

10 Famous Examples of Longitudinal Studies
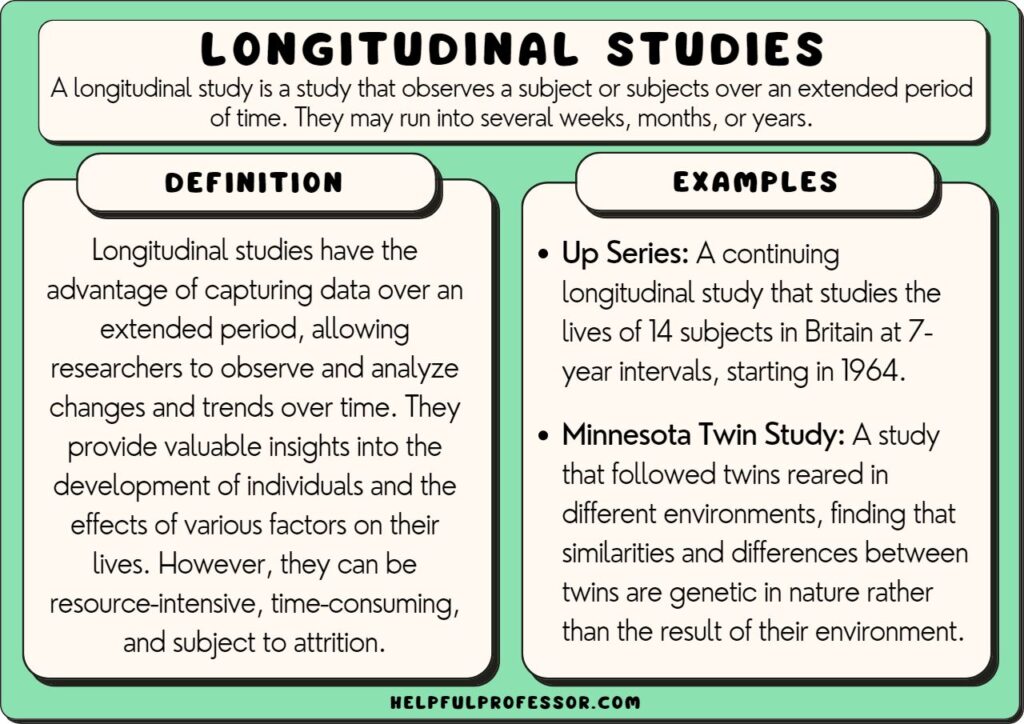
A longitudinal study is a study that observes a subject or subjects over an extended period of time. They may run into several weeks, months, or years. An examples is the Up Series which has been going since 1963.
Longitudinal studies are deployed most commonly in psychology and sociology, where the intention is to observe the changes in the subject over years, across a lifetime, and sometimes, even across generations.
There have been several famous longitudinal studies in history. Some of the most well-known examples are listed below.
Examples of Longitudinal Studies
1. up series.
Duration: 1963 to Now
The Up Series is a continuing longitudinal study that studies the lives of 14 subjects in Britain at 7-year intervals.
The study is conducted in the form of interviews in which the subjects report the changes that have occurred in their lives in the last 7 years since the last interview.
The interviews are filmed and form the subject matter of the critically acclaimed Up series of documentary films directed by Michael Apsted.
When it was first conceived, the aim of the study was to document the life progressions of a cross-section of British children through the second half of the 20th century in light of the rapid social, economic, political, and demographic changes occuring in Britain.
14 children were selected from different socio-economic backgrounds for the first study in 1963 in which all were 7 years old.
The latest installment was filmed in 2019 by which time the participants had reached 63 years of age.
The study noted that life outcomes of subjects were determined to a large extent by their socio-economic and demographic circumstances, and that chances for upward mobility remained limited in late 20th century Britain (Pearson, 2012).
2. Minnesota Twin Study
Duration: 1979 to 1990 (11 years)
Siblings who are twins not only look alike but often display similar behavioral and personality traits.
This raises an oft-asked question: how much of this similarity is genetic and how much of it is the result of the twins growing up together in a similar environment.
The Minnesota twin study was a longitudinal study that set out to find an answer to this question by studying a group of twins from 1979 to 1990 under the supervision of Thomas J Bouchard.
The study found that identical twins who were reared apart in different environments did not display any greater chances of being different from each other than twins that were raised in the same environment.
The study concluded that the similarities and differences between twins are genetic in nature, rather than being the result of their environment (Bouchard et. al., 1990).
3. Grant Study
Duration: 1942 – Present
The Grant Study is one of the most ambitious longitudinal studies. It attempts to answer a philosophical question that has been central to human existence since the beginning of time – what is the secret to living a good life? (Shenk, 2009).
It does so by studying the lives of 268 male Harvard graduates who are interrogated at least every two years with the help of questionnaires, personal interviews, and gleaning information about their physical and mental well-being from their physicians.
Begun in 1942, the study continues to this day.
The study has provided researchers with several interesting insights into what constitutes the human quality of life.
For instance:
- It reveals that the quality of our relationships is more influential than IQ when it comes to our financial success.
- It suggests that our relationships with our parents during childhood have a lasting impact on our mental and physical well-being until late into our lives.
In short, the results gleaned from the study (so far) strongly indicate that the quality of our relationships is one of the biggest factors in determining our quality of life.
4. Terman Life Cycle Study
Duration: 1921 – Present
The Terman Life-Cycle Study, also called the Genetic Studies of Genius, is one of the longest studies ever conducted in the field of psychology.
Commenced in 1921, it continues to this day, over 100 years later!
The objective of the study at its commencement in 1921 was to study the life trajectories of exceptionally gifted children, as measured by standardized intelligence tests.
Lewis Terman, the principal investigator of the study, wanted to dispel the then-prevalent notion that intellectually gifted children tended to be:
- socially inept, and
- physically deficient
To this end, Terman selected 1528 students from public schools in California based on their scores on several standardized intelligence tests such as the Stanford-Binet Intelligence scales, National Intelligence Test, and the Army Alpha Test.
It was discovered that intellectually gifted children had the same social skills and the same level of physical development as other children.
As the study progressed, following the selected children well into adulthood and in their old age, it was further discovered that having higher IQs did not affect outcomes later in life in a significant way (Terman & Oden, 1959).
5. National Food Survey
Duration: 1940 to 2000 (60 years)
The National Food Survey was a British study that ran from 1940 to 2000. It attempted to study food consumption, dietary patterns, and household expenditures on food by British citizens.
Initially commenced to measure the effects of wartime rationing on the health of British citizens in 1940, the survey was extended and expanded after the end of the war to become a comprehensive study of British dietary consumption and expenditure patterns.
After 2000, the survey was replaced by the Expenditure and Food Survey, which lasted till 2008. It was further replaced by the Living Costs and Food Survey post-2008.
6. Millennium Cohort Study
Duration: 2000 to Present
The Millennium Cohort Study (MCS) is a study similar to the Up Series study conducted by the University of London.
Like the Up series, it aims to study the life trajectories of a group of British children relative to the socio-economic and demographic changes occurring in Britain.
However, the subjects of the Millenium Cohort Study are children born in the UK in the year 2000-01.
Also unlike the Up Series, the MCS has a much larger sample size of 18,818 subjects representing a much wider ethnic and socio-economic cross-section of British society.
7. The Study of Mathematically Precocious Youths
Duration: 1971 to Present
The Study of Mathematically Precocious Youths (SMPY) is a longitudinal study initiated in 1971 at the Johns Hopkins University.
At the time of its inception, the study aimed to study children who were exceptionally gifted in mathematics as evidenced from their Scholastic Aptitude Test (SAT) scores.
Later the study shifted to Vanderbilt University and was expanded to include children who scored exceptionally high in the verbal section of the SATs as well.
The study has revealed several interesting insights into the life paths, career trajectories, and lifestyle preferences of academically gifted individuals. For instance, it revealed:
- Children with exceptionally high mathematical scores tended to gravitate towards academic, research, or corporate careers in the STEM fields.
- Children with better verbal abilities went into academic, research, or corporate careers in the social sciences and humanities .
8. Baltimore Longitudinal Study of Aging
Duration: 1958 to Present
The Baltimore Longitudinal Study of Aging (BLSA) was initiated in 1958 to study the effects of aging, making it the longest-running study on human aging in America.
With a sample size of over 3200 volunteer subjects, the study has revealed crucial information about the process of human aging.
For instance, the study has shown that:
- The most common ailments associated with the elderly such as diabetes, hypertension, and dementia are not an inevitable outcome of growing old, but rather result from genetic and lifestyle factors.
- Aging does not proceed uniformly in humans, and all humans age differently.
9. Nurses’ Health Study
Duration: 1976 to Present
The Nurses’ Health Study began in 1976 to study the effects of oral contraceptives on women’s health.
The first commercially available birth control pill was approved by the Food and Drug Administration (FDA) in 1960, and the use of such pills rapidly spread across the US and the UK.
At the same time, a lot of misinformation prevailed about the perceived harmful effects of using oral contraceptives.
The nurses’ health study aimed to study the long-term effects of the use of these pills by researching a sample composed of female nurses.
Nurses were specially chosen for the study because of their medical awareness and hence the ease of data collection that this enabled.
Over time, the study expanded to include not just oral contraceptives but also smoking, exercise, and obesity within the ambit of its research.
As its scope widened, so did the sample size and the resources required for continuing the research.
As a result, the study is now believed to be one of the largest and the most expensive observational health studies in history.
10. The Seattle 500 Study
Duration: 1974 to Present
The Seattle 500 Study is a longitudinal study being conducted by the University of Washington.
It observes a cohort of 500 individuals in the city of Seattle to determine the effects of prenatal habits on human health.
In particular, the study attempts to track patterns of substance abuse and mental health among the subjects and correlate them to the prenatal habits of the parents.
From the examples above, it is clear that longitudinal studies are essential because they provide a unique perspective into certain issues which can not be acquired through any other method .
Especially in research areas that study developmental or life span issues, longitudinal studies become almost inevitable.
A major drawback of longitudinal studies is that because of their extended timespan, the results are likely to be influenced by epochal events.
For instance, in the Genetic Studies of Genius described above, the life prospects of all the subjects would have been impacted by events such as the Great Depression and the Second World War.
The female participants in the study, despite their intellectual precocity, spent their lives as home makers because of the cultural norms of the era. Thus, despite their scale and scope, longitudinal studies do not always succeed in controlling background variables.
Bouchard, T. J. Jr, Lykken, D. T., McGue, M., Segal, N. L., & Tellegen, A. (1990). Sources of human psychological differences: the Minnesota study of twins reared apart. Science , 250 (4978), 223–228. doi: https://doi.org/10.1126/science.2218526
Pearson, A. (2012, May) Seven Up!: A tale of two Englands that, shamefully, still exist The Telegraph https://www.telegraph.co.uk/comment/columnists/allison-pearson/9269805/Seven-Up-A-tale-of-two-Englands-that-shamefully-still-exist.html
Shenk, J.W. (2009, June) What makes us happy? The Atlantic https://www.theatlantic.com/magazine/archive/2009/06/what-makes-us-happy/307439/
Terman, L. M. & Oden, M. (1959). The Gifted group at mid-Life: Thirty-five years’ follow-up of the superior child . Genetic Studies of Genius Volume V . Stanford University Press.
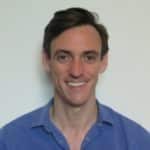
Chris Drew (PhD)
Dr. Chris Drew is the founder of the Helpful Professor. He holds a PhD in education and has published over 20 articles in scholarly journals. He is the former editor of the Journal of Learning Development in Higher Education. [Image Descriptor: Photo of Chris]
- Chris Drew (PhD) https://helpfulprofessor.com/author/chris-drew-phd/ Forest Schools Philosophy & Curriculum, Explained!
- Chris Drew (PhD) https://helpfulprofessor.com/author/chris-drew-phd/ Montessori's 4 Planes of Development, Explained!
- Chris Drew (PhD) https://helpfulprofessor.com/author/chris-drew-phd/ Montessori vs Reggio Emilia vs Steiner-Waldorf vs Froebel
- Chris Drew (PhD) https://helpfulprofessor.com/author/chris-drew-phd/ Parten’s 6 Stages of Play in Childhood, Explained!
Leave a Comment Cancel Reply
Your email address will not be published. Required fields are marked *
Longitudinal Study Design
Julia Simkus
Editor at Simply Psychology
BA (Hons) Psychology, Princeton University
Julia Simkus is a graduate of Princeton University with a Bachelor of Arts in Psychology. She is currently studying for a Master's Degree in Counseling for Mental Health and Wellness in September 2023. Julia's research has been published in peer reviewed journals.
Learn about our Editorial Process
Saul Mcleod, PhD
Editor-in-Chief for Simply Psychology
BSc (Hons) Psychology, MRes, PhD, University of Manchester
Saul Mcleod, PhD., is a qualified psychology teacher with over 18 years of experience in further and higher education. He has been published in peer-reviewed journals, including the Journal of Clinical Psychology.
Olivia Guy-Evans, MSc
Associate Editor for Simply Psychology
BSc (Hons) Psychology, MSc Psychology of Education
Olivia Guy-Evans is a writer and associate editor for Simply Psychology. She has previously worked in healthcare and educational sectors.
A longitudinal study is a type of observational and correlational study that involves monitoring a population over an extended period of time. It allows researchers to track changes and developments in the subjects over time.
What is a Longitudinal Study?
In longitudinal studies, researchers do not manipulate any variables or interfere with the environment. Instead, they simply conduct observations on the same group of subjects over a period of time.
These research studies can last as short as a week or as long as multiple years or even decades. Unlike cross-sectional studies that measure a moment in time, longitudinal studies last beyond a single moment, enabling researchers to discover cause-and-effect relationships between variables.
They are beneficial for recognizing any changes, developments, or patterns in the characteristics of a target population. Longitudinal studies are often used in clinical and developmental psychology to study shifts in behaviors, thoughts, emotions, and trends throughout a lifetime.
For example, a longitudinal study could be used to examine the progress and well-being of children at critical age periods from birth to adulthood.
The Harvard Study of Adult Development is one of the longest longitudinal studies to date. Researchers in this study have followed the same men group for over 80 years, observing psychosocial variables and biological processes for healthy aging and well-being in late life (see Harvard Second Generation Study).
When designing longitudinal studies, researchers must consider issues like sample selection and generalizability, attrition and selectivity bias, effects of repeated exposure to measures, selection of appropriate statistical models, and coverage of the necessary timespan to capture the phenomena of interest.
Panel Study
- A panel study is a type of longitudinal study design in which the same set of participants are measured repeatedly over time.
- Data is gathered on the same variables of interest at each time point using consistent methods. This allows studying continuity and changes within individuals over time on the key measured constructs.
- Prominent examples include national panel surveys on topics like health, aging, employment, and economics. Panel studies are a type of prospective study .
Cohort Study
- A cohort study is a type of longitudinal study that samples a group of people sharing a common experience or demographic trait within a defined period, such as year of birth.
- Researchers observe a population based on the shared experience of a specific event, such as birth, geographic location, or historical experience. These studies are typically used among medical researchers.
- Cohorts are identified and selected at a starting point (e.g. birth, starting school, entering a job field) and followed forward in time.
- As they age, data is collected on cohort subgroups to determine their differing trajectories. For example, investigating how health outcomes diverge for groups born in 1950s, 1960s, and 1970s.
- Cohort studies do not require the same individuals to be assessed over time; they just require representation from the cohort.
Retrospective Study
- In a retrospective study , researchers either collect data on events that have already occurred or use existing data that already exists in databases, medical records, or interviews to gain insights about a population.
- Appropriate when prospectively following participants from the past starting point is infeasible or unethical. For example, studying early origins of diseases emerging later in life.
- Retrospective studies efficiently provide a “snapshot summary” of the past in relation to present status. However, quality concerns with retrospective data make careful interpretation necessary when inferring causality. Memory biases and selective retention influence quality of retrospective data.
Allows researchers to look at changes over time
Because longitudinal studies observe variables over extended periods of time, researchers can use their data to study developmental shifts and understand how certain things change as we age.
High validation
Since objectives and rules for long-term studies are established before data collection, these studies are authentic and have high levels of validity.
Eliminates recall bias
Recall bias occurs when participants do not remember past events accurately or omit details from previous experiences.
Flexibility
The variables in longitudinal studies can change throughout the study. Even if the study was created to study a specific pattern or characteristic, the data collection could show new data points or relationships that are unique and worth investigating further.
Limitations
Costly and time-consuming.
Longitudinal studies can take months or years to complete, rendering them expensive and time-consuming. Because of this, researchers tend to have difficulty recruiting participants, leading to smaller sample sizes.
Large sample size needed
Longitudinal studies tend to be challenging to conduct because large samples are needed for any relationships or patterns to be meaningful. Researchers are unable to generate results if there is not enough data.
Participants tend to drop out
Not only is it a struggle to recruit participants, but subjects also tend to leave or drop out of the study due to various reasons such as illness, relocation, or a lack of motivation to complete the full study.
This tendency is known as selective attrition and can threaten the validity of an experiment. For this reason, researchers using this approach typically recruit many participants, expecting a substantial number to drop out before the end.
Report bias is possible
Longitudinal studies will sometimes rely on surveys and questionnaires, which could result in inaccurate reporting as there is no way to verify the information presented.
- Data were collected for each child at three-time points: at 11 months after adoption, at 4.5 years of age and at 10.5 years of age. The first two sets of results showed that the adoptees were behind the non-institutionalised group however by 10.5 years old there was no difference between the two groups. The Romanian orphans had caught up with the children raised in normal Canadian families.
- The role of positive psychology constructs in predicting mental health and academic achievement in children and adolescents (Marques Pais-Ribeiro, & Lopez, 2011)
- The correlation between dieting behavior and the development of bulimia nervosa (Stice et al., 1998)
- The stress of educational bottlenecks negatively impacting students’ wellbeing (Cruwys, Greenaway, & Haslam, 2015)
- The effects of job insecurity on psychological health and withdrawal (Sidney & Schaufeli, 1995)
- The relationship between loneliness, health, and mortality in adults aged 50 years and over (Luo et al., 2012)
- The influence of parental attachment and parental control on early onset of alcohol consumption in adolescence (Van der Vorst et al., 2006)
- The relationship between religion and health outcomes in medical rehabilitation patients (Fitchett et al., 1999)
Goals of Longitudinal Data and Longitudinal Research
The objectives of longitudinal data collection and research as outlined by Baltes and Nesselroade (1979):
- Identify intraindividual change : Examine changes at the individual level over time, including long-term trends or short-term fluctuations. Requires multiple measurements and individual-level analysis.
- Identify interindividual differences in intraindividual change : Evaluate whether changes vary across individuals and relate that to other variables. Requires repeated measures for multiple individuals plus relevant covariates.
- Analyze interrelationships in change : Study how two or more processes unfold and influence each other over time. Requires longitudinal data on multiple variables and appropriate statistical models.
- Analyze causes of intraindividual change: This objective refers to identifying factors or mechanisms that explain changes within individuals over time. For example, a researcher might want to understand what drives a person’s mood fluctuations over days or weeks. Or what leads to systematic gains or losses in one’s cognitive abilities across the lifespan.
- Analyze causes of interindividual differences in intraindividual change : Identify mechanisms that explain within-person changes and differences in changes across people. Requires repeated data on outcomes and covariates for multiple individuals plus dynamic statistical models.
How to Perform a Longitudinal Study
When beginning to develop your longitudinal study, you must first decide if you want to collect your own data or use data that has already been gathered.
Using already collected data will save you time, but it will be more restricted and limited than collecting it yourself. When collecting your own data, you can choose to conduct either a retrospective or prospective study .
In a retrospective study, you are collecting data on events that have already occurred. You can examine historical information, such as medical records, in order to understand the past. In a prospective study, on the other hand, you are collecting data in real-time. Prospective studies are more common for psychology research.
Once you determine the type of longitudinal study you will conduct, you then must determine how, when, where, and on whom the data will be collected.
A standardized study design is vital for efficiently measuring a population. Once a study design is created, researchers must maintain the same study procedures over time to uphold the validity of the observation.
A schedule should be maintained, complete results should be recorded with each observation, and observer variability should be minimized.
Researchers must observe each subject under the same conditions to compare them. In this type of study design, each subject is the control.
Methodological Considerations
Important methodological considerations include testing measurement invariance of constructs across time, appropriately handling missing data, and using accelerated longitudinal designs that sample different age cohorts over overlapping time periods.
Testing measurement invariance
Testing measurement invariance involves evaluating whether the same construct is being measured in a consistent, comparable way across multiple time points in longitudinal research.
This includes assessing configural, metric, and scalar invariance through confirmatory factor analytic approaches. Ensuring invariance gives more confidence when drawing inferences about change over time.
Missing data
Missing data can occur during initial sampling if certain groups are underrepresented or fail to respond.
Attrition over time is the main source – participants dropping out for various reasons. The consequences of missing data are reduced statistical power and potential bias if dropout is nonrandom.
Handling missing data appropriately in longitudinal studies is critical to reducing bias and maintaining power.
It is important to minimize attrition by tracking participants, keeping contact info up to date, engaging them, and providing incentives over time.
Techniques like maximum likelihood estimation and multiple imputation are better alternatives to older methods like listwise deletion. Assumptions about missing data mechanisms (e.g., missing at random) shape the analytic approaches taken.
Accelerated longitudinal designs
Accelerated longitudinal designs purposefully create missing data across age groups.
Accelerated longitudinal designs strategically sample different age cohorts at overlapping periods. For example, assessing 6th, 7th, and 8th graders at yearly intervals would cover 6-8th grade development over a 3-year study rather than following a single cohort over that timespan.
This increases the speed and cost-efficiency of longitudinal data collection and enables the examination of age/cohort effects. Appropriate multilevel statistical models are required to analyze the resulting complex data structure.
In addition to those considerations, optimizing the time lags between measurements, maximizing participant retention, and thoughtfully selecting analysis models that align with the research questions and hypotheses are also vital in ensuring robust longitudinal research.
So, careful methodology is key throughout the design and analysis process when working with repeated-measures data.
Cohort effects
A cohort refers to a group born in the same year or time period. Cohort effects occur when different cohorts show differing trajectories over time.
Cohort effects can bias results if not accounted for, especially in accelerated longitudinal designs which assume cohort equivalence.
Detecting cohort effects is important but can be challenging as they are confounded with age and time of measurement effects.
Cohort effects can also interfere with estimating other effects like retest effects. This happens because comparing groups to estimate retest effects relies on cohort equivalence.
Overall, researchers need to test for and control cohort effects which could otherwise lead to invalid conclusions. Careful study design and analysis is required.
Retest effects
Retest effects refer to gains in performance that occur when the same or similar test is administered on multiple occasions.
For example, familiarity with test items and procedures may allow participants to improve their scores over repeated testing above and beyond any true change.
Specific examples include:
- Memory tests – Learning which items tend to be tested can artificially boost performance over time
- Cognitive tests – Becoming familiar with the testing format and particular test demands can inflate scores
- Survey measures – Remembering previous responses can bias future responses over multiple administrations
- Interviews – Comfort with the interviewer and process can lead to increased openness or recall
To estimate retest effects, performance of retested groups is compared to groups taking the test for the first time. Any divergence suggests inflated scores due to retesting rather than true change.
If unchecked in analysis, retest gains can be confused with genuine intraindividual change or interindividual differences.
This undermines the validity of longitudinal findings. Thus, testing and controlling for retest effects are important considerations in longitudinal research.
Data Analysis
Longitudinal data involves repeated assessments of variables over time, allowing researchers to study stability and change. A variety of statistical models can be used to analyze longitudinal data, including latent growth curve models, multilevel models, latent state-trait models, and more.
Latent growth curve models allow researchers to model intraindividual change over time. For example, one could estimate parameters related to individuals’ baseline levels on some measure, linear or nonlinear trajectory of change over time, and variability around those growth parameters. These models require multiple waves of longitudinal data to estimate.
Multilevel models are useful for hierarchically structured longitudinal data, with lower-level observations (e.g., repeated measures) nested within higher-level units (e.g., individuals). They can model variability both within and between individuals over time.
Latent state-trait models decompose the covariance between longitudinal measurements into time-invariant trait factors, time-specific state residuals, and error variance. This allows separating stable between-person differences from within-person fluctuations.
There are many other techniques like latent transition analysis, event history analysis, and time series models that have specialized uses for particular research questions with longitudinal data. The choice of model depends on the hypotheses, timescale of measurements, age range covered, and other factors.
In general, these various statistical models allow investigation of important questions about developmental processes, change and stability over time, causal sequencing, and both between- and within-person sources of variability. However, researchers must carefully consider the assumptions behind the models they choose.
Longitudinal vs. Cross-Sectional Studies
Longitudinal studies and cross-sectional studies are two different observational study designs where researchers analyze a target population without manipulating or altering the natural environment in which the participants exist.
Yet, there are apparent differences between these two forms of study. One key difference is that longitudinal studies follow the same sample of people over an extended period of time, while cross-sectional studies look at the characteristics of different populations at a given moment in time.
Longitudinal studies tend to require more time and resources, but they can be used to detect cause-and-effect relationships and establish patterns among subjects.
On the other hand, cross-sectional studies tend to be cheaper and quicker but can only provide a snapshot of a point in time and thus cannot identify cause-and-effect relationships.
Both studies are valuable for psychologists to observe a given group of subjects. Still, cross-sectional studies are more beneficial for establishing associations between variables, while longitudinal studies are necessary for examining a sequence of events.
1. Are longitudinal studies qualitative or quantitative?
Longitudinal studies are typically quantitative. They collect numerical data from the same subjects to track changes and identify trends or patterns.
However, they can also include qualitative elements, such as interviews or observations, to provide a more in-depth understanding of the studied phenomena.
2. What’s the difference between a longitudinal and case-control study?
Case-control studies compare groups retrospectively and cannot be used to calculate relative risk. Longitudinal studies, though, can compare groups either retrospectively or prospectively.
In case-control studies, researchers study one group of people who have developed a particular condition and compare them to a sample without the disease.
Case-control studies look at a single subject or a single case, whereas longitudinal studies are conducted on a large group of subjects.
3. Does a longitudinal study have a control group?
Yes, a longitudinal study can have a control group . In such a design, one group (the experimental group) would receive treatment or intervention, while the other group (the control group) would not.
Both groups would then be observed over time to see if there are differences in outcomes, which could suggest an effect of the treatment or intervention.
However, not all longitudinal studies have a control group, especially observational ones and not testing a specific intervention.
Baltes, P. B., & Nesselroade, J. R. (1979). History and rationale of longitudinal research. In J. R. Nesselroade & P. B. Baltes (Eds.), (pp. 1–39). Academic Press.
Cook, N. R., & Ware, J. H. (1983). Design and analysis methods for longitudinal research. Annual review of public health , 4, 1–23.
Fitchett, G., Rybarczyk, B., Demarco, G., & Nicholas, J.J. (1999). The role of religion in medical rehabilitation outcomes: A longitudinal study. Rehabilitation Psychology, 44, 333-353.
Harvard Second Generation Study. (n.d.). Harvard Second Generation Grant and Glueck Study. Harvard Study of Adult Development. Retrieved from https://www.adultdevelopmentstudy.org.
Le Mare, L., & Audet, K. (2006). A longitudinal study of the physical growth and health of postinstitutionalized Romanian adoptees. Pediatrics & child health, 11 (2), 85-91.
Luo, Y., Hawkley, L. C., Waite, L. J., & Cacioppo, J. T. (2012). Loneliness, health, and mortality in old age: a national longitudinal study. Social science & medicine (1982), 74 (6), 907–914.
Marques, S. C., Pais-Ribeiro, J. L., & Lopez, S. J. (2011). The role of positive psychology constructs in predicting mental health and academic achievement in children and adolescents: A two-year longitudinal study. Journal of Happiness Studies: An Interdisciplinary Forum on Subjective Well-Being, 12( 6), 1049–1062.
Sidney W.A. Dekker & Wilmar B. Schaufeli (1995) The effects of job insecurity on psychological health and withdrawal: A longitudinal study, Australian Psychologist, 30: 1,57-63.
Stice, E., Mazotti, L., Krebs, M., & Martin, S. (1998). Predictors of adolescent dieting behaviors: A longitudinal study. Psychology of Addictive Behaviors, 12 (3), 195–205.
Tegan Cruwys, Katharine H Greenaway & S Alexander Haslam (2015) The Stress of Passing Through an Educational Bottleneck: A Longitudinal Study of Psychology Honours Students, Australian Psychologist, 50:5, 372-381.
Thomas, L. (2020). What is a longitudinal study? Scribbr. Retrieved from https://www.scribbr.com/methodology/longitudinal-study/
Van der Vorst, H., Engels, R. C. M. E., Meeus, W., & Deković, M. (2006). Parental attachment, parental control, and early development of alcohol use: A longitudinal study. Psychology of Addictive Behaviors, 20 (2), 107–116.
Further Information
- Schaie, K. W. (2005). What can we learn from longitudinal studies of adult development?. Research in human development, 2 (3), 133-158.
- Caruana, E. J., Roman, M., Hernández-Sánchez, J., & Solli, P. (2015). Longitudinal studies. Journal of thoracic disease, 7 (11), E537.

Related Articles
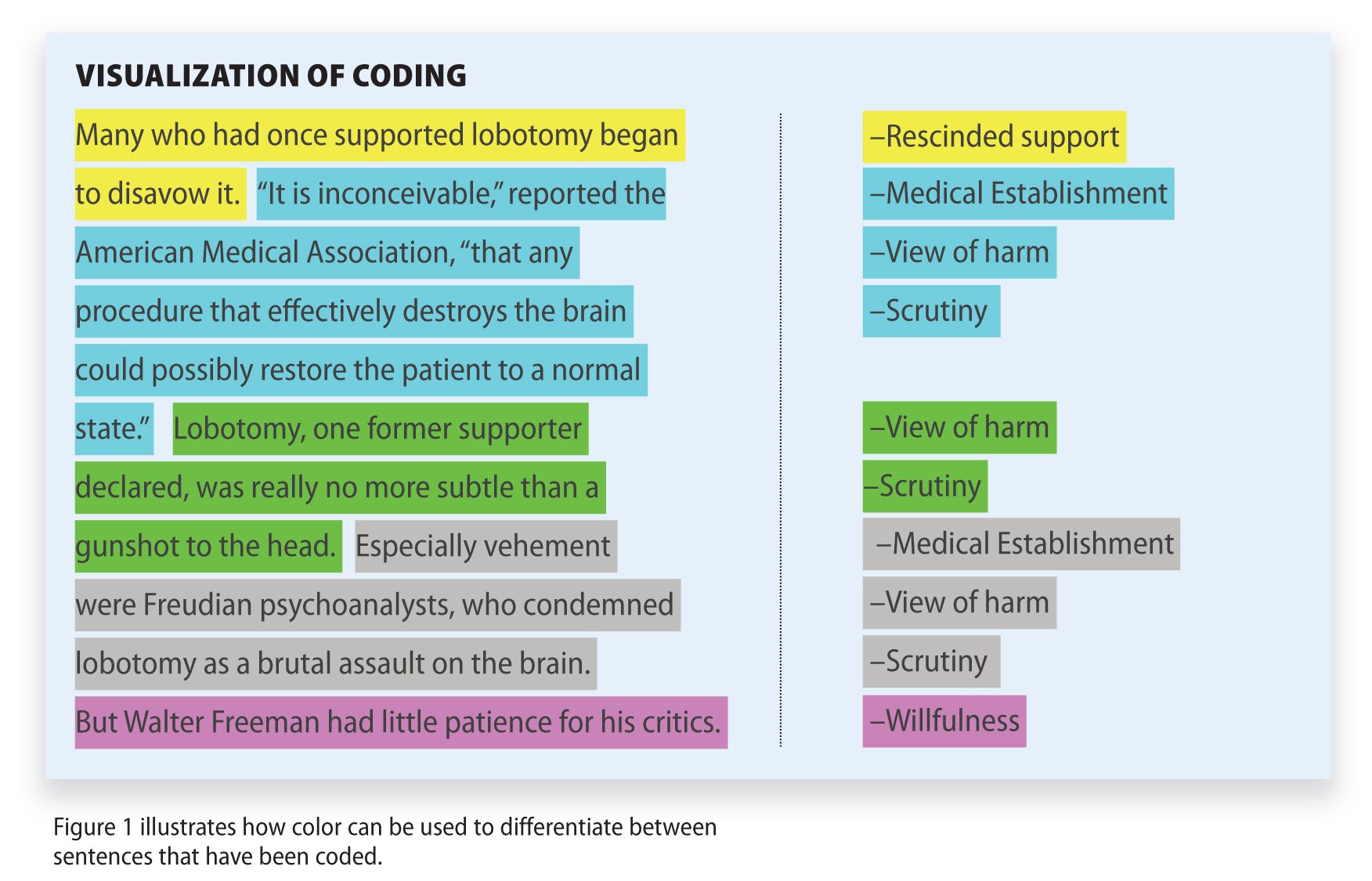
Research Methodology
Qualitative Data Coding
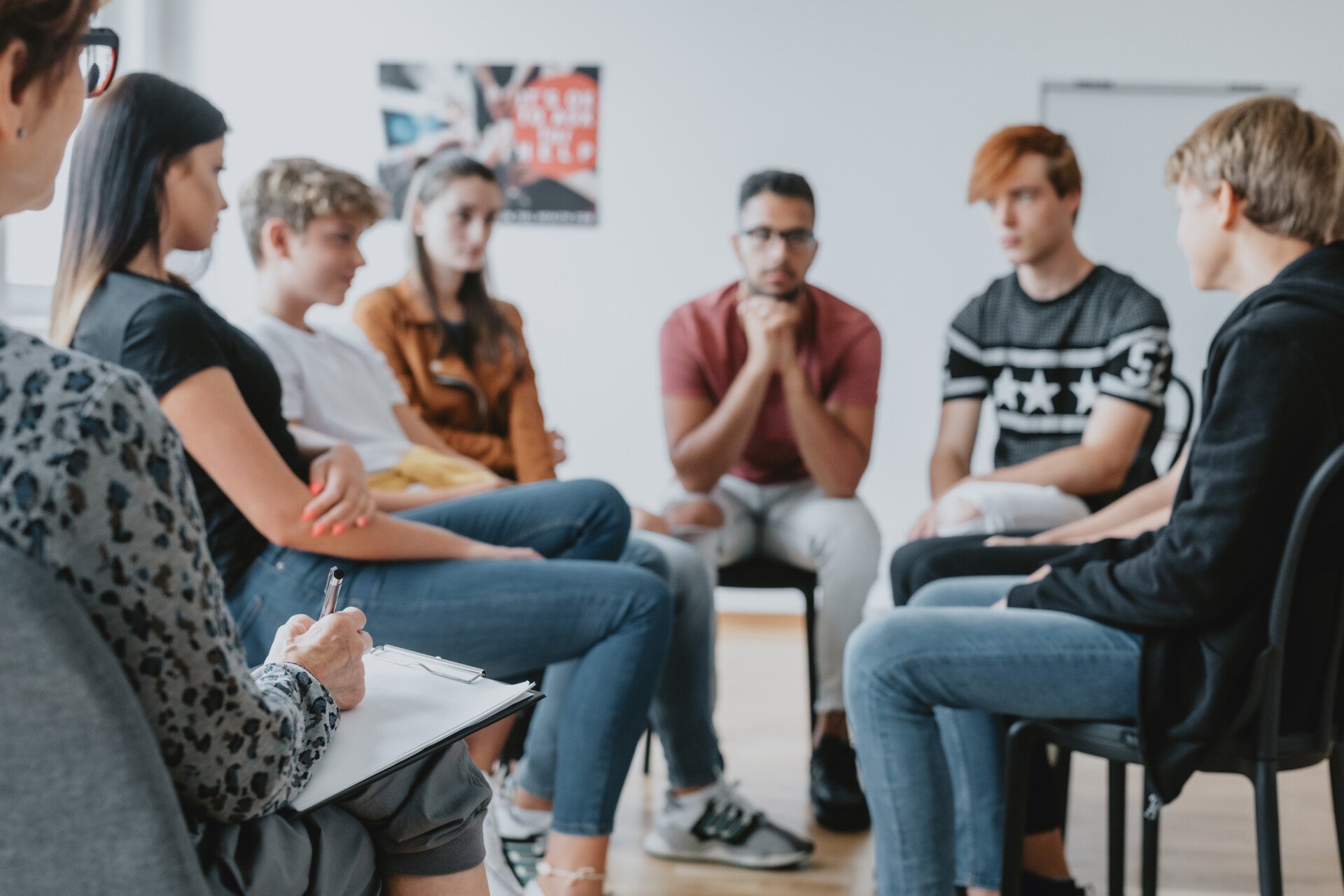
What Is a Focus Group?
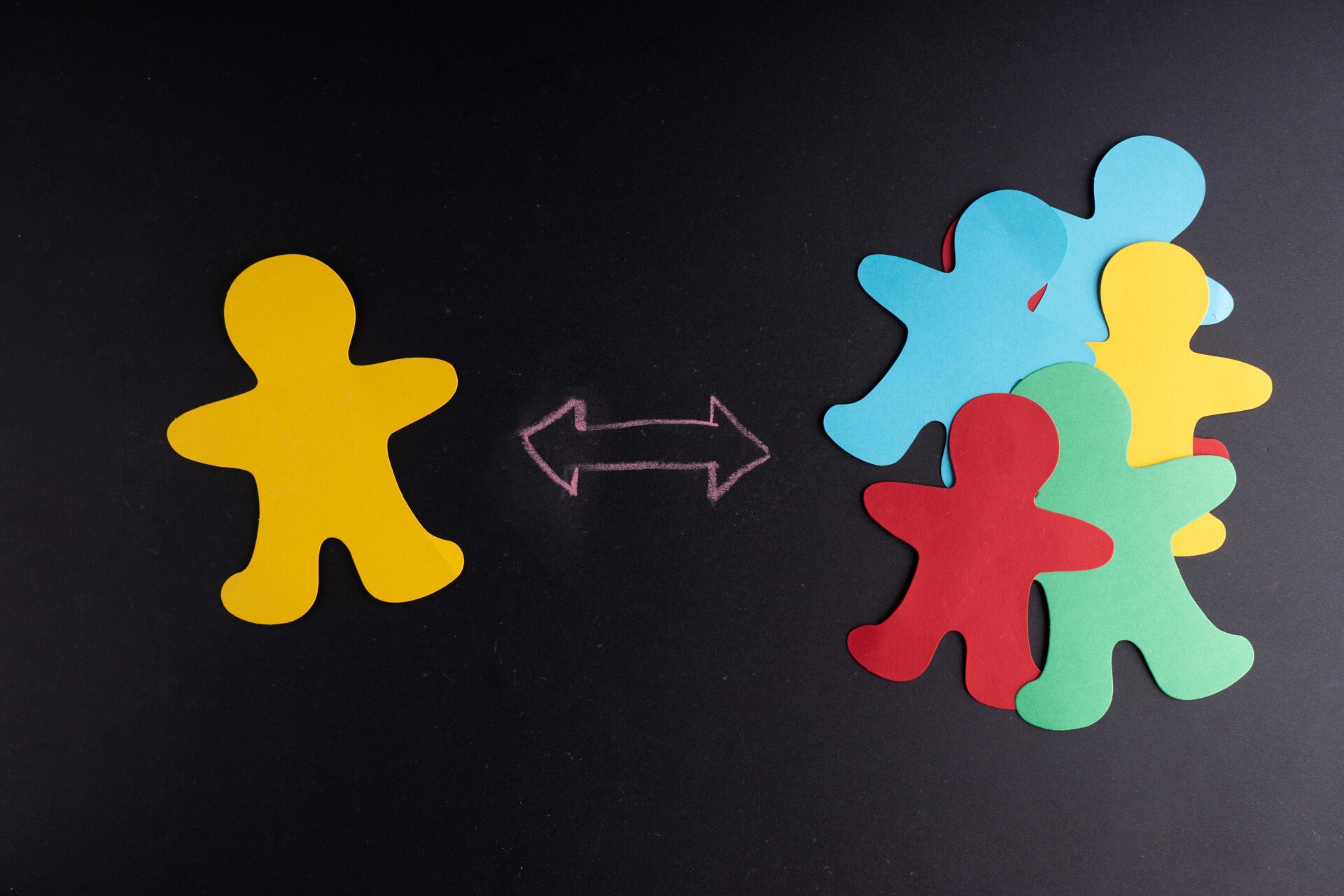
Cross-Cultural Research Methodology In Psychology
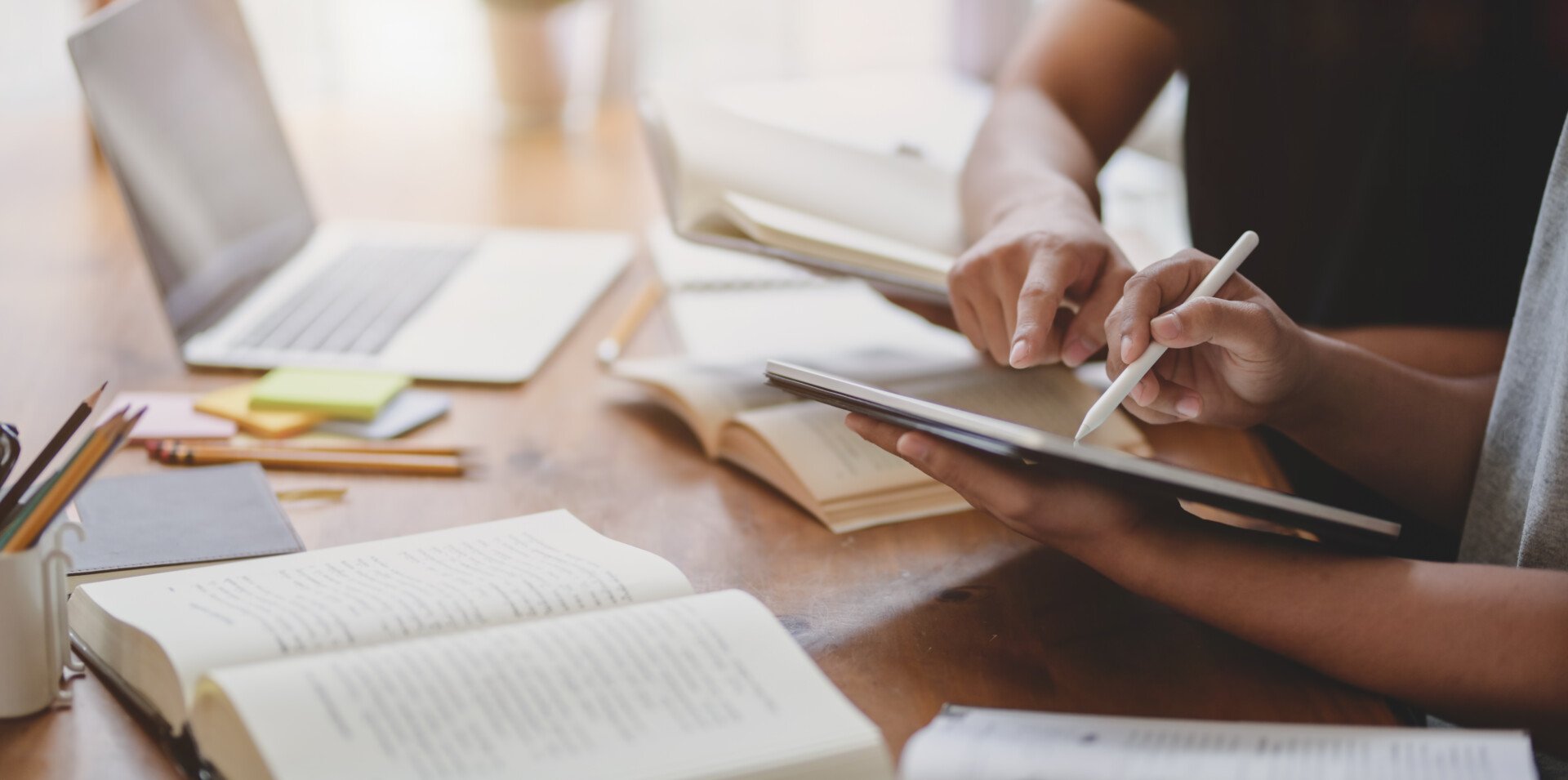
What Is Internal Validity In Research?
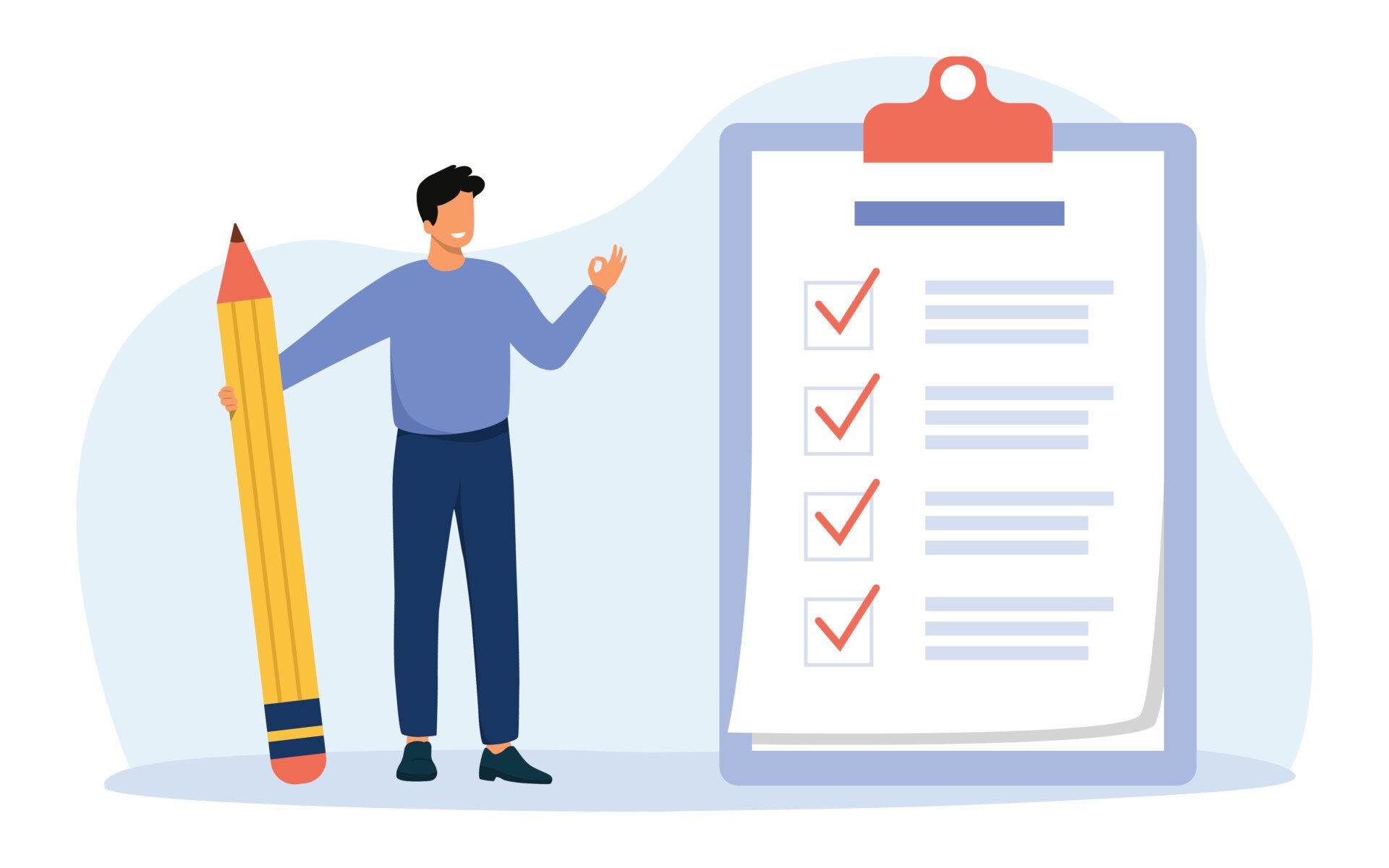
Research Methodology , Statistics
What Is Face Validity In Research? Importance & How To Measure
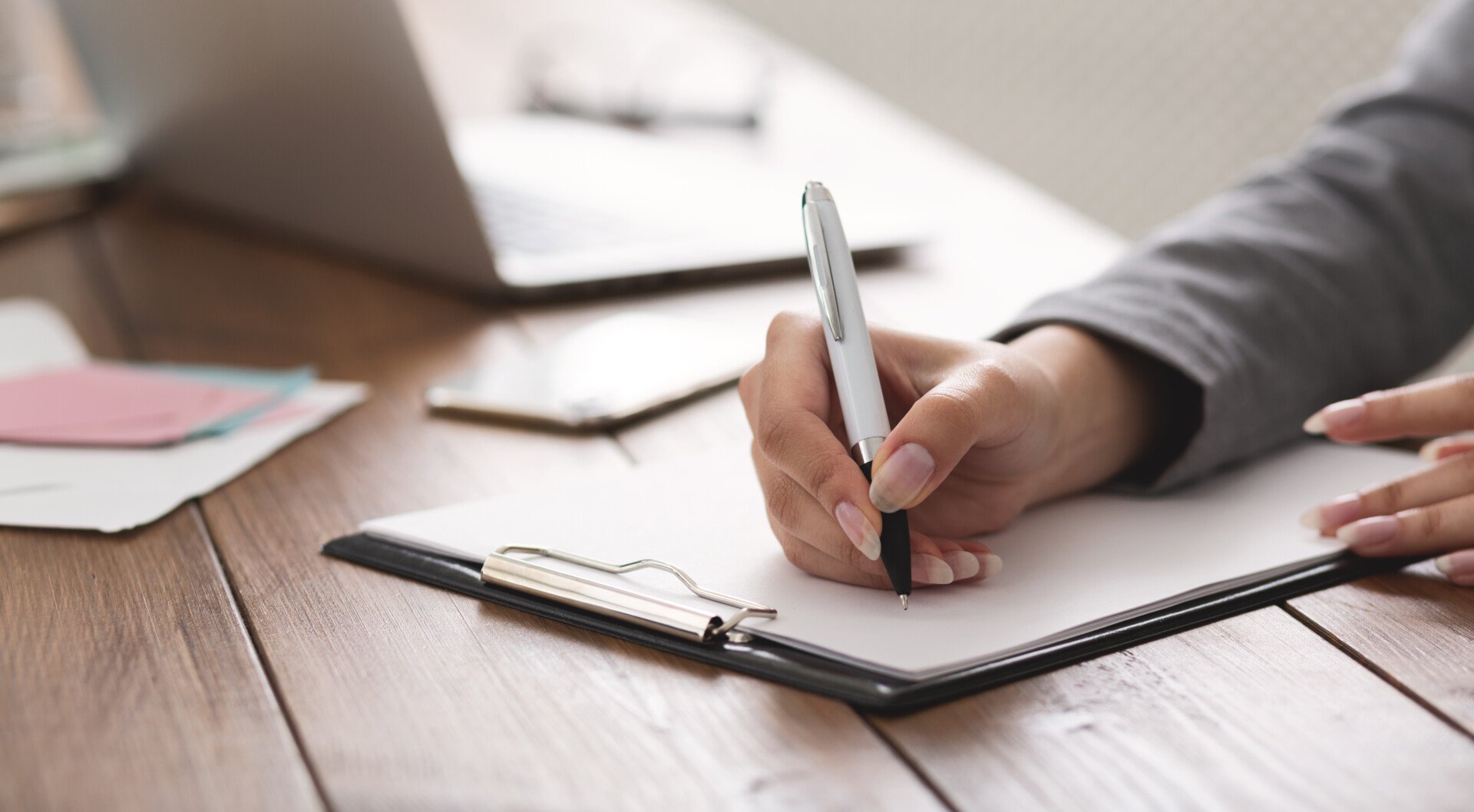
Criterion Validity: Definition & Examples
- What’s a Longitudinal Study? Types, Uses & Examples

Research can take anything from a few minutes to years or even decades to complete. When a systematic investigation goes on for an extended period, it’s most likely that the researcher is carrying out a longitudinal study of the sample population. So how does this work?
In the most simple terms, a longitudinal study involves observing the interactions of the different variables in your research population, exposing them to various causal factors, and documenting the effects of this exposure. It’s an intelligent way to establish causal relationships within your sample population.
In this article, we’ll show you several ways to adopt longitudinal studies for your systematic investigation and how to avoid common pitfalls.
What is a Longitudinal Study?
A longitudinal study is a correlational research method that helps discover the relationship between variables in a specific target population. It is pretty similar to a cross-sectional study , although in its case, the researcher observes the variables for a longer time, sometimes lasting many years.
For example, let’s say you are researching social interactions among wild cats. You go ahead to recruit a set of newly-born lion cubs and study how they relate with each other as they grow. Periodically, you collect the same types of data from the group to track their development.
The advantage of this extended observation is that the researcher can witness the sequence of events leading to the changes in the traits of both the target population and the different groups. It can identify the causal factors for these changes and their long-term impact.
Characteristics of Longitudinal Studies
1. Non-interference: In longitudinal studies, the researcher doesn’t interfere with the participants’ day-to-day activities in any way. When it’s time to collect their responses , the researcher administers a survey with qualitative and quantitative questions .
2. Observational: As we mentioned earlier, longitudinal studies involve observing the research participants throughout the study and recording any changes in traits that you notice.
3. Timeline: A longitudinal study can span weeks, months, years, or even decades. This dramatically contrasts what is obtainable in cross-sectional studies that only last for a short time.
Cross-Sectional vs. Longitudinal Studies
- Definition
A cross-sectional study is a type of observational study in which the researcher collects data from variables at a specific moment to establish a relationship among them. On the other hand, longitudinal research observes variables for an extended period and records all the changes in their relationship.
Longitudinal studies take a longer time to complete. In some cases, the researchers can spend years documenting the changes among the variables plus their relationships. For cross-sectional studies, this isn’t the case. Instead, the researcher collects information in a relatively short time frame and makes relevant inferences from this data.
While cross-sectional studies give you a snapshot of the situation in the research environment, longitudinal studies are better suited for contexts where you need to analyze a problem long-term.
- Sample Data
Longitudinal studies repeatedly observe the same sample population, while cross-sectional studies are conducted with different research samples.
Because longitudinal studies span over a more extended time, they typically cost more money than cross-sectional observations.
Types of Longitudinal Studies
The three main types of longitudinal studies are:
- Panel Study
- Retrospective Study
- Cohort Study
These methods help researchers to study variables and account for qualitative and quantitative data from the research sample.
1. Panel Study
In a panel study, the researcher uses data collection methods like surveys to gather information from a fixed number of variables at regular but distant intervals, often spinning into a few years. It’s primarily designed for quantitative research, although you can use this method for qualitative data analysis .
When To Use Panel Study
If you want to have first-hand, factual information about the changes in a sample population, then you should opt for a panel study. For example, medical researchers rely on panel studies to identify the causes of age-related changes and their consequences.
Advantages of Panel Study
- It helps you identify the causal factors of changes in a research sample.
- It also allows you to witness the impact of these changes on the properties of the variables and information needed at different points of their existing relationship.
- Panel studies can be used to obtain historical data from the sample population.
Disadvantages of Panel Studies
- Conducting a panel study is pretty expensive in terms of time and resources.
- It might be challenging to gather the same quality of data from respondents at every interval.
2. Retrospective Study
In a retrospective study, the researcher depends on existing information from previous systematic investigations to discover patterns leading to the study outcomes. In other words, a retrospective study looks backward. It examines exposures to suspected risk or protection factors concerning an outcome established at the start of the study.
When To Use Retrospective Study
Retrospective studies are best for research contexts where you want to quickly estimate an exposure’s effect on an outcome. It also helps you to discover preliminary measures of association in your data.
Medical researchers adopt retrospective study methods when they need to research rare conditions.
Advantages of Retrospective Study
- Retrospective studies happen at a relatively smaller scale and do not require much time to complete.
- It helps you to study rare outcomes when prospective surveys are not feasible.
Disadvantages of Retrospective Study
- It is easily affected by recall bias or misclassification bias.
- It often depends on convenience sampling, which is prone to selection bias.
3. Cohort Study
A cohort study entails collecting information from a group of people who share specific traits or have experienced a particular occurrence simultaneously. For example, a researcher might conduct a cohort study on a group of Black school children in the U.K.
During cohort study, the researcher exposes some group members to a specific characteristic or risk factor. Then, she records the outcome of this exposure and its impact on the exposed variables.
When To Use Cohort Study
You should conduct a cohort study if you’re looking to establish a causal relationship within your data sets. For example, in medical research, cohort studies investigate the causes of disease and establish links between risk factors and effects.
Advantages of Cohort Studies
- It allows you to study multiple outcomes that can be associated with one risk factor.
- Cohort studies are designed to help you measure all variables of interest.
Disadvantages of Cohort Studies
- Cohort studies are expensive to conduct.
- Throughout the process, the researcher has less control over variables.
When Would You Use a Longitudinal Study?
If you’re looking to discover the relationship between variables and the causal factors responsible for changes, you should adopt a longitudinal approach to your systematic investigation. Longitudinal studies help you to analyze change over a meaningful time.
How to Perform a Longitudinal Study?
There are only two approaches you can take when performing a longitudinal study. You can either source your own data or use previously gathered data.
1. Sourcing for your own data
Collecting your own data is a more verifiable method because you can trust your own data. The way you collect your data is also heavily dependent on the type of study you’re conducting.
If you’re conducting a retrospective study, you’d have to collect data on events that have already happened. An example is going through records to find patterns in cancer patients.
For a prospective study, you collect the data in real-time. This means finding a sample population, following them, and documenting your findings over the course of your study.
Irrespective of what study type you’d be conducting, you need a versatile data collection tool to help you accurately record your data. One we strongly recommend is Formplus . Signup here for free.
2. Using previously gathered data
Governmental and research institutes often carry out longitudinal studies and make the data available to the public. So you can pick up their previously researched data and use them for your own study. An example is the UK data service website .
Using previously gathered data isn’t just easy, they also allow you to carry out research over a long period of time.
The downside to this method is that it’s very restrictive because you can only use the data set available to you. You also have to thoroughly examine the source of the data given to you.
Advantages of a Longitudinal Study
- Longitudinal studies help you discover variable patterns over time, leading to more precise causal relationships and research outcomes.
- When researching developmental trends, longitudinal studies allow you to discover changes across lifespans and arrive at valid research outcomes.
- They are highly flexible, which means the researcher can adjust the study’s focus while it is ongoing.
- Unlike other research methods, longitudinal studies collect unique, long-term data and highlight relationships that cannot be discovered in a short-term investigation.
- You can collect additional data to study unexpected findings at any point in your systematic investigation.
Disadvantages and Limitations of a Longitudinal Study
- It’s difficult to predict the results of longitudinal studies because of the extended time frame. Also, it may take several years before the data begins to produce observable patterns or relationships that can be monitored.
- It costs lots of money to sustain a research effort for years. You’ll keep incurring costs every year compared to other forms of research that can be completed in a smaller fraction of the time.
- Longitudinal studies require a large sample size which might be challenging to achieve. Without this, the entire investigation will have little or no impact.
- Longitudinal studies often experience panel attrition. This happens when some members of the research sample are unable to complete the study due to several reasons like changes in contact details, refusal, incapacity, and even death.
Longitudinal Studies Examples
How does a longitudinal study work in the real world? To answer this, let’s consider a few typical scenarios.
A researcher wants to know the effects of a low-carb diet on weight loss. So, he gathers a group of obese men and kicks off the systematic investigation using his preferred longitudinal study method. He records information like how much they weigh, the number of carbs in their diet, and the like at different points. All these data help him to arrive at valid research outcomes.
Use for Free: Macros Calories Diet Plan Template
A researcher wants to know if there’s any relationship between children who drink milk before school and high classroom performance . First, he uses a sampling technique to gather a large research population.
Then, he conducts a baseline survey to establish the premise of the research for later comparison. Next, the researcher gives a log to each participant to keep track of predetermined research variables .
Example 3
You decide to study how a particular diet affects athletes’ performance over time. First, you gather your sample population , establish a baseline for the research, and observe and record the required data.
Longitudinal Studies Frequently Asked Questions (FAQs)
- Are Longitudinal Studies Quantitative or Qualitative?
Longitudinal studies are primarily a qualitative research method because the researcher observes and records changes in variables over an extended period. However, it can also be used to gather quantitative data depending on your research context.
- What Is Most Likely the Biggest Problem with Longitudinal Research?
The biggest challenge with longitudinal research is panel attrition. Due to the length of the research process, some variables might be unable to complete the study for one reason or the other. When this happens, it can distort your data and research outcomes.
- What is Longitudinal Data Collection?
Longitudinal data collection is the process of gathering information from the same sample population over a long period. Longitudinal data collection uses interviews, surveys, and observation to collect the required information from research sources.
- What is the Difference Between Longitudinal Data and a Time Series Analysis?
Because longitudinal studies collect data over a long period, they are often mistaken for time series analysis. So what’s the real difference between these two concepts?
In a time series analysis, the researcher focuses on a single individual at multiple time intervals. Meanwhile, longitudinal data focuses on multiple individuals at various time intervals.
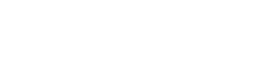
Connect to Formplus, Get Started Now - It's Free!
- cohort study
- cross-sectional study
- longitudinal study
- longitudinal study faq
- panel study
- retrospective cohort study
- sample data
- busayo.longe
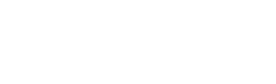
You may also like:
Selection Bias in Research: Types, Examples & Impact
In this article, we’ll discuss the effects of selection bias, how it works, its common effects and the best ways to minimize it.

11 Retrospective vs Prospective Cohort Study Differences
differences between retrospective and prospective cohort studies in definitions, examples, data collection, analysis, advantages, sample...
Cross-Sectional Studies: Types, Pros, Cons & Uses
In this article, we’ll look at what cross-sectional studies are, how it applies to your research and how to use Formplus to collect...
What is Pure or Basic Research? + [Examples & Method]
Simple guide on pure or basic research, its methods, characteristics, advantages, and examples in science, medicine, education and psychology
Formplus - For Seamless Data Collection
Collect data the right way with a versatile data collection tool. try formplus and transform your work productivity today..
- Skip to main content
- Skip to primary sidebar
- Skip to footer
- QuestionPro

- Solutions Industries Gaming Automotive Sports and events Education Government Travel & Hospitality Financial Services Healthcare Cannabis Technology Use Case NPS+ Communities Audience Contactless surveys Mobile LivePolls Member Experience GDPR Positive People Science 360 Feedback Surveys
- Resources Blog eBooks Survey Templates Case Studies Training Help center

Home Market Research
What is a Longitudinal Study?: Definition and Explanation
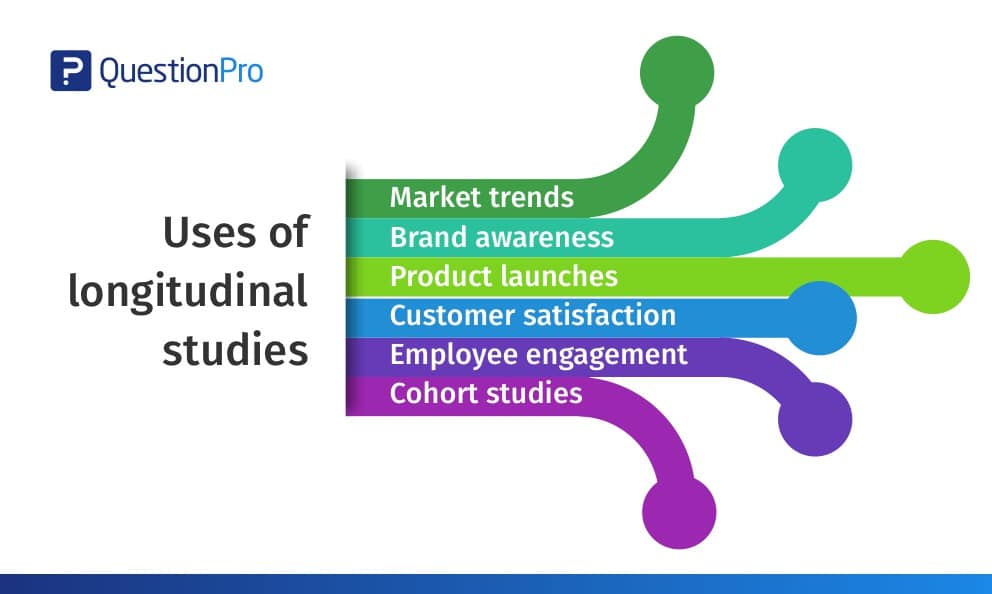
In this article, we’ll cover all you need to know about longitudinal research.
Let’s take a closer look at the defining characteristics of longitudinal studies, review the pros and cons of this type of research, and share some useful longitudinal study examples.
Content Index
What is a longitudinal study?
Types of longitudinal studies, advantages and disadvantages of conducting longitudinal surveys.
- Longitudinal studies vs. cross-sectional studies
Types of surveys that use a longitudinal study
Longitudinal study examples.
A longitudinal study is a research conducted over an extended period of time. It is mostly used in medical research and other areas like psychology or sociology.
When using this method, a longitudinal survey can pay off with actionable insights when you have the time to engage in a long-term research project.
Longitudinal studies often use surveys to collect data that is either qualitative or quantitative. Additionally, in a longitudinal study, a survey creator does not interfere with survey participants. Instead, the survey creator distributes questionnaires over time to observe changes in participants, behaviors, or attitudes.
Many medical studies are longitudinal; researchers note and collect data from the same subjects over what can be many years.
LEARN ABOUT: Action Research
Longitudinal studies are versatile, repeatable, and able to account for quantitative and qualitative data . Consider the three major types of longitudinal studies for future research:
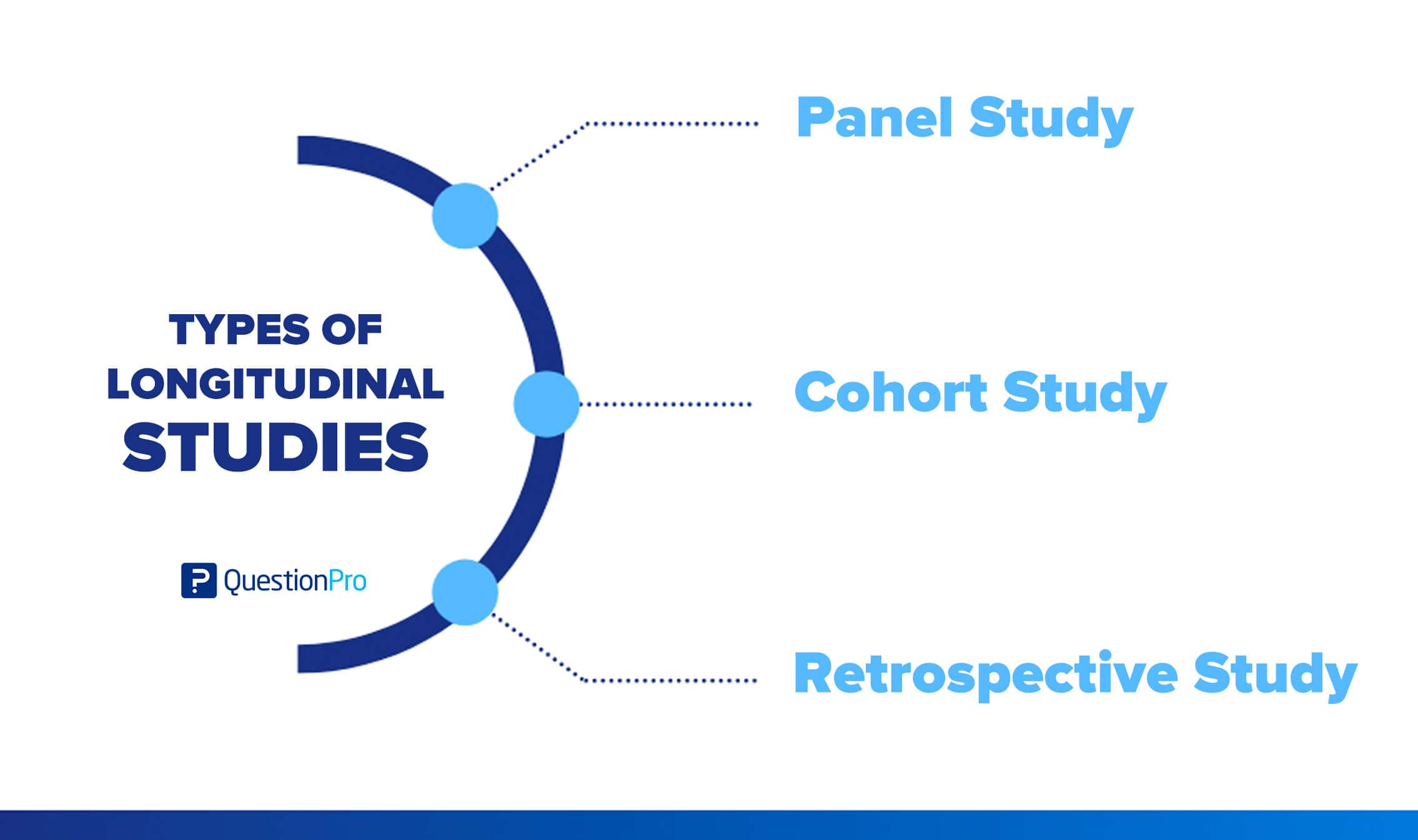
Panel study: A panel survey involves a sample of people from a more significant population and is conducted at specified intervals for a more extended period.
One of the panel study’s essential features is that researchers collect data from the same sample at different points in time. Most panel studies are designed for quantitative analysis , though they may also be used to collect qualitative data and unit of analysis .
LEARN ABOUT: Level of Analysis
Cohort Study: A cohort study samples a cohort (a group of people who typically experience the same event at a given point in time). Medical researchers tend to conduct cohort studies. Some might consider clinical trials similar to cohort studies.
In cohort studies, researchers merely observe participants without intervention, unlike clinical trials in which participants undergo tests.
Retrospective study: A retrospective study uses already existing data, collected during previously conducted research with similar methodology and variables.
While doing a retrospective study, the researcher uses an administrative database, pre-existing medical records, or one-to-one interviews.
As we’ve demonstrated, a longitudinal study is useful in science, medicine, and many other fields. There are many reasons why a researcher might want to conduct a longitudinal study. One of the essential reasons is, longitudinal studies give unique insights that many other types of research fail to provide.
Advantages of longitudinal studies
- Greater validation: For a long-term study to be successful, objectives and rules must be established from the beginning. As it is a long-term study, its authenticity is verified in advance, which makes the results have a high level of validity.
- Unique data: Most research studies collect short-term data to determine the cause and effect of what is being investigated. Longitudinal surveys follow the same principles but the data collection period is different. Long-term relationships cannot be discovered in a short-term investigation, but short-term relationships can be monitored in a long-term investigation.
- Allow identifying trends: Whether in medicine, psychology, or sociology, the long-term design of a longitudinal study enables trends and relationships to be found within the data collected in real time. The previous data can be applied to know future results and have great discoveries.
- Longitudinal surveys are flexible: Although a longitudinal study can be created to study a specific data point, the data collected can show unforeseen patterns or relationships that can be significant. Because this is a long-term study, the researchers have a flexibility that is not possible with other research formats.
Additional data points can be collected to study unexpected findings, allowing changes to be made to the survey based on the approach that is detected.
Disadvantages of longitudinal studies
- Research time The main disadvantage of longitudinal surveys is that long-term research is more likely to give unpredictable results. For example, if the same person is not found to update the study, the research cannot be carried out. It may also take several years before the data begins to produce observable patterns or relationships that can be monitored.
- An unpredictability factor is always present It must be taken into account that the initial sample can be lost over time. Because longitudinal studies involve the same subjects over a long period of time, what happens to them outside of data collection times can influence the data that is collected in the future. Some people may decide to stop participating in the research. Others may not be in the correct demographics for research. If these factors are not included in the initial research design, they could affect the findings that are generated.
- Large samples are needed for the investigation to be meaningful To develop relationships or patterns, a large amount of data must be collected and extracted to generate results.
- Higher costs Without a doubt, the longitudinal survey is more complex and expensive. Being a long-term form of research, the costs of the study will span years or decades, compared to other forms of research that can be completed in a smaller fraction of the time.
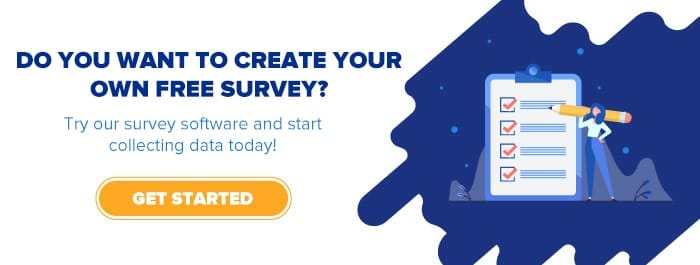
Longitudinal studies vs. Cross-sectional studies
Longitudinal studies are often confused with cross-sectional studies. Unlike longitudinal studies, where the research variables can change during a study, a cross-sectional study observes a single instance with all variables remaining the same throughout the study. A longitudinal study may follow up on a cross-sectional study to investigate the relationship between the variables more thoroughly.
The design of the study is highly dependent on the nature of the research questions . Whenever a researcher decides to collect data by surveying their participants, what matters most are the questions that are asked in the survey.
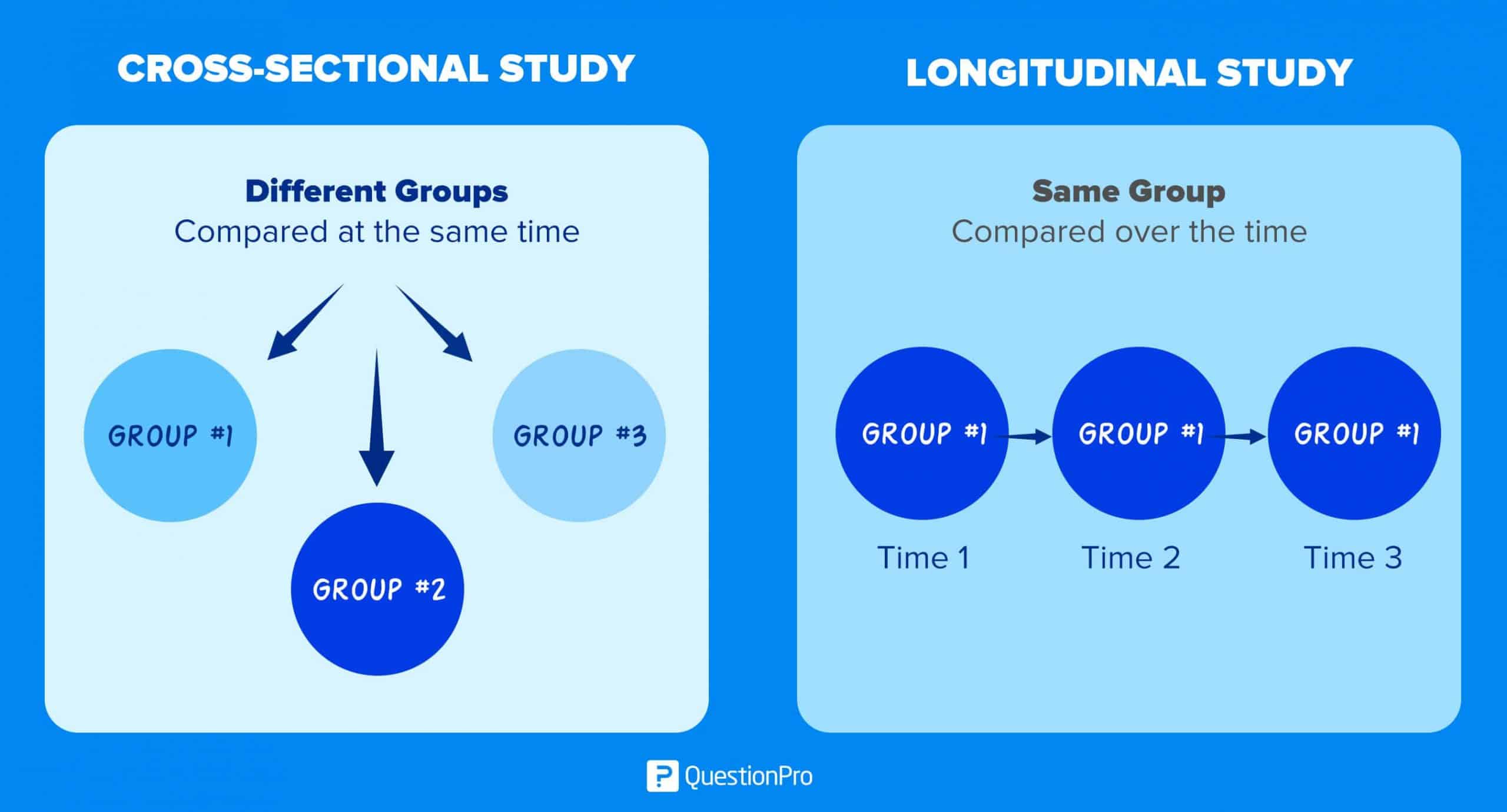
Knowing what information a study should gather is the first step in determining how to conduct the rest of the study.
With a longitudinal study, you can measure and compare various business and branding aspects by deploying surveys. Some of the classic examples of surveys that researchers can use for longitudinal studies are:
Market trends and brand awareness: Use a market research survey and marketing survey to identify market trends and develop brand awareness. Through these surveys, businesses or organizations can learn what customers want and what they will discard. This study can be carried over time to assess market trends repeatedly, as they are volatile and tend to change constantly.
Product feedback: If a business or brand launches a new product and wants to know how it is faring with consumers, product feedback surveys are a great option. Collect feedback from customers about the product over an extended time. Once you’ve collected the data, it’s time to put that feedback into practice and improve your offerings.
Customer satisfaction: Customer satisfaction surveys help an organization get to know the level of satisfaction or dissatisfaction among its customers. A longitudinal survey can gain feedback from new and regular customers for as long as you’d like to collect it, so it’s useful whether you’re starting a business or hoping to make some improvements to an established brand.
Employee engagement: When you check in regularly over time with a longitudinal survey, you’ll get a big-picture perspective of your company culture. Find out whether employees feel comfortable collaborating with colleagues and gauge their level of motivation at work.
Now that you know the basics of how researchers use longitudinal studies across several disciplines let’s review the following examples:
Example 1: Identical twins
Consider a study conducted to understand the similarities or differences between identical twins who are brought up together versus identical twins who were not. The study observes several variables, but the constant is that all the participants have identical twins.
In this case, researchers would want to observe these participants from childhood to adulthood, to understand how growing up in different environments influences traits, habits, and personality.
LEARN MORE ABOUT: Personality Survey
Over many years, researchers can see both sets of twins as they experience life without intervention. Because the participants share the same genes, it is assumed that any differences are due to environmental analysis , but only an attentive study can conclude those assumptions.
Example 2: Violence and video games
A group of researchers is studying whether there is a link between violence and video game usage. They collect a large sample of participants for the study. To reduce the amount of interference with their natural habits, these individuals come from a population that already plays video games. The age group is focused on teenagers (13-19 years old).
The researchers record how prone to violence participants in the sample are at the onset. It creates a baseline for later comparisons. Now the researchers will give a log to each participant to keep track of how much and how frequently they play and how much time they spend playing video games. This study can go on for months or years. During this time, the researcher can compare video game-playing behaviors with violent tendencies. Thus, investigating whether there is a link between violence and video games.
Conducting a longitudinal study with surveys is straightforward and applicable to almost any discipline. With our survey software you can easily start your own survey today.
GET STARTED
MORE LIKE THIS
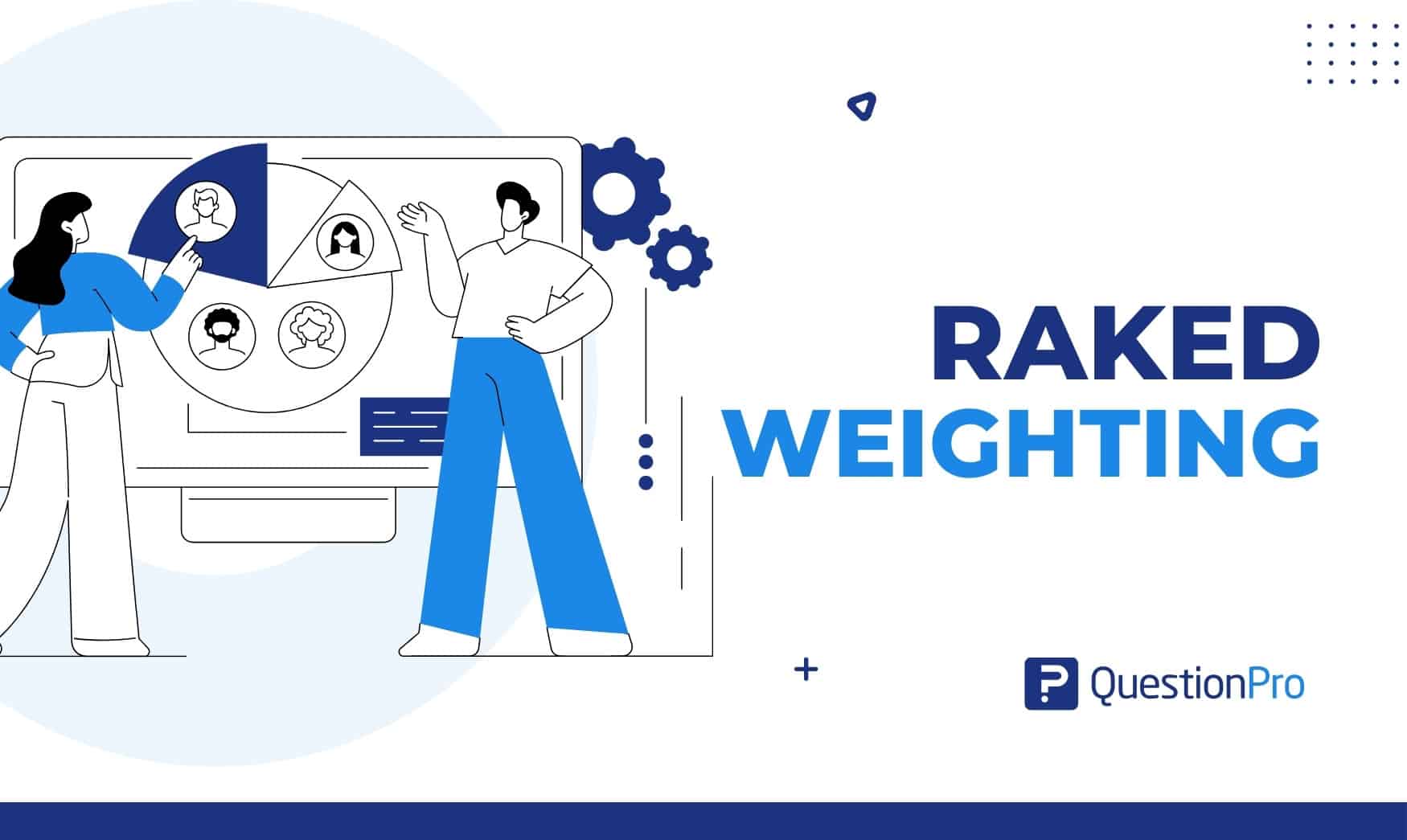
Raked Weighting: A Key Tool for Accurate Survey Results
May 31, 2024
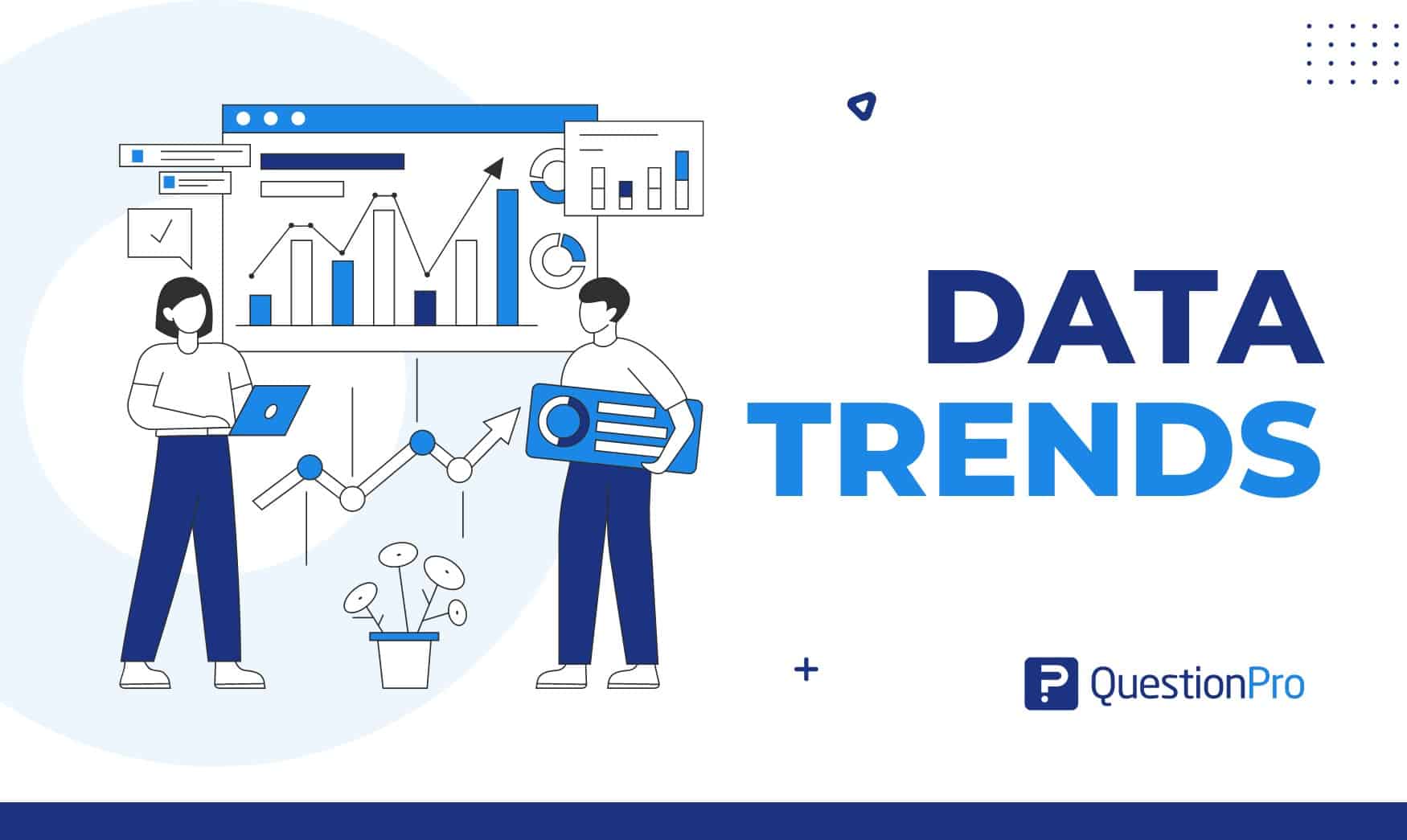
Top 8 Data Trends to Understand the Future of Data
May 30, 2024
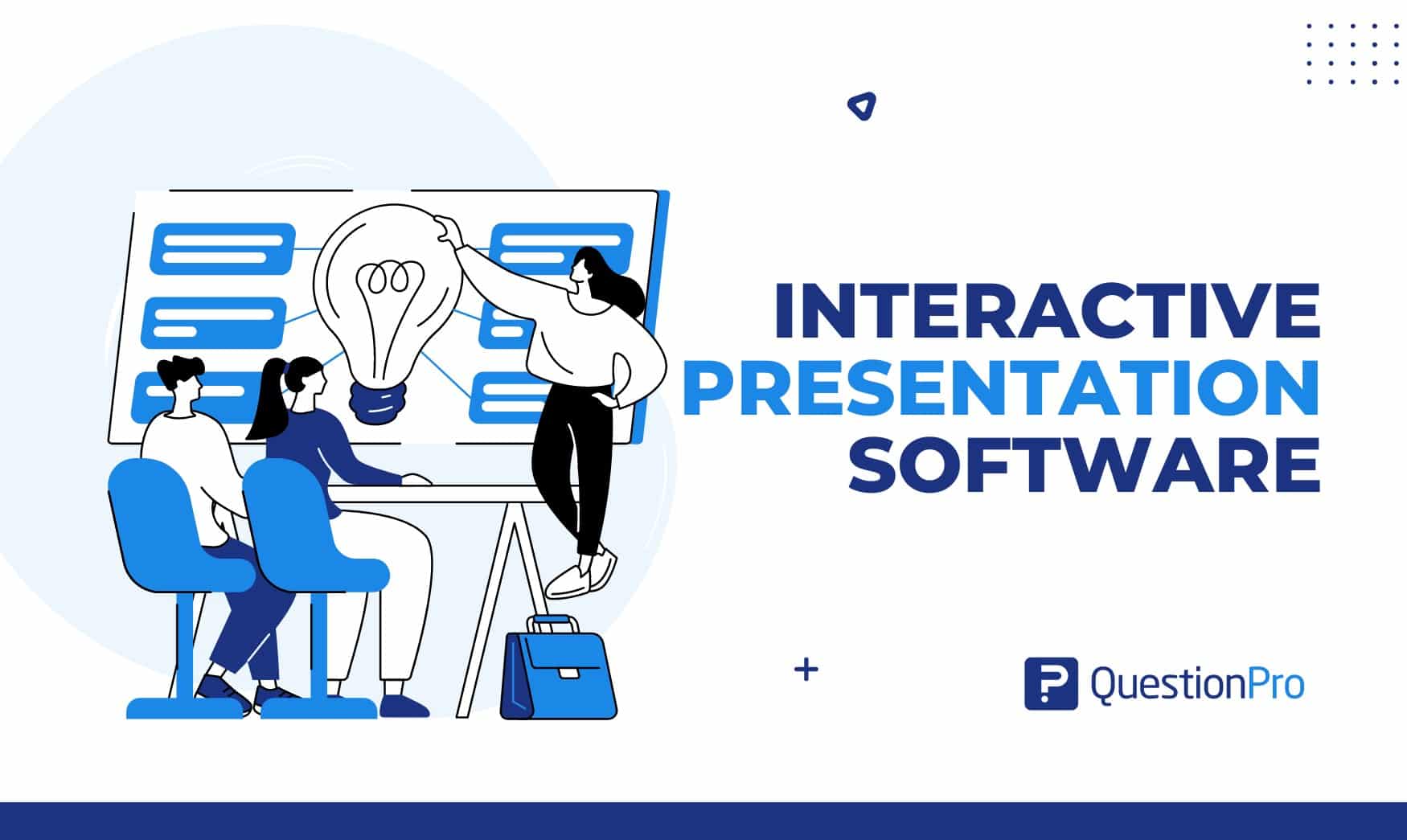
Top 12 Interactive Presentation Software to Engage Your User
May 29, 2024
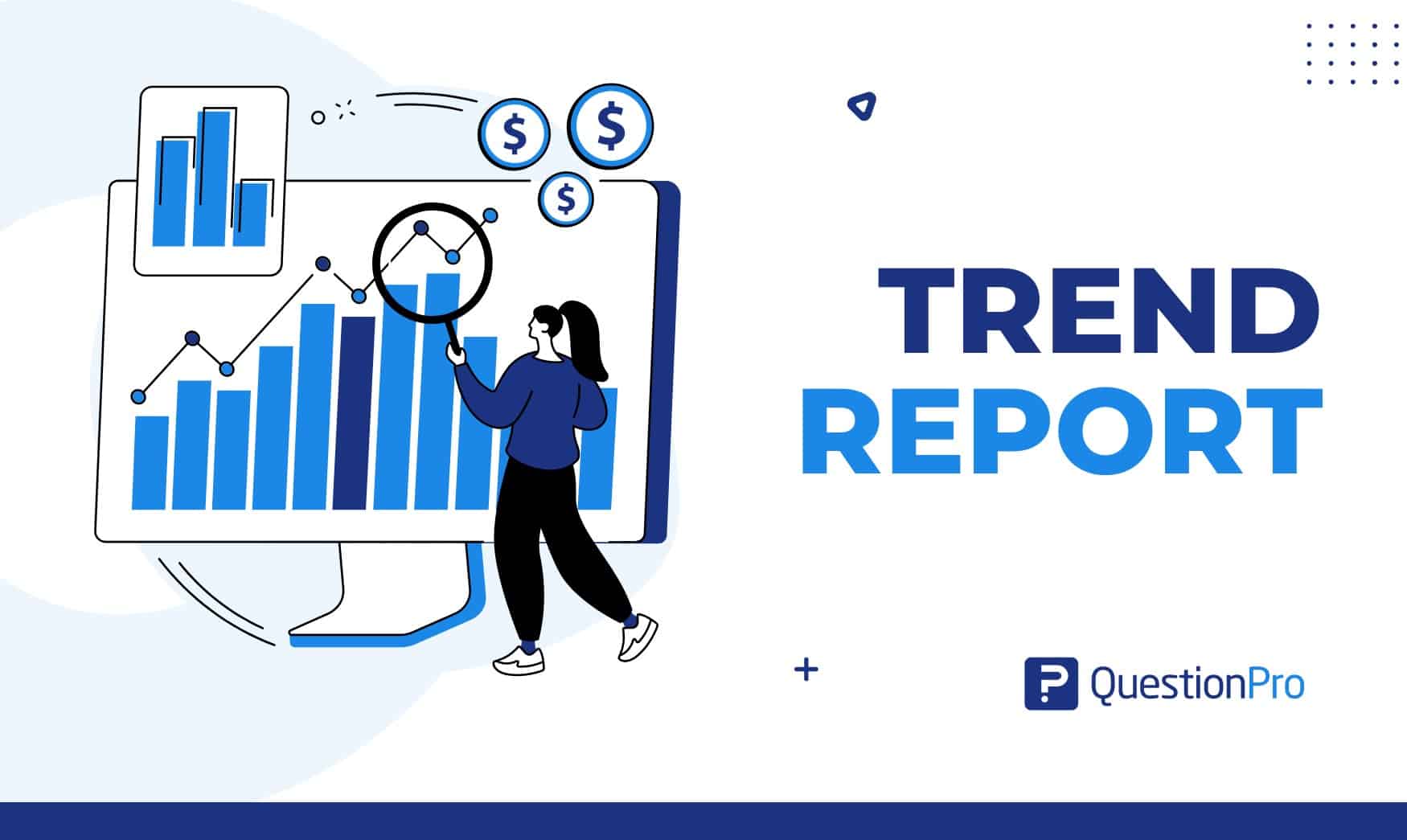
Trend Report: Guide for Market Dynamics & Strategic Analysis
Other categories.
- Academic Research
- Artificial Intelligence
- Assessments
- Brand Awareness
- Case Studies
- Communities
- Consumer Insights
- Customer effort score
- Customer Engagement
- Customer Experience
- Customer Loyalty
- Customer Research
- Customer Satisfaction
- Employee Benefits
- Employee Engagement
- Employee Retention
- Friday Five
- General Data Protection Regulation
- Insights Hub
- Life@QuestionPro
- Market Research
- Mobile diaries
- Mobile Surveys
- New Features
- Online Communities
- Question Types
- Questionnaire
- QuestionPro Products
- Release Notes
- Research Tools and Apps
- Revenue at Risk
- Survey Templates
- Training Tips
- Uncategorized
- Video Learning Series
- What’s Coming Up
- Workforce Intelligence
- Bipolar Disorder
- Therapy Center
- When To See a Therapist
- Types of Therapy
- Best Online Therapy
- Best Couples Therapy
- Best Family Therapy
- Managing Stress
- Sleep and Dreaming
- Understanding Emotions
- Self-Improvement
- Healthy Relationships
- Student Resources
- Personality Types
- Guided Meditations
- Verywell Mind Insights
- 2024 Verywell Mind 25
- Mental Health in the Classroom
- Editorial Process
- Meet Our Review Board
- Crisis Support
What Is a Longitudinal Study?
Tracking Variables Over Time
Kendra Cherry, MS, is a psychosocial rehabilitation specialist, psychology educator, and author of the "Everything Psychology Book."
:max_bytes(150000):strip_icc():format(webp)/IMG_9791-89504ab694d54b66bbd72cb84ffb860e.jpg)
Amanda Tust is a fact-checker, researcher, and writer with a Master of Science in Journalism from Northwestern University's Medill School of Journalism.
:max_bytes(150000):strip_icc():format(webp)/Amanda-Tust-1000-ffe096be0137462fbfba1f0759e07eb9.jpg)
Steve McAlister / The Image Bank / Getty Images
The Typical Longitudinal Study
Potential pitfalls, frequently asked questions.
A longitudinal study follows what happens to selected variables over an extended time. Psychologists use the longitudinal study design to explore possible relationships among variables in the same group of individuals over an extended period.
Once researchers have determined the study's scope, participants, and procedures, most longitudinal studies begin with baseline data collection. In the days, months, years, or even decades that follow, they continually gather more information so they can observe how variables change over time relative to the baseline.
For example, imagine that researchers are interested in the mental health benefits of exercise in middle age and how exercise affects cognitive health as people age. The researchers hypothesize that people who are more physically fit in their 40s and 50s will be less likely to experience cognitive declines in their 70s and 80s.
Longitudinal vs. Cross-Sectional Studies
Longitudinal studies, a type of correlational research , are usually observational, in contrast with cross-sectional research . Longitudinal research involves collecting data over an extended time, whereas cross-sectional research involves collecting data at a single point.
To test this hypothesis, the researchers recruit participants who are in their mid-40s to early 50s. They collect data related to current physical fitness, exercise habits, and performance on cognitive function tests. The researchers continue to track activity levels and test results for a certain number of years, look for trends in and relationships among the studied variables, and test the data against their hypothesis to form a conclusion.
Examples of Early Longitudinal Study Design
Examples of longitudinal studies extend back to the 17th century, when King Louis XIV periodically gathered information from his Canadian subjects, including their ages, marital statuses, occupations, and assets such as livestock and land. He used the data to spot trends over the years and understand his colonies' health and economic viability.
In the 18th century, Count Philibert Gueneau de Montbeillard conducted the first recorded longitudinal study when he measured his son every six months and published the information in "Histoire Naturelle."
The Genetic Studies of Genius (also known as the Terman Study of the Gifted), which began in 1921, is one of the first studies to follow participants from childhood into adulthood. Psychologist Lewis Terman's goal was to examine the similarities among gifted children and disprove the common assumption at the time that gifted children were "socially inept."
Types of Longitudinal Studies
Longitudinal studies fall into three main categories.
- Panel study : Sampling of a cross-section of individuals
- Cohort study : Sampling of a group based on a specific event, such as birth, geographic location, or experience
- Retrospective study : Review of historical information such as medical records
Benefits of Longitudinal Research
A longitudinal study can provide valuable insight that other studies can't. They're particularly useful when studying developmental and lifespan issues because they allow glimpses into changes and possible reasons for them.
For example, some longitudinal studies have explored differences and similarities among identical twins, some reared together and some apart. In these types of studies, researchers tracked participants from childhood into adulthood to see how environment influences personality , achievement, and other areas.
Because the participants share the same genetics , researchers chalked up any differences to environmental factors . Researchers can then look at what the participants have in common and where they differ to see which characteristics are more strongly influenced by either genetics or experience. Note that adoption agencies no longer separate twins, so such studies are unlikely today. Longitudinal studies on twins have shifted to those within the same household.
As with other types of psychology research, researchers must take into account some common challenges when considering, designing, and performing a longitudinal study.
Longitudinal studies require time and are often quite expensive. Because of this, these studies often have only a small group of subjects, which makes it difficult to apply the results to a larger population.
Selective Attrition
Participants sometimes drop out of a study for any number of reasons, like moving away from the area, illness, or simply losing motivation . This tendency, known as selective attrition , shrinks the sample size and decreases the amount of data collected.
If the final group no longer reflects the original representative sample , attrition can threaten the validity of the experiment. Validity refers to whether or not a test or experiment accurately measures what it claims to measure. If the final group of participants doesn't represent the larger group accurately, generalizing the study's conclusions is difficult.
The World’s Longest-Running Longitudinal Study
Lewis Terman aimed to investigate how highly intelligent children develop into adulthood with his "Genetic Studies of Genius." Results from this study were still being compiled into the 2000s. However, Terman was a proponent of eugenics and has been accused of letting his own sexism , racism , and economic prejudice influence his study and of drawing major conclusions from weak evidence. However, Terman's study remains influential in longitudinal studies. For example, a recent study found new information on the original Terman sample, which indicated that men who skipped a grade as children went on to have higher incomes than those who didn't.
A Word From Verywell
Longitudinal studies can provide a wealth of valuable information that would be difficult to gather any other way. Despite the typical expense and time involved, longitudinal studies from the past continue to influence and inspire researchers and students today.
A longitudinal study follows up with the same sample (i.e., group of people) over time, whereas a cross-sectional study examines one sample at a single point in time, like a snapshot.
A longitudinal study can occur over any length of time, from a few weeks to a few decades or even longer.
That depends on what researchers are investigating. A researcher can measure data on just one participant or thousands over time. The larger the sample size, of course, the more likely the study is to yield results that can be extrapolated.
Piccinin AM, Knight JE. History of longitudinal studies of psychological aging . Encyclopedia of Geropsychology. 2017:1103-1109. doi:10.1007/978-981-287-082-7_103
Terman L. Study of the gifted . In: The SAGE Encyclopedia of Educational Research, Measurement, and Evaluation. 2018. doi:10.4135/9781506326139.n691
Sahu M, Prasuna JG. Twin studies: A unique epidemiological tool . Indian J Community Med . 2016;41(3):177-182. doi:10.4103/0970-0218.183593
Almqvist C, Lichtenstein P. Pediatric twin studies . In: Twin Research for Everyone . Elsevier; 2022:431-438.
Warne RT. An evaluation (and vindication?) of Lewis Terman: What the father of gifted education can teach the 21st century . Gifted Child Q. 2018;63(1):3-21. doi:10.1177/0016986218799433
Warne RT, Liu JK. Income differences among grade skippers and non-grade skippers across genders in the Terman sample, 1936–1976 . Learning and Instruction. 2017;47:1-12. doi:10.1016/j.learninstruc.2016.10.004
Wang X, Cheng Z. Cross-sectional studies: Strengths, weaknesses, and recommendations . Chest . 2020;158(1S):S65-S71. doi:10.1016/j.chest.2020.03.012
Caruana EJ, Roman M, Hernández-Sánchez J, Solli P. Longitudinal studies . J Thorac Dis . 2015;7(11):E537-E540. doi:10.3978/j.issn.2072-1439.2015.10.63
By Kendra Cherry, MSEd Kendra Cherry, MS, is a psychosocial rehabilitation specialist, psychology educator, and author of the "Everything Psychology Book."
Insights AI
Table of Content
What is a longitudinal study definition, types, and examples.
Is your long-term research strategy unclear? Learn how longitudinal studies decode complexity. Read on for insights.
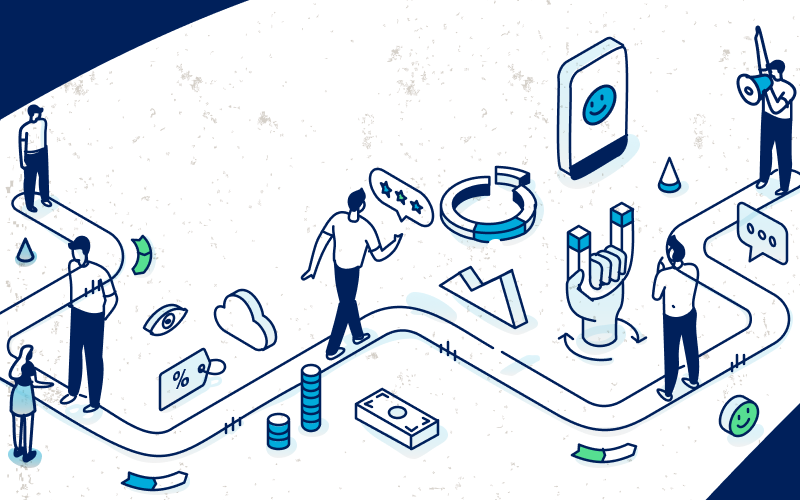
Have you ever wondered why certain questions can only be answered by going back in time?
Imagine being able to predict the long-term success of a new marketing campaign—not necessarily just from the first click-through rates, but from tracking the customer journey month after month or even year after year. This might include tracking brand awareness , purchase behavior, and customer satisfaction at appropriate intervals. This is where longitudinal studies, like time travelers, enter the research world to decode change and development across individuals, populations, and entire societies.
In this blog, we not only define what longitudinal studies are but also explore the different types and find real-world examples that prove the advantage of this research approach.
What is a Longitudinal Study?
A longitudinal study is a type of research where the scope of the study is observed and studied in the same set of people over a long period of time. This could be from a few weeks to many years.
They are most often found in different fields like health, economics, and medicine. They serve to give knowledge of the passage of events without knowing what is happening.
A company may conduct a study to observe how things change with time without interfering with what's happening. For example, an e-commerce company may ask the same questions to the same people every few months or years to determine whether the advertisement is working or whether more people are falling in love with their products.
Types of Longitudinal Studies
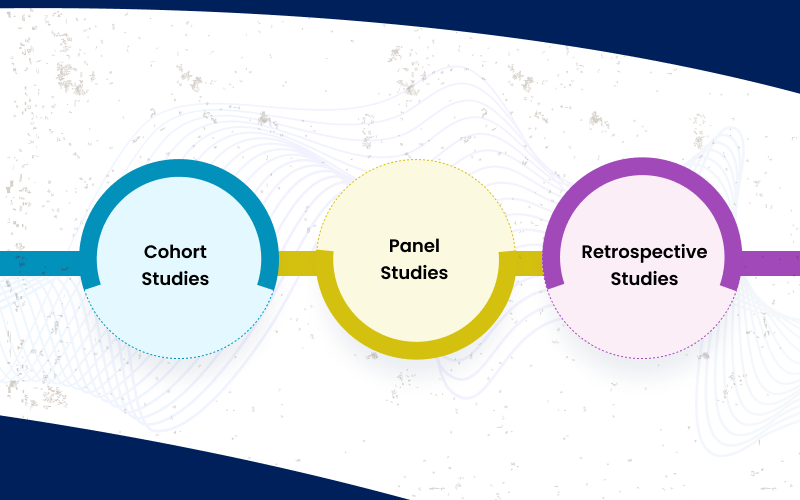
Cohort Studies
Cohort studies follow specific groups, or cohorts, of individuals over time. These groups usually share a common characteristic, such as being born in the same year or living in the same area. Researchers observe how this group changes and develops, often focusing on the impact of certain exposures or events on their health, behavior, or other outcomes.
Key Points:
Selection: Participants are chosen based on a shared characteristic.
Focus: Studying the effects of exposures or experiences on the group.
Example: The Nurses' Health Study , a large prospective cohort study launched in 1976, has followed over 100,000 female nurses to investigate various risk factors for chronic diseases like heart disease, cancer, and dementia. By observing their health and lifestyle choices over decades, researchers have gained valuable insights into the long-term impact of different factors on health outcomes.
Panel Studies
Panel studies involve collecting data from the same group of individuals at multiple time points. Unlike cohort studies, panel studies focus on the same people rather than forming groups based on shared characteristics. This allows researchers to examine individual-level changes over time.
Selection: Representative sample of a larger population.
Focus: Observing general trends and changes within the sample.
Example: The American National Election Studies (ANES) is a long-running panel study that surveys a representative sample of the US population every two years. This allows researchers to track changes in public opinion on various political and social issues over time, revealing trends in voter preferences and societal attitudes.
Retrospective Studies
Retrospective studies look back in time to collect data on past events or behaviors. Researchers gather information from participants about their past experiences and then follow up with them to track outcomes. These studies are useful for investigating long-term effects or rare events.
Data source: Existing records, medical charts, surveys, etc.
Focus: Analyzing past data to identify trends and associations.
Example: The Danish National Birth Cohort study utilizes existing data from national registries, following all individuals born in Denmark since 1996. Researchers can analyze their health records, educational attainment, and socioeconomic data to identify risk factors for various health conditions. By analyzing historical data over a long period, researchers can investigate the long-term consequences of early-life exposures on health outcomes later in life. This can inform preventative measures and interventions during critical developmental stages.
Pros & Cons of Longitudinal Studies
Advantages of longitudinal studies, understanding change.
They can give some of the most valuable insights into the way people, population, or phenomena change over time, enabling a researcher to trace trends, patterns, and causal relations that would be invisible in a snapshot view.
Cause-and-effect Insights
Longitudinal studies—though not conclusive—can add to the understanding of potential cause-and-effect relationships by tracing how changes in one variable herald changes in another.
Rare Events
They can trace events that are rare and that, in a snapshot study, might not be seen. It provides data about rare occurrences.
Generalizability
Longitudinal studies can yield generalizable results depending on sample size and ways of selecting the sample.
Disadvantages of Longitudinal Studies
Time and resource intensive.
Conducting longitudinal studies can take years and usually requires hundreds of hours of time, resources, and sustained participant engagement. Thus, this is often the major barrier, particularly for long-term studies.
Participants who drop out of a study can also affect generalizability and introduce bias. The researcher will need to develop strategies to minimize attrition and control for possible biases.
Taking repeated data from the same participants can be expensive and will require a substantial amount of funding and logistical planning.
Delayed Results
Due to its extended duration, it may take years to notice meaningful changes and to obtain definitive results. This must be braved with challenges, while the research area requires an immediate solution.
Longitudinal studies have significant advantages in the study of change and development through time. However, there are substantial challenges in the design and conduct of such research that need to be pondered carefully.
Ways to Collect Longitudinal Study Data
When you're planning a study that follows people over time, you have to decide where to get your information from. There are two main options: using data that's already been collected by someone else or collecting your own data.
Using data from other sources means you can access information that's already been gathered by previous studies. This saves you time and money because you don't have to collect it yourself. But the downside is that you're limited to the information that was collected before, and it might not cover everything you're interested in.
If you can't find data that fits your study, you'll have to collect your own. This means gathering information yourself, which can make sure it's exactly what you need. The methods you use to collect data depend on the type of study you're doing. You can use live interviews, surveys, focus group discussions, etc, to collect data.
The key to getting good data is using the right tools to collect it. This helps you get the information quickly and accurately.
Use Cases of Longitudinal Studies
Here are some compelling use cases for longitudinal studies in consumer research:
Tracking Brand Loyalty and Customer Satisfaction
Panel Study: A company can recruit a representative sample of their customers and conduct regular surveys over time. This enables them to track changes in brand awareness, satisfaction levels, and purchase behavior. By seeing how these metrics change, companies can identify trends in customer loyalty, pinpoint areas for improvement, and measure the effectiveness of marketing campaigns.
Understanding Consumer Behavior and Preferences
Cohort Study: A company may focus on a specific customer segment defined by demographics, purchasing habits, or product usage. By following this cohort over time, they are able to observe how preferences, needs, and behaviors change with changing life stages, economic situations, or technological advancements. This helps companies adapt their products, services, and marketing strategies to stay relevant to their target audience.
Measuring the Long-term Impact of Marketing Initiatives
Prospective Cohort Study: A company can introduce a new marketing campaign and recruit a group of customers exposed to it. By following this cohort over time and comparing their behavior to a control group, the company can measure the long-term impact of the campaign on brand awareness, purchase behavior, and customer lifetime value. This allows for evidence-based decisions on future marketing investment and campaign optimization.
Identifying Emerging Trends and Predicting Future Needs
Retrospective and Panel Studies: With the help of historical customer data along with current trends, companies can identify emerging patterns in consumer behavior. This can inform product development, service innovation, and marketing strategies to stay ahead of the curve and anticipate future customer needs.
Personalization and Customer Relationship Management:
Longitudinal Data Gathering: A business that works on continuous data collection on customers' tastes and purchase history and their association with the brand can be better in personalized marketing messages, product recommendations, and other customer service interactions. This facilitates the building of more intimate relationships and, hence, better customer satisfaction and retention.
Monitoring Product Usage and User Engagement
Panel Study: Enlist a sample of users representing the population and monitor usage patterns over some period. This enables users to follow the frequency of use for specific features, how users engage with the product over time, and many other factors. This information can help in the product design, pointing out areas for improvement and tailoring the user experience.
Understanding User Needs and Preferences
Cohort Study: Focus on a specific subset of users defined by demographics, usage patterns, or some other dimension. By following this cohort over time, a company can monitor how their needs, preferences, and expectations are changing as they become experienced users. This information may help in product updates, feature development, and marketing strategies in order to match user needs.
Evaluating the Long-Term Effectiveness of Product Updates
Prospective Cohort Study: Make a specific product update or feature available to one group of users and expose the control group to the product without receiving the update. By monitoring the changes in usage patterns, satisfaction levels, and task completion rates over time, a company may know how effective the update is and what should be improved.
Feature Adoption and User Behavior Trends
Retrospective and Panel Studies: The analysis of historical usage data and current trends brings the emerging patterns of interaction of users with the product. This would help drive future product roadmaps and feature development priorities and even predict emerging user needs.
Personalization and User Experience Optimization
Longitudinal Data Collection: Constant data gathering with respect to user behavior, preferences, and feedback allows for personal product recommendations, feature suggestions, and in-app guidance. This leads to a more engaged and customized user experience; hence, it increases the satisfaction and retention of users.
Longitudinal Studies with Decode Diary Studies
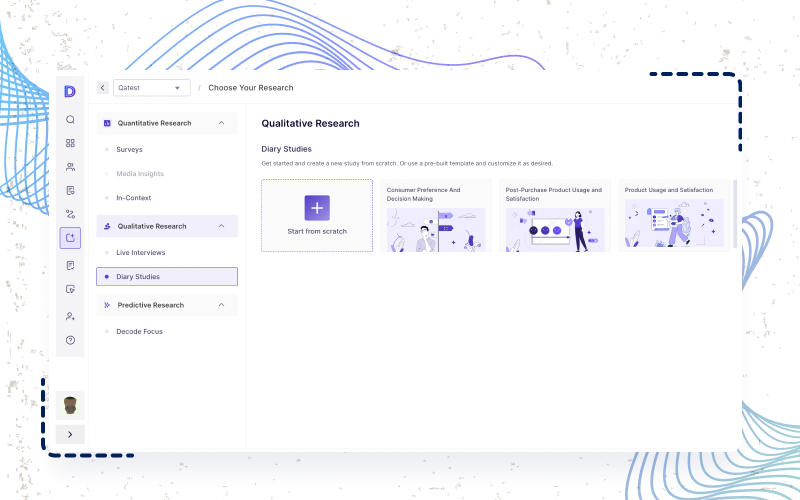
Decode provides a powerful DIY platform that makes it quite easy for researchers to conduct longitudinal studies, providing such rich tools to capture the experiences and behavior of users over long periods. Decode Diary studies allow researchers to conduct longitudinal research using longitudinal surveys, video responses, and image responses, improving the longitudinal research design.
{{cta-white}}
Frequently Asked Questions
What is a longitudinal study in research.
In a longitudinal study, researchers keep checking the same people over time to see if anything changes. These studies are like watching from the sidelines without trying to change anything, just to see how things naturally evolve.
What is the difference between longitudinal and cross-sectional studies?
The big difference is that cross-sectional studies talk to new groups of people every time they happen, while longitudinal studies stick with the same group of people and watch how they change over time.
What is an example of a longitudinal study?
A company tracking the career progression and performance of employees participating in a leadership development program over several years. By regularly collecting data on metrics like job performance, promotions, and job satisfaction, the company can evaluate the program's long-term impact on grooming future leaders and improving organizational success.
{{cta-button}}
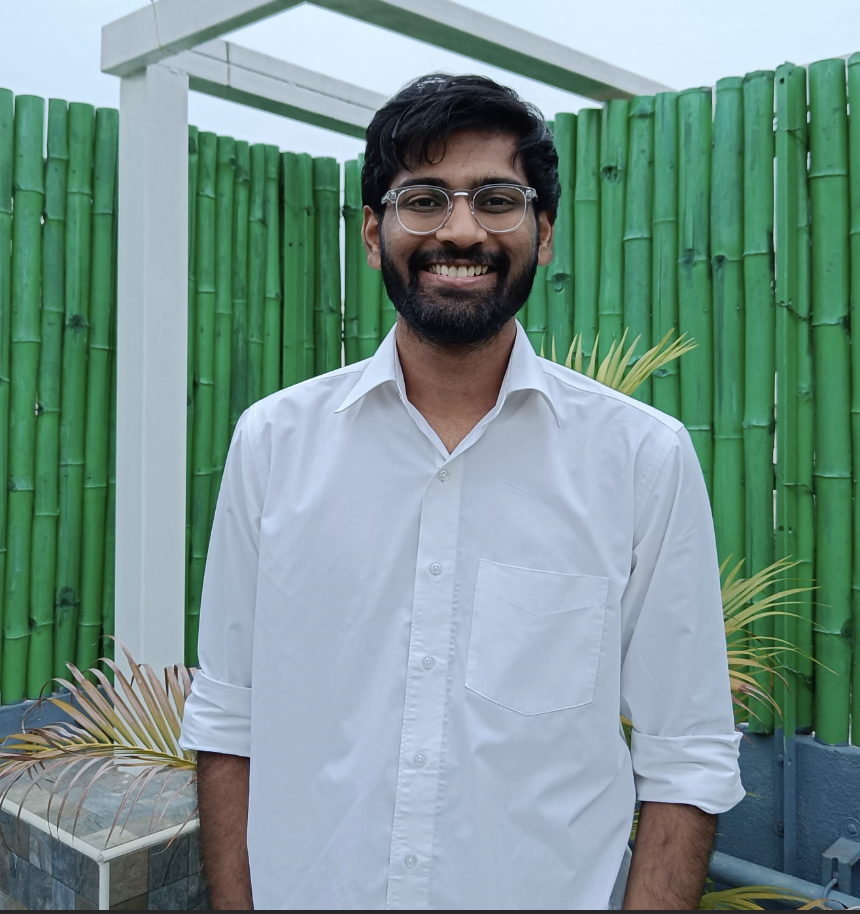
Get your Product Pack Design tested against competitors
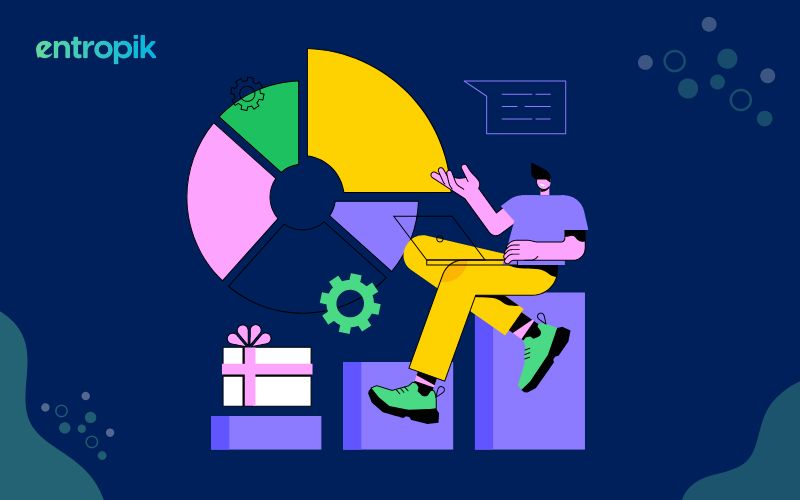
Got a question? Check out our FAQ’s
Book a demo.
- This is some text inside of a div block.
- Chapter 7. Longitudinal studies
Clinical follow up studies
- Chapter 1. What is epidemiology?
- Chapter 2. Quantifying disease in populations
- Chapter 3. Comparing disease rates
- Chapter 4. Measurement error and bias
- Chapter 5. Planning and conducting a survey
- Chapter 6. Ecological studies
- Chapter 8. Case-control and cross sectional studies
- Chapter 9. Experimental studies
- Chapter 10. Screening
- Chapter 11. Outbreaks of disease
- Chapter 12. Reading epidemiological reports
- Chapter 13. Further reading
Follow us on
Content links.
- Collections
- Health in South Asia
- Women’s, children’s & adolescents’ health
- News and views
- BMJ Opinion
- Rapid responses
- Editorial staff
- BMJ in the USA
- BMJ in South Asia
- Submit your paper
- BMA members
- Subscribers
- Advertisers and sponsors
Explore BMJ
- Our company
- BMJ Careers
- BMJ Learning
- BMJ Masterclasses
- BMJ Journals
- BMJ Student
- Academic edition of The BMJ
- BMJ Best Practice
- The BMJ Awards
- Email alerts
- Activate subscription
Information
Frequently asked questions
What is an example of a longitudinal study.
The 1970 British Cohort Study , which has collected data on the lives of 17,000 Brits since their births in 1970, is one well-known example of a longitudinal study .
Frequently asked questions: Methodology
Quantitative observations involve measuring or counting something and expressing the result in numerical form, while qualitative observations involve describing something in non-numerical terms, such as its appearance, texture, or color.
To make quantitative observations , you need to use instruments that are capable of measuring the quantity you want to observe. For example, you might use a ruler to measure the length of an object or a thermometer to measure its temperature.
Scope of research is determined at the beginning of your research process , prior to the data collection stage. Sometimes called “scope of study,” your scope delineates what will and will not be covered in your project. It helps you focus your work and your time, ensuring that you’ll be able to achieve your goals and outcomes.
Defining a scope can be very useful in any research project, from a research proposal to a thesis or dissertation . A scope is needed for all types of research: quantitative , qualitative , and mixed methods .
To define your scope of research, consider the following:
- Budget constraints or any specifics of grant funding
- Your proposed timeline and duration
- Specifics about your population of study, your proposed sample size , and the research methodology you’ll pursue
- Any inclusion and exclusion criteria
- Any anticipated control , extraneous , or confounding variables that could bias your research if not accounted for properly.
Inclusion and exclusion criteria are predominantly used in non-probability sampling . In purposive sampling and snowball sampling , restrictions apply as to who can be included in the sample .
Inclusion and exclusion criteria are typically presented and discussed in the methodology section of your thesis or dissertation .
The purpose of theory-testing mode is to find evidence in order to disprove, refine, or support a theory. As such, generalisability is not the aim of theory-testing mode.
Due to this, the priority of researchers in theory-testing mode is to eliminate alternative causes for relationships between variables . In other words, they prioritise internal validity over external validity , including ecological validity .
Convergent validity shows how much a measure of one construct aligns with other measures of the same or related constructs .
On the other hand, concurrent validity is about how a measure matches up to some known criterion or gold standard, which can be another measure.
Although both types of validity are established by calculating the association or correlation between a test score and another variable , they represent distinct validation methods.
Validity tells you how accurately a method measures what it was designed to measure. There are 4 main types of validity :
- Construct validity : Does the test measure the construct it was designed to measure?
- Face validity : Does the test appear to be suitable for its objectives ?
- Content validity : Does the test cover all relevant parts of the construct it aims to measure.
- Criterion validity : Do the results accurately measure the concrete outcome they are designed to measure?
Criterion validity evaluates how well a test measures the outcome it was designed to measure. An outcome can be, for example, the onset of a disease.
Criterion validity consists of two subtypes depending on the time at which the two measures (the criterion and your test) are obtained:
- Concurrent validity is a validation strategy where the the scores of a test and the criterion are obtained at the same time
- Predictive validity is a validation strategy where the criterion variables are measured after the scores of the test
Attrition refers to participants leaving a study. It always happens to some extent – for example, in randomised control trials for medical research.
Differential attrition occurs when attrition or dropout rates differ systematically between the intervention and the control group . As a result, the characteristics of the participants who drop out differ from the characteristics of those who stay in the study. Because of this, study results may be biased .
Criterion validity and construct validity are both types of measurement validity . In other words, they both show you how accurately a method measures something.
While construct validity is the degree to which a test or other measurement method measures what it claims to measure, criterion validity is the degree to which a test can predictively (in the future) or concurrently (in the present) measure something.
Construct validity is often considered the overarching type of measurement validity . You need to have face validity , content validity , and criterion validity in order to achieve construct validity.
Convergent validity and discriminant validity are both subtypes of construct validity . Together, they help you evaluate whether a test measures the concept it was designed to measure.
- Convergent validity indicates whether a test that is designed to measure a particular construct correlates with other tests that assess the same or similar construct.
- Discriminant validity indicates whether two tests that should not be highly related to each other are indeed not related. This type of validity is also called divergent validity .
You need to assess both in order to demonstrate construct validity. Neither one alone is sufficient for establishing construct validity.
Face validity and content validity are similar in that they both evaluate how suitable the content of a test is. The difference is that face validity is subjective, and assesses content at surface level.
When a test has strong face validity, anyone would agree that the test’s questions appear to measure what they are intended to measure.
For example, looking at a 4th grade math test consisting of problems in which students have to add and multiply, most people would agree that it has strong face validity (i.e., it looks like a math test).
On the other hand, content validity evaluates how well a test represents all the aspects of a topic. Assessing content validity is more systematic and relies on expert evaluation. of each question, analysing whether each one covers the aspects that the test was designed to cover.
A 4th grade math test would have high content validity if it covered all the skills taught in that grade. Experts(in this case, math teachers), would have to evaluate the content validity by comparing the test to the learning objectives.
Content validity shows you how accurately a test or other measurement method taps into the various aspects of the specific construct you are researching.
In other words, it helps you answer the question: “does the test measure all aspects of the construct I want to measure?” If it does, then the test has high content validity.
The higher the content validity, the more accurate the measurement of the construct.
If the test fails to include parts of the construct, or irrelevant parts are included, the validity of the instrument is threatened, which brings your results into question.
Construct validity refers to how well a test measures the concept (or construct) it was designed to measure. Assessing construct validity is especially important when you’re researching concepts that can’t be quantified and/or are intangible, like introversion. To ensure construct validity your test should be based on known indicators of introversion ( operationalisation ).
On the other hand, content validity assesses how well the test represents all aspects of the construct. If some aspects are missing or irrelevant parts are included, the test has low content validity.
- Discriminant validity indicates whether two tests that should not be highly related to each other are indeed not related
Construct validity has convergent and discriminant subtypes. They assist determine if a test measures the intended notion.
The reproducibility and replicability of a study can be ensured by writing a transparent, detailed method section and using clear, unambiguous language.
Reproducibility and replicability are related terms.
- A successful reproduction shows that the data analyses were conducted in a fair and honest manner.
- A successful replication shows that the reliability of the results is high.
- Reproducing research entails reanalysing the existing data in the same manner.
- Replicating (or repeating ) the research entails reconducting the entire analysis, including the collection of new data .
Snowball sampling is a non-probability sampling method . Unlike probability sampling (which involves some form of random selection ), the initial individuals selected to be studied are the ones who recruit new participants.
Because not every member of the target population has an equal chance of being recruited into the sample, selection in snowball sampling is non-random.
Snowball sampling is a non-probability sampling method , where there is not an equal chance for every member of the population to be included in the sample .
This means that you cannot use inferential statistics and make generalisations – often the goal of quantitative research . As such, a snowball sample is not representative of the target population, and is usually a better fit for qualitative research .
Snowball sampling relies on the use of referrals. Here, the researcher recruits one or more initial participants, who then recruit the next ones.
Participants share similar characteristics and/or know each other. Because of this, not every member of the population has an equal chance of being included in the sample, giving rise to sampling bias .
Snowball sampling is best used in the following cases:
- If there is no sampling frame available (e.g., people with a rare disease)
- If the population of interest is hard to access or locate (e.g., people experiencing homelessness)
- If the research focuses on a sensitive topic (e.g., extra-marital affairs)
Stratified sampling and quota sampling both involve dividing the population into subgroups and selecting units from each subgroup. The purpose in both cases is to select a representative sample and/or to allow comparisons between subgroups.
The main difference is that in stratified sampling, you draw a random sample from each subgroup ( probability sampling ). In quota sampling you select a predetermined number or proportion of units, in a non-random manner ( non-probability sampling ).
Random sampling or probability sampling is based on random selection. This means that each unit has an equal chance (i.e., equal probability) of being included in the sample.
On the other hand, convenience sampling involves stopping people at random, which means that not everyone has an equal chance of being selected depending on the place, time, or day you are collecting your data.
Convenience sampling and quota sampling are both non-probability sampling methods. They both use non-random criteria like availability, geographical proximity, or expert knowledge to recruit study participants.
However, in convenience sampling, you continue to sample units or cases until you reach the required sample size.
In quota sampling, you first need to divide your population of interest into subgroups (strata) and estimate their proportions (quota) in the population. Then you can start your data collection , using convenience sampling to recruit participants, until the proportions in each subgroup coincide with the estimated proportions in the population.
A sampling frame is a list of every member in the entire population . It is important that the sampling frame is as complete as possible, so that your sample accurately reflects your population.
Stratified and cluster sampling may look similar, but bear in mind that groups created in cluster sampling are heterogeneous , so the individual characteristics in the cluster vary. In contrast, groups created in stratified sampling are homogeneous , as units share characteristics.
Relatedly, in cluster sampling you randomly select entire groups and include all units of each group in your sample. However, in stratified sampling, you select some units of all groups and include them in your sample. In this way, both methods can ensure that your sample is representative of the target population .
When your population is large in size, geographically dispersed, or difficult to contact, it’s necessary to use a sampling method .
This allows you to gather information from a smaller part of the population, i.e. the sample, and make accurate statements by using statistical analysis. A few sampling methods include simple random sampling , convenience sampling , and snowball sampling .
The two main types of social desirability bias are:
- Self-deceptive enhancement (self-deception): The tendency to see oneself in a favorable light without realizing it.
- Impression managemen t (other-deception): The tendency to inflate one’s abilities or achievement in order to make a good impression on other people.
Response bias refers to conditions or factors that take place during the process of responding to surveys, affecting the responses. One type of response bias is social desirability bias .
Demand characteristics are aspects of experiments that may give away the research objective to participants. Social desirability bias occurs when participants automatically try to respond in ways that make them seem likeable in a study, even if it means misrepresenting how they truly feel.
Participants may use demand characteristics to infer social norms or experimenter expectancies and act in socially desirable ways, so you should try to control for demand characteristics wherever possible.
A systematic review is secondary research because it uses existing research. You don’t collect new data yourself.
Ethical considerations in research are a set of principles that guide your research designs and practices. These principles include voluntary participation, informed consent, anonymity, confidentiality, potential for harm, and results communication.
Scientists and researchers must always adhere to a certain code of conduct when collecting data from others .
These considerations protect the rights of research participants, enhance research validity , and maintain scientific integrity.
Research ethics matter for scientific integrity, human rights and dignity, and collaboration between science and society. These principles make sure that participation in studies is voluntary, informed, and safe.
Research misconduct means making up or falsifying data, manipulating data analyses, or misrepresenting results in research reports. It’s a form of academic fraud.
These actions are committed intentionally and can have serious consequences; research misconduct is not a simple mistake or a point of disagreement but a serious ethical failure.
Anonymity means you don’t know who the participants are, while confidentiality means you know who they are but remove identifying information from your research report. Both are important ethical considerations .
You can only guarantee anonymity by not collecting any personally identifying information – for example, names, phone numbers, email addresses, IP addresses, physical characteristics, photos, or videos.
You can keep data confidential by using aggregate information in your research report, so that you only refer to groups of participants rather than individuals.
Peer review is a process of evaluating submissions to an academic journal. Utilising rigorous criteria, a panel of reviewers in the same subject area decide whether to accept each submission for publication.
For this reason, academic journals are often considered among the most credible sources you can use in a research project – provided that the journal itself is trustworthy and well regarded.
In general, the peer review process follows the following steps:
- First, the author submits the manuscript to the editor.
- Reject the manuscript and send it back to author, or
- Send it onward to the selected peer reviewer(s)
- Next, the peer review process occurs. The reviewer provides feedback, addressing any major or minor issues with the manuscript, and gives their advice regarding what edits should be made.
- Lastly, the edited manuscript is sent back to the author. They input the edits, and resubmit it to the editor for publication.
Peer review can stop obviously problematic, falsified, or otherwise untrustworthy research from being published. It also represents an excellent opportunity to get feedback from renowned experts in your field.
It acts as a first defence, helping you ensure your argument is clear and that there are no gaps, vague terms, or unanswered questions for readers who weren’t involved in the research process.
Peer-reviewed articles are considered a highly credible source due to this stringent process they go through before publication.
Many academic fields use peer review , largely to determine whether a manuscript is suitable for publication. Peer review enhances the credibility of the published manuscript.
However, peer review is also common in non-academic settings. The United Nations, the European Union, and many individual nations use peer review to evaluate grant applications. It is also widely used in medical and health-related fields as a teaching or quality-of-care measure.
Peer assessment is often used in the classroom as a pedagogical tool. Both receiving feedback and providing it are thought to enhance the learning process, helping students think critically and collaboratively.
- In a single-blind study , only the participants are blinded.
- In a double-blind study , both participants and experimenters are blinded.
- In a triple-blind study , the assignment is hidden not only from participants and experimenters, but also from the researchers analysing the data.
Blinding is important to reduce bias (e.g., observer bias , demand characteristics ) and ensure a study’s internal validity .
If participants know whether they are in a control or treatment group , they may adjust their behaviour in ways that affect the outcome that researchers are trying to measure. If the people administering the treatment are aware of group assignment, they may treat participants differently and thus directly or indirectly influence the final results.
Blinding means hiding who is assigned to the treatment group and who is assigned to the control group in an experiment .
Explanatory research is a research method used to investigate how or why something occurs when only a small amount of information is available pertaining to that topic. It can help you increase your understanding of a given topic.
Explanatory research is used to investigate how or why a phenomenon occurs. Therefore, this type of research is often one of the first stages in the research process , serving as a jumping-off point for future research.
Exploratory research is a methodology approach that explores research questions that have not previously been studied in depth. It is often used when the issue you’re studying is new, or the data collection process is challenging in some way.
Exploratory research is often used when the issue you’re studying is new or when the data collection process is challenging for some reason.
You can use exploratory research if you have a general idea or a specific question that you want to study but there is no preexisting knowledge or paradigm with which to study it.
To implement random assignment , assign a unique number to every member of your study’s sample .
Then, you can use a random number generator or a lottery method to randomly assign each number to a control or experimental group. You can also do so manually, by flipping a coin or rolling a die to randomly assign participants to groups.
Random selection, or random sampling , is a way of selecting members of a population for your study’s sample.
In contrast, random assignment is a way of sorting the sample into control and experimental groups.
Random sampling enhances the external validity or generalisability of your results, while random assignment improves the internal validity of your study.
Random assignment is used in experiments with a between-groups or independent measures design. In this research design, there’s usually a control group and one or more experimental groups. Random assignment helps ensure that the groups are comparable.
In general, you should always use random assignment in this type of experimental design when it is ethically possible and makes sense for your study topic.
Clean data are valid, accurate, complete, consistent, unique, and uniform. Dirty data include inconsistencies and errors.
Dirty data can come from any part of the research process, including poor research design , inappropriate measurement materials, or flawed data entry.
Data cleaning takes place between data collection and data analyses. But you can use some methods even before collecting data.
For clean data, you should start by designing measures that collect valid data. Data validation at the time of data entry or collection helps you minimize the amount of data cleaning you’ll need to do.
After data collection, you can use data standardisation and data transformation to clean your data. You’ll also deal with any missing values, outliers, and duplicate values.
Data cleaning involves spotting and resolving potential data inconsistencies or errors to improve your data quality. An error is any value (e.g., recorded weight) that doesn’t reflect the true value (e.g., actual weight) of something that’s being measured.
In this process, you review, analyse, detect, modify, or remove ‘dirty’ data to make your dataset ‘clean’. Data cleaning is also called data cleansing or data scrubbing.
Data cleaning is necessary for valid and appropriate analyses. Dirty data contain inconsistencies or errors , but cleaning your data helps you minimise or resolve these.
Without data cleaning, you could end up with a Type I or II error in your conclusion. These types of erroneous conclusions can be practically significant with important consequences, because they lead to misplaced investments or missed opportunities.
Observer bias occurs when a researcher’s expectations, opinions, or prejudices influence what they perceive or record in a study. It usually affects studies when observers are aware of the research aims or hypotheses. This type of research bias is also called detection bias or ascertainment bias .
The observer-expectancy effect occurs when researchers influence the results of their own study through interactions with participants.
Researchers’ own beliefs and expectations about the study results may unintentionally influence participants through demand characteristics .
You can use several tactics to minimise observer bias .
- Use masking (blinding) to hide the purpose of your study from all observers.
- Triangulate your data with different data collection methods or sources.
- Use multiple observers and ensure inter-rater reliability.
- Train your observers to make sure data is consistently recorded between them.
- Standardise your observation procedures to make sure they are structured and clear.
Naturalistic observation is a valuable tool because of its flexibility, external validity , and suitability for topics that can’t be studied in a lab setting.
The downsides of naturalistic observation include its lack of scientific control , ethical considerations , and potential for bias from observers and subjects.
Naturalistic observation is a qualitative research method where you record the behaviours of your research subjects in real-world settings. You avoid interfering or influencing anything in a naturalistic observation.
You can think of naturalistic observation as ‘people watching’ with a purpose.
Closed-ended, or restricted-choice, questions offer respondents a fixed set of choices to select from. These questions are easier to answer quickly.
Open-ended or long-form questions allow respondents to answer in their own words. Because there are no restrictions on their choices, respondents can answer in ways that researchers may not have otherwise considered.
You can organise the questions logically, with a clear progression from simple to complex, or randomly between respondents. A logical flow helps respondents process the questionnaire easier and quicker, but it may lead to bias. Randomisation can minimise the bias from order effects.
Questionnaires can be self-administered or researcher-administered.
Self-administered questionnaires can be delivered online or in paper-and-pen formats, in person or by post. All questions are standardised so that all respondents receive the same questions with identical wording.
Researcher-administered questionnaires are interviews that take place by phone, in person, or online between researchers and respondents. You can gain deeper insights by clarifying questions for respondents or asking follow-up questions.
In a controlled experiment , all extraneous variables are held constant so that they can’t influence the results. Controlled experiments require:
- A control group that receives a standard treatment, a fake treatment, or no treatment
- Random assignment of participants to ensure the groups are equivalent
Depending on your study topic, there are various other methods of controlling variables .
An experimental group, also known as a treatment group, receives the treatment whose effect researchers wish to study, whereas a control group does not. They should be identical in all other ways.
A true experiment (aka a controlled experiment) always includes at least one control group that doesn’t receive the experimental treatment.
However, some experiments use a within-subjects design to test treatments without a control group. In these designs, you usually compare one group’s outcomes before and after a treatment (instead of comparing outcomes between different groups).
For strong internal validity , it’s usually best to include a control group if possible. Without a control group, it’s harder to be certain that the outcome was caused by the experimental treatment and not by other variables.
A questionnaire is a data collection tool or instrument, while a survey is an overarching research method that involves collecting and analysing data from people using questionnaires.
A Likert scale is a rating scale that quantitatively assesses opinions, attitudes, or behaviours. It is made up of four or more questions that measure a single attitude or trait when response scores are combined.
To use a Likert scale in a survey , you present participants with Likert-type questions or statements, and a continuum of items, usually with five or seven possible responses, to capture their degree of agreement.
Individual Likert-type questions are generally considered ordinal data , because the items have clear rank order, but don’t have an even distribution.
Overall Likert scale scores are sometimes treated as interval data. These scores are considered to have directionality and even spacing between them.
The type of data determines what statistical tests you should use to analyse your data.
A research hypothesis is your proposed answer to your research question. The research hypothesis usually includes an explanation (‘ x affects y because …’).
A statistical hypothesis, on the other hand, is a mathematical statement about a population parameter. Statistical hypotheses always come in pairs: the null and alternative hypotheses. In a well-designed study , the statistical hypotheses correspond logically to the research hypothesis.
A hypothesis states your predictions about what your research will find. It is a tentative answer to your research question that has not yet been tested. For some research projects, you might have to write several hypotheses that address different aspects of your research question.
A hypothesis is not just a guess. It should be based on existing theories and knowledge. It also has to be testable, which means you can support or refute it through scientific research methods (such as experiments, observations, and statistical analysis of data).
Cross-sectional studies are less expensive and time-consuming than many other types of study. They can provide useful insights into a population’s characteristics and identify correlations for further research.
Sometimes only cross-sectional data are available for analysis; other times your research question may only require a cross-sectional study to answer it.
Cross-sectional studies cannot establish a cause-and-effect relationship or analyse behaviour over a period of time. To investigate cause and effect, you need to do a longitudinal study or an experimental study .
Longitudinal studies and cross-sectional studies are two different types of research design . In a cross-sectional study you collect data from a population at a specific point in time; in a longitudinal study you repeatedly collect data from the same sample over an extended period of time.
Longitudinal studies are better to establish the correct sequence of events, identify changes over time, and provide insight into cause-and-effect relationships, but they also tend to be more expensive and time-consuming than other types of studies.
Longitudinal studies can last anywhere from weeks to decades, although they tend to be at least a year long.
A correlation reflects the strength and/or direction of the association between two or more variables.
- A positive correlation means that both variables change in the same direction.
- A negative correlation means that the variables change in opposite directions.
- A zero correlation means there’s no relationship between the variables.
A correlational research design investigates relationships between two variables (or more) without the researcher controlling or manipulating any of them. It’s a non-experimental type of quantitative research .
A correlation coefficient is a single number that describes the strength and direction of the relationship between your variables.
Different types of correlation coefficients might be appropriate for your data based on their levels of measurement and distributions . The Pearson product-moment correlation coefficient (Pearson’s r ) is commonly used to assess a linear relationship between two quantitative variables.
Controlled experiments establish causality, whereas correlational studies only show associations between variables.
- In an experimental design , you manipulate an independent variable and measure its effect on a dependent variable. Other variables are controlled so they can’t impact the results.
- In a correlational design , you measure variables without manipulating any of them. You can test whether your variables change together, but you can’t be sure that one variable caused a change in another.
In general, correlational research is high in external validity while experimental research is high in internal validity .
The third variable and directionality problems are two main reasons why correlation isn’t causation .
The third variable problem means that a confounding variable affects both variables to make them seem causally related when they are not.
The directionality problem is when two variables correlate and might actually have a causal relationship, but it’s impossible to conclude which variable causes changes in the other.
As a rule of thumb, questions related to thoughts, beliefs, and feelings work well in focus groups . Take your time formulating strong questions, paying special attention to phrasing. Be careful to avoid leading questions , which can bias your responses.
Overall, your focus group questions should be:
- Open-ended and flexible
- Impossible to answer with ‘yes’ or ‘no’ (questions that start with ‘why’ or ‘how’ are often best)
- Unambiguous, getting straight to the point while still stimulating discussion
- Unbiased and neutral
Social desirability bias is the tendency for interview participants to give responses that will be viewed favourably by the interviewer or other participants. It occurs in all types of interviews and surveys , but is most common in semi-structured interviews , unstructured interviews , and focus groups .
Social desirability bias can be mitigated by ensuring participants feel at ease and comfortable sharing their views. Make sure to pay attention to your own body language and any physical or verbal cues, such as nodding or widening your eyes.
This type of bias in research can also occur in observations if the participants know they’re being observed. They might alter their behaviour accordingly.
A focus group is a research method that brings together a small group of people to answer questions in a moderated setting. The group is chosen due to predefined demographic traits, and the questions are designed to shed light on a topic of interest. It is one of four types of interviews .
The four most common types of interviews are:
- Structured interviews : The questions are predetermined in both topic and order.
- Semi-structured interviews : A few questions are predetermined, but other questions aren’t planned.
- Unstructured interviews : None of the questions are predetermined.
- Focus group interviews : The questions are presented to a group instead of one individual.
An unstructured interview is the most flexible type of interview, but it is not always the best fit for your research topic.
Unstructured interviews are best used when:
- You are an experienced interviewer and have a very strong background in your research topic, since it is challenging to ask spontaneous, colloquial questions
- Your research question is exploratory in nature. While you may have developed hypotheses, you are open to discovering new or shifting viewpoints through the interview process.
- You are seeking descriptive data, and are ready to ask questions that will deepen and contextualise your initial thoughts and hypotheses
- Your research depends on forming connections with your participants and making them feel comfortable revealing deeper emotions, lived experiences, or thoughts
A semi-structured interview is a blend of structured and unstructured types of interviews. Semi-structured interviews are best used when:
- You have prior interview experience. Spontaneous questions are deceptively challenging, and it’s easy to accidentally ask a leading question or make a participant uncomfortable.
- Your research question is exploratory in nature. Participant answers can guide future research questions and help you develop a more robust knowledge base for future research.
The interviewer effect is a type of bias that emerges when a characteristic of an interviewer (race, age, gender identity, etc.) influences the responses given by the interviewee.
There is a risk of an interviewer effect in all types of interviews , but it can be mitigated by writing really high-quality interview questions.
A structured interview is a data collection method that relies on asking questions in a set order to collect data on a topic. They are often quantitative in nature. Structured interviews are best used when:
- You already have a very clear understanding of your topic. Perhaps significant research has already been conducted, or you have done some prior research yourself, but you already possess a baseline for designing strong structured questions.
- You are constrained in terms of time or resources and need to analyse your data quickly and efficiently
- Your research question depends on strong parity between participants, with environmental conditions held constant
More flexible interview options include semi-structured interviews , unstructured interviews , and focus groups .
When conducting research, collecting original data has significant advantages:
- You can tailor data collection to your specific research aims (e.g., understanding the needs of your consumers or user testing your website).
- You can control and standardise the process for high reliability and validity (e.g., choosing appropriate measurements and sampling methods ).
However, there are also some drawbacks: data collection can be time-consuming, labour-intensive, and expensive. In some cases, it’s more efficient to use secondary data that has already been collected by someone else, but the data might be less reliable.
Data collection is the systematic process by which observations or measurements are gathered in research. It is used in many different contexts by academics, governments, businesses, and other organisations.
A mediator variable explains the process through which two variables are related, while a moderator variable affects the strength and direction of that relationship.
A confounder is a third variable that affects variables of interest and makes them seem related when they are not. In contrast, a mediator is the mechanism of a relationship between two variables: it explains the process by which they are related.
If something is a mediating variable :
- It’s caused by the independent variable
- It influences the dependent variable
- When it’s taken into account, the statistical correlation between the independent and dependent variables is higher than when it isn’t considered
Including mediators and moderators in your research helps you go beyond studying a simple relationship between two variables for a fuller picture of the real world. They are important to consider when studying complex correlational or causal relationships.
Mediators are part of the causal pathway of an effect, and they tell you how or why an effect takes place. Moderators usually help you judge the external validity of your study by identifying the limitations of when the relationship between variables holds.
You can think of independent and dependent variables in terms of cause and effect: an independent variable is the variable you think is the cause , while a dependent variable is the effect .
In an experiment, you manipulate the independent variable and measure the outcome in the dependent variable. For example, in an experiment about the effect of nutrients on crop growth:
- The independent variable is the amount of nutrients added to the crop field.
- The dependent variable is the biomass of the crops at harvest time.
Defining your variables, and deciding how you will manipulate and measure them, is an important part of experimental design .
Discrete and continuous variables are two types of quantitative variables :
- Discrete variables represent counts (e.g., the number of objects in a collection).
- Continuous variables represent measurable amounts (e.g., water volume or weight).
Quantitative variables are any variables where the data represent amounts (e.g. height, weight, or age).
Categorical variables are any variables where the data represent groups. This includes rankings (e.g. finishing places in a race), classifications (e.g. brands of cereal), and binary outcomes (e.g. coin flips).
You need to know what type of variables you are working with to choose the right statistical test for your data and interpret your results .
Determining cause and effect is one of the most important parts of scientific research. It’s essential to know which is the cause – the independent variable – and which is the effect – the dependent variable.
You want to find out how blood sugar levels are affected by drinking diet cola and regular cola, so you conduct an experiment .
- The type of cola – diet or regular – is the independent variable .
- The level of blood sugar that you measure is the dependent variable – it changes depending on the type of cola.
No. The value of a dependent variable depends on an independent variable, so a variable cannot be both independent and dependent at the same time. It must be either the cause or the effect, not both.
Yes, but including more than one of either type requires multiple research questions .
For example, if you are interested in the effect of a diet on health, you can use multiple measures of health: blood sugar, blood pressure, weight, pulse, and many more. Each of these is its own dependent variable with its own research question.
You could also choose to look at the effect of exercise levels as well as diet, or even the additional effect of the two combined. Each of these is a separate independent variable .
To ensure the internal validity of an experiment , you should only change one independent variable at a time.
To ensure the internal validity of your research, you must consider the impact of confounding variables. If you fail to account for them, you might over- or underestimate the causal relationship between your independent and dependent variables , or even find a causal relationship where none exists.
A confounding variable is closely related to both the independent and dependent variables in a study. An independent variable represents the supposed cause , while the dependent variable is the supposed effect . A confounding variable is a third variable that influences both the independent and dependent variables.
Failing to account for confounding variables can cause you to wrongly estimate the relationship between your independent and dependent variables.
There are several methods you can use to decrease the impact of confounding variables on your research: restriction, matching, statistical control, and randomisation.
In restriction , you restrict your sample by only including certain subjects that have the same values of potential confounding variables.
In matching , you match each of the subjects in your treatment group with a counterpart in the comparison group. The matched subjects have the same values on any potential confounding variables, and only differ in the independent variable .
In statistical control , you include potential confounders as variables in your regression .
In randomisation , you randomly assign the treatment (or independent variable) in your study to a sufficiently large number of subjects, which allows you to control for all potential confounding variables.
In scientific research, concepts are the abstract ideas or phenomena that are being studied (e.g., educational achievement). Variables are properties or characteristics of the concept (e.g., performance at school), while indicators are ways of measuring or quantifying variables (e.g., yearly grade reports).
The process of turning abstract concepts into measurable variables and indicators is called operationalisation .
In statistics, ordinal and nominal variables are both considered categorical variables .
Even though ordinal data can sometimes be numerical, not all mathematical operations can be performed on them.
A control variable is any variable that’s held constant in a research study. It’s not a variable of interest in the study, but it’s controlled because it could influence the outcomes.
Control variables help you establish a correlational or causal relationship between variables by enhancing internal validity .
If you don’t control relevant extraneous variables , they may influence the outcomes of your study, and you may not be able to demonstrate that your results are really an effect of your independent variable .
‘Controlling for a variable’ means measuring extraneous variables and accounting for them statistically to remove their effects on other variables.
Researchers often model control variable data along with independent and dependent variable data in regression analyses and ANCOVAs . That way, you can isolate the control variable’s effects from the relationship between the variables of interest.
An extraneous variable is any variable that you’re not investigating that can potentially affect the dependent variable of your research study.
A confounding variable is a type of extraneous variable that not only affects the dependent variable, but is also related to the independent variable.
There are 4 main types of extraneous variables :
- Demand characteristics : Environmental cues that encourage participants to conform to researchers’ expectations
- Experimenter effects : Unintentional actions by researchers that influence study outcomes
- Situational variables : Eenvironmental variables that alter participants’ behaviours
- Participant variables : Any characteristic or aspect of a participant’s background that could affect study results
The difference between explanatory and response variables is simple:
- An explanatory variable is the expected cause, and it explains the results.
- A response variable is the expected effect, and it responds to other variables.
The term ‘ explanatory variable ‘ is sometimes preferred over ‘ independent variable ‘ because, in real-world contexts, independent variables are often influenced by other variables. This means they aren’t totally independent.
Multiple independent variables may also be correlated with each other, so ‘explanatory variables’ is a more appropriate term.
On graphs, the explanatory variable is conventionally placed on the x -axis, while the response variable is placed on the y -axis.
- If you have quantitative variables , use a scatterplot or a line graph.
- If your response variable is categorical, use a scatterplot or a line graph.
- If your explanatory variable is categorical, use a bar graph.
A correlation is usually tested for two variables at a time, but you can test correlations between three or more variables.
An independent variable is the variable you manipulate, control, or vary in an experimental study to explore its effects. It’s called ‘independent’ because it’s not influenced by any other variables in the study.
Independent variables are also called:
- Explanatory variables (they explain an event or outcome)
- Predictor variables (they can be used to predict the value of a dependent variable)
- Right-hand-side variables (they appear on the right-hand side of a regression equation)
A dependent variable is what changes as a result of the independent variable manipulation in experiments . It’s what you’re interested in measuring, and it ‘depends’ on your independent variable.
In statistics, dependent variables are also called:
- Response variables (they respond to a change in another variable)
- Outcome variables (they represent the outcome you want to measure)
- Left-hand-side variables (they appear on the left-hand side of a regression equation)
Deductive reasoning is commonly used in scientific research, and it’s especially associated with quantitative research .
In research, you might have come across something called the hypothetico-deductive method . It’s the scientific method of testing hypotheses to check whether your predictions are substantiated by real-world data.
Deductive reasoning is a logical approach where you progress from general ideas to specific conclusions. It’s often contrasted with inductive reasoning , where you start with specific observations and form general conclusions.
Deductive reasoning is also called deductive logic.
Inductive reasoning is a method of drawing conclusions by going from the specific to the general. It’s usually contrasted with deductive reasoning, where you proceed from general information to specific conclusions.
Inductive reasoning is also called inductive logic or bottom-up reasoning.
In inductive research , you start by making observations or gathering data. Then, you take a broad scan of your data and search for patterns. Finally, you make general conclusions that you might incorporate into theories.
Inductive reasoning is a bottom-up approach, while deductive reasoning is top-down.
Inductive reasoning takes you from the specific to the general, while in deductive reasoning, you make inferences by going from general premises to specific conclusions.
There are many different types of inductive reasoning that people use formally or informally.
Here are a few common types:
- Inductive generalisation : You use observations about a sample to come to a conclusion about the population it came from.
- Statistical generalisation: You use specific numbers about samples to make statements about populations.
- Causal reasoning: You make cause-and-effect links between different things.
- Sign reasoning: You make a conclusion about a correlational relationship between different things.
- Analogical reasoning: You make a conclusion about something based on its similarities to something else.
It’s often best to ask a variety of people to review your measurements. You can ask experts, such as other researchers, or laypeople, such as potential participants, to judge the face validity of tests.
While experts have a deep understanding of research methods , the people you’re studying can provide you with valuable insights you may have missed otherwise.
Face validity is important because it’s a simple first step to measuring the overall validity of a test or technique. It’s a relatively intuitive, quick, and easy way to start checking whether a new measure seems useful at first glance.
Good face validity means that anyone who reviews your measure says that it seems to be measuring what it’s supposed to. With poor face validity, someone reviewing your measure may be left confused about what you’re measuring and why you’re using this method.
Face validity is about whether a test appears to measure what it’s supposed to measure. This type of validity is concerned with whether a measure seems relevant and appropriate for what it’s assessing only on the surface.
Statistical analyses are often applied to test validity with data from your measures. You test convergent validity and discriminant validity with correlations to see if results from your test are positively or negatively related to those of other established tests.
You can also use regression analyses to assess whether your measure is actually predictive of outcomes that you expect it to predict theoretically. A regression analysis that supports your expectations strengthens your claim of construct validity .
When designing or evaluating a measure, construct validity helps you ensure you’re actually measuring the construct you’re interested in. If you don’t have construct validity, you may inadvertently measure unrelated or distinct constructs and lose precision in your research.
Construct validity is often considered the overarching type of measurement validity , because it covers all of the other types. You need to have face validity , content validity, and criterion validity to achieve construct validity.
Construct validity is about how well a test measures the concept it was designed to evaluate. It’s one of four types of measurement validity , which includes construct validity, face validity , and criterion validity.
There are two subtypes of construct validity.
- Convergent validity : The extent to which your measure corresponds to measures of related constructs
- Discriminant validity: The extent to which your measure is unrelated or negatively related to measures of distinct constructs
Attrition bias can skew your sample so that your final sample differs significantly from your original sample. Your sample is biased because some groups from your population are underrepresented.
With a biased final sample, you may not be able to generalise your findings to the original population that you sampled from, so your external validity is compromised.
There are seven threats to external validity : selection bias , history, experimenter effect, Hawthorne effect , testing effect, aptitude-treatment, and situation effect.
The two types of external validity are population validity (whether you can generalise to other groups of people) and ecological validity (whether you can generalise to other situations and settings).
The external validity of a study is the extent to which you can generalise your findings to different groups of people, situations, and measures.
Attrition bias is a threat to internal validity . In experiments, differential rates of attrition between treatment and control groups can skew results.
This bias can affect the relationship between your independent and dependent variables . It can make variables appear to be correlated when they are not, or vice versa.
Internal validity is the extent to which you can be confident that a cause-and-effect relationship established in a study cannot be explained by other factors.
There are eight threats to internal validity : history, maturation, instrumentation, testing, selection bias , regression to the mean, social interaction, and attrition .
A sampling error is the difference between a population parameter and a sample statistic .
A statistic refers to measures about the sample , while a parameter refers to measures about the population .
Populations are used when a research question requires data from every member of the population. This is usually only feasible when the population is small and easily accessible.
Systematic sampling is a probability sampling method where researchers select members of the population at a regular interval – for example, by selecting every 15th person on a list of the population. If the population is in a random order, this can imitate the benefits of simple random sampling .
There are three key steps in systematic sampling :
- Define and list your population , ensuring that it is not ordered in a cyclical or periodic order.
- Decide on your sample size and calculate your interval, k , by dividing your population by your target sample size.
- Choose every k th member of the population as your sample.
Yes, you can create a stratified sample using multiple characteristics, but you must ensure that every participant in your study belongs to one and only one subgroup. In this case, you multiply the numbers of subgroups for each characteristic to get the total number of groups.
For example, if you were stratifying by location with three subgroups (urban, rural, or suburban) and marital status with five subgroups (single, divorced, widowed, married, or partnered), you would have 3 × 5 = 15 subgroups.
You should use stratified sampling when your sample can be divided into mutually exclusive and exhaustive subgroups that you believe will take on different mean values for the variable that you’re studying.
Using stratified sampling will allow you to obtain more precise (with lower variance ) statistical estimates of whatever you are trying to measure.
For example, say you want to investigate how income differs based on educational attainment, but you know that this relationship can vary based on race. Using stratified sampling, you can ensure you obtain a large enough sample from each racial group, allowing you to draw more precise conclusions.
In stratified sampling , researchers divide subjects into subgroups called strata based on characteristics that they share (e.g., race, gender, educational attainment).
Once divided, each subgroup is randomly sampled using another probability sampling method .
Multistage sampling can simplify data collection when you have large, geographically spread samples, and you can obtain a probability sample without a complete sampling frame.
But multistage sampling may not lead to a representative sample, and larger samples are needed for multistage samples to achieve the statistical properties of simple random samples .
In multistage sampling , you can use probability or non-probability sampling methods.
For a probability sample, you have to probability sampling at every stage. You can mix it up by using simple random sampling , systematic sampling , or stratified sampling to select units at different stages, depending on what is applicable and relevant to your study.
Cluster sampling is a probability sampling method in which you divide a population into clusters, such as districts or schools, and then randomly select some of these clusters as your sample.
The clusters should ideally each be mini-representations of the population as a whole.
There are three types of cluster sampling : single-stage, double-stage and multi-stage clustering. In all three types, you first divide the population into clusters, then randomly select clusters for use in your sample.
- In single-stage sampling , you collect data from every unit within the selected clusters.
- In double-stage sampling , you select a random sample of units from within the clusters.
- In multi-stage sampling , you repeat the procedure of randomly sampling elements from within the clusters until you have reached a manageable sample.
Cluster sampling is more time- and cost-efficient than other probability sampling methods , particularly when it comes to large samples spread across a wide geographical area.
However, it provides less statistical certainty than other methods, such as simple random sampling , because it is difficult to ensure that your clusters properly represent the population as a whole.
If properly implemented, simple random sampling is usually the best sampling method for ensuring both internal and external validity . However, it can sometimes be impractical and expensive to implement, depending on the size of the population to be studied,
If you have a list of every member of the population and the ability to reach whichever members are selected, you can use simple random sampling.
The American Community Survey is an example of simple random sampling . In order to collect detailed data on the population of the US, the Census Bureau officials randomly select 3.5 million households per year and use a variety of methods to convince them to fill out the survey.
Simple random sampling is a type of probability sampling in which the researcher randomly selects a subset of participants from a population . Each member of the population has an equal chance of being selected. Data are then collected from as large a percentage as possible of this random subset.
Sampling bias occurs when some members of a population are systematically more likely to be selected in a sample than others.
In multistage sampling , or multistage cluster sampling, you draw a sample from a population using smaller and smaller groups at each stage.
This method is often used to collect data from a large, geographically spread group of people in national surveys, for example. You take advantage of hierarchical groupings (e.g., from county to city to neighbourhood) to create a sample that’s less expensive and time-consuming to collect data from.
In non-probability sampling , the sample is selected based on non-random criteria, and not every member of the population has a chance of being included.
Common non-probability sampling methods include convenience sampling , voluntary response sampling, purposive sampling , snowball sampling , and quota sampling .
Probability sampling means that every member of the target population has a known chance of being included in the sample.
Probability sampling methods include simple random sampling , systematic sampling , stratified sampling , and cluster sampling .
Samples are used to make inferences about populations . Samples are easier to collect data from because they are practical, cost-effective, convenient, and manageable.
While a between-subjects design has fewer threats to internal validity , it also requires more participants for high statistical power than a within-subjects design .
Advantages:
- Prevents carryover effects of learning and fatigue.
- Shorter study duration.
Disadvantages:
- Needs larger samples for high power.
- Uses more resources to recruit participants, administer sessions, cover costs, etc.
- Individual differences may be an alternative explanation for results.
In a factorial design, multiple independent variables are tested.
If you test two variables, each level of one independent variable is combined with each level of the other independent variable to create different conditions.
Yes. Between-subjects and within-subjects designs can be combined in a single study when you have two or more independent variables (a factorial design). In a mixed factorial design, one variable is altered between subjects and another is altered within subjects.
Within-subjects designs have many potential threats to internal validity , but they are also very statistically powerful .
- Only requires small samples
- Statistically powerful
- Removes the effects of individual differences on the outcomes
- Internal validity threats reduce the likelihood of establishing a direct relationship between variables
- Time-related effects, such as growth, can influence the outcomes
- Carryover effects mean that the specific order of different treatments affect the outcomes
Quasi-experimental design is most useful in situations where it would be unethical or impractical to run a true experiment .
Quasi-experiments have lower internal validity than true experiments, but they often have higher external validity as they can use real-world interventions instead of artificial laboratory settings.
In experimental research, random assignment is a way of placing participants from your sample into different groups using randomisation. With this method, every member of the sample has a known or equal chance of being placed in a control group or an experimental group.
A quasi-experiment is a type of research design that attempts to establish a cause-and-effect relationship. The main difference between this and a true experiment is that the groups are not randomly assigned.
In a between-subjects design , every participant experiences only one condition, and researchers assess group differences between participants in various conditions.
In a within-subjects design , each participant experiences all conditions, and researchers test the same participants repeatedly for differences between conditions.
The word ‘between’ means that you’re comparing different conditions between groups, while the word ‘within’ means you’re comparing different conditions within the same group.
A confounding variable , also called a confounder or confounding factor, is a third variable in a study examining a potential cause-and-effect relationship.
A confounding variable is related to both the supposed cause and the supposed effect of the study. It can be difficult to separate the true effect of the independent variable from the effect of the confounding variable.
In your research design , it’s important to identify potential confounding variables and plan how you will reduce their impact.
Triangulation can help:
- Reduce bias that comes from using a single method, theory, or investigator
- Enhance validity by approaching the same topic with different tools
- Establish credibility by giving you a complete picture of the research problem
But triangulation can also pose problems:
- It’s time-consuming and labour-intensive, often involving an interdisciplinary team.
- Your results may be inconsistent or even contradictory.
There are four main types of triangulation :
- Data triangulation : Using data from different times, spaces, and people
- Investigator triangulation : Involving multiple researchers in collecting or analysing data
- Theory triangulation : Using varying theoretical perspectives in your research
- Methodological triangulation : Using different methodologies to approach the same topic
Experimental designs are a set of procedures that you plan in order to examine the relationship between variables that interest you.
To design a successful experiment, first identify:
- A testable hypothesis
- One or more independent variables that you will manipulate
- One or more dependent variables that you will measure
When designing the experiment, first decide:
- How your variable(s) will be manipulated
- How you will control for any potential confounding or lurking variables
- How many subjects you will include
- How you will assign treatments to your subjects
Exploratory research explores the main aspects of a new or barely researched question.
Explanatory research explains the causes and effects of an already widely researched question.
The key difference between observational studies and experiments is that, done correctly, an observational study will never influence the responses or behaviours of participants. Experimental designs will have a treatment condition applied to at least a portion of participants.
An observational study could be a good fit for your research if your research question is based on things you observe. If you have ethical, logistical, or practical concerns that make an experimental design challenging, consider an observational study. Remember that in an observational study, it is critical that there be no interference or manipulation of the research subjects. Since it’s not an experiment, there are no control or treatment groups either.
These are four of the most common mixed methods designs :
- Convergent parallel: Quantitative and qualitative data are collected at the same time and analysed separately. After both analyses are complete, compare your results to draw overall conclusions.
- Embedded: Quantitative and qualitative data are collected at the same time, but within a larger quantitative or qualitative design. One type of data is secondary to the other.
- Explanatory sequential: Quantitative data is collected and analysed first, followed by qualitative data. You can use this design if you think your qualitative data will explain and contextualise your quantitative findings.
- Exploratory sequential: Qualitative data is collected and analysed first, followed by quantitative data. You can use this design if you think the quantitative data will confirm or validate your qualitative findings.
Triangulation in research means using multiple datasets, methods, theories and/or investigators to address a research question. It’s a research strategy that can help you enhance the validity and credibility of your findings.
Triangulation is mainly used in qualitative research , but it’s also commonly applied in quantitative research . Mixed methods research always uses triangulation.
Operationalisation means turning abstract conceptual ideas into measurable observations.
For example, the concept of social anxiety isn’t directly observable, but it can be operationally defined in terms of self-rating scores, behavioural avoidance of crowded places, or physical anxiety symptoms in social situations.
Before collecting data , it’s important to consider how you will operationalise the variables that you want to measure.
Hypothesis testing is a formal procedure for investigating our ideas about the world using statistics. It is used by scientists to test specific predictions, called hypotheses , by calculating how likely it is that a pattern or relationship between variables could have arisen by chance.
There are five common approaches to qualitative research :
- Grounded theory involves collecting data in order to develop new theories.
- Ethnography involves immersing yourself in a group or organisation to understand its culture.
- Narrative research involves interpreting stories to understand how people make sense of their experiences and perceptions.
- Phenomenological research involves investigating phenomena through people’s lived experiences.
- Action research links theory and practice in several cycles to drive innovative changes.
There are various approaches to qualitative data analysis , but they all share five steps in common:
- Prepare and organise your data.
- Review and explore your data.
- Develop a data coding system.
- Assign codes to the data.
- Identify recurring themes.
The specifics of each step depend on the focus of the analysis. Some common approaches include textual analysis , thematic analysis , and discourse analysis .
In mixed methods research , you use both qualitative and quantitative data collection and analysis methods to answer your research question .
Methodology refers to the overarching strategy and rationale of your research project . It involves studying the methods used in your field and the theories or principles behind them, in order to develop an approach that matches your objectives.
Methods are the specific tools and procedures you use to collect and analyse data (e.g. experiments, surveys , and statistical tests ).
In shorter scientific papers, where the aim is to report the findings of a specific study, you might simply describe what you did in a methods section .
In a longer or more complex research project, such as a thesis or dissertation , you will probably include a methodology section , where you explain your approach to answering the research questions and cite relevant sources to support your choice of methods.
The research methods you use depend on the type of data you need to answer your research question .
- If you want to measure something or test a hypothesis , use quantitative methods . If you want to explore ideas, thoughts, and meanings, use qualitative methods .
- If you want to analyse a large amount of readily available data, use secondary data. If you want data specific to your purposes with control over how they are generated, collect primary data.
- If you want to establish cause-and-effect relationships between variables , use experimental methods. If you want to understand the characteristics of a research subject, use descriptive methods.
Ask our team
Want to contact us directly? No problem. We are always here for you.
- Chat with us
- Email [email protected]
- Call +44 (0)20 3917 4242
- WhatsApp +31 20 261 6040
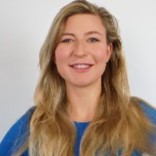
Our support team is here to help you daily via chat, WhatsApp, email, or phone between 9:00 a.m. to 11:00 p.m. CET.
Our APA experts default to APA 7 for editing and formatting. For the Citation Editing Service you are able to choose between APA 6 and 7.
Yes, if your document is longer than 20,000 words, you will get a sample of approximately 2,000 words. This sample edit gives you a first impression of the editor’s editing style and a chance to ask questions and give feedback.
How does the sample edit work?
You will receive the sample edit within 24 hours after placing your order. You then have 24 hours to let us know if you’re happy with the sample or if there’s something you would like the editor to do differently.
Read more about how the sample edit works
Yes, you can upload your document in sections.
We try our best to ensure that the same editor checks all the different sections of your document. When you upload a new file, our system recognizes you as a returning customer, and we immediately contact the editor who helped you before.
However, we cannot guarantee that the same editor will be available. Your chances are higher if
- You send us your text as soon as possible and
- You can be flexible about the deadline.
Please note that the shorter your deadline is, the lower the chance that your previous editor is not available.
If your previous editor isn’t available, then we will inform you immediately and look for another qualified editor. Fear not! Every Scribbr editor follows the Scribbr Improvement Model and will deliver high-quality work.
Yes, our editors also work during the weekends and holidays.
Because we have many editors available, we can check your document 24 hours per day and 7 days per week, all year round.
If you choose a 72 hour deadline and upload your document on a Thursday evening, you’ll have your thesis back by Sunday evening!
Yes! Our editors are all native speakers, and they have lots of experience editing texts written by ESL students. They will make sure your grammar is perfect and point out any sentences that are difficult to understand. They’ll also notice your most common mistakes, and give you personal feedback to improve your writing in English.
Every Scribbr order comes with our award-winning Proofreading & Editing service , which combines two important stages of the revision process.
For a more comprehensive edit, you can add a Structure Check or Clarity Check to your order. With these building blocks, you can customize the kind of feedback you receive.
You might be familiar with a different set of editing terms. To help you understand what you can expect at Scribbr, we created this table:
View an example
When you place an order, you can specify your field of study and we’ll match you with an editor who has familiarity with this area.
However, our editors are language specialists, not academic experts in your field. Your editor’s job is not to comment on the content of your dissertation, but to improve your language and help you express your ideas as clearly and fluently as possible.
This means that your editor will understand your text well enough to give feedback on its clarity, logic and structure, but not on the accuracy or originality of its content.
Good academic writing should be understandable to a non-expert reader, and we believe that academic editing is a discipline in itself. The research, ideas and arguments are all yours – we’re here to make sure they shine!
After your document has been edited, you will receive an email with a link to download the document.
The editor has made changes to your document using ‘Track Changes’ in Word. This means that you only have to accept or ignore the changes that are made in the text one by one.
It is also possible to accept all changes at once. However, we strongly advise you not to do so for the following reasons:
- You can learn a lot by looking at the mistakes you made.
- The editors don’t only change the text – they also place comments when sentences or sometimes even entire paragraphs are unclear. You should read through these comments and take into account your editor’s tips and suggestions.
- With a final read-through, you can make sure you’re 100% happy with your text before you submit!
You choose the turnaround time when ordering. We can return your dissertation within 24 hours , 3 days or 1 week . These timescales include weekends and holidays. As soon as you’ve paid, the deadline is set, and we guarantee to meet it! We’ll notify you by text and email when your editor has completed the job.
Very large orders might not be possible to complete in 24 hours. On average, our editors can complete around 13,000 words in a day while maintaining our high quality standards. If your order is longer than this and urgent, contact us to discuss possibilities.
Always leave yourself enough time to check through the document and accept the changes before your submission deadline.
Scribbr is specialised in editing study related documents. We check:
- Graduation projects
- Dissertations
- Admissions essays
- College essays
- Application essays
- Personal statements
- Process reports
- Reflections
- Internship reports
- Academic papers
- Research proposals
- Prospectuses
Calculate the costs
The fastest turnaround time is 24 hours.
You can upload your document at any time and choose between four deadlines:
At Scribbr, we promise to make every customer 100% happy with the service we offer. Our philosophy: Your complaint is always justified – no denial, no doubts.
Our customer support team is here to find the solution that helps you the most, whether that’s a free new edit or a refund for the service.
Yes, in the order process you can indicate your preference for American, British, or Australian English .
If you don’t choose one, your editor will follow the style of English you currently use. If your editor has any questions about this, we will contact you.

- Basics of Research Process
- Methodology
Longitudinal Research Design: Methods and Examples
- Speech Topics
- Basics of Essay Writing
- Essay Topics
- Other Essays
- Main Academic Essays
- Research Paper Topics
- Basics of Research Paper Writing
- Miscellaneous
- Chicago/ Turabian
- Data & Statistics
- Admission Writing Tips
- Admission Advice
- Other Guides
- Student Life
- Studying Tips
- Understanding Plagiarism
- Academic Writing Tips
- Basics of Dissertation & Thesis Writing

- Essay Guides
- Research Paper Guides
- Formatting Guides
- Admission Guides
- Dissertation & Thesis Guides

Table of contents

Use our free Readability checker

You may also like

A longitudinal study is a research method used to investigate changes in a group of subjects over an extended period of time. Unlike cross-sectional studies that capture data at a single point in time, longitudinal studies follow participants over a prolonged period. This allows researchers to examine how variables gradually evolve or affect individuals.
In case your research revolves around observing the same group of participants, you need to know well how to conduct longitudinal study. Today we’ll focus on this type of research data collection and find out which scientific areas require it. Its peculiar features and differences from other research types will also be examined. This article can help a lot with planning and organizing a research project over a long time period. Below you’ll find some tips on completing such work as well as a few helpful examples from a college paper writing service . Feel free to go on in case you aim to complete such work.

Or just unsure of where to start from? No worries, you’ve come to the right place – just check out our writing services . We are a team of skilled authors with significant experience in various academic areas. Just let us know how we can help you and you’ll get your paper completed in time!
Joe Eckel is an expert on Dissertations writing. He makes sure that each student gets precious insights on composing A-grade academic writing.
Let’s define ‘ longitudinal study ’ to begin with. This is an approach when data from the same respondents’ group is gathered repeatedly over a period of time. The reason why the same individuals are continuously observed over an extended period is to find changes and trends which can be analyzed. This approach is essentially observational as you aren’t expected to influence the group’s parameters you are monitoring in any way. It is typically used in scope of correlational research which means collecting data about variables without assuming any dependencies. Let’s find out more about its usage and how much time it could take.
How long is a longitudinal study? It depends on your topic and research goals. In case characteristics of the subject are changing fast, it might be enough to take just a few measurements one by one. Otherwise, one might have to wait for a long time before measuring again. So, such projects can take weeks or months but they also can extend over years or even decades. Studies like that are common in medicine, psychology and sociology, where it is important to observe how participants’ characteristics evolve.
Before actively engaging in longitudinal research, it is important to understand well what your next steps should be. Let’s define study subtypes that can be used for such research. They are:
Each of these subtypes has certain pros and cons. Gathering data yourself usually gives more confidence but it might be hard to contact the right individuals. Let’s discuss each point in detail. Likewise, you can pay someone to write my research paper .
When doing longitudinal studies of a certain group over a long period, you might find available data about them left from other researchers. Make sure to carefully examine sources of each dataset you decide to reuse. Otherwise previous researchers’ mistakes or bias may influence your results after you’ve analyzed that data. However this approach could be very efficient in case the subject has already been investigated by different researchers. Their results could be compared and gaps or bias could be easier to eliminate. As a result, much time and effort could be saved.
When doing longitudinal studies without any significant predecessors’ works available, using your own data is the only reasonable way. This data is collected through surveys, measurement or observations. Thus you have more confidence in these results however this approach requires more time and effort. You need proper research design methods prior to starting the collection process. If you choose such an approach, keep in mind that it has two major subtypes:
A longitudinal study can be applied to a wide range of cases. You need to adjust your approach, depending on a specific situation, subject’s peculiarities and your research goals. There are three major research types you can use for continuous observation:
Let’s take a closer look at each type’s definition with our coursework writing service . Dive deep to learn how data is collected and what impact is made on results.
A cohort longitudinal study involves selecting a group based on some unique event which unifies them all. It can be their birth date, geographic location, or historical experience. So there are special relationships between that group’s members which play significant roles for the entire research process. Such a peculiarity is to be carefully selected when doing test design and planning your test steps. Sometimes one unifying event may be more relevant or convenient than another.
This approach takes a special place among longitudinal studies as it involves conducting some historical investigations. As we’ve already mentioned above, during a retrospective, researchers have to make observations and measurements of past events. Collecting historical data and analyzing changes might be easier than tracking live data. However the development of such research design must include checking the credibility of datasets that were used for it.
A panel study involves sampling a cross-section of individuals. This approach is often used for collecting medical data. Such a study when performed continuously is considered more reliable compared to a regular cross sectional study and allows using smaller sample sizes, while still being representative. However, there are various problems that may occur during such studies, especially those which go on for decades. Particularly, such samples can be eventually eroded because of deaths, migration, fatigue, or even by development of response bias.
Longitudinal study design requires some serious planning to complete it properly. Keep in mind that your purpose is to directly address some individual change and variation cases. The target population should be chosen carefully so that results achieved through this study would be accurate enough. Another key element is deciding about proper timing. For example you would need bigger intervals to ensure you detect important changes. At the same time, dissertation writers suggest that the intervals shouldn’t be too big. Otherwise, you might lose track of the actual trends within your target population.
Let’s review longitudinal study advantages and disadvantages. Better wrap your head around this information if you are still choosing an optimal approach for your own project. Any study that involves complicated planning and extensive techniques can have some downsides. It is common for them to come together with benefits. So pay close attention to the information below before deciding what method to choose to observe your research subject.
These are the benefits of longitudinal study:
This is the disadvantages of longitudinal study:
Let’s review some longitudinal study example which would be helpful for illustrating the above information.
In this article we’ve explored the longitudinal research notion and reviewed its main characteristics:
Frequently Asked Questions About Longitudinal Studies
1. is a longitudinal study quantitative or qualitative.
According to the definition of a longitudinal study, quantitative methods don’t play any significant role in the process. This approach includes extended case studies, observing individuals over long periods and gaining additional insights thanks to the possibility to analyze changes over time. Since these observations and resulting assumptions mostly consist of descriptions of trends, changes and influences, we can say that it is a purely qualitative approach.
2. Are longitudinal studies more reliable?
Longitudinal studies in general have similar amounts of problems and risks as other studies do. This includes:
These factors can decrease reliability of this study type and must be taken into account when selecting such an approach.
3. Is attrition a limitation of longitudinal studies?
Depending on how big is the period they take, longitudinal studies may suffer more or less for the attrition factor. It can deteriorate generalizability of findings if participants who stay in a study are significantly different from those who drop out. In case a particular study takes many years, researchers need to see the attrition factor as a serious problem and to develop some ways to counter its negative effect.
4. What is longitudinal data collection?
Longitudinal data collection occurs sequentially from the same respondents over time. This is the core element of this study type. Repeated collection of data allows researchers to see temporal changes and understand what trends are there in this population. It allows viewing it from some new angles and thus to obtain new insights about it. There are certain limitations to such data collection, particularly when the target group tends to change over time.
- Collecting and analyzing your own data.
- Finding data already collected by some other researcher and analyzing it.
- retrospective research: collecting data about past events.
- prospective research: observing ongoing events, making measurements in more or less real time.
Longitudinal Cohort Study
Retrospective longitudinal study, longitudinal panel study.
- it can provide unique insight that might not be available any other way. Particularly, it is the only way to investigate lifespan issues. It allows researchers to track changes across the entire generation . Let’s suppose the task is to track the percentage of farms which pass from parents to children in a certain location. Obtaining such information requires using historical records.
- such observational approach shows dynamics in respondent’s data and thus allows to model trends and understand their influence. Collecting data once provides only a snapshot of your group’s current state. Doing it continuously allows you to observe this group from some new angles. For example, you would get more information about your respondents’ habits if you observe them at least several times.
- it can be quite expensive since numerous repeated measurements require enormous amounts of time and effort. Imagine you need to collect data about a certain group for 10 years. Processing this data alone would require a lot of resources.
- such high costs may induce another problem: researchers might decide to use lesser samples in order to cut the expenditures. Consequently, results of such studies may not be representative enough.
- its participants tend to drop out eventually. The reasons may vary: moving to another location, illness, death or just loss of motivation to participate further. As a result, a sample is shrinking and thus decreasing the amount of data collection in research . This process is called selective attrition. A typical example is observing the life of some neighborhood in a big city: numerous people would move in and out so it would be hard to find a single individual who is available for a long time.
- conducting observations and measurements continuously over a long period of time
- some particular new insights which can be obtained by prolonged studies
- prospective advantages and disadvantages for researchers.
- survey aging and period effects.
- delayed results.
- achieving continuity in funding and research direction.
- cumulative attrition.
What Is a Longitudinal Study: Definition
How long are longitudinal studies, how to perform longitudinal research, longitudinal study: data from other sources, longitudinal design: own data, longitudinal study types, longitudinal research design, advantages and disadvantages of longitudinal study, advantages of longitudinal study, longitudinal study disadvantages, longitudinal study examples, longitudinal: final thoughts.
Longitudinal research example A famous longitudinal case is The Terman Study of the Gifted also known previously as Genetic Studies of Genius. Its founder and the main researcher, Lewis Terman, aimed to investigate how highly intelligent children developed into adulthood. He was also going to disprove the then-prevalent belief that gifted children were typically delicate physically and also socially inept. Initial observations began in 1921, at Stanford University. Eventually it led to confirming that gifted children were not significantly different from their peers in terms of physical development and social skills. The results of this study were still being compiled during the 2000s which makes it the oldest and longest-running longitudinal study in the world. Such a huge period of data collection made it possible to obtain some really unique knowledge, not only about children’s development but about the history of education as well.
Thank you for visiting nature.com. You are using a browser version with limited support for CSS. To obtain the best experience, we recommend you use a more up to date browser (or turn off compatibility mode in Internet Explorer). In the meantime, to ensure continued support, we are displaying the site without styles and JavaScript.
- View all journals
- My Account Login
- Explore content
- About the journal
- Publish with us
- Sign up for alerts
- Open access
- Published: 30 May 2024
Longitudinal qualitative study on the psychological experiences of COVID-19 patients based on timing it right framework
- Liangyan Zhang 1 ,
- Chen Zhang 2 ,
- Kesang Li 1 &
- Yan Zhang 3
Scientific Reports volume 14 , Article number: 12409 ( 2024 ) Cite this article
60 Accesses
Metrics details
- Health care
Timing it right framework was used as a framework to explore the illness experiences of patients infected with COVID-19 and to analyze the patients' perceptions of the disease and their true inner feelings to provide a reference for the control of infectious diseases. This research adopted a phenomenological research approach to develop a longitudinal qualitative study. A purposive sampling method was used to select participants and 37 patients were recruited. Depending on the principle that participants should have maximum variation and sampling should cease when interviews content saturation is achieved, 16 COVID-19 patients in an isolation ward in Ningbo City, Zhejiang Province were finally included. Data were collected using semi-structured interviews, and the content of the interviews was analyzed by Colaizzi’s 7-step method. The themes of COVID-19 patients’ experiences at various phase were presented as follows: multiple emotions intertwined at the time of diagnosis (anxiety, stressful panic, facing the diagnosis calmly), multiple pressures during the hospitalization period (concerns about the disease, unable to adapt to the ward environment, worrying about future hardship), growth of positive illness experience during the isolation and observation period (sublimated outlook on life, affirmation of the government's anti-epidemic policy, more concerned about their own health), adjustment after returning to society (stigma, loss of previous living environment, problems caused by nucleic acid testing), and adaptation to social life (return to normal life, avoidance of illness experience, post-covid-19 syndrome). The illness experience of COVID-19 patients changed dynamically with time, but a sense of shame and uncertainty about recovery was present throughout the process. Interventions should be developed according to the needs of the patients at different times to inform subsequent optimization of care and management of infectious diseases.
Similar content being viewed by others
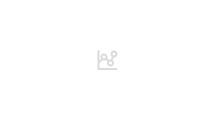
Evaluation of experiences of the patients discharged from the COVID-19 intensive care unit: a qualitative research
The impact of covid-19 on belgian mental health care: a delphi study among psychosocial health professionals, patients, and informal caretakers.
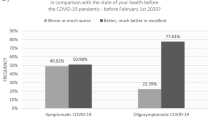
COVID-19 pandemic influence on self-reported health status and well-being in a society
Introduction.
The ongoing global epidemic of coronavirus disease 2019 (COVID-19) has had a significant impact on human society, primarily in terms of public health. As of 2 February 2023, the reported cumulative number of confirmed cases worldwide is approximately 753 million, with approximately 6,814,900 deaths 1 . Importantly, the actual number of infections worldwide may be higher than the reported data due to factors such as limited testing capacity. The Omicron and Delta strains are variants of coronaviruses with high loads, high infectivity, short incubation times, and antigenic escape 2 . Patients experience long viral nucleic acid conversion times and are more likely to develop severe and critical illnesses, with long periods of hospital isolation and medical observation 3 . Studies have shown 4 that patients with COVID-19 who stay in isolation are prone to psychological problems such as anxiety, depression, and fear. The study 5 showed that infected patients had problems such as fatigue, sleep difficulties, and anxiety after discharge from the hospital, and those with severe lung damage during hospitalization were even more likely to be in the target population for long-term rehabilitation interventions after discharge; however, the study was conducted on patients at the beginning of the 2019 outbreak, and the characteristics of the coronavirus variants can cause various psychological experiences in infected patients. There are currently few studies exploring mild COVID-19 patients' experiences during hospitalization and after reintegration into society 6 . The Timing it Right (TIR) framework was proposed by Cameron et al. 7 in the study of stroke care; it divides the process of disease development into five phases: diagnosis, stabilization, preparation, implementation and adaptation. At present, a number of scholars have used different research methods to study the dynamic needs of patients and caregivers with the TIR framework, and have achieved initial results 8 . Based on the TIR framework, it is possible to understand the changes in the experience of COVID-19 patients at different stages of illness, and formulate corresponding support plans, so that the intervention timing and intervention content can match the needs of patients at the current phase of disease 9 , 10 . Therefore, based on the five phases of disease development in the TIR framework, this research explore the illness experiences of COVID-19 patients in a longitudinal study to provide a reference for the subsequent care of patients with infectious diseases and the development of epidemic prevention and control strategies.
Design and participants
This study utilized a phenomenological research approach to develop a longitudinal qualitative study using the TIR framework. To conduct a longitudinal qualitative study, it is necessary to select specific time points for data collection, and the chosen time frame should be adequate for observing changes in the phenomenon under investigation 11 . In this study, the time frame for data collection was established through two interviews. The first interview (T1) took place during the period when the infected individuals were isolated, and the second interview (T2) occurred 6 months after their reintegration into society. The study employed a purposive sampling method to select patients who had been diagnosed with COVID-19 and were admitted to an isolation ward in Ningbo City, Zhejiang Province, between January and October 2022. The inclusion criteria were as follows: (1) confirmed COVID-19 infection; (2) age above 18 years; and (3) voluntary participation in this study and providing informed consent. The exclusion criteria were as follows: (1) those who were seriously ill and unable to communicate and (2) those with serious psychological or cognitive dysfunction. Those who automatically withdrew or were lost to follow-up during the interview were dropped from the study. A total of 37 patients were recruited for the study, and the sample size of the study was determined based on information saturation of the interviewees 12 . The specificity of the sampling was measured by demographic characteristics and the Self-Rating Depression Scale (SDS) 13 . Sampling ceased when interviews content saturation was achieved. Finally, 16 cases of representative infected patients were included in the study, including patients with no depression (9 cases, 56%), suspected mild depression (1 case, 6%), and suspected moderate depression (6 cases, 37.5%), numbered "N1" to "N16". In this study, two in-depth interviews were conducted with the infected patients, one during their isolation period and another after 6 months of reintegrating into society. Notably, during the second interview, two of the participants exhibited evasive behavior. For example, one participant was very talkative in the first interview, and he was able to talk more about his true feelings and describe them in more details based on the experience of the illness. However, in the second interview, he spoke less and only answered yes, no or fine, showing an evasive attitude. In order to fully understand the thoughts of the participants and enhance the integrity and continuity of the study, after obtaining informed consent, the interviewer supplemented the interview content by contacting their relatives, and returned the transcript of the interview content to the participants for confirmation, so as to ensure the authenticity of the content. The basic information of the study participants is shown in Table 1 .
Data collection
The participants provided informed consent and completed the demographic scale and SDS scale assessment before the interview. The interview outline was designed according to the five phases of diagnosis, stabilization, preparation, implementation and adaptation in the TIR framework. Longitudinal qualitative studies require researchers to use the insights gained from previous interviews to inform the focus of subsequent data collection 14 . The researcher preinterviewed two infected individuals prior to the formal interview and amended the interview outline. The interview outline was as follows. During Phase 1 (T1), the following questions were asked: ① How did you feel when you were diagnosed with COVID-19? ② What was your initial feeling after arriving at the isolation ward? ③ What are your needs and feelings during hospitalization? ④ How do you feel when you go to the quarantine point for medical observation? ⑤ How will the Spring Festival affect you? ⑥ If you are cured and returned to society, what expectations or concerns do you have? During Phase 2 (T2), the following questions were asked: ① What was your mood at the beginning of reintegration? Give an example of 1–2 things that were particularly impressive at that time. ② How do you feel now? How has your life changed? ③ Is there anything particularly troubling you at present? How do you solve these troubles? Do you have any hopes or suggestions? Due to the requirements of epidemic prevention and control, the interview was conducted by telephone, and the interview time was 15–60 min.
Data analysis
The interview content was analyzed by Colaizzi’s 7-step method. The steps are as follows: (1) two researchers carefully read all the original data; (2) they independently identify significant statements that were repeated by multiple participants and were important and meaningful to the research question; (3) they code repetitive and meaningful views by temporarily "suspending" their assumptions and value judgments; (4) they gather ideas after coding to form the prototype of the theme; (5) they write a detailed typical original description; (6) they identify similar ideas and condense them into themes; and (7) they return the topic structure to the participants for verification. The results of the SDS questionnaire were descriptive statistical analyzed by SPSS 25.0.
Quality control
SDS questionnaire collection: during hospitalization, a researcher issued and collected the questionnaire, explained the questions appropriately, and checked the completeness of the answers on the spot. (2) Interview data collection: all researchers in the study received professional training in qualitative research, and all interview data collection was completed by the first author (isolation ward nurse). The researcher kept in touch with the participants during the follow-up period to establish trust and friendly relationships. The researcher used the daily chat information as supplementary information. At the end of each interview, the researcher transcribed the audio-recorded information within 24 h and recorded the tone of voice, pitch change and other information of the participants. (3) Data analysis: data collection and analysis were carried out in parallel, with each of the 2 researchers listening to the audio-recordings and reading the textual information repeatedly, independently summarizing statements of significance, and summarizing the text of the statements. And the themes and subthemes extracted from the collected data were repeated to the participants by instant messaging software to further confirm whether they had the same feeling or experience and record any possible supplemental information. It was explained to the participants at the first interview that the preliminary results would be reported back to them for verification.
This study was reviewed and approved by the Ethics Committee of Ningbo No.2 Hospital (YJ-NBEY-KY-2022-102-01). All methods were performed in accordance with the relevant guidelines and regulations such as ethical standards of the institutional ethics committee and with the Declaration of Helsinki. All participants signed informed consent forms.
An interpretive understanding of the illness experiences of patients infected with COVID-19 was constructed in this study. The analysis yielded five categories and fifteen sub-categories (Table 2 ).
Theme 1: multiple emotions intertwined at the time of diagnosis
Due to different personality characteristics and understanding of the disease, infected patients showed the following emotional changes in the early stage of diagnosis.
Anxiety: since most infected patients learned about their infection suddenly, most of them had significant fidgeting, irritability, insomnia, and felt inexplicably nervous and worried when they were diagnosed or preparing for medical isolation. N5-T1: "I was so anxious when I was diagnosed that I felt like the sky was falling." N9-T1: "Since that time I have not been able to sleep well at night, I wake up after an hour of sleep." N2-T1: "I felt like it was going by so slowly and every day felt like years. Before the quarantine, I was worried that I wouldn't eat or sleep well, and that was true." Some of the infected patients felt upset when they were first diagnosed, as they received many calls for epidemiological investigations from various departments and organizations. N6-T1: "The initial phase has been very busy. I was the first to be infected, and there was a particular focus on me. At that time, my sleep was disturbed."
Stressful panic: the knowledge that there was no effective treatment, the uncertainty of the sequelae and the possibility of recurrence all put the infected patients in fear of the disease at the time of diagnosis, with three infected patients displaying more pronounced despair. N12-T1: "At that time, I was lying in bed for two days feeling like I was going to die. It was bad anyway. I was scared, scared of the after-effects, scared for my life (emotional)." N9-T1: "[Messages on my mobile phone] made me think nonsense. If the virus in my body had stayed, I would have been locked up forever. It gave me a feeling of panic."
Facing the diagnosis calmly: some of the infected patients were not so afraid of the diagnosis because people around them had already been infected. N1-T1: "The mood was a bit panicky, not particularly panicky. Because my in-laws have already been diagnosed, I already have a general idea in my mind." Some infected patients had a more comprehensive understanding of the corresponding symptoms of infection and were more receptive. N11-T1: "Not afraid of the disease. I have read the news that most of the foreign countries are infected, so I am calm." N3-T1: "It was mild… and curable, so I just didn't have much stress anyway."
Theme 2: multiple pressures during the hospitalization period
When patients were hospitalized, the disease itself, positivity for 2019-nCoV nucleic acid, and the specificity of the isolation environment made them feel deeply stressed.
Concerns about the disease: the threat to COVID-19 patients' lives and safety during hospitalization was stressful for those infected, either because of their own experiences of repositivity or because they witnessed others experiencing repositivity. N11-T1: "The biggest concern was that I didn't know how long it would take to be discharged from the hospital, how long it would take to recover … I didn't know if there were any after effects and whether there would be a relapse." N5-T1: "(After being repositive for the third time) I cried all the time during those 2 days when I was isolated again. The last 2 days were fine, (my mood) calmed down, and today I'm a little bit annoyed again, my wife and my youngest daughter they don't seem to have a good nucleic acid result. That aspect is affecting me a lot, a lot of stress (speaking faster)." N14-T1: "There is a feeling of fear in my heart. This is the first time I've had a repositive, and I've heard that there are a lot of repositive people, and I'm scared in my heart." Due to the special nature of COVID-19 patients, the vast majority of them chose to conceal their condition to avoid worrying about their families. N3-T1: "I didn't dare to make a video call to my mother. I was afraid that if my mother knew I was inside the hospital, she would have to worry about me."
Unable to adapt to the ward environment: most infected patients were transferred to isolation wards for medical observation and treatment, and the unfamiliar and confined environment often made them feel uncomfortable. N4-T1: "It feels unreal to be here, the air is treated and the rooms are airtight." Meanwhile, due to the shortage of beds, most isolation wards housed many people, to which some infected patients are not accustomed, and some infected patients were deeply stressed about secondary infections. N2-T2: "I don't like to sleep with other people in the same room. But, since I was a child, I have been timid and I am afraid to sleep alone. I am not used to sleeping in the hospital." N12-T1: "I was worried about my problems and whether sleeping in the same ward would spread the virus to each other, so I never took off my mask." Isolation also prevents infected patients from spending time with their families. During the festive season, which symbolizes reunion, they had to spend time alone. N10-T1: "The arrival of Chinese New Year had an impact on me. There are so many things I can't do because (I can't) be reunited with my family."
Worrying about future hardship: because the whole family was quarantined or because they themselves were the main laborer in the family, most infected patients reported that the long period of isolation had affected their financial income and were worried about the future. N5-T1: "There has been no income for a few months now, which means that life may be hard in the future." Due to the release of information from epidemiological surveys, infected patients are often worried about having the disease known to their acquaintances. N5-T2: "[The flow survey information] would be better to change that column where the surname is written to a number. Because we are the only ones infected in the factory, it is easy for others to guess." Infectious patients worry about social discrimination brought about by the disease and about whether they will still be able to have a suitable learning environment, job, and residence in the future. N5-T1: "I worry about whether it will affect my youngest daughter's ability to go to a better kindergarten." N10-T1: "I feel that I will not be able to find a job if people know about my disease. … I am sure I will be treated differently." "N2-T1: "We are renting a house outside, the landlord knows about it and may not let us stay in this place. What should we do?"
Theme 3: growth of positive illness experience during the isolation and observation period
Patients were under medical isolation and observation during this period. There is deeper thinking after enduring pressure from all sides, and some patients gain growth.
Sublimated outlook on life. Some infected patients were grateful for the dedication of healthcare workers. N1-T1: "Really, I feel that you are all very good. Doctors have it so hard. I didn't feel it before." Some patients thanked the teachers for caring for their children during the infection. N1-T2: "The teachers and classmates are very caring for my daughter. In particular, her class teacher was really nice. There was no one to look after her when she first came out (family members were quarantined for infection). My daughter was alone at home, and I was worried. Her teacher let my daughter go to her house. The teacher said I am not afraid." Some infected patients also said that during the isolation period, they had time to calm down and question their souls and thought more about life. N6-T1: "Actually, when I was in the isolation ward, I had more time and space to think, and then I might think more deeply about something, like the meaning of life. I would find so many things that I just naturally realized."
Affirmation of the government's anti-epidemic policy. N6-T1: "I think our government departments are very committed because our national situation is different from that of foreign countries, with a large population. If our country really doesn't care about controlling the epidemic at all, the medical resources can't keep up." N9-T1: "I fully comply with the rules and regulations of the isolation ward. The country invests a lot of resources. We eat and live medically for free."
More concerned about their own health. The experience of falling ill has made more patients pay more attention to their own health, and they actively participate in sports and pay attention to physical checkups and rest. N11-T1: "It's better to have a medical checkup to see if this virus has any other effects on your body. In the future, I will have regular medical check-ups once every six months or a year. Now, I also started to run (during the quarantine phase), I didn't run much before."
Theme 4: adjustment after returning to society
The infected often return to society in a state of fear and with a deep sense of stigma. Some infected patients have lost their former jobs and living environments, and their finances and lives have been greatly affected. At the same time, the nucleic acid test also brought troubles to their lives after returning to society.
Stigma: infected patients often have a heavy self-psychological burden when they return to society. They are afraid of being rejected and treated differently. The main manifestations are fear of stigma and concealment of medical history. N1-T2: "We definitely have to report to the community when we go back. We have to explain the situation. After explaining the situation, the people in the village will be scared to death, they will ostracize them (brother, parents), and then it will definitely have an impact on my parents and my brother's lives, so we didn't dare to go back." Some infected patients were worried that they would cause others to become infected, causing them to be quarantined as well. N16-T1: "I'm worried that if I go back to being positive in the future, I will infect others, even if it doesn't hurt, will I have to quarantine people for so many days?" With the shame of the disease, many infected patients were unable to go home to their families. N1-T2: "I would love to go back to my hometown. Because my parents are also 70 years old, and I can't take care of them when I'm out of town. But, now I can't go back to my hometown."
Loss of previous living environment. Some infected patients lost their original living and working environments after returning to society. Some COVID-19 patients moved away from their original environments of their own initiative because they were afraid that others would know that they had been infected by COVID-19. N1-T2: "We moved home. But, we are still afraid of bumping into people we know: "Some infected patients were asked not to go to work or to change their working environments for fear of mutual infection in their workplaces. N2-T2: "We were not allowed to go to work in the factory at that time because the other employees didn't agree to let us go to work." N9-T2: "She used to work in Department 5, but the original department didn't want her anymore." A new environment means a new start, which leads to greater pressure on the lives of infected patients. N1-T2: The pressure on my husband's life is too great. I also went to look for a job. After looking for a few days, I felt very sad and found that I could only do more flexible work like takeaway. Life is difficult, we've borrowed a lot of money now, and there's a lot of financial pressure and mental stress."
Problems caused by nucleic acid testing. Because of previous infections, policies require infected patients to have a separate nucleic acid test. This often meant that they had to spend time and effort looking for a separate site where they could do a separate nucleic acid test. N1-T2: "My child can't do a separate nucleic acid test at school anyway, so we have to find a nucleic acid test site by ourselves." When doing the nucleic acid test alone, they were split into two teams, separate from the people doing the mixed nucleic acid test, and the people in their team were faced with more inquiries about infection, which put more pressure on them." N1-T2: "When we went to do the nucleic acid test, the security guard at the door asked once, then the person who checked the identity after the questioning asked again, and the sampler asked again. I had nightmares every day." In addition, they were concerned that doing the nucleic acid test alone would increase the risk of reinfection. N5-T2: "When we do the nucleic acid test alone, we are with people who are at high risk. The book they have registered in we touch it again, register it again and go with them. If I get infected again, I reckon I'm going to go mad." Because of the fear of repositivity, infected patients are extremely concerned about the results of their own and their family's nucleic acid tests." N3-T2: "To be honest, some people, like my wife, have repeated repositive and go to quarantine sites over and over again. Very upset and depressed. I'm worried about my own and my wife's nucleic acid test results."
Theme 5: adaptation to social life
Six months after returning to society, most of the infected patients returned to normal life with the support and tolerance of society, but some of them still avoided infection and concealed their status as recovered persons. Some of the infected patients developed post-COVID-19 syndrome.
Return to normal life. Some infected patients integrated into society with the tolerance of colleagues and friends around them. N2-T2: "Still working as normal. My friends and I still play well and sit together for meals." N8-T2: "I was a bit afraid that my colleagues would laugh at me in various ways before I came to work. But, none of them did." Nucleic acid testing is also gradually becoming less frequent. N7-T2: "In the beginning, it was a single test, now it's all mixed." Some infected patients said that they no longer avoided the fact that they were infected under self-regulation and gradually returned to normal life. T12-N2: "When I first came out of quarantine, whenever people talked about this stuff, I wanted to avoid it and didn't want to hear about it. Now, when people talk about doing nucleic acids, I seem to slowly be able to accept it."
Avoidance of illness experience. Some infected patients still avoided infection and concealed their identities as a recovered person. N16-T2 Husband added: "Whoever mentions this, she is anxious about it. Some old folks called me and asked about this, and she was also a little anxious in her heart. There is nervousness in her heart, and she doesn't want to talk about it anymore." During the interview with N5-T2, the infected patient herself said, "I don't feel anything, it doesn't affect me at the moment. Everything is fine. Don't want to think about it." However, interviewing his lover, he said, "He used to look like a child, he used to laugh and joke around, but now he looks sad every day. He doesn't talk much in his free time." This experience has changed his personality.
Post-COVID-19 syndrome. Some infected patients still have residual weakness, chest tightness, loss of smell, sleep disorders, etc. N14-T2: "The body is a bit different anyway. I'm more tired than I used to be at work, and I'm not as fit as I used to be. Sleep has been bad, easy to wake up. I have no sense of smell." N15-T2: "I feel a bit breathless sometimes when I walk a few steps. I feel tired."
This study conducted a longitudinal exploration of COVID-19 patients' experience based on the TIR framework. In general, the TIR framework has played a good guiding role in the exploration of COVID-19 patients' illness experience. During the diagnosis phase, COVID-19 patients were usually very concerned about their own health problems, and due to great pressure caused by isolation, they were prone to psychological problems. The stabilization and preparation phase of COVID-19 patients were in the long isolation treatment. Patients gradually adapted to isolation treatment and started to plan their life after discharge. They were worried about discrimination and worried that they would never go back to the original life. In addition, because COVID-19 patients were kept in isolation for a long time, some patients began to reflect on the experience of the disease and the past life status, and thus gained growth. The stigma of COVID-19 patients was felt throughout the course of the illness, but it was particularly evident during the implementation and adaptation phase. Due to the stigma and discrimination, some patients actually made great changes to their work and life during this two phase. At this time, social support was particularly important for patients to reintegrate into society. The following discussion provides detailed analysis of the above phenomena.
The results of this study showed that all infected patients diagnosed for the first time had psychological problems such as anxiety, fear, and sleep disorders, and seven patients (43.75%) were suspected of having mild to moderate depression according to the SDS scores, which is similar to the results of the study by Deng et al. 15 . This may be due to the highly contagious nature of COVID-19 and the lack of specific treatment, resulting in infected patients being prone to excessive stress, panic, and even psychological stress disorder at the early stage of diagnosis 16 . At the same time, 14 cases (87.5%) of the infected patients expressed concern about the possibility of "repositivity" after recovery and the existence of sequelae. A related study found that noninfectious viral RNA persisted in most of the "repositive" cases, which may be due to slow disease regression 17 . A positive test does not always mean that the patient is infectious, as it may detect fragments of viral nucleic acid 18 . However, most patients know little about this and develop more anxiety and fear. In contrast, those patients who have knowledge of the new coronavirus tend to have less fear and worry. Cheng Hualing et al. 19 meta-integrated the psychological experience of COVID-19 patients in China and found that more information provided by medical personnel to infected patients about the treatment and prognosis of the disease could eliminate the anxiety caused by the patients' lack of knowledge. Therefore, health promotion should be strengthened, and patients should be encouraged to acquire knowledge of the disease in various aspects, including transmission channels, protective measures, symptom classification, prognosis, etc. Patients’ questions should be patiently answered based on their own conditions, and they should be encouraged to obtain correct information through official authoritative channels to avoid "information panic" 20 .
Four infected patients (25%) in this study indicated that the relatively confined environment and instruments in the isolation area caused feelings of loneliness and helplessness. The isolation treatment measures separated the patients from the outside world, as they left their familiar working and living environments to enter a state of isolation, confinement and monotony. The patients' original lifestyles were completely disrupted, and certain financial losses were incurred. The unfamiliar environment and medical equipment increased the patients' fear and loneliness while also hindering the discharge of their negative emotions. This is consistent with the findings of Wang et al. 20 and Shaban et al. 21 . A retrospective study 22 found that isolation may cause unexpected mental trauma for patients and may even lead to self-injurious behaviors such as suicide. These effects persisted 3 years after desegregation. Psychological disorders can lead to low immunity and reduced motivation for treatment, thus negatively affecting disease recovery 23 . Currently, the treatment and care of patients in isolation wards are mainly focused on the disease itself, with relatively little attention given to the physical and psychological effects of isolation. Improving the treatment environment in isolation areas, providing as much stimulation as possible during the normal routine of work and rest, establishing a good lifestyle, and diversifying hospital activities are of great significance in relieving emotional tension, breaking psychological barriers, and promoting disease recovery 24 . Studies have shown that having sufficient sunlight is particularly effective for psychological relief 25 . Isolation areas should be set up to face south, and lighting measures should be strengthened. Public corridors can be set up in the isolation area so that infected patients can stagger their activities appropriately. Healthcare workers who are fully "armed" can paste their names on their protective clothing to improve communication between doctors and patients and reduce patients' sense of isolation. There are also studies 26 suggesting the use of the Rosenthal effect, that is, using praise, trust and expectation, and other psychological hints to help patients regain confidence and obtain positive motivation to change their own behaviors. By communicating with patients to understand their psychological needs, sources of negative emotions and specific factors affecting their emotions, healthcare professionals, in collaboration with teams from various disciplines, can encourage infected patients to cope positively and provide social support and psychological guidance to improve their quality of life 27 . The patients in this study were often in a state of confusion and worry before discharge and were also often unable to adjust to a good rhythm of life within a short period after discharge. Healthcare professionals can help patients develop a postdischarge transition plan during inpatient isolation so that they can quickly return to their original lives. At the same time, a scale of social reintegration behavior of infected patients with infectious diseases that is suitable for our country should be developed to prospectively investigate the current status of social reintegration of infected patients and to understand the changes in social reintegration behaviors in different periods to take corresponding measures to help such people adapt to their situations as soon as possible.
As a contagious disease, COVID-19 can lead to fear among the population as well as stigma and discrimination against specific groups of people 28 , and patients develop a sense of shame about their illness as a result. The results of this study show that patients often want to keep their experience of the disease confidential. The vast majority of infected patients in this study chose to conceal their illness from their family members. Due to the existence of a sense of shame, patients not only kept their disease experience secret but also often consciously reduced their mobility after discharge from the hospital to avoid infecting others. Patients may actively distance themselves from their friends and relatives, resulting in the loss of an important source of social support and a sense of isolation 29 . There were also patients who did not actively conceal their condition, but friends and colleagues of the infected patients intentionally chose to distance themselves from the infected patients out of fear and rejection after learning of their condition. Six patients (37.5%) in this study were thus forced to leave their original places of residence or work, causing them to develop more negative emotions. On the other hand, the infected patients who indicated that their colleagues and friends treated them no differently than before reported that they resumed normal life more quickly after returning to society. Yuan et al. 19 showed that the social support system of infected patients is an important factor in their posttraumatic growth. Social support based on kinship is the main way for most Chinese people to obtain social support. If this basic relationship is damaged, it prevents infected patients from obtaining the understanding and support of others, which can have a great impact on their physical and mental health 30 . The establishment of a good social support system will enhance psychological health; in contrast, the accumulation of negative emotions will lead to a variety of psychological problems 31 . Healthcare workers in the diagnosis and treatment of COVID-19 patients not only need to give the necessary treatment measures but also need to comprehensively assess the degree of understanding of the disease and social support system of infected patients. Healthcare workers should encourage infected patients to inform their families of their illnesses through daily communication, collaborate with their families to provide relevant psychological care, and improve the level of posttraumatic growth of patients 32 . In addition, the limitations of the public's knowledge of the disease will increase the individual's self-psychological burden, which will deepen the self-perception and experience of public stigma, resulting in the internalization of stigma 33 . Therefore, it is necessary to strengthen the information dissemination of infectious diseases, set up relevant policies for social groups such as communities and companies to avoid the public's rough treatment of infected patients returning to society, to protect the normal work and lives of infected patients and to reduce economic losses. This study shows that the policy benefits given by the government make infected patients believe in the national epidemic prevention policy and thus have confidence in the diagnosis and treatment of the disease. Therefore, state policy supports the reintegration of COVID-19 patients into society through macrocontrol.
Six months after returning to the community, some of the patients in this study still had sequelae of COVID-19, such as malaise, insomnia, chest tightness, and loss of smell. A recent study published in The Lancet 34 also confirmed this phenomenon. Similar studies have shown that the acute phase of COVID-19 and subsequent health damage involves multiple systems, such as the respiratory, neurological, and cardiovascular systems 18 . Given that infected patients at this stage need professional guidance to avoid delaying their illness, we call for greater collaboration among scholars from different countries to share experiences in the treatment of the disease to improve the physical and mental health of the population in the face of the postinfection syndrome caused by the global pandemic of COVID-19 patients.
This study found that there is a lack of clarity in the division of labor between departments and duplication of investigations by various departments in the process of epidemiological investigation.. It is recommended that the relevant departments should strengthen the integration and sharing of information by using big data and increase training in epidemiological investigation to improve efficiency 26 . Knowing the benefits of epidemiological investigation and personal information protection can reduce the uneasiness of infected patients, it is recommended that the media increase the scientific knowledge of epidemiological investigations and, at the same time, hide patients’ last names in the publication of epidemiological investigation information to protect the privacy of infected patients, and prohibit malicious human searches and other behaviors.
Limitations
While the study has its merits, it also has its limitations. First, this study interviewed COVID-19 patients only in Ningbo and did not include patients from multiple regions and centers. Second, this study was conducted during the control phase of the epidemic in China, and except for filling out the SDS scale, which was face-to-face, both interviews were conducted over the phone, and preventing the use of visual aids.
Conclusions
The objective of this study was to explore the perceptions and comprehension of patients infected with COVID-19 during the Chinese new coronavirus epidemic. COVID-19 patients encounter numerous psychological challenges while simultaneously experiencing physical discomfort, isolation, a sense of shame, and uncertainty regarding recovery. While some patients eventually adapt to their circumstances, not all are able to do so. Therefore, it is crucial for healthcare providers and families to provide support in order to facilitate patient adjustment to normal life. Interventions should be tailored according to the specific needs of patients at different stages, informing subsequent optimization of care and management strategies for infectious diseases.
Data availability
The datasets generated and analysed during the current study are not publicly available due privacy protection but are available from the corresponding author on reasonable request.
World health organization (WHO). Coronavirus (COVID-19) dashboard [DB/OL] . https://covid19.who.int/ . 2023-02-03.
Chen, W. X. et al. Comparison of three experimental methods for detecting antibody levels after immunization with COVID-19 vaccine [J]. Chin. J. Microbiol. Immunol. 41 (6), 423–426 (2021).
MathSciNet Google Scholar
Zhou, X. Y., Wang, H., & Hang, H. R., et al. Epidemiological and clinical characteristics of SARS-CoV-2 Delta variant infected patients with COVID-19 in Gansu province [J/OL]. Nurs. J. Chin PLA 1–14 (2022).
Chen, H. L., & He, Q. A meta synthesis of qualitative research on the psychological experience of patients with COVID-19 infection in China. J. Southw. Jiaotong Univ. 2022 (06), 73–82 (2023). http://kns.cnki.net/kcms/detail/51.1586.c.20221123.1638.018.html .
Hanson, S. W. et al. Global burden of disease long COVID collaborators; Estimated global proportions of individuals with persistent fatigue, cognitive, and respiratory symptom clusters following symptomatic COVID-19 in 2020 and 2021 [J]. JAMA. 328 (16), 1604–1615. https://doi.org/10.1001/jama.2022.18931 (2022).
Article Google Scholar
Guo, M., Kong, M., Shi, W., Wang, M. & Yang, H. Listening to COVID-19 survivors: What they need after early discharge from hospital—A qualitative study [J]. Int. J. Qual. Stud. Health Well-being. 17 (1), 2030001. https://doi.org/10.1080/17482631.2022.2030001 (2022).
Article PubMed PubMed Central Google Scholar
Cameron, J. I. & Gigrac, M. A. “Timing It Right”: A conceptual framework for addressing the support needs of family caregivers to stroke survivors from the hospital to the home [J]. Patient Educ. Couns. 70 (3), 305–314. https://doi.org/10.1016/j.pec.2007.10.020 (2008).
Article PubMed Google Scholar
Liang, L. L. et al. Research progress on application of timing theory in disease nursing [J]. Chin. Nurs. Res. 30 (05), 524–525 (2016).
Google Scholar
Chu, H. L. et al. Sample size was determined for qualitative interviews based on the information efficacy model [J]. Chin. Gen. Pract. 24 (10), 1274–1276. https://doi.org/10.12114/j.issn.1007-9572.2020.00.415 (2021).
Ding, X. & Yao, J. Peer education intervention on adolescents’ anxiety, depression, and sleep disorder during the COVID-19 pandemic [J]. Psychiatr. Danub. 32 (3–4), 527–535. https://doi.org/10.24869/psyd.2020.527 (2020).
Balmer, D. F. & Richards, B. F. Conducting qualitative research through time: how might theory be useful in longitudinal qualitative research? [J]. Adv. Health Sci. Educ. Theory Pract. 27 (1), 277–288. https://doi.org/10.1007/s10459-021-10068-5 (2022).
Audulv, Å. et al. Qualitative longitudinal research in health research: A method study [J]. BMC Med. Res. Methodol. 22 (1), 255. https://doi.org/10.1186/s12874-022-01732-4 (2022).
Sun, J. et al. Psychological anxiety of patients with novel pneumonia during isolation period [J]. Hosp. Admin. J. Chin. People’s Liberation Army 28 (8), 758–760 (2021).
Zhang, W. et al. To summarize the experience of psychological intervention and rehabilitation of patients with COVID-19 in mobile cabin hospital [J]. Chin. J. Nurs. 55 (S1), 603–605. https://doi.org/10.3761/j.issn.0254-1769.2020.S1.231 (2020).
Article CAS Google Scholar
Deng, C. Y. et al. To analyze the depression level and its influencing factors in patients with COVID-19 [J]. Tianjin J. Nurs. 30 (04), 400–403. https://doi.org/10.3969/j.issn.1006-9143.2022.04.005 (2022).
Yao, L. Y. et al. To evaluate the mental health status and nursing of 45 patients injured in earthquake [J]. Chin. J. Nurs. 43 (12), 3. https://doi.org/10.3761/j.issn.0254-1769.2008.12.003 (2008).
Gidari, A. et al. Is recurrence possible in coronavirus disease 2019 (COVID-19)? Case series and systematic review of literature [J]. Eur. J. Clin. Microbiol. Infect. Dis. 40 (1), 1–12. https://doi.org/10.1007/s10096-020-04057-6 (2021).
Article CAS PubMed Google Scholar
Chen, H. et al. To explore the effects of life intervention on anxiety, depression and quality of life in patients with COVID-19 [J]. J. Nurs. Sci. 35 (09), 27–29 (2020).
Yuan, Z. F. et al. Current status and influencing factors of post-traumatic growth in patients with COVID-19 [J]. Chin. J. Nurs. 55 (S1), 587–589. https://doi.org/10.3761/j.issn.0254-1769.2020.S1.225 (2020).
Wang, J. Y. et al. A qualitative study on disease experience of 15 patients with COVID-19 [J]. J. Nurs. 27 (14), 63–67. https://doi.org/10.16460/j.issn1008-9969.2020.14.063 (2020).
Shaban, R. Z. et al. SARS-CoV-2 infection and COVID-19: The lived experience and perceptions of patients in isolation and care in an Australian healthcare setting [J]. Am. J. Infect. Control. 48 (12), 1445–1450. https://doi.org/10.1016/j.ajic.2020.08.032 (2020).
Brooks, S. K. et al. The psychological impact of quarantine and how to reduce it:rapid review of the evidence [J]. Lancet 395 (10227), 912–920 (2020).
Article CAS PubMed PubMed Central Google Scholar
Li, S. & Zhang, X. L. To analyze the effect of psychological nursing on elderly patients with chronic obstructive pulmonary disease [J]. China Rural Health 11 (24), 55 (2019).
Chen, J. G. et al. Study on the influencing factors of psychological status of confirmed COVID-19 patients and quarantine visitors [J]. J. Nurs. Admin. 20 (04), 247–251 (2020).
Korkut, S. Research of the coronavirus anxiety, post-traumatic stress, generalized anxiety disorder, quality of life, and stress coping styles in COVID-19 survivor [J]. Psychol. Rep. 125 (6), 3069–3083. https://doi.org/10.1177/00332941221129131 (2022).
Zhang, S. S. & Xie, W. Y. To explore the effect of psychological nursing based on Rosenthal effect on negative emotions of patients with suspected COVID-19 [J]. J. Nurs. Sci. 35 (09), 24–26 (2020).
Yuan, K. et al. A systematic review and meta-analysis on the prevalence of stigma in infectious diseases, including COVID-19: a call to action [J]. Mol. Psychiatry. 27 (1), 19–33. https://doi.org/10.1038/s41380-021-01295-8 (2022).
Huang, L. X. et al. Health outcomes in people 2 years after surviving hospitalization with COVID-19: A longitudinal cohort study [J]. Lancet Respir. Med. 10 (9), 863–876. https://doi.org/10.1016/S2213-2600(22)00126-6 (2022).
Wang, T. et al. Current status and influencing factors of stigma in patients after COVID-19 recovery [J]. Shanghai Nurs. 20 (10), 28–31 (2020).
Zhen, X. Y. Quality of life and related psychosocial factors in people living with HIV/AIDS [D] (Shanxi Medical University, 2011).
Zhou, S. J. et al. Common psychological conflicts and coping strategies of COVID-19 patients [J]. Chin. Mental Health J. 34 (03), 248–250. https://doi.org/10.3969/j.issn1000-6729.2020.3.021 (2020).
Nan, F. F. et al. Remote psychological crisis intervention service under the COVID-19 epidemic in Zhejiang province [J]. China J. Health Psychol. 29 (03), 367–370. https://doi.org/10.13342/j.cnki.cjhp.2021.03.010 (2021).
Li, R. J. et al. Qualitative research on psychological experience of pregnant women with COVID-19 [J]. Milit. Nurs. 39 (09), 49–52 (2022).
Huang, L. et al. 1-year outcomes in hospital survivors with COVID-19: A longitudinal cohort study [J]. Lancet. 398 (10302), 747–758. https://doi.org/10.1016/S0140-6736(21)01755-4 (2021).
Download references
This research was supported by Medical Scientific Research Foundation of Zhejiang Province under Grant No. 2023KY1091; Zhejiang Provincial Natural Science Foundation of China under Grant No. LY22H160006.
Author information
Authors and affiliations.
Hemtology Department, Ningbo No. 2 Hospital, Ningbo, Zhejiang, China
Liangyan Zhang & Kesang Li
Intensive Care Unit, Ningbo No. 2 Hospital, Ningbo, Zhejiang, China
Infection Department, Ningbo No. 2 Hospital, Ningbo, Zhejiang, China
You can also search for this author in PubMed Google Scholar
Contributions
Liangyan Zhang organized interviews and collected the data. Liangyan Zhang and Chen Zhang analyzed the data and wrote the main manuscript text. All authors reviewed the manuscript.
Corresponding author
Correspondence to Chen Zhang .
Ethics declarations
Competing interests.
The authors declare no competing interests.

Additional information
Publisher's note.
Springer Nature remains neutral with regard to jurisdictional claims in published maps and institutional affiliations.
Rights and permissions
Open Access This article is licensed under a Creative Commons Attribution 4.0 International License, which permits use, sharing, adaptation, distribution and reproduction in any medium or format, as long as you give appropriate credit to the original author(s) and the source, provide a link to the Creative Commons licence, and indicate if changes were made. The images or other third party material in this article are included in the article's Creative Commons licence, unless indicated otherwise in a credit line to the material. If material is not included in the article's Creative Commons licence and your intended use is not permitted by statutory regulation or exceeds the permitted use, you will need to obtain permission directly from the copyright holder. To view a copy of this licence, visit http://creativecommons.org/licenses/by/4.0/ .
Reprints and permissions
About this article
Cite this article.
Zhang, L., Zhang, C., Li, K. et al. Longitudinal qualitative study on the psychological experiences of COVID-19 patients based on timing it right framework. Sci Rep 14 , 12409 (2024). https://doi.org/10.1038/s41598-024-63215-4
Download citation
Received : 17 December 2023
Accepted : 27 May 2024
Published : 30 May 2024
DOI : https://doi.org/10.1038/s41598-024-63215-4
Share this article
Anyone you share the following link with will be able to read this content:
Sorry, a shareable link is not currently available for this article.
Provided by the Springer Nature SharedIt content-sharing initiative
- Longitudinal qualitative study
- Timing it right framework
By submitting a comment you agree to abide by our Terms and Community Guidelines . If you find something abusive or that does not comply with our terms or guidelines please flag it as inappropriate.
Quick links
- Explore articles by subject
- Guide to authors
- Editorial policies
Sign up for the Nature Briefing newsletter — what matters in science, free to your inbox daily.

Sex differences in health-related quality of life and psychological distress among colorectal cancer patients: a 2-year longitudinal study
- Open access
- Published: 24 May 2024
Cite this article
You have full access to this open access article
- Cynthia S. Bonhof 1 , 2 ,
- Belle H. de Rooij 1 , 2 ,
- Dounya Schoormans 1 , 2 ,
- Dareczka K. Wasowicz 3 ,
- Gerard Vreugdenhil 4 &
- Floortje Mols 1 , 2
130 Accesses
1 Altmetric
Explore all metrics
While sex differences in the incidence and mortality of colorectal cancer (CRC) are well documented, less is known about sex differences in patients’ health-related quality of life (HRQoL) and psychological distress. To enhance patient-tailored care, we aimed to longitudinally examine sex differences in HRQoL and psychological distress among CRC patients from diagnosis up until 2-year follow-up.
Newly diagnosed CRC patients from four Dutch hospitals were eligible for participation. Patients ( N = 334) completed questions on HRQoL (EORTC QLQ-C30) and psychological distress (HADS) before initial treatment (baseline), 4 weeks after surgery, and at 1 and 2 years after diagnosis. Also, HRQoL and psychological distress were assessed in a sex- and age-matched reference population.
When directly comparing female ( N = 126, 38%) and male ( N = 208, 62%) CRC patients, female patients reported significantly worse HRQoL, such as more insomnia at baseline, worse physical and role functioning 4 weeks after surgery, more diarrhea at 1 year, and more pain and constipation at 2-year follow-up. However, a comparison with the reference population revealed larger differences between patients and reference in males than in females. For example, at 1- and 2-year follow-up, male patients reported significantly worse cognitive and social functioning, more insomnia, and more anxiety compared with a reference population.
Conclusions
Especially male CRC patients reported worse HRQoL and more psychological distress when compared with a reference population.
Implications for cancer survivors
Knowledge of sex-specific differences in HRQoL and psychological distress among CRC patients may help healthcare providers anticipate and appropriately address patients’ unique healthcare needs.
Similar content being viewed by others
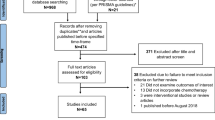
A scoping review evaluating physical and cognitive functional outcomes in cancer survivors treated with chemotherapy: charting progress since the 2018 NCI think tank on cancer and aging phenotypes
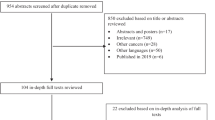
Health-related quality of life in breast cancer patients: review of reviews from 2008 to 2018
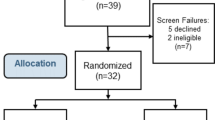
A whole food, plant-based randomized controlled trial in metastatic breast cancer: feasibility, nutrient, and patient-reported outcomes
Avoid common mistakes on your manuscript.
Introduction
Colorectal cancer (CRC) is a major health concern worldwide. It is currently the second most commonly diagnosed cancer in women, with 866,000 new cases each year, and the third in men, with 1,066,000 new cases each year [ 1 , 2 ]. Overall, due to earlier diagnosis and improvements in treatment, mortality rates have decreased in the past three decades, but remain substantially higher for males [ 3 ]. Possible explanations for the differences in incidence and mortality include both physiological and behavioral factors. For example, sex hormones may play a role, as estrogenic hormone use among women has been associated with a reduced risk for CRC and improved survival [ 4 , 5 ]. Overall, men also more often engage in lifestyle-related risk factors (e.g., smoking, alcohol consumption) [ 6 ], participate less in cancer screening programs [ 7 , 8 ], and are less likely to seek medical care [ 9 ].
Studies have shown that the health-related quality of life (HRQoL) of CRC patients is reduced in the first year after treatment, but—in the absence of disease recurrence or progression—gradually improves during the years thereafter [ 10 , 11 , 12 , 13 ]. However, while CRC survivors can reach HRQoL levels that are comparable to those of the general population, some of them may continue to face ongoing problems related to the cancer and its treatment, resulting in low HRQoL and high psychological distress (i.e., mood disorders and symptoms of anxiety and depression) [ 14 , 15 , 16 ]. In previous studies among CRC patients, differences in HRQoL were found between male and female patients [ 17 , 18 , 19 ]. In a longitudinal study among rectal cancer patients from before surgery up until 2-year follow-up, women reported worse HRQoL, including worse global HRQoL, physical functioning, and sleep quality, and more diarrhea and fatigue compared with men [ 17 ]. In another longitudinal study, also among rectal cancer patients, male patients reported worse social functioning, but only at 12 months after sphincter-saving surgery [ 18 ] compared with women. To date, no longitudinal studies on sex differences in HRQoL have included colon cancer patients. Regarding sex differences in psychological distress, studies among CRC patients have yielded conflicting findings [ 15 , 20 ], with male CRC patients reporting more distress compared to females in a 5-year longitudinal study [ 20 ] and less psychological distress in a longitudinal study among long-term CRC survivors [ 15 ].
Sex differences in HRQoL and psychological distress are already present in the general population, with men reporting better functioning and less symptoms and psychological distress compared with women [ 21 , 22 ]. To account for these inherent differences, several cross-sectional studies in CRC patients have included a comparison with the general population when examining sex differences in HRQoL and psychological distress [ 13 , 23 , 24 ]. For instance, a German study assessing HRQOL 1 year after CRC diagnosis revealed no sex-specific differences in HRQOL between CRC patients and population controls [ 13 ], whereas a cross-sectional secondary analyses of a Dutch study showed that in long-term CRC survivors, sex differences were found between CRC patients and a normative population across multiple domains [ 23 ]. Specifically, when male and female patients were directly compared, females reported worse physical and emotional functioning and more nausea and vomiting, insomnia, and anxiety. However, when compared with a reference population, symptoms such as fatigue, dyspnea, anxiety, and depression were found to be more frequent in male patients [ 23 ].
Given the increasing emphasis on the importance of providing patient-tailored follow-up care in cancer treatment, identifying sex differences in HRQoL and psychological distress is important. To our knowledge, no longitudinal studies on sex differences in HRQoL have been done among colon cancer patients, and there is a lack of longitudinal studies on sex differences in psychological distress among CRC patients. In addition, no longitudinal study has included a reference population. Therefore, the aim of this prospective study among CRC patients is to first examine the course of HRQoL and psychological distress among male and female patients from diagnosis to 2 years thereafter. Secondly, we aim to directly examine sex differences in longitudinal measurements of HRQoL and psychological distress and compare male and female CRC patients to a reference population, to gain better insight into the relative impact on potential impairments in HRQoL and psychological distress.
Setting, participants, and data collection
This study is based on data from the PROCORE study, a prospective population-based study among CRC patients aimed to examine the impact of CRC and its treatment on patient-reported outcomes. Patient inclusion took place between January 2016 and January 2019 in 4 Dutch hospitals. All eligible patients newly diagnosed with CRC as a primary tumor were invited to participate. Exclusion criteria were (1) previous diagnosis with a different carcinoma, except for basal cell carcinoma of the skin, (2) cognitive impairments, and (3) inability to read or write Dutch. The PROCORE study was approved by the certified Medical Ethic Committee of Medical research Ethics Committees United (registration number NL51119.060.14).
Details of the data collection have previously been described [ 25 ]. Data was collected through PROFILES ( www.profilesregistry.nl ), a registry for the physical and psychosocial impact of cancer and its treatment [ 26 ]. PROFILES is directly linked to the Netherlands Cancer Registry (NCR) that collects data from all newly diagnosed cancer patients in the Netherlands [ 27 ]. Shortly after diagnosis, and before start of initial treatment, eligible patients were invited to participate via their research nurse or case manager. They received an information package containing an information letter, informed consent form, and the baseline questionnaire. Follow-up questionnaires were sent at 4 weeks after surgery (when applicable), 1 year after diagnosis, and 2 years after diagnosis.
Sociodemographic and clinical characteristics
Patients’ sociodemographic (i.e., age and sex) and clinical (i.e., cancer type, clinical stage, treatment) information was available from the NCR[ 27 ]. Comorbidity was assessed with the adapted Self-Administered Comorbidity Questionnaire (SCQ) [ 28 ].While the SCQ also includes the comorbidity “depression,” we excluded this comorbidity as this is also one of our outcome measures. Questions on partner status and educational level were added to the questionnaire.
- Health-related quality of life
HRQoL was assessed with the EORTC QLQ-C30 (Version 3.0) [ 29 ]. It contains five functioning scales (i.e., physical, role, cognitive, emotional, and social functioning), a global QoL scale, three symptom scales (i.e., fatigue, pain, and nausea and vomiting), and six single items (i.e., dyspnea, insomnia, appetite loss, constipation, diarrhea, and financial impact). Each item is scored on a four-point Likert scale ranging from 1 (not at all) to 4 (very much), except for the global QoL items, which range from 1 (very poor) to 7 (excellent). Scores were linearly transformed to a 0–100 scale [ 30 ]. Higher scores on the functioning scales and global QoL indicate better functioning and QoL, whereas higher scores on the symptom scales indicate more complaints.
- Psychological distress
Anxiety and depressive symptoms were assessed with the Hospital Anxiety and Depression Scale (HADS) [ 31 ]. It consists of 14 items, seven items assess anxiety symptoms (HADS-A), and the other seven assess depressive symptoms (HADS-D). Items are answered on a four-point Likert scale. Total scores for both the anxiety and depressive symptom scale range from 0 to 21 with higher scores representing more anxiety and depressive symptoms.
Reference population
Sociodemographics (i.e., age, sex, marital status), clinical data (i.e., comorbidity), and data on HRQoL (EORTC QLQ-C30) and psychological distress (HADS) from the reference population were obtained from CentERpanel, an online household panel representative of the Dutch-speaking population in the Netherlands [ 32 ]. Details of the annual data collection have previously been described [ 33 ]. For this study, data from 2011 was used. To ensure the independence of observations, one cancer-free member per household ( N = 1401) was selected. In addition, as participants in the household panel are relatively young compared with the CRC patients, the oldest person of the household was selected. Of this sample, a random sex- and age-matched reference sample was selected (< 30, 30–34, 35–39, 40–44, 45–49, 50–54, 55–59, 60–64, 65–69, 60–64, 65–69, 70–74, 75–80, ≥ 80), reflecting the distribution of the patient sample. This resulted in a final reference sample of 334 panel members (ratio norm population/cancer patients is 1:1).
Statistical analyses
NCR data on sociodemographic and clinical characteristics enabled us to compare eligible patients and respondents, using t tests for continuous variables and chi-squared (or Fisher’s exact) tests for categorical variables. We also compared differences in baseline sociodemographic and clinical characteristics between (1) male and female CRC patients, (2) male CRC patients and the male reference population, and (3) female CRC patients and the female reference population in the same way.
For our main analyses, we first examined the courses of each HRQoL and psychological distress subscale separately among male and female CRC patients using linear mixed models (LMMs), with maximum likelihood estimation and an unstructured covariance matrix with a 2-level structure (i.e., repeated time points [lower level], patients [higher level]). Time was analyzed as a regular categorical predictor with four levels (i.e., four time points). These analyses were adjusted for age, partner status, educational level, tumor type, cancer stage, and number of comorbidities. As cancer stage and cancer treatment are closely related and we want to avoid multicollinearity, we opted to include cancer stage as a covariate, in line with our previous study [ 34 ]. Second, differences in HRQoL and psychological distress between male and female CRC patients at each time point were examined similarly, but without time as a predictor. Additionally, differences in HRQoL and psychological distress between (1) male CRC patients and the male reference population and (2) female CRC patients and the female reference population were also examined in a similar way. However, analyses were adjusted for covariates that were available in both patient and norm samples: age, partner status, educational level, and number of comorbidities.
Analyses were performed using SPSS (IBM SPSS Statistics for Windows, Version 24.0 Armonk, NY: IBM Corps USA). A p value < 0.05 was considered statistically significant.
Patient characteristics
Of the 713 CRC patients invited for the study, 68% ( n = 483) completed the questionnaire at baseline, 52% ( n = 374) at 1-year follow-up, and 49% ( n = 347) at 2-year follow-uA full flow chart of the study has previously been published [ 25 ]. Compared with all eligible patients, respondents were significantly younger, more often male, more likely to receive chemotherapy, and less likely to undergo surgery. In addition, they were significantly less often diagnosed with rectosigmoid cancer, they more often had stage III cancer, and less often stage IV cancer (data not shown).
Of the 397 patients who completed the baseline and at least the questionnaire at 1- or 2-year follow-up, 58 patients were excluded as they were previously diagnosed with cancer and/or had already started treatment at time of baseline. Additionally, five patients were excluded because of missing data on our outcome measures. The final sample consisted of 334 patients, 208 males (62%) and 126 females (38%) (Table 1 ). Male patients more often had a partner compared with female patients. No other differences in sociodemographic and clinical characteristics were found between male and female patients. Several differences were found between CRC patients and the reference population. Compared with the male reference population ( n = 208), male CRC patients more often had a partner (92% vs. 85%; p = 0.02), more often received medium education (62% vs. 52%; p = 0.03), and less often received high education (30% vs. 44%; p = 0.004). Female CRC patients more often had a partner (73% vs. 50%; p < 0.001) and more often received a low education (14% vs. 6%; p = 0.03) compared with the female reference population ( n = 126).
Course of HRQoL and psychological distress
In both sexes, global QoL and all functional scales except for emotional functioning had significantly declined 4 weeks after surgery (Fig. 1 ). Role and social functioning returned to baseline level at 1-year follow-up, and global QoL increased to a level significantly above baseline. In contrast, physical functioning remained significantly below baseline during the entire follow-up period, while emotional functioning was at its lowest level during baseline and significantly improved during follow-uCognitive functioning remained stable from baseline to follow-up among female patients, but decreased significantly below baseline during the entire follow-up period in male patients.
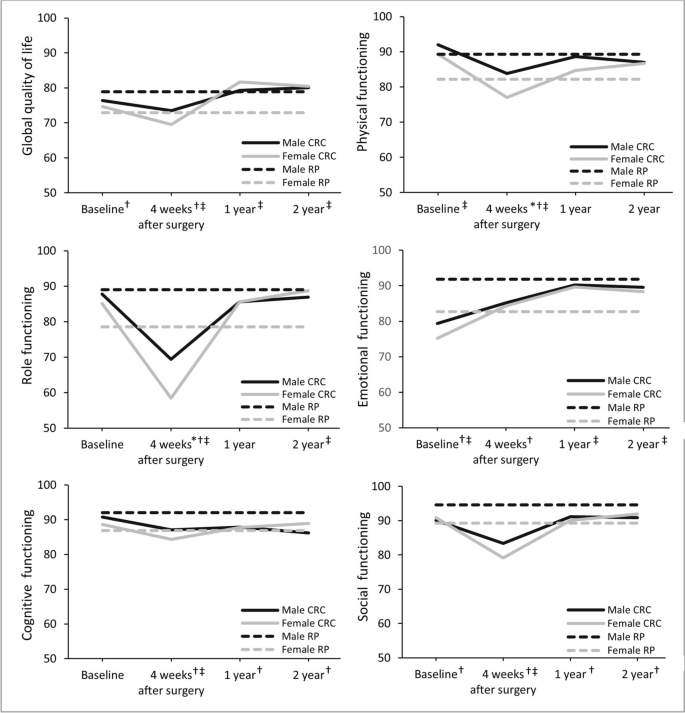
Course of European Organisation for Research and Treatment of Cancer (EORTC) Quality of Life (QoL) Questionnaire Core 30 functioning scores and global QoL for CRC patients and the reference population, stratified by sex. CRC, colorectal cancer; RP, reference population. A higher score on the scales indicates better functioning. The scales in this figure range from 50 to 100 for clear visibility, while scores of the EORTC QLQ-C30 range from 0–100. *Significant difference between male and female CRC patients; †significant difference between male CRC patients and the male reference population, ‡significant difference between female CRC patients and the female reference population
Regarding symptoms, an increase at 4 weeks after surgery with a return to baseline level at 1-year follow-up was found for fatigue, pain, and nausea and vomiting, with the latter only present in female patients (Fig. 2 ). Additionally, dyspnea remained stable from baseline to follow-up, and appetite loss increased at 4 weeks after surgery, but significantly decreased to baseline level at 1-year follow-up and below baseline level at 2-year follow-uIn female patients, insomnia was at its highest level during baseline and decreased to below baseline level at 1- and 2-year follow-up, while both insomnia and nausea and vomiting remained stable among males. In male patients, diarrhea and constipation symptoms decreased to below baseline level at 1- and 2-year follow-up, while financial difficulties increased at 4 weeks after surgery, decreased to baseline level at 1-year follow-up, and significantly increased to above baseline level at 2-year follow-uIn contrast, diarrhea, constipation, and financial difficulties remained at baseline level during follow-up among female patients. Finally, anxiety symptoms significantly improved among both sexes at 4 weeks after surgery and remained below baseline during follow-up, while depressive symptoms remained at baseline level.
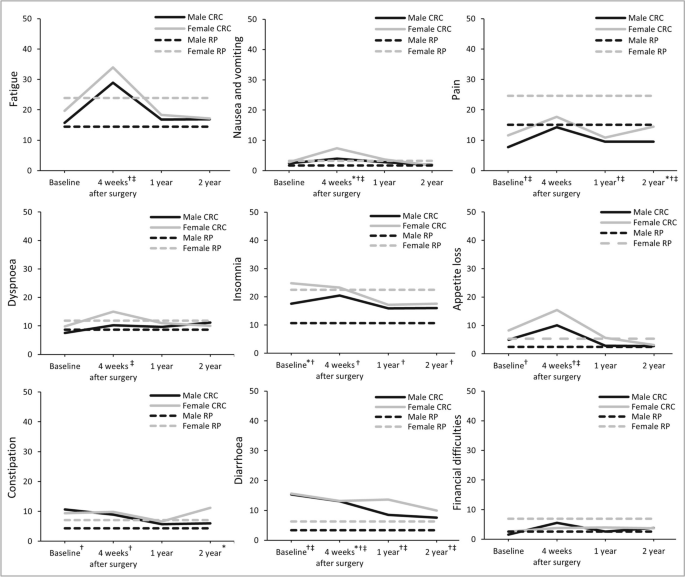
Course of European Organisation for Research and Treatment of Cancer (EORTC) Quality of Life (QoL) Questionnaire Core 30 symptom scores for CRC patients and the reference population, stratified by sex. CRC, colorectal cancer; RP, reference population. A higher score on the scales indicates more symptoms. The scales in this figure range from 0 to 50 for clear visibility, while scores of the EORTC QLQ-C30 range from 0 to 100. *Significant difference between male and female CRC patients; †significant difference between male CRC patients and the male reference population, ‡significant difference between female CRC patients and the female reference population
Male vs female CRC patients
Compared with male patients, female patients reported more insomnia at baseline, a worse physical and role functioning and more nausea and vomiting at 4 weeks after surgery, more diarrhea at 1-year follow-up, and more pain and constipation at 2-year follow-up (Figs. 1 and 2 ; Table 2 ). No other differences in HRQoL or psychological distress were found between the two sexes.
Comparison with the reference population
Both sexes reported most differences at 4 weeks after surgery when compared with the reference population (Figs. 1 and 2 ; Table 3 ). Specifically, both male and female patients reported worse global quality of life and worse physical, role, cognitive, and social functioning. Male patients also reported worse emotional functioning compared with the male reference population, while no differences were found in their female counterparts. Furthermore, while male patients reported worse cognitive and social functioning at 1- and 2-year follow-up, female patients reported better global quality of life and emotional functioning at 1- and 2-year follow-up and better role functioning at 2-year follow-up.
Regarding symptoms, both sexes reported less pain but more diarrhea at baseline compared with the reference population, while only male patients reported more insomnia, appetite loss, and constipation (Table 3 ). At 4 weeks after surgery, both sexes reported more fatigue, nausea and vomiting, appetite loss, and diarrhea. In addition, male patients reported more constipation and insomnia, and female patients more dyspnea compared with the reference population. At 1- and 2-year follow-up, both sexes reported less pain but more diarrhea, while male patients still reported more insomnia. Finally, male patients reported more anxiety symptoms at all time points, while no differences were found among females.
In this prospective study among CRC patients, we first showed that, among both sexes, HRQoL was generally reduced at 4 weeks after surgery but had improved at 1- and 2-year follow-uSome outcome measures improved to a level significantly better than baseline, such as global quality of life and anxiety symptoms. In contrast, physical functioning remained significantly below baseline during follow-uThese results are consistent with other studies among CRC patients which showed that HRQoL is reduced in the first year after treatment, but—in the absence of disease recurrence of progression—gradually improves during follow-up [ 10 , 11 , 12 , 13 ].
Comparing female CRC patients directly to male CRC patients showed that female patients reported more insomnia at baseline, worse physical and role functioning and more pain 4 weeks after surgery, more diarrhea at 1-year follow-up, and more pain and constipation at 2-year follow-uWhen compared with a sex- and age-matched reference population, more sex differences came to light. Interestingly, it was actually the male patients who reported worse functioning and more symptoms when compared with the male reference population. For example, male patients reported worse cognitive and social functioning, and more insomnia, diarrhea, and anxiety during the entire follow-up period. Interestingly, female patients reported a better global QOL, emotional functioning, and role functioning during follow-up compared with the female reference population.
The finding that female patients reported worse functioning and more symptoms when directly compared with male patients is in line with a study among rectal cancer patients from before surgery up until 2-year follow-up in which women reported worse HRQoL, including worse global HRQoL, physical functioning, and sleep quality, and more diarrhea and fatigue compared with men [ 17 ]. In our study, the comparison with a reference population resulted in different findings than the direct comparison between male and female patients: a worse functioning and more symptoms were reported more frequently by male patients than female patients when compared with the reference population. These findings are in contrast with a cross-sectional study among CRC patients 1 year after diagnosis, in which no sex differences were found when compared with a reference population [13]. However, our findings are in line with a cross-sectional study among long-term CRC patients in which female patients reported worse physical and emotional functioning and more nausea and vomiting, insomnia, and anxiety when directly compared with male patients, while symptoms such as fatigue, dyspnea, anxiety, and depression were more frequent in male patients when compared with a reference population [23].
The results of our study first show that a comparison with a reference population is very valuable when assessing sex differences in HRQoL and psychological distress among CRC patients. As sex differences in HRQoL and psychological distress are already present in the general population, with men reporting better functioning and less symptoms and psychological distress compared with women [ 21 , 22 ], a direct comparison between male and female CRC patients does not adequately capture sex differences in HRQoL and psychological distress. In addition, as we seek to provide patient-tailored and personalized (follow-up) care, the found sex differences in HRQoL and psychological distress should not be ignored. First, appropriate (sex-specific) information provision provided by healthcare professionals or via websites or flyers may help patients feel more confident in dealing with their health-related issues and may help them better adjust to symptoms [ 35 , 36 ]. Second, knowledge of sex-specific differences in HRQoL and psychological distress among CRC patients may help health care providers anticipate and appropriately address patients’ unique healthcare needs. Particularly male CRC patients seem to be in need of (sex-specific) follow-up care, as they especially reported worse HRQoL and more psychological distress when compared with the reference population. However, men are generally less likely to seek help for physical and mental difficulties [ 37 ]. This might be one of the reasons why the recovery of HRQoL and psychological distress takes longer after diagnosis and treatment among males compared to females. Methods to both provide information and diminish effects on HRQoL and psychological distress should thus be explored in a sex-specific way.
It is not completely clear why the worse functioning and more symptoms found among male patients in comparison with a reference population was, aside from more diarrhea, not found among female patients and why female patients reported better global quality of life and better role and emotional functioning compared with the reference population. Post-traumatic growth, a positive psychological change that can occur from a struggle with a highly challenging adverse life event like cancer [ 38 ] may be a possible explanation as studies in cancer and other traumatic events suggest that women have higher levels of post-traumatic growth than men [ 39 , 40 ]. Reasons behind this difference are not fully understood, but could be due to differences in coping, appraisal of stressor, or cultural expectations [ 41 , 42 ].
Several limitations should be acknowledged. First, eligible patients and respondents differed in some sociodemographic and clinical characteristics. Generalization of our results to the general population of CRC patients should thus be done with caution. Second, respondents of observational studies generally have a better survival, and their HRQoL may be systematically higher compared with non-participants, and therefore, they represent a healthier population [ 43 ]. In addition, it is unclear why patients were lost to follow-uIt could be that patients stopped participating in our study due to a poor HRQoL or a high level of psychological distress [ 44 ]. Both limitations could have led to an underestimation of the results in male CRC patients and an overestimation of the results in female CRC patients.
Despite these limitations, this study is, to our knowledge, the first prospective study to show sex differences in HRQoL and psychological distress among patients with CRC in comparison with a reference population. A major strength of this study is the comparison with a sex- and age-matched reference population, which revealed sex differences in HRQoL and psychological distress that otherwise would not have been observed. Other strengths are the prospective design and the baseline measurement prior to treatment which enabled us to examine sex-specific changes in HRQoL and psychological distress over time and to examine whether these changes return to baseline level after treatment, or even improve beyond that.
In conclusion, this prospective study among CRC patients from diagnosis up until 2-year follow-up showed sex-specific differences in HRQoL and psychological distress. Although overall female patients reported worse functioning and more symptoms compared to male patients, male patients reported worse HRQoL and more psychological distress when compared with a reference population. As we seek to provide patient-tailored and personalized (follow-up) care, knowledge of sex-specific differences in HRQoL and psychological distress among CRC patients should be utilized to help health care providers anticipate and appropriately address patients’ sex-specific healthcare needs. Methods to both provide information and diminish effects on HRQoL and psychological distress should be explored in a sex-specific way.
Data availability
The data that support the findings of this study are available upon request from the profiles registry (www.profilesregistry.nl).
Abbreviations
- Colorectal cancer
Hospital Anxiety and Depression Scale (HADS)
Linear mixed models
Netherlands Cancer Registry
Patient Reported Outcomes Following Initial Treatment and Long Term Evaluation of Survivorship
Self-Administered Comorbidity Questionnaire
Sung H, et al. Global Cancer Statistics 2020: GLOBOCAN estimates of incidence and mortality worldwide for 36 cancers in 185 countries. CA Cancer J Clin. 2021;71(3):209–49.
Article PubMed Google Scholar
World Health Organization/International Agency for Research on Cancer. Global Cancer Observatory. [cited 2023 November 9]; Available from: https://gco.iarc.fr/today/online-analysis-table .
Siegel RL, et al. Colorectal cancer statistics, 2020. CA Cancer J Clin. 2020;70(3):145–64.
Lin KJ, Cheung WY, Lai JY, Giovannucci EL. The effect of estrogen vs. combined estrogen-progestogen therapy on the risk of colorectal cancer. Int J Cancer. 2012;130(2):419–30.
Article CAS PubMed Google Scholar
Jang YC, Huang HL, Leung CY. Association of hormone replacement therapy with mortality in colorectal cancer survivor: a systematic review and meta-analysis. BMC Cancer. 2019;19(1):1199.
Article CAS PubMed PubMed Central Google Scholar
Huxley RR, et al. The impact of dietary and lifestyle risk factors on risk of colorectal cancer: a quantitative overview of the epidemiological evidence. Int J Cancer. 2009;125(1):171–80.
Christy SM, Mosher CE, Rawl SM. Integrating men’s health and masculinity theories to explain colorectal cancer screening behavior. Am J Mens Health. 2014;8(1):54–65.
Kapidzic A, et al. Gender differences in fecal immunochemical test performance for early detection of colorectal neoplasia. Clin Gastroenterol Hepatol. 2015;13(8):1464–71.e4.
van Osch L, et al. Passive cancer detection and medical help seeking for cancer symptoms: (In)adequate behavior and psychosocial determinants. Eur J Cancer Prev. 2007;16(3):266–74.
Russell L, et al. Psychological distress, quality of life, symptoms and unmet needs of colorectal cancer survivors near the end of treatment. J Cancer Surviv. 2015;9(3):462–70.
Tsunoda A, et al. Prospective analysis of quality of life in the first year after colorectal cancer surgery. Acta Oncol. 2007;46(1):77–82.
Kopp I, Bauhofer A, Koller M. Understanding quality of life in patients with colorectal cancer: comparison of data from a randomised controlled trial, a population based cohort study and the norm reference population. Inflamm Res. 2004;53(Suppl 2):S130–5.
CAS PubMed Google Scholar
Arndt V, et al. Quality of life in patients with colorectal cancer 1 year after diagnosis compared with the general population: a population-based study. J Clin Oncol. 2004;22(23):4829–36.
Jansen L, Koch L, Brenner H, Arndt V. Quality of life among long-term (⩾5years) colorectal cancer survivors—systematic review. Eur J Cancer. 2010;46(16):2879–88.
Mols F, et al. Symptoms of anxiety and depression among colorectal cancer survivors from the population-based, longitudinal PROFILES Registry: Prevalence, predictors, and impact on quality of life. Cancer. 2018;124(12):2621–8.
Qaderi SM, et al. Trajectories of health-related quality of life and psychological distress in patients with colorectal cancer: a population-based study. Eur J Cancer. 2021;158:144–55.
Schmidt CE, et al. Gender differences in quality of life of patients with rectal cancer. A five-year prospective study. World J Surg. 2005;29(12):1630–41.
Kinoshita Y, et al. A longitudinal study of gender differences in quality of life among Japanese patients with lower rectal cancer treated with sphincter-saving surgery: a 1-year follow-up. World J Surg Oncol. 2015;13:91.
Article PubMed PubMed Central Google Scholar
Laghousi D, et al. Gender differences in health-related quality of life among patients with colorectal cancer. J Gastrointest Oncol. 2019;10(3):453–61.
Dunn J, et al. Trajectories of psychological distress after colorectal cancer. Psychooncology. 2013;22(8):1759–65.
Nolte S, et al. General population normative data for the EORTC QLQ-C30 health-related quality of life questionnaire based on 15,386 persons across 13 European countries, Canada and the Unites States. Eur J Cancer. 2019;107:153–63.
Kessler RC, et al. Lifetime and 12-month prevalence of DSM-III-R psychiatric disorders in the United States: Results from the national comorbidity survey. Arch Gen Psychiatry. 1994;51(1):8–19.
Oertelt-Prigione S, et al. Sex-differences in symptoms and functioning in >5000 cancer survivors: results from the PROFILES registry. Eur J Cancer. 2021;156:24–34.
Giesinger J, et al. Are gender-associated differences in quality of life in colorectal cancer patients disease-specific? Qual Life Res. 2009;18(5):547–55.
Bonhof CS, et al. Association between peripheral neuropathy and sleep quality among colorectal cancer patients from diagnosis until 2-year follow-up: results from the PROFILES registry. J. Cancer Surviv. 2021;
van de Poll-Franse LV, et al. The patient reported outcomes following initial treatment and long term evaluation of survivorship registry: scope, rationale and design of an infrastructure for the study of physical and psychosocial outcomes in cancer survivorship cohorts. Eur J Cancer. 2011;47(14):2188–94.
Nederlandse Kankerregistratie. Cijfers over Kanker. [cited 2021 Dec 2]; Available from: http://www.cijfersoverkanker.nl/ .
Sangha O, et al. The self-administered comorbidity questionnaire: a new method to assess comorbidity for clinical and health services research. Arthritis Rheum. 2003;49(2):156–63.
Postma TJ, et al. The development of an EORTC quality of life questionnaire to assess chemotherapy-induced peripheral neuropathy: the QLQ-CIPN20. Eur J Cancer. 2005;41(8):1135–9.
Fayers PM, et al. EORTC QLQ-C30 Scoring Manual (3rd edition). Brussels: EORTC; 2001.
Google Scholar
Zigmond AS, Snaith R. The hospital anxiety and depression scale. Acta Psychiatr Scand. 1983;67(6):361–70.
CentERpanel. [cited 2021 December 2]; Available from: https://www.website.centerpanel.nl/ .
Mols F, et al. Reference data of the EORTC QLQ-C30 questionnaire: five consecutive annual assessments of approximately 2000 representative Dutch men and women. Acta Oncol. 2018;57(10):1381–91.
Bonhof CS, Mols F, Widdershoven JW, Schoormans D. Colorectal cancer and cardiovascular disease: double the burden when it comes to your health-related quality of life? Acta Oncol. 2023;62(7):737–43.
Arora NK, et al. Barriers to information access, perceived health competence, and psychosocial health outcomes: test of a mediation model in a breast cancer sample. Patient Educ Couns. 2002;47(1):37–46.
Tsuchiya M, Horn S, Ingham R. Information provision and problem-solving processes in Japanese breast cancer survivors with lymphoedema symptoms. Scand J Caring Sci. 2012;26(1):53–60.
Thompson AE, et al. The influence of gender and other patient characteristics on health care-seeking behaviour: A QUALICOPC study. BMC Fam Pract. 2016;17(1):38.
Tedeschi RG, Calhoun LG. TARGET ARTICLE: "Posttraumatic Growth: Conceptual Foundations and Empirical Evidence". Psychol Inq. 2004;15(1):1–18.
Article Google Scholar
Sharp L, et al. Posttraumatic growth in head and neck cancer survivors: Is it possible and what are the correlates? Psycho-Oncology. 2018;27(6):1517–23.
Vishnevsky T, et al. Gender differences in self-reported posttraumatic growth: a meta-analysis. Psychol Women Q. 2010;34(1):110–20.
Tamres LK, Janicki D, Helgeson VS. Sex differences in coping behavior: a meta-analytic review and an examination of relative coping. Personal Soc Psychol Rev. 2002;6(1):2–30.
Menger F, Mohammed Halim NA, Rimmer B, Sharp L. Post-traumatic growth after cancer: a scoping review of qualitative research. Support Care Cancer. 2021;29(11):7013–27.
de Rooij BH, et al. Cancer survivors not participating in observational patient-reported outcome studies have a lower survival compared to participants: the population-based PROFILES registry. Qual Life Res. 2018;27(12):3313–24.
Ramsey I, et al. Cancer survivors who fully participate in the PROFILES registry have better health-related quality of life than those who drop out. J Cancer Surviv. 2019;13(6):829–39.
Download references
Acknowledgements
We would like to thank all patients and their doctors for their participation in PROCORE. Special thanks goes to C. Rolf, MD, and F. van Heest, MD, who were willing to function as independent advisors and to answer questions of patients. In addition, we want to thank the following hospitals for their collaboration: Elisabeth-TweeSteden hospital, Tilburg; Catharina hospital, Eindhoven; Elkerliek hospital, Helmond; Máxima Medical Centre, Eindhoven and Veldhoven.
The present research was supported by the Center of Research on Psychological Disorders and Somatic Diseases (C o RPS), Tilburg University, The Netherlands; The Netherlands Comprehensive Cancer Organization, Utrecht, The Netherlands; and an Investment Subsidy Large (2016/04981/ZONMW-91101002) of the Netherlands Organization for Scientific Research (The Hague, The Netherlands).
Author information
Authors and affiliations.
CoRPS—Center of Research on Psychological Disorders and Somatic diseases, Department of Medical and Clinical Psychology, Tilburg University, Tilburg, The Netherlands
Cynthia S. Bonhof, Belle H. de Rooij, Dounya Schoormans & Floortje Mols
Department of Research, Netherlands Comprehensive Cancer Organisation (IKNL), Utrecht, The Netherlands
Department of Surgery, Elisabeth-Twee Steden hospital, Tilburg, The Netherlands
Dareczka K. Wasowicz
Department of Internal Medicine, Máxima Medical Centre, Eindhoven and Veldhoven, The Netherlands
Gerard Vreugdenhil
You can also search for this author in PubMed Google Scholar
Contributions
C.B, D.W., G.V., and F.M. collected the data. B.R. matched the data. C.B. did the formal analyses, wrote the main manuscript text and prepared the tables and figures. All authors reviewed the manuscript.
Corresponding author
Correspondence to Floortje Mols .
Ethics declarations
Ethics approval.
The PROCORE study was approved by the certified Medical Ethic Committee of Medical Research Ethics Committees United (registration number NL51119.060.14).
Consent to participate
Informed consent was obtained from all individual participants included in the study.
Consent for publication
Patients signed informed consent regarding publishing their data.
Conflict of interest
The authors declare no competing interests.
Additional information
Publisher’s note.
Springer Nature remains neutral with regard to jurisdictional claims in published maps and institutional affiliations.
Rights and permissions
Open Access This article is licensed under a Creative Commons Attribution 4.0 International License, which permits use, sharing, adaptation, distribution and reproduction in any medium or format, as long as you give appropriate credit to the original author(s) and the source, provide a link to the Creative Commons licence, and indicate if changes were made. The images or other third party material in this article are included in the article's Creative Commons licence, unless indicated otherwise in a credit line to the material. If material is not included in the article's Creative Commons licence and your intended use is not permitted by statutory regulation or exceeds the permitted use, you will need to obtain permission directly from the copyright holder. To view a copy of this licence, visit http://creativecommons.org/licenses/by/4.0/ .
Reprints and permissions
About this article
Bonhof, C.S., de Rooij, B.H., Schoormans, D. et al. Sex differences in health-related quality of life and psychological distress among colorectal cancer patients: a 2-year longitudinal study. J Cancer Surviv (2024). https://doi.org/10.1007/s11764-024-01616-0
Download citation
Received : 24 January 2024
Accepted : 11 May 2024
Published : 24 May 2024
DOI : https://doi.org/10.1007/s11764-024-01616-0
Share this article
Anyone you share the following link with will be able to read this content:
Sorry, a shareable link is not currently available for this article.
Provided by the Springer Nature SharedIt content-sharing initiative
- General population
- Find a journal
- Publish with us
- Track your research
- Open access
- Published: 25 May 2024
Perinatal posttraumatic stress disorder as a predictor of mother-child bonding quality 8 months after childbirth: a longitudinal study
- Maria Vega-Sanz ORCID: orcid.org/0000-0002-0852-6264 1 ,
- Ana Berastegui ORCID: orcid.org/0000-0002-8554-1791 1 &
- Alvaro Sanchez-Lopez ORCID: orcid.org/0000-0003-2815-7803 2
BMC Pregnancy and Childbirth volume 24 , Article number: 389 ( 2024 ) Cite this article
217 Accesses
Metrics details
Negative childbirth experiences can be related to the onset of perinatal post-traumatic stress symptomatology (P-PTSS), which significantly impacts the mother and the infant. As a response in the face of the discomfort caused by P-PTSS, maladaptive emotion regulation strategies such as brooding can emerge, contributing to the consolidation of post-partum depressive symptoms. Ultimately, both types of symptomatology, P-PTSS and post-partum depression, can act as risk factors for developing mother-child bonding difficulties. Still, this full set of temporal paths has to date remained untested. The present longitudinal study aimed to analyze the risk factors associated with the appearance of P-PTSS after post-partum and to test a path model considering the role of P-PTSS as an indirect predictor of bonding difficulties at eight months of postpartum.
An initial sample of pregnant women in the third trimester of gestation ( N = 594) participated in a longitudinal study comprising two follow-ups at two and eight months of postpartum. The mothers completed online evaluations that included socio-demographic data and measures of psychological variables. A two-step linear regression model was performed to assess the predictive role of the variables proposed as risk factors for P-PTSS, and a path model was formulated to test the pathways of influence of P-PTSS on bonding difficulties.
A history of psychopathology of the mother, the presence of depression during pregnancy, the presence of medical complications in the mother, and the occurrence of traumatic birth experiences all acted as significant predictors of P-PTSS, explaining 29.5% of its variance. Furthermore, the path model tested further confirmed an indirect effect of P-PTSS, triggered by a negative childbirth experience, on subsequent bonding difficulties eight months after labor through its association with higher levels of brooding and, ultimately, postpartum depression levels. A further path showed that bonding difficulties at two months postpartum can persist at eight months postpartum due to the onset of brooding and postpartum depression symptoms.
We identified a set of robust predictors of P-PTSS: the mother’s previous history of depression, perinatal depression during pregnancy, the presence of medical complications in the mother and the occurrence of traumatic birth experiences, which has important implications for prevention. This is particularly relevant, as P-PTSS, when triggered by a negative childbirth experience, further indirectly predicted the development of mother-child bonding difficulties through the mediation of higher use of brooding and symptoms of postpartum depression. These findings can serve as a basis for developing new longitudinal studies to further advance the understanding of perinatal mechanisms of mental health.
Peer Review reports
The childbirth experience may sometimes be associated with different uncertainties and complications, making childbirth a potentially threatening situation for some women, especially first-time mothers [ 1 ]. This can sometimes lead to experiencing childbirth as a traumatic event, being even associated with the subsequent development of perinatal post-traumatic stress symptomatology in the mother after childbirth (P-PTSS) [ 2 ]. In this study we aimed to provide an integrative analysis of the risk factors that contribute to the onset of P-PTSS and its subsequent contribution to the emergence of further crucial problems such as the emergence of mother-child bonding difficulties at post-partum.
Traumatic childbirth and its relation to perinatal post-traumatic stress symptoms
The stress of the intervention, the perceived threat to the mother’s or baby’s life, and early separation from the baby [ 3 ] can all make childbirth a traumatic experience, impacting the mother, her baby, and the whole family [ 4 , 5 , 6 , 7 , 8 ]. The experience of childbirth as a traumatic event has been consistently linked to P-PTSS due to the negative psychological impact of the childbirth experience for some women [ 9 , 10 , 11 ]. It is estimated that around 4–16% of women may present symptoms clinically compatible with a P-PTSS after giving birth and that 30% would even meet diagnostic criteria for this disorder [ 12 ]. P-PTSS is primarily characterized by nightmares, flashbacks, irritability, guilt, and attempts to avoid thinking or talking about the birth experience [ 4 , 8 ]. Some of the most prominent risk factors for the onset of P-PTSS are the presence of a previous history of depression, as well as the occurrence of depression [ 13 ], medical complications [ 12 ] and the experience of stressful life events [ 14 ] during pregnancy. The quality of medical care received during childbirth [ 12 , 14 , 15 , 16 ], negative subjective experiences of childbirth [ 11 , 12 , 13 , 15 , 17 – 18 ] and medical complications in the mother [ 12 ] as in the newborn [ 14 ], such as preterm birth [ 11 , 19 ], also stand out from the moment of birth itself as contributors to P-PTSS.
As it can be seen, although studies on risk factors for P-PTSS are numerous and identify multiple associated factors, these studies lack the inclusion of an integrative analysis considering this series of factors as a whole and do not take into consideration the occurrence of different risk factors that can emerge at different times (i.e., previous history, pregnancy period, time of childbirth) through longitudinal designs. Therefore, this was our first aim in this study. As a second aim, once we established the specific contribution of each of this series of known risk factors for the occurrence of P-PTSS, we aimed to establish its own contribution to further central problems of post-partum, such as the emergence of mother-child bonding difficulties, considering its influence in other individual processes of emotional management, such as maternal ruminative response styles (specifically brooding) that may facilitate the emergence of these problems.
Ruminative response style as a strategy to regulate discomfort related to perinatal post-traumatic stress symptomatology
The cognitive model of PTSD proposed by Ehlers et al. [ 20 ] states that rumination is used as a strategy to regulate the distress generated by intrusive memories of the traumatic experience. Ruminations may comprise focusing on the traumatic event (i.e., its meaning, consequences, including intrusions, and what life would be like if the event had not occurred), with the aim of understanding it and working through it [ 21 ]. However, this form of response style would not be helpful for the person to make a coherent narrative of the trauma, rather making it challenging to integrate it [ 20 ], and thus increasing and maintaining the presence of PTSD and its severity [ 22 ].
Moreover, maintaining a rumination-focused emotion regulation style exacerbates negative emotions in the long term [ 20 ]. Specifically, depressive rumination or “brooding”, namely repetitively thinking in response to a sad mood, focusing on past problems [ 23 ], is associated with an increased vulnerability to a depressive episode [ 24 ], as well as with increases in the duration of depressive episodes [ 25 ], which makes its study particularly relevant as a possible mechanism for the maintenance of PTSD [ 22 ], but also as an origin of depressive symptomatology in the postpartum period [ 26 ].
Appropriate screening for P-PTSS risk markers may therefore have the added value of identifying mothers at increased risk for developing mood problems. However, the specific mechanisms by which the onset of P-PTSS may lead to depression and related problems in the postpartum period remain unclear, making the study of associated brooding response styles as an intervening mechanism particularly relevant.
P-PTSS, response style, and postpartum depression
Some studies indicate that 48–55% of people diagnosed with PTSD would also meet criteria for depressive symptomatology [ 27 ]. The relationship between both pathologies could be explained by a causal relationship in which people with PTSD symptoms have a negative view of themselves, others, and the world, corresponding to the cognitive triad usually present in depression [ 28 ], which would facilitate the appearance of depressive symptomatology [ 29 ]. Specifically, P-PTSS can also act as a risk factor related to the occurrence of depressive symptomatology during the pos-partum [ 30 ]. Some studies indicate that the risk for developing postpartum depression when a woman has a single symptom of P-PTSD is 11.1 times higher than in women who do not have P-PTSS and 9.7 times higher if all PTSD criteria are met [ 12 ].
Looking at individual psychological processes, the relationship and high comorbidity between both symptomologies could be explained through the presence of common factors such as negative thoughts, which are symptoms in both clinical conditions [ 11 ]. In that way, brooding has been identified as a further predictor of postpartum depressive symptomatology during the puerperium [ 26 ]. It could therefore be argued that P-PTSS may facilitate postpartum depressive symptomatology through a higher use of ruminative brooding as a maladaptive emotion regulation strategy to cope with distress (see Fig. 1 ).

Proposed relationship between the variables P-PTSS, brooding and postpartum depression symptomatology
Importantly, several authors point out how the presence of these two types of symptomatology in the mother during the postpartum period can significantly influence the quality of mother-child bonding, which is a fundamental aspect for the correct development of the baby [ 31 , 32 , 33 ]. In this study, we thus finally aimed to consider how P-PTSS and post-partum depression, and through which paths, contribute to mother-child bonding difficulties across the post-partum period.
Mother-child bonding difficulties
Mother-child bonding comprises the mother’s mental representation of her child and her caring behaviors towards her child [ 34 ]. The consequences of difficulties in mother-child bonding are problems in emotional self-regulation, problems in stress regulation and social adaptation, alterations in the infant temperament, and difficulties in cognitive and social development from the age of three months [ 31 ].
Symptomatology of P-PTSS, brooding [ 33 ], as well as postpartum depression [ 31 ], have all been found to be related to a poorer quality of mother-child bonding across separate studies. In the case of P-PTSS, mothers who experience P-PTSS tend to have less experiences of positive emotions toward the infant [ 35 ], difficulties in caregiving tasks, and problems establishing quality bonding [ 36 – 37 ]. In addition, mothers with P-PTSS also frequently experience emotions of anger towards the infant [ 36 ] and a decrease in their perceived self-efficacy [ 3 ]. It has also been reported in the literature that, in these cases, the newborn may act as a reminder of the traumatic event, producing feelings of rejection of the mother towards her child in the initial moment [ 13 , 38 – 39 ] Moreover, the experience of P-PTSS by the mother acts as a risk factor for her child to exhibit poorer emotion regulation at 6 months [ 39 ], lower cognitive development at 17 months [ 40 ], and worse socio-emotional development at 2 years [ 41 ].
Some previous research on P-PTSS and its relationship with bonding quality suggests that this relationship might be mediated by the presence of postpartum depression symptoms [ 40 , 41 ]. verall PTSD symptomatology directly impacted bonding quality but also indirectly through depressive symptomatology [ 11 ]. The relationship and high comorbidity between both symptomologies could be explained through the presence of common factors such as negative thoughts, which are symptoms in both clinical conditions [ 11 ]. Additionally, the relationship between P-PTSS and the presence of depressive symptoms in the postpartum period could be mediated using brooding as a maladaptive emotion regulation strategy to cope with distress [ 26 ]. Moreover, brooding has been related to a poorer quality of mother-child bonding in the postpartum period [ 33 ] because depletes the mother’s cognitive resources, making it difficult for her to manage the new cohabitation with a newborn, and limiting her ability to respond to the baby’s needs in a responsive, rapid, and congruent manner [ 33 ]. It that way, a higher use of brooding may facilitate postpartum depressive symptomatology. When the mother presents depressive symptomatology during the postpartum period, she tends not to bond adequately with her child, which may cause her to emotionally distance herself from her child, experiencing fewer positive feelings towards her baby, isolating herself from the baby’s emotional demands and attending only to his/her basic needs [ 32 ]. Furthermore, it has been found that children of mothers with postpartum depression exhibit poorer cognitive and socioemotional functioning in preschool and middle childhood [ 42 – 43 ].
Overall, it is crucial to continue expanding research in this area [ 44 ] Specifically, it is necessary to clarify the possible influence of P-PTSS on lower-quality bonding during the postpartum period through its indirect impact on the use of brooding and associated depressive symptomatology. Hence, the second aim of this study was to formulate and test an empirically informed and integrative path model, with this being particularly relevant to know the processes through which P-PTSS, after childbirth, may ultimately lead to the appearance of bonding problems at eight months of postpartum, by the hypothetical mediation of brooding and postpartum depression symptoms (see Fig. 2 below).

Proposed path model
Finally, the experience of childbirth, the birth itself, should be seen as an event that takes place in each socio-cultural and health care environment, which influences the experience itself. In terms of the socio-cultural context, in Spain there are different healthcare services: public health service, private medical insurance and entirely private healthcare. The latter two options offer greater flexibility in choosing the hospital and doctor. Most deliveries take place in public or private hospitals, with the option of using birthing centers being very uncommon, and home births being extremely rare. The delivery can be either vaginal or by cesarean section, depending on the circumstances and the preferences of the healthcare professionals. During labor, women are attended to by midwives and obstetricians, but the figure of the doula is not yet established. The presence of a companion is usually allowed, except for cesarean deliveries, which varies by hospital. Options for pain control are offered, with pharmacological methods being the most prevalent nowadays, especially the use of epidurals.
In terms of the health context, the health crisis caused by the COVID-19 pandemic was still occurring at the time of the present study. Women in this study gave birth between November 2020 and May 2021. During these months, the COVID-19 pandemic in Spain was at its peak, triggering the implementation of many new protocols in the hospital context. Despite the challenges posed by the pandemic, a combination of childbirth options was maintained, and the presence of a companion continued to be valued, although temporary restrictions could vary depending on hospital policies and health conditions. Similarly, despite the limited number of community services for parents during the postpartum period in Spain, efforts were made to provide telephone and online follow-ups to address potential difficulties in the early stages.
In this study, we considered women’s negative childbirth experiences and how they may sometimes lead to the development of Perinatal Post Traumatic Stress symptoms (P-PTSS), as well as the specific paths of contribution of this problem to subsequent mother-child bonding difficulties across the postpartum. Therefore, two main aims were defined, and were addressed through an extensive longitudinal study with multiple time assessments across the pregnancy and post-partum period. First, we conducted a detailed analysis of the risk factors occurring before and during pregnancy, and at childbirth, identified by previous empirical research [ 12 , 13 , 14 , 15 , 16 , 17 , 18 ], that can predict a higher presence of P-PTSS after childbirth. Specifically, to address this first aim, we conducted an integrative study of predictors for P-PTSS that have been separately identified as risk factor in the previous literature, including pre-pregnancy (previous history of depression; 13), during pregnancy (presence of perinatal depression symptoms, generalized anxiety symptoms, the experience of stressful life events and medical complications; 12–14), as well as childbirth factors (quality of medical care received, birth experience and infant complications during childbirth; 12–18). To study the relationship between all these factors and P-PTSS, a first section of the longitudinal design was formulated to integrate them and consider their occurrence at different times (pre-pregnancy, during-pregnancy, and childbirth-related predictors of P-PTSS at eight months of postpartum).
As for the second aim, we formulated a path model to test the hypothesis that P-PTSS has an indirect predictive role in bonding difficulties at eight months of postpartum through its direct influence on brooding and associated post-partum depressive symptomatology (see Fig. 2 for more details). Thus, based on the reviewed literature, it was expected that a higher presence of P-PTSS after childbirth would predict subsequent higher levels of brooding [ 20 , 21 , 22 ], which would be associated with higher postpartum depressive symptomatology levels [ 3 , 12 ], and that in turn, these problems would be associated with higher increases of mother-child bonding difficulties across the postpartum period [ 31 , 33 ].
Design and participants
A longitudinal study with three data collection phases was designed. A non-discriminatory exponential chain demonstration (i.e., snowball sampling technique) was performed to recruit the sample. The inclusion criteria for the study were being a woman over 18 years of age and in the third trimester of pregnancy. The first evaluation (T1) comprised recruiting an initial sample of women of N = 594 in their third trimester of pregnancy. This phase was conducted from November 2020 to February 2021. The mean week of gestation in women recruited at T1 was 33.37 (SD = 3.77). The second evaluation (T2) consisted of reassessing all T1 participants two months after childbirth. A sub-sample of N = 326 was obtained from T1. This phase was conducted between January and July 2021. The average week of gestation at which participants went into labour was 39.33 (SD = 1.57). Finally, in the third evaluation (T3), the longitudinal follow-up of T2 participants continued. This T3 evaluation was completed eight months after giving birth and six months after the previous assessment at T2. This final sample was the one used in this study as all T3 participants had completed the longitudinal follow-up of all three phases. This phase was conducted between July 2021 and January 2022.
The mean age of the final sample was 32.16 (SD = 4.18), 74% of the participants had completed university studies, 95% had a couple or were married at the time of the first evaluation. The average number of children of the participants was 0.33 (SD = 0.55), and 68% followed up on their pregnancy by the public health system.
The 26% of the women reported psychotherapy during the first eight months postpartum and 6.8% reported using anxiolytics during that time. Descriptive socio-demographic data are shown in Table 1 . All participating women signed informed consent. The Ethics Committees of Universidad Pontificia Comillas approved the study protocol .
Instruments
Data were collected through online evaluation surveys using Google Forms. First, the surveys included questions on socio-demographic data: age, type of medical care, marital status, educational level, and type of delivery. The surveys also included questions testing the psychological impact of variables such as medical complications for the mother during pregnancy (i.e., ‘Did you have any medical complications during your pregnancy?‘) and during childbirth (i.e., ‘Did you have any medical complications during childbirth?‘), and the baby afterward during delivery (i.e., ‘Did your baby have any medical complications during childbirth?‘) all based on a yes/no answer option. If mothers answered ‘yes’ to any of these questions, they were asked another question about the type of complication they experienced (e.g., ‘Could you indicate what type of complication/s it was/were?‘). The quality of medical care received and the type of birth experience (i.e., whether distressing or even traumatic) was assessed through single questions formulated by the researchers (i.e., “In relation to the medical care received during the birth, please indicate how you experienced it: satisfactory, adequate, negative or threatening”; “In relation to your birth experience, please indicate whether you experienced it as a satisfactory birth, a difficult but satisfactory birth or a traumatic birth”).
Secondly, the following standardized questionnaires were used to assess risk factors for P-PTSS, as well as the variables used to test the relationship between P-PTSS and subsequent brooding, depression and mother-child bonding.
Perinatal posttraumatic stress symptoms . PCL-5, PTSD Checklist for DMS-5. The Post-traumatic Stress Disorder Symptom Checklist [ 45 ] comprises 20 items and a Likert-type response scale (5 response options). It was specified that mothers should complete this questionnaire based on their birth experience. The internal consistency in this study at eight months postpartum was excellent α = 0.93. This questionnaire was administered at T3.
Previous history of depression . The Centre for Epidemiological Studies Depression Scale, CESD-8 [ 46 ], is an 8-item scale that can be used to detect the occurrence of depressive episodes at different periods. This study assessed the degree of depression levels experienced in the worst period of the previous life identified by the participants before the third semester of pregnancy. The items are rated on a 4-point Likert scale. The scale shows a Cronbach’s alpha of 0.82 in Spanish validation [ 47 ]. The internal consistency in this study was good α = 0.89. A cut-off score ≥ 9 was used to indicate the existence of a previous history of depression. Participants were divided into two groups according to the above cut-off for the total score obtained on this questionnaire. Those who scored below the cut-off point were assigned to group 0 (i.e., no previous history of depression), and those who scored at or above the cut-off point were assigned to group 1 (i.e., presence of a prior history of depression). This questionnaire was administered at T1.
Stressful life events. Participants were asked to indicate whether they had experienced any stressful life events from the following pre-specified list of life stressors [ 48 ] during their pregnancy: separation or divorce, death in the family, job loss, domestic violence, or unwanted pregnancy. Participants who did not select any stressful life events were assigned to group 0 (i.e., no significant stressors occurred), and those who did were assigned to group 1 (i.e., significant stressors occurred). This questionnaire was administered at T1.
General anxiety. The Generalised Anxiety Scale, GAD-7 [ 49 ], is a 7-item scale rated on a 4-point Likert scale. A cut-off score ≥ 10 was used to identify clinical levels of anxious symptomatology at the time of assessment [ 49 ]. This scale shows a Cronbach’s alpha of 0.93 in its Spanish validation [ 50 ]. The internal consistency in this study, in the third trimester of pregnancy, was good α = 0.87. This questionnaire was administered at T1.
Perinatal and post-natal depression : The Edinburgh Postnatal Depression Scale, EDPS [ 51 ], has been validated in the perinatal and postnatal stages. It is a 10-item scale, and items are rated on a 4-point Likert scale. The cut-off score ≥ 13 indicates a high risk of depression during pregnancy and ≥ ten postpartum. The internal consistency of the Spanish adaptation of the instrument was 0.91 [ 52 ]. Internal consistency in this study, in the third trimester of pregnancy, was good, α = 0.86 and α = 0.88 at eight months postpartum. This questionnaire was administered at T1, T2, and T3.
Ruminative Response. The Ruminative Response Scale, RRS [ 53 ], was used to assess individual styles of ruminative response, differentiating between the use of reflection (reflective rumination) and brooding (depressive rumination). It is a 22-item scale. The items are rated on a 4-point Likert scale. The Spanish adaptation shows a Cronbach’s alpha of 0.93 for the full scale [ 54 ]. At eight months postpartum, the internal consistency in this study was excellent α = 0.94 for the total scale, and good α = 0.82 for the brooding subscale. This questionnaire was administered at T3.
Postpartum bonding difficulties. The Postpartum Bonding Questionnaire [ 55 ] was used to detect the presence of bonding difficulties. It provides a total score of the quality of bonding. It comprises 25 items with a Likert-type response scale (5 response options). The adaptation to Spanish shows a Cronbach’s alpha of 0.90 on the full scale. The internal consistency in this study, at the two postpartum assessment periods was good, α = 0.83, at two postpartum months and α = 0.86 at eight postpartum months. This questionnaire was administered at T2 and T3.
Complementarily, further measures of other variables not relevant to the aims of this study were also collected in the study. The full set of measures relevant to this study, collected at each assessment time, are summarized in Table 2 .
Statistical analyses
Statistical analyses were performed using IBM SPSS Statistics version 22 software. Initially, descriptive analyses of socio-demographic data and psychological measures of the participants were performed. In addition, a preliminary analysis with t-tests for independent samples was carried out on the sample’s representativeness.
Subsequently, Pearson bivariate correlation analyses were carried out on the association between the risk factors proposed and P-PTSS (i.e., aim 1) and the principal variables of the subsequent path model (i.e., aim 2: perinatal post-traumatic stress symptom score → brooding → depressive symptomatology → bonding difficulties). Moreover, t-tests for independent samples were carried out for differences in the dimensional variables by other risk dichotomous variables. Following Cohen’s [ 56 ] criteria, the following magnitudes will be followed to interpret the results: between +/- 0.10 and +/- 0.29 low; between +/- 0.30 and +/- 0.49 medium or moderate; between +/- 0.50 and +/- 1.0 high correlation.
As for the first aim, to determine the percentage of explained variance of the dependent variable (P-PTSS at T3) by the variables considered as predictors, a linear regression analysis was performed in three steps, considering the contribution of antenatal variables (T1; step 1) pregnancy variables (T1; step 2) and partum variables (T2; step 3).
As for the second aim, we tested the initial hypothesized path model (see Fig. 2 ) using a structural equation that included the complete set of significantly correlated variables. In that model, P-PTSS (T3) acted as an exogenous variable, predicting bonding difficulties directly (T3) and indirectly through the influence of brooding (T3) (i.e., mediator 1) and postpartum depressive symptomatology (T3) (i.e., mediator 3). For this purpose, bonding difficulties at two months postpartum (T2) were controlled (i.e., regressed on bonding difficulties at eight months postpartum, T2), which allowed to establish the change in bonding that was solely due to the proposed predictor variables in the model. The estimation of standardized parameters of the path model followed the full information maximum likelihood (FIML) estimation method. Adjustment of our model we tested using standard criteria [ 57 ]: (a) χ2: non-significant value; (b) χ2 / gl: values lower than 2;c) comparative fit index and Tucker-Lewis index, a value ≥ 0.95 indicates a good fit ; d) root mean square error of approximation, a value ≤ 0.05 indicates a good fit; e) standardized root mean square, a smaller value indicates a better fit between the observed data and the tested model; and f) Akaike information criterion, a lower value indicates the preference for selecting a model when compared to another model [ 58 – 59 ]. Moreover, we used the Mardia coefficient for assessing multivariate normality (a value ≤ 70 indicates the possibility to assume multivariate normality) [ 60 ]. Hypothesized mediation pathways (i.e., P-PTSS → brooding → depressive symptomatology → bonding difficulties) with the variable of previous levels of bonding difficulties (T2) being a controlled, providing a measure of temporal change of bonding difficulties (Fig. 3 ), were tested via the estimation of indirect effects within the full path model. The model was then reformulated following modification indices and considering the predictive role of childbirth complications at T2 in P-PPTSS levels as T3 as the predictor (see the Results section for full details on this final model). The final model’s adjustment and its mediational pathways on T2-T3 changes in bonding difficulties were tested following the same criteria and steps as detailed above. These structural equation models and the analyses of the resulting path analyses were conducted using AMOS v18.0 (SPSS).
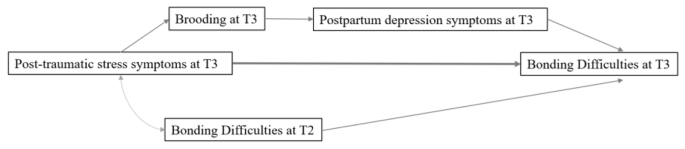
Initial proposed path model
Note. T2 = two months of postpartum; T3 = eight months of postpartum
Descriptive data of socio-demographic data and psychological impact variables of the participants in the study are shown in Table 1 above. Descriptive data of dichotomous psychological measures, and further mean and standard deviations of dimensional psychological measurements are depicted in Table 2 below.
Representativeness of the sample
For psychological measures that were collected multiple times, no significant differences were found in the variables collected in T1 between completers and non-completers at T2 (perinatal depression t= -0.998; bonding difficulties t= -0.495; brooding t = 0.650; all p’s > 0.05) and completers and non-completers at T3 (perinatal depression t= -0.335; bonding difficulties t= -0.501; brooding t = 1.407; all p’s > 0.05). No differences were neither found in variables collected in T2 between completers and non-completers at T3 (postpartum depression t= -0.402; bonding difficulties t= -0.870; brooding t= -0.845; all p’s > 0.05).
Risk factors of post-traumatic stress symptoms at eight months of postpartum
Bivariate correlations and t-tests for independent samples.
Bivariate correlation analyses showed significant correlations between post-traumatic stress symptoms and risk factors assessed to test the first aim. Specifically, P-PTSS (T3) were significantly moderate and positively related to perinatal depression (T1; r = 0.448; p = 0.001), moderate and positively related to general anxiety (T1; r = 0.388; p = 0.001), low and positively related to quality of care received (T2; r = 0.196; p = 0.018) and low and positively related to childbirth experience (T2; r = 0.277; p = 0.001).
The t-tests for independent samples indicated that women with a history of depression (T1) had, in comparison to those without a previous depression episode, higher P-PTSS (T3), t(148) = − 4.897; p = 0.001. There were no statistically significant differences in P-PTSS (T3) as a function of differences in the experience of a stressful life event during pregnancy (T1), t(36.815) = 0.748; p = 0.459, the presence of complications in the mother during the pregnancy (T1), t (148) = 1.678; p = 0.095, and during the childbirth (T2), t (148) = 0.460; p = 0.646,or the presence of complications in the baby during childbirth(T2), t (77.300) = − 0.532; p = 0.596.
Main analyses (Aim 1): Linear regression model considering three-step predictors of P-PSTD
The linear regression model was composed of three steps. In the first step [ 1 ] pre-pregnancy predictor variables were entered: previous history of depression (assessed retrospectively at T1). In the second step [ 2 ], psychological predictor variables present during the third trimester of pregnancy: perinatal depressive symptomatology, generalized anxiety symptomatology, the experience of stressful life events, and complications during pregnancy (T1) were entered (assessed at T1). In the third step [ 3 ], predictor variables specific to the childbirth were entered in the equation: quality of medical care received, birth experience, and complications in the baby after delivery (assessed retrospectively at T2, two months after labor). The outcome variable of the model was P-PTSS, assessed eight months after childbirth (T3). The results of the linear regression model are shown in Table 3 .
As can be seen in the table, 29.5% of the variance in P-PTSS (T3) at eight months postpartum was explained by the model. The significant positive predictive power of the a previous history of depression (Step 1) explained 13.7% of the variance in P-PTSS in the eighth month of postpartum. A further 11.1% of variance in P-PTSS was accounted by predictors referred to the pregnancy period (Step 2), from which perinatal depression was the only significant rpedictor. Finally, a further 4.7% of the variance in P-PTSS was predicted by predictors referred to the childbirth (Step 3), among which medical complications in the mother during childbirth and the birth experience as traumatic acted as the significant predictors. Thus, more threatening, and traumatic women’s appraisal of their birth experience accounted for greater levels of P-PTSS at eight months of postpartum.
Predictive role of post-traumatic stress symptoms at eight months of postpartum on bonding difficulties
Bivariate correlations.
Preliminary bivariate correlation analyses supported significant correlations between the main variables under study (aim 2). All variables were significantly related. Postpartum depression (T3) was significantly moderate and positively related to brooding (T3), r = 0.670; p = 0.001, bonding difficulties (T3), r = 0.517; p = 0.001, and P-PTSS (T3), r = 0.641; p = 0.001. Brooding was significantly moderate and positively related to bonding difficulties at T3, r = 0.526; p = 0.001, and P-PTSS at T3, r = 0.613; p = 0.001. Finally, P-PTSS was significantly moderate and positively related to bonding difficulties at T3, r = 0.482; p = 0.001.
Main analyses (Aim 2): path model of P-PTSS as an indirect predictor of mother-child bonding
Based on the previous bivariate correlation analysis we tested an equation model where bonding difficulties at eight months of postpartum (T3) variables were predicted by P-PTSS (at eight months of postpartum; T3) directly and/or indirectly through the use of brooding (T3) as emotional regulation strategy and the development of postpartum depressive symptoms (T3), with the variable of previous levels of bonding difficulties (T2) being a controlled, providing a measure of temporal change of bonding difficulties. All the goodness-of-fit indices are shown in Table 4 .
As shown in Table 4 , the goodness-of-fit indices for the initial hypothesized path model were not good. Moreover, the Mardia coefficient yielded a value of 15.94 which is far for the critical value (± 5). Since the fit of our initial model (Model 1) was poor, re-specification was carried out following Wald and Lagrange multiplier tests [ 61 ]. All paths with nonsignificant P values were removed consecutively. Only the path P-PTSS to bonding difficulties, both at 8 months of postpartum was removed. The variable ‘birth experience,’ assessed at 2 months postpartum (T2), was further introduced as a predictor of the onset of P-PTSS at 8 months postpartum (T3), and an additional path was included in the model, through which bonding difficulties at 2 months of postpartum predicted brooding at 8 months of postpartum (Fig. 4 ). The final respecified model (Model 1R) showed a very good fit in all the indices (Table 4 ). Despite the Mardia coefficient remaining slightly elevated at 13.67, it improved compared to Model 1, and it would be appropriate to use maximum likelihood estimation as it did not exceed the value of 70 [ 60 ].
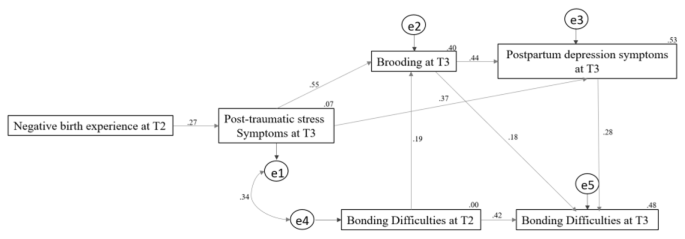
The respecified model (Model 1R) with standardized regression weights
Note. T2: 2 months postpartum. T3: 8 months postpartum
Indirect effects were tested using bias-corrected bootstrap estimations (2000 bootstrap samples with 95% CI). First, the hypothesized path was significant, with an indirect effect of P-PTSS triggered by a negative birth experience at T2 on bonding difficulties’ changes from T2 to T3, through individual differences in P-PTSS in ruminative brooding, subsequently leading to higher postpartum depression symptom levels and ultimately resulting in lower bonding ( p = 0.001; SE = 1.375; 95% CI 3.759 to 8.281), supporting the hypothesized path. Additionally, a further indirect effect of P-PTSS after a negative birth experience on bonding difficulties, exclusively through individual differences in brooding after controlling for depression levels was also statistically significant ( p = 0.001; SE = 1.388; 95% CI 3.053 to 7.606). There was also a significant indirect effect of P-PTSS after a negative birth experience on bonding difficulties, exclusively through postpartum depression symptoms after controlling for brooding levels ( p = 0.001; SE = 1.370; 95% CI 3.062 to 7.575). Furthermore, the indirect effect of bonding difficulties at two months (T2) on bonding difficulties at eight months postpartum (T3) through individual differences in ruminative brooding, subsequently leading to higher postpartum depression symptom levels and ultimately resulting in lower bonding, was significant ( p = 0.001; SE = 0.161; 95% CI 0.917 to 1.449). Additionally, the significant indirect effect of low-quality bonding (T2) on bonding difficulties (T3) through individual differences in brooding, after controlling for depression levels, was also statistically significant ( p = 0.015; SE = 0.192; 95% CI 0.182 to 0.821).
The present study considers the type of birth experience for the mother as a bridging event between pregnancy and postpartum psychological experiences, whose relevance is of great importance in the postpartum period, not only for the psychological well-being of the mother but also for the bond with her child [ 4 , 5 , 6 , 7 , 8 ]. Sometimes childbirth is not experienced as a positive but as a negative or even traumatic event [ 12 ], which can lead to P-PTSS [ 9 – 10 ]. Previous research has been focused on comprehensively studying the risk factors that may influence P-PTSS development after childbirth. Yet, these previous studies did not typically consider all possible predictors in an integrative manner, neither used different temporal assessments of relevant risk factors at different relevant periods (before and during pregnancy, and at childbirth). The first aim of the present study was thus to assess the risk factors identified in previous literature for the development of P-PTSS, but integrating all the factors identified in different studies into the model, while also taking into account their occurrence at different times (i.e., prior to pregnancy, pregnancy period, time of childbirth), through a rigorous and extensive longitudinal design.
Results indicated that the presence of an history of depression before pregnancy as well as the higher presence of depressive symptoms during the third trimester of gestation both stood out as significant predictors of P-PTSS eight months after childbirth. These results align with evidence from previous studies [ 13 ] and show the impact of the mother’s life history and current psychological well-being during pregnancy on her childbirth experience. Moreover, experiencing medical complications during childbirth by the mother, as well as perceiving the childbirth experience as threatening or traumatic, were both identified as additional significant risk factors for the subsequent development of P-PTSS. Thus, the more complex and traumatic the mothers’ childbirth experience, the greater the probability of developing P-PTSS. These results align with evidence from previous research on this issue [ 12 , 14 – 15 , 17 ], while being obtained in an integrative model that consider all these variables at different temporal stages of pregnancy and childbirth.
In contrast, generalized anxiety symptomatology during pregnancy, the experience of stressful life events and complications during pregnancy, the quality of medical care received, and the complications in the baby during birth were not found to be significant predictors of P-PTSS above and beyond the mentioned main risk factors. These results apparently contrast to the ones from other studies [ 12 , 13 , 14 , 15 ]. Nonetheless, the absence of predictive power of these other variables could be due to the lack of representativeness and variability of these variables in the present sample. The participants’ mean anxiety level in this study was below the cut-off point on the generalized anxiety symptomatology questionnaire during pregnancy, 84% did not report to have experienced stressful life events, and 81% did not report to have experienced medical complications during pregnancy. Similarly, 72% did no have medical complications after delivery. Finally, the medical quality variable could not be a predictor due to limitations in the assessment method, as it was only assessed through a single question that did not show sufficient sensitivity.
Overall, using an integrative and extensive longitudinal design, the present study allowed to determine the main risk factors contributing to higher levels of P-PTSS. This seems essential, as this problematic has been identified as a core source of dysfunction for some mothers itself but also a further predictor of additional central problems at postpartum, such as the appearance of difficulties in mother-child bonding. Yet, the specific paths for this influence remained to date unclear.
Thus, the second aim of the study was establishing the indirect impact of P-PTSS symptomatology on mothers’ bonding with the newborn through other identified regulatory and mental health issues emerging during postpartum (i.e., brooding, postpartum depression). The initial path model formulated (see Fig. 3 for more details) did not show good fit indices, so we followed modification indices and introduced the variable ‘birth experience’ into the model as the initial predictor, creating a new revised model (Model 1R, see Fig. 4 for more detail). This new path model would allow us to see two pathways of influence towards the presence of bonding difficulties at 8 months postpartum. Results showed that, on the one hand, the birth experience would act as a risk factor predicting the subsequent appearance of P-PTSS, in response to which the mother would use emotional regulation strategies based on the use of brooding, which in turn would trigger depressive symptomatology, ultimately impacting on the quality of bonding. For P-PTSS to impact the quality of bonding, brooding (a maladaptive emotion regulation strategy associated with P-PTSS) and postpartum depression symptomatology (comorbid to P-PTSS and usually caused by this type of brooding ruminative response style) must be considered as potential intervening meditators. Our analyses empirically confirmed these paths and are discussed into detail below. Moreover, in the final model, no direct effect of a negative birth experience on bonding difficulties was found, and it only indirectly influenced it through the above-mentioned path. This suggests that women may have a more difficult or potentially traumatic birth, but this would not necessarily affect her ability to bond with her baby. A negative birth experience would only impact the bond only if postpartum PTSD symptoms is subsequently developed. Thus, it would not be the experience itself but the traumatic interpretation and processing of that experience what would influence the appearance of bonding difficulties.
As suggested by other authors, ruminative response styles would be habitually used by women with P-PTSS as a maladaptive regulation strategy of their experienced distress [ 20 , 62 ]. A higher use of brooding would not promote trauma integration [ 20 ] but instead increase the severity of P-PTSS [ 20 , 22 ], generating long-term negative emotions [ 20 ], among others, being associated to postpartum depressive symptoms. For this reason, in line with our results, P-PTSS should be considered a risk factor for developing postpartum depressive symptomatology, as is the case with general PTSS and depressive symptoms [ 29 – 30 ]. The literature indicates that the causal relationship between PTSD and depressive symptomatology would be due to that people with PTSD have a negative view of themselves, others, and the world, which corresponds to the cognitive triad usually promoting risk for the onset and maintenance of depressive symptomatology [ 28 ].
Considering the postpartum stage, the presence of P-PTSS is also related to symptoms of postpartum depression, with the prevalence of this symptomatology at two months of postpartum being between 10 and 20% of women and increasing to 12–25% in new mothers [ 31 ]. In addition, postpartum depression tends to be preceded by brooding [ 26 ] which comprises a higher attention to negative cognitions referred to the cognitive triad (63. In the final supported model in this study, P-PTSS would predict a ruminative style as a maladaptive regulation strategy of distress, mainly shaped by brooding, being then related to higher postpartum depressive symptom levels.
The results also indicate that postpartum depressive symptomatology impacts the quality of mother-child interactions, favoring higher levels of bonding difficulties [ 32 ]. In addition, a higher use of brooding itself, which is responsible for facilitating depressive symptomatology, would also partly directly impact the development of poorer quality mother-child bonding [ 33 ]. This may be because brooding drains the mother’s cognitive resources, preventing her from having her baby in mind and thus limiting her ability to respond to her newborn’s needs in a responsive, rapid, and congruent manner [ 33 ]. Therefore, the occurrence of P-PTSS would play a further specific indirect role in the generation of bonding difficulties through both ruminative mechanisms as well as through higher associated levels of depressive symptomatology in the postpartum period.
Moreover, the presence of bonding difficulties at two months postpartum would predict, by itself, the continuation of these difficulties at eight months postpartum, both directly and through the emergence of brooding and postpartum depression symptoms. As mentioned earlier, the use of brooding, in this case as rumination in response to difficulties a mother may be experiencing in feeling connected to her baby, would generate long-term negative emotions in the mother [ 20 ], implicating a higher attention to negative cognitions referred to the cognitive triad [ 63 ], which is associated with postpartum depressive symptoms. This, in turn, impacts the quality of mother-child interactions, leading to higher levels of bonding difficulties [ 32 ].
Taken together, these results have several implications that should be considered. The results suggest the importance of developing preventive strategies in the postpartum, including identifying mothers with a previous history of depression and reducing avoidable stress during childbirth to reduce the risk of onset of P-PTSS.
In this line, it is essential to develop training programs for health personnel so that they can learn what aspects can turn childbirth into a traumatic event. By doing so, they can identify those mothers with whom they should work to prevent the development of a traumatic interpretation of childbirth and, consequently, avoid the onset of P-PTSS. In addition, programs focusing on screening and intervening to redirect mothers’ ruminative patterns and depressive symptoms in the early postpartum period would be very helpful in reducing their influence in the appearance of bonding disorders [ 64 ].
Limitations and strengths
Although, as commented above, the present results have several interesting implications for promoting mothers’ mental health and reducing bonding difficulties during the postpartum, a series of limitations must be considered. First, regarding the risk factors for P-PTSS development that were studied, it was only possible to collect pre-birth aspects such as the presence of psychopathology, as well as a broader view of the medical quality received by the health personnel and the birth experience, being based on a general self-report of the mother, assessed with a single question designed for the study that did not show sufficient sensitivity. Future studies should use more precise assessments of these variables and consider other potential risk factors informed by previous literature [ 12 , 13 , 18 ].
Furthermore, the diagnostic criteria established for detecting P-PTSS or postpartum depression state that the assessment should be carried out six months after presenting symptoms [ 65 ], but in the present study, it was assessed at eight months postpartum. Similarly, the assessment of P-PTSS was carried out using a PTSD scale designed for its use in the general population due to the lack of validated and standardized questionnaires specifically designed for the perinatal population. Moreover, as for the path model testing longitudinal changes in bonding difficulties, although our model considered theoretical sound paths among variables, by design, most variables needed to be assessed within the same time period, as sociodemographic variables. Thus, further intensive longitudinal research might add value by modelling how each of our supported variables predict such longitudinal changes when modelled across different time points. Similarly, these results refer to a specific socio-cultural (Spain) and health (COVID-19 pandemic) context, which, despite their relevance to the study, have not been controlled for, so it is necessary to be able to replicate this research in other contexts.
Despite these limitations, a series of strengths of the study must be highlighted. First, online recruitment, as the one used in this study, provided an opportunity to obtain larger samples of participants than most of previous studies, and to recruit more women who experience postpartum PTSD or depression, which is useful when studying patho-mechanisms of bonding problems. In this study we were able to conduct a longitudinal study at multiple phases of 150 mothers, which is not typically common, and helped to provide reliable and consistent tests of the hypotheses of our study. Further, the study of factors involved in the onset and maintenance of P-PTSS, as well as its comorbidities and subsequent influences, is a very novel adding of this research, as there are numerous studies about PTSD in the general population [ 20 , 21 , 22 ], but not in the perinatal population. Specifically, this study has aimed to provide more extensive evidence about individual cognitive processes, such as a ruminative response style, in studying P-PTSS and its relationship with postpartum depression, that are not typically integrated within this line of research. A limited number of studies have previously explored the relationship between these variables [ 20 ] and always in the general population. Furthermore, despite what has been stated about the comorbidity between P-PTSS and depressive symptomatology and the difficulties that this comprises to separate the statistical influence of one on each other [ 66 ], the specific nature of the instruments used to assess P-PTSS symptomatology and postpartum depression, made it possible to reduce the covariance of both variables at the statistical level and to isolate the effects of one on each other. Finally, this research highlights the importance of studying the presence of psychopathology during the perinatal stage, specifically in moments of great relevance but less studied, such as the childbirth stage. To this end, the longitudinal approach used in this study highlights the importance of the mother’s life and the pregnancy experience for the understanding the development of problems of mother-child bonding.
Conclusions
The present study investigated risk factors for P-PTSS in the eighth month of postpartum and the possible mechanisms for its contribution on further bonding problems. The results allow to conclude that previous history of depression, depressive symptomatology during pregnancy, presence of medical complications in the mother, and a negative childbirth experience may be risk factors predicting P-PTSS in the postpartum period. This symptomatology, triggered by a negative childbirth experience, could, in turn, lead to difficulties in mother-child bonding. This relationship would be mediated by the presence of brooding and the development of postpartum depression. P-PTSS would predict a brooding coping style in the mother, increasing the likelihood of depressive symptoms. Moreover, mothers who already had bonding issues at two months postpartum, these could persist at eight months postpartum due to the onset of brooding and postpartum depression symptoms.
These findings are highly informative and may serve as a basis for the development of new future longitudinal studies that continue advancing the understanding of these mechanisms of perinatal mental health. Studies aimed at replicating and extending the relationships between these variables will improve knowledge about the risk factors that are present during the mothers’ life history, her pregnancy, and their influence on their childbirth experience, as well as on their subsequent postpartum mental health, and the quality of mother-child bonding.
Data availability
the dataset analysed during the current study is available from the corresponding author on reasonable request.
De Vicente A, González H, Capilla P, Santamaría P. Estrés postraumático en El período perinatal. En Rodríguez Muñoz MF, coordinador. Psicología perinatal, Teoría Y Práctica. Ediciones Pirámide; 2019. pp. 101–24.
Hergüner S, Çiçek E, Annagür A, Hergüner A, Örs R. Association of delivery type with postpartum depression perceived social support and maternal attachment. J Psychiatry Neurosci. 2014;27:15–20.
Google Scholar
Ayers S. Thoughts and emotions during traumatic birth: a qualitative study. Birth. 2007;34(3):253–63.
Article PubMed Google Scholar
Ayers S. Birth trauma and post-traumatic stress disorder: the importance of risk and resilience. J Reprod Infant Psychol. 2017;35:427–30.
Gellhorn S. Other types of maternal mental health difficulties. Ed. Postnatal depression and Maternal Mental Health, British Library. West Sussex, UK: Pavilion Publishing; 2017.
Jacques N, de Mola CL, Joseph G, Mesenburg MA, da Silveira MF. Prenatal and postnatal maternal depression and infant hospitalization and mortality in the first year of life: a systematic review and meta-analysis. J Affect Disord. 2019;15:201–8.
Article Google Scholar
Kendall-Tackett K. Birth trauma: the causes and consequences of childbirth‐related trauma and PTSD. ed. Women’s Reproductive Mental Health Across the Lifespan. In: Basel, Switzerland: Springer Publishing; 2015.
Stramrood C, Slade P. A women afraid of becoming pregnant again: post-traumatic stress disorder following childbirth. eds. Bio-Psycho‐Social Obstetricsand Gynecology. In: Paarlberg KM, Van de Wiel HBM, Basel, Switzerland: Springer; 2017:33‐5.
Olza I. El síndrome de estrés postraumático como secuela obstétrica. Información para profesionales de la atención al parto. Estrategia de Atención al Parto Normal en el Sistema Nacional de Salud. 2007.
Bydlowski M, Raoul-Duval A. Un avatar psychique meconnu de la puerperality: La nervrose traumatique post-obstetricale. Perspect Psychiatry. 1978;4:321–8.
Radoš SN, Matijaš M, Anđelinović M, Čartolovni A, Ayers S. The role of posttraumatic stress and depression symptoms in mother-infant bonding. J Affect Disord. 2020;268:134–40.
Bingol FB, Bal MD. The risk factors for postpartum post-traumatic stress disorder and depression. Perspect Psyc Care J. 2020;56(4):851–7.
Ayers S, Bond R, Bertullies S, Wijma K. The etiology of post-traumatic stress following childbirth: a meta-analysis and theoretical framework. Psychol Med. 2016;46:1121–34.
Article CAS PubMed Google Scholar
Cohen MM, Ansara D, Schei B, et al. Post-traumatic stress disorder after pregnancy, labor, and delivery. J Womens Health (Larchmt). 2004;13:315–24.
Menage J. Post-traumatic stress disorder in women who have undergone obstetric and/or gynecological procedures. A consecutive series of 30 cases of PTSD. J Reprod Infant Psychol. 1993;11:221–8.
Wijma K, So¨derquist J, Wijma B. Post-traumatic stress disorder after childbirth: a cross-sectional study. J Anxiety Disord. 1997;11(6):587–97.
Allen S. A qualitative analysis of the process, mediating variables and impact of traumatic childbirth. J Reprod Infant Psychol. 1998;16:107–13.
Czarnocka J, Slade P. Prevalence and predictors of post-traumatic stress symptoms following childbirth. Br J Clin Psychol. 2003;9:35–51.
Sommerlad S, Schermelleh-Engel K, La Rosa VL, Louwen F, Oddo-Sommerfeld S. (2021). Trait anxiety and unplanned delivery mode enhance the risk for childbirth-related post-traumatic stress disorder symptoms in women with and without risk of preterm birth: a multi sample path analysis. PLoS ONE, 16(8), e0256681.
Ehlers A, Clark DM. A cognitive model of post-traumatic stress disorder. Behav Res Ther. 2000;38(4):319–45.
Moulds ML, Bisby MA, Wild J, Bryant RA. Rumination in post-traumatic stress disorder: a systematic review. Clin Psychol Rev. 2020;82:101910.
Ehring T, Frank S, Ehlers A. The role of rumination and reduced concreteness in the maintenance of post-traumatic stress disorder and depression following trauma. Cognit Ther Res. 2008;32:488–506.
Nolen-Hoeksema S. Responses to depression and their effects on the duration of depressive episodes. J Abnorm Psychol. 1991;100:569–82.
Nolen-Hoeksema S. The role of rumination in depressive disorders and mixed anxiety/depressive symptoms. J Abnorm Psychol. 2000;109(3):504.
Just N, Alloy LB. The response styles theory of Depression: tests and an extension of the theory. J Abnorm Psychol. 1997;106:221–9.
Nolen-Hoeksema S, Wisco BE, Lyubomirsky S. Rethinking rumination. Perspect Psychol Sci. 2008;3(5):400–24.
Elhai JD, Grubaugh AL, Kashdan TB, Frueh BC. An empirical examination of a proposed refinement to DSM-IV post-traumatic stress disorder symptom criteria using the National Comorbidity Survey replication data. J Clin Psychiatry. 2008;69(4):597.
Iverson KM, Gradus JL, Resick PA, Suvak MK, Smith KF, Monson CM. Cognitive–behavioral therapy for PTSD and depression symptoms reduces the risk for future intimate partner violence among interpersonal trauma survivors. J Consult Clin Psychol. 2011;79(2):193.
Article PubMed PubMed Central Google Scholar
Stander VA, Thomsen CJ, Highfill-McRoy RM. Etiology of depression comorbidity in combat-related PTSD: a review of the literature. Clin Psychol Rev. 2014;34(2):87–98.
Shahar G, Herishanu-Gilutz S, Holcberg G, Kofman O. In first-time mothers, post-partum depressive symptoms prospectively predict symptoms of post-traumatic stress. J Affect Disord. 2015;186:168–70.
Krauskopf V, Valenzuela P. Depresión perinatal: detección, diagnóstico y estrategias de tratamiento. Rev méd Clín Las Condes. 2020;31(2):139–49.
Martins C, Gaffan EA. Effects of early maternal depression on patterns of infant–mother attachment: a meta-analytic investigation. J Child Psychol Psychiatry Allied Discip. 2000;41(6):737–46.
Article CAS Google Scholar
Müller D, Teismann T, Havemann B, Michalak J, Seehagen S. Ruminative thinking as a predictor of perceived postpartum mother–infant bonding. Cognit Ther Res. 2013;37:89–96.
Raphael-Leff J. Women in the history of psychoanalysis: issues of gender, generation, and the genesis of the Committee on women and psychoanalysis (COWAP). Psychoanalysis & Psychotherapy; 2001.
Taghizadeh Z, Irajpour A, Arbabi M. Mothers’ response to psychological birth trauma: a qualitative study. Iran Red Crescent Med J. 2013;15(10):10572.
Beck CT. Post-traumatic stress disorder due to childbirth: the aftermath. Nurs Res. 2004;53(4):216–24.
Nicholls K, Ayers S. Childbirth-related post-traumatic stress disorder in couples: a qualitative study. Br J Health Psychol. 2007;12(4):491–509.
Ayers S, Eagle A, Waring H. The effects of childbirth-related post-traumatic stress disorder on women and their relationships: a qualitative study. Psychol Health Med. 2006;11(4):389–98.
Bosquet Enlow M, Kitts RL, Blood E, Bizarro A, Hofmeister M, Wright RJ. Maternal posttraumatic stress symptoms and infant emotional reactivity and emotion regulation. Infant Behav Dev. 2011;34:487–503. https://doi.org/10.1016/j.infbeh.2011 . 07.007.).
Parfitt Y, Pike A, Ayers S. Infant developmental outcomes: a family systems perspective. Infant Child Dev. 2014;23:353–73. https://doi.org/10.1002/icd.1830
Garthus-Niegel S, Ayers S, Martini J, von Soest T, Eberhard-Gran M. The impact of postpartum post-traumatic stress disorder symptoms on child development: a population-based, 2-year follow-up study. Psychol Med. 2017;47:161–70. https://doi.org/10.1017/S003329171600235X.)
Murray L, Hipwell A, Woolgar M, Cooper P. Cognitive vulnerability to depression in 5-year-old children of depressed mothers. J Child Psychol Psychiatry Allied Discipl. 2001;42:891–9.
Pawlby S, Sharp D, Hay D, O’Keane V. Postnatal depression and child outcome at 11 years: the importance of accurate diagnosis. J Affect Disord. 2008;107:241–5. https://doi.org/10.1016/j.jad.2007.08.002
McKenzie-McHarg K, Ayers S, Ford E, Horsch A, Jomeen J, Sawyer A, Slade P. Post-traumatic stress disorder following childbirth: an update of current issues and recommendations for future research. J Reproductive Infant Psychol. 2015;33(3):219–37.
Weathers FW, Litz BT, Keane TM, Palmieri PA, Marx BP, Schnurr PP. The PTSD checklist for DSM-5 (PCL-5). 2013; 206.
Radloff LS. The CES-D scale: a self-report depression scale for research in the general population. Appl Psychol Meas. 1997;1(3):385–401.
Herrero J, Gracia E. Una medida breve de la sintomatología depresiva (CESD-7). Salud Ment. 2007;30(5):40–6.
Holmes TH, Rahe RH. The social readjustment rating scale. J Psychosom Res. 1967;11(2):213–8.
Spitzer RL, Kroenke K, Williams JB, Löwe B. A brief measure for assessing generalized anxiety disorder: the GAD-7. Arch Intern Med. 2006;166(10):1092–7.
García-Campayo J, Zamorano E, Ruiz MA, Pardo A, Pérez-Páramo M, López-Gómez V, Freire O, Rejas J. Cultural adaptation into Spanish of the generalized anxiety disorder-7 (GAD-7) scale as a screening tool. Health Qual Life Outcomes. 2010;8(1):1–11.
Murray D, Cox JL. Screening for depression during pregnancy with the Edinburgh Depression Scale (EDDS). J Reprod Infant Psychol. 1990;8(2):99–107.
Alvarado R, Jadresic E, Guajardo V, Rojas G. First validation of a spanish-translated version of the Edinburgh postnatal depression scale (EPDS) for use in pregnant women. A Chilean study. Arch Womens Ment Health. 2015;18(4):607–12.
Nolen-Hoeksema S, Morrow J. A prospective study of depression and posttraumatic stress symptoms after a natural disaster. The 1989 Loma Prieta earth-quake. J Pers Soc Psychol. 1991;21:115–51.
Hervás G. Adaptacion Al Castellano De Un instrumento para evaluar El Estilo Rumiativo: La Escala De Respuestas Rumiativas. Revista De psicopatología y psicología clínica. 2008;13(2).
Brockington IF, Oates J, George S, Turner D, Vostanis P, Sullivan M et al. A screening questionnaire for mother-infant bonding disorders. Arch Womens Ment Healt.
Cohen J. Statistical power analysis for the behavioral sciences. 2nd ed. Hillsdale. NJ: Erlbaum; 1988.
Weston R, Gore PA. A brief guide to structural equation modeling. Couns Psychol. 2006;34(5):719–51.
Hu LT, Bentler PM. Cutoff criteria for fit indexes in covariance structure analysis: conventional criteria versus new alternatives. Struct Equ Model. 1999;6(1):1–55.
Ruiz MA, Pardo A, San Martín R. Modelos De ecuaciones estructurales. Papeles Del psicólogo. 2010;31(1):34–45.
Noel Rodríguez, Ayán M, Ruiz Díaz MÁ. Atenuación de la asimetría y de la curtosis de las puntuaciones observadas mediante transformaciones de variables: Incidencia Sobre La Estructura Factorial. Psicológica. Valencia; 2008. pp. 205–77. Ed. impr.).
Kline RB. Principles and practice of structural equation modeling. New York, NY: The Guilford; 2015.
Hirsch CR, Meeten F, Gordon C, Newby JM, Bick D, Moulds ML. Repetitive negative thinking and interpretation Bias in pregnancy. Clin Psychol Europe. 2020;2(4):1–17.
De Jong M, Lazar SW, Hug K, Mehling WE, Hölzel BK, Sack AT et al. Effects of mindfulness-based cognitive therapy on body awareness in patients with chronic pain and comorbid depression. Front Psychol. 2016; 967.
Palacios-Hernández B. Mother-infant bonding disorders: prevalence, risk factors, diagnostic criteria, and assessment strategies. Revista De La Universidad Industrial De Santander Salud. 2016;48(2):164–76.
Asociación Americana de Psiquiatría (APA). Manual diagnóstico y estadístico de Los Trastornos mentales, texto revisados: DSM V. ed Médica Panamericana. Washington DC: Masson; 2014.
Contractor AA, Greene T, Dolan M, Elhai JD. Relations between PTSD and depression symptom clusters in samples differentiated by PTSD diagnostic status. J Anxiety Disord. 2018;59:17–26.
Download references
Acknowledgements
Not applicable.
this research is part of the research project “Psychological well-being in the perinatal stage and quality of the mother-baby bond"of the call for funding of own research projects 2022 financed by Universidad Pontificia Comillas.
Author information
Authors and affiliations.
University Institute of Family Studies, Pontifical Comillas University, Madrid, Spain
Maria Vega-Sanz & Ana Berastegui
Department of Personality, Evaluation and Psychological Treatments, Complutense University of Madrid, Madrid, Spain
Alvaro Sanchez-Lopez
You can also search for this author in PubMed Google Scholar
Contributions
Maria Vega-Sanz: Conceptualization, Methodology, Investigation, Formal analysis, Data curation, Writing-original draft. Ana Berástegui: Conceptualization, Methodology, Writing-review, and editing. Álvaro Sánchez-López: Conceptualization, Methodology, Writing-review, and editing.
Corresponding author
Correspondence to Maria Vega-Sanz .
Ethics declarations
Ethics approval and consent to participate.
All participating women signed informed consent. The Ethics Committees of Comillas Pontifical University of Madrid approved the study protocol (ref. no. 2022/41). The dataset analyzed during the current study is available from the corresponding author upon reasonable request. All methods were carried out following relevant guidelines and regulations.
Consent for publication
Competing interests.
The authors declare no competing interests.
Additional information
Publisher’s note.
Springer Nature remains neutral with regard to jurisdictional claims in published maps and institutional affiliations.
Rights and permissions
Open Access This article is licensed under a Creative Commons Attribution 4.0 International License, which permits use, sharing, adaptation, distribution and reproduction in any medium or format, as long as you give appropriate credit to the original author(s) and the source, provide a link to the Creative Commons licence, and indicate if changes were made. The images or other third party material in this article are included in the article’s Creative Commons licence, unless indicated otherwise in a credit line to the material. If material is not included in the article’s Creative Commons licence and your intended use is not permitted by statutory regulation or exceeds the permitted use, you will need to obtain permission directly from the copyright holder. To view a copy of this licence, visit http://creativecommons.org/licenses/by/4.0/ . The Creative Commons Public Domain Dedication waiver ( http://creativecommons.org/publicdomain/zero/1.0/ ) applies to the data made available in this article, unless otherwise stated in a credit line to the data.
Reprints and permissions
About this article
Cite this article.
Vega-Sanz, M., Berastegui, A. & Sanchez-Lopez, A. Perinatal posttraumatic stress disorder as a predictor of mother-child bonding quality 8 months after childbirth: a longitudinal study. BMC Pregnancy Childbirth 24 , 389 (2024). https://doi.org/10.1186/s12884-024-06570-4
Download citation
Received : 23 June 2023
Accepted : 07 May 2024
Published : 25 May 2024
DOI : https://doi.org/10.1186/s12884-024-06570-4
Share this article
Anyone you share the following link with will be able to read this content:
Sorry, a shareable link is not currently available for this article.
Provided by the Springer Nature SharedIt content-sharing initiative
- Postpartum depression
BMC Pregnancy and Childbirth
ISSN: 1471-2393
- Submission enquiries: [email protected]
- General enquiries: [email protected]

Research Question
Ai generator.
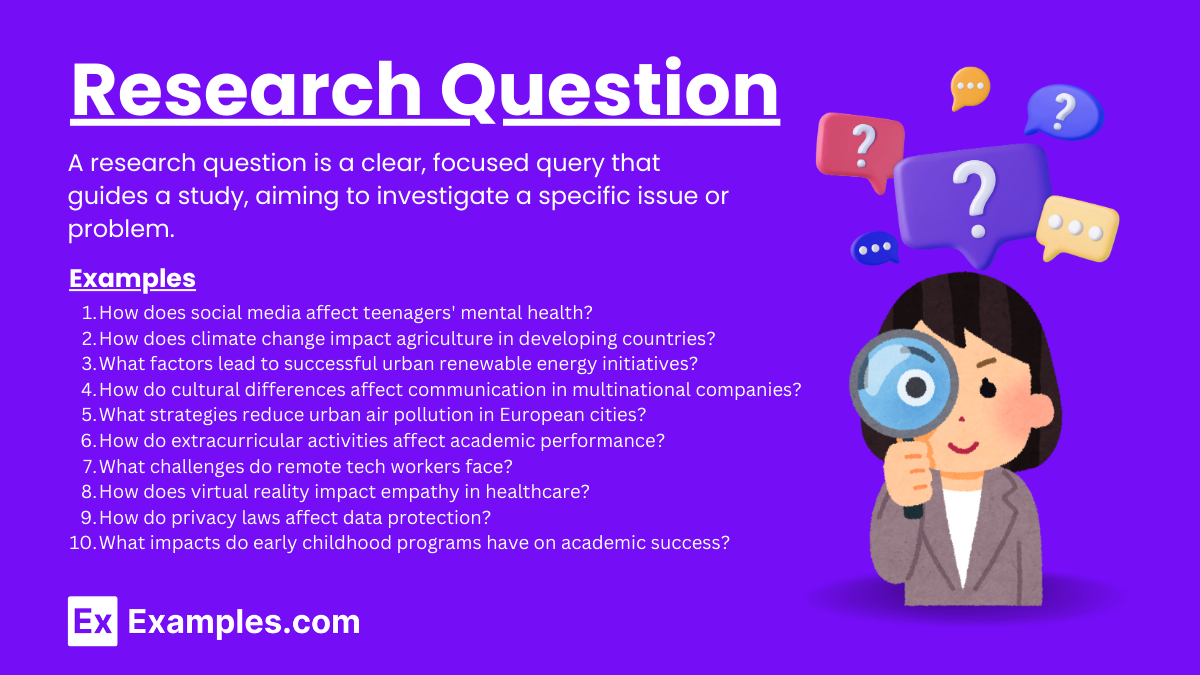
A research question serves as the foundation of any academic study, driving the investigation and framing the scope of inquiry. It focuses the research efforts, ensuring that the study addresses pertinent issues systematically. Crafting a strong research question is essential as it directs the methodology, data collection, and analysis, ultimately shaping the study’s conclusions and contributions to the field.
What is a Research Question?
A research question is the central query that guides a study, focusing on a specific problem or issue. It defines the purpose and direction of the research, influencing the methodology and analysis. A well-crafted research question ensures the study remains relevant, systematic, and contributes valuable insights to the field.
Types of Research Questions
Research questions are a crucial part of any research project. They guide the direction and focus of the study. Here are the main types of research questions:
1. Descriptive Research Questions
These questions aim to describe the characteristics or functions of a specific phenomenon or group. They often begin with “what,” “who,” “where,” “when,” or “how.”
- What are the common symptoms of depression in teenagers?
2. Comparative Research Questions
These questions compare two or more groups or variables to identify differences or similarities.
- How do the academic performances of students in private schools compare to those in public schools?
3. Correlational Research Questions
These questions seek to identify the relationships between two or more variables. They often use terms like “relationship,” “association,” or “correlation.”
- Is there a relationship between social media usage and self-esteem among adolescents?
4. Causal Research Questions
These questions aim to determine whether one variable causes or influences another. They are often used in experimental research.
- Does a new teaching method improve student engagement in the classroom?
5. Exploratory Research Questions
These questions are used when the researcher is exploring a new area or seeking to understand a complex phenomenon. They are often open-ended.
- What factors contribute to the success of start-up companies in the tech industry?
6. Predictive Research Questions
These questions aim to predict future occurrences based on current or past data. They often use terms like “predict,” “forecast,” or “expect.”
- Can high school GPA predict college success?
7. Evaluative Research Questions
These questions assess the effectiveness or impact of a program, intervention, or policy .
- How effective is the new community outreach program in reducing homelessness?
8. Ethnographic Research Questions
These questions are used in qualitative research to understand cultural phenomena from the perspective of the participants.
- How do cultural beliefs influence healthcare practices in rural communities?
9. Case Study Research Questions
These questions focus on an in-depth analysis of a specific case, event, or instance.
- What were the critical factors that led to the failure of Company X?
10. Phenomenological Research Questions
These questions explore the lived experiences of individuals to understand a particular phenomenon.
- What is the experience of living with chronic pain?
Research Question Format
A well-formulated research question is essential for guiding your study effectively. Follow this format to ensure clarity and precision:
- Begin with a broad subject area.
- Example: “Education technology”
- Define a specific aspect or variable.
- Example: “Impact of digital tools”
- Decide if you are describing, comparing, or investigating relationships.
- Example: “Effectiveness”
- Identify who or what is being studied.
- Example: “High school students”
- Formulate the complete question.
- Example: “How effective are digital tools in enhancing the learning experience of high school students?”
Sample Format: “How [specific aspect] affects [target population] in [context]?” Example: “How does the use of digital tools affect the academic performance of high school students in urban areas?”
Research Question Examples
Research questions in business.
- “What are the primary factors influencing customer loyalty in the retail industry?”
- “How does employee satisfaction differ between remote work and in-office work environments in tech companies?”
- “What is the relationship between social media marketing and brand awareness among small businesses?”
- “How does implementing a four-day workweek impact productivity in consulting firms?”
- “What are the emerging trends in consumer behavior post-COVID-19 in the e-commerce sector?”
- “Why do some startups succeed in attracting venture capital while others do not?”
- “How effective is corporate social responsibility in enhancing brand reputation for multinational companies?”
- “How do decision-making processes in family-owned businesses differ from those in publicly traded companies?”
- “What strategies do successful entrepreneurs use to scale their businesses in competitive markets?”
- “How does supply chain management affect the operational efficiency of manufacturing firms?”
Research Questions in Education
- “What are the most common challenges faced by first-year teachers in urban schools?”
- “How do student achievement levels differ between traditional classrooms and blended learning environments?”
- “What is the relationship between parental involvement and student academic performance in elementary schools?”
- “How does the implementation of project-based learning affect critical thinking skills in middle school students?”
- “What are the emerging trends in the use of artificial intelligence in education?”
- “Why do some students perform better in standardized tests than others despite similar instructional methods?”
- “How effective is the flipped classroom model in improving student engagement and learning outcomes in high school science classes?”
- “How do teachers’ professional development programs impact teaching practices and student outcomes in rural schools?”
- “What strategies can be employed to reduce the dropout rate among high school students in low-income areas?”
- “How does classroom size affect the quality of teaching and learning in elementary schools?”
Research Questions in Health Care
- “What are the most common barriers to accessing mental health services in rural areas?”
- “How does patient satisfaction differ between telemedicine and in-person consultations in primary care?”
- “What is the relationship between diet and the incidence of type 2 diabetes in adults?”
- “How does regular physical activity influence the recovery rate of patients with cardiovascular diseases?”
- “What are the emerging trends in the use of wearable technology for health monitoring?”
- “Why do some patients adhere to their medication regimen while others do not despite similar health conditions?”
- “How effective are community-based health interventions in reducing obesity rates among children?”
- “How do interdisciplinary team meetings impact patient care in hospitals?”
- “What strategies can be implemented to reduce the spread of infectious diseases in healthcare settings?”
- “How does nurse staffing level affect patient outcomes in intensive care units?”
Research Questions in Computer Science
- “What are the key features of successful machine learning algorithms used in natural language processing?”
- “How does the performance of quantum computing compare to classical computing in solving complex optimization problems?”
- “What is the relationship between software development methodologies and project success rates in large enterprises?”
- “How does the implementation of cybersecurity protocols impact the frequency of data breaches in financial institutions?”
- “What are the emerging trends in blockchain technology applications beyond cryptocurrency?”
- “Why do certain neural network architectures outperform others in image recognition tasks?”
- “How effective are different code review practices in reducing bugs in open-source software projects?”
- “How do agile development practices influence team productivity and product quality in software startups?”
- “What strategies can improve the scalability of distributed systems in cloud computing environments?”
- “How does the choice of programming language affect the performance and maintainability of enterprise-level software applications?”
Research Questions in Psychology
- “What are the most common symptoms of anxiety disorders among adolescents?”
- “How does the level of job satisfaction differ between remote workers and in-office workers?”
- “What is the relationship between social media use and self-esteem in teenagers?”
- “How does cognitive-behavioral therapy (CBT) affect the severity of depression symptoms in adults?”
- “What are the emerging trends in the treatment of post-traumatic stress disorder (PTSD)?”
- “Why do some individuals develop resilience in the face of adversity while others do not?”
- “How effective are mindfulness-based interventions in reducing stress levels among college students?”
- “How does group therapy influence the social skills development of children with autism spectrum disorder?”
- “What strategies can improve the early diagnosis of bipolar disorder in young adults?”
- “How do sleep patterns affect cognitive functioning and academic performance in high school students?”
More Research Question Examples
Research question examples for students.
- “What are the primary study habits of high-achieving college students?”
- “How do academic performances differ between students who participate in extracurricular activities and those who do not?”
- “What is the relationship between time management skills and academic success in high school students?”
- “How does the use of technology in the classroom affect students’ engagement and learning outcomes?”
- “What are the emerging trends in online learning platforms for high school students?”
- “Why do some students excel in standardized tests while others struggle despite similar study efforts?”
- “How effective are peer tutoring programs in improving students’ understanding of complex subjects?”
- “How do different teaching methods impact the learning process of students with learning disabilities?”
- “What strategies can help reduce test anxiety among middle school students?”
- “How does participation in group projects affect the development of collaboration skills in university students?”
Research Question Examples for College Students
- “What are the most common stressors faced by college students during final exams?”
- “How does academic performance differ between students who live on campus and those who commute?”
- “What is the relationship between part-time employment and GPA among college students?”
- “How does participation in study abroad programs impact cultural awareness and academic performance?”
- “What are the emerging trends in college students’ use of social media for academic purposes?”
- “Why do some college students engage in academic dishonesty despite awareness of the consequences?”
- “How effective are university mental health services in addressing students’ mental health issues?”
- “How do different learning styles affect the academic success of college students in online courses?”
- “What strategies can be employed to improve retention rates among first-year college students?”
- “How does participation in extracurricular activities influence leadership skills development in college students?”
Research Question Examples in Statistics
- “What are the most common statistical methods used in medical research?”
- “How does the accuracy of machine learning models compare to traditional statistical methods in predicting housing prices?”
- “What is the relationship between sample size and the power of a statistical test in clinical trials?”
- “How does the use of random sampling affect the validity of survey results in social science research?”
- “What are the emerging trends in the application of Bayesian statistics in data science?”
- “Why do some datasets require transformation before applying linear regression models?”
- “How effective are bootstrapping techniques in estimating the confidence intervals of small sample data?”
- “How do different imputation methods impact the results of analyses with missing data?”
- “What strategies can improve the interpretation of interaction effects in multiple regression analysis?”
- “How does the choice of statistical software affect the efficiency of data analysis in academic research?”
Research Question Examples in Socialogy
- “What are the primary social factors contributing to urban poverty in major cities?”
- “How does the level of social integration differ between immigrants and native-born citizens in urban areas?”
- “What is the relationship between educational attainment and social mobility in different socioeconomic classes?”
- “How does exposure to social media influence political participation among young adults?”
- “What are the emerging trends in family structures and their impact on child development?”
- “Why do certain communities exhibit higher levels of civic engagement than others?”
- “How effective are community policing strategies in reducing crime rates in diverse neighborhoods?”
- “How do socialization processes differ in single-parent households compared to two-parent households?”
- “What strategies can be implemented to reduce racial disparities in higher education enrollment?”
- “How does the implementation of public housing policies affect the quality of life for low-income families?”
Research Question Examples in Biology
- “What are the primary characteristics of the various stages of mitosis in eukaryotic cells?”
- “How do the reproductive strategies of amphibians compare to those of reptiles?”
- “What is the relationship between genetic diversity and the resilience of plant species to climate change?”
- “How does the presence of pollutants in freshwater ecosystems impact the growth and development of aquatic organisms?”
- “What are the emerging trends in the use of CRISPR technology for gene editing in agricultural crops?”
- “Why do certain bacteria develop antibiotic resistance more rapidly than others?”
- “How effective are different conservation strategies in protecting endangered species?”
- “How do various environmental factors influence the process of photosynthesis in marine algae?”
- “What strategies can enhance the effectiveness of reforestation programs in tropical rainforests?”
- “How does the method of seed dispersal affect the spatial distribution and genetic diversity of plant populations?”
Research Question Examples in History
- “What were the key social and economic factors that led to the Industrial Revolution in Britain?”
- “How did the political systems of ancient Athens and ancient Sparta differ in terms of governance and citizen participation?”
- “What is the relationship between the Renaissance and the subsequent scientific revolution in Europe?”
- “How did the Treaty of Versailles contribute to the rise of Adolf Hitler and the onset of World War II?”
- “What are the emerging perspectives on the causes and impacts of the American Civil Rights Movement?”
- “Why did the Roman Empire decline and eventually fall despite its extensive power and reach?”
- “How effective were the New Deal programs in alleviating the effects of the Great Depression in the United States?”
- “How did the processes of colonization and decolonization affect the political landscape of Africa in the 20th century?”
- “What strategies did the suffragette movement use to secure voting rights for women in the early 20th century?”
- “How did the logistics and strategies of the D-Day invasion contribute to the Allied victory in World War II?”
Importance of Research Questions
Research questions are fundamental to the success and integrity of any study. Their importance can be highlighted through several key aspects:
- Research questions provide a clear focus and direction for the study, ensuring that the researcher remains on track.
- Example: “How does online learning impact student engagement in higher education?”
- They establish the boundaries of the research, determining what will be included or excluded.
- Example: “What are the effects of air pollution on respiratory health in urban areas?”
- Research questions dictate the choice of research design, methodology, and data collection techniques.
- Example: “What is the relationship between physical activity and mental health in adolescents?”
- They make the objectives of the research explicit, providing clarity and precision to the study’s goals.
- Example: “Why do some startups succeed in securing venture capital while others fail?”
- Well-crafted research questions emphasize the significance and relevance of the study, justifying its importance.
- Example: “How effective are public health campaigns in increasing vaccination rates among young adults?”
- They enable a systematic approach to inquiry, ensuring that the study is coherent and logically structured.
- Example: “What are the social and economic impacts of remote work on urban communities?”
- Research questions offer a framework for analyzing and interpreting data, guiding the researcher in making sense of the findings.
- Example: “How does social media usage affect self-esteem among teenagers?”
- By addressing specific gaps or exploring new areas, research questions ensure that the study contributes meaningfully to the existing body of knowledge.
- Example: “What are the emerging trends in the use of artificial intelligence in healthcare?”
- Clear and precise research questions increase the credibility and reliability of the research by providing a focused approach.
- Example: “How do educational interventions impact literacy rates in low-income communities?”
- They help in clearly communicating the purpose and findings of the research to others, including stakeholders, peers, and the broader academic community.
- Example: “What strategies are most effective in reducing youth unemployment in developing countries?”
Research Question vs. Hypothesis
Chracteristics of research questions.
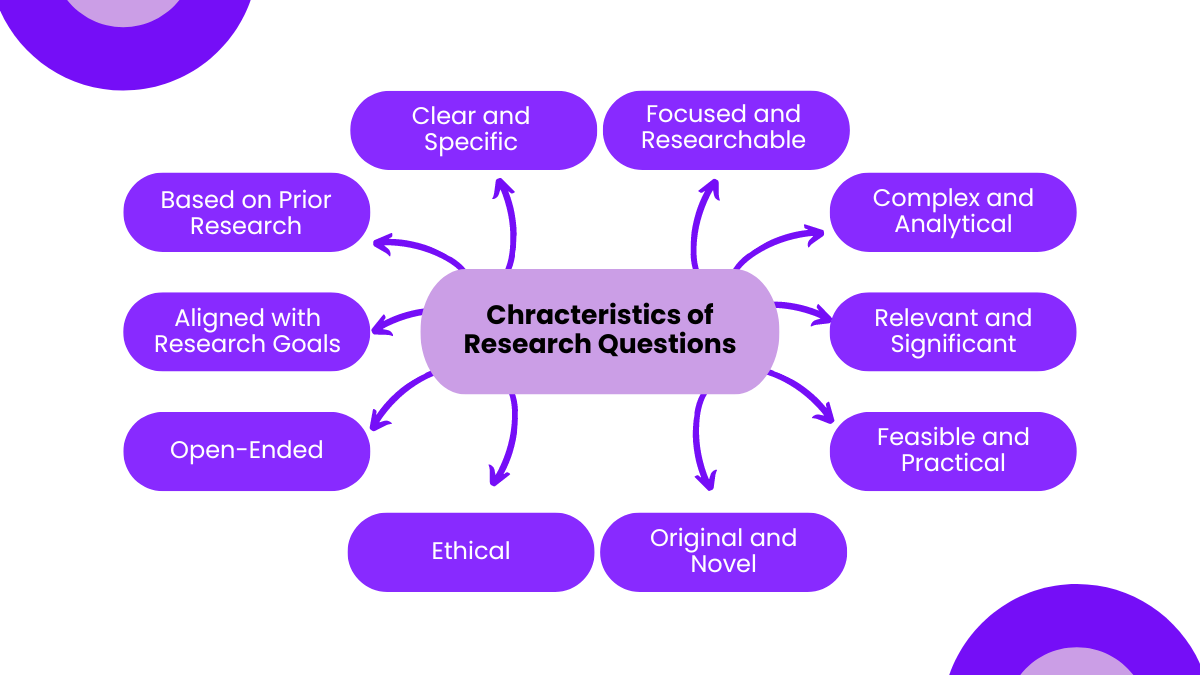
Research questions are fundamental to the research process as they guide the direction and focus of a study. Here are the key characteristics of effective research questions:
1. Clear and Specific
- The question should be clearly articulated and specific enough to be understood without ambiguity.
- Example: “What are the effects of social media on teenagers’ mental health?” rather than “How does social media affect people?”
2. Focused and Researchable
- The question should be narrow enough to be answerable through research and data collection.
- Example: “How does participation in extracurricular activities impact academic performance in high school students?” rather than “How do activities affect school performance?”
3. Complex and Analytical
- The question should require more than a simple yes or no answer and should invite analysis and discussion.
- Example: “What factors contribute to the success of renewable energy initiatives in urban areas?” rather than “Is renewable energy successful?”
4. Relevant and Significant
- The question should address an important issue or problem in the field of study and contribute to knowledge or practice.
- Example: “How does climate change affect agricultural productivity in developing countries?” rather than “What is climate change?”
5. Feasible and Practical
- The question should be feasible to answer within the constraints of time, resources, and access to information.
- Example: “What are the challenges faced by remote workers in the tech industry during the COVID-19 pandemic?” rather than “What are the challenges of remote work?”
6. Original and Novel
- The question should offer a new perspective or explore an area that has not been extensively studied.
- Example: “How do virtual reality technologies influence empathy in healthcare training?” rather than “What is virtual reality?”
- The question should be framed in a way that ensures the research can be conducted ethically.
- Example: “What are the impacts of privacy laws on consumer data protection in the digital age?” rather than “How can we collect personal data more effectively?”
8. Open-Ended
- The question should encourage detailed responses and exploration, rather than limiting answers to a simple yes or no.
- Example: “In what ways do cultural differences affect communication styles in multinational companies?” rather than “Do cultural differences affect communication?”
9. Aligned with Research Goals
- The question should align with the overall objectives of the research project or study.
- Example: “How do early childhood education programs influence long-term academic achievement?” if the goal is to understand educational impacts.
10. Based on Prior Research
- The question should build on existing literature and research, identifying gaps or new angles to explore.
- Example: “What strategies have proven effective in reducing urban air pollution in European cities?” after reviewing current studies on air pollution strategies.
Benefits of Research Question
Research questions are fundamental to the research process and offer numerous benefits, which include the following:
1. Guides the Research Process
A well-defined research question provides a clear focus and direction for your study. It helps in determining what data to collect, how to collect it, and how to analyze it.
Benefit: Ensures that the research stays on track and addresses the specific issue at hand.
2. Clarifies the Purpose of the Study
Research questions help to articulate the purpose and objectives of the study. They make it clear what the researcher intends to explore, describe, compare, or test.
Benefit: Helps in communicating the goals and significance of the research to others, including stakeholders and funding bodies.
3. Determines the Research Design
The type of research question informs the research design, including the choice of methodology, data collection methods, and analysis techniques.
Benefit: Ensures that the chosen research design is appropriate for answering the specific research question, enhancing the validity and reliability of the results.
4. Enhances Literature Review
A well-crafted research question provides a framework for conducting a thorough literature review. It helps in identifying relevant studies, theories, and gaps in existing knowledge.
Benefit: Facilitates a comprehensive understanding of the topic and ensures that the research is grounded in existing literature.
5. Focuses Data Collection
Research questions help in identifying the specific data needed to answer them. This focus prevents the collection of unnecessary data and ensures that all collected data is relevant to the study.
Benefit: Increases the efficiency of data collection and analysis, saving time and resources.
6. Improves Data Analysis
Having a clear research question aids in the selection of appropriate data analysis methods. It helps in determining how the data will be analyzed to draw meaningful conclusions.
Benefit: Enhances the accuracy and relevance of the findings, making them more impactful.
7. Facilitates Hypothesis Formation
In quantitative research, research questions often lead to the development of hypotheses that can be tested statistically.
Benefit: Provides a basis for hypothesis testing, which is essential for establishing cause-and-effect relationships.
8. Supports Result Interpretation
Research questions provide a lens through which the results of the study can be interpreted. They help in understanding what the findings mean in the context of the research objectives.
Benefit: Ensures that the conclusions drawn from the research are aligned with the original aims and objectives.
9. Enhances Reporting and Presentation
A clear research question makes it easier to organize and present the research findings. It helps in structuring the research report or presentation logically.
Benefit: Improves the clarity and coherence of the research report, making it more accessible and understandable to the audience.
10. Encourages Critical Thinking
Formulating research questions requires critical thinking and a deep understanding of the subject matter. It encourages researchers to think deeply about what they want to investigate and why.
Benefit: Promotes a more thoughtful and analytical approach to research, leading to more robust and meaningful findings.
How to Write a Research Question
Crafting a strong research question is crucial for guiding your study effectively. Follow these steps to write a clear and focused research question:
Identify a Broad Topic:
Start with a general area of interest that you are passionate about or that is relevant to your field. Example: “Climate change”
Conduct Preliminary Research:
Explore existing literature and studies to understand the current state of knowledge and identify gaps. Example: “Impact of climate change on agriculture”
Narrow Down the Topic:
Focus on a specific aspect or issue within the broad topic to make the research question more manageable. Example: “Effect of climate change on crop yields”
Consider the Scope:
Ensure the question is neither too broad nor too narrow. It should be specific enough to be answerable but broad enough to allow for thorough exploration. Example: “How does climate change affect corn crop yields in the Midwest United States?”
Determine the Research Type:
Decide whether your research will be descriptive, comparative, relational, or causal, as this will shape your question. Example: “How does climate change affect corn crop yields in the Midwest United States over the past decade?”
Formulate the Question:
Write a clear, concise question that specifies the variables, population, and context. Example: “What is the impact of increasing temperatures and changing precipitation patterns on corn crop yields in the Midwest United States from 2010 to 2020?”
Ensure Feasibility:
Make sure the question can be answered within the constraints of your resources, time, and data availability. Example: “How have corn crop yields in the Midwest United States been affected by climate change-related temperature increases and precipitation changes between 2010 and 2020?”
Review and Refine:
Evaluate the question for clarity, focus, and relevance. Revise as necessary to ensure it is well-defined and researchable. Example: “What are the specific impacts of temperature increases and changes in precipitation patterns on corn crop yields in the Midwest United States from 2010 to 2020?”
What is a research question?
A research question is a specific query guiding a study’s focus and objectives, shaping its methodology and analysis.
Why is a research question important?
It provides direction, defines scope, ensures relevance, and guides the methodology of the research.
How do you formulate a research question?
Identify a topic, narrow it down, conduct preliminary research, and ensure it is clear, focused, and researchable.
What makes a good research question?
Clarity, specificity, feasibility, relevance, and the ability to guide the research effectively.
Can a research question change?
Yes, it can evolve based on initial findings, further literature review, and the research process.
What is the difference between a research question and a hypothesis?
A research question guides the study; a hypothesis is a testable prediction about the relationship between variables.
How specific should a research question be?
It should be specific enough to provide clear direction but broad enough to allow for comprehensive investigation.
What are examples of good research questions?
Examples include: “How does social media affect academic performance?” and “What are the impacts of climate change on agriculture?”
Can a research question be too broad?
Yes, a too broad question can make the research unfocused and challenging to address comprehensively.
What role does a research question play in literature reviews?
It helps identify relevant studies, guides the search for literature, and frames the review’s focus.
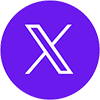
Text prompt
- Instructive
- Professional
10 Examples of Public speaking
20 Examples of Gas lighting
- Open access
- Published: 27 May 2024
Predicting higher child BMI z-score and obesity incidence in Malaysia: a longitudinal analysis of a dynamic cohort study
- Ruth Salway 1 , 2 ,
- Miranda Armstrong 1 ,
- Jeevitha Mariapun 3 ,
- Daniel D Reidpath 4 ,
- Sophia Brady 1 ,
- Mohamed Shajahan Yasin 3 ,
- Tin Tin Su 5 na1 &
- Laura Johnson 2 na1
BMC Public Health volume 24 , Article number: 1408 ( 2024 ) Cite this article
114 Accesses
Metrics details
To target public health obesity prevention, we need to predict who might become obese i.e. predictors of increasing Body Mass Index (BMI) or obesity incidence. Predictors of incidence may be distinct from more well-studied predictors of prevalence, therefore we explored parent, child and sociodemographic predictors of child/adolescent BMI z-score and obesity incidence over 5 years in Malaysia.
The South East Asia Community Observatory in Segamat, Malaysia, provided longitudinal data on children and their parents ( n = 1767). Children were aged 6–14 years at baseline (2013-14) and followed up 5 years later. Linear multilevel models estimated associations with child BMI z-score at follow-up, adjusting for baseline BMI z-score and potential confounders. Predictors included parent cardiometabolic health (overweight/obesity, central obesity, hypertension, hyperglycaemia), and socio-demographics (ethnicity, employment, education). Logistic multilevel models explored predictors of obesity incidence.
Higher baseline BMI z-score predicted higher follow-up BMI z-score both in childhood to late adolescence (0.60; 95% CI: 0.55, 0.65) and early to late adolescence (0.76; 95% CI: 0.70, 0.82). There was inconsistent evidence of association between child BMI z-score at follow-up with parent cardiometabolic risk factors independent of baseline child BMI z-score. For example, maternal obesity, but not overweight, predicted a higher BMI z-score in childhood to early adolescence (overweight: 0.16; 95% CI: -0.03, 0.36, obesity: 0.41; 95% CI: 0.20, 0.61), and paternal overweight, but not obesity, predicted a higher BMI z-score in early to late adolescence (overweight: 0.22; 95% CI: 0.01, 0.43, obesity: 0.16; 95% CI: -0.10, 0.41). Parental obesity consistently predicted five-year obesity incidence in early to late adolescence, but not childhood to early adolescence. An adolescent without obesity at baseline with parents with obesity, had 3–4 times greater odds of developing obesity during follow-up (incidence OR = 3.38 (95% CI: 1.14–9.98, mother) and OR = 4.37 (95% CI 1.34–14.27, father) respectively).
Conclusions
Having a higher BMI z-score at baseline was a stronger predictor of a higher BMI z-score at follow-up than any parental or sociodemographic factor. Targeting prevention efforts based on parent or sociodemographic factors is unwarranted but early childhood remains a key period for universal obesity prevention.
Peer Review reports
Obesity is a major public health concern, which increases the risk of developing non-communicable diseases (NCDs) such as diabetes, stroke and cardiovascular disease [ 1 ]. Children and adolescents with obesity are five times more likely to become adults with obesity, with approximately 80% of adolescents with obesity remaining so in adulthood [ 2 ]. Obesity in childhood and adolescence is independently associated with the development of NCDs later in life [ 3 ]. To date, no childhood obesity treatments show long-term success [ 4 , 5 ], so preventing new incidence of obesity in childhood and adolescence is vital for long-term NCD prevention. Prevention is particularly important in low-and-middle-income countries (LMICs) where the prevalence of NCDs is lower but increasing rapidly [ 6 ]. In Malaysia, NCDs are the most common cause of death [ 7 ], and obesity prevalence in children and adolescents has more than doubled between 2011 and 2019 (6% and 15% respectively) [ 8 , 9 ], pointing to a need to understand new incidence of obesity. Obesity rates differ by ethnicity, with higher rates among Malay and Indian and lower among Chinese ethnicities [ 9 , 10 ]. Increasing obesity prevalence in Asian LMICs is generally attributed to changes in dietary and physical activity patterns caused by economic factors and urbanisation [ 11 ]. These include shifts towards more calorie-dense westernized foods and an increase in sedentary, indoor behaviours driven by a lack of open spaces or neighbourhood safety [ 12 , 13 ]. Universal prevention efforts to improve eating and activity behaviours are generally ineffective, often because long-term behaviour change requires intensive and sustained interventions. In resource poor settings, targeting prevention strategies at populations subgroups most likely to develop obesity could be more cost-effective. Thus understanding predictors of obesity incidence as well as prevalence is essential to identifying such subgroups before they develop obesity.
Parent obesity is consistently associated with child obesity prevalence, but less is known about associations with incidence. Two meta-analyses estimated the odds of childhood obesity for a child with parents with overweight/obesity to be double that of a child with parents of healthy weight [ 14 , 15 ]. Cross-sectional associations are also seen between parent and child body mass index (BMI), with stronger associations for those children with higher BMIs, suggesting an intergenerational transmission of risk for a high BMI [ 16 , 17 ]. Maternal pre-pregnancy BMI is prospectively associated with offspring BMI in both childhood and adulthood [ 18 ], but it is not clear whether associations are gender-specific, with some studies showing a stronger maternal association [ 17 , 19 ] while others show no differences between mothers and fathers [ 14 , 20 , 21 ]. Most studies of obesity prevalence are in high income countries, and systematic reviews suggest weaker associations in LMICs [ 14 , 15 ]. In Malaysia, a previous cross-sectional analysis found a two-fold higher obesity prevalence among children with one or more parents with obesity or central obesity [ 22 ], and the prevalence of overweight mother-child pairs increased from 15 to 22% between 2006 and 2015 [ 23 ]. While prevalence studies identify groups in need of treatment, understanding predictors of greater BMI gain over time and obesity incidence could identify targets for prevention. Annual obesity incidence estimates decrease with age, from 3.2% in 5–13 year-olds to 1.8% in 13–18 year-olds in the US [ 24 ] with similar patterns in other high-income countries [ 25 , 26 ]. However, obesity incidence is often lower in LMICs [ 27 ], and less is known about how incidence is related to parental risk factors in these countries. Identifying those children who may be at risk of developing obesity in future, based on current parental risk factors may allow intervention before obesity develops and thus reduce new incidence and future prevalence.
Few studies have explored the relationship between broader parental cardiometabolic risk factors, such as hypertension and hyperglycaemia, with child obesity although associations have been reported with parent cardiovascular health [ 28 ]and diabetes in parents [ 29 , 30 ]. Intergenerational transmission of risk of NCDs could be via genetic or lifestyle mechanisms, and parents with hypertension or hyperglycaemia may have poorer diets and lower physical activity that may be shared with their children [ 31 , 32 ]. To our knowledge, no studies have described differences in BMI or obesity incidence among children in Malaysia and little is known about the factors associated with higher gains in BMI and the development of obesity in this population. Therefore, we aimed to explore how parental weight status and cardiometabolic risk factors are longitudinally associated with child BMI z-score (follow-up adjusted for baseline) and obesity incidence.
Analysis is reported following STROBE guidelines (Supplement). Data are from two health surveys from the South East Asia Community Observatory (SEACO) health and demographic surveillance system cohort in Malaysia [ 33 ], which undertakes annual enumeration of households within five of 11 sub-districts of the Segamat district. Population-wide individual level health surveys of participants aged 5 years and above were undertaken in 2013–14 and 2018-19, and collected questionnaire and biophysical measurement data on around 25,000 participants in each round (55–56% response rate of the total SEACO population), with around 10,000 participating at both timepoints.
We analysed individual data on children aged 6–14 years at baseline (11–19 years at follow-up) and their parents from the SEACO health surveys, using household structure information taken from the enumeration in 2013-14 and 2018-19 to match parents and children [ 33 ]. Participants and households were linked across surveys using a unique SEACO participant ID. The analysis sample consisted of all children who had data at both timepoints and were matched to at least one parent with data. Fig. 1 shows the flow of participants from baseline to main analysis. We treated data for 59 mothers who were pregnant as missing and excluded one pregnant adolescent. Of the 4,388 children available at baseline, 1,855 (42%) had data at follow-up, with 1,768 (95%; 40% of the baseline cohort) matched to at least one parent, and 1,341 (72%; 31% of the baseline) having two parents identified. The final analysis sample size after multiple imputation (see below) was 1,768 (40% of baseline cohort).
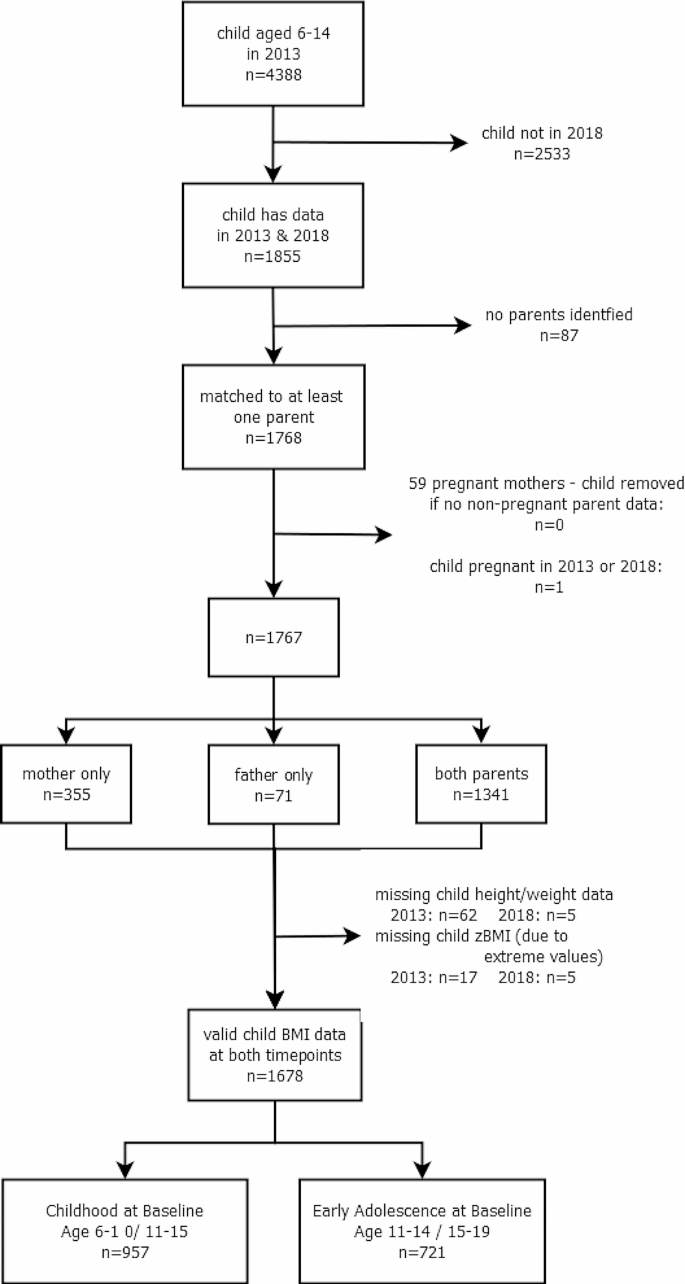
Flow diagram of participants
Ethical considerations
Ethics approval for both surveys were obtained through the Monash University Human Research Ethics Committee: MUHREC (3837) for the Health Round Survey 2013 and MUHREC (13,242) for the Health Round Survey 2018. All participants gave informed consent which allows for secondary analysis without additional consent, and data was provided in anonymised form. As part of the SEACO Health Round surveys, adult participants received free health screenings (blood pressure, blood glucose, BMI and waist circumference), with referral letters provided to high-risk respondents for future health check-ups at local clinics.
Height and weight were measured without footwear or head gear (except a light scarf or veil) using a Transtek digital weighing scale with height gauge, (model GBS-721) by trained data collectors, with one measurement taken following the SEACO Standard Operating Protocol (SOP). Child BMI was calculated from weight and height and converted to age-adjusted standardised z-scores (BMI z-score) using the sex-specific World Health Organization (WHO) 2007 BMI reference for children aged 5–19. Children were classified with thinness, overweight or obesity according to WHO definitions [ 34 ] if the standardised BMI z-score was <-2 standard deviations (SD), >1SD and > 2SD, respectively, with all remaining children classified as healthy weight.
Parent risk factors
Anthropometric measurements of all participating parents (mothers and fathers) were taken by trained data collectors in the participant’s home, with parent height and weight measured in the same way as for children, following the SEACO SOP. Waist circumference was measured using an AccuFitness Myotape, with the measurement taken at the midpoint between the lower margin of the tip of the rib and the upper point of the iliac crest (hip bone), following the WHO STEPS protocol [ 35 ]. Blood pressure was recorded after participants had been sitting for at least 15 min. Three blood pressure measurements were taken using an Omron automated blood pressure monitor (HEM-7203) with 30–60 s between subsequent measurements, and the mean of the final two readings used, according to the SEACO SOP. Participants were asked how long since they had taken food or drink (other than water), and random non-fasting blood glucose was measured using a finger-prick Omron blood glucose monitoring system, (HGM-111). BMI (kg/m 2 ) was calculated from height and weight and classified with thinness (< 18 kg/m 2 ), overweight (> 25 kg/m 2 and < 30 kg/m 2 ), obesity (≥ 30 kg/m 2 ), and of healthy weight otherwise [ 36 ], in line with previous analyses of the SEACO data [ 22 ]. Central obesity was defined using International Diabetes Federation (IDF) recommendations for Asian populations [ 37 ], as waist circumference ≥ 90 cm in men and ≥ 80 cm in women. Hypertension was defined as either systolic blood pressure ≥ 140 mmHg or diastolic blood pressure ≥ 90 mmHg [ 38 ]. Hyperglycaemia was defined as random non-fasting blood glucose ≥ 11.1 mmol/l [ 39 ].
Confounders
Parent and child age, gender and ethnicity were self-reported in the main SEACO census. Inconsistencies between the two timepoints were checked using date of birth and date of data collection with precedence given to age at baseline in 2013. We grouped children into two age groups, corresponding to Malaysian primary and secondary school ages: childhood at baseline (aged 6–10 years at baseline, aged 11–15 at follow-up) and adolescence at baseline (aged 11–14 years at baseline, aged 15–19 at follow-up)). Ethnicity was recoded into four groups: Malay, Chinese, Indian and Other (comprising indigenous (Orang Asli) and Other, grouped due to low numbers). Missing child ethnicity was derived from parent ethnicity, with preference given to 2013 data. Parental working status was classified as Working (part-time, full-time, casual) or Not Working (unemployed, housewife, student, retired). Highest parental education (up to primary, secondary, tertiary) was recorded for each parent.
Statistical analysis
Baseline sample characteristics (child: age, gender, ethnicity, BMI z-score and weight category, and parent: age, gender, highest education, employment status, weight category, central obesity, hypertension and hyperglycaemia) were described using means and SDs, or percentages, as appropriate, by child gender and age group (results provided in main document). Child and Head of Household characteristics were compared for baseline and analysis samples, and for children with zero, one and two identified parents (see Appendix). Five-year incidence rate of obesity was calculated as the percentage without obesity at baseline with obesity at follow-up. Similarly, five-year remission rates were calculated as the percentage of those with obesity who were without obesity at follow-up, and five-year persistence rate as the percentage who remained with obesity (main document).
To investigate associations between parental risk factors (overweight/obesity, central obesity, hypertension and hyperglycaemia) and change in child BMI z-score, we modelled child BMI z-score in 2018 adjusted for baseline [ 40 ]. While concerns have been raised around the use of BMI z-scores for assessing change in child BMI for children with extreme BMIs, in particular those with severe obesity [ 41 ], this study focuses on estimating associations for the general population where BMI z-score has been found to be suitable for assessing change [ 42 , 43 ] and so distortion in the extremes will have less of an impact, although associations will be underestimated for children with very high BMI. We fitted a linear multilevel model for children nested within parents, with child BMI z-score at follow-up as the outcome, adjusted for time to follow-up and baseline child BMI z-score; this assumes a linear change between baseline and follow-up. We first fitted models for each parental risk factor separately. We then fitted adjusted models, controlling for child characteristics (gender, age, ethnicity) and parent sociodemographic characteristics (age, working status and education Associations with parent hypertension and hyperglycaemia models were additionally adjusted for parent BMI category. The adjusted estimates are illustrated in Fig. 3 in the main document; estimates for unadjusted and adjusted models are given in the Appendix. We also fit models for the association between follow-up BMI z-score adjusted for baseline BMI z-score, and sociodemographic variables, specifically ethnicity, parental age, education and employment status. In a post-hoc analysis, we investigated associations between parental risk factors and obesity incidence, which focuses on children with higher BMI moving to a weight status of higher risk, and does not suffer from the same issues as using BMI z-score. We used logistic multilevel models, with a binary outcome for child with obesity at follow-up, restricted to those who were without obesity at baseline, and adjusted for time to follow-up, child characteristics, parent sociodemographic characteristics, and other parental risk factors as described above (main document). All analyses were stratified by age group, with separate models for mothers and fathers. A test for gender interactions did not support the need for stratification by gender, therefore results are reported for the whole sample combined. Adjusted models excluded child ethnicity ’Other’ due to small numbers. Descriptive summaries were performed in Stata v17 [ 44 ] and multilevel models were run in MLwiN v3.05 via the Stata command runmlwin, using restrictive iterative generalised least squares. Sensitivity analyses compared adjusted model estimates using imputed data with complete case analyses, and we repeated the analysis, first using Asian population BMI cut-offs for parental overweight (> 23 kg/m 2 and < 25 kg/m 2 ) and obesity (> 25 kg/m 2 ) and second excluding children with thinness, as for these children an increase in BMI might be considered beneficial thus potentially violating the linearity assumption (Appendix).
Missing data
We restricted analysis to children with BMI data at both timepoints who could be matched to at least one parent ( n = 1678; 38% of baseline). Missing data on gender, age and ethnicity for children and parents was taken from the other timepoint where available. Multiple imputation was used for parent risk factors to maximise the information used in the study and increase the precision of estimates [ 45 ]. We imputed missing parent data if they were included for at least one timepoint; note we did not impute child data at missing timepoints or BMI z-score.
Missing data was imputed using the jomo package in R (v4.0.2) via a simulation-based approach with a multivariate normal model to account for the multilevel structure. All analysis variables were included, and variables associated with missingness: total size of household, number of children and household structure (children/ parents, children/grandparents, and multi-generational/complex households). Continuous parental risk factors were imputed on a log scale, and parent BMI, central obesity, hypertension and hyperglycaemia were calculated from these. Twenty imputation datasets were created for each age group separately, using a burn-in of 500 iterations and 500 iterations between imputation datasets, selected by visual inspection. Model estimates were combined across the imputation datasets using Rubin’s rules [ 46 ].
There were 1,678 children included at both timepoints, with valid child BMI data, were not pregnant and were matched to at least one parent (Fig. 1 ). At baseline, 957 were aged 6–10 years (childhood at baseline) and 721 aged 11–14 (adolescence at baseline). Of these, 68% were Malay, 16% Chinese, 13% Indian and 2% Other, and 56% were female (Table 1 ). At baseline, 16% had obesity, 16% overweight and 16% thinness, with similar prevalences at follow-up. Compared to those excluded owing to missing child BMI data at a single timepoint, the children in the longitudinal analysis sample were a year younger, had lower baseline BMI z-score, were less likely to be male or Chinese, and came from households where the Head of Household had higher education and was less likely to be Indian (Table S1 ). Children with matched parents had higher BMI z-scores, were more likely to be male and were less likely to be Chinese (Table S2 ). Missing data varied between 8 and 27% for maternal and 20–36% for paternal variables (Table S3 ), primarily due to missing hypertension and hyperglycaemia. We imputed data on 9% of mothers and 24% of fathers; baseline characteristics were broadly similar between collected data and imputed data (Table S3 ). The average age of mothers was 41 years, with father slightly older at 45 years. Most parents (70%) were educated to secondary level, with most fathers in employment (91%) while mothers less likely to be employed (31%).
BMI z-score was higher at follow-up compared to baseline for girls, the childhood age-group at baseline. Whereas BMI z-score was lower at follow-up compared to baseline for the adolescent age-group at baseline (Table 1 ). Obesity prevalence was 16–18%, with movement between weight categories over time (Fig. 2 ). Five-year obesity incidence was higher in childhood (at baseline) than adolescence (at baseline) (10.8% and 6.1% respectively; Table 2 ). Five-year obesity remission rates of 42% were observed (Table 2 ). Of those participants with a healthy weight at baseline, 19% developed overweight or obesity at follow-up, compared to 7% developing thinness.
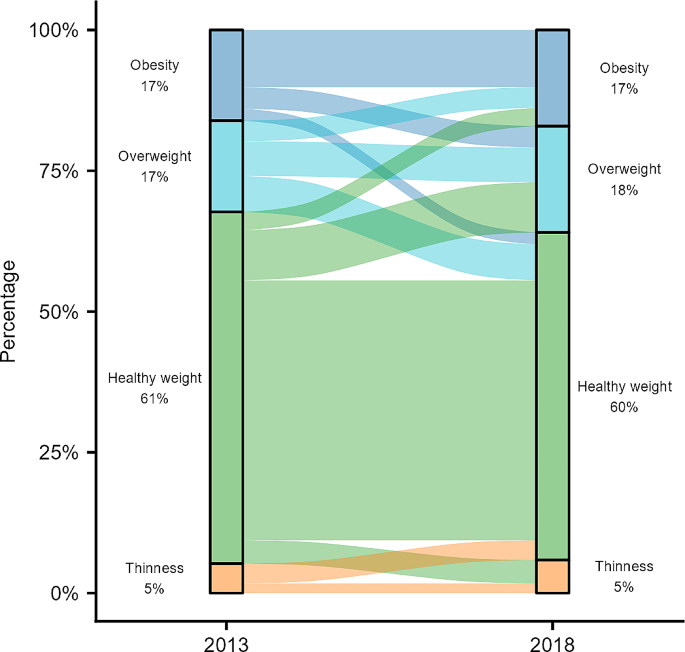
Change in child BMI category between 2013 and 2018
Fig. 3 (and Table S4 & S5 ) shows modelled associations between parent cardiometabolic health and child BMI z-score at follow-up. For all models, higher child BMI z-score at baseline was the strongest predictor of a higher child BMI z-score at follow-up. Each unit of baseline BMI z-score was associated with a 0.60 (95% CI: 0.55, 0.65) higher follow-up BMI z-score for childhood to early adolescence and 0.76 (95% CI: 0.70, 0.82) higher for early to late adolescence. Associations with parental cardiometabolic risk factors were much smaller with weak or no evidence of association. In childhood to early adolescence, compared to healthy maternal weight, obesity (B = 0.41 (95% CI: 0.20, 0.61)), but not overweight (B = 0.16 (95% CI: -0.03, 0.36)) was associated with an increase in child BMI z-score of at follow-up (Table S4 ). While not associated with higher BMI z-score among younger participants (childhood to early adolescence), compared to a healthy paternal weight, overweight (B = 0.22 (95% CI: 0.01, 0.43)) but not obesity (B = 0.16 (95% CI: -0.10, 0.41)) showed small associations with a higher BMI z-score in older participants (early to late adolescence) (Table S4 ). Parental BMI and waist circumference were strongly correlated (0.73–0.75) and so associations with central obesity followed the same patterns as parental overweight/obesity. Associations between child BMI z-score at follow-up and baseline parent hypertension and hyperglycaemia (Table S4 ), ethnicity, parent employment status or educational attainment (Table S5 ) were small with weak or no evidence of association.
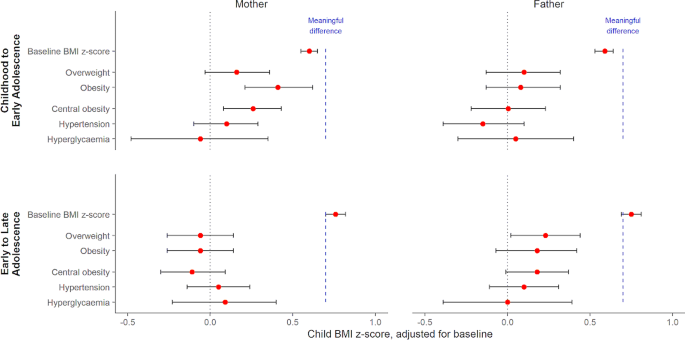
Association between parental risk factors and baseline-adjusted child BMI z-score at follow-up
Model estimates and 95% confidence intervals by age strata and parent (see also Table S4 ). Models adjusted for baseline BMI z-score, time interval, child (gender, age, ethnicity) and relevant parent characteristics (age, education, other cardiometabolic risk factors). The dashed blue line represents the minimum change in BMI z-score for a meaningful clinical impact on child and adolescent lipids and blood pressure, based on a recent meta-analysis (El-Medany et al., 2020).
Childhood to early adolescence group were aged 6–10 at baseline (11–15 at follow-up) and early to late adolescence group aged 11–14 at baseline (16–19 at follow-up).
We saw similar patterns for obesity incidence, with stronger maternal associations in childhood to early adolescence, and paternal associations in early to late adolescence (Table 3 ). In childhood to early adolescence, the odds of obesity incidence were similar among those with a parent with overweight vs. a healthy weight parent (paternal OR = 0.67 (95% CI 0.33–1.37); maternal OR = 1.33 (95% CI: 0.71–2.48)). Obesity incidence odds were higher among the older age group, especially for those with a parent with obesity at 3–4 times higher (paternal OR = 4.37 (95% CI 1.34–14.27); maternal OR = 3.38 (95% CI: 1.14–9.98)). Associations with parental central obesity followed similar patterns, especially for maternal central obesity, where odds were higher than for BMI-based overweight/obesity (Table 3 ). There were no marked associations with parent hypertension or hyperglycaemia (ORs between 0.5 and 1.5 with wide confidence intervals).
Complete case analysis showed similar associations (Table S6 ), with slightly stronger associations for mothers with overweight. Sensitivity analyses were run for the association between child BMI z-score at follow-up and parental weight category using the overweight/obesity definition for Asian populations, which has lower BMI thresholds (Table S7 ). We saw similar patterns of associations to before, but with parental obesity rather than parental overweight; specifically associations with maternal obesity for those in childhood to early adolescence, and associations with paternal obesity for those in early to late adolescence. As the linearity assumption between baseline and follow-up BMI z-score did not hold for low values of baseline BMI z-score (Figure S1 )., we repeated the analysis excluding children with thinness (Table S8 ) but found no difference in the reported associations between child BMI z-score at follow-up and parental cardiometabolic risk factors.
We have reported how parental cardiometabolic factors (overweight, obesity, central obesity, hypertension and hyperglycaemia) are associated with child BMI z-score at five-year follow-up, and the development of obesity in Malaysian children. Five-year increases in BMI z-score depended on child age at baseline, with larger increases between childhood and early adolescence compared to early and late adolescence. Previous cross-sectional analyses highlight associations between parental weight status and sociodemographic factors and child BMI z-score at a single timepoint [ 21 , 22 ]. We found parental obesity was weakly associated with child BMI z-score differences, below values considered clinically meaningful, but associated with high odds of developing obesity, suggesting that targeted childhood obesity prevention strategies may need to focus on children of parents with obesity who may not be immediately at risk. For example, existing adult weight management programmes, such as ‘My body is fit and fabulous’ in Malaysia aimed at housewives [ 47 ], could also include advice and support aimed at the whole family to target children in childhood and early adolescence before they develop obesity.
In Segamat, obesity prevalence was estimated at 16% in 6–10 year olds, 18% in 10–14 year olds and 16% in those aged 15 or more, with overweight prevalence at 15%, 20% and 15% respectively. This is consistent with overweight and obesity prevalences of 15% across ages 5–18 reported for Malaysian children in the National Health and Morbidity Survey 2019 and elsewhere [ 9 , 21 , 23 ], but highlights variation with age, with higher rates during the early adolescent period. While obesity prevalence was relatively stable, this masked substantial change. Over five years, new obesity incidence was 8.8%, while 42% of those with obesity at baseline were without obesity at follow-up. Both the incidence and remission rates are slightly lower than those reported in high-income countries [ 24 , 25 ] and we did not observe higher remission rates among younger children as other studies have reported [ 48 , 49 , 50 ]. In general, the majority of children who shift between BMI categories tend to do so at the boundaries. While some of the remission may be attributable to measurement error or regression to the mean, it may also be due to individual behaviour change, timing of maturation [ 48 ], or lower odds of remission associated with low birthweight [ 50 ].
Malaysia has a double burden of both thinness and overweight/obesity but we found more children with healthy weight at baseline developing overweight/obesity than thinness at follow-up (19% and 7% respectively). Our findings may reflect differences in LMICs where obesity rates are still increasing, with higher incidence in childhood not balanced by higher remission rates, leading to an increasing obesity prevalence over time, although conclusions are limited by having only two time points.
In childhood to early adolescence, maternal overweight/obesity were associated with higher mean BMI z-score at follow-up compared to mothers of healthy weight, whilst in early to late adolescence the associations of higher offspring BMI z-score were strongest with paternal overweight and obesity. Note that we have used WHO international BMI thresholds for parental overweight/obesity, but we found similar maternal and paternal patterns by child age group when using lower risk thresholds suggested for Asian populations, with the key difference being associations with parental obesity but not overweight, reflecting the shift in the thresholds. We observed similar patterns for central obesity, which is in line with the strong correlation between BMI and waist circumference. A recent meta-analysis [ 51 ] indicates a minimum mean increase in BMI z-score for a clinical impact on lipid profiles and blood pressure in 4–19 year olds is 0.7 z-scores. The associations we observed are over five years, and estimates (and confidence interval bounds) are much smaller than 0.7 and thus do not suggest associations are substantial enough to alter metabolic health in a clinically-relevant way. While larger cross-sectional associations between parental overweight/obesity and children’s BMI z-score [ 14 , 15 , 22 ] may be due to genetic predisposition to obesity, common obesogenic lifestyles, including diet and physical activity, due to shared living environment and/or behavioural factors passed down from parent to child [ 14 ], our analysis suggests these factors have a far smaller impact on the five-year difference in BMI z-score. We note, however, that our results may underestimate the association for those children with very high BMIs, and so further research on associations for children with severe obesity may be warranted.
There were no marked associations with parental hypertension, hyperglycaemia, or socioeconomic status, thus our study suggests that childhood obesity prevention strategies may be best targeted at those who have parents with overweight or obesity. The strongest associations observed were with BMI z-score at baseline, as children with higher initial BMI experienced larger five-year increases than expected compared to WHO references.
While increasing BMI z-scores can indicate growth in excess of normal expectations for a given age and sex, higher-than-average BMI is not in itself a cause for concern if children remain on the same trajectories into adolescence, because they will stay within the healthy BMI range. Both BMI z-score and overweight/obesity definitions in children are based on comparison to a historic cross-sectional reference population (in this study, the WHO reference population) and thus differences we observe may be a result of different underlying characteristics of populations, such as the timing of pubertal growth spurts, rather than necessarily cause for concern. Figure S2 (see Appendix) shows that median BMI was higher than the WHO growth reference at all ages for boys and girls, with the rate of change in BMI temporally shifted, so the fastest rate of increase occurred 1–2 years younger. The strong associations with baseline BMI z-score suggest that excessive growth trajectories are initiated earlier in childhood and are perpetuated into adolescence [ 52 , 53 , 54 ]. Thus, the small associations between parental risk factors and offspring BMI z-score may be because those children predisposed to obesity, having already lived with overweight or obesity from a young age. However, these difficulties in assessing change in BMI in childhood make it challenging to interpret results.
Five-year obesity incidence was higher between childhood and early adolescence than between early and late adolescence (10.8% and 6.1% respectively), consistent with patterns elsewhere of higher incidence at younger ages [ 24 , 25 ], and in line with the theory that excessive growth tends to occur earlier in childhood. Associations between parental overweight/obesity and child obesity incidence were larger in early to late adolescence than in childhood to early adolescence with parental overweight associated with a doubling in odds of five-year obesity incidence and parental obesity associated with a 3–4 times higher odds. Note that BMI changes considerably during puberty with a growth spurt and the adiposity rebound, which will occur predominantly within the childhood to early adolescence group, and may account for the weaker associations within this group. In contrast, the stronger associations in the early to late adolescence group, once puberty is more established, highlights that a child with one or both parents with obesity may not develop obesity until late adolescence, and so may not have been identified as at risk when younger. This is of concern because associations with obesity in adulthood are stronger for those with obesity in adolescence than in childhood [ 2 ], and thus indicate that this group of children may require targeting at a younger age even if they do not yet have obesity. Associations between parental obesity and adolescent obesity incidence but not BMI z-score may indicate a differential importance of parent weight for child BMI across the distribution of child BMI [ 16 , 17 ]. For example, children with higher baseline BMI z-scores would need a smaller increase in BMI z-score (such as is associated with having a parent with obesity) to move into the overweight or obesity category. Thus, parental obesity may have a stronger association for children closer to the cut-off for obesity at baseline, especially in early adolescence.
We saw some evidence of different associations by parent gender, with maternal weight dominating in childhood to early adolescence and paternal weight in early to late adolescence. Maternal obesity before and during pregnancy (a key developmental period) is associated with offspring BMI in childhood, potentially driven by intrauterine, genetic or lifestyle factors such as smoking [ 53 , 55 ]. Some cross-sectional studies have found stronger maternal associations [ 17 , 19 ] and our results suggest that associations may continue into childhood, possibly reflecting the common role of the mother as primary caregiver in younger children, especially in more traditional communities, with more influence over lifestyle factors such as diet and physical activity. However, we found this was replaced by a weaker paternal association in early to late adolescence. Thus maternal associations in childhood may thus result in earlier puberty [ 56 ], and an earlier adolescent increase in BMI, while paternal weight is associated with higher BMI at the end of adolescence. However, all these associations were small and other evidence is inconclusive, especially for fathers. While much of the current evidence focuses on maternal associations, fathers are under-represented, and more research is needed to determine the paternal role throughout childhood and adolescence. Understanding further our findings of a stronger paternal association in early to late adolescence is of particular importance as this is a critical time for BMI trajectories into adulthood.
Strengths and limitations
This study uses prospective data with a robust sampling design in the SEACO cohort, resulting in high levels of parental data, especially for fathers who are typically under-represented, with most studies focusing on mothers [ 23 ]. Parental cardiometabolic risk factors were measured objectively rather than using self-report measures. However, because data were collected at household level, matching children to parents was difficult especially with multiple families in a household and we were unable to distinguish between biological and non-biological parents. We also excluded a substantial number of children due to missing data at follow-up, and did not have data on lifestyle factors such as diet or physical activity. While multiple imputation maximises the available information in the data, this is under a missing at random assumption. Exploring changes in BMI in this age group is challenging due to puberty and childhood growth patterns, and so care should be taken in interpreting results. Furthermore, Segamat is a semi-rural region so is not generalisable to the wider Malaysian population, although overweight/obesity estimates are similar [ 23 ]. Finally, our analysis focuses on the general population; estimates of associations may be underestimated for those children with severe obesity.
In this Malaysian cohort, child/adolescent obesity prevalence was stable at 16–18%, with a five-year incidence of obesity at 8.8%. Parental overweight/obesity was prospectively associated with slightly higher child BMI z-score after adjusting for baseline, but the largest follow-up BMI z-scores were among children with a higher baseline BMI z-score. These findings support the importance of childhood as a key period for obesity prevention, rather than later intervention based on parent cardiometabolic risk. However, those in early adolescence with higher BMI z-score and at least one parent with obesity may be at an increased risk of becoming obese during late adolescence.
Data availability
Data are from an ongoing prospective cohort study and are available from SEACO by completion of a data application form to: https://www.monash.edu.my/seaco/research-and-training/how-to-collaborate-with-seaco.
Abbreviations
Body mass index
International Diabetes Federation
Low-and-middle-income country
Non-communicable disease
South East Asia Community Observatory
World Health Organization
World Health Organization. Global Health Risks: Mortality and burden of disease attributable to selected major risks. In. Geneva: World Health Organization; 2009.
Simmonds M, Llewellyn A, Owen CG, Woolacott N. Predicting adult obesity from childhood obesity: a systematic review and meta-analysis. Obes Rev. 2016;17(2):95–107.
Article CAS PubMed Google Scholar
Lin X, Xu Y, Xu J, Pan X, Song X, Shan L, Zhao Y, Shan PF. Global burden of noncommunicable disease attributable to high body mass index in 195 countries and territories, 1990–2017. Endocrine. 2020;69(2):310–20.
Mead E, Brown T, Rees K, Azevedo LB, Whittaker V, Jones D, Olajide J, Mainardi GM, Corpeleijn E, O’Malley C, et al. Diet, physical activity and behavioural interventions for the treatment of overweight or obese children from the age of 6 to 11 years. Cochrane Database Syst Rev. 2017;6:CD012651.
PubMed Google Scholar
Al-Khudairy L, Loveman E, Colquitt JL, Mead E, Johnson RE, Fraser H, Olajide J, Murphy M, Velho RM, O’Malley C, et al. Diet, physical activity and behavioural interventions for the treatment of overweight or obese adolescents aged 12 to 17 years. Cochrane Database Syst Rev. 2017;6:CD012691.
Miranda JJ, Barrientos-Gutierrez T, Corvalan C, Hyder AA, Lazo-Porras M, Oni T, Wells JCK. Understanding the rise of cardiometabolic diseases in low- and middle-income countries. Nat Med. 2019;25(11):1667–79.
Low WY, Lee YK, Samy AL. Non-communicable diseases in the Asia-Pacific region: prevalence, risk factors and community-based prevention. Int J Occup Med Environ Health. 2015;28(1):20–6.
Institute for Public Health (IPH). National Health and Morbidity Survey 2015 (NHMS 2015). Vol. II: Non-Communicable Diseases, Risk Factors & Other Health Problems. In. Malaysia; 2015.
Institute for Public Health: National Health and Morbidity Survey (NHMS). 2019: Vol. I: NCDs – Non-Communicable Diseases: Risk Factors and other Health Problems. In. Malaysia: Institute for Public Health, National Institutes of Health, Ministry of Health; 2020.
Rampal L, Rampal S, Khor GL, Zain AM, Ooyub SB, Rahmat RB, FGhani S, N, Krishnan J. A national study on the prevalence of obesity among 16,127 malaysians. Asia Pac J Clin Nutr. 2007;16(3):561–6.
Li M, Dibley M, Baur L, Twigg S, Magnusson R. Child and adolescent obesity in Asia. A modern epidemic: Expert perspectives on obesity and diabetes. Sydney, NSW: Sydney University; 2012.
Google Scholar
Gupta N, Goel K, Shah P, Misra A. Childhood obesity in developing countries: epidemiology, determinants, and prevention. Endocr Rev. 2012;33(1):48–70.
Misra A, Khurana L. Obesity and the metabolic syndrome in developing countries. J Clin Endocrinol Metab. 2008;93(11 Suppl 1):S9–30.
Wang Y, Min J, Khuri J, Li M. A systematic examination of the Association between parental and child obesity across Countries. Adv Nutr. 2017;8(3):436–48.
Article PubMed PubMed Central Google Scholar
Lee JS, Jin MH, Lee HJ. Global relationship between parent and child obesity: a systematic review and meta-analysis. Clin Exp Pediatr. 2022;65(1):35–46.
Article PubMed Google Scholar
Dolton P, Xiao M. The intergenerational transmission of body mass index across countries. Econ Hum Biol. 2017;24:140–52.
Johnson PCD, Logue J, McConnachie A, Abu-Rmeileh NME, Hart C, Upton MN, Lean M, Sattar N, Watt G. Intergenerational change and familial aggregation of body mass index. Eur J Epidemiol. 2011;27(1):53–61.
Heslehurst N, Vieira R, Akhter Z, Bailey H, Slack E, Ngongalah L, Pemu A, Rankin J. The association between maternal body mass index and child obesity: a systematic review and meta-analysis. PLoS Med. 2019;16(6):e1002817.
Whitaker RC, Wright JA, Pepe MS, Seidel KD, Dietz WH. Predicting obesity in Young Adulthood from Childhood and parental obesity. New Eng J Med 1997(13):869–73.
Davey Smith G, Steer C, Leary S, Ness A. Is there an intrauterine influence on obesity? Evidence from parent child associations in the Avon Longitudinal Study of parents and children (ALSPAC). Arch Dis Child. 2007;92(10):876–80.
Ahmad A, Zulaily N, Shahril MR, Wafa SW, Mohd Amin R, Piernas C, Ahmed A. Obesity determinants among Malaysian 12-year old school adolescents: findings from the HAT study. BMC Pediatr. 2021;21(1):418.
Partap U, Young EH, Allotey P, Sandhu MS, Reidpath DD. Anthropometric and cardiometabolic risk factors in parents and child obesity in Segamat, Malaysia. Int J Epidemiol. 2017;46(5):1523–32.
Mohamed NN, Rohana AJ, Hamid NAA, Hu FB, Malik VS, Mohd Yusoff MF, Aris T, The Global N. Epidemiologic transition Initiative G: Intergenerational Transmission of Obesity from mothers to their offspring: Trends and Associated factors derived from the Malaysian National Health and Morbidity Survey (NHMS). Nutrients 2022, 14(11).
Cheung PC, Cunningham SA, Narayan KM, Kramer MR. Childhood Obesity Incidence in the United States: a systematic review. Child Obes. 2016;12(1):1–11.
Hughes AR, Sherriff A, Lawlor DA, Ness AR, Reilly JJ. Incidence of obesity during childhood and adolescence in a large contemporary cohort. Prev Med. 2011;52(5):300–4.
Ikeda N, Nishi N. First incidence and associated factors of overweight and obesity from preschool to primary school: longitudinal analysis of a national cohort in Japan. Int J Obes (Lond). 2019;43(4):751–60.
Do LM, Tran TK, Eriksson B, Petzold M, Ascher H. Prevalence and incidence of overweight and obesity among Vietnamese preschool children: a longitudinal cohort study. BMC Pediatr. 2017;17(1):150.
Muchira JM, Gona PN, Mogos MF, Stuart-Shor E, Leveille SG, Piano MR, Hayman LL. Temporal trends and Familial Clustering of Ideal Cardiovascular Health in parents and offspring over the Life Course: An Investigation using the Framingham Heart Study. J Am Heart Assoc. 2020;9(12):e016292.
Article CAS PubMed PubMed Central Google Scholar
Linares Segovia B, Gutierrez Tinoco M, Izquierdo Arrizon A, Guizar Mendoza JM, Amador Licona N. Long-term consequences for offspring of paternal diabetes and metabolic syndrome. Exp Diabetes Res. 2012;2012:684562.
Kawasaki M, Arata N, Miyazaki C, Mori R, Kikuchi T, Ogawa Y, Ota E. Obesity and abnormal glucose tolerance in offspring of diabetic mothers: a systematic review and meta-analysis. PLoS ONE. 2018;13(1):e0190676.
Niermann CYN, Spengler S, Gubbels JS. Physical activity, screen time, and dietary intake in families: a cluster-analysis with Mother-Father-Child triads. Front Public Health. 2018;6:276.
Bogl LH, Silventoinen K, Hebestreit A, Intemann T, Williams G, Michels N, Molnar D, Page AS, Pala V, Papoutsou S et al. Familial resemblance in Dietary intakes of children, adolescents, and parents: does Dietary Quality play a role? Nutrients 2017, 9(8).
Partap U, Young EH, Allotey P, Soyiri IN, Jahan N, Komahan K, Devarajan N, Sandhu MS, Reidpath DD. HDSS Profile: the South East Asia Community Observatory Health and Demographic Surveillance System (SEACO HDSS). Int J Epidemiol. 2017;46(5):1370–g1371.
WHO. Growth Reference 5–19 Years - Application Tools. In. Geneva: World Health Organization; 2014.
STEPwise approach to NCD risk factor surveillance (STEPS). https://www.who.int/teams/noncommunicable-diseases/surveillance/systems-tools/steps.
World Health Organization. Obesity and overweight. In. Geneva: World Health Organization; 2015.
IDF. The IDF Consensus on the Definition of the Metabolic Syndrome in Children and Adolescents. In. Brussels: International Diabetes Federation; 2007.
Whitworth J. World Health Organization (WHO)/International Society of Hypertension (ISH) statement on management of hypertension. J Hypertensb. 2003;21:1983–92.
Article Google Scholar
WHO, IDF. Definition and diagnosis of diabetes Mellitus and Intermediate Hyperglycemia. In. Geneva: World Health Organization; 2006.
Tennant PWG, Arnold KF, Ellison GTH, Gilthorpe MS. Analyses of ‘change scores’ do not estimate causal effects in observational data. Int J Epidemiol. 2022;51(5):1604–15.
Freedman DS, Berenson GS. Tracking of BMI z scores for severe obesity. Pediatrics. 2017;140(3):e20171072.
Kakinami L, Henderson M, Chiolero A, Cole TJ, Paradis G. Identifying the best body mass index metric to assess adiposity change in children. Arch Dis Child. 2014;99(11):1020–4.
Inokuchi M, Matsuo N, Takayama JI, Hasegawa T. BMI z-score is the optimal measure of annual adiposity change in elementary school children. Ann Hum Biol. 2011;38(6):747–51.
StataCorp.: Stata Statistical Software: Release 17. In. College Station, TX: StataCorp LLC; 2021.
Dong Y, Peng C-Y. Principled missing data methods for researchers. SpringerPlus. 2013;2:222.
Rubin D. Multiple imputation after 18 years. J Am Stat Assoc. 1996;91(434):473–89.
Ambak R, Mohamad Nor NS, Puteh N, Mohd Tamil A, Omar MA, Shahar S, Ahmad NA, Aris T. The effect of weight loss intervention programme on health-related quality of life among low income overweight and obese housewives in the MyBFF@home study. BMC Womens Health. 2018;18(Suppl 1):111.
Kim J, Must A, Fitzmaurice GM, Gillman MW, Chomitz V, Kramer E, McGowan R, Peterson KE. Incidence and remission rates of overweight among children aged 5 to 13 years in a district-wide school surveillance system. Am J Public Health. 2005;95(9):1588–94.
von Kries R, Beyerlein A, Muller MJ, Heinrich J, Landsberg B, Bolte G, Chmitorz A, Plachta-Danielzik S. Different age-specific incidence and remission rates in pre-school and primary school suggest need for targeted obesity prevention in childhood. Int J Obes (Lond). 2012;36(4):505–10.
Luan D, Mezuk B, Bauer KW. Remission of obesity among a nationally representative sample of US children. Pediatr Obes 2019, 14(1).
El-Medany A, Birch L, Hunt L, Matson R, Chong A, Beynon R, Hamilton-Shield J, Perry R. What change in body Mass Index is required to Improve Cardiovascular outcomes in Childhood and adolescent obesity through Lifestyle interventions: a Meta-regression. Child Obes. 2020;16(7):449–78.
Dos Santos CS, Picoito J, Nunes C, Loureiro I. Early individual and Family Predictors of Weight Trajectories from Early Childhood to adolescence: results from the Millennium Cohort Study. Front Pediatr. 2020;8:417.
Nedelec R, Miettunen J, Mannikko M, Jarvelin MR, Sebert S. Maternal and infant prediction of the child BMI trajectories; studies across two generations of Northern Finland birth cohorts. Int J Obes (Lond). 2021;45(2):404–14.
Magarey AM, Daniels LA, Boulton TJ, Cockington RA. Predicting obesity in early adulthood from childhood and parental obesity. Int J Obes. 2003;27(4):505–13.
Article CAS Google Scholar
Bond TA, Karhunen V, Wielscher M, Auvinen J, Mannikko M, Keinanen-Kiukaanniemi S, Gunter MJ, Felix JF, Prokopenko I, Yang J, et al. Exploring the role of genetic confounding in the association between maternal and offspring body mass index: evidence from three birth cohorts. Int J Epidemiol. 2020;49(1):233–43.
Chen YC, Fan HY, Yang C, Hsieh RH, Pan WH, Lee YL. Assessing causality between childhood adiposity and early puberty: a bidirectional mendelian randomization and longitudinal study. Metabolism. 2019;100:153961.
Download references
Acknowledgements
The authors would like to express their appreciation to the SEACO Field Teams and survey participants. The research described in this paper was supported by the South East Asia Community Observatory (SEACO, https://www.monash.edu.my/seaco ). The views, however, are those of the authors and there is no real or implied endorsement by SEACO.
This work was supported by funding from UK Medical Research Council and the Malaysian Ministry of Higher Education/UK-MY Joint Partnership on Non-Communicable Diseases 2019/MR/T018984/1. Monash University funds the SEACO health and demographic surveillance system. Co-authors of this study are also supported by the National Institute for Health and Care Research Bristol Biomedical Research Centre (MA). The views expressed are those of the author(s) and not necessarily those of the NIHR or the Department of Health and Social Care. The funders of the study had no role in study design, data collection, data analysis, data interpretation, or writing of the report.
Author information
Tin Tin Su and Laura Johnson share the joint senior authorship.
Authors and Affiliations
Centre for Exercise, Nutrition & Health Sciences, School for Policy Studies, University of Bristol, 8 Priory Road, Bristol, BS8 1TZ, UK
Ruth Salway, Miranda Armstrong & Sophia Brady
Population Health Sciences, Bristol Medical School, University of Bristol, Canynge Hall, Bristol, BS8 2PN, UK
Ruth Salway & Laura Johnson
Clinical School Johor Bahru, Jeffrey Cheah School of Medicine and Health Sciences, Monash University Malaysia, Subang Jaya, Malaysia
Jeevitha Mariapun & Mohamed Shajahan Yasin
Institute for Global Health and Development, Queen Margaret University, Edinburgh, EH21 6UU, Scotland
Daniel D Reidpath
South East Asia Community Observatory (SEACO), and Global Public Health, Jeffrey Cheah School of Medicine and Health Sciences, Monash University Malaysia, Subang Jaya, Malaysia
You can also search for this author in PubMed Google Scholar
Contributions
The study was conceived by LJ and TTS, and funding was obtained by LJ, TTS, MA, DR and MY. The analyses were planned by LJ and RS, who wrote the analysis plan with input from TTS, JM, DR, MY and MA. LJ supervised the analysis and drafting and RS undertook the analysis and wrote the first draft of the article. All authors critically reviewed the article and approved the final manuscript.
Corresponding author
Correspondence to Ruth Salway .
Ethics declarations
Ethics approval and consent to participate.
Ethics approval for both surveys were obtained through the Monash University Human Research Ethics Committee: MUHREC (3837) for the Health Round Survey 2013 and MUHREC (13242) for the Health Round Survey 2018. All participants gave informed consent which allows for secondary analysis without additional consent, and data was provided in anonymised form.
Consent for publication
Not applicable.
Competing interests
The authors declare that they have no competing interests. For transparency: LJ has received, for research unrelated to the current paper, institutional funding from UKRI, World Cancer Research Fund, National Institute for Health Research UK, Joint Programs Initiative EU FP7, Alpro foundation, Danone Baby Nutrition, Kellogg Europe, and the Wellcome Trust. MA has received, for research unrelated to the current paper, institutional funding from the Centre for Aging Better, NIHR and Cancer Research UK.
Additional information
Publisher’s note.
Springer Nature remains neutral with regard to jurisdictional claims in published maps and institutional affiliations.
Electronic supplementary material
Below is the link to the electronic supplementary material.
Supplementary Material 1
Rights and permissions.
Open Access This article is licensed under a Creative Commons Attribution 4.0 International License, which permits use, sharing, adaptation, distribution and reproduction in any medium or format, as long as you give appropriate credit to the original author(s) and the source, provide a link to the Creative Commons licence, and indicate if changes were made. The images or other third party material in this article are included in the article’s Creative Commons licence, unless indicated otherwise in a credit line to the material. If material is not included in the article’s Creative Commons licence and your intended use is not permitted by statutory regulation or exceeds the permitted use, you will need to obtain permission directly from the copyright holder. To view a copy of this licence, visit http://creativecommons.org/licenses/by/4.0/ . The Creative Commons Public Domain Dedication waiver ( http://creativecommons.org/publicdomain/zero/1.0/ ) applies to the data made available in this article, unless otherwise stated in a credit line to the data.
Reprints and permissions
About this article
Cite this article.
Salway, R., Armstrong, M., Mariapun, J. et al. Predicting higher child BMI z-score and obesity incidence in Malaysia: a longitudinal analysis of a dynamic cohort study. BMC Public Health 24 , 1408 (2024). https://doi.org/10.1186/s12889-024-18917-9
Download citation
Received : 20 November 2023
Accepted : 21 May 2024
Published : 27 May 2024
DOI : https://doi.org/10.1186/s12889-024-18917-9
Share this article
Anyone you share the following link with will be able to read this content:
Sorry, a shareable link is not currently available for this article.
Provided by the Springer Nature SharedIt content-sharing initiative
- Adolescents
- Intergenerational obesity
- Cardiometabolic risk factors
BMC Public Health
ISSN: 1471-2458
- General enquiries: [email protected]
AI on Trial: Legal Models Hallucinate in 1 out of 6 (or More) Benchmarking Queries
A new study reveals the need for benchmarking and public evaluations of AI tools in law.
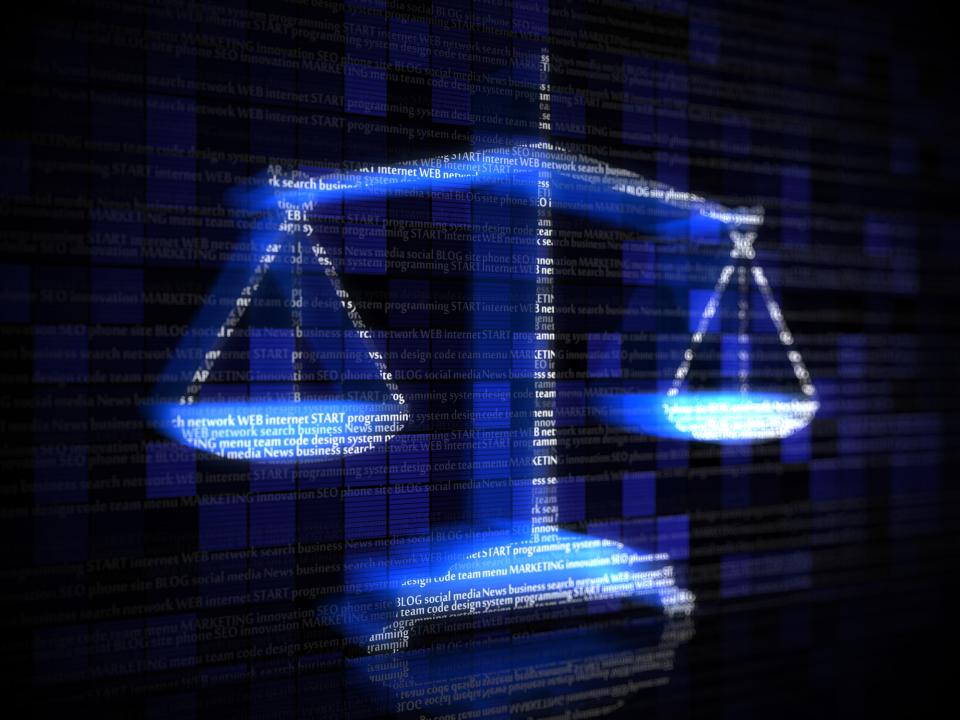
Artificial intelligence (AI) tools are rapidly transforming the practice of law. Nearly three quarters of lawyers plan on using generative AI for their work, from sifting through mountains of case law to drafting contracts to reviewing documents to writing legal memoranda. But are these tools reliable enough for real-world use?
Large language models have a documented tendency to “hallucinate,” or make up false information. In one highly-publicized case, a New York lawyer faced sanctions for citing ChatGPT-invented fictional cases in a legal brief; many similar cases have since been reported. And our previous study of general-purpose chatbots found that they hallucinated between 58% and 82% of the time on legal queries, highlighting the risks of incorporating AI into legal practice. In his 2023 annual report on the judiciary , Chief Justice Roberts took note and warned lawyers of hallucinations.
Across all areas of industry, retrieval-augmented generation (RAG) is seen and promoted as the solution for reducing hallucinations in domain-specific contexts. Relying on RAG, leading legal research services have released AI-powered legal research products that they claim “avoid” hallucinations and guarantee “hallucination-free” legal citations. RAG systems promise to deliver more accurate and trustworthy legal information by integrating a language model with a database of legal documents. Yet providers have not provided hard evidence for such claims or even precisely defined “hallucination,” making it difficult to assess their real-world reliability.
AI-Driven Legal Research Tools Still Hallucinate
In a new preprint study by Stanford RegLab and HAI researchers, we put the claims of two providers, LexisNexis (creator of Lexis+ AI) and Thomson Reuters (creator of Westlaw AI-Assisted Research and Ask Practical Law AI)), to the test. We show that their tools do reduce errors compared to general-purpose AI models like GPT-4. That is a substantial improvement and we document instances where these tools provide sound and detailed legal research. But even these bespoke legal AI tools still hallucinate an alarming amount of the time: the Lexis+ AI and Ask Practical Law AI systems produced incorrect information more than 17% of the time, while Westlaw’s AI-Assisted Research hallucinated more than 34% of the time.
Read the full study, Hallucination-Free? Assessing the Reliability of Leading AI Legal Research Tools
To conduct our study, we manually constructed a pre-registered dataset of over 200 open-ended legal queries, which we designed to probe various aspects of these systems’ performance.
Broadly, we investigated (1) general research questions (questions about doctrine, case holdings, or the bar exam); (2) jurisdiction or time-specific questions (questions about circuit splits and recent changes in the law); (3) false premise questions (questions that mimic a user having a mistaken understanding of the law); and (4) factual recall questions (questions about simple, objective facts that require no legal interpretation). These questions are designed to reflect a wide range of query types and to constitute a challenging real-world dataset of exactly the kinds of queries where legal research may be needed the most.
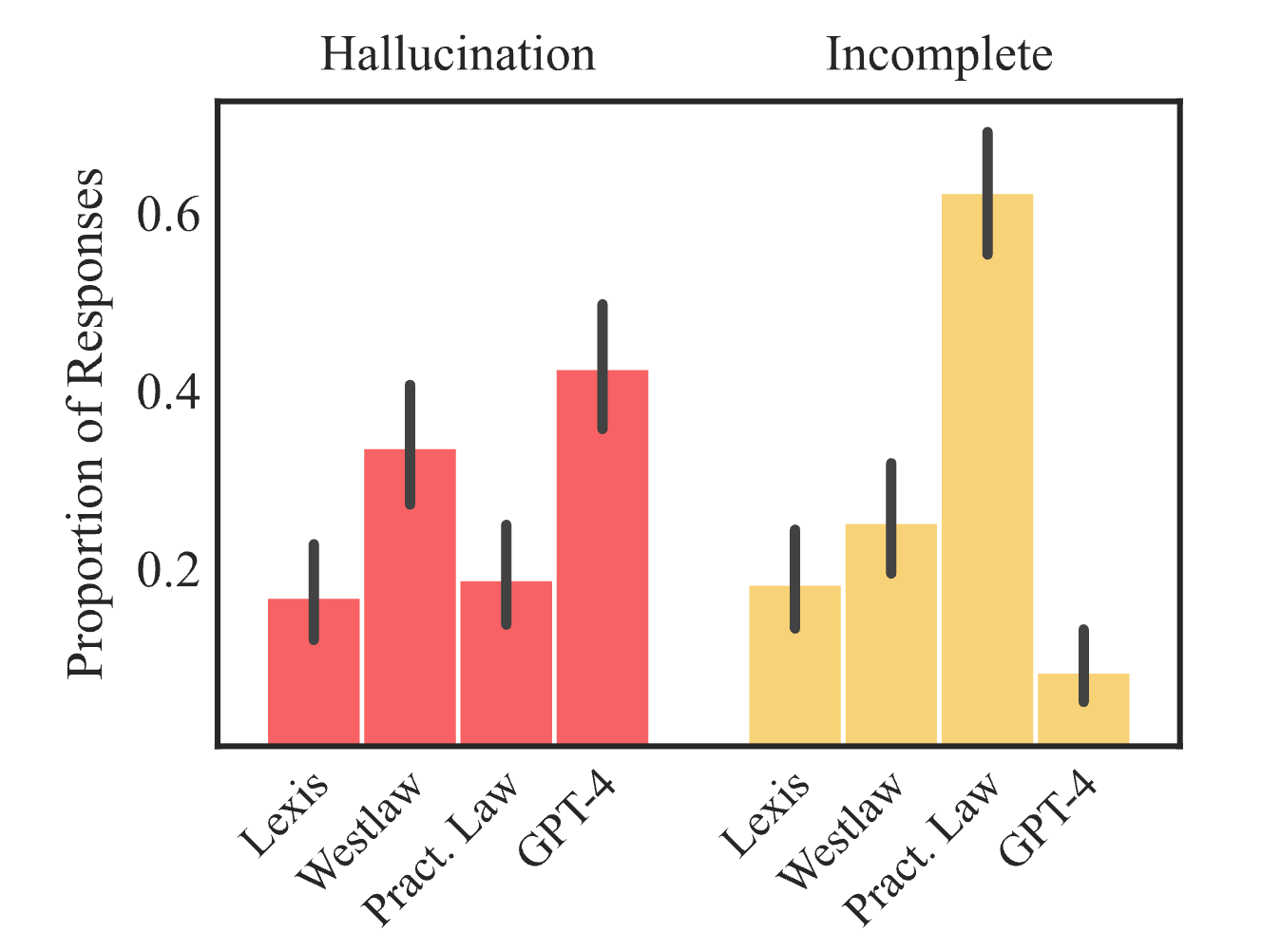
Figure 1: Comparison of hallucinated (red) and incomplete (yellow) answers across generative legal research tools.
These systems can hallucinate in one of two ways. First, a response from an AI tool might just be incorrect —it describes the law incorrectly or makes a factual error. Second, a response might be misgrounded —the AI tool describes the law correctly, but cites a source which does not in fact support its claims.
Given the critical importance of authoritative sources in legal research and writing, the second type of hallucination may be even more pernicious than the outright invention of legal cases. A citation might be “hallucination-free” in the narrowest sense that the citation exists , but that is not the only thing that matters. The core promise of legal AI is that it can streamline the time-consuming process of identifying relevant legal sources. If a tool provides sources that seem authoritative but are in reality irrelevant or contradictory, users could be misled. They may place undue trust in the tool's output, potentially leading to erroneous legal judgments and conclusions.
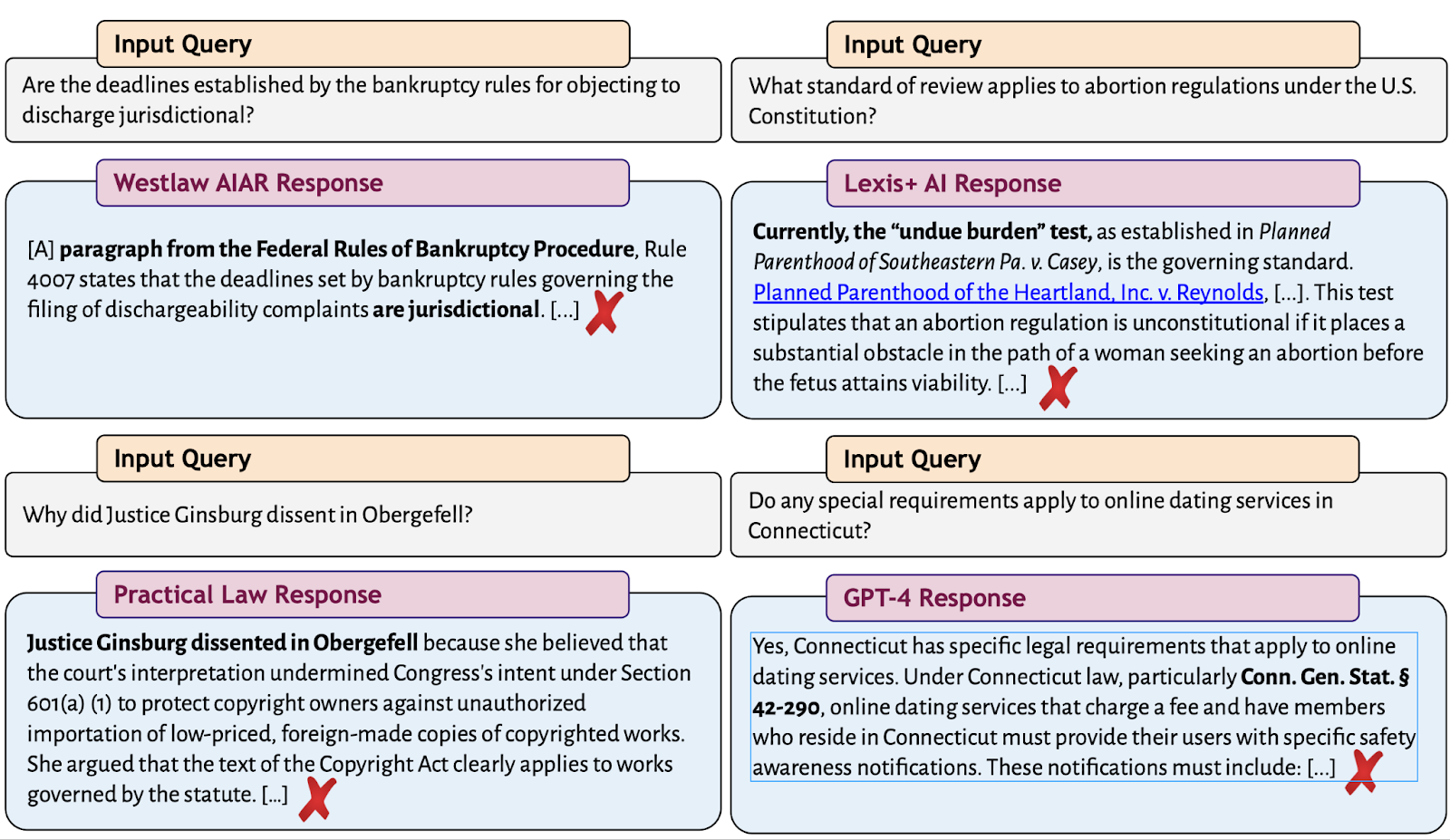
Figure 2: Top left: Example of a hallucinated response by Westlaw's AI-Assisted Research product. The system makes up a statement in the Federal Rules of Bankruptcy Procedure that does not exist (and Kontrick v. Ryan, 540 U.S. 443 (2004) held that a closely related bankruptcy deadline provision was not jurisdictional). Top right: Example of a hallucinated response by LexisNexis's Lexis+ AI. Casey and its undue burden standard were overruled by the Supreme Court in Dobbs v. Jackson Women's Health Organization, 597 U.S. 215 (2022); the correct answer is rational basis review. Bottom left: Example of a hallucinated response by Thomson Reuters's Ask Practical Law AI. The system fails to correct the user’s mistaken premise—in reality, Justice Ginsburg joined the Court's landmark decision legalizing same-sex marriage—and instead provides additional false information about the case. Bottom right: Example of a hallucinated response from GPT-4, which generates a statutory provision that has not been codified.
RAG Is Not a Panacea
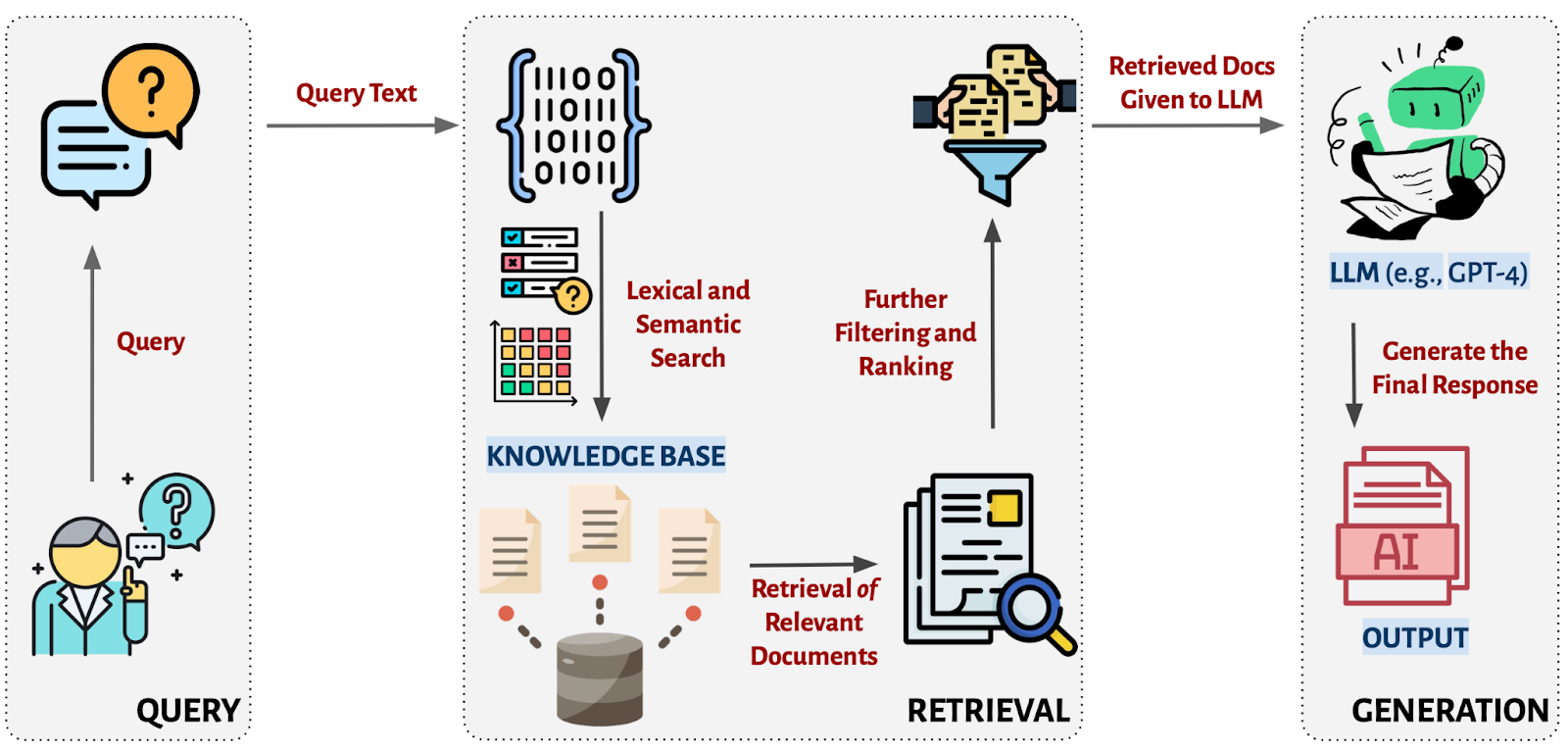
Figure 3: An overview of the retrieval-augmentation generation (RAG) process. Given a user query (left), the typical process consists of two steps: (1) retrieval (middle), where the query is embedded with natural language processing and a retrieval system takes embeddings and retrieves the relevant documents (e.g., Supreme Court cases); and (2) generation (right), where the retrieved texts are fed to the language model to generate the response to the user query. Any of the subsidiary steps may introduce error and hallucinations into the generated response. (Icons are courtesy of FlatIcon.)
Under the hood, these new legal AI tools use retrieval-augmented generation (RAG) to produce their results, a method that many tout as a potential solution to the hallucination problem. In theory, RAG allows a system to first retrieve the relevant source material and then use it to generate the correct response. In practice, however, we show that even RAG systems are not hallucination-free.
We identify several challenges that are particularly unique to RAG-based legal AI systems, causing hallucinations.
First, legal retrieval is hard. As any lawyer knows, finding the appropriate (or best) authority can be no easy task. Unlike other domains, the law is not entirely composed of verifiable facts —instead, law is built up over time by judges writing opinions . This makes identifying the set of documents that definitively answer a query difficult, and sometimes hallucinations occur for the simple reason that the system’s retrieval mechanism fails.
Second, even when retrieval occurs, the document that is retrieved can be an inapplicable authority. In the American legal system, rules and precedents differ across jurisdictions and time periods; documents that might be relevant on their face due to semantic similarity to a query may actually be inapposite for idiosyncratic reasons that are unique to the law. Thus, we also observe hallucinations occurring when these RAG systems fail to identify the truly binding authority. This is particularly problematic as areas where the law is in flux is precisely where legal research matters the most. One system, for instance, incorrectly recited the “undue burden” standard for abortion restrictions as good law, which was overturned in Dobbs (see Figure 2).
Third, sycophancy—the tendency of AI to agree with the user's incorrect assumptions—also poses unique risks in legal settings. One system, for instance, naively agreed with the question’s premise that Justice Ginsburg dissented in Obergefell , the case establishing a right to same-sex marriage, and answered that she did so based on her views on international copyright. (Justice Ginsburg did not dissent in Obergefell and, no, the case had nothing to do with copyright.) Notwithstanding that answer, here there are optimistic results. Our tests showed that both systems generally navigated queries based on false premises effectively. But when these systems do agree with erroneous user assertions, the implications can be severe—particularly for those hoping to use these tools to increase access to justice among pro se and under-resourced litigants.
Responsible Integration of AI Into Law Requires Transparency
Ultimately, our results highlight the need for rigorous and transparent benchmarking of legal AI tools. Unlike other domains, the use of AI in law remains alarmingly opaque: the tools we study provide no systematic access, publish few details about their models, and report no evaluation results at all.
This opacity makes it exceedingly challenging for lawyers to procure and acquire AI products. The large law firm Paul Weiss spent nearly a year and a half testing a product, and did not develop “hard metrics” because checking the AI system was so involved that it “makes any efficiency gains difficult to measure.” The absence of rigorous evaluation metrics makes responsible adoption difficult, especially for practitioners that are less resourced than Paul Weiss.
The lack of transparency also threatens lawyers’ ability to comply with ethical and professional responsibility requirements. The bar associations of California , New York , and Florida have all recently released guidance on lawyers’ duty of supervision over work products created with AI tools. And as of May 2024, more than 25 federal judges have issued standing orders instructing attorneys to disclose or monitor the use of AI in their courtrooms.
Without access to evaluations of the specific tools and transparency around their design, lawyers may find it impossible to comply with these responsibilities. Alternatively, given the high rate of hallucinations, lawyers may find themselves having to verify each and every proposition and citation provided by these tools, undercutting the stated efficiency gains that legal AI tools are supposed to provide.
Our study is meant in no way to single out LexisNexis and Thomson Reuters. Their products are far from the only legal AI tools that stand in need of transparency—a slew of startups offer similar products and have made similar claims , but they are available on even more restricted bases, making it even more difficult to assess how they function.
Based on what we know, legal hallucinations have not been solved.The legal profession should turn to public benchmarking and rigorous evaluations of AI tools.
This story was updated on Thursday, May 30, 2024, to include analysis of a third AI tool, Westlaw’s AI-Assisted Research.
Paper authors: Varun Magesh is a research fellow at Stanford RegLab. Faiz Surani is a research fellow at Stanford RegLab. Matthew Dahl is a joint JD/PhD student in political science at Yale University and graduate student affiliate of Stanford RegLab. Mirac Suzgun is a joint JD/PhD student in computer science at Stanford University and a graduate student fellow at Stanford RegLab. Christopher D. Manning is Thomas M. Siebel Professor of Machine Learning, Professor of Linguistics and Computer Science, and Senior Fellow at HAI. Daniel E. Ho is the William Benjamin Scott and Luna M. Scott Professor of Law, Professor of Political Science, Professor of Computer Science (by courtesy), Senior Fellow at HAI, Senior Fellow at SIEPR, and Director of the RegLab at Stanford University.
More News Topics

COMMENTS
10 Famous Examples of Longitudinal Studies. A longitudinal study is a study that observes a subject or subjects over an extended period of time. They may run into several weeks, months, or years. An examples is the Up Series which has been going since 1963. Longitudinal studies are deployed most commonly in psychology and sociology, where the ...
Revised on June 22, 2023. In a longitudinal study, researchers repeatedly examine the same individuals to detect any changes that might occur over a period of time. Longitudinal studies are a type of correlational research in which researchers observe and collect data on a number of variables without trying to influence those variables.
Panel Study. A panel study is a type of longitudinal study design in which the same set of participants are measured repeatedly over time. Data is gathered on the same variables of interest at each time point using consistent methods. This allows studying continuity and changes within individuals over time on the key measured constructs.
2. Observational: As we mentioned earlier, longitudinal studies involve observing the research participants throughout the study and recording any changes in traits that you notice. 3. Timeline: A longitudinal study can span weeks, months, years, or even decades. This dramatically contrasts what is obtainable in cross-sectional studies that ...
A longitudinal study requires an investigator to observe the participants at different time intervals. A cross-sectional study is conducted over a specified period of time. Longitudinal studies can offer researchers a cause and effect relationship. Cross-sectional studies cannot offer researchers a cause-and-effect relationship.
Longitudinal studies also allow repeated observations of the same individual over time. This means any changes in the outcome variable cannot be attributed to differences between individuals. Example: Individual differences. You decide to study how a particular weight-training program affects athletic performance.
Longitudinal studies, a type of correlational research, are usually observational, in contrast with cross-sectional research. Longitudinal research involves collecting data over an extended time, whereas cross-sectional research involves collecting data at a single point. To test this hypothesis, the researchers recruit participants who are in ...
For example, "when is an athlete able to return to play after a forearm fracture?" is a question that can be answered with a longitudinal study. In this chapter, we will define the three main types of longitudinal studies, analyze examples of prominent longitudinal studies in the literature, and offer advice on the steps needed to perform a ...
A longitudinal study is an experimental design that takes repeated measurements of the same subjects over time. These studies can span years or even decades. Unlike cross-sectional studies, which analyze data at a single point, longitudinal studies track changes and developments, producing a more dynamic assessment.
A longitudinal study (or longitudinal survey, or panel study) is a research design that involves repeated observations of the same variables (e.g., people) over long periods of time (i.e., uses longitudinal data).It is often a type of observational study, although it can also be structured as longitudinal randomized experiment.. Longitudinal studies are often used in social-personality and ...
A longitudinal study is observational and involves the continuous and repeated measurements of selected individuals followed over a period of time. Quantitative and qualitative data is gathered on "any combination of exposures and outcome." For instance, longitudinal studies are useful for observing relationships between the risk factors, development, and treatment outcomes of disease for ...
A longitudinal study is a study that repeatedly measures observations (collects data) over time. It often involves following up with patients for a prolonged period, such as years, and measuring both explanatory and outcome variables at multiple points, usually more than two, of follow-up. Longitudinal studies are most commonly observational ...
Major Developments in Longitudinal Family Research Methods and Longitudinal Datasets. Family scientists' interest in within-individual change has led to a heavy focus over the past half century on panel surveys (Menaghan & Godwin, 1993 ). Over the past 30 years, panel studies have exploded. Today, many large, often publicly available ...
A longitudinal study is a type of research where the scope of the study is observed and studied in the same set of people over a long period of time. This could be from a few weeks to many years. They are most often found in different fields like health, economics, and medicine. They serve to give knowledge of the passage of events without ...
For example, the longitudinal study of the Office of Population Censuses and Surveys prospectively follows a 1% sample of the British population that was initially identified at the 1971 census. Outcomes such as mortality and incidence of cancer have been related to employment status, housing, and other variables measured at successive censuses ...
A research hypothesis is your proposed answer to your research question. The research hypothesis usually includes an explanation ('x affects y because …'). A statistical hypothesis, on the other hand, is a mathematical statement about a population parameter. Statistical hypotheses always come in pairs: the null and alternative hypotheses.
That's the kind of thing that longitudinal research design measures. As for a formal definition, a longitudinal study is a research method that involves repeated observations of the same variable (e.g. a set of people) over some time. The observations over a period of time might be undertaken in the form of an online survey.
A longitudinal study can be applied to a wide range of cases. You need to adjust your approach, depending on a specific situation, subject's peculiarities and your research goals. There are three major research types you can use for continuous observation: Longitudinal Cohort Study; Retrospective Longitudinal Study; Longitudinal Panel Study
Attrition refers to participants leaving a study. It always happens to some extent—for example, in randomized controlled trials for medical research. Differential attrition occurs when attrition or dropout rates differ systematically between the intervention and the control group.As a result, the characteristics of the participants who drop out differ from the characteristics of those who ...
1. Sample repeated measures data based on subject IDs for cross validation of a GENMOD model, 2. Bootstrap a dataset because of a highly skewed outcome measure recorded in an unbalanced long ...
This research adopted a phenomenological research approach to develop a longitudinal qualitative study. A purposive sampling method was used to select participants and 37 patients were recruited.
Nurses' Health Study. One famous example of a cohort study is the Nurses' Health Study. This was a large, long-running analysis of female health that began in 1976. It investigated the ...
For example, at 1- and 2-year follow-up, male patients reported significantly worse cognitive and social functioning, more insomnia, and more anxiety compared with a reference population. ... In a longitudinal study among rectal cancer patients from before surgery up until 2-year follow-up, ... Questions on partner status and educational level ...
A longitudinal study with three data collection phases was designed. A non-discriminatory exponential chain demonstration (i.e., snowball sampling technique) was performed to recruit the sample. The inclusion criteria for the study were being a woman over 18 years of age and in the third trimester of pregnancy.
A longitudinal study follows subjects over a certain time period and collects data at specific intervals. Longitudinal studies are powerful study designs. They are particularly useful in medicine as they enable researchers to determine important associations and answer questions regarding prognosis. For example, "when is an athlete able to ...
The present study aimed to characterize profiles of cognitive aging and how these can be predicted from interindividual differences in demographic, lifestyle, health, and genetic factors. The participants were 1,966 older adults (mean baseline age = 71.6 years; 62.9% female), free from dementia at baseline and with at least two cognitive assessments over the 15-year follow-up, from the ...
A well-formulated research question is essential for guiding your study effectively. Follow this format to ensure clarity and precision: Specify the Topic: Begin with a broad subject area. Example: "Education technology". Narrow the Focus: Define a specific aspect or variable. Example: "Impact of digital tools".
Compared to those excluded owing to missing child BMI data at a single timepoint, the children in the longitudinal analysis sample were a year younger, had lower baseline BMI z-score, were less likely to be male or Chinese, and came from households where the Head of Household had higher education and was less likely to be Indian (Table S1).
Broadly, we investigated (1) general research questions (questions about doctrine, case holdings, or the bar exam); (2) jurisdiction or time-specific questions (questions about circuit splits and recent changes in the law); (3) false premise questions (questions that mimic a user having a mistaken understanding of the law); and (4) factual ...
Methods and analysis: DTG RESIST is a multicentre longitudinal study of adults and adolescents living with HIV in sub-Saharan Africa, Asia, and South and Central America who experienced virologic failure on dolutegravir-based ART. ... and that any patient/participant/sample identifiers included were not known to anyone (e.g., hospital staff ...