- Data Center
- Applications
- Open Source
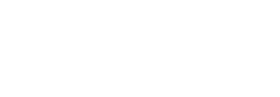

What is Data-Driven Decision-Making? 6 Key Steps (+ Examples)
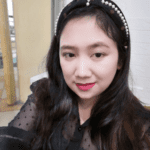
Datamation content and product recommendations are editorially independent. We may make money when you click on links to our partners. Learn More .
Data-driven decision-making is an approach that emphasizes using data and analysis instead of intuition to inform business decisions. It involves leveraging such data sources as customer feedback, market trends, and financial data to guide decision-making processes.
To completely embrace data-driven decision-making, organizations need to establish a culture that promotes critical thinking and curiosity at all levels to make decisions based on evidence and insights derived from comprehensive data analysis. The goal is to encourage all employees to question and investigate information, leading to the discovery of valuable insights that drive action.
When your business fully practices data-driven decision-making, all choices are supported by credible data and the probability of similar events recurring, leading to faster, more accurate, cost-effective decisions and eliminating bias and second-guessing.
Table of Contents
6 Key Steps of Data-Driven Decision-Making
The data-driven decision-making (DDDM) process involves six key steps: defining objectives, identifying and collecting data aligned with those objectives, organizing and exploring data, performing analysis on that data, drawing conclusions about what it says, and implementing and evaluating a plan based on those conclusions. As your organization increasingly relies on data to inform business strategies, mastering these steps can help you make well-informed choices.

1. Define Objectives
Begin by gaining a thorough understanding and articulating your company’s vision and goals. Define specific problems or decisions that require attention using data-driven insights. This sets the stage for a focused and effective approach tailored to your organizational needs.
2. Identify and Collect Data
Survey your business teams to unveil areas and data sources aligned with your objectives. Use appropriate tools to systematically collect necessary data, making sure you are well-equipped for insightful analysis that caters to your needs.
3. Organize and Explore Data
Organize your enterprise data for effective visualization and exploration. Structure it to create a foundation for seamless exploration for a deeper understanding of relevant information tailored to your specific context.
4. Perform Data Analysis
Analyze the data using reporting tools and analytical methods to uncover patterns, trends, and correlations and extract actionable insights for your decision-making. Transform raw data into meaningful ones for informed choices that match your organizational goals.
5. Draw Conclusions
Draw clear conclusions from your data analysis and communicate the implications effectively by creating a narrative around the data for shared understanding within your team. Aim to make data accessible and impactful.
6. Implement and Evaluate
Develop and deploy a plan based on your drawn conclusions. Monitor the impact and effectiveness of this plan on your defined objectives. Through iterative evaluation, refine strategies for continuous improvement.
Data-Driven Decision-Making Examples
While DDDM is widely used across industries and sectors with a wide range of applications, here’s a look at a few real-world examples of how businesses, healthcare institutions, and educational organizations are using data to back their decisions and enhance their operations.
Data-Driven Decision-Making In Business
Different types of businesses use data for targeted marketing, inventory management, personalized recommendations, and preventing customer churn:
- Amazon: Uses data to segment customers based on location, demographics, and buying behavior to build targeted marketing campaigns.
- Walmart: Uses historical data and predictive analytics to strategically place holiday items across stores, optimizing the entire shopping experience.
- Netflix: Uses data for customized suggestions, minimizing customer churn and boosting retention rates.
Data-Driven Decision-Making In Education
Educational institutions use data for analysis to gauge performance, catch warning signs of failing students, and develop curriculum:
- Purdue University: Uses a predictive analytics tool called Course Signals to monitor performance and predict students at risk of not successfully completing a course.
- Indiana University: Uses data analytics to make sure course offerings match student demand.
Data-Driven Decision-Making In Healthcare
Healthcare organizations use data to refine patient care, prevent diseases, and conduct research:
- Cleveland Clinic: Leverages data to examine the impact of factors outside of the health system on a patient’s health. It also uses analytics to identify patients that would recover successfully at home following surgery.
- Centers for Disease Control and Prevention (CDC): Uses data to build informed decisions and establish systems for emergency operations and response.
- The Broad Institute: Uses big data analytics to advance drug discovery .
5 Benefits of Data-Driven Decision-Making
Businesses can transform their operations and position themselves for long-term success by adopting a data-driven decision-making model, improving the experience for their customers and cutting-costs in the process.
Improved Customer Experience
Data-driven enterprises are more customer-focused and gain a deeper understanding of customer journeys. DDDM elevates customer experience by giving insights into customer behavior and needs. It helps you tailor your business offerings, improve services, and address customer issues effectively. This leads to personalized experiences, stronger customer relationships, and higher revenue.
Better Strategic Planning
By using data to inform our choices, we can set achievable goals and stay ahead of the competition. DDDM promotes collaboration by creating a common understanding across departments, supporting communication, and encouraging a shared commitment to achieving organizational goals.
Growth Opportunities
DDDM enables you to identify new business opportunities and areas for improvement. It helps uncover trends and patterns for spotting new market opportunities. It also lets your business swiftly uncover bottlenecks and areas you need to work on.
Increased Operational Efficiency and Optimized Costs
Relying on data can uncover productivity bottlenecks and optimize resource use, resulting in better business efficiency. Furthermore, it facilitates accurate demand forecasting, leading to significant cost savings.
More Accurate Forecasting
Since data-driven decisions allow for more accurate forecasts and predictions about the future, it brings about more efficient operations and cost reductions. For instance, AI-driven forecasting in supply chain management can reduce errors, translating into a decrease in lost sales.
5 Pro Tips For Making Data-Driven Decisions
Harnessing the power of data can lead to more informed decisions, driving enterprise success. Here are some pro tips to help you make effective, data-driven decisions, emphasizing the importance of clarity, accuracy, culture, context, and continuous improvement:
- Define Clear Objectives: Clearly define your business objectives before diving into data analysis. Understanding what you want to achieve helps in finding the relevant data to analyze and guarantees that your decisions are aligned with strategic goals.
- Prioritize Accuracy and Reliability: Inaccurate or unreliable data can lead to flawed insights and misguided decisions, so make sure that your data sources are accurate and updated. In addition, conduct regular data validation and cleansing to ensure data quality.
- Combine Data with Context: While data provides valuable insights, it’s crucial to combine it with a contextual understanding of your business environment to gain a holistic approach to decision-making. Consider industry trends, market dynamics, and qualitative factors.
- Promote a Data-Driven Culture: Foster a culture within your organization that encourages and values data-driven decision-making. This means offering training and resources for employees to enrich their data literacy, making data accessible, and promoting the use of data in discussions and decision processes.
- Iterate and Learn from Insights: Think of data-driven decision-making as a learning journey. Keep reviewing and adjusting your strategies based on what you learn and changes in the situation. Don’t just celebrate successes, but also learn from failures to keep improving your approach.
Common Challenges and Misconceptions about Data-Driven Decision-Making
Businesses in many industries turn to data-driven decision-making for precision and efficiency. However, the journey to harnessing data’s full potential isn’t without its challenges and misconceptions. Navigating these hurdles calls for a keen understanding of the common pitfalls associated with data-driven approaches.

Neglecting Data Quality
When it comes to making decisions based on data, one of the challenges is checking the data’s quality. Poor data quality can lead to inaccurate and unreliable insights, resulting in flawed decision-making. Good data management practices involve ensuring the quality of the data
A common misconception related to this is thinking that more data is always better, but the real trick is having high-quality, complete, and accurate data to make decisions you can trust. Having too much data— especially if it’s irrelevant or not properly analyzed—can overwhelm decision-makers and lead to information paralysis.
Scattered Data
Disorganized data scattered across different departments are like pieces of a puzzle that just won’t fit together. It makes collaboration difficult and decisions end up being all over the place. Some might think that advanced analytics tools can fix this, but in reality, getting everyone on the same page and smoothing out the processes are just as indispensable for making it work.
Data Illiteracy
DDDM isn’t just for data specialists—everyone from C-Suite to line staff should have a fundamental understanding of data and data management basics. Data illiteracy can lead to ineffective communication between data professionals and non-technical stakeholders. It can also hinder the successful implementation of a data-driven culture.
Understanding data and different data management types is necessary in addressing data illiteracy and fostering effective communication across teams, contributing to successful integration of a data-driven culture.
Historical Data Overreliance
Relying too much on the past can also be a stumbling block. It’s like assuming last year’s fashion trends will always be in style—markets change, trends evolve, and decisions based solely on historical data might miss what’s currently happening.
Confirmation Bias
Confirmation bias is another significant challenge in data-driven decision-making as it involves favoring information that aligns with pre-existing beliefs or hypotheses while dismissing or downplaying data that contradicts them. Decision-makers may selectively focus on evidence that confirms their expectations, leading to skewed interpretations and reinforcing existing biases.
Poor Communication of Data Insights
Even with accurate data, there can be a breakdown in communication between data analysts and decision-makers, leading to misunderstandings. It’s vital for those involved in data management to communicate findings in a clear, understandable manner, highlighting the relevance of the insights to business objectives.
Frequently Asked Questions (FAQs)
What companies are data driven.
Today, numerous global corporations rely on data for decision-making:
- Google turns to data to drive internal performance by pinpointing the qualities of the most effective managers. This enabled the company to support managers, increase their engagement and performance, and encourage them to stay working for Google.
- Tesla uses big data to drive decision-making, improve vehicle performance, and enhance the overall customer experience.
- Uber uses data, matching algorithms, and prediction models to directly estimate the driving time and allocates the optimal driver through a process.
- Starbucks uses data analytics to know their customers’ preferences and gather details about their purchasing habits.
- Coca-Cola uses data for customer retention and marketing.
What Tools are Used for Data-Driven Decision-Making?
There are many tools and software solutions specifically designed to support DDDM in various industries. These tools let organizations collect, analyze, and visualize data:
- Business intelligence (BI) Tools for data visualization and interactive reporting allow you to create dashboards and analyze data trends.
- Data analytics platforms deliver advanced analytical capabilities, including predictive analytics and machine learning.
- Data warehousing solutions consolidate and store large volumes of structured and unstructured data for smooth data retrieval and analysis.
- Big data platforms handle and process massive volumes of structured and unstructured data for enterprises dealing with large datasets and complex data analytics tasks.
- Machine learning solutions facilitate building and deploying machine learning models that are necessary for predictive analytics and automating decision-making processes.
- Data governance and quality tools ensure data quality, security, and compliance, so your organization can establish and enforce data management policies.
Bottom Line: Data-Driven Decision-Making Is Important
Data-driven decision-making can empower your organization to make informed, objective, and evidence-based choices. It eliminates biases, promoting fair and balanced decisions supported by credible data. By following the steps to data-driven decision-making, your business can establish a systematic and structured process that instills trust in stakeholders and leads to positive outcomes.
Additionally, keep in mind that data management is essential to the success of DDDM, as it guarantees the quality and reliability of your enterprise data. The value of DDDM lies in its ability to transform your decision-making processes, making sure that your business choices are grounded in evidence, analyzed comprehensively, and aligned with organizational goals. If you’re not using data to drive your decisions, you’re missing out on a valuable opportunity to improve your business.
Data management practices are designed to support the effective use of data in decision-making, ensuring that decisions contribute to the achievement of strategic objectives. Read our 10 Best Practices for Effective Data Management article to fully leverage your enterprise data as a strategic asset.
Subscribe to Data Insider
Learn the latest news and best practices about data science, big data analytics, artificial intelligence, data security, and more.
Similar articles
8 best data analytics tools: gain data-driven advantage in 2024, common data visualization examples: transform numbers into narratives, what is data management a guide to systems, processes, and tools, get the free newsletter.
Subscribe to Data Insider for top news, trends & analysis
Latest Articles
Exploring multi-tenant architecture: a..., 8 best data analytics..., common data visualization examples:..., what is data management....
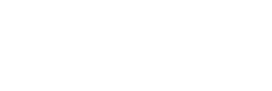
Published: 23 July 2024 Contributor: Tim Mucci
Data-driven decision-making (DDDM) is an approach that emphasizes using data and analysis instead of intuition to inform business decisions. It involves leveraging data sources such as customer feedback, market trends and financial data to guide the decision-making process. By collecting, analyzing and interpreting data, organizations can make better decisions that more closely align with business goals and objectives.
Humanity generates over 402.74 million terabytes of data daily 1 (link resides outside ibm.com). This abundance of data, if collected and processed, enables businesses to make effective decisions that serve business goals and provide good customer experiences. Data-driven decision-making allows businesses to generate real-time insights and predictions, optimize performance and test new strategies. Such informed decisions lead to sustainable growth and profitability, whereas relying on gut feelings can result in the opposite. Data provides a solid foundation for making decisions, reducing uncertainty and increasing confidence.
Discover how to harness the power of analytics and business intelligence to enhance business planning and forecasting.
Organizations that adopt a data-driven culture see benefits such as improved customer satisfaction, better strategic planning and more.
A global online retailer uses customer data extensively to build targeted marketing campaigns and enhance its recommendation engine. Analyzing this data allows the organization to create personalized shopping experiences and highly targeted marketing campaigns.
In addition to personalizing product recommendations, the organization uses customer data for dynamic pricing strategies. By monitoring competitor prices, market trends and customer demand in real time, the organization adjusts its prices to remain competitive and optimize revenue.
A popular online streaming service uses data to personalize recommendations and reduce customer churn. The platform uses vast amounts of customer data, including viewing history, ratings and even the amount of time spent watching specific content, to tailor its recommendations. This personalization is driven by sophisticated algorithms that analyze user behavior to suggest content that aligns with individual preferences.
The organization employs several strategies to further retain customers and reduce churn. One of the most effective methods is its recommendation algorithm, which continually presents users with content that matches their tastes. This algorithm both recommends what to watch next and also customizes the visual presentation of titles to appeal specifically to different viewers. By keeping users engaged with content they are likely to enjoy, they minimize the risk of subscribers canceling their service.
Predictive analytics allow businesses to anticipate trends or challenges and take preemptive actions. Financial institutions use advanced machine learning (ML) algorithms to detect and prevent fraud. A proactive approach to fighting fraud protects customers from financial loss and enhances trust in the organization.
Utility companies employ machine learning and data analytics to predict energy consumption patterns accurately. Analyzing large volumes of real-time data involves developing predictive analytics that consider factors such as the time of day, the day of the week and historical energy loads. Organizations can use the same process in manufacturing and supply chain for real-time, on-demand forecasting.
Data insight helps formulate realistic strategic plans. A global coffee brand optimizes its site selection strategy by using geographic information system (GIS) technology. This technology allows it to analyze local demographics, traffic patterns and other relevant data—this precision site selection strategy results in improved performance and higher sales for new stores.
E-commerce retailers who analyze and understand market dynamics and customer preferences can identify untapped customer segments and develop innovative products and services to identify new markets, customer segments and product opportunities.
The iterative data-driven decision-making approach enables businesses to refine their strategies and remain competitive in a rapidly changing environment. A major streaming video service uses data analytics to inform aspects of its content creation and market expansion process.
A multinational retailer uses data to manage its inventory, particularly in preparation for natural disasters. By analyzing historical sales data, the organization discovered that certain products had a significant sales spike before hurricanes. This insight, derived from mining trillions of bytes of sales data, allowed leaders to stock these items heavily in anticipation of storms, to meet higher customer demand.
Additionally, the retailer employs predictive analytics to forecast demand for various products based on historical data, weather patterns and other external factors. So, the company can dynamically adjust its inventory levels to make sure essential items are available when customers need them. Their use of big data and analytics extends to optimizing supply chain operations, where real-time data helps the business manage inventory levels across its stores and distribution centers efficiently.
Data-driven decisions minimize personal bias and safeguard objectivity. A US-based energy company implemented various debiasing techniques as part of its decision-making process. The organization established programs to raise awareness of cognitive biases among its leadership and employees. These strategies protect different perspectives and certify that decisions are not influenced by hierarchical or confirmation biases.
Every organization can benefit from taking six steps to integrate data-based decisions throughout the company. By adopting these best practices, strategies derived from data analysis can be implemented and their impact measured.
- Define objectives: This step involves clearly articulating the organization's goals. After objectives are defined, the company can make a focused and purposeful effort to meet them.
- Identify, prepare and collect data: In this step, the organization sets clear objectives, determines data needs, evaluates and prepares data sources and then systematically gathers and validates the data.
- Organize and explore: Here, the data is structured to uncover new patterns, trends and valuable insights. Cleaning data protects its accuracy and reliability. Visualizing the data helps identify patterns, outliers and trends that are not immediately obvious from raw data.
- Perform data analysis: This step transforms raw data into actionable insights by using various techniques and methodologies to uncover patterns, correlations and trends that inform business strategy. By performing data analysis, organizations can drive strategic decisions and improve overall performance.
- Draw conclusions: Here, key data analysis findings are reviewed and placed in the correct business context to form actionable insights and recommendations. Practical conclusions lead to meaningful actions that drive business success.
- Implement and evaluate: This final step is crucial for validating insights and measuring outcomes. Action plans are developed based on data-driven recommendations, resources are allocated and the initiative's progress is continuously monitored. Outcomes are measured against predefined key performance indicators (KPIs), results are analyzed, feedback is gathered and based on that information, adjustments are made in a continuous cycle of monitoring and iterative improvement. This step provides a basis for making informed adjustments to strategies and plans based on empirical evidence and feedback.
When implementing data-driven decision-making, several challenges arise that organizations need to navigate effectively. One significant issue is neglecting data quality. Poor-quality data can lead to inaccurate analyses and misguided decisions, undermining the value of data-driven strategies.
In many organizations, data resides in disparate systems and formats across departments, making it difficult to consolidate and analyze it comprehensively. Implementing data integration solutions is essential to overcoming this hurdle and enabling holistic decision-making.
Data illiteracy is often another critical challenge. Employees may lack the necessary skills to interpret and use data effectively, leading to misinterpretations and sub optimal decisions. Providing ongoing training and fostering a data-literate culture is vital for maximizing the benefits of data-driven approaches.
Overreliance on historical data can also be problematic. While past data is valuable, it might not accurately predict future trends, especially in rapidly changing environments. Balancing historical data with real-time analytics and forward-looking indicators is essential for relevant and timely decision-making.
Confirmation bias can skew data analysis. Decision-makers might selectively interpret data to support preconceived notions, leading to biased outcomes. Encouraging objective analysis and critical thinking can help mitigate this bias. Also, poor communication of insights is a similar challenge; even the most accurate data can be ineffective if not communicated clearly and compellingly to stakeholders.
Neglecting data security poses risks. Protecting data from breaches and certifying compliance with data privacy regulations is paramount to maintain trust and avoid legal repercussions.
Businesses can benefit from understanding the types of business analytics that can inform their decision-making processes.
Descriptive analysis aims to describe and summarize historical data through data aggregation and mining, providing insights into past performance. This type of analysis is used to create monthly sales reports, customer satisfaction surveys and website traffic analyses.
Diagnostic analysis focuses on determining why certain events occurred. It involves data discovery, mining and identifying correlations to uncover the root causes of trends or incidents, such as a drop in sales or a surge in customer complaints.
Predictive analysis forecasts future trends or outcomes based on historical data. Businesses can more effectively predict sales and customer behavior and manage risks by using statistical models, machine learning and forecasting techniques.
Prescriptive analysis goes a step further by recommending actions based on data. This type combines predictive analytics with optimization algorithms to suggest the best course of action, aiding in supply chain optimization, marketing campaign strategies and resource allocation decisions.
Exploratory analysis is used to discover patterns, relationships or anomalies in data without specific hypotheses. Data visualization , clustering and dimensionality reduction techniques help identify new market segments, explore customer demographics and uncover unexpected correlations.
Inferential analysis uses a data sample to make inferences about a population. It employs statistical tests such as regression analysis, hypothesis testing and confidence intervals and is useful in market research studies, product testing and consumer preference surveys.
Qualitative analysis focuses on non-numeric data to understand concepts, opinions or experiences. Methods such as content analysis, thematic analysis and text mining are employed to analyze customer feedback and social media sentiment and conduct market research interviews.
Quantitative analysis analyzes numeric data to quantify variables and uncover patterns using statistical analysis, mathematical modeling and computational techniques. This type of analysis is essential for financial modeling, operational metrics analysis and performance measurement.
Real-time analysis involves analyzing data as it is generated, providing immediate insights. Using streaming analytics, real-time dashboards and event processing, businesses can detect fraud, manage inventory in real-time and monitor live customer support.
For organizations looking to invest in data-driven decision-making tools, several advanced technologies and platforms are available to help collect, analyze and use data effectively.
Business intelligence tools
BI tools provide data visualization capabilities that allow users to create interactive and shareable dashboards. They connect to multiple data sources and are widely used for data analysis.
Data warehousing solutions
Cloud-based data warehouses offer scalability and flexibility and support various data integration and analytics tools.
Machine learning and AI platforms
These platforms provide a comprehensive suite of machine learning tools and services, such as AutoML, which allows users to build customized ML models without extensive coding experience. These platforms often offer cloud-based training environments and integrate with various analytics services.
Data integration and ETL (extract, transform, load) tools
These tools help organizations manage and transform data from various sources into a unified format. They often support data governance and cloud data management for complex integration needs.
Data analytics and visualization
These tools offer data visualizations and support associative data indexing so users can uncover hidden insights. They can also connect directly to databases to allow for real-time data analysis and reporting.
Big data processing frameworks
There are many open-source big data processing frameworks. Organizations that need to use large amounts of data need to consider a platform that supports batch and stream processing for speed and the ability to handle large-scale analytics.
Data governance tools
Governance platforms help organizations manage data quality, lineage and compliance. They provide tools for data stewardship and metadata management.
In a data-driven organization, several key data science roles are essential for using data effectively and creating a data-driven culture. In addition to data analysts, data managers and data scientists, there are other important roles that guide an organization toward success in its data-driven decision-making initiatives.
Data engineers design, build and maintain the infrastructure and systems required for data collection, storage and processing. Data engineers safeguard data pipelines so they are efficient, scalable and reliable, enabling smooth data flow from various sources to analytical platforms.
Data architects are responsible for designing and implementing an organization's overall data architecture. They create blueprints for data management systems, so data is organized, integrated and accessible.
Business intelligence (BI) developers create and manage BI solutions, such as dashboards and reporting systems. They transform raw data into meaningful insights through visualization tools, to help stakeholders make informed decisions.
Machine learning engineers are specialists who build, deploy and maintain machine learning models. They work closely with data scientists to implement algorithms that can predict outcomes and automate decision-making processes.
Chief data officer (CDO) is an executive role that oversees an organization's data strategy and governance. They ensure that data initiatives align with business objectives, compliance standards and best practices.
Chief artificial intelligence officer ( CAIO ) is an executive role that guides the organization through the complexities of AI adoption. They provide strategic leadership and oversee the development, strategy and implementation of AI technologies.
Data analysts are professionals who analyze and interpret complex datasets to provide actionable insights. They use statistical methods and tools to identify trends, patterns and correlations.
Database administrators (DBAs) manage and maintain database systems. They protect data and confirm it is stored securely, backed up regularly and retrievable efficiently. DBAs also optimize database performance and resolve any data-related issues.
Data privacy officers are responsible for ensuring that data handling practices comply with privacy laws and regulations. They develop policies and practices to protect sensitive information and maintain customer trust.
AI/ML operations ( MLOps ) engineers manage the deployment, monitoring and maintenance of machine learning models in production environments. MLOps engineers guarantee that models operate efficiently and are updated as needed.
Learn how IBM® Cognos® Analytics uses AI automation to enhance data visualization, prediction and reporting. The platform helps users uncover hidden trends, create interactive dashboards and integrate advanced analytics into daily operations.
See how IBM Planning Analytics uses AI forecasting and real-time data analysis to enhance business planning and decision-making. Planning Analytics offers tools for scenario planning, data integration and collaboration across departments, making it suitable for finance, supply chain, HR and IT management.
Explore how IBM watsonx™ BI Assistant provides AI-powered business analysis and advice, offering clear explanations for confident decision-making. Watsonx BI Assistant enables users to uncover trends, predict outcomes and take action based on personalized metrics and intelligent alerts.
Integrating AI-powered tools helps improve financial targets, operational capacity and sales goals, while eliminating data silos and empowering users to make informed decisions.
Learn how IBM Consulting® works with ESPN to enhance the fantasy football experience using AI-insights and data analysis.
Planning, budgeting and forecasting solutions integrate AI and data analytics to enhance financial planning processes.
IBM Cognos Analytics is a self-service business intelligence and data visualization tool. The platform integrates cognitive computing technology, including artificial intelligence and machine learning, so organizations can visualize data, share new insights and encourage data-driven decision-making.
1 https://explodingtopics.com/blog/data-generated-per-day#how-much (link resides outside ibm.com)
- Product overview
- All features
- Latest feature release
- App integrations
CAPABILITIES
- project icon Project management
- Project views
- Custom fields
- Status updates
- goal icon Goals and reporting
- Reporting dashboards
- asana-intelligence icon Asana AI
- workflow icon Workflows and automation
- portfolio icon Resource management
- Capacity planning
- Time tracking
- my-task icon Admin and security
- Admin console
- Permissions
- list icon Personal
- premium icon Starter
- briefcase icon Advanced
- Goal management
- Organizational planning
- Project intake
- Resource planning
- Product launches
- View all uses arrow-right icon
Featured Reads
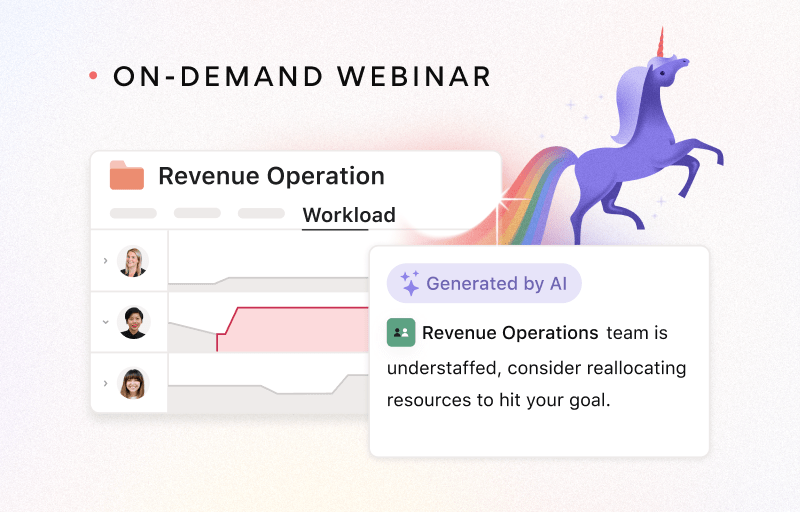
- Work management resources Discover best practices, watch webinars, get insights
- Customer stories See how the world's best organizations drive work innovation with Asana
- Help Center Get lots of tips, tricks, and advice to get the most from Asana
- Asana Academy Sign up for interactive courses and webinars to learn Asana
- Developers Learn more about building apps on the Asana platform
- Community programs Connect with and learn from Asana customers around the world
- Events Find out about upcoming events near you
- Partners Learn more about our partner programs
- Asana for nonprofits Get more information on our nonprofit discount program, and apply.
- Project plans
- Team goals & objectives
- Team continuity
- Meeting agenda
- View all templates arrow-right icon
- Project management |
- Data-driven decision making: A step-by- ...
Data-driven decision making: A step-by-step guide
Data-driven decision making is the process of collecting data based on your company’s key performance indicators (KPIs) and transforming that data into actionable insights. This process is a crucial element of modern business strategy. In this article, we’ll discuss the benefits of data-driven decision making and provide tips so you can make informed decisions at work.
If there’s a looming decision ahead of you at work, it’s often hard to know which direction to go. If you go with your gut feeling, you may feel more confident in your choices, but will those choices be right for your team members? When you use facts to make decisions, you can feel more at ease knowing your choices are based on data and meant to maximize business impact.
Whether outshining competitors or increasing profitability, data-driven decision making is a crucial part of business strategy in the modern world. Below, we dive into the benefits of data-driven decision making and provide tips for making these decisions at work.
Decision-making tools for agile businesses
In this ebook, learn how to equip employees to make better decisions—so your business can pivot, adapt, and tackle challenges more effectively than your competition.
What is data-driven decision making (DDDM)?
You can use business intelligence (BI) reporting tools during this process, which make big data collection fast and fruitful. These tools simplify data visualization, making data analytics accessible to those without advanced technical know-how.
What does being data-driven mean?
In short, the concept of being data-driven refers to using facts, or data, to find patterns, inferences, and insights to inform your decision making process.
Essentially, being data-driven means that you try to make decisions without bias or emotion. As a result, you can ensure that your company’s goals and roadmap are based on evidence and the patterns you’ve extracted from it, rather than what you like or dislike.
Why is data-driven decision making important?
Data-driven decision making is important because it helps you make decisions based on facts instead of biases. If you’re in a leadership position, making objective decisions is the best way to remain fair and balanced.
The most informed decisions stem from data that measure your business goals and populates in real time. You can aggregate the data you need to see patterns and make predictions with reporting software .
Some decisions you can make with support from data include:
How to drive profits and sales
How to establish good management behavior
How to optimize operations
How to improve team performance
While not every decision will have data to back it up, many of the most important decisions will.
5 steps for making data-driven decisions
Making data-driven decisions takes practice. If you want to improve your leadership skills , then you’ll need to know how to turn raw data into actionable steps that work toward your company initiatives. The following steps can help you make better decisions when analyzing data.
1. Know your vision
Before you can make informed decisions, you need to understand your company’s vision for the future . This helps you use both data and strategy to form your decisions. Graphs and figures have little meaning without context to support them.
Tip: Use your company’s yearly objectives and key results ( OKRs ) or quarterly team KPIs to make data-backed decisions.
2. Find data sources
Once you’ve identified the goal you’re working towards, you can start collecting data.
The tools and data sources you use will depend on the type of data you’re collecting. If your goal is to analyze data sets pertaining to internal company processes, use a universal reporting tool. Reporting tools offer a single point of reference for keeping track of how work across your organization is progressing. Some reporting tools like Microsoft’s Power BI let you gather data from various external sources. If you want to analyze marketing trends or competitor metrics, you can use one of those tools.
Some general success metrics you may want to measure include:
Gross profit margin: Gross profit margin is measured by subtracting the cost of goods sold from the company's net sales.
Return on investment (ROI): The ratio between the income and investment, ROI is commonly used to decide whether or not an initiative is worth investing time or money in. When used as a business metric, it often tracks how well an investment is performing.
Productivity: This is the measurement of how efficiently your company is producing goods or services. You can calculate this by dividing the total output by the total input.
Total number of customers: This is a simple but effective metric to track. The more paid customers, the more money earned for the business.
Recurring revenue : Commonly used by SaaS companies, this is the amount of revenue generated by all of your current active subscribers during a specific period. It's commonly measured either monthly or annually.
You can measure a variety of other data sets based on your job role and the vision you’re working toward. Machine learning makes aggregating real time data simpler than ever before.
Tip: Try to create a connected story through these metrics. If revenue is down, look at productivity and see if you can draw a connection. Keep digging through these metrics until you find a “why” for whatever problem you’re trying to solve.
3. Organize your data
Organizing your data to improve data visualization is crucial for making effective business decisions. If you can’t see all your relevant data in one place and understand how it connects, then it’s difficult to ensure you’re making the most informed decisions.
Tip: One way to organize your data is with an executive dashboard . An executive dashboard is a customizable interface that usually comes as a feature of your universal reporting tool. This dashboard will display the data that’s most critical to achieving your goals, whether those goals are strategic, tactical, analytical, or operational.
4. Perform data analysis
Once you’ve organized your data, you can begin your data-driven analysis. This is when you’ll extract actionable insights from your data that will help you in the decision-making process .
Depending on your goals, you may want to analyze the data from your executive dashboard in tandem with user research such as case studies, surveys, or testimonials so your conclusions include the customer experience.
Does your team want to improve their SEO tools to make it more competitive with other options on the market? The data sets you can use to determine necessary improvements may include:
Competitors’ performance data
Current SEO software performance data
Current customer satisfaction data
User research on a variety of SEO/marketing tools
While some of this information will come from your organization, you may need to obtain some of it from external sources. Analyzing these data sets as a whole can be helpful because you’ll draw a different conclusion than you would if you were to analyze each data set individually.
Tip: Share your analytics tools with your whole team or organization. Just like any collaborative effort, data analysis is most effective when viewed from many perspectives. While you may notice one pattern in the data, it’s entirely possible a teammate may see something completely different.
5. Draw conclusions
As you perform your data analysis, you’ll likely begin to draw conclusions about what you see. However, your conclusions deserve their own section because it’s important to flesh out what you see in the data so you can share your findings with others.
The main questions to ask yourself when drawing conclusions include:
What am I seeing that I already knew about this data?
What new information did I learn from this data?
How can I use the information I’ve gained to meet my business goals?
Once you can answer these questions, you’ve successfully performed data analysis and should be ready to make data-driven decisions for your business.
Tip: A natural next step after data analysis is writing down some SMART goals . Now that you’ve dug into the facts, you can establish achievable goals based on what you’ve learned.
Tools and technologies for data-driven decision making
Effective data-driven decision making (DDDM) in the modern business landscape requires leveraging the right tools and technologies. Organizations can use these tools to collect, analyze, and interpret large amounts of data. This allows them to transform raw information into actionable insights that drive their business strategy.
Business intelligence software
Business intelligence (BI) software plays a pivotal role in data-driven decision-making processes. These powerful platforms aggregate data from various data sources, providing decision-makers with comprehensive dashboards and reports. Popular BI tools like Tableau, Power BI, and Looker offer robust data visualization capabilities, allowing users to create interactive charts, graphs, and maps that make complex datasets more understandable.
By using BI software, organizations can:
Monitor key performance indicators (KPIs) in real-time
Identify trends and patterns in business data
Generate automated reports for stakeholders
Enhance collaboration among teams through shared insights
Data analytics tools
While BI software focuses on reporting and visualization, data analytics tools dive deeper into the data to uncover hidden patterns and correlations. These tools employ sophisticated statistical methods and algorithms to analyze both structured and unstructured data.
Popular data analytics tools include:
R and Python for statistical analysis and modeling
SAS for advanced analytics and machine learning
Apache Spark for processing large-scale data
Excel for basic data analysis and manipulation
These tools enable data analysts and data scientists to perform various types of analysis, such as:
Descriptive analytics to understand what happened
diagnostic analytics to determine why it happened
predictive analytics to forecast future trends
Prescriptive analytics to recommend actions
Machine learning and AI in DDDM
Data-driven decision making has taken a significant leap forward in analytical capabilities with the integration of machine learning and artificial intelligence (AI). These technologies process vast amounts of data at incredible speeds, identifying patterns and insights that might be impossible for humans to discern.
Key applications of machine learning and AI in DDDM include:
Predictive modeling for forecasting future outcomes
Sentiment analysis for understanding customer opinions
Recommendation engines for personalized marketing
Anomaly detection for identifying fraud or errors
Natural language processing for analyzing text data
Companies like Amazon use ML algorithms to optimize their supply chain, predict customer behavior, and personalize product recommendations, demonstrating the power of these technologies in driving business decisions.
Measuring the impact of data-driven decision making
To truly understand the benefits of data-driven decision-making, organizations must establish robust methods for measuring its impact on business performance.
Key Performance Indicators (KPIs) for DDDM
KPIs are essential metrics that help organizations track the effectiveness of their data-driven approach. Choosing KPIs for DDDM requires careful consideration of indicators that align with business goals and offer valuable insights into the decision-making process.
Some important KPIs for measuring the impact of DDDM include:
Revenue growth: This KPI measures the impact of data-driven decisions on the company's bottom line. It quantifies financial gains from DDDM initiatives, such as data-driven marketing campaigns and data-informed pricing strategies.
Operational efficiency: This KPI assesses process improvements resulting from data-driven insights. It may include metrics like reduced cycle times or increased output per employee, such as tracking production downtime reductions through predictive maintenance.
Customer satisfaction: This KPI measures how data-driven strategies affect customer experience and loyalty. Metrics can include NPS, retention rates, or customer lifetime value. It tracks the impact of using customer data for product development and personalized experiences.
Decision quality and speed: This KPI focuses on enhancing the decision-making process. It measures improvements in decision speed and quality by comparing the outcomes of choices made using data analytics versus intuition and assessing time-to-decision reductions enabled by real-time data.
By consistently tracking these KPIs, organizations can quantify the valuable insights gained from their data-driven decision-making process and demonstrate the tangible impact on their bottom line.
Data-driven decision making examples
While the data analysis itself happens behind the scenes, the way data-driven decisions affect the consumer is very apparent. Some examples of data-driven decision making in different industries include:
E-commerce
Have you ever been shopping online and wondered why you’re getting certain recommendations? Well, it’s probably because you bought something similar in the past or clicked on a certain product.
Online marketplaces like Amazon track customer journeys and use metrics like click-through rate and bounce rate to identify what items you’re engaging with most. Using this data, retailers are able to show you what you might want without you having to search for it.
In the medical field, data-driven decision making is revolutionizing patient care and treatment strategies. Hospitals and clinics utilize electronic health records (EHRs) to analyze patterns in patient data, helping doctors make more informed diagnosis and treatment plans. For instance, by examining historical data on symptoms, treatments, and outcomes, healthcare providers can predict which patients are at higher risk for certain conditions.
Additionally, pharmaceutical companies leverage big data to streamline drug discovery processes. By analyzing vast amounts of genetic and clinical trial data, researchers can identify promising drug candidates more quickly and efficiently.
Financial institutions use data in a multitude of different ways, ranging from assessing risk to customer segmentation. Risk is especially prevalent in the financial sector, so it’s important that companies are able to determine the risk factor before making any significant decisions. Historical data is the best way to understand potential risks, threats, and the likelihood they occur.
Financial institutions also use customer data to determine their target market. By grouping consumers based on socioeconomic status, spending habits, and more, financial companies can infer what consumers have the greatest lifetime value and target them.
Transportation
Data science additionally plays a huge role in determining safe transportation. The U.S. Department of Transportation’s Safety Data Initiative underscores the role that data plays in improving transportation safety.
The report pulls data from all types of motor crashes and evaluates factors like weather and road conditions to discover the source of problems. Using the hard facts, the department can work toward implementing more safety measures.
Benefits of data-driven decision making
Analytics-based decision making is more than just a helpful skill—it’s a crucial one if you want to lead by example and foster a data-driven culture.
When you use data to make decisions, you can ensure your business remains fair, goal-oriented, and focused on improvement.
Make confident decisions
The businesses that outlast their competitors do so because they’re confident in their ability to succeed. If the decision-makers within a business waiver in their choices, it can lead to mistakes, high team member turnover, and poor risk management .
When you use data to make the most important business decisions, you’ll feel confident in those decisions, which will push you and your team forward. Confidence can lead to higher team morale and better performance.
Guard against biases
Using data to make decisions will guard against any biases among business leaders. While you may not be aware of your biases, having internal favoritism or values can affect the way you make decisions.
Making decisions directly based on the facts and numbers keeps your decisions objective and fair. It also means you have something to back up your decisions when team members or stakeholders ask why you chose to do what you did.
Find unresolved questions
Without using data, there are many questions that go unanswered. There may also be questions you didn’t know you had until your data sets revealed them. Any amount of data can benefit your team by providing better visualization into areas you can’t see without statistics, graphs, and charts.
When you bring those questions to the surface, you can feel confident knowing your decisions were made by considering every bit of relevant information.
Set measurable goals
Using data is one of the simplest ways to set measurable goals for your team and successfully meet those goals. By looking at internal data on past performance, you can determine what you need to improve and get as granular as possible with your targets. For example, your team may use data to identify the following goals:
Increase number of customers by 20% year over year
Reduce overall budget spend by $20,000 each quarter
Reduce project budget spend by $500
Increase hiring by 10 team members each quarter
Reduce cost per hire by $500
Without data, it would be difficult for your company to see where they’re spending their money and where they’d like to cut costs. Setting measurable goals ultimately leads to data-driven decisions because once these goals are set, you’ll determine how to reduce the overall budget or increase the number of customers.
Improve company processes
There are ways to improve company processes without using data, but when you observe trends in team member performance using numbers or analyzing company spending patterns with graphs, the process improvements you make will be based on more than observation alone.
Processes you can improve with data may include:
Risk management based on financial data
Cost estimation based on market pricing data
Team member onboarding based on new hire performance data
Customer service based on customer feedback data
Changing a company process can be difficult if you aren’t sure about the result, but you can be confident in your decisions when the facts are in front of you.
Challenges in implementing data-driven decision making
While the benefits of DDDM are clear, organizations often face several challenges when implementing this approach. Understanding and addressing these challenges is crucial for the successful adoption of a data-driven culture.
Data quality and accuracy
The foundation of effective data-driven decision making lies in the quality and accuracy of the data used. Poor data quality can lead to flawed analysis and, consequently, misguided decisions.
On the other hand, good data management ensures accurate and complete information for quantitative analysis. This involves standardized collection, regular audits, and addressing data gaps. With reliable data, organizations can make informed decisions and avoid costly mistakes.
Ensuring data security and privacy
Data security and privacy are paramount concerns as organizations collect and analyze increasing amounts of data. Compliance with regulations like GDPR, CCPA, and HIPAA is critical. Companies must implement security measures, including data encryption, strong access controls, and regular system updates. Safeguarding customer rights and maintaining transparency in data usage are also necessary.
Overcoming resistance to change
Resistance to change often emerges when implementing data-driven decision making. This cultural shift requires effective change management strategies. Clear communication of benefits, the involvement of key stakeholders, and addressing concerns openly can help overcome resistance. Additionally, equipping employees with necessary skills through training and mentorship programs is vital for fostering a data-driven culture.
Scaling data management
Managing large data sets presents both opportunities and challenges. Big data requires storage solutions such as cloud-based systems, data lakes, or hybrid models. Efficient processing of these large datasets is key to timely decision-making. Techniques like parallel processing, in-memory computing, and stream processing can help organizations handle vast amounts of data effectively.
By addressing these challenges head-on, organizations can create a foundation for data-driven decision making, enabling them to harness the full power of their data and drive business success.
Tips for becoming more data-driven
Data-driven organizations are able to parse through the numbers and charts and find the meaning behind them. Creating a more data-driven culture starts with simply using data more often. However, this is easier said than done. If you’re ready to get started, try these tips to become more data-driven.
Find the story
The key to analyzing data, numbers, and charts is to look for the story. Without the “why,” the data itself isn’t much help, and the decision process is far more difficult. If you’re trying to become more data-driven in your decision making, look for the story the data is telling. This will be integral in making the right decisions.
Consult the data
Before making any organizational decision, ask yourself: Does the data support this? Data is everywhere and can be applied to any major decision. So why not consult it when making tough choices? Data is so helpful because it’s naturally void of bias, so make sure you’re consulting the facts before any decision.
Learn data visualization
Finding the story behind the data becomes easier when you’re able to visualize it clearly. While learning how to visualize data is often the toughest aspect of establishing a data-driven culture, it’s the best way to recognize patterns and discrepancies in the data.
Familiarize yourself with different tools and techniques for data visualization. Try to get creative with the different ways to present data. If you’re well-versed in data visualization, your data storytelling skills will skyrocket.
Make data-driven decisions easy with reporting software
You’ll need the right data in front of you to make meaningful decisions for your team. Universal reporting software aggregates data from your company and presents it on your executive dashboard so you can view it in an organized and graphical way.
Related resources
Gartner® Magic Quadrant™ for Adaptive Project Management and Reporting
How Asana drives impactful product launches in 3 steps
Waterfall, Agile, Kanban, and Scrum: What’s the difference?
New site openings: How to reduce costs and delays
- Business Essentials
- Leadership & Management
- Credential of Leadership, Impact, and Management in Business (CLIMB)
- Entrepreneurship & Innovation
- Digital Transformation
- Finance & Accounting
- Business in Society
- For Organizations
- Support Portal
- Media Coverage
- Founding Donors
- Leadership Team
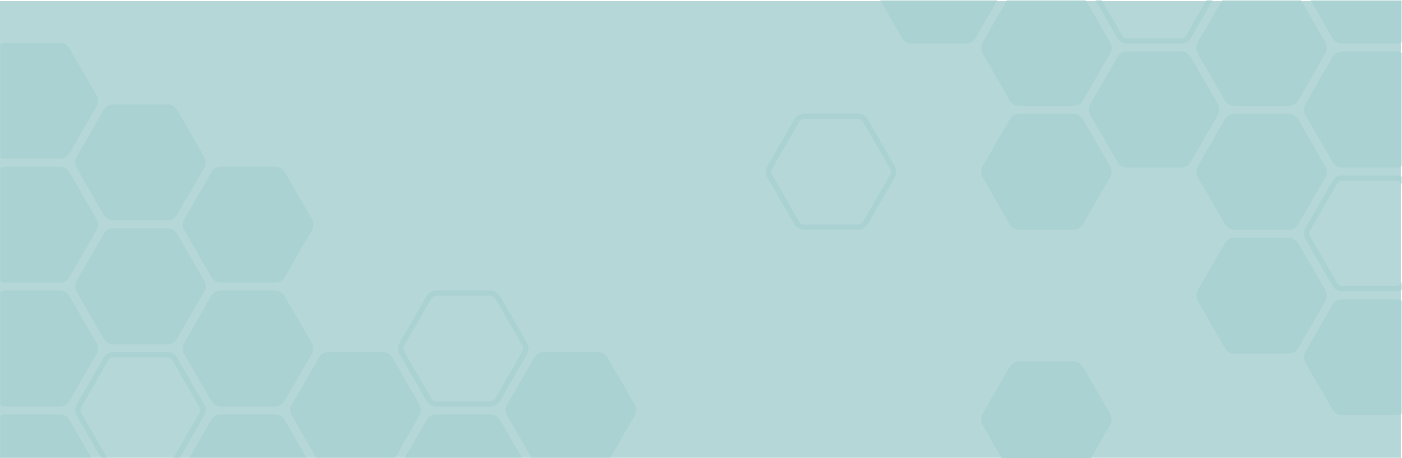
- Harvard Business School →
- HBS Online →
- Business Insights →
Business Insights
Harvard Business School Online's Business Insights Blog provides the career insights you need to achieve your goals and gain confidence in your business skills.
- Career Development
- Communication
- Decision-Making
- Earning Your MBA
- Negotiation
- News & Events
- Productivity
- Staff Spotlight
- Student Profiles
- Work-Life Balance
- AI Essentials for Business
- Alternative Investments
- Business Analytics
- Business Strategy
- Business and Climate Change
- Creating Brand Value
- Design Thinking and Innovation
- Digital Marketing Strategy
- Disruptive Strategy
- Economics for Managers
- Entrepreneurship Essentials
- Financial Accounting
- Global Business
- Launching Tech Ventures
- Leadership Principles
- Leadership, Ethics, and Corporate Accountability
- Leading Change and Organizational Renewal
- Leading with Finance
- Management Essentials
- Negotiation Mastery
- Organizational Leadership
- Power and Influence for Positive Impact
- Strategy Execution
- Sustainable Business Strategy
- Sustainable Investing
- Winning with Digital Platforms
The Advantages of Data-Driven Decision-Making
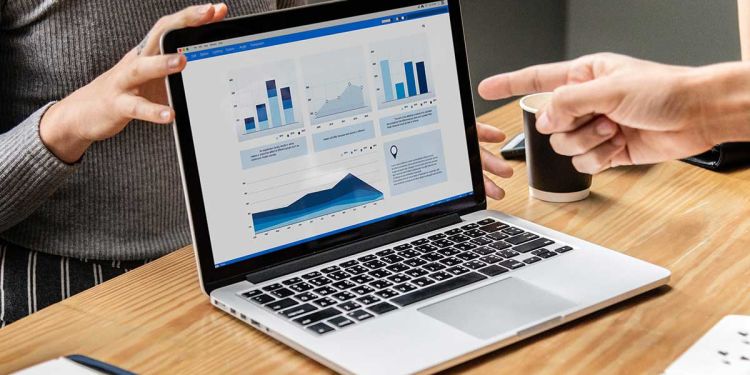
- 26 Aug 2019
Society has imbued the concept of “intuition”—of simply knowing when something is right or wrong—with a tremendous amount of prestige, importance, and influence.
In fact, according to some studies, more than half of Americans rely on their “gut” in order to decide what to believe, even when they are confronted with evidence that speaks to the contrary.
The concept of intuition has become so romanticized in modern life that it's now a part of how many people talk about and understand the “geniuses” of our generation. In science, for example, Albert Einstein is often quoted as saying, “The intuitive mind is a sacred gift," and in business, Steve Jobs is quoted as saying, “Have the courage to follow your heart and intuition; they somehow already know what you want to become.”
Though intuition can be a helpful tool , it would be a mistake to base all decisions around a mere gut feeling.
While intuition can provide a hunch or spark that starts you down a particular path, it's through data that you verify, understand, and quantify. According to a survey of more than 1,000 senior executives conducted by PwC, highly data-driven organizations are three times more likely to report significant improvements in decision-making compared to those who rely less on data.
Are you interested in learning how data-driven decision-making can enable you to be a more effective entrepreneur or member of your organization? Below is information about the benefits of becoming more data-driven, as well as a number of steps you can take to become more analytical in your processes.
Access your free e-book today.
What Is Data-Driven Decision-Making?
Data-driven decision-making (sometimes abbreviated as DDDM) is the process of using data to inform your decision-making process and validate a course of action before committing to it.
In business, this is seen in many forms. For example, a company might:
- Collect survey responses to identify products, services, and features their customers would like
- Conduct user testing to observe how customers are inclined to use their product or services and to identify potential issues that should be resolved prior to a full release
- Launch a new product or service in a test market in order to test the waters and understand how a product might perform in the market
- Analyze shifts in demographic data to determine business opportunities or threats
How exactly data can be incorporated into the decision-making process will depend on a number of factors, such as your business goals and the types and quality of data you have access to.
The collection and analysis of data have long played an important role in enterprise-level corporations and organizations. But as humanity generates more than 2.5 quintillion bytes of data each day, it's never been easier for businesses of all sizes to collect, analyze, and interpret data into real, actionable insights. Though data-driven decision-making has existed in business in one form or another for centuries, it’s a truly modern phenomenon.
Examples of Data-Driven Decision-Making
Today’s largest and most successful organizations use data to their advantage when making high-impact business decisions. To better understand how your organization can incorporate data analytics into its decision-making process, consider the success stories of these well-known businesses.
1. Leadership Development at Google
Google maintains a heavy focus on what it refers to as “people analytics.” As part of one of its well-known people analytics initiatives, Project Oxygen, Google mined data from more than 10,000 performance reviews and compared the data with employee retention rates. Google used the information to identify common behaviors of high-performing managers and created training programs to develop these competencies. These efforts boosted median favorability scores for managers from 83 percent to 88 percent .
2. Real Estate Decisions at Starbucks
After hundreds of Starbucks locations were closed in 2008, then-CEO Howard Schultz promised that the company would take a more analytical approach to identifying future store locations.
Starbucks now partners with a location-analytics company to pinpoint ideal store locations using data like demographics and traffic patterns. The organization also considers input from its regional teams before making decisions. Starbucks uses this data to determine the likelihood of success for a particular location before taking on a new investment.
3. Driving Sales at Amazon
Amazon uses data to decide which products they should recommend to customers based on their prior purchases and patterns in search behavior. Rather than blindly suggesting a product, Amazon uses data analytics and machine learning to drive its recommendation engine. McKinsey estimated that, in 2017, 35 percent of Amazon’s consumer purchases could be tied back to the company’s recommendation system.
Benefits of Data-Driven Decision-Making
1. you’ll make more confident decisions.
Once you begin collecting and analyzing data, you’re likely to find that it’s easier to reach a confident decision about virtually any business challenge, whether you’re deciding to launch or discontinue a product, adjust your marketing message , branch into a new market, or something else entirely.
Data performs multiple roles. On the one hand, it serves to benchmark what currently exists, which allows you to better understand the impact that any decision you make will have on your business.
Beyond this, data is logical and concrete in a way that gut instinct and intuition simply aren’t. By removing the subjective elements from your business decisions, you can instill confidence in yourself and your company as a whole. This confidence allows your organization to commit fully to a particular vision or strategy without being overly concerned that the wrong decision has been made.
Just because a decision is based on data doesn’t mean it will always be correct. While the data might show a particular pattern or suggest a certain outcome, if the data collection process or interpretation is flawed, then any decision based on the data would be inaccurate. This is why the impact of every business decision should be regularly measured and monitored.
Related: 3 Examples of Business Analytics in Action
2. You’ll Become More Proactive
When you first implement a data-driven decision-making process, it’s likely to be reactionary in nature. The data tells a story, which you and your organization must then react to.
While this is valuable in its own right, it’s not the only role that data and analysis can play within your business. Given enough practice and the right types and quantities of data, it’s possible to leverage it in a more proactive way—for example, by identifying business opportunities before your competition does, or by detecting threats before they grow too serious.
3. You Can Realize Cost Savings
There are many reasons a business might choose to invest in a big data initiative and aim to become more data-driven in its processes. According to a recent survey of Fortune 1,000 executives conducted by NewVantage Partners for the Harvard Business Review , these initiatives vary in their rates of success.
One of the most impactful initiatives, according to the survey, is using data to decrease expenses. Of the organizations which began projects designed to decrease expenses, more than 49 percent have seen value from their projects. Other initiatives have shown more mixed results.
“Big data is already being used to improve operational efficiency,” said Randy Bean, CEO and managing partner of consultancy firm NewVantage Partners, when announcing the results of the survey. “And the ability to make informed decisions based on the very latest up-to-the-moment information is rapidly becoming the mainstream norm.”
How to Become More Data-Driven
If you have a goal of becoming more data-driven in your approach to business, there are many steps you can take to reach that goal. Here's a look at some of the ways you can approach your daily tasks with an analytical mindset.
1. Look for Patterns Everywhere
Data analysis is, at its heart, an attempt to find a pattern within, or correlation between, different data points. It’s from these patterns and correlations that insights and conclusions can be drawn.
The first step in becoming more data-driven is making a conscious decision to be more analytical —both in business as well as in your personal life. While this might seem simple, it’s something that takes practice.
Whether you’re in the office pouring over financial statements , standing in line at the grocery store, or commuting on the train, look for patterns in the data around you. Once you have noticed those patterns, practice extrapolating insights and try to draw conclusions as to why they exist. This simple exercise can help you train yourself to become more data-driven in other areas of your life.
2. Tie Every Decision Back to the Data
Whenever you’re presented with a decision, whether business-related or personal in nature, do your best to avoid relying on gut instinct or past behavior when determining a course of action. Instead, make a conscious effort to apply an analytical mindset.
Identify what data you have available that can be used to inform your decision. If no data exists, consider ways in which you could collect it on your own. Once you have the data, analyze it, and use any insights to help you make your decision. As with the pattern-spotting exercise, the idea is to give yourself enough practice that analysis becomes a natural part of your decision-making process .
3. Visualize the Meaning Behind the Data
Data visualization is a huge part of the data analysis process. It’s nearly impossible to derive meaning from a table of numbers. By creating engaging visuals in the form of charts and graphs, you’ll be able to quickly identify trends and make conclusions about the data.
Familiarize yourself with popular data visualization techniques and tools , and practice creating visualizations with any form of data you have readily available. This can be as simple as creating a graph to visualize your monthly spending habits and drawing conclusions from the visualization. You can then use these insights to make a personal budget for the next month. After completing that exercise, you’ll have successfully made a data-driven decision.
4. Consider Furthering Your Education
If you’re uncomfortable with the idea of learning how to incorporate data into your decision-making process on your own, there are a number of educational options you can pursue to develop the data science skills needed to succeed.
Which option makes the most sense will depend on your personal and professional goals. For example, individuals considering a serious career change might decide to pursue a master’s degree with an emphasis on data analytics or data science. But for everyone else, simply taking an online business analytics or data science course could be enough to lay the foundation necessary for success.

Using Data to Answer Critical Questions
While there are many benefits to data-driven decision-making, it’s important to note that you don’t need to take an all-or-nothing approach to get there. By starting small, benchmarking your performance, documenting everything, and adjusting as you go, you can become more data-driven and thrive at your organization.
Do you want to learn more about how to use data to inform business decisions at your organization? Download our Beginner’s Guide to Data & Analytics to learn how you can leverage the power of data for professional and organizational success. This post was updated on February 19, 2021. It was originally published on August 26, 2019.

About the Author
InfoQ Software Architects' Newsletter
A monthly overview of things you need to know as an architect or aspiring architect.
View an example
We protect your privacy.
QCon San Francisco (Nov 18-22): Get assurance you’re adopting the right software practices. Register Now
Facilitating the Spread of Knowledge and Innovation in Professional Software Development
- English edition
- Chinese edition
- Japanese edition
- French edition
Back to login
Login with:
Don't have an infoq account, helpful links.
- About InfoQ
- InfoQ Editors
- Write for InfoQ
- About C4Media
Choose your language
Get clarity from senior software practitioners on today's critical dev priorities. Register Now.
Level up your software skills by uncovering the emerging trends you should focus on. Register now.
Discover emerging trends, insights, and real-world best practices in software development & tech leadership. Join now.
Your monthly guide to all the topics, technologies and techniques that every professional needs to know about. Subscribe for free.
InfoQ Homepage Presentations Data-Driven Decision Making
Data-Driven Decision Making
Zoe Vance and Denise Yu describe how to design the indicators to build an understanding of a product, how to monitor those metrics over time, and how to build feedback loops.
Zoe Vance is Senior Product Manager, Pivotal. Denise Yu is Software Engineer, Pivotal.
About the conference
Pivotal Training offers a series of hands-on, technical courses prior to SpringOne Platform. Classes are scheduled two full days before the conference and provide you and your team an opportunity to receive in-depth, lab-based training across some of the latest Pivotal technologies.
INFOQ EVENTS

The Architect’s Guide to Elasticity
Presented by: Jonas Bonér - Co-founder and CTO at Lightbend
Vance: We are talking about data-driven decision making. I am Zoe Vance. I'm the Product Manager for RabbitMQ for PCF. I've managed many products throughout my career. The motivation for this talk was, there are so many tools and frameworks that PMs use for products that are actually really helpful when thinking about other problems that the team faces. This talk was a little bit about how can we take some of the tools and frameworks that PMs use and expose them so that others can use them in their day-to-day work?
Yu: My name is Denise. I'm actually a software engineer. A few months ago, I was asked to fill in for a product manager who had recently left Pivotal Cloud Foundry, and I was like, "Sure. I can do it for a couple of weeks." That turned into six months. I accidentally found myself product managing for longer than I expected. Along the way, I found it really overwhelming to learn the entire toolset that product managers use and to figure out how to apply them to the specific and very technical domain that I was in charge of. My motivation for collaborating with Zoe on this talk is to hopefully unearth some of the things that we both learned along the way and give you some more tools, in your own toolset to make better and more data-driven decisions.
Product Team Velocity
The first thing that we want to talk about is why this topic matters. As product people, hopefully, as entire teams, everybody on a product team, we spend a lot of time thinking about velocity. We spend a lot of time thinking about how to go faster. Thinking about how to measure velocity. Is it the number of stories you've completed? Is it the number of features that you've delivered? Whatever it is. At Pivotal, we like to think of velocity as a vector, which means that it's a function of both speed and direction. It doesn't really matter how fast you run, if you're running towards the wrong goal, if you're working towards something that's not actually going to add value to your team or to your customers. Throughout, we're going to try to give you some tools to assess whether the direction that you're moving in is the right one for your team and for your customers.
Data in Service of Decision Making
Vance: This talk is called data-driven decision making. What we really mean by that is data in service of decision making with the emphasis on the decisions that you're enabled to make. Data tends to be glorified sometimes, but it doesn't matter if it's not helping you make better decisions and take better actions as a team. Given that the end goal is decision making, we're going to be talking about a framework that lets you take a problem, break it down, quantify it, understand it, and then make the decision that you need to be improving your teams and your work.
When we talk about quantitative versus qualitative data, which we will during the talk, by quantitative we mean things that are to do with quantity. It's where the word comes from. Numbers, percentages of what is happening, so sales increased 10%. Whereas on the qualitative side, it's more about the qualities and the characteristics. It's important because the what is as important as the why. The why really lets you understand what's happening, why does it matter?
Known Knowns
Yu: The way that we're going to frame this is we're going to tell you three different stories based on our own experiences working in product management. We think that when you're trying to choose the best tools to use, it's really important to understand the level of context that you're working in. How much do you really know about the world? How much does your team know about the world? I'm going to begin by telling you a story where we had many known knowns. What I mean by that is, we were in this situation on the team where everybody on the team knew and agreed that there was a problem, but we lacked consensus on how to tackle that problem.
Who has seen Concourse before? Concourse is an automation tool that many of our teams use to reduce toil. Concourse will do things like run our unit tests, run our integration tests, build our code, upload the build artifact to our remote storage, our remote buckets, that kind of thing, so that engineers are freed up to do more interesting types of problem solving. One problem that I've experienced on many of the teams I've worked on both as an engineer and a product manager is that the length of time it took for a pipeline to run could be very unpredictable, and it could take a really long time. On one of the teams that I worked on, everyone on the team knew that our pipelines were taking way too long. Everyone knew that this was painful. It came up time and again in weekly retrospectives. We weren't really equipped to act on it because we didn't know just how bad it was. We didn't have anything to point to, to evaluate, should we address this now or should we do more feature work?
What did we do? We decided to start applying data to this problem. We treated our nebulous pain on the team as if it was a valid product decision, a valid product problem that we could apply our existing toolset to. We took the last few dozen times that the pipeline ran, we exported all the data from Concourse, we plugged it into a spreadsheet that we then visualized using some other tools. We started measuring both the overall time that each pipeline took as well as the amount of time that discrete tasks took. By doing this, we were able to, first of all, put a number to this nebulous pain that we were all feeling. Second of all, identify where the bottlenecks were, and where the most unreliable tasks were.

Team Alignment
By being more metric driven about it, by being more data driven about it, we built alignment on the whole team. We were able to answer the question, how bad of a pain is this? How much time is it costing us? What is the cost of this delay compared to other things that the team could be doing? By building this alignment, we sat down as a team. We actually created an entire track of work for this. It wasn't considered chore work, or valueless work, it was prioritized the same way as we would prioritize feature work, because this is something that was actively costing the team. When we had these numbers, and we had these concrete pieces of work laid out, the entire team was able to engage in creative problem solving, by treating this problem, elevating it to the same hierarchy as a customer problem, we were able to have the whole team focus on moving that number.
The key takeaways for our team were that applying data to this nebulous problem enabled a decision to be made. It's really hard to reason about problems when all you have is these nebulous feelings and sadness in your heart when you have retros. When you have a number, that gives you a target, and that gives you a goal to focus on and to come around.
Known Unknowns
Vance: The second situation we're going to talk about is a situation where you have known unknowns. What we mean by that is that maybe there's some vague awareness of a problem lying under the surface, but there's no real consensus around it or agreement on what exactly it is. I'm actually going to speak to when I was Product Manager at a company called Kimono. It was a startup that created a tool for developers and allowed anybody to create an API from any web page. Being a hip Silicon Valley startup, we read "The Lean Startup," we read "Lean Analytics," and we started tracking the numbers that were recommended. We started looking at active users over given periods of time: daily, weekly, monthly. We defined active as users who were making a call to an API created with Kimono in that given period.
What we started to realize is that we had no idea what that meant. We would have these goals, sometimes we would hit them, sometimes we would miss them. We started to have conversations about, what does it mean that we're tracking active users? What is an active user? We broke down the problem. We looked at the quantitative user patterns, who made up those active users, and we realized there are actually many different types of activities that were contributing many different types of behavioral patterns to be counted as active. We then followed that up with qualitative interviews to understand, why do you have the pattern that you have? We realized that we basically had two very distinctive user groups in our active user number. There were the users who were calling APIs from many different websites and creating amazing things like visualizations, new apps, analyses. Then we had another group of users who were calling large amounts of data infrequently. They were essentially just stealing other people's information, essentially.
This raised a bunch of existential questions for the company. What do we want to be? Who are we building for? Why are we building it? It allowed us to then actually prioritize features and prioritize bugs, prioritize user stories based on who is the user we're actually trying to solve? The takeaway from here with this situation where we thought we had it under control, we had an active user number, was that, once we probed deeper, once we broke it down even more and applied quantitative and the qualitative framing, it helped us really understand who we were as a company. Where we were going. Why we were going there.
Unknown Unknowns
The final situation where we're going to talk about this framework of breaking a problem down, quantifying it, understanding it, making a decision is unknown unknowns. Here we really just mean very nebulous, nobody really knows that this thing even exists. Maybe nobody has talked about it. The example we're going to use is the Spotify team health check. I highly recommend the exercise. We do it as a team every couple months. The idea is you take a couple categories that are critical to teamwork, such as easy to release, fun delivery. You have a conversation with all the team members about how they feel the team is performing. This then gives you amazing insights into things that you maybe wouldn't have taken the time to talk about or think about. One thing that we realized at the back of it is we had these amazing conversations, but then we didn't do anything. You raise these great points, but they're not clean things you can just tick off. We started instituting a monthly experiment off the back of the Spotify health check that would help us actually improve on those metrics.
The experiment I'm going to talk about is learning. When the team discussed learning, the feeling was that our product surface area was so large, and we were always so busy that it was hard to stay on top of all the tools and technologies we needed to be successful. The reason why we chose learning and the reason we chose the health check for this story is because those are fundamentally unquantifiable things. It's not about the data, it's about the decisions. We took learning, how do you quantify something like learning? The first thing we did is we wrote down all the tools and technologies that we needed to understand to be effective at our jobs. This is a very abbreviated list, just the things that I could be bothered to put on a slide.
The second thing we did to quantify it is say, how important is the tool to our day-to-day success? Finally, where are we now as a team? That helped us quantifying it, in these broken down smaller parts, helped us choose the most important thing that we wanted to learn. From there, we actually step back. I don't want to tell team members how they should be learning. It became, here's the goal. Here's what we're trying to achieve. Take a day, a week and do whatever you need to feel like you are improving your learning and your knowledge on this topic. Unsurprisingly, because this data is fuzzy, the number went up. The point wasn't the number, the point was, what works for us? What ritual should we be building into our day-to-day? How do we involve more learning in our processes? The takeaway here just being, we can become a stronger team by taking even nebulous, qualitative things, breaking it down, thinking about a way to measure it to gain alignment, and then making a better decision as a group.
Frameworks to Being More Data Driven
Yu: We're going to close out the anecdotal section of this talk by explaining to you a framework that you can use to bring some of these lessons pulled out of these stories into your day-to-day work. We're going to give you a framework for how you can start to be more data driven. This also applies no matter what level of context you're working in. Although if you have already high context and high alignment, you might be able to skip the first few steps.
The first thing to do is to identify the problems that you want to solve. At Pivotal, within the product practice, we have an exercise called stinky fish, which the facilitator will ask every member on the team to identify some problem area. The way that you frame it is, what problem, if we continue to ignore it will give us the greatest amount of grief down the line? You can set timelines, in two weeks, in two months, in six months, in one year. You can customize this to whatever works for your team. The reason why we call it stinky fish is because if you leave a fish in the back of your refrigerator for two weeks, you're probably not going to want to open your refrigerator and deal with it, the longer that time goes on. This is the process of moving things from either the complete unknowns or the not well-organized known unknowns into the area of known knowns where you can begin to address them. Where you can begin to build alignment on why we should be tackling this problem.
Once you have a shortlist of problems to solve, the next step is to choose probably the biggest problem. You can choose the problem that has the greatest number of people in the team identified or you can run some sorting exercise like a two by two or affinity mapping. The process of breaking down and framing a problem is to turn something that's big and nebulous and inaccessible into something that's small, accessible, and addressable.
Once you've decided on the problem to solve, the next step is to choose what you're going to measure. As we've talked about throughout the three stories, I hope it's clear by now that neither of us are experts at this, neither of us are all-knowing. The thing that you choose to measure today is probably not going to be the same thing that you will measure on day 30 or day 100. That's a good thing. The point that we want to make here is that whatever you choose to measure doesn't have to be perfect. You don't need to read every product management handbook out there to figure out what to measure. What you do choose should be good enough that it provokes the team to have conversations, and it provokes the team to learn. You're not comparing yourself against the platonic ideal of a measurement, you're comparing this against the complete absence of any data. Something is always better than nothing.
As you learn more about the solution space, you'll discover whether you need that measurement to be greater fidelity, or whether a finger in the air, just like a sense, is enough to make a decision and move on. Of course, whatever you choose to measure, you should introspect on that. You should always question whether that's still the right thing to measure. In Zoe's story, they completely changed the way that they were analyzing customer engagement. I think that that's a healthy thing. That's actively a good thing. Hopefully, we've all seen build-measure-learn before. We should be iterating when it comes to product vision and product roadmaps. We think that when it comes to the process of measuring the things that we collect, measuring data, we should also be iterative about that.
Lean Product Development
What we've been talking about this entire time is actually just the core principles of lean product development. Dan North, said a few months ago that when we take the ideas of lean and we apply them to lean manufacturing, or lean supply, they look very different in practice than when we apply them to product development. In lean product development, we always want to be optimizing for learning. That means making mistakes, because mistakes will surface more information. They will empower you to act differently in the future. The data that you collect when you're starting to become more data driven about the day-to-day things that we do, should also be in service of the goal of discovering more.
Data driven practices from the world of product management can and should be applied more broadly to every operation on a product team, or perhaps on a consulting team, not necessarily a product team. The framework that we gave you was to figure out your hardest problems, figure out the things that are causing you the most pain, frame it, break it down, and then measure it, and understand it. Of course, always be iterating and learning.
See more presentations with transcripts
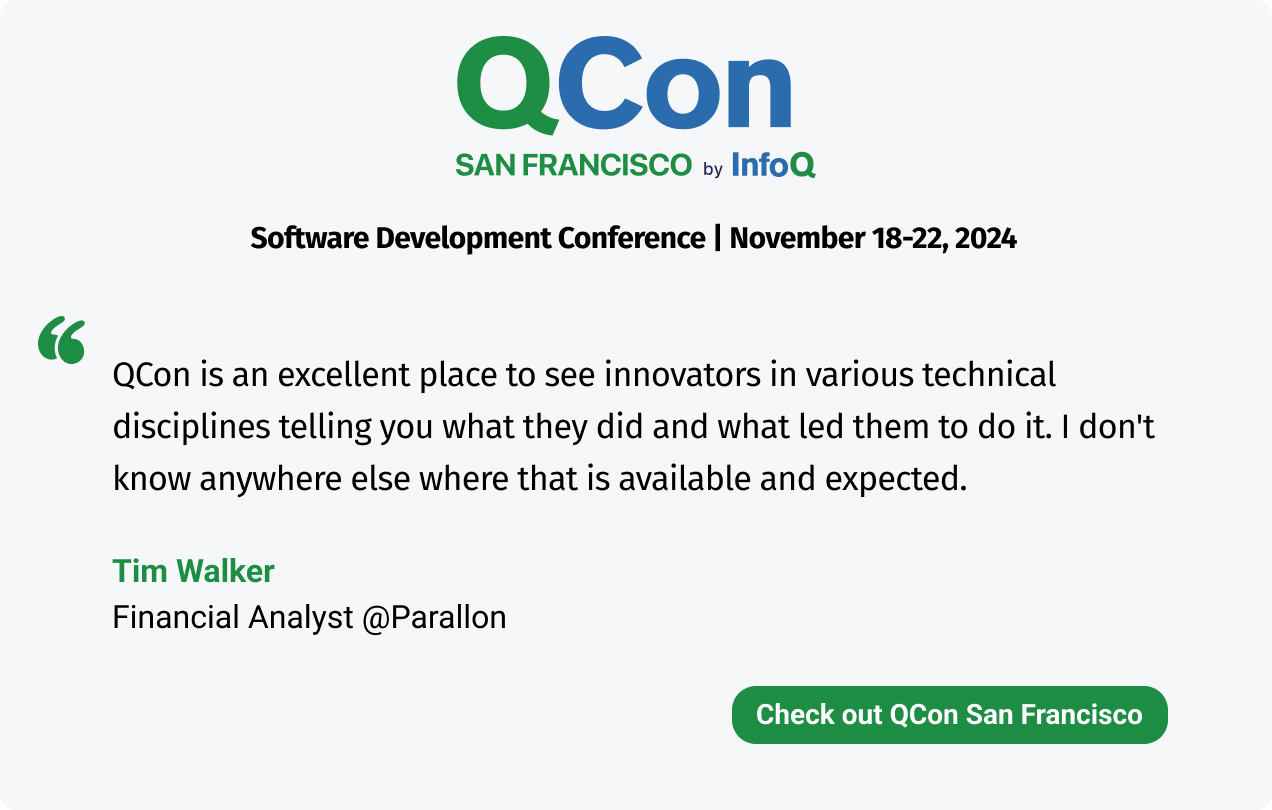
Recorded at:
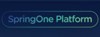
Feb 12, 2019
Related Sponsored Content
[ebook] api security for the modern enterprise, related sponsor.
/filters:no_upscale()/sponsorship/topic/ab4e0ce7-864a-4131-b009-638fe135de28/CurityLogoRSB-1712304212846.png)
Protect Identities. Secure Digital Services. Enable scalable and secure user access to web and mobile applications. Start free trial .
This content is in the Culture & Methods topic
Related topics:.
- Culture & Methods
- SpringOne Platform 2018
- Project Management
- Transcripts
Related Editorial
Popular across infoq, optimizing continuous deployment at uber: automating microservices in large monorepos, how aws well-architected framework supports frugal architecture, cloudflare introduces automatic ssl/tls to secure and simplify origin server connectivity, tiktok releases tool to improve monorepo performance, microsoft launches open-source phi-3.5 models for advanced ai development, lessons learned from the crowdstrike incident: infoq dev summit munich 2024 preview.
- Future Students
- Parents/Families
- Alumni/Friends
- Current Students
- Faculty/Staff
- MyOHIO Student Center
- Visit Athens Campus
- Regional Campuses
- OHIO Online
- Faculty/Staff Directory
Online MBAn
College of Business
- About the College
- Fast Facts & Rankings
- Diversity, Equity & Inclusion
- News & Events
- Accreditation
- College Leadership
- Advisory Boards
- Strategic Plan
- Centers & Institutes
- Faculty Research
- Plan Your Visit
- Faculty & Staff Directory
- Undergraduate Majors
- Undergraduate Minors
- Undergraduate Certificates
- Graduate Degrees
- Graduate Certificates
- Online Business Degrees
- Executive Education
- Accelerated Pathways
- Selective Programs
- Copeland Core
- Student Organizations
- Integrated Business Cluster
- International Experiences
- Academic Advising
- Career Management
- Graduate Student Career Management
- Alumni Relations
- Employer Relations
- Admission Requirements
- Tuition and Fees
- Scholarships and Aid
- Transfer Students
- International Students
- Student Ambassadors
- Faculty and Staff
Helpful Links
Navigate OHIO
Connect With Us
Essentials for Implementing Data-Driven Decision Making
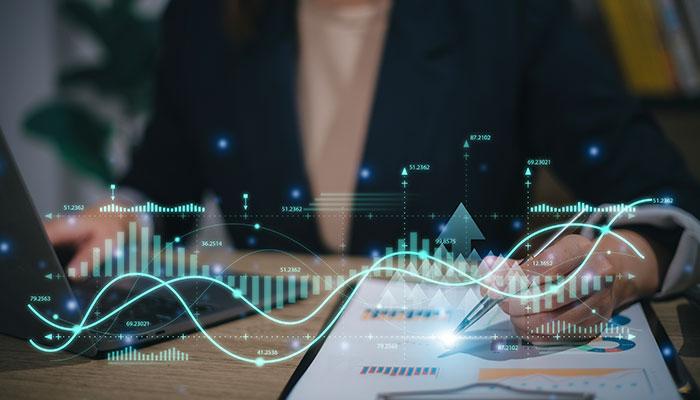
The impact of data on the modern business world cannot be overstated. As a recent article from Forbes explains, "it is critical for any organization looking to succeed in today's business landscape to prioritize the quality of data used to make important business decisions. 1 " What is data-driven decision making (DDDM), and how can an effective DDDM process yield better results for your company? In this article, we explore a five-step DDDM strategy and examine prime examples of how data analytics can guide strategic decision making.
What is Data-Driven Decision Making?
Data-driven decision making is just that – the use of data to evaluate options and make strategic business decisions. As humans, we are often tempted to make choices based on emotion, intuition or a "gut" feeling. However, when it comes to making business decisions, analytics and data can help provide more reliable, measurable and actionable results.
Over the last few decades, increased access to technology and the internet has driven a sharp rise in the amount of data available to decision-makers. For this reason, DDDM has quickly become a top skill for anyone entering the world of business. And that's just where new business professionals have the opportunity to set themselves apart – anyone with access to data can say they're using it to make decisions, but professionals need a solid grasp on analytics and the ability to identify the most valuable data in order to produce the results top employers are looking for.
With a seemingly endless supply of consumer, supply chain, marketing and other types of data, DDDM can quickly become overwhelming. It's up to skilled professionals to block out the noise, identify the most valuable data available and lead teams in making the right decisions. Utilized correctly, data can help a company improve customer satisfaction, increase profits, engage in effective problem solving and increase efficiency. 2
Earn a Master of Business Analytics from OHIO
View the Online MBAN
Five Steps for Implementing Data-Driven Decision Making
Data is essential to effective decision making in today's business world. However, even the best data is useless to business professionals who don't know how to use it. The DDDM process below can help you utilize analytics and make decisions that yield high outcomes.
Determine Business Questions or Issues
The first step in effective DDDM is to determine the appropriate questions you want to answer or issues you want to solve with data. Begin by discussing the following with your team:
- What does the company want to accomplish?
- Is the company trying to assess an opportunity or diagnose a problem?
- Which areas are most important to achieving the company's overall strategy?
Strategize and Identify Goals
Based on the questions or issues you've identified, establish a clear analytical objective for your DDDM process. Consider the following:
- What can you realistically accomplish with data?
- Who will oversee the collection and data analysis?
- What personnel will you need for the project?
- What is the ultimate end goal for this project?
- How will you measure the success of this project?
Target Data
Once you identify the strategic goals of your project, it's time to identify the data that will be most useful for you in this DDDM process. Consider the following questions in deciding which data to collect and how to do so:
- Will qualitative or quantitative data be most useful to this project?
- Is it feasible to convene focus groups to collect data?
- Would online tracking and social media monitoring reveal meaningful data analytics?
- Of the data you can access, which is most valuable to your goals?
Collect and Analyze Data
Now that you know the type of data you will target in this DDDM process, you need to identify the correct processes and personnel to gather and manage the data. Consider the following:
- Does your company already have some of the data it needs in-house?
- Will you need to purchase access to an existing data set?
- Which routes will you need to utilize to collect data (computer software, online software, personnel, cameras, etc.)?
After you have collected the necessary data, it needs to be analyzed. Consider:
- What insights can you gain from this data?
- Is it what you expected? What stands out?
- What big data platforms or tools can you access to assist you in this analysis?
Make Decisions Regarding Findings
The last step in the DDDM process is, of course, to make the data-driven decision. Leaders and other key decision makers can use the analytical insights gained from the process to take actionable steps needed for the success of a project.
How Data-Driven Decisions Shape Business
Data-driven decisions shape businesses in many ways. Big data drives most of the top tech giants, including Google, Amazon and Meta. However, DDDM isn't unique to the world of technology. The aviation company Lufthansa, for example, centralized its data collection and analysis practices in a recent attempt to improve business across its more than 550 subsidiaries. This move led to more strategic, data-driven decisions and a 30% increase in company-wide efficiency. 3 , 4
Many retail companies also use data-driven decision making to produce more positive business outcomes. One example is Nike, which has used valuable data to improve its supply chain efficiency. By understanding data regarding demand, material availability and distribution, Nike was able to both reduce costs and cut delivery times to increase customer satisfaction and loyalty. 5
What Positions Utilize Data-Driven Decision Making?
Sound, ethical and innovative data governance is essential, no matter the business sector, company or goal. DDDM requires leaders who can strike a balance between using analytics and managerial instincts, and who can set the standard for best practices. Below are a few positions that utilize DDDM in their everyday tasks.
- Data Analyst: As their title suggests, data analysts process large amounts of data to glean important findings that can guide impactful decisions. Data analysts also maintain databases, use visualization to communicate their findings and forecast trends based on their collected data. 6
- Data Engineer: Data engineers work with businesses to build stable, optimized systems that aid in the collection and analysis of data. Professionals in this role also conduct research and look for patterns in data to help forecast trends. 6
- Database Administrator: Database administrators are responsible for keeping data safe and correctly stored. People who work as database administrators manage data, implement security measures and often design the databases themselves. 6
- Business Analyst: Business analysts use data to evaluate businesses and make recommendations for improving a company's performance. Those who work in this position may use the DDDM process to make high-impact actionable recommendations to decision-makers. 6
- Data Scientist: Data scientists analyze data in order to offer business solutions and valuable insights based on their findings. They collect and process data using various data science techniques in order to offer well-founded advice to major decision-makers. Professionals in this role design data collection processes, look for trends using statistical methodologies and communicate their findings to stakeholders. 6
Effective data-driven decision making begins with the right education. The Ohio University Online Master of Business Analytics is designed to prepare students for data-related careers and teach them how to implement data-driven decisions in different types of businesses. For an introduction to data analytics and DDDM, Ohio University also offers ten Online Graduate Business Certificates , including the Business Analytics Certificate. These certificates can also be applied to a graduate business degree.
White, Jeff. "Why High-Quality And Relevant Data Is Essential In Today's Business Landscape." Forbes. Apr. 17, 2023. Retrieved Nov. 2, 2023 from https://www.forbes.com/sites/forbestechcouncil/2023/04/17/why-high-quality-and-relevant-data-is-essential-in-todays-business-landscape/
Indeed Editorial Team. "What Is Data in Business? (Plus Importance and Examples)." Indeed. Mar. 10, 2023. Retrieved Nov. 2, 2023 from https://www.indeed.com/career-advice/career-development/data-in-business#
Tableau. "A Guide To Data Driven Decision Making: What It Is, Its Importance, & How To Implement It." Retrieved Nov. 2, 2023 from https://www.tableau.com/learn/articles/data-driven-decision-making
Grant, Devin. "What is Data-Driven Decision Making? (And Why It's So Important)." Drive Research. Mar. 27, 2023. Retrieved Nov. 2, 2023 from https://www.driveresearch.com/market-research-company-blog/data-driven-decision-making-ddm/
Thought Spot. "6 Retail Big Data analytics use cases and examples." Retrieved Nov. 2, 2023 from https://www.thoughtspot.com/solutions/retail-analytics/retail-big-data-analytics-examples-and-use-cases
Chatterjee, Maria. "Top 9 Job Roles in the World of Data Science for 2024." My Great Learning. Nov. 8, 2023. Retrieved Nov. 9, 2023 from https://www.mygreatlearning.com/blog/different-data-science-jobs-roles-industry/
Your Future Starts Here
Online Programs
740.924.5725
to speak with a knowledgeable Enrollment Counselor.
Newly Launched - AI Presentation Maker
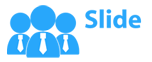
AI PPT Maker
Powerpoint Templates
Icon Bundle
Kpi Dashboard
Professional
Business Plans
Swot Analysis
Gantt Chart
Business Proposal
Marketing Plan
Project Management
Business Case
Business Model
Cyber Security
Business PPT
Digital Marketing
Digital Transformation
Human Resources
Product Management
Artificial Intelligence
Company Profile
Acknowledgement PPT
PPT Presentation
Reports Brochures
One Page Pitch
Interview PPT
All Categories

Introduction To Data Driven Decision Making Training Ppt
This slide depicts data-driven decision-making information. It emphasizes that data-driven decision-making uses data points, metrics, and facts to guide strategic business decisions. It also mentions that business intelligence tools gives you data, accessible to everyone, that enable decisions to be made.

- Add a user to your subscription for free
You must be logged in to download this presentation.
PowerPoint presentation slides
Presenting Introduction to Data-Driven Decision Making. These slides are 100 percent made in PowerPoint and are compatible with all screen types and monitors. They also support Google Slides. Premium Customer Support is available. Suitable for use by managers, employees, and organizations. These slides are easily customizable. You can edit the color, text, icon, and font size to suit your requirements.

People who downloaded this PowerPoint presentation also viewed the following :
- Business Slides , Digital Transformation
- Digital Transformation ,
- Digital Strategy ,
- Digital Organization ,
- Digital Roadmap ,
Introduction To Data Driven Decision Making Training Ppt with all 16 slides:
Use our Introduction To Data Driven Decision Making Training Ppt to effectively help you save your valuable time. They are readymade to fit into any presentation structure.
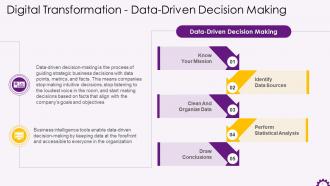
Ratings and Reviews
by Doug Carroll
December 9, 2022
by Dominick Pierce

JavaScript seems to be disabled in your browser. For the best experience on our site, be sure to turn on Javascript in your browser.

- My Wish List
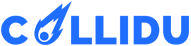
- Compare Products
- Presentations
Data-Driven Decision-Making
You must be logged in to download this file*
item details (6 Editable Slides)
(6 Editable Slides)
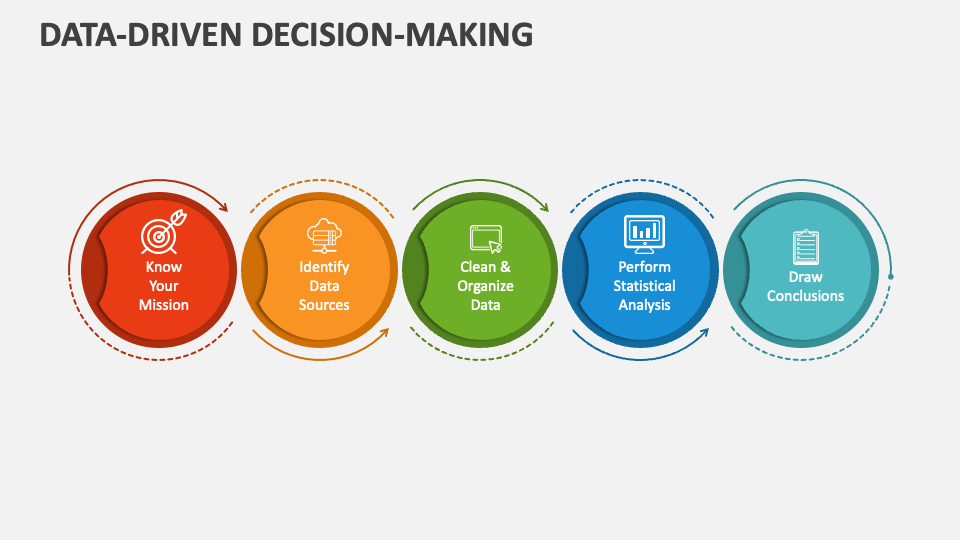
Related Products
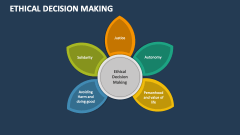
Want to add a touch of creativity and personalization to your presentations with finely-crafted visual elements? Download our appealing Data-Driven Decision-Making presentation template, compatible with MS PowerPoint and Google Slides, to describe the approach to using data, information, analytics, facts, and metrics to make informed business decisions. You can also discuss this approach's benefits over the traditional decision-making process, like better efficiency, increased accountability, etc.
Strategists, entrepreneurs, data scientists, and statisticians can leverage these impactful slides to explain the steps, advantages, and benefits of data-driven decision-making. Moreover, you can shed light on the challenges of data-driven decision-making, including dependence on IT, real-time insights, poor data visibility, etc. The combination of simple yet impressive and modern graphics in this deck will let you leave a long-lasting impact on your audience.
Sizing Charts
Size | XS | S | S | M | M | L |
---|---|---|---|---|---|---|
EU | 32 | 34 | 36 | 38 | 40 | 42 |
UK | 4 | 6 | 8 | 10 | 12 | 14 |
US | 0 | 2 | 4 | 6 | 8 | 10 |
Bust | 79.5cm / 31" | 82cm / 32" | 84.5cm / 33" | 89.5cm / 35" | 94.5cm / 37" | 99.5cm / 39" |
Waist | 61.5cm / 24" | 64cm / 25" | 66.5cm / 26" | 71.5cm / 28" | 76.5cm / 30" | 81.5cm / 32" |
Hip | 86.5cm / 34" | 89cm / 35" | 91.5cm / 36" | 96.5cm / 38" | 101.5cm / 40" | 106.5cm / 42" |
Size | XS | S | M | L | XL | XXL |
---|---|---|---|---|---|---|
UK/US | 34 | 36 | 38 | 40 | 42 | 44 |
Neck | 37cm / 14.5" | 38cm /15" | 39.5cm / 15.5" | 41cm / 16" | 42cm / 16.5" | 43cm / 17" |
Chest | 86.5cm / 34" | 91.5cm / 36" | 96.5cm / 38" | 101.5cm / 40" | 106.5cm / 42" | 111.5cm / 44" |
Waist | 71.5cm / 28" | 76.5cm / 30" | 81.5cm / 32" | 86.5cm / 34" | 91.5cm / 36" | 96.5cm / 38" |
Seat | 90cm / 35.4" | 95cm / 37.4" | 100cm / 39.4" | 105cm / 41.3" | 110cm / 43.3" | 115cm / 45.3" |

10 Best PowerPoint Templates for Presenting Decision-Making Models
Decision-making is an intricate process that involves choosing the best alternative from the available ones, taking into account and balancing both intellectual and emotional factors. To reduce the complexity of the process and help individuals and teams make effective decisions, several decision-making models have been proposed. These models guide how to choose the right course of action in a particular situation.
You can educate your audience about these models and facilitate high-quality decision-making using pre-designed PowerPoint templates. You will get many templates online; however, in this article, we have listed the top 10 decks that are 100% customizable and reduce your presentation creation time and effort. Let’s take a look!
Templates for Delivering Amazing Presentations on Decision-Making Models
1. rapid decision-making model.
Using this template, you can explain the RAPID (Recommend, Agree, Perform, Input, and Decide) model of decision-making. You can clarify who will be responsible for providing inputs, shaping and making the decision, and carrying out or executing the decision once it is taken/made. You can further explain how this model helps foster clarity and accountability, empowering organizations to broaden the scope of decision-making and involve clients, business partners, internal teams, and other relevant stakeholders in the process.
2. Vroom Yetton Decision-Making Model
This template lets you explain the Vroom Yetton Decision-Making Model, which highlights three crucial factors (i.e., decision quality, time constraints, and team commitment) to consider while choosing the best alternative. You can also showcase and describe 5 style guides, i.e., Autocratic Type 1, Autocratic Type 2, Consultative Type 1, Consultative Type 2, and Group-based Type 2, that help groups make apt decisions based on the level of subordinates’ involvement and situation.
3. Carnegie Model of Decision-Making
Leveraging this template, you can educate the audience about the model that helps make decisions in uncertain (limited information and other constraints) and conflicting situations (diverse opinions, goals, experiences, and values). You can explain how this model assists decision-makers in forming coalitions and achieving the maximum problem consensus. Further, you can explain the applicability of this model in creating decisions where time is limited and chaos is prevalent among the team/group.
4. Data-Driven Decision-Making
This pre-designed template is the best pick to educate the audience about the model that facilitates decision-making based on data, metrics, and facts. You can also explain how this model helps guide business strategies, improve operational efficiency and agility, and enhance overall performance. Additionally, you can represent the steps involved in this decision-making model.
5. Rational Decision-Making
This 100% editable template is the best visual aid to present the stepwise process of making rational and logical decisions leveraging objective knowledge. You can explain how this model focuses on evaluating risks, costs, and benefits associated with each option. You can further highlight the problems, assumptions, and limitations of this model. The spellbinding design of this deck will enthrall your audience and keep them engaged throughout the presentation.
6. Evidence-Based Decision-Making
Explain to your audience the decision-making model that considers qualitative and quantitative data, rather than instinct or guesswork, for choosing the best alternative. You can showcase the sources of evidence for making well-informed decisions. Moreover, you can describe the application of this model in healthcare, IT, finance, etc., in a visually impressive manner through this template.
7. Agile Decision-Making
Project managers can use this aesthetically pleasing template to demonstrate how this decision-making model helps speed up the software development cycle by improving project transparency, facilitating early identification of risks, and optimizing resource utilization. If you prefer simplicity, clarity, and elegance in your slides, you will love this PPT, which is all about minimalism.
8. Ethical Decision-Making
Ethical decision-making involves eliminating unethical alternatives and choosing the best ethical option. This process is guided by ethical values and principles. Using this professionally designed template, you can depict the steps to ensure the fairness and ethical aspects of the decision made at the workplace. Besides this, you can describe various models associated with this decision-making, such as Bowen’s Model for Strategic Decision-Making, TARES Ethical Persuasion, and Potter’s Box for Decision-Making.
9. Shared Decision-Making
Explain the application of a shared decision-making model in the healthcare field in an engaging and easy-to-understand manner, leveraging this remarkable template. You can explain how this collaborative approach brings together patients and care providers to make decisions on tests and treatment plans based on the patient’s preferences and evidence. You can also shed light on the importance, benefits, and steps of shared decision-making.
10. Consumer Decision-Making Process
Sales and marketing professionals will find this template useful in demonstrating to their team members the stages consumers go through while making a buying decision. You can also explain strategies to be implemented in each stage to help consumers move to the next stage without friction and close the deal. Using this deck, you can also describe various consumer buying behavior types based on the degree of buyer involvement and differences between brands.
So, this is our list of top 10 PowerPoint templates for presenting decision-making models that will infuse life and dynamism into your presentations. Each slide in these decks is created thoughtfully and with great attention to detail to maximize visual impact and audience engagement. Download all those that best suit your requirements!
Leave a Response Cancel reply
Save my name, email, and website in this browser for the next time I comment.
Please enter an answer in digits: twenty − 11 =
Ashish Arora
You might also like.
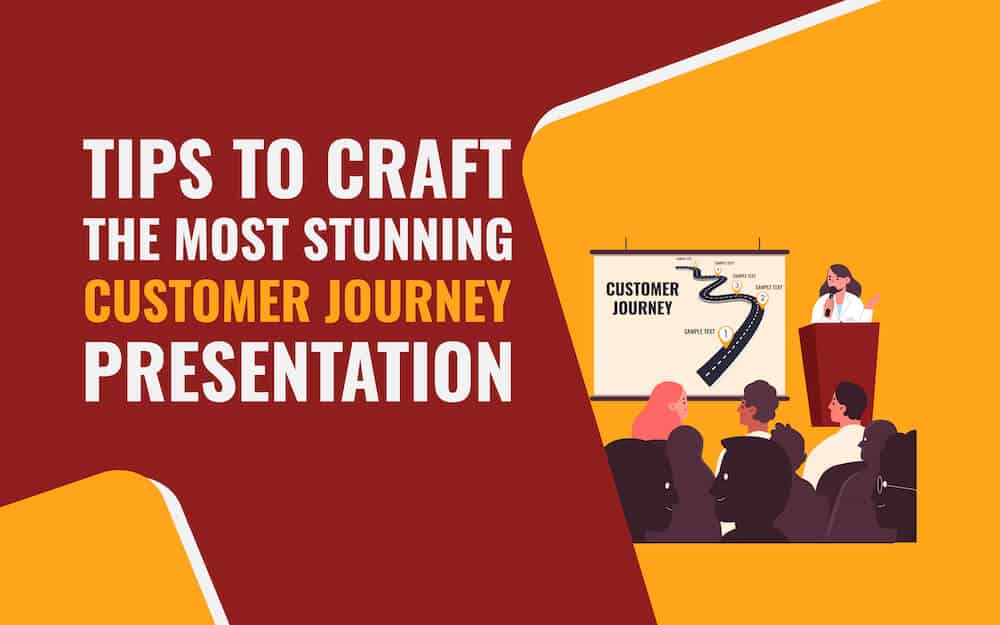
A Detailed Guide to Craft the Most Stunning Customer Journey Presentation
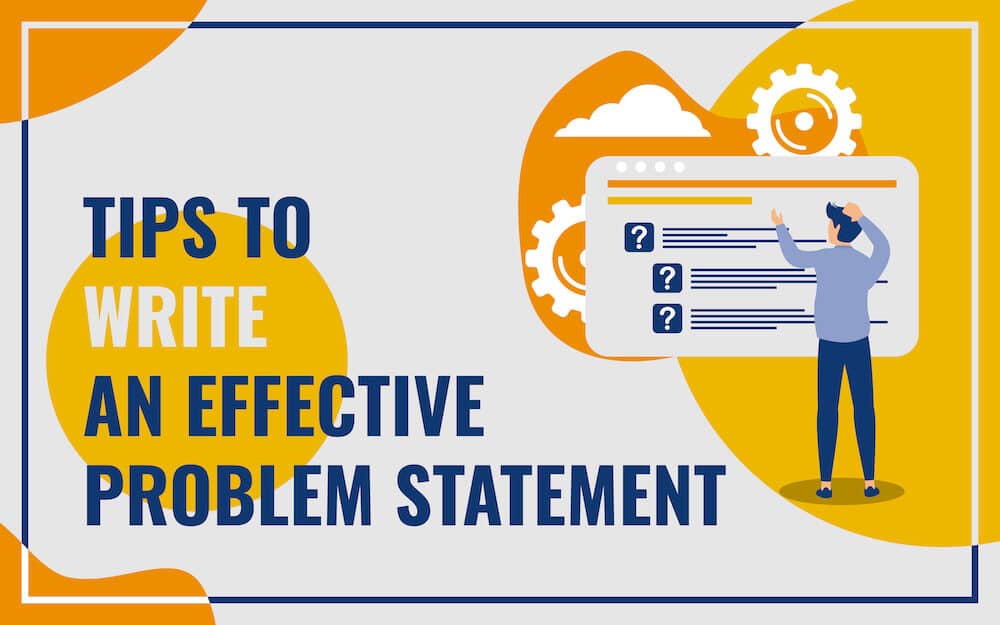
A Complete Guide to Writing a Clear and Concise Problem Statement
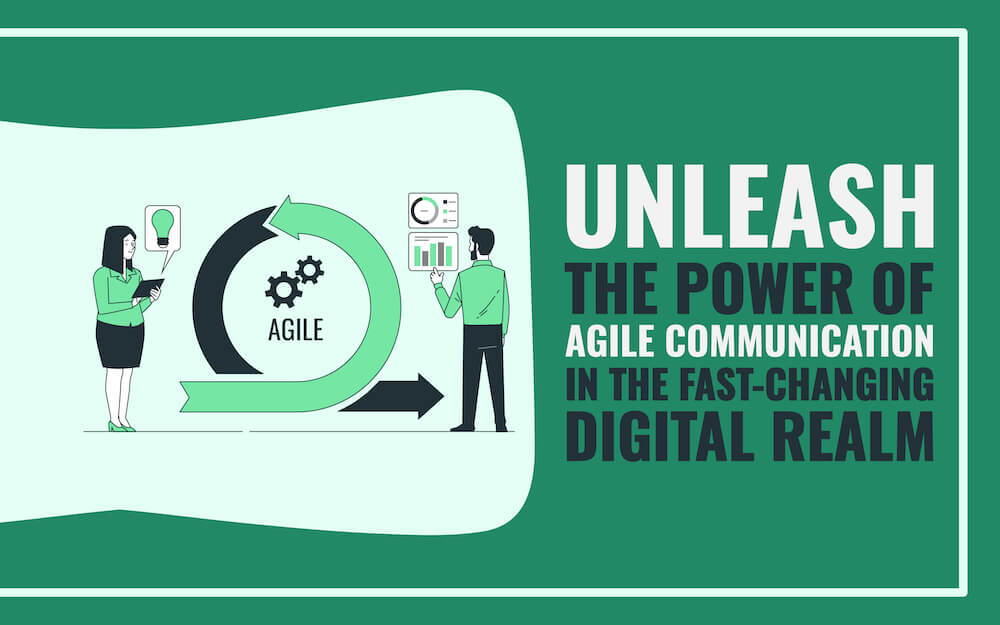
Unleash the Power of Agile Communication in the Fast-Changing Digital Realm
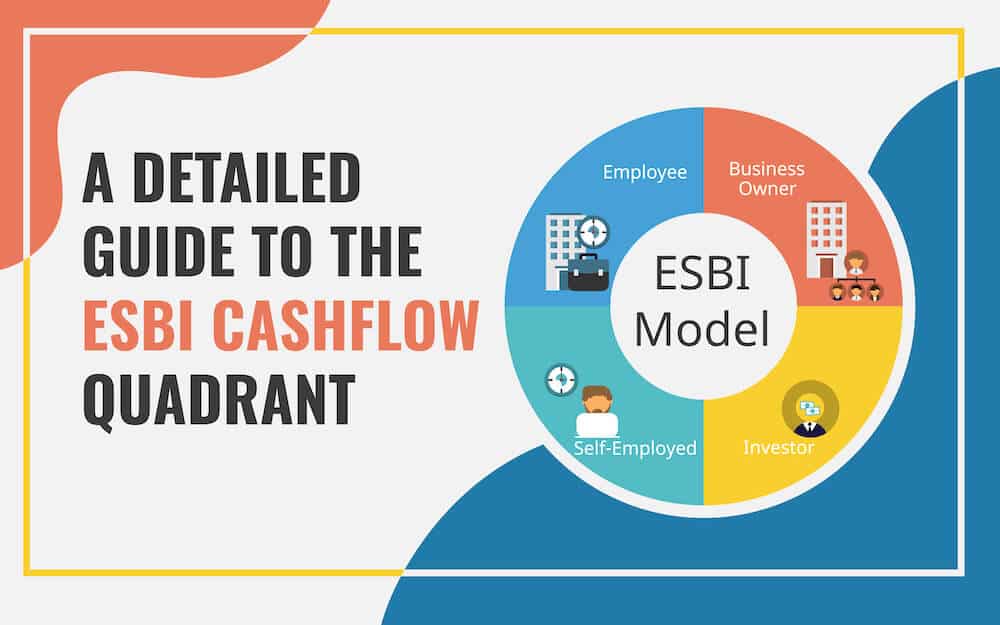
A Detailed Guide to the ESBI CashFlow Quadrant
Your Quick-Start Guide to Data-Driven Decision Making
By Joe Weller | January 22, 2019
- Share on Facebook
- Share on LinkedIn
Link copied
In business, you constantly have to make decisions — from how much raw material to order to how to optimize retail traffic for changing weather. In days gone by, you might have consulted the person who had been around the longest for their best guess; for a more scientific approach, you might have also looked at sales records. Today, companies are finding that the best answers to these questions come from another source entirely: large amounts of data and computer-driven analysis that you rigorously leverage to make predictions. This is called data-driven decision making (DDDM).
To quickly get you up to speed with data-driven decision making, we’ve pulled together this action-oriented guide that explains the technology and includes expert tips as well as easy, step-by-step how-tos.
What Does Data-Based Decision Making Mean? What Is DDDM?
Data-driven decision making (DDDM) is the practice of collecting data, analyzing it, and basing decisions on insights derived from the information. This process contrasts sharply with making decisions based on gut feeling, instinct, tradition, or theory. Data-driven decisions are more objective and can be easily evaluated according to their impact on metrics.
For example, a manufacturer that wants to increase production might time the assembly line, noting any delays. After introducing a solution to a bottleneck, the manager would time the process again to see if the change results in time savings. They might also try another solution and see which one actually speeds up production more.
The process becomes more sophisticated when you use data about the past to predict what will happen in the future.
This approach goes by many names, including big data, data analytics, business intelligence, diagnostic analytics, data analysis, data modeling, and online analytical processing.
Underlying DDDM is the belief that better data makes for better decisions. Without data, people run a much greater risk of being swayed by biases or acting on false assumptions. As the legendary engineer W. Edwards Deming put it, “Without data, you’re just another person with an opinion.”
Data-driven decision making is generating a lot of buzz in the business world as well as in the spheres of education, healthcare, and government policymaking.
But of course, the success of data-driven decision making depends on the quality of data collected and the methods used to sift through it. Data-driven decision making is heavily quantitative — historically, its use has been limited due to the need to perpetually collect statistics and crunch numbers. But the spread of moderately priced computing systems powerful enough to analyze large data sets — so-called big data — has made DDDM much more accessible.
The Benefits of Data-Driven Decision Making
DDDM is not confined to big companies and government bodies with vast resources. Organizations of any size can use data-driven decision making to transform their processes.
With DDDM, organizations become more agile, detect new business opportunities sooner, and respond to market changes more quickly. With near-real-time data collection, managers can swiftly measure results and create a fast feedback loop. These abilities make data-driven companies exceptionally customer focused and more competitive. The same is true of organizations and service providers, such as public education agencies.
Data-driven decision making leads to greater transparency and accountability, and this approach can improve teamwork and staff engagement. DDDM policies make clear that whims or fads are not driving the organization, and morale improves because people see that objective data backs up management decisions.
In organizations that prioritize data-driven decision making, goals are concrete, and results are measured. Team members often feel a greater sense of control because they can see the goal posts clearly. The tenor of interactions may become more positive because discussions are fact-based, rather than driven by ego and personality.
Data-driven analysis can pay for itself through cost savings and higher revenues. Most organizations collect data, usually for record-keeping and compliance, but many don’t do anything with this information. Often, they incur storage costs for keeping the data. So, why not sift through it and see if it tells any stories?
What Is a Data-Driven Approach?
The term “data-driven” may seem redundant, as people who make decisions might already rely on data. But in the case of data-driven decision making, companies collect data methodically and analyze it rigorously, so that the information represents reality much more accurately.
Business managers typically rely on both qualitative and quantitative sources of information to make their decisions. The difference between this approach and data-driven decision making is how the data is collected and processed.
In the absence of a systematic process to gather and analyze data, what we may perceive as reliable data is actually a hodgepodge of anecdotal evidence, personal impressions, and selective information. This “data” consists partly of intuition and experience, and is not very scientific.
Of course, intuition does have its place in business. But, as an organization grows in scale and the dollar amounts at stake become larger, relying on gut instinct becomes very risky. As mentioned above, with data-driven decision making, the processes of data collection and analysis become methodical and rigorous, so that the information yields a far more accurate reflection of reality.
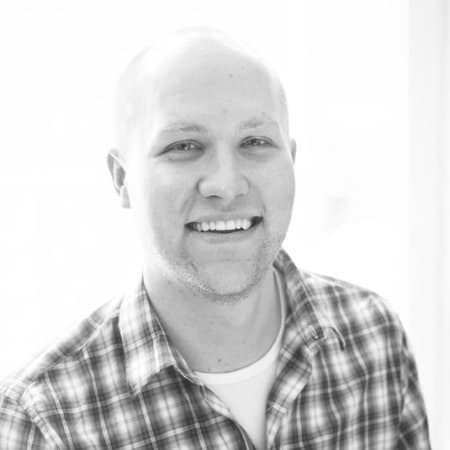
“A big error we see businesses committing is going in 100 percent for data and throwing intuition by the wayside. While data is fantastic, it, by nature, can't think outside of the box,” notes Brandon Andersen, Chief Strategist at marketing analytics firm Ceralytics . “That's up to humans. But, data is a great way to show whether or not what you're doing is working. And, it recognizes patterns that humans may be too busy to see. Data is a tool — an essential tool — but it's not the only tool for decision making,” he says.
Common sense is important too, especially when bearing in mind that correlation does not equal causation. To wit, sage investor Warren Buffett once famously quipped about his fondness for junk food: “I checked the actuarial tables, and the lowest death rate is among six-year-olds. So, I decided to eat like a six-year-old.”
When we talk about big data, eyes inevitably start glazing over. Numbers, unfortunately, give some people anxiety, so the idea of data analytics can deter them.
Business intelligence tools alleviate some of this pain by automating data collection and hiding analytics under the hood. But simply acquiring these tools won’t foster a culture of data-driven decision making any more than a paint-by-numbers kit will nurture artistic ability.
Creating a data-driven culture requires a long-term commitment to educating all members of an organization and championing this effort from the very top. Even then, expect the evolution toward this new way of doing business to take time.
This evolution toward a data-driven culture usually follows five stages:
Data Denial: The organization starts with an active distrust of data and does not use it.
Data Indifference: The company has no interest in whether data is collected or used.
Data Aware: The business is collecting data and may use it for monitoring, but the organization does not base decisions on it.
Data Informed: Managers use data selectively to aid decision making.
Data Driven: Data plays a central role in as many decisions as possible across the organization.
(Note: Some strategists consider “data informed” to be the optimal state because it retains a bigger role for human judgment and balances the use of data with that of personal expertise and insights.)
As companies mature in the data-driven stage, they typically progress in their use of analytics from descriptive to diagnostic, predictive, and prescriptive.
Data-driven organizations share some key attributes:
An emphasis on data collection
An investment in tools and skills to make sense of data
A commitment to making data widely accessible
A willingness to consider data-driven ideas that arise at any level of the organization
A dedication to ongoing improvement
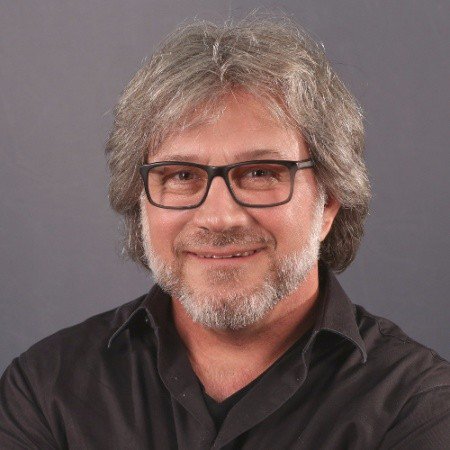
Veteran Data Scientist Paco Nathan of Derwen.ai says that breaking down barriers is an important cultural shift for businesses as they transform into data-driven organizations. “The most common mistake is that organizations tend to react to complex problems by creating silos of people, processes, data, etc.,” he says.
“Silos are the enemy for data-driven business. They tend to create tech debt and other friction, which prevents firms from even beginning to work with data at scale. Getting effective data infrastructure in place, paying down the tech debt to do so, and removing the silos — those are the table stakes in this game,” Nathan continues.
In organizations, change management is becoming increasingly data driven, and analytics using complex data sets are playing an important role in effectively managing organizational change, Harvard Business School professor Michael Tushman and his colleagues have written.
This phenomenon is becoming more evident as big data facilitates continuous improvement processes in which companies implement incremental changes, monitor key metrics, and then make further adjustments based on the findings. Data-driven change management offers some benefits over traditional models. DDDM is less dependent on the expertise of a few key leaders and delivers higher-quality decision making based on facts, a greater capacity to scale changes, agility in modeling change scenarios, and the potential for rapid implementation.
Who Uses Data-Driven Decision Making? Examples and Case Studies
DDDM can work for an organization of any size, from multinational behemoths to family businesses, as long as there’s a commitment to the method’s principles.
Big tech companies have pioneered and perfected DDDM. These firms possess a unique mix of analytical minds, technical expertise, and open culture that is conducive to data-driven decision making.
Facebook : Facebook discovered early that democratizing access to data — that is, making it widely available — enabled the company to be much more agile and responsive to market changes and product development. In one example of the impact of this approach, Facebook looked at how many people started to use a feature through which they could ask a friend to take down a photo of them, but then abandoned that request when they realized they had to write a message to the friend explaining why. Facebook analysts found that if they auto-populated a sample message, the number of users who completed the request rose to 60 percent from 20 percent . That data drove the decision to make the auto-populated message an official part of the tool.
Netflix : A fascinating example of data-driven decision making comes from video-streaming service Netflix, a leader in original programming. (According to a 2018 Morgan Stanley study, 39 percent of U.S. consumers say Netflix has the best original programming — close to three times as many as their nearest rival, HBO.) What’s Netflix’s secret? The company used data analytics to build a highly detailed picture of consumers’ tastes in videos. Then, they invested heavily in developing content that ticked off the viewer preference boxes. The result? House of Cards .
Google : Then, there’s Google’s Project Oxygen, an initiative started in 2008 that mined data from performance appraisals, surveys, and other sources, with the aim of developing better managers. The project looked at the differences between the best and worst bosses in the company based on more than 100 variables. Google used the results to create training programs for managers and identified the eight most important behaviors of high-performing managers, starting with good coaching. The result was an improvement in management and outcomes, such as staff retention and satisfaction. (Several years later, Google updated the research, and in 2018, they refreshed the list of manager behaviors and added two more.)
Southwest Airlines : More traditional businesses have also learned how to harness the power of analytics. For example, Southwest Airlines found that it could use analytics to save on jet fuel. The company also discovered that it could determine which airport gates were open to receive aircrafts so that customers would have to spend as little time waiting as possible.
Walmart : Sometimes, the results of data-driven decision making are a little less glamorous, but nonetheless constitute home runs for a business. In 2004, for example, Walmart used product purchasing data from areas where hurricanes had struck in order to find out what people bought when they stocked up before a storm. The company wanted to use predictive analytics to determine how to supply stores ahead of future storms. They found that in addition to staples, like flashlights, stores saw heavy demand for unexpected items. Sales of strawberry Pop-Tarts jumped seven-fold as a tasty nonperishable that doesn’t require cooking, and beer was the top-selling item. The retailer began sending trucks loaded with these items to stores in areas where hurricanes were forecast, and sales were brisk.
Amazon.com : Under Jeff Bezos, Amazon uses a few simple guiding principles for management. To start, the e-commerce giant constantly tracks key performance indicators (KPIs) to measure its performance. The company also makes it easy for its people at every level (not just executives) to access data related to their roles. And when it comes to making decisions, Amazon’s culture prioritizes data and evidence over seniority and personal influence. Bezos has also realized that the company needs to use data wisely if that data is to supplement the decision-making process, rather than bog it down. As such, he advocates classifying a decision as non-reversible (Type 1) or reversible (Type 2) and devoting only as many resources to each type of decision as necessary. Bezos also suggests that trying to collect all the data needed to make a decision, especially if it’s a Type 2, is unrealistic and expensive. Instead, he says, a preponderance of evidence (70 percent) is enough to get started. You can always correct course later. He accepts that even with DDDM, complete consensus on a decision is not always a given. So, he argues that managers should allow people to gamble on Type 2 decisions and learn from the aftermath.
City of Boston : The city of Boston, Massachusetts has embraced data-driven management with a program called CityScore. The initiative consists of an online dashboard that shows how the government is performing relative to its goals in 24 key areas, such as responding to emergency calls and collecting garbage. Sensors automatically record much of the data, and city workers record information on mobile apps when they complete an activity. The system makes problems apparent and helps ensure the city allocates resources where they will have the most impact for citizens.
A Snapshot of Data-Driven Decision Making in Business
Despite these success stories, the potential for data-driven decision making to make a bigger positive impact remains large for many companies. About six in 10 companies responding to the survey said that their companies make a majority of decisions based on gut feel and experience, instead of data and information. Forty percent of best-in-class companies make decisions based on gut feel or experience, while, among laggards, the figure rises to 70 percent.
Less than half of the companies agree that information is “highly valued for decision making” or “treated as an asset in their organization” today. However, a full two thirds expect that it will be in the future. Only one third of enterprises use data to identify new business opportunities and predict market shifts. Again, though, most of the other two thirds say they want to do those things going forward. And on average, only about half of all available information is used for making decisions.
Andrew McAfee and Erik Brynjolfsson, professors at the MIT Sloan School of Management, conducted a survey and found that the companies that were mostly data driven had six percent higher profits and four percent higher productivity than average. While those might sound like small differences, the impact on results is substantial. Eighty-three percent of respondents to a survey by The Economist said that using data had made their existing offerings more profitable .
Key Terms in Data-Driven Decision Making
Before we discuss how to get started with DDDM at your organization, you’ll need to be familiar with some terminology.
DataOps : A methodology for managing data and streamlining data analytics that combines data quality with integrity management, data security, and data engineering.
Data Cleaning or Cleansing : The process of finding and either fixing or deleting data set records that are inaccurate, incomplete, or corrupt.
Data Governance : Overseeing the integrity and security of data owned and used by an organization, as well as managing that data’s availability and type of use.
Data Warehouse : An integrated, centralized store of all the data an organization collects and uses for keeping records, reporting, and performing analytics.
Data Mart : A subunit of the data warehouse that deals with a single department at an organization.
Descriptive Analytics : The preliminary stage of data processing in which one extracts historical insights from data and prepares it for more advanced forms of analysis.
Diagnostic Analytics : The branch of data analytics that focuses on determining the causes of phenomena.
Predictive Analytics : The branch of data analytics that focuses on extracting patterns from historical data with the aim of predicting future events.
Prescriptive Analytics : The branch of data analytics that focuses on using data to determine the most appropriate course of action when there’s a decision to make.
Four Vs of Big Data : The four Vs are the four critical characteristics of big data. Volume refers to the amount of data. Velocity is the speed at which data is collected and/or analyzed. Variety is the number of different sources from which data is accumulated. And, veracity is a measure of how trustworthy the data is.
Database : An organized collection of data records that can be accessed through a computer system.
Relational Database : This is a type of database that can recognize pieces of data in relation to other pieces of data in the same database. This type is of limited utility for big data sets, as its need for structured data means that performance slows down as the size of the data increases.
NoSQL : A big data-friendly database that, thanks to its unstructured nature, can maintain high performance when dealing with very large data sets.
Hadoop : This is an Apache software ecosystem that enables parallel computing on a very large scale by allowing data to be spread across a large number of servers. Hadoop can radically reduce data-processing times.
CAP Theorem : This refers to the idea that a distributed database can only have two of the following three qualities: consistency, availability, and partition tolerance. There are a couple of alternative philosophies for data system design that illustrate this principle: ACID prioritizes consistency, while BASE prioritizes availability.
Data Point : A measurement derived from a single member of the units being observed (in other words, a fact).
Data Visualization : A data presentation technique that uses visual tools to help people understand data in context.
Data Gap Analysis : A technique that uses analyses of existing data to determine where an organization is failing to collect or analyze other potentially useful data.
Key Performance Indicator (KPI) : A defined measurement, collected regularly, that offers a snapshot of organizational performance in a specific area.
Data Model : A data model defines how various points of data within a database are connected, processed, and stored in relation to one another.
Metadata : This refers to data about data. Metadata records information about other data.
Ad Hoc Analysis : An analytics process built to answer a specific business question.
Application Programming Interface (API) : A software mechanism that allows applications to access features of other services, such as operating systems and other applications.
Artificial Intelligence : The constructed ability of machines to simulate human intelligence.
Machine Learning : A branch of artificial intelligence in which computers have the capacity to learn by figuring out patterns in data.
Contextual Data : Data that allows people, items, or other entities to be considered in context (as part of a bigger picture).
Extract, Transform, Load (ETL) : A combined set of database functions that extract data from one database and put it into another data repository.
Hierarchy : The organization of data points into a systematic structure.
Slice and Dice : The process of breaking up data into its constituent parts to facilitate the extraction of information.
Zero-Latency Enterprise : This refers to data architecture with the ability to exchange information across technical (OSs, DBMSs, programming languages) and organizational boundaries in near-real time, with negligible delays in transmission. Latency is the delay between an instruction for the reception of a transfer and the sending of a transfer.
Insight Platforms-as-a-Service (IPaaS) – This refers to the cloud-hosted big data services that are intended to make data analytics more widely accessible. They provide the tools needed to parse insights from data.
Translytics : A category of databases that can maintain high performance when dealing with both transactions and analytics.
A Step-by-Step Guide to Start Data-Driven Decision Making
Now that you’ve learned where and why organizations are using DDDM, you may want to start implementing this approach at your own enterprise. Large amounts of data can quickly become overwhelming, so follow these steps to get going without headaches.
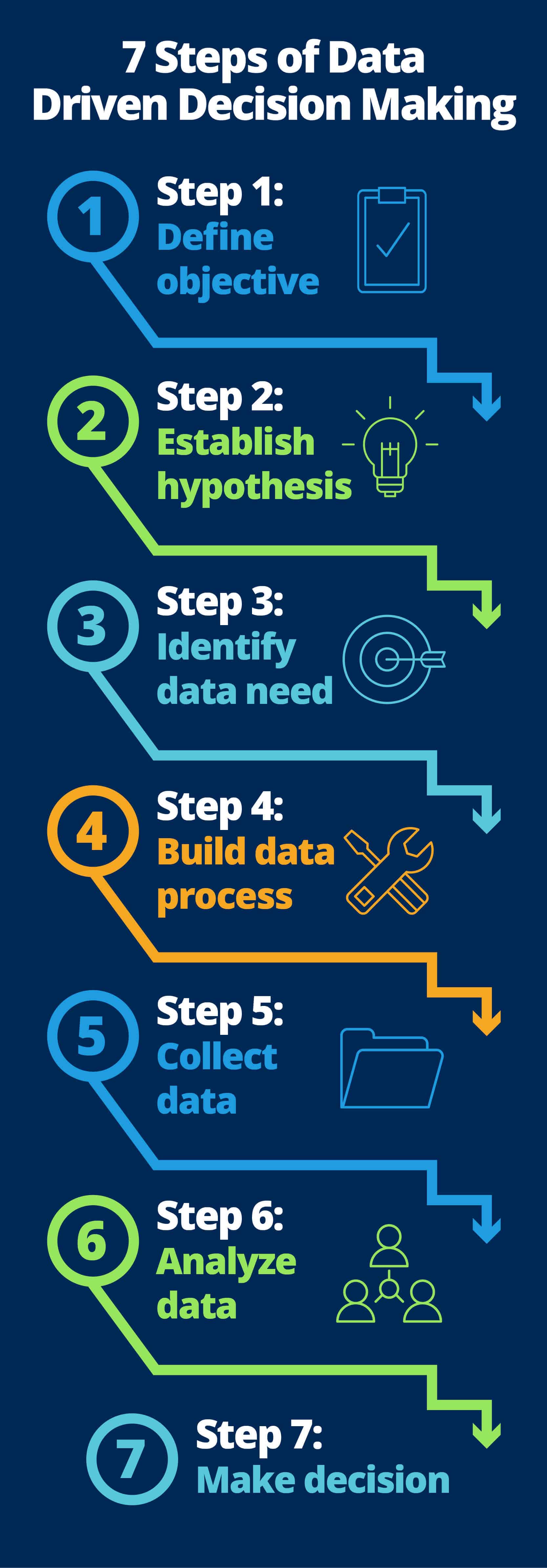
Use this downloadable checklist to help guide your process. The sheet walks you through important steps, such as forming your team and communicating your decisions.
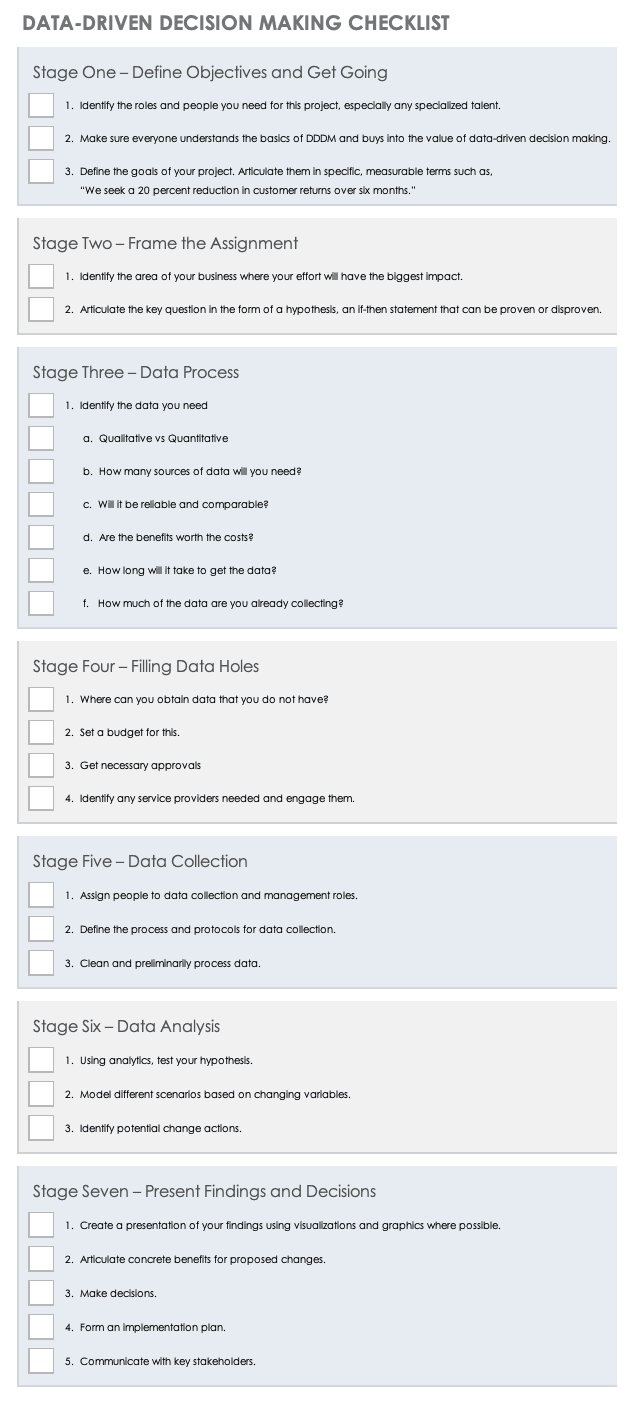
Download Data-Driven Decision Making Checklist
Excel | PDF
Steps to Implement Data-Driven Decision Making at Your Organization
Following are the seven steps to implement data-driven decision making at your organization:
Step 1: Start by Defining the Objective and by Fostering a Culture : First, figure out what goals your business hopes to achieve through DDDM. Identify your business goals, and then build a broad strategy around them. At this stage, it’s fine if the strategy isn’t completely defined. But, you do need to know how you think big data will help achieve your targeted outcomes. For example, if you own a big chain of grocery stores, you can examine customers’ fruit purchases with the goals of improving the supply chain, the placement of products in the store, the pricing, and even the packaging of store-brand offerings. Articulating these goals will shape your data collection and analytics strategies from the outset. Also at this stage, you’ll want to lay the groundwork for success by communicating to all members of your organization your commitment to data-driven decision making. This shared value and culture is just as important as having the infrastructure and tools in place. By committing to using data, you’re acknowledging its effectiveness — and you have to do so in a way that breeds confidence in everyone else. Determine whether your goals require using real-world data for analytics or experimental data for simulations or modeling. Real-world data is what you’re collecting and analyzing in the grocery store example, where you’re trying to figure out what is happening in an existing business. Experimental data , on the other hand, is collected from a controlled environment with the aim of figuring out what business practices work best.
Step 2: Focus on a Specific Business Area and Define Questions : Determine which area of your business needs the most attention. Typically, the answer is customers, finances, or operations (or some mixture of the three). Which do you suspect will bring the most benefits in terms of business growth? Prioritize the area that will have the biggest impact. Your next step is to identify the specific business questions you want to answer. Which of these are related to the business goals we talked about in step one? Formulate this thought in the form of a hypothesis, a proposed if-then statement that can be proven or disproven and that you can use as a starting point for investigation. For example, “If we add a self-service portal to the website, we will decrease the number of after-hours emergency trouble calls.” Now, you’ll also know the specific data sources you need to focus on and which data you can hope to collect from them. When you identify in advance the specific data you need to collect and how you’re going to collect it, you spend much less money, time, and stress than if you were simply to collect everything and try to figure out what to do with it later.
Step 3: Identify the Data You Need : The data you examine needs to be relevant to your business question. To build on our grocery store example, customer payment receipt data would be useful; ambient store temperatures, not so much. There are two broad categories of data, and since each informs decision making, you’ll want to collect both:
Qualitative Data : This kind of data is non-numeric and subjective. It is observed, rather than measured. For example, having a store worker ask people a few questions about their shopping experience will generate qualitative data. The shoppers may say things like, “The meat section is too far from the wine section” or “I have to make my trips super short because my toddler won’t sit still in this shopping cart seat.”
Quantitative Data : This kind of data is numerical and objective and is measured, not observed. Quantitative data is what people think about when they think of big data: rows upon rows of data concerning who purchased what, how much they spent on it, when they bought it, how often they buy it, and so on. Not all data will be suitable for use. The data must measure something meaningful, and must also be accurate, formatted correctly, and not contain duplicates. For these reasons, data collection methods often undergo a pilot test before they’re rolled out in force. If you’re working from existing data, you may need to “cleanse” the data before conducting analytics, which involves verifying information, fixing errors, and removing redundancies. To get a sense of your data’s quality, here are some key questions to answer:
Who collected the data, and is it reliable? Reliability requires that it measure what it claims to, that all data was collected using the same parameters, and that it does not contain false responses (such as a fake email address).
How was the data sampled? Is the data representative of reality? Or, were only certain customers asked to respond?
Does the data include outliers (exceptionally high or low measurements)? How are these affecting the overall data distribution?
Are relationships that you see as causal truly causal? Or, are confounding factors and intermediate variables giving an inaccurate impression of causality?
What are the assumptions underlying your data, and do they hold up to scrutiny? For example, we might assume that people buying groceries are buying them mainly for themselves, but we don’t know how true that is, since shoppers are often running errands for other people.
And, most crucially, why have you decided to analyze data one way and not another? Even if you end up ruling out some data, you’re likely to find that you have several internal data sources; one survey found that the median number of data sources for companies is five . Companies on average have three external data sources. What’s more, half of the people who work in data-driven businesses say the number of data sources they use is growing. Having multiple sources of data means that you can choose the best option for collecting data, based on whichever is fastest, cheapest, or least onerous.
Step 4: Determine How You Will Get the Data You Do Not Have : You may find that you already collect some of the data you need. If this is the case, count yourself lucky: You will save on data collection costs, and you can begin analysis sooner. If you do not already have the data, you have to figure out how to get it. Think of the four Vs of big data: How can you optimize volume, velocity, variety, and veracity? Is it by collecting the data yourself or by getting it from an external source? Remember that holes in the data are more likely to crop up when different departments use different systems to collect, manage, and report that data. Some systems, which cross-analyze data that comes from different sources, can help solve this problem. Having a variety of data sources is problematic if the data does not use common variables, which enable you to integrate those sources. If the data does not use common variables, Northeastern University instructor Joel Schwartz encourages you to consider whether the data could be useful in other future projects. If so, it may be worth the investment of time and resources to solve these data disparities now. Like any investment decision, big data demands that you have a clear case that weighs the costs against the expected benefits. Data is cheap, and it’s getting cheaper. But, it’s not free, especially if you’re collecting it yourself, and storing it incurs costs well into the future. That’s why it’s important that you focus only on the data that stands to deliver meaningful value. And, that process requires some homework. If obtaining and analyzing the data is going to cost more than the potential benefit, it’s a bad business decision. Here’s a helpful tip: There are ways to reduce data costs, such as by looking at alternate sources or alternate data collection methods. However, if you’ve reached this stage without considering how you’re going to benefit, even cheap data isn’t going to turn a bad investment into a good one.
Step 5: Collect the Data : When we talk about big data, we’re usually talking about the automated data collection of thousands, millions, or even more cases. But, data analytics can also work with smaller quantities of data that are collected by people. In either scenario, there are three things to be done:
Assign People to Data Collection and Data Management Roles : These people are the first — and sometimes the only — check on the veracity of the data.
Define the Processes and Protocols of Data Collection : This is a critical step, because the methods of data collection have a direct bearing on the four Vs of the data. Identify data sources and spell out the precise methods of data collection — that is, whether the data is being recorded by a machine or by a human. You should also create a data dictionary to catalog and define each of the recorded variables.
Clean and Do a Preliminary Processing of the Data : Cleaning data is arduous but vital work to ensure integrity and usability. This process looks for duplicated, incorrect, or corrupted data. The analyst may also run preliminary descriptive analyses, such as data distributions, which will inform more advanced analytics. Data is useful when you have a variety of it, and that means gathering data from different sources. Data from a single source, or even data from similar sources, is unidimensional, offering only a limited number of ways to think about the data. You can also find or buy pre-cleaned data sets. These are of variable utility, depending primarily on the data’s relevance to your goals. “The most common error that we see in implementing data-driven decisions is neglecting to validate the data before drawing conclusions. It's so easy, when the data is available and ready, to assume it's correct and immediately actionable, but this is often not the case,” explains Sam Underwood, Vice President of Business Strategy at data analytics firm Futurety . “Especially in organizations that have not previously relied heavily on data to drive action, this organizational data may not have undergone a quality control or governance process, and it is likely to be incorrect in many cases. We estimate that when we inherit our clients’ data, around 50 percent of it has major accuracy issues. We often build in a two-to-four-month data-cleaning phase before committing to any deliverables that use the data,” Underwood says.
Step 6: Analyze the Data : You are finally getting close to realizing insights from your data. There are a number of platforms available for big data analysis, and people with a strong knowledge of Excel can use some of them. But, more advanced and potentially more valuable analytics call for a trained specialist. Add that to the costs of dabbling in big data. Here’s a helpful tip: Even if you do not have the skill to do this analysis yourself, it’s still a good idea to know what type of analytics you need (such as diagnostic or predictive), because analyzing different data types calls for different skill sets. Dealing with quantitative data is relatively straightforward, but qualitative data, like images, video, speech, and text, calls for different conceptual knowledge and technical skills.
Step 7: Present the Findings and Make Decisions : Presenting the findings is a surprisingly difficult part of data analytics. After someone has crunched the numbers to come up with insights, you must deliver these findings to the right people at the right time in the right way. Luckily, there’s no shortage of interesting ways to present data. Even complex ideas can be easily communicated through good writing, clear presentation, and helpful graphics or visualizations. The aim is to connect the insights you gained to actions that will benefit the business. Remember to clearly portray both the reward and the risk of big data-driven decisions. As David Ritter writes for Harvard Business Review, if the risk of acting on something and being wrong is too high , acting on even firm insights can be a mistake.
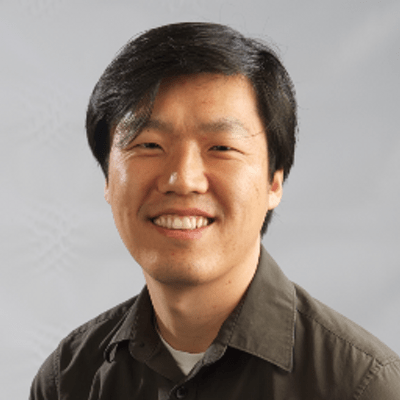
Based on your findings, formulate action recommendations and an implementation strategy. A common pitfall is “not setting clear expectations around the decision- making process,” says James Horey, CEO of Reviewbox , an application that helps vendors manage product information on e-commerce sites. He continues, “For example, what do they expect team members to bring to the table? How will decisions actually be made? When will there be ‘enough’ data to make the decision? The best way to overcome this is to come up with, document, and communicate this process to all the relevant team members.” He adds, “As the data becomes easier to collect, the temptation increases to continually pass the buck and conduct more research, which leads to decision paralysis. Effective organizations understand that most decisions need to be made in a timely manner, and they, therefore, often employ an 80 percent rule: We'll make the decision once we're confident that we have about 80 percent of the available information.”
Managing Team Members Who Are Data Averse
Despite your enthusiasm for data, you will probably find people in your organization who remain reluctant to embrace it — and not always for the wrong reasons. Why are people averse to big data, and what can executives and managers do to overcome this resistance?
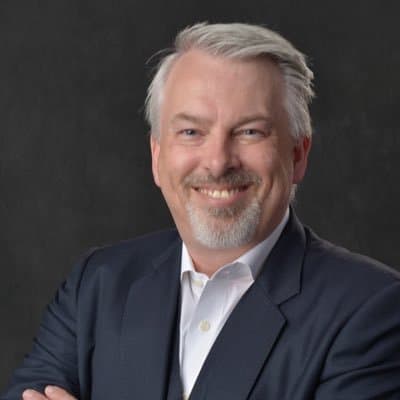
“Frequently, upper-level management can have a negative effect on the right use of big data,” says Carl Hillier, Head of Product Marketing for Ephesoft software, which uses machine learning to capture unstructured data. He adds, “These managers may resist making decisions based on data, which can undermine your data-related investments. That's why it's important to encourage team members and peers to use data to test intuition and solidify ideas.”
Thomas C. Redman, President of Data Quality Solutions, says that people aren’t apathetic toward big data as much as they are afraid of it , as analytics and data-driven predictions move from specialist areas into the mainstream. Data now feels pervasive and is transforming work and power structures, causing many people to fear for their jobs. This reaction is perhaps not surprising, considering that people like venture capitalist Mark Kvamme have predicted that 20 million jobs will disappear from the United States as a result of artificial intelligence.
Others fear that big data is a false hope because the expertise to realize its potential is not sufficiently available at many companies. Some naysayers, remembering times when data led them astray, fear catastrophic mistakes.
Moreover, there are a number of less laudable concerns. Big data generally accompanies a shift toward greater accountability for results, and that may shine an uncomfortable spotlight on people who try to get by with the bare minimum of work.
Managers and business leaders can allay many of those more reasonable fears from the outset, so that DDDM does not provoke resistance. Here are some essential tips:
Don’t Frame Data Knowledge as an Arcane Skill Set : If you do, you foster a belief that data is strictly the domain of gifted mathematicians and quants. Instead, invest in teaching people about analytics, and encourage them to use data to answer simple questions. (For example, “how often do team meetings start on time?”) The more they learn about analytics, the less likely they will be to fear data. Lead by example and expand your own skills. Read a book on the subject, such as A Practitioner’s Guide to Business Analytics by Randy Bartlett or Keeping Up with the Quants by Thomas Davenport. Enroll in one of the classes listed at the end of this article. Practice collecting some simple data, learning how to graph it, and performing some basic statistical calculations, such as figuring out the mean, median, mode, variance, standard deviation, correlation, and probability.
Don’t Overpromise : With both the C-suite and front-line staff, you want to set realistic expectations about what you can accomplish with data-driven decision making and how long it will take to get there. Present data as a tool, albeit a powerful one, not an earth-shattering paradigm shift. Manage expectations.
Show Data in Action : Look for opportunities to show how data not only helps the business make more money, but also helps individuals do their jobs better by saving time and resources or decreasing stress. (For example, data shows how much traffic a store has gotten on past Super Bowl Sundays, so you can schedule enough people to provide good service, but not so many that staff are idle.) Publicize successful use cases. Don’t forget to nurture champions for data — those individuals who act as leaders on your journey toward DDDM.
Common Pitfalls in Data-Driven Decision Making and How to Avoid Them
As using data has helped businesses make better decisions, enthusiasm for DDDM has grown. Your information is an asset and a resource, but it’s crucial to remember that data is only as valuable as the benefits you derive from it.
The key to making data worth its costs is identifying how you hope to use data and then figuring out what data you need. Working backward by gathering data and then trying to find a use for it is wasteful at best and useless at worst. Unfortunately, one of the leading complaints is that decisions were made without data because the right data was not available.
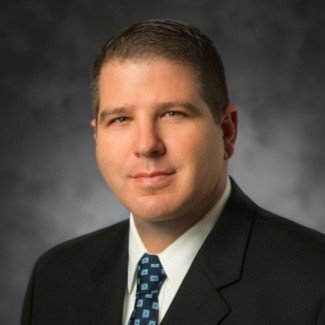
Derek Wilson, CEO of predictive analytics firm CDO Advisors , says that companies new to DDDM can run into problems by jumping into the deep end. “Start with easy-to-understand problems and solutions. Team members need to be able to understand the data and decisions,” he urges. A common mistake, he says, is “businesses that start with too many process changes at once. Implement data-driven decisions one by one and get quick wins to build confidence in the team.”
Another pitfall involves the inherent complexities of big data. Collecting massive amount of information, integrating it, extracting insights, and turning those insights into action-oriented recommendations in real time present challenges. So, expect to put a lot of work into designing your process, and realize that errors can arise at any stage. Failure to spot them can result in problems or poor decisions, so even with analytics, you can’t work on autopilot.
Human nature also confounds our attempts to make decisions based on data, as we have a maddening tendency to favor our guts over the numbers. While this can sometimes lead to home runs, the tendency subverts the intention of DDDM.
Human cognitive biases can muddy the waters further. We may cherry-pick numbers, falling victim to confirmation bias. Or, the highest paid person’s opinion (the delightfully named HiPPO) may sway us, or we may subscribe to the same assumptions about the data as everyone else (groupthink).
There’s a litany of other phenomena that skews our thinking, from loss aversion and the sunk cost fallacy (making a decision based on not wanting to waste resources that have already been spent and cannot be recovered) to the Texas sharpshooter fallacy (when differences in data are ignored, but similarities are stressed, leading to false conclusions) and the IKEA effect (a cognitive bias in which we place an irrationally high value on things we had a hand in creating).
As a final caution, critics say that over-reliance on data may go too far in removing human judgment from decisions or may dehumanize the subjects of the data, turning staff and customers into “just a number.” Many people also have privacy concerns around large-scale data collection and storage.
Building a Data-Driven Business Culture
Organizations that have successfully built a culture founded on data-driven decision making say the most important thing to remember is that your company will not transform overnight. As Klipfolio’s Marketing Director Jonathan Milne says, you have to “be patient, take your time, and start small.”
To create the conditions for a data-driven culture, you must do the following: collect high-quality data within a useful timeframe; make sure the costs of your information architecture are reasonable; and be sure the data is easily accessible. Some experts urge companies to begin collecting data as early as possible, so they have benchmarks, while others caution against a scattershot approach.
Moreover, you need to know how to present, visualize, and talk about data. Create an internal data dictionary that outlines the content, format, and structure of your databases. Make sure that managers have at least basic statistics training.
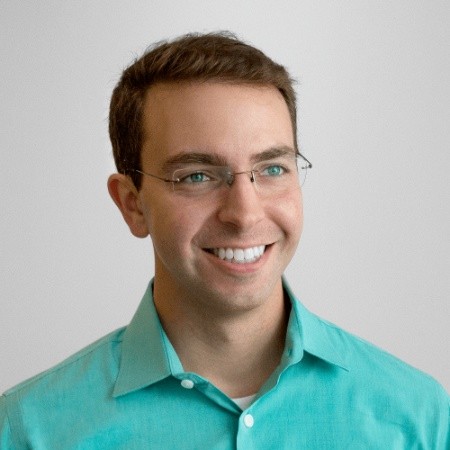
Tom O’Neill, CTO and Founder of business intelligence platform Periscope Data , has worked with more than 1,000 teams of data scientists and offers some advice based on the most successful teams:
Train everyone at your company to use and interpret data accurately. Recognize that no one is born data literate. All employees should know how to determine if they're using metrics, goals, and conclusions correctly.
Analysts must work tightly with their business counterparts and drive change together. Intentionally include different levels of experience and perspectives throughout the process to reduce the biases that homogenous groups exhibit. Using this mix of people will minimize blind spots and maximize potential solutions.
Encourage curiosity in your culture. Everyone should be empowered (and expected) to ask a lot of questions, and there need to be resources available to provide answers. You should regularly dissect KPIs into their components to provide new perspectives on what the non-curious might see as a simple sum or projection.
Use data to make testable, tactical predictions. Then, take actions based on those predictions and feed the results back into your process to improve future decisions. The data-driven decision engine looks a lot like the scientific method. Historical analysis should be focused on learning why something happened, not just reporting what happened.
Your management style must also change as your culture evolves. Data scientists Jeff Bladt and Bob Filbin say that in organizations making the transition to being data driven, there are always people who attempt to subvert the change and those who embrace it. People who adopt the data-driven approach do so to improve either their perceived or their actual performance, Bladt and Filbin say. People who have low organizational prestige but are high performers will welcome the use of data, believing that it will make their contributions more evident. With encouragement, these individuals can turn into data champions.
Conversely, highly regarded team members who are actually poor performers remain distrustful of data, which they fear will reveal their shortcomings. Many of these people are unlikely to change their views, and, over the long run, they will probably need to leave the company for it to become truly data driven.
Data-driven decision making needs to be embraced at the upper levels if it’s going to take root in the organization. Given that the successful implementation of DDDM requires particular qualities, such as a collaborative style and an openness to solutions that may come from anywhere in the ranks, the process itself demands practitioners who model from the top down and across departments.
Encourage consistent metric tracking (such as KPIs) throughout your organization as a way to get people to focus on the relevance of data to business objectives. Building a dashboard that gives you a fast snapshot of those KPIs can aid this effort.
In addition, you may be wondering where in your organization responsibility for data-driven business intelligence should reside. (This responsibility is also referred to as data governance .) Cross-departmental data “competency centers” are much more likely to be responsible for data in best-in-class companies (52 percent) than they are in average companies (40 percent) and stragglers (28 percent), according to a survey regarding corporate data governance . When asked, “Who manages and governs data for decision making?” 60 percent of respondents said it was the finance department, while 41 percent said it was the IT department, and, again, 41 percent said it was a competency center.
Strong data governance assists DDDM. You can support that governance by fostering agile IT infrastructure, cross-departmental data management, a collaborative decision culture, and the use of KPIs throughout your organization.
The Origin of Data-Driven Management and the Technologies behind It
DDDM became possible with the advent of databases that were capable of collecting and storing large amounts of information and the arrival of systems that could crunch this data. The emergence of networked computers and the internet has also played a major supporting role in the growth of big data.
The term big data began circulating around 2005, but the concept of using data to track and control operations has been around since the first entrepreneurial activities of ancient Egypt. Systems more akin to what we have today arose in the late 1930s, when IBM developed a punch-card reading machine to keep records for the new Social Security System. In the 1960s, electronic records storage began.
In the early 2000s, big data was in its infancy, and analytics consisted primarily of ad hoc data manipulation summarized in reports and KPIs. As the volume of data grew, the quantity of information became overwhelming, and data analysts bumped up against limitations. They found themselves unable to drill down to see which branches were underperforming or to compare performance to historical trends.
The introduction of Hadoop in 2005 marked a major milestone in the development of big data. Hadoop is an open-source operating system that performs the distributed parallel processing of vast quantities of data. Hadoop was invented to empower the analysis of large data sets; its ultimate goal was to be able to index the World Wide Web.
Hadoop became the backbone of big data operations and gave rise to sophisticated analytics operations that included interactive dashboards and data warehouses. Inspired by Agile software techniques and DevOps , DataOps began to spread around 2017 (a methodology to improve the quality and speed of data analytics). Simultaneous with these improvements, the volume of information has continued to explode. As of mid-2018, an estimated 4.4 trillion gigabytes of digital information has been stored and made accessible to web users, with 90 percent of that having been created in the preceding two years.
Analytics has changed a lot in a short time. Today, the process is much more user friendly. Data collection is largely automated, and, while setting up that automation is still a specialist’s job, it is far easier than it used to be. Dashboards and data visualizations make data much easier to grasp, and generating a report is much less laborious and complex than it used to be.
Cloud solutions are making data analytics more accessible to organizations without traditional data expertise or experience.
To understand how we got here, you need to trace the developments of databases and data warehouses since the turn of the millennium. Early on, data would be stored in structures called operational databases , or online transaction processing (OLTP) databases , which were designed for transactional systems , such as the withdrawal of money from a bank account.
But, these transactional databases didn’t work well for analytics, so data warehouses arose. A data warehouse, or online analytical processing (OLAP) database , is a database that is layered on top of an OLTP. This additional layer allows analytics to run efficiently. To move data from OLTPs to OLAPs, a process called extract , transform , load (ETL) comes into play. It extracts data from a source, transforms it into an analysis-suited format, and loads it into the data warehouse.
But, ETL was cumbersome, and the problem grew as the 4 Vs of data began expanding. Older database structures, called SQL or relational databases , couldn’t handle the increased demands. As data turned into big data, we needed storage and analytical technologies that could keep up with the growth.
The answer came in the form of NoSQL databases and Apache Hadoop open-source software utilities for the distributed storing and processing of data. SQL databases only work with predictable, structured data, and to crunch more data, you need more computing power. NoSQL databases work with unstructured data, and you can handle more data by distributing the load over more ordinary servers. Also, during transaction processing, SQL databases prioritize properties summarized by the acronym ACID, whereas NoSQL databases work on the BASE model. While the intricacies are complicated, the main takeaway is that ACID is exceedingly structured and rigid. In addition, this model does not work well with big data, distributed computing systems, and unstructured data. The BASE model provides the needed flexibility.
With NoSQL came Hadoop, which, in simple terms, is a processing framework that allows for parallel computing. In other words, it has the ability to solve massive computations in parallel over a network of computers. Hadoop, in conjunction with NoSQL, is the superhero of big data analytics: This combination is cheaper than data warehousing, it’s not limited to structured data, and it’s suited for both storage and computation.
As it played out, however, an even better solution turned out to be a combination of Hadoop and data warehousing: Hadoop provided the capacity to store incredibly large quantities of data, and for analytics, ETL moved data from Hadoop into warehouses.
As data began to amass in NoSQL databases, the ETL process for analyzing it became less and less efficient, so analytics for NoSQL were developed. When these ran against constraints, the workaround became a combination of SQL and NoSQL for storage and analytics. A more recent development is translytics , database solutions that handle both operations and analytics with ease.
We can now do more with our data. As big data has matured, we are moving along the continuum from descriptive to diagnostic and predictive analytics, with prescriptive analytics increasingly within reach. The most familiar big data application is predictive analytics: the use of historical data to detect patterns and forecast data. Predictive analytics’ combination of increased storage and increased computational power allows us to use machine learning.
Tech analyst Rob Enderle says , “Firms that can change their practices to make decisions data driven will, as these practices shift, quickly surpass those organizations that do not.”
If your organization has been slow to start with DDDM, perhaps you’re concerned that your company will be left behind. But, there is a way to jumpstart your effort — by using cloud-based analytics. These platforms, called IPaaS (insight platforms as a service), have recently proliferated. These applications are designed to handle the integration of data and the generation of intelligent insights. Outsourced cloud solutions are not as customized as something designed in house, but they are less costly. And, if you are struggling to get going, these tools are efficient. Embedded analytics , so named because the analytics functionalities are built into business applications, are another option. Both of these enable you to harness data at a low starting cost and with minimal data expertise.
A final technological development to be aware of is the attempt to make analytics work in real time, minimizing the lag between the generation of data and the generation of insights. In-memory processing , also called streaming , tackles this problem by using large-space RAM and flash memory, since the processing of data that’s in memory is faster than the processing of data that’s stored in disk. And, faster hardware — processors and chips — is in development.
What’s the Future of Data-Driven Decision Making?
DDDM has come a long way in a short time and is likely to continue evolving at a breakneck pace. Particularly for tech companies, data acquisition is driving ever-faster innovation cycles as well as response times to market conditions. These trends raise interesting questions about the future of DDDM.
Tech companies have been leaders in pushing the limits of DDDM. In early 2018, Twitter CEO Jack Dorsey said the network had received questions about whether it was possible to measure the “health” of conversations on Twitter; the company began exploring data solutions that could make these judgments.
The impact of DDDM on the work experience and satisfaction of humans is receiving increased attention because of the larger social implications. A study by researchers at Carnegie Mellon University found that people whose work assignments were driven by algorithms — in this case, drivers for ride-share apps — experienced dissatisfaction when the assignments didn’t make sense to them or made them feel they did not have enough control. The drivers felt that some algorithmically driven decisions “failed to accommodate their abilities, emotion, and motivation.”
The rise of automation in DDDM, a trend that is accelerating due to artificial intelligence, is also stirring debate. A good example of automated data-driven decision making is ride-sharing app Uber’s dynamic pricing, which puts demand data into an algorithm to determine when premium fares will prevail.
Automation removes the possibility of human bias and human error, but also takes away the soft skills that humans provide. Automated price surges at Uber have occasionally gone into effect at inopportune times, such as after a terrorist attack in London in 2017. The company suspended the fare increase when it became aware of the incident, but consumers had already lashed out at the company on social media, accusing it of profiting from the attack.
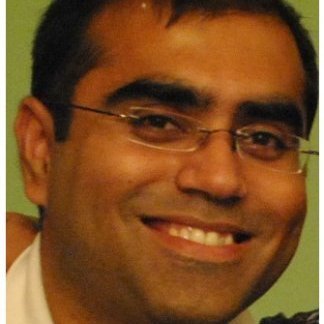
As Ketan Kapoor, CEO and Co-Founder of human resources technology company Mettl, says, “You can certainly automate tasks that have clearly defined input parameters and no grey areas. If estimates or grey areas are involved anywhere in the process, human intervention is required.”
He continues, “But, with the advent of AI, as you keep feeding the system with complex-data points, systems in the future will … deliver accurate inferences from the supplied data, irrespective of how complex the data is … It will take time to shape up. However, the day is not far off when human intervention will be merely a redundant layer in the DDDM.”
Nathan of Derwen.ai recommends viewing machine-led and human-led decision making as a continuum: “Every business problem should now be viewed as something to be solved by teams of people plus machines. In some cases, there will be mostly people, while in others, there will be mostly machines. Still, you should always start from a baseline of managing teams of both.”
In human resources, analytics allows companies to examine things like headcount trends and worker demographics across units, departments, and organizations. By cross-referencing this data with desirable outcomes, such as productivity, analysts can look for relationships between demographics and performance.
Education is a growing focus for DDDM. Schools amass mountains of student performance data, using quarterly report cards and standardized testing. Some educators are analyzing this data for indications of which specific instructional methods (or teachers) have the greatest positive impact on learning. Then, these teachers are adjusting their teaching practices accordingly. These analyses can be simple to conduct if a school system is willing to invest in data expertise. Some helpful studies on DDDM in education include one by Michael J. Donhost and Vincent A. Anfara Jr. in Middle School Journal and another by Marlow Ediger in College Student Journal .
With many schools providing students with tablets, which can function as data trackers, analysts have the potential to look at numerous variables, in addition to test scores. Educators can map personalized data and examine associations, such as whether the time spent on certain activities predicts performance improvements for low-achieving students or which instructional materials engage students the most, based on how many times they look away from the screen.
Data-Driven Decision Making Increases Demand for Data Scientists and Specialists
As data-driven decision making takes hold, there is an increasing demand for people who have trained in data analysis tools and techniques.
Most major U.S. cities have a shortage of people with data science skills. In fact, as of late 2018, there was a national shortage of more than 150,000 people, according to LinkedIn. The shortage is especially acute in New York City and the San Francisco Bay Area. The U.S. Bureau of Labor Statistics predicts that by 2026, 11.5 million more jobs will be created in data science and analytics.
Website KDNuggets, which has an audience of data scientists, estimates that in 2018, there were already between 200,000 and 1 million people worldwide doing data science-related work. However, a 2015 poll of its members found that most expected expert-level predictive analytics and data science tasks to be automated by 2025. To remain in demand, data specialists should focus on cultivating skills that are hard to automate, like business understanding and storytelling.
According to InsideBigData.com, 88 percent of data scientists have master’s degrees, and 46 percent have doctoral degrees. The talent shortage is exacerbated by a lack of university programs in data science, and that is prompting 63 percent of the companies that need these skills to provide in-house training.
Even if you aren’t ready to embark on a degree program in data science, you can improve your DDDM expertise with a variety of courses and certificate programs. Check out some of the leading options below, including online, self-paced, free, and classroom alternatives.
Course Name | Platform | Taught by | Free/Paid |
---|---|---|---|
Coursera (online) | Alex Mannella, PwC | Paid (but financial aid available) | |
EdX (online) | John W. Byers, Chris Dellarocas, Andrei Lapets, Boston University | Free (without certificate, paid for certificate) | |
Management Concepts (live classroom, virtual classroom) | - | Paid | |
Hyper Island In Person (international, in person) | - | Paid (with waivers available) | |
Northeastern University (live classroom, online) | - | Paid | |
Learn@Forbes (online) | Frank Kane, Sundog Education | Paid | |
Duke’s Fuqua School of Business (in person) | Alexandre Belloni, Peng Sun, Saša Pekeč | Paid | |
Udemy (online) | Douglas Clark | Paid | |
Cleveland State University (online) | - | Paid | |
NYU Stern School of Business (in person) | J.P. Eggers | Paid | |
Michigan Virtual | - | Paid | |
MIT (online) | Devavrat Shah, Philippe Rigollet, et al. | Paid | |
Cornell (online) | Donna Haeger | Paid |
Improve Data-Driven Decision Making with Smartsheet for Project Management
Empower your people to go above and beyond with a flexible platform designed to match the needs of your team — and adapt as those needs change.
The Smartsheet platform makes it easy to plan, capture, manage, and report on work from anywhere, helping your team be more effective and get more done. Report on key metrics and get real-time visibility into work as it happens with roll-up reports, dashboards, and automated workflows built to keep your team connected and informed.
When teams have clarity into the work getting done, there’s no telling how much more they can accomplish in the same amount of time. Try Smartsheet for free, today.
Discover why over 90% of Fortune 100 companies trust Smartsheet to get work done.
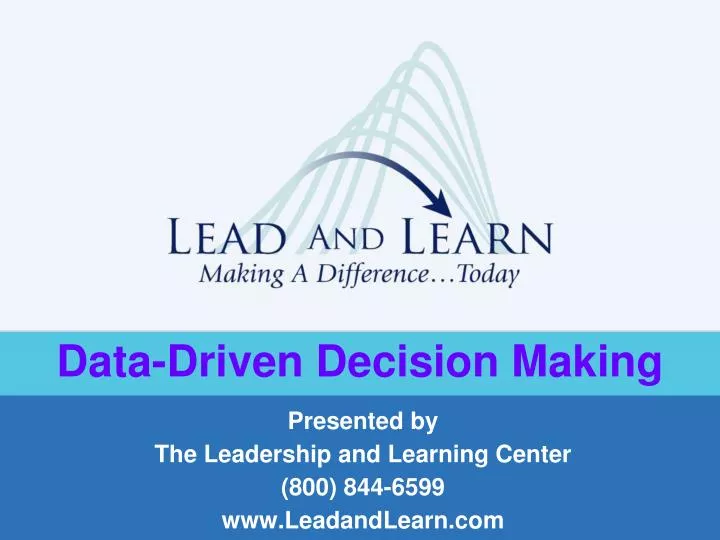
Data-Driven Decision Making
Oct 01, 2014
760 likes | 1.38k Views
Data-Driven Decision Making. Presented by The Leadership and Learning Center (800) 844-6599 www.LeadandLearn.com. Part One: Objectives. Develop a deeper understanding of the Decision Making for Results: Data-Driven Decision Making process
Share Presentation
- student achievement
- data driven
- effects results data
- step 2 analyze data
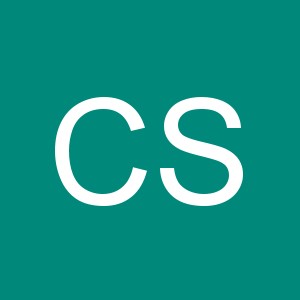
Presentation Transcript
Data-Driven Decision Making Presented by The Leadership and Learning Center (800) 844-6599 www.LeadandLearn.com
Part One: Objectives • Develop a deeper understanding of the Decision Making for Results: Data-Driven Decision Making process • Increase awareness of the relevance of data and its impact on leadership, teaching, and learning • Reinforce the importance of collecting both cause and effect data
Why are We Here? • To offer a process/framework for using data as it relates to School Improvement Planning and • To make informed data-driven decisions that lead to improved classroom practices
CONSENSOGRAM Activity Directions: • Respond to each of the consensogam questions individually using a scale of 0-100. • Place your response on a post-it note, one per question. • Place your post-its on the prepared charts in the form of a bar graph.
Essential Questions • How is your school or district doing as a learning institution? • Are all students learning? • In what ways do your community, district office administrators, principals, and classroom teachers collect and use data to make decisions?
What do you expect students to know and be able to do by the end of each year? Do you know why you are getting the results you currently have? What is the best way to assess student knowledge and application of skills? Essential Questions
Seminar Overview • Introduction • Building the Foundation • Process and Application • Action Planning
Becoming Data-Driven How are you currently embracing a data-driven decision-making process that leads to results?
Results-Driven Schools • Where’s the Proof? • 90/90/90 Schools, Reeves 2003 • Education Trust, 2002 • NCREL, 2000 • Consortium for Policy Research in Education, 2000 • EdSource, 2005 • Northern Illinois University Center for Governmental Studies, 2004 Data Driven Decisions
Reflection “The value of the data emerges only when analysis provides insights that direct decisions for students.” White, S. (2005). Beyond the numbers. Englewood, CO: Advanced Learning Press.
Part Two: Building the Foundation • Cause data and effect data • Continuous improvement cycle • Principles and processes of Decision Making for Results: Data-Driven Decision Making
“Only by evaluating both causesand effects in a comprehensive accountability system can leaders, teachers, and policymakers understand the complexities of student achievement and the efficacy of teaching and leadership practices.” Reeves, D. B. (2006). The learning leader. Alexandria, VA: ASCD.
Effect data: outcomes or results Cause data: professional practices that create specific effects or results Definitions and Examples
The Leadership/Learning Matrix (L2 Matrix) Effects/Results Data Leadership/Teaching Practices
Part Three:Process and Application
Ocean View Elementary School – a look at collaboration
The Process for Results Treasure Hunt Analyze to Prioritize Inquiry;: Develop Questions Monitor & Evaluate Results SMART Goals Results Indicators Specific Strategies
Inquiry “Data-driven decision making begins by asking fundamental questions.” D. Reeves • What questions do you have about leadership, teaching, and learning in your school or district? • What data sources are you using to gather the specific information?
Step 1: Conduct a Treasure Hunt • Why? – To gather and organize data in order to gain insights about leadership, teaching, and learning practices Considerations: • Measures of data • Disaggregation • Triangulation • Reflection
Measures of Data • Student learning • Demographics • Perceptions • School processes – behaviors within our control: instructional and leadership strategies, programs and resources, and organization
Disaggregation • To separate something into its component parts, or break apart • “Disaggregation is not a problem-solving strategy. It is a problem-finding strategy.” Victoria Bernhardt, Data Analysis, 1998 Think, pair, share: What data do you disaggregate and how do you use the information?
Triangulation – A Look at Learning
Case Study • Read case study • Part 1: How did they categorize the different data sets and record their observations? • Part 2: What did they discover?
Conduct a Treasure Hunt Application • Review inquiry questions • Conduct a “Treasure Hunt” • Organize data on templates • Use rubric to monitor and evaluate your work
Find the Data • Search for multiple measures of data: student achievement, perception, processes, demographics • Organize the data to include content areas, subskills, student population, gender, grades, and year of achievement • Disaggregate data where needed • Enter group information when examining longitudinal trends and patterns.
Can you identify with this? “It is not so much a lack of data, but an absence of analysis, and an even greater absence of actions driven by the data.” White, S. (2005). Beyond the numbers. Englewood, CO: Advanced Learning Press.
Step 2: Analyze Data to Prioritize Needs Data Analysis at Northside Middle School
Step 2: Analyze Data to Prioritize Needs • Why? – To identify causes for celebration and to identify areas of concern • Considerations • Strengths • Needs • Behavior • Rationale
“Stoplight” Highlighting Illuminating data patterns • Blue = Wow! Beyond Expectations • Green = Good – Meets Expectations • Yellow = Caution – Below Expectations • Pink = Urgent! In need of immediate improvement!
Define Your (Cutoff) Colors • Assign colors to your levels of expectations. What percent of students DO YOU WANT TO SEE at each levels? Is 80% of your students at proficient and above adequate in light of SRBI? • Make acolor keynext to your Data Table
Stoplight Highlighting(Sample Cutoffs) • Blue 80 – 100% • Green 60 – 79% • Yellow 40 – 59% • Pink 0 – 39%
Quality Prioritization • Why? To take immediate action on the most urgent needs • Quality prioritization requires a thorough understanding of: • Student population • Curriculum and Power/Priority Standards (leverage, readiness) • Antecedents affecting student achievement • Quality of program implementation White, S. (2005). Beyond the numbers. Englewood, CO: Advanced Learning Press.
Case Study • Review case study • What insights did you gain after reading analysis of student performance? • Make a recommendation: What is the most urgent need?
Review, Analyze, and Prioritize Application • Review data from Step 1 • Conduct analysis using the guiding questions • Prioritize urgent needs using the suggested criteria and your highlighting • Record your work on the templates
Step 3: Establish SMART Goals • Why? – To identify our most critical goals for student achievement based on the challenges that were identified through the inquiry process • Specific, measurable, achievable, relevant, timely
Goals - Application • Review prioritized needs • Review Treasure Hunt baseline data • Apply SMART goal formula, use templates to record your work • Use rubric to monitor and evaluate your work
Step 4: Select SpecificStrategies Let’s watch Lake Taylor High School as they dialogue about strategies.
Step 4: Select Specific Strategies • Why? • Adult actions will impact student achievement • Strategies are: • Action-oriented • Measurable/accountable • Specific • Research-based • Considerations: instructional, organizational, leadership, programmatic
Research-Based Strategies • 90/90/90 Schools, Reeves 2003 • Ten Things High Schools Can Do Right Now to Improve Student Achievement, Reeves, 2006 • What’s Happening in Schools? or Not, Learning 24/7 Observation Study, 2005
Additional Evidence in Support of Research-Based Strategies • Best Practice, Zemelman-Daniels, Hyde, 1998 • Art & Science, Marzano 2007 • The Kids Left Behind, Barr & Parrett, 2003 • School Leadership That Works, Marzano, Waters, McNulty, 2005
Let’s Do It! Guided Practice
Select Your Specific Strategies • Revisit your prioritized needs. • Research the best possible strategies to meet the learner needs • Group by type of strategy: instructional, organizational, programmatic, and leadership • Use rubric to monitor and evaluate your work
Step 5: Determine Results Indicators • Why? To monitor the degree of implementation and evaluate the effectiveness of the strategies
Results Indicators • Considerations • Serve as an interim measurement • Used to determine effective implementation of a strategy • Used to determine if strategy is having the desired impact • Help to determine midcourse corrections
Results Indicator Application • Revisit strategies (Step 4) • Develop results indicators • Use rubric to monitor and evaluate your work
“Improvement cycles require leadership follow-up and relentless efforts to maintain the focus on data if decisions are truly going to be driven by informed data.” White, S. (2005). Beyond the numbers. Englewood, CO: Advanced Learning Press.
Step 6: Monitor and Evaluate Results Why? To engage in a continuous improvement cycle that • Identifies midcourse corrections where needed • Adjusts strategies to assure fidelity of implementation
Develop Your Monitoring Plan • Review your work from developing questions to determining results indicators then determine how you will monitor the strategies. When you create your monitoring plan consider: • Teacher or administrator teams • Monitoring cycles • Goals • Strategies • Impact on student and adult behavior • Ability to make midcourse corrections
- More by User
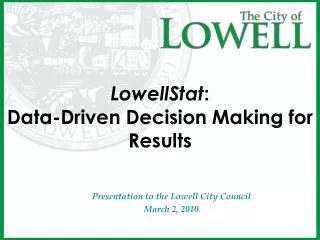
LowellStat : Data-Driven Decision Making for Results
LowellStat : Data-Driven Decision Making for Results Presentation to the Lowell City Council March 2, 2010 “ You Can ’ t Manage What You Can ’ t Measure ” Peter Drucker PerformanceStat – What Is It?
767 views • 26 slides
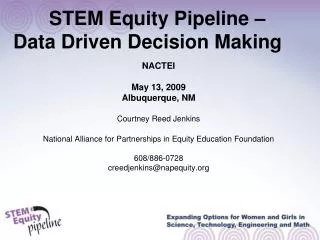
STEM Equity Pipeline – Data Driven Decision Making
STEM Equity Pipeline – Data Driven Decision Making . NACTEI May 13, 2009 Albuquerque, NM Courtney Reed Jenkins National Alliance for Partnerships in Equity Education Foundation 608/886-0728 [email protected]. Agenda. Data analysis of STEM in academics and CTE
1.03k views • 79 slides
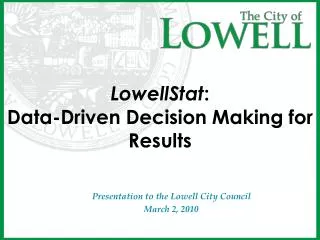
LowellStat : Data-Driven Decision Making for Results. Presentation to the Lowell City Council March 2, 2010. “ You Can ’ t Manage What You Can ’ t Measure ” Peter Drucker. PerformanceStat – What Is It?.
416 views • 26 slides
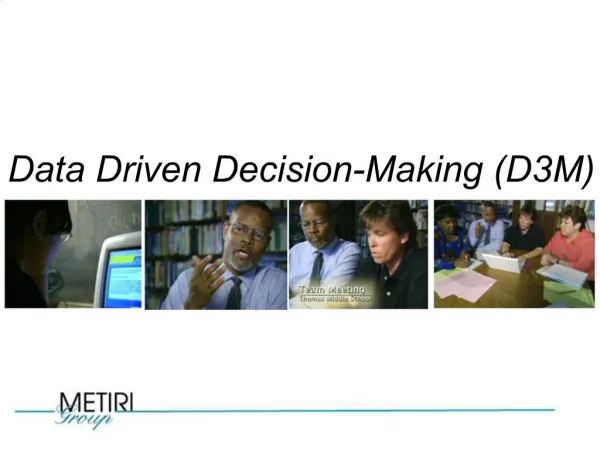
Data Driven Decision-Making D3M
493 views • 33 slides
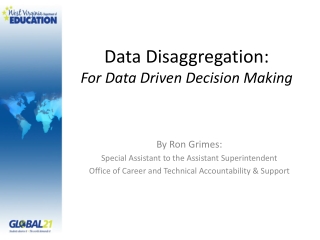
Data Disaggregation: For Data Driven Decision Making
Data Disaggregation: For Data Driven Decision Making. By Ron Grimes: Special Assistant to the Assistant Superintendent Office of Career and Technical Accountability & Support. What is “data disaggregation” and why should we use it?. Simple definition:
702 views • 24 slides
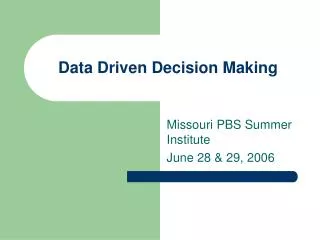
Data Driven Decision Making
Data Driven Decision Making. Missouri PBS Summer Institute June 28 & 29, 2006. Purpose. Provide guidelines for using data for team planning Provide guidelines for using data for on-going problem solving Apply guidelines to examples. Improving Decision Making. From . Problem. Solution.
377 views • 15 slides
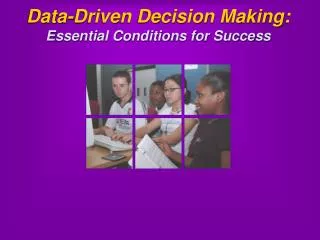
Data-Driven Decision Making: Essential Conditions for Success
Data-Driven Decision Making: Essential Conditions for Success. Context. Standards driven reform Assessments tied to standards Raised expectations for all students Technology tools Accountability mandates in NCLB Act. Moving Beyond the Mandate. NCLB is an opportunity to:
470 views • 35 slides
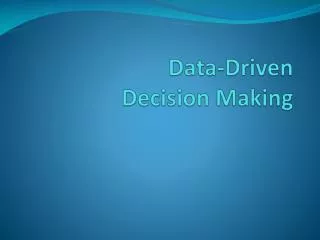
Data-Driven Decision Making. Homeroom. What Is Homeroom ?. A data management system that pulls information from Skyward and OSPI. A user-friendly method for viewing data. Another storage system for state, district, grade level and classroom assessment scores.
1.12k views • 57 slides
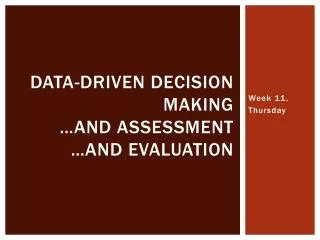
Data-Driven Decision Making …and assessment …And evaluation
Data-Driven Decision Making …and assessment …And evaluation. Week 11, Thursday. Today’s Flocks. Flock #1: Katie F., Jacob, and Jennifer Flock #2: Andi , Kim, and Josh Flock #3: Kristen, Natalie, and Brittany Flock #4: Katie B, Jordan, and Tom Flock #5: Katelin, Scott, and Grace.
492 views • 29 slides
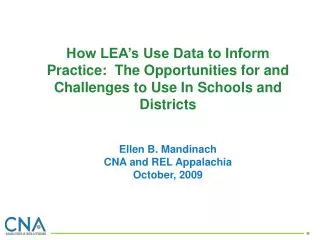
Why is Data-Driven Decision Making Important
How LEA’s Use Data to Inform Practice: The Opportunities for and Challenges to Use In Schools and Districts Ellen B. Mandinach CNA and REL Appalachia October, 2009. Why is Data-Driven Decision Making Important. Mandates from the new administration. NCLB continues.
686 views • 30 slides
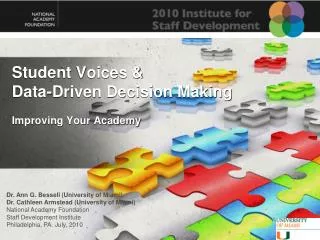
Student Voices & Data-Driven Decision Making
Student Voices & Data-Driven Decision Making . Improving Your Academy. Dr. Ann G. Bessell (University of Miami) Dr. Cathleen Armstead (University of Miami) National Academy Foundation Staff Development Institute Philadelphia, PA. July, 2010. Agenda. Overview and Purpose
462 views • 28 slides
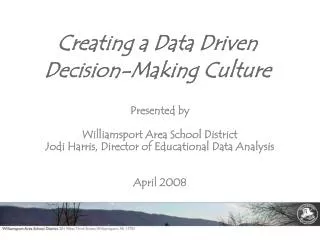
Creating a Data Driven Decision-Making Culture
Creating a Data Driven Decision-Making Culture. Presented by Williamsport Area School District Jodi Harris, Director of Educational Data Analysis April 2008. Williamsport ASD Profile. WASD enrollment - 5,800, 30% Minority, 55% ECD, 23% Special Needs
455 views • 27 slides
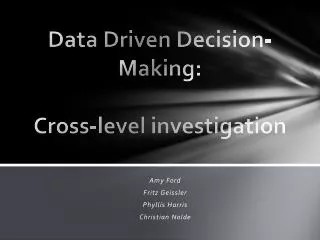
Data Driven Decision-Making: Cross-level investigation
Data Driven Decision-Making: Cross-level investigation. Amy Ford Fritz Geissler Phyllis Harris Christian Nolde. Process for action-research:. Information collected across four levels: Central Office High School Middle School Elementary School Information collected cross three areas:
229 views • 11 slides
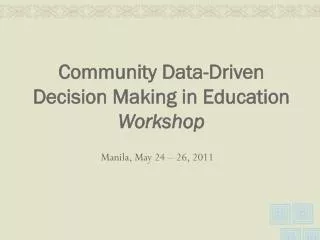
Community Data-Driven Decision Making in Education Workshop
Community Data-Driven Decision Making in Education Workshop. Manila, May 24 – 26, 2011. Purposes of Workshop. To promote data-driven education decision making at the community level
651 views • 51 slides
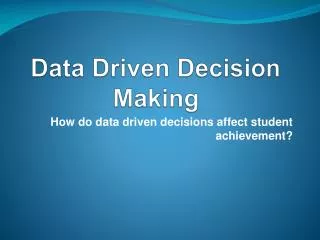
Data Driven Decision Making. How do data driven decisions affect student achievement?. Four Basic Questions for Instructional Decision-Making. What do I want my students to know and be able to do? How will I know they know it and can do it? How do I account for the students’ performance?
898 views • 35 slides
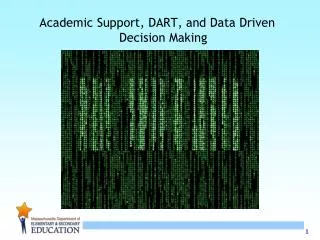
Academic Support, DART, and Data Driven Decision Making
Academic Support, DART, and Data Driven Decision Making. Data?. Data can be simple or complex Data can be quantitative (lots of numbers) or qualitative (more narrative) Data can be used to justify many different positions, sometimes using the same numbers Data is information
258 views • 11 slides
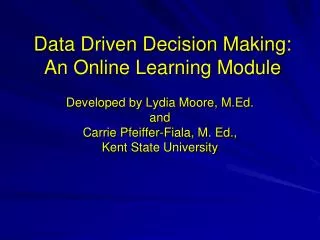
Data Driven Decision Making: An Online Learning Module
Data Driven Decision Making: An Online Learning Module. Developed by Lydia Moore, M.Ed. and Carrie Pfeiffer- Fiala , M. Ed., Kent State University. Purpose of the Product.
241 views • 6 slides
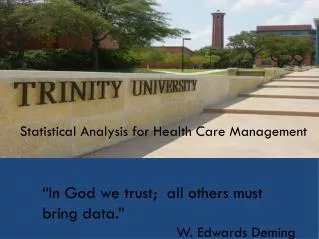
Data-Driven Decision Making Ed Schumacher, Ph.D.
Data-Driven Decision Making Ed Schumacher, Ph.D. Statistical Analysis for Health Care Management. CENTENE-TRINITY-OLIN. “In God we trust; all others must bring data.” W. Edwards Deming. Creative Destruction of Medicine. New Medicine (individual-based). Consumerism. Social Networking.
726 views • 51 slides
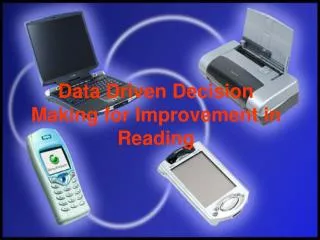
Data Driven Decision Making for Improvement in Reading
Data Driven Decision Making for Improvement in Reading. Data Driven Decision Making for Improvement in Reading. Darlene Higginbotham Mike Melton Chad Hudson. Goal. Improvement in Reading Scores for Special Education Students on PACT. Teaching Strategies. Direct Instruction (10 years)
351 views • 17 slides
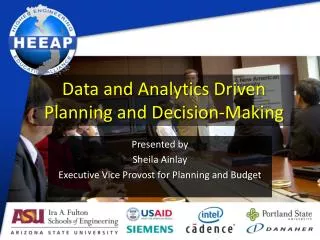
Data and Analytics Driven Planning and Decision-Making
Data and Analytics Driven Planning and Decision-Making. Presented by Sheila Ainlay Executive Vice Provost for Planning and Budget. Collaboration across units on enrollment planning analytics. Enrollment Management University Registrar Undergraduate Admissions Student Financial Assistance
297 views • 15 slides
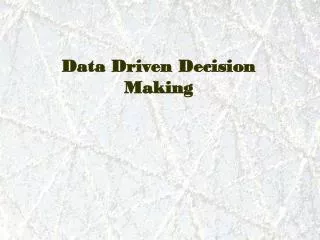
Data Driven Decision Making. Systemic way of looking at what you do to:. 1. What is working 2. What is not 3. Try to understand what is affecting A journey of discovery. 2 Roles. Researcher Participant. Micro vs Macro. Analysis Intervention Change.
202 views • 6 slides
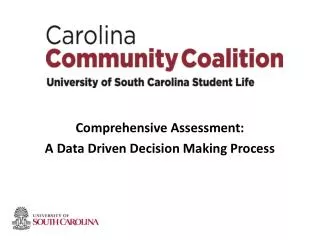
Comprehensive Assessment: A Data Driven Decision Making Process
Comprehensive Assessment: A Data Driven Decision Making Process. University of South Carolina Student Life. A caring community united for a safer Carolina.
556 views • 42 slides

Data-Driven Decisions: A Game-Changer for Small Businesses Before They Scale (Insights from CTO, Tarsem Singh)
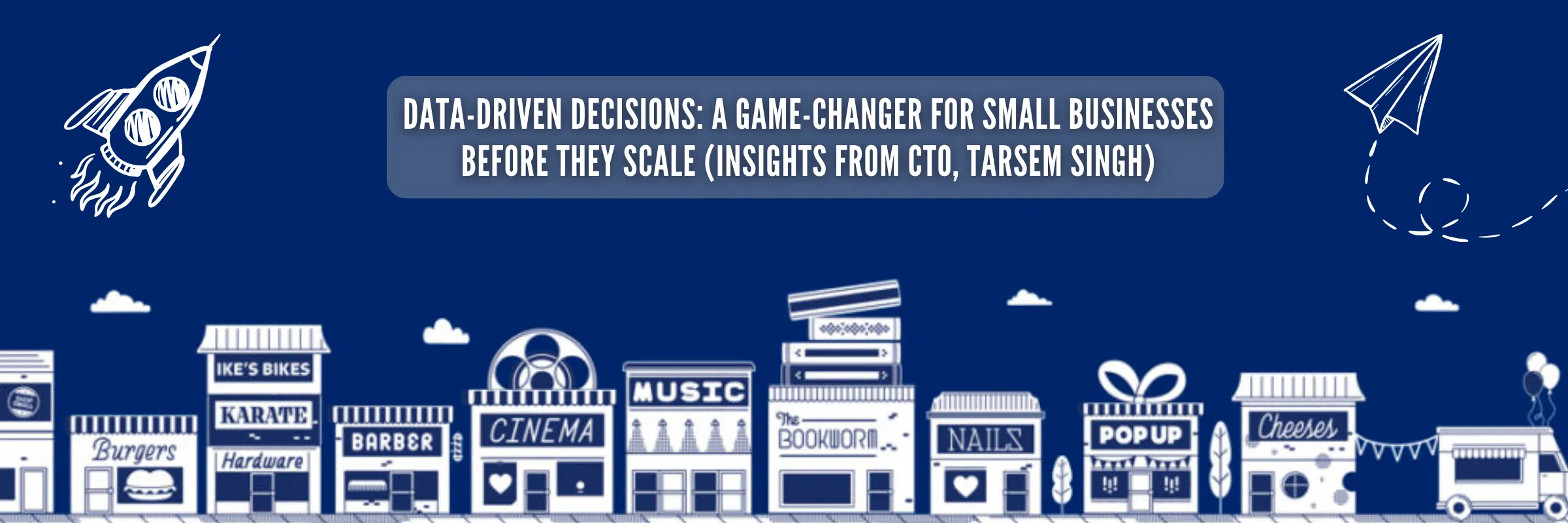
ScaleupAlly | September 8, 2024 , 10 min read
Table Of Content
- What is DDDM aka Data-Driven Decision Making?
When Should Small Businesses Make an attempt at Data-Driven Decision Making?
Why should small business owners adopt data driven decision making, tools or resources would you suggest for a small business diving into data driven business decisions, what are the essential components of a data infrastructure that a small business should consider investing in, how should small businesses measure the success of their data driven initiatives, example of how data driven decision making has made a difference for a small business, key metrics to track before scaling, final thoughts.
“Data-driven decisions are the future, and the future is already here,” quoted by Masayoshi Son, a Japanese billionaire, technology entrepreneur, investor and philanthropist. The usual rule of thumb is, to go with one’s intuition.
There is enough literature and studies on how intuitive instincts have shaped the world. The hunch may guide you, however according to a survey done by PwC, the chances of hitting the right chord, i.e., a performative decision is three times more effective with a data driven roadmap.
It is now implicit, how the data is the game changer for the businesses. “The catch is to give shape to these scattered data points, to more directional destinations, where all of the growth leads its way right through it.
From intuitive to rationalized decision making, business life comes full circle,” endorses Tarsem Singh.
Let’s see what the Data says, shall we name it Mr.Data?
- There are 23% of chances to acquire new customers/clients with DDDM (Data Driven Decision Making), and six times the probability of retaining the existing customers/clients, and 19% more profitable, according to Mckinsey Global Institute.
- BARC studies suggest DDDM (Data Driven Decision Making) accommodated by organizations saw a 10% reduction in their operational costing.
- Forbes Magazine says, 88% of the organizations have been using DDDM (Data Driven Decision Making) for improved understanding of their customer behaviour, to introduce more features and products.
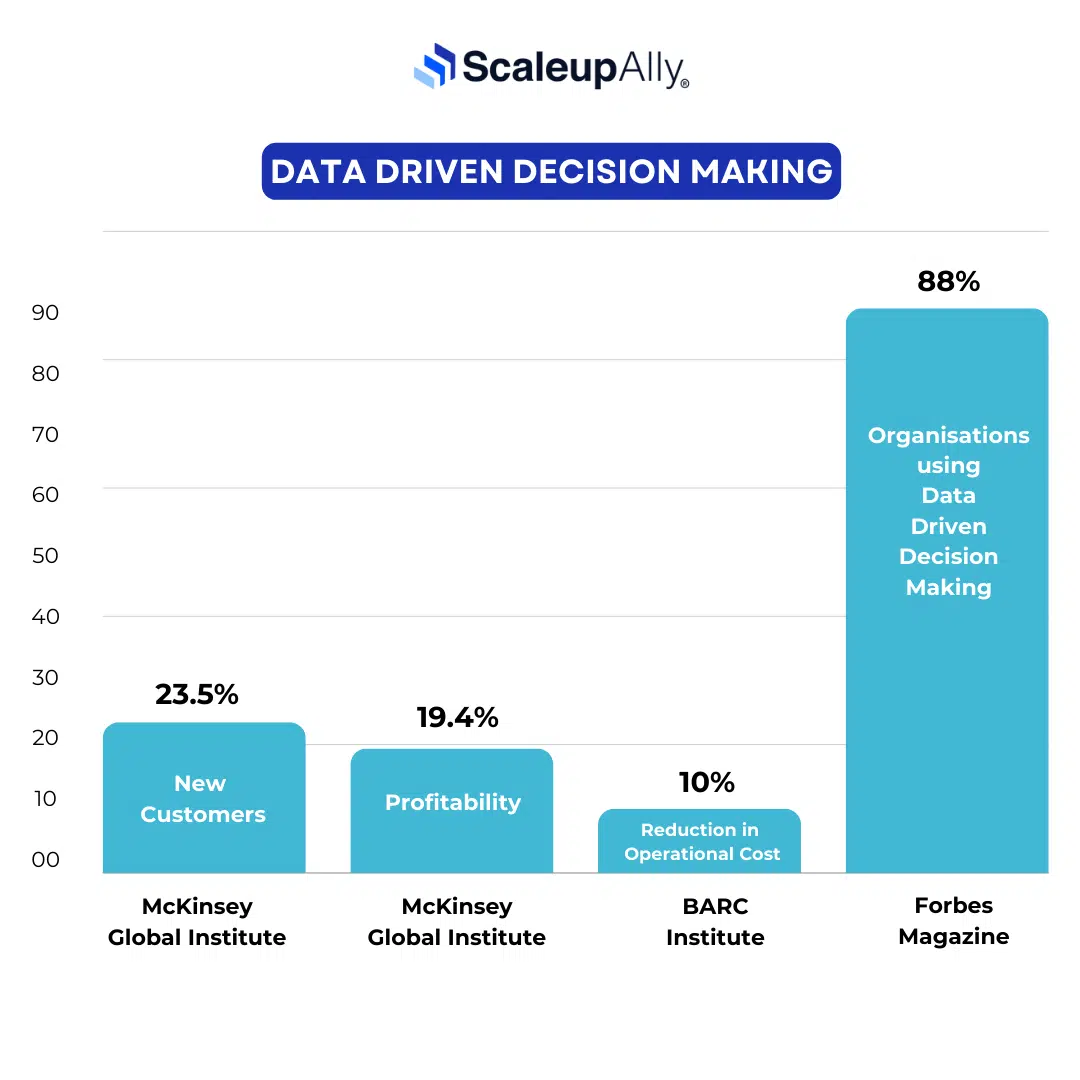
At its very core, DDDM (Data Driven Decision Making) is about using relevant data to take charge of the business choices rather than relying on intuition alone. The entire chain involves collecting, analyzing, and interpreting data to make room for informed decisions that are grounded in facts rather than assumptions. It is an empirical evidence to shape strategic plans.
Tarsem recollects, he read in a 2019 Score report, for about 51% of small businesses place firm faith in the significance of big data. What’s more, companies that do take advantage of data analytics tools enjoy 15% more in sales than those that don’t.
Although, as hard as it is to get hold of the tech lead for a discussion, we jumped at the first opportunity we got. In an attempt to pick his brains, we exchanged views on DDDM and how irrefutably it is a game changer for small businesses before they scale, with the forward thinking CTO of ScaleupAlly, Tarsem Singh. Let the stat out of the bag!
How do you define DDDM within the context of Small Businesses?
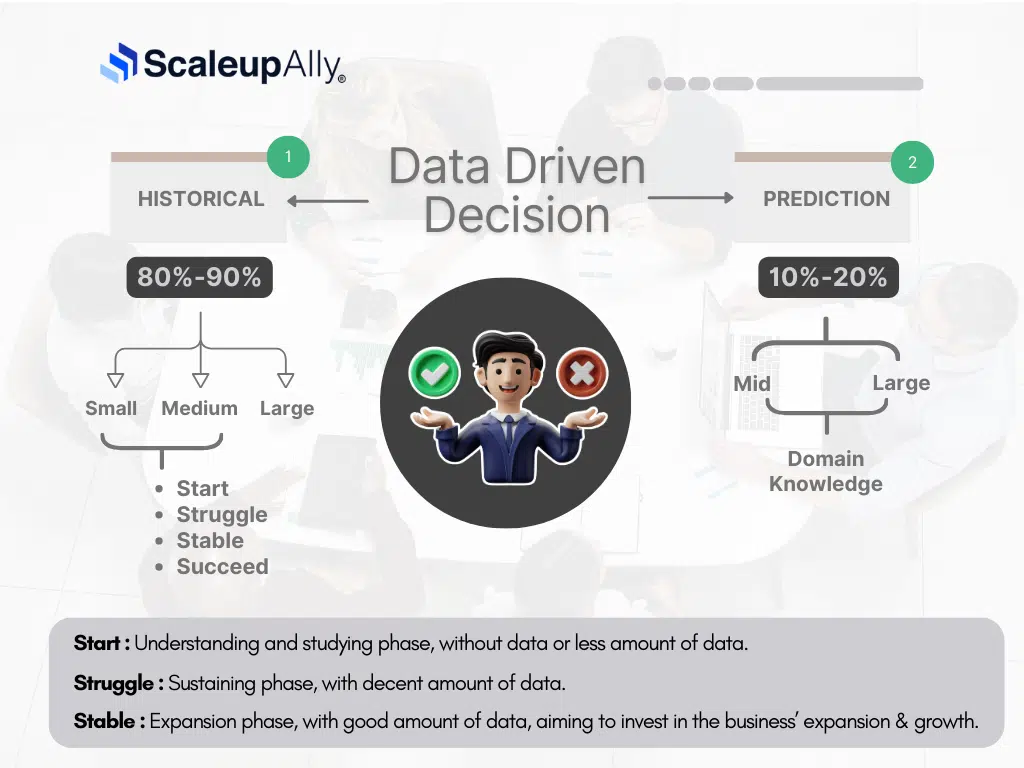
DDDM is not very technical, but mostly creative. It may look technical as it requires coding, some statistics and math to do the calculation, Tarsem affirms, “It is creativity at work with Data.” So when should a business think about it?
- Growth Hits a Snag: If your business was cruising along smoothly but recently hit a growth plateau, it might be time for some data driven business decisions.
- Operations Feel Clunky: Inefficiencies or rising costs? Let’s say you’re running a logistics company and are noticing issues with delayed deliveries. Now your customers are growing impatient! DDDM comes handy by looking at production data, analyzing route data, spot choke points and pinpointing exactly where things are going wrong. This insight lets you even out any operational bottlenecks and better customer satisfaction with timely deliveries.
- Eyeing New Market Opportunities: If exploring new markets or products is in the pipelines, DDDM spots the right opportunities. For instance, if you run a fitness apparel brand and want to explore new product lines, analyzing market trends, better insights on emerging trends, competitor offerings and customer preferences can give you hints to areas with high demand.
- Want to Optimize Marketing Efforts: Running several marketing campaigns, spending hefty amounts, without seeing the light of the day. Waste! By analyzing campaign data, customer acquisition costs, and conversion rates, you can figure out which strategies are hitting the mark for best ROI and adjust your marketing budget smartly.
- Customer Service is not Servicing: In case the customer service team is getting a lot of angry complaints and you’re not sure why, sorted data gets to the root of the problem. For example, analyzing call center data and complaint trends can reveal common issues and help you make targeted improvements.
For small business owners, the stakes are high, and every decision, even minor ones can have the butterfly effect which might affect the business in the hindsight, but invisible in the immediate scheme of things. The CTO of ScaleupAlly highlights a few key reasons why DDDM is a must for small businesses:
- Increased Efficiency and Understanding: Data can reveal inefficiencies in operations, which could break the camel’s back. For instance, analyzing sales data might highlight inventory management issues or identify the most profitable products with untapped potential. DDDM lets you ideate better with sales data, consumer feedback and market trends. One may hit the sweet spot with a product with moderate sales, but with appropriate adjustments in pricing, smart marketing, or adding new features, may fetch a higher ROI. From underperforming to outstanding sales, DDDM is that magic wand.
- Smart Decision Making: “Small businesses often operate with limited margins and need to be agile. Data helps them make smarter decisions quickly,” explains the CTO. Let us take an example of a retail store. Make observations on what products aren’t making the cut and why? Using DDDM, study purchasing patterns, customer demographics, and seasonal trends. Now you are armed, finding gaps using DDDM, allows you to adjust your inventory and marketing strategy. This is smart decision making in action.
- Personalized Customer Experience: Once the data is cleaned, and arranged, marketing and services to meet the needs of their customers becomes a cake walk. “This gains customer loyalty,” the Tarsem notes. An online clothing store, for example, can analyze in great detail, customer data, browsing history, past purchases, preferences. This study of customer behaviour lets you position yourself better.
- Risk Management: Saving sudden stock loss, due to unanticipated events, where DDDM can easily mitigate the issue. Case in point, Warehouse stock management. The Suez canal crisis happened, we all know the supply chain was acutely disrupted. And similarly, sudden unexpected stock shortages may occur, here only DDDM can rescue! Historical data on inventory levels, supplier reliability, potential market risks, all this can help one develop a contingency plan. Continuity, and minimal losses keep the boat floating.
- Competitive Advantage: In a competitive market, having an edge can make all the difference. For instance, small businesses can study competitors, their strategies, customer preferences, identify gaps in the market, and create competitive offerings. “This way Small businesses can position themselves better in the market using DDDM,” says Tarsem.
- Better Financial Management: Since the inventory management gets sorted, risk has been mitigated, time has also been freed for core decisions, all of these prevent fund leakages, and DDDM predictions allow businesses to save on extra costs.
- Stability and Growth: DDDM is an ally of the business ecosystem, once it gets assimilated with the business’s skeleton, and we know nothing provides the stability like backbone. For example, Netflix uses DDDM to personalize content recommendations by analyzing user viewing habits. This data-driven approach increases customer retention and satisfaction, while also guiding content investment decisions. As a result, Netflix achieves stability through consistent engagement and growth by expanding its library with highly demanded content.
Tarsem suggests, “for basic analytics, platforms like Google Analytics or HubSpot can be very useful. If you’re looking for more in-depth insights, consider CRM systems like Salesforce or data visualization tools like Power BI, Tableau. The key is to choose tools that are user-friendly and fit your specific needs.”
Tarsem emphasizes, to invest in tools which are secure, for others with poor security may compromise your data and kill your business from the ongoing competition. Many concerns like sensitive data security, data leakages may have a snowball effect, which could prove disastrous.
“Simple! Check your Profits or Traction.” smiles Tarsem.
“Absolutely. One of our ( ScaleupAlly ) clients, Soha Living, is a local retail store based in Thailand. They were struggling with inventory management. By thoroughly analyzing their sales data, we identified patterns in customer purchases and seasonal trends.
With these insights, they were able to adjust their inventory levels, reduce overstock, and ensure they had the right products on hand at the right times. This not only improved their sales but also reduced waste and increased profitability.” Tarsem shared.
Keeping an eye on these benchmarks show a detailed view of the business’s performance and where it’s headed. Tracking these metrics helps in identifying opportunities and adapting to changing variables to keep the growth trajectory on track.
- Revenue Growth: This metric highlights how much money the business is bringing in compared to previous periods, indicating whether the business is growing or shrinking, or stuck in stagnancy.
- Net Profit Margin: This calls attention to what percentage of our revenue is left after all business expenses are paid. A higher net profit margin means a more profitable business.
- Cash Conversion Cycle (CCC): For product-based businesses, this gives an idea of how long it takes for money to move through the business, considering inventory selling time, receivables collection, and bill payment.
- Customer Acquisition Cost (CAC): Measures how much it costs to bring in new customers and how smartly we’re acquiring them.
- Customer Lifetime Value (CLV): The total value of a customer throughout our relationship, factoring in variables like repeat purchase rate, CAC, and average order value, is what CLV tells.
- Customer Retention Rate: Important one! Acquiring new customers costs more than retaining existing ones. It tells the percentage of customers/clients who return to do business after making an initial purchase.
- Customer Churn Rate: Gives the percentage of customers who leave our business over a given period, indicating how sticky our customer base is.
- Average Order Processing Time: Evaluates the efficiency of the order processing system.
- Order Accuracy: Reflects how well the business is fulfilling customer/clients orders.
- Employee Efficiency: Measures the team’s productivity levels.
- Website Traffic: Shows the number of visitors to the website, giving insight into how well the online presence is performing.
“Data isn’t just numbers and charts; it’s a storehouse of insights waiting to be uncovered. For small businesses, data helps you understand your market, track performance, and make informed decisions,” nods Tarsem.
For the readers, a few reading suggestions by Tarsem, which he personally likes to read or watch for updates on the subject: Bernard Marr, Data Science Central, Business Insights from Harvard Business School Online.
Tarsem casts our mind to the Grofers Story. Once was a small business, with a humble beginning in 2013, it started with on demand pick-ups and drop services from peripheral areas, for pharmacy, grocery, restaurants etc. Now, it has expanded and soon to be an Unicorn company.
The subtle nudge theory in action using DDDM is how the model works. They study their customer’s activity data, understand demand with much accuracy, saving bucks. They optimise routes of delivery, stock management with better predictions as to when and what products their customer may order.
They study time and time’s relationship with customers and their business system. And we all know, time is money and money is time. Don’t we?
- PwC Market Stats 1 Market Stats 2 Score Report
Share this article
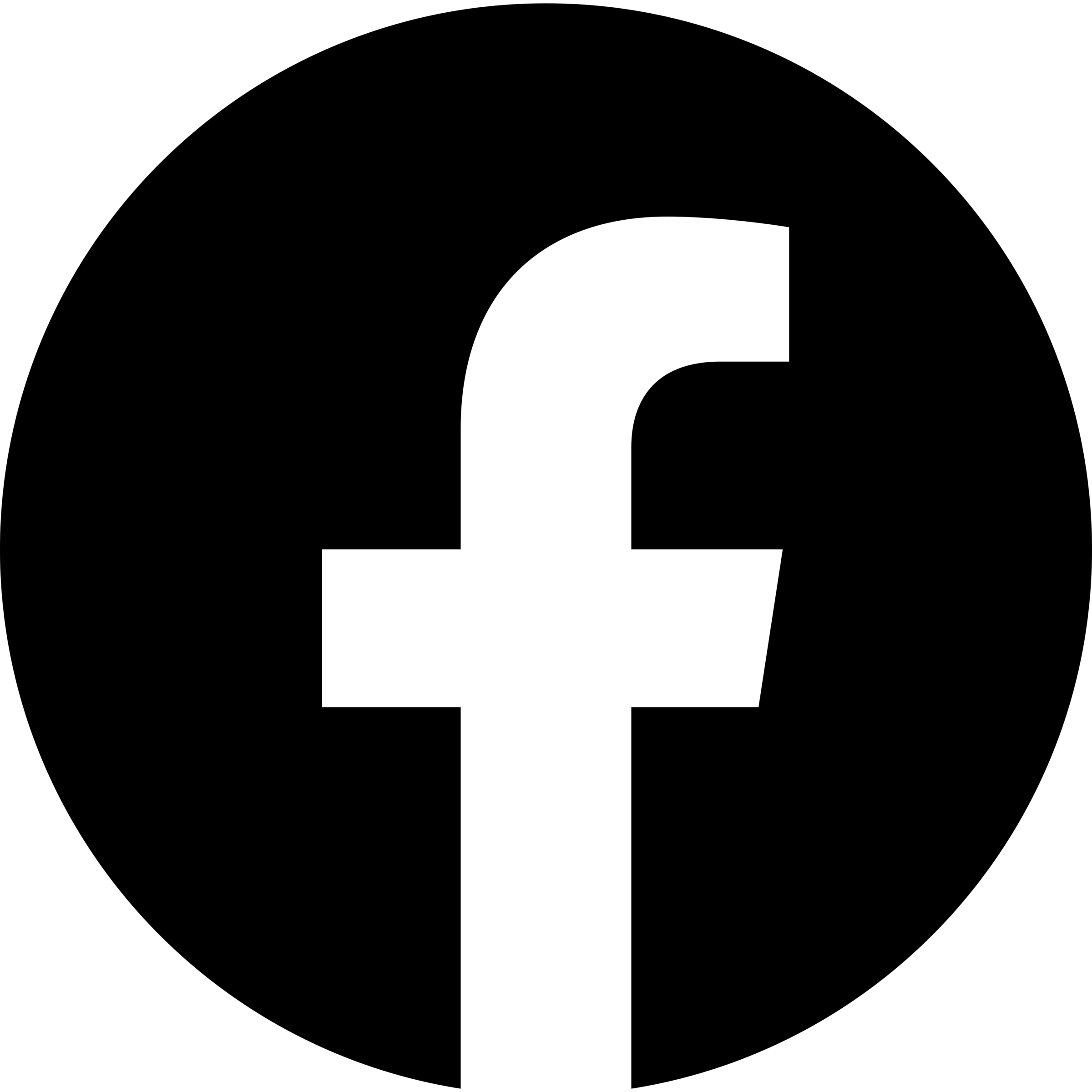
Related Blogs
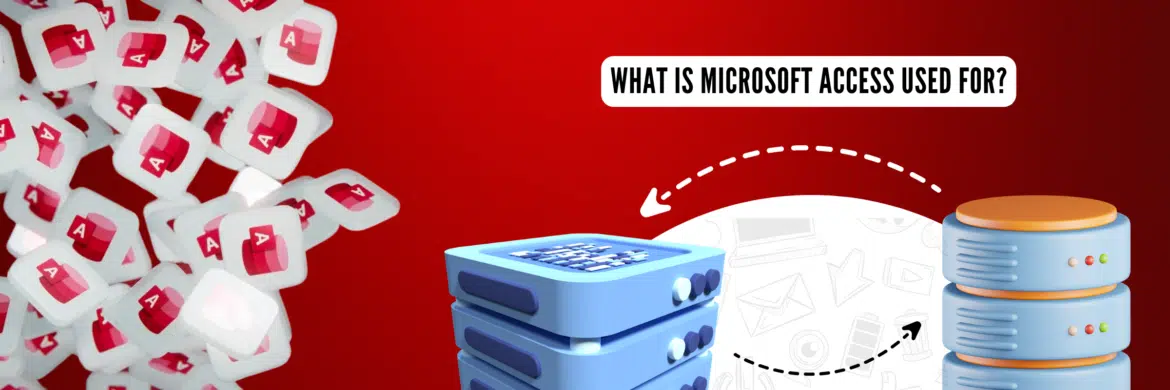
What is Microsoft Access Used For?
Discover what Microsoft Access is used for and how it can transform your data handling, from simple databases to complex reporting.
Tarsem Singh Sep 3 , 10 min read
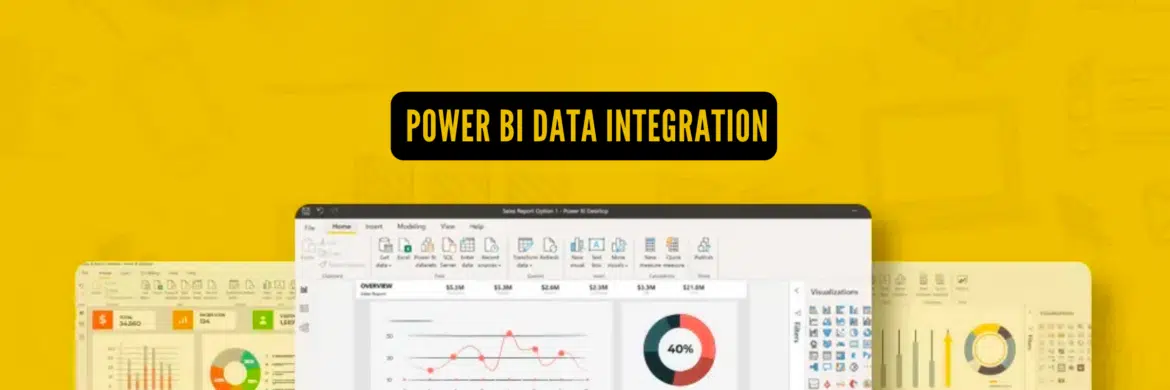
Power BI Data Integration: Everything You Need To Know
Explore how Power BI integrates with various data sources to deliver robust analytics and insights. Learn about its key features, use cases, and best practices for effective data integration.
Pranay Agrawal Sep 2 , 14 min read
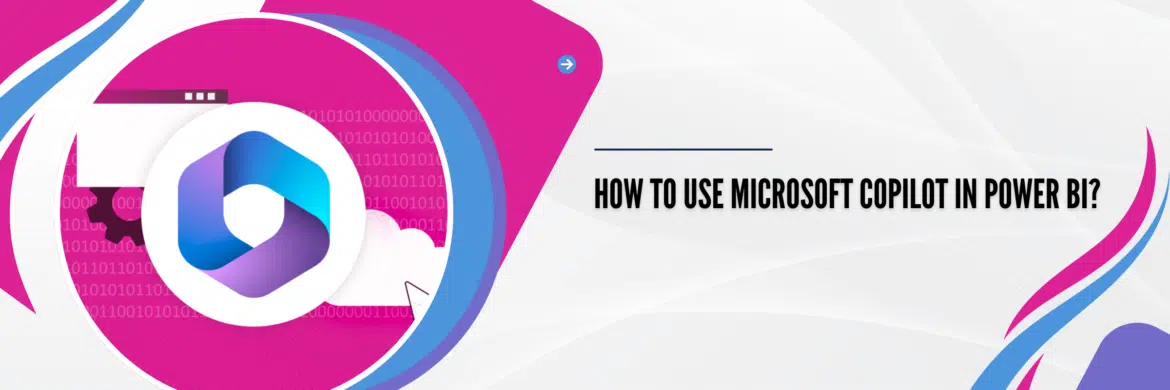
How To Use Microsoft Copilot in Power BI?
Discover how Copilot can be used in Power BI to automatically generate reports and empower anyone to become a data analysis pro.
Pranay Agrawal Sep 2 , 8 min read
Our Promise
We believe in turning ideas into impactful products.
Ready to Discuss Your Idea?
Connect with our experts, talk to our experts.

- Resources / Blog
Top Data-Driven Decision Examples: Showcasing Success
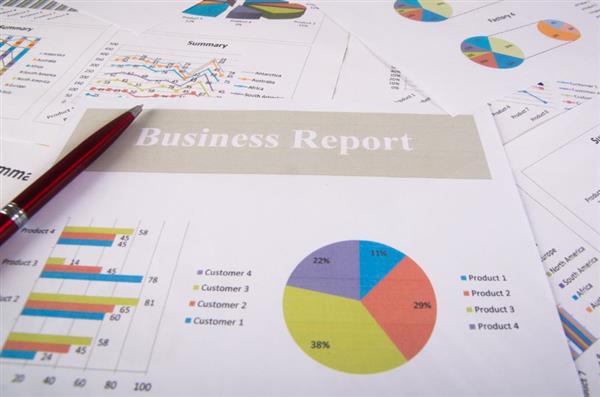
Thanks to the consistent evolution of technology, organizations now have vast oceans of data that can provide deep insights into their products, services, and customer needs. However, it is easy to drown in data if it isn’t properly managed and analyzed. Without the proper skills and tools to work with data, an old literary quote sums up an organization’s dilemma: “Water, water everywhere, and not a drop to drink.”
The amount of data generated today is staggering, with some estimates showing that 90% of the world's data has been created in just the last few years. Data’s explosive growth presents immense opportunities for organizations to solve more significant problems. The first step in using data is to collect raw data ethically and responsibly. Once collected, data must be transformed to fully harness its power to provide the actionable insights needed to make better decisions.
While organizations can collect individual data points, they remain meaningless without proper interpretation. Turning data into valuable insights involves adding meaning and narrative that leads to effective actions. To help you understand the power of data, we will illustrate how organizations across various sectors transform data insights into action, leading to substantial improvements and innovations.
On this page:
In-Depth Examples of Data-Driven Decisions
Benefits of data-driven decisions, challenges in implementing data-driven decisions, retail sector: walmart's inventory management.
Walmart uses big data analytics for inventory management, optimizing stock levels based on real-time data from its point of sale (POS) systems, weather forecasts, and social media trends. This approach helps Walmart to:
- Reduce waste by not stocking excess products
- Raise customer satisfaction scores by ensuring products are in stock
- Adjust inventory dynamically to meet anticipated demand increases
Leveraging consumer data analysis allows Walmart to make informed decisions that minimize costs and maximize sales and process efficiency. Like many organizations, Walmart gathers data through customer surveys, reviews, and social media interactions that explain product preferences and demand trends. Analyzing browsing patterns, cart abandonment rates, and time spent on product pages also provides insights into customers’ interests and potential demand.
Walmart’s customer data also helps it create personalized marketing strategies. In addition to behavioral data, Walmart collects demographic information such as age, gender, location, and income level to create customer profiles and loyalty programs. Segmenting customers based on purchasing habits and demographics enables highly personalized marketing efforts at scale, making it possible for Walmart to reach each customer group with relevant and appealing content.
Healthcare Industry: Mayo Clinic's Data-Driven Patient Care
As a world-renowned healthcare provider, the Mayo Clinic is on the cutting edge of patient care. Today, it can connect millions of data sets from patient records, surgeries, lab tests, pharmacies, images, treatment plans, and recovery data. Doctors analyze this data to determine whether patients become healthier based on the care they receive.
Taking it a step further, The Mayo Clinic has created a predictive analytics database it intends to use to advance preventative healthcare through genomics. Ultimately, by using historical patient data, The Mayo Clinic can develop models that predict the likelihood of disease onset by analyzing patterns in medical records, lab results, and genetic data. From there, physicians can identify patients at risk of developing specific complications, allowing for early intervention and personalized care plans.
Financial Services: American Express's Fraud Detection & Credit Risk Assessment
American Express (AmEx) monitors over $1.2 trillion in transactions yearly for fraudulent activity. Every time a customer swipes their card, AmEx collects data such as the transaction amount, location, merchant, time, and frequency. Thanks to advanced machine learning algorithms, AmEx can sort through and analyze massive volumes of transactional data in real-time to identify fraudulent activity patterns. It can then generate a fraud decision in milliseconds every time a customer uses their American Express card. This approach saves customers millions of dollars annually by protecting their accounts from unauthorized transactions.
Data is also central to how AmEx runs credit risk assessments for customers. It gathers a range of customer information that includes:
- Credit history, such as reports from credit bureaus that include a customer’s payment history, outstanding debts, and previous credit inquiries,
- Transactional data such as spending patterns and account balances
- Financial profile (employment status, income, and assets)
- Demographic data
AmEx then uses data modeling techniques like logistic regression to calculate the probability of default based on what they know about a customer and segment them into the appropriate risk category. Credit scores, interest rates, and limits are assigned based on the customer’s category.
Manufacturing: Caterpillar Predictive Maintenance and Operational Efficiency
Caterpillar, a renowned heavy equipment manufacturer, began using data analytics in the early 2010s to implement predictive maintenance across its machinery. By equipping its machines with sensors and utilizing data analytics, Caterpillar could predict potential failures before they occurred.
By 2014, Caterpillar had integrated this technology into its operations and began offering customers enhanced equipment service options using its Cat Connect platform, which included predictive maintenance. This approach significantly reduced unplanned customer downtime, increasing machine uptime and customer satisfaction. Additionally, by using data to optimize maintenance schedules, Caterpillar reduced maintenance costs and extended the lifespan of its equipment. Caterpillar’s data-driven initiative opened new revenue streams through service contracts and analytics-driven offerings. [BK1] [BK2]
Public Sector and Governance: New York City's Fire Risk Inspection and Urban Planning
As stewards of taxpayer funds, local governments must analyze community data to determine how to allocate resources for public services best. Local governments can collect data through surveys, town hall meetings, and online platforms that allow residents to provide feedback on how they use public services. Additionally, they can use data such as library visits, park usage, and public transportation ridership figures.
An excellent example is how New York City’s Fire Department uses data for the public good. The city developed an algorithm that ranks buildings based on fire risk. It generates a score using historical data on past fires (e.g., locations and building types), which it gives to the Fire Department, prioritizing those most at risk. This example shows how data-driven decision-making can bolster public safety and ensure the effective use of resources.
Another way data serves the public is by creating "smart cities" that use IoT devices to gather data about traffic patterns . Congestion is a significant problem in many cities worldwide. However, there is an opportunity to alleviate the problem by installing sensors on roads, traffic signals, and public transportation to collect real-time traffic data. Cities that collect and analyze traffic data will better understand why and where congestion occurs so they can plan better routes and add additional transportation options for commuters.
The examples above show how data is a powerful tool for decision-making. Whether it’s Walmart using data to optimize stock levels based on real-time data, the Mayo Clinic tailoring treatments based on past effectiveness to augment patient outcomes, Caterpillar providing customers with predictive maintenance data, AmEx protecting customers from unauthorized transactions, or local governments deciding how to allocate resources best, leveraging data analytics leads to smarter decisions, streamlined operations, and happier customers and communities.
Accountability
Data allows everyone to see how their actions contribute to organizational strategic goals. When an organization establishes a culture of accountability, the highest and lowest-performing employees and teams can get the recognition or help they deserve.
Business leaders need a roadmap to success that relies on more than intuition. High-performing organizations recognize that data is the best way to help their workforce perform at its best. Leaders who collect, analyze, and interpret data can identify inefficiencies in their processes and adjust as needed. Data-driven decision-making is the key to streamlining operations and eliminating wasteful, unnecessary procedures.
Customer Satisfaction
Understanding customer behavior and preferences allows organizations to offer personalized services and products. Using predictive analytics to forecast potential problems will enable organizations to step in and course-correct before they occur. Timely interventions, whether in healthcare, financial services, manufacturing, or retail, help to meet customer needs, often before they even know there is an issue.
Cost Savings
These benefits, taken together, show that data-driven initiatives lead to significant cost reductions. Organizations using data will waste less time and money on wasteful processes and resources, directly impacting the bottom line.
Data analysis pinpoints bottlenecks and highlights opportunities for improvement. The more data you have, the better your decisions will be, no matter the field.
Because of the sheer volume of data available, it's no surprise that harnessing and using it remains a big challenge for many organizations. There are several reasons for this:
Data Privacy and Ethics
The regulatory landscape is constantly changing to keep pace with evolving technology, and organizations must comply with stringent data protection regulations such as GDPR in Europe and CCPA in California. Collecting, storing, and analyzing vast amounts of data raises concerns about unauthorized access, data breaches, and misuse of personal information. Organizations need strong cybersecurity measures and comprehensive data governance policies to protect sensitive data and maintain customer trust.
Another top concern among regulators is transparency. Consumers deserve the right to know how companies collect data and how they are using it. As AI becomes increasingly popular, there are growing ethical concerns about how biases are baked into data, leading to unfair treatment of certain groups. For example, biased algorithms in hiring processes can perpetuate discrimination, so organizations must proactively pursue fairness and transparency by regularly auditing their data practices. Otherwise, they can unknowingly drive inequality.
Technological Gaps
Harnessing data's full potential requires integrating advanced technological infrastructure, which can be costly and complex. Integrating multiple data sources into a unified system is a significant challenge, often requiring substantial investment in new technologies and IT infrastructure. Additionally, organizations must contend with the high demand for skilled data professionals. Because of the lack of data professionals, attracting and retaining them can be challenging.
Data Quality
Organizations amassing such large volumes of data must maintain its reliability and accuracy. If data isn't reliable, it will corrupt analyses and lead to misguided decisions. Organizations need well-planned data management practices, including regular data cleaning, validation, and verification processes to keep data reliable and useful.
Communication
An organization's most significant challenge is communicating data and metrics to stakeholders. Data is only valuable if stakeholders understand the insights it provides.
Analysts need to translate and communicate information to stakeholders in ways they can easily understand. Some methods to communicate data insights include:
- Reports are static collections of data that provide high-level insights. They are quick to design and easy to use. However, they must be regularly updated since they do not display live data.
- Dashboards contain and monitor live data, allowing interaction and filtering and providing access to up-to-date information essential for real-time decision-making.
Data and its insights must always align with organizational goals. Presenting the correct data using the right tools and strategies will help stakeholders understand your analysis and make impactful data-driven decisions.
Becoming a data-driven organization is challenging, but the rewards are worth it. The valuable insights that data can add to your decision-making can be discovered when you utilize the right tools, align your decisions with the larger goals of your organization or community, and communicate transparently with your stakeholders.
If you’re ready to take a more proactive role as a data analyst in your organization, we invite you to check out New Horizon’s data and analytics training solutions. We have partnered with the creators of the world's leading data tools, including Microsoft and Amazon, to provide training solutions to help you produce results for your company.
- Expand/Collapse Microsoft Office 39 RSS
- Expand/Collapse Training Trends 107 RSS
- Expand/Collapse CyberSecurity 59 RSS
- Expand/Collapse DevOps 2 RSS
- Expand/Collapse Modern Workplace 43 RSS
- Expand/Collapse Cloud 28 RSS
- Expand/Collapse Programming 11 RSS
- Expand/Collapse Artificial Intelligence (AI) 18 RSS
- Expand/Collapse ITIL 21 RSS
- Expand/Collapse Data & Analytics 32 RSS
- Expand/Collapse Business Analyst 13 RSS
- Expand/Collapse Training By Job Role 6 RSS
- Expand/Collapse Leadership and Professional Development 14 RSS
- Expand/Collapse Managed Learning Services 3 RSS
- Expand/Collapse Design & Multi-Media 1 RSS
Three keys to building a data-driven strategy
Big data and analytics have climbed to the top of the corporate agenda. Together, they promise to transform the way companies do business, delivering the kind of performance gains last seen in the 1990s, when organizations redesigned their core processes. And as data-driven strategies take hold, they will become an increasingly important point of competitive differentiation.
In our work with dozens of companies in six data-rich industries, we have found that fully exploiting data and analytics requires three mutually supportive capabilities. First, companies must be able to identify, combine, and manage multiple sources of data. Second, they need the capability to build advanced-analytics models for predicting and optimizing outcomes. Third, and most critical, management must possess the muscle to transform the organization so that the data and models actually yield better decisions. Two important features underpin those competencies: a clear strategy for how to use data and analytics to compete and the deployment of the right technology architecture and capabilities.
Just as important, a clear vision of the desired business impact must shape the integrated approach to data sourcing, model building, and organizational transformation. That helps you avoid the common trap of starting by asking what the data can do for you. Leaders should invest sufficient time and energy in aligning managers across the organization in support of the mission.
1. Choose the right data
The universe of data and modeling has changed vastly over the past few years. The volume of information is growing rapidly, while opportunities to expand insights by combining data are accelerating. Bigger and better data give companies both more panoramic and more granular views of their business environment. The ability to see what was previously invisible improves operations, customer experiences, and strategy. That means upping your game in two areas.
Source data creatively
Often, companies already have the data they need to tackle business problems, but managers simply don’t know how they can use this information to make key decisions. Operations executives, for instance, might not grasp the potential value of the daily or hourly factory and customer-service data they possess. Companies can encourage a more comprehensive look at data by being specific about the business problems and opportunities they need to address.
Managers also need to get creative about the potential of external and new sources of data. Social media generates terabytes of nontraditional, unstructured data in the form of conversations, photos, and video. Add to that the streams of data flowing in from sensors, monitored processes, and external sources ranging from local demographics to weather forecasts. One way to prompt broader thinking about potential data is to ask, “What decisions could we make if we had all the information we need?”
Get the necessary IT support
Legacy IT structures may hinder new types of data sourcing, storage, and analysis. Existing IT architectures may prevent the integration of siloed information, and managing unstructured data often remains beyond traditional IT capabilities. Fully resolving these issues often takes years. However, business leaders can address short-term big-data needs by working with CIOs to prioritize requirements. This means quickly identifying and connecting the most important data for use in analytics and then mounting a cleanup operation to synchronize and merge overlapping data and to work around missing information.
2. Build models that predict and optimize business outcomes
Data are essential, but performance improvements and competitive advantage arise from analytics models that allow managers to predict and optimize outcomes. More important, the most effective approach to building a model usually starts, not with the data, but with identifying a business opportunity and determining how the model can improve performance. We have found that such hypothesis-led modeling generates faster outcomes and roots models in practical data relationships that are more broadly understood by managers.
Remember, too, that any modeling exercise has inherent risk. Although advanced statistical methods indisputably make for better models, statistics experts sometimes design models that are too complex to be practical and may exhaust most organizations’ capabilities. Companies should repeatedly ask, “What’s the least complex model that would improve our performance?”
3. Transform your company’s capabilities
The lead concern senior executives express to us is that their managers don’t understand or trust big data–based models and, consequently, don’t use them.
Such problems often arise because of a mismatch between an organization’s existing culture and capabilities and emerging tactics to exploit analytics successfully. The new approaches either don’t align with how companies actually arrive at decisions or fail to provide a clear blueprint for realizing business goals. Tools seem to be designed for experts in modeling rather than for people on the front lines, and few managers find the models engaging enough to champion their use—a key failing if companies want the new methods to permeate the organization. Bottom line: using big data requires thoughtful organizational change, and three areas of action can get you there.
Develop business-relevant analytics that can be put to use
Many initial implementations of big data and analytics fail because they aren’t in sync with a company’s day-to-day processes and decision-making norms. Model designers need to understand the types of business judgments that managers make to align their actions with broader company goals. Conversations with frontline managers will ensure that analytics and tools complement existing decision processes, so companies can manage a range of trade-offs effectively.
Embed analytics in simple tools for the front lines
Managers need transparent methods for using the new models and algorithms on a daily basis. By necessity, terabytes of data and sophisticated modeling are required to sharpen marketing, risk management, and operations. The key is to separate the statistics experts and software developers from the managers who use the data-driven insights. The goal: to give frontline managers intuitive tools and interfaces that help them with their jobs.
Develop capabilities to exploit big data
Even with simple and usable models, most organizations will need to upgrade their analytical skills and literacy. To make analytics part of the fabric of daily operations, managers must view it as central to solving problems and identifying opportunities. Efforts will vary, depending on a company’s goals and desired time line. Adjusting cultures and mind-sets typically requires a multifaceted approach that includes training, role modeling by leaders, and incentives and metrics to reinforce behavior. Adult learners, for instance, often benefit from a “field and forum” approach, in which they participate in real-world, analytics-based workplace decisions that allow them to learn by doing.
Our experience suggests that executives should act now to implement big data and analytics. But rather than undertaking massive change, executives should concentrate on targeted efforts to source data, build models, and transform the organizational culture. Such efforts help maintain flexibility. That’s essential, since the information itself—along with the technology for managing and analyzing it—will continue to grow and change, yielding new opportunities. As more companies learn the core skills of using big data, building superior capabilities will become a decisive competitive asset.
For more, see the full Harvard Business Review article, “ Making advanced analytics work for you ,” from which this summary is drawn (registration required).
Dominic Barton, based in McKinsey’s London office, is the firm’s global managing director. David Court, based in the Dallas office, leads the firm’s advanced-analytics practice.
Explore a career with us
Related articles.

Big data: What’s your plan?
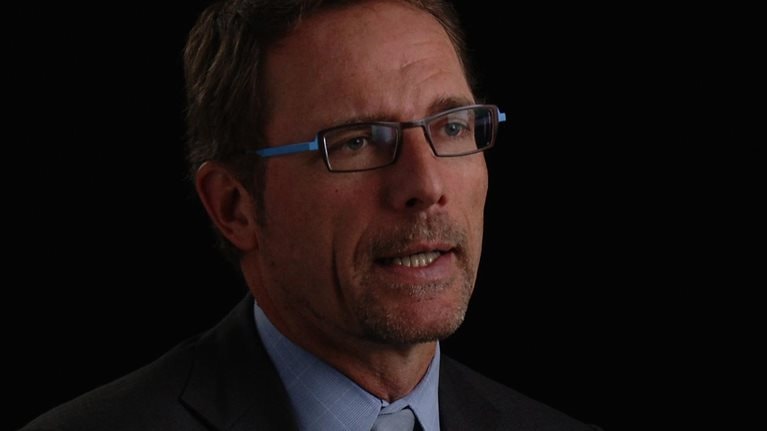
Making data analytics work: Three key challenges
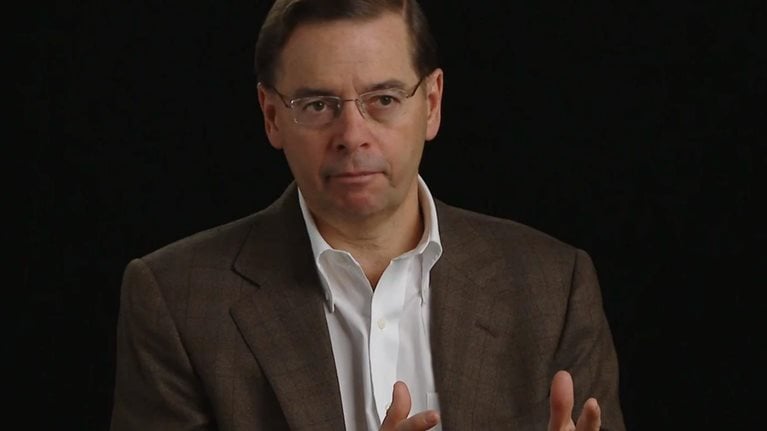
Putting big data and advanced analytics to work

IMAGES
VIDEO
COMMENTS
A Guide To Data-Driven Decision Making
Data-driven Decision Making Course by PwC
The Process of Data-Driven Decision Making. Through the process of DDDM, data are used to assess, test, and improve a program, activity, or strategy. These activities occur through four iterative stages, which are summarized below and in exhibit 1-1 on the following page. Formulating key questions.
Template 6. This data-driven decision making template is a must-have tool for every analyst, manager, and business owner as it explains analytical, heuristic, expertise, among other processes of decision making, with ease. You can use the information gathered from your analysis to make the right choice.
Data-driven decision making refers to a school's ongoing process of collecting and analyzing multiple sources of formative and summative data, such as demographic, student achievement, Georgia Milestones, End-of-Pathway, ACCESS, attendance (student & staff), staff turnover, discipline/behavior, engagement, common assessments, school climate ...
Data-Driven Decision-Making: 6 Key Steps (+ Examples)
Data-driven decision-making (DDDM) is an approach that emphasizes using data and analysis instead of intuition to inform business decisions. It involves leveraging data sources such as customer feedback, market trends and financial data to guide the decision-making process. By collecting, analyzing and interpreting data, organizations can make ...
What Is Data-Driven Decision-Making (DDDM)?
Data-Driven Decision Making: A Step-by-Step Guide [2024]
The Advantages of Data-Driven Decision-Making - HBS Online
Vance: This talk is called data-driven decision making. What we really mean by that is data in service of decision making with the emphasis on the decisions that you're enabled to make. Data tends ...
Specialization - 3 course series. This specialization explains why it is important to leverage data when contemplating organizational choices, and supplies the tools at the heart of data-driven decision making (DDDM). The three-course series explores how technology enables the collection and organization of unprecedented amounts of data, and ...
Data-driven decision making is just that - the use of data to evaluate options and make strategic business decisions. As humans, we are often tempted to make choices based on emotion, intuition or a "gut" feeling. However, when it comes to making business decisions, analytics and data can help provide more reliable, measurable and actionable ...
Data Driven Decision Making - Free download as Powerpoint Presentation (.ppt), PDF File (.pdf), Text File (.txt) or view presentation slides online. This document provides guidelines for using data to improve decision making. It discusses collecting accurate and easy-to-gather data, presenting it visually, and ensuring it is available when decisions need to be made.
Presenting Introduction to Data-Driven Decision Making. These slides are 100 percent made in PowerPoint and are compatible with all screen types and monitors. They also support Google Slides. Premium Customer Support is available. Suitable for use by managers, employees, and organizations. These slides are easily customizable.
Download our appealing Data-Driven Decision-Making presentation template, compatible with MS PowerPoint and Google Slides, to describe the approach to using data, information, analytics, facts, and metrics to make informed business decisions. You can also discuss this approach's benefits over the traditional decision-making process, like better ...
Templates for Delivering Amazing Presentations on Decision-Making Models 1. ... Data-Driven Decision-Making. This pre-designed template is the best pick to educate the audience about the model that facilitates decision-making based on data, metrics, and facts. You can also explain how this model helps guide business strategies, improve ...
Quick Guide to Data-Driven Management
Presentation Transcript. Data-Driven Decision Making Presented by The Leadership and Learning Center (800) 844-6599 www.LeadandLearn.com. Part One: Objectives • Develop a deeper understanding of the Decision Making for Results: Data-Driven Decision Making process • Increase awareness of the relevance of data and its impact on leadership ...
Data Analysis and Presentation Skills: the PwC Approach ...
BARC studies suggest DDDM (Data Driven Decision Making) accommodated by organizations saw a 10% reduction in their operational costing. Forbes Magazine says, 88% of the organizations have been using DDDM (Data Driven Decision Making) for improved understanding of their customer behaviour, to introduce more features and products.
Presenting the correct data using the right tools and strategies will help stakeholders understand your analysis and make impactful data-driven decisions. Conclusion. Becoming a data-driven organization is challenging, but the rewards are worth it. The valuable insights that data can add to your decision-making can be discovered when you ...
Bottom line: using big data requires thoughtful organizational change, and three areas of action can get you there. Develop business-relevant analytics that can be put to use. Many initial implementations of big data and analytics fail because they aren't in sync with a company's day-to-day processes and decision-making norms.