- Methodology
- Open access
- Published: 11 October 2016

Reviewing the research methods literature: principles and strategies illustrated by a systematic overview of sampling in qualitative research
- Stephen J. Gentles 1 , 4 ,
- Cathy Charles 1 ,
- David B. Nicholas 2 ,
- Jenny Ploeg 3 &
- K. Ann McKibbon 1
Systematic Reviews volume 5 , Article number: 172 ( 2016 ) Cite this article
54k Accesses
27 Citations
13 Altmetric
Metrics details
Overviews of methods are potentially useful means to increase clarity and enhance collective understanding of specific methods topics that may be characterized by ambiguity, inconsistency, or a lack of comprehensiveness. This type of review represents a distinct literature synthesis method, although to date, its methodology remains relatively undeveloped despite several aspects that demand unique review procedures. The purpose of this paper is to initiate discussion about what a rigorous systematic approach to reviews of methods, referred to here as systematic methods overviews , might look like by providing tentative suggestions for approaching specific challenges likely to be encountered. The guidance offered here was derived from experience conducting a systematic methods overview on the topic of sampling in qualitative research.
The guidance is organized into several principles that highlight specific objectives for this type of review given the common challenges that must be overcome to achieve them. Optional strategies for achieving each principle are also proposed, along with discussion of how they were successfully implemented in the overview on sampling. We describe seven paired principles and strategies that address the following aspects: delimiting the initial set of publications to consider, searching beyond standard bibliographic databases, searching without the availability of relevant metadata, selecting publications on purposeful conceptual grounds, defining concepts and other information to abstract iteratively, accounting for inconsistent terminology used to describe specific methods topics, and generating rigorous verifiable analytic interpretations. Since a broad aim in systematic methods overviews is to describe and interpret the relevant literature in qualitative terms, we suggest that iterative decision making at various stages of the review process, and a rigorous qualitative approach to analysis are necessary features of this review type.
Conclusions
We believe that the principles and strategies provided here will be useful to anyone choosing to undertake a systematic methods overview. This paper represents an initial effort to promote high quality critical evaluations of the literature regarding problematic methods topics, which have the potential to promote clearer, shared understandings, and accelerate advances in research methods. Further work is warranted to develop more definitive guidance.
Peer Review reports
While reviews of methods are not new, they represent a distinct review type whose methodology remains relatively under-addressed in the literature despite the clear implications for unique review procedures. One of few examples to describe it is a chapter containing reflections of two contributing authors in a book of 21 reviews on methodological topics compiled for the British National Health Service, Health Technology Assessment Program [ 1 ]. Notable is their observation of how the differences between the methods reviews and conventional quantitative systematic reviews, specifically attributable to their varying content and purpose, have implications for defining what qualifies as systematic. While the authors describe general aspects of “systematicity” (including rigorous application of a methodical search, abstraction, and analysis), they also describe a high degree of variation within the category of methods reviews itself and so offer little in the way of concrete guidance. In this paper, we present tentative concrete guidance, in the form of a preliminary set of proposed principles and optional strategies, for a rigorous systematic approach to reviewing and evaluating the literature on quantitative or qualitative methods topics. For purposes of this article, we have used the term systematic methods overview to emphasize the notion of a systematic approach to such reviews.
The conventional focus of rigorous literature reviews (i.e., review types for which systematic methods have been codified, including the various approaches to quantitative systematic reviews [ 2 – 4 ], and the numerous forms of qualitative and mixed methods literature synthesis [ 5 – 10 ]) is to synthesize empirical research findings from multiple studies. By contrast, the focus of overviews of methods, including the systematic approach we advocate, is to synthesize guidance on methods topics. The literature consulted for such reviews may include the methods literature, methods-relevant sections of empirical research reports, or both. Thus, this paper adds to previous work published in this journal—namely, recent preliminary guidance for conducting reviews of theory [ 11 ]—that has extended the application of systematic review methods to novel review types that are concerned with subject matter other than empirical research findings.
Published examples of methods overviews illustrate the varying objectives they can have. One objective is to establish methodological standards for appraisal purposes. For example, reviews of existing quality appraisal standards have been used to propose universal standards for appraising the quality of primary qualitative research [ 12 ] or evaluating qualitative research reports [ 13 ]. A second objective is to survey the methods-relevant sections of empirical research reports to establish current practices on methods use and reporting practices, which Moher and colleagues [ 14 ] recommend as a means for establishing the needs to be addressed in reporting guidelines (see, for example [ 15 , 16 ]). A third objective for a methods review is to offer clarity and enhance collective understanding regarding a specific methods topic that may be characterized by ambiguity, inconsistency, or a lack of comprehensiveness within the available methods literature. An example of this is a overview whose objective was to review the inconsistent definitions of intention-to-treat analysis (the methodologically preferred approach to analyze randomized controlled trial data) that have been offered in the methods literature and propose a solution for improving conceptual clarity [ 17 ]. Such reviews are warranted because students and researchers who must learn or apply research methods typically lack the time to systematically search, retrieve, review, and compare the available literature to develop a thorough and critical sense of the varied approaches regarding certain controversial or ambiguous methods topics.
While systematic methods overviews , as a review type, include both reviews of the methods literature and reviews of methods-relevant sections from empirical study reports, the guidance provided here is primarily applicable to reviews of the methods literature since it was derived from the experience of conducting such a review [ 18 ], described below. To our knowledge, there are no well-developed proposals on how to rigorously conduct such reviews. Such guidance would have the potential to improve the thoroughness and credibility of critical evaluations of the methods literature, which could increase their utility as a tool for generating understandings that advance research methods, both qualitative and quantitative. Our aim in this paper is thus to initiate discussion about what might constitute a rigorous approach to systematic methods overviews. While we hope to promote rigor in the conduct of systematic methods overviews wherever possible, we do not wish to suggest that all methods overviews need be conducted to the same standard. Rather, we believe that the level of rigor may need to be tailored pragmatically to the specific review objectives, which may not always justify the resource requirements of an intensive review process.
The example systematic methods overview on sampling in qualitative research
The principles and strategies we propose in this paper are derived from experience conducting a systematic methods overview on the topic of sampling in qualitative research [ 18 ]. The main objective of that methods overview was to bring clarity and deeper understanding of the prominent concepts related to sampling in qualitative research (purposeful sampling strategies, saturation, etc.). Specifically, we interpreted the available guidance, commenting on areas lacking clarity, consistency, or comprehensiveness (without proposing any recommendations on how to do sampling). This was achieved by a comparative and critical analysis of publications representing the most influential (i.e., highly cited) guidance across several methodological traditions in qualitative research.
The specific methods and procedures for the overview on sampling [ 18 ] from which our proposals are derived were developed both after soliciting initial input from local experts in qualitative research and an expert health librarian (KAM) and through ongoing careful deliberation throughout the review process. To summarize, in that review, we employed a transparent and rigorous approach to search the methods literature, selected publications for inclusion according to a purposeful and iterative process, abstracted textual data using structured abstraction forms, and analyzed (synthesized) the data using a systematic multi-step approach featuring abstraction of text, summary of information in matrices, and analytic comparisons.
For this article, we reflected on both the problems and challenges encountered at different stages of the review and our means for selecting justifiable procedures to deal with them. Several principles were then derived by considering the generic nature of these problems, while the generalizable aspects of the procedures used to address them formed the basis of optional strategies. Further details of the specific methods and procedures used in the overview on qualitative sampling are provided below to illustrate both the types of objectives and challenges that reviewers will likely need to consider and our approach to implementing each of the principles and strategies.
Organization of the guidance into principles and strategies
For the purposes of this article, principles are general statements outlining what we propose are important aims or considerations within a particular review process, given the unique objectives or challenges to be overcome with this type of review. These statements follow the general format, “considering the objective or challenge of X, we propose Y to be an important aim or consideration.” Strategies are optional and flexible approaches for implementing the previous principle outlined. Thus, generic challenges give rise to principles, which in turn give rise to strategies.
We organize the principles and strategies below into three sections corresponding to processes characteristic of most systematic literature synthesis approaches: literature identification and selection ; data abstraction from the publications selected for inclusion; and analysis , including critical appraisal and synthesis of the abstracted data. Within each section, we also describe the specific methodological decisions and procedures used in the overview on sampling in qualitative research [ 18 ] to illustrate how the principles and strategies for each review process were applied and implemented in a specific case. We expect this guidance and accompanying illustrations will be useful for anyone considering engaging in a methods overview, particularly those who may be familiar with conventional systematic review methods but may not yet appreciate some of the challenges specific to reviewing the methods literature.
Results and discussion
Literature identification and selection.
The identification and selection process includes search and retrieval of publications and the development and application of inclusion and exclusion criteria to select the publications that will be abstracted and analyzed in the final review. Literature identification and selection for overviews of the methods literature is challenging and potentially more resource-intensive than for most reviews of empirical research. This is true for several reasons that we describe below, alongside discussion of the potential solutions. Additionally, we suggest in this section how the selection procedures can be chosen to match the specific analytic approach used in methods overviews.
Delimiting a manageable set of publications
One aspect of methods overviews that can make identification and selection challenging is the fact that the universe of literature containing potentially relevant information regarding most methods-related topics is expansive and often unmanageably so. Reviewers are faced with two large categories of literature: the methods literature , where the possible publication types include journal articles, books, and book chapters; and the methods-relevant sections of empirical study reports , where the possible publication types include journal articles, monographs, books, theses, and conference proceedings. In our systematic overview of sampling in qualitative research, exhaustively searching (including retrieval and first-pass screening) all publication types across both categories of literature for information on a single methods-related topic was too burdensome to be feasible. The following proposed principle follows from the need to delimit a manageable set of literature for the review.
Principle #1:
Considering the broad universe of potentially relevant literature, we propose that an important objective early in the identification and selection stage is to delimit a manageable set of methods-relevant publications in accordance with the objectives of the methods overview.
Strategy #1:
To limit the set of methods-relevant publications that must be managed in the selection process, reviewers have the option to initially review only the methods literature, and exclude the methods-relevant sections of empirical study reports, provided this aligns with the review’s particular objectives.
We propose that reviewers are justified in choosing to select only the methods literature when the objective is to map out the range of recognized concepts relevant to a methods topic, to summarize the most authoritative or influential definitions or meanings for methods-related concepts, or to demonstrate a problematic lack of clarity regarding a widely established methods-related concept and potentially make recommendations for a preferred approach to the methods topic in question. For example, in the case of the methods overview on sampling [ 18 ], the primary aim was to define areas lacking in clarity for multiple widely established sampling-related topics. In the review on intention-to-treat in the context of missing outcome data [ 17 ], the authors identified a lack of clarity based on multiple inconsistent definitions in the literature and went on to recommend separating the issue of how to handle missing outcome data from the issue of whether an intention-to-treat analysis can be claimed.
In contrast to strategy #1, it may be appropriate to select the methods-relevant sections of empirical study reports when the objective is to illustrate how a methods concept is operationalized in research practice or reported by authors. For example, one could review all the publications in 2 years’ worth of issues of five high-impact field-related journals to answer questions about how researchers describe implementing a particular method or approach, or to quantify how consistently they define or report using it. Such reviews are often used to highlight gaps in the reporting practices regarding specific methods, which may be used to justify items to address in reporting guidelines (for example, [ 14 – 16 ]).
It is worth recognizing that other authors have advocated broader positions regarding the scope of literature to be considered in a review, expanding on our perspective. Suri [ 10 ] (who, like us, emphasizes how different sampling strategies are suitable for different literature synthesis objectives) has, for example, described a two-stage literature sampling procedure (pp. 96–97). First, reviewers use an initial approach to conduct a broad overview of the field—for reviews of methods topics, this would entail an initial review of the research methods literature. This is followed by a second more focused stage in which practical examples are purposefully selected—for methods reviews, this would involve sampling the empirical literature to illustrate key themes and variations. While this approach is seductive in its capacity to generate more in depth and interpretive analytic findings, some reviewers may consider it too resource-intensive to include the second step no matter how selective the purposeful sampling. In the overview on sampling where we stopped after the first stage [ 18 ], we discussed our selective focus on the methods literature as a limitation that left opportunities for further analysis of the literature. We explicitly recommended, for example, that theoretical sampling was a topic for which a future review of the methods sections of empirical reports was justified to answer specific questions identified in the primary review.
Ultimately, reviewers must make pragmatic decisions that balance resource considerations, combined with informed predictions about the depth and complexity of literature available on their topic, with the stated objectives of their review. The remaining principles and strategies apply primarily to overviews that include the methods literature, although some aspects may be relevant to reviews that include empirical study reports.
Searching beyond standard bibliographic databases
An important reality affecting identification and selection in overviews of the methods literature is the increased likelihood for relevant publications to be located in sources other than journal articles (which is usually not the case for overviews of empirical research, where journal articles generally represent the primary publication type). In the overview on sampling [ 18 ], out of 41 full-text publications retrieved and reviewed, only 4 were journal articles, while 37 were books or book chapters. Since many books and book chapters did not exist electronically, their full text had to be physically retrieved in hardcopy, while 11 publications were retrievable only through interlibrary loan or purchase request. The tasks associated with such retrieval are substantially more time-consuming than electronic retrieval. Since a substantial proportion of methods-related guidance may be located in publication types that are less comprehensively indexed in standard bibliographic databases, identification and retrieval thus become complicated processes.
Principle #2:
Considering that important sources of methods guidance can be located in non-journal publication types (e.g., books, book chapters) that tend to be poorly indexed in standard bibliographic databases, it is important to consider alternative search methods for identifying relevant publications to be further screened for inclusion.
Strategy #2:
To identify books, book chapters, and other non-journal publication types not thoroughly indexed in standard bibliographic databases, reviewers may choose to consult one or more of the following less standard sources: Google Scholar, publisher web sites, or expert opinion.
In the case of the overview on sampling in qualitative research [ 18 ], Google Scholar had two advantages over other standard bibliographic databases: it indexes and returns records of books and book chapters likely to contain guidance on qualitative research methods topics; and it has been validated as providing higher citation counts than ISI Web of Science (a producer of numerous bibliographic databases accessible through institutional subscription) for several non-biomedical disciplines including the social sciences where qualitative research methods are prominently used [ 19 – 21 ]. While we identified numerous useful publications by consulting experts, the author publication lists generated through Google Scholar searches were uniquely useful to identify more recent editions of methods books identified by experts.
Searching without relevant metadata
Determining what publications to select for inclusion in the overview on sampling [ 18 ] could only rarely be accomplished by reviewing the publication’s metadata. This was because for the many books and other non-journal type publications we identified as possibly relevant, the potential content of interest would be located in only a subsection of the publication. In this common scenario for reviews of the methods literature (as opposed to methods overviews that include empirical study reports), reviewers will often be unable to employ standard title, abstract, and keyword database searching or screening as a means for selecting publications.
Principle #3:
Considering that the presence of information about the topic of interest may not be indicated in the metadata for books and similar publication types, it is important to consider other means of identifying potentially useful publications for further screening.
Strategy #3:
One approach to identifying potentially useful books and similar publication types is to consider what classes of such publications (e.g., all methods manuals for a certain research approach) are likely to contain relevant content, then identify, retrieve, and review the full text of corresponding publications to determine whether they contain information on the topic of interest.
In the example of the overview on sampling in qualitative research [ 18 ], the topic of interest (sampling) was one of numerous topics covered in the general qualitative research methods manuals. Consequently, examples from this class of publications first had to be identified for retrieval according to non-keyword-dependent criteria. Thus, all methods manuals within the three research traditions reviewed (grounded theory, phenomenology, and case study) that might contain discussion of sampling were sought through Google Scholar and expert opinion, their full text obtained, and hand-searched for relevant content to determine eligibility. We used tables of contents and index sections of books to aid this hand searching.
Purposefully selecting literature on conceptual grounds
A final consideration in methods overviews relates to the type of analysis used to generate the review findings. Unlike quantitative systematic reviews where reviewers aim for accurate or unbiased quantitative estimates—something that requires identifying and selecting the literature exhaustively to obtain all relevant data available (i.e., a complete sample)—in methods overviews, reviewers must describe and interpret the relevant literature in qualitative terms to achieve review objectives. In other words, the aim in methods overviews is to seek coverage of the qualitative concepts relevant to the methods topic at hand. For example, in the overview of sampling in qualitative research [ 18 ], achieving review objectives entailed providing conceptual coverage of eight sampling-related topics that emerged as key domains. The following principle recognizes that literature sampling should therefore support generating qualitative conceptual data as the input to analysis.
Principle #4:
Since the analytic findings of a systematic methods overview are generated through qualitative description and interpretation of the literature on a specified topic, selection of the literature should be guided by a purposeful strategy designed to achieve adequate conceptual coverage (i.e., representing an appropriate degree of variation in relevant ideas) of the topic according to objectives of the review.
Strategy #4:
One strategy for choosing the purposeful approach to use in selecting the literature according to the review objectives is to consider whether those objectives imply exploring concepts either at a broad overview level, in which case combining maximum variation selection with a strategy that limits yield (e.g., critical case, politically important, or sampling for influence—described below) may be appropriate; or in depth, in which case purposeful approaches aimed at revealing innovative cases will likely be necessary.
In the methods overview on sampling, the implied scope was broad since we set out to review publications on sampling across three divergent qualitative research traditions—grounded theory, phenomenology, and case study—to facilitate making informative conceptual comparisons. Such an approach would be analogous to maximum variation sampling.
At the same time, the purpose of that review was to critically interrogate the clarity, consistency, and comprehensiveness of literature from these traditions that was “most likely to have widely influenced students’ and researchers’ ideas about sampling” (p. 1774) [ 18 ]. In other words, we explicitly set out to review and critique the most established and influential (and therefore dominant) literature, since this represents a common basis of knowledge among students and researchers seeking understanding or practical guidance on sampling in qualitative research. To achieve this objective, we purposefully sampled publications according to the criterion of influence , which we operationalized as how often an author or publication has been referenced in print or informal discourse. This second sampling approach also limited the literature we needed to consider within our broad scope review to a manageable amount.
To operationalize this strategy of sampling for influence , we sought to identify both the most influential authors within a qualitative research tradition (all of whose citations were subsequently screened) and the most influential publications on the topic of interest by non-influential authors. This involved a flexible approach that combined multiple indicators of influence to avoid the dilemma that any single indicator might provide inadequate coverage. These indicators included bibliometric data (h-index for author influence [ 22 ]; number of cites for publication influence), expert opinion, and cross-references in the literature (i.e., snowball sampling). As a final selection criterion, a publication was included only if it made an original contribution in terms of novel guidance regarding sampling or a related concept; thus, purely secondary sources were excluded. Publish or Perish software (Anne-Wil Harzing; available at http://www.harzing.com/resources/publish-or-perish ) was used to generate bibliometric data via the Google Scholar database. Figure 1 illustrates how identification and selection in the methods overview on sampling was a multi-faceted and iterative process. The authors selected as influential, and the publications selected for inclusion or exclusion are listed in Additional file 1 (Matrices 1, 2a, 2b).
Literature identification and selection process used in the methods overview on sampling [ 18 ]
In summary, the strategies of seeking maximum variation and sampling for influence were employed in the sampling overview to meet the specific review objectives described. Reviewers will need to consider the full range of purposeful literature sampling approaches at their disposal in deciding what best matches the specific aims of their own reviews. Suri [ 10 ] has recently retooled Patton’s well-known typology of purposeful sampling strategies (originally intended for primary research) for application to literature synthesis, providing a useful resource in this respect.
Data abstraction
The purpose of data abstraction in rigorous literature reviews is to locate and record all data relevant to the topic of interest from the full text of included publications, making them available for subsequent analysis. Conventionally, a data abstraction form—consisting of numerous distinct conceptually defined fields to which corresponding information from the source publication is recorded—is developed and employed. There are several challenges, however, to the processes of developing the abstraction form and abstracting the data itself when conducting methods overviews, which we address here. Some of these problems and their solutions may be familiar to those who have conducted qualitative literature syntheses, which are similarly conceptual.
Iteratively defining conceptual information to abstract
In the overview on sampling [ 18 ], while we surveyed multiple sources beforehand to develop a list of concepts relevant for abstraction (e.g., purposeful sampling strategies, saturation, sample size), there was no way for us to anticipate some concepts prior to encountering them in the review process. Indeed, in many cases, reviewers are unable to determine the complete set of methods-related concepts that will be the focus of the final review a priori without having systematically reviewed the publications to be included. Thus, defining what information to abstract beforehand may not be feasible.
Principle #5:
Considering the potential impracticality of defining a complete set of relevant methods-related concepts from a body of literature one has not yet systematically read, selecting and defining fields for data abstraction must often be undertaken iteratively. Thus, concepts to be abstracted can be expected to grow and change as data abstraction proceeds.
Strategy #5:
Reviewers can develop an initial form or set of concepts for abstraction purposes according to standard methods (e.g., incorporating expert feedback, pilot testing) and remain attentive to the need to iteratively revise it as concepts are added or modified during the review. Reviewers should document revisions and return to re-abstract data from previously abstracted publications as the new data requirements are determined.
In the sampling overview [ 18 ], we developed and maintained the abstraction form in Microsoft Word. We derived the initial set of abstraction fields from our own knowledge of relevant sampling-related concepts, consultation with local experts, and reviewing a pilot sample of publications. Since the publications in this review included a large proportion of books, the abstraction process often began by flagging the broad sections within a publication containing topic-relevant information for detailed review to identify text to abstract. When reviewing flagged text, the reviewer occasionally encountered an unanticipated concept significant enough to warrant being added as a new field to the abstraction form. For example, a field was added to capture how authors described the timing of sampling decisions, whether before (a priori) or after (ongoing) starting data collection, or whether this was unclear. In these cases, we systematically documented the modification to the form and returned to previously abstracted publications to abstract any information that might be relevant to the new field.
The logic of this strategy is analogous to the logic used in a form of research synthesis called best fit framework synthesis (BFFS) [ 23 – 25 ]. In that method, reviewers initially code evidence using an a priori framework they have selected. When evidence cannot be accommodated by the selected framework, reviewers then develop new themes or concepts from which they construct a new expanded framework. Both the strategy proposed and the BFFS approach to research synthesis are notable for their rigorous and transparent means to adapt a final set of concepts to the content under review.
Accounting for inconsistent terminology
An important complication affecting the abstraction process in methods overviews is that the language used by authors to describe methods-related concepts can easily vary across publications. For example, authors from different qualitative research traditions often use different terms for similar methods-related concepts. Furthermore, as we found in the sampling overview [ 18 ], there may be cases where no identifiable term, phrase, or label for a methods-related concept is used at all, and a description of it is given instead. This can make searching the text for relevant concepts based on keywords unreliable.
Principle #6:
Since accepted terms may not be used consistently to refer to methods concepts, it is necessary to rely on the definitions for concepts, rather than keywords, to identify relevant information in the publication to abstract.
Strategy #6:
An effective means to systematically identify relevant information is to develop and iteratively adjust written definitions for key concepts (corresponding to abstraction fields) that are consistent with and as inclusive of as much of the literature reviewed as possible. Reviewers then seek information that matches these definitions (rather than keywords) when scanning a publication for relevant data to abstract.
In the abstraction process for the sampling overview [ 18 ], we noted the several concepts of interest to the review for which abstraction by keyword was particularly problematic due to inconsistent terminology across publications: sampling , purposeful sampling , sampling strategy , and saturation (for examples, see Additional file 1 , Matrices 3a, 3b, 4). We iteratively developed definitions for these concepts by abstracting text from publications that either provided an explicit definition or from which an implicit definition could be derived, which was recorded in fields dedicated to the concept’s definition. Using a method of constant comparison, we used text from definition fields to inform and modify a centrally maintained definition of the corresponding concept to optimize its fit and inclusiveness with the literature reviewed. Table 1 shows, as an example, the final definition constructed in this way for one of the central concepts of the review, qualitative sampling .
We applied iteratively developed definitions when making decisions about what specific text to abstract for an existing field, which allowed us to abstract concept-relevant data even if no recognized keyword was used. For example, this was the case for the sampling-related concept, saturation , where the relevant text available for abstraction in one publication [ 26 ]—“to continue to collect data until nothing new was being observed or recorded, no matter how long that takes”—was not accompanied by any term or label whatsoever.
This comparative analytic strategy (and our approach to analysis more broadly as described in strategy #7, below) is analogous to the process of reciprocal translation —a technique first introduced for meta-ethnography by Noblit and Hare [ 27 ] that has since been recognized as a common element in a variety of qualitative metasynthesis approaches [ 28 ]. Reciprocal translation, taken broadly, involves making sense of a study’s findings in terms of the findings of the other studies included in the review. In practice, it has been operationalized in different ways. Melendez-Torres and colleagues developed a typology from their review of the metasynthesis literature, describing four overlapping categories of specific operations undertaken in reciprocal translation: visual representation, key paper integration, data reduction and thematic extraction, and line-by-line coding [ 28 ]. The approaches suggested in both strategies #6 and #7, with their emphasis on constant comparison, appear to fall within the line-by-line coding category.
Generating credible and verifiable analytic interpretations
The analysis in a systematic methods overview must support its more general objective, which we suggested above is often to offer clarity and enhance collective understanding regarding a chosen methods topic. In our experience, this involves describing and interpreting the relevant literature in qualitative terms. Furthermore, any interpretative analysis required may entail reaching different levels of abstraction, depending on the more specific objectives of the review. For example, in the overview on sampling [ 18 ], we aimed to produce a comparative analysis of how multiple sampling-related topics were treated differently within and among different qualitative research traditions. To promote credibility of the review, however, not only should one seek a qualitative analytic approach that facilitates reaching varying levels of abstraction but that approach must also ensure that abstract interpretations are supported and justified by the source data and not solely the product of the analyst’s speculative thinking.
Principle #7:
Considering the qualitative nature of the analysis required in systematic methods overviews, it is important to select an analytic method whose interpretations can be verified as being consistent with the literature selected, regardless of the level of abstraction reached.
Strategy #7:
We suggest employing the constant comparative method of analysis [ 29 ] because it supports developing and verifying analytic links to the source data throughout progressively interpretive or abstract levels. In applying this approach, we advise a rigorous approach, documenting how supportive quotes or references to the original texts are carried forward in the successive steps of analysis to allow for easy verification.
The analytic approach used in the methods overview on sampling [ 18 ] comprised four explicit steps, progressing in level of abstraction—data abstraction, matrices, narrative summaries, and final analytic conclusions (Fig. 2 ). While we have positioned data abstraction as the second stage of the generic review process (prior to Analysis), above, we also considered it as an initial step of analysis in the sampling overview for several reasons. First, it involved a process of constant comparisons and iterative decision-making about the fields to add or define during development and modification of the abstraction form, through which we established the range of concepts to be addressed in the review. At the same time, abstraction involved continuous analytic decisions about what textual quotes (ranging in size from short phrases to numerous paragraphs) to record in the fields thus created. This constant comparative process was analogous to open coding in which textual data from publications was compared to conceptual fields (equivalent to codes) or to other instances of data previously abstracted when constructing definitions to optimize their fit with the overall literature as described in strategy #6. Finally, in the data abstraction step, we also recorded our first interpretive thoughts in dedicated fields, providing initial material for the more abstract analytic steps.
Summary of progressive steps of analysis used in the methods overview on sampling [ 18 ]
In the second step of the analysis, we constructed topic-specific matrices , or tables, by copying relevant quotes from abstraction forms into the appropriate cells of matrices (for the complete set of analytic matrices developed in the sampling review, see Additional file 1 (matrices 3 to 10)). Each matrix ranged from one to five pages; row headings, nested three-deep, identified the methodological tradition, author, and publication, respectively; and column headings identified the concepts, which corresponded to abstraction fields. Matrices thus allowed us to make further comparisons across methodological traditions, and between authors within a tradition. In the third step of analysis, we recorded our comparative observations as narrative summaries , in which we used illustrative quotes more sparingly. In the final step, we developed analytic conclusions based on the narrative summaries about the sampling-related concepts within each methodological tradition for which clarity, consistency, or comprehensiveness of the available guidance appeared to be lacking. Higher levels of analysis thus built logically from the lower levels, enabling us to easily verify analytic conclusions by tracing the support for claims by comparing the original text of publications reviewed.
Integrative versus interpretive methods overviews
The analytic product of systematic methods overviews is comparable to qualitative evidence syntheses, since both involve describing and interpreting the relevant literature in qualitative terms. Most qualitative synthesis approaches strive to produce new conceptual understandings that vary in level of interpretation. Dixon-Woods and colleagues [ 30 ] elaborate on a useful distinction, originating from Noblit and Hare [ 27 ], between integrative and interpretive reviews. Integrative reviews focus on summarizing available primary data and involve using largely secure and well defined concepts to do so; definitions are used from an early stage to specify categories for abstraction (or coding) of data, which in turn supports their aggregation; they do not seek as their primary focus to develop or specify new concepts, although they may achieve some theoretical or interpretive functions. For interpretive reviews, meanwhile, the main focus is to develop new concepts and theories that integrate them, with the implication that the concepts developed become fully defined towards the end of the analysis. These two forms are not completely distinct, and “every integrative synthesis will include elements of interpretation, and every interpretive synthesis will include elements of aggregation of data” [ 30 ].
The example methods overview on sampling [ 18 ] could be classified as predominantly integrative because its primary goal was to aggregate influential authors’ ideas on sampling-related concepts; there were also, however, elements of interpretive synthesis since it aimed to develop new ideas about where clarity in guidance on certain sampling-related topics is lacking, and definitions for some concepts were flexible and not fixed until late in the review. We suggest that most systematic methods overviews will be classifiable as predominantly integrative (aggregative). Nevertheless, more highly interpretive methods overviews are also quite possible—for example, when the review objective is to provide a highly critical analysis for the purpose of generating new methodological guidance. In such cases, reviewers may need to sample more deeply (see strategy #4), specifically by selecting empirical research reports (i.e., to go beyond dominant or influential ideas in the methods literature) that are likely to feature innovations or instructive lessons in employing a given method.
In this paper, we have outlined tentative guidance in the form of seven principles and strategies on how to conduct systematic methods overviews, a review type in which methods-relevant literature is systematically analyzed with the aim of offering clarity and enhancing collective understanding regarding a specific methods topic. Our proposals include strategies for delimiting the set of publications to consider, searching beyond standard bibliographic databases, searching without the availability of relevant metadata, selecting publications on purposeful conceptual grounds, defining concepts and other information to abstract iteratively, accounting for inconsistent terminology, and generating credible and verifiable analytic interpretations. We hope the suggestions proposed will be useful to others undertaking reviews on methods topics in future.
As far as we are aware, this is the first published source of concrete guidance for conducting this type of review. It is important to note that our primary objective was to initiate methodological discussion by stimulating reflection on what rigorous methods for this type of review should look like, leaving the development of more complete guidance to future work. While derived from the experience of reviewing a single qualitative methods topic, we believe the principles and strategies provided are generalizable to overviews of both qualitative and quantitative methods topics alike. However, it is expected that additional challenges and insights for conducting such reviews have yet to be defined. Thus, we propose that next steps for developing more definitive guidance should involve an attempt to collect and integrate other reviewers’ perspectives and experiences in conducting systematic methods overviews on a broad range of qualitative and quantitative methods topics. Formalized guidance and standards would improve the quality of future methods overviews, something we believe has important implications for advancing qualitative and quantitative methodology. When undertaken to a high standard, rigorous critical evaluations of the available methods guidance have significant potential to make implicit controversies explicit, and improve the clarity and precision of our understandings of problematic qualitative or quantitative methods issues.
A review process central to most types of rigorous reviews of empirical studies, which we did not explicitly address in a separate review step above, is quality appraisal . The reason we have not treated this as a separate step stems from the different objectives of the primary publications included in overviews of the methods literature (i.e., providing methodological guidance) compared to the primary publications included in the other established review types (i.e., reporting findings from single empirical studies). This is not to say that appraising quality of the methods literature is not an important concern for systematic methods overviews. Rather, appraisal is much more integral to (and difficult to separate from) the analysis step, in which we advocate appraising clarity, consistency, and comprehensiveness—the quality appraisal criteria that we suggest are appropriate for the methods literature. As a second important difference regarding appraisal, we currently advocate appraising the aforementioned aspects at the level of the literature in aggregate rather than at the level of individual publications. One reason for this is that methods guidance from individual publications generally builds on previous literature, and thus we feel that ahistorical judgments about comprehensiveness of single publications lack relevance and utility. Additionally, while different methods authors may express themselves less clearly than others, their guidance can nonetheless be highly influential and useful, and should therefore not be downgraded or ignored based on considerations of clarity—which raises questions about the alternative uses that quality appraisals of individual publications might have. Finally, legitimate variability in the perspectives that methods authors wish to emphasize, and the levels of generality at which they write about methods, makes critiquing individual publications based on the criterion of clarity a complex and potentially problematic endeavor that is beyond the scope of this paper to address. By appraising the current state of the literature at a holistic level, reviewers stand to identify important gaps in understanding that represent valuable opportunities for further methodological development.
To summarize, the principles and strategies provided here may be useful to those seeking to undertake their own systematic methods overview. Additional work is needed, however, to establish guidance that is comprehensive by comparing the experiences from conducting a variety of methods overviews on a range of methods topics. Efforts that further advance standards for systematic methods overviews have the potential to promote high-quality critical evaluations that produce conceptually clear and unified understandings of problematic methods topics, thereby accelerating the advance of research methodology.
Hutton JL, Ashcroft R. What does “systematic” mean for reviews of methods? In: Black N, Brazier J, Fitzpatrick R, Reeves B, editors. Health services research methods: a guide to best practice. London: BMJ Publishing Group; 1998. p. 249–54.
Google Scholar
Cochrane handbook for systematic reviews of interventions. In. Edited by Higgins JPT, Green S, Version 5.1.0 edn: The Cochrane Collaboration; 2011.
Centre for Reviews and Dissemination: Systematic reviews: CRD’s guidance for undertaking reviews in health care . York: Centre for Reviews and Dissemination; 2009.
Liberati A, Altman DG, Tetzlaff J, Mulrow C, Gotzsche PC, Ioannidis JPA, Clarke M, Devereaux PJ, Kleijnen J, Moher D. The PRISMA statement for reporting systematic reviews and meta-analyses of studies that evaluate healthcare interventions: explanation and elaboration. BMJ. 2009;339:b2700–0.
Barnett-Page E, Thomas J. Methods for the synthesis of qualitative research: a critical review. BMC Med Res Methodol. 2009;9(1):59.
Article PubMed PubMed Central Google Scholar
Kastner M, Tricco AC, Soobiah C, Lillie E, Perrier L, Horsley T, Welch V, Cogo E, Antony J, Straus SE. What is the most appropriate knowledge synthesis method to conduct a review? Protocol for a scoping review. BMC Med Res Methodol. 2012;12(1):1–1.
Article Google Scholar
Booth A, Noyes J, Flemming K, Gerhardus A. Guidance on choosing qualitative evidence synthesis methods for use in health technology assessments of complex interventions. In: Integrate-HTA. 2016.
Booth A, Sutton A, Papaioannou D. Systematic approaches to successful literature review. 2nd ed. London: Sage; 2016.
Hannes K, Lockwood C. Synthesizing qualitative research: choosing the right approach. Chichester: Wiley-Blackwell; 2012.
Suri H. Towards methodologically inclusive research syntheses: expanding possibilities. New York: Routledge; 2014.
Campbell M, Egan M, Lorenc T, Bond L, Popham F, Fenton C, Benzeval M. Considering methodological options for reviews of theory: illustrated by a review of theories linking income and health. Syst Rev. 2014;3(1):1–11.
Cohen DJ, Crabtree BF. Evaluative criteria for qualitative research in health care: controversies and recommendations. Ann Fam Med. 2008;6(4):331–9.
Tong A, Sainsbury P, Craig J. Consolidated criteria for reportingqualitative research (COREQ): a 32-item checklist for interviews and focus groups. Int J Qual Health Care. 2007;19(6):349–57.
Article PubMed Google Scholar
Moher D, Schulz KF, Simera I, Altman DG. Guidance for developers of health research reporting guidelines. PLoS Med. 2010;7(2):e1000217.
Moher D, Tetzlaff J, Tricco AC, Sampson M, Altman DG. Epidemiology and reporting characteristics of systematic reviews. PLoS Med. 2007;4(3):e78.
Chan AW, Altman DG. Epidemiology and reporting of randomised trials published in PubMed journals. Lancet. 2005;365(9465):1159–62.
Alshurafa M, Briel M, Akl EA, Haines T, Moayyedi P, Gentles SJ, Rios L, Tran C, Bhatnagar N, Lamontagne F, et al. Inconsistent definitions for intention-to-treat in relation to missing outcome data: systematic review of the methods literature. PLoS One. 2012;7(11):e49163.
Article CAS PubMed PubMed Central Google Scholar
Gentles SJ, Charles C, Ploeg J, McKibbon KA. Sampling in qualitative research: insights from an overview of the methods literature. Qual Rep. 2015;20(11):1772–89.
Harzing A-W, Alakangas S. Google Scholar, Scopus and the Web of Science: a longitudinal and cross-disciplinary comparison. Scientometrics. 2016;106(2):787–804.
Harzing A-WK, van der Wal R. Google Scholar as a new source for citation analysis. Ethics Sci Environ Polit. 2008;8(1):61–73.
Kousha K, Thelwall M. Google Scholar citations and Google Web/URL citations: a multi‐discipline exploratory analysis. J Assoc Inf Sci Technol. 2007;58(7):1055–65.
Hirsch JE. An index to quantify an individual’s scientific research output. Proc Natl Acad Sci U S A. 2005;102(46):16569–72.
Booth A, Carroll C. How to build up the actionable knowledge base: the role of ‘best fit’ framework synthesis for studies of improvement in healthcare. BMJ Quality Safety. 2015;24(11):700–8.
Carroll C, Booth A, Leaviss J, Rick J. “Best fit” framework synthesis: refining the method. BMC Med Res Methodol. 2013;13(1):37.
Carroll C, Booth A, Cooper K. A worked example of “best fit” framework synthesis: a systematic review of views concerning the taking of some potential chemopreventive agents. BMC Med Res Methodol. 2011;11(1):29.
Cohen MZ, Kahn DL, Steeves DL. Hermeneutic phenomenological research: a practical guide for nurse researchers. Thousand Oaks: Sage; 2000.
Noblit GW, Hare RD. Meta-ethnography: synthesizing qualitative studies. Newbury Park: Sage; 1988.
Book Google Scholar
Melendez-Torres GJ, Grant S, Bonell C. A systematic review and critical appraisal of qualitative metasynthetic practice in public health to develop a taxonomy of operations of reciprocal translation. Res Synthesis Methods. 2015;6(4):357–71.
Article CAS Google Scholar
Glaser BG, Strauss A. The discovery of grounded theory. Chicago: Aldine; 1967.
Dixon-Woods M, Agarwal S, Young B, Jones D, Sutton A. Integrative approaches to qualitative and quantitative evidence. In: UK National Health Service. 2004. p. 1–44.
Download references
Acknowledgements
Not applicable.
There was no funding for this work.
Availability of data and materials
The systematic methods overview used as a worked example in this article (Gentles SJ, Charles C, Ploeg J, McKibbon KA: Sampling in qualitative research: insights from an overview of the methods literature. The Qual Rep 2015, 20(11):1772-1789) is available from http://nsuworks.nova.edu/tqr/vol20/iss11/5 .
Authors’ contributions
SJG wrote the first draft of this article, with CC contributing to drafting. All authors contributed to revising the manuscript. All authors except CC (deceased) approved the final draft. SJG, CC, KAB, and JP were involved in developing methods for the systematic methods overview on sampling.
Authors’ information
Competing interests.
The authors declare that they have no competing interests.
Consent for publication
Ethics approval and consent to participate, author information, authors and affiliations.
Department of Clinical Epidemiology and Biostatistics, McMaster University, Hamilton, Ontario, Canada
Stephen J. Gentles, Cathy Charles & K. Ann McKibbon
Faculty of Social Work, University of Calgary, Alberta, Canada
David B. Nicholas
School of Nursing, McMaster University, Hamilton, Ontario, Canada
Jenny Ploeg
CanChild Centre for Childhood Disability Research, McMaster University, 1400 Main Street West, IAHS 408, Hamilton, ON, L8S 1C7, Canada
Stephen J. Gentles
You can also search for this author in PubMed Google Scholar
Corresponding author
Correspondence to Stephen J. Gentles .
Additional information
Cathy Charles is deceased
Additional file
Additional file 1:.
Submitted: Analysis_matrices. (DOC 330 kb)
Rights and permissions
Open Access This article is distributed under the terms of the Creative Commons Attribution 4.0 International License ( http://creativecommons.org/licenses/by/4.0/ ), which permits unrestricted use, distribution, and reproduction in any medium, provided you give appropriate credit to the original author(s) and the source, provide a link to the Creative Commons license, and indicate if changes were made. The Creative Commons Public Domain Dedication waiver ( http://creativecommons.org/publicdomain/zero/1.0/ ) applies to the data made available in this article, unless otherwise stated.
Reprints and permissions
About this article
Cite this article.
Gentles, S.J., Charles, C., Nicholas, D.B. et al. Reviewing the research methods literature: principles and strategies illustrated by a systematic overview of sampling in qualitative research. Syst Rev 5 , 172 (2016). https://doi.org/10.1186/s13643-016-0343-0
Download citation
Received : 06 June 2016
Accepted : 14 September 2016
Published : 11 October 2016
DOI : https://doi.org/10.1186/s13643-016-0343-0
Share this article
Anyone you share the following link with will be able to read this content:
Sorry, a shareable link is not currently available for this article.
Provided by the Springer Nature SharedIt content-sharing initiative
- Systematic review
- Literature selection
- Research methods
- Research methodology
- Overview of methods
- Systematic methods overview
- Review methods
Systematic Reviews
ISSN: 2046-4053
- Submission enquiries: Access here and click Contact Us
- General enquiries: [email protected]
Criteria for Good Qualitative Research: A Comprehensive Review
- Regular Article
- Open access
- Published: 18 September 2021
- Volume 31 , pages 679–689, ( 2022 )
Cite this article
You have full access to this open access article
- Drishti Yadav ORCID: orcid.org/0000-0002-2974-0323 1
102k Accesses
48 Citations
70 Altmetric
Explore all metrics
This review aims to synthesize a published set of evaluative criteria for good qualitative research. The aim is to shed light on existing standards for assessing the rigor of qualitative research encompassing a range of epistemological and ontological standpoints. Using a systematic search strategy, published journal articles that deliberate criteria for rigorous research were identified. Then, references of relevant articles were surveyed to find noteworthy, distinct, and well-defined pointers to good qualitative research. This review presents an investigative assessment of the pivotal features in qualitative research that can permit the readers to pass judgment on its quality and to condemn it as good research when objectively and adequately utilized. Overall, this review underlines the crux of qualitative research and accentuates the necessity to evaluate such research by the very tenets of its being. It also offers some prospects and recommendations to improve the quality of qualitative research. Based on the findings of this review, it is concluded that quality criteria are the aftereffect of socio-institutional procedures and existing paradigmatic conducts. Owing to the paradigmatic diversity of qualitative research, a single and specific set of quality criteria is neither feasible nor anticipated. Since qualitative research is not a cohesive discipline, researchers need to educate and familiarize themselves with applicable norms and decisive factors to evaluate qualitative research from within its theoretical and methodological framework of origin.
Similar content being viewed by others
Good Qualitative Research: Opening up the Debate
Beyond qualitative/quantitative structuralism: the positivist qualitative research and the paradigmatic disclaimer.
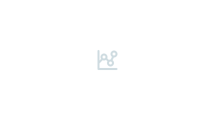
What is Qualitative in Qualitative Research
Avoid common mistakes on your manuscript.
Introduction
“… It is important to regularly dialogue about what makes for good qualitative research” (Tracy, 2010 , p. 837)
To decide what represents good qualitative research is highly debatable. There are numerous methods that are contained within qualitative research and that are established on diverse philosophical perspectives. Bryman et al., ( 2008 , p. 262) suggest that “It is widely assumed that whereas quality criteria for quantitative research are well‐known and widely agreed, this is not the case for qualitative research.” Hence, the question “how to evaluate the quality of qualitative research” has been continuously debated. There are many areas of science and technology wherein these debates on the assessment of qualitative research have taken place. Examples include various areas of psychology: general psychology (Madill et al., 2000 ); counseling psychology (Morrow, 2005 ); and clinical psychology (Barker & Pistrang, 2005 ), and other disciplines of social sciences: social policy (Bryman et al., 2008 ); health research (Sparkes, 2001 ); business and management research (Johnson et al., 2006 ); information systems (Klein & Myers, 1999 ); and environmental studies (Reid & Gough, 2000 ). In the literature, these debates are enthused by the impression that the blanket application of criteria for good qualitative research developed around the positivist paradigm is improper. Such debates are based on the wide range of philosophical backgrounds within which qualitative research is conducted (e.g., Sandberg, 2000 ; Schwandt, 1996 ). The existence of methodological diversity led to the formulation of different sets of criteria applicable to qualitative research.
Among qualitative researchers, the dilemma of governing the measures to assess the quality of research is not a new phenomenon, especially when the virtuous triad of objectivity, reliability, and validity (Spencer et al., 2004 ) are not adequate. Occasionally, the criteria of quantitative research are used to evaluate qualitative research (Cohen & Crabtree, 2008 ; Lather, 2004 ). Indeed, Howe ( 2004 ) claims that the prevailing paradigm in educational research is scientifically based experimental research. Hypotheses and conjectures about the preeminence of quantitative research can weaken the worth and usefulness of qualitative research by neglecting the prominence of harmonizing match for purpose on research paradigm, the epistemological stance of the researcher, and the choice of methodology. Researchers have been reprimanded concerning this in “paradigmatic controversies, contradictions, and emerging confluences” (Lincoln & Guba, 2000 ).
In general, qualitative research tends to come from a very different paradigmatic stance and intrinsically demands distinctive and out-of-the-ordinary criteria for evaluating good research and varieties of research contributions that can be made. This review attempts to present a series of evaluative criteria for qualitative researchers, arguing that their choice of criteria needs to be compatible with the unique nature of the research in question (its methodology, aims, and assumptions). This review aims to assist researchers in identifying some of the indispensable features or markers of high-quality qualitative research. In a nutshell, the purpose of this systematic literature review is to analyze the existing knowledge on high-quality qualitative research and to verify the existence of research studies dealing with the critical assessment of qualitative research based on the concept of diverse paradigmatic stances. Contrary to the existing reviews, this review also suggests some critical directions to follow to improve the quality of qualitative research in different epistemological and ontological perspectives. This review is also intended to provide guidelines for the acceleration of future developments and dialogues among qualitative researchers in the context of assessing the qualitative research.
The rest of this review article is structured in the following fashion: Sect. Methods describes the method followed for performing this review. Section Criteria for Evaluating Qualitative Studies provides a comprehensive description of the criteria for evaluating qualitative studies. This section is followed by a summary of the strategies to improve the quality of qualitative research in Sect. Improving Quality: Strategies . Section How to Assess the Quality of the Research Findings? provides details on how to assess the quality of the research findings. After that, some of the quality checklists (as tools to evaluate quality) are discussed in Sect. Quality Checklists: Tools for Assessing the Quality . At last, the review ends with the concluding remarks presented in Sect. Conclusions, Future Directions and Outlook . Some prospects in qualitative research for enhancing its quality and usefulness in the social and techno-scientific research community are also presented in Sect. Conclusions, Future Directions and Outlook .
For this review, a comprehensive literature search was performed from many databases using generic search terms such as Qualitative Research , Criteria , etc . The following databases were chosen for the literature search based on the high number of results: IEEE Explore, ScienceDirect, PubMed, Google Scholar, and Web of Science. The following keywords (and their combinations using Boolean connectives OR/AND) were adopted for the literature search: qualitative research, criteria, quality, assessment, and validity. The synonyms for these keywords were collected and arranged in a logical structure (see Table 1 ). All publications in journals and conference proceedings later than 1950 till 2021 were considered for the search. Other articles extracted from the references of the papers identified in the electronic search were also included. A large number of publications on qualitative research were retrieved during the initial screening. Hence, to include the searches with the main focus on criteria for good qualitative research, an inclusion criterion was utilized in the search string.
From the selected databases, the search retrieved a total of 765 publications. Then, the duplicate records were removed. After that, based on the title and abstract, the remaining 426 publications were screened for their relevance by using the following inclusion and exclusion criteria (see Table 2 ). Publications focusing on evaluation criteria for good qualitative research were included, whereas those works which delivered theoretical concepts on qualitative research were excluded. Based on the screening and eligibility, 45 research articles were identified that offered explicit criteria for evaluating the quality of qualitative research and were found to be relevant to this review.
Figure 1 illustrates the complete review process in the form of PRISMA flow diagram. PRISMA, i.e., “preferred reporting items for systematic reviews and meta-analyses” is employed in systematic reviews to refine the quality of reporting.
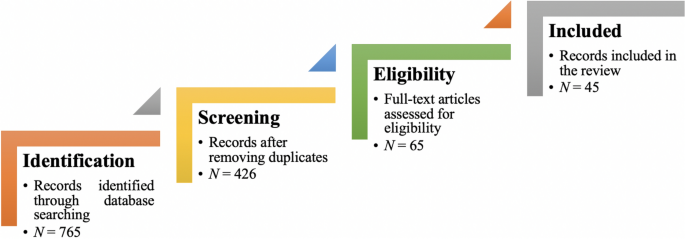
PRISMA flow diagram illustrating the search and inclusion process. N represents the number of records
Criteria for Evaluating Qualitative Studies
Fundamental criteria: general research quality.
Various researchers have put forward criteria for evaluating qualitative research, which have been summarized in Table 3 . Also, the criteria outlined in Table 4 effectively deliver the various approaches to evaluate and assess the quality of qualitative work. The entries in Table 4 are based on Tracy’s “Eight big‐tent criteria for excellent qualitative research” (Tracy, 2010 ). Tracy argues that high-quality qualitative work should formulate criteria focusing on the worthiness, relevance, timeliness, significance, morality, and practicality of the research topic, and the ethical stance of the research itself. Researchers have also suggested a series of questions as guiding principles to assess the quality of a qualitative study (Mays & Pope, 2020 ). Nassaji ( 2020 ) argues that good qualitative research should be robust, well informed, and thoroughly documented.
Qualitative Research: Interpretive Paradigms
All qualitative researchers follow highly abstract principles which bring together beliefs about ontology, epistemology, and methodology. These beliefs govern how the researcher perceives and acts. The net, which encompasses the researcher’s epistemological, ontological, and methodological premises, is referred to as a paradigm, or an interpretive structure, a “Basic set of beliefs that guides action” (Guba, 1990 ). Four major interpretive paradigms structure the qualitative research: positivist and postpositivist, constructivist interpretive, critical (Marxist, emancipatory), and feminist poststructural. The complexity of these four abstract paradigms increases at the level of concrete, specific interpretive communities. Table 5 presents these paradigms and their assumptions, including their criteria for evaluating research, and the typical form that an interpretive or theoretical statement assumes in each paradigm. Moreover, for evaluating qualitative research, quantitative conceptualizations of reliability and validity are proven to be incompatible (Horsburgh, 2003 ). In addition, a series of questions have been put forward in the literature to assist a reviewer (who is proficient in qualitative methods) for meticulous assessment and endorsement of qualitative research (Morse, 2003 ). Hammersley ( 2007 ) also suggests that guiding principles for qualitative research are advantageous, but methodological pluralism should not be simply acknowledged for all qualitative approaches. Seale ( 1999 ) also points out the significance of methodological cognizance in research studies.
Table 5 reflects that criteria for assessing the quality of qualitative research are the aftermath of socio-institutional practices and existing paradigmatic standpoints. Owing to the paradigmatic diversity of qualitative research, a single set of quality criteria is neither possible nor desirable. Hence, the researchers must be reflexive about the criteria they use in the various roles they play within their research community.
Improving Quality: Strategies
Another critical question is “How can the qualitative researchers ensure that the abovementioned quality criteria can be met?” Lincoln and Guba ( 1986 ) delineated several strategies to intensify each criteria of trustworthiness. Other researchers (Merriam & Tisdell, 2016 ; Shenton, 2004 ) also presented such strategies. A brief description of these strategies is shown in Table 6 .
It is worth mentioning that generalizability is also an integral part of qualitative research (Hays & McKibben, 2021 ). In general, the guiding principle pertaining to generalizability speaks about inducing and comprehending knowledge to synthesize interpretive components of an underlying context. Table 7 summarizes the main metasynthesis steps required to ascertain generalizability in qualitative research.
Figure 2 reflects the crucial components of a conceptual framework and their contribution to decisions regarding research design, implementation, and applications of results to future thinking, study, and practice (Johnson et al., 2020 ). The synergy and interrelationship of these components signifies their role to different stances of a qualitative research study.
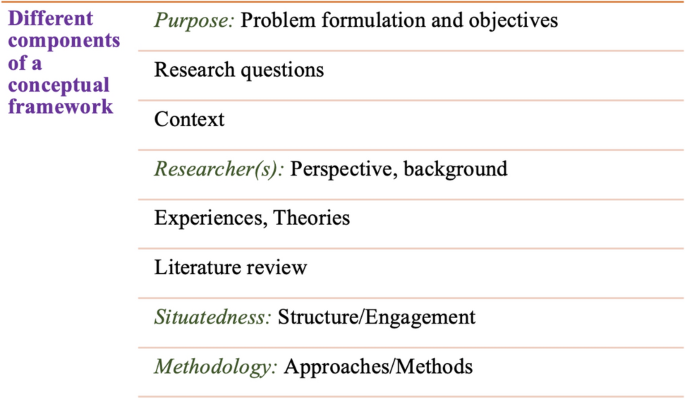
Essential elements of a conceptual framework
In a nutshell, to assess the rationale of a study, its conceptual framework and research question(s), quality criteria must take account of the following: lucid context for the problem statement in the introduction; well-articulated research problems and questions; precise conceptual framework; distinct research purpose; and clear presentation and investigation of the paradigms. These criteria would expedite the quality of qualitative research.
How to Assess the Quality of the Research Findings?
The inclusion of quotes or similar research data enhances the confirmability in the write-up of the findings. The use of expressions (for instance, “80% of all respondents agreed that” or “only one of the interviewees mentioned that”) may also quantify qualitative findings (Stenfors et al., 2020 ). On the other hand, the persuasive reason for “why this may not help in intensifying the research” has also been provided (Monrouxe & Rees, 2020 ). Further, the Discussion and Conclusion sections of an article also prove robust markers of high-quality qualitative research, as elucidated in Table 8 .
Quality Checklists: Tools for Assessing the Quality
Numerous checklists are available to speed up the assessment of the quality of qualitative research. However, if used uncritically and recklessly concerning the research context, these checklists may be counterproductive. I recommend that such lists and guiding principles may assist in pinpointing the markers of high-quality qualitative research. However, considering enormous variations in the authors’ theoretical and philosophical contexts, I would emphasize that high dependability on such checklists may say little about whether the findings can be applied in your setting. A combination of such checklists might be appropriate for novice researchers. Some of these checklists are listed below:
The most commonly used framework is Consolidated Criteria for Reporting Qualitative Research (COREQ) (Tong et al., 2007 ). This framework is recommended by some journals to be followed by the authors during article submission.
Standards for Reporting Qualitative Research (SRQR) is another checklist that has been created particularly for medical education (O’Brien et al., 2014 ).
Also, Tracy ( 2010 ) and Critical Appraisal Skills Programme (CASP, 2021 ) offer criteria for qualitative research relevant across methods and approaches.
Further, researchers have also outlined different criteria as hallmarks of high-quality qualitative research. For instance, the “Road Trip Checklist” (Epp & Otnes, 2021 ) provides a quick reference to specific questions to address different elements of high-quality qualitative research.
Conclusions, Future Directions, and Outlook
This work presents a broad review of the criteria for good qualitative research. In addition, this article presents an exploratory analysis of the essential elements in qualitative research that can enable the readers of qualitative work to judge it as good research when objectively and adequately utilized. In this review, some of the essential markers that indicate high-quality qualitative research have been highlighted. I scope them narrowly to achieve rigor in qualitative research and note that they do not completely cover the broader considerations necessary for high-quality research. This review points out that a universal and versatile one-size-fits-all guideline for evaluating the quality of qualitative research does not exist. In other words, this review also emphasizes the non-existence of a set of common guidelines among qualitative researchers. In unison, this review reinforces that each qualitative approach should be treated uniquely on account of its own distinctive features for different epistemological and disciplinary positions. Owing to the sensitivity of the worth of qualitative research towards the specific context and the type of paradigmatic stance, researchers should themselves analyze what approaches can be and must be tailored to ensemble the distinct characteristics of the phenomenon under investigation. Although this article does not assert to put forward a magic bullet and to provide a one-stop solution for dealing with dilemmas about how, why, or whether to evaluate the “goodness” of qualitative research, it offers a platform to assist the researchers in improving their qualitative studies. This work provides an assembly of concerns to reflect on, a series of questions to ask, and multiple sets of criteria to look at, when attempting to determine the quality of qualitative research. Overall, this review underlines the crux of qualitative research and accentuates the need to evaluate such research by the very tenets of its being. Bringing together the vital arguments and delineating the requirements that good qualitative research should satisfy, this review strives to equip the researchers as well as reviewers to make well-versed judgment about the worth and significance of the qualitative research under scrutiny. In a nutshell, a comprehensive portrayal of the research process (from the context of research to the research objectives, research questions and design, speculative foundations, and from approaches of collecting data to analyzing the results, to deriving inferences) frequently proliferates the quality of a qualitative research.
Prospects : A Road Ahead for Qualitative Research
Irrefutably, qualitative research is a vivacious and evolving discipline wherein different epistemological and disciplinary positions have their own characteristics and importance. In addition, not surprisingly, owing to the sprouting and varied features of qualitative research, no consensus has been pulled off till date. Researchers have reflected various concerns and proposed several recommendations for editors and reviewers on conducting reviews of critical qualitative research (Levitt et al., 2021 ; McGinley et al., 2021 ). Following are some prospects and a few recommendations put forward towards the maturation of qualitative research and its quality evaluation:
In general, most of the manuscript and grant reviewers are not qualitative experts. Hence, it is more likely that they would prefer to adopt a broad set of criteria. However, researchers and reviewers need to keep in mind that it is inappropriate to utilize the same approaches and conducts among all qualitative research. Therefore, future work needs to focus on educating researchers and reviewers about the criteria to evaluate qualitative research from within the suitable theoretical and methodological context.
There is an urgent need to refurbish and augment critical assessment of some well-known and widely accepted tools (including checklists such as COREQ, SRQR) to interrogate their applicability on different aspects (along with their epistemological ramifications).
Efforts should be made towards creating more space for creativity, experimentation, and a dialogue between the diverse traditions of qualitative research. This would potentially help to avoid the enforcement of one's own set of quality criteria on the work carried out by others.
Moreover, journal reviewers need to be aware of various methodological practices and philosophical debates.
It is pivotal to highlight the expressions and considerations of qualitative researchers and bring them into a more open and transparent dialogue about assessing qualitative research in techno-scientific, academic, sociocultural, and political rooms.
Frequent debates on the use of evaluative criteria are required to solve some potentially resolved issues (including the applicability of a single set of criteria in multi-disciplinary aspects). Such debates would not only benefit the group of qualitative researchers themselves, but primarily assist in augmenting the well-being and vivacity of the entire discipline.
To conclude, I speculate that the criteria, and my perspective, may transfer to other methods, approaches, and contexts. I hope that they spark dialog and debate – about criteria for excellent qualitative research and the underpinnings of the discipline more broadly – and, therefore, help improve the quality of a qualitative study. Further, I anticipate that this review will assist the researchers to contemplate on the quality of their own research, to substantiate research design and help the reviewers to review qualitative research for journals. On a final note, I pinpoint the need to formulate a framework (encompassing the prerequisites of a qualitative study) by the cohesive efforts of qualitative researchers of different disciplines with different theoretic-paradigmatic origins. I believe that tailoring such a framework (of guiding principles) paves the way for qualitative researchers to consolidate the status of qualitative research in the wide-ranging open science debate. Dialogue on this issue across different approaches is crucial for the impending prospects of socio-techno-educational research.
Amin, M. E. K., Nørgaard, L. S., Cavaco, A. M., Witry, M. J., Hillman, L., Cernasev, A., & Desselle, S. P. (2020). Establishing trustworthiness and authenticity in qualitative pharmacy research. Research in Social and Administrative Pharmacy, 16 (10), 1472–1482.
Article Google Scholar
Barker, C., & Pistrang, N. (2005). Quality criteria under methodological pluralism: Implications for conducting and evaluating research. American Journal of Community Psychology, 35 (3–4), 201–212.
Bryman, A., Becker, S., & Sempik, J. (2008). Quality criteria for quantitative, qualitative and mixed methods research: A view from social policy. International Journal of Social Research Methodology, 11 (4), 261–276.
Caelli, K., Ray, L., & Mill, J. (2003). ‘Clear as mud’: Toward greater clarity in generic qualitative research. International Journal of Qualitative Methods, 2 (2), 1–13.
CASP (2021). CASP checklists. Retrieved May 2021 from https://casp-uk.net/casp-tools-checklists/
Cohen, D. J., & Crabtree, B. F. (2008). Evaluative criteria for qualitative research in health care: Controversies and recommendations. The Annals of Family Medicine, 6 (4), 331–339.
Denzin, N. K., & Lincoln, Y. S. (2005). Introduction: The discipline and practice of qualitative research. In N. K. Denzin & Y. S. Lincoln (Eds.), The sage handbook of qualitative research (pp. 1–32). Sage Publications Ltd.
Google Scholar
Elliott, R., Fischer, C. T., & Rennie, D. L. (1999). Evolving guidelines for publication of qualitative research studies in psychology and related fields. British Journal of Clinical Psychology, 38 (3), 215–229.
Epp, A. M., & Otnes, C. C. (2021). High-quality qualitative research: Getting into gear. Journal of Service Research . https://doi.org/10.1177/1094670520961445
Guba, E. G. (1990). The paradigm dialog. In Alternative paradigms conference, mar, 1989, Indiana u, school of education, San Francisco, ca, us . Sage Publications, Inc.
Hammersley, M. (2007). The issue of quality in qualitative research. International Journal of Research and Method in Education, 30 (3), 287–305.
Haven, T. L., Errington, T. M., Gleditsch, K. S., van Grootel, L., Jacobs, A. M., Kern, F. G., & Mokkink, L. B. (2020). Preregistering qualitative research: A Delphi study. International Journal of Qualitative Methods, 19 , 1609406920976417.
Hays, D. G., & McKibben, W. B. (2021). Promoting rigorous research: Generalizability and qualitative research. Journal of Counseling and Development, 99 (2), 178–188.
Horsburgh, D. (2003). Evaluation of qualitative research. Journal of Clinical Nursing, 12 (2), 307–312.
Howe, K. R. (2004). A critique of experimentalism. Qualitative Inquiry, 10 (1), 42–46.
Johnson, J. L., Adkins, D., & Chauvin, S. (2020). A review of the quality indicators of rigor in qualitative research. American Journal of Pharmaceutical Education, 84 (1), 7120.
Johnson, P., Buehring, A., Cassell, C., & Symon, G. (2006). Evaluating qualitative management research: Towards a contingent criteriology. International Journal of Management Reviews, 8 (3), 131–156.
Klein, H. K., & Myers, M. D. (1999). A set of principles for conducting and evaluating interpretive field studies in information systems. MIS Quarterly, 23 (1), 67–93.
Lather, P. (2004). This is your father’s paradigm: Government intrusion and the case of qualitative research in education. Qualitative Inquiry, 10 (1), 15–34.
Levitt, H. M., Morrill, Z., Collins, K. M., & Rizo, J. L. (2021). The methodological integrity of critical qualitative research: Principles to support design and research review. Journal of Counseling Psychology, 68 (3), 357.
Lincoln, Y. S., & Guba, E. G. (1986). But is it rigorous? Trustworthiness and authenticity in naturalistic evaluation. New Directions for Program Evaluation, 1986 (30), 73–84.
Lincoln, Y. S., & Guba, E. G. (2000). Paradigmatic controversies, contradictions and emerging confluences. In N. K. Denzin & Y. S. Lincoln (Eds.), Handbook of qualitative research (2nd ed., pp. 163–188). Sage Publications.
Madill, A., Jordan, A., & Shirley, C. (2000). Objectivity and reliability in qualitative analysis: Realist, contextualist and radical constructionist epistemologies. British Journal of Psychology, 91 (1), 1–20.
Mays, N., & Pope, C. (2020). Quality in qualitative research. Qualitative Research in Health Care . https://doi.org/10.1002/9781119410867.ch15
McGinley, S., Wei, W., Zhang, L., & Zheng, Y. (2021). The state of qualitative research in hospitality: A 5-year review 2014 to 2019. Cornell Hospitality Quarterly, 62 (1), 8–20.
Merriam, S., & Tisdell, E. (2016). Qualitative research: A guide to design and implementation. San Francisco, US.
Meyer, M., & Dykes, J. (2019). Criteria for rigor in visualization design study. IEEE Transactions on Visualization and Computer Graphics, 26 (1), 87–97.
Monrouxe, L. V., & Rees, C. E. (2020). When I say… quantification in qualitative research. Medical Education, 54 (3), 186–187.
Morrow, S. L. (2005). Quality and trustworthiness in qualitative research in counseling psychology. Journal of Counseling Psychology, 52 (2), 250.
Morse, J. M. (2003). A review committee’s guide for evaluating qualitative proposals. Qualitative Health Research, 13 (6), 833–851.
Nassaji, H. (2020). Good qualitative research. Language Teaching Research, 24 (4), 427–431.
O’Brien, B. C., Harris, I. B., Beckman, T. J., Reed, D. A., & Cook, D. A. (2014). Standards for reporting qualitative research: A synthesis of recommendations. Academic Medicine, 89 (9), 1245–1251.
O’Connor, C., & Joffe, H. (2020). Intercoder reliability in qualitative research: Debates and practical guidelines. International Journal of Qualitative Methods, 19 , 1609406919899220.
Reid, A., & Gough, S. (2000). Guidelines for reporting and evaluating qualitative research: What are the alternatives? Environmental Education Research, 6 (1), 59–91.
Rocco, T. S. (2010). Criteria for evaluating qualitative studies. Human Resource Development International . https://doi.org/10.1080/13678868.2010.501959
Sandberg, J. (2000). Understanding human competence at work: An interpretative approach. Academy of Management Journal, 43 (1), 9–25.
Schwandt, T. A. (1996). Farewell to criteriology. Qualitative Inquiry, 2 (1), 58–72.
Seale, C. (1999). Quality in qualitative research. Qualitative Inquiry, 5 (4), 465–478.
Shenton, A. K. (2004). Strategies for ensuring trustworthiness in qualitative research projects. Education for Information, 22 (2), 63–75.
Sparkes, A. C. (2001). Myth 94: Qualitative health researchers will agree about validity. Qualitative Health Research, 11 (4), 538–552.
Spencer, L., Ritchie, J., Lewis, J., & Dillon, L. (2004). Quality in qualitative evaluation: A framework for assessing research evidence.
Stenfors, T., Kajamaa, A., & Bennett, D. (2020). How to assess the quality of qualitative research. The Clinical Teacher, 17 (6), 596–599.
Taylor, E. W., Beck, J., & Ainsworth, E. (2001). Publishing qualitative adult education research: A peer review perspective. Studies in the Education of Adults, 33 (2), 163–179.
Tong, A., Sainsbury, P., & Craig, J. (2007). Consolidated criteria for reporting qualitative research (COREQ): A 32-item checklist for interviews and focus groups. International Journal for Quality in Health Care, 19 (6), 349–357.
Tracy, S. J. (2010). Qualitative quality: Eight “big-tent” criteria for excellent qualitative research. Qualitative Inquiry, 16 (10), 837–851.
Download references
Open access funding provided by TU Wien (TUW).
Author information
Authors and affiliations.
Faculty of Informatics, Technische Universität Wien, 1040, Vienna, Austria
Drishti Yadav
You can also search for this author in PubMed Google Scholar
Corresponding author
Correspondence to Drishti Yadav .
Ethics declarations
Conflict of interest.
The author declares no conflict of interest.
Additional information
Publisher's note.
Springer Nature remains neutral with regard to jurisdictional claims in published maps and institutional affiliations.
Rights and permissions
Open Access This article is licensed under a Creative Commons Attribution 4.0 International License, which permits use, sharing, adaptation, distribution and reproduction in any medium or format, as long as you give appropriate credit to the original author(s) and the source, provide a link to the Creative Commons licence, and indicate if changes were made. The images or other third party material in this article are included in the article's Creative Commons licence, unless indicated otherwise in a credit line to the material. If material is not included in the article's Creative Commons licence and your intended use is not permitted by statutory regulation or exceeds the permitted use, you will need to obtain permission directly from the copyright holder. To view a copy of this licence, visit http://creativecommons.org/licenses/by/4.0/ .
Reprints and permissions
About this article
Yadav, D. Criteria for Good Qualitative Research: A Comprehensive Review. Asia-Pacific Edu Res 31 , 679–689 (2022). https://doi.org/10.1007/s40299-021-00619-0
Download citation
Accepted : 28 August 2021
Published : 18 September 2021
Issue Date : December 2022
DOI : https://doi.org/10.1007/s40299-021-00619-0
Share this article
Anyone you share the following link with will be able to read this content:
Sorry, a shareable link is not currently available for this article.
Provided by the Springer Nature SharedIt content-sharing initiative
- Qualitative research
- Evaluative criteria
- Find a journal
- Publish with us
- Track your research
- Open access
- Published: 27 May 2020
How to use and assess qualitative research methods
- Loraine Busetto ORCID: orcid.org/0000-0002-9228-7875 1 ,
- Wolfgang Wick 1 , 2 &
- Christoph Gumbinger 1
Neurological Research and Practice volume 2 , Article number: 14 ( 2020 ) Cite this article
777k Accesses
369 Citations
90 Altmetric
Metrics details
This paper aims to provide an overview of the use and assessment of qualitative research methods in the health sciences. Qualitative research can be defined as the study of the nature of phenomena and is especially appropriate for answering questions of why something is (not) observed, assessing complex multi-component interventions, and focussing on intervention improvement. The most common methods of data collection are document study, (non-) participant observations, semi-structured interviews and focus groups. For data analysis, field-notes and audio-recordings are transcribed into protocols and transcripts, and coded using qualitative data management software. Criteria such as checklists, reflexivity, sampling strategies, piloting, co-coding, member-checking and stakeholder involvement can be used to enhance and assess the quality of the research conducted. Using qualitative in addition to quantitative designs will equip us with better tools to address a greater range of research problems, and to fill in blind spots in current neurological research and practice.
The aim of this paper is to provide an overview of qualitative research methods, including hands-on information on how they can be used, reported and assessed. This article is intended for beginning qualitative researchers in the health sciences as well as experienced quantitative researchers who wish to broaden their understanding of qualitative research.
What is qualitative research?
Qualitative research is defined as “the study of the nature of phenomena”, including “their quality, different manifestations, the context in which they appear or the perspectives from which they can be perceived” , but excluding “their range, frequency and place in an objectively determined chain of cause and effect” [ 1 ]. This formal definition can be complemented with a more pragmatic rule of thumb: qualitative research generally includes data in form of words rather than numbers [ 2 ].
Why conduct qualitative research?
Because some research questions cannot be answered using (only) quantitative methods. For example, one Australian study addressed the issue of why patients from Aboriginal communities often present late or not at all to specialist services offered by tertiary care hospitals. Using qualitative interviews with patients and staff, it found one of the most significant access barriers to be transportation problems, including some towns and communities simply not having a bus service to the hospital [ 3 ]. A quantitative study could have measured the number of patients over time or even looked at possible explanatory factors – but only those previously known or suspected to be of relevance. To discover reasons for observed patterns, especially the invisible or surprising ones, qualitative designs are needed.
While qualitative research is common in other fields, it is still relatively underrepresented in health services research. The latter field is more traditionally rooted in the evidence-based-medicine paradigm, as seen in " research that involves testing the effectiveness of various strategies to achieve changes in clinical practice, preferably applying randomised controlled trial study designs (...) " [ 4 ]. This focus on quantitative research and specifically randomised controlled trials (RCT) is visible in the idea of a hierarchy of research evidence which assumes that some research designs are objectively better than others, and that choosing a "lesser" design is only acceptable when the better ones are not practically or ethically feasible [ 5 , 6 ]. Others, however, argue that an objective hierarchy does not exist, and that, instead, the research design and methods should be chosen to fit the specific research question at hand – "questions before methods" [ 2 , 7 , 8 , 9 ]. This means that even when an RCT is possible, some research problems require a different design that is better suited to addressing them. Arguing in JAMA, Berwick uses the example of rapid response teams in hospitals, which he describes as " a complex, multicomponent intervention – essentially a process of social change" susceptible to a range of different context factors including leadership or organisation history. According to him, "[in] such complex terrain, the RCT is an impoverished way to learn. Critics who use it as a truth standard in this context are incorrect" [ 8 ] . Instead of limiting oneself to RCTs, Berwick recommends embracing a wider range of methods , including qualitative ones, which for "these specific applications, (...) are not compromises in learning how to improve; they are superior" [ 8 ].
Research problems that can be approached particularly well using qualitative methods include assessing complex multi-component interventions or systems (of change), addressing questions beyond “what works”, towards “what works for whom when, how and why”, and focussing on intervention improvement rather than accreditation [ 7 , 9 , 10 , 11 , 12 ]. Using qualitative methods can also help shed light on the “softer” side of medical treatment. For example, while quantitative trials can measure the costs and benefits of neuro-oncological treatment in terms of survival rates or adverse effects, qualitative research can help provide a better understanding of patient or caregiver stress, visibility of illness or out-of-pocket expenses.
How to conduct qualitative research?
Given that qualitative research is characterised by flexibility, openness and responsivity to context, the steps of data collection and analysis are not as separate and consecutive as they tend to be in quantitative research [ 13 , 14 ]. As Fossey puts it : “sampling, data collection, analysis and interpretation are related to each other in a cyclical (iterative) manner, rather than following one after another in a stepwise approach” [ 15 ]. The researcher can make educated decisions with regard to the choice of method, how they are implemented, and to which and how many units they are applied [ 13 ]. As shown in Fig. 1 , this can involve several back-and-forth steps between data collection and analysis where new insights and experiences can lead to adaption and expansion of the original plan. Some insights may also necessitate a revision of the research question and/or the research design as a whole. The process ends when saturation is achieved, i.e. when no relevant new information can be found (see also below: sampling and saturation). For reasons of transparency, it is essential for all decisions as well as the underlying reasoning to be well-documented.
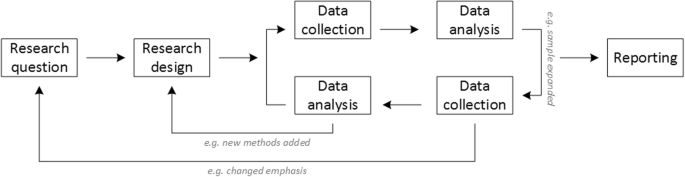
Iterative research process
While it is not always explicitly addressed, qualitative methods reflect a different underlying research paradigm than quantitative research (e.g. constructivism or interpretivism as opposed to positivism). The choice of methods can be based on the respective underlying substantive theory or theoretical framework used by the researcher [ 2 ].
Data collection
The methods of qualitative data collection most commonly used in health research are document study, observations, semi-structured interviews and focus groups [ 1 , 14 , 16 , 17 ].
Document study
Document study (also called document analysis) refers to the review by the researcher of written materials [ 14 ]. These can include personal and non-personal documents such as archives, annual reports, guidelines, policy documents, diaries or letters.
Observations
Observations are particularly useful to gain insights into a certain setting and actual behaviour – as opposed to reported behaviour or opinions [ 13 ]. Qualitative observations can be either participant or non-participant in nature. In participant observations, the observer is part of the observed setting, for example a nurse working in an intensive care unit [ 18 ]. In non-participant observations, the observer is “on the outside looking in”, i.e. present in but not part of the situation, trying not to influence the setting by their presence. Observations can be planned (e.g. for 3 h during the day or night shift) or ad hoc (e.g. as soon as a stroke patient arrives at the emergency room). During the observation, the observer takes notes on everything or certain pre-determined parts of what is happening around them, for example focusing on physician-patient interactions or communication between different professional groups. Written notes can be taken during or after the observations, depending on feasibility (which is usually lower during participant observations) and acceptability (e.g. when the observer is perceived to be judging the observed). Afterwards, these field notes are transcribed into observation protocols. If more than one observer was involved, field notes are taken independently, but notes can be consolidated into one protocol after discussions. Advantages of conducting observations include minimising the distance between the researcher and the researched, the potential discovery of topics that the researcher did not realise were relevant and gaining deeper insights into the real-world dimensions of the research problem at hand [ 18 ].
Semi-structured interviews
Hijmans & Kuyper describe qualitative interviews as “an exchange with an informal character, a conversation with a goal” [ 19 ]. Interviews are used to gain insights into a person’s subjective experiences, opinions and motivations – as opposed to facts or behaviours [ 13 ]. Interviews can be distinguished by the degree to which they are structured (i.e. a questionnaire), open (e.g. free conversation or autobiographical interviews) or semi-structured [ 2 , 13 ]. Semi-structured interviews are characterized by open-ended questions and the use of an interview guide (or topic guide/list) in which the broad areas of interest, sometimes including sub-questions, are defined [ 19 ]. The pre-defined topics in the interview guide can be derived from the literature, previous research or a preliminary method of data collection, e.g. document study or observations. The topic list is usually adapted and improved at the start of the data collection process as the interviewer learns more about the field [ 20 ]. Across interviews the focus on the different (blocks of) questions may differ and some questions may be skipped altogether (e.g. if the interviewee is not able or willing to answer the questions or for concerns about the total length of the interview) [ 20 ]. Qualitative interviews are usually not conducted in written format as it impedes on the interactive component of the method [ 20 ]. In comparison to written surveys, qualitative interviews have the advantage of being interactive and allowing for unexpected topics to emerge and to be taken up by the researcher. This can also help overcome a provider or researcher-centred bias often found in written surveys, which by nature, can only measure what is already known or expected to be of relevance to the researcher. Interviews can be audio- or video-taped; but sometimes it is only feasible or acceptable for the interviewer to take written notes [ 14 , 16 , 20 ].
Focus groups
Focus groups are group interviews to explore participants’ expertise and experiences, including explorations of how and why people behave in certain ways [ 1 ]. Focus groups usually consist of 6–8 people and are led by an experienced moderator following a topic guide or “script” [ 21 ]. They can involve an observer who takes note of the non-verbal aspects of the situation, possibly using an observation guide [ 21 ]. Depending on researchers’ and participants’ preferences, the discussions can be audio- or video-taped and transcribed afterwards [ 21 ]. Focus groups are useful for bringing together homogeneous (to a lesser extent heterogeneous) groups of participants with relevant expertise and experience on a given topic on which they can share detailed information [ 21 ]. Focus groups are a relatively easy, fast and inexpensive method to gain access to information on interactions in a given group, i.e. “the sharing and comparing” among participants [ 21 ]. Disadvantages include less control over the process and a lesser extent to which each individual may participate. Moreover, focus group moderators need experience, as do those tasked with the analysis of the resulting data. Focus groups can be less appropriate for discussing sensitive topics that participants might be reluctant to disclose in a group setting [ 13 ]. Moreover, attention must be paid to the emergence of “groupthink” as well as possible power dynamics within the group, e.g. when patients are awed or intimidated by health professionals.
Choosing the “right” method
As explained above, the school of thought underlying qualitative research assumes no objective hierarchy of evidence and methods. This means that each choice of single or combined methods has to be based on the research question that needs to be answered and a critical assessment with regard to whether or to what extent the chosen method can accomplish this – i.e. the “fit” between question and method [ 14 ]. It is necessary for these decisions to be documented when they are being made, and to be critically discussed when reporting methods and results.
Let us assume that our research aim is to examine the (clinical) processes around acute endovascular treatment (EVT), from the patient’s arrival at the emergency room to recanalization, with the aim to identify possible causes for delay and/or other causes for sub-optimal treatment outcome. As a first step, we could conduct a document study of the relevant standard operating procedures (SOPs) for this phase of care – are they up-to-date and in line with current guidelines? Do they contain any mistakes, irregularities or uncertainties that could cause delays or other problems? Regardless of the answers to these questions, the results have to be interpreted based on what they are: a written outline of what care processes in this hospital should look like. If we want to know what they actually look like in practice, we can conduct observations of the processes described in the SOPs. These results can (and should) be analysed in themselves, but also in comparison to the results of the document analysis, especially as regards relevant discrepancies. Do the SOPs outline specific tests for which no equipment can be observed or tasks to be performed by specialized nurses who are not present during the observation? It might also be possible that the written SOP is outdated, but the actual care provided is in line with current best practice. In order to find out why these discrepancies exist, it can be useful to conduct interviews. Are the physicians simply not aware of the SOPs (because their existence is limited to the hospital’s intranet) or do they actively disagree with them or does the infrastructure make it impossible to provide the care as described? Another rationale for adding interviews is that some situations (or all of their possible variations for different patient groups or the day, night or weekend shift) cannot practically or ethically be observed. In this case, it is possible to ask those involved to report on their actions – being aware that this is not the same as the actual observation. A senior physician’s or hospital manager’s description of certain situations might differ from a nurse’s or junior physician’s one, maybe because they intentionally misrepresent facts or maybe because different aspects of the process are visible or important to them. In some cases, it can also be relevant to consider to whom the interviewee is disclosing this information – someone they trust, someone they are otherwise not connected to, or someone they suspect or are aware of being in a potentially “dangerous” power relationship to them. Lastly, a focus group could be conducted with representatives of the relevant professional groups to explore how and why exactly they provide care around EVT. The discussion might reveal discrepancies (between SOPs and actual care or between different physicians) and motivations to the researchers as well as to the focus group members that they might not have been aware of themselves. For the focus group to deliver relevant information, attention has to be paid to its composition and conduct, for example, to make sure that all participants feel safe to disclose sensitive or potentially problematic information or that the discussion is not dominated by (senior) physicians only. The resulting combination of data collection methods is shown in Fig. 2 .

Possible combination of data collection methods
Attributions for icons: “Book” by Serhii Smirnov, “Interview” by Adrien Coquet, FR, “Magnifying Glass” by anggun, ID, “Business communication” by Vectors Market; all from the Noun Project
The combination of multiple data source as described for this example can be referred to as “triangulation”, in which multiple measurements are carried out from different angles to achieve a more comprehensive understanding of the phenomenon under study [ 22 , 23 ].
Data analysis
To analyse the data collected through observations, interviews and focus groups these need to be transcribed into protocols and transcripts (see Fig. 3 ). Interviews and focus groups can be transcribed verbatim , with or without annotations for behaviour (e.g. laughing, crying, pausing) and with or without phonetic transcription of dialects and filler words, depending on what is expected or known to be relevant for the analysis. In the next step, the protocols and transcripts are coded , that is, marked (or tagged, labelled) with one or more short descriptors of the content of a sentence or paragraph [ 2 , 15 , 23 ]. Jansen describes coding as “connecting the raw data with “theoretical” terms” [ 20 ]. In a more practical sense, coding makes raw data sortable. This makes it possible to extract and examine all segments describing, say, a tele-neurology consultation from multiple data sources (e.g. SOPs, emergency room observations, staff and patient interview). In a process of synthesis and abstraction, the codes are then grouped, summarised and/or categorised [ 15 , 20 ]. The end product of the coding or analysis process is a descriptive theory of the behavioural pattern under investigation [ 20 ]. The coding process is performed using qualitative data management software, the most common ones being InVivo, MaxQDA and Atlas.ti. It should be noted that these are data management tools which support the analysis performed by the researcher(s) [ 14 ].
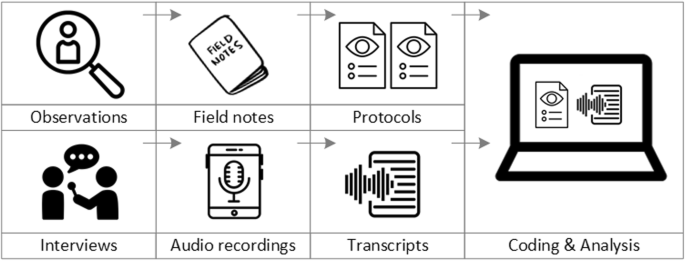
From data collection to data analysis
Attributions for icons: see Fig. 2 , also “Speech to text” by Trevor Dsouza, “Field Notes” by Mike O’Brien, US, “Voice Record” by ProSymbols, US, “Inspection” by Made, AU, and “Cloud” by Graphic Tigers; all from the Noun Project
How to report qualitative research?
Protocols of qualitative research can be published separately and in advance of the study results. However, the aim is not the same as in RCT protocols, i.e. to pre-define and set in stone the research questions and primary or secondary endpoints. Rather, it is a way to describe the research methods in detail, which might not be possible in the results paper given journals’ word limits. Qualitative research papers are usually longer than their quantitative counterparts to allow for deep understanding and so-called “thick description”. In the methods section, the focus is on transparency of the methods used, including why, how and by whom they were implemented in the specific study setting, so as to enable a discussion of whether and how this may have influenced data collection, analysis and interpretation. The results section usually starts with a paragraph outlining the main findings, followed by more detailed descriptions of, for example, the commonalities, discrepancies or exceptions per category [ 20 ]. Here it is important to support main findings by relevant quotations, which may add information, context, emphasis or real-life examples [ 20 , 23 ]. It is subject to debate in the field whether it is relevant to state the exact number or percentage of respondents supporting a certain statement (e.g. “Five interviewees expressed negative feelings towards XYZ”) [ 21 ].
How to combine qualitative with quantitative research?
Qualitative methods can be combined with other methods in multi- or mixed methods designs, which “[employ] two or more different methods [ …] within the same study or research program rather than confining the research to one single method” [ 24 ]. Reasons for combining methods can be diverse, including triangulation for corroboration of findings, complementarity for illustration and clarification of results, expansion to extend the breadth and range of the study, explanation of (unexpected) results generated with one method with the help of another, or offsetting the weakness of one method with the strength of another [ 1 , 17 , 24 , 25 , 26 ]. The resulting designs can be classified according to when, why and how the different quantitative and/or qualitative data strands are combined. The three most common types of mixed method designs are the convergent parallel design , the explanatory sequential design and the exploratory sequential design. The designs with examples are shown in Fig. 4 .

Three common mixed methods designs
In the convergent parallel design, a qualitative study is conducted in parallel to and independently of a quantitative study, and the results of both studies are compared and combined at the stage of interpretation of results. Using the above example of EVT provision, this could entail setting up a quantitative EVT registry to measure process times and patient outcomes in parallel to conducting the qualitative research outlined above, and then comparing results. Amongst other things, this would make it possible to assess whether interview respondents’ subjective impressions of patients receiving good care match modified Rankin Scores at follow-up, or whether observed delays in care provision are exceptions or the rule when compared to door-to-needle times as documented in the registry. In the explanatory sequential design, a quantitative study is carried out first, followed by a qualitative study to help explain the results from the quantitative study. This would be an appropriate design if the registry alone had revealed relevant delays in door-to-needle times and the qualitative study would be used to understand where and why these occurred, and how they could be improved. In the exploratory design, the qualitative study is carried out first and its results help informing and building the quantitative study in the next step [ 26 ]. If the qualitative study around EVT provision had shown a high level of dissatisfaction among the staff members involved, a quantitative questionnaire investigating staff satisfaction could be set up in the next step, informed by the qualitative study on which topics dissatisfaction had been expressed. Amongst other things, the questionnaire design would make it possible to widen the reach of the research to more respondents from different (types of) hospitals, regions, countries or settings, and to conduct sub-group analyses for different professional groups.
How to assess qualitative research?
A variety of assessment criteria and lists have been developed for qualitative research, ranging in their focus and comprehensiveness [ 14 , 17 , 27 ]. However, none of these has been elevated to the “gold standard” in the field. In the following, we therefore focus on a set of commonly used assessment criteria that, from a practical standpoint, a researcher can look for when assessing a qualitative research report or paper.
Assessors should check the authors’ use of and adherence to the relevant reporting checklists (e.g. Standards for Reporting Qualitative Research (SRQR)) to make sure all items that are relevant for this type of research are addressed [ 23 , 28 ]. Discussions of quantitative measures in addition to or instead of these qualitative measures can be a sign of lower quality of the research (paper). Providing and adhering to a checklist for qualitative research contributes to an important quality criterion for qualitative research, namely transparency [ 15 , 17 , 23 ].
Reflexivity
While methodological transparency and complete reporting is relevant for all types of research, some additional criteria must be taken into account for qualitative research. This includes what is called reflexivity, i.e. sensitivity to the relationship between the researcher and the researched, including how contact was established and maintained, or the background and experience of the researcher(s) involved in data collection and analysis. Depending on the research question and population to be researched this can be limited to professional experience, but it may also include gender, age or ethnicity [ 17 , 27 ]. These details are relevant because in qualitative research, as opposed to quantitative research, the researcher as a person cannot be isolated from the research process [ 23 ]. It may influence the conversation when an interviewed patient speaks to an interviewer who is a physician, or when an interviewee is asked to discuss a gynaecological procedure with a male interviewer, and therefore the reader must be made aware of these details [ 19 ].
Sampling and saturation
The aim of qualitative sampling is for all variants of the objects of observation that are deemed relevant for the study to be present in the sample “ to see the issue and its meanings from as many angles as possible” [ 1 , 16 , 19 , 20 , 27 ] , and to ensure “information-richness [ 15 ]. An iterative sampling approach is advised, in which data collection (e.g. five interviews) is followed by data analysis, followed by more data collection to find variants that are lacking in the current sample. This process continues until no new (relevant) information can be found and further sampling becomes redundant – which is called saturation [ 1 , 15 ] . In other words: qualitative data collection finds its end point not a priori , but when the research team determines that saturation has been reached [ 29 , 30 ].
This is also the reason why most qualitative studies use deliberate instead of random sampling strategies. This is generally referred to as “ purposive sampling” , in which researchers pre-define which types of participants or cases they need to include so as to cover all variations that are expected to be of relevance, based on the literature, previous experience or theory (i.e. theoretical sampling) [ 14 , 20 ]. Other types of purposive sampling include (but are not limited to) maximum variation sampling, critical case sampling or extreme or deviant case sampling [ 2 ]. In the above EVT example, a purposive sample could include all relevant professional groups and/or all relevant stakeholders (patients, relatives) and/or all relevant times of observation (day, night and weekend shift).
Assessors of qualitative research should check whether the considerations underlying the sampling strategy were sound and whether or how researchers tried to adapt and improve their strategies in stepwise or cyclical approaches between data collection and analysis to achieve saturation [ 14 ].
Good qualitative research is iterative in nature, i.e. it goes back and forth between data collection and analysis, revising and improving the approach where necessary. One example of this are pilot interviews, where different aspects of the interview (especially the interview guide, but also, for example, the site of the interview or whether the interview can be audio-recorded) are tested with a small number of respondents, evaluated and revised [ 19 ]. In doing so, the interviewer learns which wording or types of questions work best, or which is the best length of an interview with patients who have trouble concentrating for an extended time. Of course, the same reasoning applies to observations or focus groups which can also be piloted.
Ideally, coding should be performed by at least two researchers, especially at the beginning of the coding process when a common approach must be defined, including the establishment of a useful coding list (or tree), and when a common meaning of individual codes must be established [ 23 ]. An initial sub-set or all transcripts can be coded independently by the coders and then compared and consolidated after regular discussions in the research team. This is to make sure that codes are applied consistently to the research data.
Member checking
Member checking, also called respondent validation , refers to the practice of checking back with study respondents to see if the research is in line with their views [ 14 , 27 ]. This can happen after data collection or analysis or when first results are available [ 23 ]. For example, interviewees can be provided with (summaries of) their transcripts and asked whether they believe this to be a complete representation of their views or whether they would like to clarify or elaborate on their responses [ 17 ]. Respondents’ feedback on these issues then becomes part of the data collection and analysis [ 27 ].
Stakeholder involvement
In those niches where qualitative approaches have been able to evolve and grow, a new trend has seen the inclusion of patients and their representatives not only as study participants (i.e. “members”, see above) but as consultants to and active participants in the broader research process [ 31 , 32 , 33 ]. The underlying assumption is that patients and other stakeholders hold unique perspectives and experiences that add value beyond their own single story, making the research more relevant and beneficial to researchers, study participants and (future) patients alike [ 34 , 35 ]. Using the example of patients on or nearing dialysis, a recent scoping review found that 80% of clinical research did not address the top 10 research priorities identified by patients and caregivers [ 32 , 36 ]. In this sense, the involvement of the relevant stakeholders, especially patients and relatives, is increasingly being seen as a quality indicator in and of itself.
How not to assess qualitative research
The above overview does not include certain items that are routine in assessments of quantitative research. What follows is a non-exhaustive, non-representative, experience-based list of the quantitative criteria often applied to the assessment of qualitative research, as well as an explanation of the limited usefulness of these endeavours.
Protocol adherence
Given the openness and flexibility of qualitative research, it should not be assessed by how well it adheres to pre-determined and fixed strategies – in other words: its rigidity. Instead, the assessor should look for signs of adaptation and refinement based on lessons learned from earlier steps in the research process.
Sample size
For the reasons explained above, qualitative research does not require specific sample sizes, nor does it require that the sample size be determined a priori [ 1 , 14 , 27 , 37 , 38 , 39 ]. Sample size can only be a useful quality indicator when related to the research purpose, the chosen methodology and the composition of the sample, i.e. who was included and why.
Randomisation
While some authors argue that randomisation can be used in qualitative research, this is not commonly the case, as neither its feasibility nor its necessity or usefulness has been convincingly established for qualitative research [ 13 , 27 ]. Relevant disadvantages include the negative impact of a too large sample size as well as the possibility (or probability) of selecting “ quiet, uncooperative or inarticulate individuals ” [ 17 ]. Qualitative studies do not use control groups, either.
Interrater reliability, variability and other “objectivity checks”
The concept of “interrater reliability” is sometimes used in qualitative research to assess to which extent the coding approach overlaps between the two co-coders. However, it is not clear what this measure tells us about the quality of the analysis [ 23 ]. This means that these scores can be included in qualitative research reports, preferably with some additional information on what the score means for the analysis, but it is not a requirement. Relatedly, it is not relevant for the quality or “objectivity” of qualitative research to separate those who recruited the study participants and collected and analysed the data. Experiences even show that it might be better to have the same person or team perform all of these tasks [ 20 ]. First, when researchers introduce themselves during recruitment this can enhance trust when the interview takes place days or weeks later with the same researcher. Second, when the audio-recording is transcribed for analysis, the researcher conducting the interviews will usually remember the interviewee and the specific interview situation during data analysis. This might be helpful in providing additional context information for interpretation of data, e.g. on whether something might have been meant as a joke [ 18 ].
Not being quantitative research
Being qualitative research instead of quantitative research should not be used as an assessment criterion if it is used irrespectively of the research problem at hand. Similarly, qualitative research should not be required to be combined with quantitative research per se – unless mixed methods research is judged as inherently better than single-method research. In this case, the same criterion should be applied for quantitative studies without a qualitative component.
The main take-away points of this paper are summarised in Table 1 . We aimed to show that, if conducted well, qualitative research can answer specific research questions that cannot to be adequately answered using (only) quantitative designs. Seeing qualitative and quantitative methods as equal will help us become more aware and critical of the “fit” between the research problem and our chosen methods: I can conduct an RCT to determine the reasons for transportation delays of acute stroke patients – but should I? It also provides us with a greater range of tools to tackle a greater range of research problems more appropriately and successfully, filling in the blind spots on one half of the methodological spectrum to better address the whole complexity of neurological research and practice.
Availability of data and materials
Not applicable.
Abbreviations
Endovascular treatment
Randomised Controlled Trial
Standard Operating Procedure
Standards for Reporting Qualitative Research
Philipsen, H., & Vernooij-Dassen, M. (2007). Kwalitatief onderzoek: nuttig, onmisbaar en uitdagend. In L. PLBJ & H. TCo (Eds.), Kwalitatief onderzoek: Praktische methoden voor de medische praktijk . [Qualitative research: useful, indispensable and challenging. In: Qualitative research: Practical methods for medical practice (pp. 5–12). Houten: Bohn Stafleu van Loghum.
Chapter Google Scholar
Punch, K. F. (2013). Introduction to social research: Quantitative and qualitative approaches . London: Sage.
Kelly, J., Dwyer, J., Willis, E., & Pekarsky, B. (2014). Travelling to the city for hospital care: Access factors in country aboriginal patient journeys. Australian Journal of Rural Health, 22 (3), 109–113.
Article Google Scholar
Nilsen, P., Ståhl, C., Roback, K., & Cairney, P. (2013). Never the twain shall meet? - a comparison of implementation science and policy implementation research. Implementation Science, 8 (1), 1–12.
Howick J, Chalmers I, Glasziou, P., Greenhalgh, T., Heneghan, C., Liberati, A., Moschetti, I., Phillips, B., & Thornton, H. (2011). The 2011 Oxford CEBM evidence levels of evidence (introductory document) . Oxford Center for Evidence Based Medicine. https://www.cebm.net/2011/06/2011-oxford-cebm-levels-evidence-introductory-document/ .
Eakin, J. M. (2016). Educating critical qualitative health researchers in the land of the randomized controlled trial. Qualitative Inquiry, 22 (2), 107–118.
May, A., & Mathijssen, J. (2015). Alternatieven voor RCT bij de evaluatie van effectiviteit van interventies!? Eindrapportage. In Alternatives for RCTs in the evaluation of effectiveness of interventions!? Final report .
Google Scholar
Berwick, D. M. (2008). The science of improvement. Journal of the American Medical Association, 299 (10), 1182–1184.
Article CAS Google Scholar
Christ, T. W. (2014). Scientific-based research and randomized controlled trials, the “gold” standard? Alternative paradigms and mixed methodologies. Qualitative Inquiry, 20 (1), 72–80.
Lamont, T., Barber, N., Jd, P., Fulop, N., Garfield-Birkbeck, S., Lilford, R., Mear, L., Raine, R., & Fitzpatrick, R. (2016). New approaches to evaluating complex health and care systems. BMJ, 352:i154.
Drabble, S. J., & O’Cathain, A. (2015). Moving from Randomized Controlled Trials to Mixed Methods Intervention Evaluation. In S. Hesse-Biber & R. B. Johnson (Eds.), The Oxford Handbook of Multimethod and Mixed Methods Research Inquiry (pp. 406–425). London: Oxford University Press.
Chambers, D. A., Glasgow, R. E., & Stange, K. C. (2013). The dynamic sustainability framework: Addressing the paradox of sustainment amid ongoing change. Implementation Science : IS, 8 , 117.
Hak, T. (2007). Waarnemingsmethoden in kwalitatief onderzoek. In L. PLBJ & H. TCo (Eds.), Kwalitatief onderzoek: Praktische methoden voor de medische praktijk . [Observation methods in qualitative research] (pp. 13–25). Houten: Bohn Stafleu van Loghum.
Russell, C. K., & Gregory, D. M. (2003). Evaluation of qualitative research studies. Evidence Based Nursing, 6 (2), 36–40.
Fossey, E., Harvey, C., McDermott, F., & Davidson, L. (2002). Understanding and evaluating qualitative research. Australian and New Zealand Journal of Psychiatry, 36 , 717–732.
Yanow, D. (2000). Conducting interpretive policy analysis (Vol. 47). Thousand Oaks: Sage University Papers Series on Qualitative Research Methods.
Shenton, A. K. (2004). Strategies for ensuring trustworthiness in qualitative research projects. Education for Information, 22 , 63–75.
van der Geest, S. (2006). Participeren in ziekte en zorg: meer over kwalitatief onderzoek. Huisarts en Wetenschap, 49 (4), 283–287.
Hijmans, E., & Kuyper, M. (2007). Het halfopen interview als onderzoeksmethode. In L. PLBJ & H. TCo (Eds.), Kwalitatief onderzoek: Praktische methoden voor de medische praktijk . [The half-open interview as research method (pp. 43–51). Houten: Bohn Stafleu van Loghum.
Jansen, H. (2007). Systematiek en toepassing van de kwalitatieve survey. In L. PLBJ & H. TCo (Eds.), Kwalitatief onderzoek: Praktische methoden voor de medische praktijk . [Systematics and implementation of the qualitative survey (pp. 27–41). Houten: Bohn Stafleu van Loghum.
Pv, R., & Peremans, L. (2007). Exploreren met focusgroepgesprekken: de ‘stem’ van de groep onder de loep. In L. PLBJ & H. TCo (Eds.), Kwalitatief onderzoek: Praktische methoden voor de medische praktijk . [Exploring with focus group conversations: the “voice” of the group under the magnifying glass (pp. 53–64). Houten: Bohn Stafleu van Loghum.
Carter, N., Bryant-Lukosius, D., DiCenso, A., Blythe, J., & Neville, A. J. (2014). The use of triangulation in qualitative research. Oncology Nursing Forum, 41 (5), 545–547.
Boeije H: Analyseren in kwalitatief onderzoek: Denken en doen, [Analysis in qualitative research: Thinking and doing] vol. Den Haag Boom Lemma uitgevers; 2012.
Hunter, A., & Brewer, J. (2015). Designing Multimethod Research. In S. Hesse-Biber & R. B. Johnson (Eds.), The Oxford Handbook of Multimethod and Mixed Methods Research Inquiry (pp. 185–205). London: Oxford University Press.
Archibald, M. M., Radil, A. I., Zhang, X., & Hanson, W. E. (2015). Current mixed methods practices in qualitative research: A content analysis of leading journals. International Journal of Qualitative Methods, 14 (2), 5–33.
Creswell, J. W., & Plano Clark, V. L. (2011). Choosing a Mixed Methods Design. In Designing and Conducting Mixed Methods Research . Thousand Oaks: SAGE Publications.
Mays, N., & Pope, C. (2000). Assessing quality in qualitative research. BMJ, 320 (7226), 50–52.
O'Brien, B. C., Harris, I. B., Beckman, T. J., Reed, D. A., & Cook, D. A. (2014). Standards for reporting qualitative research: A synthesis of recommendations. Academic Medicine : Journal of the Association of American Medical Colleges, 89 (9), 1245–1251.
Saunders, B., Sim, J., Kingstone, T., Baker, S., Waterfield, J., Bartlam, B., Burroughs, H., & Jinks, C. (2018). Saturation in qualitative research: Exploring its conceptualization and operationalization. Quality and Quantity, 52 (4), 1893–1907.
Moser, A., & Korstjens, I. (2018). Series: Practical guidance to qualitative research. Part 3: Sampling, data collection and analysis. European Journal of General Practice, 24 (1), 9–18.
Marlett, N., Shklarov, S., Marshall, D., Santana, M. J., & Wasylak, T. (2015). Building new roles and relationships in research: A model of patient engagement research. Quality of Life Research : an international journal of quality of life aspects of treatment, care and rehabilitation, 24 (5), 1057–1067.
Demian, M. N., Lam, N. N., Mac-Way, F., Sapir-Pichhadze, R., & Fernandez, N. (2017). Opportunities for engaging patients in kidney research. Canadian Journal of Kidney Health and Disease, 4 , 2054358117703070–2054358117703070.
Noyes, J., McLaughlin, L., Morgan, K., Roberts, A., Stephens, M., Bourne, J., Houlston, M., Houlston, J., Thomas, S., Rhys, R. G., et al. (2019). Designing a co-productive study to overcome known methodological challenges in organ donation research with bereaved family members. Health Expectations . 22(4):824–35.
Piil, K., Jarden, M., & Pii, K. H. (2019). Research agenda for life-threatening cancer. European Journal Cancer Care (Engl), 28 (1), e12935.
Hofmann, D., Ibrahim, F., Rose, D., Scott, D. L., Cope, A., Wykes, T., & Lempp, H. (2015). Expectations of new treatment in rheumatoid arthritis: Developing a patient-generated questionnaire. Health Expectations : an international journal of public participation in health care and health policy, 18 (5), 995–1008.
Jun, M., Manns, B., Laupacis, A., Manns, L., Rehal, B., Crowe, S., & Hemmelgarn, B. R. (2015). Assessing the extent to which current clinical research is consistent with patient priorities: A scoping review using a case study in patients on or nearing dialysis. Canadian Journal of Kidney Health and Disease, 2 , 35.
Elsie Baker, S., & Edwards, R. (2012). How many qualitative interviews is enough? In National Centre for Research Methods Review Paper . National Centre for Research Methods. http://eprints.ncrm.ac.uk/2273/4/how_many_interviews.pdf .
Sandelowski, M. (1995). Sample size in qualitative research. Research in Nursing & Health, 18 (2), 179–183.
Sim, J., Saunders, B., Waterfield, J., & Kingstone, T. (2018). Can sample size in qualitative research be determined a priori? International Journal of Social Research Methodology, 21 (5), 619–634.
Download references
Acknowledgements
no external funding.
Author information
Authors and affiliations.
Department of Neurology, Heidelberg University Hospital, Im Neuenheimer Feld 400, 69120, Heidelberg, Germany
Loraine Busetto, Wolfgang Wick & Christoph Gumbinger
Clinical Cooperation Unit Neuro-Oncology, German Cancer Research Center, Heidelberg, Germany
Wolfgang Wick
You can also search for this author in PubMed Google Scholar
Contributions
LB drafted the manuscript; WW and CG revised the manuscript; all authors approved the final versions.
Corresponding author
Correspondence to Loraine Busetto .
Ethics declarations
Ethics approval and consent to participate, consent for publication, competing interests.
The authors declare no competing interests.
Additional information
Publisher’s note.
Springer Nature remains neutral with regard to jurisdictional claims in published maps and institutional affiliations.
Rights and permissions
Open Access This article is licensed under a Creative Commons Attribution 4.0 International License, which permits use, sharing, adaptation, distribution and reproduction in any medium or format, as long as you give appropriate credit to the original author(s) and the source, provide a link to the Creative Commons licence, and indicate if changes were made. The images or other third party material in this article are included in the article's Creative Commons licence, unless indicated otherwise in a credit line to the material. If material is not included in the article's Creative Commons licence and your intended use is not permitted by statutory regulation or exceeds the permitted use, you will need to obtain permission directly from the copyright holder. To view a copy of this licence, visit http://creativecommons.org/licenses/by/4.0/ .
Reprints and permissions
About this article
Cite this article.
Busetto, L., Wick, W. & Gumbinger, C. How to use and assess qualitative research methods. Neurol. Res. Pract. 2 , 14 (2020). https://doi.org/10.1186/s42466-020-00059-z
Download citation
Received : 30 January 2020
Accepted : 22 April 2020
Published : 27 May 2020
DOI : https://doi.org/10.1186/s42466-020-00059-z
Share this article
Anyone you share the following link with will be able to read this content:
Sorry, a shareable link is not currently available for this article.
Provided by the Springer Nature SharedIt content-sharing initiative
- Qualitative research
- Mixed methods
- Quality assessment
Neurological Research and Practice
ISSN: 2524-3489
- Submission enquiries: Access here and click Contact Us
- General enquiries: [email protected]
REVIEW article
The use of research methods in psychological research: a systematised review.
- 1 Community Psychosocial Research (COMPRES), School of Psychosocial Health, North-West University, Potchefstroom, South Africa
- 2 WorkWell Research Institute, North-West University, Potchefstroom, South Africa
Research methods play an imperative role in research quality as well as educating young researchers, however, the application thereof is unclear which can be detrimental to the field of psychology. Therefore, this systematised review aimed to determine what research methods are being used, how these methods are being used and for what topics in the field. Our review of 999 articles from five journals over a period of 5 years indicated that psychology research is conducted in 10 topics via predominantly quantitative research methods. Of these 10 topics, social psychology was the most popular. The remainder of the conducted methodology is described. It was also found that articles lacked rigour and transparency in the used methodology which has implications for replicability. In conclusion this article, provides an overview of all reported methodologies used in a sample of psychology journals. It highlights the popularity and application of methods and designs throughout the article sample as well as an unexpected lack of rigour with regard to most aspects of methodology. Possible sample bias should be considered when interpreting the results of this study. It is recommended that future research should utilise the results of this study to determine the possible impact on the field of psychology as a science and to further investigation into the use of research methods. Results should prompt the following future research into: a lack or rigour and its implication on replication, the use of certain methods above others, publication bias and choice of sampling method.
Introduction
Psychology is an ever-growing and popular field ( Gough and Lyons, 2016 ; Clay, 2017 ). Due to this growth and the need for science-based research to base health decisions on ( Perestelo-Pérez, 2013 ), the use of research methods in the broad field of psychology is an essential point of investigation ( Stangor, 2011 ; Aanstoos, 2014 ). Research methods are therefore viewed as important tools used by researchers to collect data ( Nieuwenhuis, 2016 ) and include the following: quantitative, qualitative, mixed method and multi method ( Maree, 2016 ). Additionally, researchers also employ various types of literature reviews to address research questions ( Grant and Booth, 2009 ). According to literature, what research method is used and why a certain research method is used is complex as it depends on various factors that may include paradigm ( O'Neil and Koekemoer, 2016 ), research question ( Grix, 2002 ), or the skill and exposure of the researcher ( Nind et al., 2015 ). How these research methods are employed is also difficult to discern as research methods are often depicted as having fixed boundaries that are continuously crossed in research ( Johnson et al., 2001 ; Sandelowski, 2011 ). Examples of this crossing include adding quantitative aspects to qualitative studies ( Sandelowski et al., 2009 ), or stating that a study used a mixed-method design without the study having any characteristics of this design ( Truscott et al., 2010 ).
The inappropriate use of research methods affects how students and researchers improve and utilise their research skills ( Scott Jones and Goldring, 2015 ), how theories are developed ( Ngulube, 2013 ), and the credibility of research results ( Levitt et al., 2017 ). This, in turn, can be detrimental to the field ( Nind et al., 2015 ), journal publication ( Ketchen et al., 2008 ; Ezeh et al., 2010 ), and attempts to address public social issues through psychological research ( Dweck, 2017 ). This is especially important given the now well-known replication crisis the field is facing ( Earp and Trafimow, 2015 ; Hengartner, 2018 ).
Due to this lack of clarity on method use and the potential impact of inept use of research methods, the aim of this study was to explore the use of research methods in the field of psychology through a review of journal publications. Chaichanasakul et al. (2011) identify reviewing articles as the opportunity to examine the development, growth and progress of a research area and overall quality of a journal. Studies such as Lee et al. (1999) as well as Bluhm et al. (2011) review of qualitative methods has attempted to synthesis the use of research methods and indicated the growth of qualitative research in American and European journals. Research has also focused on the use of research methods in specific sub-disciplines of psychology, for example, in the field of Industrial and Organisational psychology Coetzee and Van Zyl (2014) found that South African publications tend to consist of cross-sectional quantitative research methods with underrepresented longitudinal studies. Qualitative studies were found to make up 21% of the articles published from 1995 to 2015 in a similar study by O'Neil and Koekemoer (2016) . Other methods in health psychology, such as Mixed methods research have also been reportedly growing in popularity ( O'Cathain, 2009 ).
A broad overview of the use of research methods in the field of psychology as a whole is however, not available in the literature. Therefore, our research focused on answering what research methods are being used, how these methods are being used and for what topics in practice (i.e., journal publications) in order to provide a general perspective of method used in psychology publication. We synthesised the collected data into the following format: research topic [areas of scientific discourse in a field or the current needs of a population ( Bittermann and Fischer, 2018 )], method [data-gathering tools ( Nieuwenhuis, 2016 )], sampling [elements chosen from a population to partake in research ( Ritchie et al., 2009 )], data collection [techniques and research strategy ( Maree, 2016 )], and data analysis [discovering information by examining bodies of data ( Ktepi, 2016 )]. A systematised review of recent articles (2013 to 2017) collected from five different journals in the field of psychological research was conducted.
Grant and Booth (2009) describe systematised reviews as the review of choice for post-graduate studies, which is employed using some elements of a systematic review and seldom more than one or two databases to catalogue studies after a comprehensive literature search. The aspects used in this systematised review that are similar to that of a systematic review were a full search within the chosen database and data produced in tabular form ( Grant and Booth, 2009 ).
Sample sizes and timelines vary in systematised reviews (see Lowe and Moore, 2014 ; Pericall and Taylor, 2014 ; Barr-Walker, 2017 ). With no clear parameters identified in the literature (see Grant and Booth, 2009 ), the sample size of this study was determined by the purpose of the sample ( Strydom, 2011 ), and time and cost constraints ( Maree and Pietersen, 2016 ). Thus, a non-probability purposive sample ( Ritchie et al., 2009 ) of the top five psychology journals from 2013 to 2017 was included in this research study. Per Lee (2015) American Psychological Association (APA) recommends the use of the most up-to-date sources for data collection with consideration of the context of the research study. As this research study focused on the most recent trends in research methods used in the broad field of psychology, the identified time frame was deemed appropriate.
Psychology journals were only included if they formed part of the top five English journals in the miscellaneous psychology domain of the Scimago Journal and Country Rank ( Scimago Journal & Country Rank, 2017 ). The Scimago Journal and Country Rank provides a yearly updated list of publicly accessible journal and country-specific indicators derived from the Scopus ® database ( Scopus, 2017b ) by means of the Scimago Journal Rank (SJR) indicator developed by Scimago from the algorithm Google PageRank™ ( Scimago Journal & Country Rank, 2017 ). Scopus is the largest global database of abstracts and citations from peer-reviewed journals ( Scopus, 2017a ). Reasons for the development of the Scimago Journal and Country Rank list was to allow researchers to assess scientific domains, compare country rankings, and compare and analyse journals ( Scimago Journal & Country Rank, 2017 ), which supported the aim of this research study. Additionally, the goals of the journals had to focus on topics in psychology in general with no preference to specific research methods and have full-text access to articles.
The following list of top five journals in 2018 fell within the abovementioned inclusion criteria (1) Australian Journal of Psychology, (2) British Journal of Psychology, (3) Europe's Journal of Psychology, (4) International Journal of Psychology and lastly the (5) Journal of Psychology Applied and Interdisciplinary.
Journals were excluded from this systematised review if no full-text versions of their articles were available, if journals explicitly stated a publication preference for certain research methods, or if the journal only published articles in a specific discipline of psychological research (for example, industrial psychology, clinical psychology etc.).
The researchers followed a procedure (see Figure 1 ) adapted from that of Ferreira et al. (2016) for systematised reviews. Data collection and categorisation commenced on 4 December 2017 and continued until 30 June 2019. All the data was systematically collected and coded manually ( Grant and Booth, 2009 ) with an independent person acting as co-coder. Codes of interest included the research topic, method used, the design used, sampling method, and methodology (the method used for data collection and data analysis). These codes were derived from the wording in each article. Themes were created based on the derived codes and checked by the co-coder. Lastly, these themes were catalogued into a table as per the systematised review design.
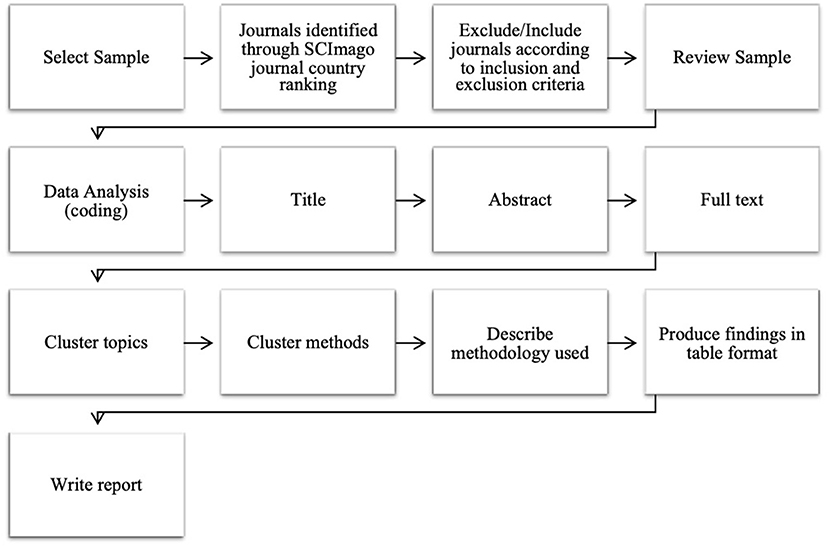
Figure 1 . Systematised review procedure.
According to Johnston et al. (2019) , “literature screening, selection, and data extraction/analyses” (p. 7) are specifically tailored to the aim of a review. Therefore, the steps followed in a systematic review must be reported in a comprehensive and transparent manner. The chosen systematised design adhered to the rigour expected from systematic reviews with regard to full search and data produced in tabular form ( Grant and Booth, 2009 ). The rigorous application of the systematic review is, therefore discussed in relation to these two elements.
Firstly, to ensure a comprehensive search, this research study promoted review transparency by following a clear protocol outlined according to each review stage before collecting data ( Johnston et al., 2019 ). This protocol was similar to that of Ferreira et al. (2016) and approved by three research committees/stakeholders and the researchers ( Johnston et al., 2019 ). The eligibility criteria for article inclusion was based on the research question and clearly stated, and the process of inclusion was recorded on an electronic spreadsheet to create an evidence trail ( Bandara et al., 2015 ; Johnston et al., 2019 ). Microsoft Excel spreadsheets are a popular tool for review studies and can increase the rigour of the review process ( Bandara et al., 2015 ). Screening for appropriate articles for inclusion forms an integral part of a systematic review process ( Johnston et al., 2019 ). This step was applied to two aspects of this research study: the choice of eligible journals and articles to be included. Suitable journals were selected by the first author and reviewed by the second and third authors. Initially, all articles from the chosen journals were included. Then, by process of elimination, those irrelevant to the research aim, i.e., interview articles or discussions etc., were excluded.
To ensure rigourous data extraction, data was first extracted by one reviewer, and an independent person verified the results for completeness and accuracy ( Johnston et al., 2019 ). The research question served as a guide for efficient, organised data extraction ( Johnston et al., 2019 ). Data was categorised according to the codes of interest, along with article identifiers for audit trails such as authors, title and aims of articles. The categorised data was based on the aim of the review ( Johnston et al., 2019 ) and synthesised in tabular form under methods used, how these methods were used, and for what topics in the field of psychology.
The initial search produced a total of 1,145 articles from the 5 journals identified. Inclusion and exclusion criteria resulted in a final sample of 999 articles ( Figure 2 ). Articles were co-coded into 84 codes, from which 10 themes were derived ( Table 1 ).
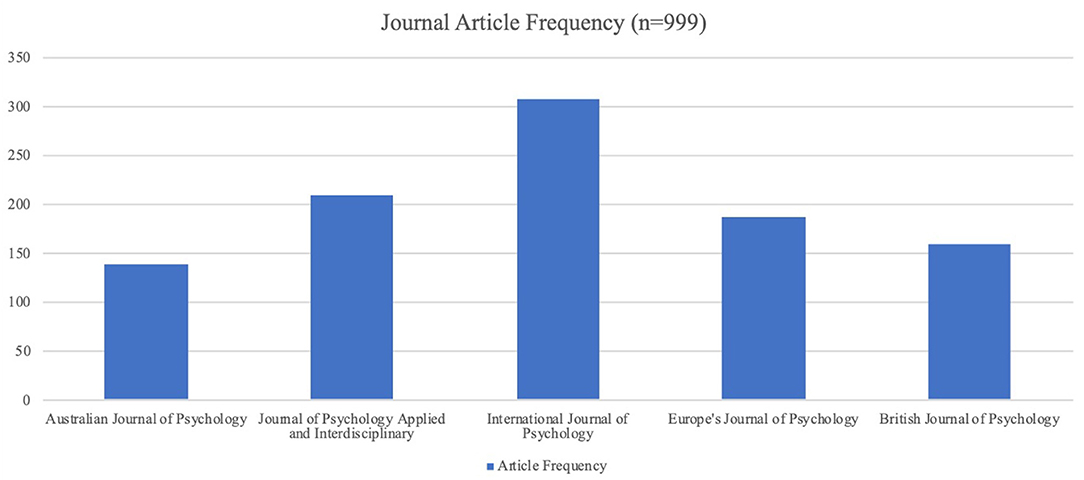
Figure 2 . Journal article frequency.
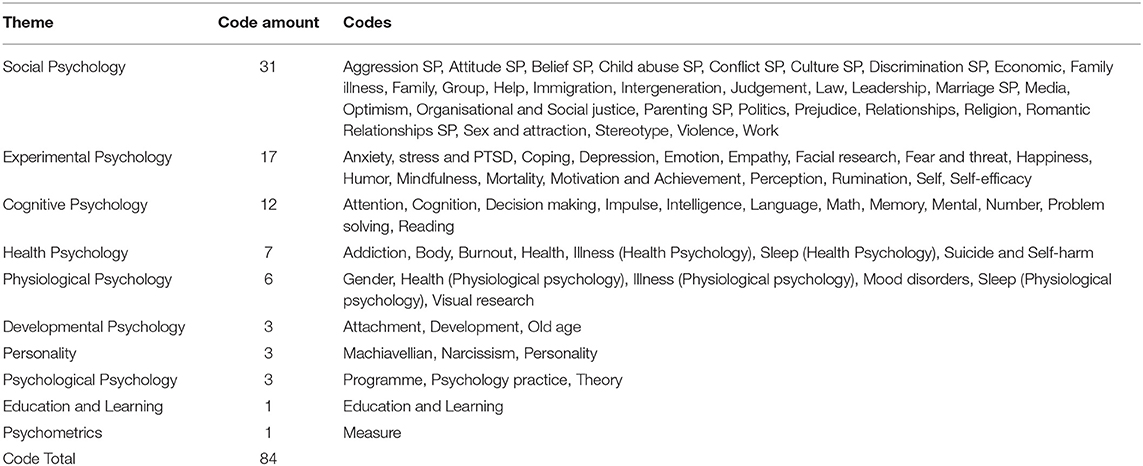
Table 1 . Codes used to form themes (research topics).
These 10 themes represent the topic section of our research question ( Figure 3 ). All these topics except, for the final one, psychological practice , were found to concur with the research areas in psychology as identified by Weiten (2010) . These research areas were chosen to represent the derived codes as they provided broad definitions that allowed for clear, concise categorisation of the vast amount of data. Article codes were categorised under particular themes/topics if they adhered to the research area definitions created by Weiten (2010) . It is important to note that these areas of research do not refer to specific disciplines in psychology, such as industrial psychology; but to broader fields that may encompass sub-interests of these disciplines.
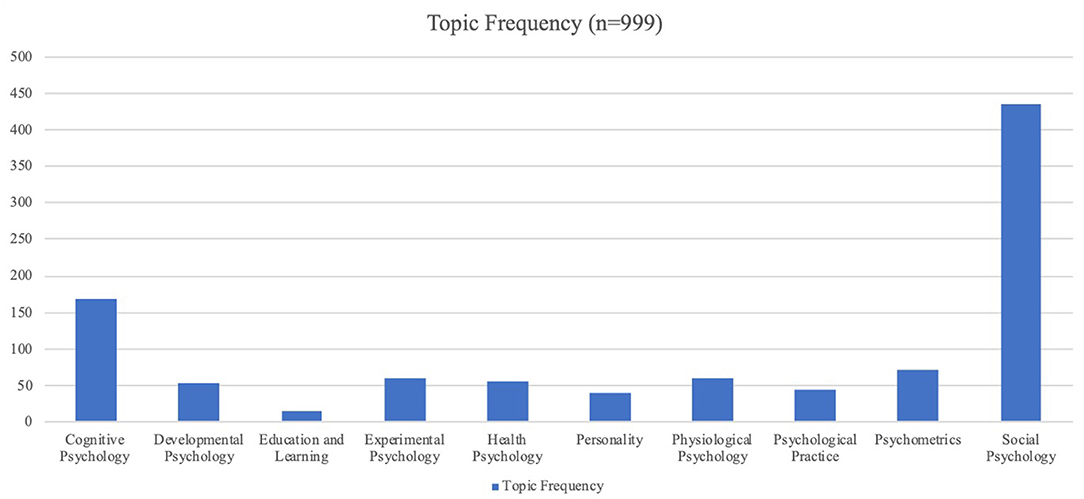
Figure 3 . Topic frequency (international sample).
In the case of developmental psychology , researchers conduct research into human development from childhood to old age. Social psychology includes research on behaviour governed by social drivers. Researchers in the field of educational psychology study how people learn and the best way to teach them. Health psychology aims to determine the effect of psychological factors on physiological health. Physiological psychology , on the other hand, looks at the influence of physiological aspects on behaviour. Experimental psychology is not the only theme that uses experimental research and focuses on the traditional core topics of psychology (for example, sensation). Cognitive psychology studies the higher mental processes. Psychometrics is concerned with measuring capacity or behaviour. Personality research aims to assess and describe consistency in human behaviour ( Weiten, 2010 ). The final theme of psychological practice refers to the experiences, techniques, and interventions employed by practitioners, researchers, and academia in the field of psychology.
Articles under these themes were further subdivided into methodologies: method, sampling, design, data collection, and data analysis. The categorisation was based on information stated in the articles and not inferred by the researchers. Data were compiled into two sets of results presented in this article. The first set addresses the aim of this study from the perspective of the topics identified. The second set of results represents a broad overview of the results from the perspective of the methodology employed. The second set of results are discussed in this article, while the first set is presented in table format. The discussion thus provides a broad overview of methods use in psychology (across all themes), while the table format provides readers with in-depth insight into methods used in the individual themes identified. We believe that presenting the data from both perspectives allow readers a broad understanding of the results. Due a large amount of information that made up our results, we followed Cichocka and Jost (2014) in simplifying our results. Please note that the numbers indicated in the table in terms of methodology differ from the total number of articles. Some articles employed more than one method/sampling technique/design/data collection method/data analysis in their studies.
What follows is the results for what methods are used, how these methods are used, and which topics in psychology they are applied to . Percentages are reported to the second decimal in order to highlight small differences in the occurrence of methodology.
Firstly, with regard to the research methods used, our results show that researchers are more likely to use quantitative research methods (90.22%) compared to all other research methods. Qualitative research was the second most common research method but only made up about 4.79% of the general method usage. Reviews occurred almost as much as qualitative studies (3.91%), as the third most popular method. Mixed-methods research studies (0.98%) occurred across most themes, whereas multi-method research was indicated in only one study and amounted to 0.10% of the methods identified. The specific use of each method in the topics identified is shown in Table 2 and Figure 4 .

Table 2 . Research methods in psychology.
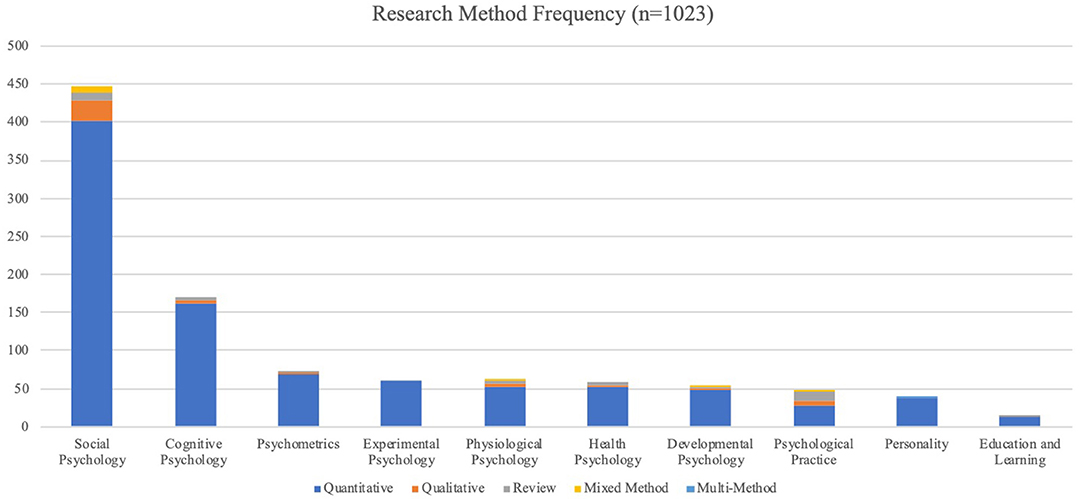
Figure 4 . Research method frequency in topics.
Secondly, in the case of how these research methods are employed , our study indicated the following.
Sampling −78.34% of the studies in the collected articles did not specify a sampling method. From the remainder of the studies, 13 types of sampling methods were identified. These sampling methods included broad categorisation of a sample as, for example, a probability or non-probability sample. General samples of convenience were the methods most likely to be applied (10.34%), followed by random sampling (3.51%), snowball sampling (2.73%), and purposive (1.37%) and cluster sampling (1.27%). The remainder of the sampling methods occurred to a more limited extent (0–1.0%). See Table 3 and Figure 5 for sampling methods employed in each topic.
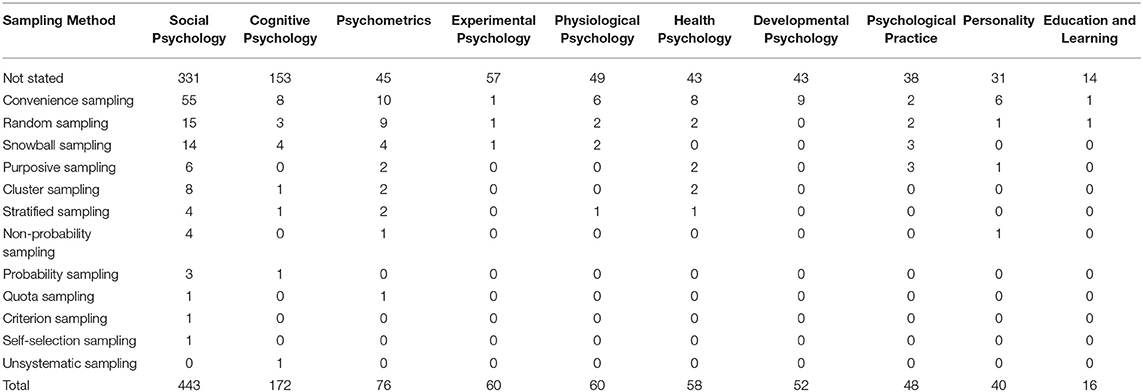
Table 3 . Sampling use in the field of psychology.
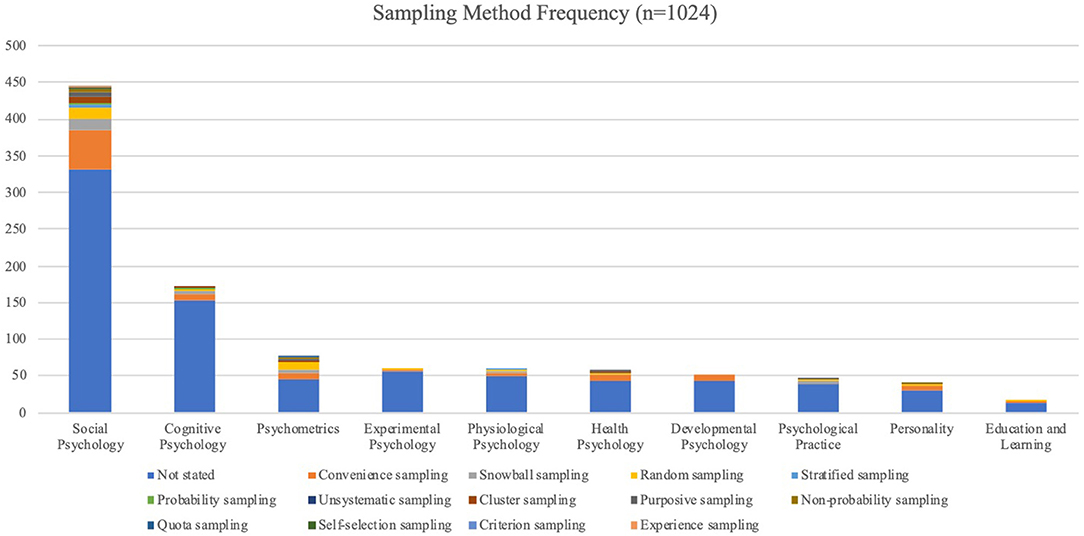
Figure 5 . Sampling method frequency in topics.
Designs were categorised based on the articles' statement thereof. Therefore, it is important to note that, in the case of quantitative studies, non-experimental designs (25.55%) were often indicated due to a lack of experiments and any other indication of design, which, according to Laher (2016) , is a reasonable categorisation. Non-experimental designs should thus be compared with experimental designs only in the description of data, as it could include the use of correlational/cross-sectional designs, which were not overtly stated by the authors. For the remainder of the research methods, “not stated” (7.12%) was assigned to articles without design types indicated.
From the 36 identified designs the most popular designs were cross-sectional (23.17%) and experimental (25.64%), which concurred with the high number of quantitative studies. Longitudinal studies (3.80%), the third most popular design, was used in both quantitative and qualitative studies. Qualitative designs consisted of ethnography (0.38%), interpretative phenomenological designs/phenomenology (0.28%), as well as narrative designs (0.28%). Studies that employed the review method were mostly categorised as “not stated,” with the most often stated review designs being systematic reviews (0.57%). The few mixed method studies employed exploratory, explanatory (0.09%), and concurrent designs (0.19%), with some studies referring to separate designs for the qualitative and quantitative methods. The one study that identified itself as a multi-method study used a longitudinal design. Please see how these designs were employed in each specific topic in Table 4 , Figure 6 .
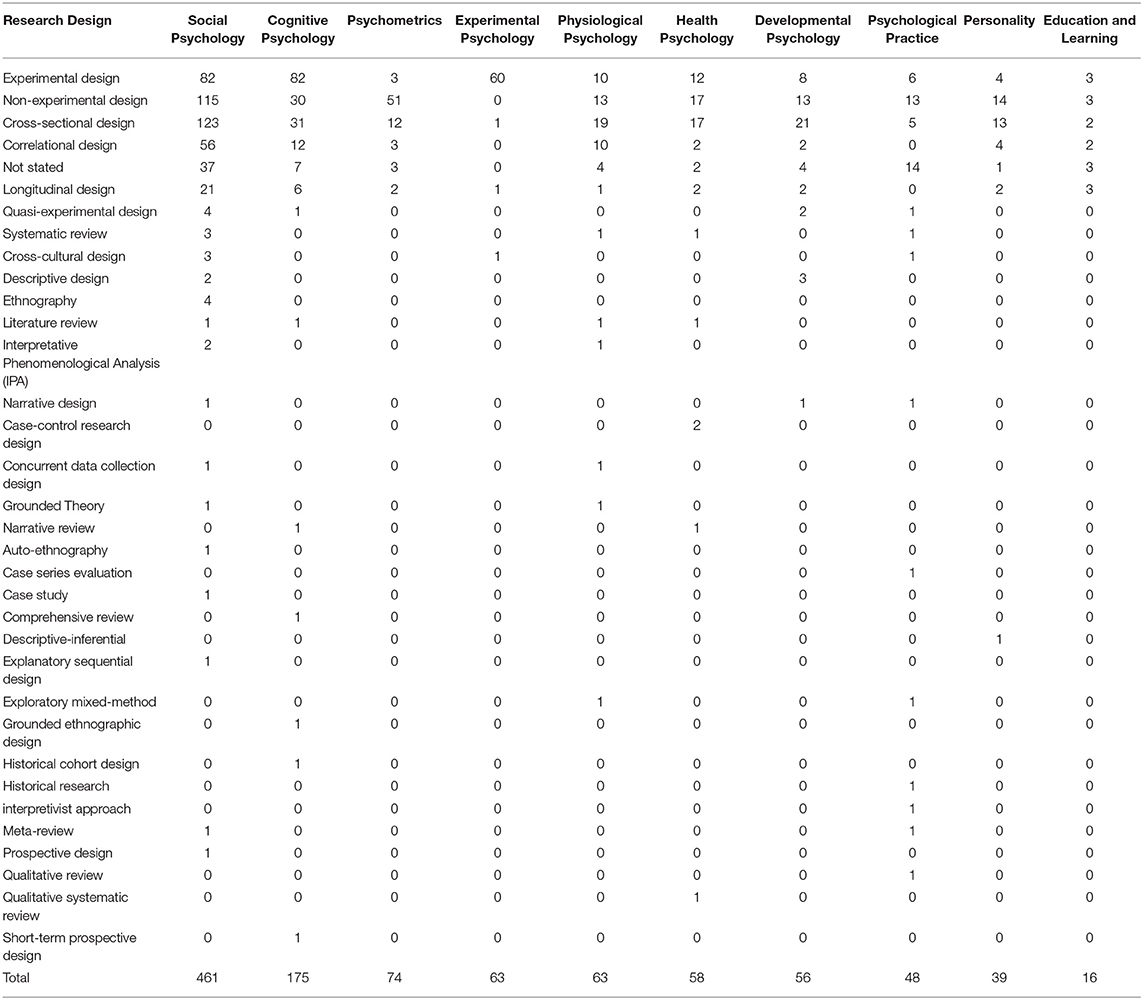
Table 4 . Design use in the field of psychology.
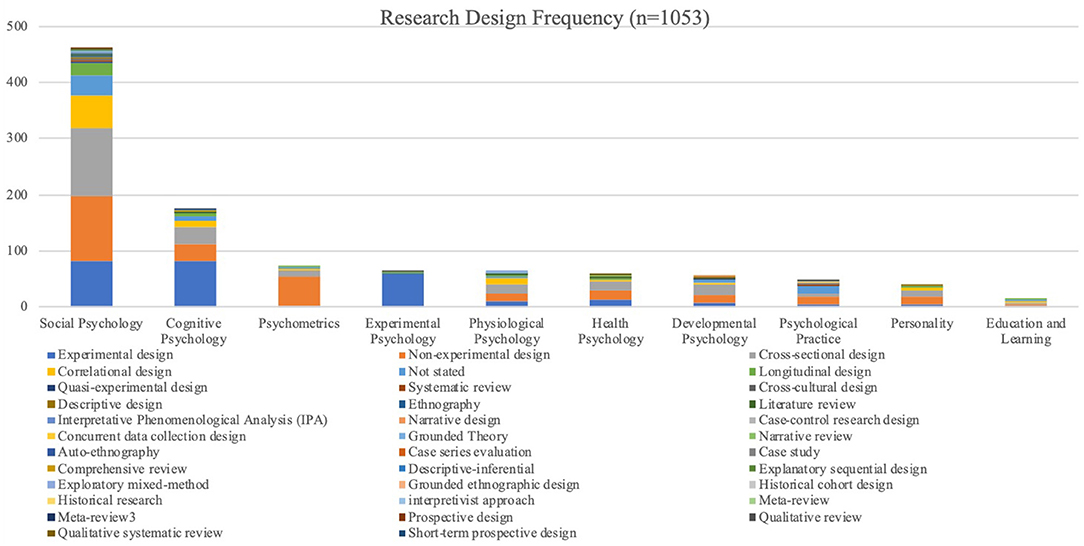
Figure 6 . Design frequency in topics.
Data collection and analysis —data collection included 30 methods, with the data collection method most often employed being questionnaires (57.84%). The experimental task (16.56%) was the second most preferred collection method, which included established or unique tasks designed by the researchers. Cognitive ability tests (6.84%) were also regularly used along with various forms of interviewing (7.66%). Table 5 and Figure 7 represent data collection use in the various topics. Data analysis consisted of 3,857 occurrences of data analysis categorised into ±188 various data analysis techniques shown in Table 6 and Figures 1 – 7 . Descriptive statistics were the most commonly used (23.49%) along with correlational analysis (17.19%). When using a qualitative method, researchers generally employed thematic analysis (0.52%) or different forms of analysis that led to coding and the creation of themes. Review studies presented few data analysis methods, with most studies categorising their results. Mixed method and multi-method studies followed the analysis methods identified for the qualitative and quantitative studies included.
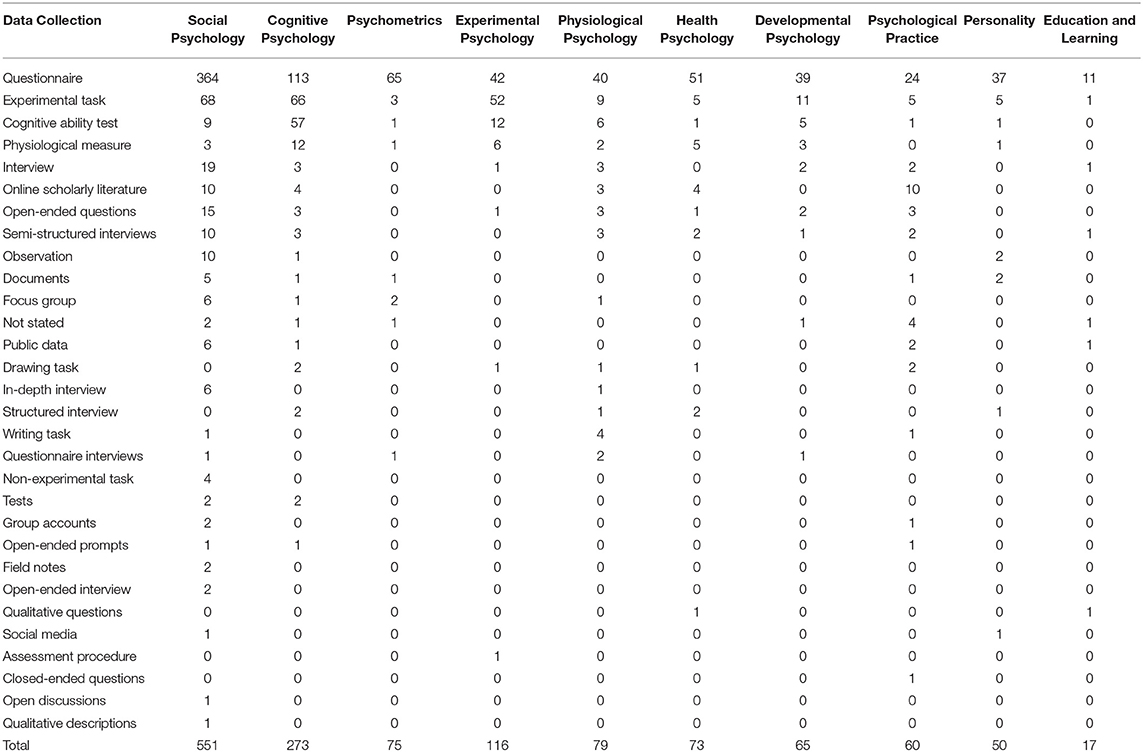
Table 5 . Data collection in the field of psychology.
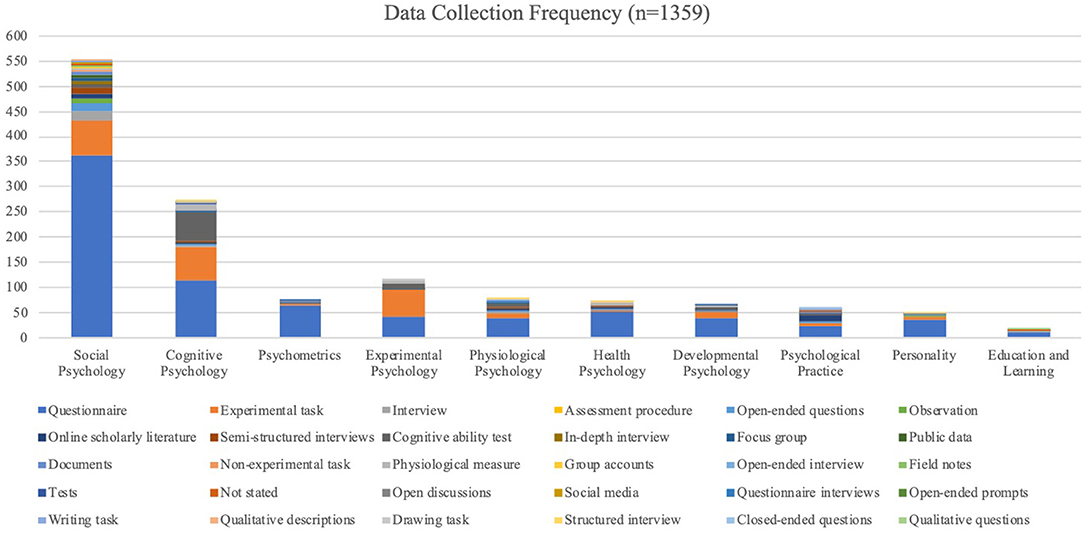
Figure 7 . Data collection frequency in topics.

Table 6 . Data analysis in the field of psychology.
Results of the topics researched in psychology can be seen in the tables, as previously stated in this article. It is noteworthy that, of the 10 topics, social psychology accounted for 43.54% of the studies, with cognitive psychology the second most popular research topic at 16.92%. The remainder of the topics only occurred in 4.0–7.0% of the articles considered. A list of the included 999 articles is available under the section “View Articles” on the following website: https://methodgarden.xtrapolate.io/ . This website was created by Scholtz et al. (2019) to visually present a research framework based on this Article's results.
This systematised review categorised full-length articles from five international journals across the span of 5 years to provide insight into the use of research methods in the field of psychology. Results indicated what methods are used how these methods are being used and for what topics (why) in the included sample of articles. The results should be seen as providing insight into method use and by no means a comprehensive representation of the aforementioned aim due to the limited sample. To our knowledge, this is the first research study to address this topic in this manner. Our discussion attempts to promote a productive way forward in terms of the key results for method use in psychology, especially in the field of academia ( Holloway, 2008 ).
With regard to the methods used, our data stayed true to literature, finding only common research methods ( Grant and Booth, 2009 ; Maree, 2016 ) that varied in the degree to which they were employed. Quantitative research was found to be the most popular method, as indicated by literature ( Breen and Darlaston-Jones, 2010 ; Counsell and Harlow, 2017 ) and previous studies in specific areas of psychology (see Coetzee and Van Zyl, 2014 ). Its long history as the first research method ( Leech et al., 2007 ) in the field of psychology as well as researchers' current application of mathematical approaches in their studies ( Toomela, 2010 ) might contribute to its popularity today. Whatever the case may be, our results show that, despite the growth in qualitative research ( Demuth, 2015 ; Smith and McGannon, 2018 ), quantitative research remains the first choice for article publication in these journals. Despite the included journals indicating openness to articles that apply any research methods. This finding may be due to qualitative research still being seen as a new method ( Burman and Whelan, 2011 ) or reviewers' standards being higher for qualitative studies ( Bluhm et al., 2011 ). Future research is encouraged into the possible biasness in publication of research methods, additionally further investigation with a different sample into the proclaimed growth of qualitative research may also provide different results.
Review studies were found to surpass that of multi-method and mixed method studies. To this effect Grant and Booth (2009) , state that the increased awareness, journal contribution calls as well as its efficiency in procuring research funds all promote the popularity of reviews. The low frequency of mixed method studies contradicts the view in literature that it's the third most utilised research method ( Tashakkori and Teddlie's, 2003 ). Its' low occurrence in this sample could be due to opposing views on mixing methods ( Gunasekare, 2015 ) or that authors prefer publishing in mixed method journals, when using this method, or its relative novelty ( Ivankova et al., 2016 ). Despite its low occurrence, the application of the mixed methods design in articles was methodologically clear in all cases which were not the case for the remainder of research methods.
Additionally, a substantial number of studies used a combination of methodologies that are not mixed or multi-method studies. Perceived fixed boundaries are according to literature often set aside, as confirmed by this result, in order to investigate the aim of a study, which could create a new and helpful way of understanding the world ( Gunasekare, 2015 ). According to Toomela (2010) , this is not unheard of and could be considered a form of “structural systemic science,” as in the case of qualitative methodology (observation) applied in quantitative studies (experimental design) for example. Based on this result, further research into this phenomenon as well as its implications for research methods such as multi and mixed methods is recommended.
Discerning how these research methods were applied, presented some difficulty. In the case of sampling, most studies—regardless of method—did mention some form of inclusion and exclusion criteria, but no definite sampling method. This result, along with the fact that samples often consisted of students from the researchers' own academic institutions, can contribute to literature and debates among academics ( Peterson and Merunka, 2014 ; Laher, 2016 ). Samples of convenience and students as participants especially raise questions about the generalisability and applicability of results ( Peterson and Merunka, 2014 ). This is because attention to sampling is important as inappropriate sampling can debilitate the legitimacy of interpretations ( Onwuegbuzie and Collins, 2017 ). Future investigation into the possible implications of this reported popular use of convenience samples for the field of psychology as well as the reason for this use could provide interesting insight, and is encouraged by this study.
Additionally, and this is indicated in Table 6 , articles seldom report the research designs used, which highlights the pressing aspect of the lack of rigour in the included sample. Rigour with regards to the applied empirical method is imperative in promoting psychology as a science ( American Psychological Association, 2020 ). Omitting parts of the research process in publication when it could have been used to inform others' research skills should be questioned, and the influence on the process of replicating results should be considered. Publications are often rejected due to a lack of rigour in the applied method and designs ( Fonseca, 2013 ; Laher, 2016 ), calling for increased clarity and knowledge of method application. Replication is a critical part of any field of scientific research and requires the “complete articulation” of the study methods used ( Drotar, 2010 , p. 804). The lack of thorough description could be explained by the requirements of certain journals to only report on certain aspects of a research process, especially with regard to the applied design (Laher, 20). However, naming aspects such as sampling and designs, is a requirement according to the APA's Journal Article Reporting Standards (JARS-Quant) ( Appelbaum et al., 2018 ). With very little information on how a study was conducted, authors lose a valuable opportunity to enhance research validity, enrich the knowledge of others, and contribute to the growth of psychology and methodology as a whole. In the case of this research study, it also restricted our results to only reported samples and designs, which indicated a preference for certain designs, such as cross-sectional designs for quantitative studies.
Data collection and analysis were for the most part clearly stated. A key result was the versatile use of questionnaires. Researchers would apply a questionnaire in various ways, for example in questionnaire interviews, online surveys, and written questionnaires across most research methods. This may highlight a trend for future research.
With regard to the topics these methods were employed for, our research study found a new field named “psychological practice.” This result may show the growing consciousness of researchers as part of the research process ( Denzin and Lincoln, 2003 ), psychological practice, and knowledge generation. The most popular of these topics was social psychology, which is generously covered in journals and by learning societies, as testaments of the institutional support and richness social psychology has in the field of psychology ( Chryssochoou, 2015 ). The APA's perspective on 2018 trends in psychology also identifies an increased amount of psychology focus on how social determinants are influencing people's health ( Deangelis, 2017 ).
This study was not without limitations and the following should be taken into account. Firstly, this study used a sample of five specific journals to address the aim of the research study, despite general journal aims (as stated on journal websites), this inclusion signified a bias towards the research methods published in these specific journals only and limited generalisability. A broader sample of journals over a different period of time, or a single journal over a longer period of time might provide different results. A second limitation is the use of Excel spreadsheets and an electronic system to log articles, which was a manual process and therefore left room for error ( Bandara et al., 2015 ). To address this potential issue, co-coding was performed to reduce error. Lastly, this article categorised data based on the information presented in the article sample; there was no interpretation of what methodology could have been applied or whether the methods stated adhered to the criteria for the methods used. Thus, a large number of articles that did not clearly indicate a research method or design could influence the results of this review. However, this in itself was also a noteworthy result. Future research could review research methods of a broader sample of journals with an interpretive review tool that increases rigour. Additionally, the authors also encourage the future use of systematised review designs as a way to promote a concise procedure in applying this design.
Our research study presented the use of research methods for published articles in the field of psychology as well as recommendations for future research based on these results. Insight into the complex questions identified in literature, regarding what methods are used how these methods are being used and for what topics (why) was gained. This sample preferred quantitative methods, used convenience sampling and presented a lack of rigorous accounts for the remaining methodologies. All methodologies that were clearly indicated in the sample were tabulated to allow researchers insight into the general use of methods and not only the most frequently used methods. The lack of rigorous account of research methods in articles was represented in-depth for each step in the research process and can be of vital importance to address the current replication crisis within the field of psychology. Recommendations for future research aimed to motivate research into the practical implications of the results for psychology, for example, publication bias and the use of convenience samples.

Ethics Statement
This study was cleared by the North-West University Health Research Ethics Committee: NWU-00115-17-S1.
Author Contributions
All authors listed have made a substantial, direct and intellectual contribution to the work, and approved it for publication.
Conflict of Interest
The authors declare that the research was conducted in the absence of any commercial or financial relationships that could be construed as a potential conflict of interest.
Aanstoos, C. M. (2014). Psychology . Available online at: http://eds.a.ebscohost.com.nwulib.nwu.ac.za/eds/detail/detail?sid=18de6c5c-2b03-4eac-94890145eb01bc70%40sessionmgr4006&vid$=$1&hid$=$4113&bdata$=$JnNpdGU9ZWRzL~WxpdmU%3d#AN$=$93871882&db$=$ers
Google Scholar
American Psychological Association (2020). Science of Psychology . Available online at: https://www.apa.org/action/science/
Appelbaum, M., Cooper, H., Kline, R. B., Mayo-Wilson, E., Nezu, A. M., and Rao, S. M. (2018). Journal article reporting standards for quantitative research in psychology: the APA Publications and Communications Board task force report. Am. Psychol. 73:3. doi: 10.1037/amp0000191
PubMed Abstract | CrossRef Full Text | Google Scholar
Bandara, W., Furtmueller, E., Gorbacheva, E., Miskon, S., and Beekhuyzen, J. (2015). Achieving rigor in literature reviews: insights from qualitative data analysis and tool-support. Commun. Ass. Inform. Syst. 37, 154–204. doi: 10.17705/1CAIS.03708
CrossRef Full Text | Google Scholar
Barr-Walker, J. (2017). Evidence-based information needs of public health workers: a systematized review. J. Med. Libr. Assoc. 105, 69–79. doi: 10.5195/JMLA.2017.109
Bittermann, A., and Fischer, A. (2018). How to identify hot topics in psychology using topic modeling. Z. Psychol. 226, 3–13. doi: 10.1027/2151-2604/a000318
Bluhm, D. J., Harman, W., Lee, T. W., and Mitchell, T. R. (2011). Qualitative research in management: a decade of progress. J. Manage. Stud. 48, 1866–1891. doi: 10.1111/j.1467-6486.2010.00972.x
Breen, L. J., and Darlaston-Jones, D. (2010). Moving beyond the enduring dominance of positivism in psychological research: implications for psychology in Australia. Aust. Psychol. 45, 67–76. doi: 10.1080/00050060903127481
Burman, E., and Whelan, P. (2011). Problems in / of Qualitative Research . Maidenhead: Open University Press/McGraw Hill.
Chaichanasakul, A., He, Y., Chen, H., Allen, G. E. K., Khairallah, T. S., and Ramos, K. (2011). Journal of Career Development: a 36-year content analysis (1972–2007). J. Career. Dev. 38, 440–455. doi: 10.1177/0894845310380223
Chryssochoou, X. (2015). Social Psychology. Inter. Encycl. Soc. Behav. Sci. 22, 532–537. doi: 10.1016/B978-0-08-097086-8.24095-6
Cichocka, A., and Jost, J. T. (2014). Stripped of illusions? Exploring system justification processes in capitalist and post-Communist societies. Inter. J. Psychol. 49, 6–29. doi: 10.1002/ijop.12011
Clay, R. A. (2017). Psychology is More Popular Than Ever. Monitor on Psychology: Trends Report . Available online at: https://www.apa.org/monitor/2017/11/trends-popular
Coetzee, M., and Van Zyl, L. E. (2014). A review of a decade's scholarly publications (2004–2013) in the South African Journal of Industrial Psychology. SA. J. Psychol . 40, 1–16. doi: 10.4102/sajip.v40i1.1227
Counsell, A., and Harlow, L. (2017). Reporting practices and use of quantitative methods in Canadian journal articles in psychology. Can. Psychol. 58, 140–147. doi: 10.1037/cap0000074
Deangelis, T. (2017). Targeting Social Factors That Undermine Health. Monitor on Psychology: Trends Report . Available online at: https://www.apa.org/monitor/2017/11/trend-social-factors
Demuth, C. (2015). New directions in qualitative research in psychology. Integr. Psychol. Behav. Sci. 49, 125–133. doi: 10.1007/s12124-015-9303-9
Denzin, N. K., and Lincoln, Y. (2003). The Landscape of Qualitative Research: Theories and Issues , 2nd Edn. London: Sage.
Drotar, D. (2010). A call for replications of research in pediatric psychology and guidance for authors. J. Pediatr. Psychol. 35, 801–805. doi: 10.1093/jpepsy/jsq049
Dweck, C. S. (2017). Is psychology headed in the right direction? Yes, no, and maybe. Perspect. Psychol. Sci. 12, 656–659. doi: 10.1177/1745691616687747
Earp, B. D., and Trafimow, D. (2015). Replication, falsification, and the crisis of confidence in social psychology. Front. Psychol. 6:621. doi: 10.3389/fpsyg.2015.00621
Ezeh, A. C., Izugbara, C. O., Kabiru, C. W., Fonn, S., Kahn, K., Manderson, L., et al. (2010). Building capacity for public and population health research in Africa: the consortium for advanced research training in Africa (CARTA) model. Glob. Health Action 3:5693. doi: 10.3402/gha.v3i0.5693
Ferreira, A. L. L., Bessa, M. M. M., Drezett, J., and De Abreu, L. C. (2016). Quality of life of the woman carrier of endometriosis: systematized review. Reprod. Clim. 31, 48–54. doi: 10.1016/j.recli.2015.12.002
Fonseca, M. (2013). Most Common Reasons for Journal Rejections . Available online at: http://www.editage.com/insights/most-common-reasons-for-journal-rejections
Gough, B., and Lyons, A. (2016). The future of qualitative research in psychology: accentuating the positive. Integr. Psychol. Behav. Sci. 50, 234–243. doi: 10.1007/s12124-015-9320-8
Grant, M. J., and Booth, A. (2009). A typology of reviews: an analysis of 14 review types and associated methodologies. Health Info. Libr. J. 26, 91–108. doi: 10.1111/j.1471-1842.2009.00848.x
Grix, J. (2002). Introducing students to the generic terminology of social research. Politics 22, 175–186. doi: 10.1111/1467-9256.00173
Gunasekare, U. L. T. P. (2015). Mixed research method as the third research paradigm: a literature review. Int. J. Sci. Res. 4, 361–368. Available online at: https://ssrn.com/abstract=2735996
Hengartner, M. P. (2018). Raising awareness for the replication crisis in clinical psychology by focusing on inconsistencies in psychotherapy Research: how much can we rely on published findings from efficacy trials? Front. Psychol. 9:256. doi: 10.3389/fpsyg.2018.00256
Holloway, W. (2008). Doing intellectual disagreement differently. Psychoanal. Cult. Soc. 13, 385–396. doi: 10.1057/pcs.2008.29
Ivankova, N. V., Creswell, J. W., and Plano Clark, V. L. (2016). “Foundations and Approaches to mixed methods research,” in First Steps in Research , 2nd Edn. K. Maree (Pretoria: Van Schaick Publishers), 306–335.
Johnson, M., Long, T., and White, A. (2001). Arguments for British pluralism in qualitative health research. J. Adv. Nurs. 33, 243–249. doi: 10.1046/j.1365-2648.2001.01659.x
Johnston, A., Kelly, S. E., Hsieh, S. C., Skidmore, B., and Wells, G. A. (2019). Systematic reviews of clinical practice guidelines: a methodological guide. J. Clin. Epidemiol. 108, 64–72. doi: 10.1016/j.jclinepi.2018.11.030
Ketchen, D. J. Jr., Boyd, B. K., and Bergh, D. D. (2008). Research methodology in strategic management: past accomplishments and future challenges. Organ. Res. Methods 11, 643–658. doi: 10.1177/1094428108319843
Ktepi, B. (2016). Data Analytics (DA) . Available online at: https://eds-b-ebscohost-com.nwulib.nwu.ac.za/eds/detail/detail?vid=2&sid=24c978f0-6685-4ed8-ad85-fa5bb04669b9%40sessionmgr101&bdata=JnNpdGU9ZWRzLWxpdmU%3d#AN=113931286&db=ers
Laher, S. (2016). Ostinato rigore: establishing methodological rigour in quantitative research. S. Afr. J. Psychol. 46, 316–327. doi: 10.1177/0081246316649121
Lee, C. (2015). The Myth of the Off-Limits Source . Available online at: http://blog.apastyle.org/apastyle/research/
Lee, T. W., Mitchell, T. R., and Sablynski, C. J. (1999). Qualitative research in organizational and vocational psychology, 1979–1999. J. Vocat. Behav. 55, 161–187. doi: 10.1006/jvbe.1999.1707
Leech, N. L., Anthony, J., and Onwuegbuzie, A. J. (2007). A typology of mixed methods research designs. Sci. Bus. Media B. V Qual. Quant 43, 265–275. doi: 10.1007/s11135-007-9105-3
Levitt, H. M., Motulsky, S. L., Wertz, F. J., Morrow, S. L., and Ponterotto, J. G. (2017). Recommendations for designing and reviewing qualitative research in psychology: promoting methodological integrity. Qual. Psychol. 4, 2–22. doi: 10.1037/qup0000082
Lowe, S. M., and Moore, S. (2014). Social networks and female reproductive choices in the developing world: a systematized review. Rep. Health 11:85. doi: 10.1186/1742-4755-11-85
Maree, K. (2016). “Planning a research proposal,” in First Steps in Research , 2nd Edn, ed K. Maree (Pretoria: Van Schaik Publishers), 49–70.
Maree, K., and Pietersen, J. (2016). “Sampling,” in First Steps in Research, 2nd Edn , ed K. Maree (Pretoria: Van Schaik Publishers), 191–202.
Ngulube, P. (2013). Blending qualitative and quantitative research methods in library and information science in sub-Saharan Africa. ESARBICA J. 32, 10–23. Available online at: http://hdl.handle.net/10500/22397 .
Nieuwenhuis, J. (2016). “Qualitative research designs and data-gathering techniques,” in First Steps in Research , 2nd Edn, ed K. Maree (Pretoria: Van Schaik Publishers), 71–102.
Nind, M., Kilburn, D., and Wiles, R. (2015). Using video and dialogue to generate pedagogic knowledge: teachers, learners and researchers reflecting together on the pedagogy of social research methods. Int. J. Soc. Res. Methodol. 18, 561–576. doi: 10.1080/13645579.2015.1062628
O'Cathain, A. (2009). Editorial: mixed methods research in the health sciences—a quiet revolution. J. Mix. Methods 3, 1–6. doi: 10.1177/1558689808326272
O'Neil, S., and Koekemoer, E. (2016). Two decades of qualitative research in psychology, industrial and organisational psychology and human resource management within South Africa: a critical review. SA J. Indust. Psychol. 42, 1–16. doi: 10.4102/sajip.v42i1.1350
Onwuegbuzie, A. J., and Collins, K. M. (2017). The role of sampling in mixed methods research enhancing inference quality. Köln Z Soziol. 2, 133–156. doi: 10.1007/s11577-017-0455-0
Perestelo-Pérez, L. (2013). Standards on how to develop and report systematic reviews in psychology and health. Int. J. Clin. Health Psychol. 13, 49–57. doi: 10.1016/S1697-2600(13)70007-3
Pericall, L. M. T., and Taylor, E. (2014). Family function and its relationship to injury severity and psychiatric outcome in children with acquired brain injury: a systematized review. Dev. Med. Child Neurol. 56, 19–30. doi: 10.1111/dmcn.12237
Peterson, R. A., and Merunka, D. R. (2014). Convenience samples of college students and research reproducibility. J. Bus. Res. 67, 1035–1041. doi: 10.1016/j.jbusres.2013.08.010
Ritchie, J., Lewis, J., and Elam, G. (2009). “Designing and selecting samples,” in Qualitative Research Practice: A Guide for Social Science Students and Researchers , 2nd Edn, ed J. Ritchie and J. Lewis (London: Sage), 1–23.
Sandelowski, M. (2011). When a cigar is not just a cigar: alternative perspectives on data and data analysis. Res. Nurs. Health 34, 342–352. doi: 10.1002/nur.20437
Sandelowski, M., Voils, C. I., and Knafl, G. (2009). On quantitizing. J. Mix. Methods Res. 3, 208–222. doi: 10.1177/1558689809334210
Scholtz, S. E., De Klerk, W., and De Beer, L. T. (2019). A data generated research framework for conducting research methods in psychological research.
Scimago Journal & Country Rank (2017). Available online at: http://www.scimagojr.com/journalrank.php?category=3201&year=2015
Scopus (2017a). About Scopus . Available online at: https://www.scopus.com/home.uri (accessed February 01, 2017).
Scopus (2017b). Document Search . Available online at: https://www.scopus.com/home.uri (accessed February 01, 2017).
Scott Jones, J., and Goldring, J. E. (2015). ‘I' m not a quants person'; key strategies in building competence and confidence in staff who teach quantitative research methods. Int. J. Soc. Res. Methodol. 18, 479–494. doi: 10.1080/13645579.2015.1062623
Smith, B., and McGannon, K. R. (2018). Developing rigor in quantitative research: problems and opportunities within sport and exercise psychology. Int. Rev. Sport Exerc. Psychol. 11, 101–121. doi: 10.1080/1750984X.2017.1317357
Stangor, C. (2011). Introduction to Psychology . Available online at: http://www.saylor.org/books/
Strydom, H. (2011). “Sampling in the quantitative paradigm,” in Research at Grass Roots; For the Social Sciences and Human Service Professions , 4th Edn, eds A. S. de Vos, H. Strydom, C. B. Fouché, and C. S. L. Delport (Pretoria: Van Schaik Publishers), 221–234.
Tashakkori, A., and Teddlie, C. (2003). Handbook of Mixed Methods in Social & Behavioural Research . Thousand Oaks, CA: SAGE publications.
Toomela, A. (2010). Quantitative methods in psychology: inevitable and useless. Front. Psychol. 1:29. doi: 10.3389/fpsyg.2010.00029
Truscott, D. M., Swars, S., Smith, S., Thornton-Reid, F., Zhao, Y., Dooley, C., et al. (2010). A cross-disciplinary examination of the prevalence of mixed methods in educational research: 1995–2005. Int. J. Soc. Res. Methodol. 13, 317–328. doi: 10.1080/13645570903097950
Weiten, W. (2010). Psychology Themes and Variations . Belmont, CA: Wadsworth.
Keywords: research methods, research approach, research trends, psychological research, systematised review, research designs, research topic
Citation: Scholtz SE, de Klerk W and de Beer LT (2020) The Use of Research Methods in Psychological Research: A Systematised Review. Front. Res. Metr. Anal. 5:1. doi: 10.3389/frma.2020.00001
Received: 30 December 2019; Accepted: 28 February 2020; Published: 20 March 2020.
Reviewed by:
Copyright © 2020 Scholtz, de Klerk and de Beer. This is an open-access article distributed under the terms of the Creative Commons Attribution License (CC BY) . The use, distribution or reproduction in other forums is permitted, provided the original author(s) and the copyright owner(s) are credited and that the original publication in this journal is cited, in accordance with accepted academic practice. No use, distribution or reproduction is permitted which does not comply with these terms.
*Correspondence: Salomé Elizabeth Scholtz, 22308563@nwu.ac.za
Disclaimer: All claims expressed in this article are solely those of the authors and do not necessarily represent those of their affiliated organizations, or those of the publisher, the editors and the reviewers. Any product that may be evaluated in this article or claim that may be made by its manufacturer is not guaranteed or endorsed by the publisher.
- Interlibrary Loan and Scan & Deliver
- Course Reserves
- Purchase Request
- Collection Development & Maintenance
- Current Negotiations
- Ask a Librarian
- Instructor Support
- Library How-To
- Research Guides
- Research Support
- Study Rooms
- Research Rooms
- Partner Spaces
- Loanable Equipment
- Print, Scan, Copy
- 3D Printers
- Poster Printing
- OSULP Leadership
- Strategic Plan
Scholarly Articles: How can I tell?
- Journal Information
- Literature Review
- Author and affiliation
- Introduction
- Specialized Vocabulary
Methodology
- Research sponsors
- Peer-review
The methodology section or methods section tells you how the author(s) went about doing their research. It should let you know a) what method they used to gather data (survey, interviews, experiments, etc.), why they chose this method, and what the limitations are to this method.
The methodology section should be detailed enough that another researcher could replicate the study described. When you read the methodology or methods section:
- What kind of research method did the authors use? Is it an appropriate method for the type of study they are conducting?
- How did the authors get their tests subjects? What criteria did they use?
- What are the contexts of the study that may have affected the results (e.g. environmental conditions, lab conditions, timing questions, etc.)
- Is the sample size representative of the larger population (i.e., was it big enough?)
- Are the data collection instruments and procedures likely to have measured all the important characteristics with reasonable accuracy?
- Does the data analysis appear to have been done with care, and were appropriate analytical techniques used?
A good researcher will always let you know about the limitations of his or her research.
- << Previous: Specialized Vocabulary
- Next: Results >>
- Last Updated: Apr 15, 2024 3:26 PM
- URL: https://guides.library.oregonstate.edu/ScholarlyArticle
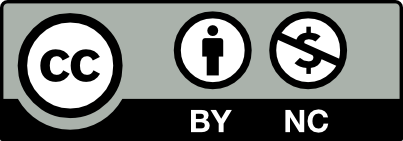
Contact Info
121 The Valley Library Corvallis OR 97331–4501
Phone: 541-737-3331
Services for Persons with Disabilities
In the Valley Library
- Oregon State University Press
- Special Collections and Archives Research Center
- Undergrad Research & Writing Studio
- Graduate Student Commons
- Tutoring Services
- Northwest Art Collection
Digital Projects
- Oregon Explorer
- Oregon Digital
- ScholarsArchive@OSU
- Digital Publishing Initiatives
- Atlas of the Pacific Northwest
- Marilyn Potts Guin Library  
- Cascades Campus Library
- McDowell Library of Vet Medicine

Have a language expert improve your writing
Run a free plagiarism check in 10 minutes, generate accurate citations for free.
- Knowledge Base
Methodology
Research Methods | Definitions, Types, Examples
Research methods are specific procedures for collecting and analyzing data. Developing your research methods is an integral part of your research design . When planning your methods, there are two key decisions you will make.
First, decide how you will collect data . Your methods depend on what type of data you need to answer your research question :
- Qualitative vs. quantitative : Will your data take the form of words or numbers?
- Primary vs. secondary : Will you collect original data yourself, or will you use data that has already been collected by someone else?
- Descriptive vs. experimental : Will you take measurements of something as it is, or will you perform an experiment?
Second, decide how you will analyze the data .
- For quantitative data, you can use statistical analysis methods to test relationships between variables.
- For qualitative data, you can use methods such as thematic analysis to interpret patterns and meanings in the data.
Table of contents
Methods for collecting data, examples of data collection methods, methods for analyzing data, examples of data analysis methods, other interesting articles, frequently asked questions about research methods.
Data is the information that you collect for the purposes of answering your research question . The type of data you need depends on the aims of your research.
Qualitative vs. quantitative data
Your choice of qualitative or quantitative data collection depends on the type of knowledge you want to develop.
For questions about ideas, experiences and meanings, or to study something that can’t be described numerically, collect qualitative data .
If you want to develop a more mechanistic understanding of a topic, or your research involves hypothesis testing , collect quantitative data .
Qualitative | to broader populations. . | |
---|---|---|
Quantitative | . |
You can also take a mixed methods approach , where you use both qualitative and quantitative research methods.
Primary vs. secondary research
Primary research is any original data that you collect yourself for the purposes of answering your research question (e.g. through surveys , observations and experiments ). Secondary research is data that has already been collected by other researchers (e.g. in a government census or previous scientific studies).
If you are exploring a novel research question, you’ll probably need to collect primary data . But if you want to synthesize existing knowledge, analyze historical trends, or identify patterns on a large scale, secondary data might be a better choice.
Primary | . | methods. |
---|---|---|
Secondary |
Descriptive vs. experimental data
In descriptive research , you collect data about your study subject without intervening. The validity of your research will depend on your sampling method .
In experimental research , you systematically intervene in a process and measure the outcome. The validity of your research will depend on your experimental design .
To conduct an experiment, you need to be able to vary your independent variable , precisely measure your dependent variable, and control for confounding variables . If it’s practically and ethically possible, this method is the best choice for answering questions about cause and effect.
Descriptive | . . | |
---|---|---|
Experimental |
Receive feedback on language, structure, and formatting
Professional editors proofread and edit your paper by focusing on:
- Academic style
- Vague sentences
- Style consistency
See an example
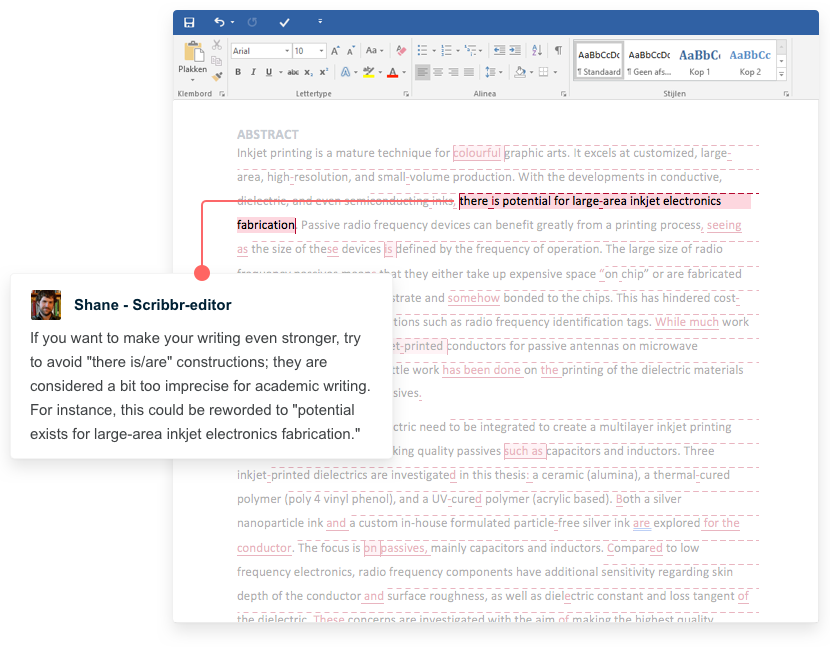
Research method | Primary or secondary? | Qualitative or quantitative? | When to use |
---|---|---|---|
Primary | Quantitative | To test cause-and-effect relationships. | |
Primary | Quantitative | To understand general characteristics of a population. | |
Interview/focus group | Primary | Qualitative | To gain more in-depth understanding of a topic. |
Observation | Primary | Either | To understand how something occurs in its natural setting. |
Secondary | Either | To situate your research in an existing body of work, or to evaluate trends within a research topic. | |
Either | Either | To gain an in-depth understanding of a specific group or context, or when you don’t have the resources for a large study. |
Your data analysis methods will depend on the type of data you collect and how you prepare it for analysis.
Data can often be analyzed both quantitatively and qualitatively. For example, survey responses could be analyzed qualitatively by studying the meanings of responses or quantitatively by studying the frequencies of responses.
Qualitative analysis methods
Qualitative analysis is used to understand words, ideas, and experiences. You can use it to interpret data that was collected:
- From open-ended surveys and interviews , literature reviews , case studies , ethnographies , and other sources that use text rather than numbers.
- Using non-probability sampling methods .
Qualitative analysis tends to be quite flexible and relies on the researcher’s judgement, so you have to reflect carefully on your choices and assumptions and be careful to avoid research bias .
Quantitative analysis methods
Quantitative analysis uses numbers and statistics to understand frequencies, averages and correlations (in descriptive studies) or cause-and-effect relationships (in experiments).
You can use quantitative analysis to interpret data that was collected either:
- During an experiment .
- Using probability sampling methods .
Because the data is collected and analyzed in a statistically valid way, the results of quantitative analysis can be easily standardized and shared among researchers.
Research method | Qualitative or quantitative? | When to use |
---|---|---|
Quantitative | To analyze data collected in a statistically valid manner (e.g. from experiments, surveys, and observations). | |
Meta-analysis | Quantitative | To statistically analyze the results of a large collection of studies. Can only be applied to studies that collected data in a statistically valid manner. |
Qualitative | To analyze data collected from interviews, , or textual sources. To understand general themes in the data and how they are communicated. | |
Either | To analyze large volumes of textual or visual data collected from surveys, literature reviews, or other sources. Can be quantitative (i.e. frequencies of words) or qualitative (i.e. meanings of words). |
If you want to know more about statistics , methodology , or research bias , make sure to check out some of our other articles with explanations and examples.
- Chi square test of independence
- Statistical power
- Descriptive statistics
- Degrees of freedom
- Pearson correlation
- Null hypothesis
- Double-blind study
- Case-control study
- Research ethics
- Data collection
- Hypothesis testing
- Structured interviews
Research bias
- Hawthorne effect
- Unconscious bias
- Recall bias
- Halo effect
- Self-serving bias
- Information bias
Quantitative research deals with numbers and statistics, while qualitative research deals with words and meanings.
Quantitative methods allow you to systematically measure variables and test hypotheses . Qualitative methods allow you to explore concepts and experiences in more detail.
In mixed methods research , you use both qualitative and quantitative data collection and analysis methods to answer your research question .
A sample is a subset of individuals from a larger population . Sampling means selecting the group that you will actually collect data from in your research. For example, if you are researching the opinions of students in your university, you could survey a sample of 100 students.
In statistics, sampling allows you to test a hypothesis about the characteristics of a population.
The research methods you use depend on the type of data you need to answer your research question .
- If you want to measure something or test a hypothesis , use quantitative methods . If you want to explore ideas, thoughts and meanings, use qualitative methods .
- If you want to analyze a large amount of readily-available data, use secondary data. If you want data specific to your purposes with control over how it is generated, collect primary data.
- If you want to establish cause-and-effect relationships between variables , use experimental methods. If you want to understand the characteristics of a research subject, use descriptive methods.
Methodology refers to the overarching strategy and rationale of your research project . It involves studying the methods used in your field and the theories or principles behind them, in order to develop an approach that matches your objectives.
Methods are the specific tools and procedures you use to collect and analyze data (for example, experiments, surveys , and statistical tests ).
In shorter scientific papers, where the aim is to report the findings of a specific study, you might simply describe what you did in a methods section .
In a longer or more complex research project, such as a thesis or dissertation , you will probably include a methodology section , where you explain your approach to answering the research questions and cite relevant sources to support your choice of methods.
Is this article helpful?
Other students also liked, writing strong research questions | criteria & examples.
- What Is a Research Design | Types, Guide & Examples
- Data Collection | Definition, Methods & Examples
More interesting articles
- Between-Subjects Design | Examples, Pros, & Cons
- Cluster Sampling | A Simple Step-by-Step Guide with Examples
- Confounding Variables | Definition, Examples & Controls
- Construct Validity | Definition, Types, & Examples
- Content Analysis | Guide, Methods & Examples
- Control Groups and Treatment Groups | Uses & Examples
- Control Variables | What Are They & Why Do They Matter?
- Correlation vs. Causation | Difference, Designs & Examples
- Correlational Research | When & How to Use
- Critical Discourse Analysis | Definition, Guide & Examples
- Cross-Sectional Study | Definition, Uses & Examples
- Descriptive Research | Definition, Types, Methods & Examples
- Ethical Considerations in Research | Types & Examples
- Explanatory and Response Variables | Definitions & Examples
- Explanatory Research | Definition, Guide, & Examples
- Exploratory Research | Definition, Guide, & Examples
- External Validity | Definition, Types, Threats & Examples
- Extraneous Variables | Examples, Types & Controls
- Guide to Experimental Design | Overview, Steps, & Examples
- How Do You Incorporate an Interview into a Dissertation? | Tips
- How to Do Thematic Analysis | Step-by-Step Guide & Examples
- How to Write a Literature Review | Guide, Examples, & Templates
- How to Write a Strong Hypothesis | Steps & Examples
- Inclusion and Exclusion Criteria | Examples & Definition
- Independent vs. Dependent Variables | Definition & Examples
- Inductive Reasoning | Types, Examples, Explanation
- Inductive vs. Deductive Research Approach | Steps & Examples
- Internal Validity in Research | Definition, Threats, & Examples
- Internal vs. External Validity | Understanding Differences & Threats
- Longitudinal Study | Definition, Approaches & Examples
- Mediator vs. Moderator Variables | Differences & Examples
- Mixed Methods Research | Definition, Guide & Examples
- Multistage Sampling | Introductory Guide & Examples
- Naturalistic Observation | Definition, Guide & Examples
- Operationalization | A Guide with Examples, Pros & Cons
- Population vs. Sample | Definitions, Differences & Examples
- Primary Research | Definition, Types, & Examples
- Qualitative vs. Quantitative Research | Differences, Examples & Methods
- Quasi-Experimental Design | Definition, Types & Examples
- Questionnaire Design | Methods, Question Types & Examples
- Random Assignment in Experiments | Introduction & Examples
- Random vs. Systematic Error | Definition & Examples
- Reliability vs. Validity in Research | Difference, Types and Examples
- Reproducibility vs Replicability | Difference & Examples
- Reproducibility vs. Replicability | Difference & Examples
- Sampling Methods | Types, Techniques & Examples
- Semi-Structured Interview | Definition, Guide & Examples
- Simple Random Sampling | Definition, Steps & Examples
- Single, Double, & Triple Blind Study | Definition & Examples
- Stratified Sampling | Definition, Guide & Examples
- Structured Interview | Definition, Guide & Examples
- Survey Research | Definition, Examples & Methods
- Systematic Review | Definition, Example, & Guide
- Systematic Sampling | A Step-by-Step Guide with Examples
- Textual Analysis | Guide, 3 Approaches & Examples
- The 4 Types of Reliability in Research | Definitions & Examples
- The 4 Types of Validity in Research | Definitions & Examples
- Transcribing an Interview | 5 Steps & Transcription Software
- Triangulation in Research | Guide, Types, Examples
- Types of Interviews in Research | Guide & Examples
- Types of Research Designs Compared | Guide & Examples
- Types of Variables in Research & Statistics | Examples
- Unstructured Interview | Definition, Guide & Examples
- What Is a Case Study? | Definition, Examples & Methods
- What Is a Case-Control Study? | Definition & Examples
- What Is a Cohort Study? | Definition & Examples
- What Is a Conceptual Framework? | Tips & Examples
- What Is a Controlled Experiment? | Definitions & Examples
- What Is a Double-Barreled Question?
- What Is a Focus Group? | Step-by-Step Guide & Examples
- What Is a Likert Scale? | Guide & Examples
- What Is a Prospective Cohort Study? | Definition & Examples
- What Is a Retrospective Cohort Study? | Definition & Examples
- What Is Action Research? | Definition & Examples
- What Is an Observational Study? | Guide & Examples
- What Is Concurrent Validity? | Definition & Examples
- What Is Content Validity? | Definition & Examples
- What Is Convenience Sampling? | Definition & Examples
- What Is Convergent Validity? | Definition & Examples
- What Is Criterion Validity? | Definition & Examples
- What Is Data Cleansing? | Definition, Guide & Examples
- What Is Deductive Reasoning? | Explanation & Examples
- What Is Discriminant Validity? | Definition & Example
- What Is Ecological Validity? | Definition & Examples
- What Is Ethnography? | Definition, Guide & Examples
- What Is Face Validity? | Guide, Definition & Examples
- What Is Non-Probability Sampling? | Types & Examples
- What Is Participant Observation? | Definition & Examples
- What Is Peer Review? | Types & Examples
- What Is Predictive Validity? | Examples & Definition
- What Is Probability Sampling? | Types & Examples
- What Is Purposive Sampling? | Definition & Examples
- What Is Qualitative Observation? | Definition & Examples
- What Is Qualitative Research? | Methods & Examples
- What Is Quantitative Observation? | Definition & Examples
- What Is Quantitative Research? | Definition, Uses & Methods
What is your plagiarism score?
- Research Process
- Manuscript Preparation
- Manuscript Review
- Publication Process
- Publication Recognition
- Language Editing Services
- Translation Services
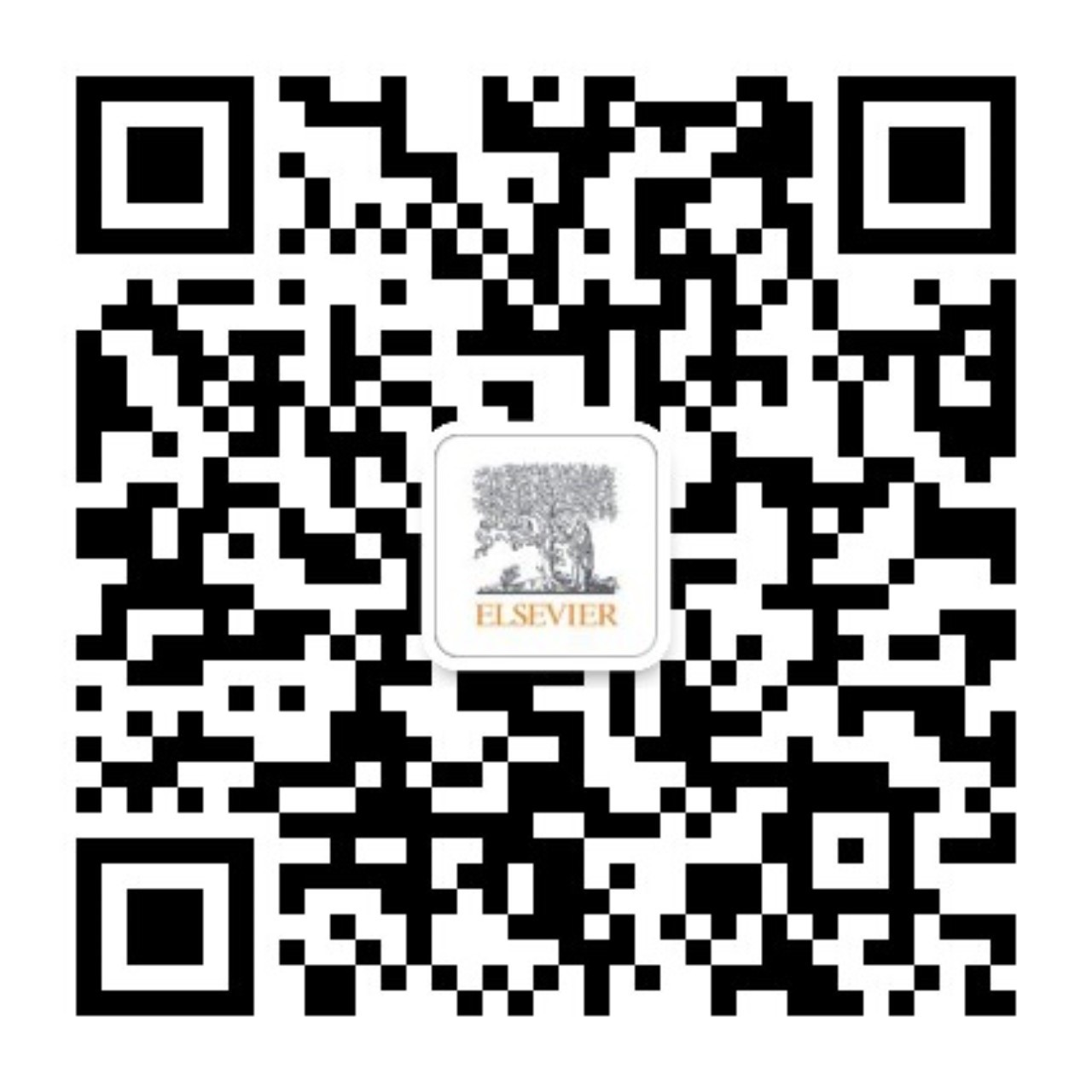
Choosing the Right Research Methodology: A Guide for Researchers
- 3 minute read
- 52.2K views
Table of Contents
Choosing an optimal research methodology is crucial for the success of any research project. The methodology you select will determine the type of data you collect, how you collect it, and how you analyse it. Understanding the different types of research methods available along with their strengths and weaknesses, is thus imperative to make an informed decision.
Understanding different research methods:
There are several research methods available depending on the type of study you are conducting, i.e., whether it is laboratory-based, clinical, epidemiological, or survey based . Some common methodologies include qualitative research, quantitative research, experimental research, survey-based research, and action research. Each method can be opted for and modified, depending on the type of research hypotheses and objectives.
Qualitative vs quantitative research:
When deciding on a research methodology, one of the key factors to consider is whether your research will be qualitative or quantitative. Qualitative research is used to understand people’s experiences, concepts, thoughts, or behaviours . Quantitative research, on the contrary, deals with numbers, graphs, and charts, and is used to test or confirm hypotheses, assumptions, and theories.
Qualitative research methodology:
Qualitative research is often used to examine issues that are not well understood, and to gather additional insights on these topics. Qualitative research methods include open-ended survey questions, observations of behaviours described through words, and reviews of literature that has explored similar theories and ideas. These methods are used to understand how language is used in real-world situations, identify common themes or overarching ideas, and describe and interpret various texts. Data analysis for qualitative research typically includes discourse analysis, thematic analysis, and textual analysis.
Quantitative research methodology:
The goal of quantitative research is to test hypotheses, confirm assumptions and theories, and determine cause-and-effect relationships. Quantitative research methods include experiments, close-ended survey questions, and countable and numbered observations. Data analysis for quantitative research relies heavily on statistical methods.
Analysing qualitative vs quantitative data:
The methods used for data analysis also differ for qualitative and quantitative research. As mentioned earlier, quantitative data is generally analysed using statistical methods and does not leave much room for speculation. It is more structured and follows a predetermined plan. In quantitative research, the researcher starts with a hypothesis and uses statistical methods to test it. Contrarily, methods used for qualitative data analysis can identify patterns and themes within the data, rather than provide statistical measures of the data. It is an iterative process, where the researcher goes back and forth trying to gauge the larger implications of the data through different perspectives and revising the analysis if required.
When to use qualitative vs quantitative research:
The choice between qualitative and quantitative research will depend on the gap that the research project aims to address, and specific objectives of the study. If the goal is to establish facts about a subject or topic, quantitative research is an appropriate choice. However, if the goal is to understand people’s experiences or perspectives, qualitative research may be more suitable.
Conclusion:
In conclusion, an understanding of the different research methods available, their applicability, advantages, and disadvantages is essential for making an informed decision on the best methodology for your project. If you need any additional guidance on which research methodology to opt for, you can head over to Elsevier Author Services (EAS). EAS experts will guide you throughout the process and help you choose the perfect methodology for your research goals.
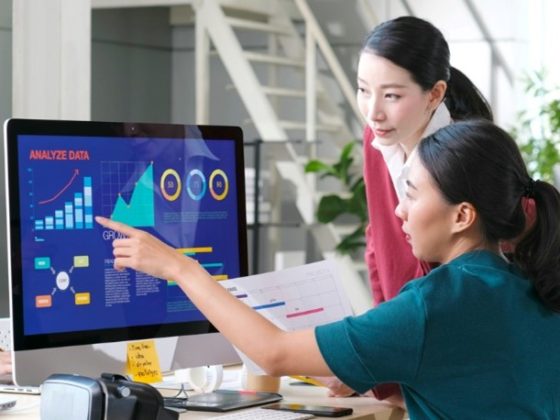
Why is data validation important in research?
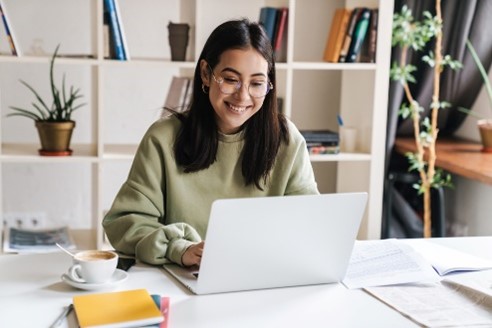
When Data Speak, Listen: Importance of Data Collection and Analysis Methods
You may also like.
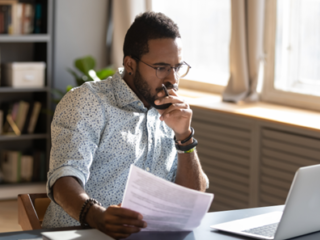
Descriptive Research Design and Its Myriad Uses
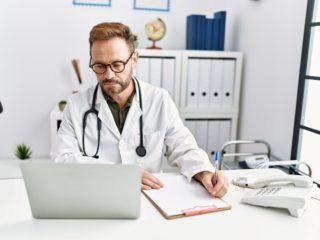
Five Common Mistakes to Avoid When Writing a Biomedical Research Paper
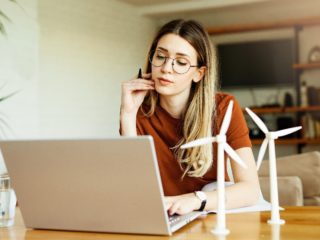
Making Technical Writing in Environmental Engineering Accessible
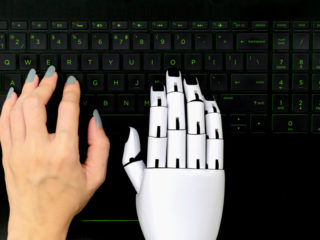
To Err is Not Human: The Dangers of AI-assisted Academic Writing
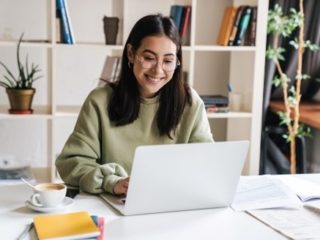
Writing a good review article
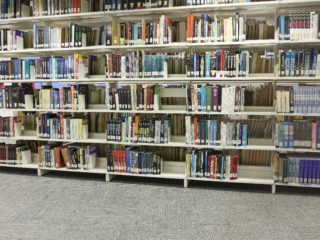
Scholarly Sources: What are They and Where can You Find Them?
Input your search keywords and press Enter.
- Open access
- Published: 16 September 2024
Artificial intelligence (AI) -integrated educational applications and college students’ creativity and academic emotions: students and teachers’ perceptions and attitudes
- Haozhuo Lin 1 &
- Qiu Chen 2
BMC Psychology volume 12 , Article number: 487 ( 2024 ) Cite this article
Metrics details
Integrating Artificial Intelligence (AI) in educational applications is becoming increasingly prevalent, bringing opportunities and challenges to the learning environment. While AI applications have the potential to enhance structured learning, they may also significantly impact students’ creativity and academic emotions.
This study aims to explore the effects of AI-integrated educational applications on college students’ creativity and academic emotions from the perspectives of both students and teachers. It also assessed undergraduate students’ and faculty’s attitudes to AI-integrated applications.
Methodology
A mixed-method research design was used. In the first phase, a qualitative research approach was employed, utilizing theoretical sampling to select informants. Data were collected through in-depth interviews with undergraduate students and university lecturers to gain comprehensive insights into their experiences and perceptions. A scale was developed, validated, and administered to 120 students and faculty in the quantitative phase. Descriptive statistics was used to analyze the data.
The study revealed that AI applications often impose rigid frameworks that constrain creative thinking and innovation, leading to emotional disengagement due to AI interactions’ repetitive and impersonal nature. Additionally, constant AI assessments heightened performance anxiety, and technical frustrations disrupted the learning process. Conversely, AI applications stimulated creativity by introducing new ideas and problem-solving techniques, enhanced engagement through interactive elements, provided personalized feedback, and supported emotional well-being with gamified elements and constant availability. Quantitative data also verified that teachers and students have positive attitudes toward the benefits and challenges of these applications.
Conclusions
AI integration in educational applications has a dual-edged impact on college students’ creativity and academic emotions. While there are notable benefits in stimulating creativity and enhancing engagement, significant challenges such as creativity constraints, emotional disengagement, and performance anxiety must be addressed. Balancing these factors requires thoughtful implementation and continuous evaluation to optimize the role of AI in education.
Peer Review reports
Introduction
Artificial Intelligence (AI) represents a subdivision of computer science that employs algorithms and machine learning techniques to emulate or mimic human intelligence [ 1 ]. AI is categorized into three types: narrow AI, general AI, and artificial superintelligence. Narrow AI, the most prevalent and developed form of AI to date, is highly goal-oriented and utilizes machine learning techniques to accomplish specific objectives or tasks, such as image and facial recognition or virtual assistants like Siri and Alexa. General AI, also known as deep AI, possesses capabilities comparable to human intelligence, including understanding the needs and emotions of other intelligent beings. In contrast, artificial superintelligence surpasses human capabilities in all respects, resembling portrayals of AI in science fiction that exceed human intelligence [ 2 ].
In the educational context, the development of AI is likely to remain within the scope of narrow AI. Current educational technologies encompass speech semantic recognition, image recognition, augmented reality/virtual reality, machine learning, brain neuroscience, quantum computing, and blockchain. These technologies are increasingly being integrated into classrooms. Many AI-based educational products are being implemented in K-12 education [ 3 ]. Research indicates that AI technology in education has been applied in at least ten areas: automatic grading systems, interval reminders, teacher feedback, virtual teachers, personalized learning, adaptive learning, augmented reality/virtual reality, precise reading, intelligent campuses, and distance learning [ 3 ]. The Artificial Intelligence in Education (AIED) community focuses on developing systems as effective as one-on-one human tutoring [ 4 ]. Significant advancements toward this goal have been made over the past 25 years. However, prioritizing the human tutor or teacher as the benchmark, AIED practices typically involve students working with computers to solve step-based problems centred on domain-specific knowledge in subjects such as science and mathematics [ 5 ]. This approach needs to fully account for recent educational practices and theory developments, including emphasizing 21st-century competencies. The 21st-century competency approach to education highlights the importance of general skills and competencies such as creativity. Modern classrooms aim to incorporate authentic practices using real-world problems in collaborative learning environments. To remain relevant and enhance its impact, the field of AIED must adapt to these evolving educational paradigms.
The impact of AI applications on various aspects of education has garnered significant attention in recent years. While research has delved into its effects on different variables, one area deserving deeper exploration is its influence on students’ creativity [ 6 , 7 , 8 , 9 , 10 , 11 , 12 , 13 , 14 , 15 ]. Creativity is a multifaceted construct crucial for problem-solving, innovation, and adaptability in an ever-evolving society. Traditional educational paradigms often need help to fully nurture and assess creativity due to their structured nature and emphasis on standardized assessments. However, AI-integrated educational applications possess the potential to revolutionize this landscape [ 6 , 7 , 8 , 9 , 10 , 11 , 12 , 13 , 14 , 15 ].
AI applications can provide personalized learning experiences tailored to students’ unique cognitive profiles, preferences, and learning styles. By offering adaptive feedback, generating diverse learning materials, and facilitating interactive learning environments, AI can foster a conducive atmosphere for creativity to flourish. Through algorithms that analyze students’ performance, identify patterns, and suggest novel approaches, AI empowers learners to explore unconventional solutions, think critically, and engage in creative problem-solving processes [ 16 , 17 , 18 , 19 , 20 , 21 , 22 ].
Moreover, AI technologies can facilitate collaborative and interdisciplinary learning experiences, exposing students to diverse perspectives, ideas, and methodologies. Virtual reality simulations, augmented reality tools, and intelligent tutoring systems can immerse students in interactive learning environments where they can experiment, innovate, and co-create content. By transcending the constraints of physical classrooms and textbooks, AI-enabled platforms offer limitless possibilities for creative expression and exploration [ 23 , 24 , 25 , 26 , 27 , 28 , 29 , 30 ].
Furthermore, AI’s ability to curate and recommend relevant resources from vast repositories of educational content enhances students’ exposure to diverse sources of inspiration and knowledge. By leveraging natural language processing algorithms, sentiment analysis, and recommendation systems, AI can identify content aligned with students’ interests, passions, and learning objectives, nurturing intrinsic motivation and curiosity-driven exploration [ 31 , 32 , 33 ]. In addition to creativity, another crucial aspect of the educational experience that AI-integrated applications may influence is academic emotions. These are the emotions experienced by students and educators in educational settings. These emotions are directly linked to academic activities like learning, teaching, studying, and taking exams. They can be positive (e.g., enjoyment, pride, and hope) or negative (e.g., anxiety, frustration, and boredom) and significantly impact motivation, learning strategies, cognitive resources, and academic performance [ 34 ]. Academic emotions encompass a spectrum of affective states, including motivation, engagement, anxiety, boredom, and satisfaction, significantly impacting students’ learning outcomes, perseverance, and overall well-being. Traditional educational approaches often overlook the complex interplay between cognitive processes and emotional experiences, resulting in suboptimal learning environments and outcomes [ 1 , 2 , 3 , 4 , 5 , 35 ].
However, AI technologies offer unprecedented opportunities to monitor, analyze, and respond to students’ academic emotions in real time [ 4 ]. By employing affective computing techniques, sentiment analysis algorithms, and facial recognition technology, AI can detect subtle cues indicative of students’ emotional states and adjust learning experiences accordingly [ 1 ]. For instance, adaptive tutoring systems can dynamically adapt to the difficulty level of tasks, provide scaffolding support, or offer motivational prompts based on students’ emotional responses and performance metrics [ 5 ]. Moreover, AI-integrated learning platforms can incorporate gamification elements, immersive storytelling, and personalized avatars to enhance students’ emotional engagement and investment in learning activities [ 4 ]. By fostering a supportive and inclusive learning environment that acknowledges and addresses students’ diverse emotional needs, AI can promote positive academic emotions, such as curiosity, excitement, and self-efficacy, while mitigating negative ones, such as frustration, anxiety, and disengagement.
Furthermore, AI-driven analytics and data visualization tools empower educators to gain deeper insights into students’ emotional trajectories, identify at-risk individuals, and implement timely interventions. By harnessing predictive analytics and machine learning algorithms, educators can anticipate students’ emotional responses to various instructional strategies, anticipate potential challenges, and proactively implement personalized interventions to foster resilience, motivation, and emotional well-being. In line with the existing gap, the following research questions were raised:
How do teachers and students perceive the challenges of using AI applications in the students’ creativity and academic emotions?
How do teachers and students perceive the merits of using AI applications in the students’ creativity and academic emotions?
What are the teachers and students’ attitudes to AI-integrated educational applications?
Artificial intelligence and higher education
21st-century higher education is rapidly changing due to globalization, technological advancements, and student demographics [ 16 ]. Online learning platforms have become widely accessible, enabling universities to offer fully online courses and degree programs, expanding access to education and providing flexibility in learning [ 17 ]. The growing diversity of the educational field, with students from various backgrounds, highlights the significance of global citizenship and intercultural understanding. Universities are playing a significant role in promoting innovation and research as technology advancements pick up speed [ 18 ], encouraging industry-academia cooperation and placing a focus on commercialization and entrepreneurship. The emphasis is shifting toward skills-based learning patterns for practical, career-focused skills, as evidenced by recent recruitment trends favouring graduates with particular skills [ 19 ].
To enhance the quality of higher education, the industry is exploring various strategies to meet stakeholders’ requirements [ 20 ]. Artificial intelligence (AI) integration is one particularly hopeful solution [ 21 ]. As technology advances, artificial intelligence (AI) in education has enormous potential to change the teaching and learning environment [ 22 ]. AI is significantly improving the quality of higher education in several ways [ 23 ]. Artificial intelligence (AI)--powered learning strategies evaluate students’ performance, pinpoint their advantages and disadvantages and offer individualized learning experiences. With the help of this strategy, students can acquire knowledge and produce more valuable results in the real world [ 24 ].
Chatbots, virtual assistants, and adaptive learning systems are examples of AI-based technology providing immersive and exciting learning environments while actively enabling students to investigate complicated ideas [ 25 ]. Artificial intelligence (AI) helps with assessment and feedback by helping with assignment grading, tracking student participation, giving quicker and more accurate feedback, and freeing up teachers’ time for other teaching responsibilities [ 26 ]. Chatbots with artificial intelligence (AI) provide quick, individualized support by evaluating student data to identify individuals who may be at risk and enabling early interventions for academic success—various AI applications and platforms, including Bit. AI, Mendeley, Turnitin, elinik. Io and Coursera tools support higher education research by analyzing large datasets, generating insights, and identifying patterns challenging for human researchers to detect [ 27 ]. We expect even more cutting-edge AI applications in education due to continued technological advancement, giving students individualized, engaging, and productive learning experiences [ 28 ].
The exciting development of AI dramatically improves both the effectiveness and engagement of instructors in postsecondary education. Adopting AI helps educators free up time for more meaningful activities by automating administrative duties like tracking attendance and grading assignments [ 29 ]. Additionally, AI helps educators pinpoint areas in which they can grow by offering individualized opportunities for professional development [ 30 ]. Solutions to enduring problems in modern higher education are needed, such as limited inclusivity and unequal access [ 31 ]. Traditional teaching methods often fail to engage students with diverse learning preferences, hindering active participation and critical thinking skills [ 32 ]. The inability of conventional assessment techniques to capture thorough understanding makes using AI necessary. AI algorithms analyze individual learning patterns, tailor coursework, and predict at-risk students, enabling timely interventions [ 33 ]. Content delivery is revolutionized by AI-driven systems that adjust to students’ learning styles, pace, and knowledge gaps.
In conclusion, adopting AI in higher education empowers the system by addressing challenges and enhancing the quality of education. Ongoing research aims to understand faculty members’ awareness of AI’s applicability and impact on learning experiences, work engagement, and productivity in higher education. This research provides insights for institutional policymakers to facilitate the adoption of new technologies and overcome specific challenges. Despite the increasing integration of technology and artificial intelligence (AI) in education, there is a notable gap in understanding how AI-empowered technology educational apps specifically influence undergraduate students’ academic emotions and test anxiety. While various studies have explored the general impact of technology on education and student emotions, there is a need for focused research on the unique effects of AI-powered educational apps. Understanding the dynamics between these technologies and students’ emotional experiences can provide valuable insights into the efficacy of AI applications in promoting positive emotions and reducing test anxiety.
AI applications and the students’ creativity
Students should be aware of AI’s potential to bolster their creativity and learning processes. Modern educational methodologies prioritize problem-solving approaches, underscoring the significance of nurturing children’s creative thinking abilities. However, extensive research corroborates the existence of a decline in creativity among younger individuals across various disciplines [ 6 , 7 ]. One explanation for this decline is attributed to the overly structured nature of school curricula and a shortage of play-based learning activities within educational frameworks [ 8 ].
Emerging research indicates how AI can enhance skills commonly associated with creativity, such as curiosity [ 9 ], perseverance, and attentiveness [ 10 ]. The potential of AI to support creativity is also under investigation. Kafai and Burke assert that AI in education aims to foster problem-solving and creativity skills through collaborative interactions with AI systems rather than solely focusing on knowledge acquisition within specific domains [ 11 ]. They suggest that AI can facilitate the unfolding of creativity, thus being intertwined with the creative process. Additionally, Ryu and Han examine Korean educators’ perceptions of AI in education, noting that experienced teachers acknowledge AI’s potential to enhance creativity [ 12 ]. Hence, AI in education could address concerns related to the decline of creativity, particularly by emphasizing the creative process. This may aid in enhancing students’ creative thinking abilities and comfort level with utilizing AI, thereby adequately preparing them for the contemporary workforce [ 13 , 14 , 15 ].
To effectively merge AI and creativity, it is imperative to gain a deeper understanding of how students perceive the relationship between these concepts. Situating AI within prevailing creativity theories, such as the 4 C model of creativity, can further enrich this understanding.
Creativity and AI in an educational setting can be analyzed through the lens of the 4 C model [ 8 ]. Mini-Q, or ‘personal creativity,’ encapsulates creativity’s subjective and developmental facets. Mini-X pertains to individualized creative discoveries that may not be recognized as such by others. For instance, a slight variation on a well-known recipe could exemplify mini-c creativity. Little-c, also known as ‘everyday creativity,’ refers to creative outputs acknowledged by others, like inventing a new recipe. Pro-c, or ‘professional creativity,’ involves becoming an expert in a particular field or discipline, akin to the chef Gordon Ramsay. Big-C, or ‘legendary creativity,’ epitomizes eminent creativity that leaves a lasting legacy, as seen in figures like August Escoffier, who revolutionized the culinary landscape [ 15 ].
AI can support creativity at the pro-c and potentially Big-C levels by extending expertise in specific domains. However, its role in fostering mini-c and little-c contributions is less apparent, as the focus in these levels lies more on the process of self-discovery than on the creative output itself. Therefore, it is crucial to understand when and where AI is most beneficial, particularly in delineating the narrow domains where AI is most apt for educational purposes and how it can encourage mini-c and little-c contributions. This study aims to explore students’ perceptions of AI and creativity and the interplay between the two.
Studies on academic emotions
Lei and Cui [ 36 ] defined academic emotions as students’ emotional experiences related to the academic processes of teaching and learning, including enjoyment, hopelessness, boredom, anxiety, anger, and pride. Based on arousal and enjoyment concepts, academic emotions have been divided into four categories: positive low-arousal, negative low-arousal, and negative high-arousal [ 37 ]. It is also argued that achievement emotions include prospective emotions, such as fear of failure, and retrospective emotions, e.g., shame, which learners experience after they receive feedback on their achievements.
Academic accomplishment serves as a commonly employed criterion for evaluating the effectiveness of educational systems, teachers, schools, and the success or failure of students. Consequently, scholars in this field have conducted empirical investigations to explore the causal link between students’ academic emotions and academic achievements, as evidenced by a body of practical studies [ 38 ]. However, the findings from these studies could be more consistent. In general, there is an anticipation that positive emotions will forecast favorable outcomes in academic settings, including high grades and commendable performance in both local and large-scale educational assessments [ 39 , 40 ]. Conversely, it is hypothesized that negative emotions will correlate with adverse consequences, such as lower grades and compromised performance in classroom activities and standardized examinations [ 41 ].
Results of the meta-analysis study undertaken by Lei and Cui [ 36 ] developed the Chinese version of the Academic Emotions Questionnaire, which was employed to evaluate the academic emotions of adolescents. Academic emotions have been linked to various variables, including cognitive activity, learning motivation, and strategies. Lei and Cui’s [ 36 ] meta-analysis study revealed positive correlations between positive high-arousal, positive low-arousal, and academic achievement and negative correlations between negative high-arousal, negative low-arousal, and academic achievement. The study suggested that factors such as the student’s age, regional location, and gender could moderate the effects of epistemic cognition on academic achievement.
Positive correlations between positive high-arousal, positive low-arousal, and academic achievement and negative correlations between negative high-arousal, negative low arousal, and academic achievement. The authors suggested that the student’s age, regional location, and gender moderated the effects of epistemic cognition on academic achievement [ 42 ].
Currently, domestically and internationally scholars are directing their attention towards analyzing academic emotions in distance learners, resulting in noteworthy research outcomes [ 43 ]. Research conducted by Thelwall et al. [ 44 ] delved into the impact of screen time on emotion regulation and student performance. The study involved over 400 children over four years, examining their usage of smartphones and tablets. The research analyzed the correlation between these behaviours, emotions, and academic performance, concurrently evaluating students’ abilities and educational achievements. Similarly [ 45 ], investigated the influence of early childhood emotions on academic preparation and social-emotional issues. Emotion regulation, identified as the process of managing emotional arousal and expression, plays a crucial role in determining children’s adaptation to the school environment.
Building on the perspectives of the previously mentioned scholars, Sakulwichitsintu [ 46 ] integrated connectionist learning theory to devise an innovative distance education model. This model introduced educational content that was aligned with emotional education objectives and implemented the Mu class teaching mode, establishing a distance learning community and humanized network courses to address emotional shortcomings in the distance education process. Ensuring effectiveness, Pekrun et al. [ 35 ] developed a hybrid reality virtual intelligent classroom system incorporating television broadcasting and interactive space technology to create a networked teaching environment. Teachers utilized diverse techniques, including video, audio, and text, to foster engagement and enhance communication between educators and students during the network teaching phase.
In addition to the earlier scholars, Fang et al. [ 47 ] introduced an emotion recognition algorithm based on facial expression scale-invariant feature transformation. This algorithm captures the facial expressions of distance learners, employing SIFT feature extraction and expression recognition to address emotional gaps in the learning phase of distance education. Simultaneously, Méndez López [ 48 ] developed a learner emotion prediction model for an intelligent learning environment utilizing a fuzzy cognitive map. This model facilitated the extraction and prediction of distance learners’ emotional states, allowing real-time adjustments to the teaching approach based on predicted emotions. Huang and Bo [ 49 ] contributed to the field by introducing the distance learner emotion self-assessment scale, defining essential emotion variables, and establishing an early warning model.
Drawing inspiration from the valuable contributions of the scholars mentioned earlier, Zembylas [ 50 ] examined the online academic emotions experienced by adults. This investigation involved the analysis of diverse influencing factors and the exploration of an environmental factor model within the online learning community, specifically focusing on academic emotional tendencies. Building upon the insights derived from these scholars, our objective is to delve into the academic emotions of distance learners. We plan to achieve this through the analysis of online learning behaviour data, with the anticipation of uncovering meaningful findings in this domain.
This study used mixed-method research (qualitative-quantitative). The following sections describe each phase.
Qualitative method
Sampling and design.
This study employs a qualitative research design to explore the impact of AI-integrated educational applications on undergraduate students’ creativity and academic emotions from the perspectives of both students and university faculties. The research was conducted at Wenzhou University, leveraging theoretical sampling to ensure a comprehensive understanding of the phenomena under investigation. The informants were selected using theoretical sampling, a technique where participants are chosen based on their potential to contribute to the development of emerging theories, ensuring that the sample is rich in information pertinent to the research questions. A total of 23 participants were included in the study, comprising 15 students and eight teachers. The decision to interview these specific numbers was driven by the principle of data saturation, which refers to the point at which no new information or themes are observed in the data. Data saturation was achieved after interviewing the 15th student and the 8th teacher, indicating that the sample size was sufficient to capture the full range of perspectives necessary for the research. The criterion for including the participants in the study was their familiarity with the components of AI. AI-integrated educational applications. These components include Adaptive Learning Systems, Intelligent Tutoring Systems (ITS), Natural Language Processing (NLP) applications, AI-enhanced collaborative Learning Platforms, and Predictive Analytics.
To evaluate the impact of AI-integrated educational applications on students’ creativity and academic emotions, we focused on several key components of AI applied to educational processes. These components include Adaptive Learning Systems, which personalize learning experiences by adjusting content and pace based on individual student performance and preferences, enhancing creativity through personalized challenges and immediate feedback. Intelligent Tutoring Systems (ITS) offer personalized tutoring and feedback, fostering creative problem-solving skills and reducing negative emotions such as anxiety and frustration. Natural Language Processing (NLP) applications facilitate interaction between computers and humans using natural language, enhancing creativity through brainstorming sessions and interactive writing assistance while monitoring changes in academic emotions. AI-enhanced collaborative Learning Platforms support and enhance collaborative learning experiences with features like intelligent grouping, real-time feedback, and automated moderation, impacting group creativity and collective emotional states. Predictive Analytics analyze data to predict student performance, engagement, and emotional states, informing instructional decisions and personalized interventions to enhance creativity and mitigate negative academic emotions.
Data collection
Data collection was carried out through semi-structured interviews, a method well-suited to qualitative research. This method allows for in-depth exploration of participants’ experiences and perceptions while providing some level of structure to ensure that all relevant topics are covered. The semi-structured format includes predefined questions but also allows for flexibility in probing deeper into interesting or unexpected responses.
Interviews were conducted in a quiet and comfortable setting within the university premises to ensure participants felt at ease, thereby facilitating open and honest communication. Each interview lasted approximately 45 to 60 min. For the student participants, the interview questions focused on their experiences using AI-integrated educational applications, perceived impacts on their creativity, and any changes in their academic emotions (e.g., motivation, anxiety, enjoyment). Teacher participants were asked about their observations of students’ engagement and creativity, as well as their own experiences and attitudes towards integrating AI applications in their teaching practices.
Before the interviews, informed consent was obtained from all participants, ensuring they were aware of the study’s purpose, their rights to confidentiality, and their freedom to withdraw from the study at any point without any repercussions. The interviews were audio-recorded with participants’ permission to ensure accurate data capture and were later transcribed verbatim for analysis.
Data analysis
The data analysis process began with the transcription of the audio-recorded interviews, followed by a thorough reading of the transcripts to gain an initial understanding of the data. Thematic analysis was employed to identify, analyze, and report patterns (themes) within the data. This method is particularly effective in qualitative research as it provides a systematic approach to handling large volumes of text and can reveal complex patterns in participants’ narratives.
The thematic analysis was conducted in several steps. First, open coding was performed, where the transcripts were examined line-by-line, and initial codes were generated to capture significant statements and ideas. These codes were then grouped into broader categories based on similarities and relationships. For instance, codes related to students’ enhanced engagement and creativity when using AI applications were grouped under a category labelled “positive impacts on creativity.” Next, the categories were reviewed and refined into overarching themes. This involved constant comparison within and between the data to ensure the themes accurately represented the participants’ perspectives. Themes were then defined and named, providing a clear and concise description of each theme’s essence. Open themes were then classified into two main categories: Challenges and Merits of AI-integrated applications.
Research quality
To ensure research quality, several rigorous steps were undertaken. The transcription of audio-recorded interviews was done verbatim to preserve the original meaning and nuances, maintaining data integrity. Researchers immersed themselves in the data by reading the transcripts multiple times, allowing for a deep understanding. Thematic analysis was systematically employed to identify, analyze, and report patterns, facilitating the uncovering of complex themes. Open coding involved line-by-line examination and initial coding to capture significant statements and ideas, ensuring comprehensive data consideration. Codes were then grouped into broader categories, organizing data meaningfully and aiding in the identification of overarching themes.
Peer debriefing sessions with colleagues provided external validation, enhancing credibility by identifying potential biases and ensuring balanced interpretations. Triangulation was used to confirm consistency and validity by comparing data from multiple sources, reinforcing the reliability of the themes. Detailed documentation of the analytical process ensured transparency and created an audit trail, allowing verification of the research steps and findings. Finally, researchers engaged in reflexivity, continuously reflecting on potential biases to ensure objectivity and accurate representation of participants’ voices, further contributing to the study’s reliability.
Quantitative method
The quantitative phase explored teachers’ and students’ attitudes towards AI applications in education. The sample consisted of 120 undergraduate students and 30 teachers. Participants were selected using a convenience sampling method, ensuring a diverse representation of experiences and perspectives within the educational environment.
Participants were asked to complete a survey that included statements related to the perceived challenges and benefits of AI applications in education. The survey featured a series of Likert scale questions where respondents indicated their level of agreement with each statement on a scale of 1 to 5, where one represented “Strongly Disagree,” 2 represented “Disagree,” 3 represented “Neutral,” 4 represented “Agree,” and five represented “Strongly Agree. The construct validity. It was estimated using exploratory factor analysis, and the items were reduced to factors: challenges and merits. All items had loading factors above 0.70, indicating that the scale enjoyed acceptable construct validity. The reliability of the scale was estimated using Cronbach’s alpha. The internal consistency of the factors of the scale were 0.85 and 0.89, respectively, and the reliability of the total scale was 0.90, which verifies the reliability of the scale (See Appendix).
The survey was divided into two sections: Constraints of AI Applications and Merits of AI Applications. The Constraints section included statements about creativity constraints, emotional disengagement, performance anxiety, technical frustration, over-reliance on AI, the digital divide, and ethical concerns. The Merits section included statements about stimulated creativity, increased engagement, personalized feedback, emotional support, collaborative creativity, accessible learning resources, and enhanced academic emotions.
Data were collected through an online survey platform, ensuring anonymity and confidentiality for all respondents. Descriptive statistics, specifically percentages, were used to summarize the responses. The rate of respondents in each agreement category (Strongly Disagree, Disagree, Neutral, Agree, Strongly Agree) was calculated for each statement. The results were then tabulated separately for teachers and students to identify any significant differences or similarities in their perceptions. This approach provided a clear overview of the collective attitudes of both groups towards AI applications in education, facilitating a detailed comparative analysis. Finally, the findings were interpreted to understand the broader implications of these attitudes on the integration of AI in educational settings. This comprehensive methodology ensured that the study captured a wide range of perspectives, providing valuable insights into how AI is perceived in the context of teaching and learning.
Qualitative findings
The interviews with participants were analyzed, resulting in two selective codes: Challenges and Merits. Each code consists of seven main themes related to students’ creativity and academic emotions. Below, each theme is explained in detail and followed by quotations from both students and teachers to exemplify these findings.
Challenges of AI-applications
Interviews with the informants were thematically analyzed, and different themes were extracted. The interviews highlighted challenges of AI applications in education, including creativity constraints, emotional disengagement, performance anxiety, technical frustration, over-reliance on AI, the digital divide, and ethical concerns. These issues affect students’ creativity, engagement, stress levels, and equitable access to technology. Each sub-theme is explained as follows.
Creativity constraints
The first challenge identified was creativity constraints. Participants noted that some AI applications impose rigid frameworks and lack the flexibility needed to foster creative thinking. These constraints can hinder students’ ability to think outside the box and explore innovative solutions. The following quotations exemplify this finding:
Student 1: “Sometimes the AI applications don’t allow much room for creativity because they follow a strict format.” Teacher 2: “I’ve noticed that some students feel boxed in by the structure imposed by the AI, hindering their creative expression.”
Emotional disengagement
Another challenge was emotional disengagement. The repetitive nature of AI interactions and the absence of a human touch were found to diminish emotional connection and motivation among students. This lack of engagement can detract from the overall learning experience. The following quotations exemplify this finding:
Student 10: “Interacting with AI can get monotonous, and I miss the personal interaction with my teachers.” Teacher 8: “There’s a certain emotional warmth in human interactions that AI can’t replicate, which some students really miss.”
Performance anxiety
Performance anxiety was a significant challenge, with students experiencing heightened stress due to constant monitoring and frequent AI assessments. This pressure can make students more fearful of making mistakes, impacting their academic emotions negatively. The following quotations exemplify this finding:
Student: “The AI assessments are so frequent that I constantly feel pressured to perform well, which makes me anxious.” Teacher: “I’ve observed that some students become overly anxious about their performance because they know the AI is always evaluating them.”
Technical frustration
Technical frustration was a common issue, with frequent glitches and difficult-to-navigate interfaces disrupting the learning process and causing frustration among students. This negatively impacted their creativity and emotional state. The following quotations exemplify this finding:
Student 8: “When the app glitches, it disrupts my workflow and frustrates me, killing my creative vibe.” Teacher 6: “Technical problems often leave students frustrated, which can stifle their creativity and motivation.”
Over-reliance on AI
Over-reliance on AI applications was another challenge, leading to reduced critical thinking and self-initiative among students. This dependency can hinder the development of essential problem-solving skills. The following quotations exemplify this finding:
Student 11: “I sometimes rely too much on the AI for answers instead of trying to figure things out myself.” Teacher 9: “There’s a danger that students may become too dependent on AI, which can hinder their ability to think critically and independently.”
Digital divide
The digital divide posed a significant challenge, with inequitable access to technology and varying levels of technological literacy affecting students’ ability to engage fully and creatively. This disparity can exacerbate existing educational inequalities. The following quotations exemplify this finding:
Student 12: “Not everyone has the same access to the necessary technology, which can be limiting for those who don’t.” Teacher 4: “Students with limited tech skills or access are at a disadvantage, impacting their ability to participate fully and creatively.”
Ethical concerns
Participants raised ethical concerns about biases in AI algorithms and the ethical use of AI in education. These concerns are related to fairness and equity in academic evaluations and the potential for AI to perpetuate existing biases. The following quotations exemplify this finding:
Student: “I’m concerned that the AI might have biases that affect how it evaluates my work.” Teacher: “There are significant ethical questions about how AI is used and whether it treats all students fairly, which can impact their academic emotions and creativity.”
Teachers and students’ perceptions of the merits of AI-applications
Teachers and students believe that AI-integrated educational applications stimulate creativity, increase engagement, provide personalized feedback, offer emotional support, facilitate collaborative creativity, and make learning resources more accessible. These benefits enhance students’ academic emotions and foster innovative approaches to learning, as illustrated by student and teacher testimonials. Each of these themes is explained and exemplified in detail as follows.
Stimulated creativity
On the positive side, AI applications were found to stimulate creativity by presenting new ideas and enhancing problem-solving skills. This allowed students to explore innovative approaches to learning. The following quotations exemplify this finding:
Student 6: “The AI applications introduce me to new ideas that I wouldn’t have thought of on my own, boosting my creativity.” Teacher 8: “I’ve seen students come up with innovative solutions and creative projects thanks to the AI applications.”
Increased engagement
Increased engagement was another significant benefit, with the interactive nature of AI applications making learning more enjoyable and keeping students motivated. This positive engagement enhanced both creativity and academic emotions. The following quotations exemplify this finding:
Student 9: “The interactive features make learning more enjoyable and keep me engaged.” Teacher 5: “Students are more engaged and seem to enjoy the learning process more when using AI applications.”
Personalized feedback
Personalized feedback provided by AI applications offered tailored guidance and immediate responses, helping students improve their work and boosting their confidence. This customised approach supported their creative and emotional development. The following quotations exemplify this finding:
Student 5: “The AI gives me personalized feedback that really helps me understand where I can improve.” Teacher 3: “The immediate, tailored feedback from AI applications helps students feel more confident and supported in their learning.”
Emotional support
AI applications also provide emotional support by reducing anxiety through their constant availability and increasing motivation with gamified elements and positive reinforcement. This support helped maintain a positive emotional state conducive to learning. The following quotations exemplify this finding:
Student 9: “The AI apps reduce my anxiety by being available whenever I need help, and the gamified elements keep me motivated.” Teacher 6: “Students seem less anxious and more motivated when they use AI applications that provide continuous support and positive feedback.”
Collaborative creativity
Collaborative creativity was facilitated by AI, which supported group projects and peer interactions, fostering a sense of community and collective problem-solving. This collaborative environment enhanced creative outcomes. The following quotations exemplify this finding:
Student 13: “AI applications make group projects easier and more creative by allowing us to collaborate effectively.” Teacher 9: “The AI applications encourage peer interaction and collaboration, leading to more creative and well-rounded projects.”
Accessible learning resources
The accessibility of a wide range of learning resources through AI applications supported continuous learning and inspired creativity. Students could explore diverse materials anytime, enhancing their educational experience. The following quotations exemplify this finding:
Student 8: “Having access to a wide range of resources anytime I need them inspires me to be more creative in my studies.” Teacher 7: “The vast array of resources available through AI applications encourages students to explore topics more deeply and creatively.”
Enhanced academic emotions
Finally, AI applications enhance academic emotions by creating positive learning experiences and building emotional resilience through adaptive learning paths and supportive environments. This improvement in emotional well-being positively influenced students’ academic performance. The following quotations exemplify this finding:
Student 4: “The AI apps make learning a more positive experience, which helps me stay emotionally resilient.” Teacher 5: “I’ve seen students develop greater emotional resilience and have more positive learning experiences with the support of AI applications.”
These findings illustrate a nuanced view of AI-integrated educational applications, highlighting both the challenges and benefits in terms of students’ creativity and academic emotions. While there are significant obstacles to overcome, the potential for enhancing creativity and emotional well-being is substantial.
Quantitative findings
To present teachers’ and students’ attitudes towards AI applications in education, we used descriptive statistics to summarize their responses to the statements provided. Tables 1 and 2 include the percentage of respondents in each category of agreement (Strongly Disagree, Disagree, Neutral, Agree, Strongly Agree) for teachers and students, respectively.
Both groups were concerned about AI applications imposing rigid frameworks that could hinder creative thinking, with 25% of both teachers and students agreeing and 15% strongly agreeing. A similar percentage disagreed, with 20% of teachers and 25% of students, while 10% of teachers and 15% of students strongly disagreed. Teachers were more neutral, with 30% compared to 20% of students.
Emotional disengagement due to AI was also a concern, with 35% of both teachers and students agreeing that AI interactions lack a personal touch. An additional 20% of teachers and 15% of students strongly agreed. Neutral responses were common, with 25% of teachers and 20% of students, while fewer disagreed (15% of teachers and 20% of students) or strongly disagreed (5% of teachers and 10% of students).
Performance anxiety caused by frequent AI assessments was another shared concern, with 25% of teachers and 20% of students agreeing and 15% of teachers and 20% of students strongly agreeing. Neutral responses were common, with 20% of teachers and 15% of students, while 25% of both teachers and students disagreed and 15% of teachers and 20% of students strongly disagreed.
Both teachers and students expressed concern over technical issues in AI applications that could disrupt the learning process. A quarter (25%) of each group agreed with this sentiment, while 15% strongly agreed. Neutral responses were quite common, with 30% of teachers and 25% of students expressing no strong opinion. A smaller proportion of participants disagreed (20% of both groups) or strongly disagreed (10% of teachers and 15% of students). There was also a shared recognition among both groups about the potential drawbacks of excessive reliance on AI, as 35% of teachers and 30% of students agreed that AI could diminish critical thinking and self-initiative, with 20% of teachers and 15% of students strongly agreeing. Neutral responses were frequent (25% for both groups), while a minority disagreed (15% of teachers and 20% of students) or strongly disagreed (5% of teachers and 10% of students).
Both groups similarly acknowledged the impact of the digital divide, with 30% of teachers and 25% of students agreeing, and 20% of both groups strongly agree. Neutral responses were common (20% for both groups), while a smaller number disagreed (20% of teachers and 15% of students) or strongly disagreed (10% of teachers and 15% of students). Ethical concerns regarding biases in AI algorithms were also similarly perceived. Agreement was noted among 30% of teachers and students, with 15% strongly agreeing. Neutral responses were pretty common (25% of teachers and 30% of students), and fewer respondents disagreed (20% of teachers and 15% of students) or strongly disagreed (10% from each group).
Both teachers and students had a favourable view of AI’s capacity to enhance problem-solving skills and creativity. 40% of both groups agreed with this perspective, and a notable number strongly agreed (25% of teachers and 20% of students). Neutral responses were less frequent (20% of teachers and 25% of students), while disagreement was relatively uncommon (10% from each group), as was strong disagreement (5% from each group). Furthermore, both groups acknowledged that AI could increase the enjoyment of learning, with 30% of teachers and 35% of students agreeing and 20% from each group strongly agreeing. Neutral responses were moderate (25% of teachers and 20% of students), while fewer participants disagreed (15% from both groups) or strongly disagreed (10% from each group).
The benefits of AI in providing personalized feedback were highly recognized, with 35% of teachers and students agreeing and a substantial proportion strongly agreeing (30% of teachers and 35% of students). Neutral responses were moderate (20% of teachers and 15% of students), while fewer respondents disagreed (10% from each group) or strongly disagreed (5% from each group). AI’s role in reducing anxiety through constant availability was similarly viewed, with 25% of teachers and 30% of students agreeing and 15% from each group strongly agreeing. Neutral responses were moderate (25% from both groups), with some disagreement (20% of teachers and 15% of students) and strong disagreement (15% of teachers and 10% of students).
Both groups positively perceived AI’s facilitation of group projects, with 35% of teachers and students agreeing and 25% from each group strongly agreeing. Neutral responses were common (25% of teachers and 20% of students), with fewer participants disagreeing (10% of teachers and 15% of students) or strongly disagreeing (5% from each group). The accessibility of a wide range of learning resources through AI was highly valued, with 35% of teachers and students agreeing and a notable portion strongly agreeing (30% of teachers and 25% of students). Neutral responses were moderate (20% of teachers and 25% of students), while fewer disagreed (10% from each group) or strongly disagreed (5% from each group). Lastly, both groups acknowledged AI’s role in fostering positive learning experiences, with 30% of teachers and students agreeing and 20% strongly agreeing. Neutral responses were moderate (25% from each group), while fewer participants disagreed (15% from both groups) or strongly disagreed (10% from each group).
The integration of AI in educational applications presents several significant challenges that impact students’ creativity and academic emotions. One major issue is the creativity constraints imposed by AI applications. Specifically, the rigid frameworks and lack of flexibility in some applications limit students’ ability to think creatively and explore innovative solutions. This finding aligns with previous research indicating that while AI can facilitate structured learning, it can also stifle creative thinking by enforcing rigid paths [ 51 , 52 ]. Moreover, another significant challenge is emotional disengagement. The repetitive nature of AI interactions and the lack of a human touch can lead to emotional detachment, reducing students’ motivation and engagement. This phenomenon is supported by studies showing that human interaction plays a crucial role in maintaining student engagement and emotional connection [ 53 , 54 ].
Additionally, technical frustration due to frequent glitches and complicated interfaces further hampers the learning experience. This frustration can disrupt creative processes and negatively affect academic emotions [ 55 ]. This issue is highlighted by research showing that technical difficulties are a common barrier to effective AI implementation in education [ 56 ].
Another concern is the over-reliance on AI applications, which can reduce critical thinking and self-initiative among students. This dependency can hinder the development of essential problem-solving skills. Zhai et al. [ 56 ] emphasized the importance of balancing AI use with opportunities for independent thought and critical reasoning.
The digital divide remains a significant challenge, with inequitable access to technology and varying levels of technological literacy among students creating disparities. This issue is well-documented, with recent studies highlighting how unequal access to digital applications can exacerbate existing educational inequalities [ 57 ].
Lastly, ethical concerns regarding biases in AI algorithms and the ethical use of AI in education were prominent. Participants worried about the fairness and equity of AI evaluations, consistent with findings from Bogina et al. [ 58 ], who discussed the potential for AI to perpetuate existing biases and inequalities in educational settings.
Despite these challenges, the integration of AI in educational applications also presents numerous merits that positively impact students’ creativity and academic emotions. One significant benefit is the stimulation of creativity. AI applications can introduce new ideas and enhance problem-solving skills, fostering innovative approaches to learning. This finding is supported by studies showing that AI can provide diverse perspectives and problem-solving techniques that stimulate creative thinking [ 59 , 60 ]. Additionally, increased engagement is another notable merit, with AI’s interactive nature making learning more enjoyable and motivating for students. This enhanced engagement is consistent with research indicating that interactive AI applications can significantly boost student motivation and participation [ 61 ]. Moreover, personalized feedback provided by AI applications offers tailored guidance and immediate responses, helping students improve their work and boosting their confidence. This personalized approach is crucial for supporting students’ creative and emotional development, as noted by Chang et al. [ 62 ], who found that personalized AI feedback enhances learning outcomes and student confidence.
Furthermore, emotional support is another significant benefit, with AI applications reducing anxiety through their constant availability and increasing motivation with gamified elements and positive reinforcement. Studies have shown that such support mechanisms are effective in maintaining a positive emotional state conducive to learning [ 63 ]. In addition, collaborative creativity facilitated by AI applications supports group projects and peer interactions, fostering a sense of community and collective problem-solving. This collaborative environment aligns with findings from Graesser et al. [ 64 ], who emphasized the role of technology in enhancing collaborative learning and creativity.
The provision of accessible learning resources by AI applications supports continuous learning and inspires creativity by allowing students to explore diverse materials anytime. This accessibility is crucial for fostering an inclusive learning environment, as highlighted by Yenduri et al. [ 65 ], who noted that diverse and readily available resources enhance educational equity and creativity. Finally, enhanced academic emotions resulting from AI integration create positive learning experiences and build emotional resilience. Adaptive learning paths and supportive environments provided by AI applications contribute to improved emotional well-being and academic performance. This is supported by research indicating that adaptive learning technologies positively impact student emotions and resilience [ 5 , 6 , 7 , 8 , 9 ].
The integration of AI in education has elicited varied responses from both teachers and students, reflecting a complex interplay of benefits and challenges. One prominent concern is the potential for AI applications to impose rigid frameworks that may stifle creativity. This apprehension aligns with the notion that while AI can provide structured guidance, it may also limit the spontaneous and divergent thinking essential for creative processes. This balance between structure and freedom is critical, as noted in the literature on educational methodologies and creativity development [ 1 , 2 , 3 ].
Emotional disengagement emerges as another significant issue, with both groups expressing that AI interactions often lack the personal touch necessary for effective learning experiences. The importance of human elements in education is well-documented, with studies emphasizing the role of personal connection in fostering student engagement and motivation [ 4 , 5 ]. This emotional component is vital, as AI systems, despite their capabilities, may only partially replicate the nuanced and empathetic interactions provided by human educators [ 6 , 7 ].
Performance anxiety due to frequent AI assessments is another shared concern. AI’s ability to provide continuous and immediate feedback can be a double-edged sword, potentially leading to increased stress and anxiety among students. This is consistent with findings that highlight the psychological impact of constant monitoring and assessment, which can detract from the learning experience and affect student well-being [ 8 , 9 ].
Technical issues associated with AI applications also pose significant challenges. Both teachers and students have reported frustrations with technical glitches disrupting the learning process. These disruptions can hinder the seamless integration of AI into educational environments, underscoring the need for robust and reliable technology infrastructure [ 10 , 11 ].
Despite these concerns, both groups recognize the benefits of AI, particularly in enhancing creativity and engagement. AI’s ability to stimulate problem-solving skills and foster creativity is acknowledged as a significant advantage. This aligns with research suggesting that AI can catalyze creative thinking by providing novel applications and approaches to problem-solving [ 12 , 13 , 14 ]. Additionally, the literature supports AI’s potential to increase student engagement through interactive and personalized learning experiences [ 15 , 16 ].
The role of AI in providing personalized feedback is highly valued, with both teachers and students appreciating its capacity to tailor educational experiences to individual needs. Customised learning, facilitated by AI, can address diverse learning styles and paces, thereby enhancing educational outcomes [ 17 , 18 ]. This personalization is crucial in meeting the unique needs of each student, fostering a more inclusive and effective learning environment [ 19 , 20 ].
AI’s contribution to collaborative creativity and accessible learning resources is also positively viewed. AI’s ability to facilitate group projects and provide a wide range of learning materials supports collaborative learning and resource accessibility, which are essential components of a modern educational framework [ 21 , 22 , 23 ]. Moreover, the enhancement of academic emotions through AI-driven learning experiences highlights AI’s potential to create positive and engaging educational environments [ 24 , 25 ].
In conclusion, the attitudes of teachers and students towards AI in education reflect a balanced perspective that acknowledges both its limitations and advantages. While there are valid concerns about emotional disengagement, ethical issues, and performance anxiety, the benefits of enhanced creativity, engagement, and personalized feedback cannot be overlooked. This underscores the need for thoughtful and strategic integration of AI in educational settings, ensuring that its deployment maximizes benefits while mitigating potential drawbacks. As AI continues to evolve, ongoing research and dialogue will be essential in navigating its role in education and optimizing its impact on teaching and learning [ 26 , 27 , 28 ].
Conclusions and implications
The integration of AI in educational applications presents a complex landscape characterized by significant challenges and notable benefits impacting students’ creativity and academic emotions. On the downside, AI applications often impose rigid frameworks that constrain creative thinking and innovation, echoing previous research on the stifling effects of structured learning paths. Emotional disengagement is another critical issue, as the repetitive and impersonal nature of AI interactions can diminish student motivation and engagement. This phenomenon underscores the importance of human interaction for maintaining emotional connections in learning. Additionally, the constant monitoring and assessments by AI applications heighten performance anxiety, negatively affecting student well-being. Technical frustrations due to frequent glitches and complex interfaces further disrupt the learning process. At the same time, an over-reliance on AI can reduce critical thinking and self-initiative, hindering essential problem-solving skills. The digital divide exacerbates educational disparities, highlighting the need for equitable access to technology. Ethical concerns about biases in AI algorithms also raise questions about fairness and equity in educational evaluations.
Conversely, AI integration offers substantial benefits, including the stimulation of creativity and enhanced engagement. AI applications can introduce new ideas and improve problem-solving skills, fostering innovative learning approaches. Their interactive nature makes learning more enjoyable and motivating, significantly boosting student participation. Personalized feedback from AI applications offers tailored guidance and immediate responses, helping students improve their work and build confidence. AI applications also provide emotional support, reducing anxiety through constant availability and enhancing motivation with gamified elements and positive reinforcement. They facilitate collaborative creativity, fostering a sense of community and collective problem-solving. Additionally, AI applications offer accessible learning resources, supporting continuous learning and inspiring creativity, which is crucial for educational equity. Adaptive learning paths and supportive environments provided by AI applications improve emotional well-being and academic performance, fostering positive learning experiences and building emotional resilience. Balancing these benefits with the challenges requires thoughtful implementation and continuous evaluation to optimize AI’s role in education.
Limitations and suggestions for further studies
Despite the merits and rich data, this study has some limitations which need to be mentioned. Firstly, the exclusive use of interviews for data collection limits the breadth of perspectives gathered. Interviews may reflect individual viewpoints rather than broader trends or consensus among participants. Additionally, the absence of focus groups in data collection further restricts the depth of insights obtained, as group dynamics and interactions that could reveal shared experiences or divergent opinions are not explored. Moreover, the study lacks detailed demographic information about participants, such as their majors, teaching experience (for teachers), or other relevant characteristics. This omission must include a nuanced understanding of how these factors might influence perceptions of AI-integrated educational applications.
Furthermore, the study’s small sample size raises concerns about the generalizability of findings. With a limited number of participants, the variability in perceptions and attitudes towards AI in education may need to be adequately captured. Additionally, a comparative analysis between teachers’ and students’ perceptions and attitudes needs to be conducted to uncover potential differences or similarities that could provide richer insights into the impact of AI on educational experiences from both perspectives.
Suggestions for future research include employing mixed-methods approaches that combine interviews with other qualitative methods, such as focus groups. This would allow for a more comprehensive exploration of diverse perspectives and enable researchers to triangulate findings for greater validity. Moreover, expanding the sample size and ensuring diversity among participants in terms of academic disciplines, teaching experience, and student backgrounds could provide a more robust basis for generalizing findings. Additionally, conducting comparative analyses between different stakeholder groups (e.g., teachers vs. students) would deepen understanding of how AI-integrated educational applications affect various participants differently. Finally, longitudinal studies could track changes in perceptions and attitudes over time as AI technologies in education continue to evolve, offering insights into the long-term impacts and adaptations within educational settings. These methodological enhancements and research directions would contribute to a more comprehensive understanding of the complex interactions between AI technology and educational practices.
Data availability
Data is provided within the manuscript or supplementary information files.
Helm JM, Swiergosz AM, Haeberle HS, Karnuta JM, Schaffer JL, Krebs VE, Spitzer AI, Ramkumar PN. Machine learning and artificial intelligence: definitions, applications, and future directions. Curr Rev Musculoskelet Med. 2020;13:69–76. https://doi.org/10.1007/s12178-020-09620-7 .
Article PubMed PubMed Central Google Scholar
Hassani H, Silva ES, Unger S, TajMazinani M, Mac Feely S. Artificial Intelligence (AI) or Intelligence Augmentation (IA): What Is the Future? AI. 2020;1:143–155. https://doi.org/10.3390/ai1020011
Yufeia L, Salehb S, Jiahuic H, Syed Mohamad Syed S. Review of the application of Artificial Intelligence in Education. Int J Innov Create Change. 2020;12:548–62.
Google Scholar
VanLehn K. The relative effectiveness of human tutoring, intelligent tutoring systems, and other tutoring systems. Educ Psychol. 2011;46:197–221.
Article Google Scholar
Trilling B, Fadel C. 21st Century skills: learning for life in our Times. San Francisco: Wiley; 2009.
Torrance EP. A longitudinal examination of the fourth-grade slump in creativity. Gifted Child Q. 1968;12:195–9.
Tubb AL, Cropley DH, Marrone RL, Patston T, Kaufman JC. The development of mathematical creativity across high school: increasing, decreasing, or both? Think Skills Creativity. 2020;35:100634.
Alves-Oliveira P, Arriaga P, Paiva A, Hoffman G, Design. Yolo, a robot for creativity: A co-design study with children. In: Proceedings of the and Children; June 27–30, 2017; Stanford, CA, USA. ACM; 2017:423–429.
Gordon G, Breazeal C, Engel S. Can children catch curiosity from a social robot? In: Proceedings of the Tenth Annual ACM/IEEE International Conference on Human-Robot Interaction; March 2–5, 2015; Portland, OR, USA. ACM; 2015:91–98.
Belpaeme T, Kennedy J, Ramachandran A, Scassellati B, Tanaka F. Social robots for education: a review. Sci Robot. 2018;3.
Kafai YB, Burke Q. Connected code: why children need to learn programming. Cambridge: MIT Press; 2014.
Book Google Scholar
Ryu M, Han S. The educational perception on artificial intelligence by elementary school teachers. J Korean Assoc Inf Educ. 2018;22:317–24.
Kaufman JC, Beghetto RA. Beyond big and little: the four C model of creativity. Rev Gen Psychol. 2009;13:1–12.
Beghetto RA, Kaufman JC, Hatcher R. Applying creativity research to cooking. J Creat Behav. 2016;50:171–7.
Marrone R, Taddeo V, Hill G. Creativity and Artificial Intelligence—A Student Perspective. J Intell. 2022;10(3):65. https://doi.org/10.3390/jintelligence10030065 .
Johnson L, Becker A, Estrada S, V., Freeman A. NMC Horizon Report: 2015 higher Education Edition. Austin, Texas: The New Media Consortium; 2015.
Allen IE, Seaman J. Digital Learning Compass: Distance Education Enrollment Report 2017. Babson Survey Research Group, e-Literate, and WCET; 2017.
Banks JA. Cultural Diversity and Education: foundations, curriculum, and teaching. Routledge; 2015.
Etzkowitz H, Leydesdorff L. The dynamics of innovation: from National systems and Mode 2 to a Triple Helix of university–industry–government relations. Res Policy. 2000;29(2):109–23.
Carnevale AP, Smith N, Strohl J. Recovery: Job Growth and Education requirements through 2020. Georgetown University Center on Education and the Workforce; 2013.
Altbach PG, Reisberg L, Rumbley LE. (2009). Trends in Global Higher Education: Tracking an Academic Revolution . A report prepared for the UNESCO 2009 World Conference on Higher Education.
Luckin R, Holmes W, Griffiths M, Forcier LB. Intelligence unleashed: an argument for AI in Education. Pearson; 2016.
Cope B, Kalantzis M. Interpreting evidence-of-learning: Educational research in the era of big data. Open Rev Educational Res. 2015;2(1):218–39.
Roll I, Wylie R. Evolution and revolution in artificial intelligence in education. Int J Artif Intell Educ. 2016;26(2):582–99.
Siemens G, Long P. Penetrating the fog: Analytics in learning and education. EDUCAUSE Rev. 2011;46(5):30–2.
Warschauer M. Technology and social inclusion: rethinking the digital divide. MIT Press; 2004.
Barocas S, Hardt M. Fairness and Abstraction in Sociotechnical Systems. IEEE Data Eng Bull. 2019;42(3):56–68.
Amornkitpinyo T, Yoosomboon S, Sopapradit S, Amornkitpinyo P. The structural equation model of actual use of Cloud Learning for Higher Education students in the 21st Century. J e-Learn Knowl Soc. 2021;17(1):72–80. https://doi.org/10.20368/1971-8829/1135300 .
Koçak O, Koçak ÖE, Younis MZ. The psychological consequences of COVID-19 fear and the moderator effects of individuals’ underlying illness and witnessing infected friends and family. Int J Environ Res Public Health. 2021;18(4):1836. https://doi.org/10.3390/ijerph18041836 .
Khan S, Zaman SI, Rais M. Measuring student satisfaction through overall quality at business schools: a structural equation modelling: student satisfaction and quality of education. South Asian J Soc Rev. 2022;1(2):34–55.
Chedrawi C, Howayeck P, Tarhini A. CSR and legitimacy in higher education accreditation programs, an isomorphic approach of Lebanese business schools. Qual Assur Educ. 2019;27(1):70–81. https://doi.org/10.1108/QAE-04-2018-0053 .
Hu J. The challenge of Traditional Teaching Approach: a study on the path to Improve Classroom Teaching Effectiveness based on secondary School Students’ psychology. Lecture Notes Educ Psychol Public Media. 2024;50(1):213–9. https://doi.org/10.54254/2753-7048/50/20240945 .
Ali SS, Choi BJ. State-of-the-art artificial intelligence techniques for distributed smart grids: a review. Electronics. 2020;9(6):1030. https://doi.org/10.3390/electronics9061030 .
Derry SJ. Learning strategies for acquiring useful knowledge. Dimensions of thinking and cognitive instruction. Routledge; 2013. pp. 347–79.
Pekrun R, Goetz T, Titz W, Perry RP. Academic emotions in students’ self-regulated learning and achievement: a program of qualitative and quantitative research. Educ Psychol. 2002;37(2):91–105.
Lei H, Cui Y. Effects of academic emotions on achievement among mainland Chinese students: a meta-analysis. Soc Behav Pers. 2016;44(9):1541–54. https://doi.org/10.2224/sbp.2016.44.9.1541 .
Artino AR, Jones KD. Exploring the complex relations between achievement emotions and self-regulated learning behaviours in online learning. Internet High Educ. 2012;15(3):170–5. https://doi.org/10.1016/j.iheduc.2012.01.006 .
Cocoradă E. Achievement emotions and performance among university students. Bull Transilv Univ Braşov. 2016;9(2–Suppl):119–28.
Lam UF, Chen WW, Zhang J, Liang T. It feels good to learn where I belong: School belonging, academic emotions, and academic achievement in adolescents. School Psychol Int. 2015;36:393–409. https://doi.org/10.1177/0143034316680410 .
Villavicencio FT. Critical thinking, negative academic emotions, and achievement: a meditational analysis. Asia-Pac Educ Res. 2011;20:118–26.
Shen B, Wang Y, Yang Y, Yu X. Relationships between Chinese university EFL learners’ academic emotions and self-regulated learning strategies: a structural equation modelling analysis. Front Psychol. 2021;12:629892. https://doi.org/10.3389/fpsyg.2021.629892 .
Alam MM, Rayhan MI, Rahman MA, Imran S. The impact of teachers’ emotion regulation, students’ learning strategies, and academic emotions on students’ learning outcomes in Bangladesh. Int J Educ Pract. 2021;9(2):306–21.
Kahu ER, Stephens C, Zepke N, Leach L. Space and time to engage: mature-aged distance students’ learning experiences. High Educ Res Dev. 2013;32(5):791–804.
Thelwall M, Buckley K, Paltoglou G. Sentiment in Twitter events. J Am Soc Inf Sci Technol. 2011;62(2):406–18.
Graziano PA, Reavis RD, Keane SP, Calkins SD. The role of emotion regulation and children’s early academic success. J Sch Psychol. 2007;45(1):3–19. https://doi.org/10.1016/j.jsp.2006.09.002 . PMID: 21179384; PMCID: PMC3004175.
Sakulwichitsintu S. Mobile technology–An innovative instructional design model in distance education. Int J Interact Mob Technol. 2023;17(7).
Fang B, Li X, Han G, He J. Facial expression recognition in educational research from the perspective of machine learning: a systematic review. IEEE Access. 2023.
Méndez López MG. Emotion and language learning: An exploration of experience and motivation in a Mexican university context [doctoral dissertation]. University of Nottingham; 2011.
Huang Y, Bo D. Emotion classification and achievement of students in distance learning based on the knowledge state model. Sustainability. 2023;15(3):2367.
Zembylas M. Adult learners’ emotions in online learning. Distance Educ. 2008;29(1):71–87.
Saputra I, Astuti M, Sayuti M, Kusumastuti D. Integration of artificial intelligence in education: opportunities, challenges, threats and obstacles. A literature review. Indones J Comput Sci. 2023;12(4).
Marrone R, Taddeo V, Hill G. Creativity and artificial intelligence—A student perspective. J Intell. 2022;10(3):65.
Lavinder KW. Staff experiences with the organizational departure of chief student affairs officers: An interpretative phenomenological analysis [doctoral dissertation]. Northeastern University; 2021.
Pekrun R, Linnenbrink-Garcia L. Academic emotions and student engagement. Handbook of Research on Student Engagement. Boston, MA: Springer US; 2012. pp. 259–82.
Chapter Google Scholar
Welter VDE, Becker LB, Großschedl J. Helping learners become their own teachers: the beneficial impact of trained concept-mapping-strategy use on metacognitive regulation in learning. Educ Sci. 2022;12(5):325.
Zhai C, Wibowo S, Li LD. The effects of over-reliance on AI dialogue systems on students’ cognitive abilities: a systematic review. Smart Learn Environ. 2024;11(1):28.
Kelly MA. Bridging digital and cultural divides: TPCK for equity of access to technology. Handbook of Technological Pedagogical Content Knowledge (TPCK) for educators. Routledge; 2014. pp. 41–68.
Bogina V, Hartman A, Kuflik T, Shulner-Tal A. Educating software and AI stakeholders about algorithmic fairness, accountability, transparency and ethics. Int J Artif Intell Educ. 2022;1–26.
Urmeneta A, Romero M. Creative applications of artificial intelligence in education. Springer Nature; 2024.
Li G, Zarei MA, Alibakhshi G, Labbafi A. Teachers and educators’ experiences and perceptions of artificial-powered interventions for autism groups. BMC Psychol. 2024;12(1):199.
Huang AY, Lu OH, Yang SJ. Effects of artificial intelligence–enabled personalized recommendations on learners’ learning engagement, motivation, and outcomes in a flipped classroom. Comput Educ. 2023;194:104684.
Chang DH, Lin MPC, Hajian S, Wang QQ. Educational design principles of using AI chatbot that supports self-regulated learning in education: goal setting, feedback, and personalization. Sustainability. 2023;15(17):12921.
Li L, Gow ADI, Zhou J. The role of positive emotions in education: a neuroscience perspective. Mind Brain Educ. 2020;14(3):220–34.
Graesser AC, Fiore SM, Greiff S, Andrews-Todd J, Foltz PW, Hesse FW. Advancing the science of collaborative problem-solving. Psychol Sci Public Interest. 2018;19(2):59–92.
Article PubMed Google Scholar
Yenduri G, Kaluri R, Rajput DS, Lakshmanna K, Gadekallu TR, Mahmud M, Brown DJ. From assistive technologies to metaverse—technologies in inclusive higher education for students with specific learning difficulties: a review. IEEE Access. 2023;11:64907–27.
Download references
Acknowledgements
The author would like to thank all the participants.
2024 Higher Education Research Project (KT2024176), ZAHE, the Zhejiang Provincial Association of Higher Education.
Author information
Authors and affiliations.
School of Marxism, Wenzhou University of Technology, Wenzhou, Zhejiang, 32500, China
Haozhuo Lin
School of Humanities, Wenzhou University, Wenzhou, Zhejiang, 32500, China
You can also search for this author in PubMed Google Scholar
Contributions
HL and QC designed the study. HL and QC collected the data. HL and QC analyzed and interpreted the data. HL and QC drafted the manuscript. HL and QC proofread the paper. HL and QC agreed to be accountable and verified the submitted version.
Corresponding author
Correspondence to Qiu Chen .
Ethics declarations
Ethics approval and consent to participate.
The Institutional Review Board of Wenzhou approved this study and issued a letter indicating that it had no side effects on the participants. All experiments and methods were carried out in accordance with relevant guidelines and regulations. Informed consent was obtained from all subjects.
Consent for publication
Not applicable .
Competing interests
The authors declare no competing interests.
Additional information
Publisher’s note.
Springer Nature remains neutral with regard to jurisdictional claims in published maps and institutional affiliations.
Electronic supplementary material
Below is the link to the electronic supplementary material.
Supplementary Material 1
Rights and permissions.
Open Access This article is licensed under a Creative Commons Attribution 4.0 International License, which permits use, sharing, adaptation, distribution and reproduction in any medium or format, as long as you give appropriate credit to the original author(s) and the source, provide a link to the Creative Commons licence, and indicate if changes were made. The images or other third party material in this article are included in the article’s Creative Commons licence, unless indicated otherwise in a credit line to the material. If material is not included in the article’s Creative Commons licence and your intended use is not permitted by statutory regulation or exceeds the permitted use, you will need to obtain permission directly from the copyright holder. To view a copy of this licence, visit http://creativecommons.org/licenses/by/4.0/ .
Reprints and permissions
About this article
Cite this article.
Lin, H., Chen, Q. Artificial intelligence (AI) -integrated educational applications and college students’ creativity and academic emotions: students and teachers’ perceptions and attitudes. BMC Psychol 12 , 487 (2024). https://doi.org/10.1186/s40359-024-01979-0
Download citation
Received : 20 May 2024
Accepted : 03 September 2024
Published : 16 September 2024
DOI : https://doi.org/10.1186/s40359-024-01979-0
Share this article
Anyone you share the following link with will be able to read this content:
Sorry, a shareable link is not currently available for this article.
Provided by the Springer Nature SharedIt content-sharing initiative
- Artificial intelligence
- Academic emotions
- AI-empowered applications
- Chinese students
BMC Psychology
ISSN: 2050-7283
- General enquiries: [email protected]

An official website of the United States government
The .gov means it’s official. Federal government websites often end in .gov or .mil. Before sharing sensitive information, make sure you’re on a federal government site.
The site is secure. The https:// ensures that you are connecting to the official website and that any information you provide is encrypted and transmitted securely.
- Publications
- Account settings
The PMC website is updating on October 15, 2024. Learn More or Try it out now .
- Advanced Search
- Journal List
- BMC Med Res Methodol

A tutorial on methodological studies: the what, when, how and why
Lawrence mbuagbaw.
1 Department of Health Research Methods, Evidence and Impact, McMaster University, Hamilton, ON Canada
2 Biostatistics Unit/FSORC, 50 Charlton Avenue East, St Joseph’s Healthcare—Hamilton, 3rd Floor Martha Wing, Room H321, Hamilton, Ontario L8N 4A6 Canada
3 Centre for the Development of Best Practices in Health, Yaoundé, Cameroon
Daeria O. Lawson
Livia puljak.
4 Center for Evidence-Based Medicine and Health Care, Catholic University of Croatia, Ilica 242, 10000 Zagreb, Croatia
David B. Allison
5 Department of Epidemiology and Biostatistics, School of Public Health – Bloomington, Indiana University, Bloomington, IN 47405 USA
Lehana Thabane
6 Departments of Paediatrics and Anaesthesia, McMaster University, Hamilton, ON Canada
7 Centre for Evaluation of Medicine, St. Joseph’s Healthcare-Hamilton, Hamilton, ON Canada
8 Population Health Research Institute, Hamilton Health Sciences, Hamilton, ON Canada
Associated Data
Data sharing is not applicable to this article as no new data were created or analyzed in this study.
Methodological studies – studies that evaluate the design, analysis or reporting of other research-related reports – play an important role in health research. They help to highlight issues in the conduct of research with the aim of improving health research methodology, and ultimately reducing research waste.
We provide an overview of some of the key aspects of methodological studies such as what they are, and when, how and why they are done. We adopt a “frequently asked questions” format to facilitate reading this paper and provide multiple examples to help guide researchers interested in conducting methodological studies. Some of the topics addressed include: is it necessary to publish a study protocol? How to select relevant research reports and databases for a methodological study? What approaches to data extraction and statistical analysis should be considered when conducting a methodological study? What are potential threats to validity and is there a way to appraise the quality of methodological studies?
Appropriate reflection and application of basic principles of epidemiology and biostatistics are required in the design and analysis of methodological studies. This paper provides an introduction for further discussion about the conduct of methodological studies.
The field of meta-research (or research-on-research) has proliferated in recent years in response to issues with research quality and conduct [ 1 – 3 ]. As the name suggests, this field targets issues with research design, conduct, analysis and reporting. Various types of research reports are often examined as the unit of analysis in these studies (e.g. abstracts, full manuscripts, trial registry entries). Like many other novel fields of research, meta-research has seen a proliferation of use before the development of reporting guidance. For example, this was the case with randomized trials for which risk of bias tools and reporting guidelines were only developed much later – after many trials had been published and noted to have limitations [ 4 , 5 ]; and for systematic reviews as well [ 6 – 8 ]. However, in the absence of formal guidance, studies that report on research differ substantially in how they are named, conducted and reported [ 9 , 10 ]. This creates challenges in identifying, summarizing and comparing them. In this tutorial paper, we will use the term methodological study to refer to any study that reports on the design, conduct, analysis or reporting of primary or secondary research-related reports (such as trial registry entries and conference abstracts).
In the past 10 years, there has been an increase in the use of terms related to methodological studies (based on records retrieved with a keyword search [in the title and abstract] for “methodological review” and “meta-epidemiological study” in PubMed up to December 2019), suggesting that these studies may be appearing more frequently in the literature. See Fig. 1 .

Trends in the number studies that mention “methodological review” or “meta-
epidemiological study” in PubMed.
The methods used in many methodological studies have been borrowed from systematic and scoping reviews. This practice has influenced the direction of the field, with many methodological studies including searches of electronic databases, screening of records, duplicate data extraction and assessments of risk of bias in the included studies. However, the research questions posed in methodological studies do not always require the approaches listed above, and guidance is needed on when and how to apply these methods to a methodological study. Even though methodological studies can be conducted on qualitative or mixed methods research, this paper focuses on and draws examples exclusively from quantitative research.
The objectives of this paper are to provide some insights on how to conduct methodological studies so that there is greater consistency between the research questions posed, and the design, analysis and reporting of findings. We provide multiple examples to illustrate concepts and a proposed framework for categorizing methodological studies in quantitative research.
What is a methodological study?
Any study that describes or analyzes methods (design, conduct, analysis or reporting) in published (or unpublished) literature is a methodological study. Consequently, the scope of methodological studies is quite extensive and includes, but is not limited to, topics as diverse as: research question formulation [ 11 ]; adherence to reporting guidelines [ 12 – 14 ] and consistency in reporting [ 15 ]; approaches to study analysis [ 16 ]; investigating the credibility of analyses [ 17 ]; and studies that synthesize these methodological studies [ 18 ]. While the nomenclature of methodological studies is not uniform, the intents and purposes of these studies remain fairly consistent – to describe or analyze methods in primary or secondary studies. As such, methodological studies may also be classified as a subtype of observational studies.
Parallel to this are experimental studies that compare different methods. Even though they play an important role in informing optimal research methods, experimental methodological studies are beyond the scope of this paper. Examples of such studies include the randomized trials by Buscemi et al., comparing single data extraction to double data extraction [ 19 ], and Carrasco-Labra et al., comparing approaches to presenting findings in Grading of Recommendations, Assessment, Development and Evaluations (GRADE) summary of findings tables [ 20 ]. In these studies, the unit of analysis is the person or groups of individuals applying the methods. We also direct readers to the Studies Within a Trial (SWAT) and Studies Within a Review (SWAR) programme operated through the Hub for Trials Methodology Research, for further reading as a potential useful resource for these types of experimental studies [ 21 ]. Lastly, this paper is not meant to inform the conduct of research using computational simulation and mathematical modeling for which some guidance already exists [ 22 ], or studies on the development of methods using consensus-based approaches.
When should we conduct a methodological study?
Methodological studies occupy a unique niche in health research that allows them to inform methodological advances. Methodological studies should also be conducted as pre-cursors to reporting guideline development, as they provide an opportunity to understand current practices, and help to identify the need for guidance and gaps in methodological or reporting quality. For example, the development of the popular Preferred Reporting Items of Systematic reviews and Meta-Analyses (PRISMA) guidelines were preceded by methodological studies identifying poor reporting practices [ 23 , 24 ]. In these instances, after the reporting guidelines are published, methodological studies can also be used to monitor uptake of the guidelines.
These studies can also be conducted to inform the state of the art for design, analysis and reporting practices across different types of health research fields, with the aim of improving research practices, and preventing or reducing research waste. For example, Samaan et al. conducted a scoping review of adherence to different reporting guidelines in health care literature [ 18 ]. Methodological studies can also be used to determine the factors associated with reporting practices. For example, Abbade et al. investigated journal characteristics associated with the use of the Participants, Intervention, Comparison, Outcome, Timeframe (PICOT) format in framing research questions in trials of venous ulcer disease [ 11 ].
How often are methodological studies conducted?
There is no clear answer to this question. Based on a search of PubMed, the use of related terms (“methodological review” and “meta-epidemiological study”) – and therefore, the number of methodological studies – is on the rise. However, many other terms are used to describe methodological studies. There are also many studies that explore design, conduct, analysis or reporting of research reports, but that do not use any specific terms to describe or label their study design in terms of “methodology”. This diversity in nomenclature makes a census of methodological studies elusive. Appropriate terminology and key words for methodological studies are needed to facilitate improved accessibility for end-users.
Why do we conduct methodological studies?
Methodological studies provide information on the design, conduct, analysis or reporting of primary and secondary research and can be used to appraise quality, quantity, completeness, accuracy and consistency of health research. These issues can be explored in specific fields, journals, databases, geographical regions and time periods. For example, Areia et al. explored the quality of reporting of endoscopic diagnostic studies in gastroenterology [ 25 ]; Knol et al. investigated the reporting of p -values in baseline tables in randomized trial published in high impact journals [ 26 ]; Chen et al. describe adherence to the Consolidated Standards of Reporting Trials (CONSORT) statement in Chinese Journals [ 27 ]; and Hopewell et al. describe the effect of editors’ implementation of CONSORT guidelines on reporting of abstracts over time [ 28 ]. Methodological studies provide useful information to researchers, clinicians, editors, publishers and users of health literature. As a result, these studies have been at the cornerstone of important methodological developments in the past two decades and have informed the development of many health research guidelines including the highly cited CONSORT statement [ 5 ].
Where can we find methodological studies?
Methodological studies can be found in most common biomedical bibliographic databases (e.g. Embase, MEDLINE, PubMed, Web of Science). However, the biggest caveat is that methodological studies are hard to identify in the literature due to the wide variety of names used and the lack of comprehensive databases dedicated to them. A handful can be found in the Cochrane Library as “Cochrane Methodology Reviews”, but these studies only cover methodological issues related to systematic reviews. Previous attempts to catalogue all empirical studies of methods used in reviews were abandoned 10 years ago [ 29 ]. In other databases, a variety of search terms may be applied with different levels of sensitivity and specificity.
Some frequently asked questions about methodological studies
In this section, we have outlined responses to questions that might help inform the conduct of methodological studies.
Q: How should I select research reports for my methodological study?
A: Selection of research reports for a methodological study depends on the research question and eligibility criteria. Once a clear research question is set and the nature of literature one desires to review is known, one can then begin the selection process. Selection may begin with a broad search, especially if the eligibility criteria are not apparent. For example, a methodological study of Cochrane Reviews of HIV would not require a complex search as all eligible studies can easily be retrieved from the Cochrane Library after checking a few boxes [ 30 ]. On the other hand, a methodological study of subgroup analyses in trials of gastrointestinal oncology would require a search to find such trials, and further screening to identify trials that conducted a subgroup analysis [ 31 ].
The strategies used for identifying participants in observational studies can apply here. One may use a systematic search to identify all eligible studies. If the number of eligible studies is unmanageable, a random sample of articles can be expected to provide comparable results if it is sufficiently large [ 32 ]. For example, Wilson et al. used a random sample of trials from the Cochrane Stroke Group’s Trial Register to investigate completeness of reporting [ 33 ]. It is possible that a simple random sample would lead to underrepresentation of units (i.e. research reports) that are smaller in number. This is relevant if the investigators wish to compare multiple groups but have too few units in one group. In this case a stratified sample would help to create equal groups. For example, in a methodological study comparing Cochrane and non-Cochrane reviews, Kahale et al. drew random samples from both groups [ 34 ]. Alternatively, systematic or purposeful sampling strategies can be used and we encourage researchers to justify their selected approaches based on the study objective.
Q: How many databases should I search?
A: The number of databases one should search would depend on the approach to sampling, which can include targeting the entire “population” of interest or a sample of that population. If you are interested in including the entire target population for your research question, or drawing a random or systematic sample from it, then a comprehensive and exhaustive search for relevant articles is required. In this case, we recommend using systematic approaches for searching electronic databases (i.e. at least 2 databases with a replicable and time stamped search strategy). The results of your search will constitute a sampling frame from which eligible studies can be drawn.
Alternatively, if your approach to sampling is purposeful, then we recommend targeting the database(s) or data sources (e.g. journals, registries) that include the information you need. For example, if you are conducting a methodological study of high impact journals in plastic surgery and they are all indexed in PubMed, you likely do not need to search any other databases. You may also have a comprehensive list of all journals of interest and can approach your search using the journal names in your database search (or by accessing the journal archives directly from the journal’s website). Even though one could also search journals’ web pages directly, using a database such as PubMed has multiple advantages, such as the use of filters, so the search can be narrowed down to a certain period, or study types of interest. Furthermore, individual journals’ web sites may have different search functionalities, which do not necessarily yield a consistent output.
Q: Should I publish a protocol for my methodological study?
A: A protocol is a description of intended research methods. Currently, only protocols for clinical trials require registration [ 35 ]. Protocols for systematic reviews are encouraged but no formal recommendation exists. The scientific community welcomes the publication of protocols because they help protect against selective outcome reporting, the use of post hoc methodologies to embellish results, and to help avoid duplication of efforts [ 36 ]. While the latter two risks exist in methodological research, the negative consequences may be substantially less than for clinical outcomes. In a sample of 31 methodological studies, 7 (22.6%) referenced a published protocol [ 9 ]. In the Cochrane Library, there are 15 protocols for methodological reviews (21 July 2020). This suggests that publishing protocols for methodological studies is not uncommon.
Authors can consider publishing their study protocol in a scholarly journal as a manuscript. Advantages of such publication include obtaining peer-review feedback about the planned study, and easy retrieval by searching databases such as PubMed. The disadvantages in trying to publish protocols includes delays associated with manuscript handling and peer review, as well as costs, as few journals publish study protocols, and those journals mostly charge article-processing fees [ 37 ]. Authors who would like to make their protocol publicly available without publishing it in scholarly journals, could deposit their study protocols in publicly available repositories, such as the Open Science Framework ( https://osf.io/ ).
Q: How to appraise the quality of a methodological study?
A: To date, there is no published tool for appraising the risk of bias in a methodological study, but in principle, a methodological study could be considered as a type of observational study. Therefore, during conduct or appraisal, care should be taken to avoid the biases common in observational studies [ 38 ]. These biases include selection bias, comparability of groups, and ascertainment of exposure or outcome. In other words, to generate a representative sample, a comprehensive reproducible search may be necessary to build a sampling frame. Additionally, random sampling may be necessary to ensure that all the included research reports have the same probability of being selected, and the screening and selection processes should be transparent and reproducible. To ensure that the groups compared are similar in all characteristics, matching, random sampling or stratified sampling can be used. Statistical adjustments for between-group differences can also be applied at the analysis stage. Finally, duplicate data extraction can reduce errors in assessment of exposures or outcomes.
Q: Should I justify a sample size?
A: In all instances where one is not using the target population (i.e. the group to which inferences from the research report are directed) [ 39 ], a sample size justification is good practice. The sample size justification may take the form of a description of what is expected to be achieved with the number of articles selected, or a formal sample size estimation that outlines the number of articles required to answer the research question with a certain precision and power. Sample size justifications in methodological studies are reasonable in the following instances:
- Comparing two groups
- Determining a proportion, mean or another quantifier
- Determining factors associated with an outcome using regression-based analyses
For example, El Dib et al. computed a sample size requirement for a methodological study of diagnostic strategies in randomized trials, based on a confidence interval approach [ 40 ].
Q: What should I call my study?
A: Other terms which have been used to describe/label methodological studies include “ methodological review ”, “methodological survey” , “meta-epidemiological study” , “systematic review” , “systematic survey”, “meta-research”, “research-on-research” and many others. We recommend that the study nomenclature be clear, unambiguous, informative and allow for appropriate indexing. Methodological study nomenclature that should be avoided includes “ systematic review” – as this will likely be confused with a systematic review of a clinical question. “ Systematic survey” may also lead to confusion about whether the survey was systematic (i.e. using a preplanned methodology) or a survey using “ systematic” sampling (i.e. a sampling approach using specific intervals to determine who is selected) [ 32 ]. Any of the above meanings of the words “ systematic” may be true for methodological studies and could be potentially misleading. “ Meta-epidemiological study” is ideal for indexing, but not very informative as it describes an entire field. The term “ review ” may point towards an appraisal or “review” of the design, conduct, analysis or reporting (or methodological components) of the targeted research reports, yet it has also been used to describe narrative reviews [ 41 , 42 ]. The term “ survey ” is also in line with the approaches used in many methodological studies [ 9 ], and would be indicative of the sampling procedures of this study design. However, in the absence of guidelines on nomenclature, the term “ methodological study ” is broad enough to capture most of the scenarios of such studies.
Q: Should I account for clustering in my methodological study?
A: Data from methodological studies are often clustered. For example, articles coming from a specific source may have different reporting standards (e.g. the Cochrane Library). Articles within the same journal may be similar due to editorial practices and policies, reporting requirements and endorsement of guidelines. There is emerging evidence that these are real concerns that should be accounted for in analyses [ 43 ]. Some cluster variables are described in the section: “ What variables are relevant to methodological studies?”
A variety of modelling approaches can be used to account for correlated data, including the use of marginal, fixed or mixed effects regression models with appropriate computation of standard errors [ 44 ]. For example, Kosa et al. used generalized estimation equations to account for correlation of articles within journals [ 15 ]. Not accounting for clustering could lead to incorrect p -values, unduly narrow confidence intervals, and biased estimates [ 45 ].
Q: Should I extract data in duplicate?
A: Yes. Duplicate data extraction takes more time but results in less errors [ 19 ]. Data extraction errors in turn affect the effect estimate [ 46 ], and therefore should be mitigated. Duplicate data extraction should be considered in the absence of other approaches to minimize extraction errors. However, much like systematic reviews, this area will likely see rapid new advances with machine learning and natural language processing technologies to support researchers with screening and data extraction [ 47 , 48 ]. However, experience plays an important role in the quality of extracted data and inexperienced extractors should be paired with experienced extractors [ 46 , 49 ].
Q: Should I assess the risk of bias of research reports included in my methodological study?
A : Risk of bias is most useful in determining the certainty that can be placed in the effect measure from a study. In methodological studies, risk of bias may not serve the purpose of determining the trustworthiness of results, as effect measures are often not the primary goal of methodological studies. Determining risk of bias in methodological studies is likely a practice borrowed from systematic review methodology, but whose intrinsic value is not obvious in methodological studies. When it is part of the research question, investigators often focus on one aspect of risk of bias. For example, Speich investigated how blinding was reported in surgical trials [ 50 ], and Abraha et al., investigated the application of intention-to-treat analyses in systematic reviews and trials [ 51 ].
Q: What variables are relevant to methodological studies?
A: There is empirical evidence that certain variables may inform the findings in a methodological study. We outline some of these and provide a brief overview below:
- Country: Countries and regions differ in their research cultures, and the resources available to conduct research. Therefore, it is reasonable to believe that there may be differences in methodological features across countries. Methodological studies have reported loco-regional differences in reporting quality [ 52 , 53 ]. This may also be related to challenges non-English speakers face in publishing papers in English.
- Authors’ expertise: The inclusion of authors with expertise in research methodology, biostatistics, and scientific writing is likely to influence the end-product. Oltean et al. found that among randomized trials in orthopaedic surgery, the use of analyses that accounted for clustering was more likely when specialists (e.g. statistician, epidemiologist or clinical trials methodologist) were included on the study team [ 54 ]. Fleming et al. found that including methodologists in the review team was associated with appropriate use of reporting guidelines [ 55 ].
- Source of funding and conflicts of interest: Some studies have found that funded studies report better [ 56 , 57 ], while others do not [ 53 , 58 ]. The presence of funding would indicate the availability of resources deployed to ensure optimal design, conduct, analysis and reporting. However, the source of funding may introduce conflicts of interest and warrant assessment. For example, Kaiser et al. investigated the effect of industry funding on obesity or nutrition randomized trials and found that reporting quality was similar [ 59 ]. Thomas et al. looked at reporting quality of long-term weight loss trials and found that industry funded studies were better [ 60 ]. Kan et al. examined the association between industry funding and “positive trials” (trials reporting a significant intervention effect) and found that industry funding was highly predictive of a positive trial [ 61 ]. This finding is similar to that of a recent Cochrane Methodology Review by Hansen et al. [ 62 ]
- Journal characteristics: Certain journals’ characteristics may influence the study design, analysis or reporting. Characteristics such as journal endorsement of guidelines [ 63 , 64 ], and Journal Impact Factor (JIF) have been shown to be associated with reporting [ 63 , 65 – 67 ].
- Study size (sample size/number of sites): Some studies have shown that reporting is better in larger studies [ 53 , 56 , 58 ].
- Year of publication: It is reasonable to assume that design, conduct, analysis and reporting of research will change over time. Many studies have demonstrated improvements in reporting over time or after the publication of reporting guidelines [ 68 , 69 ].
- Type of intervention: In a methodological study of reporting quality of weight loss intervention studies, Thabane et al. found that trials of pharmacologic interventions were reported better than trials of non-pharmacologic interventions [ 70 ].
- Interactions between variables: Complex interactions between the previously listed variables are possible. High income countries with more resources may be more likely to conduct larger studies and incorporate a variety of experts. Authors in certain countries may prefer certain journals, and journal endorsement of guidelines and editorial policies may change over time.
Q: Should I focus only on high impact journals?
A: Investigators may choose to investigate only high impact journals because they are more likely to influence practice and policy, or because they assume that methodological standards would be higher. However, the JIF may severely limit the scope of articles included and may skew the sample towards articles with positive findings. The generalizability and applicability of findings from a handful of journals must be examined carefully, especially since the JIF varies over time. Even among journals that are all “high impact”, variations exist in methodological standards.
Q: Can I conduct a methodological study of qualitative research?
A: Yes. Even though a lot of methodological research has been conducted in the quantitative research field, methodological studies of qualitative studies are feasible. Certain databases that catalogue qualitative research including the Cumulative Index to Nursing & Allied Health Literature (CINAHL) have defined subject headings that are specific to methodological research (e.g. “research methodology”). Alternatively, one could also conduct a qualitative methodological review; that is, use qualitative approaches to synthesize methodological issues in qualitative studies.
Q: What reporting guidelines should I use for my methodological study?
A: There is no guideline that covers the entire scope of methodological studies. One adaptation of the PRISMA guidelines has been published, which works well for studies that aim to use the entire target population of research reports [ 71 ]. However, it is not widely used (40 citations in 2 years as of 09 December 2019), and methodological studies that are designed as cross-sectional or before-after studies require a more fit-for purpose guideline. A more encompassing reporting guideline for a broad range of methodological studies is currently under development [ 72 ]. However, in the absence of formal guidance, the requirements for scientific reporting should be respected, and authors of methodological studies should focus on transparency and reproducibility.
Q: What are the potential threats to validity and how can I avoid them?
A: Methodological studies may be compromised by a lack of internal or external validity. The main threats to internal validity in methodological studies are selection and confounding bias. Investigators must ensure that the methods used to select articles does not make them differ systematically from the set of articles to which they would like to make inferences. For example, attempting to make extrapolations to all journals after analyzing high-impact journals would be misleading.
Many factors (confounders) may distort the association between the exposure and outcome if the included research reports differ with respect to these factors [ 73 ]. For example, when examining the association between source of funding and completeness of reporting, it may be necessary to account for journals that endorse the guidelines. Confounding bias can be addressed by restriction, matching and statistical adjustment [ 73 ]. Restriction appears to be the method of choice for many investigators who choose to include only high impact journals or articles in a specific field. For example, Knol et al. examined the reporting of p -values in baseline tables of high impact journals [ 26 ]. Matching is also sometimes used. In the methodological study of non-randomized interventional studies of elective ventral hernia repair, Parker et al. matched prospective studies with retrospective studies and compared reporting standards [ 74 ]. Some other methodological studies use statistical adjustments. For example, Zhang et al. used regression techniques to determine the factors associated with missing participant data in trials [ 16 ].
With regard to external validity, researchers interested in conducting methodological studies must consider how generalizable or applicable their findings are. This should tie in closely with the research question and should be explicit. For example. Findings from methodological studies on trials published in high impact cardiology journals cannot be assumed to be applicable to trials in other fields. However, investigators must ensure that their sample truly represents the target sample either by a) conducting a comprehensive and exhaustive search, or b) using an appropriate and justified, randomly selected sample of research reports.
Even applicability to high impact journals may vary based on the investigators’ definition, and over time. For example, for high impact journals in the field of general medicine, Bouwmeester et al. included the Annals of Internal Medicine (AIM), BMJ, the Journal of the American Medical Association (JAMA), Lancet, the New England Journal of Medicine (NEJM), and PLoS Medicine ( n = 6) [ 75 ]. In contrast, the high impact journals selected in the methodological study by Schiller et al. were BMJ, JAMA, Lancet, and NEJM ( n = 4) [ 76 ]. Another methodological study by Kosa et al. included AIM, BMJ, JAMA, Lancet and NEJM ( n = 5). In the methodological study by Thabut et al., journals with a JIF greater than 5 were considered to be high impact. Riado Minguez et al. used first quartile journals in the Journal Citation Reports (JCR) for a specific year to determine “high impact” [ 77 ]. Ultimately, the definition of high impact will be based on the number of journals the investigators are willing to include, the year of impact and the JIF cut-off [ 78 ]. We acknowledge that the term “generalizability” may apply differently for methodological studies, especially when in many instances it is possible to include the entire target population in the sample studied.
Finally, methodological studies are not exempt from information bias which may stem from discrepancies in the included research reports [ 79 ], errors in data extraction, or inappropriate interpretation of the information extracted. Likewise, publication bias may also be a concern in methodological studies, but such concepts have not yet been explored.
A proposed framework
In order to inform discussions about methodological studies, the development of guidance for what should be reported, we have outlined some key features of methodological studies that can be used to classify them. For each of the categories outlined below, we provide an example. In our experience, the choice of approach to completing a methodological study can be informed by asking the following four questions:
- What is the aim?
A methodological study may be focused on exploring sources of bias in primary or secondary studies (meta-bias), or how bias is analyzed. We have taken care to distinguish bias (i.e. systematic deviations from the truth irrespective of the source) from reporting quality or completeness (i.e. not adhering to a specific reporting guideline or norm). An example of where this distinction would be important is in the case of a randomized trial with no blinding. This study (depending on the nature of the intervention) would be at risk of performance bias. However, if the authors report that their study was not blinded, they would have reported adequately. In fact, some methodological studies attempt to capture both “quality of conduct” and “quality of reporting”, such as Richie et al., who reported on the risk of bias in randomized trials of pharmacy practice interventions [ 80 ]. Babic et al. investigated how risk of bias was used to inform sensitivity analyses in Cochrane reviews [ 81 ]. Further, biases related to choice of outcomes can also be explored. For example, Tan et al investigated differences in treatment effect size based on the outcome reported [ 82 ].
Methodological studies may report quality of reporting against a reporting checklist (i.e. adherence to guidelines) or against expected norms. For example, Croituro et al. report on the quality of reporting in systematic reviews published in dermatology journals based on their adherence to the PRISMA statement [ 83 ], and Khan et al. described the quality of reporting of harms in randomized controlled trials published in high impact cardiovascular journals based on the CONSORT extension for harms [ 84 ]. Other methodological studies investigate reporting of certain features of interest that may not be part of formally published checklists or guidelines. For example, Mbuagbaw et al. described how often the implications for research are elaborated using the Evidence, Participants, Intervention, Comparison, Outcome, Timeframe (EPICOT) format [ 30 ].
Sometimes investigators may be interested in how consistent reports of the same research are, as it is expected that there should be consistency between: conference abstracts and published manuscripts; manuscript abstracts and manuscript main text; and trial registration and published manuscript. For example, Rosmarakis et al. investigated consistency between conference abstracts and full text manuscripts [ 85 ].
In addition to identifying issues with reporting in primary and secondary studies, authors of methodological studies may be interested in determining the factors that are associated with certain reporting practices. Many methodological studies incorporate this, albeit as a secondary outcome. For example, Farrokhyar et al. investigated the factors associated with reporting quality in randomized trials of coronary artery bypass grafting surgery [ 53 ].
Methodological studies may also be used to describe methods or compare methods, and the factors associated with methods. Muller et al. described the methods used for systematic reviews and meta-analyses of observational studies [ 86 ].
Some methodological studies synthesize results from other methodological studies. For example, Li et al. conducted a scoping review of methodological reviews that investigated consistency between full text and abstracts in primary biomedical research [ 87 ].
Some methodological studies may investigate the use of names and terms in health research. For example, Martinic et al. investigated the definitions of systematic reviews used in overviews of systematic reviews (OSRs), meta-epidemiological studies and epidemiology textbooks [ 88 ].
In addition to the previously mentioned experimental methodological studies, there may exist other types of methodological studies not captured here.
- 2. What is the design?
Most methodological studies are purely descriptive and report their findings as counts (percent) and means (standard deviation) or medians (interquartile range). For example, Mbuagbaw et al. described the reporting of research recommendations in Cochrane HIV systematic reviews [ 30 ]. Gohari et al. described the quality of reporting of randomized trials in diabetes in Iran [ 12 ].
Some methodological studies are analytical wherein “analytical studies identify and quantify associations, test hypotheses, identify causes and determine whether an association exists between variables, such as between an exposure and a disease.” [ 89 ] In the case of methodological studies all these investigations are possible. For example, Kosa et al. investigated the association between agreement in primary outcome from trial registry to published manuscript and study covariates. They found that larger and more recent studies were more likely to have agreement [ 15 ]. Tricco et al. compared the conclusion statements from Cochrane and non-Cochrane systematic reviews with a meta-analysis of the primary outcome and found that non-Cochrane reviews were more likely to report positive findings. These results are a test of the null hypothesis that the proportions of Cochrane and non-Cochrane reviews that report positive results are equal [ 90 ].
- 3. What is the sampling strategy?
Methodological reviews with narrow research questions may be able to include the entire target population. For example, in the methodological study of Cochrane HIV systematic reviews, Mbuagbaw et al. included all of the available studies ( n = 103) [ 30 ].
Many methodological studies use random samples of the target population [ 33 , 91 , 92 ]. Alternatively, purposeful sampling may be used, limiting the sample to a subset of research-related reports published within a certain time period, or in journals with a certain ranking or on a topic. Systematic sampling can also be used when random sampling may be challenging to implement.
- 4. What is the unit of analysis?
Many methodological studies use a research report (e.g. full manuscript of study, abstract portion of the study) as the unit of analysis, and inferences can be made at the study-level. However, both published and unpublished research-related reports can be studied. These may include articles, conference abstracts, registry entries etc.
Some methodological studies report on items which may occur more than once per article. For example, Paquette et al. report on subgroup analyses in Cochrane reviews of atrial fibrillation in which 17 systematic reviews planned 56 subgroup analyses [ 93 ].
This framework is outlined in Fig. 2 .

A proposed framework for methodological studies
Conclusions
Methodological studies have examined different aspects of reporting such as quality, completeness, consistency and adherence to reporting guidelines. As such, many of the methodological study examples cited in this tutorial are related to reporting. However, as an evolving field, the scope of research questions that can be addressed by methodological studies is expected to increase.
In this paper we have outlined the scope and purpose of methodological studies, along with examples of instances in which various approaches have been used. In the absence of formal guidance on the design, conduct, analysis and reporting of methodological studies, we have provided some advice to help make methodological studies consistent. This advice is grounded in good contemporary scientific practice. Generally, the research question should tie in with the sampling approach and planned analysis. We have also highlighted the variables that may inform findings from methodological studies. Lastly, we have provided suggestions for ways in which authors can categorize their methodological studies to inform their design and analysis.
Acknowledgements
Abbreviations.
CONSORT | Consolidated Standards of Reporting Trials |
EPICOT | Evidence, Participants, Intervention, Comparison, Outcome, Timeframe |
GRADE | Grading of Recommendations, Assessment, Development and Evaluations |
PICOT | Participants, Intervention, Comparison, Outcome, Timeframe |
PRISMA | Preferred Reporting Items of Systematic reviews and Meta-Analyses |
SWAR | Studies Within a Review |
SWAT | Studies Within a Trial |
Authors’ contributions
LM conceived the idea and drafted the outline and paper. DOL and LT commented on the idea and draft outline. LM, LP and DOL performed literature searches and data extraction. All authors (LM, DOL, LT, LP, DBA) reviewed several draft versions of the manuscript and approved the final manuscript.
This work did not receive any dedicated funding.
Availability of data and materials
Ethics approval and consent to participate.
Not applicable.
Consent for publication
Competing interests.
DOL, DBA, LM, LP and LT are involved in the development of a reporting guideline for methodological studies.
Publisher’s Note
Springer Nature remains neutral with regard to jurisdictional claims in published maps and institutional affiliations.

IMAGES
VIDEO
COMMENTS
While many books and articles guide various qualitative research methods and analyses, there is currently no concise resource that explains and differentiates among the most common qualitative approaches. We believe novice qualitative researchers, students planning the design of a qualitative study or taking an introductory qualitative research course, and faculty teaching such courses can ...
Hence, mixed methods methodology does not provide a conceptual unification of the two approaches. Lacking a common methodological background, qualitative and quantitative research methodologies have developed rather distinct standards with regard to the aims and scope of empirical science (Freeman et al., 2007). These different standards affect ...
The lack of rigorous account of research methods in articles was represented in-depth for each step in the research process and can be of vital importance to address the current replication crisis within the field of psychology. ... A review of a decade's scholarly publications (2004-2013) in the South African Journal of Industrial Psychology ...
This is why the literature review as a research method is more relevant than ever. Traditional literature reviews often lack thoroughness and rigor and are conducted ad hoc, rather than following a specific methodology. Therefore, questions can be raised about the quality and trustworthiness of these types of reviews.
Forrest S (2017) Teaching social science research methods to undergraduate medical students: The state of the art and opportunities for practice and curriculum development. Teaching Public Administration 35(3): 280-300. Crossref. Google Scholar.
Abstract. This paper aims to provide an overview of the use and assessment of qualitative research methods in the health sciences. Qualitative research can be defined as the study of the nature of phenomena and is especially appropriate for answering questions of why something is (not) observed, assessing complex multi-component interventions ...
Background Overviews of methods are potentially useful means to increase clarity and enhance collective understanding of specific methods topics that may be characterized by ambiguity, inconsistency, or a lack of comprehensiveness. This type of review represents a distinct literature synthesis method, although to date, its methodology remains relatively undeveloped despite several aspects that ...
This review aims to synthesize a published set of evaluative criteria for good qualitative research. The aim is to shed light on existing standards for assessing the rigor of qualitative research encompassing a range of epistemological and ontological standpoints. Using a systematic search strategy, published journal articles that deliberate criteria for rigorous research were identified. Then ...
Observation in qualitative research "is one of the oldest and most fundamental research methods approaches. This approach involves collecting data using one's senses, especially looking and listening in a systematic and meaningful way" (McKechnie, 2008, p. 573).Similarly, Adler and Adler (1994) characterized observations as the "fundamental base of all research methods" in the social ...
This paper aims to provide an overview of the use and assessment of qualitative research methods in the health sciences. Qualitative research can be defined as the study of the nature of phenomena and is especially appropriate for answering questions of why something is (not) observed, assessing complex multi-component interventions, and focussing on intervention improvement. The most common ...
Secondly, in the case of how these research methods are employed, our study indicated the following.. Sampling−78.34% of the studies in the collected articles did not specify a sampling method.From the remainder of the studies, 13 types of sampling methods were identified. These sampling methods included broad categorisation of a sample as, for example, a probability or non-probability sample.
The greatest strength of the qualitative research approach lies in the richness and depth of the healthcare exploration and description it makes. In health research, these methods are considered as the most humanistic and person-centered way of discovering and uncovering thoughts and actions of human beings. Table 1.
This paper proposes a research plan to investigate the research methods issues (i.e. research design, sampling methods, data collection methods, data analysis techniques, measurement scales, and ...
The methodology section or methods section tells you how the author (s) went about doing their research. It should let you know a) what method they used to gather data (survey, interviews, experiments, etc.), why they chose this method, and what the limitations are to this method. The methodology section should be detailed enough that another ...
Sage Research Methods & Evaluation is at the forefront of research and scholarship, providing classic and cutting-edge books, video collections, reference materials, cases built on real research, key journals, and our online platform for the community Methodspace.. Download special issues, collections, and a selection of most read articles.Browse our journal portfolio.
Abstract and Figures. Research is an interesting field of study which every student or scholar has to encounter in the course of time. The objective of this paper is to explore the various ...
INTRODUCTION. The use of research methods and statistics in psychology is fundamental to the education of budding psychological researchers and clinicians alike (Jones & Mehr, 2007; Prayoga & Abraham, 2017).However, courses that involve the development of research methods and statistical knowledge and skills present as a considerable challenge for many undergraduate psychology students (Barry ...
Research methods are specific procedures for collecting and analyzing data. Developing your research methods is an integral part of your research design. When planning your methods, there are two key decisions you will make. First, decide how you will collect data. Your methods depend on what type of data you need to answer your research question:
Choosing an optimal research methodology is crucial for the success of any research project. The methodology you select will determine the type of data you collect, how you collect it, and how you analyse it. Understanding the different types of research methods available along with their strengths and weaknesses, is thus imperative to make an ...
Survey research is defined as "the collection of information from a sample of individuals through their responses to questions" (Check & Schutt, 2012, p. 160). This type of research allows for a variety of methods to recruit participants, collect data, and utilize various methods of instrumentation. Survey research can use quantitative research ...
The following multidisciplinary indexes cover popular and scholarly articles in a range of subject areas. Use the option for limiting your search results to scholarly peer-reviewed journals. QuickSearch. Use QuickSearch to find materials available from the Rutgers Libraries, including books, journals, articles, music, movies, and more.
This study aims to explore the effects of AI-integrated educational applications on college students' creativity and academic emotions from the perspectives of both students and teachers. It also assessed undergraduate students' and faculty's attitudes to AI-integrated applications. A mixed-method research design was used.
Even though methodological studies can be conducted on qualitative or mixed methods research, this paper focuses on and draws examples exclusively from quantitative research. ... Authors who would like to make their protocol publicly available without publishing it in scholarly journals, could deposit their study protocols in publicly available ...
A case study is one of the most commonly used methodologies of social research. This article attempts to look into the various dimensions of a case study research strategy, the different epistemological strands which determine the particular case study type and approach adopted in the field, discusses the factors which can enhance the effectiveness of a case study research, and the debate ...
SAGE Research Methods. Book chapter . Research Design Pitfalls. Show details Hide details. Clifford J. Drew and more... Designing and Conducting Research in Education. 2008. SAGE Knowledge. Entry . Quasi-Experimental Research. Show details Hide details. Geneva D. Haertel. Encyclopedia of Curriculum Studies. 2010.
People also read lists articles that other readers of this article have read. Recommended articles lists articles that we recommend and is powered by our AI driven recommendation engine. Cited by lists all citing articles based on Crossref citations. Articles with the Crossref icon will open in a new tab.
We believe that a fuller recognition of the embeddedness of research practices like PR does have some broadly practical implications, which we shall expand upon in the present article. Phenomenology is a branch of philosophy dedicated to the description and analysis of phenomena, that is, the way things, in the broadest sense of the word ...
Some experts believe that students with a higher EI level have a better chance of succeeding in the nursing study program and are more fully prepared for professional practice, which is currently characterized by creating a holistic therapeutic relationship with the patient and their family that takes into account social, emotional, and spiritual needs (Christodoulakis et al., 2023; Pienimaa ...