Academia.edu no longer supports Internet Explorer.
To browse Academia.edu and the wider internet faster and more securely, please take a few seconds to upgrade your browser .
Enter the email address you signed up with and we'll email you a reset link.
- We're Hiring!
- Help Center
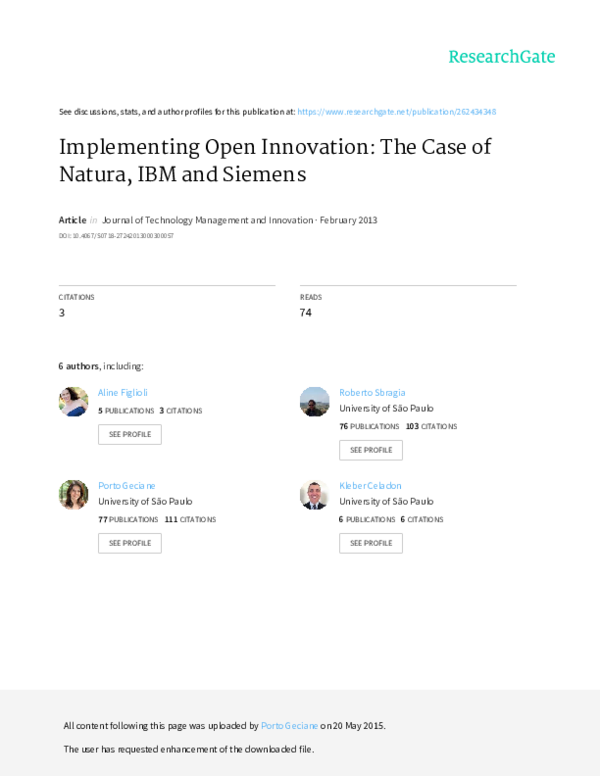

Implementing Open Innovation: The Case of Natura, IBM and Siemens

2013, Journal of technology management & innovation
Related Papers
book chapter
Giulio Ferrigno
Several approaches have been implemented by companies to elaborate open innovation (OI) 2.0 strategies. In this contribution we focus on the factors and the conditions of success that lead companies to formulate OI2 strategies starting from OI. Specifically in this study we note that three key factors enable the transition from OI to OI2 strategies: (1) technological pivot as a result of OI; (2) the presence of a clear appropriation strategy; and (3) the ability to orchestrate a rich ecosystem. Additionally, we observe four key managerial approaches, relevant for OI strategy, that are also important in the transition to an OI2 strategy: (1) carefully balancing internal and external resources; (2) leveraging organisational culture; (3) developing a sound business model; and (4) human resources management.
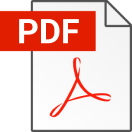
Sustainability
Open innovation has been found to have many benefits and tangible results for those who partake in it. This study aims to showcase the importance of open innovation, and through a theoretical example present how an organization (university, research center, company, firm, etc.) can take action to implement open innovation guidelines. In this paper, firstly, a demonstration showing how open innovation can work with multiple partners is shown. Secondly, a model is presented that shows the steps an organization must follow to successfully implement open innovation. This model covers the introduction of an organization to open innovation from the initial interest to the implementation of the final product. Several success stories are also presented to demonstrate how these steps have been used by major organizations during several collaborations as well as the results produced from implementing open innovation.
Acta Oeconomica Pragensia
Marek Jemala
An open technology innovation strategy can be characterised as a proactive combination of intra-institutional strengths and external opportunities of a market in order to create more complex technology solutions by exploiting capacities, capabilities and know-how ofanother institution (another company, university, research institution, etc.). This strategy may also postulate new market, industry, region, or culture penetration and concurrently has torequire new business models. The main research questions of this article are stated as follows: What are the main drivers, key barriers, successful factors, main questions and basic typologyof OI strategies in general? The issue of global innovation networks is outlined at the end of the article. The research was based on an extensive literature review, discussion with experts and several case studies (Apple, Nintendo, Nokia, etc.).
Technovation
Tim Minshall
Asia Pacific Journal of Innovation and Entrepreneurship
negar jalilian
Purpose Nowadays, to develop innovative activities in research and development units, it is desirable to rely on the concept of open innovation to take actions towards the identification of external capabilities of an organization and external knowledge acquisition. Therefore, this study aims to evaluate the impact of external technology acquisition (ETA), external technology exploitation (ETE) and culture of innovation (IC) on open innovation (OI) using SEM approach and then examine the amount of the impact of open innovation on organizational performance (OP) and value creation (VC). Design/methodology/approach This study was an applied survey in terms of research purpose and data collection method. The statistical population included all companies in Yazd Science and Technology Park (STP). To collect the data, 109 questionnaires were distributed. The content validity of the questionnaire was confirmed by experts’ comments, and Cronbach’s alpha coefficient was calculated equal to ...
World Journal of Science, Technology and Sustainable Development
Mokter Hossain
Valentina Lazzarotti
Series on Technology Management
Max von Zedtwitz
HAL (Le Centre pour la Communication Scientifique Directe)
Faiz Gallouj
Loading Preview
Sorry, preview is currently unavailable. You can download the paper by clicking the button above.
RELATED PAPERS
Journal of Promotion Management
Pejvak Oghazi
Journal of Technology Management & Innovation
Carlos Juiz
Wim Vanhaverbeke
Henry Chesbrough , Wim Vanhaverbeke
JISTEM Journal of Information Systems and Technology Management
Emerson Maccari
European Journal of Innovation Management
Francesco Galati
International Journal of Technology Management
Marina Dabić
Journal of Innovation & Knowledge
Emmanuel Adamides
Jaakko Paasi
Strategic Management Review
Teppo Felin
2012 20th Telecommunications Forum (TELFOR)
Miroslav Rogic
International Journal of Entrepreneurship and Innovation Management
International Journal of Technology Intelligence and Planning
Tobias Fredberg
International journal of innovation and knowledge management in the Middle East and North Africa
Dr Siham El-Kafafi
Convegno Sinergie-Sima 2018 “Transformative business strategies and new patterns for value creation”
Francesca Cabiddu
Journal of Knowledge Management
Nicola Bellantuono , Pierpaolo Pontrandolfo
Technology Analysis & Strategic Management
Brendan Galbraith
Journal of technology management & innovation
Susanne Ollila
EUROPEAN RESEARCH STUDIES JOURNAL
José López Rodríguez
Aysun Kanbur
Open Innovation
Gabriela Salas
RELATED TOPICS
- We're Hiring!
- Help Center
- Find new research papers in:
- Health Sciences
- Earth Sciences
- Cognitive Science
- Mathematics
- Computer Science
- Academia ©2024
- Corpus ID: 113487999
Driving Open Innovation in the Front End
- Ellen Enkel , O. Gassmann
- Published 16 May 2007
- Business, Engineering, Computer Science
51 Citations
Organizing open innovation in practice: a case study of an environmental innovation project in the automotive industry, implementation of open innovation practices in swedish manufacturing industry.
- Highly Influenced
Relational Capital and Open Innovation: Two Cases of Successful Italian Companies
Open innovation paradigm: making indian businesses competitive, exploring prerequisites for innovation at company x investigating the role of purchasing in the innovation challenge, open source as an innovation enabler: case study of an indian sme, open r&d and open innovation: exploring the phenomenon, an empirical multivariate examination of the performance impact of open and collaborative innovation strategies, exploring openness in information technology (it) innovation projects, linking entities in knowledge transfer: the innovation intermediaries, related papers.
Showing 1 through 3 of 0 Related Papers

Implementing Open Innovation: The Case of Natura, IBM and Siemens
- Cely Ades Faculdade de Economia, Administração e Contabilidade - Universidade de São Paulo. CCSA - Universidade Presbiteriana Mackenzie
- Aline Figlioli Faculdade de Economia, Administração e Contabilidade - Universidade de São Paulo
- Roberto Sbragia Faculdade de Economia, Administração e Contabilidade - Universidade de São Paulo
- Geciane Porto Faculdade de Economia, Administração e Contabilidade de Ribeirão Preto - Universidade de São Paulo
- Guilherme Ary Plonski Faculdade de Economia, Administração e Contabilidade - Universidade de São Paulo
- Kleber Celadon CENTRIM - University of Brighton - Great Britain
CHRISTENSEN, C. A inovação e a empresa madura. HSM Management. São Paulo, 23/ano4, nov/dez. 2000.
CHESBROUGH, H. W. Why companies should have Open Business Models. MIT Sloan, winter 2007.
______. The Era of Open Innovation. MIT Sloan Management Review, Spring 2003.
CHESBROUGH, H CROWTHER, AK. Beyond high tech: early adopters of open innovation. R&D Management, Vol 36, Nro. 3., pp. 229-236, Junho/2006.
DRUCKER, P. Inovação e o espírito empreendedor: prática e princípios. São Paulo: Pioneira Thomson, 2003.
EISENHARDT, K. M.; GRAEBNER, M. E. Theory Building From Cases: Opportunities and Challenges. Academy of Management Journal, 2007, Vol. 50, No. 1, 25–32.
ENKEL, E.; GASSMANN, O.; CHESBROUGH, H. Open R&D and open innovation. R&D Management, 39, 4, 2009.
FIGUEIREDO, P. Gestão da Inovação: conceitos, métricas e experiências de empresas no Brasil. 1ª. Ed. Rio de Janeiro: LTC, 2009
KNUDSEN, L.G.Opening the Black Box of open innovation: organizational practice, employee competences, ans knowledge processes. Druid-Dime Academy Winter 2007. Phd Conference on Geografy, Innovation ans Industrial Dynamics. Aalborg, Denmark, January, 25-27, 2007.
LEFÈVRE, F.; LEFÈVRE, A. M. C. O discurso do sujeito coletivo: um novo enfoque em pesquisa qualitativa (desdobramentos). Caxias do Sul: UDUCS, 2005.
MORTARA, L. et. al. How to implement open innovation: lessos from studying large multinational companies. University of Cambridge, IFM: 2009.
MOORE, G. . Solução para o insolúvel. HSM Management. São Paulo, 67, mar/abr. 2008.
RICHARDSON, R. Pesquisa social: métodos e técnicas. 3ª. ed. São Paulo: Atlas, 1999.
ROHRBECK, R.; HÖLZLE, K.; GEMÜNDEN, H. G. Opening up for competitive advantage – How Deutsche Telekom creates an open innovation ecosystem. R&D Management 39, 4, 2009.
RUIZ, J.A. Metodologia científica. 3ª. ed. São Paulo: Atlas, 1992.
SAMPIERI, R. H.; COLLADO, C. H.; LUCIO, P. B. Metodologia de pesquisa. 3ª edição. São Paulo: McGraw-Hill, 2006.
TAKAHASHI, S.; TAKAHASHI, V.P.Gestão de inovação de produtos: estratégia, processo, organização e conhecimento. Rio de Janeiro: Elsevier, 2007.
TIDD, J. et al. Gestão da Inovação. 3ª. Ed. Porto Alegre: Bookman, 2008.
VISKARI, S.; SALMI, P.; TORKKELI, M . Implementation of open innovation paradigm – cases: Cisco Systems, DuPont, IBM, Intel, Lucent, P&G, Philips and Sun Microsystems. Research Report. Department of Industrial Management. Lappeenranta University of Technology. Finland, 2007.
WEST, J.; GALLAGHER, S. Challenges of open innovation: the paradox of firm investment in open-source software. R&D Management 36, 3, 2006.
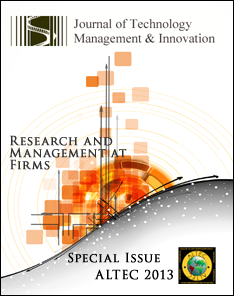
Additional Files
How to cite.
- Endnote/Zotero/Mendeley (RIS)
Copyright (c) 2013 Journal of Technology Management & Innovation

This work is licensed under a Creative Commons Attribution-ShareAlike 4.0 International License .
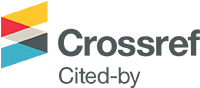
Most read articles by the same author(s)
- Ivete Rodrigues, Roberto Sbragia, The Cultural Challenges of Managing Global Project Teams: A Study of Brazilian Multinationals , Journal of Technology Management & Innovation: Vol. 8 (2013): Special Issue ALTEC: Social and Sustainable Project, Knowledge and Innovation Management
- Paulo Figueiredo, Gutenberg Silveira, Roberto Sbragia, Risk Sharing Partnerships With Suppliers: The Case of EMBRAER , Journal of Technology Management & Innovation: Vol. 3 No. 1 (2008)
- Sidirley Fabiani, Roberto Sbragia, Tax Incentives for Technological Business Innovation in Brazil: The Use of the Good Law - Lei do Bem (Law No. 11196/2005) , Journal of Technology Management & Innovation: Vol. 9 No. 4 (2014): Technical Change and Innovation Policy in Latin America: Lessons learned.
- Marcos Paixão Garcez, Roberto Sbragia, The Selection of Partners in Technological Alliances Projects , Journal of Technology Management & Innovation: Vol. 8 (2013): Special Issue ALTEC: Innovation, Territory and Vinculation Systems
- Manuel Heitor, Hugo Horta, Rosario Castañón, Roberto Sbragia, Alejandro Jiménez, Can Latin America Move Forward after a Lost Decade in Technical Change? …Looking at Opportunities for Knowledge-based Change in Times of Increasing Uncertainty , Journal of Technology Management & Innovation: Vol. 9 No. 4 (2014): Technical Change and Innovation Policy in Latin America: Lessons learned.
- Domingo González, Roberto Sbragia, Oscar Galante, Rocío Soto, Renato Valdivieso, ALTEC y la Gestión Tecnológica en Iberoamérica: Gestión de Proyectos, Conocimiento e Innovación Social y Sustentable , Journal of Technology Management & Innovation: Vol. 8 (2013): Special Issue ALTEC: Social and Sustainable Project, Knowledge and Innovation Management
- Domingo González, Roberto Sbragia, Oscar Galante, Rocío Soto, Renato Valdivieso, ALTEC y la Gestión Tecnológica en Iberoamérica: Sistemas de Innovación, Territorio y Vinculación , Journal of Technology Management & Innovation: Vol. 8 (2013): Special Issue ALTEC: Innovation, Territory and Vinculation Systems
- Domingo González González, Roberto Sbragia, Oscar Galante, Rocío Soto, Renato Valdivieso, ALTEC y la Gestión Tecnológica en Iberoamérica: Investigación y Gestión en las Empresas , Journal of Technology Management & Innovation: Vol. 8 (2013): Special Issue ALTEC: Research and Management at Firms
- Márcia May Gomel, Roberto Sbragia, A Capacitação Tecnológica e o Desempenho Exportador da Indústria Brasileira de Software: O Papel dos Investimentos em P&D , Journal of Technology Management & Innovation: Vol. 1 No. 3 (2006)
Similar Articles
- Wakhid Slamet Ciptono, Hargo Utomo, Tria Putri Noviasari, Maria Pampa Kumalaningrum, Review of Research on Management Innovation and Innovation Management: A Bibliometric Analysis , Journal of Technology Management & Innovation: Vol. 19 No. 2 (2024)
- René Pellissier, A Conceptual Framework for the Alignment of Innovation and Technology , Journal of Technology Management & Innovation: Vol. 3 No. 3 (2008)
- Serdal Temel, Anne Laure Mention, Marko Torkkeli, The Impact of Cooperation on Firms’ Innovation Propensity in Emerging Economies , Journal of Technology Management & Innovation: Vol. 8 No. 1 (2013)
- Jasmin Robertson, Tamma Sorbello, Kerrie Unsworth, Innovation Implementation: The Role of Technology Diffusion Agencies , Journal of Technology Management & Innovation: Vol. 3 No. 3 (2008)
- Irina Savitskaya, Pekka Salmi, Marko Torkkeli, Barriers to Open Innovation: Case China , Journal of Technology Management & Innovation: Vol. 5 No. 4 (2010)
- Adriana Bin, Cecilia María Gianoni, Paule Jeanne Vieira Mendes, Carolina Thaís Rio, Sergio Luiz Monteiro Salles-Filho, Luiza Maria Capanema, Organization of Research and Innovation: a Comparative Study of Public Agricultural Research Institutions , Journal of Technology Management & Innovation: Vol. 8 (2013): Special Issue ALTEC: Innovation, Territory and Vinculation Systems
- Pornpun Theinsathid, Achara Chandrachai, Suwimon Keeratipibul, Managing Bioplastics Business Innovation in Start Up Phase , Journal of Technology Management & Innovation: Vol. 4 No. 1 (2009)
- Mauricio Uriona Maldonado, Norberto Dias, Gregorio Jean Varvakis Rados, Managing Innovation in Small High-Technology Firms: A Case Study in Brazil , Journal of Technology Management & Innovation: Vol. 4 No. 2 (2009)
- Jun Young Kim, Yongtae Park, The Usefulness of Patent Stage and Sectoral Pattern in Open Innovation Licensing , Journal of Technology Management & Innovation: Vol. 3 No. 4 (2008)
- Seung-Ho Park, Yong-Gil Lee, Perspectives on Technology Transfer Strategies of Korean Companies in Point of Resource and Capability Based View , Journal of Technology Management & Innovation: Vol. 6 No. 1 (2011)
1 2 3 4 5 6 7 8 9 10 11 12 13 14 15 > >>
You may also start an advanced similarity search for this article.
Make a Submission
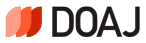
Google Scholar 2024
More details
Information
- For Readers
- For Authors
- For Librarians
2024 © Universidad Alberto Hurtado - Facultad de Economía y Negocios. Erasmo Escala 1835 - Santiago, Chile. Economic Analysis Review | Observatorio Económico | Gestión y Tendencias
Building on our history of innovation for the future of IBM
For more than a century, IBM has been rooted in the fundamental promise of technology: We believe that when we apply science to real-world problems, we can make progress — for both business and society. And as those problems have changed over time, so have we. IBM has repeatedly reinvented itself to overcome whatever obstacles stand in the way of innovation and value for our clients.
IBM scientists and engineers have been at the heart of our relentless reinvention. They have always been guided by a core principle, to deliver innovation that matters, for our company and the world.
Our commitment to research as part of our business model means we will continue to create the technologies that our clients and the world rely upon. For example, we have led US companies for decades in the number of patents received annually. Today, it was announced IBM has achieved this milestone for IBM’s total of more than 8,500 patents led the IFI Claims Patent Service 2021 rankings . 29 years in a row .
We are proud of this accomplishment and our leadership. However, the number of patents we receive has never told the full story of the innovation we drive. Our priority has always been leading the frontiers of computing and its relationship to business, science, professions, and society.
I believe that today, more than ever, we need innovation to meet the demands of many of the major challenges of our time — from models to create sustainable growth, to addressing future pandemics and climate change, to enabling energy and food security. To address them, we need faster discovery, open collaboration, efficient problem solving, and the ability to push science and business into new frontiers.
This future will be powered by a blend of high-performance computing, AI, and quantum computing, all integrated through the hybrid cloud. The confluence of these technologies represents a step change in computing, and the outcomes will surpass anything we’ve seen before. Together, these advancements can exponentially alter the speed and scale at which we can uncover solutions to complex problems. We’ve come to call this accelerated discovery.
Our priority has always been leading the frontiers of computing and its relationship to business, science, professions, and society.
But this will not happen in a vacuum. Strong innovation is built on a collaborative ecosystem, a commitment to long-term investment in hard tech challenges and fundamental materials, and the implementation of an open approach.
We have a long history of putting these principles into practice, and it’s in this spirit we undertook some of the most daunting hard technology challenges in 2021 — and delivered on them.
To name just a few: we worked with our partners to demonstrate the first 2 nm nanosheet technology for semiconductors, which will support up to 50 billion transistors on a chip the size of a fingernail and offer enormous gains in efficiency. We also collaborated with Samsung on the successful prototype of a chip that defies conventional semiconductor design, and lays the groundwork to achieve energy density and performance levels previously thought unattainable.
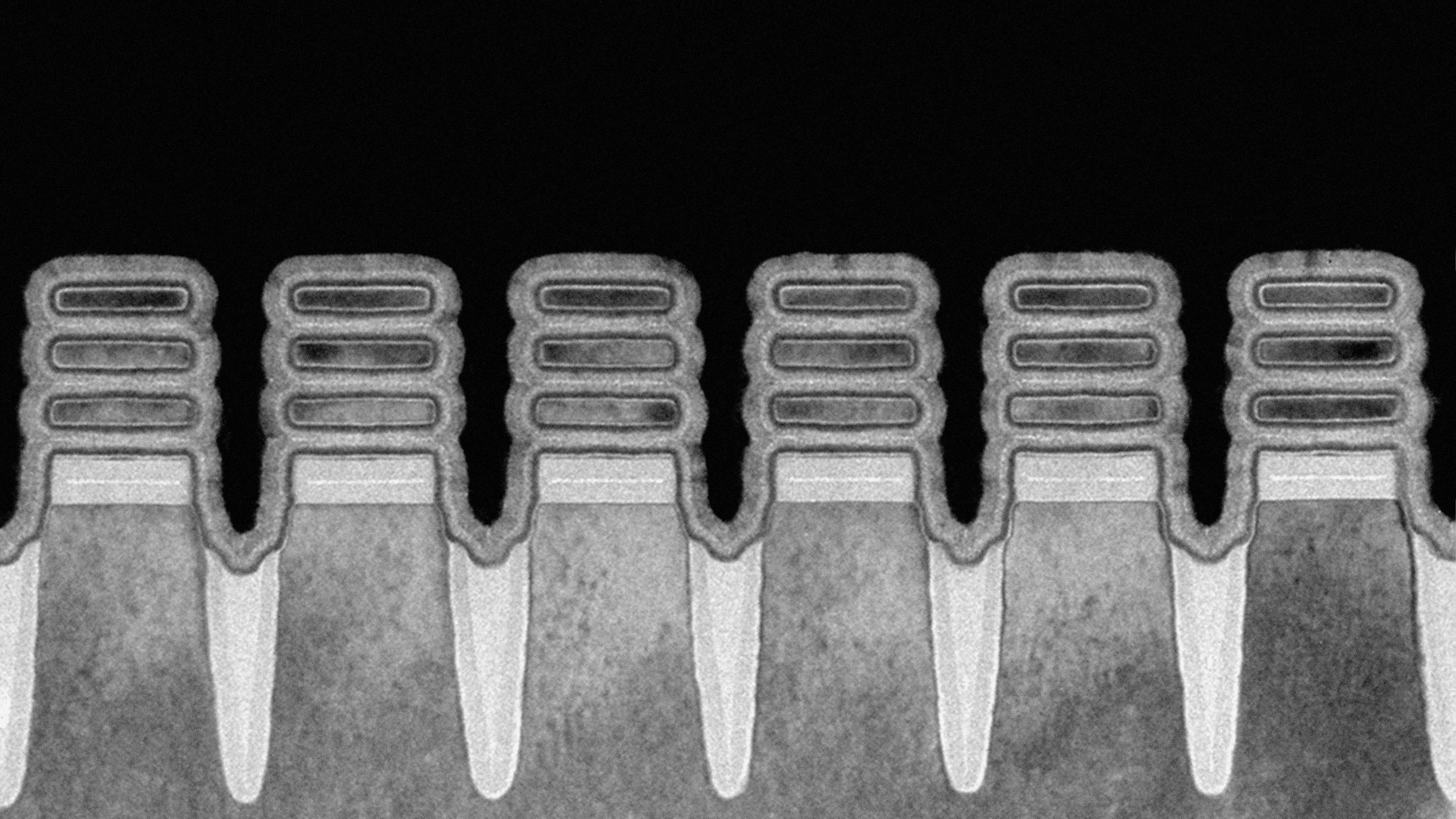
And as we lead the quest to reach practical and large-scale quantum computing, we stayed true to the ambitious roadmap we laid out in 2020 and In addition to unveiling Eagle, our 127-qubit quantum processor, and previewing the design for IBM Quantum System Two, our next-generation system that will house future quantum processors, we also introduced, Quantum Serverless, a new programming model for leveraging quantum and classical resources. Read more . delivered Eagle , our first 127-qubit processor , which will be critical to growing the nascent quantum industry IBM is pioneering.
To continue to realize a future marked by fundamental technology progress and the exploration of new scientific boundaries, we are deepening our commitment to this approach.
Building open communities for innovation
As part of our strategy, we are doubling down on our already robust and long-standing commitment to open communities. Innovation can emerge from anywhere, from a tech giant or a disruptive startup. In software, the growth of open source has redefined where innovation can come from, and how it is monetized. IBM has a long history in open source, and that continues today. Our pioneering work in serverless computing, which is quickly becoming the leading platform for the hybrid cloud industry because of the significant growth of Red Hat, is just one example of this.
We will also expand our focus to grow communities of innovation. The most successful technologies and innovations are often found when complementary institutions work together. To take one example among many, our collaboration with the The Cleveland Clinic + IBM Discovery Accelerator is a collaboration set to advance pathogen research, and foster the next-gen tech workforce for healthcare. Read more . Cleveland Clinic will bring together IBM’s technology and expertise in hybrid cloud, AI, and quantum computing to help Cleveland Clinic discover solutions to pressing issues around public health.
These sorts of collaborations will help technology to solve truly profound problems, and we hope to do so in partnership with other institutions adopting our technology, including Fraunhofer-Geselleschaft , Germany’s largest research institution, the Hartree Centre , a major AI and high-performance computing research facility in the UK, and Japan’s University of Tokyo and Keio University. Worldwide, we will continue to forge partnerships with the broader scientific community as we look to accelerate the pace of discovery.
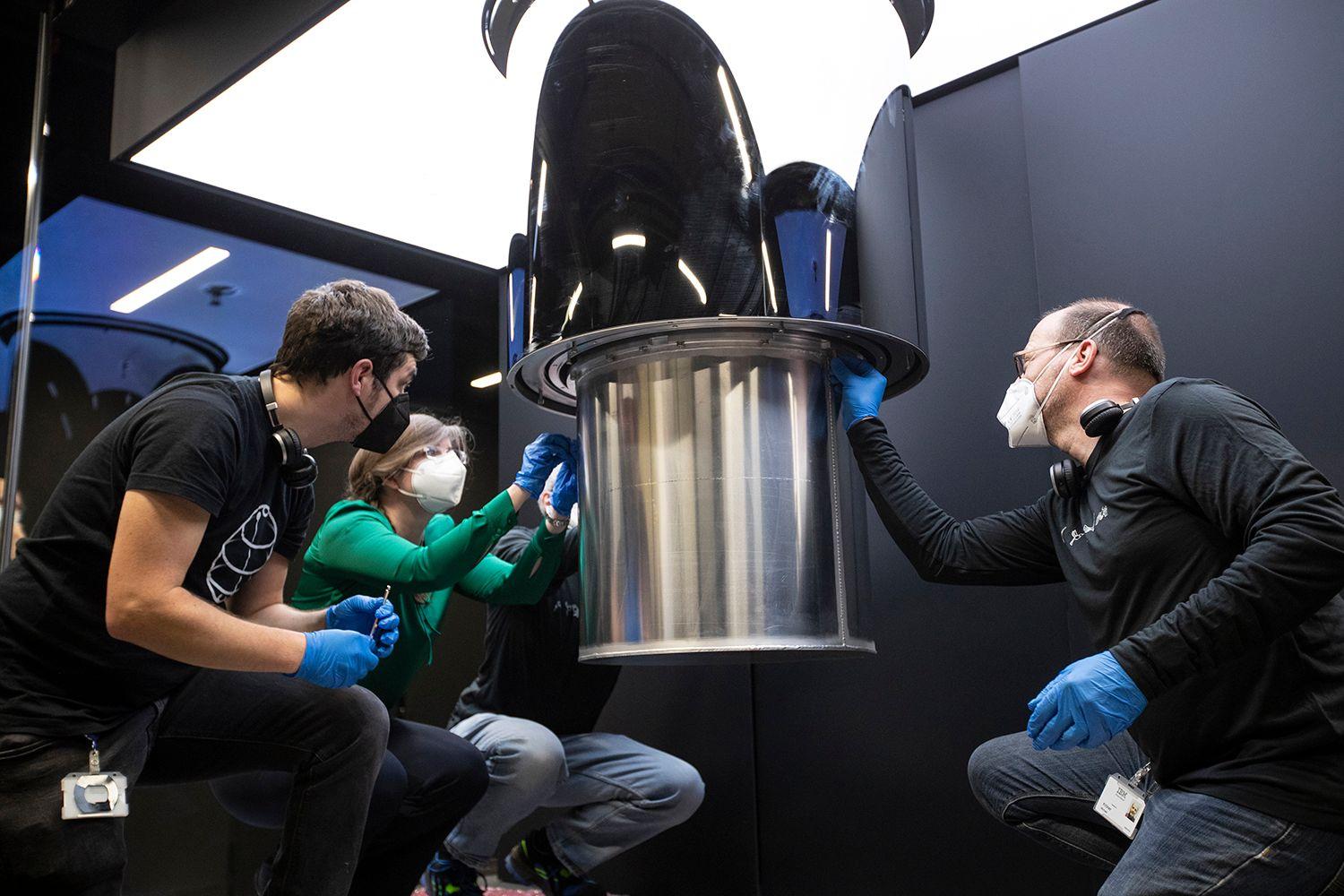
Pushing discovery beyond patent filings
Moving forward, we’re strengthening our companywide approach to focus our innovation efforts around the areas that matter most for our business and for society at large. This will include hybrid cloud, AI, quantum computing, systems and semiconductors, and security.
We believe these areas will have the most impact on our clients, industries, and the world. We also believe they’re the ones with the greatest potential for ecosystem collaboration.
While IBM will remain an intellectual property powerhouse with one of the strongest US patent portfolios, as part of our heightened focus moving forward, we’ll also take a more selective approach to patenting. We are proud of our decades-long history of topping the US patents chart, but in this new era, our position as the recipient of the most patents in any given year will not be a priority. Instead, our focus will be to prioritize growing these key technology areas of our company.
The problems the world is facing today require us to work faster than ever before. We see it as our duty to catalyze scientific progress by taking the cutting-edge technologies we’re working on, scaling them, and deploying them with partners across every industry.
Innovation is the heart and soul of IBM and serves as the engine to make our clients and the world work better. We made enormous strides in the last year, and we plan to achieve even more in 2022.
Learn more about:
Impact Science : In our impact science disciplines, we apply deep technical expertise to some of the world’s the most pressing challenges, while advancing the underlying science and creating business and societal value.
- Accelerated Discovery
- Impact Science
- Note 1 : IBM’s total of more than 8,500 patents led the IFI Claims Patent Service 2021 rankings . ↩︎
- Note 2 : In addition to unveiling Eagle, our 127-qubit quantum processor, and previewing the design for IBM Quantum System Two, our next-generation system that will house future quantum processors, we also introduced, Quantum Serverless, a new programming model for leveraging quantum and classical resources. Read more . ↩︎
- Note 3 : The Cleveland Clinic + IBM Discovery Accelerator is a collaboration set to advance pathogen research, and foster the next-gen tech workforce for healthcare. Read more . ↩︎
- Work & Careers
- Life & Arts
Case study: IBM’s reputation for innovation
Limited time offer, save 50% on standard digital, explore more offers..
Then $75 per month. Complete digital access to quality FT journalism. Cancel anytime during your trial.
Premium Digital
Complete digital access to quality FT journalism with expert analysis from industry leaders. Pay a year upfront and save 20%.
- Global news & analysis
- Expert opinion
- FT App on Android & iOS
- FT Edit app
- FirstFT: the day's biggest stories
- 20+ curated newsletters
- Follow topics & set alerts with myFT
- FT Videos & Podcasts
- 20 monthly gift articles to share
- Lex: FT's flagship investment column
- 15+ Premium newsletters by leading experts
- FT Digital Edition: our digitised print edition
FT Digital Edition
10% off your first year. The new FT Digital Edition: today’s FT, cover to cover on any device. This subscription does not include access to ft.com or the FT App.
Terms & Conditions apply
Explore our full range of subscriptions.
Why the ft.
See why over a million readers pay to read the Financial Times.
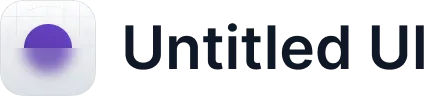
How IBM Became A Multinational Giant Through Multiple Business Transformations
Table of contents, here’s what you’ll learn from ibm’s strategy study:.
- How an accurate diagnosis of your organization’s most pressing challenge can help you form a coherent strategy to overcome it.
- How developing your strategic instinct to recognize change early and decisively transforming your business rely on unifying your organization towards a single direction.
- How focusing on short-term financial gains is putting your long-term survival and profitability in jeopardy.
IBM stands for International Business Machines Corporation and is a multinational technology corporation with over 100 years of history and multiple inventions that are prevalent today. Its headquarters are in Armonk, New York, but it operates in over 170 countries.
Institutional investors own over 55% of IBM, while around 30% belongs to mutual funds, and individual investors own less than 1%. Since 2020, IBM’s current Chairman and CEO is Arvind Krishna.
IBM’s market share and key statistics:
- Total assets of $28.999B as of September 30, 2022
- Revenue of $57.35B billion in 2021
- Total number of employees in 2022: 345,000
- Brand value of $ 96,992B in 2021
- Market Capitalization of over $130B as of December 2022

Humble beginnings: How did IBM start?
IBM was founded in 1911 as the Computing-Tabulating-Recording Company (CTR) in Endicott, New York, United States.
CTR was the product of three and a half amalgamated companies:
- The Tabulating Machine Company
- The International Time Recording Company
- The Computing Scale Company
- The Bundy Manufacturing Company
The company’s founding was very well-timed. It coincides with the profound shift of the United States’ agricultural economy to an industrial one. At that time, inventions and innovations were introduced at an unprecedented rate, evolving people’s way of life and defining our modern lifestyle.

The company’s success at these times was due to the wide range of products it offered that were in high demand in industrializing economies from time recording clocks and commercial scales to various mechanical data handling systems, tabulating machines.
But it was CTR’s corporate culture and managerial practices that enabled it to pioneer and serve that demand.
IBM’s scammy and problematic birth
The birth of IBM was the result of the vision and leadership of CTR's first president, Charles Ranlett Flint.

Flint was notorious for combining companies, creating monopolies, and having other people manage them while he simply owned stocks. That’s what he intended to do with the creation of CTR as well.
Here are the companies that formed what later became IBM:
- In 1900, Flint bought The Bundy Manufacturing Company , the inventor of the “punch card” that allowed factories to convert working hours into salaries. The company was quite successful due to increased demand from emerging factories and the founder’s acute business skills. Flint merged the company with the International Time Recorder (ITR).
- ITR was the core of CTR. By the time Flint created CTR, ITR was already a business group and an established player in selling and maintaining time recorders with an international presence. Flint had bought out almost all of his competitors, effectively creating a mini-monopoly.
- The next company that formed CTR was the Computing Scale Company . A marginally profitable company that had created a commercial scale for small merchants like butchers and cheesemakers. It was part of Flint’s vision of mechanical data handling.
- The fourth and last company that formed CTR was The Tabulating Machine Company . The main product of the company was the punch card tabulating equipment that automated parts of a manual and very labor-intensive process. It sped up “data entry,” increased accuracy, and reduced costs dramatically. It was Herman Hollerith’s invention, the second founder of CTR.
The merging of these three and a half companies didn’t make “business sense” at the time, nor was it the result of a careful business strategy.
At least not in the way we mean it today. It was a technical scheme that would allow Flint to protect his investment even if one of the companies wasn’t profitable and he had to sell it. Because, as it turns out, ITR was prosperous, and the tabulating business was slowly growing even though it required huge capital reinvestments. But the computing part of CTR was dying.
As a result, the child of this amalgamation was overvalued by twice its actual value.
This inflated value was supported by a loose argument of “economies of scale” since all these businesses were “measuring stuff.” From its very first days:
- The stock was overvalued
- The company was heavily in debt
- There were a lot of internal clashes
- The three businesses had no synergy
- There was little attention to innovation
- The board of directors only cared about profits
- The customer and employee treatment was poor
In short, IBM was born with some of the worst conditions for any company.
IBM’s coherent business strategy that got it out of the pit
Just three years after its creation, in 1914, the company changed its culture, executive team, and product line.
In ten years, it went through an astounding business transformation.
The move that initiated this transformation was the hiring of Thomas J. Watson Sr. as general manager for the company. The previous leader of the company was simply a credibility mark that Flint had implanted to draw investors. Watson’s influence on the company, however, is so monumental that he is considered the third founder of CTR, who shaped it into IBM.

Watson carried out a series of initiatives that laid the foundation for what would later become America’s largest technology company of the previous century:
- He built a mighty salesforce and a training program called “Sales School” that every salesperson had to graduate from.
- He brought clarity of purpose with frequent communication of goals and performance measures.
- He aligned daily actions with measurable targets that were part of the company’s strategy. He effectively created a culture of execution.
- He created a new line of products in the data-processing industry.
- He implemented initiatives to bring people from the three different divisions close together.
- He improved efficiencies by bringing product developers and manufacturing staff into the same building enabling cross-functional support and information exchange.
- He cultivated a system of shared beliefs and practices that empowered employees to make decisions that were consistent with the company’s priorities.
- He trained customers on how to use their products, gaining valuable feedback and ideas.
Watson spent half of his career as a valued employee of the National Cash Register Company (NCR), where he learned everything he knew about running a business. When he came to CTR, he brought all of his knowledge with him. It included: the development of the salesforce, budget and personnel practices, and even some executives that worked for his previous employer.
Watson was a highly motivated, optimistic, and conservative man of principle. During his tenure, CTR grew and consistently developed its product lines to cover a wide range of business machinery.
He took CTR from a scammy amalgamation to a respectable and healthy organization giving it a new name: International Business Machines (IBM).
Key Takeaway #1: Diagnose the challenge and tackle it with a coordinated strategy
When Watson became general manager, the company was rotten. However, he was ambitious and driven. His approach transformed the firm and affected the company’s journey for many generations. It can be summarized into two distinct steps.
When faced with a crumbling organization:
- Diagnose the most important challenge. Make an honest swot analysis . Watson found that CTR had a dying division (weakness), a profitable one (strength), and a promising one (opportunity). He based his approach on these findings.
- Devise a coherent and executable strategy. Create a strategic plan that addresses this challenge and coordinates resources. Watson applied all his expertise to developing a salesforce to seize the opportunity he found while internally reforming the company.
Watson practically transformed IBM’s culture into an improved copy of NCR’s culture. His experience fitted like a glove in IBM’s challenges. Some could argue that he happened to be a hammer who found its nail. Others that he found a nail and shaped himself into a hammer.
Whatever is true, his results were undeniable.
IBM’s Golden Period: the strategy and tactics IBM used to penetrate the computer industry
IBM went through the Great Depression and came out of it stronger, wealthier, and healthier.
It also went through World War II, which gave the company an explosive push that was hard to maintain once the war ended. IBM’s activities during WWII were plentiful and… complicated. One thing is certain, among the most important initiatives of Watson was the financial support of every IBMer’s family who went to join the fight and the promise that once the war was over, they would regain their job in the company.
As a result, once WWII ended, the company had more than 25% increased workforce at its disposal while a huge part of its revenue-generating business vanished nearly overnight: the military contracts.
Here’s how IBM faced these new challenges.
.jpeg)
IBM’s corporate strategy against an increased workforce and a vanished revenue stream
Watson recognized the problem from the beginning. His strategy may have been simple, but the flawless execution made all the difference since it wasn’t without obstacles.
The strategy had two key pillars, both focusing on technological advancement:
- Improve, marginally, current products whose demand was still high. The strategic objective was to expand sales on those product lines to generate immediate cash and keep the business floating.
- Invest in R&D of advanced electronics, a new technology that wasn’t fully understood nor ready to be commercialized. This was a necessary bet for the future of IBM.
It was obvious to Watson that the company should, one way or another, lead or at least ride a new wave of innovation and technological advancement. And that wasn’t possible with the company’s current product lines, internal structure, and culture.

The technological and business transformation that IBM went through was an undertaking that few high-tech companies have managed to pull off. Especially when so many stakeholders’ survival is dependent on the company’s well-being. Shareholders, banks that had provided loans, and employees were all highly incentivized to keep the status quo as is and fight against the transformation. “Since we’re selling, why change?” they thought.
This kind of resistance is typical when industry-reshaping technology emerges. Kodak went through the same but, unlike IBM, succumbed to stakeholder resistance, retained its status quo, and eventually died.
IBM’s strategic pivot faced a list of major challenges:
- The best minds in advanced electronics were not working at IBM.
- Advanced electronics was a relatively new industry that nobody could really understand or predict what problems it would solve and what use businesses would find in it.
- New and strong players emerged while old rivals were still actively competing. Remington Rand was an old and active foe while researchers were leaving universities to start new companies and develop systems for the U.S. Army like ENIAC. The reason was that the US government was issuing funding programs investing millions in this new technology. Whoever demonstrated enough expertise and promise was winning the funding, conducting research, innovating, and reaping the benefits.
- Sales resisted the new technology, clinging to its old and tested practices and propositions. In other words, sales and engineering weren't aligned.
The tactics IBM implemented to overcome these challenges and not only survive but also transform as a business in a record time are numerous. Since we can’t really know every single one of them, we’ll go through some of the most important events and principles that enabled the company to devise the solutions it needed.
How IBM overcame the challenges of its strategic pivot
The event that marked IBM’s transformation and sealed its strategic pivot was Watson’s son, Tom, entering the business.
Tom was a bright and ambitious young man who, with the help of his father’s influence and a series of chance events, became IBM’s Executive Vice President at the age of 33.
Tom understood the emerging new technology, and so he led that part of the business. On the other hand, Watson didn’t understand how it worked, so he focused on the more familiar, traditional and still revenue-producing product lines. The two clashed regularly and intensely on many issues. But it’s important to mention that their arguments were never focused on whether IBM needed to transform and adopt advanced electronics. They agreed on that part. They clashed only on the cadence of the transformation and the policies they put in place.
This distinction is crucial because it reveals that the company wasn't divided at its core, the direction everybody moved was the same. The clash between the old and the new was extremely productive because:
- The company started building critical mass in electronics by reinvesting earnings and rental cash flow. It didn’t rely on government funding, but rather it developed its capacity slowly and safely.
- In order to catch up with the industry’s velocity with its bootstrapped approach, IBM’s advanced electronics department had to do things differently. So it cultivated a culture of transparency and accountability where information flowed freely.
- The 604 Electronic Calculating Punch , the world's first mass-produced electronic calculator, was IBM’s first highly profitable product that came out of this approach.
- The firm used its active customer network to understand customer needs and prioritize improvements on the data processing machines. Thus it created machines with validated demand.
- The whole process enabled IBM to create “an infrastructure of knowledgeable customers, salesmen, and servicemen for electronic computers.”
As soon as the 1950s came, IBM entered the electronic computing market and became a highly competitive player. After that, it changed its strategy, took on larger computer projects, and became more dependent on federal funding to offset the associated risk.
It continued to accumulate knowledge and expertise, improving its processes and products.
Key Takeaway #2: Develop your strategic instinct and adapt fast
Develop your ability to recognize change and quickly transform your business to respond to it. To perform a successful business transformation, unite the organization towards a single direction.
If the need for change is clear at the top, it’s a matter of implementation and policies. It’s not easy, but it’s far more successful to lead a united organization than a two-headed one. So when you perform a business transformation:
- Define the direction or destination as clearly as possible.
- Align senior leadership with the desired direction.
- Build guiding policies that take you from the old to the new. Don’t simply kill the old, transform it.
- Treat the transformation as an idea worth spreading. Take advantage of your strengths and apply the Law of Diffusion of Innovations .
The decline during the last decade and IBM’s enterprise strategy to return to the top
IBM slowly but surely started shifting its business model again in the late half of the past century and the following decades.
This time, the strategic pivot was more fundamental. The company shifted from “components to infrastructure to business value.” In other words, it shifted from manufacturing computers and new technologies to offering IT consulting and integration services.
This is reflected in its revenue percentages by segment. In 1980, 90% of IBM’s revenue was generated from hardware sales. By 2015, the company was generating over 60% of its revenue from services and less than 10% from hardware sales.
However, the journey wasn’t as smooth as in past transformations.
The challenges of the consulting industry that has left IBM behind in the last decade
The shift, this time, was taking place less effectively.
The company was selling fewer and fewer pieces of hardware each year while its revenues from consulting services weren’t increasing as fast. The company wasn’t investing as much in R&D, and it entered the new era of computing with an extreme focus on financials.
In 2006 and in 2010, the company's leadership announced “Roadmap 2010” and “Roadmap 2015,” respectively. These were financial goals that were mistakenly used as strategic guiding policies. And to the company’s detriment, they dictated decision-making on every level.
Here are key facts that indicate this extreme financial focus was a terrible strategy at the worst timing:
- IBM’s new CEO, Virginia Marie “Ginni” Rometty, didn’t enjoy employee support. Due to her merciless tactics and her relentless focus to please the stakeholders, employee morale, and thus productivity, was at an all-time low.
- Revenue was decreasing year over year.
- “Rebalancing the workforce,” AKA layoffs, became a regular quarterly tactic to make the numbers.
- Current and ex-IBMers were losing faith and becoming less and less content with leadership.
- Despite the lack of growth, stocks continued rising, paying dividends and high Earnings Per Share (EPS). That was the result of “financial gimmicks” like massive stock buybacks or stashing assets and profits outside of the US to avoid taxes.
- Extreme focus on cutting back costs. Using overseas “global delivery skills,” AKA cheaper workers and even docking 10% of salaries to offer training to employees while charging high-end prices for IBM’s services.
But you can only cut costs for so much and save that much. There is a limit to how much cost-cutting you can do until you hurt operations and production. And IBM reached that limit well before 2015, the year “Roadmap 2015” was promising $20 EPS.
By the end of 2014, the company had amassed a huge debt, its hardware profitability had taken a nosedive, its margins had declined, the executive leadership forwent their personal annual incentive payments for 2013, and it abandoned the Roadmap.
To save the company, leadership had to come up with a radically different strategy. And it did. The 5 “imperatives” strategy was much more attractive to all the stakeholders and would prove to be much more effective.
IBM’s 5 imperatives and its strategy to recovering its past glory
IBM’s biggest weaknesses in the first one-and-a-half decade of the current century have been financial performance and strategic blunders.
But if it had no strengths to leverage, then it wouldn’t be alive today. And its size is one of them. IBM is huge. For example, as of 2018, the company employed around 378.000 people and commanded one of the largest collections of PhDs in computer science and technology. IBM generates over $50 billion in revenue annually with consistently large profits. In 2017, the company had over $8 billion in cash.
The “five imperatives'' were a strategy that focuses on actual performance and not financial engineering to be successful.
The five imperatives were:
- Cybersecurity
- Cloud computing
- Social networking
- Mobile technologies
The company made several acquisitions to close the competitive gap in all of those focuses while it shifted resources to support those initiatives. As a result, it surpassed its competition with analytics software and its capabilities to manage and analyze massive bodies of data. With a $2 billion acquisition of SoftLayer, it caught up with cloud computing and extended its services to include cybersecurity. A partnership with Apple Computer offered the promise of portable computing and app development platforms lodged in cloud servers. Finally, IBM offered management consulting as much as software services through its social networking focus.

IBM's Strategy has focused even more in recent years, integrating the imperatives into two major pillars: hybrid computing and Artificial Intelligence (AI).
It puts everything under the umbrella term: Digital Transformation.
IBM’s focus on digital transformation propels it into the future
IBM’s future looks promising, and its strategy is putting it back at the center of computers and technology. In January 2018, the company announced its first quarter of YoY revenue increase since 2012.

It focuses once again on delivering value to its customers by addressing the crucial challenges that accompany every digital transformation:
- Managing the increased complexity of heterogeneous enterprise IT environments.
- Extracting valuable insights from available data.
- Sustaining operational competitiveness against disruptive market changes.
- Increased cyber threats and increasing cost of cybersecurity.
- A cohesive end-to-end execution of solutions that address all of these matters.
The way IBM addresses these challenges and chooses to differentiate itself is by adopting a platform-centric hybrid cloud approach paired with advanced AI capabilities. The infrastructure relies on Linux, containers, and Kubernetes as the architectural foundation.
In layman’s terms, the value proposition of the company is the sustainable and accelerating transformation of their client’s businesses and processes through:
- Hybrid cloud that develops ability and speed.
- Tailored and trustworthy data governance respecting privacy and generating data-driven business insights.
- AI-driven decision-making that automates enterprise processes.
- Consistency, security, and compliance.
The company is rapidly growing its ecosystem, enhancing client experience while driving value and innovation with its open-source technologies.
Key Takeaway #3: To succeed long term, focus on developing business capabilities instead of financial returns
Ambitious goals and financial promises are not strategies. They might provide some returns in the short term but ultimately set the company up for future failure. Cutting costs is not an infinite-returns-yielding tactic.
When the industry changes, new trends and technologies emerge, and your competitive advantage won’t be serving you for much longer:
- Make a thorough analysis of the environment . Spot the most promising emerging trends in technology, customer expectations, and markets.
- Perform an internal analysis to define your strengths, weaknesses, and current capabilities that power your competitive advantage.
- Develop a strategy that takes advantage of your current capabilities, develops adjacent ones, and mitigates weaknesses to seize the opportunities you spot.
- Until your new strategy is performing and your competitiveness relies on it, ensure cash flow and sustainability through your current healthy lines of products.
Why is IBM so successful?
IBM’s success over its long history can’t be attributed to a single cause.
In each distinctive phase, IBM demonstrated the qualities that enabled it to thrive and pioneer in technological advancements. One consistent quality that allowed IBM to stand the test of time has been its decisive adaptability, the ability to spot new trends and transform its business in time to lead change.
Its corporate culture of respect and hard work has been the cornerstone of every single one of its achievements.
Growth by numbers
|
|
|
|
Total consolidated revenue | $104,5 b | $79,1 b | $57,3 b |
Total consolidated gross profit | $50,3 b | $36,2 b | $31,5 b |
Number of employees | 434,2 k | 366.6 k | 282.1 k |
{{cta('eed3a6a3-0c12-4c96-9964-ac5329a94a27')}}

The open innovation kaleidoscope: navigating pathways and overcoming failures
- Original Paper
- Open access
- Published: 31 August 2024
Cite this article
You have full access to this open access article
- Maral Mahdad ORCID: orcid.org/0000-0001-6612-5664 1 &
- Saeed Roshani 2
145 Accesses
Explore all metrics
In this study, we conduct a panoramic analysis of two decades of open innovation (OI), leveraging topic modeling with machine learning to map out ten critical OI pathways and their associated failure mechanisms on the micro, meso, and macro levels. Open innovation has revolutionized organizational innovation, collaboration, and competition. However, it presents complexities that require a multifaceted approach to research. Our findings, informed by interpretative thematic analysis, reveal distinct scholarly debates and three primary controversies within the OI research landscape, pointing to the need for future research to integrate these diverse narratives. By providing a comprehensive synthesis of the OI field’s evolution and current state, along with an analysis of its underlying failure mechanisms, we aim to guide strategic decision-making in OI practice, and enrich the academic discourse on its operational and strategic dimensions. Finally, we highlight several potential avenues for future research that emerge from our synthesis of the literature.
Similar content being viewed by others
Framework Linking Open Innovation Strategic Goals with Practices
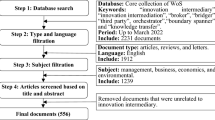
Innovation intermediaries: a review, bibliometric analysis, and research agenda
Open Innovation: Strategic Options, Actors, Tools and Tensions
Explore related subjects.
- Artificial Intelligence
Avoid common mistakes on your manuscript.
1 Introduction
In two decades of open innovation, the landscape resembles a kaleidoscope where numerous facets and aspects intersect. This gives rise to a dynamic phenomenon marked by opportunities as well as challenges (Chesbrough 2004 ). The concept of open innovation introduced by Henry Chesbrough in his seminal book (Chesbrough 2003a , b ) has reshaped the way organizations innovate and revolutionized how they collaborate, compete, and adapt in a rapidly evolving environment. Over the past two decades, OI has transformed the boundaries of theoretical constructs and scholarly research. It has embedded itself in the strategies, tactics and operations of businesses, governments, and institutions (Holgerson et al. 2022 ; McGahan et al. 2021 ; Rexhepi et al. 2019 ; West and Bogers 2017 ). However, as the open innovation landscape unfolds, it reveals a complex interplay of elements, factors and levels of analysis that require a panoramic view—a kaleidoscopic examination—to capture different pathways that characterize the spectrum. In addition, these complexities depend on a range of contextual elements in the firm and the business environment (Madanaguli et al. 2023 ).
Open innovation has proven transformative in reshaping industries (Christensen et al. 2005 ), changing competitive business environments (Bacon et al. 2020 ), and fostering opportunities for companies efficiently practicing OI (e.g. Caputo et al. 2016 ; Majchrzak et al. 2023 ). Yet, for all its promise, open innovation comes with challenges (Dabic et al. 2023 ; Madanaguli et al. 2023 ; Chaudhary et al. 2022 ). As it extends from idea generation to scalability it encompasses process-related challenges (Madanaguli et al. 2023 ). The transition from collaboration to “coopetition” introduces strategic challenges (Corbo et al. 2023 ; Gast et al. 2019 ; Kallmuenzer et al. 2021 ; Xue et al. 2023 ). Open innovation is marked by complexities and ambiguities. As organizations explore this terrain, they encounter the shadows of potential failures (Cricelli et al. 2023 ). Recognizing that the potential of a kaleidoscope lies in its ever-shifting patterns, we understand that open innovation, requires a multifaceted lens to comprehend its full potential. Creating an unbiased panoramic view through diverse literature review methods advances academic discussion and fuels our motivation. This view provides a solid foundation for our analytical exploration along research pathways (Kraus et al. 2022 ). In our research, we use topic modeling with machine learning algorithms (Hannigan et al. 2019 ) to enable the unbiased view over the two decades of OI research and increase replicability and transparency. Thereby, we incorporate extra dimensions of openness and soundness to the outcomes of traditional systematic reviews (Kraus et al. 2020 ). We add a novel abductive interpretative reasoning layer (Walton 2014 ) for comprehensively understanding open innovation failures and risks across ten open innovation pathways. In doing so, we aim to equip organizations and scholars with a well-rounded perspective that guides their strategic decision-making in practicing OI. Therefore, our research answers the question: What predominant topics have been explained by recent scholarly discourse in open innovation, and how are failure mechanisms within these topics delineated across the micro, meso, and macro organizational levels?
This multi-level system perspective was originally inspired by “Coleman’s Boat” (Coleman 1994 ), which aims to bridge macro and micro levels by pointing out the key components needed to develop robust theory. In management research, the macro level often encompasses the system, economy, industry, and society, while the micro level refers to individual and behavioral attributes (Cowen et al. 2022 ). In this context, meso-level refers to firm-level outcomes. Additionally, it is well-documented that the phenomenon of open innovation spans multiple levels, and our approach to investigating it at different levels aligns with prior literature (e.g., Bogers et al. 2017 ).
The field of open innovation, though extensively researched, continues to suffer from a fragmented understanding of its diverse and evolving nature, characterized by multiple pathways and shifting narratives. For example, Bertello et al. ( 2024 ) extensively reviewed the literature of the field. They showed that some scholars have attempted to leverage well-established theories such as a resource-based or knowledge-based view of the firms, while others have begun to renew the theoretical foundation to generate new discussions. These endeavors have enriched the field of open innovation. However, they have also increased the heterogeneity of its theoretical foundations, making it more challenging to develop coherent theories in new empirical contexts. This drives our first motivation to conduct a comprehensive examination and synthesis of open innovation research. We aim to shed light on its historical evolution and chart the path for future academic inquiries through topic modeling, a machine learning technique that uses natural language processing. Topic modeling offers a dynamic, comprehensive method for discerning hidden data patterns, surpassing traditional systematic literature reviews (SLR) in revealing emerging trends and adapting to shifts in research (Kraus et al. 2022 , 2024 ; Brem et al. 2023 ; Hannigan et al. 2019 ). Its aptitude for processing extensive datasets and the use of visualization tools simplifies the understanding of complex topic relationships (Hannigan et al. 2019 ). Objective and reflective analyses become fundamental for assessing the existing knowledge base, pinpointing knowledge gaps, and evaluating the efficacy and productivity of open innovation research This benefits both the practice and academic communities (Randhawa et al. 2016 ) and ultimately moves them forward. In fact, topic modeling techniques offer researchers the opportunity to create multi-dimensional artifacts like differentiation and novelty (Hannigan et al. 2019 ). However, its full potential is realized when an iterative and interpretive approach is integrated into the analytics. The novelty of our research comes from using interpretative thematic analysis to qualitatively examine topics identified by topic modeling techniques through the lens of failure. The diversity of OI pathways plays a pivotal role in shaping the identity and avenues of open innovation research and practice, reflecting its core concepts, paradigms, recognition mechanisms, and directions (Radziwon et al. 2022 ).
The choice of failure as a lens to examine open innovation (OI) challenges has recently received attention in OI research, although it has not been specifically carved into various OI pathways. Failure as an analytical lens was selected for two main reasons. First, the lack of studies on the downsides of OI represents a significant gap, potentially giving the misleading impression that OI is a cure-all for firms’ innovation challenges (Greco et al. 2022 ). Second, the need for alternative review techniques is necessary to gain a fine-grained understanding of open innovation failure (Chaudhary et al. 2022 ).
Our research therefore encompasses two decades of open innovation, offering a comprehensive analysis of ten critical pathways within the OI domain. In addition, it illustrates the nuanced failure mechanisms at micro, meso and macro levels that could negatively affect OI processes. Furthermore, it provides a snapshot of the current landscape and proposes prospective trajectories for the evolution of OI research based on the controversies in the field.
2 Methodology
This research improves topic modeling by integrating interpretative analysis commonly found in systematic literature reviews. This is in line with methodologies emphasized by leading experts like Kraus et al. ( 2022 ). Using an in-depth literature review conjoined with the procedure of topic modeling, the present research offers a comprehensive and categorized view of existing literature pertinent to open innovation within business and management domain. The approach combines a detailed literature review with topic modeling to offer a comprehensive perspective and categorization of existing literature on open innovation within the business and management domain. Our methodology follows a structured and transparent process, adhering to best practices for conducting systematic literature reviews (SLRs) as outlined in recent literature (Kraus et al. 2024 ). Specifically, the guidelines provided by Dhiman et al. ( 2023 ) and Rammal ( 2023 ) were instrumental in shaping our approach, ensuring that our review is thorough, systematic, and replicable. Additionally, recent studies have effectively utilized the SLR methodology to explore various aspects of management research, demonstrating its versatility and robustness. For instance, Sauer and Seuring ( 2023 ) employed an SLR to develop a comprehensive guide for conducting literature reviews in management research, emphasizing key decisions and steps. Similarly, other researchers have applied SLRs to investigate diverse topics, such as supply chain management (da Silva et al. 2024 ), absorptive capacity (Pütz et al. 2024 ), crowdsourcing and open innovation (Cricelli et al. 2022 ; Carrasco-Carvajal et al. 2023 ), the organizational, environmental, and socio-economic sustainability of digitization (Chopra et al. 2024 ), further validating the methodology’s applicability and relevance in our study.
Our methodology involves four key steps as described in Table 1 : data collection, topic modeling, topic exploration, and topic interpretation with failure as a lens. In the data collection phase, we employed a comprehensive search strategy across Web of Science, to gather relevant peer-reviewed articles. We then utilized Latent Dirichlet Allocation (LDA) for topic modeling, which allowed us to identify and categorize latent topics within the literature systematically (Blei 2012 ; Brem et al. 2023 ). The subsequent exploration and interpretation of these topics involved a critical analysis that integrates insights from the literature on systematic reviews in management research, drawing on established frameworks to ensure a comprehensive and nuanced synthesis. This robust methodological framework ensures that our study not only maps the existing body of knowledge but also identifies key gaps and future research directions in the field of open innovation, as emphasized by Rana et al. ( 2023 ), Rammal ( 2023 ) and Baltazar et al. ( 2023 ) (Fig. 1 ).
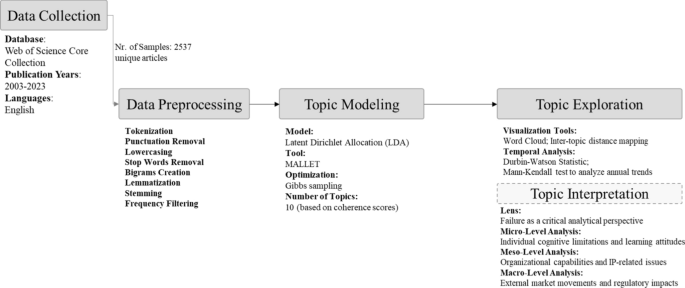
Overview of the research methodology
2.1 Data collection
To ensure a comprehensive examination of the open innovation literature, our primary data source was the Web of Science Core Collection (WoSCC) database. The WoSCC is widely recognized for its extensive coverage of high-quality research articles across various disciplines. WoS is one of the most comprehensive and widely used citation databases in the academic community. It covers more than 13,610 journals across all disciplines (Singh et al. 2021 ; Falagas et al. 2008 ). To find most relevant articles in the field, we used a search string based on Gao et al. ( 2020 ). Additionally, in recognition of the first article in this domain written by Chesbrough ( 2003a , b ), we refined our search to encompass articles from 2003 to 2023 (Gao et al. 2020 ; Kovacs et al. 2015 ). The detailed search criteria and keywords employed are presented in Table 1 .
The initial retrieval yielded a sample of 2,551 articles. Recognizing the importance of data quality in conducting rigorous research, we undertook a thorough data-cleaning process. This involved supplementing missing abstracts, removing duplicates, and discarding articles without abstracts. After this rigorous cleaning process, we were left with a final sample of 2,537 unique articles These formed the basis for our subsequent analyses.
2.2 Topic modeling
After finalizing the collection of relevant articles, we explored topic modeling, a method essential for uncovering hidden thematic patterns in large volumes of text (Blei 2012 ). This technique offers an objective perspective on dominant trends and provides a detailed understanding of the subject matter. In natural language processing and machine learning, a topic model is a statistical method for determining the “topics” in a set of documents. Latent Dirichlet Allocation (LDA) can uncover hidden semantic structures and topics in a large body of unstructured textual data using natural language processing, machine learning, and statistical algorithms (Blei et al. 2012 ; Wang and Blei 2011 ).
There are several notable advantages to using topic models. They rest on mathematically robust principles, elucidating the intricate dynamics of document generation. Moreover, they operate without needing prior categorization or labeling of documents, enabling an autonomous and expert-independent analysis. This autonomy extends to their capability to systematically organize and summarize vast swathes of documents, making them invaluable in text mining applications (Lee and Kang 2018 ). These attributes have led to an increased interest in topic models, finding successful applications across diverse text mining activities (Yan 2014 ). Several researchers in management studies have employed topic modeling techniques. Specifically, these approaches have been explored in fields such as marketing (Mustak et al. 2021 ; Amado et al. 2018 ), technology and innovation management (Lee and Kang 2018 ), information systems (Jeyaraj and Zadeh 2020 ), crisis innovation (Brem et al. 2023 ), open innovation (Lu and Chesbrough 2022 ), and human resource management (Thakral et al. 2023 ).
Before conducting topic modeling to identify relevant topics, some pre-processing procedures were required. Both the title and abstract of the articles were amalgamated to serve as the model’s input. This decision was based on the rationale that titles encapsulate the most representative terms, and the abstract delineated the study’s context, objectives, methodologies, and conclusions. We employed several steps to create a corpus that was used for topic modeling. In the initial phase, the entire text was divided into sentences and the sentences into the tokens (tokenization). Punctuation and numerical characters were subsequently excluded, and all characters were converted to lowercase. The subsequent step entailed the removal of words with fewer than three characters, including the extension of this process to eliminate structural words commonly found in abstracts, such as “aim,” “purpose,” “study,” “framework,” and “effect” (stop words). Next, word bigrams were created to link words that co-occur frequently. For instance, the combination of “business” and “model” was treated as “business_model”. Using lemmatization algorithms, words were transformed into their root forms to reduce dimensionality without loss of generality. For example, the term “industry” could manifest as “industry” or “industries” as a noun, “industrial” as an adjective, “industrialize,” “industrializes,” or “industrialized” as a verb, and “ndustrially” as an adverb. This was followed by word-stemming procedures that streamlined these words to their base forms, exemplified by the stemming of various forms of the term “industry” to “industri”. In addition, we removed all terms that occurred fewer than five times across all documents or that appeared in more than 70% of records. The final step in preprocessing was to convert the documents into a bag-of-words format. In this model, each document was depicted as a vector consisting of an unsequenced set of words. All of these tasks were implemented using gensim v. 3.8.3 (Rehurek and Sojka 2010 ), NLTK v. 3.7, and spaCy v. 3.0.0. (Honnibal et al. 2020 ).
We employed Latent Dirichlet Allocation-LDA (Blei et al. 2003 ) for topic modeling and used the Machine Learning for Language Toolkit (MALLET) for implementation. MALLET is an open-source Java-based machine learning package known for its sophisticated tools for statistical natural processing, document classification, sequence tagging, numerical optimization, and topic modeling, among others (McCullum 2002 ). One of its primary advantages is its scalable and efficient implementation of Gibbs sampling. Additionally, it provides efficient methods for document-topic hyperparameter optimization and has built-in tools for inferring topics on unseen documents using trained models. The MALLET tool is multi-threaded and optimized for performance on a single machine. However, it is worth noting its limitations. It can be memory-intensive, and handling extremely large datasets might lead to frequent garbage collection. As a result, it might not be scalable for massive datasets and can be challenging to scale across multiple nodes of a cluster (Sukhija et al. 2016 ). Despite these limitations, MALLET's robust features and efficiency, combined with the size of our data, made it a suitable choice for our study’s dataset and requirements.
Determining the optimal number of topics is a significant challenge in topic modeling. Researchers often use several measures, such as coherence and perplexity, to pinpoint the optimal number of topics. Although the perplexity measure is commonly used for this purpose (Jeong et al. 2019 ), there are no standard packages for its computation in MALLET. To address this issue, we used the topic coherence score to determine the optimal number of topics. This metric measures the quality of a given topic model by computing the semantic similarity between its highest-scoring terms. We used two specific coherence measures: c_v and u_mass. The c_v measure, rooted in a sliding window approach, uses a one-set segmentation of top words and indirect cosine similarity for confirmation. In contrast, u_mass is based on document co-occurrence counts and a one-preceding segmentation, confirming using the measure of log conditional probability (Röder et al. 2015 ). For c_v, higher values indicate better topic coherence, while for u_mass, values closer to zero suggest peak coherence (Röder et al. 2015 ) Our analysis employed both the c_v and u_mass measures. While some studies adopt statistical approaches, others rely on subjective analysis, where experts evaluate the appropriateness of number of topics (Madzík et al. 2023 ). Subsequently, we also engaged expert opinions to validate and refine our topic selection based on the coherence scores. As shown in Fig. 2 , the coherence scores, when plotted against the number of topics, provide insightful observations. The c_v scores consistently hover around their peak within the range of eight to 13 topics, reaching a peak of 0.3991 at 10 topics. This consistency suggests a stable and coherent representation of the data within this range. Meanwhile, the u_mass score indicates two topics as optimal, with scores closest to zero. However, such minimalistic categorization could potentially oversimplify our dataset, neglecting its inherent complexity and nuances. Within the range of eight to 13 topics, the u_mass scores are less negative, hinting at a semantic closeness and meaningful topic delineation. Given these findings, and for a more comprehensive perspective, we deemed it prudent to explore the topic range of eight to 13, striking a balance between coherence and detailed representation. A thorough evaluation of these topics subjectively led us to determine the optimal topic number at 10, marked by a c_v score of 0.3991 and an u_mass score of − 2.154.

Topic coherence scores
2.3 Topic exploration
Upon building the LDA model with 10 topics, we employed various techniques to further explore these topics. We visualized topics using the word_cloud library v. 1.8.1, where the size of each word within a specific topic is proportionate to its frequency within that topic. Additionally, we used the PyLDAvis library v. 2.1.2 for topic interpretation, based on the 10 topics identified earlier (Sievert and Shirley 2014 ). PyLDAvis facilitates topic visualization and offers deeper insights through its unique inter-topic distance mapping. This tool computes topic centers using Jensen–Shannon divergence (JSD) and calculates inter-topic distances using multidimensional scaling. It effectively maps multi-dimensional topic distances onto a two-dimensional plane, providing a spatial representation of topic proximity.
Subsequently, we also normalized the weight of each topic per year to analyze the annual changes in open innovation research. We used Mann–Kendall (MK) test. The null hypothesis in this nonparametric test is that the sample data are independent and randomly distributed (Hamed and Rao 1998 ). We used the pyMannKendall python package Version 1.4.2, which is a pure Python implementation of non-parametric Mann–Kendall trend analysis (Hussain and Mahmud 2019 ). However, before using the Mann–Kendall test, we needed to confirm that autocorrelation was not present in our data, as it could bias the Mann–Kendall results. For this, we employed the Durbin–Watson statistic, which tests for serial correlation between errors (Neeti and Eastman 2011 ). The Durbin–Watson test produces values that range from zero to four. A value close to two suggests no autocorrelation, while a value near zero indicates positive autocorrelation. Values near four imply negative autocorrelation. Our results shows that all values are below two, indicating the presence of positive autocorrelation in the data. In this case we used Hamed and Rao’s modification test to identify trends in topics (Hamed and Rao 1998 ). Upon further investigation, we noticed fluctuations in the data before 2002. To mitigate the potential biases these fluctuations might introduce to our trend analysis, we excluded data prior to 2002. After this adjustment, we recalculated the Mann–Kendall test to discern trends in the topics. To classify the most pertinent documents for each topic, we extracted the dominant topic for each document in our corpus. Using our LDA model, we assigned each document to the topic that had the highest contribution in that document. The “Dominant Topic” in our dataset is determined by identifying the topic number with the highest percentage contribution for that document. This is recorded as “Contribution %,” which signifies the weight of the topic in that particular document. This method ensures that each document is associated with its most relevant topic. It streamlines the process of analyzing journals and other sources based on topic dominance.
2.4 Topic interpretation and failure as a lens
Following the identification of topics, we embarked on an interpretive analysis of each one. Every article in our sample was categorized based on its predominant topic. Subsequently, to contextualize and synthesize the most pivotal contributions, we reviewed the articles with a high percentage of contribution to their associated topic. Our initial approach involved a thorough examination of the abstracts of articles with significant contributions to each topic. This facilitated the selection of works serving as exemplars. The representativeness of these chosen articles was cross-verified against the topic-related terms for accuracy. Upon this validation, we investigated these selected articles with other significant contributions within the same topic domain. As a culminating step in our analytical process, after an exhaustive review of articles within each topic, we assigned descriptive failure labels to every topic to encapsulate its essence. We adopted a failure lens for the selected articles in each topic, based on the rationale that these papers investigate OI cases representing constraints, limits, risks, or challenges for OI implementation. We then categorized the associating level as micro, meso, or macro, and included theoretical and conceptual papers in this analysis.
We collected 2,537 articles from the Web of Science database from 2003 to 2023. Figure 3 shows the number of publications in the field of open innovation from 2003 to 2023. The data shows a steady increase in the number of publications over time, with a peak in 2022 with 354 articles, followed by 276 articles in 2021 and 259 articles in 2020. The data suggests that open innovation is a rapidly growing field of research, with a high level of interest from the academic community.
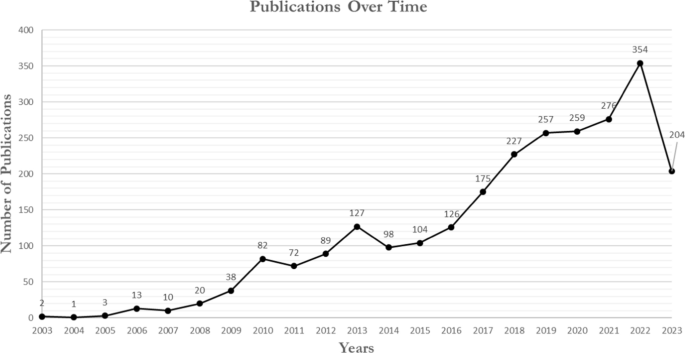
Growth of scientific publications on open innovation (Web of Science). Note : The decrease observed in 2023 can be attributed to the year not being complete at the time of analysis
Table 2 provides a detailed breakdown of the most frequently cited journals within the realm of open innovation research in business and management. “Technological Forecasting and Social Change” holds a prominent position with 133 publications and a cumulative citation count of 5,239, translating to a citation impact of 39.40. “R&D Management” and “Technology Analysis & Strategic Management” closely follow with 121 and 91 publications, amassing 10,084 and 1,748 citations, respectively. Notably, “Research Policy” has a high citation impact of 130.87, indicating its seminal contributions to this field.
3.1 Topic modeling results
The use of topic modeling enabled us to uncover latent semantic structures within the unstructured textual data we collected. Through this technique, we identified a model consisting of 10 distinct pathways, which effectively encapsulated a comprehensive range of OI-related subjects. The word-cloud analysis of the topic modeling outcomes, presented in Fig. 4 , visually portrays the word distribution within the eleven identified topics.
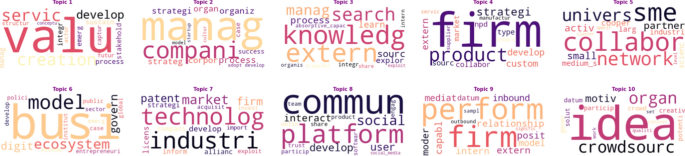
Word cloud of open innovation topics in business and management research
Table 3 provides an overview of the key terms associated with each topic, along with the corresponding count of documents and the cumulative citation count. These findings collectively underscore the diverse array of topics that span across various academic disciplines.
In this comprehensive examination of ten pivotal topics in the field of open innovation research, we present our result in each pathway implementing a dual analytical framework. Initially, we describe the principal attributes inherent in each pathway, subsequently progressing to an abductive exploration that unveils the associated failure mechanisms embedded within the open innovation landscape. This multifaceted analytical approach extends across the micro (individual), meso (organizational), and macro (system) levels of analysis, providing a holistic view of OI failures in these pathways.
3.1.1 Pathway 1: value creation and capture in OI setting
Main features :
Within the open innovation landscape, the emergence of collaborative ecosystems stands out as a pivotal theme. Central to this pathway is the intricate interplay between business models, ecosystems, and networks. Specifically, the concept of a business model as a tangible construct takes the spotlight. In open innovation contexts, business models play an indispensable role in delineating the generation and capture of value within collaborative networks. This pathway also introduces the collaborative business model concept, underscoring the significance of co-creating and capturing value that extends beyond the confines of a single firm. Furthermore, it emphasizes the imperative of network alignment and the need for well-structured, interconnected networks. These facilitate the effective creation and capture of value at a macro level. Notably, this pathway displays strong linkages with sustainability transition research. It sheds light on the role of value creation and capture mechanisms in driving sustainability-oriented open innovation.
Failures in value creation and capture in OI:
At the micro level, we found that issues related to learning, attitudes, and organizational culture can slow down value creation processes. At the individual level, resistance to change hinders creative processes related to co-creating values with other organizations. At the meso level, organizational capabilities play a pivotal role in determining the effectiveness of value capture mechanisms. Challenges such as information asymmetry, limited rationality, and opportunistic behavior can impede the realization of value from collaborative efforts. Furthermore, the uneven distribution of intellectual property (IP) ownership can lead to disputes that affect value capture mechanisms, often resulting in wasteful litigations. Our findings indicate that, on the macro level, we could not extract any factors. External factors, such as regulatory, economic, and cultural aspects, that impact how value is created and shared in the broader context, need to be further examined.
3.1.2 Pathway 2: managing the OI process
Main features:
The second pathway in our exploration phase leads to the management of open innovation processes. It includes a broad range of sub-themes, including empirical case studies on OI processes involving SMEs and LEs. An integral aspect is the emphasis on the management of knowledge flows, ensuring that valuable insights and expertise circulate efficiently in open innovation ecosystems. Furthermore, this pathway exhausts the concept of the “Liability of Smallness,” which is discussed in the context of SMEs and the unique challenges they face in the OI paradigm. Additionally, this pathway deals with the role of discovering market opportunities and the execution of collaborative research and development (R&D) efforts as two domains in managing OI processes. Additionally, assessing the strategic fit of and with partners plays a pivotal role in managing OI process.
Failure in managing the OI process:
At the micro level, individual networks may fail to contribute effectively. The “Not Invented Here” (NIH) syndrome can create barriers, leading to the dismissal of valuable external ideas. Employee competencies are critical, and misalignment with team members can result in failures. At the meso level, an organizational culture that discourages risk-taking and does not tolerate failure can stall the open innovation process. When open innovation projects lack connection and consistency with the company’s business model, underperformance and failure can occur. Risks related to asset complementarity, chain characteristics, and persistent NIH at the inter-organizational level, along with ineffective knowledge transfer, pose significant challenges. As with Pathway 1, the macro level presents no failure mechanisms to analyze in this context.
3.1.3 Pathway 3: managing knowledge inflows/outflows
This pathway leads to the dynamic processes of knowledge inflows and outflows within the context of open innovation. Central elements in this pathway include knowledge assimilation representing the internalization and integration of external knowledge within an organization, and aligning it with existing capabilities and objectives to create value. The other core sub-theme within this pathway is the role of absorptive capacity as the organization’s ability to effectively identify, acquire, and apply external knowledge, emphasizing its capacity to absorb, adapt, and leverage this knowledge for innovation and competitiveness. The pathway through many empirical examples underscores that external knowledge, while valuable, may not seamlessly align with an organization’s existing capabilities unless it is harmonized with their specific business needs and grafted onto their existing operations.
Failures in managing knowledge flows:
At the micro level, cognitive abilities and role identities can impede the effective assimilation of external knowledge within organizations. Failures can also arise from issues related to bisociative cognition and the quality of external knowledge. External knowledge that is incompatible with an individual’s existing capabilities can result in suboptimal outcomes. At the meso level, failures may stem from the inability to recognize and locate cross-border knowledge, both in terms of the search for relevant knowledge and its integration. Furthermore, the lack of organizational ambidexterity, which involves balancing the exploration and exploitation of knowledge, can hinder the effective assimilation of external knowledge. At the macro level, failure can result from an organization’s inability to reconfigure its knowledge in response to external market movements. Additionally, the knowledge climate, both locally and at a distance, can affect the efficacy of knowledge flows and their alignment with the organization’s strategic objectives.
3.1.4 Pathway 4: the fuzzy front-end of the innovation process
This pathway leads to the multifaceted process of developing new products and services. It deals with the “fuzzy front end,” the initial stage of innovation where ideas are generated and preliminary concepts take shape. The subsequent research and development (R&D) stage is a critical phase where ideas are refined and developed into tangible offerings. The research along this pathway deals with the concept of “outside in” and emphasizes the importance of considering external perspectives, such as customer feedback and market insights, in the innovation process. Moreover, it highlights the active engagement of consumers in new product development (NPD) and new service development (NSD), illustrating the growing trend of co-creation and collaboration in shaping new offerings.
Failures in the fuzzy front end of innovation:
At the micro level, limited attentional capacity can make the management of multiple issues less effective when these issues compete for the attention of top management teams during the NPD and NSD processes. This may result in crucial aspects being overlooked or underemphasized. At the meso level, failures can arise from the absence of robust legal strategies to protect intellectual property, as well as inadequate technological resources within the organization. Such failures can harm the development of innovative products and services. At the macro level, industry-level factors, such as the pace of technological change (industry-level clock), the lack of technological diversity or intensity in the market, and a scarcity of competition, along with environmental contingencies, can collectively influence the success or failure of NPD and NSD initiatives.
3.1.5 Pathway 5: the quadruple helix
Research on this pathway deals with the dynamics of collaboration between small and medium-sized enterprises (SMEs), university-industry collaboration and public partnerships. It examines the role played by entrepreneurial agents within the quadruple helix model. On this pathway the interactions among academia, industry, government, and civil society are examined as open innovation modes. Open innovation at the project level receives attention, providing a more granular perspective on collaborative network efforts and showing how individual and organizational levels interact.
Failures in the quadruple helix:
At the micro level, issues related to team openness, interpersonal skills, and leadership have been found to be critical factors for university–industry collaboration and innovation. At the project level, ambiguities surrounding project goals among partners can lead to misalignment and underperformance. Meso-level challenges encompass measurement issues and key performance indicator (KPI)-related challenges, including the need for interoperable KPIs and the potential influence of bureaucratic hurdles in different institutions. At the macro level, dilemmas related to proximity, market conditions, and governmental support can influence the success or failure of collaborations between SMEs, universities, industries, and government entities, affecting the larger innovation landscape.
3.1.6 Pathway 6: OI and resilience
This pathway leads to exploring the intricate relationship between open innovation and the concept of ecosystems. It emphasizes the interplay between resilience and the ecosystem’s health in the context of innovation. The research on this pathway deals with subjects related to exogenous shocks. An example is the impact of events like the COVID-19 pandemic on open innovation practices. Grand challenges are a focal point of this pathway, leading to discourse about how open innovation can address and contribute to overcoming societal issues. Additionally, the transformation of business models in the context of societal challenges is a key feature. Analyzing such transformations shows how open innovation can catalyze the evolution of organizational strategies and structures.
Failures in OI resilience:
At the meso level, business model failure is risky, particularly when organizations do not change their traditional business models to align with new market dynamics and innovation logic. Inconsistencies in vision regarding innovation can create challenges in navigating open innovation successfully. Resistance to change, particularly in traditional non-digital business models, and a lack of organizational agility, can stall innovation efforts. At the macro level, market turbulence is identified as a potential failure factor. The unpredictable nature of markets, particularly in the face of exogenous shocks, can pose significant challenges to open innovation initiatives. This can potentially lead to ecosystem failure. On this pathway, micro-level failure mechanisms are as yet unexplored.
3.1.7 Pathway 7: appropriation strategies
This pathway leads to intellectual property management in open innovation. It mainly deals with phenomena related to external technology acquisition. Licensing is fundamental to this pathway, as it enables organizations to grant or obtain rights to use, develop, or commercialize specific technologies. The value of licensed patents is a key discussion point, showing the value of patents as assets in open innovation. This pathway notably emphasizes the significance of technology-related strategies in open innovation practices.
Failures in appropriation strategies:
At the micro level, failure can come from a lack of meaningful interaction between individual licensees and licensors. This can impede the effective exchange of knowledge and technology. Reverse learning, where knowledge flows in an undesired direction, can also pose challenges. At the meso level, failures may stem from ineffective intellectual property (IP) strategies, as well as a lack of market knowledge, which can affect the organization’s ability to protect and leverage its technology effectively. At the macro level, the absence of market-level technology information, which involves actively seeking external technologies on a broader scale, can be a failure factor, potentially resulting in missed opportunities.
Additionally, the paradox of depth of openness and closeness in appropriation strategies is partially discussed on this pathway. The imbalance found to lead to issues such as anti-commons (resource underuse), trolling (unfair patent assertion), and the multiplication of wasteful litigations. These issues can all weaken the effective use of intellectual property in open innovation initiatives.
3.1.8 Pathway 8: platforms and communities
This pathway is characterized by a rich body of literature focused on consumer engagement as an OI mechanism. It encompasses the microfoundational aspects of engagement, including cognition and behavior, to unravel how consumers participate in various innovation communities. Usercommunities, within which tangible incentives, formal authority, and established institutions are notably absent, are central to this discourse. The pathway encompasses innovations ranging from user-led changes to open platforms. It showcases the diverse ways in which consumers contribute to innovation.
Failures in platform and communities:
At the micro level, the motivation for participation is pivotal. Failure can arise when consumers lack incentives or enthusiasm to engage actively. The diversity and effectiveness of consumers’ creativity portfolios, as well as the leadership within informal institutions, can significantly affect the outcomes of consumer engagement efforts. At the meso level, challenges can stem from a lack of ways to measure user contributions to innovative products, and thus failing to reward them effectively. At the macro level, failures may result from difficulties in adopting engagement strategies in line with socio-cultural factors and gaining collective approval. Governance failures, which pertain to the structures and processes governing consumer engagement, can weaken the effectiveness of user communities in shaping innovations. The notion of “empty bar symptoms” represents unique and noteworthy failure factors in this context. It may signify challenges related to participation, productivity, or the fulfillment of expectations within consumer engagement scenarios.
3.1.9 Pathway 9: OI activities, firm performance, and output
This pathway primarily leads to understanding how access to external technologies can affect both modular and radical forms of innovation, and ultimately a firm’s innovation performance. The Quadruple Helix model also appears here, showing the roles of customers, suppliers, and universities in shaping innovation outcomes. Notably, positive innovation output is observed when these stakeholders are involved, but cross-sector collaborative innovation may negatively affect innovation output. Additionally, the pathway explores the effects of inbound and outbound activities on innovation performance. Research on this pathway shows that inbound activities improve radical innovation performance, but impede incremental innovation performance, while focusing on outbound activities produces the opposite effects.
Failures in OI activities and performance:
Failure mechanisms in this pathway are not detailed at the micro level. At the meso level, challenges can arise from issues related to organizational capability and routines, the firm’s ability to consistently access and evaluate external knowledge resources, the lack of organizational ambidexterity, the excessive practice of open innovation, and the firm’s technological capabilities impeding organizational ability to carry out OI practices.
At the macro level, failure can result from slow changes in the technological environment, particularly in mature industries where innovation may be slower to evolve or adapt to external factors.
3.1.10 Pathway 10: crowdsourcing
This pathway leads to understanding the process of idea generation and how innovation contests facilitate it. At the microfoundational level, the focus is on motivation theory, examining what drives individuals’ participation in these contests. Social exchange theory is a central framework used in this pathway for understanding sociological and psychological factors related to the dynamic interaction between two parties. Notably, research is limited on how organizations and systems affect these processes. Most studies focus on individual and small-group dynamics.
Failures in crowdsourcing:
At the micro level, failures can occur when individuals participating in contests underinvest their efforts, leading to suboptimal solutions. Misalignment between the motivations of solvers and seekers (those seeking solutions) can hinder the success of idea generation. The lack of perspective on the ultimate impact of their solutions may also result in failures, because solvers may not fully comprehend the potential value of their contributions. Additionally, a lack of monetary reward and trust in contest platforms can act as deterrents. At the meso level, failures may stem from organizations ineffectively implementing the suggestions generated in contests. The organization’s ability to recombine knowledge, its absorptive capacity, and its ability to proactively provide and receive suggestions can affect the success of idea generation and innovation contests. Specific failure mechanisms are not detailed for the macro level in this context.
Table 4 summarizes the results of the open innovation pathways, main features, and failures.
3.2 Results of Mann–Kendall test
As previously noted, we employed the weight of each topic per year to analyze the annual shifts in open innovation research. Figure 5 visually captures the evolution of these topics from 2003 to 2023. At a glance, it appears that the publication proportion for all topics experienced fluctuations, especially between 2003 and 2005. For a more rigorous understanding of these observed trends, we applied the Mann–Kendall test. The results of this test, delineated in Table 5 , give a statistically substantiated account of the topic trajectories.
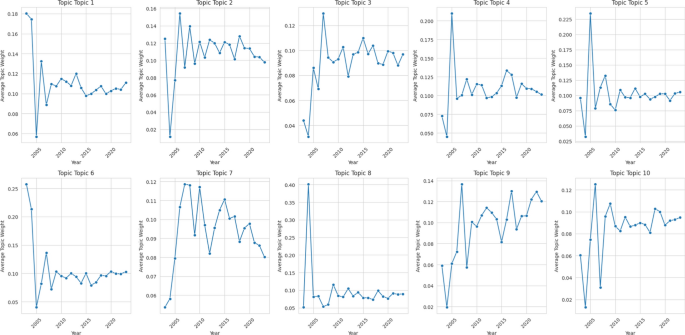
Temporal trends in open innovation research topics (1993–2023)
The Mann–Kendall Test results presented in Table 5 provide valuable insights into the annual trends of open innovation research topics over a specific time period. Each research topic has been examined for its trajectory, with particular attention paid to the direction and significance of trends, denoted by p -values and z-scores. Moreover, the tau statistic has been employed to gauge the strength and direction of these trends, offering a comprehensive perspective on the evolution of each research theme.
In our analysis, we observed that some topics have experienced a noteworthy decline in research interest. Notably, “Value Creation and Capture Process” (Topic 1) and “OI Strategic Management” (Topic 2) exhibit decreasing trends with p -values of 0.009 and < 0.000 respectively, accompanied by negative z-scores of − 2.605 and − 3.984. These findings suggest that scholarly attention to these topics has waned over the studied period.
On the other hand, certain research areas have seen a surge in interest. “Quadruple Helix” (Topic 5) and “OI and Resilience” (Topic 6) display increasing trends, with highly significant p -values of 0.034 and 0.001, and positive z-scores of 2.116 and 3.177 respectively. These results signify a growing focus on collaborative endeavors, business models, and ecosystem dynamics within the academic community. Meanwhile, “Managing Knowledge Inflows and Outflows” (Topic 3), “The Fuzzy Front-End of Innovation” (Topic 4), “Platforms and Communities” (Topic 8), and “Crowdsourcing” (Topic 10) present no significant trends, as indicated by their p -values and z-scores. These research themes have maintained a relatively stable level of attention in recent years. Finally, “OI Outputs” (Topic 9) has emerged as an area of increasing significance, shown by a significant p -value and a positive z-score of 5.324. This points to a notable uptick in scholarly focus on factors affecting firm performance within the open innovation context.
The inter-topic distance map provides valuable insights into the relationships and proximities between the identified topics in our open innovation research (Fig. 6 ). The two-dimensional visualization provides an insightful representation of the relationships between the topics in our corpus. The x and y axes represent the Inter-topic Distance Map, where each bubble represents a topic, and the distance between the bubbles indicates how distinct or similar the topics are from each other.
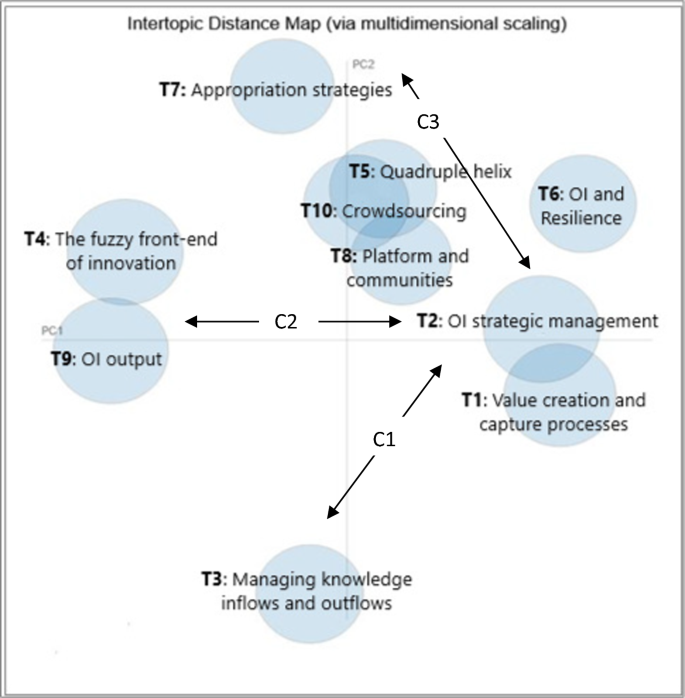
Inter-topic distance map
The map shows that Topic 10 (Crowdsourcing), Topic 5 (Quadruple Helix), and Topic 8 (Platforms and Communities) are very similar. This is likely because crowdsourcing is often enabled by platforms and communities. Topic 1 (Value Creation and Capture Process) and Topic 2 (OI Strategic Management) are also close together on the map, because the two topics are closely related. Open innovation is a process that companies use to create new value and develop new services by leveraging external resources and ideas.
“The Fuzzy Front-End of Innovation” (Topic 4) and “OI Outputs” (Topic 9) exhibit intertwined themes. The cross-linkage of terms such as firm , product , and strategy reflects the potential interplay between product development and the overarching performance of the firm. It signifies that the effectiveness of new products or service developments often translates to the overall performance metrics of firms.
However, what stands out is “Managing Knowledge Inflows and Outflows” (Topic 3). Accompanied by terms like knowledge , absorptive capacity , and transfer , this topic encompasses the mechanics of managing, assimilating, and exploiting knowledge from both internal and external sources. Topic 3 indicates that knowledge management is a broad theme that may intersect with other OI topics without being tied to particular settings. Additionally, the intertopic map reveals three distinct controversies in the open innovation scholarly research community, which we will discuss and use as guidelines for future research directions.
4 Discussion in the current landscape of OI research: pathways for future research
Intertopic distance maps serve as strategic guides in topic modeling to uncover potential research directions in the OI research field. The gaps or distances between topics on such maps can show valuable insights. They may indicate under-researched areas for future research, or point out theoretical divides that call for new or integrative theoretical frameworks. They also show visually how mature or saturated certain research areas are based on topic clustering. Additionally, the spatial separation between topics can show the need for interdisciplinary research to bridge related domains. By focusing on these distances and the relational dynamics they represent, we identify and prioritize three areas where further investigation could yield significant contributions to OI fields and topics. Then, we discuss our analytical results regarding failure in the field of open innovation. Our discussion section also informs avenues for future research.
4.1 The OI landscape
4.1.1 controversy 1: the distance between managing knowledge flows and the oi main topic cluster.
One could assume that managing knowledge flows constitutes the core of all open innovation (OI) activities and processes. The large gap between this topic and other principal OI topics has scholarly significance, requiring further understanding. We point out several reasons for this observation, looking in depth at the following topics: The literature comprising Topic 3 employs specialized terminologies tied to the knowledge management and knowledge economy sectors, mainly through the lens of the knowledge-based view (KBV) of the firm (e.g. Brunswicker and Vanhaverbeke 2015 ; Santoro et al. 2018 ; Chiang and Hung 2010 ). Conversely, the core cluster of the open innovation (OI) field is characterized by a prevalent adoption of the resource-based view (RBV) as the theoretical underpinning (e.g. Chesbrough and Crowther 2006 ; Mortara andMinshall 2011 ; Du et al. 2014 ). Thereby, it highlights a divergence in theoretical perspectives within OI research. A potential angle for future research in open innovation is to promote research using theoretical pluralism and cross-disciplinary projects. Few researchers have proposed ways to implement theoretical pluralism and combine lenses in management research. Okhuysen and Bonardi ( 2011 ) explain that the challenge in developing “multi-lens” theories lies in the conceptual closeness of the theories being integrated and the extent to which their foundational assumptions align. Their solution is a so-called “paradigm sliding.” This entails combining theoretical perspectives that are conceptually close and harmonious in terms of their fundamental premises. In general, the distance between the topics could be shortened by promoting multi-lens contributions.
4.1.2 Controversy 2: The distance between OI output and the OI main topic cluster
The open innovation concept and its research rest on the notion that collaboration positively influences innovation (Audretsch and Belitski 2024 ). Despite theoretical recognition of the crucial role external knowledge plays in enhancing a firm’s innovation and productivity through knowledge transfer or spillovers (Dahlander and Gann 2010 ; Bogers et al. 2018 ), empirical studies on OI outcomes are scarce. The complexity of such empirical research (Audretsch and Belitski 2024 ; Mention 2011 ) may contribute to the data sparsity on OI output, which, in turn, accounts for the observed research gap. Nonetheless, our findings suggest that this topic is burgeoning, so it presents an exciting avenue for future inquiry. Future research on OI could benefit from the development of new, robust structural indicators that offer a clearer measurement of OI outcomes.
4.1.3 Controversy 3: the distance between appropriation strategy and the OI main topic cluster
In the literature of strategic management and initial studies of open innovation (OI), the discussion often focused on appropriation strategies as a key means to implement OI activities, especially how firms safeguard and leverage their intellectual property, licensing, and competitive advantage. As the OI field has evolved, research has expanded to encompass the broader integration of external and internal ideas to drive innovation. This shift has led to the emergence of distinct research communities and diverse methodological approaches . The appropriation strategies topic, which typically involves technological innovation, technology procurement, and patent analysis (e.g. Guo et al. 2016 ; Noh and Li 2020 ; Klechtermans et al. 2022 ), illustrates one of the challenges in drawing the OI field’s boundaries. This subject area is often explored through econometric methods and the analysis of patent data and large technological innovation datasets. Conversely, contemporary OI research primarily examines the “how”—the practices of the OI process—with limited attention to technological valorization and IP management. This diverges from Chesbrough’s initial focus when introducing the OI concept (Chesbrough 2003a , b ). Researchers with an interest in the topic of open innovation could make a significant contribution by bridging these two research communities, renewing the focus on appropriation strategies.
4.2 OI pathways through the lens of failure
Our abductive analysis shows researchers have examined OI failure at the micro-level. Specifically, learning and culture were found to contribute to risk in the majority of topics. This aligns with Cricelli et al. ( 2023 ), who discussed elements of OI adoption resistance, “not invented here syndrome” (NIH), and similar micro-foundational issues. Our contribution to the micro-foundational elements includes the cognitive limitations of individuals and the limited attentional capacity of top management teams in managing and organizing for open innovation.
When discussing cognitive limitations, we refer to the inability of individuals to assimilate knowledge within organizations. This perspective goes beyond prior studies, which assume the success of collaboration relies on the interpretation and perception of members about themselves and others in the collaboration (e.g., Skippari et al. 2017 ). Another micro-level factor that could better inform the future of OI research is the attentional capacity of management teams toward OI activities within organizations. In a study by Sisodiya et al. ( 2013 ), it was found that managers gave different levels of attention to inbound versus outbound OI activities and their potential effects. This imbalance negatively affected OI outcomes. When examining micro-level risk factors for organizing OI activities, organizational behavior theories such as selective attention theory could enrich research on OI failure.
At the meso level, significant risks associated with OI failure include inadequate business model adjustments (e.g., Albats et al. 2023 ), IP-related failures (e.g., Grandstrand and Holgersson 2014 ), and challenges related to measuring OI performance at the organizational level (e.g., Brunswicker and Chesbrough 2018 ). Research on OI failure at the organizational level is the most developed, which is not surprising given that OI is mainly developed and practiced at this level. However, some factors remain relatively new to the field and call for further investigation.
One such factor is the excessive practice of OI, which refers to situations where an organization overinvests in building external relationships, ideas, methods, and innovations without considering the specific requirements of each investment (e.g., Greco et al. 2016 ). Research on OI failure at the meso level could benefit from exploring the question of what constitutes efficient and balanced OI activities.
At the macro level, our findings align with those of Bertello et al. ( 2024 ), indicating that this is the least developed research domain in both OI research and in addressing failure. The impact of external influences on OI has been discussed previously, particularly in the context of post-COVID-19 scenarios, where OI was used as a strategy for business continuity during crises (e.g., Liu et al. 2022 ; Bertello et al. 2022 ). These studies demonstrate that overcoming crises often necessitates open innovation practices, especially for SMEs, to mitigate the negative impacts of a large-scale crisis such as the pandemic (Markovic et al. 2021 ).
However, research has overlooked the influence of economic crises on ongoing OI activities of firms. Two research foci that we found particularly interesting and underexplored are the effects of stagnant economic growth and market turbulence on open innovation activities. It remains to be seen how a stagnant market or industry impacts the OI activities of companies and whether marginal economic growth in a sector challenges collaborative innovation. Similarly, research on OI has not sufficiently addressed the challenges posed by market turbulence, such as high variations in customer preferences and product demand (Jaworski and Kohli 1993 ), on existing OI processes.
5 Conclusions
In the pursuit of open innovation, this research presents a multifaceted analysis of ten pivotal pathways researched in the past two decades in the OI domain, proposing insights into the micro, meso, and macro levels of failure mechanisms that can affect open innovation initiatives (see Table 4 ) in addition to presenting the current state and potential future directions of the field.
Based on our results and arguments, this study proposes a way forward in the OI research domain by uncovering its pathways in the past two decades and analyzing the multifaceted mechanism of failure in each pathway. In this research we have identified key trends, patterns, and shifts that have shaped the current landscape of open innovation. This kaleidoscopic analysis allows us to pinpoint specific pathways and anticipate risk factors. Furthermore, our study unpacks the complexities of failure mechanisms and the potential for future research along different OI pathways, shedding light on their micro, meso, and macro levels.
We emphasize the lack of research on macro-level mechanisms. This analytical insight suggests that while organizations concentrate on internal and inter-organizational factors, broader contextual aspects of open innovation, such as regulatory, economic, and market influences, remain relatively unexplored. Collaboration in various forms is the backbone of OI, whether it involves other firms, universities, government entities, or consumers. Challenges related to individual/employee networks and team dynamics, intertwined with the ambiguity of collaborative project goals, have impeded open innovation efforts. Organizations should actively promote open communication, trust-building, and role clarity in collaborative networks.
Finally, the analysis of the pathway on “OI and Resilience” shows the importance of the business model as an adaptable artifact in the face of external shocks and societal challenges. Business models could act as artifacts connecting organizations to their environment and to other actors, thus embracing a panoramic view of the organization’s OI strategies.
For scholars, the pathways we have found offer a valuable roadmap for future research, guiding them to analyze the nuances of open innovation failure. Our findings emphasize the importance of considering not only individual and organizational factors, but also the broader system and the context of OI to understand how these initiatives can thrive or fail. Our analysis also leads to recommendations for organizations seeking to implement open innovation. First, by identifying and understanding the specific failure mechanisms at the micro, meso, and macro levels, organizations can develop interventions targeted at these risks. For instance, depending on the OI pathway, a firm can address cognitive limitations and attentional capacity at the micro level to improve individual and team performance in OI initiatives. At the meso level, organizations can refine their business models and IP management practices to better align with OI objectives and ensure more robust and resilient innovation processes. Furthermore, by considering the macro-level influences such as economic conditions and market turbulence, firms can adapt their OI strategies to be more responsive to external shocks and uncertainties. Ultimately, insights from our research can guide organizations in fostering more effective collaborations, optimizing resource allocation, and achieving innovation outcomes in a dynamic and complex business environment.
Finally, while we intend to show the OI pathways and the mechanisms of failure within them, our research is not without its limitations. One such limitation is the inherent dependency of our findings on the accuracy and efficiency of topic modeling algorithms. Topic modeling is a powerful tool for identifying themes and patterns within large datasets. However, the quality of its results depends on the selection of parameters, preprocessing steps, and the algorithm used (Hannigan et al. 2019 ). Furthermore, topic modeling does not account for the contextual nuances and subtleties of the text. This could result in overlooking important details and insights. The second limitation is due to the abductive analysis of the texts. While valuable for generating insights from the data, it is inherently subjective and influenced by the researchers’ perspectives and experiences. This subjectivity can introduce biases and affect the interpretation of results. The iterative nature of abductive analysis relies on the researchers’ ability to identify and make sense of patterns. This aptitude can vary significantly between individuals (Tavory and Timmermans 2014 ). Additionally, the interpretive layer added to the analytics can lead to different conclusions depending on the researchers’ backgrounds and prior knowledge. For future research, scholars in the OI field could advance their algorithms and combine multiple methods to validate the robustness of their findings. In addition, future researchers could use mixed-method approaches to reduce the risks of oversimplification in interpretative analysis.
Data availability
The data for this study has been derived from Web of Science database.
Albats E, Podmetina D, Vanhaverbeke W (2023) Open innovation in SMEs: a process view towards business model innovation. J Small Bus Manag 61(6):2519–2560
Article Google Scholar
Amado A, Cortez P, Rita P, Moro S (2018) Research trends on big data in marketing: a text mining and topic modeling based literature analysis. Eur Res Manag Bus Econ 24(1):1–7
Audretsch DB, Belitski M (2024) Knowledge collaboration, firm productivity and innovation: a critical assessment. J Bus Res 172:114412
Bacon E, Williams MD, Davies G (2020) Coopetition in innovation ecosystems: a comparative analysis of knowledge transfer configurations. J Bus Res 115:307–316
Baltazar JR, Fernandes CI, Ramadani V, Hughes M (2023) Family business succession and innovation: a systematic literature review. RMS 17(8):2897–2920
Bertello A, Bogers ML, De Bernardi P (2022) Open innovation in the face of the COVID-19 grand challenge: insights from the Pan-European hackathon ‘EUvsVirus.’ R&d Manag 52(2):178–192
Bertello A, De Bernardi P, Ricciardi F (2024) Open innovation: status quo and quo vadis-an analysis of a research field. RMS 18(2):633–683
Blei DM (2012) Probabilistic topic models. Commun ACM 55(4):77–84
Blei DM, Ng AY, Jordan MI (2003) Latent dirichlet allocation. J Mach Learn Res 3(Jan):993–1022
Google Scholar
Bogers M, Chesbrough H, Moedas C (2018) Open innovation: research, practices, and policies. Calif Manag Rev 60(2):5–16
Bogers M, Zobel AK, Afuah A, Almirall E, Brunswicker S, Dahlander L, Frederiksen L, Gawer A, Gruber M, Haefliger S, Hagedoorn J, Ter Wal AL (2017) The open innovation research landscape: established perspectives and emerging themes across different levels of analysis. Ind Innov 24(1):8–40
Brem A, Nylund PA, Roshani S (2023) Unpacking the complexities of crisis innovation: a comprehensive review of ecosystem-level responses to exogenous shocks. Rev Manag Sci 1–24
Brunswicker S, Chesbrough H (2018) The adoption of open innovation in large firms: practices, measures, and risks a survey of large firms examines how firms approach open innovation strategically and manage knowledge flows at the project level. Res Technol Manag 61(1):35–45
Brunswicker S, Vanhaverbeke W (2015) Open innovation in small and medium-sized enterprises (SMEs): external knowledge sourcing strategies and internal organizational facilitators. J Small Bus Manag 53(4):1241–1263
Caputo M, Lamberti E, Cammarano A, Michelino F (2016) Exploring the impact of open innovation on firm performances. Manag Decis 54(7):1788–1812
Carrasco-Carvajal O, Castillo-Vergara M, García-Pérez-de-Lema D (2023) Measuring open innovation in SMEs: an overview of current research. RMS 17(2):397–442
Chaudhary S, Kaur P, Talwar S, Islam N, Dhir A (2022) Way off the mark? Open innovation failures: decoding what really matters to chart the future course of action. J Bus Res 142:1010–1025
Chesbrough HW (2003a) Open innovation: the new imperative for creating and profiting from technology. Harvard Business Press
Chesbrough HW (2003b) The era of open innovation. MIT Sloan Manag Rev 44(3):35–35
Chesbrough H (2004) Managing open innovation. Res-Technol Manag 47(1):23–26
Chesbrough H, Crowther AK (2006) Beyond high tech: early adopters of open innovation in other industries. R&d Manag 36(3):229–236
Chiang YH, Hung KP (2010) Exploring open search strategies and perceived innovation performance from the perspective of inter-organizational knowledge flows. R&d Manag 40(3):292–299
Chopra R, Agrawal A, Sharma GD, Kallmuenzer A, Vasa L (2024) Uncovering the organizational, environmental, and socio-economic sustainability of digitization: evidence from existing research. RMS 18(2):685–709
Christensen JF, Olesen MH, Kjær JS (2005) The industrial dynamics of Open innovation—evidence from the transformation of consumer electronics. Res Policy 34(10):1533–1549
Coleman JS (1994) Foundations of social theory. Harvard University Press
Corbo L, Kraus S, Vlačić B, Dabić M, Caputo A, Pellegrini MM (2023) Coopetition and innovation: a review and research agenda. Technovation 122:102624
Cowen AP, Rink F, Cuypers IR, Grégoire DA, Weller I (2022) Applying Coleman’s boat in management research: opportunities and challenges in bridging macro and micro theory. Acad Manag J 65(1):1–10
Cricelli L, Mauriello R, Strazzullo S (2023) Preventing open innovation failures: a managerial framework. Technovation 127:102833
Cricelli L, Grimaldi M, Vermicelli S (2022) Crowdsourcing and open innovation: a systematic literature review, an integrated framework and a research agenda. RMS 16(5):1269–1310
Dabić M, Daim T, Bogers ML, Mention AL (2023) The limits of open innovation: failures, risks, and costs in open innovation practice and theory. Technovation 126:102786
Dahlander L, Gann DM (2010) How open is innovation? Res Policy 39(6):699–709
da Silva ADS, da Silva WV, da Silva LSCV, da Cruz NJT, Su Z, da Veiga CP (2024) Interdependence between supply chains and sustainable development: global insights from a systematic review. Rev Manag Sci 1–32
Dhiman R, Srivastava V, Srivastava A, Rajni, Uppal A (2023) How to plan and write for systematic literature review papers in management domain. Adv Methodol Conducting Lit Rev Manag Domain 37–55
Du J, Leten B, Vanhaverbeke W (2014) Managing open innovation projects with science-based and market-based partners. Res Policy 43(5):828–840
Falagas ME, Pitsouni EI, Malietzis GA, Pappas G (2008) Comparison of PubMed, Scopus, Web of Science, and Google Scholar: strengths and weaknesses. FASEB J off Publ Fed Am Soc Exp Biol 22(2):338–342
Gao H, Ding XH, Wu S (2020) Exploring the domain of open innovation: bibliometric and content analyses. J Clean Prod 275:122580
Gast J, Kallmünzer A, Kraus S, Gundolf K, Arnold J (2019) Coopetition of small-and medium-sized family enterprises: insights from an IT business network. Int J Entrep Small Bus 38(1–2):78–101
Granstrand O, Holgersson M (2014) Multinational technology and intellectual property management—is there global convergence and/or specialisation? Int J Technol Manag 64(2–4):117–147
Greco M, Grimaldi M, Cricelli L (2016) An analysis of the open innovation effect on firm performance. Eur Manag J 34(5):501–516
Greco M, Strazzullo S, Cricelli L, Grimaldi M, Mignacca B (2022) The fine line between success and failure: an analysis of open innovation projects. Eur J Innov Manag 25(6):687–715
Guo B, Li Q, Chen X (2016) Diversity of technology acquisition in technological catch-up: an industry-level analysis of Chinese manufacturing. Technol Anal Strateg Manag 28(7):755–767
Hamed KH, Rao AR (1998) A modified Mann–Kendall trend test for autocorrelated data. Jhydrol 204(1–4):182–196
Hannigan TR, Haans RF, Vakili K, Tchalian H, Glaser VL, Wang MS, Kaplan S, Jennings PD (2019) Topic modeling in management research: rendering new theory from textual data. Acad Manag Ann 13(2):586–632
Holgersson M, Baldwin CY, Chesbrough H, Bogers ML (2022) The forces of ecosystem evolution. Calif Manag Rev 64(3):5–23
Honnibal M, Montani I, Van Landeghem S, Boyd A (2020) spaCy: industrial-strength natural language processing in pythonPython. https://spacy.io/
Hussain M, Mahmud I (2019) pyMannKendall: a python package for non parametric Mann Kendall family of trend tests. J open Source Softw 4(39):1556
Jaworski BJ, Kohli AK (1993) Market orientation: antecedents and consequences. J Mark 57(3):53–70
Jeong Y, Park I, Yoon B (2019) Identifying emerging research and business development (R&BD) areas based on topic modeling and visualization with intellectual property right data. Technol Forecast Soc Chang 146:655–672
Jeyaraj A, Zadeh AH (2020) Evolution of information systems research: insights from topic modeling. Inf Manag 57(4):103207
Kallmuenzer A, Zach FJ, Wachter T, Kraus S, Salner P (2021) Antecedents of coopetition in small and medium-sized hospitality firms. Int J Hosp Manag 99:103076
Kelchtermans S, Leten B, Rabijns M, Riccaboni M (2022) Do licensors learn from out-licensing? Empirical evidence from the pharmaceutical industry. Technovation 112:102405
Kovacs A, Van Looy B, Cassiman B (2015) Exploring the scope of open innovation: a bibliometric review of a decade of research. Scientometrics 104:951–983
Kraus S, Bouncken RB, Yela Aránega A (2024) The burgeoning role of literature review articles in management research: an introduction and outlook. RMS 18(2):299–314
Kraus S, Breier M, Dasí-Rodríguez S (2020) The art of crafting a systematic literature review in entrepreneurship research. Int Entrep Manag J 16:1023–1042
Kraus S, Breier M, Lim WM, Dabić M, Kumar S, Kanbach D, Mukherjee D, Corvello V, Piñeiro-Chousa J, Liguori E, Palacios-Marqués D, Schiavone F, Ferraris A, Fernandes C, Ferreira JJ (2022) Literature reviews as independent studies: guidelines for academic practice. RMS 16(8):2577–2595
Lee H, Kang P (2018) Identifying core topics in technology and innovation management studies: a topic model approach. J Technol Transf 43:1291–1317
Liu Z, Shi Y, Yang B (2022) Open innovation in times of crisis: an overview of the healthcare sector in response to the COVID-19 Pandemic. J Open Innov Technol Market Complex 8(1):21
Lu Q, Chesbrough H (2022) Measuring open innovation practices through topic modelling: revisiting their impact on firm financial performance. Technovation 114:102434
Madanaguli A, Dhir A, Talwar S, Clauss T, Kraus S, Kaur P (2023) Diving into the uncertainties of open innovation: a systematic review of risks to uncover pertinent typologies and unexplored horizons. Technovation 119:102582
Madzík P, Falát L, Copuš L, Valeri M (2023) Digital transformation in tourism: bibliometric literature review based on machine learning approach. Eur J Innov Manag 26(7):177–205
Majchrzak A, Bogers ML, Chesbrough H, Holgersson M (2023) Creating and capturing value from open innovation: humans, firms, platforms, and ecosystems. Calif Manag Rev 65(2):5–21
Markovic S, Koporcic N, Arslanagic-Kalajdzic M, Kadic-Maglajlic S, Bagherzadeh M, Islam N (2021) Business-to-business open innovation: COVID-19 lessons for small and medium-sized enterprises from emerging markets. Technol Forecast Soc Change 170:120883
McCallum AK (2002) Mallet: a machine learning for language toolkit. http://mallet.cs.umass.edu
McGahan AM, Bogers ML, Chesbrough H, Holgersson M (2021) Tackling societal challenges with open innovation. Calif Manag Rev 63(2):49–61
Mention AL (2011) Co-operation and co-opetition as open innovation practices in the service sector: which Which influence on innovation novelty? Technovation 31(1):44–53
Mortara L, Minshall T (2011) How do large multinational companies implement open innovation? Technovation 31(10–11):586–597
Mustak M, Salminen J, Plé L, Wirtz J (2021) Artificial intelligence in marketing: topic modeling, scientometric analysis, and research agenda. J Bus Res 124:389–404
Neeti N, Eastman JR (2011) A contextual mann-kendall approach for the assessment of trend significance in image time series. Trans GIS 15(5):599–611
Noh H, Lee S (2020) What constitutes a promising technology in the era of open innovation? An investigation of patent potential from multiple perspectives. Technol Forecast Soc Chang 157:120046
Okhuysen G, Bonardi JP (2011) The challenges of building theory by combining lenses. Acad Manag Rev 36(1):6–11
Pütz L, Werner A (2024) Absorptive capacity in family firms: a systematic literature review. RMS 18(2):577–632
Radziwon A, Bogers ML, Chesbrough H, Minssen T (2022) Ecosystem effectuation: creating new value through open innovation during a pandemic. R&D Manag 52(2):376–390
Rammal HG (2023) Systematic literature reviews: steps and practical tips. In: Rana S, Singh J, Kathuria S (eds) Advancing methodologies of conducting literature review in management domain, vol 2. Emerald Publishing Limited, pp 27–35
Chapter Google Scholar
Rana S, Singh J, Kathuria S (eds) (2023) Advancing methodologies of conducting literature review in management domain. Emerald Publishing Limited
Randhawa K, Wilden R, Hohberger J (2016) A bibliometric review of open innovation: setting a research agenda. J Prod Innov Manag 33(6):750–772
Rehurek R, Sojka P (2010) Software framework for topic modelling with large corpora. In: Proceedings of the LREC 2010 workshop on new challenges for NLP frameworks
Rexhepi et al. (2019) Open innovation and entrepreneurship: an overview. Open Innovation and Entrepreneurship: impetus of growth and competitive advantages
Röder M, Both A, Hinneburg A (2015) Exploring the space of topic coherence measures. In: Proceedings of the eighth ACM international conference on web search and data mining, pp 399–408
Santoro G, Vrontis D, Thrassou A, Dezi L (2018) The Internet of Things: building a knowledge management system for open innovation and knowledge management capacity. Technol Forecast Soc Change 136:347–354
Sievert C, Shirley K (2014) LDAvis: a method for visualizing and interpreting topics. In: Proceedings of the workshop on interactive language learning, visualization, and interfaces, pp 63–70
Singh VK, Singh P, Karmakar M, Leta J, Mayr P (2021) The journal coverage of web of science, scopus and dimensions: a comparative analysis. Scientometrics 126:5113–5142
Sisodiya SR, Johnson JL, Grégoire Y (2013) Inbound open innovation for enhanced performance: enablers and opportunities. Ind Mark Manag 42(5):836–849
Skippari M, Laukkanen M, Salo J (2017) Cognitive barriers to collaborative innovation generation in supply chain relationships. Ind Mark Manag 62:108–117
Sauer PC, Seuring S (2023) How to conduct systematic literature reviews in management research: a guide in 6 steps and 14 decisions. Rev Manag Sci 17(5):1899–1933
Sukhija N, Tatineni M, Brown N, Van Moer M, Rodriguez P, Callicott S (2016) Topic modeling and visualization for big data in social sciences. In: 2016 Intl IEEE conferences on ubiquitous intelligence & computing, advanced and trusted computing, scalable computing and communications, cloud and big data computing, internet of people, and smart world congress (UIC/ATC/ScalCom/CBDCom/IoP/SmartWorld). IEEE, pp 1198–1205
Tavory I, Timmermans S (2014) Abductive analysis: theorizing qualitative research. University of Chicago Press
Book Google Scholar
Thakral P, Srivastava PR, Dash SS, Jasimuddin SM, Zhang Z (2023) Trends in the thematic landscape of HR analytics research: a structural topic modeling approach. Manag Decis 61:3665–3690
Walton D (2014) Abductive reasoning. University of Alabama Press
Wang C, Blei DM (2011) Collaborative topic modeling for recommending scientific articles. In: Proceedings of the 17th ACM SIGKDD international conference on knowledge discovery and data mining, pp 448–456
West J, Bogers M (2017) Open innovation: current status and research opportunities. Innovation 19(1):43–50
Xue J, Liu J, Geng Z, Yuan H, Chao L (2023) Why and when do paradoxical management capabilities matter to paradoxical pressure? An empirical investigation of the role of coopetition. Technovation 120:102682
Yan E (2014) Topic-based PageRank: toward a topic-level scientific evaluation. Scientomet 100(2):407–437
Download references
The authors did not receive support from any organization for the submitted work.
Author information
Authors and affiliations.
Department of Industrial Engineering and Innovation Sciences (IE&IS), Eindhoven University of Technology (TU/e), Eindhoven, The Netherlands
Maral Mahdad
Department of Management, Science and Technology, Amirkabir University of Technology, Tehran, Iran
Saeed Roshani
You can also search for this author in PubMed Google Scholar
Corresponding author
Correspondence to Maral Mahdad .
Ethics declarations
Conflict of interest.
The authors there is no conflict of interest.
Additional information
Publisher's note.
Springer Nature remains neutral with regard to jurisdictional claims in published maps and institutional affiliations.
Rights and permissions
Open Access This article is licensed under a Creative Commons Attribution 4.0 International License, which permits use, sharing, adaptation, distribution and reproduction in any medium or format, as long as you give appropriate credit to the original author(s) and the source, provide a link to the Creative Commons licence, and indicate if changes were made. The images or other third party material in this article are included in the article's Creative Commons licence, unless indicated otherwise in a credit line to the material. If material is not included in the article's Creative Commons licence and your intended use is not permitted by statutory regulation or exceeds the permitted use, you will need to obtain permission directly from the copyright holder. To view a copy of this licence, visit http://creativecommons.org/licenses/by/4.0/ .
Reprints and permissions
About this article
Mahdad, M., Roshani, S. The open innovation kaleidoscope: navigating pathways and overcoming failures. Rev Manag Sci (2024). https://doi.org/10.1007/s11846-024-00804-7
Download citation
Received : 22 January 2024
Accepted : 21 August 2024
Published : 31 August 2024
DOI : https://doi.org/10.1007/s11846-024-00804-7
Share this article
Anyone you share the following link with will be able to read this content:
Sorry, a shareable link is not currently available for this article.
Provided by the Springer Nature SharedIt content-sharing initiative
- Open innovation
- Value creation
- Topic modeling
- Holistic view
JEL Classification
- Find a journal
- Publish with us
- Track your research

Airline evolves customer experience with IBM public cloud platform and services.
UBank shrinks time to market — builds a loan app virtual assistant on IBM Cloud platform.
Filter by Industry
The American Association of Insurance Services partnered with IBM to create an open blockchain network that streamlines regulatory reporting. The network provides new insights for insurers, while also enhancing timeliness, accuracy and value for regulators.
With IBM Cloud bare metal server infrastructure hosted worldwide and the Veeam on IBM Cloud backup and restore service, Movius provides its multiline offering to enterprises around the globe.
To transform its service desk operations, banking group Creval deployed a virtual assistant, powered by IBM Watson technology, that reduced service desk calls by 80 percent and allowed staff to focus on high-value tasks that drive customer service excellence.
To help ensure clients can access the latest pricing and execute trades rapidly and accurately, online broker ActivTrades migrated its primary trading platforms from on-premises infrastructure to IBM Cloud for VMware solutions.
Allianz wanted a mobile assistant solution that worked across platforms to better serve customers. Using IBM Cloud and IBM Watson Assistant, the company created an AI-powered virtual assistant that can field 80 percent of its most frequent customer requests — for real help in real time.
IBM is helping American Airlines migrate some of its critical applications to the IBM Cloud while using new methodology to create innovative applications quickly and improve the customer experience.
To help its clients focus on strategic activities rather than low-value tasks, Contextor teamed up with IBM to augment its robotic process automation solutions with artificial intelligence capabilities.
To help state healthcare agencies address new regulations, Deloitte teamed with IBM to develop the Medicaid Enterprise Solution (MES) HealthInteractive Platform, running on IBM Cloud technology. With MES, state Medicaid programs can keep IT up to date with less effort and expense, while also aligning with federal guidance.
A Japanese airline has become the first among its competitors to develop a web-based chatbot proof of concept, generating real dialogs with 90 percent of users and confirming the company’s vision for broader cognitive applications with IBM Watson Assistant (formerly IBM Watson Conversation) and Watson Natural Language Classifier services.
Active International used the IBM Cloud to develop applications that optimize its media buying cycle and help it identify new business prospects more effectively.
Anthem partnered with IBM to drive its digital transformation and deliver an enhanced digital experience for its nearly 40 million consumers.
Assima helps employees work smarter by arming them with an intelligent application overlay hosted in the IBM Cloud and delivering AI capabilities with IBM Watson technology.
To help small and midsized businesses with liquidity management and planning, Asteria created a smart cashflow service running on scalable IBM Cloud infrastructure. With its IBM Cloud solution, the company can work in a flexible, open-source development framework while also addressing customer needs for security-rich data hosting.
Buzz Radar created a platform called the Cognitive Command Center — a digital marketing monitoring, analytics and visualization platform that harnesses IBM Watson technologies and runs in the IBM Cloud.
After working with IBM to build a cloud data warehouse and analytics architecture, Constance Hotels, Resorts & Golf can now gain data-driven insights from across its nine properties.
Building on a long track record of service innovation, ExxonMobil engaged an IBM iX team to help design and launch ExxonMobilRewards+, the industry’s first smartphone app for digital payment at the pump. The solution’s IBM Cloud public hosting platform reduces operating costs for the app by 40 percent and scales effortlessly as its user base continues to grow.
LogDNA saw a clear need to address data sprawl in the modern, cloud-native development stack. Its innovative software-as-a-service (SaaS) platform built on Kubernetes caught the attention of the IBM Cloud team, which wove it into its global framework. As both an IBM Business Partner and a client, LogDNA was able to grow and strengthen its DevOps capabilities.
Ricoh migrated its Unified Communication System operations to IBM Cloud bare metal servers, taking advantage of near-limitless scalability and capacity. This allowed the company to ensure smooth sound and vision for its rapidly expanding customer base.
UBank launched new initiatives in an IBM Cloud Platform environment, including a virtual assistant that incorporates IBM Watson technology to support the bank’s online home loan application.

IMAGES
VIDEO
COMMENTS
Open the door to open innovation | IBM. This successful new operating model harnesses the collective intelligence of stakeholders across the value chain in the pursuit of innovation and revenue growth.
Open innovation drives better business outcomes than traditional innovation. Learn what maturity looks like and what it takes to get there.
An open innovation ecosystem unleashes a significantly broader pool of AI talent, as students, academics, and existing members of the workforce can more easily access the resources necessary to acquire AI skills. This is part of the reason IBM contributes hundreds of open models and datasets to Hugging Face, the leading open-source ...
This paper analyses three case firms whose innovation management processes have been consolidated. The companies Natura, IBM (Brazilian Subsidiary) and Siemens (ChemTech/Brazil) were studied with the purpose of analysing the implementation of OI, particularly in terms of: (a) its alignment with existing corporate strategy; (b) its requirements ...
With our qualitative study of 144 companies, we identified three core open innovation processes: (1) The outside-in process: Enriching a company s own knowledge base through the integration of ...
This paper analyses three case firms whose innovation management processes have been consolidated. The companies Natura, IBM (Brazilian Subsidiary) and Siemens (ChemTech/Brazil) were studied with ...
This study aims to showcase the importance of open innovation, and through a theoretical example present how an organization (university, research center, company, firm, etc.) can take action to implement open innovation guidelines. In this paper, firstly, a demonstration showing how open innovation can work with multiple partners is shown.
An IBM case study illustrates a holistic view of open innovation management. With a qualitative study of 144 companies, this work identified three core open innovation processes: The outside-in process, the inside-out process and the coupled process, able to identify eight principle modes of innovation and four capabilities needed to ...
This paper analyses three case firms whose innovation management processes have been consolidated. The companies Natura, IBM (Brazilian Subsidiary) and Siemens (ChemTech/Brazil) were studied with ...
This paper analyses three case firms whose innovation management processes have been consolidated. The companies Natura, IBM (Brazilian Subsidiary) and Siemens (ChemTech/Brazil) were studied with the purpose of analysing the implementation of OI, particularly in terms of: (a) its alignment with existing corporate strategy; (b) its requirements such as culture, skill and motivation; (c) the ...
multiple case study analysis Giordano Pinarello, Daniel Trabucchi , ... inbound Open Innovation, which entail various degrees of integration with the external partner. There is also empirical evidence showing how firms move from a closed to an open ... IBM, Intel and Microsoft (Cusumano and Gawer, 2002). Its strategy has since significantly ...
of informational constraints. Instead, our case study of IBM suggests that inertial forces, reinforced by the "open-science" norms at the company and entrenched leadership, played a role in resisting adoption—a finding consistent with the literature on the ossifying effects of organizational inertia (Hannan and Freeman 1977, Tripsas
The IBM Institute for Business Value uses data-driven research and expert analysis to deliver thought-provoking insights to leaders on the emerging trends that will determine future success.' ... The Global C-suite Study Series. The Global C-Suite Studies, developed by the IBM Institute for Business Value (IBV), take the pulse of business ...
In software, the growth of open source has redefined where innovation can come from, and how it is monetized. IBM has a long history in open source, and that continues today. Our pioneering work in serverless computing, which is quickly becoming the leading platform for the hybrid cloud industry because of the significant growth of Red Hat, is ...
Responsible Use of Technology project, which. brings together a community of experts from civil society, governments and companies to provide practical tools for leaders for how they might: 1) educate and train their employees to think more about responsible technology; 2) design their. Don Heider.
1 Introduction. Open Innovation (OI) has become a dominant approach in innovation management over the last 10 years (Enkel et al., 2020).It was introduced by Chesbrough who popularised the idea that firms can - and should - seek out external sources of ideas and knowledge and look for new paths to market for their technologies in order to maximise their returns on their innovation efforts.
Key lessons: First, IBM is defined by innovation and cutting edge technology. Attracting top talent requires understanding the purpose of your company and fulfilling it better than anyone else ...
MakIng Open InnOvatIOn ecOSySteMS WOrk: caSe StudIeS In HealtHcare IBM center for the Business of government Foreword On behalf of the IBM Center for The Business of Government, we are pleased to present Making Open Innovation Ecosystems Work: Case Studies in Healthcare, by Donald E . Wynn, Renee M .E . Pratt, and Randy V . Bradley .
See the case study here. Frito Lay North America + IBM - With innovation built into its fabric, Frito-Lay is committed to constantly evolving its business to delight consumers. Challenge: Long before hungry consumers rip into a bag of chips, an intricate process unfolds. In part, the process is complex because of the sheer volume of business ...
IBM stands for International Business Machines Corporation and is a multinational technology corporation with over 100 years of history and multiple inventions that are prevalent today. Its headquarters are in Armonk, New York, but it operates in over 170 countries. Institutional investors own over 55% of IBM, while around 30% belongs to mutual ...
We study the nature and consequences of IBM's invention pledge program, the largest of its kind in US history. Invention pledges involve the open disclosure of patentable inventions without seeking patent protection, unlike patent pledges for which firms waive patent rights after patenting. Competing theoretical views characterize invention pledges either as low-quality inventions with poor ...
In this study, we conduct a panoramic analysis of two decades of open innovation (OI), leveraging topic modeling with machine learning to map out ten critical OI pathways and their associated failure mechanisms on the micro, meso, and macro levels. Open innovation has revolutionized organizational innovation, collaboration, and competition. However, it presents complexities that require a ...
IBM and ProMare's years of hard work and preparation came to fruition in the spring of 2021, as the Mayflower Autonomous Ship (MAS), the world's first fully autonomous vessel, embarked on its maiden voyage. Running on solar energy, captained by IBM AI, and equipped with automation and cloud technologies, MAS will explore the seas, going ...
Read the case study LogDNA. LogDNA saw a clear need to address data sprawl in the modern, cloud-native development stack. Its innovative software-as-a-service (SaaS) platform built on Kubernetes caught the attention of the IBM Cloud team, which wove it into its global framework. As both an IBM Business Partner and a client, LogDNA was able to ...