Chapter: Introduction to the Design and Analysis of Algorithms
Fundamentals of Algorithmic Problem Solving
Let us start by reiterating an important point made in the introduction to this chapter:
We can consider algorithms to be procedural solutions to problems.
These solutions are not answers but specific instructions for getting answers. It is this emphasis on precisely defined constructive procedures that makes computer science distinct from other disciplines. In particular, this distinguishes it from the-oretical mathematics, whose practitioners are typically satisfied with just proving the existence of a solution to a problem and, possibly, investigating the solution’s properties.
We now list and briefly discuss a sequence of steps one typically goes through in designing and analyzing an algorithm (Figure 1.2).
Understanding the Problem
From a practical perspective, the first thing you need to do before designing an algorithm is to understand completely the problem given. Read the problem’s description carefully and ask questions if you have any doubts about the problem, do a few small examples by hand, think about special cases, and ask questions again if needed.
There are a few types of problems that arise in computing applications quite often. We review them in the next section. If the problem in question is one of them, you might be able to use a known algorithm for solving it. Of course, it helps to understand how such an algorithm works and to know its strengths and weaknesses, especially if you have to choose among several available algorithms. But often you will not find a readily available algorithm and will have to design your own. The sequence of steps outlined in this section should help you in this exciting but not always easy task.
An input to an algorithm specifies an instance of the problem the algorithm solves. It is very important to specify exactly the set of instances the algorithm needs to handle. (As an example, recall the variations in the set of instances for the three greatest common divisor algorithms discussed in the previous section.) If you fail to do this, your algorithm may work correctly for a majority of inputs but crash on some “boundary” value. Remember that a correct algorithm is not one that works most of the time, but one that works correctly for all legitimate inputs.
Do not skimp on this first step of the algorithmic problem-solving process; otherwise, you will run the risk of unnecessary rework.
Ascertaining the Capabilities of the Computational Device
Once you completely understand a problem, you need to ascertain the capabilities of the computational device the algorithm is intended for. The vast majority of
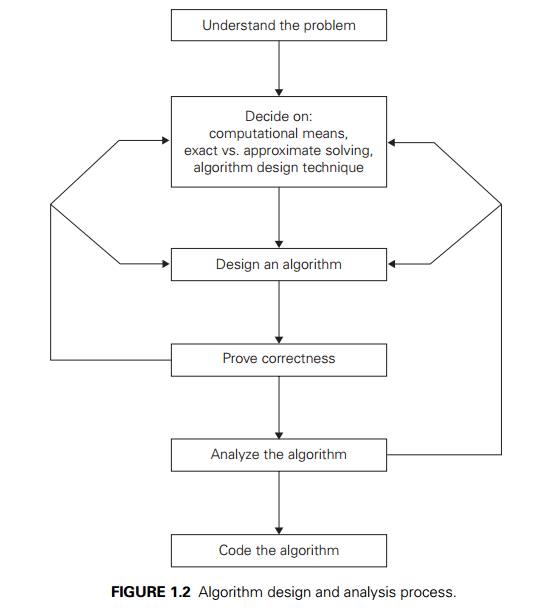
algorithms in use today are still destined to be programmed for a computer closely resembling the von Neumann machine—a computer architecture outlined by the prominent Hungarian-American mathematician John von Neumann (1903– 1957), in collaboration with A. Burks and H. Goldstine, in 1946. The essence of this architecture is captured by the so-called random-access machine ( RAM ). Its central assumption is that instructions are executed one after another, one operation at a time. Accordingly, algorithms designed to be executed on such machines are called sequential algorithms .
The central assumption of the RAM model does not hold for some newer computers that can execute operations concurrently, i.e., in parallel. Algorithms that take advantage of this capability are called parallel algorithms . Still, studying the classic techniques for design and analysis of algorithms under the RAM model remains the cornerstone of algorithmics for the foreseeable future.
Should you worry about the speed and amount of memory of a computer at your disposal? If you are designing an algorithm as a scientific exercise, the answer is a qualified no. As you will see in Section 2.1, most computer scientists prefer to study algorithms in terms independent of specification parameters for a particular computer. If you are designing an algorithm as a practical tool, the answer may depend on a problem you need to solve. Even the “slow” computers of today are almost unimaginably fast. Consequently, in many situations you need not worry about a computer being too slow for the task. There are important problems, however, that are very complex by their nature, or have to process huge volumes of data, or deal with applications where the time is critical. In such situations, it is imperative to be aware of the speed and memory available on a particular computer system.
Choosing between Exact and Approximate Problem Solving
The next principal decision is to choose between solving the problem exactly or solving it approximately. In the former case, an algorithm is called an exact algo-rithm ; in the latter case, an algorithm is called an approximation algorithm . Why would one opt for an approximation algorithm? First, there are important prob-lems that simply cannot be solved exactly for most of their instances; examples include extracting square roots, solving nonlinear equations, and evaluating def-inite integrals. Second, available algorithms for solving a problem exactly can be unacceptably slow because of the problem’s intrinsic complexity. This happens, in particular, for many problems involving a very large number of choices; you will see examples of such difficult problems in Chapters 3, 11, and 12. Third, an ap-proximation algorithm can be a part of a more sophisticated algorithm that solves a problem exactly.
Algorithm Design Techniques
Now, with all the components of the algorithmic problem solving in place, how do you design an algorithm to solve a given problem? This is the main question this book seeks to answer by teaching you several general design techniques.
What is an algorithm design technique?
An algorithm design technique (or “strategy” or “paradigm”) is a general approach to solving problems algorithmically that is applicable to a variety of problems from different areas of computing.
Check this book’s table of contents and you will see that a majority of its chapters are devoted to individual design techniques. They distill a few key ideas that have proven to be useful in designing algorithms. Learning these techniques is of utmost importance for the following reasons.
First, they provide guidance for designing algorithms for new problems, i.e., problems for which there is no known satisfactory algorithm. Therefore—to use the language of a famous proverb—learning such techniques is akin to learning to fish as opposed to being given a fish caught by somebody else. It is not true, of course, that each of these general techniques will be necessarily applicable to every problem you may encounter. But taken together, they do constitute a powerful collection of tools that you will find quite handy in your studies and work.
Second, algorithms are the cornerstone of computer science. Every science is interested in classifying its principal subject, and computer science is no exception. Algorithm design techniques make it possible to classify algorithms according to an underlying design idea; therefore, they can serve as a natural way to both categorize and study algorithms.
Designing an Algorithm and Data Structures
While the algorithm design techniques do provide a powerful set of general ap-proaches to algorithmic problem solving, designing an algorithm for a particular problem may still be a challenging task. Some design techniques can be simply inapplicable to the problem in question. Sometimes, several techniques need to be combined, and there are algorithms that are hard to pinpoint as applications of the known design techniques. Even when a particular design technique is ap-plicable, getting an algorithm often requires a nontrivial ingenuity on the part of the algorithm designer. With practice, both tasks—choosing among the general techniques and applying them—get easier, but they are rarely easy.
Of course, one should pay close attention to choosing data structures appro-priate for the operations performed by the algorithm. For example, the sieve of Eratosthenes introduced in Section 1.1 would run longer if we used a linked list instead of an array in its implementation (why?). Also note that some of the al-gorithm design techniques discussed in Chapters 6 and 7 depend intimately on structuring or restructuring data specifying a problem’s instance. Many years ago, an influential textbook proclaimed the fundamental importance of both algo-rithms and data structures for computer programming by its very title: Algorithms + Data Structures = Programs [Wir76]. In the new world of object-oriented programming, data structures remain crucially important for both design and analysis of algorithms. We review basic data structures in Section 1.4.
Methods of Specifying an Algorithm
Once you have designed an algorithm, you need to specify it in some fashion. In Section 1.1, to give you an example, Euclid’s algorithm is described in words (in a free and also a step-by-step form) and in pseudocode. These are the two options that are most widely used nowadays for specifying algorithms.
Using a natural language has an obvious appeal; however, the inherent ambi-guity of any natural language makes a succinct and clear description of algorithms surprisingly difficult. Nevertheless, being able to do this is an important skill that you should strive to develop in the process of learning algorithms.
Pseudocode is a mixture of a natural language and programming language-like constructs. Pseudocode is usually more precise than natural language, and its usage often yields more succinct algorithm descriptions. Surprisingly, computer scientists have never agreed on a single form of pseudocode, leaving textbook authors with a need to design their own “dialects.” Fortunately, these dialects are so close to each other that anyone familiar with a modern programming language should be able to understand them all.
This book’s dialect was selected to cause minimal difficulty for a reader. For the sake of simplicity, we omit declarations of variables and use indentation to show the scope of such statements as for , if , and while . As you saw in the previous section, we use an arrow “ ← ” for the assignment operation and two slashes “ // ” for comments.
In the earlier days of computing, the dominant vehicle for specifying algo-rithms was a flowchart , a method of expressing an algorithm by a collection of connected geometric shapes containing descriptions of the algorithm’s steps. This representation technique has proved to be inconvenient for all but very simple algorithms; nowadays, it can be found only in old algorithm books.
The state of the art of computing has not yet reached a point where an algorithm’s description—be it in a natural language or pseudocode—can be fed into an electronic computer directly. Instead, it needs to be converted into a computer program written in a particular computer language. We can look at such a program as yet another way of specifying the algorithm, although it is preferable to consider it as the algorithm’s implementation.
Proving an Algorithm’s Correctness
Once an algorithm has been specified, you have to prove its correctness . That is, you have to prove that the algorithm yields a required result for every legitimate input in a finite amount of time. For example, the correctness of Euclid’s algorithm for computing the greatest common divisor stems from the correctness of the equality gcd (m, n) = gcd (n, m mod n) (which, in turn, needs a proof; see Problem 7 in Exercises 1.1), the simple observation that the second integer gets smaller on every iteration of the algorithm, and the fact that the algorithm stops when the second integer becomes 0.
For some algorithms, a proof of correctness is quite easy; for others, it can be quite complex. A common technique for proving correctness is to use mathemati-cal induction because an algorithm’s iterations provide a natural sequence of steps needed for such proofs. It might be worth mentioning that although tracing the algorithm’s performance for a few specific inputs can be a very worthwhile activ-ity, it cannot prove the algorithm’s correctness conclusively. But in order to show that an algorithm is incorrect, you need just one instance of its input for which the algorithm fails.
The notion of correctness for approximation algorithms is less straightforward than it is for exact algorithms. For an approximation algorithm, we usually would like to be able to show that the error produced by the algorithm does not exceed a predefined limit. You can find examples of such investigations in Chapter 12.
Analyzing an Algorithm
We usually want our algorithms to possess several qualities. After correctness, by far the most important is efficiency . In fact, there are two kinds of algorithm efficiency: time efficiency , indicating how fast the algorithm runs, and space ef-ficiency , indicating how much extra memory it uses. A general framework and specific techniques for analyzing an algorithm’s efficiency appear in Chapter 2.
Another desirable characteristic of an algorithm is simplicity . Unlike effi-ciency, which can be precisely defined and investigated with mathematical rigor, simplicity, like beauty, is to a considerable degree in the eye of the beholder. For example, most people would agree that Euclid’s algorithm is simpler than the middle-school procedure for computing gcd (m, n) , but it is not clear whether Eu-clid’s algorithm is simpler than the consecutive integer checking algorithm. Still, simplicity is an important algorithm characteristic to strive for. Why? Because sim-pler algorithms are easier to understand and easier to program; consequently, the resulting programs usually contain fewer bugs. There is also the undeniable aes-thetic appeal of simplicity. Sometimes simpler algorithms are also more efficient than more complicated alternatives. Unfortunately, it is not always true, in which case a judicious compromise needs to be made.
Yet another desirable characteristic of an algorithm is generality . There are, in fact, two issues here: generality of the problem the algorithm solves and the set of inputs it accepts. On the first issue, note that it is sometimes easier to design an algorithm for a problem posed in more general terms. Consider, for example, the problem of determining whether two integers are relatively prime, i.e., whether their only common divisor is equal to 1. It is easier to design an algorithm for a more general problem of computing the greatest common divisor of two integers and, to solve the former problem, check whether the gcd is 1 or not. There are situations, however, where designing a more general algorithm is unnecessary or difficult or even impossible. For example, it is unnecessary to sort a list of n numbers to find its median, which is its n/ 2 th smallest element. To give another example, the standard formula for roots of a quadratic equation cannot be generalized to handle polynomials of arbitrary degrees.
As to the set of inputs, your main concern should be designing an algorithm that can handle a set of inputs that is natural for the problem at hand. For example, excluding integers equal to 1 as possible inputs for a greatest common divisor algorithm would be quite unnatural. On the other hand, although the standard formula for the roots of a quadratic equation holds for complex coefficients, we would normally not implement it on this level of generality unless this capability is explicitly required.
If you are not satisfied with the algorithm’s efficiency, simplicity, or generality, you must return to the drawing board and redesign the algorithm. In fact, even if your evaluation is positive, it is still worth searching for other algorithmic solutions. Recall the three different algorithms in the previous section for computing the greatest common divisor: generally, you should not expect to get the best algorithm on the first try. At the very least, you should try to fine-tune the algorithm you already have. For example, we made several improvements in our implementation of the sieve of Eratosthenes compared with its initial outline in Section 1.1. (Can you identify them?) You will do well if you keep in mind the following observation of Antoine de Saint-Exupery,´ the French writer, pilot, and aircraft designer: “A designer knows he has arrived at perfection not when there is no longer anything to add, but when there is no longer anything to take away.” 1
Coding an Algorithm
Most algorithms are destined to be ultimately implemented as computer pro-grams. Programming an algorithm presents both a peril and an opportunity. The peril lies in the possibility of making the transition from an algorithm to a pro-gram either incorrectly or very inefficiently. Some influential computer scientists strongly believe that unless the correctness of a computer program is proven with full mathematical rigor, the program cannot be considered correct. They have developed special techniques for doing such proofs (see [Gri81]), but the power of these techniques of formal verification is limited so far to very small programs.
As a practical matter, the validity of programs is still established by testing. Testing of computer programs is an art rather than a science, but that does not mean that there is nothing in it to learn. Look up books devoted to testing and debugging; even more important, test and debug your program thoroughly whenever you implement an algorithm.
Also note that throughout the book, we assume that inputs to algorithms belong to the specified sets and hence require no verification. When implementing algorithms as programs to be used in actual applications, you should provide such verifications.
Of course, implementing an algorithm correctly is necessary but not sufficient: you would not like to diminish your algorithm’s power by an inefficient implemen-tation. Modern compilers do provide a certain safety net in this regard, especially when they are used in their code optimization mode. Still, you need to be aware of such standard tricks as computing a loop’s invariant (an expression that does not change its value) outside the loop, collecting common subexpressions, replac-ing expensive operations by cheap ones, and so on. (See [Ker99] and [Ben00] for a good discussion of code tuning and other issues related to algorithm program-ming.) Typically, such improvements can speed up a program only by a constant factor, whereas a better algorithm can make a difference in running time by orders of magnitude. But once an algorithm is selected, a 10–50% speedup may be worth an effort.
A working program provides an additional opportunity in allowing an em-pirical analysis of the underlying algorithm. Such an analysis is based on timing the program on several inputs and then analyzing the results obtained. We dis-cuss the advantages and disadvantages of this approach to analyzing algorithms in Section 2.6.
In conclusion, let us emphasize again the main lesson of the process depicted in Figure 1.2:
As a rule, a good algorithm is a result of repeated effort and rework.
Even if you have been fortunate enough to get an algorithmic idea that seems perfect, you should still try to see whether it can be improved.
Actually, this is good news since it makes the ultimate result so much more enjoyable. (Yes, I did think of naming this book The Joy of Algorithms .) On the other hand, how does one know when to stop? In the real world, more often than not a project’s schedule or the impatience of your boss will stop you. And so it should be: perfection is expensive and in fact not always called for. Designing an algorithm is an engineering-like activity that calls for compromises among competing goals under the constraints of available resources, with the designer’s time being one of the resources.
In the academic world, the question leads to an interesting but usually difficult investigation of an algorithm’s optimality . Actually, this question is not about the efficiency of an algorithm but about the complexity of the problem it solves: What is the minimum amount of effort any algorithm will need to exert to solve the problem? For some problems, the answer to this question is known. For example, any algorithm that sorts an array by comparing values of its elements needs about n log 2 n comparisons for some arrays of size n (see Section 11.2). But for many seemingly easy problems such as integer multiplication, computer scientists do not yet have a final answer.
Another important issue of algorithmic problem solving is the question of whether or not every problem can be solved by an algorithm. We are not talking here about problems that do not have a solution, such as finding real roots of a quadratic equation with a negative discriminant. For such cases, an output indicating that the problem does not have a solution is all we can and should expect from an algorithm. Nor are we talking about ambiguously stated problems. Even some unambiguous problems that must have a simple yes or no answer are “undecidable,” i.e., unsolvable by any algorithm. An important example of such a problem appears in Section 11.3. Fortunately, a vast majority of problems in practical computing can be solved by an algorithm.
Before leaving this section, let us be sure that you do not have the misconception—possibly caused by the somewhat mechanical nature of the diagram of Figure 1.2—that designing an algorithm is a dull activity. There is nothing further from the truth: inventing (or discovering?) algorithms is a very creative and rewarding process. This book is designed to convince you that this is the case.
Exercises 1.2
Old World puzzle A peasant finds himself on a riverbank with a wolf, a goat, and a head of cabbage. He needs to transport all three to the other side of the river in his boat. However, the boat has room for only the peasant himself and one other item (either the wolf, the goat, or the cabbage). In his absence, the wolf would eat the goat, and the goat would eat the cabbage. Solve this problem for the peasant or prove it has no solution. (Note: The peasant is a vegetarian but does not like cabbage and hence can eat neither the goat nor the cabbage to help him solve the problem. And it goes without saying that the wolf is a protected species.)
New World puzzle There are four people who want to cross a rickety bridge; they all begin on the same side. You have 17 minutes to get them all across to the other side. It is night, and they have one flashlight. A maximum of two people can cross the bridge at one time. Any party that crosses, either one or two people, must have the flashlight with them. The flashlight must be walked back and forth; it cannot be thrown, for example. Person 1 takes 1 minute to cross the bridge, person 2 takes 2 minutes, person 3 takes 5 minutes, and person 4 takes 10 minutes. A pair must walk together at the rate of the slower person’s pace. (Note: According to a rumor on the Internet, interviewers at a well-known software company located near Seattle have given this problem to interviewees.)
Which of the following formulas can be considered an algorithm for comput-ing the area of a triangle whose side lengths are given positive numbers a , b , and c ?

Write pseudocode for an algorithm for finding real roots of equation ax 2 + bx + c = 0 for arbitrary real coefficients a, b, and c. (You may assume the availability of the square root function sqrt (x). )
Describe the standard algorithm for finding the binary representation of a positive decimal integer
in English.
in pseudocode.
Describe the algorithm used by your favorite ATM machine in dispensing cash. (You may give your description in either English or pseudocode, which-ever you find more convenient.)
a. Can the problem of computing the number π be solved exactly?
How many instances does this problem have?
Look up an algorithm for this problem on the Internet.
Give an example of a problem other than computing the greatest common divisor for which you know more than one algorithm. Which of them is simpler? Which is more efficient?
Consider the following algorithm for finding the distance between the two closest elements in an array of numbers.
ALGORITHM MinDistance (A [0 ..n − 1] )
//Input: Array A [0 ..n − 1] of numbers
//Output: Minimum distance between two of its elements dmin ← ∞
for i ← 0 to n − 1 do
for j ← 0 to n − 1 do
if i = j and |A[i] − A[j ]| < dmin dmin ← |A[i] − A[j ]|
return dmin
Make as many improvements as you can in this algorithmic solution to the problem. If you need to, you may change the algorithm altogether; if not, improve the implementation given.
One of the most influential books on problem solving, titled How To Solve It [Pol57], was written by the Hungarian-American mathematician George Polya´ (1887–1985). Polya´ summarized his ideas in a four-point summary. Find this summary on the Internet or, better yet, in his book, and compare it with the plan outlined in Section 1.2. What do they have in common? How are they different?
Related Topics
Privacy Policy , Terms and Conditions , DMCA Policy and Compliant
Copyright © 2018-2023 BrainKart.com; All Rights Reserved. Developed by Therithal info, Chennai.


DEV Community

Posted on Nov 8, 2023 • Updated on Nov 9, 2023
Introduction to Algorithms: What Every Beginner Should Know
Algorithms are the beating heart of computer science and programming. They are the step-by-step instructions that computers follow to solve problems and perform tasks. Whether you're a beginner or an aspiring programmer, understanding the fundamentals of algorithms is essential. In this blog post, we will introduce you to the world of algorithms, what they are, why they matter, and the key concepts every beginner should know.
What is an Algorithm?
At its core, an algorithm is a finite set of well-defined instructions for solving a specific problem or task. These instructions are executed in a predetermined sequence, often designed to transform input data into a desired output.
Think of algorithms as recipes: just as a recipe tells you how to prepare a meal, an algorithm tells a computer how to perform a particular task. But instead of cooking a delicious dish, you're instructing a computer to perform a specific computation or solve a problem.
Why Do Algorithms Matter?
Algorithms play a pivotal role in the world of computing for several reasons:
Efficiency: Well-designed algorithms can execute tasks quickly and use minimal resources, which is crucial in applications like search engines and real-time systems.
Problem Solving: Algorithms provide structured approaches to problem-solving and help us tackle complex issues more effectively.
Reusability: Once developed, algorithms can be used in various applications, promoting code reuse and reducing redundancy.
Scalability: Algorithms are essential for handling large datasets and growing computational needs in modern software.
Key Concepts in Algorithm Design
1. input and output.
Input: Algorithms take input data as their starting point. This could be a list of numbers, a text document, or any other data structure.
Output: Algorithms produce a result or output based on the input. This could be a sorted list, a specific calculation, or a solution to a problem.
2. Correctness
An algorithm is considered correct if it produces the desired output for all valid inputs. Ensuring correctness is a fundamental aspect of algorithm design and testing.
3. Efficiency
Efficiency is a critical consideration in algorithm design. It's about finding the most optimal way to solve a problem, which often involves minimizing the consumption of time and resources.
4. Scalability
Algorithms should be designed to handle input data of varying sizes efficiently. Scalable algorithms can adapt to growing data requirements without a significant decrease in performance.
5. Time Complexity and Space Complexity
Time complexity refers to the amount of time an algorithm takes to complete based on the size of the input. Space complexity concerns the amount of memory an algorithm requires. Understanding these complexities helps assess an algorithm's efficiency.
Examples of Common Algorithms
Sorting Algorithms: Algorithms like "Bubble Sort," "Quick Sort," and "Merge Sort" rearrange a list of items into a specific order.
Search Algorithms: Algorithms like "Binary Search" efficiently find a specific item in a sorted list.
Graph Algorithms: Algorithms like "Breadth-First Search" and "Dijkstra's Algorithm" navigate through networks and find optimal routes.
Dynamic Programming: Algorithms like the "Fibonacci Sequence" solver use a technique called dynamic programming to optimize problem-solving.
Algorithms are the building blocks of computer science and programming. While they might sound complex, they are at the heart of everything from internet search engines to your favorite social media platforms. As a beginner, understanding the basics of algorithms and their importance is a significant step in your coding journey.
As you dive into the world of algorithms, you'll discover that they can be both fascinating and challenging. Don't be discouraged by complex problems; instead, view them as opportunities to develop your problem-solving skills. Whether you're aiming to become a software developer, data scientist, or just a more proficient programmer, a solid grasp of algorithms is an invaluable asset. Happy coding! 🚀💡
Also, I have to tell you, this article was partially generated with the help of ChatGPT!!!
Top comments (1)
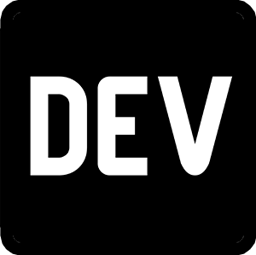
Templates let you quickly answer FAQs or store snippets for re-use.

- Location Bangkok 🇹🇭
- Joined Jun 1, 2018
Hi there. This post reads a lot like it was generated or strongly assisted by AI. If so, please consider amending it to comply with the DEV.to guidelines concerning such content...
From " The DEV Community Guidelines for AI-Assisted and -Generated Articles ":
AI-assisted and -generated articles should… Be created and published in good faith, meaning with honest, sincere, and harmless intentions. Disclose the fact that they were generated or assisted by AI in the post, either upfront using the tag #ABotWroteThis or at any point in the article’s copy (including right at the end). - For example, a conclusion that states “Surprise, this article was generated by ChatGPT!” or the disclaimer “This article was created with the help of AI” would be appropriate. Ideally add something to the conversation regarding AI and its capabilities. Tell us your story of using the tool to create content, and why!
Are you sure you want to hide this comment? It will become hidden in your post, but will still be visible via the comment's permalink .
Hide child comments as well
For further actions, you may consider blocking this person and/or reporting abuse

Functions vs Classes: When to Use Which and Why?
Sharoz Tanveer🚀 - Sep 24
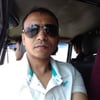
3043. Find the Length of the Longest Common Prefix
MD ARIFUL HAQUE - Sep 24

Difference Between Axios & Fetch in Javascript
pulkitgovrani - Sep 24

Advanced JavaScript Concepts Every Developer Should Know
Noor-ul-hassan - Sep 24
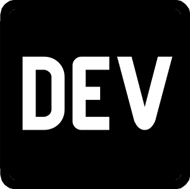
We're a place where coders share, stay up-to-date and grow their careers.
- Admiral “Amazing Grace” Hopper
Exploring the Intricacies of NP-Completeness in Computer Science
Understanding p vs np problems in computer science: a primer for beginners, understanding key theoretical frameworks in computer science: a beginner’s guide.

Learn Computer Science with Python
CS is a journey, not a destination
- Foundations
Understanding Algorithms: The Key to Problem-Solving Mastery

The world of computer science is a fascinating realm, where intricate concepts and technologies continuously shape the way we interact with machines. Among the vast array of ideas and principles, few are as fundamental and essential as algorithms. These powerful tools serve as the building blocks of computation, enabling computers to solve problems, make decisions, and process vast amounts of data efficiently.
An algorithm can be thought of as a step-by-step procedure or a set of instructions designed to solve a specific problem or accomplish a particular task. It represents a systematic approach to finding solutions and provides a structured way to tackle complex computational challenges. Algorithms are at the heart of various applications, from simple calculations to sophisticated machine learning models and complex data analysis.
Understanding algorithms and their inner workings is crucial for anyone interested in computer science. They serve as the backbone of software development, powering the creation of innovative applications across numerous domains. By comprehending the concept of algorithms, aspiring computer science enthusiasts gain a powerful toolset to approach problem-solving and gain insight into the efficiency and performance of different computational methods.
In this article, we aim to provide a clear and accessible introduction to algorithms, focusing on their importance in problem-solving and exploring common types such as searching, sorting, and recursion. By delving into these topics, readers will gain a solid foundation in algorithmic thinking and discover the underlying principles that drive the functioning of modern computing systems. Whether you’re a beginner in the world of computer science or seeking to deepen your understanding, this article will equip you with the knowledge to navigate the fascinating world of algorithms.
What are Algorithms?
At its core, an algorithm is a systematic, step-by-step procedure or set of rules designed to solve a problem or perform a specific task. It provides clear instructions that, when followed meticulously, lead to the desired outcome.
Consider an algorithm to be akin to a recipe for your favorite dish. When you decide to cook, the recipe is your go-to guide. It lists out the ingredients you need, their exact quantities, and a detailed, step-by-step explanation of the process, from how to prepare the ingredients to how to mix them, and finally, the cooking process. It even provides an order for adding the ingredients and specific times for cooking to ensure the dish turns out perfect.
In the same vein, an algorithm, within the realm of computer science, provides an explicit series of instructions to accomplish a goal. This could be a simple goal like sorting a list of numbers in ascending order, a more complex task such as searching for a specific data point in a massive dataset, or even a highly complicated task like determining the shortest path between two points on a map (think Google Maps). No matter the complexity of the problem at hand, there’s always an algorithm working tirelessly behind the scenes to solve it.
Furthermore, algorithms aren’t limited to specific programming languages. They are universal and can be implemented in any language. This is why understanding the fundamental concept of algorithms can empower you to solve problems across various programming languages.
The Importance of Algorithms
Algorithms are indisputably the backbone of all computational operations. They’re a fundamental part of the digital world that we interact with daily. When you search for something on the web, an algorithm is tirelessly working behind the scenes to sift through millions, possibly billions, of web pages to bring you the most relevant results. When you use a GPS to find the fastest route to a location, an algorithm is computing all possible paths, factoring in variables like traffic and road conditions, to provide you the optimal route.
Consider the world of social media, where algorithms curate personalized feeds based on our previous interactions, or in streaming platforms where they recommend shows and movies based on our viewing habits. Every click, every like, every search, and every interaction is processed by algorithms to serve you a seamless digital experience.
In the realm of computer science and beyond, everything revolves around problem-solving, and algorithms are our most reliable problem-solving tools. They provide a structured approach to problem-solving, breaking down complex problems into manageable steps and ensuring that every eventuality is accounted for.
Moreover, an algorithm’s efficiency is not just a matter of preference but a necessity. Given that computers have finite resources — time, memory, and computational power — the algorithms we use need to be optimized to make the best possible use of these resources. Efficient algorithms are the ones that can perform tasks more quickly, using less memory, and provide solutions to complex problems that might be infeasible with less efficient alternatives.
In the context of massive datasets (the likes of which are common in our data-driven world), the difference between a poorly designed algorithm and an efficient one could be the difference between a solution that takes years to compute and one that takes mere seconds. Therefore, understanding, designing, and implementing efficient algorithms is a critical skill for any computer scientist or software engineer.
Hence, as a computer science beginner, you are starting a journey where algorithms will be your best allies — universal keys capable of unlocking solutions to a myriad of problems, big or small.
Common Types of Algorithms: Searching and Sorting
Two of the most ubiquitous types of algorithms that beginners often encounter are searching and sorting algorithms.
Searching algorithms are designed to retrieve specific information from a data structure, like an array or a database. A simple example is the linear search, which works by checking each element in the array until it finds the one it’s looking for. Although easy to understand, this method isn’t efficient for large datasets, which is where more complex algorithms like binary search come in.
Binary search, on the other hand, is like looking up a word in the dictionary. Instead of checking each word from beginning to end, you open the dictionary in the middle and see if the word you’re looking for should be on the left or right side, thereby reducing the search space by half with each step.
Sorting algorithms, meanwhile, are designed to arrange elements in a particular order. A simple sorting algorithm is bubble sort, which works by repeatedly swapping adjacent elements if they’re in the wrong order. Again, while straightforward, it’s not efficient for larger datasets. More advanced sorting algorithms, such as quicksort or mergesort, have been designed to sort large data collections more efficiently.
Diving Deeper: Graph and Dynamic Programming Algorithms
Building upon our understanding of searching and sorting algorithms, let’s delve into two other families of algorithms often encountered in computer science: graph algorithms and dynamic programming algorithms.
A graph is a mathematical structure that models the relationship between pairs of objects. Graphs consist of vertices (or nodes) and edges (where each edge connects a pair of vertices). Graphs are commonly used to represent real-world systems such as social networks, web pages, biological networks, and more.
Graph algorithms are designed to solve problems centered around these structures. Some common graph algorithms include:
Dynamic programming is a powerful method used in optimization problems, where the main problem is broken down into simpler, overlapping subproblems. The solutions to these subproblems are stored and reused to build up the solution to the main problem, saving computational effort.
Here are two common dynamic programming problems:
Understanding these algorithm families — searching, sorting, graph, and dynamic programming algorithms — not only equips you with powerful tools to solve a variety of complex problems but also serves as a springboard to dive deeper into the rich ocean of algorithms and computer science.
Recursion: A Powerful Technique
While searching and sorting represent specific problem domains, recursion is a broad technique used in a wide range of algorithms. Recursion involves breaking down a problem into smaller, more manageable parts, and a function calling itself to solve these smaller parts.
To visualize recursion, consider the task of calculating factorial of a number. The factorial of a number n (denoted as n! ) is the product of all positive integers less than or equal to n . For instance, the factorial of 5 ( 5! ) is 5 x 4 x 3 x 2 x 1 = 120 . A recursive algorithm for finding factorial of n would involve multiplying n by the factorial of n-1 . The function keeps calling itself with a smaller value of n each time until it reaches a point where n is equal to 1, at which point it starts returning values back up the chain.
Algorithms are truly the heart of computer science, transforming raw data into valuable information and insight. Understanding their functionality and purpose is key to progressing in your computer science journey. As you continue your exploration, remember that each algorithm you encounter, no matter how complex it may seem, is simply a step-by-step procedure to solve a problem.
We’ve just scratched the surface of the fascinating world of algorithms. With time, patience, and practice, you will learn to create your own algorithms and start solving problems with confidence and efficiency.
Related Articles

Three Elegant Algorithms Every Computer Science Beginner Should Know
Hold on, while it loads...

Mastering Algorithms: A Beginner's Guide to Problem-Solving
Learn the fundamentals of algorithms and improve your problem-solving skills with this comprehensive guide. From Big O notation to dynamic programming, we've got you covered.
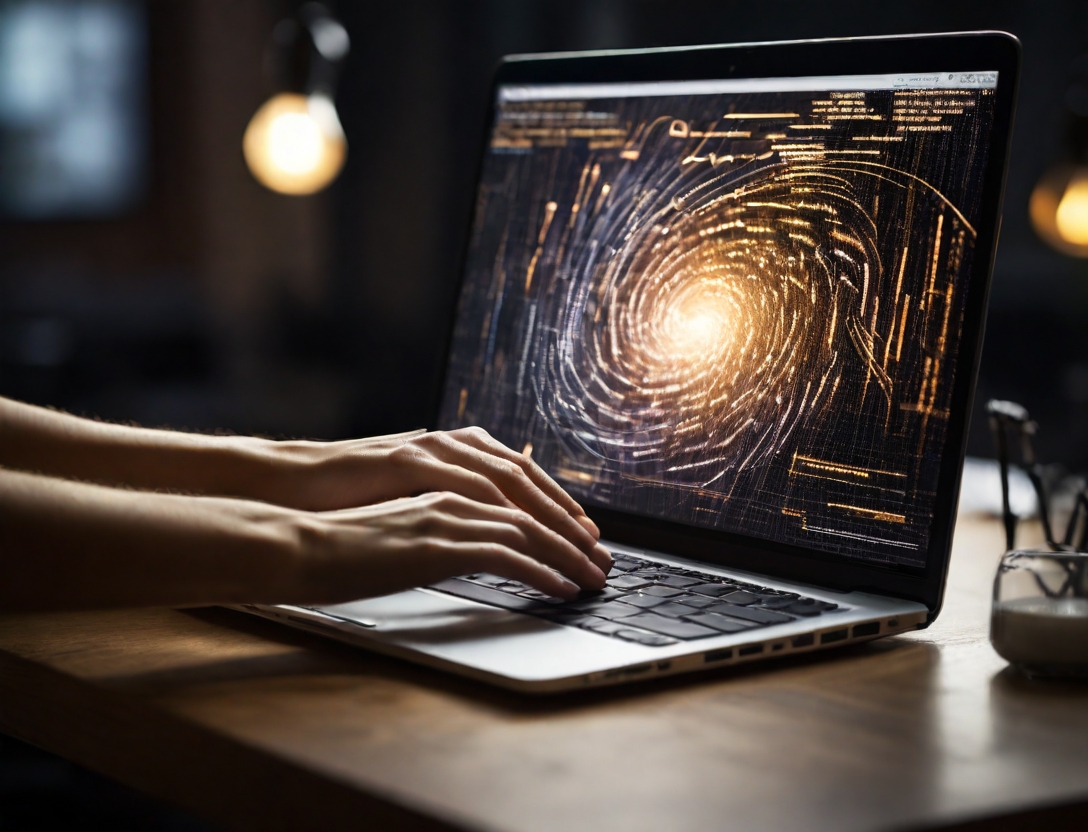
Create an image featuring JavaScript code snippets and interview-related icons or graphics. Use a color scheme of yellows and blues. Include the title '7 Essential JavaScript Interview Questions for Freshers'.
Learn Algorithms: A Comprehensive Guide to Mastering Problem-Solving
Why learn algorithms.
In the world of computer science, algorithms play a crucial role in solving complex problems efficiently. They are the heart of any computer program, and mastering them is essential for any aspiring software developer, data scientist, or engineer. Algorithms help us write efficient code, reduce computational time, and solve real-world problems. In this article, we'll delve into the world of algorithms, exploring their importance, types, and how to learn them.
What are Algorithms?
An algorithm is a well-defined procedure that takes some input and produces a corresponding output. It's a step-by-step process that solves a specific problem or performs a particular task. Algorithms can be expressed in various forms, such as natural language, flowcharts, pseudocode, or programming languages.
Characteristics of Algorithms
A good algorithm should possess the following characteristics:
- Correctness : The algorithm should produce the correct output for a given input.
- Efficiency : The algorithm should use minimal resources, such as time and memory.
- Scalability : The algorithm should be able to handle large inputs and scale well.
- Simplicity : The algorithm should be easy to understand and implement.
Types of Algorithms
Algorithms can be classified into several categories based on their purpose, complexity, and application. Here are some common types of algorithms:
1. Sorting Algorithms
Sorting algorithms arrange data in a specific order, such as alphabetical or numerical. Examples include Bubble Sort, Selection Sort, and Quick Sort.
2. Searching Algorithms
Searching algorithms find specific data within a dataset. Examples include Linear Search and Binary Search.
3. Graph Algorithms
Graph algorithms operate on graph data structures, which consist of nodes and edges. Examples include Breadth-First Search (BFS) and Depth-First Search (DFS).
4. Dynamic Programming Algorithms
Dynamic programming algorithms break down complex problems into smaller sub-problems and solve them efficiently. Examples include Fibonacci Series and Longest Common Subsequence.
5. Backtracking Algorithms
Backtracking algorithms explore all possible solutions to a problem and backtrack when a dead end is reached. Examples include N-Queens Problem and Sudoku.
How to Learn Algorithms
Learning algorithms requires practice, patience, and persistence. Here are some tips to get you started:
1. Start with the Basics
Begin with basic algorithms, such as sorting and searching. Understand the concepts, and practice implementing them in a programming language of your choice.
2. Practice, Practice, Practice
Practice is key to mastering algorithms. Websites like LeetCode, HackerRank, and CodeForces offer a vast collection of problems to solve.
3. Analyze Time and Space Complexity
Understand the time and space complexity of algorithms, which determines their efficiency.
4. Learn from Others
Study the solutions of others, and learn from their approaches.
5. Join Online Communities
Participate in online communities, such as Reddit's r/learnprogramming and r/algorithms, to connect with other learners and experts.
Common Algorithmic Concepts
Here are some essential concepts to grasp when learning algorithms:
1. Big O Notation
Big O notation measures the time and space complexity of an algorithm.
2. Trade-Offs
Trade-offs occur when optimizing one aspect of an algorithm affects another aspect.
3. Dynamic Programming
Dynamic programming solves problems by breaking them down into smaller sub-problems.
4. Greedy Algorithms
Greedy algorithms make the optimal choice at each step, hoping to find a global optimum.
5. Recursion
Recursion solves problems by breaking them down into smaller instances of the same problem.
Mastering algorithms takes time and effort, but it's a crucial skill for any aspiring software developer, data scientist, or engineer. By understanding the basics, practicing regularly, and learning from others, you can develop the problem-solving skills necessary to tackle complex problems. Remember, algorithms are the heart of computer science, and mastering them will open doors to new opportunities and challenges.
- GeeksforGeeks
- MIT OpenCourseWare: Algorithms
I hope this comprehensive guide helps you embark on your algorithmic journey!
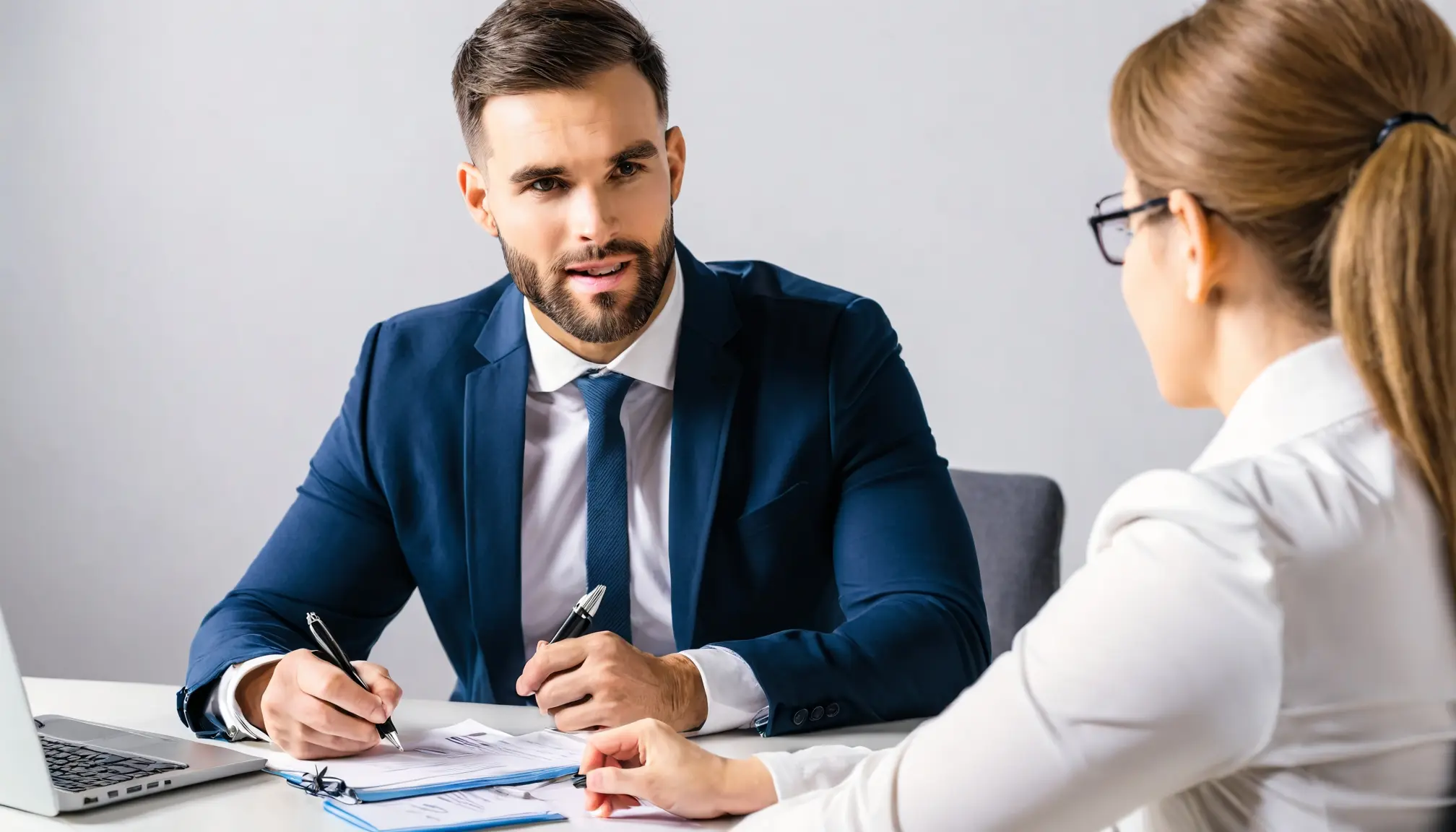
- 1. Micro-Worlds
- 2. Light-Bot in Java
- 3. Jeroos of Santong Island
- 4. Problem Solving and Algorithms
- 5. Creating Jeroo Methods
- 6. Conditionally Executing Actions
- 7. Repeating Actions
- 8. Handling Touch Events
- 9. Adding Text to the Screen
Problem Solving and Algorithms
Learn a basic process for developing a solution to a problem. Nothing in this chapter is unique to using a computer to solve a problem. This process can be used to solve a wide variety of problems, including ones that have nothing to do with computers.
Problems, Solutions, and Tools
I have a problem! I need to thank Aunt Kay for the birthday present she sent me. I could send a thank you note through the mail. I could call her on the telephone. I could send her an email message. I could drive to her house and thank her in person. In fact, there are many ways I could thank her, but that's not the point. The point is that I must decide how I want to solve the problem, and use the appropriate tool to implement (carry out) my plan. The postal service, the telephone, the internet, and my automobile are tools that I can use, but none of these actually solves my problem. In a similar way, a computer does not solve problems, it's just a tool that I can use to implement my plan for solving the problem.
Knowing that Aunt Kay appreciates creative and unusual things, I have decided to hire a singing messenger to deliver my thanks. In this context, the messenger is a tool, but one that needs instructions from me. I have to tell the messenger where Aunt Kay lives, what time I would like the message to be delivered, and what lyrics I want sung. A computer program is similar to my instructions to the messenger.
The story of Aunt Kay uses a familiar context to set the stage for a useful point of view concerning computers and computer programs. The following list summarizes the key aspects of this point of view.
A computer is a tool that can be used to implement a plan for solving a problem.
A computer program is a set of instructions for a computer. These instructions describe the steps that the computer must follow to implement a plan.
An algorithm is a plan for solving a problem.
A person must design an algorithm.
A person must translate an algorithm into a computer program.
This point of view sets the stage for a process that we will use to develop solutions to Jeroo problems. The basic process is important because it can be used to solve a wide variety of problems, including ones where the solution will be written in some other programming language.
An Algorithm Development Process
Every problem solution starts with a plan. That plan is called an algorithm.
There are many ways to write an algorithm. Some are very informal, some are quite formal and mathematical in nature, and some are quite graphical. The instructions for connecting a DVD player to a television are an algorithm. A mathematical formula such as πR 2 is a special case of an algorithm. The form is not particularly important as long as it provides a good way to describe and check the logic of the plan.
The development of an algorithm (a plan) is a key step in solving a problem. Once we have an algorithm, we can translate it into a computer program in some programming language. Our algorithm development process consists of five major steps.
Step 1: Obtain a description of the problem.
Step 2: analyze the problem., step 3: develop a high-level algorithm., step 4: refine the algorithm by adding more detail., step 5: review the algorithm..
This step is much more difficult than it appears. In the following discussion, the word client refers to someone who wants to find a solution to a problem, and the word developer refers to someone who finds a way to solve the problem. The developer must create an algorithm that will solve the client's problem.
The client is responsible for creating a description of the problem, but this is often the weakest part of the process. It's quite common for a problem description to suffer from one or more of the following types of defects: (1) the description relies on unstated assumptions, (2) the description is ambiguous, (3) the description is incomplete, or (4) the description has internal contradictions. These defects are seldom due to carelessness by the client. Instead, they are due to the fact that natural languages (English, French, Korean, etc.) are rather imprecise. Part of the developer's responsibility is to identify defects in the description of a problem, and to work with the client to remedy those defects.
The purpose of this step is to determine both the starting and ending points for solving the problem. This process is analogous to a mathematician determining what is given and what must be proven. A good problem description makes it easier to perform this step.
When determining the starting point, we should start by seeking answers to the following questions:
What data are available?
Where is that data?
What formulas pertain to the problem?
What rules exist for working with the data?
What relationships exist among the data values?
When determining the ending point, we need to describe the characteristics of a solution. In other words, how will we know when we're done? Asking the following questions often helps to determine the ending point.
What new facts will we have?
What items will have changed?
What changes will have been made to those items?
What things will no longer exist?
An algorithm is a plan for solving a problem, but plans come in several levels of detail. It's usually better to start with a high-level algorithm that includes the major part of a solution, but leaves the details until later. We can use an everyday example to demonstrate a high-level algorithm.
Problem: I need a send a birthday card to my brother, Mark.
Analysis: I don't have a card. I prefer to buy a card rather than make one myself.
High-level algorithm:
Go to a store that sells greeting cards Select a card Purchase a card Mail the card
This algorithm is satisfactory for daily use, but it lacks details that would have to be added were a computer to carry out the solution. These details include answers to questions such as the following.
"Which store will I visit?"
"How will I get there: walk, drive, ride my bicycle, take the bus?"
"What kind of card does Mark like: humorous, sentimental, risqué?"
These kinds of details are considered in the next step of our process.
A high-level algorithm shows the major steps that need to be followed to solve a problem. Now we need to add details to these steps, but how much detail should we add? Unfortunately, the answer to this question depends on the situation. We have to consider who (or what) is going to implement the algorithm and how much that person (or thing) already knows how to do. If someone is going to purchase Mark's birthday card on my behalf, my instructions have to be adapted to whether or not that person is familiar with the stores in the community and how well the purchaser known my brother's taste in greeting cards.
When our goal is to develop algorithms that will lead to computer programs, we need to consider the capabilities of the computer and provide enough detail so that someone else could use our algorithm to write a computer program that follows the steps in our algorithm. As with the birthday card problem, we need to adjust the level of detail to match the ability of the programmer. When in doubt, or when you are learning, it is better to have too much detail than to have too little.
Most of our examples will move from a high-level to a detailed algorithm in a single step, but this is not always reasonable. For larger, more complex problems, it is common to go through this process several times, developing intermediate level algorithms as we go. Each time, we add more detail to the previous algorithm, stopping when we see no benefit to further refinement. This technique of gradually working from a high-level to a detailed algorithm is often called stepwise refinement .
The final step is to review the algorithm. What are we looking for? First, we need to work through the algorithm step by step to determine whether or not it will solve the original problem. Once we are satisfied that the algorithm does provide a solution to the problem, we start to look for other things. The following questions are typical of ones that should be asked whenever we review an algorithm. Asking these questions and seeking their answers is a good way to develop skills that can be applied to the next problem.
Does this algorithm solve a very specific problem or does it solve a more general problem ? If it solves a very specific problem, should it be generalized?
For example, an algorithm that computes the area of a circle having radius 5.2 meters (formula π*5.2 2 ) solves a very specific problem, but an algorithm that computes the area of any circle (formula π*R 2 ) solves a more general problem.
Can this algorithm be simplified ?
One formula for computing the perimeter of a rectangle is:
length + width + length + width
A simpler formula would be:
2.0 * ( length + width )
Is this solution similar to the solution to another problem? How are they alike? How are they different?
For example, consider the following two formulae:
Rectangle area = length * width Triangle area = 0.5 * base * height
Similarities: Each computes an area. Each multiplies two measurements.
Differences: Different measurements are used. The triangle formula contains 0.5.
Hypothesis: Perhaps every area formula involves multiplying two measurements.
Example 4.1: Pick and Plant
This section contains an extended example that demonstrates the algorithm development process. To complete the algorithm, we need to know that every Jeroo can hop forward, turn left and right, pick a flower from its current location, and plant a flower at its current location.
Problem Statement (Step 1)
A Jeroo starts at (0, 0) facing East with no flowers in its pouch. There is a flower at location (3, 0). Write a program that directs the Jeroo to pick the flower and plant it at location (3, 2). After planting the flower, the Jeroo should hop one space East and stop. There are no other nets, flowers, or Jeroos on the island.
Start | Finish |
---|---|
Analysis of the Problem (Step 2)
The flower is exactly three spaces ahead of the jeroo.
The flower is to be planted exactly two spaces South of its current location.
The Jeroo is to finish facing East one space East of the planted flower.
There are no nets to worry about.
High-level Algorithm (Step 3)
Let's name the Jeroo Bobby. Bobby should do the following:
Get the flower Put the flower Hop East
Detailed Algorithm (Step 4)
Get the flower Hop 3 times Pick the flower Put the flower Turn right Hop 2 times Plant a flower Hop East Turn left Hop once
Review the Algorithm (Step 5)
The high-level algorithm partitioned the problem into three rather easy subproblems. This seems like a good technique.
This algorithm solves a very specific problem because the Jeroo and the flower are in very specific locations.
This algorithm is actually a solution to a slightly more general problem in which the Jeroo starts anywhere, and the flower is 3 spaces directly ahead of the Jeroo.
Java Code for "Pick and Plant"
A good programmer doesn't write a program all at once. Instead, the programmer will write and test the program in a series of builds. Each build adds to the previous one. The high-level algorithm will guide us in this process.
FIRST BUILD
To see this solution in action, create a new Greenfoot4Sofia scenario and use the Edit Palettes Jeroo menu command to make the Jeroo classes visible. Right-click on the Island class and create a new subclass with the name of your choice. This subclass will hold your new code.
The recommended first build contains three things:
The main method (here myProgram() in your island subclass).
Declaration and instantiation of every Jeroo that will be used.
The high-level algorithm in the form of comments.
The instantiation at the beginning of myProgram() places bobby at (0, 0), facing East, with no flowers.
Once the first build is working correctly, we can proceed to the others. In this case, each build will correspond to one step in the high-level algorithm. It may seem like a lot of work to use four builds for such a simple program, but doing so helps establish habits that will become invaluable as the programs become more complex.
SECOND BUILD
This build adds the logic to "get the flower", which in the detailed algorithm (step 4 above) consists of hopping 3 times and then picking the flower. The new code is indicated by comments that wouldn't appear in the original (they are just here to call attention to the additions). The blank lines help show the organization of the logic.
By taking a moment to run the work so far, you can confirm whether or not this step in the planned algorithm works as expected.
THIRD BUILD
This build adds the logic to "put the flower". New code is indicated by the comments that are provided here to mark the additions.
FOURTH BUILD (final)
Example 4.2: replace net with flower.
This section contains a second example that demonstrates the algorithm development process.
There are two Jeroos. One Jeroo starts at (0, 0) facing North with one flower in its pouch. The second starts at (0, 2) facing East with one flower in its pouch. There is a net at location (3, 2). Write a program that directs the first Jeroo to give its flower to the second one. After receiving the flower, the second Jeroo must disable the net, and plant a flower in its place. After planting the flower, the Jeroo must turn and face South. There are no other nets, flowers, or Jeroos on the island.
Jeroo_2 is exactly two spaces behind Jeroo_1.
The only net is exactly three spaces ahead of Jeroo_2.
Each Jeroo has exactly one flower.
Jeroo_2 will have two flowers after receiving one from Jeroo_1. One flower must be used to disable the net. The other flower must be planted at the location of the net, i.e. (3, 2).
Jeroo_1 will finish at (0, 1) facing South.
Jeroo_2 is to finish at (3, 2) facing South.
Each Jeroo will finish with 0 flowers in its pouch. One flower was used to disable the net, and the other was planted.
Let's name the first Jeroo Ann and the second one Andy.
Ann should do the following: Find Andy (but don't collide with him) Give a flower to Andy (he will be straight ahead) After receiving the flower, Andy should do the following: Find the net (but don't hop onto it) Disable the net Plant a flower at the location of the net Face South
Ann should do the following: Find Andy Turn around (either left or right twice) Hop (to location (0, 1)) Give a flower to Andy Give ahead Now Andy should do the following: Find the net Hop twice (to location (2, 2)) Disable the net Toss Plant a flower at the location of the net Hop (to location (3, 2)) Plant a flower Face South Turn right
The high-level algorithm helps manage the details.
This algorithm solves a very specific problem, but the specific locations are not important. The only thing that is important is the starting location of the Jeroos relative to one another and the location of the net relative to the second Jeroo's location and direction.
Java Code for "Replace Net with Flower"
As before, the code should be written incrementally as a series of builds. Four builds will be suitable for this problem. As usual, the first build will contain the main method, the declaration and instantiation of the Jeroo objects, and the high-level algorithm in the form of comments. The second build will have Ann give her flower to Andy. The third build will have Andy locate and disable the net. In the final build, Andy will place the flower and turn East.
This build creates the main method, instantiates the Jeroos, and outlines the high-level algorithm. In this example, the main method would be myProgram() contained within a subclass of Island .
This build adds the logic for Ann to locate Andy and give him a flower.
This build adds the logic for Andy to locate and disable the net.
This build adds the logic for Andy to place a flower at (3, 2) and turn South.
Smart. Open. Grounded. Inventive. Read our Ideas Made to Matter.

Which program is right for you?
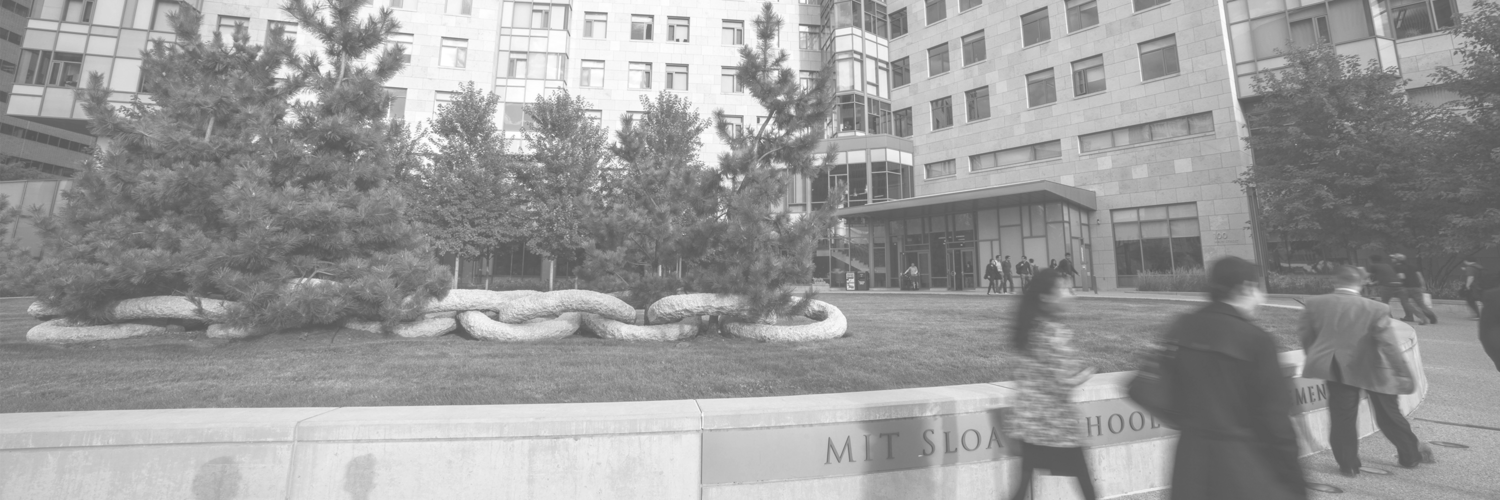
Through intellectual rigor and experiential learning, this full-time, two-year MBA program develops leaders who make a difference in the world.
Earn your MBA and SM in engineering with this transformative two-year program.
A rigorous, hands-on program that prepares adaptive problem solvers for premier finance careers.
A 12-month program focused on applying the tools of modern data science, optimization and machine learning to solve real-world business problems.
Combine an international MBA with a deep dive into management science. A special opportunity for partner and affiliate schools only.
A doctoral program that produces outstanding scholars who are leading in their fields of research.
Bring a business perspective to your technical and quantitative expertise with a bachelor’s degree in management, business analytics, or finance.
Apply now and work for two to five years. We'll save you a seat in our MBA class when you're ready to come back to campus for your degree.
Executive Programs
The 20-month program teaches the science of management to mid-career leaders who want to move from success to significance.
A full-time MBA program for mid-career leaders eager to dedicate one year of discovery for a lifetime of impact.
A joint program for mid-career professionals that integrates engineering and systems thinking. Earn your master’s degree in engineering and management.
Non-degree programs for senior executives and high-potential managers.
A non-degree, customizable program for mid-career professionals.
Generative AI enables companies to execute with speed
4 ways for data officers to take the helm on AI initiatives
In election cycles, voters tend to believe news that confirms their biases
Credit: Alejandro Giraldo
Ideas Made to Matter
How to use algorithms to solve everyday problems
Kara Baskin
May 8, 2017
How can I navigate the grocery store quickly? Why doesn’t anyone like my Facebook status? How can I alphabetize my bookshelves in a hurry? Apple data visualizer and MIT System Design and Management graduate Ali Almossawi solves these common dilemmas and more in his new book, “ Bad Choices: How Algorithms Can Help You Think Smarter and Live Happier ,” a quirky, illustrated guide to algorithmic thinking.
For the uninitiated: What is an algorithm? And how can algorithms help us to think smarter?
An algorithm is a process with unambiguous steps that has a beginning and an end, and does something useful.
Algorithmic thinking is taking a step back and asking, “If it’s the case that algorithms are so useful in computing to achieve predictability, might they also be useful in everyday life, when it comes to, say, deciding between alternative ways of solving a problem or completing a task?” In all cases, we optimize for efficiency: We care about time or space.
Note the mention of “deciding between.” Computer scientists do that all the time, and I was convinced that the tools they use to evaluate competing algorithms would be of interest to a broad audience.
Why did you write this book, and who can benefit from it?
All the books I came across that tried to introduce computer science involved coding. My approach to making algorithms compelling was focusing on comparisons. I take algorithms and put them in a scene from everyday life, such as matching socks from a pile, putting books on a shelf, remembering things, driving from one point to another, or cutting an onion. These activities can be mapped to one or more fundamental algorithms, which form the basis for the field of computing and have far-reaching applications and uses.
I wrote the book with two audiences in mind. One, anyone, be it a learner or an educator, who is interested in computer science and wants an engaging and lighthearted, but not a dumbed-down, introduction to the field. Two, anyone who is already familiar with the field and wants to experience a way of explaining some of the fundamental concepts in computer science differently than how they’re taught.
I’m going to the grocery store and only have 15 minutes. What do I do?
Do you know what the grocery store looks like ahead of time? If you know what it looks like, it determines your list. How do you prioritize things on your list? Order the items in a way that allows you to avoid walking down the same aisles twice.
For me, the intriguing thing is that the grocery store is a scene from everyday life that I can use as a launch pad to talk about various related topics, like priority queues and graphs and hashing. For instance, what is the most efficient way for a machine to store a prioritized list, and what happens when the equivalent of you scratching an item from a list happens in the machine’s list? How is a store analogous to a graph (an abstraction in computer science and mathematics that defines how things are connected), and how is navigating the aisles in a store analogous to traversing a graph?
Nobody follows me on Instagram. How do I get more followers?
The concept of links and networks, which I cover in Chapter 6, is relevant here. It’s much easier to get to people whom you might be interested in and who might be interested in you if you can start within the ball of links that connects those people, rather than starting at a random spot.
You mention Instagram: There, the hashtag is one way to enter that ball of links. Tag your photos, engage with users who tag their photos with the same hashtags, and you should be on your way to stardom.
What are the secret ingredients of a successful Facebook post?
I’ve posted things on social media that have died a sad death and then posted the same thing at a later date that somehow did great. Again, if we think of it in terms that are relevant to algorithms, we’d say that the challenge with making something go viral is really getting that first spark. And to get that first spark, a person who is connected to the largest number of people who are likely to engage with that post, needs to share it.
With [my first book], “Bad Arguments,” I spent a month pouring close to $5,000 into advertising for that project with moderate results. And then one science journalist with a large audience wrote about it, and the project took off and hasn’t stopped since.
What problems do you wish you could solve via algorithm but can’t?
When we care about efficiency, thinking in terms of algorithms is useful. There are cases when that’s not the quality we want to optimize for — for instance, learning or love. I walk for several miles every day, all throughout the city, as I find it relaxing. I’ve never asked myself, “What’s the most efficient way I can traverse the streets of San Francisco?” It’s not relevant to my objective.
Algorithms are a great way of thinking about efficiency, but the question has to be, “What approach can you optimize for that objective?” That’s what worries me about self-help: Books give you a silver bullet for doing everything “right” but leave out all the nuances that make us different. What works for you might not work for me.
Which companies use algorithms well?
When you read that the overwhelming majority of the shows that users of, say, Netflix, watch are due to Netflix’s recommendation engine, you know they’re doing something right.
Related Articles


Learn Computer Science
Explore the World Of Computer Science
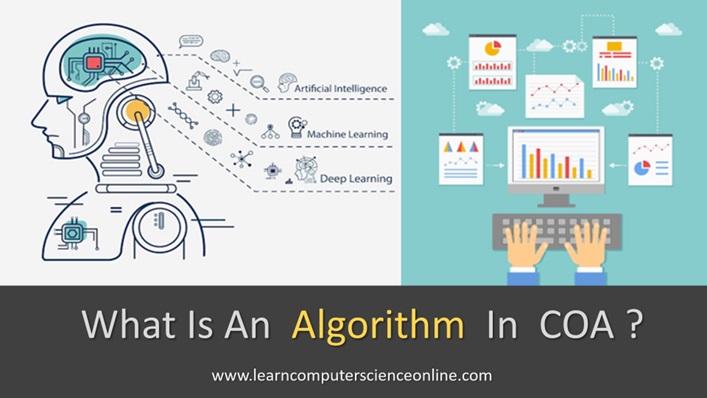
What Is An Algorithm ?
Algorithm types and features.
Beginners complete guide to the basics of algorithm , what is an algorithm, how it works, types and Characteristics.
Introduction To Algorithm
An algorithm is a well-defined set of steps that can be followed to solve a particular problem or perform a specific task. Algorithms are used in many fields, including computer science , mathematics, and engineering.
In computer science, algorithms are used to develop software and computer programs, and to solve various problems related to computation. Algorithms are step-by-step procedures for solving problems, performing computations, or carrying out tasks.
They are used in a wide range of applications, from computer science and engineering to finance, biology, and many other fields.
An algorithm can be described in many different ways, including in natural language, in a flowchart, or in pseudocode. Pseudocode is a simple programming-like language that is used to describe algorithms in a way that is easy to understand and can be translated into a programming language later.
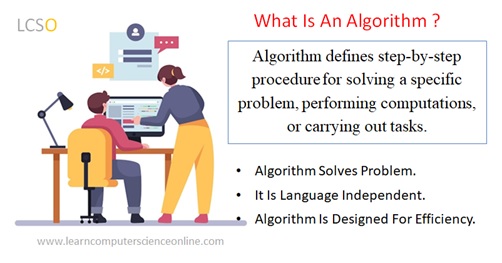
There are many different types of algorithms, including sorting algorithms, search algorithms, encryption algorithms, and compression algorithms. Each type of algorithm is designed to solve a specific problem or perform a specific task.
To analyse algorithms, computer scientists use various measures, including time complexity and space complexity. Time complexity refers to how long it takes an algorithm to run, and space complexity refers to how much memory an algorithm requires to run.
Designing and analysing algorithms is an important part of computer science and related fields. Researchers and practitioners in these fields seek to develop algorithms that are efficient, accurate, and reliable, and that can handle large volumes of data and complex problems.
Algorithms are a fundamental part of computer science and play a critical role in developing software and solving computational problems.
An algorithm is a set of well-defined instructions or steps that can be followed to solve a problem or perform a specific task. It is essentially a series of logical steps that can be executed to achieve a desired result. Algorithms can be expressed in various ways, such as natural language, flowcharts, or pseudocode.
In computer science, algorithms are used to develop software programs and solve various computational problems. For example, a search algorithm might be used to find a specific piece of information in a large database, while a sorting algorithm might be used to arrange a list of items in a specific order.
Algorithms can be classified into many different categories, including sorting algorithms, searching algorithms, graph algorithms, and optimization algorithms. Each type of algorithm is designed to solve a specific problem or perform a specific task.
Computer Programming And Algorithm
Algorithm and computer programming are closely related concepts. Algorithms are the step-by-step procedures used to solve a problem or perform a task, while computer programming involves writing code in a programming language to implement an algorithm and make it executable by a computer.
When developing a computer program, the first step is to design an algorithm that solves the problem at hand. The algorithm may be designed using pseudocode, a structured language that resembles code but is not tied to any specific programming language. Once the algorithm is designed, it can be translated into code in a specific programming language, such as Java , Python, or C++.
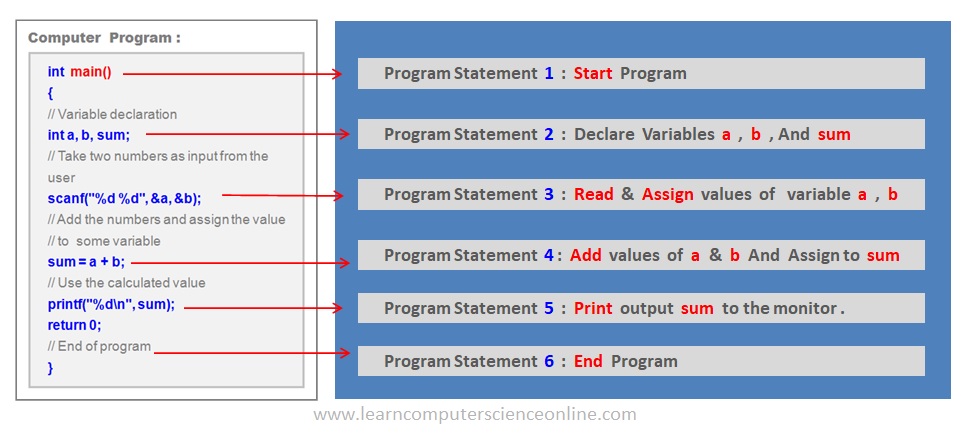
The programming language provides the syntax and structure necessary to implement the algorithm in a way that can be executed by a computer. Programmers use variables, data structures, conditional statements, loops, and other programming constructs to implement the algorithm and create a working program.
Good programming practices involve writing clear, efficient, and maintainable code that implements the algorithm correctly and handles errors and exceptions gracefully. Effective algorithms and well-written programs are essential for creating software applications that are reliable, scalable, and easy to use.
An Introduction To Computer
Importance of algorithm.
Algorithms are an essential tool for solving complex problems and making sense of large amounts of data. They play a critical role in many areas of modern life, from business and finance to scientific research and engineering.
Algorithms are important for several reasons, including:
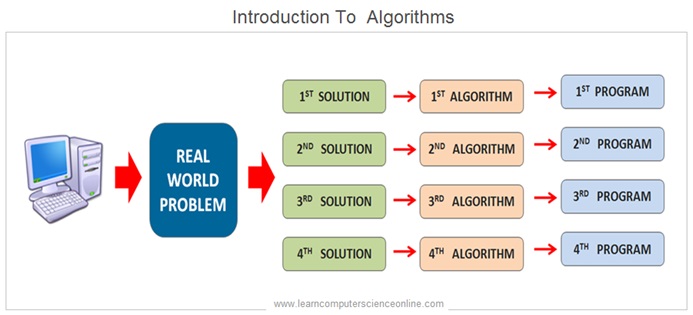
- Efficiency: Good algorithms can solve problems quickly and efficiently. This is particularly important in fields such as computer science and engineering, where large amounts of data must be processed and analysed in a timely manner.
- Scalability: Algorithms that are designed to handle large data sets and complex problems are essential in today’s world, where the amount of data being generated is increasing exponentially.
- Optimization: Many algorithms are designed to optimize a particular metric or goal, such as minimizing cost or maximizing efficiency. By using algorithms to optimize processes, companies and organizations can save time, money, and resources.
- Standardization: Algorithms provide a standardized way of solving problems, which can make it easier for people to communicate and collaborate with one another.
- Innovation: Developing new algorithms can lead to breakthroughs in technology and scientific research, as well as new applications in fields such as medicine, finance, and energy.
Characteristics Of Algorithm
The characteristics of an algorithm refer to the properties or features that an algorithm must possess to be considered a valid and effective solution for a problem. The key characteristics of an algorithm are as follows:
These characteristics ensure that an algorithm is a reliable and effective solution for a problem. By possessing these characteristics, an algorithm can be implemented and executed on a computer system to solve complex computational problems.
By following these characteristics, an algorithm can be designed to solve a particular problem or perform a specific task effectively and efficiently.
The characteristics of an algorithm are as follows:

- Well-defined : An algorithm must be well-defined, which means that each step in the algorithm must be clear and unambiguous. This ensures that the algorithm can be executed correctly and consistently.
- Input: An algorithm must have an input, which is the data or information that the algorithm will process.
- Output: An algorithm must have an output, which is the result of the algorithm’s processing of the input.
- Finite: An algorithm must terminate after a finite number of steps. This means that the algorithm must have a stopping condition, so it does not run forever.
- Correctness: An algorithm must produce the correct output for every possible input. The algorithm must be designed to handle all possible input values.
- Efficiency: An algorithm must be efficient, which means that it should use the minimum amount of time and resources necessary to produce the desired output.
- Generality: An algorithm must be general, which means that it should be applicable to a wide range of input data and not be specific to a particular data set or problem.
- Reusability: An algorithm should be designed in such a way that it can be reused in different contexts and for different applications.
Applications Of Algorithm
Algorithms are critical to solving complex problems and making sense of large amounts of data in a wide range of applications. Algorithms have a wide range of applications in various fields, some of which are as follows:
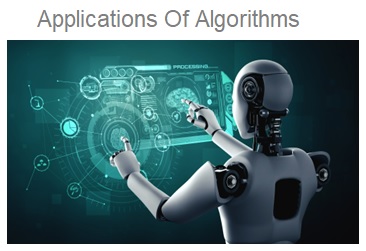
- Computer Science : Algorithms are fundamental to computer science and programming. They are used to solve various problems like sorting, searching, and graph traversal.
- Artificial Intelligence : Algorithms are extensively used in artificial intelligence and machine learning to train models, perform pattern recognition, and make predictions.
- Cryptography : Algorithms are used in cryptography to encrypt and decrypt messages, verify digital signatures, and protect information from unauthorized access.
- Computational Biology : Algorithms are used in computational biology to analyze genetic data, identify patterns, and develop predictive models for disease diagnosis and drug discovery.
- Finance : Algorithms are used in finance for risk analysis, asset allocation, and trading strategies.
- Robotics : Algorithms are used in robotics to control the movement and behavior of robots, enable navigation, and support decision-making.
- Image and video processing : Algorithms are used in image and video processing to enhance images and videos, perform object detection and tracking, and recognize faces.
- Natural language Processing : Algorithms are used in natural language processing to analyze and understand human language, perform sentiment analysis, and generate human-like responses.
Types Of Algorithm
Each type of algorithm has its strengths and weaknesses, and the choice of algorithm will depend on the specific problem at hand and the resources available. There are several types of algorithms, as follows:
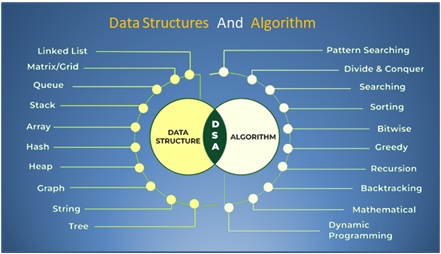
- Searching Algorithms : These algorithms are used to search for a specific value in a collection of data. Examples include linear search and binary search.
- Sorting Algorithms : These algorithms are used to sort data in a specific order, such as in ascending or descending order. Examples include bubble sort, quicksort, and merge sort.
- Graph Algorithms : These algorithms are used to traverse graphs and find paths between nodes. Examples include breadth-first search and depth-first search.
- Divide And Conquer Algorithms : These algorithms break down a problem into smaller sub-problems and solve each sub-problem independently. Examples include merge sort and binary search.
- Greedy Algorithms : These algorithms make decisions based on the current best option, without considering the long-term consequences. Examples include the knapsack problem and minimum spanning tree.
- Dynamic Programming Algorithms : These algorithms solve complex problems by breaking them down into smaller sub-problems and storing solutions to those sub-problems. Examples include the Fibonacci sequence and the longest common subsequence problem.
- Backtracking Algorithms : These algorithms explore all possible solutions to a problem by generating a tree of possibilities and backtracking when a solution is not found. Examples include the N-Queens problem and the subset sum problem.
- Randomized Algorithms : These algorithms use a random element to solve a problem, such as generating a random solution and evaluating its effectiveness. Examples include randomized quicksort and Monte Carlo algorithms.
Join The Best Seller
Computer Science Online Course
Learn computer science and programming fundamentals.
This is the most comprehensive and unique Computer Science And Programming Fundamentals course Online which will give you in depth understanding of most important fundamental concepts in computer science And Programming .
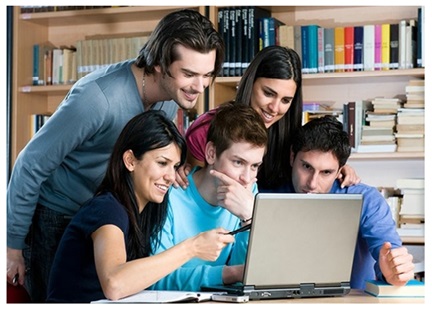
CMU 15-112: Fundamentals of Programming and Computer Science Class Notes: Algorithmic Thinking
- Optional Reading
- Problem Solving with Programming
- Programming Patterns
- Top-Down Design
- Or you can read the entire book: Polya, How to Solve It
- Wikipedia section on Common Barriers to Problem Solving
- Define the problem precisely .
- Generate test cases based on the prompt, or carefully read through test cases if they're provided.
- Make sure you can restate the problem in your own words, or explain it to someone else.
- Translate a provided algorithm into code? You can skip to the Step 3.
- Apply a known algorithm pattern to the problem? You can skip to Step 2.4.
- Generate an algorithm to solve a problem? Keep reading!
- Induction : Investigate several examples (test cases) to find a pattern that can be generalized into an algorithm.
- Top-Down Design : Break down a complex problem into several simpler problems, then solve each of the simpler problems individually. See more here
- Human Computer : Pretend that you need to carry out the task by hand. Determine the steps you would take in order to complete the task.
- Clarity : How clear is the approach to you?
- Simplicity : How straightforward will the approach be to implement?
- Efficiency : How much work will the algorithm need to do?
- Generality : How well will this algorithm adapt to new inputs?
- Future concerns (after more CS courses) : usability, accessibility, security, privacy, testability, reliability, scalability, compatibility, extensibility, durability, maintainability, portability, provability, ...
- If you need to remember a thing, give it a name- those names will later become variables.
- Write the test cases that you generated in Step 1.
- Review the relevant course materials to find a programming tool that approximately meets your need.
- Experiment with that programming tool in the interpreter until you understand how it works.
- Apply the new concept to the step in your algorithm.
- Run your function on a test input to make sure it works. If your code has a syntax or runtime error, use debugging to identify the problem and fix it.
- Run your test cases on the code to make sure they all pass. If a test case fails, use debugging to identify the algorithmic problem and fix it.
- Once all test cases pass, read over your code and make sure it makes sense based on common sense.
- (After the deadline) Discuss and compare your solution with others to learn about alternative approaches to solving the problem.
- There are many algorithmic patterns that appear in various problems, patterns which can be adapted to suit new circumstances at need. We'll see many of these patterns in class; for now, here are a few common patterns from the first weeks of class.
- nthSomething def nthSomething(n): found = 0 guess = 0 while found
- propertyTrueForAll def propertyTrueForAll(s): for c in s: if property(c) == False: return False return True
- countProperty def countProperty(s): count = 0 for c in s: if property(c) == True: count += 1 return count
- mostCommonItem def mostCommonItem(s): bestCount = 0 bestItem = None for c in s: tmpCount = count(s, c) if tmpCount > bestCount: bestCount = tmpCount bestItem = c elif tmpCount == bestCount and c > bestItem: bestItem = c return bestItem
- We often ask you to solve problems that are too complicated to be solved by one function alone. In these cases, you will need to break the problem down into sub-problems that can be mapped directly to helper functions.
- To identify a sub-problem, start writing the algorithm out. When you get to a step that seems too complicated, imagine you have a helper function that can solve that step. If you can write the function by including a call to this imaginary function, you've taken a step towards solving the problem! The process can then be repeated on the helper functions until the solution is complete.
- When testing multi-function problems, test the simplest functions first. It's important to ensure that your helper functions are working before you move on to test the main function.
- Example: nthThreeDigitPrime # First, we can write the part that we know how to do already: a basic nth function. def nthThreeDigitPrime(n): found = 0 guess = 0 while found
- In the end, the best way to learn problem solving is to practice with feedback . If you're struggling with problem solving, ask a TA or a friend to sit with you while you while you work through designing algorithms for practice problems, or ask them to demonstrate how they approach problem solving themselves. Practice should eventually lead to better understanding of how to approach problem solving in general.
- Analysis of Algorithms
- Backtracking
- Dynamic Programming
- Divide and Conquer
- Geometric Algorithms
- Mathematical Algorithms
- Pattern Searching
- Bitwise Algorithms
- Branch & Bound
- Randomized Algorithms
Design and Analysis of Algorithms
Design and Analysis of Algorithms is a fundamental aspect of computer science that involves creating efficient solutions to computational problems and evaluating their performance. DSA focuses on designing algorithms that effectively address specific challenges and analyzing their efficiency in terms of time and space complexity .
.png)
Table of Content
- What is meant by Algorithm Analysis?
- Why Analysis of Algorithms is important?
- Types of Algorithm Analysis
- Basics on Analysis of Algorithms
- Asymptotic Notations
- Some Advance topics
- Complexity Proofs
Basics on Analysis of Algorithms:
- What is algorithm and why analysis of it is important?
- Asymptotic Notation and Analysis (Based on input size) in Complexity Analysis of Algorithms
- Worst, Average and Best Case Analysis of Algorithms
- Types of Asymptotic Notations in Complexity Analysis of Algorithms
- How to Analyse Loops for Complexity Analysis of Algorithms
- How to analyse Complexity of Recurrence Relation
- Introduction to Amortized Analysis
Asymptotic Notations:
- Analysis of Algorithms | Big-O analysis
- Difference between Big Oh, Big Omega and Big Theta
- Examples of Big-O analysis
- Difference between big O notations and tilde
- Analysis of Algorithms | Big – Ω (Big- Omega) Notation
- Analysis of Algorithms | Big – Θ (Big Theta) Notation
Some Advance topics:
- Types of Complexity Classes | P, NP, CoNP, NP hard and NP complete
- Can Run Time Complexity of a comparison-based sorting algorithm be less than N logN?
- Why does accessing an Array element take O(1) time?
- What is the time efficiency of the push(), pop(), isEmpty() and peek() operations of Stacks?
Complexity Proofs:
- Proof that Clique Decision problem is NP-Complete
- Proof that Independent Set in Graph theory is NP Complete
- Prove that a problem consisting of Clique and Independent Set is NP Complete
- Prove that Dense Subgraph is NP Complete by Generalisation
- Prove that Sparse Graph is NP-Complete
Similar Reads
- Algorithms-Analysis of Algorithms
Please Login to comment...
- How to Watch NFL on NFL+ in 2024: A Complete Guide
- Best Smartwatches in 2024: Top Picks for Every Need
- Top Budgeting Apps in 2024
- 10 Best Parental Control App in 2024
- GeeksforGeeks Practice - Leading Online Coding Platform
Improve your Coding Skills with Practice
What kind of Experience do you want to share?
Have a language expert improve your writing
Check your paper for plagiarism in 10 minutes, generate your apa citations for free.
- Knowledge Base
- Using AI tools
- What Is an Algorithm? | Definition & Examples
What Is an Algorithm? | Definition & Examples
Published on August 9, 2023 by Kassiani Nikolopoulou . Revised on August 29, 2023.
An algorithm is a set of steps for accomplishing a task or solving a problem. Typically, algorithms are executed by computers, but we also rely on algorithms in our daily lives. Each time we follow a particular step-by-step process, like making coffee in the morning or tying our shoelaces, we are in fact following an algorithm.
In the context of computer science , an algorithm is a mathematical process for solving a problem using a finite number of steps. Algorithms are a key component of any computer program and are the driving force behind various systems and applications, such as navigation systems, search engines, and music streaming services.
Instantly correct all language mistakes in your text
Upload your document to correct all your mistakes in minutes
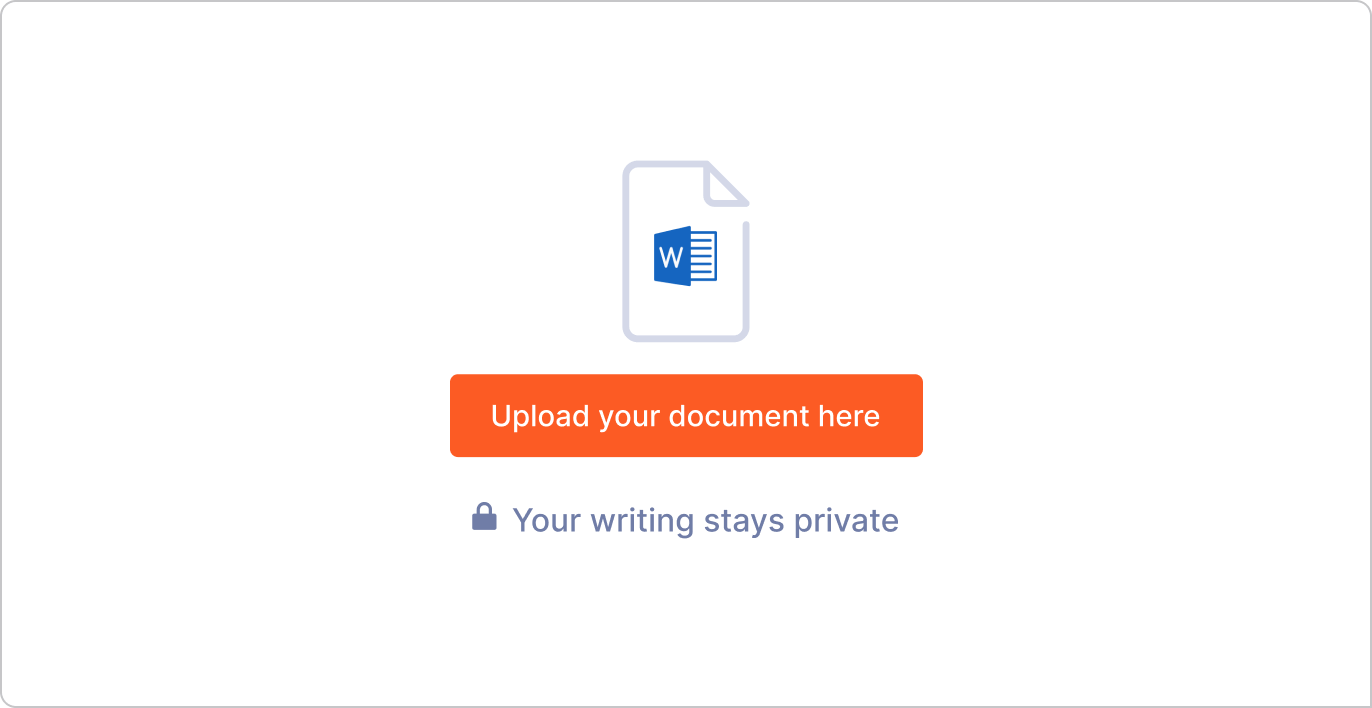
Table of contents
What is an algorithm, how do algorithms work, examples of algorithms, other interesting articles, frequently asked questions about algorithms.
An algorithm is a sequence of instructions that a computer must perform to solve a well-defined problem. It essentially defines what the computer needs to do and how to do it. Algorithms can instruct a computer how to perform a calculation, process data, or make a decision.
The best way to understand an algorithm is to think of it as a recipe that guides you through a series of well-defined actions to achieve a specific goal. Just like a recipe produces a replicable result, algorithms ensure consistent and reliable outcomes for a wide range of tasks in the digital realm.
And just like there are numerous ways to make, for example, chocolate chip cookies by following different steps or using slightly different ingredients, different algorithms can be designed to solve the same problem, with each taking a distinct approach but achieving the same result.
Algorithms are virtually everywhere around us. Examples include the following:
- Search engines rely on algorithms to find and present relevant results as quickly as possible
- Social media platforms use algorithms to prioritize the content that we see in our feeds, taking into account factors like our past behavior, the popularity of posts, and relevance.
- With the help of algorithms, navigation apps determine the most efficient route for us to reach our destination.
- It must be correct . In other words, it should take a given problem and provide the right answer or result, even if it stops working due to an error.
- It must consist of clear, practical steps that can be completed in a limited time, whether by a person or the machine that must execute the algorithm. For example, the instructions in a cookie recipe might be considered sufficiently concrete for a human cook, but they would not be specific enough for programming an automated cookie-making machine.
- There should be no confusion about which step comes next , even if choices must be made (e.g., when using “if” statements).
- It must have a set number of steps (not an infinite number) that can be managed using loops (statements describing repeated actions or iterations).
- It must eventually reach an endpoint and not get stuck in a never-ending loop.
Don't submit your assignments before you do this
The academic proofreading tool has been trained on 1000s of academic texts. Making it the most accurate and reliable proofreading tool for students. Free citation check included.
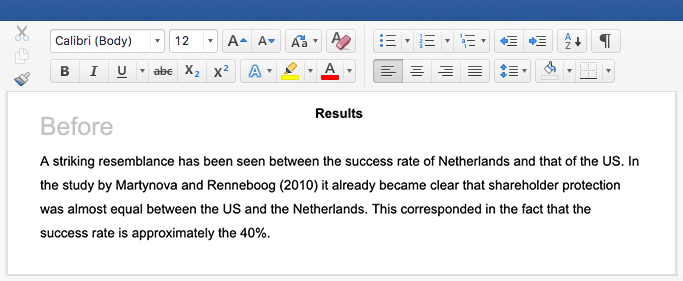
Try for free
Algorithms use a set of initial data or input , process it through a series of logical steps or rules, and produce the output (i.e., the outcome, decision, or result).
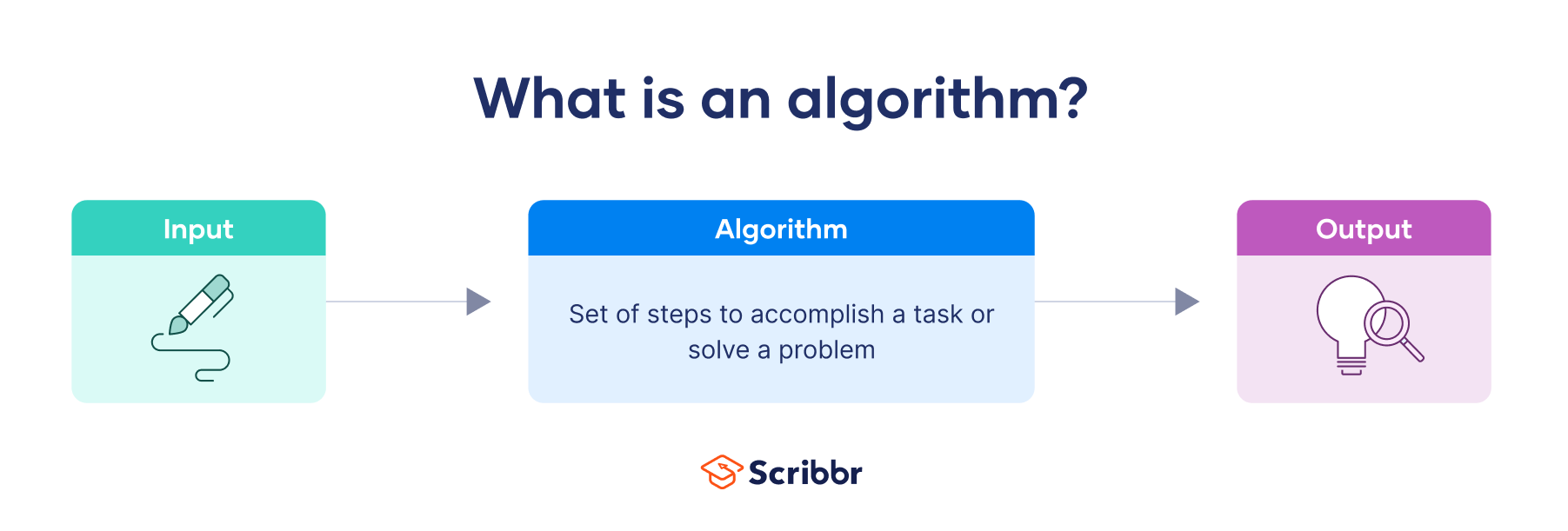
If you want to make chocolate chip cookies, for instance, the input would be the ingredients and quantities, the process would be the recipe you choose to follow, and the output would be the cookies.
Algorithms are eventually expressed in a programming language that a computer can process. However, when an algorithm is being created, it will be people, not a computer, who will need to understand it. For this reason, as a first step, algorithms are written as plain instructions.
- Input: the input data is a single-digit number (e.g., 5).
- Transformation/processing: the algorithm takes the input (number 5) and performs the specific operation (i.e., multiplies the number by itself).
- Output: the result of the calculation is the square of the input number, which, in this case, would be 25 (since 5 * 5 = 25).
We could express this as an algorithm in the following way:
Algorithm: Calculate the square of a number
- Input the number (N) whose square you want to find.
- Multiply the number (N) by itself.
- Store the result of the multiplication in a variable (result).
- Output the value of the variable (result), which represents the square of the input number.
It is important to keep in mind that an algorithm is not the same as a program or code. It is the logic or plan for solving a problem represented as a simple step-by-step description. Code is the implementation of the algorithm in a specific programming language (like C++ or Python), while a program is an implementation of code that instructs a computer on how to execute an algorithm and perform a task.
Instead of telling a computer exactly what to do, some algorithms allow computers to learn on their own and improve their performance on a specific task. These machine learning algorithms use data to identify patterns and make predictions or conduct data mining to uncover hidden insights in data that can inform business decisions.
Broadly speaking, there are three different types of algorithms:
- Linear sequence algorithms follow a specific set or steps, one after the other. Just like following a recipe, each step depends on the success of the previous one.
- For example, in the context of a cookie recipe, you would include the step “if the dough is too sticky, you might need to refrigerate it.”
- For example, a looping algorithm could be used to handle the process of making multiple cookies from a single batch of dough. The algorithm would repeat a specific set of instructions to form and bake cookies until all the dough has been used.
Algorithms are fundamental tools for problem-solving in both the digital world and many real-life scenarios. Each time we try to solve a problem by breaking it down into smaller, manageable steps, we are in fact using algorithmic thinking.
- Identify which clothes are clean.
- Consider the weather forecast for the day.
- Consider the occasion for which you are getting dressed (e.g., work or school etc.).
- Consider personal preferences (e.g., style or which items match).
In mathematics, algorithms are standard methods for performing calculations or solving equations because they are efficient, reliable, and applicable to various situations.
Suppose you want to add the numbers 345 and 278. You would follow a set of steps (i.e., the standard algorithm for addition):
- Write down the numbers so the digits align.
- Start from the rightmost digits (the ones place) and add them together: 5 + 8 = 13. Write down the 3 and carry over the 1 to the next column.
- Move to the next column (the tens place) and add the digits along with the carried-over value: 4 + 7 + 1 = 12. Write down the 2 and carry over the 1 to the next column.
- Move to the leftmost column (the hundreds place) and add the digits along with the carried-over value: 3 + 2 + 1 = 6. Write down the 6.
The final result is 623
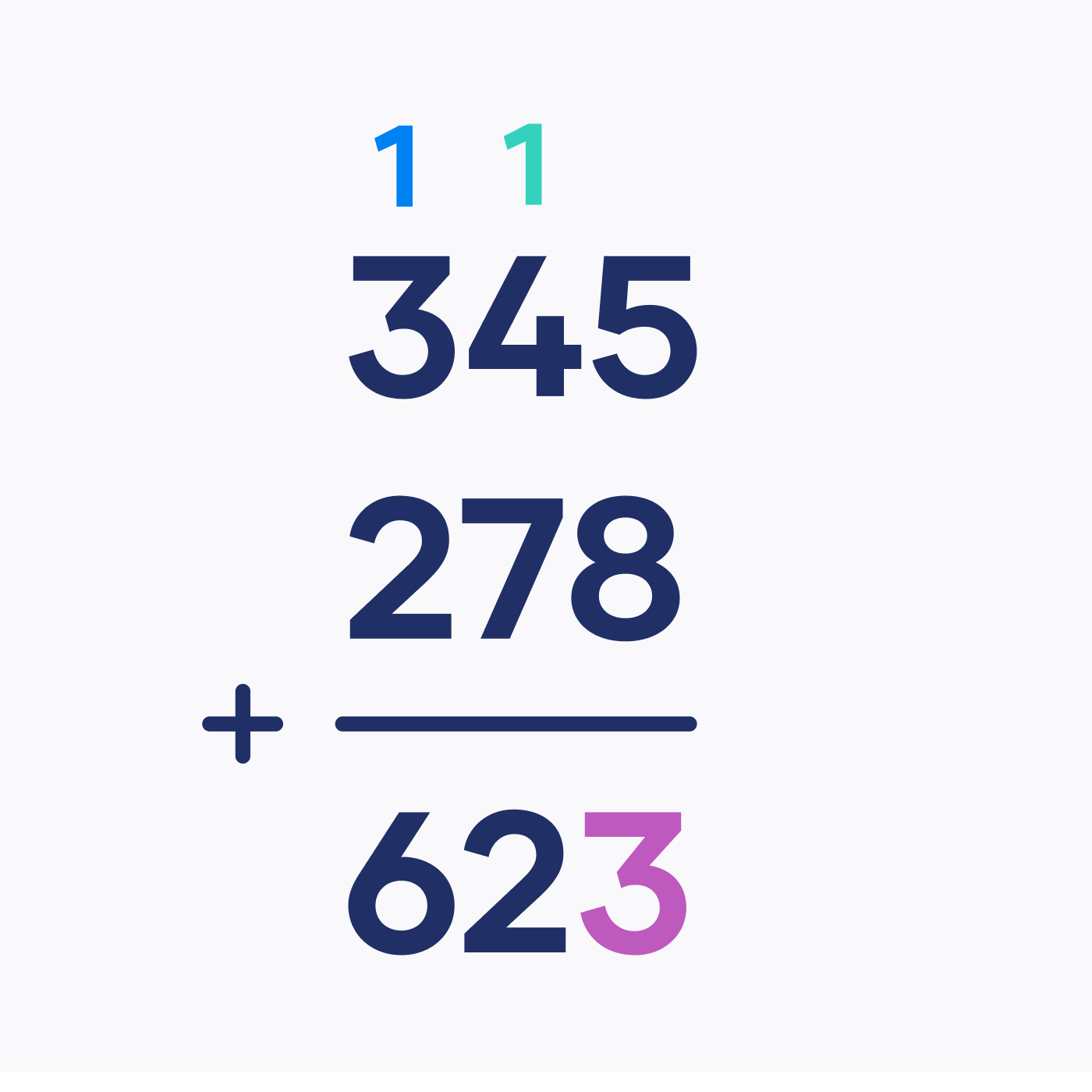
Navigation systems are another example of the use of algorithms. Such systems use algorithms to help you find the easiest and fastest route to your destination while avoiding traffic jams and roadblocks.
If you want to know more about ChatGPT, AI tools , fallacies , and research bias , make sure to check out some of our other articles with explanations and examples.
- ChatGPT vs human editor
- ChatGPT citations
- Is ChatGPT trustworthy?
- Using ChatGPT for your studies
- Sunk cost fallacy
- Straw man fallacy
- Slippery slope fallacy
- Red herring fallacy
- Ecological fallacy
- Logical fallacy
Research bias
- Implicit bias
- Framing bias
- Cognitive bias
- Optimism bias
- Hawthorne effect
- Unconscious bias
In computer science, an algorithm is a list of unambiguous instructions that specify successive steps to solve a problem or perform a task. Algorithms help computers execute tasks like playing games or sorting a list of numbers. In other words, computers use algorithms to understand what to do and give you the result you need.
Algorithms and artificial intelligence (AI) are not the same, however they are closely related.
- Artificial intelligence is a broad term describing computer systems performing tasks usually associated with human intelligence like decision-making, pattern recognition, or learning from experience.
- Algorithms are the instructions that AI uses to carry out these tasks, therefore we could say that algorithms are the building blocks of AI—even though AI involves more advanced capabilities beyond just following instructions.
Algorithms and computer programs are sometimes used interchangeably, but they refer to two distinct but interrelated concepts.
- An algorithm is a step-by-step instruction for solving a problem that is precise yet general.
- Computer programs are specific implementations of an algorithm in a specific programming language. In other words, the algorithm is the high-level description of an idea, while the program is the actual implementation of that idea.
Algorithms are valuable to us because they:
- Form the basis of much of the technology we use in our daily lives, from mobile apps to search engines.
- Power innovations in various industries that augment our abilities (e.g., AI assistants or medical diagnosis).
- Help analyze large volumes of data, discover patterns and make informed decisions in a fast and efficient way, at a scale humans are simply not able to do.
- Automate processes. By streamlining tasks, algorithms increase efficiency, reduce errors, and save valuable time.
Cite this Scribbr article
If you want to cite this source, you can copy and paste the citation or click the “Cite this Scribbr article” button to automatically add the citation to our free Citation Generator.
Nikolopoulou, K. (2023, August 29). What Is an Algorithm? | Definition & Examples. Scribbr. Retrieved September 27, 2024, from https://www.scribbr.com/ai-tools/what-is-an-algorithm/
Is this article helpful?
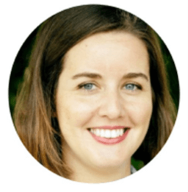
Kassiani Nikolopoulou
Other students also liked, what is deep learning | a beginner's guide, what is data mining | definition & techniques, what is machine learning | a beginner's guide, get unlimited documents corrected.
✔ Free APA citation check included ✔ Unlimited document corrections ✔ Specialized in correcting academic texts
What Is Problem Solving? How Software Engineers Approach Complex Challenges
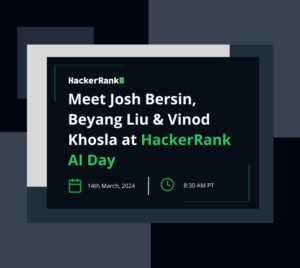
From debugging an existing system to designing an entirely new software application, a day in the life of a software engineer is filled with various challenges and complexities. The one skill that glues these disparate tasks together and makes them manageable? Problem solving .
Throughout this blog post, we’ll explore why problem-solving skills are so critical for software engineers, delve into the techniques they use to address complex challenges, and discuss how hiring managers can identify these skills during the hiring process.
What Is Problem Solving?
But what exactly is problem solving in the context of software engineering? How does it work, and why is it so important?
Problem solving, in the simplest terms, is the process of identifying a problem, analyzing it, and finding the most effective solution to overcome it. For software engineers, this process is deeply embedded in their daily workflow. It could be something as simple as figuring out why a piece of code isn’t working as expected, or something as complex as designing the architecture for a new software system.
In a world where technology is evolving at a blistering pace, the complexity and volume of problems that software engineers face are also growing. As such, the ability to tackle these issues head-on and find innovative solutions is not only a handy skill — it’s a necessity.
The Importance of Problem-Solving Skills for Software Engineers
Problem-solving isn’t just another ability that software engineers pull out of their toolkits when they encounter a bug or a system failure. It’s a constant, ongoing process that’s intrinsic to every aspect of their work. Let’s break down why this skill is so critical.
Driving Development Forward
Without problem solving, software development would hit a standstill. Every new feature, every optimization, and every bug fix is a problem that needs solving. Whether it’s a performance issue that needs diagnosing or a user interface that needs improving, the capacity to tackle and solve these problems is what keeps the wheels of development turning.
It’s estimated that 60% of software development lifecycle costs are related to maintenance tasks, including debugging and problem solving. This highlights how pivotal this skill is to the everyday functioning and advancement of software systems.
Innovation and Optimization
The importance of problem solving isn’t confined to reactive scenarios; it also plays a major role in proactive, innovative initiatives . Software engineers often need to think outside the box to come up with creative solutions, whether it’s optimizing an algorithm to run faster or designing a new feature to meet customer needs. These are all forms of problem solving.
Consider the development of the modern smartphone. It wasn’t born out of a pre-existing issue but was a solution to a problem people didn’t realize they had — a device that combined communication, entertainment, and productivity into one handheld tool.
Increasing Efficiency and Productivity
Good problem-solving skills can save a lot of time and resources. Effective problem-solvers are adept at dissecting an issue to understand its root cause, thus reducing the time spent on trial and error. This efficiency means projects move faster, releases happen sooner, and businesses stay ahead of their competition.
Improving Software Quality
Problem solving also plays a significant role in enhancing the quality of the end product. By tackling the root causes of bugs and system failures, software engineers can deliver reliable, high-performing software. This is critical because, according to the Consortium for Information and Software Quality, poor quality software in the U.S. in 2022 cost at least $2.41 trillion in operational issues, wasted developer time, and other related problems.
Problem-Solving Techniques in Software Engineering
So how do software engineers go about tackling these complex challenges? Let’s explore some of the key problem-solving techniques, theories, and processes they commonly use.
Decomposition
Breaking down a problem into smaller, manageable parts is one of the first steps in the problem-solving process. It’s like dealing with a complicated puzzle. You don’t try to solve it all at once. Instead, you separate the pieces, group them based on similarities, and then start working on the smaller sets. This method allows software engineers to handle complex issues without being overwhelmed and makes it easier to identify where things might be going wrong.
Abstraction
In the realm of software engineering, abstraction means focusing on the necessary information only and ignoring irrelevant details. It is a way of simplifying complex systems to make them easier to understand and manage. For instance, a software engineer might ignore the details of how a database works to focus on the information it holds and how to retrieve or modify that information.
Algorithmic Thinking
At its core, software engineering is about creating algorithms — step-by-step procedures to solve a problem or accomplish a goal. Algorithmic thinking involves conceiving and expressing these procedures clearly and accurately and viewing every problem through an algorithmic lens. A well-designed algorithm not only solves the problem at hand but also does so efficiently, saving computational resources.
Parallel Thinking
Parallel thinking is a structured process where team members think in the same direction at the same time, allowing for more organized discussion and collaboration. It’s an approach popularized by Edward de Bono with the “ Six Thinking Hats ” technique, where each “hat” represents a different style of thinking.
In the context of software engineering, parallel thinking can be highly effective for problem solving. For instance, when dealing with a complex issue, the team can use the “White Hat” to focus solely on the data and facts about the problem, then the “Black Hat” to consider potential problems with a proposed solution, and so on. This structured approach can lead to more comprehensive analysis and more effective solutions, and it ensures that everyone’s perspectives are considered.
This is the process of identifying and fixing errors in code . Debugging involves carefully reviewing the code, reproducing and analyzing the error, and then making necessary modifications to rectify the problem. It’s a key part of maintaining and improving software quality.
Testing and Validation
Testing is an essential part of problem solving in software engineering. Engineers use a variety of tests to verify that their code works as expected and to uncover any potential issues. These range from unit tests that check individual components of the code to integration tests that ensure the pieces work well together. Validation, on the other hand, ensures that the solution not only works but also fulfills the intended requirements and objectives.
Explore verified tech roles & skills.
The definitive directory of tech roles, backed by machine learning and skills intelligence.
Explore all roles
Evaluating Problem-Solving Skills
We’ve examined the importance of problem-solving in the work of a software engineer and explored various techniques software engineers employ to approach complex challenges. Now, let’s delve into how hiring teams can identify and evaluate problem-solving skills during the hiring process.
Recognizing Problem-Solving Skills in Candidates
How can you tell if a candidate is a good problem solver? Look for these indicators:
- Previous Experience: A history of dealing with complex, challenging projects is often a good sign. Ask the candidate to discuss a difficult problem they faced in a previous role and how they solved it.
- Problem-Solving Questions: During interviews, pose hypothetical scenarios or present real problems your company has faced. Ask candidates to explain how they would tackle these issues. You’re not just looking for a correct solution but the thought process that led them there.
- Technical Tests: Coding challenges and other technical tests can provide insight into a candidate’s problem-solving abilities. Consider leveraging a platform for assessing these skills in a realistic, job-related context.
Assessing Problem-Solving Skills
Once you’ve identified potential problem solvers, here are a few ways you can assess their skills:
- Solution Effectiveness: Did the candidate solve the problem? How efficient and effective is their solution?
- Approach and Process: Go beyond whether or not they solved the problem and examine how they arrived at their solution. Did they break the problem down into manageable parts? Did they consider different perspectives and possibilities?
- Communication: A good problem solver can explain their thought process clearly. Can the candidate effectively communicate how they arrived at their solution and why they chose it?
- Adaptability: Problem-solving often involves a degree of trial and error. How does the candidate handle roadblocks? Do they adapt their approach based on new information or feedback?
Hiring managers play a crucial role in identifying and fostering problem-solving skills within their teams. By focusing on these abilities during the hiring process, companies can build teams that are more capable, innovative, and resilient.
Key Takeaways
As you can see, problem solving plays a pivotal role in software engineering. Far from being an occasional requirement, it is the lifeblood that drives development forward, catalyzes innovation, and delivers of quality software.
By leveraging problem-solving techniques, software engineers employ a powerful suite of strategies to overcome complex challenges. But mastering these techniques isn’t simple feat. It requires a learning mindset, regular practice, collaboration, reflective thinking, resilience, and a commitment to staying updated with industry trends.
For hiring managers and team leads, recognizing these skills and fostering a culture that values and nurtures problem solving is key. It’s this emphasis on problem solving that can differentiate an average team from a high-performing one and an ordinary product from an industry-leading one.
At the end of the day, software engineering is fundamentally about solving problems — problems that matter to businesses, to users, and to the wider society. And it’s the proficient problem solvers who stand at the forefront of this dynamic field, turning challenges into opportunities, and ideas into reality.
This article was written with the help of AI. Can you tell which parts?
Get started with HackerRank
Over 2,500 companies and 40% of developers worldwide use HackerRank to hire tech talent and sharpen their skills.
- Machine Learning Decision Tree – Solved Problem (ID3 algorithm)
- Poisson Distribution | Probability and Stochastic Process
- Conditional Probability | Joint Probability
- Solved assignment problems in communicaion |online Request
- while Loop in C++
EngineersTutor
Problem solving and algorithms, problem analysis.
Problem analysis can be defined as studying a problem to arrive at a satisfactory solution. To solve a problem successfully, the first step is to understand the problem i.e, problem must be understood clearly. Also the problem must be stated clearly and accurately without any confuse. A well-defined problem and clear description of input and output are important for an effective and efficient solution. Study the outputs to be generated so that input can be specified. Design the steps, which will produce the desired result after supplying the input.
If the problem is very complex, split the problem into multiple sub-problems. Solve each sub-problem and combine the solutions of all the sub-problems to at arrive at overall solution to a large problem. This is called divide and conquer technique.
Problem solving steps
- Understand the problem and plan its logic
- Construction of the List of Variables
- Develop an algorithm
- Refine the algorithm. Refining means making changes
- Program Development
- Testing the Program (solution)
- Validating the Program (solution)
An algorithm is defined as sequence of steps to solve a problem (task) . The steps must be finite, well defined and unambiguous. Writing algorithm requires some thinking. Algorithm can also be defined as a plan to solve a problem and represents its logic. Note that an algorithm is of no use if it does not help us arrive at the desired solution
Algorithm characteristics
- It should have finite number of steps . No one can be expected to execute infinite number of steps.
- The steps must be in order and simple
- Each step should be defined clearly stated e. without un-ambiguity
- Must include all required information
- Should exhibit at least one output
For accomplishing a particular task, different algorithms can be written. Different algorithms can differ in their requirements of time and space. Programmer selects the best suited algorithm for the given task to be solved.
Algorithm for preparing two cups of tea
- Add 1.5 cups of water to the vessel
- Add 2 tea spoons of tea leaves
- Add half cup of milk
- Add some sugar
Statement 5 is an example of an ambiguous (unclear) statement. This statement doesn’t clearly state the amount of sugar to be added.
Algorithm design
- Design an algorithm that is easy to understand code and debug. Debugging is the process finding and fixing errors in a program
- Design an algorithm that makes use of resource such as space (memory) and time efficiently
- ← Merge Sort Algorithm with example
- Flowchart and Algorithm →
Gopal Krishna
Hey Engineers, welcome to the award-winning blog,Engineers Tutor. I'm Gopal Krishna. a professional engineer & blogger from Andhra Pradesh, India. Notes and Video Materials for Engineering in Electronics, Communications and Computer Science subjects are added. "A blog to support Electronics, Electrical communication and computer students".
You May Also Like
Solved assignment problems in c++ (with algorithm and flowchart), linear search algorithm, examples of algorithms and flowcharts in c, leave a reply cancel reply.
Your email address will not be published. Required fields are marked *

IMAGES
VIDEO
COMMENTS
An input to an algorithm specifies an instance of the problem the algorithm solves. It is very important to specify exactly the set of instances the algorithm needs to handle. (As an example, recall the variations in the set of instances for the three greatest common divisor algorithms discussed in the previous section.)
Algorithmic problem solving is the art of formulating efficient methods that solve problems of a mathematical nature. From the many numerical algo-rithms developed by the ancient Babylonians to the founding of graph theory by Euler, algorithmic problem solving has been a popular intellectual pursuit during the last few thousand years.
Algorithm is a step-by-step procedure for solving a problem or accomplishing a task. In the context of data structures and algorithms, it is a set of well-defined instructions for performing a specific computational task. Algorithms are fundamental to computer science and play a very important role in designing efficient solutions for various ...
Example: Consider the example to add three numbers and print the sum. Step 1: Fulfilling the pre-requisites . As discussed above, to write an algorithm, its prerequisites must be fulfilled. The problem that is to be solved by this algorithm: Add 3 numbers and print their sum.; The constraints of the problem that must be considered while solving the problem: The numbers must contain only digits ...
steps. An algorithm, whose characteristics will be discussed later, is a form that embeds the complete logic of the solution. Its formal written version is called a program, or code. Thus, algorithmic problem solving actually comes in two phases: derivation of an algorithm that solves the problem, and conversion of the algorithm into code.
an algorithm is a sequence of steps, not a program. same algorithm can be used in different programs, or the same algorithm can be expressed in different languages, because an algorithm is an entity that is abstracted from implementation details. An algorithm can be expressed in the following ways: human language; pseudo code; flow chart
3. Efficiency. Efficiency is a critical consideration in algorithm design. It's about finding the most optimal way to solve a problem, which often involves minimizing the consumption of time and resources. 4. Scalability. Algorithms should be designed to handle input data of varying sizes efficiently.
Algorithms are at the heart of various applications, from simple calculations to sophisticated machine learning models and complex data analysis. Understanding algorithms and their inner workings is crucial for anyone interested in computer science. They serve as the backbone of software development, powering the creation of innovative ...
An algorithm is a well-defined procedure that takes some input and produces a corresponding output. It's a step-by-step process that solves a specific problem or performs a particular task. Algorithms can be expressed in various forms, such as natural language, flowcharts, pseudocode, or programming languages.
Let's take some examples of algorithms for computer science problems. Example 1. Swap two numbers with a third variable. Step 1: Start. Step 2: Take 2 numbers as input. Step 3: Declare another variable as "temp". Step 4: Store the first variable to "temp". Step 5: Store the second variable to the First variable.
The development of an algorithm (a plan) is a key step in solving a problem. Once we have an algorithm, we can translate it into a computer program in some programming language. Our algorithm development process consists of five major steps. Step 1: Obtain a description of the problem. Step 2: Analyze the problem.
2. FUNDAMENTALS OF ALGORITHMIC PROBLEM SOLVING A sequence of steps involved in designing and analyzing an algorithm is shown in the figure below. FIGURE 1.2.1 Algorithm design and analysis process. (i) Understanding the Problem • This is the first step in designing of algorithm. • Read the problem's description carefully to understand the ...
My approach to making algorithms compelling was focusing on comparisons. I take algorithms and put them in a scene from everyday life, such as matching socks from a pile, putting books on a shelf, remembering things, driving from one point to another, or cutting an onion. These activities can be mapped to one or more fundamental algorithms ...
1.1: Activity 1 - Introduction to Algorithms and Problem Solving. In this learning activity section, the learner will be introduced to algorithms and how to write algorithms to solve tasks faced by learners or everyday problems. Examples of the algorithm are also provided with a specific application to everyday problems that the learner is ...
An algorithm is a set of well-defined instructions or steps that can be followed to solve a problem or perform a specific task. It is essentially a series of logical steps that can be executed to achieve a desired result. Algorithms can be expressed in various ways, such as natural language, flowcharts, or pseudocode.
Here are three common programming strategies: Induction: Investigate several examples (test cases) to find a pattern that can be generalized into an algorithm. Top-Down Design: Break down a complex problem into several simpler problems, then solve each of the simpler problems individually. See more here.
Design and Analysis of Algorithms. Design and Analysis of Algorithms is a fundamental aspect of computer science that involves creating efficient solutions to computational problems and evaluating their performance. DSA focuses on designing algorithms that effectively address specific challenges and analyzing their efficiency in terms of time ...
an algorithm is a sequence of steps, not a program. same algorithm can be used in different programs, or the same algorithm can be expressed in different languages, because an algorithm is an entity that is abstracted from implementation details. An algorithm can be expressed in the following ways: human language; pseudo code; flow chart
An algorithm is a sequence of instructions that a computer must perform to solve a well-defined problem. It essentially defines what the computer needs to do and how to do it. Algorithms can instruct a computer how to perform a calculation, process data, or make a decision. The best way to understand an algorithm is to think of it as a recipe ...
Problem solving, in the simplest terms, is the process of identifying a problem, analyzing it, and finding the most effective solution to overcome it. For software engineers, this process is deeply embedded in their daily workflow. It could be something as simple as figuring out why a piece of code isn't working as expected, or something as ...
An algorithm is defined as sequence of steps to solve a problem (task). The steps must be finite, well defined and unambiguous. Writing algorithm requires some thinking. Algorithm can also be defined as a plan to solve a problem and represents its logic. Note that an algorithm is of no use if it does not help us arrive at the desired solution.