- Search Menu

Sign in through your institution
- Advance articles
- Author Guidelines
- Submission Site
- Open Access
- About Interacting with Computers
- About the BCS, The Chartered Institute for IT
- About Interaction
- Editorial Board
- Advertising and Corporate Services
- Journals Career Network
- Self-Archiving Policy
- Dispatch Dates
- Journals on Oxford Academic
- Books on Oxford Academic

- < Previous
The Effects of Task Difficulty and Multitasking on Performance
- Article contents
- Figures & tables
- Supplementary Data
Rachel F. Adler, Raquel Benbunan-Fich, The Effects of Task Difficulty and Multitasking on Performance, Interacting with Computers , Volume 27, Issue 4, July 2015, Pages 430–439, https://doi.org/10.1093/iwc/iwu005
- Permissions Icon Permissions
Multitasking is prevalent during computer-mediated work. Users tend to switch between multiple ongoing computer-based tasks either due to a personal decision to break from the current task (self-interruption) or due to an external interruption, such as an electronic notification. To examine how different types of multitasking, along with subjective task difficulty, influence performance, we conducted a controlled experiment using a custom-developed multitasking environment. A total of 636 subjects were randomly assigned into one of the three conditions: discretionary , where they were allowed to decide when and how often to switch tasks; mandatory , where they were forced to switch tasks at specific times; and sequential , where they had to perform tasks in sequence, without switching. The experimental environment featured a primary problem-solving task and five secondary tasks. The results show that when the primary task was considered difficult, subjects forced to multitask had significantly lower performance compared with not only the subjects who did not multitask but also the subjects who were able to multitask at their discretion. Conversely, when the primary task was considered easy, subjects forced to multitask had significantly higher performance than both the subjects who did not multitask and the subjects who multitasked at their discretion.
Personal account
- Sign in with email/username & password
- Get email alerts
- Save searches
- Purchase content
- Activate your purchase/trial code
- Add your ORCID iD
Institutional access
Sign in with a library card.
- Sign in with username/password
- Recommend to your librarian
- Institutional account management
- Get help with access
Access to content on Oxford Academic is often provided through institutional subscriptions and purchases. If you are a member of an institution with an active account, you may be able to access content in one of the following ways:
IP based access
Typically, access is provided across an institutional network to a range of IP addresses. This authentication occurs automatically, and it is not possible to sign out of an IP authenticated account.
Choose this option to get remote access when outside your institution. Shibboleth/Open Athens technology is used to provide single sign-on between your institution’s website and Oxford Academic.
- Click Sign in through your institution.
- Select your institution from the list provided, which will take you to your institution's website to sign in.
- When on the institution site, please use the credentials provided by your institution. Do not use an Oxford Academic personal account.
- Following successful sign in, you will be returned to Oxford Academic.
If your institution is not listed or you cannot sign in to your institution’s website, please contact your librarian or administrator.
Enter your library card number to sign in. If you cannot sign in, please contact your librarian.
Society Members
Society member access to a journal is achieved in one of the following ways:
Sign in through society site
Many societies offer single sign-on between the society website and Oxford Academic. If you see ‘Sign in through society site’ in the sign in pane within a journal:
- Click Sign in through society site.
- When on the society site, please use the credentials provided by that society. Do not use an Oxford Academic personal account.
If you do not have a society account or have forgotten your username or password, please contact your society.
Sign in using a personal account
Some societies use Oxford Academic personal accounts to provide access to their members. See below.
A personal account can be used to get email alerts, save searches, purchase content, and activate subscriptions.
Some societies use Oxford Academic personal accounts to provide access to their members.
Viewing your signed in accounts
Click the account icon in the top right to:
- View your signed in personal account and access account management features.
- View the institutional accounts that are providing access.
Signed in but can't access content
Oxford Academic is home to a wide variety of products. The institutional subscription may not cover the content that you are trying to access. If you believe you should have access to that content, please contact your librarian.
For librarians and administrators, your personal account also provides access to institutional account management. Here you will find options to view and activate subscriptions, manage institutional settings and access options, access usage statistics, and more.
Short-term Access
To purchase short-term access, please sign in to your personal account above.
Don't already have a personal account? Register
Month: | Total Views: |
---|---|
December 2016 | 3 |
January 2017 | 13 |
February 2017 | 20 |
March 2017 | 30 |
April 2017 | 52 |
May 2017 | 43 |
June 2017 | 54 |
July 2017 | 42 |
August 2017 | 33 |
September 2017 | 65 |
October 2017 | 70 |
November 2017 | 210 |
December 2017 | 476 |
January 2018 | 686 |
February 2018 | 829 |
March 2018 | 1,191 |
April 2018 | 1,387 |
May 2018 | 1,243 |
June 2018 | 928 |
July 2018 | 914 |
August 2018 | 1,181 |
September 2018 | 1,244 |
October 2018 | 1,078 |
November 2018 | 1,380 |
December 2018 | 869 |
January 2019 | 93 |
February 2019 | 89 |
March 2019 | 83 |
April 2019 | 108 |
May 2019 | 92 |
June 2019 | 47 |
July 2019 | 23 |
August 2019 | 34 |
September 2019 | 21 |
October 2019 | 74 |
November 2019 | 74 |
December 2019 | 65 |
January 2020 | 60 |
February 2020 | 29 |
March 2020 | 34 |
April 2020 | 24 |
May 2020 | 11 |
June 2020 | 36 |
July 2020 | 23 |
August 2020 | 16 |
September 2020 | 28 |
October 2020 | 40 |
November 2020 | 38 |
December 2020 | 13 |
January 2021 | 17 |
February 2021 | 21 |
March 2021 | 34 |
April 2021 | 23 |
May 2021 | 27 |
June 2021 | 12 |
July 2021 | 6 |
August 2021 | 18 |
September 2021 | 39 |
October 2021 | 19 |
November 2021 | 32 |
December 2021 | 31 |
January 2022 | 28 |
February 2022 | 25 |
March 2022 | 32 |
April 2022 | 45 |
May 2022 | 26 |
June 2022 | 17 |
July 2022 | 13 |
August 2022 | 18 |
September 2022 | 20 |
October 2022 | 27 |
November 2022 | 25 |
December 2022 | 10 |
January 2023 | 13 |
February 2023 | 38 |
March 2023 | 28 |
April 2023 | 28 |
May 2023 | 11 |
June 2023 | 5 |
July 2023 | 43 |
August 2023 | 26 |
September 2023 | 37 |
October 2023 | 49 |
November 2023 | 63 |
December 2023 | 32 |
January 2024 | 34 |
February 2024 | 77 |
March 2024 | 42 |
April 2024 | 99 |
May 2024 | 66 |
June 2024 | 114 |
July 2024 | 111 |
August 2024 | 19 |
Email alerts
Citing articles via.
- Recommend to your Library
Affiliations
- Online ISSN 1873-7951
- Copyright © 2024 British Computer Society
- About Oxford Academic
- Publish journals with us
- University press partners
- What we publish
- New features
- Open access
- Rights and permissions
- Accessibility
- Advertising
- Media enquiries
- Oxford University Press
- Oxford Languages
- University of Oxford
Oxford University Press is a department of the University of Oxford. It furthers the University's objective of excellence in research, scholarship, and education by publishing worldwide
- Copyright © 2024 Oxford University Press
- Cookie settings
- Cookie policy
- Privacy policy
- Legal notice
This Feature Is Available To Subscribers Only
Sign In or Create an Account
This PDF is available to Subscribers Only
For full access to this pdf, sign in to an existing account, or purchase an annual subscription.

An official website of the United States government
The .gov means it’s official. Federal government websites often end in .gov or .mil. Before sharing sensitive information, make sure you’re on a federal government site.
The site is secure. The https:// ensures that you are connecting to the official website and that any information you provide is encrypted and transmitted securely.
- Publications
- Account settings
Preview improvements coming to the PMC website in October 2024. Learn More or Try it out now .
- Advanced Search
- Journal List
- BMC Res Notes

Effects of task difficulty during practice on learning a dynamic balance task in healthy young adults: An intervention study
Simon schedler.
Division of Movement and Training Sciences/Biomechanics of Sport, University of Duisburg-Essen, Gladbecker Str. 182, 45141 Essen, Germany
Pascal Leifeld
Dennis brueckner, thomas muehlbauer, associated data.
The data generated and analyzed during the present study are available from the corresponding author upon reasonable request.
Cross-sectional studies reported increased postural sway during balance tasks with a high (e.g., unipedal stance on foam ground) compared to a low (e.g., unipedal stance on firm ground) level of task difficulty. Therefore, practicing/training balance tasks using high compared to low stimuli seems to be beneficial as it addresses larger adaptive reserves. Thus, the present study was performed to investigate the role of task difficulty during practice on learning a dynamic balance task in healthy young adults.
During acquisition, both practice groups (“Easy” or “Difficult” task condition) significantly improved their performance (i.e., time in balance). Further, the statistical analysis of post-practice performance revealed a significant main effect of test (i.e., better performance under easy compared to difficult test conditions, irrespective of group) but not of group. Additionally, the Group × Test interaction did not reach the level of significance, indicating that learning a dynamic balance task did not depend on the practiced task condition.
Introduction
Several cross-sectional studies have revealed that balance performance is affected by task-difficulty (e.g., variation of stance conditions; manipulation of sensory input; restriction of compensatory movements) [ 1 – 4 ]. For example, Barbado Murillo et al. [ 1 ] reported increasing sway amplitudes when participants balanced on a stable, medium stable, and unstable base, respectively. Further, Donath et al. [ 2 ] observed increased sway paths following the manipulation of stance condition from bipedal to step stance as well as following alterations to the base of support from firm to foam and the deprivation of visual information from eyes open to eyes closed. Lastly, Gebel et al. [ 3 ] were able to demonstrate that reducing the base of support diameter from 14 to 4 cm resulted in increased sway path. To summarize, increasing the level of balance task difficulty results in larger postural sway, indicating that the postural control system is more challenged. Practicing/training balance tasks with a high compared to a low level of task difficulty may therefore induce larger adaptations and thus be beneficial to improve balance performance.
However, so far only a few studies [ 5 , 6 ] have addressed this issue by repetitively and consecutively using different levels of task difficulty throughout interventions aiming to improve balance performance. Yet, they reported varying results as Blasco et al. [ 5 ] did not find significant differences following balance training with an easy compared to a difficult task level, whereas Schedler et al. [ 6 ] in parts (i.e., measures of proactive balance) reported larger improvements in the group training with a difficult task level during exercises, indicating the use of a larger adaptive range.
Based on these results, the present study aimed to investigate the effects of different levels of task difficulty during practice on learning a dynamic balance task. We expected that both practice conditions would result in enhanced balance performance. Due to higher stimuli involving the use of larger adaptive reserves during training with a high compared to low level of task difficulty we further hypothesize that the former (i.e., “Difficult task group”) will induce learning effects when performing the practiced (difficult) as well as the unpracticed (easy) task condition. Contrary, the latter (i.e., “Easy task group”) is expected to elicit learning effects only when performing the practiced (easy) but not the unpracticed (difficult) task condition.
Participants
With the use of G*Power 3.1.9.2 [ 7 ], the a priori power analysis ( f = 0.25, α = 0.05, 1- β = 0.80, number of groups: n = 2, number of measurements: n = 2, correlation among repeated measure: r = 0.55) yielded a total sample size of N = 32 participants (i.e., n = 16 participants per group). Therefore, thirty-two healthy young adults (16 females, 16 males) recruited from the local university were randomly assigned to either an “Easy task group” ( n = 16; age: 26.4 ± 2.4 years, body height: 175.6 ± 8.7 cm) or a “Difficult task group” ( n = 16; age: 25.6 ± 2.6 years, body height: 174.1 ± 7.6 cm). All participants had no prior experience with the motor task and were not aware of the specific purpose of this study. While the examiner was not blinded to group allocation, the participants were only aware of their own training condition (i.e., “easy” or “difficult” task), but did not know how other participants trained.
Experimental procedures
After the assessment of body height, participants were instructed to balance on a swinging wooden stability platform (Lafayette Instrument, Model 16030, Lafayette, LA, USA) in order to keep the platform horizontal (± 3 degrees) [ 8 ]. The platform (stabilometer) allowed a maximum deviation of ± 15 degrees (i.e., “Easy” task condition) or ± 25 degrees (i.e., “Difficult” task condition). During pre-practice testing (day 1) and post-practice testing (day 3), the participants of both groups performed one trial per task condition. In between, the acquisition phase occurred and included seven trials of practice on day 1 and day 2 under the respective task condition. Each trial lasted 90 s and was separated by a 90-s rest period. Participants performed the assessments and the practicing on three consecutive calendar days. Knowledge of result (i.e., time in balance) was provided after each trial during acquisition but not during pre- and post-practice testing.
Statistical analysis
Descriptive statistics were presented as group means ± standard deviations. Normal distribution was examined using the Shapiro–Wilk test ( p > 0.05) and homogeneity of variances using the Levene test ( p > 0.05). A 2 (group: “Easy”, “Difficult”) × 2 (test: easy, difficult) analysis of variance (ANOVA) with repeated measures on test was used to detect differences during pre- and post-practice testing. Further, a 2 (group: “Easy”, “Difficult”) × 2 (day: day 1 and 2) × 7 (trial: trial 1 to 7) ANOVA with repeated measures on day and trial was performed to assess group discrepancies during the acquisition phases. In addition, the partial eta-squared ( η p 2 ) was used as an effect size measure and classified as small (0.02 ≤ η p 2 ≤ 0.12), medium (0.13 ≤ η p 2 ≤ 0.25), and large ( η p 2 ≥ 0.26) [ 9 ]. All analyses were performed using the Statistical Package for Social Sciences version 27.0 and the significance level was set at p < 0.05.
Pre-practice testing (day 1)
The Group × Test ANOVA showed a significant main effect of test ( F (1, 30) = 31.177, p < 0.001, η p 2 = 0.510) but not of group ( F (1, 28) = 0.251, p = 0.620, η p 2 = 0.008), indicating better balance performance under easy compared to difficult task test conditions (Fig. 1 ). The Group × Test interaction ( F (1, 32) = 0.077, p = 0.784, η p 2 = 0.003) did not reach the level of significance, indicating that irrespective of the tested task the initial balance performance did not differ between groups.

Time in balance [i.e., ± 3 degrees of the horizontal plane] (s) during pre-practice testing (Day 1), acquisition phases (Day 1 and Day 2), and post-practice testing (Day 3) for the “Easy task group” (unfilled circles) compared to the “Difficult task group” (filled circles) (Values represent means and standard deviations. E = easy task test condition; D = difficult task test condition; # indicates significant (p < .001) differences between the two test conditions)
Acquisition phase (day 1 and 2)
Figure 1 illustrates that both the “Easy task group” and the “Difficult task group” enhanced their balance performance over the two days of practice. The Group × Day × Trial ANOVA yielded a statistically significant main effect of day ( F (1, 30) = 80.397, p < 0.001, η p 2 = 0.728) and trial ( F (6, 180) = 34.609, p < 0.001, η p 2 = 0.536) but not of group ( F (1, 30) = 2.462, p = 0.127, η p 2 = 0.076), indicating performance enhancements across days and trials. The Group × Day × Trial interaction ( F (6, 180) = 1.501, p = 0.180, η p 2 = 0.048) and the Day × Trial interaction ( F (6, 180) = 0.635, p = 0.702, η p 2 = 0.021) did not reach the level of significance, indicating that balance improvements did not depend on the practiced task condition.
Post-practice testing (day 3)
The Group × Test ANOVA revealed a significant main effect of test ( F (1, 30) = 52.482, p < 0.001, η p 2 = 0.636) but not of group ( F (1, 30) = 0.171, p = 0.682, η p 2 = 0.006), again indicating better balance performance during easy compared to difficult task test conditions (Fig. 1 ). The Group × Test interaction ( F (1, 32) = 0.761, p = 0.390, η p 2 = 0.025) did not reach the level of significance, indicating that learning a dynamic balance task was independent of the practiced task condition, irrespective of the tested task.
In contrast to our hypothesis, we did not find a significant Group × Test interaction during post-practice testing. This indicates that practicing under the easy compared to the difficult task condition did not result in group-specific learning improvements. Our finding is in line with the results of Blasco et al. [ 5 ] but in contrast to the work of Schedler et al. [ 6 ]. More specifically, Blasco and colleagues [ 5 ] examined the effects of different stability conditions on balance performance in young adults. In this regard, their participants trained on stable ground (corresponds to our “Easy task group”) or on unstable surfaces (corresponds to our “Difficult task group”). After three weeks of training, they observed improvements in several measures of balance performance (i.e., Emery test, Functional Reach test, Y Balance test) that were irrespective of group allocation. On the other hand, Schedler et al. [ 6 ] investigated the effects of balance training conducted under a low level (corresponds to our “Easy task group”) vs. a high level (corresponds to our “Difficult task group”) of task difficulty on balance performance. Besides others, they detected partially larger improvements in several balance outcomes (i.e., Functional Reach test, Y Balance test) in favor of the group that used a high level of balance task difficulty during training.
The discrepancy between our findings and Blasco et al. [ 5 ] compared to those of Schedler et al. [ 6 ] may be explained by methodological differences. First, the applied treatment period was considerably shorter in the present study (i.e., 2 days) and in the work of Blasco and colleagues (i.e., 3 weeks) when compared to Schedler and co-workers (i.e., 7 weeks). Therefore, it may be assumed that a longer practicing/training period is required to elicit adaptations according to the level of task difficulty. Second, different levels of task difficulty were applied but the same basic exercise per group was used in the current study (i.e., balancing on a stabilometer) and in the study by Blasco et al. [ 5 ] (i.e., bi-/unipedal stance). Contrary, in the study by Schedler et al. [ 6 ] the level of task difficulty as well as parts of the basic exercise (i.e., walking forward vs. backward; tandem stance vs. unipedal stance) differed between groups. Therefore, practicing-/training-programs should combine both alternatives (i.e., different tasks and different difficulty levels) rather than use them individually. Third, the present study and the study by Blasco et al. [ 5 ] investigated young adults, whereas Schedler et al. [ 6 ] examined adolescents. As the postural control system has not fully matured in adolescents [ 10 , 11 ], they might possess larger adaptive reserves than young adults. Therefore, a high level of task difficulty may be a sufficient stimulus in adolescents, but not provide potential for practice-/training-related improvements in young adults with a mature postural control system. Consequently, it should be investigated whether in adults other training modalities as for instance frequency, intensity or complexity of balance training are more effective to elicit adaptations.
We investigated the effects of task difficulty during practice on learning a dynamic balance task in healthy young adults. Irrespective of the practice regime, we did not detect a significant Group × Test interaction during post-practice testing. This is contrary to our hypothesis of better performances under the practiced and unpracticed condition for the “Difficult task group” but not for the “Easy task group” and indicates that in healthy young adults the learning of a dynamic balance task is independent of the applied level of task difficulty during practice.
Limitations
Using the variable “time in balance”, we analyzed the performance on a behavioral level but not the underlying neurophysiological domain. Therefore, it remains unclear whether the applied levels of task difficulty elicited adaptations on a neuronal (i.e., functional/structural brain changes) and/or muscular (i.e., changes in muscle activity) basis. Additionally, balancing on the stabilometer is a task with high internal but low external/ecological validity, which limits the transferability of the present results on balance tasks of various difficulties performed during everyday life or sports.
Acknowledgements
Not applicable.
Abbreviations
ANOVA | Analysis of variance |
D | Difficult task test condition |
E | Easy task test condition |

Authors’ contributions
SS, DB, and TM designed the study. DB, PL, and TS planned and supervised the testing and acquisition phases. DB, PL, and TS conducted the testings and data collections. SS, DB, and TM analyzed the data. SS and TM wrote the main parts of the manuscript. All the authors contributed to critical review of draft manuscripts and approved the final manuscript. All authors read and approved the final manuscript.
We acknowledge support by the Open Access Publication Fund of the University of Duisburg-Essen. The funding body is independent of the design of the study and collection, analysis, and interpretation of data and in writing the manuscript. Open access funding enabled and organized by the project DEAL.
Availability of data and materials
Declarations.
Ethical permission was given by the Human Ethics Committee at the University of Duisburg-Essen, Faculty of Educational Sciences. All procedures performed were in accordance with the ethical standards of the institutional and/or national research committee and with the 1964 Helsinki declaration and its later amendments or comparable ethical standards. Written informed consent was obtained from all participants included in this study.
The authors declare that they have no competing interests.
Publisher's Note
Springer Nature remains neutral with regard to jurisdictional claims in published maps and institutional affiliations.
ORIGINAL RESEARCH article
The effect of task difficulty and self-contribution on fairness consideration: an event-related potential study.
- 1 College of Physical Education, Yangzhou University, Yangzhou, China
- 2 Institute of Sports, Exercise and Brain, Yangzhou University, Yangzhou, China
Self-contribution may be an influential factor in fairness consideration and consequent behavioral decisions. Few studies have investigated simultaneous effects of task difficulty and self-contribution on fairness consideration outcomes and associated neurophysiological responses. To elucidate modulation effects of task difficulty and self-contribution on fairness consideration, 30 recruited participants played a modified ultimatum game (UG) while undergoing event-related potential measurements. A 2 (task difficulty: hard vs. easy) × 3 (contribution: other-contribution vs. both-contribution vs. self-contribution) × 2 (fairness type: fair vs. unfair) within-subject design was adopted. A significant interaction between fairness type and contribution was observed in the behavioral data, with unfair offers being more acceptable in the other-contribution condition than in the self-contribution or both-contribution conditions. In the early processing time window, feedback-related negative magnitudes were greater in the hard condition than in the easy condition. P300 responses were more pronounced when participants contributed equally to the proposer than in the self- and other-contribution conditions. These results demonstrated that individuals’ decisions are influenced by their own effort contributions relative to those of others in cooperative contexts.
Introduction
Typically, people show strong motivation for sustaining fairness in their interpersonal interactions. When confronted with unfairness, fairness consideration may lead people to sacrifice some financial benefit to themselves to punish others ( Fehr and Gachter, 2002 ; Hu et al., 2014 ; Fortin et al., 2020 ; Nai et al., 2020 ). Although this punitive behavior may not seem beneficial, from an evolutionary perspective, this pattern of behavior can benefit interpersonal interactions in the long term while reducing the probability of similar harm in the future when faced with the same situation ( Barclay, 2006 ; Krasnow and Delton, 2016 ).
Fairness consideration does not depend solely on direct trade-offs between oneself and others. Contextual factors, such as social distance ( Yu et al., 2015 ; Li et al., 2020 ), facial attractiveness ( Wu et al., 2011 ; Ma et al., 2017 ), other’s intentions ( Sutter, 2007 ; Guroglu et al., 2011 ), self-contribution ( Guo et al., 2014 ; Bland et al., 2017 ), and task difficulty can influence behavior related to fairness consideration. Among these contextual factors, each individual’s contribution is particularly important. A low-contributing individual may become less likely to be selected as a cooperator. In various real-world settings, allocation based on individual contribution is considered a common and fair approach. The natural desert theory predicts that people expect that their compensation should be based on their own efforts and contributions to collective resources ( Hoffman and Spitzer, 1985 ; Barker et al., 2012 ). Indeed, in neurophysiological studies, when participants played a more important part in earning activities, they were more likely to reject unfair offers; and greater self-contribution was associated with greater activity in the anterior insula, anterior cingulate cortex, dorsolateral prefrontal cortex, and temporoparietal junction when ( Guo et al., 2014 ; Feng et al., 2015 , 2019 ).
Task difficulty is a somewhat controversial putative factor in fairness consideration. Response time and error rate are the most common indices of task difficulty ( Gilbert et al., 2012 ). For example, by manipulating response time, Wang (2017) found that participants were more likely to make fair decisions in proportion to their contribution when working in an easy condition (neat arrangement of experimental materials) than when working in a hard condition (cluttered arrangement of experimental materials; Wang, 2017 ). Conversely, when Gilbert et al. (1988) controlled task difficulty by manipulating error rates, participants assigned to a hard memory task (memorizing a seven-digit number) had higher rejection rates for unfairness than participants assigned to an easy memory condition (memorizing a three-digit number; Gilbert et al., 1988 ). This apparent inconsistency might be due to the use of different control methods for task difficulty across studies or differences in the behavioral task used to assess fairness consideration. Thus, the findings of these studies should be interpreted with caution.
Although the aforementioned findings are suggestive of an important role of self-contribution and task difficulty in fairness consideration, few studies have manipulated these factors within one experiment. Typically, researchers have analyzed these two factors separately. In real life, both factors are considered and fairness is unlikely to be achieved if individuals consider only relative contribution regardless of difficulty. Thus, the ecological validity of a task or the assessment metric can better be achieved when both factors are considered together, which also would be expected to produce results that are more valuable with respect to solving real-life allocation problems.
The ultimatum game (UG) was adopted in this study to assess the effects of both a contribution factor and a difficulty factor on fairness consideration ( Güth et al., 1982 ; Camerer and Thaler, 1995 ; Fehr and Fischbacher, 2003 ). The UG is a widely used paradigm for exploring social decision-making on fairness consideration with key indicators of fairness-related decision-making. In a typical UG, one participant plays the role of a proposer while a second participants acts as the recipient. In each round, the two players are given a certain amount of money (e.g., 50 yuan). The proposer suggests how the money should be distributed, and the recipient accepts or rejects the offer (e.g., 45 yuan to proposer and 5 yuan to recipient). If the recipient accepts the offer, the money is distributed accordingly. If the recipient rejects the offer, nothing is given to either player. Fairness consideration underlies the players choices. In the present study, task difficulty and contribution were manipulated simultaneously in a modified UG task similar to that described in a prior study ( Guo et al., 2014 ).
High temporal resolution event-related potentials (ERPs) can show the time course of brain processing, including processes related to cognition, emotion, and decision-making ( Hillyard and Picton, 2011 ; Luck, 2014 ). Previous electroencephalogram (EEG) studies investigating the neurophysiological characteristics of fairness consideration in UG decision-making have suggested that unfair offers may induce more negative FRN (feedback-related negativity) amplitudes than fair offers, particularly in recipients with high fairness concerns ( Ma et al., 2017 ; Long et al., 2018 ; Jin et al., 2020 ). The FRN is a negative deflection component that appears 200 ~ 350 ms after stimulus onset ( Ma et al., 2015 ; Massi and Luhmann, 2015 ; Sambrook and Goslin, 2015 ). FRN amplitude has been related to people’s responses to negative events that violated fairness norms ( Boksem and De Cremer, 2010 ). Source localization analysis has shown that the FRN is derived mainly from the anterior cingulate or medial frontal cortex, a region associated with cognitive control, behavioral decision-making, and conflict monitoring ( Gehring and Willoughby, 2002 ; Polezzi et al., 2010 ). Some researchers have suggested that the FRN component might reflect conflict between cognition and emotional motivation ( Sanfey et al., 2003 ), especially in response to negative events, such as unfair offers in the UG task ( Boksem and De Cremer, 2010 ; Zhou and Wu, 2011 ).
The P300 ERP component—which has long been related to higher-order cognitive operations such as selective attention and resource allocation ( Polich and Kok, 1995 ; Hajcak et al., 2010 ; Massi and Luhmann, 2015 ; Zheng et al., 2015 ), especially in response to unexpected stimuli ( Olofsson et al., 2008 )—has also been related to fairness consideration. The P300 is a late positive peak that occurs 300 ~ 700 ms after the onset of feedback. It has been suggested that the P300 may be sensitive to a top-down outcome evaluation process, in which factors related to the allocation of attentional resources come into play, including reward valence, reward magnitude, and magnitude expectancy ( Hajcak et al., 2007 ; Leng and Zhou, 2010 ; Zhou and Wu, 2011 ).
Previous studies implicating self-contribution in fairness consideration have used mostly functional magnetic resonance imaging ( Guo et al., 2014 ). To our knowledge, this study is the first ERP-based fairness consideration study to investigate self-contribution and task difficulty simultaneously. We hypothesized that different degrees of self-contribution and task difficulty would shift individuals’ fairness consideration outcomes. Behaviorally, we predicted that there will be lower acceptance of unfair offers under high self-contribution and hard conditions. Moreover, we predicted that different neural response EEG patterns, including FRN and P300 amplitudes, would be associated with different levels of task difficulty and self-contribution. Specifically, we predicted that FRN amplitude may be more sensitive to fairness concern in a hard condition than in an easy condition whereas P300 amplitude may be more positive in the self-contribution condition than in other-contribution and both-contribution conditions.
Materials and Methods
Participants.
A sample size calculation performed in G*Power (version 3.1) suggested that 24 participants would ensure 80% statistical power in the case of a medium effect size (effect size of 0.25; alpha level of 0.05) ( Faul et al., 2007 ). We used convenience sampling to recruit research participants through notices posted on Yangzhou University campus. A total of 30 undergraduate and graduate students were enrolled as participants. Three were excluded, including two who reported in the post-experimental questionnaire that they did not believe the setup at all and one whose EEG recording had excessive artifacts. The remaining 27 participants (13 females) included in our statistical analyses had a mean (±standard deviation, SD) age of 21 (±2.4) years.
The participants completed the Chinese version of the Justice Sensitivity Inventory ( Schmitt et al., 2010 ), which contains four subscales (0 = not at all, 5 = very strongly): perpetrator sensitivity, victim sensitivity, beneficiary sensitivity, and observer sensitivity. The participants’ mean scores (±SD) on these subscales were 30.5 (±6.6), 25.6 (±5.5), 27.0 (±6.8), and 23.4 (±3.6), respectively.
All participants were right-handed and had normal or corrected to normal vision. They had no history of any neurological or psychiatric disorders. Written informed consent was obtained from each participant prior to the experiment. All participants were compensated for their participation with a base payment of 30 Chinese yuan and were informed that additional monetary rewards would be paid according to their performance in the task. The base payment was implemented to attract participants and the additional rewards were set to support task completion. In reality, all participants were paid an extra 20 Chinese yuan on top of the base payment.
The experimental procedure adhered to the Declaration of Helsinki and was approved by the Ethical Committee of Yangzhou University. All participants provided informed consent for participating in the study.
Design and Procedure
The experiment had a 2 × 3 × 2 within-subject design, with the first factor being task difficulty (hard vs. easy), the second being contribution (other-contribution vs. both-contribution vs. self-contribution), and the third being fairness type (fair vs. unfair). Task difficulty was set by manipulating the proportion of correct options in a ball-guessing game. In the easy and hard levels, participants selected which box contains a ball among two boxes (one-in-two) and among four boxes (one-in-four), respectively. Manipulating error rates in this way has been shown previously to alter difficulty ( Gilbert et al., 2012 ). Contribution was set by manipulating hit combinations by participants and partners. Fairness type was defined by offer size with 25:25 being defined as a fair offer and 5:45 and 15:35 being unfair offers.
The experiment was conducted with a 22-inch Lenovo desktop computer as a stimulus presentation device. The computer was equipped with a mouse and a screen with 1,024 × 768 resolution (horizontal and visual angles <5°). The participants were instructed about the rules of the experiment task through an explanation of the written instructions. The experimental preparation, stimulus presentation, and data collection were conducted in E-prime 3 software.
The experimental task consisted of nine 100-trial blocks. At the beginning of each trial, two (easy condition) or four (hard condition) opaque boxes were displayed for 5,000 ms on a computer screen against a grey background, with a small ball hidden in one of the boxes. The participant used their mouse to select the box where the small ball might be, while the partner made an offer decision. Outcomes of participants and partners were presented for 4,000 ms with indications of the respective contribution levels of each participant and their partner. If at least one person in the participant–partner pair guessed the ball location correctly, 50 points were awarded. If neither of them guessed correctly, they received nothing (reward points were converted into payment at the end of experiment). After the presentation of a black fixation point for 400–600 ms, the participant acting in the recipient role would receive a fair or unfair offer (e.g., “Partner: 45, You: 5”) in black Song font (size 32) in the center of the screen. The recipient had to decide to accept or reject each offer. If it was accepted, the recipient and proposer were paid accordingly. Both got nothing if the offer was rejected. Subsequently, the final proposal results were displayed on the screen for 2000 ms. The next trial began after a 200–300 ms blank screen. The trial time course presented in Figure 1 .
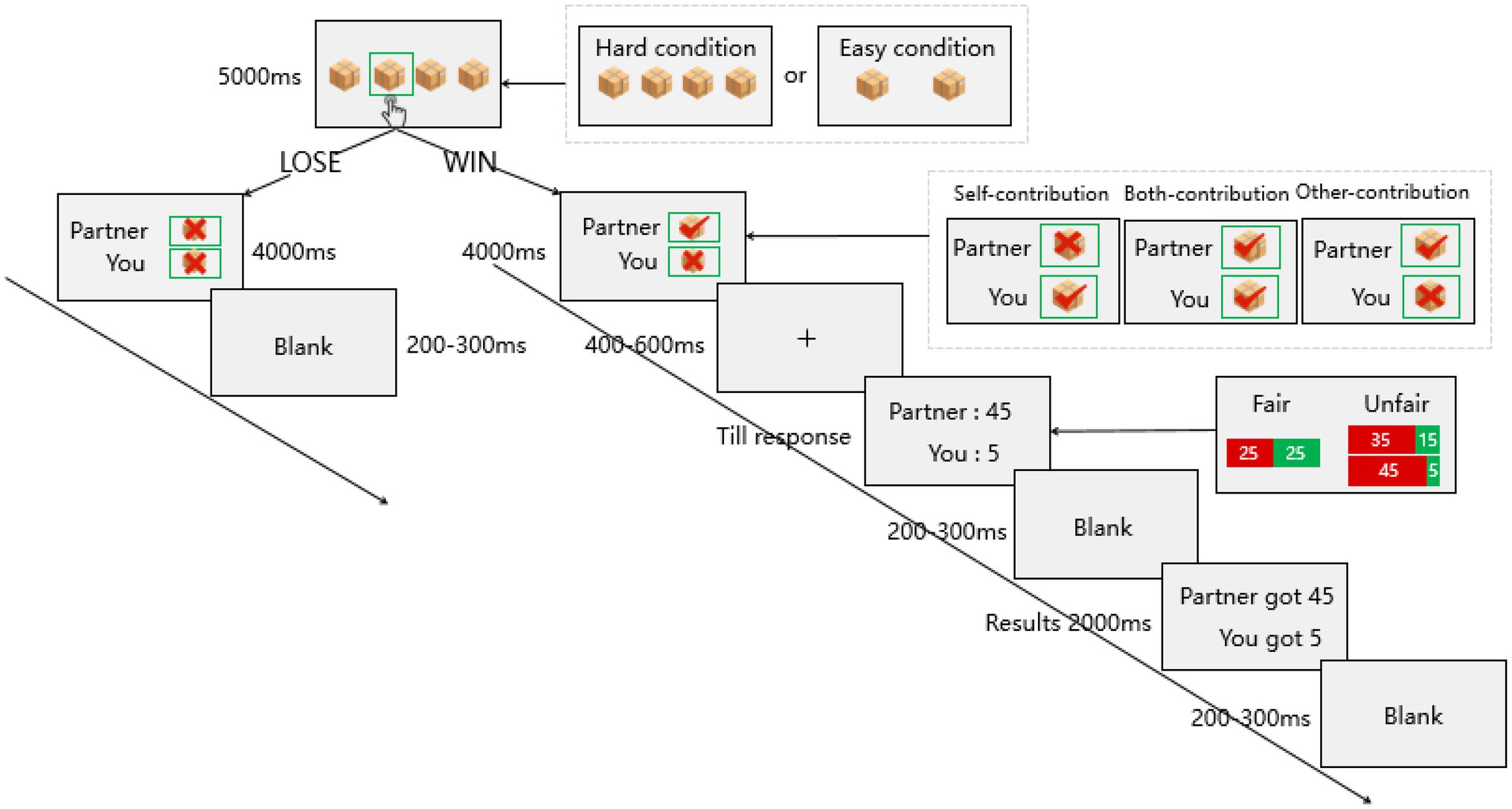
Figure 1 . Sequence of events within each trial.
From the perspective of each participant, the procedure started with meeting one’s “partner” (played by one of our experimental assistants) in the laboratory. To exclude the potential influence of gender and familiarity on fairness consideration, participants were paired with a same-gender assistants ( Eckel and Grossman, 1996 ; Flinkenflogel et al., 2017 ). Each participant was photographed with their partner standing against the wall with a digital camera. The photographs were used to make the experimental setup more realistic and to ensure that the participants’ believed they were interacting with real offers from real people. Subsequently, the participants in each pair were taken to separate rooms where they worked through a computer network to complete the entire task. The real participants were brought into a dimly lit, electromagnetically shielded room, seated comfortably at a viewing distance of 100 cm from the computer screen. During all trials, participants were instructed to direct their eyes to the center of the computer screen, where a plus sign (+) served as a central fixation point.
To facilitate procedural familiarization, the participants were required to perform 40 practice trials. After the practice trials, the participants entered the formal experiment, in which all proposer’s offers were pre-determined by a computer program and were presented in a pseudo-randomized and balanced.
EEG Recordings
EEGs were recorded from 64 scalp sites via tin electrodes mounted in elastic caps (Neuroscan Inc., Herndon, VA) according to the International 10–20 system. To monitor eye movements and blinks, a vertical electrooculogram (EOG) was recorded with left supraorbital and infraorbital electrodes and a horizontal EOG was recorded by electrodes placed 1.5 cm lateral to the right and left external mastoid. The EEGs and EOGs were amplified by SynAmps2 amplifiers; raw EEG data were referenced online using reference and ground electrodes. All electrode recordings were referenced offline relative to a mean value placed on the left and right mastoid. Continuous sampling was conducted at 1000 Hz/channel for offline analysis with electrode impedances maintained mainly below 5KΩ.
The EEG data were preprocessed with a 0.1–20 Hz (24 Db/oct) band-pass filter and analyzed in MATLAB 2020b with the EEGLAB toolkit. Blink, eye movement, electromyography artifacts were removed from the EEG signals. EEG epochs of 1,000 ms (with a 200 ms pre-stimulus baseline) were extracted offline and time-locked to the onset of each allocation offer. All trials with EEG voltages exceeding a ± 75-μv threshold range during the recording period were excluded from further analysis.
ERPs were identified based on visual inspection and previous ERP waveform studies. The FRN was measured as the peak amplitude in the 120–200-ms time window after the allocation offer onset. The P300 was measured as the peak in the 450–700-ms time window on each electrode. We conducted ERP response analysis on data from electrodes showing the predominant deflections for each ERP: the frontocentral electrode FCz for FRN responses and the parietal electrode Pz for P300 responses.
Data Analysis
All statistical analyses were conducted in SPSS software (version 22). The behavioral parameters of accepted choice percentage and accepted mean response time were subjected to repeated measures ANOVAs with three within-subjects factors: task difficulty (hard vs. easy), contribution (other-contributed vs. both-contributed vs. self-contributed), and fairness type (fair vs. unfair). If the ANOVAs yielded significant ( p < 0.05) interactions among task difficulty, self-contribution, and fairness type, then further F -tests were conducted to test for simple effects. When significant interactions were observed, interaction analysis was used. The Greenhouse–Geisser correction for violation of the sphericity assumption was applied where appropriate and the Bonferroni correction was used for multiple comparisons.
Acceptance rates for each allocation offer category are reported in Figure 2A . A repeated measures ANOVA revealed a significant main effect of fairness type [ F (1.26) = 228.03; p < 0.01; η p 2 = 0.89], indicating that the acceptance rate for fair offers (81 ± 2.4%) was higher than that for unfair offers (17 ± 2.0%). Although there was no main effect of contribution [ F (2.52) = 1.85; p = 0.16; η p 2 = 0.06], there was a significant interaction between fairness type and contribution [ F (2.52) = 37.84; p < 0.01; η p 2 = 0.59]. The interaction analysis revealed that unfair offers were more acceptable when the recipient was under the other-contribution condition (33 ± 3.2%) than when the recipient was under the self-contribution (7 ± 2.1%) or both-contribution (10 ± 2.2%) condition ( p s < 0.01). In the fair condition, acceptance rates for fair offers did not differ significantly between the both- and self-contribution conditions ( p = 0.21) and there was not a significant main effect of task difficulty [ F (1.26) = 1.02; p = 0.32; η p 2 = 0.03]. The other two-way interactions [task difficulty × fairness type: F (1.26) = 3.09; p = 0.09; η p 2 = 0.10; contribution × task difficulty: F (2.52) = 1.02; p = 0.36; η p 2 = 0.03] and the three-way interaction [task difficulty × contribution × fairness type: F (2.52) = 0.32; p = 0.72; η p 2 = 0.01] failed to reach statistical significance.
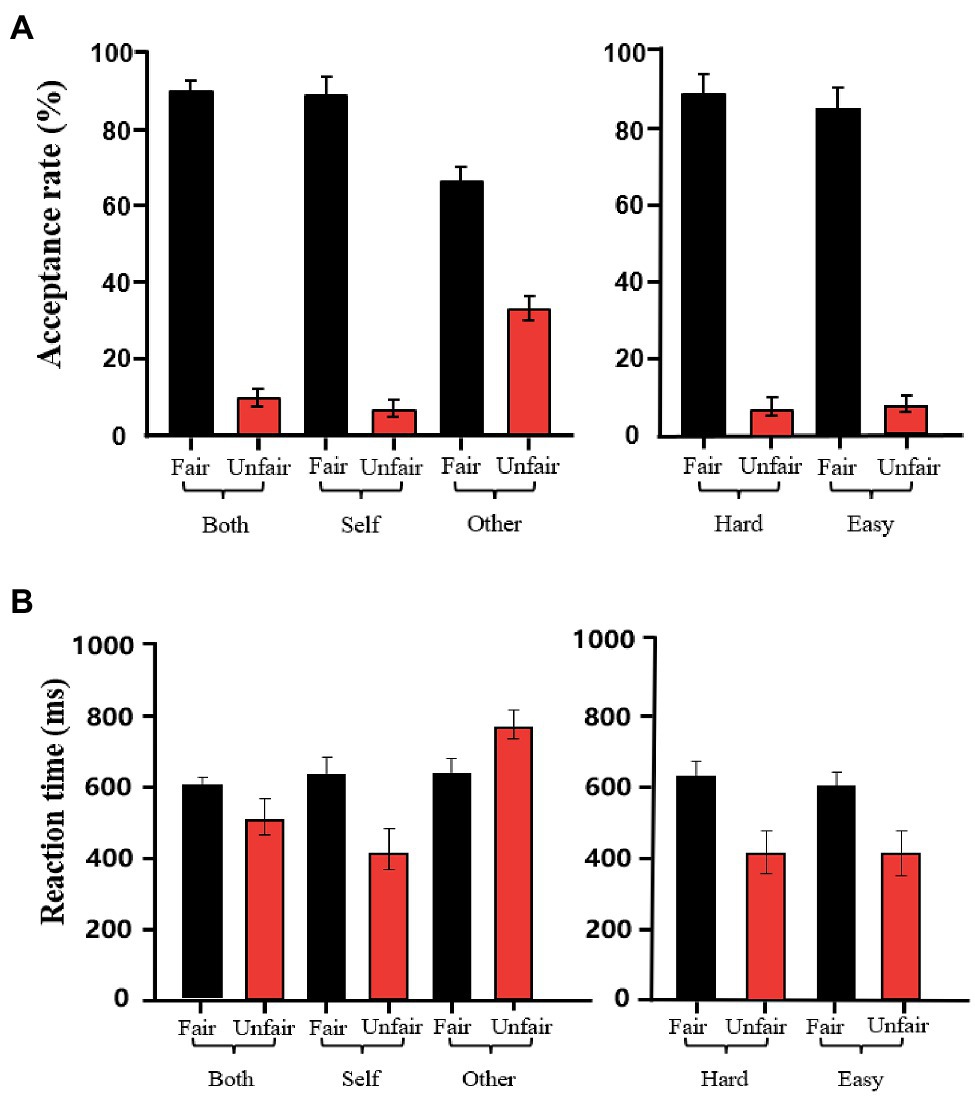
Figure 2 . Comparisons of behavioral parameters across conditions. (A) Acceptance rates. (B) Reaction times. All data are presented as the mean ± SEM.
Mean reaction times for each allocation offer category are shown in Figure 2B . A repeated measures ANOVA revealed a significant main effect of contribution [ F (2.52) = 18.39; p < 0.01; η p 2 = 0.28]. Post-hoc analysis showed that the recipients exhibited faster mean reaction times in the self-contribution condition (527 ± 33 ms) than in the other-contribution (720 ± 32 ms; p = 0.01) or both-contribution (567 ± 21 ms; p < 0.01) conditions. Reaction times for the both- and other-contribution conditions were similar ( p = 0.54). There was also a significant main effect of fairness type [ F (1.26) = 6.18; p = 0.04; η p 2 = 0.12], with recipients exhibiting a faster reaction time in the unfair condition (572 ± 27 ms) than in the fair condition (638 ± 23 ms). There was a significant interaction between fairness type and contribution [ F (2.52) =18.57; p < 0.01; η p 2 = 0.30]. The interaction analysis revealed that the reaction time difference between fair and unfair offers was greater in the self-contribution condition (215 ± 11 ms) than in the other-contribution (137 ± 5 ms) and both-contribution (101 ± 23 ms) conditions ( p s < 0.05). However, no significant main effect of task difficulty was found [ F (1.26) = 2.10; p = 0.16; η p 2 = 0.07]. No interaction was found between the contribution and task difficulty [ F (2.52) = 0.88; p = 0.42; η p 2 = 0.03], nor between task difficulty and fairness type [ F (1.26) = 0.74; p = 0.39; η p 2 = 0.02]. No significant three-way interaction was found among task difficulty, contribution, and fairness type [ F (2.52) = 0.45; p = 0.63; η p 2 = 0.04].
We also allocated participants into high-score and low-score groups based on their scores on the justice sensitivity inventory. There were no significant differences between these two justice sensitivity groups on UG task ( t = 0.68, p = 0.50), with 91.99 ± 10.8% acceptance rate of fair offers in high-score group, and 88.57 ± 12.87% in low-score group, demonstrating that our results should not be sensitive to justice sensitivity.
Event-Related Potentials
At least 50 averaged EEGs were obtained in each condition for each of the 27 participants. Grand waveforms obtained with 0.1–20-Hz band-pass filtering are shown for each experimental condition in Figures 3A , B , and the FRN amplitudes are shown in Figures 4A , B . There were not significant main effects of task difficulty [ F (1.26) = 0.28; p = 0.59; η p 2 = 0.11], contribution [ F (2.52) = 0.56; p = 0.57; η p 2 = 0.02] or fairness type [ F (1.26) = 8.61; p = 0.24; η p 2 = 0.07]. There was a significant interaction between task difficulty and fairness type [ F (1.26) = 6.52; p = 0.003; η p 2 = 0.29], but no other significant interactions [task difficulty × contribution F (2.52) = 1.81, p = 0.17, η p 2 = 0.06; contribution × fairness type F (2.52) = 2.11, p = 0.13, η p 2 = 0.07; fairness type × task difficulty × contribution F (2.52) = 5.98, p = 0.18, η p 2 = 0.05]. The interaction analysis revealed that the FRN effect (unfair minus fair) differed between the hard condition (0.20 ± 0.03 μv) and the easy condition (−2.31 ± 0.38 μv), t(26) = 0.95, p = 0.03.
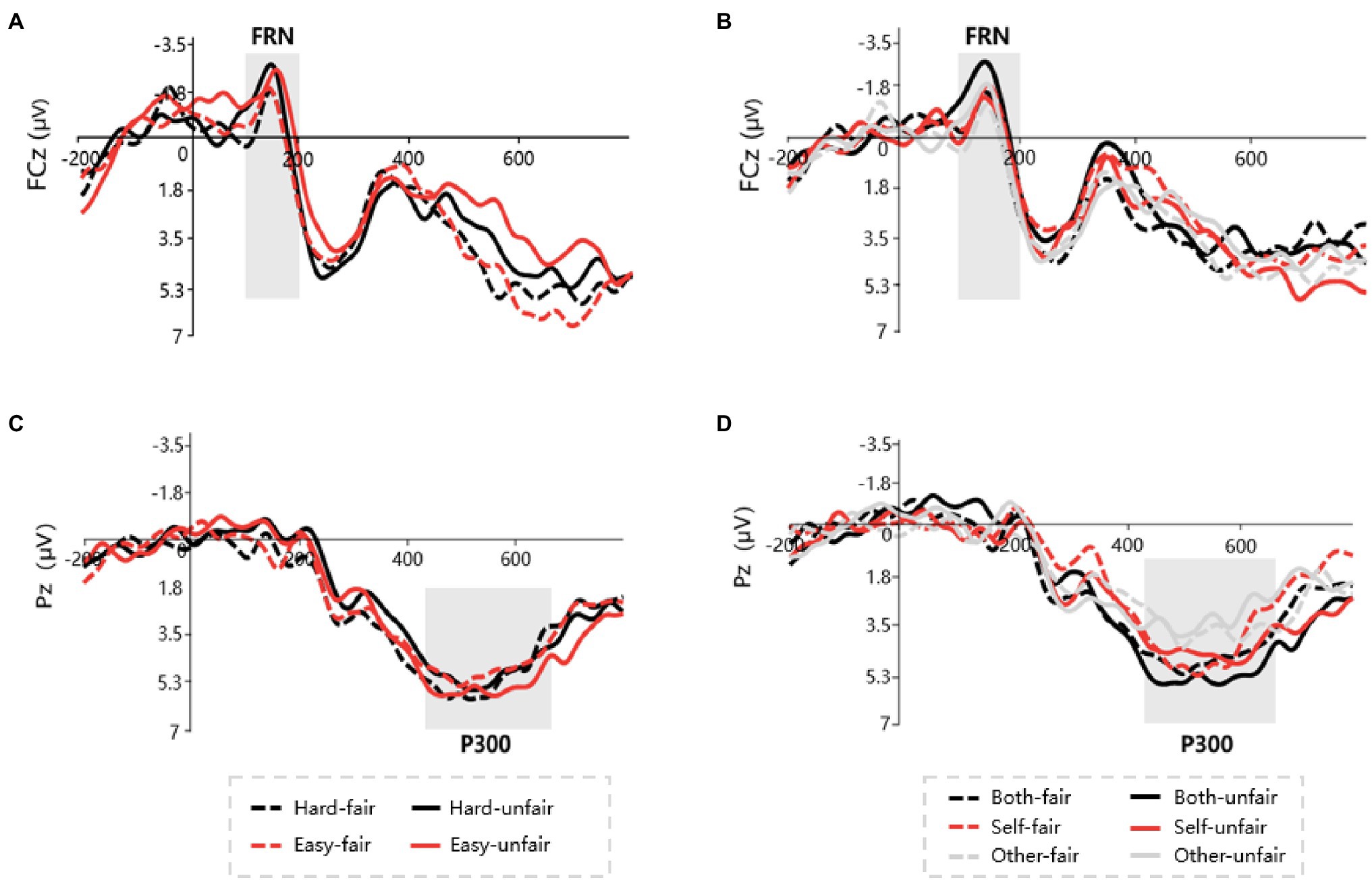
Figure 3 . ERP grand average waveforms. (A) FRN waveforms across difficulty and fairness conditions. (B) FRN waveforms across contribution and fairness conditions. (C) P300 waveforms across difficulty and fairness conditions. (D) P300 waveforms across contribution and fairness conditions.
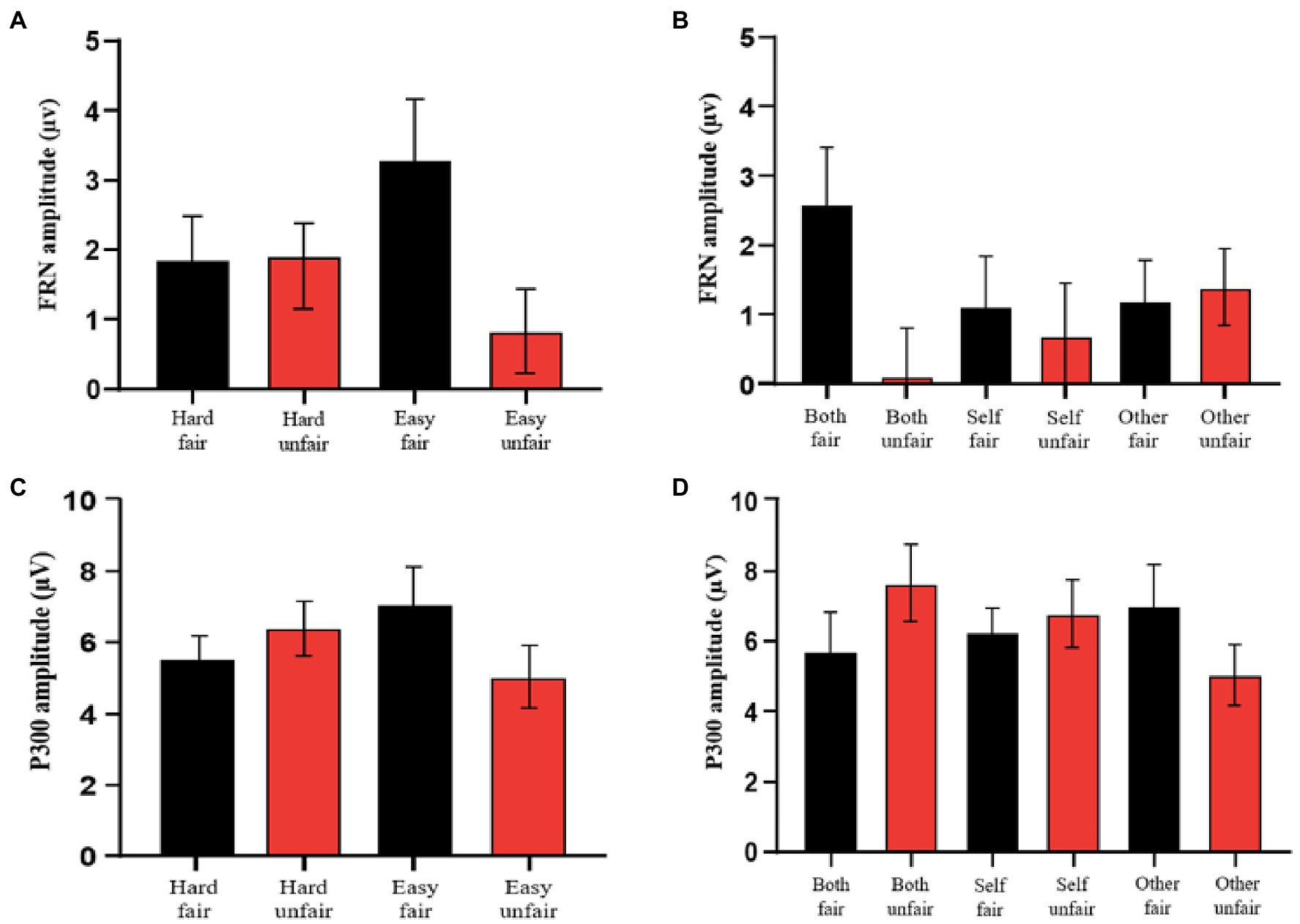
Figure 4 . ERP amplitudes. (A) Comparison of FRN amplitudes across difficulty and fairness conditions. (B) Comparison of FRN amplitudes across contribution and fairness conditions. (C) Comparison of P300 amplitudes across difficulty and fairness conditions. (D) Comparison of P300 amplitudes across contribution and fairness conditions.
Grand mean waveforms obtained for each experimental condition after 20-Hz low-pass filtering are shown in Figures 3C , D , and the P300 amplitudes are shown in Figures 4C , D . A repeated measures ANOVA of P300 amplitude (central posterior electrode) with task difficulty, contribution, and fairness type as within-subjects variables revealed a significant interaction between contribution and fairness type [ F (2.52) = 10.85; p < 0.001; η p 2 = 0.294]. The interaction analysis revealed that the P300 effect (unfair minus fair) was larger in the both-contribution condition (1.95 ± 0.03 μv) than in the self-contribution condition (0.52 ± 0.26 μv) or other-contribution condition (−1.98 ± 0.27 μv; p s < 0.01). There were no other significant main effects [task difficulty: F (1.26) = 2.75; p = 0.10; η p 2 = 0.09; contribution: F (2.52) = 0.62; p = 0.54; η p 2 = 0.02; fairness type: F (1.26) = 0.19; p = 0.66; η p 2 = 0.007] or interactions [task difficulty × contribution F (2.52) = 1.21; p = 0.35; η p 2 = 0.03; task difficulty × fairness type F (1.26) = 0.27; p = 0.60; η p 2 = 0.01; task difficulty × contribution × fairness type F (2.52) = 4.57; p = 0.15; η p 2 = 0.05].
In the present study, we investigated whether one’s own contribution and task difficulty moderate fairness consideration behaviorally or influence neurophysiological responses during performance of the UG. Behaviorally, the participants were more likely to reject unfair offers in the self-contribution condition than in the both- and other-contribution conditions. Neurophysiologically, unfair offers allocated in hard condition trials induced more negative-going FRN responses in the early time window than similar offers in easy condition trials. More positive P300 potentials were observed in the both-contribution condition than in the self- and other-contribution conditions.
Our behavioral results are similar to classic UG findings in that people tended to reject unfair offers and are further consistent with Guo et al.’s (2014) findings in indicating that self-contribution make participants less likely to accept unfair offers than when they are in other-contribution or both-contribution trials ( Guo et al., 2014 ). Hence, it appears that the participants tended to accept offers when they were in line with their own contribution proportions. Otherwise, they would often punish the proposer, apparently as a form of retaliation and defiance, by refusing unfair offers in agreement with the pattern of the distributive justice theory proposed by Hoffman and Spitzer ( Hoffman and Spitzer, 1985 ). Unfair treatment by a low-contributing partner may induce negative emotions that would reinforce the participants’ willingness to enact punishment, resulting in punitive decisions and thus rejection of unfair offers.
Interestingly, although we did not find behavioral effects of task difficulty on fairness decisions, we did see effects of task difficulty on our EEG results. This disassociation may be due to neurophysiological responses were sensitive to difficulty level. Another significant issue is that we did not observe a significant interaction of task difficulty and contribution in our experiment. There are a number of potential, attemptable methods of setting task difficulty that might better differentiate levels of difficulty ( Gilbert et al., 2012 ). It might be that the complexity of the experimental design in terms of multiple conditions masked difficulty effects to some extent.
Importantly, our results revealed that unfair offers induced larger FRNs in hard condition trials than in easy condition trials. It has been suggested that FRN amplitudes may be heavily dependent on how concerned subjects are about the decision outcome, especially in the context of social interactions ( Luo et al., 2011 ). In other words, the FRN may reflect the magnitude of outcome value. Indeed, previously, unfair offers have been reported to result in significantly larger FRN amplitudes than fair ones, especially in individuals with a high-level of concern for fairness norms ( Boksem and De Cremer, 2010 ). In fact, FRN components originating from the anterior cingulate cortex have previously been associated with conflict monitoring ( Botvinick et al., 1999 ; MacDonald et al., 2000 ). Some researchers have argued that this conflict may reflect a contradiction between the desire to accept monetary benefits and an aversion to unfair treatment ( Van Lange et al., 2013 ). In our experiment, when participants encountered unfair offers in easy condition trials, the expression of aversive emotions may have needed to be somewhat inhibited to enable them to accept an unfair benefit allocation. However, such conflicts would be suppressed in the hard condition in order to protect one’s personal interests.
The present finding of a more positive P300 response in the both-contribution condition than in the self- and other-contribution conditions was unexpected. We speculate that this result might be attributed to participants’ level of involvement in the cooperative process such that when the two contributions were balanced, both partners showed a well-coordinated and equivalent performance. Such dynamic engagement is essential to maintaining partner cooperation. Alternatively, this surprising result could be related to our use of different evaluation criteria relative to prior studies. Previously, P300 has been reported to be associated with higher-order cognitive operations, such as attentional resource allocation and motivational/affective evaluation ( Gray et al., 2004 ; Linden, 2005 ). Participants exhibiting more positive choices in the both-contribution condition might be driven by external motivations, such as maintaining a good image in front of the researcher. Choices under the other two contributions were more likely to be motivated by self-interest. Alternatively, P300 differences may reflect differences in top-down outcome evaluation processes ( Yeung and Sanfey, 2004 ; Sato et al., 2005 ). Stronger P300 responses in the both-contribution condition, than in the self- and other-contribution conditions, may indicate that more attentional resources were invested in sustaining relationships in the both-contribution condition.
Despite the significant results obtained in the present study, there are some limitations. Firstly, we did not observe significant effects of task difficulty on behavioral responses, indicating that the task difficulty shift lacked salience. Secondly, we examined fairness consideration with a single task, UG, which has been a popular paradigm for characterizing fairness-related decision-making. Future research could incorporate more paradigms (e.g., dictator games or impunity games) to further explore fairness consideration. Thirdly, the fact that only healthy university students were included as subjects may limit the representativeness of the present results. Future studies should consider including a greater diversity of participants, including people of different ages (e.g., elderly and children) and neurology patients (e.g., brain injury patients). Finally, because this study was conducted in the context of a highly collectivist culture, the results might not be generalizable to participants from other cultural backgrounds. In future research, accounting for this variable may yield more accurate and in-depth findings regarding how factors affect fairness consideration.
The present findings underscore the influence of self-contribution on fairness consideration and demonstrate neurophysiological responses during fairness consideration over the FRN and P300 time phases. Participants were inclined to make more altruistic choices when their contributions to the reward were relatively small compared to those of the proposer. As expected, task difficulty influenced neurophysiological responses during offer evaluation in addition to other criteria in fairness consideration. Unfair offers in a hard task may be judged as more violative of expectations than in an easy condition, thereby inducing more pronounced FRN amplitudes early in the stimulus processing period. At a later stage, larger P300 amplitudes were observed in both-contribution condition trials than in self-contribution or other-contribution condition trials. Our findings provide new evidence for understanding and explaining the effects of task difficulty and self-contribution on fairness evaluation in cooperative contexts.
Data Availability Statement
The raw data supporting the conclusions of this article will be made available by the authors, without undue reservation.
Ethics Statement
The study was reviewed and approved by the Ethics Committee of Yangzhou University. The participants provided their written informed consent to participate in this study.
Author Contributions
WG and BW conceived and designed the experiments. LX and BW performed the experiments and analyzed the data. LX and WG wrote the manuscript. All authors contributed to the article and approved the submitted version.
This work was supported by the National Social Science Foundation of China (Grant No. 18CTY014).
Conflict of Interest
The authors declare that the research was conducted in the absence of any commercial or financial relationships that could be construed as a potential conflict of interest.
Publisher’s Note
All claims expressed in this article are solely those of the authors and do not necessarily represent those of their affiliated organizations, or those of the publisher, the editors and the reviewers. Any product that may be evaluated in this article, or claim that may be made by its manufacturer, is not guaranteed or endorsed by the publisher.
Barclay, P. (2006). Reputational benefits for altruistic punishment. Evol. Hum. Behav . 27, 325–344. doi: 10.1016/j.evolhumbehav.2006.01.003
CrossRef Full Text | Google Scholar
Barker, J. L., Barclay, P., and Reeve, H. K. (2012). Within-group competition reduces cooperation and payoffs in human groups. Behav. Ecol . 23, 735–741. doi: 10.1093/beheco/ars020
Bland, A. R., Roiser, J. P., Mehta, M. A., Schei, T., Sahakian, B. J., Robbins, T. W., et al. (2017). Cooperative behavior in the ultimatum game and prisoner’s dilemma depends on players. contributions. Front. Psychol . 8:1017. doi: 10.3389/fpsyg.2017.01017
PubMed Abstract | CrossRef Full Text | Google Scholar
Boksem, M. A., and De Cremer, D. (2010). Fairness concerns predict medial frontal negativity amplitude in ultimatum bargaining. Soc. Neurosci . 5, 118–128. doi: 10.1080/17470910903202666
Botvinick, M., Nystrom, L. E., Fissell, K., Carter, C. S., and Cohen, J. D. (1999). Conflict monitoring versus selection-for-action in anterior cingulate cortex. Nature 402, 179–181. doi: 10.1038/46035
Camerer, C., and Thaler, R. (1995). Anomalies: ultimatums, dictators and manners. J. Econ. Perspect . 9, 209–219. doi: 10.1257/jep.9.2.209
Eckel, C. C., and Grossman, P. J. (1996). The relative price of fairness: gender differences in a punishment game. J. Econ. Behav. Organ . 30, 143–158. doi: 10.1016/S0167-2681(96)00854-2
Faul, F., Erdfelder, E., Lang, A. G., and Buchner, A. (2007). G*power 3: a flexible statistical power analysis program for the social, behavioral, and biomedical sciences. Behav. Res. Methods 39, 175–191. doi: 10.3758/bf03193146
Fehr, E., and Fischbacher, U. (2003). The nature of human altruism. Nature 425, 785–791. doi: 10.1038/nature02043
Fehr, E., and Gachter, S. (2002). Altruistic punishment in humans. Nature 415, 137–140. doi: 10.1038/415137a
Feng, C., Feng, X., Wang, L., Wang, L., Gu, R., Ni, A., et al. (2019). The neural signatures of egocentric bias in normative decision-making. Brain Imaging Behav . 13, 685–698. doi: 10.1007/s11682-018-9893-1
Feng, C., Luo, Y. J., and Krueger, F. (2015). Neural signatures of fairness-related normative decision making in the ultimatum game: a coordinate-based meta-analysis. Hum. Brain Mapp . 36, 591–602. doi: 10.1002/hbm.22649
Flinkenflogel, N., Novin, S., Huizinga, M., and Krabbendam, L. (2017). Gender moderates the influence of self-construal priming on fairness considerations. Front. Psychol . 8:503. doi: 10.3389/fpsyg.2017.00503
Fortin, M., Cropanzano, R., Cuguero-Escofet, N., Nadisic, T., and Van Wagoner, H. (2020). How do people judge fairness in supervisor and peer relationships? Another assessment of the dimensions of justice. Hum. Relat . 73, 1632–1663. doi: 10.1177/0018726719875497
Gehring, W. J., and Willoughby, A. R. (2002). The medial frontal cortex and the rapid processing of monetary gains and losses. Science 295, 2279–2282. doi: 10.1126/science.1066893
Gilbert, S., Bird, G., Frith, C., and Burgess, P. (2012). Does “task difficulty” explain “task-induced deactivation?”. Front. Psychol . 3:125. doi: 10.3389/fpsyg.2012.00125
Gilbert, D. T., Pelham, B. W., and Krull, D. S. (1988). On cognitive busyness: when person perceivers meet persons perceived. J. Pers. Soc. Psychol . 54, 733–740. doi: 10.1037/0022-3514.54.5.733
Gray, H. M., Ambady, N., Lowenthal, W. T., and Deldin, P. (2004). P300 as an index of attention to self-relevant stimuli. J. Exp. Soc. Psychol . 40, 216–224. doi: 10.1016/S0022-1031(03)00092-1
Guo, X., Zheng, L., Cheng, X., Chen, M., Zhu, L., Li, J., et al. (2014). Neural responses to unfairness and fairness depend on self-contribution to the income. Soc. Cogn. Affect. Neurosci . 9, 1498–1505. doi: 10.1093/scan/nst131
Guroglu, B., van den Bos, W., van Dijk, E., Rombouts, S. A. R. B., and Crone, E. A. (2011). Dissociable brain networks involved in development of fairness considerations: understanding intentionality behind unfairness. NeuroImage 57, 634–641. doi: 10.1016/j.neuroimage.2011.04.032
Güth, W., Schmittberger, R., and Schwarze, B. (1982). An experimental analysis of ultimatum bargaining. J. Econ. Behav. Organ . 3, 367–388. doi: 10.1016/0167-2681(82)90011-7
Hajcak, G., MacNamara, A., and Olvet, D. M. (2010). Event-related potentials, emotion, and emotion regulation: an integrative review. Dev. Neuropsychol . 35, 129–155. doi: 10.1080/87565640903526504
Hajcak, G., Moser, J. S., Holroyd, C. B., and Simons, R. F. (2007). It’s worse than you thought: the feedback negativity and violations of reward prediction in gambling tasks. Psychophysiology 44, 905–912. doi: 10.1111/j.1469-8986.2007.00567.x
Hillyard, S., and Picton, T. (2011). “Electrophysiology of cognition,” in The Handbook of physiology . ed. F. Plum (Baltimore, MD: Waverly Press), 519–584.
Google Scholar
Hoffman, E., and Spitzer, M. L. (1985). Entitlements, rights, and fairness: an experimental examination of subjects’ concepts of distributive justice. J. Empir. Legal. Stud . 14, 259–297. doi: 10.1086/467773
Hu, J., Cao, Y., Blue, P. R., and Zhou, X. L. (2014). Low social status decreases the neural salience of unfairness. Front. Behav. Neurosci . 8:402. doi: 10.3389/fnbeh.2014.00402
Jin, J., Wang, A. L., Liu, J. Y., Pan, J., and Lyu, D. (2020). How does monetary loss empathy modulate generosity in economic sharing behavior? An ERPs study. Neuropsychologia . 141:107407. doi: 10.1016/j.neuropsychologia.2020.107407
Krasnow, M. M., and Delton, A. W. (2016). Are humans too generous and too punitive? Using psychological principles to further debates about human social evolution. Front. Psychol . 7:799. doi: 10.3389/fpsyg.2016.00799
Leng, Y., and Zhou, X. J. N. (2010). Modulation of the brain activity in outcome evaluation by interpersonal relationship: an ERP study. Neuropsychologia 48, 448–455. doi: 10.1016/j.neuropsychologia.2009.10.002
Li, J., Sun, Y., Li, M., Li, H., Fan, W., and Zhong, Y. (2020). Social distance modulates prosocial behaviors in the gain and loss contexts: an event-related potential (ERP) study. Int. J. Psychophysiol . 150, 83–91. doi: 10.1016/j.ijpsycho.2020.02.003
Linden, D. E. J. (2005). The P300: where in the brain is it produced and what does it tell us? Neuroscientist 11, 563–576. doi: 10.1177/1073858405280524
Long, C., Sun, Q., Jia, S., Li, P., and Chen, A. (2018). Give me a chance! Sense of opportunity inequality affects brain responses to outcome evaluation in a social competitive context: an event-related potential study. Front. Hum. Neurosci . 12:135. doi: 10.3389/fnhum.2018.00135
Luck, S. J. (2014). An Introduction to the Event-Related Potential Technique . London: MIT Press.
Luo, Q. L., Wang, Y., and Qu, C. (2011). The near-miss effect in slot-machine gambling: modulation of feedback-related negativity by subjective value. Neuroreport 22, 989–993. doi: 10.1097/WNR.0b013e32834da8ae
Ma, Q., Meng, L., Zhang, Z., Xu, Q., Wang, Y., and Shen, Q. (2015). You did not mean it: perceived good intentions alleviate sense of unfairness. Int. J. Psychophysiol . 96, 183–190. doi: 10.1016/j.ijpsycho.2015.03.011
Ma, Q., Qian, D., Hu, L., and Wang, L. (2017). Hello handsome! Male’s facial attractiveness gives rise to female’s fairness bias in ultimatum game scenarios-An ERP study. PLoS One 12:e0180459. doi: 10.1371/journal.pone.0180459
MacDonald, A. W., Cohen, J. D., Stenger, V. A., and Carter, C. S. (2000). Dissociating the role of the dorsolateral prefrontal and anterior cingulate cortex in cognitive control. Science 288, 1835–1838. doi: 10.1126/science.288.5472.1835
Massi, B., and Luhmann, C. C. (2015). Fairness influences early signatures of reward-related neural processing. Cogn. Affect. Behav. Neurosci . 15, 768–775. doi: 10.3758/s13415-015-0362-7
Nai, J., Kotha, R., Narayanan, J., and Puranam, P. (2020). Transparency and fairness in organizational decisions: an experimental investigation using the paired ultimatum game. Strateg. Sci . 5, 55–70. doi: 10.1287/stsc.2019.0100
Olofsson, J. K., Nordin, S., Sequeira, H., and Polich, J. (2008). Affective picture processing: an integrative review of ERP findings. Biol. Psychol . 77, 247–265. doi: 10.1016/j.biopsycho.2007.11.006
Polezzi, D., Sartori, G., Rumiati, R., Vidotto, G., and Daum, I. (2010). Brain correlates of risky decision-making. NeuroImage 49, 1886–1894. doi: 10.1016/j.neuroimage.2009.08.068
Polich, J., and Kok, A. (1995). Cognitive and biological determinants of P300: an integrative review. Biol. Psychol . 41, 103–146. doi: 10.1016/0301-0511(95)05130-9
Sambrook, T. D., and Goslin, J. (2015). A neural reward prediction error revealed by a meta-analysis of ERPs using great grand averages. Psychol. Bull . 141, 213–235. doi: 10.1037/bul0000006
Sanfey, A. G., Rilling, J. K., Aronson, J. A., Nystrom, L. E., and Cohen, J. D. (2003). The neural basis of economic decision-making in the ultimatum game. Science 300, 1755–1758. doi: 10.1126/science.1082976
Sato, A., Yasuda, A., Ohira, H., Miyawaki, K., Nishikawa, M., Kumano, H., et al. (2005). Effects of value and reward magnitude on feedback negativity and P300. Neuroreport 16, 407–411. doi: 10.1097/00001756-200503150-00020
Schmitt, M., Baumert, A., Gollwitzer, M., and Maes, J. (2010). The justice sensitivity inventory: factorial validity, location in the personality facet space, demographic pattern, and normative data. Soc. Justice Res 23, 211–238. doi: 10.1007/s11211-010-0115-2
Sutter, M. (2007). Outcomes versus intentions: on the nature of fair behavior and its development with age. J. Econ. Psychol . 28, 69–78. doi: 10.1016/j.joep.2006.09.001
Van Lange, P. A. M., Joireman, J., Parks, C. D., and Van Dijk, E. (2013). The psychology of social dilemmas: a review. Organ. Behav. Hum. Dec . 120, 125–141. doi: 10.1016/j.obhdp.2012.11.003
Wang, L. (2017). From Knowledge to Behavior: Affecting factors and early development of equity principle for 3-to 5-year-old children. dissertation/doctor’s thesis. Shanghai: East China Normal University.
Wu, Y., Leliveld, M. C., and Zhou, X. L. (2011). Social distance modulates recipient’s fairness consideration in the dictator game: an ERP study. Biol. Psychol . 88, 253–262. doi: 10.1016/j.biopsycho.2011.08.009
Yeung, N., and Sanfey, A. G. (2004). Independent coding of reward magnitude and valence in the human brain. J. Neurosci . 24, 6258–6264. doi: 10.1523/JNEUROSCI.4537-03.2004
Yu, R. J., Hu, P., and Zhang, P. (2015). Social distance and anonymity modulate fairness consideration: an ERP study. Sci. Rep-Uk . 5:13452. doi: 10.1038/srep13452
Zheng, Y., Tan, F., Xu, J., Chang, Y., Zhang, Y. Y., and Shen, H. J. (2015). Diminished P300 to physical risk in sensation seeking. Biol. Psychol . 107, 44–51. doi: 10.1016/j.biopsycho.2015.03.003
Zhou, X., and Wu, Y. (2011). Sharing losses and sharing gains: increased demand for fairness under adversity. J. Exp. Soc. Psychol . 47, 582–588. doi: 10.1016/j.jesp.2010.12.017
Keywords: self-contribution, task difficulty, fairness consideration, feedback-related negativity, P300
Citation: Xu L, Wang B and Guo W (2022) The Effect of Task Difficulty and Self-Contribution on Fairness Consideration: An Event-Related Potential Study. Front. Psychol . 13:709310. doi: 10.3389/fpsyg.2022.709310
Received: 13 May 2021; Accepted: 27 January 2022; Published: 03 March 2022.
Reviewed by:
Copyright © 2022 Xu, Wang and Guo. This is an open-access article distributed under the terms of the Creative Commons Attribution License (CC BY) . The use, distribution or reproduction in other forums is permitted, provided the original author(s) and the copyright owner(s) are credited and that the original publication in this journal is cited, in accordance with accepted academic practice. No use, distribution or reproduction is permitted which does not comply with these terms.
*Correspondence: Wei Guo, [email protected]
Disclaimer: All claims expressed in this article are solely those of the authors and do not necessarily represent those of their affiliated organizations, or those of the publisher, the editors and the reviewers. Any product that may be evaluated in this article or claim that may be made by its manufacturer is not guaranteed or endorsed by the publisher.
Assessing task difficulty for other people: when deeper evaluation means “it’s more about me!”
- Published: 15 September 2016
- Volume 19 , pages 865–877, ( 2016 )
Cite this article
- Ann Krispenz 1 ,
- Oliver Dickhäuser 1 &
- Marc-André Reinhard 2
370 Accesses
4 Citations
2 Altmetric
Explore all metrics
Empirical studies have revealed that teachers face problems when assessing task difficulty for their students. By drawing on research that focuses on how individuals assess what others know, we argue that these difficulties are a consequence of the imputation of one’s own knowledge to others (i.e., social projection). In particular, we tested the assumption that individuals impute more of their own knowledge to others, the more they elaborate what these others might know. In a first experiment, students were asked to judge task difficulty for their best friend. In the second experiment, teacher trainees were asked to assess task difficulty for 9th graders. Results revealed that individuals, who deeply elaborated when assessing task difficulty for another person, more closely relied on their own rating of task difficulty than individuals with a lower elaboration depth. These findings support the notion that social projection becomes stronger, the deeper individuals elaborate.
This is a preview of subscription content, log in via an institution to check access.
Access this article
Subscribe and save.
- Get 10 units per month
- Download Article/Chapter or eBook
- 1 Unit = 1 Article or 1 Chapter
- Cancel anytime
Price includes VAT (Russian Federation)
Instant access to the full article PDF.
Rent this article via DeepDyve
Institutional subscriptions
Similar content being viewed by others
Negatively or positively biased dependencies of teachers’ judgments and expectations based on students’ ethnic and social backgrounds.
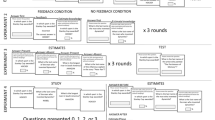
The “curse of knowledge” when predicting others’ knowledge
Agency attributions of mental effort during self-regulated learning, explore related subjects.
- Artificial Intelligence
Ames, D. R. (2004). Strategies for social inference: A similar contingency model of projection and stereotyping in attribute prevalence estimates. Journal of Personality and Social Psychology, 87 (5), 573–585.
Article Google Scholar
Brenner, L., & Bilgin, B. (2011). Preferences, projection, and packing: Support theory models of judgments of others’ preferences. Organizational Behavior and Human Decision Processes, 115 (1), 121–132.
Cho, J. C., & Knowles, E. D. (2013). I’m like you and you’re like me: Social projection and self-stereotyping both help explain self-other correspondence. Journal of Personality and Social Psychology, 104 (3), 444–456.
Cohen, J. (1988). Statistical power analysis for the behavioral sciences . Hillsdale, New Jersey: Lawrence Erlbaum Associates.
Google Scholar
Cohen, J., Cohen, P., West, S. G., & Aiken, L. S. (2003). Applied multiple regression/correlation analysis for the behavioral science . Mahaw, NJ: Erlbaum.
Faul, F., Erdfelder, E., Buchner, A., & Lang, A.-G. (2009). Statistical power analyses using G*Power 3.1: Tests for correlation and regression analyses. Behavior Research Methods, 41 (4), 1149–1160.
Helmke, A., & Schrader, F.-W. (1987). Interactional effects of instructional quality and teacher judgement accuracy on achievement. Teaching and Teacher Education, 3 (2), 91–98.
Impara, J. C., & Plake, B. S. (1998). Teachers’ ability to estimate item difficulty: A test of the assumptions in the Angoff standard setting method. Journal of Educational Measurement, 35 (1), 69–81.
Kawada, C. L. K., Oettinger, G., Gollwitzer, P. M., & Bargh, J. A. (2004). The projection of implicit and explicit goals. Journal of Personality and Social Psychology, 86 (4), 545–559.
Kelley, C. M., & Jacoby, L. L. (1996). Adult egocentrism: Subjective experience vs. analytic bases for judgment. Journal of Memory and Language, 35 (2), 157–175.
Marks, G., & Miller, N. (1987). Ten years of research on the false-consensus effect: An empirical and theoretical review. Psychological Bulletin, 102 (1), 72–90.
Neugebauer, M., Helbig, M., & Landmann, A. (2011). Unmasking the myth of the same-sex teacher advantage. European Sociological Review, 27 (5), 669–689.
Nickerson, R. S. (1999). How we know—and sometimes misjudge—what others know: Imputing one’s own knowledge to others. Psychological Bulletin, 125 (6), 737–759.
Nickerson, R. S., Baddeley, A., & Freeman, B. (1987). Are people’s estimates of what other people know influenced by what they themselves know? Acta Psychologica, 64 (3), 245–259.
PISA-Konsortium Deutschland. (2003). PISA 2003: Der Bildungsstand der Jugendlichen in Deutschland—Ergebnisse des zweiten internationalen Vergleichs . Münster u.a: Waxmann.
PISA-Konsortium Deutschland, (2006). PISA 2006: Die Ergebnisse der dritten internationalen Vergleichs . Münster u.a: Waxmann.
Stanley, J. C. (1971). Reliability. In R. L. Thorndike (Ed.), Educational measurement (pp. 356–442). Washington, DC: American Council on Education.
Van de Watering, G., & van de Rijt, J. (2006). Teachers’ and students’ perceptions of assessments: A review and a study into the ability and accuracy of estimating the difficulty levels of assessment items. Educational Research Review, 1 (2), 133–147.
Wood, W. (1982). Retrieval of attitude-relevant information from memory: Effects of susceptibility to persuasion and on intrinsic motivation. Journal of Personality and Social Psychology, 42 (5), 798–810.
Download references
Studies reported in this article were supported by a grant from the German Research Foundation (Deutsche Forschungsgemeinschaft) to the second (DI 929/2_2) and third author (RE 2218/1_2).
Author information
Authors and affiliations.
Department of Psychology, School of Social Sciences, Chair of Educational Psychology, University of Mannheim, A 5, 68131, Mannheim, Germany
Ann Krispenz & Oliver Dickhäuser
Human Sciences, Department of Psychology, University of Kassel, Holländische Str. 36-38, 34127, Kassel, Germany
Marc-André Reinhard
You can also search for this author in PubMed Google Scholar
Corresponding author
Correspondence to Ann Krispenz .
Ethics declarations
Conflict of interest.
The authors declare that they have no conflict of interest.
Additional information
Ann Krispenz, Oliver Dickhäuser and Marc-André Reinhard have contributed equally to this work.
Rights and permissions
Reprints and permissions
About this article
Krispenz, A., Dickhäuser, O. & Reinhard, MA. Assessing task difficulty for other people: when deeper evaluation means “it’s more about me!”. Soc Psychol Educ 19 , 865–877 (2016). https://doi.org/10.1007/s11218-016-9341-2
Download citation
Received : 16 June 2015
Accepted : 18 April 2016
Published : 15 September 2016
Issue Date : December 2016
DOI : https://doi.org/10.1007/s11218-016-9341-2
Share this article
Anyone you share the following link with will be able to read this content:
Sorry, a shareable link is not currently available for this article.
Provided by the Springer Nature SharedIt content-sharing initiative
- Task difficulty
- Working model of other’s knowledge
- Social projection
- Elaboration depth
- Find a journal
- Publish with us
- Track your research

IMAGES
VIDEO
COMMENTS
In an experiment examining the effects of task difficulty (easy/hard) for men and women, the factors are ____. a. easy and hard b. male and female c. male, female, easy, and hard d. difficulty and gender. d. difficulty and gender. In a within-subjects research study, factors that change over time, such as history and maturation, can be threats ...
How many factors are in the experiment? a. one b. two c. three d. six, In an experiment examining the effects of task difficulty (easy/hard) for men and women, the factors are _____. a. male and female b. easy and hard c. male, female, easy, and hard d. difficulty and gender and more.
Study with Quizlet and memorize flashcards containing terms like How many main effects are there in a 2´3 factorial design?, In an experiment examining the effects of task difficulty (easy/hard) for men and women, the factors are, If the results of an experiment show that the judge's decisions are affected when smiling occurs in a defendant who is attractive (as opposed to when the defendant ...
The purpose of this study was to explore whether RPG produces different effects due to task difficulty and ASE. In Experiment 1, participants studied tasks with varying difficulty levels, some of which were guided. Results showed that RPG could enhance learning through increased retrieval practice, and participants engaged in more retrieval for ...
The purpose of this research was to examine how rewards affected motivation and performance when students were rewarded for succeeding at an easy task versus a moderately difficult task. As part of a more general design, the experiment reported here was a 2x2 factorial with two levels of reward (reward, no reward) and two levels of task difficulty (easy, difficult). Seventy-three undergraduate ...
The current study extends these findings by examining individuals' internal conditions in terms of how learners' perceptions of task understanding and task difficulty change through collaborative learning. ... The categorization for task difficulty resulted in 86 learning sessions where learners perceived the collaborative task as more ...
For example, when examining changes from the first to the second out of four time periods, an increase in performance was associated with an increased heart rate. ... anxiety related to participation to the experiment, or effects related to sitting still. ... While effects of task difficulty on different physiological variables have been ...
The word learning task was identical to that in Experiment 1, except that during the intervening task, participants completed easy or difficult blocks of the CVOE task (pure or mixed, respectively). For each ITD, participants completed 8 repetition trials and 8 single-presentation trials for each level of difficulty, yielding a total of 32 trials.
The Effects of Task Difficulty and Multitasking on Performance ... types of multitasking, along with subjective task difficulty, influence performance, we conducted a controlled experiment using a custom-developed multitasking environment. ... 'Hi Chatbot, let's Talk about Politics!' Examining the Impact of Verbal Anthropomorphism in ...
Why are some tasks more difficult to learn than others? Hoffman et al. (Accelerated expertise: training for high proficiency in a complex world. Psychology Press, New York, 2014) hypothesized that certain task characteristics—termed "dimensions of difficulty"—hindered learning and performance. Previously, we tested two dimensions: consistent vs. variably mapped and static vs. dynamic ...
This study presents secondary analyses of an experiment that was originally designed to examine the effects of note-taking instructions and structured notes on multiple text task performance. Students were randomly assigned to one of four experimental conditions, differing in the note-taking instructions that students received and the note ...
A two-factor study comparing task performance for males versus females (factor 1) for three different levels of task difficulty (factor 2) would be described as a 2 x 3 design. True. A researcher who is examining the effects of temperature and humidity on the eating behavior of rats uses a factorial experiment comparing three different ...
Multitasking is prevalent during computer-mediated work. Users tend to switch between multiple ongoing computer-based tasks either due to a personal decision to break from the current task (self-interruption) or due to an external interruption, such as an electronic notification. To examine how different types of multitasking, along with subjective task difficulty, influence performance, we ...
Introduction. Several cross-sectional studies have revealed that balance performance is affected by task-difficulty (e.g., variation of stance conditions; manipulation of sensory input; restriction of compensatory movements) [1-4].For example, Barbado Murillo et al. [] reported increasing sway amplitudes when participants balanced on a stable, medium stable, and unstable base, respectively.
In Experiment 1 task interest and difficulty were varied in a two by two experimental design. Tasks were given to 100 subjects and procrastina- tion time was recorded. The only reliable result was that easy-interesting tasks produced less variability in behavior than any other task type.
1 College of Physical Education, Yangzhou University, Yangzhou, China; 2 Institute of Sports, Exercise and Brain, Yangzhou University, Yangzhou, China; Self-contribution may be an influential factor in fairness consideration and consequent behavioral decisions. Few studies have investigated simultaneous effects of task difficulty and self-contribution on fairness consideration outcomes and ...
Q-Chat. Study with Quizlet and memorize flashcards containing terms like If neither Variable A nor Variable B has a main effect you can have, In an experiment examining the effects of task difficulty (easy/hard) for men and women, the factors are, In the simplest case of factorial design, ____________ are tested and more.
Empirical studies have revealed that teachers face problems when assessing task difficulty for their students. By drawing on research that focuses on how individuals assess what others know, we argue that these difficulties are a consequence of the imputation of one's own knowledge to others (i.e., social projection). In particular, we tested the assumption that individuals impute more of ...
The difficulty of the tasks is possibly increasing when the amount of information provided in the material is huge, and the relationship within the information is comparatively high. It may lead ...
In an experiment examining the effects of task difficulty (easy or hard) for left-handed people compared to right-handed people, the factors are _____.left-handedness and right-handedness, easy and harddifficulty and handednesseasy and hardleft-handedness and right-handedness
In an experiment examining the effects of task difficulty (easy/hard) for men and women, the factors are ____. a. male and female b. easy and hard c. male, female, easy, and hard d. difficulty and gender. difficulty and gender. The advantage of a two-factor design compared to two single-factor designs is the ability to ____. a. save time b.
Task complexity and the effects of interruptions When examining performance research, task complexity has become an important factor [2]. Gillie and Broadbent [3] found that the type of the interruption (i.e., the similarity of the primary task) and the difficulty level of the interruption are factors which determine whether interruptions ...
How many factors are in the experiment? two. In an experiment examining the effects of task difficulty for men and women, the factors are: difficulty and gender. The advantage of a two-factor design compared to two single-factor designs is the ability to: evaluate the interaction.