
- SUGGESTED TOPICS
- The Magazine
- Newsletters
- Managing Yourself
- Managing Teams
- Work-life Balance
- The Big Idea
- Data & Visuals
- Reading Lists
- Case Selections
- HBR Learning
- Topic Feeds
- Account Settings
- Email Preferences

Research: How Socioeconomic Status Impacts the Way We Network
- Tanya Menon,
- Leigh Thompson,
- Edward “Ned” Smith

The pandemic is putting the differences into stark relief.
People need to connect with others, especially in crisis. When facing something as stressful as a job loss, personal networks — friends, family, colleagues, and acquaintances — can protect us from financial and emotional freefall.
Research conducted prior to the pandemic examined how high and low socioeconomic status people vary in activating their networks when they faced job threats. They usually choose one of two approaches: “widening,” expanding networks beyond one’s inner circle; or “winnowing,” drawing inwards to smaller, tighter networks. When high and low socioeconomic status people experienced job threats, lower socioeconomic status people tended to winnow (reporting smaller, more constrained networks), whereas high status people tended to widen (reporting larger, less constrained networks). Both groups reported that losing a job felt equally threatening, but turned to different subsections of their networks in response.
Why do these distinct responses matter? In crises, we all need comfort from our inner circles. But when higher socioeconomic status individuals widen to contacts with more novel information and opportunity, they position themselves to rebound from threats.
In these difficult times, we’ve made a number of our coronavirus articles free for all readers. To get all of HBR’s content delivered to your inbox, sign up for the Daily Alert newsletter.
It’s been said that Covid-19 places us in the same storm, but in different boats. That is, the pandemic is deadlier to some more than others physically, economically, and psychologically. Some of us may live in poorer or minority communities which have endured a disproportionate share of deaths and devastation. Some of us may be essential workers, who are physically at risk and emotionally depleted. Others may be furloughed service workers fighting for economic survival. Still more may be senior managers who are unexpectedly experiencing job insecurity. For others, the pandemic has changed life only a little.

- Tanya Menon is a professor of Management and Human Resources at the Ohio State University’s Fisher College of Business. She is the coauthor of Stop Spending, Start Managing: Strategies to Transform Wasteful Habits (Harvard Business Review Press, 2016).
- Leigh Thompson is the J. Jay Gerber Distinguished Professor of Dispute Resolution and Organizations at the Kellogg School of Management, Northwestern University. Her books include Negotiating the Sweet Spot: The Art of Leaving Nothing on the Table , Creative Conspiracy: The New Rules of Breakthrough Collaboration , Making the Team , The Mind and Heart of the Negotiator , The Truth about Negotiations , and Stop Spending, Start Managing . Visit her website at leighthompson.com .
- ES Edward “Ned” Smith is an Associate Professor of Management and Organizations at Northwestern’s Kellogg School of Management. Ned’s research examines how people and organizations come to utilize (and sometimes squander) the resources available to them in their surrounding social networks. Ned is the co-director of Kellogg’s executive program on change management and teaches additional course on organizational design, strategic alliances, and causal inference.
Partner Center

An official website of the United States government
The .gov means it’s official. Federal government websites often end in .gov or .mil. Before sharing sensitive information, make sure you’re on a federal government site.
The site is secure. The https:// ensures that you are connecting to the official website and that any information you provide is encrypted and transmitted securely.
- Publications
- Account settings
Preview improvements coming to the PMC website in October 2024. Learn More or Try it out now .
- Advanced Search
- Journal List
- Elsevier - PMC COVID-19 Collection

Socio-economic status and COVID-19–related cases and fatalities
R.b. hawkins.
a Division of Thoracic and Cardiovascular Surgery, University of Virginia, Charlottesville, VA, USA
b Center for Health Policy, University of Virginia, Charlottesville, VA, USA
E.J. Charles
J.h. mehaffey, associated data.
The United States has the highest number of coronavirus disease 2019 (COVID-19) in the world, with high variability in cases and mortality between communities. We aimed to quantify the associations between socio-economic status and COVID-19–related cases and mortality in the U.S.
Study design
The study design includes nationwide COVID-19 data at the county level that were paired with the Distressed Communities Index (DCI) and its component metrics of socio-economic status.
Severely distressed communities were classified by DCI>75 for univariate analyses. Adjusted rate ratios were calculated for cases and fatalities per 100,000 persons using hierarchical linear mixed models.
This cohort included 1,089,999 cases and 62,298 deaths in 3127 counties for a case fatality rate of 5.7%. Severely distressed counties had significantly fewer deaths from COVID-19 but higher number of deaths per 100,000 persons. In risk-adjusted analysis, the two socio-economic determinants of health with the strongest association with both higher cases per 100,000 persons and higher fatalities per 100,000 persons were the percentage of adults without a high school degree (cases: RR 1.10; fatalities: RR 1.08) and proportion of black residents (cases and fatalities: Relative risk(RR) 1.03). The percentage of the population aged older than 65 years was also highly predictive for fatalities per 100,000 persons (RR 1.07).
Lower education levels and greater percentages of black residents are strongly associated with higher rates of both COVID-19 cases and fatalities. Socio-economic factors should be considered when implementing public health interventions to ameliorate the disparities in the impact of COVID-19 on distressed communities.
- • Socio-economic factors play an important role in coronavirus disease 2019 (COVID-19) prevalence and mortality.
- • Lower education level was the strongest association with both cases and fatalities.
- • The higher proportion of Black residents was also associated with cases and fatalities.
- • The poverty rate and median income were also associated with COVID-19 cases.
- • Median income and change in employment were also associated with COVID-19 fatalities.
Introduction
The coronavirus disease 2019 (COVID-19) pandemic is a worldwide public health crisis on a scale not witnessed in modern times. The United States has the highest number of COVID-19 cases and fatalities in the world. 1 There is wide variability in COVID-19–related mortality across countries and between communities within the U.S. While research has identified a number of risk factors for mortality such as age and comorbid disease, there are new data suggesting social determinants of health also influence outcomes. 2 , 3 Data from the Centers for Disease Control and Prevention suggest strong racial disparities in both COVID-19 prevalence and outcomes. 4 This point has been highlighted by the U.S. Surgeon General, the lay press, and recent publications. 4 , 5 , 6
Socio-economic factors impact all facets of human functioning, including health-related quality of life. While a few publications have speculated on the impact of socio-economic status on the COVID-19 pandemic, there is limited quantitative analysis available. 7 , 8 , 9 , 10 A unique opportunity for investigation lies with the Distressed Communities Index (DCI). This metric was developed by the Economic Innovation Group and is a comprehensive estimate of socio-economic status by geographic location. 11 The DCI is a composite socio-economic ranking that accounts for unemployment, education level, poverty rate, median income, business growth, and housing vacancies. Previous studies have correlated a higher DCI score (lower socio-economic status) with worse health-related outcomes. 12 , 13 , 14 The objective of this study was to quantify the association between socio-economic status, both the composite metric of DCI and its individual component measures, and COVID-19 outcomes, accounting for other risk factors such as age and chronic diseases. We hypothesized that communities with lower socio-economic status (higher DCI scores) would have disproportionately higher COVID-19 prevalence and mortality.
Data sources and definitions
COVID-19–related cases and mortality were extracted from a publicly available data set of aggregated county sources (USAFacts) on May 2, 2020. 15 In addition, county-level estimates for chronic diseases were obtained from the Centers for Disease Control and Prevention. Specifically, the Centers for Disease Control and Prevention (CDC) COVID-19 response team used data from the 2018 Behavioral Risk Factor Surveillance System and U.S. Census population data to model prevalence of chronic diseases potentially associated with COVID-19 risk. 16 The model estimates for chronic kidney disease, chronic obstructive pulmonary disease, heart disease, obesity, and diabetes at the county level were included for risk adjustment. The data for rates of uninsured individuals under age 65, percent of population black, and percent of population age over 65 were obtained from the American Communities Survey 5-year Estimates. The United States is geographically and governmentally organized into 50 states. The states are then subdivided into counties with their own local governance structures. There is wide variability in the population and size of the states and counties, with the number of counties per state ranging from 3 (Delaware) to 254 (Texas). There is a total of 3143 counties or county-equivalent areas (parishes in Louisiana and boroughs in Alaska). At the time of writing, the most granular COVID-19–related data for the United States were available at the county level.
Socio-economic status was measured using the DCI and its components. A data use agreement is in place with the Economic Innovation Group. 11 The DCI score is available for 99% of the United States population and is derived from the American Communities Survey 5-year Estimates and Census Bureau County and Zip Code Business Patterns. The score is composed from the following data points: ‘percentage adults with no high school degree, housing vacancy rate, percentage of adults not working, poverty rate, median income ratio, percentage change in employment, and percentage change in business establishments.’ Detailed data definitions are available in Supplemental Table 1 . The score ranges from 0 (no distress) to 100 (most distress) and is available on the zip code, county and state level. Severely distressed communities were defined by a DCI score >75. This study conformed to the principles embodied in the Declaration of Helsinki and was exempt from review by the University of Virginia Institutional Review Board due to the deidentified nature of the publicly available data sets.
Counties were included for analysis if both COVID-19 data and DCI score were available. Individual cases and deaths were excluded from the analysis if they were not attributed to a specific county.
Statistical analysis
Categorical variables are presented as counts (%) and continuous variables as median [25th, 75th percentile]. Counties were stratified by severely distressed (DCI>75) versus less distressed (DCI ≤75) for univariate analysis using the Mann-Whitney U test. Adjusted effects of socio-economic status were approximated using hierarchical linear mixed models with Laplace approximation and a negative binomial distribution. Socio-economic effects were adjusted for county-level prevalence of elderly residents, uninsured adults, and the following comorbidities: chronic kidney disease, chronic obstructive pulmonary disease, heart disease, diabetes, and obesity. A random effect was used for clustering at the state level. Temporal bias was accounted for with a variable of days since first COVID-19 case. Regression results were exponentiated to construct adjusted rate ratios. Statistical analyses were carried out using SAS, version 9.4 (SAS Institute, Cary, NC), with some graphical representation performed with Prism 8 (GraphPad, San Diego, CA). A P -value <0.05 determined statistical significance.
Cohort description
At the time of data extraction on May 2, 2020, there were 1,096,706 cases and 64,066 deaths in the United States. There were 3143 counties with COVID-19 data available. Twenty-four states had cases not allocated to counties for a total of 6465 cases (0.6%), while 14 states had unallocated deaths for a total of 1754 deaths (2.7%), with 1445 being unallocated in Tennessee. A DCI score was available for 3127 counties with COVID-19 data. This cohort included 1,089,999 cases (99%) and 62,298 deaths (97%) for a case fatality rate of 5.7%. A consort diagram depicting the exclusions leading to the final cohort is shown in Fig. 1 .

Consort diagram of included and excluded counties, COVID-19 cases, and COVID-19 fatalities. COVID-19 = coronavirus disease 2019.
Differences by the DCI
A total of 781 counties were designated as severely distressed (DCI >75), accounting for 68,741 (6.3%) COVID-19 cases and 3811 (6.1%) COVID-19 fatalities. When compared with less distressed counties, those classified as severely distressed had significantly lower median COVID-19 cases (13 [3–39] vs 21 [4–117], P < 0.0001) and fatalities (0 [0–1] vs 0 [0–4], P < 0.0001). Owing to the rural nature of many distressed counties, after accounting for county population size, there was no difference in median cases per 100,000 persons and a reverse in the trend with higher median fatalities per 100,000 persons in severely distressed counties ( Table 1 ). The median percentage of black Americans was significantly higher in severely distressed counties compared with less distressed counties (7.3% vs 1.8%, P < 0.0001). Other significant differences included severely distressed counties having higher rates of elderly residents, uninsured individuals, and individuals with chronic kidney disease, chronic obstructive pulmonary disease, heart disease, diabetes, and obesity. However, severely distressed communities were hit later in the pandemic with lower median days since first case (34 vs 38, P < 0.0001).
Table 1
Univariate analysis by severely versus less distressed counties.
Variable | Severely distressed | Less distressed | -value |
---|---|---|---|
Cases per 100,000 persons | 61 [22–176] | 63 [27–145] | 0.813 |
Deaths per 100,000 persons | 0 [0–7.1] | 0 [0–5.4] | 0.028 |
% of population aged older than 65 years | 17.4% [15.4–19.5] | 16.8% [14.1–19.6] | <0.0001 |
% of population Black | 7.3% [1.1–33.1] | 1.8% [0.6–6.7] | <0.0001 |
% of uninsured population aged younger than 65 years | 14.9% [11.1–17.5] | 10.0% [7.0–14.0] | <0.0001 |
Chronic kidney disease prevalence | 4.0% [3.7–4.3] | 3.3% [3.0–3.5] | <0.0001 |
COPD prevalence | 11.0% [9.8–12.3] | 8.3% [7.0–9.8] | <0.0001 |
Heart disease prevalence | 10.1% [9.3–11.0] | 8.2% [7.1–9.1] | <0.0001 |
Diabetes prevalence | 15.8% [14.5–17.3] | 12.0% [10.8–13.5] | <0.0001 |
Obesity prevalence | 38.1% [35.7–40.5] | 34.6% [31.7–36.9] | <0.0001 |
Days since first case | 34 [25–39] | 38 [29–44] | <0.0001 |
All values expressed as median [interquartile range]; COPD = chronic obstructive pulmonary disease.
Risk adjusted analyses of socio-economic status
Cases of COVID-19 by county were associated with multiple socio-economic factors and days since first infection but not health-related comorbidities. The adjusted risk ratios can be visualized in Fig. 2 , with complete model results in Table 2 . A higher number of cases were associated with lower education level, higher proportion of black Americans, higher income, and lower poverty rate. The specific covariates with significant associations with cases per 100,000 persons were the percentage of adults without a high school degree (RR 1.10), proportion of black residents (RR 1.03), median income ratio (RR 1.01), and poverty rate (RR 0.98). Fig. 3 shows risk-adjusted predicted cases per 100,000 persons for the two strongest associations, the percentage of adults without a high school degree and proportion of black residents. No comorbid medical conditions were associated with COVID-19 cases at the county level.

Forrest plot of adjusted rate ratio for covariates significantly associated with either COVID-19 cases or fatalities per 100,000 persons. COVID-19 = coronavirus disease 2019.
Table 2
Adjusted rate ratios for COVID-19–related cases and fatalities per 100,000 persons.
Variable | Cases per 100,000 persons | Fatalities per 100,000 persons | ||||
---|---|---|---|---|---|---|
Rate ratio | 95% CI | -value | Rate ratio | 95% CI | -value | |
Housing vacancy rate | 1.01 | 1.00–1.02 | 0.1382 | 0.98 | 0.95–1.01 | 0.1274 |
Median income ratio | 1.01 | 1.01–1.01 | <0.0001 | 1.01 | 1.00–1.02 | 0.0094 |
Poverty rate | 0.98 | 0.96–0.99 | 0.0019 | 1.00 | 0.97–1.02 | 0.8511 |
% change in employment | 1.00 | 1.00–1.00 | 0.2358 | 0.99 | 0.99–1.00 | 0.0521 |
% change in establishments | 1.00 | 0.99–1.01 | 0.7611 | 1.00 | 0.99–1.02 | 0.7516 |
% of adults not working | 0.99 | 0.98–1.00 | 0.1496 | 0.98 | 0.96–1.00 | 0.0266 |
% of adults w/o a high school degree | 1.10 | 1.09–1.11 | <0.0001 | 1.08 | 1.05–1.11 | <0.0001 |
% population in distressed zip codes | 1.00 | 1.00–1.00 | 0.7609 | 1.00 | 0.99–1.00 | 0.2284 |
% of population aged older than 65 years | 1.01 | 0.98–1.04 | 0.410 | 1.07 | 1.01–1.13 | 0.0216 |
% of population Black | 1.03 | 1.02–1.04 | <0.0001 | 1.03 | 1.02–1.05 | <0.0001 |
% of uninsured population aged younger than 65 years | 1.01 | 1.00–1.02 | 0.1665 | 0.99 | 0.96–1.01 | 0.2239 |
Chronic kidney disease prevalence | 0.81 | 0.47–1.37 | 0.4266 | 0.84 | 0.34–2.09 | 0.7136 |
COPD prevalence | 1.00 | 0.89–1.13 | 0.967 | 1.15 | 0.95–1.41 | 0.1576 |
Heart disease prevalence | 1.03 | 0.81–1.32 | 0.7833 | 0.89 | 0.58–1.37 | 0.5919 |
Obesity prevalence | 1.00 | 0.98–1.02 | 0.7464 | 0.96 | 0.93–1.00 | 0.0358 |
Diabetes prevalence | 0.98 | 0.89–1.09 | 0.7132 | 1.06 | 0.89–1.26 | 0.5221 |
Days since first case | 1.06 | 1.06–1.07 | <0.0001 | 1.09 | 1.08–1.09 | <0.0001 |
CI = confidence interval; COPD = chronic obstructive pulmonary disease; COVID-19 = coronavirus disease 2019.

Adjusted predicted COVID-19 cases per 100,000 persons for the two strongest socio-economic factors. (A) Percentage of adults without a high school degree and (B) percentage of population Black. Estimates are shown holding all other predictors at mean values ( Supplemental Table 1 ). COVID-19 = coronavirus disease 2019.
Higher COVID-19 mortality was associated with higher income but lower education, higher employment rate, higher proportion of black Americans, older residents, and less obesity ( Table 2 ). The significant socio-economic associations were the percentage of adults without a high school degree (RR 1.08), median income ratio (RR 1.01), and percentage of adults not working (RR 0.98). Significant demographic and health-related associations included the percentage of population aged older than 65 years (RR 1.07), proportion of black residents (RR 1.03), and obesity prevalence (RR 0.96). Fig. 4 shows the risk-adjusted predicted fatalities per 100,000 persons for the two strongest socio-economic associations, the percentage of adults without a high school degree and proportion of black residents.

Adjusted predicted COVID-19 fatalities per 100,000 persons for the three strongest socio-economic factors. (A) Percentage of adults without a high school degree and (B) percentage of population Black. Estimates are shown holding all other predictors at mean values ( Supplemental Table 1 ). COVID-19 = coronavirus disease 2019.
The present study highlights community-level socio-economic disparities in COVID-19 prevalence and mortality within the United States. Severely distressed communities account for a disproportionately higher number of deaths per 100,000 persons. However, poverty and unemployment were protective against contracting COVID-19, highlighting the unique risks of a pandemic and limitations of composite metrics of socio-economic status. Risk-adjusted analysis identified two socio-economic determinants of health as having the strongest association with both population-adjusted cases and fatalities: lower education level and higher proportion of black residents. Although age, comorbidities, and overall health status also appear to impact COVID-19 prevalence and outcomes, socio-economic factors have higher risk ratios and represent the strongest associated factors with both number of cases and fatalities.
Using the composite DCI metric, which includes seven different socio-economic factors, we found that counties with lower socio-economic status (higher DCI score) have higher COVID-19 death rates per 100,000 persons compared with non-distressed counties. Rates of high mortality in distressed communities are multifactorial, likely accounting for differences in age, number of chronic medical conditions per person, and socio-economic status. One of the strongest predictors of mortality from COVID-19 is age, where in Italy the case fatality rate in octogenarians was more than 20%. 3 , 17 Distressed communities are rapidly aging and have a greater proportion of elderly residents. 18 In addition, lower socio-economic status has been associated with a higher burden of chronic medical conditions and comorbidities. 13 These underlying medical comorbidities have also been shown to increase COVID-19–related mortality. 2 However, in the present data, we demonstrate a stronger association between socio-economic factors than underlying medical conditions with only obesity inversely associated with COVID-19 mortality. Obesity has traditionally been considered protective against acute respiratory distress syndrome–related mortality, but preliminary data on COVID-19 suggest possible increased risk. 19 , 20 There are significant overlaps in socio-economic status and obesity that were not accounted for in other studies, and the independent effect of obesity of COVID-19–related cases and mortality requires further study.
Distressed communities tend to be disproportionately comprising black Americans, and these individuals have higher COVID-19 mortality rates. 4 , 5 , 6 , 21 A study not yet published found that communities with a higher proportion of black residents had increased COVID-19 cases (relative risk 1.24) and deaths (relative risk 1.18). 22 Our study corroborates these findings where a higher proportion of black residents were associated with both increased cases and fatalities per 100,000 persons. The exact reasons to explain these associations cannot be determined from the current data sets and analysis, and a more thorough understanding of racial inequities requires nationwide disaggregated data. The underlying etiology for the racial inequity likely reflects trends of economic, geographic, and health disparities seen with socioeconomically disadvantaged populations. In addition, the impact of systemic flaws in the structural organization of American society with racism, access to health care, and community-level resources should not be underestimated.
The COVID-19 pandemic in America is becoming increasingly a story of economics. Significant attention has focused on how public health measures to save lives (social distancing, closure of all non-essential business, stopping the delivery of all non-urgent/emergent health care) have resulted in one of the largest increases in unemployment in American history. However, our data focus on the opposite relationship, how economics is affecting who contracts and dies from COVID-19. 23 Those individuals in frontline jobs who maintain employment are at increased risk of contracting coronavirus, particularly with concerns related to asymptomatic spread in the setting of inadequate testing. 24 Our data are supportive of this theory in two ways. First, higher income and less poverty both were associated with higher rates of COVID-19 cases while higher unemployment was associated with fewer COVID-19 fatalities. These findings suggesting a significant amount of transmission is through the workplace. Second, lower education levels were also associated with contracting COVID-19. This could be due to frontline jobs being disproportionately low paying and without a requirement of an advanced degree. 23 The education relationship is relatively strong (RR 1.10) compared with other metrics, which may be due to the highest paying jobs being more amenable to remote working, making employment and income imperfect markers of risk.
This public health trope of economics underpinning health inequalities is true not only in America but also was highlighted in 1980 in Britain with the Black Report. 25 One of the expectations of establishing the National Health Service with universal healthcare coverage was to eliminate health disparities. However, thirty years after, it was made clear that economic divisions (employment, income, education, housing, and so on) were only widening health disparities in the United Kingdom. The next few decades saw focused efforts to identify the causes of morbidity and mortality differences, develop better metrics to gauge true health disparities, and quantify those differences. 26 , 27 , 28 COVID-19 represents a rare disruptive opportunity to refocus our efforts on using this knowledge to reverse health disparities.
Now is the time to be proactive in the management of this pandemic and focus allocation of federal and state-level resources to these distressed communities. As society attempts to safely reverse restrictive public health measures such as stay at home orders, other more resource-intensive tools including testing and contact tracing will be required. The DCI may help to guide public health interventions to areas most in need. Clear and decisive actions are needed to help safeguard vulnerable populations who are at the highest risk for complications and death. 29
This study is limited by the lack of epidemiologic data available at the zip code level or widespread detailed demographic information, representing systemic failures of the healthcare system which limits our ability to adequately respond in an equitable manner. Socio-economic factors are interrelated, and collinearity limits the utility of an epidemiologic study design such as this. Individual-level SES analyses are required to better understand the interaction of all aspects of Socioeconomic status on COVID-19. In addition, the delay in testing availability and narrow testing criteria vastly overestimate the case fatality rate, but it is unclear how this varies across the United States. Better racial data are needed regarding COVID-19 to truly understand the disparities by race beyond a rough estimation for black Americans. Finally, the DCI only accounts for a small portion of variability in case fatality rates, suggesting social determinants of health are only one factor in determining COVID-19–related outcomes.
In summary, socio-economic determinants of health are associated with COVID-19 prevalence and mortality. Severely distressed counties with low socio-economic status have higher rates of both COVID-19 cases and fatalities than communities with higher socio-economic status. The socio-economic influence is broad, with many components of the DCI (education level, income, and poverty rates) being associated with COVID-19 cases. However, due to the transmission patterns of COVID-19 in the United States, higher income but lower education levels were associated with COVID-19 cases and fatalities. A higher proportion of black residents were also one of the strongest associations with COVID-19 cases and fatalities. Socio-economic factors and the DCI could help allocate appropriate public health resources in areas with lower socio-economic status, a critical step toward an equitable fight against COVID-19.
Author statements
Ethical approval.
This study is exempt from IRB review.
No funding was utilized for this research.
Competing interests
The authors report no conflicts of interest.
Appendix A Supplementary data to this article can be found online at https://doi.org/10.1016/j.puhe.2020.09.016 .
Appendix A. Supplementary data
The following is the Supplementary data to this article:
Thank you for visiting nature.com. You are using a browser version with limited support for CSS. To obtain the best experience, we recommend you use a more up to date browser (or turn off compatibility mode in Internet Explorer). In the meantime, to ensure continued support, we are displaying the site without styles and JavaScript.
- View all journals
- Explore content
- About the journal
- Publish with us
- Sign up for alerts
- Open access
- Published: 23 March 2018
Achievement at school and socioeconomic background—an educational perspective
- Sue Thomson 1
npj Science of Learning volume 3 , Article number: 5 ( 2018 ) Cite this article
222k Accesses
89 Citations
272 Altmetric
Metrics details
INTRODUCTION
Educational achievement, and its relationship with socioeconomic background, is one of the enduring issues in educational research. The influential Coleman Report 1 concluded that schools themselves did little to affect a student’s academic outcomes over and above what the students themselves brought to them to school—‘the inequalities imposed on children by their home, neighbourhood and peer environment are carried along to become the inequalities with which they confront adult life at the end of school’ (p. 325). Over the intervening 50 years, much has been added to the research literature on this topic, including several high-quality meta-analyses. It has become ubiquitous in research studies to use a student’s socioeconomic background, and that of the school they attend, as contextual variables when seeking to investigate potential influences on achievement.
The two articles in this issue of Science of Learning touch on aspects of this discussion rarely included in the educational research literature. The article by Smith–Wooley et al. 2 asks whether whether it is the influence of the student socioeconomic background that is the greater influence or whether the parents are passing down intellectually advantageous genes to their offspring. In contrast, the article by van Dongen et al. 3 suggests that that it is likely a combination of genetics and socioeconomic background, and they examine the effect of environment on the epigenetic status of genes that are involved in learning and memory.
What do we mean by socioeconomic background?
The definition of socioeconomic background used varies widely, even across educational research. In the Organisation for Economic Cooperation and Development’s (OECD) rigorous large-scale international assessment of more than 70 countries over 15 years, the Programme for International Student Assessment (PISA), socioeconomic background is represented by the index of Economic, Social and Cultural Status, which is a composite score derived by principal components analysis and is comprised of the International Socioeconomic Index of Occupational Status; the highest level of education of the student’s parents, converted into years of schooling; the PISA index of family wealth; the PISA index of home educational resources; and the PISA index of possessions related to 'classical' culture in the family home. 4
However, examining Sirin’s 5 meta-analysis of the research into socioeconomic status and academic achievement finds that many studies use a combination of one or more of parental education, occupation and income, others include parental expectations, and many simply use whether the student gets a free or reduced-price lunch. The latter factor is most commonly used as it is readily available from school records rather than having to ask questions about occupation and education of students or parents, yet Hauser 6 as well as Sirin have argued that it is conceptually problematic and should not be used. Other studies have used family structure, 7 , 8 family size, 9 and even residential mobility. 10
Sirin’s meta-analysis, however, found that the traditional definitions of socioeconomic background were not as strongly related to educational outcomes for students from different ethnic backgrounds, for those from rural areas, or for migrants. Its use in developing countries is particularly problematic. For example, in examining the effect of household wealth on educational achievement, Filmer & Pritchett 11 found that many poor children in developing countries either never enrol in school or attend to the end of first grade only. Even within developing countries, the gap in enrolment and achievement between rich and poor was found to be only a year or two, in other countries 9 or 10 years. Often in developing countries low achievement and enrolment is attributable to the physical unavailability of schools.
Similarly education achievement is measured in many ways—achievement on a set test in certain subject areas, completion of numbers of years of schooling, entrance to university, for example.
What does this mean for educators when they are reviewing the research? It means that they need to exercise some caution. The results and the conclusions will obviously vary, as the research is, essentially, looking at different influencers and not the same influence each time. So, when the argument is made that the relationship is not stable, this may well be because the variable under consideration is different.
School-level socioeconomic background
While the Coleman Report concluded that schools themselves added little to effect outcomes, the school environment, in particular the social background of a student’s peers at the school, has certainly been found to be positively related to student achievement. On average, a student who attends a school in which the average socioeconomic status is high enjoys better educational outcomes compared to a student attending a school with a lower average peer socioeconomic level. 12 , 13
Relationship between achievement and student socioeconomic background
There is some discussion about the size of the effect, however the relationship between a student’s socioeconomic background and their educational achievement seems enduring and substantial. Using data from PISA, the OECD have concluded that 'while many disadvantaged students succeed at school … socioeconomic status is associated with significant differences in performance in most countries and economies that participate in PISA. Advantaged students tend to outscore their disadvantaged peers by large margins' (p. 214). 14 The strength of the relationship varies from very strong to moderate across participating countries, but the relationship does exist in each country. In Australia, students from the highest quartile of socioeconomic background perform, on average, at a level about 3 years higher than their counterparts from the lowest quartile. 15 Over the 15 years of PISA data currently available, the size of this relationship, on average, has changed little, and over the now 50 years since the publication of the Coleman Report, the gap between advantaged and disadvantaged students remains.
How are these effects transmitted?
What the continued gap between advantaged and disadvantaged students highlights is that despite all the research, it is still unclear how socioeconomic background influences student attainment.
There are those that argue that the relationships between socioeconomic background and educational achievement are only moderate and the effects of SES are quite small when taking into account cognitive ability or prior achievement. 16 Cognitive ability is deemed to be a genetic quality and its effects only influenced to a small degree by schools. Much of the body of research, particularly that generated from large-scale international studies, would seem to contradict this reasoning.
Others have argued that students from low socioeconomic level homes are at a disadvantage in schools because they lack an academic home environment, which influences their academic success at school. In particular, books in the home has been found over many years in many of the large-scale international studies, to be one of the most influential factors in student achievement. 15 From the beginning, parents with higher socioeconomic status are able to provide their children with the financial support and home resources for individual learning. As they are likely to have higher levels of education, they are also more likely to provide a more stimulating home environment to promote cognitive development. Parents from higher socioeconomic backgrounds may also provide higher levels of psychological support for their children through environments that encourage the development of skills necessary for success at school. 17
The issue of how school-level socioeconomic background effects achievement is also of interest. Clearly one way is in lower levels of physical and educational resourcing, but other less obvious ways include lower expectations of teachers and parents, and lower levels of student self-efficacy, enjoyment and other non-cognitive outcomes. 15 There is also some evidence that opportunity to learn (particularly in mathematics) is more restricted for lower socioeconomic students, with ‘systematically weaker content offered to lower-income students [so that] rather than ameliorating educational inequalities, schools were exacerbating them’. 18
Conclusions
If the role of education is not simply to reproduce inequalities in society then we need to understand what the role of socioeconomic background more clearly. While much research has been undertaken in the past 50 years, and we are fairly certain that socioeconomic background does have an effect on educational achievement, we are no closer to understanding how this effect is transmitted. Until we are, it will remain difficult to address. In this edition of Science of Learning, two further contributions to this body of knowledge have been added—and perhaps indicate new paths that need to be followed to develop this understanding.
Coleman, J. S. et al. Equality of Educational Opportunity (US Government Printing Office, 1966).
Smith-Woolley, E. et al. Differences in exam performance between pupils attending selective and non-selective schools mirror the genetic differences between them. npj Sci. Learn.
van Dongen, J. et al. DNA methylation signatures of educational attainment. npj Sci. Learn.
Organisation for Economic Cooperation and Development (OECD). Scaling Procedures and Construct Validation of Context Questionnaire Data . Ch. 16 (OECD Publishing, 2017).
Sirin, S. R. Socioeconomic status and academic achievement: a meta-analytic review of research. Rev. Educ. Res. 75 , 417–453 (2005).
Article Google Scholar
Hauser, R. M. Measuring socioeconomic status in studies of child development. Child. Dev. 65 , 1541–1545 (1994).
Article CAS PubMed Google Scholar
Bogges, S. Family structure, economic status, and educational attainment. J. Popul. Econ. 11 , 205–222 (1998).
Krein, S. F. & Beller, A. H. Educational attainment of children from single-parent families: differences by exposure, gender and race. Demography 25 , 221–234 (1988).
Downey, D. B. When bigger is not better: family size, parental resources, and children’s educational performance. Am. Sociol. Rev. 60 , 746–761 (1995).
Scanlon, E. & Devine, K. Residential mobility and youth well-being: research, policy, and practice issues. J. Sociol. Soc. Welf. 28 , 119–138 (2001).
Google Scholar
Filmer, D., & Pritchett, L. The effect of household wealth on educational attainment: evidence from 35 countries. Popul. Dev. Rev . 25 , 85–120 (1999).
Palardy, G. J. Differential school effects among low, middle and high social class composition schools: a multi-group, multilevel latent growth curve analysis. Sch. Eff. Sch. Improv. 19 , 21–49 (2008).
Perry, L. B. & McConney, A. Does the SES of the school matter? An examination of socioeconomic status and student achievement using PISA 2003. Teach. Coll. Rec. 112 , 1137–1162 (2010).
Organisation for Economic Cooperation and Development (OECD). PISA2015 results . Vol I : Excellence and Equity in Education (OECD, 2016).
Thomson, S., De Bortoli, L., & Underwood, C. PISA 2015: reporting Australia’s results (ACER Press, 2017).
Marks, G. N. Is SES really that important for educational outcomes in Australia? A review and some recent evidence. Aust. Educ. Res. 44 , 191–211 (2017).
Evans, M. D. R., Kelley, J., Sikora, J., Treiman, D. J. Family scholarly culture and educational success: Books and schooling in 27 nations. Research in Social Stratification and Mobility . https://doi.org/10.1016/j.rssm.2010.01.002 (2010).
Schmidt, W. H., Burroughs, N. A., Zoido, P., & Houang, R. T. The role of schooling in perpetuating educational inequality: an international perspective. Educ. Res. 44 , https://doi.org/10.3102/0013189X15603982 (2015).
Download references
Author information
Authors and affiliations.
Australian Council for Educational Research, Camberwell, VIC, Australia
Sue Thomson
You can also search for this author in PubMed Google Scholar
Corresponding author
Correspondence to Sue Thomson .
Ethics declarations
Competing interests.
The authors declare no competing interests.
Additional information
Publisher's note: Springer Nature remains neutral with regard to jurisdictional claims in published maps and institutional affiliations.
Rights and permissions
Open Access This article is licensed under a Creative Commons Attribution 4.0 International License, which permits use, sharing, adaptation, distribution and reproduction in any medium or format, as long as you give appropriate credit to the original author(s) and the source, provide a link to the Creative Commons license, and indicate if changes were made. The images or other third party material in this article are included in the article’s Creative Commons license, unless indicated otherwise in a credit line to the material. If material is not included in the article’s Creative Commons license and your intended use is not permitted by statutory regulation or exceeds the permitted use, you will need to obtain permission directly from the copyright holder. To view a copy of this license, visit http://creativecommons.org/licenses/by/4.0/ .
Reprints and permissions
About this article
Cite this article.
Thomson, S. Achievement at school and socioeconomic background—an educational perspective. npj Science Learn 3 , 5 (2018). https://doi.org/10.1038/s41539-018-0022-0
Download citation
Received : 19 January 2018
Revised : 31 January 2018
Accepted : 13 February 2018
Published : 23 March 2018
DOI : https://doi.org/10.1038/s41539-018-0022-0
Share this article
Anyone you share the following link with will be able to read this content:
Sorry, a shareable link is not currently available for this article.
Provided by the Springer Nature SharedIt content-sharing initiative
This article is cited by
No substantive effects of school socioeconomic composition on student achievement in australia: a response to sciffer, perry and mcconney.
- Gary N. Marks
Large-scale Assessments in Education (2024)
Math items about real-world content lower test-scores of students from families with low socioeconomic status
- Marjolein Muskens
- Willem E. Frankenhuis
- Lex Borghans
npj Science of Learning (2024)
Can We Talk for a Minute? Understanding Asset-Based Mechanisms for Academic Achievement from the Voices of High-Achieving Black Students
- Beverly J. Webb
- Sara C. Lawrence
The Urban Review (2024)
Teachers’ efficacy, trust, and students’ features: Internal and external forces affecting teachers’ teachability perceptions
- Lennart Van Eycken
- Jannick Demanet
- Mieke Van Houtte
Social Psychology of Education (2024)
The effect of classroom environment on literacy development
- Richard C. Dowell
- Dani Tomlin
npj Science of Learning (2023)
Quick links
- Explore articles by subject
- Guide to authors
- Editorial policies
Sign up for the Nature Briefing newsletter — what matters in science, free to your inbox daily.

Click through the PLOS taxonomy to find articles in your field.
For more information about PLOS Subject Areas, click here .
Loading metrics
Open Access
Peer-reviewed
Research Article
Socio-economic status, resilience, and vulnerability of households under COVID-19: Case of village-level data in Sichuan province
Roles Conceptualization, Methodology, Writing – original draft
Affiliation Center for Trans-Himalaya Studies, Leshan Normal University, Leshan, Sichuan, China
Roles Conceptualization, Data curation, Methodology, Writing – review & editing
Affiliation School of Economics and Management, Leshan Normal University, Leshan, Sichuan, China
Roles Conceptualization, Methodology, Writing – review & editing
Roles Conceptualization, Methodology, Supervision, Writing – review & editing
* E-mail: [email protected]

- Imran Ur Rahman,
- Deng Jian,
- Liu Junrong,
- Mohsin Shafi
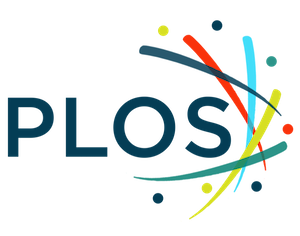
- Published: April 29, 2021
- https://doi.org/10.1371/journal.pone.0249270
- Reader Comments
This paper investigates economic impacts of COVID-19 on households based on differences in the socio-economic status (SES). We determine the household-level effects of the COVID-19 shock using income sources, types of industries, communities’ resilience, household susceptibility, and relevant policy measures. For this purpose, we used primary data of 555 households collected through snowball sampling technique using an online survey questionnaire from different villages mostly located in Sichuan Province, China. Using step-wise binary logistic regression analysis, we estimated and validated the model. Results suggest the use of SES as a better measure for understanding the impacts of COVID-19 on different households. We find that households with low SES tend to depend more on farmland income and transfer payments from the government. Contrarily, high SES households focus more on business and local employment as sources of income generation. Poor households were less resilient and more likely to fall back into poverty due to COVID-19, while the opposite stands true for non-poor households with high SES. Based on the estimations, policies encouraging employment and businesses complemented with loans on lower interest rates are recommended, which may increase the SES, thus minimizing vulnerability and enhancing the households’ resilience towards poverty alleviation and economic shocks.
Citation: Ur Rahman I, Jian D, Junrong L, Shafi M (2021) Socio-economic status, resilience, and vulnerability of households under COVID-19: Case of village-level data in Sichuan province. PLoS ONE 16(4): e0249270. https://doi.org/10.1371/journal.pone.0249270
Editor: Md Nazirul Islam Sarker, Neijiang Normal University, CHINA
Received: January 5, 2021; Accepted: March 16, 2021; Published: April 29, 2021
Copyright: © 2021 Ur Rahman et al. This is an open access article distributed under the terms of the Creative Commons Attribution License , which permits unrestricted use, distribution, and reproduction in any medium, provided the original author and source are credited.
Data Availability: The data used in this article is a part of a broader study undertaken at national level which was availed after approval from the government of China. The data used in this study is a small section of the study for which approval was granted by the Director of Leshan Economic Studies Center, Leshan Normal University for analysis of this research only. Since the data is owned by a third party, we are unable to share it. Data request may be sent directly to the following: Prof. Ren Wenju Director of Leshan Economic Studies Center, Leshan Normal University. [email protected] The authors did not have any special privileges in accessing the data and other researchers will be able to access the data in the same manner as the authors.
Funding: The study is undertaken under the provincial government fund to Leshan Normal University titled “Major Project of Soft Science in Sichuan Province (Code:2020JDR0166)".
Competing interests: The authors have declared that no competing interests exist.
Introduction
Ever since the beginning of 2020, the world economy has been shocked by a new form of coronavirus termed as Novel Coronavirus Pneumonia (NCP) or COVID-19 [ 1 ]. COVID-19 has affected the socio-economic conditions throughout the world, especially in the case of developing economies and poverty. Poor households and households with low socio-economic conditions are suffering the most because of the pandemic. There seems a disparity in the impacts of COVID-19 at household levels due to differences in the socio-economic indicators of the households. Therefore, it is necessary to study the effects of external economic shocks on different households and the response of the households to such shocks. The economic factors may provide a better understanding of the differences in the factors and relevant policy implications for households.
The impacts of COVID-19 are diverse and spread across different sectors and regions. According to estimates of Sumner et al [ 2 ]. COVID-19 will impact the poor mostly in the regions of South Asia, Sub-Saharan Africa, and East Asia. Among Asian nations, China was also affected by the outbreak and faced various economic downturns in the first two quarters with lower production and growth rate. According to the national bureau of statistics of China, the income and consumption trends varied across rural and urban areas. Although the per capita disposable income of the rural households in the poverty-stricken areas showed a nominal increase of 2.7%, the real growth rate had decreased by 3.0% after measuring for price factors influence. In terms of the sources of income, including wages, salaries, and business, there is a slight increase in the nominal growth while net transfer income increases greatly by 9.2% due to the provision of the government. The net income from property declined by 0.5% in the first quarter as a result of the shock [ 3 ]. Table 1 shows the income trends.
- PPT PowerPoint slide
- PNG larger image
- TIFF original image
https://doi.org/10.1371/journal.pone.0249270.t001
Similarly, Fig 1A and 1B ) shows the difference in the per capita disposable income of the Chinese residents in the first quarter of 2020 compared to the first quarter of 2019. The downward sloping lines clearly depict the decline in the median growth rates. Although there is a slight increase in the absolute and nominal value 8,561 Yuan, which shows an increase of 0.8% as compared to 2019; however, in terms of real value after adjusting for price factors, the income has declined by 3.9%, which can be attributed to the effects of COVID-19 on the households. For rural households, the actual decrease was 4.7%, which is higher than the urban areas due to the socio-economic status (SES) of the households in the rural areas.
( A ) Median & Absolute Level of income; ( B ) Median and average growth rate of income. (Source 2 NBS China [ 4 ]).
https://doi.org/10.1371/journal.pone.0249270.g001
The decline in the income in the case of the rural and urban areas also influenced the consumption patterns. The overall per consumption expenditure in nominal terms declined by 8.2% and in real terms by 12.5% in the first quarter. The rural and urban nominal and real per capita consumption expenditures also show downward trends. The real per capita consumption expenditure for rural households has been decreased by 10.7%; in the case of urban households it is decreased by 13.5% [ 4 ]. In the second quarter, the trends are expected to increase due to the re-opening of the main industries and the resumption of economic activities throughout China.
Although the spread of COVID-19 has impacted the majority of households in China, it is observed that some of the households are better-off during the pandemic as compared to the other households. Similarly, the poverty situation and socio-economic conditions of the households vary in outcomes and resilience towards external factors like COVID-19, with some poor and vulnerable households are assumed to have suffered more severely. These differences provide an opportunity for understanding why some households are affected insignificantly while others are affected significantly. Economic theories also provide various frameworks in estimating the effects of shocks on households based on income and consumption levels. Based on a similar assumption, the study of household’s socio-economic status (SES) for the estimation of the effect of COVID-19 on households may provide comprehensive and favorable outcomes in this case.
As various sectors of the economy are hit hard by COVID-19 but the poor and vulnerable communities are affected the most. Poor households and people living under poverty are considered to suffer due to the lockdown and closures of markets and business operations. Most of these people work on daily wages and may be unemployed with no alternate source of income. Governments across the world have stepped up relief and provision of subsidies to their citizens still the challenge for these vulnerable communities to recover hang in the air even after the pandemic is over [ 5 ]. In terms of developing countries, people living in low-income households face dual misfortunes: income-generating difficulties and vulnerability to socio-economics crises [ 6 ]. Poor households and people who are unprepared to cope with such shocks seem to be hit hardest by recession, inflation, disease and civil unrest. Similar trends are observed in terms of the impacts of COVID-19 on communities of different income groups. On contrary, rich households have greater access and a broader asset base to insurance and credit services, which will help cushion them against the effects of an external shock [ 7 ]. Thus, vulnerability and resilience of households to shocks or external factors can have diverse impacts based on the livelihood of the poor and non-poor communities. However, there is still a gap and dire need to address this issue and come up with a better and sustainable approach especially designed for protecting and supporting the poor and vulnerable communities. Based on these observations, it is also essential to understand and study the impacts of the COVID-19 on the different households and the main causes that highlight the disparities in the likelihood of the households to be in higher or lower SES level. However, there is still a gap and dire need to address this issue and come up with a better and sustainable approach especially designed for protecting and supporting the poor and vulnerable communities. Based on these observations, it is also important to understand and study the impacts of the COVID-19 on the different households and the main causes that highlight the disparities in the likelihood of the households to be in higher or lower SES level.
In this paper, we focus on the impact of COVID-19 on the household focusing on the SES differences in the villages of Sichuan Province of China. Our main objective is to determine the economics factors of households in response to COVID-19 based on SES differences. We also check the disparities in the level of resilience towards COVID-19 and households ability to counter falling under the absolute poverty by assessing the likelihood of the households SES conditions. The paper can contribute in providing better overview of the dynamics of the vulnerable households and suitable responses in fighting against external economic shocks. The outcomes may contribute in devising specific policies relevant to poor and vulnerable households.
The outline of the paper follows general rule. After introduction, the paper first summarizes the literature studies on impacts of pandemics and epidemics on different sectors and communities followed by vulnerability and resilience. Subsequently, the paper then provides the methodology adopted for estimations, followed by the main findings of the study and discussion on the output tables. Fnally the paper concludes and recommends policy implications.
Literature review
The differences in the impact of a pandemic or an epidemic based on different aspects of the human life are evident from both historical and present-day perspectives. Similarly, in terms of COVID-19, the effects of the outbreak have shown variability across different regions and conditions of the world. At household level, the effects of the economic shocks have been adverse in terms of the economic status, resilience, and vulnerability of the households. At present due to the continuous spread of the virus, there are very few literature works that have focused on the impacts of COVID-19 shocks on poor household and poverty [ 8 , 9 ]. Most of the poor live in developing nations and are more vulnerable to COVID-19 shocks and their effects on the status of the communities and households. Studies show 14–22 million people may fall below absolute poverty line due to 1% decline in global GDP [ 9 ]. Therefore, it is necessary to analyze the diverse impacts of COVID-19 on the status and livelihoods of different communities and households.
Socio-economic status
Socio-economic status (SES) of a household is an important factor in signifying the outcomes related to shocks and vulnerabilities. It can affect the results of an economic phenomenon in different ways. According to the American Psychological Association, the Socio-economic status (SES) is a person or group’s social class or status which is often calculated by combining education, income and occupation [ 10 ]. As stated by Adler & Snibble [ 11 ] households with higher SES have better access to health care, housing, knowledge and nutrition as compared to lower SES households. Several research studies have focused on the impacts of different shocks on households based on the status. Some have highlighted the impacts of economic shocks such as joblessness or income decline and their effects on the household’s status [ 12 , 13 ]. While others have argued on the health shocks effects on individuals health and in turn the productivity of the workers finally leading to decline in income level of the households [ 14 ]. There is still very few literature articles related to the impacts of shocks on the socio-economic status of different households and communities in the developing world.
Some researchers have highlighted the relationship between household status and shocks [ 15 ]. Other researchers argue that the effects of economic shocks on households are greater in households that are poor due to insufficient income to cushion against the external shock [ 16 ]. In terms of effects on communities and household, shocks at village level are usually not considered to affect the permanent income of the individual households. Others argued that household consumption declines due to negative shocks that impact the permanent income of the households [ 17 , 18 ].
Economists have formalized different form of models for the estimation of household response towards risks and shocks. Consumption response to income shock is one such model which focuses on use of combination of consumption, income or wealth in response to shocks [ 19 , 20 ]. Kruger and Perii [ 21 ] used consumption and wealth as household response towards the income shock. Nevertheless, the issue of which model fits the household response remains unclear. For this reason, this paper attempts to use the SES for the estimation purposes as it present a better proxy and a good indicator of the household’s economic situation.
Vulnerability and resilience
The concepts of vulnerability and resilience are found in various disciplines and subjects. A body of literature has emerged in the past few decades that have focused on different aspects of vulnerability. The notion of vulnerability has become an influential theoretical method for defining the condition of susceptibility to risk, inadequacy, and marginality of both physical and social institutions, and for influencing normative study of behavior to increase quality of life through risk mitigation [ 22 ]. The term vulnerability, according to the World Bank [ 23 ], is “the likelihood that a shock will result in a decline in well-being”, while some researcher have described vulnerability as a state of defenselessness and exposure to shocks [ 24 ]. This definition can be further explained that any external shock that can lead to the decline of the livelihood of households including income and other socio-economic factors, determines the vulnerability of the households.
Some studies focused on presenting evidence of vulnerability by examining the methods used by rural households in low-income countries to deal with HIV/AIDS [ 25 ]. A study by Ndirangu explores how farm households in rural Kenya react to shocks and the impact on quality of life of household risk management strategies. The research explores two primary causes of rural vulnerability: the weather and AIDS. The study shows strategies for coping with shock includes dependence on collaborative opportunities such as casual loans and gifts [ 6 ]. The impact of vulnerability in our case can be assessed and analyzed empirically through the addition of vulnerability of the households against COVID-19 in the model. We check the vulnerability and attributes of it relevant to poor households. By doing, useful understanding and insights can be obtained that can enable policy makers in formulating better policies to avoid the risk related to poor households in case of shock.
Similarly, the concept of resilience has gained much attention in recent years especially in terms of poor households [ 26 ]. Resilience refers to the ability of households to cope with basic necessities of livelihood in case of emergency or shock [ 27 , 28 ]. Resilience has been implemented by different scholars in diverse perspectives [ 29 – 31 ]. There is a broad consensus that resilience is not stagnant, but that it continues to evolve [ 29 , 32 ], that needs further analysis and examination.
Previous studies have focused on the resilience of household in case of natural disasters while others have focused on resilience of households to the socio-economic shocks [ 33 – 35 ]. Resilience of households has also been studied in terms of ecological and environmental effects [ 36 , 37 ]. Although different qualitative approaches have been undertaken in terms of analyzing the resilience but the studies based on quantitative resilience are scarce and limited [ 38 , 39 ]. The resilience of households taking into consideration the livelihood of the households is also an important factor. Several studies have focused on this component in terms of external shocks [ 40 , 41 ]. However, studies have focused on different aspects and factors linking resilience to household income and assets, yet there is a dire need to better understand the connection between these components in terms of rural households. This study will attempt to fill this gap and contribute to the empirical aspect of the literature.
In terms of measuring vulnerability and resilience, economists have argued on various economic factors including household income, status, capital, and access to social security nets [ 42 – 44 ]. In terms of understanding the response of household towards a certain external shock like the COVID-19 pandemic, resilience and vulnerability can be good measures. The impacts of COVID-19 on households are unprecedented and the responses are observed to vary from household to household due to the differences in the SES of the households. Similar, observations are reported in case of China. The assumption can be drawn that households with higher SES may have better coping strategy and resilience as compared to household with lower SES. In this paper, we analyze the difference in the households resilience based on SES using the survey data.
For empirical and quantitative analysis, some authors measured vulnerability based on its impacts on indicators of welfare of the households [ 45 , 46 ]. Others estimated vulnerability in relationship to the poverty dynamics of the households. In this paper, we follow the approach of Vulnerability as Expected Poverty (VEP). The VEP approach is defined as the probability that the expected Income expenditure of a household will fall into poverty in the future [ 47 ]. This approach was also adapted in terms of poor households in developing countries by other scholars [ 7 , 48 , 49 ]. In case of previous studies, the vulnerability was estimated in case of poverty status of the households, which stood above the absolute poverty line. These measures did not consider the effects in case of households below the poverty level, which may provide a deeper understanding, and vulnerability effects on poverty status of households. Chaudhuri [ 47 ] pointed out that welfare status of the households tend to decline where the households poverty status is below poverty line as compared to households above poverty line due to the increase in vulnerability and risk. Based on this notion, we build our model and apply it in case of household status both above and below the poverty line, which will provide a better, and in-depth measure of the vulnerability of different households as compared to previous ones.
Additionally, some past literatures have also focused on different coping strategies across multiple domains including access to credit, government support, and help from family and friends, use of savings, and other such strategies [ 50 , 51 ]. While, the coping strategies related to the SES of household especially poor households, have not been clearly observed [ 16 ]. Coping strategies towards shocks may differ and their impacts may vary based on the status of the households. In this paper, we observe a coping strategy specifically based on the significance of the policy for both poor and non-poor household status, which in this case are the loans provision on lower interest for business and its impacts on different households. There are very few research on the poor households susceptibility towards pandemic [ 52 ], there is still more to explore in terms of COVID-19 and its impacts on poor households and the response of the communities.
This paper attempts to provide the impacts of COVID-19 on household based on the SES of the households. Additionally, the paper further identifies the differences in the impacts of the outbreak taking into consideration the SES of the households by dividing the households into two groups (poor and non-poor households). Based on these differences, the resilience of the households towards a shock like COVID19 and the vulnerability of the households in face of coping with the outbreak are also analyzed and estimated for a deeper understanding of the differentiated effects of COVID19.
Methodology
Sample and data collection.
For the empirical estimations and predictions of our model, we use both primary and secondary data from different sources and methods. For the collection of primary data, an online survey was developed. The online questionnaire was shared using different social media platforms, which made it easier for data collection. The data was collected from village representatives (potential key respondents). The questionnaire was filled by different households with majority from the villages of Sichuan province (475 in Sichuan while 80 from other provinces). A total of 555 questionnaires were completely filled through the online channels over a period of two weeks. The province includes least, middle, and highly developed regions similar to mainland China. Due to measures taken by the Chinese government to contain the spread of the virus, including social-distancing and travel restrictions, the data was collected through administering an online questionnaire, which is also consistent with previous studies [ 53 , 54 ]. In case of Sichuan Province, there are about 183 counties that are different in terms of geo-political and socio-economic indicators [ 55 ]. For secondary data, online official websites were utilized. Health data and reports were collected from WHO website [ 56 , 57 ], while data on Chinese villages was collected from National Bureau of Statistics China website [ 58 ].
The data was collected through the snowball sampling technique due to its time and resources-saving advantages, as argued by various researchers [ 59 , 60 ]. The data was collected during mid of April 2020 for two weeks; at that time COVID-19 pandemic had already affected a large number of local people in terms of socio-economic and health effects of COVID-19.
We sought permission from the relevant community leader of every village prior to administering the questionnaire. Besides, the consent of each village representative was obtained prior to partaking in the survey. Each respondent was allowed not to answer any question accordingly. The current research was approved by the Ethics Committee of Leshan Normal University. Prior to taking part in the online questionnaire, informed consent was obtained from each participant. The purpose and content of the study were first introduced to the potential participants before partaking in the survey. After assuring respondents of complete anonymity, confidentiality, and other ethical considerations, they filled the online questionnaire. There was no electronic record of the participant’s consent, but all respondents agreed on the purpose of the research and took part in the online survey voluntarily.
A questionnaire was developed to study and investigate the economic impacts of COVID-19 on households based on differences in the socio-economic status (SES). In this study we attempt to determine the household-level effects of the COVID-19 shock using different socio-economic factors. For this purpose, we asked several questions from participants such as SES level, household level (poor or non-poor), number of community groups in the village, per-capita annual disposable income (in normal times), main sources of income, industry involvement, agricultural supply level, cost of livestock breading (after COVID-19 outbreak), impact of COVID-19 on rural households to fall back into poverty, vulnerability, and resilience level of households, among others. For more details about the survey questions, see S1 File .
In case of the measurement some researchers argue that although the consumption patterns and disposable income are currently applied for the evaluation of the welfare status and living standards of the households [ 61 ] but these are not the only proxy variables for representation of welfare and status of the households. The variables like welfare and living standards are somewhat hard to observe directly. Instead, different proxy variables are utilized for the indirect measurement of such values. Therefore, it is possible to estimate new proxy variables as suggested by Massari [ 61 ] which can best define the changes and effects on households. Using the Bank of Italy SHIW data, he included a new proxy variable “real assets” in addition to disposable income and consumption expenditure. Similarly, in this paper we assume the SES of the household may provide a better measure as a proxy representing the welfare of the households.
We develop a simple model based on step-wise binary logistic regression in order to estimate our variables. As we used an online survey (questionnaire) for the collection of the data, so some variables in our data are coded as binary variables while others in categorical form. Based on the design of the questionnaires, the responses and the data, it is convenient to use the logistic regression approach for better estimates, good model-fit and unbiased estimates [ 62 , 63 ].
The model predicts the impact of different sources of income, industries, and policies under COVID-19 based on the SES in the villages of Sichuan province, China. As mentioned earlier, the households are divided into poor/low SES and non-poor/high SES household based on the survey data. The household level SES is based on village representative’s identification of the average SES level of the households in the villages (high SES or low SES village) and the reply of the respondent’s in the questionnaire. Based on this fact, we assume SES to be a better indicator for the indirect impacts of COVID-19 in case of different SES levels of the households.
Where, Y i is the linear function and the dependent variable, X i denotes the independent regressors and β measures the coefficients of the regressors in the model.
Where, Y i is the dependent variable representing SES of households ‘i’ whereas X i , Z i and N i are the independent regressors in our model. X i which is divided into the four main sources of income in village ‘i’, Z i represents the two main industries in village ‘i’, and N i denotes the two impact variables of Covid-19 on households in village ‘i’. All the independent variables are chosen based on the socio-economic features of the villages and in accordance with the national statistics of China. The variables are further explained in Eq 4 . Moreover, α 0 is the constant in the model while β i , γ i and δ i are the relevant coefficient measures. μ i is the overall error term in the model.
yfarm i : Income from farmland in the household ‘i’
ybusn i : Income from doing business in household ‘i’
yemp i : Income from local employment in household ‘i’
ygov i : Income from Government Transfer payments in the household ‘i’
indagri i : Household member associated with the agricultural industry
indlivst i : Household member associated with the livestock industry
sagri i : Supply of agricultural products during the pandemic
clivestk i : Cost of livestock feed during the pandemic
res i : Resilience of household ‘i’ against COVID-19
pov i : Ability of household ‘i’ to counter falling to poverty during the pandemic
policyloan i : Desired policy of loans for business on lower interest rate by household ‘i’
The new variable res i represents the resilience of the household ‘i’ against COVID-19, Pov i shows the susceptibility of the household ‘i’ falling into poverty and policyloan i denotes the desired policy measures by the households in county ‘i’. ε i , ρ i and τ i are the respective coefficients measures of the newly added regressors.
We estimated our model using step-wise binary logistic regression. As our independent variable is binary coded and independent variables are categorical, it is an appropriate method for estimation. The model was also tested for reliability and validity through tests for consistency and goodness of fit for the factors. The results show significant estimates and in line with our assumptions and research questions. The outcomes of the analysis are reported in the next part.
Results and findings
Sample characteristics and descriptive summary.
In our sample, most respondents belong to high SES status level (74%). In terms of per capital annual disposable income during normal years, most participants reported less than RMB 13000 (61%) while only 12% of participants had income higher than RMB 20000. Further, in terms of main income sources, most survey participants were dependent on government transfer payment (89%), followed by business (66%), local employment or wage income (41%), and family farming (25%). Additionally, 59% reported that they are mainly involved in agricultural industry. When asked about supply of agricultural materials (in comparison to last year), 61% respondents reported that they are receiving adequate supply of materials. However, only 1% pointed out that there is severe shortage.
Additionally, 67% of participants said that due to COVID-19 outbreak, the cost of livestock breeding has increased. Similarly, when we asked survey participants to express the likelihood of the rural households to fall into poverty due to COVID-19 pandemic, 15% reported very high likelihood followed by high likelihood (46%). Likewise, when respondents were asked to express their resilience and ability to prevent returning to poverty independently without any external support, 46% reported that they possess strong resilience capability, while only 17% reported that they have no resilience capability.
Table 2 shows the statistical summary of the dependent and independent variables. We collected the data through an online questionnaire. A total of 555 households were observed and surveyed. Most of the variables including the dependent variable ‘SESi’, are binary coded with values of 0 or 1. Some variables including ‘hhres’, ‘fallpov’ and ‘sagri’ are ordinal or ranked assuming values of 0, 1, 2 or 3.
https://doi.org/10.1371/journal.pone.0249270.t002
The mean value of the variables lies between 0 and 2 with minimum of 0.26 for the dependent variable ‘SES’. For ‘sagri’, the maximum mean value is 1.87. The standard errors of mean are low for all the variables and ‘ygov’ shows the lowest values of 0.013. The standard deviation for all the main variables is between 0 and 1. ‘hhres’ has the highest standard deviation of 0.933 followed by ‘fallpov’ 0.843. For the maximum and minimum values of the survey responses of the households, the codes range between 0 and 3. In case of the dependent variable, the code is in reverse order assuming 0 for positive response and 1 for negative response. The codes for independent variables present 0 for low level and 1, 2 or 3 for higher categories of responses. Overall, the data shows clear statistical values for the variables used in the estimation method.
Fig 2 shows the difference in the SES of the households in percentages and the response of the households in case of socio-economic changes. Household with better socio-economic status rely on income from business (68% of high SES households) and local employment (39% high SES households) while households having low SES are more dependent on transfer payments from the government (19% of low SES households) and farm output (93% of low SES households).
https://doi.org/10.1371/journal.pone.0249270.g002
Similarly, low SES households are more vulnerable (71%) and external shocks can drive them further into poverty (31%). In contrast, households with higher SES are more resilient (47%) and can cope with external shocks like COVID-19 regardless of external support. It also shows 35% and 55% of low SES households are engaged in agricultural and livestock industries respectively.
Reliability and validity statistics
Cronbach’s alpha..
The value of Cronbach’s alpha is calculated for the reliability or internal consistency of the estimations or scale. Cronbach’s alpha was first introduced by Lee Cronbach in 1951 in order to check the internal consistency of test [ 65 ]. In order for the results to be reliable and acceptable, the threshold value of Cronbach’s alpha should be between 0.7 and 0.95 [ 66 , 67 ]. In our case, Cronbach’s alpha values for our models are above the threshold value of 0.7. The value implies that the variables used are reliable and consistent internally. The table 3 contains the Cronbach’s alpha values for the factors in each of our models.
https://doi.org/10.1371/journal.pone.0249270.t003
For testing of convergent validity, we use Factor Analysis for the four main components of all our factors, including 4 income factors in the first component, 2 industry factors as the second component, 2 cost factors as the third component, and the fourth component consists of 3 factors that have diverse loadings. The test is undertaken to check if the factors load are acceptable and distinct or not for each component. Table 4 shows the loading of the main components.
https://doi.org/10.1371/journal.pone.0249270.t004
Table 4 clearly depicts that the factors load distinctly for the four groups and confirms the validity of convergence among the main factors in each of the groups. The average factors loading for all the groups are also estimated to be higher than 0.5 threshold level and most of the groups average above 0.7 which is a good determinant of validity and also suggests convergent validity.
Goodness of fit.
After fitting the model, it is important to analyze the fitness of the data in the model. There are different methods of calculating the goodness of fit like Pearson’s Chi-Square [ 68 ] and Likelihood ratio test [ 59 , 60 ], but based on our data set, Hosmer-Lemeshow test for goodness of fit is considered a good way of presenting the fitness of our data into our model [ 69 ]. In the case of the Hosmer and Lemeshow test, the significant value should be above 0.05 for selection of the model. A lower significance value suggests the rejection of the model [ 70 ]. The results of the test are reported in Table 5 .
https://doi.org/10.1371/journal.pone.0249270.t005
According to Hosmer [ 69 ], the value of significance has to be above the threshold of 0.05% in order to determine a good fit. The null hypothesis assumes that the data is a good fit for the model. In our case, Table 5 clearly shows that the data fits our model, and our model has the goodness of fit as the significance of Hosmer-Lemeshow test estimates are above 0.05, which means we are unable to reject the model and our data fits the model. In addition, for the overall significance of the model, the Omnibus test of the Model coefficients also proved that coefficients of the independent regressors used in the model are significant. Table 6 presents the Omnibus test outcomes of the final estimations of the model.
https://doi.org/10.1371/journal.pone.0249270.t006
Main findings and discussions
Table 7 shows the stepwise binary logistic regression trends for our model based on the survey of households in different villages of Sichuan Province.
https://doi.org/10.1371/journal.pone.0249270.t007
As our dependent variable ‘SES’ assumes binary values (0, 1), therefore, binary logistic regression analysis is adopted for the estimation of the coefficients in our model. The binary logit regression provides better estimations and is recommended in case the dependent variable consists of binary values. The use of SES as the representation of the households proved to be a better indicator of showing the impacts of shock on different households’ and their response to an external shock (COVID-19) as it takes into account the main economic factors relevant to households. All the independent variables are regressed and estimated for the effects on the dependent variables.
The table also shows the coefficients ‘B’ and the exponential form of the coefficients ‘Exp (B)’ of all the independent variables in the model. Exp (B) interprets the odds of the effects of the independent variables in case of the binary dependent variable.
Income effect.
The variables ‘Yfarm’, ‘Ylemp’, ‘YBusn’, and ‘YGovt’ depict different household income sources in the villages. The significant income effect on the households can be seen from the Table 7 as all the variables are significant. Yfarm and YGovt are negative in relationship while Ylemp and YGovt attain positive sign concerning the dependent variable. The negative sign explains the odds of the independent variables to be less likely being the poor household while positive sign denotes higher odds of the effects on poor households. The Exp(B) values 3.056 and 4.440 for Yfarm and YGovt respectively demonstrate the odds of income earnings from farmland is 3.056% more for poor households while odds of depending on transfer payments from the Government are about 4.44% higher in poor households as compared to households that are non-poor with high SES.
The difference indicated by these estimations clearly suggests that poor households are more vulnerable with subsistence source as farmland income and thus more dependent on support from the government for income and livelihood while non-poor household is less vulnerable due to better and continuous income sources like a business and local employment. The differences suggest that non-poor and higher SES households are characterized by income from business and employment. Business and Employment show a key and significant difference between the low and high SES households. Such means and sources of income are estimated to have a positive impact on the SES of the households.
Effect on prices and costs.
The result provided the changes in prices and costs of agricultural products and the impacts of such changes on the different households. The significant results for agricultural industry (-0.704) and supply of agricultural products (-0.609) suggest that the odds of the poor household member working in the agricultural industry and the effect on supply of agricultural products are less likely compared to non-poor households. There is a clear distinction as working in an agricultural industry may provide higher income opportunities, and thus, may increase the SES. Similarly, the agricultural supply may be increase for non-poor households due to the shock as non-poor household demand for agricultural products may increase due to the fear of pandemic. These findings can be attributed to the fact that non-poor households depend on the supply of agricultural products, and thus, the effect on the supply of agricultural products may be higher in non-poor households. The possible explanation may be that China’s agricultural sector has been on the verge of modernization, which can be focused on future policy in giving opportunities for low-income families to be part of the agricultural industry. In addition, without the necessary source of income, the poor family may only end up decreasing marginal income with the higher opportunity cost of migration work in non-agricultural sector in town or else; while a non-poor family is in an opposite situation of its counterpart. Moreover, if the poor’s situation cannot be reversed in the long run, the rural population would be separated into the Agri-business group and the city worker group, which may be the natural selection process.
In contrast, household members working in the livestock industry and higher livestock cost are most likely to happen in the case of poor households. The finding also highlights that poor household members are about 2% more likely to be associated with livestock industry than non-poor households. It can be attributed to the fact that most of the poor households depend on livestock and products from livestock for daily consumption and livelihood. As a result of the shock, the dependence of poor households on livestock’s increase and thus the costs for feed rise.
Resilience and vulnerability on SES.
Further, estimates for the resilience of community (-1.167) in tackling the pandemic explain that the odds of households to be resilient against pandemic are less likely to be in low SES households, which can be extended as households in non-poor households tend to be more resilient towards COVID-19 due to better sources of income. Similarly, the odds of countering falling into poverty are less in poor households as compared to non-poor households. Low SES households mostly depend on income from farms and support from the government. It is unlikely for the poor households to overcome any external shock or show resilience without the support of the government. Once such households are affected by an outbreak, then the chances of these households falling into poverty increases as these households depend on subsistence sources of income and government support and assistance.
Policy effect.
In terms of the policy, the provision of loans on lower interest rates for business based on the coefficient value of -0.580 explains the odds of households desiring business loans on low-interest rates are less likely to in poor households. Non-poor households tend to establish business and earnings from employment, while households with low SES are less likely to be engaged in business and employment activities. These factors can enable households to earn higher income which in this case, the higher desire for loans for business purposes in non-poor households proves the difference between poor households and non-poor households.
The overall significance of the coefficients and the goodness of fit for variables in the model were explained previously. For explanation and predictive power of the independent variables, the binary logistic regression calculates Nagelkerke R Squared values. The value for Nagelkerke R Squared in our final model is 56%, which shows the independent variables in our model are good in specification and estimations.
For further analysis and checking of the model, we sort the data and estimate the variables in categorical forms. The categorical estimations of the model provide significant outcomes as compared to the regression analysis of the overall effects of the variables. The estimates of the coefficients in our model are presented in Table 8 .
https://doi.org/10.1371/journal.pone.0249270.t008
The estimations in the table show different categorical estimates for our main variables in the model. The results are similar to the non-categorical estimations with a few changes suggesting robust outcomes in our main model. The income effect and industrial effects of the variables show similar signs as the previous model. Similarly, the Nagelkerke R Square value is also high at 70.1%, which shows a higher predictive power of the variables. As in the case of Binary logistic regression, the Nagelkerke R Square values loosely define the effect of independent variables, therefore, we also calculated the correlation value of the probabilities of our independent variables and the dependent variable using the Pearson Correlation test. The value of the Pearson Correlation test is 61%, which is also high and shows a good choice of our independent variables in explaining the dependent variable.
The resilience has an overall significant impact in determining the odds of non-poor households to be more resilient as compared to poor households. The first category representing resilience in the table suggests that there is no resilience. Based on the estimation value of 1.941 it can be interpreted, as the odds of households with no resilience are about 1.9 times more likely to be poor and low SES households. Based on exp(B) value of 6.965 it can also be explained that a household with no resilience is approx. 6.96% more likely to be poor, which is highly significant. On the contrary, the resilience coefficients for 2 nd and 3 rd category we obtain the odds ratios of 0.166 and 0.018. Thus, the odds of a household to be resilient or highly resilient are less likely to be poor households.
Similar trends are represented in terms of countering poverty. The signs are negative showing less likeliness towards poor households, and exponential coefficients for the three categories assume values of 0.455, 0.121, and 0.020, respectively. All the category estimations were negative, predicting less likeliness of being poor or low SES households. The estimates were significant except for the first category. The results suggested that the odds of households countering poverty strongly or very strongly are less likely to be a poor household. It can be explained that a household with low SES is more vulnerable to the impacts of COVID-19 and may fall back below the poverty line, while the high SES households show more resilience and less vulnerability towards the pandemic. The results further highlight that the number of new poor may increase in the future if the pandemic continues as these households are less resilient and less likely to counter falling back into poverty.
For further explanation of the model, we estimated the predicted probabilities of the variables against the dependent variable. Fig 3 shows the predicted probabilities of the independent variables against the values of dependent variables. Although some values overlap, the majority of the distribution provides a good prediction of the model. It predicts that households with low SES are likely to fall on the positive side while a household with high SES over the negative side. The same outcomes are also presented in Fig 4 .
https://doi.org/10.1371/journal.pone.0249270.g003
Piling of the data responses and predicted probabilities on both sides of the figure explains a good outcome related to the variables and the model.
https://doi.org/10.1371/journal.pone.0249270.g004
This paper examined the difference in impacts of COVID-19 on households based on the socio-economic differences in the villages of Sichuan province, China. Our main goal was to identify the economic factors influencing households’ responses to COVID-19 based on socio-economic differences. The SES provides a better and deeper knowledge of the changes in household economic conditions and indicators as a result of an outbreak or health crisis like COVID-19. This study also assesses the likelihood of the households’ SES conditions to see if there are any disparities in the level of resilience to COVID-19 and households’ ability to avoid falling into absolute poverty.
The outcomes presented that households are less likely to be affected or the impact of COVID-19 on non-poor households is lower as compared to households that are poor with lower SES. Similarly, Ndirangu [ 6 ] argued that in the case of developing economies, people living in low-income households face dual misfortunes: income-generating difficulties and vulnerability to socio-economics crises. Likewise, Jalan & Ravallion [ 7 ] also argues that households with higher SES have greater access and a broader asset base to insurance and credit services, which will help cushion them against the effects of an external shock, like COVID-19. The chances of falling back into the poverty of a poor household are significantly higher than the Non-poor household, which suggests that the numbers of new poor as mentioned in recent articles are estimated to increase more due to the pandemic [ 71 ]. Further, Adler & Snibble [ 11 ] argue that in comparison to households with lower SES, higher SES households have better access to health care, housing, knowledge, and nutrition.
In the case of resilience, response, and tackling the economic influence of COVID-19, the estimate predicts that the odds of non-poor households (high SES) to be resilient against COVID-19 are higher in contrast to low SES households. Business and employment may be better sources of income, and industry plays key roles in determining the SES of the households, which can affect the resilience of the communities towards recovery during the pandemic.
Implications
Based on the outcomes of the paper and literature studies, it is observed that poor households and people having dependent on farm income are affected more and are more dependent on external support; therefore, it is necessary to devise policies for supporting and creating new jobs and income generating opportunities for them. Focusing on agricultural sector modernization and providing opportunities in the agricultural industry may contribute both at the household and national level. Small scales agricultural industries can be set up according to the needs of the community or county are expected to be of higher significance. Loans on lower interest rates for businesses can increase resilience and decrease the vulnerability of the poor households with access to funds. These policies can have better and long-lasting impacts on changing the livelihoods and SES level of the poor communities. At a general level, the government bodies and institutions provide relief and support to the poor households and vulnerable communities with low income and low employability [ 2 ]. Such policies and support should be temporary, as households depending on government support have a higher chance of falling or staying in the low SES category and may not divulge into other income opportunities.
Limitations
Despite insightful implications, this research is not without limitations. Primarily, this study was cross-sectional in nature which does not allow to make causal inferences. To make causal inferences, longitudinal studies are needed. Furthermore, the sample was obtained through the snowball sampling technique, which provides room for further research. Concerning limitations during the course of the research, there are some areas that may be improved in future research articles. The sample we collected was based on a single province due to time restraints; other researchers can increase the data set and collect a larger sample, a country-level data, which will give outcomes that are more robust. Similarly, since the lockdown policies and pandemic are ongoing; more research and post-pandemic scenario will provide better research opportunities and unbiased estimates for future researchers.
Supporting information
https://doi.org/10.1371/journal.pone.0249270.s001
Acknowledgments
We are grateful to the support of the members of Center for Trans-Himalaya Studies (CTHS) and the administration of Leshan Normal University (LSNU)). We highly acknowledge the assistance from the faculty of School of Economics -LSNU during the course of the research.
- 1. World Health Organization. Naming the coronavirus disease (COVID-19) and the virus that causes it. WHO Technincal Guidance [Internet]. 2020 [cited 2020 Feb 20]; Available from: https://www.who.int/emergencies/diseases/novel-coronavirus-2019/technical-guidance/naming-the-coronavirus-disease-(covid-2019)-and-the-virus-that-causes-it .
- 2. Sumner A, Hoy C, Ortiz-Juarez E. Estimates of the impact of COVID-19 on global poverty [Internet]. Helsinki; 2020. (WIDER Working Paper). Report No.: 2020/43. Available from: https://doi.org/10.35188/UNU-WIDER/2020/800-9 .
- 3. National Bureau of Statistics of China. Income of Rural Residents in Poor Areas in the First Quarter of 2020 [Internet]. Press Release. 2020 [cited 2020 Jun 20]. p. 1. Available from: http://www.stats.gov.cn/english/PressRelease/202005/t20200505_1742976.html .
- 4. National Bureau of Statistics of China. Households’ Income and Consumption Expenditure in the First Quarter of 2020 [Internet]. Press Release. 2020 [cited 2020 Jun 20]. p. 1. Available from: http://www.stats.gov.cn/english/PressRelease/202004/t20200420_1739771.html .
- 5. Bartik A, Bertrand M, Cullen Z, Glaeser EL, Luca M, Stanton C. How are Small Businesses Adjusting to COVID-19? Early Evidence from a Survey [Internet]. SSRN Electronic Journal. Chicago; 2020. (HKS Working Paper). Report No.: RWP20-012. Available from: https://papers.ssrn.com/sol3/papers.cfm?abstract_id=3574741 .
- View Article
- Google Scholar
- 9. Vos R, Martin W, Laborde D. How much will global poverty increase because of COVID-19? International Food Policy Research Institute IFPRI Blog [Internet]. 2020 Mar; Available from: https://www.foodsecurityportal.org/blog/how-much-will-global-poverty-increase-because-covid-19 .
- 10. American Psychological Association Task Force on Socioeconomic Status. Report of the APA task force on socioeconomic status. Washington, DC.; 2007.
- PubMed/NCBI
- 23. Bank World. World Development Report 2000 / 2001 Attacking Poverty [Internet]. Oxford University Press. New York; 2000. Available from: https://openknowledge.worldbank.org/handle/10986/11856 .
- 25. Gillespie S ed. AIDS, Poverty, and Hunger: Challenges and Responses: Highlights of the International Conference on HIV/AIDS and Food and Nutrition Security, Durban, South Africa, April 14–16, 2005. International Food Policy Research Institute. Washington, D.C.; 2006. 376 p.
- 30. Carpenter S, Walker B, Anderies JM, Abel N. From Metaphor to Measurement: Resilience of What to What? In: Ecosystems. 2001. p. 765–81.
- 33. Ciani F. A Resilience-Based Approach to Food Insecurity: The Impact of Mitch Hurricane on Rural Households in Nicaragua. … of Florence, Department of economics, PhD …. 2012;.
- 36. Berkes F, Folke C. Linking social and ecological systems for resilience and sustainability. In: Linking Social and Ecological Systems. New York: Cambridge University Press; 1998.
- 41. FAO. Resilient livelihoods- disaster risk reduction for food and nutrition security framework [Internet]. Rome; 2013. Available from: http://www.fao.org/3/i3270e/i3270e.pdf .
- 42. Jones L, Tanner T. Measuring “Subjective Resilience”: Using Peoples’ Perceptions to Quantify Household Resilience [Internet]. SSRN Electronic Journal. London; 2015. Report No.: 423. Available from: https://ssrn.com/abstract = 2643420.
- 45. Chaudhuri S, Jalan J, Suryahadi A. Assessing Household Vulnerability to Poverty from Cross-sectional Data: A Methodology and Estimates from Indonesia [Internet]. Department of Economics Discussion Papers. New York; 2002. (Discussion Paper Series). Report No.: 0102–52. Available from: https://academiccommons.columbia.edu/doi/10.7916/D85149GF .
- 47. Chaudhuri S. Assessing vulnerability to poverty: concepts, empirical methods and illustrative examples [Internet]. Department of Economics. New York; 2003. Available from: http://econdse.org/wp-content/uploads/2012/02/vulnerability-assessment.pdf .
- 48. Mansuri G, Healy A. Vulnerability prediction in rural Pakistan. IFPRI-World Bank Conference on Risk and Vulnerability: Estimation and Policy Implications, Washington, DC. 2002;.
- 49. Pritchett L, Suryahadi A, Sumarto S. Quantifying Vulnerability to Poverty: A Proposed Measure, with Application to Indonesia [Internet]. Policy Research Working Paper. Washington, DC.; 2000. (Working Papers). Report No.: 2437. Available from: https://elibrary.worldbank.org/doi/abs/10.1596/1813-9450-2437 .
- 50. Morduch J, Ogden T, Schneider R. An Invisible Finance Sector: How Households Use Financial Tools of Their Own Making [Internet]. U.S. Financial Diaries. New York; 2014. (Issue 3). Available from: www.usfinancialdiaries.org .
- 51. Andersen V, Austin S, Doucette J, Drazkowski A, Wood S. Addressing income volatility of low income populations. In: Workshop in Public Affairs [Internet]. University of Wisconsin–Madison: Robert M. La Follette School of Public Affairs; 2015. p. 1–37. Available from: https://lafollette.wisc.edu/images/publications/workshops/2015-income.pdf .
- 55. Baoxing. Baoxing County China [Internet]. Webpage. 2020. Available from: http://www.baoxing.gov.cn/en/about.html .
- 58. NBS China. National Bureau of Statistics of China Webiste [Internet]. 2020. Available from: http://www.stats.gov.cn/enGliSH/ .
- 63. King JE. Binary Logistic Regression. In: Osborne, J Best Practices in Quantitative Methods. CA: SAGE Publications, Inc.; 2008. p. 358–84.
- 67. DeVellis RF. Scale Development: Theory and Applications 3rd Edition. SAGE Open. Thousand Okas, CA: SAGE publication Ltd; 2003.
ORIGINAL RESEARCH article
Socio-economic development and mental health: case study of the spanish region of aragon (2010–20).
- 1 Department of Geography and Territorial Planning, University of Zaragoza, Zaragoza, Spain
- 2 Department of Microbiology, Pediatrics, Radiology and Public Health, University of Zaragoza, Zaragoza, Spain
- 3 Aragon Health Research Institute, Zaragoza, Spain
- 4 Aragon Health Service, Zaragoza, Spain
Introduction: Considering health as a cross-cutting element of all public policies leads to rethinking its interactions with the environment in which people live. The collection of large volumes of data by public administrations offers the opportunity to monitor and analyze the possible associations between health and territory. The increase in the incidence and prevalence of mental health diseases, particularly depression, justifies the need to develop studies that seek to identify links with the socioeconomic and environmental setting.
Objective: The objective of this study is to explain the behavior of the depression in a mediterranean region of Northeastern Spain from an ecological and diachronic perspective.
Methods: We conducted a correlation and multivariate logistic regression analysis to identify explanatory factors of the prevalence of depression in 2010 and 2020 and in the variation rate. Potential explanatory factors are related to the socioeconomic status and to the territorial development level.
Results: The regression models retained both socioeconomic and territorial development variables as predictors of the prevalence in both years and in the variation rate. Rural areas seem to play a protective role against the prevalence.
Conclusion: It is under the territorial prism that epidemiological studies could offer useful guidelines for proactive decision-making. The integration of data on diseases and territory must be considered when developing policies for the creation of healthier environments and for directing health services with more specific resources to where they may be needed.
Introduction
Advancing upon our current understanding of “health” raises the need to consider it beyond the mere lack of discomfort including the overall wellbeing of the individual as well as social welfare ( Santoro-Lamelas, 2016 ). Mental health conditions, and specifically depression-related ones, are the main cause of disability in western countries ( WHO, 2017 ). The increasing provision of services linked to economic growth is often associated to wellbeing and, in parallel, good health. Indeed, economic development has fostered medical advances and increasing access to health services, which ultimately contribute to welfare ( Jorm and Ryan, 2014 ). However, economic growth does not necessarily improve mental health and several studies support the notion that psychiatric disorders and depression go hand in hand with industrialization and urbanization, as it favors income inequality ( Gupta et al., 2016 ; Yu and Wang, 2016 ; Ribeiro et al., 2017 ). There is a growing interest in monitoring and reducing inequalities both from the approach of the UN SDGs and the OECD, from the assertion that inequality affects the social fabric and harms long-term economic growth ( OECD, 2015 ; United Nations, 2015 ). The drivers of depression are not yet fully understood, being it attributed to different factors such as genetic predisposition, personality traits, as well as impaired social interactions ( Dunn et al., 2015 ; Gariépy et al., 2016 ; Cai et al., 2020 ). The socioeconomic status, gender or the residential environment could also pose a risk of depression ( Freeman et al., 2016 ; Kuehner, 2017 ; Razzak et al., 2019 ). Other risk factors could be specifically manifested in rural or urban settings, especially in terms of diagnosis, treatment and use of health services ( Wang, 2004 ; Fortney et al., 2010 ; Purtle et al., 2019 ).
The Determinants of Health (DH) approach and the Health in All Policies [HiAP; ( WHO, 2014 )], stress the importance of policies, actions and interventions outside the direct remits of the healthcare sector, e.g., regional planning or economic development ( Puska, 2007 ; Ollila, 2011 ). These approaches advocate for assessing the wide array of protective and/or risk factors mediating health conditions, i.e., individual lifestyles, social and community networks, food production systems, the socioeconomic and residential environment, the access to health services or policies in place ( Dahlgren and Whitehead, 2007 ; Gnanapragasam et al., 2021 ). Parallel to the inception and evolution of the HiAP and DH approaches, the “information revolution” has enabled compiling and cataloging large amounts of data with increasing complexity in their structures and interrelationships (the so-called Big Data). Today, public administrations collect large amounts of data on a regular basis that can be put at the service of healthcare and resource management.
We hypothesize that an uneven territorial development and the environmental settings influence health status and, specifically, depression and mental conditions. Hence, landscape and urban planning may play a role in improving mental health. It is in this context of necessary interaction between health, environment, and data availability to satisfy the pressing need for management that this study is proposed. We investigate the link between socioeconomic and territorial indicators, and the prevalence of depression in Aragon. We leverage official data sources to conduct a diachronic analysis of their association. The socioeconomic factors are expressed through deprivation indicators referring to the demographic structure, the work environment, and the level of education. Those referring to the territorial development illustrate the accessibility to facilities and services and to the transportation network, the condition of residential buildings and the quality of the landscape. The hypothesis of this study is that a greater vulnerability to the prevalence of depression corresponds to a higher socioeconomic deprivation and lower levels of territorial development.
Materials and Methods
The methodological process was divided into three phases. First, a spatial database was generated on depression prevalence (as dependent variable) and indicators on socioeconomic environment and territorial development (as independent variables). Second, the variables were aggregated at the Basic Health Area (BHA) level to prepare the cartographic representation and statistical analysis. Third, a correlation and logistic regression analysis was performed to explore associations between the variables.
Study Population and Sample Size
The target population of our study are all individuals inside the National Health System registered in Aragon (Spain) during 2010 or 2020. The sample consists of patients with a digital medical record being 18 years and older, including elderly people (over 65), having been diagnosed with Depression, according to the International Classification in Primary Care (ICPC) of the World Organization of Family Doctors (WONCA) (ICPC 2 – P76). to the universal nature of the health system and the absence of other health care data providers, the sample analyzed in this study can be considered as representative of practically the 100% of the adult population diagnosed with depression.
The study area is the Autonomous Community of Aragon. The territorial administrative unit for primary health care in Aragon is the BHA. BHAs are groupings of municipalities except in the case of the three main towns, Zaragoza, Huesca and Teruel, which group census tracts. BHAs are grouped into eight health sectors: Huesca, Barbastro, Zaragoza I, Zaragoza II, Zaragoza III, Calatayud, Teruel, and Alcañiz. The region covers an area of 47.720 square km with a population density of 27.8 inhabitants per square km (in 2021). The multivariable map in Figure 1 illustrates the large contrasts that characterize this region in terms of population distribution, territorial development, and socioeconomic conditions. We find the main population concentrations in the middle and mid-west of the region. These areas are characterized by a high level of territorial development that in many cases, is accompanied by a high socioeconomic deprivation (dark cyan tones). The BHAs displayed in purple illustrate intermediate territorial development. These regions host health-care centers assigned to people in the surrounding settlements. Populations living in these areas are even young or aged and the deprivation conditions can be high (dark purple tones). In contrast with previous ones, we found numerous BHAs that show a smaller population size, especially in the north and south of the region. These are characterized by lower levels of territorial development and an overaged demographic structure. These disadvantages can be accompanied by socioeconomic deprivation (intense magenta tones). These contrasts in the settlement distribution are derived from physical factors such as the arrangement of the main relief units which ultimately explain climate conditions and land cover. There is a gradient from wet and cool conditions in the two surrounding mountain ranges (Pyrenees, 3,400 m.a.s.l. and Iberian System, 2,300 m.a.s.l.) to warm and dry situations in the central corridor of the River Ebro Valley.
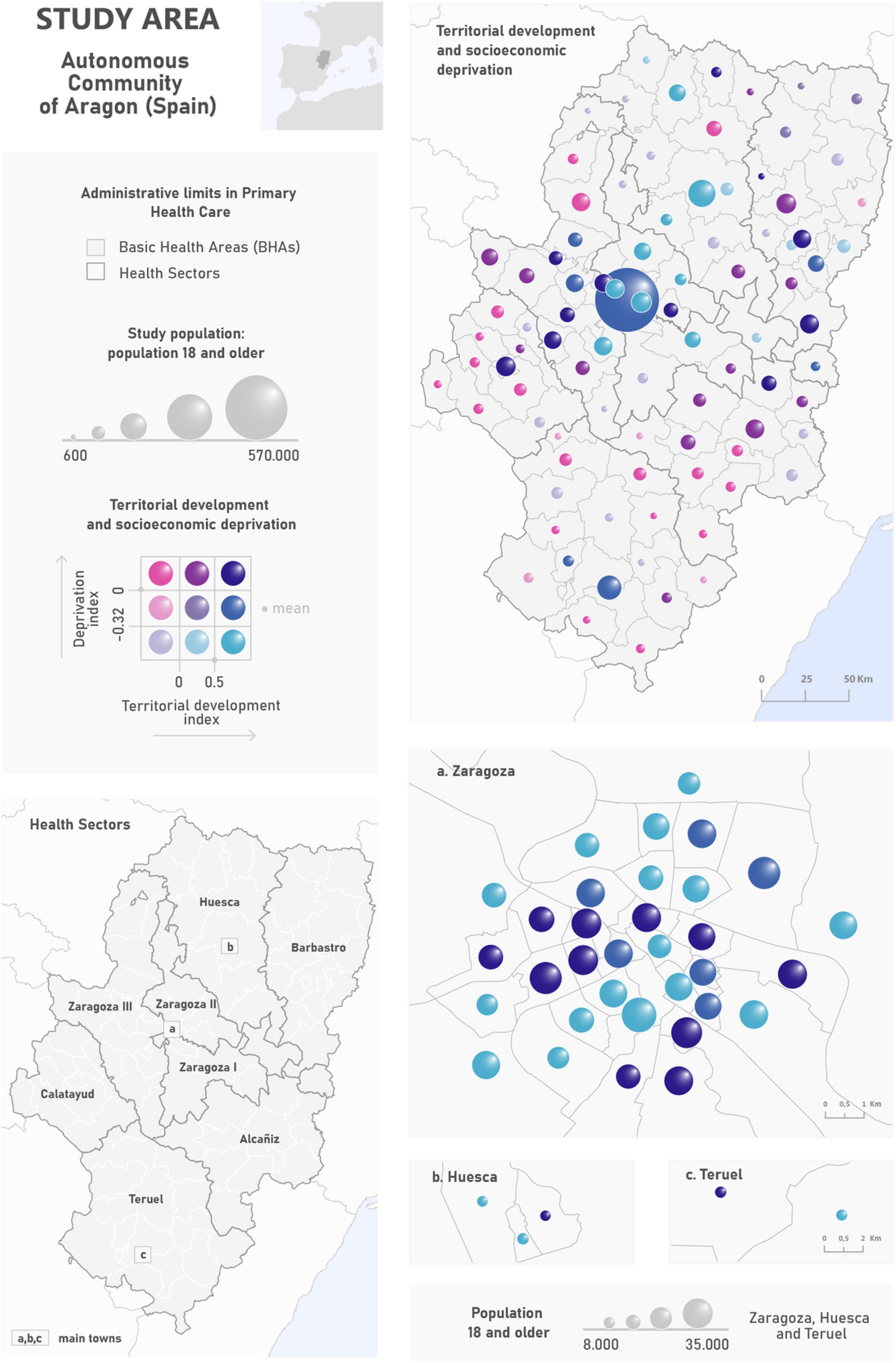
Figure 1. Study area and spatial distribution of the privation and territorial development indexes by BHA.
Variables and Data Collection
The dependent variable of this study is the prevalence rate of depression. The Aragon Health Sciences Institute of the University of Zaragoza provided standardized rates by gender and age at BHA level in the years 2010 and 2020. As independent variables, we retrieved and compiled a comprehensive set of socioeconomic and demographic indicators from official datasets and databases in Aragon ( Table 1 ).
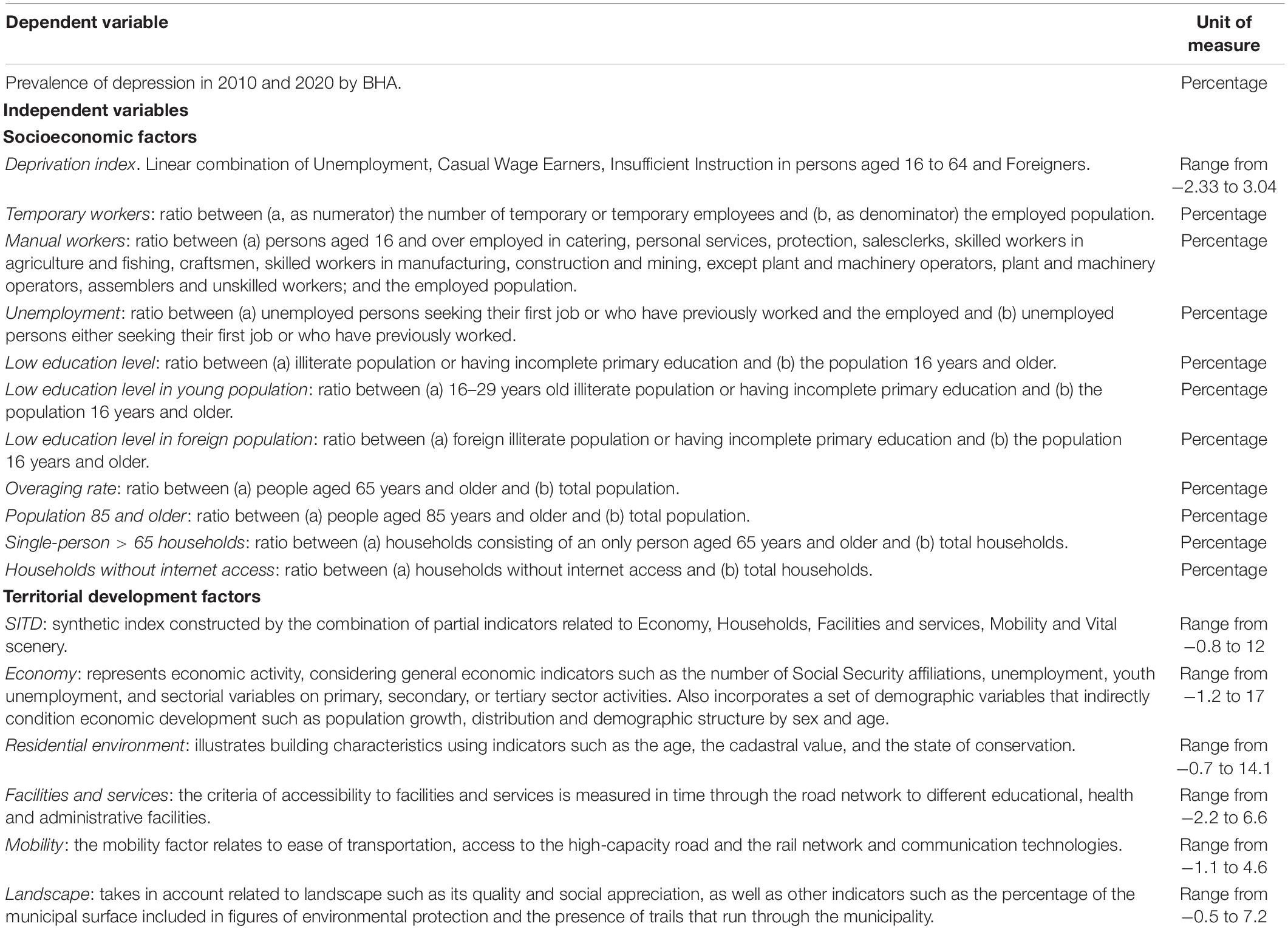
Table 1. Variables of the study, indicators, and units of measure.
The core information for our analyses comes from the Aragon’s Deprivation index ( Compés Dea et al., 2018 ). The database was created by the General Directorate of Public Health of the Government of Aragon, the Aragon Statistics Institute (ASI) and the Department of Microbiology, Preventive Medicine and Public Health of the University of Zaragoza. They developed a cross-sectional study linking mortality and deprivation, taking the BHA as the unit of analysis, aggregating data from all the inhabitants of Aragon as of January the 1st 2011 (Spanish Population Census of 2011). Out of the 26 indicators that make up the Deprivation index, we selected the following: unemployment, casual wage earners, insufficient instruction and Foreigners. In this work, we analyze both the Deprivation Index and a selection of the original indicators.
We included a second set of indicators related to territorial development, collected from the spatial database of the Synthetic Index of Territorial Development of Aragon (SITD). The SITD was created by the General Directorate of Territorial Integration, Mobility and Housing of the Government of Aragon. It consists of a combination of factors that illustrate different development axes: Economy, Residential environment, Facilities and services, Mobility and Landscape.
Statistical Analysis
To analyze the association between the prevalence of depression and the socioeconomic and territorial variables, we calculated the Spearman’s or Person’s R correlation coefficient, retrieving both the coefficient value and its significance level ( p -value). Pearson was calculated when data were normally distributed, while Spearman was used if at least one covariate did not follow a normal distribution. To further investigate potential relationships, we conducted a multivariate regression analysis. Since the assumption of normality does not hold for all covariates, we leaned toward logit regression. We constructed a separate binary response variable for 2010 and 2020, classifying as “1” all records above the median prevalence rate (6.37 in 2010 and 10.6 in 2020) and as “0” otherwise. Additionally, we analyzed the change in the prevalence rate, calculating the variation rate between 2010 and 2020. Again, the resulting variable was recoded as 0–1 using the median as separation threshold. Three separate models were evaluated (2010, 2020 and change). For each one, we calibrated a null model containing all variables as candidate predictors. We discarded those found to be collinear (VIF > 5), subsequently retaining significant predictors through a backwards stepwise procedure minimizing the AIC. Each “final” model was submitted to the Hosmer Lemeshow goodness of fit test and the Likelihood ratio test. Additionally, we computed the Nagelkerke pseudo-R2 coefficient to inform about the performance of the model.
In 2010, 80,030 adult individuals were diagnosed with depression in Aragon, a figure that rose to 123,174 in 2020, increasing by 53% during the period. Conversely, the total population in the same age group slightly decreased from 1,111,812 in 2010 to 1,096,878 in 2020. The highest prevalence rates in 2010 were observed around Zaragoza and its metropolitan area while the lowest were observed in the southern and northern ends of the region, especially in Teruel ( Figure 2 ). All socioeconomic factors -aside from wage earners, unemployment, and the Deprivation index itself- attained significant and negative correlations in both 2010 and 2020. Territorial indicators showed stronger associations, being all significant and positively correlated but landscape quality, which aroused a negative correlation ( Table 2 ). This implies that BHAs with an overaged population, low instruction level, which are less advantageous in terms of economic development and access to infrastructures/equipment are less prone to depression; meaning that rural enclaves may exert a sheltering effect. But the same indicators -either socioeconomic or territorial- were found significantly associated with the change in the prevalence rate between 2010 and 2020. However, the direction of the association was reversed in all cases. Hence, regardless of the suggested protective effect of rural areas, it is precisely these settings that are increasing faster in relative terms. The results from the logit regression models support the behavior from the correlation analyses ( Table 3 ). The 2010 and 2020 models retained the same variables as predictors of the prevalence of depression, i.e., overaging rate, manual workers, and landscape quality. In both cases it means that a lower level of over-aging, a lower proportion of manual workers and a lower quality of the landscape are associated with an increased probability of depression. In the case of the variation rate the model identified three variables with explanatory capacity (though only one was significant p < 0.05): low level of education in the young and foreign population and the overaging rate. The direction of the relationship suggests a greater probability of finding a higher increase in prevalence by 2020 in areas with an overaged demographic structure accompanied -to a lesser extent- by a lower level of education in the foreign population. The results from the goodness of fit tests are favorable in all cases (HL > 0.05 and LR < 0.05). However, the performance of the models was higher in the case of 2010, followed by the 2020 and the variance models.
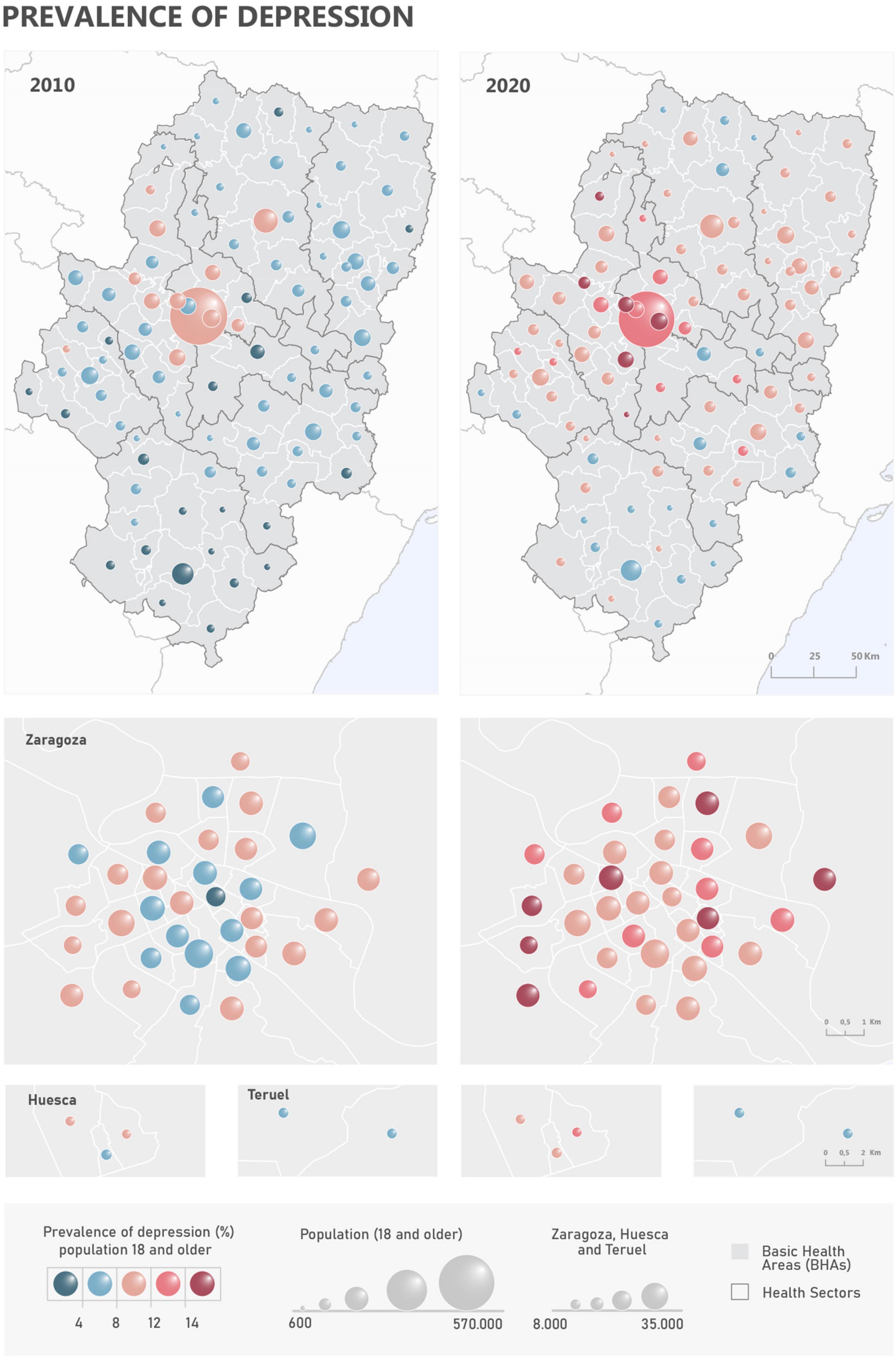
Figure 2. Spatial distribution of the prevalence of depression in 2010 and 2020.
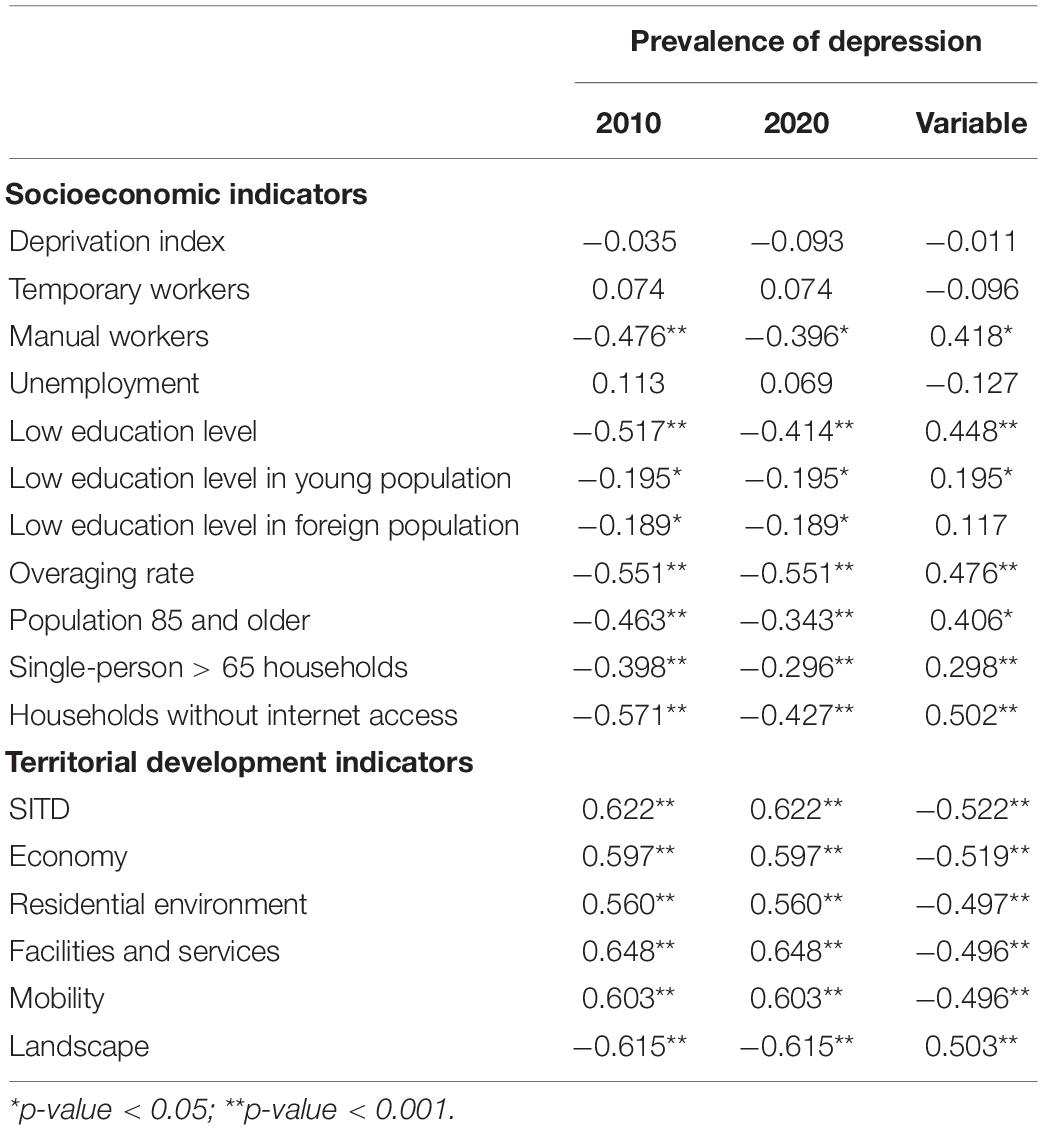
Table 2. Correlations between the prevalence of depression and the variables studied.
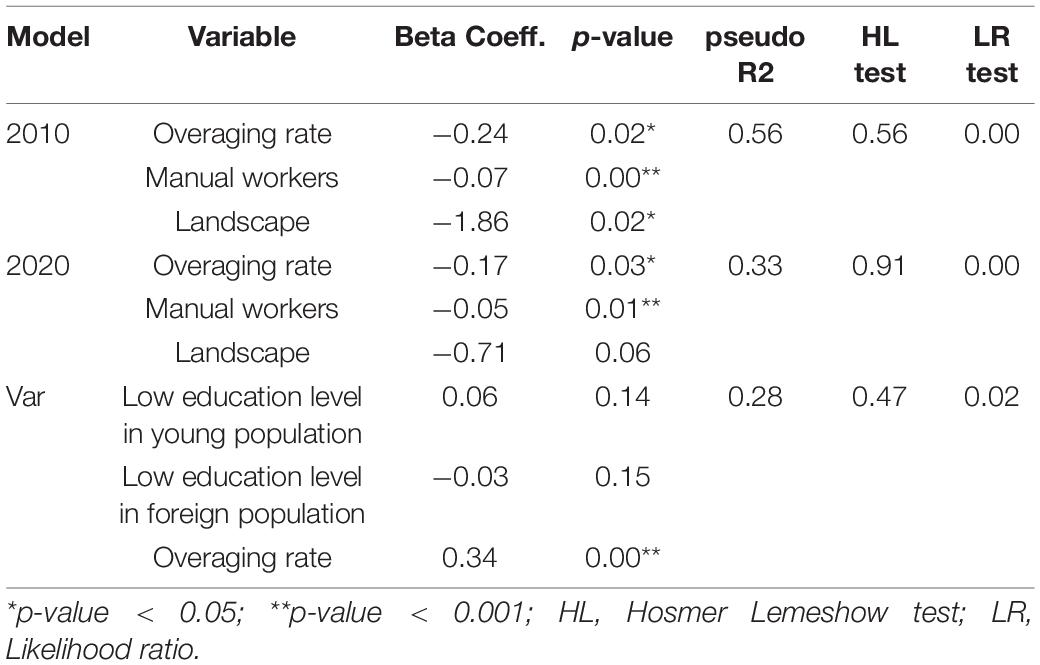
Table 3. Summary of logit regression models.
In this study we explore the spatial distribution of the prevalence of depression from a socioeconomic and territorial approach. Our findings reinforce the notion that the circumstances in which people live are linked to the variability in the prevalence of depression ( Lopizzo et al., 2015 ; Rautio et al., 2018 ). The rural-to-urban continuum seems to be the underlying driver of the spatial distribution of the prevalence rates. However, the observed associations and relationships revealed manifold nuances. The initial hypothesis about a greater vulnerability expected in areas with greater socioeconomic deprivation and lower levels of territorial development, can only be partially accepted.
The BHAs of the city of Zaragoza, its metropolitan area and those of the central-east sector of the region correspond to predominantly urban areas where high rates of depression have been observed in 2010 and 2020. The explanatory factors identified by the regression models (higher probability of prevalence with lower overaging, proportion of manual workers and landscape quality) lead to reconsideration of these areas. The positive correlations between the prevalence and the territorial development factors (except for the quality of the landscape) support such reexamination, leading us to qualify the initial hypothesis. We observe that an advantageous situation in terms of territorial development does not necessarily protect against the prevalence of depression. In urban areas, the social ties are less solid than in rural environments, the feeling of belonging to the community is lower, the urban way of life is associated with greater stress, and the enjoyment of natural spaces compared to rural areas is less affordable. In contrast, rural enclaves are usually located in natural environments attaining higher landscape quality than their urban counterparts. In addition, social relationships are strong and derive to a large extent from the existence of a sense of attachment to the place of residence. In this regard, the seemingly sheltering effect of rural livelihoods ( Ayuso-Mateos et al., 2001 ) could be explained by the social support networks ( Rubio-Aranda et al., 2012 ; Gariépy et al., 2016 ), the greater sense of belonging to the community ( Kim et al., 2004 ; Romans et al., 2010 ) and the positive effects of rural landscapes on mental health ( Velarde et al., 2007 ).
Regarding socioeconomic status (SES), there is a scientific consensus on the link between SES and mental health ( Ostler et al., 2001 ; Patel et al., 2018 ). However, in the particular case of depression, a greater heterogeneity in the results and controversy exists due to the way the psychiatric disorder and the SES is measured as well as to the contextual background ( Lorant et al., 2003 ). It is noteworthy that in our study the composite indicator of deprivation did not explain the prevalence of depression, while some partial index factors did. The explanatory factors of the variance model revealed a greater probability of an increase in the prevalence in overaged areas with lower educational attainment. This factor has been highlighted in previous studies for its protective role against the prevalence of depression ( Zimmerman and Katon, 2005 ; Bjelland et al., 2008 ; Freeman et al., 2016 ), especially in the elderly ( Miech and Shanahan, 2000 ; Domènech-Abella et al., 2018 ). In turn, other SES measurements such as the marital status or the income level have proven to be more significant than educational level ( Inaba et al., 2005 ). In other studies, these associations also vary depending on population characteristics such as sex and age ( Elliott, 2001 ; Back and Lee, 2011 ; Arias-de la Torre et al., 2018 ).
We consider that in addition to the educational attainment, there are other factors that may contribute to explain the increase in the prevalence of depression in rural areas in Aragon. The median prevalence of depression in Aragon was 6.37% in 2010 and 10.6% in 2020. The prevalence from primary care consultations offered by the PREDICT study was 12.2% ( King et al., 2006 ) and 10.6% in the ESEMED-Spain project ( Gabilondo et al., 2010 ). These figures reveal a possible under-diagnosis in the prevalence of depression in 2010 ( Llorente et al., 2018 ), a frequent situation, especially in the elderly ( Faisal-Cury et al., 2022 ). The regression results revealed an increased prevalence of depression in areas with aged population ( Kyu-man et al., 2019 ; Linder, 2019 ). This implies that, although the elderly population living in rural areas may enjoy protective environmental factors against mental health conditions, they still deserve special attention from the healthcare system. In this context, it should be noted that between 2010 and 2020, the healthcare sector in Aragon has improved the mechanisms of diagnosis and the follow-up of the prevalence through the so-called “Aragon’s mental health plan 2017–2021.” This effort has been translated into a higher integration of mental health centers in the BHAs and the incorporation of the figure of the consultant psychiatrist for primary care. In addition, screening tests for geriatric depression have been incorporated into medical history follow-up programs, such as the Yesavage test ( Yesavage, 1988 ). Therefore, the increase in prevalence in rural settings could be linked to the increased accuracy in the diagnosis capability.
In summary, this study illustrates an assessment based on the integration of official healthcare, socioeconomic and territorial information to analyze the prevalence of diseases from the DH approach. Our findings encourage the incorporation of Geographical Information Systems (GIS) to support decision-making ( Wang, 2020 ). In addition to broad patterns and relationships, GIS-based analyses offer interesting capabilities. An illustrative example is the possible underdiagnosis of depression in the elderly mentioned above. If such underdiagnosis is to be confirmed in a population group or in a given area, actions can be developed to this end.
It should also be mentioned the limitations of the study derived from the databases used. In mental diseases, the process of diagnosis, registration and treatment is subject to greater subjectivity comparted to the non-mental ones. The health inventory protocol of Aragon contains specific codes for mental pathologies that allow their unequivocal compilation (e.g., depressive disorder, anxiety disorder). However, the clinical tools for diagnosis, highly diverse and mainly based on clinical interviews, are subject to variability in medical practice ( WHO, 2004 ; Muñoz and Jaramillo, 2015 ). Actions aimed at homogenizing the diagnostic tools may minimize the bias in the information derived from this variability, though they are not in place yet. There is also a temporal mismatch between prevalence data and the socioeconomic indicators, referred to 2011 as reported in the Spanish Population and Housing Census. Despite offering an adequate spatial coverage, their frequency and update timing hinders the multitemporal analysis.
Likewise, the suite of selected indicators was able to unravel broad relationships but might be lacking “thematic sensitivity,” holding limited capability to provide further insights. It would be of great interest to incorporate in future studies variables specifically related with structural processes that take place on a regional scale but with profound effects on DH manifestations at the local and the individual level. For instance, covariates linked to economic recession, prevailing situation in Spain at the beginning of the study period. These processes impact the labor and economic environments, leading to situations of precarious, temporary employment or unemployment; to migrant workers, to situations of lack of funds that ultimately result in sadness, despair, and isolation. This information related to individual factors is recorded by health administrations in patients’ medical records. Consequently, the identification of cause-effect relationships between structural and individual factors would lead to future studies, preferably of the observational type. In the case of the ecological type, a greater level of spatial and temporal disaggregation of the data will be sought, which will require joint work and continuous feedback between the scientific community and the public administrations.
The interaction between depression prevalence and HD should not be reduced to the socioeconomic environment. There are other factors such as territorial development and organizational aspects of the health system that can support the explanation of the spatial distribution of depression prevalence from the DH approach. Public administrations offer information that, when integrated with that generated in the health system, can be used for research and decision-making aimed at reducing social and health inequalities. This demonstration case is focused on the prevalence of depression, one of the most prevalent and complex diseases in diagnosis and treatment in primary care. However, our approach can be easily adapted to other diseases and regions elsewhere.
Data Availability Statement
The data analyzed in this study is subject to the following licenses/restrictions: Data on the prevalence of depression have been provided by the Research Ethics Committee of the Autonomous Community of Aragón (BIGAN database). Data on HD have been collected from official and public sources of the Government of Aragon: (i) https://idearagon.aragon.es/atlas/# (ii) https://www.aragon.es/-/indice-sintetico-desarrollo-territorial . Requests to access these datasets should be directed to CB-M, [email protected] .
Ethics Statement
The studies involving human participants were reviewed and approved by the Research Ethics Committee of the Autonomous Community of Aragon: CEICA. The ethics committee waived the requirement of written informed consent for participation.

Author Contributions
CB-M: conceptualization, methodology, investigation, data curation, writing – original draft, and visualization. MR: conceptualization, methodology, investigation, software, validation, formal analysis, writing – review and editing, and supervision. RG-FL and JL: conceptualization, and writing – review and editing. MZ-A: conceptualization, methodology, investigation, resources, writing – review and editing, supervision, project administration, and funding acquisition. All authors contributed to the article and approved the submitted version.
This project was partially funded by the Young Research Program of the University of Zaragoza (JIUZ-2019-SOC-23): “Integration of cartographic tools and spatial analysis in health information systems: Design, validation and application to the study of the prevalence of depression, asthma, COPD and diabetes in Aragon.” Ibercaja Foundation. Group of Studies in Territorial Planning financed in the call for research groups of the Government of Aragon. Government of Aragon Predoctoral Fellowship granted to CB-M.
Conflict of Interest
The authors declare that the research was conducted in the absence of any commercial or financial relationships that could be construed as a potential conflict of interest.
Publisher’s Note
All claims expressed in this article are solely those of the authors and do not necessarily represent those of their affiliated organizations, or those of the publisher, the editors and the reviewers. Any product that may be evaluated in this article, or claim that may be made by its manufacturer, is not guaranteed or endorsed by the publisher.
Acknowledgments
We thank the Health Research Institute of Aragon.
Arias-de la Torre, J., Vilagut, G., Martín, V., Molina, A. J., and Alonso, J. (2018). Prevalence of major depressive disorder and association with personal and socio-economic factors. Results for Spain of the European Health Interview Survey 2014–2015. J. Affect. Disord. 239, 203–207. doi: 10.1016/j.jad.2018.06.051
PubMed Abstract | CrossRef Full Text | Google Scholar
Ayuso-Mateos, J. L., Vázquez-Barquero, J. L., Dowrick, C., Lehtinen, V., Dalgard, O. S., Casey, P., et al. (2001). Depressive disorders in Europe: prevalence figures from the ODIN study. Br. J. Psychiatry 179, 308–316. doi: 10.1192/bjp.179.4.308
Back, J. H., and Lee, Y. (2011). Gender differences in the association between socioeconomic status (SES) and depressive symptoms in older adults. Arch. Gerontol. Geriatr. 52, e140–e144. doi: 10.1016/j.archger.2010.09.012
Bjelland, I., Krokstad, S., Mykletun, A., Dahl, A. A., Tell, G. S., and Tambs, K. (2008). Does a higher educational level protect against anxiety and depression? The HUNT study. Soc. Sci. Med. 66, 1334–1345. doi: 10.1016/j.socscimed.2007.12.019
Cai, N., Choi, K. W., and Fried, E. I. (2020). Reviewing the genetics of heterogeneity in depression : operationalizations, manifestations and etiologies. Hum. Mol. Genet. 29, 10–18. doi: 10.1093/hmg/ddaa115
Compés Dea, M., Olivan Bellido, E., Feja Solana, C., Aguilar Palacio, I., García-Carpintero Romero del Hombrebueno, G., and Adiego Sancho, B. (2018). Construcción de un índice de privación por zona básica de salud en Aragón a partir de datos de censo de 2011. Rev. Esp. Salud Publica 92:10.
Google Scholar
Dahlgren, G., and Whitehead, M. (2007). Concepts and Principles for Tackling Social Inequities in Health: Levelling up Part 2. Copenhagen: WHO regional office for europe.
Domènech-Abella, J., Mundó, J., Leonardi, M., Chatterji, S., Tobiasz-Adamczyk, B., Koskinen, S., et al. (2018). The association between socioeconomic status and depression among older adults in Finland, Poland and Spain: a comparative cross-sectional study of distinct measures and pathways. J. Affect. Disord. 241, 311–318. doi: 10.1016/j.jad.2018.08.077
Dunn, E. C., Brown, R. C., Dai, Y., Rosand, J., Nugent, N. R., Amstadter, A. B., et al. (2015). Genetic determinants of depression: recent findings and future directions. Harv. Rev. Psychiatry 23, 1–18. doi: 10.1097/HRP.0000000000000054
Elliott, M. (2001). Gender differences in causes of depression. Women Health 33, 183–198. doi: 10.1300/J013v33n03_11
CrossRef Full Text | Google Scholar
Faisal-Cury, A., Ziebold, C., Rodrigues, D. M. O., and Matijasevich, A. (2022). Depression underdiagnosis: prevalence and associated factors. A population-based study. J. Psychiatr. Res. 151, 157–165. doi: 10.1016/j.jpsychires.2022.04.025
Fortney, J. C., Harman, J. S., Xu, S., and Dong, F. (2010). The association between rural residence and the use, type, and quality of depression care. J. Rural Health 26, 205–213. doi: 10.1111/j.1748-0361.2010.00290.x
Freeman, A., Tyrovolas, S., Koyanagi, A., Chatterji, S., Leonardi, M., Ayuso-Mateos, J. L., et al. (2016). The role of socio-economic status in depression: results from the COURAGE (aging survey in Europe). BMC Public Health 16:1098. doi: 10.1186/s12889-016-3638-0
Gabilondo, A., Rojas-Farreras, S., Vilagut, G., Haro, J. M., Fernández, A., Pinto-Meza, A., et al. (2010). Epidemiology of major depressive episode in a southern European country: results from the ESEMeD-Spain project. J. Affect. Disord. 120, 76–85. doi: 10.1016/j.jad.2009.04.016
Gariépy, G., Honkaniemi, H., and Quesnel-Vallée, A. (2016). Social support and protection from depression: systematic review of current findings in Western countries. Br. J. Psychiatry 209, 284–293. doi: 10.1192/bjp.bp.115.169094
Gnanapragasam, S. N., Astill Wright, L., Pemberton, M., and Bhugra, D. (2021). Outside/inside: social determinants of mental health. Ir. J. Psychol. Med. 1–11. doi: 10.1017/ipm.2021.49
Gupta, S., Methuen, C., Kent, P., Chatain, G., Christie, D., Torales, J., et al. (2016). Economic development does not improve public mental health spending. Int. Rev. Psychiatry 28, 415–419. doi: 10.1080/09540261.2016.1210582
Inaba, A., Thoits, P. A., Ueno, K., Gove, W. R., Evenson, R. J., and Sloan, M. (2005). Depression in the United States and Japan: gender, marital status, and SES patterns. Soc. Sci. Med. 61, 2280–2292. doi: 10.1016/j.socscimed.2005.07.014
Jorm, A. F., and Ryan, S. M. (2014). Cross-national and historical differences in subjective well-being. Int. J. Epidemiol. 43, 330–340. doi: 10.1093/ije/dyt188
Kim, J.-M., Stewart, R., Shin, I.-S., Yoon, J.-S., and Lee, H.-Y. (2004). Lifetime urban/rural residence, social support and late-life depression in Korea. Int. J. Geriatr. Psychiatry 19, 843–851. doi: 10.1002/gps.1175
King, M., Weich, S., Torres-González, F., Svab, I., Maaroos, H.-I., Neeleman, J., et al. (2006). Prediction of depression in European general practice attendees: the PREDICT study. BMC Public Health 6:6. doi: 10.1186/1471-2458-6-6
Kuehner, C. (2017). Why is depression more common among women than among men? Lancet Psychiatry 4, 146–158. doi: 10.1016/S2215-0366(16)30263-2
Kyu-man, H., Jisoon, C., Ho-kyoung, Y., Young-hoon, K., and Byung-joo, H. (2019). Relationships between hand-grip strength, socioeconomic status, and depressive symptoms in community-dwelling older adults. J. Affect. Disord. 252, 263–270. doi: 10.1016/j.jad.2019.04.023
Linder, A. (2019). Inequalities in the economic consequences of depression and anxiety in Europe : a systematic scoping review. Eur. J. Public Health 30, 767–777. doi: 10.1093/eurpub/ckz127
Llorente, J. M., Oliván-Blázquez, B., Zuñiga-Antón, M., Masluk, B., Andrés, E., García-Campayo, J., et al. (2018). Variability of the prevalence of depression in function of sociodemographic and environmental factors: ecological model. Front. Psychol. 9:2182. doi: 10.3389/fpsyg.2018.02182
Lopizzo, N., Bocchio Chiavetto, L., Cattane, N., Plazzotta, G., Tarazi, F. I., Pariante, C. M., et al. (2015). Gene–environment interaction in major depression: focus on experience-dependent biological systems. Front. Psychiatry 6:68. doi: 10.3389/fpsyt.2015.00068
Lorant, V., Deliège, D., Eaton, W., Robert, A., Philippot, P., and Ansseau, M. (2003). Socioeconomic inequalities in depression: a meta-analysis. Am. J. Epidemiol. 157, 98–112. doi: 10.1093/aje/kwf182
Miech, R. A., and Shanahan, M. J. (2000). Socioeconomic status and depression over the life course. J. Health Soc. Behav. 41, 162–176. doi: 10.2307/2676303
Muñoz, L. F., and Jaramillo, L. E. (2015). DSM-5: ¿Cambios significativos? Rev. Asoc. Española Neuropsiquiatría 35, 111–121.
OECD (2015). ¿Por qué Reducir la Desigualdad nos Beneficia?.en España. Paris: OECD.
Ollila, E. (2011). Health in All Policies: from rhetoric to action. Scand. J. Public Health 39, 11–18. doi: 10.1177/1403494810379895
Ostler, K., Thompson, C., Kinmonth, A.-L. K., Peveler, R. C., Stevens, L., and Stevens, A. (2001). Influence of socio-economic deprivation on the prevalence and outcome of depression in primary care: the Hampshire Depression Project. Br. J. Psychiatry 178, 12–17. doi: 10.1192/bjp.178.1.12
Patel, V., Burns, J. K., Dhingra, M., Tarver, L., Kohrt, B. A., and Lund, C. (2018). Income inequality and depression: a systematic review and meta-analysis of the association and a scoping review of mechanisms. World Psychiatry 17, 76–89. doi: 10.1002/wps.20492
Purtle, J., Nelson, K. L., Yang, Y., Langellier, B., Stankov, I., and Roux, A. V. D. (2019). Urban - rural differences in older adult depression: a systematic review and meta-analysis of comparative studies. Am. J. Prev. Med. 56, 603–613. doi: 10.1016/j.amepre.2018.11.008
Puska, P. (2007). Health in all policies. Eur. J. Public Health 17:328. doi: 10.1093/eurpub/ckm048
Rautio, N., Filatova, S., Lehtiniemi, H., and Miettunen, J. (2018). Living environment and its relationship to depressive mood: a systematic review. Int. J. Soc. Psychiatry 64, 92–103. doi: 10.1177/0020764017744582
Razzak, H. A., Harbi, A., and Ahli, S. (2019). Depression: prevalence and associated risk factors in the united arab emirates. Oman Med. J. 34, 274–282. doi: 10.5001/omj.2019.56
Ribeiro, W. S., Bauer, A., Andrade, M. C. R., York-Smith, M., Pan, P. M., Pingani, L., et al. (2017). Income inequality and mental illness-related morbidity and resilience: a systematic review and meta-analysis. Lancet Psychiatry 4, 554–562. doi: 10.1016/S2215-0366(17)30159-1
Romans, S. E., Cohen, M., and Forte, T. (2010). Rates of depression and anxiety in urban and rural Canada. Soc. Psychiatry Psychiatr. Epidemiol. 46, 567–575. doi: 10.1007/s00127-010-0222-2
Rubio-Aranda, E., Blasco-Montón, G., Comín-Comín, M., Martínez-Terrer, T., Magallón-Botaya, R., and García-Campayo, J. (2012). Mental health and social relations in older rural population. Actas Esp. Psiquiatr. 40, 239–247.
PubMed Abstract | Google Scholar
Santoro-Lamelas, V. (2016). La salud pública en el continuo salud-enfermedad: un análisis desde la mirada profesional. Rev. Salud Publica 18, 530–542. doi: 10.15446/rsap.v18n4.47854
United Nations (2015). UN General Assembly, Transforming Our World : the 2030 Agenda for Sustainable Development, 21 October 2015, A/RES/70/1. 16301. New York, NY: United Nations, 1–35.
Velarde, M. D., Fry, G. L. A., and Tveit, M. S. (2007). Health effects of viewing landscapes – Landscape types in environmental psychology. Urban For. Urban Green. 6, 199–212. doi: 10.1007/s12110-021-09396-7
Wang, F. (2020). Why public health needs GIS: a methodological overview. Ann. GIS 26, 1–12. doi: 10.1080/19475683.2019.1702099
Wang, J. L. (2004). Rural–urban differences in theprevalence of major depression and associatedimpairment. Soc. Psychiatry Psychiatr. Epidemiol. 39, 19–25. doi: 10.1007/s00127-004-0698-8
WHO (2004). ICD-10 : International Statistical Classification of Diseases and Related Health Problems: Tenth Revision , 2nd Edn. Geneva: World Health Organization.
WHO (2014). Health in All Policies (HiAP) Framework for Country Action. Geneva: World Health Organization, doi: 10.1093/heapro/dau035
WHO (2017). Depression and Other Common Mental Disorders: Global Health Estimates. Geneva: World Health Organization.
Yesavage, J. A. (1988). Geriatric depression scale. Psychopharmacol. Bull. 24, 709–711.
Yu, Z., and Wang, F. (2016). Socio-economic development and emotion-health connection revisited: a multilevel modeling analysis using data from 162 counties in China. BMC Public Health 16:257. doi: 10.1186/s12889-016-2926-z
Zimmerman, F. J., and Katon, W. (2005). Socioeconomic status, depression disparities, and financial strain: what lies behind the income-depression relationship? Health Econ. 14, 1197–1215. doi: 10.1002/hec.1011
Keywords : primary health care, depression, social determinants of health, territorial development, decision making, socioeconomic status
Citation: Bentué-Martínez C, Rodrigues M, García-Foncillas López R, Llorente González JM and Zúñiga-Antón M (2022) Socio-Economic Development and Mental Health: Case Study of the Spanish Region of Aragon (2010–20). Front. Psychol. 13:899278. doi: 10.3389/fpsyg.2022.899278
Received: 18 March 2022; Accepted: 23 May 2022; Published: 10 June 2022.
Reviewed by:
Copyright © 2022 Bentué-Martínez, Rodrigues, García-Foncillas López, Llorente González and Zúñiga-Antón. This is an open-access article distributed under the terms of the Creative Commons Attribution License (CC BY) . The use, distribution or reproduction in other forums is permitted, provided the original author(s) and the copyright owner(s) are credited and that the original publication in this journal is cited, in accordance with accepted academic practice. No use, distribution or reproduction is permitted which does not comply with these terms.
*Correspondence: Carmen Bentué-Martínez, [email protected]
Disclaimer: All claims expressed in this article are solely those of the authors and do not necessarily represent those of their affiliated organizations, or those of the publisher, the editors and the reviewers. Any product that may be evaluated in this article or claim that may be made by its manufacturer is not guaranteed or endorsed by the publisher.
Cookies on GOV.UK
We use some essential cookies to make this website work.
We’d like to set additional cookies to understand how you use GOV.UK, remember your settings and improve government services.
We also use cookies set by other sites to help us deliver content from their services.
You have accepted additional cookies. You can change your cookie settings at any time.
You have rejected additional cookies. You can change your cookie settings at any time.
Socio-economic case studies
Examples of projects that are supported by the NDA group socio-economic programme.
As a responsible organisation and under a legal obligation in the Energy Act (2014), the NDA takes due regard of the impact of its activities in the communities around its sites.
The NDA group socio-economic funding is intended to help local communities who are or will be impacted by the changing levels of economic activity at our sites. If local communities are particularly reliant on nuclear sites, we work with local partners to help develop alternative economic opportunities.
We also fund projects that develop local skills and help businesses take advantage of the economic opportunities from decommissioning. Funding is allocated based on an assessment of local needs and on the basis of a competitive process of projects and organisations meeting local needs.
The case studies in this collection are examples of such projects.
- 17 April 2018
- 3 April 2018
- 23 March 2018
- 30 October 2017
- 14 January 2020
- 13 April 2018
- 4 December 2019
Updates to this page
Related content, is this page useful.
- Yes this page is useful
- No this page is not useful
Help us improve GOV.UK
Don’t include personal or financial information like your National Insurance number or credit card details.
To help us improve GOV.UK, we’d like to know more about your visit today. Please fill in this survey (opens in a new tab) .
- Open access
- Published: 02 April 2022
Socio-economic correlates of quality of life in single and married urban individuals: a Polish case study
- Daniel Puciato 1 ,
- Michał Rozpara 2 ,
- Marek Bugdol 3 &
- Barbara Mróz-Gorgoń 4
Health and Quality of Life Outcomes volume 20 , Article number: 58 ( 2022 ) Cite this article
6533 Accesses
6 Citations
Metrics details
A Correction to this article was published on 03 May 2022
This article has been updated
One of key current social trends is the increasing number of single people. It has multiple implications as single individuals often live and behave differently than those living in relationships. Marital status and socioeconomic status may also be significant quality of life factors for single persons. The aim of this study is to identify relationships between quality of life and selected indicators of socioeconomic status in single and married respondents from the Wrocław metropolitan area in Poland.
4460 respondents took part in the study (1828 single, 2632 married). The study was cross-sectional based on a diagnostic survey. Data was gathered on respondents’ sex, age, education, marital status, occupational status and financial situation as well as their quality of life and perceived health condition. Frequencies (f) and relative frequencies (rf) of categories of dependent and independent variables were determined. The chi-squared test (χ 2 ) and odds ratio (OR) statistics were applied. The level of statistical significance was set at α = .05.
A stochastic dependence (p ≤ .05) between marital status and perceived health condition and quality of life in the social domain was found among the respondents. Male sex, higher education, being an entrepreneur, college student or white-collar worker, and good financial status were associated with the highest assessments of quality of life and perceived health condition. The directions of quality of life modifications determined by socioeconomic status were similar in single and married urban respondents; however, the strength of these modifications was greater in the latter.
Conclusions
It is recommended to target respondents with public health programs aimed at lifestyle improvement, tailored to the needs of single and married individuals. Public policies directed at improving education and material situation of respondents are also worth considering, as they may be essential for modeling their quality of life. In addition, research on quality of life should be continued, which is particularly relevant in a pandemic situation.
Introduction
The issue of quality of life for people of working age is of major research and practical significance. Yet there has been relatively limited previous research on the quality of life of single people. In fact, between 1980 and 2015, the number of single-person households worldwide increased from about 118 million to 300 million. It is expected to rise by another 120 million by 2030. In 2019, single-person households were the most common household type in Europe, accounting for 32.9% of all households in Europe (24.1% in Poland) [ 1 ].
Moreover, research results indicate significant differences between single and married people in such matters as consumer behaviors [ 2 ], healthcare expenditure [ 3 ], or health behaviors [ 4 , 5 ]. Consequently, it should be assumed that marital status is also relevant for modelling the quality of life of people of working age. The results of previous studies, however, are not conclusive, as they indicate different directions and strengths of relationships between quality of life and marital status. Wahl et al. [ 6 ], Kim and Kim [ 7 ], and Kowalska et al. [ 8 ] observed lower quality of life scores in working-age single individuals compared to married persons. Similar findings have been reported among older adults [ 9 , 10 , 11 ] and patients [ 12 , 13 ]. Emrani et al. [ 14 ], Rezaei et al. [ 15 ] and Nayir et al. [ 16 ], however, reported higher quality of life scores in people living alone than in people in relationships. On the other hand, Raymakers et al. [ 17 ] found no significant relationships of quality of life with marital status in their study. Therefore, the problem of quality of life assessment in single people remains open and is addressed in the current study.
Another important issue is the relationship of the quality of life of single people with their socio-economic status. Such a relationship can be assumed to exist as one-person households differ from multi-person households in terms of number of income sources, access to certain social programs, or the size and structure of expenses. Lim's [ 18 ] study of U.S. single-person households showed, for example, that single people work and save more than married people of similar socioeconomic status. However, earlier studies on socio-economic determinants of the quality of life of single people of working age have been scarce and concerned only narrow groups of respondents. It was observed that among single people of working age, male gender [ 19 ] and younger age [ 20 ] may be predictors of higher quality of life. Kim and Kim [ 7 ] reported positive associations of quality of life of single mothers with educational level and material situation. Chen et al. [ 21 ] and Liu et al. [ 22 ], on the other hand, observed that education level and economic status may positively affect the quality of life of single post-working age individuals.
The presented literature review therefore indicates the existence of several research gaps. Firstly, it is still an open research problem whether the quality of life assessment in single people is higher or lower than in married people. Secondly, it is still unclear whether socioeconomic status moderates the quality of life of working-age single individuals. Thirdly, whether socioeconomic modifiers of quality of life are similar for single and married individuals is also an interesting and open research problem. Bridging the indicated research gaps is the key task of the present study.
The aim of this study is to identify relationships of overall quality of life, perceived health condition and health-related quality of life with selected indices of socioeconomic status in single and married adults from the metropolitan area of Wrocław, Poland. The following research questions were addressed in the study:
What is the assessment of overall quality of life, health status, and health-related quality of life by single and married respondents?
Do sex, age, education level, occupational status, having a steady source of income, per capita income, savings and debt modify respondents’ assessment of their overall quality of life, perceived health condition and health-related quality of life?
Are the potential effects of socioeconomic correlates of quality of life similar among single and married respondents?
Study design
The research project had been approved by the Commission of Bioethics of the University School of Physical Education in Wrocław. The study had a cross-sectional survey design. The method of a diagnostic survey with the questionnaire technique was applied. A flowchart of study stages is shown in Fig. 1 .
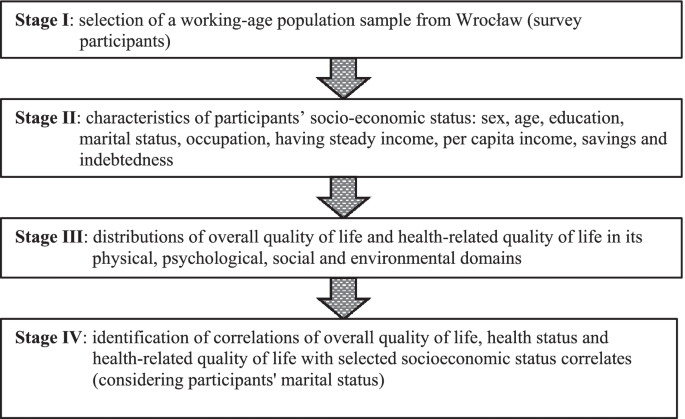
Source : author’s own
Flowchart of study stages.
Sample selection and size
The study was carried out in the Wrocław urban area. Wrocław is a city in south-western Poland, located close to the border with the Czech Republic and Germany, with a population of approximately 638,000. Wrocław is the fastest growing Polish city and has repeatedly been ranked among the top cities in the European Union in socio-economic development rankings. Research results also indicate that Wrocław has some of the highest values of quality of life indices in Poland [ 23 ]. These premises were the most important factors behind the choice of Wrocław as a research area.
The study population consisted of 4460 persons (2331 women and 2129 men) of working age 18–64 years living in Wrocław. Among them 1828 people were single, including 1000 women and 828 men, and 2632 were married, including 1331 women and 1301 men. The division of respondents into single and married persons was based on the extended definition of de facto marital status used in European official statistics [ 24 ]. The group of single respondents included single, widowed, divorced, separated, and legally separated persons as well as persons not living in a consensual union with another person, legally married persons but not forming a de facto marriage and not living in a consensual union with another person. The group of married respondents included married persons and persons living with their partners.
The sample size was estimated according to the following formula for a finite population [ 25 ]:
where N —the number of Wrocław inhabitants as of December 31, 2013 ( N = 632,067); p —the proportion of Wrocław working age population as of December 31, 2013 ( p = 0.63); E —the margin of error ( E = 0.015); z —the z-score associated with a 95% confidence interval ( z = 1.96).
The sample size was increased by 15% from the estimated sample size to account for potential refusal to participate in the survey.
The selection of the sample was multistage and mixed (random and purposive). In the first stage, using a random number table, 10 housing estates in Wrocław were drawn. In the second stage, using the same random mechanism, 3 streets were selected from each of the 10 housing estates. In the last stage, from among passers-by encountered in the selected streets, every fourth person was asked to participate in the survey.
The inclusion criteria were address of residence in one of the selected streets and working age (18–64 years). The exclusion criteria included chronic diseases, e.g. cancer, diabetes, arterial hypertension, osteoarthritis, osteoporosis. All respondents were informed about the purpose and course of the survey and their voluntary participation. They were asked to provide their informed consent to participate. Out of 4548 individuals who were asked to participate, 88 declined to take part in the survey.
Socioeconomic background
Data were obtained on the empirical distributions of selected socio-economic indicators, i.e. sex (woman, man), age (under 34 years, 35–44 years, 45 and more years), education (primary, secondary, university), occupation (laborer, white collar worker, entrepreneur, student, unemployed), having steady income (YES, NO), per capita income (under USD 260, USD 260–400, over USD 400), having money savings (YES, NO), and being in debt (YES, NO) as independent variables; and marital status (single, married) as the stratifying variable.
The World Health Organization Quality of Life (WHOQOL BREF) [ 26 , 27 ] questionnaire was used to assess respondents’ quality of life. It is a tool commonly used in population-based quality of life research. Jaracz et al. [ 28 ] and Kowalska et al. [ 29 ] demonstrated that the Polish adaptation of the questionnaire is a reliable instrument of quality of life assessment also in adults and economically active people.
The questionnaire consisted of 26 closed questions with answers on a five-level Likert scale. Answers to particular questionnaire items were used in accordance with the accepted data processing key to determine the following indicators: overall quality of life (1–5 pts.); perceived health condition (1–5 pts.); and health-related quality of life in four domains: physical (7–35 pts.), psychological (6–30 pts.), social (3–15 pts.), and environmental (8–40 pts.). Quality of life and perceived health condition indices were converted into an ordinal scale. The 33rd and 66th percentiles were used as division points, where: < 33th percentile indicated low quality of life, 33–66th percentile—average quality of life, and > 66th percentile—high quality of life. In the present study, quality of life and perceived health condition were regarded as dependent variables.
Statistical analysis
The collected data were subjected to statistical analysis, following which the frequencies (f) and relative frequencies (rf) of categories of considered dependent and independent variables were determined. Pearson's chi-squared test (χ 2 ) was used to assess the differences in the distributions of these variables in groups of respondents divided by their marital status [ 30 ]. Multinomial logistic regression, including odds ratio (OR) and the confidence interval (CI) [ 31 , 32 ], was used to assess the relationships between: overall quality of life, perceived health condition and health-related quality of life in the physical, psychological, social and environmental domains, and socioeconomic status, when stratifying respondents by their marital status. The statistical significance of the difference between ORs in the groups of single and married respondents was verified using z = δ/SE(δ), where: δ is the absolute difference between the natural logarithm of OR in each group, and SE(δ) is the standard error of this difference [ 32 ]. The level of statistical significance was set at α = 0.05. All statistical calculations were made using IBM SPSS Statistics 26 (IBM Corporation, Armonk, NY, USA).
Participants
The respondents’ characteristics are shown in Table 1 .
Quality of life and perceived health condition assessment
Table 2 shows the characteristics of the respondents’ quality of life and perceived health condition. The highest percentage of single respondents (47.4%) and married respondents (48.3%) rated their overall quality of life as average. Low overall quality of life was reported by about 36% of respondents regardless of their marital status, while high quality of life was stated by 16.3% of single and 15.3% of married respondents. The single Wrocław residents were significantly more likely (p ≤ 0.05) than married residents to rate their perceived health condition as average (52.8% versus 43.3%) or high (14.7% versus 9.9%). In contrast, 32.4% of single and 46.8% of married respondents rated their perceived health condition as low. There were no significant differences (p > 0.05) in health-related quality of life in the physical, psychological, and environmental domains between single and married respondents. In both groups, the highest percentage of respondents assessed their health-related quality of life as average. On the other hand, the study results revealed a stochastic dependence (p ≤ 0.05) between health-related quality of life in the social domain and marital status. It was rated as average by 40.2% of single and 51.3% of married respondents, and low by 30.3% and 27.4% of respondents, respectively. High quality of life in the social domain was reported by 29.5% of single and 21.4% of married respondents (Table 2 ).
Overall quality of life in terms of socio-economic status
Table 3 presents the results of multinomial logistic regression illustrating the relationships of overall quality of life and perceived health condition (dependent variables) with selected socio-economic status indicators (independent variables), considering the respondents' marital status as the stratifying variable. The odds of high as compared to low overall quality of life were about 35% (OR 0.66, CI 0.50–0.87) lower in single women and 25% (OR 0.75, CI 0.59–0.95) lower in married women than in men. In respondents in the youngest age group, the odds of average versus low overall quality of life were more than four times (OR 4.36, CI 3.26–5.83) higher in single respondents and more than two times (OR 2.02, CI 1.60–2.57) higher in married respondents than in those in the oldest age group. The odds ratio differences in single and married individuals were statistically significant (p ≤ 0.05). Also, the odds of reporting high versus average overall quality of life were more than threefold (OR 3.12) higher in the single respondents and nearly twofold (OR 1.62) higher in married respondents for those aged 35–44 compared to those aged 45 and older. The z-test values also indicated significant differences (p ≤ 0.05) in OR between the groups of respondents of different marital status. The conditional probability of high and not low assessment of overall quality of life was almost three times (OR 2.99, CI 2.05–4.36) higher in the group of respondents with a higher education and almost seven times (OR 6.90, CI 5.12–9.29) higher in the group of married people than in those with a primary education. Also, respondents with a secondary education had higher odds of high versus low overall quality of life scores (OR 1.71 in single respondents, and OR 1.41 in married respondents) than those with a primary education. In both cases, the odds ratio values were significantly different in groups with different marital status (p ≤ 0.05). Occupational status was also a significant modifier of overall quality of life among the respondents. Compared to the reference group, i.e. the unemployed, the highest odds of reporting high rather than low overall quality of life were found among single entrepreneurs (OR 5.05) and married entrepreneurs (OR 48.21), single college students (OR 3.04), married students (OR 16.38), and single white-collar workers (OR 2.08) and married white-collar workers (OR 11.95). All differences in OR values between those single and married respondents were statistically significant (p ≤ 0.05) (Table 3 ).
The assessment of the overall quality of life was also significantly modified by economic factors. The odds of high versus low assessment of overall quality of life were more than 50% higher in the single respondents (OR 1.52, CI 1.11–2.08) and more than nine times higher in the married respondents (OR 9.33, CI 5.26–16.53) with a steady income than those without a regular income. The odds of high versus low and average versus low overall quality of life also rose with increasing per capita income. In addition, the respondents with savings had higher odds of high versus low (OR 5.78 for single respondents, OR 12.37 for married respondents) and high versus average (OR 2.35 for single respondents and OR 4.21 for married respondents) overall quality of life scores. The modifying effect of economic factors on respondents' overall quality of life was significantly higher (p ≤ 0.05) in married individuals than in single ones. The odds of high versus low and average versus low overall quality of life assessment were also higher in those without debt than in those with debt (Table 3 ).
Perceived health condition in terms of socio-economic status
Among the single respondents, the odds of a high versus low assessment of perceived health condition were almost twice as low among women than men (OR 0.51, CI 0.38–0.68). The odds of a high versus low assessment of perceived health condition were more than five times higher (OR 5.24 in single individuals and OR 5.10 in married individuals) among the respondents aged 35–44 years, and more than three times lower among those under 34 years of age (OR 3.34), compared to those aged 45 and over. The highest, compared to the control group (the unemployed) odds of high vs. low perceived health condition were found among single entrepreneurs (OR 4.15), and among students living in relationships (OR 63.84). Conversely, the odds of a high versus low perceived health condition assessment were more than twice as high among single labourers (OR 2.54, CI 1.56–4.15) and more than 30 times as high among married workers (OR 30.28, CI 9.50–96.52) than among the unemployed. Also, married blue-collar workers had nearly fourteen times higher odds of high versus low perceived health condition scores compared to the reference group (OR 13.91, CI 4.29–45.06). The odds ratio values were significantly higher (p ≤ 0.05) in married than in single respondents (Table 3 ).
Having a steady source of income was related to perceived health condition only among married individuals. Respondents with a steady income were more than six times as likely as those without to report high versus low perceived health condition (OR 6.45, CI 3.47–11.97). The odds ratio of having a high versus low perceived health condition was more than four times higher among single respondents (OR 4.11, CI 2.77–6.09) and almost eight times higher among married respondents (OR 7.72, CI 4.88–12.23) than among those with a per capita income of ˃ USD 400 than among those with an income < USD 260. The odds ratio values in the two groups were significantly different (p ≤ 0.05). The odds of high versus low perceived health condition were also higher in those with incomes of USD 260–400 than those with incomes < USD 260 (OR 2.95, CI 1.88–4.61 in single and OR 2.43, CI 1.42–4.14 in married individuals). Respondents with savings were more than three times more likely to have high versus low (OR 3.58 in single and OR 3.05 in married individuals) and nearly twice as likely to have an average versus low (OR 1.82—single and OR 1.70—married) perceived health condition. The conditional probability of high versus low and average versus low perceived health condition was also lower in those with debt than those without debt (Table 3 ).
Health-related quality of life in the physical, psychological, social, and environmental domains with regard to socio-economic status
Tables 4 and 5 present the results of multinomial logistic regression demonstrating the relationships of health-related quality of life in the physical, psychological, social, and environmental domains (dependent variables) with selected factors of socioeconomic status (independent variables), and respondents’ marital status as the stratifying variable.
The odds of a high versus low assessment of health-related quality of life in the physical domain were lower in female respondents than in male respondents, regardless of their marital status. Single respondents from the youngest age group were nearly 50% less likely to have a high versus low health-related quality of life in the physical domain than the oldest respondents (OR 0.48, CI 0.34–0.67). Married Wrocław residents with a college education were more than twice (OR 2.04, CI 1.59–2.61) more likely to have a high versus low assessment of health-related quality of life in the physical domain than those with a primary education. On the other hand, among the single respondents, the conditional probability of a high versus low health-related quality of life was lower among those with a secondary education compared to those with a primary education (OR 0.66, CI 0.51–0.87). Health-related quality of life in the physical domain was further associated with occupational status, but only in married respondents. The conditional probability of high versus low health-related quality of life was more than seven times higher in white-collar workers (OR 7.13), blue-collar workers more than five times higher (OR 5.07), while entrepreneurs and students were more than four times more likely to have high versus low scores (OR 4.25 and OR 4.03), compared to the unemployed. The odds of high versus low health-related quality of life in the physical domain were higher in those with a steady source of income compared to those without it (OR 1.57, CI 1.2–2.04 in single respondents; OR 6.12, CI 4.19–8.93 in married respondents). The differences between the odds ratio values in both groups of respondents were statistically significant (p ≤ 0.05). The odds of high versus low health-related quality of life in the physical domain were more than four times higher (OR 4.05, CI 3.13–5.25) in the highest earning married individuals than in the lowest earning respondents. Higher odds of having a high versus low health-related quality of life, compared with the lowest earners, were also reported by single respondents with per capita incomes between USD 260 and USD 400 (OR 1.43, CI 1.05–1.95). In both study groups, the conditional probability of high versus low and average versus low health-related quality of life scores in the physical domain was furthermore higher in those with savings than those without, and in those not in debt than those in debt (Table 4 ).
The women, irrespective of their marital status, were characterized by a lower rating of health-related quality of life in the psychological domain than men. Among the married men from Wrocław, the odds of high versus low health-related quality of life in the psychological domain was higher in those aged 35–44 (OR 2.24, CI 1.78–2.83) and under 35 (OR 1.82, CI 1.39–2.38), compared to individuals aged 45 and over. In both single and married respondents, the odds of high versus low and average versus low health-related quality of life in the psychological domain increased with the level of education. Moreover, compared to the unemployed, the highest odds of high versus low rating of this domain of health-related quality of life were found among entrepreneurs (single: OR 3.01; married: OR 9.83), white-collar workers (single: OR 1.56; married: OR 7.67) and students (single: OR 1.50; married: OR 4.41). Statistically significantly higher odds ratio values (p ≤ 0.05) were noted among married persons as compared to single persons. In both groups of respondents, the conditional probability of high vs. low and average vs. low scores for health-related quality of life in the psychological domain was higher in people with a steady income compared to those without it; those with savings vs. those without savings; and those without debt vs. those with debt. The odds of high versus low and average versus low quality of life in this domain also increased with the rise in per capita household income (Table 4 ).
Among respondents in the youngest age group, the odds of a high versus low and average versus low assessment of health-related quality of life in the social domain were higher than those in the oldest age group. Regardless of respondents' marital status, the conditional probability of a high versus low and average versus low rating of health-related quality of life in the social domain was higher for those with a university education compared to those with a primary education. Among student respondents, regardless of their marital status, there was a higher conditional probability of high versus low rating of health-related quality of life in the social domain, compared to those who were unemployed (OR 1.66, CI 1.14–2.43 in single respondents and OR 5.21, CI 2.91–9.31 in married respondents). In addition, among the married respondents, statistically significantly higher odds of high versus low scores were observed in entrepreneurs (OR 4.75, CI 3.20–7.04), white-collar workers (OR 2.67, CI 1.89–3.77), and laborers (OR 1.97, CI 1.37–2.84) compared to the unemployed. Among the married respondents, having a steady income was also associated with higher odds of high versus low (OR 6.12, CI 4.19–8.93) and average versus low (OR 1.84, CI 1.44–2.36) health-related quality of life scores in the social domain compared to those who did not have one. These odds also increased with the rise in per capita income in the households of married respondents. The odds of high versus low health-related quality of life scores in the social domain were higher in those with savings (single: OR 1.53, CI 1.2–1.94; married: OR 4.74, CI 3.74–6.01), compared to those without savings. Statistically significantly higher odds ratio values were reported by married respondents compared to single respondents (p ≤ 0.05). The odds of high versus low and average versus low health-related quality of life in the social domain were also, regardless of marital status, lower in people with debt compared to those without debt (Table 5 ).
Female sex was related to lower odds of high versus low and average versus low of health-related quality of life in the environmental domain in all respondents, regardless of their marital status. The conditional probability of high versus low rating of health-related quality of life in the environmental domain was higher among respondents from both marital status groups in those aged up to 44 years compared to those aged 45 years and older. Among single and married respondents, the odds of high versus low and average versus low ratings of health-related quality of life in the environmental domain also increased with an education level. Among the respondents, quality of life in the environmental domain was also significantly modified by their occupational status. Entrepreneurs (single: OR 10.93, CI 5.64–21.16; married: OR 8.81, CI 6.11–12.69), students (single: OR 5.69, CI 3.56–9.09; married: OR 6.86, CI 3.75–12.55) and white-collar workers (single: OR 4.35, CI 2.70–7.02; married: OR 4.43, CI 3.16–6.21). The odds of high versus low health-related quality of life scores in the environmental domain was almost twofold in the group of single respondents (OR 1.96, CI 1.50–2.56) and threefold in the group of married respondents (OR 2.86, CI 2.10–3.89), and higher in those with a steady source of income compared to those without it. The odds also increased with per capita income, regardless of respondents' marital status. The single respondents with savings, compared to those without, were also nearly four times as likely to report high versus low health-related quality of life in the environmental domain (OR 3.99, CI 3.10–5.13) and nine times as likely to report high versus low health-related quality of life if they were married (OR 8.93, CI 7.08–11.25). These odds were also nearly four times lower in the single respondents in debt (OR 0.26) and more than two times lower in married respondents in debt (OR 0.46), compared to the non-indebted respondents. The effect of economic factors on the assessment of health-related quality of life in the environmental domain was significantly (p ≤ 0.05) greater in the married respondents than in the single respondents (Table 5 ).
The study attempted to address three main research questions. The first concerned the assessment of quality of life of single respondents as compared to married respondents. The study results revealed higher assessment levels of perceived health condition and quality of life in the social domain among the single adults. However, in the case of other quality of life correlates, there were no significant differences between the single and married respondents.
The results of previous studies of relationships of quality of life with marital status have been inconclusive. Wang et al. [ 33 ] reported higher overall quality of life, perceived health condition, and health-related quality of life assessments in married Chinese men aged 28–65 years, compared to single men. On the other hand, Kim and Kim [ 7 ] found higher quality of life levels in married mothers than in single mothers. Kowalska et al. [ 8 ] in her analysis of health-related quality of life in the environmental domain in adults from the Upper Silesia region of Poland observed that married respondents were characterized, on average, by a higher assessment of this domain, than single respondents. Also Wahl et al. [ 6 ] and Sarla et al. [ 11 ] noted a positive effect of relationships on quality of life. Researchers emphasize, however, that it is not so much the fact of mere being in a relationship that is important in the context of modeling quality of life, but its quality, such as the strength of the relationship or the possibility of receiving necessary support. The indicated attributes of relationship quality can positively affect health and well-being, moderate the negative effects of stress, and be strong determinants of mental health improvement and happy living [ 34 , 35 ]. However, some studies [ 36 ] also suggest that social relationships may negatively affect individuals because they may generate pressure, conflict, frustration, or ineffective support. It should also be emphasized that high quality of interpersonal relationships and the possibility of social support do not have to be experienced only by married individuals, but may also apply to those who are single and have good relationships with family, friends or acquaintances. Similarly, authors of some previous studies [ 14 , 15 ] reported higher overall quality of life assessments among single individuals than married individuals. Moreover, higher health-related quality of life in the physical and environmental domains in single respondents from Turkey, compared to married respondents, was reported by Nayir et al. [ 16 ]. On the other hand, Raymakers et al. [ 17 ] found no significant associations of quality of life with marital status. The issue of the relationship between quality of life assessment and marital status, therefore, remains open.
The second research problem addressed in the study was the relationship of quality of life of single people with their socioeconomic status. Respondents from the working-age population of Wrocław indicated that such factors as sex, level of education, occupational status, and financial situation significantly affect their quality of life.
The present study has revealed that women exhibited lower quality of life scores than men. The available literature tends to confirm this observation. Higher quality of life scores for men, compared to women, were also observed in Iranian [ 14 ], South Korean [ 37 , 38 ], and Croatian [ 39 ] populations. In addition, Nayir et al. [ 16 ] reported higher health-related quality of life levels in the physical, psychological, social, and environmental domains among Turkish men compared to women. Aghamolaei et al. [ 40 ], on the other hand, after considering the potential impact of other socio-demographic factors, demonstrated that female sex may be independently associated with lower health-related quality of life in the physical and psychological domains. Kowalska et al. [ 8 ] also noted a similar effect with respect to health-related quality of life in the environmental domain. Only Wahl et al. [ 6 ] in their study on adult residents of Norway observed that women rated their quality of life higher than men.
In the case of the Wrocław respondents, positive correlations between quality of life and level of education were also noted. Similar consistencies were also reported by Wang et al. [ 33 ], Rezaei et al. [ 15 ], Wahl et al. [ 6 ], Han et al. [ 41 ], Song et al. [ 38 ] and Emrani et al. [ 14 ]. Nayir et al. [ 16 ] reported an increase in health-related quality of life in the physical, psychological, social, and environmental domains as the level of education rose. A similar observation regarding health-related quality of life in the environmental domain was made by Kowalska et al. [ 8 ]. The reason for the noted patterns may be, on average, higher health awareness in better educated individuals, which has been empirically documented [ 42 ]. Well-educated people are more likely than less-educated people to implement the so-called pro-healthy lifestyle by undertaking physical activity, eating rationally, or avoiding risky health behaviors [ 43 ]. Higher levels of optimism were also found in well-educated individuals [ 44 ], which may again be important for quality of life evaluation.
The quality of life of working-age respondents was most strongly influenced by their occupational status. Entrepreneurs, students and white-collar workers rated their quality of life as the highest, while the unemployed assessed it the lowest. Some previous studies also indicated a high quality of life for entrepreneurs compared to representatives of other occupational groups [ 45 , 46 , 47 ]. The main reasons for the entrepreneurs' high quality of life include good material situation [ 48 ], opportunity for development [ 46 ] and financial independence [ 49 ]. On the other hand, higher values of the health-related quality of life in the environmental domain in white-collar workers, than in blue-collar workers, were reported by Kowalska et al. [ 8 ]. In a study of an Iranian population, Emrani et al. [ 14 ] found significantly higher quality of life levels in students and employed people, compared to the unemployed and housewives. Higher health-related quality of life in the physical and psychological domains among the employed Turks, compared to the unemployed, was also observed by Nayir et al. [ 16 ]. Also, Wahl et al. [ 6 ] noticed the lowest quality of life measures in unemployed respondents. In addition, Norstrom et al. [ 50 ] documented a deterioration in the quality of life and perceived health condition of jobless Swedish adults.
The results of the present study also reveal a positive relationship between quality of life and financial situation. Similarly, in the study by Wang et al. [ 33 ], the respondents' quality of life was significantly and positively associated with the level of annual income. An increase in the values of health-related quality of life with the improvement of material situation, mainly the income level, was also reported by Nayir et al. [ 16 ], Kulik et al. [ 51 ], Rėklaitienė et al. [ 52 ], Kooi-Yau Chean et al. [ 53 ], Povey et al. [ 54 ], Han et al. [ 41 ], Huang et al. [ 55 ] and Song et al. [ 38 ]. The highest quality of life assessment in people with the highest income was found by Rezaei et al. [ 17 ], while the lowest quality of life assessment in people with the lowest income by Zhang et al. [ 56 ]. Yasartürk et al. [ 57 ] reported positive correlations between quality of life and leisure satisfaction and personal and family income in college students. Whereas the significant relevance of financial security, including family income and medical insurance coverage, to health-related quality of life was also confirmed by Chiu and Yang [ 58 ]. The study of the Greek population by Kokaliari [ 59 ] provides interesting insights. The author showed that for modeling the quality of life, not only the household material situation is important, but also the state of the national economy. He also found that the quality of life of people deteriorates significantly, for example, due to the incidence of economic crises. This is supported by Wong [ 60 ] who found that the economic deterioration of a country and the stress associated with it are almost always associated with lower quality of life and sometimes even with an increased mortality rate in citizens. Some authors also indicate particularly strong, positive relationships between quality of life and income situation in countries with a medium level of economic development, which also includes Poland. Frijters et al. [ 61 ] demonstrated, for example, that higher real household income levels resulted in a significant increase in life satisfaction among the residents of East Germany following reunification.
The third research problem explored in this study was whether socioeconomic modifiers of quality of life were similar in single and married respondents. Among the Wrocław residents of working age, the relationships between quality of life and socio-economic factors were similar among people of different marital status, but the strength of their impact was higher in married than in single respondents. The differences were particularly significant with respect to education, occupational status, and financial situation. This research problem has not been addressed in previous studies. However, Asakawa et al. [ 62 ] in their analysis of a Canadian population noted that socioeconomic variables such as education and material status were important predictors of quality of life scores in working-age individuals. However, at later ages, lifestyle factors become more important than socioeconomic status in older adults. This observation confirms the relationships between quality of life and socioeconomic factors in working-age individuals found in the present study. It can also be assumed the lifestyle played a more important role in shaping the quality of life in single people than any other socioeconomic factors. For example, the lack of a steady source of income does not have to have such a strong negative impact on quality of life as it does in the case of people with families with children. In addition, single persons have more leisure time on average than those in relationships. They can also allocate some of this surplus leisure time for health behaviors such as undertaking physical activity, following a rational and balanced diet, or for preventive health care. Higher levels of health behaviors in single individuals compared to those in relationships have already been documented [ 63 ]. Engaging in such health behaviors may directly and positively affect one's health status and, consequently, quality of life, which was reflected in the present study. In addition, the unmarried respondents from Wrocław were also on average younger than those in relationships, which may also have some relevance to their health status and quality of life, since, for example, the level of physical activity often decreases with age [ 43 ].
Study strengths and limitations
The article has its strengths and limitations. One strength is the assessment of socioeconomic modifiers of quality of life, conducted separately for single and married individuals. This is particularly important as the study reports significant differences in the strength of the modeling of quality of life by socioeconomic factors in both groups of respondents. It is also noteworthy that the survey was conducted among healthy individuals of working age, as previous studies have focused on the elderly and sick. The study also includes a research sample representative for the population of the Wrocław metropolitan area. Potential modifiers of quality of life such as having a steady source of income, income per capita, savings or debt have not been considered so far. The limitation of the study is its spatial scope as it was confined to one city only. This makes it impossible to apply the results of the study to the general population. Future research should consider a research group representative for Poland as a whole and even for other Central and Eastern European countries. Cross-sectional surveys should also be replaced by more continuous surveys, e.g. cohort-based, in order to take into account the effects of changes in marital status on quality of life. The dichotomous division of respondents into single and married ones is another limitation. Future research should consider subcategories of single respondents, i.e.: unmarried, widows and widowers, divorced, as well as of married respondents, e.g. married and in partnerships. An interesting research question in the context of the quality of life of single people may also be whether living alone is the result of a conscious, voluntary choice or results from the life situation. Similarly, with regard to people in relationships, future research should consider an assessment of the quality of those relationships.
The findings of the study permit the formulation of three research conclusions:
Among the Wrocław respondents of working age, the single adults on average rated their health status and quality of life in the social domain higher than married adults.
Male gender, higher education, being an entrepreneur, student, or white-collar worker, and good financial situation were associated with the highest ratings of quality of life among single respondents.
The directions of quality of life modeled by socioeconomic status were similar in both groups of respondents, but the strength of the socioeconomic status impact was greater in married individuals than in single individuals.
The findings of the study have some practical implications. First, despite the respondents' generally good assessment of their quality of life, about one-third of the single and almost half of the married respondents rated their health poorly. It is therefore appropriate to recommend targeting respondents from both groups with public health programs aimed at improving their lifestyles. In addition, these programs should be tailored to the needs of their recipients, as, for example, the preferred forms of physical activity by single and married individuals may differ. The second practical implication to be formulated in response to the research findings concerns the respondents’ socio-economic determinants of quality of life. It is postulated that economic and social policies aimed at improving the educational level and material condition of society may have a role in modeling the quality of life of respondents, particularly strong in the case of those in married relationships. However, the implementation of such policies is often associated with the problem of coordination of activities aimed at quality of life improvement carried out by various bodies, such as state administration, local government, or businesses. In this context, it is important to integrate these activities and support them in accordance with the principles of public management, following the conclusions of quality of life diagnosis. This is the rationale for the third practical recommendation, since the results of the present study also confirm a need for more detailed assessment of different life situations of single and married adults. In order to improve their quality of life, further diagnostic research is essential, which can be used in social policy programmes covering differences between single and married adults in terms of quality of life domains but also behavioural problems (e.g. the impact of extra responsibilities resulting from raising children or caring for the elderly) and the effects of socioeconomic variables, health status, disability, and employment on quality of life. Further in-depth research on how quality of life is affected by the pandemic situation is also important. In this area, research findings regarding quality of life in the workplace (as this topic has been studied mainly in terms of membership in an organization) and quality of life in relation to interpersonal relationships are crucial for social policy programs.
Availability of data and materials
The datasets used and/or analysed in the current study are available from the corresponding author on reasonable request.
Change history
03 may 2022.
A Correction to this paper has been published: https://doi.org/10.1186/s12955-022-01984-0
Abbreviations
The World Health Organization Quality of Life
Number of private households by household composition, number of children and age of youngest child. Luxembourg: Eurostat; 2019.
Dąbrowska A, Janoś-Kresło M, Lubowiecki-Vikuk A, Słaby T. Consumer behavior of singles in Poland. Warsaw: Warsaw School of Economics; 2018.
Google Scholar
Tur-Sinai A, Magnezi R, Grinvald-Fogel H. Assessing the determinants of healthcare expenditures in single-person households. Isr J Health Policy Res. 2018;7:48.
Article PubMed PubMed Central Google Scholar
Kim S, Lee K, Lee Y. Selection attributes of home meal replacement by food-related lifestyles of single-person households in South Korea. Food Qual Prefer. 2018;66:44–51.
Article Google Scholar
Young M, Lachapelle U. Transportation behaviours of the growing Canadian single-person households. Transp Policy. 2017;57:41–50.
Wahl A, Rustoen T, Hanestad B, Lerdal A, Moum T. Quality of life in the general Norwegian population, measured by the Quality of Life Scale (QOLS-N). Qual Life Res. 2004;13(3):1001–9.
Article PubMed Google Scholar
Kim G, Kim E. Factors affecting the quality of life of single mothers compared to married mothers. BMC Psychiatry. 2020;20:169.
Kowalska M, Danso F, Humeniuk M, Kulak E, Arasiewicz H. Determinants of environmental domain of quality of life in economically active population living in Silesian Agglomeration, Poland. Int J Occup Med Environ Health. 2013;26(1):132–43.
Bilotta C, Bowling A, Nicolini P, Case A, Vergani C. Quality of life in older outpatients living alone in the community in Italy. Health Soc Care Community. 2012;20(1):32–41.
Harada K, Masumoto K, Katagiri K, Fukuzawa A, Chogahara M, Kondo N, Okada S. Frequency of going outdoors and health-related quality of life among older adults: Examining the moderating role of living alone and employment status. Geriatr Gerontol Int. 2018;18(4):640–7.
Sarla E, Lambrinou E, Galanis P, Kalokairinou A, Sourtzi P. Factors that influence the relationship between social support and health-related quality of life of older people living in the community. Gerontol Geriatr Med. 2020;6:2333721420911474.
Kement M, Gezen C, Aydin H, Haksal M, Can U, Aksakal N, Ocel M. Descriptive survey study to evaluate the relationship between socio-demographic factors and quality of life in patients with a permanent colostomy. Ostomy Wound Manag. 2012;60(10):18–23.
Rastenyte D, Kranciukaite-Butylkiniene D, Baceviciene M, Luksiene D, Kranciukas R. The relationship of quality of life with social and demographic factors among stroke survivors. Med Lith. 2010;46(12):851–8.
Emrani Z, Sari A, Zeraati H, Olyaeemanesh A, Daroudi R. Health-related quality of life measured using the EQ-5D-5 L: population norms for the capital of Iran. Health Qual Life Outcomes. 2020;18(1):e108.
Rezaei S, Hajizadeh M, Kazemi A, Khosravipour M, Khosravi F, Rezaeian S. Determinants of health-related quality of life in Iranian adults: evidence from a cross-sectional study. Epidemiol Health. 2017;39:e2017038.
Nayir T, Uskun E, Yurekli M, Devran H, Celik A, Okyay R. Does body image affect quality of life?: A population based study. PLoS ONE. 2016;11(9):e0163290.
Raymakers A, Gillespie P, O’Hara M, Griffi D, Dinneen S. Factors influencing health-related quality of life in patients with Type 1 diabetes. Health Qual Life Outcomes. 2018;16:27.
Article CAS PubMed PubMed Central Google Scholar
Lim T. The rise of single-person households and the macroeconomic consequences. Hitotsubashi J Econ. 2019;60:189–98.
Bolton K, Kremer P, Rossthorn N, Moodie M, Gibbs L, Waters E, Swinburn B, de Silva A. The effect of gender and age on the association between weight status and health-related quality of life in Australian adolescents. BMC Public Health. 2014;14:898.
Midttun N, Gostautas A, Zekas R. Age dependent changes in self reported health, quality of life and health related behaviour in Lithuania. Psychol Health. 2013;28:223.
Chen Y, Hicks A, While A. Validity and reliability of the modified Chinese version of the Older People’s Quality of Life Questionnaire (OPQOL) in older people living alone in China. Int J Older People Nurs. 2014;9(4):306–16.
Liu N, Zeng L, Li Z, Wang J. Health-related quality of life and long-term care needs among elderly individuals living alone: a cross-sectional study in rural areas of Shaanxi Province. China BMC Public Health. 2013;13:313.
Nowak P. Regional variety in quality of life in Poland. Oecon Copernic. 2018;9(3):381–401.
Instructions on methodology for the 2011 National Population and Housing Census. Warsaw: GUS; 2011.
Wayne WD. Biostatistics: a foundation for analysis in the health sciences. 7th ed. Hoboken: Wiley; 1999.
WHOQOL-BREF: introduction, administration, scoring and generic version of the assessment: field trial version. Geneva: World Health Organization; 1996.
Agresti A. An introduction to categorical data analysis. Hoboken: Wiley; 2018.
Jaracz K, Kalfoss M, Górna K, Baczyk G. Quality of life in Polish respondents: psychometric properties of the Polish WHOQOL-Bref. Scand J Caring Sci. 2006;20(3):251–60.
Kowalska M, Skrzypek M, Danso F, Humeniuk M. Assessment of reliability of the WHOQOL-BREF questionnaire in a study of quality of life among adults, the economically active population of the Silesian Agglomeration. Przegl Epidemiol. 2012;66:531–7.
PubMed Google Scholar
Frątczak E. Zaawansowane metody analizy statystycznych (Advanced methods of statistical analysis). Warsaw: Warsaw School of Economics; 2012.
Hosmer D, Lemeshow S, Sturdivant R. Applied logistic regression. Hoboken: Wiley; 2013.
Book Google Scholar
Stanisz A. Modele regresji logistycznej. Zastosowanie w medycynie, naukach przyrodniczych i społecznych (Logistic regression models. Applications in medicine, life and social sciences). Kraków: StatSoft Polska; 2016.
Wang S, Yang X, Attané I. Social support networks and quality of life of rural men in a context of marriage squeeze in China. Am J Mens Health. 2018;12(4):706–19.
Kroenke C, Kwan M, Neugut A, Ergas I, Wright J, Caan B, Kushi L. Social networks, social support mechanisms, and quality of life after breast cancer diagnosis. Breast Cancer Res Treat. 2013;139(2):515–27.
Li J. Social support and quality of life of the elderly in China. J Popul Res. 2007;31(3):50–60.
Helgeson V. Social support and quality of life. Qual Life Res. 2003;12(1):25–31.
Noh J, Kim J, Park J, Kim H, Kwon Y. Gender difference in relationship between health-related quality of life and work status. PLoS ONE. 2015;10(12):e0143579.
Song H, Park S, Kwon J. Quality of life of middle-aged adults in single households in South Korea. Qual Life Res. 2018;27(8):2117–25.
Samardzic S, Marvinac G. Health related quality of life of smokers in Croatia. Coll Antropol. 2009;33(1):107–14.
Aghamolaei T, Tavafian S, Zare S. Determinants of health related quality of life on people living in Bandar Abbas. Iran Iran J Public Health. 2011;40(3):128–35.
CAS PubMed Google Scholar
Han K, Park E, Kim J, Kim S, Park S. Is marital status associated with quality of life? Health Qual Life Outcomes. 2014;12:e109.
Tanvir S, Sajjad S, Roshan R. Awareness levels of prevention of cardiac diseases in general population of Rawalpindi and requirement of health education. Public Health. 2018;57(1):107–10.
Puciato D. Socioeconomic determinants of physical activity among manual workers from Wroclaw. Appl Econ Lett. 2016;23(12):831–4.
Krypel M, Henderson-King D. Stress, coping styles, and optimism: are they related to meaning of education in students’ lives? Soc Psychol Educ. 2010;13(3):409–24.
Litvin A, Petrascu S. Improvement of the investment climate as a main condition for the enhancement of the quality of life in rural areas. Sci Proc Manag Econ Eng Agric Rural Dev. 2016;16(4):205–11.
Woodside A, Megehee C, Isaksson L, Ferguson L. Consequences of national cultures and motivations on entrepreneurship, innovation, ethical behavior, and quality-of-life. J Bus Ind Mark. 2020;35(1):40–60.
Vargas-Hernández J. Women entrepreneurship to improve the quality of life through best practices in rural microbusiness. Econ Mark Commun Rev. 2016;6(1):48–65.
Zbierowski P. Well-being of entrepreneurs—international comparison based on gem data. J Posit Manag. 2014;5(4):89–100.
Charles-Leija H, Rodríguez R, Ramírez A, Aguirre J. Entrepreneurs in Mexico, an approach to their quality of life. Dimensión Empresarial. 2019;17(3):29–39.
Norstrom F, Waenerlund A, Lindholm L, Nygren R, Sahlen K, Brydsten A. Does unemployment contribute to poorer health-related quality of life among Swedish adults? BMC Public Health. 2019;19:e457.
Kulik A, Neyaskina Y, Frizen M, Shiryaeva O, Surikova Y. Unique nature of the quality of life in the context of extreme climatic, geographical and specific socio-cultural living conditions. Int J Environ Sci Educ. 2016;11(14):6581–91.
Rėklaitienė R, Bacevičienė M, Andrijauskas K. Quality of life in Lithuanian population: the impact of country residence and socio-economic status. Cent Eur J Med. 2009;4(4):476–82.
Kooi-Yau C, Surajudeen A, Mei-Wai C, Kean-Chye T. Comparative study of respiratory quality of life among firefighters, traffic police and other occupations in Malaysia. Int J Occup Environ Med. 2019;10(4):203–15.
Povey J, Boreham P, Tomaszewski W. The development of a new multi-faceted model of social wellbeing: does income level make a difference? J Sociol. 2016;52(2):155–72.
Huang W, Yu H, Liu C, Liu G, Wu Q, Zhou J, Zhang X, Zhao X, Shi L, Xu X. Assessing health-related quality of life of Chinese adults in Heilongjiang using EQ-5D-3L. Int J Environ Res Public Health. 2017;14:224.
Article PubMed Central Google Scholar
Zhang Y, Ou F, Gao S, Gao Q, Hu L, Liu Y. Effect of low income on health-related quality of life: A cross-sectional study in Northeast China. Asia Pac J Public Health. 2015;27(2):1013–25.
Yasartürk F, Akyüz H, Gönülates S. The investigation of the relationship between university students’ levels of life quality and leisure satisfaction. Univers J Educ Res. 2019;7(3):739–45.
Chiu S, Yang Z. Influence of family income and medical insurance coverage on health-related quality of life and optimism in cancer patients at a Hong Kong private hospital: A cross-sectional study. Psychooncology. 2019;28(10):1971–7.
Kokaliari E. Quality of life, anxiety, depression, and stress among adults in Greece following the global financial crisis. Int Soc Work. 2016;28:1–15.
Wong H. The quality of life of Hong Kong’s poor households in the 1990s: Levels of expenditure, income security and poverty. Soc Indic Res. 2005;71(1–3):411.
Frijters P, Haisken-DeNew J, Shields M. Money does matter! Evidence from increasing real income and life satisfaction in East Germany following reunification. Am Econ Rev. 2004;94(3):730–40.
Asakawa K, Senthilselvan A, Feeny D, Johnson J, Rolfson D. Trajectories of health-related quality of life differ by age among adults: results from an eight-year longitudinal study. J Health Econ. 2012;31(1):207–18.
Drygas W, Saklak W, Kwasniewska M, Bandosz P, Rutkowski M, Bielecki W, Rebowska E, Prusik K, Zdrojewski T. Epidemiology of physical activity in adult Polish population in the second decade of the 21st century. Results of the NATPOL 2011 study. Int J Occup Med Environ Health. 2013;26(6):846–55.
Download references
Acknowledgements
Not applicable.
The project is financed by the Ministry of Science and Higher Education in Poland under the programme "Regional Initiative of Excellence" 2019–2022 project number 015/RID/2018/19 total funding amount 10 721 040,00 PLN.
Author information
Authors and affiliations.
Faculty of Finance and Management, WSB University in Wrocław, ul. Fabryczna 29-31, 53-609, Wrocław, Poland
Daniel Puciato
Institute of Sport Sciences, The Jerzy Kukuczka Academy of Physical Education, ul. Mikołowska 72A, 40-065, Katowice, Poland
Michał Rozpara
Faculty of Management and Social Communication, Jagiellonian University, ul. prof. St. Łojasiewicza 4, 30-348, Kraków, Poland
Marek Bugdol
Faculty of Business and Management, Wroclaw University of Economics and Business, ul. Komandorska 118-120, 53-345, Wrocław, Poland
Barbara Mróz-Gorgoń
You can also search for this author in PubMed Google Scholar
Contributions
Study design and conduct—DP, statistical analysis—MR, literature analysis—MB, BGM. All authors contributed to data interpretation and editing of the manuscript. All authors read and approved the final manuscript.
Corresponding author
Correspondence to Daniel Puciato .
Ethics declarations
Ethics approval and consent to participate.
No person-identifying information was collected. All respondents were informed about the purpose and course of the survey and about their voluntary participation. They were asked to provide their informed consent to participate. The research project had been given a positive opinion by the Commission of Bioethics of the University School of Physical Education in Wrocław.
Consent for publication
Competing interests.
The authors declare that they have no competing interests.
Additional information
Publisher's note.
Springer Nature remains neutral with regard to jurisdictional claims in published maps and institutional affiliations.
Rights and permissions
Open Access This article is licensed under a Creative Commons Attribution 4.0 International License, which permits use, sharing, adaptation, distribution and reproduction in any medium or format, as long as you give appropriate credit to the original author(s) and the source, provide a link to the Creative Commons licence, and indicate if changes were made. The images or other third party material in this article are included in the article's Creative Commons licence, unless indicated otherwise in a credit line to the material. If material is not included in the article's Creative Commons licence and your intended use is not permitted by statutory regulation or exceeds the permitted use, you will need to obtain permission directly from the copyright holder. To view a copy of this licence, visit http://creativecommons.org/licenses/by/4.0/ . The Creative Commons Public Domain Dedication waiver ( http://creativecommons.org/publicdomain/zero/1.0/ ) applies to the data made available in this article, unless otherwise stated in a credit line to the data.
Reprints and permissions
About this article
Cite this article.
Puciato, D., Rozpara, M., Bugdol, M. et al. Socio-economic correlates of quality of life in single and married urban individuals: a Polish case study. Health Qual Life Outcomes 20 , 58 (2022). https://doi.org/10.1186/s12955-022-01966-2
Download citation
Received : 27 November 2021
Accepted : 15 March 2022
Published : 02 April 2022
DOI : https://doi.org/10.1186/s12955-022-01966-2
Share this article
Anyone you share the following link with will be able to read this content:
Sorry, a shareable link is not currently available for this article.
Provided by the Springer Nature SharedIt content-sharing initiative
- Quality of life
- Socioeconomic status
- Marital status
Health and Quality of Life Outcomes
ISSN: 1477-7525
- Submission enquiries: [email protected]
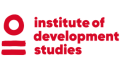
Smallholder Dairy Production and Marketing in Zimbabwe: a Socio-Economic Study of the Gokwe, Rusitu and Marirangwe Dairy Development Projects
The field study, analysis and publication of this article was made possible by generous financial and other forms of logistical support from the zimbabwe farmers union and the friedrich ebert stiftung., ids item types, copyright holder, usage metrics.
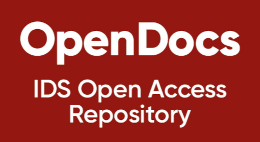
Drivers of land use land cover change and livelihood coping strategies in Sheka biosphere reserve; a case of Shato forest, Southwest Ethiopia
- Open access
- Published: 21 August 2024
- Volume 5 , article number 208 , ( 2024 )
Cite this article
You have full access to this open access article
- Workaferahu Ameneshewa 1 ,
- Yechale Kebede 1 ,
- Abiyot Legesse 2 &
- Dikaso Unbushe 3
It is evident that the means of subsistence of the community have a significant impact on the management of natural resources. This study examined the socio-economic drivers of LULCC and assessed the impacts of such changes on rural livelihoods in Shato forest, southwest Ethiopia. To map the land use and land cover, supervised classifications were used. The data were collected from 358 household heads through semi-structured questionnaires. A logistic regression model was employed to investigate the dependence of rural households on forest resources. LULC analysis results showed that about 308.29 ha of wetland and 3215.6 ha of natural forest were converted to other land use types during the last 30 years. The findings reveal that a household’s education level, household size, distance from the market, total land owned, skills and social network significantly affect their dependency on forest resources. Respondents gave high rankings to covariates such as erratic rainfall (1.70), market price (1.53), low crop output (1.28), and inadequate infrastructure (1.24). These covariates force rural communities of the study area into two major livelihood diversification strategies. These were crop and income diversification. The study comes to conclude that, the extensive and imprudent use of natural resources is a result of changes in livelihood strategies to cope up with the aforementioned shocks. Thus, the decreasing nature of forest resources results in covariate shocks in the study area and needs serious intervention mechanisms to tackle this trajectory of catastrophe.
Avoid common mistakes on your manuscript.
1 Introduction
Throughout human history, the interaction between humans and nature has been described and conceptualized in a variety of ways, with significant cultural variation continuing to this day [ 1 , 2 , 3 ]. Land-use and land-cover change (LULC) is a complex process driven by social, economic, and environmental factors [ 4 ]. These changes can have significant impacts on ecosystems, biodiversity, and human livelihoods [ 5 , 6 ].
In recent years, there has been increasing concern about the conservation of ecosystems in semiarid regions [ 7 ], which resulted in the creation of conservation units [ 8 ]. However, in some situations, the creation of reserve areas can affect the livelihoods of local populations, who must find new ways to survive [ 9 ]. In addition, when natural resources are banned from being used, those rural residents who depend on them may become more socioeconomically vulnerable, which could lead to conflicts between the environment and social institutions [ 10 ].
In developing countries like Ethiopia, where a large portion of the population relies on agriculture and natural resources for subsistence, understanding the drivers of LULC is crucial [ 11 ]. Therefore, a more thorough comprehension of the intricate relationships between changing land use and land cover, rural livelihoods, and coping mechanisms is essential for making decisions [ 12 ]. High dependence on the forest may affect the strategies that the residents execute to collect resources. In humid tropical forests, local people usually prefer to use plant species from forests near their settlements [ 13 ].
Accurate mapping and measurements of land cover conversions are necessary to quantify the dynamics of land use and land cover, and doing so also helps in understanding the contribution of terrestrial ecosystems to the global carbon pool [ 5 ]. To understand the impact of changes in land use and cover on biodiversity, fine-resolution, spatially explicit data on landscape fragmentation were required [ 14 , 15 ]. The impact of land use changes on biodiversity, the feedback on livelihood strategies from dynamics of LULC, and the susceptibility of places and people to changes in land use and cover all depend on a thorough understanding of the dynamic human–environment interactions related to land use change [ 16 , 17 ].
The multitude of issues contributing to the intricate interplay between social, political, economic, technological, and biophysical factors can contribute to LULCC [ 4 ]. As a result, extensive studies have been done to determine the factors contributing to LULCC: from land use change processes in urban areas [ 18 ], to deforestation in tropical regions [ 19 , 20 ], and to Changes in land use and expansion of agriculture in mountainous regions [ 21 , 22 ].
In developing nations like Africa, a majority of population rely on agriculture both commercial and subsistence farming and the production of charcoal to make a living [ 11 , 23 ]. The main drivers of LULC change in Ethiopia were the expansion of agriculture and settlement into forested areas, logging, the production of charcoal, and the collection of fuel wood [ 24 ]. And, the socioeconomic drivers of LULC dynamics are multi faced and intricate in space and time, demanding more investigation in Ethiopia [ 25 , 26 ]. The degree to which households rely on forests is determined by several factors, including the distance between the household's homestead and the forest patch, the distance between the household’s homestead and market, skill, income, household size, and the head of the household's educational attainment [ 27 ].
Ethiopia is among the countries characterized by diverse vegetation zones [ 28 ]. But the country’s forests are deteriorating and becoming less plentiful as a result of the increased demand for agricultural land brought on by population growth [ 29 , 30 ]. A decrease in natural vegetation, such as forests, shrub lands, and woods, has been shown in most recent studies. This drop is attributed to the conversion of forests into agricultural and grazing lands, which has released settlement areas around the nation [ 31 , 32 ].
To alleviate poverty and improve living standards, rural households in developing countries engage in a variety of activities [ 33 ]. As defined by [ 34 ], livelihood diversification: is the process by which households combine a variety of activities and social support systems to mitigate shocks and improve their welfare. It's acknowledged as a strategy for dealing with the diverse idiosyncratic shocks people face [ 33 ]. Research on livelihood diversification in the past has primarily focused on measuring the extent and drivers as well as analyzing the primary sources of income for various sets of livelihood activities [ 33 , 35 , 36 ]. Several studies have been carried out to determine the livelihood diversification activities pursued by rural communities [ 36 , 37 , 38 ]. However, more recent research has gone beyond measurement and analysis to distinguish between two main types of livelihood diversification; distressed diversification, which encourages impoverished families to fend off shocks brought on by LULC dynamics [ 34 , 37 ], activities frequently linked to gathering or making use of natural resources like wild foods, fisheries, or hunting [ 39 ]. During difficult times, people turn to low-skill nonfarm activities in an attempt to secure their financial future [ 40 ]. Progressive diversification, is usually thought of as an ex-ante strategy implemented by merely well-off households [ 41 ], also contribute to the total income of richer households [ 42 ]
Rural communities in forested regions rely on forest resources for their livelihoods, including food, medicine, and construction materials [ 43 ]. Alterations in land use patterns can disrupt these livelihood strategies by limiting access to essential resources and undermining traditional practices [ 44 ]. The influence of the community on natural resource management is displayed through their interaction with the environment which can be clear from an analysis of their livelihood. Increasing dependence on forest resources could put additional pressure on it, which would result in excessive use, and ultimately lead to deforestation and land degradation [ 45 ].
LSAI is abbreviation for large scale agricultural investment. There isn’t a single, widely recognized definition for the term “LSAI” because, it can be determined a variety of contextual factors as well as the parties’ interests [ 46 ]. In this article, we conceptualized LSAI as a commercial agricultural investment that is mechanized and undertaken on a land area larger than 200 hectares by either domestic or foreign investors [ 47 ].
In Southwestern Ethiopia, Masha district ( Shato area in particular), where this study was conducted, human-induced changes in land use/land cover are often observed [ 48 , 49 ]. Major land uses in the area included wetlands, natural forests, tea and coffee plantations, crops, and settlements [ 50 ].
The researcher was observed that, in addition to the efforts made by various researchers on the causes of LULCC that have been done in the Sheka zone; high deforestation rates of 36% for tea plantations had also been observed between the periods of 1987 and 2005 in four areas in Sheka zone [ 51 , 52 , 53 ]. There were trends of increasing tea and coffee plantations, rural settlement, and decreasing forest land in the area. The result showed that from the year 1991–2021, about 20% of natural forest in the study area was converted to other LU/LC types while plantation and rural settlement increased by 2, 234.3 ha and 1289.6 ha. Shato core area covers 5023.3 ha (25.5%) of the study area, and currently, only 3541 ha (18%) are left [ 54 ]. The major proximate drivers of LU/LCC were an expansion of subsistence and commercial agriculture and unsustainable exploitations of forest products.
The primary source of income for the local community of Masha district's are cattle production and crop farming [ 49 ]. Furthermore, non-farm pursuits like beekeeping and non-timber forest products provide additional revenue streams and means of subsistence for the locals [ 55 ]. One of the main drivers of the LULC changes in the Masha district ( Shato core area), was the expansion of large-scale commercial coffee production and tea plantations.
Analyses of the Shakichos livelihood or means of subsistence indicated how a current change in their ecological resource management contributes to environmental degradation [ 48 ]. Little is known about the impacts of changes in land use and land cover as a function of rural livelihood coping strategies in the communities found around Shato forest of Sheka biosphere reserve. This is even though the region is one of the most favorable for large-scale agriculture particularly cash crop cultivation in Ethiopia.
In addition, the livelihood of the rural population in the area around the forest depends on the forests near their homes, forest-related items, and traditional small-scale farming practices [ 56 ]. Therefore, the degradation of the natural forest of Masha woreda , in addition to its global impact has had a more prominent negative impact on the livelihoods of residents living in the area or adjacent areas [ 27 ]. However, no studies have been undertaken to explore socioeconomic drivers of LULC dynamics: identifying short-term idiosyncratic shocks induced by LULC dynamics, and analyzing rural communities’ decision to pursue different livelihood strategies in Sheka Zone, Southwest Ethiopia. The results of the research serve to foster the development of sound policies and management strategies for the sustainable use and management of the natural resources of the area [ 26 ]. Therefore, this study examined the socio-economic drivers of land use and land cover change and assesses the relationship between socio-economic drivers and rural livelihoods in Sheka Biosphere Reserve, A case of Shato forest, Southwest Ethiopia.
2 Theoretical framework
We address the study of the link between nature and society from a more balanced perspective by concentrating on “cultural-human ecology.” To do this, we utilize systems theory and ecology to offer thorough explanations of the intricate relationships that exist between individuals and their biophysical surroundings [ 57 ], and to analyze the ways in which human communities and cultures adapt to the particulars of their local ecosystem through livelihood patterns [ 58 ].
The idea of adaptation, which refers to the continuous process of adjustment humans go through to deal with both internal and external impulses, is significant in the majority of studies [ 59 ]. The basic function of adaptation is ‘to maintain a balance between population, resources and productivity’ [ 60 ]. It is acknowledged that our theoretical explanation of land-use change has certain limitations. Researchers argue, for example, that cultural-human ecology falls in explaining the social transformation processes that have an impact on the environment and in missing to consider the power dynamics that both facilitate and propel social struggles against traditional social categories [ 61 , 62 ]. Also, it does not take into consideration the class-based disparities that result in unequal choices for all members of a system, particularly those related to the environment. Additionally, the viewpoint of cultural-human ecology is “historical and does not account for the fact that decisions made in specific social systems and locational settings are the product of environmental transformations.” [ 63 ]. In the study context, the drivers of land-use change and community perceptions of land-use change are explained using the theoretical paradigm discussed above.
3 Methods and materials
3.1 description of the study area.
This study (Fig. 1 ) was conducted in four Kebeles of Masha Woreda which share a common boundary with Shato forest (core area), Sheka zone, southwest Ethiopia. It extends 7° 45′ 0″ to 7° 52′ 30″ N and 35° 25′ 0″ to 35° 35′ 0″ E. Rugged topography conditions from gentle to very steep slopes and its elevation ranging from 1639 to 2376 m. The primary source of income for about 90% of the households in the subject area is a mixed agricultural and livestock system used for subsistence.
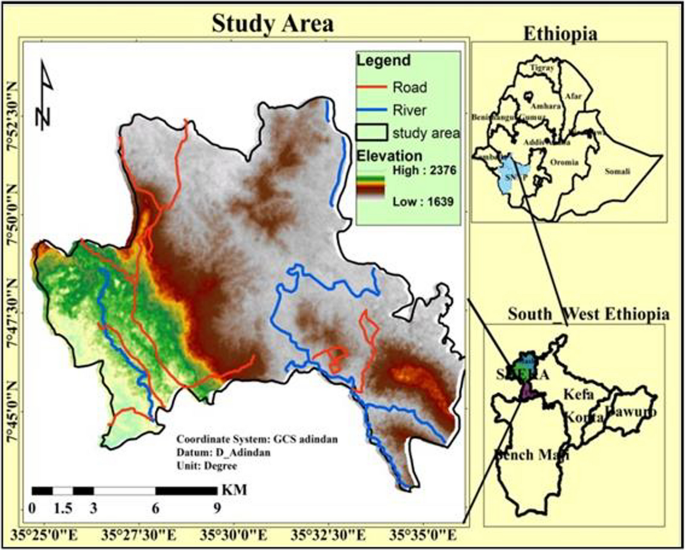
Map of the study area
3.2 Research approach and data sources
The study used an inductive approach and employed a mixed research design dominantly quantitative method supplemented by qualitative data (QUAN/Qual). And, investigated the socioeconomic drivers of LULC dynamics on the livelihoods of rural communities and the households cope up the consequence of land use/land cover change. The researcher analyzed data generated from satellite images, questionnaires, focus group discussions, participant observation, and document review. Responses by the respondents regarding the socioeconomic drivers of LULC dynamics and coping strategies were ranked using a Likert scale. The responses of respondents were coded and analyzed in SPSS V. 23. A thematic analysis was also conducted during FGDs and key informant interviews. It is led by a skilled facilitator and involves six to twelve participants who have shared characteristics relevant to the specific discussion topic [ 64 ].
Common characteristics may be related to a specific issue, occupation, age, socioeconomic class, place of residence, or experience adopting or not adopting a behavior that a project has advocated. Scholars discern recurring themes or trends within the conversations pertaining to a particular subject matter or research. This allows for a deeper understanding of the insights gained from FGDs. In order to uncover and interpret themes in qualitative data that was gathered, such as interviews, thematic analysis was also used. Data collected through FGDs and key informant interviews were qualitatively analyzed in alignment with the quantitative techniques.
3.3 Data sources
Primary and secondary data were the two major categories of data sources widely used in this study. Primary data instances were from questionnaires, focus group discussions and in-depth interviews with key informants, while secondary data were acquired from both published and unpublished materials.
3.4 Sampling procedure and sample size determination
Due to the nature of vulnerability to changes in cultural practice, livelihood system, and land use land cover change, Masha Woreda was selected purposively. This was confirmed during the field observation, and supported by a study of [ 65 , 66 ], Masha Woreda was exposed to all causes of land use land cover change ranging from forest conversion to agriculture by smallholder farmers to a large-scale coffee and tea plantations of their first kind in the country. Four kebeles ( Keja-chewaqa, Welo, Beto, and Yepho ) were selected as a sample of the study from a total of 19 kebeles, based on four reasons; its vicinity to huge investment (i.e. covering over 3000 ha) and number of Agri-investments available in the woreda (pilot survey result) , population size [ 67 ], indigenous cultural practices of forest conservation and management and high vulnerability for LULC dynamics during the last 30 years (1991–2021). Four FGDs were held having eight members each (inclusion in the group was based on role, gender, and age).
To select the sampled households, the total list of households living within the sampled kebeles was obtained from the local administration office. According to projected demographic estimates from the Sheka zone Finance and Economic Development Department (2022), the total population of sampled kebeles were 11,404 with household size of 3427. The sample size of the respondents was determined using the method developed by Yamane [ 68 ] and [ 69 ]. The formula was reasonably selected because, a population is finite and whose size can be determined [ 70 ].
The researcher employed a simple random sampling technique to reduce the risk of bias or inaccuracy within the data being collected [ 71 ].
where n is the sample size, N is the population size, e is the precision level (5–10%). The precision level in this research is 5% (0.05). The total sample size of the respondents was 358. The number of sample respondents from each kebele was selected by using proportional allocation formula [ 72 ]. By using the formula (Eq. 2 ), 119, 93, 76, and 70 respondents were selected for Welo, Keja-Chewaqa, Yepho and Beto Kebeles respectively.
ni is the sample size for Kebele I; Ni is the population size of sample Kebele I; N is the total population size of all sample kebele; n is the total sample size.
According to methodological flow chart (Fig. 2 ), landsat images of the study area from 1991, 2006, and 2021 as well as socioeconomic data from sample households were used in the research. After classifying the Landsat images, maps of land use and cover were generated and 358 sample households were selected randomly for the study. These sample respondents, along with four focus group participants, identified and ranked livelihood shocks, coping mechanisms, and socioeconomic drivers of LULCC. Lastly, a binary regression model was utilized to examine the relationship between socioeconomic factor causes LULC dynamics and the livelihoods of the rural community of the research area.
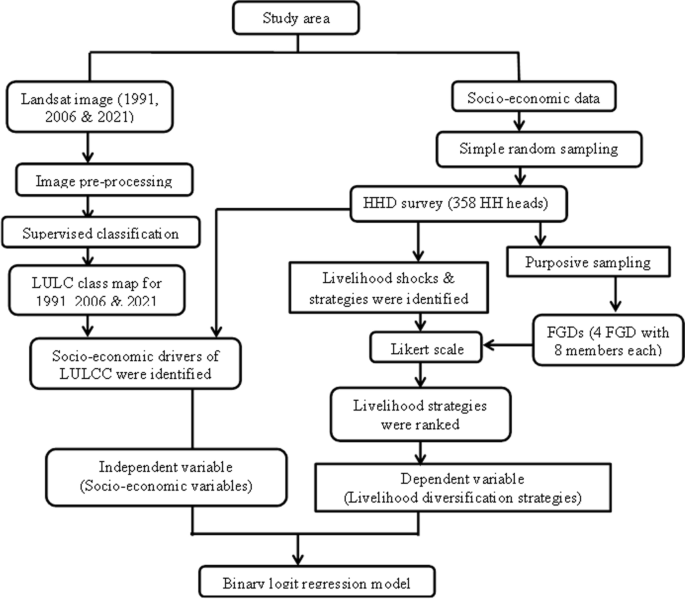
(Source: Author, 2023/24)
Methodological flow chart of drivers of LULCC and rural livelihoods
3.4.1 Method of data collection and analysis
Data for socioeconomic drivers of LULCC and livelihood coping strategies were collected through semi-structured questionnaires both open and close-ended. This was done to meet the requirements of the main drivers of land use and land cover change, socioeconomic data should be integrated [ 73 ].
The questionnaires were developed based on literature and expert discussions. By using the methods, we were able to employ cross-reference and confirm the answers, which ultimately increased the validity and consistency of the results. Questionnaires were distributed to 358 households identified through simple random sampling. Questionnaire data were processed and coded using the SPSS V.20 and subjected to further analysis. Likert scale was also used to measure (rank) the major socioeconomic drivers and shocks as a response to land use land cover dynamics. Pearson’s Chi-square analysis was used to determine associations between socioeconomic variables and diversification strategies.
A series of logistic regression was fitted to determine the effect of the predictors on the livelihood coping strategies, from which the estimated odds ratios (y) were generated. This allowed for the identification of variables that were independent predictors (socio-economic variable) for main coping strategies (dependent variable). Odds ratios were used to measure the magnitude of the strength of association between two binary data values. In this case, we quantitatively assessed the relative importance of several predictor variables. Content analysis was used to analyze qualitative data, whereby the discussions from the focus group were objectively and subjectively analyzed [ 74 ]. Based on similarities, codes are grouped into categories, which are then further examined and integrated to find overarching themes that reflect key ideas, patterns, or meanings in the data related with study objectives.
For land use land cover classification MSS Landsat imagers for the years 1991, 2006, and 2021 were used (selection of band resampling). After doing all image pre-processing steps, the researchers used maximum likelihood classification techniques in conjunction with supervised image classification using the Non-Parametric Rule. The primary benefit of supervised learning is that it enables the involvement of experts who can gather data or generate data output based on prior knowledge and who have a precise understanding of the classes present in the training data [ 75 ]. LULC classification and layout were done in ERDAS imagine V.2015 and Arc gis v10.8, respectively. Finally accuracy assessment was made to cross-check LULC classes prepared in software with spatial features found on the ground using ground truth data.
3.4.2 Measurement of variables
This section was aimed at collecting data to characterize rural livelihoods that are impacted by changes in land use and land cover in the study area. The questionnaires were pretested and revised before the actual interviews of the sampled households. The standardized questionnaire was written in English and was translated into Amharic languages and then Shekinonoo (local language) in which respondents were conversant during the interview. Respondents to the survey were not identified either by name or by location to maintain confidentiality (i.e., the researchers made respondents name and location anonymous). In each household, data on the socio-demographics, shocks, and livelihood strategies were collected using a questionnaire. The survey was administered in the period March–April and September 2023.
3.4.3 Model specification
Logistic regression has been widely used in several studies to examine the relationship between rural livelihood and forest resources dependency [ 76 , 77 , 78 ]. When analyzing dichotomous outcome variables, the binary logit has an advantage over the probit due to its great flexibility and ease of use, even though both produce equal parameter estimates [ 79 ]. Moreover, it changes the focus from forecasting probabilities inside the interval (0, 1) to predict the odds of an event occurring inside the real line [ 80 ]. Thus, a binary logit regression model was applied to show the relationship between independents (Socioeconomic variable that aggravates LULC dynamics) and outcome or dependent variables (livelihoods coping strategies of communities) of the biosphere reserve, a case of Shato forest (core area).
To identify the determinants of the rural household decision to employ various livelihood coping strategies to the changes in land use and land cover. It was assumed that a rational rural household could choose among the two mutually exclusive livelihood coping strategies that offer the maximum utility. For each of the coping options, the households were categorized as either equal to 1 if that household had opted for the livelihood coping option and 0 if that household had not opted for the livelihood coping option. Therefore, in this study, each indicator was taken as a binary outcome and used logistic regression to model several explanatory variables including (1) gender; (2) age; (3) education; (4) social network (yes;1, no;0). The age variables were categorized as follows: age: 0–29, 30–45 and above 45 [ 81 , 82 ]. And, education: illiterate (not able to read & write) and literate (able to read and write) [ 83 ]; the distance between the household and the forest in Km; 0–3; 4–8 and 9–12 and the distance between the household and market place in km; 0–3; 4–8, 9–12. The same standardizations were done for the rest continuous variables (total land owned, family size, and annual income).
In the logistic regression analyses, dummy variables were constructed for these categories with the last category used as a reference (Table 1 ). Before that, the response of the respondents was classified into dummy responses (Are you diversifying your livelihoods to cope up with shocks?), and the responses were, yes (1) and no (2). The respondents who said “yes” were further asked (i.e. which one is their major livelihood diversification strategy? The Chi-square test at a = 0.05 significance level was used to assess the goodness of fit of the models. Coping strategies such as selling fuel wood did nothing and other was not included in the logistic regression model because they were mentioned by few respondents, which made them insufficient for inclusion in the model.
The functional form of the binary logistic regression model used in the present study for estimation was specified following [ 84 ], as:
For ease of exposition, the probability that a given household will be apply livelihood diversification strategy to shocks (environmental vulnerability) is expressed as
where βo is an intercept and β 1 , β 2 …and β 11 are slopes of the equation in the model, and X 1 , X 2 … and X 11 are vectors of relevant household characteristics.
4 Results and discussion
4.1 results, 4.1.1 land use land cover dynamics.
Five major LULC categories, namely Forest, Rural settlement, settlement, wetland, and plantation were analyzed based on Landsat imagery for the years 1991, 2006, and 2021. The accuracy assessment is performed by using the random sampling method, a total of 155 points were selected from different LULC classes in ArcGIS v10.8.
The most terrible disaster that happened to natural forests in the study area was almost all deforestation occurred in and around the core area of a biosphere reserve. The following map (Fig. 4 ) shows clearly the severity of forest cover change in Shato core area. This study has quantified the dynamics of LU/LCC and its drivers in the Shato forest (core area) of the Sheka Biosphere Reserve southwestern Ethiopia. The result showed that about 20% of natural forest in the study area was converted to other LU/LC types while plantation and rural settlement increased by 2, 234.3 ha (10.2%) and 1289.6 ha (6.6%). Shato core area covers 5023.3 ha (25.5%) of the study area, and currently, only 3,541 ha (18%) are left. The rapid decline in forest resources occurred between 1991 and 2021 (Figs. 3 and 4 ).
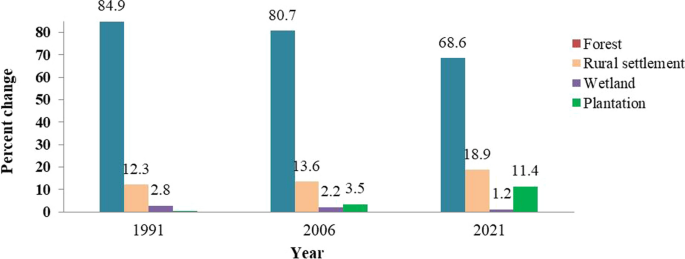
LULCC of the study area for 1991, 2006 & 2021. Time period from 1991 to 2021
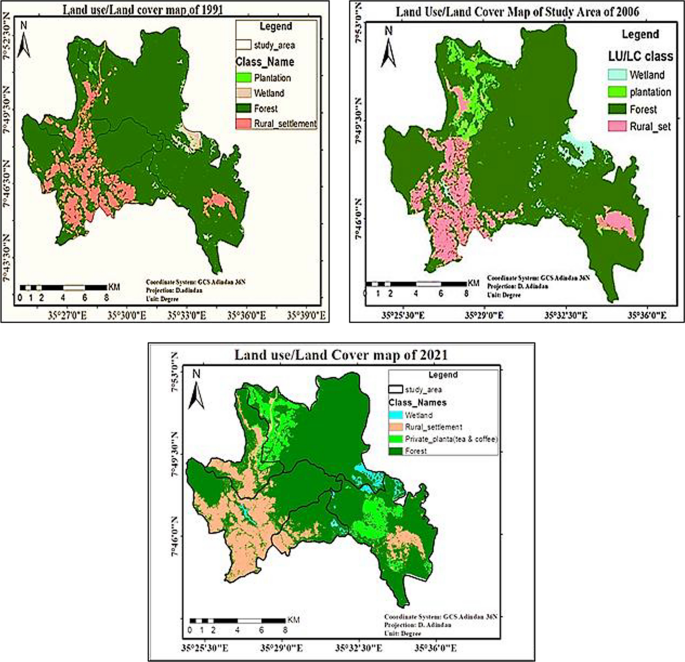
LULC map of the study area for the year 1991, 2006 & 2021. Time period from 1991 to 2021
The accuracy assessment of the finding was 74%, 81%, and 81.2%, respectively, for the images from 1991, 2006, and 2021, according to the data acquired using the random sample technique.
The producer's accuracy ranged from 60 to 91%, whereas the user's accuracy varied from 60 to 88% for all classifications. An accuracy value greater than 70% is considered to be acceptable and the Kappa value ranging from 0.40 to 0.85 represents good correspondence [ 85 ]. Because, of the quality of + ETM 1991 image and cloud coverage, the kappa coefficient result of the 1991 accuracy assessment is 0.74 (which falls in good), while MSS 2006 & 2021 accuracy assessment results are 0.81 and 0.812 respectively.
4.1.2 Demographic and socioeconomic characteristics of the respondents
The sample survey result indicates an average rural household in the study area consists of 358 households. Of the sample respondents, 12.3% (n = 44) of Household heads were female while males accounted for 87.7% (n = 314) of the sample respondents. Based on the responses of households, 69.8% (n = 250) can read and write.
However, 30.2% (n = 108) of the respondents never attended school. Almost, 78.2% (n = 280) respondents (heads of households) were between 30–45 years and the rest 21.8% (n = 78) household heads were between 0–29 and above 45 years. Most heads of households 89.7% (n = 321) reportedly use firewood for cooking and heating and the rest 10.3% (n = 37) use charcoal. The majority of the supply of fuelwood for heating and cooking was covered by females and children 80.4% (n = 288), self-15.4% (n = 55), and purchase 4.2% (n = 15). The role of heads of households 80.4% (n = 288) were farmers, 4.2% (n = 15) were engaged in fuelwood collection, 5.3% (n = 19) worked at private investment, 3.9% (n = 14) engaged in marketing products; 3.1% (n = 11) also involved in weeding & herding, and the rest 3.1% (n = 11) had involved in sowing and herding. Regarding mitigation measures for forest degradation, 59.8% (n = 214) responded that they apply customary forest management and conservation measures whereas 40.2% (n = 144) regularly plant trees. The survey result has also shown that major causes for deforestation in the study area were the need for fertile land 185(51.7%); the need for additional farmland 88 (24.6%) and illegal expansions of investment responded by 85 (23.7%) respectively.
4.1.3 Driving forces of land use/land cover dynamics
Land use and land cover change is a complex phenomenon that directly and indirectly is influenced by multiple socioeconomic and biophysical driving forces that operate over different scales. A checklist of possible drivers of LULC Changes was developed from the literature [ 86 ]. FGDs, analysis discovered that the main factors that aggravate LULCC (deforestation) were extensive agriculture, specifically the development of coffee and tea plantations in and around the core area; population growth and settlement expansion were the dominant drivers. Using the Relative Importance Index (RII) in Likert scale analysis from survey responses discovered that, from ten socioeconomic variables identified, and ranked by respondents, five major socioeconomic variables analyzed and interpreted as follows respectively: land size (0.86), family size (0.82), educational status (0.79), skills (0.70) and age (0.62) which directly or indirectly linked with the drivers of LULC dynamics in the study area (Table 2 ).
The above mean ranks were further elaborated by FGDs conducted in September 2023 at Welo and Yepho Kebele with communities; they responded that because of large-scale agricultural investment in the area, new villages were established in and around the core area, which is not recommended for agricultural activities. And, subsequently, the settlement expansion took place toward forest (induced by LSAI in the study area forces households to expand and increase the size of the land (86% of respondents listed as primary drivers). Followed by family size (82%), the increment of family size leads to the establishment of new settlements in and around forest areas, which leads to LULC change.
Educational status ranked as the third major driver with a mean rank of (0.79), majority of respondents replied that land (forest) is major source on which their livelihood depends. The more members of the household engage in formal education, the more they tend to diversify their livelihood strategy and the less the family relies on forest resources. Education can also influence the ability of households to cope with changes in land use and livelihood opportunities [ 87 , 88 ]. Skills related to forest product extraction with a particular emphasis on the widespread problem of illegal logging, beehive preparation, collecting fuelwood, and producing charcoal scored 0.70.
Besides, the area’s LULC dynamics (declining forest resources) can be attributed to several reasons, including age (ranked at 0.54), distance to market (0.53), and distance to the forest (rated at 0.50) (Table 2 ). Older age is also expected to reduce dependence on forests (that is, older adults spend less time and physical energy on forestry activities [ 89 ]. However, according to [ 83 ], the majority of household heads (respondents) in the study area found between 30–45 years (active age for agricultural activities).
For the first analysis of the relationship between dependent variables (livelihood coping strategies) and socioeconomic variables (age, educational status, family size, land size, distance from biosphere reserve, distance to market, social network, skills, land question, and annual income), correlation analysis was applied before modeling continued (Table 3 ).
From eleven socioeconomic variables, seven of them (Table 3 ) show significant correlations with dependent variables (i.e., educational status, land-holding size, family size, distance to market(km), skills, social network, and annual income) were analyzed to determine the relationship with livelihood coping strategies.
According to [ 90 ], Pearson correlation analysis can be performed to describe the relationship between the dependent variable and the independent socioeconomic variables before modeling analysis. Similarly, the Pearson correlation coefficient indicates positive and negative correlations. While a negative correlation suggests that the dependent variable decreases as the value of the independent socioeconomic variable rises, a positive correlation shows that the dependent variable (in this case, livelihood diversification) increases with the value of the independent socioeconomic variable.
The independent variable distance to market negatively correlated with the livelihood diversification strategies. The correlation result depicts that distance to market increases, the livelihood diversification strategy decreases. As furthest the market place; the less they diversify their livelihoods. According to our research, market distance has an impact on the livelihood possibilities for rural HHDs and is well substantiated by [ 91 ], but differs from the results of [ 92 ], market distance had a positive influence on the choice of non-farm activities. In our discussion with the FGDs, there is no transportation access between village and nearby market place, majority of items were transported by human power (bare foot) this impedes the frequents movement of HHDs to market place to sell their products and decrease their income source(decrease livelihood diversification). This was supported by [ 93 ], revealed that access to infrastructure would help households to diversify to more remunerative strategies. However, it was discovered that certain goods, like charcoal and chat, may have brought in more money when they were sold directly to buyers in distant marketplaces [ 94 ].
In the case of landholding size, as the number of landless respondent’s increases, the drivers of land use/land cover dynamics increase, while as the number of landless respondent’s decreases, the drivers of land use/land cover dynamics also decrease.
Shocks are an important source of the vulnerability of rural households and were reported for the previous 5 consecutive years during the study (according to ranked survey results and FGDs). In the present study, covariate shocks (exist for a short period) such as irregular rainfall (1.70), market price (1.53), low crop yield (1.28%), and poor infrastructure (1.24) were the most highly ranked shocks by respondents (Fig. 5 ).
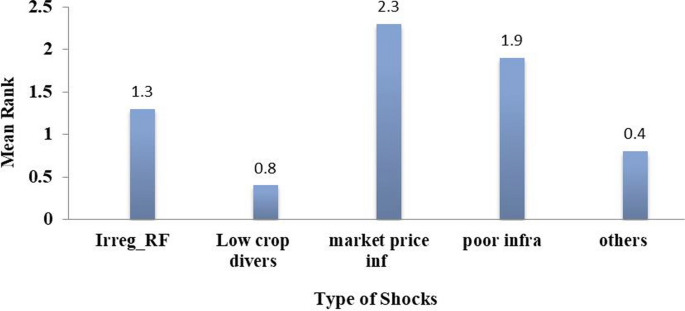
Types of shocks ranked by Community. NB: Irreg_RF: Irregular rainfall, Low crop divers: low crop diversification; market price inf: market price inflation, Poor infra: Poor infrastructure. Time period from 2019 to 2024
4.2.1 Livelihood coping strategies
Rural households in the study area engage in various livelihood coping strategies for short-term shocks. Virtually, all households rely on a combination of these livelihood coping strategies as a means of survival. Depending on the survey results obtained the prominent coping strategies were crop and income diversification. The rural communities from the study area were engaged in different livelihood coping strategies to counter the shocks they faced due to LULC changes during the study period.
The results have revealed that the most prominent coping strategies used in response to LULC change-related shocks included crop diversification (planting inset), income diversification (selling livestock, crop stock, forest products, borrowing from relatives), and others. Rural communities in the study area tend to employ the following major Livelihood coping strategies to cope up with shocks driven by socioeconomic-related LULC dynamics. Crop diversification ranks first with 0.516 followed by income diversification with a mean value of 0.41 and the rest of the respondents (0.07) did nothing in response to shocks induced by LULC dynamics.
The independent variables that explain the diversification of rural families to irregular shocks (vulnerability) were family size, age, educational status, land size, social network, distance to market, distance to forest, skills, source of income, land acquisitions, and annual income (Table 4 ). Concerning the tendency of respondents to choose a diversity of income sources, the influence of gender was significant (p\0.05).
About 29% of female respondents ranked agriculture as the priority source of income compared to their male counterparts (71%). The results further revealed that livestock rearing is an important source of income for men (71%) and less important for women (29%). In addition, 63% of women respondents considered off-farm activities as an important source of income compared to their male counterparts (37%). The dominant off-farm activities in the study area include selling charcoal & firewood, collecting honey, preparing and selling beehives, collecting traditional medicine, selling poles…etc.
Table 5 displays the correlation between the predictor variables and the outcome as well as the regression coefficients (B), Wald statistics (for assessing statistical significance), and the most significant odds ratios (Exp (B) for each variable category. The result for educational status is very significant at (Wald = 5.696, df = 2, p < 0 0.005), The variable family size has an Exp (b) of 0.621 and exp (b) of 0.621 times more likely than a households with no family. People with a large family size have a 62.1% higher chance of diversifying their livelihood to participate in LULC dynamics than households without a family.
With more family members, households may have the workforce needed to pursue diverse income-generating activities simultaneously, such as farming, off-farm employment, or small-scale businesses [ 95 ].
B (Beta) is the expected change in log chances for a unit change in the ratio between the odds (Table 5 ). The regression model considers the response of the survey result (i.e. dummy) “Is household apply livelihood coping strategy for covariates (shocks) caused by LULC dynamics in your study area?” The outcome variable of the model is categorical and takes a value equal to 1 if a rural household uses a livelihood diversification strategy, 0 if not, and is included in the model (Table 5 ). Compared to households with no education (illiterate), households having an education are significantly more likely to use crop diversification and income diversification. The output of the logistic regression model revealed that after adjusting for the effect of the factors, there is a significant influence of education and coping strategies (p\0.01) and was associated with high uncertainty (y [1]).
The age of the head of the household showed no significant influence when choosing a coping strategy (p[0.5) and had a negative impact on livelihood diversification. Compared to households with a market distance of above 3 km, households with a distance of below 3 are more likely to use both crop and income diversification.
4.2.2 Discussion
Rural communities in the study area have traditional practices and cultural ties to forests that are integral to their way of life. Changes in forest cover disrupted these practices, leading to a loss of cultural identity and traditional knowledge, which can have a profound impact on the livelihoods and well-being of local communities. A decrease in forest cover can lead to a reduction in the availability of these resources, affecting the livelihoods of communities that depend on them for their income and sustenance [ 96 ]. The remote sensing, rural household interview, and FGD results confirmed that land use and land cover changes have been ongoing during the study period, but reached a noticeable peak. The magnitude of the changes in land use and land cover reported here is likely to be influenced by the highly heterogeneous, mosaic, and complex spatio-temporal characteristics of land use and land cover of the study area.
LULC analysis results showed that about 308.29 ha (56.7%) of wetland and 3215.6 ha (19.6%) of natural forest were converted to other land use types in the last 30 years. Plantation and rural settlement increased by 2234.3 ha (10.2%) and 1289.6 ha (6.6%) respectively from 1991–2021(Fig. 4 ). While these responses do not identify specific changes in the day-to-day activities of focus group members, they point to changes in their livelihood coping strategies among their households as a result of the changes in land use and land cover. One of the female respondents said ‘‘If my community has no availability of natural resources, I will need to take up other companies to ensure my children's food and education”. The perceived expansion of forest land also confirms the findings from the analysis of satellite images. A set of predictor variables and the extent of rural household livelihood diversification were compared using the binary logistic regression model (Table 3 ). It was selected because it can be employed with mixed continuous, discrete, and dichotomous variables [ 97 ]. Rural household livelihood diversification status is a dichotomous outcome variable of the binary logistic regression model used in this analysis. It is assigned a value of 1 if a rural household has diversified its livelihood and 0 otherwise.
The likelihood ratio tests (Table 5 ) of this study measure how the log-likelihood improves if a predictor is added as an explanatory variable. Of the total 11 variables included in the study, six were significant at 5% and 10%. These results reveal that family size, education, the distance to the market in kilometers, total land, social network, and household skills are all six strongly significantly contributing to the explanation of variation in livelihood diversification strategies (**). Age is weakly significantly contributing to the explanation of the variation in livelihood diversification strategies (*).
Distance to the forest, land acquisitions, annual income, and source of income do not significantly contribute to the explanation of the variation in livelihood diversification strategies. The significant variables were education (p = 0.014, at 5% significant), family size (p = 0.035, at 5%, significant), distance from the market place in km (p = 0.025 at 5%), total land owned (p = 0.054, at 10% significant), skills (p = 0.049, 5% significant) and social network (P = 0.04, 5% significant) (Table 5 ). Even if land size and distance from the market were significant in the regression model, both have a negative relationship with outcome (dependent variables).
In this analysis, land size was found to have a negative impact on rural household livelihood diversification. Its influence on livelihood diversification was statistically significant at p < 0.05 probability level. In relation to rural households with smaller land sizes, the negative coefficient shows that rural families with relatively larger land sizes are less likely to diversify their livelihood options. Assuming all other factors remain unchanged, an increase of one unit in the land-holding sizes of rural households results in 0.841 reductions in the odds ratio favoring the diversification of rural livelihood options. The probability of a rural household pursuing varied livelihood strategies decreases as the amount of their land holding grows. This is because households with relatively larger land holdings are more willing to attempt new ventures such as extra farming [ 97 , 98 ]. This finding is in agreement with that of [ 38 , 98 , 99 , 100 ], because households cannot sustain themselves solely from agricultural produce on a smaller plot of cultivated land, they must engage in additional non-farm income-generating ventures. Diversification reduces risk by distributing it among several crops or enterprises, but it also creates uncertainty. Due to their lower risks, smaller landowners might be more open to trying out new crops or pursuits [ 101 ]. Furthermore, rather than pursuing diversification of their means of subsistence, rural families with larger land holdings are forced to pursue extensification of agriculture [ 102 ].
The variable educational status has an exp (b) of 1.72 times more likely than an uneducated person. Education people have a 72% higher chance of choosing a livelihood diversification strategy than those illiterate (income diversification). Education is regarded as one of the most important contributors to more remunerative salaried and skilled employment in rural Africa [ 103 , 104 ]. Therefore, education opens up the potential for better-paying jobs that demand formal education. According to our study, regular employment prospects that provide a steady monthly income were not regarded as major sources of livelihood. Based on the logistic regression results, the odds ratio increased for education level. This is so that people can learn how to cope and diversify their sources of income to live better lives. One explanation for this might be that the sample households' average level of education is insufficient to qualify them for formal employment. This is consistent with the findings by [ 91 ] for all of sub-Saharan Africa.
The study revealed a statistically significant and inverse relationship between the distance traveled to reach the closest marketing area and the diversification of livelihood strategies, with a significance level of less than 5%. This indicates a rural family’s chances of diversifying livelihood decreases with distance from the marketing area increase. All other variables being equal, for every one unit increase in market distance, the odds ratio in favor of diversifying rural livelihoods decreases by a factor of 0.331. The study’s findings are consistent with those of [ 36 , 105 ], who revealed that the distance traveled to reach the marketing place had an adverse impact on the state of rural households’ ability to diversify their livelihoods.
However, Age, distance from the nearest biosphere reserve, land acquisitions, and major source of income were not significant in explaining those dependent variables. Moreover, results shown in (Table 5 ) also illustrate that the independent variables of the study have a significant association with livelihood strategies. Similarly, 0.75 Pseudo R2 was formed by the model; moreover, 75% correct prediction percentage signifies the rationality of the study model’s (logistic regression model) descriptive power. The likelihood ratio test (Table 5 ), reveals that the model with explanatory variables is significantly better able to predict the variation in livelihood diversification strategy than the model without explanatory variables (intercept only, Chi-square (11 = 17.69, p = 0.049). The insignificant Pearson’s Goodness-of-Fit test means that predicted probabilities are in line with the observed probabilities, as the binomial distribution predicts. This implies a good model fit and the result of Goodness-of-Fit model of this study was good. The Hosmer and Lemeshow test statistic indicates a poor fit if the significance value is less than 0.05 [ 106 ]. In this test, the Hosmer and Lemeshow model has a good fit, as the chi-square value is 9.2, p = 0.31, which is greater than 0.05. The model states there is a relationship between socio-economic variables and livelihood diversification strategies. LULC dynamics in the study area have created socioeconomic impacts on rural livelihoods and the community tends to diversify their livelihoods to overcome short-term shocks.
In order to maximize benefits and minimize negative effects, the study result is expected to facilitate formulation of better policies, programs and intervention mechanisms for sustainable management of natural resource and sustainable livelihood options in the study area. It helps to bridge the knowledge gaps by creating awareness on the concerned to prioritize the issue of Shato forest (core area), Sheka Biosphere Reserve for intervention planning. Thus, this study undoubtedly viable to serve as source of information for further research activities wider both in scope and depth.
5 Conclusions and recommendations
Land is the major natural resource that economic, social, infrastructure, and other human activities are undertaken. Hence, changes in land use/land cover have occurred at all times in the past, are presently ongoing, and are likely to continue in the future. However, changes in the condition and composition of land use/land cover affect the livelihood of rural communities directly or indirectly. The Sheka Zone has greater potential for Large-scale agricultural investment (LSAI) and has attracted many LSAIs such as the East African Tea Plantation farm project and the Haile and Alem coffee plantation. In total, both companies have received above 5000 hectares of land in and around Shato forest of Sheka Biosphere Reserve. In Ethiopia, especially in the Southwest Ethiopia regional state, the impact of large scale agri-investment on the improvement of local people's livelihoods has not received the attention it deserves due to a lack of reliable data. Hence, the majority of the local community in the study area depends on small-scale and family agriculture for survival.
LULC analysis results showed that decrease in wetland and natural forests, and an increase in LSAI (Plantation) and rural settlement from the year 1991–2021. LSAI in general and LSAI-induced rural settlement expansions, in particular, were the trigger factors for the land use land cover dynamics in the study area. Additionally, the livelihoods of the locals have been significantly influenced by LULC dynamics, which has forced rural communities to search for various diversification options. The FGDs and survey questions responses reveal that LULC dynamics lead to the occurrence of covariate shocks (existing for a short period); such as irregular rainfall, market price, low crop yield, and poor infrastructure were the most highly ranked shocks by respondents. The socioeconomic drivers of land use and land cover dynamics in the study area were also identified by this study; the most prevalent ones were an expansion of land owned by household, a lack of formal education, and an increase in family sizes, and skills.
The binary logistic regression model examined the relationship between the livelihood diversification strategy (dependent) and the socioeconomic (independent) variables. As demonstrated by the likelihood ratio test, the model with explanatory variables considerably outperforms the model without explanatory factors in predicting the variation in livelihood diversification strategy. The model showed that the educational status of the household head, land size in the household, family size, social network, distance traveled to arrive at the nearest marketing area, and skills were among the critical factors determining rural livelihood diversification. Policymakers and stakeholders should consider these variables while designing intervention mechanisms. Only a few variables were examined in this study due to financial and time constraints; other factors, such as agricultural income, on-farm income, and off-farm income, should be taken into account in future research. In order to promote equitable development and livelihood diversification strategies, sustainable land management techniques that put the interests and rights of rural communities need to be taken as crucial agenda. Future studies ought to focus on the interaction between the agricultural value chain and the LSAI, as well as the implications of the LSAI for gender. Future studies are advised to dealt on the functioning of the biotic and ecosystem of the biosphere reserve. Our analysis was restricted to the relationship between LULC dynamics and livelihoods of the rural community.
Data availability
Data is provided within the manuscript.
Russell R, Guerry AD, Balvanera P, Gould RK, Basurto X, Chan KM, et al. Humans and nature: How knowing and experiencing nature affect well-being. Annu Rev Environ Resour. 2013;38:473–502.
Article Google Scholar
Vasseur L, Horning D, Thornbush M, Cohen-Shacham E, Andrade A, Barrow E, et al. Complex problems and unchallenged solutions: bringing ecosystem governance to the forefront of the UN sustainable development goals. Ambio. 2017;46:731–42.
Yeung HW. Rethinking relational economic geography. Trans Inst Br Geogr. 2005;30(1):37–51.
Geist HJ, Lambin EF. Proximate causes and underlying driving forces of tropical deforestation: Tropical forests are disappearing as the result of many pressures, both local and regional, acting in various combinations in different geographical locations. Bioscience. 2002;52(2):143–50.
Lambin EF, Geist HJ, Lepers E. Dynamics of land-use and land-cover change in tropical regions. Annu Rev Environ Resour. 2003;28(1):205–41.
Maitima JM, Olson J, Mugatha S, Mugisha S, Mutie I. Land use changes, impacts and options for sustaining productivity and livelihoods in the basin of lake Victoria. J Sustain Dev Afr. 2010;12(3):1520–5509.
Google Scholar
Cao S. Impact of China’s large-scale ecological restoration program on the environment and society in arid and semiarid areas of China: achievements, problems, synthesis, and applications. Crit Rev Environ Sci Technol. 2011;41(4):317–35.
Article CAS Google Scholar
Neri M, Jameli D, Bernard E, Melo FP. Green versus green? Adverting potential conflicts between wind power generation and biodiversity conservation in Brazil. Perspect Ecol Conserv. 2019;17(3):131–5.
Gonçalves PHS, de Cunha Melo CVS, de Assis Andrade C, de Oliveira DVB, de Moura Brito Junior V, Rito KF, et al. Livelihood strategies and use of forest resources in a protected area in the Brazilian semiarid. Environ Dev Sustain. 2021;24:1–21.
Schwarz AM, Béné C, Bennett G, Boso D, Hilly Z, Paul C, et al. Vulnerability and resilience of remote rural communities to shocks and global changes: empirical analysis from Solomon Islands. Glob Environ Change. 2011;21(3):1128–40.
Sedano F, Mizu-Siampale A, Duncanson L, Liang M. Influence of charcoal production on forest degradation in Zambia: a remote sensing perspective. Remote Sens. 2022;14(14):3352.
Scoones I. Livelihoods perspectives and rural development. In: Scoones I, editor. Critical perspectives in rural development studies. Routledge; 2013. p. 159–84.
Angelsen A, Wunder S. Exploring the forest-poverty link. CIFOR Occas Pap. 2003;40:1–20.
De Chazal J, Rounsevell MD. Land-use and climate change within assessments of biodiversity change: a review. Glob Environ Change. 2009;19(2):306–15.
Castillo CP, Jacobs-Crisioni C, Diogo V, Lavalle C. Modelling agricultural land abandonment in a fine spatial resolution multi-level land-use model: an application for the EU. Environ Model Softw. 2021;136: 104946.
Moges DM, Bhat HG. An insight into land use and land cover changes and their impacts in Rib watershed, north-western highland Ethiopia. Land Degrad Dev. 2018;29(10):3317–30.
Munthali M, Mustak S, Adeola A, Botai J, Singh S, Davis N. Modelling land use and land cover dynamics of Dedza district of Malawi using hybrid Cellular Automata and Markov model. Remote Sens Appl Soc Environ. 2020;17: 100276.
Seto KC, Kaufmann RK. Modeling the drivers of urban land use change in the Pearl River Delta, China: integrating remote sensing with socioeconomic data. Land Econ. 2003;79(1):106–21.
DeFries RS, Rudel T, Uriarte M, Hansen M. Deforestation driven by urban population growth and agricultural trade in the twenty-first century. Nat Geosci. 2010;3(3):178–81.
Houghton R. Carbon emissions and the drivers of deforestation and forest degradation in the tropics. Curr Opin Environ Sustain. 2012;4(6):597–603.
Alexander P, Rounsevell MD, Dislich C, Dodson JR, Engström K, Moran D. Drivers for global agricultural land use change: the nexus of diet, population, yield and bioenergy. Glob Environ Change. 2015;35:138–47.
Mottet A, Ladet S, Coqué N, Gibon A. Agricultural land-use change and its drivers in mountain landscapes: a case study in the Pyrenees. Agric Ecosyst Environ. 2006;114(2–4):296–310.
Wang J, Bretz M, Dewan MAA, Delavar MA. Machine learning in modelling land-use and land cover-change (LULCC): current status, challenges and prospects. Sci Total Environ. 2022;822: 153559.
Negassa MD, Mallie DT, Gemeda DO. Forest cover change detection using Geographic Information Systems and remote sensing techniques: a spatio-temporal study on Komto Protected forest priority area, East Wollega Zone, Ethiopia. Environ Syst Res. 2020;9:1–14.
Reid RS, Kruska RL, Muthui N, Taye A, Wotton S, Wilson CJ, et al. Land-use and land-cover dynamics in response to changes in climatic, biological and socio-political forces: the case of southwestern Ethiopia. Landsc Ecol. 2000;15:339–55.
Tolessa T, Dechassa C, Simane B, Alamerew B, Kidane M. Land use/land cover dynamics in response to various driving forces in Didessa sub-basin. Ethiopia GeoJournal. 2020;85:747–60.
Seyoum A. Economic value of afromontane natural forest in Sheka Zone, southwest Ethiopia. Forests of Sheka. MELCA Mahiber Afr Biodivers Netw. 2007;183–218.
Teketay D, Lemenih M, Bekele T, Yemshaw Y, Feleke S, Tadesse W, et al. Forest resources and challenges of sustainable forest management and conservation in Ethiopia. In: Degraded forests in Eastern Africa. Routledge; 2010. p. 31–75.
Berhanu A, Woldu Z, Demissew S, Melesse S. Temporal vegetation cover dynamics in Northwestern Ethiopia: status and trends. Ethiop J Biol Sci. 2019;18(2):123–43.
Zegeye H. Major drivers and consequences of deforestation in Ethiopia: implications for forest conservation. Asian J Sci Technol. 2017;8(8):5166–75.
Mesfin D, Simane B, Belay A, Recha JW, Taddese H. Woodland cover change in the Central Rift Valley of Ethiopia. Forests. 2020;11(9):916.
Wassie SB. Natural resource degradation tendencies in Ethiopia: a review. Environ Syst Res. 2020;9:1–29.
Alobo LS. Rural livelihood diversification in sub-Saharan Africa: a literature review. J Dev Stud. 2015;51(9):1125–38.
Ellis F. Rural livelihoods and diversity in developing countries. Oxford University Press; 2000.
Book Google Scholar
Babatunde R, Olagunju F, Fakayode S, Adejobi A. Determinants of participation in off-farm employment among small-holder farming households in Kwara State. Nigeria Prod Agric Technol. 2010;6(2):1–14.
Kassie GW, Kim S, Fellizar FP Jr. Determinant factors of livelihood diversification: evidence from Ethiopia. Cogent Soc Sci. 2017;3(1):1369490.
Ellis F, Freeman HA. Rural livelihoods and poverty reduction strategies in four African countries. J Dev Stud. 2004;40(4):1–30.
Abera A, Yirgu T, Uncha A. Determinants of rural livelihood diversification strategies among Chewaka resettlers’ communities of Southwestern Ethiopia. Agric Food Secur. 2021;10(1):30.
Rubiyanto CW, Hirota I. A review on livelihood diversification: dynamics, measurements and case studies in Montane mainland Southeast Asia. Rev Agric Sci. 2021;9:128–42.
Mcelwee PD. Forest environmental income in Vietnam: household socioeconomic factors influencing forest use. Environ Conser. 2008;35(2):147–59.
Assan JK, Beyene FR. Livelihood impacts of environmental conservation programmes in the Amhara region of Ethiopia. J Sustain Dev. 2013;6(10):87.
Vedeld P, Angelsen A, Sjaastad E, Kobugabe Berg G. Counting on the environment: forest incomes and the rural poor. World Bank; 2004.
Rasmussen LV, Watkins C, Agrawal A. Forest contributions to livelihoods in changing agriculture-forest landscapes. For Policy Econ. 2017;84:1–8.
Wunder S, Angelsen A, Belcher B. Forests, livelihoods, and conservation: broadening the empirical base. World Dev. 2014;64:S1-11.
Kamwi JM, Chirwa PW, Manda SO, Graz PF, Kätsch C. Livelihoods, land use and land cover change in the Zambezi Region, Namibia. Popul Environ. 2015;37:207–30.
Guyalo AK, Alemu EA, Degaga DT. Impact of large-scale agricultural investments on the food security status of local community in Gambella region, Ethiopia. Agric Food Secur. 2022;11(1):43.
Matrix L. Land Matrix: Ethiopia. Ethiop Land Matrix. 2016.
Woldemariam T, Fetene M. Forests of Sheka: Ecological, social, legal and economic dimensions of recent land use/land cover changes, overview and synthesis. For Sheka Multidiscip Case Stud Impacts Land UseLand Cover Chang Melca-Mahiber Addis Ababa Viii 231 pp. 2007;1–21.
Berhanu Y, Dalle G, Sintayehu DW, Kelboro G, Nigussie A. Woody species dynamics in Sheka Forest Biosphere Reserve, Southwest Ethiopia. For Ecol Manag. 2022;519: 120313.
Ameneshewa W. Spatio-Temporal forest cover change detection using remote sensing and GIS techniques: in the case of Masha Woreda, Sheka Zone, SNNPRS of Ethiopia; 2015;
Bedru S. Land-use/land-cover changes in Andracha and Masha Woredas of Sheka Zone, SNNP Regional State. For Sheka Ecol Soc Leg Econ Dimens Recent Land-UseLand-Cover Chang Overv Synth Multidiscip Case Stud Impact Land-UseLand-Cover Chang South West Ethiop. 2007;21–56.
Mahiber M. Communal forest ownership: an option to address the underlying causes of deforestation and forest degradation in Ethiopia; 2008.
Girma HM, Hassan RM, Hertzler G. Forest conservation versus conversion under uncertain market and environmental forest benefits in Ethiopia: the case of Sheka forest. For Policy Econ. 2012;21:101–7.
Ameneshewa W, Kebede Y, Unbushe D, Legesse A. Correction: Trends of land use land cover dynamics of sheka biosphere reserve, a case of Shato Core Area, Southwest Ethiopia. PLoS ONE. 2023;18(12): e0296112.
Belay A. Sheka forest biosphere reserve beekeeping practices and characteristics of Schefflera abyssinica honey Ethiopia. Environ Dev Sustain. 2021;23:11818–36.
Fekadu A, Soromessa T, Warkineh DB. Role of forest provisioning services to local livelihoods: based on relative forest income (RFI) approach in southwest Ethiopia coffee forest. Environ Syst Res. 2021;10:1–15.
Galaz V, Olsson P, Hahn T, Folke C, Svedin U. The problem of fit among biophysical systems, environmental and resource regimes, and broader governance systems: insights and emerging challenges. 2008.
Steward JH. The concept and method of cultural ecology. Anthropol Theory Issues Epistemol. 2005;Sep 12:100–6.
Smith CA, Lazarus RS. Emotion and adaptation. Handb Personal Theory Res. 1990;21:609–37.
Briassoulis H. Analysis of land use change: theoretical and modeling approaches. Regional Research Institute, West Virginia University; 2020.
Campbell B. Beyond cultural models of the environment: linking subjectivities of dwelling and power. In: Culture and the environment in the Himalaya. Routledge; 2009. p. 204–21.
Campbell EK. Beyond anthropocentrism. J Hist Behav Sci. 1983;19(1):54–67.
Raphael John L, Hambati H, Ato AF. An intensity analysis of land-use and land-cover change in Karatu District, Tanzania: community perceptions and coping strategies. Afr Geogr Rev. 2014;33(2):150–73.
Scheelbeek PF, Hamza YA, Schellenberg J, Hill Z. Improving the use of focus group discussions in low income settings. BMC Med Res Methodol. 2020;20:1–10.
Girma A. Plant communities, species diversity, seedling bank and resprouting in Nandi forests, Kenya. 2012.
Taddesse YY. Mathematical modeling on assessing rate of deforestation in the Sheka Forest South West Ethiopia. Int J Sci Acad Res. 2022;2(2):1–7.
Genet A. Population growth and land use land cover change scenario in Ethiopia. Int J Environ Prot Policy. 2020;8(4):77–85.
Yamane T. Statistics: An introductory analysis 1973.
Nanjundeswaraswamy T, Divakar S. Determination of sample size and sampling methods in applied research. Proc Eng Sci. 2021;3(1):25–32.
Singh AS, Masuku MB. Sampling techniques & determination of sample size in applied statistics research: an overview. Int J Econ Commer Manag. 2014;2(11):1–22.
Burns TJ, Kick EL, Davis BL. Theorizing and rethinking linkages between the natural environment and the modern world-system: deforestation in the late 20th century. J World-Syst Res. 2003. https://doi.org/10.5195/jwsr.2003.237 .
Nguyen T, Vojnovic M. Weighted proportional allocation. ACM SIGMETRICS Perform Eval Rev. 2011;39(1):133–44.
Hailu A, Mammo S, Kidane M. Dynamics of land use, land cover change trend and its drivers in Jimma Geneti District, Western Ethiopia. Land Use Policy. 2020;99: 105011.
Hsieh HF, Shannon SE. Three approaches to qualitative content analysis. Qual Health Res. 2005;15(9):1277–88.
Richards JA. Supervised classification techniques. In: Remote sensing digital image analysis. Springer; 2013. p. 247–318.
Chapter Google Scholar
Shakibaie A, Firozjaie EL, Firozjaie HL, Khatami S. Estimated value of forest conservation of Gazu forest in Mazandaran. Am-Eur J Agric Environ Sci. 2013;13(7):1007–11.
Hussain J, Zhou K, Akbar M, Raza G, Ali S, Hussain A, et al. Dependence of rural livelihoods on forest resources in Naltar Valley, a dry temperate mountainous region, Pakistan. Glob Ecol Conserv. 2019;20: e00765.
Ali N, Hu X, Hussain J. The dependency of rural livelihood on forest resources in Northern Pakistan’s Chaprote Valley. Glob Ecol Conserv. 2020;22: e01001.
Menard SW. Logistic regression: from introductory to advanced concepts and applications. Sage; 2010.
Hosmer DW Jr, Lemeshow S, May S. Applied survival analysis: regression modeling of time-to-event data, vol. 618. John Wiley & Sons; 2008.
Yeboah FK, Jayne TS, Muyanga M, Chamberlin J. Youth access to land, migration and employment opportunities: evidence from sub-Saharan Africa. 2019.
Yigezu WG. The challenges and prospects of Ethiopian agriculture. Cogent Food Agric. 2021;7(1):1923619.
Gidey E, Dikinya O, Sebego R, Segosebe E, Zenebe A, Mussa S, et al. Land use and land cover change determinants in Raya Valley, Tigray, Northern Ethiopian Highlands. Agriculture. 2023;13(2):507.
Bekabil UT, Bedemo A. Dynamics of farmers’ participation in conservation agriculture: binary logistic regression analysis. Dynamics. 2015;13:74–83.
Congalton RG. A review of assessing the accuracy of classifications of remotely sensed data. Remote Sens Environ. 1991;37(1):35–46.
Lambin EF, Geist HJ. Land-use and land-cover change: local processes and global impacts. Springer Science & Business Media; 2008.
Paavola J. Livelihoods, vulnerability and adaptation to climate change in Morogoro, Tanzania. Environ Sci Policy. 2008;11(7):642–54.
Uberhuaga P, Smith-Hall C, Helles F. Forest income and dependency in lowland Bolivia. Environ Dev Sustain. 2012;14(1):3–23.
Lepetu J, Alavalapati J, Nair P. Forest dependency and its implication for protected areas management: a case study from Kasane Forest Reserve, Botswana. Int J Environ Res. 2009;3(4):525–36.
Chen P, Shi X. Dynamic evaluation of China’s ecological civilization construction based on target correlation degree and coupling coordination degree. Environ Impact Assess Rev. 2022;93: 106734.
Ellis F, Bahiigwa G. Livelihoods and rural poverty reduction in Uganda. World Dev. 2003;31(6):997–1013.
Kung JK, Fai LY. So what if there is income inequality? The distributive consequence of nonfarm employment in rural China. Econ Dev Cult Change. 2001;50(1):19–46.
Jiao X, Pouliot M, Walelign SZ. Livelihood strategies and dynamics in rural Cambodia. World Dev. 2017;97:266–78.
Teshager Abeje M, Tsunekawa A, Adgo E, Haregeweyn N, Nigussie Z, Ayalew Z, et al. Exploring drivers of livelihood diversification and its effect on adoption of sustainable land management practices in the Upper Blue Nile Basin, Ethiopia. Sustainability. 2019;11(10):2991.
Reardon T, Berdegué J, Escobar G. Rural nonfarm employment and incomes in Latin America: overview and policy implications. World Dev. 2001;29(3):395–409.
Vedeld P, Angelsen A, Bojö J, Sjaastad E, Berg GK. Forest environmental incomes and the rural poor. For Policy Econ. 2007;9(7):869–79.
Gecho Y, Ayele G, Lemma T, Alemu D. Rural household livelihood strategies: Options and determinants in the case of Wolaita Zone, Southern Ethiopia. Soc Sci. 2014;3(3):92–104.
Tamerat T. Livelihood resources and determinants in Tigray Region of Ethiopia. Int J Lean Think. 2016;7(2):57–66.
Adugna E. Livelihood strategies and food security in Wolayta, Southern Ethiopia: the case of Boloso Sore district. MSc Thesis Submitt Sch Grad Stud Haramaya Univ; 2008.
Yizengaw YS, Okoyo EN, Beyene F. Determinants of livelihood diversification strategies: the case of smallholder rural farm households in Debre Elias Woreda, East Gojjam Zone, Ethiopia. Afr J Agric Res. 2015;10(19):1998–2013.
Adnan KM, Ying L, Ayoub Z, Sarker SA, Menhas R, Chen F, et al. Risk management strategies to cope catastrophic risks in agriculture: the case of contract farming, diversification and precautionary savings. Agriculture. 2020;10(8):351.
Anshiso D, Shiferaw M. Determinants of rural livelihood diversification: The case of rural households in Lemmo district, Hadiyya Zone of Southern Ethiopia. J Econ Sustain Dev. 2016;7(5):32–9.
Barrett CB, Bezuneh M, Clay DC, Reardon T. Heterogeneous Contraints, Incentives, and Income Diversification Strategies in Rural Africa. 2001.
Belay Wondim G. Livelihood Diversification among pastoralists and its effect on poverty: the case of Amibara District, Zone Three of Afar National Regional State. 2017.
Debele B, Desta G. Livelihood diversification: strategies, determinants and challenges for pastoral and agro-pastoral communities of Bale Zone, Ethiopia. Int Rev Soc Sci Humanit. 2016;11(2):37–51.
Nattino G, Pennell ML, Lemeshow S. Assessing the goodness of fit of logistic regression models in large samples: a modification of the Hosmer-Lemeshow test. Biometrics. 2020;76(2):549–60.
Download references
Author information
Authors and affiliations.
Department of Geography and Environmental Studies, College of Social Science, Arbaminch University, Arbaminch, Ethiopia
Workaferahu Ameneshewa & Yechale Kebede
Department of Geography and Environmental Studies, College of Social Science, Dilla University, Dilla, Ethiopia
Abiyot Legesse
Department of Biology, College of Natural and Computational Science, Wolaita Sodo University, Sodo, Ethiopia
Dikaso Unbushe
You can also search for this author in PubMed Google Scholar
Contributions
The first author and corresponding author did analysis, map, graph, table preparation, edition, and overall write up. Co-authors supports with supervision and edition.
Corresponding author
Correspondence to Workaferahu Ameneshewa .
Ethics declarations
Ethics approval and consent to participate.
This study involved a questionnaire-based survey of households and the study protocol was conducted in accordance with the 1964 Helsinki Declaration and approved by Arbaminch University, research and extension office based on Helsinki guidelines. Informed consent was obtained from all individual participants included in the study.
Competing interests
The authors declare no competing interests.
Additional information
Publisher's note.
Springer Nature remains neutral with regard to jurisdictional claims in published maps and institutional affiliations.
Rights and permissions
Open Access This article is licensed under a Creative Commons Attribution-NonCommercial-NoDerivatives 4.0 International License, which permits any non-commercial use, sharing, distribution and reproduction in any medium or format, as long as you give appropriate credit to the original author(s) and the source, provide a link to the Creative Commons licence, and indicate if you modified the licensed material. You do not have permission under this licence to share adapted material derived from this article or parts of it. The images or other third party material in this article are included in the article’s Creative Commons licence, unless indicated otherwise in a credit line to the material. If material is not included in the article’s Creative Commons licence and your intended use is not permitted by statutory regulation or exceeds the permitted use, you will need to obtain permission directly from the copyright holder. To view a copy of this licence, visit http://creativecommons.org/licenses/by-nc-nd/4.0/ .
Reprints and permissions
About this article
Ameneshewa, W., Kebede, Y., Legesse, A. et al. Drivers of land use land cover change and livelihood coping strategies in Sheka biosphere reserve; a case of Shato forest, Southwest Ethiopia. Discov Sustain 5 , 208 (2024). https://doi.org/10.1007/s43621-024-00415-y
Download citation
Received : 09 June 2024
Accepted : 12 August 2024
Published : 21 August 2024
DOI : https://doi.org/10.1007/s43621-024-00415-y
Share this article
Anyone you share the following link with will be able to read this content:
Sorry, a shareable link is not currently available for this article.
Provided by the Springer Nature SharedIt content-sharing initiative
- Shato Forest
- Crop diversification
- Sheka biosphere
- Find a journal
- Publish with us
- Track your research

IMAGES
COMMENTS
Research conducted prior to the pandemic examined how high and low socioeconomic status people vary in activating their networks when they faced job threats. They usually choose one of two ...
The impact of socioeconomic factors on mental health and the case for collective action ... findings from the Global Burden of Disease Study 2010. ... Racial differences in physical and mental ...
In this context, the article, based on the analysis of unit level data of NSS 77th round, argues that while differences in socio-economic status based on land ownership, caste, religion, education, gender, etc., which are indicative of differential well-being conditions of households, are also instrumental in inducing some other relative ...
Results. This cohort included 1,089,999 cases and 62,298 deaths in 3127 counties for a case fatality rate of 5.7%. Severely distressed counties had significantly fewer deaths from COVID-19 but higher number of deaths per 100,000 persons. In risk-adjusted analysis, the two socio-economic determinants of health with the strongest association with ...
In the Organisation for Economic Cooperation and Development's ... Hauser, R. M. Measuring socioeconomic status in studies of child development. Child. Dev. 65, 1541-1545 (1994).
The population of Ethiopia is 64 million and is estimated to be growing at a rate of 2.76% per annum. Fifty percent of the population is between the ages of 15 and 64 and the. male/female ratio of this age group is 1.01. Forty-seven percent of the population is under 15. Two ethnic groups dominate Ethiopia.
We use the Serengeti as an exemplar case-study in which the relative economic and social benefits of a road can be assessed against the ecological impacts. Specifically, we compare three possible transportation routes and ask which route maximizes the socio-economic returns for the people while minimizing the ecological costs.
The second phase was a more detailed socio-eco-nomic study. It concentrated on a representative subset of the communities identified in the first phase, and employed questionnaires, personal interviews and focus-group studies to delve more deeply into the socio-economic profiles of fisher households and the nature of resources harvested by them.
This paper investigates economic impacts of COVID-19 on households based on differences in the socio-economic status (SES). We determine the household-level effects of the COVID-19 shock using income sources, types of industries, communities' resilience, household susceptibility, and relevant policy measures. For this purpose, we used primary data of 555 households collected through snowball ...
Socio-Economic Development and Mental Health: Case Study of the Spanish Region of Aragon (2010-20) ... Socio-economic development and emotion-health connection revisited: a multilevel modeling analysis using data from 162 counties in China. BMC Public Health 16:257. doi: 10.1186/s12889-016-2926-z. PubMed Abstract | CrossRef Full Text | Google ...
The first one of these is the precise assessment of individual-level socio-economic development (Nadeem et al., Citation 2021 and Saydaliyev et al., Citation 2020). The second is the impact of financial inclusion, through microfinance, on this individual-level socio-economic development, which will be addressed in this study.
In this case study, we have collected five successive admission years' records (from 2006-2007 to 2010-21011) of the 257 students. ... K., Ratnoo, S., Bajaj, A. (2022). Impact of Socio-Economic Factors on Students' Academic Performance: A Case Study of Jawahar Navodaya Vidyalaya. In: Abraham, A., et al. Innovations in Bio-Inspired ...
Abstract. The NEDUM-2D model is used to downscale four global socio-economic scenarios at city scale and simulate the evolution of the Paris urban area between 1900 and 2100. It is based on a dynamic extension of the classical urban economic theory, to explain the spatial distribution of land and real estate values, dwelling surfaces ...
The construction of roads has become very popular in our age due to heightened modernization, industrialization and the various benefits reaped from proper road systems. However, construction processes massively contribute to environmental pollution and negatively impact socio-economic activities, necessitating this study. The aim of this paper is to assess the impact of road construction and ...
Socio-economic case studies ... The case studies in this collection are examples of such projects. England. West Cumbria Works: a socio-economic investment project. 17 April 2018
in interdisciplinary development studies and development economics, for policymakers and ... Socio-economic consequences of population growth 172 5.6.1. Pessimistic and optimistic perspectives 172 5.6.2. Malthusian analyses 174 5.6.3. The Neo-Malthusian trap 174 5.6.4. Growth of national income, growth of per capita income and the
socioeconomic factors caused for farmers' suicide. Socio factors such as, literacy rate, family size, caste, victim's cultivation experience, opinion on seeds. fertilizers and pesticides were ...
Series title and vol / issues. Socio-economic studies, 12; ISBN
The study utilizes a two-fold methodology for gathering data, incorporating previous literature and case studies to thoroughly examine the process of social enterprise transformation and its socio-economic effects in chosen developed and developing nations.
The condition of women is more miserable in the rural India with respect to various socio-economic aspects. Poverty: Rural poverty is one of the important characteristics of India and nearly 45 percent of rural people are below poverty line. Most of them are just surviving with day to day earnings.Under such circumstances, within the family ...
The aim of this study is to identify relationships between quality of life and selected indicators of socioeconomic status in single and married respondents from the Wrocław metropolitan area in Poland. 4460 respondents took part in the study (1828 single, 2632 married). The study was cross-sectional based on a diagnostic survey.
positive tourism impacts relat ing to the social w e ll-being of. the community as the stimulation of infrastructure. development (road s, communications, healthcare, educat ion, public transport ...
This report presents the main findings from a socio-economic impact study of Diamond Light Source ("Diamond"), which was undertaken by Technopolis in 2018-2019. ... 2019 and involved a range of surveys, interviews, case studies and desk-based assessments. The study set out to measure and demonstrate Diamond's scientific, technological ...
Focus on agricultural development in Sub-Saharan Africa has seen a shift towards the smallholder sector, which is home and employment provider to more than 70 percent of the population. However, on-the-ground realities and the viability status of enterprises within this sector remain largely un-probed research areas. This paper, which is * based on case studies of three dairy development ...
It is evident that the means of subsistence of the community have a significant impact on the management of natural resources. This study examined the socio-economic drivers of LULCC and assessed the impacts of such changes on rural livelihoods in Shato forest, southwest Ethiopia. To map the land use and land cover, supervised classifications were used. The data were collected from 358 ...