

Centre for Big Data Research in Health
Enhancing the health and wellbeing of all, by maximising the productive use of all possible sources of health big data in medical research.

PhD Top-Up Scholarships
The Centre for Big Data Research in Health (CBDRH) is excited to launch Top-Up Scholarships for high-achieving domestic and international candidates seeking to start a PhD in 2025.

Data-driven health solutions
We are Australia’s first research centre dedicated to health research using big data. Our research is collaborative, involving codesign and coproduction methods with consumers, communities and health care providers. Together, we aim to facilitate long term translation and implementation into health policy, service and programs.
We are privileged to have many enthusiastic partners including government agencies, health organisations from the public, private and not-for-profit sectors, research funders, clinicians, health consumers and community members.

We are Australia’s first research centre dedicated to health research using large-scale electronic data spanning the biomedical, clinical, health services and public health domains.

Clinical registries systematically collect health-related information to monitor and improve quality of care, support evidence-based research, and inform patients and healthcare professionals about specific clinical areas or treatments.

The Machine Learning in Health Club is a semi-formal weekly seminar hosted by the Centre for Big Data Research in Health.

Learn more about the student experience at the UNSW Medicine & Health Centre for Big Data Research in Health (CBDRH).
Thank you for visiting nature.com. You are using a browser version with limited support for CSS. To obtain the best experience, we recommend you use a more up to date browser (or turn off compatibility mode in Internet Explorer). In the meantime, to ensure continued support, we are displaying the site without styles and JavaScript.
- View all journals
- Explore content
- About the journal
- Publish with us
- Sign up for alerts
Collection 16 June 2021
Conceptualising health research participation in the era of big data
The rise of big data in health care research, particularly when incorporated into health care delivery, presents a complex landscape where the role, status and value of the patient or citizen as a research subject is configured in numerous ways. Social science scholars have drawn attention to the potential for health research participation to constitute exploitation, empowerment or even a form of contemporary citizenship. Others have considered the results of participation in terms of the (bio)value attached to bodily samples through, for example, commodity exchange or the assetisation of patients, samples and/or data. Emerging big data research practices add another dimension to these issues. They raise questions about how we make sense of health research participation in the change towards datafication of human health, and the automation of data agglomeration and analysis. Such practices also raise questions about their governance by prompting us to ask whether existing local and centralised ethical regimes are fit for purpose.
Considerations of related discourses, practices and oversight are vital as ‘participation’ in health research has multiple forms, takes place in diverse settings, and is sponsored by different kinds of entities. From trial subject to patient advisory group member, from biobank donor to the infinitely searchable database entry, each of these forms are affected in some way by emerging big data practices. Participation is complicated further by research itself becoming more globally collaborative and thus dealing with multiple local contexts.
This Collection seeks to examine the diverse ways big data and health research participation converge and are co-produced with local and centralised approaches to governance. Drawing from the fields of sociology, anthropology, science and technology studies, health research, empirical ethics, bioethics, and critical data studies, we ask authors to engage with these two overarching questions: How is the health research participant constituted, valued and assetised in the era of big data? What are the implications of this for health research practices and/or policy making?
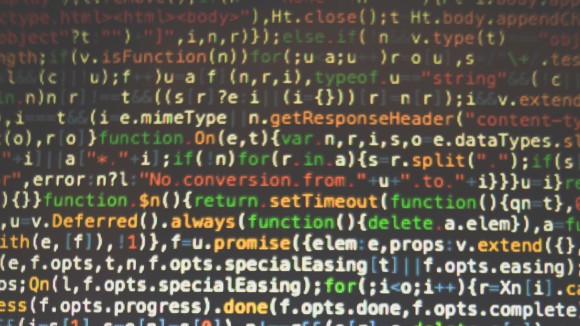
David Wyatt
King's College London, UK
Matthias Wienroth
Northmbria University, UK
Christopher McKevitt
- Collection content
- About the Guest Editors
- Collection policies
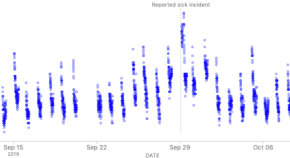
Protecting communities during the COVID-19 global health crisis: health data research and the international use of contact tracing technologies
- Toija Cinque
The participatory turn in health and medicine: The rise of the civic and the need to ‘give back’ in data-intensive medical research
- Lotje E. Siffels
- Tamar Sharon
- Andrew S. Hoffman
Common good in the era of data-intensive healthcare
- Kirsikka Grön
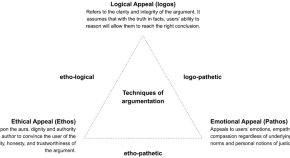
Public engagement with health data governance: the role of visuality
- Joanna Sleigh
- Effy Vayena
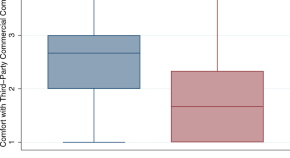
The public’s comfort with sharing health data with third-party commercial companies
- M. Grace Trinidad
- Jodyn Platt
- Sharon L. R. Kardia
Data promiscuity: how the public–private distinction shaped digital data infrastructures and notions of privacy
- Klaus Hoeyer
Quick links
- Explore articles by subject
- Guide to authors
- Editorial policies


An official website of the United States government
The .gov means it’s official. Federal government websites often end in .gov or .mil. Before sharing sensitive information, make sure you’re on a federal government site.
The site is secure. The https:// ensures that you are connecting to the official website and that any information you provide is encrypted and transmitted securely.
- Publications
- Account settings
- My Bibliography
- Collections
- Citation manager
Save citation to file
Email citation, add to collections.
- Create a new collection
- Add to an existing collection
Add to My Bibliography
Your saved search, create a file for external citation management software, your rss feed.
- Search in PubMed
- Search in NLM Catalog
- Add to Search
Risks and Opportunities to Ensure Equity in the Application of Big Data Research in Public Health
Affiliations.
- 1 Department of Epidemiology and Biostatistics, University of California, San Francisco, California, USA; email: [email protected].
- 2 Bakar Computational Health Sciences Institute, University of California, San Francisco, California, USA.
- 3 Department of Radiation Oncology, University of California, San Francisco, California, USA.
- 4 Department of Health Behavior and Health Education, School of Public Health, University of Michigan, Ann Arbor, Michigan, USA.
- 5 Department of Social, Behavioral and Population Sciences, School of Public Health and Tropical Medicine, Tulane University, New Orleans, Louisiana, USA.
- 6 Department of Medicine, University of California, San Francisco, California, USA.
- 7 Zuckerberg San Francisco General Hospital and Trauma Center, San Francisco, California, USA.
- 8 Partnerships for Research in Implementation Science for Equity (PRISE), University of California, San Francisco, California, USA.
- PMID: 34871504
- PMCID: PMC8983486
- DOI: 10.1146/annurev-publhealth-051920-110928
The big data revolution presents an exciting frontier to expand public health research, broadening the scope of research and increasing the precision of answers. Despite these advances, scientists must be vigilant against also advancing potential harms toward marginalized communities. In this review, we provide examples in which big data applications have (unintentionally) perpetuated discriminatory practices, while also highlighting opportunities for big data applications to advance equity in public health. Here, big data is framed in the context of the five Vs (volume, velocity, veracity, variety, and value), and we propose a sixth V, virtuosity, which incorporates equity and justice frameworks. Analytic approaches to improving equity are presented using social computational big data, fairness in machine learning algorithms, medical claims data, and data augmentation as illustrations. Throughout, we emphasize the biasing influence of data absenteeism and positionality and conclude with recommendations for incorporating an equity lens into big data research.
Keywords: health equity; machine learning; multilevel models; multiple systems estimation.
PubMed Disclaimer
The 6 Vs of Big…
The 6 Vs of Big Data Adapted for conceptualizing Big Data with Foregrounding…
Similar articles
- Big Data in Public Health: Terminology, Machine Learning, and Privacy. Mooney SJ, Pejaver V. Mooney SJ, et al. Annu Rev Public Health. 2018 Apr 1;39:95-112. doi: 10.1146/annurev-publhealth-040617-014208. Epub 2017 Dec 20. Annu Rev Public Health. 2018. PMID: 29261408 Free PMC article. Review.
- A digital highway for data fluidity and data equity in precision medicine. Chin L, Khozin S. Chin L, et al. Biochim Biophys Acta Rev Cancer. 2021 Aug;1876(1):188575. doi: 10.1016/j.bbcan.2021.188575. Epub 2021 May 29. Biochim Biophys Acta Rev Cancer. 2021. PMID: 34062153 Review.
- Big data, machine learning, and population health: predicting cognitive outcomes in childhood. Bowe AK, Lightbody G, Staines A, Murray DM. Bowe AK, et al. Pediatr Res. 2023 Jan;93(2):300-307. doi: 10.1038/s41390-022-02137-1. Epub 2022 Jun 9. Pediatr Res. 2023. PMID: 35681091 Free PMC article. Review.
- ASAS-NANP symposium: mathematical modeling in animal nutrition-Making sense of big data and machine learning: how open-source code can advance training of animal scientists. Brennan JR, Menendez HM 3rd, Ehlert K, Tedeschi LO. Brennan JR, et al. J Anim Sci. 2023 Jan 3;101:skad317. doi: 10.1093/jas/skad317. J Anim Sci. 2023. PMID: 37997926
- Big Data/AI in Neurocritical Care: Maybe/Summary. Suarez JI. Suarez JI. Neurocrit Care. 2022 Aug;37(Suppl 2):166-169. doi: 10.1007/s12028-021-01422-x. Epub 2021 Dec 29. Neurocrit Care. 2022. PMID: 34966957
- Inter-institutional data-driven education research: consensus values, principles, and recommendations to guide the ethical sharing of administrative education data in the Canadian medical education research context. Grierson L, Cavanagh A, Youssef A, Lee-Krueger R, McNeill K, Button B, Kulasegaram K. Grierson L, et al. Can Med Educ J. 2023 Nov 8;14(5):113-120. doi: 10.36834/cmej.75874. eCollection 2023 Nov. Can Med Educ J. 2023. PMID: 38045068 Free PMC article.
- Exploring Online Crowdfunding for Cancer-Related Costs Among LGBTQ+ (Lesbian, Gay, Bisexual, Transgender, Queer, Plus) Cancer Survivors: Integration of Community-Engaged and Technology-Based Methodologies. Waters AR, Turner C, Easterly CW, Tovar I, Mulvaney M, Poquadeck M, Johnston H, Ghazal LV, Rains SA, Cloyes KG, Kirchhoff AC, Warner EL. Waters AR, et al. JMIR Cancer. 2023 Oct 30;9:e51605. doi: 10.2196/51605. JMIR Cancer. 2023. PMID: 37902829 Free PMC article.
- The inclusion of social determinants of health into evaluations of quality and appropriateness of AI assistant-ChatGPT. Hswen Y, Nguyen TT. Hswen Y, et al. Prostate Cancer Prostatic Dis. 2024 Mar;27(1):157. doi: 10.1038/s41391-023-00720-z. Epub 2023 Sep 13. Prostate Cancer Prostatic Dis. 2024. PMID: 37704752 No abstract available.
- Community perspectives on AI/ML and health equity: AIM-AHEAD nationwide stakeholder listening sessions. Vishwanatha JK, Christian A, Sambamoorthi U, Thompson EL, Stinson K, Syed TA. Vishwanatha JK, et al. PLOS Digit Health. 2023 Jun 30;2(6):e0000288. doi: 10.1371/journal.pdig.0000288. eCollection 2023 Jun. PLOS Digit Health. 2023. PMID: 37390116 Free PMC article.
- Community engagement in the development of health-related data visualizations: a scoping review. Chau D, Parra J, Santos MG, Bastías MJ, Kim R, Handley MA. Chau D, et al. J Am Med Inform Assoc. 2024 Jan 18;31(2):479-487. doi: 10.1093/jamia/ocad090. J Am Med Inform Assoc. 2024. PMID: 37279890 Free PMC article. Review.
- Abrams MP, Torres FE, Little SJ. Biometric Registration to an HIV Research Study may Deter Participation. AIDS Behav [Internet]. 2021;25(5):1552–9. Available from: 10.1007/s10461-020-02995-y - DOI - PMC - PubMed
- Acquisti A, & Gross R. (2006). Imagined communities: Awareness, information sharing, and privacy on the Facebook. Paper presented at the International workshop on privacy enhancing technologies.
- Agarwal A, Beygelzimer A, Dud´ık M, Langford J, and Wallach H. (2018). A reductions approach to fair classification. In International Conference on Machine Learning
- Anderson MJ, Fienberg SE. Who Counts? The politics of census-taking in contemporary America. New York: Russell Sage Foundation; 1999. 119–127 p.
- Ayhan CHB, Bilgin H, Uluman OT, Sukut O, Yilmaz S, & Buzlu S. (2020). A systematic review of the discrimination against sexual and gender minority in health care settings. International Journal of Health Services, 50(1), 44–61. - PubMed
Publication types
- Search in MeSH
Grants and funding
- T32 MH019105/MH/NIMH NIH HHS/United States
- K08 EB026500/EB/NIBIB NIH HHS/United States
- 75N91020C00039/CA/NCI NIH HHS/United States
- K01 MH119910/MH/NIMH NIH HHS/United States
- K01 AI145572/AI/NIAID NIH HHS/United States
- R56 AR063705/AR/NIAMS NIH HHS/United States
- R01 DK115492/DK/NIDDK NIH HHS/United States
LinkOut - more resources
Full text sources.
- Europe PubMed Central
- Ingenta plc
- PubMed Central
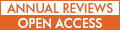
- Citation Manager
NCBI Literature Resources
MeSH PMC Bookshelf Disclaimer
The PubMed wordmark and PubMed logo are registered trademarks of the U.S. Department of Health and Human Services (HHS). Unauthorized use of these marks is strictly prohibited.
- Introduction
- Conclusions
- Article Information
This AI-readiness framework serves as a guide for data set stakeholders to consider when creating health data sets for ML research. The framework incorporates data quality expectations and provides context about the contemporary needs of ML researchers.
eMethods. Survey on Participant Demographics, Data Roles, and Role Responsibilities
eTable. Data Quality Dimensions, Elements, and Attributes for AI-Readiness Framework Development
Data Sharing Statement
See More About
Sign up for emails based on your interests, select your interests.
Customize your JAMA Network experience by selecting one or more topics from the list below.
- Academic Medicine
- Acid Base, Electrolytes, Fluids
- Allergy and Clinical Immunology
- American Indian or Alaska Natives
- Anesthesiology
- Anticoagulation
- Art and Images in Psychiatry
- Artificial Intelligence
- Assisted Reproduction
- Bleeding and Transfusion
- Caring for the Critically Ill Patient
- Challenges in Clinical Electrocardiography
- Climate and Health
- Climate Change
- Clinical Challenge
- Clinical Decision Support
- Clinical Implications of Basic Neuroscience
- Clinical Pharmacy and Pharmacology
- Complementary and Alternative Medicine
- Consensus Statements
- Coronavirus (COVID-19)
- Critical Care Medicine
- Cultural Competency
- Dental Medicine
- Dermatology
- Diabetes and Endocrinology
- Diagnostic Test Interpretation
- Drug Development
- Electronic Health Records
- Emergency Medicine
- End of Life, Hospice, Palliative Care
- Environmental Health
- Equity, Diversity, and Inclusion
- Facial Plastic Surgery
- Gastroenterology and Hepatology
- Genetics and Genomics
- Genomics and Precision Health
- Global Health
- Guide to Statistics and Methods
- Hair Disorders
- Health Care Delivery Models
- Health Care Economics, Insurance, Payment
- Health Care Quality
- Health Care Reform
- Health Care Safety
- Health Care Workforce
- Health Disparities
- Health Inequities
- Health Policy
- Health Systems Science
- History of Medicine
- Hypertension
- Images in Neurology
- Implementation Science
- Infectious Diseases
- Innovations in Health Care Delivery
- JAMA Infographic
- Law and Medicine
- Leading Change
- Less is More
- LGBTQIA Medicine
- Lifestyle Behaviors
- Medical Coding
- Medical Devices and Equipment
- Medical Education
- Medical Education and Training
- Medical Journals and Publishing
- Mobile Health and Telemedicine
- Narrative Medicine
- Neuroscience and Psychiatry
- Notable Notes
- Nutrition, Obesity, Exercise
- Obstetrics and Gynecology
- Occupational Health
- Ophthalmology
- Orthopedics
- Otolaryngology
- Pain Medicine
- Palliative Care
- Pathology and Laboratory Medicine
- Patient Care
- Patient Information
- Performance Improvement
- Performance Measures
- Perioperative Care and Consultation
- Pharmacoeconomics
- Pharmacoepidemiology
- Pharmacogenetics
- Pharmacy and Clinical Pharmacology
- Physical Medicine and Rehabilitation
- Physical Therapy
- Physician Leadership
- Population Health
- Primary Care
- Professional Well-being
- Professionalism
- Psychiatry and Behavioral Health
- Public Health
- Pulmonary Medicine
- Regulatory Agencies
- Reproductive Health
- Research, Methods, Statistics
- Resuscitation
- Rheumatology
- Risk Management
- Scientific Discovery and the Future of Medicine
- Shared Decision Making and Communication
- Sleep Medicine
- Sports Medicine
- Stem Cell Transplantation
- Substance Use and Addiction Medicine
- Surgical Innovation
- Surgical Pearls
- Teachable Moment
- Technology and Finance
- The Art of JAMA
- The Arts and Medicine
- The Rational Clinical Examination
- Tobacco and e-Cigarettes
- Translational Medicine
- Trauma and Injury
- Treatment Adherence
- Ultrasonography
- Users' Guide to the Medical Literature
- Vaccination
- Venous Thromboembolism
- Veterans Health
- Women's Health
- Workflow and Process
- Wound Care, Infection, Healing
Get the latest research based on your areas of interest.
Others also liked.
- Download PDF
- X Facebook More LinkedIn
Ng MY , Youssef A , Miner AS, et al. Perceptions of Data Set Experts on Important Characteristics of Health Data Sets Ready for Machine Learning : A Qualitative Study . JAMA Netw Open. 2023;6(12):e2345892. doi:10.1001/jamanetworkopen.2023.45892
Manage citations:
© 2024
- Permissions
Perceptions of Data Set Experts on Important Characteristics of Health Data Sets Ready for Machine Learning : A Qualitative Study
- 1 Department of Medicine (Biomedical Informatics), Stanford University School of Medicine, Stanford, California
- 2 Department of Biomedical Data Science, Stanford University School of Medicine, Stanford, California
- 3 Department of Radiology, Stanford University School of Medicine, Stanford, California
- 4 Department of Psychiatry and Behavioral Sciences, Stanford University School of Medicine, Stanford, California
- 5 Department of Pediatrics, Stanford University School of Medicine, Stanford, California
Question What makes data sets for artificial intelligence (AI) ready for health and biomedical machine learning (ML) research purposes?
Findings In this qualitative study consisting of interviews with 20 data set experts who are creators and/or ML researchers, participants largely appraised data set AI readiness with a set of intrinsic and contextual elements, described what they perceived as optimal characteristics of AI-ready data sets, and provided insights on what factors facilitate the creation of AI-ready data sets. Ethical acquisition and societal impact emerged as appraisal considerations that have not been described in prior data quality frameworks.
Meaning The findings of this study suggest that strategic updates to data set creation practices are warranted in the advent of AI and ML to better develop reliable, relevant, and ethical clinical applications for patient care.
Importance The lack of data quality frameworks to guide the development of artificial intelligence (AI)-ready data sets limits their usefulness for machine learning (ML) research in health care and hinders the diagnostic excellence of developed clinical AI applications for patient care.
Objective To discern what constitutes high-quality and useful data sets for health and biomedical ML research purposes according to subject matter experts.
Design, Setting, and Participants This qualitative study interviewed data set experts, particularly those who are creators and ML researchers. Semistructured interviews were conducted in English and remotely through a secure video conferencing platform between August 23, 2022, and January 5, 2023. A total of 93 experts were invited to participate. Twenty experts were enrolled and interviewed. Using purposive sampling, experts were affiliated with a diverse representation of 16 health data sets/databases across organizational sectors. Content analysis was used to evaluate survey information and thematic analysis was used to analyze interview data.
Main Outcomes and Measures Data set experts’ perceptions on what makes data sets AI ready.
Results Participants included 20 data set experts (11 [55%] men; mean [SD] age, 42 [11] years), of whom all were health data set creators, and 18 of the 20 were also ML researchers. Themes (3 main and 11 subthemes) were identified and integrated into an AI-readiness framework to show their association within the health data ecosystem. Participants partially determined the AI readiness of data sets using priority appraisal elements of accuracy, completeness, consistency, and fitness. Ethical acquisition and societal impact emerged as appraisal considerations in that participant samples have not been described to date in prior data quality frameworks. Factors that drive creation of high-quality health data sets and mitigate risks associated with data reuse in ML research were also relevant to AI readiness. The state of data availability, data quality standards, documentation, team science, and incentivization were associated with elements of AI readiness and the overall perception of data set usefulness.
Conclusions and Relevance In this qualitative study of data set experts, participants contributed to the development of a grounded framework for AI data set quality. Data set AI readiness required the concerted appraisal of many elements and the balancing of transparency and ethical reflection against pragmatic constraints. The movement toward more reliable, relevant, and ethical AI and ML applications for patient care will inevitably require strategic updates to data set creation practices.
Clinical artificial intelligence (AI) applications have the potential to improve patient care and advance biomedical research. Machine learning (ML) research is already producing AI models across a spectrum of disease areas. 1 - 3 Central to ML research is the data from which models are trained. To accelerate ML discoveries and meet an ethical obligation to treat health data as a public good, 4 many health data sets have been publicly released to support growing calls for open science and transparency in ML research. 5 - 7 However, ML models derived from these data continue to be criticized for lacking usefulness, reliability, and fairness. 8 , 9
Many of these challenges are inextricably attributed to the quality of data sets. Making data sets AI ready or high-quality and useful for the development of ML applications in health care is often an intensive process that requires coordination across the data preparation pipeline. 10 Most available data sets lack diversity 11 , 12 and have a paucity of high-quality labels necessary for ML, including diagnoses, demographic characteristics, and other critical elements of clinical context. Consequently, only a small fraction of open health data sets (eg, COVID-19–related data sets) contain the clinically relevant annotations to support generalizable ML research. 13 , 14
Machine learning models reflect the episodic decisions of stakeholders across the AI life cycle. 15 The proper use and reuse of AI-ready data sets by researchers is also integral to preventing harmful bias in ML models used for patient care and resource allocation. 16 - 18 Therefore, producing unbiased AI-ready data sets requires a comprehensive understanding of these issues to combat the dynamic unpredictability of ML model development.
A definition of what constitutes AI-ready data sets for ML remains elusive. We drew on existing data quality frameworks as a guiding tool for our evaluation. Despite numerous frameworks with established data quality dimensions, 19 including those specific to big data, 20 - 28 ethics, 29 and ML, 30 none fully integrate the nuances required for ML research in health care or considerations that are conducive to AI-ready data set creation in practice. The lack of frameworks to guide the development of AI-ready data sets limits their usefulness for ML research in health care and prevents us from attaining diagnostic excellence. 31 We envision an AI-readiness framework that is informed by both conventional expectations of data quality and the contemporary needs of ML researchers. Such a framework can lead to greater understanding of how to strengthen data set production and data sharing for clinical AI innovation. In this study, we explored the perspectives of data set creators and ML researchers to determine what makes health data sets AI ready.
The Stanford School of Medicine Institutional Review Board reviewed and approved this qualitative study, with a waiver of documentation of consent. Participants provided verbal consent to be interviewed and received an information sheet stating that findings/data may be published in scientific journals. Participants did not receive financial compensation. This study followed the Consolidated Criteria for Reporting Qualitative Research ( COREQ ) reporting guideline.
We conducted qualitative interviews of experts involved in the creation of data sets and/or their use for ML research. The semistructured interview gathered participant demographic characteristics, data roles, role responsibilities, and perspectives on data set AI readiness and related topics.
We identified eligible experts who were involved in the creation of publicly available health data sets or the use of these data sets for ML research. Some experts met both criteria. Starting with a list of health data sets or databases, we relied on accessible sources that included consulting respective database web pages, associated publications (scientific or media), collaborators and other experts, and the open web to identify and corroborate eligible experts and obtain contact information. Purposive sampling, or the intentional selection of information-rich individuals, 32 was used to optimize inclusion of participants from diverse data sets and organizational sectors. From August 23, 2022, to January 5, 2023, we recruited 20 participants after approaching 93 eligible experts with an email invitation; nonrespondents were sent a follow-up email. Race was documented to provide information about participants and potential perspectives that may not have been included.
Data collection occurred in 2 stages during a scheduled interview session. All interviews were conducted in English through a secure video conferencing platform by the team leader (M.Y.N.). First, participants were asked to verbally complete a survey on demographic characteristics, data roles, and role responsibilities (eMethods in Supplement 1 ). Second, the interviewer used a semistructured interview guide developed with the study team to gather participant perspectives. Interview questions focused on optimal characteristics of AI-ready data sets and their associated facilitators and barriers. The semistructured format allowed for both focused discussions and probing questions during interviews. Interviews were video and audio recorded and transcribed verbatim. Interviews were conducted until reaching thematic saturation, defined as the point where no new codes or themes emerge from the data. 33
We used quantitative content analysis to categorize and count frequencies of specific content from the survey responses. 34 , 35 Thematic analysis 36 - 38 that drew on techniques of grounded theory 39 , 40 was used to identify themes or patterns from the interview data. Interview transcripts were imported into MaxQDA 2022. 41 First, the team leader (M.Y.N.) generated initial codes from the raw interview data using inductive and deductive approaches. Deductive codes were selected to organize the interview data into broad content areas (eg, optimal characteristics, facilitators, and barriers) during initial coding. An initial codebook was created with both inductive and deductive codes. Second, team members (M.Y.N., A.Y., and D.S.) independently coded, line-by-line, a subset of transcripts with these emergent codes. Disagreements were resolved via discussion until consensus was reached. The initial codebook was iteratively refined throughout the coding process. The team leader (M.Y.N.) reviewed all coded transcripts and applied revisions where appropriate to align with the refined codebook; consensus among team members (M.Y.N., A.Y., and D.S.) was reaffirmed. In addition, identified core concepts and connections between categories were shared among the entire study team to triangulate key themes of data set AI readiness.
We endeavored to develop a framework that depicts the data set quality elements specific to ML research and relevant connections. Framework development occurred in 2 discrete steps. First, we compiled a list of possible data quality elements to consider deductively, informed by select data quality frameworks (eTable in Supplement 1 ). Second, once themes were identified, we iteratively refined and organized the most relevant themes to create a data set AI-readiness framework. The study team reviewed and approved the final framework.
A total of 20 experts in data set creation and ML research were interviewed ( Table 1 ). Of these participants, 11 individuals (55%) identified as male and 8 (40%) as female; 15 (75%) were younger than 49 years, with mean (SD) age, 42 (11) years. In terms of race, 6 individuals (30%) identified as Asian, 1 (5%) as multiracial, and 12 (60%) as White. All demographic data were self-reported; 1 (5%) participant did not provide this information. While 18 (90%) participants identified as both data creators and ML researchers, 2 (10%) identified primarily as data set creators. Participants were involved in various tasks across the data preparation pipeline, 10 with most involved in data curation (90%), data documentation (85%), and data analysis (85%). The mean (SD) duration of the interviews was 49 (11) minutes. Participants worked across diverse data sets and databases, as shown by their general characteristics and select traits relevant for clinical data reuse (eg, repository type, longitudinal observations, and research accessibility) ( Table 2 ). 42 We identified 3 themes, each with subthemes ( Table 3 ) and corresponding salient quotations ( Table 4 ).
Inherent characteristics of AI readiness that are independent of ML use case include accuracy, completeness, consistency, and ethical acquisition. These categories are most relevant to the reliability dimension of data quality, which is defined as whether a user can trust the data. 23
Participants expressed that accuracy consists of well-defined labels as well as ground truth annotations for training and testing of ML models. Participants emphasized the importance of having labels and annotations that are good measurements of what the model intends to predict ( Table 4 , quotation 1.1). Thus, the accuracy of labels and annotations are core to AI readiness. Documentation that provides supportive proof or describes how labels and annotations were generated can further enhance AI readiness.
Completeness or meeting an expectation of comprehensiveness contributes to AI readiness. Characteristics such as the size, granularity, breadth, diversity, low missingness, and temporality of data provide indications of data set completeness ( Table 4 , quotation 1.2). Data sets are considered more AI ready when they contain a comprehensive picture of the area of study (eg, patient journey). Larger data sets are hence preferred for ML research because they increase the likelihood that a desired level of data set completeness will be attained.
Consistency in data creation, acquisition, and preprocessing is an important expectation. Artificial intelligence readiness is more likely when data are generated using equivalent methods, variables are collected and coded in a similar manner, and the data are harmonized to the intended use ( Table 4 , quotation 1.3).
Ethically acquired data are also fundamental to AI readiness. A major determinant of ethical acquisition is whether informed consent was obtained from data contributors that allows for broad and originally unintended secondary use of the data. Data sets without proper permissions should not be used by ML researchers. Data sets that rectify informed consent deficiencies across its data sources are inherently more ready for ML research as it is less likely that research endeavors and integrity will be compromised ( Table 4 , quotation 1.4-1.5).
Contextual characteristics of AI readiness that depend on the ML use case include fitness and societal impact. Fitness is pertinent to the relevance dimension of data quality. 23 Societal impact is aligned with the ethical dimension of data quality, which explores the ethical implications of the use of subpar data sets.
Participants described characteristics of fitness, or whether a data set meets the requirements of a particular ML research task. Each biomedical ML research task has a unique set of requirements that dictate data set fitness. Users determine a data set’s fitness for use for ML research by assessing the alignment between the ML task requirements and the data set contents. Data set fitness requires appraisal of contextual information across the life cycle of the ML task, including its intended purpose, the target population compared with the populations represented in the data set, and the eventual deployment environment ( Table 4 , quotation 2.1)
Representativeness of the data helps users appraise data set fitness for an ML research task. Participants noted the importance that the target population in which the ML model will be deployed is represented in the data set. The heterogeneity of a data set can be measured not only on sociodemographic factor and health outcomes, but also on the diversity of health care sites, resource settings, expertise levels, and geographic locations ( Table 4 , quotation 2.2).
When determining AI readiness, participants considered the societal implications of data set use. Users feel an obligation to assess the risks, harms, or biases that may arise. Machine learning tasks or models developed for health or biomedical purposes have unique ethical, societal, and safety implications that differentially impact populations, which may be further exacerbated through the use of inappropriate or imbalanced data sets ( Table 4 , quotation 2.3).
Participants divulged contributors that affect user appraisals of data set AI readiness. These contributors include the state of data availability, data quality standards, documentation, team science, and incentivization.
Participants were supportive of making health data sets publicly available and considered it beneficial to AI readiness. Open access to data sets enables users to appraise AI readiness and identify areas for improvement. Data set shortcomings are more easily discovered through actual use and public scrutiny ( Table 4 , quotation 3.1). The recognition that data sets are not independent can further enhance AI readiness. Although a data set on its own may not be ready for a particular ML research task, it may achieve a sufficient level of AI readiness when combined with other data sets ( Table 4 , quotation 3.2). However, systemic inequities in data availability continue to hinder the creation of AI-ready data sets, which ultimately limit the potential of ML research ( Table 4 , quotation 3.3).
Data quality standards and frameworks contribute to AI readiness, but their application and use appear to be highly variable. Some teams were adamant about incorporating data quality standards during data set creation while others chose to optimize data quality elements or dimensions in an ad hoc manner. Nevertheless, one participant attributed the prolonged usefulness of their data set to the incorporation of FAIR (findability, accessibility, interoperability, and reusability) data principles, 43 a set of guidelines that help enhance the reusability of data sets ( Table 4 , quotation 3.4). Data quality standards and frameworks need to be updated to fully drive AI readiness. Current standards do not adequately convey what matters most in ML research or how data set creators can bring a host of data set debt into compliance ( Table 4 , quotation 3.5).
Participants cited documentation as a key contributor to AI readiness. The extent of documentation, especially qualitative contextual information about data provenance and data processing decisions, helps users make decisive judgments about AI readiness for their specific ML research tasks. Important accompanying documentation includes data origination, data collection circumstances, data contributor sociodemographic characteristics, intended uses, data preprocessing decisions, label and annotation generation decisions, recommended tools and resources, and other information necessary for robust and fair model development. The transparency of this information clarifies a data set’s purpose and caveats, so that users can inspect, select, and use the data set they deem most appropriate ( Table 4 , quotation 3.6). It also indicates the limitations that may arise in developing the ML model. Furthermore, data sets with comprehensive documentation shorten the learning curve for ML researchers and allow for efficient data use ( Table 4 , quotation 3.7). Documentation also needs to be up-to-date and living to account for changes to a data set after its publication date and the evolving research landscape ( Table 4 , quotation 3.8).
Participants mentioned the benefits of team science as another driver of AI readiness. The makeup of a team, including diversity of expertise, training, and experiences, contributes to more comprehensive and thoughtful construction of data sets ( Table 4 , quotation 3.9). Informed teams can yield operational decisions that enhance intrinsic and contextual elements of AI readiness. Furthermore, well-formed teams from reputable institutions can enhance the perceived trustworthiness of the data sets produced. Patients and other data contributors should be considered as part of an effective data set creation team ( Table 4 , quotation 3.10). A component of AI readiness is whether the data set contains relevant information about the needs and health outcomes of the populations they aim to serve. Keeping knowledgeable humans in the loop throughout data set creation is also considered essential for maintaining AI-ready data sets ( Table 4 , quotation 3.11).
The professional incentivization of data set work would support the creation of AI-ready data sets. Those involved in data set creation and quality maintenance have noted the increasing labor required to meet the latest demands of researchers, yet resources and funding for that work remain lacking. Professionals in academia, as expressed by one respondent, are less inclined to be invested in data quality work as the system largely rewards those who use the data for research ( Table 4 , quotation 3.12). Some participants also noted the limits of their individual effort in creating and sustaining quality data sets, since they are subject to the constraints of organizational decisions, motivations, and risk tolerance ( Table 4 , quotation 3.13). Incentivization (ie, direct benefits to funding, resources, or reputation) also needs to be aligned with the organization to compel systemic changes that facilitate AI-ready data set creation.
We mapped these themes onto a framework to show their association within the health data ecosystem ( Figure ). The framework consists of 3 core components: (1) drivers of AI-ready data sets, (2) elements of data set AI readiness, and (3) the health data ecosystem.
Our study set out to delineate what constitutes an AI-ready data set that is useful for ML research in health and does not perpetuate harm and bias. We sought perspectives from experts working with a broad spectrum of data sets. Using themes grounded in their perspectives, we developed a broadly applicable AI-readiness framework that informs data set stakeholders about the most relevant data set quality metrics for ML research and considerations to recapitulate a facilitating environment for AI-ready data set creation.
We strived to distinguish how our framework varied from existing data quality frameworks. Accuracy, completeness, consistency, and fitness were entrenched expectations and have been well described across many data quality frameworks. Machine learning researchers partially determined the AI readiness of data sets using these priority appraisal characteristics. Ethical acquisition and societal impact emerged as expectations of our participant sample that have not been described in prior frameworks. Ethical considerations permeated AI-readiness discussions, reflecting a key challenge in the ML research landscape. This increased emphasis is likely due to recent ethical controversies in ML research, including the misuse of user data, privacy and data breaches, and cases of algorithmic bias. 16 - 18 Our framework recognizes how they appraise not only the historical ethical aspects of a data set (ie, permissions allowed by original informed consent and data use agreements) but also the prospective ethical impact of data set use (ie, production of fair algorithms).
Our framework also identifies factors that drive creation of high-value health data sets and mitigate risks associated with data reuse, which may negatively affect patient care decisions, limit research potential, and waste important resources. These driving factors affect elements of AI readiness and hence ML researchers’ overall perception of data set usefulness. There are several factors that drive AI readiness. The first is availability, which aligns with the community’s call to make data sets open access or easily accessible, thereby increasing collaboration and reproducibility. 6 Open access data sets are subject to continuous public auditing, which can surface hidden biases. Despite these advantages, there remains resistance to open data set sharing. 44
Data quality standards can provide a systematic guide to data set creation and drive AI readiness. Standards are a set of aspirational recommendations that can help address common shortcomings in most contexts (eg, using a common data model). The advantages of data quality standards must be balanced by the practicality of adhering to standards in lower resourced health care settings. Data from these sources may not be considered AI ready according to some data quality standards, but their inclusion would nonetheless be valuable for addressing the biases and imbalances in data sets. These ethical trade-offs are important to consider before enforcement of data quality standards.
Documentation drives AI readiness by enhancing the transparency of the data set creation process. Documentation provides stakeholders with known data set quality information, 45 so that they may decide whether a data set meets the threshold of AI readiness sufficient for their research needs. Improving documentation for health data sets, such as with the addition of datasheets, 46 healthsheets, 47 or data set nutrition label 48 provides ML researchers with key information necessary to facilitate decision-making with model development and meet downstream model reporting guidelines. 9 , 49 Comprehensive documentation encourages an equitable ecosystem in which diverse ML researchers can more easily access, understand, and use health data sets appropriately.
Team science is another driver of AI readiness. Team science recognizes the value of diverse cross-disciplinary teams for helping solve multifaceted problems. 50 , 51 Diverse and inclusive AI teams are integral to bias mitigation, 52 with compounded benefits if implemented at the data set creation stage. For example, jury learning, in which diverse annotators make data labeling decisions, meaningfully altered classification outcomes. 53 Diverse teams can create more relevant and informed labels, further contributing to data set AI readiness.
In addition, the value of incentives to drive AI readiness points to the need for more resources invested in the data set quality workforce. Data set creation and maintenance are underappreciated by traditional metrics of academic productivity. Data set creators made decisions that impact AI readiness (eg, deciding to not carry out clinical annotations) due to the lack of resources and incentives for such tasks. While data set creators may feel a moral obligation to continue high-touch maintenance and oversight after the public sharing of a data set, they often provide this service to the detriment of their professional growth. Given the appreciating value of quality health data sets for ML research, incentives, and resources need to be aligned (eg, National Institutes of Health funding initiatives and journal requirements) for those involved in data set quality work to meet AI-readiness metrics, manage developing risks, and be recognized for their contributions.
Our study was limited by the sample size. Responses from the 20 participants may not be representative of all data set experts. Data set use and requirements for AI and ML research are also rapidly evolving and in flux. Thus, our work represents a snapshot in time. Future work will require frequent updates to data quality frameworks and the meaning of AI readiness.
The AI readiness of health data sets is a key factor in clinical AI and ML innovation. This qualitative study developed a grounded framework for AI data set quality. Our work suggests that the concept of data set AI readiness is complex and requires the concerted appraisal of many elements and the balancing of transparency and ethical reflection against pragmatic constraints. The movement toward more reliable, relevant, and ethical ML research will inevitably require strategic updates to data set creation practices.
Accepted for Publication: October 20, 2023.
Published: December 1, 2023. doi:10.1001/jamanetworkopen.2023.45892
Open Access: This is an open access article distributed under the terms of the CC-BY License . © 2023 Ng MY et al. JAMA Network Open .
Corresponding Author: Madelena Y. Ng, DrPH, MPH, Center for Biomedical Informatics Research, Stanford University School of Medicine, 3180 Porter Dr, Palo Alto, CA 94304 ( [email protected] ).
Author Contributions: Dr Ng had full access to all of the data in the study and takes responsibility for the integrity of the data and the accuracy of the data analysis. Drs Langlotz and Hernandez-Boussard contributed equally as co–last authors.
Concept and design: Ng, Youssef, Miner, Long, Larson, Hernandez-Boussard, Langlotz.
Acquisition, analysis, or interpretation of data: Ng, Youssef, Miner, Sarellano, Hernandez-Boussard, Langlotz.
Drafting of the manuscript: Ng.
Critical review of the manuscript for important intellectual content: All authors.
Statistical analysis: Ng, Long.
Obtained funding: Miner, Langlotz.
Administrative, technical, or material support: Ng, Sarellano, Larson.
Supervision: Ng, Miner, Long, Hernandez-Boussard, Langlotz.
Conflict of Interest Disclosures: Dr Larson reported being a shareholder in Bunkerhill Health. Dr Langlotz reported receiving grants from the National Institutes of Health National Institute of Biomedical Imaging and Bioengineering during the conduct of the study; holding stock from Bunkerhill Health and stock options with Whiterabbit.ai, GalileoCDS, Sirona Medical, Adra.ai, and Kheiron; personal fees from Sixth Street and Gilmartin Capital; grants from Bunkerhill Health, Carestream, CARPL, Clairity, GE Healthcare, Google Cloud, IBM, Kheiron, Lambda, Lunit, Nightingale Open Science, Philips, Siemens Healthineers, Stability.ai, Subtle Medical, VinBrain, Whiterabbit.ai, and Lowenstein Foundation; and nonfinancial support from Microsoft outside the submitted work. No other disclosures were reported.
Funding/Support: This study was funded by grants 10848 from the Gordon and Betty Moore Foundation and R01LM013362 from the National Library of Medicine.
Role of the Funder/Sponsor: The funders had no role in the design and conduct of the study; collection, management, analysis, and interpretation of the data; preparation, review, or approval of the manuscript; and decision to submit the manuscript for publication.
Data Sharing Statement: See Supplement 2 .
Additional Contributions: We acknowledge and thank our expert advisory group for providing valuable feedback at various stages of this project.
- Register for email alerts with links to free full-text articles
- Access PDFs of free articles
- Manage your interests
- Save searches and receive search alerts
Small data challenges for intelligent prognostics and health management: a review
- Open access
- Published: 23 July 2024
- Volume 57 , article number 214 , ( 2024 )
Cite this article
You have full access to this open access article
- Chuanjiang Li 1 ,
- Shaobo Li 1 ,
- Yixiong Feng 1 ,
- Konstantinos Gryllias 2 ,
- Fengshou Gu 3 &
- Michael Pecht 4
69 Accesses
Explore all metrics
Prognostics and health management (PHM) is critical for enhancing equipment reliability and reducing maintenance costs, and research on intelligent PHM has made significant progress driven by big data and deep learning techniques in recent years. However, complex working conditions and high-cost data collection inherent in real-world scenarios pose small-data challenges for the application of these methods. Given the urgent need for data-efficient PHM techniques in academia and industry, this paper aims to explore the fundamental concepts, ongoing research, and future trajectories of small data challenges in the PHM domain. This survey first elucidates the definition, causes, and impacts of small data on PHM tasks, and then analyzes the current mainstream approaches to solving small data problems, including data augmentation, transfer learning, and few-shot learning techniques, each of which has its advantages and disadvantages. In addition, this survey summarizes benchmark datasets and experimental paradigms to facilitate fair evaluations of diverse methodologies under small data conditions. Finally, some promising directions are pointed out to inspire future research.
Avoid common mistakes on your manuscript.
1 Introduction
Prognostics and health management (PHM), an increasingly important framework for realizing condition awareness and intelligent maintenance of mechanical equipment by analyzing collected monitoring data, is being applied in a growing spectrum of industries, such as aerospace (Randall 2021 ), transportation (Li et al. 2023a ), and wind turbines (Han et al. 2023 ). According to a survey conducted by the National Science Foundation (NSF) (Gray et al. 2012 ), PHM technologies have created economic benefits of $855 million over the past decade. It is the fact that PHM has such great application potential that it continues to attract sustained attention and research from different academic communities, including but not limited to reliability analysis, mechanical engineering, and computer science.
Functionally, PHM covers the entire monitoring lifecycle of an equipment, fulfilling roles across four key dimensions: anomaly detection (AD), fault diagnosis (FD), remaining useful life (RUL) prediction, and maintenance execution (ME) (Zio 2022 ). First, AD aims to discern rare events that deviate significantly from standard patterns, and the crux lies in accurately differentiating a handful of anomalous data from an extensive volume of normal data (Li et al. 2022a ). The focus of FD is to classify diverse faults, and the difficulty is to extract effective fault features under complex working conditions. RUL prediction emphasizes on estimating the time remaining before a component or system fails, and its main challenge is to construct comprehensive health indicators capable of characterizing trends in health degradation. Finally, ME optimizes maintenance decisions based on diagnostic and prognostic results (Lee and Mitici 2023 ).
Methodologically, the techniques employed to execute the PHM tasks of AD, FD, and RUL prediction can be classified into physics model-based, data-driven, and hybrid methods (Lei et al. 2018 ). Physics model-based methods utilize mathematical models to describe failure mechanisms and signal relationships, representative techniques include state observers (Choi et al. 2020 ), parameter estimation (Schmid et al. 2020 ), and some signal processing approaches (Gangsar and Tiwari 2020 ). However, data-driven methods involve manual or adaptive extraction of features from sensor signals, including statistical methods (Wang et al. 2022 ), machine learning (ML) (Huang et al. 2021 ) and deep learning (DL) (Fink et al. 2020 ). Hybrid approaches (Zhou et al. 2023a ) combine elements from both physics model-based and data-driven techniques. Among these methods, DL-based techniques have gained widespread interest in PHM tasks, spanning from AD to ME, which is attributed to their pronounced advantages over conventional techniques in automatic feature extraction and pattern recognition.
Figure 1 depicts the intelligent PHM cycle based on DL models (Omri et al. 2020 ), the steps include data collection and processing, model construction, feature extraction, task execution, and model deployment. It is evident that monitoring data forms the foundation of this cycle, its volume and quality wield decisive influence on the eventual performance of DL models in industrial contexts. However, gathering substantial datasets consisting of diverse anomaly and fault patterns with precise labels under different working conditions is time-consuming, dangerous, and costly, leading to small data problems that challenge models’ performance in PHM tasks. A recent investigation conducted by Dimensional Research underscores this quandary, revealing that 96% of companies have encountered small data issues in implementing industrial ML and DL projects (D. Research 2019 ).
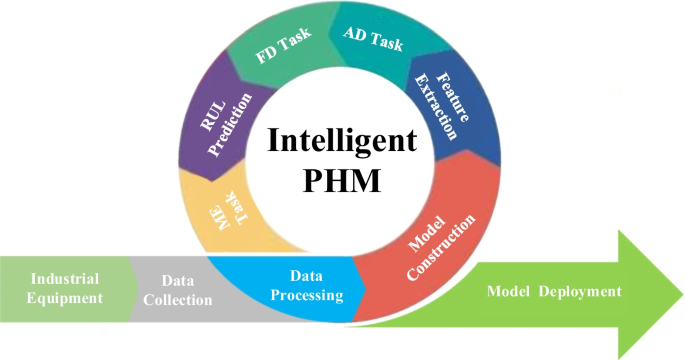
The intelligent PHM cycle based on DL models (Omri et al. 2020 )
To address the small data issues in intelligent PHM, organizations have started to shift their focus from big data to small data to enhance the efficiency and robustness of Artificial Intelligence (AI) models, which is strongly evidenced by the rapid growth of academic publications over recent years. To provide a comprehensive overview, we applied the preferred reporting items for systematic reviews and meta-analyses (PRISMA) (Huang et al. 2024 ; Kumar et al. 2023 ) method for paper investigation and selection. As shown in Fig. 2 , the PRISMA technique includes three steps: Defining the scope, databases, and keywords, screening search results, and identifying articles for analysis. At first, the search scope was limited to articles published in IEEE Xplore, Elsevier, and Web of Science databases from 2018 to 2023, and the keywords consisted of topic terms such as “small/limited/imbalanced/incomplete data”, technical terms such as “data augmentation”, “deep learning”, “transfer learning”, “few-shot learning”, “meta-learning”, and application-specific terminologies such as “intelligent PHM”, “anomaly detection”, “fault diagnosis”, “RUL prediction” etc. The second stage is to search the literature in the databases by looking for articles whose title, abstract and keywords contain the predefined keywords, resulting in 139, 1232, and 281 papers from IEEE Xplore, Elsevier, and Web of Science, respectively. In order to eliminate duplicate literature and select the most relevant literature on small data problems in PHM, the first 100 non-duplicate studies from each database were sorted (producing a sum of 300 papers) according to the inclusion and exclusion criteria as listed in Table 1 . Finally, we further refined the obtained results with thorough review and evaluation, and a total of 201 representative papers were chosen for analysis presented in this survey.
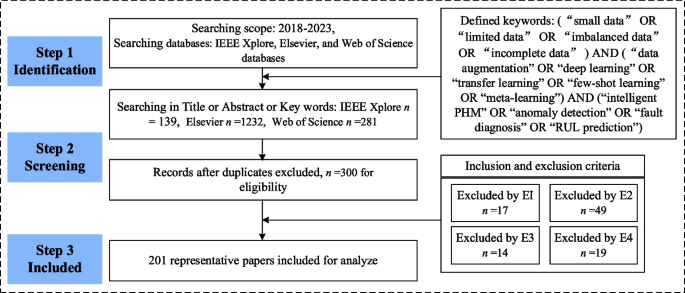
The procedure for paper investigation and selection using PRISMA method
Despite the growing number of studies, the statistics highlight that there are few review articles on the topic of small data challenges. The first related review is the report entitled “Small data’s big AI potential”, which was released by the Center for Security and Emerging Technology (CSET) at Georgetown University in September 2021 (Chahal et al. 2021 ), and it emphasized the benefits of small data and introduced some typical approaches. Then, Adadi ( 2021 ) reviewed and discussed four categories of data-efficient algorithms for tackling data-hungry problems in ML. More recently, a study (Cao et al. 2023 ) theoretically analyzed learning on small data, followed an agnostic active sampling theory and reviewed the aspects of generalization, optimization and challenges. Since 2021, scholars in the PHM community have been focusing on the small data problem in intelligent FD and have conducted some review studies. Pan et al. ( 2022 ) reviewed the applications of generative adversarial network (GAN)-based methods, Zhang et al . ( 2022a ) outlined solutions from the perspective of data processing, feature extraction, and fault classification, and Li et al. ( 2022b ) organized a comprehensive survey on transfer learning (TL) covering theoretical foundations, practical applications, and prevailing challenges.
It is worth noting that existing studies provide valuable guidance, but they have yet to delve into the foundational concepts of small data and exhibit certain limitations in the analysis. For instance, some reviews studied the small data problems from a macro perspective without considering the application characteristics of PHM tasks (Chahal et al. 2021 ; Adadi 2021 ; Cao et al. 2023 ). However, some concentrated solely on particular methodologies that were used to address small data challenges in FD tasks (Pan et al. 2022 ; Zhang et al. 2022a ; Li et al. 2022b ), lack the systematic research on the solutions to AD and RUL prediction tasks, seriously limiting the development and industrial application of intelligent PHM. Therefore, an in-depth exploration of the small data challenges in the PHM domain is necessary to provide guidance for the successful applications of intelligent models in the industry.
This review is a direct response to the contemporary demand for addressing the small data challenges in PHM, and it aims to clarify the following three key questions: (1) What is small data in PHM? (2) Why solve the small data challenges? and (3) How to address small data challenges effectively? These fundamental issues distinguish our work from existing surveys and demonstrate the major contributions:
Small data challenges for intelligent PHM are studied for the first time, and the definition, causes, and impacts are analyzed in detail.
An overview of various state-of-the-art methods for solving small data problems is presented, and the specific issues and remaining challenges for each PHM task are discussed.
The commonly used benchmark datasets and experimental settings are summarized to provide a reference for developing and evaluating data-efficient models in PHM.
Finally, promising directions are indicated to facilitate future research on small data.
Consequently, this paper is organized according to the hierarchical architecture shown in Fig. 3 . Section 2 discusses the definition of small data in the PHM domain and analyzes the corresponding causes and impacts. Section 3 provides a comprehensive overview of representative approaches—including data augmentation (DA) methods (Sect. 3.1 ), transfer learning (TL) methods (Sect. 3.2 ), and few-shot learning (FSL) methods (Sect. 3.3 ). The problems in PHM applications are discussed in Sect. 4 . Section 5 summarizes the datasets and experimental settings for model evaluation. Finally, potential research directions are given in Sect. 6 and the conclusions are drawn in Sect. 7 . In addition, the abbreviations of notations used in this paper are summarized in Table 2 .
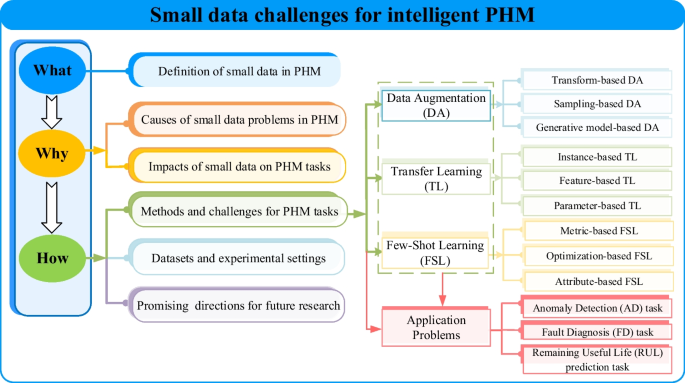
The hierarchical architecture of this review
2 Analysis of small data challenges in PHM
The excellent performance of DL models in executing PHM tasks is intricately tied to the premise of abundant and high-quality labeled data. However, this assumption is unlikely to be satisfied in industry, as small data is often the real situation, which exhibits distinct data distributions and may lead to difficulties in model learning. Therefore, this section first analyzes the definition, causes, and impacts of small data in PHM.
2.1 What is “small data”?
Before answering the question of what small data is, let us first review the relative term, “big data”, which has garnered distinct interpretations among scholars since its birth in 2012. Ward and Barker ( 1309 ) regarded big data as a phrase that “describes the storage and analysis of large or complex datasets using a series of techniques”. Another perspective, as presented in Suthaharan ( 2014 ), focused on the data’s cardinality, continuity, and complexity. Among the various definitions, the one that has been widely accepted is characterized by the “5 V” attributes: volume, variety, value, velocity, and veracity (Jin et al. 2015 ).
After long-term research, some experts have discovered the fact that big data is not ubiquitous, and the paradigm of small data has emerged as a novel area worthy of thorough investigation in the field of AI (Vapnik 2013 ; Berman 2013 ; Baeza-Yates 202 4; Kavis 2015 ). Vapnik ( 2013 ) stands among the pioneers in this pursuit, having defined small data as a scenario where “the ratio of the number of training samples to the Vapnik–Chervonenkis (VC) dimensions of a learning machine is less than 20.” Berman ( 2013 ) considered small data as being used to solve discrete questions based on limited and structured data that come from one institution. Another study defines small data as “data in a volume and format that makes it accessible, informative and actionable.” (Baeza-Yates 2024 ). In an industrial context, Kavis ( 2015 ) described small data as “The small set of specific attributes produced by the Internet of Things, these are typically a small set of sensor data such as temperature, wind speed, vibration and status”.
Considering the distinctive attributes of equipment signals within industries, a new definition for small data in PHM is given here: Small data refers to datasets consisting of equipment or system status information collected from sensors that are characterized by a limited quantity or quality of samples. Taking the FD task as an example, the corresponding mathematical expression is: Given a dataset \(D = \left\{ {F_{I} (x_{i}^{I} ,y_{i}^{I} )_{i = 1}^{{n_{I} }} } \right\}_{I = 1}^{N}\) , \(\left( {x_{i}^{I} ,y_{i}^{I} } \right)\) are the samples and labels (if any) of the I th fault \(F_{I}\) . \(N\) represents the number of fault classes in \(D,\) and each fault set has a sample size of \(n_{I}\) . Notably, the term “small” carries two connotations: (i) On a quantitative scale, “small” signifies a limited dataset volume, a limited sample size \(n_{I}\) , or a minimal total number of fault types \(N\) ; and (ii) From a qualitative perspective, “small” indicates a scarcity of valuable information within \(D\) due to a substantial proportion of anomalous, missing, unlabeled, or noisy-labeled data in \(\left( {x_{i}^{I} ,y_{i}^{I} } \right)\) . There is no fixed threshold to define “small” concerning both quantity and quality, which is an open question depending on the specific PHM task to be performed, the equipment analyzed, the chosen methodology, and the desired performance. To further understand the meaning of small data, a comprehensive comparison is conducted with big data in Table 3 .
2.2 Causes of small data problems in PHM
Rapid advances in sensors and industrial Internet technology has simplified the process of collecting monitoring data from equipment. However, only large companies currently have the ability to acquire data on a large scale. Since most of the collected data are normal samples with limited abnormal or faulty data, they cannot provide enough information for model training. As illustrated in Fig. 4 , four main causes for small data challenges in PHM are analyzed.
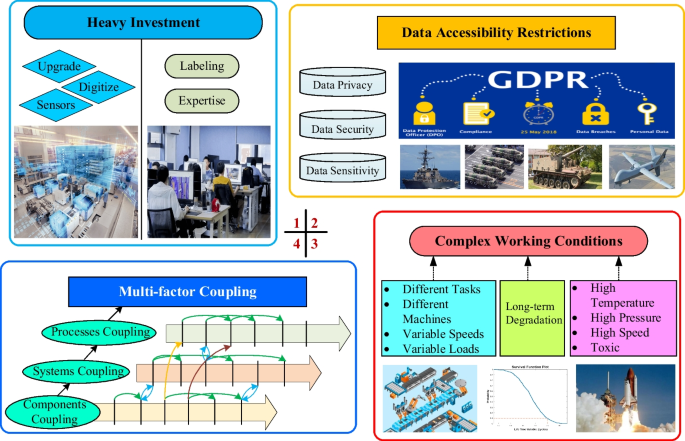
Four main causes of small data challenges in PHM
2.2.1 Heavy investment
When deploying an intelligent PHM system, Return on Investment (ROI) is the top concern of companies. The substantial investment comes from two main aspects, as shown in the first quadrant of Fig. 4 : First, factories need to digitally upgrade existing old equipment to collect monitoring data. (ii) Second, data labeling and processing requires manual operation and domain expertise. Although the costs of sensors and labeling outsourcing are relatively low today, installing sensors across numerous machines and processing terabytes of data is still beyond the reach of most manufacturers.
2.2.2 Data accessibility restrictions
Illustrated in the second quadrant, this factor is underscored by follows: (i) The sensitivity, security, or privacy of the data often leads to strict access controls, an example is data collection of military equipment. (ii) For data transfers and data sharing, individuals, corporations, and nations need to comply with laws and supervisory ordinances, especially after the release of the General Data Protection Regulation (Zarsky 2016 ).
2.2.3 Complex working conditions
The contents depicted in the third quadrant of Fig. 4 include: (i) Data distribution within PHM inherently displays significant variability across diverse production tasks, machines and operating conditions (Zhang et al. 2023 ), making it impossible to collect data under all potential conditions. (ii) Acquiring data within specialized service environments, such as high radiation, carries inherent risks. (iii) The development of equipment from a healthy state to eventual failure experiences a long process.
2.2.4 Multi-factor coupling
As equipment becomes more intricately integrated, correlation and coupling effects have undergone continuous augmentation. As shown in the fourth quadrant of Fig. 4 : Couplings exist between (i) multiple-components, (ii) multiple-systems, and (iii) diverse processes. Such interactions are commonly characterized by nonlinearity, temporal variability, and uncertain attributes, further increasing the complexity of data acquisition.
2.3 Impacts of small data on PHM tasks
The availability of labeled and high-quality data remains limited, producing some impacts on performing PHM tasks, particularly involving both data and models (Wang et al. 2020a ). As shown on the left side of Fig. 5 , the effects at the data level primarily include incomplete data and unbalanced distribution, which subsequently leads to poor generalization at the model-level. This section analyzes the impacts with corresponding evaluation metrics based on the example of FD task.
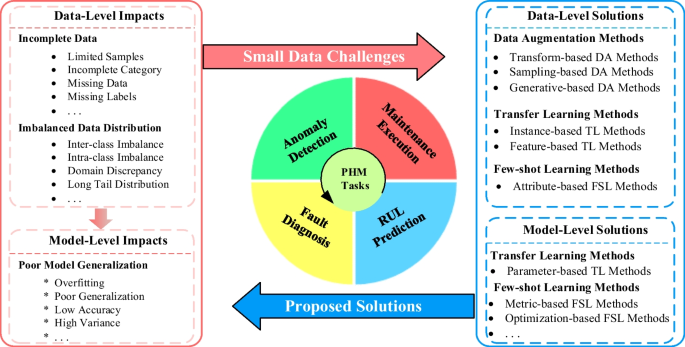
Impacts of small data problems in the PHM domain, with current mainstream approaches
2.3.1 Incomplete data
Data integrity refers to the “breadth, depth, and scope of information contained in the data” (Chen et al. 2023a ). However, the obtained small dataset often exhibits a low density of supervised information owing to restricted fault categories or sample size. Further, missing values and labels, or outliers in the incomplete data exacerbates the scarcity of valuable information. Data incompleteness in PHM can be measured by the following metrics:
where \(I_{D}\) represents the incompleteness of the dataset \(D\) , \(n_{{_{D} }}\) and \(N_{{_{D} }}\) are the number of incomplete samples and the total samples in \(D\) , respectively. Similarly, this metric can also assess the incompleteness of samples within a certain class \(C_{i}\) in line with Eq. ( 2 ). When either \(I_{D}\) or \(I_{{C_{i} }}\) approaches 0, it indicates a relatively complete dataset or class. Conversely, a higher value represents a severe degree of data incompleteness, leading to a substantial loss of information within the data.
2.3.2 Imbalanced data distribution
The second impact is the imbalanced data distribution. The fault classes containing higher or lower numbers of samples are called the majority and minority classes, respectively. Depending on the imbalance that exists between different classes or within a single class, the phenomena of inter-class imbalance or intra-class imbalance arises accordingly. Considering a dataset with two distinct fault types, each comprising two subclasses, the degrees of inter-class \(IR\) and intra-class \(IR_{{C_{i} }}\) imbalances can be quantified as (Ren et al. 2023 ):
where \(N_{{{\text{maj}}}}\) and \(N_{\min }\) represent the count of the majority and minority classes within the dataset. \(n_{{{\text{maj}}}}\) and \(n_{\min }\) signify the respective sample sizes of the two subclasses within class \(C_{i}\) . The above values span the interval [1, ∞) to describe the extent of the imbalance. A value of \(IR\) or \(IR_{{C_{i} }}\) is 1 indicates a balanced inter-class or intra-class case, whereas a value of 50 is typically thought of as a highly imbalanced task by domain experts (Triguero et al. 2015 ).
2.3.3 Poor model generalization
Technically, the principal of supervised DL is to build a model \(f\) , which learns the underlying patterns from a training set \(D_{train}\) and tries to predict the labels of previously unseen test data \(D_{test}\) . The empirical error \(E_{emp}\) on the training set and the expected error \(E_{exp}\) on the test set can be derived by calculating the discrepancy between the true labels \(Y\) and the predicted labels \(\hat{Y}\) , respectively. And the difference between these two errors, i.e., the generalization error \(G(f,D_{train} ,D_{test} )\) , is commonly used to measure the generalizability of the trained model on a test set. Generalization error is bounded by the model’s complexity \(h\) and the training data size \(P\) as follows (LeCun et al. 1998 ):
where k is a constant and α is a coefficient with a value range of [0.5, 1.0]. The equation above shows that the parameter \(P\) determines the model’s generalization. When \(P\) is large enough, \(G(f,D_{train} ,D_{test} )\) for the model with a certain \(h\) will converge towards to 0. However, the small, incomplete or unbalanced data often result in larger \(G(f,D_{train} ,D_{test} )\) and poor generalization.
3 Overview of approaches to small data challenges in PHM
This section provides a structured overview of the latest advancements in tackling small data challenges in representative PHM tasks such as AD, FD and RUL prediction. As depicted on the right-hand side of Fig. 5 , three main strategies have been extracted from the current literatures: DA, TL and FSL. In the upcoming subsections, we delve into the relevant theories and proposed methodologies for each category, followed by a brief summary.
3.1 Data augmentation methods
DA methods provide data-level solutions to address small data issues, and their efficacy has been verified in many studies. The basic principle is to improve the quantity or quality of the training dataset by creating copies or new synthetic samples of existing data (Gay et al. 2023 ). Depending on how the auxiliary data are generated, transform-based, sampling-based, and deep generative models-based DA methods are analyzed.
3.1.1 Transform-based DA
Transform-based methods is one of the earliest classes of DA, which increases the size of small datasets by employing geometric transformations to existing samples without changing labels. These transformations are so diverse and flexible that they include random cropping, vertical and horizontal flipping, and noise injection. However, most of them were initially designed for two-dimensional (2-D) images and cannot be directly applied to one-dimensional (1-D) signals of equipment (Iglesias et al. 2023 ).
Considering the sequential nature of monitoring data, scholars have devised transformation methods applicable to increase the size of 1-D data (Meng et al. 2019 ; Li et al. 2020a ; Zhao et al. 2020a ; Fu et al. 2020 ; Sadoughi et al. 2019 ; Gay et al. 2022 ). For example, Meng et al . ( 2019 ) proposed a DA approach for FD of rotating machinery, which equally divided the original sample and then randomly reorganized the two segments to form a new fault sample. In Li et al. ( 2020a ) and Zhao et al. ( 2020a ), various transformation techniques, such as Gaussian noise, random scaling, time stretching, and signal translation, are simultaneously applied, as illustrated in Fig. 6 . It is worth noting that all the aforementioned techniques are global transformations that are imposed on the entire signal, potentially overlooking the local fault properties. Consequently, some studies have combined local and global transforms (Zhang et al. 2020a ; Yu et al. 2020 , 2021a ) to change both segments and the entirety of the original signal to obtain more authentic samples. For instance, Yu et al . ( 2020 ) simultaneously used strategies of local and global signal amplification, noise addition, and data exchange to improve the diversity of fault samples.
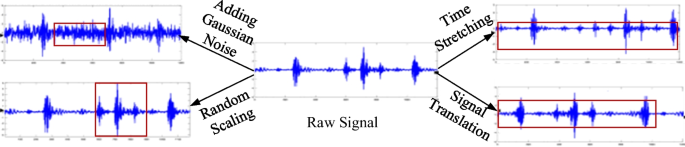
Illustration of the transformations applied in Li et al. ( 2020a ) and Zhao et al. ( 2020a ). Gaussian noise was randomly added to the raw samples, random scaling was achieved by multiplying the raw signal with a random factor, time stretching was implemented by horizontally stretching the signals along the time axis, and signal translation was done by shifting the signal forward or backwards
3.1.2 Sampling-based DA
Sampling-based DA methods are usually applied to solve data imbalance problems under small data conditions. Among them, under-sampling techniques solve data imbalance by reducing the sample size of the majority class, while over-sampling methods achieve DA by expanding samples of the minority class. Over-sampling can be further classified into random over-sampling and synthetic minority over-sampling techniques (SMOTE) (Chawla et al. 2002 ) depending on whether or not new classes are created. As shown in Fig. 7 , random over-sampling copies the data of a minority class n times to increases data size, and SMOTE creates synthetic samples by calculating the k nearest neighbors of the samples from minority classes, thus enhancing both the quantity and the diversity of samples.
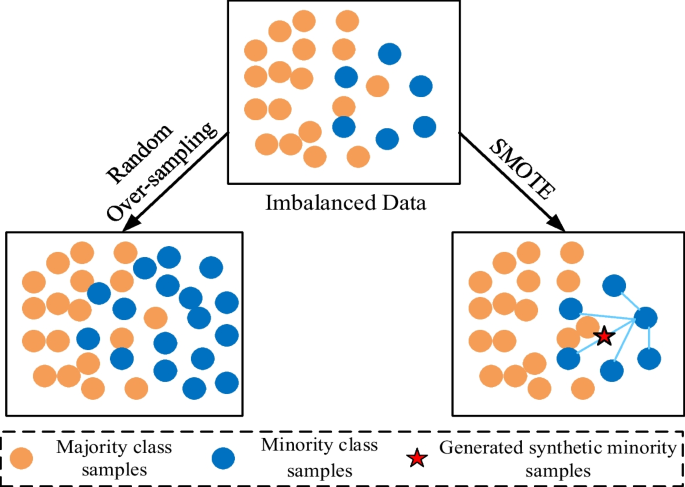
Comparison between random over-sampling and SMOTE
To address data imbalance arising from abundant healthy samples and fewer faulty samples in monitoring data, some studies (Yang et al. 2020a ; Hu et al. 2020 ) have introduced enhanced random over-sampling methods for augmentation of small data. For example, Yang et al. ( 2020a ) enhanced random over-sampling method by introducing a variable-scale sampling strategy for unbalanced and incomplete data in the FD task, and Hu et al. ( 2020 ) used resampling method to simulate data under different working conditions to decrease domain bias. In comparison, the SMOTE technique has gained widespread utilization in PHM tasks due to its inherent advantages (Hao and Liu 2020 ; Mahmoodian et al. 2021 ). Hao and Liu ( 2020 ) combined SMOTE with Euclidean distance to achieve better over-sampling of minority class samples. To address the difficulties of selecting appropriate nearest neighbors for synthetic samples, Zhu et al. ( 2022 ) calculated the Euclidean and Mahalanobis distances of the nearest neighbors, and Wang et al. ( 2023 ) used the characteristics of neighborhood distribution to equilibrate samples. Moreover, studies of (Liu and Zhu 2020 ; Fan et al. 2020 ; Dou et al. 2023 ) further improved the adaptability of SMOTE by employing weighted distributions to shift the importance of classification boundaries more toward the challenging minority classes, demonstrated effectiveness in resolving data imbalance issues.
3.1.3 Deep generative models-based DA
In addition, deep generative models have emerged as highly promising solutions to small data since 2017, autoencoder (AE) and generative adversarial network (GAN) are two prominent representatives (Moreno-Barea et al. 2020 ). AE is a special type of neural network characterized by encoding its input to the output in an unsupervised manner (Hinton and Zemel 1994 ), where the optimization goal is to learn an effective representation of the input data. The fundamental architecture of an AE, illustrated in Fig. 8 a, comprises two symmetric parts encompassing a total of five shallow layers. The first half, known as the encoder, transforms input data into a latent space, while the second half, or the decoder, deciphers this latent representation to reconstruct the data. Likewise, a GAN is composed of two fundamental components, as shown in Fig. 8 b. The first is the generator, responsible for creating fake samples based on input random noise, and the second is the discriminator for identifying the authenticity of the generated samples. These two components engage in an adversarial training process, progressively moving towards a state of Nash equilibrium.
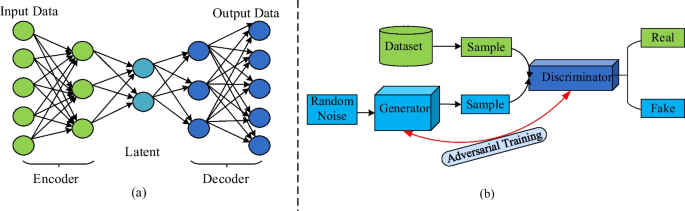
Basic architectures of a AE and b GAN
The unique advantages of GAN in generating diverse samples makes it superior to traditional over-sampling DA methods, especially in tackling data imbalance problems for PHM tasks (Behera et al. 2023 ). Various innovative models have emerged, including variational auto-encoder (VAE) (Qi et al. 2023 ), deep convolutional GAN (DCGAN) (Zheng et al. 2019 ), Wasserstein GAN (Yu et al. 2019 ), etc. These methods can be classified into two groups based on their input types. The first commonly generates data from 1-D inputs like raw signals (Zheng et al. 2019 ; Yu et al. 2019 ; Dixit and Verma 2020 ; Ma et al. 2021 ; Zhao et al. 2021a , 2020b ; Liu et al. 2022 ; Guo et al. 2020 ; Wan et al. 2021 ; Huang et al. 2020 , 2022 ; Zhang et al. 2020b ; Behera and Misra 2021 ; Wenbai et al. 2021 ; Jiang et al. 2023 ) and frequency features (Ding et al. 2019 ; Miao et al. 2021 ; Mao et al. 2019 ), which can capture the inherent temporal information in signals without complex pre-processing. For instance, Dixit and Verma ( 2020 ) proposed an improved conditional VAE to generate synthetic samples using raw vibration signals, yielding remarkable FD performance despite limited data availability. The work in Mao et al. ( 2019 ) applied the Fast Fourier Transform (FFT) to convert original signals into the frequency domain as inputs for GAN and obtained higher-quality generated samples. On the other hand, some studies (Du et al. 2019 ; Yan et al. 2022 ; Liang et al. 2020 ; Zhao and Yuan 2021 ; Zhao et al. 2022 ; Sun et al. 2021 ; Zhang et al. 2022b ; Bai et al. 2023 ) took the strengths of AEs and GANs in the image domain, aimed to generate corresponding images by utilizing 2-D time–frequency representations. For instance, Bai et al. ( 2023 ) employed an intertemporal return plot to transform time-series data to 2-D images as inputs for Wasserstein GAN, and this method reduced data imbalance and improved diagnostic accuracy of bearing faults.
3.1.4 Epilog
Table 4 summarizes the diverse DA-based solutions to addressing small data problems in PHM, covering specific issues tackled by each technique, as well as the merits and drawbacks of each technique. It is evident that DA approaches focus on mitigating small data challenges at the data-level, including problems characterized by insufficient labelled training data, class imbalance, incomplete data, and samples contaminated with noise. To tackle these, transform-based methods primarily increase the size of the training dataset by imposing transformations onto signals, but the effectiveness depends on the quality of raw signals. As for sampling-based approaches, they excel at dealing with unbalanced problems in the PHM tasks, and SMOTE methods demonstrate proficiency in both augmenting minority class samples and diversifying their composition, but refining nearest neighbor selection and bolstering adaptability to high levels of class imbalance remain open research areas. While deep generative models-based DA provides a flexible and promising tool capable of generating samples for various equipment under different working conditions, but more in-depth research is needed on integrating the characteristics of PHM tasks, quality assessment of the generated data, and efficient training of generative models.
3.2 Transfer learning methods
Traditional DL models assumes that training and test data originate from an identical domain, however, changes in operating conditions inevitably cause divergences in data distributions. TL is a new technique that eliminates the requirement for same data distribution by transferring and reusing data or knowledge from related domains, ultimately solving small data problems in the target domain. TL is defined in terms of domains and tasks, each domain \(D\) consists of a feature space and a corresponding marginal distribution, and the task \(T\) associated with each domain contains a label space and a learning function (Yao et al. 2023 ). Within the PHM context, TL can be concisely defined as: Given a source domain \(D_{S}\) and a task \(T_{S}\) , and a target domain \(D_{T}\) with a task \(T_{T}\) . The goal of TL is to exploit the knowledge of certain equipment that is learned from \(D_{S}\) and \(T_{S}\) to enhance the learning process for \(T_{T}\) within \(D_{T}\) under the setting of \(D_{S} \ne D_{T}\) or \(T_{S} \ne T_{T}\) , and the data volume of the source domain is considered much larger than that of the target domain. There is a range of categorization criteria for TL methods in the existing literature. From the perspective of “what to transfer” during the implementation phase, TL can be categorized into three types: instance-based TL, feature-based TL, and parameter-based TL. Among these categories, the former two are affiliated with solutions operating at the data level, while the latter belong to the realm of model-level approaches. These classifications are visually represented in Fig. 9 .
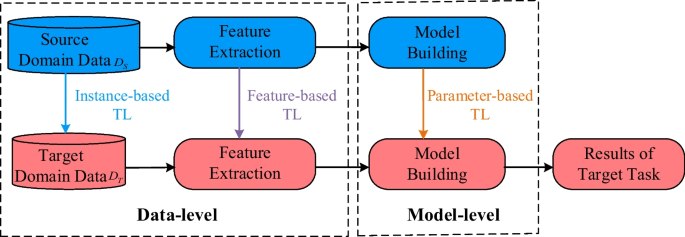
Descriptions of the main categories of TL
3.2.1 Instance-based TL
The premise of applying TL is that the source domain contains sufficient labeled data, whereas the target domain either lacks sufficient labeled data or predominantly consists of unlabeled data. Although a straightforward way is to train a model for the target domain using samples from the source domain, which proves impractical due to the inherent distribution disparities between the two domains. Therefore, finding and applying labeled instances in the source domain that have similar data distribution to the target domain is the key. For this purpose, various methods have been proposed to minimize the distribution divergence, and weighting strategies are the most widely used.
Dynamic weight adjustment (DWA) is a popular strategy, and its novelty lies in reweighting the source and target domain samples based on their contributions to the learning of the target model. Take the well-known TrAdaBoost algorithm (Dai et al. 2007 ) as an example, which increases the weights of samples that are similar to the target domain, and reduces the weights of irrelevant source instances. The effectiveness of TrAdaBoost has been validated in FD for wind turbines (Chen et al. 2021 ), bearings (Miao et al. 2020 ), and induction motors (Xiao et al. 2019 ). Evolving from foundational research, scholars also introduced multi-objective optimization (Lee et al. 2021 ) and DL theories (Jamil et al. 2022 ; Zhang et al. 2020c ) into TrAdaBoost to improve model training efficiency. However, DWA requires labeled target samples, otherwise, weight adjustment methods based on kernel mapping techniques are needed to estimate the key weight parameters, such as matching the mean of source and target domain samples in the replicated kernel Hilbert space (RKHS) (Tang et al. 2023a ). For example, Chen et al . ( 2020 ) designed a white cosine similarity criterion based on kernel principal component analysis to determine the weight parameters for data in the source and target domain, boosting the diagnostic performance for gears under limited data and varying working conditions. More research can be found in Liu and Ren ( 2020 ), Xing et al. ( 2021 ), Ruan et al. ( 2022 ).
3.2.2 Feature-based TL
Unlike instance-based TL that finds similarities between different domains in the space of raw samples, feature-based methods perform knowledge transfer within a shared feature space between source and target domains. As demonstrated in Fig. 10 , feature-based TL is widely applied in domain-adaption and domain-generalization scenarios, where the former focuses on how to migrate knowledge from the source domain to the target domain, and domain generalization aims to develop a model that is robust across multiple source domains so that it can be generalized to any new domain. The key to feature-based TL is to reduce the disparities between the marginal and conditional distributions of different domains by some operations, such as discrepancy-based methods and feature reduction methods, which eventually enable the model to achieve excellent adaptation and generalization on target tasks (Qin et al. 2023 ).
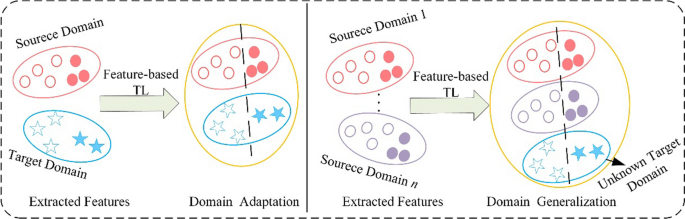
Application scenarios of feature-based TL
The main challenge for discrepancy-based methods is to accurately quantify the distributional similarity between domains, which relies on specific distance metrics. Table 5 lists the popular metrics (Borgwardt et al. 2006 ; Kullback and Leibler 1951 ; Gretton et al. 2012 ; Sun and Saenko 2016 ; Arjovsky et al. 2017 ) and the algorithms applied to PHM tasks (Yang et al. 2018 , 2019a ; Cheng et al. 2020 ; Zhao et al. 2020c ; Xia et al. 2021 ; Zhu et al. 2023a ; Li et al. 2020b , c , 2021a ; He et al. 2021 ). Maximum Mean Discrepancy (MMD) is based on the distance between instance means in the RKHS, and Wasserstein distance assesses the likeness of probability distributions by considering geometric properties, both of which are widely used. For example, Yang et al . ( 2018 ) devised a convolutional adaptation network with multicore MMD to minimize the distribution discrepancy between the feature distributions derived from both laboratory and real machines failure data. And the integration of Wasserstein distance in Cheng et al. ( 2020 ) greatly enhanced the domain adaptation capability of the proposed model. Moreover, Fan et al. ( 2023a ) proposed a domain-based discrepancy metric for domain generalization fault diagnosis under unseen conditions, which helps model balance the intra- and interdomain distances for multiple source domains. On the other hand, feature reduction approaches aim to automatically capture general representations across different domains, mainly using unsupervised methods such as clustering (Michau and Fink 2021 ; He et al. 2020a ; Mao et al. 2021 ) and AE models (Tian et al. 2020 ; Lu and Yin 2021 ; Hu et al. 2021a ; Mao et al. 2020 ). For instance, Mao et al. ( 2021 ) integrated time series clustering into TL, and used the meta-degradation information obtained from each cluster for temporal domain adaptation in bearing RUL prediction. To improve model performance for imbalanced and transferable FD, Lu and Yin ( 2021 ) designed a weakly supervised convolutional AE (CAE) model to learn representations from multi-domain data. Liao et al. ( 2020 ) presented a deep semi-supervised domain generalization network, which showed excellent generalization performance in performing rotary machinery fault diagnosis under unseen speed.
3.2.3 Parameter-based TL
The third category of TL is parameter-based TL, which supposes that the source and target tasks share certain knowledge at the model level, and the knowledge is encoded in the architecture and parameters of the model pre-trained on the source domain. It is motivated by the fact that retraining a model from scratch requires substantial data and time, while it is more efficient to directly transfer pre-trained parameters and fine-tune them in the target domain. In this way, there are basically two main implementations depending on the utilization of the transferred parameters in target model training: full fine-tuning (or freezing) and partial fine-tuning (or freezing), as shown in Fig. 11 .
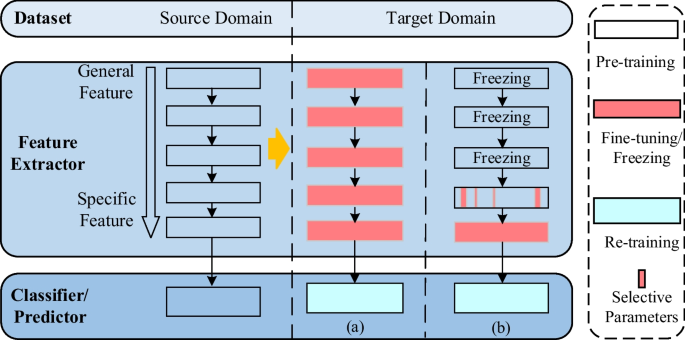
Parameter-based TL. a Full fine-tuning (or freezing), b partial fine-tuning (or freezing)
Full fine-tuning (or freezing) means that all parameters transferred from the source domain are fine-tuned with limited labelled data from the target domain, or those parameters are frozen without updating during the training of the target model. Conversely, partial fine-tuning (or freezing) is the selective fine-tuning of only specific upper layers or parameters, keeping the lower layer parameters consistent with the pre-trained model. In both cases, the classifier or predictor of the target model needs to be retrained with randomly initialized parameters to align with the number of classes or data distribution of the target task. The full fine-tuning (or freezing) approach is particularly applicable when the source and target domain samples exhibit a high degree of similarity, so that general features can be extracted from the target domain by using the pre-training parameters (Cho et al. 2020 ; He et al. 2019 , 2020b ; Zhiyi et al. 2020 ; Wu and Zhao 2020 ; Peng et al. 2021 ; Zhang et al. 2018 ; Che et al. 2020 ; Cao et al. 2018 ; Wen et al. 2020 , 2019 ). From the perspective of the size of the pre-trained model and the fine-tuning time, the full fine-tuning and full freezing strategies are suitable for small and large models, respectively. For example, He et al . (Zhiyi et al. 2020 ) proposed to achieve knowledge transfer between bearings mounted on different machines by fully fine-tuning the pre-trained parameters with few target training samples. In Wen et al. ( 2020 , 2019 ), researchers applied deep convolutional neural networks (CNN)—ResNet-50 (a 50-layer CNN) and VGG-19 (a 19-layer CNN) that was pre-trained on ImageNet as feature extractors, and train target FD models using full freezing methods. In contrast, partial fine-tuning (or freezing) strategies are more suitable for handling cases with significant domain differences (Wu et al. 2020 ; Zhang et al. 2020d ; Yang et al. 2021 ; Brusa et al. 2021 ; Li et al. 2021b ), such as transfer between complex working conditions (Wu et al. 2020 ) and multimodal data sources (Brusa et al. 2021 ). In addition, Kim and Youn ( 2019 ) introduced an innovative approach known as selective parameter freezing (SPF), where only a portion of parameters within each layer is frozen, which enables explicit selection of output-sensitive parameters from the source model, reducing the risk of overfitting the target model under limited data conditions.
3.2.4 Epilog
The TL framework breaks the assumption of homogeneous distribution of training and test data in traditional DL and compensates for the lack of labeled data in the target domain by acquiring and transferring knowledge from a large amount of easily collected data. As summarized in Table 6 , instance-based TL can be regarded as a borrowed augmentation, wherein other datasets with similar distributions are utilized to enrich the samples in the target domain. Among the techniques, DWA strategies demonstrate superiority in solving insufficient labeled target data and imbalanced data, whereas their drawbacks of high computational cost and high dependence on similar distributions need further optimization. As a comparison, feature-based TL performs knowledge transfer by learning general fault representations and has the ability to handle domain-adaption and domain-generalization tasks with large distribution differences, such as transfers between distinct working conditions (He et al. 2020a ), transfers between diverse components (Yang et al. 2019a ), or even transfers from simulated to physical processes (Li et al. 2020b ). And weakly supervised-based feature reduction techniques are capable of adaptively discovering better feature representations, and showing great potential in open domain generalization problems. Finally, parameter-based TL saves the target model from being retrained from scratch, but the effectiveness of these parameters hinges on the size and quality of the source samples, and model pre-training on multi-source domain data can be considered (Li et al. 2023b ; Tang et al. 2021 ).
3.3 Few-shot learning methods
DA and TL methods both require that training dataset has a certain number (ranging from dozens to hundreds) of labeled samples. However, in some industrial cases, samples of specific classes (such as incipient failures or compound faults) may be exceptionally rare and inaccessible, with only a handful of samples (e.g., 5–10) per category for DL model training, resulting in poor model performance on such “few-shot” problems (Song et al. 2022 ). Inspired by the human ability to learn and reuse prior knowledge from previous tasks, which Juirgen Schmidhuber initially named meta-learning (Schmidhuber 1987 ), FSL methods have been proposed to learn a model that can be trained and quickly adapted to tasks with only a few examples. As shown in Fig. 12 , there are some differences between traditional DL, TL, and FSL methods: (1) traditional DL and TL are trained and tested on data points from a single task, while FSL methods are often learning at the task level; (2) the learning of traditional DL requires large amounts of labeled training and test data, TL requires large amounts of labeled training data in source domain, while FSL methods perform meta-training and meta-test with limited data. The organization of FSL tasks follows the “ N -way K -shot Q -query” protocol (Thrun and Pratt 2012 ), where N categories are randomly selected, and K support samples and Q query samples are randomly drawn from each category for each task. The objective of FSL is to combine previously acquired knowledge from multiple tasks during meta-training with a few support samples to predict the class of query samples during meta-test. Based on the way prior knowledge is learned, metric-, optimization-, and attribute-based FSL methods are primarily discussed.

Comparison between a traditional DL, b TL, and c FSL methods
3.3.1 Metric-based FSL
Metric-based FSL is to learn priori knowledge by measuring sample similarities, which consists of two components: a feature embedding module responsible for mapping samples to feature vectors, and a metric module to compute similarity (Li et al. 2021 ). Siamese Neural Networks is one of the pioneers, initially proposed by Koch et al. in 2015 for one-shot image recognition (Koch et al. 2015 ), which used two parallel CNNs and L1 distance to determine whether paired inputs are identical. Subsequently, Vinyals et al . ( 2016 ) introducing long short-term memory (LSTM) with attention mechanisms for effective assessment of multi-class similarity, and Snell et al . ( 2017 ) developed Prototypical Networks to calculate the distance between prototype representations, and Relation Networks (Sung et al. 2018 ) utilized adaptive neural networks instead of traditional functions. Table 7 lists the differences between these representative approaches in terms of embedding modules and metric functions.
According to current studies, two forms of metric-based FSL methods are applied in PHM tasks. The first utilizes fixed metrics (e.g., cosine distance) for measuring similarity, while the second leverages learnable metrics, such as the neural network of Relation Networks. For example, Zhang et al . ( 2019 ) firstly introduced a wide-kernel deep CNN-based Siamese Networks for the FD of rolling bearings, which achieved excellent performance with limited data under different working conditions. Then, various FSL algorithms based on Siamese networks (Li et al. 2022c ; Zhao et al. 2023 ; Wang and Xu 2021 ), matching networks (Xu et al. 2020 ; Wu et al. 2023 ; Zhang et al. 2020e ) and prototypical networks (Lao et al. 2023 ; Jiang et al. 2022 ; Long et al. 2023 ; Zhang et al. 2022c ) have been developed for PHM tasks. Zhang et al. ( 2020e ) designed an iterative matching network combined with a selective signal reuse strategy for the few-shot FD of wind turbines. Jiang et al. ( 2022 ) developed a two-branch prototype network (TBPN) model, which integrated both time and frequency domain signals to enhance fault classification accuracy. While Relation Networks have shown superiority over fixed metric-based FSL methods when measuring samples from different domains, and they are therefore widely applied for cross-domain few-shot tasks (Lu et al. 2021 ; Wang et al. 2020b ; Luo et al. 2022 ; Yang et al. 2023a ; Tang et al. 2023b ). To illustrate, Lu et al . ( 2021 ) considered the FD of rotating machinery with limited data as a similarity metric learning problem, and they introduced Relation Networks into the TL framework as a solution. Luo et al. ( 2022 ) proposed a Triplet Relation Network method for performing cross-component few-shot FD tasks, and Tang et al. ( 2023b ) designed a novel lightweight relation network for performing cross-domain few-shot FD tasks with high efficiency. Furthermore, to address domain shift issues resulting from diverse working conditions, Feng et al. ( 2021 ) integrated similarity-based meta-learning network with domain-adversarial for cross-domain fault identification.
3.3.2 Optimization-based FSL
Optimization-based FSL methods adhere to the “learning to optimize” principle to solve overfitting problems arising from small samples. Specifically, these techniques learn good global initialization parameters across various tasks, allowing the model to quickly adapt to new few-shot tasks during the meta-test (Parnami and Lee 2022 ). Taking the best-known model agnostic meta-learning (MAML) (Finn et al. 2017 ) algorithm as an example, optimization-based FSL typically follows a two-loop learning process, first learning a task-specific model (base learner) for a given task in the inner loop, and then learning a meta-learner over a distribution of tasks in the outer loop, where meta-knowledge is embedded in the model parameters and then used as initialization parameters of the model for meta-test tasks. MAML is compatible with diverse models that are trained using gradient descent, allowing models to generalize well to new few-shot tasks without overfitting.
Recent literature highlights the potential of MAML in PHM, mainly focuses on meta-classification and meta-regression. For meta-classification methods, the aim is to learn an optimized classification model based on multiple meta-training tasks that can accurately classify novel classes in meta-test with a few samples as support, typically used for AD (Chen et al. 2022 ) and FD tasks (Li et al. 2021c , 2023c ; Hu et al. 2021b ; Lin et al. 2023 ; Yu et al. 2021b ; Chen et al. 2023b ; Zhang et al. 2021 ; Ren et al. 2024 ). For example, Li et al . ( 2021c ) proposed a MAML-based meta-learning FD technique for bearings under new conditions by exploiting the prior knowledge of known working conditions. To further improve meta-learning capabilities, advanced models such as task-sequencing MAML (Hu et al. 2021b ) and meta-transfer MAML (Li et al. 2023c ) have been designed for few-shot FD tasks, and a meta-learning based domain generalization framework was proposed to alleviate both low-resource and domain shift problems (Ren et al. 2024 ). On the other hand, meta-regression methods target prediction tasks in PHM, with the goal of predicting continuous variables using limited input samples based on meta-optimized models derived from analogous regression tasks (Li et al. 2019 , 2022d ; Ding et al. 2021 , 2022a ; Mo et al. 2022 ; Ding and Jia 2021 ). Li et al . ( 2019 ) first explored the application of MAML to RUL prediction with small size data in 2019, a fully connected neural network (FCNN)-based meta-regression model was designed for predicting tool wear under varying cutting conditions. In addition, MAML has also been integrated into reinforcement learning for fault control under degraded conditions, and more insights can be found in Dai et al. ( 2022 ), Yu et al. ( 2023 ).
3.3.3 Attribute-based FSL
There is also a unique paradigm of FSL known as “zero-shot learning” (Yang et al. 2022 ), where models are used to predict the classes for which no samples were seen during meta-training. In this setup, auxiliary information is necessary to bridge the information gap of unseen classes due to the absence of training data. The supplementary information must be valid, unique, and representative that can effectively differentiate various classes, such as attribute information for images in computer vision. As shown in Fig. 13 , the classes of unseen animals are inferenced by transferring the between-class attributes, such as semantic descriptions of animals’ shape, voice, or habitats, whose effectiveness has been validated in many zero-shot tasks (Zhou et al. 2023b ).
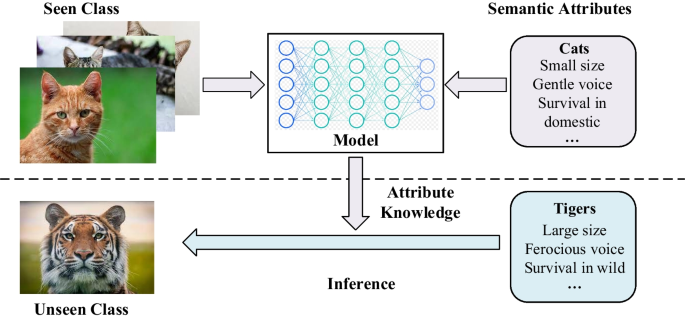
Attribute-based methods in zero-shot image identification
The idea of attributed-based FSL approach offers potential solutions to the zero-sample problem in PHM tasks. However, visual attributes cannot be used directly because they do not match the physical meaning of the sensor signals, and for this reason, scholars have worked on effective fault attributes. Given that fault-related semantic descriptions can be easily obtained from maintenance records and can be defined for specific faults in practice, semantic attributes are widely used in current research (Zhuo and Ge 2021 ; Feng and Zhao 2020 ; Xu et al. 2022 ; Chen et al. 2023c ; Xing et al. 2022 ). For example, Feng and Zhao ( 2020 ) pioneered the implementation of zero-shot FD based on the transfer of fault description attributes, which included failure position, fault causes and consequences, providing auxiliary knowledge for the target faults. Xu et al. ( 2022 ) devised a zero-shot learning framework for compound FD, and the semantic descriptor of the framework can define distinct fault semantics for singular and compound faults. Fan et al. ( 2023b ) proposed an attribute fusion transfer method for zero-shot FD with new fault modes. Despite the strides made in description-drive semantic attributes, certain limitations exist, including reliance on expert insights and inaccurate information sources. More recently, attributes without semantic information (termed non-semantic attributes) have also been explored in Lu et al. ( 2022 ), Lv et al. ( 2020 ). Lu et al. ( 2022 ) developed a zero-shot intelligent FD system by employing statistical attributes extracted from time and frequency domains of signal.
3.3.4 Epilog
FSL methods are advantageous in solving small data problems with extremely limited samples, such as giving only five, one, or even zero samples per class in each task. As listed in Table 8 , metric-based FSL methods are concise in their principles and computation, and they shift the focus from sample quantity to intrinsic similarity, but the reliance on labeled data during the training of feature embeddings constrains their applicability in supervised settings. Optimization-based FSL, particularly those underpinned by MAML, boast broader applications including fault classification, RUL prediction, and fault control, but these techniques need substantial computational resources for the gradient optimization of deep networks, and the balance between the optimization parameters and model training speed is the key (Hu et al. 2023 ). Attribute-based FSL is an emerging but promising research topic that has huge potential to significantly reduce the cost of data collection in the industry, and zero-shot learning enables model generalize to new failure modes or conditions without retraining, achieving intelligent prognostics for complex systems even with “zero” abnormal or fault sample. In industry, few-shot is often accompanied by domain shift problems caused by varying speed and load conditions, which is a more difficult problem and poses challenges for traditional FSL methods to learn enough representative fault features that can be adapted and generalized to unseen data distributions, and research in this area has recently begun (Liu et al. 2023 ).
4 Discussion of problems in PHM applications
Different PHM tasks have distinct goals and characteristics, thus producing various forms of small data problems and needs corresponding solutions. Therefore, based on the methods discussed in Sect. 3 , this section will further explore the specific issues and remaining challenges from the perspective of PHM applications. And the distribution of specific issues and corresponding methods for each task is shown in Fig. 14 .
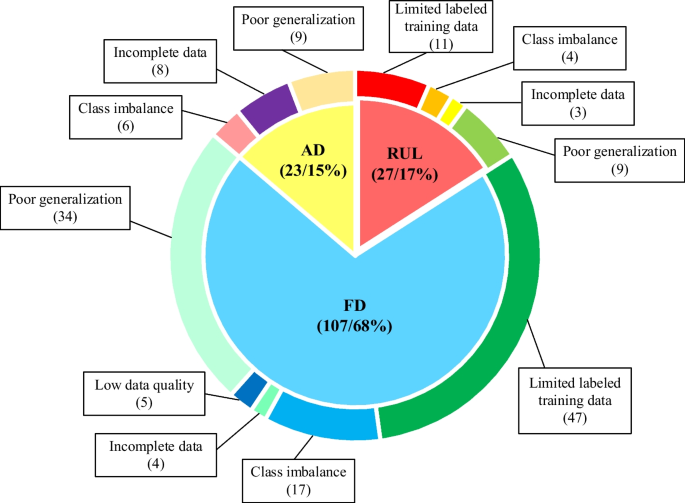
Pie chart of specific problems for each PHM task, and the numbers represent articles published on the corresponding topic
4.1 Small data problems in AD tasks
4.1.1 main problems and corresponding solutions.
In industrial applications, the amount of abnormal data is much less than normal data, which seriously hinders the development of accurate anomaly detectors. According to the statistics in Fig. 14 , current research on small data in AD tasks focuses on three core issues: class imbalance, incomplete data, and poor model generalization, which have different impacts on AD tasks. Specifically, class imbalance may cause the model to be biased towards the normal class, which reduces the sensitivity of detecting rare anomalies; incomplete data can make it difficult for the model to distinguish between normal variations and true anomalies when key features are missing; and the problem of poor model generalization may lead to false-positives or false-negatives, which reduces the overall reliability of the anomaly detection system.
To address the above class imbalance problems, existing studies demonstrate that directly increasing the samples of minority classes through DA techniques yields positive results. In our survey of the literature, two papers (Fan et al. 2020 ; Rajagopalan et al. 2023 ) introduced optimized SMOTE algorithms, and one study employed GAN-based DA methods to AD tasks of wind turbines. These methods facilitated the generation of additional anomalous samples, and enhanced model accuracy while minimizing false positive rates. Another prevalent challenge in AD tasks is incomplete data, stemming from faulty sensors, inaccurate measurements, or different sampling rates. Deep generative models, with their superior learning capabilities, have been widely used to improve information density of incomplete data (Guo et al. 2020 ; Yan et al. 2022 ). To address inadequate model generalization when confronted with limited labeled training samples, Michau and Fink ( 2021 ) proposed an unsupervised TL framework. Notably, the majority of AD methods advanced in current research are rooted in unsupervised learning models, such as AE, with wide applications involving electric motors (Rajagopalan et al. 2023 ), process equipment (Guo et al. 2020 ), and wind turbines (Liu et al. 2019 ).
4.1.2 Remaining challenges
AD is an integral and fundamental task in equipment health monitoring, where the difficulty lies in dealing with a complex set of data and various anomalies (Pang et al. 2021 ). Though existing research has provided valuable insights into addressing small data challenges, certain unresolved issues warrant further exploration.
4.1.2.1 Adaptability of detection models
The majority of AD algorithms are domain-dependent and designed for specific anomalies and conditions. However, industrial production constitutes a dynamic and nonlinear process, where changes in variables such as environment, speed, or load may lead to data drift and novel anomalies. For small datasets, even minor changes in the underlying patterns can have a pronounced impact on the dataset’s characteristics, thus degrading anomaly detection performance of models. To address these issues, it is imperative to improve the adaptability of detection models by using adaptive optimizers and learners, such as the online adaptive Recurrent Neural Network proposed in Fekri et al. ( 2021 ), which had the capability to learn from newly arriving data and adapt to novel patterns.
4.1.2.2 Real-time anomaly detection
Real-time is always a desirable property of detection models, which ensure that anomalies can be detected and reported to the operator in a timely manner, and corresponding decisions can be made quickly, and this is especially important for complex equipment, such as UAVs (Yang et al. 2023b ). The deployment of lightweight network architectures and edge computing technologies holds promise in enabling the realization of real-time detection capabilities.
4.2 Small data problems in FD tasks
4.2.1 main problems and corresponding solutions.
Accuracy is one of the most important metrics for evaluating models’ performance in classifying different types of faults, but it is strongly influenced by the size of the fault data. As shown in Fig. 14 , the small data challenge in FD has received the most extensive research attention compared to AD and RUL prediction tasks. The small data problem has also manifested itself in a richer variety of ways, including limited labeled training data, class imbalance, incomplete data, low data quality, and poor generalization. First, limited labeled training data increases the risk of model overfitting and poses a challenge in capturing variations in fault conditions, class imbalance leads to lower sensitivity to few and unseen faults, incomplete data leads to incomplete extraction of fault features, low-quality data misleads the diagnostic model to generate false positives or false negatives; and poor generalization capability limits the applicability of the model to different operating conditions and equipment.
To address the scarcity of labeled training data, two practical solutions emerge: using samples within the existing datasets, and borrowing from external data sources. The former involves employing already acquired signals to generate samples adhering to the same data distribution. Following this idea, there are five and eight surveyed papers have utilized transform-based and deep generative models-based DA methods, respectively, with 1-D vibration signals as input. The second involves three main techniques: reusing samples from other domains through sample-based TL, obtaining available features via feature-based TL, and utilizing attribute representations through attribute-based FSL. According to the statistics of the surveyed papers, feature-based approaches were employed 15 times for cross-domain scenarios, and attribute-based methods were chosen 7 times for predicting novel classes with zero training samples. Data imbalance is another common problem in FD, with 16 articles retrieved on this topic, and most of them applying deep generative models to address inter-class imbalance problems. In addition to the issues discussed above, data quality problems such as incomplete data and noisy labels have also gained attention, with two and three papers based on deep generative models being presented, respectively.
Secondly, as for the issues caused by limited data at the model level, such as overfitting, diminished accuracy, and weakened generalization, researchers also have also proposed various solutions. This includes 12 papers using parameter-based TL methods, 14 papers applying metric-based FSL methods, and 8 papers using MAML-based FSL approaches. Among these, parameter-based TL methods leverage knowledge within the structure and parameters of models to decrease training time, while metric-based FSL alleviates the requirement for sample size by learning category similarities, and MAML-based FSL achieves fast adaptation to novel FD tasks by using meta-learned knowledge. These successful applications also demonstrate the potential for integrating TL and FSL paradigms to improve model accuracy and generalizability.
4.2.2 Remaining challenges
The data-level and model-level approaches proposed above have made significant progress in solving the small data problems in FD tasks. However, there are still some challenges that need to be addressed urgently.
4.2.2.1 Quality of small data
In our survey of 107 studies on FD tasks, most focused on solving sample size problems, only five papers investigated data quality issues in small data challenges. It is important for researchers to realize that a voluminous collection of irrelevant samples is far inferior to a small yet high-quality dataset on FD tasks. And the poor quality of small data results from both samples and labels, including but not limited to missing data, noise and outliers in signal measurement, and the errors during labeling. Consequently, there is a large research gap in factor analysis, data quality assessment and data enhancement.
4.2.2.2 System-level FD with limited data
The majority of current algorithms for handling small data problems focus on component-level FD, as evidenced by their applications to bearings (Zhang et al. 2020a ; Yu et al. 2020 , 2021a ) and gears (Zhao et al. 2020b ). However, these methods cannot meet the diagnostic demands of intricate industrial systems composed of multiple components. Thus, developing intelligent models to perform system-level FD with limited data requires more exploration.
4.3 Small data problems in RUL prediction tasks
4.3.1 main problems and corresponding solutions.
The paradox inherent in RUL prediction lies in its aim to estimate degradation trends of an equipment based on historical monitoring data, whereas run-to-failure data are difficult to obtain. This paradox has motivated scholars to recognize the significance of small data issues within prognostic tasks. Among the 27 reviewed papers, the problems of limited labeled training data, class imbalance, incomplete data, and poor model generalization are mainly studied. While these issues are similar to those in the FD task, the RUL prediction task has different implications due to its continuous label space nature (Ding et al. 2023a ). Specifically, limited labeled training data makes it difficult to learn sufficiently robust representations of health indictors, class imbalance may lead to more frequent prediction of non-failure events and produce conservative estimates, missing information in incomplete data further increases prediction uncertainty, and poorer generalization capability reduces the compatibility of the model for different operating conditions or devices.
RUL prediction is a typical regression task, wherein the quantity of training data profoundly influences the feature learning and nonlinear fitting abilities of DL models. Addressing the challenges of limited labeled training data, solutions include transform-(Fu et al. 2020 ; Sadoughi et al. 2019 ; Gay et al. 2022 ) and generative model-based (Zhang et al. 2020b ) DA methods, alongside instance- (Zhang et al. 2020c ; Ruan et al. 2022 ) and feature-based (Xia et al. 2021 ; Mao et al. 2021 , 2020 ) TL methods. Among the reviewed papers, three sampling-based DA methods and one deep generative models-based DA approach have been reported to alleviate class imbalance problems. For instance, the Adaptive Synthetic over-sampling strategy was proposed in Liu and Zhu ( 2020 ) for tool wear prediction with imbalanced truncation data. Another major challenge of RUL prediction is the incomplete time-series data, which is treated as an imputation problem, the proposed GAN-based methods in Huang et al. ( 2022 ), Wenbai et al. ( 2021 ) achieved the insertion of missing data by automatically learning the correlations within time series. Model-level solutions based on TL and FSL have also been employed to enhance the generalization of predictive models across domains when faced with limited time series samples. Notably, MAML-based few-shot prognostics (Li et al. 2019 , 2022d ; Ding et al. 2021 , 2022a ; Mo et al. 2022 ; Ding and Jia 2021 ) have recently demonstrated substantial advancements within the PHM field. In addition, LSTM has become a popular benchmark model for RUL prediction tasks, due to their proficiency in capturing long-term dependencies, and the combination of LSTM with CNN has extended the capability of learning degradation patterns (Wenbai et al. 2021 ; Xia et al. 2021 ).
4.3.2 Remaining challenges
Significant strides have been made in addressing the challenges of limited data in RUL predictions. However, it is noteworthy that many of the proposed methods rely more or less on certain assumptions that might not hold in real-world conditions. In order to achieve more reliable forecasts, a number of major challenges must be addressed.
4.3.2.1 Interpretability of prognostic models
Although numerous prognostic models have shown impressive predictive performance, many are poorly interpreted. The inherent “black box” nature of DL models diminishes their desired interpretability, transparency, and causal insights about both the models and their outcomes. Consequently, within RUL prediction, interpretability is much needed to reveal the underlying degradation mechanisms hidden in the monitoring data, thus increasing the level of “trust” of intelligent models.
4.3.2.2 Uncertainty quantification in small data conditions
Uncertainty quantification (UQ) is an important dimension of the PHM framework that can improve the quality of RUL prediction through risk assessment and management. Uncertainty involved in RUL predictions can be categorized into aleatory uncertainty and epistemic uncertainty (Kiureghian and Ditlevsen 2009 ), where the first type often results from the noise inherent in data, such as the noise in signal measurements; while the second category of uncertainty is attributed to the deficiency of model knowledge, including model architecture, and model parameters. As discussed above, the impacts of small data challenge at the data level (incomplete data and unbalanced distribution) and model level (poor generalization) both further increase the uncertainty of predictive results, leading to few studies on UQ under small data conditions. The existing research on the UQ of intelligent RUL predictions mainly applies Gaussian process regression and Bayesian neural networks. For example, Ding et al. ( 2023b ) designed a Bayesian approximation enhanced probabilistic meta-learning method to reduce parameter uncertainty in few-shot prognostics. The recent study (Nemani et al. 2023 ) demonstrates that the physics-informed ML is promising for the UQ of RUL predictions in small data conditions by combining physics-based and data-driven modeling.
5 Datasets and experimental settings
There is a growing number of proposed methods for small data problems in the PHM domain, but there is a lack of corresponding unified criteria for fair and valid evaluation of the proposed methods, one of the major reasons being the complexity and variability of the equipment and working conditions under study. To this end, we analyze and distill two key elements of model evaluation in current studies—datasets and small data settings, which are summarized in this section to provide guidance for effective evaluation of existing models.
5.1 Datasets
In the past decade, the PHM community has released many diagnostic and prognostic benchmarks that cover different mechanical objects, such as bearings (Smith and Randall 2015 ; Lessmeier et al. 2016 ; Qiu et al. 2006 ; Nectoux et al. 2012 ; Wang et al. 2018a ; Bechhoefer 2013 ), gearbox (Shao et al. 2018 ; Xie et al. 2016 ), turbofan engines (Saxena et al. 2008 ), and cutting tools (Agogino and Goebel 2007 ). Table 9 lists several datasets that have been widely used in existing research to study small data problems, and the signal types, failure modes, counts of operational conditions, application to PHM tasks, and features of these datasets are outlined.
Different datasets exhibit distinct characteristics and are therefore suitable for studying various problems. Depending on how the fault data is generated, these datasets can be broadly categorized as simulated fault datasets, real fault datasets, and hybrid datasets. Among them, the simulated fault datasets (Smith and Randall 2015 ; Qiu et al. 2006 ; Wang et al. 2018a ; Shao et al. 2018 ; Xie et al. 2016 ; Saxena et al. 2008 ; Bronz et al. 2020 ; Downs and Vogel 1993 ) obtain fault samples by using artificially induced faults or simulation software, and the experimental process involves limited and human-controlled variables, so the fault characteristics and degradation modes in the data are relatively simpler, and the DL models often achieve excellent performance. A typical example is the Case Western Reserve University (CWRU) dataset (Smith and Randall 2015 ), which is a well-known simulation benchmark widely used for small data problems in AD, FD and RUL prediction tasks. The CWRU has characteristics of multiple failure modes, unbalanced classes, different bearings, and various operating conditions, which provide opportunities for studying limited labeled training data (Ding et al. 2019 ), class imbalance (Mao et al. 2019 ), incomplete data (Yang et al. 2020a ), and equipment degradation under various conditions (Kim and Youn 2019 ; Li et al. 2021c ).
However, real fault datasets (Nectoux et al. 2012 ; Agogino and Goebel 2007 ) collect failure samples from equipment during natural degradation, which is often accompanied by many uncontrollable factors from the equipment itself and the external environment, resulting in more complex data distributions. These datasets are generally used to validate the robustness of small-data solutions in practical conditions (Sadoughi et al. 2019 ). Moreover, hybrid datasets (Lessmeier et al. 2016 ; Bechhoefer 2013 ) contain both artificially damaged and real-damaged fault data, and they are used to validate the transfer between failures across objects, working conditions, and from laboratory to real environments (Wang et al. 2020b ).
Further, in terms of the types of signals contained in the datasets, vibration signals, sound signals, electric currents, and temperatures are the most common. These various signals open up avenues for developing multi-source data fusion techniques (Yan et al. 2023 ). In addition, some datasets include not only single faults but also composite faults, and these datasets facilitate the study of diagnosis and prognosis of compound faults. Moreover, as shown in Table 9 , most of the datasets collect signals from individual components, but samples from subsystems (Shao et al. 2018 ) or entire systems (Downs and Vogel 1993 ) for system-level diagnostics and prediction are required.
5.2 Experimental setups
During the execution of intelligent PHM tasks, the general process of conducting experiments for DL models is to first divide the dataset into training, validation, and test sets according to a certain ratio. However, in order to simulate limited data scenarios, designing “small data” is a conundrum. There are two popular strategies used in the current studies, as shown in Table 10 .
5.2.1 Setting a small sample size
The most direct and commonly employed setup in studying small data problems involves reducing the number of training or test samples to a few or dozens, which is achieved by selecting a tiny subset of the entire dataset. For example, 2.5% of the dataset was used for training in Xing et al. ( 2022 ), meaning only five fault samples of each class were provided to the model, which is far less than the hundreds or thousands of samples required by traditional DL methods. Due to the ease of implementation and understanding, this strategy has been widely used in AD, FD, and RUL prediction tasks with limited data, and it was notably observed in most experiments using DA and TL methods. However, the number of “small sample” is relative to the total size of the dataset and lacks a unified standard, which should be consistent when comparing various methods.
5.2.2 Following the N -way K -shot protocol
Another strategy is to treat PHM tasks under limited data conditions as a few-shot classification or regression problems. This strategy draws on the organization of the FSL method that extends the input samples from data point level to task space. As described in Sect. 3.3 , each N -way K -shot task consists of N ( N ≤ 20) classes, with each class containing K ( K ≤ 10) support samples. Creating multiple N -way K -shot subtasks can be used for the training and test of FSL models. In the case of the CWRU dataset, for example, 10-way 1/5-shot FD tasks are frequently designed. This setting better aligns the principles of the FSL framework and proves to be beneficial in detecting novel faults under unseen conditions. However, tasks need to be sampled from a sufficiently large number of categories, otherwise the tasks will be homogeneous and degrade model performance.
6 Future research directions
Currently, most of the data sizes involved in intelligent PHM tasks are still in the small data stage and will be for a long time. Various methods proposed in existing research have made significant progress, but there is still a long way to go to realize data-efficient PHM. For this reason, we propose some directions for further research on small data challenges.
6.1 Data governance
Existing research on the limited data challenge focuses on the quantity of monitoring data, with relatively little attention paid to the quality of the samples. In fact, monitoring data serves as the “raw material” for implementing PHM tasks, and its quality seriously affects the performance of intelligent models as well as the accuracy of maintenance decisions. As a result, it is imperative to research the theories and methodologies concerning the governance of industrial data, which involves the quantification, assessment, and enhancement of data quality (Karkošková 2023 ). An in-depth exploration of these ensures that the collected monitoring data meets the data quality requirements set out in the ISO/IEC 25012 standard (Gualo et al. 2021 ), thereby minimizing the adverse effects of factors such as sensor drift, measurement errors, environmental noise, and label inaccuracies. Data governance is a key component to steer the trajectory of intelligent PHM from the prevalent model-centric paradigm towards a data-centric fashion (Zha et al. 2023 ).
6.2 Multimodal learning
Multimodal learning is a novel paradigm to train models with multiple different data modalities, which provides a potential means to solve small data problems in PHM. Specifically, rich forms of monitoring data exist in industry, including but not limited to surveillance videos, equipment images, and maintenance records, these data contain a wealth of intermodal and cross-modal information, which can be fused by multimodal learning techniques (Xu et al. 2023 ) to compensate for the low information density of limited unimodal data. Meanwhile, multimodal data from different systems and equipment can help to perceive their health status more comprehensively, thus improving the intelligent diagnosis and forecasting capability for the entire fleet of equipment (Jose et al. 2023 ).
6.3 Physics-informed data-driven approaches
Existing studies have demonstrated that data-driven approaches, especially those based on DL, excel at capturing underlying patterns from multivariable data, but they are susceptible to small dataset size. While physics model-based methods incorporate mechanisms or expert knowledge during the modeling process, but have limited data processing capabilities. Considering the respective strengths and weaknesses of these two paradigms, an emerging trend is to develop hybrid frameworks that integrate domain knowledge with implicit knowledge extracted from data (Ma et al. 2023 ), which has two obvious advantages in solving small data problems. On the one hand, the introduction of physical knowledge reduces the black-box characteristics of the DL model to a certain extent and enhances the interpretability of the PHM task decision-making under small samples (Weikun et al. 2023 ); On the other hand, physical modeling takes the known physical laws and principles as priori knowledge, which can reduce the uncertainty and domain bias brought by the small-sample data under the complex working conditions, for example, Shi et al. ( 2022 ) have validated the effectiveness of introducing multibody dynamic simulation into data augmentation for robustness enhancement.
6.4 Weak-supervised learning
DL-based models have demonstrated much potential in numerous PHM tasks, but their performance relies heavily on supervised learning, and the need for abundant annotated data are significant barriers to deploying these models in the industry. As we all know, obtaining high-quality labeled data is time-intensive and expensive, but unlabeled data is more readily available in practice. This reality has spurred the exploration of techniques such as unsupervised and self-supervised learning methods to perform autonomous construction of learning models using unlabeled data. Weak-supervised strategies have been successfully employed in the fields of computer vision and natural language processing, and the application potential in PHM tasks has been explored by Zhao et al . ( 2021b ) and Ding et al . ( 2022b ), and the results illustrate that these methods excel at addressing the open-set diagnostic and prognostic problems with small data.
6.5 Federated learning
Federated learning (FL) (Yang et al. 2019b ), a promising framework for developing DL models with low resources, which adheres to the unique principle of “data stays put, models move”. FL allows the training of decentralized models using data generated by each manufacturing company separately, without the need to aggregate the data from all manufacturers into a centralized repository, resulting in two significant benefits. First, from a cost perspective, FL reduces the expenses associated with large-scale data collection, transmission, storage, and model training. Second, from a data privacy standpoint, the FL approach directly leverages locally-held data without data sharing, eliminating concerns of data owners about data sovereignty and business secrets. Moreover, the distributed training process of exchanging only partially model parameters reduces the risk of malicious attacks on PHM models in industrial applications (Arunan et al. 2023 ). At present, representative models include federated averaging (FedAvg) (McMahan et al. 2017 ), federated proximal (FedProx) (Mishchenko et al. 2022 ), federated transfer learning (Kevin et al. 2021 ), and federated meta-learning (Fallah et al. 2020 ), which provide valuable guidance in developing reliable and responsible intelligent PHM. Due to the complexity of equipment composition and working conditions, issues such as device heterogeneity and data imbalance in FL applications in PHM require more attention and research (Berghout et al. 2022 ).
6.6 Large-scale models
Since the release of GPT-3 (Brown et al. 2020 ) and ChatGPT (Scheurer et al. 2023 ), large-scale models have become a hot topic in academia and industry, triggering a new wave of innovation. Technically, large-scale models are the evolution and extension of traditional DL models that they require large amounts of data and computing resources to train the hundreds of millions of parameters, and demonstrate amazing abilities in data understanding, multi-task performing, logical reasoning, and domain generalization. Considering the remaining challenges of traditional DL models in performing PHM tasks with small data, developing large-scale models for the PHM-domain is a promising direction, the pre-trained large-scale model is first chosen based on the target PHM task and signal type, such as the pre-trained BERT is reused for RUL prediction (Zhu et al. 2023b ), and which is then fine-tuned by freezing most layers and only fine-tuning the top layers with small amounts of data, and regularization and architecture adjustment techniques may be used to alleviate overfitting during the process. The study in Li et al. ( 2023d ) have validated that the large-scale models pretrained on multi-modal data from related equipment and working conditions can be generalized to cross-task and cross-domain tasks with zero-shot.
7 Conclusions
Intelligent PHM is a key part in Industry 4.0, and it is closely linked to big data and AI models. To address the difficulties of developing DL models with limited data, we provide the first comprehensive overview of small data challenges in PHM. The definition, causes and impacts of small data are first systematically analyzed to answer the research questions of “what” and “why” of solving data scarcity problems. We then comprehensively summarize the proposed solutions along three technical lines to report how the small data issues have been addressed in existing studies. Furthermore, the problems and remaining challenges within each specific PHM task are explored. Additionally, available benchmark datasets, experimental settings, and promising directions are discussed, to offer valuable references for future research on more intelligent, data-efficient, and explainable PHM methods. Learning from small data is critical to advancing intelligent PHM, as well as contributing the development of General Industrial AI.
Adadi A (2021) A survey on data-efficient algorithms in big data era. J Big Data 8:1–54
Article Google Scholar
Agogino A, Goebel K (2007) BEST lab, UC Berkeley, Milling Data Set. NASA Ames Prognostics Data Repository, NASA Ames Research Center, Moffett Field
Arjovsky M, Chintala S, Bottou L (2017) Wasserstein generative adversarial networks. PMLR, pp 214–223
Google Scholar
Arunan A, Qin Y, Li X, Yuen C (2023) A federated learning-based industrial health prognostics for heterogeneous edge devices using matched feature extraction. IEEE Trans Autom Sci Eng. https://doi.org/10.1109/TASE.2023.3274648
Baeza-Yates R (2024) Gold blog BIG, small or right data: Which is the proper focus?
Bai G, Sun W, Cao C, Wang D, Sun Q, Sun L (2023) GAN-based bearing fault diagnosis method for short and imbalanced vibration signal. IEEE Sens J 24:1894–1904
Bechhoefer E (2013) Condition based maintenance fault database for testing diagnostics and prognostic algorithms. MFPT Data
Behera S, Misra R (2021) Generative adversarial networks based remaining useful life estimation for IIoT. Comput Electr Eng 92:107195
Behera S, Misra R, Sillitti A (2023) GAN-based multi-task learning approach for prognostics and health management of IIoT. IEEE Trans Autom Sci Eng. https://doi.org/10.1109/TASE.2023.3267860
Berghout T, Benbouzid M, Bentrcia T, Lim WH, Amirat Y (2022) Federated learning for condition monitoring of industrial processes: a review on fault diagnosis methods challenges, and prospects. Electronics 12:158
Berman JJ (2013) Principles of big data: preparing, sharing, and analyzing complex information. Newnes
Borgwardt KM, Gretton A, Rasch MJ, Kriegel H-P, Schölkopf B, Smola AJ (2006) Integrating structured biological data by kernel maximum mean discrepancy. Bioinformatics 22:e49–e57
Bronz M, Baskaya E, Delahaye D, Puechmore S (2020) Real-time fault detection on small fixed-wing UAVs using machine learning. In: 2020 AIAA/IEEE 39th Digital Avionics Systems Conference (DASC), IEEE, San Antonio, TX, USA, pp 1–10
Brown T, Mann B, Ryder N, Subbiah M, Kaplan JD, Dhariwal P, Neelakantan A, Shyam P, Sastry G, Askell A, Agarwal S, Herbert-Voss A, Krueger G, Henighan T, Child R, Ramesh A, Ziegler D, Wu J, Winter C, Hesse C, Chen M, Sigler E, Litwin M, Gray S, Chess B, Clark J, Berner C, McCandlish S, Radford A, Sutskever I, Amodei D (2020) Language models are few-shot learners. Advances in neural information processing systems. Curran Associates Inc, pp 1877–1901
Brusa E, Delprete C, Di Maggio LG (2021) Deep transfer learning for machine diagnosis: from sound and music recognition to bearing fault detection. Appl Sci 11:11663
Cao P, Zhang S, Tang J (2018) Preprocessing-free gear fault diagnosis using small datasets with deep convolutional neural network-based transfer learning. IEEE Access 6:26241–26253
Cao X, Bu W, Huang S, Zhang M, Tsang IW, Ong YS, Kwok JT (2023) A survey of learning on small data: generalization, optimization, and challenge
Chahal H, Toner H, Rahkovsky I (2021) Small data’s big AI potential. Center for Security and Emerging Technology
Book Google Scholar
Chawla NV, Bowyer KW, Hall LO, Kegelmeyer WP (2002) SMOTE: synthetic minority over-sampling technique. J Artif Intell Res 16:321–357
Che C, Wang H, Ni X, Fu Q (2020) Domain adaptive deep belief network for rolling bearing fault diagnosis. Comput Ind Eng 143:106427
Chen C, Shen F, Xu J, Yan R (2020) Domain adaptation-based transfer learning for gear fault diagnosis under varying working conditions. IEEE Trans Instrum Meas 70:1–10
Chen W, Qiu Y, Feng Y, Li Y, Kusiak A (2021) Diagnosis of wind turbine faults with transfer learning algorithms. Renew Energy 163:2053–2067
Chen J, Hu W, Cao D, Zhang Z, Chen Z, Blaabjerg F (2022) A meta-learning method for electric machine bearing fault diagnosis under varying working conditions with limited data. IEEE Trans Indus Inform 19:2552–2564
Chen X, Liu H, Nikitas N (2023a) Internal pump leakage detection of the hydraulic systems with highly incomplete flow data. Adv Eng Inform 56:101974
Chen J, Tang J, Li W (2023b) Industrial edge intelligence: federated-meta learning framework for few-shot fault diagnosis. IEEE Trans Netw Sci Eng. https://doi.org/10.1109/TNSE.2023.3266942
Article MathSciNet Google Scholar
Chen X, Zhao C, Ding J (2023c) Pyramid-type zero-shot learning model with multi-granularity hierarchical attributes for industrial fault diagnosis. Reliab Eng Syst Saf 240:109591
Cheng C, Zhou B, Ma G, Wu D, Yuan Y (2020) Wasserstein distance based deep adversarial transfer learning for intelligent fault diagnosis with unlabelled or insufficient labelled data. Neurocomputing 409:35–45
Cho SH, Kim S, Choi J-H (2020) Transfer learning-based fault diagnosis under data deficiency. Appl Sci 10:7768
Choi K, Kim Y, Kim S-K, Kim K-S (2020) Current and position sensor fault diagnosis algorithm for PMSM drives based on robust state observer. IEEE Trans Industr Electron 68:5227–5236
D Research (2019) Artificial intelligence and machine learning projects are obstructed by data issues
Dai W, Yang Q, Xue GR, Yu Y (2007) Boosting for transfer learning. 2007. In: Proceedings of the 24th International Conference on Machine Learning
Dai H, Chen P, Yang H (2022) Metalearning-based fault-tolerant control for skid steering vehicles under actuator fault conditions. Sensors 22:845
Der Kiureghian A, Ditlevsen O (2009) Aleatory or epistemic? Does it matter? Struct Saf 31:105–112
Ding P, Jia M (2021) Mechatronics equipment performance degradation assessment using limited and unlabeled data. IEEE Trans Industr Inf 18:2374–2385
Ding Y, Ma L, Ma J, Wang C, Lu C (2019) A generative adversarial network-based intelligent fault diagnosis method for rotating machinery under small sample size conditions. IEEE Access 7:149736–149749
Ding P, Jia M, Zhao X (2021) Meta deep learning based rotating machinery health prognostics toward few-shot prognostics. Appl Soft Comput 104:107211
Ding P, Jia M, Ding Y, Cao Y, Zhao X (2022a) Intelligent machinery health prognostics under variable operation conditions with limited and variable-length data. Adv Eng Inform 53:101691
Ding Y, Zhuang J, Ding P, Jia M (2022b) Self-supervised pretraining via contrast learning for intelligent incipient fault detection of bearings. Reliab Eng Syst Saf 218:108126
Ding P, Zhao X, Shao H, Jia M (2023a) Machinery cross domain degradation prognostics considering compound domain shifts. Reliab Eng Syst Saf 239:109490
Ding P, Jia M, Ding Y, Cao Y, Zhuang J, Zhao X (2023b) Machinery probabilistic few-shot prognostics considering prediction uncertainty. IEEE/ASME Trans Mechatron 29:106–118
Dixit S, Verma NK (2020) Intelligent condition-based monitoring of rotary machines with few samples. IEEE Sens J 20:14337–14346
Dou J, Wei G, Song Y, Zhou D, Li M (2023) Switching triple-weight-smote in empirical feature space for imbalanced and incomplete data. IEEE Trans Autom Sci Eng 21:1–17
Downs JJ, Vogel EF (1993) A plant-wide industrial process control problem. Comput Chem Eng 17:245–255
Du Y, Zhang W, Wang J, Wu H (2019) DCGAN based data generation for process monitoring. In: IEEE, pp 410–415
Fallah A, Mokhtari A, Ozdaglar A (2020) Personalized federated learning with theoretical guarantees: a model-agnostic meta-learning approach. Adv Neural Inf Process Syst 33:3557–3568
Fan Y, Cui X, Han H, Lu H (2020) Chiller fault detection and diagnosis by knowledge transfer based on adaptive imbalanced processing. Sci Technol Built Environ 26:1082–1099
Fan Z, Xu Q, Jiang C, Ding SX (2023a) Deep mixed domain generalization network for intelligent fault diagnosis under unseen conditions. IEEE Trans Industr Electron 71:965–974
Fan L, Chen X, Chai Y, Lin W (2023b) Attribute fusion transfer for zero-shot fault diagnosis. Adv Eng Inform 58:102204
Fekri MN, Patel H, Grolinger K, Sharma V (2021) Deep learning for load forecasting with smart meter data: online adaptive recurrent neural network. Appl Energy 282:116177
Feng L, Zhao C (2020) Fault description based attribute transfer for zero-sample industrial fault diagnosis. IEEE Trans Industr Inf 17:1852–1862
Feng Y, Chen J, Yang Z, Song X, Chang Y, He S, Xu E, Zhou Z (2021) Similarity-based meta-learning network with adversarial domain adaptation for cross-domain fault identification. Knowl-Based Syst 217:106829
Fink O, Wang Q, Svensen M, Dersin P, Lee W-J, Ducoffe M (2020) Potential, challenges and future directions for deep learning in prognostics and health management applications. Eng Appl Artif Intell 92:103678
Finn C, Abbeel P, Levine S (2017) Model-agnostic meta-learning for fast adaptation of deep networks. In: 34th International Conference on Machine Learning, ICML 2017 3:1856–1868
Fu B, Yuan W, Cui X, Yu T, Zhao X, Li C (2020) Correlation analysis and augmentation of samples for a bidirectional gate recurrent unit network for the remaining useful life prediction of bearings. IEEE Sens J 21:7989–8001
Gangsar P, Tiwari R (2020) Signal based condition monitoring techniques for fault detection and diagnosis of induction motors: a state-of-the-art review. Mech Syst Signal Process 144:106908
Gay A, Voisin A, Iung B, Do P, Bonidal R, Khelassi A (2022) Data augmentation-based prognostics for predictive maintenance of industrial system. CIRP Ann 71:409–412
Gay A, Voisin A, Iung B, Do P, Bonidal R, Khelassi A (2023) A study on data augmentation optimization for data-centric health prognostics of industrial systems. IFAC-PapersOnLine 56:1270–1275
Gray DO, Rivers D, Vermont G (2012) Measuring the economic impacts of the NSF Industry/University Cooperative Research Centers Program: a feasibility study, Arlington, Virginia
Gretton A, Sejdinovic D, Strathmann H, Balakrishnan S, Pontil M, Fukumizu K, Sriperumbudur BK (2012) Optimal kernel choice for large-scale two-sample tests. Adv Neural Inform Process Syst 25
Gualo F, Rodríguez M, Verdugo J, Caballero I, Piattini M (2021) Data quality certification using ISO/IEC 25012: industrial experiences. J Syst Softw 176:110938
Guo C, Hu W, Yang F, Huang D (2020) Deep learning technique for process fault detection and diagnosis in the presence of incomplete data. Chin J Chem Eng 28:2358–2367
Han T, Xie W, Pei Z (2023) Semi-supervised adversarial discriminative learning approach for intelligent fault diagnosis of wind turbine. Inf Sci 648:119496
Hao W, Liu F (2020) Imbalanced data fault diagnosis based on an evolutionary online sequential extreme learning machine. Symmetry 12:1204
He Z, Shao H, Zhang X, Cheng J, Yang Y (2019) Improved deep transfer auto-encoder for fault diagnosis of gearbox under variable working conditions with small training samples. IEEE Access 7:115368–115377
He Y, Hu M, Feng K, Jiang Z (2020a) An intelligent fault diagnosis scheme using transferred samples for intershaft bearings under variable working conditions. IEEE Access 8:203058–203069
He Z, Shao H, Wang P, (Jing) Lin J, Cheng J, Yang Y (2020b) Deep transfer multi-wavelet auto-encoder for intelligent fault diagnosis of gearbox with few target training samples. Knowl-Based Syst 191:105313
He J, Li X, Chen Y, Chen D, Guo J, Zhou Y (2021) Deep transfer learning method based on 1d-cnn for bearing fault diagnosis. Shock Vib 2021:1–16
Hinton GE, Zemel RS (1994) Autoencoders, minimum description length, and Helmholtz free energy. Adv Neural Inf Process Syst 6:3–10
Hu T, Tang T, Lin R, Chen M, Han S, Wu J (2020) A simple data augmentation algorithm and a self-adaptive convolutional architecture for few-shot fault diagnosis under different working conditions. Measurement 156:107539
Hu C, Zhou Z, Wang B, Zheng W, He S (2021a) Tensor transfer learning for intelligence fault diagnosis of bearing with semisupervised partial label learning. J Sens 2021:1–11
Hu Y, Liu R, Li X, Chen D, Hu Q (2021b) Task-sequencing meta learning for intelligent few-shot fault diagnosis with limited data. IEEE Trans Industr Inf 18:3894–3904
Hu Z, Shen L, Wang Z, Liu T, Yuan C, Tao D (2023) Architecture, dataset and model-scale agnostic data-free meta-learning. In: Proceedings of the IEEE/CVF Conference on Computer Vision and Pattern Recognition, pp 7736–7745
Huang N, Chen Q, Cai G, Xu D, Zhang L, Zhao W (2020) Fault diagnosis of bearing in wind turbine gearbox under actual operating conditions driven by limited data with noise labels. IEEE Trans Instrum Meas 70:1–10
Huang F, Sava A, Adjallah KH, Wang Z (2021) Fuzzy model identification based on mixture distribution analysis for bearings remaining useful life estimation using small training data set. Mech Syst Signal Process 148:107173
Huang Y, Tang Y, VanZwieten J, Liu J (2022) Reliable machine prognostic health management in the presence of missing data. Concurr Comput Pract Exp 34:e5762
Huang C, Bu S, Lee HH, Chan KW, Yung WKC (2024) Prognostics and health management for induction machines: a comprehensive review. J Intell Manuf 35:937–962
Iglesias G, Talavera E, González-Prieto Á, Mozo A, Gómez-Canaval S (2023) Data Augmentation techniques in time series domain: a survey and taxonomy. Neural Comput Appl 35:10123–10145
Jamil F, Verstraeten T, Nowé A, Peeters C, Helsen J (2022) A deep boosted transfer learning method for wind turbine gearbox fault detection. Renew Energy 197:331–341
Jiang C, Chen H, Xu Q, Wang X (2022) Few-shot fault diagnosis of rotating machinery with two-branch prototypical networks. J Intell Manuf. https://doi.org/10.1007/s10845-021-01904-x
Jiang Y, Drescher B, Yuan G (2023) A GAN-based multi-sensor data augmentation technique for CNC machine tool wear prediction. IEEE Access 11:95782–95795
Jin X, Wah BW, Cheng X, Wang Y (2015) Significance and challenges of big data research. Big Data Res 2:59–64
Jose S, Nguyen KTP, Medjaher K (2023) Multimodal machine learning in prognostics and health management of manufacturing systems. Artificial intelligence for smart manufacturing: methods, applications, and challenges. Springer, pp 167–197
Chapter Google Scholar
Karkošková S (2023) Data governance model to enhance data quality in financial institutions. Inf Syst Manag 40:90–110
Kavis M (2015) Forget big data–small data is driving the Internet of Things, https://www.Forbes.Com/Sites/Mikekavis/2015/02/25/Forget-Big-Datasmall-Data-Is-Driving-the-Internet-of-Things
Kevin I, Wang K, Zhou X, Liang W, Yan Z, She J (2021) Federated transfer learning based cross-domain prediction for smart manufacturing. IEEE Trans Industr Inf 18:4088–4096
Kim H, Youn BD (2019) A new parameter repurposing method for parameter transfer with small dataset and its application in fault diagnosis of rolling element bearings. IEEE Access 7:46917–46930
Koch G, Zemel R, Salakhutdinov R (2015) Siamese neural networks for one-shot image recognition. In: ICML Deep Learning Workshop
Kullback S, Leibler RA (1951) On information and sufficiency. Ann Math Stat 22:79–86
Kumar P, Raouf I, Kim HS (2023) Review on prognostics and health management in smart factory: from conventional to deep learning perspectives. Eng Appl Artif Intell 126:107126
Lao Z, He D, Jin Z, Liu C, Shang H, He Y (2023) Few-shot fault diagnosis of turnout switch machine based on semi-supervised weighted prototypical network. Knowl-Based Syst 274:110634
LeCun Y, Bottou L, Bengio Y, Haffner P (1998) Gradient-based learning applied to document recognition. Proc IEEE 86:2278–2324
Lee YO, Jo J, Hwang J (2017) Application of deep neural network and generative adversarial network to industrial maintenance: a case study of induction motor fault detection. In: Proceedings—2017 IEEE International Conference on Big Data, Big Data 2017 2018-Janua, pp 3248–3253
Lee J, Mitici M (2023) Deep reinforcement learning for predictive aircraft maintenance using probabilistic remaining-useful-life prognostics. Reliab Eng Syst Saf 230:108908
Lee K, Han S, Pham VH, Cho S, Choi H-J, Lee J, Noh I, Lee SW (2021) Multi-objective instance weighting-based deep transfer learning network for intelligent fault diagnosis. Appl Sci 11:2370
Lei Y, Li N, Guo L, Li N, Yan T, Lin J (2018) Machinery health prognostics: a systematic review from data acquisition to RUL prediction. Mech Syst Signal Process 104:799–834
Lessmeier C, Kimotho JK, Zimmer D, Sextro W (2016) Condition monitoring of bearing damage in electromechanical drive systems by using motor current signals of electric motors: a benchmark data set for data-driven classification, 17
Li Y, Liu C, Hua J, Gao J, Maropoulos P (2019) A novel method for accurately monitoring and predicting tool wear under varying cutting conditions based on meta-learning. CIRP Ann 68:487–490
Li X, Zhang W, Ding Q, Sun JQ (2020a) Intelligent rotating machinery fault diagnosis based on deep learning using data augmentation. J Intell Manuf 31:433–452
Li W, Gu S, Zhang X, Chen T (2020b) Transfer learning for process fault diagnosis: knowledge transfer from simulation to physical processes. Comput Chem Eng 139:106904
Li X, Zhang W, Ding Q, Li X (2020c) Diagnosing rotating machines with weakly supervised data using deep transfer learning. IEEE Trans Industr Inf 16:1688–1697
Li F, Tang T, Tang B, He Q (2021a) Deep convolution domain-adversarial transfer learning for fault diagnosis of rolling bearings. Measurement 169:108339
Li Y, Jiang W, Zhang G, Shu L (2021b) Wind turbine fault diagnosis based on transfer learning and convolutional autoencoder with small-scale data. Renew Energy 171:103–115
Li C, Li S, Zhang A, He Q, Liao Z, Hu J (2021c) Meta-learning for few-shot bearing fault diagnosis under complex working conditions. Neurocomputing 439:197–211
Li X, Yang X, Ma Z, Xue JH (2021d) Deep metric learning for few-shot image classification: a selective review, arXiv Preprint https://arXiv.org/2105.08149
Li Z, Sun Y, Yang L, Zhao Z, Chen X (2022a) Unsupervised machine anomaly detection using autoencoder and temporal convolutional network. IEEE Trans Instrum Meas 71:1–13
Li W, Huang R, Li J, Liao Y, Chen Z, He G, Yan R, Gryllias K (2022b) A perspective survey on deep transfer learning for fault diagnosis in industrial scenarios: theories, applications and challenges. Mech Syst Signal Process 167:108487
Li C, Li S, Zhang A, Yang L, Zio E, Pecht M, Gryllias K (2022c) A Siamese hybrid neural network framework for few-shot fault diagnosis of fixed-wing unmanned aerial vehicles. J Comput Design Eng 9:1511–1524
Li Y, Wang J, Huang Z, Gao RX (2022d) Physics-informed meta learning for machining tool wear prediction. J Manuf Syst 62:17–27
Li Y, Yang Y, Feng K, Zuo MJ, Chen Z (2023a) Automated and adaptive ridge extraction for rotating machinery fault detection. IEEE/ASME Trans Mechatron 28:2565
Li K, Lu J, Zuo H, Zhang G (2023b) Source-free multi-domain adaptation with fuzzy rule-based deep neural networks. IEEE Trans Fuzzy Syst. https://doi.org/10.1109/TFUZZ.2023.3276978
Li C, Li S, Wang H, Gu F, Ball AD (2023c) Attention-based deep meta-transfer learning for few-shot fine-grained fault diagnosis. Knowl-Based Syst 264:110345
Li Y-F, Wang H, Sun M (2023d) ChatGPT-like large-scale foundation models for prognostics and health management: a survey and roadmaps. Reliab Eng Syst Saf 243:109850
Liang P, Deng C, Wu J, Yang Z, Zhu J, Zhang Z (2020) Single and simultaneous fault diagnosis of gearbox via a semi-supervised and high-accuracy adversarial learning framework. Knowl-Based Syst 198:105895
Liao Y, Huang R, Li J, Chen Z, Li W (2020) Deep semisupervised domain generalization network for rotary machinery fault diagnosis under variable speed. IEEE Trans Instrum Meas 69:8064–8075
Lin J, Shao H, Zhou X, Cai B, Liu B (2023) Generalized MAML for few-shot cross-domain fault diagnosis of bearing driven by heterogeneous signals. Expert Syst Appl 230:120696
Liu J, Ren Y (2020) A general transfer framework based on industrial process fault diagnosis under small samples. IEEE Trans Industr Inf 3203:1–11
Liu C, Zhu L (2020) A two-stage approach for predicting the remaining useful life of tools using bidirectional long short-term memory. Measurement 164:108029
Liu J, Qu F, Hong X, Zhang H (2019) A small-sample wind turbine fault detection method with synthetic fault data using generative adversarial nets. IEEE Trans Industr Inf 15:3877–3888
Liu S, Jiang H, Wu Z, Li X (2022) Data synthesis using deep feature enhanced generative adversarial networks for rolling bearing imbalanced fault diagnosis. Mech Syst Signal Process 163:108139
Liu S, Chen J, He S, Shi Z, Zhou Z (2023) Few-shot learning under domain shift: attentional contrastive calibrated transformer of time series for fault diagnosis under sharp speed variation. Mech Syst Signal Process 189:110071
Long J, Chen Y, Huang H, Yang Z, Huang Y, Li C (2023) Multidomain variance-learnable prototypical network for few-shot diagnosis of novel faults. J Intell Manuf. https://doi.org/10.1007/s10845-023-02123-2
Lu N, Yin T (2021) Transferable common feature space mining for fault diagnosis with imbalanced data. Mech Syst Signal Process 156:107645
Lu N, Hu H, Yin T, Lei Y, Wang S (2021) Transfer relation network for fault diagnosis of rotating machinery with small data. IEEE Trans Cybern 52:11927–11941
Lu N, Zhuang G, Ma Z, Zhao Q (2022) A zero-shot intelligent fault diagnosis system based on EEMD. IEEE Access 10:54197–54207
Luo M, Xu J, Fan Y, Zhang J (2022) TRNet: a cross-component few-shot mechanical fault diagnosis. IEEE Trans Indus Inform. https://doi.org/10.1109/TII.2022.3204554
Lv H, Chen J, Pan T, Zhou Z (2020) Hybrid attribute conditional adversarial denoising autoencoder for zero-shot classification of mechanical intelligent fault diagnosis. Appl Soft Comput 95:106577
Ma L, Ding Y, Wang Z, Wang C, Ma J, Lu C (2021) An interpretable data augmentation scheme for machine fault diagnosis based on a sparsity-constrained generative adversarial network. Expert Syst Appl 182:115234
Ma Z, Liao H, Gao J, Nie S, Geng Y (2023) Physics-informed machine learning for degradation modelling of an electro-hydrostatic actuator system. Reliab Eng Syst Saf 229:108898
Mahmoodian A, Durali M, Saadat M, Abbasian T (2021) A life clustering framework for prognostics of gas turbine engines under limited data situations. Int J Eng Trans C: Aspects 34:728–736
Mao W, Liu Y, Ding L, Li Y (2019) Imbalanced fault diagnosis of rolling bearing based on generative adversarial network: a comparative study. IEEE Access 7:9515–9530
Mao W, He J, Zuo MJ (2020) Predicting remaining useful life of rolling bearings based on deep feature representation and transfer learning. IEEE Trans Instrum Meas 69:1594–1608
Mao W, He J, Sun B, Wang L (2021) Prediction of bearings remaining useful life across working conditions based on transfer learning and time series clustering. IEEE Access 9:135285–135303
McMahan B, Moore E, Ramage D, Hampson S, Arcas BAY (2017) Communication-efficient learning of deep networks from decentralized data. Artificial intelligence and statistics. PMLR, pp 1273–1282
Meng Z, Guo X, Pan Z, Sun D, Liu S (2019) Data segmentation and augmentation methods based on raw data using deep neural networks approach for rotating machinery fault diagnosis. IEEE Access 7:79510–79522
Miao Y, Jiang Y, Huang J, Zhang X, Han L (2020) Application of fault diagnosis of seawater hydraulic pump based on transfer learning. Shock Vib 2020:1–8
Miao J, Wang J, Zhang D, Miao Q (2021) Improved generative adversarial network for rotating component fault diagnosis in scenarios with extremely limited data. IEEE Trans Instrum Meas 71:1–13
Michau G, Fink O (2021) Unsupervised transfer learning for anomaly detection: application to complementary operating condition transfer. Knowl-Based Syst 216:106816
Mishchenko K, Khaled A, Richtárik P (2022) Proximal and federated random reshuffling. In: International Conference on Machine Learning, PMLR, pp 15718–15749
Mo Y, Li L, Huang B, Li X (2022) Few-shot RUL estimation based on model-agnostic meta-learning. J Intell Manuf 34:1–14
Moreno-Barea FJ, Jerez JM, Franco L (2020) Improving classification accuracy using data augmentation on small data sets. Expert Syst Appl 161:113696
Nectoux P, Gouriveau R, Medjaher K, Ramasso E, Chebel-Morello B, Zerhouni N, Varnier C (2012) PRONOSTIA: an experimental platform for bearings accelerated degradation tests. In: IEEE International Conference on Prognostics and Health Management, PHM’12, pp 1–8
Nemani V, Biggio L, Huan X, Hu Z, Fink O, Tran A, Wang Y, Zhang X, Hu C (2023) Uncertainty quantification in machine learning for engineering design and health prognostics: a tutorial. Mech Syst Signal Process 205:110796
Omri N, Al-Masry Z, Mairot N, Giampiccolo S, Zerhouni N (2020) Industrial data management strategy towards an SME-oriented PHM. J Manuf Syst 56:23–36
Pan T, Chen J, Zhang T, Liu S, He S, Lv H (2022) Generative adversarial network in mechanical fault diagnosis under small sample: a systematic review on applications and future perspectives. ISA Trans 128:1–10
Pang G, Cao L, Aggarwal C (2021) Deep learning for anomaly detection: challenges, methods, and opportunities, pp 1127–1130
Parnami A, Lee M (2022) Learning from few examples: a summary of approaches to few-shot learning. arXiv Preprint https://arXiv.org/2203.04291
Peng C, Li L, Chen Q, Tang Z, Gui W, He J (2021) A fault diagnosis method for rolling bearings based on parameter transfer learning under imbalance data sets. Energies 14:944
Qi L, Ren Y, Fang Y, Zhou J (2023) Two-view LSTM variational auto-encoder for fault detection and diagnosis in multivariable manufacturing processes. Neural Comput Appl 35:1–20
Qin A, Mao H, Zhong J, Huang Z, Li X (2023) Generalized transfer extreme learning machine for unsupervised cross-domain fault diagnosis with small and imbalanced samples. IEEE Sens J 23:15831–15843
Qiu H, Lee J, Lin J, Yu G (2006) Wavelet filter-based weak signature detection method and its application on rolling element bearing prognostics. J Sound Vib 289:1066–1090
Rajagopalan S, Singh J, Purohit A (2023) VMD-based ensembled SMOTEBoost for imbalanced multi-class rotor mass imbalance fault detection and diagnosis under industrial noise. J Vib Eng Technol 12:1–22
Randall RB (2021) Vibration-based condition monitoring: industrial, automotive and aerospace applications. Wiley
Ren Z, Lin T, Feng K, Zhu Y, Liu Z, Yan K (2023) A systematic review on imbalanced learning methods in intelligent fault diagnosis. IEEE Trans Instrum Meas 72:3508535
Ren L, Mo T, Cheng X (2024) Meta-learning based domain generalization framework for fault diagnosis with gradient aligning and semantic matching. IEEE Trans Ind Inf 20:754–764
Ruan D, Wu Y, Yan J, Gühmann C (2022) Fuzzy-membership-based framework for task transfer learning between fault diagnosis and RUL prediction. IEEE Trans Reliab 72:989–1002
Sadoughi M, Lu H, Hu C (2019) A deep learning approach for failure prognostics of rolling element bearings. In: IEEE, pp 1–7
Saxena A, Goebel K, Simon D, Eklund N (2008) Damage propagation modeling for aircraft engine run-to-failure simulation. In: IEEE, pp 1–9
Scheurer J, Campos JA, Korbak T, Chan JS, Chen A, Cho K, Perez E (2023) Training language models with language feedback at scale, arXiv Preprint https://arXiv.org/2303.16755
Schmid M, Gebauer E, Hanzl C, Endisch C (2020) Active model-based fault diagnosis in reconfigurable battery systems. IEEE Trans Power Electron 36:2584–2597
Schmidhuber J (1987) Evolutionary principles in self-referential learning, or on learning how to learn: the meta-meta-... hook
Shao S, McAleer S, Yan R, Baldi P (2018) Highly accurate machine fault diagnosis using deep transfer learning. IEEE Trans Industr Inf 15:2446–2455
Shi D, Ye Y, Gillwald M, Hecht M (2022) Robustness enhancement of machine fault diagnostic models for railway applications through data augmentation. Mech Syst Signal Process 164:108217
Smith WA, Randall RB (2015) Rolling element bearing diagnostics using the Case Western Reserve University data: a benchmark study. Mech Syst Signal Process 64:100–131
Snell J, Swersky K, Zemel R (2017) Prototypical networks for few-shot learning. Adv Neural Inform Process Syst 30
Song Y, Wang T, Mondal SK, Sahoo JP (2022) A comprehensive survey of few-shot learning: evolution applications, challenges, and opportunities. ACM Comput Surv 271:1–40
Sun B, Saenko K (2016) Deep coral: correlation alignment for deep domain adaptation. Springer, pp 443–450
Sun Y, Zhao T, Zou Z, Chen Y, Zhang H (2021) Imbalanced data fault diagnosis of hydrogen sensors using deep convolutional generative adversarial network with convolutional neural network. Rev Sci Instrum 92:095007
Sung F, Yang Y, Zhang L, Xiang T, Torr PH, Hospedales TM (2018) Learning to compare: relation network for few-shot learning. In: Proceedings of the IEEE Computer Society Conference on Computer Vision and Pattern Recognition, pp 1199–1208
Suthaharan S (2014) Big data classification: problems and challenges in network intrusion prediction with machine learning. ACM SIGMETRICS Perform Eval Rev 41:70–73
Tang Z, Bo L, Liu X, Wei D (2021) An autoencoder with adaptive transfer learning for intelligent fault diagnosis of rotating machinery. Meas Sci Technol 32:55110
Tang Y, Xiao X, Yang X, Lei B (2023a) Research on a small sample feature transfer method for fault diagnosis of reciprocating compressors. J Loss Prev Process Ind 85:105163
Tang T, Qiu C, Yang T, Wang J, Zhao J, Chen M, Wu J, Wang L (2023b) A novel lightweight relation network for cross-domain few-shot fault diagnosis. Measurement 213:112697
Thrun S, Pratt L (2012) Learning to learn. Springer Science Business Media
Tian Y, Tang Y, Peng X (2020) Cross-task fault diagnosis based on deep domain adaptation with local feature learning. IEEE Access 8:127546–127559
Triguero I, Del Río S, López V, Bacardit J, Benítez JM, Herrera F (2015) ROSEFW-RF: the winner algorithm for the ECBDL’14 big data competition: an extremely imbalanced big data bioinformatics problem. Knowl-Based Syst 87:69–79
Vapnik V (2013) The nature of statistical learning theory. Springer Science Business Media
Vinyals O, Blundell C, Lillicrap T, Kavukcuoglu K, Wierstra D (2016) Matching networks for one shot learning. Adv Neural Inform Process Syst 29:3637–3645
Wan W, He S, Chen J, Li A, Feng Y (2021) QSCGAN: an un-supervised quick self-attention convolutional GAN for LRE bearing fault diagnosis under limited label-lacked data. IEEE Trans Instrum Meas 70:1–16
Wang C, Xu Z (2021) An intelligent fault diagnosis model based on deep neural network for few-shot fault diagnosis. Neurocomputing 456:550–562
Wang B, Lei Y, Li N, Li N (2018a) A hybrid prognostics approach for estimating remaining useful life of rolling element bearings. IEEE Trans Reliab 69:401–412
Wang Z, Wang J, Wang Y (2018b) An intelligent diagnosis scheme based on generative adversarial learning deep neural networks and its application to planetary gearbox fault pattern recognition. Neurocomputing 310:213–222
Wang Y, Yao Q, Kwok JT, Ni LM (2020a) Generalizing from a few examples: a survey on few-shot learning. ACM Comput Surv (CSUR) 53:1–34
Wang S, Wang D, Kong D, Wang J, Li W, Zhou S (2020b) Few-shot rolling bearing fault diagnosis with metric-based meta learning. Sensors (switzerland) 20:1–15
Wang D, Zhang M, Xu Y, Lu W, Yang J, Zhang T (2021) Metric-based meta-learning model for few-shot fault diagnosis under multiple limited data conditions. Mech Syst Signal Process 155:107510
Wang Z, Yang J, Guo Y (2022) Unknown fault feature extraction of rolling bearings under variable speed conditions based on statistical complexity measures. Mech Syst Signal Process 172:108964
Wang S, Ma L, Wang J (2023) Fault diagnosis method based on CND-SMOTE and BA-SVM algorithm. J Phys Conf Ser 2493:012008
Ward JS, Barker A (2013) Undefined by data: a survey of big data definitions. arXiv Preprint https://arXiv.org/1309.5821
Weikun D, Nguyen KT, Medjaher K, Christian G, Morio J (2023) Physics-informed machine learning in prognostics and health management: state of the art and challenges. Appl Math Model 124:325–352
Wen L, Li X, Li X, Gao L (2019) A new transfer learning based on VGG-19 network for fault diagnosis. In: IEEE, pp 205–209
Wen L, Li X, Gao L (2020) A transfer convolutional neural network for fault diagnosis based on ResNet-50. Neural Comput Appl 32:6111–6124
Wenbai C, Chang L, Weizhao C, Huixiang L, Qili C, Peiliang W (2021) A prediction method for the RUL of equipment for missing data. Complexity 2021:2122655
Wu H, Zhao J (2020) Fault detection and diagnosis based on transfer learning for multimode chemical processes. Comput Chem Eng 135:106731
Wu J, Zhao Z, Sun C, Yan R, Chen X (2020) Few-shot transfer learning for intelligent fault diagnosis of machine. Measurement 166:108202
Wu K, Yukang N, Wu J, Yuanhang W (2023) Prior knowledge-based self-supervised learning for intelligent bearing fault diagnosis with few fault samples. Meas Sci Technol 34:105104
Xia P, Huang Y, Li P, Liu C, Shi L (2021) Fault knowledge transfer assisted ensemble method for remaining useful life prediction. IEEE Trans Industr Inf 18:1758–1769
Xiao D, Huang Y, Qin C, Liu Z, Li Y, Liu C (2019) Transfer learning with convolutional neural networks for small sample size problem in machinery fault diagnosis. Proc Inst Mech Eng C J Mech Eng Sci 233:5131–5143. https://doi.org/10.1177/0954406219840381
Xie J, Zhang L, Duan L, Wang J (2016) On cross-domain feature fusion in gearbox fault diagnosis under various operating conditions based on transfer component analysis. In: IEEE, pp 1–6
Xing S, Lei Y, Yang B, Lu N (2021) Adaptive knowledge transfer by continual weighted updating of filter kernels for few-shot fault diagnosis of machines. IEEE Trans Industr Electron 69:1968–1976
Xing S, Lei Y, Wang S, Lu N, Li N (2022) A label description space embedded model for zero-shot intelligent diagnosis of mechanical compound faults. Mech Syst Signal Process 162:108036
Xu J, Xu P, Wei Z, Ding X, Shi L (2020) DC-NNMN: across components fault diagnosis based on deep few-shot learning. Shock Vib 2020:3152174
Xu J, Zhou L, Zhao W, Fan Y, Ding X, Yuan X (2022) Zero-shot learning for compound fault diagnosis of bearings. Expert Syst Appl 190:116197
Xu P, Zhu X, Clifton DA (2023) Multimodal learning with transformers: a survey. IEEE Trans Pattern Anal Mach Intell. https://doi.org/10.1109/TPAMI.2023.3275156
Yan H, Wang J, Chen J, Liu Z, Feng Y (2022) Virtual sensor-based imputed graph attention network for anomaly detection of equipment with incomplete data. J Manuf Syst 63:52–63
Yan H, Liu Z, Chen J, Feng Y, Wang J (2023) Memory-augmented skip-connected autoencoder for unsupervised anomaly detection of rocket engines with multi-source fusion. ISA Trans 133:53–65
Yang B, Lei Y, Jia F, Xing S (2018) A transfer learning method for intelligent fault diagnosis from laboratory machines to real-case machines. In: IEEE, pp 35–40
Yang B, Lei Y, Jia F, Xing S (2019a) An intelligent fault diagnosis approach based on transfer learning from laboratory bearings to locomotive bearings. Mech Syst Signal Process 122:692–706
Yang Q, Liu Y, Cheng Y, Kang Y, Chen T, Yu H (2019b) Federated learning, synthesis lectures on artificial intelligence and machine learning 13, pp 1–207
Yang J, Xie G, Yang Y (2020a) An improved ensemble fusion autoencoder model for fault diagnosis from imbalanced and incomplete data. Control Eng Pract 98:104358
Yang Y, Wang H, Liu Z, Yang Z (2020b) Few-shot learning for rolling bearing fault diagnosis via Siamese two-dimensional convolutional neural network. In: Proceedings—11th International Conference on Prognostics and System Health Management, PHM-Jinan 2020, pp 373–378
Yang X, Bai M, Liu J, Liu J, Yu D (2021) Gas path fault diagnosis for gas turbine group based on deep transfer learning. Measurement 181:109631
Yang G, Ye Z, Zhang R, Huang K (2022) A comprehensive survey of zero-shot image classification: methods, implementation, and fair evaluation. ACI 2:1–31
Yang C, Zhang J, Chang Y, Zou J, Liu Z, Fan S (2023a) A novel deep parallel time-series relation network for fault diagnosis. IEEE Trans Instrum Meas 72:1–13
Yang L, Li S, Li C, Zhu C, Zhang A, Liang G (2023b) Data-driven unsupervised anomaly detection and recovery of unmanned aerial vehicle flight data based on spatiotemporal correlation. Sci China Technol Sci 66:1–13
Yao S, Kang Q, Zhou M, Rawa MJ, Abusorrah A (2023) A survey of transfer learning for machinery diagnostics and prognostics. Artif Intell Rev 56:2871–2922
Yin H, Li Z, Zuo J, Liu H, Yang K, Li F (2020) Wasserstein generative adversarial network and convolutional neural network (WG-CNN) for bearing fault diagnosis. Math Probl Eng 2020:2604191
Yu Y, Tang B, Lin R, Han S, Tang T, Chen M (2019) CWGAN: conditional Wasserstein generative adversarial nets for fault data generation. In: IEEE, pp 2713–2718
Yu K, Ma H, Lin TR, Li X (2020) A consistency regularization based semi-supervised learning approach for intelligent fault diagnosis of rolling bearing. Measurement 165:107987
Yu K, Lin TR, Ma H, Li X, Li X (2021a) A multi-stage semi-supervised learning approach for intelligent fault diagnosis of rolling bearing using data augmentation and metric learning. Mech Syst Signal Process 146:107043
Yu C, Ning Y, Qin Y, Su W, Zhao X (2021b) Multi-label fault diagnosis of rolling bearing based on meta-learning. Neural Comput Appl 33:5393–5407
Yu Q, Luo L, Liu B, Hu S (2023) Re-planning of quadrotors under disturbance based on meta reinforcement learning. J Intell Rob Syst 107:13
Zarsky TZ (2016) Incompatible: the GDPR in the age of big data. Seton Hall L Rev 47:995
Zha D, Bhat ZP, Lai K-H, Yang F, Hu X (2023) Data-centric AI: perspectives and challenges. In: Proceedings of the 2023 SIAM International Conference on Data Mining (SDM), SIAM, pp 945–948
Zhang A, Wang H, Li S, Cui Y, Liu Z, Yang G, Hu J (2018) Transfer learning with deep recurrent neural networks for remaining useful life estimation. Appl Sci 8:2416
Zhang A, Li S, Cui Y, Yang W, Dong R, Hu J (2019) Limited data rolling bearing fault diagnosis with few-shot learning. IEEE Access 7:110895–110904
Zhang Y, Ren Z, Zhou S (2020a) An intelligent fault diagnosis for rolling bearing based on adversarial semi-supervised method. IEEE Access 8:149868–149877
Zhang X, Qin Y, Yuen C, Jayasinghe L, Liu X (2020b) Time-series regeneration with convolutional recurrent generative adversarial network for remaining useful life estimation. IEEE Trans Industr Inf 17:6820–6831
Zhang L, Guo L, Gao H, Dong D, Fu G, Hong X (2020c) Instance-based ensemble deep transfer learning network: a new intelligent degradation recognition method and its application on ball screw. Mech Syst Signal Process 140:106681
Zhang H, Zhang Q, Shao S, Niu T, Yang X, Ding H (2020d) Sequential network with residual neural network for rotatory machine remaining useful life prediction using deep transfer learning. Shock Vib 2020:1–16
Zhang K, Chen J, Zhang T, He S, Pan T, Zhou Z (2020e) Intelligent fault diagnosis of mechanical equipment under varying working condition via iterative matching network augmented with selective Signal reuse strategy. J Manuf Syst 57:400–415
Zhang S, Ye F, Wang B, Habetler TG (2021) Few-shot bearing fault diagnosis based on model-agnostic meta-learning. IEEE Trans Ind Appl 57:4754–4764
Zhang T, Chen J, Li F, Zhang K, Lv H, He S, Xu E (2022a) Intelligent fault diagnosis of machines with small & imbalanced data: a state-of-the-art review and possible extensions. ISA Trans 119:152–171
Zhang X, Wu B, Zhang X, Zhou Q, Hu Y, Liu J (2022b) A novel assessable data augmentation method for mechanical fault diagnosis under noisy labels. Measurement 198:111114
Zhang X, Wang J, Han B, Zhang Z, Yan Z, Jia M, Guo L (2022c) Feature distance-based deep prototype network for few-shot fault diagnosis under open-set domain adaptation scenario. Measurement 201:111522
Zhang T, Chen J, Liu S, Liu Z (2023) Domain discrepancy-guided contrastive feature learning for few-shot industrial fault diagnosis under variable working conditions. IEEE Trans Industr Inf 19:10277–10287
Zhao B, Yuan Q (2021) Improved generative adversarial network for vibration-based fault diagnosis with imbalanced data. Measurement 169:108522
Zhao Z, Li T, Wu J, Sun C, Wang S, Yan R, Chen X (2020a) Deep learning algorithms for rotating machinery intelligent diagnosis: an open source benchmark study. ISA Trans 107:224–255
Zhao X, Jia M, Lin M (2020b) Deep Laplacian auto-encoder and its application into imbalanced fault diagnosis of rotating machinery. Measurement 152:107320
Zhao K, Jiang H, Wu Z, Lu T (2020c) A novel transfer learning fault diagnosis method based on manifold embedded distribution alignment with a little labeled data. J Intell Manuf 33:1–15
Zhao B, Niu Z, Liang Q, Xin Y, Qian T, Tang W, Wu Q (2021a) Signal-to-signal translation for fault diagnosis of bearings and gears with few fault samples. IEEE Trans Instrum Meas 70:1–10
Zhao Z, Zhang Q, Yu X, Sun C, Wang S, Yan R, Chen X (2021b) Applications of unsupervised deep transfer learning to intelligent fault diagnosis: a survey and comparative study. IEEE Trans Instrum Meas 70:1–28
Zhao K, Jiang H, Liu C, Wang Y, Zhu K (2022) A new data generation approach with modified Wasserstein auto-encoder for rotating machinery fault diagnosis with limited fault data. Knowl-Based Syst 238:107892
Zhao J, Yuan M, Cui J, Huang J, Zhao F, Dong S, Qu Y (2023) A novel hierarchical training architecture for Siamese Neural Network based fault diagnosis method under small sample. Measurement 215:112851
Zheng T, Song L, Guo B, Liang H, Guo L (2019) An efficient method based on conditional generative adversarial networks for imbalanced fault diagnosis of rolling bearing. In: IEEE, pp 1–8
Zhiyi H, Haidong S, Lin J, Junsheng C, Yu Y (2020) Transfer fault diagnosis of bearing installed in different machines using enhanced deep auto-encoder. Measurement 152:107393
Zhou K, Diehl E, Tang J (2023a) Deep convolutional generative adversarial network with semi-supervised learning enabled physics elucidation for extended gear fault diagnosis under data limitations. Mech Syst Signal Process 185:109772
Zhou L, Liu Y, Bai X, Li N, Yu X, Zhou J, Hancock ER (2023b) Attribute subspaces for zero-shot learning. Pattern Recogn 144:109869
Zhu QX, Zhang N, He YL, Xu Y (2022) Novel imbalanced fault diagnosis method based on CSMOTE integrated with LSDA and LightGBM for industrial process. In: IEEE, pp 326–331
Zhu R, Peng W, Wang D, Huang C-G (2023a) Bayesian transfer learning with active querying for intelligent cross-machine fault prognosis under limited data. Mech Syst Signal Process 183:109628
Zhu J, Long Z, Ma X, Luan F (2023b) Bearing remaining useful life prediction based on BERT fine-tuning. In: 2023 Global Reliability and Prognostics and Health Management Conference (PHM-Hangzhou), IEEE, pp 1–6
Zhuo Y, Ge Z (2021) Auxiliary information guided industrial data augmentation for any-shot fault learning and diagnosis. IEEE Trans Industr Inf 3203:1–11
Zio E (2022) Prognostics and health management (PHM): where are we and where do we (need to) go in theory and practice. Reliab Eng Syst Saf 218:108119
Download references
Acknowledgements
This work was supported in part by the National Key Research and Development Program of China [No. 2023YFB3308800]; in part by National Natural Science Foundation of China [No. 52275480]; in part by the Guizhou Province Higher Education Project [No. QJH KY [2020]005], in part by the Guizhou University Natural Sciences Special Project (Guida Tegang Hezi (2023) No.61).

Author information
Authors and affiliations.
State Key Laboratory of Public Big Data, Guizhou University, Guiyang, 550025, Guizhou, China
Chuanjiang Li, Shaobo Li & Yixiong Feng
Department of Mechanical Engineering, Flanders Make, KU Leuven, 3000, Louvain, Belgium
Konstantinos Gryllias
School of Computing and Engineering, University of Huddersfield, Huddersfield, HD1 3DH, UK
Fengshou Gu
Advanced Life Cycle Engineering, University of Maryland, College Park, MD, 20742, USA
Michael Pecht
You can also search for this author in PubMed Google Scholar
Contributions
Chuanjiang Li: Conceptualization, Investigation, Methodology, Software, Data curation, Writing-Original draft preparation. Shaobo Li: Conceptualization, Supervision, Funding support. Yixiong Feng: Investigation, Writing-review. Konstantinos Gryllias: Methodology, Writing-review. Fengshou Gu: Methodology, Writing-review & editing. Michael Pecht: Methodology, Writing-review & editing.
Corresponding author
Correspondence to Chuanjiang Li .
Ethics declarations
Conflict of interest.
The authors declare that they have no known competing financial interests or personal relationships that could have appeared to influence the work reported in this paper.
Additional information
Publisher's note.
Springer Nature remains neutral with regard to jurisdictional claims in published maps and institutional affiliations.
Rights and permissions
Open Access This article is licensed under a Creative Commons Attribution 4.0 International License, which permits use, sharing, adaptation, distribution and reproduction in any medium or format, as long as you give appropriate credit to the original author(s) and the source, provide a link to the Creative Commons licence, and indicate if changes were made. The images or other third party material in this article are included in the article's Creative Commons licence, unless indicated otherwise in a credit line to the material. If material is not included in the article's Creative Commons licence and your intended use is not permitted by statutory regulation or exceeds the permitted use, you will need to obtain permission directly from the copyright holder. To view a copy of this licence, visit http://creativecommons.org/licenses/by/4.0/ .
Reprints and permissions
About this article
Li, C., Li, S., Feng, Y. et al. Small data challenges for intelligent prognostics and health management: a review. Artif Intell Rev 57 , 214 (2024). https://doi.org/10.1007/s10462-024-10820-4
Download citation
Accepted : 28 May 2024
Published : 23 July 2024
DOI : https://doi.org/10.1007/s10462-024-10820-4
Share this article
Anyone you share the following link with will be able to read this content:
Sorry, a shareable link is not currently available for this article.
Provided by the Springer Nature SharedIt content-sharing initiative
- Prognostics and health management (PHM)
- Data augmentation
- Few-shot learning
- Transfer learning
- Find a journal
- Publish with us
- Track your research

- SUGGESTED TOPICS
- The Magazine
- Newsletters
- Managing Yourself
- Managing Teams
- Work-life Balance
- The Big Idea
- Data & Visuals
- Reading Lists
- Case Selections
- HBR Learning
- Topic Feeds
- Account Settings
- Email Preferences
Physicians Need Better Data Management Systems to Improve Patient Care
Sponsor content from Siemens Healthineers.

The health care industry produces an astonishing amount of data: nearly one-third of the world’s data volume. The amount of data health care providers generate can seem overwhelming—and it can overwhelm an organization’s ability to find valuable insights that can help its physicians and its patients.
In Innsbruck, Austria, Tirol Kliniken oversees one of the largest medical vendor-neutral sites in Europe, with a data volume of over 740 TB. This volume contains more than 1 billion objects—both digital imaging and communications in medicine (DICOM) studies and non-DICOM data—and approximately 5 TB of data enter the archive each month.
This rapid growth makes the need for a comprehensive health care data management system more urgent each day. “It would be simply impossible to manage 190 GB of data produced daily without a powerful tool,” says Andreas Nuener, head of IT special systems at Tirol Kliniken.
The growing volume of data is only one part of the challenge. Various data types stored in various formats create an additional hurdle to efficiently storing, retrieving, and sharing clinically important patient data.
Health care providers, physicians, and patients need such a data system to meet several key capabilities:
1. Interoperability. The ability to connect and share information among various IT systems, reducing administrative burden and streamlining workflows, is imperative to delivering high-quality care in the modern health care environment.
2. Flexibility. When an organization adopts one flexible enterprisewide imaging and reporting system to replace distinct software products used by different clinical departments, that solution can eliminate costly redundancies and help reduce total cost of ownership by alleviating factors such as installation, training, maintenance, and upgrades.
3. Modularity. A modular architecture enables health care providers and systems to tailor their enterprise information IT system to their specific needs, such as integrating specialist applications for reading and reporting, AI-powered functionalities, advanced visualization, and third-party tools.
4. Scalability. The solution should be designed to grow with an organization, expanding the number of servers and storage capacity as necessary.
Manage data around patients, not departments
Using information technology to minimize administrative burden and streamline workflow is imperative to delivering high-quality care in the modern health care environment.
A health care enterprise that pairs an open image and data management (IDM) system with an intuitive reading and reporting workspace can consolidate patient data in a single location instead of across multiple data silos.
The aim must be to bring imaging data, diagnostic software elements, and clinical tools together into a single, intuitive workspace for both routine and more complex cases, providing a patient-centric view with all relevant information at hand. With all data accessible and managed in one location, every clinician involved in patient care could trust the reliability of the information they access.
Enhanced patient care
Tirol Kliniken’s robust IDM consolidates patient data and connects systems across the enterprise, enabling simple, standards-compliant connectivity into existing information systems and subsystems, including the Health Information System (HIS) and Radiology Information System (RIS).
The organization’s central universal archive houses nearly all image and multimedia data acquired across five sites that comprise the health care organization and is connected with more than 250 subsystems from more than 100 vendors.
Clinicians use the front end of the IDM either holistically or via the web. Both methods allow health care professionals to access patient data from multiple sources, enabling a higher level of care.
“What I like the most is the versatility and tight integration,” says Dr. Gerhard Pierer, head of plastic, reconstructive, and aesthetic surgery at University Hospital in Innsbruck. “It allows me to have a holistic, patient-centric view on all DICOM and non-DICOM data of my patients.”
Eliminating silos and enabling clinicians to efficiently and securely access clinically relevant information helps Tirol Kliniken turn data into a strategic asset for its health care professionals and the patients they care for.
Learn how Syngo Carbon Core helps health care organizations share greater insights and support the quality of patient care
- UB Directory
- Research and Economic Development >
- Need to Know and Events >
UB announces first round of seed funding for health projects integrating AI
research news

Mary Ellen Giger, A.N. Pritzker Distinguished Service Professor of Radiology at the University of Chicago, gave the keynote talk last winter at UB’s first research in AI and health care symposium. The four funded research projects were among 12 proposals presented at the symposium. Photo: Sandra Kicman
By ELLEN GOLDBAUM
Published July 23, 2024
Drugs customized to a person’s DNA. Streamlined hospital admissions for older adults with complex medical issues. Improved language development in children who are late talkers. A way to enhance surgical skills and patient outcomes.
These four projects spanning multiple disciplines are using artificial intelligence to enhance health care. Each was awarded $50,000 in UB’s first round of competitive interdisciplinary seed funding for AI research in health care.
The funding is being provided through a collaboration between the Office of the Vice President for Research and Economic Development and the Office of the Vice President for Health Sciences.
“With UB as the home of Empire AI, our Institute for Artificial Intelligence and Data Science, and our six health sciences schools plus engineering, UB clearly has the talent and experience to lead AI in health,” says Venu Govindaraju, vice president for research and economic development. “As these four projects demonstrate, our researchers are actively innovating across all disciplines to leverage AI for the common good.”
Announced earlier this year by Gov. Kathy Hochul and approved by state lawmakers, Empire AI is a consortium of public and private higher education and philanthropic partners across the state. It aims to secure New York’s place at the forefront of artificial intelligence research with the goal of accelerating research and innovation in AI. The consortium’s computing center, to be located at UB, will be used by leading New York institutions to promote responsible research and development, create jobs and advance AI for the public good.
“As medical professionals, we are enthusiastic about harnessing the power of AI to tackle some of society’s most urgent health challenges,” says Allison Brashear, vice president for health sciences and dean of the Jacobs School of Medicine and Biomedical Sciences. “The creativity and innovation these researchers have demonstrated reflects the potential of AI to bring truly game-changing innovation to the clinic and the bedside to provide tangible benefit to patients and their caregivers. Their cross-disciplinary approaches harness the many strengths of UB and will bring forward creative and impactful solutions.”

Venu Govindaraju, vice president for research and economic development, addressed the symposium earlier this year, while Mary Ellen Giger, the keynote speaker, and Jinjun Xiong, SUNY Empire Innovation Professor, look on. Photo: Sandra Kicman
The call for AI in health research proposals generated more than 40 applications from the more than 200 UB researchers who are working on AI innovations in drug discovery, medicine, robotics and throughout health care and beyond. A requirement for submissions was that proposals needed to include faculty members from different academic units to ensure the teams reflected cross-disciplinary expertise.
Of the 40 submissions, 12 research proposals were presented at UB’s first research in AI and health care symposium last winter, where they received feedback from university leadership. Mary Ellen Giger, A.N. Pritzker Distinguished Service Professor of Radiology at the University of Chicago, gave the keynote talk.
The goal of this first round of pilot funding is to provide UB researchers with the opportunity to generate preliminary results and eventually attract more significant funding from the National Institutes of Health and other federal agencies. Seed funding for projects like these is essential to allow researchers to collect preliminary data, especially in emerging fields.
The four teams will present their preliminary findings at the next health and AI symposium sponsored by the Office of the Vice President for Health Sciences on Oct. 4, when David C. Rhew, global chief medical officer and vice president of health care for Microsoft, will be the guest speaker.
The principal investigators describe their projects below:
- SWAXSFold: A New AI Tool to Determine Protein Structures, Thomas D. Grant, assistant professor of structural biology, Jacobs School.
“SWAXSFold brings together the latest advances in AI modeling with powerful experimental X-ray scattering to model protein structures with higher accuracy than ever before,” Grant says. “In the future, this will enable a new generation of targeted, precision medicine, where drugs are designed for individual patients based on their own DNA.”
- AI to Identify Protective Factors for Children with Delayed Language Development, Federica Bulgarelli, assistant professor of psychology, College of Arts and Sciences.
“In this project, we are hoping to test whether some aspects of what parents say to kids can serve as naturally protective factors for later language delay diagnoses,” says Bulgarelli. “If we are able to identify these potentially protective factors, not only may we be able to identify children who are at risk earlier, but we may also be able to encourage parents to shift their language to focus more on these protective properties of speech.”
- Leveraging AI for Clinical Summaries in Hospital Care, Sabrina Cascucci, assistant professor of industrial and systems engineering, School of Engineering and Applied Sciences.
“Our team is extremely excited to develop new AI methods and health information exchange solutions for generating care summaries from longitudinal, multisource, multimodal historical care records at the time of hospital admission,” says Cascucci. “This is a critical challenge in the care of complex older adults as developing an accurate and meaningful understanding of community-based care and understanding how these factors impact readmission risk can have a significant impact on hospital-based care and post-hospital health outcomes for these vulnerable patients.”
- SurgiVdoNet: A Digital Common for Surgical Videos, Gene Yang, clinical assistant professor of surgery, Jacobs School.
“SurgiVdoNet is a digital common for annotated surgical video providing a data repository for ethical surgical artificial intelligence development,” Yang says. “Blockchain ledger technology provides secure and robust tracking to provide permanent ownership and attribution for all stakeholders including patients, hospitals and physicians. SurgiVdoNet provides a rich source of high quality, annotated surgical video data for training, testing and development of state-of-the-art, generalizable AI models to democratize surgical skill and improve patient outcomes.”
The state of AI in early 2024: Gen AI adoption spikes and starts to generate value
If 2023 was the year the world discovered generative AI (gen AI) , 2024 is the year organizations truly began using—and deriving business value from—this new technology. In the latest McKinsey Global Survey on AI, 65 percent of respondents report that their organizations are regularly using gen AI, nearly double the percentage from our previous survey just ten months ago. Respondents’ expectations for gen AI’s impact remain as high as they were last year , with three-quarters predicting that gen AI will lead to significant or disruptive change in their industries in the years ahead.
About the authors
This article is a collaborative effort by Alex Singla , Alexander Sukharevsky , Lareina Yee , and Michael Chui , with Bryce Hall , representing views from QuantumBlack, AI by McKinsey, and McKinsey Digital.
Organizations are already seeing material benefits from gen AI use, reporting both cost decreases and revenue jumps in the business units deploying the technology. The survey also provides insights into the kinds of risks presented by gen AI—most notably, inaccuracy—as well as the emerging practices of top performers to mitigate those challenges and capture value.
AI adoption surges
Interest in generative AI has also brightened the spotlight on a broader set of AI capabilities. For the past six years, AI adoption by respondents’ organizations has hovered at about 50 percent. This year, the survey finds that adoption has jumped to 72 percent (Exhibit 1). And the interest is truly global in scope. Our 2023 survey found that AI adoption did not reach 66 percent in any region; however, this year more than two-thirds of respondents in nearly every region say their organizations are using AI. 1 Organizations based in Central and South America are the exception, with 58 percent of respondents working for organizations based in Central and South America reporting AI adoption. Looking by industry, the biggest increase in adoption can be found in professional services. 2 Includes respondents working for organizations focused on human resources, legal services, management consulting, market research, R&D, tax preparation, and training.
Also, responses suggest that companies are now using AI in more parts of the business. Half of respondents say their organizations have adopted AI in two or more business functions, up from less than a third of respondents in 2023 (Exhibit 2).

Future frontiers: Navigating the next wave of tech innovations
Join Lareina Yee and Roger Roberts on Tuesday, July 30, at 12:30 p.m. EDT/6:30 p.m. CET as they discuss the future of these technological trends, the factors that will fuel their growth, and strategies for investing in them through 2024 and beyond.
Gen AI adoption is most common in the functions where it can create the most value
Most respondents now report that their organizations—and they as individuals—are using gen AI. Sixty-five percent of respondents say their organizations are regularly using gen AI in at least one business function, up from one-third last year. The average organization using gen AI is doing so in two functions, most often in marketing and sales and in product and service development—two functions in which previous research determined that gen AI adoption could generate the most value 3 “ The economic potential of generative AI: The next productivity frontier ,” McKinsey, June 14, 2023. —as well as in IT (Exhibit 3). The biggest increase from 2023 is found in marketing and sales, where reported adoption has more than doubled. Yet across functions, only two use cases, both within marketing and sales, are reported by 15 percent or more of respondents.
Gen AI also is weaving its way into respondents’ personal lives. Compared with 2023, respondents are much more likely to be using gen AI at work and even more likely to be using gen AI both at work and in their personal lives (Exhibit 4). The survey finds upticks in gen AI use across all regions, with the largest increases in Asia–Pacific and Greater China. Respondents at the highest seniority levels, meanwhile, show larger jumps in the use of gen Al tools for work and outside of work compared with their midlevel-management peers. Looking at specific industries, respondents working in energy and materials and in professional services report the largest increase in gen AI use.
Investments in gen AI and analytical AI are beginning to create value
The latest survey also shows how different industries are budgeting for gen AI. Responses suggest that, in many industries, organizations are about equally as likely to be investing more than 5 percent of their digital budgets in gen AI as they are in nongenerative, analytical-AI solutions (Exhibit 5). Yet in most industries, larger shares of respondents report that their organizations spend more than 20 percent on analytical AI than on gen AI. Looking ahead, most respondents—67 percent—expect their organizations to invest more in AI over the next three years.
Where are those investments paying off? For the first time, our latest survey explored the value created by gen AI use by business function. The function in which the largest share of respondents report seeing cost decreases is human resources. Respondents most commonly report meaningful revenue increases (of more than 5 percent) in supply chain and inventory management (Exhibit 6). For analytical AI, respondents most often report seeing cost benefits in service operations—in line with what we found last year —as well as meaningful revenue increases from AI use in marketing and sales.
Inaccuracy: The most recognized and experienced risk of gen AI use
As businesses begin to see the benefits of gen AI, they’re also recognizing the diverse risks associated with the technology. These can range from data management risks such as data privacy, bias, or intellectual property (IP) infringement to model management risks, which tend to focus on inaccurate output or lack of explainability. A third big risk category is security and incorrect use.
Respondents to the latest survey are more likely than they were last year to say their organizations consider inaccuracy and IP infringement to be relevant to their use of gen AI, and about half continue to view cybersecurity as a risk (Exhibit 7).
Conversely, respondents are less likely than they were last year to say their organizations consider workforce and labor displacement to be relevant risks and are not increasing efforts to mitigate them.
In fact, inaccuracy— which can affect use cases across the gen AI value chain , ranging from customer journeys and summarization to coding and creative content—is the only risk that respondents are significantly more likely than last year to say their organizations are actively working to mitigate.
Some organizations have already experienced negative consequences from the use of gen AI, with 44 percent of respondents saying their organizations have experienced at least one consequence (Exhibit 8). Respondents most often report inaccuracy as a risk that has affected their organizations, followed by cybersecurity and explainability.
Our previous research has found that there are several elements of governance that can help in scaling gen AI use responsibly, yet few respondents report having these risk-related practices in place. 4 “ Implementing generative AI with speed and safety ,” McKinsey Quarterly , March 13, 2024. For example, just 18 percent say their organizations have an enterprise-wide council or board with the authority to make decisions involving responsible AI governance, and only one-third say gen AI risk awareness and risk mitigation controls are required skill sets for technical talent.
Bringing gen AI capabilities to bear
The latest survey also sought to understand how, and how quickly, organizations are deploying these new gen AI tools. We have found three archetypes for implementing gen AI solutions : takers use off-the-shelf, publicly available solutions; shapers customize those tools with proprietary data and systems; and makers develop their own foundation models from scratch. 5 “ Technology’s generational moment with generative AI: A CIO and CTO guide ,” McKinsey, July 11, 2023. Across most industries, the survey results suggest that organizations are finding off-the-shelf offerings applicable to their business needs—though many are pursuing opportunities to customize models or even develop their own (Exhibit 9). About half of reported gen AI uses within respondents’ business functions are utilizing off-the-shelf, publicly available models or tools, with little or no customization. Respondents in energy and materials, technology, and media and telecommunications are more likely to report significant customization or tuning of publicly available models or developing their own proprietary models to address specific business needs.
Respondents most often report that their organizations required one to four months from the start of a project to put gen AI into production, though the time it takes varies by business function (Exhibit 10). It also depends upon the approach for acquiring those capabilities. Not surprisingly, reported uses of highly customized or proprietary models are 1.5 times more likely than off-the-shelf, publicly available models to take five months or more to implement.
Gen AI high performers are excelling despite facing challenges
Gen AI is a new technology, and organizations are still early in the journey of pursuing its opportunities and scaling it across functions. So it’s little surprise that only a small subset of respondents (46 out of 876) report that a meaningful share of their organizations’ EBIT can be attributed to their deployment of gen AI. Still, these gen AI leaders are worth examining closely. These, after all, are the early movers, who already attribute more than 10 percent of their organizations’ EBIT to their use of gen AI. Forty-two percent of these high performers say more than 20 percent of their EBIT is attributable to their use of nongenerative, analytical AI, and they span industries and regions—though most are at organizations with less than $1 billion in annual revenue. The AI-related practices at these organizations can offer guidance to those looking to create value from gen AI adoption at their own organizations.
To start, gen AI high performers are using gen AI in more business functions—an average of three functions, while others average two. They, like other organizations, are most likely to use gen AI in marketing and sales and product or service development, but they’re much more likely than others to use gen AI solutions in risk, legal, and compliance; in strategy and corporate finance; and in supply chain and inventory management. They’re more than three times as likely as others to be using gen AI in activities ranging from processing of accounting documents and risk assessment to R&D testing and pricing and promotions. While, overall, about half of reported gen AI applications within business functions are utilizing publicly available models or tools, gen AI high performers are less likely to use those off-the-shelf options than to either implement significantly customized versions of those tools or to develop their own proprietary foundation models.
What else are these high performers doing differently? For one thing, they are paying more attention to gen-AI-related risks. Perhaps because they are further along on their journeys, they are more likely than others to say their organizations have experienced every negative consequence from gen AI we asked about, from cybersecurity and personal privacy to explainability and IP infringement. Given that, they are more likely than others to report that their organizations consider those risks, as well as regulatory compliance, environmental impacts, and political stability, to be relevant to their gen AI use, and they say they take steps to mitigate more risks than others do.
Gen AI high performers are also much more likely to say their organizations follow a set of risk-related best practices (Exhibit 11). For example, they are nearly twice as likely as others to involve the legal function and embed risk reviews early on in the development of gen AI solutions—that is, to “ shift left .” They’re also much more likely than others to employ a wide range of other best practices, from strategy-related practices to those related to scaling.
In addition to experiencing the risks of gen AI adoption, high performers have encountered other challenges that can serve as warnings to others (Exhibit 12). Seventy percent say they have experienced difficulties with data, including defining processes for data governance, developing the ability to quickly integrate data into AI models, and an insufficient amount of training data, highlighting the essential role that data play in capturing value. High performers are also more likely than others to report experiencing challenges with their operating models, such as implementing agile ways of working and effective sprint performance management.
About the research
The online survey was in the field from February 22 to March 5, 2024, and garnered responses from 1,363 participants representing the full range of regions, industries, company sizes, functional specialties, and tenures. Of those respondents, 981 said their organizations had adopted AI in at least one business function, and 878 said their organizations were regularly using gen AI in at least one function. To adjust for differences in response rates, the data are weighted by the contribution of each respondent’s nation to global GDP.
Alex Singla and Alexander Sukharevsky are global coleaders of QuantumBlack, AI by McKinsey, and senior partners in McKinsey’s Chicago and London offices, respectively; Lareina Yee is a senior partner in the Bay Area office, where Michael Chui , a McKinsey Global Institute partner, is a partner; and Bryce Hall is an associate partner in the Washington, DC, office.
They wish to thank Kaitlin Noe, Larry Kanter, Mallika Jhamb, and Shinjini Srivastava for their contributions to this work.
This article was edited by Heather Hanselman, a senior editor in McKinsey’s Atlanta office.
Explore a career with us
Related articles.

Moving past gen AI’s honeymoon phase: Seven hard truths for CIOs to get from pilot to scale
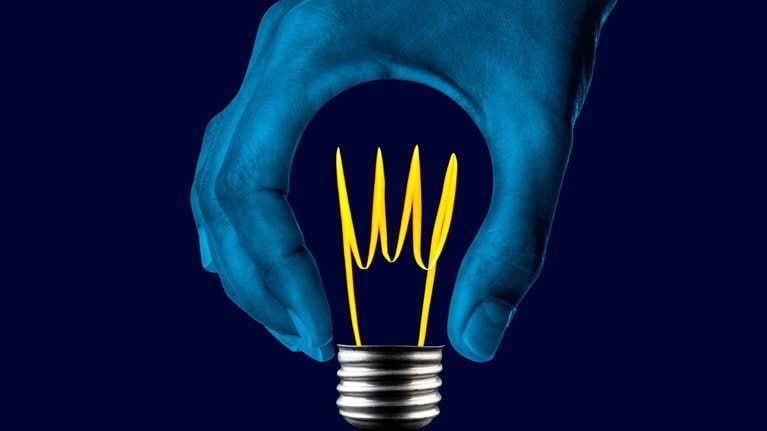
A generative AI reset: Rewiring to turn potential into value in 2024

Implementing generative AI with speed and safety

Why Jury Consultants Are Now Essential in High-Stakes Trials
By David Lat

Are reports of the jury trial’s death greatly exaggerated? While jury trials are less common than they were decades ago, we’ve seen a surprising number of them in 2024: E. Jean Carroll’s civil case against former President Donald Trump for sexual abuse and defamation, Manhattan district attorney Alvin Bragg’s criminal case against Donald Trump over hush-money payments, gun prosecutions of Hunter Biden and Alec Baldwin, and the corruption case against US Sen. Bob Menendez.
With the exception of the Baldwin case, which was dismissed mid-trial, all these cases went to verdict. And here’s something else they probably shared in common: trial or jury consultants.
“There’s been a big shift in the legal industry over the past 20 years,” said Eric Rudich, managing partner of Blueprint Trial Consulting. “In the early 2000s, senior partners might say, ‘Why do I need a consultant? I’ve done a million cases.’ But now they say, ‘I need a trial consultant to see how I should try this case.’”
“I have not gone to trial in 15 years without a jury consultant,” said Gibson Dunn’s Orin Snyder, one of the nation’s top trial lawyers. “Trying cases is a team sport, and even if there’s a captain or quarterback, an integral part of the team in modern jury practice is a highly skilled and effective jury consultant.”
Trial consultants provide a wide range of services. They conduct community research surveys, convene focus groups, hold mock trials, assist with jury selection, and prepare demonstrative exhibits or visual aids to educate the jury. Trial lawyers and their clients can select particular options, depending on their needs and budget.
This requires consulting firms to have “a lot of disciplines under one roof,” according to Renato Stabile of Dubin Research & Consulting. Stabile and DRC’s founder, Josh Dubin, are lawyers by training. But their firm’s staff of approximately 60 also includes experts in psychology, data science, statistics, graphics, and technology. Experts with Ph.D. degrees in social psychology or communications are not uncommon in jury consulting.
Turning to jury selection, for which trial consulting firms are most well-known, the process more aptly would be named “jury deselection,” according to Stabile.
“You can only get rid of people from the jury, either for cause or using peremptory strikes,” he explained. “Don’t fall in love with any particular person because if the other side is doing their job, that individual won’t make it onto the jury.”
And in high-profile cases, weeding out so-called “nightmare jurors” is easier said than done. Lawyers must watch out for what Stabile called “stealth jurors,” who don’t reveal their true feelings about the relevant issues because they want to be part of the case. As an example, he cited the Trump hush money case, where certain people in the jury pool claimed they could be fair and impartial—until postings on their social media accounts suggested otherwise.
Stabile and his colleagues perform extensive research to unearth and expose potential biases among jury candidates. Before trial, they might conduct a community attitude survey, surveying thousands of jury-eligible individuals in the jurisdiction where the trial will take place.
This might be followed by a mock trial lasting two to four days. Lawyers or consultants present key evidence and arguments to perhaps 50 to 100 mock jurors—jury-eligible individuals who live in the jurisdiction and are paid for their time. Throughout the presentation, jurors use iPads to answer questions and provide reactions in real time.
After the presentation is over, the jurors are divided into smaller panels to deliberate for several hours, just like real juries. Provided with jury instructions and a verdict form, they’re asked to reach a decision. The lawyers and consultants watch the deliberations, either through one-way glass or recordings. The consultants write up their findings in research reports.
This research can inform what lawyers advance as key themes of the case; which witnesses they call, and how they prep them; which evidence gets used, and how it’s presented; how the lawyers write their opening and closing statements; what questions they ask of potential jurors, in questionnaires and voir dire; and which jurors the lawyers strike.
Hiring a jury consulting firm isn’t cheap—it can cost tens of thousands of dollars, all the way into the millions, to have a consulting firm join a case early, assist in guiding discovery, work on developing litigation strategy, and help execute that strategy during a months-long trial.
In light of the cost, it’s important to manage client expectations about jury consultants. As David Oscar Markus of Markus/Moss, one of the country’s leading criminal defense attorneys, told me, “When clients are spending all this money on a trial consultant, we have to be clear with them: hiring a consultant is just another tool in the toolbox. Nobody is a magician or a miracle worker. We are hiring a consultant to maximize the chance of a good result.”
Good results aren’t guaranteed. Just ask Donald Trump, who hired consultants in the E. Jean Carroll and hush-money cases—and lost both.
So is hiring a consultant worth it? Snyder of Gibson Dunn thinks so: “Yes, it’s expensive—but if you have a client who can afford it, in every instance it’s a great investment.”
Jury and trial expert Robert Hirschhorn learned about the value of jury consulting in 1984, when he was going to trial in what he thought was an unwinnable case. He hired pioneering trial consultant Cathy “Cat” Bennett, a psychologist who started advising lawyers on jury selection as early as 1972.
After she revamped his case and he won, Hirschhorn was hooked on jury consulting. He went on to work with (and later marry) Bennett, and they picked juries in several famous cases—including the 1991 criminal trial of William Kennedy Smith, in which their client was acquitted. A year later, in 1992, Bennett died of cancer —but more than 30 years later, Hirschhorn’s consulting firm still proudly bears her name.
Most jury consultants today rely heavily on empirical research. As Stabile of DRC told me, “We are big believers in following the data, even when it’s counterintuitive.”
Hirschhorn takes a more old-school approach. Although he also conducts focus groups—which he claims are accurate in civil cases, on the issue of liability, more than 80% of the time—he believes there’s no substitute for looking a potential juror in the eye, in open court, and relying on your intuition.
“A lot of people want to make you think it’s all science,” he told me. “At least the way I do it, maybe 20 or 30% of it is science. It’s really instinct, with a little science sprinkled in there. I let my heart and gut lead the way.”
Hirschhorn has been picking juries this way for 40 years. But he doesn’t think jury consulting will last forever.
“Consultants will eventually be replaced by AI,” he predicted. “In the beginning, the AI tools will just crunch data and produce demographic profiles, so they won’t replace us. But once AI progresses to the point where it can evaluate nonverbal communication, recognize emotion, and detect empathy, that’s when the game will change.”
“I don’t know if that will take 20, 30, 40, or 50 years. But it will happen—eventually.”
David Lat , a lawyer turned writer, publishes Original Jurisdiction . He founded Above the Law and Underneath Their Robes, and is author of the novel “Supreme Ambitions.”
Read More Exclusive Jurisdiction
To contact the editors responsible for this story: Alison Lake at [email protected] ; Rebecca Baker at [email protected]
Learn more about Bloomberg Law or Log In to keep reading:
Learn about bloomberg law.
AI-powered legal analytics, workflow tools and premium legal & business news.
Already a subscriber?
Log in to keep reading or access research tools.
Numbers, Facts and Trends Shaping Your World
Read our research on:
Full Topic List
Regions & Countries
- Publications
- Our Methods
- Short Reads
- Tools & Resources
Read Our Research On:
Key facts about Americans and guns
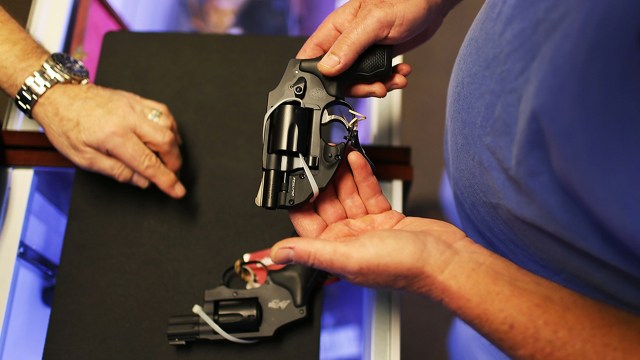
Guns are deeply ingrained in American society and the nation’s political debates.
The Second Amendment to the United States Constitution guarantees the right to bear arms, and about a third of U.S. adults say they personally own a gun. At the same time, in response to concerns such as rising gun death rates and mass shootings , the U.S. surgeon general has taken the unprecedented step of declaring gun violence a public health crisis .
Here are some key findings about Americans’ views of gun ownership, gun policy and other subjects, drawn from Pew Research Center surveys.
Pew Research Center conducted this analysis to summarize key facts about Americans’ relationships with guns. We used data from recent Center surveys to provide insights into Americans’ views on gun policy and how those views have changed over time, as well as to examine the proportion of adults who own guns and their reasons for doing so.
The Center survey questions used in this analysis, and more information about the surveys’ methodologies, and can be found at the links in the text.
Measuring gun ownership in the United States comes with unique challenges. Unlike many demographic measures, there is not a definitive data source from the government or elsewhere on how many American adults own guns.
The Pew Research Center survey conducted June 5-11, 2023, on the Center’s American Trends Panel, used two separate questions to measure personal and household ownership. About a third of adults (32%) say they own a gun, while another 10% say they do not personally own a gun but someone else in their household does. These shares have changed little from surveys conducted in 2021 and 2017 . In each of those surveys, 30% reported they owned a gun.
These numbers are largely consistent with rates of gun ownership reported by Gallup and those reported by NORC’s General Social Survey .
The FBI maintains data on background checks on individuals attempting to purchase firearms in the United States. The FBI reported a surge in background checks in 2020 and 2021, during the coronavirus pandemic, but FBI statistics show that the number of federal background checks declined in 2022 and 2023. This pattern seems to be continuing so far in 2024. As of June, fewer background checks have been conducted than at the same point in 2023, according to FBI statistics.
About four-in-ten U.S. adults say they live in a household with a gun, including 32% who say they personally own one, according to a Center survey conducted in June 2023 . These numbers are virtually unchanged since the last time we asked this question in 2021.
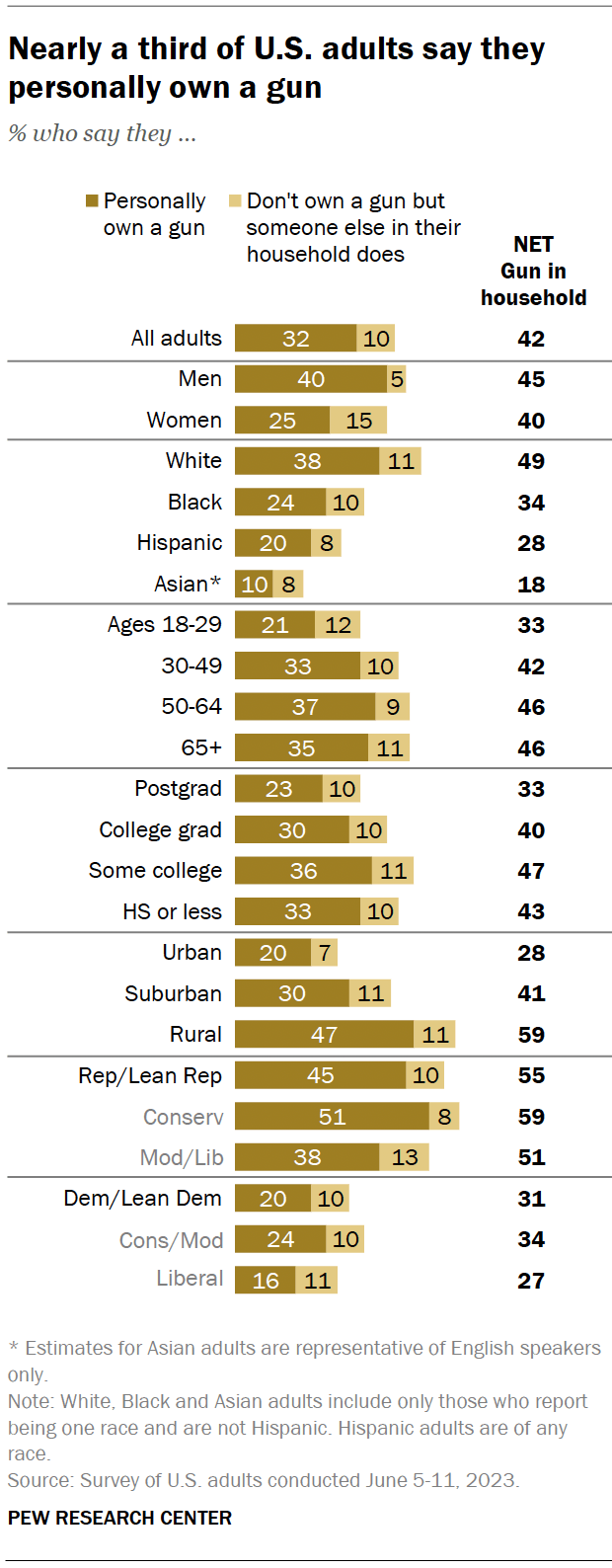
There are differences in gun ownership rates by political affiliation, gender, community type and other factors.
- Party: 45% of Republicans and GOP-leaning independents say they personally own a gun, compared with 20% of Democrats and Democratic leaners.
- Gender: 40% of men say they own a gun, versus 25% of women.
- Community type: 47% of adults living in rural areas report owning a firearm, as do smaller shares of those who live in suburbs (30%) or urban areas (20%).
- Race and ethnicity: 38% of White Americans own a gun, compared with smaller shares of Black (24%), Hispanic (20%) and Asian (10%) Americans.
Personal protection tops the list of reasons gun owners give for having a firearm. About seven-in-ten gun owners (72%) say protection is a major reason they own a gun. Considerably smaller shares say that a major reason they own a gun is for hunting (32%), for sport shooting (30%), as part of a gun collection (15%) or for their job (7%).
Americans’ reasons behind gun ownership have changed only modestly since we fielded a separate survey about these topics in spring 2017. At that time, 67% of gun owners cited protection as a major reason they had a firearm.
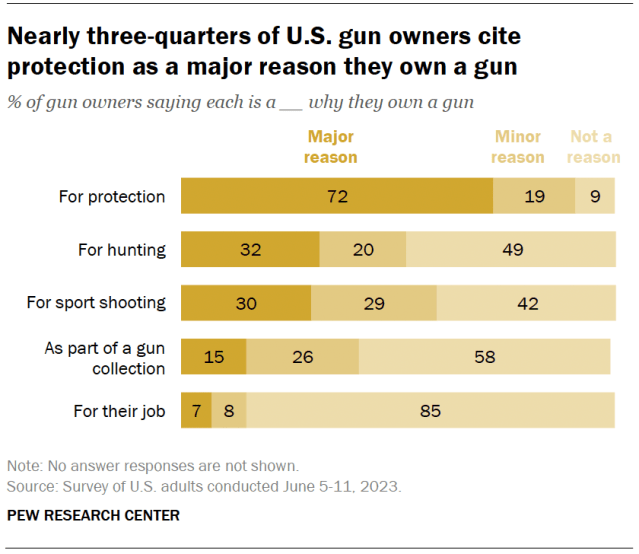
Gun owners tend to have much more positive feelings about having a gun in the house than nonowners who live with them do. For instance, 71% of gun owners say they enjoy owning a gun – but just 31% of nonowners living in a household with a gun say they enjoy having one in the home. And while 81% of gun owners say owning a gun makes them feel safer, a narrower majority of nonowners in gun households (57%) say the same. Nonowners are also more likely than owners to worry about having a gun at home (27% vs. 12%).
Feelings about gun ownership also differ by political affiliation, even among those who personally own a firearm. Republican gun owners are more likely than Democratic owners to say owning one gives them feelings of safety and enjoyment, while Democratic owners are more likely to say they worry about having a gun in the home.
Non-gun owners are split on whether they see themselves owning a firearm in the future. About half of Americans who don’t own a gun (52%) say they could never see themselves owning one, while nearly as many (47%) could imagine themselves as gun owners in the future.
Among those who currently do not own a gun, attitudes about owning one in the future differ by party and other factors.
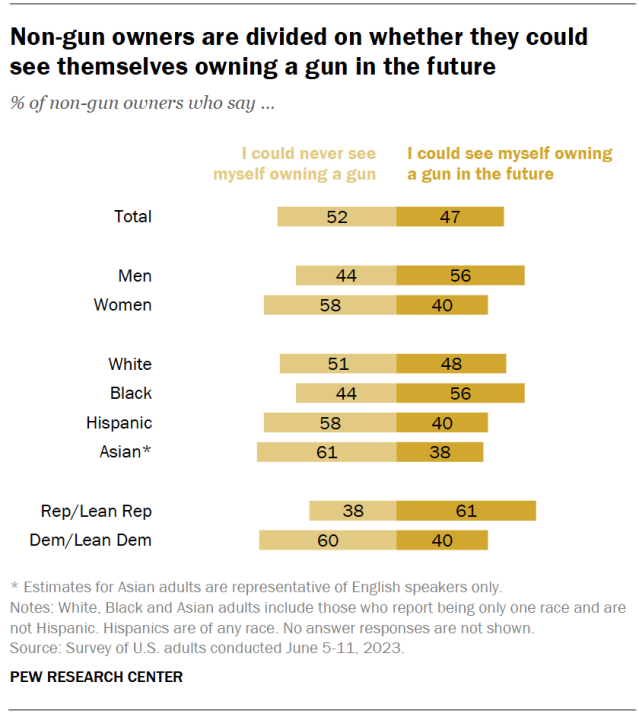
- Party: 61% of Republicans who don’t own a gun say they could see themselves owning one in the future, compared with 40% of Democrats.
- Gender: 56% of men who don’t own a gun say they could see themselves owning one someday; 40% of women nonowners say the same.
- Race and ethnicity: 56% of Black nonowners say they could see themselves owning a gun one day, compared with smaller shares of White (48%), Hispanic (40%) and Asian (38%) nonowners.
A majority of Americans (61%) say it is too easy to legally obtain a gun in this country, according to the June 2023 survey. Far fewer (9%) say it is too hard, while another 30% say it’s about right.
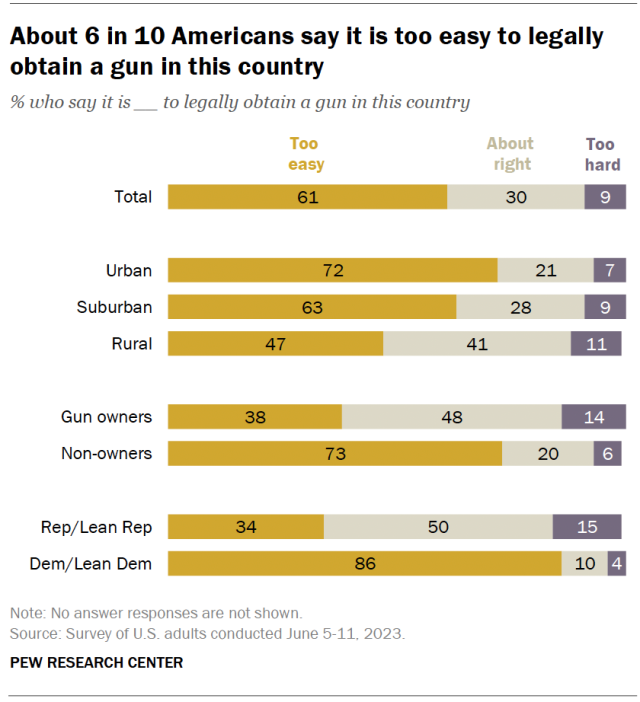
Non-gun owners are nearly twice as likely as gun owners to say it is too easy to legally obtain a gun (73% vs. 38%). Gun owners, in turn, are more than twice as likely as nonowners to say the ease of obtaining a gun is about right (48% vs. 20%).
There are differences by party and community type on this question, too. While 86% of Democrats say it is too easy to obtain a gun legally, far fewer Republicans (34%) say the same. Most urban (72%) and suburban (63%) residents say it’s too easy to legally obtain a gun, but rural residents are more divided: 47% say it is too easy, 41% say it is about right and 11% say it is too hard.
About six-in-ten U.S. adults (58%) favor stricter gun laws. Another 26% say that U.S. gun laws are about right, while 15% favor less strict gun laws.
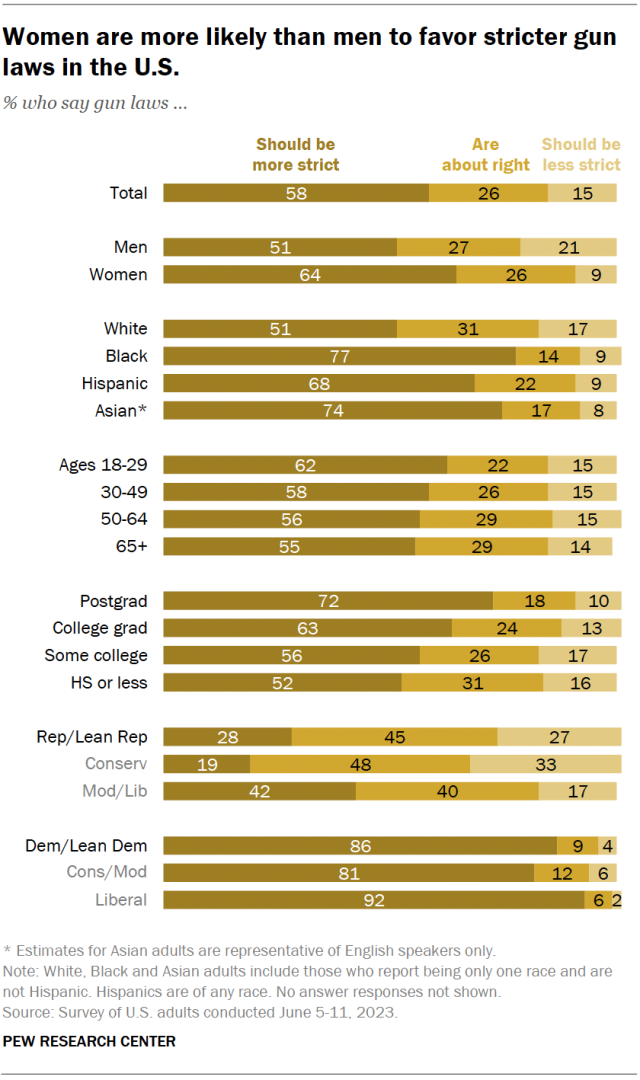
There is broad partisan agreement on some gun policy proposals, but most are politically divisive. Majorities of U.S. adults in both partisan coalitions somewhat or strongly favor two policies that would restrict gun access: preventing those with mental illnesses from purchasing guns (88% of Republicans and 89% of Democrats support this) and increasing the minimum age for buying guns to 21 years old (69% of Republicans, 90% of Democrats). Majorities in both parties also oppose allowing people to carry concealed firearms without a permit (60% of Republicans and 91% of Democrats oppose this).
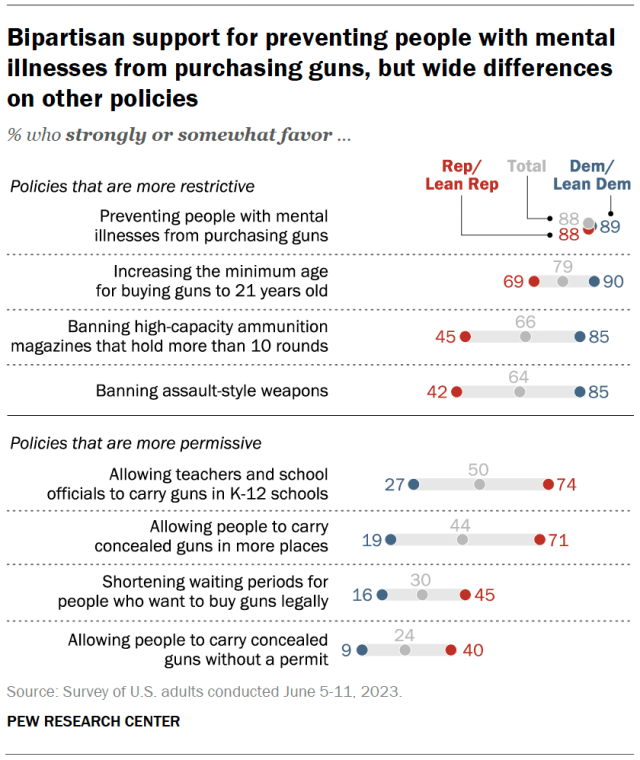
Republicans and Democrats differ on several other proposals. While 85% of Democrats favor banning both assault-style weapons and high-capacity ammunition magazines that hold more than 10 rounds, majorities of Republicans oppose these proposals (57% and 54%, respectively).
Most Republicans, on the other hand, support allowing teachers and school officials to carry guns in K-12 schools (74%) and allowing people to carry concealed guns in more places (71%). These proposals are supported by just 27% and 19% of Democrats, respectively.
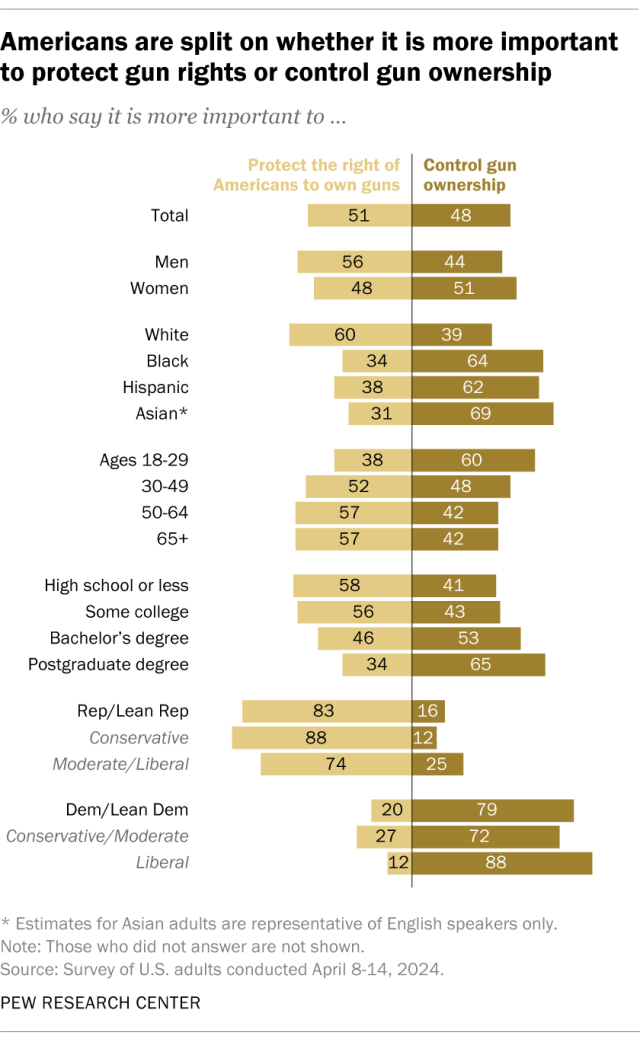
The public remains closely divided over whether it’s more important to protect gun rights or control gun ownership, according to an April 2024 survey . Overall, 51% of U.S. adults say it’s more important to protect the right of Americans to own guns, while a similar share (48%) say controlling gun ownership is more important.
Views have shifted slightly since 2022, when we last asked this question. That year, 47% of adults prioritized protecting Americans’ rights to own guns, while 52% said controlling gun ownership was more important.
Views on this topic differ sharply by party. In the most recent survey, 83% of Republicans say protecting gun rights is more important, while 79% of Democrats prioritize controlling gun ownership.
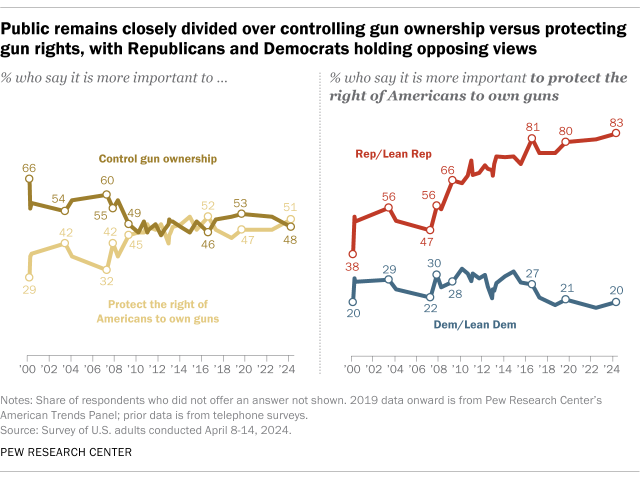
Americans are slightly more likely to say gun ownership does more to increase safety than to decrease it. Around half of Americans (52%) say gun ownership does more to increase safety by allowing law-abiding citizens to protect themselves, while a slightly smaller share (47%) say gun ownership does more to reduce safety by giving too many people access to firearms and increasing misuse. Views were evenly divided (49% vs. 49%) when we last asked in 2023.
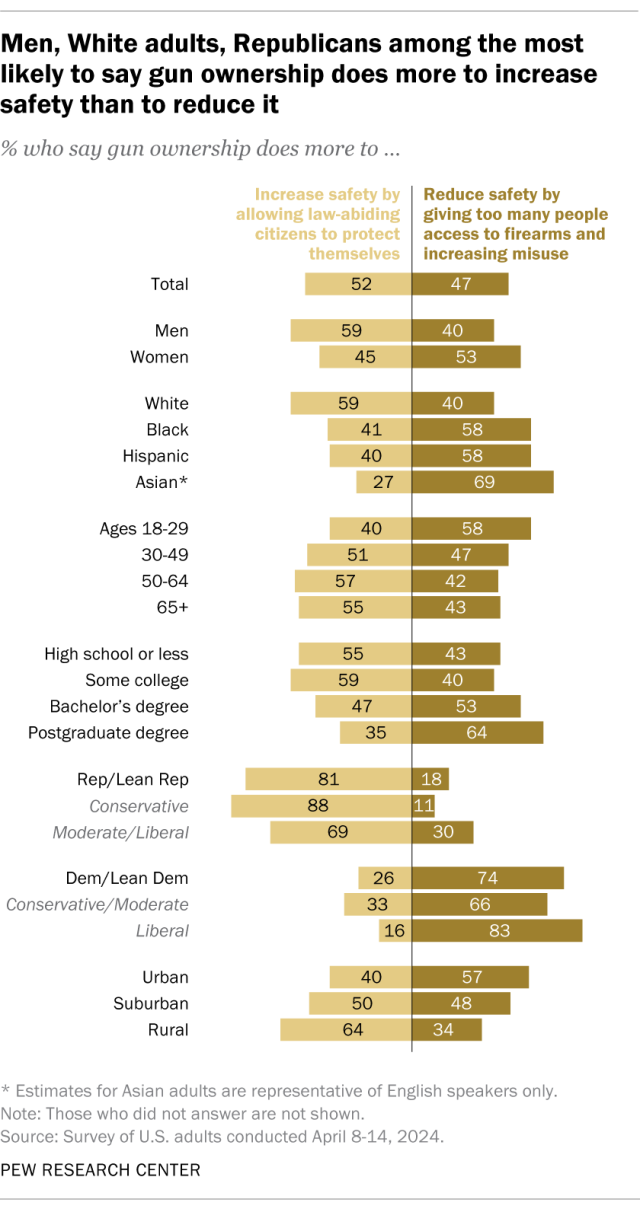
Republicans and Democrats differ widely on this question: 81% of Republicans say gun ownership does more to increase safety, while 74% of Democrats say it does more to reduce safety.
Rural and urban Americans also have starkly different views. Among adults who live in rural areas, 64% say gun ownership increases safety, while among those in urban areas, 57% say it reduces safety. Those living in the suburbs are about evenly split in their views.
More than half of U.S. adults say an increase in the number of guns in the country is bad for society, according to the April 2024 survey. Some 54% say, generally, this is very or somewhat bad for society. Another 21% say it is very or somewhat good for society, and a quarter say it is neither good nor bad for society.
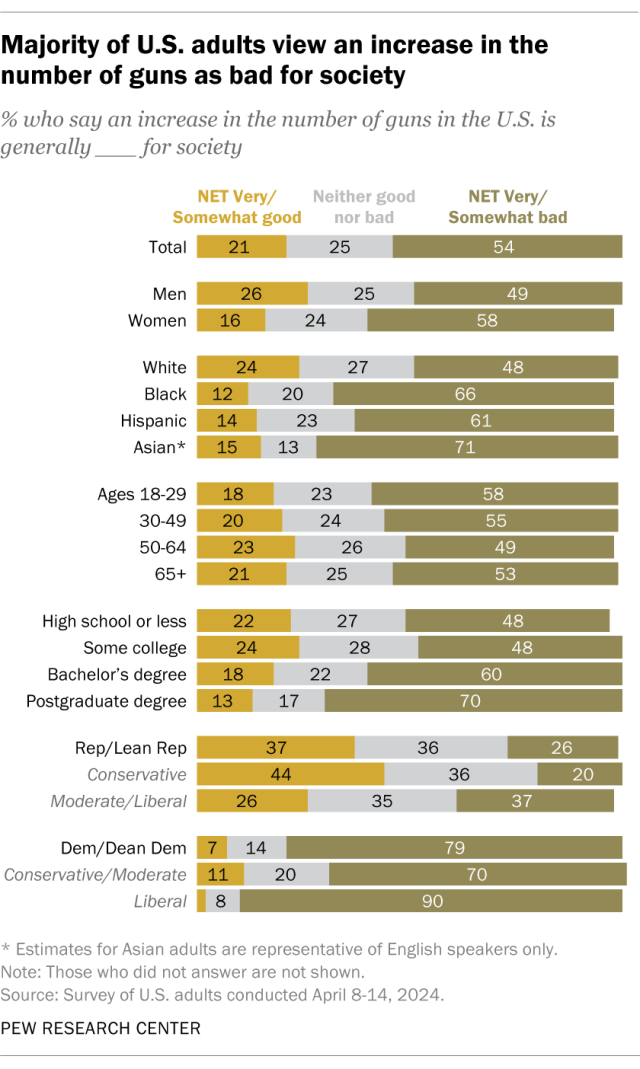
About half of Americans (49%) see gun violence as a major problem, according to a May 2024 survey. This is down from 60% in June 2023, but roughly on par with views in previous years. In the more recent survey, 27% say gun violence is a moderately big problem, and about a quarter say it is either a small problem (19%) or not a problem at all (4%).
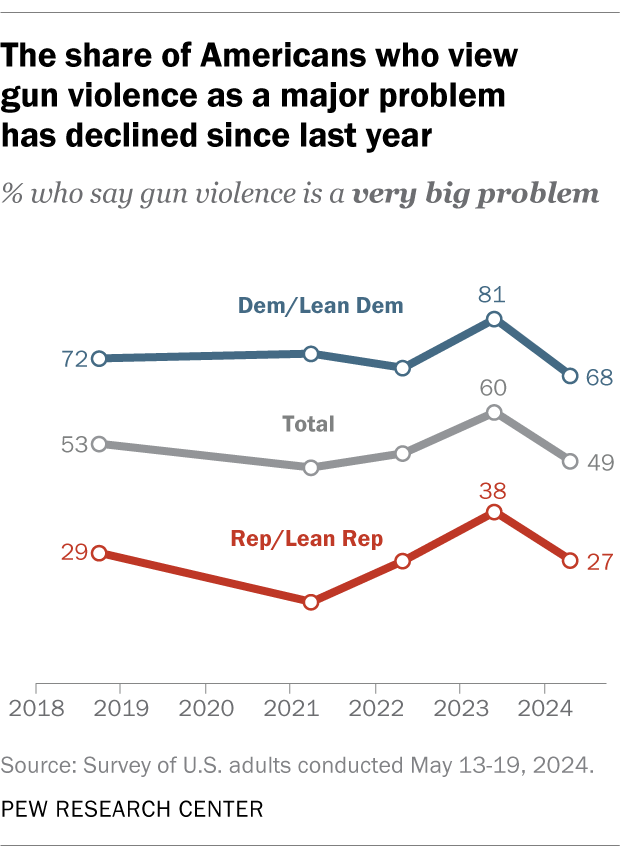
A majority of public K-12 teachers (59%) say they are at least somewhat worried about the possibility of a shooting ever happening at their school, including 18% who are very or extremely worried, according to a fall 2023 Center survey of teachers . A smaller share of teachers (39%) say they are not too or not at all worried about a shooting occurring at their school.
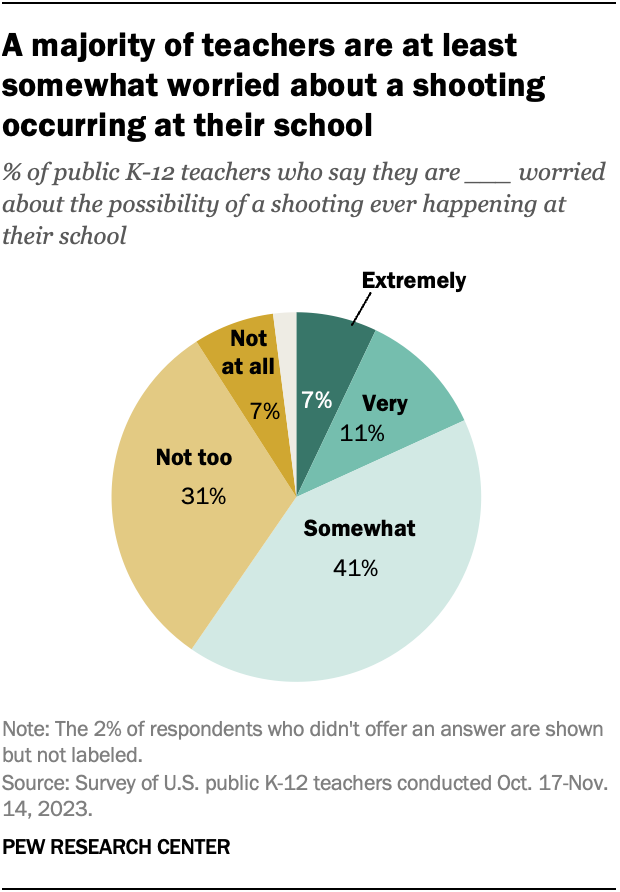
School shootings are a concern for K-12 parents as well: 32% say they are very or extremely worried about a shooting ever happening at their children’s school, while 37% are somewhat worried, according to a fall 2022 Center survey of parents with at least one child younger than 18 who is not homeschooled. Another 31% of K-12 parents say they are not too or not at all worried about this.
Note: This is an update of a post originally published on Jan. 5, 2016 .
- Partisanship & Issues
- Political Issues
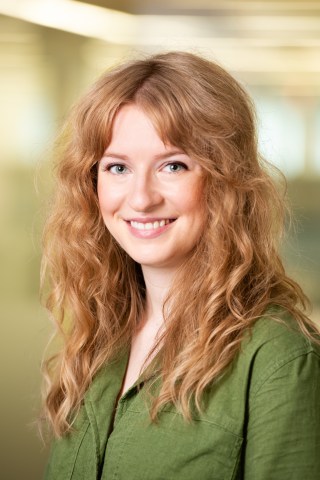
Katherine Schaeffer is a research analyst at Pew Research Center .
Americans’ Extreme Weather Policy Views and Personal Experiences
U.s. adults under 30 have different foreign policy priorities than older adults, many adults in east and southeast asia support free speech, are open to societal change, nato seen favorably in member states; confidence in zelenskyy down in europe, u.s., same-sex marriage around the world, most popular.
901 E St. NW, Suite 300 Washington, DC 20004 USA (+1) 202-419-4300 | Main (+1) 202-857-8562 | Fax (+1) 202-419-4372 | Media Inquiries
Research Topics
- Email Newsletters
ABOUT PEW RESEARCH CENTER Pew Research Center is a nonpartisan fact tank that informs the public about the issues, attitudes and trends shaping the world. It conducts public opinion polling, demographic research, media content analysis and other empirical social science research. Pew Research Center does not take policy positions. It is a subsidiary of The Pew Charitable Trusts .
© 2024 Pew Research Center

An official website of the United States government
The .gov means it’s official. Federal government websites often end in .gov or .mil. Before sharing sensitive information, make sure you’re on a federal government site.
The site is secure. The https:// ensures that you are connecting to the official website and that any information you provide is encrypted and transmitted securely.
- Publications
- Account settings
Preview improvements coming to the PMC website in October 2024. Learn More or Try it out now .
- Advanced Search
- Journal List
- Front Public Health
Big Data’s Role in Precision Public Health
Shawn dolley.
1 Cloudera, Inc., Palo Alto, CA, United States
Precision public health is an emerging practice to more granularly predict and understand public health risks and customize treatments for more specific and homogeneous subpopulations, often using new data, technologies, and methods. Big data is one element that has consistently helped to achieve these goals, through its ability to deliver to practitioners a volume and variety of structured or unstructured data not previously possible. Big data has enabled more widespread and specific research and trials of stratifying and segmenting populations at risk for a variety of health problems. Examples of success using big data are surveyed in surveillance and signal detection, predicting future risk, targeted interventions, and understanding disease. Using novel big data or big data approaches has risks that remain to be resolved. The continued growth in volume and variety of available data, decreased costs of data capture, and emerging computational methods mean big data success will likely be a required pillar of precision public health into the future. This review article aims to identify the precision public health use cases where big data has added value, identify classes of value that big data may bring, and outline the risks inherent in using big data in precision public health efforts.
Introduction
This review article aims to identify the precision public health use cases where big data has added value, identify classes of value that big data may bring, and outline the risks inherent in using big data in precision public health efforts. This article focuses on surveying current practice, with a breadth of examples. The article does not include a critical review of the methods included in the big data and precision public health published research. It is hoped this article may pave the way for future researchers to measure the strengths and weaknesses, robustness, and validity of individual studies, interventions and outcomes. With the breadth of practice defined here, such follow-on in-depth critical review could identify precision public health best practices in design, methods, implementation, and analysis.
The terms “big data” and “precision public health”—two relatively new disciplines—often do not appear in the nomenclature of contemporary public health interventions and studies. Searching for the terms “big data” or “precision public health” returns a small fraction of the actual activity. Based on the lack of existing reviews and the complexity in identifying the intersection of precision public health and big data, the rationale of this narrative review article is to find examples of the use of big data in implementations of precision public health published in peer-reviewed academic journals. The author (a) reviewed a large number of public health studies to look for precision and big data, as well as related and follow-on studies, (b) identified and searched for specific types of big data being applied to public health, and (c) searched for uses of data in precision public health to identify big vs. small data—always using the definition of these terms rather than relying on the presence of the terms “big data” or “precision public health.”
Searches were performed using Google Scholar and Google. Examples of public health implementations—with and without big data—and precision public health implementations—with and without big data—only qualified for this article if they were published in peer-reviewed journals. In the presence of multiple qualifying examples, best attempts were made to limit examples to a single citation. In the presence of multiple examples, to reduce risk of bias and attempt to identify the most robust examples, the examples selected were those with the (a) most clearly identifiable public health use case, (b) clearest use of big data, (c) most “precision,” (d) in journals with the highest impact factor, that were (e) the most recent—and in that order of priority. Searches were concluded by July 20, 2017.
Search terms used were as follows:
- For identifying implementations using big data volume, the term “public health” and each of the following: “big data,” “gene-wide,” “genome,” “genomic,” “germline,” “GWAS,” “imaging,” “molecular,” “multi-omic,” “pan-omic,” “phenome,” “PWAS,” “translational,” “video,” “whole exome,” and “whole genome.”
- For identifying implementations using big data variety, the term “public health” and each of the following: “big data,” “drone,” “Facebook,” “Instagram,” “IoT,” “internet of things,” “linked,” “linked data,” “patient-centered,” “patient generated,” “mobile,” “mobile phone,” “registry,” “registries,” “secondary use,” “semantic,” “sensors,” “social media,” “surveys,” “Twitter,” “UAV,” “unmanned aerial vehicle,” “variety,” and “wearable.”
- For identifying implementations using big data velocity, the term “public health” and each of the following: “big data,” “continuous,” “monitor,” “real-time,” “sensor,” “streams,” “streaming,” “velocity,” and “video.”
- For identifying public health implementations—including programs, trials, innovations and experiments—using big data, the term “big data” and each of the following: “adverse drug event,” “ADE,” “adverse event,” “cohort,” “epidemic,” “epidemiology,” “health intervention,” “health risk,” “heterogeneous,” “homogeneous,” “human movement,” “outcomes,” “pandemic,” “pharmaco-epidemiology,” “population health,” “precision public health,” “prevention,” “public health,” “signal detection,” “surveillance,” “targeted intervention,” “tracking,” “vaccine,” “vector,” and “virus.”
Google Scholar also provides lists of more recent studies which have cited the current study. These lists were reviewed to identify if more recent studies existed that provided better examples of pertinent characteristics.
This method has a number of limitations. Google Scholar has limitations, including relying on the end user to discriminate which studies returned are from peer-reviewed journals. No review protocol exists independent of this review article. No study selection or summary measures were collected, and no meta-analysis was performed. No study characteristics were collected. No assessment of the validity of included studies was performed beyond their inclusion in peer-reviewed academic journals. No assessment of cumulative level bias risk was performed. No additional analysis methods were used. The selection of studies included was not independently reviewed. The scope of this narrative review precludes enumerating additional limitations. Limitations aside, the result of these methods is a collection of studies or programs where big data and precision public health—as these terms are defined in this article—are being used together. Through implementing these methods, this review article is the first to identify the scope and scale of big data’s role in precision public health, highlight classes of innovation, and identify the risks of using big data in this field.
Precision Public Health
“Precision public health is a new field driven by technological advances that enable more precise descriptions and analyzes of individuals and population groups, with a view to improving the overall health of populations” ( 1 ). The term was coined in Australia by Dr. Tarun Weeramanthri in 2013, and first found in print in 2014 ( 2 ). Dr. Muin Khoury and Dr. Sandro Galea describe precision public health as “improving the ability to prevent disease, promote health, and reduce health disparities in populations by applying emerging methods and technologies for measuring disease, pathogens, exposures, behaviors, and susceptibility in populations; and developing policies and targeted implementation programs to improve health” ( 3 ). Precision public health leverages big data and its enabling technologies to achieve a previously impossible level of targeting or speed ( 4 ). The Bill & Melinda Gates Foundation adds that precision public health “requires robust primary surveillance data, rapid application of sophisticated analytics to track the geographical distribution of disease, and the capacity to act on such information” ( 5 ). Precision public health works because “more-accurate methods for measuring disease, pathogens, exposures, behaviors, and susceptibility could allow better assessment of population health and development of policies and targeted programs for preventing disease” ( 4 ). Arnett & Claas add “Precision public health is characterized by discovering, validating, and optimizing care strategies for well-characterized population strata” ( 6 ). As for the size of the strata, Colijn et al. state “precision approaches must act at the right scale, which will often be intermediate—between “one size fits all” medicine and fully individualized therapies” ( 7 ).
The prominence of the term “precision” in the new practices of precision medicine and precision public health will invariably raise questions about their similarity. While precision medicine requires genetic, lifestyle, and environmental data to meet goals of more customized and potentially individualized clinical treatments, precision public health is about increased accuracy and granularity in defining public cohorts and delivering target interventions of many types ( 4 – 6 ). Precision medicine and precision public health are independent.
Big Data in Healthcare and Public Health
Big data has recently become a ubiquitous approach to driving insights, innovation and new interventions across economic sectors ( 8 , 9 ). The United States National Institute of Standards and Technology defines big data as follows: “Big Data consists of extensive datasets—primarily in the characteristics of volume, variety, velocity, and/or variability—that require a scalable architecture for efficient storage, manipulation, and analysis,” ( 10 ). Decreases in costs of technology enabled the big data phenomenon to emerge ( 11 ). Data of “such a high volume, velocity and variety to require specific technology and analytical methods for its transformation into value” has a symbiotic relationship with the technology innovation on which it relies; the term big data often conflates the actual physical data with the unique technologies required to use it ( 12 , 13 ).
In patient-specific healthcare, big data technology has helped enable greater scales of volume, variety and velocity ( 14 , 15 ). Usable data volume has significantly increased in areas such as genomics ( 16 , 17 ), molecular research ( 18 , 19 ), medical image mining ( 20 ), and population health ( 21 , 22 ). Enabling a variety of data to be integrated, for a more complete view of patient or population, has occurred in areas including air quality ( 23 , 24 ), wearables ( 25 , 26 ), patient generated content via the web ( 27 ), patient or physician movement ( 28 , 29 ), medical studies ( 30 ), and critical care ( 31 ). Big data enabling increased velocity in healthcare was one of the earliest uses, in areas such as clinical prediction ( 32 , 33 ), and diagnostics ( 15 , 33 ). Current examples and future vision for use of big data exists in multiple and varying pathologies, including cancer ( 34 ), cardiology ( 35 ), epilepsy ( 36 ), family medicine ( 37 ), gastroenterology ( 38 ), nursing ( 39 ), pediatric ophthalmology ( 40 ), psychiatry ( 41 , 42 ), and women’s health ( 43 ) as examples.
Barrett et al. state succinctly: “Big data can play a key role in both research and intervention activities and accelerate progress in disease prevention and population health” ( 44 ). Big data shows utility across the entire spectrum of public health disciplines. This capability ranges from “monitoring population health in real-time” to building “definitive extents and databases on the occurrence of many diseases” ( 45 ). Public health subject areas that include examples of the use of big data include community health ( 46 ), environmental health science ( 24 , 47 ), epidemiology ( 48 ), infectious disease ( 45 ), maternal and child health ( 49 ), occupational health and safety ( 50 ), and nutrition ( 51 ). There is optimism and evidence for big data’s value in public health, both in research and in intervention ( 52 ).
Big Data in Precision Public Health
Today, use of big data has been shown to improve precision in select disciplines of public health. These areas include performing disease surveillance and signal detection ( 53 , 54 ), predicting risk ( 55 , 56 ), targeting interventions ( 6 ), and understanding disease ( 57 ). Research and proofs-of-concept with this data for these applications have been performed around the world. With the pace of technology innovation, and the speed at which precision health practitioners have embraced big data, there will likely be more public health disciplines, practices, approaches, and interventions implemented in the future or that are beyond the scope of this article ( 58 , 59 ).
Performing Disease Surveillance and Signal Detection
Disease surveillance and signal detection are among the most commonly cited and revolutionary of the big data use cases in precision public health ( 45 , 60 – 62 ). Precision signal detection or disease surveillance using big data has shown efficacy in air pollution ( 23 , 24 ), antibiotic resistance ( 63 ), cholera ( 64 ), dengue ( 65 , 66 ), drowning ( 67 ), drug safety ( 68 , 69 ), electromagnetic field exposure ( 70 ), Influenza A H1N1 ( 71 ), Lyme disease ( 72 ), monitoring food intake ( 73 ), and whooping cough ( 74 ).
Disease surveillance often includes tracking affected individuals, i.e., human carriers, patients, or victims ( 75 ). Stoddard et al. stated in 2009: “Human movement is a critical, understudied behavioral component underlying the transmission dynamics of many vector-borne pathogens” ( 76 ). In the effort to track disease spread by human vectors, a premium is placed on information that is more recent and granular ( 77 , 78 ). Thus, access to huge volumes of streaming real-time data generated by humans seems at once an ideal signal repository for identifying and tracking affected individuals, and definitionally big data ( 78 ).
Indeed, big data supports alternate and in some ways superior methods to track affected individuals ( 45 , 62 ). Because affected individuals move so quickly and at such a wide range, the real-time capabilities of big data and big data technology are now critical in this discipline ( 79 , 80 ). Studies have shown efficacy using mobile phone data in tracking movement in cholera ( 81 ), dengue ( 82 ), Ebola ( 83 ), human immunodeficiency virus (HIV) ( 84 ), malaria ( 85 ), rubella ( 85 ), and schistosomiasis ( 86 ). Other mechanisms that have shown efficacy or promise in tracking movement of affected individuals include air travel data ( 87 ), GPS data-loggers ( 88 ), magnetometers ( 89 ), Twitter ( 71 ), and web searches ( 65 ).
Predicting Risk
Effective signal detection often leads to attempts to predict future signals ( 90 , 91 ). Predicting public health risk leads to a chance to implement preventive interventions ( 56 , 92 ). Models predicting either disease spread or outcomes, using traditional or non-big data sources, have been developed across the spectrum of public health crises, including dengue ( 93 ), HIV ( 94 ), influenza ( 95 ), malaria ( 96 ), Rift Valley Fever ( 97 ), and tuberculosis ( 98 ).
One early example of using big data for public health prediction, Google Flu Trends, was a well-publicized failure ( 99 ). Since that episode, approaches to predicting risk using the internet and social media have shown special care to include merging big data with non-social media data sources, avoid overfitting models with relatively few cases, and being conscious of the risks of big data ( 56 , 100 ).
Big data has been used for risk prediction of spread or outcomes in public health topics such as air pollution ( 101 ), antibiotic resistance ( 102 ), avian influenza A ( 103 ), blood lead levels ( 104 ), child abuse ( 49 ), diabetes ( 105 ), Ebola ( 106 ), HIV ( 107 ), malaria ( 108 ), gestational diabetes ( 109 ), smoking progression ( 110 ), West Nile ( 111 ), and Zika ( 86 , 112 , 113 ).
Targeting Treatment Interventions
Applying treatment interventions to homogeneous cohorts within a larger heterogeneous population has been advocated since Lalonde’s seminal report “A New Perspective on the Health of Canadians” in 1974 ( 114 ). Historical examples of adding precision to public health treatment populations include gonorrhea in the 1980s ( 115 ), HIV in the 1990s ( 116 ), breast cancer in the 2000s ( 117 ), and malaria in the 2010s ( 118 ). In 2010, the US Department of Health and Human Services said of those citizens with multiple chronic conditions: “Indeed, developing means for determining homogeneous subgroups among this heterogeneous population is viewed as an important step in the effort to improve the health status of the total population” ( 119 ).
Big data was leveraged in public health research identifying finer-grain treatment interventions in childhood asthma ( 120 ), childhood obesity ( 121 ), diarrhea ( 122 ), Hepatitis C ( 123 ), HIV ( 124 ), injectable drug use ( 125 ), malaria ( 126 ), opioid medication misuse ( 127 ), use of smokeless tobacco ( 128 ), and the Zika virus ( 129 ).
One clinical example at the intersection of identifying subpopulations for effective interventions and big data is personalized vaccinology or “vaccinomics” ( 130 ). Most vaccines today are applied in a one-size fits all model: the typical implementation assumes a homogenous population, uses the same vaccine and dosages for all patients, ignores replicated, empirical realities of a heterogeneous population, and does not use sophisticated genomic capabilities at hand ( 131 , 132 ). While today’s vaccines are applied homogeneously, the results are individual: “The response to a vaccine is the cumulative result of non-random interactions with host genes, epigenetic phenomena, metagenomics and the microbiome, gene dominance, complementarity, epistasis, coinfections, and other factors” ( 133 ). Vaccinomics would focus on homogeneous subpopulations treated with vaccines, dosages and approaches that would “hold the promise of moving away from one standard vaccine against all human populations…to one where vaccines can be relatively easily tailor-fitted to individual, community and population specificity” ( 134 ).
Understanding Disease
Data volume and variety in epidemiology have grown consistently over time well before the age of big data ( 135 – 137 ). Contemporary exponential increases in data sizes, and perhaps more importantly increases in variety of data sources, make big data a valuable addition to the epidemiologist’s toolkit ( 64 , 138 ). Glymour states “We recommend that social epidemiologists take advantage of recent revolutionary improvements in data availability and computing power to examine new hypotheses and expand our repertoire of study designs” ( 139 ). Big data may have added relevance in study designs that are patient-centric and precision-oriented ( 140 ).
“Person-oriented approaches, in contrast, focus on differences between individuals as characterized by configurations and patterns of variables. This is well in line with a precision-medicine approach to understanding disease risk, resilience, and treatment response in subpopulations of individuals” ( 140 ).
Big data is a component in studies that have shown new precision characteristics of such public health concerns as cholera ( 141 ), chikungunya ( 142 ), diabetes ( 143 , 144 ), diarrhea ( 145 ), heatwave ( 146 ), influenza ( 147 ), opioid epidemic ( 148 , 149 ), preterm birth ( 150 ), stunting ( 151 ), and Zika ( 152 ).
Table Table1 1 summarizes the public health crises cited previously for which exists peer-reviewed research in at least two of the four precision public health disciplines. While the precision health research in Table Table1 1 and in this article has peer-reviewed and exhaustive methods, there are some opportunity gaps that future research should consider and include. Table Table2 2 lists critical gaps that occasionally exist in the research, grouped by precision public health discipline.
Precision public health research leveraging big data.
Precision public health discipline | ||||
---|---|---|---|---|
Public health crisis | Performing disease surveillance and signal detection | Predicting risk | Targeting treatment interventions | Understanding disease |
Air pollution | ( , ) | ( ) | ||
Antibiotic resistance | ( ) | ( ) | ||
Diabetes | ( , ) | ( , ) | ||
Diarrhea | ( ) | ( ) | ||
Ebola | ( ) | ( ) | ||
HIV | ( ) | ( ) | ( ) | |
Influenza (multiple) | ( ) | ( ) | ( ) | |
Malaria | ( ) | ( ) | ( ) | |
Opioid epidemic | ( ) | ( , ) | ||
Zika | ( , , ) | ( ) | ( ) |
Research studies (by citation) applying precision with the help of big data to a public health crisis. Public health crises are only included if big data in precision public health examples exist in more than one precision public health discipline .
Potential gaps in research methods in precision public health using big data.
Precision public health discipline | ||||
---|---|---|---|---|
Study attribute | Performing disease surveillance and signal detection | Predicting risk | Targeting treatment interventions | Understanding disease |
Data | electronic health records or detailed clinical data | to validate homogeneity of precision subgroups | ||
Subjects | ” in the high risk areas limits validity measure results at subject or molecular levels | ,” cannot attain high confidence levels, with no guidance for future alternatives to increase confidence levels | ,” cannot attain high confidence levels, with no guidance for future alternatives to increase confidence levels | |
Geography | ||||
Scaling | rather than as a result of testing multiple methods, limiting potential to scale the approach forward |
Critical features sometimes missing from precision public health studies leveraging big data, shown by public health discipline type .
Contributions of Big Data
Big data offers special contributions to precision public health in enabling a wider view of health variables through linking disparate or novel data ( 44 , 153 , 154 ) and enabling large study populations with volumes of multiomic data to identify “molecular cohorts” ( 155 ).
The technologies behind big data make it much easier to integrate a variety of data within a study ( 156 ). For example, because big data does not require investment in an a priori data schema, users can bring together a variety of different data and link it when the analytics are created ( 157 ). This enables researchers to link a mélange of unstructured disease and outcome data ( 158 , 159 ). In their 2017 study, Harry Hemingway, in their completion of 33 studies using linked data with a total population of two million patients, said “Our findings clearly show that research using one of the NHS greatest assets—its data—is vital to innovate improvements in disease prevention, to make earlier diagnoses and to give the best treatments” ( 160 ). The inclusion of data variety increases the number of independent variables; one novel variable—or a combination of as yet uncompared variables—could end up being significant in defining relevant precision subpopulations ( 161 , 162 ).
Examples of data that has been linked to help identify more precise cohorts of populations include: longitudinal health claims data ( 163 , 164 ); secondary use anonymized electronic health records ( 159 , 165 ); cohort studies, health surveys, and registries ( 166 – 168 ); environmental variables ( 104 ); molecular data such as from the genome, exposome, microbiome, or transcriptome ( 169 – 172 ); “mhealth” wearable and sensor data ( 173 ); mobile phone sensing data and self-reports ( 174 ); online patient generated content ( 175 ); and the semantic web ( 176 ).
The explosion of new volumes of genomic “big data” helped make possible the precision medicine movement ( 177 ). One of precision medicine’s promises was to lead to development of new treatments for subpopulations defined by their similarities at the molecular level ( 178 , 179 ). Currently, translational efforts in precision medicine often work by identifying cohorts of patients who have or lack specific genomic or molecular biomarkers ( 132 , 180 ). Since today’s precision medicine works at the granularity of disease subtypes and population strata and not at the “n of one” level, contemporary precision medicine really is—when applied to community crises—an example of precision public health ( 2 ).
Researchers agree that only by using very large sample sizes will genomic studies have the proper statistical power ( 181 , 182 ). “These large case–control studies are essential for boosting the statistical power needed to detect the genetic variants responsible for rare diseases and can provide the necessary knowledge for use in the clinical setting,” ( 183 ). Big data has been a necessary component in the scale-up of genomic sample sizes, enabled by the decrease in cost of gene sequencing ( 183 ). Future versions of sovereign genomics programs in over ten countries have the potential to create data sets with millions of samples ( 184 – 186 ). These databases should be ideal platforms for research such as genome wide association studies, which have been used with over ten thousand cases per study in public health diseases such as Alzheimer’s disease (25,000+ cases), autism (16,000 cases), high blood pressure (200,000+ cases), posttraumatic stress disorder (10,000+ cases), and smoking (50,000+ cases) ( 187 – 191 ).
The most sophisticated precision approaches to public health today at once include data from multiple omic disciplines, can make use of linked phenotype data, and leverage novel or recent types of computation ( 7 , 132 , 192 , 193 ). In targeting interventions, de novo or improved computational methods like geospatial risk modeling, latent class modeling, social molecular pathological epidemiology, and agent-based modeling simulation all benefit from big data to better identify these “intermediate” subpopulations ( 49 , 122 , 126 , 193 – 196 ).
More work needs to be done both enumerating and evaluating the risks and challenges of using big data in precision public health.
- Individuals could be stigmatized, even when not singularly identified, when they are stratified into small, observable cohorts, where they cannot maintain a “concealable stigmatized identity” ( 197 ).
- Big data could enable non-consented individuals to identify patients’ or citizens’ identities either due to small cohorts or by “drilling through” the deeper and wider set of population data ( 198 – 200 ).
- There are known drawbacks in increased reliance on a “high-risk” strategy, as originated by Rose, including ignoring population level determinants of health; taking focus away from a radical campaign that could have more sustainable positive effect for a larger population; risking missed interventions to borderline cases; or encouraging behaviors that continue to exist outside of social norms ( 201 ).
- Big data risks targeting only relatively wealthier communities where data can be collected, or where big data expertise or distribution technologies are endemic ( 72 , 202 , 203 ).
- For data collected through social media, crowdsourcing or similar channels, there may be more data about, in or from urban centers or areas of dense population, which will require additional computational governance ( 64 ).
- Prevalence of large volumes of new types of individual health information available digitally risks that it could fall into the hands of unregulated commercial enterprises, or of insurance companies ( 204 ).
- Experiencing governance gaps due to default use of existing governing legislation, rules or principles designed for data and technologies “that have now been superseded” by big data calls for more regulation ( 16 , 205 ).
- Applying novel big data without the appropriate controls, clinical interpretation, or statistical governance could lead to model overfitting, lack of accuracy, or results like Google Flu Trends, and could damage public faith in big data’s ability to add precision to public health or trust in contributing their own data ( 99 , 206 – 208 ).
- Big data brings unique challenges in data quality. Cai and Zhu created a big data quality framework with no less than 14 attributes by which any big data’s robustness should be assessed. Ignoring qualities like timeliness, accuracy, completeness or reliability leads to research weakness ( 209 ).
- Performing healthcare research that includes big data is marked by, and needs, larger teams of diverse practitioners, often including informaticians, data scientists, computer scientists, physicians, researchers, and more—potentially leading to fewer studies and the challenges inherent in collaborating in large teams ( 59 , 173 ).
- Research that includes big data with high “variety” or linked data is likely to include a higher median number of data sources, which could require increased investment in cleaning and curating the data—resulting in slower scientific progress—or could compel the challenges of analyzing high dimensional data ( 210 ). For example, the high dimensionality of data found in both molecular and linked data incurs specific risk. Alyass et al. believe this data is “prone to high rates of false-positives due to chance alone…this requires researchers to adjust for multiple testing to control for type 1 error rates…or reduce dimensionality via sparse methods” ( 211 ).
Precision public health is exciting. Today’s public health programs can achieve new levels of speed and accuracy not plausible a decade ago. Adding precision to many parts of public health engagement has led and will lead to tangible benefits. Precision can enable public health programs to maintain the same efficacy while decreasing costs, or hold costs constant while delivering better, smarter, faster, and different education, cures and interventions, saving lives.
Precision public health does not require big data. That said, the future of big data in precision public health is assured, based on its successes and acceleration of use to date. Big data and the methods created to make it useful allow precision public health practitioners to operate at the top of their license and can bring more insight to cohort membership, disease pathways and treatments. Big data enables lower costs and more precision to find, educate, track, and help each high-risk citizen. In the future, precision public health needs, imperatives, mandates and techniques will drive new capabilities into big data.
Using big data in precision public health has risks. A number of risks were identified here and future study will expand these or identify more. Protecting the dignity, privacy, security of citizens and patients, while finding truly meaningful significant outcomes in a reasonable timeframe will take effort on the part of each and every researcher in this space.
What are the calls to action? Investment has increased, but additional investment and research are needed in many areas. First, more experimentation is needed to understand how to best create and mobilize open data, open science, open source communities, and open collaboration platforms. For context, the Observational Health Data Sciences and Informatics collaborative is a thriving global open science community focused on large scale population health outcomes and prediction. If such a collaborative existed for precision public health, one imagines practitioners could leverage shared best practices, data, open software, and opportunities. Second, there are opportunity gaps in training precision public health workers in countries with a dearth of data scientists, on-premise data storage and computational assets, or access to big data. For example, communities suffering public health crises increasingly desire to “learn how to use the information and improve their ability to respond to future outbreaks in the region,” rather than having their data removed for analysis by better funded nations ( 212 ). Third, follow-on research is needed in the area of big data in precision public health. Specifically, (a) best practices in performing data quality assessment along a broad range of attributes should be enumerated, (b) existing research should be scored along these attributes as well as those studies’ compliance with statistical best practices specific to big data and high dimensionality, (c) each area of value delivery—disease surveillance, predicting risk, targeting intervention and understanding disease—needs their own full treatment with regard to methods, data sources, data management, and more, (d) some critical framework ought to be created and proposed to systematically measure precision public health studies and programs, specific to and beyond big data, and (e) as precision public health becomes more mature, emerging trends should be noticed and evaluated. Fourth, more work is needed in areas of ethics, risk, and governance. The community should be watching for overreliance on big data-driven approaches that lead to decreases in radical whole-population solutions that increase baseline health norms. Fifth, the global economic opportunity of using big data prescriptively in public health has not been systematically measured, beyond specific country or disease successes. For context, organizations such as the United Nations, the World Bank, and the United States Agency for International Development have estimated economic impacts of individual epidemics. These or other institutions could convene a task force to estimate the economic benefit of applying precision to public health responses, as well as the relative contribution of big data. Sixth, precision public health centers of excellence in universities can help. Today, leaders in schools of public health are speaking and writing about precision public health; presumably academic courses, concentrations and centers will follow in stepwise progression. Seventh, new technical innovation must continue and needs investment. For example, this could include applying deep learning to precision public health use cases, or creating a novel free and open source data science software “pipeline” for geospatial event prediction.
Future precision public health will be transformative. It will include new applications, modifications, and uses of today’s assets, including social media and communication platforms, unmanned aerial vehicles, mobile applications, mobile sequencing, self-screening, sensors, vaccine or drug internet-of-things inventions, and more. Tomorrow, we could be looking up, wondering if a high-resolution satellite is mapping our neighborhood to predict the path of an infectious disease, or if a drone is approaching with a targeted intervention. With future applications of precision public health and the speed of big data adoption, tomorrow’s new public health students and young practitioners soon won’t think of the discipline as precision public health. They will only think of it as public health.
Author Contributions
The author confirms being the sole contributor of this work and approved it for publication.
Conflict of Interest Statement
The author is employed by Cloudera, Inc., a provider of big data technology.
Featured Article
The biggest data breaches in 2024: 1 billion stolen records and rising
Thanks to unitedhealth, snowflake and at&t (twice).

We’re over halfway through 2024, and already this year we have seen some of the biggest, most damaging data breaches in recent history. And just when you think that some of these hacks can’t get any worse, they do.
From huge stores of customers’ personal information getting scraped, stolen and posted online, to reams of medical data covering most people in the United States getting stolen, the worst data breaches of 2024 to date have already surpassed at least 1 billion stolen records and rising. These breaches not only affect the individuals whose data was irretrievably exposed, but also embolden the criminals who profit from their malicious cyberattacks.
Travel with us to the not-so-distant past to look at how some of the biggest security incidents of 2024 went down, their impact and. in some cases, how they could have been stopped.
AT&T’s data breaches affect “nearly all” of its customers, and many more non-customers
For AT&T, 2024 has been a very bad year for data security. The telecoms giant confirmed not one, but two separate data breaches just months apart.
In July, AT&T said cybercriminals had stolen a cache of data that contained phone numbers and call records of “nearly all” of its customers, or around 110 million people , over a six-month period in 2022 and in some cases longer. The data wasn’t stolen directly from AT&T’s systems, but from an account it had with data giant Snowflake (more on that later).
Although the stolen AT&T data isn’t public (and one report suggests AT&T paid a ransom for the hackers to delete the stolen data ) and the data itself does not contain the contents of calls or text messages, the “metadata” still reveals who called who and when, and in some cases the data can be used to infer approximate locations. Worse, the data includes phone numbers of non-customers who were called by AT&T customers during that time. That data becoming public could be dangerous for higher-risk individuals , such as domestic abuse survivors.
That was AT&T’s second data breach this year. Earlier in March, a data breach broker dumped online a full cache of 73 million customer records to a known cybercrime forum for anyone to see, some three years after a much smaller sample was teased online.
The published data included customers’ personal information, including names, phone numbers and postal addresses, with some customers confirming their data was accurate .
But it wasn’t until a security researcher discovered that the exposed data contained encrypted passcodes used for accessing a customer’s AT&T account that the telecoms giant took action. The security researcher told TechCrunch at the time that the encrypted passcodes could be easily unscrambled, putting some 7.6 million existing AT&T customer accounts at risk of hijacks. AT&T force-reset its customers’ account passcodes after TechCrunch alerted the company to the researcher’s findings.
One big mystery remains: AT&T still doesn’t know how the data leaked or where it came from .
Change Healthcare hackers stole medical data on “substantial proportion” of people in America
In 2022, the U.S. Justice Department sued health insurance giant UnitedHealth Group to block its attempted acquisition of health tech giant Change Healthcare, fearing that the deal would give the healthcare conglomerate broad access to about “half of all Americans’ health insurance claims” each year. The bid to block the deal ultimately failed. Then, two years later, something far worse happened: Change Healthcare was hacked by a prolific ransomware gang; its almighty banks of sensitive health data were stolen because one of the company’s critical systems was not protected with multi-factor authentication .
The lengthy downtime caused by the cyberattack dragged on for weeks, causing widespread outages at hospitals, pharmacies and healthcare practices across the United States. But the aftermath of the data breach has yet to be fully realized, though the consequences for those affected are likely to be irreversible. UnitedHealth says the stolen data — which it paid the hackers to obtain a copy — includes the personal, medical and billing information on a “substantial proportion” of people in the United States.
UnitedHealth has yet to attach a number to how many individuals were affected by the breach. The health giant’s chief executive, Andrew Witty, told lawmakers that the breach may affect around one-third of Americans , and potentially more. For now, it’s a question of just how many hundreds of millions of people in the U.S. are affected.
Synnovis ransomware attack sparked widespread outages at hospitals across London
A June cyberattack on U.K. pathology lab Synnovis — a blood and tissue testing lab for hospitals and health services across the U.K. capital — caused ongoing widespread disruption to patient services for weeks. The local National Health Service trusts that rely on the lab postponed thousands of operations and procedures following the hack, prompting the declaration of a critical incident across the U.K. health sector.
A Russia-based ransomware gang was blamed for the cyberattack, which saw the theft of data related to some 300 million patient interactions dating back a “significant number” of years. Much like the data breach at Change Healthcare, the ramifications for those affected are likely to be significant and life-lasting.
Some of the data was already published online in an effort to extort the lab into paying a ransom. Synnovis reportedly refused to pay the hackers’ $50 million ransom , preventing the gang from profiting from the hack but leaving the U.K. government scrambling for a plan in case the hackers posted millions of health records online.
One of the NHS trusts that runs five hospitals across London affected by the outages reportedly failed to meet the data security standards as required by the U.K. health service in the years that ran up to the June cyberattack on Synnovis.
Ticketmaster had an alleged 560 million records stolen in the Snowflake hack
A series of data thefts from cloud data giant Snowflake quickly snowballed into one of the biggest breaches of the year, thanks to the vast amounts of data stolen from its corporate customers.
Cybercriminals swiped hundreds of millions of customer data from some of the world’s biggest companies — including an alleged 560 million records from Ticketmaster , 79 million records from Advance Auto Parts and some 30 million records from TEG — by using stolen credentials of data engineers with access to their employer’s Snowflake environments. For its part, Snowflake does not require (or enforce) its customers to use the security feature, which protects against intrusions that rely on stolen or reused passwords.
Incident response firm Mandiant said around 165 Snowflake customers had data stolen from their accounts, in some cases a “significant volume of customer data.” Only a handful of the 165 companies have so far confirmed their environments were compromised, which also includes tens of thousands of employee records from Neiman Marcus and Santander Bank , and millions of records of students at Los Angeles Unified School District . Expect many Snowflake customers to come forward.
More TechCrunch
Get the industry’s biggest tech news, techcrunch daily news.
Every weekday and Sunday, you can get the best of TechCrunch’s coverage.
Startups Weekly
Startups are the core of TechCrunch, so get our best coverage delivered weekly.
TechCrunch Fintech
The latest Fintech news and analysis, delivered every Tuesday.
TechCrunch Mobility
TechCrunch Mobility is your destination for transportation news and insight.
Here’s why David Sacks, Paul Graham and other big Silicon Valley names had a brawl on X over VC behavior
A decade-old drama involving VC David Sacks and Rippling founder Parker Conrad has blown up on X with many among the Silicon Valley elite taking sides.
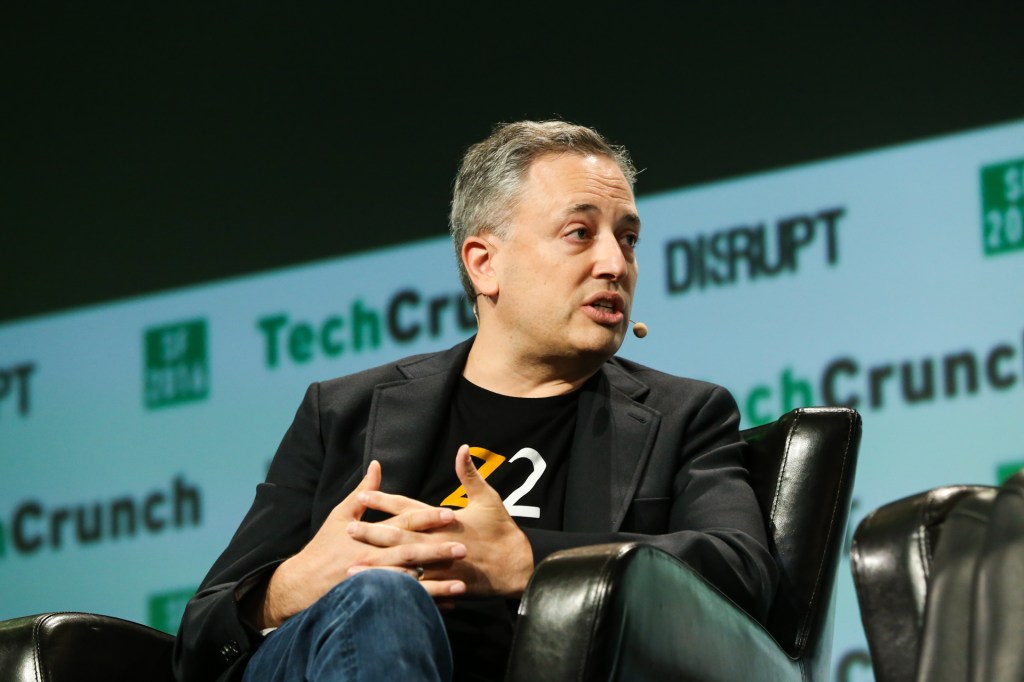
ChatGPT: Everything you need to know about the AI-powered chatbot
ChatGPT, OpenAI’s text-generating AI chatbot, has taken the world by storm since its launch in November 2022. What started as a tool to hyper-charge productivity through writing essays and code…
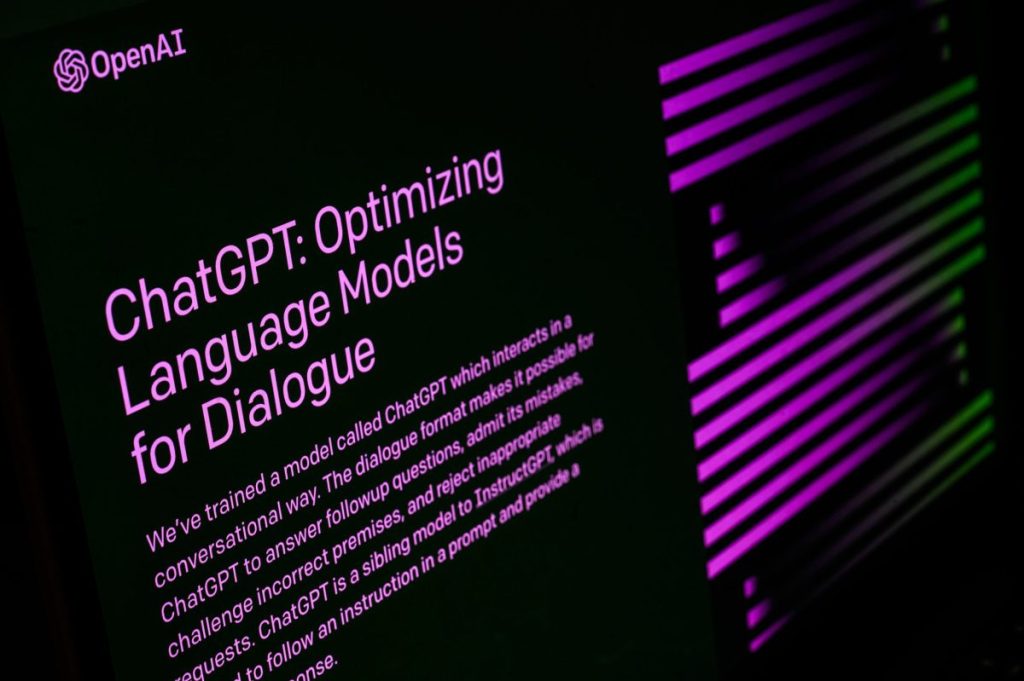
Applied Intuition closes $300M secondary four months after raising $250M
Autonomous vehicle software startup Applied Intuition has closed a $300 million secondary sale just four months after raising a $250 million Series E round, yet another sign of how white-hot…

With Google in its sights, OpenAI unveils SearchGPT
OpenAI may have designs to get into the search game — challenging not only upstarts like Perplexity, but Google and Bing, too. The company on Thursday unveiled SearchGPT, a search…

Uber, Lyft, DoorDash can continue to classify drivers as contractors in California
The California Supreme Court ruled Thursday that Proposition 22 – the ballot measure that passed in November 2020 and classified app-based gig workers as independent contractors rather than employees –…

Mark Zuckerberg says WhatsApp has 100 million monthly active users in the U.S.
WhatsApp has recently ramped up its marketing push in the U.S.

Alphabet pours $5B into Waymo, Cruise scraps the Origin and Elon’s bet on autonomy
Welcome back to TechCrunch Mobility — your central hub for news and insights on the future of transportation. Sign up here for free — just click TechCrunch Mobility! I don’t…

Archera helps customers access deep cloud discounts
In addition to insured commitments, Archera provides consulting services to help build purchasing strategies for customers to optimize their cloud usage.

Google makes its Gemini chatbot faster and more widely available
In its bid to maintain pace with generative AI rivals like Anthropic and OpenAI, Google is rolling out updates to the no-fee tier of Gemini, its AI-powered chatbot. The updates…

ZoomInfo alum raises $15M for startup that builds AI sales engineers
Until a year ago, Arjun Pillai had the comfortable yet important role of chief data officer at ZoomInfo, a B2B database company. But the serial entrepreneur was getting antsy. He…

Substack writers can now draft and publish posts in iOS app
Substack is rolling out the ability for writers to draft and publish new posts directly from their phone via its iOS app, the company announced on Thursday. Until now, users…

Disrupt 2024 Career Fair: Your gateway to top tech talent
Disrupt 2024 is the premier event where tech careers are launched, connections are forged, and the future of technology talent takes center stage. The Disrupt Career Fair is the perfect…

Hacked, leaked, exposed: Why you should never use stalkerware apps
Using stalkerware is creepy, unethical, potentially illegal, and puts your data and that of your loved ones in danger.

Endeavor CEO says long-term capital needs to be prioritized in emerging ecosystems
Venture capital has become a more global industry as the tech sector slowly decentralizes. In 2022, more than 50% of VC deployed globally was invested in startups outside the U.S., according to data available from the National Science Foundation (NSF) — a stark contrast to 20 years ago, when nearly…

Data breach exposes US spyware maker behind Windows, Mac, Android and Chromebook malware
Exclusive: The Minnesota-based spyware maker Spytech snooped on thousands of devices before it was hacked earlier this year.

Singaporean e-commerce firm Qoo10’s Korean units face probe due to payment delays to merchants
The e-commerce market in South Korea ranks as one of the largest in the world, but it’s also proving to be a precarious one. On Thursday, South Korea’s Fair Trade…
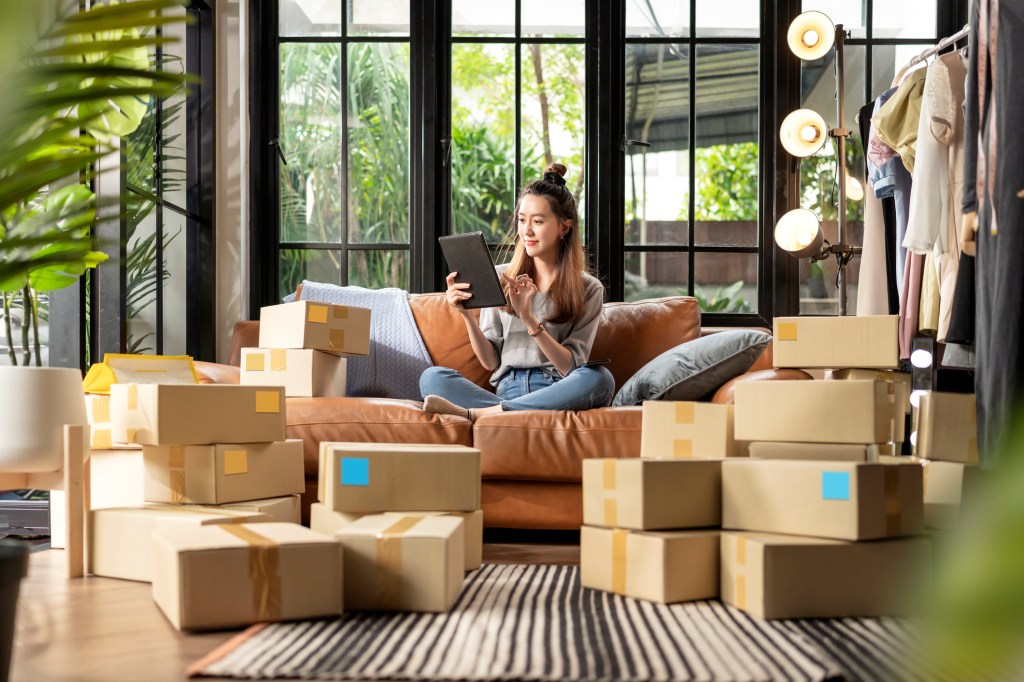
Kodiak Robotics is taking self-driving trucks off-road to reach profitability faster
Don Burnette, CEO and co-founder of self-driving truck startup Kodiak Robotics, had an “aha” moment when the company started working with the U.S. Department of Defense. Kodiak’s mission has always…

Lodestar’s robotic arm will be an orbital ‘first responder’ for satellites in need
Satellites are among our most critical infrastructure, providing everything from GPS to disaster coordination, yet their inherent inaccessibility leaves them vulnerable to relatively simple technical issues or attacks. London-based Lodestar…

Intron Health gets backing for its speech-recognition tool that recognizes African accents
Voice recognition is getting integrated in nearly all facets of modern living, but there remains a big gap: Speakers of minority languages and those with thick accents or speech disorders…

GM-backed Addionics aims to make lithium-ion batteries cheaper with wavy foil
The startup has developed a way to create copper and aluminum foils that are laced with tiny holes and riddled with undulating peaks and valleys.

Revolut receives long-awaited UK banking license
This is a significant milestone for the London-based fintech company, particularly since it has been trying to secure this license since 2021.

Oversight Board wants Meta to refine its policies around AI-generated explicit images
The Board wants Meta to change the terminology it uses for labeling explicit, AI-generated images from “derogatory” to “non-consensual.”

Google Maps adds a slew of features to entice Indian drivers, commuters and travelers
Google Maps is improving navigation through flyovers and narrow roads in India through new feature updates.

bunch raises $15.5M for its platform that simplifies investment management for VCs
Public market investors have a large variety of infrastructure and software that helps them keep track of, analyze and manage their investments, but that’s not the case for investors in…

Jio partners with Taiwan’s MediaTek to tap into two-wheeler EV market
India’s Jio has partnered with Taiwanese semiconductor giant MediaTek to launch its 4G smart dashboards for electric two-wheelers.

Hacker claims theft of Piramal Group’s employee data
A hacker claims to be selling data relating to thousands of current and former employees of India’s Piramal Group.

CRED launches personal finance manager for India’s affluent
CRED, an Indian fintech startup, has rolled out a new feature that will help its customers manage and gain deeper insights into their cash flow, as the startup seeks to…

A new Chinese video-generating model appears to be censoring politically sensitive topics
A powerful new video-generating AI model became widely available today — but there’s a catch: The model appears to be censoring topics deemed too politically sensitive by the government in…

Star Catcher wants to build a space power grid to supercharge orbital industry
Our growth as a civilization is tightly coupled to our ability to sufficiently generate ever-increasing amounts of electricity. Could the same be true in space? Star Catcher Industries, a startup…

Mistral’s Large 2 is its answer to Meta and OpenAI’s latest models
For frontier AI models, when it rains, it pours. Mistral released a fresh new flagship model on Wednesday, Large 2, which it claims to be on par with the latest…


COMMENTS
A specific definition of what Big Data means for health research was proposed by the Health Directorate of the Directorate-General for Research and Innovation of the European Commission: Big Data in health encompasses high volume, high diversity biological, clinical, environmental, and lifestyle information collected from single individuals to ...
Medicine is a major field predicted to increase the use of big data in 2025. Big data in medicine may be used by commercial, academic, government, and public sectors. It includes biologic, biometric, and electronic health data. Examples of biologic data include biobanks; biometric data may have individual wellness data from devices; electronic ...
The promise of big data has brought great hope in health care research for drug discovery, treatment innovation, personalized medicine, and optimal patient care that can reduce cost and improve patient outcomes. Billions of dollars have been invested to capture large amounts of data outlined in big initiatives that are often isolated.
Big Data initiatives in the United Kingdom. The UK Biobank is a prospective cohort initiative that is composed of individuals between the ages of 40 and 69 before disease onset (Allen et al. 2012 ...
Big data and health. The digital health revolution is here. Innovations include not only the collection and analysis of electronic health records and personal genomes, but also diverse physiological and molecular measurements in individuals at a level that has not previously been possible. Our recent studies, , in which we deep-profiled 109 ...
In the U.S., the National Institute of Health established the Big Data to Knowledge (BD2K) program designed to bring biomedical big data to researchers, clinicians, and others. Initiatives such as these will increasingly empower healthcare providers to improve patient care while simultaneously countering the unsustainable cost trajectory.
Significant research work has been reported in the domains of healthcare big data analytics. To process this vast amount of information in timely manner and identify someone's health condition ...
Big Data in health care has its own features, such as heterogeneity, incompleteness, timeliness and longevity, privacy, and ownership. These features bring a series of challenges for data storage, mining, and sharing to promote health-related research. To deal with these challenges, analysis approaches focusing on Big Data in health care need ...
A big-data revolution is under way in health care. Start with the vastly increased supply of information. Over the last decade, pharmaceutical companies have been aggregating years of research and development data into medical databases, while payors and providers have digitized their patient records. Meanwhile, the US federal government and ...
Big data-based research in health. Research based on big data can be classified thematically to studies focusing on metabolic diseases, cardiovascular conditions, oncology, mental health, neurological conditions, pulmonology, and public health. A number of these studies focus on specific big data-based methodologies, while other studies ...
Data-driven health solutions. We are Australia's first research centre dedicated to health research using big data. Our research is collaborative, involving codesign and coproduction methods with consumers, communities and health care providers. Together, we aim to facilitate long term translation and implementation into health policy ...
The cost pressure in the US system is not a new phenomenon, since healthcare expenses have been rising rapidly over the last two decades. By 2009, they represented 17.6 percent of GDP—nearly $600 billion more than the expected benchmark for a nation of the United States' size and wealth.
Problems in health data accumulation. Prior research observed several issues related to big data accumulated in healthcare, such as data quality (Sabharwal, Gupta, and Thirunavukkarasu Citation 2016) and data quantity (Gopal et al. Citation 2019). However, there is a lack of research into the types of problems that may occur during data ...
Toward Data Sense-Making in Digital Health Communication Research: Why Theory Matters in the Age of Big Data 27 February 2020 | Frontiers in Communication, Vol. 5 The meaning and enactment of ...
Research projects involving large-scale processing of health data and data linkage (so-called Big Data projects), were already well underway before the Covid-19 pandemic struck in March 2020. 1 But without question, since the pandemic has spread across the globe, projects of this nature have accelerated and received heightened attention for the benefits they can bring to science and medicine ...
The rise of big data in health care research, particularly when incorporated into health care delivery, presents a complex landscape where the role, status and value of the patient or citizen as a ...
Big data research in healthcare. Big Data refers to the mass of structured and unstructured data generated worldwide [].In healthcare, this encompasses everything from electronic medical records, to internet-connected (loT) devices like medical wearables or augmented reality diagnostic tools [16, 17].In general concepts and according to the broader literature, Big Data spans four dimensions ...
Abstract. The big data revolution presents an exciting frontier to expand public health research, broadening the scope of research and increasing the precision of answers. Despite these advances, scientists must be vigilant against also advancing potential harms toward marginalized communities. In this review, we provide examples in which big ...
The emergence of a global industry of digital health platforms operated by Big Tech corporations, and its growing entanglements with academic and pharmaceutical research networks, raise pressing questions on the capacity of current data governance models, regulatory and legal frameworks to safeguard the sustainability of the health research ecosystem.
Key Points. Question What makes data sets for artificial intelligence (AI) ready for health and biomedical machine learning (ML) research purposes?. Findings In this qualitative study consisting of interviews with 20 data set experts who are creators and/or ML researchers, participants largely appraised data set AI readiness with a set of intrinsic and contextual elements, described what they ...
Prognostics and health management (PHM) is critical for enhancing equipment reliability and reducing maintenance costs, and research on intelligent PHM has made significant progress driven by big data and deep learning techniques in recent years. However, complex working conditions and high-cost data collection inherent in real-world scenarios pose small-data challenges for the application of ...
The health care industry produces an astonishing amount of data: nearly one-third of the world's data volume. The amount of data health care providers generate can seem overwhelming—and it can ...
Each was awarded $50,000 in UB's first round of competitive interdisciplinary seed funding for AI research in health care. The funding is being provided through a collaboration between the Office of the Vice President for Research and Economic Development and the Office of the Vice President for Health Sciences.
Future research on the use of Big Data in medical facilities will concern the definition of strategies adopted by medical facilities to promote and implement such solutions, as well as the benefits they gain from the use of Big Data analysis and how the perspectives in this area are seen. ... Agrawal A, Choudhary A. Health services data: big ...
Think big here. The only limit on use cases is an organization's imagination for what can be done with the data. 3 | Expect barriers to adoption. Hospitals and health systems that want to provide actionable, real-time data at the point of care to their clinicians will face barriers to adoption before the project begins and after the go-live ...
If 2023 was the year the world discovered generative AI (gen AI), 2024 is the year organizations truly began using—and deriving business value from—this new technology.In the latest McKinsey Global Survey on AI, 65 percent of respondents report that their organizations are regularly using gen AI, nearly double the percentage from our previous survey just ten months ago.
This requires consulting firms to have "a lot of disciplines under one roof," according to Renato Stabile of Dubin Research & Consulting. Stabile and DRC's founder, Josh Dubin, are lawyers by training. But their firm's staff of approximately 60 also includes experts in psychology, data science, statistics, graphics, and technology.
Six-in-ten U.S. adults say gun violence is a very big problem in the country today, up 9 percentage points from spring 2022. ... Pew Research Center conducted this analysis to summarize key facts about Americans' relationships with guns. We used data from recent Center surveys to provide insights into Americans' views on gun policy and how ...
Big data was leveraged in public health research identifying finer-grain treatment interventions in childhood asthma , childhood obesity , diarrhea , Hepatitis C , HIV , injectable drug use , malaria , opioid medication misuse , use of smokeless tobacco , and the Zika virus .
Change Healthcare hackers stole medical data on "substantial proportion" of people in America. In 2022, the U.S. Justice Department sued health insurance giant UnitedHealth Group to block its ...