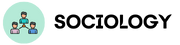

Ad hoc Hypothesis

Ad hoc hypothesis denotes a supplementary hypothesis given to a theory to prevent it from being refuted. According to Karl Popper’s philosophy of science, the only way that falsifiable intellectual systems like Marxism and Freudianism have been sustained is through the dependence on ad hoc hypotheses to fill gaps. Ad hoc hypotheses are used to account for abnormalities that the theory’s unaltered form could not foresee.
Explanation
Ad hoc theories are only acceptable if and only if their non-universal, precise nature can be shown, or, to put it another way, if their potential for direct generalization is disproven. It is the hypothesis that is embraced without any other justification in order to save a theory from refutations or criticism. This technique is deployed in sociological research studies.
The derivation of the particular conclusion in an issue may be deemed invalid if an ad hoc hypothesis was proven to be acceptable and non-universal; as a result, the specific example loses its scientific significance. The necessity of repeat testing is implied in the aforementioned working rule for the acceptance of ad hoc hypotheses, which makes this process seem all the more justifiable.
Notably, the system seems to be in question whenever the introduction of an ad hoc hypothesis is required until the acceptability of the ad hoc hypothesis appears to be established by the requisite falsification attempts. The restriction of ad hoc hypotheses and the continuity principle appear to guarantee the objectivity of falsification; in other words, a theory should only be regarded as falsified if its falsification is theoretically testable.
In addition, because it gives a preferential position to a critical evaluation or falsification, this principle of restriction serves as, in a sense, the second part of the working definition for the idea of a theoretical system’s falsification. Ad hoc hypotheses can be used to attempt to prevent falsification based on the continuity principle, but this can only be done if a different hypothesis, the generalized ad hoc hypothesis (which is also subject to the continuity principle), can also be refuted. Therefore, avoiding falsification depends on (yet another) deception.
The first falsification will take effect if the second one is unsuccessful. This methodological constraint, or the concept of the restriction of ad hoc hypothesis, has effectively eliminated the “conventionalist argument to falsifiability.” The argument that this system is, in theory, not falsifiable has been demonstrated to be inconsistent (via the principle of the restriction of ad hoc hypotheses) provided that a system enables the derivation of empirically verifiable consequences in the first place.
Since the non-falsifiability of any hypothesis (even a generalized ad hoc hypothesis) would necessitate the falsifiability of other hypotheses, this principle gives a workable definition of the term “falsification” (that is, the falsification of the original axiomatic system). This is obviously inconsistent.
The ad hoc hypothesis “This (otherwise accurate) watch showed the wrong time under such and such circumstances” is only a valid ad hoc hypothesis if the universal statement “All (otherwise accurate) watches show the wrong time under such and such circumstances” can be shown to be false, or refuted, by counterexamples.
Related Posts:
- Aggression Definition & Explanation
- Action Theory Definition & Explanation
- Analytic Induction Definition & Explanation
- Class Consciousness Definition & Explanation
- Althusserian Marxism Definition & Explanation
- Age Definition & Explanation
- Action Research Definition & Explanation
- Anarchism Definition & Explanation
- Class Definition & Explanation
- Applied Social Psychology Definition & Explanation
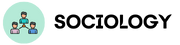

- Table of Contents
- Random Entry
- Chronological
- Editorial Information
- About the SEP
- Editorial Board
- How to Cite the SEP
- Special Characters
- Advanced Tools
- Support the SEP
- PDFs for SEP Friends
- Make a Donation
- SEPIA for Libraries
- Entry Contents
Bibliography
Academic tools.
- Friends PDF Preview
- Author and Citation Info
- Back to Top
Prediction versus Accommodation
In early philosophical literature, a ‘prediction’ was considered to be an empirical consequence of a theory that had not yet been verified at the time the theory was constructed—an ‘accommodation’ was one that had. The view that predictions are superior to accommodations in the assessment of scientific theories is known as ‘predictivism’. Commonly, however, predictivism is understood more precisely as entailing that evidence confirms theory more strongly when predicted than when accommodated. Much ink has been spilled modifying the concept of ‘prediction’ and explaining why predictivism is or is not true, and whether the history of science and, more recently, logic (Martin and Hjortland 2021) reveals that scientists are predictivist in their assessment of theories. The debate over predictivism also figures importantly in the debate about scientific realism.
1. Historical Introduction
2. ad hoc hypotheses, 3. early characterizations of novelty, 4. a predictivist taxonomy, 5. the null support thesis, 6.1 reliable discovery methods, 6.2 the fudging explanation, 6.3 arbitrary and non-arbitrary conjunctions, 6.4 severe tests, 6.5 conditional and unconditional confirmation, 6.6 the archer analogy, 6.7 the akaike approach, 6.8 endorsement novelty and the confirmation of background beliefs, 7. anti-predictivism, 8 the realist/anti-realist debate, other internet resources, related entries.
There was in the eighteenth and nineteenth centuries a passionate debate about scientific method—at stake was the ‘method of hypothesis’ which postulated hypotheses about unobservable entities which ‘saved the phenomena’ and thus were arguably true (see Laudan 1981a). Critics of this method pointed out that hypotheses could always be adjusted artificially to accommodate any amount of data. But it was noted that some such theories had the further virtue of generating specific predictions of heretofore unobserved phenomena—thus scientists like John Herschel and William Whewell argued that hypotheses that saved phenomena could be justified when they were confirmed by such ‘novel’ phenomena. Whewell maintained that predictions carry special weight because a theory that correctly predicts a surprising result cannot have done so by chance, and thus must be true (Whewell 1849 [1968: 294]). It thus appeared that predicted evidence confirmed theory more strongly than accommodated evidence. But John Stuart Mill (in his debate with Whewell) categorically denied this claim, affirming that
(s)uch predictions and their fulfilment are, indeed, well calculated to impress the ignorant vulgar, whose faith in science rests solely upon similar coincidences between its prophecies and what comes to pass. But it is strange that any considerable stress should be laid upon such a coincidence by scientific thinkers. (1843, Vol. 2, 23)
John Maynard Keynes provides a simple account of why predictivism has a misleading appearance of truth in a brief passage in his book A Treatise on Probability :
The peculiar virtue of prediction or predesignation is altogether imaginary… The plausibility of the argument [for predictivism] is derived from a different source. If a hypothesis is proposed a priori , this commonly means that there is some ground for it, arising out of our previous knowledge, apart from the purely inductive ground, and if such is the case the hypothesis is clearly stronger than one which reposes on inductive grounds only. But if it is merely a guess, the lucky fact of its preceding some or all of the cases which verify it adds nothing whatever to its value. It is the union of prior knowledge, with the inductive grounds which arise out of the immediate instances, that lends weight to any hypothesis, and not the occasion on which the hypothesis is first proposed. (1921: 305–306) [ 1 ]
By ‘the inductive ground’ for a hypothesis Keynes clearly means the data that the hypothesis fits. Keynes means that when some theorist who undertakes to test a hypothesis first proposes it, typically some other (presumably theoretical) form of support prompted the proposal. Thus hypotheses which are proposed without being built to fit the empirical data (which they are subsequently shown to entail) are typically better supported than hypotheses which are proposed merely to fit the data—for the latter lack the independent support possessed by the former. The appearance of plausibility to predictivism arises because the role of the preliminary hypothesis-inducing evidence is being suppressed.
Karl Popper is probably the most famous proponent of prediction in the history of philosophy. In his lecture “Science: Conjectures and Refutations” Popper recounts his boyhood attempt to grapple with the question “When should a theory be ranked as scientific?” (Popper 1963: 33–65). Popper had become convinced that certain popular theories of his day, including Marx’s theory of history and Freudian psychoanalysis, were pseudosciences. Popper deemed the problem of distinguishing scientific from pseudoscientific theories ‘the demarcation problem’. His solution to the demarcation problem, as is well known, was to identify the quality of falsifiability (or ‘testability’) as the mark of the scientific theory.
The pseudosciences were marked, Popper claimed, by their vast explanatory power. They could explain not only all the relevant actual phenomena the world presented, they could explain any conceivable phenomena that might fall within their domain. This was because the explanations offered by the pseudosciences were sufficiently malleable that they could always be adjusted ex post facto to explain anything. Thus the pseudosciences never ran the risk of being inconsistent with the data. By contrast, a genuinely scientific theory made specific predictions about what should be observed and thus ran the risk of falsification. Popper emphasized that what established the scientific character of relativity theory was that it ‘stuck its neck out’ in a way that pseudosciences never did.
Like Whewell and Herschel, Popper appeals to the predictions a theory makes as a way of separating the illegitimate uses of the method of hypothesis from its legitimate uses. But while Whewell and Herschel pointed to predictive success as a necessary condition for the acceptability of a theory that had been generated by the method of hypothesis, Popper focuses in his solution to the demarcation problem not on the success of a prediction but on the fact that the theory made the prediction at all. Of course, there was for Popper an important difference between scientific theories whose predictions were confirmed and those whose prediction were falsified. Falsified theories were to be rejected, whereas theories that survived testing were to be ‘tentatively accepted’ until falsified. Popper did not hold, with Whewell and Hershel, that successful predictions could constitute legitimate proof of a theory—in fact Popper held that it was impossible to show that a theory was even probable based on the evidence, for he embraced Hume’s critique of inductive logic that made evidential support for the truth of theories impossible. Thus, one should ascribe to Popper a commitment to predictivism only in the broad sense that he held predictions to be superior to accommodations—he did not hold that predictions confirmed theory more strongly than accommodations. It would ultimately prove impossible for Popper to reconcile his claim that a theory which enjoyed predictive success ought to be ‘tentatively accepted’ with his anti-inductivism (see, e.g., Salmon 1981).
Imre Lakatos (1970, 1971) proposed an account of scientific method in the form of his ‘methodology of scientific research programmes’ which was a development of Popper’s approach. A scientific research program was constituted by a ‘hard core’ of propositions which were retained throughout the life of that programme together with a ‘protective belt’ which was constituted by auxiliary hypotheses that were adjusted so as to reconcile the hard core with the empirical data. The attempt on the part of the proponents of the research programme to reconcile the programme to empirical data produced a series of theories \(T_1\), \(T_2\),… \(T_n\) where, at least in some cases, \(T_{i+1}\) serves to explain some data that is anomalous for \(T_i\). Lakatos held that a research programme was ‘theoretically progressive’ insofar as each new theory predicts some novel hitherto unexpected fact. A research programme is ‘empirically progressive’ to the extent that its novel empirical content was corroborated, that is, if each new theory leads to the discovery of “some new fact” (Lakatos 1970: 118). Lakatos thus offered a new solution to the demarcation problem: a research programme was pseudoscientific to the extent that it was not theoretically progressive. Theory evaluation is construed in terms of competing research programmes: a research programme defeats a rival programme by proving more empirically progressive over the long run.
According to Merriam-Webster’s Collegiate Dictionary, [ 2 ] something is ‘ad hoc’ if it is ‘formed or used for specific or immediate problems or needs’. An ad hoc hypothesis then is one formed to address a specific problem—such as the problem of immunizing a particular theory from falsification by anomalous data (and thereby accommodating that data). Consequently what makes a hypothesis ad hoc, in the ordinary English sense of the term, has nothing to do with the content of the hypothesis but simply with the motivation of the scientist who proposes it—and it is unclear why there would be anything suspicious about such a motivation. Nonetheless, ad hoc hypotheses have long been suspect in discussions of scientific method, a suspicion that resonates with the predictivist’s skepticism about accommodation.
For Popper, a conjecture is ad hoc “if it is introduced…to explain a particular difficulty, but…cannot be tested independently” (Popper 1974: 986). Thus Popper’s conception of ad hocness added to the ordinary English meaning a further requirement—in the case of an ad hoc hypothesis that was simply introduced to explain a single phenomenon, the ad hoc hypothesis has no testable consequences other than that phenomenon. In the case of an ad hoc theory modification introduced to resolve an anomaly for a theory, the modified theory had no testable consequences other than those of the original theory.
Popper offered two explications of why ad hoc hypotheses were suspect. One was that if we offer T as an explanation of f , but then cite f as the only reason we have to believe T , Popper claims that we have engaged in reasoning that is suspicious for reasons of circularity (Popper 1972: 192–3). This was arguably fallacious on Popper’s part—a circular proof would offer one proposition, p , in support of a second proposition q , when q has already been offered in support of p . But in the above example, while f is offered as evidence for T , T is offered as an explanation of (not as evidence for) f —and thus there is no circular reasoning (Bamford 1993: 338).
Popper’s other explanation of why ad hoc hypotheses were regarded with suspicion was that they ran counter to the aim of science, which for Popper included the proposal of theories with increasing empirical content, viz., increasing falsifiability. Ad hoc hypotheses, for Popper, suffer from a lack of independent testability and thus reduce (or at least fail to increase) the testability of the theories they modify (cf. above). However, Popper’s claim that the process of modifying a theory ad hoc tends to lead to insufficient falsifiability and is ‘unscientific practice’ has been challenged (e.g., Bamford 1993: 350).
Subsequent authors argued that a hypothesis proposed for the sake of immunizing a theory from falsification could be ‘suspicious’ for various reasons, and thus could be ‘ad hoc’ in various ways. Zahar (1973) argued that a hypothesis was ad hoc 1 if it had no novel consequences as compared with its predecessor (i.e. was not independently testable), ad hoc 2 if none of its novel predictions have actually been verified (either because it has not yet been tested or has been falsified), and ad hoc 3
if it is obtained from its predecessor through a modification of the auxiliary hypotheses which does not accord with the spirit of the heuristic of the programme. (1973: 101)
Beyond Popper’s criterion of a lack of independent testability then, a hypothesis introduced to accommodate some datum could be ad hoc because it was simply unconfirmed (ad hoc 2 ) or because it failed to cohere with the basic commitments of the research programme in which it is proposed (ad hoc 3 ).
Another approach proposes that a hypothesis H introduced into a theory T in response to an experimental result E is ad hoc if it is generally unsupported and appears to be a superficial attempt to paper over deep problems with a theory that is actually in need of substantive revision. Thus to level the charge of ad hocness against a hypothesis was actually to direct serious skepticism toward the theory the hypothesis was meant to rescue. This concept of ad hocness arguably makes sense of Einstein’s critique of the Lorentz-Fitzgerald contraction hypothesis as ‘ad hoc’ as a supplementary hypothesis to the aether theory, and Pauli’s postulation of the neutrino as an ad hoc rescue of classical quantum mechanics (Leplin 1975, 1982; for further discussion see Grünbaum 1976).
It seems clearly true that the scientific community’s judgment about whether a hypothesis is ad hoc can change. Given this revisability, and the aesthetic dimension of theory evaluation (which leaves assessment to some degree ‘in the eye of the beholder’) there may be no particular point to embracing a theory of ad hocness, if by the term ‘ad hoc’ we mean ‘illegitimately proposed’ (Hunt 2012).
Popper wrote that
Confirmations should count only if they are the result of risky predictions; that is to say, if, unenlightened by the theory in question, we should have expected an event which was incompatible with the theory in question, we should have expected an event which was incompatible with the theory—an event which would have refuted the theory. (1963: 36)
Popper (and subsequently Lakatos) thereby endorsed a temporal condition of novelty—a prediction counts as novel is if it is not known to be true (or is expected to prove false) at the time the theory is constructed. But it was fairly obvious that this made important questions of confirmation turn implausibly on the time at which certain facts were known.
Thus Zahar proposed that a fact is novel “if it did not belong to the problem-situation which governed the construction of the hypothesis” (1973: 103). This form of novelty has been deemed ‘problem-novelty’ (Gardner 1982: 2). But in the same paper Zahar purports to exemplify this concept of novelty by referring to the case in which Einstein did not use the known behavior of Mercury’s perihelion in constructing his theory of relativity. [ 3 ] Gardner notes that this latter conception of novelty, which he deemed ‘use-novelty’, is distinct from problem-novelty (Gardner 1982: 3). Evidence is use-novel for T if T was not built to fit that evidence (whether or not it was part of the relevant ‘problem-situation’ the theory was intended to address). In subsequent literature, the so-called heuristic conception of novelty has been identified with use-novelty—it was further articulated in Worrall 1978 and 1985. [ 4 ]
Another approach argues that a novel consequence of a theory is one that was not known to the theorist at the time she formulated the theory—this seems like a version of the temporal conception, but this point appeals implicitly to the heuristic conception: if a theorist knew of a result prior to constructing a theory which explains it, it may be difficult to determine whether that theorist somehow tailored the theory to fit the fact (e.g., she may have done so unconsciously). A knowledge-based conception is thus the best that we can do to handle this difficulty (Gardner 1982). [ 5 ]
The heuristic conception is, however, deeply controversial—because it makes the epistemic assessment of theories curiously dependent on the mental life of their constructors, specifically on the knowledge and intentions of the theorist to build a theory that accommodated certain data rather than others. Leplin’s comment is typical:
The theorist’s hopes, expectations, knowledge, intentions, or whatever, do not seem to relate to the epistemic standing of his theory in a way that can sustain a pivotal role for them…. (1997: 54)
(For similar comments see Gardner 1982: 6; Thomason 1992: 195; Schlesinger 1987: 33; Achinstein 2001: 210–230; and Collins 1994.)
Another approach notes that scientists operate with competing theories and that the role of novel confirmations is to decide between them. Thus, a consequence of a theory T is a ‘novel prediction’ if it is not a consequence of the best available theory actually present in the field other than T (e.g., the prediction of the Mercury perihelion by Einstein’s relativity theory constituted a novel prediction because it was not a (straightforward) consequence of Newtonian mechanics; Musgrave 1974: 18). Operating in a Lakatosian framework, Frankel claims a consequence was novel with respect to a theory and its research programme if it is not similar to a fact which already has been used by members of the same research program to support a theory designed to solve the same problems as the theory in question (1979: 25). Also in a Lakatosian framework, Nunan claims that a consequence is novel if it has not already been used to support, or cannot readily be explained in terms of, a theory entertained in some rival research program (1984: 279). [ 6 ]
There are clearly multiple forms of novelty and it is generally recognized that a fact could be ‘novel’ in multiple senses—as we will see, some carry more epistemic weight than others (Murphy 1989).
Global predictivism holds that predictions are always superior to accommodations, while local predictivism holds that this only holds in certain cases. Strong predictivism asserts that prediction is intrinsically superior to accommodation, whereas weak predictivism holds that predictive success is epistemically relevant because it is symptomatic of other features that have epistemic import. The distinction between strong and weak predictivism cross classifies with the distinctions between different types of novelty. For example, one could maintain that temporal predictions are intrinsically superior to temporal accommodations (strong temporal predictivism) or that temporal predictions were symptomatic of some other good-making feature of theories (weak temporal predictivism; Hitchcock and Sober 2004: 3–5). These distinctions will be further illustrated below.
A version of global strong heuristic predictivism is the null support thesis that holds that theories never receive confirmation from evidence they were built to fit—precisely because of how they were built. This thesis has been attributed to Bacon and Descartes (Howson 1990: 225). Popper and Lakatos also subscribe to this thesis, though it is important to remember that they do not recognize any form of confirmational support—even from successful predictions. But others who maintained that successful predictions do confirm theories nonetheless endorsed the null support hypothesis. Giere provides the following argument:
If the known facts were used in constructing the model and were thus built into the resulting hypothesis…then the fit between these facts and the hypothesis provides no evidence that the hypothesis is true [since] these facts had no chance of refuting the hypothesis. (1984: 161; Glymour 1980: 114 and Zahar 1983: 245 offer similar arguments)
The idea is that the way the theory was built provided an illegitimate protection against falsification by the facts—hence the facts cannot support the theory. Others however find this argument specious, noting that since the content of the hypothesis is fixed, it makes no sense to think of any facts as having a ‘chance’ to falsify the theory. The theory says what it says, and any particular fact refutes it or it doesn’t.
Giere has confused what is in effect a random variable (the experimental setup or data source E together with its set of distinct possible outcomes) with one of its values (the outcome e )…Moreover, it makes perfectly good sense to say that E might well have produced an outcome other than the one, e , it did as a matter of fact produce. (Howson 1990: 229; see also Collins 1994: 220)
Thus Giere’s argument collapses.
Howson argued in a series of papers (1984, 1988, 1990) that the null support thesis is falsified using simple examples, such as the following:
An urn contains an unknown number of black and white tickets, where the proportion p of black tickets is also unknown. The data consists simply in a report of the relative frequency \(r/k\) of black tickets in a large number k of draws with replacement from the urn. In the light of the data we propose the hypothesis that \(p = (r/k)+\epsilon\) for some suitable \(\epsilon\) depending on k . This hypothesis is, according to standard statistical lore, very well supported by the data from which it is clearly constructed. (1990: 231)
In this case there is, Howson notes, a background theory that supplies a model of the experiment (it is a sequence of Bernoulli trials, viz., a sequence of trials with two outcomes in which the probability of getting either outcome is the same on each trial; it leaves only a single parameter to be evaluated). As long as we have good reason to believe that this model applies, our inference to the high probability of the hypothesis is a matter of standard statistical methodology, and the null support thesis is refuted.
It has been argued that one of the limitations of Bayesianism is that it is fatally committed to the (clearly false) null support thesis (Glymour 1980). The standard Bayesian condition by which evidence e supports h is given by the inequality \(p(h\mid e) \gt p(h)\). But where e is known (and thus \(p(e) = 1\)), we have \(p(h\mid e) = p(h)\). This came to be known as the ‘Bayesian problem of old evidence’. Howson (1984) noted that this problem could be overcome by selecting a probability function \(p^*\) based on the assumption that e was not known—thus even if \(p(h\mid e) = p(h)\), it could still hold that \({p^*}(h\mid e) \gt {p^*}(h)\). Thus followed an extensive literature on the old evidence problem which will not be summarized here (see, e.g., Christiansen 1999; Eells & Fitelson 2000; Barnes 1999, 2008: Ch. 7; and Hartmann & Fitelson 2015).
6 Contemporary Theories of Predictivism
Patrick Maher (1988, 1990, 1993) presented a seminal thought experiment and a Bayesian analysis of its predictivist implications.
The thought experiment contained two scenarios: in the first scenario, a subject (the accommodator) is presented with E , a sequence of 99 coin flips. E forms an apparently random sequence of heads and tails. The accommodator is then instructed to tell us the outcome of the first 100 flips—he responds by reciting E and then adding the prediction that the 100 th toss will be heads—the conjunction of E and this last toss is T . In the other scenario, another subject (the predictor) is asked to predict the first 100 flip outcomes without witnessing any outcomes—the predictor endorses theory T . Thereafter the coin is flipped 99 times, E is established, and the predictor’s first 99 predictions are confirmed. The question is in which of these two scenarios is T better confirmed. It is strongly intuitive that T is better confirmed in the predictor’s scenario than in the accommodator’s scenario, suggesting that predictivism holds true in this case. If we allow ‘ O ’ to assert that evidence E was input into the construction of T , predictivism asserts:
Maher argues that the successful prediction of the initial 99 flips constitutes persuasive evidence that the predictor ‘has a reliable method’ for making predictions of coin flip outcomes. T ’s consistency with E in the case of the accommodator provides no particular evidence that the accommodator’s method of prediction is reliable—thus we have no particular reason to endorse his prediction about the 100 th flip. Allowing R to assert that the method in question is reliable, and \(M_T\) that method M generated hypothesis T , this amounts to:
Maher’s (1988) provides a rigorous proof of (2), which is shown to entail (1) on various assumptions.
Maher’s (1988) makes the simplifying assumption that any method of prediction used by a predictor is either completely reliable (this is the claim abbreviated by ‘ R ’) or is no better than a random method (\(\neg R\)). (Maher [1990] shows that this assumption can be surrendered and a continuum of degrees of reliability of scientific methods assumed; the predictivist result is still generated.) In qualitative terms, where M generates T (and thus predicts E ) without input of evidence E , we should infer that it is much more likely that the method that generated E is reliable than that E just happened to turn out true though R was no better than a random method. In other words, we judge that we are much more likely to stumble on a subject using a reliable method M of coin flip prediction than we are to stumble on a sequence of 99 true flip predictions that were merely lucky guesses—because
Maher has articulated a weak heuristic predictivism because he claims that predictive success is symptomatic of the use of a reliable discovery method. [ 7 ]
For critical discussion of Maher’s theory of predictivism see Howson and Franklin 1991 (and Maher’s 1993 reply); Barnes 1996a,b; Lange 2001; Harker 2006; and Worrall 2014. [ 8 ]
It was noted above that ad hoc hypotheses stand under suspicion for various reasons, one of which was that a hypothesis that was proposed to resolve a particular difficulty may not cohere well with the theory it purports to save or relevant background beliefs. [ 9 ] This could result from the fact that there is no obvious way to resolve the difficulty in a way that is wholly ‘natural’ from the standpoint of the theory itself or operative criteria of theory choice. For example, the phlogiston theory claimed that substances emitted phlogiston while burning. However, it was established that some substances actually gained weight while burning. To accommodate the latter phenomenon it was proposed that phlogiston had negative weight—but the latter hypothesis was clearly ad hoc in the sense of failing to cohere with the background belief that substances simply do not have negative weight, and with the knowledge that many objects lost weight when burned (Partington & McKie 1938a: 33–38).
Thus the ‘fudging explanation’ defends predictivism by pointing out that the process of accommodation lends itself to the proposal of hypotheses that do not cohere naturally with operative constraints on theory choice, while successful predictions are immune from this worry (Lipton 1990, 1991: Ch. 8). Of course, it is an important question whether scientists actually rely on the fact that evidence was predicted (or accommodated) in their assessment of theories—if a theory was fudged to accommodate some datum, couldn’t the scientist simply note that the fudged theory suffers a defect of coherence and pay no attention to whether the data was accommodated or predicted? Some argue, however that scientists are imperfect judges of such coherence—a scientist who accommodates some datum may think his accommodation is fully coherent, while his peers may have a more accurate and objective view that it is not. The scientist’s ‘assessed support’ of his proposed accommodation may thus fail to coincide with its ‘objective support’, and the scientist might rely on the fact that his evidence was accommodated as evidence that it was fudged (or conversely, that his evidence was predicted as evidence that it was not fudged; Lipton 1991: 150f).
Lange (2001) offers an alternate interpretation of the coin flip example that claims that the process of accommodation (unlike prediction) tends to generate theories that are not strongly supported by confirming data. He imagines a ‘tweaked’ version of the coin flip example in which the initial 99 outcomes form a strict alternating sequence ‘tails heads tails heads…’ (instead of forming the ‘apparently random sequence’ of outcomes provided in the original case). Again we imagine a predictor who correctly predicts 99 outcomes in advance and an accommodator who witnesses them. Both the predictor and the accommodator predict that the 100 th outcome will be tails. Now there is little or no difference in our assessed probability that the subject will correctly predicted the 100 th outcome.
This suggests that the intuitive difference between Maher’s original pair of examples does not reflect a difference between prediction and accommodation per se. (Lange 2001: 580)
Lange’s analysis appeals to what Goodman called an ‘arbitrary conjunction’—the mark of which is that
establishment of one component endows the whole statement with no credibility that is transmitted to other component statements. (1983: 68–9)
An example of an arbitrary conjunction is “The sun is made of helium and August 3 rd 2017 falls on a Thursday and 17 is a prime number”. In the original coin flip case, we judge that H is weakly supported in the accommodator’s scenario because we judge that the apparently random sequence of outcomes is probably an arbitrary conjunction—thus the fact that the initial 99 conjuncts are confirmed implies almost nothing about what the 100 th outcome will be. But the success of the predictor in predicting the initial 99 outcomes strongly implies that the sequence is not an arbitrary conjunction after all:
(w)e now believe it more likely that the agent was led to posit this particular sequence by way of something we have not noticed that ties the sequence together—that would keep it from being a coincidence that the hypothesis is accurate to the 100 th toss…. (Lange 2001: 581)
Having judged it not to be an arbitrary conjunction, we are now prepared to recognize the first 99 outcomes as strongly confirming the prediction in the 100 th case. What accounts for the difference between the two scenarios, in other words, is not primarily whether E was predicted or accommodated, but whether we judge H to be an arbitrary conjunction, and thus whether E provides support for the remaining portion of H .
Thus in Lange’s tweaked case, the non-existence of the predictivist effect is due to the fact that it is clear from the initial 99 flips that the sequence is not an arbitrary conjunction—thus E confirms H equally strongly in both scenarios.
Lange goes on to suggest that in actual science the practice of constructing a hypothesis by way of accommodating known evidence has a tendency to generate arbitrary conjunctions. Thus Lorentz’s contraction hypothesis, when appended to his electrodynamics to accommodate the failure to detect optically any motion with respect to the aether, resulted in an arbitrary conjunction (since evidence that supported the contraction hypothesis did not support the electrodynamics, or vice versa)—essentially for this reason, Lange argues, it was rejected by Einstein as ad hoc. When evidence is predicted by a theory, by contrast, this is typically because the theory is not an arbitrary conjunction. The evidential significance of prediction and accommodation for Lange is that they tend to be correlated (negatively and positively) with the construction of theories that are arbitrary conjunctions. Lange’s view might thus be classed as a weak heuristic predictivism, though Lange never takes a stand on whether scientists actually rely on such correlations in assessing theories.
For critical discussion of Lange’s theory see Worrall 2014: 59–61 and Harker 2006: 317f.
Deborah Mayo has argued (particularly in Mayo 1991, 1996, and 2014) that the intuition that predictivism is true derives from a premium on severe tests of hypotheses. A test of a hypothesis H is severe to the extent that H is unlikely to pass that test if H is false. Intuitively, if a novel consequence N is shown to follow from H , and the probability of N on the assumption \({\sim}H\) is very low (for the reason of its being novel), then testing for N would seem to count as a severe test of H , and a positive outcome should strongly support H . Here novelty and severity appear to coincide—but Mayo observes that there are cases in which they come apart. For example, it has seemed to many that if H is built to fit some body of evidence E then the fact that H fits E does not support H because this fit does not constitute H ’s having survived a severe test (or a test at all). One of Mayo’s central objectives is to expose the fallacies that this latter reasoning involves.
Giere (1984: 161, 163) affirms that evidence H was built to fit cannot support H because, given how H was built, it was destined to fit that evidence. Mayo summarizes his reasoning as follows:
- (1) If H is use-constructed, then a successful fit is assured no matter what.
But Mayo notes that ‘no matter what’ can be interpreted in two ways: (a) no matter what the data are, and (b) no matter whether H is true or false. (1) is true when interpreted as (a), but in order to establish that accommodated evidence fails to support H (as Giere intends) (1) must be interpreted as (b). However, (1) is false when so interpreted. Mayo (1996: 271) illustrates this with a simple example: let the evidence e be a list of SAT scores from students in a particular class. Use this evidence to compute the average score x , and set h = the mean SAT score for these students is x . Now of course h has been use-constructed from e . It is true that whatever mean score was computed would fit the data no matter what the data are—but hardly true that h would have fit the evidence no matter whether h was true or false. If h were false it would not fit the data, because the data will inevitably fit only a true hypothesis. Thus h has passed a maximally severe test: it is virtually impossible for h to fit the data if h is false—despite the fact that h is built to fit e .
Mayo gives an additional example of how a use-constructed hypothesis can count as having survived a severe test that pertains to the famous 1919 Eddington eclipse experiment of Einstein’s General Theory of Relativity. GTR predicted that starlight that passed by the sun would be bent to a specific degree (specifically 1.75 arcseconds). There were actually two expeditions carried out during the eclipse—one to Sobral in Northern Brazil and the other to the island of Principe in the Gulf of Guinea. Each expedition generated a result that supported GTR, but there was a third result generated by the Sobral expedition that appeared to refute GTR. This result was however disqualified because it was determined that a mirror used to acquire the images of the stars’ position had been damaged by the heat of the sun. While one might worry that such dismissing of anomalous evidence was the kind of ad hoc adjustment that Popper warned against, Mayo notes that this is instead a perfectly legitimate case of using evidence to support a hypothesis (that the third result was unreliable) that amounted to that hypothesis having passed a severe test. Mayo concludes that a general prohibition on use-constructed hypothesis “fails to distinguish between problematic and unproblematic use-constructions (or double countings)” (1996: 285). However, Hudson (2003) argues that there is historical evidence that suggests there was legitimate reason to question the hypothesis that the third result was unreliable (he uses this point to support his own contention that the fact that a hypothesis was use-constructed is prima facie evidence that the hypothesis is suspect). Mayo (2003) replies that insofar as the third result was nonetheless suspect the physicists involved were right to discard it.
Mayo (1996: Ch. 9) defends a predictivist-like position attributed to Neyman-Pearson statistical methods—the prohibition on after-trial constructions of hypotheses. To illustrate: Kish (1959) describes a study that investigated the statistical relationship between a large number of infant training experiences (nursing, toilet training, weaning, etc.) and subsequent personality and behavioral traits (e.g., school adjustment, nail biting, etc.) The study found a number of high correlations between certain training experience and later traits. The problem was that the study investigated so many training experiences that it was quite likely that some correlations would appear in the data simply by chance—even if there would ultimately prove to be no such correlation. An investigator who studied many possible correlations thus could survey that data and simply look for statistically significant differences and proclaim evidence for correlations despite such evidence being misleading—thus engaging in the dubious practice of the ‘after-trial construction of hypothesis’. [ 10 ] Mayo notes that such hypotheses should not count as having passed a severe test, thus she endorses the Neyman-Pearson prohibition on such construction. Hitchcock and Sober (2004) note that Mayo’s definition of severity as applied in this case differs from the one she employs in dealing with cases like her SAT example; Mayo (2008) replies at length to their criticism and argues that while she does employ two versions of the severity definition they nonetheless reflect a unified conception of severity.
For critical discussion of Mayo’s account see Iseda 1999 and Worrall 2006: 56–60, 2010: 145–153—see also Mayo’s (1996: 265f, 2010) replies to Worrall.
John Worrall has been an important contributor to the predictivism literature from the 1970s until the present time. He was, along with Elie Zahar, one of the early proponents of the significance of heuristic novelty (e.g., Worrall 1978, 1985). In his more recent work (cf. his 1989, 2002, 2005, 2006, 2010, 2014; also Scerri & Worrall 2001) Worrall has laid out a detailed theory of predictivism that, while sometimes presented in heuristic terms, is “at root a logical theory of confirmation” (2005: 819)—it is thus a weak heuristic account that takes use-novelty of evidence to be symptomatic of underlying logical features that establish strong confirmation of theory.
Worrall’s mature account is based on a view of scientific theories that he credits to Duhem—which claims that a scientific theory is naturally thought of as consisting of a core claim together with some set of more specific auxiliary claims. It is commonly the case that the core theory will leave undetermined certain ‘free parameters’ and the auxiliary claims fix values for such parameters. To cite an example Worrall often uses, the wave theory of light consists of the core theory that light is a periodic disturbance transmitted though some sort of elastic medium. This core claim by itself leaves open various free parameters concerning the wavelengths of particular types of monochromatic light. Worrall proposes to understand the diminished status of evidential support associated with accommodation as follows: when evidence e is ‘used’ in the construction of a theory, it is typically used to establish the value of a free parameter in some core theory T . The fixed version will be a specific version \(T'\) of T . e serves to confirm \(T'\), then, only on the condition that there is independent support for T —thus accommodation provides only ‘conditional confirmation’. Importantly, evidence e that is used in this way will by itself typically provide no evidence for core theory T . Worrall (2002: 201) offers as an illustration the support offered to the wave theory of light ( W ) by the two slit experiment using light from a sodium arc—the data will consist of various alternating light and dark ‘fringes’. The fringe data can be used to compute the wavelength of sodium light—and thus used to generate a more specific version of the wave theory of light \(W'\)—one which conjoins W with a claim about the wavelength of this particular sort of light. But the data offer merely conditional support to \(W'\)—that is the data support \(W'\) only on the condition that there is independent evidence for W .
Predicted evidence for Worrall is thus evidence that is not used to fix free parameters. Worrall cites two forms that predictions can take: one is when a particular evidential consequence falls ‘immediately out of the core’, i.e., is a consequence of the core, together with ‘natural auxiliaries’, and the other is when it is a consequence of a specific version of a theory whose free parameters have been fixed using other data. To illustrate the first: retrograde motion [ 11 ] was a natural consequence of the Copernican core (the claim that the earth and planets orbit the sun) because observation of the planets was carried out on a moving observatory that periodically passed other planets—however it could only be accommodated by Ptolemaic astronomy by proposing and adjusting auxiliary hypotheses that supposed the planet to move on an epicycle (retrograde motion did not follow naturally from the Ptolemaic core idea that the Sun, stars and planets orbit the earth). Thus retrograde motion was predicted by the Copernican theory and thus offered unconditional support to that theory, while it offered only conditional confirmation to the Ptolemaic theory. The second form of prediction is one which follows from a specific version of a theory but was not used to fix a parameter—imagine \(W'\) in the preceding paragraph makes a new prediction p (say for another experiment, such as the one slit experiment)— p offers unconditional confirmation of \(W'\) (and W ; Worrall 2002: 203).
However it is important to understand that Worrall’s repeated expression of his position in terms of the heuristic conception of novelty (particularly after his 1985) does not amount to an endorsement of strong heuristic predictivism. Worrall clarifies this in his 1989 article that focuses on the evidential significance of the ‘white spot’ confirmation of Fresnel’s version of the wave theory of light. The reason the white spot datum carried such important weight is not ultimately that it was not used by Fresnel in the construction of the theory but because this datum followed naturally from the core theory that light is a wave. The reason the fringe data that was used to compute the wavelength of sodium light (cf. above) did not carry such weight is that it is not a consequence of this core idea (nor has the wavelength of sodium light been fixed by some other data). Thus d is novel for T when “there is a heuristic path to [ T ] that does not presuppose [d’s] existence” (Scerri & Worrall 2001: 418). As Worrall sometimes puts it, whether d carries unconditional confirmation for T does not depend on whether d was actually used in constructing T , but whether it was ‘needed’ to construct T (e.g., 1989: 149–151). Thus Worrall is actually a proponent of ‘essential use-novelty’ (Alai 2014: 304). For Worrall, facts about heuristic prediction and accommodation serve to track underlying facts about the logical relationship between theory and evidence. Thus Worrall is ultimately a proponent of weak (not strong) heuristic predictivism. Worrall categorically rejects temporal predictivism, arguing that the fact that the white spot was a temporally novel consequence in itself was of no epistemic importance.
For further discussion of Worrall’s theory of predictivism see Mayo 2010: 155f; Schurz 2014; Votsis 2014; and Douglas & Magnus 2013: 587–8.
Scerri and Worrall 2001 contains a detailed rendering of the historical episode of the scientific community’s assessment of Mendeleev’s theory of the periodic law—it is argued that this story ultimately vindicates Worrall’s theory of predictivism.
For discussion of Scerri and Worrall see Akeroyd 2003; Barnes 2005b (and replies from Worrall 2005 and Scerri 2005); Schindler 2008, 2014; Brush 2007; and Sereno 2020.
A common argument for predictivism is that we should avoid inferring that a theory T is true on the basis of evidence E that it is built to fit because we can explain why T entails E by simply noting how T was built—but if T was not built to fit E then only the truth of T can explain the fact that T fits E . Various philosophers have noted that this reasoning is fallacious. As noted above it makes no sense to offer an explanation (for example, in terms of how the theory was built) for the fact that T entails E —for this latter fact is a logical fact for which no causal explanation can be given. Insofar as there is an explanandum in need of an explanans here it is rather the fact that the theorist managed to construct or ‘choose’ a theory (which turned out to be T ) that correctly entailed E (Collins 1994; Barnes 2002)—that explanandum could be explained by noting that the theorist built a theory (which turned out to be T ) to fit E , or endorsed it because it fit E .
White (2003) offers a theory of predictivism that begins with this same insight—the relevant explanandum is:
- (ES) The theorist selected a datum-entailing theory.
This explanandum could be explained in one of two ways:
- (DS) The theorist designed her theory to entail the datum.
- (RA) The theorist’s selection of her theory was reliably aimed at the truth.
White explains that (RA) means “roughly that the mechanisms which led to her selection of a theory gave her a good chance of arriving at the truth” (2003: 664). (Thus White analogizes the theorist to an ‘archer’ who is more or less reliable in ‘aiming’ at the truth in selecting a theory.) Then White offers a simple argument for predictivism: assuming ~DS, ES provides evidence for RA. But assuming DS, ES provides no evidence for RA. Thus, heuristic predictivism is true.
Interestingly, White bills his account as a strong heuristic account. In making this claim he is claiming that the epistemic advantage of prediction would not be entirely erased for an observer who was completely aware of all relevant evidence and background knowledge possessed by the scientific community at the relevant point in time. This is because the degree to which theorizing is reliable depends upon principles of evidence assessment and causal relations (including the reliability of our perceptual faculties, accuracy of measuring instruments, etc.) that are not entirely “transparent” to us. [ 12 ] Insofar as fully informed scientists may not be fully convinced of just how reliable these principles and relations are, evidence that they lead to the endorsement of theories which are predictively successful continues to redound to their assessed reliability. Thus, White concludes, strong heuristic predictivism is vindicated (2003: 671–4).
Hitchcock and Sober (2004) provide an original theory of weak heuristic predictivism that is based on a particular worry about accommodation. On the assumption that data are noisy (i.e. imbued with observational error), a good theory will almost never fit the data perfectly. To construct a theory that fits the data better than a good theory should, given noisy data, is to be guilty of “overfitting”—if we know a theorist built her theory to accommodate data, we may well worry that she has overfit the data and thus constructed a flawed theory. If we know however that a theorist built her theory without access to such data, or without using it in the process of theory construction, we need not worry that overfitting that data has occurred. When such a theory goes on to make successful predictions, Hitchcock and Sober moreover argue, this provides us with evidence that the data on which the theory was initially based were not overfit in the process of constructing the theory.
Hitchcock and Sober’s approach derives from a particular solution to the curve-fitting problem presented in Forster and Sober 1994. The curve fitting problem is how to select an optimally supported curve on the basis of a given body of data (e.g., a set of \([X,Y]\) points plotted on a coordinate graph). A well-supported curve will feature both ‘goodness of fit’ with the data and simplicity (intuitively, avoiding highly bumpy or irregular patterns). Solving the curve-fitting problem requires some precise way of characterizing a curve’s simplicity, a way of characterizing goodness of fit, and a method of balancing simplicity against goodness of fit to identify an optimal curve.
Forster and Sober cite Akaike’s (1973) result that an unbiased estimate of the predictive accuracy of a model can be computed by assessing both its goodness of fit and its simplicity as measured by the number of adjustable parameters it contains. A model is a statement (a polynomial, in the case of a proposed curve) that contains at least one adjustable parameter. For any particular model M , a given data set, and identifying \(L(M)\) as the likeliest (i.e. best data fitting) curve from M , Akaike showed that the following expression describes an unbiased estimate of the predictive accuracy of model M:
This estimate is deemed a model’s ‘Akaike Information Criterion’ (AIC) score—it measures goodness of fit in terms of the log likelihood of the data on the assumption of \(L(M)\). The simplicity of the model is inversely proportion to k , the number of adjustable parameters in the model. The intuitive idea is that models with a high k value will provide a large variety of curves that will tend to fit data more closely than models with a lower k value—and thus large k values are more prone to overfitting than small k values. So the AIC score assesses a model’s likely predictive accuracy in a way that balances both goodness of fit and simplicity, and the curve-fitting problem is arguably solved.
Hitchcock and Sober (2004) consider a hypothetical example involving two scientists, Penny Predictor and Annie Accommodator. Working independently, they acquire the same set of data D —Penny proposes theory Tp while Annie proposes Ta . The critical difference however was that Penny proposed Tp on the basis of an initial segment of the data D 1—thereafter she predicted the remaining data D 2 to a high degree of accuracy \((D = D1 \cup D2)\). Annie however was in possession of all the data in D prior to proposing Ta and in proposing this theory accommodated D . Hitchcock and Sober ask whether there might be reason to suspect that Penny’s theory will be more predictively accurate in the future, and in this precise sense be better confirmed.
Hitchcock and Sober argue that there is no one answer to this question—and then present a series of several cases. Insofar as predictivism holds in some and not others, their account of predictivism is clearly a local (rather than global) account. In cases in which Penny and Annie propose the same theory, or propose theories whose AIC scores can be computed and directly compared, there is no reason to regard facts about how they built the theory to carry further significance. But if we do not know which theories were proposed, or by what method they were constructed, the fact that Penny predicted data that Annie accommodated can argue for Penny’s theory having a higher AIC score than Annie’s, and thus carry an epistemic advantage.
Insofar as predictivism holds in some cases but not the others, the question whether predictivism holds in actual episodes of science depends on which cases such actual episodes tend to resemble, but Hitchcock and Sober “take no stand on how often the various cases arise” (2004: 21).
Although their account of predictivism is tailored initially to the curve-fitting problem, it is by no means limited to such cases. They note that it is natural to think of a model as analogous to the ontological framework of a scientific theory where the various ontological commitments can function as ‘adjustable parameters’—for example, the Ptolemaic and Copernican world pictures both begin with a claim that a certain entity (the sun or the earth) is at the center, and these models are articulated by producing models with adjustable parameters.
For critical discussion of Sober and Hitchcock’s account, see Lee 2012, 2013 and Douglas & Magnus 2013: 582–584. Peterson (2019) argues that Sober and Hitchcock's approach can be extended to issue methodological recommendations involving methods of cross validation and replication in psychology.
Barnes (2005a, 2008) maintains that predictivism is frequently a manifestation of a phenomenon he calls ‘epistemic pluralism’. A ‘ T -evaluator’ (a scientist who assigns some probability to theory T ) is an epistemic pluralist insofar as she regards one form of evidence to be the probabilities posted (i.e. publicly presented) by other scientists for and against T and other relevant claims (she is an epistemic individualist if she does not do this but considers only the scientific evidence ‘on her own’). One form of pluralistic evidence is the event in which a reputable scientist endorses a theory—this takes place when a scientist posts a probability for T that is (1) no lower than the evaluator’s probability and (2) high enough that subsequent predictive confirmation of T would redound to the scientist’s credibility (2008: 2.2).
Barnes rejects the heuristic conception of novelty on the grounds that it is a mistake to think that what matters epistemically is the process by which the theory was constructed—what matters is on what basis the theory was endorsed (2008: 33f) . In the example above, confirmation of N (a consequence of T ) could carry special weight for an evaluator who learned that the theorist endorsed the theory without appeal to observational evidence for N (irrespective of how the theory was constructed). He proposes to replace the heuristic conception with his endorsement conception of novelty: N (a known consequence of T ) counts as a novel confirmation of T relative to agent X insofar as X posts an endorsement-level probability for T that is based on a body of evidence that does not include observation-based evidence for N .
Barnes claims that the notion of endorsement novelty has several advantages over the heuristic conception—one is that endorsement novelty can account for the fact that prediction is a matter of degree: the more strongly the theorist endorses T , the more strongly its consequence N is predicted (and thus the more evidence for T for pluralist evaluators who trust the endorser). Another is that the orthodox distinction between the context of discovery and the context of justification is preserved. According to the latter distinction, it does not matter for purposes of theory evaluation how a theory was discovered. But this turns out not to be true on the heuristic conception given the central importance it accords to how a theory was built (cf. Leplin 1987). Endorsement novelty respects the irrelevance of the process by which theories are discovered (Barnes 2008: 37–8).
One claim central to this account is that confirmation is a three-way relation between theory, evidence, and background belief (cf. Good 1967). Barnes distinguishes between two types of theory endorser: (1) virtuous endorsers post probabilities for theories that cohere with their evidence and background beliefs and (2) unvirtuous endorsers who post probabilities that do not so cohere. A common way of explaining the predictivist intuition is to note that accommodators tend to be viewed with a certain suspicion—their endorsement of T based on accommodated evidence may reflect a kind of social pressure to endorse T whatever its merits (cf. the ‘fudging explanation’ above). Such an endorser may post a probability for T that is too high given her total evidence and background belief—predictivism thus becomes a strategy by which pluralist endorsers protect themselves from unvirtuous accommodators (Barnes 2008: 61–69).
Barnes then presents a theory of predictivism that is designed to apply to virtuous endorsers. Virtuous predictivism has two roots: (1) the prediction per se, which is constituted by an endorser’s posting an endorsement level probability for T that entails empirical consequence N on a basis that does not include observation-based evidence for T , and (2) predictive success, constituted by the empirical demonstration that N is true. The prediction per se carries epistemic significance for a pluralist endorser because it implies that the predictor possesses reason R (consisting of background beliefs) that supports T . If the endorser views the predictor as credible, this simple act of prediction carries epistemic weight. Predictive success then confirms the truth of R , which thereby counts as evidence for T . Novel confirmation thus has the special virtue of confirming the background beliefs of the predictor—accommodative confirmation lacks this virtue.
Barnes presents two Bayesian thought experiments that purport to establish virtuous predictivism. In each experiment an evaluator Eva faces two scenarios—one in which she confronts Peter who posts an endorsement probability for T without appeal to N -supporting observations (thus Peter predicts N ) and another in which she confronts Alex who posts an endorsement probability for T on a basis that includes observations that establish N (thus Alex accommodates N ). The idea behind both thought experiments is to make the scenarios otherwise as similar as possible—Barnes makes a number of ceteris paribus assumption that render the probability functions of Peter and Alex maximally similar. However it turns out that there is more than one way to keep the scenarios maximally similar: in the first experiment, Peter and Alex have the same likelihood ratio but have different posteriors for T . In the second scenario they have the same posteriors but different likelihood ratios. Barnes demonstrates that Eva’s posterior probability is higher in the predictor scenario in both experiments—thus vindicating virtuous predictivism (2008: 69–80).
Although his defense of virtuous predictivism is the centerpiece of his account, Barnes claims that predictivism can hold true of actual theory evaluation in a variety of ways. He maintains that the position deemed ‘weak predictivism’ is actually ambiguous—it could refer to the claim that scientists actually rely on knowledge that evidence was (or was not) predicted because prediction is symptomatic of a some other feature(s) of theories that is epistemically important (‘tempered predictivism’ [ 13 ] ) or simply to the fact that there is a correlation between prediction and this other feature(s) (‘thin predictivism’). The distinction between tempered and thin predictivism cross classifies with the distinction between virtuous and unvirtuous predictivism to produce four varieties of weak predictivism. Barnes then turns to the case of Mendeleev’s periodic law and argues that all four varieties can be distinguished in the scientific community’s reaction to Mendeleev’s theory of the elements (2008: 82–122). In particular, he argues that it was specifically Mendeleev’s predicted evidence, not his accommodated evidence, that had the power to confirm his scientific and methodological background beliefs from the standpoint of the scientific community.
Critical responses to Barnes’s account are presented in Glymour 2008; Leplin 2009; and Harker 2011. Barnes 2014 responds to these. See also Magnus 2011 and Alai 2016.
It was noted in Section 1 that John Maynard Keynes rejected predictivism—he argued that when a theory T is first constructed it is usually the case that there are reasons R that favor T . If T goes on to generate successful novel predictions E then those reasons combine with R to support T —but if some \(T'\) is constructed ‘merely because it fit E ’ then \(T'\) will be less supported than T . This has been deemed the “Keynesian dissolution of the paradox of predictivism” (Barnes 2008: 15–18)
Colin Howson cites with approval the Keynesian dissolution (1988: 382) and provides the following illustration: consider h and \(h'\) which are rival explanatory frameworks. \(h'\) independently predicts e ; h does not entail e but has a free parameter which is fixed on the basis of e to produce \(h(a_{0})\)—this latter hypothesis thus entails e . So \(h'\) predicts e while \(h(a_{0})\) merely accommodates e . Let us assume that the prior probabilities of h and \(h'\) are equal (i.e., \(p(h) = p(h')\)). Now it stands to reason that \(p(h(a_0)) \lt p(h)\) since \(h(a_{0})\) entails h but not vice versa—thus Howson shows it follows that the effect of e ’s confirmation will be to leave \(h'\) no less probable—and quite possibly more probable—than \(h(a_{0})\) (1990: 236–7). Thus predictivism appears true but the operating factor is the role of unequal prior probabilities. [ 14 ]
The argument from Keynes and Howson against predictivism holds that the evidence which appears to support predictivism is illusory—they are clearly asserting that strong predictivism is false, presumably in its temporal and heuristic forms.
However, it is important to note that the arguments of Keynes and Howson cited above predate the injection of the concept of ‘weak predictivism’ into the literature. [ 15 ] It is thus unclear what stand Keynes or Howson would take on weak predictivism. Likewise, Collins’ 1994 paper “Against the Epistemic Value of Prediction” strongly rejects predictivism, but what he is clearly denying is what has since been deemed strong heuristic predictivism. He might endorse weak heuristic predictivism as he concedes that
all sides to the debate agree that knowing that a theory predicted, instead of accommodated, a set of data can give us an additional reason for believing it is true by telling us something about the structural/relational features of a theory. (1994: 213)
Similarly Harker argues that “it is time to leave predictivism behind” but also concedes that “some weak predictivist theses may be correct” (2008: 451); Harker worries that proclaiming weak predictivism may mislead some into thinking that predictive success is somehow more important than other epistemic indicators (such as endorsement by reliable scientists). White goes so far as to claim that weak predictivism “is not controversial” (2003: 656).
Stephen Brush is the author of a body of historical work much of which purports to show that temporal predictivism does not hold in various episodes of the history of science. [ 16 ] These include the case of starlight bending in the assessment of the General Theory of Relativity (Brush 1989), Alfvén’s theories of space plasma phenomena (Brush 1990), and the revival of big bang cosmology (Brush 1993). However, Brush (1996) argues that temporal novelty did play a role in the acceptance of Mendeleev’s Periodic Table based on Mendeleev’s predictions. Scerri and Worrall (2001) presents considerable historical detail about the assessment of Mendeleev’s theory and dispute Brush’s claim that temporal novelty played an important role in the acceptance of the theory (2001: 428–436). (See also Brush 2007.) Steele and Werndl (2013) argue that predictivism fails to hold in assessing models of climate change, while Frish (2015) affirms that it displays weak predictivism.
Another form of anti-predictivism holds that accommodations are superior to predictions in theory confirmation. “The information that the data was accommodated rather than predicted suggests that the data is less likely to have been manipulated or fabricated, which in turn increases the likelihood that the hypothesis is correct in light of the data” (Dellsen forthcoming).
Scientific realism holds that there is sufficient evidence to believe that the theories of the ‘mature sciences’ are at least approximately true. Appeals to novelty have been important in formulating two arguments for realism—these are the ‘no miracle argument’ and the realist reply to the so-called ‘pessimistic induction’. [ 17 ]
The no-miracle argument for scientific realism holds that realism is the only account that does not make the success of science a miracle (Putnam 1975: 73). ‘The success of science’ here refers to the myriad verified empirical consequences of the theories of the mature sciences—but as we have seen there is a long standing tendency to regard with suspicion those verified empirical consequences the theory was built to fit. Thus the ‘ultimate argument for scientific realism’ refers to a version of the no miracle argument that focuses just on the verified novel consequences of theories—it would be a miracle, this argument proclaims, if a theory managed to have a sustained record of successful novel predictions if the theory were not at least approximately true. Thus, assuming there are no competing theories with comparable records of novel success, we ought to infer that such theories are at least approximately true (Musgrave 1988). [ 18 ]
Insofar as the ultimate argument for realism clearly emphasizes a special role for novel successes, the nature of novelty has been an important focus in the realist account. Leplin 1997 is a book length articulation of the ultimate argument for realism; Leplin proposes a sufficient condition for novelty consisting of two conditions:
An observational result O is novel for T if:
- Independence Condition: There is a minimally adequate reconstruction of the reasoning leading to T that does not cite any qualitative generalization of O .
- Uniqueness Condition: There is some qualitative generalization of O that T explains and predicts, and of which, at the time that T first does so, no alternative theory provides a viable reason to expect instances. (Leplin 1997: 77).
Leplin clarifies that a ‘minimally adequate reconstruction’ of such reasoning will be a valid deduction D of the ‘basic identifying hypotheses’ of T from independently warranted background assumptions—the premises of D cannot be weakened or simplified while preserving D ’s validity. Thus for Leplin what establishes whether O is a novel consequence of T is not whether O was actually used in the construction of T , but rather whether it was ‘needed’ for T ’s construction. As with Worrall’s mature ‘essential use’ conception of novelty, what matters is whether there is a heuristic path to T that does not appeal to O , whether or not O was used in constructing T . The Uniqueness Condition helps bolster the argument for the truth of theories with true novel consequences, for if there were another theory \(T'\) (incompatible with T ) that also provides a viable explanation of O , the imputation of truth could not explain the novel success of both T and \(T'\). The success of at least one would have to be due to chance, but if chance could explain one such success it could explain the other as well.
Both of these conditions for novelty have been questioned. Given the Independence Condition, it is unclear that any observational result O will count as novel for any theory, for it may always be true that the logically weakest set of premises that entail T (which will be cited in a minimally adequate reconstruction of the reasoning that led to T ) will include O as a disjunct of one of the premises (Healey 2001: 779). The Uniqueness Condition insists that there be no available alternative explanation of O at the time T first explains O —but clearly, theories that explain O could be subsequently proposed and would threaten the imputation of truth to T no less. This condition seems arbitrarily to privilege theories depending on when they were proposed (Sarkar 1998: 206–8; Ladyman 1999: 184).
Another conception of novelty whose purpose is to bolster the ultimate argument for realism is ‘functional novelty’ (Alai 2014). A datum d is ‘functionally novel’ for theory T if (1) d was not used essentially in constructing T (viz., there is a heuristic path to T and related auxiliary hypotheses that does not cite d ), (2) d is a priori improbable, and (3) d is heterogeneous with respect to data that is used in constructing T and related auxiliary hypotheses (i.e. d is qualitatively different from such data). Functional novelty is a ‘gradual’ concept insofar as a priori improbability and data heterogeneity come in degrees. If there is more than one theory for which d is functionally novel then the dispute between these theories cannot be settled by the ultimate argument (Alai 2014: 306).
Anti-realists have argued that insofar as we adopt a naturalistic philosophy of science, the same standards should be used for assessing philosophical theories as scientific theories. Consequently, if novel confirmations are necessary for inferring a theory’s truth then scientific realism should not be accepted as true, as the latter thesis has no novel confirmations to its credit (Frost-Arnold 2010, Mizrahi 2012).
Another component of the realist/anti-realist debate in which appeals to novel success figure importantly is the debate over the ‘pessimistic induction’ (or ‘pessimistic meta-induction’). According to this argument, the history of science is almost entirely a history of theories that were judged empirically successful in their day only to be shown subsequently to be entirely false. There is no reason to think that currently accepted theories are any different in this regard (Laudan 1981b).
In response some realists have defended ‘selective realism’ which concedes that while the majority of theories from the history of science have proven false, some of them have components that were retained in subsequent theories—these tend to be the components that were responsible for novel successes. Putative examples of this phenomenon are the caloric theory of heat and nineteenth century optical theories (Psillos 1999: Ch. 6), both of which were ultimately rejected as false but which had components that were retained in subsequent theories; these were the portions that were responsible for their novel confirmations. [ 19 ] So in line with the ultimate argument the claim is made that novel successes constitute a serious argument for the truth of the theory component which generates them. However, antirealists have responded by citing cases of theoretical claims that were subsequently determined to be entirely false but which managed nonetheless to generate impressive records of novel predictions. These include certain key claims made by Johannes Kepler in his Mysterium Cosmographicum (1596), assumptions used by Adams and Leverrier in the prediction of the planet Neptune’s existence and location (Lyons 2006), and Ptolemaic astronomy (Carman & Díez 2015). Leconte (2017) maintains that predictive success legitimates only sceptical realism – the claim that some part of a theory is true, but it is not known which part.
- Achinstein, Peter, 1994, “Explanation vs. Prediction: Which Carries More Weight”, PSA: Proceedings of the Biennial Meeting of the Philosophy of Science Association , 1994(2): 156–164. doi:10.1086/psaprocbienmeetp.1994.2.192926
- –––, 2001, The Book of Evidence , Oxford: Oxford University Press. doi:10.1093/0195143892.001.0001
- Akaike, Hirotugu, 1973, “Information Theory as an Extension of the Maximum Likelihood Principle”, in B.N. Petrov and F. Csaki, (eds.) Second International Symposium on Information Theory , Budapest, Akademiai Kiado, pp. 267–281.
- Akeroyd, F. Michael, 2003, “Prediction and the Periodic Table: A Response to Scerri and Worrall”, Journal for General Philosophy of Science , 34(2): 337–355. doi:10.1023/B:JGPS.0000005277.60641.ca
- Alai, Mario, 2014, “Novel Predictions and the No Miracle Argument”, Erkenntnis , 79(2): 297–326. doi:10.1007/s10670-013-9495-7
- –––, 2016, “The No Miracle Argument and Strong Predictivism vs. Barnes”, in Lorenzo Magnini and Claudia Casadio (eds.), Model Based Reasoning in Science and Technology , (Studies in Applied Philosophy, Epistemology and Rational Ethics, 27), Switzerland: Springer International Publishing, pp.541–556. doi:10.1007/978-3-319-38983-7_30
- Bamford, Greg, 1993, “Popper’s Explication of Ad Hoc ness: Circularity, Empirical Content, and Scientific Practice”, British Journal for the Philosophy of Science , 44(2): 335–355. doi:10.1093/bjps/44.2.335
- Barnes, Eric Christian, 1996a, “Discussion: Thoughts on Maher’s Predictivism”, Philosophy of Science , 63: 401–10. doi:10.1086/289918
- –––, 1996b, “Social Predictivism”, Erkenntnis , 45(1): 69–89. doi:10.1007/BF00226371
- –––, 1999, “The Quantitative Problem of Old Evidence”, British Journal for the Philosophy of Science , 50(2): 249–264. doi:10.1093/bjps/50.2.249
- –––, 2002, “Neither Truth Nor Empirical Adequacy Explain Novel Success”, Australasian Journal of Philosophy , 80(4): 418–431. doi:10.1080/713659528
- –––, 2005a, “Predictivism for Pluralists”, British Journal for the Philosophy of Science , 56(3): 421–450. doi:10.1093/bjps/axi131
- –––, 2005b, “On Mendeleev’s Predictions: Comment on Scerri and Worrall”, Studies in the History and Philosophy of Science , 36(4): 801–812. doi:10.1016/j.shpsa.2005.08.005
- –––, 2008, The Paradox of Predictivism , Cambridge: Cambridge University Press. doi:10.1017/CBO9780511487330
- –––, 2014, “The Roots of Predictivism”, Studies in the History and Philosophy of Science , 45: 46–53. doi:10.1016/j.shpsa.2013.10.002
- Brush, Stephen G., 1989, “Prediction and Theory Evaluation: The Case of Light Bending”, Science , 246(4934): 1124–1129. doi:10.1126/science.246.4934.1124
- –––, 1990, “Prediction and Theory Evaluation: Alfvén on Space Plasma Phenomena”, Eos , 71(2): 19–33. doi:10.1029/EO071i002p00019
- –––, 1993, “Prediction and Theory Evaluation: Cosmic Microwaves and the Revival of the Big Bang”, Perspectives on Science , 1(4):: 565–601.
- –––, 1994, “Dynamics of Theory Change: The Role of Predictions”, PSA: Proceedings of the Biennial Meeting of the Philosophy of Science Association , 1994(2): 133–145. doi:10.1086/psaprocbienmeetp.1994.2.192924
- –––, 1996, “The Reception of Mendeleev’s Periodic Law in America and Britain”, Isis , 87(4): 595–628. doi:10.1086/357649
- –––, 2007, “Predictivism and the Periodic Table”, Studies in the History and Philosophy of Science Part A , 38(1): 256–259. doi:10.1016/j.shpsa.2006.12.007
- Campbell, Richmond and Thomas Vinci, 1983, “Novel Confirmation”, British Journal for the Philosophy of Science , 34(4): 315–341. doi:10.1093/bjps/34.4.315
- Carman, Christián and José Díez, 2015, “Did Ptolemy Make Novel Predictions? Launching Ptolemaic Astronomy into the Scientific Realism Debate”, Studies in the History and Philosophy of Science , 52: 20–34. doi:10.1016/j.shpsa.2015.04.002
- Carrier, Martin, 2014, “Prediction in context: On the comparative epistemic merit of predictive success”, Studies in the History and Philosophy of Science , 45: 97–102. doi:10.1016/j.shpsa.2013.10.003
- Chang, Hasok, 2003, “Preservative Realism and Its Discontents: Revisiting Caloric”, Philosophy of Science , 70(5): 902–912. doi:10.1086/377376
- Christiansen, David, 1999, “Measuring Confirmation”, Journal of Philosophy , 96(9): 437–461. doi:10.2307/2564707
- Collins, Robin, 1994, “Against the Epistemic Value of Prediction over Accommodation”, Noûs , 28(2): 210–224. doi:10.2307/2216049
- Dawid, R. and Stephan Hartmann, 2017, “The No Miracles Argument without the Base-Rate Fallacy”, Synthese . doi:10.1007/s11229-017-1408-x
- Dellsen, Finnur, (forthcoming), “An Epistemic Advantage of Accommodation Over Prediction”, Philosophers' Imprint
- Dicken, P., 2013, “Normativity, the Base-Rate Fallacy, and Some Problems for Retail Realism”, Studies in the History and Philosophy of Science Part A, 44 (4): 563–570.
- Douglas, Heather and P.D. Magnus, 2013, “State of the Field: Why Novel Prediction Matters”, Studies in the History and Philosophy of Science , 44(4): 580–589. doi:10.1016/j.shpsa.2013.04.001
- Eells, Ellery and Branden Fitelson, 2000, “Measuring Confirmation and Evidence”, Journal of Philosophy , 97(12): 663–672. doi:10.2307/2678462
- Forster, Malcolm R., 2002, “Predictive Accuracy as an Achievable Goal of Science”, Philosophy of Science , 69(S3): S124–S134. doi:10.1086/341840
- Forster, Malcolm and Elliott Sober, 1994, “How to Tell when Simpler, More Unified, or Less Ad Hoc Theories Will Provide More Accurate Predictions”, British Journal for the Philosophy of Science , 45(1): 1–35. doi:10.1093/bjps/45.1.1
- Frankel, Henry, 1979, “The Career of Continental Drift Theory: An application of Imre Lakatos’ analysis of scientific growth to the rise of drift theory”, Studies in the History and Philosophy of Science , 10(1): 21–66. doi:10.1016/0039-3681(79)90003-7
- Frisch, Mathias, 2015, “Predictivism and Old Evidence: A Critical Look at Climate Model Tuning”, European Journal for the Philosophy of Science , 5(2): 171–190. doi:10.1007/s13194-015-0110-4
- Frost-Arnold, Greg, 2010, “The No-Miracles Argument for Scientific Realism: Inference to an Unacceptable Explanation”, Philosophy of Science , 77(1): 35–58. doi:10.1086/650207
- Gardner, Michael R., 1982, “Predicting Novel Facts”, British Journal for the Philosophy of Science , 33(1): 1–15. doi:10.1093/bjps/33.1.1
- Giere, Ronald N., 1984, Understanding Scientific Reasoning , second edition, New York: Holt, Rinehart, and Winston. First edition 1979.
- Glymour, Clark N., 1980, Theory and Evidence , Princeton, NJ: Princeton University Press.
- –––, 2008, “Review: The Paradox of Predictivism by Eric Christian Barnes”, Notre Dame Philosophical Reviews , 2008.06.13. [ Glymour 2008 available online ]
- Good, I.J. 1967, “The White Shoe is a Red Herring”, British Journal for the Philosophy of Science , 17(4): 322. doi:10.1093/bjps/17.4.322
- Goodman, Nelson, 1983, Fact, Fiction and Forecast , fourth edition, Cambridge, MA: Harvard University Press. First edition 1950.
- Grünbaum, Adolf, 1976, “ Ad Hoc Auxiliary Hypotheses and Falsificationism”, British Journal for the Philosophy of Science , 27(4): 329–362. doi:10.1093/bjps/27.4.329
- Hacking, Ian, 1979, “Imre Lakatos’s Philosophy of Science”, British Journal for the Philosophy of Science , 30(4): 381–410. doi:10.1093/bjps/30.4.381
- Harker, David, 2006, “Accommodation and Prediction: The Case of the Persistent Head”, British Journal for the Philosophy of Science , 57(2): 309–321. doi:10.1093/bjps/axl004
- –––, 2008, “The Predilections for Predictions”, British Journal for the Philosophy of Science , 59(3): 429–453. doi:10.1093/bjps/axn017
- –––, 2010, “Two Arguments for Scientific Realism Unified”, Studies in the History and Philosophy of Science , 41(2): 192–202. doi:10.1016/j.shpsa.2010.03.006
- –––, 2011, “ Review: The Paradox of Predictivism by Eric Christian Barnes”, British Journal for the Philosophy of Science , 62(1): 219–223. doi:10.1093/bjps/axq027
- Hartman, Stephan and Branden Fitelson, 2015, “A New Garber-Style Solution to the Problem of Old Evidence”, Philosophy of Science , 82(4): 712–717. doi:10.1086/682916
- Healey, Richard, 2001, “Review: A Novel Defense of Scientific Realism by Jarrett Leplin”, Mind , 110(439): 777–780. doi:10.1093/mind/110.439.777
- Henderson, Leah, 2017, “The No Miracles Argument and the Base-Rate Fallacy”, Synthese (4): 1295–1302.
- Hitchcock, Christopher and Elliott Sober, 2004, “Prediction versus Accommodation and the Risk of Overfitting”, British Journal for the Philosophy of Science , 55(1): 1–34. doi:10.1093/bjps/55.1.1
- Holton, Gerald, 1988, Thematic Origins of Scientific Thought: Kepler to Einstein , revised edition, Cambridge, MA and London, England: Harvard University Press. First edition 1973.
- Howson, Colin, 1984, “Bayesianism and Support by Novel Facts”, British Journal for the Philosophy of Science , 35(3): 245–251. doi:10.1093/bjps/35.3.245
- –––, 1988, “Accommodation, Prediction and Bayesian Confirmation Theory”, PSA: Proceedings of the Biennial Meeting of the Philosophy of Science Association, 1988 , 2: 381–392. doi:10.1086/psaprocbienmeetp.1988.2.192899
- –––, 1990, “Fitting Your Theory to the Facts: Probably Not Such a Bad Thing After All”, in Scientific Theories , ( Minnesota Studies in the Philosophy of Science , Vol. XIV), C. Wade Savage (ed.), Minneapolis: University of Minnesota Press, pp. 224–244. [ Howson 1990 available online ]
- Howson, Colin and Allan Franklin, 1991, “Maher, Mendeleev and Bayesianism”, Philosophy of Science , 58(4): 574–585. doi:10.1086/289641
- Hudson, Robert G., 2003, “Novelty and the 1919 Eclipse Experiments”, Studies in the History and Philosophy of Modern Physics , 34(1): 107–129. doi:10.1016/S1355-2198(02)00082-5
- –––, 2007, “What’s Really at Issue with Novel Predictions?” Synthese , 155(1): 1–20. doi:10.1007/s11229-005-6267-1
- Hunt, J. Christopher, 2012, “On Ad Hoc Hypotheses”, Philosophy of Science , 79(1): 1–14. doi:10.1086/663238
- Iseda, Tetsuji, 1999, “Use-Novelty, Severity, and a Systematic Neglect of Relevant Alternatives”, Philosophy of Science , 66: S403–S413. doi:10.1086/392741
- Kahn, J.A., S.E. Landsberg, and A.C. Stockman, 1990, “On Novel Confirmation”, British Journal for the Philosophy of Science , 43, 503–516.
- Keynes, John Maynard, 1921, A Treatise on Probability , London: Macmillan.
- Kish, Leslie, 1959, “Some Statistical Problems in Research Design”, American Sociological Review , 24(3): 328–338; reprinted in Denton E. Morrison and Ramon E. Henkel (eds.), The Significance Test Controversy: A Reader , Chicago: Aldine, pp. 127–141. doi:10.2307/2089381
- Kitcher, Philip, 1993, The Advancement of Science: Science without Legend, Objectivity without Illusions , Oxford: Oxford University Press.
- Ladyman, James, 1999, “Review: Jarrett Leplin, A Novel Defense of Scientific Realism ”, British Journal for the Philosophy of Science , 50(1): 181–188. doi:10.1093/bjps/50.1.181
- Lakatos, Imre, 1970, “Falsification and the Methodology of Scientific Research Programmes”, in Imre Lakatos and Alan Musgrave (eds.), Criticism and the Growth of Knowledge: Proceedings of the International Colloquium in the Philosophy of Science, London, 1965 , Cambridge: Cambridge University Press, pp. 91–196. doi:10.1017/CBO9781139171434.009
- –––, 1971, “History of Science and its Rational Reconstructions”, in Roger C. Buck and Robert S. Cohen (eds.), PSA 1970 , ( Boston Studies in the Philosophy of Science , 8), Dordrecht: Springer Netherlands, pp. 91–135. doi:10.1007/978-94-010-3142-4_7
- Lange, Marc, 2001, “The Apparent Superiority of Prediction to Accommodation: a Reply to Maher”, British Journal for the Philosophy of Science , 52(3): 575–588. doi:10.1093/bjps/52.3.575
- Laudan, Larry, 1981a, “The Epistemology of Light: Some Methodological Issues in the Subtle Fluids Debate”, in Science and Hypothesis: Historical Essays on Scientific Methodology (University of Western Ontario Series in Philosophy of Science, 19), Dordrecht: D. Reidel, pp. 111–140.
- –––, 1981b, “A Confutation of Convergent Realism”, Philosophy of Science , 48(1): 19–49. doi:10.1086/288975
- Leconte, Gauvain, 2017, “Predictive Success, Partial Truth, and Duhemian Realism”, Synthese , 194(9): 3245–3265. doi:10.1007/s11229-016-1305-8
- Lee, Wang-Yen, 2012, “Hitchcock and Sober on Weak Predictivism”, Philosophia , 40(3): 553–562. doi:10.1007/s11406-011-9331-8
- –––, 2013, “Akaike’s Theorem and Weak Predictivism in Science” Studies in the History and Philosophy of Science Part A , 44(4): 594–599. doi:10.1016/j.shpsa.2013.06.001
- Leplin, Jarrett, 1975, “The Concept of an Ad Hoc Hypothesis”, Studies in History and Philosophy of Science , 5 No. 3: 309–345. doi:10.1016/0039-3681(75)90006-0
- –––, 1982, “The Assessment of Auxiliary Hypotheses”, British Journal for the Philosophy of Science , 33(3): 235–249. doi:10.1093/bjps/33.3.235
- –––, 1987, “The Bearing of Discovery on Justification”, Canadian Journal of Philosophy , 17: 805–814. doi:10.1080/00455091.1987.10715919
- –––, 1997, A Novel Defense of Scientific Realism , New York, Oxford: Oxford University Press.
- –––, 2009, “Review: The Paradox of Predictivism by Eric Christian Barnes”, The Review of Metaphysics , 63(2): 455–457.
- Lipton, Peter 1990, “Prediction and Prejudice”, International Studies in the Philosophy of Science , 4(1): 51–65. doi:10.1080/02698599008573345
- –––, 1991, Inference to the Best Explanation , London/New York: Routledge.
- Lyons, Timothy D., 2006, “Scientific Realism and the Strategema de Divide et Impera”, British Journal for the Philosophy of Science , 57(3): 537–560. doi:10.1093/bjps/axl021
- Magnus, P.D., 2011, “Miracles, trust, and ennui in Barnes’ Predictivism”, Logos & Episteme , 2(1): 103–115. doi:10.5840/logos-episteme20112152
- Magnus, P.D. and Craig Callender, 2004, “Realist Ennui and the Base Rate Fallacy”, Philosophy of Science , 71(3): 320–338. doi:10.1086/421536
- Maher, Patrick, 1988, “Prediction, Accommodation, and the Logic of Discovery”, PSA: Proceedings of the Biennial Meeting of the Philosophy of Science Association 1988 , 1: 273–285. doi:10.1086/psaprocbienmeetp.1988.1.192994
- –––, 1990, “How Prediction Enhances Confirmation”, in J. Michael Dunn and Anil Gupta (eds.), Truth or Consequences: Essays in Honor of Nuel Belnap , Dordrecht: Kluwer, pp. 327–343.
- –––, 1993, “Howson and Franklin on Prediction”, Philosophy of Science , 60(2): 329–340. doi:10.1086/289736
- Martin, Ben and Ole Hjortland, 2021, “Logical Predictivism”, Journal of Philosophical Logic , 50: 285–318.
- Mayo, Deborah G., 1991, “Novel Evidence and Severe Tests”, Philosophy of Science , 58(4): 523–552. doi:10.1086/289639
- –––, 1996, Error and the Growth of Experimental Knowledge , Chicago and London: University of Chicago Press.
- –––, 2003, “Novel Work on the Problem of Novelty? Comments on Hudson”, Studies in the History and Philosophy of Modern Physics , 34: 131–134. doi:10.1016/S1355-2198(02)00083-7
- –––, 2008, “How to Discount Double-Counting When It Counts: Some Clarifications”, British Journal for the Philosophy of Science , 59(4): 857–879. doi:10.1093/bjps/axn034
- –––, 2010, “An Ad Hoc Save of a Theory of Adhocness? Exchanges with John Worrall” in Mayo and Spanos 2010: 155–169.
- –––, 2014, “Some surprising facts about (the problem of) surprising facts (from the Dusseldorf Conference, February 2011)”, Studies in the History and Philosophy of Science , 45: 79–86. doi:10.1016/j.shpsa.2013.10.005
- Mayo, Deborah G. and Aris Spanos (eds.), Error and Inference: Recent Exchanges on Experimental Reasoning, Reliability, and the Objectivity and Rationality of Science , Cambridge: Cambridge University Press. doi:10.1017/CBO9780511657528
- McCain, Kevin, 2012, “A Predictivist Argument Against Skepticism” Analysis , 72(4): 660–665. doi:10.1093/analys/ans109
- McIntyre, Lee, 2001, “Accommodation, Prediction, and Confirmation”, Perspectives on Science , 9(3): 308–328. doi:10.1162/10636140160176161
- Menke, C., 2014, “Does the Miracle Argument Embody a Base-Rate Fallacy?”, Studies in the History and Philosophy of Science Part A, 45: 103–108.
- Mill, John Stuart, 1843, A System of Logic, Ratiocinative and Inductive: Being a Connected View of the Principles of Evidence and the Methods of Scientific Investigation , Vol. 2, London: John W. Parker.
- Mizrahi, Moti, 2012, “Why the Ultimate Argument for Scientific Realism Fails”, Studies in the History and Philosophy of Science , 43(1): 132–138. doi:10.1016/j.shpsa.2011.11.001
- Murphy, Nancey, 1989, “Another Look at Novel Facts”, Studies in the History and Philosophy of Science , 20(3): 385–388. doi:10.1016/0039-3681(89)90014-9
- Musgrave, Alan, 1974, “Logical versus Historical Theories of Confirmation”, British Journal for the Philosophy of Science , 25(1): 1–23. doi:10.1093/bjps/25.1.1
- –––, 1988, “The Ultimate Argument for Scientific Realism”, in Robert Nola (ed.), Relativism and Realism in Science , Dordrecht: Kluwer Academic Publishers, pp. 229–252. doi:10.1007/978-94-009-2877-0_10
- Nunan, Richard, 1984, “Novel Facts, Bayesian Rationality, and the History of Continental Drift”, Studies in the History and Philosophy of Science , 15(4): 267–307. doi:10.1016/0039-3681(84)90013-X
- 1937, “I. The Levity of Phlogiston”, 2(4): 361–404, doi:10.1080/00033793700200691
- 1938a, “II. The Negative Weight of Phlogiston”, 3(1): 1–58, doi:10.1080/00033793800200781
- 1938b, “III. Light and Heat in Combustion”, 3(4): 337–371, doi:10.1080/00033793800200951
- Peterson, Clayton, 2019, “Accommodation, Prediction, and Replication: Model Selection in Scale Construction”, Synthese , 196: 4329–4350.
- Popper, Karl, 1963, Conjectures and Refutations: The Growth of Scientific Knowledge , New York and Evanston: Harper and Row.
- –––, 1972, Objective Knowledge , Oxford: Clarendon Press.
- –––, 1974, “Replies to my critics”, in Paul Arthur Schilpp (ed.), The Philosophy of Karl Popper , Book II, 961–1197, La Salle, Illinois: Open Court.
- Psillos, Stathis, 1999, Scientific Realism: How Science Tracks the Truth , London and New York: Routledge.
- Putnam, Hilary, 1975, Philosophical Papers,Vol. 1, Mathematics, Matter, and Method , Cambridge: Cambridge University Press.
- Redhead, Michael, 1978, “Adhocness and the Appraisal of Theories”, British Journal for the Philosophy of Science , 29: 355–361.
- Salmon, Wesley C., 1981, “Rational Prediction”, British Journal for the Philosophy of Science , 32(2): 115–125. doi:10.1093/bjps/32.2.115
- Sarkar, Husain, 1998, “Review of A Novel Defense of Scientific Realism by Jarrett Leplin”, Journal of Philosophy , 95(4): 204–209. doi:10.2307/2564685
- Scerri, Eric R., 2005, “Response to Barnes’s critique of Scerri and Worrall”, Studies in the History and Philosophy of Science , 36(4): 813–816. doi:10.1016/j.shpsa.2005.08.006
- Scerri, Eric R. and John Worrall, 2001, “Prediction and the Periodic Table”, Studies in the History and Philosophy of Science , 32(3): 407–452. doi:10.1016/S0039-3681(01)00023-1
- Schindler, Samuel, 2008, “Use Novel Predictions and Mendeleev’s Periodic Table: Response to Scerri and Worrall (2001)”, Studies in the History and Philosophy of Science Part A , 39(2): 265–269. doi:10.1016/j.shpsa.2008.03.008
- –––, 2014, “Novelty, coherence, and Mendeleev’s periodic table”, Studies in the History and Philosophy of Science Part A , 45: 62–69. doi:10.1016/j.shpsa.2013.10.007
- Schlesinger, George N., 1987, “Accommodation and Prediction”, Australasian Journal of Philosophy , 65(1): 1 33–42. doi:10.1080/00048408712342751
- Schurz, Gerhard, 2014, “Bayesian Pseudo-Confirmation, Use-Novelty, and Genuine Confirmation”, Studies in History and Philosophy of Science Part A , 45: 87–96. doi:10.1016/j.shpsa.2013.10.008
- Sereno, Sergio Gabriele Maria, 2020, “Prediction, Accommodation, and the Periodic Table: A Reappraisal”, Foundations of Chemistry , 22: 477–488.
- Stanford, P. Kyle, 2006, Exceeding Our Grasp: Science, History, and the Problem of Unconceived Altenatives , Oxford: Oxford University Press. doi:10.1093/0195174089.001.0001
- Steele, Katie and Charlotte Werndl, 2013, “Climate Models, Calibration, and Confirmation”, The British Journal for the Philosophy of Science , 64 (30): 609–635.
- Swinburne, Richard, 2001, Epistemic Justification , Oxford: Oxford University Press. doi:10.1093/0199243794.001.0001
- Thomason, Neil, 1992, “Could Lakatos, Even with Zahar’s Criterion of Novel Fact, Evaluate the Copernican Research Programme?”, British Journal for the Philosophy of Science , 43(2): 161–200. doi:10.1093/bjps/43.2.161
- Votsis, Ioannis, 2014, “Objectivity in Confirmation: Post Hoc Monsters and Novel Predictions”, Studies in the History and Philosophy of Science Part A , 45: 70–78. doi:10.1016/j.shpsa.2013.10.009
- Whewell, William, 1849 [1968], “Mr. Mill’s Logic”, originally published 1849, reprinted in Robert E. Butts (ed.), William Whewell’s Theory of Scientific Method , Pittsburgh, PA: University of Pittsburgh Press, pp. 265–308.
- White, Roger, 2003, “The Epistemic Advantage of Prediction over Accommodation”, Mind , 112(448): 653–683. doi:10.1093/mind/112.448.653
- Worrall, John, 1978, “The Ways in Which the Methodology of Scientific Research Programmes Improves Upon Popper’s Methodology”, in Gerard Radnitzky and Gunnar Andersson (eds.) Progress and Rationality in Science , (Boston studies in the philosophy of science, 58), Dordrecht: D. Reidel, pp. 45–70. doi:10.1007/978-94-009-9866-7_3
- –––, 1985, “Scientific Discovery and Theory-Confirmation”, in Joseph C. Pitt (ed.), Change and Progress in Modern Science: Papers Related to and Arising from the Fourth International Conference on History and Philosophy of Science, Blacksburg, Virginia, November 1982 , Dordrecht: D. Reidel, pp. 301–331. doi:10.1007/978-94-009-6525-6_11
- –––, 1989, “Fresnel, Poisson and the White Spot: The Role of Successful Predictions in the Acceptance of Scientific Theories”, in David Gooding, Trevor Pinch, and Simon Schaffer (eds.), The Uses of Experiment: Studies in the Natural Sciences , Cambridge: Cambridge University Press, pp. 135–157.
- –––, 2002, “New Evidence for Old”, in Peter Gärdenfors, Jan Wolenski, and K. Kijania-Placek (eds.), In the Scope of Logic, Methodology and Philosophy of Science: Volume One of the 11th International Congress of Logic, Methodology and Philosophy of Science, Cracow, August 1999 , Dordrecht: Kluwer Academic Publishers, pp. 191–209.
- –––, 2005, “Prediction and the ‘Periodic Law’: A Rejoinder to Barnes”, Studies in the History and Philosophy of Science , 36(4): 817–826. doi:10.1016/j.shpsa.2005.08.007
- –––, 2006, “Theory-Confirmation and History”, in Colin Cheyne and John Worrall. (eds.), Rationality and Reality: Conversations with Alan Musgrave , Dordrecht: Springer, pp. 31–61. doi:10.1007/1-4020-4207-8_4
- –––, 2010, “Errors, Tests, and Theory Confirmation”, in Mayo. and Spanos 2010: 125–154.
- –––, 2014, “Prediction and Accommodation Revisited”, Studies in History and Philosophy of Science Part A , 45: 54–61. doi:10.1016/j.shpsa.2013.10.001
- Wright, John, 2012, Explaining Science’s Success: Understanding How Scientific Knowledge Works , Durham, England: Acumen.
- Zahar, Elie, 1973, “Why did Einstein’s Programme supersede Lorentz’s? (I)”, British Journal for the Philosophy of Science , 24(2): 95–123. doi:10.1093/bjps/24.2.95
- –––, 1983, Einstein’s Revolution: A Study In Heuristic , La Salle, IL: Open Court.
How to cite this entry . Preview the PDF version of this entry at the Friends of the SEP Society . Look up topics and thinkers related to this entry at the Internet Philosophy Ontology Project (InPhO). Enhanced bibliography for this entry at PhilPapers , with links to its database.
[Please contact the author with suggestions.]
confirmation | epistemology: Bayesian | Lakatos, Imre | Mill, John Stuart | Popper, Karl | realism: and theory change in science | scientific discovery | scientific explanation | scientific method | scientific realism | Whewell, William
Copyright © 2022 by Eric Christian Barnes < ebarnes @ smu . edu >
- Accessibility
Support SEP
Mirror sites.
View this site from another server:
- Info about mirror sites
The Stanford Encyclopedia of Philosophy is copyright © 2023 by The Metaphysics Research Lab , Department of Philosophy, Stanford University
Library of Congress Catalog Data: ISSN 1095-5054
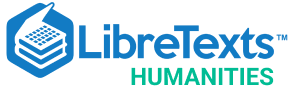
- school Campus Bookshelves
- menu_book Bookshelves
- perm_media Learning Objects
- login Login
- how_to_reg Request Instructor Account
- hub Instructor Commons
Margin Size
- Download Page (PDF)
- Download Full Book (PDF)
- Periodic Table
- Physics Constants
- Scientific Calculator
- Reference & Cite
- Tools expand_more
- Readability
selected template will load here
This action is not available.
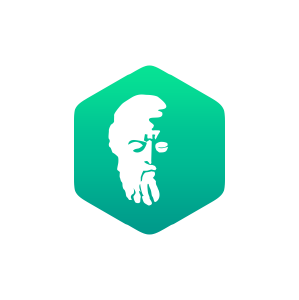
9.1: Hypothetical Reasoning
- Last updated
- Save as PDF
- Page ID 223910
\( \newcommand{\vecs}[1]{\overset { \scriptstyle \rightharpoonup} {\mathbf{#1}} } \)
\( \newcommand{\vecd}[1]{\overset{-\!-\!\rightharpoonup}{\vphantom{a}\smash {#1}}} \)
\( \newcommand{\id}{\mathrm{id}}\) \( \newcommand{\Span}{\mathrm{span}}\)
( \newcommand{\kernel}{\mathrm{null}\,}\) \( \newcommand{\range}{\mathrm{range}\,}\)
\( \newcommand{\RealPart}{\mathrm{Re}}\) \( \newcommand{\ImaginaryPart}{\mathrm{Im}}\)
\( \newcommand{\Argument}{\mathrm{Arg}}\) \( \newcommand{\norm}[1]{\| #1 \|}\)
\( \newcommand{\inner}[2]{\langle #1, #2 \rangle}\)
\( \newcommand{\Span}{\mathrm{span}}\)
\( \newcommand{\id}{\mathrm{id}}\)
\( \newcommand{\kernel}{\mathrm{null}\,}\)
\( \newcommand{\range}{\mathrm{range}\,}\)
\( \newcommand{\RealPart}{\mathrm{Re}}\)
\( \newcommand{\ImaginaryPart}{\mathrm{Im}}\)
\( \newcommand{\Argument}{\mathrm{Arg}}\)
\( \newcommand{\norm}[1]{\| #1 \|}\)
\( \newcommand{\Span}{\mathrm{span}}\) \( \newcommand{\AA}{\unicode[.8,0]{x212B}}\)
\( \newcommand{\vectorA}[1]{\vec{#1}} % arrow\)
\( \newcommand{\vectorAt}[1]{\vec{\text{#1}}} % arrow\)
\( \newcommand{\vectorB}[1]{\overset { \scriptstyle \rightharpoonup} {\mathbf{#1}} } \)
\( \newcommand{\vectorC}[1]{\textbf{#1}} \)
\( \newcommand{\vectorD}[1]{\overrightarrow{#1}} \)
\( \newcommand{\vectorDt}[1]{\overrightarrow{\text{#1}}} \)
\( \newcommand{\vectE}[1]{\overset{-\!-\!\rightharpoonup}{\vphantom{a}\smash{\mathbf {#1}}}} \)
Suppose I’m going on a picnic and I’m only selecting items that fit a certain rule. You want to find out what rule I’m using, so you offer up some guesses at items I might want to bring:
An Egg Salad Sandwich
A grape soda
Suppose now that I tell you that I’m okay with the first two, but I won’t bring the third. Your next step is interesting: you look at the first two, figure out what they have in common, and then you take a guess at the rule I’m using. In other words, you posit a hypothesis. You say something like
Do you only want to bring things that are yellow or tan?
Notice how at this point your hypothesis goes way beyond the evidence. Bananas and egg salad sandwiches have so much more in common than being yellow/tan objects. This is how hypothetical reasoning works: you look at the evidence, add a hypothesis that makes sense of that evidence (one among many hypotheses available), and then check to be sure that your hypothesis continues to make sense of new evidence as it is collected.
Suppose I now tell you that you haven’t guessed the right rule. So, you might throw out some more objects:
A key lime pie
A jug of orange juice
I then tell you that the first two are okay, but again the last item is not going with me on this picnic.
It’s solid items! Solid items are okay, but liquid items are not.
Again, not quite. Try another set of items. You are still convinced that it has to do with the soda and the juice being liquid, so you try out an interesting tactic:
An ice cube
Some liquid water
Some water Vapor
The first and last items are okay, but not the middle one. Now you think you’ve got me. You guess that the rule is “anything but liquids,” but I refuse to tell you whether you got it right. You’re pretty confident at this point, but perhaps you’re not certain . In principle, there could always be more evidence that upsets your hypothesis. I might say that the ocean is okay but a fresh water lake isn’t, and that would be very confusing for you. You’ll never be quite certain that you’ve guessed my rule correctly because it’s always in principle possible that I have a super complex rule that is more complex than your hypothesis.
So in hypothetical reasoning what we’re doing is making a leap from the evidence we have available to the rule or principle or theory which explains that evidence. The hypothesis is the link between the two. We have some finite evidence available to us, and we hypothesize an explanation. The explanation we posit either is or is not the true explanation, and so we’re using the hypothesis as a bridge to get onto the true explanation of what is happening in the world.
The hypothetical method has four stages. Let’s illustrate each with an example. You are investigating a murder and have collected a lot of evidence but do not yet have a guess as to who the killer might be.
1. The occurrence of a problem
Example \(\PageIndex{1}\)
Someone has been murdered and we need to find out who the killer is so that we might bring them to justice.
2. Formulating a hypothesis
Example \(\PageIndex{2}\)
After collecting some evidence, you weigh the reasons in favor of thinking that each suspect is indeed the murderer, and you decide that the spouse is responsible.
3. Drawing implications from the hypothesis
Example \(\PageIndex{3}\)
If the spouse was the murderer, then a number of things follow. The spouse must have a weak alibi or their alibi must rest on some falsehood. There is likely to be some evidence on their property or among their belongings that links the spouse to the murder. The spouse likely had motive. etc., etc., etc.
We can go on for ages, but the basic point is that once we’ve got an idea of what the explanation for the murder is (in this case, the hypothesis is that the spouse murdered the victim), we can ask ourselves what the world would have to be like for that to have been true. Then we move onto the final step:
4. Test those implications.
Example \(\PageIndex{4}\)
We can search the murder scene, try to find a murder weapon, run DNA analysis on the organic matter left at the scene, question the spouse about their alibi and possible motives, check their bank accounts, talk to friends and neighbors, etc. Once we have a hypothesis, in other words, that hypothesis drives the search for new evidence—it tells us what might be relevant and what irrelevant and therefore what is worth our time and what is not.
The Logic of Hypothetical Reasoning
If the spouse did it, then they must have a weak alibi. Their alibi is only verifiable by one person: the victim. So they do have a weak alibi. Therefore...they did it? Not quite.
Just because they have a weak alibi doesn’t mean they did it. If that were true, anyone with a weak alibi would be guilty for everything bad that happened when they weren’t busy with a verifiable activity.
Similarly, if your car’s battery is dead, then it won’t start. This doesn’t mean that whenever your car doesn’t start, the battery is dead. That would be a wild and bananas claim to make (and obviously false), but the original conditional (the first sentence in this paragraph) isn’t wild and bananas. In fact, it’s a pretty normal claim to make and it seems obviously true.
Let’s talk briefly about the logic of hypothetical reasoning so we can discover an important truth.
If the spouse did it, then their alibi will be weak
Their alibi is weak
So, the spouse did it
This is bad reasoning. How do we know? Well, here’s the logical form:
If A, then B
Therefore, A
This argument structure—called “affirming the consequent”—is invalid because there are countless instances of this general structure that have true premises and a false conclusion. Consider the following examples:
Example \(\PageIndex{5}\)
If I cook, I eat well
I ate well tonight, so I cooked.
Example \(\PageIndex{6}\)
If Eric runs for student president, he’ll become more popular.
Eric did become more popular, so he must’ve run for student president.
Maybe I ate well because I’m at the finest restaurant in town. Maybe I ate well because my brother cooked for me. Any of these things is possible, which is the root problem with this argument structure. It infers that one of the many possible antecedents to the conditional is the true antecedent without giving any reason for choosing or preferring this antecedent.
More concretely, affirming the consequent is the structure of an argument that states that a) one thing will explain an event, and b) that the event in question in fact occurred, and then concludes that c) the one thing that would’ve explained the event is the correct explanation of the event.
More concretely still, here’s yet another example of affirming the consequent:
Example \(\PageIndex{7}\)
My being rich would explain my being popular
I am in fact popular,
Therefore I am in fact rich
I might be popular without having a penny to my name. People sometimes root for underdogs, or respond to the right kind of personality regardless of their socioeconomic standing, or respect a good sense of humor or athletic prowess.
If I were rich, though, that would be one potential explanation for my being popular. Rich people have nice clothes, cool cars, nice houses, and get to have the kinds of experiences that make someone a potentially popular person because everyone wants to hear the cool stories or be associated with the exciting life they lead. Perhaps, people often seem to think, they’ll get to participate in the next adventure if they cozy up to the rich people. Rich kids in high school can also throw the best parties (if we’re honest, and that’s a great source of popularity).
But If I’m not rich, that doesn’t mean I’m not popular. It only means that I’m not popular because I’m rich .
Okay, so we’ve established that hypothetical reasoning has the logical structure of affirming the consequent. We’ve further established that affirming the consequent is an invalid deductive argumentative structure. Where does this leave us? Is the hypothetical method bad reasoning ?!?!?!? Nope! Luckily not all reasoning is deductive reasoning.
Remember that we’re discussing inductive reasoning in this chapter. Inductive reasoning doesn’t obey the rules of deductive logic. So it’s no crime for a method of inductive reasoning to be deductively invalid. The crime against logic would be to claim that we have certain knowledge when we only use inductive reasoning to justify that knowledge. The upshot? Science doesn’t produce certain knowledge—it produces justified knowledge, knowledge to a more or less high degree of certitude, knowledge that we can rely on and build bridges on, knowledge that almost certainly won’t let us down (but it doesn’t produce certain knowledge).
We can, though, with deductive certainty, falsify a hypothesis. Consider the murder case: if the spouse did it, then they’d have a weak alibi. That is, if the spouse did it, then they wouldn’t have an airtight alibi because they’d have to be lying about where they were when the murder took place. If it turns out that the spouse does have an airtight alibi, then your hypothesis was wrong.
Let’s take a look at the logic of falsification:
If the spouse did it, then they won’t have an airtight alibi
They have an airtight alibi
So the spouse didn’t do it
Now it’s possible that the conditional premise (the first premise) isn’t true, but we’ll assume it’s true for the sake of the illustration. The hypothesis was that the spouse did it and so the spouse’s alibi must have some weakness.
It’s also possible that our detective work hasn’t been thorough enough and so the second premise is false. These are important possibilities to keep in mind. Either way, here’s the logical form (a bit cleaned up and simplified):
Therefore not A
This is what argument pattern? That’s right! You’re so smart! It’s modus tollens or “the method of denying”. It’s a type of argument where you deny the implications of something and thereby deny that very thing. It’s a deductively valid argument form (remember from our unit on natural deduction?), so we can falsify hypotheses with deductive certainty: if your hypothesis implies something with necessity, and that something doesn’t come to pass, then your hypothesis is wrong.
Your hypothesis is wrong. That is, your hypothesis as it stands was wrong. You might be like one of those rogue and dogged detectives in the television shows that never gives up on a hunch and ultimately discovers the truth through sheer stubbornness and determination. You might think that the spouse did it, even though they’ve got an airtight alibi. In that case, you’ll have to alter your hypothesis a bit.
The process of altering a hypothesis to react to potentially falsifying evidence typically involves adding extra hypotheses onto your original hypothesis such that the original hypothesis no longer has the troubling implications which turned out not to be true. These extra hypotheses are called ad hoc hypotheses.
As an example, Newton’s theory of gravity had one problem: it made a sort of wacky prediction. So the idea was that gravity was an instantaneous attractive force exerted by all massive bodies on all other bodies. That is, all bodies attract all other bodies regardless of distance or time. The result of this should be that all massive bodies should smack into each other over time (after all, they still have to travel towards one another). But we don’t witness this. We should see things crashing towards the center of gravity of the universe at incredible speeds, but that’s not what’s happening. So, by the logic of falsification, Newton’s theory is simply false.
But Newton had a trick up his sleeve: he claimed that God arranged things such that the heavenly bodies are so far apart from one another that they are prevented from crashing into one another. Problem solved! God put things in the right spatial orientation such that the theory of gravity is saved: they won’t crash into each other because they’re so far apart! Newton employed an ad hoc hypothesis to save his theory from falsification.
Abductive Reasoning
There’s one more thing to discuss while we’re still on the topic of hypothetical reasoning or reasoning using hypotheses. ‘Abduction’ is a fancy word for a process or method sometimes called “inference to the best explanation. The basic idea is that we have a bunch of evidence, we try to explain it, and we find that we could explain it in multiple ways. Then we find the “best” explanation or hypothesis and infer that this is the true explanation.
For example, say we’re playing a game that’s sort of like the picnic game from before. I give you a series of numbers, and then you give me more series of numbers so that I can confirm or deny that each meets the rule I have in mind. So I say:
And then you offer the following series (serieses?):
60, 90, 120
Each of these series tests a particular hypothesis. The first tests whether the important thing is that the numbers start with 2, 3, and 4. The second tests whether the rule is to add 10 each successive number in the series. The third tests a more complicated hypothesis: add half of the first number to itself to get the second number, then add one third of the second number to itself to get the third number.
Now let’s say I tell you that only the third series is acceptable. What now?
Well, our hypothesis was pretty complex, but it seems pretty good. I can infer that this is the correct rule. Alternatively, I might look at other hypotheses which fit the evidence equally well: 1x, 1.5x, 2x? or maybe it’s 2x, 3x, 4x? What about x, 1.5x, x\(^2\)? These all make sense of the data, but are they equal apart from that?
Let’s suppose we can’t easily get more data with which to test our various hypotheses. We’ve got 4 to choose from and nothing in the evidence suggests that one of the hypotheses is better than the others—they all fit the evidence perfectly. What do we do?
One thing we could do is choose which hypothesis is best for reasons other than fit with the evidence. Maybe we want a simpler hypothesis, or maybe we want a more elegant hypothesis, or one which suggests more routes for investigation. These are what we might call “theoretical virtues”—they’re the things we want to see in a theory. The process of abduction is the process of selecting the hypothesis that has the most to offer in terms of theoretical virtues: the simplest, most elegant, most fruitful, most general, and so on.
In science in particular, we value a few theoretical virtues over others: support by the empirical evidence available, replicability of the results in a controlled setting by other scientists, ideally mathematical precision or at least a lack of vagueness, and parsimony or simplicity in terms of the sorts of things the hypothesis requires us to believe in.
Confirmation Bias
This is a great opportunity to discuss confirmation bias, or the natural tendency we have to seek out evidence which supports our beliefs and to ignore evidence which gets in the way of our beliefs. We’ll discuss cognitive biases more in Chapter 10, but since we’re dealing with the relationship between evidence and belief, this seems like a good spot to pause and reflect on how our minds work.
The way our minds work naturally, it seems, is to settle on a belief and then work hard to maintain that belief whatever happens. We come to believe that global warming is anthropogenic—is caused by human activities—and then we’re happy to accept a wide variety of evidence for the claim. If the evidence supports our belief, in other words, we don’t take the time or energy to really investigate exactly how convincing that evidence is. If we already believe the conclusion of an inference, in other words, we are much less likely to test or analyze the inference.
Alternatively, when we see pieces of evidence or arguments that appear to point to the contrary, we are either more skeptical of that evidence or more critical of that argument. For instance, if someone notes that the Earth goes through normal cycles of warming and ice ages and warming again, we immediately will look for ways to explain how this warming period is different than others in the past. Or we might look at the period of the cycles to find out if this is happening at the “right” time in our geological history for it not to be caused by humankind. In other words, we’re more skeptical of arguments or evidence that would defeat or undermine our beliefs, but we’re less skeptical and critical of arguments and evidence that supports our beliefs.
Here are some questions to reflect on as you try to decide how guilty you are of confirmation bias in your own reasoning:
Questions for Reflection:
1. Which news sources do you trust? Why?
2. What’s your process for exploring a topic—say a political or scientific or news topic?
3. How do you decide what to believe about a new subject?
4. When other people express an opinion about someone you don’t know, do you withhold judgment? How well do you do so?
5. Are you harder on arguments and evidence that would shake up your beliefs?
- Subscriber Services
- For Authors
- Publications
- Archaeology
- Art & Architecture
- Bilingual dictionaries
- Classical studies
- Encyclopedias
- English Dictionaries and Thesauri
- Language reference
- Linguistics
- Media studies
- Medicine and health
- Names studies
- Performing arts
- Science and technology
- Social sciences
- Society and culture
- Overview Pages
- Subject Reference
- English Dictionaries
- Bilingual Dictionaries
Recently viewed (0)
- Save Search
- Share This Facebook LinkedIn Twitter
Related Content
More like this.
Show all results sharing this subject:
ad hoc hypothesis
Quick reference.
Hypothesis adopted purely for the purpose of saving a theory from difficulty or refutation, but without any independent rationale.
From: ad hoc hypothesis in The Oxford Dictionary of Philosophy »
Subjects: Philosophy
Related content in Oxford Reference
Reference entries.
View all related items in Oxford Reference »
Search for: 'ad hoc hypothesis' in Oxford Reference »
- Oxford University Press
PRINTED FROM OXFORD REFERENCE (www.oxfordreference.com). (c) Copyright Oxford University Press, 2023. All Rights Reserved. Under the terms of the licence agreement, an individual user may print out a PDF of a single entry from a reference work in OR for personal use (for details see Privacy Policy and Legal Notice ).
date: 04 June 2024
- Cookie Policy
- Privacy Policy
- Legal Notice
- Accessibility
- [66.249.64.20|81.177.180.204]
- 81.177.180.204
Character limit 500 /500
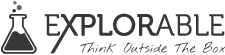
- Foundations
- Write Paper
Search form
- Experiments
- Anthropology
- Self-Esteem
- Social Anxiety
- Experiments >
Ad Hoc Analysis
An ad hoc analysis is an extra type of hypothesis added to the results of an experiment to try to explain away contrary evidence.
This article is a part of the guide:
- Significance 2
- Sample Size
- Experimental Probability
- Cronbach’s Alpha
- Systematic Error
Browse Full Outline
- 1 Inferential Statistics
- 2.1 Bayesian Probability
- 3.1.1 Significance 2
- 3.2 Significant Results
- 3.3 Sample Size
- 3.4 Margin of Error
- 3.5.1 Random Error
- 3.5.2 Systematic Error
- 3.5.3 Data Dredging
- 3.5.4 Ad Hoc Analysis
- 3.5.5 Regression Toward the Mean
- 4.1 P-Value
- 4.2 Effect Size
- 5.1 Philosophy of Statistics
- 6.1.1 Reliability 2
- 6.2 Cronbach’s Alpha
The scientific method dictates that, if a hypothesis is rejected, then that is final. The research needs to be redesigned or refined before the hypothesis can be tested again.
Amongst pseudo-scientists, an ad hoc hypothesis is often appended, in an attempt to justify why the expected results were not obtained.
An often quoted example of an ad hoc analysis is of a paranormal investigator investigating psychic waves, under scientific conditions. Upon finding that the experiment did not give positive results, they blame the negative brain waves given out by others.
The oft-quoted example of an ad hoc analysis is of a paranormal investigator investigating psychic waves, under scientific conditions. Upon finding that the experiment did not give positive results, they blame the negative brain waves given out by others.
This is simply trying to deflect criticism and failure by throwing out other, completely random reasons. This ad hoc analysis would need the brain waves of the onlookers to be also tested and eliminated, moving the goalpost and creating a fallacy.
The idea of biorhythms, where the body and mind are affected by deep and regular cycles unrelated to biological circadian rhythms, has long been viewed with skepticism. Every time that scientific research debunks the theory, the adherents move the goal posts, inventing some other underlying reason to explain the results.
Often, astrologers presented with contrary evidence will blame the results upon some ‘unknown’ astrological phenomenon. This, of course, is impossible to prove and so the ad hoc analysis conveniently removes the pseudo-science from the debate.
The insanely stupid Water4Gas scam works along the same principles – when researchers pointed out that the whole idea revolves around the principle of perpetual motion, they invented another ad hoc hypothesis to explain where the ‘money saving’ energy came from.
Ad hoc analysis is not always a bad thing, and can often be part of the process of refining research.
Imagine, for example, that a research group was conducting an experiment into water turbulence, but kept receiving strange results, disproving their hypothesis. Whilst attempting to eliminate any potential confounding variables, they discover that the air conditioning unit is faulty, transmitting vibrations through the lab. This is switched off when the experiment is running and they retest the hypothesis.
This is part of the normal scientific process, and is part of refining the research design rather than trying to move the goalposts.
Ad hoc analysis is only a problem when a non-testable ad hoc hypothesis is added to the results to justify failure and deflect criticisms.
The air conditioning unit hypothesis can be tested very easily, simply by switching it off, and was a result of experimental flaw. Negative brainwaves cannot be easily tested, and therefore the deflection causes a fallacy.
- Psychology 101
- Flags and Countries
- Capitals and Countries
Martyn Shuttleworth (Nov 17, 2008). Ad Hoc Analysis. Retrieved Jun 04, 2024 from Explorable.com: https://explorable.com/ad-hoc-analysis
You Are Allowed To Copy The Text
The text in this article is licensed under the Creative Commons-License Attribution 4.0 International (CC BY 4.0) .
This means you're free to copy, share and adapt any parts (or all) of the text in the article, as long as you give appropriate credit and provide a link/reference to this page.
That is it. You don't need our permission to copy the article; just include a link/reference back to this page. You can use it freely (with some kind of link), and we're also okay with people reprinting in publications like books, blogs, newsletters, course-material, papers, wikipedia and presentations (with clear attribution).
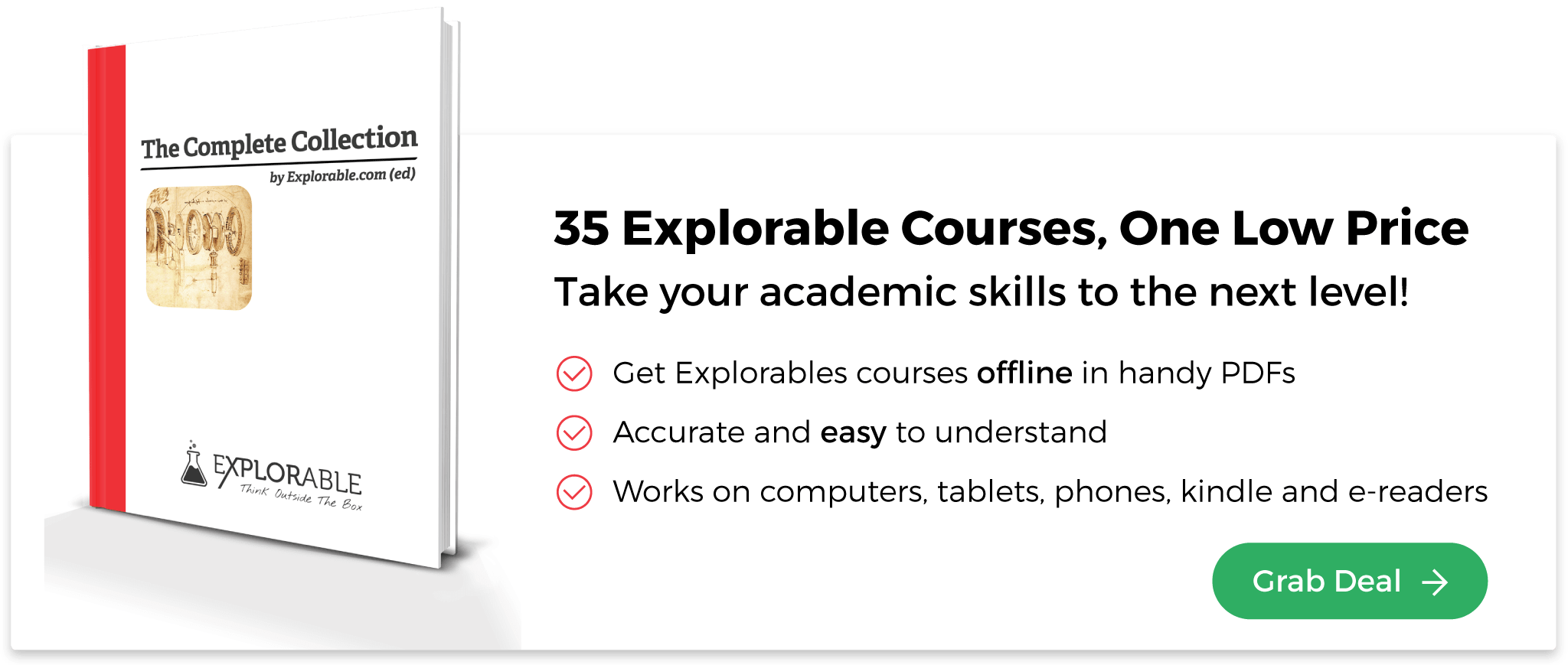
Related articles
Experimental Research
Philosophy of Science
Want to stay up to date? Follow us!
Save this course for later.
Don't have time for it all now? No problem, save it as a course and come back to it later.
Footer bottom
- Privacy Policy
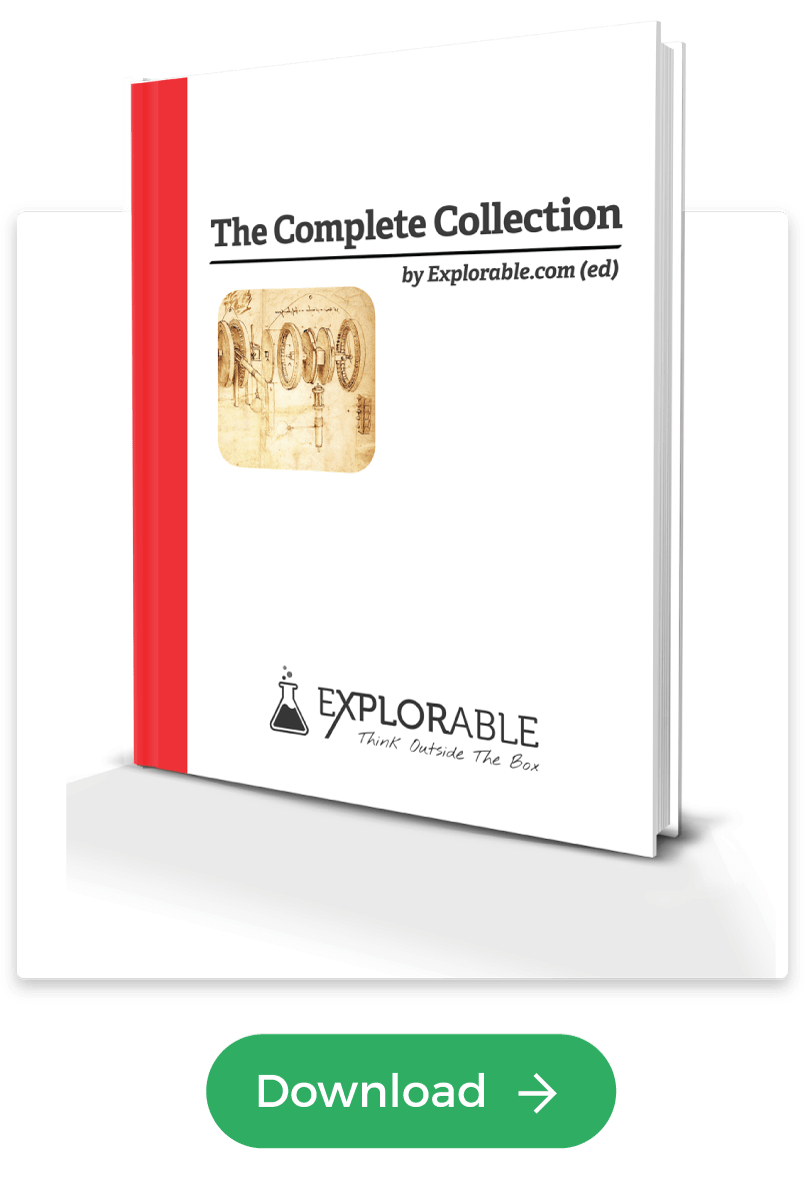
- Subscribe to our RSS Feed
- Like us on Facebook
- Follow us on Twitter
What is the Problem of Ad Hoc Hypotheses?
- Published: July 1999
- Volume 8 , pages 375–386, ( 1999 )
Cite this article
- Greg Bamford 1
623 Accesses
6 Citations
Explore all metrics
The received view of an ad hochypothesis is that it accounts for only the observation(s) it was designed to account for, and so non-ad hocness is generally held to be necessary or important for an introduced hypothesis or modification to a theory. Attempts by Popper and several others to convincingly explicate this view, however, prove to be unsuccessful or of doubtful value, and familiar and firmer criteria for evaluating the hypotheses or modified theories so classified are characteristically available. These points are obscured largely because the received view fails to adequately separate psychology from methodology or to recognise ambiguities in the use of 'ad hoc_'.
This is a preview of subscription content, log in via an institution to check access.
Access this article
Price includes VAT (Russian Federation)
Instant access to the full article PDF.
Rent this article via DeepDyve
Institutional subscriptions
Similar content being viewed by others
Ad Hoc Hypotheses and the Monsters Within
Bayesian inference for psychology. part i: theoretical advantages and practical ramifications.
The Replacement for Hypothesis Testing
Asimov, I.: 1975, Eyes on the Universe: A History of the Telescope , Houghton Mifflin, Boston.
Google Scholar
Bamford, G.S.: 1989, Popper, Refutation, and 'Avoidance' of Refutation , Ph.D. thesis, The University of Queensland, Brisbane.
Bamford, G.S.: 1993, 'Popper's Explications of Ad Hoc ness: Circularity, Empirical Content, and Scientific Practice', The British Journal for the Philosophy of Science 44 , 335–55.
Bamford, G.S.: 1996, 'Popper and his Commentators on the Discovery of Neptune: A Close Shave for the Law of Gravitation?', Studies in History and Philosophy of Science 27 (2), 207–32.
Chalmers, A.F.: 1982, What is This Thing Called Science: An Assessment of the Nature and Status of Science and its Methods? (second edition), University of Queensland Press, St. Lucia, Queensland.
Drake, S.: 1957, Introduction to Discoveries and Opinions of Galileo , by Galileo, trans. S. Drake, Anchor Books, New York.
Drake, S.: 1978, Galileo at Work: His Scientific Biography , University of Chicago Press, Chicago.
Fetzer, J.H. and Almeder, R.F.: 1993, s.v. 'Ad hoc/ad hocness/ad hoc hypotheses', Glossary of Epistemology/Philosophy of Science , Paragon House, New York.
Galileo, G.: 1929–39, Le Opere di Galileo Galilei , 20 vols. in 21, vol. 11, Barbera, Firenze.
Gillies, D.: 1990, 'Bayesianism Versus Falsificationism', Ratio (New Series) III , 82–98.
Grant, R.: 1966, History of Physical Astronomy , The Sources of Science, no. 38, Johnson Reprint Corporation, New York.
Grosser, M.: 1962, The Discovery of Neptune , Harvard University Press, Cambridge, Mass.
Grünbaum, A.: 1976, ' Ad Hoc Auxiliary Hypotheses and Falsificationism', The British Journal for the Philosophy of Science 27 , 329–62.
Hempel, C.G.: 1966, Philosophy of Natural Science , Foundations of Philosophy Series, Prentice Hall, Englewood Cliffs, N.J.
Holton, G.: 1969, 'Einstein, Michelson, and the Crucial Experiment', Isis 60 (Summer), 133–97.
Howson, C. and Urbach, P.: 1989, Scientific Reasoning: The Bayesian Approach , Open Court, La Salle, Illinois.
Klüber, H. von.: 1960, 'The Determination of Einstein's Light Deflection in the Gravitational Field of the Sun', in A. Beer (ed.), Vistas in Astronomy 3 , Pergamon Press, London, 47–77.
Lakatos, I.: 1970, 'Falsification and the Methodology of Scientific Research Programmes'. In I. Lakatos and A. Musgrave (eds.), Criticism and the Growth of Knowledge , Cambridge University Press, Cambridge, 91–195.
Laudan, L.: 1977, Progress and its Problems: Towards a Theory of Scientific Growth , University of California Press, Berkeley.
Leplin, J.: 1982, 'The Assessment of Auxiliary Hypotheses', The British Journal for the Philosophy of Science 33 , 235–49.
Mason, S.F.: 1956, Main Currents of Scientific Thought: A History of the Sciences , Routledge and Kegan Paul, London.
Maxwell, G.C.: 1974, 'Corroboration without Demarcation', in Schilpp (ed.), 292–321.
Miller, D.W.: 1981, s.v. ' Ad-Hoc Hypotheses', in W. Bynum, E. Browne and R. Porter (eds.), Dictionary of the History of Science , Princeton University Press, Princeton.
Musgrave, A.E.: 1973, 'Falsification and its Critics', in P. Suppes, L. Henkin, A. Joga, and Gr. Moisil (eds.), Logic, Methodology and Philosophy of Science IV: Proceedings of the Fourth International Congress for Logic, Methodology, and Philosophy of Science, Bucharest, 1971 , North Holland Publishing Co., Amsterdam, 393–406.
Musgrave, A.E.: 1974, 'Logical versus Historical Theories of Confirmation', The British Journal for the Philosophy of Science 25 , 1–23.
Musgrave, A.E.: 1976, 'Method or Madness? Can the Methodology of Scientific Research Programmes be Rescued from Epistemological Anarchism?', in R. Cohen, P. Feyerabend, and M. Wartofsky (eds.), Essays in Memory of Imre Lakatos , Boston Studies in the Philosophy of Science, vol. 39, D. Reidel, Dordrecht, 457–91.
Musgrave, A.E.: 1978, 'Evidential Support, Falsification, Heuristics, and Anarchism'. In G. Radnitzky and G. Andersson (eds.), Progress and Rationality in Science , Boston Studies in the Philosophy of Science, vol. 58, D. Reidel, Dordrecht, 181–201.
Newcomb, S.: 1929, 'Discordances in the Secular Variations of the Inner Planets', in H. Shapely and H. Howarth (eds.), A Source Book in Astronomy , Source Books in the History of Science, McGraw Hill, New York, 330–38.
Newcomb, S.: 1911, 'Neptune', Encyclopedia Britannica (eleventh edition), vol. XIX, Cambridge University Press, Cambridge, 385–87.
Popper, K.R.: 1972, Conjectures and Refutations: The Growth of Scientific Knowledge (fourth revised edition), Routledge and Kegan Paul, London.
Popper, K.R.: 1974, 'Replies to My Critics', in Schilpp (ed.), 961–1197.
Popper, K.R.: 1975, The Logic of Scientific Discovery (eighth impression), Hutchinson and Co., London.
Popper, K.R.: 1976, Unended Quest: An Intellectual Autobiography , Fontana/Collins, London.
Quine, W.V. and Ullian, J.S.: 1980, 'Hypothesis', in E. Klemke, R. Hollinger and A. Kline (eds.), Introductory Readings in the Philosophy of Science , Prometheus Books, New York, 196–206.
Radnitzky, G.: 1981, 'Progress and Rationality in Research', in M. Grmek, R. Cohen and G. Cimino (eds.), On Scientific Discovery: The Erice Lectures 1977 , D. Reidel, Dordrecht, 43–102.
Salmon, W.C.: 1990, 'Rationality and Objectivity in Science, or Tom Kuhn Meets Tom Bayes', in C. Savage (ed.), Scientific Theories , Minnesota Studies in the Philosophy of Science, vol. 14, University of Minneapolis Press, Minneapolis, 175–204.
Schilpp, P.A. (ed.), 1974, The Philosophy of Karl Popper , The Library of Living Philosophers, vol. 14, Open Court, La Salle, Illinois.
Schlesinger, G.: 1987, 'Accommodation and Prediction', Australasian Journal of Philosophy 65 , 33–42.
Sprott, W.F.: 1936, 'Review of A Dynamical Theory of Personality ', by K. Lewin, Mind 45 , 246–51.
Sutton, C.: 1992, Spaceship Neutrino , Cambridge University Press, Cambridge.
The Shorter Oxford English Dictionary: 1973, s.v. 'ad hoc', (third revised edition).
Download references
Author information
Authors and affiliations.
Department of Architecture, The University of Queensland, Brisbane, Queensland, Australia, 4072
Greg Bamford
You can also search for this author in PubMed Google Scholar
Rights and permissions
Reprints and permissions
About this article
Bamford, G. What is the Problem of Ad Hoc Hypotheses?. Science & Education 8 , 375–386 (1999). https://doi.org/10.1023/A:1008633808051
Download citation
Issue Date : July 1999
DOI : https://doi.org/10.1023/A:1008633808051
Share this article
Anyone you share the following link with will be able to read this content:
Sorry, a shareable link is not currently available for this article.
Provided by the Springer Nature SharedIt content-sharing initiative
- Receive View
- Firm Criterion
- Find a journal
- Publish with us
- Track your research
Ad Hoc Explanations, Causes, and Rationalization
Faulty Causation Fallacy
- Belief Systems
- Key Figures in Atheism
- M.A., Princeton University
- B.A., University of Pennsylvania
Fallacy Name: Ad Hoc
Alternative Names: Questionable Cause Questionable Explanation
Category: Faulty Causation
Explanation of the Ad Hoc Fallacy
Strictly speaking, an ad hoc fallacy probably shouldn't really be considered a fallacy because it occurs when a faulty explanation is given for some event rather than as faulty reasoning in an argument. However, such explanations are usually designed to look like arguments, and as such, they need to be addressed - especially here, since they purport to identify causes of events.
The Latin ad hoc means "for this [special purpose]." Almost any explanation could be considered "ad hoc" if we define the concept broadly enough because every hypothesis is designed to account for some observed event. However, the term is normally used more narrowly to refer to some explanation which exists for no other reason but to save a favored hypothesis. It is thus not an explanation which is supposed to help us better understand a general class of events.
Typically, you will see statements referred to as "ad hoc rationalizations" or "ad hoc explanations" when someone's attempt to explain an event is effectively disputed or undermined and so the speaker reaches for some way to salvage what he can. The result is an "explanation" which is not very coherent, does not really "explain" anything at all, and which has no testable consequences - even though to someone already inclined to believe it, it certainly looks valid.
Examples and Discussion
Here is a commonly cited example of an ad hoc explanation or rationalization:
I was healed from cancer by God! Really? Does that mean that God will heal all others with cancer? Well... God works in mysterious ways.
A key characteristic of ad hoc rationalizations is that the "explanation" offered is only expected to apply to the one instance in question. For whatever reason, it is not applied any other time or place where similar circumstances exist and is not offered as a general principle which might be applied more broadly. Note in the above that God's " miraculous powers of healing " are not applied to everyone who has cancer, never mind to everyone who is suffering from a serious or deadly illness, but only this one at this time, for this one person, and for reasons which are completely unknown.
Another key characteristic of an ad hoc rationalization is that it contradicts some other basic assumption - and often an assumption which is was either explicit or implicit in the original explanation itself. In other words, it's an assumption which the person originally accepted - implicitly or explicitly - but which they are now attempting to abandon. That is why, usually, an ad hoc statement is only applied in one instance and then quickly forgotten. Because of this, ad hoc explanations are often cited as an example of the fallacy of Special Pleading. In the above conversation, for example, the idea that not everyone will be healed by God contradicts the common belief that God loves everyone equally.
A third characteristic is the fact that the "explanation" has no testable consequences. What could possibly be done to test to see if God is working in "mysterious ways" or not? How could we tell when it is happening and when it is not? How could we differentiate between a system where God has acted in a "mysterious way" and one where the results are due to chance or some other cause? Or, to put it more simply, what could we possibly do in order to determine if this alleged explanation really does explain anything at all?
The fact of the matter is, we can't - the "explanation" offered above provides us with nothing to test, something which is a direct consequence of having failed to provide a better understanding of the circumstances at hand. That, of course, is what an explanation is supposed to do, and why an ad hoc explanation is a defective explanation.
Thus, most ad hoc rationalizations do not really "explain" anything at all. The claim that "God works in mysterious ways" does not tell us how or why this person was healed, much less how or why others will not be healed. A genuine explanation makes events more understandable, but if anything the above rationalization makes the situation less understandable and less coherent.
- According to Science, God Does Not Exist
- Gods' Contradictory Characteristics: Making God Impossible to Exist
- Argument From Miracles: Do Miracles Prove God Exists?
- Atheism vs. Freethought
- Is Astrology a Pseudoscience?
- Is There Proof of Creationism?
- Mercy vs. Justice: a Clash of Virtues
- The Coherence Theory of Truth
- What Is Religion?
- The Criteria for Science and Scientific Theories
- What Is Existentialism?
- Axiological Arguments from Morals and Values
- God is Eternal
- What Is Open Mindedness in Critical Thinking?
- Top Conversation Killers for Atheists
- Why Does Religion Exist?
- Daily Crossword
- Word Puzzle
- Word Finder
- Word of the Day
- Synonym of the Day
- Word of the Year
- Language stories
- All featured
- Gender and sexuality
- All pop culture
- Writing hub
- Grammar essentials
- Commonly confused
- All writing tips
- Pop culture
- Writing tips
Advertisement
[ ad hok ; Latin ahd hohk ]
a committee formed ad hoc to deal with the issue.
The ad hoc committee disbanded after making its final report.
/ æd ˈhɒk /
an ad hoc committee
an ad hoc decision
- A phrase describing something created especially for a particular occasion: “We need an ad hoc committee to handle this new problem immediately.” From Latin , meaning “toward this (matter).”
Discover More
Word history and origins.
Origin of ad hoc 1
Idioms and Phrases
Example sentences.
A number of ad hoc initiatives currently do this work, but it’s a patchwork and insufficient system.
It adds process, and checks and balances, to what is currently an ad hoc authority.
Williams’ case is a signal to stop the ad hoc adoption of facial recognition before an injustice occurs that cannot be undone.
When a report of abuse comes in, an ad hoc team of up to 10 NSO employees is assembled to investigate.
Technology has offered a ready solution for some types of ad hoc conversations during the pandemic.
Congress keeps funding it ad hoc—but when the GOP takes over the Senate next year, who knows.
An ad hoc network, Bibles, Badges & Business, represents the diversity of the pro-reform lobby.
The ad hoc granular alliances described in Unstoppable promise less but may achieve more.
During the dozen years or so since the R2P concept was formulated, its application has been complicated and ad hoc.
The stones had been pulled up to create ad-hoc fortifications around the Maidan.
Francisco Manrique de Lara, Episcopo, ex vetere ad hoc templum facta translatio xxv.
An ad hoc bipartisan conference called a session of the Senate and the Senate elected a new president.
The witnesses against him were two forgers, released ad hoc from prison, his own witnesses were hundreds.
No elaboration of statute law can forestall variant cases and the need of interpretation ad hoc.
To establish an international court ad hoc, in the middle of the war, and ask it to settle the new questions as they arise?
Related Words
- provisional
More About Ad Hoc
What does ad hoc mean.
Something ad hoc is put together on the fly for one narrow, pressing, or special purpose. For example, a government committee arranged to address one specific problem would be an ad hoc committee . More loosely, it can mean “spontaneous,” “unplanned,” or “on the spot.”
Ad hoc is one of those Latin phrases commonly found in academic, law, and government contexts. It literally means “for this (thing).”
Where does ad hoc come from?
English borrowed the Latin phrase ad hoc in the mid 1500s, when the expression was quickly being adopted into legal and judicial writings.
Ad hoc spreads as a term in such contexts in the 1800s. A Louisiana Code of Practice for civil law from 1839, for example, lists the various situations where a person, such as a minor, may be assigned what is called a curator ad hoc , a “caretaker for this purpose.” An 1869 judicial report from the state of New York, as another instance, describes forming ad hoc committees by the courts to investigate specific matters.
Around the same time, ad hoc was spreading to other areas. The phrase ad hoc hypothesis began to appear in scientific writing. An ad hoc hypothesis is basically a scientific excuse, a logical fallacy . It’s when someone makes up a new complication to brush off evidence against their claim—like if you said there’s a little green alien following you around, and when everyone asked where it was, you said that only you could see it.
Of course, not all ad hoc hypotheses are out of this world. An 1894 article on color perception points out how two of the common theories of the time relied on an extra, unproven ad hoc hypothesis about the vibration of light waves. Today, there’s even a festival dedicated to ad hoc hypotheses , where scientists can blow off steam by making stuff up.
In 1970, Alvin Toffler, the author of Future Shock , proposed that ad hoc organizations had some real benefits. Riffing on political terms like democracy , Toffler popularized the word adhocracy (from a slightly earlier coinage in 1966) to describe a kind of flexible organizational structure that could replace bureaucracy.
Six years later, adhocracy was discussed in a business book aimed at administrators. An entire book on the subject followed in 1990, and the topic became popular again in 2015 as an organizational model for structuring businesses.
In computing, an ad hoc network is a network of computers temporarily connected directly to other computers without a router or hub. Ad hoc networks were discussed in a communications journal in 1994, and there is currently an entire journal dedicated to the topic.
How is ad hoc used in real life?
You’re often going to see ad hoc describing government committees and judges, which are formed for very special purposes. Most often you’ll see it preceding what it modifies, e.g., an ad hoc judge , but especially in legal settings, following it: judges ad hoc .
Nakuru County Governor Lee Kinyanjui appearing before Senate Ad-hoc Committee investigating the #SolaiDam tragedy engage our senior Parliament reporter @edkabasa for more updates ^MK pic.twitter.com/rM1WylPlwx — KBC Channel1 News (@KBCChannel1) July 18, 2018
You’ll also see ad hoc in everyday settings, like an ad hoc train stop (unscheduled), an ad hoc job (working as needed), or an ad hoc movie set (improvised).
Any Manchester based freelance web designers out there? Get in touch with @bamboo_mcr if you're looking for some ad hoc project work 💻 — Freelance Folk (@FreelanceFolk) July 17, 2018
Ad hoc can be used to criticize an organization or event for being a little too loose or improvisational, though. The criticism is that it’s unstructured and wasn’t thought out.
More examples of ad hoc :
“The Registrar of Delhi University said on Monday that no assurance had been given or could be given by the Vice-Chancellor regarding the continuation of ad hoc teachers in the new session.”
—The Hindu , June 2018
“Mammals sleep because they hate themselves. Human intelligence evolved thanks to alcohol. Fish are stupid because they’d be too sad if they knew how boring their lives were. These are a few of the asinine arguments from BAHfest, the festival of bad ad hoc hypotheses—or as the organizers put it “a celebration of well-argued and thoroughly researched but completely incorrect scientific theories.”
—David Shultz, Science , October 2017
Definitions and idiom definitions from Dictionary.com Unabridged, based on the Random House Unabridged Dictionary, © Random House, Inc. 2023
Idioms from The American Heritage® Idioms Dictionary copyright © 2002, 2001, 1995 by Houghton Mifflin Harcourt Publishing Company. Published by Houghton Mifflin Harcourt Publishing Company.
- More from M-W
- To save this word, you'll need to log in. Log In

Definition of ad hoc
(Entry 1 of 2)
Definition of ad hoc (Entry 2 of 2)
Did you know?
In Latin ad hoc literally means "for this," and in English it describes anything that can be thought of as existing "for this purpose only." For example, an ad hoc committee is generally authorized to look into a single matter of limited scope, not to pursue any issue of interest. Ad hoc can also be used as an adverb meaning "for the particular end or case at hand without consideration of wider application," as in "decisions were made ad hoc."
- down and dirty
- extemporaneous
- extemporary
- improvisational
- off-the-cuff
- spur-of-the-moment
- unconsidered
- unpremeditated
- unrehearsed
Examples of ad hoc in a Sentence
These examples are programmatically compiled from various online sources to illustrate current usage of the word 'ad hoc.' Any opinions expressed in the examples do not represent those of Merriam-Webster or its editors. Send us feedback about these examples.
Word History
borrowed from Latin, "for this"
derivative of ad hoc entry 1
1639, in the meaning defined above
1879, in the meaning defined at sense 1a
Articles Related to ad hoc
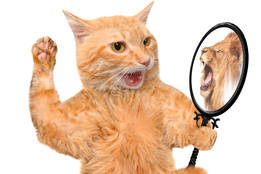
8 Latin Phrases That Mean Something...
8 Latin Phrases That Mean Something Different in English
Same Latin, different meaning
Get Word of the Day delivered to your inbox!
Dictionary Entries Near ad hoc
Cite this entry.
“Ad hoc.” Merriam-Webster.com Dictionary , Merriam-Webster, https://www.merriam-webster.com/dictionary/ad%20hoc. Accessed 4 Jun. 2024.
Legal Definition
Legal definition of ad hoc.
Legal Definition of ad hoc (Entry 2 of 2)
Latin, for this
More from Merriam-Webster on ad hoc
Nglish: Translation of ad hoc for Spanish Speakers
Britannica English: Translation of ad hoc for Arabic Speakers
Subscribe to America's largest dictionary and get thousands more definitions and advanced search—ad free!
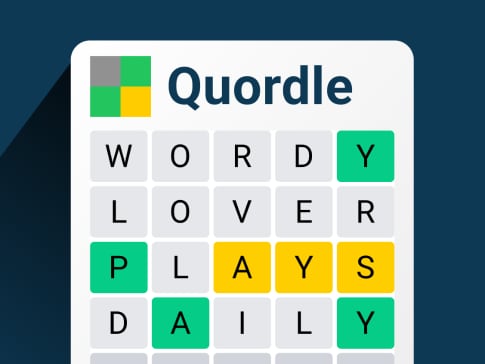
Can you solve 4 words at once?
Word of the day.
See Definitions and Examples »
Get Word of the Day daily email!
Popular in Grammar & Usage
More commonly misspelled words, commonly misspelled words, how to use em dashes (—), en dashes (–) , and hyphens (-), absent letters that are heard anyway, how to use accents and diacritical marks, popular in wordplay, the words of the week - may 31, pilfer: how to play and win, 9 superb owl words, 10 words for lesser-known games and sports, etymologies for every day of the week, games & quizzes.
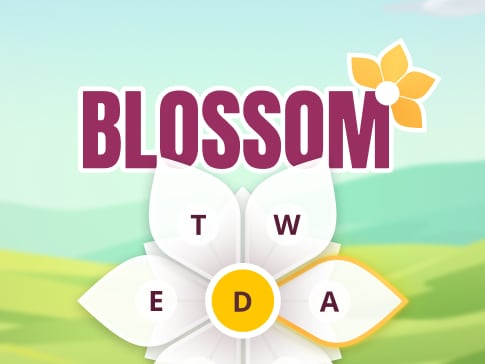
What is Ad Hoc Analysis and Reporting? Process, Examples
Appinio Research · 26.03.2024 · 33min read
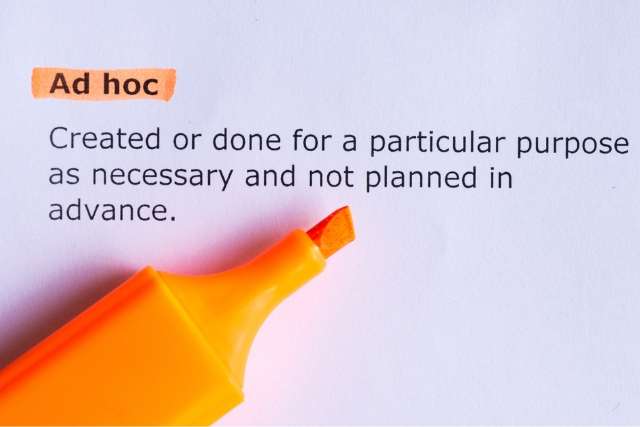
Have you ever needed to find quick answers to pressing questions or solve unexpected problems in your business? Enter ad hoc analysis, a powerful approach that allows you to dive into your data on demand, uncover insights, and make informed decisions in real time. In today's fast-paced world, where change is constant and uncertainties abound, having the ability to explore data flexibly and adaptively is invaluable. Whether you're trying to understand customer behavior , optimize operations, or mitigate risks, ad hoc analysis empowers you to extract actionable insights from your data swiftly and effectively. It's like having a flashlight in the dark, illuminating hidden patterns and revealing opportunities that may have otherwise gone unnoticed.
What is Ad Hoc Analysis?
Ad hoc analysis is a dynamic process that involves exploring data to answer specific questions or address immediate needs. Unlike routine reporting, which follows predefined formats and schedules, ad hoc analysis is driven by the need for timely insights and actionable intelligence. Its purpose is to uncover hidden patterns, trends, and relationships within data that may not be readily apparent, enabling organizations to make informed decisions and respond quickly to changing circumstances.
Ad hoc analysis involves the flexible and on-demand exploration of data to gain insights or solve specific problems. It allows analysts to dig deeper into datasets, ask ad hoc questions, and derive meaningful insights that may not have been anticipated beforehand. The term "ad hoc" is derived from Latin and means "for this purpose," emphasizing the improvised and opportunistic nature of this type of analysis.
Purpose of Ad Hoc Analysis
The primary purpose of ad hoc analysis is to support decision-making by providing timely and relevant insights into complex datasets. It allows organizations to:
- Identify emerging trends or patterns that may impact business operations.
- Investigate anomalies or outliers to understand their underlying causes .
- Explore relationships between variables to uncover opportunities or risks.
- Generate hypotheses and test assumptions in real time.
- Inform strategic planning, resource allocation, and risk management efforts.
By enabling analysts to explore data in an iterative and exploratory manner, ad hoc analysis empowers organizations to adapt to changing environments, seize opportunities, and mitigate risks effectively.
Importance of Ad Hoc Analysis in Decision Making
Ad hoc analysis plays a crucial role in decision-making across various industries and functions. Here are some key reasons why ad hoc analysis is important:
- Flexibility : Ad hoc analysis offers flexibility and agility, allowing organizations to respond quickly to evolving business needs and market dynamics. It enables decision-makers to explore new ideas, test hypotheses, and adapt strategies in real time.
- Customization : Unlike standardized reports or dashboards, ad hoc analysis allows for customization and personalization. Analysts can tailor their analyses to specific questions or problems, ensuring that insights are directly relevant to decision-makers needs.
- Insight Generation : Ad hoc analysis uncovers insights that may not be captured by routine reporting or predefined metrics. Analysts can uncover hidden patterns, trends, and correlations that drive innovation and competitive advantage by delving into data with a curious and open-minded approach.
- Risk Management : In today's fast-paced and uncertain business environment, proactive risk management is essential. Ad hoc analysis enables organizations to identify and mitigate risks by analyzing historical data, monitoring key indicators, and anticipating potential threats.
- Opportunity Identification : Ad hoc analysis helps organizations identify new opportunities for growth, innovation, and optimization. Analysts can uncover untapped markets, customer segments, or product offerings that drive revenue and profitability by exploring data from different angles and perspectives.
- Continuous Improvement : Ad hoc analysis fosters a culture of constant improvement and learning within organizations. By encouraging experimentation and exploration, organizations can drive innovation, refine processes, and stay ahead of the competition.
Ad hoc analysis is not just a tool for data analysis—it's a mindset and approach that empowers organizations to harness the full potential of their data, make better decisions, and achieve their strategic objectives.
Understanding Ad Hoc Analysis
Ad hoc analysis is a dynamic process that involves digging into your data to answer specific questions or solve immediate problems. Let's delve deeper into what it entails.
Ad Hoc Analysis Characteristics
At its core, ad hoc analysis refers to the flexible and on-demand examination of data to gain insights or address specific queries. Unlike routine reporting, which follows predetermined schedules, ad hoc analysis is triggered by the need to explore a particular issue or opportunity.
Its characteristics include:
- Flexibility : Ad hoc analysis adapts to the ever-changing needs of businesses, allowing analysts to explore data as new questions arise.
- Timeliness : It offers timely insights, enabling organizations to make informed decisions quickly in response to emerging issues or opportunities.
- Unstructured Nature : Ad hoc analysis often deals with unstructured or semi-structured data, requiring creativity and resourcefulness in data exploration.
Ad Hoc Analysis vs. Regular Reporting
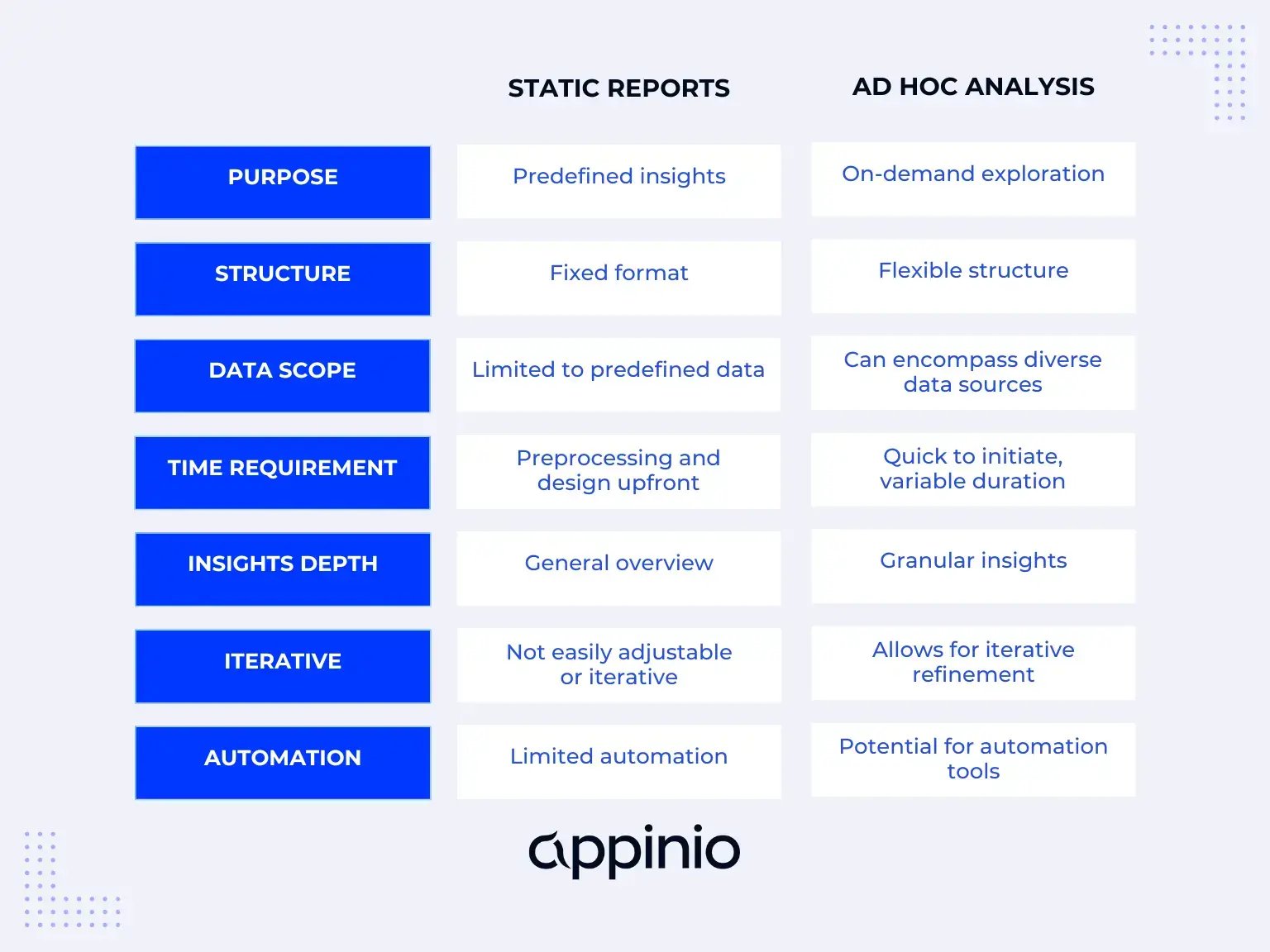
- Purpose : Regular reporting aims to track key performance indicators (KPIs) over time, while ad hoc analysis seeks to uncover new insights or address specific questions.
- Frequency : Regular reporting occurs at regular intervals (e.g., daily, weekly, monthly), whereas ad hoc analysis occurs on an as-needed basis.
- Scope : Regular reporting focuses on predefined metrics and reports, whereas ad hoc analysis explores a wide range of data sources and questions.
Types of Ad Hoc Analysis
Ad hoc analysis encompasses various types, each serving distinct purposes in data exploration and decision-making. These types include:
- Exploratory Analysis : This type involves exploring data to identify patterns, trends, or relationships without predefined hypotheses. It's often used in the initial stages of data exploration.
- Diagnostic Analysis : Diagnostic analysis aims to uncover the root causes of observed phenomena or issues. It delves deeper into data to understand why specific outcomes occur.
- Predictive Analysis : Predictive analysis leverages historical data to forecast future trends, behaviors, or events. It employs statistical modeling and machine learning algorithms to make predictions based on past patterns.
Common Data Sources
Ad hoc analysis can draw upon a wide array of data sources, depending on the nature of the questions being addressed and the data availability. Common data sources include:
- Structured Data : This includes data stored in relational databases, spreadsheets, and data warehouses, typically organized in rows and columns.
- Unstructured Data : Unstructured data sources, such as text documents, social media feeds, and multimedia content, require specialized techniques for analysis.
- External Data : Organizations may also tap into external data sources, such as market research reports, government databases, or third-party APIs, to enrich their analyses.
Organizations can gain comprehensive insights and make more informed decisions by leveraging diverse data sources. Understanding these foundational aspects of ad hoc analysis is crucial for conducting effective data exploration and driving actionable insights.
How to Prepare for Ad Hoc Analysis?
Before diving into ad hoc analysis, it's crucial to lay a solid foundation by preparing adequately. This involves defining your objectives, gathering and organizing data, selecting the right tools, and ensuring data quality. Let's explore these steps in detail.
Defining Objectives and Questions
The first step in preparing for ad hoc analysis is to clearly define your objectives and formulate the questions you seek to answer.
- Identify Key Objectives : Determine the overarching goals of your analysis. What are you trying to achieve? Are you looking to optimize processes, identify growth opportunities, or solve a specific problem?
- Formulate Relevant Questions : Break down your objectives into specific, actionable questions. What information do you need to answer these questions? What insights are you hoping to uncover?
By defining clear objectives and questions, you can focus your analysis efforts and ensure that you gather the necessary data to address your specific needs.
Data Collection and Organization
Once you have defined your objectives and questions, the next step is to gather relevant data and organize it in a format conducive to analysis.
- Identify Data Sources : Determine where your data resides. This may include internal databases, third-party sources, or even manual sources such as surveys or interviews.
- Extract and Collect Data : Extract data from the identified sources and collect it in a central location. This may involve using data extraction tools, APIs, or manual data entry.
- Clean and Preprocess Data : Before conducting analysis, it's essential to clean and preprocess the data to ensure its quality and consistency. This may involve removing duplicates, handling missing values, and standardizing formats.
Organizing your data in a systematic manner will streamline the analysis process and ensure that you can easily access and manipulate the data as needed. For a streamlined data collection process that complements your ad hoc analysis needs, consider leveraging Appinio .
With its intuitive platform and robust capabilities, Appinio simplifies data collection from diverse sources, allowing you to gather real-time consumer insights effortlessly. By incorporating Appinio into your data collection strategy, you can expedite the process and focus on deriving actionable insights to drive your business forward.
Ready to experience the power of rapid data collection? Book a demo today and see how Appinio can revolutionize your ad hoc analysis workflow.
Book a Demo
Tools and Software
Choosing the right tools and software is critical for conducting ad hoc analysis efficiently and effectively.
- Analytical Capabilities : Choose tools that offer a wide range of analytical capabilities, including data visualization, statistical analysis , and predictive modeling .
- Ease of Use : Look for user-friendly and intuitive tools, especially if you're not a seasoned data analyst. This will reduce the learning curve and enable you to get up and running quickly.
- Compatibility : Ensure the tools you choose are compatible with your existing systems and data sources. This will facilitate seamless integration and data exchange.
- Scalability : Consider the tools' scalability, especially if your analysis needs are likely to grow over time. Choose tools that can accommodate larger datasets and more complex analyses.
Popular tools for ad hoc analysis include Microsoft Excel and Python with libraries like Pandas and NumPy, R, and business intelligence platforms like Tableau and Power BI.
Data Quality Assurance
Ensuring the quality of your data is paramount for obtaining reliable insights and making informed decisions. To assess and maintain data quality:
- Data Validation : Perform data validation checks to ensure the data is accurate, complete, and consistent. This may involve verifying data against predefined rules or business logic.
- Data Cleansing : Cleanse the data by removing duplicates, correcting errors, and standardizing formats. This will help eliminate discrepancies and ensure uniformity across the dataset.
- Data Governance : Implement data governance policies and procedures to maintain data integrity and security. This may include access controls, data encryption, and regular audits.
- Continuous Monitoring : Continuously monitor data quality metrics and address any issues that arise promptly. This will help prevent data degradation over time and ensure your analyses are based on reliable information.
By prioritizing data quality assurance, you can enhance the accuracy and reliability of your ad hoc analyses, leading to more confident decision-making and better outcomes.
How to Perform Ad Hoc Analysis?
Now that you've prepared your data and defined your objectives, it's time to conduct ad hoc analysis. This involves selecting appropriate analytical techniques, exploring your data, applying advanced statistical methods, visualizing your findings, and validating hypotheses.
Choosing Analytical Techniques
Selecting the proper analytical techniques is crucial for extracting meaningful insights from your data.
- Nature of the Data : Assess the nature of your data, including its structure, size, and complexity. Different techniques may be more suitable for structured versus unstructured data or small versus large datasets.
- Objectives of Analysis : Align the choice of techniques with your analysis objectives. Are you trying to identify patterns, relationships, anomalies, or trends? Choose techniques that are well-suited to address your specific questions.
- Expertise and Resources : Consider your team's knowledge and the availability of resources, such as computational power and software tools. Choose techniques that your team is comfortable with and that can be executed efficiently.
Standard analytical techniques include descriptive statistics, inferential statistics, machine learning algorithms, and data mining techniques.
Exploratory Data Analysis (EDA)
Exploratory Data Analysis (EDA) is a critical step in ad hoc analysis that involves uncovering patterns, trends, and relationships within your data. Here's how to approach EDA:
- Summary Statistics : Calculate summary statistics such as mean, median, mode, variance, and standard deviation to understand the central tendencies and variability of your data.
- Data Visualization : Visualize your data using charts, graphs, and plots to identify patterns and outliers. Popular visualization techniques include histograms, scatter plots, box plots, and heat maps .
- Correlation Analysis : Explore correlations between variables to understand how they are related to each other. Use correlation matrices and scatter plots to visualize relationships.
- Dimensionality Reduction : If working with high-dimensional data, consider using dimensionality reduction techniques such as principal component analysis (PCA) or t-distributed stochastic neighbor embedding (t-SNE) to visualize and explore the data in lower dimensions.
Advanced Statistical Methods
For more in-depth analysis, consider applying advanced statistical methods to your data. These methods can help uncover hidden insights and relationships. Some advanced statistical methods include:
- Regression Analysis : Use regression analysis to model the relationship between dependent and independent variables. Linear regression, logistic regression, and multivariate regression are common techniques.
- Hypothesis Testing : Conduct hypothesis tests to assess the statistical significance of observed differences or relationships. Standard tests include t-tests, chi-square tests, ANOVA, and Mann-Whitney U tests.
- Time Series Analysis : If working with time series data, apply time-series analysis techniques to understand patterns and trends over time. This may involve methods such as autocorrelation, seasonal decomposition, and forecasting.
Data Visualization
Visualizing your findings is essential for communicating insights effectively to stakeholders.
- Choose the Right Visualizations : Select visualizations that best represent your data and convey your key messages. Consider factors such as the type of data, the relationships you want to highlight, and the audience's preferences .
- Use Clear Labels and Titles : Ensure that your visualizations are easy to interpret by using clear labels, titles, and legends. Avoid clutter and unnecessary decorations that may distract from the main message.
- Interactive Visualizations : If possible, create interactive visualizations allowing users to explore the data interactively. This can enhance engagement and enable users to gain deeper insights by drilling down into specific data points.
- Accessibility : Make your visualizations accessible to all users, including those with visual impairments. Use appropriate color schemes, font sizes, and contrast ratios to ensure readability.
Iterative Approach and Hypothesis Testing
Adopting an iterative approach to analysis allows you to refine your hypotheses and validate your findings through hypothesis testing.
- Formulate Hypotheses : Based on your initial explorations, formulate hypotheses about the relationships or patterns in the data that you want to test.
- Design Experiments : Design experiments or tests to evaluate your hypotheses. This may involve collecting additional data or conducting statistical tests.
- Evaluate Results : Analyze the results of your experiments and assess whether they support or refute your hypotheses. Consider factors such as statistical significance , effect size, and practical significance.
- Iterate as Needed : If the results are inconclusive or unexpected, iterate on your analysis by refining your hypotheses and conducting further investigations. This iterative process helps ensure that your conclusions are robust and reliable.
By following these steps and techniques, you can perform ad hoc analysis effectively, uncover valuable insights, and make informed decisions based on data-driven evidence.
Ad Hoc Analysis Examples
To better understand how ad hoc analysis can be applied in real-world scenarios, let's explore some examples across different industries and domains:
1. Marketing Campaign Optimization
Imagine you're a marketing analyst tasked with optimizing a company's digital advertising campaigns . Through ad hoc analysis, you can delve into various metrics such as click-through rates, conversion rates, and return on ad spend (ROAS) to identify trends and patterns. For instance, you may discover that certain demographic segments or ad creatives perform better than others. By iteratively testing and refining different campaign strategies based on these insights, you can improve overall campaign performance and maximize ROI.
2. Supply Chain Optimization
In the realm of supply chain management, ad hoc analysis can play a critical role in identifying inefficiencies and optimizing processes. For example, you might analyze historical sales data, inventory levels, and production schedules to identify bottlenecks or excess inventory. Through exploratory analysis, you may uncover seasonal demand patterns or supply chain disruptions that impact operations. Armed with these insights, supply chain managers can make data-driven decisions to streamline operations, reduce costs, and improve customer satisfaction.
3. Financial Risk Assessment
Financial institutions leverage ad hoc analysis to assess and mitigate various types of risks, such as credit risk, market risk, and operational risk. For example, a bank may analyze loan performance data to identify factors associated with loan defaults or delinquencies. By applying advanced statistical methods such as logistic regression or decision trees , analysts can develop predictive models to assess creditworthiness and optimize lending strategies. This enables banks to make informed decisions about loan approvals, pricing, and risk management.
4. Retail Merchandising Analysis
In the retail industry, ad hoc analysis is used to optimize merchandising strategies, pricing decisions, and inventory management. Retailers may analyze sales data, customer demographics , and market trends to identify product preferences and purchasing behaviors . Through segmentation analysis, retailers can tailor their merchandising efforts to specific customer segments and optimize product assortments. By monitoring key performance indicators (KPIs) such as sell-through rates and inventory turnover, retailers can make data-driven decisions to maximize sales and profitability.
How to Report Ad Hoc Analysis Findings?
After conducting ad hoc analysis, effectively communicating your findings is essential for driving informed decision-making within your organization. Let's explore how to structure your report, interpret and communicate results, tailor reports to different audiences, incorporate visual aids, and document methods and assumptions.
1. Structure the Report
Structuring your report in a clear and logical manner enhances readability and ensures that your findings are presented in a cohesive manner.
- Executive Summary : Provide a brief overview of your analysis, including the objectives, key findings, and recommendations. This section should concisely summarize the main points of your report.
- Introduction : Introduce the purpose and scope of the analysis, as well as any background information or context that is relevant to understanding the findings.
- Methodology : Describe the methods and techniques used in the analysis, including data collection , analytical approaches, and any assumptions made.
- Findings : Present the main findings of your analysis, organized in a logical sequence. Use headings, subheadings, and bullet points to enhance clarity and readability.
- Discussion : Interpret the findings in the context of the objectives and provide insights into their implications. Discuss any patterns, trends, or relationships observed in the data.
- Recommendations : Based on the analysis findings, provide actionable recommendations. Clearly outline the steps to address any issues or capitalize on opportunities identified.
- Conclusion : Summarize the main findings and recommendations, reiterating their importance and potential impact on the organization.
- References : Include a list of references or citations for any sources of information or data used in the analysis.
2. Interpret and Communicate Results
Interpreting and communicating the results of your analysis effectively is crucial for ensuring that stakeholders understand the implications and can make informed decisions.
- Use Plain Language : Avoid technical jargon and complex terminology that may confuse or alienate non-technical stakeholders. Use plain language to explain concepts and findings in a clear and accessible manner.
- Provide Context : Help stakeholders understand the significance of the findings by providing relevant context and background information. Explain why the analysis was conducted and how the findings relate to broader organizational goals or objectives.
- Highlight Key Insights : Focus on the most important insights and findings rather than overwhelming stakeholders with excessive detail. Use visual aids, summaries, and bullet points to highlight key takeaways.
- Address Implications : Discuss the implications of the findings and their potential impact on the organization. Consider both short-term and long-term implications and any risks or uncertainties.
- Encourage Dialogue : Foster open communication and encourage stakeholders to ask questions and seek clarification. Be prepared to engage in discussions and provide additional context or information as needed.
3. Tailor Reports to Different Audiences
Different stakeholders may have varying levels of expertise and interests, so it's essential to tailor your reports to meet their specific needs and preferences.
- Executive Summary for Decision Makers : Provide a concise executive summary highlighting key findings and recommendations for senior leaders and decision-makers who may not have time to review the full report.
- Detailed Analysis for Analysts : Include more thorough analysis, methodologies , and supporting data for analysts or technical stakeholders who require a deeper understanding of the analysis process and results.
- Customized Dashboards or Visualizations : Create customized dashboards or visualizations for different audiences, allowing them to interact with the data and explore insights relevant to their areas of interest.
- Personalized Presentations : Deliver personalized presentations or briefings to different stakeholder groups, focusing on the aspects of the analysis most relevant to their roles or responsibilities.
By tailoring your reports to different audiences, you can ensure that each stakeholder receives the information they need in a meaningful and actionable format.
4. Incorporate Visual Aids
Visual aids such as charts, graphs, and diagrams can enhance the clarity and impact of your reports by making complex information more accessible and engaging.
- Choose Appropriate Visualizations : Select visualizations that best represent the data and convey the key messages of your analysis. Choose from various chart types, including bar charts, line charts, pie charts, scatter plots, and heat maps.
- Simplify Complex Data : Use visualizations to simplify complex data and highlight trends, patterns, or relationships. Avoid clutter and unnecessary detail that may detract from the main message.
- Ensure Readability : Use clear labels, titles, and legends to ensure that visualizations are easy to read and interpret. Use appropriate colors, fonts, and formatting to enhance readability and accessibility.
- Use Interactive Features : If possible, incorporate interactive features into your visualizations that allow stakeholders to explore the data further. This can enhance engagement and enable stakeholders to gain deeper insights by drilling down into specific data points.
- Provide Context : Provide context and annotations to help stakeholders understand the significance of the visualizations and how they relate to the analysis objectives.
By incorporating visual aids effectively, you can make your reports more engaging and persuasive, helping stakeholders better understand and act on the findings of your analysis.
5. Document Methods and Assumptions
Documenting the methods and assumptions used in your analysis is essential for transparency and reproducibility. It allows stakeholders to understand how the findings were obtained and evaluate their reliability.
- Describe Data Sources and Collection Methods : Provide details about the sources of data used in the analysis and the methods used to collect and prepare the data for analysis.
- Explain Analytical Techniques : Describe the analytical techniques and methodologies used in the analysis, including any statistical methods, algorithms, or models employed.
- Document Assumptions and Limitations : Clearly state any assumptions made during the analysis, as well as any limitations or constraints that may impact the validity of the findings. Be transparent about the uncertainties and risks associated with the analysis.
- Provide Reproducible Code or Scripts : If applicable, provide reproducible code or scripts that allow others to replicate the analysis independently. This can include programming code, SQL queries, or data manipulation scripts.
- Include References and Citations : Provide references or citations for any external sources of information or data used in the analysis, ensuring that proper credit is given and allowing stakeholders to access additional information if needed.
By documenting methods and assumptions thoroughly, you can build trust and credibility with stakeholders and facilitate collaboration and knowledge sharing within your organization.
Ad Hoc Analysis Best Practices
Performing ad hoc analysis effectively requires a combination of skills, techniques, and strategies. Here are some best practices and tips to help you conduct ad hoc analysis more efficiently and derive valuable insights:
- Define Clear Objectives : Before analyzing the data, clearly define the objectives and questions you seek to answer. This will help you focus your efforts and ensure that you stay on track.
- Start with Exploratory Analysis : Begin your analysis with exploratory techniques to gain an initial understanding of the data and identify any patterns or trends. This will provide valuable insights that can guide further analysis.
- Iterate and Refine : Adopt an iterative approach to analysis, refining your hypotheses and techniques based on initial findings. Be open to adjusting your approach as new insights emerge.
- Leverage Diverse Data Sources : Tap into diverse data sources to enrich your analysis and gain comprehensive insights. Consider both internal and external sources of data that may provide valuable context or information.
- Maintain Data Quality : Prioritize data quality assurance throughout the analysis process, ensuring your findings are based on accurate, reliable data. Cleanse, validate, and verify the data to minimize errors and inconsistencies.
- Document Processes and Assumptions : Document the methods, assumptions, and decisions made during the analysis to ensure transparency and reproducibility. This will facilitate collaboration and knowledge sharing within your organization.
- Communicate Findings Effectively : Use clear, concise language to communicate your findings and recommendations to stakeholders. Tailor your reports and presentations to the needs and preferences of different audiences.
- Stay Curious and Open-Minded : Approach ad hoc analysis with curiosity and an open mind, remaining receptive to unexpected insights and discoveries. Embrace uncertainty and ambiguity as opportunities for learning and exploration.
- Seek Feedback and Collaboration : Solicit feedback from colleagues, mentors, and stakeholders throughout the analysis process. Collaboration and peer review can help validate findings and identify blind spots or biases.
- Continuously Learn and Improve : Invest in ongoing learning and professional development to expand your analytical skills and stay abreast of emerging trends and techniques in data analysis.
Ad Hoc Analysis Challenges
While ad hoc analysis offers numerous benefits, it also presents unique challenges that analysts must navigate. Here are some common challenges associated with ad hoc analysis:
- Data Quality Issues : Poor data quality, including missing values, errors, and inconsistencies, can hinder the accuracy and reliability of ad hoc analysis results. Addressing data quality issues requires careful data cleansing and validation.
- Time Constraints : Ad hoc analysis often needs to be performed quickly to respond to immediate business needs or opportunities. Time constraints can limit the depth and thoroughness of analysis, requiring analysts to prioritize key insights.
- Resource Limitations : Limited access to data, tools, or expertise can pose challenges for ad hoc analysis. Organizations may need to invest in training, infrastructure, or external resources to support effective analysis.
- Complexity of Unstructured Data : Dealing with unstructured or semi-structured data, such as text documents or social media feeds, can be challenging. Analysts must employ specialized techniques and tools to extract insights from these data types.
- Overcoming Analytical Bias : Analysts may inadvertently introduce biases into their analysis, leading to skewed or misleading results. It's essential to remain vigilant and transparent about potential biases and take steps to mitigate them.
By recognizing and addressing these challenges, analysts can enhance the effectiveness and credibility of their ad hoc analysis efforts, ultimately driving more informed decision-making within their organizations.
Conclusion for Ad Hioc Analysis
Ad hoc analysis is a versatile tool that empowers organizations to navigate the complexities of data and make informed decisions quickly. By enabling analysts to explore data on demand, ad hoc analysis provides a flexible and adaptive approach to problem-solving, allowing organizations to respond effectively to changing circumstances and capitalize on opportunities. From marketing campaign optimization to supply chain management, healthcare outcomes analysis, financial risk assessment, and retail merchandising analysis, the applications of ad hoc analysis are vast and varied. By embracing the principles of ad hoc analysis and incorporating best practices into their workflows, organizations can unlock the full potential of their data and drive business success. In today's data-driven world, the ability to extract actionable insights from data is more critical than ever. Ad hoc analysis offers a pathway to deeper understanding and better decision-making, enabling organizations to stay agile, competitive, and resilient in the face of uncertainty. By harnessing the power of ad hoc analysis, organizations can gain a competitive edge, optimize processes, mitigate risks, and uncover new opportunities for growth and innovation. As technology continues to evolve and data volumes grow exponentially, the importance of ad hoc analysis will only continue to increase. So, whether you're a seasoned data analyst or just beginning your journey into data analysis, embracing ad hoc analysis can lead to better outcomes and brighter futures for your organization.
How to Quickly Collect Data for Ad Hoc Analysis?
Introducing Appinio , your gateway to lightning-fast market research within the realm of ad hoc analysis. As a real-time market research platform, Appinio specializes in delivering immediate consumer insights, empowering companies to make swift, data-driven decisions.
With Appinio, conducting your own market research becomes a breeze:
- Lightning-fast Insights: From questions to insights in mere minutes, Appinio accelerates the pace of ad hoc analysis, ensuring you get the answers you need precisely when you need them.
- Intuitive Platform: No need for a PhD in research—Appinio's platform is designed to be user-friendly and accessible to all, allowing anyone to conduct sophisticated market research effortlessly.
- Global Reach: With access to over 90 countries and the ability to define precise target groups from 1200+ characteristics, Appinio enables you to gather insights from diverse demographics worldwide, all with an average field time of under 23 minutes for 1,000 respondents.
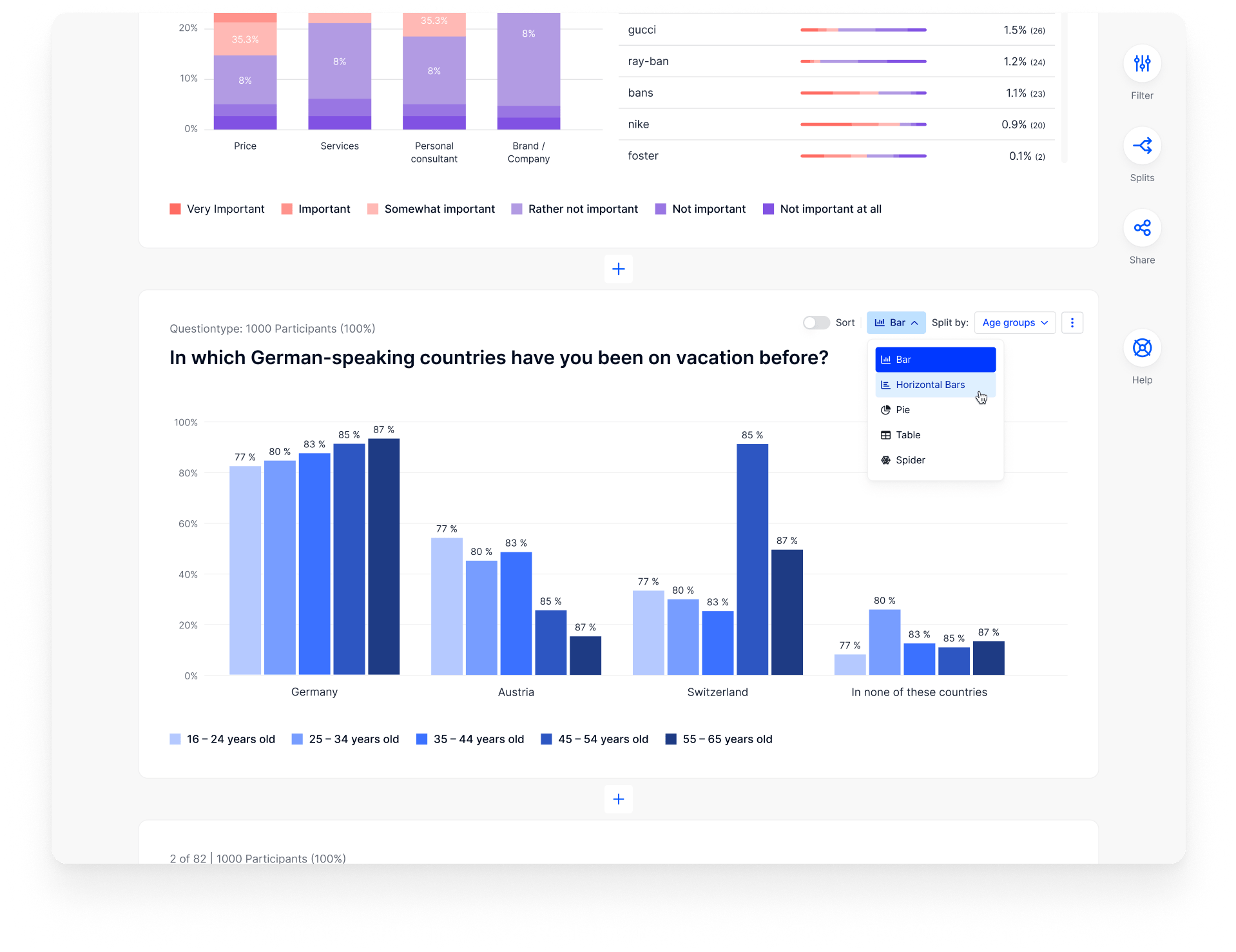
Get free access to the platform!
Join the loop 💌
Be the first to hear about new updates, product news, and data insights. We'll send it all straight to your inbox.
Get the latest market research news straight to your inbox! 💌
Wait, there's more
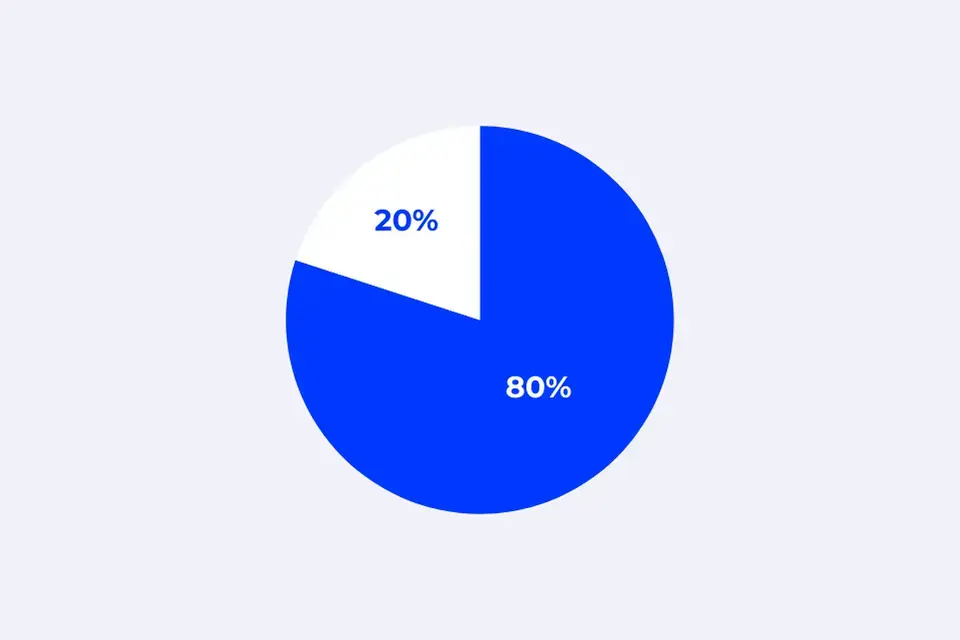
30.05.2024 | 29min read
Pareto Analysis: Definition, Pareto Chart, Examples
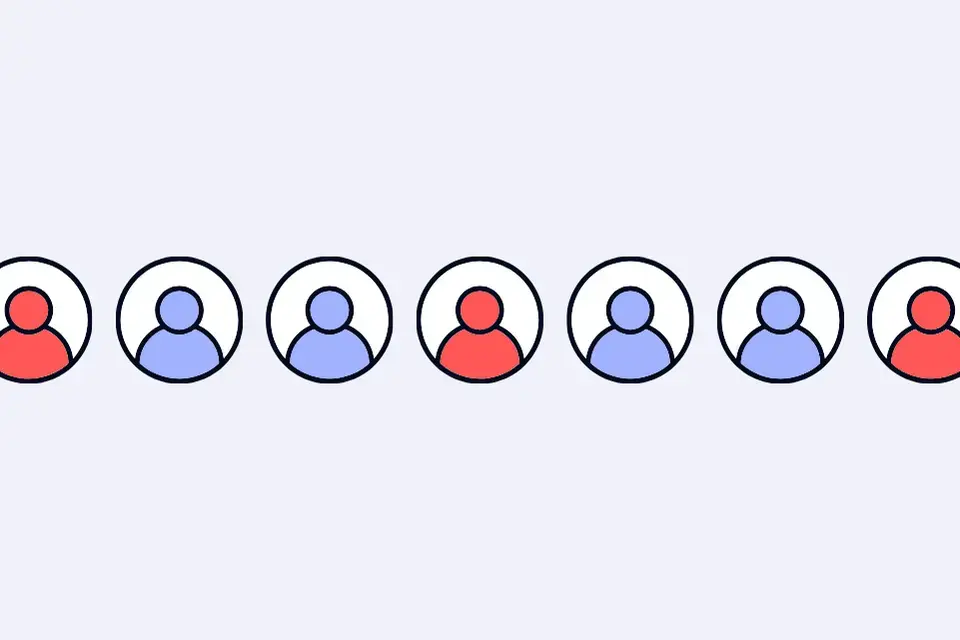
28.05.2024 | 32min read
What is Systematic Sampling? Definition, Types, Examples
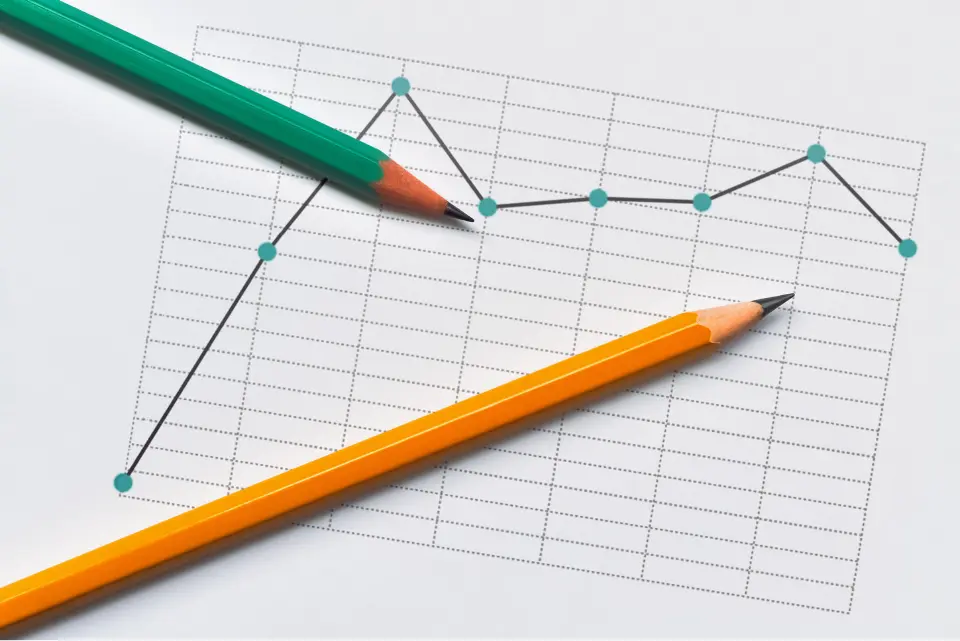
16.05.2024 | 30min read
Time Series Analysis: Definition, Types, Techniques, Examples

( Photo-illustration by Realtor.com; Source: Getty Images (3) )
Local Home Prices in Swing States Could Result in ‘Vote-Switching’ in 2024 Race
Home prices could play a subtle but important role in the 2024 presidential election, according to a recent first-of-its kind study.
The academic study, Housing Performance and the Electorate , analyzed home prices and election results at the county level for each of the six presidential elections from 2000 to 2020.
The authors found that local home price performance significantly affects voting in presidential elections at the county level. Counties with superior gains in home prices in the four years preceding an election were more likely to “vote-switch” to the incumbent party’s presidential candidate.
Conversely, counties with relatively inferior home price performance leading up to an election were more likely to flip their vote to support the candidate challenging the incumbent party.
In other words, quickly rising home prices tend to favor the incumbent president’s party, whether it be the Democrats or Republicans. The study found that the relationship is strongest in the years closest to an election, and that home prices were most influential in the small group of “swing counties” with a history of switching party preference.
University of Alabama Associate Professor of Finance Alan Tidwell , one of the study’s co-authors, explains that the logic driving this trend is simple: For most voters, their home represents their single largest asset.
“People feel more financially wealthy if they have a lot of housing equity, relative to lower housing equity,” he says. “How financially wealthy they feel really impacts their sense of financial and economic well-being.”
For the upcoming presidential election, the new finding suggests the outcome could be partly influenced by home prices in swing counties of the seven battleground states: Arizona, Georgia, Michigan, Nevada, North Carolina, Pennsylvania, and Wisconsin.
“In the swing counties, they care about this economic factor most, and real estate is one of the main drivers of household wealth,” says lead author Eren Cifci , an assistant professor of finance at Austin Peay State University in Tennessee. “So there may be many other factors that affect how people vote, but this definitely appears to be one of the factors influencing voters when they make their decisions.”
Explaining the 'homevoter hypothesis'
The study’s finding is an extension of the “homevoter hypothesis,” which holds that homeowners tend to vote in support of policies and candidates they believe will boost their home values.
While that phenomenon is well documented in local politics, where government policies have the clearest impact on home values, the new study is the first to show evidence of homevoter behavior in national elections.
The term “homevoter” was coined in 2001 by William A. Fischel , a now-retired economics professor at Dartmouth College and expert in local government and land use regulation.
Fischel conceived the homevoter hypothesis while serving on the local zoning board in Hannover, NH. Regularly, he would hear objections and concerns about zoning changes that seemed esoteric, and noticed that the complaints were always from homeowners.
Fischel says he came to realize that homeowners are essentially shareholders in their community, similar to owners of stock in a company—but that unlike corporate shareholders, they cannot easily diversify their portfolio or liquidate their holdings.
“It's people who are voting their homes, and that's actually an old concept in economics,” says Fischel. “But also, they're very risk-averse, because so much of their assets are stuck in one stock, in one place.”
Fischel says he was surprised by the recent study linking home prices and voting in national elections, since he had always viewed homevoting as primarily a local phenomenon.
“I can see, a little bit, what a presidential election might mean for home values. But it's so indirect, I was really quite surprised at the strength of the evidence,” he says. “How did they find such a strong mechanism? But I have no reason to doubt their evidence.”
For his part, Tidwell argues that the economy plays a major role in most presidential elections, and that rising home equity has a significant impact on how voters perceive the strength of the economy.
Even if local policies, such as zoning laws and public school funding, have a bigger direct impact on local home prices, national elections are where more voters take the opportunity to weigh in with their concern or satisfaction, he says.
“Local elections don't have big turnout, and they don't have big visibility, whereas the national election has a whole bunch more turnout and a whole lot more national media exposure, especially with talk of the economy,” says Tidwell.
Home prices play the biggest role in swing counties
To conduct their study, Cifci and Tidwell, with co-authors Sherwood Clements and Andres Jauregui , looked at the voting results for every county in the continental U.S. over the past six presidential elections.
Of those counties, 77% never changed their party preference, voting for either the Democrat or the Republican in every election since 2000, which was used as the base year for analyzing the 2004 election.
But 641 counties across the country–or 23%–switched their party vote at least once across the survey period, some as many as four times. In that subset of swing counties, home prices appeared to have the biggest impact on election results, according to the study.
In swing counties, for every 1% increase in home values over the four years preceding an election, the county was 0.36% more likely to vote for the incumbent party in the next election, the study found.
As well, the data showed that each 1% increase in home prices made the county 0.19% more likely to “flip” its vote to the incumbent party’s candidate. Those figures are after the study controlled for a variety of other factors that could sway elections, such as changes in demographics, the economy, and government benefits.
“The larger the return [on home values], the more likely you are to vote for the incumbent, or to flip for the incumbent,” explains Tidwell. “For every percent of positive return, there is a percentage increase in voting for the incumbent.”
What does it mean for 2024?
Home prices have risen rapidly across the country over the past four years, including in the seven swing states.
From March 2020 to March 2024, national home values rose 46.4%, according to the Freddie Mac Home Price Index. Of the swing states, North Carolina, Arizona, Georgia, and Wisconsin all outperformed the national average, with four-year price gains greater than 50%.
The study suggests that trend would tend broadly to favor the incumbent, President Joe Biden , as he seeks reelection, particularly in the areas that have seen the strongest home price gains. But the authors caution that their finding only demonstrates a statistical nudge in one direction or the other. They warn that there are many other variables at play in an election.
“It's just one of many factors,” Tidwell says of home price performance. “It's not really a forecast on its own.”
As well, voter turnout in counties that are reliably Democratic or Republican can be just as important to the state-level results in swing states as the marginal shifts in counties that flip from one party to another.
But in an election that is increasingly focused on the housing market, the new findings provide an interesting twist on the role of home prices in voter decision making.
Donald Trump , the presumptive Republican nominee, and his allies have recently levied attacks against Biden over rising home prices, pointing to the challenges raised for prospective first-time homebuyers.
“Under President Biden, home prices have risen almost 50%, making it nearly impossible for millennials to buy their first home and driving the American Dream further and further out of reach,” wrote Sen. Tim Scott , a South Carolina Republican and staunch Trump supporter, on the social media platform X.
On his own Truth Social platform, Trump himself recently wrote: “Crooked Joe has made it impossible for millions of Americans, especially YOUNG Americans, to buy a home.” (Conversely, Trump has also accused Biden of trying to “destroy your property values” by abolishing single-family zoning in the suburbs. The two arguments seem difficult to reconcile.)
It’s true that rising home prices, along with high mortgage rates, are key factors in a national housing crisis that has pushed ownership out of reach for many prospective homebuyers. But on the flip side, most voters are already homeowners. The U.S. homeownership rate is about 66%, and homeowners are significantly more likely to vote than renters.
For existing homeowners, rising home prices mean more equity and higher household net worth, the same as what rising stock prices mean for shareholders.
It suggests that for Republicans, attacking Biden over rising home prices might not carry the same weight with voters as criticism over inflation for goods such as gasoline and groceries.
“When you go to the grocery store or restaurant or the gas pump, I think maybe people feel a little bit different pain than if they own a house and they see their house price going up,” says Tidwell.
On the other hand, the study found evidence that, for swing counties, the economically rational choice might be to always flip to the non-incumbent party, which in 2024 would be the Republicans.
The study found that counties that flipped their vote to an incumbent party candidate were not rewarded with superior home price returns in the four years after the election.
However, counties that flipped to vote for the non-incumbent did experience “positive and significant post-election housing returns” if that candidate won. The authors speculate that this might be due to the winning party rewarding new supporters by increasing investment in those areas after regaining the White House.
“The counties that make the national results flip parties, they do well,” says Clements, a collegiate assistant professor of real estate at Virginia Tech. “Whatever counties voted for Biden last time and vote for Trump this time, if you believe our research, they’re going to have home prices rising if Trump wins.”
Editor's note: This article is part of a special Realtor.com series on the housing market and the swing states in the 2024 presidential election. For additional coverage in this series, click here .
Keith Griffith is a journalist at Realtor.com. He covers the housing market and real estate trends.
- Related Articles
Share this Article

IMAGES
VIDEO
COMMENTS
Ad hoc. hypothesis. In science and philosophy, an ad hoc hypothesis is a hypothesis added to a theory in order to save it from being falsified. Often, ad hoc hypothesizing is employed to compensate for anomalies not anticipated by the theory in its unmodified form. For example, a person that wants to believe in leprechauns can avoid ever being ...
Definition. Ad hoc hypothesis denotes a supplementary hypothesis given to a theory to prevent it from being refuted. According to Karl Popper's philosophy of science, the only way that falsifiable intellectual systems like Marxism and Freudianism have been sustained is through the dependence on ad hoc hypotheses to fill gaps.
An ad hoc hypothesis then is one formed to address a specific problem—such as the problem of immunizing a particular theory from falsification by anomalous data (and thereby accommodating that data). ... Thus Popper's conception of ad hocness added to the ordinary English meaning a further requirement—in the case of an ad hoc hypothesis ...
The process of altering a hypothesis to react to potentially falsifying evidence typically involves adding extra hypotheses onto your original hypothesis such that the original hypothesis no longer has the troubling implications which turned out not to be true. These extra hypotheses are called ad hoc hypotheses.
ad hoc hypothesis. in The Oxford Dictionary of Philosophy (2 rev) Length: 22 words. Hypothesis adopted purely for the purpose of saving a theory from difficulty or refutation, but without any independent rationale.
Here, an ad hoc hypothesis is one that does not allow for the generation of new, falsifiable predictions. Popper gives the example of Marxism, which he argues had originally made definite predictions about the evolution of society: the capitalist, free-market system would self-destruct and be replaced by joint ownership of the means of ...
Abstract. In this article I review attempts to define the term "ad hoc hypothesis," focusing on the efforts of, among others, Karl Popper, Jarrett Leplin, and Gerald Holton. I conclude that the term is unhelpful; what is "ad hoc" seems to be a judgment made by particular scientists not on the basis of any well-established definition but ...
Philosophers of science were once very interested in specifying the epistemic meaning of ad hocness; mostly in the 1960-1980s. The still by far most popular epistemic account of ad hocness is the view that associates ad hocness with a lack of independent support. According to that view, a hypothesis is ad hoc when it has no empirical support ...
Accounts that spell out ad hocness as the lack of testability, as the lack of independent support, as the lack of unifiedness, or as mere subjective projections are all unsatisfactory. Instead, this chapter proposes that ad hocness has to do with the lack of coherence between the hypothesis in question and (i) the theory which the hypothesis is ...
would be non-ad hoc under Popper's definition. Hempel then suggested a modification of the definition of ad hoc: "An auxiliary [hypothesis] which enables a theory . . . to explain an [embarrassing] result in conjunction with [the hypothesis] is ad hoc if it does not have any observational con-
In Leplin's words, an "ad hoc hypothesis is one introduced in response to an experiment that provides its only support" (Leplin 1975, p. 319). Footnote 6 We consider this condition the central condition of ad hocness, carrying the actual meaning of the Latin expression "ad hoc" (="for this"):
An ad hoc analysis is an extra type of hypothesis added to the results of an experiment to try to explain away contrary evidence. The scientific method dictates that, if a hypothesis is rejected, then that is final. The research needs to be redesigned or refined before the hypothesis can be tested again. Amongst pseudo-scientists, an ad hoc ...
The connection between 'ad hocness' and the falsifiability requirement has undergone an elaborate evolution of qualifications and distinctions.See, for example. Grunbaum and Lakatos each introduce three different senses of 'ad hoc', only one of these six departing substantially from the connection with falsifiability.More recently. has proposed refinements in Lakatos' distinctions.
The LFC hypothesis was one of Popper's main examples of an ad hoc hypothesis (Reference Popper 1959: 83), and it is now a litmus test for any definition of 'ad hoc solution'. The LFC states that an object contracts in its direction of travel.
On Ad Hoc Hypotheses*. In this article I review attempts to define the term "ad hoc hypothesis," focusing on the efforts of, among others, Karl Popper, Jarrett Leplin, and Gerald Holton. I conclude that the term is unhelpful; what is "ad hoc" seems to be a judgment made by particular scientists not on the basis of any well-established ...
Ad hoc means "for a particular purpose only.". Ad Hoc Analysis is used in business intelligence to answer a specific question at a specific time; In other words, on an "as needed basis.". The result is a graph, report or other summary that helps you to make a business decision. Ad hoc analysis can be facilitated with use of a dashboard.
The received view of an ad hochypothesis is that it accounts for only the observation(s) it was designed to account for, and so non-ad hocness is generally held to be necessary or important for an introduced hypothesis or modification to a theory. Attempts by Popper and several others to convincingly explicate this view, however, prove to be unsuccessful or of doubtful value, and familiar and ...
Almost any explanation could be considered "ad hoc" if we define the concept broadly enough because every hypothesis is designed to account for some observed event. However, the term is normally used more narrowly to refer to some explanation which exists for no other reason but to save a favored hypothesis. It is thus not an explanation which ...
Ad hoc is a Latin phrase meaning literally ' for this '.In English, it typically signifies a solution for a specific purpose, problem, or task rather than a generalized solution adaptable to collateral instances (compare with a priori).. Common examples are ad hoc committees and commissions created at the national or international level for a specific task, and the term is often used to ...
Ad hoc definition: for the special purpose or end presently under consideration. See examples of AD HOC used in a sentence.
Ad-hoc testing is a style of informal, unstructured software testing that seeks to break the testing process to discover potential flaws or faults at the earliest. It is performed randomly and is typically an unplanned activity that does not adhere to test design principles or documentation when writing test cases.
ad hoc: [adverb] for the particular end or case at hand without consideration of wider application.
Ad hoc analysis is a dynamic process that involves digging into your data to answer specific questions or solve immediate problems. Let's delve deeper into what it entails. Ad Hoc Analysis Characteristics. At its core, ad hoc analysis refers to the flexible and on-demand examination of data to gain insights or address specific queries.
Explaining the 'homevoter hypothesis' The study's finding is an extension of the "homevoter hypothesis," which holds that homeowners tend to vote in support of policies and candidates they ...