- Original article
- Open access
- Published: 13 July 2021

Assisting you to advance with ethics in research: an introduction to ethical governance and application procedures
- Shivadas Sivasubramaniam 1 ,
- Dita Henek Dlabolová 2 ,
- Veronika Kralikova 3 &
- Zeenath Reza Khan 3
International Journal for Educational Integrity volume 17 , Article number: 14 ( 2021 ) Cite this article
18k Accesses
12 Citations
4 Altmetric
Metrics details
Ethics and ethical behaviour are the fundamental pillars of a civilised society. The focus on ethical behaviour is indispensable in certain fields such as medicine, finance, or law. In fact, ethics gets precedence with anything that would include, affect, transform, or influence upon individuals, communities or any living creatures. Many institutions within Europe have set up their own committees to focus on or approve activities that have ethical impact. In contrast, lesser-developed countries (worldwide) are trying to set up these committees to govern their academia and research. As the first European consortium established to assist academic integrity, European Network for Academic Integrity (ENAI), we felt the importance of guiding those institutions and communities that are trying to conduct research with ethical principles. We have established an ethical advisory working group within ENAI with the aim to promote ethics within curriculum, research and institutional policies. We are constantly researching available data on this subject and committed to help the academia to convey and conduct ethical behaviour. Upon preliminary review and discussion, the group found a disparity in understanding, practice and teaching approaches to ethical applications of research projects among peers. Therefore, this short paper preliminarily aims to critically review the available information on ethics, the history behind establishing ethical principles and its international guidelines to govern research.
The paper is based on the workshop conducted in the 5th International conference Plagiarism across Europe and Beyond, in Mykolas Romeris University, Lithuania in 2019. During the workshop, we have detailed a) basic needs of an ethical committee within an institution; b) a typical ethical approval process (with examples from three different universities); and c) the ways to obtain informed consent with some examples. These are summarised in this paper with some example comparisons of ethical approval processes from different universities. We believe this paper will provide guidelines on preparing and training both researchers and research students in appropriately upholding ethical practices through ethical approval processes.
Introduction
Ethics and ethical behaviour (often linked to “responsible practice”) are the fundamental pillars of a civilised society. Ethical behaviour with integrity is important to maintain academic and research activities. It affects everything we do, and gets precedence with anything that would include/affect, transform, or impact upon individuals, communities or any living creatures. In other words, ethics would help us improve our living standards (LaFollette, 2007 ). The focus on ethical behaviour is indispensable in certain fields such as medicine, finance, or law, but is also gaining recognition in all disciplines engaged in research. Therefore, institutions are expected to develop ethical guidelines in research to maintain quality, initiate/own integrity and above all be transparent to be successful by limiting any allegation of misconduct (Flite and Harman, 2013 ). This is especially true for higher education organisations that promote research and scholarly activities. Many European institutions have developed their own regulations for ethics by incorporating international codes (Getz, 1990 ). The lesser developed countries are trying to set up these committees to govern their academia and research. World Health Organization has stated that adhering to “ ethical principles … [is central and important]... in order to protect the dignity, rights and welfare of research participants ” (WHO, 2021 ). Ethical guidelines taught to students can help develop ethical researchers and members of society who uphold values of ethical principles in practice.
As the first European-wide consortium established to assist academic integrity (European Network for Academic Integrity – ENAI), we felt the importance of guiding those institutions and communities that are trying to teach, research, and include ethical principles by providing overarching understanding of ethical guidelines that may influence policy. Therefore, we set up an advisory working group within ENAI in 2018 to support matters related to ethics, ethical committees and assisting on ethics related teaching activities.
Upon preliminary review and discussion, the group found a disparity in understanding, practice and teaching approaches to ethical applications among peers. This became the premise for this research paper. We first carried out a literature survey to review and summarise existing ethical governance (with historical perspectives) and procedures that are already in place to guide researchers in different discipline areas. By doing so, we attempted to consolidate, document and provide important steps in a typical ethical application process with example procedures from different universities. Finally, we attempted to provide insights and findings from practical workshops carried out at the 5th International Conference Plagiarism across Europe and Beyond, in Mykolas Romeris University, Lithuania in 2019, focussing on:
• highlighting the basic needs of an ethical committee within an institution,
• discussing and sharing examples of a typical ethical approval process,
• providing guidelines on the ways to teach research ethics with some examples.
We believe this paper provides guidelines on preparing and training both researchers and research students in appropriately upholding ethical practices through ethical approval processes.
Background literature survey
Responsible research practice (RRP) is scrutinised by the aspects of ethical principles and professional standards (WHO’s Code of Conduct for responsible Research, 2017). The Singapore statement on research integrity (The Singapore Statement on Research integrity, 2010) has provided an internationally acceptable guidance for RRP. The statement is based on maintaining honesty, accountability, professional courtesy in all aspects of research and maintaining fairness during collaborations. In other words, it does not simply focus on the procedural part of the research, instead covers wider aspects of “integrity” beyond the operational aspects (Israel and Drenth, 2016 ).
Institutions should focus on providing ethical guidance based on principles and values reflecting upon all aspects/stages of research (from the funding application/project development stage upto or beyond project closing stage). Figure 1 summarizes the different aspects/stages of a typical research and highlights the needs of RRP in compliance with ethical governance at each stage with examples (the figure is based on Resnik, 2020 ; Žukauskas et al., 2018 ; Anderson, 2011 ; Fouka and Mantzorou, 2011 ).
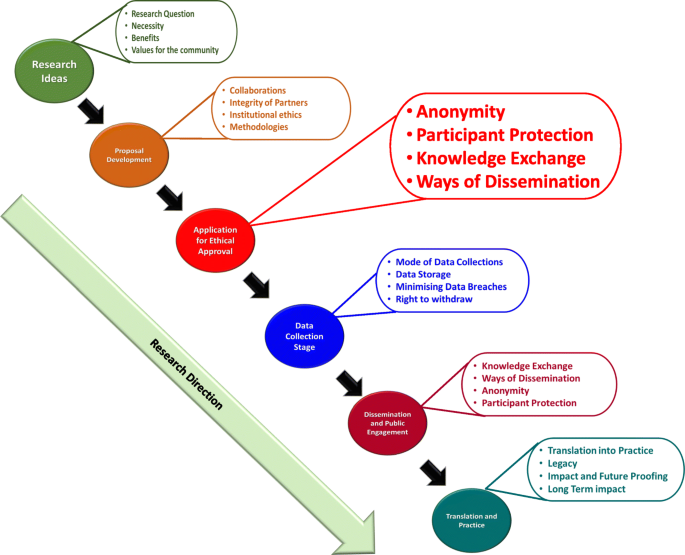
Summary of the enabling ethical governance at different stages of research. Note that it is imperative for researchers to proactively consider the ethical implications before, during and after the actual research process. The summary shows that RRP should be in line with ethical considerations even long before the ethical approval stage
Individual responsibilities to enhance RRP
As explained in Fig. 1 , a successfully governed research should consider ethics at the planning stages prior to research. Many international guidance are compatible in enforcing/recommending 14 different “responsibilities” that were first highlighted in the Singapore Statement (2010) for researchers to follow and achieve competency in RRP. In order to understand the purpose and the expectation of these ethical guidelines, we have carried out an initial literature survey on expected individual responsibilities. These are summarised in Table 1 .
By following these directives, researchers can carry out accountable research by maximising ethical self-governance whilst minimising misconducts. In our own experiences of working with many researchers, their focus usually revolves around ethical “clearance” rather than behaviour. In other words, they perceive this as a paper exercise rather than trying to “own” ethical behaviour in everything they do. Although the ethical principles and responsibilities are explicitly highlighted in the majority of international guidelines [such as UK’s Research Governance Policy (NICE, 2018 ), Australian Government’s National Statement on Ethical Conduct in Human Research (Difn website a - National Statement on Ethical Conduct in Human Research (NSECHR), 2018 ), the Singapore Statement (2010) etc.]; and the importance of holistic approach has been argued in ethical decision making, many researchers and/or institutions only focus on ethics linked to the procedural aspects.
Studies in the past have also highlighted inconsistencies in institutional guidelines pointing to the fact that these inconsistencies may hinder the predicted research progress (Desmond & Dierickx 2021 ; Alba et al., 2020 ; Dellaportas et al., 2014 ; Speight 2016 ). It may also be possible that these were and still are linked to the institutional perceptions/expectations or the pre-empting contextual conditions that are imposed by individual countries. In fact, it is interesting to note many research organisations and HE institutions establish their own policies based on these directives.
Research governance - origins, expectations and practices
Ethical governance in clinical medicine helps us by providing a structure for analysis and decision-making. By providing workable definitions of benefits and risks as well as the guidance for evaluating/balancing benefits over risks, it supports the researchers to protect the participants and the general population.
According to the definition given by National Institute of Clinical care Excellence, UK (NICE 2018 ), “ research governance can be defined as the broad range of regulations, principles and standards of good practice that ensure high quality research ”. As stated above, our literature-based research survey showed that most of the ethical definitions are basically evolved from the medical field and other disciplines have utilised these principles to develop their own ethical guidance. Interestingly, historical data show that the medical research has been “self-governed” or in other words implicated by the moral behaviour of individual researchers (Fox 2017 ; Shaw et al., 2005 ; Getz, 1990 ). For example, early human vaccination trials conducted in 1700s used the immediate family members as test subjects (Fox, 2017 ). Here the moral justification might have been the fact that the subjects who would have been at risk were either the scientists themselves or their immediate families but those who would reap the benefits from the vaccination were the general public/wider communities. However, according to the current ethical principles, this assumption is entirely not acceptable.
Historically, ambiguous decision-making and resultant incidences of research misconduct have led to the need for ethical research governance in as early as the 1940’s. For instance, the importance of an international governance was realised only after the World War II, when people were astonished to note the unethical research practices carried out by Nazi scientists. As a result of this, in 1947 the Nuremberg code was published. The code mainly focussed on the following:
Informed consent and further insisted the research involving humans should be based on prior animal work,
The anticipated benefits should outweigh the risk,
Research should be carried out only by qualified scientists must conduct research,
Avoiding physical and mental suffering and.
Avoiding human research that would result in which death or disability.
(Weindling, 2001 ).
Unfortunately, it was reported that many researchers in the USA and elsewhere considered the Nuremberg code as a document condemning the Nazi atrocities, rather than a code for ethical governance and therefore ignored these directives (Ghooi, 2011 ). It was only in 1964 that the World Medical Association published the Helsinki Declaration, which set the stage for ethical governance and the implementation of the Institutional Review Board (IRB) process (Shamoo and Irving, 1993 ). This declaration was based on Nuremberg code. In addition, the declaration also paved the way for enforcing research being conducted in accordance with these guidelines.
Incidentally, the focus on research/ethical governance gained its momentum in 1974. As a result of this, a report on ethical principles and guidelines for the protection of human subjects of research was published in 1979 (The Belmont Report, 1979 ). This report paved the way to the current forms of ethical governance in biomedical and behavioural research by providing guidance.
Since 1994, the WHO itself has been providing several guidance to health care policy-makers, researchers and other stakeholders detailing the key concepts in medical ethics. These are specific to applying ethical principles in global public health.
Likewise, World Organization for Animal Health (WOAH), and International Convention for the Protection of Animals (ICPA) provide guidance on animal welfare in research. Due to this continuous guidance, together with accepted practices, there are internationally established ethical guidelines to carry out medical research. Our literature survey further identified freely available guidance from independent organisations such as COPE (Committee of Publication Ethics) and ALLEA (All European Academics) which provide support for maintaining research ethics in other fields such as education, sociology, psychology etc. In reality, ethical governance is practiced differently in different countries. In the UK, there is a clinical excellence research governance, which oversees all NHS related medical research (Mulholland and Bell, 2005 ). Although, the governance in other disciplines is not entirely centralised, many research funding councils and organisations [such as UKRI (UK-Research and Innovation; BBSC (Biotechnology and Biological Sciences Research Council; MRC (Medical Research Council); EPSRC (Economic and Social Research Council)] provide ethical governance and expect institutional adherence and monitoring. They expect local institutional (i.e. university/institutional) research governance for day-to-day monitoring of the research conducted within the organisation and report back to these funding bodies, monthly or annually (Department of Health, 2005). Likewise, there are nationally coordinated/regulated ethics governing bodies such as the US Office for Human Research Protections (US-OHRP), National Institute of Health (NIH) and the Canadian Institutes for Health Research (CIHR) in the USA and Canada respectively (Mulholland and Bell, 2005 ). The OHRP in the USA formally reviews all research activities involving human subjects. On the other hand, in Canada, CIHR works with the Natural Sciences and Engineering Research Council (NSERC), and the Social Sciences and Humanities Research Council (SSHRC). They together have produced a Tri-Council Policy Statement (TCPS) (Stephenson et al., 2020 ) as ethical governance. All Canadian institutions are expected to adhere to this policy for conducting research. As for Australia, the research is governed by the Australian code for the responsible conduct of research (2008). It identifies the responsibilities of institutions and researchers in all areas of research. The code has been jointly developed by the National Health and Medical Research Council (NHMRC), the Australian Research Council (ARC) and Universities Australia (UA). This information is summarized in Table 2 .
Basic structure of an institutional ethical advisory committee (EAC)
The WHO published an article defining the basic concepts of an ethical advisory committee in 2009 (WHO, 2009 - see above). According to this, many countries have established research governance and monitor the ethical practice in research via national and/or regional review committees. The main aims of research ethics committees include reviewing the study proposals, trying to understand the justifications for human/animal use, weighing the merits and demerits of the usage (linking to risks vs. potential benefits) and ensuring the local, ethical guidelines are followed Difn website b - Enago academy Importance of Ethics Committees in Scholarly Research, 2020 ; Guide for Research Ethics - Council of Europe, 2014 ). Once the research has started, the committee needs to carry out periodic surveillance to ensure the institutional ethical norms are followed during and beyond the study. They may also be involved in setting up and/or reviewing the institutional policies.
For these aspects, IRB (or institutional ethical advisory committee - IEAC) is essential for local governance to enhance best practices. The advantage of an IRB/EEAC is that they understand the institutional conditions and can closely monitor the ongoing research, including any changes in research directions. On the other hand, the IRB may be overly supportive to accept applications, influenced by the local agenda for achieving research excellence, disregarding ethical issues (Kotecha et al., 2011 ; Kayser-Jones, 2003 ) or, they may be influenced by the financial interests in attracting external funding. In this respect, regional and national ethics committees are advantageous to ensure ethical practice. Due to their impartiality, they would provide greater consistency and legitimacy to the research (WHO, 2009 ). However, the ethical approval process of regional and national ethics committees would be time consuming, as they do not have the local knowledge.
As for membership in the IRBs, most of the guidelines [WHO, NICE, Council of Europe, (2012), European Commission - Facilitating Research Excellence in FP7 ( 2013 ) and OHRP] insist on having a variety of representations including experts in different fields of research, and non-experts with the understanding of local, national/international conflicts of interest. The former would be able to understand/clarify the procedural elements of the research in different fields; whilst the latter would help to make neutral and impartial decisions. These non-experts are usually not affiliated to the institution and consist of individuals representing the broader community (particularly those related to social, legal or cultural considerations). IRBs consisting of these varieties of representation would not only be in a position to understand the study procedures and their potential direct or indirect consequences for participants, but also be able to identify any community, cultural or religious implications of the study.
Understanding the subtle differences between ethics and morals
Interestingly, many ethical guidelines are based on society’s moral “beliefs” in such a way that the words “ethics”‘and “morals” are reciprocally used to define each other. However, there are several subtle differences between them and we have attempted to compare and contrast them herein. In the past, many authors have interchangeably used the words “morals”‘and “ethics”‘(Warwick, 2003 ; Kant, 2018 ; Hazard, GC (Jr)., 1994 , Larry, 1982 ). However, ethics is linked to rules governed by an external source such as codes of conduct in workplaces (Kuyare et al., 2014 ). In contrast, morals refer to an individual’s own principles regarding right and wrong. Quinn ( 2011 ) defines morality as “ rules of conduct describing what people ought and ought not to do in various situations … ” while ethics is “... the philosophical study of morality, a rational examination into people’s moral beliefs and behaviours ”. For instance, in a case of parents demanding that schools overturn a ban on use of corporal punishment of children by schools and teachers (Children’s Rights Alliance for England, 2005 ), the parents believed that teachers should assume the role of parent in schools and use corporal or physical punishment for children who misbehaved. This stemmed from their beliefs and what they felt were motivated by “beliefs of individuals or groups”. For example, recent media highlights about some parents opposing LGBT (Lesbian, Gay, Bisexual, and Transgender) education to their children (BBC News, 2019 ). One parent argued, “Teaching young children about LGBT at a very early stage is ‘morally’ wrong”. She argued “let them learn by themselves as they grow”. This behaviour is linked to and governed by the morals of an ethnic community. Thus, morals are linked to the “beliefs of individuals or group”. However, when it comes to the LGBT rights these are based on ethical principles of that society and governed by law of the land. However, the rights of children to be protected from “inhuman and degrading” treatment is based on the ethical principles of the society and governed by law of the land. Individuals, especially those who are working in medical or judicial professions have to follow an ethical code laid down by their profession, regardless of their own feelings, time or preferences. For instance, a lawyer is expected to follow the professional ethics and represent a defendant, despite the fact that his morals indicate the defendant is guilty.
In fact, we as a group could not find many scholarly articles clearly comparing or contrasting ethics with morals. However, a table presented by Surbhi ( 2015 ) (Difn website c ) tries to differentiate these two terms (see Table 3 ).
Although Table 3 gives some insight on the differences between these two terms, in practice many use these terms as loosely as possible mainly because of their ambiguity. As a group focussed on the application of these principles, we would recommend to use the term “ethics” and avoid “morals” in research and academia.
Based on the literature survey carried out, we were able to identify the following gaps:
there is some disparity in existing literature on the importance of ethical guidelines in research
there is a lack of consensus on what code of conduct should be followed, where it should be derived from and how it should be implemented
The mission of ENAI’s ethical advisory working group
The Ethical Advisory Working Group of ENAI was established in 2018 to promote ethical code of conduct/practice amongst higher educational organisations within Europe and beyond (European Network for Academic Integrity, 2018 ). We aim to provide unbiased advice and consultancy on embedding ethical principles within all types of academic, research and public engagement activities. Our main objective is to promote ethical principles and share good practice in this field. This advisory group aims to standardise ethical norms and to offer strategic support to activities including (but not exclusive to):
● rendering advice and assistance to develop institutional ethical committees and their regulations in member institutions,
● sharing good practice in research and academic ethics,
● acting as a critical guide to institutional review processes, assisting them to maintain/achieve ethical standards,
● collaborating with similar bodies in establishing collegiate partnerships to enhance awareness and practice in this field,
● providing support within and outside ENAI to develop materials to enhance teaching activities in this field,
● organising training for students and early-career researchers about ethical behaviours in form of lectures, seminars, debates and webinars,
● enhancing research and dissemination of the findings in matters and topics related to ethics.
The following sections focus on our suggestions based on collective experiences, review of literature provided in earlier sections and workshop feedback collected:
a) basic needs of an ethical committee within an institution;
b) a typical ethical approval process (with examples from three different universities); and
c) the ways to obtain informed consent with some examples. This would give advice on preparing and training both researchers and research students in appropriately upholding ethical practices through ethical approval processes.
Setting up an institutional ethical committee (ECs)
Institutional Ethical Committees (ECs) are essential to govern every aspect of the activities undertaken by that institute. With regards to higher educational organisations, this is vital to establish ethical behaviour for students and staff to impart research, education and scholarly activities (or everything) they do. These committees should be knowledgeable about international laws relating to different fields of studies (such as science, medicine, business, finance, law, and social sciences). The advantages and disadvantages of institutional, subject specific or common (statutory) ECs are summarised in Fig. 2 . Some institutions have developed individual ECs linked to specific fields (or subject areas) whilst others have one institutional committee that overlooks the entire ethical behaviour and approval process. There is no clear preference between the two as both have their own advantages and disadvantages (see Fig. 2 ). Subject specific ECs are attractive to medical, law and business provisions, as it is perceived the members within respective committees would be able to understand the subject and therefore comprehend the need of the proposed research/activity (Kadam, 2012 ; Schnyder et al., 2018 ). However, others argue, due to this “ specificity ”, the committee would fail to forecast the wider implications of that application. On the other hand, university-wide ECs would look into the wider implications. Yet they find it difficult to understand the purpose and the specific applications of that research. Not everyone understands dynamics of all types of research methodologies, data collection, etc., and therefore there might be a chance of a proposal being rejected merely because the EC could not understand the research applications (Getz, 1990 ).
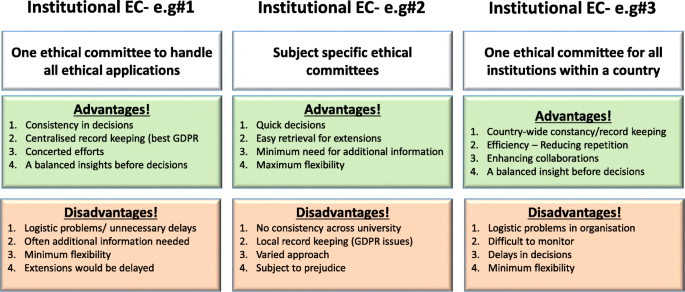
Summary of advantages and disadvantages of three different forms of ethical committees
[N/B for Fig. 2 : Examples of different types of ethical application procedures and forms used were discussed with the workshop attendees to enhance their understanding of the differences. GDPR = General Data Protection Regulation].
Although we recommend a designated EC with relevant professional, academic and ethical expertise to deal with particular types of applications, the membership (of any EC) should include some non-experts who would represent the wider community (see above). Having some non-experts in EC would not only help the researchers to consider explaining their research in layperson’s terms (by thinking outside the box) but also would ensure efficiency without compromising participants/animal safety. They may even help to address the common ethical issues outside research culture. Some UK universities usually offer this membership to a clergy, councillor or a parliamentarian who does not have any links to the institutions. Most importantly, it is vital for any EC members to undertake further training in addition to previous experience in the relevant field of research ethics.
Another issue that raises concerns is multi-centre research, involving several institutions, where institutionalised ethical approvals are needed from each partner. In some cases, such as clinical research within the UK, a common statutory EC called National Health Services (NHS) Research Ethics Committee (NREC) is in place to cover research ethics involving all partner institutions (NHS, 2018 ). The process of obtaining approval from this type of EC takes time, therefore advanced planning is needed.
Ethics approval forms and process
During the workshop, we discussed some anonymised application forms obtained from open-access sources for qualitative and quantitative research as examples. Considering research ethics, for the purpose of understanding, we arbitrarily divided this in two categories; research based on (a) quantitative and (b) qualitative methodologies. As their name suggests their research approach is extremely different from each other. The discussion elicited how ECs devise different types of ethical application form/questions. As for qualitative research, these are often conducted as “face-to-face” interviews, which would have implications on volunteer anonymity.
Furthermore, discussions posited when the interviews are replaced by on-line surveys, they have to be administered through registered university staff to maintain confidentiality. This becomes difficult when the research is a multi-centre study. These types of issues are also common in medical research regarding participants’ anonymity, confidentially, and above all their right to withdraw consent to be involved in research.
Storing and protecting data collected in the process of the study is also a point of consideration when applying for approval.
Finally, the ethical processes of invasive (involving human/animals) and non-invasive research (questionnaire based) may slightly differ from one another. Following research areas are considered as investigations that need ethical approval:
research that involves human participants (see below)
use of the ‘products’ of human participants (see below)
work that potentially impacts on humans (see below)
research that involves animals
In addition, it is important to provide a disclaimer even if an ethical approval is deemed unnecessary. Following word cloud (Fig. 3 ) shows the important variables that need to be considered at the brainstorming stage before an ethical application. It is worth noting the importance of proactive planning predicting the “unexpected” during different phases of a research project (such as planning, execution, publication, and future directions). Some applications (such as working with vulnerable individuals or children) will require safety protection clearance (such as DBS - Disclosure and Barring Service, commonly obtained from the local police). Please see section on Research involving Humans - Informed consents for further discussions.
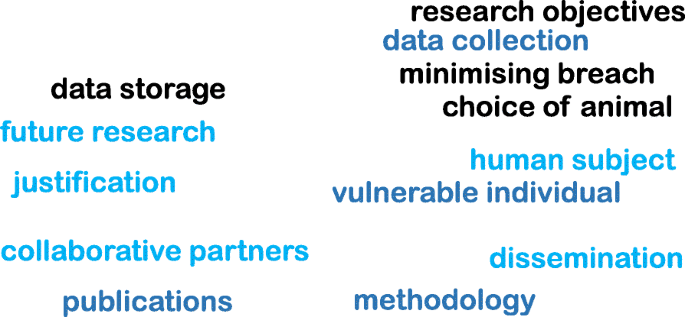
Examples of important variables that need to be considered for an ethical approval
It is also imperative to report or re-apply for ethical approval for any minor or major post-approval changes to original proposals made. In case of methodological changes, evidence of risk assessments for changes and/or COSHH (Control of Substances Hazardous to Health Regulations) should also be given. Likewise, any new collaborative partners or removal of researchers should also be notified to the IEAC.
Other findings include:
in case of complete changes in the project, the research must be stopped and new approval should be seeked,
in case of noticing any adverse effects to project participants (human or non-human), these should also be notified to the committee for appropriate clearance to continue the work, and
the completion of the project must also be notified with the indication whether the researchers may restart the project at a later stage.
Research involving humans - informed consents
While discussing research involving humans and based on literature review, findings highlight the human subjects/volunteers must willingly participate in research after being adequately informed about the project. Therefore, research involving humans and animals takes precedence in obtaining ethical clearance and its strict adherence, one of which is providing a participant information sheet/leaflet. This sheet should contain a full explanation about the research that is being carried out and be given out in lay-person’s terms in writing (Manti and Licari 2018 ; Hardicre 2014 ). Measures should also be in place to explain and clarify any doubts from the participants. In addition, there should be a clear statement on how the participants’ anonymity is protected. We provide below some example questions below to help the researchers to write this participant information sheet:
What is the purpose of the study?
Why have they been chosen?
What will happen if they take part?
What do they have to do?
What happens when the research stops?
What if something goes wrong?
What will happen to the results of the research study?
Will taking part be kept confidential?
How to handle “vulnerable” participants?
How to mitigate risks to participants?
Many institutional ethics committees expect the researchers to produce a FAQ (frequently asked questions) in addition to the information about research. Most importantly, the researchers also need to provide an informed consent form, which should be signed by each human participant. The five elements identified that are needed to be considered for an informed consent statement are summarized in Fig. 4 below (slightly modified from the Federal Policy for the Protection of Human Subjects ( 2018 ) - Diffn website c ).
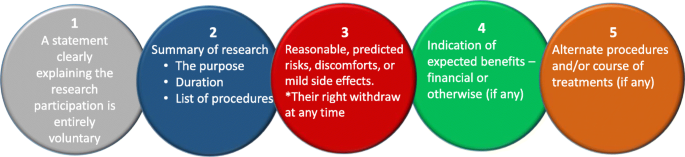
Five basic elements to consider for an informed consent [figure adapted from Diffn website c ]
The informed consent form should always contain a clause for the participant to withdraw their consent at any time. Should this happen all the data from that participant should be eliminated from the study without affecting their anonymity.
Typical research ethics approval process
In this section, we provide an example flow chart explaining how researchers may choose the appropriate application and process, as highlighted in Fig. 5 . However, it is imperative to note here that these are examples only and some institutions may have one unified application with separate sections to demarcate qualitative and quantitative research criteria.
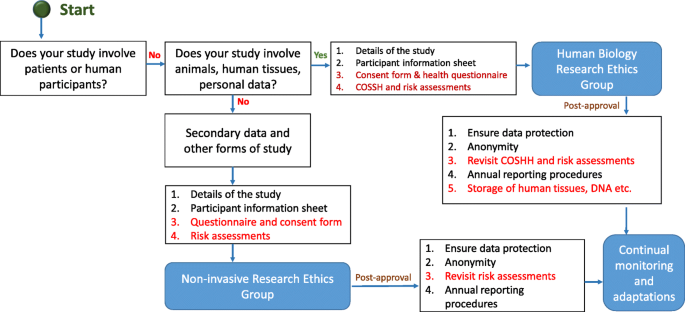
Typical ethical approval processes for quantitative and qualitative research. [N/B for Fig. 5 - This simplified flow chart shows that fundamental process for invasive and non-invasive EC application is same, the routes and the requirements for additional information are slightly different]
Once the ethical application is submitted, the EC should ensure a clear approval procedure with distinctly defined timeline. An example flow chart showing the procedure for an ethical approval was obtained from University of Leicester as open-access. This is presented in Fig. 6 . Further examples of the ethical approval process and governance were discussed in the workshop.
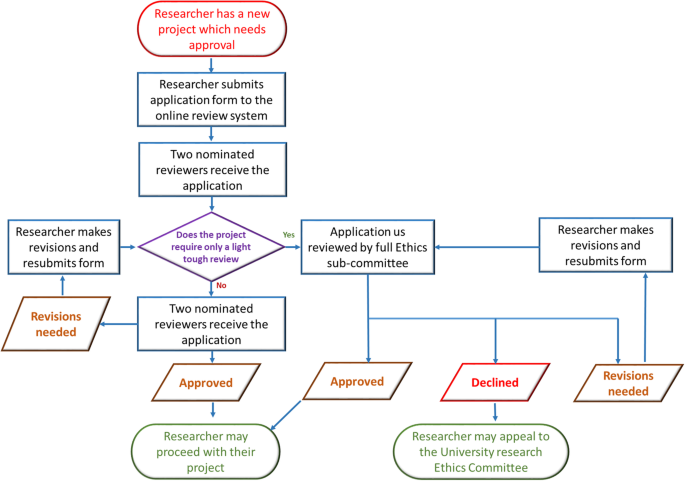
An example ethical approval procedures conducted within University of Leicester (Figure obtained from the University of Leicester research pages - Difn website d - open access)
Strategies for ethics educations for students
Student education on the importance of ethics and ethical behaviour in research and scholarly activities is extremely essential. Literature posits in the area of medical research that many universities are incorporating ethics in post-graduate degrees but when it comes to undergraduate degrees, there is less appetite to deliver modules or even lectures focussing on research ethics (Seymour et al., 2004 ; Willison and O’Regan, 2007 ). This may be due to the fact that undergraduate degree structure does not really focus on research (DePasse et al., 2016 ). However, as Orr ( 2018 ) suggested, institutions should focus more on educating all students about ethics/ethical behaviour and their importance in research, than enforcing punitive measures for unethical behaviour. Therefore, as an advisory committee, and based on our preliminary literature survey and workshop results, we strongly recommend incorporating ethical education within undergraduate curriculum. Looking at those institutions which focus on ethical education for both under-and postgraduate courses, their approaches are either (a) a lecture-based delivery, (b) case study based approach or (c) a combined delivery starting with a lecture on basic principles of ethics followed by generating a debate based discussion using interesting case studies. The combined method seems much more effective than the other two as per our findings as explained next.
As many academics who have been involved in teaching ethics and/or research ethics agree, the underlying principles of ethics is often perceived as a boring subject. Therefore, lecture-based delivery may not be suitable. On the other hand, a debate based approach, though attractive and instantly generates student interest, cannot be effective without students understanding the underlying basic principles. In addition, when selecting case studies, it would be advisable to choose cases addressing all different types of ethical dilemmas. As an advisory group within ENAI, we are in the process of collating supporting materials to help to develop institutional policies, creating advisory documents to help in obtaining ethical approvals, and teaching materials to enhance debate-based lesson plans that can be used by the member and other institutions.
Concluding remarks
In summary, our literature survey and workshop findings highlight that researchers should accept that ethics underpins everything we do, especially in research. Although ethical approval is tedious, it is an imperative process in which proactive thinking is essential to identify ethical issues that might affect the project. Our findings further lead us to state that the ethical approval process differs from institution to institution and we strongly recommend the researchers to follow the institutional guidelines and their underlying ethical principles. The ENAI workshop in Vilnius highlighted the importance of ethical governance by establishing ECs, discussed different types of ECs and procedures with some examples and highlighted the importance of student education to impart ethical culture within research communities, an area that needs further study as future scope.
Declarations
The manuscript was entirely written by the corresponding author with contributions from co-authors who have also taken part in the delivery of the workshop. Authors confirm that the data supporting the findings of this study are available within the article. We can also confirm that there are no potential competing interests with other organisations.
Availability of data and materials
Authors confirm that the data supporting the findings of this study are available within the article.
Abbreviations
ALL European academics
Australian research council
Biotechnology and biological sciences research council
Canadian institutes for health research
Committee of publication ethics
Ethical committee
European network of academic integrity
Economic and social research council
International convention for the protection of animals
institutional ethical advisory committee
Institutional review board
Immaculata university of Pennsylvania
Lesbian, gay, bisexual, and transgender
Medical research council)
National health services
National health services nih national institute of health (NIH)
National institute of clinical care excellence
National health and medical research council
Natural sciences and engineering research council
National research ethics committee
National statement on ethical conduct in human research
Responsible research practice
Social sciences and humanities research council
Tri-council policy statement
World Organization for animal health
Universities Australia
UK-research and innovation
US office for human research protections
Alba S, Lenglet A, Verdonck K, Roth J, Patil R, Mendoza W, Juvekar S, Rumisha SF (2020) Bridging research integrity and global health epidemiology (BRIDGE) guidelines: explanation and elaboration. BMJ Glob Health 5(10):e003237. https://doi.org/10.1136/bmjgh-2020-003237
Article Google Scholar
Anderson MS (2011) Research misconduct and misbehaviour. In: Bertram Gallant T (ed) Creating the ethical academy: a systems approach to understanding misconduct and empowering change in higher education. Routledge, pp 83–96
BBC News. (2019). Birmingham school LGBT LESSONS PROTEST investigated. March 8, 2019. Retrieved February 14, 2021, available online. URL: https://www.bbc.com/news/uk-england-birmingham-47498446
Children’s Rights Alliance for England. (2005). R (Williamson and others) v Secretary of State for Education and Employment. Session 2004–05. [2005] UKHL 15. Available Online. URL: http://www.crae.org.uk/media/33624/R-Williamson-and-others-v-Secretary-of-State-for-Education-and-Employment.pdf
Council of Europe. (2014). Texts of the Council of Europe on bioethical matters. Available Online. https://www.coe.int/t/dg3/healthbioethic/Texts_and_documents/INF_2014_5_vol_II_textes_%20CoE_%20bio%C3%A9thique_E%20(2).pdf
Dellaportas S, Kanapathippillai S, Khan, A and Leung, P. (2014). Ethics education in the Australian accounting curriculum: a longitudinal study examining barriers and enablers. 362–382. Available Online. URL: https://doi.org/10.1080/09639284.2014.930694 , 23, 4, 362, 382
DePasse JM, Palumbo MA, Eberson CP, Daniels AH (2016) Academic characteristics of orthopaedic surgery residency applicants from 2007 to 2014. JBJS 98(9):788–795. https://doi.org/10.2106/JBJS.15.00222
Desmond H, Dierickx K (2021) Research integrity codes of conduct in Europe: understanding the divergences. https://doi.org/10.1111/bioe.12851
Difn website a - National Statement on Ethical Conduct in Human Research (NSECHR). (2018). Available Online. URL: https://www.nhmrc.gov.au/about-us/publications/australian-code-responsible-conduct-research-2018
Difn website b - Enago academy Importance of Ethics Committees in Scholarly Research (2020, October 26). Available online. URL: https://www.enago.com/academy/importance-of-ethics-committees-in-scholarly-research/
Difn website c - Ethics vs Morals - Difference and Comparison. Retrieved July 14, 2020. Available online. URL: https://www.diffen.com/difference/Ethics_vs_Morals
Difn website d - University of Leicester. (2015). Staff ethics approval flowchart. May 1, 2015. Retrieved July 14, 2020. Available Online. URL: https://www2.le.ac.uk/institution/ethics/images/ethics-approval-flowchart/view
European Commission - Facilitating Research Excellence in FP7 (2013) https://ec.europa.eu/research/participants/data/ref/fp7/89888/ethics-for-researchers_en.pdf
European Network for Academic Integrity. (2018). Ethical advisory group. Retrieved February 14, 2021. Available online. URL: http://www.academicintegrity.eu/wp/wg-ethical/
Federal Policy for the Protection of Human Subjects. (2018). Retrieved February 14, 2021. Available Online. URL: https://www.federalregister.gov/documents/2017/01/19/2017-01058/federal-policy-for-the-protection-of-human-subjects#p-855
Flite, CA and Harman, LB. (2013). Code of ethics: principles for ethical leadership Perspect Health Inf Mana; 10(winter): 1d. PMID: 23346028
Fouka G, Mantzorou M (2011) What are the major ethical issues in conducting research? Is there a conflict between the research ethics and the nature of nursing. Health Sci J 5(1) Available Online. URL: https://www.hsj.gr/medicine/what-are-the-major-ethical-issues-in-conducting-research-is-there-a-conflict-between-the-research-ethics-and-the-nature-of-nursing.php?aid=3485
Fox G (2017) History and ethical principles. The University of Miami and the Collaborative Institutional Training Initiative (CITI) Program URL https://silo.tips/download/chapter-1-history-and-ethical-principles # (Available Online)
Getz KA (1990) International codes of conduct: An analysis of ethical reasoning. J Bus Ethics 9(7):567–577
Ghooi RB (2011) The nuremberg code–a critique. Perspect Clin Res 2(2):72–76. https://doi.org/10.4103/2229-3485.80371
Hardicre, J. (2014) Valid informed consent in research: an introduction Br J Nurs 23(11). https://doi.org/10.12968/bjon.2014.23.11.564 , 567
Hazard, GC (Jr). (1994). Law, morals, and ethics. Yale law school legal scholarship repository. Faculty Scholarship Series. Yale University. Available Online. URL: https://digitalcommons.law.yale.edu/cgi/viewcontent.cgi?referer=https://www.google.com/&httpsredir=1&article=3322&context=fss_papers
Israel, M., & Drenth, P. (2016). Research integrity: perspectives from Australia and Netherlands. In T. Bretag (Ed.), Handbook of academic integrity (pp. 789–808). Springer, Singapore. https://doi.org/10.1007/978-981-287-098-8_64
Kadam R (2012) Proactive role for ethics committees. Indian J Med Ethics 9(3):216. https://doi.org/10.20529/IJME.2012.072
Kant I (2018) The metaphysics of morals. Cambridge University Press, UK https://doi.org/10.1017/9781316091388
Kayser-Jones J (2003) Continuing to conduct research in nursing homes despite controversial findings: reflections by a research scientist. Qual Health Res 13(1):114–128. https://doi.org/10.1177/1049732302239414
Kotecha JA, Manca D, Lambert-Lanning A, Keshavjee K, Drummond N, Godwin M, Greiver M, Putnam W, Lussier M-T, Birtwhistle R (2011) Ethics and privacy issues of a practice-based surveillance system: need for a national-level institutional research ethics board and consent standards. Can Fam physician 57(10):1165–1173. https://europepmc.org/article/pmc/pmc3192088
Kuyare, MS., Taur, SR., Thatte, U. (2014). Establishing institutional ethics committees: challenges and solutions–a review of the literature. Indian J Med Ethics. https://doi.org/10.20529/IJME.2014.047
LaFollette, H. (2007). Ethics in practice (3rd edition). Blackwell
Larry RC (1982) The teaching of ethics and moral values in teaching. J High Educ 53(3):296–306. https://doi.org/10.1080/00221546.1982.11780455
Manti S, Licari A (2018) How to obtain informed consent for research. Breathe (Sheff) 14(2):145–152. https://doi.org/10.1183/20734735.001918
Mulholland MW, Bell J (2005) Research Governance and Research Funding in the USA: What the academic surgeon needs to know. J R Soc Med 98(11):496–502. https://doi.org/10.1258/jrsm.98.11.496
National Institute of Health (NIH) Ethics in Clinical Research. n.d. Available Online. URL: https://clinicalcenter.nih.gov/recruit/ethics.html
NHS (2018) Flagged Research Ethics Committees. Retrieved February 14, 2021. Available online. URL: https://www.hra.nhs.uk/about-us/committees-and-services/res-and-recs/flagged-research-ethics-committees/
NICE (2018) Research governance policy. Retrieved February 14, 2021. Available online. URL: https://www.nice.org.uk/Media/Default/About/what-we-do/science-policy-and-research/research-governance-policy.pdf
Orr, J. (2018). Developing a campus academic integrity education seminar. J Acad Ethics 16(3), 195–209. https://doi.org/10.1007/s10805-018-9304-7
Quinn, M. (2011). Introduction to Ethics. Ethics for an Information Age. 4th Ed. Ch 2. 53–108. Pearson. UK
Resnik. (2020). What is ethics in Research & why is it Important? Available Online. URL: https://www.niehs.nih.gov/research/resources/bioethics/whatis/index.cfm
Schnyder S, Starring H, Fury M, Mora A, Leonardi C, Dasa V (2018) The formation of a medical student research committee and its impact on involvement in departmental research. Med Educ Online 23(1):1. https://doi.org/10.1080/10872981.2018.1424449
Seymour E, Hunter AB, Laursen SL, DeAntoni T (2004) Establishing the benefits of research experiences for undergraduates in the sciences: first findings from a three-year study. Sci Educ 88(4):493–534. https://doi.org/10.1002/sce.10131
Shamoo AE, Irving DN (1993) Accountability in research using persons with mental illness. Account Res 3(1):1–17. https://doi.org/10.1080/08989629308573826
Shaw, S., Boynton, PM., and Greenhalgh, T. (2005). Research governance: where did it come from, what does it mean? Research governance framework for health and social care, 2nd ed. London: Department of Health. https://doi.org/10.1258/jrsm.98.11.496 , 98, 11, 496, 502
Book Google Scholar
Speight, JG. (2016) Ethics in the university |DOI: https://doi.org/10.1002/9781119346449 scrivener publishing LLC
Stephenson GK, Jones GA, Fick E, Begin-Caouette O, Taiyeb A, Metcalfe A (2020) What’s the protocol? Canadian university research ethics boards and variations in implementing tri-Council policy. Can J Higher Educ 50(1)1): 68–81
Surbhi, S. (2015). Difference between morals and ethics [weblog]. March 25, 2015. Retrieved February 14, 2021. Available Online. URL: http://keydifferences.com/difference-between-morals-and-ethics.html
The Belmont Report (1979). Ethical Principles and Guidelines for the Protection of Human Subjects of Research. The National Commission for the Protection of Human Subjects of Biomedical and Behavioral Research. Retrieved February 14, 2021. Available online. URL: https://www.hhs.gov/ohrp/sites/default/files/the-belmont-report-508c_FINAL.pdf
The Singapore Statement on Research Integrity. (2020). Nicholas Steneck and Tony Mayer, Co-chairs, 2nd World Conference on Research Integrity; Melissa Anderson, Chair, Organizing Committee, 3rd World Conference on Research Integrity. Retrieved February 14, 2021. Available online. URL: https://wcrif.org/documents/327-singapore-statement-a4size/file
Warwick K (2003) Cyborg morals, cyborg values, cyborg ethics. Ethics Inf Technol 5(3):131–137. https://doi.org/10.1023/B:ETIN.0000006870.65865.cf
Weindling P (2001) The origins of informed consent: the international scientific commission on medical war crimes, and the Nuremberg code. Bull Hist Med 75(1):37–71. https://doi.org/10.1353/bhm.2001.0049
WHO. (2009). Research ethics committees Basic concepts for capacity-building. Retrieved February 14, 2021. Available online. URL: https://www.who.int/ethics/Ethics_basic_concepts_ENG.pdf
WHO. (2021). Chronological list of publications. Retrieved February 14, 2021. Available online. URL: https://www.who.int/ethics/publications/year/en/
Willison, J. and O’Regan, K. (2007). Commonly known, commonly not known, totally unknown: a framework for students becoming researchers. High Educ Res Dev 26(4). 393–409. https://doi.org/10.1080/07294360701658609
Žukauskas P, Vveinhardt J, and Andriukaitienė R. (2018). Research Ethics In book: Management Culture and Corporate Social Responsibility Eds Jolita Vveinhardt IntechOpenEditors DOI: https://doi.org/10.5772/intechopen.70629 , 2018
Download references
Acknowledgements
Authors wish to thank the organising committee of the 5th international conference named plagiarism across Europe and beyond, in Vilnius, Lithuania for accepting this paper to be presented in the conference.
Not applicable as this is an independent study, which is not funded by any internal or external bodies.
Author information
Authors and affiliations.
School of Human Sciences, University of Derby, DE22 1, Derby, GB, UK
Shivadas Sivasubramaniam
Department of Informatics, Mendel University in Brno, Zemědělská, 1665, Brno, Czechia
Dita Henek Dlabolová
Centre for Academic Integrity in the UAE, Faculty of Engineering & Information Sciences, University of Wollongong in Dubai, Dubai, UAE
Veronika Kralikova & Zeenath Reza Khan
You can also search for this author in PubMed Google Scholar
Contributions
The manuscript was entirely written by the corresponding author with contributions from co-authors who have equally contributed to presentation of this paper in the 5th international conference named plagiarism across Europe and beyond, in Vilnius, Lithuania. Authors have equally contributed for the information collection, which were then summarised as narrative explanations by the Corresponding author and Dr. Zeenath Reza Khan. Then checked and verified by Dr. Dlabolova and Ms. Králíková. The author(s) read and approved the final manuscript.
Corresponding author
Correspondence to Shivadas Sivasubramaniam .
Ethics declarations
Competing interests.
We can also confirm that there are no potential competing interest with other organisations.
Additional information
Publisher’s note.
Springer Nature remains neutral with regard to jurisdictional claims in published maps and institutional affiliations.
Rights and permissions
Open Access This article is licensed under a Creative Commons Attribution 4.0 International License, which permits use, sharing, adaptation, distribution and reproduction in any medium or format, as long as you give appropriate credit to the original author(s) and the source, provide a link to the Creative Commons licence, and indicate if changes were made. The images or other third party material in this article are included in the article's Creative Commons licence, unless indicated otherwise in a credit line to the material. If material is not included in the article's Creative Commons licence and your intended use is not permitted by statutory regulation or exceeds the permitted use, you will need to obtain permission directly from the copyright holder. To view a copy of this licence, visit http://creativecommons.org/licenses/by/4.0/ . The Creative Commons Public Domain Dedication waiver ( http://creativecommons.org/publicdomain/zero/1.0/ ) applies to the data made available in this article, unless otherwise stated in a credit line to the data.
Reprints and permissions
About this article
Cite this article.
Sivasubramaniam, S., Dlabolová, D.H., Kralikova, V. et al. Assisting you to advance with ethics in research: an introduction to ethical governance and application procedures. Int J Educ Integr 17 , 14 (2021). https://doi.org/10.1007/s40979-021-00078-6
Download citation
Received : 17 July 2020
Accepted : 25 April 2021
Published : 13 July 2021
DOI : https://doi.org/10.1007/s40979-021-00078-6
Share this article
Anyone you share the following link with will be able to read this content:
Sorry, a shareable link is not currently available for this article.
Provided by the Springer Nature SharedIt content-sharing initiative
- Higher education
- Ethical codes
- Ethics committee
- Post-secondary education
- Institutional policies
- Research ethics
International Journal for Educational Integrity
ISSN: 1833-2595
- Submission enquiries: Access here and click Contact Us
- General enquiries: [email protected]
Have a language expert improve your writing
Run a free plagiarism check in 10 minutes, automatically generate references for free.
- Knowledge Base
- Methodology
- Ethical Considerations in Research | Types & Examples
Ethical Considerations in Research | Types & Examples
Published on 7 May 2022 by Pritha Bhandari .
Ethical considerations in research are a set of principles that guide your research designs and practices. Scientists and researchers must always adhere to a certain code of conduct when collecting data from people.
The goals of human research often include understanding real-life phenomena, studying effective treatments, investigating behaviours, and improving lives in other ways. What you decide to research and how you conduct that research involve key ethical considerations.
These considerations work to:
- Protect the rights of research participants
- Enhance research validity
- Maintain scientific integrity
Table of contents
Why do research ethics matter, getting ethical approval for your study, types of ethical issues, voluntary participation, informed consent, confidentiality, potential for harm, results communication, examples of ethical failures, frequently asked questions about research ethics.
Research ethics matter for scientific integrity, human rights and dignity, and collaboration between science and society. These principles make sure that participation in studies is voluntary, informed, and safe for research subjects.
You’ll balance pursuing important research aims with using ethical research methods and procedures. It’s always necessary to prevent permanent or excessive harm to participants, whether inadvertent or not.
Defying research ethics will also lower the credibility of your research because it’s hard for others to trust your data if your methods are morally questionable.
Even if a research idea is valuable to society, it doesn’t justify violating the human rights or dignity of your study participants.
Prevent plagiarism, run a free check.
Before you start any study involving data collection with people, you’ll submit your research proposal to an institutional review board (IRB) .
An IRB is a committee that checks whether your research aims and research design are ethically acceptable and follow your institution’s code of conduct. They check that your research materials and procedures are up to code.
If successful, you’ll receive IRB approval, and you can begin collecting data according to the approved procedures. If you want to make any changes to your procedures or materials, you’ll need to submit a modification application to the IRB for approval.
If unsuccessful, you may be asked to re-submit with modifications or your research proposal may receive a rejection. To get IRB approval, it’s important to explicitly note how you’ll tackle each of the ethical issues that may arise in your study.
There are several ethical issues you should always pay attention to in your research design, and these issues can overlap with each other.
You’ll usually outline ways you’ll deal with each issue in your research proposal if you plan to collect data from participants.
Voluntary participation means that all research subjects are free to choose to participate without any pressure or coercion.
All participants are able to withdraw from, or leave, the study at any point without feeling an obligation to continue. Your participants don’t need to provide a reason for leaving the study.
It’s important to make it clear to participants that there are no negative consequences or repercussions to their refusal to participate. After all, they’re taking the time to help you in the research process, so you should respect their decisions without trying to change their minds.
Voluntary participation is an ethical principle protected by international law and many scientific codes of conduct.
Take special care to ensure there’s no pressure on participants when you’re working with vulnerable groups of people who may find it hard to stop the study even when they want to.
Informed consent refers to a situation in which all potential participants receive and understand all the information they need to decide whether they want to participate. This includes information about the study’s benefits, risks, funding, and institutional approval.
- What the study is about
- The risks and benefits of taking part
- How long the study will take
- Your supervisor’s contact information and the institution’s approval number
Usually, you’ll provide participants with a text for them to read and ask them if they have any questions. If they agree to participate, they can sign or initial the consent form. Note that this may not be sufficient for informed consent when you work with particularly vulnerable groups of people.
If you’re collecting data from people with low literacy, make sure to verbally explain the consent form to them before they agree to participate.
For participants with very limited English proficiency, you should always translate the study materials or work with an interpreter so they have all the information in their first language.
In research with children, you’ll often need informed permission for their participation from their parents or guardians. Although children cannot give informed consent, it’s best to also ask for their assent (agreement) to participate, depending on their age and maturity level.
Anonymity means that you don’t know who the participants are and you can’t link any individual participant to their data.
You can only guarantee anonymity by not collecting any personally identifying information – for example, names, phone numbers, email addresses, IP addresses, physical characteristics, photos, and videos.
In many cases, it may be impossible to truly anonymise data collection. For example, data collected in person or by phone cannot be considered fully anonymous because some personal identifiers (demographic information or phone numbers) are impossible to hide.
You’ll also need to collect some identifying information if you give your participants the option to withdraw their data at a later stage.
Data pseudonymisation is an alternative method where you replace identifying information about participants with pseudonymous, or fake, identifiers. The data can still be linked to participants, but it’s harder to do so because you separate personal information from the study data.
Confidentiality means that you know who the participants are, but you remove all identifying information from your report.
All participants have a right to privacy, so you should protect their personal data for as long as you store or use it. Even when you can’t collect data anonymously, you should secure confidentiality whenever you can.
Some research designs aren’t conducive to confidentiality, but it’s important to make all attempts and inform participants of the risks involved.
As a researcher, you have to consider all possible sources of harm to participants. Harm can come in many different forms.
- Psychological harm: Sensitive questions or tasks may trigger negative emotions such as shame or anxiety.
- Social harm: Participation can involve social risks, public embarrassment, or stigma.
- Physical harm: Pain or injury can result from the study procedures.
- Legal harm: Reporting sensitive data could lead to legal risks or a breach of privacy.
It’s best to consider every possible source of harm in your study, as well as concrete ways to mitigate them. Involve your supervisor to discuss steps for harm reduction.
Make sure to disclose all possible risks of harm to participants before the study to get informed consent. If there is a risk of harm, prepare to provide participants with resources, counselling, or medical services if needed.
Some of these questions may bring up negative emotions, so you inform participants about the sensitive nature of the survey and assure them that their responses will be confidential.
The way you communicate your research results can sometimes involve ethical issues. Good science communication is honest, reliable, and credible. It’s best to make your results as transparent as possible.
Take steps to actively avoid plagiarism and research misconduct wherever possible.
Plagiarism means submitting others’ works as your own. Although it can be unintentional, copying someone else’s work without proper credit amounts to stealing. It’s an ethical problem in research communication because you may benefit by harming other researchers.
Self-plagiarism is when you republish or re-submit parts of your own papers or reports without properly citing your original work.
This is problematic because you may benefit from presenting your ideas as new and original even though they’ve already been published elsewhere in the past. You may also be infringing on your previous publisher’s copyright, violating an ethical code, or wasting time and resources by doing so.
In extreme cases of self-plagiarism, entire datasets or papers are sometimes duplicated. These are major ethical violations because they can skew research findings if taken as original data.
You notice that two published studies have similar characteristics even though they are from different years. Their sample sizes, locations, treatments, and results are highly similar, and the studies share one author in common.
Research misconduct
Research misconduct means making up or falsifying data, manipulating data analyses, or misrepresenting results in research reports. It’s a form of academic fraud.
These actions are committed intentionally and can have serious consequences; research misconduct is not a simple mistake or a point of disagreement about data analyses.
Research misconduct is a serious ethical issue because it can undermine scientific integrity and institutional credibility. It leads to a waste of funding and resources that could have been used for alternative research.
Later investigations revealed that they fabricated and manipulated their data to show a nonexistent link between vaccines and autism. Wakefield also neglected to disclose important conflicts of interest, and his medical license was taken away.
This fraudulent work sparked vaccine hesitancy among parents and caregivers. The rate of MMR vaccinations in children fell sharply, and measles outbreaks became more common due to a lack of herd immunity.
Research scandals with ethical failures are littered throughout history, but some took place not that long ago.
Some scientists in positions of power have historically mistreated or even abused research participants to investigate research problems at any cost. These participants were prisoners, under their care, or otherwise trusted them to treat them with dignity.
To demonstrate the importance of research ethics, we’ll briefly review two research studies that violated human rights in modern history.
These experiments were inhumane and resulted in trauma, permanent disabilities, or death in many cases.
After some Nazi doctors were put on trial for their crimes, the Nuremberg Code of research ethics for human experimentation was developed in 1947 to establish a new standard for human experimentation in medical research.
In reality, the actual goal was to study the effects of the disease when left untreated, and the researchers never informed participants about their diagnoses or the research aims.
Although participants experienced severe health problems, including blindness and other complications, the researchers only pretended to provide medical care.
When treatment became possible in 1943, 11 years after the study began, none of the participants were offered it, despite their health conditions and high risk of death.
Ethical failures like these resulted in severe harm to participants, wasted resources, and lower trust in science and scientists. This is why all research institutions have strict ethical guidelines for performing research.
Ethical considerations in research are a set of principles that guide your research designs and practices. These principles include voluntary participation, informed consent, anonymity, confidentiality, potential for harm, and results communication.
Scientists and researchers must always adhere to a certain code of conduct when collecting data from others .
These considerations protect the rights of research participants, enhance research validity , and maintain scientific integrity.
Research ethics matter for scientific integrity, human rights and dignity, and collaboration between science and society. These principles make sure that participation in studies is voluntary, informed, and safe.
Anonymity means you don’t know who the participants are, while confidentiality means you know who they are but remove identifying information from your research report. Both are important ethical considerations .
You can only guarantee anonymity by not collecting any personally identifying information – for example, names, phone numbers, email addresses, IP addresses, physical characteristics, photos, or videos.
You can keep data confidential by using aggregate information in your research report, so that you only refer to groups of participants rather than individuals.
These actions are committed intentionally and can have serious consequences; research misconduct is not a simple mistake or a point of disagreement but a serious ethical failure.
Cite this Scribbr article
If you want to cite this source, you can copy and paste the citation or click the ‘Cite this Scribbr article’ button to automatically add the citation to our free Reference Generator.
Bhandari, P. (2022, May 07). Ethical Considerations in Research | Types & Examples. Scribbr. Retrieved 31 May 2024, from https://www.scribbr.co.uk/research-methods/ethical-considerations/
Is this article helpful?
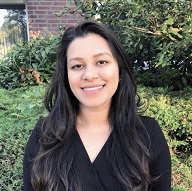
Pritha Bhandari
Other students also liked, a quick guide to experimental design | 5 steps & examples, data collection methods | step-by-step guide & examples, how to avoid plagiarism | tips on citing sources.
- Privacy Policy

Home » Ethical Considerations – Types, Examples and Writing Guide
Ethical Considerations – Types, Examples and Writing Guide
Table of Contents

Ethical Considerations
Ethical considerations in research refer to the principles and guidelines that researchers must follow to ensure that their studies are conducted in an ethical and responsible manner. These considerations are designed to protect the rights, safety, and well-being of research participants, as well as the integrity and credibility of the research itself
Some of the key ethical considerations in research include:
- Informed consent: Researchers must obtain informed consent from study participants, which means they must inform participants about the study’s purpose, procedures, risks, benefits, and their right to withdraw at any time.
- Privacy and confidentiality : Researchers must ensure that participants’ privacy and confidentiality are protected. This means that personal information should be kept confidential and not shared without the participant’s consent.
- Harm reduction : Researchers must ensure that the study does not harm the participants physically or psychologically. They must take steps to minimize the risks associated with the study.
- Fairness and equity : Researchers must ensure that the study does not discriminate against any particular group or individual. They should treat all participants equally and fairly.
- Use of deception: Researchers must use deception only if it is necessary to achieve the study’s objectives. They must inform participants of the deception as soon as possible.
- Use of vulnerable populations : Researchers must be especially cautious when working with vulnerable populations, such as children, pregnant women, prisoners, and individuals with cognitive or intellectual disabilities.
- Conflict of interest : Researchers must disclose any potential conflicts of interest that may affect the study’s integrity. This includes financial or personal relationships that could influence the study’s results.
- Data manipulation: Researchers must not manipulate data to support a particular hypothesis or agenda. They should report the results of the study objectively, even if the findings are not consistent with their expectations.
- Intellectual property: Researchers must respect intellectual property rights and give credit to previous studies and research.
- Cultural sensitivity : Researchers must be sensitive to the cultural norms and beliefs of the participants. They should avoid imposing their values and beliefs on the participants and should be respectful of their cultural practices.
Types of Ethical Considerations
Types of Ethical Considerations are as follows:
Research Ethics:
This includes ethical principles and guidelines that govern research involving human or animal subjects, ensuring that the research is conducted in an ethical and responsible manner.
Business Ethics :
This refers to ethical principles and standards that guide business practices and decision-making, such as transparency, honesty, fairness, and social responsibility.
Medical Ethics :
This refers to ethical principles and standards that govern the practice of medicine, including the duty to protect patient autonomy, informed consent, confidentiality, and non-maleficence.
Environmental Ethics :
This involves ethical principles and values that guide our interactions with the natural world, including the obligation to protect the environment, minimize harm, and promote sustainability.
Legal Ethics
This involves ethical principles and standards that guide the conduct of legal professionals, including issues such as confidentiality, conflicts of interest, and professional competence.
Social Ethics
This involves ethical principles and values that guide our interactions with other individuals and society as a whole, including issues such as justice, fairness, and human rights.
Information Ethics
This involves ethical principles and values that govern the use and dissemination of information, including issues such as privacy, accuracy, and intellectual property.
Cultural Ethics
This involves ethical principles and values that govern the relationship between different cultures and communities, including issues such as respect for diversity, cultural sensitivity, and inclusivity.
Technological Ethics
This refers to ethical principles and guidelines that govern the development, use, and impact of technology, including issues such as privacy, security, and social responsibility.
Journalism Ethics
This involves ethical principles and standards that guide the practice of journalism, including issues such as accuracy, fairness, and the public interest.
Educational Ethics
This refers to ethical principles and standards that guide the practice of education, including issues such as academic integrity, fairness, and respect for diversity.
Political Ethics
This involves ethical principles and values that guide political decision-making and behavior, including issues such as accountability, transparency, and the protection of civil liberties.
Professional Ethics
This refers to ethical principles and standards that guide the conduct of professionals in various fields, including issues such as honesty, integrity, and competence.
Personal Ethics
This involves ethical principles and values that guide individual behavior and decision-making, including issues such as personal responsibility, honesty, and respect for others.
Global Ethics
This involves ethical principles and values that guide our interactions with other nations and the global community, including issues such as human rights, environmental protection, and social justice.
Applications of Ethical Considerations
Ethical considerations are important in many areas of society, including medicine, business, law, and technology. Here are some specific applications of ethical considerations:
- Medical research : Ethical considerations are crucial in medical research, particularly when human subjects are involved. Researchers must ensure that their studies are conducted in a way that does not harm participants and that participants give informed consent before participating.
- Business practices: Ethical considerations are also important in business, where companies must make decisions that are socially responsible and avoid activities that are harmful to society. For example, companies must ensure that their products are safe for consumers and that they do not engage in exploitative labor practices.
- Environmental protection: Ethical considerations play a crucial role in environmental protection, as companies and governments must weigh the benefits of economic development against the potential harm to the environment. Decisions about land use, resource allocation, and pollution must be made in an ethical manner that takes into account the long-term consequences for the planet and future generations.
- Technology development : As technology continues to advance rapidly, ethical considerations become increasingly important in areas such as artificial intelligence, robotics, and genetic engineering. Developers must ensure that their creations do not harm humans or the environment and that they are developed in a way that is fair and equitable.
- Legal system : The legal system relies on ethical considerations to ensure that justice is served and that individuals are treated fairly. Lawyers and judges must abide by ethical standards to maintain the integrity of the legal system and to protect the rights of all individuals involved.
Examples of Ethical Considerations
Here are a few examples of ethical considerations in different contexts:
- In healthcare : A doctor must ensure that they provide the best possible care to their patients and avoid causing them harm. They must respect the autonomy of their patients, and obtain informed consent before administering any treatment or procedure. They must also ensure that they maintain patient confidentiality and avoid any conflicts of interest.
- In the workplace: An employer must ensure that they treat their employees fairly and with respect, provide them with a safe working environment, and pay them a fair wage. They must also avoid any discrimination based on race, gender, religion, or any other characteristic protected by law.
- In the media : Journalists must ensure that they report the news accurately and without bias. They must respect the privacy of individuals and avoid causing harm or distress. They must also be transparent about their sources and avoid any conflicts of interest.
- In research: Researchers must ensure that they conduct their studies ethically and with integrity. They must obtain informed consent from participants, protect their privacy, and avoid any harm or discomfort. They must also ensure that their findings are reported accurately and without bias.
- In personal relationships : People must ensure that they treat others with respect and kindness, and avoid causing harm or distress. They must respect the autonomy of others and avoid any actions that would be considered unethical, such as lying or cheating. They must also respect the confidentiality of others and maintain their privacy.
How to Write Ethical Considerations
When writing about research involving human subjects or animals, it is essential to include ethical considerations to ensure that the study is conducted in a manner that is morally responsible and in accordance with professional standards. Here are some steps to help you write ethical considerations:
- Describe the ethical principles: Start by explaining the ethical principles that will guide the research. These could include principles such as respect for persons, beneficence, and justice.
- Discuss informed consent : Informed consent is a critical ethical consideration when conducting research. Explain how you will obtain informed consent from participants, including how you will explain the purpose of the study, potential risks and benefits, and how you will protect their privacy.
- Address confidentiality : Describe how you will protect the confidentiality of the participants’ personal information and data, including any measures you will take to ensure that the data is kept secure and confidential.
- Consider potential risks and benefits : Describe any potential risks or harms to participants that could result from the study and how you will minimize those risks. Also, discuss the potential benefits of the study, both to the participants and to society.
- Discuss the use of animals : If the research involves the use of animals, address the ethical considerations related to animal welfare. Explain how you will minimize any potential harm to the animals and ensure that they are treated ethically.
- Mention the ethical approval : Finally, it’s essential to acknowledge that the research has received ethical approval from the relevant institutional review board or ethics committee. State the name of the committee, the date of approval, and any specific conditions or requirements that were imposed.
When to Write Ethical Considerations
Ethical considerations should be written whenever research involves human subjects or has the potential to impact human beings, animals, or the environment in some way. Ethical considerations are also important when research involves sensitive topics, such as mental health, sexuality, or religion.
In general, ethical considerations should be an integral part of any research project, regardless of the field or subject matter. This means that they should be considered at every stage of the research process, from the initial planning and design phase to data collection, analysis, and dissemination.
Ethical considerations should also be written in accordance with the guidelines and standards set by the relevant regulatory bodies and professional associations. These guidelines may vary depending on the discipline, so it is important to be familiar with the specific requirements of your field.
Purpose of Ethical Considerations
Ethical considerations are an essential aspect of many areas of life, including business, healthcare, research, and social interactions. The primary purposes of ethical considerations are:
- Protection of human rights: Ethical considerations help ensure that people’s rights are respected and protected. This includes respecting their autonomy, ensuring their privacy is respected, and ensuring that they are not subjected to harm or exploitation.
- Promoting fairness and justice: Ethical considerations help ensure that people are treated fairly and justly, without discrimination or bias. This includes ensuring that everyone has equal access to resources and opportunities, and that decisions are made based on merit rather than personal biases or prejudices.
- Promoting honesty and transparency : Ethical considerations help ensure that people are truthful and transparent in their actions and decisions. This includes being open and honest about conflicts of interest, disclosing potential risks, and communicating clearly with others.
- Maintaining public trust: Ethical considerations help maintain public trust in institutions and individuals. This is important for building and maintaining relationships with customers, patients, colleagues, and other stakeholders.
- Ensuring responsible conduct: Ethical considerations help ensure that people act responsibly and are accountable for their actions. This includes adhering to professional standards and codes of conduct, following laws and regulations, and avoiding behaviors that could harm others or damage the environment.
Advantages of Ethical Considerations
Here are some of the advantages of ethical considerations:
- Builds Trust : When individuals or organizations follow ethical considerations, it creates a sense of trust among stakeholders, including customers, clients, and employees. This trust can lead to stronger relationships and long-term loyalty.
- Reputation and Brand Image : Ethical considerations are often linked to a company’s brand image and reputation. By following ethical practices, a company can establish a positive image and reputation that can enhance its brand value.
- Avoids Legal Issues: Ethical considerations can help individuals and organizations avoid legal issues and penalties. By adhering to ethical principles, companies can reduce the risk of facing lawsuits, regulatory investigations, and fines.
- Increases Employee Retention and Motivation: Employees tend to be more satisfied and motivated when they work for an organization that values ethics. Companies that prioritize ethical considerations tend to have higher employee retention rates, leading to lower recruitment costs.
- Enhances Decision-making: Ethical considerations help individuals and organizations make better decisions. By considering the ethical implications of their actions, decision-makers can evaluate the potential consequences and choose the best course of action.
- Positive Impact on Society: Ethical considerations have a positive impact on society as a whole. By following ethical practices, companies can contribute to social and environmental causes, leading to a more sustainable and equitable society.
About the author
Muhammad Hassan
Researcher, Academic Writer, Web developer
You may also like

How to Cite Research Paper – All Formats and...

Data Collection – Methods Types and Examples

Delimitations in Research – Types, Examples and...

Research Paper Format – Types, Examples and...

Research Process – Steps, Examples and Tips

Research Design – Types, Methods and Examples
- PRO Courses Guides New Tech Help Pro Expert Videos About wikiHow Pro Upgrade Sign In
- EDIT Edit this Article
- EXPLORE Tech Help Pro About Us Random Article Quizzes Request a New Article Community Dashboard This Or That Game Popular Categories Arts and Entertainment Artwork Books Movies Computers and Electronics Computers Phone Skills Technology Hacks Health Men's Health Mental Health Women's Health Relationships Dating Love Relationship Issues Hobbies and Crafts Crafts Drawing Games Education & Communication Communication Skills Personal Development Studying Personal Care and Style Fashion Hair Care Personal Hygiene Youth Personal Care School Stuff Dating All Categories Arts and Entertainment Finance and Business Home and Garden Relationship Quizzes Cars & Other Vehicles Food and Entertaining Personal Care and Style Sports and Fitness Computers and Electronics Health Pets and Animals Travel Education & Communication Hobbies and Crafts Philosophy and Religion Work World Family Life Holidays and Traditions Relationships Youth
- Browse Articles
- Learn Something New
- Quizzes Hot
- This Or That Game
- Train Your Brain
- Explore More
- Support wikiHow
- About wikiHow
- Log in / Sign up
- Education and Communications
- College University and Postgraduate
- Academic Writing
How to Write an Ethics Paper
Last Updated: May 16, 2023 Approved
This article was co-authored by Emily Listmann, MA . Emily Listmann is a Private Tutor and Life Coach in Santa Cruz, California. In 2018, she founded Mindful & Well, a natural healing and wellness coaching service. She has worked as a Social Studies Teacher, Curriculum Coordinator, and an SAT Prep Teacher. She received her MA in Education from the Stanford Graduate School of Education in 2014. Emily also received her Wellness Coach Certificate from Cornell University and completed the Mindfulness Training by Mindful Schools. wikiHow marks an article as reader-approved once it receives enough positive feedback. In this case, 100% of readers who voted found the article helpful, earning it our reader-approved status. This article has been viewed 252,571 times.
Writing an ethics paper can present some unique challenges. For the most part, the paper will be written like any other essay or research paper, but there are some key differences. An ethics paper will generally require you to argue for a specific position rather than simply present an overview of an issue. Arguing this position will also involve presenting counterarguments and then refuting them. Finally, ensuring that your reasoning is valid and sound and citing the appropriate sources will allow you to write an ethics paper that will satisfy any critic.
Getting Started

- What is the main objective of the assignment?
- What specific things do you need to do in order to get a good grade?
- How much time will you need to complete the assignment?

- For example, you might begin with a topic of "ethical problems of euthanasia." This is very broad, and so forms a good starting point.

- Remember, you may refine your topic even further after you have begun writing your paper. This is perfectly acceptable, and is part of the advantage of writing a paper in multiple drafts.

- For example, you might include issues such as: "describing specifically what is meant by 'extreme, constant pain.' "Other issues might include, "the rights and responsibilities of physicians regarding euthanasia," and "voluntary versus involuntary euthanasia."
- After making this list, group or order them in some way. For example, you might imagine yourself taking the position that euthanasia is acceptable in this circumstance, and you could order the issues based on how you would draw supporting evidence and build your claim.
Developing Your Thesis Statement

- In your thesis, you should take a specific stand on the ethical issue. For example, you might write your thesis as follows: "Euthanasia is an immoral option even when patients are in constant, extreme pain."

- For example, this thesis statement is ambiguous: "Patients should not undergo euthanasia even when suffering constant, extreme pain." With how it's worded, it's unclear whether you mean that euthanasia should be outlawed or that it is morally wrong.
- Clarify your position to create a strong thesis: "Euthanasia is an immoral option even when patients are in constant, extreme pain."

- For example, in the thesis, "It is immoral for patients to choose euthanasia even when suffering constant, extreme pain," the moral burden is on the patient's actions. The author of this thesis would need to make sure to focus on the patient in the essay and not to focus on the moral implications of the doctor's actions.
- If the thesis you have written does not reflect what you want to argue in your paper, start over and draft a new thesis statement.
Conducting Research

- Ask a librarian for help finding sources if you are not sure how to access your library’s databases.
- A simple way to strengthen your argument through citations is by incorporating some relevant statistics. Simple statistics can have a major impact if presented after you've made a bold assertion. For instance, you may claim that the patient's family members would be unduly traumatized if the patient chose euthanasia, and then cite a university study that catalogued a majority of families reporting trauma or stress in this situation.
- Another helpful citation is one in which the broad issue itself is discussed. For instance, you might cite a prominent ethicist's position on your issue to strengthen your position.

- The author and his or her credentials. Does the source provide the author’s first and last name and credentials (M.D., Ph.D, etc.)? Steer clear of sources without an author attached to them or that lack credentials when credentials seem crucial, such as in an article about a medical subject. [3] X Research source
- Type of publication. Is the publication a book, journal, magazine, or website? Is the publisher an academic or educational institution? Does the publisher have a motive other than education? Who is the intended audience? Ask yourself these questions to determine if this source is reliable. For example, a university or government website might be reliable, but a site that sells items may be biased toward what they're selling.
- Citations. How well has the author researched his or her topic? Check the author’s bibliography or works cited page. If the author has not provided any sources, then you may want to look for a different source. [4] X Research source
- Bias. Has the author presented an objective, well-reasoned account of the topic? If the sources seems skewed towards one side of the argument, then it may not be a good choice. [5] X Research source
- Publication date. Does this source present the most up to date information on the subject? If the sources is outdated, then try to find something more recent. [6] X Research source

- To check for comprehension after reading a source, try to summarize the source in your own words and generate a response to the author’s main argument. If you cannot do one or both of these things, then you may need to read the source again.
- Creating notecards for your sources may also help you to organize your ideas. Write the citation for the source on the top of the notecard, then write a brief summary and response to the article in the lined area of the notecard. [7] X Research source

- Remember to indicate when you have quoted a source in your notes by putting it into quotation marks and including information about the source such as the author’s name, article or book title, and page number. [8] X Research source
Writing and Revising Your Ethics Paper

- To expand on your outline, write a couple of sentences describing and/or explaining each of the items in your outline. Include a relevant source for each item as well.

- Check your outline to see if you have covered each of these items in this order. If not, you will need to add a section and use your sources to help inform that section.

- In your first draft, focus on the quality of the argument, rather than the quality of the prose. If the argument is structured well and each conclusion is supported by your reasoning and by cited evidence, you will be able to focus on the writing itself on the second draft.
- Unless major revisions are needed to your argument (for example, if you have decided to change your thesis statement), use the second draft to strengthen your writing. Focus on sentence lengths and structures, vocabulary, and other aspects of the prose itself.

- Try to allow yourself a few days or even a week to revise your paper before it is due. If you do not allow yourself enough time to revise, then you will be more prone to making simple mistakes and your grade may suffer as a result. [10] X Research source

- Does my paper fulfill the requirements of the assignment? How might it score according to the rubric provided by my instructor?
- What is your main point? How might you clarify your main point?
- Who is your audience? Have you considered their needs and expectations?
- What is your purpose? Have you accomplished your purpose with this paper?
- How effective is your evidence? How might your strengthen your evidence?
- Does every part of your paper relate back to your thesis? How might you improve these connections?
- Is anything confusing about your language or organization? How might your clarify your language or organization?
- Have you made any errors with grammar, punctuation, or spelling? How can you correct these errors?
- What might someone who disagrees with you say about your paper? How can you address these opposing arguments in your paper? [11] X Research source

- As you read your paper out loud, highlight or circle any errors and revise as necessary before printing your final copy.
Community Q&A

- If at all possible, have someone else read through your paper before submitting it. They can provide valuable feedback on style as well as catching grammatical errors. Thanks Helpful 0 Not Helpful 1

Things You'll Need
- Word-processing software
- Access to your library’s databases
- Pencil and highlighter
You Might Also Like

- ↑ https://owl.english.purdue.edu/owl/resource/688/1/
- ↑ https://owl.english.purdue.edu/owl/resource/553/03/
- ↑ http://guides.jwcc.edu/content.php?pid=65900&sid=538553
- ↑ http://www.writing.utoronto.ca/advice/reading-and-researching/notes-from-research
- ↑ https://owl.english.purdue.edu/owl/resource/658/05/
- ↑ https://owl.english.purdue.edu/owl/resource/561/05/
About This Article

To write an ethics paper, start by researching the issue you want to write about and evaluating your sources for potential bias and trustworthiness. Next, develop a thesis statement that takes a specific stand on the issue and create an outline that includes the key arguments. As you write, avoid using words like “could” or “might,” which will seem ambiguous to the reader. Once you’ve finished your paper, take a break for a few days so your mind is clear, then go back and revise what you wrote, focusing on the quality of your argument. For tips from our Education reviewer on how to annotate source material as you research, read on! Did this summary help you? Yes No
- Send fan mail to authors
Reader Success Stories

Kristal Okeke
Apr 3, 2017
Did this article help you?

Tumelo Ratladi
Aug 9, 2016

Timela Crutcher
Dec 11, 2016

Aug 29, 2016

Jimm Hopper
Apr 18, 2018

Featured Articles

Trending Articles

Watch Articles

- Terms of Use
- Privacy Policy
- Do Not Sell or Share My Info
- Not Selling Info
Get all the best how-tos!
Sign up for wikiHow's weekly email newsletter
Writing Ethical Papers: Top Tips to Ace Your Assignment
17 August, 2021
13 minutes read
Author: Kate Smith
Writing a complex essay paper can be a tough task for any student, especially for those who do not have their skills developed well or do not have enough time for lengthy assignments. At the same time, the majority of college students need to keep their grades high to maintain their right to receive merit-based scholarships and continue their studies the next year. To help you with your ethical papers writing, we created this guide. Below, you will find out what an ethical paper is, how to structure it and write it efficiently.
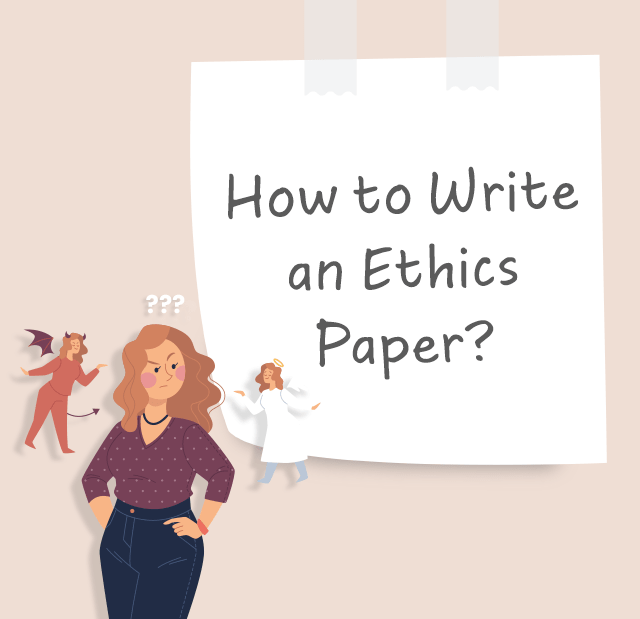
What is an Ethical Paper?
An ethics paper is a type of an argumentative assignment that deals with a certain ethical problem that a student has to describe and solve. Also, it can be an essay where a certain controversial event or concept is elaborated through an ethical lens (e.g. moral rules and principles), or a certain ethical dilemma is explained. Since ethics is connected to moral concepts and choices, a student needs to have a fair knowledge of philosophy and get ready to answer questions related to relationships, justice, professional and social duties, the origin of good and evil, etc., to write a quality paper. Also, writing an ethics paper implies that a student should process a great amount of information regarding their topic and analyze it according to paper terms.
General Aspects of Writing an Ethics Paper
Understanding the ethical papers’ features.
Every essay has differences and features that make it unique. Writing ethical papers implies that a student will use their knowledge of morality and philosophy to resolve a certain ethical dilemma or solve a situation. It can also be a paper in which a student needs to provide their reasoning on ethical or legal circumstances that follow a social issue. Finally, it can be an assignment in which an ethical concept and its application are described. On the contrary, a history essay deals with events that took place somewhen earlier, while a narrative essay is a paper where students demonstrate their storytelling skills, etc.
Defining What Type of Essay Should Be Written
Most of the time, ethical paper topics imply that a student will write an argumentative essay; however, ethics essays can also be descriptive and expository. Each of these essay types has different guidelines for writing, so be sure you know them before you start writing your papers on ethics. In case you missed this step in your ethical paper preparation stage, you would end up writing a paper that misses many important points.
Studying the Ethical Paper Guidelines
Once you get your ethical paper assignment, look through the guidelines that your instructor provided to you. If you receive them during the class, don’t hesitate to pose any questions immediately to remove any misunderstanding before writing an ethics paper outline, or ask for references that you need to use. When you are about to write your first draft, don’t rush: read the paper instructions once again to make sure you understand what is needed from you.
Paying Attention to the Paper Topic
The next thing you need to pay attention to is the ethical paper topic: once you are given one, make sure it falls into the scope of your educational course. After that, consider what additional knowledge may be needed to elaborate on your topic and think about what courses of your program could be helpful for it. Once you are done, read through your topic again to recheck whether you understand your assignment right.
Understanding the Notions of Ethical Arguments, Ethical and Legal Implications, and Ethical Dilemma
Last but not least, another important factor is that a student has to understand the basic terms of the assignment to write a high-quality paper. Ethical arguments are a set of moral rules that are used to defend your position on an ethical issue stated in your essay topic. We refer to ethical versus legal implications when we think about the compensation for certain ethical dilemma outcomes and whether it should be a moral punishment or legal judgment. An ethical dilemma itself refers to a problem or situation which makes an individual doubt what position to take: e.g, abortion, bribery, corruption, etc.
Writing Outline and Structure of an Ethics Paper
Every essay has a structure that makes it a solid piece of writing with straight reasoning and argumentation, and an ethics paper is not an exclusion. This paper has an introduction, body paragraphs, and conclusion. Below, we will describe how each part of ethical papers should be organized and what information they should contain.
First comes the introduction. It is the opening part of your paper which helps a reader to get familiar with your topic and understand what your paper will be about. Therefore, it should contain some information on your ethics paper topics and a thesis statement, which is a central statement of your paper.
The essay body is the most substantive part of your essay where all the reasoning and arguments should be presented. Each paragraph should contain an argument that supports or contradicts your thesis statement and pieces of evidence to support your position. Pick at least three arguments to make your position clear in your essay, and then your paper will be considered well-structured.
The third part of an ethics paper outline is a conclusion, which is a finishing essay part. Its goal is to wrap up the whole essay and make the author’s position clear for the last time. The thoughtful formulation in this essay part should be especially clear and concise to demonstrate the writer’s ability to make conclusions and persuade readers.
Also, don’t forget to include the works cited page after your writing. It should mention all the reference materials that you used in your paper in the order of appearance or in the alphabetical one. This page should be formatted according to the assigned formatting style. Most often, the most frequently used format for ethical papers is APA.
20 Examples of Ethical Paper Topics
- Are there any issues in the 21st century that we can consider immoral and why?
- What is corporate ethics?
- Why is being selfish no longer an issue in 2023?
- Euthanasia: pros and cons
- Marijuana legalization: should it be allowed all over the world?
- Is abortion an ethical issue nowadays?
- Can we invent a universal religion appropriate for all?
- Is the church necessary to pray to God?
- Can we forgive infidelity and should we do it?
- How to react if you are witnessing high school bullying?
- What are the ways to respond to a family abusing individual?
- How to demand your privacy protection in a digital world?
- The history of the American ethical thought
- Can war be ethical and what should the conflicting sides do to make it possible?
- Ethical issues of keeping a zoo in 2023
- Who is in charge of controlling the world’s population?
- How to achieve equality in the world’s rich and poor gap?
- Is science ethical?
- How ethical is genetic engineering?
- Why many countries refuse to go back to carrying out the death penalty?
Ethical Papers Examples
If you still have no idea about how to write an ethics paper, looking through other students’ successful examples is always a good idea. Below, you can find a relevant ethics paper example that you can skim through and see how to build your reasoning and argumentation in your own paper.
https://www.currentschoolnews.com/education-news/ethics-essay-examples/
https://sites.psu.edu/academy/2014/11/18/essay-2-personal-ethics-and-decision-making/
Ethical Papers Writing Tips
Choose a topic that falls into the ethics course program.
In case you were not given the ethics paper topic, consider choosing it yourself. To do that, brainstorm the ethical issues that fascinate you enough to do research. List all these issues on a paper sheet and then cross out those that are too broad or require expertise that you don’t have. The next step you need to take is to choose three or four ethical topics for papers from the list and try to do a quick search online to find out whether these topics are elaborated enough to find sources and reference materials on them. Last, choose one topic that you like the most and find the most relevant one in terms of available data for reference.
Do your research
Once the topic is chosen and organized, dive deeper into it to find the most credible, reliable, and trusted service. Use your university library, online scientific journals, documentaries, and other sources to get the information from. Remember to take notes while working with every new piece of reference material to not forget the ideas that you will base your argumentation on.
Follow the guidelines for a paper outline
During the preparation for your ethical paper and the process of writing it, remember to follow your professor’s instructions (e.g. font, size, spacing, citation style, etc.). If you neglect them, your grade for the paper will decrease significantly.
Write the essay body first
Do not rush to start writing your ethics papers from the very beginning; to write a good essay, you need to have your outline and thesis statement first. Then, go to writing body paragraphs to demonstrate your expertise on the issue you are writing about. Remember that one supporting idea should be covered in one paragraph and should be followed by the piece of evidence that confirms it.
Make sure your introduction and conclusion translate the same message
After your essay body is done, write a conclusion and an introduction for your paper. The main tip regarding these ethics paper parts is that you should make them interrelated: your conclusion has to restate your introduction but not repeat it. Also, a conclusion should wrap up your writing and make it credible for the audience.
Add citations
Every top-quality paper has the works cited page and citations to demonstrate that the research on the topic has been carried out. Therefore, do not omit this point when formatting your paper: add all the sources to the works cited page and pay attention to citing throughout the text. The latter should be done according to the formatting style indicated in your instructions.
Edit your paper
Last but not least is the editing and proofreading stage that you need to carry out before you submit your paper to your instructor. Consider keeping your first draft away from sight for a day or two to have a rest, and then go back to check it for errors and redundant phrases. Don’t rush to change anything immediately after finishing your writing since you are already tired and less focused, so some mistakes may be missed.
Writing Help by Handmadewriting
If you feel that you need help with writing an ethics paper in view of its chellnging nature, you can contact us and send an order through a respective button. You can add your paper details by following all steps of the order placing process that you will find on the website. Once your order is placed, we will get back to you as soon as possible. You will be able to contact your essay writer and let them know all your wishes regarding your ethical paper.
Our writers have expertise in writing ethical papers including, so you don’t need to worry about the quality of the essay that you will receive. Your assignment will be delivered on time and at a reasonable price. Note that urgent papers will cost slightly more than assignments with a postponed deadline, so do not wait too long to make your order. We will be glad to assist you with your writing and guarantee 24/7 support until you receive your paper.
Lastly, remember that no paper can be written overnight, so if you intend to complete your paper in a few hours, you can end up writing only a first draft with imperfections. If you have only half a day before your task is due, feel free to place an urgent order, and we will deliver it in just three hours.
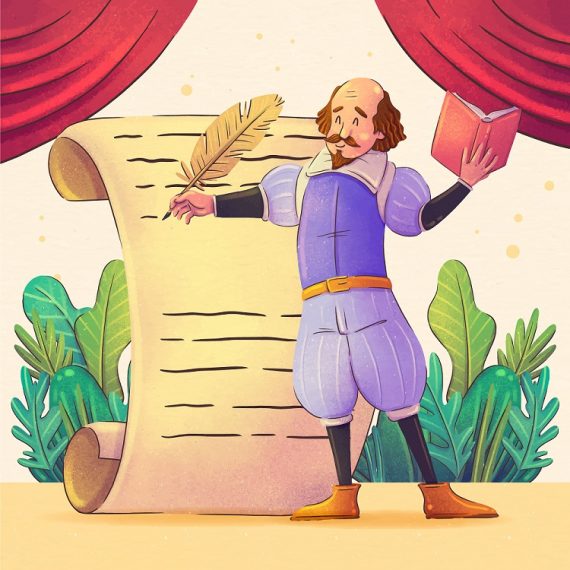
A life lesson in Romeo and Juliet taught by death
Due to human nature, we draw conclusions only when life gives us a lesson since the experience of others is not so effective and powerful. Therefore, when analyzing and sorting out common problems we face, we may trace a parallel with well-known book characters or real historical figures. Moreover, we often compare our situations with […]
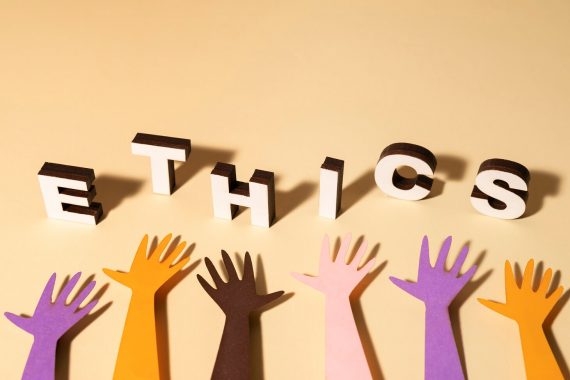
Ethical Research Paper Topics
Writing a research paper on ethics is not an easy task, especially if you do not possess excellent writing skills and do not like to contemplate controversial questions. But an ethics course is obligatory in all higher education institutions, and students have to look for a way out and be creative. When you find an […]
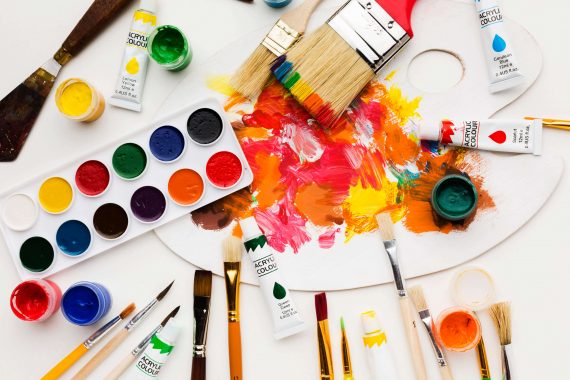
Art Research Paper Topics
Students obtaining degrees in fine art and art & design programs most commonly need to write a paper on art topics. However, this subject is becoming more popular in educational institutions for expanding students’ horizons. Thus, both groups of receivers of education: those who are into arts and those who only get acquainted with art […]
Home / Guides / Citation Guides / How to Cite Sources
How to Cite Sources
Here is a complete list for how to cite sources. Most of these guides present citation guidance and examples in MLA, APA, and Chicago.
If you’re looking for general information on MLA or APA citations , the EasyBib Writing Center was designed for you! It has articles on what’s needed in an MLA in-text citation , how to format an APA paper, what an MLA annotated bibliography is, making an MLA works cited page, and much more!
MLA Format Citation Examples
The Modern Language Association created the MLA Style, currently in its 9th edition, to provide researchers with guidelines for writing and documenting scholarly borrowings. Most often used in the humanities, MLA style (or MLA format ) has been adopted and used by numerous other disciplines, in multiple parts of the world.
MLA provides standard rules to follow so that most research papers are formatted in a similar manner. This makes it easier for readers to comprehend the information. The MLA in-text citation guidelines, MLA works cited standards, and MLA annotated bibliography instructions provide scholars with the information they need to properly cite sources in their research papers, articles, and assignments.
- Book Chapter
- Conference Paper
- Documentary
- Encyclopedia
- Google Images
- Kindle Book
- Memorial Inscription
- Museum Exhibit
- Painting or Artwork
- PowerPoint Presentation
- Sheet Music
- Thesis or Dissertation
- YouTube Video
APA Format Citation Examples
The American Psychological Association created the APA citation style in 1929 as a way to help psychologists, anthropologists, and even business managers establish one common way to cite sources and present content.
APA is used when citing sources for academic articles such as journals, and is intended to help readers better comprehend content, and to avoid language bias wherever possible. The APA style (or APA format ) is now in its 7th edition, and provides citation style guides for virtually any type of resource.
Chicago Style Citation Examples
The Chicago/Turabian style of citing sources is generally used when citing sources for humanities papers, and is best known for its requirement that writers place bibliographic citations at the bottom of a page (in Chicago-format footnotes ) or at the end of a paper (endnotes).
The Turabian and Chicago citation styles are almost identical, but the Turabian style is geared towards student published papers such as theses and dissertations, while the Chicago style provides guidelines for all types of publications. This is why you’ll commonly see Chicago style and Turabian style presented together. The Chicago Manual of Style is currently in its 17th edition, and Turabian’s A Manual for Writers of Research Papers, Theses, and Dissertations is in its 8th edition.
Citing Specific Sources or Events
- Declaration of Independence
- Gettysburg Address
- Martin Luther King Jr. Speech
- President Obama’s Farewell Address
- President Trump’s Inauguration Speech
- White House Press Briefing
Additional FAQs
- Citing Archived Contributors
- Citing a Blog
- Citing a Book Chapter
- Citing a Source in a Foreign Language
- Citing an Image
- Citing a Song
- Citing Special Contributors
- Citing a Translated Article
- Citing a Tweet
6 Interesting Citation Facts
The world of citations may seem cut and dry, but there’s more to them than just specific capitalization rules, MLA in-text citations , and other formatting specifications. Citations have been helping researches document their sources for hundreds of years, and are a great way to learn more about a particular subject area.
Ever wonder what sets all the different styles apart, or how they came to be in the first place? Read on for some interesting facts about citations!
1. There are Over 7,000 Different Citation Styles
You may be familiar with MLA and APA citation styles, but there are actually thousands of citation styles used for all different academic disciplines all across the world. Deciding which one to use can be difficult, so be sure to ask you instructor which one you should be using for your next paper.
2. Some Citation Styles are Named After People
While a majority of citation styles are named for the specific organizations that publish them (i.e. APA is published by the American Psychological Association, and MLA format is named for the Modern Language Association), some are actually named after individuals. The most well-known example of this is perhaps Turabian style, named for Kate L. Turabian, an American educator and writer. She developed this style as a condensed version of the Chicago Manual of Style in order to present a more concise set of rules to students.
3. There are Some Really Specific and Uniquely Named Citation Styles
How specific can citation styles get? The answer is very. For example, the “Flavour and Fragrance Journal” style is based on a bimonthly, peer-reviewed scientific journal published since 1985 by John Wiley & Sons. It publishes original research articles, reviews and special reports on all aspects of flavor and fragrance. Another example is “Nordic Pulp and Paper Research,” a style used by an international scientific magazine covering science and technology for the areas of wood or bio-mass constituents.
4. More citations were created on EasyBib.com in the first quarter of 2018 than there are people in California.
The US Census Bureau estimates that approximately 39.5 million people live in the state of California. Meanwhile, about 43 million citations were made on EasyBib from January to March of 2018. That’s a lot of citations.
5. “Citations” is a Word With a Long History
The word “citations” can be traced back literally thousands of years to the Latin word “citare” meaning “to summon, urge, call; put in sudden motion, call forward; rouse, excite.” The word then took on its more modern meaning and relevance to writing papers in the 1600s, where it became known as the “act of citing or quoting a passage from a book, etc.”
6. Citation Styles are Always Changing
The concept of citations always stays the same. It is a means of preventing plagiarism and demonstrating where you relied on outside sources. The specific style rules, however, can and do change regularly. For example, in 2018 alone, 46 new citation styles were introduced , and 106 updates were made to exiting styles. At EasyBib, we are always on the lookout for ways to improve our styles and opportunities to add new ones to our list.

Why Citations Matter
Here are the ways accurate citations can help your students achieve academic success, and how you can answer the dreaded question, “why should I cite my sources?”
They Give Credit to the Right People
Citing their sources makes sure that the reader can differentiate the student’s original thoughts from those of other researchers. Not only does this make sure that the sources they use receive proper credit for their work, it ensures that the student receives deserved recognition for their unique contributions to the topic. Whether the student is citing in MLA format , APA format , or any other style, citations serve as a natural way to place a student’s work in the broader context of the subject area, and serve as an easy way to gauge their commitment to the project.
They Provide Hard Evidence of Ideas
Having many citations from a wide variety of sources related to their idea means that the student is working on a well-researched and respected subject. Citing sources that back up their claim creates room for fact-checking and further research . And, if they can cite a few sources that have the converse opinion or idea, and then demonstrate to the reader why they believe that that viewpoint is wrong by again citing credible sources, the student is well on their way to winning over the reader and cementing their point of view.
They Promote Originality and Prevent Plagiarism
The point of research projects is not to regurgitate information that can already be found elsewhere. We have Google for that! What the student’s project should aim to do is promote an original idea or a spin on an existing idea, and use reliable sources to promote that idea. Copying or directly referencing a source without proper citation can lead to not only a poor grade, but accusations of academic dishonesty. By citing their sources regularly and accurately, students can easily avoid the trap of plagiarism , and promote further research on their topic.
They Create Better Researchers
By researching sources to back up and promote their ideas, students are becoming better researchers without even knowing it! Each time a new source is read or researched, the student is becoming more engaged with the project and is developing a deeper understanding of the subject area. Proper citations demonstrate a breadth of the student’s reading and dedication to the project itself. By creating citations, students are compelled to make connections between their sources and discern research patterns. Each time they complete this process, they are helping themselves become better researchers and writers overall.
When is the Right Time to Start Making Citations?
Make in-text/parenthetical citations as you need them.
As you are writing your paper, be sure to include references within the text that correspond with references in a works cited or bibliography. These are usually called in-text citations or parenthetical citations in MLA and APA formats. The most effective time to complete these is directly after you have made your reference to another source. For instance, after writing the line from Charles Dickens’ A Tale of Two Cities : “It was the best of times, it was the worst of times…,” you would include a citation like this (depending on your chosen citation style):
(Dickens 11).
This signals to the reader that you have referenced an outside source. What’s great about this system is that the in-text citations serve as a natural list for all of the citations you have made in your paper, which will make completing the works cited page a whole lot easier. After you are done writing, all that will be left for you to do is scan your paper for these references, and then build a works cited page that includes a citation for each one.
Need help creating an MLA works cited page ? Try the MLA format generator on EasyBib.com! We also have a guide on how to format an APA reference page .
2. Understand the General Formatting Rules of Your Citation Style Before You Start Writing
While reading up on paper formatting may not sound exciting, being aware of how your paper should look early on in the paper writing process is super important. Citation styles can dictate more than just the appearance of the citations themselves, but rather can impact the layout of your paper as a whole, with specific guidelines concerning margin width, title treatment, and even font size and spacing. Knowing how to organize your paper before you start writing will ensure that you do not receive a low grade for something as trivial as forgetting a hanging indent.
Don’t know where to start? Here’s a formatting guide on APA format .
3. Double-check All of Your Outside Sources for Relevance and Trustworthiness First
Collecting outside sources that support your research and specific topic is a critical step in writing an effective paper. But before you run to the library and grab the first 20 books you can lay your hands on, keep in mind that selecting a source to include in your paper should not be taken lightly. Before you proceed with using it to backup your ideas, run a quick Internet search for it and see if other scholars in your field have written about it as well. Check to see if there are book reviews about it or peer accolades. If you spot something that seems off to you, you may want to consider leaving it out of your work. Doing this before your start making citations can save you a ton of time in the long run.
Finished with your paper? It may be time to run it through a grammar and plagiarism checker , like the one offered by EasyBib Plus. If you’re just looking to brush up on the basics, our grammar guides are ready anytime you are.
How useful was this post?
Click on a star to rate it!
We are sorry that this post was not useful for you!
Let us improve this post!
Tell us how we can improve this post?
Citation Basics
Harvard Referencing
Plagiarism Basics
Plagiarism Checker
Upload a paper to check for plagiarism against billions of sources and get advanced writing suggestions for clarity and style.
Get Started
The ethics of using artificial intelligence in scientific research: new guidance needed for a new tool
- Original Research
- Open access
- Published: 27 May 2024
Cite this article
You have full access to this open access article
- David B. Resnik ORCID: orcid.org/0000-0002-5139-9555 1 &
- Mohammad Hosseini 2 , 3
404 Accesses
Explore all metrics
Using artificial intelligence (AI) in research offers many important benefits for science and society but also creates novel and complex ethical issues. While these ethical issues do not necessitate changing established ethical norms of science, they require the scientific community to develop new guidance for the appropriate use of AI. In this article, we briefly introduce AI and explain how it can be used in research, examine some of the ethical issues raised when using it, and offer nine recommendations for responsible use, including: (1) Researchers are responsible for identifying, describing, reducing, and controlling AI-related biases and random errors; (2) Researchers should disclose, describe, and explain their use of AI in research, including its limitations, in language that can be understood by non-experts; (3) Researchers should engage with impacted communities, populations, and other stakeholders concerning the use of AI in research to obtain their advice and assistance and address their interests and concerns, such as issues related to bias; (4) Researchers who use synthetic data should (a) indicate which parts of the data are synthetic; (b) clearly label the synthetic data; (c) describe how the data were generated; and (d) explain how and why the data were used; (5) AI systems should not be named as authors, inventors, or copyright holders but their contributions to research should be disclosed and described; (6) Education and mentoring in responsible conduct of research should include discussion of ethical use of AI.
Similar content being viewed by others
Biases and Ethical Considerations for Machine Learning Pipelines in the Computational Social Sciences
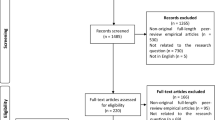
Publics’ views on ethical challenges of artificial intelligence: a scoping review
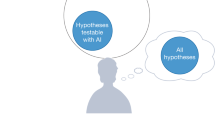
Artificial intelligence and illusions of understanding in scientific research
Avoid common mistakes on your manuscript.
1 Introduction: exponential growth in the use of artificial intelligence in scientific research
In just a few years, artificial intelligence (AI) has taken the world of scientific research by storm. AI tools have been used to perform or augment a variety of scientific tasks, including Footnote 1 :
Analyzing data and images [ 34 , 43 , 65 , 88 , 106 , 115 , 122 , 124 , 149 , 161 ].
Interpreting data and images [ 13 , 14 , 21 , 41 ].
Generating hypotheses [ 32 , 37 , 41 , 107 , 149 ].
Modelling complex phenomena [ 32 , 41 , 43 , 122 , 129 ].
Designing molecules and materials [ 15 , 37 , 43 , 205 ].
Generating data for use in validation of hypotheses and models [ 50 , 200 ].
Searching and reviewing the scientific literature [ 30 , 72 ].
Writing and editing scientific papers, grant proposals, consent forms, and institutional review board applications [ 3 , 53 , 54 , 82 , 163 ].
Reviewing scientific papers and other research outputs [ 53 , 54 , 98 , 178 , 212 ].
The applications of AI in scientific research appears to be limitless, and in the next decade AI is likely to completely transform the process of scientific discovery and innovation [ 6 , 7 , 8 , 9 , 105 , 201 ].
Although using AI in scientific research has steadily grown, ethical guidance has lagged far behind. With the exception of using AI to draft or edit scientific papers (see discussion in Sect. 7.6 ), most codes and policies do not explicitly address ethical issues related to using AI in scientific research. For example, the 2023 revision of the European Code of Conduct for Research Integrity [ 4 ] briefly discusses the importance of transparency. The code stipulates that researchers should report “their results and methods including the use of external services or AI and automated tools” (Ibid., p. 7) and considers “hiding the use of AI or automated tools in the creation of content or drafting of publications” as a violation of research integrity (Ibid. p. 10). One of the most thorough and up-to-date institutional documents, the National Institutes of Health Guidelines and Policies for the Conduct of Research provides guidance for using AI to write and edit manuscripts but not for other tasks [ 158 ]. Footnote 2 Codes of AI ethics, such as UNESCO’s [ 223 ] Ethics of Artificial Intelligence and the Office of Science and Technology Policy’s [ 168 , 169 ] Blueprint for an AI Bill of Rights, provide useful guidance for the development and use of AI in general without including specific guidance concerning the development and use of AI in scientific research [ 215 ].
There is therefore a gap in ethical and policy guidance concerning AI use in scientific research that needs to be filled to promote its appropriate use. Moreover, the need for guidance is urgent because using AI raises novel epistemological and ethical issues related to objectivity, reproducibility, transparency, accountability, responsibility, and trust in science [ 9 , 102 ]. In this paper, we will examine important questions related to AI’s impact on ethics of science. We will argue that while the use of AI does not require a radical change in the ethical norms of science, it will require the scientific community to develop new guidance for the appropriate use of AI. To defend this thesis, we will provide an overview of AI and an account of ethical norms of science, and then we will discuss the implications of AI for ethical norms of science and offer recommendations for its appropriate use.
2 What is AI?
AI can be defined as “a technical and scientific field devoted to the engineered system that generates outputs such as content, forecasts, recommendations or decisions for a given set of human-defined objectives [ 114 ].” AI is a subfield within the discipline of computer science [ 144 ]. However, the term ‘AI’ is also commonly used to refer to technologies (or tools) that can perform human tasks that require intelligence, such as perception, judgment, reasoning, or decision-making. We will use both senses of ‘AI’ in this paper, depending on the context.
While electronic calculators, cell phone apps, and programs that run on personal computers can perform functions associated with intelligence, they are not generally considered to be AI because they do not “learn” from the data [ 108 ]. As discussed below, AI systems can learn from the data insofar as they can adapt their programming in response to input data. While applying the term ‘learning’ to a machine may seem misleadingly anthropomorphic, it does make sense to say that a machine can learn if learning is regarded as a change in response to information about the environment [ 151 ]. Many different entities can learn in this sense of the term, including the immune system, which changes after being exposed to molecular information about pathogens, foreign objects, and other things that provoke an immune response.
This paper will focus on what is commonly referred to as narrow (or weak) AI, which is already being extensively used in science. Narrow AI has been designed and developed to do a specific task, such as playing chess, modelling complex phenomena, or identifying possible brain tumors in diagnostic images [ 151 ]. See Fig. 1 . Footnote 3 Other types of AI discussed in the literature include broad AI (also known as artificial general intelligence or AGI), which is a machine than can perform multiple tasks requiring human-like intelligence; and artificial consciousness (AC), which is a form of AGI with characteristics widely considered to be essential for consciousness [ 162 , 219 ]. Because there are significant technical and conceptual obstacles to developing AGI and AC, it may be years before machines have this degree of human-like intelligence [ 206 , 227 ]. Footnote 4
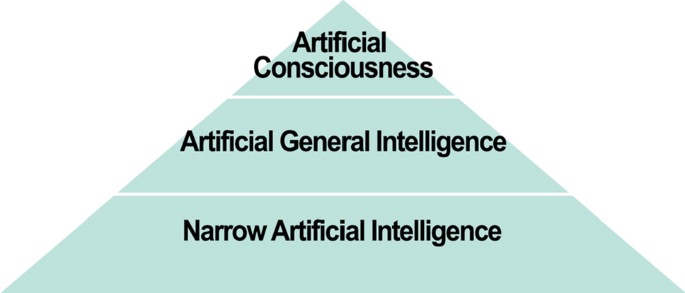
Levels of Artificial Intelligence, according to Turing [ 219 ]
3 What is machine learning?
Machine learning (ML) can be defined as a branch of AI “that focuses on the using data and algorithms to enable AI to imitate the way that humans learn, gradually improving its accuracy [ 112 ].” There are several types of ML, including support vector machines, decisions trees, and neural networks. In this paper we will focus on ML that uses artificial neural networks (ANNs).
An ANN is composed of artificial neurons, which are modelled after biological neurons. An artificial neuron receives a series of computational inputs, Footnote 5 applies a function, and produces an output. The inputs have different weightings. In most applications, a specific output is generated only when a certain threshold value for the inputs is reached. In the example below, an output of ‘1’ would be produced if the threshold is reached; otherwise, the output would be ‘0’. See Fig. 2 . A pair statements describing how a very simple artificial neuron processes inputs could be as follows:
where x1, x2, x3, and x4 are inputs; w1, w2, w3, and w4 are weightings, T is a threshold value; and U is an output value (1 or 0). An artificial neuron is represented schematically in Fig. 2 , below.
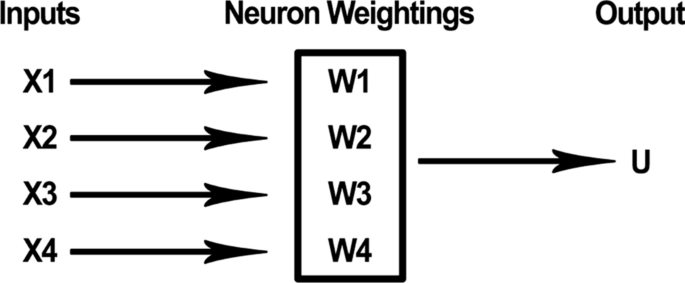
Artificial neuron
A single neuron may have dozens of inputs. An ANN may consist of thousands of interconnected neurons. In a deep learning ANN, there may be many hidden layers of neurons between the input and output layers. See Fig. 3 .
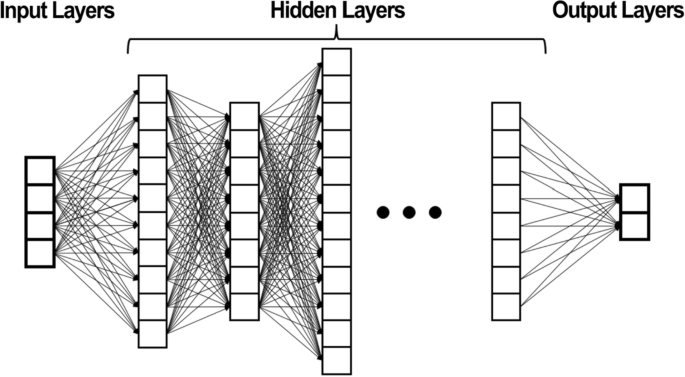
Deep learning artificial neural network [ 38 ]
Training (or reinforcement) occurs when the weightings on inputs are changed in response to system’s output. Changes in the weightings are based on their contribution to the neuron’s error, which can be understood as the difference between the output value and the correct value as determined by the human trainers (see discussion of error in Sect. 5 ). Training can occur via supervised or unsupervised learning. In supervised learning, the ANN works with labelled data and becomes adept at correctly representing structures in the data recognized by human trainers. In unsupervised learning, the ANN works with unlabeled data and discovers structures inherent in the data that might not have been recognized by humans [ 59 , 151 ]. For example, to use supervised learning to train an ANN to recognize dogs, human beings could present the system with various images and evaluate the accuracy of its output accordingly. If the ANN labels an image a “dog” that human beings recognize as a dog, then its output would be correct, otherwise, it would be incorrect (see discussion of error in Sects. 5.1 and 5.5). In unsupervised learning, the ANN would be presented with images and would be reinforced for accurately modelling structures inherent in the data, which may or may not correspond to patterns, properties, or relationships that humans would recognize or conceive of.
For an example of the disconnect between ML and human processing of information, consider research conducted by Roberts et al. [ 195 ]. In this study, researchers trained an ML system on radiologic images from hospital patients so that it would learn to identify patients with COVID-19 and predict the course of their illness. Since the patients who were sicker tended to laying down when their images were taken, the ML system identified laying down as a diagnostic criterion and disease predictor [ 195 ]. However, laying down is a confounding factor that has nothing to do with the likelihood of having COVID-19 or getting very sick from it [ 170 ]. The error occurred because the ML system did not account for this fundamental fact of clinical medicine.
Despite problems like the one discovered by Roberts et al. [ 195 ], the fact that ML systems process and analyze data differently from human beings can be a great benefit to science and society because these systems may be able to identify useful and innovative structures, properties, patterns, and relationships that human beings would not recognize. For example, ML systems have been able to design novel compounds and materials that human beings might not be able to conceive of [ 15 ]. That said, the disconnect between AI/ML and human information processing can also make it difficult to anticipate, understand, control, and reduce errors produced by ML systems. (See discussion of error in Sects. 5.1–5.5).
Training ANNs is a resource-intensive activity that involves gigabytes of data, thousands of computers, and hundreds of thousands of hours of human labor [ 182 , 229 ]. A system can continue to learn after the initial training period as it processes new data [ 151 ]. ML systems can be applied to any dataset that has been properly prepared for manipulation by computer algorithms, including digital images, audio and video recordings, natural language, medical records, chemical formulas, electromagnetic radiation, business transactions, stock prices, and games [ 151 ].
One of the most impressive feats accomplished by ML systems is their contribution to solving the protein folding problem [ 41 ]. See Fig. 4 . A protein is composed of one or more long chains of amino acids known as polypeptides. The three-dimensional (3-D) structure of the protein is produced by folding of the polypeptide(s), which is caused by the interplay of hydrogen bonds, Van der Waals attractive forces, and conformational entropy between different parts of the polypeptide [ 2 ]. Molecular biologists and biochemists have been trying to develop rules for predicting the 3-D structures of proteins from amino acid sequences since the 1960s, but this is, computationally speaking, a very hard problem, due to the immense number of possible ways that polypeptides can fold [ 52 , 76 ]. Tremendous progress on the protein-folding problem was made in 2022, when scientists demonstrated that an ML system, DeepMind’s AlphaFold, can predict 3-D structures from amino acid sequences with 92.4% accuracy [ 118 , 204 ]. AlphaFold, which built upon available knowledge of protein chemistry [ 176 ], was trained on thousands of amino acids sequences and their corresponding 3-D structures. Although human researchers still needed to test and refine AlphaFold’s output to ensure that the proposed structure is 100% accurate, the ML system greatly improves the efficiency of protein chemistry research [ 216 ]. Recently developed ML systems can generate new proteins by going in the opposite direction and predicting amino acids sequences from 3-D protein structures [ 156 ]. Since proteins play a key role in the structure and function of all living things, these advances in protein science are likely to have important applications in different areas of biology and medicine [ 204 ].
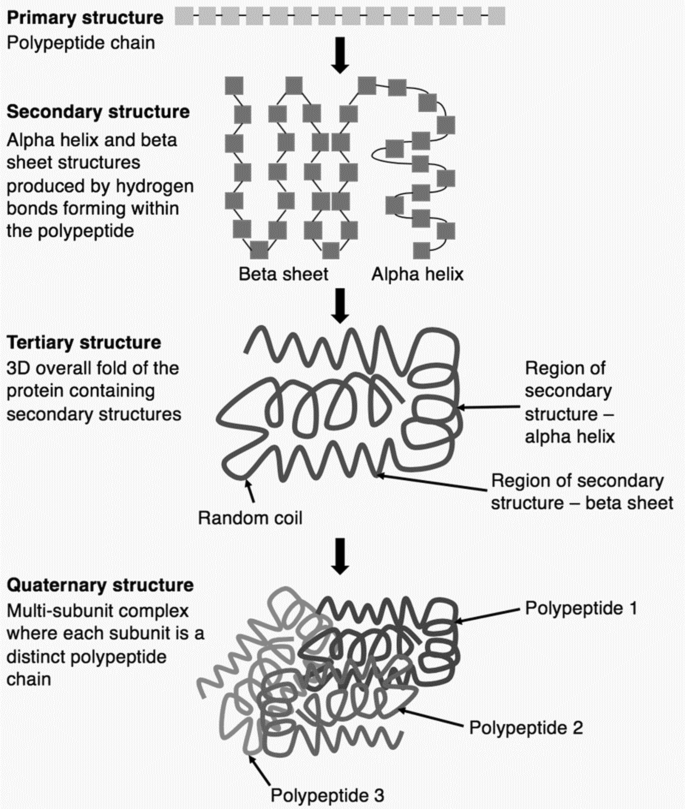
Protein folding. CC BY-SA 4.0 DEED [ 45 ]
4 What is generative AI?
Not only can ML image processing systems recognize patterns in the data that correspond to objects (e.g., cat, dog, car), when coupled with appropriate algorithms they can also generate images in response to visual or linguistic prompts [ 87 ]. The term ‘generative AI’ refers to “deep-learning models that can generate high-quality text, images, and other content based on the data they were trained on” [ 111 ].
Perhaps the most well-known types of generative AI are those that are based on large language models (LLMs), such as chatbots like OpenAI’s ChatGPT and Google’s Gemini, which analyze, paraphrase, edit, translate, and generate text, images and other types of content. LLMs are statistical algorithms trained on huge sets of natural language data, such as text from the internet, books, journal articles, and magazines. By processing this data, LLMs can learn to estimate probabilities associated with possible responses to text and can rank responses according to the probability that they will be judged to be correct by human beings [ 151 ]. In just a few years, some types of generative AI, such as ChatGPT, have become astonishingly proficient at responding to text data. ChatGPT has passed licensing exams for medicine and law and scored in the 93rd percentile on the Scholastic Aptitude Test reading exam and in the 89th percentile on the math exam [ 133 , 138 , 232 ]. Some researchers have used ChatGPT to write scientific papers and have even named them as authors [ 48 , 53 , 54 , 167 ]. Footnote 6 Some LLMs are so adept at mimicking the type of discourse associated with conscious thought that computer scientists, philosophers, and cognitive psychologists are updating the Turing test (see Fig. 5 ) to more reliably distinguish between humans and machines [ 5 , 22 ].
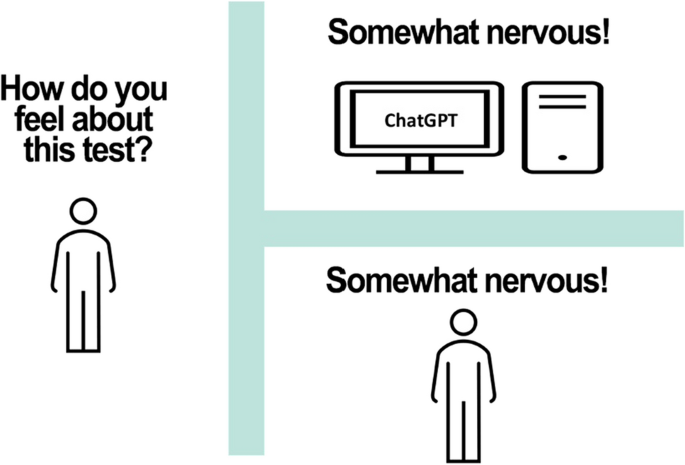
The Turing test. Computer scientist Alan Turing [ 220 ] proposed a famous test for determining whether a machine can think. The test involves a human interrogating another person, and a computer. The interrogator poses questions to the interviewees, who are in different rooms, so that interrogator cannot see where the answers are coming from. If the interrogator cannot distinguish between answers to questions given by another person and answers provided by a computer, then the computer passes the Turing test
5 Challenges of using AI
It has been long known that any AI systems are not error-free. To understand this topic, it is important to define ‘error’ and distinguish between systemic errors and random errors. The word ‘error’ has various meanings: we speak of grammatical errors, reasoning errors, typographical errors, measurement errors, etc. What these different senses of ‘error’ have in common is (1) errors involve divergence from a standard of correctness; and (2) errors, when committed by conscious beings, are unintentional; that is, they are accidents or mistakes and different from frauds, deceptions, or jokes.
If we set aside questions related to intent on the grounds that AI systems are not moral agents (see discussion in Sect. 7.6), we can think of AI error as the difference between the output of an AI system and the correct output . The difference between an AI output and the correct output can be measured quantitatively or qualitatively, depending on what is being measured and the purpose of the measurement [ 151 ]. For example, if a ML image recognition tool is presented with 50 images of wolves and 50 images of dogs, and it labels 98 of them correctly, we could measure its error quantitatively (i.e., 2%). In other cases, we might measure (or describe) error qualitatively. For example, if we ask ChatGPT to write a 12-line poem about a microwave oven in the style Edgar Allan Poe, we could rate its performance as ‘excellent,’ ‘very good,’ ‘good,’ ‘fair,’ or ‘poor.’ We could also assign numbers to these ratings to convert qualitative measurements into quantitative assessments (e.g., 5 = excellent, 4 = very good).
The correct output of an AI system is ultimately defined by its users and others who may be affected. For example, radiologists define correctness for reading diagnostic images; biochemists define the standard for modeling proteins; and attorneys, judges, clients, and law professors define the standard for writing legal briefs. In some contexts, such as testing hypotheses or reading radiologic images, ‘correct’ may mean ‘true’; in other contexts, such as generating text or creating models, it may simply mean ‘acceptable’ or ‘desirable.’ Footnote 7 While AI systems can play a key role in providing information that is used to define correct outputs (for example, when a system is used to discover new chemical compounds or solve complex math problems), human beings are ultimately responsible for determining whether outputs are correct (see discussion of moral agency in Sect. 7.6 ).
5.2 Random versus systemic errors ( Bias )
We can use an analogy with target shooting to think about the difference between random and systemic errors [ 94 ]. If error is understood as the distance of a bullet hole from a target, then random error would be a set of holes distributed randomly around the target without a discernable pattern (Fig. 6 A), while systemic error (or bias) would be a set of holes with a discernable pattern, for example holes skewed in a particular direction (Fig. 6 B). The accuracy of a set of bullet holes would be a function of their distance from the target, while their precision would be a function of their distance from each other [ 27 , 172 , 184 ].
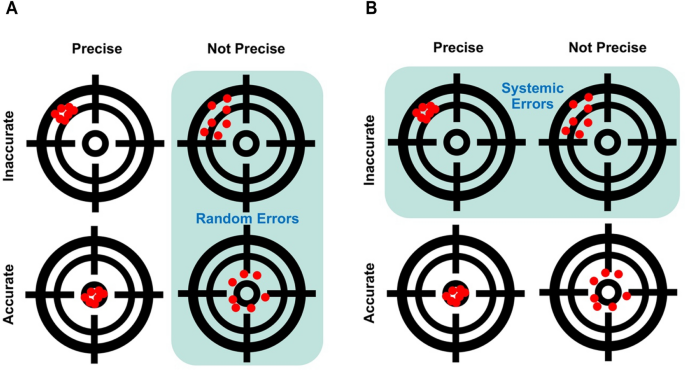
Random errors versus systemic errors
The difference between systemic and random errors can be ambiguous because errors that appear to be random may be shown to be systemic when one acquires more information about how they were generated or once a pattern is discerned. Footnote 8 Nevertheless, the distinction is useful. Systemic errors are often more detrimental to science and society than random ones, because they may negatively affect many different decisions involving people, projects, and paradigms. For example, racist biases distorted most research on human intelligence from the 1850s to the 1960s, including educational policies based on the applications of intelligence research. As will be discussed below, AI systems can make systemic and random errors [ 70 , 174 ].
5.3 AI biases
Since AI systems are designed to accurately represent the data on which they are trained, they can reproduce or even amplify racial, ethnic, gender, political, or other biases in the training data and subsequent data received [ 131 ]. The computer science maxim “garbage in, garbage out” applies here. Studies have shown that racial and ethnic biases impact the use of AI/ML in medical imaging, diagnosis, and prognosis due to biases in healthcare databases [ 78 , 154 ]. Bias is also a problem in using AI systems to find relationships between genomics and disease due to racial and ethnic prejudices in genomic databases [ 55 ]. LLMs are also impacted by various biases inherent in their training data, and when used in generative AI models like ChatGPT, can propagate biases related to race, ethnicity, nationality, gender, sexuality, age, and politics [ 25 , 171 ]. Footnote 9
Because scientific theories, hypotheses, and models are based on human perceptual categories, concepts, and assumptions, bias-free research is not possible [ 121 , 125 , 137 ]. Nevertheless, scientists can (and should) take steps to understand sources of bias and control them, especially those that can lead to discrimination, stigmatization, harm, or injustice [ 89 , 154 , 188 ]. Indeed, bias reduction and management is essential to promoting public trust in AI (discussed in Sects. 5.5 and 5.7 ).
Scientists have dealt with bias in research for years and have developed methods and strategies for minimizing and controlling bias in experimental design, data analysis, model building, and theory construction [ 79 , 89 , 104 ]. However, bias related to using AI in science can be subtle and difficult to detect due to the size and complexity of research data and interactions between data, algorithms, and applications [ 131 ]. See Fig. 7 . Scientists who use AI systems in research should take appropriate steps to anticipate, identify, control, and minimize biases by ensuring that datasets reflect the diversity of the investigated phenomena and disclosing the variables, algorithms, models, and parameters used in data analysis [ 56 ]. Managing bias related to the use of AI should involve continuous testing of the outputs in real world applications and adjusting systems accordingly [ 70 , 131 ]. For example, if a ML tool is used to read radiologic images, software developers, radiologists, and other stakeholders should continually evaluate the tool and its output to improve accuracy and precision.
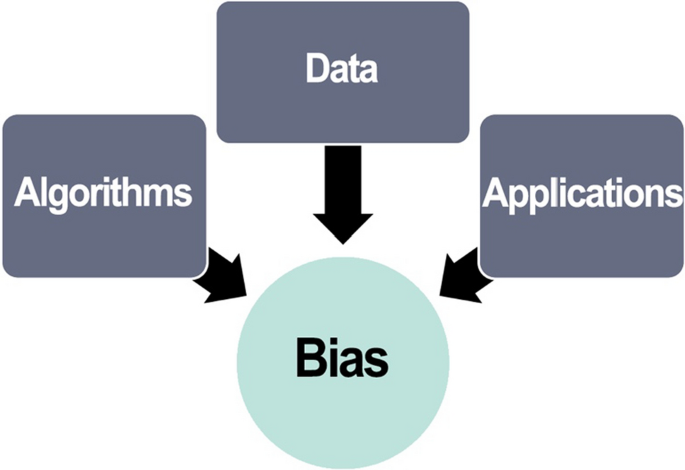
Sources of bias in AI/ML
5.4 Random errors in AI
AI systems can make random errors even after extensive training [ 51 , 151 ]. Nowhere has this problem been more apparent and concerning than in the use of LLMs in business, law, and scientific research. ChatGPT, for example, is prone to making random factual and citation errors. For example, Bhattacharyya et al. [ 24 ] used ChatGPT 3.5 to generate 30 short papers (200 words or less) on medical topics. 47% of the references produced by the chatbot were fabricated, 46% were authentic but inaccurately used, and only 7% were correct. Although ChatGPT 4.0 performs significantly better than ChatGPT 3.5, it still produces fabricated and inaccurate citations [ 230 ]. Another example of a random error was seen in a now-retracted paper published in Frontiers in Cell Development and Biology , which included an AI-generated image of a rat with unreal genitals [ 179 ]. Concerns raised by researchers led to OpenAI [ 173 ] warning users that “ChatGPT may produce inaccurate information about people, places, or facts.” The current interface includes the following disclaimer underneath the input box “ChatGPT can make mistakes. Consider checking important information.” Two US lawyers learned this lesson the hard way after a judge fined them $5000 for submitting court filing prepared by ChatGPT that included fake citations. The judge said that there was nothing improper about using ChatGPT but that the lawyers should exhibit due care in checking its work for accuracy [ 150 ].
An example of random errors made by generative AI discussed in the literature pertains to fake citations. Footnote 10 One reason why LLM-based systems, such as ChatGPT produce fake, but realistic-looking citations is that they process text data differently from human beings. Researchers produce citations by reading a specific text and citating it, but ChatGPT produces citations by processing a huge amount of text data and generating a highly probable response to a request for a citation. Software developers at OpenAI, Google, and other chatbot companies have been trying to fix this problem, but it is not easy to solve, due to differences between human and LLM processing of language [ 24 , 230 ]. AI companies advise users to use context-specific GPTs installed on top of ChatGPT. For instance, by using the Consensus.ai GPT ( https://consensus.app/ ), which claims to be connected to “200M + scientific papers”, users can ask for specific citations for a given input (e.g., “coffee is good for human health”). While the offered citations are likely to be correct bibliometrically, errors and biases may not be fully removed because it is unclear how these systems come to their conclusions and offer specific citations (see discussion of the black box problem in Sect. 5.7 ). Footnote 11
5.5 Prospects for reducing AI errors
If AI systems follow the path taken by most other technologies, it is likely that errors will decrease over time as improvements are made [ 151 ]. For example, early versions of ChatGPT were very bad at solving math problems but newer versions are much better math because they include special GPTs for performing this task [ 210 ]. AI systems also make errors in reading, classifying, and reconstructing radiological images, but the error rate is decreasing, and AI systems will soon outperform humans in terms of speed and accuracy of image reading [ 12 , 17 , 103 , 228 ]. However, it is also possible that AI systems will make different types of errors as they evolve or that there will be limits to their improvement. For example, newer versions of ChatGPT are prone to reasoning errors associated with intuitive thinking but older versions did not make these errors [ 91 ]. Also, studies have shown that LLMs are not good at self-correcting and need human supervision and fine-tuning to perform this task well [ 61 ].
Some types of errors may be difficult to eliminate due to differences between human perception/understanding and AI data processing. As discussed previously, AI systems, such as the system that generated the implausible hypothesis that laying down when having a radiologic image taken is a COVID-19 risk factor, make errors because they process information differently from humans. The AI system made this implausible inference because it did not factor basic biological and medical facts that would be obvious to doctors and scientists [ 170 ]. Another salient example of this phenomenon occurred when an image recognition AI was trained to distinguish between wolves and huskies, but it had difficulty recognizing huskies in the snow or wolves on the grass, because it had learned to distinguish between wolves and huskies by attending to the background of the images [ 222 ]. Humans are less prone to this kind of error because they use concepts to process perceptions and can therefore recognize objects in different settings. Consider, for example, captchas (Completely Automated Public Turing test to tell Computers and Humans Apart), which are used by many websites for security purposes and take advantage of some AI image processing deficiencies to authenticate whether a user is human [ 109 ]. Humans can pass Captchas tests because they learn to recognize images in various contexts and can apply what they know to novel situations [ 23 ].
Some of the factual and reasoning errors made by LLM-based systems occur because they lack human-like understanding of language [ 29 , 135 , 152 , 153 ]. ChatGPT, for example, can perform well when it comes to processing language that has already been curated by humans, such as describing the organelles in a cell or explaining known facts about photosynthesis, but they may perform sub-optimally (and sometimes very badly) when dealing with novel text that requires reasoning and problem-solving because it does do not have a human-like understanding of language. When a person processes language, they usually form a mental model that provides meaning and context for the words [ 29 ]. This mental model is based on implicit facts and assumptions about the natural world, human psychology, society, and culture, or what we might call commonsense [ 119 , 152 , 153 , 197 ]. LLMs do not do this,they only process symbols and predict the most likely string of symbols from linguistic prompts. Thus, to perform optimally, LLMs often need human supervision and input to provide the necessary meaning and context for language [ 61 ].
As discussed in Sect. 4 , because AI systems do not process information in the way that humans do, it can be difficult to anticipate, understand and detect the errors these tools make. For this reason, continual monitoring of AI performance in real-world applications, including feedback from end-users, developers, and other stakeholders, is essential to AI quality control and quality improvement and public trust in AI [ 131 , 174 ].
5.6 Lack of moral agency
As mentioned in Sect. 2 , narrow AI systems, such as LLMs, lack the capacities regarded as essential for moral agency, such as consciousness, self-concepts, personal memory, life experiences, goals, and emotions [ 18 , 139 , 151 ]. While this is not a problem for most technologies, it is for AI systems because they may be used to perform activities with significant moral and social consequences, such as reading radiological images or writing scientific papers (see discussion in Sect. 7.6 ), even though AI cannot be held morally or legally responsible or accountable. The lack of moral agency, when combined with other AI limitations, such as lack of a meaningful and human-like connection to the physical world, can produce dangerous results. For example, in 2021, Alexa, Amazon’s LLM-based voice-assistant, instructed a 10-year-old girl to stick a penny into an electric outlet when she asked it for a challenge to do [ 20 ]. In 2023, the widow of a Belgian man who committed suicide claimed that he had been depressed and was chatting with an LLM that encouraged him to kill himself [ 44 , 69 ]). OpenAI and other companies have tried to put guardrails in place to prevent their systems from giving dangerous advice, but this is not easy to fix. A recent study found that while ChatGPT can pass medical boards, it can give dangerous medical advice due to its tendency to make factual errors and its lack of understanding of the meaning and context of language [ 51 ].
5.7 The black box problem
Suppose ChatGPT produces erroneous output, and a computer scientist or engineer wants to know why. As a first step, they could examine the training data and algorithms to determine the source of the problem. Footnote 12 However, to fully understand what ChatGPT is doing they need to probe deeply into the system and examine not only the code but also the weightings attached to inputs in the ANN layers and the mathematical computations produced from the inputs. While an expert computer scientist or engineer could troubleshoot the code, they will not be able to interpret the thousands of numbers used in the weightings and the billions of calculations from those numbers [ 110 , 151 , 199 ]. This is what is meant when an AI system is described as a “black box.” See Fig. 8 . Trying to understand the meaning of the weightings and calculations in ML is very different from trying to understand other types of computer programs, such as those used in most cell phones or personal computers, in which an expert could examine the system (as a whole) to determine what it is doing and why [ 151 , 199 ]. Footnote 13
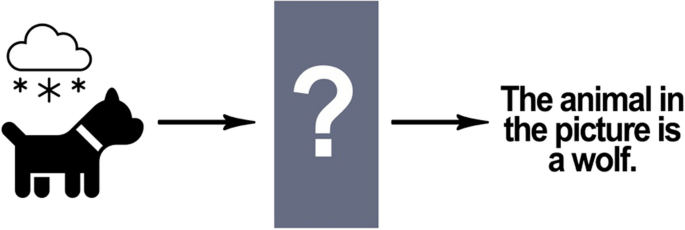
The black box: AI incorrectly labels a picture of a dog as a picture of a wolf but a complete investigation of this error is not possible due to a “black box” in the system
The opacity of AI systems is ethically problematic because one might argue that we should not use these devices if we cannot trust them, and we cannot trust them if even the best experts do not completely understand how they work [ 6 , 7 , 39 , 47 , 63 , 186 ]. Trust in a technology is partially based on understanding that technology. If we do not understand how a telescope works, then we should not trust in what we see with it. Footnote 14 Likewise, if computer experts do not completely understand how an AI/ML system works, then perhaps we should not use them for important tasks, such as making hiring decisions, diagnosing diseases, analyzing data, or generating scientific hypotheses or theories [ 63 , 74 ].
The black box problem raises important ethical issues for science (discussed further in Sect. 7.4 ), because it can undermine public trust in science, which is already in decline, due primarily to the politicization of topics with significant social implications, such as climate change, COVID-19 vaccines and public health measures [ 123 , 189 ].
One way of responding to the black box problem is to argue that we do not need to completely understand AI systems to trust them; what matters is an acceptably low rate of error [ 136 , 186 ]. Proponents of this view draw an analogy between using AI systems and using other artifacts, such as using aspirin for pain relief, without fully understanding how they work. All that really matters for trusting a machine or tool is that we have evidence that it works well for our purposes, not that we completely understand how it works. This line of argument implies that it is justifiable to use AI systems to read radiological images, model the 3-D structures of proteins, or write scientific papers provided that we have evidence that they perform these tasks as well as human beings [ 136 ].
This response to the black box problem does not solve the problem but simply tells us not to worry about it [ 63 ]. Footnote 15 There are several reasons to be concerned about the black box problem. First, if something goes wrong with a tool or technology, regulatory agencies, injured parties, insurers, politicians, and others want to know precisely how it works to prevent similar problems in the future and hold people and organizations legally accountable [ 141 ]. For example, when the National Transportation Safety Board [ 160 ] investigates an airplane crash, they want to know what precisely went wrong. Was the crash due to human error? Bad weather? A design flaw? A defective part? The NTSB will not be satisfied with an explanation that appeals to a mysterious technology within the airplane.
Second, when regulatory agencies, such as the Food and Drug Administration (FDA), make decisions concerning the approval of new products, they want to know how the products work, so they can make well-informed, publicly-defendable decisions and inform the consumers about risks. To obtain FDA approval for a new drug, a manufacturer must submit a vast amount of information to the agency, including information about the drug’s chemistry, pharmacology, and toxicology; the results of pre-clinical and clinical trials; processes for manufacturing the drug; and proposed labelling and advice to healthcare providers [ 75 ]. Indeed, dealing with the black box problem has been a key issue in FDA approval of medical devices that use AI/ML [ 74 , 183 ].
Third, end-users of technologies, such as consumers, professionals, researchers, government officials, and business leaders may not be satisfied with black boxes. Although most laypeople comfortably use technologies without fully understanding their innerworkings, they usually assume that experts who understand how these technologies work have assessed them and deemed them to be safe. End-users may become highly dissatisfied with a technology when it fails to perform its function, especially when not even the experts can explain why. Public dissatisfaction with responses to the black box problem may undermine the adoption of AI/ML technologies, especially when these technologies cause harm, invade privacy, or produce biased claims and results [ 60 , 85 , 134 , 175 ].
5.8 Explainable AI
An alternative to the non-solution approach is to make AI explainable [ 11 , 96 , 151 , 186 ]. The basic idea behind explainability is to develop “processes and methods that allows human users to comprehend and trust the results and output created by machine learning algorithms” [ 110 ]. Transparency of algorithms, models, parameters, and data is essential to making AI explainable, so that users can understand an AI system’s accuracy and precision and the types of errors it is prone to making. Explainable AI does not attempt to “peer inside” the black box, but it can make AI behavior more understandable to developers, users, and other stakeholders. Explainability, according to proponents of this approach, helps to promote trust in AI because it allows users and other stakeholders to make rational and informed decisions about it [ 77 , 83 , 110 , 186 ].
While the explainable AI approach is preferable to the non-solution approach, it still has some shortcomings. First, it is unclear whether making AI explainable will satisfy non-experts because considerable expertise in computer science and/or data analytics may be required to understand what is being explained [ 120 , 186 ]. For transparency to be effective, it must address the audience’s informational needs [ 68 ]. Explainable AI, at least in its current form, may not address the informational needs of laypeople, politicians, professionals, or scientists because the information is too technical [ 58 ]. To be explainable to non-experts, the information should be expressed in plain, jargon-free language that describes what the AI did and why [ 96 ].
Second, it is unclear whether explainable AI completely solves issues related to accountability and legal liability because we are yet to witness how legal systems will deal with AI lawsuits in which information pertaining to explainability (or lack thereof) is used as evidence in a court [ 141 ]. However, it is conceivable that the information conveyed to make AI explainable will satisfy the courts in some cases and set judicial precedent, so that legal doctrines and practices related to liability for AI-caused harms will emerge, much in the same way that doctrines and practices for medical technologies emerged.
Third, there is also the issue of whether explainable AI will satisfy the requirements of regulatory agencies, such as the FDA. However, regulatory agencies have been making some progress toward addressing the black box problem and explainability is likely to play a key role in these efforts [ 183 ].
Fourth, private companies uninterested in sharing information about their systems may not comply with explainable AI requirements or they may “game” the requirements to resemble compliance without actually complying. ChatGPT, for example, is a highly opaque system that is yet to disclose its training data and it is unclear whether/when OpenAI would open up its technology to external scrutiny [ 28 , 66 , 130 ].
Despite these shortcomings, the explainable AI approach is a reasonable way of dealing with transparency issues, and we encourage its continued development and application to AI/ML systems.
6 Ethical norms of science
With this overview of AI in mind, we can now consider how using AI in research impacts the ethical norms of science. But first, we need to describe these norms. Ethical norms of science are principles, values, or virtues that are essential for conducting good research [ 147 , 180 , 187 , 191 ]. See Table 1 . These norms apply to various practices, including research design; experimentation and testing; modelling; concept formation; data collection and storage; data analysis and interpretation; data sharing; publication; peer review; hypothesis/theory formulation and acceptance; communication with the public; as well as mentoring and education [ 207 ]. Many of these norms are expressed in codes of conduct, professional guidelines, institutional or journal policies, or books and papers on scientific methodology [ 4 , 10 , 113 , 235 ]. Others, like collegiality, might not be codified but are implicit in the practice of science. Some norms, such as testability, rigor, and reproducibility, are primarily epistemic, while others, such as fair attribution of credit, protection of research subjects, and social responsibility, are primarily moral (when enshrined in law, like instance of fraud, these norms become legal but here we only focus on ethical norms). There are also some like honesty, openness, and transparency, which have both epistemic and moral dimensions [ 191 , 192 ].
Scholars from different fields, including philosophy, sociology, history, logic, decision theory, and statistics have studied ethical norms of science [ 84 , 89 , 104 , 125 , 128 , 137 , 147 , 180 , 208 , 209 , 237 ]. Sociologists such as Merton [ 147 ] and Shapin [ 208 ], tend to view ethical norms as generalizations that accurately describe the practice of science, while philosophers, such as Kitcher [ 125 ] and Haack [ 89 ], conceive of these norms as prescriptive standards that scientists ought to follow. These approaches need not be mutually exclusive, and both can offer useful insights about ethical norms of science. Clearly, the study of norms must take the practice of science as its starting point, otherwise our understanding of norms would have no factual basis. However, one cannot simply infer the ethical norms of science from the practice of science because scientists may endorse and defend norms without always following them. For example, most scientists would agree that they should report data honestly, disclose significant conflicting interests, and keep good research records, but evidence indicates that they sometimes fail to do so [ 140 ].
One way of bridging the gap between descriptive and prescriptive accounts of ethical norms of science is to reflect on the social and epistemological foundations (or justifications) of these norms. Ethical norms of science can be justified in at least three ways [ 191 ].
First, these norms help the scientific community achieve its epistemic and practical goals, such as understanding, predicting, and controlling nature. It is nearly impossible to understand how a natural or social process works or make accurate predictions about it without standards pertaining to honesty, logical consistency, empirical support, and reproducibility of data and results. These and other epistemic standards distinguish science form superstition, pseudoscience, and sophistry [ 89 ].
Second, ethical norms promote trust among scientists, which is essential for collaboration, peer review, publication, sharing of data and resources, mentoring, education, and other scientific activities. Scientists need to be able to trust that the data and results reported in papers have not been fabricated, falsified, or manipulated; that reviewers for journals and funding agencies will maintain confidentiality; that colleagues or mentors will not steal their ideas and other forms of intellectual property; and that credit for collaborative work will be distributed fairly [ 26 , 233 ].
Third, ethical norms are important for fostering public support for science. The public is not likely to financially, legally, or socially support research that is perceived as corrupt, incompetent, untrustworthy, or unethical [ 191 ]. Taken together, these three modes of justification link ethical norms to science’s social foundations; that is, ethical norms are standards that govern the scientific community, which itself operates within and interacts with a larger community, namely society [ 137 , 187 , 209 ].
Although vital for conducting science, ethical norms are not rigid rules. Norms sometimes conflict, and when they do, scientists must make decisions concerning epistemic or moral priorities [ 191 ]. For example, model-building in science may involve tradeoffs among various epistemic norms, including generality, precision, realism, simplicity, and explanatory power [ 143 ]. Research with human subjects often involves tradeoffs between rigor and protection of participants. For example, placebo control groups are not used in clinical trials when receiving a placebo instead of an effective treatment would cause serious harm to the participant [ 207 ].
Although the norms can be understood as guidelines, some have higher priority than others. For example, honesty is the hallmark of good science, and there are very few situations in which scientists are justified in deviating from this norm. Footnote 16 Openness, on the other hand, can be deemphasized to protect research participants’ privacy, intellectual property, classified information, or unpublished research [ 207 ].
Finally, science’s ethical norms have changed over time, and they are likely to continue to evolve [ 80 , 128 , 147 , 237 ]. While norms such as empiricism, objectivity, and consistency originated in ancient Greek science, others, such as reproducibility and openness, developed during the 1500s; and many, such as protection of research subjects and social responsibility, did not emerge as formalized norms until the twentieth century. This evolution is in response to changes in science’s social, institutional, economic, and political environment and advancements in scientific instruments, tools, and methods [ 100 ]. For example, the funding of science by private companies and their requirements concerning data access and release policies have led to changes in norms related to open sharing of data and materials [ 188 ]. The increased presence of women and racial and ethnic minorities in science has led to the development of policies for preventing sexual and other forms of harassment [ 185 ]. The use of computer software to analyze large sets of complex data has challenged traditional views about norms related to hypothesis testing [ 193 , 194 ].
7 AI and the ethical norms of science
We will divide our discussion of AI and the ethics of science into six topics corresponding to the problems and issues previously identified in this paper and seventh topic related to scientific education. While these topics may seem somewhat disconnected, they all involve ethical issues that scientists who use AI in research are currently dealing with.
7.1 AI biases and the ethical norms of science
Bias can undermine the quality and trustworthiness of science and its social impacts [ 207 ]. While reducing and managing bias are widely recognized as essential to good scientific methodology and practice [ 79 , 89 ], they become crucial when AI is employed in research because AI can reproduce and amplify biases inherent in the data and generate results that lend support to policies that are discriminatory, unfair, harmful, or ineffective [ 16 , 202 ]. Moreover, by taking machines’ disinterestedness in findings as a necessary and sufficient condition of objectivity, users of AI in research may overestimate the objectivity of their findings. AI biases in medical research have generated considerable concern, since biases related to race, ethnicity, gender, sexuality, age, nationality, and socioeconomic status in health-related datasets can perpetuate health disparities by supporting biased hypotheses, models, theories, and policies [ 177 , 198 , 211 ]. Biases also negatively impact areas of science outside the health sphere, including ecology, forestry, urban planning, economics, wildlife management, geography, and agriculture [ 142 , 164 , 165 ].
OpenAI, Google, and other generative AI developers have been using filters that prevent their systems from generating text that is outright racist, sexist, homophobic, pornographic, offensive, or dangerous [ 93 ]. While bias reduction is a necessary step to make AI safe for human use, there are reasons to be skeptical of the idea that AI can be appropriately sanitized. First, the biases inherent in data are so pervasive that no amount of filtering can remove all of them [ 44 , 69 ]. Second, AI systems may also have political and social biases that are difficult to identify or control [ 19 ]. Even in the case of generative AI models where some filtering has happened, changing the inputted prompt may simply confuse and push a system to generate biased content anyway [ 98 ].
Third, by removing, reducing and controlling some biases, AI developers may create other biases, which are difficult to anticipate, identify or describe at this point. For example, LLMs have been trained using data gleaned from the Internet, scholarly articles and Wikipedia [ 90 ], all of which consist of the broad spectrum of human behavior and experience, from good to bad and virtuous to sinister. If we try to weed undesirable features of this data, we will eliminate parts of our language and culture, and ultimately, parts of us. Footnote 17 If we want to use LLMs to make sound moral and political judgments, sanitizing their data processing and output may hinder their ability to excel at this task, because the ability to make sound moral judgements or anticipate harm may depend, in part, on some familiarity with immoral choices and the darker side of humanity. It is only by understanding evil that we can freely and rationally choose the good [ 40 ]. We admit this last point is highly speculative, but it is worth considering. Clearly, the effects of LLM bias-management bear watching.
While the problem of AI bias does not require a radical revision of scientific norms, it does imply that scientists who use AI systems in research have special obligations to identify, describe, reduce, and control bias [ 132 ]. To fulfill these obligations, scientists must not only attend to matters of research design, data analysis and interpretation, but also address issues related to data diversity, sampling, and representativeness [ 70 ]. They must also realize that they are ultimately accountable for AI biases, both to other scientists and to members of the public. As such, they should only use AI in contexts where their expertise and judgement are sufficient to identify and remove biases [ 97 ]. This is important because given the accessibility of AI systems and the fact that they can exploit our cognitive shortcomings, they are creating an illusion of understanding [ 148 ].
Furthermore, to build public trust in AI and promote transparency and accountability, scientists who use AI should engage with impacted populations, communities and other stakeholders to address their needs and concerns and seek their assistance in identifying and reducing potential biases [ 132 , 181 , 202 ]. Footnote 18 During the engagement process, researchers should help populations and communities understand how their AI system works, why they are using it, and how it may produce bias. To address the problem of AI bias, the Biden Administration recently signed an executive order that directs federal agencies to identify and reduce bias and protect the public from algorithmic discrimination [ 217 ].
7.2 AI random errors and the ethical norms of science
Like bias, random errors can undermine the validity and reliability of scientific knowledge and have disastrous consequences for public health, safety, and social policy [ 207 ]. For example, random errors in the processing of radiologic images in a clinical trial of a new cancer drug could harm patients in the trial and future patients who take an approved drug, and errors related to the modeling of the transmission of an infectious disease could undermine efforts to control an epidemic. Although some random errors are unavoidable in science, an excessive amount when using AI could be considered carelessness or recklessness when using AI (see discussion of misconduct in Sect. 7.3 ).
Reduction of random errors, like reduction of bias, is widely recognized as essential to good scientific methodology and practice [ 207 ]. Although some random errors are unavoidable in research, scientists have obligations to identify, describe, reduce, and correct them because they are ultimately accountable for both human and AI errors. Scientists who use AI in their research should disclose and discuss potential limitations and (known) AI-related errors. Transparency about these is important for making research trustworthy and reproducible [ 16 ].
Strategies for reducing errors in science include time-honored quality assurance and quality improvement techniques, such as auditing data, instruments, and systems; validating and testing instruments that analyze or process data; and investigating and analyzing errors [ 1 ]. Replication of results by independent researchers, journal peer review, and post-publication peer review also play a major role in error reduction [ 207 ]. However, given that content generated by AI systems is not always reproducible [ 98 ], identifying and adopting measures to reduce errors is extremely complicated. Either way, accountability requires that scientists take responsibility for errors produced by AI/ML systems, that they can explain why errors have occurred, and that they transparently share their limitations of their knowledge related to these errors.
7.3 AI and research misconduct
Failure to appropriately control AI-related errors could make scientists liable for research misconduct, if they intentionally, knowingly, or recklessly disseminate false data or plagiarize [ 207 ]. Footnote 19 Although most misconduct regulations and policies distinguish between misconduct and honest error, scientists may still be liable for misconduct due to recklessness [ 42 , 193 , 194 ], which may have consequences for using AI. Footnote 20 For example, a person who uses ChatGPT to write a paper without carefully checking its output for errors or plagiarism could be liable for research misconduct for reckless use of AI. Potential liability for misconduct is yet another reason why using AI in research requires taking appropriate steps to minimize and control errors.
It is also possible that some scientists will use AI to fabricate data or images presented in scientific papers, grant proposals, or other documents. This unethical use of AI is becoming increasingly likely since generative models can be used to create synthetic datasets from scratch or make alternative versions of existing datasets [ 50 , 155 , 200 , 214 ]. Synthetic data are playing an increasingly important role in some areas of science. For example, researchers can use synthetic data to develop and validate models and enhance statistical analysis. Also, because synthetic data are similar to but not the same as real data, they can be used to eliminate or mask personal identifiers and protect the confidentiality of human participants [ 31 , 81 , 200 ].
Although we do not know of any cases where scientists have been charged with research misconduct for presenting synthetic data as real data, it is only a matter of time until this happens, given the pressures to produce results, publish, and obtain grants, and the temptations to cheat or cut corners. Footnote 21 This speculation is further corroborated by the fact that a small proportion of scientists deliberately fabricate or falsify data at some point in their careers [ 73 , 140 ]. Also, using synthetic data in research, even appropriately, may blur the line between real and fake data and undermine data integrity. Researchers who use synthetic data should (1) indicate which parts of data are synthetic, (2) describe how the data were generated; (3) explain how and why they were used [ 221 ].
7.4 The black box problem and the ethical norms of science
The black box problem presents significant challenges to the trustworthiness and transparency of research that use AI because some of the steps in the scientific process will not be fully open and understandable to humans, including AI experts. An important implication of the black box problem is that scientists who use AI are obligated to make their use of the technology explainable to their peers and the public. While precise details concerning what makes an AI system explainable may vary across disciplines and contexts, some baseline requirements for transparency may include:
The type, name, and version of AI system used.
What task(s) the system was used for.
How, when and by which contributor a system was used.
Why a certain system was used instead of alternatives (if available).
What aspects of a system are not explainable (e.g., weightings).
Technical details related to model’s architecture, training data and optimization procedures, influential features involved in model’s decisions, the reliability and accuracy of the system (if known).
Whether inferences drawn by the AI system are supported by currently accepted scientific theories, principles, or concepts.
This information should be expressed in plain language to allow non-experts to understand the whos, whats, hows, and whys related to the AI system. Ideally, this information would become a standard part of reported research that used AI. The information could be reported in the materials and methods section or in supplemental material, much that same way that information about statistical methods and software is currently reported.
As mentioned previously, making AI explainable does not completely solve the black box problem but it can play a key role in promoting transparency, accountability, and trust [ 7 , 9 ]. While there seems to be an emerging consensus on the utility and importance of making AI explainable, there is very little agreement about what explainability means in practice, because what makes AI explainable depends on the context of its use [ 58 ]. Clearly, this is a topic where more empirical research and ethical/policy analysis is needed.
7.5 AI and confidentiality
Using AI in research, especially generative AI models, raises ethical issues related to data privacy and confidentiality. ChatGPT, for example, stores the information submitted by users, including data submitted in initial prompts and subsequent interactions. Unless users opt out, this information could be used for training and other purposes. The data could potentially include personal and confidential information, such as information contained in drafts of scientific papers, grant proposals, experimental protocols, or institutional policies; computer code; legal strategies; business plans; and private information about human research participants [ 67 , 85 ]. Due to concerns about breaches of confidentiality, the National Institutes of Health (NIH) recently prohibited the use of generative AI technologies, such as LLMs, in grant peer review [ 159 ]. Footnote 22 Some US courts now require lawyers to disclose their use of generative AI in preparing legal documents and make assurances that they have taken appropriate steps to protect confidentiality [ 146 ].
While we are not suggesting that concerns about confidentiality justify prohibiting generative AI use in science, we think that considerable caution is warranted. Researchers who use generative AI to edit or review a document should assume that the material contained in it will not be kept confidential, and therefore, should not use these systems to edit or review anything containing confidential or personal information.
It is worth noting that technological solutions to the confidentiality problem may be developed in due course. For example, if an organization operates a local application of an LLM and places the technology behind a secure firewall, its members can use the technology safely. Electronic medical records, for example, have this type of security [ 127 ]. Some universities have already begun experimenting with operating their own AI systems for use by students, faculty, and administrators [ 225 ]. Also, as mentioned in Sect. 7.3 , the use of synthetic data may help to protect confidentiality.
7.6 AI and moral agency
The next issue we will discuss is whether AI can be considered a moral agent that participates in an epistemic community, that is, as a partner in knowledge generation. This became a major issue for the ethical norms of science in the winter of 2022–2023, when some researchers listed ChatGPT as authors on papers [ 102 ]. These publications initiated a vigorous debate in the research community, and journals scrambled to develop policies to deal with LLMs’ use in research. On one end of the spectrum, Jenkins and Lin [ 116 ] argued that AI systems can be authors if they make a substantial contribution to the research, and on the other end, Thorp [ 218 ] argued that AI systems cannot be named as authors and should not be used at all in preparing manuscripts. Currently, there seems to be an emerging consensus that falls in between these two extremes position, namely, that AI systems can be used in preparing manuscripts but that their use should be appropriately disclosed and discussed, [ 4 , 102 ]. In 2023, the International Committee of Medical Journal Editors (ICMJE), a highly influential organization with over 4,500 member journals, released the following statement about AI and authorship:
At submission, the journal should require authors to disclose whether they used artificial intelligence (AI)assisted technologies (such as Large Language Models [LLMs], chatbots, or image creators) in the production of submitted work. Authors who use such technology should describe, in both the cover letter and the submitted work, how they used it. Chatbots (such as ChatGPT) should not be listed as authors because they cannot be responsible for the accuracy, integrity, and originality of the work, and these responsibilities are required for authorship (see Section II.A.1). Therefore, humans are responsible for any submitted material that included the use of AI-assisted technologies. Authors should carefully review and edit the result because AI can generate authoritative-sounding output that can be incorrect, incomplete, or biased. Authors should not list AI and AI assisted technologies as an author or co-author, nor cite AI as an author. Authors should be able to assert that there is no plagiarism in their paper, including in text and images produced by the AI. Humans must ensure there is appropriate attribution of all quoted material, including full citations [ 113 ].
We agree with the ICMJE’s position, which mirrors views we defended in print before the ICMJE released its guidance [ 101 , 102 ].
Authorship on scientific papers is based not only on making a substantial contribution, but also on being accountable for the work [ 207 ]. Because authorship implies significant epistemic and ethical responsibilities, one should not be named as an author on a work if one cannot be accountable for one’s contribution to the work. If questions arise about the work after publication, one needs to be able to answer those questions intelligibly and if deemed liable, face possible legal, financial, or social consequences for one’s actions.
AI systems cannot be held accountable for their actions for two reasons: (1) they cannot provide intelligible explanations for what they did, (2) they cannot be held morally responsible for their actions, (3) they cannot suffer consequences nor can be sanctioned. The first reason has to do with the previously discussed black box problem. Although current proposals for making AI explainable may help to deal with this issue, they still fall far short of humanlike accountability, because these proposals do not require that the AI system, itself , should provide an explanation. Regarding the second reason, when we hold humans accountable, we expect them to explain their behavior in clear and intelligible language. Footnote 23 If a principal investigator wonders why a graduate student did not report all the data related to experiment, the investigator expects the student to explain why they did what they did. Current AI systems cannot do this. In some cases, someone else may be able to provide an explanation of how they work and what they do, but this not the same as the AI providing the explanation, which is a prerequisite for accountability. The third reason has to do with the link between accountabilities and sanctions. If an AI system makes a mistake which harms others, it cannot be sanctioned. These systems do not have interests, values, reputation and feelings in the same way that humans do and cannot be punished by law enforcement.
Even if an AI can intelligibly explain itself in the future, this does not imply that it can be morally responsible. While the concept of moral agency, like the concept of consciousness, is controversial, there is general agreement that moral agency requires the capacity to perform intentional (or purposeful) actions, understand moral norms, and make decisions based on moral norms. These capacities also presuppose additional capacities, such as consciousness, self-awareness, personal memory, perception, general intelligence, and emotions [ 46 , 95 , 213 ]. While computer scientists are making some progress on developing AI systems that have quasi-moral agency, that is, AI systems that can make decisions based on moral norms [ 71 , 196 , 203 ], they are still a long way from developing AGI or AC (see definitions of these terms in Sect. 2 ), which would seem to be required for genuine moral agency.
Moreover, other important implications follow from current AI’s lack of moral agency. First, AI systems cannot be named as inventors on patents, because inventorship also implies moral agency [ 62 ]. Patents are granted to individuals, i.e., persons, but since AI systems lack moral agency, they do not qualify as persons under the patent laws adopted by most countries. Second, AI systems cannot be copyright holders, because to own a copyright, one must be a person [ 49 ]. Copyrights, under US law, are granted only to people [ 224 ].
Although AI systems should not be named as authors or inventors, it is still important to appropriately recognize their contributions. Recognition should be granted not only to promote honesty and transparency in research but also to prevent human authors from receiving undue credit. For example, although many scientists and engineers deserve considerable accolades for solving the protein folding problem [ 118 , 176 ], failing to mention the role of AlphaFold in this discovery would be giving human contributors more credit than they deserve.
7.7 AI and research ethics education
The last topic we will address in this section has to do with education and mentoring in responsible conduct of research (RCR), which is widely recognized as essential to promoting ethical judgment, reasoning, and behavior in science [ 207 ]. In the US, the NIH and National Science Foundation (NSF) require RCR education for funded students and trainees, and many academic institutions require some form of RCR training for all research faculty [ 190 ]. Topics typically covered in RCR courses, seminars, workshops, or training sessions include data fabrication and falsification, plagiarism, investigation of misconduct, scientific record keeping, data management, rigor and reproducibility, authorship, peer review, publication, conflict of interest, mentoring, safe research environment, protection and human and animal subjects, and social responsibility [ 207 ]. As demonstrated in this paper, the use of AI in research has a direct bearing on most of these topics, but especially on authorship, rigor and reproducibility, peer review, and social responsibility. We recommend, therefore, that RCR education and training incorporate discussion of the use of AI in research, wherever relevant.
8 Conclusion
Using AI in research benefits science and society but also creates some novel and complex ethical issues that affect accountability, responsibility, transparency, trustworthiness, reproducibility, fairness, and objectivity, and other important values in research. Although scientists do not need to radically revise their ethical norms to deal with these issues, they do need new guidance for the appropriate use of AI in research. Table 2 provides a summary of our recommendations for this guidance. Since AI continues to advance rapidly, scientists, academic institutions, funding agencies and publishers, should continue to discuss AI’s impact on research and update their knowledge, ethical guidelines and policies accordingly. Guidance should be periodically revised as AI becomes woven into the fabric of scientific practice (or normalized) and researchers learn about it, adapt to it, and use it in novel ways. Since science has significant impacts on society, public engagement in such discussions is crucial for responsible the use, development, and AI in research [ 234 ].
In closing, we will observe that many scholars, including ourselves, assume that today’s AI systems lack the capacities necessary for moral agency. This assumption has played a key role in our analysis of ethical uses of AI in research and has informed our recommendations. We realize that a day may arrive, possibly sooner than many would like to believe, when AI will advance to the point that this assumption will need to be revised, and that society will need to come to terms with the moral rights and responsibilities of some types of AI systems. Perhaps AI systems will one day participate in science as full partners in discovery and innovation [ 33 , 126 ]. Although we do not view this as a matter that now demands immediate attention, we remain open to further discussion of this issue in the future.
There is not sufficient space in this paper to conduct a thorough review of all the ways that AI is being used in scientific research. For a review of the information, see Wang et al. [ 231 ] and Krenn et al. [ 126 ].
However, the National Institutes of Health has prohibited the use of AI to review grants (see Sect. 7.5 ).
This a simplified taxonomy of AI that we have found useful to frame the research ethics issues. For more detailed taxonomy, see Graziani et al. [ 86 ].
See Krenn et al. [ 126 ] for a thoughtful discussion of the possible role of AGI in scientific research.
We will use the term ‘input’ in a very general sense to refer to data which are routed into the system, such as numbers, text, or image pixels.
It is important to note that the [ 167 ] paper was corrected to remove ChatGPT as an author because the tool did not meet the journal’s authorship criteria. See O’Connor [ 166 ].
There are important, philosophical issues at stake here concerning whether AI users should regard an output as ‘acceptable’ or ‘true’, but these questions are beyond the scope of our paper.
The question of whether true randomness exists in nature is metaphysically controversial because some physicists and philosophers argue that nothing happens by pure chance [ 64 ]. We do not need to delve into this issue here, since most people agree that the distinction can be viewed as epistemic and not metaphysical, that is, an error is systemic or random relative to our knowledge about the generation of the error.
Some of the most well-known cases of bias involved the use of AI systems by private companies. For example, Amazon stopped using an AI hiring tool in 2018 after it discovered that the tool was biased against women [ 57 ]. In 2021, Facebook faced public ridicule and shame for using image recognition software the labelled images of African American men as non-human primates [ 117 ]. In 2021, Zillow lost hundreds of millions of dollars because its algorithm systematically overestimated the market value of homes the company purchased [ 170 ].
Fake citations and factual errors made by LLMs are often referred to as ‘hallucinations.’ We prefer not to use this term because it ascribes mental states to AI.
An additional, and perhaps more concerning, issue is that using chatbots to review the literature contributes to the deskilling of humanity because it involves trusting an AI’s interpretation and synthesis of the literature instead of reading it and thinking about it for oneself. Since deskilling is a problem with many different applications of AI, we will not explore it in depth here. See Vallor [ 226 ].
We are assuming here that the engineer or scientist has access to the computer code and training data, which private companies may to be loath to provide. For example, developers at OpenAI and Google have not provided the public with access to their training data and code [ 130 ].
Although our discussion of the black box problem focuses on ML, in theory this problem could arise in any type of AI in which its workings cannot be understood by human beings.
Galileo had to convince his critics that his telescope could be trusted to convey reliable information about heavenly bodies, such as the moon and Jupiter. Explaining how the telescope works and comparing it to the human eye played an important role in his defense of the instrument [ 36 ].
This response may also conflate trust with verification. According to some theories of trust, if you trust something, you do not need to continually verify it. If I trust someone to tell me the truth, I do not need to continually verify that they are telling the trust. Indeed, it seems that we verify because we do not trust. For further discussion, see McLeod [ 145 ].
One could argue that deviation from honesty might be justified to protect human research subjects in some situations. For example, pseudonyms are often used in qualitative social/behavioral research to refer to participants or communities in order to protect their privacy [ 92 ].
Sanitizing LLMs is a form of censorship, which may be necessary in some cases, but also carries significant risks for freedom of expression [ 236 ].
While public, community, and stakeholder engagement is widely accepted as important for promoting trust in science and technology but can be difficult to implement, especially since publics, communities, and stakeholders can be difficult to identify and may have conflicting interests [ 157 ].
US federal policy defines research misconduct as data fabrication or falsification or plagiarism [ 168 ].
While the difference between recklessness and negligence can be difficult to ascertain, one way of thinking of recklessness is that it involves an indifference to or disregard for the veracity or integrity of research. Although almost all misconduct findings claim that the accused person (or respondent) acted intentionally, knowingly, or recklessly, there have been a few cases in which the respondent was found only to have acted recklessly [ 42 , 193 , 194 ].
The distinction between synthetic and real data raises some interesting and important philosophical and policy issues that we will examine in more depth in future work.
Some editors and publishers have been using AI to review and screen journal submissions [ 35 , 212 ]. For a discussion of issues raised by using AI in peer review, see Hosseini and Horbach [ 98 , 99 ].
This issue reminds us of the scene in 2001: A Space Odyssey in which the human astronauts ask the ship’s computer, HAL, to explain why it incorrectly diagnosed a problem with the AE-35 unit. HAL responds that HAL 9000 computers have never made an error so the misdiagnosis must be due to human error.
Aboumatar, H., Thompson, C., Garcia-Morales, E., Gurses, A.P., Naqibuddin, M., Saunders, J., Kim, S.W., Wise, R.: Perspective on reducing errors in research. Contemp. Clin. Trials Commun. 23 , 100838 (2021)
Article Google Scholar
Alberts, B., Johnson, A., Lewis, J., Raff, M., Roberts, K., Walters, P.: Molecular Biology of the Cell, 4th edn. Garland Science, New York and London (2002)
Google Scholar
Ali, R., Connolly, I.D., Tang, O.Y., Mirza, F.N., Johnston, B., Abdulrazeq, H.F., Galamaga, P.F., Libby, T.J., Sodha, N.R., Groff, M.W., Gokaslan, Z.L., Telfeian, A.E., Shin, J.H., Asaad, W.F., Zou, J., Doberstein, C.E.: Bridging the literacy gap for surgical consents: an AI-human expert collaborative approach. NPJ Digit. Med. 7 (1), 63 (2024)
All European Academies.: The European Code of Conduct for Research Integrity, Revised Edition 2023 (2023). https://allea.org/code-of-conduct/
Allyn, B.: The Google engineer who sees company's AI as 'sentient' thinks a chatbot has a soul. NPR (2022). https://www.npr.org/2022/06/16/1105552435/google-ai-sentient
Alvarado, R.: Should we replace radiologists with deep learning? Bioethics 36 (2), 121–133 (2022)
Alvarado, R.: What kind of trust does AI deserve, if any? AI Ethics (2022). https://doi.org/10.1007/s43681-022-00224-x
Alvarado, R.: Computer simulations as scientific instruments. Found. Sci. 27 (3), 1183–1205 (2022)
Article MathSciNet Google Scholar
Alvarado, R.: AI as an epistemic technology. Sci. Eng. Ethics 29 , 32 (2023)
American Society of Microbiology.: Code of Conduct (2021). https://asm.org/Articles/Ethics/COEs/ASM-Code-of-Ethics-and-Conduct
Ankarstad, A.: What is explainable AI (XAI)? Towards Data Science (2020). https://towardsdatascience.com/what-is-explainable-ai-xai-afc56938d513
Antun, V., Renna, F., Poon, C., Adcock, B., Hansen, A.C.: On instabilities of deep learning in image reconstruction and the potential costs of AI. Proc. Natl. Acad. Sci. U.S.A. 117 (48), 30088–30095 (2020)
Assael, Y., Sommerschield, T., Shillingford, B., Bordbar, M., Pavlopoulos, J., Chatzipanagiotou, M., Androutsopoulos, I., Prag, J., de Freitas, N.: Restoring and attributing ancient texts using deep neural networks. Nature 603 , 280–283 (2022)
Babu, N.V., Kanaga, E.G.M.: Sentiment analysis in social media data for depression detection using artificial intelligence: a review. SN Comput. Sci. 3 , 74 (2022)
Badini, S., Regondi, S., Pugliese, R.: Unleashing the power of artificial intelligence in materials design. Materials 16 (17), 5927 (2023). https://doi.org/10.3390/ma16175927
Ball, P.: Is AI leading to a reproducibility crisis in science? Nature 624 , 22–25 (2023)
Barrera, F.J., Brown, E.D.L., Rojo, A., Obeso, J., Plata, H., Lincango, E.P., Terry, N., Rodríguez-Gutiérrez, R., Hall, J.E., Shekhar, S.: Application of machine learning and artificial intelligence in the diagnosis and classification of polycystic ovarian syndrome: a systematic review. Front. Endocrinol. (2023). https://doi.org/10.3389/fendo.2023.1106625
Bartosz, B.B., Bartosz, J.: Can artificial intelligences be moral agents? New Ideas Psychol. 54 , 101–106 (2019)
Baum, J., Villasenor, J.: The politics of AI: ChatGPT and political biases. Brookings (2023). https://www.brookings.edu/articles/the-politics-of-ai-chatgpt-and-political-bias/
BBC News.: Alexa tells 10-year-old girl to touch live plug with penny. BBC News (2021). https://www.bbc.com/news/technology-59810383
Begus, G., Sprouse, R., Leban, A., Silva, M., Gero, S.: Vowels and diphthongs in sperm whales (2024). https://doi.org/10.31219/osf.io/285cs
Bevier, C.: ChatGPT broke the Turing test—the race is on for new ways to assess AI. Nature (2023). https://www.nature.com/articles/d41586-023-02361-7
Bevier, C.: The easy intelligence test that AI chatbots fail. Nature 619 , 686–689 (2023)
Bhattacharyya, M., Miller, V.M., Bhattacharyya, D., Miller, L.E.: High rates of fabricated and inaccurate references in ChatGPT-generated medical content. Cureus 15 (5), e39238 (2023)
Biddle, S.: The internet’s new favorite AI proposes torturing Iranians and surveilling mosques. The Intercept (2022). https://theintercept.com/2022/12/08/openai-chatgpt-ai-bias-ethics/
Bird, S.J., Housman, D.E.: Trust and the collection, selection, analysis and interpretation of data: a scientist’s view. Sci. Eng. Ethics 1 (4), 371–382 (1995)
Biology for Life.: n.d. https://www.biologyforlife.com/error-analysis.html
Blumauer, A.: How ChatGPT works and the problems with non-explainable AI. Pool Party (2023). https://www.poolparty.biz/blogposts/how-chat-gpt-works-non-explainable-ai#:~:text=ChatGPT%20is%20the%20antithesis%20of,and%20explainability%20are%20critical%20requirements
Bogost, I.: ChatGPT is dumber than you think. The Atlantic (2022). https://www.theatlantic.com/technology/archive/2022/12/chatgpt-openai-artificial-intelligencewriting-ethics/672386/
Bolanos, F., Salatino, A., Osborne, F., Motta, E.: Artificial intelligence for literature reviews: opportunities and challenges (2024). arXiv:2402.08565
Bordukova, M., Makarov, N., Rodriguez-Esteban, P., Schmich, F., Menden, M.P.: Generative artificial intelligence empowers digital twins in drug discovery and clinical trials. Expert Opin. Drug Discov. 19 (1), 33–42 (2024)
Borowiec, M.L., Dikow, R.B., Frandsen, P.B., McKeeken, A., Valentini, G., White, A.E.: Deep learning as a tool for ecology and evolution. Methods Ecol. Evol. 13 (8), 1640–1660 (2022)
Bostrom, N.: Superintelligence: Paths, Dangers, Strategies. Oxford University Press, Oxford (2014)
Bothra, A., Cao, Y., Černý, J., Arora, G.: The epidemiology of infectious diseases meets AI: a match made in heaven. Pathogens 12 (2), 317 (2023)
Brainard, J.: As scientists face a flood of papers, AI developers aim to help. Science (2023). https://www.science.org/content/article/scientists-face-flood-papers-ai-developers-aim-help
Brown, H.I.: Galileo on the telescope and the eye. J. Hist. Ideas 46 (4), 487–501 (1985)
Brumfiel, G.: New proteins, better batteries: Scientists are using AI to speed up discoveries. NPR (2023). https://www.npr.org/sections/health-shots/2023/10/12/1205201928/artificial-intelligence-ai-scientific-discoveries-proteins-drugs-solar
Brunello, N.: Example of a deep neural network (2021). https://commons.wikimedia.org/wiki/File:Example_of_a_deep_neural_network.png
Burrell, J.: How the machine ‘thinks’: understanding opacity in machine learning algorithms. Big Data Soc. 3 (1), 2053951715622512 (2016)
Calder, T.: The concept of evil. Stanford Encyclopedia of Philosophy (2022). https://plato.stanford.edu/entries/concept-evil/#KanTheEvi
Callaway, A.: ‘The entire protein universe’: AI predicts shape of nearly every known protein. Nature 608 , 14–16 (2022)
Caron, M.M., Dohan, S.B., Barnes, M., Bierer, B.E.: Defining "recklessness" in research misconduct proceedings. Accountability in Research, pp. 1–23 (2023)
Castelvecchi, D.: AI chatbot shows surprising talent for predicting chemical properties and reactions. Nature (2024). https://www.nature.com/articles/d41586-024-00347-7
CBS News.: ChatGPT and large language model bias. CBS News (2023). https://www.cbsnews.com/news/chatgpt-large-language-model-bias-60-minutes-2023-03-05/
CC BY-SA 4.0 DEED.: Amino-acid chains, known as polypeptides, fold to form a protein (2020). https://en.wikipedia.org/wiki/AlphaFold#/media/File:Protein_folding_figure.png
Cervantes, J.A., López, S., Rodríguez, L.F., Cervantes, S., Cervantes, F., Ramos, F.: Artificial moral agents: a survey of the current status. Sci. Eng. Ethics 26 (2), 501–532 (2020)
Chan, B.: Black-box assisted medical decisions: AI power vs. ethical physician care. Med. Health Care Philos. 26 , 285–292 (2023)
ChatGPT, Zhavoronkov, A.: Rapamycin in the context of Pascal’s Wager: generative pre-trained transformer perspective. Oncoscience 9 , 82–84 (2022)
Chatterjee, M.: AI cannot hold copyright, federal judge rules. Politico (2023). https://www.politico.com/news/2023/08/21/ai-cannot-hold-copyright-federal-judge-rules-00111865#:~:text=Friday's%20ruling%20will%20be%20a%20critical%20component%20in%20future%20legal%20fights.&text=Artificial%20intelligence%20cannot%20hold%20a,a%20federal%20judge%20ruled%20Friday
Chen, R.J., Lu, M.Y., Chen, T.Y., Williamson, D.F., Mahmood, F.: Synthetic data in machine learning for medicine and healthcare. Nat. Biomed. Eng. 5 , 493–497 (2021)
Chen, S., Kann, B.H., Foote, M.B., Aerts, H.J.W.L., Savova, G.K., Mak, R.H., Bitterman, D.S.: Use of artificial intelligence chatbots for cancer treatment information. JAMA Oncol. 9 (10), 1459–1462 (2023)
Cyrus, L.: How to fold graciously. In: Mossbauer Spectroscopy in Biological Systems: Proceedings of a Meeting Held at Allerton House, Monticello, Illinois, pp. 22–24 (1969)
Conroy, G.: Scientists used ChatGPT to generate an entire paper from scratch—but is it any good? Nature 619 , 443–444 (2023)
Conroy, G.: How ChatGPT and other AI tools could disrupt scientific publishing. Nature (2023). https://www.nature.com/articles/d41586-023-03144-w
Dai, B., Xu, Z., Li, H., Wang, B., Cai, J., Liu, X.: Racial bias can confuse AI for genomic studies. Oncologie 24 (1), 113–130 (2022)
Daneshjou, R., Smith, M.P., Sun, M.D., Rotemberg, V., Zou, J.: Lack of transparency and potential bias in artificial intelligence data sets and algorithms: a scoping review. JAMA Dermatol. 157 (11), 1362–1369 (2021)
Dastin, J.: Amazon scraps secret AI recruiting tool that showed bias against women. Reuters (2018). https://www.reuters.com/article/us-amazon-com-jobs-automation-insight-idUSKCN1MK08G
de Bruijn, H., Warnier, M., Janssen, M.: The perils and pitfalls of explainable AI: strategies for explaining algorithmic decision-making. Gov. Inf. Q. 39 (2), 101666 (2022)
Delua, J.: Supervised vs. unsupervised learning: What’s the difference? IBM (2021). https://www.ibm.com/blog/supervised-vs-unsupervised-learning/
Dhinakaran, A.: Overcoming AI’s transparency paradox. Forbes (2021). https://www.forbes.com/sites/aparnadhinakaran/2021/09/10/overcoming-ais-transparency-paradox/?sh=6c6b18834b77
Dickson, B.: LLMs can’t self-correct in reasoning tasks, DeepMind study finds. Tech Talks (2023). https://bdtechtalks.com/2023/10/09/llm-self-correction-reasoning-failures
Dunlap, T.: Artificial intelligence (AI) as an inventor? Dunlap, Bennett and Ludwig (2023). https://www.dbllawyers.com/artificial-intelligence-as-an-inventor/
Durán, J.M., Jongsma, K.R.: Who is afraid of black box algorithms? On the epistemological and ethical basis of trust in medical AI. J. Med. Ethics 47 (5), 329–335 (2021)
Einstein, A.: Letter to Max Born. Walker and Company, New York (1926). Published in: Irene Born (translator), The Born-Einstein Letters (1971)
Eisenstein, M.: Teasing images apart, cell by cell. Nature 623 , 1095–1097 (2023)
Eliot, L.: Nobody can explain for sure why ChatGPT is so good at what it does, troubling AI ethics and AI Law. Forbes (2023). https://www.forbes.com/sites/lanceeliot/2023/04/17/nobody-can-explain-for-sure-why-chatgpt-is-so-good-at-what-it-does-troubling-ai-ethics-and-ai-law/?sh=334c95685041
Eliot, L.: Generative AI ChatGPT can disturbingly gobble up your private and confidential data, forewarns AI ethics and AI law. Forbes (2023). https://www.forbes.com/sites/lanceeliot/2023/01/27/generative-ai-chatgpt-can-disturbingly-gobble-up-your-private-and-confidential-data-forewarns-ai-ethics-and-ai-law/?sh=592b16547fdb
Elliott, K.C., Resnik, D.B.: Making open science work for science and society. Environ. Health Perspect. 127 (7), 75002 (2019)
Euro News.: Man ends his life after an AI chatbot 'encouraged' him to sacrifice himself to stop climate change. Euro News (2023). https://www.euronews.com/next/2023/03/31/man-ends-his-life-after-an-ai-chatbot-encouraged-him-to-sacrifice-himself-to-stop-climate
European Agency for Fundamental Rights.: Data quality and Artificial Intelligence—Mitigating Bias and Error to Protect Fundamental Rights (2019). https://fra.europa.eu/sites/default/files/fra_uploads/fra-2019-data-quality-and-ai_en.pdf
Evans, K., de Moura, N., Chauvier, S., Chatila, R., Dogan, E.: Ethical decision making in autonomous vehicles: the AV ethics project. Sci. Eng. Ethics 26 , 3285–3312 (2020)
Extance, A.: How AI technology can tame the scientific literature. Nature (2018). https://www.nature.com/articles/d41586-018-06617-5
Fanelli, D.: How many scientists fabricate and falsify research? A systematic review and meta-analysis of survey data. PLoS ONE 4 (5), e5738 (2009)
Food and Drug Administration.: Artificial intelligence (AI) and machine learning (ML) in medical devices (2020). https://www.fda.gov/media/142998/download
Food and Drug Administration.: Development and approval process: drugs (2023). https://www.fda.gov/drugs/development-approval-process-drugs
Fraenkel, A.S.: Complexity of protein folding. Bull. Math. Biol. 55 (6), 1199–1210 (1993)
Fuhrman, J.D., Gorre, N., Hu, Q., Li, H., El Naqa, I., Giger, M.L.: A review of explainable and interpretable AI with applications in COVID-19 imaging. Med. Phys. 49 (1), 1–14 (2022)
Garin, S.P., Parekh, V.S., Sulam, J., Yi, P.H.: Medical imaging data science competitions should report dataset demographics and evaluate for bias. Nat. Med. 29 (5), 1038–1039 (2023)
Giere, R., Bickle, J., Maudlin, R.F.: Understanding Scientific Reasoning, 5th edn. Wadsworth, Belmont (2005)
Gillispie, C.C.: The Edge of Objectivity. Princeton University Press, Princeton (1960)
Giuffrè, M., Shung, D.L.: Harnessing the power of synthetic data in healthcare: innovation, application, and privacy. NPJ Digit. Med. 6 , 186 (2023)
Godwin, R.C., Bryant, A.S., Wagener, B.M., Ness, T.J., DeBerryJJ, H.L.L., Graves, S.H., Archer, A.C., Melvin, R.L.: IRB-draft-generator: a generative AI tool to streamline the creation of institutional review board applications. SoftwareX 25 , 101601 (2024)
Google.: Responsible AI practices (2023). https://ai.google/responsibility/responsible-ai-practices/
Goldman, A.I.: Liaisons: philosophy meets the cognitive and social sciences. MIT Press, Cambridge (2003)
Grad, P.: Trick prompts ChatGPT to leak private data. TechXplore (2023). https://techxplore.com/news/2023-12-prompts-chatgpt-leak-private.html
Graziani, M., Dutkiewicz, L., Calvaresi, D., Amorim, J.P., Yordanova, K., Vered, M., Nair, R., Abreu, P.H., Blanke, T., Pulignano, V., Prior, J.O., Lauwaert, L., Reijers, W., Depeursinge, A., Andrearczyk, V., Müller, H.: A global taxonomy of interpretable AI: unifying the terminology for the technical and social sciences. Artif. Intell. Rev. 56 , 3473–3504 (2023)
Guinness, H.: The best AI image generators in 2023. Zappier (2023). https://zapier.com/blog/best-ai-image-generator/
Gulshan, V., Peng, L., Coram, M., Stumpe, M.C., Wu, D., Narayanaswamy, A., Venugopalan, S., Widner, K., Madams, T., Cuadros, J., Kim, R., Raman, R., Nelson, P.C., Mega, J.L., Webster, D.R.: Development and validation of a deep learning algorithm for detection of diabetic retinopathy in retinal fundus photographs. JAMA 316 (22), 2402–2410 (2016)
Haack, S.: Defending Science within Reason. Prometheus Books, New York (2007)
Hackernoon.: (2024). https://hackernoon.com/the-times-v-microsoftopenai-unauthorized-reproduction-of-times-works-in-gpt-model-training-10
Hagendorff, T., Fabi, S., Kosinski, M.: Human-like intuitive behavior and reasoning biases emerged in large language models but disappeared in ChatGPT. Nat. Comput. Sci. (2023). https://doi.org/10.1038/s43588-023-00527-x
Heaton, J.: “*Pseudonyms are used throughout”: a footnote, unpacked. Qual. Inq. 1 , 123–132 (2022)
Heikkilä, M.: How OpenAI is trying to make ChatGPT safer and less biased. The Atlantic (2023). https://www.technologyreview.com/2023/02/21/1068893/how-openai-is-trying-to-make-chatgpt-safer-and-less-biased/
Helmenstine, A.: Systematic vs random error—differences and examples. Science Notes (2021). https://sciencenotes.org/systematic-vs-random-error-differences-and-examples/
Himma, K.E.: Artificial agency, consciousness, and the criteria for moral agency: what properties must an artificial agent have to be a moral agent? Ethics Inf. Technol. 11 , 19–29 (2009)
Holzinger, A., Langs, G., Denk, H., Zatloukal, K., Müller, H.: Causability and explainability of artificial intelligence in medicine. Wires (2019). https://doi.org/10.1002/widm.1312
Hosseini, M., Holmes, K.: Is it ethical to use generative AI if you can’t tell whether it is right or wrong? [Blog Post]. Impact of Social Sciences(2024). https://blogs.lse.ac.uk/impactofsocialsciences/2024/03/15/is-it-ethical-to-use-generative-ai-if-you-cant-tell-whether-it-is-right-or-wrong/
Hosseini, M., Horbach, S.P.J.M.: Fighting reviewer fatigue or amplifying bias? Considerations and recommendations for use of ChatGPT and other large language models in scholarly peer review. Res. Integr. Peer Rev. 8 (1), 4 (2023)
Hosseini, M., Horbach, S.P.J.M.: Can generative AI add anything to academic peer review? [Blog Post] Impact of Social Sciences(2023). https://blogs.lse.ac.uk/impactofsocialsciences/2023/09/26/can-generative-ai-add-anything-to-academic-peer-review/
Hosseini, M., Senabre Hidalgo, E., Horbach, S.P.J.M., Güttinger, S., Penders, B.: Messing with Merton: the intersection between open science practices and Mertonian values. Accountability in Research, pp. 1–28 (2022)
Hosseini, M., Rasmussen, L.M., Resnik, D.B.: Using AI to write scholarly publications. Accountability in Research, pp. 1–9 (2023)
Hosseini, M., Resnik, D.B., Holmes, K.: The ethics of disclosing the use of artificial intelligence in tools writing scholarly manuscripts. Res. Ethics (2023). https://doi.org/10.1177/17470161231180449
Hosny, A., Parmar, C., Quackenbush, J., Schwartz, L.H., Aerts, H.J.W.L.: Artificial intelligence in radiology. Nat. Rev. Cancer 18 (8), 500–510 (2018)
Howson, C., Urbach, P.: Scientific Reasoning: A Bayesian Approach, 3rd edn. Open Court, New York (2005)
Humphreys, P.: Extending Ourselves: Computational Science, Empiricism, and Scientific Method. Oxford University Press, New York (2004)
Book Google Scholar
Huo, T., Li, L., Chen, X., Wang, Z., Zhang, X., Liu, S., Huang, J., Zhang, J., Yang, Q., Wu, W., Xie, Y., Wang, H., Ye, Z., Deng, K.: Artificial intelligence-aided method to detect uterine fibroids in ultrasound images: a retrospective study. Sci. Rep. 13 (1), 3714 (2023)
Hutson. M.: Hypotheses devised by AI could find ‘blind spots’ in research. Nature (2023). https://www.nature.com/articles/d41586-023-03596
IBM.: What is AI? (2023). https://www.ibm.com/topics/artificial-intelligence
IBM.: What is a Captcha? (2023). https://www.ibm.com/topics/captcha
IBM.: Explainable AI (2023). https://www.ibm.com/topics/explainable-ai
IBM.: What is generative AI? (2023). https://research.ibm.com/blog/what-is-generative-AI
IBM.: What is ML? (2024). https://www.ibm.com/topics/machine-learning
International Committee of Medical Journal Editors.: Recommendations for the Conduct, Reporting, Editing, and Publication of Scholarly work in Medical Journals (2023). https://www.icmje.org/icmje-recommendations.pdf
International Organization for Standardization.: What is AI? (2024). https://www.iso.org/artificial-intelligence/what-is-ai#:~:text=Artificial%20intelligence%20is%20%E2%80%9Ca%20technical,%2FIEC%2022989%3A2022%5D
Janowicz, K., Gao, S., McKenzie, G., Hu, Y., Bhaduri, B.: GeoAI: spatially explicit artificial intelligence techniques for geographic knowledge discovery and beyond. Int. J. Geogr. Inf. Sci. 34 (4), 625–636 (2020)
Jenkins, R., Lin, P.:. AI-assisted authorship: How to assign credit in synthetic scholarship. SSRN Scholarly Paper No. 4342909 (2023). https://doi.org/10.2139/ssrn.4342909
Jones, D.: Facebook apologizes after its AI labels black men as 'primates'. NPR (2021). https://www.npr.org/2021/09/04/1034368231/facebook-apologizes-ai-labels-black-men-primates-racial-bias
Jumper, J., Evans, R., Pritzel, A., Green, T., Figurnov, M., Ronneberger, O., Tunyasuvunakool, K., Bates, R., Žídek, A., Potapenko, A., Bridgland, A., Meyer, C., Kohl, S.A.A., Ballard, A.J., Cowie, A., Romera-Paredes, B., Nikolov, S., Jain, R., Adler, J., Back, T., Petersen, S., Reiman, D., Clancy, E., Zielinski, M., Steinegger, M., Pacholska, M., Berghammer, T., Bodenstein, S., Silver, D., Vinyals, O., Senior, A.W., Kavukcuoglu, K., Kohli, P., Hassabis, D.: Highly accurate protein structure prediction with AlphaFold. Nature 596 (7873), 583–589 (2021)
Junction AI.: What is ChatGPT not good at? Junction AI (2023). https://junction.ai/what-is-chatgpt-not-good-at/
Kahn, J.: What wrong with “explainable A.I.” Fortune (2022). https://fortune.com/2022/03/22/ai-explainable-radiology-medicine-crisis-eye-on-ai/
Kahneman, D.: Thinking, Fast and Slow. Farrar, Straus, Giroux, New York (2011)
Kembhavi, A., Pattnaik, R.: Machine learning in astronomy. J. Astrophys. Astron. 43 , 76 (2022)
Kennedy, B., Tyson, A., Funk, C.: Americans’ trust in scientists, other groups declines. Pew Research Center (2022). https://www.pewresearch.org/science/2022/02/15/americans-trust-in-scientists-other-groups-declines/
Kim, I., Kang, K., Song, Y., Kim, T.J.: Application of artificial intelligence in pathology: trends and challenges. Diagnostics (Basel) 12 (11), 2794 (2022)
Kitcher, P.: The Advancement of Knowledge. Oxford University Press, New York (1993)
Krenn, M., Pollice, R., Guo, S.Y., Aldeghi, M., Cervera-Lierta, A., Friederich, P., Gomes, G.P., Häse, F., Jinich, A., Nigam, A., Yao, Z., Aspuru-Guzik, A.: On scientific understanding with artificial intelligence. Nat. Rev. Phys. 4 , 761–769 (2022)
Kruse, C.S., Smith, B., Vanderlinden, H., Nealand, A.: Security techniques for the electronic health records. J. Med. Syst. 41 (8), 127 (2017)
Kuhn, T.S.: The Essential Tension. University of Chicago Press, Chicago (1977)
Lal, A., Pinevich, Y., Gajic, O., Herasevich, V., Pickering, B.: Artificial intelligence and computer simulation models in critical illness. World Journal of Critical Care Medicine 9 (2), 13–19 (2020)
La Malfa, E., Petrov, A., Frieder, S., Weinhuber, C., Burnell, R., Cohn, A.G., Shadbolt, N., Woolridge, M.: The ARRT of language-models-as-a-service: overview of a new paradigm and its challenges (2023). arXiv: 2309.16573
Larkin, Z.: AI bias—what Is it and how to avoid it? Levity (2022). https://levity.ai/blog/ai-bias-how-to-avoid
Lee, N.T., Resnick, P., Barton, G.: Algorithmic Bias Detection and Mitigation: Best Practices and Policies to Reduce Consumer Harms. Brookings Institute, Washington, DC (2019)
Leswing, K.: OpenAI announces GPT-4, claims it can beat 90% of humans on the SAT. CNBC (2023). https://www.cnbc.com/2023/03/14/openai-announces-gpt-4-says-beats-90percent-of-humans-on-sat.html
Licht, K., Licht, J.: Artificial intelligence, transparency, and public decision-making: Why explanations are key when trying to produce perceived legitimacy. AI Soc. 35 , 917–926 (2020)
Lipenkova, J.: Overcoming the limitations of large language models: how to enhance LLMs with human-like cognitive skills. Towards Data Science (2023). https://towardsdatascience.com/overcoming-the-limitations-of-large-language-models-9d4e92ad9823
London, A.J.: Artificial intelligence and black-box medical decisions: accuracy versus explainability. Hastings Cent. Rep. 49 (1), 15–21 (2019)
Longino, H.: Science as Social Knowledge. Princeton University Press, Princeton (1990)
Lubell, J.: ChatGPT passed the USMLE. What does it mean for med ed? AMA (2023). https://www.ama-assn.org/practice-management/digital/chatgpt-passed-usmle-what-does-it-mean-med-ed
Martinho, A., Poulsen, A., Kroesen, M., Chorus, C.: Perspectives about artificial moral agents. AI Ethics 1 , 477–490 (2021)
Martinson, B.C., Anderson, M.S., de Vries, R.: Scientists behaving badly. Nature 435 (7043), 737–738 (2005)
Martins, C., Padovan, P., Reed, C.: The role of explainable AI (XAI) in addressing AI liability. SSRN (2020). https://ssrn.com/abstract=3751740
Matta, V., Bansal, G., Akakpo, F., Christian, S., Jain, S., Poggemann, D., Rousseau, J., Ward, E.: Diverse perspectives on bias in AI. J. Inf. Technol. Case Appl. Res. 24 (2), 135–143 (2022)
Matthewson, J.: Trade-offs in model-building: a more target-oriented approach. Stud. Hist. Philos. Sci. Part A 42 (2), 324–333 (2011)
McCarthy, J.: What is artificial intelligence? (2007). https://www-formal.stanford.edu/jmc/whatisai.pdf
McLeod, C.: Trust. Stanford Encyclopedia of Philosophy (2020). https://plato.stanford.edu/entries/trust/
Merken, S.: Another US judge says lawyers must disclose AI use. Reuters (2023). https://www.reuters.com/legal/transactional/another-us-judge-says-lawyers-must-disclose-ai-use-2023-06-08/
Merton, R.: The Sociology of Science. University of Chicago Press, Chicago (1973)
Messeri, L., Crockett, M.J.: Artificial intelligence and illusions of understanding in scientific research. Nature (2024). https://doi.org/10.1038/s41586-024-07146-0
Mieth, B., Rozier, A., Rodriguez, J.A., Höhne, M.M., Görnitz, N., Müller, R.K.: DeepCOMBI: explainable artificial intelligence for the analysis and discovery in genome-wide association studies. NAR Genom. Bioinform. 3 (3), lqab065 (2021)
Milmo, D.: Two US lawyers fined for submitting fake court citations from ChatGPT. The Guardian (2023). https://www.theguardian.com/technology/2023/jun/23/two-us-lawyers-fined-submitting-fake-court-citations-chatgpt
Mitchell, M.: Artificial Intelligence. Picador, New York (2019)
Mitchell, M.: What does it mean for AI to understand? Quanta Magazine (2021). https://www.quantamagazine.org/what-does-it-mean-for-ai-to-understand-20211216/
Mitchell, M.: AI’s challenge of understanding the world. Science 382 (6671), eadm8175 (2023)
Mittermaier, M., Raza, M.M., Kvedar, J.C.: Bias in AI-based models for medical applications: challenges and mitigation strategies. NPJ Digit. Med. 6 , 113 (2023)
Naddaf, M.: ChatGPT generates fake data set to support scientific hypothesis. Nature (2023). https://www.nature.com/articles/d41586-023-03635-w#:~:text=Researchers%20say%20that%20the%20model,doesn't%20pass%20for%20authentic
Nahas, K.: Now AI can be used to generate proteins. The Scientist (2023). https://www.the-scientist.com/news-opinion/now-ai-can-be-used-to-design-new-proteins-70997
National Academies of Sciences, Engineering, and Medicine: Gene Drives on the Horizon: Advancing Science, Navigating Uncertainty, and Aligning Research with Public Values. National Academies Press, Washington, DC (2016)
National Institutes of Health.: Guidelines for the Conduct of Research in the Intramural Program of the NIH (2023). https://oir.nih.gov/system/files/media/file/2023-11/guidelines-conduct_research.pdf
National Institutes of Health.: The use of generative artificial intelligence technologies is prohibited for the NIH peer review process. NOT-OD-23-149 (2023). https://grants.nih.gov/grants/guide/notice-files/NOT-OD-23-149.html
National Transportation and Safety Board.: Investigations (2023). https://www.ntsb.gov/investigations/Pages/Investigations.aspx
Nawaz, M.S., Fournier-Viger, P., Shojaee, A., Fujita, H.: Using artificial intelligence techniques for COVID-19 genome analysis. Appl. Intell. (Dordrecht) 51 (5), 3086–3103 (2021)
Ng, G.W., Leung, W.C.: Strong artificial intelligence and consciousness. J. Artif. Intell. Conscious. 7 (1), 63–72 (2020)
Nordling, L.: How ChatGPT is transforming the postdoc experience. Nature 622 , 655–657 (2023)
Nost, E., Colven, E.: Earth for AI: a political ecology of data-driven climate initiatives. Geoforum 130 , 23–34 (2022)
Ntoutsi, E., Fafalios, P., Gadiraju, U., Iosifidis, V., Nejdl, W., Vidal, M.E., Ruggieri, S., Turini, F., Papadopoulos, S., Krasanakis, E., Kompatsiaris, I., Kinder-Kurlanda, K., Wagner, C., Karimi, F., Fernandez, M., Alani, H., Berendt, B., Kruegel, T., Heinze, C., Broelemann, K., Kasneci, K., Tiropanis, T., Staab, S.: Bias in data-driven artificial intelligence systems—an introductory survey. Wires (2020). https://doi.org/10.1002/widm
O’Connor, S.: Corrigendum to “Open artificial intelligence platforms in nursing education: tools for academic progress or abuse?” [Nurse Educ. Pract. 66 (2023) 103537]. Nurse Educ. Pract. 67 , 103572 (2023)
O’Connor, S., ChatGPT: Open artificial intelligence platforms in nursing education: tools for academic progress or abuse? Nurse Educ. Pract. 66 , 103537 (2023)
Office of Science and Technology Policy: Federal research misconduct policy. Fed. Reg. 65 (235), 76260–76264 (2000)
Office and Science and Technology Policy.: Blueprint for an AI Bill of Rights (2022). https://www.whitehouse.gov/ostp/ai-bill-of-rights/
Olavsrud, T.: 9 famous analytics and AI disasters. CIO (2023). https://www.cio.com/article/190888/5-famous-analytics-and-ai-disasters.html
Omiye, J.A., Lester, J.C., Spichak, S., Rotemberg, V., Daneshjou, R.: Large language models propagate race-based medicine. NPJ Digit. Med. 6 , 195 (2023)
Oncology Medical Physics.: Accuracy, precision, and error (2024). https://oncologymedicalphysics.com/quantifying-accuracy-precision-and-error/
OpenAI.: (2023). https://openai.com/chatgpt
Osoba, O., Welser, W.: An Intelligence in Our Image: The Risks of Bias and Errors in Artificial Intelligence. Rand Corporation (2017). https://www.rand.org/content/dam/rand/pubs/research_reports/RR1700/RR1744/RAND_RR1744.pdf
Othman, K.: Public acceptance and perception of autonomous vehicles: a comprehensive review. AI Ethics 1 , 355–387 (2021)
Ovchinnikov, S., Park, H., Varghese, N., Huang, P.S., Pavlopoulos, G.A., Kim, D.E., Kamisetty, H., Kyrpides, N.C., Baker, D.: Protein structure determination using metagenome sequence data. Science 355 (6322), 294–298 (2017)
Parikh, R.B., Teeple, S., Navathe, A.S.: Addressing bias in artificial intelligence in health care. J. Am. Med. Assoc. 322 (24), 2377–2378 (2019)
Parrilla, J.M.: ChatGPT use shows that the grant-application system is broken. Nature (2023). https://www.nature.com/articles/d41586-023-03238-5
Pearson, J.: Scientific Journal Publishes AI-Generated Rat with Gigantic Penis In Worrying Incident [Internet]. Vice (2024). https://www.vice.com/en/article/dy3jbz/scientific-journal-frontiers-publishes-ai-generated-rat-with-gigantic-penis-in-worrying-incident
Pennock, R.T.: An Instinct for Truth: Curiosity and the Moral Character of Science. MIT Press, Cambridge (2019)
Perni, S., Lehmann, L.S., Bitterman, D.S.: Patients should be informed when AI systems are used in clinical trials. Nat. Med. 29 (8), 1890–1891 (2023)
Perrigo, B.: OpenAI used Kenyan workers on less than $2 per hour to make ChatGPT less toxic. Time Magazine (2023). https://time.com/6247678/openai-chatgpt-kenya-workers/
Pew Charitable Trust.: How FDA regulates artificial intelligence in medical products. Issue brief (2021). https://www.pewtrusts.org/en/research-and-analysis/issue-briefs/2021/08/how-fda-regulates-artificial-intelligence-in-medical-products
Raeburn, A.: What’s the difference between accuracy and precision? Asana (2023). https://asana.com/resources/accuracy-vs-precision
Rasmussen, L.: Why and how to incorporate issues of race/ethnicity and gender in research integrity education. Accountability in Research (2023)
Ratti, E., Graves, M.: Explainable machine learning practices: opening another black box for reliable medical AI. AI Ethics 2 , 801–814 (2022)
Resnik, D.B.: Social epistemology and the ethics of research. Stud. Hist. Philos. Sci. 27 , 566–586 (1996)
Resnik, D.B.: The Price of Truth: How Money Affects the Norms of Science. Oxford University Press, New York (2007)
Resnik, D.B.: Playing Politics with Science: Balancing Scientific Independence and Government Oversight. Oxford University Press, New York (2009)
Resnik, D.B., Dinse, G.E.: Do U.S. research institutions meet or exceed federal mandates for instruction in responsible conduct of research? A national survey. Acad. Med. 87 , 1237–1242 (2012)
Resnik, D.B., Elliott, K.C.: Value-entanglement and the integrity of scientific research. Stud. Hist. Philos. Sci. 75 , 1–11 (2019)
Resnik, D.B., Elliott, K.C.: Science, values, and the new demarcation problem. J. Gen. Philos. Sci. 54 , 259–286 (2023)
Resnik, D.B., Elliott, K.C., Soranno, P.A., Smith, E.M.: Data-intensive science and research integrity. Account. Res. 24 (6), 344–358 (2017)
Resnik, D.B., Smith, E.M., Chen, S.H., Goller, C.: What is recklessness in scientific research? The Frank Sauer case. Account. Res. 24 (8), 497–502 (2017)
Roberts, M., Driggs, D., Thorpe, M., Gilbey, J., Yeung, M., Ursprung, S., Aviles-Rivero, A.I., Etmann, C., McCague, C., Beer, L., Weir-McCall, J.R., Teng, Z., Gkrania-Klotsas, E., AIX-COVNET, Rudd, J.H.F., Sala, E., Schönlieb, C.B.: Common pitfalls and recommendations for using machine learning to detect and prognosticate for COVID-19 using chest radiographs and CT scans. Nat. Mach. Intell. 3 , 199–217 (2021)
Rodgers, W., Murray, J.M., Stefanidis, A., Degbey, W.Y., Tarba, S.: An artificial intelligence algorithmic approach to ethical decision-making in human resource management processes. Hum. Resour. Manag. Rev. 33 (1), 100925 (2023)
Romero, A.: AI won’t master human language anytime soon. Towards Data Science (2021). https://towardsdatascience.com/ai-wont-master-human-language-anytime-soon-3e7e3561f943
Röösli, E., Rice, B., Hernandez-Boussard, T.: Bias at warp speed: how AI may contribute to the disparities gap in the time of COVID-19. J. Am. Med. Inform. Assoc. 28 (1), 190–192 (2021)
Savage, N.: Breaking into the black box of artificial intelligence. Nature (2022). https://www.nature.com/articles/d41586-022-00858-1
Savage, N.: Synthetic data could be better than real data. Nature (2023). https://www.nature.com/articles/d41586-023-01445-8
Schmidt, E.: This is how AI will transform the way science gets done. MIT Technology Review (2023). https://www.technologyreview.com/2023/07/05/1075865/eric-schmidt-ai-will-transform-science/#:~:text=AI%20can%20also%20spread%20the,promising%20candidates%20for%20new%20drugs
Schwartz, R., Vassilev, A., Greene, K., Perine, L., Burt, A., Hal, P.: Towards a standard for identifying and managing bias in artificial intelligence. National Institute of Standards and Technology (2022). https://view.ckcest.cn/AllFiles/ZKBG/Pages/264/c914336ac0e68a6e3e34187adf9dd83bb3b7c09f.pdf
Semler, J.: Artificial quasi moral agency. In: AIES '22: Proceedings of the 2022 AAAI/ACM Conference on AI, Ethics, and Society (2022). https://doi.org/10.1145/3514094.3539549
Service RF: The game has changed. AI trumphs at protein folding. Science 370 (6521), 1144–1145 (2022)
Service R.: Materials-predicting AI from DeepMind could revolutionize electronics, batteries, and solar cells. Science (2023). https://www.science.org/content/article/materials-predicting-ai-deepmind-could-revolutionize-electronics-batteries-and-solar
Seth, A.: Being You: A New Science of Consciousness. Faber and Faber, London (2021)
Shamoo, A.E., Resnik, D.B.: Responsible Conduct of Research, 4th edn. Oxford University Press, New York (2022)
Shapin, S.: Here and everywhere: sociology of scientific knowledge. Ann. Rev. Sociol. 21 , 289–321 (1995)
Solomon, M.: Social Empiricism. MIT Press, Cambridge (2007)
Southern, M.G.: ChatGPT update: Improved math capabilities. Search Engine Journal (2023). https://www.searchenginejournal.com/chatgpt-update-improved-math-capabilities/478057/
Straw, I., Callison-Burch, C.: Artificial Intelligence in mental health and the biases of language based models. PLoS ONE 15 (12), e0240376 (2020)
Swaak, T.: ‘We’re all using it’: Publishing decisions are increasingly aided by AI. That’s not always obvious. The Chronicle of Higher Education (2023). https://deal.town/the-chronicle-of-higher-education/academe-today-publishing-decisions-are-increasingly-aided-by-ai-but-thats-not-always-obvious-PK2J5KUC4
Talbert, M.: Moral responsibility. Stanford Encyclopedia of Philosophy (2019). https://plato.stanford.edu/entries/moral-responsibility/
Taloni, A., Scorcia, V., Giannaccre, G.: Large language model advanced data analysis abuse to create a fake data set in medical research. JAMA Ophthalmol. (2023). https://jamanetwork.com/journals/jamaophthalmology/fullarticle/2811505
Tambornino, L., Lanzerath, D., Rodrigues, R., Wright, D.: SIENNA D4.3: survey of REC approaches and codes for Artificial Intelligence & Robotics (2019). https://zenodo.org/records/4067990
Terwilliger, T.C., Liebschner, D., Croll, T.I., Williams, C.J., McCoy, A.J., Poon, B.K., Afonine, P.V., Oeffner, R.D., Richardson, J.S., Read, R.J., Adams, P.D.: AlphaFold predictions are valuable hypotheses and accelerate but do not replace experimental structure determination. Nat. Methods (2023). https://doi.org/10.1038/s41592-023-02087-4
The White House.: Biden-Harris administration secures voluntary commitments from leading artificial intelligence companies to manage the risks posed by AI (2023). https://www.whitehouse.gov/briefing-room/statements-releases/2023/07/21/fact-sheet-biden-harris-administration-secures-voluntary-commitments-from-leading-artificial-intelligence-companies-to-manage-the-risks-posed-by-ai/#:~:text=President%20Biden%20signed%20an%20Executive,the%20public%20from%20algorithmic%20discrimination
Thorp, H.H.: ChatGPT is fun, but not an author. Science 379 (6630), 313 (2023)
Turing.: Complete analysis of artificial intelligence vs artificial consciousness (2023). https://www.turing.com/kb/complete-analysis-of-artificial-intelligence-vs-artificial-consciousness
Turing, A.: Computing machinery and intelligence. Mind 59 (236), 433–460 (1950)
UK Statistic Authority.: Ethical considerations relating to the creation and use of synthetic data (2022). https://uksa.statisticsauthority.gov.uk/publication/ethical-considerations-relating-to-the-creation-and-use-of-synthetic-data/pages/2/
Unbable.: Why AI fails in the wild. Unbable (2019). https://resources.unbabel.com/blog/artificial-intelligence-fails
UNESCO.: Ethics of Artificial Intelligence (2024). https://www.unesco.org/en/artificial-intelligence/recommendation-ethics
US Copyright Office: Copyright registration guidance: works containing material generated by artificial intelligence. Fed. Reg. 88 (51), 16190–16194 (2023)
University of Michigan.: Generative artificial intelligence (2023). https://genai.umich.edu/
Vallor, S.: Moral deskilling and upskilling in a new machine age: reflections on the ambiguous future of character. Philos. Technol. 28 , 107–124 (2015)
Van Gulick, R.: Consciousness. Stanford Encyclopedia of Philosophy (2018). https://plato.stanford.edu/entries/consciousness/
Varoquaux, G., Cheplygina, V.: Machine learning for medical imaging: methodological failures and recommendations for the future. NPJ Digit. Med. 5 , 48 (2022)
Vanian, J., Leswing, K.: ChatGPT and generative AI are booming, but the costs can be extraordinary. CNBC (2023). https://www.cnbc.com/2023/03/13/chatgpt-and-generative-ai-are-booming-but-at-a-very-expensive-price.html
Walters, W.H., Wilder, E.I.: Fabrication and errors in the bibliographic citations generated by ChatGPT. Sci. Rep. 13 , 14045 (2023)
Wang, H., Fu, T., Du, Y., Gao, W., Huang, K., Liu, Z., Chandak, P., Liu, S., Van Katwyk, P., Deac, A., Anandkumar, A., Bergen, K., Gomes, C.P., Ho, S., Kohli, P., Lasenby, J., Leskovec, J., Liu, T.Y., Manrai, A., Marks, D., Ramsundar, B., Song, L., Sun, J., Tang, J., Veličković, P., Welling, M., Zhang, L., Coley, C.W., Bengio, Y., Zitnik, M.: Scientific discovery in the age of artificial intelligence. Nature 620 (7972), 47–60 (2023)
Weiss, D.C.: Latest version of ChatGPT aces bar exam with score nearing 90th percentile. ABA J. (2023). https://www.abajournal.com/web/article/latest-version-of-chatgpt-aces-the-bar-exam-with-score-in-90th-percentile
Whitbeck, C.: Truth and trustworthiness in research. Sci. Eng. Ethics 1 (4), 403–416 (1995)
Wilson, C.: Public engagement and AI: a values analysis of national strategies. Gov. Inf. Q. 39 (1), 101652 (2022)
World Conference on Research Integrity.: Singapore Statement (2010). http://www.singaporestatement.org/statement.html
Zheng, S.: China’s answers to ChatGPT have a censorship problem. Bloomberg (2023). https://www.bloomberg.com/news/newsletters/2023-05-02/china-s-chatgpt-answers-raise-questions-about-censoring-generative-ai
Ziman, J.: Real Science. Cambridge University Press, Cambridge (2000)
Download references
Open access funding provided by the National Institutes of Health. Funding was provided by Foundation for the National Institutes of Health (Grant number: ziaes102646-10).
Author information
Authors and affiliations.
National Institute of Environmental Health Sciences, Durham, USA
David B. Resnik
Department of Preventive Medicine, Northwestern University Feinberg School of Medicine, Chicago, IL, USA
Mohammad Hosseini
Galter Health Sciences Library and Learning Center, Northwestern University Feinberg School of Medicine, Chicago, IL, USA
You can also search for this author in PubMed Google Scholar
Corresponding author
Correspondence to David B. Resnik .
Ethics declarations
Conflict of interest.
On behalf of all authors, the corresponding author states that there is no conflict of interest.
Additional information
Publisher's note.
Springer Nature remains neutral with regard to jurisdictional claims in published maps and institutional affiliations.
Rights and permissions
Open Access This article is licensed under a Creative Commons Attribution 4.0 International License, which permits use, sharing, adaptation, distribution and reproduction in any medium or format, as long as you give appropriate credit to the original author(s) and the source, provide a link to the Creative Commons licence, and indicate if changes were made. The images or other third party material in this article are included in the article's Creative Commons licence, unless indicated otherwise in a credit line to the material. If material is not included in the article's Creative Commons licence and your intended use is not permitted by statutory regulation or exceeds the permitted use, you will need to obtain permission directly from the copyright holder. To view a copy of this licence, visit http://creativecommons.org/licenses/by/4.0/ .
Reprints and permissions
About this article
Resnik, D.B., Hosseini, M. The ethics of using artificial intelligence in scientific research: new guidance needed for a new tool. AI Ethics (2024). https://doi.org/10.1007/s43681-024-00493-8
Download citation
Received : 14 December 2023
Accepted : 07 May 2024
Published : 27 May 2024
DOI : https://doi.org/10.1007/s43681-024-00493-8
Share this article
Anyone you share the following link with will be able to read this content:
Sorry, a shareable link is not currently available for this article.
Provided by the Springer Nature SharedIt content-sharing initiative
- Artificial intelligence
- Transparency
- Accountability
- Explainability
- Social responsibility
- Find a journal
- Publish with us
- Track your research

An official website of the United States government
The .gov means it’s official. Federal government websites often end in .gov or .mil. Before sharing sensitive information, make sure you’re on a federal government site.
The site is secure. The https:// ensures that you are connecting to the official website and that any information you provide is encrypted and transmitted securely.
- Publications
- Account settings
Preview improvements coming to the PMC website in October 2024. Learn More or Try it out now .
- Advanced Search
- Journal List
- Malays Fam Physician
- v.1(2-3); 2006
Common Ethical Issues In Research And Publication
Introduction.
Research is the pillar of knowledge, and it constitutes an integral part of progress. In the fast-expanding field of biomedical research, this has improved the quality and quantity of life. Historically, medical doctors have been in the privileged position to carry out research, especially in clinical research which involves people. They are able to control “life and death” of patients and have free access to their confidential information. Moreover, medical researchers have also enjoyed immunity from accountability due to high public regard for science and medicine. This has resulted in some researchers conducting unethical researches. For instance, in World War II, medical doctors had conducted unethical experiments on human in the name of science, resulting in harm and even death in some cases. 1 More recently, the involvement of pharmaceutical industry in clinical trials have raised issues about how to safeguard patient’s care and to ensure the published research findings are objective. 2
In the light of these ethical controversies, the Declaration of Helsinki was established to inform biomedical researchers the principles of clinical research. 3 This declaration highlighted a tripartite guidelines for good clinical practice which include respect for the dignity of the person; research should not override the health, well-being and care of subjects; principles of justice. Committee on Publication Ethics (COPE) was also founded in 1997 to address the breaches of research and publication ethics. 4
How do we apply all these principles in our daily conduct of research? This paper will discuss different ethical issues in research, including study design and ethical approval, data analysis, authorship, conflict of interest and redundant publication and plagiarism. I have also included two case scenarios in this paper to illustrate common ethical issues in research and publication.
ETHICAL ISSUES IN RESEARCH
1. study design and ethics approval.
According to COPE, “good research should be well adjusted, well-planned, appropriately designed, and ethically approved. To conduct research to a lower standard may constitute misconduct.” 3 This may appear to be a stringent criterion, but it highlights the basic requirement of a researcher is to conduct a research responsibly. To achieve this, a research protocol should be developed and adhered to. It must be carefully agreed to by all contributors and collaborators, and the precise roles of each team member should be spelled out early, including matters of authorship and publications. Research should seek to answer specific questions, rather than just collect data.
It is essential to obtain approval from the Institutional Review Board, or Ethics Committee, of the respective organisations for studies involving people, medical records, and anonymised human tissues. The research proposal should discuss potential ethical issues pertaining to the research. The researchers should pay special attention to vulnerable subjects to avoid breech of ethical codes (e.g. children, prisoners, pregnant women, mentally challenged, educationally and economically disadvantaged). Patient information sheet should be given to the subjects during recruitment, detailing the objectives, procedures, potential benefits and harms, as well as rights to refuse participation in the research. Consent should be explained and obtained from the subjects or guardians, and steps should be taken to ensure confidentiality of information provided by the subjects.
2. Data analysis
It is the responsibility of the researcher to analyse the data appropriately. Although inappropriate analysis does not necessarily amount to misconduct, intentional omission of result may cause misinterpretation and mislead the readers. Fabrication and falsification of data do constitute misconduct. For example, in a clinical trial, if a drug is found to be ineffective, this study should be reported. There is a tendency for the researchers to under-report negative research findings, 5 and this is partly contributed by pressure from the pharmaceutical industry which funds the clinical trial.
To ensure appropriate data analysis, all sources and methods used to obtain and analyse data should be fully disclosed. Failure to do so may lead the readers to misinterpret the results without considering possibility of the study being underpowered. The discussion section of a paper should mention any issues of bias, and explain how they have been dealt with in the design and interpretation of the study.
3. Authorship
There is no universally agreed definition of authorship. 6 It is generally agreed that an author should have made substantial contribution to the intellectual content, including conceptualising and designing the study; acquiring, analysing and interpreting the data. The author should also take responsibility to certify that the manuscript represents valid work and take public responsibility for the work. Finally, an author is usually involved in drafting or revising the manuscript, as well as approving the submitted manuscript. Data collection, editing of grammar and language, and other routine works by itself, do not deserve an authorship.
It is crucial to decide early on in the planning of a research who will be credited as authors, as contributors, and who will be acknowledged. It is also advisable to read carefully the “Advice to Authors” of the target journal which may serve as a guide to the issue of authorship.
4. Conflicts of interest
This happens when researchers have interests that are not fully apparent and that may influence their judgments on what is published. These conflicts include personal, commercial, political, academic or financial interest. Financial interests may include employment, research funding, stock or share ownership, payment for lecture or travel, consultancies and company support for staff. This issue is especially pertinent in biomedical research where a substantial number of clinical trials are funded by pharmaceutical company.
Such interests, where relevant, should be discussed in the early stage of research. The researchers need to take extra effort to ensure that their conflicts of interest do not influence the methodology and outcome of the research. It would be useful to consult an independent researcher, or Ethics Committee, on this issue if in doubt. When publishing, these conflicts of interest should be declared to editors, and readers will judge for themselves whether the research findings are trustworthy.
5. Redundant publication and plagiarism
Redundant publication occurs when two or more papers, without full cross reference, share the same hypothesis, data, discussion points, or conclusions. However, previous publication of an abstract during the proceedings of meetings does not preclude subsequent submission for publication, but full disclosure should be made at the time of submission. This is also known as self-plagiarism. In the increasing competitive environment where appointments, promotions and grant applications are strongly influenced by publication record, researchers are under intense pressure to publish, and a growing minority is seeking to bump up their CV through dishonest means. 7
On the other hand, plagiarism ranges from unreferenced use of others’ published and unpublished ideas, including research grant applications to submission under “new” authorship of a complete paper, sometimes in different language.
Therefore, it is important to disclose all sources of information, and if large amount of other people’s written or illustrative materials is to be used, permission must be sought.
It is the duty of the researcher to ensure that research is conducted in an ethical and responsible manner from planning to publication. Researchers and authors should familiarise themselves with these principles and follows them strictly. Any potential ethical issues in research and publication should be discussed openly within the research team. If in doubt, it is advisable to consult the respective institutional review board (IRB) for their expert opinions.
Case Scenario 1:
“A community survey on prevalence of domestic violence among secondary school students.”
- To conduct this study, we need to seek approval from the Ministry of Education and permission from the school principal. However, consent should be taken from parents, who are the legal guardians of the students.
- These ethical issues should be discussed at the proposal stage, and the participants/guardians should be informed about the decision to report to the police while taking the consent. This will potentially affect the response rate; but this is also the responsibility of the researcher to protect the participants and their families.
- Yes, you can. However, you need to declare to the publisher that you have presented the paper in the conference. Redundant publication happens when an author has submitted two papers with similar objective, methodology and results, without cross referencing.
- Yes, you can. However, you have to declare to the publisher that you have published an identical paper in a different language.
Case Scenario 2:
“Does HRT improve vasomotor symptoms among menopausal women in a Malaysian primary care clinic?”
- HRT has been proven to be effective in relieving vasomotor symptoms in many well-designed studies. It is inappropriate for the researcher to repeat an established therapy which may potentially cause harm to them (e.g. deep vein thrombosis and breast cancer). However, it is appropriate to repeat research if the researchers feel that it may yield a different outcome in the local setting based on a firm theoretical basis.
- Yes, even though it is part of our normal practice, all research involving human subjects, especially when it involves drugs, should be subjected to ethics approval. (E.g. “How did the researchers ensure that they explain to the patients fully about the potential harm of HRT?”)
- As mentioned earlier, it is the duty of the researcher to ensure that the participant understands the benefits and risks of the treatment. The information should be conveyed in an objectively manner in the patient information sheet. Any queries from the patient should be answered truthfully, and it is the patient’s rights to refuse to participate in the research.
- It is acceptable to quote sentences from a paper as long as they are duly referenced.
Cookies on GOV.UK
We use some essential cookies to make this website work.
We’d like to set additional cookies to understand how you use GOV.UK, remember your settings and improve government services.
We also use cookies set by other sites to help us deliver content from their services.
You have accepted additional cookies. You can change your cookie settings at any time.
You have rejected additional cookies. You can change your cookie settings at any time.

Register to vote Register by 18 June to vote in the General Election on 4 July.
- Government reform
- Civil service reform
- Election guidance for civil servants
- Cabinet Office
- Civil Service
General election guidance 2024: guidance for civil servants (HTML)
Updated 23 May 2024
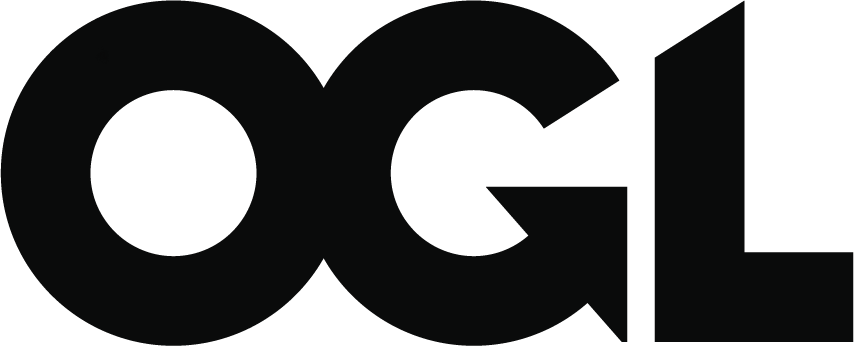
© Crown copyright 2024
This publication is licensed under the terms of the Open Government Licence v3.0 except where otherwise stated. To view this licence, visit nationalarchives.gov.uk/doc/open-government-licence/version/3 or write to the Information Policy Team, The National Archives, Kew, London TW9 4DU, or email: [email protected] .
Where we have identified any third party copyright information you will need to obtain permission from the copyright holders concerned.
This publication is available at https://www.gov.uk/government/publications/election-guidance-for-civil-servants/general-election-guidance-2024-guidance-for-civil-servants-html
1. General elections have a number of implications for the work of departments and civil servants. These arise from the special character of government business during an election campaign, and from the need to maintain, and be seen to maintain, the impartiality of the Civil Service, and to avoid any criticism of an inappropriate use of official resources. This guidance takes effect from 00:01 on 25 May 2024 at which point the ‘election period’ begins. The Prime Minister will write separately to Ministers advising them of the need to adhere to this guidance and to uphold the impartiality of the Civil Service.
2. This guidance applies to all UK civil servants, and the board members and staff of Non-Departmental Public Bodies (NDPBs) and other arms’ length bodies.
General Principles
3. During the election period, the Government retains its responsibility to govern, and Ministers remain in charge of their departments. Essential business (which includes routine business necessary to ensure the continued smooth functioning of government and public services) must be allowed to continue. However, it is customary for Ministers to observe discretion in initiating any new action of a continuing or long term character. Decisions on matters of policy on which a new government might be expected to want the opportunity to take a different view from the present government should be postponed until after the election, provided that such postponement would not be detrimental to the national interest or wasteful of public money.
4. Advice on handling such issues is set out in this guidance. This guidance will not cover every eventuality, but the principles should be applied to the particular circumstances.
5. The principles underlying the conduct of civil servants in a general election are an extension of those that apply at all times, as set out in the Civil Service Code
- The basic principle for civil servants is not to undertake any activity that could call into question their political impartiality or that could give rise to criticism that public resources are being used for party political purposes. This principle applies to all staff working in departments.
- Departmental and NDPB activity should not be seen to compete with the election campaign for public attention. The principles and conventions set out in this guidance also apply to public bodies.
- It is also a requirement of the Ministerial Code that Ministers must not use government resources for party political purposes and must uphold the political impartiality of the Civil Service.
Election queries
6. For any detailed queries on this guidance, or other questions, officials should in the first instance seek guidance from their line management chain, and, where necessary, escalate to their Permanent Secretary who may consult the Cabinet Secretary, or the Propriety and Ethics Team in the Cabinet Office.
7. The Propriety and Ethics Team handle general queries relating to conduct during the election period, provide advice on the handling of enquiries and any necessary co-ordination where enquiries raise issues that affect a number of departments (through their Permanent Secretary).
8. In dealing with queries, the Propriety and Ethics Team will function most effectively if it is in touch with relevant developments in departments.
Departments should therefore:
- draw to their attention, for advice or information, any approach or exchange that raises issues that are likely to be of interest to other departments; and
- seek advice before a Minister makes a significant Ministerial statement during the election period.
Section A: Enquiries, Briefing, Requests for Information and attending events
1. This note gives guidance on:
- the handling by departments and agencies of requests for information and other enquiries during a general election campaign;
- briefing of Ministers during the election period;
- the handling of constituency letters received from Members of Parliament before dissolution, and of similar letters from parliamentary candidates during the campaign; and
- the handling of FOI requests.
2. At a general election, the government of the day is expected to defend its policies to the electorate. By convention, the governing party is entitled to check with departments that statements made on its behalf are factually correct and consistent with government policy. As at all times, however, government departments and their staff must not engage in, or appear to engage in, party politics or be used for party ends. They should provide consistent factual information on request to candidates of all parties, as well as to organisations and members of the public, and should in all instances avoid becoming involved or appearing to become involved, in a partisan way, in election issues.
Requests for Factual Information
3. Departments and agencies should provide any parliamentary candidate, organisation or member of the public with information in accordance with the Freedom of Information Act 2000. Local and regional offices should deal similarly with straightforward enquiries, referring doubtful cases through their line management chain and, where necessary to their Permanent Secretary for decision.
4. Other requests for information will range from enquiries about existing government policy that are essentially factual in nature, to requests for justification and comment on existing government policy. All requests for information held by departments must be dealt with in accordance with the requirements of the Freedom of Information Act 2000. The handling of press enquiries is covered in Section I.
5. Where the enquiry concerns the day-to-day management of a non-ministerial department or executive agency and the chief executive would normally reply, he or she should do so in the usual way, taking special care to avoid becoming involved in any matters of political controversy.
6. Enquiries concerning policies newly announced in a party manifesto or for a comparison of the policies of different parties are for the political party concerned. Civil servants should not provide any assistance on these matters. See also paragraph 14.
7. Officials should draft replies, whether for official or Ministerial signature, with particular care to avoid party political controversy, especially criticism of the policies of other parties. Ministers may decide to amend draft replies to include a party political context. Where this is the case, Ministers should be advised to issue the letter on party notepaper. The guiding principle is whether the use of departmental resources, including headed paper, would be a proper use of public funds for governmental as opposed to party political purposes, and could be defended as such.
Speed of Response
8. The circumstances of a general election demand the greatest speed in dealing with enquiries. In particular, the aim should be to answer enquiries from parliamentary candidates or from any of the political parties’ headquarters within 24 hours. All candidates should be treated equally.
9. Where a request will take longer to deal with, the requester should be advised of this as he/she may wish to submit a refined request.
FOI requests
10. Requests that would normally be covered by the Freedom of Information Act (FOIA) must be handled in accordance with the requirements of the Act and the deadlines set therein. Where the application of the public interest balance requires more time, that is permitted under the Act but there is no general power to defer a decision.
11. Where a request needs to be considered under FOIA it will not normally be possible to get back to the parliamentary candidate, or others, within 24 hours and he or she should be advised of this as they may wish to submit a request more in line with paragraph 8 above.
Role of Ministers in FOIA decisions
12. Ministers have a number of statutory functions in relation to requests for information. They are the qualified person for the purpose of using section 36 of the FOI Act for their departments. During the general election period, Ministers will be expected to carry out these functions.
13. Where there is any doubt, requests should be referred to the FOI Policy team in the Cabinet Office.
Briefing and Support for Ministers
14. Ministers continue to be in charge of departments. It is reasonable for departments to continue to provide support for any necessary governmental functions, and receive any policy advice or factual briefing necessary to resolve issues that cannot be deferred until after the election.
15. Departments can check statements for factual accuracy and consistency with established government policy. Officials should not, however, be asked to devise new arguments or cost policies for use in the election campaign. Departments should not undertake costings or analysis of Opposition policies during the election period.
Officials attending public or stakeholder events
16. Officials should decline invitations to events where they may be asked to respond on questions about future government policy or on matters of public controversy.
Constituency Correspondence
17. During the election period, replies to constituency letters received from Members of Parliament before the dissolution, or to similar letters from parliamentary candidates, should take into account the fact that if they become public knowledge they will do so in the more politically-charged atmosphere of an election and are more likely to become the subject of political comment. Outstanding correspondence should be cleared quickly. Letters may be sent to former MPs at the House of Commons after dissolution, to be picked up or forwarded. Departments and agencies whose staff routinely deal directly with MPs’ enquiries should ensure that their regional and local offices get early guidance on dealing with questions from parliamentary candidates. Such guidance should reflect the following points:
a. Once Parliament is dissolved, a Member of Parliament’s constitutional right to represent his or her constituents’ grievances to government disappears, and all candidates for the election are on an equal footing. This doctrine should be applied in a reasonable way. In general, replies should be sent by Ministers to constituency letters that were written by MPs before dissolution. Where there is a pressing need for Ministers to reply to letters on constituency matters written after the dissolution by former Members, this should be handled in a way that avoids any preferential treatment or the appearance of preferential treatment between letters from the governing party and those from other candidates. It will normally be appropriate to send a Private Secretary reply to letters on constituency matters from prospective parliamentary candidates who were not Members before the dissolution.
b. The main consideration must be to ensure that the citizen’s interests are not prejudiced. But it is possible that a personal case may become politically controversial during the election period. Departments should therefore make particular efforts to ensure, so far as possible, that letters are factual, straightforward and give no room for misrepresentation.
c. Replies to constituency correspondence to be sent after polling day should, where there has been a change of MP, normally be sent direct to the constituent concerned. It should be left to the constituent to decide whether or not to copy the letter to any new MP. Where there is no change in MP, correspondence should be returned to the MP in the normal way.
Section B: Special Advisers
1. Special Advisers must agree with the Cabinet Office the termination of their contracts on or before 30 May (except for a small number of Special Advisers who may remain in post, where the express agreement of their appointing Minister and the Prime Minister to continue in post has been given).
2. An exception to this is where a Special Adviser has been publicly identified as a candidate or prospective candidate for election to the UK Parliament, in which case they must instead resign at the start of the short campaign period ahead of the election.
3. Special Advisers who leave government for any reason will no longer have preferential access to papers and officials. Any request for advice from a former Special Adviser will be treated in the same way as requests from other members of the public.
4. On leaving government, Special Advisers should return all departmental property e.g. mobile phones, remote access and other IT equipment. Special Advisers may leave a voicemail message or out of office reply on departmental IT with forwarding contact details.
5. Special Advisers receive severance pay when their appointment is terminated, but not where they resign. Severance pay for Special Advisers is taxable as normal income and will be paid as a lump sum. The amount an individual is entitled to will be determined by their length of service as set out in the Model Contract for Special Advisers. Special Advisers are required to agree that if they are reappointed, they will repay any amount above that which they would have been paid in salary had they remained in post. Any excess severance will be reclaimed automatically through payroll on reappointment.
6. If the Prime Minister agrees exceptionally that a Special Adviser should remain in post during the election period, their appointment will be automatically terminated the day after polling day. In those cases, Special Advisers may continue to give advice on government business to their Ministers as before. They must continue to adhere to the requirements of the Code of Conduct for Special Advisers and may not take any public part in the campaign. Section A is also relevant in relation to the commissioning of briefing.
7. Different arrangements can be made for Special Advisers on, or about to begin, maternity leave when a UK general election is called. These arrangements are set out in the Maternity Policy for Special Advisers, and Special Adviser HR are best placed to advise on specific circumstances.
8. If there is no change of government following the election, a Special Adviser may be reappointed. The Prime Minister’s approval will be required before any commitments are made, and a new contract issued, including for any advisers who have stayed in post.
Section C: Contacts with the Opposition Party
1. The Prime Minister has authorised pre-election contact between the main opposition parties and Permanent Secretaries from 11 January 2024. These contacts are strictly confidential and are designed to allow Opposition spokespeople to inform themselves of factual questions of departmental organisation and to inform civil servants of any organisational or policy changes likely in the event of a change of government.
2. Separate guidance on handling such contacts is set out in the Cabinet Manual.
Section D: Contact with Select Committees
1. House of Commons Select Committees set up by Standing Order continue in existence, technically, until that Standing Order is amended or rescinded. In practice, when Parliament is dissolved pending a general election, membership of committees lapses and work on their inquiries ceases.
2. House of Lords Select Committees are not set up by Standing Orders and technically cease to exist at the end of each session.
3. The point of contact for departments continues to be the Committee Clerk who remains in post to process the basic administrative work of the committee (and prepare for the re-establishment of the Committee in the next Parliament).
4. Departments should continue to work, on a contingency basis, on any outstanding evidence requested by the outgoing committee and on any outstanding government responses to committee reports. It will be for any newly-appointed Ministers to approve the content of any response. It will be for the newly-appointed committee to decide whether to continue with its predecessor committee’s inquiries and for the incoming administration to review the terms of draft responses before submitting to the newly appointed committee.
5. It is for the newly-appointed committee to decide whether to publish government responses to its predecessor reports. There may be some delay before the committee is reconstituted, and an incoming government may well wish to publish such responses itself by means of a Command Paper. In this event, the department should consult the Clerk of the Committee before publication of the report response.
Section E: Political Activities of Civil Servants
1. Permanent Secretaries will wish to remind staff of the general rules governing national political activities. These are set out in the Civil Service Management Code and departmental staff handbooks.
2. For this purpose, the Civil Service is divided into three groups:
a. the “politically free” – industrial and non-office grades;
b. the “politically restricted” – members of the Senior Civil Service, civil servants in Grades 6 and 7 (or equivalent) and members of the Fast Stream Development Programme; and
c. civil servants outside the “politically free” and “politically restricted” groups
3. Civil servants on secondment to outside organisations (or who are on any form of paid or unpaid leave) remain civil servants and the rules relating to political activity continue to apply to them. Departments should seek to contact individuals on secondment outside the civil service to remind them of this. Individuals seconded into the Civil Service are also covered by these rules for the duration of their appointment.
Civil Servants Standing for Parliament
4. All civil servants are disqualified from election to Parliament (House of Commons Disqualification Act 1975) and must resign from the Civil Service before standing for election. Individuals must resign from the Civil Service on their formal adoption as a prospective parliamentary candidate, and must complete their last day of service before their adoption papers are completed. If the adoption process does not reasonably allow for the individual to give full notice, departments and agencies may at their discretion pay an amount equivalent to the period of notice that would normally be given.
Other Political Activity
5. “Politically restricted” civil servants are prohibited from any participation in national political activities.
6. All other civil servants may engage in national political activities with the permission of the department, which may be subject to certain conditions.
7. Where, on a case by case basis, permission is given by departments, civil servants must still act in accordance with the requirements of the Civil Service Code, including ensuring that they meet the Code’s values and standards of behaviour about impartiality and political impartiality. Notwithstanding any permission to engage in national political activities, they must ensure that their actions (and the perception of those actions) are compatible with the requirements to:
- serve the government, whatever its political persuasion, to the best of their ability in a way which maintains political impartiality and is in line with the requirements of the Code, no matter what their own political beliefs are; and
- act in a way which deserves and retains the confidence of ministers, while at the same time ensuring that they will be able to establish the same relationship with those whom they may be required to serve in some future government.
Reinstatement
8. Departments and agencies must reinstate former civil servants who have resigned from “politically free” posts to stand for election and whose candidature has proved unsuccessful, provided they apply within a week of declaration day.
9. Departments and agencies have discretion to reinstate all other former civil servants who have resigned to stand for election and whose candidature has proved unsuccessful. Former civil servants in this category seeking reinstatement should apply within a week of declaration day if they are not elected. Departments are encouraged to consider all applications sympathetically and on their merits. For some individuals, it may not be possible to post them back to their former area of employment because, for instance, of the sensitivity of their work and/or because their previous job is no longer vacant. In these cases, every effort should be made to post these staff to other areas rather than reject their applications.
Section F: Cabinet and Official Documents
1. In order to enable Ministers to fulfil their continuing responsibilities as members of the Government during the election period, departments should retain the Cabinet documents issued to them. Cabinet documents refers to all papers, minutes and supplementary materials relating to Cabinet and its committees. This is applicable to meetings of and correspondence to Cabinet and its committees.
2. If there is no change of government after the election, Ministers who leave office or who move to another Ministerial position must surrender any Cabinet or Cabinet committee papers or minutes (including electronic copies) and they should be retained in the department in line with guidance issued by the Cabinet Office. Ministers who leave office or move to another Ministerial position should also not remove or destroy papers that are the responsibility of their former department: that is, those papers that are not personal, party or constituency papers.
3. If a new government is formed, all Cabinet and Cabinet committee documents issued to Ministers should be destroyed. Clearly no instructions can be given to this effect until the result of the election is known, but Permanent Secretaries may wish to alert the relevant Private Secretaries.
4. The conventions regarding the access by Ministers and Special Advisers to papers of a previous Administration are explained in more detail in the Cabinet Manual. Further guidance to departments will be issued by the Cabinet Office once the outcome of the election is known.
5. More detailed guidance on managing records in the event of a change of administration will be held by your Departmental Records Officer. The Head of Public Records and Archives in the Cabinet Office can also provide further advice and written guidance can be found here:
Guidance management of private office information and records
Section G: Government Decisions
1. During an election campaign the Government retains its responsibility to govern and Ministers remain in charge of their departments. Essential business (including routine business necessary to ensure the continued smooth functioning of government and public services) must be carried on. Cabinet committees are not expected to meet during the election period, nor are they expected to consider issues by correspondence. However there may be exceptional circumstances under which a collective decision of Ministers is required. If something requires collective agreement and cannot wait until after the General Election, the Cabinet Secretary should be consulted.
2. However, it is customary for Ministers to observe discretion in initiating any action of a continuing or long term character. Decisions on matters of policy, and other issues such as large and/or contentious commercial contracts, on which a new government might be expected to want the opportunity to take a different view from the present government, should be postponed until after the election, provided that such postponement would not be detrimental to the national interest or wasteful of public money.
Statutory Instruments
3. The principles outlined above apply to making statutory instruments.
Departmental lawyers can advise in more detail, in conjunction with the Statutory Instrument Hub.
4. The general principle that Ministers should observe discretion in initiating any new action of a continuing or long-term character applies to the making of commencement orders, which during the election period should be exceptional. As is usual practice, statutory instruments are required to go through the Parliamentary Business and Legislation Committee process before they can be laid.
Section H: Public and Senior Civil Service Appointments
1. All appointments requiring approval by the Prime Minister, and other Civil Service and public appointments likely to prove sensitive (including those where Ministers have delegated decisions to officials or other authorities) should be frozen until after the election, except in exceptional circumstances (further detail below). This includes appointments where a candidate has already accepted a written offer (and the appointment has been announced before the election period), but where the individual is not due to take up post until after the election. The individual concerned should be told that the appointment will be subject to confirmation by the new Administration after the election.
2. It is recognised that this may result in the cancellation (or delay) of an appointment by the new Administration, and that the relevant department could be vulnerable to legal action by a disappointed candidate. To reduce the risk of this, departments might wish to:
- recommend to their Secretary of State the advisability of bringing forward or delaying key stages in the process, where an appointment would otherwise likely take effect just before or after an election;
- issue a conditional offer letter, making it clear that the formal offer of the appointment will need to be confirmed by a new Administration.
3. In cases where an appointment is due to end between dissolution and election day, and no announcement has been made concerning the new appointment, it will normally be possible for the post to be left vacant or the current term extended until incoming Ministers have been able to take a decision either about reappointment of the existing appointee or the appointment of a new person. This situation is also likely to apply to any appointments made by Letters Patent, or otherwise requiring royal approval, since it would not be appropriate to invite His Majesty to make a conditional appointment.
4. In exceptional cases where it is not possible to apply these temporary arrangements and there is an essential need to make an appointment during the election period, departments may wish to advise their Ministers about consulting the Opposition before a final decision is taken. Departments should consult the Public Appointments Policy Team in the Cabinet Office.
5. In the case of public and Senior Civil Service appointments, departments should delay the launch of any open competition during an election period, to give any incoming Administration the option of deciding whether to follow the existing approach.
6. In those cases where an appointment is required to be made, it is acceptable, in the case of sensitive Senior Civil Service positions, to allow temporary promotion.
Section I: Communication Activities during a General Election
1. The general principle governing communication activities during a general election is to do everything possible to avoid competition with parliamentary candidates for the attention of the public, and not to undertake any activity that could call into question civil servants’ political impartiality or that could give rise to criticism that public resources are being used for party political purposes. Special care must be taken during the course of an election since material produced with complete impartiality, which would be accepted as objective in ordinary times, may generate criticism during an election period when feelings are running high. All communication activity should be conducted in line with Government Communication Service (GCS) guidance on propriety and propriety in digital and social media .
2. Departmental communications staff may properly continue to discharge their normal function during the election period, to the extent of providing factual explanation of current government policy, statements and decisions. They must be particularly careful not to become involved in a partisan way in election issues.
3. During the election period, access to departmental briefing systems will be restricted to permanent civil servants who will produce briefing, and answer requests for information, in line with the principles set out in Section A of the election guidance. Any updating of lines to take should be confined to matters of fact and explanations of existing government policy in order to avoid criticism of serving, or appearing to serve, a party political purpose.
News Media
4. In response to questions departments should, where possible, provide factual information by reference to published material, including that on websites. Specific requests for unpublished material should be handled in accordance with the requirements of the Freedom of Information Act.
5. Routine factual press notices may continue to be issued – for example statistics that are issued on a regular basis or reports of publicly-owned bodies, independent committees etc., which a department is required to publish.
6. There would normally be no objection to issuing routine factual publications, for example health and safety advice, but these should be decided on a case by case basis, in consultation with the Director or Head of Communications, who should take account of the subject matter and the intended audience. A similar approach should apply to blogs and social media.
7. Press releases and other material normally sent to Members of Parliament should cease at the point at which this guidance comes into effect.
8. Statements that refer to the future intentions of the Government should not be handled by a department and should be treated as party political statements. Where a Minister considers it necessary to hold a governmental press conference to make clear the Government’s existing policies on a particular subject prior to the election, then his or her department should provide facilities and give guidance. Ultimately, each case must be judged on its merits, including consideration of whether an announcement needs to be made, in consultation with the Director or Head of Communications.
9. The Propriety and Ethics Team in the Cabinet Office must be consulted before a Minister makes an official Ministerial statement during the election period.
10. Statements or comments referring to the policies, commitments or perceived intentions of Opposition parties should not be handled by departments.
Press Articles, Interviews, and Broadcasts and Webcasts by Ministers
11. During the election period, arrangements for newspaper articles, interviews and broadcasts by Ministers, including online, will normally be made on the political network. Care should be taken by communications staff in arranging any press interviews for Ministers during this period because of the possibility that such interviews would have a strong political content. They should not arrange broadcasts through official channels unless they are satisfied there is a need to do so and that the Minister is speaking in a government, not party, capacity.
Paid Media
12. Advertising, including partnership and influencer marketing. New campaigns will in general be postponed and live campaigns will be paused (across all advertising and marketing channels). A very small number of campaigns (for example, relating to essential recruitment, or public health, such as blood and organ donation or health and safety) may be approved by the Permanent Secretary, in consultation with GCS and the Propriety and Ethics Team.
a. International activity. Where marketing is delivered outside the UK and targeting non-UK citizens, the campaign can continue during the election period, subject to Permanent Secretary approval and as long as consideration has been given to the potential for the campaign to garner interest within the UK and to reach UK diaspora. If continuing the campaign is likely to generate domestic interest, it should be paused.
b. Official radio ‘fillers’ will be reviewed and withdrawn unless essential.
13. Films, videos and photographs from departmental libraries or sources should not be made available for use by political parties.
14. Printed material should not normally be given any fresh distribution in the United Kingdom during the election period, in order to avoid any competition with the flow of election material. The effect on departments that distribute posters and leaflets to the public is as follows:
a. Posters. The normal display of existing posters on official premises may continue but efforts should not be made to seek display elsewhere. Specific requests by employers, trade unions etc for particular posters may, however, be met in the ordinary way.
b. Leaflets. Small numbers of copies of leaflets may be issued on request to members of the public and to parliamentary candidates, in consultation with the Director or Head of Communications, who should take account of the subject matter and the intended audience. Bulk supplies should not be issued to any individuals or organisations without appropriate approval.
c. Export promotion stories and case studies for overseas use may continue to be sought in the UK but it must be made clear on each occasion that this information is needed for use abroad, and permission must be sought from the Permanent Secretary before proceeding.
d. The use of public buildings for communication purposes is covered in Section L.
15. Exhibitions. Official exhibitions on a contentious policy or proposal should not be kept open or opened during the election period. Official exhibitions that form part of a privately sponsored exhibition do not have to be withdrawn unless they are contentious, in which case they should be withdrawn.
Social Media and Digital Channels
16. Official websites and social media channels will be scrutinised closely by news media and political parties during the election period. All content must be managed in accordance with GCS propriety guidance.
Publishing content online
17. Content Design: planning, writing and managing content guidance should be consulted when publishing any online content.
18. Material that has already been published in accordance with the rules on propriety and that is part of the public domain record can stand. It may also be updated for factual accuracy, for example a change of address. However, while it can be referred to in handling media enquiries and signposting in response to enquiries from the public, nothing should be done to draw further attention to it.
19. Updating the public with essential factual information may continue (e.g. transport delays) but social media and blogs that comment on government policies and proposals should not be updated for the duration of the election period.
20. Ministers’ biographies and details of their responsibilities can remain on sites, no additions should be made. Social media profiles should not be updated during this period.
21. Site maintenance and planned functional and technical development for existing sites can continue, but this should not involve new campaigns or extending existing campaigns.
22. News sections of websites and blogs must comply with the advice on press releases. News tickers and other mechanisms should be discontinued for the election period.
23. In the event of an emergency, digital channels can be used as part of Crisis Communication activity in the normal way.
Further Guidance
24. In any case of doubt about the application of this guidance in a particular case, communications staff should consult their Director or Head of Communications in the first instance, then, if necessary, the Chief Executive, Government Communication Service, Chief Operating Officer, Government Communication Service, or the departmental Permanent Secretary who will liaise with the Propriety and Ethics Team in the Cabinet Office.
Section J: Guidance on Consultations during an election period
1. In general, new public consultations should not be launched during the election period. If there are exceptional circumstances where launching a consultation is considered essential (for example, safeguarding public health), permission should be sought from the Propriety and Ethics Team in the Cabinet Office.
2. If a consultation is on-going at the time this guidance comes into effect, it should continue as normal. However, departments should not take any steps during an election period that will compete with parliamentary candidates for the public’s attention. This effectively means a ban on publicity for those consultations that are still in process.
3. As these restrictions may be detrimental to a consultation, departments are advised to decide on steps to make up for that deficiency while strictly observing the guidance. That can be done, for example, by:
a. prolonging the consultation period; and
b. putting out extra publicity for the consultation after the election in order to revive interest (following consultation with any new Minister).
4. Some consultations, for instance those aimed solely at professional groups, and that carry no publicity, will not have the impact of those where a very public and wide-ranging consultation is required. Departments need, therefore, to take into account the circumstances of each consultation. Some may need no remedial action – but this is a practical rather than propriety question so long as departments observe the broader guidance here.
5. During the election period, departments may continue to receive and analyse responses with a view to putting proposals to the incoming government but they should not make any statement or generate publicity during this period.
Section K: Statistical Activities during a General Election
1. This note gives guidance on the conduct of statistical activities across government during a general election period. [footnote 1]
2. The same principles apply to social research and other government analytical services.
3. Under the terms of the Statistics and Registration Service Act 2007, the UK Statistics Authority, headed by the National Statistician, is responsible for promoting and safeguarding the integrity of official statistics. It should be consulted in any cases of doubt about the application of this guidance.
Key Principles
4. Statistical activities should continue to be conducted in accordance with the Code of Practice for Official Statistics and the UK Government’s Prerelease Access to Official Statistics Order 2008, taking great care, in each case, to avoid competition with parliamentary candidates for the attention of the public.
Statistical publications, releases, etc.
5. The greatest care must continue to be taken to ensure that information is presented impartially and objectively.
6. Regular pre-announced statistical releases (e.g. press notices, bulletins, publications or electronic releases) will continue to be issued and published. Any other ad hoc statistical releases should be released only in exceptional circumstances and with the approval of the National Statistician, consulting with the Propriety and Ethics Team in the Cabinet Office where appropriate. Where a pre-announcement has specified that the information would be released during a specified period (e.g. a week, or longer time period), but did not specify a precise day, releases should not be published within the election period. The same applies to social research publications
Requests for information
7. Any requests for unpublished statistics, including from election candidates, should be handled in an even-handed manner, in accordance with the Freedom of Information Act. Guidance on handling FOI requests can be found in Section A.
Commentary and Briefing
8. Special care must be taken in producing commentary for inclusion in announcements of statistical publications issued during the election period. Commentary that would be accepted as impartial and objective analysis or interpretation at ordinary times, may attract criticism during an election. Commentary by civil servants should be restricted to the most basic factual clarification during this period. Ultimately the content of the announcement is left to the discretion of the departmental Head of Profession, seeking advice from the National Statistician as appropriate.
9. Pre-election arrangements for statistics, whereby pre-release access for briefing purposes is given to Ministers or chief executives (and their appropriate briefing officials) who have policy responsibility for a subject area covered by a particular release, should continue, in accordance with the principles embodied in the UK Government’s Pre-release Access to Official Statistics Order 2008.
10. In general, during this period, civil servants involved in the production of official statistics will not provide face to face briefing to Ministers. Only if there is a vital operational need for information, (e.g. an out of the ordinary occurrence of market-sensitive results with significant implications for the economy, or some new management figures with major implications for the running of public services), should such briefing be provided. Any such briefing should be approved by the National Statistician.
11. Requests for advice on the interpretation or analysis of statistics should be handled with care, and in accordance with the guidance in paragraphs 6 and 7.
12. Requests for factual guidance on methodology should continue to be met.
13. Requests for small numbers of copies of leaflets, background papers or free publications that were available before the election period may continue to be met but no bulk issues to individuals or organisations should be made without appropriate approval. Regular mailings of statistical bulletins to customers on existing mailing lists may continue.
Censuses, Surveys and other forms of quantitative or qualitative research enquiry
14. Regular, continuous and on-going censuses and surveys of individuals, households, businesses or other organisations may continue. Ad hoc surveys and other forms of research that are directly related to and in support of a continuing statistical series may also continue. Ad hoc surveys and other forms of research that may give rise to controversy or be related to an election issue should be postponed or abandoned.
Consultations
15. Statistical consultations that are on-going at the point at which Parliament dissolves should continue as normal, but any publicity for such consultations should cease. New public consultations, even if preannounced, should not be launched but should be delayed until after the result of the election is officially declared.
Further Advice
16. If officials working on statistics in any area across government are unsure about any matters relating to their work during the election period, they should seek the advice of their Head of Profession in the first instance. Heads of Profession should consult the National Statistician in any cases of doubt. Queries relating to social research, or other analytical services should similarly be referred to the relevant Head of Profession or departmental lead and Permanent Secretary’s office in the first instance. Further advice can be sought from the Propriety and Ethics Team in the Cabinet Office.
Section L: Use of Government Property
1. Neither Ministers, nor any other parliamentary candidates, should involve government establishments in the general election campaign by visiting them for electioneering purposes.
2. In the case of NHS property, decisions are for the relevant NHS Trust but should visits be permitted to, for example, hospitals, the Department of Health and Social Care advise that there should be no disruption to services and the same facilities should be offered to other candidates. In any case, it is advised that election meetings should not be permitted on NHS premises. NHS England publishes its own information to NHS organisations about the pre-election period.
3. Decisions on the use of other public sector and related property must be taken by those legally responsible for the premises concerned – for example, for schools, the Governors or the Local Education Authority or Trust Board, and so on. If those concerned consult departments, they should be told that the decision is left to them but that they will be expected to treat the candidates of all parties in an even-handed way, and that there should be no disruption to services. The Department for Education will provide advice to schools on the use of school premises and resources.
4. It is important that those legally responsible for spending public funds or the use of public property ensure that there is no misuse, or the perception of misuse, for party political purposes. Decision-makers must respect the Seven Principles of Public Life when considering the use of public funds or property during the election period. The principles include an expectation that public office holders take decisions impartially, fairly and on merit and maintain their accountability to the public for their decisions and actions.
Section M: International Business
1. This guidance specifically addresses the principles that will apply to international business.
2. International business will continue as normal during the period of the general election.
International meetings
3. Decisions on Ministerial attendance and representation at international meetings will continue to be taken on a case by case basis by the lead UK Minister. For example, Ministers will be entitled to attend international summits (such as meetings of the G20).
4. When Ministers speak at international meetings, they are fully entitled to pursue existing UK Government policies. All Ministers, whether from the UK Government or the Devolved Administrations, should avoid exploiting international engagements for electoral purposes. Ministers should observe discretion on new initiatives and before stating new positions or making new commitments (see Section G for further advice on Government decision-making).
5. Where a Minister is unable to attend an international meeting that has been assessed as of significant interest to the UK, the UK may be represented by a senior official. In this case, where an item is likely to be pressed to a decision (a legislative decision, or some other form of commitment, e.g. a resolution, conclusions), officials should engage in negotiations and vote in line with the cleared UK position and in line with a detailed brief cleared by the lead UK Minister. Officials should engage actively where there will be a general discussion or orientation debate, but should seek to avoid taking high profile decisions on issues of domestic political sensitivity. If decisions fall to be taken at an international summit that risk being controversial between the UK political parties, departments should consult their Permanent Secretary about the line to follow who may in turn wish to consult the Cabinet Secretary.
Changes to International Negotiating Positions
6. There may be an unavoidable need for changes to a cleared UK position that require the collective agreement of Ministers. This may arise, for example, through the need for officials to have sufficiently clear negotiating instructions or as a result of the agreed UK position coming under pressure in the closing stages of negotiation. If collective agreement is required, the Cabinet Secretary should be consulted (see Section G). The Cabinet Secretariat can advise departments where they are unsure whether an issue requires further collective agreement.
7. Departments should note that the reduced availability of Ministers during the election period means that it will be necessary to allow as much time as possible for Ministers to consider an issue.
Relations with the Press
8. Departmental Communication staff may properly continue to discharge, during the election period, their normal function only to the extent of providing factual explanation of current government policy, statements and decisions. They must be particularly careful not to become involved in a partisan way in election issues.
9. Ministers attending international meetings will no doubt wish to brief the press afterwards in the normal manner. But where officials attend meetings in place of Ministers, they should be particularly circumspect in responding to the press on any decision or discussion in the meeting that could be regarded as touching on matters of domestic political sensitivity. If departments wish to issue press notices following international meetings on the discussions or decisions that took place, they should be essentially factual. Any comment, especially on items of domestic sensitivity, should be made by Ministers. In doing so, consideration will need to be given as to whether such comment should be handled by the department or the party. This must be agreed in advance with the Permanent Secretary.
International Appointments
10. The UK should not normally make nominations or put forward candidates for senior international appointments until after the election. It remains possible to make nominations or put forward candidates for other positions. Departments should consult their Permanent Secretary and the Propriety and Ethics Team in Cabinet Office on appointments that risk being controversial between the UK political parties.
Section N: The Devolved Administrations
1. The general election does not affect the devolved administrations in the same way. The devolved legislatures are elected separately to the House of Commons. Devolved Ministers in Scotland, Wales and Northern Ireland will continue to carry out their devolved functions in those countries as usual.
2. Under the Civil Service Code, which also applies to all civil servants, civil servants in the devolved administrations serve Ministers elected through elections in Scotland, Wales and Northern Ireland and do not report to the UK Government. Accordingly, this guidance does not apply to them. They will continue to support their Ministers in their work.
3. However, the devolved administrations acknowledge that their activities could have a bearing on the general election campaign. While the devolved administrations will continue largely as normal, they are aware of the need to avoid any action that is, or could be construed as being, party political or likely to have a direct bearing on the general election. Staff in the devolved administrations will continue to refer requests for information about reserved issues from MPs, parliamentary candidates and political parties to the relevant UK department. Requests for information about devolved issues will be handled in accordance with relevant FOI legislation, taking account of the need for prompt responses in the context of an election period.
4. Officials in the devolved administrations are subject to the rules in Section E as regards their personal political activities, in the same way as UK Government officials.
5. Discussions with the devolved administrations during the election period should be conducted in this context. For more general details on how best to work with the devolved administrations see the Cabinet Office guidance: Devolution guidance for civil servants
Section O: Public Bodies
1. The general principles and conventions set out in this guidance apply to the board members and staff of all NDPBs and similar public bodies. Some NDPBs and ALBs employ civil servants.
2. NDPBs and other public sector bodies must be, and be seen to be, politically impartial. They should avoid becoming involved in party political controversy. Decisions on individual matters are for the bodies concerned in consultation with their sponsor department who will wish to consider whether proposed activities could reflect adversely on the work or reputation of the NDPB or public body in question.
This includes departments and their agencies and other relevant public bodies including all public bodies deemed to be producers of official statistics by dint of an Order in Parliament. ↩
Is this page useful?
- Yes this page is useful
- No this page is not useful
Help us improve GOV.UK
Don’t include personal or financial information like your National Insurance number or credit card details.
To help us improve GOV.UK, we’d like to know more about your visit today. Please fill in this survey (opens in a new tab) .

IMAGES
VIDEO
COMMENTS
According to Resnik (2011), many people think of ethics as a set of rules distinguishing right from wrong, but actually the term "ethics" refers to norms of conduct or of action and in disciplines of study. Research ethics or norms promote the "knowledge, truth, and avoidance of error" (p. 1) and protect against "fabricating ...
Revised on May 9, 2024. Ethical considerations in research are a set of principles that guide your research designs and practices. Scientists and researchers must always adhere to a certain code of conduct when collecting data from people. The goals of human research often include understanding real-life phenomena, studying effective treatments ...
INTRODUCTION. Fostering scientific advancement requires strict adherence to ethical guidelines for research and scientific writing. Several professional organizations have policies to address the ethics associated with scientific writing and publishing, including the Committee on Publication Ethics and the International Council of Medical Journal Editors (ICMJE); the majority of medical ...
Abstract. Published articles in scientific journals are a key method for knowledge-sharing. Researchers can face the pressures to publish and this can sometimes lead to a breach of ethical values, whether consciously or unconsciously. The prevention of such practices is achieved by the application of strict ethical guidelines applicable to ...
A Guide to Writing in Ethical Reasoning 15 | page 1 Introduction This guide is intended to provide advice for students writing the papers in Ethical Reasoning 15. Most of the paper assignments for the course can be approached flexibly and creatively — there is no single recipe for writing successful papers in the course.
Conducting research without informed consent or ethics approval and not maintaining data confidentiality is a form of scientific misconduct. Editors or publication houses do take disciplinary action as per COPE recommendations against scientific misconduct. ... research, analysis, or writing of a paper. Often guest or gift authors are well ...
Abstract. Scientific writing is the process of putting information and thinking into a final permanent report, so it can be read and used by other people. For any given research study, there are innumerable various ways to legitimately write that report (depending on what exactly the authors want to say and how).
Research ethics are moral principles that need to be adhered to when conducting a research study as well as when writing a scientific article, with the prime aim of avoiding deception or intent to harm study's participants, the scientific community, and society. Practicing and adhering to research ethics is essential for personal integrity as ...
The Ethics of Research, Writing, and Publication. Jaynelle F. Stichler, DNS, RN, NEA-BC, EDAC, FACHE, FAAN. Nothing is more exciting than seeing your name in print as the author of a well-written article in a respected, peer-reviewed, scholarly jour-nal. A published article is the ultimate goal of a research project, an evidence-based design ...
Ethics and ethical behaviour are the fundamental pillars of a civilised society. The focus on ethical behaviour is indispensable in certain fields such as medicine, finance, or law. In fact, ethics gets precedence with anything that would include, affect, transform, or influence upon individuals, communities or any living creatures. Many institutions within Europe have set up their own ...
In any case, a course in research ethics can be useful in helping to prevent deviations from norms even if it does not prevent misconduct. Education in research ethics is can help people get a better understanding of ethical standards, policies, and issues and improve ethical judgment and decision making.
Publication of scientific paper is critical for modern science evolution, and professional advancement. However, it comes with many responsibilities. An author must be aware of good publication practices. While refraining from scientific misconduct or research frauds, authors should adhere to Good Publication Practices (GPP). Publications which draw conclusions from manipulated or fabricated ...
Ethical considerations in research are a set of principles that guide your research designs and practices. Scientists and researchers must always adhere to a certain code of conduct when collecting data from people. The goals of human research often include understanding real-life phenomena, studying effective treatments, investigating ...
Describing all the different project phases based on writing within a research project, the paper distinguishes different influences on the distribution of power that emerge through a focus on written communication. The focus of the present paper is to illuminate the issues of ethics, power and the dimensions of hierarchy, physical location and ...
How to Write Ethical Considerations. When writing about research involving human subjects or animals, it is essential to include ethical considerations to ensure that the study is conducted in a manner that is morally responsible and in accordance with professional standards. Here are some steps to help you write ethical considerations:
s Ethics Toolkit contains introductory materials to help you get started, and you can visit the Ethics in Re Publication website at ethics .com and download the files mentioned in this T lus you will also find more tools including: webinars hosted by the experts -depth personal interviews, topical videos, white papers and timely ethics
PRINCIPLES OF WRITING A SCIENTIFIC PAPER. Scientific research demands precision.[2,3,4] Scientific writing should respect this precision in the form of clarity.Unfortunately, a glance at almost any scientific journal will reveal that the above-stated ideal is often not attained in the real world of scholarly publication.[5,6] Indeed, many of the accusations by nonscientific of "obscurity ...
Abstract. Ethics, as an integral component of human decision-making, undeniably shape the landscape of scientific research. This article delves deeply into the nuanced realm of ethical ...
2. Choose a topic for your ethics paper. If you're writing the paper as a class assignment, the topic may already be given to you. If not, choose a topic that is both interesting to you and that you know a good deal about. Your topic should be very broad at first, after which you can develop it into a specific inquiry.
An ethics paper is a type of an argumentative assignment that deals with a certain ethical problem that a student has to describe and solve. Also, it can be an essay where a certain controversial event or concept is elaborated through an ethical lens (e.g. moral rules and principles), or a certain ethical dilemma is explained.
Permanence—Published articles are included in a permanent, searchable knowledge base, that is, the "knowledge system". Perfection-editing, reviewing, revising etc. the process of modification. Broadness—available to a large number of readers. Reliability—The published article has the QUALITY ASSURANCE mark because it is recognized by many ...
Introduction. Ethics are a guiding principle that shapes the conduct of researchers. It influences both the process of discovery and the implications and applications of scientific findings 1.Ethical considerations in research include, but are not limited to, the management of data, the responsible use of resources, respect for human rights, the treatment of human and animal subjects, social ...
The Chicago/Turabian style of citing sources is generally used when citing sources for humanities papers, and is best known for its requirement that writers place bibliographic citations at the bottom of a page (in Chicago-format footnotes) or at the end of a paper (endnotes). The Turabian and Chicago citation styles are almost identical, but ...
Using artificial intelligence (AI) in research offers many important benefits for science and society but also creates novel and complex ethical issues. While these ethical issues do not necessitate changing established ethical norms of science, they require the scientific community to develop new guidance for the appropriate use of AI. In this article, we briefly introduce AI and explain how ...
This paper will discuss different ethical issues in research, including study design and ethical approval, data analysis, authorship, conflict of interest and redundant publication and plagiarism. I have also included two case scenarios in this paper to illustrate common ethical issues in research and publication. Go to:
This guidance takes effect from 00:01 on 25 May 2024 at which point the 'election period' begins. The Prime Minister will write separately to Ministers advising them of the need to adhere to ...