Thank you for visiting nature.com. You are using a browser version with limited support for CSS. To obtain the best experience, we recommend you use a more up to date browser (or turn off compatibility mode in Internet Explorer). In the meantime, to ensure continued support, we are displaying the site without styles and JavaScript.
- View all journals
- Explore content
- About the journal
- Publish with us
- Sign up for alerts
- Open access
- Published: 29 March 2019

Over-exploitation of natural resources is followed by inevitable declines in economic growth and discount rate
- Adam Lampert ORCID: orcid.org/0000-0001-8115-6688 1 , 2
Nature Communications volume 10 , Article number: 1419 ( 2019 ) Cite this article
96k Accesses
76 Citations
15 Altmetric
Metrics details
- Environmental economics
- Sustainability
A major challenge in environmental policymaking is determining whether and how fast our society should adopt sustainable management methods. These decisions may have long-lasting effects on the environment, and therefore, they depend critically on the discount factor, which determines the relative values given to future environmental goods compared to present ones. The discount factor has been a major focus of debate in recent decades, and nevertheless, the potential effect of the environment and its management on the discount factor has been largely ignored. Here we show that to maximize social welfare, policymakers need to consider discount factors that depend on changes in natural resource harvest at the global scale. Particularly, the more our society over-harvests today, the more policymakers should discount the near future, but the less they should discount the far future. This results in a novel discount formula that implies significantly higher values for future environmental goods.
Similar content being viewed by others
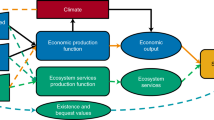
Use and non-use value of nature and the social cost of carbon
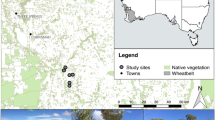
Balancing the books of nature by accounting for ecosystem condition following ecological restoration
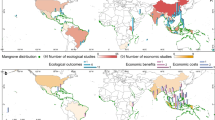
A meta-analysis of the ecological and economic outcomes of mangrove restoration
Introduction.
The exploitation of ecosystems by humans has long-lasting consequences for the future provision of natural resources and ecosystem services 1 , 2 . This may negatively affect the provision of food, increase health hazards and risks of natural disasters, and more. Degraded ecosystems may be slow to recover or may not recover naturally even after their exploitation stops 3 , 4 , 5 . Consequently, the availability of natural resources such as food, clean air, and other ecosystem services, may be adversely impacted for extended periods if the ecosystems providing these resources become degraded. For example, the emission of greenhouse gases may affect the global climate for centuries 6 , 7 ; invasive species and diseases may irreversibly damage ecosystems 8 , 9 ; and the non-sustainable harvest of fisheries and forests may leave these systems degraded for decades 2 , 4 , or even lead to their irreversible and permanent degradation 3 , 10 . Since natural resources are limited, it has been widely recognized that a transition to sustainable harvest is necessary 11 . What the optimal pathway and speed are for this transition, however, constitute the focus of an ongoing debate. For example, it has been suggested that an abrupt transition may slow economic growth in developing countries and may negatively affect production 12 , and that rapid emission cuts may create energy deficits before we manage to develop viable substitutes 13 .
Determining the optimal strategy for the adoption of sustainable management over time requires cost-benefit analyses. A common approach is to consider a social planner whose objective is to maximize social welfare 14 , 15 , 16 . This is often formalized as maximizing a net present value,
where B ( t ) is the benefit minus the cost (in units of consumption) due to both the management and the environment at time t , and Δ( t ) is the cumulative discount. In turn, the discount factor, exp(−Δ( t )), is the number of units of some good or currency needed at present to compensate for the lack of one unit at time t . The rationale behind discounting is that the objective of our society is to maximize welfare rather than net consumption. In turn, if society is going to be wealthier in the future, then one unit of consumed goods in the future may add less to welfare than the same unit today 14 , 15 , 16 .
Accurate discounting is particularly important for environmental policies in which the resultant damages are long-term, such as policies concerning climate change and provision of natural resources 6 , 17 , 18 . Specifically, a small difference in the discount may lead to a large difference in estimates of long-term environmental cost. For example, consider no changes in prices and a constant annual discount rate, δ ≡ dΔ/d t . Then, if the cost due to losing some good today is $1M, then the cost due to losing the exact same good (no depreciation) 100 years from now is ~$50K if δ = 3%, and only ~$2.5K if δ = 6%. Therefore, even the best estimates of environmental damages may lead to an inadequate policy if we are unable to accurately convert future costs to their present-equivalent dollar value.
The central role that discounting plays in the valuation of natural resources has led to extensive debates over the value that policymakers should use for the discount rate and over how this value varies over time. Specifically, the small values given to future environmental goods due to discounting may contradict our intuition that our society should sustain our planet’s ecosystems for future generations. One major debate followed the publication of the Stern report 6 , which used a discount rate that is smaller than those used in previous major assessments, and consequently, argued for radical emission cuts. The bulk of the criticism 19 has focused on which discount rate should policymakers use (not on the comprehensive cost assessments). Also, several authors 16 , 20 , 21 , 22 , 23 proposed that policymakers should use a discount rate that declines over time, and they showed that this is justified if future economic growth is uncertain. Another mechanism that could affect the discount rate is a large perturbation that significantly affects social welfare 24 , 25 , such as an environmental degradation that may occur due to climate change or over-harvesting 26 , 27 , 28 , 29 . Particularly, several authors showed that global changes in the provision of non-substitutable natural resources might affect their relative prices 30 , 31 and the discount rate 25 , 32 . Nevertheless, these authors considered the changes in the provision of natural resources as given, while the long-term consequences of harvesting on economic growth and discount rate remain largely unknown.
In this paper, we examine how the discount rate and factor are affected by large changes in the harvest methods used at the global scale, such as the transition from over-harvesting to harvesting sustainably. Specifically, the decline in the provision of natural resources due to the future transition might be so large that it will significantly affect social welfare and economic growth. In turn, since discount rates depend on welfare and growth, this means that the discount rate itself could be affected. Revealing harvest-induced changes in the discount will provide policymakers with better evaluations of long-term benefits and costs, thereby enabling them to improve long-term environmental policies. We focus on the harvest of renewable resources in a broad sense, where non-sustainable harvest suppresses the future provision of the resource or the ecosystem service. Examples include the over-harvesting of fish and timber that degrades fisheries and forests 10 , and non-sustainable agriculture and land-use that make future land-use less effective 33 , 34 . We show that over-harvesting temporarily keeps the discount rate higher, but is followed by a period of lower discount rates during the same period in which society makes the transition to sustainable harvesting. Specifically, during the transition, the rates of economic growth and discount could be much lower than their rates before and after the transition. Therefore, the more our society over-harvests natural resources today, the more policymakers should discount the near future, but the less they should discount the far future. Furthermore, we prove a theorem implying that postponing or slowing the transition to sustainable harvesting cannot prevent the ultimate declines in the cumulative discount. Accordingly, we develop a discount formula that incorporates the changes in the harvest methods, which, in turn, dictates significantly higher net costs due to long-lasting environmental damages.
Theoretical framework
We consider a social welfare function, U T , that depends on the provision of some natural resource at the global scale, f ( t ), and on the consumption of the other goods, including manufactured goods, c ( t ) (Methods, Eq. 4 ). In turn, the dynamics of the c ( t ) and f ( t ), together with U T , determine the social rate of discount, δ ( t ), which specifies the rate at which goods should be discounted by a social planner whose objective is to maximize social welfare 15 , 16 , 35 . To define the social rate of discount (hereafter, the discount rate), we adopt a well-established framework 12 , 14 , 16 , 32 , 36 , 37 and we assume that it is given by the rate of decline in the marginal contribution of consumption to social welfare (consumption rate of discount). Specifically, we consider a given currency unit, a dollar, that enables the consumption of exactly με units of the natural resource and (1 − μ ) ε units of the other goods, where 0 ≤ μ ≤ 1 and ε is very small. Accordingly, the discount factor at time t is given by the number of dollars needed at present to compensate for a lack of one dollar at time t . Note that the choice of μ does not affect the value given to future goods, and therefore, it does not affect the policy and/or the management decisions; rather, μ determines the units and it affects only the relative role of the discount factor and the prices in determining the value of future goods 36 , 37 . In turn, we show that this implies that the discount rate, δ ( t ), and the cumulative discount \({\mathrm{\Delta }}(t) = {\int}_0^t \delta \left( {t\prime } \right){\mathrm{d}}t\prime\) , are given by Eq. 5 , and the prices of the natural resource and of the other goods are given by Eq. A10 (Methods and Supplementary Note 1). Specifically, the discount rate and the prices depend on the substitutability of the natural resource and the other goods, which is incorporated in the social welfare function. In Supplementary Note 2, we derive specific expressions for the discount rate and for the prices in two cases, one in which the natural resource and the other goods are non-substitutable (Eqs. B5 , B9 ), and one in which they are partially substitutable (Eqs. B12 , B15 , B16 ).
In turn, the novel part of this study comes from endogenizing the dynamics of c ( t ) and f ( t ) by modeling how they depend on the harvest methods used globally (see Methods). This allows us to examine how the discount factor and the prices depend on changes in harvest methods. We assume that, if the harvest methods do not change, then c ( t ) and f ( t ) increase exponentially at fixed rates, g c and g f , respectively, due to exogenous factors such as technological developments and exogenous environmental changes; however, changes in the patterns of harvest may affect c ( t ) and f ( t ), thereby affecting the discount rate over time (see Methods). This approach builds on and generalizes previous studies that considered f ( t ) and c ( t ) that grows exponentially irrespective of the harvest 32 , 37 . Specifically, note that c ( t ) and f ( t ) characterize the total provision of the goods at the global scale, and accordingly, we consider a large ecosystem that comprises a large number of distinct regions (Fig. 1 ). This ecosystem may be, for example, the entire planet’s aquatic ecosystem, where each region is some local fishery providing fish; the forest area on a given continent, where each region is a single forest providing timber; or the area that can be used for agriculture worldwide, where each region is a local geographic area comprised of agricultural fields. We are interested in the long-lasting effects of harvesting on the provision of the natural resource, and therefore, we focus on irreversible degradations of the ecosystem, rather than on temporary fluctuations of the resource stock. These degradations may occur, for example, if some ecosystem services are permanently lost 5 or if the ecosystem that provides the renewable resource collapses or undergoes an irreversible regime shift in some of its regions, such as occurs in eutrophication and deforestation 3 , 4 , 10 . We assume that higher rates of non-sustainable harvest (higher H n ) result in a greater provision of the natural resource at the time of harvest but also result in a higher degradation of the ecosystem (Eq. 6 , see Methods). Specifically, we assume that a given portion of the global ecosystem, H ( t ), is being harvested in year t , while some portion of the ecosystem, H n ( t ), becomes degraded during that year due to non-sustainable harvest, and cannot be used for harvest thereafter (Fig. 1 ). For example, H n ( t ) may characterize the portion of the global fish or timber stock that is lost due to the collapse of fisheries or the irreversible degradation of forests worldwide in year t 38 . For another example, H n ( t ) may characterize the persistent reduction in the yield of crop caused by the degradation of vital ecosystem services and the increase in the persistence of pests 33 , 34 . In turn, H ( t ) and H n ( t ) are determined by the various harvest methods used in the system (see Methods).
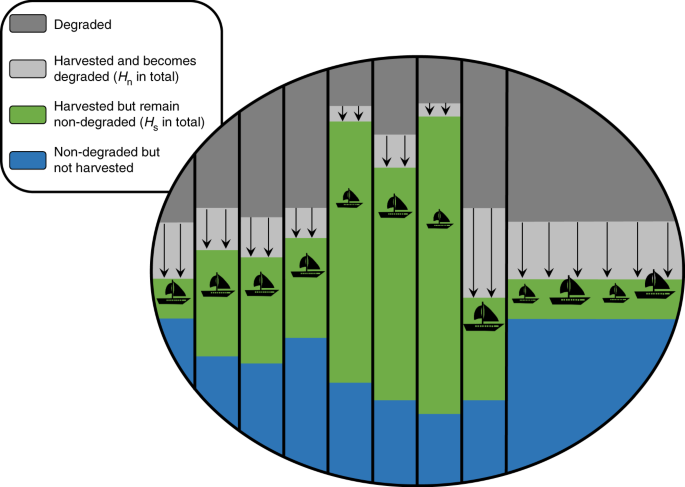
Schematic illustration of the model. Demonstrated is the state of the system at the global scale (e.g., the entire planet’s marine area, forest area, or agricultural area) in a given year. The dark-gray area characterizes the part of the system that is degraded due to former non-sustainable harvesting. The light gray area with the arrows characterizes the part of the system that is being harvested non-sustainably and will be degraded starting next year (total dark-gray area is given by H n ). The green area with the fishing vessels characterizes the part of the system that is being harvested sustainably and will remain non-degraded next year (total green area is given by H s ). (Note that the total area under harvest, H , is given by the green and the light gray areas combined, H = H n + H s ). The blue area characterizes the part of the system that is not degraded but is still not being harvested. We assume that the spatial scale of the system is very large, and therefore, the recovery of the degraded areas due to migrating biota from other regions is negligible and the total degraded area increases over time. Each year, H n and H s are determined by the aggregate management by all the managers. We assume that managers may be subject to different externalities in distinct regions, e.g., some regions are managed by a single manager that dictates the harvest method, while some regions are shared (open-access), and all managers are free to harvest in them (rightmost region). The variables x 1 and x 2 (Eqs. 7 and 8 ) characterize the total non-degraded areas (blue, green, and light gray) in the managed and in the shared regions, respectively
To examine the effect of over-harvesting on the natural resource and on the discount rate, we compare scenarios in which over-harvesting occurs to scenarios in which it does not. We consider two approaches. First, we consider a competitive market approach in which we compare the optimal solution that maximizes social welfare with the solution that emerges in a model of a perfectly competitive market with externalities (Figs. 2 and 3 ). Specifically, the competitive market includes managed regions that have a single manager (e.g., landowner, government), and shared regions in which multiple managers are free to harvest (e.g., open-access) (see Methods). Second, we consider a more general approach in which we compare the dynamics that emerge when the harvest is entirely sustainable with the dynamics that emerge following various ad hoc choices of non-sustainable harvest functions (Theorem and Fig. 4 ).
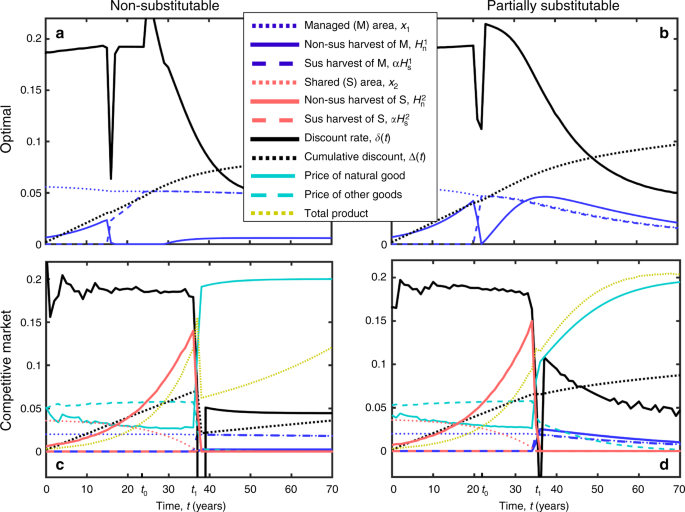
Over-harvesting extends the period during which the discount rate is high, but it is followed by sharp declines in the discount rate and the cumulative discount. Panels a and b demonstrate the optimal harvest of the natural resource from a social planner’s perspective, where the natural resource and the other goods are either non-substitutable ( a , Eq. B2 ) or partially substitutable ( b , Eq. B10 ). In the early stages, harvesting activity increases exponentially and the discount rate is high. Approximately at time t 0 , when harvesting is occurring in the whole system ( H s + H n = x 1 + x 2 ), the total harvest stops increasing and the discount rate decreases. Next, panels c and d demonstrate harvesting in a competitive market in which some of the regions are shared. The parameters and utility functions used in panels c and d are identical to those used in panels a and b , respectively. The period during which the discount rate is high is extended until t = t 1 due to over-harvesting of the natural resource in the shared regions (compare panel a with panel c , and compare panel b with panel d ). However, this period is followed by a rebound in which harvesting declines and the discount rate and the cumulative discount drop. In addition, around t = t 1 , the price of the natural resource increases and the total product decreases. Note that, in accordance with the theorem, the cumulative discount approaches lower values if the harvest is determined by the market. Scaling: the harvest rates are given in (years) −1 , the total non-degraded areas are given in units showing the maximal annual sustainable yield ( ax 1 and ax 2 ), and Δ is given by 100 times the value on the y -axis. The parameter values used are within their realistic ranges (Methods). Parameter values and Source data are provided as a Source Data file
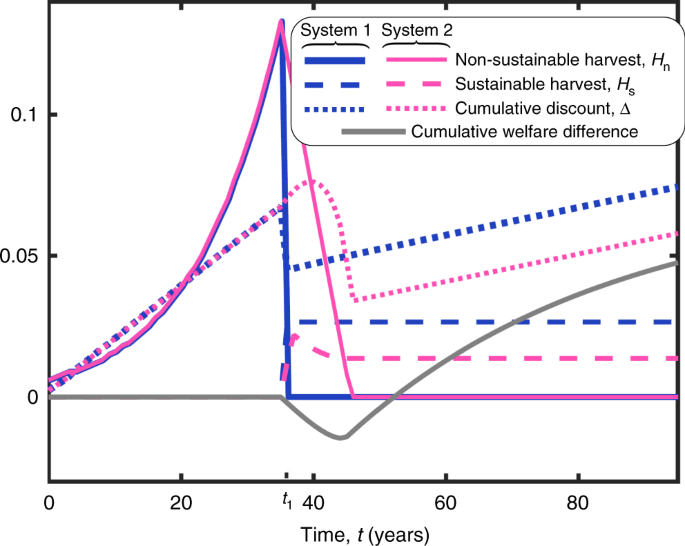
Social welfare and the cumulative discount are ultimately lower if the transition to sustainable harvest is more gradual. Demonstrated are the aggregate non-sustainable harvest, H n ( t ) (solid lines); the aggregate sustainable harvest, H s ( t ) (dashed lines); and the cumulative discount, Δ (dotted lines), for two systems. System 1 (blue) follows the market solution, in which society abruptly stops harvesting non-sustainably at t = t 1 . System 2 (purple) follows the same dynamics until t = t 1 , but then, society gradually shifts to sustainable harvest. The gradual transition postpones the decline in the cumulative discount, but ultimately, it declines to an even lower value than its value in system 1. Moreover, the cumulative welfare, U t , in system 1 is initially smaller, but it ultimately becomes greater compared to system 2 (gray). Harvest rates are given in units of (years) −1 , and Δ is given by 100 times the value on the y -axis. The parameters are the same as in Fig. 2c (Parameter values and Source data are provided as a Source Data file)
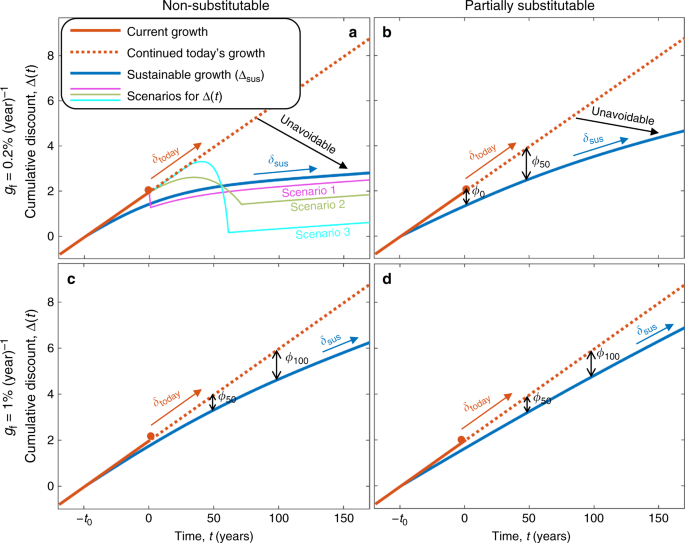
The decline in the cumulative discount is unavoidable (demonstration of the theorem). At some point in time, − t 0 , some planetary boundaries for harvest have been approached, and the rate of discount that would have occurred if managers used only sustainable harvesting has decreased from δ today to δ sus (blue lines, Δ sus ). Nevertheless, due to over-harvesting, the economy grew faster and the cumulative discount, \({\mathrm{\Delta }}(t) = {\int}_0^t \delta (t{\prime}){\mathrm{d}}t{\prime}\) , continued to grow at a higher rate, δ today (solid orange lines), at least until today ( t = 0). In turn, the future value of Δ( t ) depends on the future harvest patterns. If over-harvesting continues, the discount rate might remain close to δ today for several years or decades (dotted orange lines). But in the longer run, according to the theorem, Δ has to decrease below the blue curve that characterizes Δ sus , regardless of how the resource is being harvested. This is also demonstrated for three scenarios in panel a : In scenario 1, the non-sustainable harvest stops today, while in scenarios 2 and 3, the non-sustainable harvest continues for a few decades and then declines gradually. Also, note that Δ sus increases at a rate δ sus , so if one assumes that the discount rate remains δ today for the next τ years and becomes δ sus afterward, then he/she needs to subtract at least ϕ τ to obtain the correct Δ (Eqs. 2 and 3 ). (The value of ϕ τ is demonstrated in Fig. 5 .) We assume that u ( c , f ) is given by Eq. B5 (non-substitutable goods) in panels a and c , and by Eq. B12 (partially substitutable goods) in panels b and d . In turn, the scenarios are calculated for three different choices of H n ( t ), where the dynamics follow Eqs. 6 – 9 with H ( t ) = x 1 ( t ) + x 2 ( t ) for all t . The parameter values used are within their realistic ranges (Methods). Parameter values and Source data are provided as a Source Data file
Over-harvesting is followed by declines in the discount rate
Following the optimal solution in which the harvest functions maximize social welfare, two phases emerge along the time axis (Fig. 2a, b ). In the first phase ( t < t 0 ), c ( t ) is initially small, and the harvest rates are limited due to the direct cost of harvesting (Methods, Eq. 9 ). Over time, as c ( t ) increases, the direct cost plays a less significant role, and the harvest rates increase. Consequently, f ( t ) increases at a rate that is greater than g f , and the discount rate approximately follows Ramsey’s formula. In the second phase ( t > t 0 ), the entire ecosystem is under harvest (either sustainable or non-sustainable). Therefore, the society cannot increase f via harvesting without increasing the non-sustainable harvest (i.e., increasing H n ), which would negatively affect the resource’s future provision. Consequently, the non-sustainable harvest decreases exponentially and c ( t ) and f ( t ) increase at approximately the rates of their technological developments, namely, \(\dot c/c \approx g_{\mathrm{c}}\) and \(\dot f/f \approx g_{\mathrm{f}}\) . This implies that, if g f < g c , the discount rate in the second phase is lower than it was in the first phase (Eqs. B6 , B13 , Supplementary Note 2 ). Note that the optimal solution comprises non-sustainable harvest ( H n > 0) because an increase in f at a given time has a greater effect on welfare than the same increase at a later time; the lower the discount rate, the lower the rate of non-sustainable harvest.
In turn, in the competitive market solution (see Methods), the rate of non-sustainable harvest is higher than the socially optimal rate, namely, the solution exhibits over-harvesting (Fig. 2c, d ). Specifically, the harvest is still primarily sustainable in the managed regions but is non-sustainable in the shared regions. The total area under (non-sustainable) harvest in the shared regions increases over time, and consequently, f ( t ) continues to increase over an extended period of time, which postpones the decline in the discount rate. Eventually, however, at time t = t 1 (Fig. 2 ), the shared regions become entirely degraded and the total rate of non-sustainable harvest declines. In turn, the period during which managers over-harvest ( t < t 1 ) is followed by declines in the discount rate, the cumulative discount (Δ), total production (Eq. A11 ), and the price of manufactured goods (Eq. A10 ). These declines are greater if the magnitude and/or duration of the over-harvesting are greater (e.g., if more regions are shared), and also if the natural and the manufactured goods are non-substitutable. Note that the optimal solution exhibits no declines in economic growth or in Δ because the social planner plans for the forthcoming constraints on the harvest by avoiding over-harvesting in the early stages ( t < t 0 ); in the market solution, managers also take into account the forthcoming decline in f and avoid non-sustainable harvesting in the managed regions prior to time t = t 1 , but they still over-harvest in the shared regions. Also note that, in both the optimal and the market solutions, the harvest functions, as well as c ( t ) and f ( t ), do not depend on μ (only the discount and the prices do).
Decline in the cumulative discount is unavoidable (theorem)
More generally, the following theorem shows that over-harvesting may result in an increase in Δ in the short run, but ultimately, Δ would return to a lower value than it would have had if managers used optimal harvesting or only sustainable harvesting (see proof in Supplementary Note 3 and demonstration in Figs. 3 and 4 ). Specifically, a more gradual transition to using sustainable harvest methods may result in a more gradual decline in Δ, but the ultimate magnitude of the decline must exceed that of the incline in Δ that occurred formerly due to the over-harvesting (Figs. 3 and 4a ). In particular, the theorem shows that the result is robust and does not depend on specific assumptions and parameters. It applies not only in the competitive market model but also in the more general case in which non-sustainable harvest is used instead of more sustainable harvest.
Theorem . Assume that the social welfare, U T , is given by Eq. 4 , where f(t) is given by Eq. 6 , and c(t) is given by Eq. 9 with C 1 = C 2 = constant (Methods). Also, assume that u(c,f) is monotonically increasing and twice differentiable with respect to both of c and f, and all of its second partial derivatives are non-positive (namely, an increase in c or f does not cause another increase to be more beneficial). In addition, we consider g f = 0 and assume that as c \(\rightarrow\) ∞ while f remains fixed, u c /u f \(\rightarrow\) 0 (the price of c approaches 0), u cc /u ff \(\rightarrow\) 0 and u cf /u ff \(\rightarrow\) 0. (Alternatively, we consider g f > 0 and assume that u satisfies the conditions of Lemmas 2B and 3). Finally, we assume that, for sufficiently large t, cu fc /u f and fu ff /u f are monotone with respect to t. (All these assumptions are satisfied if u is given by Eqs. B2 , B10 with η > 1, or various other standard forms 32 , 37 .)
Denote Δ opt as the cumulative discount (Eq. 5 ) that emerges following the optimal harvest. Namely, the non-negative harvest functions maximize social welfare (max U T subject to Eqs. 6 – 9 where T \(\rightarrow\) ∞; see Methods). Next, denote Δ market as the cumulative discount that emerges where the harvest functions are determined if each manager aims to maximize her/his own profit and the non-sustainable harvest may be higher than its socially optimal level (Methods). Then, there exists a time t c such that Δ market ≤ Δ opt for all t ≥ t c . Furthermore, denote Δ sus as the cumulative discount that emerges following optimal harvest while excluding non-sustainable harvest (H n = 0). Then, for any Δ that emerges if non-sustainable harvest occurs (H n (t) > 0) between times t 0 and t 1 , there exists t c > t 1 such that Δ(t c ) ≤ Δ sus (t c ) .
A new discount formula
The theorem shows that an upper bound on Δ( t ) in the long run is given by Δ sus ( t ), the cumulative discount that would have occurred if managers used only sustainable harvest, which increases at a rate given by δ sus (Fig. 4 and Supplementary Note 2). Also, the present value of Δ sus is below Δ because over-harvesting already has occurred prior to today. Specifically, ϕ 0 = Δ(0) − Δ sus (0) reflects the negative shock to Δ that must occur during the transition to sustainable harvest methods due to the prior over-harvesting. It follows that, if t is sufficiently large and δ sus is constant, then
Particularly, if the discount rate has been δ today > δ sus due to non-sustainable harvest during the last t 0 years, and if δ today and δ sus have been constants, then ϕ 0 = ( δ today − δ sus ) t 0 .
The correction to the value of future goods is significant
Next, we calculate the correction to the value of future natural goods as dictated from Eq. 2 . Specifically, we compare the value dictated by the formula to the value dictated by a benchmark policy that assumes that the rate of increase in the provision of the natural resource would remain g c for the next τ years and decrease to g f thereafter 12 . Namely, this benchmark policy ignores the negative shock and simply uses a discount rate given by δ ( t ) = δ today if t ≤ τ and δ ( t ) = δ sus if t > τ . In turn, we would like to calculate the correction to that policy due to the negative shock to Δ. Note that the inevitable decline in the future value of the cumulative discount, ϕ 0 (Eq. 2 ), is what policymakers need to incorporate due to the over-harvesting that has already occurred before t = 0. But if the discount rate remained δ today for the next τ years, until t = τ , then the lower bound on the negative shock, ϕ τ , would be greater than ϕ 0 and given by (Fig. 4 )
This greater shock would compensate for the τ years with the higher discount, such that, in the long run, Δ( t ) would still satisfy Eq. 2 . Note that the shock may be gradual and spread over many years, but this decline in Δ( t ) eventually occurs (Theorem, Figs. 3 and 4 ).
Therefore, this shock implies that the correct discount factor should be greater by a factor of at least exp( ϕ τ ) compared to the one implied by the benchmark policy. Namely, ignoring this shock and simply considering the benchmark policy would result in underestimating the value of future natural goods by a factor of at least exp( ϕ τ ) (Fig. 5 ). In turn, the magnitude of ϕ τ depends on the substitutability of the natural resource and the other goods, as well as on the exogenous growth rates, g c and g f (Supplementary Note 2). For example, if the natural resource is non-substitutable (Eq. B2 ), then δ sus is given by Eq. B5 and ( δ today − δ sus ) \(\rightarrow\) η ( g c − g f ) as t \(\rightarrow\) ∞ (Eq. B7 ). Expressions that result from other utility functions are given in Supplementary Note 2 and in the literature 32 , 37 . These expressions enable us to quantify exp( ϕ τ ) and examine how it depends on the parameters (Fig. 5 ). For example, if g f = 1% year −1 , g c = 2% year −1 36 and τ = 50 years, then the value of future goods before the adjustment is underestimated by a factor greater than two (exp( ϕ τ ) > 2), and this factor is greater if g f is smaller or if τ is larger.
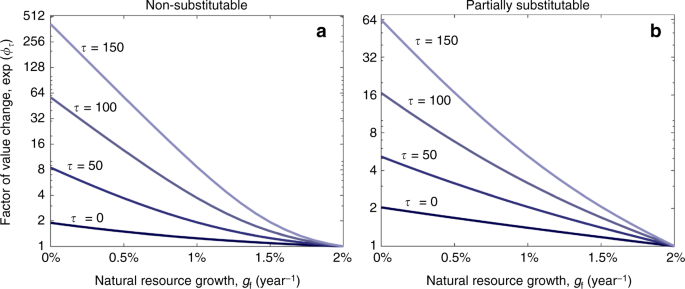
Endogenizing changes in harvest patterns implies a larger discount factor and higher values for future environmental goods. If a policymaker considers a gradual transition to sustainable harvest that would occur within τ years, then he/she may consider a sustainable discount rate, δ sus , starting from year τ . In addition, however, he/she needs to add to Δ another factor, ϕ τ , that accounts for the decline in the cumulative discount that will follow due to over-harvesting prior to time τ (Eqs. 2 , 3 and Fig. 4 ). This factor may impose significantly higher values on future goods, e.g., over two times higher if τ = 50 years and g f = 1% year −1 (exp( ϕ τ ) > 2 in both panels a and b ) and even significantly higher for higher values of τ or lower values of g f . However, if the long-term provision of the natural resource continues to increase at the same rate as the other goods, i.e., g f = g c = 2% year −1 , then δ sus = δ today and ϕ τ = 0 (Eq. 3 ). The other parameter values are the same as in Fig. 4 (Parameter values and Source data are provided as a Source Data file)
After over-harvesting for decades, many societies around the world are beginning to transition to sustainable environmental management practices and sustainable harvest methods 11 . Our study shows that the transition to sustainable harvest methods after a period of over-harvesting is expected to result in a decline in social welfare, economic growth, and the discount rate. In particular, we show that the discount rate, or the social rate of discount, does not decline gradually to its sustainable asymptotic rate; rather, the transition to sustainable harvest may include a period during which the discount rate is far below its asymptotic level (Figs. 2 – 4 and Theorem). Note that several studies suggested that policymakers need to consider discount rates that decline gradually over time due to various mechanisms, including uncertainty in technological growth 16 , 20 , 21 , 22 , 23 , slowdown in technological development due to environmental degradation 27 , 28 , and declining production due to decline in the exploitation of natural resources 12 . In contrast, we showed here that the transition to sustainable harvest imposes a sharper, non-gradual decline in the cumulative discount (Figs. 2 – 4 ). The mechanism underlying this sharper decline is that the rate of increase in the provision of natural resources not only slows down, but must at some point become lower than it would be if over-harvesting had never occurred. In turn, social welfare depends on the provision of natural resources, and therefore, a decline in their provision implies a lower discount rate.
Our results also suggest that the calculations of the discount factor in the long run should not rely on simple extrapolations of the discount rates in the short run. Specifically, over-harvesting might continue for a couple of decades, which may keep the provision of natural resources high in the short run, but will ultimately result in an even lower provision of these resources. Therefore, continued over-harvesting may justify considering higher discount rates in the short run, but it also necessitates discounting the long run less (Fig. 4a ). Ignoring the harvest-induced decline in the discount rate not only falsifies cost-benefit analyses, it also creates a bias: Over-harvesting increases the discount rate in the short run, which might unjustifiably bias the expectations of policymakers to anticipate higher future discount rates, which, in turn, is used to justify further exploitation. (This may also explain why policymakers should consider lower discount rates in the long run although there is no clear evidence that the rate of return on capital will decline during the next 30–40 years 15 .)
To correct for this bias and account for the future decline in the cumulative discount, we developed a new discount formula (Eqs. 2 and 3 ), which provides a simple way to estimate the increase in the present value of future goods due to the transition to sustainable harvest methods. Specifically, policymakers need to consider a cumulative discount, Δ( t ) (Eq. 1 ), that is lower in the long run due to its decline during the transition to sustainable harvest. Although further over-harvesting may postpone the timing of the decline, we prove in the theorem that the decline eventually comes with a rebound as Δ( t ) decreases even further: The more our society over-harvests, the lower Δ( t ) ultimately becomes. Therefore, the expected decline in the cumulative discount must be at least as large as its former increase due to over-harvesting (Eq. 2 , Fig. 4 ). In turn, this former increase is given by Eq. 3 . The correction to discounting suggested by our formula is significant (Fig. 5 ), where adjustments of the order of magnitude implied by the formula suggest significant changes in climate policy, including significant emission cuts 6 , 36 .
Note that the effect of harvest on discounting should be considered in addition to (not instead of) changes dictated by various other mechanisms and considerations. In particular, there is a controversy over the value of the rate of pure time preference, ρ , that should be used in environmental policies; some authors argue that policymakers should determine ρ based on individual’s preferences ( ρ ≈ 3% year −1 ), but others argue that policymakers should use ρ ≈ 0 based on considerations of intergenerational equity 6 , 16 , 19 , 39 . The value of ϕ τ , however, does not depend on the value of ρ and should be subtracted from Δ regardless of that choice. Similarly, uncertainty about technological development may imply that policymakers need to consider δ sus that declines over time 16 , 20 , 21 , 22 , 23 , which implies another decline in the cumulative discount on top of the one suggested here. Also, note that the future values of natural resources do not depend on the proportion given to their consumption in the currency unit, μ . Specifically, their future values do not depend on whether they are accounted for as market or as non-market goods. Nevertheless, μ does affect the relative weights given to the discount factor and to the prices of natural resources in determining the resources’ future values 32 , 36 , 37 . Specifically, ignoring the role of non-market natural resources in economic growth (considering a small μ ) would imply that a change in the provision of these resources has a larger effect on their prices but a smaller effect on the discount factor (Supplementary Note 1). Therefore, focusing on the inevitable increase in the price of natural resources following their over-harvesting would result in the same conclusions and present an alternative approach to the one presented here. In particular, the adjustment exp( ϕ τ ) (Fig. 5 ) is due to the change in the discount factor, while the complementary change in the price (Fig. 2 ) introduces another adjustment to the future value of natural resources 36 . The total adjustment due to changes in both discount and prices does not depend on the choice of μ , and would be ≥exp( ϕ τ ). The significant effect that the global transition to sustainable harvest has on the future value of natural resources suggests that climate policies should be determined jointly with other environmental policies.
Model overview
We begin with describing a well-established framework 32 , 36 , 37 that specifies how social welfare and the discount rate depend on the provision of the natural resource over time, f ( t ), and on the consumption of other goods over time, c ( t ). Next, we specify how harvest at the global scale affects the dynamics of f ( t ) and c ( t ) (which would grow exponentially if the harvest functions are fixed). We complete the model by describing how the harvest strategies are determined by the various managers in a competitive market.
Model of social welfare and the discount rate
We consider a social welfare function that is given by the widely-used form 12 , 32 , 36 , 37
where u ( c , f ) is the instantaneous utility that increases as c and f increase (Table 1 ), ρ is a constant rate of pure time preference, and T is a time horizon (we are interested in the limit T \(\rightarrow\) ∞). The distinction between the provision or consumption of the natural resource, f ( t ), and that of the other goods, c ( t ) is necessary here because, if the natural resource and the other goods are not entirely substitutable and the ratio between them varies over time, then social welfare depends on the ratio between c and f over time and cannot be written as a function of a single variable 29 . In turn, the substitutability is determined by the form of u 12 , 29 , 37 . For example, the goods may be non-substitutable, characterized by separable utility functions (Supplementary Note 2, Eq. B2 ), if one good cannot compensate for the lack of the other good (e.g., many cars cannot compensate for a lack of food). Alternatively, the goods may be partially substitutable (Eq. B10 ) if a sufficient amount of one good may compensate for the lack of the other good (e.g., many carrots can compensate for the lack of fish).
In turn, note that there are several candidates for quantifying the social rate of discount 15 , including the consumption rate of discount and the social and private rates of return to investment. These three quantities are closely-related, and, in a perfectly competitive market, they become equal and reflect the marginal productivity of capital. In this study, as in numerous related studies 12 , 14 , 16 , 32 , 36 , 37 , the focus is on the consumption rate of discount, which is the rate of decline in the marginal contribution of consumption to social welfare. In other words, the corresponding discount factor specifies how many units of consumption added at present would have the same effect on social welfare as a single unit added at time t . In turn when the welfare depends on multiple goods, the discount may depend on the particular good that the policymaker considers 31 , 36 , 37 . (This simply reflects the relative price changes of the goods.) Therefore, to define discount in our system, we consider a small, marginal perturbation to both c and f . Specifically, we consider a given currency unit, a dollar, that allows the consumption of exactly με units of the natural resource and (1 − μ ) ε units of the other goods, where 0 ≤ μ ≤ 1 and ε ≪ c (0), f (0). Accordingly, we define the discount factor at time t as the number of dollars needed at present to compensate for a lack of one dollar at time t . This implies that the discount rate, δ ( t ), is given by (Supplementary Note 1)
where subscripts in this equation denote partial derivatives and the discount factor is given by exp(−Δ). The right side of Eq. 5 , without the term ρ , is due to the change in the marginal contribution of c and f to social welfare. (Note that, if μ = 0 and d c / d t = cg c , then Eq. 5 becomes the Ramsey’s discount formula 14 , 16 , δ ( t ) = ηg c + ρ , where η ≡ cu c / u cc .) In turn, if μ reflects the portion in society’s basket of goods allocated to consumption of the natural resource, then our definition is consistent with the way the marginal productivity of capital is measured, and the total product (e.g., GDP) is proportional to the total value of all the goods (Supplementary Note 1 , Eq. A11 ). Alternatively, if we are interested in discounting some climate damage, then we can chose μ to be proportional to the cost that is due to the damage to the natural resource. Note, however, that the choice of μ only determines the units and does not affect the value given to future goods. Specifically, if the proportion of damages to the natural resource differs from μ , then one should consider the changes in relative prices in addition to discounting 36 , 37 . For example, several authors 37 considered a dual discounting framework in which the natural resource is discounted with μ = 0 and the manufactured goods with μ = 1, where the change in the relative price accounts for the difference; this approach is equivalent to the one presented here.
Model of the dynamics and management of the natural resource
Next, we specify how the harvest methods of the renewable natural resource at the global scale determine the dynamics of c ( t ) and f ( t ) (Fig. 1). Note that the aggregate harvest functions at the global scale are determined by the various harvest methods used at the local scale. In turn, at the local scale, a non-sustainable harvest in a given area during a given year yields β units of the natural resource per unit area, but the ecosystem in that area becomes degraded and ceases to yield resources thereafter. In turn, sustainable harvest in a given area yields less resource ( αβ units, where 0 < α < 1 is a constant), but the area remains fully functional for future use. For example, non-sustainable harvest may include aggressive fishing methods that inflict irreversible damage on fish populations and their habitats, while sustainable harvest implies sustaining fish populations and harvest at the fish growth rate, while also using methods that preserve the habitat and the age and size structures of the fish 38 . In turn, the productivity of the natural resource per unit area, β , may increase due to technological developments but may also decrease due to other environmental changes, such as climate change. Accordingly, we assume that β ( t ) = β 0 exp( g f t ), where β 0 = β (0) and g f is the rate of change in productivity. It follows that the total amount of the natural resource harvested globally at time t is given by
where H n is the area that is non-sustainably harvested in year t (becomes degraded and cannot be harvested thereafter), and H s ( t ) is the area that is being sustainably harvested and remain non-degraded in year t ( H s ( t ) = H ( t ) − H n ( t )).
In turn, we distinguish two types of regions: those that have a single manager, and those that are shared such that all managers are free to harvest. Ultimately, the harvest methods used by all managers determine the total areas that become degraded at time t in the managed and in the shared regions at the global scale, \(H_{\mathrm{n}}^1(t)\) and \(H_{\mathrm{n}}^2(t)\) , respectively \(\left( {H_{\mathrm{n}} = H_{\mathrm{n}}^1 + H_{\mathrm{n}}^2} \right)\) . Accordingly, the total non-degraded areas in all managed regions, x 1 , and in all shared regions, x 2 , decrease due to non-sustainable harvest as follows:
Moreover, the harvest functions are constrained by the non-degraded areas:
for all t , where \(H_{\mathrm{s}} = H_{\mathrm{s}}^1 + H_{\mathrm{s}}^2\) .
In turn, we assume that harvest comes with a direct cost as more labor and resources are directed toward harvesting. We incorporate this direct cost as a reduction in c ( t ), which would otherwise grow exponentially at an exogenous rate g c due to technological developments. Specifically, we assume that c ( t ) is given by
where C 1 and C 2 are the direct costs of harvesting (in units of c ), and λ is the ratio between the direct costs of non-sustainable and sustainable harvest.
Model of the competitive market
It remains to specify how the harvest strategies of the managers at the local scale are determined, and how these strategies determine the harvest functions at the global scale, \(H_{\mathrm{s}}^1(t),\hskip 4ptH_{\mathrm{s}}^2(t),\hskip 4ptH_{\mathrm{n}}^1\) , and \(H_{\mathrm{n}}^2(t)\) . We are interested in comparing two types of solutions: The optimal solution that maximizes the social welfare, and the market solution that emerges in a competitive market. The optimal solution is found via the maximization of the social welfare (Eq. 4 ) subject to the constraints given in Eqs. 6 – 9 . In turn, to define the market solution, we consider a competitive market in which each manager aims to maximize her/his own utility. Specifically, we consider a well-established framework in which the market is perfectly competitive, such that, if property rights are defined everywhere and there are no externalities, the market solution coincides with the optimal solution 12 , 14 , 31 , 40 , 41 , 42 . In turn, the market solution depends on the form of the externalities for the various managers, namely, it depends on how non-sustainable harvest by a given manager affects the ecosystem in regions managed by other managers.
To define the externalities, we distinguish between managed regions and shared regions (Fig. 1 ). Each managed region is managed by a single manager who determines the harvest method, which may vary anywhere between using only sustainable methods and using only non-sustainable methods. In turn, the harvest method in a given region determines the portion of the region that is harvested and the rate at which the region becomes degraded (Fig. 1 ). We assume that the management in a given managed region has no externalities as it affects only the degradation level in that region. In turn, the shared regions are managed by a very large number of managers, each of whom is free to harvest without restrictions there. Specifically, we assume that each manager ignores the effect of her/his actions on the future provision of the resource in the shared regions and considers only her/his instantaneous benefit and cost from the harvest. Consequently, the managers have the incentive to increase non-sustainable harvest in the shared regions until the price of the natural resource equals the direct cost of the harvest. These considerations enable us to find the market solution that is given by the unique Nash equilibrium (see the section Numerical methods). In particular, the perfectly competitive market assumption implies that the management in the managed regions is socially optimal under the constraint given by the management in the shared regions. Note that, without shared regions ( x 2 = 0), there are no externalities and the market solution coincides with the optimal solution.
Numerical methods
The numerical results showing the optimal and market solutions are demonstrated in Figs. 2 and 3 , system 1. The optimal solution is given by the unique set of non-negative aggregate harvest functions, \(H_{\mathrm{s}}^1(t),\hskip 4ptH_{\mathrm{s}}^2(t),\hskip 4ptH_{\mathrm{n}}^1\) , and \(H_{\mathrm{n}}^2(t)\) , that maximize social welfare: max U T (Eq. 4 ) in the limit T \(\rightarrow\) ∞, where c ( t ) and f ( t ) are given by Eqs. 6 and 9 , subject to the constraint given in Eqs. 7 and 8 . (Note that using the social welfare function given in Eq. 4 with a constant ρ , and considering deterministic dynamics of c and f , guarantee that the optimization problem is time consistent and has a unique solution 12 , 37 .) In turn, the market solution is determined by a perfectly competitive market where each manager maximizes her/his own profit. Specifically, consider the set of non-negative harvest functions that maximize utility, max U T (Eq. 4 ) as T \(\rightarrow\) ∞, subject to the constraint given by Eqs. 7a and 8 and the constraint d x 2 /d t = X ( t ). Then, the market harvest is given by the unique solution that satisfies \(X(t) = H_{\mathrm{n}}^2(t)\) (consisteny criterion).
We used algorithms that find the exact solutions provided that the resolutions are sufficiently fine. Specifically, to find the optimal solution numerically, our algorithm uses Stochastic Programming with backward induction (Supplementary Note 4) 43 , 44 . (Note that the model’s dynamics are deterministic but the general method is still called stochastic.) To find the market solution, our algorithm also uses Stochastic Programming to solve for a given value of X . But it finds a solution multiple times, each time for a different value of X , until it finds the solution that satisfies the consisteny criterion. These algorithms are coded in C/C++ and are described in detail in Supplementary Note 4.
In turn, in the results shown in Fig. 3 , system 2, as well as in Figs. 4 and 5 and in the graphical tool, we assume that the dynamics of c and f follow Eqs. 6 – 9 , but we consider harvest functions that are not given by either the optimal solution or the market solution. In Fig. 3 , system 2, we consider harvest functions that follow the market solution until t = t 1 and after t = t 1 + 10, but between these times, the non-sustainable harvest decreases gradually from its maximal level to zero. In Fig. 4 , we calculate Δ sus , which is the cumulative discount that emerges if the harvest is entirely sustainable, namely, H n = 0 and H s = x 1 + x 2 if t > 0. Also, in Fig. 4a , we consider three scenarios in which the non-sustainable harvest is higher in the beginning but eventually approaches zero, while H n + H s = x 1 + x 2 .
After we determine the harvest functions, the functions c ( t ) and f ( t ) are calculated according to Eqs. 6 and 9 . In turn, we calculate the discount rate and the cumulative discount according to Eq. 5 (where the cumulative discount is the integral over time of the discount rate). Specifically, for the case in which only sustainable harvest is used (Δ sus in Fig. 4 ), the discount rates are calculated in Supplementary Note 2 and are given by Eqs. B5 and B12 . The prices are given by Eq. A10 , and the total product is given by Eq. A11 . All of these equations are derived in Supplementary Notes 1 , 2 .
Choice of parameters
The parameter values used for all of the numerical simulations, which are given in the Source Data file, are within their realistic ranges. The rate of technological growth is around 1.5–2.0% year −1 in developed countries and is higher in some developing countries 16 , 45 . In turn, the rate of growth in the yield per unit of sustainable harvest, g f , depends on the specific natural resource, where values that were considered in the literature vary from g c down to much lower (even negative) values 32 , 37 . Next, the value of 0 ≤ a ≤ 1 (unitless) also depends on the particular system. In a fishery, for example, if non-sustainable harvest would imply catching all the fish and sustainable harvest would imply keeping the fish population size fixed, then a would be the growth rate of the fish (i.e., 2% year −1 for large fish and higher rates for smaller fish) 38 ; In agriculture, sustainable management implies the use of environmentally friendly pest control methods and effective water management, which may result in a comparable crop yield ( α ≲ 1), but may be more expensive ( λ > 1) 33 , 34 . In turn, the ratio between c ( t ) and the direct costs, C 1 and C 2 (Eq. 9 ), determines the relative portion of c that is needed per unit of harvest. Specifically, c (and thus the ratio) is initially small but increases due to technological changes. Also, C 1 and C 2 may vary with x 1 and x 2 if the cost varies among regions (e.g., if near-shore regions are depleted, the average direct cost of harvest may increase). Next, note that 0 ≤ μ ≤ 1 (unitless) can be chosen arbitrarily by the policymaker, as it does not affect the harvest strategy and the future value of the natural resource; rather, it determines the currency unit, which, in turn, determines the relative role of the discount and the price in determining the future value of the natural resource. A reasonable choice would be the portion in the basket of goods of the natural resource (e.g., the portion of agricultural products in consumption is ~5% in the United States and is higher in various developing countries), but μ may be higher if non-market goods are incorporated. Finally, a variety of utility functions that incorporate both c and f were suggested in the literature 12 , 32 , 37 , including the two that are used here (Eqs. B2 , B10 ) 12 , where estimates of η vary between 1 and 3 (unitless) 16 , 41 , 45 , and suggested values for ρ varies between 0 and 3% (year −1 ) 6 , 16 , 19 , 45 .
Analytical and theoretical analysis
The general discount formula (Eq. 5 ) is derived in Supplementary Note 1. The discount formulas for the special cases presented in the figures are derived in Supplementary Note 2. The proof of the theorem is given in Supplementary Note 3.
Data availability
No datasets were generated or analyzed during the current study. All the data needed to reproduce the results is given in the paper. In particular, the parameter values used for each figure are given in the Source Data file. These parameter values are taken from the references that are cited in the Methods section.
Code availability
The algorithm that we used for finding the optimal and market solutions (Figs. 2 and 3 ) is described in Supplementary Note 4. The C/C++ code used for generating Figs. 2 and 3 as well as the Matlab code used for generating Figs. 4 and 5 are available as a supplementary code.
Assessment, M. E. Ecosystems and Human Well-being (Island Press, Washington, DC, 2005).
Groom, M. J., Meffe, G. K. & Carroll, C. R. Principles of Conservation Biology (Sinauer Associates, Sunderland, 2006).
Scheffer, M. & Carpenter, S. R. Catastrophic regime shifts in ecosystems: linking theory to observation. Trends Ecol. & Evol. 18 , 648–656 (2003).
Article Google Scholar
Folke, C. et al. Regime shifts, resilience, and biodiversity in ecosystem management. Annu. Rev. Ecol., Evol., Syst. 35 , 557–581 (2004).
Moreno-Mateos, D. et al. Anthropogenic ecosystem disturbance and the recovery debt. Nat. Commun. 8 , 14163 (2017).
Article ADS CAS Google Scholar
Stern, N. H. The Economics of Climate Change: the Stern Review . (Cambridge University Press, Cambridge, 2007).
Solomon, S., Plattner, G.-K., Knutti, R. & Friedlingstein, P. Irreversible climate change due to carbon dioxide emissions. Proc. Natl Acad. Sci. USA 106 , 1704–1709 (2009).
Coomes, D. A., Allen, R. B., Forsyth, D. M. & Lee, W. G. Factors preventing the recovery of New Zealand forests following control of invasive deer. Conserv. Biol. 17 , 450–459 (2003).
Hulme, P. E. Beyond control: wider implications for the management of biological invasions. J. Appl. Ecol. 43 , 835–847 (2006).
Barnosky, A. D. et al. Approaching a state shift in Earth’s biosphere. Nature 486 , 52–58 (2012).
Bringezu, S. & Bleischwitz, R. Sustainable Resource Management: Global Trends, Visions and Policies (Routledge, New York, 2009).
Xepapadeas, A. in Handbook of Environmental Economics (ed. Vincent, J. R. and Mäler, K. G.). (Elsevier, North Holland, 2005).
Chu, S. & Majumdar, A. Opportunities and challenges for a sustainable energy future. Nature 488 , 294–303 (2012).
Ramsey, F. P. A mathematical theory of saving. Econ. J. 38 , 543–559 (1928).
Groom, B., Hepburn, C., Koundouri, P. & Pearce, D. Declining discount rates: the long and the short of it. Environ. Resour. Econ. 32 , 445–493 (2005).
Arrow, K. J. et al. Should governments use a declining discount rate in project analysis? Rev. Environ. Econ. Policy 8 , 145–163 (2014).
Nordhaus, W. D. To slow or not to slow: the economics of the greenhouse effect. Econ. J. 101 , 920–937 (1991).
Arrow, K. J. et al. Determining benefits and costs for future generations. Science 341 , 349–350 (2013).
Nordhaus, W. D. A review of the Stern review on the economics of climate change. J. Econ. Lit. 45 , 686–702 (2007).
Weitzman, M. L. Why the far-distant future should be discounted at its lowest possible rate. J. Environ. Econ. Manag. 36 , 201–208 (1998).
Weitzman, M. L. Gamma discounting. Am. Econ. Rev. 90 , 260–271 (2001).
Weitzman, M. L. A review of the Stern Review on the economics of climate change. J. Econ. Lit. 45 , 703–724 (2007).
Gollier, C. Pricing the Planet’s Future: the Economics of Discounting in an Uncertain World (Princeton University Press, Princeton, 2013).
Van der Zwaan, B. C., Gerlagh, R. & Schrattenholzer, L. Endogenous technological change in climate change modelling. Energy Econ. 24 , 1–19 (2002).
Dietz, S. & Hepburn, C. Benefit–cost analysis of non-marginal climate and energy projects. Energy Econ. 40 , 61–71 (2013).
Dell, M., Jones, B. F. & Olken, B. A. Temperature shocks and economic growth: evidence from the last half century. Am. Econ. J.: Macroecon. 4 , 66–95 (2012).
Google Scholar
Moore, F. C. & Diaz, D. B. Temperature impacts on economic growth warrant stringent mitigation policy. Nat. Clim. Change 5 , 127–131 (2015).
Article ADS Google Scholar
Dietz, S. & Stern, N. Endogenous growth, convexity of damage and climate risk: how Nordhaus’ framework supports deep cuts in carbon emissions. Econ. J. 125 , 574–620 (2015).
Drupp, M. A. Limits to substitution between ecosystem services and manufactured goods and implications for social discounting. Environ. Resour. Econ. 69 , 135–158 (2018).
Neumayer, E. Global warming: discounting is not the issue, but substitutability is. Energy Policy 27 , 33–43 (1999).
Neumayer, E. Weak Versus Strong Sustainability: Exploring the Limits of Two Opposing Paradigms (Edward Elgar Publishing, Cheltenham, 2003).
Hoel, M. & Sterner, T. Discounting and relative prices. Clim. Change 84 , 265–280 (2007).
Brewer, M. J. & Goodell, P. B. Approaches and incentives to implement integrated pest management that addresses regional and environmental issues. Annu. Rev. Entomol. 57 , 41–59 (2012).
Article CAS Google Scholar
Lefebvre, M., Langrell, S. R. & Gomez-y-Paloma, S. Incentives and policies for integrated pest management in Europe: a review. Agron. Sustain. Dev. 35 , 27–45 (2015).
Lind, R. C. in Discounting for Time and Risk in Energy Policy (eds Lind, R. C. et al.) (Resources for the Future, Washington, DC, 1982).
Sterner, T. & Persson, U. M. An even sterner review: Introducing relative prices into the discounting debate. Rev. Environ. Econ. Policy 2 , 61–76 (2008).
Traeger, C. P. Sustainability, limited substitutability, and non-constant social discount rates. J. Environ. Econ. Manag. 62 , 215–228 (2011).
Clark, C. W. Mathematical Bioeconomics: the Mathematics of Conservation (John Wiley & Sons, New Jersey, 2010).
Stern, N. Ethics, equity and the economics of climate change paper 2. Econ. Philos. 30 , 445–501 (2014).
Becker, R. A. & Boyd III, J. H. Capital Theory, Equilibrium Analysis, and Recursive Utility (Wiley-Blackwell, New Jersey, 1997).
Evans, D. J. The elasticity of marginal utility of consumption: estimates for 20 OECD countries. Fisc. Stud. 26 , 197–224 (2005).
Romer D. Advanced Macroeconomics , 4th edn (Mcgraw-Hill, New York, 2011).
Conrad, J. M. & Clark, C. W. Natural Resource Economics: Notes and Problems (Cambridge University Press, Cambridge, 1987).
Clark, C. W. & Mangel, M. Dynamic State Variable Models in Ecology: Methods and Applications (Oxford University Press, Oxford, 2000).
Nordhaus, W. D. Managing the Global Commons: the Economics of Climate Change . (MIT press, Cambridge, MA, 1994).
Download references
Acknowledgements
The author sincerely thanks Charles Perrings for his valuable comments on the paper. The author thanks SAL MCMSC, CLAS and SHESC, ASU, for funding (no. DN5-1057).
Author information
Authors and affiliations.
School of Human Evolution and Social Change, Arizona State University, Tempe, AZ, 85287, USA
Adam Lampert
Simon A. Levin Mathematical, Computational and Modeling Science Center, Arizona State University, Tempe, AZ, 85287, USA
You can also search for this author in PubMed Google Scholar
Contributions
A.L. designed the research, composed the model, conducted the research, and wrote the paper.
Corresponding author
Correspondence to Adam Lampert .
Ethics declarations
Competing interests.
The author declares no competing interests.
Additional information
Journal peer review information : Nature Communications thanks the anonymous reviewers for their contribution to the peer review of this work. Peer reviewer reports are available.
Publisher’s note: Springer Nature remains neutral with regard to jurisdictional claims in published maps and institutional affiliations.
Supplementary information
Supplementary information, peer review file, source data, source data, rights and permissions.
Open Access This article is licensed under a Creative Commons Attribution 4.0 International License, which permits use, sharing, adaptation, distribution and reproduction in any medium or format, as long as you give appropriate credit to the original author(s) and the source, provide a link to the Creative Commons license, and indicate if changes were made. The images or other third party material in this article are included in the article’s Creative Commons license, unless indicated otherwise in a credit line to the material. If material is not included in the article’s Creative Commons license and your intended use is not permitted by statutory regulation or exceeds the permitted use, you will need to obtain permission directly from the copyright holder. To view a copy of this license, visit http://creativecommons.org/licenses/by/4.0/ .
Reprints and permissions
About this article
Cite this article.
Lampert, A. Over-exploitation of natural resources is followed by inevitable declines in economic growth and discount rate. Nat Commun 10 , 1419 (2019). https://doi.org/10.1038/s41467-019-09246-2
Download citation
Received : 08 August 2018
Accepted : 25 February 2019
Published : 29 March 2019
DOI : https://doi.org/10.1038/s41467-019-09246-2
Share this article
Anyone you share the following link with will be able to read this content:
Sorry, a shareable link is not currently available for this article.
Provided by the Springer Nature SharedIt content-sharing initiative
This article is cited by
A comprehensive analysis of the inter-relationships of impact between automotive industry, economic growth, natural resources and environmental degradation: morocco as an example.
- Mohammed El-Khodary
- Amine El Kadri
- Sara Dassouli
Environment, Development and Sustainability (2024)
Impact of LULC changes on hydrological flow regimes and runoff coefficient in Upper Jhelum Basin, India
- Shahid Ul Islam
- Sumedha Chakma
Sustainable Water Resources Management (2024)
Selection mechanism of water saving service company based on multi-attribute bidding and auction
- Honghua Shi
- Zhengrong Cheng
Urban water-energy-food nexus in the kitchen and social practices of diet and cooking: implications for household sustainability
- Abubakari Ahmed
Industrial output, services and carbon emissions: the role of information and communication technologies and economic freedom in Africa
- Chinazaekpere Nwani
- Festus Victor Bekun
- Ekpeno L. Effiong
Environment, Development and Sustainability (2023)
By submitting a comment you agree to abide by our Terms and Community Guidelines . If you find something abusive or that does not comply with our terms or guidelines please flag it as inappropriate.
Quick links
- Explore articles by subject
- Guide to authors
- Editorial policies
Sign up for the Nature Briefing newsletter — what matters in science, free to your inbox daily.


- Goals & Strategies
- Find a Technical Expert
- Academic Program Accreditation
- Alpha Epsilon Honor Society
- STEM Resources
- ASABE Global Initiative
- Soil Erosion Research Priorities
- Policy Statement on Diversity
- Resource Magazine
- Latest News
- Inside ASABE
- The Lead with ASABE
- Alliance for Modernizing African Agrifood Systems
- Circular Bioeconomy Systems Institute
- The ASABE Career Center
- Order of the Engineer
- Choosing a Program
- Accredited Academic Programs in Ag/Bio Engineering & Technology
- Celebrate Engineering
- Ag & Bio Professional Licensure
- Grade of Fellow
- Standards Development Awards
- Educational Aids Blue Ribbon Awards
- ITSC Paper Awards
- AE50 Award Winners
- Initiative Fund
- Giving Back Fund
- Pinches Schwab Fund
- Policy on Awards & Recognition
- Undergraduate Competitions, Awards, and Scholarships
- Presenters & Moderators
- Event Organizers
- International Travelers
- Committee Overviews
- Sections & Districts
- ASABE Foundation
- From the ISB President
- Student Newsletter – Spring 2024
- Young Professionals
- Google Advanced Search
- Google Scholar Search
- Site Licenses
- Special Collections
- Technical Library Help
- ISO US TAG TC 347 Data-driven Agrifood Systems
- Standards Balloting
- ASABE Standards Committees
- Published Standards
- Standards Key Word Index
- ASABE Standards Patent Release Information
- Standards Updates
- US Recognized Documents
- Canadian Recognized Standards
- Job Postings
- Guide To Consultants
- Journal of the ASABE
- Applied Engineering in Agriculture
- Journal of Natural Resources and Agricultural Ecosystems
- Journal of Agricultural Safety and Health
- Transactions of the ASABE
- Editors & Reviewers
- Instructors & Students
- Order Publications
Editorial Board ITSC and PAFS Community Editor Call for Nominations )-->
Associate Editors
This new open access peer reviewed engineering journal began publishing in 2023. The NRES Community Editor will also serve as the editor for this journal.
This engineering journal includes a variety of article types (i.e., research articles, research briefs, review articles, frontier articles, and perspective articles) on the topics of hydrological, evapotranspiration, soil erosion, water quality, and biogeochemical processes; drainage, irrigation, conveyance, and reservoir infrastructures; agricultural, forest, peri-urban, stream, wetland, and coastal systems; microbial, organism, plot, field, farm, watershed, ecosystem, continental, and global scales and interactions; sustainability, resiliency, circularity, management, mitigation, recovery, and restoration approaches; and disciplinary, interdisciplinary, and transdisciplinary perspectives, which integrate engineering and physical sciences related to natural and environmental systems. The journal encourages articles that consider systems thinking using a variety of multidisciplinary approaches including field and laboratory experimentation, measurement techniques, modeling, data analysis, decision support, and case studies. The journal invites articles from an international audience.
ASABE publishes Journal of Natural Resources and Agricultural Ecosystems four times a year. Articles will be published online as they are completed.
The number of published pages for articles are restricted to:
- four for research brief, frontier, and perspectives articles,
- eight for research articles,
- 15 for review articles, and
- eight pages or fewer for special collection articles.
Read our journal descriptions to see which one is best for your content. Article types include research, research briefs, review, frontier, editorial, and perspective. You may also initiate a special collection or participate in an existing open special collection .
Why publish with us? Meet our previous Editor in Chief .
Read about publishing in Navigating the Publication Process: An ASABE Journals’ Perspective . Review the 2019 journal survey results in Changes to ASABE Journals Guided by Member Feedback .

Community Editor Nominations Due June 30 for ITSC and PAFS
ASABE is currently seeking nominations for a new Community Editor for the Information Technology, Sensors, and Controls technical community and for the Plant, Animal, and Facility Systems (PAFS) technical community, as Dr. as Naiqian Zhang and Dr. Jun Zhu will be completing their term and have decided to step down after many years of outstanding service.
Discount for Publishing Your AIM 2024 Presentation in Our Journals
Further develop your AIM non-refereed presentation into a high-quality peer reviewed manuscript, submit to the ASABE journals, and receive a page charge discount of $100 or 10%, if over 10 pages for the final layout. You must be registered for the annual meeting, present your work at the AIM, and submit your manuscript to ScholarOne by October 1, 2024. Note that all manuscripts must go through our high-quality review process to be published in the ASABE journals. When uploading to ScholarOne, at the end of Step 1: Type, Title, & Abstract , be sure to select the special collection Annual International Meeting (AIM) Discount and include in your cover letter your AIM paper number. Step 1: Type, Title, & Abstract , be sure to select the special collection Annual International Meeting (AIM) Discount and include in your cover letter your 2023 AIM paper number.-->
Literature Review Service (paused until new EIC is appointed)
Interested in saving some time and making sure that you cite relevant articles from the society's publications? Complete this Google form to receive a list of key articles to assist with your manuscript preparation. Note there is no obligation to incorporate suggested citations into your article. If you have questions about this optional, free service, please email [email protected] . We look forward to helping you publish your research!
Article Processing Charges
The charges per article for this journal shown below are different than our other journals. See our article type descriptions for more details.
- $1500 - four pages or fewer research briefs, frontier, and perspective
- $2100 - eight pages or fewer research article
- $2400 - 15 pages or fewer review article
- $1800 - eight pages or fewer special collection article
If page count limit for the article type is exceeded, the additional fee is $100 per page.
In general, the page count is about half of the double-spaced template that we provide. Tables and images can change that depending on the sizes needed. If you do not use our template, the final page count may be more.
Call for Papers
The following collections are open to submissions of manuscripts from anyone with relevant content. Please include a cover letter file with your submission that states the name of the collection chosen. Also, choose the special collection name from the list in ScholarOne Step 1.
Climate Change Impacts on Natural Resource and Agricultural Systems
Young Gu Her, Guest Editor and Coordinator Kati Migliaccio, Community Editor, Natural Resources & Environmental Systems, ASABE Journals
See the Call for Papers flyer for more details and contact information. Articles will be published in the 2025 issues of our open access Journal of Natural Resources and Agricultural Ecosystems .
This Special Collection of peer-reviewed papers invites studies that 1) evaluate climate change impacts on natural resources and agricultural systems, 2) address the connection of climate change to water supply and greater socio-economic implications, 2) develop mitigation and adaptation strategies/plans to address the impacts of climate change, 3) propose new methodology and frameworks to improve the precision and accuracy of climate change impact assessment, 4) provide reviews on state-of-the-art findings and technologies and the context for future climate change research related to natural resources and agricultural systems and 5) demonstrate climate change impacts and successful mitigation/adaptation actions for a case study.
Manuscript submission | 12/30/2024 |
First decision | 2/1/2025 |
Final acceptance | 4/1/2025 |

Regenerative Agriculture
Srinivasulu Ale, Meetpal Kukal, and Xiaoyu Feng, Associate Guest Editors Kati Migliaccio, Community Editor, Natural Resources & Environmental Systems, ASABE Journals Garey Fox, Editor-In-Chief, ASABE Journals
See the Call for Papers flyer for more details and contact information. Articles will be published in the 2024 issues of our open access Journal of Natural Resources and Agricultural Ecosystems or hybrid Journal of the ASABE and Applied Engineering in Agriculture . Review our journal descriptions before submitting.
This special collection welcomes papers from a wide range of topics and community contributions related to evaluation of multidimensional impacts of regenerative agricultural practices, including, but not limited to, (1) biophysical impacts, i.e., on soil-plant-atmospheric continuum, resource use efficiency, etc.; (2) environmental, economic, and social outcomes; and (3) technological innovations that streamline and promote adoption of such practices. Experts from within ASABE as well as other synergistic disciplines are invited to participate.
Manuscript submission | 12/31/2024 (extended) |
First decision | 3/15/2025 |
Final acceptance | 6/15/2025 |
Constructed Wetlands
Aaron Mittelstet and Tiffany Messer, Associate Guest Editors Kati Migliaccio, Community Editor, Natural Resources & Environmental Systems, ASABE Journals Garey Fox, Editor-In-Chief, ASABE Journals
See the Call for Papers flyer for more details and contact information. Articles will be published in the 2024 issues of our open access Journal of Natural Resources and Agricultural Ecosystems . Review our journal descriptions before submitting.
This special collection welcomes papers from a wide range of topics related to the design, effectiveness and challenges of constructed wetlands, including floating treatment wetlands and their impact on the local ecosystem. Experts from within ASABE as well as other synergistic disciplines are invited to participate.
Manuscript submission | 9/15/2024 |
First decision | 11/30/2024 |
Final acceptance | 12/31/2024 |
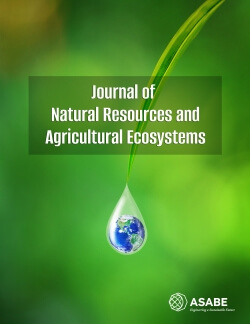
Journal Author Links
- Article Processing Charge (APC)
- Copyright FAQ
- Cover Letters
- English-Language Editing
- Journal Article Types
- Journal Author FAQ
- Journal Descriptions
- Journal Peer-Review Process
- Journal Submissions
- Keywords List
- Literature Review Service
- ScholarOne Manuscripts
- Supplemental Information
- Technical Community Descriptions
- Templates for Word
- Volunteer to Review
Peer-Reviewed Journals

An official website of the United States government
Here's how you know
Official websites use .gov A .gov website belongs to an official government organization in the United States.
Secure .gov websites use HTTPS A lock ( Lock A locked padlock ) or https:// means you’ve safely connected to the .gov website. Share sensitive information only on official, secure websites.

Pacific Northwest Research Station
The Pacific Northwest (PNW) Research Station is a leader in the scientific study of natural resources. We generate and communicate impartial knowledge to help people understand and make informed choices about natural resource management and sustainability.

Anderson to retire as Director of Pacific Northwest Research Station

The Forests of Palau: A Closer Look
Bsal and Beyond: Task Force Helps Stave Off Amphibian Disease Threat
New publications, latest products.
Beyond the battlefield: Public lands as healing spaces for military veterans
21st century wildlife monitoring – a case study of spotted owls and artificial intelligence.

Time tells the story: Concerns for long-term species resilience with habitat changes

Riparian and Aquatic Ecosystem Vulnerability Evaluation (RAEVEN) Data Library

Western Oregon WeT DRy model (WOWTDR)

Upstream Regional LiDAR Model of Trout (UPRLIMET) Dashboard
Enterprise Risk Management: Its Drivers and Value in the North American Energy and Natural Resources Sector
25 Pages Posted: 2 Jul 2024
Organization for Environmental Research and Green Innovation
Keith Mogola Khupe Bothongo
Organisation for Environmental Research and Green Innovation
Date Written: May 2, 2024
This study examines the drivers of Enterprise Risk Management (ERM) adoption and its impact on firm value in the North American energy and natural resources sector. We used a survey to collect data on the current state of ERM from 119 firms listed on the NYSE and NASDAQ. Data on Firms’ characteristics was collected from companies’ databases and annual reports. Our ordinal logistic regression results revealed that the presence of a Chief Risk Officer (CRO), board of directors monitoring, and risk culture significantly and positively impact ERM adoption, while leverage hinders its implementation. Also, findings from our Stepwise regression indicated that ERM is value-creating. To the best of our knowledge, this is the first study to examine the drivers and value of ERM in the North American energy and natural resources sectors despite the high-risk exposure of firms in this sector. The study utilizes a quantitative multi-method design and investigates new ERM drivers that have received little attention in the literature, such as Risk Culture and Board of Directors. The study provides actionable insights for policymakers, practitioners, and investors in North American publicly traded energy and natural resources companies.
Keywords: Enterprise Risk Management, Board of Directors, Chief Risk Officer, Firm Size, Corporate Governance, Risk Culture
Suggested Citation: Suggested Citation
Ali Kinyar (Contact Author)
Organization for environmental research and green innovation ( email ).
53 Linceslade Grove Loughton Milton Keynes, MK5 8DJ United Kingdom
Organisation for Environmental Research and Green Innovation ( email )
53 Linceslade Grove, Loughton, Milton Keynes Milton Keynes, Buckinghamshire MK5 8DJ United Kingdom MK5 8DJ (Fax)
Do you have a job opening that you would like to promote on SSRN?
Paper statistics, related ejournals, risk management ejournal.
Subscribe to this fee journal for more curated articles on this topic
Corporate Governance: Disclosure, Internal Control, & Risk-Management eJournal
Corporate governance practice series ejournal.
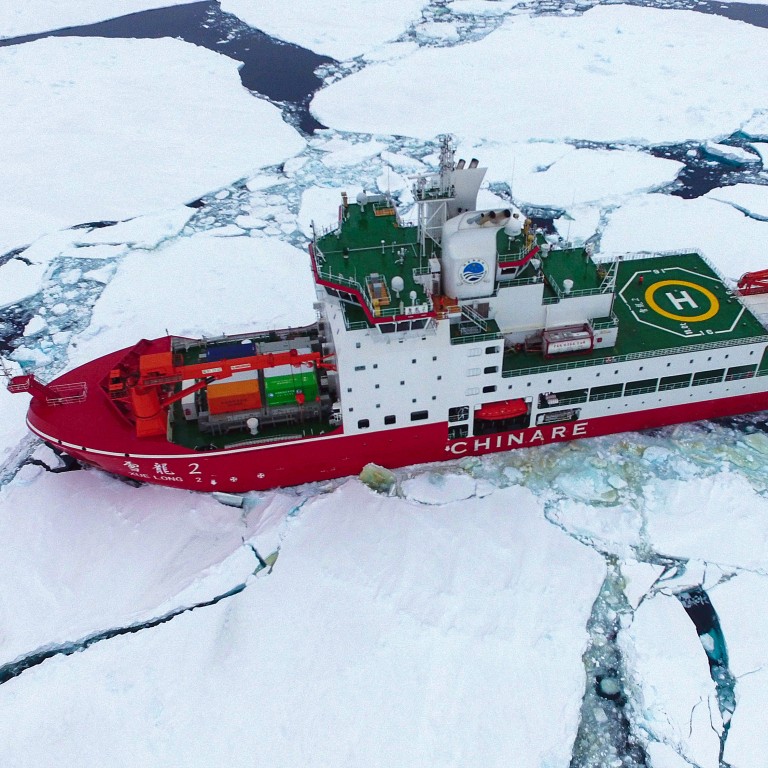
China to boost basic research in natural resources on its path to tech self-reliance
- Ministry of Natural Resources guidelines reveal aim to work in global community, explore deep environments and boost scientist incentives

The objectives are among the Ministry of Natural Resources’ new policy guidelines to strengthen fundamental research released by the ministry on its official social media accounts on Wednesday.
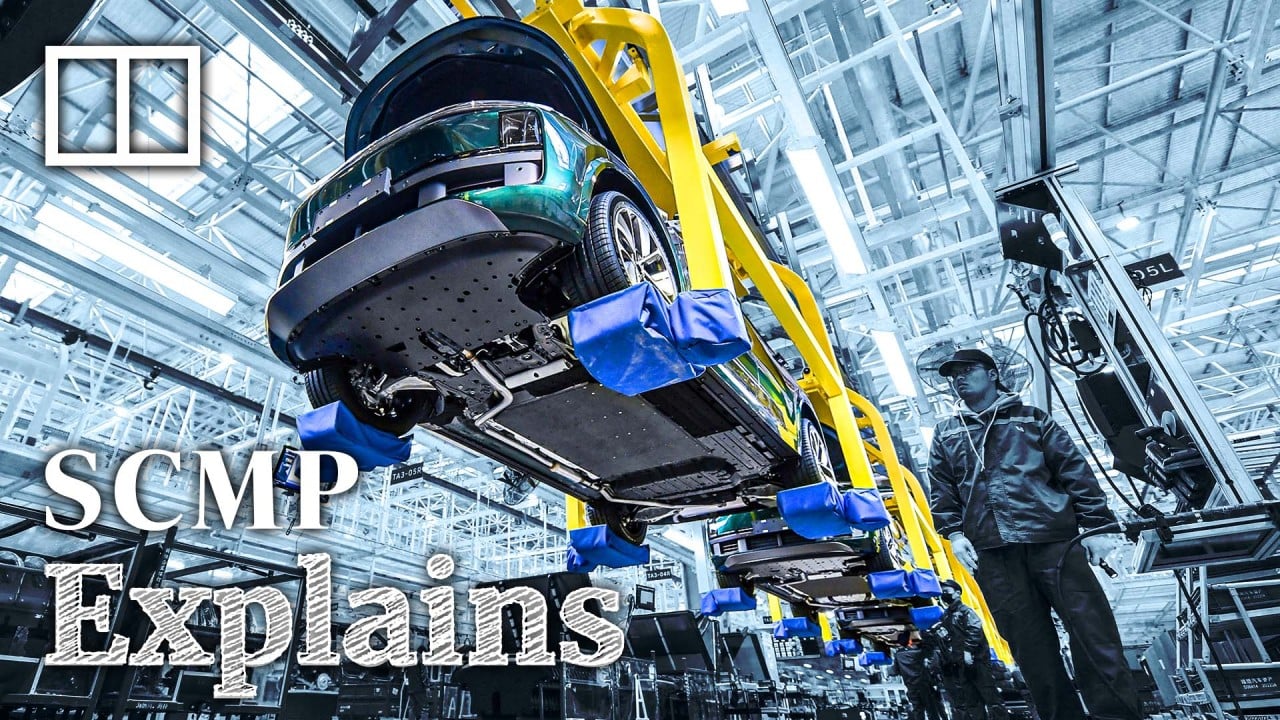
‘Overtaking on a bend’: how China’s EV industry charged ahead to dominate the global market
The ministry vows to actively work with the global basic research innovation community by taking part in major international projects, such as Digital Earth, research on negative ocean emissions technology, deep-sea habitats and seamless forecasts for the ocean and climate.
It said China would actively take part in important international science programmes – such as the International Continental Scientific Drilling Programme, the International Ocean Discovery Programme as well as joint laboratories and research centres – and would support international organisations and well-known scientific research institutions to set up branches in China.
The ministry would also encourage regular exchanges and joint work on research projects with scientists from Hong Kong, Macau and Taiwan.
On the mainland, the ministry committed to focusing on important research directions in natural resources, such as the mineralisation pattern of strategic resources, exploring and exploiting deep-earth resources, investigating deep-sea abyss systems and ensuring the security of resource and environmental in seas in the polar regions.
It has pledged to direct a strategic basic research system that will be the source of disruptive technologies, although it did not elaborate on this goal.
The ministry’s focus on human resources includes China’s commitment to nurture a group of “strategic” scientists in the field of natural resources, boost incentives for scientific talent and optimise their appraisal system so they could focus on research.
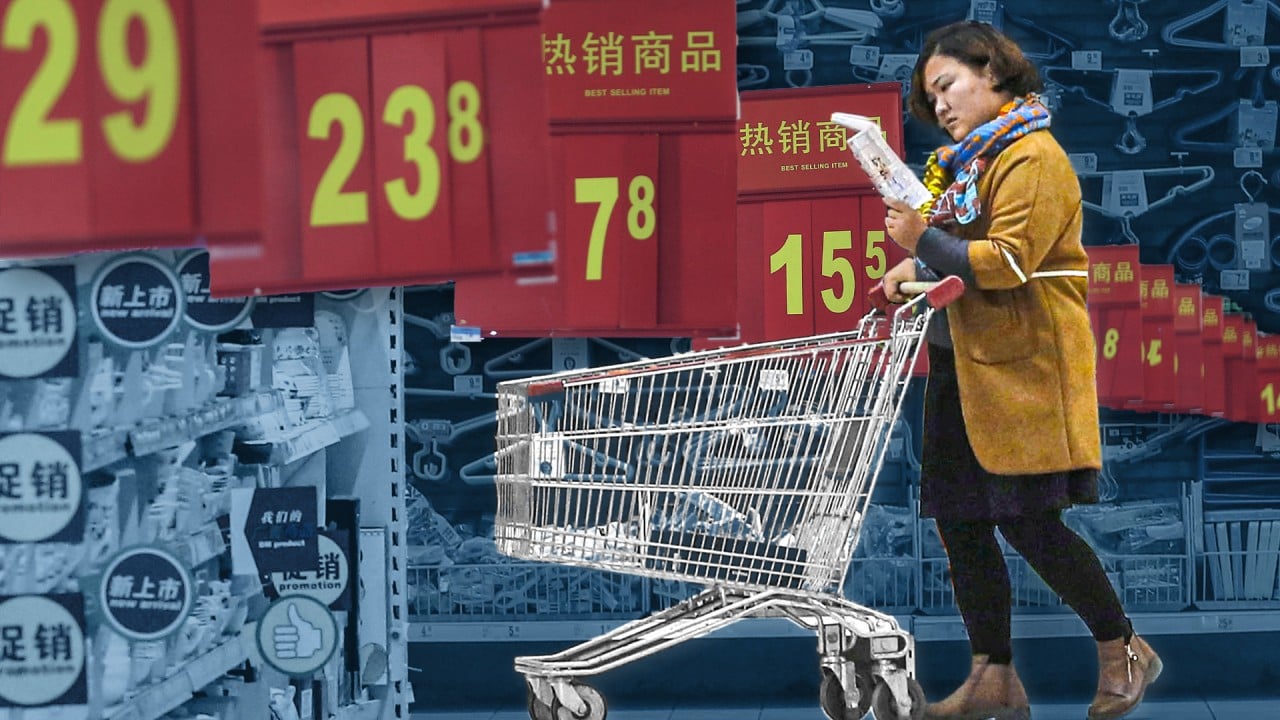
What does it mean for the world when Chinese consumers tighten their belts?
The ministry called for more intelligent surveying and mapping tools to be developed to help take stock of China’s resources and improve geographic information security.
It sought better understanding of ecological systems, including various landscapes, the identification of land degradation and prevention methods and development of modelling and early warning systems to detect disasters on land and at sea.
Information
- Author Services
Initiatives
You are accessing a machine-readable page. In order to be human-readable, please install an RSS reader.
All articles published by MDPI are made immediately available worldwide under an open access license. No special permission is required to reuse all or part of the article published by MDPI, including figures and tables. For articles published under an open access Creative Common CC BY license, any part of the article may be reused without permission provided that the original article is clearly cited. For more information, please refer to https://www.mdpi.com/openaccess .
Feature papers represent the most advanced research with significant potential for high impact in the field. A Feature Paper should be a substantial original Article that involves several techniques or approaches, provides an outlook for future research directions and describes possible research applications.
Feature papers are submitted upon individual invitation or recommendation by the scientific editors and must receive positive feedback from the reviewers.
Editor’s Choice articles are based on recommendations by the scientific editors of MDPI journals from around the world. Editors select a small number of articles recently published in the journal that they believe will be particularly interesting to readers, or important in the respective research area. The aim is to provide a snapshot of some of the most exciting work published in the various research areas of the journal.
Original Submission Date Received: .
- Active Journals
- Find a Journal
- Proceedings Series
- For Authors
- For Reviewers
- For Editors
- For Librarians
- For Publishers
- For Societies
- For Conference Organizers
- Open Access Policy
- Institutional Open Access Program
- Special Issues Guidelines
- Editorial Process
- Research and Publication Ethics
- Article Processing Charges
- Testimonials
- Preprints.org
- SciProfiles
- Encyclopedia
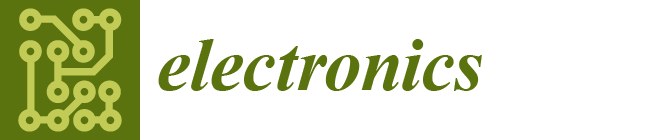
Article Menu
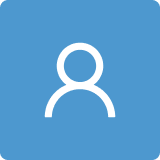
- Subscribe SciFeed
- Recommended Articles
- Google Scholar
- on Google Scholar
- Table of Contents
Find support for a specific problem in the support section of our website.
Please let us know what you think of our products and services.
Visit our dedicated information section to learn more about MDPI.
JSmol Viewer
Towards sustainable cloud computing: load balancing with nature-inspired meta-heuristic algorithms.
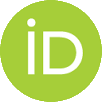
1. Introduction
- Nature-inspired meta-heuristic focus: Unlike other research primarily examining traditional load-balancing solutions, this study delves further into nature-inspired meta-heuristic algorithms. This study examines the benefits, distinctive characteristics, and present use of cloud computing, providing a fresh viewpoint.
- Comparative performance evaluation: Our approach involves surveying current meta-heuristic algorithms, conducting a thorough study, and comparing their performance using actual data obtained from case studies and experiments. This technique allows us to determine which algorithms are most suited for certain cloud resource load-balancing situations we have established.
- Integration of heuristic initial solutions: Our study emphasizes the significance of using typical heuristic methods to provide initial solutions for meta-heuristics to enhance the overall optimization process. This hybrid technique has received little attention in the existing literature and represents a novel addition to the discipline.
2. Background
2.1. cloud computing characteristics, 2.2. role of load balancing in cloud computing, 2.3. load-balancing challenges, 2.4. load-balancing policies, 2.5. meta-heuristic algorithms, 2.6. classification of load-balancing algorithms, 3. meta-heuristic algorithms for cloud load balancing, 3.1. ant colony optimization algorithm, 3.2. artificial bee colony algorithm, 3.3. genetic algorithm, 3.4. particle swarm optimization algorithm, 3.5. bat algorithm, 3.6. whale optimization algorithm, 3.7. simulated annealing algorithm, 3.8. biogeography-based optimization algorithm, 3.9. firefly algorithm, 3.10. grey wolf optimizer, 4. discussion.
- Complex optimization: Load balancing in cloud computing involves distributing tasks and workloads across multiple servers or VMs to ensure efficient resource utilization and reduced response times. This task is often a complex optimization problem that requires finding optimal or near-optimal solutions. Nature-inspired algorithms provide powerful optimization techniques to tackle these challenges.
- Global search: Cloud environments can have numerous variables and constraints, making it challenging to find the best solution. Nature-inspired algorithms, such as genetic algorithms, particle swarm optimization, and ant colony optimization, are designed to perform global searches in the solution space, helping to find solutions that traditional algorithms might miss.
- Flexibility and adaptability: Nature-inspired algorithms are often designed to adapt and evolve, mimicking the ability of natural systems to adapt to changing environments. In cloud computing, workloads and resource availability can vary dynamically. These algorithms can help adapt load-balancing strategies to changing conditions effectively.
- Parallelism and scalability: Cloud environments are inherently parallel and scalable. Many nature-inspired algorithms can be easily parallelized, allowing them to leverage the distributed nature of cloud computing resources. This makes them well-suited for addressing load-balancing challenges in large-scale cloud environments.
- Multi-objective optimization: Load balancing often involves optimizing multiple objectives simultaneously, such as minimizing response time, maximizing resource utilization, and minimizing energy consumption. Nature-inspired algorithms can handle multi-objective optimization, allowing cloud administrators to find trade-offs among different goals.
- Dynamic nature: Some nature-inspired algorithms, like particle swarm optimization, mimic the behavior of particles moving through a solution space. This dynamic nature aligns well with the dynamic nature of load balancing in cloud computing, where workloads and resources change over time.
- Exploration and exploitation: Nature-inspired algorithms strike a balance between exploration (searching for new and unexplored areas of the solution space) and exploitation (refining solutions in promising regions). This is vital for finding optimal or near-optimal solutions to load-balancing problems.
- Heuristic solutions: Load-balancing problems are often NP-hard, meaning that finding an optimal solution in a reasonable amount of time is practically impossible. Nature-inspired algorithms provide heuristic solutions that can efficiently find good solutions even for highly complex and large-scale load-balancing instances.
- Domain-agnostic: Nature-inspired algorithms are generally domain-agnostic and can be applied to various problems, including load balancing in cloud computing. They can adapt to different system architectures and characteristics.
- Earliest Deadline First (EDF): Tasks are prioritized according to their deadlines, with the tasks with the earliest dates given more priority. This strategy is efficient in time-sensitive situations where fulfilling deadlines is essential.
- Least Laxity First (LLF): Similar to EDF, LLF arranges jobs according to the amount of time available before their deadlines, known as slack time or laxity. Tasks with the lowest amount of flexibility are assigned more importance, guaranteeing prompt completion.
- First-Fit Decreasing (FFD): The tasks are arranged in descending order based on their size, then assigned to the first available resource to accommodate them. This strategy optimally allocates jobs within restricted resources, minimizes fragmentation, and enhances resource use.
- Best-Fit Decreasing (BFD): Like FFD, tasks are assigned to the resource that has the lowest remaining capacity following the assignment. The objective of this strategy is to reduce the amount of unused space and enhance the efficiency of packing.
- Greedy algorithms: These algorithms use local, optimal decisions at each stage in the expectation of discovering a global optimum. For instance, a greedy load balancer may allocate each incoming job to the server with the lowest current load, with the objective of gradually achieving load balance.
- Dynamic policy selection: The scheduler assesses many policies in real time and selects the one that most effectively aligns with the present workload and system condition. This flexibility improves efficiency and the usage of resources.
- Policy portfolio: The portfolio comprises a varied range of scheduling policies, including round-robin, least-connection, and FCFS. This enables the scheduler to seamlessly transition between policies as required in order to optimize performance.
5. Open Issues and Future Directions
6. conclusions, author contributions, data availability statement, conflicts of interest.
- Pourghebleh, B.; Anvigh, A.A.; Ramtin, A.R.; Mohammadi, B. The importance of nature-inspired meta-heuristic algorithms for solving virtual machine consolidation problem in cloud environments. Clust. Comput. 2021 , 24 , 2673–2696. [ Google Scholar ] [ CrossRef ]
- Hayyolalam, V.; Pourghebleh, B.; Chehrehzad, M.R.; Kazem, A.A.P. Single-objective service composition methods in cloud manufacturing systems: Recent techniques, classification, and future trends. Concurr. Comput. Pract. Exp. 2022 , 34 , e6698. [ Google Scholar ] [ CrossRef ]
- Pourghebleh, B.; Hayyolalam, V. A comprehensive and systematic review of the load balancing mechanisms in the Internet of Things. Clust. Comput. 2019 , 23 , 641–661. [ Google Scholar ] [ CrossRef ]
- Afzal, S.; Kavitha, G. Load balancing in cloud computing—A hierarchical taxonomical classification. J. Cloud Comput. 2019 , 8 , 22. [ Google Scholar ] [ CrossRef ]
- Kumar, P.; Kumar, R. Issues and challenges of load balancing techniques in cloud computing: A survey. ACM Comput. Surv. (CSUR) 2019 , 51 , 1–35. [ Google Scholar ] [ CrossRef ]
- Shafiq, D.A.; Jhanjhi, N.Z.; Abdullah, A.; Alzain, M.A. A load balancing algorithm for the data centres to optimize cloud computing applications. IEEE Access 2021 , 9 , 41731–41744. [ Google Scholar ] [ CrossRef ]
- Agrawal, N.; Tapaswi, S. Defense mechanisms against DDoS attacks in a cloud computing environment: State-of-the-art and research challenges. IEEE Commun. Surv. Tutor. 2019 , 21 , 3769–3795. [ Google Scholar ] [ CrossRef ]
- Zhang, W.-Z.; Elgendy, I.A.; Hammad, M.; Iliyasu, A.M.; Du, X.; Guizani, M.; El-Latif, A.A.A. Secure and optimized load balancing for multitier IoT and edge-cloud computing systems. IEEE Internet Things J. 2020 , 8 , 8119–8132. [ Google Scholar ] [ CrossRef ]
- Mazumder, A.M.R.; Uddin, K.A.; Arbe, N.; Jahan, L.; Whaiduzzaman, M. Dynamic task scheduling algorithms in cloud computing. In Proceedings of the 2019 3rd International conference on Electronics, Communication and Aerospace Technology (ICECA), Coimbatore, India, 12–14 June 2019; IEEE: Piscataway, NJ, USA; pp. 1280–1286. [ Google Scholar ]
- Saeik, F.; Avgeris, M.; Spatharakis, D.; Santi, N.; Dechouniotis, D.; Violos, J.; Leivadeas, A.; Athanasopoulos, N.; Mitton, N.; Papavassiliou, S. Task offloading in Edge and Cloud Computing: A survey on mathematical, artificial intelligence and control theory solutions. Comput. Netw. 2021 , 195 , 108177. [ Google Scholar ] [ CrossRef ]
- Hayyolalam, V.; Pourghebleh, B.; Kazem, A.A.P. Trust management of services (TMoS): Investigating the current mechanisms. Trans. Emerg. Telecommun. Technol. 2020 , 31 , e4063. [ Google Scholar ] [ CrossRef ]
- Zhang, W.; Chen, L.; Luo, J.; Liu, J. A two-stage container management in the cloud for optimizing the load balancing and migration cost. Future Gener. Comput. Syst. 2022 , 135 , 303–314. [ Google Scholar ] [ CrossRef ]
- Tawfeeg, T.M.; Yousif, A.; Hassan, A.; Alqhtani, S.M.; Hamza, R.; Bashir, M.B.; Ali, A. Cloud Dynamic Load Balancing and Reactive Fault Tolerance Techniques: A Systematic Literature Review (SLR). IEEE Access 2022 , 10 , 71853–71873. [ Google Scholar ] [ CrossRef ]
- Kong, L.; Mapetu, J.P.B.; Chen, Z. Heuristic load balancing based zero imbalance mechanism in cloud computing. J. Grid Comput. 2020 , 18 , 123–148. [ Google Scholar ] [ CrossRef ]
- Kumar, K. P2BED-C: A novel peer to peer load balancing and energy efficient technique for data-centers over cloud. Wirel. Pers. Commun. 2022 , 123 , 311–324. [ Google Scholar ] [ CrossRef ]
- Liang, B.; Dong, X.; Wang, Y.; Zhang, X. A low-power task scheduling algorithm for heterogeneous cloud computing. J. Supercomput. 2020 , 76 , 7290–7314. [ Google Scholar ] [ CrossRef ]
- Raza, M.S.; Wei, J.; Muslam, M.M.A. A Succinct Review of Intelligent Computational Techniques in Green Cloud Computing. In Proceedings of the 2020 International Conference on Communications, Signal Processing, and their Applications (ICCSPA, Sharjah, United Arab Emirates, 16–18 March 2021; IEEE: Piscataway, NJ, USA, 2021; pp. 1–4. [ Google Scholar ]
- Li, C.; Sun, H.; Tang, H.; Luo, Y. Adaptive resource allocation based on the billing granularity in edge-cloud architecture. Comput. Commun. 2019 , 145 , 29–42. [ Google Scholar ] [ CrossRef ]
- Ebrahim, M.; Hafid, A. Resilience and load balancing in Fog networks: A Multi-Criteria Decision Analysis approach. Microprocess. Microsyst. 2023 , 101 , 104893. [ Google Scholar ] [ CrossRef ]
- Kumar, Y.; Kaul, S.; Hu, Y.-C. Machine learning for energy-resource allocation, workflow scheduling and live migration in cloud computing: State-of-the-art survey. Sustain. Comput. Inform. Syst. 2022 , 36 , 100780. [ Google Scholar ] [ CrossRef ]
- Motlagh, A.A.; Movaghar, A.; Rahmani, A.M. Task scheduling mechanisms in cloud computing: A systematic review. Int. J. Commun. Syst. 2020 , 33 , e4302. [ Google Scholar ] [ CrossRef ]
- Neelakantan, P.; Yadav, N.S. An Optimized Load Balancing Strategy for an Enhancement of Cloud Computing Environment. Wirel. Pers. Commun. 2023 , 131 , 1745–1765. [ Google Scholar ] [ CrossRef ]
- Li, K.; Chang, C.; Yun, K.; Zhang, J. Research on Container Migration Mechanism of Power Edge Computing on Load Balancing. In Proceedings of the 2021 IEEE 6th International Conference on Cloud Computing and Big Data Analytics (ICCCBDA), Chengdu, China, 24–26 April 2021; IEEE: Piscataway, NJ, USA; pp. 386–390. [ Google Scholar ]
- Guo, X.-Q.; Chen, W.-N.; Wei, F.-F.; Mao, W.-T.; Hu, X.-M.; Zhang, J. Edge–Cloud Co-Evolutionary Algorithms for Distributed Data-Driven Optimization Problems. IEEE Trans. Cybern. 2022 , 53 , 6598–6611. [ Google Scholar ] [ CrossRef ] [ PubMed ]
- Kannan, K.; Sunitha, G.; Deepa, S.; Babu, D.V.; Avanija, J. A multi-objective load balancing and power minimization in cloud using bio-inspired algorithms. Comput. Electr. Eng. 2022 , 102 , 108225. [ Google Scholar ] [ CrossRef ]
- Qawqzeh, Y.; Alharbi, M.T.; Jaradat, A.; Sattar, K.N.A. A review of swarm intelligence algorithms deployment for scheduling and optimization in cloud computing environments. PeerJ Comput. Sci. 2021 , 7 , e696. [ Google Scholar ] [ CrossRef ] [ PubMed ]
- Sridharan, S.; Subramanian, R.K.; Srirangan, A.K. Physics based meta heuristics in manufacturing. Mater. Today Proc. 2021 , 39 , 805–811. [ Google Scholar ] [ CrossRef ]
- Camacho-Villalón, C.L.; Dorigo, M.; Stützle, T. Exposing the grey wolf, moth-flame, whale, firefly, bat, and antlion algorithms: Six misleading optimization techniques inspired by bestial metaphors. Int. Trans. Oper. Res. 2023 , 30 , 2945–2971. [ Google Scholar ] [ CrossRef ]
- Gao, R.; Wu, J. Dynamic load balancing strategy for cloud computing with ant colony optimization. Future Internet 2015 , 7 , 465–483. [ Google Scholar ] [ CrossRef ]
- Muteeh, A.; Sardaraz, M.; Tahir, M. MrLBA: Multi-resource load balancing algorithm for cloud computing using ant colony optimization. Clust. Comput. 2021 , 24 , 3135–3145. [ Google Scholar ] [ CrossRef ]
- Xu, P.; He, G.; Li, Z.; Zhang, Z. An efficient load balancing algorithm for virtual machine allocation based on ant colony optimization. Int. J. Distrib. Sens. Netw. 2018 , 14 , 1550147718793799. [ Google Scholar ] [ CrossRef ]
- Gabhane, J.P.; Pathak, S.; Thakare, N.M. A novel hybrid multi-resource load balancing approach using ant colony optimization with Tabu search for cloud computing. Innov. Syst. Softw. Eng. 2023 , 19 , 81–90. [ Google Scholar ] [ CrossRef ]
- Bui, K.T.; Pham, T.V.; Tran, H.C. A load balancing game approach for VM provision cloud computing based on ant colony optimization. In Proceedings of the Context-Aware Systems and Applications: 5th International Conference, ICCASA 2016, Thu Dau Mot, Vietnam, 24–25 November 2016; Proceedings 5. Springer: Berlin/Heidelberg, Germany, 2017; pp. 52–63. [ Google Scholar ]
- Ragmani, A.; Elomri, A.; Abghour, N.; Moussaid, K.; Rida, M. An improved hybrid fuzzy-ant colony algorithm applied to load balancing in cloud computing environment. Procedia Comput. Sci. 2019 , 151 , 519–526. [ Google Scholar ] [ CrossRef ]
- Mohammadian, V.; Navimipour, N.J.; Hosseinzadeh, M.; Darwesh, A. LBAA: A novel load balancing mechanism in cloud environments using ant colony optimization and artificial bee colony algorithms. Int. J. Commun. Syst. 2023 , 36 , e5481. [ Google Scholar ] [ CrossRef ]
- Raghav, Y.Y.; Vyas, V. ACBSO: A hybrid solution for load balancing using ant colony and bird swarm optimization algorithms. Int. J. Inf. Technol. 2023 , 15 , 2847–2857. [ Google Scholar ] [ CrossRef ]
- Minarolli, D. A Distributed Task Scheduling Approach for Cloud Computing Based on Ant Colony Optimization and Queue Load Information. In Proceedings of the International Conference on P2P, Parallel, Grid, Cloud and Internet Computing, Tirana, Albania, 27–29 October 2022; Springer: Berlin/Heidelberg, Germany, 2022; pp. 13–24. [ Google Scholar ]
- Amer, D.A.; Attiya, G.; Ziedan, I. An efficient multi-objective scheduling algorithm based on spider monkey and ant colony optimization in cloud computing. Clust. Comput. 2023 , 27 , 1799–1819. [ Google Scholar ] [ CrossRef ]
- Kruekaew, B.; Kimpan, W. Multi-objective task scheduling optimization for load balancing in cloud computing environment using hybrid artificial bee colony algorithm with reinforcement learning. IEEE Access 2022 , 10 , 17803–17818. [ Google Scholar ] [ CrossRef ]
- Kruekaew, B.; Kimpan, W. Enhancing of artificial bee colony algorithm for virtual machine scheduling and load balancing problem in cloud computing. Int. J. Comput. Intell. Syst. 2020 , 13 , 496–510. [ Google Scholar ] [ CrossRef ]
- Kumar, R.; Chaturvedi, A. Improved cuckoo search with artificial bee colony for efficient load balancing in cloud computing environment. In Proceedings of the Smart Innovations in Communication and Computational Sciences: Proceedings of ICSICCS 2020, Dallas, TX, USA; Springer: Berlin/Heidelberg, Germany, 2021; pp. 123–131. [ Google Scholar ]
- Janakiraman, S.; Priya, M.D. Improved artificial bee colony using monarchy butterfly optimization algorithm for load balancing (IABC-MBOA-LB) in cloud environments. J. Netw. Syst. Manag. 2021 , 29 , 39. [ Google Scholar ] [ CrossRef ]
- Milan, S.T.; Navimipour, N.J.; Bavil, H.L.; Yalcin, S. A QoS-based technique for load balancing in green cloud computing using an artificial bee colony algorithm. J. Exp. Theor. Artif. Intell. 2023 , 1–36. [ Google Scholar ] [ CrossRef ]
- Sefati, S.S.; Halunga, S. A hybrid service selection and composition for cloud computing using the adaptive penalty function in genetic and artificial bee colony algorithm. Sensors 2022 , 22 , 4873. [ Google Scholar ] [ CrossRef ] [ PubMed ]
- Makasarwala, H.A.; Hazari, P. Using genetic algorithm for load balancing in cloud computing. In Proceedings of the 2016 8th International Conference on Electronics, Computers and Artificial Intelligence (ECAI), Ploiesti, Romania, 30 June–2 July 2016; IEEE: Piscataway, NJ, USA, 2016; pp. 1–6. [ Google Scholar ]
- Saadat, A.; Masehian, E. Load balancing in cloud computing using genetic algorithm and fuzzy logic. In Proceedings of the 2019 International Conference on Computational Science and Computational Intelligence (CSCI), Las Vegas, NV, USA, 5–7 December 2019; IEEE: Piscataway, NJ, USA, 2019; pp. 1435–1440. [ Google Scholar ]
- Gulbaz, R.; Siddiqui, A.B.; Anjum, N.; Alotaibi, A.A.; Althobaiti, T.; Ramzan, N. Balancer genetic algorithm—A novel task scheduling optimization approach in cloud computing. Appl. Sci. 2021 , 11 , 6244. [ Google Scholar ] [ CrossRef ]
- Pradhan, A.; Bisoy, S.K. A novel load balancing technique for cloud computing platform based on PSO. J. King Saud Univ.-Comput. Inf. Sci. 2022 , 34 , 3988–3995. [ Google Scholar ] [ CrossRef ]
- Alguliyev, R.M.; Imamverdiyev, Y.N.; Abdullayeva, F.J. PSO-based load balancing method in cloud computing. Autom. Control Comput. Sci. 2019 , 53 , 45–55. [ Google Scholar ] [ CrossRef ]
- Mapetu, J.P.B.; Chen, Z.; Kong, L. Low-time complexity and low-cost binary particle swarm optimization algorithm for task scheduling and load balancing in cloud computing. Appl. Intell. 2019 , 49 , 3308–3330. [ Google Scholar ] [ CrossRef ]
- Malik, M.; Suman. Lateral Wolf Based Particle Swarm Optimization (LW-PSO) for Load Balancing on Cloud Computing. Wirel. Pers. Commun. 2022 , 125 , 1125–1144. [ Google Scholar ] [ CrossRef ]
- Sharma, S.; Luhach, A.K.; Sinha, S. An optimal load balancing technique for cloud computing environment using bat algorithm. Indian J. Sci. Technol. 2016 , 9 , 1–4. [ Google Scholar ] [ CrossRef ]
- Ullah, A.; Chakir, A. Improvement for tasks allocation system in VM for cloud datacenter using modified bat algorithm. Multimed. Tools Appl. 2022 , 81 , 29443–29457. [ Google Scholar ] [ CrossRef ] [ PubMed ]
- Zheng, J.; Wang, Y. A hybrid multi-objective bat algorithm for solving cloud computing resource scheduling problems. Sustainability 2021 , 13 , 7933. [ Google Scholar ] [ CrossRef ]
- Ramya, K.; Ayothi, S. Hybrid dingo and whale optimization algorithm-based optimal load balancing for cloud computing environment. Trans. Emerg. Telecommun. Technol. 2023 , 34 , e4760. [ Google Scholar ] [ CrossRef ]
- Strumberger, I.; Bacanin, N.; Tuba, M.; Tuba, E. Resource scheduling in cloud computing based on a hybridized whale optimization algorithm. Appl. Sci. 2019 , 9 , 4893. [ Google Scholar ] [ CrossRef ]
- Ni, L.; Sun, X.; Li, X.; Zhang, J. GCWOAS2: Multiobjective task scheduling strategy based on Gaussian cloud-whale optimization in cloud computing. Comput. Intell. Neurosci. 2021 , 2021 , 5546758. [ Google Scholar ] [ CrossRef ]
- Sabar, N.R.; Song, A. Grammatical evolution enhancing simulated annealing for the load balancing problem in cloud computing. in Proc. Genet. Evol. Comput. Conf. 2016 , 2016 , 997–1003. [ Google Scholar ]
- Hanine, M.; Benlahmar, E.-H. A load-balancing approach using an improved simulated annealing algorithm. J. Inf. Process. Syst. 2020 , 16 , 132–144. [ Google Scholar ]
- Kumar, S.; Singh, G.; Prakash, S. A Performance Improvement Model for Cloud Computing Using Simulated Annealing Algorithm. Int. J. Softw. Innov. (IJSI) 2022 , 10 , 1–17. [ Google Scholar ]
- Ghobaei-Arani, M. A workload clustering based resource provisioning mechanism using Biogeography based optimization technique in the cloud based systems. Soft Comput. 2021 , 25 , 3813–3830. [ Google Scholar ] [ CrossRef ]
- Bouhank, A.; Daoudi, M. Non-dominated ranking biogeography based optimization algorithm for virtual machine placement in cloud Computing. In Intelligent Computing: Proceedings of the 2021 Computing Conference ; Springer: Berlin/Heidelberg, Germany, 2022; Volume 1, pp. 423–438. [ Google Scholar ]
- Devaraj, A.F.S.; Elhoseny, M.; Dhanasekaran, S.; Lydia, E.L.; Shankar, K. Hybridization of firefly and improved multi-objective particle swarm optimization algorithm for energy efficient load balancing in cloud computing environments. J. Parallel Distrib. Comput. 2020 , 142 , 36–45. [ Google Scholar ] [ CrossRef ]
- RM, S.P.; Bhattacharya, S.; Maddikunta, P.K.R.; Somayaji, S.R.K.; Lakshmanna, K.; Kaluri, R.; Hussien, A.; Gadekallu, T.R. Load balancing of energy cloud using wind driven and firefly algorithms in internet of everything. J. Parallel Distrib. Comput. 2020 , 142 , 16–26. [ Google Scholar ] [ CrossRef ]
- Sekaran, K.; Khan, M.S.; Patan, R.; Gandomi, A.H.; Krishna, P.V.; Kallam, S. Improving the response time of m-learning and cloud computing environments using a dominant firefly approach. IEEE Access 2019 , 7 , 30203–30212. [ Google Scholar ] [ CrossRef ]
- Gohil, B.N.; Patel, D.R. A hybrid GWO-PSO algorithm for load balancing in cloud computing environment. In Proceedings of the 2018 Second International Conference on Green Computing and Internet of Things (ICGCIoT), Bangalore, India, 16–18 August 2018; IEEE: Piscataway, NJ, USA, 2018; pp. 185–191. [ Google Scholar ]
- Sefati, S.; Mousavinasab, M.; Farkhady, R.Z. Load balancing in cloud computing environment using the Grey wolf optimization algorithm based on the reliability: Performance evaluation. J. Supercomput. 2022 , 78 , 18–42. [ Google Scholar ] [ CrossRef ]
- Sekaran, R.; Munnangi, A.K.; Rajeyyagari, S.; Ramachandran, M.; Al-Turjman, F. Ant colony resource optimization for Industrial IoT and CPS. Int. J. Intell. Syst. 2021 , 37 , 10513–10532. [ Google Scholar ] [ CrossRef ]
- Karaboga, D.; Akay, B. A comparative study of artificial bee colony algorithm. Appl. Math. Comput. 2009 , 214 , 108–132. [ Google Scholar ] [ CrossRef ]
- Zhao, F.; Zeng, X. Simulated annealing–genetic algorithm for transit network optimization. J. Comput. Civ. Eng. 2006 , 20 , 57–68. [ Google Scholar ] [ CrossRef ]
- Shami, T.M.; El-Saleh, A.A.; Alswaitti, M.; Al-Tashi, Q.; Summakieh, M.A.; Mirjalili, S. Particle swarm optimization: A comprehensive survey. IEEE Access 2022 , 10 , 10031–10061. [ Google Scholar ] [ CrossRef ]
- Yang, X.S.; Gandomi, A.H. Bat algorithm: A novel approach for global engineering optimization. Eng. Comput. 2012 , 29 , 464–483. [ Google Scholar ] [ CrossRef ]
- Mirjalili, S.; Lewis, A. The whale optimization algorithm. Adv. Eng. Softw. 2016 , 95 , 51–67. [ Google Scholar ] [ CrossRef ]
- Amine, K. Multiobjective simulated annealing: Principles and algorithm variants. Adv. Oper. Res. 2019 , 2019 , 8134674. [ Google Scholar ] [ CrossRef ]
- Simon, D. Biogeography-based optimization. IEEE Trans. Evol. Comput. 2008 , 12 , 702–713. [ Google Scholar ] [ CrossRef ]
- Yang, X.-S.; He, X. Firefly algorithm: Recent advances and applications. Int. J. Swarm Intell. 2013 , 1 , 36–50. [ Google Scholar ] [ CrossRef ]
- Mirjalili, S.; Mirjalili, S.M.; Lewis, A. Grey wolf optimizer. Adv. Eng. Softw. 2014 , 69 , 46–61. [ Google Scholar ] [ CrossRef ]
Click here to enlarge figure
Reference | Main Objective | Targeted Issue | Key Contributions |
---|---|---|---|
Gao and Wu [ ] | Optimal resource utilization and load avoidance | Task distribution and coordination in cloud computing | Efficient load balancing in cloud computing using ACO with improved network performance. |
Muteeh et al. [ ] | Efficient resource utilization and load balancing | Load balancing in cloud computing | Significant reduction in execution time and cost in cloud resource utilization. |
Xu et al. [ ] | Achieving load balancing and enhancing resource utilization | Multidimensional resource load balancing across physical machines | Improved resource utilization and load balancing in cloud computing through ACO-based VM allocation. |
Gabhane et al. [ ] | Enhancing multi-resource load balancing | Multi-resource load balancing | Outperformance of existing optimization methods in terms of data delivery and processing. |
Bui et al. [ ] | Balancing the interests of service providers and customers | VM provisioning and load balancing | Use of coefficients for achieving load balancing in VM provisioning. |
Ragmani et al. [ ] | Enhancing load balancing and response time | Load balancing in the cloud | Superior load balancing and response time using Fuzzy-ACO. |
Mohammadian et al. [ ] | Evenly distributing the workload across systems | Load balancing in data centers | Improved response time, imbalance degree, makespan, and resource utilization. |
Raghav and Vyas [ ] | Hybrid approach for load balancing | Load balancing in cloud computing | Improved performance compared to standalone ACO and bird swarm optimization. |
Minarolli [ ] | Distributed task scheduling using swarm intelligence | Task allocation during high-load conditions | Superior outcomes compared to distributed scheduling based solely on ACO or queue load information. |
Amer et al. [ ] | Efficient resource allocation and cloud performance enhancement | Multi-objective scheduling challenges | Efficient resource allocation, cloud performance enhancement, and increased profits. |
Reference | Main Objective | Targeted Issue | Key Contributions |
---|---|---|---|
Kruekaew and Kimpan [ ] | Optimizing task scheduling and resource utilization | Scheduling optimization and load balancing in cloud computing | Improved makespan, cost reduction, load balancing, increased throughput, and resource utilization. |
Kruekaew and Kimpan [ ] | Enhanced VM scheduling | VM scheduling in cloud computing | Superior VM scheduling in both homogeneous and heterogeneous environments. |
Kumar and Chaturvedi [ ] | Load balancing for efficient VM scheduling | Load distribution across VMs in cloud computing | Superior average VM load distribution, high accuracy, and low complexity compared to existing methods. |
Janakiraman and Priya [ ] | Optimizing resource allocation in cloud environments | Resource allocation challenges in cloud computing | Minimizing load variance, makespan, connection deviations, imbalance degree, and maximizing throughput. |
Tabagchi Milan et al. [ ] | Improving QoS and reducing energy consumption | QoS and energy efficiency in green computing | Enhanced QoS, reduced makespan, and minimized energy usage compared to alternatives. |
Sefati and Halunga [ ] | Optimized service selection in cloud computing | Service selection and allocation optimization in cloud computing | Improvements in reliability, availability, and cost-effectiveness in service selection and allocation. |
Reference | Main Objective | Targeted Issue | Key Contributions |
---|---|---|---|
Makasarwala and Hazari [ ] | Enhancing real-world applicability of load balancing | Cloud computing load balancing | Incorporation of time-based request priority for improved real-world relevance and superior performance. |
Saadat and Masehian [ ] | Swift optimization and user satisfaction improvement | Load balancing in cloud computing | Achieving superior solutions faster, enhancing user satisfaction, and elevating cloud computing load balancing. |
Gulbaz et al. [ ] | Simultaneous improvement in makespan and load balancing | Load balancing in computing systems | An effective load-balancing mechanism considers the actual VM load and significantly improves makespan, throughput, and load balancing. |
Reference | Main Objective | Targeted Issue | Key Contributions |
---|---|---|---|
Pradhan and Bisoy [ ] | Optimizing task scheduling in cloud environments | Task scheduling and resource utilization in the cloud | Superior performance in minimizing makespan and maximizing resource utilization. |
Alguliyev et al. [ ] | Task-based load balancing in the cloud | Load balancing and task migration in cloud computing | Achieves optimal task scheduling, equitable task distribution, and reduced time consumption for task-to-VM assignments. |
Mapetu et al. [ ] | Efficient task scheduling and load balancing in cloud computing | Task scheduling and load balancing | Outperforms existing heuristic and meta-heuristic algorithms in enhancing task scheduling and load distribution. |
Malik and Suman [ ] | Optimal load distribution and task scheduling in cloud computing | Task scheduling and VM load balancing | Balanced VM loads, reduced response times, and superior performance over existing systems in task scheduling and load distribution. |
Reference | Main Objective | Targeted Issue | Key Contributions |
---|---|---|---|
Sharma et al. [ ] | Fulfilling load balancer objectives using the bat algorithm | Load balancing and its impact on response time | Acknowledged the impact of load balancing on response time and aims for future work on job migration algorithm development. |
Ullah and Chakir [ ] | Enhancing task distribution within cloud computing’s VMs | Task distribution and load balancing in cloud computing | Outperforms standard techniques, significantly boosting the accuracy and efficiency of cloud data centers. |
Zheng and Wang [ ] | Enhancing cloud computing service quality through a hybrid multi-objective bat algorithm | Service quality improvement in cloud computing | Superior performance over multiple optimization algorithms, particularly regarding makespan, imbalance degree, throughput, and cost. |
Reference | Main Objective | Targeted Issue | Key Contributions |
---|---|---|---|
Ramya and Ayothi [ ] | Enhancing cloud performance through load balancing | Cloud performance optimization | Improved throughput, reliability, makespan, and resource allocation in CloudSim experiments. |
Strumberger et al. [ ] | Tackling cloud resource scheduling challenges | Cloud resource scheduling | Consistently outperforms the original whale optimization algorithm and other heuristics and meta-heuristics in enhancing cloud resource scheduling. |
Ni et al. [ ] | Multi-objective task scheduling in cloud computing | Task scheduling, resource utilization, and load balancing | Improved task completion time, VM load balance, and resource utilization compared to other meta-heuristic algorithms. |
Reference | Main Objective | Targeted Issue | Key Contributions |
---|---|---|---|
Sabar and Song [ ] | Novel load-balancing technique combining simulated annealing (SA) with grammatical evolution (GE) | Load balancing and parameter tuning in SA | Superiority over state-of-the-art algorithms in achieving load balancing, particularly for the Google machine reassignment problem. |
Hanine and Benlahmar [ ] | Achieving workload balance among VMs | Workload balance among VMs | Improved task allocation with fewer iterations compared to standard SA. |
Kumar et al. [ ] | Minimizing execution time and ensuring load balance in job scheduling | Job scheduling and load balancing | Optimal solutions outperform various algorithms and significantly reduce job schedule execution times. |
Reference | Main Objective | Targeted Issue | Key Contributions |
---|---|---|---|
Ghobaei-Arani [ ] | Optimizing cloud application execution through workload clustering and resource provisioning | Workload clustering and QoS-aware resource provisioning | Reduction in delay, SLA violations, cost, and energy consumption compared to alternatives, confirming superiority in optimizing cloud application execution. |
Bouhank and Daoudi [ ] | Minimizing resource wastage and power consumption during VM placement | Resource optimization in VM placement | Improved efficiency, convergence, and solution coverage compared to other multi-objective approaches for VM placement. |
Reference | Main Objective | Targeted Issue | Key Contributions |
---|---|---|---|
Devaraj et al. [ ] | Balancing load distribution, enhancing resource utilization, and reducing task response times | Load balancing in cloud computing | Achieves balanced load distribution, enhanced resource utilization, and reduced task response times, outperforming alternatives in simulations. |
RM et al. [ ] | Integrating domains for energy-efficient Internet of Everything (IoE) services | Energy efficiency and traffic reduction in IoT networks | Superiority in extending IoT network lifetimes and significantly reducing traffic burdens compared to state-of-the-art techniques. |
Sekaran et al. [ ] | Optimizing task distribution for improved mobile learning system accuracy | Load balancing in cloud servers for m-learning | Potential to boost throughput and response times in mobile and cloud environments by addressing load imbalance in cloud servers for m-learning. |
Reference | Main Objective | Targeted Issue | Key Contributions |
---|---|---|---|
Gohil and Patel [ ] | Enhancing system performance and resource utilization equity in cloud computing | Load balancing in cloud computing | Enhanced convergence rates and implementation simplicity compared to other optimization techniques, promising potential for advancing cloud load balancing. |
Sefati et al. [ ] | Achieving effective load balancing with resource reliability consideration | Load balancing and resource allocation in cloud computing | Superior performance over alternatives, with reduced costs, response times, and optimal solutions in CloudSim-based simulations, addressing cloud-based load-balancing challenges effectively. |
The statements, opinions and data contained in all publications are solely those of the individual author(s) and contributor(s) and not of MDPI and/or the editor(s). MDPI and/or the editor(s) disclaim responsibility for any injury to people or property resulting from any ideas, methods, instructions or products referred to in the content. |
Share and Cite
Li, P.; Wang, H.; Tian, G.; Fan, Z. Towards Sustainable Cloud Computing: Load Balancing with Nature-Inspired Meta-Heuristic Algorithms. Electronics 2024 , 13 , 2578. https://doi.org/10.3390/electronics13132578
Li P, Wang H, Tian G, Fan Z. Towards Sustainable Cloud Computing: Load Balancing with Nature-Inspired Meta-Heuristic Algorithms. Electronics . 2024; 13(13):2578. https://doi.org/10.3390/electronics13132578
Li, Peiyu, Hui Wang, Guo Tian, and Zhihui Fan. 2024. "Towards Sustainable Cloud Computing: Load Balancing with Nature-Inspired Meta-Heuristic Algorithms" Electronics 13, no. 13: 2578. https://doi.org/10.3390/electronics13132578
Article Metrics
Article access statistics, further information, mdpi initiatives, follow mdpi.
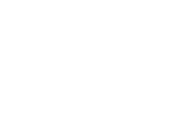
Subscribe to receive issue release notifications and newsletters from MDPI journals
Geoecological Assessment of the City of Vidnoe Using Multifractal Analysis
- RESEARCH TECHNIQUES
- Published: 04 July 2024
- Volume 45 , pages 94–100, ( 2024 )
Cite this article
- B. I. Kochurov 1 &
- M. A. Movchan 1
Modern scientific research in the field of geoecology, urban ecology, and urban planning focuses on the issues of geoecological assessment and the management of sustainable development of urban systems (urban geosystems). This research paper highlights both traditional methods of geoecological assessment, including environmental risk assessment and a comparison of MPC and MPL indicators, as well as modern methods such as remote sensing of the earth (analysis of satellite images) and modeling—a fractal analysis of urban geosystems. In addition, the article uses data from state statistics and environmental monitoring of the city and data from its own field research. It has been revealed that, in order to solve the problems of urban planning and management of territories based on the principles of sustainable development, such a system of geoecological assessment is required that would reflect the degree of development of the structure of urban geosystems, including the deviation of the development of urban geosystems from the optimum in conditions of multicomponent anthropogenic loads and risks of disruption of the sustainable functioning of the urban geosystem. Using the fractal modeling of the impact of anthropogenic factors, data on the deficit or redundancy of their impact were calculated. The advantage of territorial planning and the proposal of measures for the sustainable development of the city of Vidnoe based on fractal analysis consists of justifying the optimal degree of construction and the development of the transport network, reducing the risk of chaotic sprawl of the district and problems (first and foremost, traffic congestion and the pollution of environmental components), and achieving a minimum level of spatial fragmentation of the urban environment.
This is a preview of subscription content, log in via an institution to check access.
Access this article
Subscribe and save.
- Get 10 units per month
- Download Article/Chapter or Ebook
- 1 Unit = 1 Article or 1 Chapter
- Cancel anytime
Price includes VAT (Russian Federation)
Instant access to the full article PDF.
Rent this article via DeepDyve
Institutional subscriptions
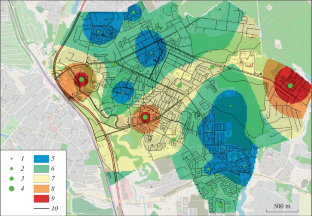
Iudin, D.I., Chechin, A.V., and Kashchenko, O.V., Application of fractal analysis methods and geoinformation technologies for the analysis of the road network of urbanized territories, Geoinf.: Inf. Kosm. , 2014, no. 1, pp. 84–87.
Mandel’brot, B., Fraktal’naya geometriya prirody (Fractal Geometry of Nature), Moscow: Inst. Komp’yut. Issled., 2002.
Guidelines for assessing the risk to public health from exposure to chemicals that pollute the environment. R 2.1.10.1920-04. https://normativ.kontur.ru/document? moduleId=1&-documentId=85628#h1595. Cited November 2, 2022.
Turchin, R., Evolution in population dynamics, Nature , 2003, vol. 424, pp. 257–258.
Article CAS Google Scholar
Lu, Y. and Tang, J., Fractal dimension of a transportation network and its relationship with urban growth: A study of the Dallas-Fort worth area, Environ. Plan. B: Plan. Design , 2004, vol. 31, pp. 895–911.
Article Google Scholar
Administration of the urban settlement of Vidnoye, Leninsky municipal district, Moscow oblast. Official website. http://vidnoe.adm-vidnoe.ru/?show=o_g._vidnoe. Cited November 5, 2022.
Moskovskaya oblast’. Atlas (Moscow Oblast. Atlas), Fedosova, M.A., Gryunberg, G.Yu., and Galiullina, E.G., Eds., Moscow: Glavn. Upravl. Geodez. Kartogr., 1976.
Google Scholar
Copernicus Open Access Hub. Space Imagery Open Access Center. https://scihub.copernicus.eu/dhus/#/ home. Cited November 10, 2022.
Earth Observing System. EOS Crop Monitoring. https://eos.com/es/ndsi. Cited November 12, 2022.
Sobol’, S.V., Fraktal’nye parametry vodnykh ob’’ektov: monografiya (Fractal Parameters of Water Bodies: Monograph), Nizhny Novgorod: Nizhegorod. Gos. Arkhitekt.-Stroit. Univ., 2019.
General plan of the urban settlement Vidnoye, Leninsky municipal district, Moscow oblast, part 2, 2022. http://vidnoe24.ru/news/images/2012/vidnoe_shemi_ utv_part.pdf. Cited December 1, 2022.
Kahramanova, Sh.Sh., Modeling in urban planning and ecology, Vestn. Tomsk. Gos. Arkhitekt.-Stroit. Univ. , 2012, no. 1, pp. 28–40.
Methodological Recommendations 2.1.10: The State of Health of the Population in Connection with the State of the Environment and Living Conditions of the Population. Assessing the Risk to Public Health from Exposure to Traffic Noise , Moscow: Federal. Tsentr Gigiyeny Epidemiol. Rospotrebnadzora, 2011.
Sokolskaya, E.V., Methodology for geoecological assessment of the quality of the urban environment based on multifactor modeling, Extended Abstract of Cand. Sci. (Gepgr.) Dissertation , Renaissance LLC, Tiraspol, 2019.
Download references
This study was carried out on the topic of the State Task of the Institute of Geography of the Russian Academy of Sciences (FMGE-2019-0007 AAAA-A19-119021990093-8) “Assessment of Physical-Geographical, Hydrological, and Biotic Changes in the Environment and Their Consequences for Creating the Foundations for Sustainable Environmental Management.”
Author information
Authors and affiliations.
Institute of the Geography, Russian Academy of Sciences, 119017, Moscow, Russia
B. I. Kochurov & M. A. Movchan
You can also search for this author in PubMed Google Scholar
Corresponding authors
Correspondence to B. I. Kochurov or M. A. Movchan .
Ethics declarations
The authors of this work declare that they have no conflicts of interest.
Additional information
Translated by L. Solovyova
Publisher’s Note.
Pleiades Publishing remains neutral with regard to jurisdictional claims in published maps and institutional affiliations.
Rights and permissions
Reprints and permissions
About this article
Kochurov, B.I., Movchan, M.A. Geoecological Assessment of the City of Vidnoe Using Multifractal Analysis. Geogr. Nat. Resour. 45 , 94–100 (2024). https://doi.org/10.1134/S1875372824700148
Download citation
Received : 28 December 2022
Revised : 11 September 2023
Accepted : 23 November 2023
Published : 04 July 2024
Issue Date : March 2024
DOI : https://doi.org/10.1134/S1875372824700148
Share this article
Anyone you share the following link with will be able to read this content:
Sorry, a shareable link is not currently available for this article.
Provided by the Springer Nature SharedIt content-sharing initiative
- urban geosystem
- territory planning
- environmental risk
- fractal approach
- Vidnoe city
- sustainability
- Find a journal
- Publish with us
- Track your research

IMAGES
VIDEO
COMMENTS
This journal publishes quantitative studies of natural (mainly but not limited to mineral) resources exploration, evaluation and exploitation, including environmental and risk-related aspects. Typical articles use geoscientific data or analyses to assess, test, or compare resource-related aspects. NRR covers a wide variety of resources ...
Full article: Sustaining natural resources in a changing environment: evidence, policy and impact. Contemporary Social Science. Journal of the Academy of Social Sciences. Volume 13, 2018 - Issue 1: Sustaining natural resources in a changing environment: Guest edited by Linda Hantrais, Ruth Kattumuri and Ashley Thomas Lenihan. Free access.
1. Introduction. Natural resource scarcity is a growing concern in many parts of the world. Rapid population growth and increasing industrialization are placing considerable pressure on the world's finite resources, leading to a shortage in many areas (Rinkesh, 2020).This is particularly true for essential resources such as water, soil, and energy.
Natural Resources Research. Articles. Natural Resources Research. Official Journal of the International Association for Mathematical Geosciences. Publishing model: Hybrid. ... Original Paper Open access 10 May 2024 Pages: 1439 - 1469 Identification of Geochemical Anomalies Using an End-to-End Transformer. Shuyan Yu; Hao Deng; Xiancheng Mao ...
The exploitation of ecosystems by humans has long-lasting consequences for the future provision of natural resources and ecosystem services 1,2.This may negatively affect the provision of food ...
Natural resources are the materials and substances that occur in nature and are valuable to humans for various purposes. They form the foundation of human societies, supporting our survival, economic activities, and overall well-being. Understanding the concept of natural resources is essential for recognizing their importance, managing them ...
This research has contributions to the literature for the following directions: (1) it is the first paper analysing the natural resource curse for these top wealthy P5 + 1 large economies of the world, which can lead to designing some important policy implications for the natural resources management in these countries, especially in the ...
Explore the latest full-text research PDFs, articles, conference papers, preprints and more on NATURAL RESOURCES. Find methods information, sources, references or conduct a literature review on ...
Studies on critical natural resources have grown in number over the last decade out of concern for resource availability and its potential impacts. Nonetheless, only a handful of studies explicitly define criticality for natural resources. Through a systematic literature review, we identified four main perspectives in the descriptions of critical natural resources: (1) economic importance is ...
The research was supported by the Innovation Research 2035 Pilot Plan of Southwest University (SWUPilotPlan026), ... This paper examines the natural resources hindrance and the ecological footprint nexus in the United States from 1971 to 2019, using mineral rents, energy use, and electricity production from coal as moderator variables. ...
Management of natural resources is pivotal for sustained economic growth—the increasing ecological footprints causing biocapacity deficit threaten the resource conversation agenda. The study identified the potential causes and consequences of natural resource depletion in a broad cross-section of 138 countries. Ecological footprints, international migrant stocks, industrial value-added, and ...
cularity; and guarantee green public procurement. Natural resources are central to human wellbeing. We cannot live without the clean air we. breathe, the plants we eat, or the water we drink. We need natural resour. es to put roofs over our heads and hea. non-living elements of the Earth system, includingplants, f.
ASABE publishes Journal of Natural Resources and Agricultural Ecosystems four times a year. Articles will be published online as they are completed. The number of published pages for articles are restricted to: four for research brief, frontier, and perspectives articles, eight for research articles, 15 for review articles, and.
The Special Issue Community, Natural Resources, and Sustainability seeks to engage in an interdisciplinary and international dialogue on the interrelationships of society, natural resources, and sustainability at the community level. In addition to introducing the twelve research articles published in this collection, we provide an overview of the existing literature on community and natural ...
Gender research in AMJ: An overview of five decades of research and call to action. Academy of Management Journal, 58: 1459-1475.Link, Google Scholar; Kassinis G., Vafeas N. 2006. Stakeholder pressures and environmental performance. Academy of Management Journal, 49: 145-159.Link, Google Scholar; King A. 2015. Why It Pays to Become a Rule ...
Volume 30 February - December 2021. Issue 6 December 2021. Issue 5 October 2021. Issue 4 August 2021. Special Issue on the ISME-XV: Toward Sustainable Society with Natural Resources - Development of Resource Exploration Technology in the Past Thirty Years and for the Future. Issue 3 June 2021.
renewable and nonrenewable. A resource is any natural or arti cial substance or energy which can be. used for the bene ts of mankind. Natural resources are those which exist. in the environment ...
The key. aspect of natural resources is that they determine the survival of humans and other life. forms on earth. These resources include land, rocks, forests (vegetation), water (ocean, lakes, s ...
Abstract. Natural resources and their rents have become critical as explanatory factors in economic research, most popularly with measures made available by the World Bank. However, the used definition of natural resources seems to miss out on the two natural resources: water and fertile land. This paper argues the importance of these two ...
The Pacific Northwest (PNW) Research Station is a leader in the scientific study of natural resources. We generate and communicate impartial knowledge to help people understand and make informed choices about natural resource management and sustainability.
Petrophysical Characterization of the Turonian and Cenomanian Intervals in the Abu Gharadig Field, Western Desert, Egypt: Inferences on Reservoir Quality and Resource Development. Sherif Farouk. Souvik Sen. Mohamed Mahmoud Elhossainy. Original Paper 02 May 2022 Pages: 1793 - 1824. Volume 31, issue 3 articles listing for Natural Resources Research.
The study utilizes a quantitative multi-method design and investigates new ERM drivers that have received little attention in the literature, such as Risk Culture and Board of Directors. The study provides actionable insights for policymakers, practitioners, and investors in North American publicly traded energy and natural resources companies.
This research paper aims to bring ... by heavy reliance on natural resources, thus putting extensive pressure on resources leading to increased costs, higher rate of forest degradation and reduced ...
On the mainland, the ministry committed to focusing on important research directions in natural resources, such as the mineralisation pattern of strategic resources, exploring and exploiting deep ...
Growth of publication in Natural Resources and Indigenous Communities (1979-2020) A total number of 1258 publications (TP), retrieved from the Scopus database about Natural Resources and Indigenous Communities (NR&IC) research, was divided into 10 document types for the period from 1979 to 2020, as presented in Fig. 1.It was observed that journal articles presented a total of 898 documents ...
The field of research focuses on optimizing algorithms for training large language models (LLMs), which are essential for understanding and generating human language. These models are critical for various applications, including natural language processing and artificial intelligence. Training LLMs requires significant computational resources and memory, making optimizing these processes a ...
Cloud computing is considered suitable for organizations thanks to its flexibility and the provision of digital services via the Internet. The cloud provides nearly limitless computing resources on demand without any upfront costs or long-term contracts, enabling organizations to meet their computing needs more economically. Furthermore, cloud computing provides higher security, scalability ...
Natural resources are resources that come from the natural environment and can be harnessed to meet the needs of man and other living things. ... Research should be carried out to identify microbes of nutritional benefits to livestock and affordable ways to adopt them. ... Watershed development adaptation strategy for climate change. Paper ...
Abstract Modern scientific research in the field of geoecology, urban ecology, and urban planning focuses on the issues of geoecological assessment and the management of sustainable development of urban systems (urban geosystems). This research paper highlights both traditional methods of geoecological assessment, including environmental risk assessment and a comparison of MPC and MPL ...