- U.S. Department of Health & Human Services

- Virtual Tour
- Staff Directory
- En Español

You are here
Science, health, and public trust.
September 8, 2021
Explaining How Research Works
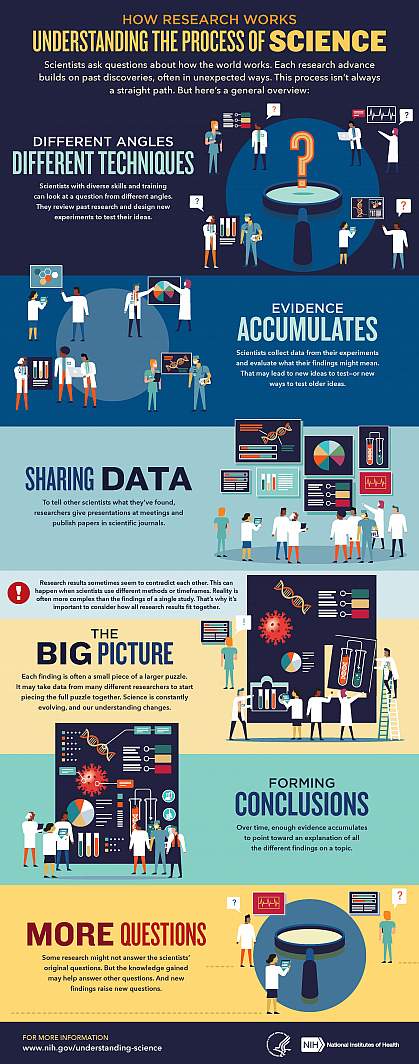
We’ve heard “follow the science” a lot during the pandemic. But it seems science has taken us on a long and winding road filled with twists and turns, even changing directions at times. That’s led some people to feel they can’t trust science. But when what we know changes, it often means science is working.

Explaining the scientific process may be one way that science communicators can help maintain public trust in science. Placing research in the bigger context of its field and where it fits into the scientific process can help people better understand and interpret new findings as they emerge. A single study usually uncovers only a piece of a larger puzzle.
Questions about how the world works are often investigated on many different levels. For example, scientists can look at the different atoms in a molecule, cells in a tissue, or how different tissues or systems affect each other. Researchers often must choose one or a finite number of ways to investigate a question. It can take many different studies using different approaches to start piecing the whole picture together.
Sometimes it might seem like research results contradict each other. But often, studies are just looking at different aspects of the same problem. Researchers can also investigate a question using different techniques or timeframes. That may lead them to arrive at different conclusions from the same data.
Using the data available at the time of their study, scientists develop different explanations, or models. New information may mean that a novel model needs to be developed to account for it. The models that prevail are those that can withstand the test of time and incorporate new information. Science is a constantly evolving and self-correcting process.
Scientists gain more confidence about a model through the scientific process. They replicate each other’s work. They present at conferences. And papers undergo peer review, in which experts in the field review the work before it can be published in scientific journals. This helps ensure that the study is up to current scientific standards and maintains a level of integrity. Peer reviewers may find problems with the experiments or think different experiments are needed to justify the conclusions. They might even offer new ways to interpret the data.
It’s important for science communicators to consider which stage a study is at in the scientific process when deciding whether to cover it. Some studies are posted on preprint servers for other scientists to start weighing in on and haven’t yet been fully vetted. Results that haven't yet been subjected to scientific scrutiny should be reported on with care and context to avoid confusion or frustration from readers.
We’ve developed a one-page guide, "How Research Works: Understanding the Process of Science" to help communicators put the process of science into perspective. We hope it can serve as a useful resource to help explain why science changes—and why it’s important to expect that change. Please take a look and share your thoughts with us by sending an email to [email protected].
Below are some additional resources:
- Discoveries in Basic Science: A Perfectly Imperfect Process
- When Clinical Research Is in the News
- What is Basic Science and Why is it Important?
- What is a Research Organism?
- What Are Clinical Trials and Studies?
- Basic Research – Digital Media Kit
- Decoding Science: How Does Science Know What It Knows? (NAS)
- Can Science Help People Make Decisions ? (NAS)
Connect with Us
- More Social Media from NIH
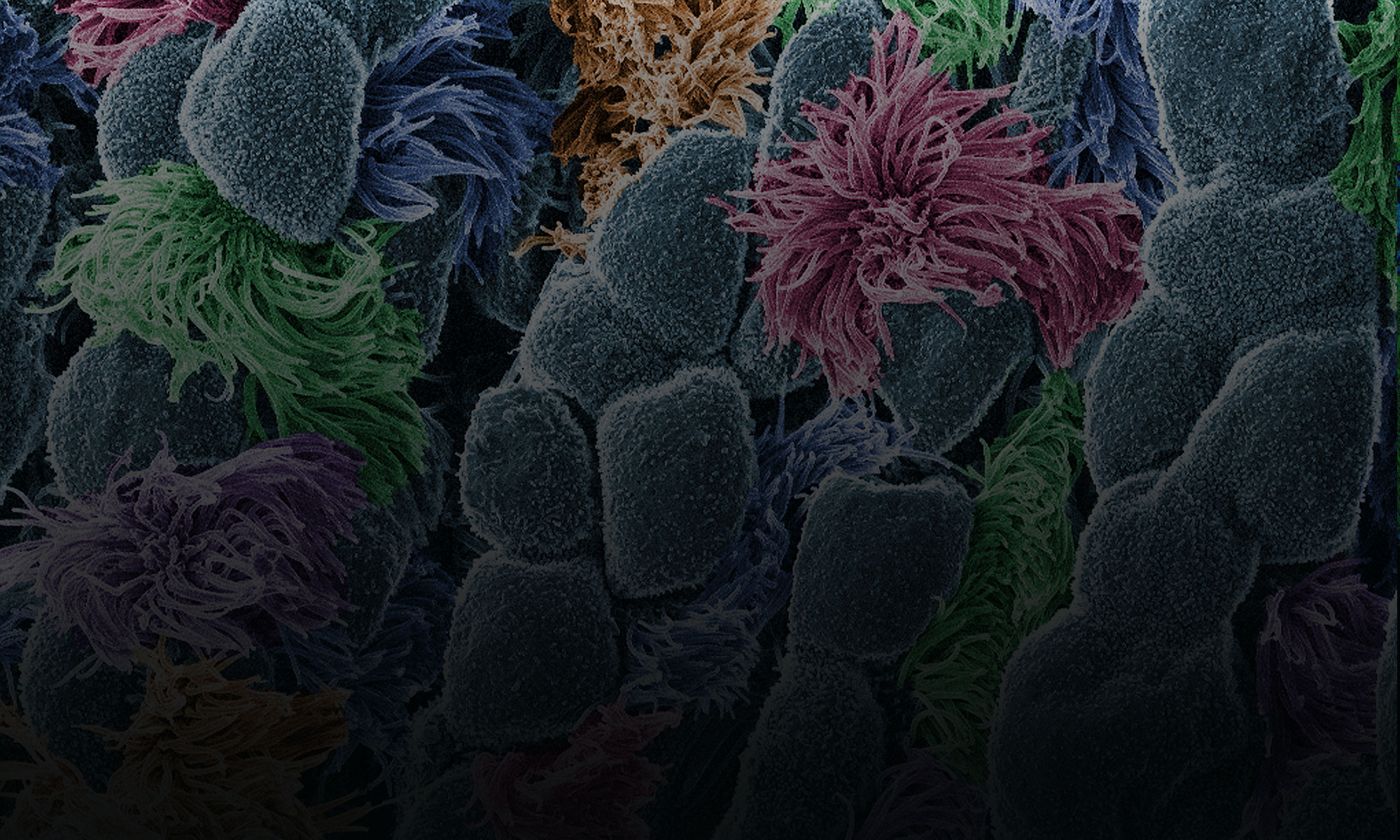
Biomedical Beat Blog – National Institute of General Medical Sciences
Follow the process of discovery
Search this blog
How research works: understanding the process of science.
Have you ever wondered how research works? How scientists make discoveries about our health and the world around us? Whether they’re studying plants, animals, humans, or something else in our world, they follow the scientific method. But this method isn’t always—or even usually—a straight line, and often the answers are unexpected and lead to more questions. Let’s dive in to see how it all works.
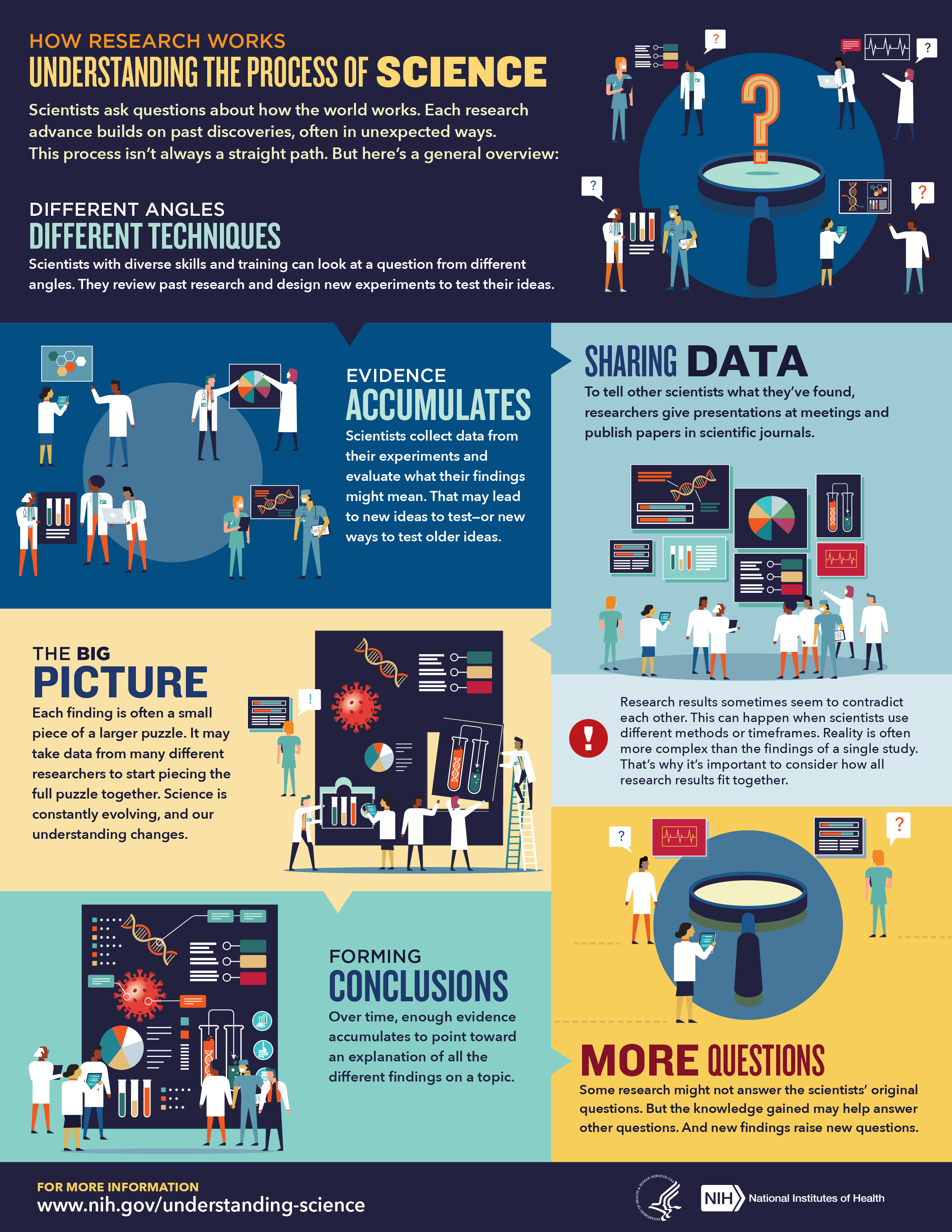
The Question Scientists start with a question about something they observe in the world. They develop a hypothesis, which is a testable prediction of what the answer to their question will be. Often their predictions turn out to be correct, but sometimes searching for the answer leads to unexpected outcomes.
The Techniques To test their hypotheses, scientists conduct experiments. They use many different tools and techniques, and sometimes they need to invent a new tool to fully answer their question. They may also work with one or more scientists with different areas of expertise to approach the question from other angles and get a more complete answer to their question.
The Evidence Throughout their experiments, scientists collect and analyze their data. They reach conclusions based on those analyses and determine whether their results match the predictions from their hypothesis. Often these conclusions trigger new questions and new hypotheses to test.
Researchers share their findings with one another by publishing papers in scientific journals and giving presentations at meetings. Data sharing is very important for the scientific field, and although some results may seem insignificant, each finding is often a small piece of a larger puzzle. That small piece may spark a new question and ultimately lead to new findings.
Sometimes research results seem to contradict each other, but this doesn’t necessarily mean that the results are wrong. Instead, it often means that the researchers used different tools, methods, or timeframes to obtain their results. The results of a single study are usually unable to fully explain the complex systems in the world around us. We must consider how results from many research studies fit together. This perspective gives us a more complete picture of what’s really happening.
Even if the scientific process doesn’t answer the original question, the knowledge gained may help provide other answers that lead to new hypotheses and discoveries.
Learn more about the importance of communicating how this process works in the NIH News in Health article, “ Explaining How Research Works .”
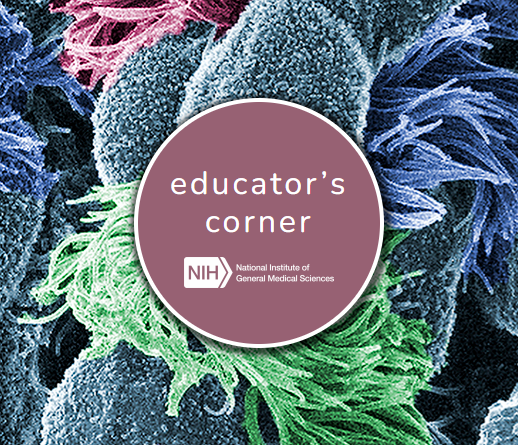
This post is a great supplement to Pathways: The Basic Science Careers Issue.
Pathways introduces the important role that scientists play in understanding the world around us, and all scientists use the scientific method as they make discoveries—which is explained in this post.
Learn more in our Educator’s Corner .
2 Replies to “How Research Works: Understanding the Process of Science”
Nice basic explanation. I believe informing the lay public on how science works, how parts of the body interact, etc. is a worthwhile endeavor. You all Rock! Now, we need to spread the word ‼️❗️‼️ Maybe eith a unique app. And one day, with VR and incentives to read & answer a couple questions.
As you know, the importance of an informed population is what will keep democracy alive. Plus it will improve peoples overall wellness & life outcomes.
Thanks for this clear explanation for the person who does not know science. Without getting too technical or advanced, it might be helpful to follow your explanation of replication with a reference to meta-analysis. You might say something as simple as, “Meta-analysis is a method for doing research on all the best research; meta-analytic research confirms the overall trend in results, even when the best studies show different results.”
Comments are closed.
Subscribe to Biomedical Beat
Get our latest blog posts delivered straight to your inbox! Sign Up Here
- Skip to main content
- Skip to primary sidebar
- Skip to footer
- QuestionPro

- Solutions Industries Gaming Automotive Sports and events Education Government Travel & Hospitality Financial Services Healthcare Cannabis Technology Use Case AskWhy Communities Audience Contactless surveys Mobile LivePolls Member Experience GDPR Positive People Science 360 Feedback Surveys
- Resources Blog eBooks Survey Templates Case Studies Training Help center

Home Market Research
What is Research: Definition, Methods, Types & Examples
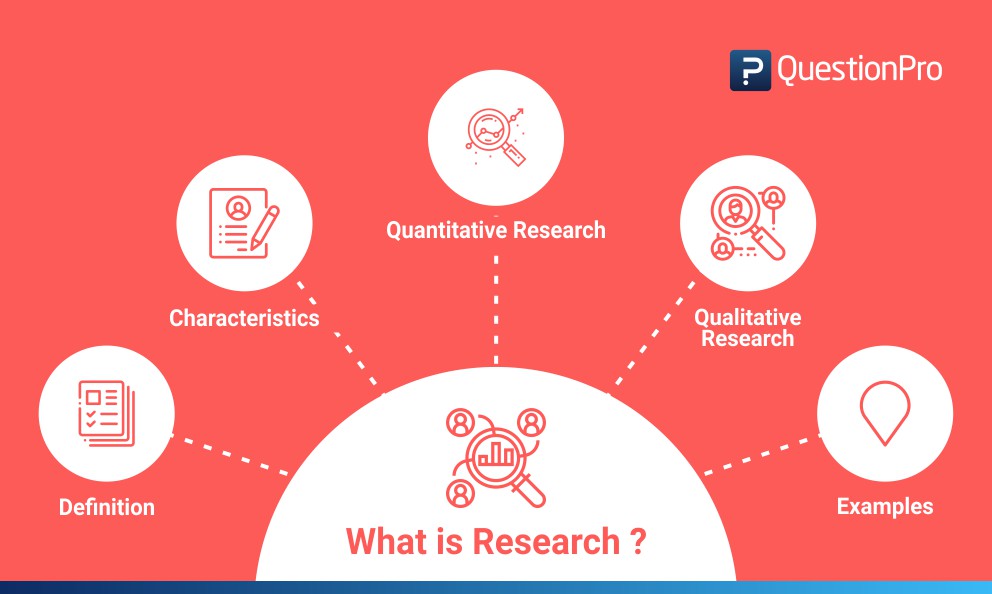
The search for knowledge is closely linked to the object of study; that is, to the reconstruction of the facts that will provide an explanation to an observed event and that at first sight can be considered as a problem. It is very human to seek answers and satisfy our curiosity. Let’s talk about research.
Content Index
What is Research?
What are the characteristics of research.
- Comparative analysis chart
Qualitative methods
Quantitative methods, 8 tips for conducting accurate research.
Research is the careful consideration of study regarding a particular concern or research problem using scientific methods. According to the American sociologist Earl Robert Babbie, “research is a systematic inquiry to describe, explain, predict, and control the observed phenomenon. It involves inductive and deductive methods.”
Inductive methods analyze an observed event, while deductive methods verify the observed event. Inductive approaches are associated with qualitative research , and deductive methods are more commonly associated with quantitative analysis .
Research is conducted with a purpose to:
- Identify potential and new customers
- Understand existing customers
- Set pragmatic goals
- Develop productive market strategies
- Address business challenges
- Put together a business expansion plan
- Identify new business opportunities
- Good research follows a systematic approach to capture accurate data. Researchers need to practice ethics and a code of conduct while making observations or drawing conclusions.
- The analysis is based on logical reasoning and involves both inductive and deductive methods.
- Real-time data and knowledge is derived from actual observations in natural settings.
- There is an in-depth analysis of all data collected so that there are no anomalies associated with it.
- It creates a path for generating new questions. Existing data helps create more research opportunities.
- It is analytical and uses all the available data so that there is no ambiguity in inference.
- Accuracy is one of the most critical aspects of research. The information must be accurate and correct. For example, laboratories provide a controlled environment to collect data. Accuracy is measured in the instruments used, the calibrations of instruments or tools, and the experiment’s final result.
What is the purpose of research?
There are three main purposes:
- Exploratory: As the name suggests, researchers conduct exploratory studies to explore a group of questions. The answers and analytics may not offer a conclusion to the perceived problem. It is undertaken to handle new problem areas that haven’t been explored before. This exploratory data analysis process lays the foundation for more conclusive data collection and analysis.
LEARN ABOUT: Descriptive Analysis
- Descriptive: It focuses on expanding knowledge on current issues through a process of data collection. Descriptive research describe the behavior of a sample population. Only one variable is required to conduct the study. The three primary purposes of descriptive studies are describing, explaining, and validating the findings. For example, a study conducted to know if top-level management leaders in the 21st century possess the moral right to receive a considerable sum of money from the company profit.
LEARN ABOUT: Best Data Collection Tools
- Explanatory: Causal research or explanatory research is conducted to understand the impact of specific changes in existing standard procedures. Running experiments is the most popular form. For example, a study that is conducted to understand the effect of rebranding on customer loyalty.
Here is a comparative analysis chart for a better understanding:
Approach used | Unstructured | Structured | Highly structured |
Conducted through | Asking questions | Asking questions | By using hypotheses. |
Time | Early stages of decision making | Later stages of decision making | Later stages of decision making |
It begins by asking the right questions and choosing an appropriate method to investigate the problem. After collecting answers to your questions, you can analyze the findings or observations to draw reasonable conclusions.
When it comes to customers and market studies, the more thorough your questions, the better the analysis. You get essential insights into brand perception and product needs by thoroughly collecting customer data through surveys and questionnaires . You can use this data to make smart decisions about your marketing strategies to position your business effectively.
To make sense of your study and get insights faster, it helps to use a research repository as a single source of truth in your organization and manage your research data in one centralized data repository .
Types of research methods and Examples
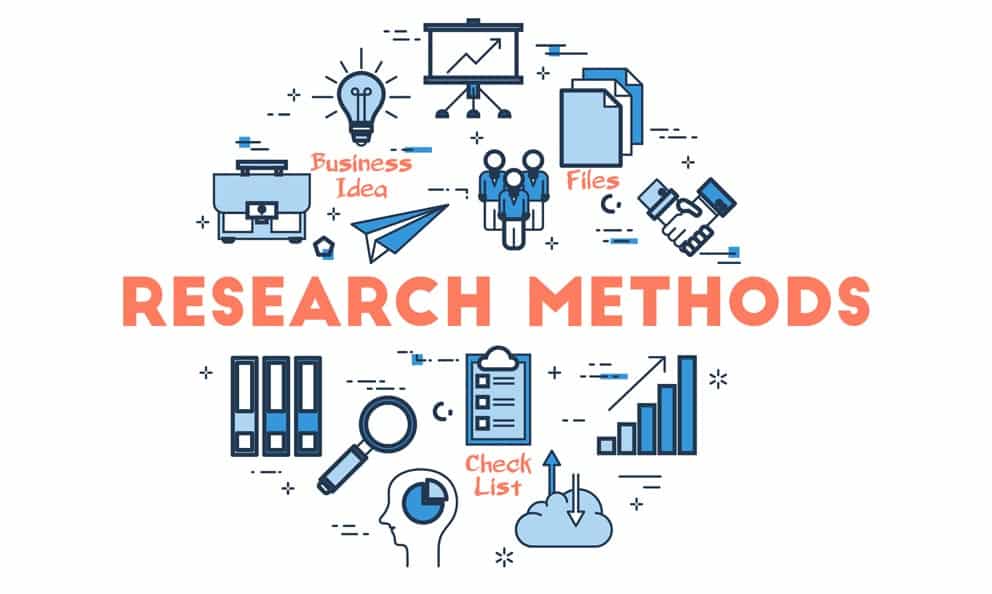
Research methods are broadly classified as Qualitative and Quantitative .
Both methods have distinctive properties and data collection methods .
Qualitative research is a method that collects data using conversational methods, usually open-ended questions . The responses collected are essentially non-numerical. This method helps a researcher understand what participants think and why they think in a particular way.
Types of qualitative methods include:
- One-to-one Interview
- Focus Groups
- Ethnographic studies
- Text Analysis
Quantitative methods deal with numbers and measurable forms . It uses a systematic way of investigating events or data. It answers questions to justify relationships with measurable variables to either explain, predict, or control a phenomenon.
Types of quantitative methods include:
- Survey research
- Descriptive research
- Correlational research
LEARN MORE: Descriptive Research vs Correlational Research
Remember, it is only valuable and useful when it is valid, accurate, and reliable. Incorrect results can lead to customer churn and a decrease in sales.
It is essential to ensure that your data is:
- Valid – founded, logical, rigorous, and impartial.
- Accurate – free of errors and including required details.
- Reliable – other people who investigate in the same way can produce similar results.
- Timely – current and collected within an appropriate time frame.
- Complete – includes all the data you need to support your business decisions.
Gather insights
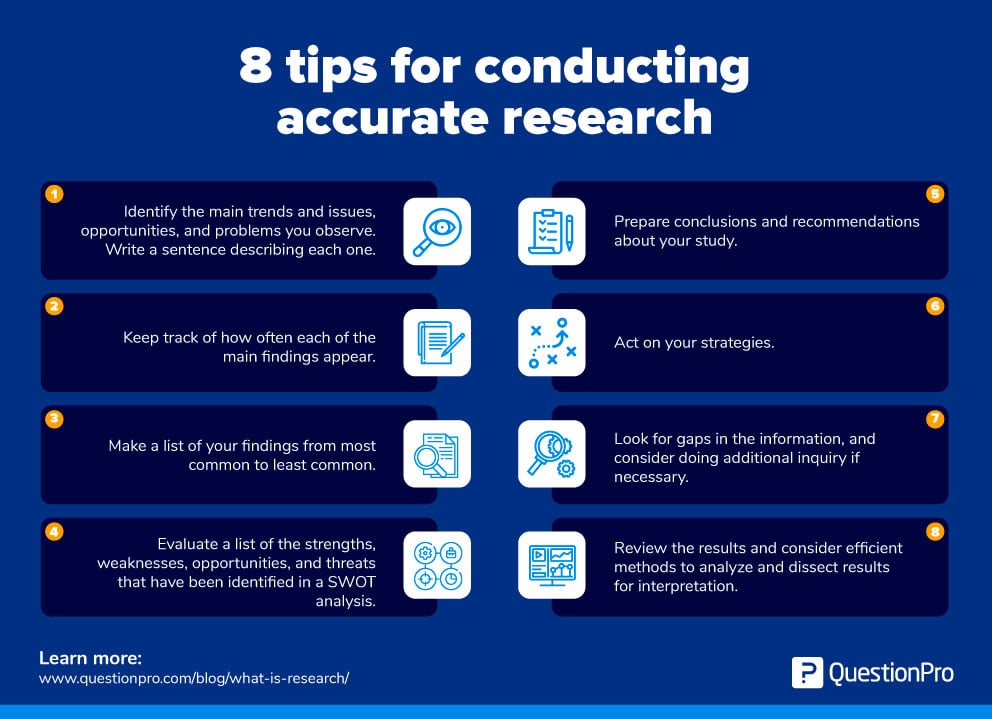
- Identify the main trends and issues, opportunities, and problems you observe. Write a sentence describing each one.
- Keep track of the frequency with which each of the main findings appears.
- Make a list of your findings from the most common to the least common.
- Evaluate a list of the strengths, weaknesses, opportunities, and threats identified in a SWOT analysis .
- Prepare conclusions and recommendations about your study.
- Act on your strategies
- Look for gaps in the information, and consider doing additional inquiry if necessary
- Plan to review the results and consider efficient methods to analyze and interpret results.
Review your goals before making any conclusions about your study. Remember how the process you have completed and the data you have gathered help answer your questions. Ask yourself if what your analysis revealed facilitates the identification of your conclusions and recommendations.
LEARN MORE ABOUT OUR SOFTWARE FREE TRIAL
MORE LIKE THIS
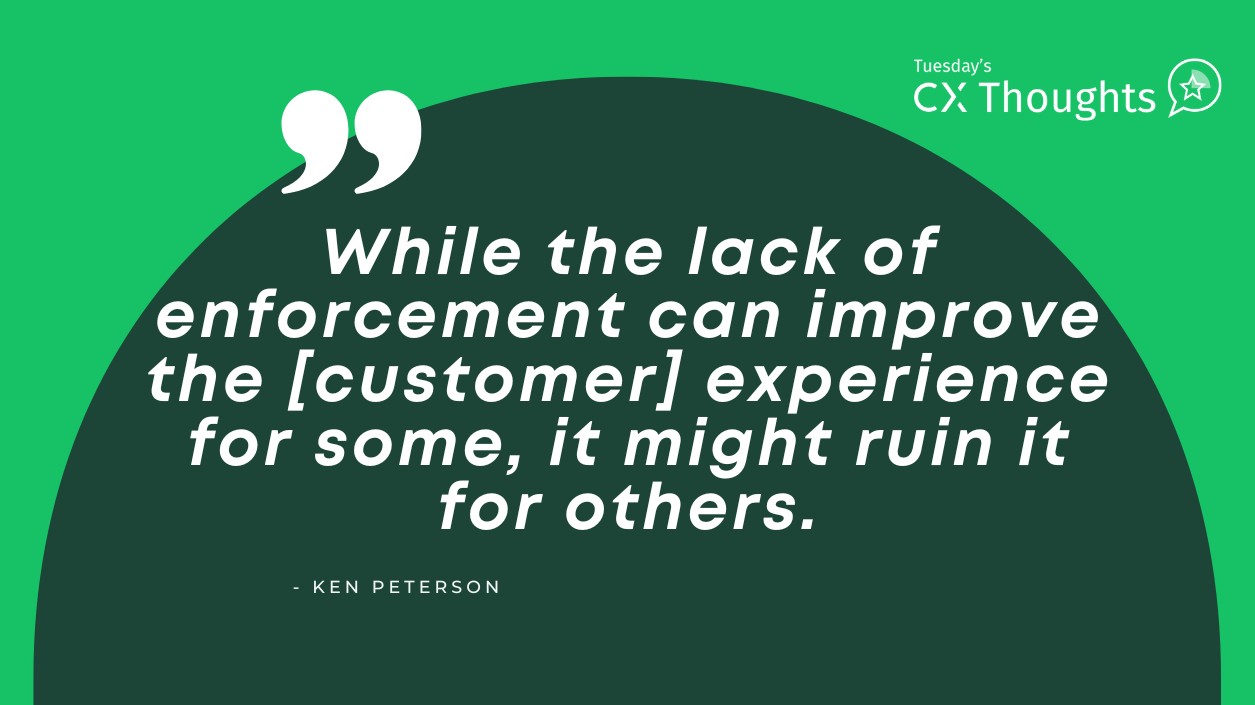
Was The Experience Memorable? (Part II) — Tuesday CX Thoughts
Sep 17, 2024
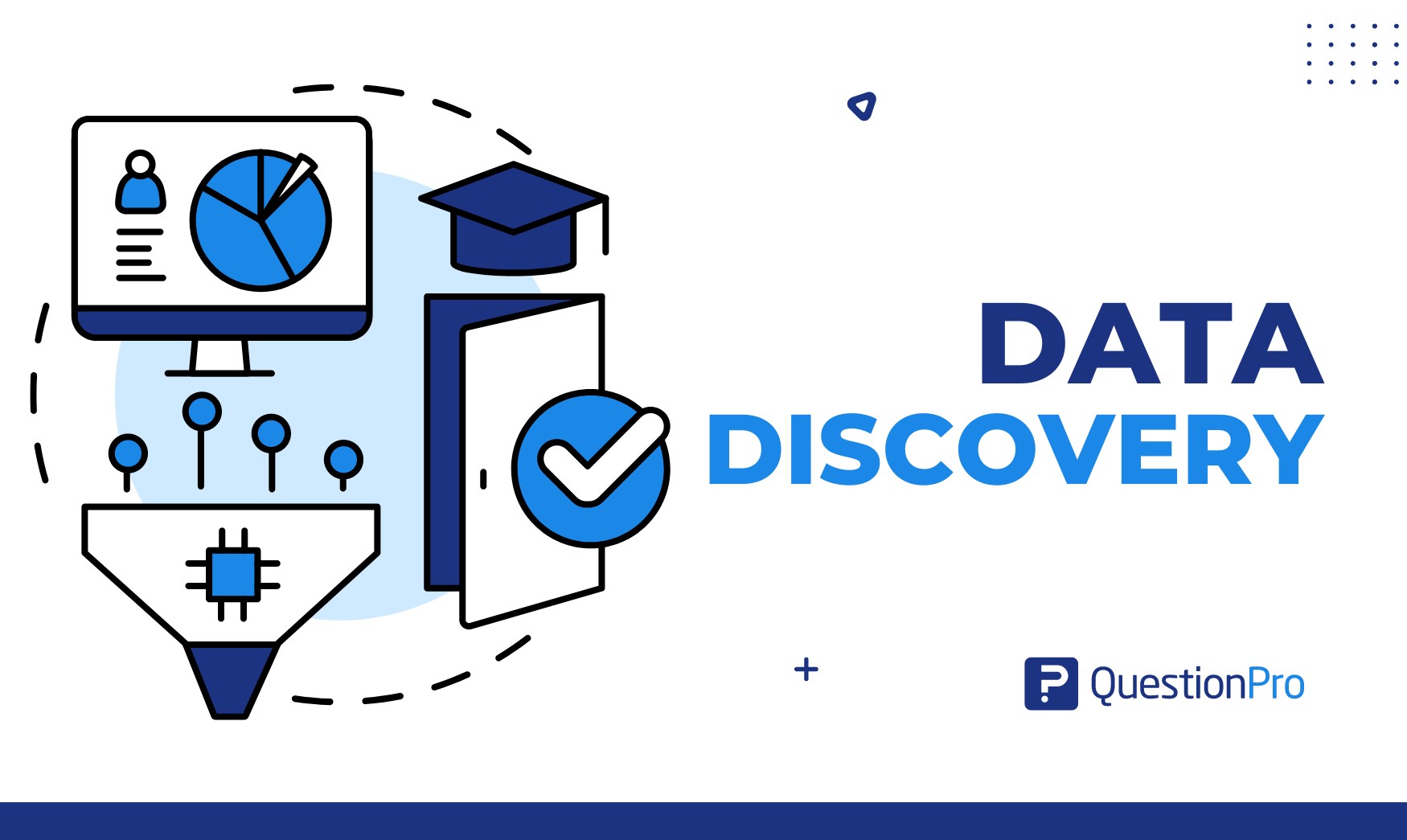
Data Discovery: What it is, Importance, Process + Use Cases
Sep 16, 2024
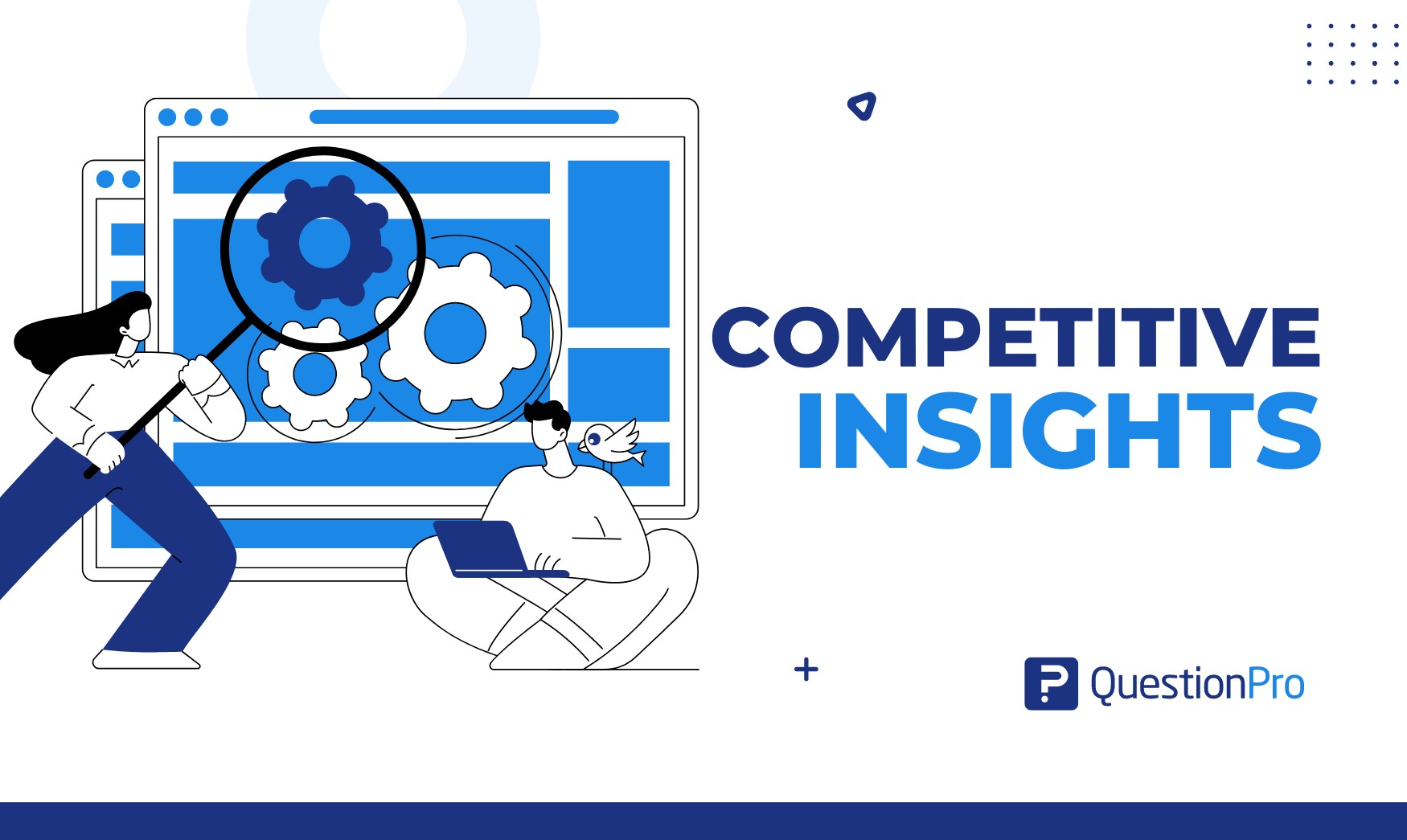
Competitive Insights : Importance, How to Get + Usage
Sep 13, 2024
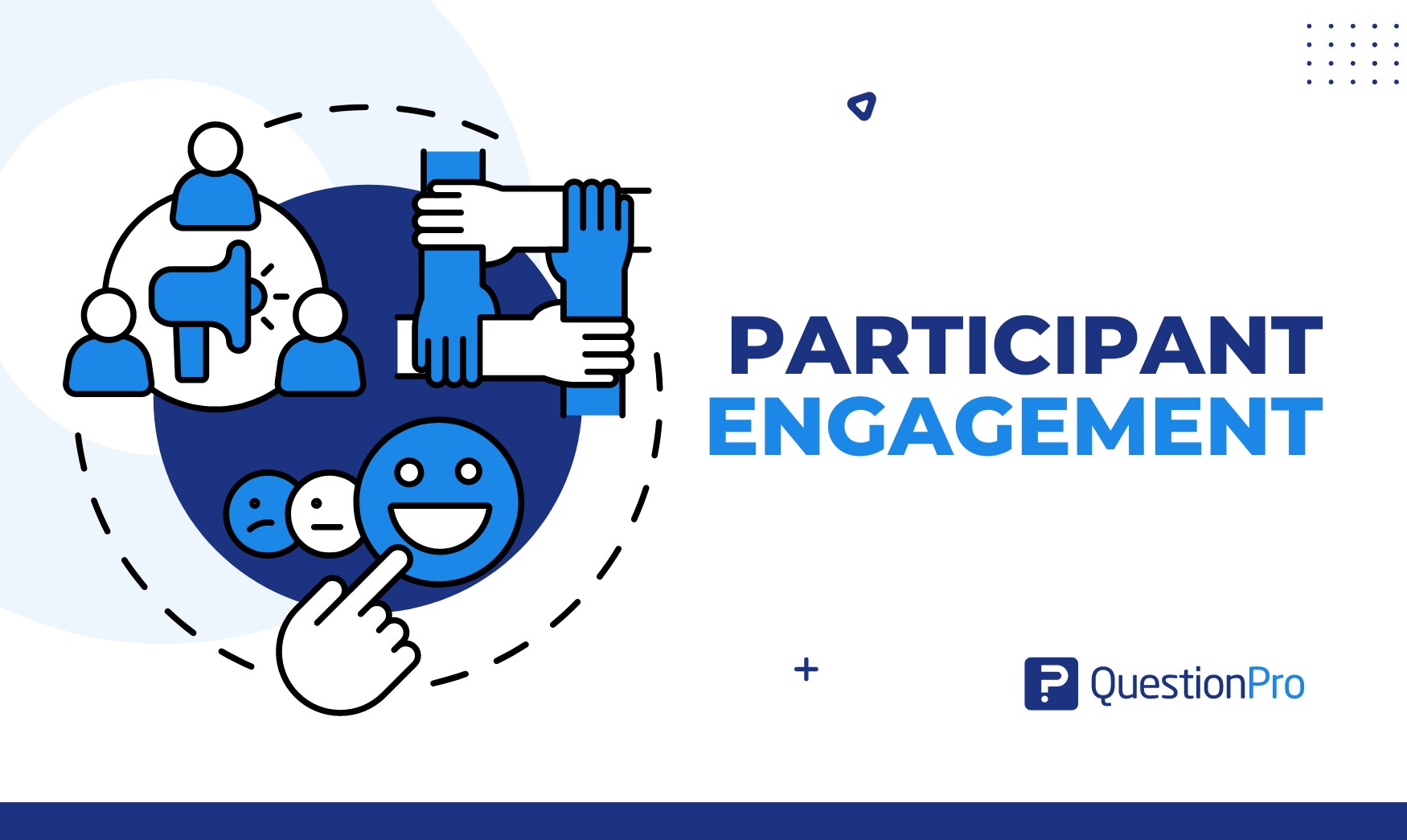
Participant Engagement: Strategies + Improving Interaction
Sep 12, 2024
Other categories
- Academic Research
- Artificial Intelligence
- Assessments
- Brand Awareness
- Case Studies
- Communities
- Consumer Insights
- Customer effort score
- Customer Engagement
- Customer Experience
- Customer Loyalty
- Customer Research
- Customer Satisfaction
- Employee Benefits
- Employee Engagement
- Employee Retention
- Friday Five
- General Data Protection Regulation
- Insights Hub
- Life@QuestionPro
- Market Research
- Mobile diaries
- Mobile Surveys
- New Features
- Online Communities
- Question Types
- Questionnaire
- QuestionPro Products
- Release Notes
- Research Tools and Apps
- Revenue at Risk
- Survey Templates
- Training Tips
- Tuesday CX Thoughts (TCXT)
- Uncategorized
- What’s Coming Up
- Workforce Intelligence
Educational resources and simple solutions for your research journey

What is Research? Definition, Types, Methods, and Examples
Academic research is a methodical way of exploring new ideas or understanding things we already know. It involves gathering and studying information to answer questions or test ideas and requires careful thinking and persistence to reach meaningful conclusions. Let’s try to understand what research is.
Table of Contents
Why is research important?
Whether it’s doing experiments, analyzing data, or studying old documents, research helps us learn more about the world. Without it, we rely on guesswork and hearsay, often leading to mistakes and misconceptions. By using systematic methods, research helps us see things clearly, free from biases. (1)
What is the purpose of research?
In the real world, academic research is also a key driver of innovation. It brings many benefits, such as creating valuable opportunities and fostering partnerships between academia and industry. By turning research into products and services, science makes meaningful improvements to people’s lives and boosts the economy. (2)(3)
What are the characteristics of research?
The research process collects accurate information systematically. Logic is used to analyze the collected data and find insights. Checking the collected data thoroughly ensures accuracy. Research also leads to new questions using existing data.
Accuracy is key in research, which requires precise data collection and analysis. In scientific research, laboratories ensure accuracy by carefully calibrating instruments and controlling experiments. Every step is checked to maintain integrity, from instruments to final results. Accuracy gives reliable insights, which in turn help advance knowledge.
Types of research
The different forms of research serve distinct purposes in expanding knowledge and understanding:
- Exploratory research ventures into uncharted territories, exploring new questions or problem areas without aiming for conclusive answers. For instance, a study may delve into unexplored market segments to better understand consumer behaviour patterns.
- Descriptive research delves into current issues by collecting and analyzing data to describe the behaviour of a sample population. For instance, a survey may investigate millennials’ spending habits to gain insights into their purchasing behaviours.
- Explanatory research, also known as causal research, seeks to understand the impact of specific changes in existing procedures. An example might be a study examining how changes in drug dosage over some time improve patients’ health.
- Correlational research examines connections between two sets of data to uncover meaningful relationships. For instance, a study may analyze the relationship between advertising spending and sales revenue.
- Theoretical research deepens existing knowledge without attempting to solve specific problems. For example, a study may explore theoretical frameworks to understand the underlying principles of human behaviour.
- Applied research focuses on real-world issues and aims to provide practical solutions. An example could be a study investigating the effectiveness of a new teaching method in improving student performance in schools. (4)
Types of research methods
- Qualitative Method: Qualitative research gathers non-numerical data through interactions with participants. Methods include one-to-one interviews, focus groups, ethnographic studies, text analysis, and case studies. For example, a researcher interviews cancer patients to understand how different treatments impact their lives emotionally.
- Quantitative Method: Quantitative methods deal with numbers and measurable data to understand relationships between variables. They use systematic methods to investigate events and aim to explain or predict outcomes. For example, Researchers study how exercise affects heart health by measuring variables like heart rate and blood pressure in a large group before and after an exercise program. (5)
Basic steps involved in the research process
Here are the basic steps to help you understand the research process:
- Choose your topic: Decide the specific subject or area that you want to study and investigate. This decision is the foundation of your research journey.
- Find information: Look for information related to your research topic. You can search in journals, books, online, or ask experts for help.
- Assess your sources: Make sure the information you find is reliable and trustworthy. Check the author’s credentials and the publication date.
- Take notes: Write down important information from your sources that you can use in your research.
- Write your paper: Use your notes to write your research paper. Broadly, start with an introduction, then write the body of your paper, and finish with a conclusion.
- Cite your sources: Give credit to the sources you used by including citations in your paper.
- Proofread: Check your paper thoroughly for any errors in spelling, grammar, or punctuation before you submit it. (6)
How to ensure research accuracy?
Ensuring accuracy in research is a mix of several essential steps:
- Clarify goals: Start by defining clear objectives for your research. Identify your research question, hypothesis, and variables of interest. This clarity will help guide your data collection and analysis methods, ensuring that your research stays focused and purposeful.
- Use reliable data: Select trustworthy sources for your information, whether they are primary data collected by you or secondary data obtained from other sources. For example, if you’re studying climate change, use data from reputable scientific organizations with transparent methodologies.
- Validate data: Validate your data to ensure it meets the standards of your research project. Check for errors, outliers, and inconsistencies at different stages, such as during data collection, entry, cleaning, or analysis.
- Document processes: Documenting your data collection and analysis processes is essential for transparency and reproducibility. Record details such as data collection methods, cleaning procedures, and analysis techniques used. This documentation not only helps you keep track of your research but also enables others to understand and replicate your work.
- Review results: Finally, review and verify your research findings to confirm their accuracy and reliability. Double-check your analyses, cross-reference your data, and seek feedback from peers or supervisors. (7)
Research is crucial for better understanding our world and for social and economic growth. By following ethical guidelines and ensuring accuracy, researchers play a critical role in driving this progress, whether through exploring new topics or deepening existing knowledge.
References:
- Why is Research Important – Introductory Psychology – Washington State University
- The Role Of Scientific Research In Driving Business Innovation – Forbes
- Innovation – Royal Society
- Types of Research – Definition & Methods – Bachelor Print
- What Is Qualitative vs. Quantitative Study? – National University
- Basic Steps in the Research Process – North Hennepin Community College
- Best Practices for Ensuring Data Accuracy in Research – LinkedIn
Researcher.Life is a subscription-based platform that unifies the best AI tools and services designed to speed up, simplify, and streamline every step of a researcher’s journey. The Researcher.Life All Access Pack is a one-of-a-kind subscription that unlocks full access to an AI writing assistant, literature recommender, journal finder, scientific illustration tool, and exclusive discounts on professional publication services from Editage.
Based on 21+ years of experience in academia, Researcher.Life All Access empowers researchers to put their best research forward and move closer to success. Explore our top AI Tools pack, AI Tools + Publication Services pack, or Build Your Own Plan. Find everything a researcher needs to succeed, all in one place – Get All Access now starting at just $17 a month !
Related Posts

Join Us for Peer Review Week 2024

How Editage All Access is Boosting Productivity for Academics in India

* Research Basics *
- Introduction
So What Do We Mean By “Formal Research?”
- Guide License
- Types of Research
- Secondary Research | Literature Review
- Developing Your Topic
- Using and Evaluating Sources
- Ethics & Responsible Conduct of Research
- More Information
Paul V. Galvin Library

email: [email protected]
Chat with us:
Make a research appointment:, search our faq:.
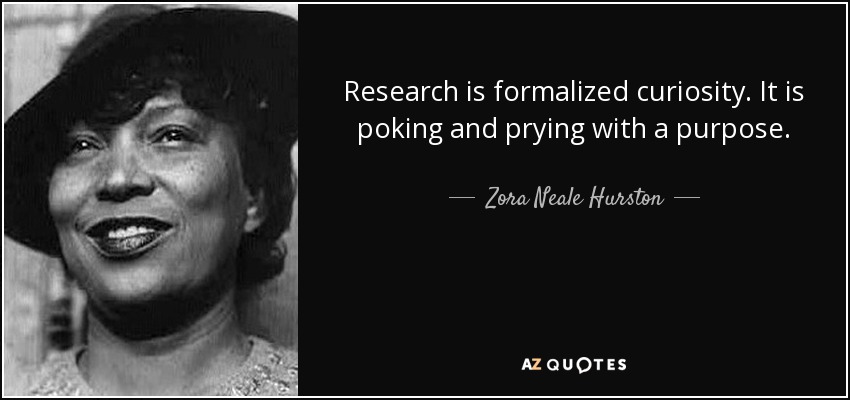
A good working definition of research might be:
Research is the deliberate, purposeful, and systematic gathering of data, information, facts, and/or opinions for the advancement of personal, societal, or overall human knowledge.
Based on this definition, we all do research all the time. Most of this research is casual research. Asking friends what they think of different restaurants, looking up reviews of various products online, learning more about celebrities; these are all research.
Formal research includes the type of research most people think of when they hear the term “research”: scientists in white coats working in a fully equipped laboratory. But formal research is a much broader category that just this. Most people will never do laboratory research after graduating from college, but almost everybody will have to do some sort of formal research at some point in their careers.
Casual research is inward facing: it’s done to satisfy our own curiosity or meet our own needs, whether that’s choosing a reliable car or figuring out what to watch on TV. Formal research is outward facing. While it may satisfy our own curiosity, it’s primarily intended to be shared in order to achieve some purpose. That purpose could be anything: finding a cure for cancer, securing funding for a new business, improving some process at your workplace, proving the latest theory in quantum physics, or even just getting a good grade in your Humanities 200 class.
What sets formal research apart from casual research is the documentation of where you gathered your information from. This is done in the form of “citations” and “bibliographies.” Citing sources is covered in the section "Citing Your Sources."
Formal research also follows certain common patterns depending on what the research is trying to show or prove. These are covered in the section “Types of Research.”

- Next: TL;DR >>
- Last Updated: Jul 24, 2024 4:33 PM
- URL: https://guides.library.iit.edu/research_basics
What Is Research, and Why Do People Do It?
- Open Access
- First Online: 03 December 2022
Cite this chapter
You have full access to this open access chapter
- James Hiebert 6 ,
- Jinfa Cai 7 ,
- Stephen Hwang 7 ,
- Anne K Morris 6 &
- Charles Hohensee 6
Part of the book series: Research in Mathematics Education ((RME))
23k Accesses
Abstractspiepr Abs1
Every day people do research as they gather information to learn about something of interest. In the scientific world, however, research means something different than simply gathering information. Scientific research is characterized by its careful planning and observing, by its relentless efforts to understand and explain, and by its commitment to learn from everyone else seriously engaged in research. We call this kind of research scientific inquiry and define it as “formulating, testing, and revising hypotheses.” By “hypotheses” we do not mean the hypotheses you encounter in statistics courses. We mean predictions about what you expect to find and rationales for why you made these predictions. Throughout this and the remaining chapters we make clear that the process of scientific inquiry applies to all kinds of research studies and data, both qualitative and quantitative.
You have full access to this open access chapter, Download chapter PDF
Part I. What Is Research?
Have you ever studied something carefully because you wanted to know more about it? Maybe you wanted to know more about your grandmother’s life when she was younger so you asked her to tell you stories from her childhood, or maybe you wanted to know more about a fertilizer you were about to use in your garden so you read the ingredients on the package and looked them up online. According to the dictionary definition, you were doing research.
Recall your high school assignments asking you to “research” a topic. The assignment likely included consulting a variety of sources that discussed the topic, perhaps including some “original” sources. Often, the teacher referred to your product as a “research paper.”
Were you conducting research when you interviewed your grandmother or wrote high school papers reviewing a particular topic? Our view is that you were engaged in part of the research process, but only a small part. In this book, we reserve the word “research” for what it means in the scientific world, that is, for scientific research or, more pointedly, for scientific inquiry .
Exercise 1.1
Before you read any further, write a definition of what you think scientific inquiry is. Keep it short—Two to three sentences. You will periodically update this definition as you read this chapter and the remainder of the book.
This book is about scientific inquiry—what it is and how to do it. For starters, scientific inquiry is a process, a particular way of finding out about something that involves a number of phases. Each phase of the process constitutes one aspect of scientific inquiry. You are doing scientific inquiry as you engage in each phase, but you have not done scientific inquiry until you complete the full process. Each phase is necessary but not sufficient.
In this chapter, we set the stage by defining scientific inquiry—describing what it is and what it is not—and by discussing what it is good for and why people do it. The remaining chapters build directly on the ideas presented in this chapter.
A first thing to know is that scientific inquiry is not all or nothing. “Scientificness” is a continuum. Inquiries can be more scientific or less scientific. What makes an inquiry more scientific? You might be surprised there is no universally agreed upon answer to this question. None of the descriptors we know of are sufficient by themselves to define scientific inquiry. But all of them give you a way of thinking about some aspects of the process of scientific inquiry. Each one gives you different insights.
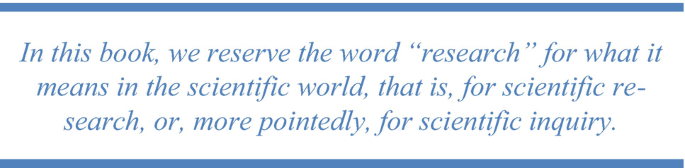
Exercise 1.2
As you read about each descriptor below, think about what would make an inquiry more or less scientific. If you think a descriptor is important, use it to revise your definition of scientific inquiry.
Creating an Image of Scientific Inquiry
We will present three descriptors of scientific inquiry. Each provides a different perspective and emphasizes a different aspect of scientific inquiry. We will draw on all three descriptors to compose our definition of scientific inquiry.
Descriptor 1. Experience Carefully Planned in Advance
Sir Ronald Fisher, often called the father of modern statistical design, once referred to research as “experience carefully planned in advance” (1935, p. 8). He said that humans are always learning from experience, from interacting with the world around them. Usually, this learning is haphazard rather than the result of a deliberate process carried out over an extended period of time. Research, Fisher said, was learning from experience, but experience carefully planned in advance.
This phrase can be fully appreciated by looking at each word. The fact that scientific inquiry is based on experience means that it is based on interacting with the world. These interactions could be thought of as the stuff of scientific inquiry. In addition, it is not just any experience that counts. The experience must be carefully planned . The interactions with the world must be conducted with an explicit, describable purpose, and steps must be taken to make the intended learning as likely as possible. This planning is an integral part of scientific inquiry; it is not just a preparation phase. It is one of the things that distinguishes scientific inquiry from many everyday learning experiences. Finally, these steps must be taken beforehand and the purpose of the inquiry must be articulated in advance of the experience. Clearly, scientific inquiry does not happen by accident, by just stumbling into something. Stumbling into something unexpected and interesting can happen while engaged in scientific inquiry, but learning does not depend on it and serendipity does not make the inquiry scientific.
Descriptor 2. Observing Something and Trying to Explain Why It Is the Way It Is
When we were writing this chapter and googled “scientific inquiry,” the first entry was: “Scientific inquiry refers to the diverse ways in which scientists study the natural world and propose explanations based on the evidence derived from their work.” The emphasis is on studying, or observing, and then explaining . This descriptor takes the image of scientific inquiry beyond carefully planned experience and includes explaining what was experienced.
According to the Merriam-Webster dictionary, “explain” means “(a) to make known, (b) to make plain or understandable, (c) to give the reason or cause of, and (d) to show the logical development or relations of” (Merriam-Webster, n.d. ). We will use all these definitions. Taken together, they suggest that to explain an observation means to understand it by finding reasons (or causes) for why it is as it is. In this sense of scientific inquiry, the following are synonyms: explaining why, understanding why, and reasoning about causes and effects. Our image of scientific inquiry now includes planning, observing, and explaining why.

We need to add a final note about this descriptor. We have phrased it in a way that suggests “observing something” means you are observing something in real time—observing the way things are or the way things are changing. This is often true. But, observing could mean observing data that already have been collected, maybe by someone else making the original observations (e.g., secondary analysis of NAEP data or analysis of existing video recordings of classroom instruction). We will address secondary analyses more fully in Chap. 4 . For now, what is important is that the process requires explaining why the data look like they do.
We must note that for us, the term “data” is not limited to numerical or quantitative data such as test scores. Data can also take many nonquantitative forms, including written survey responses, interview transcripts, journal entries, video recordings of students, teachers, and classrooms, text messages, and so forth.
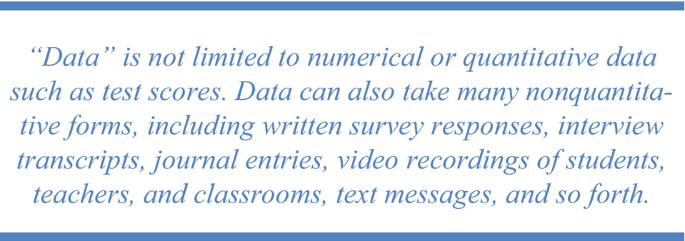
Exercise 1.3
What are the implications of the statement that just “observing” is not enough to count as scientific inquiry? Does this mean that a detailed description of a phenomenon is not scientific inquiry?
Find sources that define research in education that differ with our position, that say description alone, without explanation, counts as scientific research. Identify the precise points where the opinions differ. What are the best arguments for each of the positions? Which do you prefer? Why?
Descriptor 3. Updating Everyone’s Thinking in Response to More and Better Information
This descriptor focuses on a third aspect of scientific inquiry: updating and advancing the field’s understanding of phenomena that are investigated. This descriptor foregrounds a powerful characteristic of scientific inquiry: the reliability (or trustworthiness) of what is learned and the ultimate inevitability of this learning to advance human understanding of phenomena. Humans might choose not to learn from scientific inquiry, but history suggests that scientific inquiry always has the potential to advance understanding and that, eventually, humans take advantage of these new understandings.
Before exploring these bold claims a bit further, note that this descriptor uses “information” in the same way the previous two descriptors used “experience” and “observations.” These are the stuff of scientific inquiry and we will use them often, sometimes interchangeably. Frequently, we will use the term “data” to stand for all these terms.
An overriding goal of scientific inquiry is for everyone to learn from what one scientist does. Much of this book is about the methods you need to use so others have faith in what you report and can learn the same things you learned. This aspect of scientific inquiry has many implications.
One implication is that scientific inquiry is not a private practice. It is a public practice available for others to see and learn from. Notice how different this is from everyday learning. When you happen to learn something from your everyday experience, often only you gain from the experience. The fact that research is a public practice means it is also a social one. It is best conducted by interacting with others along the way: soliciting feedback at each phase, taking opportunities to present work-in-progress, and benefitting from the advice of others.
A second implication is that you, as the researcher, must be committed to sharing what you are doing and what you are learning in an open and transparent way. This allows all phases of your work to be scrutinized and critiqued. This is what gives your work credibility. The reliability or trustworthiness of your findings depends on your colleagues recognizing that you have used all appropriate methods to maximize the chances that your claims are justified by the data.
A third implication of viewing scientific inquiry as a collective enterprise is the reverse of the second—you must be committed to receiving comments from others. You must treat your colleagues as fair and honest critics even though it might sometimes feel otherwise. You must appreciate their job, which is to remain skeptical while scrutinizing what you have done in considerable detail. To provide the best help to you, they must remain skeptical about your conclusions (when, for example, the data are difficult for them to interpret) until you offer a convincing logical argument based on the information you share. A rather harsh but good-to-remember statement of the role of your friendly critics was voiced by Karl Popper, a well-known twentieth century philosopher of science: “. . . if you are interested in the problem which I tried to solve by my tentative assertion, you may help me by criticizing it as severely as you can” (Popper, 1968, p. 27).
A final implication of this third descriptor is that, as someone engaged in scientific inquiry, you have no choice but to update your thinking when the data support a different conclusion. This applies to your own data as well as to those of others. When data clearly point to a specific claim, even one that is quite different than you expected, you must reconsider your position. If the outcome is replicated multiple times, you need to adjust your thinking accordingly. Scientific inquiry does not let you pick and choose which data to believe; it mandates that everyone update their thinking when the data warrant an update.
Doing Scientific Inquiry
We define scientific inquiry in an operational sense—what does it mean to do scientific inquiry? What kind of process would satisfy all three descriptors: carefully planning an experience in advance; observing and trying to explain what you see; and, contributing to updating everyone’s thinking about an important phenomenon?
We define scientific inquiry as formulating , testing , and revising hypotheses about phenomena of interest.
Of course, we are not the only ones who define it in this way. The definition for the scientific method posted by the editors of Britannica is: “a researcher develops a hypothesis, tests it through various means, and then modifies the hypothesis on the basis of the outcome of the tests and experiments” (Britannica, n.d. ).

Notice how defining scientific inquiry this way satisfies each of the descriptors. “Carefully planning an experience in advance” is exactly what happens when formulating a hypothesis about a phenomenon of interest and thinking about how to test it. “ Observing a phenomenon” occurs when testing a hypothesis, and “ explaining ” what is found is required when revising a hypothesis based on the data. Finally, “updating everyone’s thinking” comes from comparing publicly the original with the revised hypothesis.
Doing scientific inquiry, as we have defined it, underscores the value of accumulating knowledge rather than generating random bits of knowledge. Formulating, testing, and revising hypotheses is an ongoing process, with each revised hypothesis begging for another test, whether by the same researcher or by new researchers. The editors of Britannica signaled this cyclic process by adding the following phrase to their definition of the scientific method: “The modified hypothesis is then retested, further modified, and tested again.” Scientific inquiry creates a process that encourages each study to build on the studies that have gone before. Through collective engagement in this process of building study on top of study, the scientific community works together to update its thinking.
Before exploring more fully the meaning of “formulating, testing, and revising hypotheses,” we need to acknowledge that this is not the only way researchers define research. Some researchers prefer a less formal definition, one that includes more serendipity, less planning, less explanation. You might have come across more open definitions such as “research is finding out about something.” We prefer the tighter hypothesis formulation, testing, and revision definition because we believe it provides a single, coherent map for conducting research that addresses many of the thorny problems educational researchers encounter. We believe it is the most useful orientation toward research and the most helpful to learn as a beginning researcher.
A final clarification of our definition is that it applies equally to qualitative and quantitative research. This is a familiar distinction in education that has generated much discussion. You might think our definition favors quantitative methods over qualitative methods because the language of hypothesis formulation and testing is often associated with quantitative methods. In fact, we do not favor one method over another. In Chap. 4 , we will illustrate how our definition fits research using a range of quantitative and qualitative methods.
Exercise 1.4
Look for ways to extend what the field knows in an area that has already received attention by other researchers. Specifically, you can search for a program of research carried out by more experienced researchers that has some revised hypotheses that remain untested. Identify a revised hypothesis that you might like to test.
Unpacking the Terms Formulating, Testing, and Revising Hypotheses
To get a full sense of the definition of scientific inquiry we will use throughout this book, it is helpful to spend a little time with each of the key terms.
We first want to make clear that we use the term “hypothesis” as it is defined in most dictionaries and as it used in many scientific fields rather than as it is usually defined in educational statistics courses. By “hypothesis,” we do not mean a null hypothesis that is accepted or rejected by statistical analysis. Rather, we use “hypothesis” in the sense conveyed by the following definitions: “An idea or explanation for something that is based on known facts but has not yet been proved” (Cambridge University Press, n.d. ), and “An unproved theory, proposition, or supposition, tentatively accepted to explain certain facts and to provide a basis for further investigation or argument” (Agnes & Guralnik, 2008 ).
We distinguish two parts to “hypotheses.” Hypotheses consist of predictions and rationales . Predictions are statements about what you expect to find when you inquire about something. Rationales are explanations for why you made the predictions you did, why you believe your predictions are correct. So, for us “formulating hypotheses” means making explicit predictions and developing rationales for the predictions.
“Testing hypotheses” means making observations that allow you to assess in what ways your predictions were correct and in what ways they were incorrect. In education research, it is rarely useful to think of your predictions as either right or wrong. Because of the complexity of most issues you will investigate, most predictions will be right in some ways and wrong in others.
By studying the observations you make (data you collect) to test your hypotheses, you can revise your hypotheses to better align with the observations. This means revising your predictions plus revising your rationales to justify your adjusted predictions. Even though you might not run another test, formulating revised hypotheses is an essential part of conducting a research study. Comparing your original and revised hypotheses informs everyone of what you learned by conducting your study. In addition, a revised hypothesis sets the stage for you or someone else to extend your study and accumulate more knowledge of the phenomenon.
We should note that not everyone makes a clear distinction between predictions and rationales as two aspects of hypotheses. In fact, common, non-scientific uses of the word “hypothesis” may limit it to only a prediction or only an explanation (or rationale). We choose to explicitly include both prediction and rationale in our definition of hypothesis, not because we assert this should be the universal definition, but because we want to foreground the importance of both parts acting in concert. Using “hypothesis” to represent both prediction and rationale could hide the two aspects, but we make them explicit because they provide different kinds of information. It is usually easier to make predictions than develop rationales because predictions can be guesses, hunches, or gut feelings about which you have little confidence. Developing a compelling rationale requires careful thought plus reading what other researchers have found plus talking with your colleagues. Often, while you are developing your rationale you will find good reasons to change your predictions. Developing good rationales is the engine that drives scientific inquiry. Rationales are essentially descriptions of how much you know about the phenomenon you are studying. Throughout this guide, we will elaborate on how developing good rationales drives scientific inquiry. For now, we simply note that it can sharpen your predictions and help you to interpret your data as you test your hypotheses.
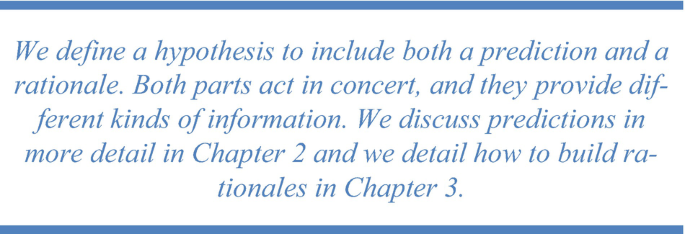
Hypotheses in education research take a variety of forms or types. This is because there are a variety of phenomena that can be investigated. Investigating educational phenomena is sometimes best done using qualitative methods, sometimes using quantitative methods, and most often using mixed methods (e.g., Hay, 2016 ; Weis et al. 2019a ; Weisner, 2005 ). This means that, given our definition, hypotheses are equally applicable to qualitative and quantitative investigations.
Hypotheses take different forms when they are used to investigate different kinds of phenomena. Two very different activities in education could be labeled conducting experiments and descriptions. In an experiment, a hypothesis makes a prediction about anticipated changes, say the changes that occur when a treatment or intervention is applied. You might investigate how students’ thinking changes during a particular kind of instruction.
A second type of hypothesis, relevant for descriptive research, makes a prediction about what you will find when you investigate and describe the nature of a situation. The goal is to understand a situation as it exists rather than to understand a change from one situation to another. In this case, your prediction is what you expect to observe. Your rationale is the set of reasons for making this prediction; it is your current explanation for why the situation will look like it does.
You will probably read, if you have not already, that some researchers say you do not need a prediction to conduct a descriptive study. We will discuss this point of view in Chap. 2 . For now, we simply claim that scientific inquiry, as we have defined it, applies to all kinds of research studies. Descriptive studies, like others, not only benefit from formulating, testing, and revising hypotheses, but also need hypothesis formulating, testing, and revising.
One reason we define research as formulating, testing, and revising hypotheses is that if you think of research in this way you are less likely to go wrong. It is a useful guide for the entire process, as we will describe in detail in the chapters ahead. For example, as you build the rationale for your predictions, you are constructing the theoretical framework for your study (Chap. 3 ). As you work out the methods you will use to test your hypothesis, every decision you make will be based on asking, “Will this help me formulate or test or revise my hypothesis?” (Chap. 4 ). As you interpret the results of testing your predictions, you will compare them to what you predicted and examine the differences, focusing on how you must revise your hypotheses (Chap. 5 ). By anchoring the process to formulating, testing, and revising hypotheses, you will make smart decisions that yield a coherent and well-designed study.
Exercise 1.5
Compare the concept of formulating, testing, and revising hypotheses with the descriptions of scientific inquiry contained in Scientific Research in Education (NRC, 2002 ). How are they similar or different?
Exercise 1.6
Provide an example to illustrate and emphasize the differences between everyday learning/thinking and scientific inquiry.
Learning from Doing Scientific Inquiry
We noted earlier that a measure of what you have learned by conducting a research study is found in the differences between your original hypothesis and your revised hypothesis based on the data you collected to test your hypothesis. We will elaborate this statement in later chapters, but we preview our argument here.
Even before collecting data, scientific inquiry requires cycles of making a prediction, developing a rationale, refining your predictions, reading and studying more to strengthen your rationale, refining your predictions again, and so forth. And, even if you have run through several such cycles, you still will likely find that when you test your prediction you will be partly right and partly wrong. The results will support some parts of your predictions but not others, or the results will “kind of” support your predictions. A critical part of scientific inquiry is making sense of your results by interpreting them against your predictions. Carefully describing what aspects of your data supported your predictions, what aspects did not, and what data fell outside of any predictions is not an easy task, but you cannot learn from your study without doing this analysis.
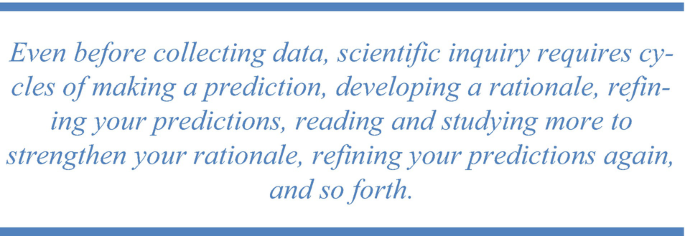
Analyzing the matches and mismatches between your predictions and your data allows you to formulate different rationales that would have accounted for more of the data. The best revised rationale is the one that accounts for the most data. Once you have revised your rationales, you can think about the predictions they best justify or explain. It is by comparing your original rationales to your new rationales that you can sort out what you learned from your study.
Suppose your study was an experiment. Maybe you were investigating the effects of a new instructional intervention on students’ learning. Your original rationale was your explanation for why the intervention would change the learning outcomes in a particular way. Your revised rationale explained why the changes that you observed occurred like they did and why your revised predictions are better. Maybe your original rationale focused on the potential of the activities if they were implemented in ideal ways and your revised rationale included the factors that are likely to affect how teachers implement them. By comparing the before and after rationales, you are describing what you learned—what you can explain now that you could not before. Another way of saying this is that you are describing how much more you understand now than before you conducted your study.
Revised predictions based on carefully planned and collected data usually exhibit some of the following features compared with the originals: more precision, more completeness, and broader scope. Revised rationales have more explanatory power and become more complete, more aligned with the new predictions, sharper, and overall more convincing.
Part II. Why Do Educators Do Research?
Doing scientific inquiry is a lot of work. Each phase of the process takes time, and you will often cycle back to improve earlier phases as you engage in later phases. Because of the significant effort required, you should make sure your study is worth it. So, from the beginning, you should think about the purpose of your study. Why do you want to do it? And, because research is a social practice, you should also think about whether the results of your study are likely to be important and significant to the education community.
If you are doing research in the way we have described—as scientific inquiry—then one purpose of your study is to understand , not just to describe or evaluate or report. As we noted earlier, when you formulate hypotheses, you are developing rationales that explain why things might be like they are. In our view, trying to understand and explain is what separates research from other kinds of activities, like evaluating or describing.
One reason understanding is so important is that it allows researchers to see how or why something works like it does. When you see how something works, you are better able to predict how it might work in other contexts, under other conditions. And, because conditions, or contextual factors, matter a lot in education, gaining insights into applying your findings to other contexts increases the contributions of your work and its importance to the broader education community.
Consequently, the purposes of research studies in education often include the more specific aim of identifying and understanding the conditions under which the phenomena being studied work like the observations suggest. A classic example of this kind of study in mathematics education was reported by William Brownell and Harold Moser in 1949 . They were trying to establish which method of subtracting whole numbers could be taught most effectively—the regrouping method or the equal additions method. However, they realized that effectiveness might depend on the conditions under which the methods were taught—“meaningfully” versus “mechanically.” So, they designed a study that crossed the two instructional approaches with the two different methods (regrouping and equal additions). Among other results, they found that these conditions did matter. The regrouping method was more effective under the meaningful condition than the mechanical condition, but the same was not true for the equal additions algorithm.
What do education researchers want to understand? In our view, the ultimate goal of education is to offer all students the best possible learning opportunities. So, we believe the ultimate purpose of scientific inquiry in education is to develop understanding that supports the improvement of learning opportunities for all students. We say “ultimate” because there are lots of issues that must be understood to improve learning opportunities for all students. Hypotheses about many aspects of education are connected, ultimately, to students’ learning. For example, formulating and testing a hypothesis that preservice teachers need to engage in particular kinds of activities in their coursework in order to teach particular topics well is, ultimately, connected to improving students’ learning opportunities. So is hypothesizing that school districts often devote relatively few resources to instructional leadership training or hypothesizing that positioning mathematics as a tool students can use to combat social injustice can help students see the relevance of mathematics to their lives.
We do not exclude the importance of research on educational issues more removed from improving students’ learning opportunities, but we do think the argument for their importance will be more difficult to make. If there is no way to imagine a connection between your hypothesis and improving learning opportunities for students, even a distant connection, we recommend you reconsider whether it is an important hypothesis within the education community.
Notice that we said the ultimate goal of education is to offer all students the best possible learning opportunities. For too long, educators have been satisfied with a goal of offering rich learning opportunities for lots of students, sometimes even for just the majority of students, but not necessarily for all students. Evaluations of success often are based on outcomes that show high averages. In other words, if many students have learned something, or even a smaller number have learned a lot, educators may have been satisfied. The problem is that there is usually a pattern in the groups of students who receive lower quality opportunities—students of color and students who live in poor areas, urban and rural. This is not acceptable. Consequently, we emphasize the premise that the purpose of education research is to offer rich learning opportunities to all students.
One way to make sure you will be able to convince others of the importance of your study is to consider investigating some aspect of teachers’ shared instructional problems. Historically, researchers in education have set their own research agendas, regardless of the problems teachers are facing in schools. It is increasingly recognized that teachers have had trouble applying to their own classrooms what researchers find. To address this problem, a researcher could partner with a teacher—better yet, a small group of teachers—and talk with them about instructional problems they all share. These discussions can create a rich pool of problems researchers can consider. If researchers pursued one of these problems (preferably alongside teachers), the connection to improving learning opportunities for all students could be direct and immediate. “Grounding a research question in instructional problems that are experienced across multiple teachers’ classrooms helps to ensure that the answer to the question will be of sufficient scope to be relevant and significant beyond the local context” (Cai et al., 2019b , p. 115).
As a beginning researcher, determining the relevance and importance of a research problem is especially challenging. We recommend talking with advisors, other experienced researchers, and peers to test the educational importance of possible research problems and topics of study. You will also learn much more about the issue of research importance when you read Chap. 5 .
Exercise 1.7
Identify a problem in education that is closely connected to improving learning opportunities and a problem that has a less close connection. For each problem, write a brief argument (like a logical sequence of if-then statements) that connects the problem to all students’ learning opportunities.
Part III. Conducting Research as a Practice of Failing Productively
Scientific inquiry involves formulating hypotheses about phenomena that are not fully understood—by you or anyone else. Even if you are able to inform your hypotheses with lots of knowledge that has already been accumulated, you are likely to find that your prediction is not entirely accurate. This is normal. Remember, scientific inquiry is a process of constantly updating your thinking. More and better information means revising your thinking, again, and again, and again. Because you never fully understand a complicated phenomenon and your hypotheses never produce completely accurate predictions, it is easy to believe you are somehow failing.
The trick is to fail upward, to fail to predict accurately in ways that inform your next hypothesis so you can make a better prediction. Some of the best-known researchers in education have been open and honest about the many times their predictions were wrong and, based on the results of their studies and those of others, they continuously updated their thinking and changed their hypotheses.
A striking example of publicly revising (actually reversing) hypotheses due to incorrect predictions is found in the work of Lee J. Cronbach, one of the most distinguished educational psychologists of the twentieth century. In 1955, Cronbach delivered his presidential address to the American Psychological Association. Titling it “Two Disciplines of Scientific Psychology,” Cronbach proposed a rapprochement between two research approaches—correlational studies that focused on individual differences and experimental studies that focused on instructional treatments controlling for individual differences. (We will examine different research approaches in Chap. 4 ). If these approaches could be brought together, reasoned Cronbach ( 1957 ), researchers could find interactions between individual characteristics and treatments (aptitude-treatment interactions or ATIs), fitting the best treatments to different individuals.
In 1975, after years of research by many researchers looking for ATIs, Cronbach acknowledged the evidence for simple, useful ATIs had not been found. Even when trying to find interactions between a few variables that could provide instructional guidance, the analysis, said Cronbach, creates “a hall of mirrors that extends to infinity, tormenting even the boldest investigators and defeating even ambitious designs” (Cronbach, 1975 , p. 119).
As he was reflecting back on his work, Cronbach ( 1986 ) recommended moving away from documenting instructional effects through statistical inference (an approach he had championed for much of his career) and toward approaches that probe the reasons for these effects, approaches that provide a “full account of events in a time, place, and context” (Cronbach, 1986 , p. 104). This is a remarkable change in hypotheses, a change based on data and made fully transparent. Cronbach understood the value of failing productively.
Closer to home, in a less dramatic example, one of us began a line of scientific inquiry into how to prepare elementary preservice teachers to teach early algebra. Teaching early algebra meant engaging elementary students in early forms of algebraic reasoning. Such reasoning should help them transition from arithmetic to algebra. To begin this line of inquiry, a set of activities for preservice teachers were developed. Even though the activities were based on well-supported hypotheses, they largely failed to engage preservice teachers as predicted because of unanticipated challenges the preservice teachers faced. To capitalize on this failure, follow-up studies were conducted, first to better understand elementary preservice teachers’ challenges with preparing to teach early algebra, and then to better support preservice teachers in navigating these challenges. In this example, the initial failure was a necessary step in the researchers’ scientific inquiry and furthered the researchers’ understanding of this issue.
We present another example of failing productively in Chap. 2 . That example emerges from recounting the history of a well-known research program in mathematics education.
Making mistakes is an inherent part of doing scientific research. Conducting a study is rarely a smooth path from beginning to end. We recommend that you keep the following things in mind as you begin a career of conducting research in education.
First, do not get discouraged when you make mistakes; do not fall into the trap of feeling like you are not capable of doing research because you make too many errors.
Second, learn from your mistakes. Do not ignore your mistakes or treat them as errors that you simply need to forget and move past. Mistakes are rich sites for learning—in research just as in other fields of study.
Third, by reflecting on your mistakes, you can learn to make better mistakes, mistakes that inform you about a productive next step. You will not be able to eliminate your mistakes, but you can set a goal of making better and better mistakes.
Exercise 1.8
How does scientific inquiry differ from everyday learning in giving you the tools to fail upward? You may find helpful perspectives on this question in other resources on science and scientific inquiry (e.g., Failure: Why Science is So Successful by Firestein, 2015).
Exercise 1.9
Use what you have learned in this chapter to write a new definition of scientific inquiry. Compare this definition with the one you wrote before reading this chapter. If you are reading this book as part of a course, compare your definition with your colleagues’ definitions. Develop a consensus definition with everyone in the course.
Part IV. Preview of Chap. 2
Now that you have a good idea of what research is, at least of what we believe research is, the next step is to think about how to actually begin doing research. This means how to begin formulating, testing, and revising hypotheses. As for all phases of scientific inquiry, there are lots of things to think about. Because it is critical to start well, we devote Chap. 2 to getting started with formulating hypotheses.
Agnes, M., & Guralnik, D. B. (Eds.). (2008). Hypothesis. In Webster’s new world college dictionary (4th ed.). Wiley.
Google Scholar
Britannica. (n.d.). Scientific method. In Encyclopaedia Britannica . Retrieved July 15, 2022 from https://www.britannica.com/science/scientific-method
Brownell, W. A., & Moser, H. E. (1949). Meaningful vs. mechanical learning: A study in grade III subtraction . Duke University Press..
Cai, J., Morris, A., Hohensee, C., Hwang, S., Robison, V., Cirillo, M., Kramer, S. L., & Hiebert, J. (2019b). Posing significant research questions. Journal for Research in Mathematics Education, 50 (2), 114–120. https://doi.org/10.5951/jresematheduc.50.2.0114
Article Google Scholar
Cambridge University Press. (n.d.). Hypothesis. In Cambridge dictionary . Retrieved July 15, 2022 from https://dictionary.cambridge.org/us/dictionary/english/hypothesis
Cronbach, J. L. (1957). The two disciplines of scientific psychology. American Psychologist, 12 , 671–684.
Cronbach, L. J. (1975). Beyond the two disciplines of scientific psychology. American Psychologist, 30 , 116–127.
Cronbach, L. J. (1986). Social inquiry by and for earthlings. In D. W. Fiske & R. A. Shweder (Eds.), Metatheory in social science: Pluralisms and subjectivities (pp. 83–107). University of Chicago Press.
Hay, C. M. (Ed.). (2016). Methods that matter: Integrating mixed methods for more effective social science research . University of Chicago Press.
Merriam-Webster. (n.d.). Explain. In Merriam-Webster.com dictionary . Retrieved July 15, 2022, from https://www.merriam-webster.com/dictionary/explain
National Research Council. (2002). Scientific research in education . National Academy Press.
Weis, L., Eisenhart, M., Duncan, G. J., Albro, E., Bueschel, A. C., Cobb, P., Eccles, J., Mendenhall, R., Moss, P., Penuel, W., Ream, R. K., Rumbaut, R. G., Sloane, F., Weisner, T. S., & Wilson, J. (2019a). Mixed methods for studies that address broad and enduring issues in education research. Teachers College Record, 121 , 100307.
Weisner, T. S. (Ed.). (2005). Discovering successful pathways in children’s development: Mixed methods in the study of childhood and family life . University of Chicago Press.
Download references
Author information
Authors and affiliations.
School of Education, University of Delaware, Newark, DE, USA
James Hiebert, Anne K Morris & Charles Hohensee
Department of Mathematical Sciences, University of Delaware, Newark, DE, USA
Jinfa Cai & Stephen Hwang
You can also search for this author in PubMed Google Scholar
Rights and permissions
Open Access This chapter is licensed under the terms of the Creative Commons Attribution 4.0 International License ( http://creativecommons.org/licenses/by/4.0/ ), which permits use, sharing, adaptation, distribution and reproduction in any medium or format, as long as you give appropriate credit to the original author(s) and the source, provide a link to the Creative Commons license and indicate if changes were made.
The images or other third party material in this chapter are included in the chapter's Creative Commons license, unless indicated otherwise in a credit line to the material. If material is not included in the chapter's Creative Commons license and your intended use is not permitted by statutory regulation or exceeds the permitted use, you will need to obtain permission directly from the copyright holder.
Reprints and permissions
Copyright information
© 2023 The Author(s)
About this chapter
Hiebert, J., Cai, J., Hwang, S., Morris, A.K., Hohensee, C. (2023). What Is Research, and Why Do People Do It?. In: Doing Research: A New Researcher’s Guide. Research in Mathematics Education. Springer, Cham. https://doi.org/10.1007/978-3-031-19078-0_1
Download citation
DOI : https://doi.org/10.1007/978-3-031-19078-0_1
Published : 03 December 2022
Publisher Name : Springer, Cham
Print ISBN : 978-3-031-19077-3
Online ISBN : 978-3-031-19078-0
eBook Packages : Education Education (R0)
Share this chapter
Anyone you share the following link with will be able to read this content:
Sorry, a shareable link is not currently available for this article.
Provided by the Springer Nature SharedIt content-sharing initiative
- Publish with us
Policies and ethics
- Find a journal
- Track your research

An official website of the United States government
The .gov means it’s official. Federal government websites often end in .gov or .mil. Before sharing sensitive information, make sure you’re on a federal government site.
The site is secure. The https:// ensures that you are connecting to the official website and that any information you provide is encrypted and transmitted securely.
- Publications
- Account settings
The PMC website is updating on October 15, 2024. Learn More or Try it out now .
- Advanced Search
- Journal List
- HHS Author Manuscripts

How to Conduct Responsible Research: A Guide for Graduate Students
Alison l. antes.
1 Department of Medicine, Division of General Medical Sciences, Washington University School of Medicine, St. Louis, Missouri, 314-362-6006
Leonard B. Maggi, Jr.
2 Department of Medicine, Division of Molecular Oncology, Siteman Cancer Center, Washington University School of Medicine, St. Louis, Missouri, 314-362-4102
Researchers must conduct research responsibly for it to have an impact and to safeguard trust in science. Essential responsibilities of researchers include using rigorous, reproducible research methods, reporting findings in a trustworthy manner, and giving the researchers who contributed appropriate authorship credit. This “how-to” guide covers strategies and practices for doing reproducible research and being a responsible author. The article also covers how to utilize decision-making strategies when uncertain about the best way to proceed in a challenging situation. The advice focuses especially on graduate students but is appropriate for undergraduates and experienced researchers. The article begins with an overview of the responsible conduct of research, research misconduct, and ethical behavior in the scientific workplace. The takeaway message is that responsible conduct of research requires a thoughtful approach to doing research to ensure trustworthy results and conclusions and that researchers receive fair credit.
INTRODUCTION
Doing research is stimulating and fulfilling work. Scientists make discoveries to build knowledge and solve problems, and they work with other dedicated researchers. Research is a highly complex activity, so it takes years for beginning researchers to learn everything they need to know to do science well. Part of this large body of knowledge is learning how to do research responsibly. Our purpose in this article is to provide graduate students a guide for how to perform responsible research. Our advice is also relevant to undergraduate researchers and for principal investigators (PIs), postdocs, or other researchers who mentor beginning researchers and wish to share our advice.
We begin by introducing some fundamentals about the responsible conduct of research (RCR), research misconduct, and ethical behavior. We focus on how to do reproducible science and be a responsible author. We provide practical advice for these topics and present scenarios to practice thinking through challenges in research. Our article concludes with decision-making strategies for addressing complex problems.
What is the responsible conduct of research?
To be committed to RCR means upholding the highest standards of honesty, accuracy, efficiency, and objectivity ( Steneck, 2007 ). Each day, RCR requires engaging in research in a conscientious, intentional fashion that yields the best science possible ( “Research Integrity is Much More Than Misconduct,” 2019 ). We adopt a practical, “how-to” approach, discussing the behaviors and habits that yield responsible research. However, some background knowledge about RCR is helpful to frame our discussion.
The scientific community uses many terms to refer to ethical and responsible behavior in research: responsible conduct of research, research integrity, scientific integrity, and research ethics ( National Academies of Science, 2009 ; National Academies of Sciences Engineering and Medicine, 2017 ; Steneck, 2007 ). A helpful way to think about these concepts is “doing good science in a good manner” ( DuBois & Antes, 2018 ). This means that the way researchers do their work, from experimental procedures to data analysis and interpretation, research reporting, and so on, leads to trustworthy research findings and conclusions. It also includes respectful interactions among researchers both within research teams (e.g., between peers, mentors and trainees, and collaborators) and with researchers external to the team (e.g., peer reviewers). We expand on trainee-mentor relationships and interpersonal dynamics with labmates in a companion article ( Antes & Maggi, 2021 ). When research involves human or animal research subjects, RCR includes protecting the well-being of research subjects.
We do not cover all potential RCR topics but focus on what we consider fundamentals for graduate students. Common topics covered in texts and courses on RCR include the following: authorship and publication; collaboration; conflicts of interest; data management, sharing, and ownership; intellectual property; mentor and trainee responsibilities; peer review; protecting human subjects; protecting animal subjects; research misconduct; the role of researchers in society; and laboratory safety. A number of topics prominently discussed among the scientific community in recent years are also relevant to RCR. These include the reproducibility of research ( Baker, 2016 ; Barba, 2016 ; Winchester, 2018 ), diversity and inclusion in science ( Asplund & Welle, 2018 ; Hofstra et al., 2020 ; Meyers, Brown, Moneta-Koehler, & Chalkley, 2018 ; National Academies of Sciences Engineering and Medicine, 2018a ; Roper, 2019 ), harassment and bullying ( Else, 2018 ; National Academies of Sciences Engineering and Medicine, 2018b ; “ No Place for Bullies in Science,” 2018 ), healthy research work environments ( Norris, Dirnagl, Zigmond, Thompson-Peer, & Chow, 2018 ; “ Research Institutions Must Put the Health of Labs First,” 2018 ), and the mental health of graduate students ( Evans, Bira, Gastelum, Weiss, & Vanderford, 2018 ).
The National Institutes of Health (NIH) ( National Institutes of Health, 2009 ) and the National Science Foundation ( National Science Foundation, 2017 ) have formal policies indicating research trainees must receive education in RCR. Researchers are accountable to these funding agencies and the public which supports research through billions in tax dollars annually. The public stands to benefit from, or be harmed by, research. For example, the public may be harmed if medical treatments or social policies are based on untrustworthy research findings. Funding for research, participation in research, and utilization of the fruits of research all rely on public trust ( Resnik, 2011 ). Trustworthy findings are also essential for good stewardship of scarce resources ( Emanuel, Wendler, & Grady, 2000 ). Researchers are further accountable to their peers, colleagues, and scientists more broadly. Trust in the work of other researchers is essential for science to advance. Finally, researchers are accountable for complying with the rules and policies of their universities or research institutions, such as rules about laboratory safety, bullying and harassment, and the treatment of animal research subjects.
What is research misconduct?
When researchers intentionally misrepresent or manipulate their results, these cases of scientific fraud often make the news headlines ( Chappell, 2019 ; O’Connor, 2018 ; Park, 2012 ), and they can seriously undermine public trust in research. These cases also harm trust within the scientific community.
The U.S. defines research misconduct as fabrication, falsification, and plagiarism (FFP) ( Department of Health and Human Services, 2005 ). FFP violate the fundamental ethical principle of honesty. Fabrication is making up data, and falsification is manipulating or changing data or results so they are no longer truthful. Plagiarism is a form of dishonesty because it includes using someone’s words or ideas and portraying them as your own. When brought to light, misconduct involves lengthy investigations and serious consequences, such as ineligibility to receive federal research funding, loss of employment, paper retractions, and, for students, withdrawal of graduate degrees.
One aspect of responsible behavior includes addressing misconduct if you observe it. We suggest a guide titled “Responding to Research Wrongdoing: A User-Friendly Guide” that provides advice for thinking about your options if you think you have observed misconduct ( Keith-Spiegel, Sieber, & Koocher, 2010 ). Your university will have written policies and procedures for investigating allegations of misconduct. Making an allegation is very serious. As Keith-Spiegel et al.’s guide indicates, it is important to know the evidence that supports your claim, and what to expect in the process. We encourage, if possible, talking to the persons involved first. For example, one of us knew of a graduate student who reported to a journal editor their suspicion of falsified data in a manuscript. It turned out that the student was incorrect. Going above the PI directly to the editor ultimately led to the PI leaving the university, and the student had a difficult time finding a new lab to complete their degree. If the student had first spoken to the PI and lab members, they could have learned that their assumptions about the data in the paper were wrong. In turn, they could have avoided accusing the PI of a serious form of scientific misconduct—making up data—and harming everyone’s scientific career.
What shapes ethical behavior in the scientific workplace?
Responsible conduct of research and research misconduct are two sides of a continuum of behavior—RCR upholds the ideals of research and research misconduct violates them. Problematic practices that fall in the middle but are not defined formally as research misconduct have been labeled as detrimental research practices ( National Academies of Sciences Engineering and Medicine, 2017 ). Researchers conducting misleading statistical analyses or PIs providing inadequate supervision are examples of the latter. Research suggests that characteristics of individual researchers and research environments explain (un)ethical behavior in the scientific workplace ( Antes et al., 2007 ; Antes, English, Baldwin, & DuBois, 2018 ; Davis, Riske-Morris, & Diaz, 2007 ; DuBois et al., 2013 ).
These two influences on ethical behavior are helpful to keep in mind when thinking about your behavior. When people think about their ethical behavior, they think about their personal values and integrity and tend to overlook the influence of their environment. While “being a good person” and having the right intentions are essential to ethical behavior, the environment also has an influence. In addition, knowledge of standards for ethical research is important for ethical behavior, and graduate students new to research do not yet know everything they need to. They also have not fully refined their ethical decision-making skills for solving professional problems. We discuss strategies for ethical decision-making in the final section of this article ( McIntosh, Antes, & DuBois, 2020 ).
The research environment influences ethical behavior in a number of ways. For example, if a research group explicitly discusses high standards for research, people will be more likely to prioritize these ideals in their behavior ( Plemmons et al., 2020 ). A mentor who sets a good example is another important factor ( Anderson et al., 2007 ). Research labs must also provide individuals with adequate training, supervision and feedback, opportunities to discuss data, and the psychological safety to feel comfortable communicating about problems, including mistakes ( Antes, Kuykendall, & DuBois, 2019a , 2019b ). On the other hand, unfair research environments, inadequate supervision, poor communication, and severe stress and anxiety may undermine ethical decision-making and behavior; particularly when many of these factors exist together. Thus, (un)ethical behavior is a complex interplay of individual factors (e.g., personality, stress, decision-making skills) and the environment.
For graduate students, it is important to attend to what you are learning and how the environment around you might influence your behavior. You do not know what you do not know, and you necessarily rely on others to teach you responsible practices. So, it is important to be aware. Ultimately, you are accountable for your behavior. You cannot just say “I didn’t know.” Rather, just like you are curious about your scientific questions, maintain a curiosity about responsible behavior as a researcher. If you feel uncomfortable with something, pay attention to that feeling, speak to someone you trust, and seek out information about how to handle the situation. In what follows, we cover key tips for responsible behavior in the areas of reproducibility and authorship that we hope will help you as you begin.
HOW TO DO REPRODUCIBLE SCIENCE
The foremost responsibility of scientists is to ensure they conduct research in such a manner that the findings are trustworthy. Reproducibility is the ability to duplicate results ( Goodman, Fanelli, & Ioannidis, 2016 ). The scientific community has called for greater openness, transparency, and rigor as key remedies for lack of reproducibility ( Munafò et al., 2017 ). As a graduate student, essential to fostering reproducibility is the rigor of your approach to doing experiments and handling data. We discuss how to utilize research protocols, document experiments in a lab notebook, and handle data responsibly.
Utilize research protocols
1. learn and utilize the lab’s protocols.
Research protocols describe the step-by-step procedures for doing an experiment. They are critical for the quality and reproducibility of experiments. Lab members must learn and follow the lab’s protocols with the understanding that they may need to make adjustments based on the requirements of a specific experiment.
Also, it is important to distinguish between the experiment you are performing and analyzing the data from that experiment. For example, the experiment you want to perform might be to determine if loss of a gene blocks cell growth. Several protocols, each with pros and cons, will allow you to examine “cell growth.” Using the wrong experimental protocol can produce data that leads to muddled conclusions. In this example, the gene does block cell growth, but the experiment used to produce the data that you analyze to understand cell growth is wrong, thus giving a result that is a false negative.
When first joining a lab, it is essential to commit to learning the protocols necessary for your assigned research project. Researchers must ensure they are proficient in executing a protocol and can perform their experiments reliably. If you do not feel confident with a protocol, you should do practice runs if possible. Repetition is the best way to work through difficulties with protocols. Often it takes several attempts to work through the steps of a protocol before you will be comfortable performing it. Asking to watch another lab member perform the protocol is also helpful. Be sure to watch closely how steps are performed, as often there are minor steps taken that are not written down. Also, experienced lab members may do things as second nature and not think to explicitly mention them when working through the protocol. Ask questions of other lab members so that you can improve your knowledge and gain confidence with a protocol. It is better to ask a question than potentially ruin a valuable or hard-to-get sample.
Be cautious of differences in the standing protocols in the lab and how you actually perform the experiment. Even the most minor deviations can seriously impact the results and reproducibility of an experiment. As mentioned above, often there are minor things that are done that might not be listed in the protocol. Paying attention and asking questions are the best ways to learn, in addition to adding notes to the protocol if you find minor details are missing.
2. Develop your own protocols
Often you will find that a project requires a protocol that has not been performed in the lab. If performing a new experiment in the lab and no protocol exists, find a protocol and try it. Protocols can be obtained from many different sources. A great source is other labs on campus, as you can speak directly to the person who performs the experiment. There are many journal sources as well, such as Current Protocols, Nature Protocols, Nature Methods, and Cell STAR Methods . These methods journals provide the most detailed protocols for experiments often with troubleshooting tips. Scientific papers are the most common source of protocols. However, keep in mind that due to the common brevity of methods sections, they often omit crucial details or reference other papers that may not contain a complete description of the protocol.
3. Handle mistakes or problems promptly
At some point, everyone encounters problems with a protocol, or realizes they made a mistake. You should be prepared to handle this situation by being able to detail exactly how you performed the experiment. Did you skip a step? Shorten or lengthen a time point? Did you have to make a new buffer or borrow a labmate’s buffer? There are too many ways an experiment can go wrong to list here but being able to recount all the steps you performed in detail will help you work through the problem. Keep in mind that often the best way to understand how to perform an experiment is learning from when something goes wrong. This situation requires you to critically think through what was done and understand the steps taken. When everything works perfectly, it is easy to pay less attention to the details, which can lead to problems down the line.
It is up to you to be attentive and meticulous in the lab. Paying attention to the details may feel like a pain at first, or even seem overwhelming. Practice and repetition will help this focus on details become a natural part of your lab work. Ultimately, this skill will be essential to being a responsible scientist.
Document experiments in a lab notebook
1. recognize the importance of a lab notebook.
Maintaining detailed documentation in a lab notebook allows researchers to keep track of their experiments and generation of data. This detailed documentation helps you communicate about your research with others in the lab, and serves as a basis for preparing publications. It also provides a lasting record for the lab that exists beyond your time in the lab. After graduate students leave the lab, sometimes it is necessary to go back to the results of older experiments. A complete and detailed notebook is essential, or all of the time, effort, and resources are lost.
2. Learn the note-keeping practices in your lab
When you enter a new lab, it is important to understand how the lab keeps notebooks and the expectations for documentation. Being conscientious about documentation will make you a better scientist. In some labs, the PI might routinely examine your notebook, while in other labs you may be expected to maintain a notebook, but it may not be regularly viewed by others. It is tempting to become relaxed in documentation if you think your notebook may not be reviewed. Avoid this temptation; documentation of your ideas and process will improve your ability to think critically about research. Further, even if the PI or lab members do not physically view your notebook, you will need to communicate with them about your experiments. This documentation is necessary to communicate effectively about your work.
3. Organize your lab notebook
Different labs use different formats; some use electronic notebooks while others handwritten notebooks. The contents of a good notebook include the purpose of the experiment, the details of the experimental procedure, the data, and thoughts about the results. To effectively document your experiment, there are 5 critical questions that the information you record should be able to answer.
- Why I am doing this experiment? (purpose)
- What did I do to perform the experiment? (protocol)
- What are the results of what I did? (data, graphs)
- What do I think about the results?
- What do I think are the next steps?
We also recommend a table of contents. It will make the information more useful to you and the lab in the future. The table of contents should list the title of the experiment, the date(s) it was performed, and the page numbers on which it is recorded. Also, make sure that you write clearly and provide a legend or explanation of any shorthand or non-standard abbreviation you use. Often labs will have a combination of written lab notebooks and electronic data. It is important to reference where electronic data are located that go with each experiment. The idea is to make it as easy as possible to understand what you did and where to find all the data (electronic and hard copy) that accompanies your experiment.
Keeping a lab notebook becomes easier with practice. It can be thought of almost like journaling about your experiment. Sometimes people think of it as just a place to paste their protocol and a graph or data. We strongly encourage you to include your thoughts about why you made the decisions you made when conducting the experiment and to document your thoughts about next steps.
4. Commit to doing it the right way
A common reason to become lax in documentation is feeling rushed for time. Although documentation takes time, it saves time in the long-run and fosters good science. Without good notes, you will waste time trying to recall precisely what you did, reproduce your findings, and remember what you thought would be important next steps. The lab notebook helps you think about your research critically and keep your thoughts together. It can also save you time later when writing up results for publication. Further, well-documented data will help you draft a cogent and rigorous dissertation.
Handle data responsibly
1. keep all data.
Data are the product of research. Data include raw data, processed data, analyzed data, figures, and tables. Many data today are electronic, but not all. Generating data requires a lot of time and resources and researchers must treat data with care. The first essential tip is to keep all data. Do not discard data just because the experiment did not turn out as expected. A lot of experiments do not turn out to yield publishable data, but the results are still important for informing next steps.
Always keep the original, raw data. That is, as you process and analyze data, always maintain an unprocessed version of the original data.
Universities and funding agencies have data retention policies. These policies specify the number of years beyond a grant that data must be kept. Some policies also indicate researchers need to retain original data that served as the basis for a publication for a certain number of years. Therefore, your data will be important well beyond your time in graduate school. Most labs require you to keep samples for reanalysis until a paper is published, then the analyzed data are enough. If you leave a lab before a paper is accepted for publication, you are responsible for ensuring your data and original samples are well documented for others to find and use.
2. Document all data
In addition to keeping all data, data must be well-organized and documented. This means that no matter the way you keep your data (e.g., electronic or in written lab notebooks), there is a clear guide—in your lab notebook, a binder, or on a lab hard drive—to finding the data for a particular experiment. For example, it must be clear which data produced a particular graph. Version control of data is also critical. Your documentation should include “metadata” (data about your data) that tracks versions of the data. For example, as you edit data for a table, you should save separate versions of the tables, name the files sequentially, and note the changes that were made to each version.
3. Backup your data
You should backup electronic data regularly. Ideally, your lab has a shared server or cloud storage to backup data. If you are supposed to put your data there, make sure you do it! When you leave the lab, it must be possible to find your data.
4. Perform data analysis honestly and competently
Inappropriate use of statistics is a major concern in the scientific community, as the results and conclusions will be misleading if done incorrectly ( DeMets, 1999 ). Some practices are clearly an abuse of statistics, while other inappropriate practices stem from lack of knowledge. For example, a practice called “p-hacking” describes when researchers “collect or select data or statistical analyses until nonsignificant results become significant” ( Head, Holman, Lanfear, Kahn, & Jennions, 2015 ). In addition to avoiding such misbehavior, it is essential to be proficient with statistics to ensure you do statistical procedures appropriately. Learning statistical procedures and analyzing data takes many years of practice, and your statistics courses may only cover the basics. You will need to know when to consult others for help. In addition to consulting members in your lab or your PI, your university may have statistical experts who can provide consultations.
5. Master pressure to obtain favored results
When you conduct an experiment, the results are the results. As a beginning researcher, it is important to be prepared to manage the frustration of experiments not turning out as expected. It is also important to manage the real or perceived pressure to produce favored results. Investigators can become wedded to a hypothesis, and they can have a difficult time accepting the results. Sometimes you may feel this pressure coming from yourself; for example, if you want to please your PI, or if you want to get results for a certain publication. It is important to always follow the data no matter where it leads.
If you do feel pressure, this situation can be uncomfortable and stressful. If you have been meticulous and followed the above recommendations, this can be one great safeguard. You will be better able to confidently communicate your results to the PI because of your detailed documentation, and you will be more confident in your procedures if the possibility of error is suggested. Typically, with enough evidence that the unexpected results are real, the PI will concede. We recommend seeking the support of friends or colleagues to vent and cope with stress. In the rare case that the PI does not relent, you could turn to an advisor outside the lab if you need advice about how to proceed. They can help you look at the data objectively and also help you think about the interpersonal aspects of navigating this situation.
6. Communicate about your data in the lab
A critical element of reproducible research is communication in the lab. Ideally, there are weekly or bi-weekly meetings to discuss data. You need to develop your communication skills for writing and speaking about data. Often you and your labmates will discuss experimental issues and results informally during the course of daily work. This is an excellent way to hone critical thinking and communication skills about data.
Scenario 1 – The Protocol is Not Working
At the beginning of a rotation during their first year, a graduate student is handed a lab notebook and a pen and is told to keep track of their work. There does not appear to be a specific format to follow. There are standard lab protocols that everyone follows, but minor tweaks to the protocols do not seem to be tracked from experiment to experiment in the standard lab protocol nor in other lab notebooks. After two weeks of trying to follow one of the standard lab protocols, the student still cannot get the experiment to work. The student has included the appropriate positive and negative controls which are failing, making the experiment uninterpretable. After asking others in the lab for help, the graduate student learns that no one currently in the lab has performed this particular experiment. The former lab member who had performed the experiment only lists the standard protocol in their lab notebook.
How should the graduate student start to solve the problem?
Speaking to the PI would be the next logical step. As a first-year student in a lab rotation, the PI should expect this type of situation and provide additional troubleshooting guidance. It is possible that the PI may want to see how the new graduate student thinks critically and handles adversity in the lab. Rather than giving an answer, the PI might ask the student to work through the problem. The PI should give guidance, but it may not be an immediate fix for the problem. If the PI’s suggestions fail to correct the problem, asking a labmate or the PI for the contact information of the former lab member who most recently performed the experiment would be a reasonable next step. The graduate student’s conversations with the PI and labmates in this situation will help them learn a lot about how the people in the lab interact.
Most of the answers for these types of problems will require you as a graduate student to take the initiative to answer. They will require your effort and ingenuity to talk to other lab members, other labs at the university, and even scour the literature for alternatives. While labs have standard protocols, there are multiple ways to do many experiments, and working out an alternative will teach you more than when everything works. Having to troubleshoot problems will result in better standard protocols in the lab and better science.
HOW TO BE A RESPONSIBLE AUTHOR
Researchers communicate their findings via peer-reviewed publications, and publications are important for advancing in a research career. Many graduate students will first author or co-author publications in graduate school. For good advice on how to write a research manuscript, consult the Current Protocols article “How to write a research manuscript” ( Frank, 2018 ). We focus on the issues of assigning authors and reporting your findings responsibly. First, we describe some important basics: journal impact factors, predatory journals, and peer review.
What are journal impact factors?
It is helpful to understand journal impact factors. There is criticism about an overemphasis on impact factors for evaluating the quality or importance of researchers’ work ( DePellegrin & Johnston, 2015 ), but they remain common for this purpose. Journal impact factors reflect the average number of times articles in a journal were cited in the last two years. Higher impact factors place journals at a higher rank. Approximately 2% of journals have an impact factor of 10 or higher. For example, Cell, Science, and Nature have impact factors of approximately 39, 42, and 43, respectively. Journals can be great journals but have lower impact factors; often this is because they focus on a smaller specialty field. For example, Journal of Immunology and Oncogene are respected journals, but their impact factors are about 4 and 7, respectively.
Research trainees often want to publish in journals with the highest possible impact factor because they expect this to be viewed favorably when applying to future positions. We encourage you to bear in mind that many different journals publish excellent science and focus on publishing where your work will reach the desired audience. Also, keep in mind that while a high impact factor can direct you to respectable, high-impact science, it does not guarantee that the science in the paper is good or even correct. You must critically evaluate all papers you read no matter the impact factor.
What are predatory journals?
Predatory journals have flourished over the past few years as publishing science has moved online. An international panel defined predatory journals as follows ( Grudniewicz et al., 2019 ):
Predatory journals and publishers are entities that prioritize self-interest at the expense of scholarship and are characterized by false or misleading information, deviation from best editorial and publication practices, a lack of transparency, and/or the use of aggressive and indiscriminate solicitation practices. (p. 211)
Often young researchers receive emails soliciting them to submit their work to a journal. There are typically small fees (around $99 US) requested but these fees will be much lower than open access fees of reputable journals (often around $2000 US). A warning sign of a predatory journal is outlandish promises, such as 24-hour peer review or immediate publication. You can find a list of predatory journals created by a postdoc in Europe at BeallsList.net ( “Beall’s List of Potential Predatory Journals and Publishers,” 2020 ).
What is peer review?
Peer reviewers are other scientists who have the expertise to evaluate a manuscript. Typically 2 or 3 reviewers evaluate a manuscript. First, an editor performs an initial screen of the manuscript to ensure its appropriateness for the journal and that it meets basic quality standards. At this stage, an editor can decide to reject the manuscript and not send it to review. Not sending a paper for peer review is common in the highest impact journals that receive more submissions per year than can be reviewed and published. For average-impact journals and specialty journals, typically your paper will be sent for peer review.
In general, peer review focuses on three aspects of a manuscript: research design and methods, validity of the data and conclusions, and significance. Peer reviewers assess the merit and rigor of the research design and methodology, and they evaluate the overall validity of the results, interpretations, and conclusions. Essentially, reviewers want to ensure that the data support the claims. Additionally, reviewers evaluate the overall significance, or contribution, of the findings, which involves the novelty of the research and the likelihood that the findings will advance the field. Significance standards vary between journals. Some journals are open to publishing findings that are incremental advancements in a field, while others want to publish only what they deem as major advancements. This feature can distinguish the highest impact journals which seek the most significant advancements and other journals that tend to consider a broader range of work as long as it is scientifically sound. It is important to keep in mind that determining at the stage of review and publication whether a paper is “high impact” is quite subjective. In reality, this can only really be determined in retrospect.
The key ethical issues in peer review are fairness, objectivity, and confidentiality ( Shamoo & Resnik, 2015 ). Peer reviewers are to evaluate the manuscript on its merits and not based on biases related to the authors or the science itself. If reviewers have a conflict of interest, this should be disclosed to the editor. Confidentiality of peer review means that the reviewers should keep private the information; they should not share the information with others or use it to their benefit. Reviewers can ultimately recommend that the manuscript is rejected, revised, and resubmitted (major or minor revisions), or accepted. The editor evaluates the reviewers’ feedback and makes a judgment about rejecting, accepting, or requesting a revision. Sometimes PIs will ask experienced graduate students to assist with peer reviewing a manuscript. This is a good learning opportunity. The PI should disclose to the editor that they included a trainee in preparing the review.
Assign authorship fairly
Authorship gives credit to the people who contributed to the research. This includes thinking of the ideas, designing and performing experiments, interpreting the results, and writing the paper. Two key questions regarding authorship include: 1 - Who will be an author? 2 - What will be the order in which authors are listed? These seem simple on the surface but can get quite complex.
1. Know authorship guidelines
Authorship guidelines published by journals, professional societies, and universities communicate key principles of authorship and standards for earning authorship. The core ethical principle of assigning authorship is fairness in who receives credit for the work. The people who contributed to the work should get credit for it. This seems simply enough, but determining authorship can (and often does) create conflict.
Many universities have authorship guidelines, and you should know the policies at your university. The International Committee of Medical Journal Editors (ICMJE) provides four criteria for determining who should be an author ( International Committee of Medical Journal Editors, 2020 ). These criteria indicate that an author should do all of the following: 1) make “substantial contributions” to the development of the idea or research design, or to acquiring, analyzing, or interpreting the data, 2) write the manuscript or revise it a substantive way, 3) give approval of the final manuscript (i.e., before it is submitted for review, and after it is revised, if necessary), and 4) agree to be responsible for any questions about the accuracy or integrity of the research.
Several types of authorship violate these guidelines and should be avoided. Guest authorship is when respected researchers are added out of appreciation, or to have the manuscript be perceived more favorably to get it published or increase its impact. Gift authorship is giving authorship to reward an individual, or as a favor. Ghost authorship is when someone made significant contributions to the paper but is not listed as an author. To increase transparency, some journals require authors to indicate how each individual contributed to the research and manuscript.
2. Apply the guidelines
Conflicts often arise from disagreements about how much people contributed to the research and whether those contributions merit authorship. The best approach is an open, honest, and ongoing discussion about authorship, which we discuss in #3 below. To have effective, informed conversations about authorship, you must understand how to apply the guidelines to your specific situation. The following is a simple rule of thumb that indicates there are three components of authorship. We do not list giving final approval of the manuscript and agreeing to be accountable, but we do consider these essentials of authorship.
- Thinking – this means contributing to the ideas leading to the hypothesis of the work, designing experiments to address the hypothesis, and/or analyzing the results in the larger context of the literature in the field.
- Doing – this means performing and analyzing the experiments.
- Writing – this means editing a draft, or writing the entire paper. The first author often writes the entire first draft.
In our experience, a first author would typically do all three. They also usually coordinate the writing and editing process. Co-authors are typically very involved in at least two of the three, and are somewhat involved in the other. The PI, who oversees and contributes to all three, is often the last, or “senior author.” The “senior author” is typically the “corresponding author”—the person listed as the individual to contact about the paper. The other co-authors are listed between the first and senior author either alphabetically, or more commonly, in order from the largest to smallest contribution.
Problems in assigning authorship typically arise due to people’s interpretations of #1 (thinking) and #2 (doing)—what and how much each individual contributed to a project’s design, execution, and analysis. Different fields or PIs may have their own slight variations on these guidelines. The potential conflicts associated with assigning authorship lead to the most common recommendation for responsibly assigning authorship: discuss authorship expectations early and revisit them during the project.
3. Discuss authorship with your collaborators
Publications are important for career advancement, so you can see why people might be worried about fairness in assigning authorship. If the problem arises from a lack of a shared understanding about contributions to the research, the only way to clarify this is an open discussion. This discussion should ideally take place very early at the beginning of a project, and should be ongoing. Hopefully you work in a laboratory that makes these discussions a natural part of the research process; this makes it much easier to understand the expectations upfront.
We encourage you to speak up about your interest in making a contribution that would merit authorship, especially if you want to earn first authorship. Sometimes norms about authoring papers in a lab make it clear you are expected to first and co-author publications, but it is best to communicate your interest in earning authorship. If the project is not yours, but you wish to collaborate, you can inquire what you may be able to contribute that would merit authorship.
If it is not a norm in your lab to discuss authorship throughout the life of projects, then as a graduate student you may feel reluctant to speak up. You could initiate a conversation with a more senior graduate student, a postdoc, or your PI, depending on the dynamics in the group. You could ask generally about how the lab approaches assignment of authorship, but discussing a specific project and paper may be best. It may feel awkward to ask, but asking early is less uncomfortable than waiting until the end of the project. If the group is already drafting a manuscript and you are told that your contribution is insufficient for authorship, this situation is much more discouraging than if you had asked earlier about what is expected to earn authorship.

How to report findings responsibly
The most significant responsibility of authors is to present their research accurately and honestly. Deliberately presenting misleading information is clearly unethical, but there are significant judgment calls about how to present your research findings. For example, an author can mislead by overstating the conclusions given what the data support.
1. Commit to presenting your findings honestly
Any good scientific manuscript writer will tell you that you need to “tell a good story.” This means that your paper is organized and framed to draw the reader into the research and convince them of the importance of the findings. But, this story must be sound and justified by the data. Other authors are presenting their findings in the best, most “publishable” light, so it is a balancing act to be persuasive but also responsible in presenting your findings in a trustworthy manner. To present your findings honestly, you must be conscious of how you interpret your data and present your conclusions so that they are accurate and not overstated.
One misbehavior known as “HARKing,” Hypothesis After the Results are Known, occurs when hypotheses are created after seeing the results of an experiment, but they are presented as if they were defined prior to collecting the data ( Munafò et al., 2017 ). This practice should be avoided. HARKing may be driven, in part, by a concern in scientific publishing known as publication bias. This bias is a preference that reviewers, editors, and researchers have for papers describing positive findings instead of negative findings ( Carroll, Toumpakari, Johnson, & Betts, 2017 ). This preference can lead to manipulating one’s practices, such as by HARKing, so that positive findings can be reported.
It is important to note that in addition to avoiding misbehaviors such as HARKing, all researchers are susceptible to a number of more subtle traps in judgment. Even the most well-intentioned researcher may jump to conclusions, discount alternative explanations, or accept results that seem correct without further scrutiny ( Nuzzo, 2015 ). Therefore, researchers must not only commit to presenting their findings honestly but consider how they can counteract such traps by slowing down and increasing their skepticism towards their findings.
2. Provide an appropriate amount of detail
Providing enough detail in a manuscript can be a challenge with the word limits imposed by most journals. Therefore, you will need to determine what details to include and which to exclude, or potentially include in the supplemental materials. Methods sections can be long and are often the first to be shortened, but complete methods are important for others to evaluate the research and to repeat the methods in other studies. Even more significant is making decisions about what experimental data to include and potentially exclude from the manuscript. Researchers must determine what data is required to create a complete scientific story that supports the central hypothesis of the paper. On the other hand, it is not necessary or helpful to include so much data in the manuscript, or in supplemental material, that the central point of the paper is difficult to discern. It is a tricky balance.
3. Follow proper citation practices
Of course, responsible authorship requires avoiding plagiarism. Many researchers think that plagiarism is not a concern for them because they assume it is always done intentionally by “copying and pasting” someone else’s words and claiming them as your own. Sometimes poor writing practices, such as taking notes from references without distinguishing between direct quotes and paraphrased material, can lead to including material that is not quoted properly. More broadly, proper citation practices include accurately and completely referencing prior studies to provide appropriate context for your manuscript.
4. Attend to the other important details
The journal will require several pieces of additional information, such as disclosure of sources of funding and potential conflicts of interest. Typically, graduate students do not have relationships that constitute conflicts of interest, but a PI who is a co-author may. In submitting a manuscript, also make sure to acknowledge individuals not listed as authors but who contributed to the work.
5. Share data and promote transparency
Data sharing is a key facet of promoting transparency in science ( Nosek et al., 2015 ). It will be important to know the expectations of the journals in which you wish to publish. Many top journals now require data sharing; for example, sharing your data files in an online repository so others have access to the data for secondary use. Funding agencies like NIH also increasingly require data sharing. To further foster transparency and public trust in research, researchers must deposit their final peer-reviewed manuscripts that report on research funded by NIH to PubMed Central. PubMed makes biomedical and life science research publicly accessible in a free, online database.
Scenario 2 – Authors In Conflict
To prepare a manuscript for publication, a postdoc’s data is added to a graduate student’s thesis project. After working together to combine the data and write the paper, the postdoc requests co-first authorship on the paper. The graduate student balks at this request on the basis that it is their thesis project. In a weekly meeting with the lab’s PI to discuss the status of the paper, the graduate student states that they should divide the data between the authors as a way to prove that the graduate student should be the sole first author. The PI agrees to this attempt to quantify how much data each person contributed to the manuscript. All parties agree the writing and thinking were equally shared between them. After this assessment, the graduate student sees that the postdoc actually contributed more than half of the data presented in the paper. The graduate student and a second graduate student contributed the remaining data; this means the graduate student contributed much less than half of the data in the paper. However, the graduate student is still adamant that they must be the sole first author of the paper because it is their thesis project.
Is the graduate student correct in insisting that it is their project, so they are entitled to be the sole first author?
Co-first authorship became popular about 10 years ago as a way to acknowledge shared contributions to a paper in which authors worked together and contributed equally. If the postdoc contributed half of the data and worked with the graduate student to combine their interpretations and write the first draft of the paper, then the postdoc did make a substantial contribution. If the graduate student wrote much of the first draft of the paper, contributed significantly to the second half of data, and played a major role in the thesis concept and design, this is also a major contribution. We summarized authorship requirements as contributing to thinking, doing, and writing, and we noted that a first author usually contributes to all of these. The graduate student has met all 3 elements to claim first authorship. However, it appears that the postdoc has also met these 3 requirements. Thus, it is at least reasonable for the postdoc to ask about co-first authorship.
The best way to move forward is to discuss their perspectives openly. Both the graduate student and postdoc want first authorship on papers to advance their careers. The postdoc feels they contributed more to the overall concept and design than the graduate student is recognizing, and the postdoc did contribute half of the data. This is likely frustrating and upsetting for the postdoc. On the other hand, perhaps the postdoc is forgetting how much a thesis becomes like “your baby,” so to speak. The work is the graduate student’s thesis, so it is easy to see why the graduate student would feel a sense of ownership of it. Given this fact, it may be hard for the graduate student to accept the idea that they would share first-author recognition for the work. Yet, the graduate student should consider that the manuscript would not be possible without the postdoc’s contribution. Further, if the postdoc was truly being unreasonable, then the postdoc could make the case for sole first authorship based on contributing the most data to the paper, in addition to contributing ideas and writing the paper. The graduate student should consider that the postdoc may be suggesting co-first authorship in good faith.
As with any interpersonal conflict, clear communication is key. While it might be temporarily uncomfortable to voice their views and address this disagreement, it is critical to avoiding permanent damage to their working relationship. The pair should consider each other’s perspectives and potential alternatives. For example, if the graduate student is first author and the postdoc second, at a minimum they could include an author note in the manuscript that describes the contribution of each author. This would make it clear the scope of the postdoc’s contribution, if they decided not to go with co-first authorship. Also, the graduate student should consider their assumptions about co-first authorship. Maybe they assume it makes it appear they contributed less, but instead, perhaps co-first authorship highlights their collaborative approach to science. Collaboration is a desirable quality many (although arguably not all) research organizations look for when they are hiring.
They will also need to speak with others for advice. The pair should definitely speak with the PI who could provide input about how these cases have been handled in the past. Ultimately, if they cannot reach an agreement, the PI, who is likely to be the last or “senior” author, may make the final decision. They should also speak to the other graduate student who is an author.
If either individual is upset with the situation, they will want to discuss it when they have had time to cool down. This might mean taking a day before discussing, or speaking with someone outside of the lab for support. Ideally, all authors on this paper would have initiated this conversation earlier, and the standards in the lab for first authorship would be discussed routinely. Clear communication may have avoided the conflict.
HOW TO USE DECISION-MAKING STRATEGIES TO NAVIGATE CHALLENGES
We have provided advice on some specific challenges you might encounter in research. This final section covers our overarching recommendation that you adopt a set of ethical decision-making strategies. These strategies help researchers address challenges by helping them think through a problem and possible alternatives ( McIntosh et al., 2020 ). The strategies encourage you to gather information, examine possible outcomes, consider your assumptions, and address emotional reactions before acting. They are especially helpful when you are uncertain how to proceed, face a new problem, or when the consequences of a decision could negatively impact you or others. The strategies also help people be honest with themselves, such as when they are discounting important factors or have competing goals, by encouraging them to identify outside perspectives and test their motivations. You can remember the strategies using the acronym SMART .
1. S eek Help
Obtain input from others who can be objective and that you trust. They can assist you with assessing the situation, predicting possible outcomes, and identifying potential options. They can also provide you with support. Individuals to consult may be peers, other faculty, or people in your personal life. It is important that you trust the people you talk with, but it is also good when they challenge your perspective, or encourage you to think in a new way about a problem. Keep in mind that people such as program directors and university ombudsmen are often available for confidential, objective advice.
2. M anage Emotions
Consider your emotional reaction to the situation and how it might influence your assessment of the situation, and your potential decisions and actions. In particular, identify negative emotions, like frustration, anxiety, fear, and anger, as they particularly tend to diminish decision-making and the quality of interactions with others. Take time to address these emotions before acting, for example, by exercising, listening to music, or simply taking a day before responding.
3. A nticipate Consequences
Think about how the situation could turn out. This includes for you, for the research team, and anyone else involved. Consider the short, middle-term, and longer-term impacts of the problem and your potential approach to addressing the situation. Ideally, it is possible to identify win-win outcomes. Often, however, in tough professional situations, you may need to select the best option from among several that are not ideal.
4. R ecognize Rules and Context
Determine if any ethical principles, professional policies, or rules apply that might help guide your choices. For instance, if the problem involves an authorship dispute, consider the authorship guidelines that apply. Recognizing the context means considering the situational factors that could impact your options and how you proceed. For example, factors such as the reality that ultimately the PI may have the final decision about authorship.
5. T est Assumptions and Motives
Examine your beliefs about the situation and whether any of your thoughts may not be justified. This includes critically examining the personal motivations and goals that are driving your interpretation of the problem and thoughts about how to resolve it.
These strategies do not have to be engaged in order, and they are interrelated. For example, seeking help can help you manage emotions, test assumptions, and anticipate consequences. Go back to the scenarios and our advice throughout this article, and you will see many of our suggestions align with these strategies. Practice applying SMART strategies when you encounter a problem and they will become more natural.
Learning practices for responsible research will be the foundation for your success in graduate school and your career. We encourage you to be reflective and intentional as you learn and hope that our advice helps you along the way.
ACKNOWLEDGEMENTS
This work was supported by the National Human Genome Research Institute (Antes, K01HG008990) and the National Center for Advancing Translational Sciences (UL1 TR002345).
LITERATURE CITED
- Anderson MS, Horn AS, Risbey KR, Ronning EA, De Vries R, & Martinson BC (2007). What Do Mentoring and Training in the Responsible Conduct of Research Have To Do with Scientists’ Misbehavior? Findings from a National Survey of NIH-Funded Scientists . Academic Medicine , 82 ( 9 ), 853–860. doi: 10.1097/ACM.0b013e31812f764c [ PubMed ] [ CrossRef ] [ Google Scholar ]
- Antes AL, Brown RP, Murphy ST, Waples EP, Mumford MD, Connelly S, & Devenport LD (2007). Personality and Ethical Decision-Making in Research: The Role of Perceptions of Self and Others . Journal of Empirical Research on Human Research Ethics , 2 ( 4 ), 15–34. doi: 10.1525/jer.2007.2.4.15 [ PubMed ] [ CrossRef ] [ Google Scholar ]
- Antes AL, English T, Baldwin KA, & DuBois JM (2018). The Role of Culture and Acculturation in Researchers’ Perceptions of Rules in Science . Science and Engineering Ethics , 24 ( 2 ), 361–391. doi: 10.1007/s11948-017-9876-4 [ PMC free article ] [ PubMed ] [ CrossRef ] [ Google Scholar ]
- Antes AL, Kuykendall A, & DuBois JM (2019a). The Lab Management Practices of “Research Exemplars” that Foster Research Rigor and Regulatory Compliance: A Qualitative Study of Successful Principal Investigators . PloS One , 14 ( 4 ), e0214595. doi: 10.1371/journal.pone.0214595 [ PMC free article ] [ PubMed ] [ CrossRef ] [ Google Scholar ]
- Antes AL, Kuykendall A, & DuBois JM (2019b). Leading for Research Excellence and Integrity: A Qualitative Investigation of the Relationship-Building Practices of Exemplary Principal Investigators . Accountability in Research , 26 ( 3 ), 198–226. doi: 10.1080/08989621.2019.1611429 [ PMC free article ] [ PubMed ] [ CrossRef ] [ Google Scholar ]
- Antes AL, & Maggi LB Jr. (2021). How to Navigate Trainee-Mentor Relationships and Interpersonal Dynamics in the Lab . Current Protocols Essential Laboratory Techniques. [ PMC free article ] [ PubMed ] [ Google Scholar ]
- Asplund M, & Welle CG (2018). Advancing Science: How Bias Holds Us Back . Neuron , 99 ( 4 ), 635–639. doi: 10.1016/j.neuron.2018.07.045 [ PubMed ] [ CrossRef ] [ Google Scholar ]
- Baker M (2016). Is There a Reproducibility Crisis? Nature , 533 , 452–454. doi: 10.1038/533452a [ PubMed ] [ CrossRef ] [ Google Scholar ]
- Barba LA (2016). The Hard Road to Reproducibility . Science , 354 ( 6308 ), 142. doi: 10.1126/science.354.6308.142 [ PubMed ] [ CrossRef ] [ Google Scholar ]
- Beall’s List of Potential Predatory Journals and Publishers . (2020). Retrieved from https://beallslist.net/#update [ Google Scholar ]
- Carroll HA, Toumpakari Z, Johnson L, & Betts JA (2017). The Perceived Feasibility of Methods to Reduce Publication Bias . PloS One , 12 ( 10 ), e0186472–e0186472. doi: 10.1371/journal.pone.0186472 [ PMC free article ] [ PubMed ] [ CrossRef ] [ Google Scholar ]
- Chappell B (2019). Duke Whistleblower Gets More Than $33 Million in Research Fraud Settlement . NPR. Retrieved from https://www.npr.org/2019/03/25/706604033/duke-whistleblower-gets-more-than-33-million-in-research-fraud-settlement [ Google Scholar ]
- Davis MS, Riske-Morris M, & Diaz SR (2007). Causal Factors Implicated in Research Misconduct: Evidence from ORI Case Files . Science and Engineering Ethics , 13 ( 4 ), 395–414. doi: 10.1007/s11948-007-9045-2 [ PubMed ] [ CrossRef ] [ Google Scholar ]
- DeMets DL (1999). Statistics and Ethics in Medical Research . Science and Engineering Ethics , 5 ( 1 ), 97–117. doi: 10.1007/s11948-999-0059-9 [ PubMed ] [ CrossRef ] [ Google Scholar ]
- Department of Health and Human Services. (2005). 42 CFR Parts 50 and 93 Public Health Service Policies on Research Misconduct; Final Rule. Retrieved from https://ori.hhs.gov/sites/default/files/42_cfr_parts_50_and_93_2005.pdf [ Google Scholar ]
- DePellegrin TA, & Johnston M (2015). An Arbitrary Line in the Sand: Rising Scientists Confront the Impact Factor . Genetics , 201 ( 3 ), 811–813. [ PMC free article ] [ PubMed ] [ Google Scholar ]
- DuBois JM, Anderson EE, Chibnall J, Carroll K, Gibb T, Ogbuka C, & Rubbelke T (2013). Understanding Research Misconduct: A Comparative Analysis of 120 Cases of Professional Wrongdoing . Account Res , 20 ( 5–6 ), 320–338. doi: 10.1080/08989621.2013.822248 [ PMC free article ] [ PubMed ] [ CrossRef ] [ Google Scholar ]
- DuBois JM, & Antes AL (2018). Five Dimensions of Research Ethics: A Stakeholder Framework for Creating a Climate of Research Integrity . Academic Medicine , 93 ( 4 ), 550–555. doi: 10.1097/ACM.0000000000001966 [ PMC free article ] [ PubMed ] [ CrossRef ] [ Google Scholar ]
- Else H (2018). Does Science have a Bullying Problem? Nature , 563 , 616–618. doi: 10.1038/d41586-018-07532-5 [ PubMed ] [ CrossRef ] [ Google Scholar ]
- Emanuel EJ, Wendler D, & Grady C (2000). What Makes Clinical Research Ethical ? Journal of the American Medical Association , 283 ( 20 ), 2701–2711. doi:jsc90374 [pii] [ PubMed ] [ Google Scholar ]
- Evans TM, Bira L, Gastelum JB, Weiss LT, & Vanderford NL (2018). Evidence for a Mental Health Crisis in Graduate Education . Nature Biotechnology , 36 ( 3 ), 282–284. doi: 10.1038/nbt.4089 [ PubMed ] [ CrossRef ] [ Google Scholar ]
- Frank DJ (2018). How to Write a Research Manuscript . Current Protocols Essential Laboratory Techniques , 16 ( 1 ), e20. doi: 10.1002/cpet.20 [ CrossRef ] [ Google Scholar ]
- Goodman SN, Fanelli D, & Ioannidis JPA (2016). What Does Research Reproducibility Mean? Science Translational Medicine , 8 ( 341 ), 341ps312. doi: 10.1126/scitranslmed.aaf5027 [ PubMed ] [ CrossRef ] [ Google Scholar ]
- Grudniewicz A, Moher D, Cobey KD, Bryson GL, Cukier S, Allen K, … Lalu MM (2019). Predatory journals: no definition, no defence . Nature , 576 ( 7786 ), 210–212. doi: 10.1038/d41586-019-03759-y [ PubMed ] [ CrossRef ] [ Google Scholar ]
- Head ML, Holman L, Lanfear R, Kahn AT, & Jennions MD (2015). The Extent and Consequences of P-Hacking in Science . PLoS Biology , 13 ( 3 ), e1002106. doi: 10.1371/journal.pbio.1002106 [ PMC free article ] [ PubMed ] [ CrossRef ] [ Google Scholar ]
- Hofstra B, Kulkarni VV, Munoz-Najar Galvez S, He B, Jurafsky D, & McFarland DA (2020). The Diversity–Innovation Paradox in Science . Proceedings of the National Academy of Sciences , 117 ( 17 ), 9284. doi: 10.1073/pnas.1915378117 [ PMC free article ] [ PubMed ] [ CrossRef ] [ Google Scholar ]
- International Committee of Medical Journal Editors. (2020). Defining the Role of Authors and Contributors . Retrieved from http://www.icmje.org/recommendations/browse/roles-and-responsibilities/defining-the-role-of-authors-and-contributors.html
- Keith-Spiegel P, Sieber J, & Koocher GP (2010). Responding to Research Wrongdoing: A User-Friendly Guide . Retrieved from http://users.neo.registeredsite.com/1/4/0/20883041/assets/RRW_11-10.pdf
- McIntosh T, Antes AL, & DuBois JM (2020). Navigating Complex, Ethical Problems in Professional Life: A Guide to Teaching SMART Strategies for Decision-Making . Journal of Academic Ethics . doi: 10.1007/s10805-020-09369-y [ PMC free article ] [ PubMed ] [ CrossRef ] [ Google Scholar ]
- Meyers LC, Brown AM, Moneta-Koehler L, & Chalkley R (2018). Survey of Checkpoints along the Pathway to Diverse Biomedical Research Faculty . PloS One , 13 ( 1 ), e0190606–e0190606. doi: 10.1371/journal.pone.0190606 [ PMC free article ] [ PubMed ] [ CrossRef ] [ Google Scholar ]
- Munafò MR, Nosek BA, Bishop DVM, Button KS, Chambers CD, Percie du Sert N, … Ioannidis JPA (2017). A manifesto for reproducible science . Nature Human Behaviour , 1 ( 1 ), 0021. doi: 10.1038/s41562-016-0021 [ PMC free article ] [ PubMed ] [ CrossRef ] [ Google Scholar ]
- National Academies of Science. (2009). On Being a Scientist: A Guide to Responsible Conduct in Research . Washington DC: National Academics Press. [ PubMed ] [ Google Scholar ]
- National Academies of Sciences Engineering and Medicine. (2017). Fostering Integrity in Research . Washington, DC: The National Academies Press [ PubMed ] [ Google Scholar ]
- National Academies of Sciences Engineering and Medicine. (2018a). An American Crisis: The Growing Absence of Black Men in Medicine and Science: Proceedings of a Joint Workshop . Washington, DC: The National Academies Press. [ PubMed ] [ Google Scholar ]
- National Academies of Sciences Engineering and Medicine. (2018b). Sexual harassment of women: climate, culture, and consequences in academic sciences, engineering, and medicine : National Academies Press. [ PubMed ] [ Google Scholar ]
- National Institutes of Health. (2009). Update on the Requirement for Instruction in the Responsible Conduct of Research . NOT-OD-10-019 . Retrieved from https://grants.nih.gov/grants/guide/notice-files/NOT-OD-10-019.html
- National Science Foundation. (2017). Important Notice No. 140 Training in Responsible Conduct of Research – A Reminder of the NSF Requirement . Retrieved from https://www.nsf.gov/pubs/issuances/in140.jsp
- No Place for Bullies in Science. (2018). Nature , 559 ( 7713 ), 151. doi: 10.1038/d41586-018-05683-z [ PubMed ] [ CrossRef ] [ Google Scholar ]
- Norris D, Dirnagl U, Zigmond MJ, Thompson-Peer K, & Chow TT (2018). Health Tips for Research Groups . Nature , 557 , 302–304. doi: 10.1038/d41586-018-05146-5 [ PubMed ] [ CrossRef ] [ Google Scholar ]
- Nosek BA, Alter G, Banks GC, Borsboom D, Bowman SD, Breckler SJ, … Yarkoni T (2015). Scientific Standards . Promoting an Open Research Culture. Science , 348 ( 6242 ), 1422–1425. doi: 10.1126/science.aab2374 [ PMC free article ] [ PubMed ] [ CrossRef ] [ Google Scholar ]
- Nuzzo R (2015). How Scientists Fool Themselves - and How They Can Stop . Nature , 526 , 182–185. [ PubMed ] [ Google Scholar ]
- O’Connor A (2018). More Evidence that Nutrition Studies Don’t Always Add Up . The New York Times. Retrieved from https://www.nytimes.com/2018/09/29/sunday-review/cornell-food-scientist-wansink-misconduct.html [ Google Scholar ]
- Park A (2012). Great Science Frauds . Time. Retrieved from https://healthland.time.com/2012/01/13/great-science-frauds/slide/the-baltimore-case/ [ Google Scholar ]
- Plemmons DK, Baranski EN, Harp K, Lo DD, Soderberg CK, Errington TM, … Esterling KM (2020). A Randomized Trial of a Lab-embedded Discourse Intervention to Improve Research Ethics . Proceedings of the National Academy of Sciences , 117 ( 3 ), 1389. doi: 10.1073/pnas.1917848117 [ PMC free article ] [ PubMed ] [ CrossRef ] [ Google Scholar ]
- Research Institutions Must Put the Health of Labs First. (2018). Nature , 557 ( 7705 ), 279–280. doi: 10.1038/d41586-018-05159-0 [ PubMed ] [ CrossRef ] [ Google Scholar ]
- Research Integrity is Much More Than Misconduct . (2019). ( 570 ). doi: 10.1038/d41586-019-01727-0 [ PubMed ] [ CrossRef ] [ Google Scholar ]
- Resnik DB (2011). Scientific Research and the Public Trust . Science and Engineering Ethics , 17 ( 3 ), 399–409. doi: 10.1007/s11948-010-9210-x [ PMC free article ] [ PubMed ] [ CrossRef ] [ Google Scholar ]
- Roper RL (2019). Does Gender Bias Still Affect Women in Science? Microbiology and Molecular Biology Reviews , 83 ( 3 ), e00018–00019. doi: 10.1128/MMBR.00018-19 [ PMC free article ] [ PubMed ] [ CrossRef ] [ Google Scholar ]
- Shamoo AE, & Resnik DB (2015). Responsible Conduct of Research (3rd ed.). New York: Oxford University Press. [ Google Scholar ]
- Steneck NH (2007). ORI Introduction to the Responsible Conduct of Research (Updated ed.). Washington, D.C.: U.S. Government Printing Office. [ Google Scholar ]
- Winchester C (2018). Give Every Paper a Read for Reproducibility . Nature , 557 , 281. doi: 10.1038/d41586-018-05140-x [ PubMed ] [ CrossRef ] [ Google Scholar ]
- Privacy Policy

Home » Research Summary – Structure, Examples and Writing Guide
Research Summary – Structure, Examples and Writing Guide
Table of Contents

Research Summary
Definition:
A research summary is a brief and concise overview of a research project or study that highlights its key findings, main points, and conclusions. It typically includes a description of the research problem, the research methods used, the results obtained, and the implications or significance of the findings. It is often used as a tool to quickly communicate the main findings of a study to other researchers, stakeholders, or decision-makers.
Structure of Research Summary
The Structure of a Research Summary typically include:
- Introduction : This section provides a brief background of the research problem or question, explains the purpose of the study, and outlines the research objectives.
- Methodology : This section explains the research design, methods, and procedures used to conduct the study. It describes the sample size, data collection methods, and data analysis techniques.
- Results : This section presents the main findings of the study, including statistical analysis if applicable. It may include tables, charts, or graphs to visually represent the data.
- Discussion : This section interprets the results and explains their implications. It discusses the significance of the findings, compares them to previous research, and identifies any limitations or future directions for research.
- Conclusion : This section summarizes the main points of the research and provides a conclusion based on the findings. It may also suggest implications for future research or practical applications of the results.
- References : This section lists the sources cited in the research summary, following the appropriate citation style.
How to Write Research Summary
Here are the steps you can follow to write a research summary:
- Read the research article or study thoroughly: To write a summary, you must understand the research article or study you are summarizing. Therefore, read the article or study carefully to understand its purpose, research design, methodology, results, and conclusions.
- Identify the main points : Once you have read the research article or study, identify the main points, key findings, and research question. You can highlight or take notes of the essential points and findings to use as a reference when writing your summary.
- Write the introduction: Start your summary by introducing the research problem, research question, and purpose of the study. Briefly explain why the research is important and its significance.
- Summarize the methodology : In this section, summarize the research design, methods, and procedures used to conduct the study. Explain the sample size, data collection methods, and data analysis techniques.
- Present the results: Summarize the main findings of the study. Use tables, charts, or graphs to visually represent the data if necessary.
- Interpret the results: In this section, interpret the results and explain their implications. Discuss the significance of the findings, compare them to previous research, and identify any limitations or future directions for research.
- Conclude the summary : Summarize the main points of the research and provide a conclusion based on the findings. Suggest implications for future research or practical applications of the results.
- Revise and edit : Once you have written the summary, revise and edit it to ensure that it is clear, concise, and free of errors. Make sure that your summary accurately represents the research article or study.
- Add references: Include a list of references cited in the research summary, following the appropriate citation style.
Example of Research Summary
Here is an example of a research summary:
Title: The Effects of Yoga on Mental Health: A Meta-Analysis
Introduction: This meta-analysis examines the effects of yoga on mental health. The study aimed to investigate whether yoga practice can improve mental health outcomes such as anxiety, depression, stress, and quality of life.
Methodology : The study analyzed data from 14 randomized controlled trials that investigated the effects of yoga on mental health outcomes. The sample included a total of 862 participants. The yoga interventions varied in length and frequency, ranging from four to twelve weeks, with sessions lasting from 45 to 90 minutes.
Results : The meta-analysis found that yoga practice significantly improved mental health outcomes. Participants who practiced yoga showed a significant reduction in anxiety and depression symptoms, as well as stress levels. Quality of life also improved in those who practiced yoga.
Discussion : The findings of this study suggest that yoga can be an effective intervention for improving mental health outcomes. The study supports the growing body of evidence that suggests that yoga can have a positive impact on mental health. Limitations of the study include the variability of the yoga interventions, which may affect the generalizability of the findings.
Conclusion : Overall, the findings of this meta-analysis support the use of yoga as an effective intervention for improving mental health outcomes. Further research is needed to determine the optimal length and frequency of yoga interventions for different populations.
References :
- Cramer, H., Lauche, R., Langhorst, J., Dobos, G., & Berger, B. (2013). Yoga for depression: a systematic review and meta-analysis. Depression and anxiety, 30(11), 1068-1083.
- Khalsa, S. B. (2004). Yoga as a therapeutic intervention: a bibliometric analysis of published research studies. Indian journal of physiology and pharmacology, 48(3), 269-285.
- Ross, A., & Thomas, S. (2010). The health benefits of yoga and exercise: a review of comparison studies. The Journal of Alternative and Complementary Medicine, 16(1), 3-12.
Purpose of Research Summary
The purpose of a research summary is to provide a brief overview of a research project or study, including its main points, findings, and conclusions. The summary allows readers to quickly understand the essential aspects of the research without having to read the entire article or study.
Research summaries serve several purposes, including:
- Facilitating comprehension: A research summary allows readers to quickly understand the main points and findings of a research project or study without having to read the entire article or study. This makes it easier for readers to comprehend the research and its significance.
- Communicating research findings: Research summaries are often used to communicate research findings to a wider audience, such as policymakers, practitioners, or the general public. The summary presents the essential aspects of the research in a clear and concise manner, making it easier for non-experts to understand.
- Supporting decision-making: Research summaries can be used to support decision-making processes by providing a summary of the research evidence on a particular topic. This information can be used by policymakers or practitioners to make informed decisions about interventions, programs, or policies.
- Saving time: Research summaries save time for researchers, practitioners, policymakers, and other stakeholders who need to review multiple research studies. Rather than having to read the entire article or study, they can quickly review the summary to determine whether the research is relevant to their needs.
Characteristics of Research Summary
The following are some of the key characteristics of a research summary:
- Concise : A research summary should be brief and to the point, providing a clear and concise overview of the main points of the research.
- Objective : A research summary should be written in an objective tone, presenting the research findings without bias or personal opinion.
- Comprehensive : A research summary should cover all the essential aspects of the research, including the research question, methodology, results, and conclusions.
- Accurate : A research summary should accurately reflect the key findings and conclusions of the research.
- Clear and well-organized: A research summary should be easy to read and understand, with a clear structure and logical flow.
- Relevant : A research summary should focus on the most important and relevant aspects of the research, highlighting the key findings and their implications.
- Audience-specific: A research summary should be tailored to the intended audience, using language and terminology that is appropriate and accessible to the reader.
- Citations : A research summary should include citations to the original research articles or studies, allowing readers to access the full text of the research if desired.
When to write Research Summary
Here are some situations when it may be appropriate to write a research summary:
- Proposal stage: A research summary can be included in a research proposal to provide a brief overview of the research aims, objectives, methodology, and expected outcomes.
- Conference presentation: A research summary can be prepared for a conference presentation to summarize the main findings of a study or research project.
- Journal submission: Many academic journals require authors to submit a research summary along with their research article or study. The summary provides a brief overview of the study’s main points, findings, and conclusions and helps readers quickly understand the research.
- Funding application: A research summary can be included in a funding application to provide a brief summary of the research aims, objectives, and expected outcomes.
- Policy brief: A research summary can be prepared as a policy brief to communicate research findings to policymakers or stakeholders in a concise and accessible manner.
Advantages of Research Summary
Research summaries offer several advantages, including:
- Time-saving: A research summary saves time for readers who need to understand the key findings and conclusions of a research project quickly. Rather than reading the entire research article or study, readers can quickly review the summary to determine whether the research is relevant to their needs.
- Clarity and accessibility: A research summary provides a clear and accessible overview of the research project’s main points, making it easier for readers to understand the research without having to be experts in the field.
- Improved comprehension: A research summary helps readers comprehend the research by providing a brief and focused overview of the key findings and conclusions, making it easier to understand the research and its significance.
- Enhanced communication: Research summaries can be used to communicate research findings to a wider audience, such as policymakers, practitioners, or the general public, in a concise and accessible manner.
- Facilitated decision-making: Research summaries can support decision-making processes by providing a summary of the research evidence on a particular topic. Policymakers or practitioners can use this information to make informed decisions about interventions, programs, or policies.
- Increased dissemination: Research summaries can be easily shared and disseminated, allowing research findings to reach a wider audience.
Limitations of Research Summary
Limitations of the Research Summary are as follows:
- Limited scope: Research summaries provide a brief overview of the research project’s main points, findings, and conclusions, which can be limiting. They may not include all the details, nuances, and complexities of the research that readers may need to fully understand the study’s implications.
- Risk of oversimplification: Research summaries can be oversimplified, reducing the complexity of the research and potentially distorting the findings or conclusions.
- Lack of context: Research summaries may not provide sufficient context to fully understand the research findings, such as the research background, methodology, or limitations. This may lead to misunderstandings or misinterpretations of the research.
- Possible bias: Research summaries may be biased if they selectively emphasize certain findings or conclusions over others, potentially distorting the overall picture of the research.
- Format limitations: Research summaries may be constrained by the format or length requirements, making it challenging to fully convey the research’s main points, findings, and conclusions.
- Accessibility: Research summaries may not be accessible to all readers, particularly those with limited literacy skills, visual impairments, or language barriers.
About the author
Muhammad Hassan
Researcher, Academic Writer, Web developer
You may also like

Research Gap – Types, Examples and How to...

What is a Hypothesis – Types, Examples and...

Research Techniques – Methods, Types and Examples

Research Contribution – Thesis Guide

Purpose of Research – Objectives and Applications

Assignment – Types, Examples and Writing Guide
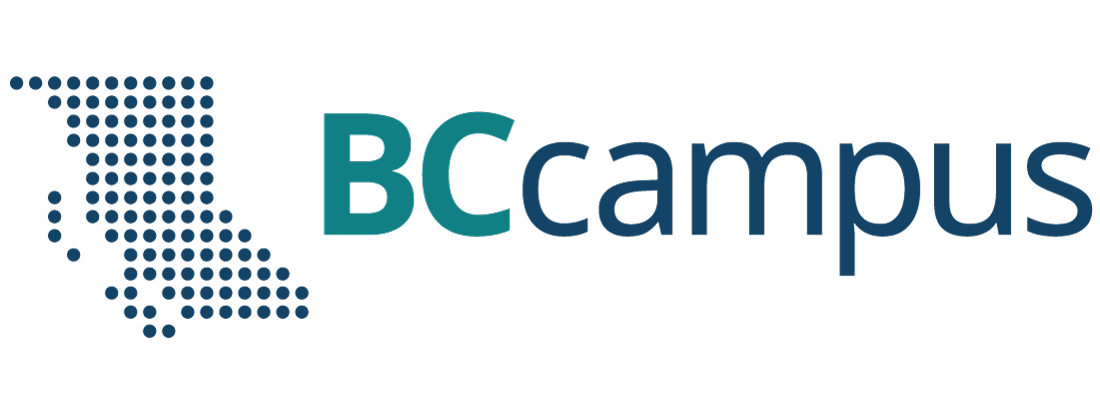
Want to create or adapt books like this? Learn more about how Pressbooks supports open publishing practices.
Chapter 3: Developing a Research Question
3.2 Exploration, Description, Explanation
As you can see, there is much to think about and many decisions to be made as you begin to define your research question and your research project. Something else you will need to consider in the early stages is whether your research will be exploratory, descriptive, or explanatory. Each of these types of research has a different aim or purpose, consequently, how you design your research project will be determined in part by this decision. In the following paragraphs we will look at these three types of research.
Exploratory Research
Researchers conducting exploratory research are typically at the early stages of examining their topics. These sorts of projects are usually conducted when a researcher wants to test the feasibility of conducting a more extensive study; he or she wants to figure out the lay of the land with respect to the particular topic. Perhaps very little prior research has been conducted on this subject. If this is the case, a researcher may wish to do some exploratory work to learn what method to use in collecting data, how best to approach research participants, or even what sorts of questions are reasonable to ask. A researcher wanting to simply satisfy his or her own curiosity about a topic could also conduct exploratory research. Conducting exploratory research on a topic is often a necessary first step, both to satisfy researcher curiosity about the subject and to better understand the phenomenon and the research participants in order to design a larger, subsequent study. See Table 2.1 for examples.
Descriptive Research
Sometimes the goal of research is to describe or define a particular phenomenon. In this case, descriptive research would be an appropriate strategy. A descriptive may, for example, aim to describe a pattern. For example, researchers often collect information to describe something for the benefit of the general public. Market researchers rely on descriptive research to tell them what consumers think of their products. In fact, descriptive research has many useful applications, and you probably rely on findings from descriptive research without even being aware that that is what you are doing. See Table 3.1 for examples.
Explanatory Research
The third type of research, explanatory research, seeks to answer “why” questions. In this case, the researcher is trying to identify the causes and effects of whatever phenomenon is being studied. An explanatory study of college students’ addictions to their electronic gadgets, for example, might aim to understand why students become addicted. Does it have anything to do with their family histories? Does it have anything to do with their other extracurricular hobbies and activities? Does it have anything to do with the people with whom they spend their time? An explanatory study could answer these kinds of questions. See Table 3.1 for examples.
Degree of Problem Definition | Key variables not define | Key variables not define | Key variables not define |
“The quality of service is declining and we don’t know why.” | “What have been the trends in organizational downsizing over the past ten years?” | “Which of two training programs is more effective for reducing labour turnover? | |
“Would people be interested in our new product idea? | “Did last year’s product recall have an impact on our company’s share price?” | “Can I predict the value of energy stocks if I know the current dividends and growth rates of dividends?” | |
“How important is business process reengineering as a strategy? | “Has the average merger rate for financial institutions increased in the past decade?” | “Do buyers prefer our product in a new package?” |
Research Methods for the Social Sciences: An Introduction Copyright © 2020 by Valerie Sheppard is licensed under a Creative Commons Attribution-NonCommercial-ShareAlike 4.0 International License , except where otherwise noted.
Share This Book
- Affiliate Program

- UNITED STATES
- 台灣 (TAIWAN)
- TÜRKIYE (TURKEY)
- Academic Editing Services
- - Research Paper
- - Journal Manuscript
- - Dissertation
- - College & University Assignments
- Admissions Editing Services
- - Application Essay
- - Personal Statement
- - Recommendation Letter
- - Cover Letter
- - CV/Resume
- Business Editing Services
- - Business Documents
- - Report & Brochure
- - Website & Blog
- Writer Editing Services
- - Script & Screenplay
- Our Editors
- Client Reviews
- Editing & Proofreading Prices
- Wordvice Points
- Partner Discount
- Plagiarism Checker
- APA Citation Generator
- MLA Citation Generator
- Chicago Citation Generator
- Vancouver Citation Generator
- - APA Style
- - MLA Style
- - Chicago Style
- - Vancouver Style
- Writing & Editing Guide
- Academic Resources
- Admissions Resources
How to Write the Rationale of the Study in Research (Examples)
What is the Rationale of the Study?
The rationale of the study is the justification for taking on a given study. It explains the reason the study was conducted or should be conducted. This means the study rationale should explain to the reader or examiner why the study is/was necessary. It is also sometimes called the “purpose” or “justification” of a study. While this is not difficult to grasp in itself, you might wonder how the rationale of the study is different from your research question or from the statement of the problem of your study, and how it fits into the rest of your thesis or research paper.
The rationale of the study links the background of the study to your specific research question and justifies the need for the latter on the basis of the former. In brief, you first provide and discuss existing data on the topic, and then you tell the reader, based on the background evidence you just presented, where you identified gaps or issues and why you think it is important to address those. The problem statement, lastly, is the formulation of the specific research question you choose to investigate, following logically from your rationale, and the approach you are planning to use to do that.
Table of Contents:
How to write a rationale for a research paper , how do you justify the need for a research study.
- Study Rationale Example: Where Does It Go In Your Paper?
The basis for writing a research rationale is preliminary data or a clear description of an observation. If you are doing basic/theoretical research, then a literature review will help you identify gaps in current knowledge. In applied/practical research, you base your rationale on an existing issue with a certain process (e.g., vaccine proof registration) or practice (e.g., patient treatment) that is well documented and needs to be addressed. By presenting the reader with earlier evidence or observations, you can (and have to) convince them that you are not just repeating what other people have already done or said and that your ideas are not coming out of thin air.
Once you have explained where you are coming from, you should justify the need for doing additional research–this is essentially the rationale of your study. Finally, when you have convinced the reader of the purpose of your work, you can end your introduction section with the statement of the problem of your research that contains clear aims and objectives and also briefly describes (and justifies) your methodological approach.
When is the Rationale for Research Written?
The author can present the study rationale both before and after the research is conducted.
- Before conducting research : The study rationale is a central component of the research proposal . It represents the plan of your work, constructed before the study is actually executed.
- Once research has been conducted : After the study is completed, the rationale is presented in a research article or PhD dissertation to explain why you focused on this specific research question. When writing the study rationale for this purpose, the author should link the rationale of the research to the aims and outcomes of the study.
What to Include in the Study Rationale
Although every study rationale is different and discusses different specific elements of a study’s method or approach, there are some elements that should be included to write a good rationale. Make sure to touch on the following:
- A summary of conclusions from your review of the relevant literature
- What is currently unknown (gaps in knowledge)
- Inconclusive or contested results from previous studies on the same or similar topic
- The necessity to improve or build on previous research, such as to improve methodology or utilize newer techniques and/or technologies
There are different types of limitations that you can use to justify the need for your study. In applied/practical research, the justification for investigating something is always that an existing process/practice has a problem or is not satisfactory. Let’s say, for example, that people in a certain country/city/community commonly complain about hospital care on weekends (not enough staff, not enough attention, no decisions being made), but you looked into it and realized that nobody ever investigated whether these perceived problems are actually based on objective shortages/non-availabilities of care or whether the lower numbers of patients who are treated during weekends are commensurate with the provided services.
In this case, “lack of data” is your justification for digging deeper into the problem. Or, if it is obvious that there is a shortage of staff and provided services on weekends, you could decide to investigate which of the usual procedures are skipped during weekends as a result and what the negative consequences are.
In basic/theoretical research, lack of knowledge is of course a common and accepted justification for additional research—but make sure that it is not your only motivation. “Nobody has ever done this” is only a convincing reason for a study if you explain to the reader why you think we should know more about this specific phenomenon. If there is earlier research but you think it has limitations, then those can usually be classified into “methodological”, “contextual”, and “conceptual” limitations. To identify such limitations, you can ask specific questions and let those questions guide you when you explain to the reader why your study was necessary:
Methodological limitations
- Did earlier studies try but failed to measure/identify a specific phenomenon?
- Was earlier research based on incorrect conceptualizations of variables?
- Were earlier studies based on questionable operationalizations of key concepts?
- Did earlier studies use questionable or inappropriate research designs?
Contextual limitations
- Have recent changes in the studied problem made previous studies irrelevant?
- Are you studying a new/particular context that previous findings do not apply to?
Conceptual limitations
- Do previous findings only make sense within a specific framework or ideology?
Study Rationale Examples
Let’s look at an example from one of our earlier articles on the statement of the problem to clarify how your rationale fits into your introduction section. This is a very short introduction for a practical research study on the challenges of online learning. Your introduction might be much longer (especially the context/background section), and this example does not contain any sources (which you will have to provide for all claims you make and all earlier studies you cite)—but please pay attention to how the background presentation , rationale, and problem statement blend into each other in a logical way so that the reader can follow and has no reason to question your motivation or the foundation of your research.
Background presentation
Since the beginning of the Covid pandemic, most educational institutions around the world have transitioned to a fully online study model, at least during peak times of infections and social distancing measures. This transition has not been easy and even two years into the pandemic, problems with online teaching and studying persist (reference needed) .
While the increasing gap between those with access to technology and equipment and those without access has been determined to be one of the main challenges (reference needed) , others claim that online learning offers more opportunities for many students by breaking down barriers of location and distance (reference needed) .
Rationale of the study
Since teachers and students cannot wait for circumstances to go back to normal, the measures that schools and universities have implemented during the last two years, their advantages and disadvantages, and the impact of those measures on students’ progress, satisfaction, and well-being need to be understood so that improvements can be made and demographics that have been left behind can receive the support they need as soon as possible.
Statement of the problem
To identify what changes in the learning environment were considered the most challenging and how those changes relate to a variety of student outcome measures, we conducted surveys and interviews among teachers and students at ten institutions of higher education in four different major cities, two in the US (New York and Chicago), one in South Korea (Seoul), and one in the UK (London). Responses were analyzed with a focus on different student demographics and how they might have been affected differently by the current situation.
How long is a study rationale?
In a research article bound for journal publication, your rationale should not be longer than a few sentences (no longer than one brief paragraph). A dissertation or thesis usually allows for a longer description; depending on the length and nature of your document, this could be up to a couple of paragraphs in length. A completely novel or unconventional approach might warrant a longer and more detailed justification than an approach that slightly deviates from well-established methods and approaches.
Consider Using Professional Academic Editing Services
Now that you know how to write the rationale of the study for a research proposal or paper, you should make use of Wordvice AI’s free AI Grammar Checker , or receive professional academic proofreading services from Wordvice, including research paper editing services and manuscript editing services to polish your submitted research documents.
You can also find many more articles, for example on writing the other parts of your research paper , on choosing a title , or on making sure you understand and adhere to the author instructions before you submit to a journal, on the Wordvice academic resources pages.
Thank you for visiting nature.com. You are using a browser version with limited support for CSS. To obtain the best experience, we recommend you use a more up to date browser (or turn off compatibility mode in Internet Explorer). In the meantime, to ensure continued support, we are displaying the site without styles and JavaScript.
- View all journals
- Explore content
- About the journal
- Publish with us
- Sign up for alerts
- Published: 16 September 2024
From whole-slide image to biomarker prediction: end-to-end weakly supervised deep learning in computational pathology
- Omar S. M. El Nahhas ORCID: orcid.org/0000-0002-2542-2117 1 , 2 ,
- Marko van Treeck 1 ,
- Georg Wölflein ORCID: orcid.org/0000-0002-0407-7617 3 ,
- Michaela Unger 1 ,
- Marta Ligero 1 ,
- Tim Lenz 1 ,
- Sophia J. Wagner 4 , 5 ,
- Katherine J. Hewitt 1 ,
- Firas Khader 2 , 6 ,
- Sebastian Foersch 7 ,
- Daniel Truhn 2 , 6 &
- Jakob Nikolas Kather ORCID: orcid.org/0000-0002-3730-5348 1 , 2 , 8 , 9
Nature Protocols ( 2024 ) Cite this article
1 Altmetric
Metrics details
- Bioinformatics
- Cancer imaging
- Image processing
Hematoxylin- and eosin-stained whole-slide images (WSIs) are the foundation of diagnosis of cancer. In recent years, development of deep learning-based methods in computational pathology has enabled the prediction of biomarkers directly from WSIs. However, accurately linking tissue phenotype to biomarkers at scale remains a crucial challenge for democratizing complex biomarkers in precision oncology. This protocol describes a practical workflow for solid tumor associative modeling in pathology (STAMP), enabling prediction of biomarkers directly from WSIs by using deep learning. The STAMP workflow is biomarker agnostic and allows for genetic and clinicopathologic tabular data to be included as an additional input, together with histopathology images. The protocol consists of five main stages that have been successfully applied to various research problems: formal problem definition, data preprocessing, modeling, evaluation and clinical translation. The STAMP workflow differentiates itself through its focus on serving as a collaborative framework that can be used by clinicians and engineers alike for setting up research projects in the field of computational pathology. As an example task, we applied STAMP to the prediction of microsatellite instability (MSI) status in colorectal cancer, showing accurate performance for the identification of tumors high in MSI. Moreover, we provide an open-source code base, which has been deployed at several hospitals across the globe to set up computational pathology workflows. The STAMP workflow requires one workday of hands-on computational execution and basic command line knowledge.
STAMP (solid tumor associative modeling in pathology) is a practical workflow for end-to-end weakly supervised deep learning in computational pathology, enabling prediction of biomarkers directly from whole-slide images.
This protocol differentiates itself from others by providing a collaborative framework through which clinical researchers can work with engineers to set up a complete computational pathology project.
This is a preview of subscription content, access via your institution
Access options
Access Nature and 54 other Nature Portfolio journals
Get Nature+, our best-value online-access subscription
24,99 € / 30 days
cancel any time
Subscribe to this journal
Receive 12 print issues and online access
251,40 € per year
only 20,95 € per issue
Buy this article
- Purchase on SpringerLink
- Instant access to full article PDF
Prices may be subject to local taxes which are calculated during checkout
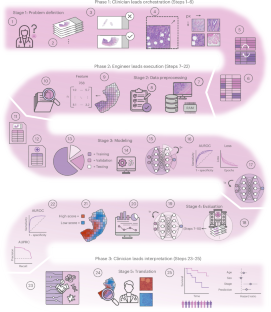
Similar content being viewed by others
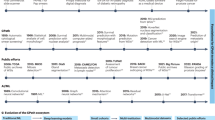
Artificial intelligence for digital and computational pathology
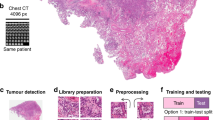
Deep learning in cancer pathology: a new generation of clinical biomarkers
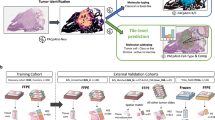
Pacpaint: a histology-based deep learning model uncovers the extensive intratumor molecular heterogeneity of pancreatic adenocarcinoma
Data availability.
Histopathology slides and genomics data from TCGA and CPTAC were used to train and validate the models. The slides for TCGA are available at https://portal.gdc.cancer.gov/ . The slides for CPTAC are available at https://proteomics.cancer.gov/data-portal . The molecular and clinical data for TCGA and CPTAC used in the experiments are available at https://github.com/KatherLab/cancer-metadata . Source data are provided with this paper.
Code availability
The open-source STAMP software for the implementation of the MSI experiments is available on GitHub ( https://github.com/KatherLab/STAMP ).
Shmatko, A., Ghaffari Laleh, N., Gerstung, M. & Kather, J. N. Artificial intelligence in histopathology: enhancing cancer research and clinical oncology. Nat. Cancer 3 , 1026–1038 (2022).
Article PubMed Google Scholar
Ghaffari Laleh, N. et al. Benchmarking weakly-supervised deep learning pipelines for whole slide classification in computational pathology. Med. Image Anal. 79 , 102474 (2022).
Foersch, S. et al. Deep learning for diagnosis and survival prediction in soft tissue sarcoma. Ann. Oncol. 32 , 1178–1187 (2021).
Article CAS PubMed Google Scholar
Klein, C. et al. Artificial intelligence for solid tumour diagnosis in digital pathology. Br. J. Pharmacol. 178 , 4291–4315 (2021).
Woerl, A.-C. et al. Deep learning predicts molecular subtype of muscle-invasive bladder cancer from conventional histopathological slides. Eur. Urol. 78 , 256–264 (2020).
Hong, R., Liu, W., DeLair, D., Razavian, N. & Fenyö, D. Predicting endometrial cancer subtypes and molecular features from histopathology images using multi-resolution deep learning models. Cell Rep. Med. 2 , 100400 (2021).
Article CAS PubMed PubMed Central Google Scholar
Kather, J. N. et al. Predicting survival from colorectal cancer histology slides using deep learning: a retrospective multicenter study. PLoS Med. 16 , e1002730 (2019).
Article PubMed PubMed Central Google Scholar
Ghaffari Laleh, N. et al. Deep Learning for interpretable end-to-end survival (E-ESurv) prediction in gastrointestinal cancer histopathology. Proceedings of the MICCAI Workshop on Computational Pathology . PMLR 156 , 81–93 (2021).
Foersch, S. et al. Multistain deep learning for prediction of prognosis and therapy response in colorectal cancer. Nat. Med. 29 , 430–439 (2023).
Wang, C.-W. et al. Weakly supervised deep learning for prediction of treatment effectiveness on ovarian cancer from histopathology images. Comput. Med. Imaging Graph. 99 , 102093 (2022).
Ghaffari Laleh, N., Ligero, M., Perez-Lopez, R. & Kather, J. N. Facts and hopes on the use of artificial intelligence for predictive immunotherapy biomarkers in cancer. Clin. Cancer Res. 29 , 316–323 (2023).
Kather, J. N. et al. Pan-cancer image-based detection of clinically actionable genetic alterations. Nat. Cancer 1 , 789–799 (2020).
Kanavati, F. et al. Weakly-supervised learning for lung carcinoma classification using deep learning. Sci. Rep. 10 , 9297 (2020).
Wang, X. et al. Weakly supervised deep learning for whole slide lung cancer image analysis. IEEE Trans. Cybern. 50 , 3950–3962 (2020).
Kather, J. N. et al. Deep learning can predict microsatellite instability directly from histology in gastrointestinal cancer. Nat. Med. 25 , 1054–1056 (2019).
Bilal, M. et al. Development and validation of a weakly supervised deep learning framework to predict the status of molecular pathways and key mutations in colorectal cancer from routine histology images: a retrospective study. Lancet Digit. Health 3 , e763–e772 (2021).
Schrammen, P. L. et al. Weakly supervised annotation-free cancer detection and prediction of genotype in routine histopathology. J. Pathol. 256 , 50–60 (2022).
Echle, A. et al. Clinical-grade detection of microsatellite instability in colorectal tumors by deep learning. Gastroenterology 159 , 1406–1416.e11 (2020).
Zeng, Q. et al. Artificial intelligence predicts immune and inflammatory gene signatures directly from hepatocellular carcinoma histology. J. Hepatol. 77 , 116–127 (2022).
Jaroensri, R. et al. Deep learning models for histologic grading of breast cancer and association with disease prognosis. NPJ Breast Cancer 8 , 113 (2022).
Li, C. et al. Weakly supervised mitosis detection in breast histopathology images using concentric loss. Med. Image Anal. 53 , 165–178 (2019).
Zheng, Q. et al. A weakly supervised deep learning model and human-machine fusion for accurate grading of renal cell carcinoma from histopathology slides. Cancers (Basel) 15 , 3198 (2023).
Muti, H. S. et al. The Aachen Protocol for Deep Learning Histopathology: A Hands-on Guide for Data Preprocessing. Available at https://oa.mg/work/10.5281/zenodo.3694994 (2020).
Graziani, M. et al. Attention-based interpretable regression of gene expression in histology. Interpretability of Machine Intelligence in Medical Image Computing: 5th International Workshop, iMIMIC 2022, Held in Conjunction with MICCAI 2022, Singapore, Singapore, September 22, 2022, Proceedings 44–60 (Springer-Verlag, 2022).
Campanella, G. et al. Clinical-grade computational pathology using weakly supervised deep learning on whole slide images. Nat. Med. 25 , 1301–1309 (2019).
Schmauch, B. et al. A deep learning model to predict RNA-Seq expression of tumours from whole slide images. Nat. Commun. 11 , 1–15 (2020).
Article Google Scholar
Lu, M. Y. et al. AI-based pathology predicts origins for cancers of unknown primary. Nature 594 , 106–110 (2021).
Wagner, S. J. et al. Built to last? Reproducibility and reusability of deep learning algorithms in computational pathology. Mod. Pathol. 37 , 100350 (2023).
Veldhuizen, G. P. et al. Deep learning-based subtyping of gastric cancer histology predicts clinical outcome: a multi-institutional retrospective study. Gastric Cancer 26 , 708–720 (2023).
Muti, H. S. et al. Deep learning trained on lymph node status predicts outcome from gastric cancer histopathology: a retrospective multicentric study. Eur. J. Cancer 194 , 113335 (2023).
Saldanha, O. L. et al. Direct prediction of genetic aberrations from pathology images in gastric cancer with swarm learning. Gastric Cancer 26 , 264–274 (2023).
Niehues, J. M. et al. Generalizable biomarker prediction from cancer pathology slides with self-supervised deep learning: a retrospective multi-centric study. Cell Rep. Med. 4 , 100980 (2023).
Wagner, S. J. et al. Transformer-based biomarker prediction from colorectal cancer histology: a large-scale multicentric study. Cancer Cell 41 , 1650–1661.e4 (2023).
Jiang, X. et al. End-to-end prognostication in colorectal cancer by deep learning: a retrospective, multicentre study. Lancet Digit. Health 6 , e33–e43 (2024).
Chatterji, S. et al. Prediction models for hormone receptor status in female breast cancer do not extend to males: further evidence of sex-based disparity in breast cancer. NPJ Breast Cancer 9 , 91 (2023).
Hewitt, K. J. et al. Direct image to subtype prediction for brain tumors using deep learning. Neurooncol. Adv. 5 , vdad139 (2023).
PubMed PubMed Central Google Scholar
Saldanha, O. L. et al. Self-supervised attention-based deep learning for pan-cancer mutation prediction from histopathology. NPJ Precis. Oncol. 7 , 35 (2023).
Loeffler, C. M. L. et al. Direct prediction of Homologous Recombination Deficiency from routine histology in ten different tumor types with attention-based Multiple Instance Learning: a development and validation study. Preprint at https://www.medrxiv.org/content/10.1101/2023.03.08.23286975v1 (2023).
El Nahhas, O. S. M. et al. Regression-based Deep-Learning predicts molecular biomarkers from pathology slides. Nat. Commun. 15 , 1–253 (2024).
Google Scholar
Wang, X. et al. Transformer-based unsupervised contrastive learning for histopathological image classification. Med. Image Anal. 81 , 102559 (2022).
Causality in digital medicine. Nat. Commun . 12 , 5471 (2021).
Wölflein, G. et al. Benchmarking pathology feature extractors for whole slide image classification. Preprint at https://arxiv.org/abs/2311.11772 (2023).
Goode, A., Gilbert, B., Harkes, J., Jukic, D. & Satyanarayanan, M. OpenSlide: a vendor-neutral software foundation for digital pathology. J. Pathol. Inform. 4 , 27 (2013).
Bankhead, P. et al. QuPath: open source software for digital pathology image analysis. Sci. Rep. 7 , 16878 (2017).
Ghassemi, M., Oakden-Rayner, L. & Beam, A. L. The false hope of current approaches to explainable artificial intelligence in health care. Lancet Digit. Health 3 , e745–e750 (2021).
Paszke, A. et al. PyTorch: An imperative style, high-performance deep learning library. In Advances in Neural Information Processing Systems (eds Wallach, H. et al.) Vol. 32 (Curran Associates, Inc., 2019).
Jorge Cardoso, M. et al. MONAI: an open-source framework for deep learning in healthcare. Preprint at https://arxiv.org/abs/2211.02701 (2022).
Martinez, K. & Cupitt, J. VIPS - a highly tuned image processing software architecture. In IEEE International Conference on Image Processing 2005 . Genova, Italy II–574 (IEEE, 2005).
Janowczyk, A., Zuo, R., Gilmore, H., Feldman, M. & Madabhushi, A. HistoQC: an open-source quality control tool for digital pathology slides. JCO Clin. Cancer Inform. 3 , 1–7 (2019).
Pedersen, A. et al. FastPathology: an open-source platform for deep learning-based research and decision support in digital pathology. IEEE Access 9 , 58216–58229 (2021).
Pocock, J. et al. TIAToolbox as an end-to-end library for advanced tissue image analytics. Commun. Med. (Lond.) 2 , 120 (2022).
Lu, M. Y. et al. Data-efficient and weakly supervised computational pathology on whole-slide images. Nat. Biomed. Eng. 5 , 555–570 (2021).
Verghese, G. et al. Computational pathology in cancer diagnosis, prognosis, and prediction—present day and prospects. J. Pathol. 260 , 551–563 (2023).
Saillard, C. et al. Validation of MSIntuit as an AI-based pre-screening tool for MSI detection from colorectal cancer histology slides. Nat. Commun. 14 , 6695 (2023).
Greenson, J. K. et al. Pathologic predictors of microsatellite instability in colorectal cancer. Am. J. Surg. Pathol. 33 , 126–133 (2009).
Cancer Genome Atlas Network. Comprehensive molecular characterization of human colon and rectal cancer. Nature 487 , 330–337 (2012).
Ellis, M. J. et al. Connecting genomic alterations to cancer biology with proteomics: the NCI Clinical Proteomic Tumor Analysis Consortium. Cancer Discov. 3 , 1108–1112 (2013).
Macenko, M. et al. A method for normalizing histology slides for quantitative analysis. In 2009 IEEE International Symposium on Biomedical Imaging: From Nano to Macro 1107–1110 (IEEE, 2009).
Howard, F. M. et al. The impact of site-specific digital histology signatures on deep learning model accuracy and bias. Nat. Commun. 12 , 4423 (2021).
Canny, J. A computational approach to edge detection. IEEE Trans. Pattern Anal. Mach. Intell. 8 , 679–698 (1986).
Comes, M. C. et al. A deep learning model based on whole slide images to predict disease-free survival in cutaneous melanoma patients. Sci. Rep. 12 , 20366 (2022).
Jiang, S., Suriawinata, A. A. & Hassanpour, S. MHAttnSurv: multi-head attention for survival prediction using whole-slide pathology images. Comput. Biol. Med. 158 , 106883 (2023).
Sounderajah, V. et al. Developing a reporting guideline for artificial intelligence-centred diagnostic test accuracy studies: the STARD-AI protocol. BMJ Open 11 , e047709 (2021).
Collins, G. S. et al. Protocol for development of a reporting guideline (TRIPOD-AI) and risk of bias tool (PROBAST-AI) for diagnostic and prognostic prediction model studies based on artificial intelligence. BMJ Open 11 , e048008 (2021).
Trautmann, K. et al. Chromosomal instability in microsatellite-unstable and stable colon cancer. Clin. Cancer Res. 12 , 6379–6385 (2006).
Lin, E. I. et al. Mutational profiling of colorectal cancers with microsatellite instability. Oncotarget 6 , 42334–42344 (2015).
Boland, C. R. & Goel, A. Microsatellite instability in colorectal cancer. Gastroenterology 138 , 2073–2087.e3 (2010).
Battaglin, F., Naseem, M., Lenz, H.-J. & Salem, M. E. Microsatellite instability in colorectal cancer: overview of its clinical significance and novel perspectives. Clin. Adv. Hematol. Oncol. 16 , 735–745 (2018).
Selvaraju, R. R. et al. Grad-CAM: visual explanations from deep networks via gradient-based localization. In 2017 IEEE International Conference on Computer Vision (ICCV) 618–626 (IEEE, 2017).
Pataki, B. Á. et al. HunCRC: annotated pathological slides to enhance deep learning applications in colorectal cancer screening. Sci. Data 9 , 370 (2022).
Cheng, J. et al. Computational analysis of pathological images enables a better diagnosis of TFE3 Xp11.2 translocation renal cell carcinoma. Nat. Commun. 11 , 1778 (2020).
Download references
Acknowledgements
We thank the testers of the protocol, S. Sainath, O. L. Saldanha, L. Žigutytė, C. Kummer, G. Serna, K. Boehm and L. Shaktah, who executed the STAMP protocol on various systems at cancer centers around the world. O.S.M.E.N. is supported by the German Federal Ministry of Education and Research (BMBF) through grant 1IS23070, Software Campus 3.0 (TU Dresden), as part of the Software Campus project ’MIRACLE-AI’. J.N.K. is supported by the German Federal Ministry of Health (DEEP LIVER, ZMVI1-2520DAT111), the German Cancer Aid (DECADE, 70115166), the German Federal Ministry of Education and Research (PEARL, 01KD2104C; CAMINO, 01EO2101; SWAG, 01KD2215A; TRANSFORM LIVER, 031L0312A; TANGERINE, 01KT2302 through ERA-NET Transcan), the German Academic Exchange Service (SECAI, 57616814), the German Federal Joint Committee (TransplantKI, 01VSF21048), the European Union’s Horizon Europe and innovation programme (ODELIA, 101057091; GENIAL, 101096312) and the National Institute for Health and Care Research (NIHR, NIHR213331) Leeds Biomedical Research Centre. G.W. is supported by Lothian NHS. D.T. is supported by the German Federal Ministry of Education and Research (SWAG, 01KD2215A; TRANSFORM LIVER) and the European Union’s Horizon Europe and innovation programme (ODELIA, 101057091). S.F. is supported by the German Federal Ministry of Education and Research (SWAG, 01KD2215A), the German Cancer Aid (DECADE, 70115166) and the German Research Foundation (504101714). S.J.W. was supported by the Helmholtz Association under the joint research school ‘Munich School for Data Science – MUDS’ and the Add-on Fellowship of the Joachim Herz Foundation. The views expressed are those of the author(s) and not necessarily those of the NHS, the NIHR or the Department of Health and Social Care. This work was funded by the European Union. Views and opinions expressed are however those of the author(s) only and do not necessarily reflect those of the European Union. Neither the European Union nor the granting authority can be held responsible for them.
Author information
Authors and affiliations.
Else Kroener Fresenius Center for Digital Health, Medical Faculty Carl Gustav Carus, Technical University Dresden, Dresden, Germany
Omar S. M. El Nahhas, Marko van Treeck, Michaela Unger, Marta Ligero, Tim Lenz, Katherine J. Hewitt & Jakob Nikolas Kather
StratifAI GmbH, Dresden, Germany
Omar S. M. El Nahhas, Firas Khader, Daniel Truhn & Jakob Nikolas Kather
School of Computer Science, University of St Andrews, St Andrews, UK
Georg Wölflein
Helmholtz Munich–German Research Center for Environment and Health, Munich, Germany
Sophia J. Wagner
School of Computation, Information and Technology, Technical University of Munich, Munich, Germany
Department of Diagnostic and Interventional Radiology, University Hospital Aachen, Aachen, Germany
Firas Khader & Daniel Truhn
Institute of Pathology–University Medical Center Mainz, Mainz, Germany
Sebastian Foersch
Medical Oncology, National Center for Tumor Diseases (NCT), University Hospital Heidelberg, Heidelberg, Germany
Jakob Nikolas Kather
Department of Medicine 1, University Hospital and Faculty of Medicine Carl Gustav Carus, Technical University Dresden, Dresden, Germany
You can also search for this author in PubMed Google Scholar
Contributions
O.S.M.E.N. and J.N.K. designed the protocol. O.S.M.E.N., M.v.T., G.W. and T.L. developed the software and wrote technical documentation. O.S.M.E.N., M.v.T., G.W., T.L., M.L., M.U., S.J.W., F.K., S.F. and D.T. tested the software. O.S.M.E.N., J.N.K. and K.J.H. interpreted and analyzed the data. All authors wrote and reviewed the protocol and approved the final version for submission.
Corresponding author
Correspondence to Jakob Nikolas Kather .
Ethics declarations
Competing interests.
O.S.M.E.N., F.K. and D.T. hold shares in StratifAI GmbH. J.N.K. declares consulting services for Owkin, France; DoMore Diagnostics, Norway; Panakeia, UK,; Scailyte, Switzerland; Mindpeak, Germany; and Histofy, UK; furthermore, he holds shares in StratifAI GmbH, Germany, and has received honoraria for lectures by AstraZeneca, Bayer, Eisai, MSD, BMS, Roche, Pfizer and Fresenius. D.T. received honoraria for lectures by Bayer and holds shares in StratifAI GmbH, Germany. S.F. has received honoraria from MSD and BMS.
Peer review
Peer review information.
Nature Protocols thanks the anonymous reviewers for their contribution to the peer review of this work.
Additional information
Publisher’s note Springer Nature remains neutral with regard to jurisdictional claims in published maps and institutional affiliations.
Related links
Key references using this protocol
Wagner, S. J. et al. Cancer Cell 41 , 1650–1661.e4 (2023): https://doi.org/10.1016/j.ccell.2023.08.002
El Nahhas, O. S. M. et al. Nat. Commun . 15 , 1253 (2024): https://doi.org/10.1038/s41467-024-45589-1
Jiang, X. et al. Lancet Digit. Health 6 , e33–e43 (2024): https://doi.org/10.1016/S2589-7500(23)00208-X
Hewitt, K. J. et al. Neurooncol. Adv . 5 , vdad139 (2023): https://doi.org/10.1093/noajnl/vdad139
Saldanha, O. L. et al. npj Precis. Onc . 7 , 35 (2023): https://doi.org/10.1038/s41698-023-00365-0
Supplementary information
Supplementary information.
Supplementary Text 1, Table 1 and Fig. 1
Source data
Rights and permissions
Springer Nature or its licensor (e.g. a society or other partner) holds exclusive rights to this article under a publishing agreement with the author(s) or other rightsholder(s); author self-archiving of the accepted manuscript version of this article is solely governed by the terms of such publishing agreement and applicable law.
Reprints and permissions
About this article
Cite this article.
El Nahhas, O.S.M., van Treeck, M., Wölflein, G. et al. From whole-slide image to biomarker prediction: end-to-end weakly supervised deep learning in computational pathology. Nat Protoc (2024). https://doi.org/10.1038/s41596-024-01047-2
Download citation
Received : 17 December 2023
Accepted : 04 July 2024
Published : 16 September 2024
DOI : https://doi.org/10.1038/s41596-024-01047-2
Share this article
Anyone you share the following link with will be able to read this content:
Sorry, a shareable link is not currently available for this article.
Provided by the Springer Nature SharedIt content-sharing initiative
By submitting a comment you agree to abide by our Terms and Community Guidelines . If you find something abusive or that does not comply with our terms or guidelines please flag it as inappropriate.
Quick links
- Explore articles by subject
- Guide to authors
- Editorial policies
Sign up for the Nature Briefing: Cancer newsletter — what matters in cancer research, free to your inbox weekly.

Popular Searches
- Master’s of AI Engineering
- Engineering Magazine
- graduate programs
- Manufacturing Futures Institute
- student organizations
- Rethink the Rink
Social Media
- @CMUEngineering
- CMUEngineering
- College of Engineering
A novel neural explanation for choking under pressure
by Sara Pecchia
New research from Carnegie Mellon University and the University of Pittsburgh reveals a first-of-its-kind neural explanation for choking under pressure: a deficit in motor preparation induced by an overly large potential “jackpot” payoff.
- Research sheds new light on decreased performance under pressure
- How does learning something new not overwrite what we know?
- Connecting the dots between engagement and learning
Every professional who functions at a high level of performance knows the value of keeping things loose during harrowing tasks. Choking under pressure, or being unable to perform to one’s highest standard when it matters most, is an undesirable alternative. While athletes are often associated with this phenomenon, people choke under pressure in many settings, for example, test-taking, giving presentations, puzzle-solving, and beyond. New research from Carnegie Mellon University and the University of Pittsburgh reveals a first-of-its-kind neural explanation for choking under pressure: a deficit in motor preparation induced by an overly large potential “jackpot” payoff.
To study how motor performance is impacted by choking under pressure, researchers recorded the spiking activity of hundreds of motor control neurons in Rhesus monkeys, who were trained to perform a challenging task to earn rewards of varying sizes. When an unusually large jackpot reward was at stake, the animals underperformed, leading the group to examine how cued rewards modulated neural population activity during movement preparation.
“By looking at the activity of populations of neurons in the motor cortex, we found a signature of choking under pressure, that at the precision of 100s of milliseconds, was indicative of whether or not a subject would fail in an upcoming trial,” explained Adam Smoulder, a graduate student at Carnegie Mellon and first author of the Neuron paper . “Through a series of three hypotheses, we sought a more mechanistic explanation of choking under pressure. We found that rewards interact with target preparation signals to drive neural activity toward a region associated with improved reach execution, and then, at the highest rewards, spread away from this region. So, it seems that increasing motivation by offering larger rewards can improve the discriminability of the neural signals, but only up to a point. Beyond that point, we actually see a collapse in neural information, and that’s tightly correlated with when the animals choke under pressure.”
This nuts-and-bolts level of explanation differs from previous, more holistic work, due to its high-resolution nature, and ability to consider the activity of populations of neurons, versus either the aggregate activity which can be seen with fMRI, or prior work in which only the activity of individual neurons was available.
“It’s hard work to take something that everybody has an intuition about and relate it to neural activity,” noted Aaron Batista, professor of bioengineering at the University of Pittsburgh. “Our data indicates that subjects seemed to become overcautious, self-monitoring to their detriment when the jackpots were offered. If people trying to avoid choking under pressure were to benefit from our study, we suggest they could beat it by finding the right balance between self-awareness and self-control, and just generally keeping it loose when the stakes go up, even if there is a natural tendency to clamp down.”
If people trying to avoid choking under pressure were to benefit from our study, we suggest they could beat it by finding the right balance between self-awareness and self-control, and just generally keeping it loose when the stakes go up. Aaron Batista , professor of bioengineering , University of Pittsburgh
In terms of helpful application, the group believes that knowing what’s going on in your brain can help people with coping and mitigating the risks of choking under pressure.
“Choking under pressure is a really interesting example of when the brain gets it wrong,” added Steve Chase, professor of biomedical engineering at Carnegie Mellon and the Neuroscience Institute. “Now that we understand a little bit about how the brain is failing under these high reward situations, we want to try and correct it. One way to do this would be to design techniques that leverage our combined brain-computer interface (BCI) experience to encourage the brain not to do those things and ultimately, rescue the behavior.”
The group’s work is ongoing and done in collaboration with the Center for Neural Basis of Cognition, a cross-university research and educational program between Carnegie Mellon and the University of Pittsburgh that leverages each institution’s strengths to investigate the cognitive and neural mechanisms that give rise to biological intelligence and behavior.
Have a language expert improve your writing
Run a free plagiarism check in 10 minutes, generate accurate citations for free.
- Knowledge Base
Methodology
- Explanatory Research | Definition, Guide, & Examples
Explanatory Research | Definition, Guide, & Examples
Published on December 3, 2021 by Tegan George and Julia Merkus. Revised on November 20, 2023.
Explanatory research is a research method that explores why something occurs when limited information is available. It can help you increase your understanding of a given topic, ascertain how or why a particular phenomenon is occurring, and predict future occurrences.
Explanatory research can also be explained as a “cause and effect” model, investigating patterns and trends in existing data that haven’t been previously investigated. For this reason, it is often considered a type of causal research .
Table of contents
When to use explanatory research, explanatory research questions, explanatory research data collection, explanatory research data analysis, step-by-step example of explanatory research, explanatory vs. exploratory research, advantages and disadvantages of explanatory research, other interesting articles, frequently asked questions about explanatory research.
Explanatory research is used to investigate how or why a phenomenon takes place. Therefore, this type of research is often one of the first stages in the research process, serving as a jumping-off point for future research. While there is often data available about your topic, it’s possible the particular causal relationship you are interested in has not been robustly studied.
Explanatory research helps you analyze these patterns, formulating hypotheses that can guide future endeavors. If you are seeking a more complete understanding of a relationship between variables, explanatory research is a great place to start. However, keep in mind that it will likely not yield conclusive results.
You analyzed their final grades and noticed that the students who take your course in the first semester always obtain higher grades than students who take the same course in the second semester.
Receive feedback on language, structure, and formatting
Professional editors proofread and edit your paper by focusing on:
- Academic style
- Vague sentences
- Style consistency
See an example
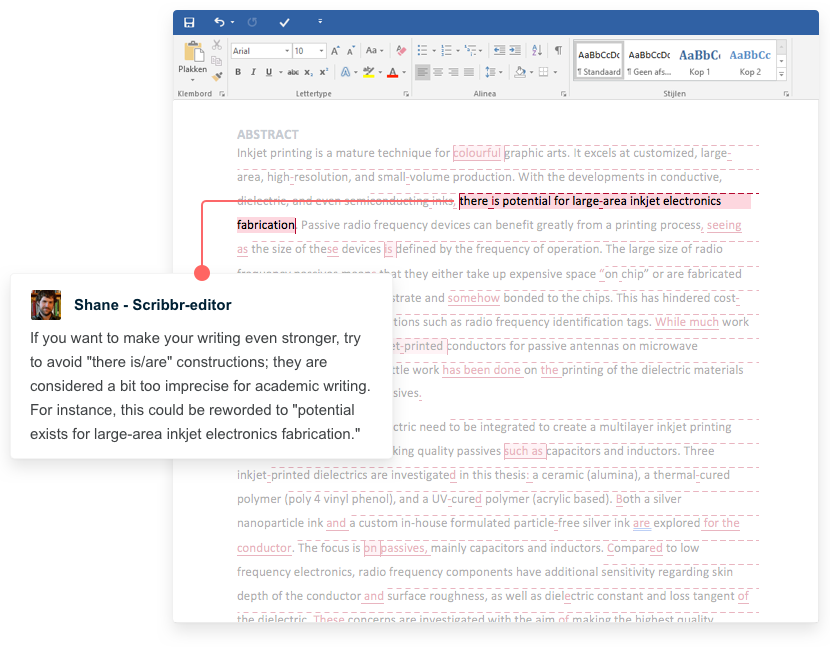
Explanatory research answers “why” and “how” questions, leading to an improved understanding of a previously unresolved problem or providing clarity for related future research initiatives.
Here are a few examples:
- Why do undergraduate students obtain higher average grades in the first semester than in the second semester?
- How does marital status affect labor market participation?
- Why do multilingual individuals show more risky behavior during business negotiations than monolingual individuals?
- How does a child’s ability to delay immediate gratification predict success later in life?
- Why are teens more likely to litter in a highly littered area than in a clean area?
After choosing your research question, there is a variety of options for research and data collection methods to choose from.
A few of the most common research methods include:
- Literature reviews
- Interviews and focus groups
- Pilot studies
- Observations
- Experiments
The method you choose depends on several factors, including your timeline, budget, and the structure of your question. If there is already a body of research on your topic, a literature review is a great place to start. If you are interested in opinions and behavior, consider an interview or focus group format. If you have more time or funding available, an experiment or pilot study may be a good fit for you.
In order to ensure you are conducting your explanatory research correctly, be sure your analysis is definitively causal in nature, and not just correlated.
Always remember the phrase “correlation doesn’t mean causation.” Correlated variables are merely associated with one another: when one variable changes, so does the other. However, this isn’t necessarily due to a direct or indirect causal link.
Causation means that changes in the independent variable bring about changes in the dependent variable. In other words, there is a direct cause-and-effect relationship between variables.
Causal evidence must meet three criteria:
- Temporal : What you define as the “cause” must precede what you define as the “effect.”
- Variation : Intervention must be systematic between your independent variable and dependent variable.
- Non-spurious : Be careful that there are no mitigating factors or hidden third variables that confound your results.
Correlation doesn’t imply causation, but causation always implies correlation. In order to get conclusive causal results, you’ll need to conduct a full experimental design .
Your explanatory research design depends on the research method you choose to collect your data . In most cases, you’ll use an experiment to investigate potential causal relationships. We’ll walk you through the steps using an example.
Step 1: Develop the research question
The first step in conducting explanatory research is getting familiar with the topic you’re interested in, so that you can develop a research question .
Let’s say you’re interested in language retention rates in adults.
You are interested in finding out how the duration of exposure to language influences language retention ability later in life.
Step 2: Formulate a hypothesis
The next step is to address your expectations. In some cases, there is literature available on your subject or on a closely related topic that you can use as a foundation for your hypothesis . In other cases, the topic isn’t well studied, and you’ll have to develop your hypothesis based on your instincts or on existing literature on more distant topics.
You phrase your expectations in terms of a null (H 0 ) and alternative hypothesis (H 1 ):
- H 0 : The duration of exposure to a language in infancy does not influence language retention in adults who were adopted from abroad as children.
- H 1 : The duration of exposure to a language in infancy has a positive effect on language retention in adults who were adopted from abroad as children.
Step 3: Design your methodology and collect your data
Next, decide what data collection and data analysis methods you will use and write them up. After carefully designing your research, you can begin to collect your data.
You compare:
- Adults who were adopted from Colombia between 0 and 6 months of age.
- Adults who were adopted from Colombia between 6 and 12 months of age.
- Adults who were adopted from Colombia between 12 and 18 months of age.
- Monolingual adults who have not been exposed to a different language.
During the study, you test their Spanish language proficiency twice in a research design that has three stages:
- Pre-test : You conduct several language proficiency tests to establish any differences between groups pre-intervention.
- Intervention : You provide all groups with 8 hours of Spanish class.
- Post-test : You again conduct several language proficiency tests to establish any differences between groups post-intervention.
You made sure to control for any confounding variables , such as age, gender, proficiency in other languages, etc.
Step 4: Analyze your data and report results
After data collection is complete, proceed to analyze your data and report the results.
You notice that:
- The pre-exposed adults showed higher language proficiency in Spanish than those who had not been pre-exposed. The difference is even greater for the post-test.
- The adults who were adopted between 12 and 18 months of age had a higher Spanish language proficiency level than those who were adopted between 0 and 6 months or 6 and 12 months of age, but there was no difference found between the latter two groups.
To determine whether these differences are significant, you conduct a mixed ANOVA. The ANOVA shows that all differences are not significant for the pre-test, but they are significant for the post-test.
Step 5: Interpret your results and provide suggestions for future research
As you interpret the results, try to come up with explanations for the results that you did not expect. In most cases, you want to provide suggestions for future research.
However, this difference is only significant after the intervention (the Spanish class.)
You decide it’s worth it to further research the matter, and propose a few additional research ideas:
- Replicate the study with a larger sample
- Replicate the study for other maternal languages (e.g. Korean, Lingala, Arabic)
- Replicate the study for other language aspects, such as nativeness of the accent
It can be easy to confuse explanatory research with exploratory research. If you’re in doubt about the relationship between exploratory and explanatory research, just remember that exploratory research lays the groundwork for later explanatory research.
Exploratory research questions often begin with “what”. They are designed to guide future research and do not usually have conclusive results. Exploratory research is often utilized as a first step in your research process, to help you focus your research question and fine-tune your hypotheses.
Explanatory research questions often start with “why” or “how”. They help you study why and how a previously studied phenomenon takes place.
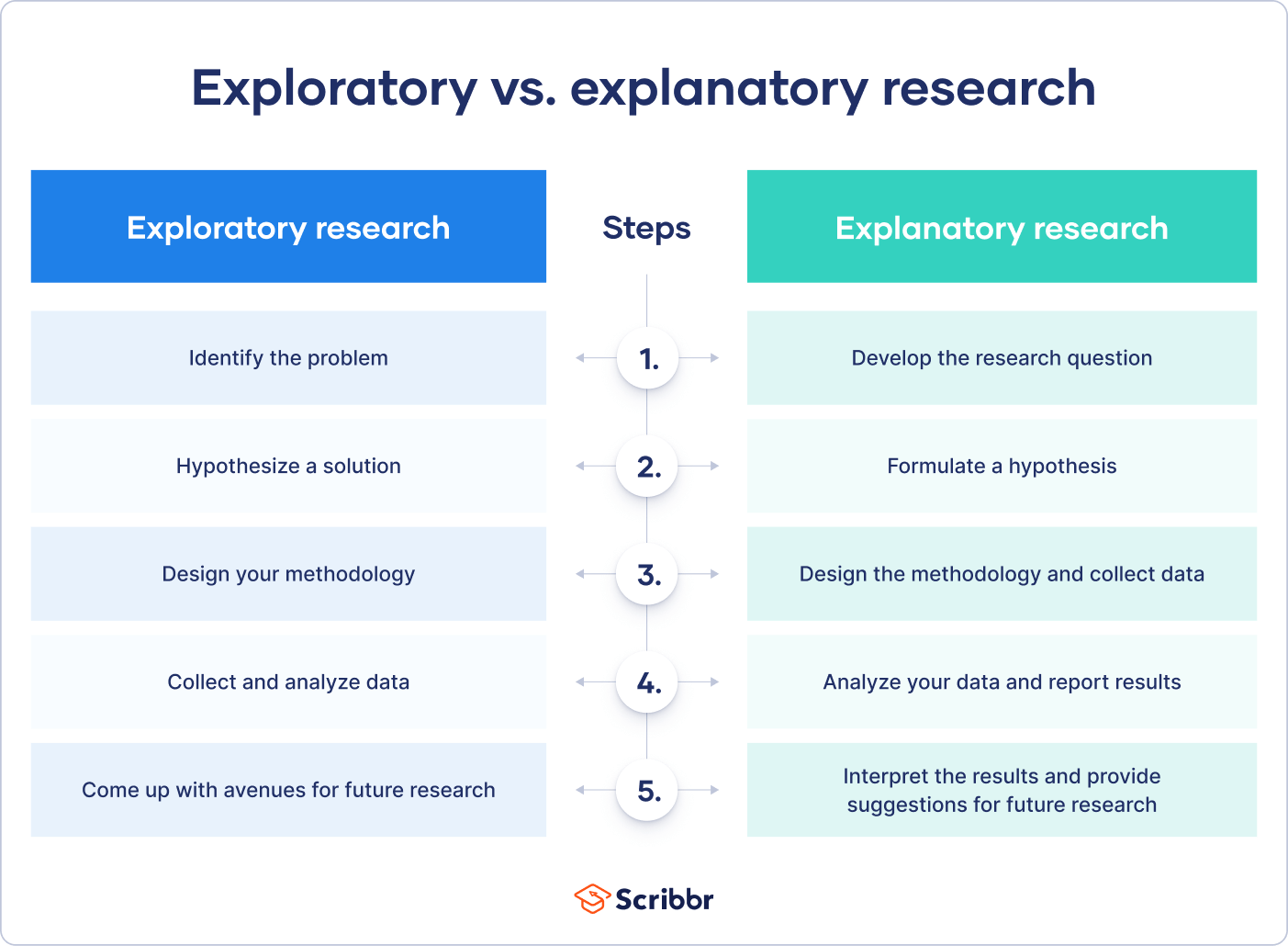
Like any other research design , explanatory research has its trade-offs: while it provides a unique set of benefits, it also has significant downsides:
- It gives more meaning to previous research. It helps fill in the gaps in existing analyses and provides information on the reasons behind phenomena.
- It is very flexible and often replicable , since the internal validity tends to be high when done correctly.
- As you can often use secondary research, explanatory research is often very cost- and time-effective, allowing you to utilize pre-existing resources to guide your research prior to committing to heavier analyses.
Disadvantages
- While explanatory research does help you solidify your theories and hypotheses, it usually lacks conclusive results.
- Results can be biased or inadmissible to a larger body of work and are not generally externally valid . You will likely have to conduct more robust (often quantitative ) research later to bolster any possible findings gleaned from explanatory research.
- Coincidences can be mistaken for causal relationships , and it can sometimes be challenging to ascertain which is the causal variable and which is the effect.
If you want to know more about statistics , methodology , or research bias , make sure to check out some of our other articles with explanations and examples.
- Normal distribution
- Degrees of freedom
- Null hypothesis
- Discourse analysis
- Control groups
- Mixed methods research
- Non-probability sampling
- Quantitative research
- Ecological validity
Research bias
- Rosenthal effect
- Implicit bias
- Cognitive bias
- Selection bias
- Negativity bias
- Status quo bias
Explanatory research is a research method used to investigate how or why something occurs when only a small amount of information is available pertaining to that topic. It can help you increase your understanding of a given topic.
Exploratory research aims to explore the main aspects of an under-researched problem, while explanatory research aims to explain the causes and consequences of a well-defined problem.
Explanatory research is used to investigate how or why a phenomenon occurs. Therefore, this type of research is often one of the first stages in the research process , serving as a jumping-off point for future research.
Quantitative research deals with numbers and statistics, while qualitative research deals with words and meanings.
Quantitative methods allow you to systematically measure variables and test hypotheses . Qualitative methods allow you to explore concepts and experiences in more detail.
Cite this Scribbr article
If you want to cite this source, you can copy and paste the citation or click the “Cite this Scribbr article” button to automatically add the citation to our free Citation Generator.
George, T. & Merkus, J. (2023, November 20). Explanatory Research | Definition, Guide, & Examples. Scribbr. Retrieved September 16, 2024, from https://www.scribbr.com/methodology/explanatory-research/
Is this article helpful?
Tegan George
Other students also liked, exploratory research | definition, guide, & examples, what is a research design | types, guide & examples, qualitative vs. quantitative research | differences, examples & methods, "i thought ai proofreading was useless but..".
I've been using Scribbr for years now and I know it's a service that won't disappoint. It does a good job spotting mistakes”
- Undergraduate Admission
- Student Affairs
- Events Calendar
- George W. Bush Presidential Center
- Prospective Students
- Current Students
- Information for Faculty & Staff
What is a Digital Twin?
SMU Lyle’s new Center for Digital and Human-Augmented Manufacturing explores how digital twin technology will shape the future of manufacturing – but what is it, how does it work, and what are the benefits?

- X (formerly Twitter)
Imagine if you could create a digital copy of yourself. The virtual replica – or digital twin – would be like you in every way, except with a superpower: any mistakes you make would have no real-life impact. You could simply study the consequences and learn from them.
Decision making in your life would suddenly become a whole lot easier.
Now imagine owning a business and applying that same concept to your operations. You could run simulations, better understand and optimize processes, and make predictions about the future – all in a risk-free environment. Add artificial intelligence (AI) and machine learning into the equation, and now your digital twin can be fed real-time data and transform into a living, dynamic model that helps you infinitely improve.
This is the power of a digital twin, or more simply stated, a virtual replica of a real-world object, system, or process that can evolve in real time through data. While not a brand-new concept, digital twins are quickly gaining traction and becoming more powerful though increased sophistication in AI. Seventy percent of C-suite technology executives at large enterprises are already exploring and investing in digital twins to drive more agile business operations.
“The future of manufacturing is digital connectedness and moving toward total automation – that’s where the digital twin comes in,” said Chris Colaw, the Co-Executive Director of SMU Lyle’s new Center for Digital and Human-Augmented Manufacturing and Associate Professor of Practice in Mechanical Engineering. “You can gain a competitive edge in industry by integrating digital technologies that reduce costs, improve efficiencies, optimize workflows and give you enhanced insight into your manufacturing processes through sensors and data.”
Learn more about SMU Lyle's new Center for Digital and Human-Augmented Manufacturing
Colaw – an SMU Lyle alum – has spent his career at Lockheed Martin exploring how digital transformation and automation can revolutionize manufacturing. His efforts have culminated in more than $200M in cost savings for the company and improved safety and efficiency.
At SMU Lyle, Colaw is now helping students, researchers and industry professionals better understand how to harness digitalization and data-driven decision-making for the future of manufacturing. SMU is uniquely positioned to support this research through its NVIDIA DGX SuperPOD™ that provides high-performance AI supercomputing power.
“Pushing the limits of Industry 4.0 capabilities and showing the world how you can integrate these technologies is a valuable skill, especially for an emerging workforce of future-ready, resilient engineers at SMU Lyle,” Colaw said.
What is a digital twin, and how does it work?
Digital twins are not like traditional, static 3D models. They’re continuously learning, updating, and optimizing through data from the real-world object, system, or process they’re “twinning” with.
The real-world object is outfitted with sensors that produce data, which is applied to the digital twin. The digital model then can be used to conduct simulations, predict problems before they happen, explore new opportunities, and plan for the future. Users can interact with the digital model through augmented and virtual reality.
For example, SMU Lyle’s Mustang Rocketry Club used digital twin technology to project the trajectory of their rocket in the Spaceport America Cup rocket engineering competition. Alexander Brandt, Ph.D. student in Mechanical Engineering , created Mustang Rocketry’s digital twin using a computer-aided design model that could be visualized to scale with virtual and augmented reality, along with simulation software regarding the rocket's kinetics and kinematics.
“It’s more than just a simulation,” Brandt said. “It allows us to accurately represent something in the physical world and know cohesively that this rocket is going to do what we think it’s going to do. I have the data from the first launch that I can now bring back to the digital twin and update to continuously improve the model with subsequent launches.”
Colaw is also researching how to digitize human-based inspection of aircraft. Typically, a mechanic would check every crevice for foreign objects or defects with an inspector following behind. With digital twin technology, workers could be alerted immediately when an AI camera detects a foreign object or defect, and the virtual model would indicate its exact location.
“You need to-the-millimeter precision on the location of aircraft defects,” Colaw said. “That’s where the digital twin comes in. You can also simulate how a foreign object migrated into the aircraft. Objects that should not be there can have serious consequences – like blocking a fuel filter and causing a crash.”
Digital twin benefits
The possibilities for digital twin technology are vast and exciting, including:
- Monitoring equipment : tracking performance and predicting failures before they happen
- Designing products: testing product designs in a virtual environment before production
- Personalizing medicine: developing individualized treatments and predict outcomes
- Managing infrastructure: maintaining roads, bridges, and utilities
- Optimizing the energy grid: simulating natural disasters and predicting loads and failures
- Testing vehicle designs: evaluating performance virtually before manufacturing
- Creating virtual classrooms: developing digital environments for immersive learning
“We’re excited to bring digital twin research to SMU and the Dallas area through our Center for Digital and Human-Augmented Manufacturing,” said Adel Alaeddini, Ph.D., the center’s Executive Director of Research Innovation and professor of Mechanical Engineering at SMU Lyle. “Engineers in our future workforce will need to think and work much differently than they do today, integrating AI and machine learning with traditional mechanical engineering. Our center will provide a space to explore the possibilities.”
About the Bobby Lyle School of Engineering SMU's Lyle School of Engineering thrives on innovation that transcends traditional boundaries. We strongly believe in the power of externally funded, industry-supported research to drive progress and provide exceptional students with valuable industry insights. Our mission is to lead the way in digital transformation within engineering education, all while ensuring that every student graduates as a confident leader. Founded in 1925, SMU Lyle is one of the oldest engineering schools in the Southwest, offering undergraduate and graduate programs, including master’s and doctoral degrees.
About SMU SMU is the nationally ranked global research university in the dynamic city of Dallas. SMU’s alumni, faculty and nearly 12,000 students in eight degree-granting schools demonstrate an entrepreneurial spirit as they lead change in their professions, community and the world.
Primary navigation menu
Search the smu website, popular searches.
Request info
Schedule a visit

IMAGES
VIDEO
COMMENTS
Placing research in the bigger context of its field and where it fits into the scientific process can help people better understand and interpret new findings as they emerge. A single study usually uncovers only a piece of a larger puzzle. Questions about how the world works are often investigated on many different levels.
Research is defined as a meticulous and systematic inquiry process designed to explore and unravel specific subjects or issues with precision. This methodical approach encompasses the thorough collection, rigorous analysis, and insightful interpretation of information, aiming to delve deep into the nuances of a chosen field of study.
Research is "creative and systematic work undertaken to increase the stock of knowledge". [1] It involves the collection, organization, and analysis of evidence to increase understanding of a topic, ... Another definition of research is given by John W. Creswell, who states that "research is a process of steps used to collect and analyze ...
They may also work with one or more scientists with different areas of expertise to approach the question from other angles and get a more complete answer to their question. ... Nice basic explanation. I believe informing the lay public on how science works, how parts of the body interact, etc. is a worthwhile endeavor. ... "Meta-analysis is ...
Research is the careful consideration of study regarding a particular concern or research problem using scientific methods. According to the American sociologist Earl Robert Babbie, "research is a systematic inquiry to describe, explain, predict, and control the observed phenomenon. It involves inductive and deductive methods.".
Research methods are specific procedures for collecting and analyzing data. Developing your research methods is an integral part of your research design. When planning your methods, there are two key decisions you will make. First, decide how you will collect data. Your methods depend on what type of data you need to answer your research question:
A research design is a strategy for answering your research question using empirical data. Creating a research design means making decisions about: Your overall research objectives and approach. Whether you'll rely on primary research or secondary research. Your sampling methods or criteria for selecting subjects. Your data collection methods.
Definition, Types, Methods, and Examples. Academic research is a methodical way of exploring new ideas or understanding things we already know. It involves gathering and studying information to answer questions or test ideas and requires careful thinking and persistence to reach meaningful conclusions. Let's try to understand what research is.
Step 4: Create a research design. The research design is a practical framework for answering your research questions. It involves making decisions about the type of data you need, the methods you'll use to collect and analyze it, and the location and timescale of your research. There are often many possible paths you can take to answering ...
Research is the deliberate, purposeful, and systematic gathering of data, information, facts, and/or opinions for the advancement of personal, societal, or overall human knowledge. Based on this definition, we all do research all the time. Most of this research is casual research. Asking friends what they think of different restaurants, looking ...
Definition: Research Process is a systematic and structured approach that involves the collection, analysis, and interpretation of data or information to answer a specific research question or solve a particular problem. ... to provide a theoretical framework for the research and also ensures that the research is not duplicating previous work ...
INTRODUCTION. Scientific research is usually initiated by posing evidenced-based research questions which are then explicitly restated as hypotheses.1,2 The hypotheses provide directions to guide the study, solutions, explanations, and expected results.3,4 Both research questions and hypotheses are essentially formulated based on conventional theories and real-world processes, which allow the ...
Research conducted for the purpose of contributing towards science by the systematic collection, interpretation and evaluation of data and that, too, in a planned manner is called scientific research: a researcher is the one who conducts this research. The results obtained from a small group through scientific studies are socialised, and new ...
Abstractspiepr Abs1. Every day people do research as they gather information to learn about something of interest. In the scientific world, however, research means something different than simply gathering information. Scientific research is characterized by its careful planning and observing, by its relentless efforts to understand and explain ...
Definition: Research Paper is a written document that presents the author's original research, analysis, and interpretation of a specific topic or issue. It is typically based on Empirical Evidence, and may involve qualitative or quantitative research methods, or a combination of both. The purpose of a research paper is to contribute new ...
Doing research is stimulating and fulfilling work. Scientists make discoveries to build knowledge and solve problems, and they work with other dedicated researchers. Research is a highly complex activity, so it takes years for beginning researchers to learn everything they need to know to do science well.
Example: A researcher examines if and how employee satisfaction changes in the same employees after one year, three years and five years with the same company. 16. Mixed research. Mixed research includes both qualitative and quantitative data. The results are often presented as a mix of graphs, words and images.
Research Summary. Definition: A research summary is a brief and concise overview of a research project or study that highlights its key findings, main points, and conclusions. It typically includes a description of the research problem, the research methods used, the results obtained, and the implications or significance of the findings.
Choose a research paper topic. Conduct preliminary research. Develop a thesis statement. Create a research paper outline. Write a first draft of the research paper. Write the introduction. Write a compelling body of text. Write the conclusion. The second draft.
In fact, descriptive research has many useful applications, and you probably rely on findings from descriptive research without even being aware that that is what you are doing. See Table 3.1 for examples. Explanatory Research. The third type of research, explanatory research, seeks to answer "why" questions.
If you want to learn how to write your own plan for your research project, consider the following seven steps: 1. Define the project purpose. The first step to creating a research plan for your project is to define why and what you're researching. Regardless of whether you're working with a team or alone, understanding the project's purpose can ...
The rationale of the study is the justification for taking on a given study. It explains the reason the study was conducted or should be conducted. This means the study rationale should explain to the reader or examiner why the study is/was necessary. It is also sometimes called the "purpose" or "justification" of a study.
The protocol consists of five main stages that have been successfully applied to various research problems: formal problem definition, data preprocessing, modeling, evaluation and clinical ...
The group's work is ongoing and done in collaboration with the Center for Neural Basis of Cognition, a cross-university research and educational program between Carnegie Mellon and the University of Pittsburgh that leverages each institution's strengths to investigate the cognitive and neural mechanisms that give rise to biological ...
Explanatory Research | Definition, Guide, & Examples. Published on December 3, 2021 by Tegan George and Julia Merkus. Revised on November 20, 2023. Explanatory research is a research method that explores why something occurs when limited information is available. It can help you increase your understanding of a given topic, ascertain how or why a particular phenomenon is occurring, and predict ...
Imagine if you could create a digital copy of yourself. The virtual replica - or digital twin - would be like you in every way, except with a superpower: any mistakes you make would have no real-life impact. You could simply study the consequences and learn from them. Decision making in your ...
Despite an increasing interest in understanding the mindset of entrepreneurs, little consensus exists on what an entrepreneurial mindset (EM) is, how it is developed, or its precise outcomes. Given the fragmented nature of the multidisciplinary study of EM, we review prior work in an effort to enhance scholarly progress. To this end, we identify and review 61 publications on the topic and ...