
An official website of the United States government
The .gov means it’s official. Federal government websites often end in .gov or .mil. Before sharing sensitive information, make sure you’re on a federal government site.
The site is secure. The https:// ensures that you are connecting to the official website and that any information you provide is encrypted and transmitted securely.
- Publications
- Account settings
The PMC website is updating on October 15, 2024. Learn More or Try it out now .
- Advanced Search
- Journal List
- J Korean Med Sci
- v.37(16); 2022 Apr 25


A Practical Guide to Writing Quantitative and Qualitative Research Questions and Hypotheses in Scholarly Articles
Edward barroga.
1 Department of General Education, Graduate School of Nursing Science, St. Luke’s International University, Tokyo, Japan.
Glafera Janet Matanguihan
2 Department of Biological Sciences, Messiah University, Mechanicsburg, PA, USA.
The development of research questions and the subsequent hypotheses are prerequisites to defining the main research purpose and specific objectives of a study. Consequently, these objectives determine the study design and research outcome. The development of research questions is a process based on knowledge of current trends, cutting-edge studies, and technological advances in the research field. Excellent research questions are focused and require a comprehensive literature search and in-depth understanding of the problem being investigated. Initially, research questions may be written as descriptive questions which could be developed into inferential questions. These questions must be specific and concise to provide a clear foundation for developing hypotheses. Hypotheses are more formal predictions about the research outcomes. These specify the possible results that may or may not be expected regarding the relationship between groups. Thus, research questions and hypotheses clarify the main purpose and specific objectives of the study, which in turn dictate the design of the study, its direction, and outcome. Studies developed from good research questions and hypotheses will have trustworthy outcomes with wide-ranging social and health implications.
INTRODUCTION
Scientific research is usually initiated by posing evidenced-based research questions which are then explicitly restated as hypotheses. 1 , 2 The hypotheses provide directions to guide the study, solutions, explanations, and expected results. 3 , 4 Both research questions and hypotheses are essentially formulated based on conventional theories and real-world processes, which allow the inception of novel studies and the ethical testing of ideas. 5 , 6
It is crucial to have knowledge of both quantitative and qualitative research 2 as both types of research involve writing research questions and hypotheses. 7 However, these crucial elements of research are sometimes overlooked; if not overlooked, then framed without the forethought and meticulous attention it needs. Planning and careful consideration are needed when developing quantitative or qualitative research, particularly when conceptualizing research questions and hypotheses. 4
There is a continuing need to support researchers in the creation of innovative research questions and hypotheses, as well as for journal articles that carefully review these elements. 1 When research questions and hypotheses are not carefully thought of, unethical studies and poor outcomes usually ensue. Carefully formulated research questions and hypotheses define well-founded objectives, which in turn determine the appropriate design, course, and outcome of the study. This article then aims to discuss in detail the various aspects of crafting research questions and hypotheses, with the goal of guiding researchers as they develop their own. Examples from the authors and peer-reviewed scientific articles in the healthcare field are provided to illustrate key points.
DEFINITIONS AND RELATIONSHIP OF RESEARCH QUESTIONS AND HYPOTHESES
A research question is what a study aims to answer after data analysis and interpretation. The answer is written in length in the discussion section of the paper. Thus, the research question gives a preview of the different parts and variables of the study meant to address the problem posed in the research question. 1 An excellent research question clarifies the research writing while facilitating understanding of the research topic, objective, scope, and limitations of the study. 5
On the other hand, a research hypothesis is an educated statement of an expected outcome. This statement is based on background research and current knowledge. 8 , 9 The research hypothesis makes a specific prediction about a new phenomenon 10 or a formal statement on the expected relationship between an independent variable and a dependent variable. 3 , 11 It provides a tentative answer to the research question to be tested or explored. 4
Hypotheses employ reasoning to predict a theory-based outcome. 10 These can also be developed from theories by focusing on components of theories that have not yet been observed. 10 The validity of hypotheses is often based on the testability of the prediction made in a reproducible experiment. 8
Conversely, hypotheses can also be rephrased as research questions. Several hypotheses based on existing theories and knowledge may be needed to answer a research question. Developing ethical research questions and hypotheses creates a research design that has logical relationships among variables. These relationships serve as a solid foundation for the conduct of the study. 4 , 11 Haphazardly constructed research questions can result in poorly formulated hypotheses and improper study designs, leading to unreliable results. Thus, the formulations of relevant research questions and verifiable hypotheses are crucial when beginning research. 12
CHARACTERISTICS OF GOOD RESEARCH QUESTIONS AND HYPOTHESES
Excellent research questions are specific and focused. These integrate collective data and observations to confirm or refute the subsequent hypotheses. Well-constructed hypotheses are based on previous reports and verify the research context. These are realistic, in-depth, sufficiently complex, and reproducible. More importantly, these hypotheses can be addressed and tested. 13
There are several characteristics of well-developed hypotheses. Good hypotheses are 1) empirically testable 7 , 10 , 11 , 13 ; 2) backed by preliminary evidence 9 ; 3) testable by ethical research 7 , 9 ; 4) based on original ideas 9 ; 5) have evidenced-based logical reasoning 10 ; and 6) can be predicted. 11 Good hypotheses can infer ethical and positive implications, indicating the presence of a relationship or effect relevant to the research theme. 7 , 11 These are initially developed from a general theory and branch into specific hypotheses by deductive reasoning. In the absence of a theory to base the hypotheses, inductive reasoning based on specific observations or findings form more general hypotheses. 10
TYPES OF RESEARCH QUESTIONS AND HYPOTHESES
Research questions and hypotheses are developed according to the type of research, which can be broadly classified into quantitative and qualitative research. We provide a summary of the types of research questions and hypotheses under quantitative and qualitative research categories in Table 1 .
Quantitative research questions | Quantitative research hypotheses |
---|---|
Descriptive research questions | Simple hypothesis |
Comparative research questions | Complex hypothesis |
Relationship research questions | Directional hypothesis |
Non-directional hypothesis | |
Associative hypothesis | |
Causal hypothesis | |
Null hypothesis | |
Alternative hypothesis | |
Working hypothesis | |
Statistical hypothesis | |
Logical hypothesis | |
Hypothesis-testing | |
Qualitative research questions | Qualitative research hypotheses |
Contextual research questions | Hypothesis-generating |
Descriptive research questions | |
Evaluation research questions | |
Explanatory research questions | |
Exploratory research questions | |
Generative research questions | |
Ideological research questions | |
Ethnographic research questions | |
Phenomenological research questions | |
Grounded theory questions | |
Qualitative case study questions |
Research questions in quantitative research
In quantitative research, research questions inquire about the relationships among variables being investigated and are usually framed at the start of the study. These are precise and typically linked to the subject population, dependent and independent variables, and research design. 1 Research questions may also attempt to describe the behavior of a population in relation to one or more variables, or describe the characteristics of variables to be measured ( descriptive research questions ). 1 , 5 , 14 These questions may also aim to discover differences between groups within the context of an outcome variable ( comparative research questions ), 1 , 5 , 14 or elucidate trends and interactions among variables ( relationship research questions ). 1 , 5 We provide examples of descriptive, comparative, and relationship research questions in quantitative research in Table 2 .
Quantitative research questions | |
---|---|
Descriptive research question | |
- Measures responses of subjects to variables | |
- Presents variables to measure, analyze, or assess | |
What is the proportion of resident doctors in the hospital who have mastered ultrasonography (response of subjects to a variable) as a diagnostic technique in their clinical training? | |
Comparative research question | |
- Clarifies difference between one group with outcome variable and another group without outcome variable | |
Is there a difference in the reduction of lung metastasis in osteosarcoma patients who received the vitamin D adjunctive therapy (group with outcome variable) compared with osteosarcoma patients who did not receive the vitamin D adjunctive therapy (group without outcome variable)? | |
- Compares the effects of variables | |
How does the vitamin D analogue 22-Oxacalcitriol (variable 1) mimic the antiproliferative activity of 1,25-Dihydroxyvitamin D (variable 2) in osteosarcoma cells? | |
Relationship research question | |
- Defines trends, association, relationships, or interactions between dependent variable and independent variable | |
Is there a relationship between the number of medical student suicide (dependent variable) and the level of medical student stress (independent variable) in Japan during the first wave of the COVID-19 pandemic? |
Hypotheses in quantitative research
In quantitative research, hypotheses predict the expected relationships among variables. 15 Relationships among variables that can be predicted include 1) between a single dependent variable and a single independent variable ( simple hypothesis ) or 2) between two or more independent and dependent variables ( complex hypothesis ). 4 , 11 Hypotheses may also specify the expected direction to be followed and imply an intellectual commitment to a particular outcome ( directional hypothesis ) 4 . On the other hand, hypotheses may not predict the exact direction and are used in the absence of a theory, or when findings contradict previous studies ( non-directional hypothesis ). 4 In addition, hypotheses can 1) define interdependency between variables ( associative hypothesis ), 4 2) propose an effect on the dependent variable from manipulation of the independent variable ( causal hypothesis ), 4 3) state a negative relationship between two variables ( null hypothesis ), 4 , 11 , 15 4) replace the working hypothesis if rejected ( alternative hypothesis ), 15 explain the relationship of phenomena to possibly generate a theory ( working hypothesis ), 11 5) involve quantifiable variables that can be tested statistically ( statistical hypothesis ), 11 6) or express a relationship whose interlinks can be verified logically ( logical hypothesis ). 11 We provide examples of simple, complex, directional, non-directional, associative, causal, null, alternative, working, statistical, and logical hypotheses in quantitative research, as well as the definition of quantitative hypothesis-testing research in Table 3 .
Quantitative research hypotheses | |
---|---|
Simple hypothesis | |
- Predicts relationship between single dependent variable and single independent variable | |
If the dose of the new medication (single independent variable) is high, blood pressure (single dependent variable) is lowered. | |
Complex hypothesis | |
- Foretells relationship between two or more independent and dependent variables | |
The higher the use of anticancer drugs, radiation therapy, and adjunctive agents (3 independent variables), the higher would be the survival rate (1 dependent variable). | |
Directional hypothesis | |
- Identifies study direction based on theory towards particular outcome to clarify relationship between variables | |
Privately funded research projects will have a larger international scope (study direction) than publicly funded research projects. | |
Non-directional hypothesis | |
- Nature of relationship between two variables or exact study direction is not identified | |
- Does not involve a theory | |
Women and men are different in terms of helpfulness. (Exact study direction is not identified) | |
Associative hypothesis | |
- Describes variable interdependency | |
- Change in one variable causes change in another variable | |
A larger number of people vaccinated against COVID-19 in the region (change in independent variable) will reduce the region’s incidence of COVID-19 infection (change in dependent variable). | |
Causal hypothesis | |
- An effect on dependent variable is predicted from manipulation of independent variable | |
A change into a high-fiber diet (independent variable) will reduce the blood sugar level (dependent variable) of the patient. | |
Null hypothesis | |
- A negative statement indicating no relationship or difference between 2 variables | |
There is no significant difference in the severity of pulmonary metastases between the new drug (variable 1) and the current drug (variable 2). | |
Alternative hypothesis | |
- Following a null hypothesis, an alternative hypothesis predicts a relationship between 2 study variables | |
The new drug (variable 1) is better on average in reducing the level of pain from pulmonary metastasis than the current drug (variable 2). | |
Working hypothesis | |
- A hypothesis that is initially accepted for further research to produce a feasible theory | |
Dairy cows fed with concentrates of different formulations will produce different amounts of milk. | |
Statistical hypothesis | |
- Assumption about the value of population parameter or relationship among several population characteristics | |
- Validity tested by a statistical experiment or analysis | |
The mean recovery rate from COVID-19 infection (value of population parameter) is not significantly different between population 1 and population 2. | |
There is a positive correlation between the level of stress at the workplace and the number of suicides (population characteristics) among working people in Japan. | |
Logical hypothesis | |
- Offers or proposes an explanation with limited or no extensive evidence | |
If healthcare workers provide more educational programs about contraception methods, the number of adolescent pregnancies will be less. | |
Hypothesis-testing (Quantitative hypothesis-testing research) | |
- Quantitative research uses deductive reasoning. | |
- This involves the formation of a hypothesis, collection of data in the investigation of the problem, analysis and use of the data from the investigation, and drawing of conclusions to validate or nullify the hypotheses. |
Research questions in qualitative research
Unlike research questions in quantitative research, research questions in qualitative research are usually continuously reviewed and reformulated. The central question and associated subquestions are stated more than the hypotheses. 15 The central question broadly explores a complex set of factors surrounding the central phenomenon, aiming to present the varied perspectives of participants. 15
There are varied goals for which qualitative research questions are developed. These questions can function in several ways, such as to 1) identify and describe existing conditions ( contextual research question s); 2) describe a phenomenon ( descriptive research questions ); 3) assess the effectiveness of existing methods, protocols, theories, or procedures ( evaluation research questions ); 4) examine a phenomenon or analyze the reasons or relationships between subjects or phenomena ( explanatory research questions ); or 5) focus on unknown aspects of a particular topic ( exploratory research questions ). 5 In addition, some qualitative research questions provide new ideas for the development of theories and actions ( generative research questions ) or advance specific ideologies of a position ( ideological research questions ). 1 Other qualitative research questions may build on a body of existing literature and become working guidelines ( ethnographic research questions ). Research questions may also be broadly stated without specific reference to the existing literature or a typology of questions ( phenomenological research questions ), may be directed towards generating a theory of some process ( grounded theory questions ), or may address a description of the case and the emerging themes ( qualitative case study questions ). 15 We provide examples of contextual, descriptive, evaluation, explanatory, exploratory, generative, ideological, ethnographic, phenomenological, grounded theory, and qualitative case study research questions in qualitative research in Table 4 , and the definition of qualitative hypothesis-generating research in Table 5 .
Qualitative research questions | |
---|---|
Contextual research question | |
- Ask the nature of what already exists | |
- Individuals or groups function to further clarify and understand the natural context of real-world problems | |
What are the experiences of nurses working night shifts in healthcare during the COVID-19 pandemic? (natural context of real-world problems) | |
Descriptive research question | |
- Aims to describe a phenomenon | |
What are the different forms of disrespect and abuse (phenomenon) experienced by Tanzanian women when giving birth in healthcare facilities? | |
Evaluation research question | |
- Examines the effectiveness of existing practice or accepted frameworks | |
How effective are decision aids (effectiveness of existing practice) in helping decide whether to give birth at home or in a healthcare facility? | |
Explanatory research question | |
- Clarifies a previously studied phenomenon and explains why it occurs | |
Why is there an increase in teenage pregnancy (phenomenon) in Tanzania? | |
Exploratory research question | |
- Explores areas that have not been fully investigated to have a deeper understanding of the research problem | |
What factors affect the mental health of medical students (areas that have not yet been fully investigated) during the COVID-19 pandemic? | |
Generative research question | |
- Develops an in-depth understanding of people’s behavior by asking ‘how would’ or ‘what if’ to identify problems and find solutions | |
How would the extensive research experience of the behavior of new staff impact the success of the novel drug initiative? | |
Ideological research question | |
- Aims to advance specific ideas or ideologies of a position | |
Are Japanese nurses who volunteer in remote African hospitals able to promote humanized care of patients (specific ideas or ideologies) in the areas of safe patient environment, respect of patient privacy, and provision of accurate information related to health and care? | |
Ethnographic research question | |
- Clarifies peoples’ nature, activities, their interactions, and the outcomes of their actions in specific settings | |
What are the demographic characteristics, rehabilitative treatments, community interactions, and disease outcomes (nature, activities, their interactions, and the outcomes) of people in China who are suffering from pneumoconiosis? | |
Phenomenological research question | |
- Knows more about the phenomena that have impacted an individual | |
What are the lived experiences of parents who have been living with and caring for children with a diagnosis of autism? (phenomena that have impacted an individual) | |
Grounded theory question | |
- Focuses on social processes asking about what happens and how people interact, or uncovering social relationships and behaviors of groups | |
What are the problems that pregnant adolescents face in terms of social and cultural norms (social processes), and how can these be addressed? | |
Qualitative case study question | |
- Assesses a phenomenon using different sources of data to answer “why” and “how” questions | |
- Considers how the phenomenon is influenced by its contextual situation. | |
How does quitting work and assuming the role of a full-time mother (phenomenon assessed) change the lives of women in Japan? |
Qualitative research hypotheses | |
---|---|
Hypothesis-generating (Qualitative hypothesis-generating research) | |
- Qualitative research uses inductive reasoning. | |
- This involves data collection from study participants or the literature regarding a phenomenon of interest, using the collected data to develop a formal hypothesis, and using the formal hypothesis as a framework for testing the hypothesis. | |
- Qualitative exploratory studies explore areas deeper, clarifying subjective experience and allowing formulation of a formal hypothesis potentially testable in a future quantitative approach. |
Qualitative studies usually pose at least one central research question and several subquestions starting with How or What . These research questions use exploratory verbs such as explore or describe . These also focus on one central phenomenon of interest, and may mention the participants and research site. 15
Hypotheses in qualitative research
Hypotheses in qualitative research are stated in the form of a clear statement concerning the problem to be investigated. Unlike in quantitative research where hypotheses are usually developed to be tested, qualitative research can lead to both hypothesis-testing and hypothesis-generating outcomes. 2 When studies require both quantitative and qualitative research questions, this suggests an integrative process between both research methods wherein a single mixed-methods research question can be developed. 1
FRAMEWORKS FOR DEVELOPING RESEARCH QUESTIONS AND HYPOTHESES
Research questions followed by hypotheses should be developed before the start of the study. 1 , 12 , 14 It is crucial to develop feasible research questions on a topic that is interesting to both the researcher and the scientific community. This can be achieved by a meticulous review of previous and current studies to establish a novel topic. Specific areas are subsequently focused on to generate ethical research questions. The relevance of the research questions is evaluated in terms of clarity of the resulting data, specificity of the methodology, objectivity of the outcome, depth of the research, and impact of the study. 1 , 5 These aspects constitute the FINER criteria (i.e., Feasible, Interesting, Novel, Ethical, and Relevant). 1 Clarity and effectiveness are achieved if research questions meet the FINER criteria. In addition to the FINER criteria, Ratan et al. described focus, complexity, novelty, feasibility, and measurability for evaluating the effectiveness of research questions. 14
The PICOT and PEO frameworks are also used when developing research questions. 1 The following elements are addressed in these frameworks, PICOT: P-population/patients/problem, I-intervention or indicator being studied, C-comparison group, O-outcome of interest, and T-timeframe of the study; PEO: P-population being studied, E-exposure to preexisting conditions, and O-outcome of interest. 1 Research questions are also considered good if these meet the “FINERMAPS” framework: Feasible, Interesting, Novel, Ethical, Relevant, Manageable, Appropriate, Potential value/publishable, and Systematic. 14
As we indicated earlier, research questions and hypotheses that are not carefully formulated result in unethical studies or poor outcomes. To illustrate this, we provide some examples of ambiguous research question and hypotheses that result in unclear and weak research objectives in quantitative research ( Table 6 ) 16 and qualitative research ( Table 7 ) 17 , and how to transform these ambiguous research question(s) and hypothesis(es) into clear and good statements.
Variables | Unclear and weak statement (Statement 1) | Clear and good statement (Statement 2) | Points to avoid |
---|---|---|---|
Research question | Which is more effective between smoke moxibustion and smokeless moxibustion? | “Moreover, regarding smoke moxibustion versus smokeless moxibustion, it remains unclear which is more effective, safe, and acceptable to pregnant women, and whether there is any difference in the amount of heat generated.” | 1) Vague and unfocused questions |
2) Closed questions simply answerable by yes or no | |||
3) Questions requiring a simple choice | |||
Hypothesis | The smoke moxibustion group will have higher cephalic presentation. | “Hypothesis 1. The smoke moxibustion stick group (SM group) and smokeless moxibustion stick group (-SLM group) will have higher rates of cephalic presentation after treatment than the control group. | 1) Unverifiable hypotheses |
Hypothesis 2. The SM group and SLM group will have higher rates of cephalic presentation at birth than the control group. | 2) Incompletely stated groups of comparison | ||
Hypothesis 3. There will be no significant differences in the well-being of the mother and child among the three groups in terms of the following outcomes: premature birth, premature rupture of membranes (PROM) at < 37 weeks, Apgar score < 7 at 5 min, umbilical cord blood pH < 7.1, admission to neonatal intensive care unit (NICU), and intrauterine fetal death.” | 3) Insufficiently described variables or outcomes | ||
Research objective | To determine which is more effective between smoke moxibustion and smokeless moxibustion. | “The specific aims of this pilot study were (a) to compare the effects of smoke moxibustion and smokeless moxibustion treatments with the control group as a possible supplement to ECV for converting breech presentation to cephalic presentation and increasing adherence to the newly obtained cephalic position, and (b) to assess the effects of these treatments on the well-being of the mother and child.” | 1) Poor understanding of the research question and hypotheses |
2) Insufficient description of population, variables, or study outcomes |
a These statements were composed for comparison and illustrative purposes only.
b These statements are direct quotes from Higashihara and Horiuchi. 16
Variables | Unclear and weak statement (Statement 1) | Clear and good statement (Statement 2) | Points to avoid |
---|---|---|---|
Research question | Does disrespect and abuse (D&A) occur in childbirth in Tanzania? | How does disrespect and abuse (D&A) occur and what are the types of physical and psychological abuses observed in midwives’ actual care during facility-based childbirth in urban Tanzania? | 1) Ambiguous or oversimplistic questions |
2) Questions unverifiable by data collection and analysis | |||
Hypothesis | Disrespect and abuse (D&A) occur in childbirth in Tanzania. | Hypothesis 1: Several types of physical and psychological abuse by midwives in actual care occur during facility-based childbirth in urban Tanzania. | 1) Statements simply expressing facts |
Hypothesis 2: Weak nursing and midwifery management contribute to the D&A of women during facility-based childbirth in urban Tanzania. | 2) Insufficiently described concepts or variables | ||
Research objective | To describe disrespect and abuse (D&A) in childbirth in Tanzania. | “This study aimed to describe from actual observations the respectful and disrespectful care received by women from midwives during their labor period in two hospitals in urban Tanzania.” | 1) Statements unrelated to the research question and hypotheses |
2) Unattainable or unexplorable objectives |
a This statement is a direct quote from Shimoda et al. 17
The other statements were composed for comparison and illustrative purposes only.
CONSTRUCTING RESEARCH QUESTIONS AND HYPOTHESES
To construct effective research questions and hypotheses, it is very important to 1) clarify the background and 2) identify the research problem at the outset of the research, within a specific timeframe. 9 Then, 3) review or conduct preliminary research to collect all available knowledge about the possible research questions by studying theories and previous studies. 18 Afterwards, 4) construct research questions to investigate the research problem. Identify variables to be accessed from the research questions 4 and make operational definitions of constructs from the research problem and questions. Thereafter, 5) construct specific deductive or inductive predictions in the form of hypotheses. 4 Finally, 6) state the study aims . This general flow for constructing effective research questions and hypotheses prior to conducting research is shown in Fig. 1 .

Research questions are used more frequently in qualitative research than objectives or hypotheses. 3 These questions seek to discover, understand, explore or describe experiences by asking “What” or “How.” The questions are open-ended to elicit a description rather than to relate variables or compare groups. The questions are continually reviewed, reformulated, and changed during the qualitative study. 3 Research questions are also used more frequently in survey projects than hypotheses in experiments in quantitative research to compare variables and their relationships.
Hypotheses are constructed based on the variables identified and as an if-then statement, following the template, ‘If a specific action is taken, then a certain outcome is expected.’ At this stage, some ideas regarding expectations from the research to be conducted must be drawn. 18 Then, the variables to be manipulated (independent) and influenced (dependent) are defined. 4 Thereafter, the hypothesis is stated and refined, and reproducible data tailored to the hypothesis are identified, collected, and analyzed. 4 The hypotheses must be testable and specific, 18 and should describe the variables and their relationships, the specific group being studied, and the predicted research outcome. 18 Hypotheses construction involves a testable proposition to be deduced from theory, and independent and dependent variables to be separated and measured separately. 3 Therefore, good hypotheses must be based on good research questions constructed at the start of a study or trial. 12
In summary, research questions are constructed after establishing the background of the study. Hypotheses are then developed based on the research questions. Thus, it is crucial to have excellent research questions to generate superior hypotheses. In turn, these would determine the research objectives and the design of the study, and ultimately, the outcome of the research. 12 Algorithms for building research questions and hypotheses are shown in Fig. 2 for quantitative research and in Fig. 3 for qualitative research.

EXAMPLES OF RESEARCH QUESTIONS FROM PUBLISHED ARTICLES
- EXAMPLE 1. Descriptive research question (quantitative research)
- - Presents research variables to be assessed (distinct phenotypes and subphenotypes)
- “BACKGROUND: Since COVID-19 was identified, its clinical and biological heterogeneity has been recognized. Identifying COVID-19 phenotypes might help guide basic, clinical, and translational research efforts.
- RESEARCH QUESTION: Does the clinical spectrum of patients with COVID-19 contain distinct phenotypes and subphenotypes? ” 19
- EXAMPLE 2. Relationship research question (quantitative research)
- - Shows interactions between dependent variable (static postural control) and independent variable (peripheral visual field loss)
- “Background: Integration of visual, vestibular, and proprioceptive sensations contributes to postural control. People with peripheral visual field loss have serious postural instability. However, the directional specificity of postural stability and sensory reweighting caused by gradual peripheral visual field loss remain unclear.
- Research question: What are the effects of peripheral visual field loss on static postural control ?” 20
- EXAMPLE 3. Comparative research question (quantitative research)
- - Clarifies the difference among groups with an outcome variable (patients enrolled in COMPERA with moderate PH or severe PH in COPD) and another group without the outcome variable (patients with idiopathic pulmonary arterial hypertension (IPAH))
- “BACKGROUND: Pulmonary hypertension (PH) in COPD is a poorly investigated clinical condition.
- RESEARCH QUESTION: Which factors determine the outcome of PH in COPD?
- STUDY DESIGN AND METHODS: We analyzed the characteristics and outcome of patients enrolled in the Comparative, Prospective Registry of Newly Initiated Therapies for Pulmonary Hypertension (COMPERA) with moderate or severe PH in COPD as defined during the 6th PH World Symposium who received medical therapy for PH and compared them with patients with idiopathic pulmonary arterial hypertension (IPAH) .” 21
- EXAMPLE 4. Exploratory research question (qualitative research)
- - Explores areas that have not been fully investigated (perspectives of families and children who receive care in clinic-based child obesity treatment) to have a deeper understanding of the research problem
- “Problem: Interventions for children with obesity lead to only modest improvements in BMI and long-term outcomes, and data are limited on the perspectives of families of children with obesity in clinic-based treatment. This scoping review seeks to answer the question: What is known about the perspectives of families and children who receive care in clinic-based child obesity treatment? This review aims to explore the scope of perspectives reported by families of children with obesity who have received individualized outpatient clinic-based obesity treatment.” 22
- EXAMPLE 5. Relationship research question (quantitative research)
- - Defines interactions between dependent variable (use of ankle strategies) and independent variable (changes in muscle tone)
- “Background: To maintain an upright standing posture against external disturbances, the human body mainly employs two types of postural control strategies: “ankle strategy” and “hip strategy.” While it has been reported that the magnitude of the disturbance alters the use of postural control strategies, it has not been elucidated how the level of muscle tone, one of the crucial parameters of bodily function, determines the use of each strategy. We have previously confirmed using forward dynamics simulations of human musculoskeletal models that an increased muscle tone promotes the use of ankle strategies. The objective of the present study was to experimentally evaluate a hypothesis: an increased muscle tone promotes the use of ankle strategies. Research question: Do changes in the muscle tone affect the use of ankle strategies ?” 23
EXAMPLES OF HYPOTHESES IN PUBLISHED ARTICLES
- EXAMPLE 1. Working hypothesis (quantitative research)
- - A hypothesis that is initially accepted for further research to produce a feasible theory
- “As fever may have benefit in shortening the duration of viral illness, it is plausible to hypothesize that the antipyretic efficacy of ibuprofen may be hindering the benefits of a fever response when taken during the early stages of COVID-19 illness .” 24
- “In conclusion, it is plausible to hypothesize that the antipyretic efficacy of ibuprofen may be hindering the benefits of a fever response . The difference in perceived safety of these agents in COVID-19 illness could be related to the more potent efficacy to reduce fever with ibuprofen compared to acetaminophen. Compelling data on the benefit of fever warrant further research and review to determine when to treat or withhold ibuprofen for early stage fever for COVID-19 and other related viral illnesses .” 24
- EXAMPLE 2. Exploratory hypothesis (qualitative research)
- - Explores particular areas deeper to clarify subjective experience and develop a formal hypothesis potentially testable in a future quantitative approach
- “We hypothesized that when thinking about a past experience of help-seeking, a self distancing prompt would cause increased help-seeking intentions and more favorable help-seeking outcome expectations .” 25
- “Conclusion
- Although a priori hypotheses were not supported, further research is warranted as results indicate the potential for using self-distancing approaches to increasing help-seeking among some people with depressive symptomatology.” 25
- EXAMPLE 3. Hypothesis-generating research to establish a framework for hypothesis testing (qualitative research)
- “We hypothesize that compassionate care is beneficial for patients (better outcomes), healthcare systems and payers (lower costs), and healthcare providers (lower burnout). ” 26
- Compassionomics is the branch of knowledge and scientific study of the effects of compassionate healthcare. Our main hypotheses are that compassionate healthcare is beneficial for (1) patients, by improving clinical outcomes, (2) healthcare systems and payers, by supporting financial sustainability, and (3) HCPs, by lowering burnout and promoting resilience and well-being. The purpose of this paper is to establish a scientific framework for testing the hypotheses above . If these hypotheses are confirmed through rigorous research, compassionomics will belong in the science of evidence-based medicine, with major implications for all healthcare domains.” 26
- EXAMPLE 4. Statistical hypothesis (quantitative research)
- - An assumption is made about the relationship among several population characteristics ( gender differences in sociodemographic and clinical characteristics of adults with ADHD ). Validity is tested by statistical experiment or analysis ( chi-square test, Students t-test, and logistic regression analysis)
- “Our research investigated gender differences in sociodemographic and clinical characteristics of adults with ADHD in a Japanese clinical sample. Due to unique Japanese cultural ideals and expectations of women's behavior that are in opposition to ADHD symptoms, we hypothesized that women with ADHD experience more difficulties and present more dysfunctions than men . We tested the following hypotheses: first, women with ADHD have more comorbidities than men with ADHD; second, women with ADHD experience more social hardships than men, such as having less full-time employment and being more likely to be divorced.” 27
- “Statistical Analysis
- ( text omitted ) Between-gender comparisons were made using the chi-squared test for categorical variables and Students t-test for continuous variables…( text omitted ). A logistic regression analysis was performed for employment status, marital status, and comorbidity to evaluate the independent effects of gender on these dependent variables.” 27
EXAMPLES OF HYPOTHESIS AS WRITTEN IN PUBLISHED ARTICLES IN RELATION TO OTHER PARTS
- EXAMPLE 1. Background, hypotheses, and aims are provided
- “Pregnant women need skilled care during pregnancy and childbirth, but that skilled care is often delayed in some countries …( text omitted ). The focused antenatal care (FANC) model of WHO recommends that nurses provide information or counseling to all pregnant women …( text omitted ). Job aids are visual support materials that provide the right kind of information using graphics and words in a simple and yet effective manner. When nurses are not highly trained or have many work details to attend to, these job aids can serve as a content reminder for the nurses and can be used for educating their patients (Jennings, Yebadokpo, Affo, & Agbogbe, 2010) ( text omitted ). Importantly, additional evidence is needed to confirm how job aids can further improve the quality of ANC counseling by health workers in maternal care …( text omitted )” 28
- “ This has led us to hypothesize that the quality of ANC counseling would be better if supported by job aids. Consequently, a better quality of ANC counseling is expected to produce higher levels of awareness concerning the danger signs of pregnancy and a more favorable impression of the caring behavior of nurses .” 28
- “This study aimed to examine the differences in the responses of pregnant women to a job aid-supported intervention during ANC visit in terms of 1) their understanding of the danger signs of pregnancy and 2) their impression of the caring behaviors of nurses to pregnant women in rural Tanzania.” 28
- EXAMPLE 2. Background, hypotheses, and aims are provided
- “We conducted a two-arm randomized controlled trial (RCT) to evaluate and compare changes in salivary cortisol and oxytocin levels of first-time pregnant women between experimental and control groups. The women in the experimental group touched and held an infant for 30 min (experimental intervention protocol), whereas those in the control group watched a DVD movie of an infant (control intervention protocol). The primary outcome was salivary cortisol level and the secondary outcome was salivary oxytocin level.” 29
- “ We hypothesize that at 30 min after touching and holding an infant, the salivary cortisol level will significantly decrease and the salivary oxytocin level will increase in the experimental group compared with the control group .” 29
- EXAMPLE 3. Background, aim, and hypothesis are provided
- “In countries where the maternal mortality ratio remains high, antenatal education to increase Birth Preparedness and Complication Readiness (BPCR) is considered one of the top priorities [1]. BPCR includes birth plans during the antenatal period, such as the birthplace, birth attendant, transportation, health facility for complications, expenses, and birth materials, as well as family coordination to achieve such birth plans. In Tanzania, although increasing, only about half of all pregnant women attend an antenatal clinic more than four times [4]. Moreover, the information provided during antenatal care (ANC) is insufficient. In the resource-poor settings, antenatal group education is a potential approach because of the limited time for individual counseling at antenatal clinics.” 30
- “This study aimed to evaluate an antenatal group education program among pregnant women and their families with respect to birth-preparedness and maternal and infant outcomes in rural villages of Tanzania.” 30
- “ The study hypothesis was if Tanzanian pregnant women and their families received a family-oriented antenatal group education, they would (1) have a higher level of BPCR, (2) attend antenatal clinic four or more times, (3) give birth in a health facility, (4) have less complications of women at birth, and (5) have less complications and deaths of infants than those who did not receive the education .” 30
Research questions and hypotheses are crucial components to any type of research, whether quantitative or qualitative. These questions should be developed at the very beginning of the study. Excellent research questions lead to superior hypotheses, which, like a compass, set the direction of research, and can often determine the successful conduct of the study. Many research studies have floundered because the development of research questions and subsequent hypotheses was not given the thought and meticulous attention needed. The development of research questions and hypotheses is an iterative process based on extensive knowledge of the literature and insightful grasp of the knowledge gap. Focused, concise, and specific research questions provide a strong foundation for constructing hypotheses which serve as formal predictions about the research outcomes. Research questions and hypotheses are crucial elements of research that should not be overlooked. They should be carefully thought of and constructed when planning research. This avoids unethical studies and poor outcomes by defining well-founded objectives that determine the design, course, and outcome of the study.
Disclosure: The authors have no potential conflicts of interest to disclose.
Author Contributions:
- Conceptualization: Barroga E, Matanguihan GJ.
- Methodology: Barroga E, Matanguihan GJ.
- Writing - original draft: Barroga E, Matanguihan GJ.
- Writing - review & editing: Barroga E, Matanguihan GJ.

An official website of the United States government
Here’s how you know
Official websites use .gov A .gov website belongs to an official government organization in the United States.
Secure .gov websites use HTTPS A lock ( A locked padlock ) or https:// means you’ve safely connected to the .gov website. Share sensitive information only on official, secure websites.
- Heart-Healthy Living
- High Blood Pressure
- Sickle Cell Disease
- Sleep Apnea
- Information & Resources on COVID-19
- The Heart Truth®
- Learn More Breathe Better®
- Blood Diseases & Disorders Education Program
- Publications and Resources
- Clinical Trials
- Blood Disorders and Blood Safety
- Sleep Science and Sleep Disorders
- Lung Diseases
- Health Disparities and Inequities
- Heart and Vascular Diseases
- Precision Medicine Activities
- Obesity, Nutrition, and Physical Activity
- Population and Epidemiology Studies
- Women’s Health
- Research Topics
- All Science A-Z
- Grants and Training Home
- Policies and Guidelines
- Funding Opportunities and Contacts
- Training and Career Development
- Email Alerts
- NHLBI in the Press
- Research Features
- Ask a Scientist
- Past Events
- Upcoming Events
- Mission and Strategic Vision
- Divisions, Offices and Centers
- Advisory Committees
- Budget and Legislative Information
- Jobs and Working at the NHLBI
- Contact and FAQs
- NIH Sleep Research Plan
- < Back To Health Topics
Study Quality Assessment Tools
In 2013, NHLBI developed a set of tailored quality assessment tools to assist reviewers in focusing on concepts that are key to a study’s internal validity. The tools were specific to certain study designs and tested for potential flaws in study methods or implementation. Experts used the tools during the systematic evidence review process to update existing clinical guidelines, such as those on cholesterol, blood pressure, and obesity. Their findings are outlined in the following reports:
- Assessing Cardiovascular Risk: Systematic Evidence Review from the Risk Assessment Work Group
- Management of Blood Cholesterol in Adults: Systematic Evidence Review from the Cholesterol Expert Panel
- Management of Blood Pressure in Adults: Systematic Evidence Review from the Blood Pressure Expert Panel
- Managing Overweight and Obesity in Adults: Systematic Evidence Review from the Obesity Expert Panel
While these tools have not been independently published and would not be considered standardized, they may be useful to the research community. These reports describe how experts used the tools for the project. Researchers may want to use the tools for their own projects; however, they would need to determine their own parameters for making judgements. Details about the design and application of the tools are included in Appendix A of the reports.
Quality Assessment of Controlled Intervention Studies - Study Quality Assessment Tools
Criteria | Yes | No | Other (CD, NR, NA)* |
---|---|---|---|
1. Was the study described as randomized, a randomized trial, a randomized clinical trial, or an RCT? | |||
2. Was the method of randomization adequate (i.e., use of randomly generated assignment)? | |||
3. Was the treatment allocation concealed (so that assignments could not be predicted)? | |||
4. Were study participants and providers blinded to treatment group assignment? | |||
5. Were the people assessing the outcomes blinded to the participants' group assignments? | |||
6. Were the groups similar at baseline on important characteristics that could affect outcomes (e.g., demographics, risk factors, co-morbid conditions)? | |||
7. Was the overall drop-out rate from the study at endpoint 20% or lower of the number allocated to treatment? | |||
8. Was the differential drop-out rate (between treatment groups) at endpoint 15 percentage points or lower? | |||
9. Was there high adherence to the intervention protocols for each treatment group? | |||
10. Were other interventions avoided or similar in the groups (e.g., similar background treatments)? | |||
11. Were outcomes assessed using valid and reliable measures, implemented consistently across all study participants? | |||
12. Did the authors report that the sample size was sufficiently large to be able to detect a difference in the main outcome between groups with at least 80% power? | |||
13. Were outcomes reported or subgroups analyzed prespecified (i.e., identified before analyses were conducted)? | |||
14. Were all randomized participants analyzed in the group to which they were originally assigned, i.e., did they use an intention-to-treat analysis? |
Quality Rating (Good, Fair, or Poor) |
---|
Rater #1 initials: |
Rater #2 initials: |
Additional Comments (If POOR, please state why): |
*CD, cannot determine; NA, not applicable; NR, not reported
Guidance for Assessing the Quality of Controlled Intervention Studies
The guidance document below is organized by question number from the tool for quality assessment of controlled intervention studies.
Question 1. Described as randomized
Was the study described as randomized? A study does not satisfy quality criteria as randomized simply because the authors call it randomized; however, it is a first step in determining if a study is randomized
Questions 2 and 3. Treatment allocation–two interrelated pieces
Adequate randomization: Randomization is adequate if it occurred according to the play of chance (e.g., computer generated sequence in more recent studies, or random number table in older studies). Inadequate randomization: Randomization is inadequate if there is a preset plan (e.g., alternation where every other subject is assigned to treatment arm or another method of allocation is used, such as time or day of hospital admission or clinic visit, ZIP Code, phone number, etc.). In fact, this is not randomization at all–it is another method of assignment to groups. If assignment is not by the play of chance, then the answer to this question is no. There may be some tricky scenarios that will need to be read carefully and considered for the role of chance in assignment. For example, randomization may occur at the site level, where all individuals at a particular site are assigned to receive treatment or no treatment. This scenario is used for group-randomized trials, which can be truly randomized, but often are "quasi-experimental" studies with comparison groups rather than true control groups. (Few, if any, group-randomized trials are anticipated for this evidence review.)
Allocation concealment: This means that one does not know in advance, or cannot guess accurately, to what group the next person eligible for randomization will be assigned. Methods include sequentially numbered opaque sealed envelopes, numbered or coded containers, central randomization by a coordinating center, computer-generated randomization that is not revealed ahead of time, etc. Questions 4 and 5. Blinding
Blinding means that one does not know to which group–intervention or control–the participant is assigned. It is also sometimes called "masking." The reviewer assessed whether each of the following was blinded to knowledge of treatment assignment: (1) the person assessing the primary outcome(s) for the study (e.g., taking the measurements such as blood pressure, examining health records for events such as myocardial infarction, reviewing and interpreting test results such as x ray or cardiac catheterization findings); (2) the person receiving the intervention (e.g., the patient or other study participant); and (3) the person providing the intervention (e.g., the physician, nurse, pharmacist, dietitian, or behavioral interventionist).
Generally placebo-controlled medication studies are blinded to patient, provider, and outcome assessors; behavioral, lifestyle, and surgical studies are examples of studies that are frequently blinded only to the outcome assessors because blinding of the persons providing and receiving the interventions is difficult in these situations. Sometimes the individual providing the intervention is the same person performing the outcome assessment. This was noted when it occurred.
Question 6. Similarity of groups at baseline
This question relates to whether the intervention and control groups have similar baseline characteristics on average especially those characteristics that may affect the intervention or outcomes. The point of randomized trials is to create groups that are as similar as possible except for the intervention(s) being studied in order to compare the effects of the interventions between groups. When reviewers abstracted baseline characteristics, they noted when there was a significant difference between groups. Baseline characteristics for intervention groups are usually presented in a table in the article (often Table 1).
Groups can differ at baseline without raising red flags if: (1) the differences would not be expected to have any bearing on the interventions and outcomes; or (2) the differences are not statistically significant. When concerned about baseline difference in groups, reviewers recorded them in the comments section and considered them in their overall determination of the study quality.
Questions 7 and 8. Dropout
"Dropouts" in a clinical trial are individuals for whom there are no end point measurements, often because they dropped out of the study and were lost to followup.
Generally, an acceptable overall dropout rate is considered 20 percent or less of participants who were randomized or allocated into each group. An acceptable differential dropout rate is an absolute difference between groups of 15 percentage points at most (calculated by subtracting the dropout rate of one group minus the dropout rate of the other group). However, these are general rates. Lower overall dropout rates are expected in shorter studies, whereas higher overall dropout rates may be acceptable for studies of longer duration. For example, a 6-month study of weight loss interventions should be expected to have nearly 100 percent followup (almost no dropouts–nearly everybody gets their weight measured regardless of whether or not they actually received the intervention), whereas a 10-year study testing the effects of intensive blood pressure lowering on heart attacks may be acceptable if there is a 20-25 percent dropout rate, especially if the dropout rate between groups was similar. The panels for the NHLBI systematic reviews may set different levels of dropout caps.
Conversely, differential dropout rates are not flexible; there should be a 15 percent cap. If there is a differential dropout rate of 15 percent or higher between arms, then there is a serious potential for bias. This constitutes a fatal flaw, resulting in a poor quality rating for the study.
Question 9. Adherence
Did participants in each treatment group adhere to the protocols for assigned interventions? For example, if Group 1 was assigned to 10 mg/day of Drug A, did most of them take 10 mg/day of Drug A? Another example is a study evaluating the difference between a 30-pound weight loss and a 10-pound weight loss on specific clinical outcomes (e.g., heart attacks), but the 30-pound weight loss group did not achieve its intended weight loss target (e.g., the group only lost 14 pounds on average). A third example is whether a large percentage of participants assigned to one group "crossed over" and got the intervention provided to the other group. A final example is when one group that was assigned to receive a particular drug at a particular dose had a large percentage of participants who did not end up taking the drug or the dose as designed in the protocol.
Question 10. Avoid other interventions
Changes that occur in the study outcomes being assessed should be attributable to the interventions being compared in the study. If study participants receive interventions that are not part of the study protocol and could affect the outcomes being assessed, and they receive these interventions differentially, then there is cause for concern because these interventions could bias results. The following scenario is another example of how bias can occur. In a study comparing two different dietary interventions on serum cholesterol, one group had a significantly higher percentage of participants taking statin drugs than the other group. In this situation, it would be impossible to know if a difference in outcome was due to the dietary intervention or the drugs.
Question 11. Outcome measures assessment
What tools or methods were used to measure the outcomes in the study? Were the tools and methods accurate and reliable–for example, have they been validated, or are they objective? This is important as it indicates the confidence you can have in the reported outcomes. Perhaps even more important is ascertaining that outcomes were assessed in the same manner within and between groups. One example of differing methods is self-report of dietary salt intake versus urine testing for sodium content (a more reliable and valid assessment method). Another example is using BP measurements taken by practitioners who use their usual methods versus using BP measurements done by individuals trained in a standard approach. Such an approach may include using the same instrument each time and taking an individual's BP multiple times. In each of these cases, the answer to this assessment question would be "no" for the former scenario and "yes" for the latter. In addition, a study in which an intervention group was seen more frequently than the control group, enabling more opportunities to report clinical events, would not be considered reliable and valid.
Question 12. Power calculation
Generally, a study's methods section will address the sample size needed to detect differences in primary outcomes. The current standard is at least 80 percent power to detect a clinically relevant difference in an outcome using a two-sided alpha of 0.05. Often, however, older studies will not report on power.
Question 13. Prespecified outcomes
Investigators should prespecify outcomes reported in a study for hypothesis testing–which is the reason for conducting an RCT. Without prespecified outcomes, the study may be reporting ad hoc analyses, simply looking for differences supporting desired findings. Investigators also should prespecify subgroups being examined. Most RCTs conduct numerous post hoc analyses as a way of exploring findings and generating additional hypotheses. The intent of this question is to give more weight to reports that are not simply exploratory in nature.
Question 14. Intention-to-treat analysis
Intention-to-treat (ITT) means everybody who was randomized is analyzed according to the original group to which they are assigned. This is an extremely important concept because conducting an ITT analysis preserves the whole reason for doing a randomized trial; that is, to compare groups that differ only in the intervention being tested. When the ITT philosophy is not followed, groups being compared may no longer be the same. In this situation, the study would likely be rated poor. However, if an investigator used another type of analysis that could be viewed as valid, this would be explained in the "other" box on the quality assessment form. Some researchers use a completers analysis (an analysis of only the participants who completed the intervention and the study), which introduces significant potential for bias. Characteristics of participants who do not complete the study are unlikely to be the same as those who do. The likely impact of participants withdrawing from a study treatment must be considered carefully. ITT analysis provides a more conservative (potentially less biased) estimate of effectiveness.
General Guidance for Determining the Overall Quality Rating of Controlled Intervention Studies
The questions on the assessment tool were designed to help reviewers focus on the key concepts for evaluating a study's internal validity. They are not intended to create a list that is simply tallied up to arrive at a summary judgment of quality.
Internal validity is the extent to which the results (effects) reported in a study can truly be attributed to the intervention being evaluated and not to flaws in the design or conduct of the study–in other words, the ability for the study to make causal conclusions about the effects of the intervention being tested. Such flaws can increase the risk of bias. Critical appraisal involves considering the risk of potential for allocation bias, measurement bias, or confounding (the mixture of exposures that one cannot tease out from each other). Examples of confounding include co-interventions, differences at baseline in patient characteristics, and other issues addressed in the questions above. High risk of bias translates to a rating of poor quality. Low risk of bias translates to a rating of good quality.
Fatal flaws: If a study has a "fatal flaw," then risk of bias is significant, and the study is of poor quality. Examples of fatal flaws in RCTs include high dropout rates, high differential dropout rates, no ITT analysis or other unsuitable statistical analysis (e.g., completers-only analysis).
Generally, when evaluating a study, one will not see a "fatal flaw;" however, one will find some risk of bias. During training, reviewers were instructed to look for the potential for bias in studies by focusing on the concepts underlying the questions in the tool. For any box checked "no," reviewers were told to ask: "What is the potential risk of bias that may be introduced by this flaw?" That is, does this factor cause one to doubt the results that were reported in the study?
NHLBI staff provided reviewers with background reading on critical appraisal, while emphasizing that the best approach to use is to think about the questions in the tool in determining the potential for bias in a study. The staff also emphasized that each study has specific nuances; therefore, reviewers should familiarize themselves with the key concepts.
Quality Assessment of Systematic Reviews and Meta-Analyses - Study Quality Assessment Tools
Criteria | Yes | No | Other (CD, NR, NA)* |
---|---|---|---|
1. Is the review based on a focused question that is adequately formulated and described? | |||
2. Were eligibility criteria for included and excluded studies predefined and specified? | |||
3. Did the literature search strategy use a comprehensive, systematic approach? | |||
4. Were titles, abstracts, and full-text articles dually and independently reviewed for inclusion and exclusion to minimize bias? | |||
5. Was the quality of each included study rated independently by two or more reviewers using a standard method to appraise its internal validity? | |||
6. Were the included studies listed along with important characteristics and results of each study? | |||
7. Was publication bias assessed? | |||
8. Was heterogeneity assessed? (This question applies only to meta-analyses.) |
Guidance for Quality Assessment Tool for Systematic Reviews and Meta-Analyses
A systematic review is a study that attempts to answer a question by synthesizing the results of primary studies while using strategies to limit bias and random error.424 These strategies include a comprehensive search of all potentially relevant articles and the use of explicit, reproducible criteria in the selection of articles included in the review. Research designs and study characteristics are appraised, data are synthesized, and results are interpreted using a predefined systematic approach that adheres to evidence-based methodological principles.
Systematic reviews can be qualitative or quantitative. A qualitative systematic review summarizes the results of the primary studies but does not combine the results statistically. A quantitative systematic review, or meta-analysis, is a type of systematic review that employs statistical techniques to combine the results of the different studies into a single pooled estimate of effect, often given as an odds ratio. The guidance document below is organized by question number from the tool for quality assessment of systematic reviews and meta-analyses.
Question 1. Focused question
The review should be based on a question that is clearly stated and well-formulated. An example would be a question that uses the PICO (population, intervention, comparator, outcome) format, with all components clearly described.
Question 2. Eligibility criteria
The eligibility criteria used to determine whether studies were included or excluded should be clearly specified and predefined. It should be clear to the reader why studies were included or excluded.
Question 3. Literature search
The search strategy should employ a comprehensive, systematic approach in order to capture all of the evidence possible that pertains to the question of interest. At a minimum, a comprehensive review has the following attributes:
- Electronic searches were conducted using multiple scientific literature databases, such as MEDLINE, EMBASE, Cochrane Central Register of Controlled Trials, PsychLit, and others as appropriate for the subject matter.
- Manual searches of references found in articles and textbooks should supplement the electronic searches.
Additional search strategies that may be used to improve the yield include the following:
- Studies published in other countries
- Studies published in languages other than English
- Identification by experts in the field of studies and articles that may have been missed
- Search of grey literature, including technical reports and other papers from government agencies or scientific groups or committees; presentations and posters from scientific meetings, conference proceedings, unpublished manuscripts; and others. Searching the grey literature is important (whenever feasible) because sometimes only positive studies with significant findings are published in the peer-reviewed literature, which can bias the results of a review.
In their reviews, researchers described the literature search strategy clearly, and ascertained it could be reproducible by others with similar results.
Question 4. Dual review for determining which studies to include and exclude
Titles, abstracts, and full-text articles (when indicated) should be reviewed by two independent reviewers to determine which studies to include and exclude in the review. Reviewers resolved disagreements through discussion and consensus or with third parties. They clearly stated the review process, including methods for settling disagreements.
Question 5. Quality appraisal for internal validity
Each included study should be appraised for internal validity (study quality assessment) using a standardized approach for rating the quality of the individual studies. Ideally, this should be done by at least two independent reviewers appraised each study for internal validity. However, there is not one commonly accepted, standardized tool for rating the quality of studies. So, in the research papers, reviewers looked for an assessment of the quality of each study and a clear description of the process used.
Question 6. List and describe included studies
All included studies were listed in the review, along with descriptions of their key characteristics. This was presented either in narrative or table format.
Question 7. Publication bias
Publication bias is a term used when studies with positive results have a higher likelihood of being published, being published rapidly, being published in higher impact journals, being published in English, being published more than once, or being cited by others.425,426 Publication bias can be linked to favorable or unfavorable treatment of research findings due to investigators, editors, industry, commercial interests, or peer reviewers. To minimize the potential for publication bias, researchers can conduct a comprehensive literature search that includes the strategies discussed in Question 3.
A funnel plot–a scatter plot of component studies in a meta-analysis–is a commonly used graphical method for detecting publication bias. If there is no significant publication bias, the graph looks like a symmetrical inverted funnel.
Reviewers assessed and clearly described the likelihood of publication bias.
Question 8. Heterogeneity
Heterogeneity is used to describe important differences in studies included in a meta-analysis that may make it inappropriate to combine the studies.427 Heterogeneity can be clinical (e.g., important differences between study participants, baseline disease severity, and interventions); methodological (e.g., important differences in the design and conduct of the study); or statistical (e.g., important differences in the quantitative results or reported effects).
Researchers usually assess clinical or methodological heterogeneity qualitatively by determining whether it makes sense to combine studies. For example:
- Should a study evaluating the effects of an intervention on CVD risk that involves elderly male smokers with hypertension be combined with a study that involves healthy adults ages 18 to 40? (Clinical Heterogeneity)
- Should a study that uses a randomized controlled trial (RCT) design be combined with a study that uses a case-control study design? (Methodological Heterogeneity)
Statistical heterogeneity describes the degree of variation in the effect estimates from a set of studies; it is assessed quantitatively. The two most common methods used to assess statistical heterogeneity are the Q test (also known as the X2 or chi-square test) or I2 test.
Reviewers examined studies to determine if an assessment for heterogeneity was conducted and clearly described. If the studies are found to be heterogeneous, the investigators should explore and explain the causes of the heterogeneity, and determine what influence, if any, the study differences had on overall study results.
Quality Assessment Tool for Observational Cohort and Cross-Sectional Studies - Study Quality Assessment Tools
Criteria | Yes | No | Other (CD, NR, NA)* |
---|---|---|---|
1. Was the research question or objective in this paper clearly stated? | |||
2. Was the study population clearly specified and defined? | |||
3. Was the participation rate of eligible persons at least 50%? | |||
4. Were all the subjects selected or recruited from the same or similar populations (including the same time period)? Were inclusion and exclusion criteria for being in the study prespecified and applied uniformly to all participants? | |||
5. Was a sample size justification, power description, or variance and effect estimates provided? | |||
6. For the analyses in this paper, were the exposure(s) of interest measured prior to the outcome(s) being measured? | |||
7. Was the timeframe sufficient so that one could reasonably expect to see an association between exposure and outcome if it existed? | |||
8. For exposures that can vary in amount or level, did the study examine different levels of the exposure as related to the outcome (e.g., categories of exposure, or exposure measured as continuous variable)? | |||
9. Were the exposure measures (independent variables) clearly defined, valid, reliable, and implemented consistently across all study participants? | |||
10. Was the exposure(s) assessed more than once over time? | |||
11. Were the outcome measures (dependent variables) clearly defined, valid, reliable, and implemented consistently across all study participants? | |||
12. Were the outcome assessors blinded to the exposure status of participants? | |||
13. Was loss to follow-up after baseline 20% or less? | |||
14. Were key potential confounding variables measured and adjusted statistically for their impact on the relationship between exposure(s) and outcome(s)? |
Guidance for Assessing the Quality of Observational Cohort and Cross-Sectional Studies
The guidance document below is organized by question number from the tool for quality assessment of observational cohort and cross-sectional studies.
Question 1. Research question
Did the authors describe their goal in conducting this research? Is it easy to understand what they were looking to find? This issue is important for any scientific paper of any type. Higher quality scientific research explicitly defines a research question.
Questions 2 and 3. Study population
Did the authors describe the group of people from which the study participants were selected or recruited, using demographics, location, and time period? If you were to conduct this study again, would you know who to recruit, from where, and from what time period? Is the cohort population free of the outcomes of interest at the time they were recruited?
An example would be men over 40 years old with type 2 diabetes who began seeking medical care at Phoenix Good Samaritan Hospital between January 1, 1990 and December 31, 1994. In this example, the population is clearly described as: (1) who (men over 40 years old with type 2 diabetes); (2) where (Phoenix Good Samaritan Hospital); and (3) when (between January 1, 1990 and December 31, 1994). Another example is women ages 34 to 59 years of age in 1980 who were in the nursing profession and had no known coronary disease, stroke, cancer, hypercholesterolemia, or diabetes, and were recruited from the 11 most populous States, with contact information obtained from State nursing boards.
In cohort studies, it is crucial that the population at baseline is free of the outcome of interest. For example, the nurses' population above would be an appropriate group in which to study incident coronary disease. This information is usually found either in descriptions of population recruitment, definitions of variables, or inclusion/exclusion criteria.
You may need to look at prior papers on methods in order to make the assessment for this question. Those papers are usually in the reference list.
If fewer than 50% of eligible persons participated in the study, then there is concern that the study population does not adequately represent the target population. This increases the risk of bias.
Question 4. Groups recruited from the same population and uniform eligibility criteria
Were the inclusion and exclusion criteria developed prior to recruitment or selection of the study population? Were the same underlying criteria used for all of the subjects involved? This issue is related to the description of the study population, above, and you may find the information for both of these questions in the same section of the paper.
Most cohort studies begin with the selection of the cohort; participants in this cohort are then measured or evaluated to determine their exposure status. However, some cohort studies may recruit or select exposed participants in a different time or place than unexposed participants, especially retrospective cohort studies–which is when data are obtained from the past (retrospectively), but the analysis examines exposures prior to outcomes. For example, one research question could be whether diabetic men with clinical depression are at higher risk for cardiovascular disease than those without clinical depression. So, diabetic men with depression might be selected from a mental health clinic, while diabetic men without depression might be selected from an internal medicine or endocrinology clinic. This study recruits groups from different clinic populations, so this example would get a "no."
However, the women nurses described in the question above were selected based on the same inclusion/exclusion criteria, so that example would get a "yes."
Question 5. Sample size justification
Did the authors present their reasons for selecting or recruiting the number of people included or analyzed? Do they note or discuss the statistical power of the study? This question is about whether or not the study had enough participants to detect an association if one truly existed.
A paragraph in the methods section of the article may explain the sample size needed to detect a hypothesized difference in outcomes. You may also find a discussion of power in the discussion section (such as the study had 85 percent power to detect a 20 percent increase in the rate of an outcome of interest, with a 2-sided alpha of 0.05). Sometimes estimates of variance and/or estimates of effect size are given, instead of sample size calculations. In any of these cases, the answer would be "yes."
However, observational cohort studies often do not report anything about power or sample sizes because the analyses are exploratory in nature. In this case, the answer would be "no." This is not a "fatal flaw." It just may indicate that attention was not paid to whether the study was sufficiently sized to answer a prespecified question–i.e., it may have been an exploratory, hypothesis-generating study.
Question 6. Exposure assessed prior to outcome measurement
This question is important because, in order to determine whether an exposure causes an outcome, the exposure must come before the outcome.
For some prospective cohort studies, the investigator enrolls the cohort and then determines the exposure status of various members of the cohort (large epidemiological studies like Framingham used this approach). However, for other cohort studies, the cohort is selected based on its exposure status, as in the example above of depressed diabetic men (the exposure being depression). Other examples include a cohort identified by its exposure to fluoridated drinking water and then compared to a cohort living in an area without fluoridated water, or a cohort of military personnel exposed to combat in the Gulf War compared to a cohort of military personnel not deployed in a combat zone.
With either of these types of cohort studies, the cohort is followed forward in time (i.e., prospectively) to assess the outcomes that occurred in the exposed members compared to nonexposed members of the cohort. Therefore, you begin the study in the present by looking at groups that were exposed (or not) to some biological or behavioral factor, intervention, etc., and then you follow them forward in time to examine outcomes. If a cohort study is conducted properly, the answer to this question should be "yes," since the exposure status of members of the cohort was determined at the beginning of the study before the outcomes occurred.
For retrospective cohort studies, the same principal applies. The difference is that, rather than identifying a cohort in the present and following them forward in time, the investigators go back in time (i.e., retrospectively) and select a cohort based on their exposure status in the past and then follow them forward to assess the outcomes that occurred in the exposed and nonexposed cohort members. Because in retrospective cohort studies the exposure and outcomes may have already occurred (it depends on how long they follow the cohort), it is important to make sure that the exposure preceded the outcome.
Sometimes cross-sectional studies are conducted (or cross-sectional analyses of cohort-study data), where the exposures and outcomes are measured during the same timeframe. As a result, cross-sectional analyses provide weaker evidence than regular cohort studies regarding a potential causal relationship between exposures and outcomes. For cross-sectional analyses, the answer to Question 6 should be "no."
Question 7. Sufficient timeframe to see an effect
Did the study allow enough time for a sufficient number of outcomes to occur or be observed, or enough time for an exposure to have a biological effect on an outcome? In the examples given above, if clinical depression has a biological effect on increasing risk for CVD, such an effect may take years. In the other example, if higher dietary sodium increases BP, a short timeframe may be sufficient to assess its association with BP, but a longer timeframe would be needed to examine its association with heart attacks.
The issue of timeframe is important to enable meaningful analysis of the relationships between exposures and outcomes to be conducted. This often requires at least several years, especially when looking at health outcomes, but it depends on the research question and outcomes being examined.
Cross-sectional analyses allow no time to see an effect, since the exposures and outcomes are assessed at the same time, so those would get a "no" response.
Question 8. Different levels of the exposure of interest
If the exposure can be defined as a range (examples: drug dosage, amount of physical activity, amount of sodium consumed), were multiple categories of that exposure assessed? (for example, for drugs: not on the medication, on a low dose, medium dose, high dose; for dietary sodium, higher than average U.S. consumption, lower than recommended consumption, between the two). Sometimes discrete categories of exposure are not used, but instead exposures are measured as continuous variables (for example, mg/day of dietary sodium or BP values).
In any case, studying different levels of exposure (where possible) enables investigators to assess trends or dose-response relationships between exposures and outcomes–e.g., the higher the exposure, the greater the rate of the health outcome. The presence of trends or dose-response relationships lends credibility to the hypothesis of causality between exposure and outcome.
For some exposures, however, this question may not be applicable (e.g., the exposure may be a dichotomous variable like living in a rural setting versus an urban setting, or vaccinated/not vaccinated with a one-time vaccine). If there are only two possible exposures (yes/no), then this question should be given an "NA," and it should not count negatively towards the quality rating.
Question 9. Exposure measures and assessment
Were the exposure measures defined in detail? Were the tools or methods used to measure exposure accurate and reliable–for example, have they been validated or are they objective? This issue is important as it influences confidence in the reported exposures. When exposures are measured with less accuracy or validity, it is harder to see an association between exposure and outcome even if one exists. Also as important is whether the exposures were assessed in the same manner within groups and between groups; if not, bias may result.
For example, retrospective self-report of dietary salt intake is not as valid and reliable as prospectively using a standardized dietary log plus testing participants' urine for sodium content. Another example is measurement of BP, where there may be quite a difference between usual care, where clinicians measure BP however it is done in their practice setting (which can vary considerably), and use of trained BP assessors using standardized equipment (e.g., the same BP device which has been tested and calibrated) and a standardized protocol (e.g., patient is seated for 5 minutes with feet flat on the floor, BP is taken twice in each arm, and all four measurements are averaged). In each of these cases, the former would get a "no" and the latter a "yes."
Here is a final example that illustrates the point about why it is important to assess exposures consistently across all groups: If people with higher BP (exposed cohort) are seen by their providers more frequently than those without elevated BP (nonexposed group), it also increases the chances of detecting and documenting changes in health outcomes, including CVD-related events. Therefore, it may lead to the conclusion that higher BP leads to more CVD events. This may be true, but it could also be due to the fact that the subjects with higher BP were seen more often; thus, more CVD-related events were detected and documented simply because they had more encounters with the health care system. Thus, it could bias the results and lead to an erroneous conclusion.
Question 10. Repeated exposure assessment
Was the exposure for each person measured more than once during the course of the study period? Multiple measurements with the same result increase our confidence that the exposure status was correctly classified. Also, multiple measurements enable investigators to look at changes in exposure over time, for example, people who ate high dietary sodium throughout the followup period, compared to those who started out high then reduced their intake, compared to those who ate low sodium throughout. Once again, this may not be applicable in all cases. In many older studies, exposure was measured only at baseline. However, multiple exposure measurements do result in a stronger study design.
Question 11. Outcome measures
Were the outcomes defined in detail? Were the tools or methods for measuring outcomes accurate and reliable–for example, have they been validated or are they objective? This issue is important because it influences confidence in the validity of study results. Also important is whether the outcomes were assessed in the same manner within groups and between groups.
An example of an outcome measure that is objective, accurate, and reliable is death–the outcome measured with more accuracy than any other. But even with a measure as objective as death, there can be differences in the accuracy and reliability of how death was assessed by the investigators. Did they base it on an autopsy report, death certificate, death registry, or report from a family member? Another example is a study of whether dietary fat intake is related to blood cholesterol level (cholesterol level being the outcome), and the cholesterol level is measured from fasting blood samples that are all sent to the same laboratory. These examples would get a "yes." An example of a "no" would be self-report by subjects that they had a heart attack, or self-report of how much they weigh (if body weight is the outcome of interest).
Similar to the example in Question 9, results may be biased if one group (e.g., people with high BP) is seen more frequently than another group (people with normal BP) because more frequent encounters with the health care system increases the chances of outcomes being detected and documented.
Question 12. Blinding of outcome assessors
Blinding means that outcome assessors did not know whether the participant was exposed or unexposed. It is also sometimes called "masking." The objective is to look for evidence in the article that the person(s) assessing the outcome(s) for the study (for example, examining medical records to determine the outcomes that occurred in the exposed and comparison groups) is masked to the exposure status of the participant. Sometimes the person measuring the exposure is the same person conducting the outcome assessment. In this case, the outcome assessor would most likely not be blinded to exposure status because they also took measurements of exposures. If so, make a note of that in the comments section.
As you assess this criterion, think about whether it is likely that the person(s) doing the outcome assessment would know (or be able to figure out) the exposure status of the study participants. If the answer is no, then blinding is adequate. An example of adequate blinding of the outcome assessors is to create a separate committee, whose members were not involved in the care of the patient and had no information about the study participants' exposure status. The committee would then be provided with copies of participants' medical records, which had been stripped of any potential exposure information or personally identifiable information. The committee would then review the records for prespecified outcomes according to the study protocol. If blinding was not possible, which is sometimes the case, mark "NA" and explain the potential for bias.
Question 13. Followup rate
Higher overall followup rates are always better than lower followup rates, even though higher rates are expected in shorter studies, whereas lower overall followup rates are often seen in studies of longer duration. Usually, an acceptable overall followup rate is considered 80 percent or more of participants whose exposures were measured at baseline. However, this is just a general guideline. For example, a 6-month cohort study examining the relationship between dietary sodium intake and BP level may have over 90 percent followup, but a 20-year cohort study examining effects of sodium intake on stroke may have only a 65 percent followup rate.
Question 14. Statistical analyses
Were key potential confounding variables measured and adjusted for, such as by statistical adjustment for baseline differences? Logistic regression or other regression methods are often used to account for the influence of variables not of interest.
This is a key issue in cohort studies, because statistical analyses need to control for potential confounders, in contrast to an RCT, where the randomization process controls for potential confounders. All key factors that may be associated both with the exposure of interest and the outcome–that are not of interest to the research question–should be controlled for in the analyses.
For example, in a study of the relationship between cardiorespiratory fitness and CVD events (heart attacks and strokes), the study should control for age, BP, blood cholesterol, and body weight, because all of these factors are associated both with low fitness and with CVD events. Well-done cohort studies control for multiple potential confounders.
Some general guidance for determining the overall quality rating of observational cohort and cross-sectional studies
The questions on the form are designed to help you focus on the key concepts for evaluating the internal validity of a study. They are not intended to create a list that you simply tally up to arrive at a summary judgment of quality.
Internal validity for cohort studies is the extent to which the results reported in the study can truly be attributed to the exposure being evaluated and not to flaws in the design or conduct of the study–in other words, the ability of the study to draw associative conclusions about the effects of the exposures being studied on outcomes. Any such flaws can increase the risk of bias.
Critical appraisal involves considering the risk of potential for selection bias, information bias, measurement bias, or confounding (the mixture of exposures that one cannot tease out from each other). Examples of confounding include co-interventions, differences at baseline in patient characteristics, and other issues throughout the questions above. High risk of bias translates to a rating of poor quality. Low risk of bias translates to a rating of good quality. (Thus, the greater the risk of bias, the lower the quality rating of the study.)
In addition, the more attention in the study design to issues that can help determine whether there is a causal relationship between the exposure and outcome, the higher quality the study. These include exposures occurring prior to outcomes, evaluation of a dose-response gradient, accuracy of measurement of both exposure and outcome, sufficient timeframe to see an effect, and appropriate control for confounding–all concepts reflected in the tool.
Generally, when you evaluate a study, you will not see a "fatal flaw," but you will find some risk of bias. By focusing on the concepts underlying the questions in the quality assessment tool, you should ask yourself about the potential for bias in the study you are critically appraising. For any box where you check "no" you should ask, "What is the potential risk of bias resulting from this flaw in study design or execution?" That is, does this factor cause you to doubt the results that are reported in the study or doubt the ability of the study to accurately assess an association between exposure and outcome?
The best approach is to think about the questions in the tool and how each one tells you something about the potential for bias in a study. The more you familiarize yourself with the key concepts, the more comfortable you will be with critical appraisal. Examples of studies rated good, fair, and poor are useful, but each study must be assessed on its own based on the details that are reported and consideration of the concepts for minimizing bias.
Quality Assessment of Case-Control Studies - Study Quality Assessment Tools
Criteria | Yes | No | Other (CD, NR, NA)* |
---|---|---|---|
1. Was the research question or objective in this paper clearly stated and appropriate? | |||
2. Was the study population clearly specified and defined? | |||
3. Did the authors include a sample size justification? | |||
4. Were controls selected or recruited from the same or similar population that gave rise to the cases (including the same timeframe)? | |||
5. Were the definitions, inclusion and exclusion criteria, algorithms or processes used to identify or select cases and controls valid, reliable, and implemented consistently across all study participants? | |||
6. Were the cases clearly defined and differentiated from controls? | |||
7. If less than 100 percent of eligible cases and/or controls were selected for the study, were the cases and/or controls randomly selected from those eligible? | |||
8. Was there use of concurrent controls? | |||
9. Were the investigators able to confirm that the exposure/risk occurred prior to the development of the condition or event that defined a participant as a case? | |||
10. Were the measures of exposure/risk clearly defined, valid, reliable, and implemented consistently (including the same time period) across all study participants? | |||
11. Were the assessors of exposure/risk blinded to the case or control status of participants? | |||
12. Were key potential confounding variables measured and adjusted statistically in the analyses? If matching was used, did the investigators account for matching during study analysis? |
Quality Rating ( |
---|
Rater #1 Initials: |
Rater #2 Initials: |
Additional Comments (If POOR, please state why): |
Guidance for Assessing the Quality of Case-Control Studies
The guidance document below is organized by question number from the tool for quality assessment of case-control studies.
Did the authors describe their goal in conducting this research? Is it easy to understand what they were looking to find? This issue is important for any scientific paper of any type. High quality scientific research explicitly defines a research question.
Question 2. Study population
Did the authors describe the group of individuals from which the cases and controls were selected or recruited, while using demographics, location, and time period? If the investigators conducted this study again, would they know exactly who to recruit, from where, and from what time period?
Investigators identify case-control study populations by location, time period, and inclusion criteria for cases (individuals with the disease, condition, or problem) and controls (individuals without the disease, condition, or problem). For example, the population for a study of lung cancer and chemical exposure would be all incident cases of lung cancer diagnosed in patients ages 35 to 79, from January 1, 2003 to December 31, 2008, living in Texas during that entire time period, as well as controls without lung cancer recruited from the same population during the same time period. The population is clearly described as: (1) who (men and women ages 35 to 79 with (cases) and without (controls) incident lung cancer); (2) where (living in Texas); and (3) when (between January 1, 2003 and December 31, 2008).
Other studies may use disease registries or data from cohort studies to identify cases. In these cases, the populations are individuals who live in the area covered by the disease registry or included in a cohort study (i.e., nested case-control or case-cohort). For example, a study of the relationship between vitamin D intake and myocardial infarction might use patients identified via the GRACE registry, a database of heart attack patients.
NHLBI staff encouraged reviewers to examine prior papers on methods (listed in the reference list) to make this assessment, if necessary.
Question 3. Target population and case representation
In order for a study to truly address the research question, the target population–the population from which the study population is drawn and to which study results are believed to apply–should be carefully defined. Some authors may compare characteristics of the study cases to characteristics of cases in the target population, either in text or in a table. When study cases are shown to be representative of cases in the appropriate target population, it increases the likelihood that the study was well-designed per the research question.
However, because these statistics are frequently difficult or impossible to measure, publications should not be penalized if case representation is not shown. For most papers, the response to question 3 will be "NR." Those subquestions are combined because the answer to the second subquestion–case representation–determines the response to this item. However, it cannot be determined without considering the response to the first subquestion. For example, if the answer to the first subquestion is "yes," and the second, "CD," then the response for item 3 is "CD."
Question 4. Sample size justification
Did the authors discuss their reasons for selecting or recruiting the number of individuals included? Did they discuss the statistical power of the study and provide a sample size calculation to ensure that the study is adequately powered to detect an association (if one exists)? This question does not refer to a description of the manner in which different groups were included or excluded using the inclusion/exclusion criteria (e.g., "Final study size was 1,378 participants after exclusion of 461 patients with missing data" is not considered a sample size justification for the purposes of this question).
An article's methods section usually contains information on sample size and the size needed to detect differences in exposures and on statistical power.
Question 5. Groups recruited from the same population
To determine whether cases and controls were recruited from the same population, one can ask hypothetically, "If a control was to develop the outcome of interest (the condition that was used to select cases), would that person have been eligible to become a case?" Case-control studies begin with the selection of the cases (those with the outcome of interest, e.g., lung cancer) and controls (those in whom the outcome is absent). Cases and controls are then evaluated and categorized by their exposure status. For the lung cancer example, cases and controls were recruited from hospitals in a given region. One may reasonably assume that controls in the catchment area for the hospitals, or those already in the hospitals for a different reason, would attend those hospitals if they became a case; therefore, the controls are drawn from the same population as the cases. If the controls were recruited or selected from a different region (e.g., a State other than Texas) or time period (e.g., 1991-2000), then the cases and controls were recruited from different populations, and the answer to this question would be "no."
The following example further explores selection of controls. In a study, eligible cases were men and women, ages 18 to 39, who were diagnosed with atherosclerosis at hospitals in Perth, Australia, between July 1, 2000 and December 31, 2007. Appropriate controls for these cases might be sampled using voter registration information for men and women ages 18 to 39, living in Perth (population-based controls); they also could be sampled from patients without atherosclerosis at the same hospitals (hospital-based controls). As long as the controls are individuals who would have been eligible to be included in the study as cases (if they had been diagnosed with atherosclerosis), then the controls were selected appropriately from the same source population as cases.
In a prospective case-control study, investigators may enroll individuals as cases at the time they are found to have the outcome of interest; the number of cases usually increases as time progresses. At this same time, they may recruit or select controls from the population without the outcome of interest. One way to identify or recruit cases is through a surveillance system. In turn, investigators can select controls from the population covered by that system. This is an example of population-based controls. Investigators also may identify and select cases from a cohort study population and identify controls from outcome-free individuals in the same cohort study. This is known as a nested case-control study.
Question 6. Inclusion and exclusion criteria prespecified and applied uniformly
Were the inclusion and exclusion criteria developed prior to recruitment or selection of the study population? Were the same underlying criteria used for all of the groups involved? To answer this question, reviewers determined if the investigators developed I/E criteria prior to recruitment or selection of the study population and if they used the same underlying criteria for all groups. The investigators should have used the same selection criteria, except for study participants who had the disease or condition, which would be different for cases and controls by definition. Therefore, the investigators use the same age (or age range), gender, race, and other characteristics to select cases and controls. Information on this topic is usually found in a paper's section on the description of the study population.
Question 7. Case and control definitions
For this question, reviewers looked for descriptions of the validity of case and control definitions and processes or tools used to identify study participants as such. Was a specific description of "case" and "control" provided? Is there a discussion of the validity of the case and control definitions and the processes or tools used to identify study participants as such? They determined if the tools or methods were accurate, reliable, and objective. For example, cases might be identified as "adult patients admitted to a VA hospital from January 1, 2000 to December 31, 2009, with an ICD-9 discharge diagnosis code of acute myocardial infarction and at least one of the two confirmatory findings in their medical records: at least 2mm of ST elevation changes in two or more ECG leads and an elevated troponin level. Investigators might also use ICD-9 or CPT codes to identify patients. All cases should be identified using the same methods. Unless the distinction between cases and controls is accurate and reliable, investigators cannot use study results to draw valid conclusions.
Question 8. Random selection of study participants
If a case-control study did not use 100 percent of eligible cases and/or controls (e.g., not all disease-free participants were included as controls), did the authors indicate that random sampling was used to select controls? When it is possible to identify the source population fairly explicitly (e.g., in a nested case-control study, or in a registry-based study), then random sampling of controls is preferred. When investigators used consecutive sampling, which is frequently done for cases in prospective studies, then study participants are not considered randomly selected. In this case, the reviewers would answer "no" to Question 8. However, this would not be considered a fatal flaw.
If investigators included all eligible cases and controls as study participants, then reviewers marked "NA" in the tool. If 100 percent of cases were included (e.g., NA for cases) but only 50 percent of eligible controls, then the response would be "yes" if the controls were randomly selected, and "no" if they were not. If this cannot be determined, the appropriate response is "CD."
Question 9. Concurrent controls
A concurrent control is a control selected at the time another person became a case, usually on the same day. This means that one or more controls are recruited or selected from the population without the outcome of interest at the time a case is diagnosed. Investigators can use this method in both prospective case-control studies and retrospective case-control studies. For example, in a retrospective study of adenocarcinoma of the colon using data from hospital records, if hospital records indicate that Person A was diagnosed with adenocarcinoma of the colon on June 22, 2002, then investigators would select one or more controls from the population of patients without adenocarcinoma of the colon on that same day. This assumes they conducted the study retrospectively, using data from hospital records. The investigators could have also conducted this study using patient records from a cohort study, in which case it would be a nested case-control study.
Investigators can use concurrent controls in the presence or absence of matching and vice versa. A study that uses matching does not necessarily mean that concurrent controls were used.
Question 10. Exposure assessed prior to outcome measurement
Investigators first determine case or control status (based on presence or absence of outcome of interest), and then assess exposure history of the case or control; therefore, reviewers ascertained that the exposure preceded the outcome. For example, if the investigators used tissue samples to determine exposure, did they collect them from patients prior to their diagnosis? If hospital records were used, did investigators verify that the date a patient was exposed (e.g., received medication for atherosclerosis) occurred prior to the date they became a case (e.g., was diagnosed with type 2 diabetes)? For an association between an exposure and an outcome to be considered causal, the exposure must have occurred prior to the outcome.
Question 11. Exposure measures and assessment
Were the exposure measures defined in detail? Were the tools or methods used to measure exposure accurate and reliable–for example, have they been validated or are they objective? This is important, as it influences confidence in the reported exposures. Equally important is whether the exposures were assessed in the same manner within groups and between groups. This question pertains to bias resulting from exposure misclassification (i.e., exposure ascertainment).
For example, a retrospective self-report of dietary salt intake is not as valid and reliable as prospectively using a standardized dietary log plus testing participants' urine for sodium content because participants' retrospective recall of dietary salt intake may be inaccurate and result in misclassification of exposure status. Similarly, BP results from practices that use an established protocol for measuring BP would be considered more valid and reliable than results from practices that did not use standard protocols. A protocol may include using trained BP assessors, standardized equipment (e.g., the same BP device which has been tested and calibrated), and a standardized procedure (e.g., patient is seated for 5 minutes with feet flat on the floor, BP is taken twice in each arm, and all four measurements are averaged).
Question 12. Blinding of exposure assessors
Blinding or masking means that outcome assessors did not know whether participants were exposed or unexposed. To answer this question, reviewers examined articles for evidence that the outcome assessor(s) was masked to the exposure status of the research participants. An outcome assessor, for example, may examine medical records to determine the outcomes that occurred in the exposed and comparison groups. Sometimes the person measuring the exposure is the same person conducting the outcome assessment. In this case, the outcome assessor would most likely not be blinded to exposure status. A reviewer would note such a finding in the comments section of the assessment tool.
One way to ensure good blinding of exposure assessment is to have a separate committee, whose members have no information about the study participants' status as cases or controls, review research participants' records. To help answer the question above, reviewers determined if it was likely that the outcome assessor knew whether the study participant was a case or control. If it was unlikely, then the reviewers marked "no" to Question 12. Outcome assessors who used medical records to assess exposure should not have been directly involved in the study participants' care, since they probably would have known about their patients' conditions. If the medical records contained information on the patient's condition that identified him/her as a case (which is likely), that information would have had to be removed before the exposure assessors reviewed the records.
If blinding was not possible, which sometimes happens, the reviewers marked "NA" in the assessment tool and explained the potential for bias.
Question 13. Statistical analysis
Were key potential confounding variables measured and adjusted for, such as by statistical adjustment for baseline differences? Investigators often use logistic regression or other regression methods to account for the influence of variables not of interest.
This is a key issue in case-controlled studies; statistical analyses need to control for potential confounders, in contrast to RCTs in which the randomization process controls for potential confounders. In the analysis, investigators need to control for all key factors that may be associated with both the exposure of interest and the outcome and are not of interest to the research question.
A study of the relationship between smoking and CVD events illustrates this point. Such a study needs to control for age, gender, and body weight; all are associated with smoking and CVD events. Well-done case-control studies control for multiple potential confounders.
Matching is a technique used to improve study efficiency and control for known confounders. For example, in the study of smoking and CVD events, an investigator might identify cases that have had a heart attack or stroke and then select controls of similar age, gender, and body weight to the cases. For case-control studies, it is important that if matching was performed during the selection or recruitment process, the variables used as matching criteria (e.g., age, gender, race) should be controlled for in the analysis.
General Guidance for Determining the Overall Quality Rating of Case-Controlled Studies
NHLBI designed the questions in the assessment tool to help reviewers focus on the key concepts for evaluating a study's internal validity, not to use as a list from which to add up items to judge a study's quality.
Internal validity for case-control studies is the extent to which the associations between disease and exposure reported in the study can truly be attributed to the exposure being evaluated rather than to flaws in the design or conduct of the study. In other words, what is ability of the study to draw associative conclusions about the effects of the exposures on outcomes? Any such flaws can increase the risk of bias.
In critical appraising a study, the following factors need to be considered: risk of potential for selection bias, information bias, measurement bias, or confounding (the mixture of exposures that one cannot tease out from each other). Examples of confounding include co-interventions, differences at baseline in patient characteristics, and other issues addressed in the questions above. High risk of bias translates to a poor quality rating; low risk of bias translates to a good quality rating. Again, the greater the risk of bias, the lower the quality rating of the study.
In addition, the more attention in the study design to issues that can help determine whether there is a causal relationship between the outcome and the exposure, the higher the quality of the study. These include exposures occurring prior to outcomes, evaluation of a dose-response gradient, accuracy of measurement of both exposure and outcome, sufficient timeframe to see an effect, and appropriate control for confounding–all concepts reflected in the tool.
If a study has a "fatal flaw," then risk of bias is significant; therefore, the study is deemed to be of poor quality. An example of a fatal flaw in case-control studies is a lack of a consistent standard process used to identify cases and controls.
Generally, when reviewers evaluated a study, they did not see a "fatal flaw," but instead found some risk of bias. By focusing on the concepts underlying the questions in the quality assessment tool, reviewers examined the potential for bias in the study. For any box checked "no," reviewers asked, "What is the potential risk of bias resulting from this flaw in study design or execution?" That is, did this factor lead to doubt about the results reported in the study or the ability of the study to accurately assess an association between exposure and outcome?
By examining questions in the assessment tool, reviewers were best able to assess the potential for bias in a study. Specific rules were not useful, as each study had specific nuances. In addition, being familiar with the key concepts helped reviewers assess the studies. Examples of studies rated good, fair, and poor were useful, yet each study had to be assessed on its own.
Quality Assessment Tool for Before-After (Pre-Post) Studies With No Control Group - Study Quality Assessment Tools
Criteria | Yes | No | Other |
---|---|---|---|
1. Was the study question or objective clearly stated? | |||
2. Were eligibility/selection criteria for the study population prespecified and clearly described? | |||
3. Were the participants in the study representative of those who would be eligible for the test/service/intervention in the general or clinical population of interest? | |||
4. Were all eligible participants that met the prespecified entry criteria enrolled? | |||
5. Was the sample size sufficiently large to provide confidence in the findings? | |||
6. Was the test/service/intervention clearly described and delivered consistently across the study population? | |||
7. Were the outcome measures prespecified, clearly defined, valid, reliable, and assessed consistently across all study participants? | |||
8. Were the people assessing the outcomes blinded to the participants' exposures/interventions? | |||
9. Was the loss to follow-up after baseline 20% or less? Were those lost to follow-up accounted for in the analysis? | |||
10. Did the statistical methods examine changes in outcome measures from before to after the intervention? Were statistical tests done that provided p values for the pre-to-post changes? | |||
11. Were outcome measures of interest taken multiple times before the intervention and multiple times after the intervention (i.e., did they use an interrupted time-series design)? | |||
12. If the intervention was conducted at a group level (e.g., a whole hospital, a community, etc.) did the statistical analysis take into account the use of individual-level data to determine effects at the group level? |
Guidance for Assessing the Quality of Before-After (Pre-Post) Studies With No Control Group
Question 1. Study question
Question 2. Eligibility criteria and study population
Did the authors describe the eligibility criteria applied to the individuals from whom the study participants were selected or recruited? In other words, if the investigators were to conduct this study again, would they know whom to recruit, from where, and from what time period?
Here is a sample description of a study population: men over age 40 with type 2 diabetes, who began seeking medical care at Phoenix Good Samaritan Hospital, between January 1, 2005 and December 31, 2007. The population is clearly described as: (1) who (men over age 40 with type 2 diabetes); (2) where (Phoenix Good Samaritan Hospital); and (3) when (between January 1, 2005 and December 31, 2007). Another sample description is women who were in the nursing profession, who were ages 34 to 59 in 1995, had no known CHD, stroke, cancer, hypercholesterolemia, or diabetes, and were recruited from the 11 most populous States, with contact information obtained from State nursing boards.
To assess this question, reviewers examined prior papers on study methods (listed in reference list) when necessary.
Question 3. Study participants representative of clinical populations of interest
The participants in the study should be generally representative of the population in which the intervention will be broadly applied. Studies on small demographic subgroups may raise concerns about how the intervention will affect broader populations of interest. For example, interventions that focus on very young or very old individuals may affect middle-aged adults differently. Similarly, researchers may not be able to extrapolate study results from patients with severe chronic diseases to healthy populations.
Question 4. All eligible participants enrolled
To further explore this question, reviewers may need to ask: Did the investigators develop the I/E criteria prior to recruiting or selecting study participants? Were the same underlying I/E criteria used for all research participants? Were all subjects who met the I/E criteria enrolled in the study?
Question 5. Sample size
Did the authors present their reasons for selecting or recruiting the number of individuals included or analyzed? Did they note or discuss the statistical power of the study? This question addresses whether there was a sufficient sample size to detect an association, if one did exist.
An article's methods section may provide information on the sample size needed to detect a hypothesized difference in outcomes and a discussion on statistical power (such as, the study had 85 percent power to detect a 20 percent increase in the rate of an outcome of interest, with a 2-sided alpha of 0.05). Sometimes estimates of variance and/or estimates of effect size are given, instead of sample size calculations. In any case, if the reviewers determined that the power was sufficient to detect the effects of interest, then they would answer "yes" to Question 5.
Question 6. Intervention clearly described
Another pertinent question regarding interventions is: Was the intervention clearly defined in detail in the study? Did the authors indicate that the intervention was consistently applied to the subjects? Did the research participants have a high level of adherence to the requirements of the intervention? For example, if the investigators assigned a group to 10 mg/day of Drug A, did most participants in this group take the specific dosage of Drug A? Or did a large percentage of participants end up not taking the specific dose of Drug A indicated in the study protocol?
Reviewers ascertained that changes in study outcomes could be attributed to study interventions. If participants received interventions that were not part of the study protocol and could affect the outcomes being assessed, the results could be biased.
Question 7. Outcome measures clearly described, valid, and reliable
Were the outcomes defined in detail? Were the tools or methods for measuring outcomes accurate and reliable–for example, have they been validated or are they objective? This question is important because the answer influences confidence in the validity of study results.
An example of an outcome measure that is objective, accurate, and reliable is death–the outcome measured with more accuracy than any other. But even with a measure as objective as death, differences can exist in the accuracy and reliability of how investigators assessed death. For example, did they base it on an autopsy report, death certificate, death registry, or report from a family member? Another example of a valid study is one whose objective is to determine if dietary fat intake affects blood cholesterol level (cholesterol level being the outcome) and in which the cholesterol level is measured from fasting blood samples that are all sent to the same laboratory. These examples would get a "yes."
An example of a "no" would be self-report by subjects that they had a heart attack, or self-report of how much they weight (if body weight is the outcome of interest).
Question 8. Blinding of outcome assessors
Blinding or masking means that the outcome assessors did not know whether the participants received the intervention or were exposed to the factor under study. To answer the question above, the reviewers examined articles for evidence that the person(s) assessing the outcome(s) was masked to the participants' intervention or exposure status. An outcome assessor, for example, may examine medical records to determine the outcomes that occurred in the exposed and comparison groups. Sometimes the person applying the intervention or measuring the exposure is the same person conducting the outcome assessment. In this case, the outcome assessor would not likely be blinded to the intervention or exposure status. A reviewer would note such a finding in the comments section of the assessment tool.
In assessing this criterion, the reviewers determined whether it was likely that the person(s) conducting the outcome assessment knew the exposure status of the study participants. If not, then blinding was adequate. An example of adequate blinding of the outcome assessors is to create a separate committee whose members were not involved in the care of the patient and had no information about the study participants' exposure status. Using a study protocol, committee members would review copies of participants' medical records, which would be stripped of any potential exposure information or personally identifiable information, for prespecified outcomes.
Question 9. Followup rate
Higher overall followup rates are always desirable to lower followup rates, although higher rates are expected in shorter studies, and lower overall followup rates are often seen in longer studies. Usually an acceptable overall followup rate is considered 80 percent or more of participants whose interventions or exposures were measured at baseline. However, this is a general guideline.
In accounting for those lost to followup, in the analysis, investigators may have imputed values of the outcome for those lost to followup or used other methods. For example, they may carry forward the baseline value or the last observed value of the outcome measure and use these as imputed values for the final outcome measure for research participants lost to followup.
Question 10. Statistical analysis
Were formal statistical tests used to assess the significance of the changes in the outcome measures between the before and after time periods? The reported study results should present values for statistical tests, such as p values, to document the statistical significance (or lack thereof) for the changes in the outcome measures found in the study.
Question 11. Multiple outcome measures
Were the outcome measures for each person measured more than once during the course of the before and after study periods? Multiple measurements with the same result increase confidence that the outcomes were accurately measured.
Question 12. Group-level interventions and individual-level outcome efforts
Group-level interventions are usually not relevant for clinical interventions such as bariatric surgery, in which the interventions are applied at the individual patient level. In those cases, the questions were coded as "NA" in the assessment tool.
General Guidance for Determining the Overall Quality Rating of Before-After Studies
The questions in the quality assessment tool were designed to help reviewers focus on the key concepts for evaluating the internal validity of a study. They are not intended to create a list from which to add up items to judge a study's quality.
Internal validity is the extent to which the outcome results reported in the study can truly be attributed to the intervention or exposure being evaluated, and not to biases, measurement errors, or other confounding factors that may result from flaws in the design or conduct of the study. In other words, what is the ability of the study to draw associative conclusions about the effects of the interventions or exposures on outcomes?
Critical appraisal of a study involves considering the risk of potential for selection bias, information bias, measurement bias, or confounding (the mixture of exposures that one cannot tease out from each other). Examples of confounding include co-interventions, differences at baseline in patient characteristics, and other issues throughout the questions above. High risk of bias translates to a rating of poor quality; low risk of bias translates to a rating of good quality. Again, the greater the risk of bias, the lower the quality rating of the study.
In addition, the more attention in the study design to issues that can help determine if there is a causal relationship between the exposure and outcome, the higher quality the study. These issues include exposures occurring prior to outcomes, evaluation of a dose-response gradient, accuracy of measurement of both exposure and outcome, and sufficient timeframe to see an effect.
Generally, when reviewers evaluate a study, they will not see a "fatal flaw," but instead will find some risk of bias. By focusing on the concepts underlying the questions in the quality assessment tool, reviewers should ask themselves about the potential for bias in the study they are critically appraising. For any box checked "no" reviewers should ask, "What is the potential risk of bias resulting from this flaw in study design or execution?" That is, does this factor lead to doubt about the results reported in the study or doubt about the ability of the study to accurately assess an association between the intervention or exposure and the outcome?
The best approach is to think about the questions in the assessment tool and how each one reveals something about the potential for bias in a study. Specific rules are not useful, as each study has specific nuances. In addition, being familiar with the key concepts will help reviewers be more comfortable with critical appraisal. Examples of studies rated good, fair, and poor are useful, but each study must be assessed on its own.
Quality Assessment Tool for Case Series Studies - Study Quality Assessment Tools
Criteria | Yes | No | Other |
---|---|---|---|
1. Was the study question or objective clearly stated? | |||
2. Was the study population clearly and fully described, including a case definition? | |||
3. Were the cases consecutive? | |||
4. Were the subjects comparable? | |||
5. Was the intervention clearly described? | |||
6. Were the outcome measures clearly defined, valid, reliable, and implemented consistently across all study participants? | |||
7. Was the length of follow-up adequate? | |||
8. Were the statistical methods well-described? | |||
9. Were the results well-described? |
Background: Development and Use - Study Quality Assessment Tools
Learn more about the development and use of Study Quality Assessment Tools.
Last updated: July, 2021
Quality Assurance in Research
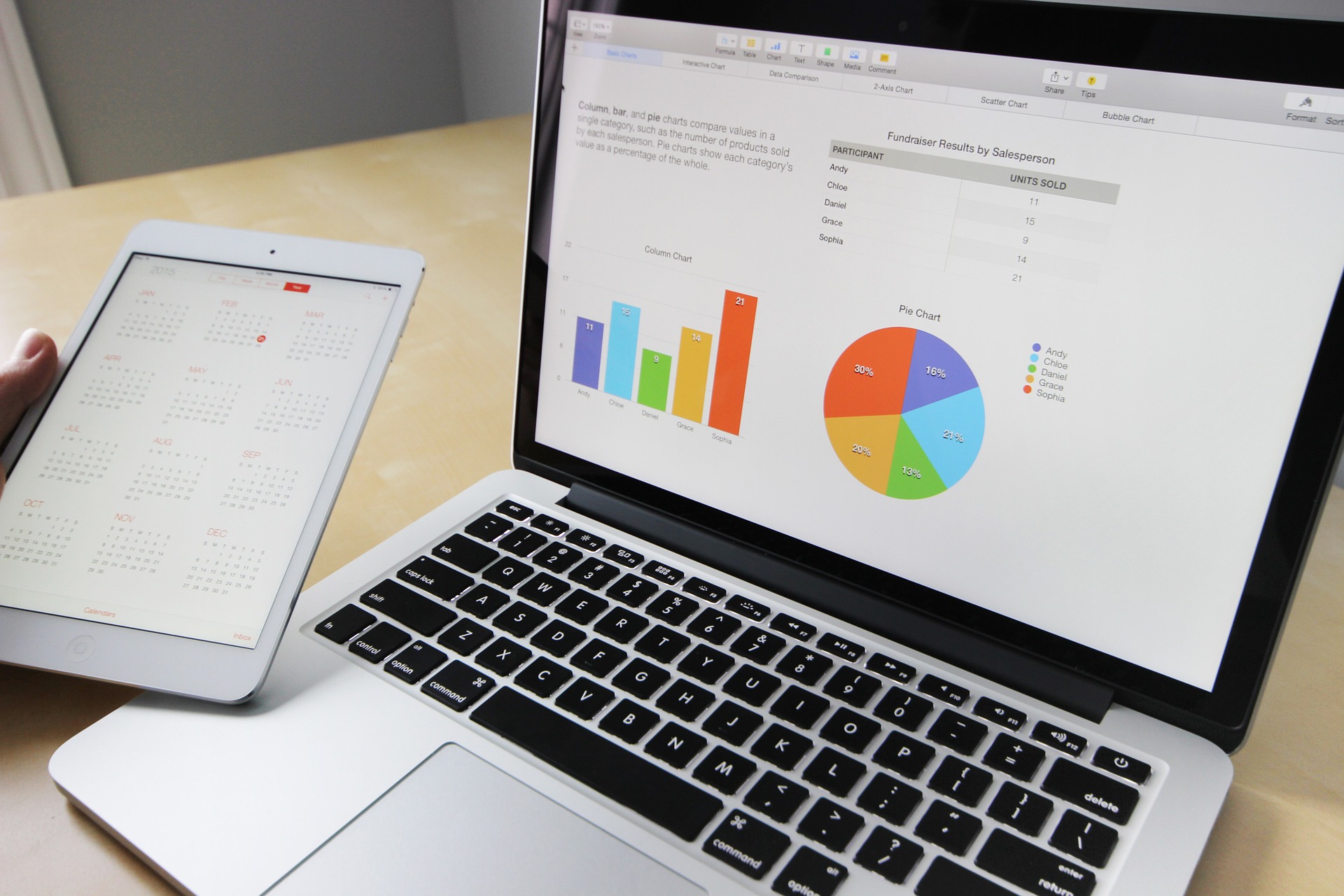
In research contexts, quality assurance (QA) refers to strategies and policies for ensuring that data integrity, quality, and reliability are maintained at every stage of the project. This includes strategies for preventing errors from entering the datasets, taking precautions before data is collected, and establishing procedures while data is used in a study.
Quality assurance is important for many reasons. The most obvious is that the whole point of research projects is to produce reliable data that yield rigorous and reproducible research results. There are other important factors as well. Internal Review Boards (IRBs), funding agencies, and other organizations that oversee research activity often require quality assurance procedures be implemented into project workflows to ensure that all policies are followed and that disbursed funds are going to well-organized and executed projects. There are also compliance issues in which research projects must be able to establish that data collection and analysis followed all protocols for human and animal subjects, privacy rules and regulations such as HIPAA and FERPA, and other safeguards that guarantee research projects are conducted in a responsible manner. In some instances, administrative audits are conducted to evaluate your project’s quality assurance and policy compliance.
Having quality assurance practices in place helps your project compliance and also helps evaluating your own research and data management practices to produce the best results possible.
Here are some steps you can take to promote quality assurance in your research:
Establishing clear data normalization protocols: Normalizing the data you record can have substantial impacts in all aspects of your research project. Normalizing means standardizing all the features and categories of data so that everyone working on the project has a clear sense for how to record it as it’s collected. Planning ahead and having clearly defined protocols for data collection before beginning the collection process means that all data that is part of the project adheres to the same standards.
Using consistent data formats and measurement standards: Using consistent format and measurement standards is part of the normalization process, and often you can find controlled vocabularies or ontologies that will provide established structural and definitional guidelines for your data based on your discipline. This will result in consistency in your data, not only within your own project, but also for others who may want to use it later on for further analysis or evaluation.
Rigorous data handling and analysis procedures: This is one of the most crucial components of quality assurance because data collection introduces significant opportunities for human error to undermine the integrity of data. At every stage of data collection in which a researcher records, transforms, or analyzes data, there is the potential for simple mistakes. Identifying those stages in data collection where errors are more likely to occur, and putting preventative measures in place can minimize those errors. Simple things such as establishing data and measurement formats can help, but also the tools you select for data collection can have significant impacts.
Select data collection and storage tools that promote data consistency: Spreadsheets for instance, are notorious for making it easy for errors to occur in data collection because they offer few controls on how it’s entered. Other tools such as databases or fillable forms provide features that allow you to control how data is entered. If you have a large team of researchers collecting data from the field or in varying contexts it’s easy for inconsistencies to arise. If the tools the researchers are using require consistency, you can be more successful at maintaining data integrity at every stage of handling data.
Metadata documenting how data was collected, recorded, and processed: Documenting how your data was handled throughout your project is good practice for a host of reasons, and it’s particularly helpful for maintaining data integrity. Making your data handling procedures explicit and formalized in the way metadata demands requires, first, that you consider these issues carefully. It also clarifies any ambiguities in your workflow so that a researcher during the project or making use of your research outputs at a later date could identify when the data is correct and where errors may have occurred.
Research staff training: Perhaps the most important thing you can do to produce consistent and reliable data is to make sure everyone working on the research project, from seasoned researchers to graduate and undergraduate project team members, have proper training in all the data collection and analysis procedures. Having everyone on the same page means that you can be confident that each person working on the project knows how their data handling tasks contribute to the overall project’s data quality goals.
Want to learn more about this topic? Check out the following resources:
The UK Data Service provides detailed information on establishing quality assurance strategies in your research.
DataOne provides guidance on how to craft a quality assurance plan that will allow you to “think systematically” as you put these protocols in place.
Thank you for visiting nature.com. You are using a browser version with limited support for CSS. To obtain the best experience, we recommend you use a more up to date browser (or turn off compatibility mode in Internet Explorer). In the meantime, to ensure continued support, we are displaying the site without styles and JavaScript.
- View all journals
- Explore content
- About the journal
- Publish with us
- Sign up for alerts
- News Feature
- Published: 27 January 2016
How quality control could save your science
- Monya Baker 1
Nature volume 529 , pages 456–458 ( 2016 ) Cite this article
6447 Accesses
42 Citations
416 Altmetric
Metrics details
- Research management
It may not be sexy, but quality assurance is becoming a crucial part of lab life.
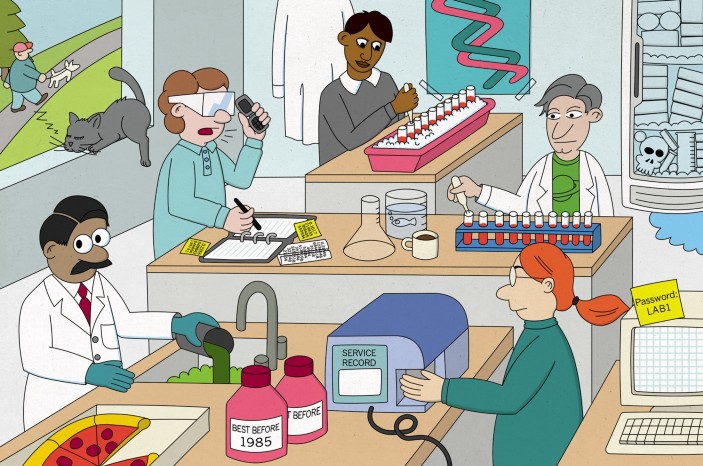
Rebecca Davies remembers a time when quality assurance terrified her. In 2007, she had been asked to lead accreditation efforts at the University of Minnesota's Veterinary Diagnostic Laboratory in Saint Paul. The lab needed to ensure that the tens of thousands of tests it conducts to monitor disease in pets, poultry, livestock and wildlife were watertight. “It was a huge task. I felt sick to my stomach,” recalls Davies, an endocrinologist at the university's College of Veterinary Medicine.
She nevertheless accepted the challenge, and soon found herself hooked on finding — and fixing — problems in the research process. She and her team tracked recurring tissue-contamination issues to how containers were being filled and stored; they traced an assay's erratic performance to whether technicians let an enzyme warm to room temperature; and they established systems to eliminate spotty data collection, malfunctioning equipment and neglected controls. Her efforts were crucial to keeping the diagnostic lab in business, but they also forced her to realize how much researchers' work could improve. “That is the beauty of quality assurance,” Davies says. “That is what we were missing out on as scientists.”
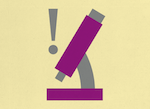
Davies wanted to spread the word. In 2009, she got permission and financial support to launch an internal consulting group for the college, to help labs with the dry but essential work of quality assurance (QA). The group, called Quality Central, now supports more than half a dozen research labs — helping them to design systems to ensure that their equipment, materials and data are up to scratch, and helping them to improve.
She is also part of a small but growing group of professionals around the world who hope to transform basic biomedical research. Many were hired by their universities to help labs to meet certain regulatory standards, but these QA consultants have a broader vision. They are not pushing for universal adoption of formal regulatory certifications. Instead, they advocate 'voluntary QA'. With the right strategies, they argue, scientists can strengthen their research and improve reproducibility.
When Davies first started proselytizing to her fellow faculty members, the responses were not encouraging. “None of them found the idea compelling at all,” Davies recalls. How important could QA be, they asked, if the US National Institutes of Health did not require it? How could anyone afford to spend money or time on non-essentials? Shouldn't they focus on the discoveries lurking in their data, and not the systems for collecting them?
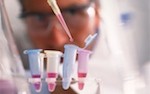
But some saw the potential, based on their own experiences. Before she had heard of Quality Central, University of Minnesota virologist Montserrat Torremorell was grateful when a colleague let her use his instruments to track transmissible disease in swine. But the results made no sense. Samples from pigs experimentally infected with influenza showed extremely low levels of the virus. It turned out that her benefactor had, like many scientists, skimped on equipment maintenance to save money. “It was a real eye-opener,” Torremorell recalls. “It just made me think that I could not rely on other people's equipment.”
Quality for all
Quality systems are an integral part of most commercial goods and services, used in manufacturing everything from planes to paint. Some labs that focus on clinical applications implement certified QA systems such as Good Clinical Practice, Good Manufacturing Practice and Good Laboratory Practice for data submitted to regulatory bodies. There have also been efforts to guide research practices outside these schemes. In 2001, the World Health Organization published guidelines for QA in basic research. And in 2006, the British Association of Research Quality Assurance (now simply the RQA) in Ipswich issued guidelines for basic biomedical research. But few academic researchers know that these standards exist (Davies certainly didn't back in 2007).
Instead, QA tends to be ad hoc in academic settings. Many scientists are taught how to keep lab notebooks by their mentors, supplemented perhaps by a perfunctory training course. Investigators often improvise ways to safeguard data, maintain equipment or catalogue and care for experimental materials. Too often, data quality is as likely to be assumed as assured.
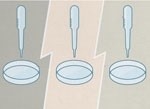
Scientific rigour has taken a drubbing in the past few years, with reports that fewer than one-third of biomedical papers can be reproduced (see Nature http://doi.org/477 ; 2015 ). Scientific culture, training and incentives have all been blamed for promoting sloppy work; a common refrain is that the status quo values publication counts over careful experimentation and documentation. “There is chaos in academia,” says Masha Fridkis-Hareli, head of ATR, a biotechnology consultancy in Worcester, Massachusetts, that also conducts laboratory work to help move basic research into industry. For every careful researcher she has encountered, there have been others who have thought nothing of scribbling data on paper towels, repeating experiments without running controls and guessing at details months after an experiment. Davies insists that plenty of scientists are doing robust work, but there is always room for improvement (see 'Solutions'). “There are easy fixes to situations that shouldn't be happening, but are,” she says.
Michael Murtaugh, a swine biologist at the University of Minnesota, had tried to establish practices to beef up the reliability of his team's lab notebooks, but the attempts that he made on his own never gained traction. Then Davies got on his case. After a year or so of her “planting seeds” — as she puts it — Murtaugh agreed to work with Quality Central and implement a low-tech but effective solution.
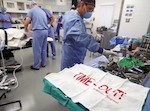
On designated Mondays, each member of Murtaugh's lab draws a name from a paper bag to determine whose notebook to audit. The scientists check that their assigned books include relevant controls for experiments, and indicate where data are stored and which particular machine generated them. The group also makes sure that any problems noted in the previous check have been addressed. It takes about ten minutes per researcher every few weeks, but that's enough to change people's habits. Graduate student Michael Rahe says that the checks ensure that he keeps his notebook legible and up to date. “I never used to put in raw data,” he says.
Albert Cirera, a technologist developing gas nanosensors at the University of Barcelona in Spain, has also embraced QA. As his lab group grew to 12 people, he found it difficult to monitor everyone's experiments, and his own efforts to implement a tracking system were inadequate. He turned to a university-based QA consulting service for help. Now, samples, equipment and their data are all linked with tracking numbers printed on stickers and recorded in individuals' notebooks, on samples and in a central tracking file. The system does not slow down experiments, and staying abreast of projects is a breeze, says Cirera. But getting to this point took about four months and frequent consultations. “It was not something that you can create from zero,” he says.
Making a market
Any scientist adopting a QA system has to wager that the up-front hassle will pay off in the future. “It is very difficult to get people to check and annotate everything, because they think it is nonsense,” says Carmen Navarro-Aragay, head of the University of Barcelona quality team that worked with Cirera. “They realize the value only when they get results that they do not understand and find that the answer is lurking somewhere in their notebooks.”
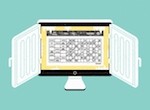
Even when experiments go as expected, quality systems can save time, says Murtaugh. Methods and data sections in papers practically write themselves, with no time wasted in frenzied hunting for missing information. There are fewer questions about how experiments were done and where data are stored, says Murtaugh. “It allows us to concentrate on biological explanations for results.”
The more difficult data are to collect, the more important a good QA system becomes. Catherine Bens, a QA manager at Colorado State University in Fort Collins, says that she remembers getting cold, wet and dirty when she had to monitor a study involving ultrasound scans and blood samples from a population of feral horses in North Dakota. Typical animal-identification practices such as ear tagging were not allowed. So, before the collection started, Bens supported researchers as they rehearsed procedures, pre-labelled tubes, made back-up labels and recruited animal photographers and park volunteers to ensure that samples would be linked to the correct animals. Even in a snow storm with winds so loud that everyone had to shout, the team made sure that each data point could be traced.
Rare samples or not, few basic researchers are clamouring to get QA systems in place. Most are unfamiliar with the discipline, says Davies. Others are hostile. “They see it as trying to constrain them, and that you're making them do more work.”
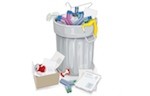
Before awarding certain grants, the Found Animals Foundation in Los Angeles, California, which funds research on animal sterilization, requires proof that instruments have been calibrated and that written plans exist for tracing data and dealing with outliers. It can be a struggle, says Shirley Johnston, scientific director of the foundation. One grant recipient argued that QA systems were unnecessary because just looking over the data would reveal their quality.
Part of the resistance may be down to how some QA professionals present themselves. “A lot of them are there to tell you what you are doing is wrong, and a lot of them are not very nice about it,” says Terry Nett, a reproductive biologist at Colorado State University who experienced this first-hand when he worked with outside consultants to incorporate Good Laboratory Practice principles in his lab. The effort was frustrating. “Instead of helping us understand, they would act like a dictator,” Nett recalls. “I just didn't want them in my lab.” A few years ago, however, the university hired its own quality managers, and things changed. The current manager, Bens, acts more like a partner, Nett says. She points out where labs are already using robust practices, and explains the reasoning behind QA practices that she introduces.
To win scientists over, Bens stresses that QA systems produce data that can withstand criticism. “You build a support system around any data point you collect,” she says. When there is a strange result, researchers have documentation to trace its provenance. That can show whether a data point is real, an outlier or a problem — for example if a blood sample was not kept cold or was stored in the wrong tube.
There are easy fixes to situations that shouldn't be happening, but are.
Scientists need to take the lead on which QA elements they incorporate, says Melissa Eitzen, director of regulatory operations at the University of Texas Medical Branch in Galveston. “You want to give them tips that they can take or not take,” she says. “If they choose it, they'll do it. If you tell them they have to do it, that's a struggle.”
Rapport is paramount, says Michael Jamieson at the University of Southern California in Los Angeles, who helps other faculty members to move research towards clinical applications. Instead of talking about quality systems, he prefers to discuss concrete behaviours, such as labelling bottles with expiry dates and storage conditions. QA jargon puts scientists off, he says. “Using the term good research practice makes most researchers want to run the other way.”
It's a lesson that many QA specialists have taken to heart. Some say 'assessment' or 'quality improvement' instead of 'audit'. Even 'research integrity' can be an inflammatory phrase, says Davies. “You have to find a way to communicate that QA is not punitive or guilt-inspiring.”
Not into temptation
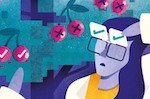
Having data that are traceable — down to who did what experiment on which machine, and where the source data are stored — has knock-on benefits for research integrity, says Nett. “You can't pick out the data that you want.” Researchers who must provide strong explanations about why they chose to leave any information out of their analysis will be less tempted to cherry-pick data . QA can also weed out digital meddling: popular spreadsheet programs such as Microsoft Excel can be vulnerable to errors or manipulation if not properly locked, but QA teams can set up instruments to store read-only files and prevent researchers from tampering with data accidentally or intentionally. “I can't help but think that QA is going to make fraud harder,” says Davies.
And good quality systems can be contagious. Melanie Graham, who studies diabetes at the University of Minnesota, often collaborates with others to test potential treatments. More than once, she says, collaborators have sent her samples in a polystyrene tube with nothing but a single letter written on it. Graham sends it back and requests a label that specifies the sample's identity and provenance, and a range of storage temperatures. 'Keep frozen' is too vague — she will not risk performing uninformative experiments because reagents stored in a standard freezer were supposed to be kept at −80 °C.
I can't help but think that QA is going to make fraud harder.
When she first sent documentation requirements to collaborators, she expected them to push back. Instead, reactions were overwhelmingly positive. “It's a relief for them,” says Graham. “They want us to handle their test article in a trusted way.”
The benefits go beyond providing solid data. In 2013, Davies worked with Torremorell and other Minnesota faculty members on a proposal to monitor and calibrate equipment used by several labs. The plan that they put in place helped them to secure US$1.8 million to build shared lab space to deal with animal pathogens, says Torremorell. “If we want to be competitive to get funding, and if we want people to believe our data, we need to be serious about the data that we generate.”
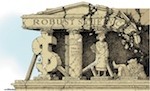
Davies is still trying to spread the word. Her invitations to give talks and review grant applications have mushroomed. She and collaborators at other institutions have been developing online training materials and offering classes to technicians, postdocs, graduate students and principal investigators. After a presentation last year, a member of the audience told her that he had reviewed a grant from one of her clients; the QA plan had made the application stand out in a positive way. Davies was delighted. “I could finally come back to my folks and say, 'It was noticed.'”
Davies knows it is still an uphill battle, but her ultimate goal is to make QA as much a part of research as peer review. It may not have the flash and dazzle of other efforts to ensure that research is robust and reproducible, but that is not the point. “A QA programme isn't sexy,” says Michael Conzemius, a veterinary researcher at the University of Minnesota and another client of Quality Central. “It's just kind of become the nuts and bolts of the scientific process for us.”
Quality assured
Scientists share their favourite tips and tricks for good quality-assurance practices.
Start from passion Put quality systems around the projects for which enthusiasm is high and researchers are more willing to put in some extra work, says Karen Dobos, who develops tuberculosis vaccines and assays at Colorado State University in Fort Collins. “Focus your quality system around the thing that you are most passionate about, and when you feel good about that, then everything else can grow around that.”
Take baby steps Researchers who adopt too many quality assurance (QA) practices too fast risk burning out. “Small steps work better than big steps,” says Marcella Sarzotti-Kelsoe, an immunologist at Duke University in Durham, North Carolina, who directs the QA programme for the US National Institutes of Health’s Centers for AIDS Research. Diagrams that map out where samples should be placed in an assay plate or images of how cells should look when they are ready for an assay can eliminate errors and provide useful reference materials. Simply signing and dating forms can help to trace data back to unusual conditions, such as equipment failure or power outage.
Customize for your lab There is nothing wrong with using forms or standard operating procedures from other labs, says Rebecca Davies, director of Quality Central at the University of Minnesota in Saint Paul. But these documents need to be adapted for individual teams’ questions and procedures. “Don't get too familiar with a routine form,” she says.
Trust your technicians Long-term lab managers and research associates can make sure that quality practices stick, and are often most willing to make time to work with quality consultants, says Michael Murtaugh, a swine biologist at the University of Minnesota. He credits a research scientist in his lab with keeping a continuous audit programme on track.
Frame QA as a hunt for uncontrolled variables Documenting details about procedures, diet, temperatures and more is not paperwork, it’s information that could identify variables that affect experimental results, says Melanie Graham, who studies diabetes at the University of Minnesota. “We look at this as real data, real scientific data, not a punitive logging error.”
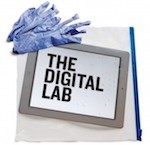
For more tips, see the Quality Assurance Toolkit .
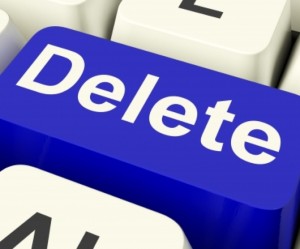
There are many things wrong with the fictitious lab shown above. But, here are six that a quality-assurance manager would identify, and how they would solve them.
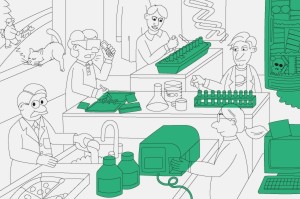
DISORGANIZED SAMPLE STORAGE Clear labelling and proper organization are important for incubators and freezers. Everyone in the lab should be able to identify a sample, where it came from, who did what to it, how old it is and how it should be stored. INADEQUATE DATA LOGGING Data should be logged in a lab notebook, not scribbled onto memo paper or other detritus and carelessly transcribed. Notebooks should be bound or digital; loose paper can too easily be lost or removed. VARIABLE EXPERIMENTS Protocols should be followed to the letter or deviations documented. If reagents need to be kept on ice while in use, each lab member must comply. UNSECURED DATA ANALYSIS Each lab member should have their own password for accessing and working with data, to make it clear who works on what, when. Some popular spreadsheet programs can be locked down so that manipulating data, even accidentally, is difficult. MISSED MAINTENANCE Instruments should be calibrated and maintained according to a regular, documented schedule. OLD AND UNDATED REAGENTS These can affect experimental results. Scientists should specify criteria for age and storage of all important reagents.
Author information
Authors and affiliations.
Monya Baker writes for Nature from San Francisco, California.,
Monya Baker
You can also search for this author in PubMed Google Scholar
Additional information
Tweet Facebook LinkedIn weibo
Related links
Related links in nature research.
Poorly designed animal experiments in the spotlight 2015-Oct-13
Biomedical researchers lax in checking for imposter cell lines 2015-Oct-12
Hospital checklists are meant to save lives — so why do they often fail? 2015-Jul-28
Reproducibility crisis: Blame it on the antibodies 2015-May-19
Nature special: Reproducibility
Related external links
Quality Assurance Toolkit
How to Start, and Keep, a Lab Notebook
Quality Systems Workbook
Rights and permissions
Reprints and permissions
About this article
Cite this article.
Baker, M. How quality control could save your science. Nature 529 , 456–458 (2016). https://doi.org/10.1038/529456a
Download citation
Published : 27 January 2016
Issue Date : 28 January 2016
DOI : https://doi.org/10.1038/529456a
Share this article
Anyone you share the following link with will be able to read this content:
Sorry, a shareable link is not currently available for this article.
Provided by the Springer Nature SharedIt content-sharing initiative
This article is cited by
Pisa-tree - a data management framework for life science research projects using a standardised directory tree.
- Marko Petek
- Maja Zagorščak
- Kristina Gruden
Scientific Data (2022)
Qresp, a tool for curating, discovering and exploring reproducible scientific papers
- Marco Govoni
- Milson Munakami
- Giulia Galli
Scientific Data (2019)
Quality assurance and best research practices for non-regulated veterinary clinical studies
- M. Conzemius
BMC Veterinary Research (2017)
Validating reference genes using minimally transformed qpcr data: findings in human cortex and outcomes in schizophrenia
- Madhara Udawela
- Elizabeth Scarr
BMC Psychiatry (2016)
Quick links
- Explore articles by subject
- Guide to authors
- Editorial policies
Sign up for the Nature Briefing newsletter — what matters in science, free to your inbox daily.

Data Use: An evaluation of quality-control questions
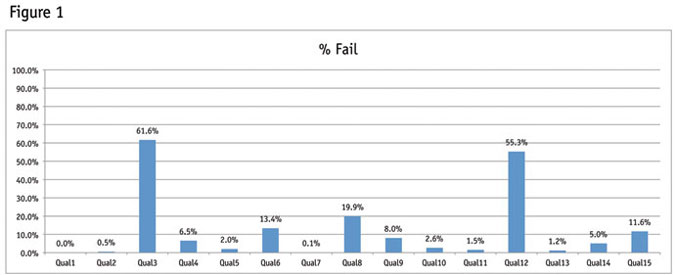
A report on a research-on-research study that examined the impact of various types of in-survey quality-control measures.
Editor's note: Keith Phillips is senior methodologist with SSI, Shelton, Conn.
How do we, as researchers, decide on quality data? We usually look at the behavior of participants within a questionnaire itself. We look at how long they took to complete the survey, what they wrote in the open-ended questions, whether they gave the same answer option through a grid question, if they gave consistent answers and we look at quality-control questions. These quality-control questions are designed to measure attentiveness and remove participants who are not paying attention.
There are different types of quality-control questions. Some are simply inserted within a grid and ask participants to select a specific punch; others measure quality by allowing participants to contradict themselves. Some intentionally misdirect participants, so that the question being asked is in the detail of a long instruction and the question is not at all what it seems to be.
Quality-control questions assume that participant misbehavior in a particular moment is indicative of misbehavior throughout the entire survey. For this reason, the data quality is improved with the omission of this participant. An alternate assumption is that a degree of inattentiveness is normal throughout a survey and participants may be attentive during the trap question but not during key measures. Conversely, those failing the trap may not have been paying attention in the moment but are contributing elsewhere, meaning many are no different than the participants that are being kept.
First hypothesis
Working at a sample provider, I see many questionnaires for a variety of industries conducting an array of research. One thing I have noticed is the variety of quality-control measurements used to validate the online self-completion surveys; in particular, the varying amount of participants being excluded due to poor data quality, which was specifically dependent on the quality-control questions.
This led to my first hypothesis: Design of the quality-control question has a large impact on the number of participants flagged for having poor data quality and it is more a measurement of question effectiveness than participant quality.
Knowing that these quality-control questions threw out such a wide range of participants, I formulated a second hypothesis: Exclusion based on quality-control measurements would result in a variety of sample compositions and some of these would be more accurate than others.
In order to test my theories, I designed an online survey that looked at 15 different quality-control measurements: 12 quality-control questions, speeding, straightlining and an open-end assessment. The survey was administered among 2,100 online participants in the U.S. The survey covered a mixture of topics including entertainment, social issues, lifestyle and general behavior questions. The survey used 12 offline benchmarks as measurements of quality to compare the data against. The median survey time was 12.5 minutes; short enough not to encourage fatigue.
The results
As illustrated in Figure 1, my first hypothesis proved true: the design of the question itself has a large impact on the amount of participants being excluded from the sample. Shown here is the percent of the same sample that failed each of the quality-control measurements. There is a large distribution that goes from 0 percent failing at Qual1 to 61.6 percent failing at Qual 3. More detail on each of these quality-control measurements will be given later in this article.
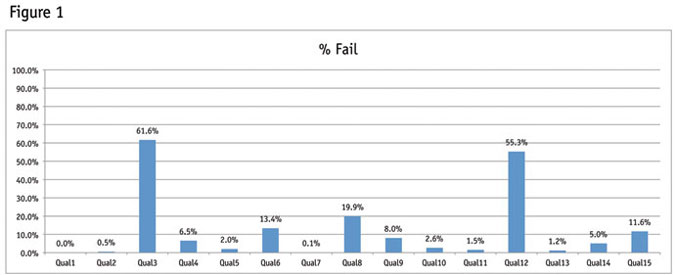
By Cheskie Rosenzweig, MS, Josef Edelman, MS & Aaron Moss, PhD
Why Are Attention Checks Used in Research?
Attention check questions are frequently used by researchers to measure data quality . The goal of such checks is to differentiate between people who provide high-quality responses and those who provide low-quality or unreliable data.
Although attention checks are an important way to measure data quality, they are only one of several data points researchers should use to isolate and quarantine bad data. Others include things like measuring inconsistency in responses, nonsense open-ended data, and extreme speeding.
In this blog, we describe what makes a good attention check and provide examples of good and bad attention check questions.
What Makes a Good Attention Check?
A good attention check question has a few core components:
- It measures attention, not other constructs related to memory, education, or cultural specific knowledge
- It has a clear, correct answer
- It is not overly stringent or too easy
- It doesn’t require participants to violate norms of human behavior
The example below contains all of these elements.
Common Mistakes Researchers Make When Writing Attention Checks
Attention check questions can be subpar for many reasons, but most often when a question does not function as it should it’s because one of the core components above has been violated.
Attention Checks That Measure Something Other Than Attention
Researchers sometimes adopt attention check questions that ask participants to think back to an earlier part of the survey and recall specific information. This kind of question certainly has something to do with attention, but it also measures memory. And often the difficulty of the recall required is unclear, as in the example below.
Even though this question has a clear and factual answer it is unclear what to conclude about someone who gets the question wrong. Did the person fail because they were not paying attention or because they forgot the story’s details? Were the number of oranges a critical piece of the story or a minor detail that people may have understandably failed to encode?
Rather than asking attention check questions that rely on memory, we recommend asking questions that check for comprehension just after critical materials are presented within the study. Then, questions that assess attention can be used solely to assess attention, regardless of where in the study they are presented.
Attention Checks Can be Unclear
Another reason attention check questions sometimes fail is because they lack a clear and correct answer. At times, this is because the question’s answer options are ambiguous.
Even though this question appears straightforward and is similar to the example of a good attention check item given above, it actually contains some ambiguity. Some participants may decide to indicate their attention by selecting strongly disagree rather than just disagree. Are these people being inattentive?
At other times, questions can be unclear because participants interpret things differently than researchers intended. Here is an example:
And another:
In both questions above, it appears there is little room for ambiguity or error. Nevertheless, research shows that attentive participants can sometimes ‘fail’ these items because they interpret them differently than researchers intend ( Curran & Hauser, 2019 ). For instance, participants asked to explain why they agreed with the statements above said things like even though they were not sure what parabanjology is, it sounds interesting, and they did not necessarily want to rule it out. In response to the second statement, participants may agree that they are paid bi-weekly, just not by leprechauns.
Instead of the items above, we recommend asking participants to “strongly agree” or “strongly disagree” with items, or asking questions that are short, simple, and hard to reason around.
Attention Checks Vary in Difficulty and Can Require Participants to Violate Behavioral Norms
Sometimes an attention check isn’t overly difficult because of the content it is asking participants to provide or recall, but because it is designed to only be answerable by participants who are highly attentive to every minute detail or who follow every single word in a survey. Questions like these end up excluding participants who are otherwise high-quality respondents, but are not extraordinarily attentive and conscientious:
Attention checks shouldn’t require participants to ignore direct questions that appear straightforward. Instead, questions like this should reference that the instructions are important and ensure that the response participants are expected to give is clear, like the example below.
The above examples are reasons why we recommend that researchers use attention check questions that have been previously tested and validated as good checks of attention. When researchers create attention checks based on personal intuition, the checks they use often lack validity ( see Berinsky, Margolis, & Sances, 2014 ).
Automate Attention Checks and Ensure Better Data With Sentry® By CloudResearch
Rooted in these best practices in developing attention checks and other measurements of data quality, CloudResearch has undertaken several large scale initiatives aimed at improving data quality in online surveys. We have cleaned up data on one platform often used for online research: Mechanical Turk . We also have data quality solutions that can be applied to any and all sample sources: our Sentry system. Sentry is an all encompassing data quality solution that ensures top notch respondents through multiple behavioral and technological analyses, including attention checks. L earn more about Sentry today!
Related Articles
New solutions dramatically improve research data quality on mturk.
Amazon Mechanical Turk (MTurk) is a microtask platform that has been used for social science research for nearly a decade. During this time,
Clean Data: the Key to Accurate Conclusions
Considering how much time and effort goes into designing, fielding, and analyzing online surveys, it is critical that the responses researchers collect are high quality. When companies make decisions based...
SUBSCRIBE TO RECEIVE UPDATES
2024 Grant Application Form
Personal and institutional information.
- Full Name * First Last
- Position/Title *
- Affiliated Academic Institution or Research Organization *
Detailed Research Proposal Questions
- Project Title *
- Research Category * - Antisemitism Islamophobia Both
- Objectives *
- Methodology (including who the targeted participants are) *
- Expected Outcomes *
- Significance of the Study *
Budget and Grant Tier Request
- Requested Grant Tier * - $200 $500 $1000 Applicants requesting larger grants may still be eligible for smaller awards if the full amount requested is not granted.
- Budget Justification *
Research Timeline
- Projected Start Date * MM slash DD slash YYYY Preference will be given to projects that can commence soon, preferably before September 2024.
- Estimated Completion Date * MM slash DD slash YYYY Preference will be given to projects that aim to complete within a year.
- Project Timeline *
- Email This field is for validation purposes and should be left unchanged.
- Name * First Name Last Name
- I would like to request a demo of the Sentry platform
- Name This field is for validation purposes and should be left unchanged.
- Name * First name Last name
- Name * First Last
- Name * First and Last
- Please select the best time to discuss your project goals/details to claim your free Sentry pilot for the next 60 days or to receive 10% off your first Managed Research study with Sentry.
- Comments This field is for validation purposes and should be left unchanged.
- Phone This field is for validation purposes and should be left unchanged.
- Email * Enter Email Confirm Email
- Organization
- Job Title *

Child Care and Early Education Research Connections
Assessing research quality: key questions to ask.
This guidance is designed to help you think about the usefulness of research when considering policy and practice decisions. There are three sections in this resource to help you consider the quality of the research for your needs.
Is the research relevant?
Is the research credible?
Is the research rigorous.
These guiding questions are meant to give you a broad understanding of questions to ask when reviewing research, and it is not meant to be a comprehensive resource. Keep in mind there may be other factors to consider as you use research to guide your practice and policymaking decisions. If you can’t find the answers to most of these questions, you may want to search for additional research that is relevant, credible, and rigorous.
Is the research relevant to my needs?
Relevance refers to the extent to which the research is useful to understanding your policy and practice needs and how the research connects to your interests in ways that add value.
Key questions:
Is the research useful to you? Does it reflect a policy or practice that you are interested in learning more about? Some research describes how an intervention should work under ideal conditions to produce positive results. However, even if the study did not produce the intended results, you may be able to learn more about the relationships between the activities and outcomes that can inform your work.
Is it recent and important? Look to see when the research was conducted and if any unusual circumstances may have occurred during the time the research was conducted. For example, look at the time of when the data was collected. Did the research happen during COVID, and could that have impacted its relevance to you?
Is research useful in contexts other than the one studied? Look to see if the research was carried out in an area (urban, rural, suburban, etc.) that aligns with your interests. Even if the research took place in a different context than the one you are interested in, there might be information that the researcher shares that could be useful to you. For example, if an intervention showed promising findings for dual-language children in a rural preschool setting, does the research discuss how the intervention might work in other contexts? This information might be found in the literature review or discussion of the findings.
Is it relevant and beneficial to the current populations you're working with or interested in? Look to see if the research was conducted with populations (Head Start families, dual language learners, infant and toddler teachers, rural families, etc.) that align with your interests. Think about cultural considerations as you review the research and how it aligns with your needs, such as diversity of race, ethnicity, language, and/or country of origin of the research participants. If you work with or are designing policies for children and families that represent different demographics, make sure the research represents these populations.
Credibility refers to the extent to which you can trust the information from the resource, the author, or the organization that produced the research. It is also important to understand the bias that some authors or organizations may have on a particular topic; this might influence how the study was conducted or the conclusions that are made.
Keep in mind that new or more immediate questions may have less available evidence, or the evidence may be mixed. This may require some judgment regarding whether the evidence is strong enough to use for decision-making.
Does the research relate to what is already known on the subject? Research should present existing evidence on a topic and discuss any gaps in knowledge or understanding. This information is usually found in the literature review section.
Are policy or practice recommendations supported by the research? Not all research-based resources include policy recommendations. If recommendations are presented, it should be tied to the evidence presented and not based on the author’s opinions.
Does the research discuss the limitations of its findings? Acknowledgment of research limitations is crucial. Every study has constraints, and being forthright about them helps you better understand the usefulness of the research to your needs.
Are there financial or other considerations that could influence the author’s professional judgment? Many academic journals require authors to disclose conflicts of interest. Information on who or what organization funded the research might be shared in a footnote or acknowledgement at the end of the resource.
Does the author or organization have any stated advocacy goals that might impact their ability to be independent? The existence of conflicts of interest, such as advocacy goals, does not inherently mean that a resource is not objective or credible. Instead, these characteristics can be considered along with the overall quality of the resource in making decisions on whether the research fits your needs. Look for language or statements where the author may use words or phrases that try to persuade you about the topic or if the author spends more time presenting only one viewpoint on the topic.
Does the author or the organization discuss social or cultural factors that might contribute to differences in the findings? Understanding these factors may help you understand what practices or policies work for different groups of people. For example, if language barriers affect families’ ability to access child care subsidies, look for a discussion on how a practice or policy in addressing language barriers might have impacted study findings.
Research rigor refers to whether a research resource used appropriate methods to answer the research questions and whether the researcher adhered to established procedures and protocols. Research rigor is described differently in quantitative, qualitative, and mixed methods research. Each approach has its unique strengths and is best at answering certain types of research questions. One is not necessarily better than the other, and they serve different purposes. Some research resources may describe their methods in highly technical terms. You may want to ask someone who has training in research methods to help you assess this.
Is the methodology appropriate to answer the research questions? The research questions should influence the methods used to gather information to answer the research questions. For example, a quantitative study may help provide insights on a large group of people such as all families in the state child care subsidy system. A qualitative study may allow for exploration of topics in greater detail, such as families who receive child care subsidies and have an infant with a special need. A mixed method study uses aspects of both approaches. You may want to consult with someone who has training in research methods to help assess this if you are concerned about the methodology.
Are the study design and data collection processes described? A study design is the strategy that researchers use to answer their research questions. There are different research designs for different types of research questions, such as descriptive studies , experiments and quasi-experimental studies for quantitative studies and direct observation, participant observation, and interviews for qualitative studies. Research studies should clearly explain the procedures used to collect and analyze the data . For example, the study might include information on how the data was collected (through surveys, interviews, focus groups, using administrative data, etc.), how often it was collected, how data collectors were trained, and so on.
Did the research describe how participants were selected? You should be able to tell how the sample was selected and where the participants came from. The strategy researchers use to recruit study participants is based on the study methodology and research questions. In quantitative studies, the researcher might use random selection to ensure generalizability to ensure that the findings can be applied to a larger population. A qualitative study may use purposeful sampling to ensure that the researcher has an in-depth understanding of the phenomena and participants perspectives.
What techniques were used to analyze and interpret the data? This might be the most technical part of a research paper. Researchers should describe their data analysis plan. Quantitative research is focused on statistical analysis while qualitative analysis focuses on interpreting narrative to develop themes to better understand the phenomena. Check to see if the researchers present the data by race, ethnicity, or other social/cultural factors to help understand how the research may have different findings for different groups of people. You may want to consult with someone who has training in research methods to help assess this if you are concerned about the data analysis and interpretation of the data.
Was the research peer-reviewed? Peer reviewed research articles are reviewed by other experts in the field before they are published. Most people associate peer-review with academic journals, however reports and articles from government agencies and research organizations often go through many levels of internal review and approval before being distributed. Visiting the website of the organization, agency, or journal where the resource is published can provide additional detail about the peer-review or approval process.

Why Is Quality Control in Research So Important?
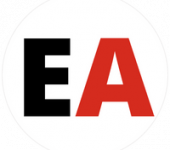
Quality control can mean the difference between good science and bad science. So, what is quality control? Very simply, it is any process that aims to monitor and maintain laboratory standards.
The processes of quality control can include detecting, reducing, and correcting any problems within a lab. Quality control can also help to make sure that the results of an experiment or method are consistent. Quality control is known as quality assurance or quality management.
Good Laboratory Practice (GLP) is one form of quality control. GLP was introduced in the field of chemical research, to try to ensure high quality, reliable test data. This was a good starting point. However, it was not perfect.
What is the Best Type of Quality Control?
The best practice for quality control is a Laboratory Quality Management (LQM) program. The design of LQM helps control factors that cause variation in a lab. By doing this, an LQM can increase the researcher’s confidence in the results of their experiments.
An LQM has six vital parts:
- Quality manual
- Labs should have a manual that describes its quality control systems in detail.
- Staff and training
- Training for all staff should be thorough and consistent.
3. Methodology
- The method must be consistent throughout the lab. They should be validated to check that they are precise and accurate.
- In-house reference materials
- This means that labs can have their control samples for standard methods. These samples will have known properties. They can be used to check that methods are working correctly.
- Record keeping
- Write down the preparation, procedure, and analysis of the experiment
- Cost vs. benefits
- The prices of setting up an LQM should be low. Benefits include confidence in results, fewer problems, and lower costs.
Tackling Problems in Quality Control
In a Nature article, a scientist describes her experiences with quality control. Rebecca Davies manages quality control at her lab for a long time. Although her task was huge, she soon became hooked on finding and fixing problems.
Once she started looking, Davies found several problems. These ranged from issues with sample storage to issues in data collection. She also discovered faulty equipment and spotted missing controls. However, these problems did not put Davies off. Instead, she realized how much the lab’s work could improve.
In 2009, Davies set up a group called Quality Central. The group helps several research labs to design proper quality control systems. Along with some other scientists, Davies believes in “voluntary” quality assurance (QA). Voluntary QA does not force quality control through regulation. Instead, it helps scientists to strengthen their research with QA.
What Happens when Quality Control is Poor
Scientific rigor is a hot topic. Over the last few years, several issues in science have caused both researchers and the public to question the scientific process. For example, some studies have found that as few as one-third of scientific papers are reproduced . Peer review and plagiarism have also been a problem. Among researchers, there is a general opinion that publications are of more value than the science itself.
Many scientists are cautious in their work. However, some are careless. Failing to record data, writing reports months after the experiment and not using controls are just a few examples. Each of these may seem like a small problem. But together, they can lead to studies that cannot be reproduced.
We can fix a lot of issues with better quality control. Unfortunately, many labs still carry out quality control in a casual, ad hoc way.
Barriers to Good Quality Control
In many labs, quality control is not seen as the best use of resources. With limited funds, other things can take priority. Some scientists explain that a lack of quality control is due to both lack of funds and lack of staff.
When Davies first set up Quality Central, she found that other researchers at her college were not interested. They thought that it was not essential, and so was a waste of time and money.
However, one scientist was interested. The researcher had used another lab’s equipment, but the results seemed odd. She discovered that to save money, the PI of the lab had not been maintaining the equipment. Equipment maintenance is one of the things that a good quality control program should check
The Benefits of Great Quality Control
Thanks to the efforts of Davies and others, researchers are starting to understand the benefits of quality control.
Quality control does not need to be complicated. Here is one example. Notebooks checking is a weekly task in a laboratory. To make sure this is fair, each member of the lab draws a name from a paper bag to decide whose notebook they will check. Notebooks include factors such as whether control was used, how and where data was recorded, and which equipment was used. Any previous problems should be fixed. This is a tremendous low-tech quality control system.
Some PI’s with large labs find it hard to check everyone’s work. It can be challenging to track samples, data, and equipment. One solution is a tracking system. This gives tracking numbers to every sample or data record. PI’s can then easily follow the progress of a study.
Often, researchers only realize the benefits of quality control when problems occur. Unexpected results can mean searching through stacks of data to try to find the cause. With reasonable quality control, this should be a rare event. Moreover, if it does happen, the reason should be easy to find.
Do you want to learn more about running a lab? Alternatively, finding and fixing problems? Why not start with this Enago article on GLP.
What are the quality control systems in your lab? Has your work ever suffered from poor quality control? Do you have any suggestions for improvement? Share your ideas in the comments below.
Rate this article Cancel Reply
Your email address will not be published.
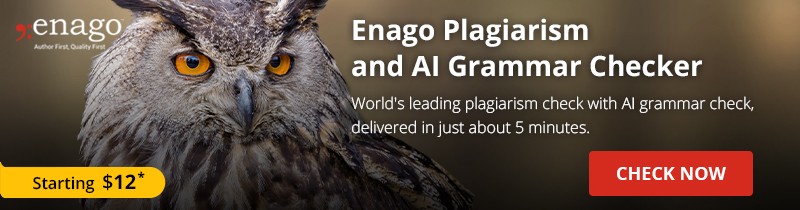
Enago Academy's Most Popular Articles
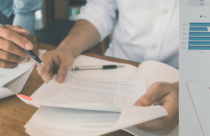
- Reporting Research
Choosing the Right Analytical Approach: Thematic analysis vs. content analysis for data interpretation
In research, choosing the right approach to understand data is crucial for deriving meaningful insights.…
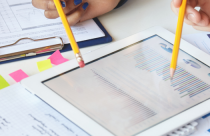
Comparing Cross Sectional and Longitudinal Studies: 5 steps for choosing the right approach
The process of choosing the right research design can put ourselves at the crossroads of…
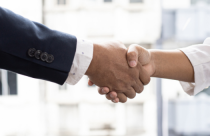
- Career Corner
Unlocking the Power of Networking in Academic Conferences
Embarking on your first academic conference experience? Fear not, we got you covered! Academic conferences…
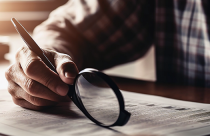
Research Recommendations – Guiding policy-makers for evidence-based decision making
Research recommendations play a crucial role in guiding scholars and researchers toward fruitful avenues of…
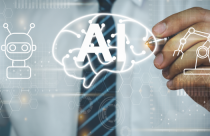
- AI in Academia
Disclosing the Use of Generative AI: Best practices for authors in manuscript preparation
The rapid proliferation of generative and other AI-based tools in research writing has ignited an…
Avoiding the AI Trap: Pitfalls of relying on ChatGPT for PhD applications
10 Ways to Help Students Restore Focus on Learning
Switching Your Major As a Researcher: Things to Consider Before Making the Decision

Sign-up to read more
Subscribe for free to get unrestricted access to all our resources on research writing and academic publishing including:
- 2000+ blog articles
- 50+ Webinars
- 10+ Expert podcasts
- 50+ Infographics
- 10+ Checklists
- Research Guides
We hate spam too. We promise to protect your privacy and never spam you.
Criteria for Good Qualitative Research: A Comprehensive Review
- Regular Article
- Open access
- Published: 18 September 2021
- Volume 31 , pages 679–689, ( 2022 )
Cite this article
You have full access to this open access article
- Drishti Yadav ORCID: orcid.org/0000-0002-2974-0323 1
103k Accesses
48 Citations
70 Altmetric
Explore all metrics
This review aims to synthesize a published set of evaluative criteria for good qualitative research. The aim is to shed light on existing standards for assessing the rigor of qualitative research encompassing a range of epistemological and ontological standpoints. Using a systematic search strategy, published journal articles that deliberate criteria for rigorous research were identified. Then, references of relevant articles were surveyed to find noteworthy, distinct, and well-defined pointers to good qualitative research. This review presents an investigative assessment of the pivotal features in qualitative research that can permit the readers to pass judgment on its quality and to condemn it as good research when objectively and adequately utilized. Overall, this review underlines the crux of qualitative research and accentuates the necessity to evaluate such research by the very tenets of its being. It also offers some prospects and recommendations to improve the quality of qualitative research. Based on the findings of this review, it is concluded that quality criteria are the aftereffect of socio-institutional procedures and existing paradigmatic conducts. Owing to the paradigmatic diversity of qualitative research, a single and specific set of quality criteria is neither feasible nor anticipated. Since qualitative research is not a cohesive discipline, researchers need to educate and familiarize themselves with applicable norms and decisive factors to evaluate qualitative research from within its theoretical and methodological framework of origin.
Similar content being viewed by others
Good Qualitative Research: Opening up the Debate
Beyond qualitative/quantitative structuralism: the positivist qualitative research and the paradigmatic disclaimer.
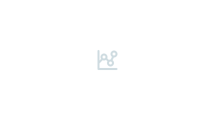
What is Qualitative in Research
Avoid common mistakes on your manuscript.
Introduction
“… It is important to regularly dialogue about what makes for good qualitative research” (Tracy, 2010 , p. 837)
To decide what represents good qualitative research is highly debatable. There are numerous methods that are contained within qualitative research and that are established on diverse philosophical perspectives. Bryman et al., ( 2008 , p. 262) suggest that “It is widely assumed that whereas quality criteria for quantitative research are well‐known and widely agreed, this is not the case for qualitative research.” Hence, the question “how to evaluate the quality of qualitative research” has been continuously debated. There are many areas of science and technology wherein these debates on the assessment of qualitative research have taken place. Examples include various areas of psychology: general psychology (Madill et al., 2000 ); counseling psychology (Morrow, 2005 ); and clinical psychology (Barker & Pistrang, 2005 ), and other disciplines of social sciences: social policy (Bryman et al., 2008 ); health research (Sparkes, 2001 ); business and management research (Johnson et al., 2006 ); information systems (Klein & Myers, 1999 ); and environmental studies (Reid & Gough, 2000 ). In the literature, these debates are enthused by the impression that the blanket application of criteria for good qualitative research developed around the positivist paradigm is improper. Such debates are based on the wide range of philosophical backgrounds within which qualitative research is conducted (e.g., Sandberg, 2000 ; Schwandt, 1996 ). The existence of methodological diversity led to the formulation of different sets of criteria applicable to qualitative research.
Among qualitative researchers, the dilemma of governing the measures to assess the quality of research is not a new phenomenon, especially when the virtuous triad of objectivity, reliability, and validity (Spencer et al., 2004 ) are not adequate. Occasionally, the criteria of quantitative research are used to evaluate qualitative research (Cohen & Crabtree, 2008 ; Lather, 2004 ). Indeed, Howe ( 2004 ) claims that the prevailing paradigm in educational research is scientifically based experimental research. Hypotheses and conjectures about the preeminence of quantitative research can weaken the worth and usefulness of qualitative research by neglecting the prominence of harmonizing match for purpose on research paradigm, the epistemological stance of the researcher, and the choice of methodology. Researchers have been reprimanded concerning this in “paradigmatic controversies, contradictions, and emerging confluences” (Lincoln & Guba, 2000 ).
In general, qualitative research tends to come from a very different paradigmatic stance and intrinsically demands distinctive and out-of-the-ordinary criteria for evaluating good research and varieties of research contributions that can be made. This review attempts to present a series of evaluative criteria for qualitative researchers, arguing that their choice of criteria needs to be compatible with the unique nature of the research in question (its methodology, aims, and assumptions). This review aims to assist researchers in identifying some of the indispensable features or markers of high-quality qualitative research. In a nutshell, the purpose of this systematic literature review is to analyze the existing knowledge on high-quality qualitative research and to verify the existence of research studies dealing with the critical assessment of qualitative research based on the concept of diverse paradigmatic stances. Contrary to the existing reviews, this review also suggests some critical directions to follow to improve the quality of qualitative research in different epistemological and ontological perspectives. This review is also intended to provide guidelines for the acceleration of future developments and dialogues among qualitative researchers in the context of assessing the qualitative research.
The rest of this review article is structured in the following fashion: Sect. Methods describes the method followed for performing this review. Section Criteria for Evaluating Qualitative Studies provides a comprehensive description of the criteria for evaluating qualitative studies. This section is followed by a summary of the strategies to improve the quality of qualitative research in Sect. Improving Quality: Strategies . Section How to Assess the Quality of the Research Findings? provides details on how to assess the quality of the research findings. After that, some of the quality checklists (as tools to evaluate quality) are discussed in Sect. Quality Checklists: Tools for Assessing the Quality . At last, the review ends with the concluding remarks presented in Sect. Conclusions, Future Directions and Outlook . Some prospects in qualitative research for enhancing its quality and usefulness in the social and techno-scientific research community are also presented in Sect. Conclusions, Future Directions and Outlook .
For this review, a comprehensive literature search was performed from many databases using generic search terms such as Qualitative Research , Criteria , etc . The following databases were chosen for the literature search based on the high number of results: IEEE Explore, ScienceDirect, PubMed, Google Scholar, and Web of Science. The following keywords (and their combinations using Boolean connectives OR/AND) were adopted for the literature search: qualitative research, criteria, quality, assessment, and validity. The synonyms for these keywords were collected and arranged in a logical structure (see Table 1 ). All publications in journals and conference proceedings later than 1950 till 2021 were considered for the search. Other articles extracted from the references of the papers identified in the electronic search were also included. A large number of publications on qualitative research were retrieved during the initial screening. Hence, to include the searches with the main focus on criteria for good qualitative research, an inclusion criterion was utilized in the search string.
From the selected databases, the search retrieved a total of 765 publications. Then, the duplicate records were removed. After that, based on the title and abstract, the remaining 426 publications were screened for their relevance by using the following inclusion and exclusion criteria (see Table 2 ). Publications focusing on evaluation criteria for good qualitative research were included, whereas those works which delivered theoretical concepts on qualitative research were excluded. Based on the screening and eligibility, 45 research articles were identified that offered explicit criteria for evaluating the quality of qualitative research and were found to be relevant to this review.
Figure 1 illustrates the complete review process in the form of PRISMA flow diagram. PRISMA, i.e., “preferred reporting items for systematic reviews and meta-analyses” is employed in systematic reviews to refine the quality of reporting.
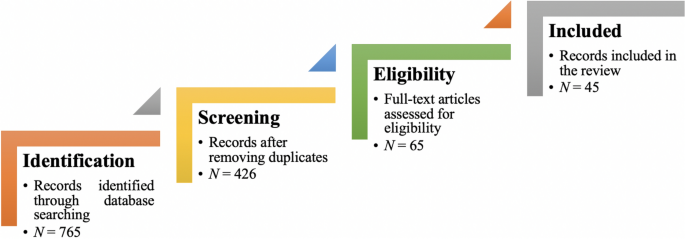
PRISMA flow diagram illustrating the search and inclusion process. N represents the number of records
Criteria for Evaluating Qualitative Studies
Fundamental criteria: general research quality.
Various researchers have put forward criteria for evaluating qualitative research, which have been summarized in Table 3 . Also, the criteria outlined in Table 4 effectively deliver the various approaches to evaluate and assess the quality of qualitative work. The entries in Table 4 are based on Tracy’s “Eight big‐tent criteria for excellent qualitative research” (Tracy, 2010 ). Tracy argues that high-quality qualitative work should formulate criteria focusing on the worthiness, relevance, timeliness, significance, morality, and practicality of the research topic, and the ethical stance of the research itself. Researchers have also suggested a series of questions as guiding principles to assess the quality of a qualitative study (Mays & Pope, 2020 ). Nassaji ( 2020 ) argues that good qualitative research should be robust, well informed, and thoroughly documented.
Qualitative Research: Interpretive Paradigms
All qualitative researchers follow highly abstract principles which bring together beliefs about ontology, epistemology, and methodology. These beliefs govern how the researcher perceives and acts. The net, which encompasses the researcher’s epistemological, ontological, and methodological premises, is referred to as a paradigm, or an interpretive structure, a “Basic set of beliefs that guides action” (Guba, 1990 ). Four major interpretive paradigms structure the qualitative research: positivist and postpositivist, constructivist interpretive, critical (Marxist, emancipatory), and feminist poststructural. The complexity of these four abstract paradigms increases at the level of concrete, specific interpretive communities. Table 5 presents these paradigms and their assumptions, including their criteria for evaluating research, and the typical form that an interpretive or theoretical statement assumes in each paradigm. Moreover, for evaluating qualitative research, quantitative conceptualizations of reliability and validity are proven to be incompatible (Horsburgh, 2003 ). In addition, a series of questions have been put forward in the literature to assist a reviewer (who is proficient in qualitative methods) for meticulous assessment and endorsement of qualitative research (Morse, 2003 ). Hammersley ( 2007 ) also suggests that guiding principles for qualitative research are advantageous, but methodological pluralism should not be simply acknowledged for all qualitative approaches. Seale ( 1999 ) also points out the significance of methodological cognizance in research studies.
Table 5 reflects that criteria for assessing the quality of qualitative research are the aftermath of socio-institutional practices and existing paradigmatic standpoints. Owing to the paradigmatic diversity of qualitative research, a single set of quality criteria is neither possible nor desirable. Hence, the researchers must be reflexive about the criteria they use in the various roles they play within their research community.
Improving Quality: Strategies
Another critical question is “How can the qualitative researchers ensure that the abovementioned quality criteria can be met?” Lincoln and Guba ( 1986 ) delineated several strategies to intensify each criteria of trustworthiness. Other researchers (Merriam & Tisdell, 2016 ; Shenton, 2004 ) also presented such strategies. A brief description of these strategies is shown in Table 6 .
It is worth mentioning that generalizability is also an integral part of qualitative research (Hays & McKibben, 2021 ). In general, the guiding principle pertaining to generalizability speaks about inducing and comprehending knowledge to synthesize interpretive components of an underlying context. Table 7 summarizes the main metasynthesis steps required to ascertain generalizability in qualitative research.
Figure 2 reflects the crucial components of a conceptual framework and their contribution to decisions regarding research design, implementation, and applications of results to future thinking, study, and practice (Johnson et al., 2020 ). The synergy and interrelationship of these components signifies their role to different stances of a qualitative research study.
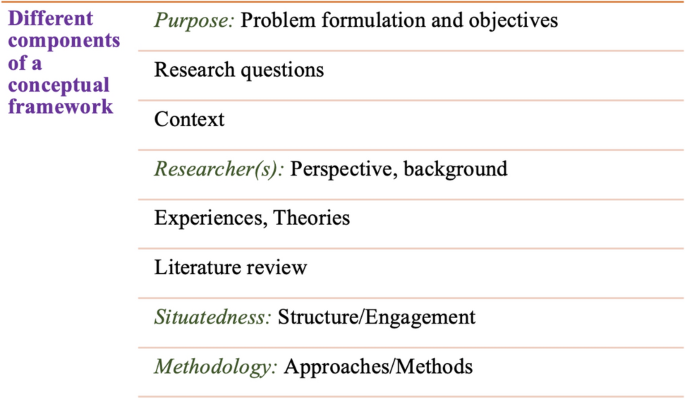
Essential elements of a conceptual framework
In a nutshell, to assess the rationale of a study, its conceptual framework and research question(s), quality criteria must take account of the following: lucid context for the problem statement in the introduction; well-articulated research problems and questions; precise conceptual framework; distinct research purpose; and clear presentation and investigation of the paradigms. These criteria would expedite the quality of qualitative research.
How to Assess the Quality of the Research Findings?
The inclusion of quotes or similar research data enhances the confirmability in the write-up of the findings. The use of expressions (for instance, “80% of all respondents agreed that” or “only one of the interviewees mentioned that”) may also quantify qualitative findings (Stenfors et al., 2020 ). On the other hand, the persuasive reason for “why this may not help in intensifying the research” has also been provided (Monrouxe & Rees, 2020 ). Further, the Discussion and Conclusion sections of an article also prove robust markers of high-quality qualitative research, as elucidated in Table 8 .
Quality Checklists: Tools for Assessing the Quality
Numerous checklists are available to speed up the assessment of the quality of qualitative research. However, if used uncritically and recklessly concerning the research context, these checklists may be counterproductive. I recommend that such lists and guiding principles may assist in pinpointing the markers of high-quality qualitative research. However, considering enormous variations in the authors’ theoretical and philosophical contexts, I would emphasize that high dependability on such checklists may say little about whether the findings can be applied in your setting. A combination of such checklists might be appropriate for novice researchers. Some of these checklists are listed below:
The most commonly used framework is Consolidated Criteria for Reporting Qualitative Research (COREQ) (Tong et al., 2007 ). This framework is recommended by some journals to be followed by the authors during article submission.
Standards for Reporting Qualitative Research (SRQR) is another checklist that has been created particularly for medical education (O’Brien et al., 2014 ).
Also, Tracy ( 2010 ) and Critical Appraisal Skills Programme (CASP, 2021 ) offer criteria for qualitative research relevant across methods and approaches.
Further, researchers have also outlined different criteria as hallmarks of high-quality qualitative research. For instance, the “Road Trip Checklist” (Epp & Otnes, 2021 ) provides a quick reference to specific questions to address different elements of high-quality qualitative research.
Conclusions, Future Directions, and Outlook
This work presents a broad review of the criteria for good qualitative research. In addition, this article presents an exploratory analysis of the essential elements in qualitative research that can enable the readers of qualitative work to judge it as good research when objectively and adequately utilized. In this review, some of the essential markers that indicate high-quality qualitative research have been highlighted. I scope them narrowly to achieve rigor in qualitative research and note that they do not completely cover the broader considerations necessary for high-quality research. This review points out that a universal and versatile one-size-fits-all guideline for evaluating the quality of qualitative research does not exist. In other words, this review also emphasizes the non-existence of a set of common guidelines among qualitative researchers. In unison, this review reinforces that each qualitative approach should be treated uniquely on account of its own distinctive features for different epistemological and disciplinary positions. Owing to the sensitivity of the worth of qualitative research towards the specific context and the type of paradigmatic stance, researchers should themselves analyze what approaches can be and must be tailored to ensemble the distinct characteristics of the phenomenon under investigation. Although this article does not assert to put forward a magic bullet and to provide a one-stop solution for dealing with dilemmas about how, why, or whether to evaluate the “goodness” of qualitative research, it offers a platform to assist the researchers in improving their qualitative studies. This work provides an assembly of concerns to reflect on, a series of questions to ask, and multiple sets of criteria to look at, when attempting to determine the quality of qualitative research. Overall, this review underlines the crux of qualitative research and accentuates the need to evaluate such research by the very tenets of its being. Bringing together the vital arguments and delineating the requirements that good qualitative research should satisfy, this review strives to equip the researchers as well as reviewers to make well-versed judgment about the worth and significance of the qualitative research under scrutiny. In a nutshell, a comprehensive portrayal of the research process (from the context of research to the research objectives, research questions and design, speculative foundations, and from approaches of collecting data to analyzing the results, to deriving inferences) frequently proliferates the quality of a qualitative research.
Prospects : A Road Ahead for Qualitative Research
Irrefutably, qualitative research is a vivacious and evolving discipline wherein different epistemological and disciplinary positions have their own characteristics and importance. In addition, not surprisingly, owing to the sprouting and varied features of qualitative research, no consensus has been pulled off till date. Researchers have reflected various concerns and proposed several recommendations for editors and reviewers on conducting reviews of critical qualitative research (Levitt et al., 2021 ; McGinley et al., 2021 ). Following are some prospects and a few recommendations put forward towards the maturation of qualitative research and its quality evaluation:
In general, most of the manuscript and grant reviewers are not qualitative experts. Hence, it is more likely that they would prefer to adopt a broad set of criteria. However, researchers and reviewers need to keep in mind that it is inappropriate to utilize the same approaches and conducts among all qualitative research. Therefore, future work needs to focus on educating researchers and reviewers about the criteria to evaluate qualitative research from within the suitable theoretical and methodological context.
There is an urgent need to refurbish and augment critical assessment of some well-known and widely accepted tools (including checklists such as COREQ, SRQR) to interrogate their applicability on different aspects (along with their epistemological ramifications).
Efforts should be made towards creating more space for creativity, experimentation, and a dialogue between the diverse traditions of qualitative research. This would potentially help to avoid the enforcement of one's own set of quality criteria on the work carried out by others.
Moreover, journal reviewers need to be aware of various methodological practices and philosophical debates.
It is pivotal to highlight the expressions and considerations of qualitative researchers and bring them into a more open and transparent dialogue about assessing qualitative research in techno-scientific, academic, sociocultural, and political rooms.
Frequent debates on the use of evaluative criteria are required to solve some potentially resolved issues (including the applicability of a single set of criteria in multi-disciplinary aspects). Such debates would not only benefit the group of qualitative researchers themselves, but primarily assist in augmenting the well-being and vivacity of the entire discipline.
To conclude, I speculate that the criteria, and my perspective, may transfer to other methods, approaches, and contexts. I hope that they spark dialog and debate – about criteria for excellent qualitative research and the underpinnings of the discipline more broadly – and, therefore, help improve the quality of a qualitative study. Further, I anticipate that this review will assist the researchers to contemplate on the quality of their own research, to substantiate research design and help the reviewers to review qualitative research for journals. On a final note, I pinpoint the need to formulate a framework (encompassing the prerequisites of a qualitative study) by the cohesive efforts of qualitative researchers of different disciplines with different theoretic-paradigmatic origins. I believe that tailoring such a framework (of guiding principles) paves the way for qualitative researchers to consolidate the status of qualitative research in the wide-ranging open science debate. Dialogue on this issue across different approaches is crucial for the impending prospects of socio-techno-educational research.
Amin, M. E. K., Nørgaard, L. S., Cavaco, A. M., Witry, M. J., Hillman, L., Cernasev, A., & Desselle, S. P. (2020). Establishing trustworthiness and authenticity in qualitative pharmacy research. Research in Social and Administrative Pharmacy, 16 (10), 1472–1482.
Article Google Scholar
Barker, C., & Pistrang, N. (2005). Quality criteria under methodological pluralism: Implications for conducting and evaluating research. American Journal of Community Psychology, 35 (3–4), 201–212.
Bryman, A., Becker, S., & Sempik, J. (2008). Quality criteria for quantitative, qualitative and mixed methods research: A view from social policy. International Journal of Social Research Methodology, 11 (4), 261–276.
Caelli, K., Ray, L., & Mill, J. (2003). ‘Clear as mud’: Toward greater clarity in generic qualitative research. International Journal of Qualitative Methods, 2 (2), 1–13.
CASP (2021). CASP checklists. Retrieved May 2021 from https://casp-uk.net/casp-tools-checklists/
Cohen, D. J., & Crabtree, B. F. (2008). Evaluative criteria for qualitative research in health care: Controversies and recommendations. The Annals of Family Medicine, 6 (4), 331–339.
Denzin, N. K., & Lincoln, Y. S. (2005). Introduction: The discipline and practice of qualitative research. In N. K. Denzin & Y. S. Lincoln (Eds.), The sage handbook of qualitative research (pp. 1–32). Sage Publications Ltd.
Google Scholar
Elliott, R., Fischer, C. T., & Rennie, D. L. (1999). Evolving guidelines for publication of qualitative research studies in psychology and related fields. British Journal of Clinical Psychology, 38 (3), 215–229.
Epp, A. M., & Otnes, C. C. (2021). High-quality qualitative research: Getting into gear. Journal of Service Research . https://doi.org/10.1177/1094670520961445
Guba, E. G. (1990). The paradigm dialog. In Alternative paradigms conference, mar, 1989, Indiana u, school of education, San Francisco, ca, us . Sage Publications, Inc.
Hammersley, M. (2007). The issue of quality in qualitative research. International Journal of Research and Method in Education, 30 (3), 287–305.
Haven, T. L., Errington, T. M., Gleditsch, K. S., van Grootel, L., Jacobs, A. M., Kern, F. G., & Mokkink, L. B. (2020). Preregistering qualitative research: A Delphi study. International Journal of Qualitative Methods, 19 , 1609406920976417.
Hays, D. G., & McKibben, W. B. (2021). Promoting rigorous research: Generalizability and qualitative research. Journal of Counseling and Development, 99 (2), 178–188.
Horsburgh, D. (2003). Evaluation of qualitative research. Journal of Clinical Nursing, 12 (2), 307–312.
Howe, K. R. (2004). A critique of experimentalism. Qualitative Inquiry, 10 (1), 42–46.
Johnson, J. L., Adkins, D., & Chauvin, S. (2020). A review of the quality indicators of rigor in qualitative research. American Journal of Pharmaceutical Education, 84 (1), 7120.
Johnson, P., Buehring, A., Cassell, C., & Symon, G. (2006). Evaluating qualitative management research: Towards a contingent criteriology. International Journal of Management Reviews, 8 (3), 131–156.
Klein, H. K., & Myers, M. D. (1999). A set of principles for conducting and evaluating interpretive field studies in information systems. MIS Quarterly, 23 (1), 67–93.
Lather, P. (2004). This is your father’s paradigm: Government intrusion and the case of qualitative research in education. Qualitative Inquiry, 10 (1), 15–34.
Levitt, H. M., Morrill, Z., Collins, K. M., & Rizo, J. L. (2021). The methodological integrity of critical qualitative research: Principles to support design and research review. Journal of Counseling Psychology, 68 (3), 357.
Lincoln, Y. S., & Guba, E. G. (1986). But is it rigorous? Trustworthiness and authenticity in naturalistic evaluation. New Directions for Program Evaluation, 1986 (30), 73–84.
Lincoln, Y. S., & Guba, E. G. (2000). Paradigmatic controversies, contradictions and emerging confluences. In N. K. Denzin & Y. S. Lincoln (Eds.), Handbook of qualitative research (2nd ed., pp. 163–188). Sage Publications.
Madill, A., Jordan, A., & Shirley, C. (2000). Objectivity and reliability in qualitative analysis: Realist, contextualist and radical constructionist epistemologies. British Journal of Psychology, 91 (1), 1–20.
Mays, N., & Pope, C. (2020). Quality in qualitative research. Qualitative Research in Health Care . https://doi.org/10.1002/9781119410867.ch15
McGinley, S., Wei, W., Zhang, L., & Zheng, Y. (2021). The state of qualitative research in hospitality: A 5-year review 2014 to 2019. Cornell Hospitality Quarterly, 62 (1), 8–20.
Merriam, S., & Tisdell, E. (2016). Qualitative research: A guide to design and implementation. San Francisco, US.
Meyer, M., & Dykes, J. (2019). Criteria for rigor in visualization design study. IEEE Transactions on Visualization and Computer Graphics, 26 (1), 87–97.
Monrouxe, L. V., & Rees, C. E. (2020). When I say… quantification in qualitative research. Medical Education, 54 (3), 186–187.
Morrow, S. L. (2005). Quality and trustworthiness in qualitative research in counseling psychology. Journal of Counseling Psychology, 52 (2), 250.
Morse, J. M. (2003). A review committee’s guide for evaluating qualitative proposals. Qualitative Health Research, 13 (6), 833–851.
Nassaji, H. (2020). Good qualitative research. Language Teaching Research, 24 (4), 427–431.
O’Brien, B. C., Harris, I. B., Beckman, T. J., Reed, D. A., & Cook, D. A. (2014). Standards for reporting qualitative research: A synthesis of recommendations. Academic Medicine, 89 (9), 1245–1251.
O’Connor, C., & Joffe, H. (2020). Intercoder reliability in qualitative research: Debates and practical guidelines. International Journal of Qualitative Methods, 19 , 1609406919899220.
Reid, A., & Gough, S. (2000). Guidelines for reporting and evaluating qualitative research: What are the alternatives? Environmental Education Research, 6 (1), 59–91.
Rocco, T. S. (2010). Criteria for evaluating qualitative studies. Human Resource Development International . https://doi.org/10.1080/13678868.2010.501959
Sandberg, J. (2000). Understanding human competence at work: An interpretative approach. Academy of Management Journal, 43 (1), 9–25.
Schwandt, T. A. (1996). Farewell to criteriology. Qualitative Inquiry, 2 (1), 58–72.
Seale, C. (1999). Quality in qualitative research. Qualitative Inquiry, 5 (4), 465–478.
Shenton, A. K. (2004). Strategies for ensuring trustworthiness in qualitative research projects. Education for Information, 22 (2), 63–75.
Sparkes, A. C. (2001). Myth 94: Qualitative health researchers will agree about validity. Qualitative Health Research, 11 (4), 538–552.
Spencer, L., Ritchie, J., Lewis, J., & Dillon, L. (2004). Quality in qualitative evaluation: A framework for assessing research evidence.
Stenfors, T., Kajamaa, A., & Bennett, D. (2020). How to assess the quality of qualitative research. The Clinical Teacher, 17 (6), 596–599.
Taylor, E. W., Beck, J., & Ainsworth, E. (2001). Publishing qualitative adult education research: A peer review perspective. Studies in the Education of Adults, 33 (2), 163–179.
Tong, A., Sainsbury, P., & Craig, J. (2007). Consolidated criteria for reporting qualitative research (COREQ): A 32-item checklist for interviews and focus groups. International Journal for Quality in Health Care, 19 (6), 349–357.
Tracy, S. J. (2010). Qualitative quality: Eight “big-tent” criteria for excellent qualitative research. Qualitative Inquiry, 16 (10), 837–851.
Download references
Open access funding provided by TU Wien (TUW).
Author information
Authors and affiliations.
Faculty of Informatics, Technische Universität Wien, 1040, Vienna, Austria
Drishti Yadav
You can also search for this author in PubMed Google Scholar
Corresponding author
Correspondence to Drishti Yadav .
Ethics declarations
Conflict of interest.
The author declares no conflict of interest.
Additional information
Publisher's note.
Springer Nature remains neutral with regard to jurisdictional claims in published maps and institutional affiliations.
Rights and permissions
Open Access This article is licensed under a Creative Commons Attribution 4.0 International License, which permits use, sharing, adaptation, distribution and reproduction in any medium or format, as long as you give appropriate credit to the original author(s) and the source, provide a link to the Creative Commons licence, and indicate if changes were made. The images or other third party material in this article are included in the article's Creative Commons licence, unless indicated otherwise in a credit line to the material. If material is not included in the article's Creative Commons licence and your intended use is not permitted by statutory regulation or exceeds the permitted use, you will need to obtain permission directly from the copyright holder. To view a copy of this licence, visit http://creativecommons.org/licenses/by/4.0/ .
Reprints and permissions
About this article
Yadav, D. Criteria for Good Qualitative Research: A Comprehensive Review. Asia-Pacific Edu Res 31 , 679–689 (2022). https://doi.org/10.1007/s40299-021-00619-0
Download citation
Accepted : 28 August 2021
Published : 18 September 2021
Issue Date : December 2022
DOI : https://doi.org/10.1007/s40299-021-00619-0
Share this article
Anyone you share the following link with will be able to read this content:
Sorry, a shareable link is not currently available for this article.
Provided by the Springer Nature SharedIt content-sharing initiative
- Qualitative research
- Evaluative criteria
- Find a journal
- Publish with us
- Track your research
Learn Quality Management
SITE-WIDE SALE IS LIVE NOW.
CLICK HERE.
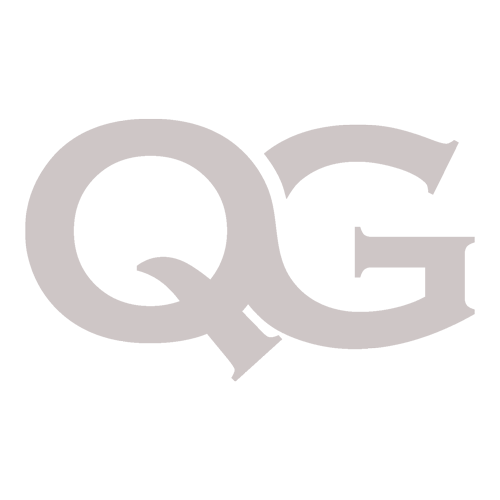
- ASQ® CQA Exam
- ASQ® CQE Exam
- ASQ® CSQP Exam
- ASQ® CSSYB Exam
- ASQ® CSSGB Exam
- ASQ® CSSBB Exam
- ASQ® CMQ/OE Exam
- ASQ® CQT Exam
- ASQ® CQPA Exam
- ASQ® CQIA Exam
- 7 Quality Tools
- Quality Gurus
- ISO 9001:2015
- Quality Cost
- Six Sigma Basics
- Risk Management
- Lean Manufacturing
- Design of Experiments
- Quality Acronyms
- Quality Awareness
- Quality Circles
- Acceptance Sampling
- Measurement System
- APQP + PPAP
- GD&T Symbols
- Project Quality (PMP)
- Full List of Quizzes >>
- Reliability Engineering
- Statistics with Excel
- Statistics with Minitab
- Multiple Regression
- Quality Function Deployment
- Benchmarking
- Statistical Process Control
- Measurement Scales
- Quality Talks >> New
- Six Sigma White Belt
- Six Sigma Yellow Belt
- Six Sigma Green Belt
- Six Sigma Black Belt
- Minitab 17 for Six Sigma
- Casio fx-991MS Calculator
- CSSYB/LSSYB Mock Exam
- CSSGB/LSSGB Mock Exam
- CSSBB/LSSBB Mock Exam
- ASQ® CCQM Preparation
- ASQ® CQA Preparation
- ASQ® CQE Preparation
- ASQ® CQPA Preparation
- ASQ® CQIA Preparation
- CQE Mock Exams
- CMQ/OE Mock Exams
- CQA Mock Exams
- CQIA Mock Exams
- CQPA Mock Exam
- CQT Mock Exam
- CQI Mock Exam
- CSQP Mock Exam
- CCQM Mock Exam
- Design of Experiments (DoE)
- Measurement System Analysis
- Statistics Using R
- Data Visualization with R
- Statistics Using Python
- Data Visualization with Python
- Regression with Minitab
- Logistic Regression
- Data Analysis Using Excel
- The Git Mindset
- Statistics Quiz
- Root Cause Analysis
- Kano Analysis
- Lean Management
- QMS Lead Auditor
- Quality Management
- ISO 9001:2015 Transition
- Project Quality Manager
- गुणवत्ता.org
- Summary Sheets
- Practice Tests
- QG Hall of Fame
- Testimonials – ASQ Exams Preparation
Blogs , ISO 9001
- Quality Control: Understanding Its Importance, Benefits, Approaches and Key Strategies
** Unlock Your Full Potential **
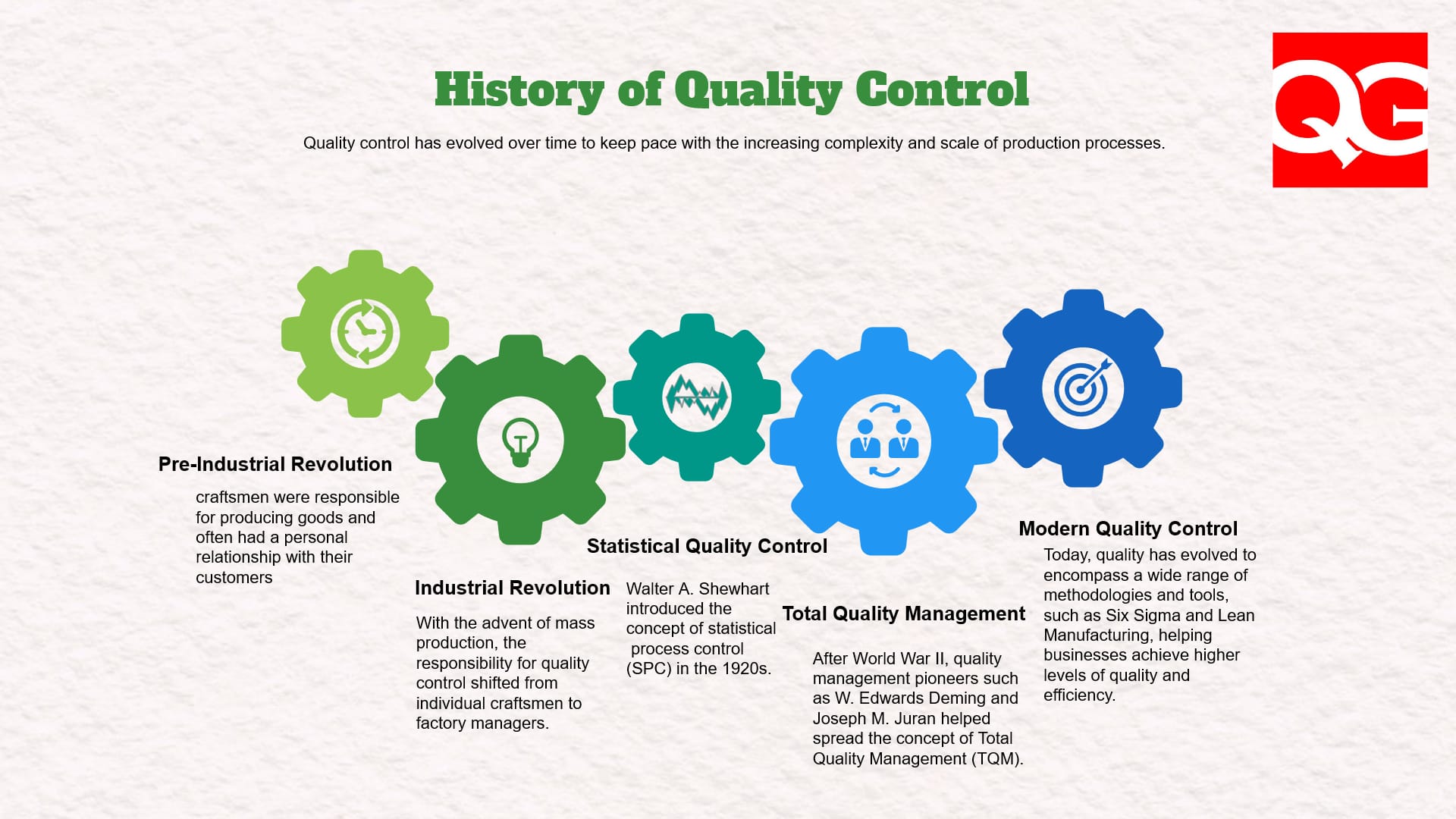
Maintaining high-quality products and services is crucial for success in today's competitive business environment. Quality control (QC) plays a critical role in ensuring that your company consistently meets customer expectations and regulatory requirements. This comprehensive guide will explore the importance of quality control, its benefits, and key strategies, with industry examples to illustrate its practical applications.
What is Quality Control?
Quality control refers to the systematic process of identifying, monitoring and correcting potential defects or deviations in products or services. This process ensures that the final output meets the established quality standards and customer requirements. QC is an essential part of the overall quality management system ( QMS ) and involves regular inspections, testing, and monitoring of various production stages.
ISO 9001:2015 defines Quality Control as “a part of quality management focused on fulfilling quality requirements.” It includes activities such as the inspection and testing of incoming raw materials, in-process products, and finished goods.
History of Quality Control
Quality control has evolved over time to keep pace with the increasing complexity and scale of production processes. Let's take a brief look at the key milestones in the history of quality control:
Craftsmanship Era (Pre-Industrial Revolution): Before the Industrial Revolution, craftsmen were responsible for producing goods and often had a personal relationship with their customers. Quality was maintained by the craftsman's reputation, skill, and pride in their work.
Industrial Revolution (Late 18th Century to Mid-19th Century): With the advent of mass production, the responsibility for quality control shifted from individual craftsmen to factory managers. Inspectors were employed to identify and segregate defective products, but the focus was on finding and fixing defects rather than preventing them.
Scientific Management (Early 20th Century): The introduction of scientific management principles by Frederick Winslow Taylor marked a significant shift in quality control. Taylor's ideas laid the groundwork for more systematic and data-driven approaches to managing production processes, paving the way for modern quality control methods.
Statistical Quality Control (Mid-20th Century): Walter A. Shewhart introduced the concept of statistical process control ( SPC ) in the 1920s. SPC allowed manufacturers to monitor and control production processes using statistical methods, enabling them to detect and correct defects more efficiently. During World War II, the U.S. military adopted statistical quality control techniques to improve the production of munitions and other equipment.
Total Quality Management (Post-WWII): After World War II, quality management pioneers such as W. Edwards Deming and Joseph M. Juran helped spread the concept of Total Quality Management ( TQM ). TQM emphasized continuous improvement, customer satisfaction, and employee involvement, transforming how companies approached quality control.
ISO 9001 and Modern Quality Control (Late 20th Century to Present): In 1987, the International Organization for Standardization (ISO) introduced the ISO 9000 quality management standards, including ISO 9001 . These standards provided a global framework for implementing effective quality management systems. Today, quality has evolved to encompass a wide range of methodologies and tools, such as Six Sigma and Lean Manufacturing, helping businesses achieve higher levels of quality and efficiency.
The history of quality control shows how the concept has evolved and adapted to the changing needs of production processes and market demands. Understanding this history can help businesses appreciate the value of quality control and implement more effective systems to ensure long-term success.
Benefits and Importance of Quality Control
- Customer Satisfaction: Consistently delivering high-quality products and services helps build customer trust and loyalty, increasing the likelihood of repeat business and positive word-of-mouth marketing.
- Regulatory Compliance: QC processes help companies adhere to industry-specific regulations and standards, preventing costly fines or sanctions.
- Brand Reputation: A strong commitment to quality control enhances a company's reputation for producing reliable, high-quality products or services.
- Cost Savings: Identifying and correcting defects early in production minimizes waste and reduces the need for expensive rework or recalls.
- Competitive Advantage: Companies with robust QC systems are better positioned to differentiate themselves from competitors and capture market share.
Key Strategies for Effective Quality Control
- Establish Clear Quality Standards: Define and communicate the specific quality criteria for each product or service, ensuring all team members understand the expectations.
- Implement Regular Inspections and Testing: Conduct routine checks at various stages of production to identify defects and deviations from quality standards.
- Invest in Employee Training: Provide ongoing training to equip employees with the necessary skills and knowledge to maintain high-quality standards.
- Utilize Statistical Process Control ( SPC ): SPC techniques can help identify trends and patterns in production data, enabling companies to predict and prevent quality issues.
- Embrace Continuous Improvement: Encourage a culture that values ongoing learning and improvement and proactively empowers employees to identify and address quality concerns.
Quality Control Approaches
Different industries and organizations may adopt various approaches to quality, depending on their specific needs and goals. Some popular QC methodologies include:
- Total Quality Management ( TQM ): A holistic approach to quality management focuses on continuous improvement, customer satisfaction, and employee involvement. It aims to integrate quality principles into all aspects of a company's operations.
- Six Sigma: Six Sigma is a data-driven quality management methodology seeking to reduce defects and process variation. The goal is to achieve a defect rate of 3.4 per million opportunities, ensuring near-perfect quality.
- Lean Manufacturing: Lean focuses on eliminating waste and optimizing processes to deliver maximum value to customers. Although not explicitly a quality control approach, Lean principles can significantly contribute to improving product quality by enhancing efficiency and reducing defects.
- ISO 9001: This international standard sets out the criteria for a quality management system . Achieving ISO 9001 certification demonstrates a company's commitment to maintaining consistent quality standards and continuously improving its processes.
Conclusion:
Quality control plays a crucial role in ensuring that businesses deliver high-quality products and services, meeting customer expectations and regulatory requirements. Companies can develop and implement effective QC systems that contribute to long-term success by understanding its importance, benefits, and key strategies.
Related Posts:
Root cause analysis and the 8d problem solving bootcamp (accredited) (online training), summary sheets – six sigma black belt, multiple regression with minitab – online training, summary sheets – six sigma green belt, certified quality process analyst (cqpa) training, unacceptable corrective actions, iso 9001:2015 unchanged, next revision iso 9001:2030, 10 strategies for reducing human error in your workplace, quality leads to sustainability.
49 Courses on SALE!
67 Quality Control Essay Topic Ideas & Examples
🏆 best quality control topic ideas & essay examples, 📌 simple & easy quality control essay titles, 👍 good essay topics on quality control.
- The Mars Company Process and Quality Control This paper discusses the Mars Company with special consideration to process and quality control of chocolate candies in a bid to discover the formula that the company applies in the distribution of the different colors […]
- C-Chart for Service Quality Control The control tool allows the organization to measure the stability of a process and track the results of improvement or deterioration.
- Comparison of Philosophies of Edward W. Deming and Joseph M. Juran in Quality Control Management Total Quality management is one of the management strategies focused on establishing a conscious and continuous supply of quality work in the entire organizational process. One of the breaking points of quality management is to […]
- Tesla’s Quality Control for Expansion to China Tesla has had to confront some issues with the quality of its batteries in the Chinese market. To take care of quality problems, Tesla ought to give precedence to safety and quality in its vehicles […]
- Quality Assurance and Quality Control – Is There a Difference? In the case of failure detection within the adopted QA process during the QC inspection test, it is clear that there was an error in the QA process being used.
- “Statistical Quality Control” by Diana Montgomery According to Montgomery, Six-Sigma is an experience of business management that is used to develop business operations in the modern world. Quality control is commonly utilized to promote quality of business products.
- Golden Age Hospital: Quality Control and Accreditation GAH’s core competencies include professionalism, evidence-based practices, effective communication, patient safety, patient-centered approaches and quality improvement.
- Quality Control of Digital Forensics The quality control over computer forensic products is necessary because of the growth of the Internet services. Thus, the investigator and the lab are challenged not only with solving a case but also providing the […]
- Going Inc.’s Quality Control & Service Improvement The steps need to be drastic and firm so that the trend of losing business over the last 20 months vanishes away and their goal of becoming the most successful airline service provider to the […]
- Modern Methods for Quality Control and Improvement The management have the additional responsibility of considering the total effectiveness of the organization in terms of its use of all resources and the environmental implications of their actions.
- Quality Control Issues in Production While one can identify numerous issues that combined to severely damage the commercial success of the product, its poor quality, as well as that of the services surrounding it, should be considered the primary causes.
- The Management and Control of Quality Managers in the contemporary world know that it is important to ensure that make sure that every process within the organization is successful because it is the individual processes that make up the entire system.
- GAH Organization Quality Control and Accreditation GAH will ensure that it meets its core objective of providing safe and beneficial healthcare to the elderly. It will also conduct regular studies and use outcomes to improve safety and quality of care to […]
- Sustainable Strategies in Water Quality Control With regards to the first strategy, it is important to touch the hearts and minds of the next generation’s leaders and policy makers. They have to see and experience the benefits of their actions.
- Indoor Air Quality (IAQ) Control In the present city life, the quality of air in the indoor setting has a substantial effect on individual’s fitness and well-being.
- Contract Financing: Audit Requirements and Quality Control When selecting a contractor, the client must evaluate the financial strength of the potential contractors based on the scope of the project.
- The Production of Beef: Quality Control, Inventory Management, Production Service Design The first characteristic that will indicate the level of quality in the cattle is the muscle and bone ratio of the cow.
- Quality Control in Traditional and Agile Project Management Approaches In the initiation phase of a project, there is an in-depth elaboration and exploration of the idea in the project with the decisions on the people to execute the project being made.
- Sunshine Enterprises Quality Control The areas of focus include the following: Customer Satisfaction It is notable that the owner of the restaurant chains has given some attention to customer satisfaction by making enquiries to customers so that she obtains […]
- Quality Control for All-repairs Mechanics The mean for the satisfaction indicates that most of the customers are satisfied with the job while that of the time taken to complete the tasks indicates that a larger percentage of jobs […]
- Quality Control: Zen And The Art Of Motorcycle Maintenance By Robert Pirsig
- When Quality Control Gets in the Way of Quality
- Prediction Based On Time Series. Applications In Quality Control
- The Organization of Contracting and Quality Control in Dairy Supply Chains in Kyrgyzstan
- The Problems, Possible Solution, Patient Positioning, Technique, Quality Control, and Alternatives of Mammography, a Breast Imaging Method
- The Use of a Six Sigma Quality Control Strategy to Improve Overall Quality of Salem Press
- Quality Control: The Challenges of Globalization to Nigerian Manufacturing Firms
- Intel For Quality Assurance And Quality Control Steps
- Sushi Restaurant Enhances Quality Control, Customer Service with RFID Technology
- Model Driven Adaptive Quality Control in Service Oriented Architectures
- On Determining the Optimal Sampling Frequency for Feedback Quality Control Systems
- The Role Of The Financial Information In The Tourism Services Quality Control
- Mathematical Programming Models for Environmental Quality Control
- The Quality Control Principles in a Christian World View
- Quality Control In Airlines And Aerospace
- Improve Quality Control In Airlines Manufacturing Sectors
- The Quality Control System For The Financial Audit In Romania And Spain
- Operational Management: Statistical Quality Control and Performance Improvement
- Quality Control through Venetian Blinds: Regulating the Swedish Auditing Industry
- Transshipments In Hazardous Environments: Cooperative Versus Noncooperative Quality Control Game
- Statistical Quality Control Limits for the Sample Mean Chart Using Robust Extreme Ranked Set Sampling
- Why Corporations Need Quality Control
- The Auditof the Quality Control Systemwithin the Information Technology Field
- Quality Control for Pharmaceutical Products
- Quality Control and Due Diligence in Project Management: Getting Decisions Right by Taking the Outside View
- Probability Weighted Moments Approach to Quality Control Charts
- Seven Basic Tools of Quality Control: An Appropriate Tools for Solving Quality Problems in the Organizations
- Multivariate Quality Control-Estimation of the Percentage Good Products
- Utility of Quality Control Tools and Statistical Process Control to Improve the Productivity and Quality in an Industry
- The Need for Quality Control in High Dose Rate Brachytherapy
- Quality Control Processes Of The Cuban Cigar Industry
- Water Quality Control from the Perspective of Water Supply System Users’ Safety
- Quality Control Failures at Johnson & Johnson
- Tosco Marketing Company Quality Control
- Joint Determination of Optimal Inventory and Quality Control Policy
- Markets Segmented by Regional – Origin Labeling with Quality Control
- The Confluence of Sociology, Statistics, and Public Policy in the Quality Control of the Food Stamps, Afdc, and Medicaid Family Assistance Programs
- Subgrouping, Quality Control, and Statistical Process Control
- Project Costing, Risk Evaluation, and Quality Control
- Quality Control and the Connection Between Total Quality Management and Reengineering
- On a Generalization of the Weibull Distribution and Its Application in Quality Control
- The Relationship Between Re-engineering And TQM In Quality Control
- Aviation Safety Research Ideas
- Civil Engineering Essay Titles
- Computers Essay Ideas
- Success Ideas
- Construction Research Ideas
- Systems Thinking Essay Ideas
- Agile Project Management Research Topics
- Performance Indicators Essay Topics
- Chicago (A-D)
- Chicago (N-B)
IvyPanda. (2024, March 1). 67 Quality Control Essay Topic Ideas & Examples. https://ivypanda.com/essays/topic/quality-control-essay-topics/
"67 Quality Control Essay Topic Ideas & Examples." IvyPanda , 1 Mar. 2024, ivypanda.com/essays/topic/quality-control-essay-topics/.
IvyPanda . (2024) '67 Quality Control Essay Topic Ideas & Examples'. 1 March.
IvyPanda . 2024. "67 Quality Control Essay Topic Ideas & Examples." March 1, 2024. https://ivypanda.com/essays/topic/quality-control-essay-topics/.
1. IvyPanda . "67 Quality Control Essay Topic Ideas & Examples." March 1, 2024. https://ivypanda.com/essays/topic/quality-control-essay-topics/.
Bibliography
IvyPanda . "67 Quality Control Essay Topic Ideas & Examples." March 1, 2024. https://ivypanda.com/essays/topic/quality-control-essay-topics/.
IvyPanda uses cookies and similar technologies to enhance your experience, enabling functionalities such as:
- Basic site functions
- Ensuring secure, safe transactions
- Secure account login
- Remembering account, browser, and regional preferences
- Remembering privacy and security settings
- Analyzing site traffic and usage
- Personalized search, content, and recommendations
- Displaying relevant, targeted ads on and off IvyPanda
Please refer to IvyPanda's Cookies Policy and Privacy Policy for detailed information.
Certain technologies we use are essential for critical functions such as security and site integrity, account authentication, security and privacy preferences, internal site usage and maintenance data, and ensuring the site operates correctly for browsing and transactions.
Cookies and similar technologies are used to enhance your experience by:
- Remembering general and regional preferences
- Personalizing content, search, recommendations, and offers
Some functions, such as personalized recommendations, account preferences, or localization, may not work correctly without these technologies. For more details, please refer to IvyPanda's Cookies Policy .
To enable personalized advertising (such as interest-based ads), we may share your data with our marketing and advertising partners using cookies and other technologies. These partners may have their own information collected about you. Turning off the personalized advertising setting won't stop you from seeing IvyPanda ads, but it may make the ads you see less relevant or more repetitive.
Personalized advertising may be considered a "sale" or "sharing" of the information under California and other state privacy laws, and you may have the right to opt out. Turning off personalized advertising allows you to exercise your right to opt out. Learn more in IvyPanda's Cookies Policy and Privacy Policy .

How to Improve Survey Data Integrity With Quality Control Questions

Including quality control questions when designing your survey questionnaire is an important step to ensure the reliability, validity, and integrity of the data you collect. Incorporating these checks into survey design not only helps maintain data quality but also enhances the efficiency of data analysis by filtering out unreliable responses early in the process. The result is cleaner data that reduces the need for extensive data cleansing , allowing researchers to focus on analysis and get insights faster. In this article, we’ll cover five types of quality control questions you can employ to enhance the reliability of your surveys.
What Are Quality Control Questions?
Quality control questions, such as attention check, authentication, or trap questions, are included when surveys are programmed to ensure respondents are paying attention and providing accurate responses. These questions are designed to catch fraudulent respondents, as well as real respondents who may be responding randomly, speeding through the survey, or not fully engaging with the questions. When a respondent fails a quality control question, they are then flagged for further review. A general rule of thumb is that if a respondent fails any three quality checks, they should automatically be removed.
5 Types of Quality Control Questions to Include in Your Survey Design
In addition to programmatic checks such as speeder or straightlining flags, you can include several quality control questions in your questionnaire.
Consistency Checks
These are questions interspersed throughout a survey that can identify whether or not a respondent is authentically providing answers that align with their personal experience. Researchers can insert a combination of opposite statements or unrealistic actions to deduce whether they’re consistently responding to very similar questions the same way.
Attention Checks
To determine whether a respondent is paying attention, researchers can use an attention check by inserting a prompt such as “Please select the number 3 for quality purposes.” If these particular exercises are incorrectly answered, researchers can rightfully suspect that the respondent is speed-clicking through the survey without regard to the content of the questions.
Knowledge Checks
It’s critical to check if a respondent is who they say they are, which can be determined with a question they should be able to answer. If the target audience is IT-related, for example, researchers could include a computer-related knowledge check that a respondent cannot quickly look up online and that the target respondent should know.
Seeking Checks
To boost their odds of qualifying for online surveys, clever respondents often exaggerate their qualifications. A seeking check is a multi-select question in a survey screener that asks about a variety of experiences or interests and includes highly uncommon activities, like helicopter shopping. Respondents who select the distractor option or an above-average number of options may immediately be disqualified or flagged as potential seekers for further review. Alternatively, researchers may present a list of known uncommon activities–buying a new car, flying to Mauritania, buying an insurance policy against bed bugs, passing your driving license exam–and set a maximum threshold that respondents must not pass.
Frequency Checks
This type of check is used to implicitly verify respondent consistency. For example, researchers can present a list of known frequent activities–drinking water, shopping for food, watching TV, using a bank account, driving–and set a minimum threshold that respondents must pass.
Considerations When Placing Quality Control Questions in Your Survey
Effective quality control questions require careful alignment with your study’s goals and objectives. The aim is for them to be subtle yet effective without disrupting the survey flow. Some factors to consider: Relevance: Always align your questions with the survey’s main objectives. Irrelevant or tangential questions may confuse respondents and compromise data quality. Placement : Integrate quality control questions throughout the questionnaire. Distributing them throughout the sections helps maintain engagement and prevents predictability. Flow: Quality control questions should be embedded seamlessly in the overall questionnaire structure. Avoid abrupt transitions or interruptions that could disrupt the respondents’ focus. Complexity: Keep questions clear and concise so they are easy to understand. Complex or ambiguous wording can confuse respondents and lead to inaccurate responses. Frequency: Balance the frequency of quality control questions to maintain data integrity without overwhelming respondents. Too many questions may increase cognitive load and decrease survey completion rates, while too few may compromise the ability to detect inaccuracies effectively.
Improving Your Data With Quality Control Questions
Investing in a high-quality questionnaire and well-written screener is paramount to the success of any survey project. Adding quality control questions will help ensure your final data set is of the highest possible standard. IntelliSurvey carefully reviews all questionnaires and provides thoughtful feedback for every project engagement. The combined strategy of using quality control questions and IntelliSurvey's propriety CheatSweep™ data cleansing algorithm makes us well-positioned to support the most challenging of fielding efforts. For more information on how we can partner to perfect your questionnaire and support your survey fielding, please get in touch.
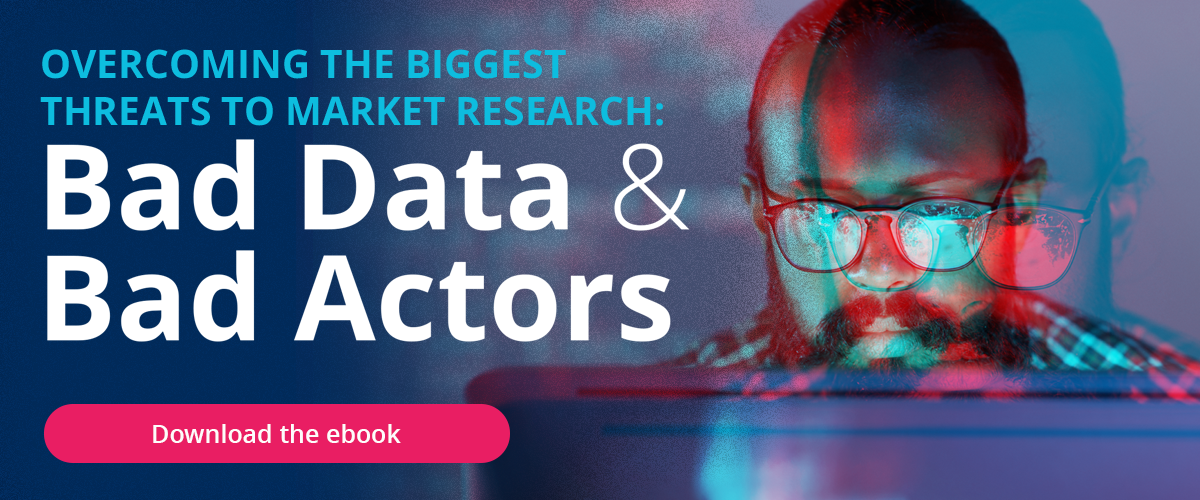
Subscribe to our Monthly Newsletter
Related posts.
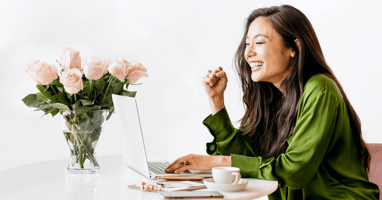
Engaging Questions, Motivated Respondents, Quality Data | IntelliSurvey
Wishing you could liven up your respondent engagement? Go beyond the typical survey question types...
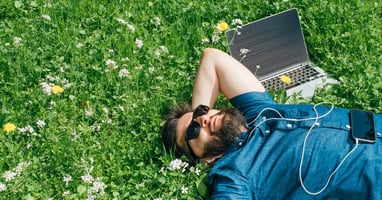
How to Write Effective Attention Checks
Ensuring your respondents are fully engaged and paying attention to your survey questions is always...
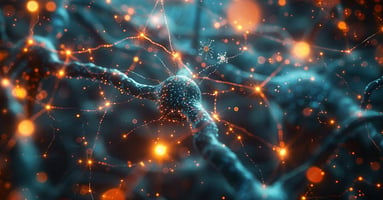
8 Ways to Use Neuroscience to Improve Your Online Survey Questionnaire
Integrating principles from neuroscience into your questionnaire design can profoundly enhance the...

A .gov website belongs to an official government organization in the United States.
A lock ( ) or https:// means you've safely connected to the .gov website. Share sensitive information only on official, secure websites.
- Prevent Adult Blood Culture Contamination
- GeT-RM Coordination Program
- Individualized Quality Control Plan
- Provider-Performed Microscopy Procedures
- Waived Tests
- Project ECHO
- The Next Generation Sequencing Quality Initiative
- The Clinical Laboratory Improvement Amendments of 1988 (CLIA) regulations require a laboratory to have quality control (QC) procedures.
- The Individualized Quality Control Plan (IQCP) option offers the laboratory flexibility for meeting regulatory QC requirements.
- Learn how to develop an IQCP.

The CLIA regulations require a laboratory to have QC procedures to monitor the accuracy and precision of the complete testing process. A QC option now allows labs to customize an IQCP for their specific testing environment and patient needs. The IQCP option offers the laboratory flexibility for meeting regulatory QC requirements appropriate for the testing performed.
IQCP is an all-inclusive approach to assuring quality. It includes practices already used by laboratories to ensure quality testing, beyond just testing QC materials at a set frequency. IQCP applies to all nonwaived testing performed, including existing and new test systems. All CLIA specialties and subspecialties except Pathology are eligible for IQCP.
The Developing an IQCP - A Step-By-Step Guide workbook assists in developing an IQCP for one or more test systems. The workbook uses an example scenario to guide development of a sustained and modified IQCP over time through a step-by-step process. You will evaluate your current quality activities and develop an IQCP worksheet which, when completed, can serve as your IQCP document. The approach outlined in this workbook is one example for documentation; it is not mandatory or the only format available.
Hard Copies
More information.
For questions, or comments, please e-mail: [email protected]
Laboratory Quality
CDC offers training and technical assistance to help clinical and public health labs achieve high-quality science and ensure the safety of laboratory professionals and their communities.

IMAGES
VIDEO
COMMENTS
8. Culture of quality and accountability: Foster a culture of quality and accountability within the laboratory. Encourage open communication, collaboration, and a commitment to continuous ...
Since publication in 2003 of a review 'Internal quality control: planning and implementation strategies,' 1 quality control (QC) has evolved as part of a comprehensive quality management system (QMS). The language of quality today is defined by International Standard Organization (ISO) in an effort to standardize terminology and quality management practices for world-wide applications.
Abstract. Attributes of rigor and quality and suggested best practices for qualitative research design as they relate to the steps of designing, conducting, and reporting qualitative research in health professions educational scholarship are presented. A research question must be clear and focused and supported by a strong conceptual framework ...
INTRODUCTION. Scientific research is usually initiated by posing evidenced-based research questions which are then explicitly restated as hypotheses.1,2 The hypotheses provide directions to guide the study, solutions, explanations, and expected results.3,4 Both research questions and hypotheses are essentially formulated based on conventional theories and real-world processes, which allow the ...
Quality Control for Scientific Research: Addressing Reproducibility, Responsiveness, and Relevance. March 2019; ... with research questions (S todden 2015;H u b b a r d 2016). The.
ABSTRACT. Efforts to address a reproducibility crisis have generated several valid proposals for improving the quality of scientific research. We argue there is also need to address the separate but related issues of relevance and responsiveness.To address relevance, researchers must produce what decision makers actually need to inform investments and public policy—that is, the probability ...
The content of an SOP is the responsibility of the qualified personnel (author) and of the approving party (management). Activity: implementation of a procedure. QC: communication and the implementation of a new SOP to applicable personnel. QA: inspection of study personnel's training and adherence to the SOP.
In 2013, NHLBI developed a set of tailored quality assessment tools to assist reviewers in focusing on concepts that are key to a study's internal validity. The tools were specific to certain study designs and tested for potential flaws in study methods or implementation. Experts used the tools during the systematic evidence review process to ...
Quality Assurance in Research. In research contexts, quality assurance (QA) refers to strategies and policies for ensuring that data integrity, quality, and reliability are maintained at every stage of the project. This includes strategies for preventing errors from entering the datasets, taking precautions before data is collected, and ...
How quality control could save your science. Monya Baker. Nature 529, 456-458 (2016) Cite this article. 6398 Accesses. 42 Citations. 416 Altmetric. Metrics. It may not be sexy, but quality ...
Quality control is an essential component of international large-scale assessment. Rigorous quality control processes help ensure high quality data and facilitate cross-country comparisons of study results. Although quality control can and does exist at all stages, including before, during, and after data collection, the term is often used ...
Quality in Research: Asking the Right Question. Joan E ... Definitions of breastfeeding: Call for the development and use of consistent definitions in research and peer-reviewed literature. Breastfeeding Medicine, 7(6), 397-402. Crossref. PubMed. Google Scholar. Moher D., Liberati A., Tetzlaff J., Altman D. G., ... Frequently asked questions ...
Quality surveys yield rich, nuanced, useful data that help answer one or more research or . evaluation. questions (see Chapter 2 for more on research and . evaluation questions). Through quality questions, quality surveys effectively engage respondents in providing accurate and useful data. But a survey is more than just a simple set of questions.
Quality-control questions assume that participant misbehavior in a particular moment is indicative of misbehavior throughout the entire survey. For this reason, the data quality is improved with the omission of this participant. An alternate assumption is that a degree of inattentiveness is normal throughout a survey and participants may be ...
Although attention checks are an important way to measure data quality, they are only one of several data points researchers should use to isolate and quarantine bad data. Others include things like measuring inconsistency in responses, nonsense open-ended data, and extreme speeding. In this blog, we describe what makes a good attention check ...
Research rigor refers to whether a research resource used appropriate methods to answer the research questions and whether the researcher adhered to established procedures and protocols. Research rigor is described differently in quantitative, qualitative, and mixed methods research. Each approach has its unique strengths and is best at ...
Quality Control. Quality control can be defined as "part of quality management focused on fulfilling quality requirements." While quality assurance relates to how a process is performed or how a product is made, quality control is more the inspection aspect of quality management. An alternate definition is "the operational techniques and ...
Quality control can also help to make sure that the results of an experiment or method are consistent. Quality control is known as quality assurance or quality management. Good Laboratory Practice (GLP) is one form of quality control. GLP was introduced in the field of chemical research, to try to ensure high quality, reliable test data.
Fundamental Criteria: General Research Quality. Various researchers have put forward criteria for evaluating qualitative research, which have been summarized in Table 3.Also, the criteria outlined in Table 4 effectively deliver the various approaches to evaluate and assess the quality of qualitative work. The entries in Table 4 are based on Tracy's "Eight big‐tent criteria for excellent ...
Quality control plays a crucial role in ensuring that businesses deliver high-quality products and services, meeting customer expectations and regulatory requirements. Companies can develop and implement effective QC systems that contribute to long-term success by understanding its importance, benefits, and key strategies. ISO 9001:2015 defines ...
Quality control is commonly utilized to promote quality of business products. Golden Age Hospital: Quality Control and Accreditation. GAH's core competencies include professionalism, evidence-based practices, effective communication, patient safety, patient-centered approaches and quality improvement.
Complexity: Keep questions clear and concise so they are easy to understand. Complex or ambiguous wording can confuse respondents and lead to inaccurate responses. Frequency: Balance the frequency of quality control questions to maintain data integrity without overwhelming respondents. Too many questions may increase cognitive load and decrease ...
This report is intended to help investigators, funders, state and local health departments, and patient advocates understand key priority research questions for health care. The prevention of healthcare-associated infections (HAIs) and antimicrobial-resistant (AR) infections is a mixed story of progress and setbacks with a long path ahead.
The Clinical Laboratory Improvement Amendments of 1988 (CLIA) regulations require a laboratory to have quality control (QC) procedures. The Individualized Quality Control Plan (IQCP) option offers the laboratory flexibility for meeting regulatory QC requirements. Learn how to develop an IQCP.