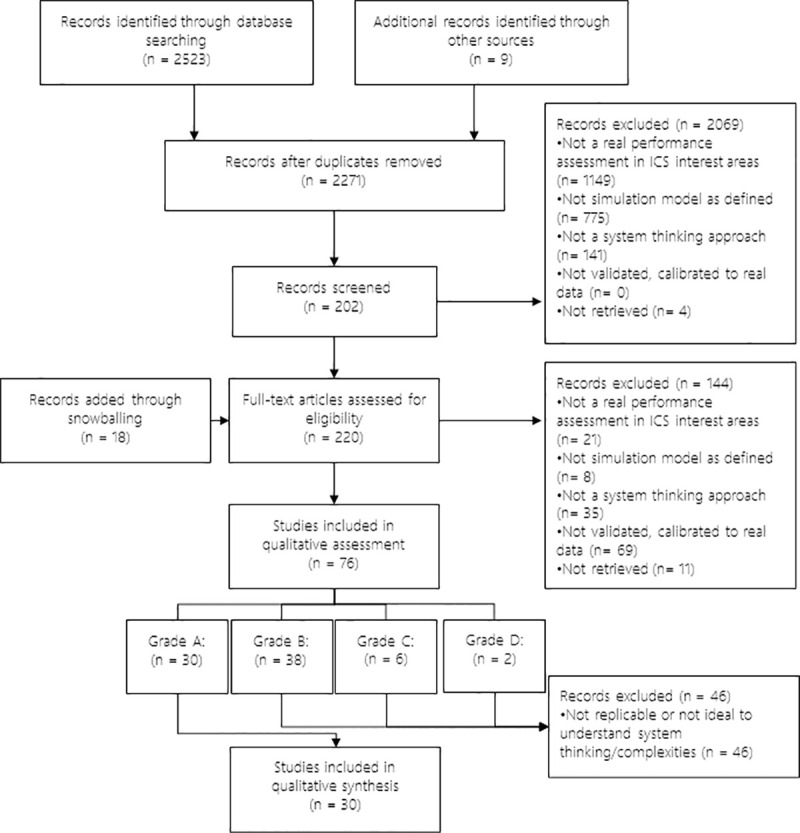
Table 1. Selected papers.
* The model has been used in several projects and the time provided corresponds the one used most recently by Vidyanti et al. 2015 [ 67 ]
3.1 IHS topics-of-interest
Eleven IHS topics-of-interest were identified and classified in four areas of assessment. The first area of assessment covers simulation models of Policy and Strategy. This comprises studies that use simulation modeling for evaluating health policies and interventions directed to change or improve the structure, assess incentives, goals, or values in the overall system; such as (1.) pay for performance incentive scheme or (2.) national health reform evaluation. The second area of assessment covers Chronic Disease Management. Studies in this area evaluated the effectiveness of interventions or the evolution of chronic conditions, such as (3.) evaluating care management and interventions of chronic conditions and (4). diabetes population dynamics. The third area of assessment addresses Lifestyle Interventions, including evaluation of interventions directed at lifestyle behavior, health risks, and social determinants of health, such as (5.) tobacco harm policies, market control, and interventions or (6.) evaluation of public health interventions. The last area of assessment addresses Health Resource Management and comprises studies that use SM for resource management or system design to optimize healthcare service flow or forecast demands. In this area topics were (7.) performance evaluation of community health, (8.) performance measures evaluation in outpatient center, (9.) health facility operations simulation, (10.) planning health force, and (11.) evaluation of information systems.
3.2 Description of simulation modeling techniques in IHS
Five simulation modeling techniques were identified in the selected articles: Two Markov Models (MM), eleven System Dynamics (SD), two Micro-Simulations (MS), twelve Discrete Event Simulations (DES), and two Agent-Based Models (ABM). Finally, one paper combined three techniques, adding a sixth, Hybrid models (HM). Supported by complementary literature, we describe each technique features and use the selected papers to exemplify their implementation in integrated care. Table 2 summarizes the simulation techniques in terms of strengths, limitations, and estimation considerations and provides references for complementary literature.
Table 2. Summary descriptions of simulation modeling techniques.
Markov models.
Markov models are state transition models. They have clearly defined, exclusive states, and transitions between states are defined as quantities per cycle. States cannot happen simultaneously for the same agent and transitions from one state to another depend only on the current state (Markovian property). Time can be continuous or discrete, but in the case of this review, both papers use discrete time. Markov models can define transition probabilities differently for each time step, allowing the inclusion of trend factors, and together with ‘tunnel states’ (states with no possibility of remaining in the said state in time) time-depending dynamism and partial influence of historic events are enabled. Laurence et al. [ 53 ] explore the complexity of state transitions by constructing a model comprised of four separate parts (demand, supply, productivity, and training) of the system determining the health force gap, a common topic on integrated care initiatives. The demand and training parts of the model define partial outcomes dependent on several variables. These outcomes are then used in a second stage for the supply and productivity parts of the model, resulting in further partial outcomes. The third stage studies the main outcome (workforce gap) influenced by the outcomes of the previous stages. The structure enables the inclusion of mediated relationships between the initial variables, their interaction with partial outcomes, and the main outcome. The SimSmoke simulation, presented in Levy et al. [ 56 ] was developed in the early 2000s to estimate the smoking population and the effects of possible lifestyle interventions. The model distinguishes a population by age and gender evolving through birth and death rates. The population is further divided into never, current, and former smokers. By differentiating models for different strata of the population and including tunnel states, the author can represent the influence of historical events, having portions of the population ‘jumping’ to the next model when an event happens.
System dynamics
The objective of system dynamics is to capture all determinant variables, causal pathways, and feedback loops of the system to be analyzed [ 48 ]. In SD structure determines performance, and the primarily goal is to evaluate the effect of an intervention over the qualitative nature of system performance (e.g. growth function, overshoot and collapse, oscillations, chaotic response, etc.) [ 27 , 68 ]. To conceptualize the structure, relevant elements and the direction and nature of their inter-relations must be known. This information is extracted from the system’s stakeholders underlying knowledge of the way the system operates [ 37 , 59 ]. This way, Homer et al. [ 48 ] and Loyo et al. [ 57 ] integrate the most important risk factors of several chronic diseases in a single model. The model calculates the expected prevalence and indirect cost effect of these diseases in the population. Milstein et al. [ 59 ] include all relevant causal pathways related to health reform policies in the US. Kang et al. [ 51 ] and Sugiyama et al. [ 65 ] use the same approach to model the care of chronic kidney disease and the effect of interventions over diabetes and dialysis. The inclusion of all known determinants and causal pathways is complemented with the possibility to include “soft” variables, enabling the exploration of aspects of a system behavior particularly relevant to integrate care such as “Gaming”, “Extrinsic motivation” [ 37 ], “Insurance complexity”, “Care coordination” [ 59 ], “Staff resistance to new policies” or “Workload pressure” [ 63 ]. This flexibility is essential to capture the influence of important variables but limits the statistical validity of the results [ 69 ]. Loyo et al. [ 57 ] undermine this limitation stating that `community decisions need to be made even though the data are disparate and incomplete’.
The model structure is represented in a causal loop diagram. There is a special focus on capturing the correct feedback loops affecting the system behavior. Feedback loops are what makes the system dynamic, by influencing the nature of the relationship between variables as the system progresses.
In the area of chronic disease management, Jones et al. [ 49 ] use causal loops to model the states of the disease itself, understanding that a key determinant in diabetes care is the reinforcement loop generated by the relation between the disease diagnosis behavior and detrimental consequences. When assessing the effect of a new nicotine product, Hill et al. [ 47 ] integrate the feedback effect of ‘normality of smoking’ to predict smoking initiation and quitting rates, while Alonge et al. [ 37 ] introduce the negative feedback loop of gaming to understand the failure of a pay for performance incentive scheme in Afghanistan.
The structure of the system is transformed into a stock-and-flow-diagram, defining the nature of the elements presented in the causal loop diagram. Stocks (elements that accumulate value) and variables that influence flows (functions that determine the growth or decline of the value in stock) are differentiated. Functions are established for flows and initial quantities are assigned to stocks, so that differential equations can be used to determine the values in the stocks over time. Ansah et al. [ 38 ] uses this structure to set up the labor market for long term care, and uses a deterministic approach to study the effect of policies to reduce unwanted market disturbances. de Andrade et al. [ 41 ] use system dynamics to represent the different stages of the maturing process related to the management of a myocardial infarction case in a hospital environment. This type of structure is known as “Aging Chains” and is useful to gather information about how long the modeled entity stays in each stage and test delays-improving policies.
Microsimulations
As Markov Models, microsimulations are also state transition models, but they describe the population dynamics at individual levels and can be used to describe interactions between policies and individual decision-making units [ 70 ]. As state transition models, they are structured by clearly defined states. Transitions between states are generated by stochastic processes out of the parametrization of transition evidence, differentiating from the rational responses following an objective of Agent-Based Models or the time to event of Discrete Event Simulations [ 70 , 71 ]. Even though the structure is similar to Markov models, they do not share some of the limitations. Besides the interaction of relevant variables, the individual approach adds the possibility of including ‘tracking variables’, to account for historical occurrences. Modeling the complexity of factors contributing to health care cost is the key objective of the “Future elderly model” created by Goldman et al. [ 45 ]. In said model, individualization and influence of historical occurrences allows for the inclusion of a multidimensional characterization of health status accounting for risk factors such as smoking, weight, age and education, along with lagged health and financial states. In their dynamic form, microsimulation models allow individuals to change their characteristics due to endogenous factors within the model [ 72 ]. In this sense, they are more suitable for modeling processes and large population dynamics, like the model Lay-Yee et al. [ 54 ] uses for estimating child health utilization. The authors modeled a child with a set of attributes as a starting point. Using equations derived from statistical analysis of real longitudinal data, they set the rules for the individual in the system and stochastically simulate changes in status over time. In other words, the model generates a set of diverse synthetic health histories for a starting sample of children. Then it uses the simulated sample as a counterfactual for estimation including the effect of interventions.
Discrete event simulation
Discrete event simulation is a process-centric simulation methodology that describes a chronological sequence of events affecting an entity. The entity (e.g., patients) carries its information, individualizing the type of relationship with each event. Vataire et al. [ 66 ] and Cooper et al. [ 40 ] use this characteristic for individualizing treatments for major depressive disorder, and to realistically assess the response to the prescription of prevention drugs for cardiovascular disease, respectively. All occurrences are registered in the entity’s information, enabling the influence of historical events in future outcomes [ 74 ]. Getsios et al. [ 44 ] use this feature to model the effect of smoking cessation attempts in tobacco-related outcomes.
Events are listed in order after random sampling over the parametrization of time-to-event evidence, rewriting the list after each occurrence. Events have their own associated time that passes when the event occurs, hence DES is best suited to model discrete processes. As events have different duration, the cycle lengths are not necessarily equal. Several authors [ 42 , 46 , 55 , 62 ] find this structure convenient for modeling the care pathway of a health facility. The timing structure of a DES model allows the assessment of multiple and competing risks, as they will be organized in the future events list by time-to-event [ 79 ], with no immediate restriction for two events to happen simultaneously [ 73 ]. Kotiadis [ 52 ] and Norouzzadeh et al. [ 61 ] take advantage of this characteristic to model different times for referrals depending on medical factors while tracing key indicators in the system. DES also allows for the status of variables in the system to affect the nature of the relationships of an individual with the rest of the system. Günal et al. [ 46 ], Oh et al. [ 62 ] and Comans [ 39 ] uses the interference feature to evaluate the queues and backlogs at different stages of the patient pathway, understanding waiting time as a change in the manner a patient interacts with a provider, given the providers’ status (e.g., ‘Occupied’). By fixing the maximum waiting time allowed in concordance with national guidelines, the authors can assess the requirements in the rest of the system to reach this goal.
As Microsimulations, Discrete Event Simulations aim at producing statistically valid estimations out of the documented behavior of a system. This rigidity poses an important trade-off compared to other techniques as it needs detailed, well-defined processes, accurate historical data, and high intellectual, computer, and data management capabilities. Standfield et al. [ 73 ] conclude that if individualization or interference is not an important driver of the performance of the system, including these characteristics would be an unnecessary over-specification and unlikely to be informative to decision-makers.
Agent-based models
Agent-based models focus on the activities of the agents composing the system. Each agent is individually defined with a set of rules and an objective, that may be described from heuristics to the optimization of a utility function. Kalton et al. [ 50 ] use this technique to model how mental patients engage with medical and social ecosystems while studying the effect of coordination capabilities. The individualization allows the agents to be influenced by their history and external variables. At the same time, agency focus allows the technique to capture emergent population phenomena [ 76 ].
The system is modeled in a simulated space, adding the possibility to include spatial variables. Nianogo et al. [ 60 ] exploit these characteristics when understanding the dynamics of the diabetes population in L.A, USA. The ‘Virtual Los Angeles Obesity’ model simulates a cohort of patients with different characteristics that interact differently with different environments. By assigning rules for the relations with the environment, the model seeks to describe the trends in obesity and diabetes out of the behavior of the agents, and at the same time test interventions by changing the environmental conditions or characteristics of said agents.
Agent-based models also allow for the inclusion of random factors to consider the bounded rationality that is present in agents’ behavior. Finally, as agents can be affected by spatial or other types of determinants, and because the rules commanding agent’s behavior can be set as thresholds, endogenous and time-dependent feedback loops are also possible. In advanced models, agents can evolve and learn with methods like neural networks and other forms of machine learning [ 29 , 77 , 78 ].
As with System Dynamics, authors use proxies and expert opinions when hard evidence is not available [ 46 ]. This flexibility makes them appropriate to test behavioral theories and understand complex population phenomena. On the other hand, statistical validity is not usually the first concern in either technique, where the usefulness of the assessment is more important.
Hybrid simulations
Hybrid simulations can combine the strengths of two or more models. Gao et al. [ 43 ] developed a tripartite model combining System Dynamics, Agent-Based Models, and Discrete Event Simulations. He uses a previously developed System Dynamics model to understand the progression of diabetes up until the early stage of renal disease. As described by Jones et al. [ 49 ], the model properly describes diabetes progression by including key feedback loops. Constructing from this model, Gao et al. [ 43 ] include two different types of hybrid relationships. First, there is an upstream-downstream relation between the original model and an Agent-Based Model for the populations that flows into a particular state (diabetes) to become individualized agents. The ABM model can study the incidence of a complication (early-stage renal disease) by simulating key behaviors in the development of the disease. In parallel, the second hybrid relation integrates DES for monitoring the different status of the patients and tracks the evolution of healthcare processes and resource availability and usage.
3.3 Complexity
To understand and compare the representation of complexity in simulation models we first compiled 13 distinct features of complex systems identified by Randall [ 80 ] and Wilenksy & Rand [ 81 ]: Undetermined or fuzzy boundaries, the possibility of being open, possibility of having nested sub subsystems, dynamism in the network of relationships with different scales of interconnectivity, emergent phenomena, nonlinear relationships, feedback loops, leverage points, memory/path dependence, sensitivity to initial conditions, robustness, diversity and heterogeneity, interconnectedness and interactions. Building from the previous section, we identified the characteristics of the described modeling techniques that can represent features of complex systems specifically related to relationships between system components. The modeled complexities were classified into one framework with definitions that could be applicable across methodologies. The exercise resulted in nine aspects of complex relations that can be represented with simulation models. We present the nine aspects of complex relations together with the characteristics in each discipline to represent them. In parenthesis, we show the number of papers modeling each complexity. Among the complexities identified, four are non-linearities (1 to 4), and they were the most commonly modeled. Table 3 summarizes the aspects of complexity enabled in each modeling technique.
Table 3. Complexity aspects enabled per simulation modeling technique.
1 The technique can incorporate dynamic changes over time, but not endogenous feedback loops.
2 Even though the ‘Markovian Property’ defines that transition probabilities will depend only on the current state and not on previous states thus eliminating the possibility of having ‘Memory’, researchers can overcome this by incorporating tunnel states and parallel models.
Interactions (30/30): We understand this complexity as the dependence of the causal effect of one component (A) to another (B) on the effect of (C) over (A). i.e. mediated effects. For MM, SD, and MS interactions are embedded in the state transitions–chain structure. For DES and ABM, interactions between components are stored in their individualized information and will affect their effect on other components [ 70 , 73 , 75 ]. Homer’s [ 69 ] model of policies aimed at chronic conditions presents a good example of interaction. The policies in question affect a risky behavior, which in time affects the status of the disease, which in time affects healthcare provision. By interacting, each component affects the final outcome according to its particular characteristics and those of the previous component.
Dynamism (23/30): Dynamism represents the circular causality of a system. If component (A) changes the nature of its relations in the system as the system progresses, then we say the system presents dynamism. Besides the dynamics produced by the passing of time, relations can be influenced by the changing conditions of any other component, producing endogenous feedback loops. In methods where estimation correspond to ordinary differential equations, the value of component (A) will be determined by a function of the state of other components (B, C) [ 74 ]. For MM the other components (B, C) can only be time, hence no endogenous feedback loops are possible [ 73 ]. For ABM, conditions ruling the behavior of agents can change depending on other components of the system or time as programmed by the modeler [ 74 ]. In Alonge’s [ 37 ] model for a pay for performance incentive scheme, dynamism is clear when understanding the effect of ‘volume of service’ over the reduction in ‘quality’ and the increase of ‘revenue’, which in time affect the ‘volume of service’ downwards and upwards respectively.
Interference (10/30): We understand interference as the dependence of the causal effect of one component (A) to another (B) on the effect of a third component (C) over (B). i.e queueing. DES handles interference by given the components of the system mutable states. The particular state will affect the relationship with other components, and at the same time mutations between states are triggered by these relations. Similarly, ABM can define different behaviors of its agents depending on current or past relations with the rest of the system [ 70 , 75 ]. The best example of interference is the change from available to occupied of rooms modeled by Günal [ 46 ]. Because a patient is occupying a room, other patients have to change their behavior to that room and wait.
(Intelligent) Adaptation (2/30): Adaptation is the ability of a component to change the nature of its behavior to contingency happening in the system. This ability presumes the intelligence of components to make decisions. ABM can integrate this complexity when specifying agents’ behavior not only as a function of other system components but also as conditions and operations in said function such as ‘ifs’ and optimization [ 14 , 75 ]. For example, in Kalton’s model [ 50 ] patients can make up to 40 decisions based on logic and preferences developed during their life process, care experience and health status. Decisions include taking their medicine, looking for employment, starting to abuse substances, etc.
Soft variables (9/30): Refers to the possibility of incorporating simplified proxies for difficult-to-measure variables. Allows the inclusion of behavioral and qualitative relations. The possibility of using soft variables in ABM [ 82 ] and SD [ 76 ] responds to each methodology obtaining outputs focusing on agents’ behavior and system structure respectively, instead of mathematical correctness to represent phenomena. A good representation of a soft variable is “Workload pressure” modeled by Rashawn et al. [ 63 ] as the ratio between the actual nurse-to-patient ratio and the standard nurse-to-patient ratio.
Individualization (17/30): Integrates the possibility of including individual-level characteristics. Comprehends the complex system features of heterogeneity and diversity. DES and MS use a sample of individual units, each with a unique set of attributes [ 73 , 75 ]. ABM can program each agent with different characteristics [ 82 ]. Individualization is notable in the model by Lay-yee et al. [ 54 ], where data is granular at patient level, with variables such as gender, ethnicity and housing status. Each of these variables affects the subject’s number of doctor visits, reading ability and conduct problems.
Simultaneity of events (5/30): Possibility of two or more events happening in parallel for the same component of the system. The concept is related to the possibility of having nested systems within a complex system. Modelers of ABM can create parallel behavior rules for the same component. Similarly, events triggering a particular state can overlap in DES, creating parallel situations for the same component. A clear example is the case of Parkison disease treatmeant as modeled by Lebcir et al. [ 55 ], where one or a combination of the diferent treatment schemes are possible for distinct patients. When a combination is chosen, the treatment sections of the model happen in parallel.
Historical occurrences / Memory (18/30): Also known as hysteresis, the concept includes path dependence. It refers to the influence of past states on the nature of the relationships of the current state. In methods that allow individualization, events can be stored in the individual’s characteristics. For SD, the influence of events is stored in the stocks. A good example is the model by Vataire et al. [ 66 ], where the number of previous depression events updates the model attributes.
Emergence (2/30): Characteristics of a system to develop new behaviors, different from those of the sum of its parts. ABM enables this characteristic by allowing agents to interact freely, only following the programmed behavior [ 82 ]. For example, in Nianogo’s model for policies to treat population obesity [ 60 ], researches realize that their agents would change non objective behaviors because of the interventions, making them ineffective. Also, agents would quickly go back to the undesirable behavior after the intervention was finished (in despite of the intervention objective), diminishing the long-term effect.
3.4 Optimization capabilities
All simulation modeling techniques used ‘ what if ? ’ scenarios, defined as to gain information about the performance of the system (or parts of the system) when simulating the change of a variable from its original value, while using as counterfactual the baseline model. Fourteen (out of 30) articles complemented the assessment with ‘ how to ? ’ scenarios, defined as fixing a variable’s value as a goal and focusing on how the other variables change from the baseline values to meet this condition.
3.5 Long term assessment
The studies had different time lengths in their assessment. While some papers had a closer look at the activities on a working day (3/30), the majority had assessments of at least 5 years (21/30). The mean number of years in the assessments was 18 years (standard deviation 20). Lifelong simulations (2/30) were considered as 60 years and working hours of a working day as 10 hours.
4. Discussion
We have characterized the use of simulation models for IHS performance assessment. First, by exposing topics of interest to IHS that can be modeled, and the techniques to model them. Second, by exposing how these techniques can implement system thinking in said topics of interest, while enabling features befitting of integrated care performance assessment.
To characterize the ability of the reviewed simulation models to implement system thinking, we have created a common framework with 9 complexity features enabled differently across modeling technique. These complexity features allow for the correct understanding of causality paths in a system’s performance. For integrated care, this means enabling accurate accountability for system components and consequently, creates a better position to guide system improvement. Accurate accountability is necessary for value-based care, and especially value-based payment schemes, two key elements of integrated care initiatives [ 4 , 11 ]. Furthermore, disentangling the complex relations between system components is the key to deal with comorbidities, identifying consumed resources, and implementing ad-hoc interventions [ 11 ]. While accurately representing the complex relations of the system is essential for the model structure, simulation models can optimize interventions by testing ‘what if?’ & ‘how to?’ scenarios. These scenarios simulate changes (or fix values, respectively) anywhere in the system and compare it to a baseline value of system performance. By doing so, SM provides an easy way to compare the value of multiple interventions, understand the value of each component and identify bottlenecks and other deficiencies in the system. At the same time, the term of assessment is manageable in function of the objective of the study. Short and long-term interventions aimed at improving efficiency, changing health behavior, and preventive care are an important part of the toolbox of IHS, and the possibility of assessing them and optimize their implementation in the correct time frame is expected when in pursuit of the Triple Aim [ 3 ].
The application areas identified in the review were in line with the findings of previous work focused on characterizing applications areas of simulation modeling in healthcare [ 18 ]. Likewise, the simulation techniques covered in this work are the most used and studied in literature. Markov Models are the simplest among simulation models, because of relatively low computer, human, and data needs. It is the preferred methodology when assessing situations with low complexity. System Dynamics models add the possibility of including feedback effects and soft variables with a population perspective, characteristics that make it more prevalent in the “Policy and Strategy” area, a realization in line with results of extensive reviews aimed at linking simulation methods and healthcare areas of application [ 22 , 25 ]. Microsimulations and Discrete event simulations extend the complexity into individual-level assessments, which in place enables the influence of past events. The main difference between the two is that Discrete Event Simulations add the possibility of including interference. This characteristic makes it more suitable to understand health processes that require queuing, a common feature in the topic of “Health Resource Management”. Furthermore, several authors coincide in that Discrete Event Simulation is the most common technique for evaluating the operation management of care facilities [ 19 , 22 , 25 ]. Agent-Based Models understand the behavior of the system out of the behavior of its agents. This simple definition allows the study of complex phenomena with a relatively simple technical construction. The technique can include all the described complexities, but the fact that works in an entirely simulated environment diminish the validity of its results.
A common characteristic of all the simulation modeling techniques is the inclusion of data from multiple sources and the possibility of a probabilistic estimation. Twenty out of the 27 papers performed a probabilistic sensitivity analysis, either with Monte Carlo simulations or other. A probabilistic estimation is not included as an aspect of complexity as we don’t consider uncertainty to be unique to complex systems, and for the same reason, Monte Carlo simulations are not included as a SM technique to assess complex systems. However, the possibility to include probabilistic estimations allows the inclusion of uncertain evidence, which is essential for the comprehensiveness of the models. Validation is key for the usefulness of the simulation results. Described in detail elsewhere [ 29 ], typically, a five steps approach is used in SM, comprising: Face validity, internal validity, cross validity, external validity, and predictive validity.
Model selection
A system is most appropriately modeled by the technique that allows the inclusion of the most important characteristics of said system. The selection of the most appropriate simulation modeling technique to assess performance must consider the characteristics of the system and the capabilities of each technique. It is important that only essential characteristics are considered so there is not an over-specification that hinders the analysis. In this line, identifying and prioritizing the complexities that rule the system to be modeled will help evaluators in selecting the most appropriate simulation model. Using our framework for complexity for this purpose, we created a conceptual map ( Fig 2 ) that aids evaluators in selecting a simulation model to produce an accurate assessment of situations where complex relations are important. The tool is a summary of the results and characterization presented in this paper. The first step is to identify the most important complexity of the system to be modeled. Following a few key questions, the tool points to the technique with fewer inputs and technical difficulties that is appropriate to model said system.
Fig 2. Visual aid to select simulation modeling technique.
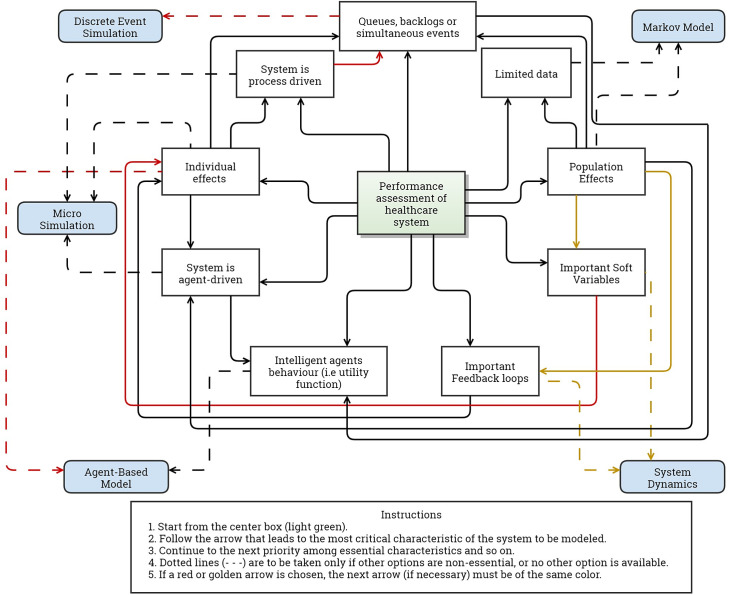
To help readers navigate the tool, we use the evaluation of a pay for performance incentive scheme by Alonge et al. [ 37 ] as an example. We start by assuming that the most important characteristics of the issue are (1.) the feedback loops that performance bonuses generate over the revenue and quality of services and (2.) the effect of “Gaming” (a soft variable) of the staff over this new payment scheme. Starting from the center and navigating through the figure we could go to either “Important feedback loops” or “Soft variables” and if individual effects are not considered essential, the tool takes us to System Dynamics—that is the approach used by the author. Another example is the evaluation of interventions for reducing waiting time in a health facility. Queues and backlogs are assumed the most important characteristic. If we consider non-essential the intelligent behavior of the agents, then the tool points to Discrete Event Simulation. Otherwise, an Agent-Based Model would be the most appropriate.
Sometimes the complexities of a system cannot be ranked according to their importance. If this is the case, evaluators should repeat the exercise starting from all the identified complexities as if each were the most important one. If the different runs result in different modeling techniques, a hybrid model is to be considered. This is the case for the paper by Gao et al. [ 43 ]. In this case the authors seek to model three elements of diabetes care. First, diabetes progression at the population level, with feedback loops being the most important complexity. Selecting important feedback loops in the figure takes you directly to System Dynamics (when individualization is not important). Second, disease complication, where individualization of risk factors and healthy behavior is crucial. After individual effects, the figure passes through agent behavior towards Agent-Based Models. Finally, the authors study the status of every patient to track the use of resources. In this case, individualization is the priority complexity, but as agent behavior is not important for this element, the user will lean in favor of simultaneity of events, arriving at Discrete Event Simulation. As selected by the authors, the tool guides each situation following the characteristics and prioritization of complexities to the appropriate modeling technique.
Limitations
By focusing only on simulation modeling, the review overlooks many analytical methods to assess complex systems. Several authors have described other analytical methods for studying different aspects of complexity in health systems, including network analysis, marginal structural models, queuing theory, Petri nets [ 22 ], and artificial intelligence [ 83 ]. Previous work by Jun et al. [ 22 ] characterizes and compares a wider set of modeling methods. However, it does not consider the distinctive characteristics of the system to be modeled or describe how do they apply system thinking. Our review focuses solely on simulation models because of the advantages they present in the assessment of integrated care systems. Network Analysis provides an assessment of the structure of the (complex) relations in a system but does not consider causal pathways. Marginal structural models and queuing theory are useful to represent time-dependent covariates and interference (as defined in this paper) respectively, but they are limited to these capacities. SM and Artificial intelligence methods, such as Machine Learning, differ in that the latter constructs a model from patterns in the data, while SM constructs from the structure of the system and then populates the model with data. Besides making the estimations more comprehensible, this characteristic of SM allows policymakers to test structure changing interventions, such as the ones in integrated care. In any case, the mentioned analytical approaches are complementary to SM, as they can provide the necessary inputs to build and populate the simulation model. Comparisons between different analytical methods, understanding their capacities to represent complex system characteristics, is scarce and should be further assessed in future research.
It is important to highlight that the approach to find IHS topics-of-interest is not the extent of subjectivity, as there are multiple definitions for integrated care [ 3 ]. In this sense, it is probable to encounter multiple other IHS topics-of-interest that can be successfully modeled with SM techniques. In the same line, our selection criteria focused on finding papers that allowed us to understand the implementation of a complex system perspective, criteria that resulted in fewer reviewed papers than previous literature linking simulation modeling and healthcare performance assessment. Nevertheless, we are confident that the selection of papers in the review together with the complementary literature used, allowed us to accurately characterize the field of simulation modeling in their ability to use system thinking in integrated healthcare.
Finally, clarify that our work does not provide an in-depth description of the different simulation modeling techniques. We acknowledge that such a task would be impossible to undertake with our study design. Instead, we provide readers with an introduction to the identified simulation modeling techniques and highlight the characteristics that allow them to implement system thinking. We encourage readers that find a solution in this work to the challenges they encounter when assessing the performance of a complex health system to learn in detail the technique that our paper has pointed towards. For this purpose, we recommend starting with the complementary literature that we include for each technique in Table 2 .
5. Conclusion
Simulation modeling techniques can use system thinking and evaluate performance emphasizing the complex relations between system components, in topics of relevance for integrated healthcare systems. By using simulation models to complement the performance assessment of integrated health systems, managers can correctly attribute causality to system components, optimize interventions, and create long term assessments. All these are important advantages over traditional assessment methods. Adding simulation models to the performance assessment tools at disposition of health authorities may be the key to understand the full value of integrated care. Selecting a simulation technique is facilitated when both the characteristics of the modeling techniques are understood, and the complexities ruling the system performance are identified and prioritized. To facilitate the use of the discipline, we consolidated complexity features of different modeling techniques into one framework and provide future performance evaluators with a visual aid to guide the selection of the most appropriate model for the assessment of complexity-enhanced systems, such as integrated healthcare.
Supporting information
Acknowledgments.
Ph.D. (Candidate) Sophie Wang (SW) contributed as a second literature reviewer and quality assessor.
Data Availability
All relevant data are within the paper and its S1 Checklist , S1 Data , and S1 – S3 Tables.
Funding Statement
This project has received funding from the European Union’s Horizon 2020 research and innovation programme under the Marie Skłodowska-Curie grant agreement No 765141. Both authors are employed at OptiMedis AG. The funder provided support in the form of salaries for authors NL & OG, but did not have any additional role in the study design, data collection and analysis, decision to publish, or preparation of the manuscript. The specific roles of these authors are articulated in the ‘author contributions’ section.
- 1. WHO Regional Office for Europe. Integrated care: An Overview. World Health Organization; 2016. [ Google Scholar ]
- 2. Tham TY, Tran TL, Prueksaritanond S, Isidro J, Setia S, Welluppillai V. Integrated health care systems in Asia: an urgent necessity. Clin Interv Aging. 2018. Dec; Volume 13:2527–38. doi: 10.2147/CIA.S185048 [ DOI ] [ PMC free article ] [ PubMed ] [ Google Scholar ]
- 3. European Commission. Directorate General for Health and Food Safety. Blocks: tools and methodologies to assess integrated care in Europe : report by the Expert Group on Health Systems Performance Assessment. [Internet]. LU: Publications Office; 2017. [cited 2021 Mar 5]. Available from: https://data.europa.eu/doi/10.2875/017891 [ Google Scholar ]
- 4. Edgren L, Barnard K. Complex adaptive systems for management of integrated care. Leadersh Health Serv. 2012. Jan 27;25[1]:39–51. [ Google Scholar ]
- 5. Berwick DM, Nolan TW, Whittington J. The Triple Aim: Care, Health, And Cost. Health Aff (Millwood). 2008. May;27[3]:759–69. doi: 10.1377/hlthaff.27.3.759 [ DOI ] [ PubMed ] [ Google Scholar ]
- 6. Optimity Advisors. Performance Assessment Framework. 2018. [EU Third Health Programme [2014–2020]].
- 7. Dates M, Lennox-Chugani N, Pereira HS, Tedeschi M. Health system performance assessment–Integrated Care Assessment (20157303 HSPA). Brussels: Public Health—European Commission; 2018. [ Google Scholar ]
- 8. Nuño Solinís R, Stein KV. Measuring Integrated Care–The Quest for Disentangling a Gordian Knot. Int J Integr Care. 2016. Oct 17;16[3]:18. doi: 10.5334/ijic.2525 [ DOI ] [ PMC free article ] [ PubMed ] [ Google Scholar ]
- 9. European Steering Group on Sustainable Healthcare. Acting Together: A Roadmap for Sustainable Healthcare. Milan: Universita Cattolica del Sacro Cuore; 2016. [ Google Scholar ]
- 10. Papanicolas I, European Observatory on Health Systems and Policies, editors. Health system performance comparison: an agenda for policy, information and research. Maidenhead, Berkshire, England: Open University Press; 2013. 384 p. [European observatory on health systems and policies series]. [ Google Scholar ]
- 11. Smith P, editor. Performance measurement for health system improvement: experiences, challenges and prospects. Cambridge ; New York: Cambridge University Press; 2009. 726 p. [The Cambridge health economics, policy and management series]. [ Google Scholar ]
- 12. Bautista MAC, Nurjono M, Lim YW, Dessers E, Vrijhoef HJ. Instruments Measuring Integrated Care: A Systematic Review of Measurement Properties: Instruments Measuring Integrated Care. Milbank Q. 2016. Dec;94[4]:862–917. doi: 10.1111/1468-0009.12233 [ DOI ] [ PMC free article ] [ PubMed ] [ Google Scholar ]
- 13. Singer SJ, Kerrissey M, Friedberg M, Phillips R. A Comprehensive Theory of Integration. Med Care Res Rev. 2020. Apr;77[2]:196–207. doi: 10.1177/1077558718767000 [ DOI ] [ PubMed ] [ Google Scholar ]
- 14. Marshall DA, Burgos-Liz L, IJzerman MJ, Osgood ND, Padula WV, Higashi MK, et al. Applying dynamic simulation modeling methods in health care delivery research-the SIMULATE checklist: report of the ISPOR simulation modeling emerging good practices task force. Value Health J Int Soc Pharmacoeconomics Outcomes Res. 2015. Jan;18[1]:5–16. doi: 10.1016/j.jval.2014.12.001 [ DOI ] [ PubMed ] [ Google Scholar ]
- 15. Petticrew M, Knai C, Thomas J, Rehfuess EA, Noyes J, Gerhardus A, et al. Implications of a complexity perspective for systematic reviews and guideline development in health decision making. BMJ Glob Health. 2019. Jan;4[Suppl 1]:e000899. doi: 10.1136/bmjgh-2018-000899 [ DOI ] [ PMC free article ] [ PubMed ] [ Google Scholar ]
- 16. Sokolowski JA, Banks CM, Hakim P. Simulation training to improve blood management: an approach to globalizing instruction in patient safety. Simul-Trans Soc Model Simul Int. 2014. Feb;90[2, SI]:133–42. [ Google Scholar ]
- 17. Roy SN, Shah BJ, Gajjar H. Application of Simulation in Healthcare Service Operations: A Review and Research Agenda. ACM Trans Model Comput Simul [Internet]. 2021. Dec;31[1]. Available from: 10.1145/3427753 [ DOI ] [ Google Scholar ]
- 18. Salleh S, Thokala P, Brennan A, Hughes R, Booth A. Simulation Modelling in Healthcare: An Umbrella Review of Systematic Literature Reviews. PharmacoEconomics. 2017. Sep;35[9]:937–49. doi: 10.1007/s40273-017-0523-3 [ DOI ] [ PubMed ] [ Google Scholar ]
- 19. Günal MM, Pidd M. Discrete event simulation for performance modelling in health care: a review of the literature. J Simul. 2010. Mar;4[1]:42–51. [ Google Scholar ]
- 20. Yousefi M, Yousefi M, Fogliatto FS. Simulation-based optimization methods applied in hospital emergency departments: A systematic review. SIMULATION. 2020. Oct;96[10]:791–806. [ Google Scholar ]
- 21. Rueckel D, Koch S. Application Areas of Predictive Analytics in Healthcare. In Boston; 2017.
- 22. Jun GT, Morris Z, Eldabi T, Harper P, Naseer A, Patel B, et al. Development of modelling method selection tool for health services management: from problem structuring methods to modelling and simulation methods. BMC Health Serv Res. 2011. May 19;11:108. doi: 10.1186/1472-6963-11-108 [ DOI ] [ PMC free article ] [ PubMed ] [ Google Scholar ]
- 23. Brailsford SC, Harper PR, Patel B, Pitt M. An analysis of the academic literature on simulation and modelling in health care. J Simul. 2009. Sep;3[3]:130–40. [ Google Scholar ]
- 24. Roy S, Prasanna Venkatesan S, Goh M. Healthcare services: A systematic review of patient-centric logistics issues using simulation. J Oper Res Soc. 2020. Aug 3;1–23. [ Google Scholar ]
- 25. Mielczarek B, Uziałko-Mydlikowska J. Application of computer simulation modeling in the health care sector: a survey. SIMULATION. 2012. Feb;88[2]:197–216. [ Google Scholar ]
- 26. Vanbrabant L, Braekers K, Ramaekers K, Van Nieuwenhuyse I. Simulation of emergency department operations: A comprehensive review of KPIs and operational improvements. Comput Ind Eng. 2019. May;131:356–81. [ Google Scholar ]
- 27. Laker LF, Torabi E, France DJ, Froehle CM, Goldlust EJ, Hoot NR, et al. Understanding Emergency Care Delivery Through Computer Simulation Modeling. Acad Emerg Med Off J Soc Acad Emerg Med. 2018. Feb;25[2]:116–27. doi: 10.1111/acem.13272 [ DOI ] [ PMC free article ] [ PubMed ] [ Google Scholar ]
- 28. Banks CM, Sokolowski JA. Handbook of real-world applications in modeling and simulation [Internet]. Hoboken, N.J.: Wiley; 2012. [cited 2021 Mar 4]. Available from: http://site.ebrary.com/id/10580204 [ Google Scholar ]
- 29. Eddy DM, Hollingworth W, Caro JJ, Tsevat J, McDonald KM, Wong JB. Model Transparency and Validation: A Report of the ISPOR-SMDM Modeling Good Research Practices Task Force–7. Med Decis Making. 2012. Sep;32[5]:733–43. doi: 10.1177/0272989X12454579 [ DOI ] [ PubMed ] [ Google Scholar ]
- 30. 30. Tyndall J. AACODS Checklist. [Internet]. Flinders University; 2010. Available from: https://dspace.flinders.edu.au/xmlui/bitstream/handle/2328/3326/AACODS_Checklist.pdf?sequence=4&isAllowed=y
- 31. Strandberg-Larsen M, Krasnik A. Measurement of integrated healthcare delivery: a systematic review of methods and future research directions. Int J Integr Care [Internet]. 2009. Feb 4 [cited 2021 Mar 5];9[1]. Available from: http://www.ijic.org/article/10.5334/ijic.305/ [ DOI ] [ PMC free article ] [ PubMed ] [ Google Scholar ]
- 32. McDonald K, Schultz E, Albin L, Pineda N, Lonhart J, Sundaram V, et al. Care Coordination Atlas Version 3 (Prepared y Stanford University under subcontract to attelle on Contract No. 290-04-0020). Rockville, MD: Agency for Healthcare Research and Quality; 2010. Nov. [AHRQ Publication No. 11-0023-EF]. [ Google Scholar ]
- 33. Fone D, Hollinghurst S, Temple M, Round A, Lester N, Weightman A, et al. Systematic review of the use and value of computer simulation modelling in population health and health care delivery. J Public Health Med. 2003. Dec;25[4]:325–35. doi: 10.1093/pubmed/fdg075 [ DOI ] [ PubMed ] [ Google Scholar ]
- 34. Crabtree BF, Miller WL, editors. Doing qualitative research. 2nd ed. Thousand Oaks, Calif: Sage Publications; 1999. 406 p. [ Google Scholar ]
- 35. Boyatzis RE. Transforming qualitative information: thematic analysis and code development. Thousand Oaks, CA: Sage Publications; 1998. 184 p. doi: 10.1080/00221329809596155 [ DOI ] [ Google Scholar ]
- 36. Peric N, Hofmarcher-Holzhacker MM, Simon J. Health system performance assessment landscape at the EU level: a structured synthesis of actors and actions. Arch Public Health Arch Belg Sante Publique. 2017;75:5. [ DOI ] [ PMC free article ] [ PubMed ] [ Google Scholar ]
- 37. Alonge O, Lin S, Igusa T, Peters DH. Improving health systems performance in low- and middle-income countries: a system dynamics model of the pay-for-performance initiative in Afghanistan. Health Policy Plan. 2017. Dec 1;32[10]:1417–26. doi: 10.1093/heapol/czx122 [ DOI ] [ PMC free article ] [ PubMed ] [ Google Scholar ]
- 38. Ansah JP, Matchar DB, Malhotra R, Love SR, Liu C, Do Y. Projecting the effects of long-term care policy on the labor market participation of primary informal family caregivers of elderly with disability: insights from a dynamic simulation model. BMC Geriatr. 2016. Mar 23;16. doi: 10.1186/s12877-016-0243-0 [ DOI ] [ PMC free article ] [ PubMed ] [ Google Scholar ]
- 39. Comans TA, Chang AT, Standfield L, Knowles D, O’Leary S, Raymer M. The development and practical application of a simulation model to inform musculoskeletal service delivery in an Australian public health service. Oper Res Health CARE. 2017. Dec;15:13–8. [ Google Scholar ]
- 40. Cooper K, Davies R, Raftery J, Roderick P. Use of a coronary heart disease simulation model to evaluate the costs and effectiveness of drugs for the prevention of heart disease. J Oper Res Soc. 2008. Sep;59[9]:1173–81. [ Google Scholar ]
- 41. de Andrade L, Lynch C, Carvalho E, Rodrigues CG, Vissoci JRN, Passos GF, et al. System dynamics modeling in the evaluation of delays of care in ST-segment elevation myocardial infarction patients within a tiered health system. PloS One. 2014;9[7]:e103577. doi: 10.1371/journal.pone.0103577 [ DOI ] [ PMC free article ] [ PubMed ] [ Google Scholar ]
- 42. Fialho AS, Oliveira MD, Sa AB. Using discrete event simulation to compare the performance of family health unit and primary health care centre organizational models in Portugal. BMC Health Serv Res. 2011. Oct 15;11:274. doi: 10.1186/1472-6963-11-274 [ DOI ] [ PMC free article ] [ PubMed ] [ Google Scholar ]
- 43. 43. Gao A, Osgood ND, An W, Dyck RF. A tripartite hybrid model architecture for investigating health and cost impacts and intervention tradeoffs for diabetic end-stage renal disease. In: Proceedings of the Winter Simulation Conference 2014 [Internet]. Savanah, GA, USA: IEEE; 2014 [cited 2018 Nov 13]. p. 1676–87. Available from: http://ieeexplore.ieee.org/document/7020018/
- 44. Getsios D, Marton JP, Revankar N, Ward AJ, Willke RJ, Rublee D, et al. Smoking cessation treatment and outcomes patterns simulation: a new framework for evaluating the potential health and economic impact of smoking cessation interventions. PharmacoEconomics. 2013. Sep;31[9]:767–80. doi: 10.1007/s40273-013-0070-5 [ DOI ] [ PubMed ] [ Google Scholar ]
- 45. 45. Goldman D, Shekelle P, Bhattacharya J, Hurd M, Joyce G, Lakdawalla D, et al. Health Status and Medical Treatment of the Future Elderly: Final Report [Internet]. RAND Corporation; 2004 [cited 2021 May 31]. Available from: https://www.rand.org/pubs/technical_reports/TR169.html
- 46. Günal MM, Pidd M. DGHPSIM:: Generic Simulation of Hospital Performance. ACM Trans Model Comput Simul. 2011. Sep;21[4]:23:1–23:22. [ Google Scholar ]
- 47. Hill A, Camacho OM. A system dynamics modelling approach to assess the impact of launching a new nicotine product on population health outcomes. Regul Toxicol Pharmacol RTP. 2017. Jun;86:265–78. doi: 10.1016/j.yrtph.2017.03.012 [ DOI ] [ PubMed ] [ Google Scholar ]
- 48. Homer J, Milstein B, Wile K, Trogdon J, Huang P, Labarthe D, et al. Simulating and evaluating local interventions to improve cardiovascular health. Prev Chronic Dis. 2010. Jan;7[1]:A18. [ PMC free article ] [ PubMed ] [ Google Scholar ]
- 49. Jones AP, Homer JB, Murphy DL, Essien JDK, Milstein B, Seville DA. Understanding diabetes population dynamics through simulation modeling and experimentation. Am J Public Health. 2006. Mar;96[3]:488–94. doi: 10.2105/AJPH.2005.063529 [ DOI ] [ PMC free article ] [ PubMed ] [ Google Scholar ]
- 50. Kalton A, Falconer E, Docherty J, Alevras D, Brann D, Johnson K. Multi-Agent-Based Simulation of a Complex Ecosystem of Mental Health Care. J Med Syst. 2016. Feb;40[2]:39. doi: 10.1007/s10916-015-0374-4 [ DOI ] [ PMC free article ] [ PubMed ] [ Google Scholar ]
- 51. Kang H, Nembhard HB, Ghahramani N, Curry W. A system dynamics approach to planning and evaluating interventions for chronic disease management. J Oper Res Soc. 2018;69[7]:987–1005. [ Google Scholar ]
- 52. 52. Kotiadis K. Extracting a conceptual model for a complex integrated system in health care. Proc Soc Two-Day Workshop SW06 235–245. 2006;
- 53. Laurence CO, Karnon J. Improving the planning of the GP workforce in Australia: a simulation model incorporating work transitions, health need and service usage. Hum Resour Health. 2016. Apr 11;14:13. doi: 10.1186/s12960-016-0110-2 [ DOI ] [ PMC free article ] [ PubMed ] [ Google Scholar ]
- 54. Lay-Yee R, Milne B, Davis P, Pearson J, McLay J. Determinants and disparities: a simulation approach to the case of child health care. Soc Sci Med 1982. 2015. Mar;128:202–11. doi: 10.1016/j.socscimed.2015.01.025 [ DOI ] [ PubMed ] [ Google Scholar ]
- 55. Lebcir R, Demir E, Ahmad R, Vasilakis C, Southern D. A discrete event simulation model to evaluate the use of community services in the treatment of patients with Parkinson’s disease in the United Kingdom. BMC Health Serv Res. 2017. Jan 18;17[1]:50. doi: 10.1186/s12913-017-1994-9 [ DOI ] [ PMC free article ] [ PubMed ] [ Google Scholar ]
- 56. Levy D, Mohlman MK, Zhang Y. Estimating the Potential Impact of Tobacco Control Policies on Adverse Maternal and Child Health Outcomes in the United States Using the SimSmoke Tobacco Control Policy Simulation Model. Nicotine Tob Res Off J Soc Res Nicotine Tob. 2016. May;18[5]:1240–9. doi: 10.1093/ntr/ntv178 [ DOI ] [ PMC free article ] [ PubMed ] [ Google Scholar ]
- 57. Loyo HK, Batcher C, Wile K, Huang P, Orenstein D, Milstein B. From model to action: using a system dynamics model of chronic disease risks to align community action. Health Promot Pract. 2013. Jan;14[1]:53–61. doi: 10.1177/1524839910390305 [ DOI ] [ PubMed ] [ Google Scholar ]
- 58. Matta ME, Patterson SS. Evaluating multiple performance measures across several dimensions at a multi-facility outpatient center. Health Care Manag Sci. 2007. May 18;10[2]:173–94. doi: 10.1007/s10729-007-9010-2 [ DOI ] [ PubMed ] [ Google Scholar ]
- 59. Milstein B, Homer J, Hirsch G. Analyzing national health reform strategies with a dynamic simulation model. Am J Public Health. 2010. May;100[5]:811–9. doi: 10.2105/AJPH.2009.174490 [ DOI ] [ PMC free article ] [ PubMed ] [ Google Scholar ]
- 60. Nianogo RA, Arah OA. Impact of Public Health Interventions on Obesity and Type 2 Diabetes Prevention: A Simulation Study. Am J Prev Med. 2018;55[6]:795–802. doi: 10.1016/j.amepre.2018.07.014 [ DOI ] [ PubMed ] [ Google Scholar ]
- 61. 61. Norouzzadeh S, Riebling N, Carter L, Conigliaro J, Doerfler ME. SIMULATION MODELING TO OPTIMIZE HEALTH CARE DELIVERY IN AN OUTPATIENT CLINIC. In: 2015 WINTER SIMULATION CONFERENCE (WSC). 2015. p. 1355–66. [Winter Simulation Conference Proceedings].
- 62. Oh C, Novotny AM, Carter PL, Ready RK, Campbell DD, Leckie MC. Use of a simulation-based decision support tool to improve emergency department throughput. Oper Res Health CARE. 2016. Jun;9:29–39. [ Google Scholar ]
- 63. 63. Rashwan W, Arisha A. Modeling behavior of nurses in clinical medical unit in university hospital: Burnout implications. In: 2015 Winter Simulation Conference (WSC) [Internet]. Huntington Beach, CA, USA: IEEE; 2015 [cited 2018 Nov 13]. p. 3880–91. Available from: http://ieeexplore.ieee.org/document/7408544/
- 64. Rejeb O, Pilet C, Hamana S, Xie X, Durand T, Aloui S, et al. Performance and cost evaluation of health information systems using micro-costing and discrete-event simulation. Health Care Manag Sci. 2018. Jun;21[2]:204–23. doi: 10.1007/s10729-017-9402-x [ DOI ] [ PubMed ] [ Google Scholar ]
- 65. Sugiyama T, Goryoda S, Inoue K, Sugiyama-Ihana N, Nishi N. Construction of a simulation model and evaluation of the effect of potential interventions on the incidence of diabetes and initiation of dialysis due to diabetic nephropathy in Japan. BMC Health Serv Res. 2017. Dec 16;17[1]:833. doi: 10.1186/s12913-017-2784-0 [ DOI ] [ PMC free article ] [ PubMed ] [ Google Scholar ]
- 66. Vataire A-L, Aballea S, Antonanzas F, Roijen LH, Lam RW, McCrone P, et al. Core discrete event simulation model for the evaluation of health care technologies in major depressive disorder. Value Health J Int Soc Pharmacoeconomics Outcomes Res. 2014. Mar;17[2]:183–95. doi: 10.1016/j.jval.2013.11.012 [ DOI ] [ PubMed ] [ Google Scholar ]
- 67. 67. Vidyanti I, Basurto-Davila R. Projecting long-term impact of modest sodium reduction in Los Angeles County. In: 2015 Winter Simulation Conference (WSC) [Internet]. Huntington Beach, CA, USA: IEEE; 2015 [cited 2018 Nov 21]. p. 1459–70. Available from: http://ieeexplore.ieee.org/document/7408268/
- 68. Eren Şenaras A. Structure And Behavior in System Dynamics: A Case Study in Logistic. J Bus Res—Turk. 2017. Dec 30;9[4]:321–40. [ Google Scholar ]
- 69. Homer JB, Hirsch GB. System dynamics modeling for public health: background and opportunities. Am J Public Health. 2006. Mar;96[3]:452–8. doi: 10.2105/AJPH.2005.062059 [ DOI ] [ PMC free article ] [ PubMed ] [ Google Scholar ]
- 70. Heard D, Dent G, Schifeling T, Banks D. Agent-Based Models and Microsimulation. Annu Rev Stat Its Appl. 2015. Apr 10;2[1]:259–72. [ Google Scholar ]
- 71. Hennessy DA, Flanagan WM, Tanuseputro P, Bennett C, Tuna M, Kopec J, et al. The Population Health Model (POHEM): an overview of rationale, methods and applications. Popul Health Metr. 2015. Dec;13[1]:24. doi: 10.1186/s12963-015-0057-x [ DOI ] [ PMC free article ] [ PubMed ] [ Google Scholar ]
- 72. O’Donoghue C. Dynamic Microsimulation: A Methodological Survey. Dep Econ Universidade Fed Pernamb. 2001. Dec; vol. 4(2). [ Google Scholar ]
- 73. Standfield L, Comans T, Scuffham P. MARKOV MODELING AND DISCRETE EVENT SIMULATION IN HEALTH CARE: A SYSTEMATIC COMPARISON. Int J Technol Assess Health Care. 2014. Apr;30[2]:165–72. doi: 10.1017/S0266462314000117 [ DOI ] [ PubMed ] [ Google Scholar ]
- 74. Marshall DA, Burgos-Liz L, IJzerman MJ, Crown W, Padula WV, Wong PK, et al. Selecting a dynamic simulation modeling method for health care delivery research-part 2: report of the ISPOR Dynamic Simulation Modeling Emerging Good Practices Task Force. Value Health J Int Soc Pharmacoeconomics Outcomes Res. 2015. Mar;18[2]:147–60. doi: 10.1016/j.jval.2015.01.006 [ DOI ] [ PubMed ] [ Google Scholar ]
- 75. Birkin M, Wu B. A Review of Microsimulation and Hybrid Agent-Based Approaches. In: Heppenstall AJ, Crooks AT, See LM, Batty M, editors. Agent-Based Models of Geographical Systems [Internet]. Dordrecht: Springer Netherlands; 2012. [cited 2021 Mar 5]. p. 51–68. Available from: http://link.springer.com/10.1007/978-90-481-8927-4_3 [ Google Scholar ]
- 76. Auchincloss AH, Garcia LMT. Brief introductory guide to agent-based modeling and an illustration from urban health research. Cad Saude Publica. 2015. Nov;31 Suppl 1:65–78. doi: 10.1590/0102-311X00051615 [ DOI ] [ PMC free article ] [ PubMed ] [ Google Scholar ]
- 77. Bonabeau E. Agent-based modeling: Methods and techniques for simulating human systems. Proc Natl Acad Sci. 2002. May 14;99[Supplement 3]:7280–7. doi: 10.1073/pnas.082080899 [ DOI ] [ PMC free article ] [ PubMed ] [ Google Scholar ]
- 78. Marshall BDL, Galea S. Formalizing the Role of Agent-Based Modeling in Causal Inference and Epidemiology. Am J Epidemiol. 2015. Jan 15;181[2]:92–9. doi: 10.1093/aje/kwu274 [ DOI ] [ PMC free article ] [ PubMed ] [ Google Scholar ]
- 79. 79. Zulkepli J, Eldabi T, Mustafee N. Hybrid Simulation for Modelling Large Systems: An Example of Integrated Care Model. In: Proceedings of the Winter Simulation Conference [Internet]. Berlin, Germany: Winter Simulation Conference; 2012. p. 68:1–68:12. [WSC ‘12]. Available from: http://dl.acm.org/citation.cfm?id=2429759.2429848
- 80. Randall A. Risk and Precaution [Internet]. Cambridge: Cambridge University Press; 2011. [cited 2021 Mar 5]. Available from: http://ebooks.cambridge.org/ref/id/CBO9780511974557 [ Google Scholar ]
- 81. Wilensky U, Rand W. An introduction to agent-based modeling: modeling natural, social, and engineered complex systems with NetLogo. Cambridge, Massachusetts: The MIT Press; 2015. 482 p. [ Google Scholar ]
- 82. 82. Rand. An introduction to Agent-Based Modeling [Internet]. Poole College of Management, North Carolina State University; Available from: https://uzay00.github.io/kahve/slides/abm1.pdf
- 83. Thesmar D, Sraer D, Pinheiro L, Dadson N, Veliche R, Greenberg P. Combining the Power of Artificial Intelligence with the Richness of Healthcare Claims Data: Opportunities and Challenges. PharmacoEconomics. 2019. Jun;37[6]:745–52. doi: 10.1007/s40273-019-00777-6 [ DOI ] [ PubMed ] [ Google Scholar ]
Decision Letter 0
Federica Angeli
22 Sep 2020
PONE-D-20-10819
A systematic review of simulation modeling to assess health system performance:
characterization of the field and visual aid to guide model selection.
Dear Dr. Larrain,
Thank you for submitting your manuscript to PLOS ONE. After careful consideration, we feel that it has merit but does not fully meet PLOS ONE’s publication criteria as it currently stands. Therefore, we invite you to submit a revised version of the manuscript that addresses the points raised during the review process.
Thank you for submitting your work to PLOS One. Although the reviewers and I see some merit in the study, there are major issue that need to be addressed before the article can be considered for publication, particularly related to the paper's contribution and theoretical positioning. The reviewers provide detailed suggestions for improvement, which I hope will guide you in revising your work.
Please submit your revised manuscript by Nov 06 2020 11:59PM. If you will need more time than this to complete your revisions, please reply to this message or contact the journal office at [email protected] . When you're ready to submit your revision, log on to https://www.editorialmanager.com/pone/ and select the 'Submissions Needing Revision' folder to locate your manuscript file.
Please include the following items when submitting your revised manuscript:
A rebuttal letter that responds to each point raised by the academic editor and reviewer(s). You should upload this letter as a separate file labeled 'Response to Reviewers'.
A marked-up copy of your manuscript that highlights changes made to the original version. You should upload this as a separate file labeled 'Revised Manuscript with Track Changes'.
An unmarked version of your revised paper without tracked changes. You should upload this as a separate file labeled 'Manuscript'.
If you would like to make changes to your financial disclosure, please include your updated statement in your cover letter. Guidelines for resubmitting your figure files are available below the reviewer comments at the end of this letter.
If applicable, we recommend that you deposit your laboratory protocols in protocols.io to enhance the reproducibility of your results. Protocols.io assigns your protocol its own identifier (DOI) so that it can be cited independently in the future. For instructions see: http://journals.plos.org/plosone/s/submission-guidelines#loc-laboratory-protocols
We look forward to receiving your revised manuscript.
Kind regards,
Academic Editor
Journal Requirements:
When submitting your revision, we need you to address these additional requirements.
1. Please ensure that your manuscript meets PLOS ONE's style requirements, including those for file naming. The PLOS ONE style templates can be found at
https://journals.plos.org/plosone/s/file?id=wjVg/PLOSOne_formatting_sample_main_body.pdf and
https://journals.plos.org/plosone/s/file?id=ba62/PLOSOne_formatting_sample_title_authors_affiliations.pdf
2. Thank you for stating the following in the Competing Interests section:
"The authors have declared that no competing interests exist."
We note that one or more of the authors are employed by a commercial company: OptiMedis AG.
2.1. Please provide an amended Funding Statement declaring this commercial affiliation, as well as a statement regarding the Role of Funders in your study. If the funding organization did not play a role in the study design, data collection and analysis, decision to publish, or preparation of the manuscript and only provided financial support in the form of authors' salaries and/or research materials, please review your statements relating to the author contributions, and ensure you have specifically and accurately indicated the role(s) that these authors had in your study. You can update author roles in the Author Contributions section of the online submission form.
Please also include the following statement within your amended Funding Statement.
“The funder provided support in the form of salaries for authors [insert relevant initials], but did not have any additional role in the study design, data collection and analysis, decision to publish, or preparation of the manuscript. The specific roles of these authors are articulated in the ‘author contributions’ section.”
If your commercial affiliation did play a role in your study, please state and explain this role within your updated Funding Statement.
2.2. Please also provide an updated Competing Interests Statement declaring this commercial affiliation along with any other relevant declarations relating to employment, consultancy, patents, products in development, or marketed products, etc.
Within your Competing Interests Statement, please confirm that this commercial affiliation does not alter your adherence to all PLOS ONE policies on sharing data and materials by including the following statement: "This does not alter our adherence to PLOS ONE policies on sharing data and materials.” (as detailed online in our guide for authors http://journals.plos.org/plosone/s/competing-interests ) . If this adherence statement is not accurate and there are restrictions on sharing of data and/or materials, please state these. Please note that we cannot proceed with consideration of your article until this information has been declared.
Please include both an updated Funding Statement and Competing Interests Statement in your cover letter. We will change the online submission form on your behalf.
Please know it is PLOS ONE policy for corresponding authors to declare, on behalf of all authors, all potential competing interests for the purposes of transparency. PLOS defines a competing interest as anything that interferes with, or could reasonably be perceived as interfering with, the full and objective presentation, peer review, editorial decision-making, or publication of research or non-research articles submitted to one of the journals. Competing interests can be financial or non-financial, professional, or personal. Competing interests can arise in relationship to an organization or another person. Please follow this link to our website for more details on competing interests: http://journals.plos.org/plosone/s/competing-interests
[Note: HTML markup is below. Please do not edit.]
Reviewers' comments:
Reviewer's Responses to Questions
Comments to the Author
1. Is the manuscript technically sound, and do the data support the conclusions?
The manuscript must describe a technically sound piece of scientific research with data that supports the conclusions. Experiments must have been conducted rigorously, with appropriate controls, replication, and sample sizes. The conclusions must be drawn appropriately based on the data presented.
Reviewer #1: Partly
Reviewer #2: Partly
2. Has the statistical analysis been performed appropriately and rigorously?
Reviewer #1: N/A
Reviewer #2: N/A
3. Have the authors made all data underlying the findings in their manuscript fully available?
The PLOS Data policy requires authors to make all data underlying the findings described in their manuscript fully available without restriction, with rare exception (please refer to the Data Availability Statement in the manuscript PDF file). The data should be provided as part of the manuscript or its supporting information, or deposited to a public repository. For example, in addition to summary statistics, the data points behind means, medians and variance measures should be available. If there are restrictions on publicly sharing data—e.g. participant privacy or use of data from a third party—those must be specified.
Reviewer #1: Yes
Reviewer #2: Yes
4. Is the manuscript presented in an intelligible fashion and written in standard English?
PLOS ONE does not copyedit accepted manuscripts, so the language in submitted articles must be clear, correct, and unambiguous. Any typographical or grammatical errors should be corrected at revision, so please note any specific errors here.
5. Review Comments to the Author
Please use the space provided to explain your answers to the questions above. You may also include additional comments for the author, including concerns about dual publication, research ethics, or publication ethics. (Please upload your review as an attachment if it exceeds 20,000 characters)
Reviewer #1: Authors introduce the topics very clearly, exploring the whole health care performance context thoroughly. They illustrate the issue the paper should contribute to address: how to keep into account complex interactions amid factors those which impact triple aim. They also specify that their review wants to explore possible simulations for improving “what if and “how to” scenario processes, listing the major limits of actual models proposed by some institutions. Final aim – presenting a visual aid to select the most appropriate simulation - is clearly expressed.
Methods section details search strategy. Inclusion ad exclusion criteria are clean.
Authors discuss data extraction and analysis which they adopted. They carefully show the process they followed, focusing how each step contributes to an integrated frame which looks coherent with paper’s aims.
The description of Areas of Assessment is readable as it connects its themes with the topics in a structured way. The PRISMA diagram contributes to clarity.
Table 1 classifies selected papers by described criteria.
The description of simulation modelling techniques starts classifying them by five categories and adding a sixth one which includes studies based on three or more models (hybrid). The section structure is coherent but it could have started explaining reasons behind authors’ choice. Even though it should be considered a minor issue, it would add value trying to adopt a specific classification for simulation models. In case criteria available do not fit due to specificity of authors aims, it could help to quickly explore the connection between this classification and those are often adopted in decision making under uncertainty (e.g see the book Kochenderfer, M.J., 2015, Decision Making Under Uncertainty (MIT Lincoln Laboratory Series) The MIT Press.).
The table offers a synthetic view which allows readers getting the big picture. Once again, as minor issue authors should have quoted the sources they based upon to state strengths and limitations of different types of models. It could be especially helpful for readers who do not have specific background.
The Complexity section is especially interesting and at the center of authors aims. Lack of complexity in evaluating health care performances is one of the issues that authors want to address, so this section is expected to be rigorous and original. The latter expectation is quite satisfied while it is not possible to evaluate the first. Authors list nine complexity features those which are present in the 27 simulation models they’d previously selected. These features sound impactful and relevant but author should quote studies to help readers see them in the broader frame of system modelling theories. It also should allow to better understand both the connection among these features and how they impact on estimating health care system performance. Some features look ambiguous if considered outside a frame of reference as in different field they are referred to different phenomena. E.g. dynamism in dynamic system theory could refer to different meanings ranging from the presence of state variables to the time-variant characteristic of the system itself or both. Again, authors connect Adaptation with intelligence as the ability to make decisions following specific rules. While it is a possible option, the definition of dynamism few rows above could bring someone wonder whether these rules must vary over time for a model showing dynamism.
In summary, while this section is relevant and innovative, authors should better explain references and help readers put these features in a unitary frame.
The discussion starts exploring why a family of models can be helpful in modelling a specific system. While the intent is correct, this part sounds a little bit narrative. Maybe a more schematic description could help to stay connected with both the nine features and the five types of models described above. This part looks as it was written through some and partial examples, so the reader could wonder why other considerations were neglected by authors and especially why.
In the second and in the third part authors discuss the core of the paper: how to improve the choice of performance simulation model for evaluating health care performance in the late of the “triple aim”.
They begin explaining how different models can cope with different health care systems, then they explain how they applied these concepts to design the visual tools, showed in fig. 2.
The visual tools is exciting for its simplicity. Authors illustrate through one example how to apply it for choosing a simulation model which fits both your needs and constraints. Nonetheless it shows a major weakness: authors do not specify how to integrate its different components. E.g. if the system under scrutiny calls for more than one relevant feature should the user follow different connectors, probably ending up in more than one loop? In this case, should users integrate different models?
Authors should be more systematic discussing the tool which should be considered like a model itself, as it offers a way to choose simulation models through matching the features proposed by authors, as a result of their review, and needs coming from triple aim approach. More examples should be proposed to help readers understand how to use the tool when more than one feature is necessary.
Limitations are expressed clearly.
Conclusion suffers the weakness posed in the discussion section.
• Relevant goals and clear expression of the issues to address
• Impactful contents
• High quality review process
• Clear language
• Clarify classification criteria
• Better connect the nine features in a common frame
• Explore and explain how to use the visual tool when health system calls for multiple features and needs
Reviewer #2: While this paper is well written, and discusses a timely topic that in principle is worthy of academic investigation, I do not think that the paper should be accepted for publication. In my view, the paper provides an interesting overview of recent simulation modeling efforts in health systems (with a special emphasis on complexity, being an important characteristic of such systems). But it does not go beyond that, and indeed more is needed to justify publication in a scholarly journal.
First, the paper misses a scientific motivation. The justification for the paper is given in lines 70-72 and can be summarized by saying that simulation models have recently gained more recognition. But this in itself is no (scholarly) motivation for reviewing the related literature. A proper motivation would include: a discussion of why and to whom such a review would be beneficial, i.e., what is the aim of doing such a review? Who would use it, and to what aim? What goes wrong when the study (review) is not done? Also, a scientific motivation would include the identification of a clear knowledge gap: what other review efforts have been published in the academic literature? What is missing in those reviews, making this review necessary? Can the health domain learn from reviews of simulation models in other fields? Especially in light of various recently published review papers (14, 24, 65) it is of paramount importance that the authors justify the need for another review paper on this topic. Without such a clear motivation, the paper reads more like a (interesting) policy report, not a scholarly article.
Second, although the paper provides an interesting overview of relevant papers and the properties of the models described in them, it does not offer as much as I hoped for, in terms of deep reflection and discussion of subtle connections between the models. This can be due to the fact that an enormously wide net is cast across a variety of modeling approaches, each of which having been studied in thousands and thousands of academic papers. Describing such canonical and grand modeling traditions in so few words inescapably leads to gross simplifications. Although understandable, this does not do justice to the subtleties and complexities of each of these models, let alone the nuanced relations between them. Similarly, the applications of the discussed models in the healthcare domain are so diverse (see column IC Topic-of-interest in Table 1), that drawing commonalities and generic lessons from them is hardly possible. What can one learn from comparing the use of model A in context X with the use of model B in context Y? For this to lead to credible results, many more data points are needed. As a consequence, the paper results in a well-structured but unavoidably shallow discussion.
These two issues together make that this reviewer, having read the paper, feels that the paper never really explained what its added value to the academic literature would be (point 1) and that it is indeed difficult to identify key lessons learned from the material that is presented (point 2). In combination, this makes the paper rather unsuitable for publication in a scholarly journal, although I can imagine that with some extensions the manuscript could be made suitable as a report for practitioners and policy makers, especially those with limited knowledge of simulation models and a wish to have a systematic, practical guide to help them navigate such models in the healthcare domain.
6. PLOS authors have the option to publish the peer review history of their article ( what does this mean? ). If published, this will include your full peer review and any attached files.
If you choose “no”, your identity will remain anonymous but your review may still be made public.
Do you want your identity to be public for this peer review? For information about this choice, including consent withdrawal, please see our Privacy Policy .
Reviewer #1: Yes: Andrea Montefusco
Reviewer #2: Yes: Caspar Chorus
[NOTE: If reviewer comments were submitted as an attachment file, they will be attached to this email and accessible via the submission site. Please log into your account, locate the manuscript record, and check for the action link "View Attachments". If this link does not appear, there are no attachment files.]
While revising your submission, please upload your figure files to the Preflight Analysis and Conversion Engine (PACE) digital diagnostic tool, https://pacev2.apexcovantage.com/ . PACE helps ensure that figures meet PLOS requirements. To use PACE, you must first register as a user. Registration is free. Then, login and navigate to the UPLOAD tab, where you will find detailed instructions on how to use the tool. If you encounter any issues or have any questions when using PACE, please email PLOS at [email protected] . Please note that Supporting Information files do not need this step.
Author response to Decision Letter 0
Collection date 2021.
19 Nov 2020
Dear reviewers and Academic Editor;
We deeply appreciate your constructive critique and valuable comments. We have extensively revised the manuscript to address each of the concerns.
Reviewer 1.
Comment 1: Authors introduce the topics very clearly, exploring the whole health care performance context thoroughly. They illustrate the issue the paper should contribute to address: how to keep into account complex interactions amid factors those which impact triple aim. They also specify that their review wants to explore possible simulations for improving “what if and “how to” scenario processes, listing the major limits of actual models proposed by some institutions. Final aim – presenting a visual aid to select the most appropriate simulation - is clearly expressed.
Authors discuss data extraction and analysis which they adopt-ed. They carefully show the process they followed, focusing how each step contributes to an integrated frame which looks coherent with paper’s aims.
Response 1: Thank you very much for your positive assessment of our methodological approach.
Comment 2: Results
The description of Areas of Assessment is readable as it connects its themes with the topics in a structured way. The PRIS-MA diagram contributes to clarity.
Response 2: The reviewer is correct in raising that the classification used for Simulation models and the structure used in the results section requires further explanation. As the reviewer mentioned, the reason is that criteria previously reported in the literature do not fit well the practical applications of our study, which we have now further specified. Furthermore, several reviews have been published with different approaches of classifying simulation models. Our structure choice purposely differentiates from these classifications in an effort to expose clearly the added value and practical implication of our paper. Nevertheless, we do agree that an explanation of our choice was necessary and was added in section 2.4, on lines 138 - 155, including the references that guided each item.
We thank the reviewer for the reference provided. In studying it we believe that its scope is more aligned with classifications responding to technical and mathematical issues (presented in a complexity progression typical of academic books) more than an exposé of usefulness for a particular area(Integrated care), like our work. We have however added a short mention to decision making under uncertainty on the Discussion section, lines 431-437 of the final manuscript.
Comment 3: The table offers a synthetic view which allows readers getting the big picture. Once again, as minor issue authors should have quoted the sources they based upon to state strengths and limitations of different types of models. It could be especially helpful for readers who do not have specific background.
Response 3: We thank the reviewer and agree with the assessment. The sources have been referenced in the table.
Comment 4: The Complexity section is especially interesting and at the center of authors aims. Lack of complexity in evaluating health care performances is one of the issues that authors want to address, so this section is expected to be rigorous and original. The latter expectation is quite satisfied while it is not possible to evaluate the first. Authors list nine complexity features those which are present in the 27 simulation models they’d previously selected. These features sound impactful and relevant but author should quote studies to help readers see them in the broader frame of system modelling theories.
Response 4: We thank the reviewer for the positive assessment of the originality of our study. In order to allow evaluation of the rigor employed, we have now added an expanded explanation of the common framework to evaluate complex relations between system components (section Results; 3.3 Complexity; lines 333-346). References for the terms and concepts in the different fields were provided.
Comment 5: It also should allow to better understand both the connection among these features and how they impact on estimating health care system performance.
Response 5: We agree and have updated the explanations for better internalization of the concepts (lines 347-391).
Comment 6: Some features look ambiguous if considered outside a frame of reference as in different field they are referred to different phenomena. E.g. dynamism in dynamic system theory could refer to different meanings ranging from the presence of state variables to the time-variant characteristic of the system itself or both. Again, authors connect Adaptation with intelligence as the ability to make decisions following specific rules. While it is a possible option, the definition of dynamism few rows above could bring someone wonder whether these rules must vary over time for a model showing dynamism.
Response 6: We thank the reviewer for raising this issue. We added references to a common frame-work to consolidate the concepts from different fields. (lines 333-391)
Comment 7: In summary, while this section is relevant and innovative, authors should better explain references and help readers put these features in a unitary frame.
Response 7: We are particularly thankful for this comment as it motivated us to present and give central importance to the complexity framework we had developed.
Comment 8: Discussion
The discussion starts exploring why a family of models can be helpful in modelling a specific system. While the intent is correct, this part sounds a little bit narrative. Maybe a more schematic description could help to stay connected with both the nine features and the five types of models described above.
This part looks as it was written through some and partial examples, so the reader could wonder why other considerations were neglected by authors and especially why.
Response 8: Based on the reviewer´s comment we have now reorganized this section and changed the discussion with two main focuses. 1st to discuss the advantages for integrated care of implementing systems thinking, including a summary of the contribution of each modeling technique and the discipline in general in this regard.
2nd On the usefulness of our research and how to use the tool that summarizes our findings.
Comment 9: In the second and in the third part authors discuss the core of the paper: how to improve the choice of performance simulation model for evaluating health care performance in the late of the “triple aim”.
Authors should be more systematic discussing the tool which should be considered like a model itself, as it offers a way to choose simulation models through matching the features pro-posed by authors, as a result of their review, and needs coming from triple aim approach. More examples should be proposed to help readers understand how to use the tool when more than one feature is necessary.
Response 9: As we said before, we thank the reviewer for pushing us to give more emphasis to our selection tool. We have added a general rationale for use of the tool (Discussion, lines 451-456) and a third example that covers how to deal with a system with multiple parallel complexities (467-479). The key to the use of the selection tool is the identification and prioritization of the complexities that rule the system’s performance.
We acknowledge the limitation of the tool to integrate several complexities with a different priority. However, after the selection of the most important complexity to be modeled, the transit options will integrate the most com-mon second or third level complexities associated with systems ruled by the selected top complexity. We believe the practical utility of our tool to orient users towards an appropriate modeling approach justifies our approach
Comment 10: Limitations are expressed clearly. Conclusion suffers the weakness posed in the discussion section.
Response 10: Based on the reviewer's comments, we have updated our conclusion in line with the new structure of the discussion.
Reviewer 2.
Comment 1: While this paper is well written, and discusses a timely topic that in principle is worthy of academic investigation, I do not think that the paper should be accepted for publication. In my view, the paper provides an interesting overview of recent simulation modeling efforts in health systems (with a special emphasis on complexity, being an important characteristic of such systems). But it does not go beyond that, and indeed more is needed to justify publication in a scholarly journal.
Response 1: We thank the reviewer for stating that we are providing an interesting overview of recent simulation modeling efforts in health systems, one of the objectives of our paper.
However, we kindly disagree that our paper does not go beyond that.
First, while we clearly state that previous reviews have been conducted in the field, some of them are quite outdated. Our review provides a thorough and up-to-date overview of current approaches in the field.
Secondly, as also pointed out by reviewer one we have presented an innovative approach to help users identify the most appropriate simulation technique, given a set of criteria. We believe that our presentation of this approach would indeed stimulate scholarly debate and may lead to further refinements of the method.
Comment 2: First, the paper misses a scientific motivation. The justification for the paper is given in lines 70-72 and can be summarized by saying that simulation models have recently gained more recognition. But this in itself is no (scholarly) motivation for reviewing the related literature. A proper motivation would include: a discussion of why and to whom such a review would be beneficial, i.e., what is the aim of doing such a review? Who would use it, and to what aim? What goes wrong when the study (review) is not done?
Response 2: We thank the reviewer for the reflection which we considered carefully. The assessment of the reviewer might be informed by the expectation of a typical PICO style presentation of the research questions, which however does not apply to our study subject. In order to respond to the points raised by the reviewer, we have re-written the Introduction to better state the scientific motivation(57-59), the knowledge gap(71-82.), our aim(83-86) and objectives and the audience of the review(86-88).
In short, the paper’s motivation relates to the lack of tools to assess integrated care as a dominant health system reform strategy. The identified problem is that system thinking is missing in integrated care performance assessment framework.
A solution to this problem is simulation modeling. The identified knowledge gap is the link between the complex system perspective of SM and the usefulness for integrated care performance assessment. We close this gap by reviewing papers that use simulation models with systems thinking for evaluations in topics relevant to integrated care systems and extracting the particular features that are useful for evaluating integrated care. We focus on the features for implementation of system thinking in a common framework and culminate our results in a tool for guiding model selection. We hope that the reviewer is satisfied with these improvements to the manuscript.
Comment 3: Also, a scientific motivation would include the identification of a clear knowledge gap: what other review efforts have been published in the academic literature? What is missing in those reviews, making this review necessary? Can the health domain learn from reviews of simulation models in other fields? Especially in light of various recently published review papers (14, 24, 65) it is of paramount importance that the authors justify the need for another review paper on this topic. Without such a clear motivation, the paper reads more like a (interesting) policy report, not a scholarly article.
Response 3: We agree with the reviewer that an articulation of the knowledge gap required strengthening. We added a paragraph referencing previous literature and stating clearly the knowledge gap that we are covering with the review. Lines 71-82.
Comment 4: Second, although the paper provides an interesting overview of relevant papers and the properties of the models described in them, it does not offer as much as I hoped for, in terms of deep reflection and discussion of subtle connections between the models. This can be due to the fact that an enormously wide net is cast across a variety of modeling approaches, each of which having been studied in thousands and thousands of academic papers. Describing such canonical and grand modeling traditions in so few words inescapably leads to gross simplifications. Although understandable, this does not do justice to the subtle-ties and complexities of each of these models, let alone the nuanced relations between them.
Response 4: We thank and acknowledge the limitation highlighted by the reviewer and have addressed it in the limitations section (lines 503-509).
Nevertheless, (as the added paragraph clarifies) we think that the provided simplifications don’t undermine the usefulness and academic value of our work. We acknowledge that an in-depth description of the identified models that would allow an analysis of the subtle connections between models would be impossible to undertake with our study design. Instead, we provide readers with an introduction to the identified simulation modeling techniques and highlight the characteristics that allow them to implement system thinking.
Furthermore, we provide a way forward by encouraging readers that find a solution in our work to the challenges they encounter when assessing the performance of a complex health system to learn in detail the technique that our paper has pointed towards. Such an approach, where an extensive literature/discipline is undertaken by a few practical applications to provide a practical way forward, is common in the academic literature and fundamental for bringing together different fields of expertise. An example is the paper by Behrendt & Groene 2016 (DOI: 10.1016/j.healthpol.2016.08.003)
Comment 5: Similarly, the applications of the discussed models in the healthcare domain are so diverse (see column IC Topic-of-interest in Table 1), that drawing commonalities and generic lessons from them is hardly possible. What can one learn from comparing the use of model A in context X with the use of model B in context Y? For this to lead to credible results, many more data points are needed. As a consequence, the paper results in a well-structured but unavoidably shallow discussion.
Response 5: In agreement with the reviewer comment, we too believe that is not possible to discern clear patterns between models and types of application in integrated healthcare with such a small sample size. However, two incipient patterns do emerge; (1.) System Dynamics for “Policy and strategy” and (2.) Discrete Event Simulations for “Health Resource Management”. This analysis is mention in the Discussion (lines 419-431).
This being said, even though finding a relation between the type of model and the different applications would be useful for integrated healthcare managers, a similar classification has already been made (Jun et al 2011) and escapes our main objective, that is to characterize simulation modeling focusing on the ability to implement system thinking.
Instead, we provide the type of applications (section 3.1, line 168) as part of a comprehensive characterization of simulation modeling in integrated care, with the purpose of informing readers of the reach of the discipline in integrated care topics. The classification also serves the purpose of grouping complexities, and we think it will help readers to identify complexities in different settings.
Comment 6: These two issues together make that this reviewer, having read the paper, feels that the paper never really explained what its added value to the academic literature would be (point 1) and that it is indeed difficult to identify key lessons learned from the material that is presented (point 2). In combination, this makes the paper rather unsuitable for publication in a scholarly journal, although I can imagine that with some extensions the manuscript could be made suitable as a report for practitioners and policy makers, especially those with limited knowledge of simulation models and a wish to have a systematic, practical guide to help them navigate such models in the healthcare domain.
Response 6: We thank the reviewer for the critique, which we have carefully considered and based on which we have substantially revised the manuscript.
With utmost respect, we provide a summarized answer to the two points raised by the reviewer.
Point 1: Exposing simulation models as a solution for the challenges of integrated healthcare systems performance assessment, focusing particularly on their ability to implement system thinking.
Point 2. A summary of key (to IHS) characteristics and complexity features in a common framework that helps in guiding the identification and prioritization of complexity, which in turn are useful to select an appropriate simulation model.
We strongly believe that our methodological approach adheres to rigorous standards and that our work goes far beyond the content expected for a policy brief or practical guide and indeed would inform scholarly debate.
• https://journals.plos.org/plosone/s/file?id=wjVg/PLOSOne_formatting_sample_main_body.pdf and
• https://journals.plos.org/plosone/s/file?id=ba62/PLOSOne_formatting_sample_title_authors_affiliations.pdf
2.2. Please also provide an updated Competing Interests Statement declaring this commercial affiliation along with any other relevant declarations relating to employment, consultancy, patents, products in development, or marketed products, etc.
Within your Competing Interests Statement, please confirm that this commercial affiliation does not alter your adherence to all PLOS ONE policies on sharing data and materials by including the following statement: "This does not alter our adherence to PLOS ONE policies on sharing data and materials.” (as detailed online in our guide for authors http://journals.plos.org/plosone/s/competing-interests ) . If this adherence statement is not accurate and there are restrictions on sharing of data and/or materials, please state these. Please note that we cannot proceed with consideration of your article until this information has been declared.
We thank the Academic Editor for your comments and guidelines. In response, we are pleased to declare the following:
1. We have formatted the manuscript as stated in the templates.
2. We have provided an amended Funding Statement in the Cover letter as recommended
3. We have provided an amended Competing Interests Statement in the Cover Letter as recommended.
Submitted filename: Response to Reviewers.docx
Decision Letter 1
Yong-hong kuo.
PONE-D-20-10819R1
A systematic review of simulation modeling to assess health system performance: Characterization of the field and visual aid to guide model selection.
Please submit your revised manuscript by Mar 21 2021 11:59PM. If you will need more time than this to complete your revisions, please reply to this message or contact the journal office at [email protected] . When you're ready to submit your revision, log on to https://www.editorialmanager.com/pone/ and select the 'Submissions Needing Revision' folder to locate your manuscript file.
Additional Editor Comments (if provided):
The referees from the last round were invited to review this revision. One of them agreed and returned the review report. The reviewer still had some comments for the authors to address in their work. The other was not available to review for this round. Thus, I have gone through his/her comments and the revision. Below is my evaluation.
1. As the reviewers suggested, the academic value of the work shall be strengthened. Currently, it is unclear about the research questions and how the state-of-the-art is advanced by this work. The scientific motivation is still missing.
2. The analysis part shall be strengthened. What are the key messages resulting from the analysis? Also, it would be nice to have this article discussing the research trends and shedding light on future research directions.
3. There have been dozens of literature review / survey papers on simulation models of healthcare applications. The position of this paper is unclear. How is this paper different from the existing review papers?
4. The number of papers on healthcare simulation is tremendous. Currently, only 27 papers were analyzed. This coverage is much narrow than those covered by the existing review papers. I suggest the authors have a more comprehensive review of the studies, particularly the recent ones. To my knowledge, the below studies are relevant to this review work. However, the list below is not exhaustive and the authors shall identify further related studies:
• Abramovich, M. N., Hershey, J. C., Callies, B., Adalja, A. A., Tosh, P. K., & Toner, E. S. (2017). Hospital influenza pandemic stockpiling needs: a computer simulation. American journal of infection control, 45(3), 272-277.
• Chen, Y., Kuo, Y. H., Balasubramanian, H., & Wen, C. (2015, December). Using simulation to examine appointment overbooking schemes for a medical imaging center. In 2015 Winter Simulation Conference (WSC) (pp. 1307-1318). IEEE.
• Gul, M., & Guneri, A. F. (2015). A comprehensive review of emergency department simulation applications for normal and disaster conditions. Computers & Industrial Engineering, 83, 327-344.
• Kuo, Y. H. (2014). Integrating simulation with simulated annealing for scheduling physicians in an understaffed emergency department. HKIE transactions, 21(4), 253-261.
• Kuo, Y. H., Leung, J. M., Graham, C. A., Tsoi, K. K., & Meng, H. M. (2018). Using simulation to assess the impacts of the adoption of a fast-track system for hospital emergency services. Journal of Advanced Mechanical Design, Systems, and Manufacturing, 12(3), JAMDSM0073-JAMDSM0073.
• Kuo, Y. H., Rado, O., Lupia, B., Leung, J. M., & Graham, C. A. (2016). Improving the efficiency of a hospital emergency department: a simulation study with indirectly imputed service-time distributions. Flexible Services and Manufacturing Journal, 28(1-2), 120-147.
• Hu, X., Barnes, S., & Golden, B. (2018). Applying queueing theory to the study of emergency department operations: a survey and a discussion of comparable simulation studies. International transactions in operational research, 25(1), 7-49.
• Moeke, D., van de Geer, R., Koole, G., & Bekker, R. (2016). On the performance of small-scale living facilities in nursing homes: a simulation approach. Operations research for health care, 11, 20-34.
• Niessner, H., Rauner, M. S., & Gutjahr, W. J. (2018). A dynamic simulation–Optimization approach for managing mass casualty incidents. Operations research for health care, 17, 82-100.
• Ordu, M., Demir, E., Tofallis, C., & Gunal, M. M. (2020). A novel healthcare resource allocation decision support tool: A forecasting-simulation-optimization approach. Journal of the operational research society, 1-16.
• Roy, S. N., Shah, B. J., & Gajjar, H. (2020). Application of Simulation in Healthcare Service Operations: A Review and Research Agenda. ACM Transactions on Modeling and Computer Simulation (TOMACS), 31(1), 1-23.
• Roy, S., Prasanna Venkatesan, S., & Goh, M. (2020). Healthcare services: A systematic review of patient-centric logistics issues using simulation. Journal of the Operational Research Society, 1-23.
• Salleh, S., Thokala, P., Brennan, A., Hughes, R., & Booth, A. (2017). Simulation modelling in healthcare: an umbrella review of systematic literature reviews. PharmacoEconomics, 35(9), 937-949.
• Vanbrabant, L., Braekers, K., Ramaekers, K., & Van Nieuwenhuyse, I. (2019). Simulation of emergency department operations: A comprehensive review of KPIs and operational improvements. Computers & Industrial Engineering, 131, 356-381.
• Vanbrabant, L., Martin, N., Ramaekers, K., & Braekers, K. (2019). Quality of input data in emergency department simulations: framework and assessment techniques. Simulation Modelling Practice and Theory, 91, 83-101.
• Weissman, G. E., Crane-Droesch, A., Chivers, C., Luong, T., Hanish, A., Levy, M. Z., ... & Halpern, S. D. (2020). Locally informed simulation to predict hospital capacity needs during the COVID-19 pandemic. Annals of internal medicine, 173(1), 21-28.
• Yousefi, M., Yousefi, M., & Fogliatto, F. S. (2020). Simulation-based optimization methods applied in hospital emergency departments: A systematic review. Simulation, 96(10), 791-806.
• Zhang, C., Grandits, T., Härenstam, K. P., Hauge, J. B., & Meijer, S. (2018). A systematic literature review of simulation models for non-technical skill training in healthcare logistics. Advances in Simulation, 3(1), 1-16.
Based on the reviewer's and my one evaluations, I recommend major revision.
Hope that the authors shall find the comments constructive. The revision will go through a rigorous review process again. Unsuccessful revision can lead to rejection of the work.
1. If the authors have adequately addressed your comments raised in a previous round of review and you feel that this manuscript is now acceptable for publication, you may indicate that here to bypass the “Comments to the Author” section, enter your conflict of interest statement in the “Confidential to Editor” section, and submit your "Accept" recommendation.
Reviewer #1: (No Response)
2. Is the manuscript technically sound, and do the data support the conclusions?
3. Has the statistical analysis been performed appropriately and rigorously?
4. Have the authors made all data underlying the findings in their manuscript fully available?
5. Is the manuscript presented in an intelligible fashion and written in standard English?
6. Review Comments to the Author
Reviewer #1: The following comments refer directly to those in the previous review and the authors’ answers. The actual review did not limit to verifying whether the authors have addressed issues but reconsidered the entire paper.
The authors profoundly modified section 2.4. While before, it explained the data analysis approach weakly, now it meets expectations. Authors fully reformulate sentences, making possible a better understanding of the paper aims too. The section specifies, step by step, the rationale behind every item’s choice. It allows the reader to navigate the paper with a clean schema. The connections between items and the research question are now clear. Though this section calls for attention, it progressively helps readers build the first representation of how items integrate each other. It results in a sort of “integrated variable space” which appears to be the real novelty of this paper.
The authors followed the suggestion and added the reference column in Table 1. Though it was a minor issue, expert readers can now connect with their knowledge while everyone could search sources directly and efficiently.
The authors rewrote section 3.3 completely and, now the reader can find proper references. It helps understand the rationale behind the nine aspects of complex relations and the way to model them. It is easy to go through authors’ statements, as the text and the table together provide a clean, integrated view.
While the authors analyzed complexity dimensions well, section 3.3 still misses why the nine dimensions impact health system performance. They go through that in session 4, Discussion. That is correct, as this way, they directly connect their framework with the research question and the final paper’s goal. It could help anticipate here straightforward examples of each complexity component's impacts on health care system performance. Although this could be considered a minor issue, nonetheless, it can improve the clarity much. Perhaps it is not easy to connect these concepts with health care system performance for those who have no in-depth knowledge of complex system theory and health care. Despite being a minor issue, this could limit the paper’s practical impact. Delivering examples in section 3.3 could increase the readability of section 4, as the readers would have examples in their minds.
See comment 4.
See comment 5. The authors hit the nail, though they could have delivered examples of impacts in this section too.
Comment 8-9
The authors provided the suggested schematic connection between the nine features and health care. The section is informative now, though it still lacks a synthesis. Maybe its presence – A draw? Flow diagramm? - could help readers generalizing the application of the frame. Although the authors commented on the examples more in-depth than in the first submission, maybe the readers could find it challenging to apply the tools to a specific case.
With their thorough revision, the authors improved the first and the second parts of the paper. The final sections get better after the revision, but it requires further work to keep the previous sections' promises.
While reading it, it is hard to focus on the research question, as authors still do not explicitly connect their results’ discussion with keywords like triple aims and system performances, for just quickly quote them in the Limitations and Conclusion sections.
Conclusion and Limitation must exploit the potential that is now shown by the previous sections. At the moment, they seem to be far weaker than the rest of the paper.
7. PLOS authors have the option to publish the peer review history of their article ( what does this mean? ). If published, this will include your full peer review and any attached files.
Author response to Decision Letter 1
20 Mar 2021
Dear reviewers and Academic Editor.
We are deeply thankful for your revisions, valuable comments, and additional clarifications. We have extensively revised the manuscript to address each of your concerns. We have taken the liberty of organizing your comments in the following list, only including the second revision and responses organized by reviewer and commentary. We recommend attending the attached "Response to reviewers" letter, that provides both revisions and responses, for a better assessment.
Reviewer 1:
1. Comment 1:
2nd revision: No comment
� Response 2nd revision: -
2. Comment 2:
2nd revision: The authors profoundly modified section 2.4. While before, it explained the data analysis approach weakly, now it meets expectations. Authors fully reformulate sentences, making possible a better understanding of the paper aims too. The section specifies, step by step, the rationale behind every item’s choice. It allows the reader to navigate the paper with a clean schema. The connections between items and the research question are now clear. Though this section calls for attention, it progressively helps readers build the first representation of how items integrate each other. It results in a sort of “integrated variable space” which appears to be the real novelty of this paper.
� Response 2nd revision: We thank the reviewer for the positive comments.
3. Comment 3:
2nd revision: The authors followed the suggestion and added the reference column in Table 1. Though it was a minor issue, expert readers can now connect with their knowledge while everyone could search sources directly and efficiently.
� Response 2nd revision: We thank the reviewer for his comments and contribution in this section.
4. Comment 4:
2nd revision: The authors rewrote section 3.3 completely and, now the reader can find proper references. It helps understand the rationale behind the nine aspects of complex relations and the way to model them. It is easy to go through authors’ statements, as the text and the table together provide a clean, integrated view.
5. Comment 5:
2nd revision: While the authors analyzed complexity dimensions well, section 3.3 still misses why the nine dimensions impact health system performance. They go through that in session 4, Discussion. That is correct, as this way, they directly connect their framework with the research question and the final paper’s goal. It could help anticipate here straightforward examples of each complexity component's impacts on health care system performance. Although this could be considered a minor issue, nonetheless, it can improve the clarity much. Perhaps it is not easy to connect these concepts with health care system performance for those who have no in-depth knowledge of complex system theory and health care. Despite being a minor issue, this could limit the paper’s practical impact. Delivering examples in section 3.3 could increase the readability of section 4, as the readers would have examples in their minds.
� Response 2nd revision: We thank the observation by the reviewer, and we concur in his assessment. We included short and clear examples for each complexity feature in section 3.3, referencing to previously explained phenomena when possible.
6. Comment 6
2nd revision: -
7. Comment 7:
2nd revision: See comment 4.
8. Comment 8:
2nd revision: See comment 5. The authors hit the nail, though they could have delivered examples of impacts in this section too.
9. Comment 9:
2nd revision: (Comment 8-9(10)) The authors provided the suggested schematic connection between the nine features and health care. The section is informative now, though it still lacks a synthesis. Maybe its presence – A draw? Flow diagram? - could help readers generalizing the application of the frame. Although the authors commented on the examples more in-depth than in the first submission, maybe the readers could find it challenging to apply the tools to a specific case.
� Response 2nd revision: We appreciate the reviewer comments. Building on the academic editor comments, we have changed the introduction, methods and analysis sections to clearly state the scientific motivation, research question and objectives so that the results and discussion are in line with what the paper promises. This being said, we have re-structured the discussion and limitations section to better explain the link we have created between SM and IHS performance assessment. We appreciated the idea of a visual aid for better understanding the characterization of SM in the field of IHS, but we think that Table 2, 3 and figure 2 comply with this role. We link the characterization of SM with triple aim and system performance assessment in lines 466-483, when stating the implications of the exposed characteristics of SM.
� We think that the best trait of the selection tool is its simplicity. We have changed the writing of the examples to highlight this trait. We hope that the reviewer is pleased with the new discussion and that the contribution of the paper is stated clearly in the conclusion.
10. Comment 10:
2nd revision: See comment 9
11. Comment 11:
Academic Editor 2nd Revision:
1. Comment 1.
As the reviewers suggested, the academic value of the work shall be strengthened. Currently, it is unclear about the research questions and how the state-of-the-art is advanced by this work. The scientific motivation is still missing.
� Response: We thank the editor for his revisions. We acknowledge that the introduction, starting with the title, invited readers into a topic that was broader than what the paper is covering. For this reason, we have strengthened the introduction to highlight the scientific motivation and the contribution of our work and clearly stating the real scope of our article. While including a more comprehensive revision of past literature, we have clearly stated the existing knowledge gap (lines 106-110) and the position of the paper.
2. Comment 2.
� Response: We thank the editor for his comment. The new introduction is a better guide into what readers can expect from the paper and goes in line with the analysis. The analysis explanation on the methods section 2.4 was corrected so that the expectations created in the introduction and the results are in line. We agree that the key messages from the analysis should be strengthen, and we do so in the changes we did to the conclusion section. To summarize: 1. SM can use system thinking to evaluate topic of relevance for IHS. 2. SM allow IHS managers to correctly attribute causality, optimize interventions, and create long term assessments. 3. Selecting a simulation technique is facilitated when both the characteristics of the modeling techniques are understood, and the complexities ruling the system performance are identified and prioritized. Our framework for assessing complexity can be used for this purpose.
� We hope that with the new introduction section (that highlights the contribution and scientific motivation of our work) it is left clearer why the current analysis is fit for the purpose.
As suggested by the editor, we have included a much broader contextualization, so we can better position the contribution of our paper to current literature.
3. Comment 3.
There have been dozens of literature review / survey papers on simulation models of healthcare applications. The position of this paper is unclear. How is this paper different from the existing review papers?
� Response: As suggested by the editor, we have included a much broader contextualization, so we can better position the contribution of our paper to current literature. (lines 76-104)
4. Comment 4.
The number of papers on healthcare simulation is tremendous. Currently, only 27 papers were analyzed. This coverage is much narrow than those covered by the existing review papers. I suggest the authors have a more comprehensive review of the studies, particularly the recent ones. To my knowledge, the below studies are relevant to this review work. However, the list below is not exhaustive, and the authors shall identify further related studies:
1. Abramovich, M. N., Hershey, J. C., Callies, B., Adalja, A. A., Tosh, P. K., & Toner, E. S. (2017). Hospital influenza pandemic stockpiling needs: a computer simulation. American journal of infection control, 45(3), 272-277.
2. Chen, Y., Kuo, Y. H., Balasubramanian, H., & Wen, C. (2015, December). Using simulation to examine appointment overbooking schemes for a medical imaging center. In 2015 Winter Simulation Conference (WSC) (pp. 1307-1318). IEEE.
3. Gul, M., & Guneri, A. F. (2015). A comprehensive review of emergency department simulation applications for normal and disaster conditions. Computers & Industrial Engineering, 83, 327-344.
4. Kuo, Y. H. (2014). Integrating simulation with simulated annealing for scheduling physicians in an understaffed emergency department. HKIE transactions, 21(4), 253-261.
5. Kuo, Y. H., Leung, J. M., Graham, C. A., Tsoi, K. K., & Meng, H. M. (2018). Using simulation to assess the impacts of the adoption of a fast-track system for hospital emergency services. Journal of Advanced Mechanical Design, Systems, and Manufacturing, 12(3), JAMDSM0073-JAMDSM0073.
6. Kuo, Y. H., Rado, O., Lupia, B., Leung, J. M., & Graham, C. A. (2016). Improving the efficiency of a hospital emergency department: a simulation study with indirectly imputed service-time distributions. Flexible Services and Manufacturing Journal, 28(1-2), 120-147.
7. Hu, X., Barnes, S., & Golden, B. (2018). Applying queueing theory to the study of emergency department operations: a survey and a discussion of comparable simulation studies. International transactions in operational research, 25(1), 7-49.
8. Moeke, D., van de Geer, R., Koole, G., & Bekker, R. (2016). On the performance of small-scale living facilities in nursing homes: a simulation approach. Operations research for health care, 11, 20-34.
9. Niessner, H., Rauner, M. S., & Gutjahr, W. J. (2018). A dynamic simulation–Optimization approach for managing mass casualty incidents. Operations research for health care, 17, 82-100.
10. Ordu, M., Demir, E., Tofallis, C., & Gunal, M. M. (2020). A novel healthcare resource allocation decision support tool: A forecasting-simulation-optimization approach. Journal of the operational research society, 1-16.
11. Roy, S. N., Shah, B. J., & Gajjar, H. (2020). Application of Simulation in Healthcare Service Operations: A Review and Research Agenda. ACM Transactions on Modeling and Computer Simulation (TOMACS), 31(1), 1-23.
12. Roy, S., Prasanna Venkatesan, S., & Goh, M. (2020). Healthcare services: A systematic review of patient-centric logistics issues using simulation. Journal of the Operational Research Society, 1-23.
13. Salleh, S., Thokala, P., Brennan, A., Hughes, R., & Booth, A. (2017). Simulation modelling in healthcare: an umbrella review of systematic literature reviews. PharmacoEconomics, 35(9), 937-949.
14. Vanbrabant, L., Braekers, K., Ramaekers, K., & Van Nieuwenhuyse, I. (2019). Simulation of emergency department operations: A comprehensive review of KPIs and operational improvements. Computers & Industrial Engineering, 131, 356-381.
15. Vanbrabant, L., Martin, N., Ramaekers, K., & Braekers, K. (2019). Quality of input data in emergency department simulations: framework and assessment techniques. Simulation Modelling Practice and Theory, 91, 83-101.
16. Weissman, G. E., Crane-Droesch, A., Chivers, C., Luong, T., Hanish, A., Levy, M. Z., ... & Halpern, S. D. (2020). Locally informed simulation to predict hospital capacity needs during the COVID-19 pandemic. Annals of internal medicine, 173(1), 21-28.
17. Yousefi, M., Yousefi, M., & Fogliatto, F. S. (2020). Simulation-based optimization methods applied in hospital emergency departments: A systematic review. Simulation, 96(10), 791-806.
18. Zhang, C., Grandits, T., Härenstam, K. P., Hauge, J. B., & Meijer, S. (2018). A systematic literature review of simulation models for non-technical skill training in healthcare logistics. Advances in Simulation, 3(1), 1-16.
� Response: As we mentioned before, starting with the title, the paper was not clear in the scope of the research. We have worked to better explain the scope of our paper, which gives reason to our much smaller sample of papers. We understand that the selection criteria was not detailed correctly, and we have worked to correct this issue (sections 2.1 & 2.2). We gave emphasis on the link between the objectives of the paper and the selection criteria, and why are these appropriate.
In short, we selected papers using a simulation technique in topics of interest to integrated healthcare system that took a system thinking perspective and had the detail and quality necessary to understand the complexities in components relations that were represented.
We also acknowledge the limitation of our systematic search in terms of the databases that we used. In particular, because articles such as 4.(Kuo 2014) ; 5.(Kuo 2018); 6.(Kuo 2016) & 8. (Moeke 2016) were not present in said databases. We will acknowledge this limitation in the corresponding section of our article(line 538).
In the same line, we acknowledge that our search strategy did not identify all relevant papers on simulation modeling, as we addressed a very specific use case which led to the exclusion of papers such as 1. (Abramovich 2017) and 2. (Chen 2015). Our search strategy with the focus on integrated healthcare was based on the work and expertise of worldwide leaders in the topic of performance assessment of integrated healthcare systems in an effort to focus the characterization of simulation modeling and their ability to implement system thinking for this particular area of research. To attend to this issue, we have strengthened the argument behind our search strategy, and we have stated a clear differentiation to what could be characterized as a comprehensive review of simulation modeling in healthcare management. (lines 126-127 147-151; 161-165; 181)
Given that the, now better explained, aim of our study is a characterization of the field of simulation modeling in areas of interest for integrated healthcare systems (particularly in the discipline’s ability to implement system thinking) and not a quantitative summary in the line of a meta-analysis, we are confident that our methodological approach allowed us to analyze a pertinent set of rigorously selected articles.
We hope that our substantive revision of the manuscript and response to the constructive comments by the reviewers and editor do now fully meet your expectations.
Decision Letter 2
19 Apr 2021
PONE-D-20-10819R2
Simulation modeling to assess performance of integrated healthcare systems: Systematic literature review to characterize the field and visual aid to guide model selection.
Please submit your revised manuscript by Jun 03 2021 11:59PM. If you will need more time than this to complete your revisions, please reply to this message or contact the journal office at [email protected] . When you're ready to submit your revision, log on to https://www.editorialmanager.com/pone/ and select the 'Submissions Needing Revision' folder to locate your manuscript file.
If applicable, we recommend that you deposit your laboratory protocols in protocols.io to enhance the reproducibility of your results. Protocols.io assigns your protocol its own identifier (DOI) so that it can be cited independently in the future. For instructions see: http://journals.plos.org/plosone/s/submission-guidelines#loc-laboratory-protocols . Additionally, PLOS ONE offers an option for publishing peer-reviewed Lab Protocol articles, which describe protocols hosted on protocols.io. Read more information on sharing protocols at https://plos.org/protocols?utm_medium=editorial-email&utm_source=authorletters&utm_campaign=protocols .
The revision has been reviewed by the reviewer from the last round. The reviewer has made a favorable recommendation.
I have gone through the revision again. I highly appreciate that the authors have seriously addressed some of the concerns. The scope of the work and research question are now clear.
I believe the work has certain degree of merit and potential to be published. However, since PLOS ONE publishes scientifically rigorous studies, there are still two major issues which have to be addressed:
1. As compared with other similar studies on simulation modeling in healthcare system, the studies reviewed in this work (only 27) are significantly fewer. The arguments and conclusions made in this study are therefore not promising. This problem is particularly clear as shown in Section 3.2. For examples, the results presented are only based on 2 studies for Markov models, 1 study for microsimulation, 2 studies for agent-based models, 1 study for hybrid simulations, etc. What were claimed in those sections are not comprehensive and convincing.
2. The problem stated in point 1 is probably caused by a subjective selection of articles (on p. 10) by only the two authors. This work is title a "systematic" review but this process systems to be non-systematic. I suggest there is a clear description of how the reviewers determine whether to include a study is included. A quality score is given to an article by the two authors; however, is this quality score reliable? (Do the authors imply that out of 2271 articles, only 27 are of quality? Others are not of quality?) Another issue is that a systematic literature review is to identify trends in the research area. It would be necessary to include studies not only based on the subjectively determined quality but need to identify the overall trends.
Reviewer #1: All comments have been addressed
Reviewer #1: Is the manuscript technically sound, and do the data support the conclusions?
The authors have progressively addressed the original version issues. Their paper now sounds informative while it illustrates its aims and methods sharply. The authors illustrate their framework clearly. Now the flow is logical, and connections between scope, data, and conclusions are evident.
Have the authors made all data underlying the findings in their manuscript fully available?
Yes, they did.
Is the manuscript presented in an intelligible fashion and written in standard English?
The authors have progressively evolved their manuscript through discourse with the reviewers. After the last revision, the paper will offer a novel and informative perspective to health care scholars and especially professionals. It will help readers look at the “Triple Aim” model with a broader framework to assess health care performances considering complexity accurately and robustly. Though the proposed tool has limits, it shows the readers the relevance of the systemic approach in evaluating a health care system. The paper starts a practical conversation that goes beyond simple improvement in health care assessment techniques. This proposal falls in the field of accurate and relevant feedback conversation (e.g, see Zollo, 2009 about superstitious learning). Despite its limits, the tool connects the diverse elements that concur in performance and allows H.C. professionals to take them and their complex interactions into account in evaluating and comparing performance.
This reviewer can't judge the English level of the manuscprit.
Author response to Decision Letter 2
Dear reviewer and Academic Editor:
We are deeply thankful for your revision. We have revised each of your comments and provide each with a response in the following text. For a more structured version of this response, please see the attached “Response to Reviewers" file.
Editor Comments, General:
- Response:
We are deeply thankful to the Editor for recognizing our work in the article. We have made extensive changes to the core of the article to address the editors’ concerns. Regarding the Editor’s second comment, in summary, we understand that the current selection of articles is deemed to be more subjective than what is usually found in systematic literature reviews. In particular, because even though the criterion for selection is clearly defined, part of the definition (Section 2.2 line 155: <<Finally, we excluded from the data extraction and analysis studies whose reporting standards were insufficient to fully understand and replicate the assessment >>) leaves room for a subjective interpretation. While even in the most rigorous systematic reviews data extractors have to introduce a certain level of interpretation in deciding which study and which data to include, we agree that our approach previously has not been optimal. In order to correct for subjectivity, we have now included all the articles with the highest quality score following our quality assessment (+3 extra articles). Furthermore, to be transparent with reader expectations, we have decided to define our article as a literature review with systematic a search . The PROSPERO record was modified stating this change. We have noted that PLOS ONE publishes all types of reviews of the literature (in terms of Grant and Booth typology), not only systematic reviews of the literature. However, by now labelling our study as literature review with systematic search we believe that our methodological approach meets all expectations a reader might have.
Editor Comments, 1.
We thank the editor for the comment. Even though we defend our article selection to be fit for purpose and aligned with the objective of the study, we recognize that the writing of section 3.2 leads readers to think that the revision of the different methodologies was based solely in the articles selected from the systematic search. This is not correct. The methodologies were extracted from the selected articles. Then, selected articles were used to explain each methodology features. Nevertheless, the features themselves were summarized using complementary literature (presented in table 2). This is explained in the methods section 2.4 (line 184-186). We have changed the first paragraph of the section 3.2 (lines 223-228) to better explain were the features explanation come from. The complementary literature used is not solely based on case articles, but mostly on review articles and books explaining the methodologies in detail.
Editor Comments, 2.
First, please see above regarding the change in the title of the document according to Grant´s and Booth’s typology.
Second, we edited the methods section, stating explicitly that articles were selected according to our appreciation of their reporting standards to allow for the objective of our paper(section 2.2). In other words, the final selection criteria relate to the ability of reviewers to fully understand and replicate the article for a complete assessment of how the complexities of the system were included in the model. The limitations section was also edited stating the limitation of the selection process.
Three extra articles were added following the correction in the selection process:
1. Ansah et al. 2016: The article was initially excluded because even though evaluated with quality A, it did not add any additional information to the analysis. Following the corrected exclusion criteria, the article is now added in the review.
2. Goldman et al. 2004: The article was not included in the original revision because even though the model has top quality, the practical application and validation of said model are found in subsequent articles. Meanwhile, articles depicting practical applications of the model lacked a detailed description of the model. Initially, we though evaluating them together as a whole was a reach of our quality assessment criteria, hence the initial exclusion.
3. Comans et al. 2017: Similarly, to number (1.), the paper was excluded because it did not add information for analysis. Following the corrected exclusion criteria, the article is now added in the review.
We included the assessment of the new articles and mention them as examples to explain system thinking where they contributed to the analysis. Nevertheless, the results, discussion and conclusion did not change (with exception of the count of optimization capabilities and time frame for assessment) with the inclusion with the new articles.
The abstract was edited showing these changes.
We understand that many systematic reviews indicate research trends, but we are certain that research trends are only reported when in line with the objectives of the study. Our article did not focus on pooled effect sizes or research trends, as the explanation of how SM methods are able to integrate system thinking is not time dependent. In the same line, the selection of a SM method is determined by identifying complexities and the overall objective and not on trends.
Furthermore, given that we have chosen to classify the article as a literature review (where reporting research trends is less common), we think that reporting research trends is less justified.
All comments by the reviewer were positive and no detailed response is needed.
We thank the reviewer for the recognition of our work, and we appreciate his positive appreciation and useful comments during the revision process.
Decision Letter 3
28 Jun 2021
Simulation modeling to assess performance of integrated healthcare systems: Literature review to characterize the field and visual aid to guide model selection.
PONE-D-20-10819R3
We’re pleased to inform you that your manuscript has been judged scientifically suitable for publication and will be formally accepted for publication once it meets all outstanding technical requirements.
Within one week, you’ll receive an e-mail detailing the required amendments. When these have been addressed, you’ll receive a formal acceptance letter and your manuscript will be scheduled for publication.
An invoice for payment will follow shortly after the formal acceptance. To ensure an efficient process, please log into Editorial Manager at http://www.editorialmanager.com/pone/ , click the 'Update My Information' link at the top of the page, and double check that your user information is up-to-date. If you have any billing related questions, please contact our Author Billing department directly at [email protected] .
If your institution or institutions have a press office, please notify them about your upcoming paper to help maximize its impact. If they’ll be preparing press materials, please inform our press team as soon as possible -- no later than 48 hours after receiving the formal acceptance. Your manuscript will remain under strict press embargo until 2 pm Eastern Time on the date of publication. For more information, please contact [email protected] .
Additional Editor Comments (optional):
Acceptance letter
Dear Dr. Larrain:
I'm pleased to inform you that your manuscript has been deemed suitable for publication in PLOS ONE. Congratulations! Your manuscript is now with our production department.
If your institution or institutions have a press office, please let them know about your upcoming paper now to help maximize its impact. If they'll be preparing press materials, please inform our press team within the next 48 hours. Your manuscript will remain under strict press embargo until 2 pm Eastern Time on the date of publication. For more information please contact [email protected] .
If we can help with anything else, please email us at [email protected] .
Thank you for submitting your work to PLOS ONE and supporting open access.
PLOS ONE Editorial Office Staff
on behalf of
Dr. Yong-Hong Kuo
Associated Data
This section collects any data citations, data availability statements, or supplementary materials included in this article.
Supplementary Materials
Data availability statement.
- View on publisher site
- PDF (1.2 MB)
- Collections
Similar articles
Cited by other articles, links to ncbi databases.
- Download .nbib .nbib
- Format: AMA APA MLA NLM
Add to Collections

COMMENTS
This paper describes a Systematic Literature Review (SLR) which returned 8070 papers (published from 2013 to 2019) by a systematic search in 4 digital libraries. After conducting this SLR, 36 Software Process Simulation Modeling (SPSM) works were selected as primary studies and were documented following a specific characterization scheme.
Software that offer simple and complete interfaces that encompass all stages of a simulation project (from design, modeling, implementation to model validation) find their place in decision making centers.
A systematic literature review, following the PRISMA guidelines, was performed to identify simulation models that could be used for training individuals in operative logistical coordination that occurs on a daily basis.
This paper describes a systematic review about Software Process Simulation Modelling (SPSM). After the conducting phase, 36 primary studies were selected and studied in depth. It is possible to conclude that SPSM continues to be a topic very addressed, but each contribution has particular goals.
A simulation model can take into account inventory, assembly, production and human resources, leading to decisions that can maintain or improve efficiency at the lowest possible cost. The data obtained through the simulation allow to test different combinations and scenarios in the virtual world.
Salleh et al. published an umbrella review including 37 reviews, that together cover articles from 1950 to 2016 and explore the wide range of applications in healthcare, software tools and data sources used in the field of healthcare simulation.