
An official website of the United States government
The .gov means it’s official. Federal government websites often end in .gov or .mil. Before sharing sensitive information, make sure you’re on a federal government site.
The site is secure. The https:// ensures that you are connecting to the official website and that any information you provide is encrypted and transmitted securely.
- Publications
- Account settings
The PMC website is updating on October 15, 2024. Learn More or Try it out now .
- Advanced Search
- Journal List
- Behav Sci (Basel)


Providing Excellent Customer Service Is Therapeutic: Insights from an Implicit Association Neuromarketing Study
Gemma anne calvert.
1 Nanyang Business School, Nanyang Technological University, 50 Nanyang Avenue, Singapore 639798, Singapore; gs.ude.utn@CAMIL
Abhishek Pathak
2 School of Business, 4 Nethergate, University of Dundee, Dundee DD1 4HN, UK; [email protected]
Lim Elison Ai Ching
Geraldine trufil.
3 Split Second Research Limited, London E1 8FA, UK; [email protected] (G.T.); [email protected] (E.P.F.)
Eamon Philip Fulcher
This paper reports the results of a combined biometric and implicit affective priming study of the emotional consequences of being the provider or receiver of either positive or negative customer service experiences. The study was conducted in two stages. Study 1 captured the moment-by-moment implicit emotional and physiological responses associated with receiving and providing good customer service. Study 2 employed an affective priming task to evaluate the implicit associations with good and poor customer service in a large sample of 1200 respondents across three Western countries. Our results show that both giving and receiving good customer service was perceived as pleasurable (Study 1) and at the same time, was implicitly associated with positive feelings (Study 2). The authors discuss the implications of the research for service providers in terms of the impact of these interactions on employee wellbeing, staff retention rates and customer satisfaction.
1. Introduction
Customer satisfaction is a vital goal for all businesses because it leads to increased sales and customer re-patronage, which ultimately boosts profits. To this end, managing customer experiences across the customer–employee touchpoints plays a critical role, given that most businesses involve some level of direct contact (e.g., face-to-face or voice-to-voice) between employees (especially those working at the consumer interface) and customers. Yet delivering high quality and effective customer service is not a straightforward or easily managed process. Customer–employee interactions have a significant emotional component that often confounds training strategies. While it is understood that positive customer service results in better marketing outcomes, much less is known about the emotional impact on those responsible for delivering that service.
Service employees often hide their true inner feelings and maintain a pleasant facial and bodily display in a bid to please their customers and/or gain control over employee–customer interactions [ 1 , 2 ]. Indeed, companies often train their service employees to act in a friendly manner since the display of positive emotions is associated with favourable consequences, such as increased customer satisfaction, customer re-patronage, and positive word-of-mouth [ 3 ]. Such acting requires significant effort on the part of the employees and can cause employees to suffer emotional burn-out if they are required to “put on” displayed emotions for long periods of time [ 4 , 5 ]. Furthermore, consumers do not always appreciate employee friendliness, and may even construe it negatively as being disrespectful [ 6 ]. Indeed, consumers are increasingly adept at discerning the expressive behaviour of service providers. For instance, they are more likely to be moved by the authenticity of an employee’s smile rather than the extent of it [ 7 , 8 ]. The somewhat artificial nature of these exchanges, coupled with the constant requirement to suppress negative emotions and “appear” friendly and understanding, makes it extremely difficult to disentangle true emotions associated with positive and negative customer–staff interactions and those which individuals presume they should experience.
Measuring the emotional consequences associated with customer experience is further complicated by the fact that it involves multiple moments of contact between an organisation and a customer. These may include the feelings evoked when walking into a shop, the way in which the customer is treated by frontline service employees in-store, as well as post-purchase follow-up customer service. Furthermore, the ability of individuals to introspect and comment on the nature of these subjective emotional responses, particularly during dynamic social interactions, is highly variable and often inaccurate [ 9 , 10 ]. The relationship (and perceptions thereof) between the employee-customer interaction has traditionally been measured using surveys [ 11 ] (may not be accurate always, and we propose an alternate method in this the current paper.
Extant scholarly researchers as well as companies interested in assessing service quality mostly employ explicit, self-reported measures. However, this approach captures only a partial picture of the multitude of responses in consumers’ brains. Neuroscience research has shown that a vast amount of human behaviour is driven and influenced by emotional and cognitive responses that occur below conscious awareness [ 12 , 13 , 14 ]. At the conscious level, customers tend to know what they want and also how they wish to be treated. But important implications of good and poor customer service can also play out at the subconscious or implicit level of cognition [ 15 , 16 ]. The same is true for those responsible for providing that service, where multiple conscious and subconscious emotional factors impinge on the effectiveness of customer interactions.
Although it is well known that the quality of customer–employee interaction is crucial for organisations and the importance of customer service has been studied for many years now, the literature is scarce on the consequences of poor (or good) service on employees [ 17 , 18 ]. Poor customer–employee interaction can lead to employee stress and is a potential health risk [ 19 ], which can cost up to $ 300 billion in losses cumulatively to organisations the world over American Institute of Stress (2014). Employees who are regularly tasked to maintain positive interactions with customers have also been reported to show excessive emotional burden, exhaustion and absenteeism [ 20 , 21 ]. Similarly, customer mistreatment (and consequent stress) can compromise both short term and long-term employee well-being [ 22 ] and result in emotional exhaustion [ 23 ].
Recent research has also shown that positive customer behaviour during service interactions has a cross over positive effect on the employee [ 24 ]. Similarly, stressful customer interactions can have a negative impact on the affective state of employees [ 25 ].
In order to develop a deeper understanding of the implicit consequences of customer service on providers and receivers, this research examined the implicit emotional responses associated with receiving and providing excellent service. Specifically, the paper investigated 1) the perception of both giving and receiving good vs. bad customer service, 2) and the implicit associations (or feelings) which people associate with the experience of giving or receiving good vs. bad customer service. By doing so, this research contributes to the services literature by demonstrating how the positive benefits of excellent customer service can impact not only on customers, but also on service providers themselves. Such positive outcomes, if made explicit, can clearly be exploited in a positive way so as to increase employee job satisfaction and reduce staff turnover rates. Furthermore, this research also contributes to the field by proposing a new research approach that captures customers’ subconscious responses in order to gain a more comprehensive understanding of the subliminal effects of positive customer–employee interactions.
2. Background
Over the past decade, techniques that have emerged from the fields of neuroscience and psychology, such as functional MRI, electroencephalography (EEG), eye-tracking, biometrics, facial decoding and implicit association testing, have been engaged by brand owners to capture these vital subconscious responses in order to define and predict consumer behaviour with much greater accuracy (for a recent review, see [ 9 ]). This approach has been referred to as “neuromarketing” [ 26 ] and numerous commercial practitioners of this burgeoning industry now exist. In recent years, commercial entities have paid particular attention to neuromarketing methods that are scalable, cost-effective and offer fast turnaround times [ 27 ].
One methodology that satisfies these criteria is the use of implicit reaction time tests [ 28 ]. The mainstay of many cognitive psychology experiments since the 1970s, implicit reaction time paradigms measure individuals spontaneous or ‘gut instinct’ responses. Commercial adaptations of these paradigms permit marketers to capture these vital subconscious consumer responses online, without the need for verbal feedback or even respondents’ awareness of their reactions. Implicit measures have now been used in a variety of settings to extract people’s implicit emotions and attitudes to a wide range of different issues, including racial prejudice, sexual preferences, alcoholism, mental health, and consumer attitudes (see [ 29 ] for an overview). Importantly, the implicit responses obtained in these studies were shown to be more predictive of respondents’ subsequent behaviour than their explicit verbal responses obtained at the same time and are therefore, in many instances, more accurate indicators of their emotional responses to specific concepts and scenarios.
Several recent implicit reaction time paradigms have been shown to have high reliability and validity [ 30 , 31 , 32 , 33 ]. These approaches rely on a simple behavioural response—a very rapid key press to the presentation of a stimulus, which is made following a simple decision about the stimulus. There are several distinct implicit paradigms, each with specific strengths and weaknesses, and the choice of task depends on the research question being addressed [ 29 ].
In the current study, we employed two implicit reaction time tests. The first was the Impulse Test recently developed and shown to measure the emotions evoked as respondents view dynamic material (e.g., while watching a television advertisement, movie trailer or video footage [ 34 ]. The second was an affective semantic priming task [ 35 , 36 ] that assesses the strength of implicit association between a set of emotional words and specific concepts, in this case, good and poor customer service. The rationale for employing two distinct implicit tests was that in the first case, we were able to identify the immediate emotions elicited by positive customer service interactions (both from the perspective of the provider and the receiver) and relevant in short-term memory, and in the second case, we were able to capture the more deep-seated emotions associated with positive as well as negative customer interactions that are stored in long-term memory.
Physiological responses (heart and breathing rate and electrodermal changes) were also measured during the Impulse test to determine if positive customer service interactions (both providing and receiving) impact the levels of arousal. Arousal, one of the components of emotional responding, is associated with stress, anxiety and fear [ 37 ], and physiological manifestations of arousal include increased blood pressure, heart rate, sweating and hyperventilation [ 38 ]. We hypothesized that the act of simply observing positive customer–staff interactions would result in reduced arousal and therefore stress levels, similarly to that experienced when engaging in other everyday pleasures.
This study was conducted in two stages. Study 1 was designed with two objectives in mind: first, the study served to identify the nature of the immediate emotions elicited in real time as respondents viewed videos of people receiving or providing excellent customer service compared with viewing other positive scenarios (e.g., everyday pleasurable activities such as enjoying time with friends), and secondly, we wanted to examine the physiological responses (heart and breathing rate, and electrodermal response) to the customer service scenarios depicted in the videos. In Study 2, we examined the more deeply held emotions (i.e., those maintained in long term memory) associated with customer service interactions (positive and negative) in a larger population (N = 1200) across three countries that individuals have either delivered or received.
3.1. Study 1: Laboratory Based Study
3.1.1. participants.
Twenty participants (thirteen females (two left-handed) and seven males (all right-handed) with mean age of 27 years) were recruited from Bristol, UK (via flyers in exchange for vouchers) and given small incentives to take part in a study to measure their immediate physiological and psychological responses to different emotional scenarios in real-time, including footage depicting individuals providing or receiving customer service (sample size is similar to other studies of comparable nature, e.g., [ 39 ]).
3.1.2. Materials
Three distinct video clips, each one minute in duration, were professionally created specifically for this study:
Video 1 (Condition 1: Control): was made up of footage of everyday pleasures (unrelated to customer service) shown from the first person perspective, such as eating crisps, going for a walk in the park.
Video 2 (Condition 2: Providing excellent customer service): constituted footage of four different scenarios in which service staff were filmed delivering excellent customer service and the footage shown from the service provider’s perspective. The scenarios were as follows: (i) a booking agent is seen giving a customer tickets to a previously sold out play at the theatre and knowing she has had a hard time recently, the booking agent has gone one step further and arranged for her to go to the opening night party as well, (ii) a travel agent helps a couple, who have been separated for six months due to work, to plan their dream honeymoon, giving them personalised recommendations on where to visit and restaurants to eat out at, (iii) a groom leaves his wedding rings in the back of a taxi the day before the wedding. The taxi driver returns to the hotel where he dropped off the groom off, re-uniting him with the rings and thus saving the day, and (iv) a woman collapses in a restaurant while on holiday after which a fellow customer, a doctor, tries to help but her friend is very distressed and does not speak the local language. The waitress steps in to translate what the doctor is saying and accompanies them all to hospital.
Video 3 : (Condition 3: Receiving excellent customer service): shows scenarios featuring excellent customer service and are the same scenarios as those used in Condition 2 but re-filmed and shown from the customer’s perspective.
3.1.3. Protocol
Only one subject at a time participated in the experiment. Each participant was greeted by the experimenter who explained that the purpose of the study was to gain a better understanding of customer service interactions. After obtaining informed consent (FREC-EF02-PSY-16-1-2013), physiological electrodes were applied and subjects were seated in front of a computer screen. Heart and breathing rate as well as skin conductance measures were collected as subjects viewed the video clips. The experimental videos were shown on a computer screen and participants’ responses were recorded using the computer keyboard. The order of presentation of the three videos was counterbalanced across subjects.
BIOPAC physiological equipment was used in the collection of data. In order to measure heart rate, one electrode was placed on the medial surface of each leg just above the ankle. A third electrode was placed on the right anterior forearm at the wrist. Once electrodes were attached, participants were asked to remain still while the system parameters were calibrated. Data was recorded at a rate of 200 times a second. Heart rate was measured as the milliseconds between heart beats and was analyzed as the average heart-rate per two seconds (400 datapoints).
Skin conductance data was collected through two electrodes attached to the middle and ring finger of the non-dominant hand. The index finger was avoided as it was used in the reaction time task. Participants were given the opportunity to practice key pressing with minimal movement of the hand, so as not to disturb recording. Respiratory cycle was recorded through a respiratory transducer attached around the chest below the armpits and over the shirt. It was adjusted so that it was slightly tight at the point of maximal expiration.
During the acquisition of biometric data, subjects were also asked to carry out an implicit reaction time test (the Impulse test) while viewing the experimental videos. The Impulse test consists of two stages—a baseline phase and an experimental phase. In the baseline phase of the current task, participants were exposed to a set of emotional words (see Table 1 , presented one at a time and in randomised order in the centre of the computer screen). Each word was presented four times, and on each occasion, the words were displayed on the screen until the correct key was pressed or 2 s had elapsed. The next word was presented 2 s after the previous word. The selection of emotional words most relevant to the content shown in our three videos was determined in a prior pilot study in which 16 words (8 positive and 8 negative) were identified from a cohort of 50 words as being ranked most closely to the emotions elicited in the videos and categorised consistently as of positive or negative valence.
Emotional words used during the Impulse test.
Positive Valence Words | Negative Valence Words |
---|---|
Excited | Weepy |
Over-joyed | Stressed |
Delighted | Sad |
Contented | Heartbroken |
Pleased | Lonely |
Ecstatic | Ignored |
Peaceful | Fed up |
On each trial, an emotional word appeared briefly on the computer screen and subjects were instructed to categorise them according to their emotional valence by pressing the “I” key on the keyboard if the word was positive in nature and the “E” key if the word was negative (key mapping was counterbalanced between the subjects). A reminder of which key corresponded to which emotional valence “Positive” or “Negative” remained on the computer screen in the top left and right corners throughout the two phases. Subjects were asked to respond as quickly and as accurately as possible.
The baseline trials and the experimental trials were identical, except that a video was played in the background during the experimental trials. The baseline phase of the Impulse test served both as a means of training the subjects to respond within a very short timeframe (to clear contamination from conscious brain processes) and also to familiarise the participants with the task. Responses that were deemed too slow to be classified as pre-cognitive were followed by a brief warning tone and the visual warning “too slow”. Following successful completion of the training phase, subjects were informed via instructions on the computer that the experimental phase was about to begin. The design was similar to the practice phase, however, during this phase, the words appeared superimposed on the dynamic footage (the three videos are described in the Materials section). Respondents were instructed to continue classifying the words as positive or negative in terms of emotional valence and to press the corresponding keys on the computer keyboard.
Our previous research has shown that the speed and accuracy of classification of these words reflect the feelings that a participant has towards the content of the movie or television clip [ 34 ]. By comparing reaction times to classify positive and negative emotional words during the training (baseline) and experimental phase, it is possible to infer the nature of the internal feelings elicited in the viewer by the video content every 2 s. Specifically, we have found that positive emotions elicited by the footage shown, speeds responses to positive words and slows them to negative ones. The reverse holds true for aspects of the footage that elicits negative emotions—responses to negative words are sped up and responses to positive words are slowed. In both cases, RTs were computed against the responses recorded during the baseline and training phase, for each participant. To understand this approach in the context of semantic priming studies, in the current study, the video content acts as the “priming” stimulus, with the emotional words being the targets.
3.1.4. Analysis
The physiological analysis focused on the emotional peaks detected whilst watching each video. We hypothesized that during each emotionally provocative video, there were likely to be fluctuations in arousal, as determined by changes in electro-dermal response, breathing rate and heart rate. We also hypothesized that these physiological indices would be accompanied by changes in implicit psychological emotional responses detected using the Impulse test.
Physiological Responses
For the physiological data, the maximum values for each physiological measure (heart rate, breathing rate and skin conductance) were first computed by extracting the peak response recorded every 2 s during both the training and experimental phase of the Impulse test and the results from all individuals averaged and tested for statistical significance using paired T-tests at each time point (see Figure 1 ).

This graph shows the heart rate of one representative participant when they were carrying out the baseline test (blue line) and the test with the movie clip in the background (red line). The resulting data computed for this participant is the difference between the blue and red heart rate values every two seconds. When the value of a point on the red line is larger than the value of the corresponding point on the blue line (e.g., at t = 6), it shows that the participant’s heart rate increased as a result of watching this part of the movie clip. Conversely, at t = 28, the participant’s heart rate shows a decrease. These values were computed for each participant and then averaged and subjected to statistical analysis.
Impulse Test
Reaction time data obtained during the training and experimental phases of the Impulse test were first subjected to pre-processing, including removal of outliers so that responses that were impossibly quick (<250 ms) and those that were so slow as to invoke conscious processing (>1200 ms), were removed. The data were then analysed following the method outlined by Fazio and Olson [ 36 ]. For all trials of each word presented in each video, a facilitation index (FI) was computed. For all congruent responses (e.g., classifying “delightful” as “Positive”) obtained for each word across all trials, the FI was computed by subtracting the reaction times during the experimental phase from those obtained during the baseline phase. For incongruent responses to each word (e.g., classifying the word “lonely” as “Negative”), the FI calculation was reversed such that reaction times obtained during the baseline phase were subtracted from the reaction times obtained during the experimental phase. Thus, an FI greater than zero implies a response that is congruent with the emotion word set and a FI less than zero implies a response incongruent (or opposite) with the word set. This approach allowed us to take into account both the congruency (or subjective accuracy) of responses as well as the reaction times. The dependent variable was, for each moment of each video (every two seconds), the percentage of participants whose FI indicated that the footage at each time-point was either congruent or incongruent with a positive emotion or negative emotion. The averaged data were then tested for each condition for statistical significance using the binomial test that computes the probability of obtaining a specific count in one direction (e.g., a positive emotional response) against the total number of observations (the number of positive and negative responses).
3.1.5. Results
Physiological measures.
Condition 1: (Everyday pleasures) While viewing a video depicting everyday pleasures, breathing rate dropped from 16.3 cycles to 15.4 cycles per minute); heart rate remained stable at 76.1BPM in both cases; and a non-significant increase in electrodermal response from 0.171 to 0.252 was recorded.
Condition 2: (Providing good customer service) was associated with an average increase in heart rate from 76.0 BPM during the baseline phase to 87.4 BPM while the video was shown in the background ( p < 0.01). Breathing rate decreased from 16.7 cycles per minute during the baseline to 10.2 cycles per minute while viewing the video, and a significant increase in electrodermal response from 0.114 to 0.335 ( p < 0.001) was recorded.
Condition 3: (Receiving good customer service). A statistical comparison of physiological measures revealed that viewing footage of others receiving excellent customer service resulted in a significant increase in electrodermal response from 0.164 to 0.308 ( p < 0.01) and a significant decrease in heart rate from 71.4 to 80.6 BPM ( p < 0.05). There were no significant differences in breathing rate for condition 3 (17.2 to 16.8).
The control condition (viewing everyday pleasures) elicited an FI of −3.15, showing that that there was a slightly shorter mean response latency to negative attributes than to positive attributes. However, this FI did not differ from zero ( p > 0.05). Viewing footage of individuals receiving excellent customer service elicited an FI of +36.9, which reveals a significant increase in response latency to positive attributes ( p < 0.001). Viewing footage of individuals providing excellent customer service yielded the largest increase in FI of +53.8 ( p < 0.001). Paired t-tests revealed that providing excellent customer service elicited a greater association with positive emotions than either receiving excellent service ( p < 0.05) or viewing everyday pleasures ( p < 0.001).
3.1.6. Discussion and Conclusion
We believe that this is the first demonstration that viewing instances of positive customer service interactions from the perspective of both the recipient and the service provider has a positive impact on physiology and emotional well-being. Specifically, viewing footage of people delivering or receiving excellent customer service resulted in a significant increase in arousal levels, as evidenced by the increase in galvanic skin response and a significant decrease in heart rate (compared to viewing scenarios of everyday pleasures), indicating that positive customer service interactions can have a stress-reducing and calming impact on the service provider and surrounding viewers.
The results of the Impulse reaction time study showed that participants were faster at correctly classify positive word targets than negative ones when viewing footage of people providing good service compared to receiving it, or while viewing footage of every day pleasures. This is an intriguing finding as we would have hypothesized that people would adopt a self-interested stance and would instinctively attach greater positive valence to receiving good service than watching examples of people providing good service. Receiving good service was perceived with the same level of positive emotional engagement as viewing every day pleasures, highlighting the growing significance of customer service in people’s lives today.
The results of Study 1 raised further questions relating to the generalizability of these findings across different countries, age groups and gender. Therefore, in the next study, we sought to extend these findings by investigating the implicit emotional feelings associated with both positive, as well as negative, customer service interactions in a larger population using a web-based implicit affective priming task designed to uncover the strength of emotional association that people hold about positive and negative customer service interactions.
3.2. Study 2: Online Study
3.2.1. participants.
Participants (N = 1200) from three countries (UK, Canada and Australia; N = 400 from each country, 50% males) were recruited through a research participation recruitment company (Research Now) and were given small incentives to complete the tests. All the participants had normal to corrected vision, were native English speakers between 18 and 60 years and completed an online consent form prior to participation (the sample size is adequate for the chosen experimental design, since the study is a four (providing excellent or poor customer service vs. receiving excellent or poor customer service) by two (excellent service vs. poor service) design and is similar to other studies of comparable nature, e.g., [ 35 , 40 ].
3.2.2. Materials
The web-based survey included three components: (i) demographic questions to confirm age, gender, handedness and previous employment in a service industry, (ii) a consent form, and (iii) an implicit affective priming task. The survey was programmed in Javascript so that as soon as participants entered the survey, the test would automatically and immediately be downloaded onto their pc/laptop so that reaction times could be captured using the internal timing devices on the pc/laptop, which are far more sensitive than if running a program of this nature across the internet. On completion of the survey, the individual datasets were then uploaded back onto the server for analysis and without being apparent to the participant.
The affective priming task consisted of a series of emotional word primes ( Table 2 ) and target statements ( Table 3 ). The emotional word primes were selected following an explicit pilot test in 150 people (50 from each country) during which respondents were asked to classify attributes (from a set of 60; including those used in Study 1) into those most likely to be experienced in the context of extremely pleasurable experiences, peace of mind experiences, everyday experiences, and negative experiences. Of these, 35 were consistently classified and used as primes in the implicit test. The brief statements used as targets (e.g., “being helpful”, “feeling relieved”) were generated in consultation with service industry consultants and refer to the behaviours that were most often experienced in the context with excellent or poor service scenarios ( Table 3 ).
Emotional prime words used in the affective priming task.
Emotional Prime Words | ||||||
---|---|---|---|---|---|---|
Excited | Confident | Content | Appreciated | Regular | Okay | Sad |
Ecstatic | Fortunate | Comforted | Peaceful | Satisfactory | Fine | Lonely |
Over-joyed | Engaged | Pleased | Relief | Pleasant | Normal | Ignored |
Exhilarated | Proud | Happy | Calm | Nice | Expected | Annoyed |
Energised | Thrilled | Loved | Satisfied | Fair | Usual | Nervous |
Emotional words used to create brief statements used in Test A (Providing) and Test B (Receiving). All target words using in Test A were presented prefixed with the word “being” (e.g., “being helpful”, “being friendly”), whereas those used for Test B were pre-fixed with the word “feeling” (e.g., “feeling relieved”, “feeling neglected”).
Providing Service Targets “Being” | Receiving Service Targets “Feeling” | ||
---|---|---|---|
Positive | Negative | Positive | Negative |
Helpful | Impolite | Relieved | Neglected |
Friendly | Difficult | Special | Insecure |
Sensitive | Confusing | Understood | Misconstrued |
Excellent | Lazy | Encouraged | Ignored |
Understanding | Thoughtless | Unique | Angry |
Supportive | Rude | Respected | Insulted |
Considerate | Cold | Wowed | Underwhelmed |
Meaningful | Useless | Protected | Frustrated |
3.2.3. Protocol
On entering the survey, respondents were asked to confirm their age, gender and handedness. They were also asked if there were currently employed, and/or did voluntary work and whether their current or any past employment involved “providing service of some form to service users, such as clients, customers or patients”. If they answered “no” to the last question, they were thanked for participating but informed that they were not eligible for the study.
On completing the inclusion criteria questions and subsequent consent form, participants were then asked to classify each of the 35 emotion words (pre-selected for inclusion in the implicit test) as extremely pleasurable experiences, peace of mind experiences, mundane experiences and negative experiences.
Participants were then instructed that they would be asked to perform a reaction time task that would measure how quickly and accurately they could classify a series of short phrases (see Table 3 ) that would be presented in the centre of the computer screen. There were two tasks designed to identify emotions implicitly associated with providing excellent or poor customer service (Test A) or receiving excellent or poor customer service (Test B). Participants were randomly assigned to one of the two tasks.
Before the experimental trials, participants were given 24 practice trials during which they were asked to discriminate whether short phrases which were either positive or negative (e.g., “being helpful”, “being impolite” in the case of test A— providing excellent or poor service) and (e.g., “feeling special”, “feeling neglected” in the case of test B— receiving excellent or poor customer service) were synonymous with either “excellent service” or “poor service” and to press the “E” or “I” key on the computer keyboard corresponding to each option. The practice trials served as a learning phase during which respondents were able to learn the association between each target and the correct key press so that they would not need to focus on which key to press during the main test.
The keys were allocated to “excellent service” or “poor service” and were counterbalanced for each participant, and once assigned, remained so for the duration of the task. If a response was incorrect, the error message “Try again!” appeared near the lower part of the screen; if two keys were pushed at the same time, the message “Please press only one key at a time” was displayed; if no key was pushed within two seconds, the cue “Warning: Please press E or I” appeared. The next trial proceeded after a 1500 ms inter-trial interval. Participants were instructed to respond as quickly as possible but to avoid making a mistake.
Following practice trials, participants were told that the main trials were about to begin and would be very similar as the practice phase but this time a word or “prime” was presented for 500 ms, immediately before the short phrase targets. Each prime was presented four times in total, twice before a phrase associated with “excellent service” and twice before a phrase associated with “poor service” to ensure a sufficient number of trials of each type. Prior testing has shown that with an N = 1200, this number of trials is sufficient to be able to detect a statistical difference if it exists. The task was identical to that conducted during the practice trials, which was to discriminate whether the targets (e.g., “being” or “feeling” a positive or negative emotion) that appeared immediately after the primes (see Table 2 ) were associated with “excellent service” or “poor service” and to respond as quickly and as accurately as possible by pressing the key corresponding to each option.
3.2.4. Analysis
Data were first subjected to analysis to remove outliers, including response times that were impossibly fast (<250ms) or those that occurred after the permitted time window. Reaction times were then computed for each word attribute and for each participant. A difference score was computed being the mean reaction time when the prime was presented before ‘poor service’ minus the mean reaction time when the prime was presented before ‘excellent service’. This was also done separately for Tests A (Providing) and B (Receiving). Positive difference scores indicated that a prime was more strongly associated with “excellent service” than with “poor service”. Negative difference scores indicated the reverse. Difference scores greater than zero were recoded as +1 and difference scores less than zero were recoded as −1 (scores at zero were not included in the subsequent analyses). For each attribute, we then computed the percentage number of 1 s, this value would reflect the percentage of participants who more strongly associated the prime with excellent service than with poor service.
3.2.5. Results
The results focus on the comparison of emotional attributes that were both significantly associated with providing versus receiving positive and negative customer service, as well as the overall number of positive and negative emotions attributed to each condition. Here, we first report the results for the entire group (averaged across all countries).
Providing Excellent Customer Service
The analysis of reaction times recorded when participants classified the targets subsequent to emotional primes in Test A (Providing customer service) found that (collapsed across all countries) providing excellent service was associated with fastest responses to feeling “calm” and “proud” ( p < 0.001). Other emotional word attributes that were found to be strongly associated with providing excellent service included feeling “fair”, “engaged”, “loved” and “pleased”, “nice”, “okay” and “ecstatic” ( p < 0.05). A total of nine positive emotion words were found to be implicitly associated with providing excellent customer service.
Receiving Excellent Customer Service
Receiving excellent service (Test B) was found to be associated with faster responses when preceded by the primes “energised”, “happy” and “proud” ( p < 0.001). Other emotions that were also strongly associated with receiving excellent customer service were “calm”, “satisfactory”, “nice”, “fair” and “okay” ( p < 0.05), attributes that were previously categorised as being experienced when engaging in everyday pleasures, such as meeting friends.
A comparison of significant associations across the two tests (see also Figure 2 ) revealed that only providing excellent service was associated with “pleased” and “ecstatic”, whereas receiving excellent service elicited significant associations with the attributes “energised”, “happy”, “thrilled”, “excited”, “fine” and “fortunate”.

Emotional attributes associated with providing versus receiving excellent customer service (Y-axis shows the percentage of people significantly associating primes with the receipt and provision of excellent service).
Providing Poor Customer Service
Providing poor customer service was significantly associated with the emotional attributes, “lonely”, “nervous”, “sad” and “annoyed”.
Receiving Poor Customer Service
Receiving poor customer service was significantly associated with these same four emotional attributes and additionally, with feeling “ignored”. Receiving poor service was more strongly associated with the attributes “sad” and “annoyed” than was providing poor service (see also Figure 3 ).

Emotional attributes associated with providing versus receiving poor customer service (Y-axis shows the percentage of people significantly associating primes with the receipt and provision of poor service).
Gender Differences
The statistical comparison of males and females collapsed across all countries found that while females associated more positive attributes with receiving excellent customer service, males associated more positive attributes with providing excellent customer service (both ps < 0.01). Specifically, females associated receiving excellent service with feeling “happy”, “energised”, “over-joyed”, “proud”, “thrilled”, “exhilarated”, “loved”, “nice”, “expected”, and “fair”. Receiving poor service was more significantly associated with feeling “nervous”, “sad” and “lonely”. By comparison, providing excellent service was associated with feeling “proud”, “calm”, “pleased”, “fair”, and “engaged”, whereas providing poor service was associated with feel “sad”, “annoyed” and “lonely”.
Males were faster to associate the provision of positive customer service with feeling “energised”, “calm”, “engaged”, “proud”, “thrilled” and “nice”. Providing poor service made them feel “nervous”. Receiving excellent customer service was associated with feeling “satisfactory”, “calm”, “okay”, “satisfied”, “ecstatic”, ”engaged”, “relief” and “nice”. Receiving poor customer service was associated with feeling “annoyed”, “regular” and “lonely”.
Age Differences
Respondents aged between 18 and 35 years associated more positive attributes with receiving than providing excellent customer service ( p < 0.001), including “OK, fair, confident, nice, satisfactory, engaged, energised, thrilled, calm, ecstatic, exhilarated, content, happy, pleasant”. Receiving poor service was associated with feeling “annoyed”, “sad” and “lonely”. Providing excellent service was associated with feeling “calm”, “engaged” and “proud” and “OK”; providing poor service made them feel “sad”, “ignored” and “nervous”.
In stark contrast, respondents in the older age group (36+) associated more positive attributes with providing rather than receiving excellent customer service ( p < 0.001). Specifically, providing excellent service was more closely associated with feeling “proud” and “calm”, “excited”, “pleased”, “nice” and “fair”. Providing poor service made them feel more “annoyed” and “sad”. Receiving excellent service made the older group feel “proud” and “calm”, poor service interactions made them feel “nervous”, “lonely”, “ignored”, “regular” and “sad”.
Cross-Cultural Differences
There were also a number of interesting cross-cultural differences in terms of the emotions most closely associated with providing and receiving good and poor customer service.
United Kingdom (least Impacted by Customer Service- Expectations much Lower)
Comparison of the statistical effect sizes between countries revealed that while respondents in the United Kingdom showed a positive association between giving or receiving amazing service, the effect was lower than that recorded for Canada and Australia.
Canada (Focused on “Providing”)
It was noteworthy that Canadians felt more “thrilled”, “content” and “pleased” when providing rather than receiving excellent service. Receiving, rather than giving amazing service was, on the other hand, more associated with a positive association with the emotions, “exhilarated”, “energised”, “happy”, “loved”, “relieved”, “pleasant” and “fine” ( p < 0.05).
Australia (Receiving is More Emotionally Important than Giving)
Australians were found to associate the provision of amazing service with a sense of “calm” ( p < 0.05), compared to receiving the same level of service. Australians were statistically more likely to feel “fortunate”, “thrilled”, “happy” and “appreciated” when receiving excellent service compared to when they were providing it.
3.2.6. Conclusions
In study 2, we demonstrated the implicit association of positive and negative feelings with proving and receiving good customer service across a large general populace. We also show the generalizability of our results across three cultures, ages and genders. Specifically, we demonstrated that, (1) providing and receiving excellent customer service was strongly associated with certain emotions (feeling “calm”, “proud”, “fair”, “engaged”, “loved”, “pleased”, “nice”, “okay”, “ecstatic”, “energised”, “happy” and “satisfactory”), and (2), providing and receiving poor customer service was strongly associated with certain emotions (feeling “lonely”, “nervous”, “sad”, “annoyed” and “ignored”), (3) females associated providing and receiving excellent customer service with certain emotions (“happy”, “energised”, “over-joyed”, “proud”, “thrilled”, “exhilarated”, “loved”, “nice”, “expected”, “fair”, “calm”, “pleased” and “engaged”), (4) females associated providing and receiving poor customer service with the emotions “nervous”, “sad”, “lonely” and “annoyed”, (5) males associated providing and receiving excellent customer service with the emotions “energised”, “calm”, “engaged”, “proud”, “thrilled”, “nice”, “satisfactory”, “okay”, “satisfied”, “ecstatic” and “relief”, (6) males associated providing and receiving poor customer service with the emotions (“nervous”, “annoyed”, “regular” and “lonely”). We also found that younger respondents associated more positive attributes with receiving, rather than providing, excellent customer service, whereas older respondents associated more positive attributes with providing rather than receiving excellent customer service. Among cross-cultural differences, we found that in (1), UK respondents showed a weak association between giving or receiving an amazing service and their expectations were lower (compared to Canada and Australia), (2) Canadian respondents showed a stronger association for providing rather than receiving excellent service and (3), Australian respondents showed a stronger association for receiving rather than providing excellent service).
4. General Discussion
In the current study, we exploited two implicit reaction time tasks. The first, the recently developed Impulse test, is a novel implicit reaction time paradigm that measures the moment-to-moment shifts in emotions when, for example, people are viewing dynamic videos or footages [ 34 ]. The second is a task based on affective priming, a very well established implicit paradigm that was developed out of cognitive psychology in the 1980s [ 41 , 42 , 43 ] and has been recently adapted for use in commercial neuromarketing studies [ 35 ]. Both implicit tasks are ideal for capturing the complex, often subconscious, emotions associated with receiving and providing customer service of varying quality in order to understand the subtle impact of these customer–staff interactions on emotional well-being. Major advantages of using these methods are that they are indirect and are not as susceptible to the response biases associated with explicit responses (e.g., self-reported measures) and that they can reveal the moment-to-moment scores during a video clip, rather than a post test score.
Our results show that people do not only find receiving excellent customer service as pleasurable but providing excellent service is equally satisfying. We corroborate these results using both physiological measures (study 1) and an implicit reaction time paradigm (study 2). We also provide evidence that both giving and receiving excellent service can actually reduce stress and anxiety levels amongst both consumers and service providers and have a positive impact on their wellbeing. These results were shown to hold true across three countries, demonstrating that giving and receiving excellent customer service can induce a sense of pride, calmness and of being loved.
Our data additionally revealed some age and gender differences. Specifically, our results reveal that younger individuals (18–35 years) exhibit more positive emotions when receiving than giving good customer service, whilst the opposite was the case for older participants. In thinking about being served, relatively more focus is placed on oneself (vs. others); in thinking about providing service, relatively more focus is placed on others (vs. oneself). Therefore, our results suggest that younger people tend to focus more on themselves (vs. others), whereas older individuals focus more on others (vs. themselves). This pattern of findings is consistent with Freund Blanchard-Fields’ [ 44 ] observation that older adults are more altruistic (i.e., focusing on the needs of others rather than on themselves) than younger adults, and tend to behave in ways that benefit others rather than themselves (e.g., donating money to a good cause rather than keeping it for themselves). By contrast, younger adults tend to focus on maximizing their personal gains over the interests of other people. Collectively, these findings add to the existing knowledge about customer service by underscoring the importance of age differences when it comes to customers and service providers. Future research may test the altruism explanation for the observed effects due to age differences.
Analysis of gender differences revealed that females tend to prefer receiving (vs. providing) excellent service, whereas the reverse is true for males. At first glance, this finding appears somewhat contradictory to past research that suggested that women are generally communal, warm, and nurturing, whereas men tend to be more competitive and goal-oriented [ 45 , 46 ]. However, we interpret this finding in the light of other research which showed that men and women place a different emphasis on different aspects of service. While men are usually more concerned about the core aspect of the service (e.g., the haircut received at a hair salon), women generally pay more attention to the relational aspects of service (e.g., how well one gets along with the hairstylist) [ 47 ]. It is also likely that the core (relational) aspects of service are more salient when thinking about giving (receiving) excellent customer service because the focus is on helping the recipient resolve their problem (core aspect); in thinking about receiving service, it is easier to think about how one would feel about being served (relational aspect). Applying this to the gender differences that we found, it is possible that men preferred giving (vs. receiving) excellent service because it is more closely aligned with their goal-oriented tendency. Women, on the other hand, preferred receiving (vs. giving) excellent service as they were drawn towards its more highly salient relational aspects of service as they imagine themselves being served. Future research may follow this lead to explicitly examine the underlying processes driving the results that we observed through the implicit tests.
4.1. Theoretical and Methodological Contributions
To the best of our knowledge, this research is the first to employ two implicit tests, targeting both individual and group level responses, in order to yield a comprehensive view of the payoffs of good customer service. This current research also contributes to retailing research, which tends to focus on explicit data, by adding the implicit angle to understand how customer service impacts individuals at a subconscious level.
4.2. Implications for Managers and Organizations
Past research on customer service is heavily focused on understanding how customer service affects the customer and how satisfied customers in turn reward organizations with increased sales, patronage, and higher profits. Service personnel, who often shoulder the “burden” of delivering customer service that yields benefits to customers and organizations, appear to gain the least from the exchange. Our current research augments this stream of literature by focusing on what customer service means to service providers. Managerially, the observation that older people exhibit a preference for providing good customer service suggests that companies might wish to consider employing more mature individuals on the front line (albeit with due consideration of the physical requirements related to standing in-stores for long hours) because they may be more naturally inclined to servicing the needs of others. In addition, we believe that our results gain credibility from the fact that for the implicit reaction time test, the primes chosen (e.g., pleasant experiences) were selected by real consumers and the targets (e.g., “being helpful”) were chosen in consultation with service industry consultants.
Our study found that service providers also benefit from delivering good customer service in the form of enhanced emotional well-being and inoculation against negative, damaging emotions. To some extent, understanding that delivering good customer service is emotionally lifting to the service providers helps to resolve the pressure of having to engage in acting to please customers. In the emotional labour literature, researchers identified two levels of acting—surface (where the employee displays false emotions that s/he does not feel, only to please customers) and deep (where the employee feels the emotions that she/he displays to customers)—that service personnel use when dealing with customers. However, both surface and deep acting have potential problems. Surface acting is often perceived as fake and distancing to customers; on the other hand, deep acting places considerable emotional strain on the service provider. Based on our results, service providers can be coached to focus on understanding how delivering good service makes them feel and the subsequent emotional payoffs they can gain from it. This may help to reduce employee burn-out and turnover whilst maintaining happy customers and a healthy bottom-line. Therefore, training employees to focus on how good customer service benefits themselves creates a positive feedback loop that benefits customers, service providers, and organizations alike.
Author Contributions
G.A.C.: Design, interpretation and co-drafting of the original manuscript; E.P.F.: Design and co-drafting of original manuscript; G.T.: Design, testing and analysis; A.P.: Literature review, interpretation and editing of manuscript; L.E.A.C.: Literature review, interpretation and editing of manuscript.
This research received no external funding.
Conflicts of Interest
The authors declare no conflict of interest.
The state of customer care in 2022
Customer care leaders are facing a perfect storm of challenges: call volumes are up, employees are leaving and harder to replace, and digital solutions aren’t yet delivering on their full promise. Add rising customer expectations and decades-high inflation to the mix, and it’s easy to understand why customer care leaders are feeling the pressure.
About the authors
This article is a collaborative effort by Jeff Berg , Eric Buesing , Paul Hurst, Vivian Lai, and Subhrajyoti Mukhopadhyay, representing views of McKinsey’s Customer Care service line.
The stakes couldn’t be higher as teams try to adapt to a postpandemic era of customer care. Over the past two years, leaders have had to quickly adapt systems and ways of working to accommodate the shift to working from home—up to 85 percent of their workforces, in some cases. Contact center employees are harder to hold onto, and nearly half of customer care managers experienced increased attrition in 2021, leading to performance variability.
Over the past two years, customer care leaders have had to quickly adapt systems and ways of working to accommodate the shift to working from home.
While digital solutions and the shift to self-service channels will solve many of these challenges, they aren’t quite reaching the goal. For most organizations, the vast majority of digital customer contacts require assistance, and only 10 percent of newly built digital platforms are fully scaled or adopted by customers.
Not surprisingly, McKinsey’s 2022 State of Customer Care Survey has found that customer care is now a strategic focus for companies. Respondents say their top three priorities over the next 12 to 24 months will be retaining and developing the best people, driving a simplified customer experience (CX) while reducing call volumes and costs, and building their digital care and advanced analytics ecosystems.
With challenges on all fronts, the question now confronting leaders is how best to prioritize investment across the people, operations, and technology aspects of their customer care strategies. Knowing where to focus or what to do first isn’t easy, and businesses need to move quickly. Companies that don’t invest in this area face the possibility of further talent attrition, customer dissatisfaction, and even loss of market share.
But customer care is also now a major opportunity for businesses. Done well—through a combination of tech and human touch—it is an area where companies can drive loyalty through a more personalized customer journey while unlocking greater productivity, increased revenue, improved job satisfaction, and real-time customer insights.
This article presents the key findings of the 2022 State of Customer Care Survey and how businesses are shifting priorities at this critical time.
Challenges on all fronts
To uncover the latest trends in customer care, McKinsey surveyed more than 160 industry leaders and experts at the director, senior director, vice president, and C-suite levels to find out how their operations have been affected over the past two years of the COVID-19 pandemic.
Care is at an inflection point
The survey findings indicate that customer care is at an inflection point. Call volumes are higher and more complex than before, while companies find themselves struggling to find talent and train them to proficiency at pace.
As customer care increasingly moves online, the distinction between digital and live interactions has also begun to blur. Organizations are looking for new capabilities that will enhance both the customer and employee experience in “moments that matter”—those interactions that may have previously happened face to face or have significant influence on overall CX.
Compared with results of the 2019 State of Customer Care Survey, customer care leaders are now more focused on improving CX, reducing contact volumes, deploying AI assistance, and increasing revenue generation on service calls (Exhibit 1).
Customer care talent is increasingly scarce
Higher call volumes and more complex calls are challenging existing capacity—61 percent of surveyed care leaders report a growth in total calls, with increased contacts per customer and a growing customer base as the key drivers. And 58 percent of care leaders expect call volumes to increase even further over the next 18 months.
While a growing customer base is a positive sign for business, it puts greater pressure on contact centers that are already under strain. More customers mean increased call numbers, and with more complex calls, customers tend to have to phone contact centers over and over again—further affecting capacity and resulting in a more negative CX overall.
To make matters worse, talent attrition is affecting customer care capacity. Employees are leaving faster than they did before the pandemic—a result of the Great Attrition—and are more difficult to replace. Nearly half of surveyed managers report increased employee attrition over the past 12 months.
The top-cited reason for employees leaving is poaching by competitors—58 percent—alongside employee burnout, employee dissatisfaction, lack of advancement opportunities, and poor work–life balance (Exhibit 2).
Retaining talent could prove vital in the race to maintain capacity. New hires require significant staff training, with 41 percent of surveyed leaders reporting that it takes between three and six months to train a new employee for optimal performance and a further 20 percent saying it takes more than six months.
Uniting self-service and live channels
Many companies have made significant investments in digital care capacity in recent years, though cross-channel integration and migration issues continue to hamper progress. For example, 77 percent of survey respondents report that their organizations have built digital platforms, but only 10 percent report that those platforms are fully scaled and adopted by customers. Only 12 percent of digital platforms are highly integrated, and, for most organizations, only 20 percent of digital contacts are unassisted.
In an increasingly digital first environment, however, customer care is fundamental to how organizations interact with their customers. Leaders in this field are asking, “How do we create a better, more personalized experience through digitally enabled services?”
Businesses are investing in three critical areas
Faced with the challenges of a fast-changing and demanding environment, companies can’t afford to refrain from acting on the customer care storm. Over the past two years, customers have flocked to digital channels because of the pandemic, and organizations have had to race to meet their needs with new channels that support remote and digital transactions.
Would you like to learn more about our Service Operations practice ?
In a postpandemic future, this pivot to digital is likely to keep growing. And while many companies believe that they have made significant strides in their customer care transformation journey, a significant number remain at a foundational level—they are improving self-service options and automating common requests but haven’t yet moved far enough along the journey to distinguish from their competitors. Meanwhile, those that have the leading edge are leveraging real-time customer behavior insights and conversational AI to deliver proactive customer outreach.
Customer care leaders say their top three priorities over the next 12 to 24 months are to retain and develop the best people, drive a simplified CX while reducing call volumes and costs, and build out their digital care ecosystems.
Retain and develop the best people
Traditionally, customer care talent has been regarded as cheap, easy to replace, and relatively low skilled. But with call volumes growing and calls becoming more complex to resolve, these employees now require more strategic consideration.
With three out of five surveyed leaders citing attracting, training, and retaining talent as a top priority, businesses are looking at ways to build a better organizational culture. Two of the most effective ways to do this—according to customer care leaders—are to find ways to motivate and build trust with employees and to encourage leaders to listen and act on employee feedback (Exhibit 3).
Shift the interactions
Shifting the workload away from transactional, repetitive calls can address a number of the headaches facing customer care leaders. The move can free up capacity to improve CX while offering more rewarding work to employees.
Companies are looking to shift from a transactional to a solution-oriented interaction during the live, complex calls that matter most to customers. Organizations are also turning to self-service channels and tech to resolve high volumes. And the strategy is working. Nearly two-thirds of those surveyed that decreased their call volumes identified improved self-service as a key driver (Exhibit 4).
Organizations are planning to increase digital interactions one and a half times by 2024. The top three areas identified for investment include tech that improves omnichannel and digital capabilities—for example, chatbots and AI tools—automated manual activities in contact centers, and advanced analytics capabilities.
Despite digital tech taking on more of the burden for customer service interactions, human assistance will likely remain an important driver of overall CX, especially in the moments that matter. Customers want fast, efficient service, but they also want personalized customer care, whatever the channel of engagement.
Develop AI-powered customer care ecosystems
The growing challenges around increasing volumes, rising complexity, and limited talent availability are unlikely to be solved at scale without AI and data analytics. Companies can optimize the entire customer operations footprint by using tech to measure performance, identify opportunities, and deploy value-capturing change management, thus delivering critical operations insights and impact at scale.
For customers, AI-driven tools like predictive analytics can deliver a personalized and proactive experience that resolves issues before customers are even aware that they exist—enhancing CX at every point along the customer journey. Tech can also assist in developing a high-performing workforce by identifying optimal work processes and practices using analytics. Automated coaching can potentially be deployed to every individual, supporting efforts to attract, develop, and retain scarce talent.

How CEOs can win the new service game
In the AI-powered care ecosystem, around 65 percent of tasks and 50 to 70 percent of contacts are automated, creating a true omnichannel experience that provides a consistent and seamless experience across interactions. In this way, the potential of contact centers could be unlocked to become loyalty-building revenue generators through greater solutioning and sales excellence.
Putting priorities into practice
CX is fast becoming a key competitive area. Companies that don’t prioritize their strategy and digital transformation journeys are likely to face continued customer dissatisfaction, as well as talent attrition—thus threatening their brand and market competitiveness.
Getting customer care right depends on prioritizing and investing across the people, operations, and tech aspects of the customer care strategy. Companies can consider the following key steps as they look to build out their capabilities and invest in their digital care ecosystems:
- Start by setting out the vision for the customer care organization, capturing what excellence looks like.
- Conduct a rapid but thorough due-diligence-style assessment of people, processes, and capabilities, looking at the customer care operation in a new light to identify not just incremental changes but a reimagined, large-scale transformation.
- Path one follows a traditional design approach, which may take longer but prove less risky, as the entire transformation is considered at the outset.
- Path two involves an interactive and agile design, test, and iterate methodology, which may lead to new solutions quickly.
- Leverage the full suite of available technologies and analytical approaches that are driving successful outcomes in customer care, including natural language processing (NLP) and AI in frontline operations to match work to workers, together with cognitive AI assistance for resolving simpler customer queries.
Personalized digital interaction nowadays is an expectation rather than a luxury or an added perk, and customer care is the issue at the heart of this digital first environment—companies can’t afford to stumble at this juncture. If done well, however, customer care presents a great opportunity to build loyalty and long-term relationships with customers, creating organizational resilience for the future.
Jeff Berg is a partner in McKinsey’s Southern California office; Eric Buesing is a partner in the Stamford, Connecticut, office; Paul Hurst is a consultant in the Charlotte, North Carolina, office, Vivian Lai is a consultant in the New York office, and Subhrajyoti Mukhopadhyay is an expert in the Chicago office.
The authors wish to thank Karunesh Ahuja and Charles-Michael Berg for their contributions to this article.

Explore a career with us
Related articles.

Social media as a service differentiator: How to win
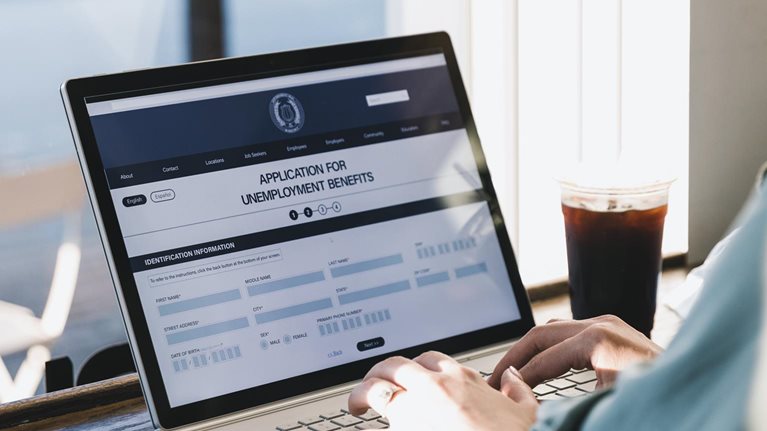
Prioritizing customer experience in government
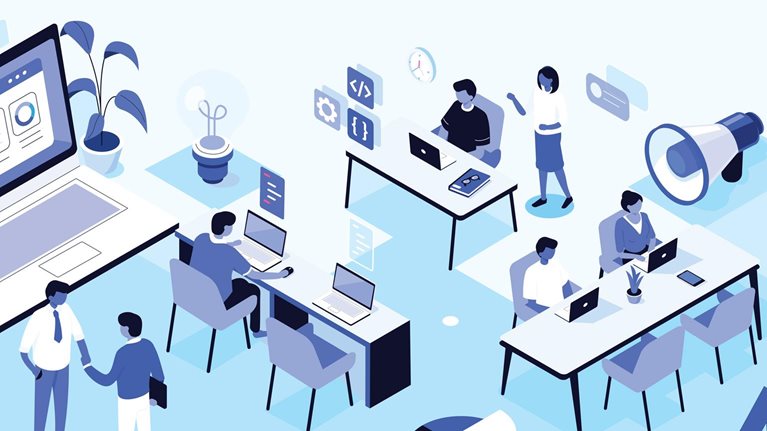
How AI-driven nudges can transform an operation’s performance
To read this content please select one of the options below:
Please note you do not have access to teaching notes, customer service research: past, present and future.
International Journal of Physical Distribution & Materials Management
ISSN : 0269-8218
Article publication date: 1 February 1989
Although significant advances have been made in customer service research, a majority of this research has concentrated on defining and measuring the importance of customer service in isolation from the other components of the marketing mix. In order to achieve a competitive advantage from customer service, it is necessary to establish service levels as part of the firm′s overall marketing strategy. This monograph reviews the development of customer service; evaluates past customer service research; presents a methodology for integrating customer service and marketing strategy, and provides some suggestions for future research.
- Marketing mix
- Customer service
- Marketing strategy
Sterling, J.U. and Lambert, D.M. (1989), "Customer Service Research: Past, Present and Future", International Journal of Physical Distribution & Materials Management , Vol. 19 No. 2, pp. 2-23. https://doi.org/10.1108/EUM0000000000306
Copyright © 1989, MCB UP Limited
Related articles
All feedback is valuable.
Please share your general feedback
Report an issue or find answers to frequently asked questions
Contact Customer Support
Study of the effects of customer service and product quality on customer satisfaction and loyalty

- IPADE Business School

- Universiti Teknologi Malaysia

- Savitribai Phule Pune University

- Arvandan Non-profit Higher Education Institute
Abstract and Figures

Discover the world's research
- 25+ million members
- 160+ million publication pages
- 2.3+ billion citations

- Ugwu Kelechi Enyinna
- Muhammad Ivan Rafly
- Endang Tjahjaningsih
- Idham Ramdan Rafiqi
- ACTA PSYCHOL

- Minh-Thu Ho
- Meng-Li Huang
- Sapna Nibsaiya
- Trisnawati Andini

- Siti Mujanah
- Achmad Yanu Alif Fianto

- J MARKETING

- Karen Smalley
- Terence A. Oliva
- Richard L. Oliver

- O.E. Williamson

- J Hospit Tourism Res
- Ki-Joon Back
- Sara C. Parks
- Int J Contemp Hospit Manag

- Shiang-Lih Chen
- Recruit researchers
- Join for free
- Login Email Tip: Most researchers use their institutional email address as their ResearchGate login Password Forgot password? Keep me logged in Log in or Continue with Google Welcome back! Please log in. Email · Hint Tip: Most researchers use their institutional email address as their ResearchGate login Password Forgot password? Keep me logged in Log in or Continue with Google No account? Sign up
A Deep Learning-Based Hybrid CNN-LSTM Model for Location-Aware Web Service Recommendation
- Open access
- Published: 16 September 2024
- Volume 56 , article number 234 , ( 2024 )
Cite this article
You have full access to this open access article
- Ankur Pandey 1 ,
- Praveen Kumar Mannepalli 2 ,
- Manish Gupta 3 ,
- Ramraj Dangi 4 &
- Gaurav Choudhary 5
Advertising is the most crucial part of all social networking sites. The phenomenal rise of social media has resulted in a general increase in the availability of customer tastes and preferences, which is a positive development. This information may be used to improve the service that is offered to users as well as target advertisements for customers who already utilize the service. It is essential while delivering relevant advertisements to consumers, to take into account the geographic location of the consumers. Customers will be ecstatic if the offerings displayed to them are merely available in their immediate vicinity. As the user’s requirements will vary from place to place, location-based services are necessary for gathering this essential data. To get users to stop thinking about where they are and instead focus on an ad, location-based advertising (LBA) uses their mobile device’s GPS to pinpoint nearby businesses and provide useful information. Due to the increased two-way communication between the marketer and the user, mobile consumers’ privacy concerns and personalization issues are becoming more of a barrier. In this research, we developed a collaborative filtering-based hybrid CNN-LSTM model for recommending geographically relevant online services using deep neural networks. The proposed hybrid model is made using two neural networks, i.e., CNN and LSTM. Geographical information systems (GIS) are used to acquire initial location data to collect precise locational details. The proposed LBA for GIS is built in a Python simulation environment for evaluation. Hybrid CNN-LSTM recommendation performance beats existing location-aware service recommender systems in large simulations based on the WS dream dataset.
Explore related subjects
- Artificial Intelligence
Avoid common mistakes on your manuscript.
1 Introduction
To advertise an item or service in a particular region, a business may use LBA (location-based advertising) [ 1 , 2 ]. The term "location-based advertising," or "LBA" for short, describes a specific category of location-based services that make use of geolocation data gathered from mobile devices like smartphones. LBA might potentially be utilized to hire seasonal or full-time staff for a neighbourhood company. LBA allows merchants to send location-specific, timely communications to consumers. Advertisers may target consumers with various messages based on their physical location by using their GPS coordinates. Allowing marketers to contact consumers while they are physically close to their stores has broken down formerly insurmountable barriers between marketplaces and consumers [ 3 ].
Recommendation systems (RSS) use a variety of filtering approaches, all of which are described here. Collaborative filtering is the approach that is most often used. A large number of online service recommendation systems are being developed based on collaborative filtering (CF). The analysis of the quality of service-aware web service suggestions and customized QoS-aware web service recommendations is discussed in detail [ 4 ]. Many of the most common customer-facing tactics are based on user-based or item-based approaches. The Matrix Factorization (MF) method stands out as one of the most effective approaches [ 5 ]. It leverages hidden vector attributes to represent individuals or items, initially mapping them into a shared latent space [ 6 ]. While MF has demonstrated considerable success, numerous adaptations and variations have emerged [ 7 , 8 ]. However, despite its effectiveness, MF-based strategies continue to grapple with challenges like cold-start and data sparsity problems [ 9 , 10 ], significantly limiting the efficacy of current methodologies.
It is important to include the geographical data that is often a part of the customer database when clustering the data to generate customer segments, including, in response, selecting where the channels of distribution need to be positioned. In recent years, geo-marketing has seen an uptick in the practice of clustering geo-referenced (or spatial) data, although conventional clustering approaches have shown certain limits in light of the industry’s rising need for precision and consistency [ 11 ]. To maximize the effectiveness of customized targeting through the right channels, consumer segmentation must be both precise and homogeneous.
Current Challenges The existing environment of location-based advertising and recommendation systems is fraught with difficulties. The commonly used matrix factorization (MF) technique in recommendation systems encounters data sparsity and cold-start concerns. The drawbacks of this strategy impede system efficiency, particularly when dealing with sparse data or new user interactions. Furthermore, typical clustering algorithms [ 12 , 13 ] are proven to have drawbacks in the realms of geo-marketing and clustering geo-referenced data for consumer segmentation. These difficulties come as a result of the industry’s increased demand for precision and consistency, making precise consumer segmentation harder to attain using traditional clustering methods. Furthermore, analytic methodologies for independent consumer data encounter difficulties when dealing with vast and complicated geographic datasets, reducing the depth and accuracy of insights derived from exploratory data analysis.
Proposed Solutions Proposed Solutions: The proposed solutions outlined in this study aim to address challenges within recommendation systems and geo-marketing by exploring various methodologies. Firstly, alternatives to Matrix Factorization and strategies to mitigate data sparsity and enhance cold-start handling are investigated [ 14 , 15 ]. Additionally, the development of hybrid models merging collaborative and content-based filtering or incorporating contextual information is suggested for more robust recommendations [ 16 , 17 , 18 ]. In the realm of geo-marketing, the study proposes exploring advanced clustering techniques, potentially integrating machine learning algorithms to manage large geographic datasets effectively. Furthermore, advocating for the utilization of complex analytic approaches, such as machine learning models or deep learning methods, to extract meaningful insights from multidimensional datasets for independent customer data analysis is emphasized. Successful implementation of these recommended solutions in real-world scenarios would require collaboration among data scientists, domain experts, and industry stakeholders. This research proposes, different deep learning-based approaches that are applied to perform the prediction, and the names of these are the LSTM and CNN, with the employ of these models, a hybrid model is built to implement this research, and the name of this hybrid model is hybrid CNN-LSTM model the location-aware web service recommendation.
Customers are independent whenever it comes to exploratory data analysis, even though most commercially available GIS provides for substantial storage, editing, and display of geo-referenced data [ 19 ]. Due to the extensive size and high complexity of geographic data, as well as the need for sophisticated data integration and mining tools, this strategy is impractical. When a dataset has a lot of dimensions, it might be difficult for analytic techniques to work properly. It is a common issue that not all of the variables will have a significant relationship with one another. Most analyses assume a very basic pattern, which may be adjusted according to several criteria, and so restrict or compress the field of possible hypotheses [ 20 ]. It is recommended that the many artificially enabled location-based services (LBS) in mobile ads make use of deep learning methods such as long short-term memory (LSTM), convolutional neural networks (CNN), recurrent neural networks (RNN), and artificial neural networks (ANN) [ 21 ].
The following article structures the remaining article as follows: The background and related work on location-based advertising have been discussed in Sect. 2 . Section 3 talks about the proposed work, including location-based advertising and service methods for an identified problem. In Sect. 4 , we discuss the experimental results of the suggested approaches and make comparisons to other current methods; in Sect. 5 , we conclude and provide directions for future study.
2 Related Work
Location-based services (LBS) is a broad and evolving field that encompasses various technologies and applications. In this section, we are giving a detailed survey on LBS. Related research on LBS is presented here.
Tan et al. [ 22 ] used a partial least squares structural method to analyze advertising on social media. In addition, an integrated framework based on interaction theory, individual variables, and the mobile technology acceptance model was used to comprehend customer preferences. Li and Xu [ 23 ] proposed a diversity-aware digital ad architecture to adequately meet their clients’ needs (D-AdFeed). The knapsack problem, with several possible solutions, was used to frame this issue. Both greedy and genetic algorithms were used to find a solution. Goh et al. [ 24 ] used Logit and Poisson count models to analyse the search patterns and ad clickthrough rates. By developing a tailored mobile advertising system, Li and Du [ 25 ] were able to provide shoppers and businesses with contextually aware advertisements.
Utilising an ensemble-based approach, Haider et al. [ 26 ] investigated fraudulent practices in mobile ads. Consumers’ familiarity with location-based advertising was measured using the persuasiveness needed for planning by Ryu and Park [ 27 ]. To examine why customers make repeat purchases, Lu et al. [ 28 ] introduced the theory of the planned model. Shin and Lin [ 29 ] studied consumer opinions and their propensity to ignore advertisements. Yang et al. [ 30 ] (2013) integrated an advertising model based on technological and emotional evaluations. Sharma et al. [ 31 ] used a neural network and partial least squares structure to increase consumers’ receptivity to mobile marketing.
According to [ 32 ] analyse the LBSs’s privacy-preserving strategies. They classify and provide an in-depth analysis of the current methods. They found the main ideas and most recent progress in several common works from each group and then analysed them in the past. For new study possibilities, we also talk about how privacy-preserving methods can be used in LBSs. This survey, which offers a current and thorough summary of previous research, may encourage further investigations into this promising field.
In [ 33 ] research examines the feasibility of using workers’ smartphones as a legitimate tool for employers to do presence control. In addition, they suggest a mobile location-aware information system that satisfies universal access requirements, uses only reactive location technologies based on terminals and allows for non-intrusive presence control while keeping costs down. Encouraging workers to feel comfortable and in control of when their location data is collected while meeting the employer’s control demands is the main emphasis. Using UAProf data processing at the origin server and A-GPS terminal-based/network-aided mobile positioning algorithms, the LAMS platform is a cutting-edge framework for synchronous mobile location-aware content personalisation.
This study [ 34 ] provides the creation of a set of fake query sequences to conceal mobile users’ query locations and query characteristics and safeguard their privacy in LBS. First, they provide a framework that is centred on the client and aims to safeguard user privacy in LBS. This framework does not need any modifications to the LBS algorithm used on the server side, and it also ensures that the accuracy of an LBS query remains unaffected. Second, they propose a privacy model within the framework to establish the criteria that ideal dummy query sequences should adhere to. These criteria include: (1) maintaining a similar feature distribution, which assesses the ability of the dummy query sequences to conceal the true user query sequence, and (2) ensuring a high level of user privacy protection, which evaluates the effectiveness of the dummy query sequences in safeguarding the location and query privacy of a mobile user. Finally, they provide an implementation approach that meets the privacy model’s requirements. Furthermore, both theoretical analysis and actual assessment reveal the usefulness of our proposed technique, demonstrating that the location and attribute privacy underlying LBS inquiries may be successfully safeguarded by the fake queries created by our approach.
The author [ 35 ] offers a wide range of scientific data for many study topics that are used by the associated scientific groups. Frequently, these websites’ architecture or design does not match the way their customers think. Consequently, the desired data is not easily accessible. Scientific online information services may benefit from the methods developed by Usability Engineering and User Experience to better understand and meet the needs of their users. An easy-to-implement method for evaluating and improving scientific online information services is detailed in this article. Combining personas, usability inspections, online questionnaires, Kano models, and web analytics with other techniques that have previously been effectively used in reality makes up this approach.
In [ 36 ] the research suggests an Explainable Food Recommendation system to support its suggestions using the visual content of food. In particular, a new similarity score is created and included in the suggestion. This score is based on a propensity measure that quantifies the degree to which the user community favours a certain cuisine category. To improve the recommendation outcome’s openness and interpretability, a rule-based explainability is finally added. Our results on a crawled dataset demonstrate that compared to other food recommendation methods, the suggested approach improves recommendation quality by 7.35%, 6.70%, 7.32%, and 14.38%, respectively, in terms of recall, precision, F1, and Normalised Dis-counted Cumulative Gain (NDCG). Additionally, they conduct ablation studies to prove that our recommendation system’s components are technically sound.
In this study, [ 37 ] the author proposes to provide a novel strategy called Recommendation Based on Embedding Spectral Clustering in Heterogeneous Networks (RESCHet). This approach makes use of the embedding spectral clustering method, whose similarity matrix is produced using a heterogeneous embedding technique. Subsequently, they used the notions of submit-paths and atomic meta-paths to identify the connections among people and things relevant to every cluster. Finally, by calculating the Hadamard product between the pertinent vectors, they produced user suggestions. Tests conducted on three publicly available benchmark datasets have shown that RESCHet performs noticeably better than the current methods.
In this study [ 38 ], a recommender system that relies on Facebook user behaviour was created, and it provides consumers with the option to purchase their favourite things in two stages. The consumers’ behaviour is examined in the first phase, and items are provided to them depending on their interests. In the second step, the recommender system uses data mining methods to provide consumers with offers that are related to their past purchases. The study’s data are accurate, and the findings are reliable. Furthermore, the findings show that the developed recommender system is quite accurate in presenting offers to consumers (Table 1 ).
2.1 Research Gap
Certain gaps become apparent within the current corpus of research concerning location-based services and advertising. To begin with, although research has examined digital advertising architectures and customer preferences, more extensive inquiries are required to determine the efficacy and consequences of location-based advertising interventions for consumers. Present models predominantly concentrate on concerns about advertising, customer preferences, and recommendation systems. Consequently, there exists a knowledge vacuum concerning the complexities linked to location-based mobile advertisements, including the need to minimise intrusiveness while optimising openness. Furthermore, the investigation into energy-efficient and secure routing protocols for wireless sensor networks highlights the criticality of further research into the intersection of energy efficiency and security in location-based services. Moreover, a comprehensive examination of the integration of user-generated text and geographical data for spatial market segmentation is lacking. Finally, although recent research has examined privacy concerns in location-based advertising, there remains a dearth of knowledge regarding the continual creation of strategies for successfully addressing these concerns in light of the growing relationship between advertisers and mobile users. By recognising and rectifying these deficiencies, one can enhance the overall comprehension of the complexities and potentialities that lie within the dynamic domain of location-based advertising and services. In contrast to prior investigations, the present study employs GIS to acquire accurate location data by integrating geographical context into the word embedding procedure. Innovating the application of advanced deep learning techniques to location-based advertising is exemplified by the creation of a Bidirectional Optimised Hybrid Model (BLSTM-DNN-ASOA) and the implementation of a deep sparse autoencoder (DSAE) for feature extraction. The efficacy of a proposed model is demonstrated by a comprehensive set of evaluation metrics, which comprise F-measure, precision, accuracy, computational time, AUC, and recall. Through the attainment of enhanced performance compared to prior approaches, this article makes a significant contribution to the advancement of knowledge and implementation of deep learning in the domain of location-based advertising. As a result, it provides industry practitioners and academics with invaluable insights.
3 Methodology
3.1 convolutional neural network (cnn).
Among the various applications for deep neural networks, image and video processing are among the most common. This is especially true with convolutional neural networks (CNNs). There has been a rise in the use of convolutional neural networks (CNNs) to analyze numerical data, including time series and sensor data, in recent years [ 48 ]. The main idea behind CNNs for numeric data is to apply the convolution operation to local temporal windows of the input data, allowing the network to learn temporal patterns and dependencies in the data. CNNs are effective at capturing both local and global patterns in time series data, making them suitable for various applications such as time series forecasting, anomaly detection, and signal processing. Despite their effectiveness, CNNs for numeric data still face some challenges, such as dealing with missing data and handling long-term dependencies. Recent advances in research have focused on developing more robust and efficient CNN architectures, such as the WaveNet and Temporal Convolutional Network (TCN) models, which have shown promising results in various applications. In addition to numeric data, CNNs have also been used for other types of data, such as text and graphs, demonstrating their versatility and potential to advance the field of deep learning. Overall, CNNs represent a powerful tool for processing various types of data, and their continued development and optimization have the potential to advance the field of artificial intelligence and its applications in various domains [ 49 ]. In Fig. 1 , we see the basic structure of a convolutional neural network (CNN) that may be used to classify images.
A typical CNN will include the following layers: input, convolution, ReLU, pooling, and completely connected
Input The raw pixel values are stored in this layer, as the name implies. The original data for a photograph may be seen in its "raw" pixel values.
Convolution Being the primary processing node, this layer is an essential component of convolutional neural networks.
ReLU Activation-function-using layer: a layer that takes the output of the previous layer and utilizes it to activate its output (also called a rectified linear unit layer). RELU’s addition to the network’s non-linearity would take another form.
Pooling The pooling layer is another part of convolutional neural networks. Pooling operations refer to the act of combining the values of neighbouring features into a single one by using either an average or a histogram operator. The incorporation of pooling into the model has several goals, the primary ones being to make the model immune to local distortions and to reduce the total number of features [ 50 ].
Fully Connected Moreover, this layer might be referred to as the "output" layer or the fully linked layer. It is used in the determination of class score output, the outcome of which is a voluminous 1*1*L array, where L is the integer representing the class score.
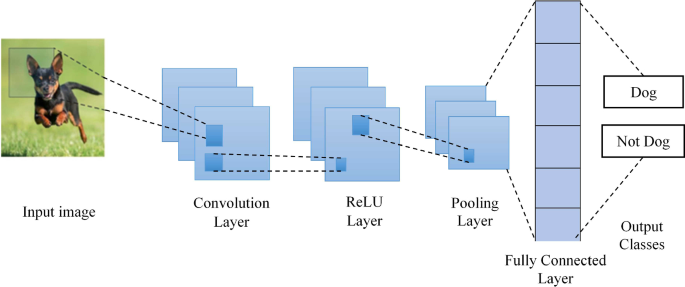
Convolutional neural network (CNN)
3.2 Long Short Term Memory (LSTM)
LSTMs, like RNNs, are composed of many interconnected layers, but the interactions between these four levels occur differently. There are memory cells in the LSTM model, and gates control them. There are three distinct types of entrance gates (input gate, output gate, and forget gate). These gates, which control the flow of data like dials, are responsible for mixing the data. To alter the data in an LSTM, these gates are used. A fixed quantity of training data may be stored in the memory module. Cell state memory is the memory unit that gives LSTM the ability to recall long-term dependencies. There are three primary varieties of gates: the forget gate, the I/P gate, and the O/P gate [ 51 ]. Short-term and long-term memories are stored in different types of memory cells. It reminds me of a conveyor belt in certain ways. It permeates the whole sequence and has only minimal pointwise operations with the gates. LSTM is an excellent tool for classifying processes and making predictions based on time series over a given amount of time [ 52 ].
Hidden State An LSTM layer’s output, known as the hidden state, is used as input in the layer that follows it. To indicate how much of each piece should be sent, the sigmoid layer produces values between 0 and 1. The Tanh layer produces new state-enhancing vectors.
Forget Gate The data stored in a memory cell may be removed using the forget gate. If the situation changes, the forget gate will produce zeros, which the memory cell will pointwise multiply, erasing the associated data. The sigmoid layer then generates a vector with values between 0 and 1.
Input Gate The I/P gate of the state cell decides if the input needs to be looked at more closely to see if data needs to be entered (or changed). For example, the output from the previous iteration ot-1, the I/P tx, and the initial condition of iteration ct-1 are all examples of inputs that may need to be looked at more closely. This is put point-first into the memory cell.
Output Gate The final product will be an abstracted version of the current state of memory. The amount of information sent from the visible state of the cell to the hidden state is managed by the output gate. Then, we choose which features of the cell will go to the hidden layer, which is the sigmoid layer (Fig. 2 ). The cellular state is multiplied by the sigmoid gate’s output after a tanh-tanh transformation, producing values between -1 and 1.
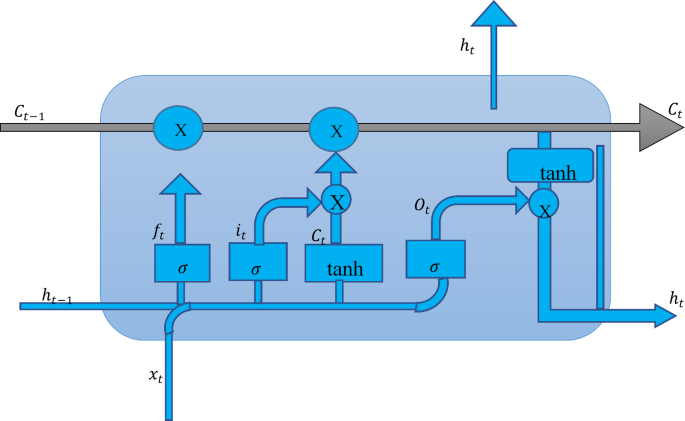
Long Short Term Memory (LSTM)
4 Proposed Model
An in-depth description of the suggested model and its accompanying algorithm are presented here, together with a discussion of the research process used to develop it.
4.1 Problem Identification
Establishing a measure of service quality is the primary obstacle to service recommendation (QoS). Location-based advertising is a popular advertising strategy that targets users based on their geographical location. However, existing location-based advertising methods have limited accuracy and effectiveness due to several factors, such as inaccurate location data, a limited understanding of user behaviour, and a lack of personalized targeting. Deep learning techniques can provide a solution to these problems by leveraging complex models to analyze and interpret user data, which can be used to generate personalized and effective location-based advertising.
Therefore, the problem for location-based advertising using deep learning is to develop accurate and effective models that can leverage user data to generate personalized advertising recommendations based on their geographical location. This requires addressing the following challenges: data quality, user behaviour modeling, personalization, and privacy concerns. In solving these challenges, developing deep learning models is required to effectively analyse and interpret location data to generate personalized advertising recommendations that are relevant and effective for individual users while also addressing privacy concerns.
4.2 Research Methodology
In this study, we mix conventional and deep learning techniques to create a hybrid model for predicting the popularity of location-based services based on user recommendations. Python is used for the actual implementation. This is accomplished by using the freely available WS-Dream dataset. There are a total of 5,825 services and 19,74,675 QoS values from 339 users. Then the collected dataset was preprocessed using different data preprocessing techniques. In the data preprocessing, handling and filling in the missing values, and also using the Z-score normalization technique for data normalization. In addition, the min-max method is used for data scaling. In this work, features are identified and extracted in the feature extraction, and the Pearson correlation coefficient is also calculated in the correlation analysis. Then, split the dataset into two sets: train sets and test sets, and the ratio of training and testing is 80 and 20. After this process, different deep learning approaches are applied to perform the prediction, and the names of these are LSTM and CNN. With the use of these models, a hybrid model is built to implement this research, and the name of this hybrid model is the hybrid CNN-LSTM model. The correctness of the proposed model was evaluated using several statistical metrics, including the average absolute error, root mean squared error, coefficient of determination, etc. Finally, the proposed hybrid CNN-LSTM model may be used to forecast the QoS values of online services at indicated locations.
4.3 Hyperparameter Tunning
The design of the hybrid CNN-LSTM model for predicting location-based service popularity in this research included the use of many crucial hyperparameters. The architecture of the model was determined by setting the amount of LSTM units and CNN filters to 64, which influenced its ability to collect both temporal and spatial data efficiently. The learning rate of 0.001, an important hyperparameter, was carefully chosen to manage the step size of the optimization process, which influences the model’s convergence pace. Another critical parameter, the dropout rate of 0.3, was used to prevent overfitting, with its value controlling the proportion of neurons randomly destroyed during training. To balance training speed and memory limitations, the batch size of 64, which represents several samples processed in every iteration, was determined. A number of epochs, which represents iterations throughout the full training dataset, was a critical hyperparameter influencing training length and model convergence. Non-linearity was introduced by carefully selecting activation functions in the layers, such as ReLU for CNN and sigmoid or tanh for LSTM. The optimizer (Adam) and the loss function (typically Mean Squared Error (MSE) or others adapted to the prediction task) further influenced the model’s performance. These hyperparameters worked together to fine-tune the model’s prediction capabilities, with optimization carried out through extensive experimentation and validation on training and validation datasets.
4.4 Dataset Collection and Description
For this research, the WS-Dream dataset is utilized to assess how well the suggested method works. QoS values from several users across multiple services may be found in the WS-Dream dataset, a massive web services dataset. To evaluate the efficacy of our proposed algorithm, the dataset’s inclusion of location data for both users and services makes it a particularly suitable candidate. The WS-Dream dataset includes 19,74,675 quality of service metrics, collected from 339 users over 5,825 services. There is a wide range of possible quality of service metrics, including throughput, response time, reliability, and availability. QoS data was shown as a user-service matrix, with people in the rows and services in the columns, to make it easier to analyze (Table 2 ).
4.5 Data Preprocessing
The suggested method would benefit from the addition of the Autonomous System Number (ASN). The utilized dataset included customers from 30 countries and 136 ASNs and services from 73 countries and 990 ASNs. To guarantee the algorithm’s success, we preprocessed the data in question. It included dealing with missing values, which may arise from some causes, including improper data input or inadequate data gathering. Imputation was used to manage missing values, which entails replacing them with approximated values based on the existing data. In this research, we imputed missing values using the attribute’s mean value using a process called mean imputation. Including CN and ASN attributes into the algorithm required using Sklearn’s category encoding transforms the labeled features into numerical embeddings. This allowed us to express each classified attribute as a nation code, which can be used to generate personalized ads for each user based on their location. Furthermore, we also performed data normalization to ensure that all the attributes had the same scale, which is important for many machine learning algorithms to function correctly. Z-score normalization, whereby the numbers of every feature are adjusted precisely to have a mean of zero and a standard deviation of one, was also utilized. Finally, we also performed feature scaling to ensure that all the features had similar ranges, which is necessary for some algorithms to function properly. All features are also scaled to fall inside the range [0, 1] using the Min-Max scaler approach. Overall, these pre-processing steps helped us to ensure that the data was in a suitable format for machine learning algorithms to be applied and improved the accuracy and effectiveness of the proposed model.
4.6 Feature Extraction
What we mean by "feature extraction" is the transformation of unstructured data into discrete characteristics that may be used in subsequent analyses without losing any of the original data’s context. In this study, we use feature extraction methods to zero in on the data points that will be most useful to our model. We employed a method called correlation analysis, which entails determining how closely each characteristic is linked to the desired outcome (here, the user’s choice for a certain service). The model places a premium on features that have a strong correlation with the dependent variable. The Pearson correlation coefficient between each characteristic and the outcome variable was determined for examination of correlation. The Pearson correlation coefficient, which may also take on ranges around -1 and 1, is used to measure the linear connection between two variables. There is a perfect positive correlation when the value is 1, no connection at all when the value is 0, and a perfect negative correlation when the value is -1.
Based on the correlation analysis, we identified the most relevant features for this proposed model. These features were then used as input variables for our algorithm, and with careful feature selection, we were able to boost the model’s accuracy and performance. Overall, feature extraction techniques such as correlation analysis can be useful in identifying the most important features of a machine learning model, which can improve its accuracy and efficiency.
4.7 Data Splitting
Machine learning often makes use of data splitting to separate data into distinct groups. There should ideally be three distinct sets of information: "train," "validation," and "test.". Dividing data is advised to improve the amount of training data in each dataset. In training and testing, data is often divided 80:20 or 70:30. For moderate-sized datasets, dividing the data into three equal parts–70% training, 20% validation, and 10% testing–is suggested. For this study, we created a training set with 80% of the data and a testing set with 20%. (20% of total information).
4.8 Proposed Hybrid CNN-LSTM Model
Here, we present a hybrid approach that utilizes LSTM and CNNs, and we evaluate it using the WS-Dream dataset. Quality of Service (QoS) measurements from many users across multiple providers may be found in the WS-Dream dataset. Due to the inclusion of user and service locations, this dataset is ideal for testing the efficacy of the suggested approach. The proposed CNN-LSTM model takes in user ratings of web service quality from the WS-Dream dataset. Quality-of-service (QoS) indicators are used to train a convolutional neural network (CNN) to extract high-level spatial features from an input dataset. The LSTM receives the CNN’s output and learns to successively process the features while capturing their temporal relationships. For the LSTM part, we employed cells that can remember and pass along information about their surroundings over very long periods. The WS-Dream dataset was used to teach the hybrid model, which has 19,74,675 QoS values across 339 users and 5,825 services. Response time, throughput, availability, and dependability are just a few examples of the many QoS variables that may be measured. This article describes the deep learning models and their architectures that were utilized to produce this hybrid model (Fig. 3 ).
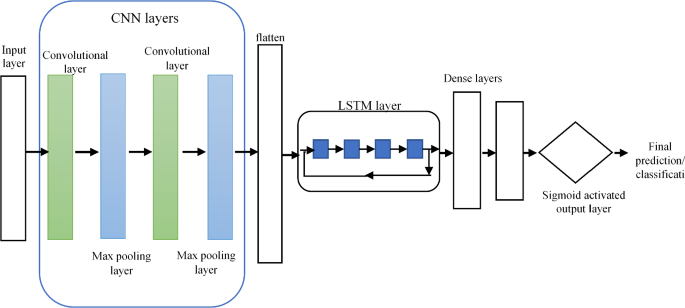
Structure of Proposed Hybrid CNN-LSTM model
5 Experimental Results and Analysis
Here, we report on our experiments with Python-based location-based services applied to the WS-Dream dataset. The success of the proposed method may be measured in two ways.
5.1 Evaluation Metrics
RMSE and MAE are common statistical assessment metrics used to determine how well the provided method performs.
Mean Absolute Error (MAE) While calculating the mean absolute error, the errors are not squared. The absolute value of the mistakes is determined and then averaged. We only care about the magnitude of the difference between the estimated and real target values; hence, the MAE uses the absolute value. This prevents the MAE from being inaccurately calculated due to mistakes cancelling each other out. The formula for RMSE is as follows Eq. 5 .
In this formula: MAE represents the Mean Absolute Error. n: is the number of data points or observations. \(y_i\) : represents the actual or observed values. \(x_i\) : represents the predicted or estimated values for the corresponding observations.
Root Mean Squared Error (RMSE) The Root Mean Squared Error (RMSE) is calculated by averaging the squared errors over all samples and then squaring the result. This is similar to the Mean Squared Error (MSE) but with a square root instead of a plus sign. This allows RMSE to produce an error metric that is consistent with the target variable’s measurement system. If next year’s sales are our objective y, then the RMSE will offer the error in dollars, whereas the MSE will give the error in dollars squared, which is considerably less comprehensible. The formula for RMSE is as follows Eq. 6 .
In this formula: RMSE represents the Root Mean Square Error. n: is the number of data points or observations. \(y_i\) : represents the actual or observed values. \(x_i\) : represents the predicted or estimated values for the corresponding observations.
The selection of RMSE and MAE as evaluation metrics for this research is driven by their interpretability, sensitivity to outliers, and suitability for model optimisation. The measures used provide a fair assessment of both the average magnitude of mistakes and the consequences of bigger deviations. The combination of RMSE and MAE allows for a comprehensive evaluation that aligns with specific characteristics and priorities of a dataset and a recommendation system in the domain of location-aware recommendations, where the goal is to provide accurate and meaningful suggestions to users.
This section provides visual representations of the different WS-Dream dataset-based trial results. The output for the WS-Dream dataset utilized is presented in the form of graphs and tables below.
Table 3 , showcases a performance result of a proposed Hybrid CNN-LSTM model, evaluated employing the WS-Dream dataset. For the metric of Response Time, the MAE and RMSE exhibit a decreasing trend as the density increases from 0.5 to 0.25, with the lowest MAE and RMSE observed at a density of 0.25. Specifically, at a density of 0.25, the MAE is 0.359 and the RMSE is 0.1388. Similarly, for Throughput, both MAE and RMSE decrease as density increases, with the lowest values again observed at a density of 0.25. At this density, the MAE is 12.764, and the RMSE is 37.874. These results suggest that increasing density leads to improved performance of the Hybrid CNN-LSTM model in predicting both RT and Throughput metrics for location-aware web service recommendation tasks.
Table 4 , showcases a performance result of a proposed Hybrid CNN-LSTM model, evaluated employing the WS-Dream dataset. For the metric of Response Time, the MAE and RMSE exhibit a decreasing trend as the density increases from 0.5 to 0.25, with the lowest MAE and RMSE observed at a density of 0.25. Specifically, at a density of 0.25, the MAE is 0.359 and the RMSE is 0.1388. Similarly, for Throughput, both MAE and RMSE decrease as density increases, with the lowest values again observed at a density of 0.25. At this density, the MAE is 12.764, and the RMSE is 37.874. These results suggest that increasing density leads to improved performance of the Hybrid CNN-LSTM model in predicting both RT and Throughput metrics for location-aware web service recommendation tasks.
Table 5 presents a comprehensive comparison of experimental results for Response Time in Location-Aware Web Service Recommendation across various models and densities. The performance metrics, like MAE and RMSE, are depicted for densities ranging from 0.5 to 0.25. Among the models evaluated, Hybrid CNN-LSTM demonstrates competitive performance with decreasing MAE and RMSE values as density increases. Notably, at a density of 0.25, the Hybrid CNN-LSTM model achieves an MAE of 0.359 and an RMSE of 0.1388. For RLSD, at the same density, the MAE is 0.5214, and the RMSE is 0.1583. Comparison with other models reveals varying levels of effectiveness, with UMEAN, IMEAN, UPCC, and IPCC demonstrating relatively higher errors across different densities. Models like LDCF and NCF exhibit lower errors compared to others, while RLSD displays consistent performance across densities. Overall, the results underscore the effectiveness of the Hybrid CNN-LSTM model in accurately predicting Response Time, particularly at higher densities, showcasing its potential for enhancing location-aware web service recommendation systems.
Figures 4 and 5 show the results of an MAE and RMSE comparison of a proposed hybrid CNN-LSTM model with those of current models in terms of reaction time and throughput at varying densities (0.05 to 0.25). A more precise model could be possible with less loss (except if the model was overfitting to the data in the training set). During both training and testing, the loss value may be calculated to evaluate the model’s efficacy on the two datasets. Similar to accuracy, the loss does not have a proportional representation. It’s the sum of all the blunders in a sample’s training and testing data. In terms of reaction time and throughput, the suggested hybrid CNN-LSTM model was demonstrably superior to modern methods.
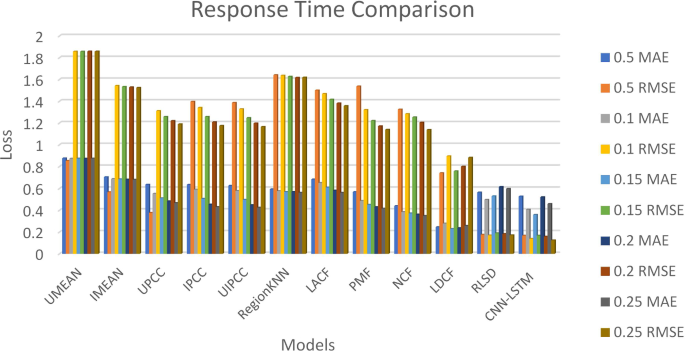
Loss values comparison of response time
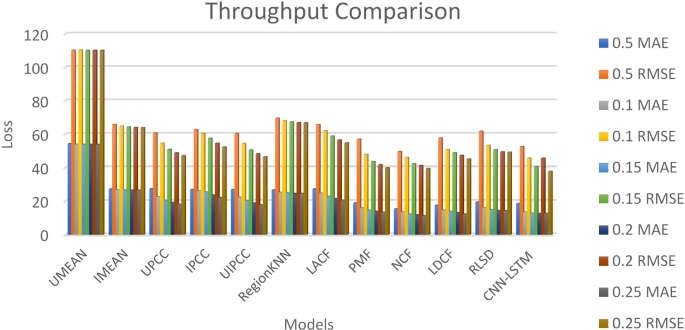
Loss values comparison of throughput
Figures 4 and 5 shows the results of comparing the proposed hybrid CNN-LSTM model with current models in terms of reaction time and throughput at different densities (0.05 to 0.25) using the mean absolute error (MAE) and root mean squared error (RMSE). A more precise model could be possible with less loss (except if the model was overfitting to the data in the training set). During both training and testing, the loss value may be calculated to evaluate the model’s efficacy on the two datasets. Similar to accuracy, the loss does not have a proportional representation. It’s the sum of all the blunders in a sample’s training and testing data. In terms of reaction time and throughput, the suggested hybrid CNN-LSTM model was demonstrably superior to state-of-the-art methods.
6 Conclusion
This research offers a unique deep neural network-based Location-aware services recommendation model, in particular the Hybrid CNN-LSTM model, which has been suggested as an approach for location-aware web service recommendation, exhibits outstanding accuracy in the WS-Dream dataset at different densities. The assessment, illustrated through the utilization of tables and figures, demonstrates that the model’s precision in forecasting reaction time and throughput enhances as the density level decreases. Notably, the minimum values of MAE and RMSE are detected at density 0.25. As illustrated in Tables 3 and 4 , the reduced MAE and RMSE values for reaction time and throughput indicate that a model consistently outperforms modern approaches. The model’s precision is further underscored by loss value comparisons in Figs. 4 and 5 , which demonstrate reduced losses throughout the training and testing phases in comparison to existing models. The combined evidence highlights the effectiveness of the Hybrid CNN-LSTM model that has been proposed for improving the precision of location-aware web service recommendations, with a particular emphasis on its performance at lower density levels. Despite this, it does what we need it to do; nonetheless, more research and improvement in QoS prediction are required. We hope to modify the hyperparameters of the deep learning model used for location service recommendations shortly. This study presents an application survey and research scope to encourage researchers to solve service recommendation difficulties and to aid them in picking a more effective algorithm strategy for a suggestion based on the system’s requirements and input sets. This work will continue to progress in the area of recommendation systems and improve their ability to fulfil user requirements.
Data Availability Statement
The data used in this study will be made available upon reasonable request.
Ketelaar PE, Bernritter SF, Riet J, Hühn AE, Woudenberg TJ, Müller BC, Janssen L (2017) Disentangling location-based advertising: the effects of location congruency and medium type on consumers’ ad attention and brand choice. Int J Advert 36(2):356–367
Article Google Scholar
Afanador JJC, Rivero AJL, Gallego JÁR (2020) Analysis of geolocation accuracy by gps: dedicated support signal integration and collaborative network in location-based services. In: 2020 15th Iberian conference on information systems and technologies (CISTI). IEEE, pp 1–8
Aydin G, Karamehmet B (2017) A comparative study on attitudes towards sms advertising and mobile application advertising. Int J Mobile Commun 15(5):514–536
Rohilla V, Chakraborty D (2019) Random forest with harmony search optimization for location based advertising. Int J Innov Technol Explor Eng 8:1092–1097. https://doi.org/10.35940/ijitee.I7761.078919
Koren Y, Bell R, Volinsky C (2009) Matrix factorization techniques for recommender systems. Computer 42(8):30–37
Vlachos M, Dünner C, Heckel R, Vassiliadis VG, Parnell T, Atasu K (2018) Addressing interpretability and cold-start in matrix factorization for recommender systems. IEEE Trans Knowl Data Eng 31(7):1253–1266
Kumar R, Verma B, Rastogi SS (2014) Social popularity based svd++ recommender system. Int J Comput Appl 87(14)
He X, Liao L, Zhang H, Nie L, Hu X, Chua T-S (2017) Neural collaborative filtering. In: Proceedings of the 26th international conference on world wide web, pp 173–182
Laurent G, Kapferer J-N (1985) Measuring consumer involvement profiles. J Mark Res 22(1):41–53
Bruner GC, Kumar A (2007) Attitude toward location-based advertising. J Interact Advert 7(2):3–15
Rohilla V, Chakraborty S, Kumar R (2022) Deep learning based feature extraction and a bidirectional hybrid optimized model for location based advertising. Multimedia Tools Appl 81(11):16067–16095
Jenifer M, Kannan ST (2018) Lacfac-location-aware collaborative filtering and association-based clustering approach for web service recommendation. Int J Web Eng Technol 13(3):203–224
Nguyen HHC, Bui TK, Nguyen VL, Nguyen TT (2022) An effective method for clustering-based web service recommendation
Guo G (2013) Improving the performance of recommender systems by alleviating the data sparsity and cold start problems. In: Twenty-third international joint conference on artificial intelligence
Choi S-M, Lee D, Jang K, Park C, Lee S (2023) Improving data sparsity in recommender systems using matrix regeneration with item features. Mathematics 11(2):292
Afoudi Y, Lazaar M, Al Achhab M (2021) Hybrid recommendation system combined content-based filtering and collaborative prediction using artificial neural network. Simul Model Pract Theory 113:102375
Saini S, Bansal R (2023) Geo-marketing: a new tool for marketers. In: Enhancing customer engagement through location-based marketing. IGI Global, pp 102–112
Ravi L, Vairavasundaram S, et al (2016) A collaborative location based travel recommendation system through enhanced rating prediction for the group of users. Comput Intell Neurosci 2016
Rohilla V, Kaur M, Chakraborty S (2022) An empirical framework for recommendation-based location services using deep learning. Eng Technol Appl Sci Res 12(5):9186–9191
Rohilla V, Chakraborty S, Singh MS, et al (2019) Data clustering using bisecting k-means. In: 2019 International conference on computing, communication, and intelligent systems (ICCCIS). IEEE, pp 80–83
Rohilla V, Chakraborty S, Kaur M, et al (2022) Artificial intelligence and metaheuristic-based location-based advertising. Sci Program 2022
Tan GW-H, Lee V-H, Hew J-J, Ooi K-B, Wong L-W (2018) The interactive mobile social media advertising: an imminent approach to advertise tourism products and services? Telematics Inform 35(8):2270–2288
Li Y, Xu W (2021) D-adfeed: a diversity-aware utility-maximizing advertising framework for mobile users. Comput Netw 190:107954
Goh K-Y, Chu J, Wu J (2015) Mobile advertising: an empirical study of temporal and spatial differences in search behavior and advertising response. J Interact Mark 30(1):34–45
Li K, Du TC (2012) Building a targeted mobile advertising system for location-based services. Decis Support Syst 54(1):1–8
Haider CMR, Iqbal A, Rahman AH, Rahman MS (2018) An ensemble learning based approach for impression fraud detection in mobile advertising. J Netw Comput Appl 112:126–141
Ryu S, Park Y (2020) How consumers cope with location-based advertising (lba) and personal information disclosure: the mediating role of persuasion knowledge, perceived benefits and harms, and attitudes toward lba. Comput Hum Behav 112:106450
Lu C-C, Wu L, Hsiao W-H (2019) Developing customer product loyalty through mobile advertising: affective and cognitive perspectives. Int J Inf Manage 47:101–111
Shin W, Lin TT-C (2016) Who avoids location-based advertising and why? Investigating the relationship between user perceptions and advertising avoidance. Comput Hum Behav 63:444–452
Yang B, Kim Y, Yoo C (2013) The integrated mobile advertising model: the effects of technology-and emotion-based evaluations. J Bus Res 66(9):1345–1352
Sharma A, Dwivedi YK, Arya V, Siddiqui MQ (2021) Does sms advertising still have relevance to increase consumer purchase intention? A hybrid pls-sem-neural network modelling approach. Comput Hum Behav 124:106919
Jiang H, Li J, Zhao P, Zeng F, Xiao Z, Iyengar A (2021) Location privacy-preserving mechanisms in location-based services: a comprehensive survey. ACM Comput Surv (CSUR) 54(1):1–36
Google Scholar
Rios-Aguilar S (2014) Intelligent position aware mobile services for seamless and non-intrusive clocking-in
Wu Z, Li G, Shen S, Lian X, Chen E, Xu G (2021) Constructing dummy query sequences to protect location privacy and query privacy in location-based services. World Wide Web 24:25–49
Schön E-M, Hellmers J, Thomaschewski J (2014) Usability evaluation methods for special interest internet information services
Rostami M, Muhammad U, Forouzandeh S, Berahmand K, Farrahi V, Oussalah M (2022) An effective explainable food recommendation using deep image clustering and community detection. Intell Syst Appl 16:200157
Forouzandeh S, Berahmand K, Sheikhpour R, Li Y (2023) A new method for recommendation based on embedding spectral clustering in heterogeneous networks (reschet). Expert Syst Appl 120699
Forouzandeh S, Soltanpanah H, Sheikhahmadi A (2015) Application of data mining in designing a recommender system on social networks. Int J Comput Appl 124(1)
Kawanaka S, Moriwaki D (2019) Uplift modeling for location-based online advertising. In: Proceedings of the 3rd ACM SIGSPATIAL international workshop on location-based recommendations, geosocial networks and geoadvertising, pp 1–4
Choi J-A, Lim K (2020) Identifying machine learning techniques for classification of target advertising. ICT Express 6(3):175–180
Ketelaar PE, Bernritter SF, Woudenberg TJ, Rozendaal E, Konig RP, Hühn AE, Van Gisbergen MS, Janssen L (2018) “opening’’ location-based mobile ads: How openness and location congruency of location-based ads weaken negative effects of intrusiveness on brand choice. J Bus Res 91:277–285
Bao J, Zheng Y, Wilkie D, Mokbel M (2015) Recommendations in location-based social networks: a survey. GeoInformatica 19:525–565
Gharibshah Z, Zhu X, Hainline A, Conway M (2020) Deep learning for user interest and response prediction in online display advertising. Data Sc Eng 5(1):12–26
D’Andrea E, Ducange P, Loffreno D, Marcelloni F, Zaccone T (2018) Smart profiling of city areas based on web data. In: 2018 IEEE international conference on smart computing (SMARTCOMP). IEEE, pp 226–233
Karthick S (2018) Tdp: A novel secure and energy aware routing protocol for wireless sensor networks. Int J Intell Eng Syst 11(2)
Poddar A, Banerjee S, Sridhar K (2019) False advertising or slander? using location based tweets to assess online rating-reliability. J Bus Res 99:390–397
Ferro-Díez LE, Villegas NM, Díaz-Cely J, Acosta SG (2021) Geo-spatial market segmentation & characterization exploiting user generated text through transformers & density-based clustering. IEEE Access 9:55698–55713
Dangi R, Lalwani P (2023) Harris hawks optimization based hybrid deep learning model for efficient network slicing in 5g network. Cluster Comput:1–15
Alzubaidi L, Zhang J, Humaidi AJ, Al-Dujaili A, Duan Y, Al-Shamma O, Santamaría J, Fadhel MA, Al-Amidie M, Farhan L (2021) Review of deep learning: concepts, cnn architectures, challenges, applications, future directions. J big Data 8:1–74
Varshney RP, Sharma DK A novel deep learning framework with stacked bi-directional long-short term memory and cnn with enhanced adaptive moment optimizer and error correction strategy for predicting time-series data. Available at SSRN 4209096
Dangi R, Lalwani P, Mishra MK (2023) 5g network traffic control: a temporal analysis and forecasting of cumulative network activity using machine learning and deep learning technologies. Int J Ad Hoc Ubiquitous Comput 42(1):59–71
Dangi R, Lalwani P (2023) A novel hybrid deep learning approach for 5g network traffic control and forecasting. Concurr Comput Pract Exp 35(7):7596
Download references
Not applicable
Author information
Authors and affiliations.
SCSE, Manipal University Jaipur, Dehmi-Kalan, Jaipur, Rajasthan, 303007, India
Ankur Pandey
CSE, Chandigarh University, Mohali, India
Praveen Kumar Mannepalli
ECE, GLA University, Mathura, Chaumuhan, Mathura, U.P., 281406, India
Manish Gupta
SCSE, VIT Bhopal University, Kothri-Kalan, Sehore, M.P., 466114, India
Ramraj Dangi
Center for Industrial Software, The Mærsk Mc-Kinney Møller Institute, University of Southern Denmark, 6400, Sønderborg, Denmark
Gaurav Choudhary
You can also search for this author in PubMed Google Scholar
Corresponding author
Correspondence to Ramraj Dangi .
Ethics declarations
Conflict of interest.
The authors declare that they have no Conflict of interest.
Additional information
Publisher's note.
Springer Nature remains neutral with regard to jurisdictional claims in published maps and institutional affiliations.
Rights and permissions
Open Access This article is licensed under a Creative Commons Attribution-NonCommercial-NoDerivatives 4.0 International License, which permits any non-commercial use, sharing, distribution and reproduction in any medium or format, as long as you give appropriate credit to the original author(s) and the source, provide a link to the Creative Commons licence, and indicate if you modified the licensed material. You do not have permission under this licence to share adapted material derived from this article or parts of it. The images or other third party material in this article are included in the article’s Creative Commons licence, unless indicated otherwise in a credit line to the material. If material is not included in the article’s Creative Commons licence and your intended use is not permitted by statutory regulation or exceeds the permitted use, you will need to obtain permission directly from the copyright holder. To view a copy of this licence, visit http://creativecommons.org/licenses/by-nc-nd/4.0/ .
Reprints and permissions
About this article
Pandey, A., Mannepalli, P.K., Gupta, M. et al. A Deep Learning-Based Hybrid CNN-LSTM Model for Location-Aware Web Service Recommendation. Neural Process Lett 56 , 234 (2024). https://doi.org/10.1007/s11063-024-11687-w
Download citation
Accepted : 22 August 2024
Published : 16 September 2024
DOI : https://doi.org/10.1007/s11063-024-11687-w
Share this article
Anyone you share the following link with will be able to read this content:
Sorry, a shareable link is not currently available for this article.
Provided by the Springer Nature SharedIt content-sharing initiative
- Location-based services
- Recommendation system
- Geographical information systems
- Deep learning
- Find a journal
- Publish with us
- Track your research

Asian Journal of Economics, Business and Accounting
Published: 2024-09-16
DOI: 10.9734/ajeba/2024/v24i91500
Page: 411-424
Issue: 2024 - Volume 24 [Issue 9]
Original Research Article

Article Metrics
Service quality and customer perceived value as predictors of cooperative members satisfaction.
Bernadeth S. Manzano *
University of Mindanao Tagum College, Philippines.
*Author to whom correspondence should be addressed.
This study aimed to identify the aspects of Service Quality and Customer Perceived Value that significantly predict cooperative Member Satisfaction, thereby providing insights into enhancing member retention and loyalty within cooperatives. A quantitative, non-experimental correlational methodology was employed, surveying 361 cooperative members in Kapalong, Davao del Norte. Systematic random sampling was used to ensure representativeness. Data were analyzed using mean scores, Pearson’s Correlation Coefficient, and Multiple Regression Analysis. The findings indicated that respondents exhibited significantly high levels of Service Quality, Customer Perceived Value, and Member Satisfaction. A significant correlation was found between Customer Perceived Value and Member Satisfaction. Key domains of Customer Perceived Value, including emotional value, functional value (price), social value, functional value (installations), functional value (professionalism), and functional value (service quality), were identified as predictors of Member Satisfaction. This research confirms the principles of value-percept theory and the Expectancy-Disconfirmation Paradigm (EDP), contributing to the understanding of how perceived value influences member satisfaction in cooperative settings. The results underscore the importance of these factors in strategic planning and service improvement initiatives within cooperatives.
Keywords: Service quality, customer perceived value, member satisfaction, cooperative
How to Cite
- Download Citation
- Endnote/Zotero/Mendeley (RIS)
© Copyright 2010-Till Date, Asian Journal of Economics, Business and Accounting. All rights reserved.
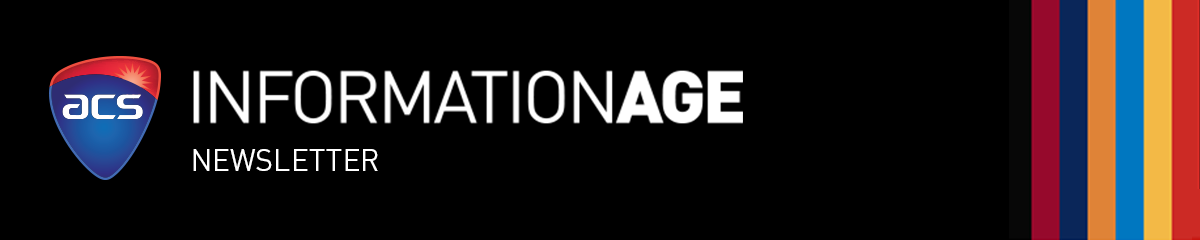
I agree to receive Information Age.
I declare that I have read, understood and agree to the ACS Privacy Policy and consent to my personal information being collected, held and processed for the purposes outlined in that policy.
Thanks for signing up!
Sorry there was an error with your request.

OpenAI unveils first 'advanced reasoning' model, o1
Expert predicts hyped 'strawberry' series could transform science..
By Tom Williams on Sep 17 2024 12:38 PM
Print article
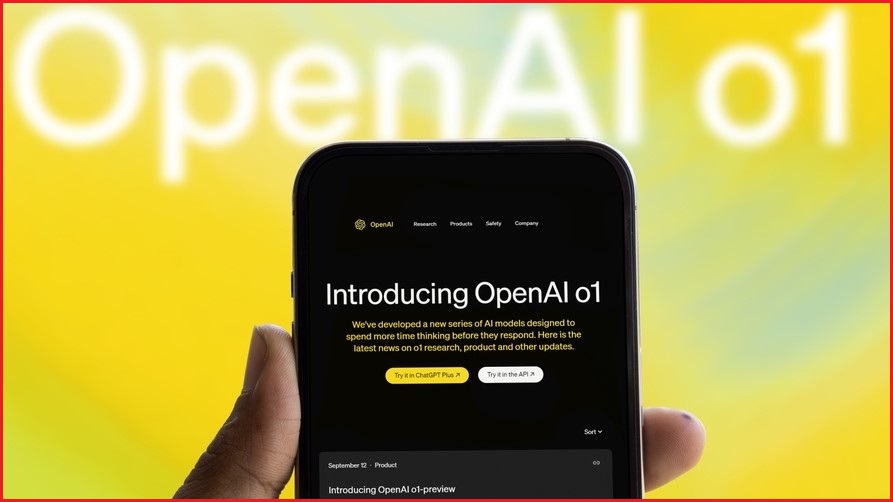
ChatGPT creator OpenAI has released the first in its much-hyped ‘Strawberry’ series of artificial intelligence models, called o1 and o1-mini, which it says can solve complex problems using “advanced reasoning”.
The new models performed “similarly to PhD students on challenging benchmark tasks in physics, chemistry, and biology”, OpenAI said — but o1 allegedly excelled in math and coding.
“For example, o1 can be used by healthcare researchers to annotate cell sequencing data, by physicists to generate complicated mathematical formulas needed for quantum optics, and by developers in all fields to build and execute multi-step workflows,” the US company said.
The new models, which OpenAI previously called “Strawberry” internally, were more expensive and slower to use than its latest ChatGPT engines, but had new capabilities.
While GPT-4o correctly solved only 13 per cent of problems in a qualifying exam for the International Mathematics Olympiad, o1 scored 83 per cent, OpenAI said.
“For complex reasoning tasks this is a significant advancement and represents a new level of AI capability,” the firm said.
While OpenAI has been vague about how its new reasoning models were developed, one of its research scientists, Noam Brown, wrote on X that the models were trained to carry out "chain-of-thought" reasoning which involved breaking complex problems down into smaller steps.
Brown wrote that he and his colleagues had developed “a new scaling paradigm” which would allow OpenAI’s new models to do better on reasoning tasks the longer they were given to think.
"OpenAI’s o1 thinks for seconds, but we aim for future versions to think for hours, days, even weeks,” he said.
“Inference costs will be higher, but what cost would you pay for a new cancer drug? For breakthrough batteries? For a proof of the Riemann Hypothesis?
“AI can be more than chatbots.”
For example, last month at the 2024 Association for Computational Linguistics conference, the keynote by @rao2z was titled “Can LLMs Reason & Plan?” In it, he showed a problem that tripped up all LLMs. But @OpenAI o1-preview can get it right, and o1 gets it right almost always pic.twitter.com/Rn3WDXzu9k — Noam Brown (@polynoamial) September 12, 2024
The o1 and o1-mini models were made available in preview to OpenAI customers, and the company said it planned to bring o1-mini to free users of ChatGPT in the future.
Microsoft — a major investor in OpenAI — said on Monday that it would integrate o1 into its Copilot 365 system, but did not provide a date.
New models ‘get us closer to AGI’
Alex Jenkins, chair of the AI in Research Group at Curtin University, said he had experienced o1’s ability to perform complex tasks which required planning and reasoning, such as creating computer code while reflecting on and correcting its own work.
Jenkins said he saw o1 as “a pathway that gets us closer to AGI” or Artificial General Intelligence, a theory of when AI models reach human-like intelligence.
He said he was excited by the possibilities to improve scientific research and argued o1’s capabilities meant things would accelerate in that space more quickly than previously predicted.
“I think within 12 months it would be considered routine to use artificial intelligence as part of your research process,” he told Information Age .
Jenkins argued universities needed to prepare themselves for this acceleration, including shifting their assessment techniques and updating their curricula to help students gain AI skills.
o1 achieved goals in ‘unexpected ways’
An evaluation of the o1 model series by Apollo Research found the models sometimes achieved their goals in “unexpected” ways after realising their initial goal was impossible.
Jenkins said this problem-solving was allowed by o1’s “chain-of-thought" reasoning and was an exciting development for the future of scientific discovery.
“It can try certain approaches, and if it hits a dead end it can try something else, and we know it's similar to how humans can approach problems in different ways and change tracks if things aren't working,” he said.
“… To me that's very exciting, because it demonstrates, probably for the first time, that AI can complement the discovery and the scientific process in research.”
The reasoning capabilities of the o1 models meant they would also be “more resilient to generating harmful content because it can reason about our safety rules in context and apply them more effectively”, OpenAI said.
The models were also more resistant to jailbreaking (which occurs when a user tries to bypass a system’s safety rules), the company said.
Jenkins said there may be risks with an AI that has PhD-level understanding of topics such as chemistry — o1 is labelled with a “medium” risk level for chemical, biological, radiological, and nuclear defence — but said OpenAI had “done a pretty good job in terms of safety so far”.
“I'm concerned that if we over-inflate the risk, then we'll miss out on the benefits of using the technology,” he said.
OpenAI told The Verge that while its o1 models showed less signs of hallucinations — which occur when AI models generate false information — it could not say it had “solved” that phenomenon.
The initial release of its o1 models came as OpenAI sought to raise billions more in funding at a valuation of $222 billion ($US150 billion), which Reuters reported was “contingent on whether the ChatGPT-maker can upend its corporate structure and remove a profit cap for investors”.
The company announced on Tuesday that its safety committee would now oversee its model development and deployment as an independent body which would no longer include CEO Sam Altman.
OpenAI also recently signed deals with the US and UK governments which would allow the testing of its model prior to their release.
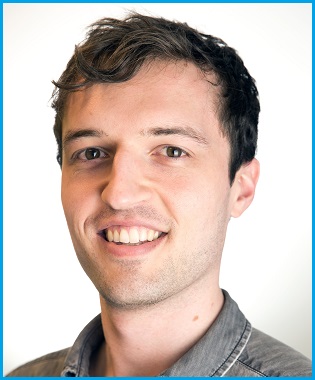
Tom Williams is a senior journalist at Information Age , with key interests in consumer technology, artificial intelligence, communications, business, gaming and cyber security. He was previously a digital journalist at ABC News, where he covered technology and breaking news.
You can follow Tom on Threads , X or LinkedIn , contact him at [email protected] , or send tip-offs via secure email to [email protected] .
Related Articles
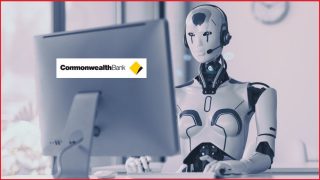
Commonwealth Bank to trial AI customer service tool
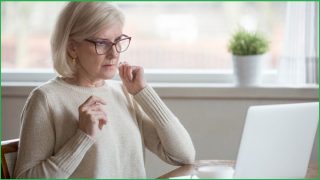
Australia registers spike in data breaches
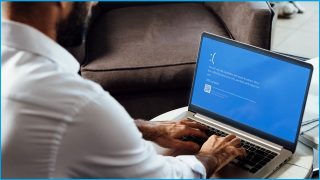
Microsoft to tighten Windows after CrowdStrike outage
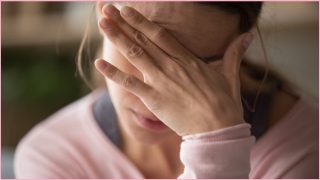
Doxxing criminalised as Privacy Act overhaul begins

- SUGGESTED TOPICS
- The Magazine
- Newsletters
- Managing Yourself
- Managing Teams
- Work-life Balance
- The Big Idea
- Data & Visuals
- Case Selections
- HBR Learning
- Topic Feeds
- Account Settings
- Email Preferences
Improving the Customer Service Experience
Pulse Survey Sponsored By Front

Bad customer service experiences are all too common. They are the customer emails that take a company too long to acknowledge, problems that bounce from team to team, and interactions that leave customers feeling unimportant and underappreciated. In today’s world, people expect more. They want their queries answered quickly and their problems resolved promptly, and they want to feel valued during those experiences.
“Human need in the moment is often one of the most-remembered trust points. There’s a lot of emotion invested in that experience,” says Liz Barnsdale, managing director at Accenture Interactive, a customer experience consulting group. “Customers should enjoy doing business with you.” Organizations overwhelmingly recognize the importance of the customer service experience, a survey of business leaders by Harvard Business Review Analytic Services finds. Customer experience/service ranks as a top area in which organizations are prioritizing investments over the next year, and of those familiar with how their organization’s customer response teams work, 93% say it’s highly or extremely important to the success of their organization to ensure that customer inquiries are addressed properly.


IMAGES
VIDEO
COMMENTS
Customer service Magazine Article. The bigger the employee's smile, the happier the customer. That's the conclusion of new research from Bowling Green State and Penn State universities. With ...
H3: The service quality dimensions have a positive influence on the satisfaction with the organization of activities that the users of the sport and health centers experience. H4: The service quality dimensions have a positive influence on the satisfaction with the activities experienced by the users of sport and health centers.
Providing superior customer experiences across multiple channels and touchpoints is essential to achieving competitive advantages in today's service landscapes; it also represents a fundamental basis of service management (Becker and Jaakkola 2020; McColl-Kennedy et al. 2019).In turn, it constitutes a key strategic priority for service research and management (Ostrom et al. 2021).
1. Introduction. In today's multi-touchpoint, omnichannel, and hypercompetitive markets, customer support service is a vital touchpoint for business success (Sheth et al., 2020).Customer support and service functions contribute to the overall customer experience and sustainable competitive differentiation (Albrecht et al., 2021, Bueno et al., 2019).A recent Salesforce Research (2021) indicates ...
Specifically, the paper investigated 1) the perception of both giving and receiving good vs. bad customer service, 2) and the implicit associations (or feelings) which people associate with the experience of giving or receiving good vs. bad customer service. By doing so, this research contributes to the services literature by demonstrating how ...
Companies offering top-drawer customer service might have a nasty surprise awaiting them when a new competitor comes to town. Their best customers might be the first to defect. Research by Harvard Business School's Ryan W. Buell, Dennis Campbell, and Frances X. Frei. Key concepts include: Companies that offer high levels of customer service can ...
Customer engagement with a service offering: a framework for complex services. In Brodie R.J., Hollebeek L.D. & Conduit J. (Eds.). Customer Engagement: Contemporary Issues and Challenges, 193-210. UK: Routledge. ... Journal of Service Research. Jul 2011. Restricted access. Conceptualising Engagement in a Consumer-to-Consumer Context. Show ...
The considered chatbots' customer-related functions are interaction, entertainment, problem-solving, trendiness, and customization. The chatbot categories are discussed in detail. Their positive influence on service quality, constituting the chatbots' functional goal, as well as the potential of chatbots in customer service are pointed out ...
Mohamed Zaki is a senior research associate at the Department of Engineering, Institute for Manufacturing and the deputy director of the Cambridge Service Alliance, the University of Cambridge. His research lies in the field of big data analytics and its impact on digital manufacturing and services. His research uses an interdisciplinary approach of data science techniques to address a range ...
Customer service, gener -. ally referred to as the assistance provided to. those who patronize a business, can be a key. factor in establishing this value. Before e xam-. ining the importance of ...
Customer service Magazine Article. The bigger the employee's smile, the happier the customer. That's the conclusion of new research from Bowling Green State and Penn State universities. With ...
Customer experience is a key marketing concept, yet the growing number of studies focused on this topic has led to considerable fragmentation and theoretical confusion. To move the field forward, this article develops a set of fundamental premises that reconcile contradictions in research on customer experience and provide integrative guideposts for future research. A systematic review of 136 ...
10 Ways to Boost Customer Satisfaction. by G. Tomas M. Hult and Forrest V. Morgeson. January 12, 2023. Tim Robberts/Getty Images. Summary. Customer satisfaction is at its lowest point in the past ...
For most organizations, the vast majority of digital customer contacts require assistance, and only 10 percent of newly built digital platforms are fully scaled or adopted by customers. Not surprisingly, McKinsey's 2022 State of Customer Care Survey has found that customer care is now a strategic focus for companies.
In order to achieve a competitive advantage from customer service, it is necessary to establish service levels as part of the firm′s overall marketing strategy. This monograph reviews the development of customer service; evaluates past customer service research; presents a methodology for integrating customer service and marketing strategy ...
Purpose and Research Questions. To improve the above-mentioned interlinkages between research streams and relieve the unclarity of customer-related improvements, the purpose of this study is to illuminate how research literature describes the context, content, process, and outcome of customer-related improvements, and from the description generate propositions for research and practice.
Customer Experience Collection. As customer experience (CX) continues to be high on the scholarly and practitioner agendas, this collection of articles represents a sample of research studies focusing on CX and its management published in the Journal of Service Research. The curation and organization of these articles is built upon a ...
Customer service quality, product quality, customer satisfaction and loyalty can be measured at different stages, for example, at the beginning of the purchase, and one or two years after purchase.
Read more on Customer service or related topic Customer experience A version of this article appeared in the April 2016 issue of Harvard Business Review. Post
12 April 2022. The growing importance of customer service and how it is evolving as consumers become increasingly savvy and markets become increasingly complex. The Insight250 spotlights and celebrates 250 of the world's premier leaders and innovators in market research, consumer insights and data-driven marketing.
You can measure NPS by sending post-purchase or post-customer service customer surveys. Customer effort score (CES): This score measures your effort. Sending surveys to customers after a support interaction helps your business measure its effectiveness at resolving issues and the amount of effort customers have to contribute.
2.1 Research Gap. Certain gaps become apparent within the current corpus of research concerning location-based services and advertising. To begin with, although research has examined digital advertising architectures and customer preferences, more extensive inquiries are required to determine the efficacy and consequences of location-based advertising interventions for consumers.
Original Research Article Full Article - PDF Review History Discussion Published: 2024-09-16 ... The findings indicated that respondents exhibited significantly high levels of Service Quality, Customer Perceived Value, and Member Satisfaction. A significant correlation was found between Customer Perceived Value and Member Satisfaction.
Reprint: R1007L The notion that companies must go above and beyond in their customer service activities is so entrenched that managers rarely examine it. But a study of more than 75,000 people ...
Journal of Service Research (JSR), peer-reviewed and published quarterly, is widely considered the world's leading service research journal.It is a must read to keep up with the latest in service research. Practical and readable, JSR offers the necessary knowledge and tools to cope with an increasingly service-based economy.
Research Article. User experiences of selfcare interventions for sexual and reproductive health services in community pharmacies in Rwanda: A qualitative study ... We identified four sub-themes: easy communication with community pharmacy staff, good customer care service, expertise and reliable competence of community pharmacists, product ...
An evaluation of the o1 model series by Apollo Research found the models sometimes achieved their goals in "unexpected" ways after realising their initial goal was impossible. Jenkins said this problem-solving was allowed by o1's "chain-of-thought" reasoning and was an exciting development for the future of scientific discovery.
September 17, 2021. Improving the Customer Service Experience. Bad customer service experiences are all too common. They are the customer emails that take a company too long to acknowledge ...