Popular searches
- How to Get Participants For Your Study
- How to Do Segmentation?
- Conjoint Preference Share Simulator
- MaxDiff Analysis
- Likert Scales
- Reliability & Validity

Request consultation
Do you need support in running a pricing or product study? We can help you with agile consumer research and conjoint analysis.
Looking for an online survey platform?
Conjointly offers a great survey tool with multiple question types, randomisation blocks, and multilingual support. The Basic tier is always free.
Research Methods Knowledge Base
- Navigating the Knowledge Base
- Foundations
- Construct Validity
- Reliability
- Levels of Measurement
- Survey Research
- Scaling in Measurement
- Qualitative Measures
- Unobtrusive Measures
- Research Design
- Table of Contents
Fully-functional online survey tool with various question types, logic, randomisation, and reporting for unlimited number of surveys.
Completely free for academics and students .
Measurement
Measurement is the process of observing and recording the observations that are collected as part of a research effort. There are two major issues that will be considered here.
First, you have to understand the fundamental ideas involved in measuring. Here we consider two of major measurement concepts. In Levels of Measurement , I explain the meaning of the four major levels of measurement: nominal, ordinal, interval and ratio. Then we move on to the reliability of measurement, including consideration of true score theory and a variety of reliability estimators.
Second, you have to understand the different types of measures that you might use in social research. We consider four broad categories of measurements. Survey research includes the design and implementation of interviews and questionnaires. Scaling involves consideration of the major methods of developing and implementing a scale. Qualitative research provides an overview of the broad range of non-numerical measurement approaches. And unobtrusive measures presents a variety of measurement methods that don’t intrude on or interfere with the context of the research.
Cookie Consent
Conjointly uses essential cookies to make our site work. We also use additional cookies in order to understand the usage of the site, gather audience analytics, and for remarketing purposes.
For more information on Conjointly's use of cookies, please read our Cookie Policy .
Which one are you?
I am new to conjointly, i am already using conjointly.
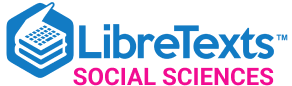
- school Campus Bookshelves
- menu_book Bookshelves
- perm_media Learning Objects
- login Login
- how_to_reg Request Instructor Account
- hub Instructor Commons
Margin Size
- Download Page (PDF)
- Download Full Book (PDF)
- Periodic Table
- Physics Constants
- Scientific Calculator
- Reference & Cite
- Tools expand_more
- Readability
selected template will load here
This action is not available.
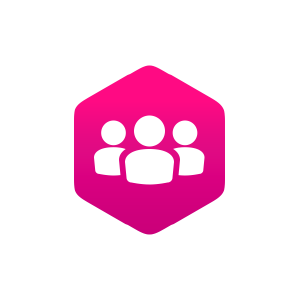
4.1: What is Measurement?
- Last updated
- Save as PDF
- Page ID 124458

\( \newcommand{\vecs}[1]{\overset { \scriptstyle \rightharpoonup} {\mathbf{#1}} } \)
\( \newcommand{\vecd}[1]{\overset{-\!-\!\rightharpoonup}{\vphantom{a}\smash {#1}}} \)
\( \newcommand{\id}{\mathrm{id}}\) \( \newcommand{\Span}{\mathrm{span}}\)
( \newcommand{\kernel}{\mathrm{null}\,}\) \( \newcommand{\range}{\mathrm{range}\,}\)
\( \newcommand{\RealPart}{\mathrm{Re}}\) \( \newcommand{\ImaginaryPart}{\mathrm{Im}}\)
\( \newcommand{\Argument}{\mathrm{Arg}}\) \( \newcommand{\norm}[1]{\| #1 \|}\)
\( \newcommand{\inner}[2]{\langle #1, #2 \rangle}\)
\( \newcommand{\Span}{\mathrm{span}}\)
\( \newcommand{\id}{\mathrm{id}}\)
\( \newcommand{\kernel}{\mathrm{null}\,}\)
\( \newcommand{\range}{\mathrm{range}\,}\)
\( \newcommand{\RealPart}{\mathrm{Re}}\)
\( \newcommand{\ImaginaryPart}{\mathrm{Im}}\)
\( \newcommand{\Argument}{\mathrm{Arg}}\)
\( \newcommand{\norm}[1]{\| #1 \|}\)
\( \newcommand{\Span}{\mathrm{span}}\) \( \newcommand{\AA}{\unicode[.8,0]{x212B}}\)
\( \newcommand{\vectorA}[1]{\vec{#1}} % arrow\)
\( \newcommand{\vectorAt}[1]{\vec{\text{#1}}} % arrow\)
\( \newcommand{\vectorB}[1]{\overset { \scriptstyle \rightharpoonup} {\mathbf{#1}} } \)
\( \newcommand{\vectorC}[1]{\textbf{#1}} \)
\( \newcommand{\vectorD}[1]{\overrightarrow{#1}} \)
\( \newcommand{\vectorDt}[1]{\overrightarrow{\text{#1}}} \)
\( \newcommand{\vectE}[1]{\overset{-\!-\!\rightharpoonup}{\vphantom{a}\smash{\mathbf {#1}}}} \)
Learning Objective
- Define measurement.
Measurement
Measurement is important. Recognizing that fact, and respecting it, will be of great benefit to you—both in research methods and in other areas of life as well. If, for example, you have ever baked a cake, you know well the importance of measurement. As someone who much prefers rebelling against precise rules over following them, I once learned the hard way that measurement matters. A couple of years ago I attempted to bake my husband a birthday cake without the help of any measuring utensils. I’d baked before, I reasoned, and I had a pretty good sense of the difference between a cup and a tablespoon. How hard could it be? As it turns out, it’s not easy guesstimating precise measures. That cake was the lumpiest, most lopsided cake I’ve ever seen. And it tasted kind of like Play-Doh. Figure 4.1 depicts the monstrosity I created, all because I did not respect the value of measurement.
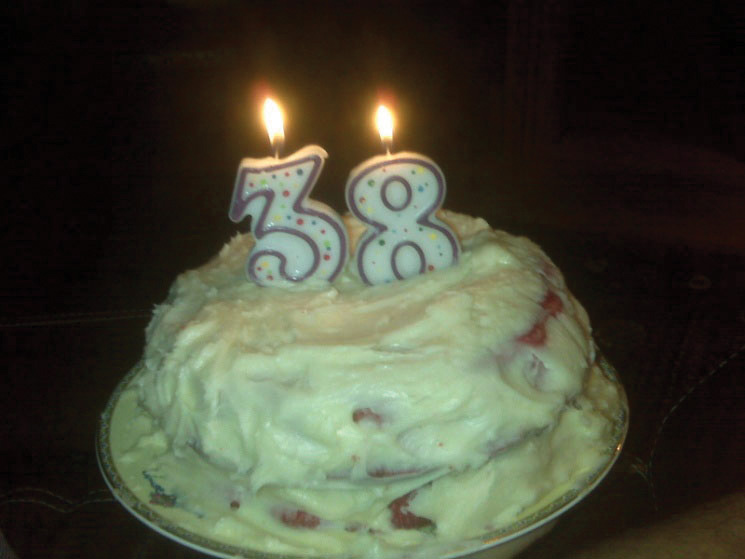
Measurement is important in baking and in research.
Just as measurement is critical to successful baking, it is as important to successfully pulling off a social scientific research project. In sociology, when we use the term measurement we mean the process by which we describe and ascribe meaning to the key facts, concepts, or other phenomena that we are investigating. At its core, measurement is about defining one’s terms in as clear and precise a way as possible. Of course, measurement in social science isn’t quite as simple as using some predetermined or universally agreed-on tool, such as a measuring cup or spoon, but there are some basic tenants on which most social scientists agree when it comes to measurement. We’ll explore those as well as some of the ways that measurement might vary depending on your unique approach to the study of your topic.
What Do Social Scientists Measure?
The question of what social scientists measure can be answered by asking oneself what social scientists study. Think about the topics you’ve learned about in other sociology classes you’ve taken or the topics you’ve considered investigating yourself. Or think about the many examples of research you’ve read about in this text. Classroom learning environments and the mental health of first grade children. Journal of Health and Social Behavior, 52 , 4–22. of first graders’ mental health. In order to conduct that study, Milkie and Warner needed to have some idea about how they were going to measure mental health. What does mental health mean, exactly? And how do we know when we’re observing someone whose mental health is good and when we see someone whose mental health is compromised? Understanding how measurement works in research methods helps us answer these sorts of questions.
As you might have guessed, social scientists will measure just about anything that they have an interest in investigating. For example, those who are interested in learning something about the correlation between social class and levels of happiness must develop some way to measure both social class and happiness. Those who wish to understand how well immigrants cope in their new locations must measure immigrant status and coping. Those who wish to understand how a person’s gender shapes their workplace experiences must measure gender and workplace experiences. You get the idea. Social scientists can and do measure just about anything you can imagine observing or wanting to study.
How Do Social Scientists Measure?
Measurement in social science is a process. It occurs at multiple stages of a research project: in the planning stages, in the data collection stage, and sometimes even in the analysis stage. Recall that previously we defined measurement as the process by which we describe and ascribe meaning to the key facts, concepts, or other phenomena that we are investigating. Once we’ve identified a research question, we begin to think about what some of the key ideas are that we hope to learn from our project. In describing those key ideas, we begin the measurement process.
Let’s say that our research question is the following: How do new college students cope with the adjustment to college? In order to answer this question, we’ll need to some idea about what coping means. We may come up with an idea about what coping means early in the research process, as we begin to think about what to look for (or observe) in our data-collection phase. Once we’ve collected data on coping, we also have to decide how to report on the topic. Perhaps, for example, there are different types or dimensions of coping, some of which lead to more successful adjustment than others. However we decide to proceed, and whatever we decide to report, the point is that measurement is important at each of these phases.
As the preceding paragraph demonstrates, measurement is a process in part because it occurs at multiple stages of conducting research. We could also think of measurement as a process because of the fact that measurement in itself involves multiple stages. From identifying one’s key terms to defining them to figuring out how to observe them and how to know if our observations are any good, there are multiple steps involved in the measurement process. An additional step in the measurement process involves deciding what elements one’s measures contain. A measure’s elements might be very straightforward and clear, particularly if they are directly observable. Other measures are more complex and might require the researcher to account for different themes or types. These sorts of complexities require paying careful attention to a concept’s level of measurement and its dimensions.
KEY TAKEAWAYS
- Measurement is the process by which we describe and ascribe meaning to the key facts, concepts, or other phenomena that we are investigating.
- Measurement occurs at all stages of research.

Want to create or adapt books like this? Learn more about how Pressbooks supports open publishing practices.
5.1 Understanding Psychological Measurement
Learning objectives.
- Define measurement and give several examples of measurement in psychology.
- Explain what a psychological construct is and give several examples.
- Distinguish conceptual from operational definitions, give examples of each, and create simple operational definitions.
- Distinguish the four levels of measurement, give examples of each, and explain why this distinction is important.
What Is Measurement?
Measurement is the assignment of scores to individuals so that the scores represent some characteristic of the individuals. This very general definition is consistent with the kinds of measurement that everyone is familiar with—for example, weighing oneself by stepping onto a bathroom scale, or checking the internal temperature of a roasting turkey by inserting a meat thermometer. It is also consistent with measurement throughout the sciences. In physics, for example, one might measure the potential energy of an object in Earth’s gravitational field by finding its mass and height (which of course requires measuring those variables) and then multiplying them together along with the gravitational acceleration of Earth (9.8 m/s 2 ). The result of this procedure is a score that represents the object’s potential energy.
Of course this general definition of measurement is consistent with measurement in psychology too. (Psychological measurement is often referred to as psychometrics .) Imagine, for example, that a cognitive psychologist wants to measure a person’s working memory capacity—his or her ability to hold in mind and think about several pieces of information all at the same time. To do this, she might use a backward digit span task, where she reads a list of two digits to the person and asks him or her to repeat them in reverse order. She then repeats this several times, increasing the length of the list by one digit each time, until the person makes an error. The length of the longest list for which the person responds correctly is the score and represents his or her working memory capacity. Or imagine a clinical psychologist who is interested in how depressed a person is. He administers the Beck Depression Inventory, which is a 21-item self-report questionnaire in which the person rates the extent to which he or she has felt sad, lost energy, and experienced other symptoms of depression over the past 2 weeks. The sum of these 21 ratings is the score and represents his or her current level of depression.
The important point here is that measurement does not require any particular instruments or procedures. It does not require placing individuals or objects on bathroom scales, holding rulers up to them, or inserting thermometers into them. What it does require is some systematic procedure for assigning scores to individuals or objects so that those scores represent the characteristic of interest.
Psychological Constructs
Many variables studied by psychologists are straightforward and simple to measure. These include sex, age, height, weight, and birth order. You can almost always tell whether someone is male or female just by looking. You can ask people how old they are and be reasonably sure that they know and will tell you. Although people might not know or want to tell you how much they weigh, you can have them step onto a bathroom scale. Other variables studied by psychologists—perhaps the majority—are not so straightforward or simple to measure. We cannot accurately assess people’s level of intelligence by looking at them, and we certainly cannot put their self-esteem on a bathroom scale. These kinds of variables are called constructs (pronounced CON-structs ) and include personality traits (e.g., extroversion), emotional states (e.g., fear), attitudes (e.g., toward taxes), and abilities (e.g., athleticism).
Psychological constructs cannot be observed directly. One reason is that they often represent tendencies to think, feel, or act in certain ways. For example, to say that a particular college student is highly extroverted (see Note 5.6 “The Big Five” ) does not necessarily mean that she is behaving in an extroverted way right now. In fact, she might be sitting quietly by herself, reading a book. Instead, it means that she has a general tendency to behave in extroverted ways (talking, laughing, etc.) across a variety of situations. Another reason psychological constructs cannot be observed directly is that they often involve internal processes. Fear, for example, involves the activation of certain central and peripheral nervous system structures, along with certain kinds of thoughts, feelings, and behaviors—none of which is necessarily obvious to an outside observer. Notice also that neither extroversion nor fear “reduces to” any particular thought, feeling, act, or physiological structure or process. Instead, each is a kind of summary of a complex set of behaviors and internal processes.
The Big Five
The Big Five is a set of five broad dimensions that capture much of the variation in human personality. Each of the Big Five can even be defined in terms of six more specific constructs called “facets” (Costa & McCrae, 1992).
The conceptual definition of a psychological construct describes the behaviors and internal processes that make up that construct, along with how it relates to other variables. For example, a conceptual definition of neuroticism (another one of the Big Five) would be that it is people’s tendency to experience negative emotions such as anxiety, anger, and sadness across a variety of situations. This definition might also include that it has a strong genetic component, remains fairly stable over time, and is positively correlated with the tendency to experience pain and other physical symptoms.
Students sometimes wonder why, when researchers want to understand a construct like self-esteem or neuroticism, they do not simply look it up in the dictionary. One reason is that many scientific constructs do not have counterparts in everyday language (e.g., working memory capacity). More important, researchers are in the business of developing definitions that are more detailed and precise—and that more accurately describe the way the world is—than the informal definitions in the dictionary. As we will see, they do this by proposing conceptual definitions, testing them empirically, and revising them as necessary. Sometimes they throw them out altogether. This is why the research literature often includes different conceptual definitions of the same construct. In some cases, an older conceptual definition has been replaced by a newer one that works better. In others, researchers are still in the process of deciding which of various conceptual definitions is the best.
Operational Definitions
An operational definition is a definition of a variable in terms of precisely how it is to be measured. These measures generally fall into one of three broad categories. Self-report measures are those in which participants report on their own thoughts, feelings, and actions, as with the Rosenberg Self-Esteem Scale. Behavioral measures are those in which some other aspect of participants’ behavior is observed and recorded. This is an extremely broad category that includes the observation of people’s behavior both in highly structured laboratory tasks and in more natural settings. A good example of the former would be measuring working memory capacity using the backward digit span task. A good example of the latter is a famous operational definition of physical aggression from researcher Albert Bandura and his colleagues (Bandura, Ross, & Ross, 1961). They let each of several children play for 20 minutes in a room that contained a clown-shaped punching bag called a Bobo doll. They filmed each child and counted the number of acts of physical aggression he or she committed. These included hitting the doll with a mallet, punching it, and kicking it. Their operational definition, then, was the number of these specifically defined acts that the child committed in the 20-minute period. Finally, physiological measures are those that involve recording any of a wide variety of physiological processes, including heart rate and blood pressure, galvanic skin response, hormone levels, and electrical activity and blood flow in the brain.

In addition to self-report and behavioral measures, researchers in psychology use physiological measures. An electroencephalograph (EEG) records electrical activity from the brain.
Wikimedia Commons – public domain.
For any given variable or construct, there will be multiple operational definitions. Stress is a good example. A rough conceptual definition is that stress is an adaptive response to a perceived danger or threat that involves physiological, cognitive, affective, and behavioral components. But researchers have operationally defined it in several ways. The Social Readjustment Rating Scale is a self-report questionnaire on which people identify stressful events that they have experienced in the past year and assigns points for each one depending on its severity. For example, a man who has been divorced (73 points), changed jobs (36 points), and had a change in sleeping habits (16 points) in the past year would have a total score of 125. The Daily Hassles and Uplifts Scale is similar but focuses on everyday stressors like misplacing things and being concerned about one’s weight. The Perceived Stress Scale is another self-report measure that focuses on people’s feelings of stress (e.g., “How often have you felt nervous and stressed?”). Researchers have also operationally defined stress in terms of several physiological variables including blood pressure and levels of the stress hormone cortisol.
When psychologists use multiple operational definitions of the same construct—either within a study or across studies—they are using converging operations . The idea is that the various operational definitions are “converging” on the same construct. When scores based on several different operational definitions are closely related to each other and produce similar patterns of results, this constitutes good evidence that the construct is being measured effectively and that it is useful. The various measures of stress, for example, are all correlated with each other and have all been shown to be correlated with other variables such as immune system functioning (also measured in a variety of ways) (Segerstrom & Miller, 2004). This is what allows researchers eventually to draw useful general conclusions, such as “stress is negatively correlated with immune system functioning,” as opposed to more specific and less useful ones, such as “people’s scores on the Perceived Stress Scale are negatively correlated with their white blood counts.”
Levels of Measurement
The psychologist S. S. Stevens suggested that scores can be assigned to individuals so that they communicate more or less quantitative information about the variable of interest (Stevens, 1946). For example, the officials at a 100-m race could simply rank order the runners as they crossed the finish line (first, second, etc.), or they could time each runner to the nearest tenth of a second using a stopwatch (11.5 s, 12.1 s, etc.). In either case, they would be measuring the runners’ times by systematically assigning scores to represent those times. But while the rank ordering procedure communicates the fact that the second-place runner took longer to finish than the first-place finisher, the stopwatch procedure also communicates how much longer the second-place finisher took. Stevens actually suggested four different levels of measurement (which he called “scales of measurement”) that correspond to four different levels of quantitative information that can be communicated by a set of scores.
The nominal level of measurement is used for categorical variables and involves assigning scores that are category labels. Category labels communicate whether any two individuals are the same or different in terms of the variable being measured. For example, if you look at your research participants as they enter the room, decide whether each one is male or female, and type this information into a spreadsheet, you are engaged in nominal-level measurement. Or if you ask your participants to indicate which of several ethnicities they identify themselves with, you are again engaged in nominal-level measurement.
The remaining three levels of measurement are used for quantitative variables. The ordinal level of measurement involves assigning scores so that they represent the rank order of the individuals. Ranks communicate not only whether any two individuals are the same or different in terms of the variable being measured but also whether one individual is higher or lower on that variable. The interval level of measurement involves assigning scores so that they represent the precise magnitude of the difference between individuals, but a score of zero does not actually represent the complete absence of the characteristic. A classic example is the measurement of heat using the Celsius or Fahrenheit scale. The difference between temperatures of 20°C and 25°C is precisely 5°, but a temperature of 0°C does not mean that there is a complete absence of heat. In psychology, the intelligence quotient (IQ) is often considered to be measured at the interval level. Finally, the ratio level of measurement involves assigning scores in such a way that there is a true zero point that represents the complete absence of the quantity. Height measured in meters and weight measured in kilograms are good examples. So are counts of discrete objects or events such as the number of siblings one has or the number of questions a student answers correctly on an exam.
Stevens’s levels of measurement are important for at least two reasons. First, they emphasize the generality of the concept of measurement. Although people do not normally think of categorizing or ranking individuals as measurement, in fact they are as long as they are done so that they represent some characteristic of the individuals. Second, the levels of measurement can serve as a rough guide to the statistical procedures that can be used with the data and the conclusions that can be drawn from them. With nominal-level measurement, for example, the only available measure of central tendency is the mode. Also, ratio-level measurement is the only level that allows meaningful statements about ratios of scores. One cannot say that someone with an IQ of 140 is twice as intelligent as someone with an IQ of 70 because IQ is measured at the interval level, but one can say that someone with six siblings has twice as many as someone with three because number of siblings is measured at the ratio level.
Key Takeaways
- Measurement is the assignment of scores to individuals so that the scores represent some characteristic of the individuals. Psychological measurement can be achieved in a wide variety of ways, including self-report, behavioral, and physiological measures.
- Psychological constructs such as intelligence, self-esteem, and depression are variables that are not directly observable because they represent behavioral tendencies or complex patterns of behavior and internal processes. An important goal of scientific research is to conceptually define psychological constructs in ways that accurately describe them.
- For any conceptual definition of a construct, there will be many different operational definitions or ways of measuring it. The use of multiple operational definitions, or converging operations, is a common strategy in psychological research.
- Variables can be measured at four different levels—nominal, ordinal, interval, and ratio—that communicate increasing amounts of quantitative information. The level of measurement affects the kinds of statistics you can use and conclusions you can draw from your data.
- Practice: Complete the Rosenberg Self-Esteem Scale and compute your overall score.
- Practice: Think of three operational definitions for sexual jealousy, decisiveness, and social anxiety. Consider the possibility of self-report, behavioral, and physiological measures. Be as precise as you can.
Practice: For each of the following variables, decide which level of measurement is being used.
- A college instructor measures the time it takes his students to finish an exam by looking through the stack of exams at the end. He assigns the one on the bottom a score of 1, the one on top of that a 2, and so on.
- A researcher accesses her participants’ medical records and counts the number of times they have seen a doctor in the past year.
- Participants in a research study are asked whether they are right-handed or left-handed.
Bandura, A., Ross, D., & Ross, S. A. (1961). Transmission of aggression through imitation of aggressive models. Journal of Abnormal and Social Psychology, 63 , 575–582.
Costa, P. T., Jr., & McCrae, R. R. (1992). Normal personality assessment in clinical practice: The NEO Personality Inventory. Psychological Assessment, 4 , 5–13.
Segerstrom, S. E., & Miller, G. E. (2004). Psychological stress and the human immune system: A meta-analytic study of 30 years of inquiry. Psychological Bulletin, 130 , 601–630.
Research Methods in Psychology Copyright © 2016 by University of Minnesota is licensed under a Creative Commons Attribution-NonCommercial-ShareAlike 4.0 International License , except where otherwise noted.
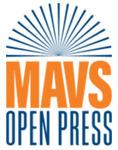
Want to create or adapt books like this? Learn more about how Pressbooks supports open publishing practices.
10.1 What is measurement?
Learning objectives.
Learners will be able to…
- Define measurement
- Explain where measurement fits into the process of designing research
- Apply Kaplan’s three categories to determine the complexity of measuring a given variable
Pre-awareness check (Knowledge)
What do you already know about measuring key variables in your research topic?
In social science, when we use the term measurement , we mean the process by which we describe and ascribe meaning to the key facts, concepts, or other phenomena that we are investigating. In this chapter, we’ll use the term “concept” to mean an abstraction that has meaning. Concepts can be understood from our own experiences or from particular facts, but they don’t have to be limited to real-life phenomenon. We can have a concept of anything we can imagine or experience such as weightlessness, friendship, or income. Understanding exactly what our concepts mean is necessary in order to measure them.
In research, measurement is a systematic procedure for assigning scores, meanings, and descriptions to concepts so that those scores represent the characteristic of interest. Social scientists can and do measure just about anything you can imagine observing or wanting to study. Of course, some things are easier to observe or measure than others.
Where does measurement fit in the process of designing research?
Table 10.1 is intended as a partial review and outlines the general process researchers can follow to get from problem formulation to data collection, including measurement. Use the drop down feature in the table to view the examples for each component of the research process. Keep in mind that this process is iterative. For example, you may find something in your literature review that leads you to refine your conceptualizations, or you may discover as you attempt to conceptually define your terms that you need to return back to the literature for further information. Accordingly, this table should be seen as a suggested path to take rather than an inflexible rule about how research must be conducted.
Table 10.1. Components of the Research Process from Problem Formulation to Data Collection. Note. Information on attachment theory in this table came from: Bowlby, J. (1978). Attachment theory and its therapeutic implications. Adolescent Psychiatry, 6 , 5-33
Categories of concepts that social scientists measure
In 1964, philosopher Abraham Kaplan (1964) [1] wrote The Conduct of Inquiry , which has been cited over 8,500 times. [2] In his text, Kaplan describes different categories of things that behavioral scientists observe. One of those categories, which Kaplan called “observational terms,” is probably the simplest to measure in social science. Observational terms are simple concepts. They are the sorts of things that we can see with the naked eye simply by looking at them. Kaplan roughly defines them as concepts that are easy to identify and verify through direct observation. If, for example, we wanted to know how the conditions of playgrounds differ across different neighborhoods, we could directly observe the variety, amount, and condition of equipment at various playgrounds.
Indirect observables , on the other hand, are less straightforward concepts to assess. In Kaplan’s framework, they are conditions that are subtle and complex that we must use existing knowledge and intuition to define. If we conducted a study for which we wished to know a person’s income, we’d probably have to ask them their income, perhaps in an interview or a survey. Thus, we have observed income, even if it has only been observed indirectly. Birthplace might be another indirect observable. We can ask study participants where they were born, but chances are good we won’t have directly observed any of those people being born in the locations they report.
Sometimes the concepts that we are interested in are more complex and more abstract than observational terms or indirect observables. Because they are complex, constructs generally consist of more than one concept. Let’s take for example, the construct “bureaucracy.” We know this term has something to do with hierarchy, organizations, and how they operate but measuring such a construct is trickier than measuring something like a person’s income because of the complexity involved. Here’s another construct: racism. What is racism? How would you measure it? The constructs of racism and bureaucracy represent constructs whose meanings we have come to agree on.
Though we may not be able to observe constructs directly, we can observe their components. In Kaplan’s categorization, constructs are concepts that are “not observational either directly or indirectly” (Kaplan, 1964, p. 55), [3] but they can be defined based on observables. An example would be measuring the construct of depression. A diagnosis of depression can be made through the DSM-V which includes diagnostic criteria of fatigue, poor concentration, etc. Each of these components of depression can be observed indirectly. We are able to measure constructs by defining them in terms of what we can observe. Though we may not be able to observe them, we can observe their components.
TRACK 1 (IF YOU ARE CREATING A RESEARCH PROPOSAL FOR THIS CLASS):
Look at the variables in your research question.
- Classify them as direct observables, indirect observables, or constructs.
- Do you think measuring them will be easy or hard?
- What are your first thoughts about how to measure each variable? No wrong answers here, just write down a thought about each variable.
TRACK 2 (IF YOU AREN’T CREATING A RESEARCH PROPOSAL FOR THIS CLASS):
You are interested in studying older adults’ social-emotional well-being. Specifically, you would like to research the impact on levels of older adult loneliness of an intervention that pairs older adults living in assisted living communities with university student volunteers for a weekly conversation.
Develop a working research question for this topic. Then, look at the variables in your research question.
- Kaplan, A. (1964). The conduct of inquiry: Methodology for behavioral science. San Francisco, CA: Chandler Publishing Company. ↵
- Earl Babbie offers a more detailed discussion of Kaplan’s work in his text. You can read it in: Babbie, E. (2010). The practice of social research (12th ed.). Belmont, CA: Wadsworth. ↵
- Kaplan, A. (1964). The conduct of inquiry: Methodology for behavioral science . San Francisco, CA: Chandler Publishing Company. ↵
The process by which we describe and ascribe meaning to the key facts, concepts, or other phenomena under investigation in a research study.
In measurement, conditions that are easy to identify and verify through direct observation.
things that require subtle and complex observations to measure, perhaps we must use existing knowledge and intuition to define.
Conditions that are not directly observable and represent states of being, experiences, and ideas.
Doctoral Research Methods in Social Work Copyright © by Mavs Open Press. All Rights Reserved.
Share This Book

- Table of Contents
- Random Entry
- Chronological
- Editorial Information
- About the SEP
- Editorial Board
- How to Cite the SEP
- Special Characters
- Advanced Tools
- Support the SEP
- PDFs for SEP Friends
- Make a Donation
- SEPIA for Libraries
- Entry Contents
Bibliography
Academic tools.
- Friends PDF Preview
- Author and Citation Info
- Back to Top
Measurement in Science
Measurement is an integral part of modern science as well as of engineering, commerce, and daily life. Measurement is often considered a hallmark of the scientific enterprise and a privileged source of knowledge relative to qualitative modes of inquiry. [ 1 ] Despite its ubiquity and importance, there is little consensus among philosophers as to how to define measurement, what sorts of things are measurable, or which conditions make measurement possible. Most (but not all) contemporary authors agree that measurement is an activity that involves interaction with a concrete system with the aim of representing aspects of that system in abstract terms (e.g., in terms of classes, numbers, vectors etc.) But this characterization also fits various kinds of perceptual and linguistic activities that are not usually considered measurements, and is therefore too broad to count as a definition of measurement. Moreover, if “concrete” implies “real”, this characterization is also too narrow, as measurement often involves the representation of ideal systems such as the average household or an electron at complete rest.
Philosophers have written on a variety of conceptual, metaphysical, semantic and epistemological issues related to measurement. This entry will survey the central philosophical standpoints on the nature of measurement, the notion of measurable quantity and related epistemological issues. It will refrain from elaborating on the many discipline-specific problems associated with measurement and focus on issues that have a general character.
1. Overview
2. quantity and magnitude: a brief history, 3.1 fundamental and derived measurement, 3.2 the classification of scales, 3.3 the measurability of sensation, 3.4 representational theory of measurement, 4. operationalism and conventionalism, 5. realist accounts of measurement, 6. information-theoretic accounts of measurement, 7.1 the roles of models in measurement, 7.2 models and measurement in economics, 7.3 psychometric models and construct validity, 8.1 standardization and scientific progress, 8.2 theory-ladenness of measurement, 8.3 accuracy and precision, other internet resources, related entries.
Modern philosophical discussions about measurement—spanning from the late nineteenth century to the present day—may be divided into several strands of scholarship. These strands reflect different perspectives on the nature of measurement and the conditions that make measurement possible and reliable. The main strands are mathematical theories of measurement, operationalism, conventionalism, realism, information-theoretic accounts and model-based accounts. These strands of scholarship do not, for the most part, constitute directly competing views. Instead, they are best understood as highlighting different and complementary aspects of measurement. The following is a very rough overview of these perspectives:
- Mathematical theories of measurement view measurement as the mapping of qualitative empirical relations to relations among numbers (or other mathematical entities).
- Operationalists and conventionalists view measurement as a set of operations that shape the meaning and/or regulate the use of a quantity-term.
- Realists view measurement as the estimation of mind-independent properties and/or relations.
- Information-theoretic accounts view measurement as the gathering and interpretation of information about a system.
- Model-based accounts view measurement as the coherent assignment of values to parameters in a theoretical and/or statistical model of a process.
These perspectives are in principle consistent with each other. While mathematical theories of measurement deal with the mathematical foundations of measurement scales, operationalism and conventionalism are primarily concerned with the semantics of quantity terms, realism is concerned with the metaphysical status of measurable quantities, and information-theoretic and model-based accounts are concerned with the epistemological aspects of measuring. Nonetheless, the subject domain is not as neatly divided as the list above suggests. Issues concerning the metaphysics, epistemology, semantics and mathematical foundations of measurement are interconnected and often bear on one another. Hence, for example, operationalists and conventionalists have often adopted anti-realist views, and proponents of model-based accounts have argued against the prevailing empiricist interpretation of mathematical theories of measurement. These subtleties will become clear in the following discussion.
The list of strands of scholarship is neither exclusive nor exhaustive. It reflects the historical trajectory of the philosophical discussion thus far, rather than any principled distinction among different levels of analysis of measurement. Some philosophical works on measurement belong to more than one strand, while many other works do not squarely fit either. This is especially the case since the early 2000s, when measurement returned to the forefront of philosophical discussion after several decades of relative neglect. This recent body of scholarship is sometimes called “the epistemology of measurement”, and includes a rich array of works that cannot yet be classified into distinct schools of thought. The last section of this entry will be dedicated to surveying some of these developments.
Although the philosophy of measurement formed as a distinct area of inquiry only during the second half of the nineteenth century, fundamental concepts of measurement such as magnitude and quantity have been discussed since antiquity. According to Euclid’s Elements , a magnitude—such as a line, a surface or a solid—measures another when the latter is a whole multiple of the former (Book V, def. 1 & 2). Two magnitudes have a common measure when they are both whole multiples of some magnitude, and are incommensurable otherwise (Book X, def. 1). The discovery of incommensurable magnitudes allowed Euclid and his contemporaries to develop the notion of a ratio of magnitudes. Ratios can be either rational or irrational, and therefore the concept of ratio is more general than that of measure (Michell 2003, 2004a; Grattan-Guinness 1996).
Aristotle distinguished between quantities and qualities. Examples of quantities are numbers, lines, surfaces, bodies, time and place, whereas examples of qualities are justice, health, hotness and paleness ( Categories §6 and §8). According to Aristotle, quantities admit of equality and inequality but not of degrees, as “one thing is not more four-foot than another” (ibid. 6.6a19). Qualities, conversely, do not admit of equality or inequality but do admit of degrees, “for one thing is called more pale or less pale than another” (ibid. 8.10b26). Aristotle did not clearly specify whether degrees of qualities such as paleness correspond to distinct qualities, or whether the same quality, paleness, was capable of different intensities. This topic was at the center of an ongoing debate in the thirteenth and fourteenth centuries (Jung 2011). Duns Scotus supported the “addition theory”, according to which a change in the degree of a quality can be explained by the addition or subtraction of smaller degrees of that quality (2011: 553). This theory was later refined by Nicole Oresme, who used geometrical figures to represent changes in the intensity of qualities such as velocity (Clagett 1968; Sylla 1971). Oresme’s geometrical representations established a subset of qualities that were amenable to quantitative treatment, thereby challenging the strict Aristotelian dichotomy between quantities and qualities. These developments made possible the formulation of quantitative laws of motion during the sixteenth and seventeenth centuries (Grant 1996).
The concept of qualitative intensity was further developed by Leibniz and Kant. Leibniz’s “principle of continuity” stated that all natural change is produced by degrees. Leibniz argued that this principle applies not only to changes in extended magnitudes such as length and duration, but also to intensities of representational states of consciousness, such as sounds (Jorgensen 2009; Diehl 2012). Kant is thought to have relied on Leibniz’s principle of continuity to formulate his distinction between extensive and intensive magnitudes. According to Kant, extensive magnitudes are those “in which the representation of the parts makes possible the representation of the whole” (1787: A162/B203). An example is length: a line can only be mentally represented by a successive synthesis in which parts of the line join to form the whole. For Kant, the possibility of such synthesis was grounded in the forms of intuition, namely space and time. Intensive magnitudes, like warmth or colors, also come in continuous degrees, but their apprehension takes place in an instant rather than through a successive synthesis of parts. The degrees of intensive magnitudes “can only be represented through approximation to negation” (1787: A 168/B210), that is, by imagining their gradual diminution until their complete absence.
Scientific developments during the nineteenth century challenged the distinction between extensive and intensive magnitudes. Thermodynamics and wave optics showed that differences in temperature and hue corresponded to differences in spatio-temporal magnitudes such as velocity and wavelength. Electrical magnitudes such as resistance and conductance were shown to be capable of addition and division despite not being extensive in the Kantian sense, i.e., not synthesized from spatial or temporal parts. Moreover, early experiments in psychophysics suggested that intensities of sensation such as brightness and loudness could be represented as sums of “just noticeable differences” among stimuli, and could therefore be thought of as composed of parts (see Section 3.3 ). These findings, along with advances in the axiomatization of branches of mathematics, motivated some of the leading scientists of the late nineteenth century to attempt to clarify the mathematical foundations of measurement (Maxwell 1873; von Kries 1882; Helmholtz 1887; Mach 1896; Poincaré 1898; Hölder 1901; for historical surveys see Darrigol 2003; Michell 1993, 2003; Cantù and Schlaudt 2013; Biagioli 2016: Ch. 4, 2018). These works are viewed today as precursors to the body of scholarship known as “measurement theory”.
3. Mathematical Theories of Measurement (“Measurement Theory”)
Mathematical theories of measurement (often referred to collectively as “measurement theory”) concern the conditions under which relations among numbers (and other mathematical entities) can be used to express relations among objects. [ 2 ] In order to appreciate the need for mathematical theories of measurement, consider the fact that relations exhibited by numbers—such as equality, sum, difference and ratio—do not always correspond to relations among the objects measured by those numbers. For example, 60 is twice 30, but one would be mistaken in thinking that an object measured at 60 degrees Celsius is twice as hot as an object at 30 degrees Celsius. This is because the zero point of the Celsius scale is arbitrary and does not correspond to an absence of temperature. [ 3 ] Similarly, numerical intervals do not always carry empirical information. When subjects are asked to rank on a scale from 1 to 7 how strongly they agree with a given statement, there is no prima facie reason to think that the intervals between 5 and 6 and between 6 and 7 correspond to equal increments of strength of opinion. To provide a third example, equality among numbers is transitive [if (a=b & b=c) then a=c] but empirical comparisons among physical magnitudes reveal only approximate equality, which is not a transitive relation. These examples suggest that not all of the mathematical relations among numbers used in measurement are empirically significant, and that different kinds of measurement scale convey different kinds of empirically significant information.
The study of measurement scales and the empirical information they convey is the main concern of mathematical theories of measurement. In his seminal 1887 essay, “Counting and Measuring”, Hermann von Helmholtz phrased the key question of measurement theory as follows:
[W]hat is the objective meaning of expressing through denominate numbers the relations of real objects as magnitudes, and under what conditions can we do this? (1887: 4)
Broadly speaking, measurement theory sets out to (i) identify the assumptions underlying the use of various mathematical structures for describing aspects of the empirical world, and (ii) draw lessons about the adequacy and limits of using these mathematical structures for describing aspects of the empirical world. Following Otto Hölder (1901), measurement theorists often tackle these goals through formal proofs, with the assumptions in (i) serving as axioms and the lessons in (ii) following as theorems. A key insight of measurement theory is that the empirically significant aspects of a given mathematical structure are those that mirror relevant relations among the objects being measured. For example, the relation “bigger than” among numbers is empirically significant for measuring length insofar as it mirrors the relation “longer than” among objects. This mirroring, or mapping, of relations between objects and mathematical entities constitutes a measurement scale. As will be clarified below, measurement scales are usually thought of as isomorphisms or homomorphisms between objects and mathematical entities.
Other than these broad goals and claims, measurement theory is a highly heterogeneous body of scholarship. It includes works that span from the late nineteenth century to the present day and endorse a wide array of views on the ontology, epistemology and semantics of measurement. Two main differences among mathematical theories of measurement are especially worth mentioning. The first concerns the nature of the relata , or “objects”, whose relations numbers are supposed to mirror. These relata may be understood in at least four different ways: as concrete individual objects, as qualitative observations of concrete individual objects, as abstract representations of individual objects, or as universal properties of objects. Which interpretation is adopted depends in large part on the author’s metaphysical and epistemic commitments. This issue will be especially relevant to the discussion of realist accounts of measurement ( Section 5 ). Second, different measurement theorists have taken different stands on the kind of empirical evidence that is required to establish mappings between objects and numbers. As a result, measurement theorists have come to disagree about the necessary conditions for establishing the measurability of attributes, and specifically about whether psychological attributes are measurable. Debates about measurability have been highly fruitful for the development of measurement theory, and the following subsections will introduce some of these debates and the central concepts developed therein.
During the late nineteenth and early twentieth centuries several attempts were made to provide a universal definition of measurement. Although accounts of measurement varied, the consensus was that measurement is a method of assigning numbers to magnitudes . For example, Helmholtz (1887: 17) defined measurement as the procedure by which one finds the denominate number that expresses the value of a magnitude, where a “denominate number” is a number together with a unit, e.g., 5 meters, and a magnitude is a quality of objects that is amenable to ordering from smaller to greater, e.g., length. Bertrand Russell similarly stated that measurement is
any method by which a unique and reciprocal correspondence is established between all or some of the magnitudes of a kind and all or some of the numbers, integral, rational or real. (1903: 176)
Norman Campbell defined measurement simply as “the process of assigning numbers to represent qualities”, where a quality is a property that admits of non-arbitrary ordering (1920: 267).
Defining measurement as numerical assignment raises the question: which assignments are adequate, and under what conditions? Early measurement theorists like Helmholtz (1887), Hölder (1901) and Campbell (1920) argued that numbers are adequate for expressing magnitudes insofar as algebraic operations among numbers mirror empirical relations among magnitudes. For example, the qualitative relation “longer than” among rigid rods is (roughly) transitive and asymmetrical, and in this regard shares structural features with the relation “larger than” among numbers. Moreover, the end-to-end concatenation of rigid rods shares structural features—such as associativity and commutativity—with the mathematical operation of addition. A similar situation holds for the measurement of weight with an equal-arms balance. Here deflection of the arms provides ordering among weights and the heaping of weights on one pan constitutes concatenation.
Early measurement theorists formulated axioms that describe these qualitative empirical structures, and used these axioms to prove theorems about the adequacy of assigning numbers to magnitudes that exhibit such structures. Specifically, they proved that ordering and concatenation are together sufficient for the construction of an additive numerical representation of the relevant magnitudes. An additive representation is one in which addition is empirically meaningful, and hence also multiplication, division etc. Campbell called measurement procedures that satisfy the conditions of additivity “fundamental” because they do not involve the measurement of any other magnitude (1920: 277). Kinds of magnitudes for which a fundamental measurement procedure has been found—such as length, area, volume, duration, weight and electrical resistance—Campbell called “fundamental magnitudes”. A hallmark of such magnitudes is that it is possible to generate them by concatenating a standard sequence of equal units, as in the example of a series of equally spaced marks on a ruler.
Although they viewed additivity as the hallmark of measurement, most early measurement theorists acknowledged that additivity is not necessary for measuring. Other magnitudes exist that admit of ordering from smaller to greater, but whose ratios and/or differences cannot currently be determined except through their relations to other, fundamentally measurable magnitudes. Examples are temperature, which may be measured by determining the volume of a mercury column, and density, which may be measured as the ratio of mass and volume. Such indirect determination came to be called “derived” measurement and the relevant magnitudes “derived magnitudes” (Campbell 1920: 275–7).
At first glance, the distinction between fundamental and derived measurement may seem reminiscent of the distinction between extensive and intensive magnitudes, and indeed fundamental measurement is sometimes called “extensive”. Nonetheless, it is important to note that the two distinctions are based on significantly different criteria of measurability. As discussed in Section 2 , the extensive-intensive distinction focused on the intrinsic structure of the quantity in question, i.e., whether or not it is composed of spatio-temporal parts. The fundamental-derived distinction, by contrast, focuses on the properties of measurement operations . A fundamentally measurable magnitude is one for which a fundamental measurement operation has been found. Consequently, fundamentality is not an intrinsic property of a magnitude: a derived magnitude can become fundamental with the discovery of new operations for its measurement. Moreover, in fundamental measurement the numerical assignment need not mirror the structure of spatio-temporal parts. Electrical resistance, for example, can be fundamentally measured by connecting resistors in a series (Campbell 1920: 293). This is considered a fundamental measurement operation because it has a shared structure with numerical addition, even though objects with equal resistance are not generally equal in size.
The distinction between fundamental and derived measurement was revised by subsequent authors. Brian Ellis (1966: Ch. 5–8) distinguished among three types of measurement: fundamental, associative and derived. Fundamental measurement requires ordering and concatenation operations satisfying the same conditions specified by Campbell. Associative measurement procedures are based on a correlation of two ordering relationships, e.g., the correlation between the volume of a mercury column and its temperature. Derived measurement procedures consist in the determination of the value of a constant in a physical law. The constant may be local, as in the determination of the specific density of water from mass and volume, or universal, as in the determination of the Newtonian gravitational constant from force, mass and distance. Henry Kyburg (1984: Ch. 5–7) proposed a somewhat different threefold distinction among direct, indirect and systematic measurement, which does not completely overlap with that of Ellis. [ 4 ] A more radical revision of the distinction between fundamental and derived measurement was offered by R. Duncan Luce and John Tukey (1964) in their work on conjoint measurement, which will be discussed in Section 3.4 .
The previous subsection discussed the axiomatization of empirical structures, a line of inquiry that dates back to the early days of measurement theory. A complementary line of inquiry within measurement theory concerns the classification of measurement scales. The psychophysicist S.S. Stevens (1946, 1951) distinguished among four types of scales: nominal, ordinal, interval and ratio. Nominal scales represent objects as belonging to classes that have no particular order, e.g., male and female. Ordinal scales represent order but no further algebraic structure. For example, the Mohs scale of mineral hardness represents minerals with numbers ranging from 1 (softest) to 10 (hardest), but there is no empirical significance to equality among intervals or ratios of those numbers. [ 5 ] Celsius and Fahrenheit are examples of interval scales: they represent equality or inequality among intervals of temperature, but not ratios of temperature, because their zero points are arbitrary. The Kelvin scale, by contrast, is a ratio scale, as are the familiar scales representing mass in kilograms, length in meters and duration in seconds. Stevens later refined this classification and distinguished between linear and logarithmic interval scales (1959: 31–34) and between ratio scales with and without a natural unit (1959: 34). Ratio scales with a natural unit, such as those used for counting discrete objects and for representing probabilities, were named “absolute” scales.
As Stevens notes, scale types are individuated by the families of transformations they can undergo without loss of empirical information. Empirical relations represented on ratio scales, for example, are invariant under multiplication by a positive number, e.g., multiplication by 2.54 converts from inches to centimeters. Linear interval scales allow both multiplication by a positive number and a constant shift, e.g., the conversion from Celsius to Fahrenheit in accordance with the formula °C × 9/5 + 32 = °F. Ordinal scales admit of any transformation function as long as it is monotonic and increasing, and nominal scales admit of any one-to-one substitution. Absolute scales admit of no transformation other than identity. Stevens’ classification of scales was later generalized by Louis Narens (1981, 1985: Ch. 2) and Luce et al. (1990: Ch. 20) in terms of the homogeneity and uniqueness of the relevant transformation groups.
While Stevens’ classification of scales met with general approval in scientific and philosophical circles, its wider implications for measurement theory became the topic of considerable debate. Two issues were especially contested. The first was whether classification and ordering operations deserve to be called “measurement” operations, and accordingly whether the representation of magnitudes on nominal and ordinal scales should count as measurement. Several physicists, including Campbell, argued that classification and ordering operations did not provide a sufficiently rich structure to warrant the use of numbers, and hence should not count as measurement operations. The second contested issue was whether a concatenation operation had to be found for a magnitude before it could be fundamentally measured on a ratio scale. The debate became especially heated when it re-ignited a longer controversy surrounding the measurability of intensities of sensation. It is to this debate we now turn.
One of the main catalysts for the development of mathematical theories of measurement was an ongoing debate surrounding measurability in psychology. The debate is often traced back to Gustav Fechner’s (1860) Elements of Psychophysics , in which he described a method of measuring intensities of sensation. Fechner’s method was based on the recording of “just noticeable differences” between sensations associated with pairs of stimuli, e.g., two sounds of different intensity. These differences were assumed to be equal increments of intensity of sensation. As Fechner showed, under this assumption a stable linear relationship is revealed between the intensity of sensation and the logarithm of the intensity of the stimulus, a relation that came to be known as “Fechner’s law” (Heidelberger 1993a: 203; Luce and Suppes 2004: 11–2). This law in turn provides a method for indirectly measuring the intensity of sensation by measuring the intensity of the stimulus, and hence, Fechner argued, provides justification for measuring intensities of sensation on the real numbers.
Fechner’s claims concerning the measurability of sensation became the subject of a series of debates that lasted nearly a century and proved extremely fruitful for the philosophy of measurement, involving key figures such as Mach, Helmholtz, Campbell and Stevens (Heidelberger 1993a: Ch. 6 and 1993b; Michell 1999: Ch. 6). Those objecting to the measurability of sensation, such as Campbell, stressed the necessity of an empirical concatenation operation for fundamental measurement. Since intensities of sensation cannot be concatenated to each other in the manner afforded by lengths and weights, there could be no fundamental measurement of sensation intensity. Moreover, Campbell claimed that none of the psychophysical regularities discovered thus far are sufficiently universal to count as laws in the sense required for derived measurement (Campbell in Ferguson et al. 1940: 347). All that psychophysicists have shown is that intensities of sensation can be consistently ordered, but order by itself does not yet warrant the use of numerical relations such as sums and ratios to express empirical results.
The central opponent of Campbell in this debate was Stevens, whose distinction between types of measurement scale was discussed above. Stevens defined measurement as the “assignment of numerals to objects or events according to rules” (1951: 1) and claimed that any consistent and non-random assignment counts as measurement in the broad sense (1975: 47). In useful cases of scientific inquiry, Stevens claimed, measurement can be construed somewhat more narrowly as a numerical assignment that is based on the results of matching operations, such as the coupling of temperature to mercury volume or the matching of sensations to each other. Stevens argued against the view that relations among numbers need to mirror qualitative empirical structures, claiming instead that measurement scales should be regarded as arbitrary formal schemas and adopted in accordance with their usefulness for describing empirical data. For example, adopting a ratio scale for measuring the sensations of loudness, volume and density of sounds leads to the formulation of a simple linear relation among the reports of experimental subjects: loudness = volume × density (1975: 57–8). Such assignment of numbers to sensations counts as measurement because it is consistent and non-random, because it is based on the matching operations performed by experimental subjects, and because it captures regularities in the experimental results. According to Stevens, these conditions are together sufficient to justify the use of a ratio scale for measuring sensations, despite the fact that “sensations cannot be separated into component parts, or laid end to end like measuring sticks” (1975: 38; see also Hempel 1952: 68–9).
In the mid-twentieth century the two main lines of inquiry in measurement theory, the one dedicated to the empirical conditions of quantification and the one concerning the classification of scales, converged in the work of Patrick Suppes (1951; Scott and Suppes 1958; for historical surveys see Savage and Ehrlich 1992; Diez 1997a,b). Suppes’ work laid the basis for the Representational Theory of Measurement (RTM), which remains the most influential mathematical theory of measurement to date (Krantz et al. 1971; Suppes et al. 1989; Luce et al. 1990). RTM defines measurement as the construction of mappings from empirical relational structures into numerical relational structures (Krantz et al. 1971: 9). An empirical relational structure consists of a set of empirical objects (e.g., rigid rods) along with certain qualitative relations among them (e.g., ordering, concatenation), while a numerical relational structure consists of a set of numbers (e.g., real numbers) and specific mathematical relations among them (e.g., “equal to or bigger than”, addition). Simply put, a measurement scale is a many-to-one mapping—a homomorphism—from an empirical to a numerical relational structure, and measurement is the construction of scales. [ 6 ] RTM goes into great detail in clarifying the assumptions underlying the construction of different types of measurement scales. Each type of scale is associated with a set of assumptions about the qualitative relations obtaining among objects represented on that type of scale. From these assumptions, or axioms, the authors of RTM derive the representational adequacy of each scale type, as well as the family of permissible transformations making that type of scale unique. In this way RTM provides a conceptual link between the empirical basis of measurement and the typology of scales. [ 7 ]
On the issue of measurability, the Representational Theory takes a middle path between the liberal approach adopted by Stevens and the strict emphasis on concatenation operations espoused by Campbell. Like Campbell, RTM accepts that rules of quantification must be grounded in known empirical structures and should not be chosen arbitrarily to fit the data. However, RTM rejects the idea that additive scales are adequate only when concatenation operations are available (Luce and Suppes 2004: 15). Instead, RTM argues for the existence of fundamental measurement operations that do not involve concatenation. The central example of this type of operation is known as “additive conjoint measurement” (Luce and Tukey 1964; Krantz et al. 1971: 17–21 and Ch. 6–7). Here, measurements of two or more different types of attribute, such as the temperature and pressure of a gas, are obtained by observing their joint effect, such as the volume of the gas. Luce and Tukey showed that by establishing certain qualitative relations among volumes under variations of temperature and pressure, one can construct additive representations of temperature and pressure, without invoking any antecedent method of measuring volume. This sort of procedure is generalizable to any suitably related triplet of attributes, such as the loudness, intensity and frequency of pure tones, or the preference for a reward, it size and the delay in receiving it (Luce and Suppes 2004: 17). The discovery of additive conjoint measurement led the authors of RTM to divide fundamental measurement into two kinds: traditional measurement procedures based on concatenation operations, which they called “extensive measurement”, and conjoint or “nonextensive” fundamental measurement. Under this new conception of fundamentality, all the traditional physical attributes can be measured fundamentally, as well as many psychological attributes (Krantz et al. 1971: 502–3).
Above we saw that mathematical theories of measurement are primarily concerned with the mathematical properties of measurement scales and the conditions of their application. A related but distinct strand of scholarship concerns the meaning and use of quantity terms. Scientific theories and models are commonly expressed in terms of quantitative relations among parameters, bearing names such as “length”, “unemployment rate” and “introversion”. A realist about one of these terms would argue that it refers to a set of properties or relations that exist independently of being measured. An operationalist or conventionalist would argue that the way such quantity-terms apply to concrete particulars depends on nontrivial choices made by humans, and specifically on choices that have to do with the way the relevant quantity is measured. Note that under this broad construal, realism is compatible with operationalism and conventionalism. That is, it is conceivable that choices of measurement method regulate the use of a quantity-term and that, given the correct choice, this term succeeds in referring to a mind-independent property or relation. Nonetheless, many operationalists and conventionalists adopted stronger views, according to which there are no facts of the matter as to which of several and nontrivially different operations is correct for applying a given quantity-term. These stronger variants are inconsistent with realism about measurement. This section will be dedicated to operationalism and conventionalism, and the next to realism about measurement.
Operationalism (or “operationism”) about measurement is the view that the meaning of quantity-concepts is determined by the set of operations used for their measurement. The strongest expression of operationalism appears in the early work of Percy Bridgman (1927), who argued that
we mean by any concept nothing more than a set of operations; the concept is synonymous with the corresponding set of operations. (1927: 5)
Length, for example, would be defined as the result of the operation of concatenating rigid rods. According to this extreme version of operationalism, different operations measure different quantities. Length measured by using rulers and by timing electromagnetic pulses should, strictly speaking, be distinguished into two distinct quantity-concepts labeled “length-1” and “length-2” respectively. This conclusion led Bridgman to claim that currently accepted quantity concepts have “joints” where different operations overlap in their domain of application. He warned against dogmatic faith in the unity of quantity concepts across these “joints”, urging instead that unity be checked against experiments whenever the application of a quantity-concept is to be extended into a new domain. Nevertheless, Bridgman conceded that as long as the results of different operations agree within experimental error it is pragmatically justified to label the corresponding quantities with the same name (1927: 16). [ 8 ]
Operationalism became influential in psychology, where it was well-received by behaviorists like Edwin Boring (1945) and B.F. Skinner (1945). Indeed, Skinner maintained that behaviorism is “nothing more than a thoroughgoing operational analysis of traditional mentalistic concepts” (1945: 271). Stevens, who was Boring’s student, was a key promoter of operationalism in psychology, and argued that psychological concepts have empirical meaning only if they stand for definite and concrete operations (1935: 517; see also Isaac 2017). The idea that concepts are defined by measurement operations is consistent with Stevens’ liberal views on measurability, which were discussed above ( Section 3.3 ). As long as the assignment of numbers to objects is performed in accordance with concrete and consistent rules, Stevens maintained that such assignment has empirical meaning and does not need to satisfy any additional constraints. Nonetheless, Stevens probably did not embrace an anti-realist view about psychological attributes. Instead, there are good reasons to think that he understood operationalism as a methodological attitude that was valuable to the extent that it allowed psychologists to justify the conclusions they drew from experiments (Feest 2005). For example, Stevens did not treat operational definitions as a priori but as amenable to improvement in light of empirical discoveries, implying that he took psychological attributes to exist independently of such definitions (Stevens 1935: 527). This suggests that Stevens’ operationalism was of a more moderate variety than that found in the early writings of Bridgman. [ 9 ]
Operationalism met with initial enthusiasm by logical positivists, who viewed it as akin to verificationism. Nonetheless, it was soon revealed that any attempt to base a theory of meaning on operationalist principles was riddled with problems. Among such problems were the automatic reliability operationalism conferred on measurement operations, the ambiguities surrounding the notion of operation, the overly restrictive operational criterion of meaningfulness, and the fact that many useful theoretical concepts lack clear operational definitions (Chang 2009). [ 10 ] In particular, Carl Hempel (1956, 1966) criticized operationalists for being unable to define dispositional terms such as “solubility in water”, and for multiplying the number of scientific concepts in a manner that runs against the need for systematic and simple theories. Accordingly, most writers on the semantics of quantity-terms have avoided espousing an operational analysis. [ 11 ]
A more widely advocated approach admitted a conventional element to the use of quantity-terms, while resisting attempts to reduce the meaning of quantity terms to measurement operations. These accounts are classified under the general heading “conventionalism”, though they differ in the particular aspects of measurement they deem conventional and in the degree of arbitrariness they ascribe to such conventions. [ 12 ] An early precursor of conventionalism was Ernst Mach, who examined the notion of equality among temperature intervals (1896: 52). Mach noted that different types of thermometric fluid expand at different (and nonlinearly related) rates when heated, raising the question: which fluid expands most uniformly with temperature? According to Mach, there is no fact of the matter as to which fluid expands more uniformly, since the very notion of equality among temperature intervals has no determinate application prior to a conventional choice of standard thermometric fluid. Mach coined the term “principle of coordination” for this sort of conventionally chosen principle for the application of a quantity concept. The concepts of uniformity of time and space received similar treatments by Henri Poincaré (1898, 1902: Part 2). Poincaré argued that procedures used to determine equality among durations stem from scientists’ unconscious preference for descriptive simplicity, rather than from any fact about nature. Similarly, scientists’ choice to represent space with either Euclidean or non-Euclidean geometries is not determined by experience but by considerations of convenience.
Conventionalism with respect to measurement reached its most sophisticated expression in logical positivism. Logical positivists like Hans Reichenbach and Rudolf Carnap proposed “coordinative definitions” or “correspondence rules” as the semantic link between theoretical and observational terms. These a priori , definition-like statements were intended to regulate the use of theoretical terms by connecting them with empirical procedures (Reichenbach 1927: 14–19; Carnap 1966: Ch. 24). An example of a coordinative definition is the statement: “a measuring rod retains its length when transported”. According to Reichenbach, this statement cannot be empirically verified, because a universal and experimentally undetectable force could exist that equally distorts every object’s length when it is transported. In accordance with verificationism, statements that are unverifiable are neither true nor false. Instead, Reichenbach took this statement to expresses an arbitrary rule for regulating the use of the concept of equality of length, namely, for determining whether particular instances of length are equal (Reichenbach 1927: 16). At the same time, coordinative definitions were not seen as replacements, but rather as necessary additions, to the familiar sort of theoretical definitions of concepts in terms of other concepts (1927: 14). Under the conventionalist viewpoint, then, the specification of measurement operations did not exhaust the meaning of concepts such as length or length-equality, thereby avoiding many of the problems associated with operationalism. [ 13 ]
Realists about measurement maintain that measurement is best understood as the empirical estimation of an objective property or relation. A few clarificatory remarks are in order with respect to this characterization of measurement. First, the term “objective” is not meant to exclude mental properties or relations, which are the objects of psychological measurement. Rather, measurable properties or relations are taken to be objective inasmuch as they are independent of the beliefs and conventions of the humans performing the measurement and of the methods used for measuring. For example, a realist would argue that the ratio of the length of a given solid rod to the standard meter has an objective value regardless of whether and how it is measured. Second, the term “estimation” is used by realists to highlight the fact that measurement results are mere approximations of true values (Trout 1998: 46). Third, according to realists, measurement is aimed at obtaining knowledge about properties and relations, rather than at assigning values directly to individual objects. This is significant because observable objects (e.g., levers, chemical solutions, humans) often instantiate measurable properties and relations that are not directly observable (e.g., amount of mechanical work, more acidic than, intelligence). Knowledge claims about such properties and relations must presuppose some background theory. By shifting the emphasis from objects to properties and relations, realists highlight the theory-laden character of measurements.
Realism about measurement should not be confused with realism about entities (e.g., electrons). Nor does realism about measurement necessarily entail realism about properties (e.g., temperature), since one could in principle accept only the reality of relations (e.g., ratios among quantities) without embracing the reality of underlying properties. Nonetheless, most philosophers who have defended realism about measurement have done so by arguing for some form of realism about properties (Byerly and Lazara 1973; Swoyer 1987; Mundy 1987; Trout 1998, 2000). These realists argue that at least some measurable properties exist independently of the beliefs and conventions of the humans who measure them, and that the existence and structure of these properties provides the best explanation for key features of measurement, including the usefulness of numbers in expressing measurement results and the reliability of measuring instruments.
For example, a typical realist about length measurement would argue that the empirical regularities displayed by individual objects’ lengths when they are ordered and concatenated are best explained by assuming that length is an objective property that has an extensive structure (Swoyer 1987: 271–4). That is, relations among lengths such as “longer than” and “sum of” exist independently of whether any objects happen to be ordered and concatenated by humans, and indeed independently of whether objects of some particular length happen to exist at all. The existence of an extensive property structure means that lengths share much of their structure with the positive real numbers, and this explains the usefulness of the positive reals in representing lengths. Moreover, if measurable properties are analyzed in dispositional terms, it becomes easy to explain why some measuring instruments are reliable. For example, if one assumes that a certain amount of electric current in a wire entails a disposition to deflect an ammeter needle by a certain angle, it follows that the ammeter’s indications counterfactually depend on the amount of electric current in the wire, and therefore that the ammeter is reliable (Trout 1998: 65).
A different argument for realism about measurement is due to Joel Michell (1994, 2005), who proposes a realist theory of number based on the Euclidean concept of ratio. According to Michell, numbers are ratios between quantities, and therefore exist in space and time. Specifically, real numbers are ratios between pairs of infinite standard sequences, e.g., the sequence of lengths normally denoted by “1 meter”, “2 meters” etc. and the sequence of whole multiples of the length we are trying to measure. Measurement is the discovery and estimation of such ratios. An interesting consequence of this empirical realism about numbers is that measurement is not a representational activity, but rather the activity of approximating mind-independent numbers (Michell 1994: 400).
Realist accounts of measurement are largely formulated in opposition to strong versions of operationalism and conventionalism, which dominated philosophical discussions of measurement from the 1930s until the 1960s. In addition to the drawbacks of operationalism already discussed in the previous section, realists point out that anti-realism about measurable quantities fails to make sense of scientific practice. If quantities had no real values independently of one’s choice of measurement procedure, it would be difficult to explain what scientists mean by “measurement accuracy” and “measurement error”, and why they try to increase accuracy and diminish error. By contrast, realists can easily make sense of the notions of accuracy and error in terms of the distance between real and measured values (Byerly and Lazara 1973: 17–8; Swoyer 1987: 239; Trout 1998: 57). A closely related point is the fact that newer measurement procedures tend to improve on the accuracy of older ones. If choices of measurement procedure were merely conventional it would be difficult to make sense of such progress. In addition, realism provides an intuitive explanation for why different measurement procedures often yield similar results, namely, because they are sensitive to the same facts (Swoyer 1987: 239; Trout 1998: 56). Finally, realists note that the construction of measurement apparatus and the analysis of measurement results are guided by theoretical assumptions concerning causal relationships among quantities. The ability of such causal assumptions to guide measurement suggests that quantities are ontologically prior to the procedures that measure them. [ 14 ]
While their stance towards operationalism and conventionalism is largely critical, realists are more charitable in their assessment of mathematical theories of measurement. Brent Mundy (1987) and Chris Swoyer (1987) both accept the axiomatic treatment of measurement scales, but object to the empiricist interpretation given to the axioms by prominent measurement theorists like Campbell (1920) and Ernest Nagel (1931; Cohen and Nagel 1934: Ch. 15). Rather than interpreting the axioms as pertaining to concrete objects or to observable relations among such objects, Mundy and Swoyer reinterpret the axioms as pertaining to universal magnitudes, e.g., to the universal property of being 5 meter long rather than to the concrete instantiations of that property. This construal preserves the intuition that statements like “the size of x is twice the size of y ” are first and foremost about two sizes , and only derivatively about the objects x and y themselves (Mundy 1987: 34). [ 15 ] Mundy and Swoyer argue that their interpretation is more general, because it logically entails all the first-order consequences of the empiricist interpretation along with additional, second-order claims about universal magnitudes. Moreover, under their interpretation measurement theory becomes a genuine scientific theory, with explanatory hypotheses and testable predictions. Building on this work, Jo Wolff (2020a) has recently proposed a novel realist account of quantities that relies on the Representational Theory of Measurement. According to Wolff’s structuralist theory of quantity, quantitative attributes are relational structures. Specifically, an attribute is quantitative if its structure has translations that form an Archimedean ordered group. Wolff’s focus on translations, rather than on specific relations such as concatenation and ordering, means that quantitativeness can be realized in multiple ways and is not restricted to extensive structures. It also means that being a quantity does not have anything special to do with numbers, as both numerical and non-numerical structures can be quantitative.
Information-theoretic accounts of measurement are based on an analogy between measuring systems and communication systems. In a simple communication system, a message (input) is encoded into a signal at the transmitter’s end, sent to the receiver’s end, and then decoded back (output). The accuracy of the transmission depends on features of the communication system as well as on features of the environment, i.e., the level of background noise. Similarly, measuring instruments can be thought of as “information machines” (Finkelstein 1977) that interact with an object in a given state (input), encode that state into an internal signal, and convert that signal into a reading (output). The accuracy of a measurement similarly depends on the instrument as well as on the level of noise in its environment. Conceived as a special sort of information transmission, measurement becomes analyzable in terms of the conceptual apparatus of information theory (Hartley 1928; Shannon 1948; Shannon and Weaver 1949). For example, the information that reading \(y_i\) conveys about the occurrence of a state \(x_k\) of the object can be quantified as \(\log \left[\frac{p(x_k \mid y_i)}{p(x_k)}\right]\), namely as a function of the decrease of uncertainty about the object’s state (Finkelstein 1975: 222; for alternative formulations see Brillouin 1962: Ch. 15; Kirpatovskii 1974; and Mari 1999: 185).
Ludwik Finkelstein (1975, 1977) and Luca Mari (1999) suggested the possibility of a synthesis between Shannon-Weaver information theory and measurement theory. As they argue, both theories centrally appeal to the idea of mapping: information theory concerns the mapping between symbols in the input and output messages, while measurement theory concerns the mapping between objects and numbers. If measurement is taken to be analogous to symbol-manipulation, then Shannon-Weaver theory could provide a formalization of the syntax of measurement while measurement theory could provide a formalization of its semantics. Nonetheless, Mari (1999: 185) also warns that the analogy between communication and measurement systems is limited. Whereas a sender’s message can be known with arbitrary precision independently of its transmission, the state of an object cannot be known with arbitrary precision independently of its measurement.
Information-theoretic accounts of measurement were originally developed by metrologists — experts in physical measurement and standardization — with little involvement from philosophers. Independently of developments in metrology, Bas van Fraassen (2008: 141–185) has recently proposed a conception of measurement in which information plays a key role. He views measurement as composed of two levels: on the physical level, the measuring apparatus interacts with an object and produces a reading, e.g., a pointer position. [ 16 ] On the abstract level, background theory represents the object’s possible states on a parameter space. Measurement locates an object on a sub-region of this abstract parameter space, thereby reducing the range of possible states (2008: 164 and 172). This reduction of possibilities amounts to the collection of information about the measured object. Van Fraassen’s analysis of measurement differs from information-theoretic accounts developed in metrology in its explicit appeal to background theory, and in the fact that it does not invoke the symbolic conception of information developed by Shannon and Weaver.
7. Model-Based Accounts of Measurement
Since the early 2000s a new wave of philosophical scholarship has emerged that emphasizes the relationships between measurement and theoretical and statistical modeling (Morgan 2001; Boumans 2005a, 2015; Mari 2005b; Mari and Giordani 2013; Tal 2016, 2017; Parker 2017; Miyake 2017). According to model-based accounts, measurement consists of two levels: (i) a concrete process involving interactions between an object of interest, an instrument, and the environment; and (ii) a theoretical and/or statistical model of that process, where “model” denotes an abstract and local representation constructed from simplifying assumptions. The central goal of measurement according to this view is to assign values to one or more parameters of interest in the model in a manner that satisfies certain epistemic desiderata, in particular coherence and consistency.
Model-based accounts have been developed by studying measurement practices in the sciences, and particularly in metrology. Metrology, officially defined as the “science of measurement and its application” (JCGM 2012: 2.2), is a field of study concerned with the design, maintenance and improvement of measuring instruments in the natural sciences and engineering. Metrologists typically work at standardization bureaus or at specialized laboratories that are responsible for the calibration of measurement equipment, the comparison of standards and the evaluation of measurement uncertainties, among other tasks. It is only recently that philosophers have begun to engage with the rich conceptual issues underlying metrological practice, and particularly with the inferences involved in evaluating and improving the accuracy of measurement standards (Chang 2004; Boumans 2005a: Chap. 5, 2005b, 2007a; Frigerio et al. 2010; Teller 2013, 2018; Riordan 2015; Schlaudt and Huber 2015; Tal 2016a, 2018; Mitchell et al. 2017; Mößner and Nordmann 2017; de Courtenay et al. 2019).
A central motivation for the development of model-based accounts is the attempt to clarify the epistemological principles underlying aspects of measurement practice. For example, metrologists employ a variety of methods for the calibration of measuring instruments, the standardization and tracing of units and the evaluation of uncertainties (for a discussion of metrology, see the previous section). Traditional philosophical accounts such as mathematical theories of measurement do not elaborate on the assumptions, inference patterns, evidential grounds or success criteria associated with such methods. As Frigerio et al. (2010) argue, measurement theory is ill-suited for clarifying these aspects of measurement because it abstracts away from the process of measurement and focuses solely on the mathematical properties of scales. By contrast, model-based accounts take scale construction to be merely one of several tasks involved in measurement, alongside the definition of measured parameters, instrument design and calibration, object sampling and preparation, error detection and uncertainty evaluation, among others (2010: 145–7).
According to model-based accounts, measurement involves interaction between an object of interest (the “system under measurement”), an instrument (the “measurement system”) and an environment, which includes the measuring subjects. Other, secondary interactions may also be relevant for the determination of a measurement outcome, such as the interaction between the measuring instrument and the reference standards used for its calibration, and the chain of comparisons that trace the reference standard back to primary measurement standards (Mari 2003: 25). Measurement proceeds by representing these interactions with a set of parameters, and assigning values to a subset of those parameters (known as “measurands”) based on the results of the interactions. When measured parameters are numerical they are called “quantities”. Although measurands need not be quantities, a quantitative measurement scenario will be supposed in what follows.
Two sorts of measurement outputs are distinguished by model-based accounts [JCGM 2012: 2.9 & 4.1; Giordani and Mari 2012: 2146; Tal 2013]:
- Instrument indications (or “readings”): these are properties of the measuring instrument in its final state after the measurement process is complete. Examples are digits on a display, marks on a multiple-choice questionnaire and bits stored in a device’s memory. Indications may be represented by numbers, but such numbers describe states of the instrument and should not be confused with measurement outcomes, which concern states of the object being measured.
- Measurement outcomes (or “results”): these are knowledge claims about the values of one or more quantities attributed to the object being measured, and are typically accompanied by a specification of the measurement unit and scale and an estimate of measurement uncertainty. For example, a measurement outcome may be expressed by the sentence “the mass of object a is 20±1 grams with a probability of 68%”.
As proponents of model-based accounts stress, inferences from instrument indications to measurement outcomes are nontrivial and depend on a host of theoretical and statistical assumptions about the object being measured, the instrument, the environment and the calibration process. Measurement outcomes are often obtained through statistical analysis of multiple indications, thereby involving assumptions about the shape of the distribution of indications and the randomness of environmental effects (Bogen and Woodward 1988: 307–310). Measurement outcomes also incorporate corrections for systematic effects, and such corrections are based on theoretical assumptions concerning the workings of the instrument and its interactions with the object and environment. For example, length measurements need to be corrected for the change of the measuring rod’s length with temperature, a correction which is derived from a theoretical equation of thermal expansion. Systematic corrections involve uncertainties of their own, for example in the determination of the values of constants, and these uncertainties are assessed through secondary experiments involving further theoretical and statistical assumptions. Moreover, the uncertainty associated with a measurement outcome depends on the methods employed for the calibration of the instrument. Calibration involves additional assumptions about the instrument, the calibrating apparatus, the quantity being measured and the properties of measurement standards (Rothbart and Slayden 1994; Franklin 1997; Baird 2004: Ch. 4; Soler et al. 2013). Another component of uncertainty originates from vagueness in the definition of the measurand, and is known as “definitional uncertainty” (Mari and Giordani 2013; Grégis 2015). Finally, measurement involves background assumptions about the scale type and unit system being used, and these assumptions are often tied to broader theoretical and technological considerations relating to the definition and realization of scales and units.
These various theoretical and statistical assumptions form the basis for the construction of one or more models of the measurement process. Unlike mathematical theories of measurement, where the term “model” denotes a set-theoretical structure that interprets a formal language, here the term “model” denotes an abstract and local representation of a target system that is constructed from simplifying assumptions. [ 17 ] The relevant target system in this case is a measurement process, that is, a system composed of a measuring instrument, objects or events to be measured, the environment (including human operators), secondary instruments and reference standards, the time-evolution of these components, and their various interactions with each other. Measurement is viewed as a set of procedures whose aim is to coherently assign values to model parameters based on instrument indications. Models are therefore seen as necessary preconditions for the possibility of inferring measurement outcomes from instrument indications, and as crucial for determining the content of measurement outcomes. As proponents of model-based accounts emphasize, the same indications produced by the same measurement process may be used to establish different measurement outcomes depending on how the measurement process is modeled, e.g., depending on which environmental influences are taken into account, which statistical assumptions are used to analyze noise, and which approximations are used in applying background theory. As Luca Mari puts it,
any measurement result reports information that is meaningful only in the context of a metrological model, such a model being required to include a specification for all the entities that explicitly or implicitly appear in the expression of the measurement result. (2003: 25)
Similarly, models are said to provide the necessary context for evaluating various aspects of the goodness of measurement outcomes, including accuracy, precision, error and uncertainty (Boumans 2006, 2007a, 2009, 2012b; Mari 2005b).
Model-based accounts diverge from empiricist interpretations of measurement theory in that they do not require relations among measurement outcomes to be isomorphic or homomorphic to observable relations among the items being measured (Mari 2000). Indeed, according to model-based accounts relations among measured objects need not be observable at all prior to their measurement (Frigerio et al. 2010: 125). Instead, the key normative requirement of model-based accounts is that values be assigned to model parameters in a coherent manner. The coherence criterion may be viewed as a conjunction of two sub-criteria: (i) coherence of model assumptions with relevant background theories or other substantive presuppositions about the quantity being measured; and (ii) objectivity, i.e., the mutual consistency of measurement outcomes across different measuring instruments, environments and models [ 18 ] (Frigerio et al. 2010; Tal 2017a; Teller 2018). The first sub-criterion is meant to ensure that the intended quantity is being measured, while the second sub-criterion is meant to ensure that measurement outcomes can be reasonably attributed to the measured object rather than to some artifact of the measuring instrument, environment or model. Taken together, these two requirements ensure that measurement outcomes remain valid independently of the specific assumptions involved in their production, and hence that the context-dependence of measurement outcomes does not threaten their general applicability.
Besides their applicability to physical measurement, model-based analyses also shed light on measurement in economics. Like physical quantities, values of economic variables often cannot be observed directly and must be inferred from observations based on abstract and idealized models. The nineteenth century economist William Jevons, for example, measured changes in the value of gold by postulating certain causal relationships between the value of gold, the supply of gold and the general level of prices (Hoover and Dowell 2001: 155–159; Morgan 2001: 239). As Julian Reiss (2001) shows, Jevons’ measurements were made possible by using two models: a causal-theoretical model of the economy, which is based on the assumption that the quantity of gold has the capacity to raise or lower prices; and a statistical model of the data, which is based on the assumption that local variations in prices are mutually independent and therefore cancel each other out when averaged. Taken together, these models allowed Jevons to infer the change in the value of gold from data concerning the historical prices of various goods. [ 19 ]
The ways in which models function in economic measurement have led some philosophers to view certain economic models as measuring instruments in their own right, analogously to rulers and balances (Boumans 1999, 2005c, 2006, 2007a, 2009, 2012a, 2015; Morgan 2001). Marcel Boumans explains how macroeconomists are able to isolate a variable of interest from external influences by tuning parameters in a model of the macroeconomic system. This technique frees economists from the impossible task of controlling the actual system. As Boumans argues, macroeconomic models function as measuring instruments insofar as they produce invariant relations between inputs (indications) and outputs (outcomes), and insofar as this invariance can be tested by calibration against known and stable facts. When such model-based procedures are combined with expert judgment, they can produce reliable measurements of economic phenomena even outside controlled laboratory settings (Boumans 2015: Chap. 5).
Another area where models play a central role in measurement is psychology. The measurement of most psychological attributes, such as intelligence, anxiety and depression, does not rely on homomorphic mappings of the sort espoused by the Representational Theory of Measurement (Wilson 2013: 3766). Instead, psychometric theory relies predominantly on the development of abstract models that are meant to predict subjects’ performance in certain tasks. These models are constructed from substantive and statistical assumptions about the psychological attribute being measured and its relation to each measurement task. For example, Item Response Theory, a popular approach to psychological measurement, employs a variety of models to evaluate the reliability and validity of questionnaires. Consider a questionnaire that is meant to assess English language comprehension (the “ability”), by presenting subjects with a series of yes/no questions (the “items”). One of the simplest models used to calibrate such questionnaires is the Rasch model (Rasch 1960). This model supposes a straightforward algebraic relation—known as the “log of the odds”—between the probability that a subject will answer a given item correctly, the difficulty of that particular item, and the subject’s ability. New questionnaires are calibrated by testing the fit between their indications and the predictions of the Rasch model and assigning difficulty levels to each item accordingly. The model is then used in conjunction with the questionnaire to infer levels of English language comprehension (outcomes) from raw questionnaire scores (indications) (Wilson 2013; Mari and Wilson 2014).
The sort of statistical calibration (or “scaling”) provided by Rasch models yields repeatable results, but it is often only a first step towards full-fledged psychological measurement. Psychologists are typically interested in the results of a measure not for its own sake, but for the sake of assessing some underlying and latent psychological attribute, e.g., English language comprehension. A good fit between item responses and a statistical model does not yet determine what the questionnaire is measuring. The process of establishing that a procedure measures the intended psychological attribute is known as “validation”. One way of validating a psychometric instrument is to test whether different procedures that are intended to measure the same latent attribute provide consistent results. Such testing belongs to a family of validation techniques known as “construct validation”. A construct is an abstract representation of the latent attribute intended to be measured, and
reflects a hypothesis […] that a variety of behaviors will correlate with one another in studies of individual differences and/or will be similarly affected by experimental manipulations. (Nunnally & Bernstein 1994: 85)
Constructs are denoted by variables in a model that predicts which correlations would be observed among the indications of different measures if they are indeed measures of the same attribute. Such models involve substantive assumptions about the attribute, including its internal structure and its relations to other attributes, and statistical assumptions about the correlation among different measures (Campbell & Fiske 1959; Nunnally & Bernstein 1994: Ch. 3; Angner 2008).
In recent years, philosophers of science have become increasingly interested in psychometrics and the concept of validity. One debate concerns the ontological status of latent psychological attributes. Denny Borsboom has argued against operationalism about latent attributes, and in favour of defining validity in a manner that embraces realism: “a test is valid for measuring an attribute if and only if a) the attribute exists, and b) variations in the attribute causally produce variations in the outcomes of the measurement procedure” (2005: 150; see also Hood 2009, 2013; Feest 2020). Elina Vessonen has defended a moderate form of operationalism about psychological attributes, and argued that moderate operationalism is compatible with a cautious type of realism (2019). Another recent discussion focuses on the justification for construct validation procedures. According to Anna Alexandrova, construct validation is in principle a justified methodology, insofar as it establishes coherence with theoretical assumptions and background knowledge about the latent attribute. However, Alexandrova notes that in practice psychometricians who intend to measure happiness and well-being often avoid theorizing about these constructs, and instead appeal to respondents’ folk beliefs. This defeats the purpose of construct validation and turns it into a narrow, technical exercise (Alexandrova and Haybron 2016; Alexandrova 2017; see also McClimans et al. 2017).
A more fundamental criticism leveled against psychometrics is that it dogmatically presupposes that psychological attributes can be quantified. Michell (2000, 2004b) argues that psychometricians have not made serious attempts to test whether the attributes they purport to measure have quantitative structure, and instead adopted an overly loose conception of measurement that disguises this neglect. In response, Borsboom and Mellenbergh (2004) argue that Item Response Theory provides probabilistic tests of the quantifiability of attributes. Psychometricians who construct a statistical model initially hypothesize that an attribute is quantitative, and then subject the model to empirical tests. When successful, such tests provide indirect confirmation of the initial hypothesis, e.g. by showing that the attribute has an additive conjoint structure (see also Vessonen 2020).
Several scholars have pointed out similarities between the ways models are used to standardize measurable quantities in the natural and social sciences. For example, Mark Wilson (2013) argues that psychometric models can be viewed as tools for constructing measurement standards in the same sense of “measurement standard” used by metrologists. Others have raised doubts about the feasibility and desirability of adopting the example of the natural sciences when standardizing constructs in the social sciences. Nancy Cartwright and Rosa Runhardt (2014) discuss “Ballung” concepts, a term they borrow from Otto Neurath to denote concepts with a fuzzy and context-dependent scope. Examples of Ballung concepts are race, poverty, social exclusion, and the quality of PhD programs. Such concepts are too multifaceted to be measured on a single metric without loss of meaning, and must be represented either by a matrix of indices or by several different measures depending on which goals and values are at play (see also Bradburn, Cartwright, & Fuller 2016, Other Internet Resources). Alexandrova (2008) points out that ethical considerations bear on questions about the validity of measures of well-being no less than considerations of reproducibility. Such ethical considerations are context sensitive, and can only be applied piecemeal. In a similar vein, Leah McClimans (2010) argues that uniformity is not always an appropriate goal for designing questionnaires, as the open-endedness of questions is often both unavoidable and desirable for obtaining relevant information from subjects. [ 20 ] The intertwining of ethical and epistemic considerations is especially clear when psychometric questionnaires are used in medical contexts to evaluate patient well-being and mental health. In such cases, small changes to the design of a questionnaire or the analysis of its results may result in significant harms or benefits to patients (McClimans 2017; Stegenga 2018, Chap. 8). These insights highlight the value-laden and contextual nature of the measurement of mental and social phenomena.
8. The Epistemology of Measurement
The development of model-based accounts discussed in the previous section is part of a larger, “epistemic turn” in the philosophy of measurement that occurred in the early 2000s. Rather than emphasizing the mathematical foundations, metaphysics or semantics of measurement, philosophical work in recent years tends to focus on the presuppositions and inferential patterns involved in concrete practices of measurement, and on the historical, social and material dimensions of measuring. The philosophical study of these topics has been referred to as the “epistemology of measurement” (Mari 2003, 2005a; Leplège 2003; Tal 2017a). In the broadest sense, the epistemology of measurement is the study of the relationships between measurement and knowledge. Central topics that fall under the purview of the epistemology of measurement include the conditions under which measurement produces knowledge; the content, scope, justification and limits of such knowledge; the reasons why particular methodologies of measurement and standardization succeed or fail in supporting particular knowledge claims, and the relationships between measurement and other knowledge-producing activities such as observation, theorizing, experimentation, modelling and calculation. In pursuing these objectives, philosophers are drawing on the work of historians and sociologists of science, who have been investigating measurement practices for a longer period (Wise and Smith 1986; Latour 1987: Ch. 6; Schaffer 1992; Porter 1995, 2007; Wise 1995; Alder 2002; Galison 2003; Gooday 2004; Crease 2011), as well as on the history and philosophy of scientific experimentation (Harré 1981; Hacking 1983; Franklin 1986; Cartwright 1999). The following subsections survey some of the topics discussed in this burgeoning body of literature.
A topic that has attracted considerable philosophical attention in recent years is the selection and improvement of measurement standards. Generally speaking, to standardize a quantity concept is to prescribe a determinate way in which that concept is to be applied to concrete particulars. [ 21 ] To standardize a measuring instrument is to assess how well the outcomes of measuring with that instrument fit the prescribed mode of application of the relevant concept. [ 22 ] The term “measurement standard” accordingly has at least two meanings: on the one hand, it is commonly used to refer to abstract rules and definitions that regulate the use of quantity concepts, such as the definition of the meter. On the other hand, the term “measurement standard” is also commonly used to refer to the concrete artifacts and procedures that are deemed exemplary of the application of a quantity concept, such as the metallic bar that served as the standard meter until 1960. This duality in meaning reflects the dual nature of standardization, which involves both abstract and concrete aspects.
In Section 4 it was noted that standardization involves choices among nontrivial alternatives, such as the choice among different thermometric fluids or among different ways of marking equal duration. These choices are nontrivial in the sense that they affect whether or not the same temperature (or time) intervals are deemed equal, and hence affect whether or not statements of natural law containing the term “temperature” (or “time”) come out true. Appealing to theory to decide which standard is more accurate would be circular, since the theory cannot be determinately applied to particulars prior to a choice of measurement standard. This circularity has been variously called the “problem of coordination” (van Fraassen 2008: Ch. 5) and the “problem of nomic measurement” (Chang 2004: Ch. 2). As already mentioned, conventionalists attempted to escape the circularity by positing a priori statements, known as “coordinative definitions”, which were supposed to link quantity-terms with specific measurement operations. A drawback of this solution is that it supposes that choices of measurement standard are arbitrary and static, whereas in actual practice measurement standards tend to be chosen based on empirical considerations and are eventually improved or replaced with standards that are deemed more accurate.
A new strand of writing on the problem of coordination has emerged in recent years, consisting most notably of the works of Hasok Chang (2001, 2004, 2007; Barwich and Chang 2015) and Bas van Fraassen (2008: Ch. 5; 2009, 2012; see also Padovani 2015, 2017; Michel 2019). These works take a historical and coherentist approach to the problem. Rather than attempting to avoid the problem of circularity completely, as their predecessors did, they set out to show that the circularity is not vicious. Chang argues that constructing a quantity-concept and standardizing its measurement are co-dependent and iterative tasks. Each “epistemic iteration” in the history of standardization respects existing traditions while at the same time correcting them (Chang 2004: Ch. 5). The pre-scientific concept of temperature, for example, was associated with crude and ambiguous methods of ordering objects from hot to cold. Thermoscopes, and eventually thermometers, helped modify the original concept and made it more precise. With each such iteration the quantity concept was re-coordinated to a more stable set of standards, which in turn allowed theoretical predictions to be tested more precisely, facilitating the subsequent development of theory and the construction of more stable standards, and so on.
How this process avoids vicious circularity becomes clear when we look at it either “from above”, i.e., in retrospect given our current scientific knowledge, or “from within”, by looking at historical developments in their original context (van Fraassen 2008: 122). From either vantage point, coordination succeeds because it increases coherence among elements of theory and instrumentation. The questions “what counts as a measurement of quantity X ?” and “what is quantity X ?”, though unanswerable independently of each other, are addressed together in a process of mutual refinement. It is only when one adopts a foundationalist view and attempts to find a starting point for coordination free of presupposition that this historical process erroneously appears to lack epistemic justification (2008: 137).
The new literature on coordination shifts the emphasis of the discussion from the definitions of quantity-terms to the realizations of those definitions. In metrological jargon, a “realization” is a physical instrument or procedure that approximately satisfies a given definition (cf. JCGM 2012: 5.1). Examples of metrological realizations are the official prototypes of the kilogram and the cesium fountain clocks used to standardize the second. Recent studies suggest that the methods used to design, maintain and compare realizations have a direct bearing on the practical application of concepts of quantity, unit and scale, no less than the definitions of those concepts (Riordan 2015; Tal 2016). The relationship between the definition and realizations of a unit becomes especially complex when the definition is stated in theoretical terms. Several of the base units of the International System (SI) — including the meter, kilogram, ampere, kelvin and mole — are no longer defined by reference to any specific kind of physical system, but by fixing the numerical value of a fundamental physical constant. The kilogram, for example, was redefined in 2019 as the unit of mass such that the numerical value of the Planck constant is exactly 6.62607015 × 10 -34 kg m 2 s -1 (BIPM 2019:131). Realizing the kilogram under this definition is a highly theory-laden task. The study of the practical realization of such units has shed new light on the evolving relationships between measurement and theory (Tal 2018; de Courtenay et al 2019; Wolff 2020b).
As already discussed above (Sections 7 and 8.1 ), theory and measurement are interdependent both historically and conceptually. On the historical side, the development of theory and measurement proceeds through iterative and mutual refinements. On the conceptual side, the specification of measurement procedures shapes the empirical content of theoretical concepts, while theory provides a systematic interpretation for the indications of measuring instruments. This interdependence of measurement and theory may seem like a threat to the evidential role that measurement is supposed to play in the scientific enterprise. After all, measurement outcomes are thought to be able to test theoretical hypotheses, and this seems to require some degree of independence of measurement from theory. This threat is especially clear when the theoretical hypothesis being tested is already presupposed as part of the model of the measuring instrument. To cite an example from Franklin et al. (1989: 230):
There would seem to be, at first glance, a vicious circularity if one were to use a mercury thermometer to measure the temperature of objects as part of an experiment to test whether or not objects expand as their temperature increases.
Nonetheless, Franklin et al. conclude that the circularity is not vicious. The mercury thermometer could be calibrated against another thermometer whose principle of operation does not presuppose the law of thermal expansion, such as a constant-volume gas thermometer, thereby establishing the reliability of the mercury thermometer on independent grounds. To put the point more generally, in the context of local hypothesis-testing the threat of circularity can usually be avoided by appealing to other kinds of instruments and other parts of theory.
A different sort of worry about the evidential function of measurement arises on the global scale, when the testing of entire theories is concerned. As Thomas Kuhn (1961) argues, scientific theories are usually accepted long before quantitative methods for testing them become available. The reliability of newly introduced measurement methods is typically tested against the predictions of the theory rather than the other way around. In Kuhn’s words, “The road from scientific law to scientific measurement can rarely be traveled in the reverse direction” (1961: 189). For example, Dalton’s Law, which states that the weights of elements in a chemical compound are related to each other in whole-number proportions, initially conflicted with some of the best known measurements of such proportions. It is only by assuming Dalton’s Law that subsequent experimental chemists were able to correct and improve their measurement techniques (1961: 173). Hence, Kuhn argues, the function of measurement in the physical sciences is not to test the theory but to apply it with increasing scope and precision, and eventually to allow persistent anomalies to surface that would precipitate the next crisis and scientific revolution. Note that Kuhn is not claiming that measurement has no evidential role to play in science. Instead, he argues that measurements cannot test a theory in isolation, but only by comparison to some alternative theory that is proposed in an attempt to account for the anomalies revealed by increasingly precise measurements (for an illuminating discussion of Kuhn’s thesis see Hacking 1983: 243–5).
Traditional discussions of theory-ladenness, like those of Kuhn, were conducted against the background of the logical positivists’ distinction between theoretical and observational language. The theory-ladenness of measurement was correctly perceived as a threat to the possibility of a clear demarcation between the two languages. Contemporary discussions, by contrast, no longer present theory-ladenness as an epistemological threat but take for granted that some level of theory-ladenness is a prerequisite for measurements to have any evidential power. Without some minimal substantive assumptions about the quantity being measured, such as its amenability to manipulation and its relations to other quantities, it would be impossible to interpret the indications of measuring instruments and hence impossible to ascertain the evidential relevance of those indications. This point was already made by Pierre Duhem (1906: 153–6; see also Carrier 1994: 9–19). Moreover, contemporary authors emphasize that theoretical assumptions play crucial roles in correcting for measurement errors and evaluating measurement uncertainties. Indeed, physical measurement procedures become more accurate when the model underlying them is de-idealized, a process which involves increasing the theoretical richness of the model (Tal 2011).
The acknowledgment that theory is crucial for guaranteeing the evidential reliability of measurement draws attention to the “problem of observational grounding”, which is an inverse challenge to the traditional threat of theory-ladenness (Tal 2016b). The challenge is to specify what role observation plays in measurement, and particularly what sort of connection with observation is necessary and/or sufficient to allow measurement to play an evidential role in the sciences. This problem is especially clear when one attempts to account for the increasing use of computational methods for performing tasks that were traditionally accomplished by measuring instruments. As Margaret Morrison (2009) and Wendy Parker (2017) argue, there are cases where reliable quantitative information is gathered about a target system with the aid of a computer simulation, but in a manner that satisfies some of the central desiderata for measurement such as being empirically grounded and backward-looking (see also Lusk 2016). Such information does not rely on signals transmitted from the particular object of interest to the instrument, but on the use of theoretical and statistical models to process empirical data about related objects. For example, data assimilation methods are customarily used to estimate past atmospheric temperatures in regions where thermometer readings are not available. Some methods do this by fitting a computational model of the atmosphere’s behavior to a combination of available data from nearby regions and a model-based forecast of conditions at the time of observation (Parker 2017). These estimations are then used in various ways, including as data for evaluating forward-looking climate models. Regardless of whether one calls these estimations “measurements”, they challenge the idea that producing reliable quantitative evidence about the state of an object requires observing that object, however loosely one understands the term “observation”. [ 23 ]
Two key aspects of the reliability of measurement outcomes are accuracy and precision. Consider a series of repeated weight measurements performed on a particular object with an equal-arms balance. From a realist, “error-based” perspective, the outcomes of these measurements are accurate if they are close to the true value of the quantity being measured—in our case, the true ratio of the object’s weight to the chosen unit—and precise if they are close to each other. An analogy often cited to clarify the error-based distinction is that of arrows shot at a target, with accuracy analogous to the closeness of hits to the bull’s eye and precision analogous to the tightness of spread of hits (cf. JCGM 2012: 2.13 & 2.15, Teller 2013: 192). Though intuitive, the error-based way of carving the distinction raises an epistemological difficulty. It is commonly thought that the exact true values of most quantities of interest to science are unknowable, at least when those quantities are measured on continuous scales. If this assumption is granted, the accuracy with which such quantities are measured cannot be known with exactitude, but only estimated by comparing inaccurate measurements to each other. And yet it is unclear why convergence among inaccurate measurements should be taken as an indication of truth. After all, the measurements could be plagued by a common bias that prevents their individual inaccuracies from cancelling each other out when averaged. In the absence of cognitive access to true values, how is the evaluation of measurement accuracy possible?
In answering this question, philosophers have benefited from studying the various senses of the term “measurement accuracy” as used by practicing scientists. At least five different senses have been identified: metaphysical, epistemic, operational, comparative and pragmatic (Tal 2011: 1084–5). In particular, the epistemic or “uncertainty-based” sense of the term is metaphysically neutral and does not presuppose the existence of true values. Instead, the accuracy of a measurement outcome is taken to be the closeness of agreement among values reasonably attributed to a quantity given available empirical data and background knowledge (cf. JCGM 2012: 2.13 Note 3; Giordani & Mari 2012; de Courtenay and Grégis 2017). Thus construed, measurement accuracy can be evaluated by establishing robustness among the consequences of models representing different measurement processes (Basso 2017; Tal 2017b; Bokulich 2020; Staley 2020).
Under the uncertainty-based conception, imprecision is a special type of inaccuracy. For example, the inaccuracy of weight measurements is the breadth of spread of values that are reasonably attributed to the object’s weight given the indications of the balance and available background knowledge about the way the balance works and the standard weights used. The imprecision of these measurements is the component of inaccuracy arising from uncontrolled variations to the indications of the balance over repeated trials. Other sources of inaccuracy besides imprecision include imperfect corrections to systematic errors, inaccurately known physical constants, and vague measurand definitions, among others (see Section 7.1 ).
Paul Teller (2018) raises a different objection to the error-based conception of measurement accuracy. He argues against an assumption he calls “measurement accuracy realism”, according to which measurable quantities have definite values in reality. Teller argues that this assumption is false insofar as it concerns the quantities habitually measured in physics, because any specification of definite values (or value ranges) for such quantities involves idealization and hence cannot refer to anything in reality. For example, the concept usually understood by the phrase “the velocity of sound in air” involves a host of implicit idealizations concerning the uniformity of the air’s chemical composition, temperature and pressure as well as the stability of units of measurement. Removing these idealizations completely would require adding infinite amount of detail to each specification. As Teller argues, measurement accuracy should itself be understood as a useful idealization, namely as a concept that allows scientists to assess coherence and consistency among measurement outcomes as if the linguistic expression of these outcomes latched onto anything in the world. Precision is similarly an idealized concept, which is based on an open-ended and indefinite specification of what counts as repetition of measurement under “the same” circumstances (Teller 2013: 194).
- Alder, K., 2002, The Measure of All Things: The Seven-Year Odyssey and Hidden Error That Transformed the World , New York: The Free Press.
- Alexandrova, A., 2008, “First Person Reports and the Measurement of Happiness”, Philosophical Psychology , 21(5): 571–583.
- –––, 2017, A Philosophy for the Science of Well-Being , Oxford: Oxford University Press.
- Alexandrova, A. and D.M. Haybron, 2016, “Is Construct Validation Valid?” Philosophy of Science , 83(5): 1098–1109.
- Angner, E., 2008, “The Philosophical Foundations of Subjective Measures of Well-Being”, in Capabilities and Happiness , L. Bruni, F. Comim, and M. Pugno (eds.), Oxford: Oxford University Press.
- –––, 2013, “Is it Possible to Measure Happiness? The argument from measurability”, European Journal for Philosophy of Science , 3: 221–240.
- Aristotle, Categories , in The Complete Works of Aristotle , Volume I, J. Barnes (ed.), Princeton: Princeton University Press, 1984.
- Baird, D., 2004, Thing Knowledge: A Philosophy of Scientific Instruments , Berkeley: University of California Press.
- Barwich, A.S., and H. Chang, 2015, “Sensory Measurements: Coordination and Standardization”, Biological Theory , 10(3): 200–211.
- Basso, A., 2017, “The Appeal to Robustness in Measurement Practice”, Studies in History and Philosophy of Science Part A , 65: 57–66.
- Biagioli, F., 2016, Space, Number, and Geometry from Helmholtz to Cassirer , Dordrecht: Springer.
- –––, 2018, “Cohen and Helmholtz on the Foundations of Measurement”, in C. Damböck (ed.), Philosophie Und Wissenschaft Bei Hermann Cohen – Philosophy and Science in Hermann Cohen , Dordrecht: Springer, 77–100.
- BIPM (Bureau International des Poids et Mesures), 2019, The International System of Units (SI Brochure), 9th Edition. [ BIPM 2019 available online ]
- Bogen, J. and J. Woodward, 1988, “Saving the Phenomena”, The Philosophical Review , 97(3): 303–352.
- Bokulich, A., 2020, “Calibration, Coherence, and Consilience in Radiometric Measures of Geologic Time”, Philosophy of Science , 87(3): 425–56.
- Boring, E.G., 1945, “The use of operational definitions in science”, in Boring et al. 1945: 243–5.
- Boring, E.G., P.W. Bridgman, H. Feigl, H. Israel, C.C Pratt, and B.F. Skinner, 1945, “Symposium on Operationism”, The Psychological Review , 52: 241–294.
- Borsboom, D., 2005, Measuring the Mind: Conceptual Issues in Contemporary Psychometrics , Cambridge: Cambridge University Press.
- Borsboom, D., and G.J. Mellenbergh, 2004, “Why psychometrics is not pathological: A comment on Michell”, Theory & Psychology , 14: 105–120.
- Boumans, M., 1999, “Representation and Stability in Testing and Measuring Rational Expectations”, Journal of Economic Methodology , 6(3): 381–401.
- –––, 2005a, How Economists Model the World into Numbers , New York: Routledge.
- –––, 2005b, “Truth versus Precision”, in Logic, Methodology and Philosophy of Science: Proceedings of the Twelfth International Congress , P. Hájek, L. Valdés-Villanueva, and D. Westerstahl (eds.), London: College Publications, pp. 257–269.
- –––, 2005c, “Measurement outside the laboratory”, Philosophy of Science , 72: 850–863.
- –––, 2006, “The difference between answering a ‘why’ question and answering a ‘how much’ question”, in Simulation: Pragmatic Construction of Reality , J. Lenhard, G Küppers, and T Shinn (eds.), Dordrecht: Springer, pp. 107–124.
- –––, 2007a, “Invariance and Calibration”, in 2007: 231–248.
- ––– (ed.), 2007b, Measurement in Economics: A Handbook , London: Elsevier.
- –––, 2009, “Grey-Box Understanding in Economics”, in Scientific Understanding: Philosophical Perspectives , H.W. de Regt, S. Leonelli, and K. Eigner, Pittsburgh: University of Pittsburgh Press, pp. 210–229.
- –––, 2012a, “Modeling Strategies for Measuring Phenomena In- and Outside the Laboratory”, in EPSA Philosophy of Science: Amsterdam 2009 (The European Philosophy of Science Association Proceedings), H.W. de Regt, S. Hartmann, and S. Okasha (eds.), Dordrecht: Springer, pp. 1–11.
- –––, 2012b, “Measurement in Economics”, in Philosophy of Economics (Handbook of the Philosophy of Science: Volume 13), University of Mäki (ed.), Oxford: Elsevier, pp. 395–423.
- –––, 2015, Science Outside the Laboratory: Measurement in Field Science and Economics , Oxford: Oxford University Press.
- Bridgman, P.W., 1927, The Logic of Modern Physics , New York: Macmillan.
- –––, 1938, “Operational Analysis”, Philosophy of Science , 5: 114–131.
- –––, 1945, “Some General Principles of Operational Analysis”, in Boring et al. 1945: 246–249.
- –––, 1956, “The Present State of Operationalism”, in Frank 1956: 74–79.
- Brillouin, L., 1962, Science and information theory , New York: Academic Press, 2nd edition.
- Byerly, H.C. and V.A. Lazara, 1973, “Realist Foundations of Measurement”, Philosophy of Science , 40(1): 10–28.
- Campbell, N.R., 1920, Physics: the Elements , London: Cambridge University Press.
- Campbell, D.T. and D.W. Fiske, 1959, “Convergent and discriminant validation by the multitrait-multimethod matrix”, Psychological Bulletin , 56(2): 81–105.
- Cantù, P. and O. Schlaudt (eds.), 2013, “The Epistemological Thought of Otto Hölder”, special issue of Philosophia Scientiæ , 17(1).
- Carnap, R., 1966, Philosophical foundations of physics , G. Martin (ed.), reprinted as An Introduction to the Philosophy of Science , NY: Dover, 1995.
- Carrier, M., 1994, The Completeness of Scientific Theories: On the Derivation of Empirical Indicators Within a Theoretical Framework: the Case of Physical Geometry , The University of Western Ontario Series in Philosophy of Science Vol. 53, Dordrecht: Kluwer.
- Cartwright, N.L., 1999, The Dappled World: A Study of the Boundaries of Science , Cambridge: Cambridge University Press.
- Cartwright, N.L. and R. Runhardt, 2014, “Measurement”, in N.L. Cartwright and E. Montuschi (eds.), Philosophy of Social Science: A New Introduction , Oxford: Oxford University Press, pp. 265–287.
- Chang, H., 2001, “Spirit, air, and quicksilver: The search for the ‘real’ scale of temperature”, Historical Studies in the Physical and Biological Sciences , 31(2): 249–284.
- –––, 2004, Inventing Temperature: Measurement and Scientific Progress , Oxford: Oxford University Press.
- –––, 2007, “Scientific Progress: Beyond Foundationalism and Coherentism”, Royal Institute of Philosophy Supplement , 61: 1–20.
- –––, 2009, “Operationalism”, The Stanford Encyclopedia of Philosophy (Fall 2009 Edition), E.N. Zalta (ed.), URL= < https://plato.stanford.edu/archives/fall2009/entries/operationalism/ >
- Chang, H. and N.L. Cartwright, 2008, “Measurement”, in The Routledge Companion to Philosophy of Science , S. Psillos and M. Curd (eds.), New York: Routledge, pp. 367–375.
- Clagett, M., 1968, Nicole Oresme and the medieval geometry of qualities and motions , Madison: University of Wisconsin Press.
- Cohen, M.R. and E. Nagel, 1934, An introduction to logic and scientific method , New York: Harcourt, Brace & World.
- Crease, R.P., 2011, World in the Balance: The Historic Quest for an Absolute System of Measurement , New York and London: W.W. Norton.
- Darrigol, O., 2003, “Number and measure: Hermann von Helmholtz at the crossroads of mathematics, physics, and psychology”, Studies in History and Philosophy of Science Part A , 34(3): 515–573.
- de Courtenay, N., O. Darrigol, and O. Schlaudt (eds.), 2019, The Reform of the International System of Units (SI): Philosophical, Historical and Sociological Issues , London and New York: Routledge.
- de Courtenay, N. and F. Grégis, 2017, “The evaluation of measurement uncertainties and its epistemological ramifications”, Studies in History and Philosophy of Science (Part A), 65: 21–32.
- Diehl, C.E., 2012, The Theory of Intensive Magnitudes in Leibniz and Kant , Ph.D. Dissertation, Princeton University. [ Diehl 2012 available online ]
- Diez, J.A., 1997a, “A Hundred Years of Numbers. An Historical Introduction to Measurement Theory 1887–1990—Part 1”, Studies in History and Philosophy of Science , 28(1): 167–185.
- –––, 1997b, “A Hundred Years of Numbers. An Historical Introduction to Measurement Theory 1887–1990—Part 2”, Studies in History and Philosophy of Science , 28(2): 237–265.
- Dingle, H., 1950, “A Theory of Measurement”, The British Journal for the Philosophy of Science , 1(1): 5–26.
- Duhem, P., 1906, The Aim and Structure of Physical Theory , P.P. Wiener (trans.), New York: Atheneum, 1962.
- Ellis, B., 1966, Basic Concepts of Measurement , Cambridge: Cambridge University Press.
- Euclid, Elements , in The Thirteen Books of Euclid’s Elements , T.L. Heath (trans.), Cambridge: Cambridge University Press, 1908.
- Fechner, G., 1860, Elements of Psychophysics , H.E. Adler (trans.), New York: Holt, Reinhart & Winston, 1966.
- Feest, U., 2005, “Operationism in Psychology: What the Debate Is About, What the Debate Should Be About”, Journal of the History of the Behavioral Sciences , 41(2): 131–149.
- –––, 2020, “Construct Validity in Psychological Tests–the Case of Implicit Social Cognition”, European Journal for Philosophy of Science , 10(1): 4.
- Ferguson, A., C.S. Myers, R.J. Bartlett, H. Banister, F.C. Bartlett, W. Brown, N.R. Campbell, K.J.W. Craik, J. Drever, J. Guild, R.A. Houstoun, J.O. Irwin, G.W.C. Kaye, S.J.F. Philpott, L.F. Richardson, J.H. Shaxby, T. Smith, R.H. Thouless, and W.S. Tucker, 1940, “Quantitative estimates of sensory events”, Advancement of Science , 2: 331–349. (The final report of a committee appointed by the British Association for the Advancement of Science in 1932 to consider the possibility of measuring intensities of sensation. See Michell 1999, Ch 6. for a detailed discussion.)
- Finkelstein, L., 1975, “Representation by symbol systems as an extension of the concept of measurement”, Kybernetes , 4(4): 215–223.
- –––, 1977, “Introductory article”, (instrument science), Journal of Physics E: Scientific Instruments , 10(6): 566–572.
- Frank, P.G. (ed.), 1956, The Validation of Scientific Theories . Boston: Beacon Press. (Chapter 2, “The Present State of Operationalism” contains papers by H. Margenau, G. Bergmann, C.G. Hempel, R.B. Lindsay, P.W. Bridgman, R.J. Seeger, and A. Grünbaum)
- Franklin, A., 1986, The Neglect of Experiment , Cambridge: Cambridge University Press.
- –––, 1997, “Calibration”, Perspectives on Science , 5(1): 31–80.
- Franklin, A., M. Anderson, D. Brock, S. Coleman, J. Downing, A. Gruvander, J. Lilly, J. Neal, D. Peterson, M. Price, R. Rice, L. Smith, S. Speirer, and D. Toering, 1989, “Can a Theory-Laden Observation Test the Theory?”, The British Journal for the Philosophy of Science , 40(2): 229–231.
- Frigerio, A., A. Giordani, and L. Mari, 2010, “Outline of a general model of measurement”, Synthese , 175(2): 123–149.
- Galison, P., 2003, Einstein’s Clocks, Poincaré’s Maps: Empires of Time , New York and London: W.W. Norton.
- Gillies, D.A., 1972, “Operationalism”, Synthese , 25(1): 1–24.
- Giordani, A., and L. Mari, 2012, “Measurement, models, and uncertainty”, IEEE Transactions on Instrumentation and Measurement , 61(8): 2144–2152.
- Gooday, G., 2004, The Morals of Measurement: Accuracy, Irony and Trust in Late Victorian Electrical Practice , Cambridge: Cambridge University Press.
- Grant, E., 1996, The foundations of modern science in the middle ages , Cambridge: Cambridge University Press.
- Grattan-Guinness, I., 1996, “Numbers, magnitudes, ratios, and proportions in Euclid’s Elements: How did he handle them?”, Historia Mathematica , 23: 355–375.
- Grégis, F., 2015, “Can We Dispense with the Notion of ‘True Value’ in Metrology?”, in Schlaudt and Huber 2015, 81–93.
- Guala, F., 2008, “Paradigmatic Experiments: The Ultimatum Game from Testing to Measurement Device”, Philosophy of Science , 75: 658–669.
- Hacking, I, 1983, Representing and Intervening , Cambridge: Cambridge University Press.
- Harré, R., 1981, Great Scientific Experiments: Twenty Experiments that Changed our View of the World , Oxford: Phaidon Press.
- Hartley, R.V., 1928, “Transmission of information”, Bell System technical journal , 7(3): 535–563.
- Heidelberger, M., 1993a, Nature from Within: Gustav Theodore Fechner and His Psychophysical Worldview , C. Klohr (trans.), Pittsburgh: University of Pittsburgh Press, 2004.
- –––, 1993b, “Fechner’s impact for measurement theory”, commentary on D.J. Murray, “A perspective for viewing the history of psychophysics”, Behavioural and Brain Sciences , 16(1): 146–148.
- von Helmholtz, H., 1887, Counting and measuring , C.L. Bryan (trans.), New Jersey: D. Van Nostrand, 1930.
- Hempel, C.G., 1952, Fundamentals of concept formation in empirical science , International Encyclopedia of Unified Science, Vol. II. No. 7, Chicago and London: University of Chicago Press.
- –––, 1956, “A logical appraisal of operationalism”, in Frank 1956: 52–67.
- –––, 1966, Philosophy of Natural Science , Englewood Cliffs, N.J.: Prentice-Hall.
- Hölder, O., 1901, “Die Axiome der Quantität und die Lehre vom Mass”, Berichte über die Verhandlungen der Königlich Sächsischen Gesellschaft der Wissenschaften zu Leipzig, Mathematische-Physische Klasse , 53: 1–64. (for an excerpt translated into English see Michell and Ernst 1996)
- Hood, S.B., 2009, “Validity in Psychological Testing and Scientific Realism”, Theory & Psychology , 19(4): 451–473.
- –––, 2013, “Psychological Measurement and Methodological Realism”, Erkenntnis , 78(4): 739–761.
- Hoover, K. and M. Dowell, 2001, “Measuring Causes: Episodes in the Quantitative Assessment of the Value of Money”, in The Age of Economic Measurement (Supplement to History of Political Economy : Volume 33), J. Klein and M. Morgan (eds.), pp. 137–161.
- Isaac, A.M. C., 2017, “Hubris to Humility: Tonal Volume and the Fundamentality of Psychophysical Quantities”, Studies in History and Philosophy of Science (Part A), 65–66: 99–111.
- Israel-Jost, V., 2011, “The Epistemological Foundations of Scientific Observation”, South African Journal of Philosophy , 30(1): 29–40.
- JCGM (Joint Committee for Guides in Metrology), 2012, International Vocabulary of Metrology—Basic and general concepts and associated terms (VIM), 3rd edition with minor corrections, Sèvres: JCGM. [ JCGM 2012 available online ]
- Jorgensen, L.M., 2009, “The Principle of Continuity and Leibniz’s Theory of Consciousness”, Journal of the History of Philosophy , 47(2): 223–248.
- Jung, E., 2011, “Intension and Remission of Forms”, in Encyclopedia of Medieval Philosophy , H. Lagerlund (ed.), Netherlands: Springer, pp. 551–555.
- Kant, I., 1787, Critique of Pure Reason , P. Guyer and A.W. Wood (trans.), Cambridge: Cambridge University Press, 1998.
- Kirpatovskii, S.I., 1974, “Principles of the information theory of measurements”, Izmeritel’naya Tekhnika , 5: 11–13, English translation in Measurement Techniques , 17(5): 655–659.
- Krantz, D.H., R.D. Luce, P. Suppes, and A. Tversky, 1971, Foundations of Measurement Vol 1: Additive and Polynomial Representations , San Diego and London: Academic Press. (For references to the two other volumes see Suppes et al. 1989 and Luce et al. 1990.)
- von Kries, J., 1882, “Über die Messung intensiver Grösse und über das sogenannte psychophysiches Gesetz”, Vierteljahrschrift für wissenschaftliche Philosophie (Leipzig), 6: 257–294.
- Kuhn, T.S., 1961, “The Function of Measurement in Modern Physical Sciences”, Isis , 52(2): 161–193.
- Kyburg, H.E. Jr., 1984, Theory and Measurement , Cambridge: Cambridge University Press.
- Latour, B., 1987, Science in Action , Cambridge: Harvard University Press.
- Leplège, A., 2003, “Epistemology of Measurement in the Social Sciences: Historical and Contemporary Perspectives”, Social Science Information , 42: 451–462.
- Luce, R.D., D.H. Krantz, P. Suppes, and A. Tversky, 1990, Foundations of Measurement (Volume 3: Representation, Axiomatization, and Invariance), San Diego and London: Academic Press. (For references to the two other volumes see Krantz et al. 1971 and Suppes et al. 1989.)
- Luce, R.D., and J.W. Tukey, 1964, “Simultaneous conjoint measurement: A new type of fundamental measurement”, Journal of mathematical psychology , 1(1): 1–27.
- Luce, R.D. and P. Suppes, 2004, “Representational Measurement Theory”, in Stevens’ Handbook of Experimental Psychology (Volume 4: Methodology in Experimental Psychology), J. Wixted and H. Pashler (eds.), New York: Wiley, 3rd edition, pp. 1–41.
- Lusk, G., 2016, “Computer simulation and the features of novel empirical data”, Studies in History and Philosophy of Science Part A , 56: 145–152.
- Mach, E., 1896, Principles of the Theory of Heat , T.J. McCormack (trans.), Dordrecht: D. Reidel, 1986.
- Mari, L., 1999, “Notes towards a qualitative analysis of information in measurement results”, Measurement , 25(3): 183–192.
- –––, 2000, “Beyond the representational viewpoint: a new formalization of measurement”, Measurement , 27: 71–84.
- –––, 2003, “Epistemology of Measurement”, Measurement , 34: 17–30.
- –––, 2005a, “The problem of foundations of measurement”, Measurement , 38: 259–266.
- –––, 2005b, “Models of the Measurement Process”, in Handbook of Measuring Systems Design , vol. 2, P. Sydenman and R. Thorn (eds.), Wiley, Ch. 104.
- Mari, L., and M. Wilson, 2014, “An introduction to the Rasch measurement approach for metrologists”, Measurement , 51: 315–327.
- Mari, L. and A. Giordani, 2013, “Modeling measurement: error and uncertainty,”, in Error and Uncertainty in Scientific Practice , M. Boumans, G. Hon, and A. Petersen (eds.), Ch. 4.
- Maxwell, J.C., 1873, A Treatise on Electricity and Magnetism , Oxford: Clarendon Press.
- McClimans, L., 2010, “A theoretical framework for patient-reported outcome measures”, Theoretical Medicine and Bioethics , 31: 225–240.
- –––, 2017, “Psychological Measures, Risk, and Values”, In Measurement in Medicine: Philosophical Essays on Assessment and Evaluation , L. McClimans (ed.), London and New York: Rowman & Littlefield, 89–106.
- McClimans, L. and P. Browne, 2012, “Quality of life is a process not an outcome”, Theoretical Medicine and Bioethics , 33: 279–292.
- McClimans, L., J. Browne, and S. Cano, 2017, “Clinical Outcome Measurement: Models, Theory, Psychometrics and Practice”, Studies in History and Philosophy of Science (Part A), 65: 67–73.
- Michel, M., 2019, “The Mismeasure of Consciousness: A Problem of Coordination for the Perceptual Awareness Scale”, Philosophy of Science , 86(5): 1239–49.
- Michell, J., 1993, “The origins of the representational theory of measurement: Helmholtz, Hölder, and Russell”, Studies in History and Philosophy of Science (Part A), 24(2): 185–206.
- –––, 1994, “Numbers as Quantitative Relations and the Traditional Theory of Measurement”, British Journal for the Philosophy of Science , 45: 389–406.
- –––, 1999, Measurement in Psychology: Critical History of a Methodological Concept , Cambridge: Cambridge University Press.
- –––, 2000, “Normal science, pathological science and psychometrics”, Theory & Psychology , 10: 639–667.
- –––, 2003, “Epistemology of Measurement: the Relevance of its History for Quantification in the Social Sciences”, Social Science Information , 42(4): 515–534.
- –––, 2004a, “History and philosophy of measurement: A realist view”, in Proceedings of the 10th IMEKO TC7 International symposium on advances of measurement science , [ Michell 2004 available online ]
- –––, 2004b, “Item response models, pathological science and the shape of error: Reply to Borsboom and Mellenbergh”, Theory & Psychology , 14: 121–129.
- –––, 2005, “The logic of measurement: A realist overview”, Measurement , 38(4): 285–294.
- Michell, J. and C. Ernst, 1996, “The Axioms of Quantity and the Theory of Measurement”, Journal of Mathematical Psychology , 40: 235–252. (This article contains a translation into English of a long excerpt from Hölder 1901.)
- Mitchell, D.J., E. Tal, and H. Chang, 2017, “The Making of Measurement: Editors’ Introduction.” Studies in History and Philosophy of Science (Part A), 65–66: 1–7.
- Miyake, T., 2017, “Uncertainty and Modeling in Seismology”, in Mößner & Nordmann (eds.) 2017, 232–244.
- Morgan, M., 2001, “Making measuring instruments”, in The Age of Economic Measurement (Supplement to History of Political Economy : Volume 33), J.L. Klein and M. Morgan (eds.), pp. 235–251.
- Morgan, M. and M. Morrison (eds.), 1999, Models as Mediators: Perspectives on Natural and Social Science , Cambridge: Cambridge University Press.
- Morrison, M., 1999, “Models as Autonomous Agents”, in Morgan and Morrison 1999: 38–65.
- –––, 2009, “Models, measurement and computer simulation: the changing face of experimentation”, Philosophical Studies , 143: 33–57.
- Morrison, M. and M. Morgan, 1999, “Models as Mediating Instruments”, in Morgan and Morrison 1999: 10–37.
- Mößner, N. and A. Nordmann (eds.), 2017, Reasoning in Measurement , London and New York: Routledge.
- Mundy, B., 1987, “The metaphysics of quantity”, Philosophical Studies , 51(1): 29–54.
- Nagel, E., 1931, “Measurement”, Erkenntnis , 2(1): 313–333.
- Narens, L., 1981, “On the scales of measurement”, Journal of Mathematical Psychology , 24: 249–275.
- –––, 1985, Abstract Measurement Theory , Cambridge, MA: MIT Press.
- Nunnally, J.C., and I.H. Bernstein, 1994, Psychometric Theory , New York: McGraw-Hill, 3rd edition.
- Padovani, F., 2015, “Measurement, Coordination, and the Relativized a Priori”, Studies in History and Philosophy of Science (Part B: Studies in History and Philosophy of Modern Physics), 52: 123–28.
- –––, 2017, “Coordination and Measurement: What We Get Wrong About What Reichenbach Got Right”, In M. Massimi, J.W. Romeijn, and G. Schurz (eds.), EPSA15 Selected Papers (European Studies in Philosophy of Science), Cham: Springer International Publishing, 49–60.
- Parker, W., 2017, “Computer Simulation, Measurement, and Data Assimilation”, British Journal for the Philosophy of Science , 68(1): 273–304.
- Poincaré, H., 1898, “The Measure of Time”, in The Value of Science , New York: Dover, 1958, pp. 26–36.
- –––, 1902, Science and Hypothesis , W.J. Greenstreet (trans.), New York: Cosimo, 2007.
- Porter, T.M., 1995, Trust in Numbers: The Pursuit of Objectivity in Science and Public Life , New Jersey: Princeton University Press.
- –––, 2007, “Precision”, in Boumans 2007b: 343–356.
- Rasch, G., 1960, Probabilistic Models for Some Intelligence and Achievement Tests , Copenhagen: Danish Institute for Educational Research.
- Reiss, J., 2001, “Natural Economic Quantities and Their Measurement”, Journal of Economic Methodology , 8(2): 287–311.
- Riordan, S., 2015, “The Objectivity of Scientific Measures”, Studies in History and Philosophy of Science (Part A), 50: 38–47.
- Reichenbach, H., 1927, The Philosophy of Space and Time , New York: Dover Publications, 1958.
- Rothbart, D. and S.W. Slayden, 1994, “The Epistemology of a Spectrometer”, Philosophy of Science , 61: 25–38.
- Russell, B., 1903, The Principles of Mathematics , New York: W.W. Norton.
- Savage, C.W. and P. Ehrlich, 1992, “A brief introduction to measurement theory and to the essays”, in Philosophical and Foundational Issues in Measurement Theory , C.W. Savage and P. Ehrlich (eds.), New Jersey: Lawrence Erlbaum, pp. 1–14.
- Schaffer, S., 1992, “Late Victorian metrology and its instrumentation: a manufactory of Ohms”, in Invisible Connections: Instruments, Institutions, and Science , R. Bud and S.E. Cozzens (eds.), Cardiff: SPIE Optical Engineering, pp. 23–56.
- Schlaudt, O. and Huber, L. (eds.), 2015, Standardization in Measurement: Philosophical, Historical and Sociological Issues , London and New York: Routledge.
- Scott, D. and P. Suppes, 1958, “Foundational aspects of theories of measurement”, Journal of Symbolic Logic , 23(2): 113–128.
- Shannon, C.E., 1948, “A Mathematical Theory of Communication”, The Bell System Technical Journal , 27: 379–423 and 623–656.
- Shannon, C.E. and W. Weaver, 1949, A Mathematical Theory of Communication , Urbana: The University of Illinois Press.
- Shapere, D., 1982, “The Concept of Observation in Science and Philosophy”, Philosophy of Science , 49(4): 485–525.
- Skinner, B.F., 1945, “The operational analysis of psychological terms”, in Boring et al. 1945: 270–277.
- Soler, L., F. Wieber, C. Allamel-Raffin, J.L. Gangloff, C. Dufour, and E. Trizio, 2013, “Calibration: A Conceptual Framework Applied to Scientific Practices Which Investigate Natural Phenomena by Means of Standardized Instruments”, Journal for General Philosophy of Science , 44(2): 263–317.
- Staley, K. W., 2020, “Securing the empirical value of measurement results”, The British Journal for the Philosophy of Science , 71(1): 87–113.
- Stegenga, J., 2018, Medical Nihilism , Oxford: Oxford University Press.
- Stevens, S.S., 1935, “The operational definition of psychological concepts”, Psychological Review , 42(6): 517–527.
- –––, 1946, “On the theory of scales of measurement”, Science , 103: 677–680.
- –––, 1951, “Mathematics, Measurement, Psychophysics”, in Handbook of Experimental Psychology , S.S. Stevens (ed.), New York: Wiley & Sons, pp. 1–49.
- –––, 1959, “Measurement, psychophysics and utility”, in Measurement: Definitions and Theories , C.W. Churchman and P. Ratoosh (eds.), New York: Wiley & Sons, pp. 18–63.
- –––, 1975, Psychophysics: Introduction to Its Perceptual, Neural and Social Prospects , New York: Wiley & Sons.
- Suppes, P., 1951, “A set of independent axioms for extensive quantities”, Portugaliae Mathematica , 10(4): 163–172.
- –––, 1960, “A Comparison of the Meaning and Uses of Models in Mathematics and the Empirical Sciences”, Synthese , 12(2): 287–301.
- –––, 1962, “Models of Data”, in Logic, methodology and philosophy of science: proceedings of the 1960 International Congress , E. Nagel (ed.), Stanford: Stanford University Press, pp. 252–261.
- –––, 1967, “What is a Scientific Theory?”, in Philosophy of Science Today , S. Morgenbesser (ed.), New York: Basic Books, pp. 55–67.
- Suppes, P., D.H. Krantz, R.D. Luce, and A. Tversky, 1989, Foundations of Measurement Vol 2: Geometrical, Threshold and Probabilistic Representations , San Diego and London: Academic Press. (For references to the two other volumes see Krantz et al. 1971 and Luce et al. 1990.)
- Swoyer, C., 1987, “The Metaphysics of Measurement”, in Measurement, Realism and Objectivity , J. Forge (ed.), Reidel, pp. 235–290.
- Sylla, E., 1971, “Medieval quantifications of qualities: The ‘Merton School’”, Archive for history of exact sciences , 8(1): 9–39.
- Tabor, D., 1970, “The hardness of solids”, Review of Physics in Technology , 1(3): 145–179.
- Tal, E., 2011, “How Accurate Is the Standard Second?”, Philosophy of Science , 78(5): 1082–96.
- –––, 2013, “Old and New Problems in Philosophy of Measurement”, Philosophy Compass , 8(12): 1159–1173.
- –––, 2016a, “Making Time: A Study in the Epistemology of Measurement”, British Journal for the Philosophy of Science , 67(1): 297–335
- –––, 2016b, “How Does Measuring Generate Evidence? The Problem of Observational Grounding”, Journal of Physics: Conference Series , 772: 012001.
- –––, 2017a, “A Model-Based Epistemology of Measurement”, in Mößner & Nordmann (eds.) 2017, 233–253.
- –––, 2017b, “Calibration: Modelling the Measurement Process”, Studies in History and Philosophy of Science (Part A), 65: 33–45.
- –––, 2018, “Naturalness and Convention in the International System of Units”, Measurement , 116: 631–643.
- Teller, P., 2013, “The concept of measurement-precision”, Synthese , 190: 189–202.
- –––, 2018, “Measurement Accuracy Realism”, in I. Peschard and B.C. van Fraassen (eds.), The Experimental Side of Modeling , Minneapolis: University of Minnesota Press, 273–98.
- Thomson, W., 1889, “Electrical Units of Measurement”, in Popular Lectures and Addresses (Volume 1), London: MacMillan, pp. 73–136.
- Trout, J.D., 1998, Measuring the intentional world: Realism, naturalism, and quantitative methods in the behavioral sciences , Oxford: Oxford University Press.
- –––, 2000, “Measurement”, in A Companion to the Philosophy of Science , W.H. Newton-Smith (ed.), Malden, MA: Blackwell, pp. 265–276.
- van Fraassen, B.C., 1980, The Scientific Image , Oxford: Clarendon Press.
- –––, 2008, Scientific Representation: Paradoxes of Perspective , Oxford: Oxford University Press.
- –––, 2009, “The perils of Perrin, in the hands of philosophers”, Philosophical Studies , 143: 5–24.
- –––, 2012, “Modeling and Measurement: The Criterion of Empirical Grounding”, Philosophy of Science , 79(5): 773–784.
- Vessonen, E., 2019. “Operationalism and Realism in Psychometrics”, Philosophy Compass , 14(10): e12624.
- –––, 2020, “The Complementarity of Psychometrics and the Representational Theory of Measurement”, The British Journal for the Philosophy of Science , 71(2): 415–442.
- Wilson, M., 2013, “Using the concept of a measurement system to characterize measurement models used in psychometrics”, Measurement , 46(9): 3766–3774.
- Wise, M.N. (ed.), 1995, The Values of Precision , NJ: Princeton University Press.
- Wise, M.N. and C. Smith, 1986, “Measurement, Work and Industry in Lord Kelvin’s Britain”, Historical Studies in the Physical and Biological Sciences , 17(1): 147–173.
- Wolff, J. E., 2020a, The Metaphysics of Quantities , Oxford: Oxford University Press.
- –––, 2020b, “Heaps of Moles? – Mediating Macroscopic and Microscopic Measurement of Chemical Substances”, Studies in History and Philosophy of Science (Part A), 80: 19–27.
How to cite this entry . Preview the PDF version of this entry at the Friends of the SEP Society . Look up topics and thinkers related to this entry at the Internet Philosophy Ontology Project (InPhO). Enhanced bibliography for this entry at PhilPapers , with links to its database.
- Bradburn,, M., Cartwright, N.L., and Fuller, J., 2016, “ A Theory of Measurement ”, CHESS Working Paper No. 2016-07 (Centre for Humanities Engaging Science and Society), Durham University. (A summary of this paper appears in R.M. Li (ed.), The Importance of Common Metrics for Advancing Social Science Theory and Research: A Workshop Summary , Washington, DC: National Academies Press, 2011, pp. 53–70.)
- Openly accessible guides to metrological terms and methods by the International Bureau of Weights and Measures (BIPM)
- Bibliography on measurement in science at PhilPapers.
Duhem, Pierre | economics: philosophy of | empiricism: logical | Helmholtz, Hermann von | Mach, Ernst | models in science | operationalism | physics: experiment in | Poincaré, Henri | quantum theory: philosophical issues in | Reichenbach, Hans | science: theory and observation in | scientific objectivity | Vienna Circle
Acknowledgments
The author would like to thank Stephan Hartmann, Wendy Parker, Paul Teller, Alessandra Basso, Sally Riordan, Jo Wolff, Conrad Heilmann and participants of the History and Philosophy of Physics reading group at the Department of History and Philosophy of Science at the University of Cambridge for helpful feedback on drafts of this entry. The author is also indebted to Joel Michell and Oliver Schliemann for useful bibliographical advice, and to John Wiley and Sons Publishers for permission to reproduce excerpt from Tal (2013). Work on this entry was supported by an Alexander von Humboldt Postdoctoral Research Fellowship and a Marie Curie Intra-European Fellowship within the 7 th European Community Framework Programme. Work on the 2020 revision of this entry was supported by an FRQSC New Academic grant, a Healthy Brains for Healthy Lives Knowledge Mobilization grant, and funding from the Canada Research Chairs program.
Copyright © 2020 by Eran Tal < eran . tal @ mcgill . ca >
- Accessibility
Support SEP
Mirror sites.
View this site from another server:
- Info about mirror sites
The Stanford Encyclopedia of Philosophy is copyright © 2023 by The Metaphysics Research Lab , Department of Philosophy, Stanford University
Library of Congress Catalog Data: ISSN 1095-5054
What Is Measurement?
- First Online: 26 March 2021
Cite this chapter
- David Torres Irribarra 2
Part of the book series: SpringerBriefs in Psychology ((BRIEFSTHEORET))
411 Accesses
This chapter reviews the central theories and perspectives of measurement that have informed its practice, including the Classical Theory of Measurement, Operationalism, the Representational Theory of Measurement, Latent Variable Modeling, and Metrology.
This is a preview of subscription content, log in via an institution to check access.
Access this chapter
- Available as EPUB and PDF
- Read on any device
- Instant download
- Own it forever
- Compact, lightweight edition
- Dispatched in 3 to 5 business days
- Free shipping worldwide - see info
Tax calculation will be finalised at checkout
Purchases are for personal use only
Institutional subscriptions
Not to be confused with the Classical Test Theory in psychometrics (Lord & Novick 1968 ), despite some overlap in terminology.
According to Michell ( 2011 ) the analysis of multitudes through frequencies is indeed quantitative, but not measurement: “Counting is a quantitative method, but not measurement (although it may be involved in processes of measurement)…” (p. 255). Footnote 1 (page 46) briefly touches on how is counting considered under the pragmatic perspective in this book.
Hölder’s original work was published in 1901 but was not translated into English until the late 1990s by Joel Michell and Catherine Ernst.
“The concept of length is therefore fixed when the operations by which length is measured are fixed: that is, the concept of length involves as much as and nothing more than the set of operations by which length is determined” (Bridgman 1927 , p. 5).
See Michell ( 1999 ) for an overview of the theoretical origins of the rtm and its departure from the classical conception of measurement in the work of Russell, Campbell, and Nagel.
Berka ( 1983 ) points out that this is not Campbell’s only definition throughout his work, characterizing it also as “the process of assigning numbers to represent qualities” (Campbell 1920 , p. 267), “the assignment of numerals to represent properties according to scientific laws” (Campbell 1928 , p. 1), and “the assignment of numerals to things so as to represent facts or conventions about them” (Campbell 1940 , p. 340).
It is worth noting that in his 1946 paper, Stevens discusses and details basic empirical operations associated with each one of the scale types. However, his summary regarding the characterization of measurement focuses exclusively in the rules of assignment and ignores completely any empirical requirements.
Stevens ( 1946 ) states at the beginning of his discussion on interval scales (after discussing both nominal and ordinal scales) that “with the interval scale we come to a form that is “quantitative” in the ordinary sense of the word.” This is one of the only three allusions to quantity in his paper, and the only one outside direct quotes to the baas report.
A detailed overview of amt is outside the scope of this book. Introductory texts to the basics of the theory can be found in Michell ( 1990 ), Narens and Luce ( 1986 ), and Narens ( 2013 ). An extensive treatment can be found in the three volumes of Foundations of Measurement (Krantz et al. 1971/2007 ; Luce et al. 1990/2007 ; Suppes et al. 1989/2007 ).
As an example of the extent to which amt is considered by some scholars to be the definition of measurement, Dawes and Smith ( 1985 ) implicitly assume that anything other than amt is effectively measurement by fiat, which they label as non-representational measurement .
The member organizations are the International Bureau of Weights and Measures (bipm), the International Electrotechnical Commission (iec), the International Federation of Clinical Chemistry and Laboratory Medicine (ifcc), the International Organization for Standardization (iso), the International Union of Pure and Applied Chemistry (iupac), the International Union of Pure and Applied Physics (iupap), the International Organization of Legal Metrology (oiml), and the International Laboratory Accreditation Cooperation (ilac).
Adams, E. W. (1965). Elements of a theory of inexact measurement. Philosophy of Science, 32 (3/4), 205–228.
Article Google Scholar
Adams, E. W. (1966). On the nature and purpose of measurement. Synthese, 16 (2), 125–169.
Adams, E. W. (1979). Measurement theory. In P. Asquith & H. Kyburg (Eds.), Current research in philosophy of science (pp. 207–227). East Lansing, MI: Philosophy of Science Association.
Google Scholar
Aristotle. (2001). Categories. In R. McKeon (Ed.), The basic works of Aristotle (pp. 7–37). New York: Modern Library.
Armstrong, D. M. (1987). Comments on Swoyer and Forge. In J. Forge (Ed.), Measurement, realism and objectivity (pp. 311–317). Holland: Reidel.
Chapter Google Scholar
Berka, K. (1983). Measurement: Its concepts, theories, and problems . Dordrecht: Reidel.
Book Google Scholar
Birnbaum, A. (1968). Some latent trait models and their use in inferring an examinee’s ability. In F. M. Lord & M. R. Novick (Eds.), Statistical theories of mental test scores (pp. 395–479). Reading, MA: Addison-Wesley.
Boring, E. G. (1921). The stimulus-error. The American Journal of Psychology, 32 (4), 449–471.
Boring, E. G. (1961). The beginning and growth of measurement in psychology. In H. Woolf (Ed.), Quantification: A history of the meaning measurement in the natural and social sciences (pp. 108–127). Indianapolis: Bobbs-Merrill.
Boumans, M. (2007). Measurement in economics: A handbook . London: Academic.
Brennan, R. L. (Ed.). (2006). Educational measurement . Westport, CT: Praeger Publishers.
Bridgman, P. W. (1927). The logic of modern physics . New York: Macmillan Co.
Bureau International des Poids et Mesures. (2014). What is Metrology? Retrieved October 12, 2014, from http://www.bipm.org/en/worldwide-metrology/
Campbell, N. R. (1920). Physics: The elements . Cambridge: University Press.
Campbell, N. R. (1928). An account of the principles of measurement and calculation . London: Longmans, Green and Co.
Campbell, N. R. (1940). Notes on Physical Measurement. The Advancement of Science, 1 (2), 340–342.
Chang, H. (2009). Operationalism. Retrieved October 12, 2014, from http://plato.stanford.edu/entries/operationalism/ .
Cliff, N. (1992). Abstract measurement theory and the revolution that never happened. Psychological Science, 3 (3), 186–190.
Crocker, L. M., & Algina, J. (1986). Introduction to classical and modern test theory . New York: Holt, Rinehart, and Winston.
Croon, M. (1990). Latent class analysis with ordered latent classes. British Journal of Mathematical and Statistical Psychology, 43 (2), 171–192.
Croon, M. (1991). Investigating Mokken scalability of dichotomous items by means of ordinal latent class analysis. British Journal of Mathematical and Statistical Psychology, 44 (2), 315–331.
Croon, M. (2002). Ordering the classes. In J. A. Hagenaars & A. L. McCutcheon (Eds.), Applied latent class analysis (pp. 137–162). New York: Cambridge University Press.
Dawes, R., & Smith, T. (1985). Attitude and opinion measurement. In G. Lindzey & E. Aronson (Eds.), The handbook of social psychology (Vol. I, pp. 509–566). Hillsdale, NJ: Lawrence Erlbaum.
de Ayala, R. J. (2009). The theory and practice of item response theory . New York: Guilford Press.
Dingle, H. (1950). A theory of measurement. The British Journal for the Philosophy of Science, 1 (1), 5–26.
Domingue, B. (2014). Evaluating the equal-interval hypothesis with test score scales. Psychometrika, 79 (1), 1–19.
Article PubMed Google Scholar
Duncan, O. D. (1984). Notes on social measurement: Historical and critical . New York: Russell Sage Foundation.
Elmes, D. G., Kantowitz, B. H., & Roediger, H. L. (2012). Research methods in psychology . Melbourne: Wadsworth Cengage Learning.
Estes, W. (1975). Some targets for mathematical psychology. Journal of Mathematical Psychology, 12 (3), 263–282.
Euclid. (1956). The thirteen books of Euclid’s Elements (T. L. Heath, Trans.). New York: Dover Publications.
Falmagne, J.-C. (1976). Random conjoint measurement and loudness summation. Psychological Review, 83 (1), 65–79.
Falmagne, J.-C. (1980). A probabilistic theory of extensive measurement. Philosophy of Science, 47 (2), 277–296.
Finkelstein, L. (2003). Widely, strongly and weakly defined measurement. Measurement, 34 (1), 39–48.
Formann, A. (1995). Linear logistic latent class analysis and the Rasch model. In G. H. Fischer & I. W. Molenaar (Eds.), Rasch models: Foundations, recent developments, and applications (pp. 239–256). New York: Springer-Verlag.
Grace, R. C. (2001). On the failure of operationism. Theory & Psychology, 11 (1), 5–33.
Green, C. D. (1992). Of immortal mythological beasts operationism in psychology. Theory & Psychology, 2 (3), 291–320.
Green, C. D. (2001). Operationism again: What did Bridgman say? What did Bridgman need? Theory & Psychology, 11 (1), 45–51.
Guilford, J. P. (1954). Psychometric methods . New York: McGraw-Hill.
Hagenaars, J. A., & McCutcheon, A. L. (2002). Applied latent class analysis . New York: Cambridge University Press.
Hand, D. J. (2004). Measurement theory and practice . London: Arnold.
Heinen, T. (1996). Latent class and discrete latent trait models: Similarities and differences . Thousand Oaks, CA: Sage.
Hölder, O. (1901). Die Axiome der Quantität und die Lehre vom Mass [The axioms of quantity and the theory of measurement]. In Berichte über die Verhandlungen der Königlich-Sächsischen Gesellschaft der Wissenschaften zu Leipzig: Mathematische-Physische Classe [Reports of the Proceedings of the Royal Saxon Society of Sciences in Leipzig: Mathematical-Physical Division] (Vol. 53, pp. 3–64).
Hornstein, G. A. (1988). Quantifying psychological phenomena: Debates, dilemmas, and implications. In J. G. Morawski (Ed.), The rise of experimentation in american psychology (pp. 1–34). New Haven: Yale University Press.
Huntington, E. V. (1902). A complete set of postulates for the theory of absolute continuous magnitude. Transactions of the American Mathematical Society, 3 (2), 264–279.
International Measurement Confederation. (2014). Table of Technical Committees . Retrieved October 12, 2014, from http://www.imeko.org
Johnson, H. M. (1936). Pseudo-mathematics in the mental and social sciences. The American Journal of Psychology, 48 (2), 342–351.
Joint Committee for Guides in Metrology. (2008). Evaluation of measurement data — Guide to the expression of uncertainty in measurement . Paris: Bureau International des Poids et Mesures.
Joint Committee for Guides in Metrology. (2012). The international vocabulary of metrology – Basic and general concepts and associated terms ( vim ) (3rd ed.). Paris: Bureau International des Poids et Mesures.
Kaarls, R. (2007). Evolving needs for metrology in trade, industry and society and the role of the bipm . Paris: Bureau International des Poids et Mesures.
Koch, S. (1992). Psychology’s Bridgman vs Bridgman’s Bridgman: An essay in reconstruction. Theory and Psychology, 2 (3), 261–290.
Krantz, D. H. (1967). Extensive measurement in semiorders. Philosophy of Science, 34 (4), 348–362.
Krantz, D. H., Luce, R. D., Suppes, P., & Tversky, A. (2007). Foundations of measurement. Volume I: Additive and polynomial representations . Mineola, NY: Dover Publications Inc. (Original work published 1971).
Langeheine, R., & Rost, J. (1988). Latent trait and latent class models . New York: Plenum Press.
Lazarsfeld, P. F. (1961). Notes on the history of quantification in sociology – Trends, sources and problems. In H. Woolf (Ed.), Quantification: A history of the meaning measurement in the natural and social sciences (pp. 147–203). Indianapolis: Bobbs-Merrill.
Lazarsfeld, P. F., & Henry, N. W. (1968). Latent structure analysis . Boston: Houghton Mifflin Company.
Lindquist, E. F., & Thorndike, R. L. (Eds.). (1951). Educational measurement . Washington: American Council on Education.
Lord, F. M., & Novick, M. R. (1968). Statistical theories of mental test scores . Reading, MA: Addison-Wesley Pub. Co.
Lubke, G., & Muthen, B. O. (2005). Investigating population heterogeneity with factor mixture models. Psychological Methods, 10 (1), 21–39.
Luce, R. D. (1956). Semiorders and a theory of utility discrimination. Econometrica, 24 (2), 178–191.
Luce, R. D., Krantz, D. H., Suppes, P., & Tversky, A. (2007). Foundations of measurement. Volume III . Mineola, NY: Dover Publications Inc. (Original work published 1990).
Luce, R. D., & Tukey, J. W. (1964). Simultaneous conjoint measurement: A new type of fundamental measurement. Journal of Mathematical Psychology, 1 (1), 1–27.
Mari, L. (2014). Evolution of 30 years of the International Vocabulary of Metrology ( vim ). Metrologia, 52 (1), R1–R10.
Markus, K. A., & Borsboom, D. (2013). Frontiers of test validity theory: Measurement, causation and meaning . New York: Routledge.
McDonald, R. P. (1999). Test theory a unified treatment . Mahwah, NJ: Lawrence Erlbaum Associates.
Messick, S. (1989). Validity. In R. Linn (Ed.), Educational measurement (pp. 13–104). New York, NY: Macmillan Publishing Company.
Michell, J. (1990). An introduction to the logic of psychological measurement . Hillsdale, NJ: Lawrence Erlbaum Associates, Inc.
Michell, J. (1993). The origins of the representational theory of measurement: Helmholtz, Hölder, and Russell. Studies in History and Philosophy of Science Part A, 24 (2), 185–206.
Michell, J. (1997a). Bertrand Russell’s 1897 critique of the traditional theory of measurement. Synthese, 110 (2), 257–276.
Michell, J. (1997b). Quantitative science and the definition of measurement in psychology. British Journal of Psychology, 88 (3), 355–383.
Michell, J. (1999). Measurement in psychology: Critical history of a methodological concept . New York: Cambridge University Press.
Michell, J. (2005). The logic of measurement: A realist overview. Measurement, 38 (4), 285–294.
Michell, J. (2011). Qualitative research meets the ghost of Pythagoras. Theory & Psychology, 21 (2), 241–259.
Michell, J. (2012b). Alfred Binet and the concept of heterogeneous orders. Frontiers in psychology, 3 (261), 1–8.
Michell, J., & Ernst, C. (1996). The axioms of quantity and the theory of measurement: Translated from Part I of Otto Hölder’s German text “Die Axiome der Quantität und die Lehre vom Mass”. Journal of Mathematical Psychology, 40 (3), 235–252.
Michell, J., & Ernst, C. (1997). The axioms of quantity and the theory of measurement: Translated from Part II of Otto Hölder’s German text “Die Axiome der Quantität und die Lehre vom Mass”. Journal of Mathematical Psychology, 41 (4), 345–356.
Muthen, B. O., & Asparouhov, T. (2006). Item response mixture modeling: Application to tobacco dependence criteria. Addictive Behaviors, 31 (6), 1050–1066.
Nagel, E. (1931). Measurement. Erkenntnis, 2 (1), 313–333.
Narens, L. (2013). Introduction to the theories of measurement and meaningfulness and the use of symmetry in science . Hove: Psychology Press.
Narens, L., & Luce, R. D. (1986). Measurement: The theory of numerical assignments. Psychological Bulletin, 99 (2), 166–180.
Narens, L., & Luce, R. D. (1993). Further comments on the “nonrevolution” arising from axiomatic measurement theory. Psychological Science, 4 (2), 127–130.
Nature Publishing Group. (1939). Quantitative estimates of sensory events. Nature, 144 (3658), 973–973.
Nestor, P. G., & Schutt, R. K. (2014). Research methods in psychology: Investigating human behavior . Thousand Oaks, CA: Sage Publications.
Perline, R., Wright, B. D., & Wainer, H. (1979). The Rasch model as additive conjoint measurement. Applied Psychological Measurement, 3 (2), 237–255.
Pfanzagl, J. (1968). Theory of measurement . New York: Wiley.
Porter, T. M. (1996). Trust in numbers: The pursuit of objectivity in science and public life . Princeton University Press.
Psychometric Society. (2021). Psychometrics & the Psychometric Society. Retrieved October 14, 2014, from https://www.psychometricsociety.org/about-us
Rasch, G. (1960/1980). Probabilistic models for some intelligence and attainment tests . Chicago: University of Chicago Press (Original work published 1960).
Russell, B. (1897). On the Relations of Number and Quantity. Mind, 6 (23), 326–341.
Russell, B. (1903). The principles of mathematics, vol. 1 . Cambridge: University Press.
Schönemann, P. H. (1994). Measurement: The reasonable ineffectiveness of mathematics in the social sciences. Trends and Perspectives in Empirical Social Research , 149–160.
Schwager, K. W. (1991). The representational theory of measurement: An assessment. Psychological Bulletin, 110 (3), 618–626.
Scott, D., & Suppes, P. (1958). Foundational aspects of theories of measurement. Journal of Symbolic Logic, 23 (2), 113–128.
Skrondal, A., & Rabe-Hesketh, S. (2004). Generalized latent variable modeling: Multilevel, longitudinal, and structural equation models . Boca Raton, FL: Chapman & Hall/CRC.
Spearman, C. (1904a). “General Intelligence,” objectively determined and measured. The American Journal of Psychology, 15 (2), 201–292.
Spearman, C. (1904b). The proof and measurement of association between two things. The American Journal of Psychology, 15 (1), 72–101.
Spengler, J. J. (1961). On the progress of quantification in economics. In H. Woolf (Ed.), Quantification: A history of the meaning measurement in the natural and social sciences (pp. 128–146). Indianapolis: Bobbs-Merrill.
Stevens, S. S. (1946). On the theory of scales of measurement. Science, 103 (2684), 677–680.
Stevens, S. S. (1958). Measurement and man. Science, 127 (3295), 383–389.
Suppes, P., Krantz, D. H., Luce, R. D., & Tversky, A. (2007). Foundations of measurement. Volume II . Mineola, NY: Dover Publications Inc. (Original work published 1989).
Suppes, P., & Zinnes, J. L. (1963). Basic measurement theory. In R. D. Luce, R. Bush, & E. Galanter (Eds.), Handbook of mathematical psychology, vol. 1 (pp. 3–76). New York: Wiley.
Torgerson, W. S. (1958). Theory and methods of scaling . New York, NY: Wiley.
Uebersax, J. (1993). Statistical modeling of expert ratings on medical treatment appropriateness. Journal of the American Statistical Association, 88 (422), 421–427.
van der Linden, W. J. (1994). Fundamental measurement and the fundamentals of Rasch measurement. In M. Wilson (Ed.), Objective measurement: Theory into practice (Vol. 2, pp. 3–24). Norwood, NJ: Ablex Pub. Corp.
Van Onna, M. (2004). Ordered latent class models in nonparametric item response theory (Unpublished doctoral dissertation). University of Groningen, The Netherlands.
Velleman, P. F., & Wilkinson, L. (1993). Nominal, ordinal, interval, and ratio typologies are misleading. The American Statistician, 47 (1), 65–72.
Weitzenhoffer, A. M. (1951). Mathematical structures and psychological measurements. Psychometrika, 16 (4), 387–406.
Wilson, M. (2005). Constructing measures: An item response modeling approach . Mahwah, NJ: Lawrence Erlbaum Associates.
Wolins, L. (1978). Interval measurement: Physics, psychophysics, and metaphysics. Educational and Psychological Measurement, 38 (1), 1–9.
Download references
Author information
Authors and affiliations.
Escuela de Psicología, Pontificia Universidad Católica de Chile, Santiago, Chile
David Torres Irribarra
You can also search for this author in PubMed Google Scholar
Rights and permissions
Reprints and permissions
Copyright information
© 2021 The Author(s), under exclusive license to Springer Nature Switzerland AG
About this chapter
Torres Irribarra, D. (2021). What Is Measurement?. In: A Pragmatic Perspective of Measurement. SpringerBriefs in Psychology(). Springer, Cham. https://doi.org/10.1007/978-3-030-74025-2_2
Download citation
DOI : https://doi.org/10.1007/978-3-030-74025-2_2
Published : 26 March 2021
Publisher Name : Springer, Cham
Print ISBN : 978-3-030-74024-5
Online ISBN : 978-3-030-74025-2
eBook Packages : Behavioral Science and Psychology Behavioral Science and Psychology (R0)
Share this chapter
Anyone you share the following link with will be able to read this content:
Sorry, a shareable link is not currently available for this article.
Provided by the Springer Nature SharedIt content-sharing initiative
- Publish with us
Policies and ethics
- Find a journal
- Track your research
Measurement Tools/Research Instruments
- Find a Specific Test
- Find Test for a Variable
- Find QOL Tests
- Find Test Reviews
Electronic Statistics Textbook

Contact Your Library Liaison for More Information
Find your library liaison or submit a question to via this comment box .
Introduction
Measurement tools are instruments used by researchers and practitioners to aid in the assessment or evaluation of subjects, clients or patients. The instruments are used to measure or collect data on a variety of variables ranging from physical functioning to psychosocial wellbeing. Types of measurement tools include scales, indexes, surveys, interviews, and informal observations.
This guide will:
- Walk you through the process for finding measurement tools.
- Demonstrate examples of commonly asked questions (scenarios).
- Highlight resources that can answer questions about measurement tools.
IMPORTANT NOTE : This is an instructional guide. It may not help you find your specific test nor is it a direct link to the full-text of tests.
This guide will provide you with strategies to:
- Find a specific test
- Find a test for a variable
- Find a test review
Assumptions About You
This guide assumes you have:
- Basic knowledge of the research terminology (e.g., variable, reliability, validity).
- General ability to read research articles.
- Some experience with database and web searching.
- Connection to UW online restricted resources. See Connect from Off-Campus to UW-Restricted Resources .
How To Proceed
Use the Table of Contents to the left as your guide. You may begin at any point. However, we recommend you start at "Paths to Information," proceed to "Scenarios," view the "Resource Table," then complete the "Summary" overview.
- Next: Overview >>
- Last Updated: Jan 31, 2024 10:32 AM
- URL: https://guides.lib.uw.edu/hsl/measure
Be boundless
1959 NE Pacific Street | T334 Health Sciences Building | Box 357155 | Seattle, WA 98195-7155 | 206-543-3390
© 2024 University of Washington | Seattle, WA

Measurements in quantitative research: how to select and report on research instruments
Affiliation.
- 1 Department of Acute and Tertiary Care in the School of Nursing, University of Pittsburgh in Pennsylvania.
- PMID: 24969252
- DOI: 10.1188/14.ONF.431-433
Measures exist to numerically represent degrees of attributes. Quantitative research is based on measurement and is conducted in a systematic, controlled manner. These measures enable researchers to perform statistical tests, analyze differences between groups, and determine the effectiveness of treatments. If something is not measurable, it cannot be tested.
Keywords: measurements; quantitative research; reliability; validity.
- Clinical Nursing Research / methods*
- Clinical Nursing Research / standards
- Fatigue / nursing*
- Neoplasms / nursing*
- Oncology Nursing*
- Quality of Life*
- Reproducibility of Results
- Member Benefits
- Communities
- Grants and Scholarships
- Student Nurse Resources
- Member Directory
- Course Login
- Professional Development
- Organizations Hub
- ONS Course Catalog
- ONS Book Catalog
- ONS Oncology Nurse Orientation Program™
- Account Settings
- Help Center
- Print Membership Card
- Print NCPD Certificate
- Verify Cardholder or Certificate Status
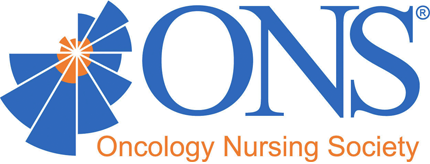
- Trouble finding what you need?
- Check our search tips.
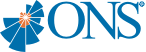
- Oncology Nursing Forum
- Number 4 / July 2014
Measurements in Quantitative Research: How to Select and Report on Research Instruments
Teresa L. Hagan
Measures exist to numerically represent degrees of attributes. Quantitative research is based on measurement and is conducted in a systematic, controlled manner. These measures enable researchers to perform statistical tests, analyze differences between groups, and determine the effectiveness of treatments. If something is not measurable, it cannot be tested.
Become a Member
Purchase this article.
has been added to your cart
Related Articles
Systematic reviews, case study research methodology in nursing research, preferred reporting items for systematic reviews and meta-analyses.

An official website of the United States government
The .gov means it's official. Federal government websites often end in .gov or .mil. Before sharing sensitive information, make sure you're on a federal government site.
The site is secure. The https:// ensures that you are connecting to the official website and that any information you provide is encrypted and transmitted securely.
- Publications
- Account settings
- Browse Titles
NCBI Bookshelf. A service of the National Library of Medicine, National Institutes of Health.
Velentgas P, Dreyer NA, Nourjah P, et al., editors. Developing a Protocol for Observational Comparative Effectiveness Research: A User's Guide. Rockville (MD): Agency for Healthcare Research and Quality (US); 2013 Jan.

Developing a Protocol for Observational Comparative Effectiveness Research: A User's Guide.
- Hardcopy Version at Agency for Healthcare Research and Quality
Chapter 6 Outcome Definition and Measurement
Priscilla Velentgas , PhD, Nancy A Dreyer , MPH, PhD, and Albert W Wu , MD, MPH.
This chapter provides an overview of considerations for the development of outcome measures for observational comparative effectiveness research (CER) studies, describes implications of the proposed outcomes for study design, and enumerates issues of bias that may arise in incorporating the ascertainment of outcomes into observational research, and means of evaluating, preventing and/or reducing these biases. Development of clear and objective outcome definitions that correspond to the nature of the hypothesized treatment effect and address the research questions of interest, along with validation of outcomes or use of standardized patient reported outcome (PRO) instruments validated for the population of interest, contribute to the internal validity of observational CER studies. Attention to collection of outcome data in an equivalent manner across treatment comparison groups is also required. Use of appropriate analytic methods suitable to the outcome measure and sensitivity analysis to address varying definitions of at least the primary study outcomes are needed to draw robust and reliable inferences. The chapter concludes with a checklist of guidance and key considerations for outcome determination and definitions for observational CER protocols.
- Introduction
The selection of outcomes to include in observational comparative effectiveness research (CER) studies involves the consideration of multiple stakeholder viewpoints (provider, patient, payer, regulatory, industry, academic and societal) and the intended use for decisionmaking of resulting evidence. It is also dependent on the level of funding and scope of the study. These studies may focus on clinical outcomes, such as recurrence-free survival from cancer or coronary heart disease mortality; general health-related quality of life measures, such as the EQ-5D and the SF-36; or disease-specific scales, like the uterine fibroid symptom and quality of life questionnaire (UFS-QOL); and/or health resource utilization or cost measures. As with other experimental and observational research studies, the hypotheses or study questions of interest must be translated to one or more specific outcomes with clear definitions.
The choice of outcomes to include in a CER study will in turn drive other important design considerations such as the data source(s) from which the required information can be obtained (see chapter 8 ), the frequency and length of followup assessments to be included in the study following initial treatment, and the sample size, which is influenced by the expected frequency of the outcome in addition to the magnitude of relative treatment effects and scale of measurement.
In this chapter, we provide an overview of types of outcomes (with emphasis on those most relevant to observational CER studies); considerations in defining outcomes; the process of outcome ascertainment, measurement and validation; design and analysis considerations; and means to evaluate and address bias that may arise.
- Conceptual Models of Health Outcomes
In considering the range of health outcomes that may be of interest to patients, health care providers, and other decisionmakers, key areas of focus are medical conditions, impact on health-related or general quality of life, and resource utilization. To address the interrelationships of these outcomes, some conceptual models have been put forth by researchers with a particular focus on health outcomes studies. Two such models are described here.
Wilson and Cleary proposed a conceptual model or taxonomy integrating concepts of biomedical patient outcomes and measures of health-related quality of life. The taxonomy is divided into five levels: biological and physiological factors, symptoms, functioning, general health perceptions, and overall quality of life. 1 The authors discuss causal relationships between traditional clinical variables and measures of quality of life that address the complex interactions of biological and societal factors on health status, as summarized in Table 6.1 .
Wilson and Cleary's taxonomy of biomedical and health-related quality of life outcomes.
An alternative model, the ECHO (Economic, Clinical, Humanistic Outcomes) Model, was developed for planning health outcomes and pharmacoeconomic studies, and goes a step further than the Wilson and Cleary model in incorporating costs and economic outcomes and their interrelationships with clinical and humanistic outcomes ( Figure 6.1 ). 2 The ECHO model does not explicitly incorporate characteristics of the patient as an individual or psychosocial factors to the extent that the Wilson and Cleary model does, however.
The ECHO model. See Kozma CM, Reeder CE, Schultz RM. Economic, clinical, and humanistic outcomes: a planning model for pharmacoeconomic research. Clin Ther. 1993;15(6):1121-32. This figure is copyrighted by Elsevier Inc. and reprinted with permission. (more...)
As suggested by the complex interrelationships between different levels and types of health outcomes, different terminology and classifications may be used, and there are areas of overlap between the major categories of outcomes important to patients. In this chapter, we will discuss outcomes according to the broad categories of clinical, humanistic, and economic and utilization outcome measures.
- Outcome Measurement Properties
The properties of outcome measures that are an integral part of an investigator's evaluation and selection of appropriate measures include reliability, validity, and variability. Reliability is the degree to which a score or other measure remains unchanged upon test and retest (when no change is expected), or across different interviewers or assessors. It is measured by statistics including kappa, and the inter- or intra-class correlation coefficient. Validity, broadly speaking, is the degree to which a measure assesses what it is intended to measure, and types of validity include face validity (the degree to which users or experts perceive that a measure is assessing what it is intended to measure), content validity (the extent to which a measure accurately and comprehensively measures what it is intended to measure), and construct validity (the degree to which an instrument accurately measures a nonphysical attribute or construct such as depression or anxiety, which is itself a means of summarizing or explaining different aspects of the entity being measured). 3 Variability usually refers to the distribution of values associated with an outcome measure in the population of interest, with a broader distribution or range of values said to show more variability.
Responsiveness is another property usually discussed in the context of patient-reported outcomes (PROs) but extendable to other measures, representing the ability of a measure to detect change in an individual over time.
These measurement properties may affect the degree of measurement error or misclassification that an outcome measure is subject to, with the consideration that the properties themselves are specific to the population and setting in which the measures are used. Issues of misclassification and considerations in reducing this type of error are discussed further in the section on “avoidance of bias in study design.”
- Clinical Outcomes
Clinical outcomes are perhaps the most common category of outcome to be considered in CER studies. Medical treatments are developed and must demonstrate efficacy in preapproval clinical trials to prevent the occurrence of undesirable outcomes such as coronary events, osteoporosis, or death; to delay disease progression such as in rheumatoid arthritis; to hasten recovery or improve survival from disease, such as in cancer or H5N1 influenza; or to manage or reduce the burden of chronic diseases including diabetes, psoriasis, Parkinson's disease, and depression. Postapproval observational CER studies are often needed to compare newer treatments against the standard of care; to obtain real-world data on effectiveness as treatments are used in different medical care settings and broader patient populations than those studied in clinical trials; and to increase understanding of the relative benefits and risks of treatments by weighing quality of life, cost, and safety outcomes alongside clinical benefits. For observational studies, this category of outcome generally focuses on clinically meaningful outcomes such as time between disease flares; number of swollen, inflamed joints; or myocardial infarction. Feasibility considerations sometimes dictate the use of intermediate endpoints, which are discussed in further detail later in the chapter.
Definitions of Clinical Outcomes
Temporal aspects.
The nature of the disease state to be treated, the mechanism, and the intended effect of the treatment under study determine whether the clinical outcomes to be identified are incident (a first or new diagnosis of the condition of interest), prevalent (existing disease), or recurrent (new occurrence or exacerbation of disease in a patient who has a previous diagnosis of that condition). The disease of interest may be chronic (a long-term or permanent condition), acute (a condition with a clearly identifiable and rapid onset), transient (a condition that comes and goes), or episodic (a condition that comes and goes in episodes), or have more than one of these aspects.
Subjective Versus Objective Assessments
Most clinical outcomes involve a diagnosis or assessment by a health care provider. These may be recorded in a patient's medical record as part of routine care, coded as part of an electronic health record (EHR) or administrative billing system using coding systems such as ICD-9 or ICD-10, or collected specifically for a given study.
While there are varying degrees of subjectivity involved in most assessments by health care providers, objective measures are those that are not subject to a large degree of individual interpretation, and are likely to be reliably measured across patients in a study, by different health care providers, and over time. Laboratory tests may be considered objective measures in most cases and can be incorporated as part of a standard outcome definition to be used for a study when appropriate. Some clinical outcomes, such as all-cause mortality, can be ascertained directly and may be more reliable than measures that are subject to interpretation by individual health care providers, such as angina or depression.
Instruments have been developed to help standardize the assessment of some conditions for which a subjective clinical assessment might introduce unwanted variability. Consider the example of a study of a new psoriasis treatment. Psoriasis is a chronic skin condition that causes lesions affecting varying amounts of body surface area, with varying degrees of severity. While a physician may be able to assess improvement within an individual patient, a quantifiable measure that would be reproducible across patients and raters improves the information value of comparative trials and observational studies of psoriasis treatment effectiveness. An outcome assessment that relies on purely subjective assessments of improvement such as, “Has the patient's condition improved a lot, a little, or not at all?” is vulnerable to measurement error that arises from subjective judgments or disagreement among clinicians about what comprises the individual categories and how to rate them, often resulting in low reproducibility or inter-rater reliability of the measure. In the psoriasis example, an improved measure of the outcome would be a standardized assessment of the severity and extent of disease expressed as percentage of affected body surface area, such as the Psoriasis Area Severity Index or PASI Score. 4 The PASI score requires rating the severity of target symptoms [erythema (E), infiltration (I), and desquamation (D)] and area of psoriatic involvement (A) for each of four main body areas [head (h), trunk (t), upper extremities (e), lower extremities (l)]. Target symptom severity is rated on a 0–4 scale; area of psoriatic involvement is rated on a 0–6 scale, with each numerical value representing a percentage of area involvement. 4 The final calculated score ranges from 0 (no disease) to 72 (severe disease), with the score contribution of each body area weighted by its percentage of total body area (10, 20, 30, and 40% of body area for head, upper extremities, trunk, and lower extremities, respectively). 4 Compared with subjective clinician assessment of overall performance, using changes in the PASI score increases reproducibility and comparability across studies that use the score.
Relatedly, the U.S. Food and Drug Administration (FDA) has provided input on types of Clinical Outcome Assessments (COAs) that may be considered for qualification for use in clinical trials, with the goals of increasing the reliability of such assessments within a specific context of use in drug development and regulatory decisionmaking to measure a specific concept with a specific interpretation. Contextual considerations include the specific disease of interest, target population, clinical trial design and objectives, regionality, and mode of administration. The types of COAs described are: 5
- Patient-reported outcome (PRO) assessment : A measurement based on a report that comes directly from the patient (i.e., the study subject) about the status of particular aspects of or events related to a patient's health condition. PROs are recorded without amendment or interpretation of the patient's response by a clinician or other observer. A PRO measurement can be recorded by the patient directly, or recorded by an interviewer, provided that the interviewer records the patient's response exactly.
- Observer-reported outcome (ObsRO) assessment : An assessment that is determined by an observer who does not have a background of professional training that is relevant to the measurement being made, i.e., a nonclinician observer such as a teacher or caregiver. This type of assessment is often used when the patient is unable to self-report (e.g., infants, young children). An ObsRO assessment should only be used in the reporting of observable concepts (e.g., signs or behaviors); ObsROs cannot be validly used to directly assess symptoms (e.g., pain) or other unobservable concepts.
- Clinician-reported outcome (ClinRO) assessment : An assessment that is determined by an observer with some recognized professional training that is relevant to the measurement being made.
Other considerations related to use of PROs for measurement of health-related quality of life and other concepts are addressed later on in this chapter.
Composite Endpoints
Some clinical outcomes are composed of a series of items, and are referred to as composite endpoints. A composite endpoint is often used when the individual events included in the score are rare, and/or when it makes biological and clinical sense to group them. The study power for a given sample size may be increased when such composite measures are used as compared with individual outcomes, since by grouping numerous types of events into a larger category, the composite endpoint will occur more frequently than any of the individual components. As desirable as this can be from a statistical point of view, challenges include interpretation of composite outcomes that incorporate both safety and effectiveness, and broader adoption of reproducible definitions that will enhance cross-study comparisons. For example, Kip and colleagues 6 point out that there is no standard definition for MACE (major adverse cardiac events), a commonly used outcome in clinical cardiology research. They conducted analyses to demonstrate that varying definitions of composite endpoints, such as MACE, can lead to substantially different results and conclusions. The investigators utilized the DEScover registry patient population, a prospective observational registry of drug-eluting stent (DES) users, to evaluate differences in 1-year risk for three definitions of MACE in comparisons of patients with and without myocardial infarction (MI), and patients with multi-lesion stenting versus single-lesion stenting (also referred to as percutaneous coronary intervention or PCI). The varying definitions of MACE included one related to safety only [composite of death, MI, and stent thrombosis (ST)], and two relating to both safety and effectiveness [composite of death, MI, ST, and either (1) target vessel revascularization (TVR) or (2) any repeat vascularization]. When comparing patients with and without acute MI, the three definitions of MACE yielded very different hazard ratios. The safety-only definition of MACE yielded a hazard ratio of 1.75 (p<0.05), indicating that patients with acute MI were at greater risk of 1-year MACE. However, for the composite of safety and effectiveness endpoints, the risk of 1-year MACE was greatly attenuated and no longer statistically significant. Additionally, when comparing patients with single versus multiple lesions treated with PCI, the three definitions also yielded different results; while the safety-only composite endpoint demonstrated that there was no difference in 1-year MACE, adding TVR to the composite endpoint definition led to a hazard ratio of 1.4 (p<0.05) for multi-lesion PCI versus single-lesion PCI. This research serves as a cautionary tale for the creation and use of composite endpoints. Not only can varying definitions of composite endpoints such as MACE lead to substantially different results and conclusions; results must also be carefully interpreted, especially in the case where safety and effectiveness endpoints are combined.
Intermediate Endpoints
The use of an intermediate or surrogate endpoint is more common in clinical trials than in observational studies. This type of endpoint is often a biological marker for the condition of interest, and may be used to reduce the followup period required to obtain results from a study of treatment effectiveness. An example would be the use of measures of serum lipids as endpoints in randomized trials of the effectiveness of statins, for which the major disease outcomes of interest to patients and physicians are a reduction in coronary heart disease incidence and mortality. The main advantages of intermediate endpoints are that the followup time required to observe possible effects of treatment on these outcomes may be substantially shorter than for the clinical outcome(s) of primary interest, and if they are measured on all patients, the number of outcomes for analysis may be larger. Much as with composite endpoints, using intermediate endpoints will increase study power for a given sample size as compared with outcomes that may be relatively rare, such as primary myocardial infarction. Surrogate or intermediate outcomes, however, may provide an incomplete picture of the benefits or risk. Treatment comparisons based on intermediate endpoints may differ in magnitude or direction from those based on major disease endpoints, as evidenced in a clinical trial of nifedipine versus placebo 7 - 8 as well as other clinical trials of antihypertensive therapy. 9 On one hand, nifedipine, a calcium channel blocker, was superior to placebo in reduction of onset of new coronary lesions; on the other hand, mortality was sixfold greater among patients who received nifedipine versus placebo. 7
Freedman and colleagues have provided recommendations regarding the use of intermediate endpoints. 10 Investigators should consider the degree to which the intermediate endpoint is reflective of the main outcome, as well as the degree to which effects of the intervention may be mediated through the intermediate endpoint. Psaty and colleagues have cautioned that because drugs have multiple effects, to the extent that a surrogate endpoint is likely to measure only a subset of those effects, results of studies based on surrogate endpoints may be a misleading substitute for major disease outcomes as a basis for choosing one therapy over another. 9
Table 6.2 Clinical outcome definitions and objective measures
View in own window
Selection of Clinical Outcome Measures
Identification of a suitable measure of a clinical outcome for an observational CER study is a process in which various aspects of the nature of the disease or condition under study should be considered along with sources of information by which the required information may be feasibly and reliably obtained.
The choice of outcome measure may follow directly from the expected biological mechanism of action of the intervention(s) under study and its impact on specific medical conditions. For example, the medications tamoxifen and raloxifene are selective estrogen receptor modulators that act through binding to estrogen receptors to block the proliferative effect of estrogen on mammary tissue and reduce the long-term risk of primary and recurrent invasive and non-invasive breast cancer. 11 Broader or narrower outcome definitions may be appropriate to specific research questions or designs. In some situations, however, the putative biologic mechanism may not be well understood. Nonetheless, studies addressing the clinical question of comparative effectiveness of treatment alternatives may still inform decisionmaking, and advances in understanding of the biological mechanism may follow discovery of an association through an observational CER study.
The selection of clinical outcome measures may be challenging when there are many clinical aspects that may be of interest, and a single measure or scale may not adequately capture the perspective of the clinician and patient. For example, in evaluating treatments or other interventions that may prolong the time between flares of systematic lupus erythematosus (SLE), researchers may use an index such as the Systemic Lupus Erythematosus Disease Activity Index (SLEDAI) which measures changes in disease activity. Or they may use the SLICC/ACR damage index, an instrument designed to assess accumulated damage since the onset of the disease. 12 - 14 This measure of disease activity has been tested in different populations and has demonstrated high reliability, evidence for validity, and responsiveness to change. 15 Yet, multiple clinical outcomes in addition to disease activity may be of interest in studying treatment effectiveness in SLE, such as reduction or increase in time to flare, reduction in corticosteroid use, or occurrence of serious acute manifestations (e.g., acute confusional state or acute transverse myelitis). 16
Interactions With the Health Care System
For any medical condition, one should first determine the source of reporting or detection that may lead to initial contact with the medical system. The manner in which the patient presents for medical attention may provide insights as to data source(s) that may be useful in studying the condition. The decision whether to collect information directly from the physician, through medical record abstraction, directly from patients, and/or through use of electronic health records (EHRs) and/or administrative claims data will follow from this. For example, general hospital medical records are unlikely to provide the key components of an outcome such as respiratory failure, which requires information about use of mechanical ventilation. In contrast, hospital medical records are useful for the study of myocardial infarction, which must be assessed and treated in a hospital setting and are nearly always accompanied by an overnight stay. General practice physician office records and emergency department records may be useful in studying the incidence of influenza A or urticaria, with selection of which of these sources depending on the severity of the condition. A prospective study may be required to collect clinical assessments of disease severity using a standard instrument, as these are not consistently recorded in medical practice and are not coded in administrative data sources. The chapter on data sources ( chapter 8 ) provides additional information on selection of appropriate sources of data for an observational CER study.
- Humanistic Outcomes
While outcomes of interest to patients generally include those of interest to physicians, payers, regulators, and others, they are often differentiated by two characteristics: (1) they are clinically meaningful with practical implications for disease recognition and management (i.e., patients generally have less interest in intermediate pathways with no clear clinical impact); and (2) they include reporting of outcomes based on a patient's unique perspective, e.g., patient-reported scales that indicate pain level, degree of functioning, etc. This section deals with measures of health-related quality of life (HRQoL) and the range of measures collectively described as patient-reported outcomes (PROs), which include measures of HRQoL. Other humanistic perspectives relevant to patients (e.g., economics, utilization of health services, etc.) are covered elsewhere.
Health-Related Quality of Life
Health-related quality of life (HRQoL) measures the impact of disease and treatment on the lives of patients and is defined as “the capacity to perform the usual daily activities for a person's age and major social role.” 17 HRQoL commonly includes physical functioning, psychological well-being, and social role functioning. This construct comprises outcomes from the patient perspective and are measured by asking the patient or surrogate reporters about them.
HRQoL is an outcome increasingly used in randomized and non-randomized studies of health interventions, and as such FDA has provided clarifying definitions of HRQoL and of improvements in HRQoL. The FDA defines HRQoL as follows:
HRQL is a multidomain concept that represents the patient's general perception of the effect of illness and treatment on physical, psychological, and social aspects of life. Claiming a statistical and meaningful improvement in HRQL implies: (1) that all HRQL domains that are important to interpreting change in how the clinical trial's population feels or functions as a result of the targeted disease and its treatment were measured; (2) that a general improvement was demonstrated; and (3) that no decrement was demonstrated in any domain. 18
Patient-Reported Outcomes
Patient-reported outcomes (PROs) include any outcomes that are based on data provided by patients or by people who can report on their behalf (proxies), as opposed to data from other sources. 19 PROs refer to patient ratings and reports about any of several outcomes, including health status, health-related quality of life, quality of life defined more broadly, symptoms, functioning, satisfaction with care, and satisfaction with treatment. Patients can also report about their health behaviors, including adherence and health habits. Patients may be asked to directly report information about clinical outcomes or health care utilization and out-of-pocket costs when these are difficult to measure through other sources. The FDA defines a PRO as “a measurement based on a report that comes directly from the patient (i.e., study subject) about the status of a patient's health condition without amendment or interpretation of the patient's response by a clinician or anyone else. A PRO can be measured by self-report or by interview provided that the interviewer records only the patient's response.” 18
In this section we focus mainly on the use of standard instruments for measurement of PROs, in domains including specific disease areas, health-related quality of life, and functioning. PRO measures may be designed to measure the current state of health of an individual or to measure a change in health state. PROs have similarities to other outcome variables measured in observational studies. They are measured with components of both random and systematic error (bias). To be most useful, it is important to have evidence about the reliability, validity, responsiveness, and interpretation of PRO measures, discussed further later in this section.
Types of Humanistic Outcome Measures
Generic measures.
Generic PRO questionnaires are measurement instruments designed to be used across different subgroups of individuals, and contain common domains that are relevant to almost all populations. They can be used to compare one population with another, or to compare scores in a specific population with normative scores. Many have been used for years, and have well established and well understood measurement properties.
Generic PRO questionnaires can focus on a comprehensive set of domains, or on a narrow range of domains such as symptoms or aspects of physical, mental, or social functioning. An example of a generic PRO measure is the Sickness Impact Profile (SIP), one of the oldest and most rigorously developed questionnaires, which measures 12 domains that are affected by illness. 20 The SIP produces two subscale scores, one for physical and one for mental health, and an overall score. Another questionnaire, the SF-36, measures eight domains including general health perceptions, pain, physical functioning, role functioning (as limited by physical health), social functioning, mental health, and vitality. 21 The SF-36 produces a Physical Component Score and a Mental Component Score. 22 The EQ-5D is another generic measure of health-related quality of life, intended for self-completion, that generates a single index score. This scale defines health in terms of 5 dimensions: mobility, self-care, usual activities, pain/discomfort, and anxiety/depression.
Each dimension has three response categories corresponding to no problem/some problem/extreme problem. Taken as a whole, the EQ-5D defines a total of 243 possible states, to which two further states (dead and unconscious) have been added. 23 Another broadly used indicator of quality of life relates to the ability to work. The Work Productivity Index (WPAI) was created as a patient-reported quantitative assessment of the amount of absenteeism, presenteeism, and daily activity impairment attributable to general health (WPAI:GH) or to a specific health problem (WPAI:SHP) (see below), in an effort to develop a quantitative approach to measuring the ability to work. 24
Examples of generic measures that assess a more restricted set of domains include the SCL-90 to measure symptoms, 25 the Index of Activities of Daily Living to measure independence in performing basic functioning, 26 the Psychological General Well-Being Index to measure psychological well-being (PGWBI), 27 and the Beck Depression Inventory. 28
Disease- or Population-Specific Measures
Specific PRO questionnaires are sometimes referred to as “disease-specific.” While a questionnaire can be disease- or condition-specific (e.g., chronic heart failure), it can also be designed for use in a specific population (e.g., pediatric, geriatric), or for use to evaluate a specific treatment (e.g., renal dialysis). Specific questionnaires may be more sensitive to symptoms that are experienced by a particular group of patients. Thus, they are thought to detect differences and changes in scores when they occur in response to interventions.
Some specific measurement instruments assess multiple domains that are affected by a condition. For example, the Arthritis Impact Measurement Scales (AIMS) includes nine subscales that assess problems specific to the health-related quality of life of patients with rheumatoid arthritis and its treatments. 29 The MOS-HIV Health Survey includes 10 domains that are salient for people with HIV and its treatments. 30
Some of these measures take a modular approach, including a core measure that is used for assessment of a broader set of conditions, accompanied by modules that are specific to disease subtypes. For example, the FACIT and EORTC families of measures for evaluating cancer therapies each include a core module that is used for all cancer patients, and specific modules for each type of cancer, such as a module pertaining specifically to breast cancer. 31 - 33
Other measures focus more narrowly on a few domains most likely to be affected by a disease, or most likely to improve with treatment. For example, the Headache Impact Test includes only six items. 34 In contrast, other popular measures focus on symptoms that are affected by many diseases, such as the Brief Pain Inventory and the M.D. Anderson Symptom Inventory (MDASI), which measure the severity of pain and other symptoms and the impact of symptoms on function, and have been developed, refined, and validated in many languages and patient subgroups over three decades. 35 - 36
It is possible, though not always advisable, to design a new PRO instrument for use in a specific study. The process of developing and testing a new PRO measure can be lengthy—generally requiring at least a year in time–and there is no guarantee that a new measure will work as well as more generic but better tested instruments. Nonetheless, it may be necessary to do so in the case of an uncommon condition for which there are no existing PRO measures, for a specific cultural context that differs from the ones that have been studied before, and/or to capture effects of new treatments that may require a different approach to measurement. However, when possible, in these cases it is still prudent to include a PRO measure with evidence for reliability and validity, ideally in the target patient population, in case the newly designed instruments fail to work as intended. This approach will allow comparisons with the new measure to assess content validity if there is some overlap of the concepts being measured.
Item Response Theory (IRT) and Computer Adaptive Testing (CAT)
Item Response Theory (IRT) is a framework for the development of tests and measurement tools, and for the assessment of how well the tools work. Computer Adaptive Testing (CAT) represents an area of innovation in measuring PROs. CAT allows items to be selected to be administered so that questions are relevant to the respondent and targeted to the specific level of the individual, with the last response determining the next question that is asked. Behind the scenes, items are selected from “item banks,” comprising collections of dozens to hundreds of questions that represent the universe of potential levels of the dimension of interest, along with an indication of the relative difficulty or dysfunction that they represent. For example, the Patient-Reported Outcomes Measurement Information System (PROMIS) item bank for physical functioning includes 124 items that range in difficulty from getting out of bed to running several miles. 37 This individualized administration can both enhance measurement precision and reduce respondent burden. 38 Computer adaptive testing is based on IRT methods of scaling items and drawing subsets of items from a larger item bank. 39 Considerations around adaptive testing involve balancing the benefit of tailoring the set of items and measurements to the specific individual with the risk of inappropriate targeting or classification if items answered incorrectly early on determine the later set of items to which a subject is able to respond. PROMIS 40 is a major NIH initiative that leverages these desirable properties for PROs in clinical research and practice applications.
Descriptive Versus Preference Format
Descriptive questionnaires ask about general or common domains and complaints, and usually provide multiple scores. Preference-based measures, generally referred to as utility measures, provide a single score, usually on a 0–1 scale, that represents the aggregate of multiple domains for an overall estimate of burden.
Most of the questionnaires familiar to clinical researchers fall into the category of descriptive measures, including all of those mentioned in the preceding paragraphs. Patients or other respondents are asked to indicate the extent to which descriptions of specific feelings, abilities, or behaviors apply to them. Utility measures are discussed further in the following section.
Other Attributes of PROs
Within each of the above options, there are several attributes of PRO instruments to consider. These include response format (numeric scales vs. verbal descriptors or visual analogue scales), the focus of what is being assessed (frequency, severity, impairment, all of the above), and recall period. Shorter, more recent recall periods more accurately capture the individual's actual experience, but may not provide as good an estimate of their typical activities or experiences. (For example, not everyone vacuums or has a headache every day.)
Content Validity
Content validity is the extent to which a PRO instrument covers the breadth and depth of salient issues for the intended group of patients. If a PRO instrument is not valid with respect to its content, then there is an increased chance that it may fail to capture adequately the impact of an intervention. For example, in a study to compare the impact of different regimens for rheumatoid arthritis, a PRO that does not assess hand function could be judged to have poor content validity, and might fail to capture differences among therapies. FDA addresses content validity as being of primary interest in assessing a PRO, with other measurement properties being secondary. and defines content validity as follows:
Evidence from qualitative research demonstrating that the instrument measures the concept of interest including evidence that the items and domains of an instrument are appropriate and comprehensive relative to its intended measurement concept, population, and use. Testing other measurement properties will not replace or rectify problems with content validity. 18
Content validity is generally assessed qualitatively rather than statistically. It is important to understand and consider the population being studied, including their usual activities and problems, the condition (especially its impact on the patient's functioning), and the interventions being evaluated (including both their positive and adverse effects).
Responsiveness and Minimally Important Difference
Responsiveness is a measure of a PRO instrument's sensitivity to changes in health status or other outcome being measured. If a PRO is not sufficiently responsive, it may not provide adequate evidence of effectiveness in observational studies or clinical trials. Related to responsiveness is the minimally important difference that a PRO measure may detect. Both the patient's and the health care provider's perspectives are needed to determine if the minimally important difference detectable by an instrument is in fact of relevance to the patient's overall health status. 41
Floor and Ceiling Effects
Poor content validity can also lead to a mismatch between the distribution of responses and the true distribution of the concept of interest in the population. For example, if questions in a PRO to assess ability to perform physical activities are too “easy” relative to the level of ability in the population, then the PRO will not reflect the true distribution. This problem can present as a “ceiling” effect, where a larger proportion of the sample reports no disability. Similarly, “floor” effects are seen when questions regarding a level of ability are skewed too difficult for the population and the responses reflect this lack of variability.
Interpretation of PRO Scores
Clinicians and clinical researchers may be unfamiliar with how to interpret PRO scores. They may not understand or have reference to the usual distribution of scores of a particular PRO in a clinical or general population. Without knowledge of normal ranges, physicians may not know what cutpoints of scoring indicate that action is warranted. Without reference values from a comparable population, researchers will not know whether an observed difference between two groups is meaningful, and whether a given change within or between groups is important. The task of understanding the meaning of scores is made more difficult by the fact that different PRO measurement tools tend to use different scoring systems. For most questionnaires, higher scores imply better health, but for some, a higher score is worse. Some scales are scored from 0 to 1, where 0=dead and 1=perfect health. Others are scores on a 0–100 scale, where 0 is simply the lowest attainable score (i.e., the respondent indicates the “worst” health state in response to all of the questions) and 100 is the highest. Still others are “normalized,” so that, for example, a score of 50 represents the mean score for the healthy or nondiseased population, with a standard deviation of 10 points. It is therefore crucial for researchers and users of PRO data to understand the scoring system being used for an instrument and the expected distribution, including the distributional properties.
For some PRO instruments, particularly generic questionnaires that have been applied to large groups of patients over many years, population norms have been collected and established. These can be used as reference points. Scoring also can be recalculated and “normalized” to a “T-score” so that a specific score (often 50 or 100) corresponds to the mean score for the population, and a specific number of points (often 5 or 10) corresponds to 1 standard deviation unit in that population.
Selection of a PRO Measure
There are a number of practical considerations to take into account when selecting PRO measures for use in a CER study. The measurement properties discussed in the preceding sections also require evaluation in all instances for the specific instrument selected, within a given population, setting, and intended purpose.
It is important to understand the target population that will be completing the PRO assessment. These may range from individuals who can self-report, to individuals requiring the assistance of a proxy or medical professional (e.g., children, mentally or cognitively limited individuals, visually impaired individuals). Some respondents may be ambulatory individuals living in the community, whereas others may be inpatients or institutionalized individuals.
If a PRO questionnaire is to be used in non–English-speaking populations or in multiple languages, it is necessary to have versions appropriately adapted to language and culture. One should have evidence for the reliability and validity of the translated and culturally adapted version, as applied to the concerned population. One also should have data showing the comparability of performance across different language and cultural groups. This is of special importance when pooling data across language versions, as in a multinational clinical trial or registry study.
It is important to match the respondent burden created by a PRO instrument to the requirements of the population being studied. Patients with greater levels of illness or disability are less able to complete lengthy questionnaires. In some cases, the content or specific questions posed in a PRO may be upsetting or otherwise unacceptable to respondents. In other cases, a PRO questionnaire may be too cognitively demanding or written at a reading level that is above that of the intended population. The total burden of study-related data collection on patients and providers must also be considered, as an excessive number of forms that must be completed are likely to reduce compliance.
Cost and Copyright
Another practical consideration is the copyright status of a PRO being considered for use. Some PRO questionnaires are entirely in the public domain and are free for use. Others are copyrighted and require permission and/or the payment of fees for use. Some scales, such as the SF-12 and SF-36, require payment of fees for scoring.
Mode and Format of Administration
As noted above, there are various options for how a questionnaire should be administered and how the data should be captured, each method having both advantages and disadvantages. A PRO questionnaire can be (1) self-administered at the time of a clinical encounter, (2) administered by an interviewer at the time of a clinical encounter, (3) administered with computer assistance at the time of a clinical encounter, (4) self-administered by mail, (5) self-administered on-line, (6) interviewer-administered by telephone, or (7) computer-administered by telephone. Self-administration at the time of a clinical encounter requires little technology or up-front cost, but requires staff for supervision and data entry and can be difficult for respondents with limited literacy or sophistication. Face-to-face administration engages respondents and reduces their burden but requires trained interviewers. Computer-assisted administration provides an intermediate solution but also requires capital investment. Mailed surveys afford more privacy to respondents, but they generate mailing expenses and do not eliminate problems with literacy. Paper-based formats require data entry, scoring, and archiving and are prone to calculation errors. Online administration is relatively inexpensive, especially for large surveys, and surveys can be completed any time, but not all individuals have Internet access. Administration by live telephone interview is engaging and allows interviewer flexibility but is also expensive. “Cold calls” to potential study participants may result in low response rates, given the increased prevalence of caller ID screening systems and widespread skepticism about “telemarketing.”
Interactive voice response systems (or IVRS) can also be used to conduct telephone interviews, but it can be tedious to respond using the telephone key pad, and this format strikes some as impersonal.
Static Versus Dynamic Questionnaires
Static forms are the type of questionnaire that employs a fixed-format set of questions and response options. They can be administered on paper, by interview, or through the Internet. Dynamic questionnaires select followup questions to administer based on the responses already obtained for previous questions. Since they are more efficient, more domains can be assessed.
Economic and Utilization Outcomes
While clinical outcomes represent the provider and professional perspective, and humanistic outcomes represent the patient perspective, economic outcomes, including measures of health resource utilization, represent the payer and societal perspective. In the United States, measures of cost and cost-effectiveness are often excluded from government-funded CER studies. However, these measures are important to a variety of important stakeholders such as payers and product manufacturers, and are routinely included in cost-effectiveness research in countries such as Australia, the United Kingdom, Canada, France, and Germany. 42
Research questions addressing issues of cost-effectiveness and resource utilization may be formulated in a number of ways. Cost identification studies measure the cost of applying a specified treatment to a population under a certain set of conditions. These studies describe the cost incurred without comparison to alternative interventions.
Some cost identification studies describe the total costs of care for a particular population, whereas others isolate costs of care related to a specific condition; this latter approach requires that each episode of care be ascribed as having been related or unrelated to the illness of interest and involves substantial review. 43 Cost-benefit studies are typically measured in dollars or other currency. These studies compare the monetary costs of an intervention against the standard of care with the cost savings that result from the benefits of that treatment. In these studies, mortality is also assigned a dollar value, although techniques for assigning value to a human life are controversial. Cost-effectiveness is a relative concept, and its analysis compares the costs of treatments and benefits of treatments in terms of a specified outcome, such as reduced mortality or morbidity, years of life saved, or infections averted.
Types of Health Resource Utilization and Cost Measures
Monetary costs.
Studies most often examine direct costs (i.e., the monetary costs of the medical treatments themselves, potentially including associated costs of administering treatment or conditions associated with treatment), but may also include measures of indirect costs (e.g., the costs of disability or loss of livelihood, both actual and potential). Multiple measures of costs are commonly included in any given study.
Health Resource Utilization
Measures of health resource utilization, such as number of inpatient or outpatient visits, total days of hospitalization in a given year, or number of days treated with IV antibiotics, are often used as efficient and easily interpretable proxies for measuring cost, since actual costs are dependent on numerous factors (e.g., institutional overhead, volume discounts) and can be difficult to obtain, since they often may be confidential, since, in part, they reflect business acumen in price negotiation. Costs may also vary by institution or location, such as the cost of a day in the hospital or a medical procedure. Resource utilization measures may be preferred when a study is intended to yield results that may be generalizable to health systems or reimbursement systems other than those under study, as they are not dependent on a particular reimbursement structure such as Medicare. Alternatively, a specific cost or reimbursement structure, such as the amount reimbursed by the Centers for Medicare and Medicaid Services (CMS) for specific treatment items, or average wholesale drug costs, may be applied to units of health resource use when conducting studies that pool data from different health systems.
Utility and Preference-Based Measures
PROs and cost analyses intersect around the calculation of cost-utility. Utility measures are derived from economic and decision theory. The term utility refers to the value placed by the individual on a particular health state. Utility is summarized as a score ranging from 0.0 representing death to 1.0 representing perfect health.
In health economic analyses, utilities are used to justify devoting resources to a treatment. There are several widely used preference-based instruments that are used to estimate utility.
Preference measures are based on the fundamental concept that individuals or groups have reliable preferences about different health states. To evaluate those preferences, individuals rate a series of health states: for example, a person with specific levels of physical functioning (able to walk one block but not climb stairs), mental health (happy most of the time), and social role functioning (not able to work due to health). The task for the individual is to directly assign a degree of preference to that state. These include the Standard Gamble and Time Tradeoff methods, 44 - 45 the EQ-5D, also referred to as the Euroqol, 23 the Health Utilities Index, 46 - 47 and the Quality of Well-Being Scale. 48
Quality-Adjusted Life Years (QALYs)
Utility scores associated with treatment can be used to weight the duration of life according to its quality, and are thereby used to generate QALYs. Utility scores are generally first ascertained directly in a sample of people with the condition in question, either cross-sectionally or over time with a clinical trial. Utility values are sometimes estimated indirectly using other sources of information about the health status of people in a population. The output produced by an intervention can be calculated as the area under the cost-utility curve.
For example, if the mean utility score for patients receiving antiretroviral treatment for HIV disease is 0.80, then the outcome for a treated group would be survival time multiplied by 0.80.
Disability-Adjusted Life Years (DALYs)
DALYs are another measure of overall disease burden expressed as the number of years lost to poor health, disability, or premature death. 49 As with QALYs, mortality and morbidity are combined in a single metric. Potential years of life lost to premature death are supplemented with years of health life lost due to less than optimal health. Whereas 1 QALY corresponds to one year of life in optimal health, 1 DALY corresponds to one year of healthy life lost.
An important aspect of the calculation of DALYs is that the value assigned to each year of life depends on age. Years lived as a young adult are valued more highly than those spent as a young child or older adult, reflecting the different capacity for work productivity during different phases of life. DALYs are therefore estimated for different chronic illnesses by first calculating the age- and sex-adjusted incidence of disease. A DALY is calculated as the sum of the average years of life lost, and the average years lived with a disability. For example, to estimate the years of healthy life lost in a region due to HIV/AIDS, one would first estimate the prevalence of the disease by age. The DALY value is calculated by summing the average of years of life lost and the average number of years lived with AIDS, discounted based on a universal set of standard weights based on expert valuations.
Selection of Resource Utilization and Cost Measures
The selection of measures of resource utilization or costs should correspond to the primary hypothesis in terms of the impact of an intervention. For example, will treatment reduce the need for hospitalization or result in a shorter length of stay? Or, will treatment or other intervention reduce complications that require hospitalization? Or, will a screening method reduce the total number of diagnostic procedures required per diagnosis?
It is useful to consider what types of costs are of interest to the investigators and to various stakeholders. Are total costs of interest, or costs associated with specific resources (e.g., prescription drug costs)? Are only direct costs being measured, or are you also interested in indirect costs such as those related to days lost from work?
When it is determined that results will be presented in terms of dollars rather than units of resources, several different methods can be applied. In the unusual case that an institution has a cost-accounting system, cost can be measured directly. In most cases, resource units are collected, and costs are assigned based on local or national average prices for the specific resources being considered, for example, reimbursement from CMS for a CT scan, or a hospital day. Application of an external standard cost system reduces variability in costs due to region, payer source, and other variables that might obscure the impact of the intervention in question.
- Study Design and Analysis Considerations
Study Period and Length of Followup
In designing a study, the required study period and length of followup are determined by the expected time frame within which an intervention may be expected to impact the outcome of interest. A study comparing traditional with minimally invasive knee replacement surgery will need to follow subjects at least for the duration of the expected recovery time of 3 to 6 months or longer. The optimal duration of a study can be problematic when studying effects that may become manifest over a long time period, such as treatments to prevent or delay the onset of chronic disease. In these cases, data sources with a high degree of turnover of patients, such as administrative claims databases from managed care organizations, may not be suitable. For example, in the case of Alzheimer's disease, a record of health care is likely to be present in health insurance claims. However, with the decline in cognitive function, patients may lose ability to work and may enter assisted care facilities, where utilization is not typically captured in large health insurance claims systems. Some studies may be undertaken for the purpose of determining how long an intervention can be expected to impact the outcome of interest. For example, various measures are used to aid in reducing obesity and in smoking cessation, and patients, health care providers, and payers are interested in knowing how long these interventions work (if at all), for whom, and in what situations.
Notwithstanding the limitations of intermediate endpoints (discussed in a preceding section), one of the main advantages of their use is the potential truncation of the required study followup period. Consider, for example, a study of the efficacy of the human papilloma virus vaccine, for which the major medical endpoint of interest is prevention of cervical cancer. The long latency period (more than 2 years, depending on the study population) and the relative infrequency of cervical cancer raise the possibility that intermediate endpoints should be used. Candidates might include new diagnoses of genital warts, or new diagnoses of the precancerous conditions cervical intraepithelial neoplasia (CIN) or vaginal intraepithelial neoplasia (VIN), which have shorter latency periods of less than 1 year or 2 years (minimum), respectively. Use of these endpoints would allow such a study to provide meaningful evidence informing the use of the HPV vaccine in a shorter timeframe, during which more patients might benefit from its use. Alternatively, if the vaccine is shown to be ineffective, this information could avoid years of unnecessary treatment and the associated costs as well as the costs of running a longer trial.
Avoidance of Bias in Study Design
Misclassification.
The role of the researcher is to understand the extent and sources of misclassification in outcome measurement, and to try to reduce these as much as possible. To ensure comparability between treatment groups with as little misclassification (also referred to as measurement error) of outcomes as possible, a clear and objective (i.e., verifiable and not subject to individual interpretation insofar as possible) definition of the outcome of interest is needed. An unclear outcome definition can lead to misclassification and bias in the measure of treatment effectiveness. When the misclassification is nondifferential, or equivalent across treatment groups, the estimate of treatment effectiveness will be biased toward the null, reducing the apparent effectiveness of treatment, which may result in an erroneous conclusion that no effect (or one smaller than the true effect size) exists. When the misclassification differs systematically between treatment groups, it may distort the estimate of treatment effectiveness in either direction.
For clinical outcomes, incorporation of an objective measure such as a validated tool that has been developed for use in clinical practice settings, or an adjudication panel for review of outcomes with regard to whether they meet the predetermined definition of an event, would both be approaches that increase the likelihood that outcomes will be measured and classified accurately and in a manner unlikely to vary according to who is doing the assessment. For PROs, measurement error can stem from several sources, including the way in which a question is worded and hence understood by a respondent, how the question is presented, the population being assessed, the literacy level of respondents, the language in which the questions are written, and elements of culture that it represents.
To avoid differential misclassification of outcomes, care must also be taken to use the same methods of ascertainment and definitions of study outcomes whenever possible. For prospective or retrospective studies with contemporaneous comparators, this is usually not an issue, since it is most straightforward to utilize the same data sources and methods of outcome ascertainment for each comparison group. A threat to validity may arise in use of a historical comparison group, which may be used in certain circumstances. For example, this occurs when a new treatment largely displaces use of an older treatment within a given indication, but further evidence is needed for the comparative effectiveness of the newer and older treatments, such as enzyme replacement for lysosomal storage disorders. In such instances, use of the same or similar data sources and equivalent outcome definitions to the extent possible will reduce the likelihood of bias due to differential outcome ascertainment.
Other situations that may give rise to issues of differential misclassification of outcomes include: when investigators are not blinded to the hypothesis of the study, and “rule-out” diagnoses are more common in those with a particular exposure of interest; when screening or detection of outcomes is more common or more aggressive in those with one treatment than another (i.e., surveillance bias, e.g., when liver function testing are preferentially performed in patients using a new drug compared to other treatments for that condition); and when loss to followup occurs that is related to the risk of experiencing the outcome. For example, once a safety signal has been identified and publicized, physicians have been alerted and then look more proactively for clinical signs and symptoms in treated patients. This situation is even greater for products that are subject to controlled distribution or Risk Evaluation and Mitigation Strategies (REMS). Consider clozapine, an anti-schizophrenia drug that is subject to controlled distribution through a “no blood, no drug” monitoring program. The blood testing program was implemented to detect early development of agranulocytemia. When comparing patients treated with clozapine with those treated with other antischizophrenics, those using clozapine may appear to have a worse safety profile with respect to this outcome.
Sensitivity analyses may be conducted in order to estimate the impact of different levels of differential or nondifferential misclassification on effect estimates from observational CER studies. These approaches are covered in detail in chapter 11 .
Validation and Adjudication
In some instances, additional information must be collected (usually from medical records) to validate the occurrence of the outcome of interest, including to exclude erroneous or “rule-out” diagnoses. This is particularly important for medical events identified in administrative claims databases, for which a diagnosis code associated with a medical encounter may represent a “rule out” diagnosis or a condition that does not map to a specific diagnosis code. For some complex diagnoses, such as unstable angina, a standard clinical definition must be applied by an adjudication panel that has access to detailed records inclusive of subjects' relevant medical history, symptomatic presentation, diagnostic work-up, and treatment. Methods of validation and adjudication of outcomes strengthen the internal validity and therefore the evidence that can be drawn from a CER study. However, they are resource-intensive.

Issues Specific to PROs
PROs are prone to several specific sources of bias. Self-reports of health status are likely to differ systematically from reports by surrogates, who, for example, are likely to report less pain than the individuals themselves. 50 Some biases may be population-dependent. For example, there may be a greater tendency of some populations to succumb to acquiescence bias (agreeing with the statements in a questionnaire) or social desirability bias (answering in a way that would cast the respondent in the best light). 51 In some situations, however, a PRO may be the most useful marker of disease activity, such as with episodic conditions that cause short-duration disease flares such as low back pain and gout, where patients may not present for health care immediately, if at all.
The goal of the researcher is to understand and reduce sources of bias, considering those most likely to apply in the specific population and topics under study. In the case of well understood systematic biases, adjustments can be made so that distributions of responses are more consistent. In other cases, redesigning items and scales, for example, by including both positively and negatively worded items, can reduce specific kinds of bias.
Missing data, an issue covered in more detail in chapter 10 , pose a particular problem with PROs, since PRO data are usually not missing at random. Instead, respondents whose health is poorer are more likely to fail to complete an assessment. Another special case of missing data occurs when a patient dies and is unable to complete an assessment. If this issue is not taken into account in the data analysis, and scores are only recorded for living patients, incorrect conclusions may be drawn. Strategies for handling this type of missing data include selection of an instrument that incorporates a score for death, such as the Sickness Impact Profile 20 , 52 or the Quality of Well-Being Scale, 48 or through an analytic strategy that allows for some missing values.
Failure to account for missing PRO data that are related to poor health or death will lead to an overestimate of the health of the population based on responses from subjects who do complete PRO forms. Therefore, in research using PROs, it is very important to understand the extent and pattern of missing data, both at the level of the individual as well as for specific items or scales on an instrument. 53
A strategy should be put in place to handle missing data when developing the study protocol and analysis plans. Such strategies that pertain to use of PROs in research are discussed in further detail in publications such as the book by Fairclough and colleagues.
Analytic Considerations
Form of outcome measure and analysis approach.
To a large extent, the form of the primary outcome of interest—that is, whether the outcome is measured and expressed as a dichotomous or polytomous categorical variable or a continuous variable, and whether it is to be measured at a single time point, measured repeatedly at fixed intervals, or measured repeatedly at varying time intervals—determines the appropriate statistical methods that may be applied in analysis. These topics are covered in detail in chapter 10 .
Sensitivity Analysis
One of the key factors to address in planned sensitivity analyses for an observational CER study is how varying definitions of the study outcome or related outcomes will affect the measures of association from the study. These investigations include assessing multiple related outcomes within a disease area; for example, assessing multiple measures of respiratory function such as FEV1, FEV1% predicted, and FVC in studies of asthma treatment effectiveness in children; assessing the effect of different cutoffs for dichotomized continuous outcome measures; for example, the use of Systemic Lupus Erythematosus Disease Activity Index-2000 scores to define active disease in lupus treatment studies, 54 or the use of different sets of diagnosis codes to capture a condition such as influenza and related respiratory conditions, in administrative data. These and other considerations for sensitivity analyses are covered in detail in chapter 11 .
Future Directions
Increased use of EHRs as a source of data for observational research, including registries, other types of observational studies, and specifically for CER, has prompted initiatives to develop standardized definitions of key outcomes and other data elements that would be used across health systems and different EHR platforms to facilitate comparisons between studies and pooling of data. The National Cardiovascular Research Infrastructure partnership between the American College of Cardiology and Duke Clinical Research Institute, which received American Recovery and Reinvestment Act funding to establish intra-operable data standards based on the National Cardiovascular Data Registry, is an example of such a current activity. 55
This chapter has provided an overview of considerations in development of outcome definitions for observational CER studies; has described implications of the nature of the proposed outcomes for the study design; and has enumerated issues of bias that may arise in incorporating the ascertainment of outcomes into observational research. It has also suggested means of preventing or reducing these biases.
Development of clear and objective outcome definitions that correspond to the nature of the hypothesized treatment effect and address the research questions of interest, along with validation of outcomes where warranted or use of standardized PRO instruments validated for the population of interest, contribute to the internal validity of observational CER studies. Attention to collection of outcome data in an equivalent manner across treatment comparison groups is also required. Use of appropriate analytic methods suitable to the outcome measure, and sensitivity analysis to address varying definitions of at least the primary study outcomes, are needed to make inferences drawn from such studies more robust and reliable.
Checklist: Guidance and key considerations for outcome selection and measurement for an observational CER protocol
Developing a Protocol for Observational Comparative Effectiveness Research: A User’s Guide is copyrighted by the Agency for Healthcare Research and Quality (AHRQ). The product and its contents may be used and incorporated into other materials on the following three conditions: (1) the contents are not changed in any way (including covers and front matter), (2) no fee is charged by the reproducer of the product or its contents for its use, and (3) the user obtains permission from the copyright holders identified therein for materials noted as copyrighted by others. The product may not be sold for profit or incorporated into any profitmaking venture without the expressed written permission of AHRQ.
- Cite this Page Velentgas P, Dreyer NA, Wu AW. Outcome Definition and Measurement. In: Velentgas P, Dreyer NA, Nourjah P, et al., editors. Developing a Protocol for Observational Comparative Effectiveness Research: A User's Guide. Rockville (MD): Agency for Healthcare Research and Quality (US); 2013 Jan. Chapter 6.
- PDF version of this title (5.8M)
In this Page
Other titles in these collections.
- AHRQ Methods for Effective Health Care
- Health Services/Technology Assessment Text (HSTAT)
Related information
- PMC PubMed Central citations
- PubMed Links to PubMed
Recent Activity
- Outcome Definition and Measurement - Developing a Protocol for Observational Com... Outcome Definition and Measurement - Developing a Protocol for Observational Comparative Effectiveness Research: A User's Guide
Your browsing activity is empty.
Activity recording is turned off.
Turn recording back on
Connect with NLM
National Library of Medicine 8600 Rockville Pike Bethesda, MD 20894
Web Policies FOIA HHS Vulnerability Disclosure
Help Accessibility Careers

6.1 Measurement
Learning objectives.
- Define measurement.
- Describe Kaplan’s three categories of the things that social scientists measure.
- Identify the stages at which measurement is important.
Measurement is important. Recognizing that fact, and respecting it, will be of great benefit to you—both in research methods and in other areas of life as well. If, for example, you have ever baked a cake, you know well the importance of measurement. As someone who much prefers rebelling against precise rules over following them, I once learned the hard way that measurement matters. A couple of years ago I attempted to bake my husband a birthday cake without the help of any measuring utensils. I’d baked before, I reasoned, and I had a pretty good sense of the difference between a cup and a tablespoon. How hard could it be? As it turns out, it’s not easy guesstimating precise measures. That cake was the lumpiest, most lopsided cake I’ve ever seen. And it tasted kind of like Play-Doh. Figure 6.1 depicts the monstrosity I created, all because I did not respect the value of measurement.

Measurement is important in baking and in research.
Just as measurement is critical to successful baking, it is as important to successfully pulling off a social scientific research project. In sociology, when we use the term measurement The process by which we describe and ascribe meaning to the key facts, concepts, or phenomena that we are investigating. we mean the process by which we describe and ascribe meaning to the key facts, concepts, or other phenomena that we are investigating. At its core, measurement is about defining one’s terms in as clear and precise a way as possible. Of course, measurement in social science isn’t quite as simple as using some predetermined or universally agreed-on tool, such as a measuring cup or spoon, but there are some basic tenants on which most social scientists agree when it comes to measurement. We’ll explore those as well as some of the ways that measurement might vary depending on your unique approach to the study of your topic.
What Do Social Scientists Measure?
The question of what social scientists measure can be answered by asking oneself what social scientists study. Think about the topics you’ve learned about in other sociology classes you’ve taken or the topics you’ve considered investigating yourself. Or think about the many examples of research you’ve read about in this text. In Chapter 2 "Linking Methods With Theory" we learned about Melissa Milkie and Catharine Warner’s study (2011) Milkie, M. A., & Warner, C. H. (2011). Classroom learning environments and the mental health of first grade children. Journal of Health and Social Behavior, 52 , 4–22. of first graders’ mental health. In order to conduct that study, Milkie and Warner needed to have some idea about how they were going to measure mental health. What does mental health mean, exactly? And how do we know when we’re observing someone whose mental health is good and when we see someone whose mental health is compromised? Understanding how measurement works in research methods helps us answer these sorts of questions.
As you might have guessed, social scientists will measure just about anything that they have an interest in investigating. For example, those who are interested in learning something about the correlation between social class and levels of happiness must develop some way to measure both social class and happiness. Those who wish to understand how well immigrants cope in their new locations must measure immigrant status and coping. Those who wish to understand how a person’s gender shapes their workplace experiences must measure gender and workplace experiences. You get the idea. Social scientists can and do measure just about anything you can imagine observing or wanting to study. Of course, some things are easier to observe, or measure, than others, and the things we might wish to measure don’t necessarily all fall into the same category of measureables.
In 1964, philosopher Abraham Kaplan (1964) Kaplan, A. (1964). The conduct of inquiry: Methodology for behavioral science . San Francisco, CA: Chandler Publishing Company. wrote what has since become a classic work in research methodology, The Conduct of Inquiry (Babbie, 2010). Earl Babbie offers a more detailed discussion of Kaplan’s work in his text. You can read it in Chapter 5 "Research Design" of the following: Babbie, E. (2010). The practice of social research (12th ed.). Belmont, CA: Wadsworth. In his text, Kaplan describes different categories of things that behavioral scientists observe. One of those categories, which Kaplan called “observational terms,” is probably the simplest to measure in social science. Observational terms Things that we can see with the naked eye simply by looking at them. are the sorts of things that we can see with the naked eye simply by looking at them. They are terms that “lend themselves to easy and confident verification” (1964, p. 54). Kaplan, A. (1964). The conduct of inquiry: Methodology for behavioral science . San Francisco, CA: Chandler Publishing Company, p. 54. If, for example, we wanted to know how the conditions of playgrounds differ across different neighborhoods, we could directly observe the variety, amount, and condition of equipment at various playgrounds.
Indirect observables Things that we cannot see with the naked eye but that require some more complex assessment. , on the other hand, are less straightforward to assess. They are “terms whose application calls for relatively more subtle, complex, or indirect observations, in which inferences play an acknowledged part. Such inferences concern presumed connections, usually causal, between what is directly observed and what the term signifies” (1964, p. 55). Kaplan, A. (1964). The conduct of inquiry: Methodology for behavioral science . San Francisco, CA: Chandler Publishing Company, p. 55. If we conducted a study for which we wished to know a person’s income, we’d probably have to ask them their income, perhaps in an interview or a survey. Thus we have observed income, even if it has only been observed indirectly. Birthplace might be another indirect observable. We can ask study participants where they were born, but chances are good we won’t have directly observed any of those people being born in the locations they report.
Sometimes the measures that we are interested in are more complex and more abstract than observational terms or indirect observables. Think about some of the concepts you’ve learned about in other sociology classes—ethnocentrism, for example. What is ethnocentrism? Well, you might know from your intro to sociology class that it has something to do with the way a person judges another’s culture. But how would you measure it? Here’s another construct: bureaucracy. We know this term has something to do with organizations and how they operate, but measuring such a construct is trickier than measuring, say, a person’s income. In both cases, ethnocentrism and bureaucracy, these theoretical notions represent ideas whose meaning we have come to agree on. Though we may not be able to observe these abstractions directly, we can observe the confluence of things that they are made up of. Kaplan referred to these more abstract things that behavioral scientists measure as constructs Abstractions that cannot be observed directly but that can be defined based on that which is observable. . Constructs are “not observational either directly or indirectly” (1964, p. 55), Kaplan, A. (1964). The conduct of inquiry: Methodology for behavioral science . San Francisco, CA: Chandler Publishing Company, p. 55. but they can be defined based on observables.
Thus far we have learned that social scientists measure what Abraham Kaplan called observational terms, indirect observables, and constructs. These terms refer to the different sorts of things that social scientists may be interested in measuring. But how do social scientists measure these things? That is the next question we’ll tackle.
How Do Social Scientists Measure?
Measurement in social science is a process. It occurs at multiple stages of a research project: in the planning stages, in the data collection stage, and sometimes even in the analysis stage. Recall that previously we defined measurement as the process by which we describe and ascribe meaning to the key facts, concepts, or other phenomena that we are investigating. Once we’ve identified a research question, we begin to think about what some of the key ideas are that we hope to learn from our project. In describing those key ideas, we begin the measurement process.
Let’s say that our research question is the following: How do new college students cope with the adjustment to college? In order to answer this question, we’ll need to some idea about what coping means. We may come up with an idea about what coping means early in the research process, as we begin to think about what to look for (or observe) in our data-collection phase. Once we’ve collected data on coping, we also have to decide how to report on the topic. Perhaps, for example, there are different types or dimensions of coping, some of which lead to more successful adjustment than others. However we decide to proceed, and whatever we decide to report, the point is that measurement is important at each of these phases.
As the preceding paragraph demonstrates, measurement is a process in part because it occurs at multiple stages of conducting research. We could also think of measurement as a process because of the fact that measurement in itself involves multiple stages. From identifying one’s key terms to defining them to figuring out how to observe them and how to know if our observations are any good, there are multiple steps involved in the measurement process. An additional step in the measurement process involves deciding what elements one’s measures contain. A measure’s elements might be very straightforward and clear, particularly if they are directly observable. Other measures are more complex and might require the researcher to account for different themes or types. These sorts of complexities require paying careful attention to a concept’s level of measurement and its dimensions. We’ll explore these complexities in greater depth at the end of this chapter, but first let’s look more closely at the early steps involved in the measurement process.
Key Takeaways
- Measurement is the process by which we describe and ascribe meaning to the key facts, concepts, or other phenomena that we are investigating.
- Kaplan identified three categories of things that social scientists measure including observational terms, indirect observables, and constructs.
- Measurement occurs at all stages of research.
- See if you can come up with one example of each of the following: an observational term, an indirect observable, and a construct. How might you measure each?

Want to create or adapt books like this? Learn more about how Pressbooks supports open publishing practices.
Understanding Psychological Measurement
Rajiv S. Jhangiani; I-Chant A. Chiang; Carrie Cuttler; and Dana C. Leighton
Learning Objectives
- Define measurement and give several examples of measurement in psychology.
- Explain what a psychological construct is and give several examples.
- Distinguish conceptual from operational definitions, give examples of each, and create simple operational definitions.
- Distinguish the four levels of measurement, give examples of each, and explain why this distinction is important.
What Is Measurement?
Measurement is the assignment of scores to individuals so that the scores represent some characteristic of the individuals. This very general definition is consistent with the kinds of measurement that everyone is familiar with—for example, weighing oneself by stepping onto a bathroom scale, or checking the internal temperature of a roasting turkey using a meat thermometer. It is also consistent with measurement in the other sciences. In physics, for example, one might measure the potential energy of an object in Earth’s gravitational field by finding its mass and height (which of course requires measuring those variables) and then multiplying them together along with the gravitational acceleration of Earth (9.8 m/s2). The result of this procedure is a score that represents the object’s potential energy.
This general definition of measurement is consistent with measurement in psychology too. (Psychological measurement is often referred to as psychometrics .) Imagine, for example, that a cognitive psychologist wants to measure a person’s working memory capacity—their ability to hold in mind and think about several pieces of information all at the same time. To do this, she might use a backward digit span task, in which she reads a list of two digits to the person and asks them to repeat them in reverse order. She then repeats this several times, increasing the length of the list by one digit each time, until the person makes an error. The length of the longest list for which the person responds correctly is the score and represents their working memory capacity. Or imagine a clinical psychologist who is interested in how depressed a person is. He administers the Beck Depression Inventory, which is a 21-item self-report questionnaire in which the person rates the extent to which they have felt sad, lost energy, and experienced other symptoms of depression over the past 2 weeks. The sum of these 21 ratings is the score and represents the person’s current level of depression.
The important point here is that measurement does not require any particular instruments or procedures. What it does require is some systematic procedure for assigning scores to individuals or objects so that those scores represent the characteristic of interest.
Psychological Constructs
Many variables studied by psychologists are straightforward and simple to measure. These include age, height, weight, and birth order. You can ask people how old they are and be reasonably sure that they know and will tell you. Although people might not know or want to tell you how much they weigh, you can have them step onto a bathroom scale. Other variables studied by psychologists—perhaps the majority—are not so straightforward or simple to measure. We cannot accurately assess people’s level of intelligence by looking at them, and we certainly cannot put their self-esteem on a bathroom scale. These kinds of variables are called constructs (pronounced CON-structs ) and include personality traits (e.g., extraversion), emotional states (e.g., fear), attitudes (e.g., toward taxes), and abilities (e.g., athleticism).
Psychological constructs cannot be observed directly. One reason is that they often represent tendencies to think, feel, or act in certain ways. For example, to say that a particular university student is highly extraverted does not necessarily mean that she is behaving in an extraverted way right now. In fact, she might be sitting quietly by herself, reading a book. Instead, it means that she has a general tendency to behave in extraverted ways (e.g., being outgoing, enjoying social interactions) across a variety of situations. Another reason psychological constructs cannot be observed directly is that they often involve internal processes. Fear, for example, involves the activation of certain central and peripheral nervous system structures, along with certain kinds of thoughts, feelings, and behaviors—none of which is necessarily obvious to an outside observer. Notice also that neither extraversion nor fear “reduces to” any particular thought, feeling, act, or physiological structure or process. Instead, each is a kind of summary of a complex set of behaviors and internal processes.
The Big Five
The Big Five is a set of five broad dimensions that capture much of the variation in human personality. Each of the Big Five can even be defined in terms of six more specific constructs called “facets” (Costa & McCrae, 1992) [1] .
Table 4.1 The Big Five Personality Dimensions
The conceptual definition of a psychological construct describes the behaviors and internal processes that make up that construct, along with how it relates to other variables. For example, a conceptual definition of neuroticism (another one of the Big Five) would be that it is people’s tendency to experience negative emotions such as anxiety, anger, and sadness across a variety of situations. This definition might also include that it has a strong genetic component, remains fairly stable over time, and is positively correlated with the tendency to experience pain and other physical symptoms.
Students sometimes wonder why, when researchers want to understand a construct like self-esteem or neuroticism, they do not simply look it up in the dictionary. One reason is that many scientific constructs do not have counterparts in everyday language (e.g., working memory capacity). More important, researchers are in the business of developing definitions that are more detailed and precise—and that more accurately describe the way the world is—than the informal definitions in the dictionary. As we will see, they do this by proposing conceptual definitions, testing them empirically, and revising them as necessary. Sometimes they throw them out altogether. This is why the research literature often includes different conceptual definitions of the same construct. In some cases, an older conceptual definition has been replaced by a newer one that fits and works better. In others, researchers are still in the process of deciding which of various conceptual definitions is the best.
Operational Definitions
An operational definition is a definition of a variable in terms of precisely how it is to be measured. These measures generally fall into one of three broad categories. Self-report measures are those in which participants report on their own thoughts, feelings, and actions, as with the Rosenberg Self-Esteem Scale (Rosenberg, 1965) [2] . Behavioral measures are those in which some other aspect of participants’ behavior is observed and recorded. This is an extremely broad category that includes the observation of people’s behavior both in highly structured laboratory tasks and in more natural settings. A good example of the former would be measuring working memory capacity using the backward digit span task. A good example of the latter is a famous operational definition of physical aggression from researcher Albert Bandura and his colleagues (Bandura, Ross, & Ross, 1961) [3] . They let each of several children play for 20 minutes in a room that contained a clown-shaped punching bag called a Bobo doll. They filmed each child and counted the number of acts of physical aggression the child committed. These included hitting the doll with a mallet, punching it, and kicking it. Their operational definition, then, was the number of these specifically defined acts that the child committed during the 20-minute period. Finally, physiological measures are those that involve recording any of a wide variety of physiological processes, including heart rate and blood pressure, galvanic skin response, hormone levels, and electrical activity and blood flow in the brain.
For any given variable or construct, there will be multiple operational definitions. Stress is a good example. A rough conceptual definition is that stress is an adaptive response to a perceived danger or threat that involves physiological, cognitive, affective, and behavioral components. But researchers have operationally defined it in several ways. The Social Readjustment Rating Scale (Holmes & Rahe, 1967) [4] is a self-report questionnaire on which people identify stressful events that they have experienced in the past year and assigns points for each one depending on its severity. For example, a man who has been divorced (73 points), changed jobs (36 points), and had a change in sleeping habits (16 points) in the past year would have a total score of 125. The Hassles and Uplifts Scale (Delongis, Coyne, Dakof, Folkman & Lazarus, 1982) [5] is similar but focuses on everyday stressors like misplacing things and being concerned about one’s weight. The Perceived Stress Scale (Cohen, Kamarck, & Mermelstein, 1983) [6] is another self-report measure that focuses on people’s feelings of stress (e.g., “How often have you felt nervous and stressed?”). Researchers have also operationally defined stress in terms of several physiological variables including blood pressure and levels of the stress hormone cortisol.
When psychologists use multiple operational definitions of the same construct—either within a study or across studies—they are using converging operations . The idea is that the various operational definitions are “converging” or coming together on the same construct. When scores based on several different operational definitions are closely related to each other and produce similar patterns of results, this constitutes good evidence that the construct is being measured effectively and that it is useful. The various measures of stress, for example, are all correlated with each other and have all been shown to be correlated with other variables such as immune system functioning (also measured in a variety of ways) (Segerstrom & Miller, 2004) [7] . This is what allows researchers eventually to draw useful general conclusions, such as “stress is negatively correlated with immune system functioning,” as opposed to more specific and less useful ones, such as “people’s scores on the Perceived Stress Scale are negatively correlated with their white blood counts.”
Levels of Measurement
The psychologist S. S. Stevens suggested that scores can be assigned to individuals in a way that communicates more or less quantitative information about the variable of interest (Stevens, 1946) [8] . For example, the officials at a 100-m race could simply rank order the runners as they crossed the finish line (first, second, etc.), or they could time each runner to the nearest tenth of a second using a stopwatch (11.5 s, 12.1 s, etc.). In either case, they would be measuring the runners’ times by systematically assigning scores to represent those times. But while the rank ordering procedure communicates the fact that the second-place runner took longer to finish than the first-place finisher, the stopwatch procedure also communicates how much longer the second-place finisher took. Stevens actually suggested four different levels of measurement (which he called “scales of measurement”) that correspond to four types of information that can be communicated by a set of scores, and the statistical procedures that can be used with the information.
The nominal level of measurement is used for categorical variables and involves assigning scores that are category labels. Category labels communicate whether any two individuals are the same or different in terms of the variable being measured. For example, if you ask your participants about their marital status, you are engaged in nominal-level measurement. Or if you ask your participants to indicate which of several ethnicities they identify themselves with, you are again engaged in nominal-level measurement. The essential point about nominal scales is that they do not imply any ordering among the responses. For example, when classifying people according to their favorite color, there is no sense in which green is placed “ahead of” blue. Responses are merely categorized. Nominal scales thus embody the lowest level of measurement [9] .
The remaining three levels of measurement are used for quantitative variables. The ordinal level of measurement involves assigning scores so that they represent the rank order of the individuals. Ranks communicate not only whether any two individuals are the same or different in terms of the variable being measured but also whether one individual is higher or lower on that variable. For example, a researcher wishing to measure consumers’ satisfaction with their microwave ovens might ask them to specify their feelings as either “very dissatisfied,” “somewhat dissatisfied,” “somewhat satisfied,” or “very satisfied.” The items in this scale are ordered, ranging from least to most satisfied. This is what distinguishes ordinal from nominal scales. Unlike nominal scales, ordinal scales allow comparisons of the degree to which two individuals rate the variable. For example, our satisfaction ordering makes it meaningful to assert that one person is more satisfied than another with their microwave ovens. Such an assertion reflects the first person’s use of a verbal label that comes later in the list than the label chosen by the second person.
On the other hand, ordinal scales fail to capture important information that will be present in the other levels of measurement we examine. In particular, the difference between two levels of an ordinal scale cannot be assumed to be the same as the difference between two other levels (just like you cannot assume that the gap between the runners in first and second place is equal to the gap between the runners in second and third place). In our satisfaction scale, for example, the difference between the responses “very dissatisfied” and “somewhat dissatisfied” is probably not equivalent to the difference between “somewhat dissatisfied” and “somewhat satisfied.” Nothing in our measurement procedure allows us to determine whether the two differences reflect the same difference in psychological satisfaction. Statisticians express this point by saying that the differences between adjacent scale values do not necessarily represent equal intervals on the underlying scale giving rise to the measurements. (In our case, the underlying scale is the true feeling of satisfaction, which we are trying to measure.)
The interval level of measurement involves assigning scores using numerical scales in which intervals have the same interpretation throughout. As an example, consider either the Fahrenheit or Celsius temperature scales. The difference between 30 degrees and 40 degrees represents the same temperature difference as the difference between 80 degrees and 90 degrees. This is because each 10-degree interval has the same physical meaning (in terms of the kinetic energy of molecules).
Interval scales are not perfect, however. In particular, they do not have a true zero point even if one of the scaled values happens to carry the name “zero.” The Fahrenheit scale illustrates the issue. Zero degrees Fahrenheit does not represent the complete absence of temperature (the absence of any molecular kinetic energy). In reality, the label “zero” is applied to its temperature for quite accidental reasons connected to the history of temperature measurement. Since an interval scale has no true zero point, it does not make sense to compute ratios of temperatures. For example, there is no sense in which the ratio of 40 to 20 degrees Fahrenheit is the same as the ratio of 100 to 50 degrees; no interesting physical property is preserved across the two ratios. After all, if the “zero” label were applied at the temperature that Fahrenheit happens to label as 10 degrees, the two ratios would instead be 30 to 10 and 90 to 40, no longer the same! For this reason, it does not make sense to say that 80 degrees is “twice as hot” as 40 degrees. Such a claim would depend on an arbitrary decision about where to “start” the temperature scale, namely, what temperature to call zero (whereas the claim is intended to make a more fundamental assertion about the underlying physical reality).
In psychology, the intelligence quotient (IQ) is often considered to be measured at the interval level. While it is technically possible to receive a score of 0 on an IQ test, such a score would not indicate the complete absence of IQ. Moreover, a person with an IQ score of 140 does not have twice the IQ of a person with a score of 70. However, the difference between IQ scores of 80 and 100 is the same as the difference between IQ scores of 120 and 140.
Finally, the ratio level of measurement involves assigning scores in such a way that there is a true zero point that represents the complete absence of the quantity. Height measured in meters and weight measured in kilograms are good examples. So are counts of discrete objects or events such as the number of siblings one has or the number of questions a student answers correctly on an exam. You can think of a ratio scale as the three earlier scales rolled up in one. Like a nominal scale, it provides a name or category for each object (the numbers serve as labels). Like an ordinal scale, the objects are ordered (in terms of the ordering of the numbers). Like an interval scale, the same difference at two places on the scale has the same meaning. However, in addition, the same ratio at two places on the scale also carries the same meaning (see Table 4.1).
The Fahrenheit scale for temperature has an arbitrary zero point and is therefore not a ratio scale. However, zero on the Kelvin scale is absolute zero. This makes the Kelvin scale a ratio scale. For example, if one temperature is twice as high as another as measured on the Kelvin scale, then it has twice the kinetic energy of the other temperature.
Another example of a ratio scale is the amount of money you have in your pocket right now (25 cents, 50 cents, etc.). Money is measured on a ratio scale because, in addition to having the properties of an interval scale, it has a true zero point: if you have zero money, this actually implies the absence of money. Since money has a true zero point, it makes sense to say that someone with 50 cents has twice as much money as someone with 25 cents.
Stevens’s levels of measurement are important for at least two reasons. First, they emphasize the generality of the concept of measurement. Although people do not normally think of categorizing or ranking individuals as measurement, in fact, they are as long as they are done so that they represent some characteristic of the individuals. Second, the levels of measurement can serve as a rough guide to the statistical procedures that can be used with the data and the conclusions that can be drawn from them. With nominal-level measurement, for example, the only available measure of central tendency is the mode. With ordinal-level measurement, the median or mode can be used as indicators of central tendency. Interval and ratio-level measurement are typically considered the most desirable because they permit for any indicators of central tendency to be computed (i.e., mean, median, or mode). Also, ratio-level measurement is the only level that allows meaningful statements about ratios of scores. Once again, one cannot say that someone with an IQ of 140 is twice as intelligent as someone with an IQ of 70 because IQ is measured at the interval level, but one can say that someone with six siblings has twice as many as someone with three because number of siblings is measured at the ratio level.
- Costa, P. T., Jr., & McCrae, R. R. (1992). Normal personality assessment in clinical practice: The NEO Personality Inventory. Psychological Assessment, 4 , 5–13. ↵
- Rosenberg, M. (1965). Society and the adolescent self-image. Princeton, NJ: Princeton University Press ↵
- Bandura, A., Ross, D., & Ross, S. A. (1961). Transmission of aggression through imitation of aggressive models. Journal of Abnormal and Social Psychology, 63 , 575–582. ↵
- Holmes, T. H., & Rahe, R. H. (1967). The Social Readjustment Rating Scale. Journal of Psychosomatic Research, 11 (2), 213-218. ↵
- Delongis, A., Coyne, J. C., Dakof, G., Folkman, S., & Lazarus, R. S. (1982). Relationships of daily hassles, uplifts, and major life events to health status. Health Psychology, 1 (2), 119-136. ↵
- Cohen, S., Kamarck, T., & Mermelstein, R. (1983). A global measure of perceived stress. Journal of Health and Social Behavior, 24, 386-396. ↵
- Segerstrom, S. E., & Miller, G. E. (2004). Psychological stress and the human immune system: A meta-analytic study of 30 years of inquiry. Psychological Bulletin, 130 , 601–630. ↵
- Stevens, S. S. (1946). On the theory of scales of measurement. Science, 103 , 677–680. ↵
- Levels of Measurement. Retrieved from http://wikieducator.org/Introduction_to_Research_Methods_In_Psychology/Theories_and_Measurement/Levels_of_Measurement ↵
Is the assignment of scores to individuals so that the scores represent some characteristic of the individuals.
A subfield of psychology concerned with the theories and techniques of psychological measurement.
Psychological variables that represent an individual's mental state or experience, often not directly observable, such as personality traits, emotional states, attitudes, and abilities.
Describes the behaviors and internal processes that make up a psychological construct, along with how it relates to other variables.
A definition of the variable in terms of precisely how it is to be measured.
Measures in which participants report on their own thoughts, feelings, and actions.
Measures in which some other aspect of participants’ behavior is observed and recorded.
Measures that involve recording any of a wide variety of physiological processes, including heart rate and blood pressure, galvanic skin response, hormone levels, and electrical activity and blood flow in the brain.
When psychologists use multiple operational definitions of the same construct—either within a study or across studies.
Four categories, or scales, of measurement (i.e., nominal, ordinal, interval, and ratio) that specify the types of information that a set of scores can have, and the types of statistical procedures that can be used with the scores.
A measurement used for categorical variables and involves assigning scores that are category labels.
A measurement that involves assigning scores so that they represent the rank order of the individuals.
A measurement that involves assigning scores using numerical scales in which intervals have the same interpretation throughout.
A measurement that involves assigning scores in such a way that there is a true zero point that represents the complete absence of the quantity.
Understanding Psychological Measurement Copyright © by Rajiv S. Jhangiani; I-Chant A. Chiang; Carrie Cuttler; and Dana C. Leighton is licensed under a Creative Commons Attribution-NonCommercial-ShareAlike 4.0 International License , except where otherwise noted.
Share This Book
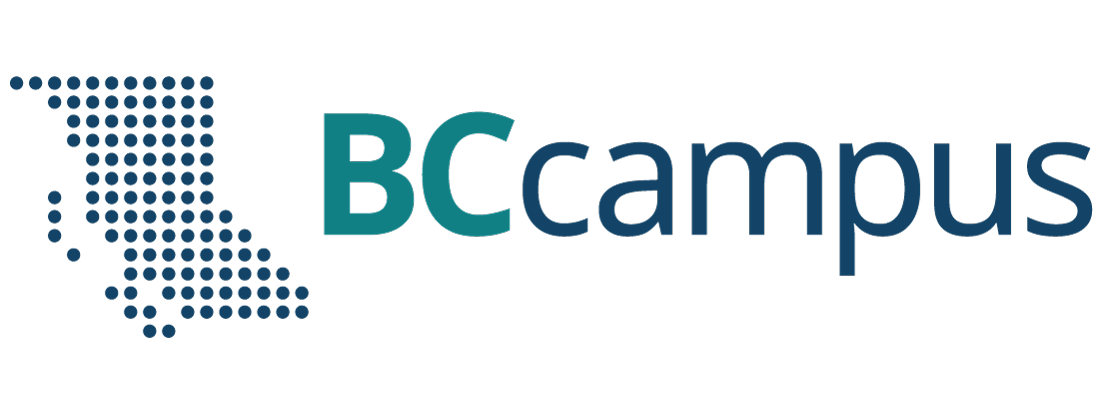
Want to create or adapt books like this? Learn more about how Pressbooks supports open publishing practices.
Chapter 5: Psychological Measurement
Reliability and Validity of Measurement
Learning Objectives
- Define reliability, including the different types and how they are assessed.
- Define validity, including the different types and how they are assessed.
- Describe the kinds of evidence that would be relevant to assessing the reliability and validity of a particular measure.
Again, measurement involves assigning scores to individuals so that they represent some characteristic of the individuals. But how do researchers know that the scores actually represent the characteristic, especially when it is a construct like intelligence, self-esteem, depression, or working memory capacity? The answer is that they conduct research using the measure to confirm that the scores make sense based on their understanding of the construct being measured. This is an extremely important point. Psychologists do not simply assume that their measures work. Instead, they collect data to demonstrate that they work. If their research does not demonstrate that a measure works, they stop using it.
As an informal example, imagine that you have been dieting for a month. Your clothes seem to be fitting more loosely, and several friends have asked if you have lost weight. If at this point your bathroom scale indicated that you had lost 10 pounds, this would make sense and you would continue to use the scale. But if it indicated that you had gained 10 pounds, you would rightly conclude that it was broken and either fix it or get rid of it. In evaluating a measurement method, psychologists consider two general dimensions: reliability and validity.
Reliability
Reliability refers to the consistency of a measure. Psychologists consider three types of consistency: over time (test-retest reliability), across items (internal consistency), and across different researchers (inter-rater reliability).
Test-Retest Reliability
When researchers measure a construct that they assume to be consistent across time, then the scores they obtain should also be consistent across time. Test-retest reliability is the extent to which this is actually the case. For example, intelligence is generally thought to be consistent across time. A person who is highly intelligent today will be highly intelligent next week. This means that any good measure of intelligence should produce roughly the same scores for this individual next week as it does today. Clearly, a measure that produces highly inconsistent scores over time cannot be a very good measure of a construct that is supposed to be consistent.
Assessing test-retest reliability requires using the measure on a group of people at one time, using it again on the same group of people at a later time, and then looking at test-retest correlation between the two sets of scores. This is typically done by graphing the data in a scatterplot and computing Pearson’s r . Figure 5.2 shows the correlation between two sets of scores of several university students on the Rosenberg Self-Esteem Scale, administered two times, a week apart. Pearson’s r for these data is +.95. In general, a test-retest correlation of +.80 or greater is considered to indicate good reliability.
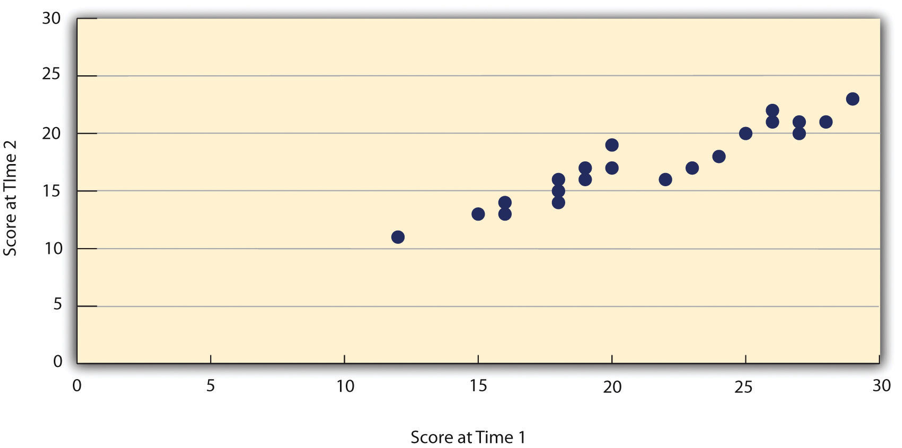
Again, high test-retest correlations make sense when the construct being measured is assumed to be consistent over time, which is the case for intelligence, self-esteem, and the Big Five personality dimensions. But other constructs are not assumed to be stable over time. The very nature of mood, for example, is that it changes. So a measure of mood that produced a low test-retest correlation over a period of a month would not be a cause for concern.
Internal Consistency
A second kind of reliability is internal consistency , which is the consistency of people’s responses across the items on a multiple-item measure. In general, all the items on such measures are supposed to reflect the same underlying construct, so people’s scores on those items should be correlated with each other. On the Rosenberg Self-Esteem Scale, people who agree that they are a person of worth should tend to agree that that they have a number of good qualities. If people’s responses to the different items are not correlated with each other, then it would no longer make sense to claim that they are all measuring the same underlying construct. This is as true for behavioural and physiological measures as for self-report measures. For example, people might make a series of bets in a simulated game of roulette as a measure of their level of risk seeking. This measure would be internally consistent to the extent that individual participants’ bets were consistently high or low across trials.
Like test-retest reliability, internal consistency can only be assessed by collecting and analyzing data. One approach is to look at a split-half correlation . This involves splitting the items into two sets, such as the first and second halves of the items or the even- and odd-numbered items. Then a score is computed for each set of items, and the relationship between the two sets of scores is examined. For example, Figure 5.3 shows the split-half correlation between several university students’ scores on the even-numbered items and their scores on the odd-numbered items of the Rosenberg Self-Esteem Scale. Pearson’s r for these data is +.88. A split-half correlation of +.80 or greater is generally considered good internal consistency.
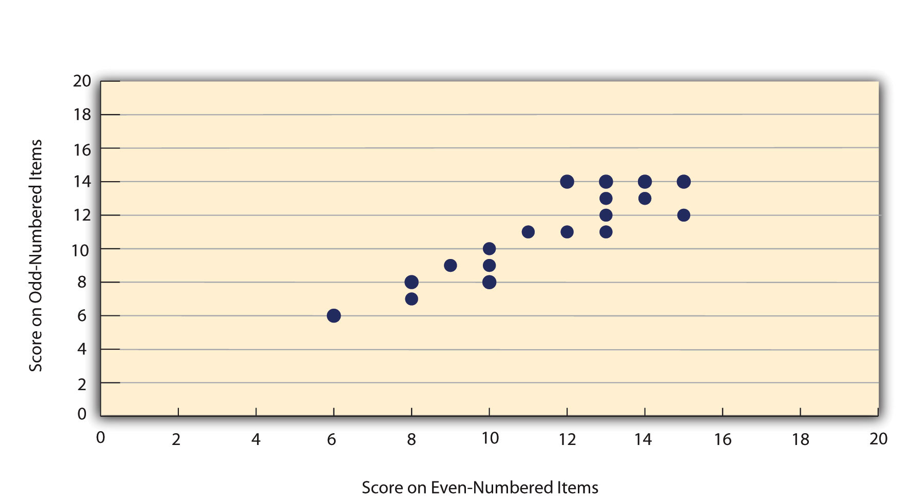
Perhaps the most common measure of internal consistency used by researchers in psychology is a statistic called Cronbach’s α (the Greek letter alpha). Conceptually, α is the mean of all possible split-half correlations for a set of items. For example, there are 252 ways to split a set of 10 items into two sets of five. Cronbach’s α would be the mean of the 252 split-half correlations. Note that this is not how α is actually computed, but it is a correct way of interpreting the meaning of this statistic. Again, a value of +.80 or greater is generally taken to indicate good internal consistency.
Interrater Reliability
Many behavioural measures involve significant judgment on the part of an observer or a rater. Inter-rater reliability is the extent to which different observers are consistent in their judgments. For example, if you were interested in measuring university students’ social skills, you could make video recordings of them as they interacted with another student whom they are meeting for the first time. Then you could have two or more observers watch the videos and rate each student’s level of social skills. To the extent that each participant does in fact have some level of social skills that can be detected by an attentive observer, different observers’ ratings should be highly correlated with each other. Inter-rater reliability would also have been measured in Bandura’s Bobo doll study. In this case, the observers’ ratings of how many acts of aggression a particular child committed while playing with the Bobo doll should have been highly positively correlated. Interrater reliability is often assessed using Cronbach’s α when the judgments are quantitative or an analogous statistic called Cohen’s κ (the Greek letter kappa) when they are categorical.
Validity is the extent to which the scores from a measure represent the variable they are intended to. But how do researchers make this judgment? We have already considered one factor that they take into account—reliability. When a measure has good test-retest reliability and internal consistency, researchers should be more confident that the scores represent what they are supposed to. There has to be more to it, however, because a measure can be extremely reliable but have no validity whatsoever. As an absurd example, imagine someone who believes that people’s index finger length reflects their self-esteem and therefore tries to measure self-esteem by holding a ruler up to people’s index fingers. Although this measure would have extremely good test-retest reliability, it would have absolutely no validity. The fact that one person’s index finger is a centimetre longer than another’s would indicate nothing about which one had higher self-esteem.
Discussions of validity usually divide it into several distinct “types.” But a good way to interpret these types is that they are other kinds of evidence—in addition to reliability—that should be taken into account when judging the validity of a measure. Here we consider three basic kinds: face validity, content validity, and criterion validity.
Face Validity
Face validity is the extent to which a measurement method appears “on its face” to measure the construct of interest. Most people would expect a self-esteem questionnaire to include items about whether they see themselves as a person of worth and whether they think they have good qualities. So a questionnaire that included these kinds of items would have good face validity. The finger-length method of measuring self-esteem, on the other hand, seems to have nothing to do with self-esteem and therefore has poor face validity. Although face validity can be assessed quantitatively—for example, by having a large sample of people rate a measure in terms of whether it appears to measure what it is intended to—it is usually assessed informally.
Face validity is at best a very weak kind of evidence that a measurement method is measuring what it is supposed to. One reason is that it is based on people’s intuitions about human behaviour, which are frequently wrong. It is also the case that many established measures in psychology work quite well despite lacking face validity. The Minnesota Multiphasic Personality Inventory-2 (MMPI-2) measures many personality characteristics and disorders by having people decide whether each of over 567 different statements applies to them—where many of the statements do not have any obvious relationship to the construct that they measure. For example, the items “I enjoy detective or mystery stories” and “The sight of blood doesn’t frighten me or make me sick” both measure the suppression of aggression. In this case, it is not the participants’ literal answers to these questions that are of interest, but rather whether the pattern of the participants’ responses to a series of questions matches those of individuals who tend to suppress their aggression.
Content Validity
Content validity is the extent to which a measure “covers” the construct of interest. For example, if a researcher conceptually defines test anxiety as involving both sympathetic nervous system activation (leading to nervous feelings) and negative thoughts, then his measure of test anxiety should include items about both nervous feelings and negative thoughts. Or consider that attitudes are usually defined as involving thoughts, feelings, and actions toward something. By this conceptual definition, a person has a positive attitude toward exercise to the extent that he or she thinks positive thoughts about exercising, feels good about exercising, and actually exercises. So to have good content validity, a measure of people’s attitudes toward exercise would have to reflect all three of these aspects. Like face validity, content validity is not usually assessed quantitatively. Instead, it is assessed by carefully checking the measurement method against the conceptual definition of the construct.
Criterion Validity
Criterion validity is the extent to which people’s scores on a measure are correlated with other variables (known as criteria ) that one would expect them to be correlated with. For example, people’s scores on a new measure of test anxiety should be negatively correlated with their performance on an important school exam. If it were found that people’s scores were in fact negatively correlated with their exam performance, then this would be a piece of evidence that these scores really represent people’s test anxiety. But if it were found that people scored equally well on the exam regardless of their test anxiety scores, then this would cast doubt on the validity of the measure.
A criterion can be any variable that one has reason to think should be correlated with the construct being measured, and there will usually be many of them. For example, one would expect test anxiety scores to be negatively correlated with exam performance and course grades and positively correlated with general anxiety and with blood pressure during an exam. Or imagine that a researcher develops a new measure of physical risk taking. People’s scores on this measure should be correlated with their participation in “extreme” activities such as snowboarding and rock climbing, the number of speeding tickets they have received, and even the number of broken bones they have had over the years. When the criterion is measured at the same time as the construct, criterion validity is referred to as concurrent validity ; however, when the criterion is measured at some point in the future (after the construct has been measured), it is referred to as predictive validity (because scores on the measure have “predicted” a future outcome).
Criteria can also include other measures of the same construct. For example, one would expect new measures of test anxiety or physical risk taking to be positively correlated with existing measures of the same constructs. This is known as convergent validity .
Assessing convergent validity requires collecting data using the measure. Researchers John Cacioppo and Richard Petty did this when they created their self-report Need for Cognition Scale to measure how much people value and engage in thinking (Cacioppo & Petty, 1982) [1] . In a series of studies, they showed that people’s scores were positively correlated with their scores on a standardized academic achievement test, and that their scores were negatively correlated with their scores on a measure of dogmatism (which represents a tendency toward obedience). In the years since it was created, the Need for Cognition Scale has been used in literally hundreds of studies and has been shown to be correlated with a wide variety of other variables, including the effectiveness of an advertisement, interest in politics, and juror decisions (Petty, Briñol, Loersch, & McCaslin, 2009) [2] .
Discriminant Validity
Discriminant validity , on the other hand, is the extent to which scores on a measure are not correlated with measures of variables that are conceptually distinct. For example, self-esteem is a general attitude toward the self that is fairly stable over time. It is not the same as mood, which is how good or bad one happens to be feeling right now. So people’s scores on a new measure of self-esteem should not be very highly correlated with their moods. If the new measure of self-esteem were highly correlated with a measure of mood, it could be argued that the new measure is not really measuring self-esteem; it is measuring mood instead.
When they created the Need for Cognition Scale, Cacioppo and Petty also provided evidence of discriminant validity by showing that people’s scores were not correlated with certain other variables. For example, they found only a weak correlation between people’s need for cognition and a measure of their cognitive style—the extent to which they tend to think analytically by breaking ideas into smaller parts or holistically in terms of “the big picture.” They also found no correlation between people’s need for cognition and measures of their test anxiety and their tendency to respond in socially desirable ways. All these low correlations provide evidence that the measure is reflecting a conceptually distinct construct.
Key Takeaways
- Psychological researchers do not simply assume that their measures work. Instead, they conduct research to show that they work. If they cannot show that they work, they stop using them.
- There are two distinct criteria by which researchers evaluate their measures: reliability and validity. Reliability is consistency across time (test-retest reliability), across items (internal consistency), and across researchers (interrater reliability). Validity is the extent to which the scores actually represent the variable they are intended to.
- Validity is a judgment based on various types of evidence. The relevant evidence includes the measure’s reliability, whether it covers the construct of interest, and whether the scores it produces are correlated with other variables they are expected to be correlated with and not correlated with variables that are conceptually distinct.
- The reliability and validity of a measure is not established by any single study but by the pattern of results across multiple studies. The assessment of reliability and validity is an ongoing process.
- Practice: Ask several friends to complete the Rosenberg Self-Esteem Scale. Then assess its internal consistency by making a scatterplot to show the split-half correlation (even- vs. odd-numbered items). Compute Pearson’s r too if you know how.
- Discussion: Think back to the last college exam you took and think of the exam as a psychological measure. What construct do you think it was intended to measure? Comment on its face and content validity. What data could you collect to assess its reliability and criterion validity?
- Cacioppo, J. T., & Petty, R. E. (1982). The need for cognition. Journal of Personality and Social Psychology, 42 , 116–131. ↵
- Petty, R. E, Briñol, P., Loersch, C., & McCaslin, M. J. (2009). The need for cognition. In M. R. Leary & R. H. Hoyle (Eds.), Handbook of individual differences in social behaviour (pp. 318–329). New York, NY: Guilford Press. ↵
The consistency of a measure.
The consistency of a measure over time.
The consistency of a measure on the same group of people at different times.
Consistency of people’s responses across the items on a multiple-item measure.
Method of assessing internal consistency through splitting the items into two sets and examining the relationship between them.
A statistic in which α is the mean of all possible split-half correlations for a set of items.
The extent to which different observers are consistent in their judgments.
The extent to which the scores from a measure represent the variable they are intended to.
The extent to which a measurement method appears to measure the construct of interest.
The extent to which a measure “covers” the construct of interest.
The extent to which people’s scores on a measure are correlated with other variables that one would expect them to be correlated with.
In reference to criterion validity, variables that one would expect to be correlated with the measure.
When the criterion is measured at the same time as the construct.
when the criterion is measured at some point in the future (after the construct has been measured).
When new measures positively correlate with existing measures of the same constructs.
The extent to which scores on a measure are not correlated with measures of variables that are conceptually distinct.
Research Methods in Psychology - 2nd Canadian Edition Copyright © 2015 by Paul C. Price, Rajiv Jhangiani, & I-Chant A. Chiang is licensed under a Creative Commons Attribution-NonCommercial-ShareAlike 4.0 International License , except where otherwise noted.
Share This Book
Measure Harmonization
Measure harmonization and alignment.
The definition of measure harmonization is standardizing specifications for related measures when they
- have the same measure focus (i.e., numerator criteria )
- have the same target population (i.e., denominator criteria)
- have elements that apply to many measures (e.g., age designation for children)
Measure developers should harmonize measures unless there is a compelling reason for not doing so that would justify keeping two or more similar appearing measures separate (e.g., significant risk variation by age, comorbidity, race). The measure developer should harmonize and standardize measure specifications so they are uniform or compatible unless the measure developer can justify differences as dictated by the evidence.
The dimensions of harmonization can include numerator, denominator, numerator and denominator exclusions, denominator exceptions, calculation, and data source and collection instructions. The extent of harmonization depends on the relationship of the measures, evidence for the specific measure focus, and differences in data sources.
The measure developer must ensure harmonization of the risk adjustment methodology of the harmonized measure with the risk adjustment methodology of the related measure or justify any differences. Measure developers should use the Blueprint content on the CMS MMS Hub as a guide to understand some of the concepts to explore during the development and assessment of the risk adjustment model. Because of the complexity of risk adjustment models, the measure developer should provide sufficient information to facilitate the understanding of the measure when vetted through CMS and its measure development partners, e.g., other federal agencies, or CMS consensus-based entity (CBE) for endorsement. For more information on risk adjustment, see the Risk Adjustment in Quality Measurement supplemental material.
The Blueprint content defines measure alignment as encouraging the use of similar standardized quality measures among government and private sector efforts. Harmonization is related to measure alignment because multiple programs and care settings may use harmonized measures of similar concepts. CMS seeks to align measures across programs, with other federal programs, and with private sector initiatives as much as is reasonable.
Alignment of quality initiatives across programs and with other federal partners and insurers helps to ensure clear information for patients and other consumers. A core set of measures increases signal for public and private recognition and payment programs ( Conway, Mostashari, & Clancy, 2013 ). When selecting harmonized measures across programs, it becomes possible to compare the provision of care in different settings. For example, if the calculation method of the influenza immunization rate measure is the same in hospitals, nursing homes, and other settings, it is possible to compare the achievement for population health across the multiple settings. If there is harmonization of functional status measurement and alignment of measure use across programs, it would be possible to compare gains across the continuum of care. Consumers and payers are enabled to choose measures based on similar calculations. In these and other ways, harmonization promotes
- comparisons of population health outcomes
- coordination across settings in the continuum of care
- clearer choices for consumers and payers
The Core Quality Measures Collaborative (CQMC) is a public-private partnership between America’s Health Insurance Plans and CMS. The membership is comprised of more than 70 organizations, including health insurance providers, primary care and specialty societies, consumer and employer groups, and other quality collaboratives. The aims of the CQMC are to
- Identify high-value, high-impact, evidenced-based measures promoting better patient outcomes and providing useful information for improvement, decision-making, and payment.
- Align measures across public and private payers to achieve congruence in the measures used for quality improvement, transparency, and payment purposes.
- Reduce the burden of quality measurement by eliminating low-value metrics, redundancies, and inconsistencies in measure specifications and quality measure reporting requirements across payors. The CQMC has core sets of quality measures in multiple categories.

CRO Platform
Test your insights. Run experiments. Win. Or learn. And then win.

eCommerce Customer Analytics Platform

Acquisition matters. But retention matters more. Understand, monitor & nurture the best customers.
- Case Studies
- Ebooks, Tools, Templates
- Digital Marketing Glossary
- eCommerce Growth Stories
- eCommerce Growth Show
- Help & Technical Documentation
CRO Guide > Chapter 3.1
Qualitative Research: Definition, Methodology, Limitation & Examples
Qualitative research is a method focused on understanding human behavior and experiences through non-numerical data. Examples of qualitative research include:
- One-on-one interviews,
- Focus groups, Ethnographic research,
- Case studies,
- Record keeping,
- Qualitative observations
In this article, we’ll provide tips and tricks on how to use qualitative research to better understand your audience through real world examples and improve your ROI. We’ll also learn the difference between qualitative and quantitative data.

Table of Contents
Marketers often seek to understand their customers deeply. Qualitative research methods such as face-to-face interviews, focus groups, and qualitative observations can provide valuable insights into your products, your market, and your customers’ opinions and motivations. Understanding these nuances can significantly enhance marketing strategies and overall customer satisfaction.
What is Qualitative Research
Qualitative research is a market research method that focuses on obtaining data through open-ended and conversational communication. This method focuses on the “why” rather than the “what” people think about you. Thus, qualitative research seeks to uncover the underlying motivations, attitudes, and beliefs that drive people’s actions.
Let’s say you have an online shop catering to a general audience. You do a demographic analysis and you find out that most of your customers are male. Naturally, you will want to find out why women are not buying from you. And that’s what qualitative research will help you find out.
In the case of your online shop, qualitative research would involve reaching out to female non-customers through methods such as in-depth interviews or focus groups. These interactions provide a platform for women to express their thoughts, feelings, and concerns regarding your products or brand. Through qualitative analysis, you can uncover valuable insights into factors such as product preferences, user experience, brand perception, and barriers to purchase.
Types of Qualitative Research Methods
Qualitative research methods are designed in a manner that helps reveal the behavior and perception of a target audience regarding a particular topic.
The most frequently used qualitative analysis methods are one-on-one interviews, focus groups, ethnographic research, case study research, record keeping, and qualitative observation.
1. One-on-one interviews
Conducting one-on-one interviews is one of the most common qualitative research methods. One of the advantages of this method is that it provides a great opportunity to gather precise data about what people think and their motivations.
Spending time talking to customers not only helps marketers understand who their clients are, but also helps with customer care: clients love hearing from brands. This strengthens the relationship between a brand and its clients and paves the way for customer testimonials.
- A company might conduct interviews to understand why a product failed to meet sales expectations.
- A researcher might use interviews to gather personal stories about experiences with healthcare.
These interviews can be performed face-to-face or on the phone and usually last between half an hour to over two hours.
When a one-on-one interview is conducted face-to-face, it also gives the marketer the opportunity to read the body language of the respondent and match the responses.
2. Focus groups
Focus groups gather a small number of people to discuss and provide feedback on a particular subject. The ideal size of a focus group is usually between five and eight participants. The size of focus groups should reflect the participants’ familiarity with the topic. For less important topics or when participants have little experience, a group of 10 can be effective. For more critical topics or when participants are more knowledgeable, a smaller group of five to six is preferable for deeper discussions.
The main goal of a focus group is to find answers to the “why”, “what”, and “how” questions. This method is highly effective in exploring people’s feelings and ideas in a social setting, where group dynamics can bring out insights that might not emerge in one-on-one situations.
- A focus group could be used to test reactions to a new product concept.
- Marketers might use focus groups to see how different demographic groups react to an advertising campaign.
One advantage that focus groups have is that the marketer doesn’t necessarily have to interact with the group in person. Nowadays focus groups can be sent as online qualitative surveys on various devices.
Focus groups are an expensive option compared to the other qualitative research methods, which is why they are typically used to explain complex processes.
3. Ethnographic research
Ethnographic research is the most in-depth observational method that studies individuals in their naturally occurring environment.
This method aims at understanding the cultures, challenges, motivations, and settings that occur.
- A study of workplace culture within a tech startup.
- Observational research in a remote village to understand local traditions.
Ethnographic research requires the marketer to adapt to the target audiences’ environments (a different organization, a different city, or even a remote location), which is why geographical constraints can be an issue while collecting data.
This type of research can last from a few days to a few years. It’s challenging and time-consuming and solely depends on the expertise of the marketer to be able to analyze, observe, and infer the data.
4. Case study research
The case study method has grown into a valuable qualitative research method. This type of research method is usually used in education or social sciences. It involves a comprehensive examination of a single instance or event, providing detailed insights into complex issues in real-life contexts.
- Analyzing a single school’s innovative teaching method.
- A detailed study of a patient’s medical treatment over several years.
Case study research may seem difficult to operate, but it’s actually one of the simplest ways of conducting research as it involves a deep dive and thorough understanding of the data collection methods and inferring the data.
5. Record keeping
Record keeping is similar to going to the library: you go over books or any other reference material to collect relevant data. This method uses already existing reliable documents and similar sources of information as a data source.
- Historical research using old newspapers and letters.
- A study on policy changes over the years by examining government records.
This method is useful for constructing a historical context around a research topic or verifying other findings with documented evidence.
6. Qualitative observation
Qualitative observation is a method that uses subjective methodologies to gather systematic information or data. This method deals with the five major sensory organs and their functioning, sight, smell, touch, taste, and hearing.
- Sight : Observing the way customers visually interact with product displays in a store to understand their browsing behaviors and preferences.
- Smell : Noting reactions of consumers to different scents in a fragrance shop to study the impact of olfactory elements on product preference.
- Touch : Watching how individuals interact with different materials in a clothing store to assess the importance of texture in fabric selection.
- Taste : Evaluating reactions of participants in a taste test to identify flavor profiles that appeal to different demographic groups.
- Hearing : Documenting responses to changes in background music within a retail environment to determine its effect on shopping behavior and mood.
Below we are also providing real-life examples of qualitative research that demonstrate practical applications across various contexts:
Qualitative Research Real World Examples
Let’s explore some examples of how qualitative research can be applied in different contexts.
1. Online grocery shop with a predominantly male audience
Method used: one-on-one interviews.
Let’s go back to one of the previous examples. You have an online grocery shop. By nature, it addresses a general audience, but after you do a demographic analysis you find out that most of your customers are male.
One good method to determine why women are not buying from you is to hold one-on-one interviews with potential customers in the category.
Interviewing a sample of potential female customers should reveal why they don’t find your store appealing. The reasons could range from not stocking enough products for women to perhaps the store’s emphasis on heavy-duty tools and automotive products, for example. These insights can guide adjustments in inventory and marketing strategies.
2. Software company launching a new product
Method used: focus groups.
Focus groups are great for establishing product-market fit.
Let’s assume you are a software company that wants to launch a new product and you hold a focus group with 12 people. Although getting their feedback regarding users’ experience with the product is a good thing, this sample is too small to define how the entire market will react to your product.
So what you can do instead is holding multiple focus groups in 20 different geographic regions. Each region should be hosting a group of 12 for each market segment; you can even segment your audience based on age. This would be a better way to establish credibility in the feedback you receive.
3. Alan Pushkin’s “God’s Choice: The Total World of a Fundamentalist Christian School”
Method used: ethnographic research.
Moving from a fictional example to a real-life one, let’s analyze Alan Peshkin’s 1986 book “God’s Choice: The Total World of a Fundamentalist Christian School”.
Peshkin studied the culture of Bethany Baptist Academy by interviewing the students, parents, teachers, and members of the community alike, and spending eighteen months observing them to provide a comprehensive and in-depth analysis of Christian schooling as an alternative to public education.
The study highlights the school’s unified purpose, rigorous academic environment, and strong community support while also pointing out its lack of cultural diversity and openness to differing viewpoints. These insights are crucial for understanding how such educational settings operate and what they offer to students.
Even after discovering all this, Peshkin still presented the school in a positive light and stated that public schools have much to learn from such schools.
Peshkin’s in-depth research represents a qualitative study that uses observations and unstructured interviews, without any assumptions or hypotheses. He utilizes descriptive or non-quantifiable data on Bethany Baptist Academy specifically, without attempting to generalize the findings to other Christian schools.
4. Understanding buyers’ trends
Method used: record keeping.
Another way marketers can use quality research is to understand buyers’ trends. To do this, marketers need to look at historical data for both their company and their industry and identify where buyers are purchasing items in higher volumes.
For example, electronics distributors know that the holiday season is a peak market for sales while life insurance agents find that spring and summer wedding months are good seasons for targeting new clients.
5. Determining products/services missing from the market
Conducting your own research isn’t always necessary. If there are significant breakthroughs in your industry, you can use industry data and adapt it to your marketing needs.
The influx of hacking and hijacking of cloud-based information has made Internet security a topic of many industry reports lately. A software company could use these reports to better understand the problems its clients are facing.
As a result, the company can provide solutions prospects already know they need.
Real-time Customer Lifetime Value (CLV) Benchmark Report
See where your business stands compared to 1,000+ e-stores in different industries.
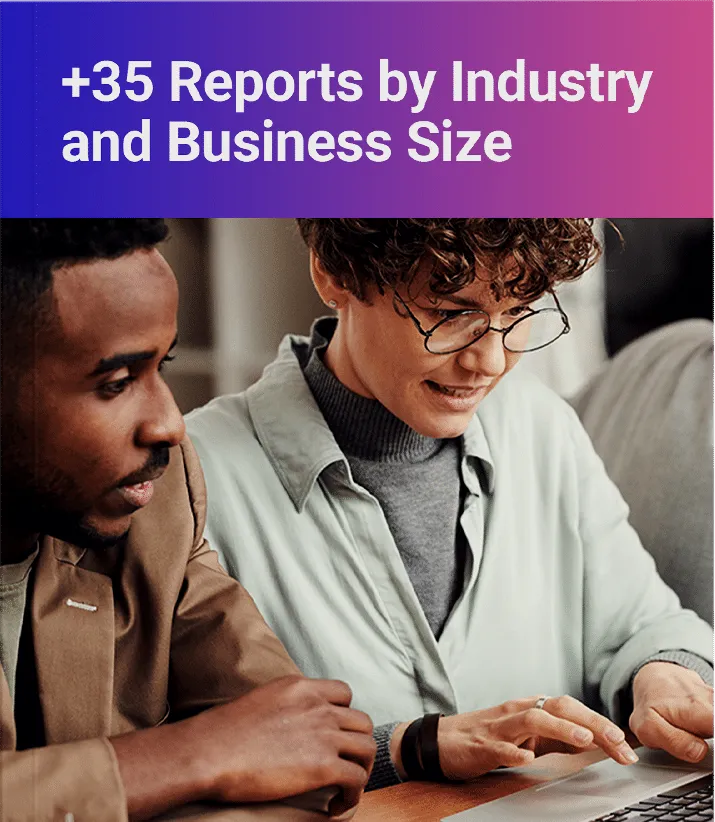
Qualitative Research Approaches
Once the marketer has decided that their research questions will provide data that is qualitative in nature, the next step is to choose the appropriate qualitative approach.
The approach chosen will take into account the purpose of the research, the role of the researcher, the data collected, the method of data analysis , and how the results will be presented. The most common approaches include:
- Narrative : This method focuses on individual life stories to understand personal experiences and journeys. It examines how people structure their stories and the themes within them to explore human existence. For example, a narrative study might look at cancer survivors to understand their resilience and coping strategies.
- Phenomenology : attempts to understand or explain life experiences or phenomena; It aims to reveal the depth of human consciousness and perception, such as by studying the daily lives of those with chronic illnesses.
- Grounded theory : investigates the process, action, or interaction with the goal of developing a theory “grounded” in observations and empirical data.
- Ethnography : describes and interprets an ethnic, cultural, or social group;
- Case study : examines episodic events in a definable framework, develops in-depth analyses of single or multiple cases, and generally explains “how”. An example might be studying a community health program to evaluate its success and impact.
How to Analyze Qualitative Data
Analyzing qualitative data involves interpreting non-numerical data to uncover patterns, themes, and deeper insights. This process is typically more subjective and requires a systematic approach to ensure reliability and validity.
1. Data Collection
Ensure that your data collection methods (e.g., interviews, focus groups, observations) are well-documented and comprehensive. This step is crucial because the quality and depth of the data collected will significantly influence the analysis.
2. Data Preparation
Once collected, the data needs to be organized. Transcribe audio and video recordings, and gather all notes and documents. Ensure that all data is anonymized to protect participant confidentiality where necessary.
3. Familiarization
Immerse yourself in the data by reading through the materials multiple times. This helps you get a general sense of the information and begin identifying patterns or recurring themes.
Develop a coding system to tag data with labels that summarize and account for each piece of information. Codes can be words, phrases, or acronyms that represent how these segments relate to your research questions.
- Descriptive Coding : Summarize the primary topic of the data.
- In Vivo Coding : Use language and terms used by the participants themselves.
- Process Coding : Use gerunds (“-ing” words) to label the processes at play.
- Emotion Coding : Identify and record the emotions conveyed or experienced.
5. Thematic Development
Group codes into themes that represent larger patterns in the data. These themes should relate directly to the research questions and form a coherent narrative about the findings.
6. Interpreting the Data
Interpret the data by constructing a logical narrative. This involves piecing together the themes to explain larger insights about the data. Link the results back to your research objectives and existing literature to bolster your interpretations.
7. Validation
Check the reliability and validity of your findings by reviewing if the interpretations are supported by the data. This may involve revisiting the data multiple times or discussing the findings with colleagues or participants for validation.
8. Reporting
Finally, present the findings in a clear and organized manner. Use direct quotes and detailed descriptions to illustrate the themes and insights. The report should communicate the narrative you’ve built from your data, clearly linking your findings to your research questions.
Limitations of qualitative research
The disadvantages of qualitative research are quite unique. The techniques of the data collector and their own unique observations can alter the information in subtle ways. That being said, these are the qualitative research’s limitations:
1. It’s a time-consuming process
The main drawback of qualitative study is that the process is time-consuming. Another problem is that the interpretations are limited. Personal experience and knowledge influence observations and conclusions.
Thus, qualitative research might take several weeks or months. Also, since this process delves into personal interaction for data collection, discussions often tend to deviate from the main issue to be studied.
2. You can’t verify the results of qualitative research
Because qualitative research is open-ended, participants have more control over the content of the data collected. So the marketer is not able to verify the results objectively against the scenarios stated by the respondents. For example, in a focus group discussing a new product, participants might express their feelings about the design and functionality. However, these opinions are influenced by individual tastes and experiences, making it difficult to ascertain a universally applicable conclusion from these discussions.
3. It’s a labor-intensive approach
Qualitative research requires a labor-intensive analysis process such as categorization, recording, etc. Similarly, qualitative research requires well-experienced marketers to obtain the needed data from a group of respondents.
4. It’s difficult to investigate causality
Qualitative research requires thoughtful planning to ensure the obtained results are accurate. There is no way to analyze qualitative data mathematically. This type of research is based more on opinion and judgment rather than results. Because all qualitative studies are unique they are difficult to replicate.
5. Qualitative research is not statistically representative
Because qualitative research is a perspective-based method of research, the responses given are not measured.
Comparisons can be made and this can lead toward duplication, but for the most part, quantitative data is required for circumstances that need statistical representation and that is not part of the qualitative research process.
While doing a qualitative study, it’s important to cross-reference the data obtained with the quantitative data. By continuously surveying prospects and customers marketers can build a stronger database of useful information.
Quantitative vs. Qualitative Research

Image source
Quantitative and qualitative research are two distinct methodologies used in the field of market research, each offering unique insights and approaches to understanding consumer behavior and preferences.
As we already defined, qualitative analysis seeks to explore the deeper meanings, perceptions, and motivations behind human behavior through non-numerical data. On the other hand, quantitative research focuses on collecting and analyzing numerical data to identify patterns, trends, and statistical relationships.
Let’s explore their key differences:
Nature of Data:
- Quantitative research : Involves numerical data that can be measured and analyzed statistically.
- Qualitative research : Focuses on non-numerical data, such as words, images, and observations, to capture subjective experiences and meanings.
Research Questions:
- Quantitative research : Typically addresses questions related to “how many,” “how much,” or “to what extent,” aiming to quantify relationships and patterns.
- Qualitative research: Explores questions related to “why” and “how,” aiming to understand the underlying motivations, beliefs, and perceptions of individuals.
Data Collection Methods:
- Quantitative research : Relies on structured surveys, experiments, or observations with predefined variables and measures.
- Qualitative research : Utilizes open-ended interviews, focus groups, participant observations, and textual analysis to gather rich, contextually nuanced data.
Analysis Techniques:
- Quantitative research: Involves statistical analysis to identify correlations, associations, or differences between variables.
- Qualitative research: Employs thematic analysis, coding, and interpretation to uncover patterns, themes, and insights within qualitative data.

Do Conversion Rate Optimization the Right way.
Explore helps you make the most out of your CRO efforts through advanced A/B testing, surveys, advanced segmentation and optimised customer journeys.

If you haven’t subscribed yet to our newsletter, now is your chance!

Like what you’re reading?
Join the informed ecommerce crowd.
We will never bug you with irrelevant info.
By clicking the Button, you confirm that you agree with our Terms and Conditions .
Continue your Conversion Rate Optimization Journey
- Last modified: January 3, 2023
- Conversion Rate Optimization , User Research
Valentin Radu

We’re a team of people that want to empower marketers around the world to create marketing campaigns that matter to consumers in a smart way. Meet us at the intersection of creativity, integrity, and development, and let us show you how to optimize your marketing.
Our Software
- > Book a Demo
- > Partner Program
- > Affiliate Program
- Blog Sitemap
- Terms and Conditions
- Privacy & Security
- Cookies Policy
- REVEAL Terms and Conditions
Featured Topics
Featured series.
A series of random questions answered by Harvard experts.
Explore the Gazette
Read the latest.
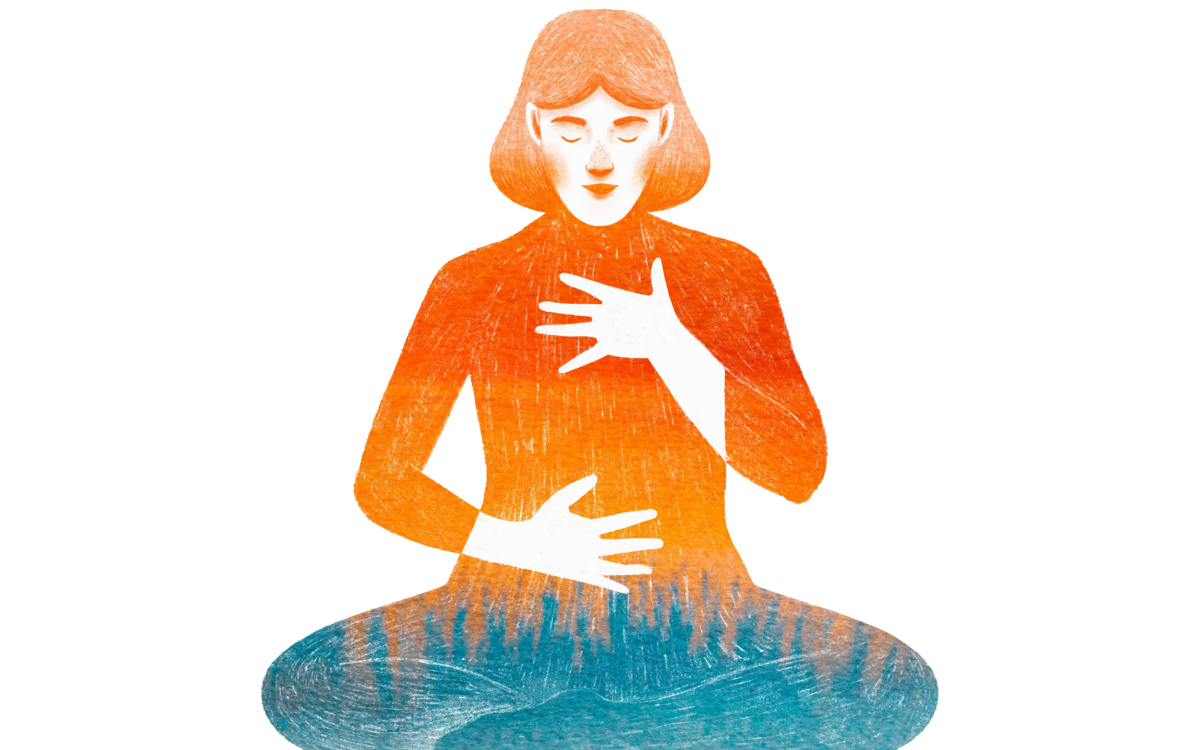
Had a bad experience meditating? You’re not alone.
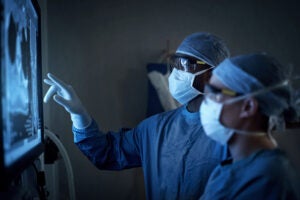
Families may remove brain-injured patients from life support too soon
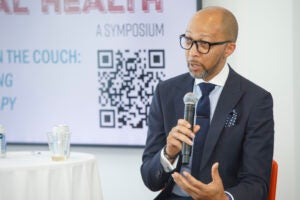
What to do about mental health crisis among Black males
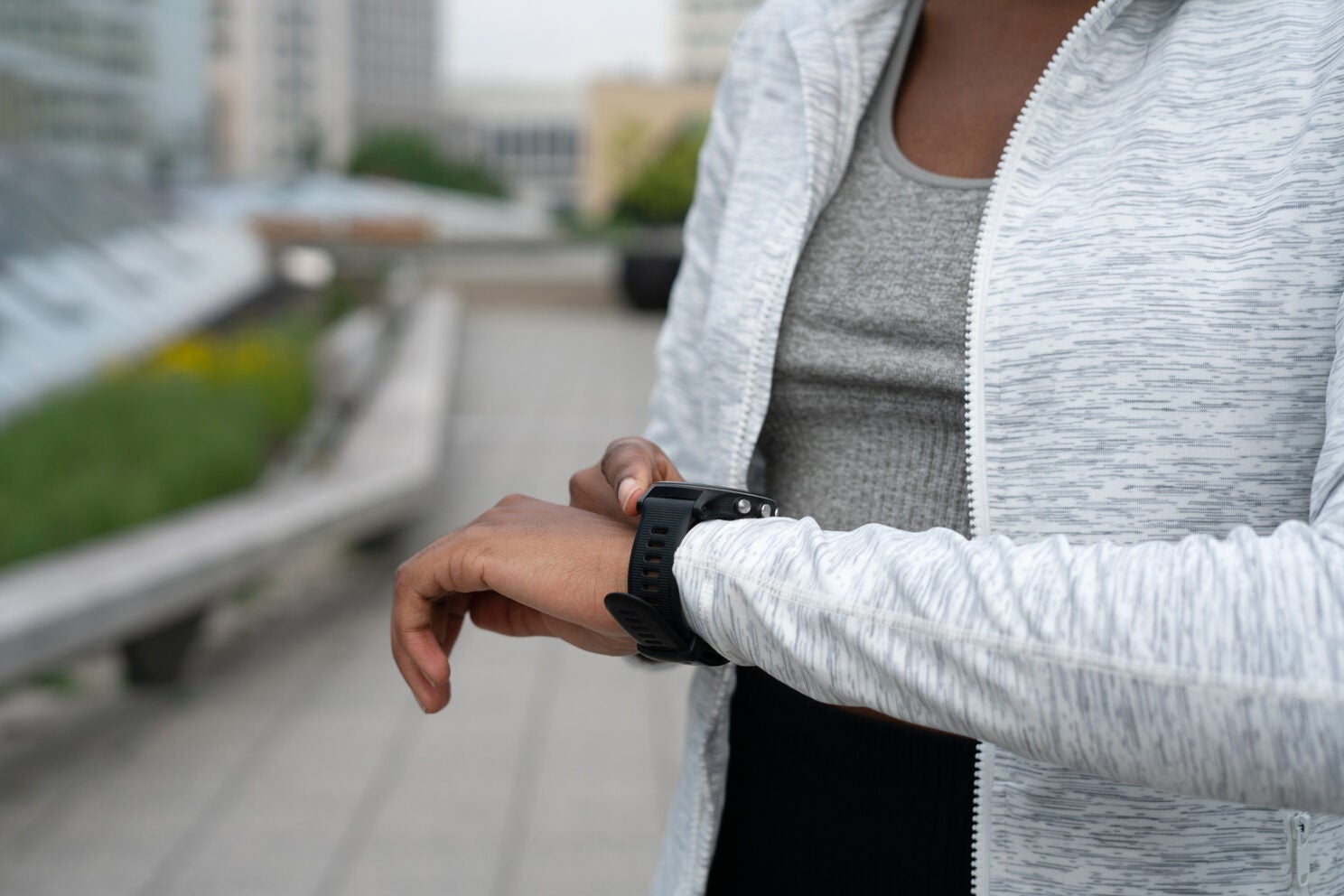
Everything counts!
Research finds step-count and time are equally valid in reducing health risks
Kira Sampson
BWH Communications
A new study suggests that both step-count and time-based exercise goals are equally effective in reducing risks of heart disease and early death.
Researchers from Harvard-affiliated Brigham and Women’s Hospital reviewed data on healthy women age 62+, who used wearable devices to record their physical activity, and then tracked their health outcomes. After a median follow-up of nine years, the researchers found higher levels of physical activity, whether in time of exercise or step counts, were associated with large risk reductions in mortality and cardiovascular disease. The most active quarter of women in the study had a 30 to 40 percent reduced risk compared to the least active quarter.
Results of the study are published in JAMA Internal Medicine .
“We recognized that existing physical activity guidelines focus primarily on activity duration and intensity but lack step-based recommendations,” said lead author Rikuta Hamaya, a researcher in the Division of Preventive Medicine at BWH. “With more people using smartwatches to measure their steps and overall health, we saw the importance of ascertaining how step-based measurements compare to time-based targets in their association with health outcomes — is one better than the other?”
“Movement looks different for everyone, and nearly all forms of movement are beneficial to our health.” Rikuta Hamaya
The current U.S. guidelines , last updated in 2018, recommend that adults engage in at least 150 minutes of moderate to vigorous physical activity (e.g., brisk walking) or 75 minutes of vigorous activity (e.g., jogging) per week. At that time, most of the existing evidence on health benefits came from studies where participants self-reported their physical activity. Few data points existed on the relationship between steps and health. Fast-forward to the present — with wearables being ubiquitous, step counts are now a popular metric among many fitness-tracking platforms. How do time-based goals stack up against step-based ones? Investigators sought to answer this question.
For this study, investigators collected data from 14,399 women who participated in the Women’s Health Study, and were healthy (free from cardiovascular disease and cancer). Between 2011 and 2015, participants aged 62 years and older were asked to wear research-grade wearables for seven consecutive days to record their physical activity levels, only removing the devices for sleep or water-related activities. Throughout the study period, annual questionnaires were administered to ascertain health outcomes of interest, in particular, death from any cause and cardiovascular disease. Investigators followed up with participants through the end of 2022.
At the time of device wear, researchers found that participants engaged in a median of 62 minutes of moderate-to-vigorous intensity physical activity per week and accumulated a median of 5,183 steps per day. During a median follow-up of nine years, approximately 9 percent of participants had passed and roughly 4 percent developed cardiovascular disease.
Higher levels of physical activity (whether assessed as step counts or time in moderate to vigorous activity) were associated with large risk reductions in death or cardiovascular disease — the most active quarter of women reduced their risk by 30-40 percent compared with the least-active quarter. Individuals in the top three quartiles of physical activity outlived those in the bottom quartile by an average of 2.22 and 2.36 months respectively, based on time and step-based measurements, at nine years of follow-up. This survival advantage persisted regardless of differences in body mass index (BMI).
While both metrics are useful in portraying health status, Hamaya explained that each has its advantages and downsides. For one, step counts may not account for differences in fitness levels. For example, if a 20-year-old and 80-year-old both walk for 30 minutes at moderate intensity, their step counts may differ significantly. Conversely, steps are straightforward to measure and less subject to interpretation compared to exercise intensity. Additionally, steps capture even sporadic movements of everyday life, not just exercise, and these kinds of daily life activities likely are those carried out by older individuals.
“For some, especially for younger individuals, exercise may involve activities like tennis, soccer, walking, or jogging, all of which can be easily tracked with steps. However, for others, it may consist of bike rides or swimming, where monitoring the duration of exercise is simpler,” said Hamaya. “That’s why it’s important for physical-activity guidelines to offer multiple ways to reach goals. Movement looks different for everyone, and nearly all forms of movement are beneficial to our health.”
The authors note that this study incorporates only a single assessment of time and step-based physical activity metrics. Further, most women included in the study were white and of higher socioeconomic status. Finally, this study was observational, and thus causal relations cannot be proven. In the future, Hamaya aims to collect more data via a randomized controlled trial to better understand the relationship between time and step-based exercise metrics and health.
“The next federal physical activity guidelines are planned for 2028,” said senior author I-Min Lee, an epidemiologist in the Division of Preventive Medicine at BWH. “Our findings further establish the importance of adding step-based targets, in order to accommodate flexibility of goals that work for individuals with differing preferences, abilities and lifestyles.”
Disclosures : Hamaya reported receiving consulting fees from DeSC Healthcare, Inc., outside of the submitted work. Co-authors Christopher Moore, Julie Buring, Kelly Evenson, and Lee reported receiving institutional support from the National Institutes of Health during the conduct of the study.
This research was supported in part by the National Institutes of Health (CA154647, CA047988, CA182913, HL043851, HL080467, and HL09935), the National Cancer Institute (5R01CA227122), Office of the Director, Office of Disease Prevention, and Office of Behavioral and Social Sciences Research; and by the extramural research program at the National Heart, Lung, and Blood Institute.
Get the best of the Gazette delivered to your inbox
By subscribing to this newsletter you’re agreeing to our privacy policy
Share this article
You might like.
Altered states of consciousness through yoga, mindfulness more common than thought and mostly beneficial, study finds — though clinicians ill-equipped to help those who struggle
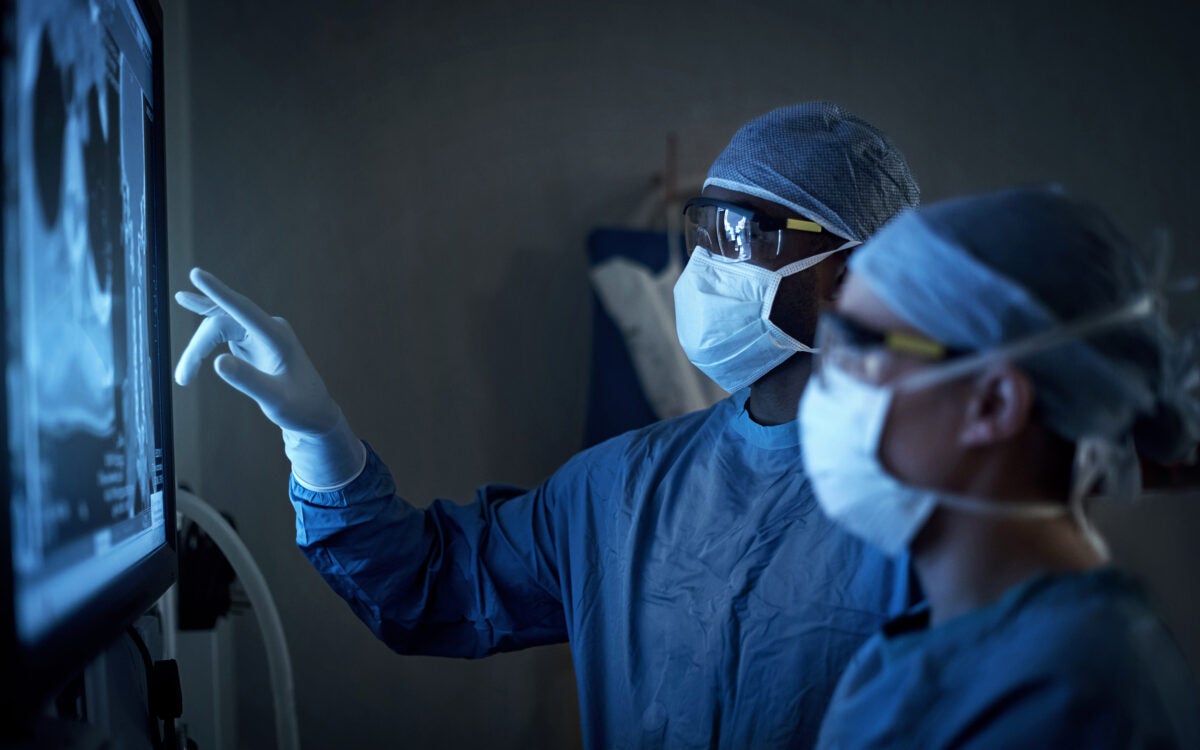
Of the survivors within one study group, more than 40% recovered at least some independence.
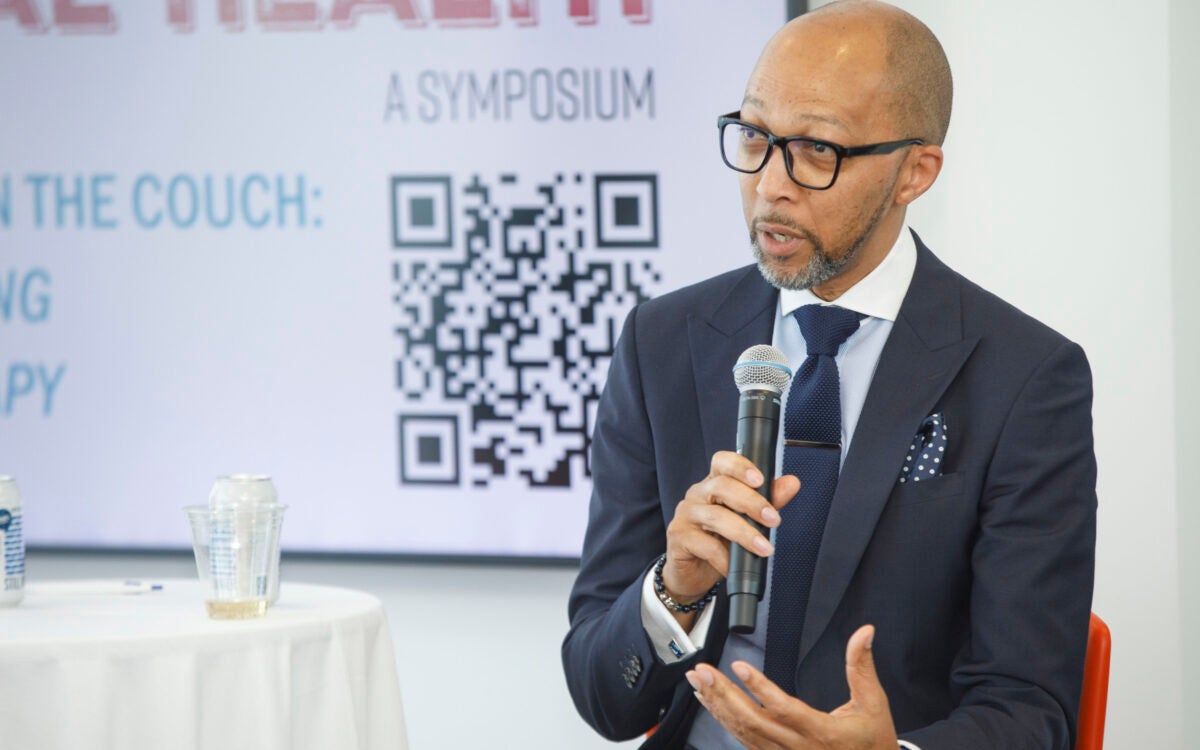
Symposium examines thorny, multifaceted dilemma from systemic racism in policing, healthcare to stigma attached to psychotherapy in community
Six receive honorary degrees
Harvard recognizes educator, conductor, theoretical physicist, advocate for elderly, writer, and Nobel laureate
New study finds step-count and time are equally valid in reducing health risks
Bridging social distance, embracing uncertainty, fighting for equity
Student Commencement speeches to tap into themes faced by Class of 2024
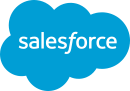
Salesforce is closed for new business in your area.

IMAGES
VIDEO
COMMENTS
lying concepts that need to be measured. This element of the definition of measurement highlights the importance of finding the most appropriate attributes to study in a research area. This element also emphasizes under-standing what these attributes really mean, that is, fully understanding the underlying concepts being measured.
Measurement is the process of observing and recording the observations that are collected as part of a research effort. There are two major issues that will be considered here. First, you have to understand the fundamental ideas involved in measuring. Here we consider two of major measurement concepts. In Levels of Measurement, I explain the ...
Measurement in social science is a process. It occurs at multiple stages of a research project: in the planning stages, in the data collection stage, and sometimes even in the analysis stage. Recall that previously we defined measurement as the process by which we describe and ascribe meaning to the key facts, concepts, or other phenomena that ...
What Is Measurement? Measurement is the assignment of scores to individuals so that the scores represent some characteristic of the individuals. This very general definition is consistent with the kinds of measurement that everyone is familiar with—for example, weighing oneself by stepping onto a bathroom scale, or checking the internal temperature of a roasting turkey by inserting a meat ...
In research, measurement is a systematic procedure for assigning scores, meanings, and descriptions to concepts so that those scores represent the characteristic of interest. Social scientists can and do measure just about anything you can imagine observing or wanting to study. Of course, some things are easier to observe or measure than others.
Measurement is an integral part of modern science as well as of engineering, commerce, and daily life. Measurement is often considered a hallmark of the scientific enterprise and a privileged source of knowledge relative to qualitative modes of inquiry. [] Despite its ubiquity and importance, there is little consensus among philosophers as to how to define measurement, what sorts of things are ...
Measurement in science begins with the activity of distinguishing groups or phenomena from one another. This process, which is generally termed classification, implies that we can place units of scientific study—such as victims, offenders, crimes, or crime places—in clearly defined categories or along some continuum.
The importance of measurement in research and technology is indisputable. Measurement is the fundamental mechanism of scientific study and development, and it allows to describe the different phenomena of the universe through the exact and general language of mathematics, without which it would be challenging to define practical or theoretical approaches from scientific investigation.
Historically, few scholars in mathematics, physics, philosophy, and psychology have striven to present a unified definition of measurement. Some, like Michell (), have turned to the history of the concept in the physical sciences to "properly define" what "scientific measurement" is.Others, like Berka (), for instance, have embraced the multiplicity of applications and have sought to ...
Measurement tools are instruments used by researchers and practitioners to aid in the assessment or evaluation of subjects, clients or patients. The instruments are used to measure or collect data on a variety of variables ranging from physical functioning to psychosocial wellbeing. Types of measurement tools include scales, indexes, surveys ...
Quantitative research is based on measurement and is conducted in a systematic, controlled manner. These measures enable researchers to perform statistical tests, analyze differences between groups, and determine the effectiveness of treatments. If something is not measurable, it cannot be tested. Keywords: measurements; quantitative research ...
Measures exist to numerically represent degrees of attributes. Quantitative research is based on measurement and is conducted in a systematic, controlled manner. These measures enable researchers to perform statistical tests, analyze differences between groups, and determine the effectiveness of treatments. If something is not measurable, it ...
measurement, the process of associating numbers with physical quantities and phenomena. Measurement is fundamental to the sciences; to engineering, construction, and other technical fields; and to almost all everyday activities. For that reason the elements, conditions, limitations, and theoretical foundations of measurement have been much ...
This chapter provides an overview of considerations for the development of outcome measures for observational comparative effectiveness research (CER) studies, describes implications of the proposed outcomes for study design, and enumerates issues of bias that may arise in incorporating the ascertainment of outcomes into observational research, and means of evaluating, preventing and/or ...
Just as measurement is critical to successful baking, it is as important to successfully pulling off a social scientific research project. In sociology, when we use the term measurement The process by which we describe and ascribe meaning to the key facts, concepts, or phenomena that we are investigating. we mean the process by which we describe and ascribe meaning to the key facts, concepts ...
Measurement is critical to successful baking as well as successful social scientific research projects. In social science, measurement refers to the process by which we describe and ascribe meaning to the key facts, concepts, or other phenomena that we are investigating. At its core, measurement is about clearly and precisely defining one's ...
There are 4 levels of measurement: Nominal: the data can only be categorized. Ordinal: the data can be categorized and ranked. Interval: the data can be categorized, ranked, and evenly spaced. Ratio: the data can be categorized, ranked, evenly spaced, and has a natural zero. Depending on the level of measurement of the variable, what you can do ...
Vulnerability. The conceptual definition of a psychological construct describes the behaviors and internal processes that make up that construct, along with how it relates to other variables. For example, a conceptual definition of neuroticism (another one of the Big Five) would be that it is people's tendency to experience negative emotions ...
Revised on June 22, 2023. Quantitative research is the process of collecting and analyzing numerical data. It can be used to find patterns and averages, make predictions, test causal relationships, and generalize results to wider populations. Quantitative research is the opposite of qualitative research, which involves collecting and analyzing ...
Reliability is consistency across time (test-retest reliability), across items (internal consistency), and across researchers (interrater reliability). Validity is the extent to which the scores actually represent the variable they are intended to. Validity is a judgment based on various types of evidence.
Reliability is about the consistency of a measure, and validity is about the accuracy of a measure.opt. It's important to consider reliability and validity when you are creating your research design, planning your methods, and writing up your results, especially in quantitative research. Failing to do so can lead to several types of research ...
Most of the measurements in Psychology a re on the interval scale. e.g. the Likert scale, RATIO MEASUREMENT. This is a further refinement in the measurement levels in that it provides us with ...
The definition of measure. The definition of measure harmonization is standardizing specifications for related measures when they. have the same measure focus (i.e., numerator criteria) have the same target population (i.e., denominator criteria); have elements that apply to many measures (e.g., age designation for children)
The definitions of the concept of systems thinking are plentiful, and the phenomena are difficult to approach; ... Previous research has validated the measurement in cross-cultural settings (Toivainen et al., 2019). The discussion of whether the associative capability is part of divergence or convergence modes of creative thinking ...
Qualitative research: Explores questions related to "why" and "how," aiming to understand the underlying motivations, beliefs, and perceptions of individuals. Data Collection Methods: Quantitative research: Relies on structured surveys, experiments, or observations with predefined variables and measures.
EDSP 725 Page 1 of 6 R ESEARCH D ESIGN S INGLE S UBJECT T EMPLATE Research Design: _Single Subject_ Part 1: Definitions Personal Definition: Definition: In the single-subject research design, the researcher takes charge of the research of the study. The researcher measures the behavior and assesses the participant in small size to find out which appropriate intervention works for the students ...
Research finds step-count and time are equally valid in reducing health risks Kira Sampson BWH Communications ... Conversely, steps are straightforward to measure and less subject to interpretation compared to exercise intensity. Additionally, steps capture even sporadic movements of everyday life, not just exercise, and these kinds of daily ...
In its most basic form, marketing automation is a set of tools designed to streamline and simplify some of the most time-consuming responsibilities of the modern marketing and sales roles. From automating the lead qualification process to creating a hub for digital campaign creation, automation is all about simplifying a business world that is growing far too complex, much too quickly.
Research methods are specific procedures for collecting and analyzing data. Developing your research methods is an integral part of your research design. When planning your methods, there are two key decisions you will make. First, decide how you will collect data. Your methods depend on what type of data you need to answer your research question:
NASA has launched the first of two research satellites to measure how much heat is lost to space from the Arctic and Antarctica. The shoebox-size satellite lifted off Saturday at 7:42 p.m. local ...