How to conduct a meta-analysis in eight steps: a practical guide
- Open access
- Published: 30 November 2021
- Volume 72 , pages 1–19, ( 2022 )

Cite this article
You have full access to this open access article
- Christopher Hansen 1 ,
- Holger Steinmetz 2 &
- Jörn Block 3 , 4 , 5
168k Accesses
56 Citations
158 Altmetric
Explore all metrics
Avoid common mistakes on your manuscript.
1 Introduction
“Scientists have known for centuries that a single study will not resolve a major issue. Indeed, a small sample study will not even resolve a minor issue. Thus, the foundation of science is the cumulation of knowledge from the results of many studies.” (Hunter et al. 1982 , p. 10)
Meta-analysis is a central method for knowledge accumulation in many scientific fields (Aguinis et al. 2011c ; Kepes et al. 2013 ). Similar to a narrative review, it serves as a synopsis of a research question or field. However, going beyond a narrative summary of key findings, a meta-analysis adds value in providing a quantitative assessment of the relationship between two target variables or the effectiveness of an intervention (Gurevitch et al. 2018 ). Also, it can be used to test competing theoretical assumptions against each other or to identify important moderators where the results of different primary studies differ from each other (Aguinis et al. 2011b ; Bergh et al. 2016 ). Rooted in the synthesis of the effectiveness of medical and psychological interventions in the 1970s (Glass 2015 ; Gurevitch et al. 2018 ), meta-analysis is nowadays also an established method in management research and related fields.
The increasing importance of meta-analysis in management research has resulted in the publication of guidelines in recent years that discuss the merits and best practices in various fields, such as general management (Bergh et al. 2016 ; Combs et al. 2019 ; Gonzalez-Mulé and Aguinis 2018 ), international business (Steel et al. 2021 ), economics and finance (Geyer-Klingeberg et al. 2020 ; Havranek et al. 2020 ), marketing (Eisend 2017 ; Grewal et al. 2018 ), and organizational studies (DeSimone et al. 2020 ; Rudolph et al. 2020 ). These articles discuss existing and trending methods and propose solutions for often experienced problems. This editorial briefly summarizes the insights of these papers; provides a workflow of the essential steps in conducting a meta-analysis; suggests state-of-the art methodological procedures; and points to other articles for in-depth investigation. Thus, this article has two goals: (1) based on the findings of previous editorials and methodological articles, it defines methodological recommendations for meta-analyses submitted to Management Review Quarterly (MRQ); and (2) it serves as a practical guide for researchers who have little experience with meta-analysis as a method but plan to conduct one in the future.
2 Eight steps in conducting a meta-analysis
2.1 step 1: defining the research question.
The first step in conducting a meta-analysis, as with any other empirical study, is the definition of the research question. Most importantly, the research question determines the realm of constructs to be considered or the type of interventions whose effects shall be analyzed. When defining the research question, two hurdles might develop. First, when defining an adequate study scope, researchers must consider that the number of publications has grown exponentially in many fields of research in recent decades (Fortunato et al. 2018 ). On the one hand, a larger number of studies increases the potentially relevant literature basis and enables researchers to conduct meta-analyses. Conversely, scanning a large amount of studies that could be potentially relevant for the meta-analysis results in a perhaps unmanageable workload. Thus, Steel et al. ( 2021 ) highlight the importance of balancing manageability and relevance when defining the research question. Second, similar to the number of primary studies also the number of meta-analyses in management research has grown strongly in recent years (Geyer-Klingeberg et al. 2020 ; Rauch 2020 ; Schwab 2015 ). Therefore, it is likely that one or several meta-analyses for many topics of high scholarly interest already exist. However, this should not deter researchers from investigating their research questions. One possibility is to consider moderators or mediators of a relationship that have previously been ignored. For example, a meta-analysis about startup performance could investigate the impact of different ways to measure the performance construct (e.g., growth vs. profitability vs. survival time) or certain characteristics of the founders as moderators. Another possibility is to replicate previous meta-analyses and test whether their findings can be confirmed with an updated sample of primary studies or newly developed methods. Frequent replications and updates of meta-analyses are important contributions to cumulative science and are increasingly called for by the research community (Anderson & Kichkha 2017 ; Steel et al. 2021 ). Consistent with its focus on replication studies (Block and Kuckertz 2018 ), MRQ therefore also invites authors to submit replication meta-analyses.
2.2 Step 2: literature search
2.2.1 search strategies.
Similar to conducting a literature review, the search process of a meta-analysis should be systematic, reproducible, and transparent, resulting in a sample that includes all relevant studies (Fisch and Block 2018 ; Gusenbauer and Haddaway 2020 ). There are several identification strategies for relevant primary studies when compiling meta-analytical datasets (Harari et al. 2020 ). First, previous meta-analyses on the same or a related topic may provide lists of included studies that offer a good starting point to identify and become familiar with the relevant literature. This practice is also applicable to topic-related literature reviews, which often summarize the central findings of the reviewed articles in systematic tables. Both article types likely include the most prominent studies of a research field. The most common and important search strategy, however, is a keyword search in electronic databases (Harari et al. 2020 ). This strategy will probably yield the largest number of relevant studies, particularly so-called ‘grey literature’, which may not be considered by literature reviews. Gusenbauer and Haddaway ( 2020 ) provide a detailed overview of 34 scientific databases, of which 18 are multidisciplinary or have a focus on management sciences, along with their suitability for literature synthesis. To prevent biased results due to the scope or journal coverage of one database, researchers should use at least two different databases (DeSimone et al. 2020 ; Martín-Martín et al. 2021 ; Mongeon & Paul-Hus 2016 ). However, a database search can easily lead to an overload of potentially relevant studies. For example, key term searches in Google Scholar for “entrepreneurial intention” and “firm diversification” resulted in more than 660,000 and 810,000 hits, respectively. Footnote 1 Therefore, a precise research question and precise search terms using Boolean operators are advisable (Gusenbauer and Haddaway 2020 ). Addressing the challenge of identifying relevant articles in the growing number of database publications, (semi)automated approaches using text mining and machine learning (Bosco et al. 2017 ; O’Mara-Eves et al. 2015 ; Ouzzani et al. 2016 ; Thomas et al. 2017 ) can also be promising and time-saving search tools in the future. Also, some electronic databases offer the possibility to track forward citations of influential studies and thereby identify further relevant articles. Finally, collecting unpublished or undetected studies through conferences, personal contact with (leading) scholars, or listservs can be strategies to increase the study sample size (Grewal et al. 2018 ; Harari et al. 2020 ; Pigott and Polanin 2020 ).
2.2.2 Study inclusion criteria and sample composition
Next, researchers must decide which studies to include in the meta-analysis. Some guidelines for literature reviews recommend limiting the sample to studies published in renowned academic journals to ensure the quality of findings (e.g., Kraus et al. 2020 ). For meta-analysis, however, Steel et al. ( 2021 ) advocate for the inclusion of all available studies, including grey literature, to prevent selection biases based on availability, cost, familiarity, and language (Rothstein et al. 2005 ), or the “Matthew effect”, which denotes the phenomenon that highly cited articles are found faster than less cited articles (Merton 1968 ). Harrison et al. ( 2017 ) find that the effects of published studies in management are inflated on average by 30% compared to unpublished studies. This so-called publication bias or “file drawer problem” (Rosenthal 1979 ) results from the preference of academia to publish more statistically significant and less statistically insignificant study results. Owen and Li ( 2020 ) showed that publication bias is particularly severe when variables of interest are used as key variables rather than control variables. To consider the true effect size of a target variable or relationship, the inclusion of all types of research outputs is therefore recommended (Polanin et al. 2016 ). Different test procedures to identify publication bias are discussed subsequently in Step 7.
In addition to the decision of whether to include certain study types (i.e., published vs. unpublished studies), there can be other reasons to exclude studies that are identified in the search process. These reasons can be manifold and are primarily related to the specific research question and methodological peculiarities. For example, studies identified by keyword search might not qualify thematically after all, may use unsuitable variable measurements, or may not report usable effect sizes. Furthermore, there might be multiple studies by the same authors using similar datasets. If they do not differ sufficiently in terms of their sample characteristics or variables used, only one of these studies should be included to prevent bias from duplicates (Wood 2008 ; see this article for a detection heuristic).
In general, the screening process should be conducted stepwise, beginning with a removal of duplicate citations from different databases, followed by abstract screening to exclude clearly unsuitable studies and a final full-text screening of the remaining articles (Pigott and Polanin 2020 ). A graphical tool to systematically document the sample selection process is the PRISMA flow diagram (Moher et al. 2009 ). Page et al. ( 2021 ) recently presented an updated version of the PRISMA statement, including an extended item checklist and flow diagram to report the study process and findings.
2.3 Step 3: choice of the effect size measure
2.3.1 types of effect sizes.
The two most common meta-analytical effect size measures in management studies are (z-transformed) correlation coefficients and standardized mean differences (Aguinis et al. 2011a ; Geyskens et al. 2009 ). However, meta-analyses in management science and related fields may not be limited to those two effect size measures but rather depend on the subfield of investigation (Borenstein 2009 ; Stanley and Doucouliagos 2012 ). In economics and finance, researchers are more interested in the examination of elasticities and marginal effects extracted from regression models than in pure bivariate correlations (Stanley and Doucouliagos 2012 ). Regression coefficients can also be converted to partial correlation coefficients based on their t-statistics to make regression results comparable across studies (Stanley and Doucouliagos 2012 ). Although some meta-analyses in management research have combined bivariate and partial correlations in their study samples, Aloe ( 2015 ) and Combs et al. ( 2019 ) advise researchers not to use this practice. Most importantly, they argue that the effect size strength of partial correlations depends on the other variables included in the regression model and is therefore incomparable to bivariate correlations (Schmidt and Hunter 2015 ), resulting in a possible bias of the meta-analytic results (Roth et al. 2018 ). We endorse this opinion. If at all, we recommend separate analyses for each measure. In addition to these measures, survival rates, risk ratios or odds ratios, which are common measures in medical research (Borenstein 2009 ), can be suitable effect sizes for specific management research questions, such as understanding the determinants of the survival of startup companies. To summarize, the choice of a suitable effect size is often taken away from the researcher because it is typically dependent on the investigated research question as well as the conventions of the specific research field (Cheung and Vijayakumar 2016 ).
2.3.2 Conversion of effect sizes to a common measure
After having defined the primary effect size measure for the meta-analysis, it might become necessary in the later coding process to convert study findings that are reported in effect sizes that are different from the chosen primary effect size. For example, a study might report only descriptive statistics for two study groups but no correlation coefficient, which is used as the primary effect size measure in the meta-analysis. Different effect size measures can be harmonized using conversion formulae, which are provided by standard method books such as Borenstein et al. ( 2009 ) or Lipsey and Wilson ( 2001 ). There also exist online effect size calculators for meta-analysis. Footnote 2
2.4 Step 4: choice of the analytical method used
Choosing which meta-analytical method to use is directly connected to the research question of the meta-analysis. Research questions in meta-analyses can address a relationship between constructs or an effect of an intervention in a general manner, or they can focus on moderating or mediating effects. There are four meta-analytical methods that are primarily used in contemporary management research (Combs et al. 2019 ; Geyer-Klingeberg et al. 2020 ), which allow the investigation of these different types of research questions: traditional univariate meta-analysis, meta-regression, meta-analytic structural equation modeling, and qualitative meta-analysis (Hoon 2013 ). While the first three are quantitative, the latter summarizes qualitative findings. Table 1 summarizes the key characteristics of the three quantitative methods.
2.4.1 Univariate meta-analysis
In its traditional form, a meta-analysis reports a weighted mean effect size for the relationship or intervention of investigation and provides information on the magnitude of variance among primary studies (Aguinis et al. 2011c ; Borenstein et al. 2009 ). Accordingly, it serves as a quantitative synthesis of a research field (Borenstein et al. 2009 ; Geyskens et al. 2009 ). Prominent traditional approaches have been developed, for example, by Hedges and Olkin ( 1985 ) or Hunter and Schmidt ( 1990 , 2004 ). However, going beyond its simple summary function, the traditional approach has limitations in explaining the observed variance among findings (Gonzalez-Mulé and Aguinis 2018 ). To identify moderators (or boundary conditions) of the relationship of interest, meta-analysts can create subgroups and investigate differences between those groups (Borenstein and Higgins 2013 ; Hunter and Schmidt 2004 ). Potential moderators can be study characteristics (e.g., whether a study is published vs. unpublished), sample characteristics (e.g., study country, industry focus, or type of survey/experiment participants), or measurement artifacts (e.g., different types of variable measurements). The univariate approach is thus suitable to identify the overall direction of a relationship and can serve as a good starting point for additional analyses. However, due to its limitations in examining boundary conditions and developing theory, the univariate approach on its own is currently oftentimes viewed as not sufficient (Rauch 2020 ; Shaw and Ertug 2017 ).
2.4.2 Meta-regression analysis
Meta-regression analysis (Hedges and Olkin 1985 ; Lipsey and Wilson 2001 ; Stanley and Jarrell 1989 ) aims to investigate the heterogeneity among observed effect sizes by testing multiple potential moderators simultaneously. In meta-regression, the coded effect size is used as the dependent variable and is regressed on a list of moderator variables. These moderator variables can be categorical variables as described previously in the traditional univariate approach or (semi)continuous variables such as country scores that are merged with the meta-analytical data. Thus, meta-regression analysis overcomes the disadvantages of the traditional approach, which only allows us to investigate moderators singularly using dichotomized subgroups (Combs et al. 2019 ; Gonzalez-Mulé and Aguinis 2018 ). These possibilities allow a more fine-grained analysis of research questions that are related to moderating effects. However, Schmidt ( 2017 ) critically notes that the number of effect sizes in the meta-analytical sample must be sufficiently large to produce reliable results when investigating multiple moderators simultaneously in a meta-regression. For further reading, Tipton et al. ( 2019 ) outline the technical, conceptual, and practical developments of meta-regression over the last decades. Gonzalez-Mulé and Aguinis ( 2018 ) provide an overview of methodological choices and develop evidence-based best practices for future meta-analyses in management using meta-regression.
2.4.3 Meta-analytic structural equation modeling (MASEM)
MASEM is a combination of meta-analysis and structural equation modeling and allows to simultaneously investigate the relationships among several constructs in a path model. Researchers can use MASEM to test several competing theoretical models against each other or to identify mediation mechanisms in a chain of relationships (Bergh et al. 2016 ). This method is typically performed in two steps (Cheung and Chan 2005 ): In Step 1, a pooled correlation matrix is derived, which includes the meta-analytical mean effect sizes for all variable combinations; Step 2 then uses this matrix to fit the path model. While MASEM was based primarily on traditional univariate meta-analysis to derive the pooled correlation matrix in its early years (Viswesvaran and Ones 1995 ), more advanced methods, such as the GLS approach (Becker 1992 , 1995 ) or the TSSEM approach (Cheung and Chan 2005 ), have been subsequently developed. Cheung ( 2015a ) and Jak ( 2015 ) provide an overview of these approaches in their books with exemplary code. For datasets with more complex data structures, Wilson et al. ( 2016 ) also developed a multilevel approach that is related to the TSSEM approach in the second step. Bergh et al. ( 2016 ) discuss nine decision points and develop best practices for MASEM studies.
2.4.4 Qualitative meta-analysis
While the approaches explained above focus on quantitative outcomes of empirical studies, qualitative meta-analysis aims to synthesize qualitative findings from case studies (Hoon 2013 ; Rauch et al. 2014 ). The distinctive feature of qualitative case studies is their potential to provide in-depth information about specific contextual factors or to shed light on reasons for certain phenomena that cannot usually be investigated by quantitative studies (Rauch 2020 ; Rauch et al. 2014 ). In a qualitative meta-analysis, the identified case studies are systematically coded in a meta-synthesis protocol, which is then used to identify influential variables or patterns and to derive a meta-causal network (Hoon 2013 ). Thus, the insights of contextualized and typically nongeneralizable single studies are aggregated to a larger, more generalizable picture (Habersang et al. 2019 ). Although still the exception, this method can thus provide important contributions for academics in terms of theory development (Combs et al., 2019 ; Hoon 2013 ) and for practitioners in terms of evidence-based management or entrepreneurship (Rauch et al. 2014 ). Levitt ( 2018 ) provides a guide and discusses conceptual issues for conducting qualitative meta-analysis in psychology, which is also useful for management researchers.
2.5 Step 5: choice of software
Software solutions to perform meta-analyses range from built-in functions or additional packages of statistical software to software purely focused on meta-analyses and from commercial to open-source solutions. However, in addition to personal preferences, the choice of the most suitable software depends on the complexity of the methods used and the dataset itself (Cheung and Vijayakumar 2016 ). Meta-analysts therefore must carefully check if their preferred software is capable of performing the intended analysis.
Among commercial software providers, Stata (from version 16 on) offers built-in functions to perform various meta-analytical analyses or to produce various plots (Palmer and Sterne 2016 ). For SPSS and SAS, there exist several macros for meta-analyses provided by scholars, such as David B. Wilson or Andy P. Field and Raphael Gillet (Field and Gillett 2010 ). Footnote 3 Footnote 4 For researchers using the open-source software R (R Core Team 2021 ), Polanin et al. ( 2017 ) provide an overview of 63 meta-analysis packages and their functionalities. For new users, they recommend the package metafor (Viechtbauer 2010 ), which includes most necessary functions and for which the author Wolfgang Viechtbauer provides tutorials on his project website. Footnote 5 Footnote 6 In addition to packages and macros for statistical software, templates for Microsoft Excel have also been developed to conduct simple meta-analyses, such as Meta-Essentials by Suurmond et al. ( 2017 ). Footnote 7 Finally, programs purely dedicated to meta-analysis also exist, such as Comprehensive Meta-Analysis (Borenstein et al. 2013 ) or RevMan by The Cochrane Collaboration ( 2020 ).
2.6 Step 6: coding of effect sizes
2.6.1 coding sheet.
The first step in the coding process is the design of the coding sheet. A universal template does not exist because the design of the coding sheet depends on the methods used, the respective software, and the complexity of the research design. For univariate meta-analysis or meta-regression, data are typically coded in wide format. In its simplest form, when investigating a correlational relationship between two variables using the univariate approach, the coding sheet would contain a column for the study name or identifier, the effect size coded from the primary study, and the study sample size. However, such simple relationships are unlikely in management research because the included studies are typically not identical but differ in several respects. With more complex data structures or moderator variables being investigated, additional columns are added to the coding sheet to reflect the data characteristics. These variables can be coded as dummy, factor, or (semi)continuous variables and later used to perform a subgroup analysis or meta regression. For MASEM, the required data input format can deviate depending on the method used (e.g., TSSEM requires a list of correlation matrices as data input). For qualitative meta-analysis, the coding scheme typically summarizes the key qualitative findings and important contextual and conceptual information (see Hoon ( 2013 ) for a coding scheme for qualitative meta-analysis). Figure 1 shows an exemplary coding scheme for a quantitative meta-analysis on the correlational relationship between top-management team diversity and profitability. In addition to effect and sample sizes, information about the study country, firm type, and variable operationalizations are coded. The list could be extended by further study and sample characteristics.

Exemplary coding sheet for a meta-analysis on the relationship (correlation) between top-management team diversity and profitability
2.6.2 Inclusion of moderator or control variables
It is generally important to consider the intended research model and relevant nontarget variables before coding a meta-analytic dataset. For example, study characteristics can be important moderators or function as control variables in a meta-regression model. Similarly, control variables may be relevant in a MASEM approach to reduce confounding bias. Coding additional variables or constructs subsequently can be arduous if the sample of primary studies is large. However, the decision to include respective moderator or control variables, as in any empirical analysis, should always be based on strong (theoretical) rationales about how these variables can impact the investigated effect (Bernerth and Aguinis 2016 ; Bernerth et al. 2018 ; Thompson and Higgins 2002 ). While substantive moderators refer to theoretical constructs that act as buffers or enhancers of a supposed causal process, methodological moderators are features of the respective research designs that denote the methodological context of the observations and are important to control for systematic statistical particularities (Rudolph et al. 2020 ). Havranek et al. ( 2020 ) provide a list of recommended variables to code as potential moderators. While researchers may have clear expectations about the effects for some of these moderators, the concerns for other moderators may be tentative, and moderator analysis may be approached in a rather exploratory fashion. Thus, we argue that researchers should make full use of the meta-analytical design to obtain insights about potential context dependence that a primary study cannot achieve.
2.6.3 Treatment of multiple effect sizes in a study
A long-debated issue in conducting meta-analyses is whether to use only one or all available effect sizes for the same construct within a single primary study. For meta-analyses in management research, this question is fundamental because many empirical studies, particularly those relying on company databases, use multiple variables for the same construct to perform sensitivity analyses, resulting in multiple relevant effect sizes. In this case, researchers can either (randomly) select a single value, calculate a study average, or use the complete set of effect sizes (Bijmolt and Pieters 2001 ; López-López et al. 2018 ). Multiple effect sizes from the same study enrich the meta-analytic dataset and allow us to investigate the heterogeneity of the relationship of interest, such as different variable operationalizations (López-López et al. 2018 ; Moeyaert et al. 2017 ). However, including more than one effect size from the same study violates the independency assumption of observations (Cheung 2019 ; López-López et al. 2018 ), which can lead to biased results and erroneous conclusions (Gooty et al. 2021 ). We follow the recommendation of current best practice guides to take advantage of using all available effect size observations but to carefully consider interdependencies using appropriate methods such as multilevel models, panel regression models, or robust variance estimation (Cheung 2019 ; Geyer-Klingeberg et al. 2020 ; Gooty et al. 2021 ; López-López et al. 2018 ; Moeyaert et al. 2017 ).
2.7 Step 7: analysis
2.7.1 outlier analysis and tests for publication bias.
Before conducting the primary analysis, some preliminary sensitivity analyses might be necessary, which should ensure the robustness of the meta-analytical findings (Rudolph et al. 2020 ). First, influential outlier observations could potentially bias the observed results, particularly if the number of total effect sizes is small. Several statistical methods can be used to identify outliers in meta-analytical datasets (Aguinis et al. 2013 ; Viechtbauer and Cheung 2010 ). However, there is a debate about whether to keep or omit these observations. Anyhow, relevant studies should be closely inspected to infer an explanation about their deviating results. As in any other primary study, outliers can be a valid representation, albeit representing a different population, measure, construct, design or procedure. Thus, inferences about outliers can provide the basis to infer potential moderators (Aguinis et al. 2013 ; Steel et al. 2021 ). On the other hand, outliers can indicate invalid research, for instance, when unrealistically strong correlations are due to construct overlap (i.e., lack of a clear demarcation between independent and dependent variables), invalid measures, or simply typing errors when coding effect sizes. An advisable step is therefore to compare the results both with and without outliers and base the decision on whether to exclude outlier observations with careful consideration (Geyskens et al. 2009 ; Grewal et al. 2018 ; Kepes et al. 2013 ). However, instead of simply focusing on the size of the outlier, its leverage should be considered. Thus, Viechtbauer and Cheung ( 2010 ) propose considering a combination of standardized deviation and a study’s leverage.
Second, as mentioned in the context of a literature search, potential publication bias may be an issue. Publication bias can be examined in multiple ways (Rothstein et al. 2005 ). First, the funnel plot is a simple graphical tool that can provide an overview of the effect size distribution and help to detect publication bias (Stanley and Doucouliagos 2010 ). A funnel plot can also support in identifying potential outliers. As mentioned above, a graphical display of deviation (e.g., studentized residuals) and leverage (Cook’s distance) can help detect the presence of outliers and evaluate their influence (Viechtbauer and Cheung 2010 ). Moreover, several statistical procedures can be used to test for publication bias (Harrison et al. 2017 ; Kepes et al. 2012 ), including subgroup comparisons between published and unpublished studies, Begg and Mazumdar’s ( 1994 ) rank correlation test, cumulative meta-analysis (Borenstein et al. 2009 ), the trim and fill method (Duval and Tweedie 2000a , b ), Egger et al.’s ( 1997 ) regression test, failsafe N (Rosenthal 1979 ), or selection models (Hedges and Vevea 2005 ; Vevea and Woods 2005 ). In examining potential publication bias, Kepes et al. ( 2012 ) and Harrison et al. ( 2017 ) both recommend not relying only on a single test but rather using multiple conceptionally different test procedures (i.e., the so-called “triangulation approach”).
2.7.2 Model choice
After controlling and correcting for the potential presence of impactful outliers or publication bias, the next step in meta-analysis is the primary analysis, where meta-analysts must decide between two different types of models that are based on different assumptions: fixed-effects and random-effects (Borenstein et al. 2010 ). Fixed-effects models assume that all observations share a common mean effect size, which means that differences are only due to sampling error, while random-effects models assume heterogeneity and allow for a variation of the true effect sizes across studies (Borenstein et al. 2010 ; Cheung and Vijayakumar 2016 ; Hunter and Schmidt 2004 ). Both models are explained in detail in standard textbooks (e.g., Borenstein et al. 2009 ; Hunter and Schmidt 2004 ; Lipsey and Wilson 2001 ).
In general, the presence of heterogeneity is likely in management meta-analyses because most studies do not have identical empirical settings, which can yield different effect size strengths or directions for the same investigated phenomenon. For example, the identified studies have been conducted in different countries with different institutional settings, or the type of study participants varies (e.g., students vs. employees, blue-collar vs. white-collar workers, or manufacturing vs. service firms). Thus, the vast majority of meta-analyses in management research and related fields use random-effects models (Aguinis et al. 2011a ). In a meta-regression, the random-effects model turns into a so-called mixed-effects model because moderator variables are added as fixed effects to explain the impact of observed study characteristics on effect size variations (Raudenbush 2009 ).
2.8 Step 8: reporting results
2.8.1 reporting in the article.
The final step in performing a meta-analysis is reporting its results. Most importantly, all steps and methodological decisions should be comprehensible to the reader. DeSimone et al. ( 2020 ) provide an extensive checklist for journal reviewers of meta-analytical studies. This checklist can also be used by authors when performing their analyses and reporting their results to ensure that all important aspects have been addressed. Alternative checklists are provided, for example, by Appelbaum et al. ( 2018 ) or Page et al. ( 2021 ). Similarly, Levitt et al. ( 2018 ) provide a detailed guide for qualitative meta-analysis reporting standards.
For quantitative meta-analyses, tables reporting results should include all important information and test statistics, including mean effect sizes; standard errors and confidence intervals; the number of observations and study samples included; and heterogeneity measures. If the meta-analytic sample is rather small, a forest plot provides a good overview of the different findings and their accuracy. However, this figure will be less feasible for meta-analyses with several hundred effect sizes included. Also, results displayed in the tables and figures must be explained verbally in the results and discussion sections. Most importantly, authors must answer the primary research question, i.e., whether there is a positive, negative, or no relationship between the variables of interest, or whether the examined intervention has a certain effect. These results should be interpreted with regard to their magnitude (or significance), both economically and statistically. However, when discussing meta-analytical results, authors must describe the complexity of the results, including the identified heterogeneity and important moderators, future research directions, and theoretical relevance (DeSimone et al. 2019 ). In particular, the discussion of identified heterogeneity and underlying moderator effects is critical; not including this information can lead to false conclusions among readers, who interpret the reported mean effect size as universal for all included primary studies and ignore the variability of findings when citing the meta-analytic results in their research (Aytug et al. 2012 ; DeSimone et al. 2019 ).
2.8.2 Open-science practices
Another increasingly important topic is the public provision of meta-analytical datasets and statistical codes via open-source repositories. Open-science practices allow for results validation and for the use of coded data in subsequent meta-analyses ( Polanin et al. 2020 ), contributing to the development of cumulative science. Steel et al. ( 2021 ) refer to open science meta-analyses as a step towards “living systematic reviews” (Elliott et al. 2017 ) with continuous updates in real time. MRQ supports this development and encourages authors to make their datasets publicly available. Moreau and Gamble ( 2020 ), for example, provide various templates and video tutorials to conduct open science meta-analyses. There exist several open science repositories, such as the Open Science Foundation (OSF; for a tutorial, see Soderberg 2018 ), to preregister and make documents publicly available. Furthermore, several initiatives in the social sciences have been established to develop dynamic meta-analyses, such as metaBUS (Bosco et al. 2015 , 2017 ), MetaLab (Bergmann et al. 2018 ), or PsychOpen CAMA (Burgard et al. 2021 ).
3 Conclusion
This editorial provides a comprehensive overview of the essential steps in conducting and reporting a meta-analysis with references to more in-depth methodological articles. It also serves as a guide for meta-analyses submitted to MRQ and other management journals. MRQ welcomes all types of meta-analyses from all subfields and disciplines of management research.
Gusenbauer and Haddaway ( 2020 ), however, point out that Google Scholar is not appropriate as a primary search engine due to a lack of reproducibility of search results.
One effect size calculator by David B. Wilson is accessible via: https://www.campbellcollaboration.org/escalc/html/EffectSizeCalculator-Home.php .
The macros of David B. Wilson can be downloaded from: http://mason.gmu.edu/~dwilsonb/ .
The macros of Field and Gillet ( 2010 ) can be downloaded from: https://www.discoveringstatistics.com/repository/fieldgillett/how_to_do_a_meta_analysis.html .
The tutorials can be found via: https://www.metafor-project.org/doku.php .
Metafor does currently not provide functions to conduct MASEM. For MASEM, users can, for instance, use the package metaSEM (Cheung 2015b ).
The workbooks can be downloaded from: https://www.erim.eur.nl/research-support/meta-essentials/ .
Aguinis H, Dalton DR, Bosco FA, Pierce CA, Dalton CM (2011a) Meta-analytic choices and judgment calls: Implications for theory building and testing, obtained effect sizes, and scholarly impact. J Manag 37(1):5–38
Google Scholar
Aguinis H, Gottfredson RK, Joo H (2013) Best-practice recommendations for defining, identifying, and handling outliers. Organ Res Methods 16(2):270–301
Article Google Scholar
Aguinis H, Gottfredson RK, Wright TA (2011b) Best-practice recommendations for estimating interaction effects using meta-analysis. J Organ Behav 32(8):1033–1043
Aguinis H, Pierce CA, Bosco FA, Dalton DR, Dalton CM (2011c) Debunking myths and urban legends about meta-analysis. Organ Res Methods 14(2):306–331
Aloe AM (2015) Inaccuracy of regression results in replacing bivariate correlations. Res Synth Methods 6(1):21–27
Anderson RG, Kichkha A (2017) Replication, meta-analysis, and research synthesis in economics. Am Econ Rev 107(5):56–59
Appelbaum M, Cooper H, Kline RB, Mayo-Wilson E, Nezu AM, Rao SM (2018) Journal article reporting standards for quantitative research in psychology: the APA publications and communications BOARD task force report. Am Psychol 73(1):3–25
Aytug ZG, Rothstein HR, Zhou W, Kern MC (2012) Revealed or concealed? Transparency of procedures, decisions, and judgment calls in meta-analyses. Organ Res Methods 15(1):103–133
Begg CB, Mazumdar M (1994) Operating characteristics of a rank correlation test for publication bias. Biometrics 50(4):1088–1101. https://doi.org/10.2307/2533446
Bergh DD, Aguinis H, Heavey C, Ketchen DJ, Boyd BK, Su P, Lau CLL, Joo H (2016) Using meta-analytic structural equation modeling to advance strategic management research: Guidelines and an empirical illustration via the strategic leadership-performance relationship. Strateg Manag J 37(3):477–497
Becker BJ (1992) Using results from replicated studies to estimate linear models. J Educ Stat 17(4):341–362
Becker BJ (1995) Corrections to “Using results from replicated studies to estimate linear models.” J Edu Behav Stat 20(1):100–102
Bergmann C, Tsuji S, Piccinini PE, Lewis ML, Braginsky M, Frank MC, Cristia A (2018) Promoting replicability in developmental research through meta-analyses: Insights from language acquisition research. Child Dev 89(6):1996–2009
Bernerth JB, Aguinis H (2016) A critical review and best-practice recommendations for control variable usage. Pers Psychol 69(1):229–283
Bernerth JB, Cole MS, Taylor EC, Walker HJ (2018) Control variables in leadership research: A qualitative and quantitative review. J Manag 44(1):131–160
Bijmolt TH, Pieters RG (2001) Meta-analysis in marketing when studies contain multiple measurements. Mark Lett 12(2):157–169
Block J, Kuckertz A (2018) Seven principles of effective replication studies: Strengthening the evidence base of management research. Manag Rev Quart 68:355–359
Borenstein M (2009) Effect sizes for continuous data. In: Cooper H, Hedges LV, Valentine JC (eds) The handbook of research synthesis and meta-analysis. Russell Sage Foundation, pp 221–235
Borenstein M, Hedges LV, Higgins JPT, Rothstein HR (2009) Introduction to meta-analysis. John Wiley, Chichester
Book Google Scholar
Borenstein M, Hedges LV, Higgins JPT, Rothstein HR (2010) A basic introduction to fixed-effect and random-effects models for meta-analysis. Res Synth Methods 1(2):97–111
Borenstein M, Hedges L, Higgins J, Rothstein H (2013) Comprehensive meta-analysis (version 3). Biostat, Englewood, NJ
Borenstein M, Higgins JP (2013) Meta-analysis and subgroups. Prev Sci 14(2):134–143
Bosco FA, Steel P, Oswald FL, Uggerslev K, Field JG (2015) Cloud-based meta-analysis to bridge science and practice: Welcome to metaBUS. Person Assess Decis 1(1):3–17
Bosco FA, Uggerslev KL, Steel P (2017) MetaBUS as a vehicle for facilitating meta-analysis. Hum Resour Manag Rev 27(1):237–254
Burgard T, Bošnjak M, Studtrucker R (2021) Community-augmented meta-analyses (CAMAs) in psychology: potentials and current systems. Zeitschrift Für Psychologie 229(1):15–23
Cheung MWL (2015a) Meta-analysis: A structural equation modeling approach. John Wiley & Sons, Chichester
Cheung MWL (2015b) metaSEM: An R package for meta-analysis using structural equation modeling. Front Psychol 5:1521
Cheung MWL (2019) A guide to conducting a meta-analysis with non-independent effect sizes. Neuropsychol Rev 29(4):387–396
Cheung MWL, Chan W (2005) Meta-analytic structural equation modeling: a two-stage approach. Psychol Methods 10(1):40–64
Cheung MWL, Vijayakumar R (2016) A guide to conducting a meta-analysis. Neuropsychol Rev 26(2):121–128
Combs JG, Crook TR, Rauch A (2019) Meta-analytic research in management: contemporary approaches unresolved controversies and rising standards. J Manag Stud 56(1):1–18. https://doi.org/10.1111/joms.12427
DeSimone JA, Köhler T, Schoen JL (2019) If it were only that easy: the use of meta-analytic research by organizational scholars. Organ Res Methods 22(4):867–891. https://doi.org/10.1177/1094428118756743
DeSimone JA, Brannick MT, O’Boyle EH, Ryu JW (2020) Recommendations for reviewing meta-analyses in organizational research. Organ Res Methods 56:455–463
Duval S, Tweedie R (2000a) Trim and fill: a simple funnel-plot–based method of testing and adjusting for publication bias in meta-analysis. Biometrics 56(2):455–463
Duval S, Tweedie R (2000b) A nonparametric “trim and fill” method of accounting for publication bias in meta-analysis. J Am Stat Assoc 95(449):89–98
Egger M, Smith GD, Schneider M, Minder C (1997) Bias in meta-analysis detected by a simple, graphical test. BMJ 315(7109):629–634
Eisend M (2017) Meta-Analysis in advertising research. J Advert 46(1):21–35
Elliott JH, Synnot A, Turner T, Simmons M, Akl EA, McDonald S, Salanti G, Meerpohl J, MacLehose H, Hilton J, Tovey D, Shemilt I, Thomas J (2017) Living systematic review: 1. Introduction—the why, what, when, and how. J Clin Epidemiol 91:2330. https://doi.org/10.1016/j.jclinepi.2017.08.010
Field AP, Gillett R (2010) How to do a meta-analysis. Br J Math Stat Psychol 63(3):665–694
Fisch C, Block J (2018) Six tips for your (systematic) literature review in business and management research. Manag Rev Quart 68:103–106
Fortunato S, Bergstrom CT, Börner K, Evans JA, Helbing D, Milojević S, Petersen AM, Radicchi F, Sinatra R, Uzzi B, Vespignani A (2018) Science of science. Science 359(6379). https://doi.org/10.1126/science.aao0185
Geyer-Klingeberg J, Hang M, Rathgeber A (2020) Meta-analysis in finance research: Opportunities, challenges, and contemporary applications. Int Rev Finan Anal 71:101524
Geyskens I, Krishnan R, Steenkamp JBE, Cunha PV (2009) A review and evaluation of meta-analysis practices in management research. J Manag 35(2):393–419
Glass GV (2015) Meta-analysis at middle age: a personal history. Res Synth Methods 6(3):221–231
Gonzalez-Mulé E, Aguinis H (2018) Advancing theory by assessing boundary conditions with metaregression: a critical review and best-practice recommendations. J Manag 44(6):2246–2273
Gooty J, Banks GC, Loignon AC, Tonidandel S, Williams CE (2021) Meta-analyses as a multi-level model. Organ Res Methods 24(2):389–411. https://doi.org/10.1177/1094428119857471
Grewal D, Puccinelli N, Monroe KB (2018) Meta-analysis: integrating accumulated knowledge. J Acad Mark Sci 46(1):9–30
Gurevitch J, Koricheva J, Nakagawa S, Stewart G (2018) Meta-analysis and the science of research synthesis. Nature 555(7695):175–182
Gusenbauer M, Haddaway NR (2020) Which academic search systems are suitable for systematic reviews or meta-analyses? Evaluating retrieval qualities of Google Scholar, PubMed, and 26 other resources. Res Synth Methods 11(2):181–217
Habersang S, Küberling-Jost J, Reihlen M, Seckler C (2019) A process perspective on organizational failure: a qualitative meta-analysis. J Manage Stud 56(1):19–56
Harari MB, Parola HR, Hartwell CJ, Riegelman A (2020) Literature searches in systematic reviews and meta-analyses: A review, evaluation, and recommendations. J Vocat Behav 118:103377
Harrison JS, Banks GC, Pollack JM, O’Boyle EH, Short J (2017) Publication bias in strategic management research. J Manag 43(2):400–425
Havránek T, Stanley TD, Doucouliagos H, Bom P, Geyer-Klingeberg J, Iwasaki I, Reed WR, Rost K, Van Aert RCM (2020) Reporting guidelines for meta-analysis in economics. J Econ Surveys 34(3):469–475
Hedges LV, Olkin I (1985) Statistical methods for meta-analysis. Academic Press, Orlando
Hedges LV, Vevea JL (2005) Selection methods approaches. In: Rothstein HR, Sutton A, Borenstein M (eds) Publication bias in meta-analysis: prevention, assessment, and adjustments. Wiley, Chichester, pp 145–174
Hoon C (2013) Meta-synthesis of qualitative case studies: an approach to theory building. Organ Res Methods 16(4):522–556
Hunter JE, Schmidt FL (1990) Methods of meta-analysis: correcting error and bias in research findings. Sage, Newbury Park
Hunter JE, Schmidt FL (2004) Methods of meta-analysis: correcting error and bias in research findings, 2nd edn. Sage, Thousand Oaks
Hunter JE, Schmidt FL, Jackson GB (1982) Meta-analysis: cumulating research findings across studies. Sage Publications, Beverly Hills
Jak S (2015) Meta-analytic structural equation modelling. Springer, New York, NY
Kepes S, Banks GC, McDaniel M, Whetzel DL (2012) Publication bias in the organizational sciences. Organ Res Methods 15(4):624–662
Kepes S, McDaniel MA, Brannick MT, Banks GC (2013) Meta-analytic reviews in the organizational sciences: Two meta-analytic schools on the way to MARS (the Meta-Analytic Reporting Standards). J Bus Psychol 28(2):123–143
Kraus S, Breier M, Dasí-Rodríguez S (2020) The art of crafting a systematic literature review in entrepreneurship research. Int Entrepreneur Manag J 16(3):1023–1042
Levitt HM (2018) How to conduct a qualitative meta-analysis: tailoring methods to enhance methodological integrity. Psychother Res 28(3):367–378
Levitt HM, Bamberg M, Creswell JW, Frost DM, Josselson R, Suárez-Orozco C (2018) Journal article reporting standards for qualitative primary, qualitative meta-analytic, and mixed methods research in psychology: the APA publications and communications board task force report. Am Psychol 73(1):26
Lipsey MW, Wilson DB (2001) Practical meta-analysis. Sage Publications, Inc.
López-López JA, Page MJ, Lipsey MW, Higgins JP (2018) Dealing with effect size multiplicity in systematic reviews and meta-analyses. Res Synth Methods 9(3):336–351
Martín-Martín A, Thelwall M, Orduna-Malea E, López-Cózar ED (2021) Google Scholar, Microsoft Academic, Scopus, Dimensions, Web of Science, and OpenCitations’ COCI: a multidisciplinary comparison of coverage via citations. Scientometrics 126(1):871–906
Merton RK (1968) The Matthew effect in science: the reward and communication systems of science are considered. Science 159(3810):56–63
Moeyaert M, Ugille M, Natasha Beretvas S, Ferron J, Bunuan R, Van den Noortgate W (2017) Methods for dealing with multiple outcomes in meta-analysis: a comparison between averaging effect sizes, robust variance estimation and multilevel meta-analysis. Int J Soc Res Methodol 20(6):559–572
Moher D, Liberati A, Tetzlaff J, Altman DG, Prisma Group (2009) Preferred reporting items for systematic reviews and meta-analyses: the PRISMA statement. PLoS medicine. 6(7):e1000097
Mongeon P, Paul-Hus A (2016) The journal coverage of Web of Science and Scopus: a comparative analysis. Scientometrics 106(1):213–228
Moreau D, Gamble B (2020) Conducting a meta-analysis in the age of open science: Tools, tips, and practical recommendations. Psychol Methods. https://doi.org/10.1037/met0000351
O’Mara-Eves A, Thomas J, McNaught J, Miwa M, Ananiadou S (2015) Using text mining for study identification in systematic reviews: a systematic review of current approaches. Syst Rev 4(1):1–22
Ouzzani M, Hammady H, Fedorowicz Z, Elmagarmid A (2016) Rayyan—a web and mobile app for systematic reviews. Syst Rev 5(1):1–10
Owen E, Li Q (2021) The conditional nature of publication bias: a meta-regression analysis. Polit Sci Res Methods 9(4):867–877
Page MJ, McKenzie JE, Bossuyt PM, Boutron I, Hoffmann TC, Mulrow CD, Shamseer L, Tetzlaff JM, Akl EA, Brennan SE, Chou R, Glanville J, Grimshaw JM, Hróbjartsson A, Lalu MM, Li T, Loder EW, Mayo-Wilson E,McDonald S,McGuinness LA, Stewart LA, Thomas J, Tricco AC, Welch VA, Whiting P, Moher D (2021) The PRISMA 2020 statement: an updated guideline for reporting systematic reviews. BMJ 372. https://doi.org/10.1136/bmj.n71
Palmer TM, Sterne JAC (eds) (2016) Meta-analysis in stata: an updated collection from the stata journal, 2nd edn. Stata Press, College Station, TX
Pigott TD, Polanin JR (2020) Methodological guidance paper: High-quality meta-analysis in a systematic review. Rev Educ Res 90(1):24–46
Polanin JR, Tanner-Smith EE, Hennessy EA (2016) Estimating the difference between published and unpublished effect sizes: a meta-review. Rev Educ Res 86(1):207–236
Polanin JR, Hennessy EA, Tanner-Smith EE (2017) A review of meta-analysis packages in R. J Edu Behav Stat 42(2):206–242
Polanin JR, Hennessy EA, Tsuji S (2020) Transparency and reproducibility of meta-analyses in psychology: a meta-review. Perspect Psychol Sci 15(4):1026–1041. https://doi.org/10.1177/17456916209064
R Core Team (2021). R: A language and environment for statistical computing . R Foundation for Statistical Computing, Vienna, Austria. URL https://www.R-project.org/ .
Rauch A (2020) Opportunities and threats in reviewing entrepreneurship theory and practice. Entrep Theory Pract 44(5):847–860
Rauch A, van Doorn R, Hulsink W (2014) A qualitative approach to evidence–based entrepreneurship: theoretical considerations and an example involving business clusters. Entrep Theory Pract 38(2):333–368
Raudenbush SW (2009) Analyzing effect sizes: Random-effects models. In: Cooper H, Hedges LV, Valentine JC (eds) The handbook of research synthesis and meta-analysis, 2nd edn. Russell Sage Foundation, New York, NY, pp 295–315
Rosenthal R (1979) The file drawer problem and tolerance for null results. Psychol Bull 86(3):638
Rothstein HR, Sutton AJ, Borenstein M (2005) Publication bias in meta-analysis: prevention, assessment and adjustments. Wiley, Chichester
Roth PL, Le H, Oh I-S, Van Iddekinge CH, Bobko P (2018) Using beta coefficients to impute missing correlations in meta-analysis research: Reasons for caution. J Appl Psychol 103(6):644–658. https://doi.org/10.1037/apl0000293
Rudolph CW, Chang CK, Rauvola RS, Zacher H (2020) Meta-analysis in vocational behavior: a systematic review and recommendations for best practices. J Vocat Behav 118:103397
Schmidt FL (2017) Statistical and measurement pitfalls in the use of meta-regression in meta-analysis. Career Dev Int 22(5):469–476
Schmidt FL, Hunter JE (2015) Methods of meta-analysis: correcting error and bias in research findings. Sage, Thousand Oaks
Schwab A (2015) Why all researchers should report effect sizes and their confidence intervals: Paving the way for meta–analysis and evidence–based management practices. Entrepreneurship Theory Pract 39(4):719–725. https://doi.org/10.1111/etap.12158
Shaw JD, Ertug G (2017) The suitability of simulations and meta-analyses for submissions to Academy of Management Journal. Acad Manag J 60(6):2045–2049
Soderberg CK (2018) Using OSF to share data: A step-by-step guide. Adv Methods Pract Psychol Sci 1(1):115–120
Stanley TD, Doucouliagos H (2010) Picture this: a simple graph that reveals much ado about research. J Econ Surveys 24(1):170–191
Stanley TD, Doucouliagos H (2012) Meta-regression analysis in economics and business. Routledge, London
Stanley TD, Jarrell SB (1989) Meta-regression analysis: a quantitative method of literature surveys. J Econ Surveys 3:54–67
Steel P, Beugelsdijk S, Aguinis H (2021) The anatomy of an award-winning meta-analysis: Recommendations for authors, reviewers, and readers of meta-analytic reviews. J Int Bus Stud 52(1):23–44
Suurmond R, van Rhee H, Hak T (2017) Introduction, comparison, and validation of Meta-Essentials: a free and simple tool for meta-analysis. Res Synth Methods 8(4):537–553
The Cochrane Collaboration (2020). Review Manager (RevMan) [Computer program] (Version 5.4).
Thomas J, Noel-Storr A, Marshall I, Wallace B, McDonald S, Mavergames C, Glasziou P, Shemilt I, Synnot A, Turner T, Elliot J (2017) Living systematic reviews: 2. Combining human and machine effort. J Clin Epidemiol 91:31–37
Thompson SG, Higgins JP (2002) How should meta-regression analyses be undertaken and interpreted? Stat Med 21(11):1559–1573
Tipton E, Pustejovsky JE, Ahmadi H (2019) A history of meta-regression: technical, conceptual, and practical developments between 1974 and 2018. Res Synth Methods 10(2):161–179
Vevea JL, Woods CM (2005) Publication bias in research synthesis: Sensitivity analysis using a priori weight functions. Psychol Methods 10(4):428–443
Viechtbauer W (2010) Conducting meta-analyses in R with the metafor package. J Stat Softw 36(3):1–48
Viechtbauer W, Cheung MWL (2010) Outlier and influence diagnostics for meta-analysis. Res Synth Methods 1(2):112–125
Viswesvaran C, Ones DS (1995) Theory testing: combining psychometric meta-analysis and structural equations modeling. Pers Psychol 48(4):865–885
Wilson SJ, Polanin JR, Lipsey MW (2016) Fitting meta-analytic structural equation models with complex datasets. Res Synth Methods 7(2):121–139. https://doi.org/10.1002/jrsm.1199
Wood JA (2008) Methodology for dealing with duplicate study effects in a meta-analysis. Organ Res Methods 11(1):79–95
Download references
Open Access funding enabled and organized by Projekt DEAL. No funding was received to assist with the preparation of this manuscript.
Author information
Authors and affiliations.
University of Luxembourg, Luxembourg, Luxembourg
Christopher Hansen
Leibniz Institute for Psychology (ZPID), Trier, Germany
Holger Steinmetz
Trier University, Trier, Germany
Erasmus University Rotterdam, Rotterdam, The Netherlands
Wittener Institut Für Familienunternehmen, Universität Witten/Herdecke, Witten, Germany
You can also search for this author in PubMed Google Scholar
Corresponding author
Correspondence to Jörn Block .
Ethics declarations
Conflict of interest.
The authors have no relevant financial or non-financial interests to disclose.
Additional information
Publisher's note.
Springer Nature remains neutral with regard to jurisdictional claims in published maps and institutional affiliations.
See Table 1 .
Rights and permissions
Open Access This article is licensed under a Creative Commons Attribution 4.0 International License, which permits use, sharing, adaptation, distribution and reproduction in any medium or format, as long as you give appropriate credit to the original author(s) and the source, provide a link to the Creative Commons licence, and indicate if changes were made. The images or other third party material in this article are included in the article's Creative Commons licence, unless indicated otherwise in a credit line to the material. If material is not included in the article's Creative Commons licence and your intended use is not permitted by statutory regulation or exceeds the permitted use, you will need to obtain permission directly from the copyright holder. To view a copy of this licence, visit http://creativecommons.org/licenses/by/4.0/ .
Reprints and permissions
About this article
Hansen, C., Steinmetz, H. & Block, J. How to conduct a meta-analysis in eight steps: a practical guide. Manag Rev Q 72 , 1–19 (2022). https://doi.org/10.1007/s11301-021-00247-4
Download citation
Published : 30 November 2021
Issue Date : February 2022
DOI : https://doi.org/10.1007/s11301-021-00247-4
Share this article
Anyone you share the following link with will be able to read this content:
Sorry, a shareable link is not currently available for this article.
Provided by the Springer Nature SharedIt content-sharing initiative
- Find a journal
- Publish with us
- Track your research
Study Design 101: Meta-Analysis
- Case Report
- Case Control Study
- Cohort Study
- Randomized Controlled Trial
- Practice Guideline
- Systematic Review
Meta-Analysis
- Helpful Formulas
- Finding Specific Study Types
A subset of systematic reviews; a method for systematically combining pertinent qualitative and quantitative study data from several selected studies to develop a single conclusion that has greater statistical power. This conclusion is statistically stronger than the analysis of any single study, due to increased numbers of subjects, greater diversity among subjects, or accumulated effects and results.
Meta-analysis would be used for the following purposes:
- To establish statistical significance with studies that have conflicting results
- To develop a more correct estimate of effect magnitude
- To provide a more complex analysis of harms, safety data, and benefits
- To examine subgroups with individual numbers that are not statistically significant
If the individual studies utilized randomized controlled trials (RCT), combining several selected RCT results would be the highest-level of evidence on the evidence hierarchy, followed by systematic reviews, which analyze all available studies on a topic.
- Greater statistical power
- Confirmatory data analysis
- Greater ability to extrapolate to general population affected
- Considered an evidence-based resource
Disadvantages
- Difficult and time consuming to identify appropriate studies
- Not all studies provide adequate data for inclusion and analysis
- Requires advanced statistical techniques
- Heterogeneity of study populations
Design pitfalls to look out for
The studies pooled for review should be similar in type (i.e. all randomized controlled trials).
Are the studies being reviewed all the same type of study or are they a mixture of different types?
The analysis should include published and unpublished results to avoid publication bias.
Does the meta-analysis include any appropriate relevant studies that may have had negative outcomes?
Fictitious Example
Do individuals who wear sunscreen have fewer cases of melanoma than those who do not wear sunscreen? A MEDLINE search was conducted using the terms melanoma, sunscreening agents, and zinc oxide, resulting in 8 randomized controlled studies, each with between 100 and 120 subjects. All of the studies showed a positive effect between wearing sunscreen and reducing the likelihood of melanoma. The subjects from all eight studies (total: 860 subjects) were pooled and statistically analyzed to determine the effect of the relationship between wearing sunscreen and melanoma. This meta-analysis showed a 50% reduction in melanoma diagnosis among sunscreen-wearers.
Real-life Examples
Goyal, A., Elminawy, M., Kerezoudis, P., Lu, V., Yolcu, Y., Alvi, M., & Bydon, M. (2019). Impact of obesity on outcomes following lumbar spine surgery: A systematic review and meta-analysis. Clinical Neurology and Neurosurgery, 177 , 27-36. https://doi.org/10.1016/j.clineuro.2018.12.012
This meta-analysis was interested in determining whether obesity affects the outcome of spinal surgery. Some previous studies have shown higher perioperative morbidity in patients with obesity while other studies have not shown this effect. This study looked at surgical outcomes including "blood loss, operative time, length of stay, complication and reoperation rates and functional outcomes" between patients with and without obesity. A meta-analysis of 32 studies (23,415 patients) was conducted. There were no significant differences for patients undergoing minimally invasive surgery, but patients with obesity who had open surgery had experienced higher blood loss and longer operative times (not clinically meaningful) as well as higher complication and reoperation rates. Further research is needed to explore this issue in patients with morbid obesity.
Nakamura, A., van Der Waerden, J., Melchior, M., Bolze, C., El-Khoury, F., & Pryor, L. (2019). Physical activity during pregnancy and postpartum depression: Systematic review and meta-analysis. Journal of Affective Disorders, 246 , 29-41. https://doi.org/10.1016/j.jad.2018.12.009
This meta-analysis explored whether physical activity during pregnancy prevents postpartum depression. Seventeen studies were included (93,676 women) and analysis showed a "significant reduction in postpartum depression scores in women who were physically active during their pregnancies when compared with inactive women." Possible limitations or moderators of this effect include intensity and frequency of physical activity, type of physical activity, and timepoint in pregnancy (e.g. trimester).
Related Terms
A document often written by a panel that provides a comprehensive review of all relevant studies on a particular clinical or health-related topic/question.
Publication Bias
A phenomenon in which studies with positive results have a better chance of being published, are published earlier, and are published in journals with higher impact factors. Therefore, conclusions based exclusively on published studies can be misleading.
Now test yourself!
1. A Meta-Analysis pools together the sample populations from different studies, such as Randomized Controlled Trials, into one statistical analysis and treats them as one large sample population with one conclusion.
a) True b) False
2. One potential design pitfall of Meta-Analyses that is important to pay attention to is:
a) Whether it is evidence-based. b) If the authors combined studies with conflicting results. c) If the authors appropriately combined studies so they did not compare apples and oranges. d) If the authors used only quantitative data.
Evidence Pyramid - Navigation
- Meta- Analysis
- Case Reports
- << Previous: Systematic Review
- Next: Helpful Formulas >>

- Last Updated: Sep 25, 2023 10:59 AM
- URL: https://guides.himmelfarb.gwu.edu/studydesign101

- Himmelfarb Intranet
- Privacy Notice
- Terms of Use
- GW is committed to digital accessibility. If you experience a barrier that affects your ability to access content on this page, let us know via the Accessibility Feedback Form .
- Himmelfarb Health Sciences Library
- 2300 Eye St., NW, Washington, DC 20037
- Phone: (202) 994-2962
- [email protected]
- https://himmelfarb.gwu.edu
Thank you for visiting nature.com. You are using a browser version with limited support for CSS. To obtain the best experience, we recommend you use a more up to date browser (or turn off compatibility mode in Internet Explorer). In the meantime, to ensure continued support, we are displaying the site without styles and JavaScript.
- View all journals
- Explore content
- About the journal
- Publish with us
- Sign up for alerts
- Review Article
- Published: 08 March 2018
Meta-analysis and the science of research synthesis
- Jessica Gurevitch 1 ,
- Julia Koricheva 2 ,
- Shinichi Nakagawa 3 , 4 &
- Gavin Stewart 5
Nature volume 555 , pages 175–182 ( 2018 ) Cite this article
56k Accesses
928 Citations
735 Altmetric
Metrics details
- Biodiversity
- Outcomes research
Meta-analysis is the quantitative, scientific synthesis of research results. Since the term and modern approaches to research synthesis were first introduced in the 1970s, meta-analysis has had a revolutionary effect in many scientific fields, helping to establish evidence-based practice and to resolve seemingly contradictory research outcomes. At the same time, its implementation has engendered criticism and controversy, in some cases general and others specific to particular disciplines. Here we take the opportunity provided by the recent fortieth anniversary of meta-analysis to reflect on the accomplishments, limitations, recent advances and directions for future developments in the field of research synthesis.
This is a preview of subscription content, access via your institution
Access options
Access Nature and 54 other Nature Portfolio journals
Get Nature+, our best-value online-access subscription
$29.99 / 30 days
cancel any time
Subscribe to this journal
Receive 51 print issues and online access
$199.00 per year
only $3.90 per issue
Buy this article
- Purchase on SpringerLink
- Instant access to full article PDF
Prices may be subject to local taxes which are calculated during checkout
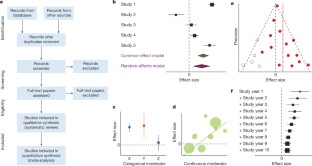
Similar content being viewed by others
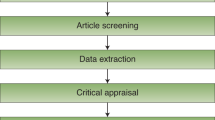
Eight problems with literature reviews and how to fix them
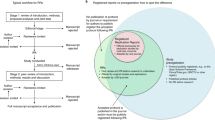
The past, present and future of Registered Reports
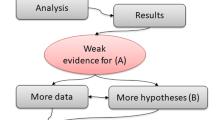
Raiders of the lost HARK: a reproducible inference framework for big data science
Jennions, M. D ., Lortie, C. J. & Koricheva, J. in The Handbook of Meta-analysis in Ecology and Evolution (eds Koricheva, J . et al.) Ch. 23 , 364–380 (Princeton Univ. Press, 2013)
Article Google Scholar
Roberts, P. D ., Stewart, G. B. & Pullin, A. S. Are review articles a reliable source of evidence to support conservation and environmental management? A comparison with medicine. Biol. Conserv. 132 , 409–423 (2006)
Bastian, H ., Glasziou, P . & Chalmers, I. Seventy-five trials and eleven systematic reviews a day: how will we ever keep up? PLoS Med. 7 , e1000326 (2010)
Article PubMed PubMed Central Google Scholar
Borman, G. D. & Grigg, J. A. in The Handbook of Research Synthesis and Meta-analysis 2nd edn (eds Cooper, H. M . et al.) 497–519 (Russell Sage Foundation, 2009)
Ioannidis, J. P. A. The mass production of redundant, misleading, and conflicted systematic reviews and meta-analyses. Milbank Q. 94 , 485–514 (2016)
Koricheva, J . & Gurevitch, J. Uses and misuses of meta-analysis in plant ecology. J. Ecol. 102 , 828–844 (2014)
Littell, J. H . & Shlonsky, A. Making sense of meta-analysis: a critique of “effectiveness of long-term psychodynamic psychotherapy”. Clin. Soc. Work J. 39 , 340–346 (2011)
Morrissey, M. B. Meta-analysis of magnitudes, differences and variation in evolutionary parameters. J. Evol. Biol. 29 , 1882–1904 (2016)
Article CAS PubMed Google Scholar
Whittaker, R. J. Meta-analyses and mega-mistakes: calling time on meta-analysis of the species richness-productivity relationship. Ecology 91 , 2522–2533 (2010)
Article PubMed Google Scholar
Begley, C. G . & Ellis, L. M. Drug development: Raise standards for preclinical cancer research. Nature 483 , 531–533 (2012); clarification 485 , 41 (2012)
Article CAS ADS PubMed Google Scholar
Hillebrand, H . & Cardinale, B. J. A critique for meta-analyses and the productivity-diversity relationship. Ecology 91 , 2545–2549 (2010)
Moher, D . et al. Preferred reporting items for systematic reviews and meta-analyses: the PRISMA statement. PLoS Med. 6 , e1000097 (2009). This paper provides a consensus regarding the reporting requirements for medical meta-analysis and has been highly influential in ensuring good reporting practice and standardizing language in evidence-based medicine, with further guidance for protocols, individual patient data meta-analyses and animal studies.
Moher, D . et al. Preferred reporting items for systematic review and meta-analysis protocols (PRISMA-P) 2015 statement. Syst. Rev. 4 , 1 (2015)
Nakagawa, S . & Santos, E. S. A. Methodological issues and advances in biological meta-analysis. Evol. Ecol. 26 , 1253–1274 (2012)
Nakagawa, S ., Noble, D. W. A ., Senior, A. M. & Lagisz, M. Meta-evaluation of meta-analysis: ten appraisal questions for biologists. BMC Biol. 15 , 18 (2017)
Hedges, L. & Olkin, I. Statistical Methods for Meta-analysis (Academic Press, 1985)
Viechtbauer, W. Conducting meta-analyses in R with the metafor package. J. Stat. Softw. 36 , 1–48 (2010)
Anzures-Cabrera, J . & Higgins, J. P. T. Graphical displays for meta-analysis: an overview with suggestions for practice. Res. Synth. Methods 1 , 66–80 (2010)
Egger, M ., Davey Smith, G ., Schneider, M. & Minder, C. Bias in meta-analysis detected by a simple, graphical test. Br. Med. J. 315 , 629–634 (1997)
Article CAS Google Scholar
Duval, S . & Tweedie, R. Trim and fill: a simple funnel-plot-based method of testing and adjusting for publication bias in meta-analysis. Biometrics 56 , 455–463 (2000)
Article CAS MATH PubMed Google Scholar
Leimu, R . & Koricheva, J. Cumulative meta-analysis: a new tool for detection of temporal trends and publication bias in ecology. Proc. R. Soc. Lond. B 271 , 1961–1966 (2004)
Higgins, J. P. T . & Green, S. (eds) Cochrane Handbook for Systematic Reviews of Interventions : Version 5.1.0 (Wiley, 2011). This large collaborative work provides definitive guidance for the production of systematic reviews in medicine and is of broad interest for methods development outside the medical field.
Lau, J ., Rothstein, H. R . & Stewart, G. B. in The Handbook of Meta-analysis in Ecology and Evolution (eds Koricheva, J . et al.) Ch. 25 , 407–419 (Princeton Univ. Press, 2013)
Lortie, C. J ., Stewart, G ., Rothstein, H. & Lau, J. How to critically read ecological meta-analyses. Res. Synth. Methods 6 , 124–133 (2015)
Murad, M. H . & Montori, V. M. Synthesizing evidence: shifting the focus from individual studies to the body of evidence. J. Am. Med. Assoc. 309 , 2217–2218 (2013)
Rasmussen, S. A ., Chu, S. Y ., Kim, S. Y ., Schmid, C. H . & Lau, J. Maternal obesity and risk of neural tube defects: a meta-analysis. Am. J. Obstet. Gynecol. 198 , 611–619 (2008)
Littell, J. H ., Campbell, M ., Green, S . & Toews, B. Multisystemic therapy for social, emotional, and behavioral problems in youth aged 10–17. Cochrane Database Syst. Rev. https://doi.org/10.1002/14651858.CD004797.pub4 (2005)
Schmidt, F. L. What do data really mean? Research findings, meta-analysis, and cumulative knowledge in psychology. Am. Psychol. 47 , 1173–1181 (1992)
Button, K. S . et al. Power failure: why small sample size undermines the reliability of neuroscience. Nat. Rev. Neurosci. 14 , 365–376 (2013); erratum 14 , 451 (2013)
Parker, T. H . et al. Transparency in ecology and evolution: real problems, real solutions. Trends Ecol. Evol. 31 , 711–719 (2016)
Stewart, G. Meta-analysis in applied ecology. Biol. Lett. 6 , 78–81 (2010)
Sutherland, W. J ., Pullin, A. S ., Dolman, P. M . & Knight, T. M. The need for evidence-based conservation. Trends Ecol. Evol. 19 , 305–308 (2004)
Lowry, E . et al. Biological invasions: a field synopsis, systematic review, and database of the literature. Ecol. Evol. 3 , 182–196 (2013)
Article PubMed Central Google Scholar
Parmesan, C . & Yohe, G. A globally coherent fingerprint of climate change impacts across natural systems. Nature 421 , 37–42 (2003)
Jennions, M. D ., Lortie, C. J . & Koricheva, J. in The Handbook of Meta-analysis in Ecology and Evolution (eds Koricheva, J . et al.) Ch. 24 , 381–403 (Princeton Univ. Press, 2013)
Balvanera, P . et al. Quantifying the evidence for biodiversity effects on ecosystem functioning and services. Ecol. Lett. 9 , 1146–1156 (2006)
Cardinale, B. J . et al. Effects of biodiversity on the functioning of trophic groups and ecosystems. Nature 443 , 989–992 (2006)
Rey Benayas, J. M ., Newton, A. C ., Diaz, A. & Bullock, J. M. Enhancement of biodiversity and ecosystem services by ecological restoration: a meta-analysis. Science 325 , 1121–1124 (2009)
Article ADS PubMed CAS Google Scholar
Leimu, R ., Mutikainen, P. I. A ., Koricheva, J. & Fischer, M. How general are positive relationships between plant population size, fitness and genetic variation? J. Ecol. 94 , 942–952 (2006)
Hillebrand, H. On the generality of the latitudinal diversity gradient. Am. Nat. 163 , 192–211 (2004)
Gurevitch, J. in The Handbook of Meta-analysis in Ecology and Evolution (eds Koricheva, J . et al.) Ch. 19 , 313–320 (Princeton Univ. Press, 2013)
Rustad, L . et al. A meta-analysis of the response of soil respiration, net nitrogen mineralization, and aboveground plant growth to experimental ecosystem warming. Oecologia 126 , 543–562 (2001)
Adams, D. C. Phylogenetic meta-analysis. Evolution 62 , 567–572 (2008)
Hadfield, J. D . & Nakagawa, S. General quantitative genetic methods for comparative biology: phylogenies, taxonomies and multi-trait models for continuous and categorical characters. J. Evol. Biol. 23 , 494–508 (2010)
Lajeunesse, M. J. Meta-analysis and the comparative phylogenetic method. Am. Nat. 174 , 369–381 (2009)
Rosenberg, M. S ., Adams, D. C . & Gurevitch, J. MetaWin: Statistical Software for Meta-Analysis with Resampling Tests Version 1 (Sinauer Associates, 1997)
Wallace, B. C . et al. OpenMEE: intuitive, open-source software for meta-analysis in ecology and evolutionary biology. Methods Ecol. Evol. 8 , 941–947 (2016)
Gurevitch, J ., Morrison, J. A . & Hedges, L. V. The interaction between competition and predation: a meta-analysis of field experiments. Am. Nat. 155 , 435–453 (2000)
Adams, D. C ., Gurevitch, J . & Rosenberg, M. S. Resampling tests for meta-analysis of ecological data. Ecology 78 , 1277–1283 (1997)
Gurevitch, J . & Hedges, L. V. Statistical issues in ecological meta-analyses. Ecology 80 , 1142–1149 (1999)
Schmid, C. H . & Mengersen, K. in The Handbook of Meta-analysis in Ecology and Evolution (eds Koricheva, J . et al.) Ch. 11 , 145–173 (Princeton Univ. Press, 2013)
Eysenck, H. J. Exercise in mega-silliness. Am. Psychol. 33 , 517 (1978)
Simberloff, D. Rejoinder to: Don’t calculate effect sizes; study ecological effects. Ecol. Lett. 9 , 921–922 (2006)
Cadotte, M. W ., Mehrkens, L. R . & Menge, D. N. L. Gauging the impact of meta-analysis on ecology. Evol. Ecol. 26 , 1153–1167 (2012)
Koricheva, J ., Jennions, M. D. & Lau, J. in The Handbook of Meta-analysis in Ecology and Evolution (eds Koricheva, J . et al.) Ch. 15 , 237–254 (Princeton Univ. Press, 2013)
Lau, J ., Ioannidis, J. P. A ., Terrin, N ., Schmid, C. H . & Olkin, I. The case of the misleading funnel plot. Br. Med. J. 333 , 597–600 (2006)
Vetter, D ., Rucker, G. & Storch, I. Meta-analysis: a need for well-defined usage in ecology and conservation biology. Ecosphere 4 , 1–24 (2013)
Mengersen, K ., Jennions, M. D. & Schmid, C. H. in The Handbook of Meta-analysis in Ecology and Evolution (eds Koricheva, J. et al.) Ch. 16 , 255–283 (Princeton Univ. Press, 2013)
Patsopoulos, N. A ., Analatos, A. A. & Ioannidis, J. P. A. Relative citation impact of various study designs in the health sciences. J. Am. Med. Assoc. 293 , 2362–2366 (2005)
Kueffer, C . et al. Fame, glory and neglect in meta-analyses. Trends Ecol. Evol. 26 , 493–494 (2011)
Cohnstaedt, L. W. & Poland, J. Review Articles: The black-market of scientific currency. Ann. Entomol. Soc. Am. 110 , 90 (2017)
Longo, D. L. & Drazen, J. M. Data sharing. N. Engl. J. Med. 374 , 276–277 (2016)
Gauch, H. G. Scientific Method in Practice (Cambridge Univ. Press, 2003)
Science Staff. Dealing with data: introduction. Challenges and opportunities. Science 331 , 692–693 (2011)
Nosek, B. A . et al. Promoting an open research culture. Science 348 , 1422–1425 (2015)
Article CAS ADS PubMed PubMed Central Google Scholar
Stewart, L. A . et al. Preferred reporting items for a systematic review and meta-analysis of individual participant data: the PRISMA-IPD statement. J. Am. Med. Assoc. 313 , 1657–1665 (2015)
Saldanha, I. J . et al. Evaluating Data Abstraction Assistant, a novel software application for data abstraction during systematic reviews: protocol for a randomized controlled trial. Syst. Rev. 5 , 196 (2016)
Tipton, E. & Pustejovsky, J. E. Small-sample adjustments for tests of moderators and model fit using robust variance estimation in meta-regression. J. Educ. Behav. Stat. 40 , 604–634 (2015)
Mengersen, K ., MacNeil, M. A . & Caley, M. J. The potential for meta-analysis to support decision analysis in ecology. Res. Synth. Methods 6 , 111–121 (2015)
Ashby, D. Bayesian statistics in medicine: a 25 year review. Stat. Med. 25 , 3589–3631 (2006)
Article MathSciNet PubMed Google Scholar
Senior, A. M . et al. Heterogeneity in ecological and evolutionary meta-analyses: its magnitude and implications. Ecology 97 , 3293–3299 (2016)
McAuley, L ., Pham, B ., Tugwell, P . & Moher, D. Does the inclusion of grey literature influence estimates of intervention effectiveness reported in meta-analyses? Lancet 356 , 1228–1231 (2000)
Koricheva, J ., Gurevitch, J . & Mengersen, K. (eds) The Handbook of Meta-Analysis in Ecology and Evolution (Princeton Univ. Press, 2013) This book provides the first comprehensive guide to undertaking meta-analyses in ecology and evolution and is also relevant to other fields where heterogeneity is expected, incorporating explicit consideration of the different approaches used in different domains.
Lumley, T. Network meta-analysis for indirect treatment comparisons. Stat. Med. 21 , 2313–2324 (2002)
Zarin, W . et al. Characteristics and knowledge synthesis approach for 456 network meta-analyses: a scoping review. BMC Med. 15 , 3 (2017)
Elliott, J. H . et al. Living systematic reviews: an emerging opportunity to narrow the evidence-practice gap. PLoS Med. 11 , e1001603 (2014)
Vandvik, P. O ., Brignardello-Petersen, R . & Guyatt, G. H. Living cumulative network meta-analysis to reduce waste in research: a paradigmatic shift for systematic reviews? BMC Med. 14 , 59 (2016)
Jarvinen, A. A meta-analytic study of the effects of female age on laying date and clutch size in the Great Tit Parus major and the Pied Flycatcher Ficedula hypoleuca . Ibis 133 , 62–67 (1991)
Arnqvist, G. & Wooster, D. Meta-analysis: synthesizing research findings in ecology and evolution. Trends Ecol. Evol. 10 , 236–240 (1995)
Hedges, L. V ., Gurevitch, J . & Curtis, P. S. The meta-analysis of response ratios in experimental ecology. Ecology 80 , 1150–1156 (1999)
Gurevitch, J ., Curtis, P. S. & Jones, M. H. Meta-analysis in ecology. Adv. Ecol. Res 32 , 199–247 (2001)
Lajeunesse, M. J. phyloMeta: a program for phylogenetic comparative analyses with meta-analysis. Bioinformatics 27 , 2603–2604 (2011)
CAS PubMed Google Scholar
Pearson, K. Report on certain enteric fever inoculation statistics. Br. Med. J. 2 , 1243–1246 (1904)
Fisher, R. A. Statistical Methods for Research Workers (Oliver and Boyd, 1925)
Yates, F. & Cochran, W. G. The analysis of groups of experiments. J. Agric. Sci. 28 , 556–580 (1938)
Cochran, W. G. The combination of estimates from different experiments. Biometrics 10 , 101–129 (1954)
Smith, M. L . & Glass, G. V. Meta-analysis of psychotherapy outcome studies. Am. Psychol. 32 , 752–760 (1977)
Glass, G. V. Meta-analysis at middle age: a personal history. Res. Synth. Methods 6 , 221–231 (2015)
Cooper, H. M ., Hedges, L. V . & Valentine, J. C. (eds) The Handbook of Research Synthesis and Meta-analysis 2nd edn (Russell Sage Foundation, 2009). This book is an important compilation that builds on the ground-breaking first edition to set the standard for best practice in meta-analysis, primarily in the social sciences but with applications to medicine and other fields.
Rosenthal, R. Meta-analytic Procedures for Social Research (Sage, 1991)
Hunter, J. E ., Schmidt, F. L. & Jackson, G. B. Meta-analysis: Cumulating Research Findings Across Studies (Sage, 1982)
Gurevitch, J ., Morrow, L. L ., Wallace, A . & Walsh, J. S. A meta-analysis of competition in field experiments. Am. Nat. 140 , 539–572 (1992). This influential early ecological meta-analysis reports multiple experimental outcomes on a longstanding and controversial topic that introduced a wide range of ecologists to research synthesis methods.
O’Rourke, K. An historical perspective on meta-analysis: dealing quantitatively with varying study results. J. R. Soc. Med. 100 , 579–582 (2007)
Shadish, W. R . & Lecy, J. D. The meta-analytic big bang. Res. Synth. Methods 6 , 246–264 (2015)
Glass, G. V. Primary, secondary, and meta-analysis of research. Educ. Res. 5 , 3–8 (1976)
DerSimonian, R . & Laird, N. Meta-analysis in clinical trials. Control. Clin. Trials 7 , 177–188 (1986)
Lipsey, M. W . & Wilson, D. B. The efficacy of psychological, educational, and behavioral treatment. Confirmation from meta-analysis. Am. Psychol. 48 , 1181–1209 (1993)
Chalmers, I. & Altman, D. G. Systematic Reviews (BMJ Publishing Group, 1995)
Moher, D . et al. Improving the quality of reports of meta-analyses of randomised controlled trials: the QUOROM statement. Quality of reporting of meta-analyses. Lancet 354 , 1896–1900 (1999)
Higgins, J. P. & Thompson, S. G. Quantifying heterogeneity in a meta-analysis. Stat. Med. 21 , 1539–1558 (2002)
Download references
Acknowledgements
We dedicate this Review to the memory of Ingram Olkin and William Shadish, founding members of the Society for Research Synthesis Methodology who made tremendous contributions to the development of meta-analysis and research synthesis and to the supervision of generations of students. We thank L. Lagisz for help in preparing the figures. We are grateful to the Center for Open Science and the Laura and John Arnold Foundation for hosting and funding a workshop, which was the origination of this article. S.N. is supported by Australian Research Council Future Fellowship (FT130100268). J.G. acknowledges funding from the US National Science Foundation (ABI 1262402).
Author information
Authors and affiliations.
Department of Ecology and Evolution, Stony Brook University, Stony Brook, 11794-5245, New York, USA
Jessica Gurevitch
School of Biological Sciences, Royal Holloway University of London, Egham, TW20 0EX, Surrey, UK
Julia Koricheva
Evolution and Ecology Research Centre and School of Biological, Earth and Environmental Sciences, University of New South Wales, Sydney, 2052, New South Wales, Australia
Shinichi Nakagawa
Diabetes and Metabolism Division, Garvan Institute of Medical Research, 384 Victoria Street, Darlinghurst, Sydney, 2010, New South Wales, Australia
School of Natural and Environmental Sciences, Newcastle University, Newcastle upon Tyne, NE1 7RU, UK
Gavin Stewart
You can also search for this author in PubMed Google Scholar
Contributions
All authors contributed equally in designing the study and writing the manuscript, and so are listed alphabetically.
Corresponding authors
Correspondence to Jessica Gurevitch , Julia Koricheva , Shinichi Nakagawa or Gavin Stewart .
Ethics declarations
Competing interests.
The authors declare no competing financial interests.
Additional information
Reviewer Information Nature thanks D. Altman, M. Lajeunesse, D. Moher and G. Romero for their contribution to the peer review of this work.
Publisher's note: Springer Nature remains neutral with regard to jurisdictional claims in published maps and institutional affiliations.
PowerPoint slides
Powerpoint slide for fig. 1, rights and permissions.
Reprints and permissions
About this article
Cite this article.
Gurevitch, J., Koricheva, J., Nakagawa, S. et al. Meta-analysis and the science of research synthesis. Nature 555 , 175–182 (2018). https://doi.org/10.1038/nature25753
Download citation
Received : 04 March 2017
Accepted : 12 January 2018
Published : 08 March 2018
Issue Date : 08 March 2018
DOI : https://doi.org/10.1038/nature25753
Share this article
Anyone you share the following link with will be able to read this content:
Sorry, a shareable link is not currently available for this article.
Provided by the Springer Nature SharedIt content-sharing initiative
This article is cited by
Accelerating evidence synthesis for safety assessment through clinicaltrials.gov platform: a feasibility study.
BMC Medical Research Methodology (2024)
Investigate the relationship between the retraction reasons and the quality of methodology in non-Cochrane retracted systematic reviews: a systematic review
- Azita Shahraki-Mohammadi
- Leila Keikha
- Razieh Zahedi
Systematic Reviews (2024)
A meta-analysis on global change drivers and the risk of infectious disease
- Michael B. Mahon
- Alexandra Sack
- Jason R. Rohr
Nature (2024)
Systematic review of the uncertainty of coral reef futures under climate change
- Shannon G. Klein
- Cassandra Roch
- Carlos M. Duarte
Nature Communications (2024)
Meta-analysis reveals weak associations between reef fishes and corals
- Pooventhran Muruga
- Alexandre C. Siqueira
- David R. Bellwood
Nature Ecology & Evolution (2024)
By submitting a comment you agree to abide by our Terms and Community Guidelines . If you find something abusive or that does not comply with our terms or guidelines please flag it as inappropriate.
Quick links
- Explore articles by subject
- Guide to authors
- Editorial policies
Sign up for the Nature Briefing newsletter — what matters in science, free to your inbox daily.

Log in using your username and password
- Search More Search for this keyword Advanced search
- Latest content
- Current issue
- BMJ Journals
You are here
- Volume 23, Issue 2
- Methodological quality and synthesis of case series and case reports
- Article Text
- Article info
- Citation Tools
- Rapid Responses
- Article metrics

- http://orcid.org/0000-0001-5502-5975 Mohammad Hassan Murad 1 ,
- Shahnaz Sultan 2 ,
- Samir Haffar 3 ,
- Fateh Bazerbachi 4
- 1 Evidence-Based Practice Center, Mayo Clinic , Rochester , Minnesota , USA
- 2 Division of Gastroenterology, Hepatology, and Nutrition , University of Minnesota, Center for Chronic Diseases Outcomes Research, Minneapolis Veterans Affairs Healthcare System , Minneapolis , Minnesota , USA
- 3 Digestive Center for Diagnosis and Treatment , Damascus , Syrian Arab Republic
- 4 Department of Gastroenterology and Hepatology , Mayo Clinic , Rochester , Minnesota , USA
- Correspondence to Dr Mohammad Hassan Murad, Evidence-Based Practice Center, Mayo Clinic, Rochester, MN 55905, USA; murad.mohammad{at}mayo.edu
https://doi.org/10.1136/bmjebm-2017-110853
Statistics from Altmetric.com
Request permissions.
If you wish to reuse any or all of this article please use the link below which will take you to the Copyright Clearance Center’s RightsLink service. You will be able to get a quick price and instant permission to reuse the content in many different ways.
- epidemiology
In 1904, Dr James Herrick evaluated a 20-year-old patient from Grenada who was studying in Chicago and suffered from anaemia and a multisystem illness. The patient was found to have ‘freakish’ elongated red cells that resembled a crescent or a sickle. Dr Herrick concluded that the red cells were not artefacts because the appearance of the cells was maintained regardless of how the smear slide was prepared. He followed the patient who had subsequently received care from other physicians until 1907 and questioned whether this was syphilis or a parasite from the tropics. Then in 1910, in a published case report, he concluded that this presentation strongly suggested a previously unrecognised change in the composition of the corpuscle itself. 1 Sickle cell disease became a diagnosis thereafter.
Case reports and case series have profoundly influenced the medical literature and continue to advance our knowledge in the present time. In 1985, the American Medical Association reprinted 51 papers from its journal that had significantly changed the science and practice of medicine over the past 150 years, and five of these papers were case reports. 2 However, concerns about weak inferences and the high likelihood of bias associated with such reports have resulted in minimal attention being devoted to developing frameworks for approaching, appraising, synthesising and applying evidence derived from case reports/series. Nevertheless, such observations remain the bread and butter of learning by pattern recognition and integral to advancing medical knowledge.
Guidance on how to write a case report is available (ie, a reporting guideline). The Case Report (CARE) guidelines 3 were developed following a three-phase consensus process and provide a 13-item checklist that can assist researchers in publishing complete and meaningful exposition of medical information. This checklist encourages the explicit presentation of patient information, clinical findings, timeline, diagnostic assessment, therapeutic interventions, follow-up and outcomes. 3 Yet, systematic reviewers appraising the evidence for decision-makers require tools to assess the methodological quality (risk of bias assessment) of this evidence.
In this guide, we present a framework to evaluate the methodological quality of case reports/series and synthesise their results, which is particularly important when conducting a systematic review of a body of evidence that consists primarily of uncontrolled clinical observations.
Definitions
In the biomedical published literature, a case report is the description of the clinical course of one individual, which may include particular exposures, symptoms, signs, interventions or outcomes. A case report is the smallest publishable unit in the literature, whereas case series report aggregates individual cases in one publication. 4
If a case series is prospective, differentiating it from a single-arm uncontrolled cohort study becomes difficult. In one clinical practice guideline, it was proposed that studies without internal comparisons can be labelled as case series unless they explicitly report having a protocol before commencement of data collection, a definition of inclusion and exclusion criteria, a standardised follow-up and clear reporting of the number of excluded patients and those lost to follow-up. 6
Evaluating methodological quality
Pierson 7 provided an approach to evaluate the validity of a case report based on five components: documentation, uniqueness, objectivity, interpretation and educational value, resulting in a score with a maximum of 10 (a score above 5 was suggested indicate a valid case report). This approach, however, was rarely used in subsequent work and seems to conflate methodological quality with other constructs. For case reports of adverse drug reactions, other systems classify an association as definite, probable, possible or doubtful based on leading questions. 8 9 These questions are derived from the causality criteria that was established in 1965 by the English epidemiologist Bradford Hills. 10 Lastly, we have adapted the Newcastle Ottawa scale 11 for cohort and case–control studies by removing items that relate to comparability and adjustment (which are not relevant to non-comparative studies) and retained items that focused on selection, representativeness of cases and ascertainment of outcomes and exposure. This tool was applied in several published systematic reviews with good inter-rater agreement. 12–16
Proposed tool
The previous criteria from Pierson, 7 Bradford Hills 10 and Newcastle Ottawa scale modifications 11 converge into eight items that can be categorised into four domains: selection, ascertainment, causality and reporting. The eight items with leading explanatory questions are summarised in table 1 .
- View inline
Tool for evaluating the methodological quality of case reports and case series
For example, a study that explicitly describes all the cases who have presented to a medical centre over a certain period of time would satisfy the selection domain. In contrast, a study that reports on several individuals with unclear selection approach leaves the reader with uncertainty to whether this is the whole experience of the researchers and suggests possible selection bias. For the domain of ascertainment, self-report (of the exposure or the outcome) is less reliable than ascertainment using administrative and billing codes, which in turn is less reliable than clinical records. For the domain of causality, we would have stronger inference in a case report of an adverse drug reaction that has resolved with cessation of the drug and reoccurred after reintroduction of the drug. Lastly, for the domain of reporting, a case report that is described with sufficient details may allow readers to apply the evidence derived from the report in their practice. On the other hand, an inadequately reported case will likely be unhelpful in the course of clinical care.
We suggest using this tool in systematic reviews of case reports/series. One option to summarise the results of this tool is to sum the scores of the eight binary responses into an aggregate score. A better option is not to use an aggregate score because numeric representation of methodological quality may not be appropriate when one or two questions are deemed most critical to the validity of a report (compared with other questions). Therefore, we suggest making an overall judgement about methodological quality based on the questions deemed most critical in the specific clinical scenario.
Synthesis of case reports/series
A single patient case report does not allow the estimation of an effect size and would only provide descriptive or narrative results. Case series of more than one patient may allow narrative or quantitative synthesis.
Narrative synthesis
A systematic review of the cases with the rare syndrome of lipodystrophy was able to suggest core and supportive clinical features and narratively summarised data on available treatment approaches. 17 Another systematic review of 172 cases of the infrequently encountered glycogenic hepatopathy was able to characterise for the first time patterns of liver enzymes and hepatic injury in this disease. 18
Quantitative synthesis
Quantitative analysis of non-comparative series does not produce relative association measures such as ORs or relative risks but can provide estimates of prevalence or event rates in the form of a proportion (with associated precision). Proportions can be pooled using fixed or random effects models by means of the various available meta-analysis software. For example, a meta-analysis of case series of patients presenting with aortic transection showed that mortality was significantly lower in patients who underwent endovascular repair, followed by open repair and non-operative management (9%, 19% and 46%, respectively, P<0.01). 19
A common challenge, however, occurs when proportions are too large or too small (close to 0 or to 1). In this situation, the variance of the proportion becomes very small leading to an inappropriately large weight in meta-analysis. One way to overcome this challenge is to transform prevalence to a variable that is not constrained to the 0–1 range and has approximately normal distribution, conduct the meta-analysis and then transform the estimate back to a proportion. 20 This is done using logit transformation or using the Freeman-Tukey double arcsine transformation, 21 with the latter being often preferred. 20
Another type of quantitative analysis that may be utilised is regression. A meta-analysis of 47 published cases of hypocalcaemia and cardiac dysfunction used univariate linear regression analysis to demonstrate that both QT interval and left ventricular ejection fraction were significantly correlated with corrected total serum calcium level. 22 Meta-regression, which is a regression in which the unit of analysis is a study, not a patient, can also be used to synthesise case series and control for study-level confounders. A meta-regression analysis of uncontrolled series of patients with uveal melanoma treated with proton beam therapy has shown that this treatment was associated with better outcomes than brachytherapy. 23 It is very important, however, to recognise that meta-regression results can be severely affected by ecological bias.
From evidence to decision
Several authors have described various important reasons to publish case reports/series ( table 2 ). 7 24 25
Role of case reports/series in the medical literature
It is paramount to recognise that a systematic review and meta-analysis of case reports/series should not be placed at the top of the hierarchy in a pyramid that depicts validity. 26 The certainty of evidence derived from a meta-analysis is contingent on the design of included studies, their risk of bias, as well as other factors such as imprecision, indirectness, inconsistency and likelihood of publication bias. 27 Commonly, certainty in evidence derived from case series/reports will be very low. Nevertheless, inferences from such reports can be used for decision-making. In the example of case series of aortic transection showing lower mortality with endovascular repair, a guideline recommendation was made stating ‘We suggest that endovascular repair be performed preferentially over open surgical repair or non-operative management’. This was graded as a weak recommendation based on low certainty evidence. 28 The strength of this recommendation acknowledged that the recommendation might not universally apply to everyone and that variability in decision-making was expected. The certainty in evidence rating of this recommendation implied that future research would likely yield different results that may change the recommendation. 28
The Grading of Recommendations, Assessment, Development and Evaluation (GRADE) approach clearly separates the certainty of evidence from the strength of recommendation. This separation allows decision-making based on lower levels of evidence. For example, despite low certainty evidence (derived from case series) regarding the association between aspirin and Reye’s syndrome in febrile children, a strong recommendation for using acetaminophen over aspirin is possible. 29 GRADE literature also describes five paradigmatic situations in which a strong recommendation can be made based on low quality evidence. 30 One of which is when the condition is life threatening. An example of which would be using hyperbaric oxygen therapy for purpura fulminans, which is only based on case reports. 31
Guideline developers and decision-makers often struggle when dealing with case reports/case series. On occasions, they ignore such evidence and focus the scope of guidelines on areas with higher quality evidence. Sometimes they label recommendations based on case reports as expert opinion. 32 We propose an approach to evaluate the methodological quality of case reports/series based on the domains of selection, ascertainment, causality and reporting and provide signalling questions to aid evidence-based practitioners and systematic reviewers in their assessment. We suggest the incorporation of case reports/series in decision-making based on the GRADE approach when no other higher level of evidence is available.
In this guide, we have made the case for publishing case reports/series and proposed synthesis of their results in systematic reviews to facilitate using this evidence in decision-making. We have proposed a tool that can be used to evaluate the methodological quality in systematic reviews that examine case reports and case series.
- Gagnier JJ ,
- Altman DG , et al
- Grimes DA ,
- Abu-Zidan FM ,
- Schünemann HJ ,
- Naranjo CA ,
- Sellers EM , et al
- 9. ↵ The World health Organization-Uppsala Monitoring Centre . The use of the WHO-UMC system for standardised case causality assessment . https://www.who-umc.org/media/2768/standardised-case-causality-assessment.pdf ( accessed 20 Sep 2017 ).
- O’Connell D , et al
- Bazerbachi F ,
- Prokop L , et al
- Vargas EJ , et al
- Watt KD , et al
- Szarka LA , et al
- Hussain MT , et al
- Farah W , et al
- Leise MD , et al
- Malgor R , et al
- Barendregt JJ ,
- Lee YY , et al
- Freeman MF ,
- Newman DB ,
- Fidahussein SS ,
- Kashiwagi DT , et al
- Schild SE , et al
- Vandenbroucke JP
- Alsawas M , et al
- Matsumura JS ,
- Mitchell RS , et al
- Guyatt GH ,
- Vist GE , et al
- Domecq JP ,
- Murad MH , et al
- Mestrovic J , et al
- Alvarez-Villalobos N ,
- Shah R , et al
- Conboy EE ,
- Mounajjed T , et al
- Gottlieb MS
- Coodin FJ ,
- Uchida IA ,
- Goldfinger SE
- Lennox BR ,
- Jones PB , et al
Contributors MHM drafted the paper and all coauthors critically revised the manuscript. All the authors contributed to conceive the idea and approved the final submitted version.
Competing interests None declared.
Provenance and peer review Not commissioned; externally peer reviewed.
Read the full text or download the PDF:
Doing a Meta-Analysis: A Practical, Step-by-Step Guide
Saul McLeod, PhD
Editor-in-Chief for Simply Psychology
BSc (Hons) Psychology, MRes, PhD, University of Manchester
Saul McLeod, PhD., is a qualified psychology teacher with over 18 years of experience in further and higher education. He has been published in peer-reviewed journals, including the Journal of Clinical Psychology.
Learn about our Editorial Process
Olivia Guy-Evans, MSc
Associate Editor for Simply Psychology
BSc (Hons) Psychology, MSc Psychology of Education
Olivia Guy-Evans is a writer and associate editor for Simply Psychology. She has previously worked in healthcare and educational sectors.
On This Page:
What is a Meta-Analysis?
Meta-analysis is a statistical procedure used to combine and synthesize findings from multiple independent studies to estimate the average effect size for a particular research question.
Meta-analysis goes beyond traditional narrative reviews by using statistical methods to integrate the results of several studies, leading to a more objective appraisal of the evidence.
This method addresses limitations like small sample sizes in individual studies, providing a more precise estimate of a treatment effect or relationship strength.
Meta-analyses are particularly valuable when individual study results are inconclusive or contradictory, as seen in the example of vitamin D supplementation and the prevention of fractures.
For instance, a meta-analysis published in JAMA in 2017 by Zhao et al. examined 81 randomized controlled trials involving 53,537 participants.
The results of this meta-analysis suggested that vitamin D supplementation was not associated with a lower risk of fractures among community-dwelling adults. This finding contradicted some earlier beliefs and individual study results that had suggested a protective effect.
What’s the difference between a meta-analysis, systematic review, and literature review?
Literature reviews can be conducted without defined procedures for gathering information. Systematic reviews use strict protocols to minimize bias when gathering and evaluating studies, making them more transparent and reproducible.
While a systematic review thoroughly maps out a field of research, it cannot provide unbiased information on the magnitude of an effect. Meta-analysis statistically combines effect sizes of similar studies, going a step further than a systematic review by weighting each study by its precision.
What is Effect Size?
Statistical significance is a poor metric in meta-analysis because it only indicates whether an effect is likely to have occurred by chance. It does not provide information about the magnitude or practical importance of the effect.
While a statistically significant result may indicate an effect different from zero, this effect might be too small to hold practical value. Effect size, on the other hand, offers a standardized measure of the magnitude of the effect, allowing for a more meaningful interpretation of the findings
Meta-analysis goes beyond simply synthesizing effect sizes; it uses these statistics to provide a weighted average effect size from studies addressing similar research questions. The larger the effect size the stronger the relationship between two variables.
If effect sizes are consistent, the analysis demonstrates that the findings are robust across the included studies. When there is variation in effect sizes, researchers should focus on understanding the reasons for this dispersion rather than just reporting a summary effect.
Meta-regression is one method for exploring this variation by examining the relationship between effect sizes and study characteristics.
T here are three primary families of effect sizes used in most meta-analyses:
- Mean difference effect sizes : Used to show the magnitude of the difference between means of groups or conditions, commonly used when comparing a treatment and control group.
- Correlation effect sizes : Represent the degree of association between two continuous measures, indicating the strength and direction of their relationship.
- Odds ratio effect sizes : Used with binary outcomes to compare the odds of an event occurring between two groups, like whether a patient recovers from an illness or not.
The most appropriate effect size family is determined by the nature of the research question and dependent variable. All common effect sizes are able to be transformed from one version to another.
Real-Life Example
Brewin, C. R., Andrews, B., & Valentine, J. D. (2000). Meta-analysis of risk factors for posttraumatic stress disorder in trauma-exposed adults. Journal of Consulting and Clinical Psychology , 68 (5), 748.
This meta-analysis of 77 articles examined risk factors for posttraumatic stress disorder (PTSD) in trauma-exposed adults, with sample sizes ranging from 1,149 to over 11,000. Several factors consistently predicted PTSD with small effect sizes (r = 0.10 to 0.19), including female gender, lower education, lower intelligence, previous trauma, childhood adversity, and psychiatric history. Factors occurring during or after trauma showed somewhat stronger effects (r = 0.23 to 0.40), including trauma severity, lack of social support, and additional life stress. Most risk factors did not predict PTSD uniformly across populations and study types, with only psychiatric history, childhood abuse, and family psychiatric history showing homogeneous effects. Notable differences emerged between military and civilian samples, and methodological factors influenced some risk factor effects. The authors concluded that identifying a universal set of pretrauma predictors is premature and called for more research to understand how vulnerability to PTSD varies across populations and contexts.
How to Conduct a Meta-Analysis
Researchers should develop a comprehensive research protocol that outlines the objectives and hypotheses of their meta-analysis.
This document should provide specific details about every stage of the research process, including the methodology for identifying, selecting, and analyzing relevant studies.
For example, the protocol should specify search strategies for relevant studies, including whether the search will encompass unpublished works.
The protocol should be created before beginning the research process to ensure transparency and reproducibility.
Research Protocol
- To estimate the overall effect of growth mindset interventions on the academic achievement of students in primary and secondary school.
- To investigate if the effect of growth mindset interventions on academic achievement differs for students of different ages (e.g., elementary school students vs. high school students).
- To examine if the duration of the growth mindset intervention impacts its effectiveness.
- Growth mindset interventions will have a small, but statistically significant, positive effect on student academic achievement.
- Growth mindset interventions will be more effective for younger students than for older students.
- Longer growth mindset interventions will be more effective than shorter interventions.
Eligibility Criteria
- Published studies in English-language journals.
- Studies must include a quantitative measure of academic achievement (e.g., GPA, course grades, exam scores, or standardized test scores).
- Studies must involve a growth mindset intervention as the primary focus (including control vs treatment group comparison).
- Studies that combine growth mindset training with other interventions (e.g., study skills training, other types of psychological interventions) should be excluded.
Search Strategy
The researchers will search the following databases:
Keywords Combined with Boolean Operators:
- (“growth mindset” OR “implicit theories of intelligence” OR “mindset theory”) AND (“intervention” OR “training” OR “program”) ” OR “educational outcomes”) * OR “pupil ” OR “learner*”)**
Additional Search Strategies:
- Citation Chaining: Examining the reference lists of included studies can uncover additional relevant articles.
- Contacting Experts: Reaching out to researchers in the field of growth mindset can reveal unpublished studies or ongoing research.
Coding of Studies
The researchers will code each study for the following information:
- Sample size
- Age of participants
- Duration of intervention
- Type of academic outcome measured
- Study design (e.g., randomized controlled trial, quasi-experiment)
Statistical Analysis
- The researchers will calculate an effect size (e.g., standardized mean difference) for each study.
- The researchers will use a random-effects model to account for variation in effect sizes across studies.
- The researchers will use meta-regression to test the hypotheses about moderators of the effect of growth mindset interventions.
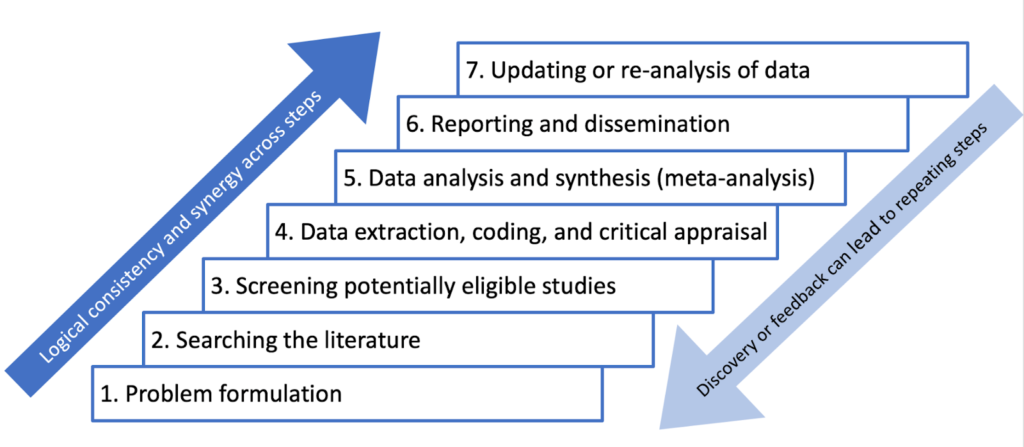
PRISMA (Preferred Reporting Items for Systematic Reviews and Meta-Analyses) is a reporting guideline designed to improve the transparency and completeness of systematic review reporting.
PRISMA was created to tackle the issue of inadequate reporting often found in systematic reviews
- Checklist : PRISMA features a 27-item checklist covering all aspects of a meta-analysis, from the rationale and objectives to the synthesis of findings and discussion of limitations. Each checklist item is accompanied by detailed reporting recommendations in an Explanation and Elaboration document .
- Flow Diagram : PRISMA also includes a flow diagram to visually represent the study selection process, offering a clear, standardized way to illustrate how researchers arrived at the final set of included studies
Step 1: Defining a Research Question
A well-defined research question is a fundamental starting point for any research synthesis. The research question should guide decisions about which studies to include in the meta-analysis, and which statistical model is most appropriate.
For example:
- How do dysfunctional attitudes and negative automatic thinking directly and indirectly impact depression?
- Do growth mindset interventions generally improve students’ academic achievement?
- What is the association between child-parent attachment and prosociality in children?
- What is the relation of various risk factors to Post Traumatic Stress Disorder (PTSD)?
Step 2: Search Strategy
Present the full search strategies for all databases, registers and websites, including any filters and limits used. PRISMA 2020 Checklist
A search strategy is a comprehensive and reproducible plan for identifying all relevant research studies that address a specific research question.
This systematic approach to searching helps minimize bias.
It’s important to be transparent about the search strategy and document all decisions for auditability. The goal is to identify all potentially relevant studies for consideration.
PRISMA (Preferred Reporting Items for Systematic reviews and Meta-Analyses) provide appropriate guidance for reporting quantitative literature searches.
Information Sources
The primary goal is to find all published and unpublished studies that meet the predefined criteria of the research question. This includes considering various sources beyond typical databases
Information sources for a meta-analysis can include a wide range of resources like scholarly databases, unpublished literature, conference papers, books, and even expert consultations.
Specify all databases, registers, websites, organisations, reference lists and other sources searched or consulted to identify studies. Specify the date when each source was last searched or consulted. PRISMA 2020 Checklist
An exhaustive, systematic search strategy is developed with the assistance of an expert librarian.
- Databases: Searches should include seven key databases: CINAHL, Medline, APA PsycArticles, Psychology and Behavioral Sciences Collection, APA PsycInfo, SocINDEX with Full Text, and Web of Science: Core Collections.
- Grey Literature : In addition to databases, forensic or ‘expansive’ searches can be conducted. This includes: grey literature database searches (e.g. OpenGrey , WorldCat , Ethos ), conference proceedings, unpublished reports, theses , clinical trial databases , searches by names of authors of relevant publications. Independent research bodies may also be good sources of material, e.g. Centre for Research in Ethnic Relations , Joseph Rowntree Foundation , Carers UK .
- Citation Searching : Reference lists often lead to highly cited and influential papers in the field, providing valuable context and background information for the review.
- Contacting Experts: Reaching out to researchers or experts in the field can provide access to unpublished data or ongoing research not yet publicly available.
It is important to note that this may not be an exhaustive list of all potential databases.
Search String Construction
It is recommended to consult topic experts on the review team and advisory board in order to create as complete a list of search terms as possible for each concept.
To retrieve the most relevant results, a search string is used. This string is made up of:
- Keywords: Search terms should be relevant to the research questions, key variables, participants, and research design. Searches should include indexed terms, titles, and abstracts. Additionally, each database has specific indexed terms, so a targeted search strategy must be created for each database.
- Synonyms: These are words or phrases with similar meanings to the keywords, as authors may use different terms to describe the same concepts. Including synonyms helps cover variations in terminology and increases the chances of finding all relevant studies. For example, a drug intervention may be referred to by its generic name or by one of its several proprietary names.
- Truncation symbols : These broaden the search by capturing variations of a keyword. They function by locating every word that begins with a specific root. For example, if a user was researching interventions for smoking, they might use a truncation symbol to search for “smok*” to retrieve records with the words “smoke,” “smoker,” “smoking,” or “smokes.” This can save time and effort by eliminating the need to input every variation of a word into a database.
- Boolean operators: The use of Boolean operators (AND/OR/NEAR/NOT) helps to combine these terms effectively, ensuring that the search strategy is both sensitive and specific. For instance, using “AND” narrows the search to include only results containing both terms, while “OR” expands it to include results containing either term.
When conducting these searches, it is important to combine browsing of texts (publications) with periods of more focused systematic searching. This iterative process allows the search to evolve as the review progresses.
It is important to note that this information may not be entirely comprehensive and up-to-date.
Studies were identified by searching PubMed, PsycINFO, and the Cochrane Library. We conducted searches for studies published between the first available year and April 1, 2009, using the search term mindfulness combined with the terms meditation, program, therapy, or intervention and anxi , depress , mood, or stress. Additionally, an extensive manual review was conducted of reference lists of relevant studies and review articles extracted from the database searches. Articles determined to be related to the topic of mindfulness were selected for further examination.
Specify the inclusion and exclusion criteria for the review. PRISMA 2020 Checklist
Before beginning the literature search, researchers should establish clear eligibility criteria for study inclusion
To maintain transparency and minimize bias, eligibility criteria for study inclusion should be established a priori. Ideally, researchers should aim to include only high-quality randomized controlled trials that adhere to the intention-to-treat principle.
The selection of studies should not be arbitrary, and the rationale behind inclusion and exclusion criteria should be clearly articulated in the research protocol.
When specifying the inclusion and exclusion criteria, consider the following aspects:
- Intervention Characteristics: Researchers might decide that, in order to be included in the review, an intervention must have specific characteristics. They might require the intervention to last for a certain length of time, or they might determine that only interventions with a specific theoretical basis are appropriate for their review.
- Population Characteristics: A meta-analysis might focus on the effects of an intervention for a specific population. For instance, researchers might choose to focus on studies that included only nurses or physicians.
- Outcome Measures: Researchers might choose to include only studies that used outcome measures that met a specific standard.
- Age of Participants: If a meta-analysis is examining the effects of a treatment or intervention for children, the authors of the review will likely choose to exclude any studies that did not include children in the target age range.
- Diagnostic Status of Participants: Researchers conducting a meta-analysis of treatments for anxiety will likely exclude any studies where the participants were not diagnosed with an anxiety disorder.
- Study Design: Researchers might determine that only studies that used a particular research design, such as a randomized controlled trial, will be included in the review.
- Control Group: In a meta-analysis of an intervention, researchers might choose to include only studies that included certain types of control groups, such as a waiting list control or another type of intervention.
- Publication status : Decide whether only published studies will be included or if unpublished works, such as dissertations or conference proceedings, will also be considered.
Studies were selected if (a) they included a mindfulness-based intervention, (b) they included a clinical sample (i.e., participants had a diagnosable psychological or physical/medical disorder), (c) they included adult samples (18 – 65 years of age), (d) the mindfulness program was not coupled with treatment using acceptance and commitment therapy or dialectical behavior therapy, (e) they included a measure of anxiety and/or mood symptoms at both pre and postintervention, and (f) they provided sufficient data to perform effect size analyses (i.e., means and standard deviations, t or F values, change scores, frequencies, or probability levels). Studies were excluded if the sample overlapped either partially or completely with the sample of another study meeting inclusion criteria for the meta-analysis. In these cases, we selected for inclusion the study with the larger sample size or more complete data for measures of anxiety and depression symptoms. For studies that provided insufficient data but were otherwise appropriate for the analyses, authors were contacted for supplementary data.
Iterative Process
The iterative nature of developing a search strategy stems from the need to refine and adapt the search process based on the information encountered at each stage.
A single attempt rarely yields the perfect final strategy. Instead, it is an evolving process involving a series of test searches, analysis of results, and discussions among the review team.
Here’s how the iterative process unfolds:
- Initial Strategy Formulation: Based on the research question, the team develops a preliminary search strategy, including identifying relevant keywords, synonyms, databases, and search limits.
- Test Searches and Refinement: The initial search strategy is then tested on chosen databases. The results are reviewed for relevance, and the search strategy is refined accordingly. This might involve adding or modifying keywords, adjusting Boolean operators, or reconsidering the databases used.
- Discussions and Iteration: The search results and proposed refinements are discussed within the review team. The team collaboratively decides on the best modifications to improve the search’s comprehensiveness and relevance.
- Repeating the Cycle: This cycle of test searches, analysis, discussions, and refinements is repeated until the team is satisfied with the strategy’s ability to capture all relevant studies while minimizing irrelevant results.
By constantly refining the search strategy based on the results and feedback, researchers can be more confident that they have identified all relevant studies.
This iterative process ensures that the applied search strategy is sensitive enough to capture all relevant studies while maintaining a manageable scope.
Throughout this process, meticulous documentation of the search strategy, including any modifications, is crucial for transparency and future replication of the meta-analysis.
Step 3: Search the Literature
Conduct a systematic search of the literature using clearly defined search terms and databases.
Applying the search strategy involves entering the constructed search strings into the respective databases’ search interfaces. These search strings, crafted using Boolean operators, truncation symbols, wildcards, and database-specific syntax, aim to retrieve all potentially relevant studies addressing the research question.
The researcher, during this stage, interacts with the database’s features to refine the search and manage the retrieved results.
This might involve employing search filters provided by the database to focus on specific study designs, publication types, or other relevant parameters.
Applying the search strategy is not merely a mechanical process of inputting terms; it demands a thorough understanding of database functionalities and a discerning eye to adjust the search based on the nature of retrieved results.
Step 4: Screening & Selecting Research Articles
Once the literature search is complete, the next step is to screen and select the studies that will be included in the meta-analysis.
This involves carefully reviewing each study to determine its relevance to the research question and its methodological quality.
The goal is to identify studies that are both relevant to the research question and of sufficient quality to contribute to a meaningful synthesis.
Studies meeting the eligibility criteria are usually saved into electronic databases, such as Endnote or Mendeley , and include title, authors, date and publication journal along with an abstract (if available).
Selection Process
Specify the methods used to decide whether a study met the inclusion criteria of the review, including how many reviewers screened each record and each report retrieved, whether they worked independently, and if applicable, details of automation tools used in the process. PRISMA 2020 Checklist
The selection process in a meta-analysis involves multiple reviewers to ensure rigor and reliability.
Two reviewers should independently screen titles and abstracts, removing duplicates and irrelevant studies based on predefined inclusion and exclusion criteria.
- Initial screening of titles and abstracts: After applying a strategy to search the literature,, the next step involves screening the titles and abstracts of the identified articles against the predefined inclusion and exclusion criteria. During this initial screening, reviewers aim to identify potentially relevant studies while excluding those clearly outside the scope of the review. It is crucial to prioritize over-inclusion at this stage, meaning that reviewers should err on the side of keeping studies even if there is uncertainty about their relevance. This cautious approach helps minimize the risk of inadvertently excluding potentially valuable studies.
- Retrieving and assessing full texts: For studies which a definitive decision cannot be made based on the title and abstract alone, reviewers need to obtain the full text of the articles for a comprehensive assessment against the predefined inclusion and exclusion criteria. This stage involves meticulously reviewing the full text of each potentially relevant study to determine its eligibility definitively.
- Resolution of Disagreements : In cases of disagreement between reviewers regarding a study’s eligibility, a predefined strategy involving consensus-building discussions or arbitration by a third reviewer should be in place to reach a final decision. This collaborative approach ensures a fair and impartial selection process, further strengthening the review’s reliability.
PRISMA Flowchart
The PRISMA flowchart is a visual representation of the study selection process within a systematic review.
The flowchart illustrates the step-by-step process of screening, filtering, and selecting studies based on predefined inclusion and exclusion criteria.
The flowchart visually depicts the following stages:
- Identification: The initial number of titles and abstracts identified through database searches.
- Screening: The screening process, based on titles and abstracts.
- Eligibility: Full-text copies of the remaining records are retrieved and assessed for eligibility.
- Inclusion: Applying the predefined inclusion criteria resulted in the inclusion of publications that met all the criteria for the review.
- Exclusion: The flowchart details the reasons for excluding the remaining records.
This systematic and transparent approach, as visualized in the PRISMA flowchart, ensures a robust and unbiased selection process, enhancing the reliability of the systematic review’s findings.
The flowchart serves as a visual record of the decisions made during the study selection process, allowing readers to assess the rigor and comprehensiveness of the review.
- How to fill a PRISMA flow diagram
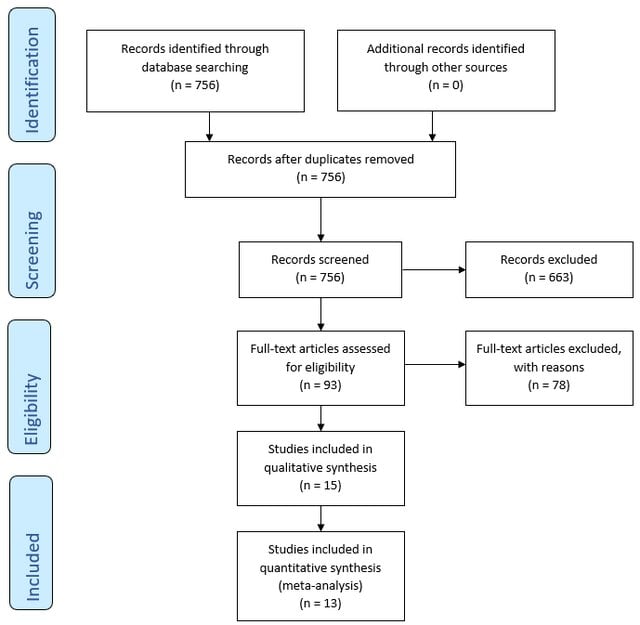
Step 5: Evaluating the Quality of Studies
Data collection process.
Specify the methods used to collect data from reports, including how many reviewers collected data from each report, whether they worked independently, any processes for obtaining or confirming data from study investigators, and if applicable, details of automation tools used in the process. PRISMA 2020 Checklist
Data extraction focuses on information relevant to the research question, such as risk or recovery factors related to a particular phenomenon.
Extract data relevant to the research question, such as effect sizes, sample sizes, means, standard deviations, and other statistical measures.
It can be useful to focus on the authors’ interpretations of findings rather than individual participant quotes, as the latter lacks the full context of the original data.
The coding of studies in a meta-analysis involves carefully and systematically extracting data from each included study in a standardized and reliable manner. This step is essential for ensuring the accuracy and validity of the meta-analysis’s findings.
This information is then used to calculate effect sizes, examine potential moderators, and draw overall conclusions.
Coding procedures typically involve creating a standardized record form or coding protocol. This form guides the extraction of data from each study in a consistent and organized manner. Two independent observers can help to ensure accuracy and minimize errors during data extraction.
Beyond basic information like authors and publication year, code crucial study characteristics relevant to the research question.
For example, if the meta-analysis focuses on the effects of a specific therapy, relevant characteristics to code might include:
- Study characteristics : Publicatrion year, authors, country of origin, publication status ( Published : Peer-reviewed journal articles and book chapters Unpublished : Government reports, websites, theses/dissertations, conference presentations, unpublished manuscripts).
- Intervention : Type (e.g., CBT), duration of treatment, frequency (e.g., weekly sessions), delivery method (e.g., individual, group, online), intention-to-treat analysis (Yes/No)
- Outcome measures : Primary vs. secondary outcomes, time points of measurement (e.g., post-treatment, follow-up).
- Moderators : Participant characteristics that might moderate the effect size. (e.g., age, gender, diagnosis, socioeconomic status, education level, comorbidities).
- Study design : Design (RCT quasi-experiment, etc.), blinding, control group used (e.g., waitlist control, treatment as usual), study setting (clinical, community, online/remote, inpatient vs. outpatient), pre-registration (yes/no), allocation method (simple randomization, block randomization, etc.).
- Sample : Recruitment method (snowball, random, etc.), sample size (total and groups), sample location (treatment & control group), attrition rate, overlap with sample(s) from another study?
- Adherence to reporting guidelines : e.g., CONSORT, STROBE, PRISMA
- Funding source : Government, industry, non-profit, etc.
- Effect Size : Comprehensive meta-analysis program is used to compute d and/or r. Include up to 3 digits after the decimal point for effect size information and internal consistency information. Also record the page number and table number from which the information is coded. This information helps when checking reliability and accuracy to ensure we are coding from the same information.
Before applying the coding protocol to all studies, it’s crucial to pilot test it on a small subset of studies. This helps identify any ambiguities, inconsistencies, or areas for improvement in the coding protocol before full-scale coding begins.
It’s common to encounter missing data in primary research articles. Develop a clear strategy for handling missing data, which might involve contacting study authors, using imputation methods, or performing sensitivity analyses to assess the impact of missing data on the overall results.
Quality Appraisal Tools
Researchers use standardized tools to assess the quality and risk of bias in the quantitative studies included in the meta-analysis. Some commonly used tools include:
- Recommended by the Cochrane Collaboration for assessing randomized controlled trials (RCTs).
- Evaluates potential biases in selection, performance, detection, attrition, and reporting.
- Used for assessing the quality of non-randomized studies, including case-control and cohort studies.
- Evaluates selection, comparability, and outcome assessment.
- Assesses risk of bias in non-randomized studies of interventions.
- Evaluates confounding, selection bias, classification of interventions, deviations from intended interventions, missing data, measurement of outcomes, and selection of reported results.
- Specifically designed for diagnostic accuracy studies.
- Assesses risk of bias and applicability concerns in patient selection, index test, reference standard, and flow and timing.
By using these tools, researchers can ensure that the studies included in their meta-analysis are of high methodological quality and contribute reliable quantitative data to the overall analysis.
Step 6: Choice of Effect Size
The choice of effect size metric is typically determined by the research question and the nature of the dependent variable.
- Odds Ratio (OR) : For instance, if researchers are working in medical and health sciences where binary outcomes are common (e.g., yes/no, failed/success), effect sizes like relative risk and odds ratio are often used.
- Mean Difference : Studies focusing on experimental or between-group comparisons often employ mean differences. The raw mean difference, or unstandardized mean difference, is suitable when the scale of measurement is inherently meaningful and comparable across studies.
- Standardized Mean Difference (SMD) : If studies use different scales or measures, the standardized mean difference (e.g., Cohen’s d) is more appropriate. When analyzing observational studies, the correlation coefficient is commonly chosen as the effect size.
- Pearson correlation coefficient (r) : A statistical measure frequently employed in meta-analysis to examine the strength of the relationship between two continuous variables.
Conversion of efect sizes to a common measure
May be necessary to convert reported findings to the chosen primary effect size. The goal is to harmonize different effect size measures to a common metric for meaningful comparison and analysis.
This conversion allows researchers to include studies that report findings using various effect size metrics. For instance, r can be approximately converted to d, and vice versa, using specific equations. Similarly, r can be derived from an odds ratio using another formula.
Many equations relevant to converting effect sizes can be found in Rosenthal (1991).
Step 7: Assessing Heterogeneity
Heterogeneity refers to the variation in effect sizes across studies after accounting for within-study sampling errors.
Heterogeneity refers to how much the results (effect sizes) vary between different studies, where no variation would mean all studies showed the same improvement (no heterogeneity), while greater variation indicates more heterogeneity.
Assessing heterogeneity matters because it helps us understand if the study intervention works consistently across different contexts and guides how we combine and interpret the results of multiple studies.
While little heterogeneity allows us to be more confident in our overall conclusion, significant heterogeneity necessitates further investigation into its underlying causes.
How to assess heterogeneity
- Homogeneity Test : Meta-analyses typically include a homogeneity test to determine if the effect sizes are estimating the same population parameter. The test statistic, denoted as Q, is a weighted sum of squares that follows a chi-square distribution. A significant Q statistic suggests that the effect sizes are heterogeneous.
- I2 Statistic : The I2 statistic is a relative measure of heterogeneity that represents the ratio of between-study variance (τ2) to the total variance (between-study variance plus within-study variance). Higher I2 values indicate greater heterogeneity.
- Prediction Interval : Examining the width of a prediction interval can provide insights into the degree of heterogeneity. A wide prediction interval suggests substantial heterogeneity in the population effect size.
Step 8: Choosing the Meta-Analytic Model
Meta-analysts address heterogeneity by choosing between fixed-effects and random-effects analytical models.
Use a random-effects model if heterogeneity is high. Use a fixed-effect model if heterogeneity is low, or if all studies are functionally identical and you are not seeking to generalize to a range of scenarios.
Although a statistical test for homogeneity can help assess the variability in effect sizes across studies, it shouldn’t dictate the choice between fixed and random effects models.
The decision of which model to use is ultimately a conceptual one, driven by the researcher’s understanding of the research field and the goals of the meta-analysis.
If the number of studies is limited, a fixed-effects analysis is more appropriate, while more studies are required for a stable estimate of the between-study variance in a random-effects model.
It is important to note that using a random-effects model is generally a more conservative approach.
Fixed-effects models
- Assumes all studies are measuring the exact same thing
- Gives much more weight to larger studies
- Use when studies are very similar
Fixed-effects models assume that there is one true effect size underlying all studies. The goal is to estimate this common effect size with the greatest precision, which is achieved by minimizing the within-study (sampling).
Consequently, studies are weighted by the inverse of their variance.
This means that larger studies, which generally have smaller variances, are assigned greater weight in the analysis because they provide more precise estimates of the common effect size
- Simplicity: The fixed-effect model is straightforward to implement and interpret, making it computationally simpler.
- Precision: When the assumption of a common effect size is met, fixed-effect models provide more precise estimates with narrower confidence intervals compared to random-effects models.
- Suitable for Conditional Inferences: Fixed-effect models are appropriate when the goal is to make inferences specifically about the studies included in the meta-analysis, without generalizing to a broader population.
- Restrictive Assumptions: The fixed-effect model assumes all studies estimate the same population parameter, which is often unrealistic, particularly with studies drawn from diverse methodologies or populations.
- Limited Generalizability: Findings from fixed-effect models are conditional on the included studies, limiting their generalizability to other contexts or populations.
- Sensitivity to Heterogeneity: Fixed-effect models are sensitive to the presence of heterogeneity among studies, and may produce misleading results if substantial heterogeneity exists.
Random-effects models
- Assumes studies might be measuring slightly different things
- Gives more balanced weight to both large and small studies
- Use when studies might vary in methods or populations
Random-effects models assume that the true effect size can vary across studies. The goal here is to estimate the mean of these varying effect sizes, considering both within-study variance and between-study variance (heterogeneity).
This approach acknowledges that each study might estimate a slightly different effect size due to factors beyond sampling error, such as variations in study populations, interventions, or designs.
This balanced weighting prevents large studies from disproportionately influencing the overall effect size estimate, leading to a more representative average effect size that reflects the distribution of effects across a range of studies.
- Realistic Assumptions: Random-effects models acknowledge the presence of between-study variability by assuming true effects are randomly distributed, making it more suitable for real-world research scenarios.
- Generalizability: Random-effects models allow for broader inferences to be made about a population of studies, enhancing the generalizability of findings.
- Accommodation of Heterogeneity: Random-effects models explicitly model heterogeneity, providing a more accurate representation of the overall effect when studies have varying effect sizes.
- Complexity: Random-effects models are computationally more complex, requiring the estimation of additional parameters, such as between-study variance.
- Reduced Precision: Confidence intervals tend to be wider compared to fixed-effect models, particularly when between-study heterogeneity is substantial.
- Requirement for Sufficient Studies: Accurate estimation of between-study variance necessitates a sufficient number of studies, making random-effects models less reliable with smaller meta-analyses.
Step 9: Perform the Meta-Analysis
This step involves statistically combining effect sizes from chosen studies. Meta-analysis uses the weighted mean of effect sizes, typically giving larger weights to more precise studies (often those with larger sample sizes).
The main function of meta-analysis is to estimate effects in a population by combining the effect sizes from multiple articles.
It uses a weighted mean of the effect sizes, typically giving larger weights to more precise studies, often those with larger sample sizes.
This weighting scheme makes statistical sense because an effect size with good sampling accuracy (i.e., likely to be an accurate reflection of reality) is weighted highly.
On the other hand, effect sizes from studies with lower sampling accuracy are given less weight in the calculations.
the process:
- Calculate weights for each study
- Multiply each study’s effect by its weight
- Add up all these weighted effects
- Divide by the sum of all weights
Estimating effect size using fixed effects
The fixed-effects model in meta-analysis operates under the assumption that all included studies are estimating the same true effect size.
This model focuses solely on within-study variance when determining the weight of each study.
The weight is calculated as the inverse of the within-study variance, which typically results in larger studies receiving substantially more weight in the analysis.
This approach is based on the idea that larger studies provide more precise estimates of the true effect.
The weighted mean effect size (M) is calculated by summing the products of each study’s effect size (ESi) and its corresponding weight (wi) and dividing that sum by the total sum of the weights:
1. Calculate weights (wi) for each study:
The weight is often the inverse of the variance of the effect size. This means studies with larger sample sizes and less variability will have greater weight, as they provide more precise estimates of the effect size
This weighting scheme reflects the assumption in a fixed-effect model that all studies are estimating the same true effect size, and any observed differences in effect sizes are solely due to sampling error. Therefore, studies with less sampling error (i.e., smaller variances) are considered more reliable and are given more weight in the analysis.
Here’s the formula for calculating the weight in a fixed-effect meta-analysis:
Wi = 1 / VYi 1
- Wi represents the weight assigned to study i.
- VYi is the within-study variance for study i.
Practical steps:
- The weight for each study is calculated as: Weight = 1 / (within-study variance)
- For example: Let’s say a study reports a within-study variance of 0.04. The weight for this study would be: 1 / 0.04 = 25
- Calculate the weight for every study included in your meta-analysis using this method.
- These weights will be used in subsequent calculations, such as computing the weighted mean effect size.
- Note : In a fixed-effects model, we do not calculate or use τ² (tau squared), which represents between-study variance. This is only used in random-effects models.
2. Multiply each study’s effect by its weight:
After calculating the weight for each study, multiply the effect size by its corresponding weight. This step is crucial because it ensures that studies with more precise effect size estimates contribute proportionally more to the overall weighted mean effect size
- For each study, multiply its effect size by the weight we just calculated.
3. Add up all these weighted effects:
Sum up all the products from step 2.
4. Divide by the sum of all weights:
- Add up all the weights we calculated in step 1.
- Divide the sum from step 3 by this total weight.
Implications of the fixed-effects model
- Larger studies (with smaller within-study variance) receive substantially more weight.
- This model assumes that differences between study results are due only to sampling error.
- It’s most appropriate when studies are very similar in methods and sample characteristics.

Estimating effect size using random effects
Random effects meta-analysis is slightly more complicated because multiple sources of differences potentially affecting effect sizes must be accounted for.
The main difference in the random effects model is the inclusion of τ² (tau squared) in the weight calculation. This accounts for between-study heterogeneity, recognizing that studies might be measuring slightly different effects.
This process results in an overall effect size that takes into account both within-study and between-study variability, making it more appropriate when studies differ in methods or populations.
The model estimates the variance of the true effect sizes (τ²). This requires a reasonable number of studies, so random effects estimation might not be feasible with very few studies.
Estimation is typically done using statistical software, with restricted maximum likelihood (REML) being a common method.
1. Calculate weights for each study:
In a random-effects meta-analysis, the weight assigned to each study (W*i) is calculated as the inverse of that study’s variance, similar to a fixed-effect model. However, the variance in a random-effects model considers both the within-study variance (VYi) and the between-studies variance (T^2).
The inclusion of T^2 in the denominator of the weight formula reflects the random-effects model’s assumption that the true effect size can vary across studies.
This means that in addition to sampling error, there is another source of variability that needs to be accounted for when weighting the studies. The between-studies variance, T^2, represents this additional source of variability.
Here’s the formula for calculating the weight in a random-effects meta-analysis:
W*i = 1 / (VYi + T^2)
- W*i represents the weight assigned to study i.
- T^2 is the estimated between-studies variance.
First, we need to calculate something called τ² (tau squared). This represents the between-study variance.
The estimation of T^2 can be done using different methods, one common approach being the method of moments (DerSimonian and Laird method).
The formula for T^2 using the method of moments is: T^2 = (Q – df) / C
- Q is the homogeneity statistic.
- df is the degrees of freedom (number of studies -1).
- C is a constant calculated based on the study weights
- The weight for each study is then calculated as: Weight = 1 / (within-study variance + τ²). This is different from the fixed effects model because we’re adding τ² to account for between-study variability.
Add up all the weights we calculated in step 1. Divide the sum from step 3 by this total weight
Implications of the random-effects model
- Weights are more balanced between large and small studies compared to the fixed-effects model.
- It’s most appropriate when studies vary in methods, sample characteristics, or other factors that might influence the true effect size.
- The random-effects model typically produces wider confidence intervals, reflecting the additional uncertainty from between-study variability.
- Results are more generalizable to a broader population of studies beyond those included in the meta-analysis.
- This model is often more realistic for social and behavioral sciences, where true effects may vary across different contexts or populations.
Step 10: Sensitivity Analysis
Assess the robustness of your findings by repeating the analysis using different statistical methods, models (fixed-effects and random-effects), or inclusion criteria. This helps determine how sensitive your results are to the choices made during the process.
Sensitivity analysis strengthens a meta-analysis by revealing how robust the findings are to the various decisions and assumptions made during the process. It helps to determine if the conclusions drawn from the meta-analysis hold up when different methods, criteria, or data subsets are used.
This is especially important since opinions may differ on the best approach to conducting a meta-analysis, making the exploration of these variations crucial.
Here are some key ways sensitivity analysis contributes to a more robust meta-analysis:
- Assessing Impact of Different Statistical Methods : A sensitivity analysis can involve calculating the overall effect using different statistical methods, such as fixed and random effects models. This comparison helps determine if the chosen statistical model significantly influences the overall results. For instance, in the meta-analysis of β-blockers after myocardial infarction, both fixed and random effects models yielded almost identical overall estimates. This suggests that the meta-analysis findings are resilient to the statistical method employed.
- Evaluating the Influence of Trial Quality and Size : By analyzing the data with and without trials of questionable quality or varying sizes, researchers can assess the impact of these factors on the overall findings.
- Examining the Effect of Trials Stopped Early : Including trials that were stopped early due to interim analysis results can introduce bias. Sensitivity analysis helps determine if the inclusion or exclusion of such trials noticeably changes the overall effect. In the example of the β-blocker meta-analysis, excluding trials stopped early had a negligible impact on the overall estimate.
- Addressing Publication Bias : It’s essential to assess and account for publication bias, which occurs when studies with statistically significant results are more likely to be published than those with null or nonsignificant findings. This can be accomplished by employing techniques like funnel plots, statistical tests (e.g., Begg and Mazumdar’s rank correlation test, Egger’s test), and sensitivity analyses.
By systematically varying different aspects of the meta-analysis, researchers can assess the robustness of their findings and address potential concerns about the validity of their conclusions.
This process ensures a more reliable and trustworthy synthesis of the research evidence.
Common Mistakes
When conducting a meta-analysis, several common pitfalls can arise, potentially undermining the validity and reliability of the findings. Sources caution against these mistakes and offer guidance on conducting methodologically sound meta-analyses.
- Insufficient Number of Studies: If there are too few primary studies available, a meta-analysis might not be appropriate. While a meta-analysis can technically be conducted with only two studies, the research community might not view findings based on a limited number of studies as reliable evidence. A small number of studies could suggest that the research field is not mature enough for meaningful synthesis.
- Inappropriate Combination of Studies : Meta-analyses should not simply combine studies indiscriminately. Avoid the “apples and oranges” problem, where studies with different research objectives, designs, measures, or samples are inappropriately combined. Such practices can obscure important differences between studies and lead to misleading conclusions.
- Misinterpreting Heterogeneity : One common mistake is using the Q statistic or p-value from a test of heterogeneity as the sole indicator of heterogeneity. While these statistics can signal heterogeneity, they do not quantify the extent of variation in effect sizes.
- Over-Reliance on Published Studies : This dependence on published literature introduces the risk of publication bias, where studies with statistically significant or favorable results are more likely to be published. Failure to acknowledge and address publication bias can lead to overestimating the true effect size.
- Neglecting Study Quality : Including studies with poor methodological quality can bias the results of a meta-analysis leading to unreliable and inaccurate effect size estimates. The decision of which studies to include should be based on predefined eligibility criteria to ensure the quality and relevance of the synthesis.
- Fixation on Statistical Significance : Placing excessive emphasis on the statistical significance of an overall effect while neglecting its practical significance is a critical mistake in meta-analysis, as is the case in primary studies. Considers both statistical and clinical or substantive significance.
- Misinterpreting Significance Testing in Subgroup Analyses : When comparing effect sizes across subgroups, merely observing that an effect is statistically significant in one subgroup but not another is insufficient. Conduct formal tests of statistical significance for the difference in effects between subgroups or to calculate the difference in effects with confidence intervals.
- Ignoring Dependence : Neglecting dependence among effect sizes, particularly when multiple effect sizes are extracted from the same study, is a mistake. This oversight can inflate Type I error rates and lead to inaccurate estimations of average effect sizes and standard errors.
- Inadequate Reporting : Failing to transparently and comprehensively report the meta-analysis process is a crucial mistake. A meta-analysis should include a detailed written protocol outlining the research question, search strategy, inclusion criteria, and analytical methods.
Reading List
- Bar-Haim, Y., Lamy, D., Pergamin, L., Bakermans-Kranenburg, M. J., & Van Ijzendoorn, M. H. (2007). Threat-related attentional bias in anxious and nonanxious individuals: a meta-analytic study . Psychological bulletin , 133 (1), 1.
- Borenstein, M., Hedges, L. V., Higgins, J. P., & Rothstein, H. R. (2021). Introduction to meta-analysis . John Wiley & Sons.
- Crits-Christoph, P. (1992). A Meta-analysis . American Journal of Psychiatry , 149 , 151-158.
- Duval, S. J., & Tweedie, R. L. (2000). A nonparametric “trim and fill” method of accounting for publication bias in meta-analysis. Journal of the American Statistical Association, 95 (449), 89–98.
- Egger, M., Davey Smith, G., Schneider, M., & Minder, C. (1997). Bias in meta-analysis detected by a simple, graphical test . BMJ, 315 (7109), 629–634.
- Egger, M., Smith, G. D., & Phillips, A. N. (1997). Meta-analysis: principles and procedures . Bmj , 315 (7121), 1533-1537.
- Field, A. P., & Gillett, R. (2010). How to do a meta‐analysis . British Journal of Mathematical and Statistical Psychology , 63 (3), 665-694.
- Hedges, L. V., & Pigott, T. D. (2004). The power of statistical tests for moderators in meta-analysis . Psychological methods , 9 (4), 426.
- Hedges, L. V., & Olkin, I. (2014). Statistical methods for meta-analysis . Academic press.
- Hofmann, S. G., Sawyer, A. T., Witt, A. A., & Oh, D. (2010). The effect of mindfulness-based therapy on anxiety and depression: A meta-analytic review . Journal of consulting and clinical psychology , 78 (2), 169.
- Littell, J. H., Corcoran, J., & Pillai, V. (2008). Systematic reviews and meta-analysis . Oxford University Press.
- Lyubomirsky, S., King, L., & Diener, E. (2005). The benefits of frequent positive affect: Does happiness lead to success? . Psychological bulletin , 131 (6), 803.
- Macnamara, B. N., & Burgoyne, A. P. (2022). Do growth mindset interventions impact students’ academic achievement? A systematic review and meta-analysis with recommendations for best practices. Psychological Bulletin .
- Polanin, J. R., & Pigott, T. D. (2015). The use of meta‐analytic statistical significance testing . Research Synthesis Methods , 6 (1), 63-73.
- Rodgers, M. A., & Pustejovsky, J. E. (2021). Evaluating meta-analytic methods to detect selective reporting in the presence of dependent effect sizes . Psychological methods , 26 (2), 141.
- Rosenthal, R. (1991). Meta-analysis: a review. Psychosomatic medicine , 53 (3), 247-271.
- Tipton, E., Pustejovsky, J. E., & Ahmadi, H. (2019). A history of meta‐regression: Technical, conceptual, and practical developments between 1974 and 2018 . Research synthesis methods , 10 (2), 161-179.
- Zhao, J. G., Zeng, X. T., Wang, J., & Liu, L. (2017). Association between calcium or vitamin D supplementation and fracture incidence in community-dwelling older adults: a systematic review and meta-analysis. Jama , 318 (24), 2466-2482.

- - Google Chrome
Intended for healthcare professionals
- My email alerts
- BMA member login
- Username * Password * Forgot your log in details? Need to activate BMA Member Log In Log in via OpenAthens Log in via your institution

Search form
- Advanced search
- Search responses
- Search blogs
- A guide to prospective...
A guide to prospective meta-analysis
- Related content
- Peer review
- Kylie E Hunter , senior project officer 1 ,
- Saskia Cheyne , senior evidence analyst 1 ,
- Davina Ghersi , senior principal research scientist, adjunct professor 1 2 ,
- Jesse A Berlin , vice president, global head of epidemiology 3 ,
- Lisa Askie , professor and director of systematic reviews and health technology assessment, manager of the Australian New Zealand Clinical Trials Registry 1
- 1 NHMRC Clinical Trials Centre, University of Sydney, Locked bag 77, Camperdown NSW 1450, Australia
- 2 National Health and Medical Research Council, Canberra, Australia
- 3 Johnson & Johnson, Titusville, NJ, USA
- Correspondence to: A L Seidler lene.seidler{at}ctc.usyd.edu.au
- Accepted 8 August 2019
In a prospective meta-analysis (PMA), study selection criteria, hypotheses, and analyses are specified before the results of the studies related to the PMA research question are known, reducing many of the problems associated with a traditional (retrospective) meta-analysis. PMAs have many advantages: they can help reduce research waste and bias, and they are adaptive, efficient, and collaborative. Despite an increase in the number of health research articles labelled as PMAs, the methodology remains rare, novel, and often misunderstood. This paper provides detailed guidance on how to address the key elements for conducting a high quality PMA with a case study to illustrate each step.
Summary points
In a prospective meta-analysis (PMA), studies are identified and determined to be eligible for inclusion before the results of the studies related to the PMA research question are known
PMAs are applicable to high priority research questions where limited previous evidence exists and where new studies are expected to emerge
Compared with standard systematic review and meta-analysis protocols, key adaptations should be made to a PMA protocol, including search methods to identify planned and ongoing studies, details of studies that have already been identified for inclusion, core outcomes to be measured by all studies, collaboration management, and publication policy
A systematic search for planned and ongoing studies should precede a PMA, including a search of clinical trial registries and medical literature databases, and contacting relevant stakeholders in the specialty
PMAs are ideally conducted by a collaboration or consortium, including a central steering and data analysis committee, and representatives from each individual study
Usually PMAs collect individual participant data, but PMAs of aggregate data are also possible. PMAs can include interventional or observational studies
PMAs can enable harmonised collection of core outcomes, which can be particularly useful for rare but important outcomes, such as adverse side effects
Adaptive forms of PRISMA (preferred reporting items for systematic reviews and meta-analyses) and quality assessment approaches such as GRADE (grading of recommendations assessment, development, and evaluation) should be used to report and assess the quality of evidence for a PMA. The development of a standardised set of reporting guidelines and PMA specific evidence rating tools is highly desirable
PMAs can help to reduce research waste and bias, and they are adaptive, efficient, and collaborative
Systematic reviews and meta-analyses of the best available evidence are widely used to inform healthcare policy and practice. 1 2 Yet the retrospective nature of traditional systematic reviews and meta-analyses can be problematic. Positive results are more likely to be reported and published (phenomena known as selective outcome reporting and publication bias), and therefore including only published results in a meta-analysis can produce misleading results 3 and pose a threat to the validity of evidence based medicine. 4 In the planning stage of a traditional meta-analysis, knowledge of individual study results can influence the study selection process as choosing the key components of the review question and eligibility criteria might be based on one or more positive studies. 2 5 Meta-analyses on the same topic can reach conflicting conclusions because of different eligibility criteria. 2 Also, inconsistencies across individual studies in outcome measurement and analyses can make the combination of data difficult. 6
Prospective meta-analyses (PMAs, see box 1) have recently been described as next generation systematic reviews 7 that reduce the problems of traditional retrospective meta-analyses. Ioannidis and others even argue that “all primary original research may be designed, executed, and interpreted as prospective meta-analyses.” 8 9 For PMAs, studies are included prospectively, meaning before any individual study results related to the PMA research question are known. 10 This reduces the risk of publication bias and selective reporting bias and can enable better harmonisation of study outcomes.
Definition of a prospective meta-analysis
The key feature of a prospective meta-analysis (PMA) is that the studies or cohorts are identified as eligible for inclusion in the meta-analysis, and hypotheses and analysis strategies are specified, before the results of the studies or cohorts related to the PMA research question are known
The number of meta-analyses described as PMAs is increasing ( fig 1 ). But the definition, methodology, and reporting of previous PMAs vary greatly, and guidance on how to conduct them is limited, outdated, and inconsistent. 11 12 With recent advancements in computing capabilities, and the ability to identify planned and ongoing studies through increased trial registration, the planning and conduct of PMAs have become more efficient and effective. For PMAs to be successfully implemented in future health research, a revised PMA definition and expanded guidance are required. In this article, we, the Cochrane PMA Methods Group, present a step by step guide on how to perform a PMA. Our aim is to provide up to date guidance on the key principles, rationale, methods, and challenges for each step, to enable more researchers to understand and use this methodology successfully. Figure 2 shows a summary of the steps needed to perform a PMA.

Number of prospective meta-analyses (PMAs) over time. Possible PMA describes studies that seem to fulfil the criteria for a PMA but not enough information was reported to make a definite decision on their status as a PMA. These data are based on a systematic search of the literature (see appendix 1 for methodology)
- Download figure
- Open in new tab
- Download powerpoint

Steps in conducting a prospective meta-analysis (PMA)
Case study: Neonatal Oxygenation Prospective Meta-analysis (NeOProM)
We will illustrate each step with an example of a PMA of randomised controlled trials conducted by the Neonatal Oxygenation Prospective Meta-analysis (NeOProM) Collaboration. 13 In this PMA, five groups prospectively planned to conduct separate, but similar, trials assessing different target ranges for oxygen saturation in preterm infants, and combine their results on completion. Although no difference was found in the composite primary outcome of death or major disability, a statistically significant reduction in the secondary outcome of death alone was found for the higher oxygen target range, but no change in major disability. This PMA resolved a major debate in neonatology.
Steps for performing a prospective meta-analysis
Step 0: deciding if a pma is the right methodology.
PMA methodology should be considered for a high priority research question for which new studies are expected to emerge and limited previous evidence exists (fig 3):
Priority research question —PMAs should be planned for research questions that are a high priority for healthcare decision makers. Ideally, these questions should be identified using priority setting methods within consumer-clinician collaborations, and/or they should address priorities identified by guideline committees, funding bodies, or clinical and research associations. Often these questions are in areas where important new treatment or prevention strategies have recently emerged, or where practice varies because of insufficient evidence.
New studies expected —PMAs are only feasible if new studies are likely to be included—for example, if the research question is an explicit priority for funding bodies or research associations. Some PMAs have been initiated after researchers learnt they were planning or conducting similar studies, and so they decided to collaborate and prospectively plan to combine their data. In other cases, a research question is posed by a consortium of investigators who then decide to plan similar studies that are combined on completion. A research team planning a PMA can play an active role in motivating other researchers to conduct similar studies addressing the same research question. A PMA can therefore be a catalyst for initiating a programme of priority research to answer important questions. 8 Initiating a PMA rather than conducting a large multicentre study can be advantageous as PMAs allow flexibility for each study to answer additional local questions, and the studies can be funded independently, which circumvents the problem of funding a mega study.
Insufficient previous evidence —A PMA should only be conducted if insufficient evidence exists to answer the research question. If sufficient evidence is available (eg, based on a retrospective meta-analysis), no further studies and no PMA should be planned, to avoid research waste.

When to conduct a prospective meta-analysis (PMA)
If evidence is available, but is insufficient for clinical decision making, a nested PMA should be considered. A nested PMA integrates prospective evidence into a retrospective meta-analysis, making best use of existing and emerging evidence while also retaining some benefits of PMAs. A nested PMA allows the assessment of publication bias and selective reporting bias by comparing prospectively included evidence with retrospective evidence in a sensitivity analysis. Studies that are prospectively included can be harmonised with other ongoing studies, and with previous related retrospective studies, to optimise evidence synthesis (see step 5).
PMA methodology was chosen to determine the optimal target range for oxygen saturation in preterm infants for several reasons:
Priority research question —oxygen has been used to treat preterm infants for more than 60 years. The different oxygen saturation target ranges used in practice have been associated with clinically important outcomes, such as mortality, disability, and blindness. Changing the oxygen saturation target range would be relatively easy to implement in clinical practice.
Insufficient previous evidence —evidence was mainly observational, with no recent, high quality randomised controlled trials available.
New studies expected— a total sample size of about 5000 infants was needed to detect an absolute difference in death or major disability of 4%. The NeOProM PMA was originally proposed as one large multicentre, multinational trial. 14 But because expensive masked pulse oximeters were needed, one funder could not support a study of sufficient sample size to reliably answer the clinical question. Instead, a PMA collaboration was initiated. Each group of NeOProM investigators obtained funding to conduct their own trial (although alone each study was underpowered to answer the main clinical question), could choose their own focus, and publish their own results, but with agreement to contribute data to the PMA to ensure sufficient combined statistical power to reliably detect differences in important outcomes.
Step 1: defining the research question and the eligibility criteria
At the start of a PMA, a research question needs to be specified. Research questions for PMAs should be formed in a similar way to traditional retrospective systematic reviews. Guidance for formulating a review question is available in the Cochrane Handbook for Systematic Reviews of Interventions . 15 For PMAs of interventional studies, the PICO system (population, intervention, comparison, outcome) should be used. To avoid selective reporting bias, the PMA research question and hypotheses need to be specified before any study results related to the PMA research questions are known.
PMAs are possible for a wide range of different study types—their applicability reaches beyond randomised controlled trials. An interventional PMA includes interventional studies (eg, randomised controlled trials or non-randomised studies of interventions). For interventional PMAs, the key inclusion criterion of “no results being known” usually means that the analyses have not been conducted in any of the trials included in the PMA.
An observational PMA includes observational studies. For observational PMAs, “no results being known” would mean that no analyses related to the PMA research question have been done. As many observational studies collect data on different outcomes, a meta-analysis can be classified as a PMA if unrelated research questions have already been analysed before inclusion in the PMA. For instance, for a PMA on the risk of lung cancer for people exposed to air pollution, observational studies where the relation between cardiovascular disease and air pollution has already been analysed can be included in the PMA, but only if the analyses on the association between lung cancer and air pollution have not been done. In this case, however, little harmonisation of outcome collection is possible (unless the investigators agree to collect additional data).
The NeOProM PMA addressed the research question, does targeting a lower oxygen saturation range in extremely preterm infants, from birth or soon after, increase or decrease the composite outcome of death or major disability in survivors by 4% or more?
The PICOS system was applied to define the eligibility criteria:
• Participants=infants born before 28 weeks’ gestation and enrolled within 24 hours of birth
• Intervention=target a lower (85-89%) oxygen saturation (SpO 2 ) range
• Comparator=target a higher (91-95%) SpO 2 range
• Outcome=composite of death or major disability at a corrected age of 18-24 months
• Study type=double blinded, randomised controlled trial (making this an interventional PMA).
Step 2: writing the protocol
Key elements of the protocol need to be finalised for the PMA before any individual study results related to the PMA research question are known. These include specification of the research questions, eligibility criteria for inclusion of studies, hypotheses, outcomes, and the statistical analysis strategy. The preferred reporting items for systematic reviews and meta-analyses extension for protocols (PRISMA-P) 16 provides some guidance on what should be included. As these reporting items were created for retrospective meta-analyses, however, key adaptations need to be made for PMA protocols (see box 2).
Key additional reporting items for a PMA protocol
For a PMA, several key items should be reported in the protocol in addition to PRISMA-P items:
Search methods
The search methods need to include how planned and ongoing studies are identified and how potential collaborators will be or have been contacted to participate (see step 3)
Study details
Details for studies already identified for inclusion should be listed, along with a statement that their results related to the PMA research question are not yet known (see step 1)
Core outcomes
Any core outcomes that will be measured by all the included studies should be specified, along with details on how and why they should be measured, to facilitate outcome harmonisation (see step 5)
Type of data collected
PMAs often collect individual participant data (that is, row by row data for each participant) but they may also collect aggregate data (that is, summary data for each study), and some combine both (see step 6)
Collaboration management and publication policy
Collaboration management and publication policy (see steps 4 and 7) should be specified, including details of any central steering and data analysis committees
An initial PMA protocol should be drafted before the search for eligible studies, but it can be amended after searching and after all studies have been included if the results of the included studies are not known when the PMA protocol is finalised. The investigators of the included studies can agree on the collection and analysis of additional rare outcomes and these outcomes can be included in a revised version of the protocol.
The final PMA protocol should be publicly available on the international prospective register of systematic reviews, PROSPERO 17 (which supports registration of PMAs), before the results (relating to the PMA research question) of any of the included studies are known. A full version of the PMA protocol can be published in a peer reviewed journal or elsewhere.
For the NeOProM PMA, an initial protocol was drafted by the lead investigators and discussed and refined by collaborators from all the included trials. The PMA protocol was registered on ClinicalTrials.gov in 2010 ( NCT01124331 ) because PROSPERO had not yet been launched. After the launch of PROSPERO in 2011, the protocol was registered (CRD42015019508). The full version of the protocol was published in BMC Pediatrics . 18
Step 3: searching for studies
After the PMA protocol is finalised, a systematic literature search is conducted, similar to that of a systematic review for a high quality meta-analysis. The main resources available for identifying planned and ongoing studies are clinical trial registries. Currently, 17 global clinical trial registries provide data to the World Health Organization’s International Clinical Trials Registry Platform. 19 Views on the best strategies for searching trial registries differ. 20 Limiting the search by date can be useful (eg, only studies registered within a reasonable time frame, taking into account the expected study duration and follow-up times) to reduce the search burden and exclude studies registered earlier that would likely be completed and thus ineligible for a PMA. Ideally, searches should be repeated on a regular basis to identify new eligible studies.
Prospective trial registration is mandated by various legislative, ethical, and regulatory bodies but compliance is not complete. 21 22 23 Observational studies are not required to be registered. Hence additional approaches to identifying planned and ongoing studies should be pursued, including searching bibliographic databases for conference abstracts, study protocols, and cohort descriptions, and approaching relevant stakeholders. The existence and possibility of joining the PMA can be publicised through the publication of PMA protocols, presentations at relevant conferences and research forums, and through an online presence (eg, a collaboration website).
For NeOProM, the Cochrane Central Register of Controlled Trials, Medline through PubMed, Embase, and CINAHL, clinical trial registries (using the WHO portal ( www.who.int/ictrp/en/ ) and ClinicalTrials.gov), conference proceedings, and the reference lists of retrieved articles were searched. Key researchers in the specialty were contacted to inquire if they were aware of additional trials. The abstracts of the relevant perinatal meetings (including the Neonatal Register and the Society for Paediatric Research) were searched using the keywords “oxygen saturation”. Five planned or ongoing trials meeting the inclusion criteria for the NeOProM PMA were identified, based in Australia, New Zealand, Canada, the United Kingdom, and the United States. The trials completed enrolment and follow-up between 2005 and 2014 and recruited a total of 4965 preterm infants born before 28 weeks’ gestation. No results for any of the trials were known at the time each trial agreed to be included in the PMA. All the NeOProM trials were identified by discussion with collaborators, and no additional trials were identified from electronic database searches.
Step 4: forming a collaboration of study investigators
Ideally, PMAs are conducted by a collaboration or consortium, including a central steering committee (leading the PMA and managing the collaboration), a data analysis committee (responsible for data management, processing, and analysis), and representatives from each study (involved in decisions on the protocol, analysis, and interpretation of the results). Regular collaboration meetings can be beneficial for achieving consensus on disagreements and in keeping study investigators involved in the PMA process. Transparent processes and a priori agreements are crucial for building and maintaining trust within a PMA collaboration.
Investigators might refuse to collaborate. Refusal to collaborate is less likely in a PMA than in a retrospective individual participant data meta-analysis as reaching agreement to share data is easier if studies are in their planning phases and can still be amended and harmonised after internal discussions. Aggregate data can be included in the PMA even if investigators refuse to collaborate, if the relevant summary data can be extracted from the resulting publications when the studies are completed. The ability to harmonise studies (step 5), however, may be limited if eligible investigators refuse to participate.
The NeOProM Collaboration comprised at least one investigator and a statistician from each of the included trials, and a steering group. All investigators and the steering group agreed on key aspects of the protocol before the results of the trials were known, and they also developed and agreed on a common data collection form, coding sheet, and detailed analysis plan. The NeOProM Collaboration met regularly by teleconference, and at least once a year face to face, to reach consensus on disagreements and to discuss the progress of individual trials, funding, data harmonisation, analysis plans, and interpretation of the PMA findings.
Step 5: harmonisation of included study population, intervention/exposure, and outcome collection
When a collaboration of investigators of planned or ongoing studies has been formed, the investigators can work together to harmonise the design, conduct, and outcome collection of the included studies to facilitate a meta-analysis and interpretation. A common problem with retrospective meta-analyses is that interventions are administered slightly differently across studies, or to different populations, and outcome collection, measurement, or reporting can differ. These differences make it difficult, and sometimes impossible, to synthesise results that are directly relevant to the study outcomes, interventions, and populations. In a PMA, studies are included as they are being planned or are ongoing, allowing researchers to agree on how to conduct their studies and collect common core outcomes. The PMA design enables the generation of evidence that is directly relevant to the research questions and thus increases confidence in the strength of the statements and recommendations derived from the PMA.
The ability to harmonise varies depending on the time when the PMA is first planned ( fig 4 ). In a de novo PMA, studies are planned as part of a PMA. For PMAs of interventional studies, a de novo PMA is similar to a multicentre trial: the included trials often share a common protocol, and usually the study population, interventions, and outcome collection are fully harmonised. In contrast, some PMAs identify studies for inclusion when data collection has already finished but no analyses related to the PMA research question have been conducted (outside of data safety monitoring committees). These types of PMAs allow little to no data harmonisation and are more similar to traditional retrospective meta-analyses. Yet they still have the advantage of reducing selection bias as the studies are deemed eligible for inclusion before their PMA specific results are known.

Different scenarios and time points when studies can be included in a prospective meta-analysis (PMA)
Harmonisation of studies in a PMA can occur for different elements of the included studies: study populations and settings; interventions or exposures (that is, independent variables); and outcomes collection. For study populations, settings, and interventions/exposures, harmonisation of studies to some degree is often beneficial to enable their successful synthesis. But some variation in the individual study protocols, populations, and interventions/exposures is often desirable to improve the generalisability (that is, external validity) of the research findings beyond one study, one form of the intervention, or narrow study specific populations. The variation in populations also enables subgroup analyses, evaluating if differences in populations between and within the studies leads to differences in treatment effects. If particular subgroups appear in more than one study, additional statistical power for subgroup analyses is also achieved.
Harmonisation of outcome collection requires careful consideration of the amount of common data needed to answer the relevant research questions. These discussions should aim to minimise unnecessary burden on participants and reduce research waste by avoiding excessive data collection, while increasing the ability to answer important research questions. Researchers can also agree to collect and analyse rare outcomes, such as severe but rare adverse events, that their individual studies would not have had the statistical power to detect. Collaborations should be specific on exactly how shared outcomes will be measured to avoid heterogeneity in outcome collection and difficulties in combining data. The COMET (core outcome measures in effectiveness trials) initiative ( www.comet-initiative.org/ ) has introduced methods for the development of core outcome sets, as detailed in its handbook. 24 These core outcome sets specify what and how outcomes should be measured by all studies of specific conditions to facilitate comparison and synthesis of the results. For health conditions with common core outcome sets, PMA collaborators should include the core outcomes, and also consider collecting other common outcomes that are particularly relevant for the specific research question posed. Not all outcomes have to be harmonised and collected by all studies: individual studies in a PMA have more autonomy than individual centres in a multicentre study and can collect study specific outcomes for their own purposes.
The improved availability of common core outcomes in a PMA has recently been shown in a PMA of childhood obesity interventions. 25 Harmonisation increased from 18% of core outcomes collected by all trials before the trial investigators agreed to collaborate, to 91% after the investigators decided to collaborate in a PMA.
Investigators of the five NeOProM trials first met in 2005 when the first trial was about to begin and the other four studies were in the early planning stages. With de novo PMA planning, all trials had the same intervention and comparator and collected similar outcome and subgroup variables. Some inconsistencies in outcome definitions and assessment methods across studies remained, however, and required substantial discussion to harmonise the final outcome collection and analyses.
Step 6: synthesising the evidence and assessing certainty of evidence
When all the individual studies have been completed, data can be synthesised in a PMA. For aggregate data PMA, results are extracted from publications or provided by the study authors. For individual participant data PMA, the line by line data from each participant in each study must be collated, harmonised, and analysed. This process is usually easier for PMAs than for traditional, retrospective individual participant data meta-analyses because if outcome collection and coding were previously harmonised, fewer inconsistencies should arise. If possible, plans to share data should be outlined in each study’s ethics application and consent form. For PMAs that are planned after the eligible studies have commenced, amendments to ethics applications may be necessary for data sharing. To assure independent data analysis, some PMAs appoint an independent data manager and statistician who have not been involved in any of the studies. The initial time intensive planning and harmonisation phase is followed by a waiting period when all the individual studies are completed before their data are made available and synthesised. During this middle period, PMAs usually demand little time and can run alongside other projects.
For studies where data safety monitoring committees are appropriate, it might be sensible for the committees to communicate and plan joint interim analyses to take account of all the available evidence when making recommendations to continue or stop a study. The PMA collaboration should consider establishing a joint data monitoring committee to synthesise data from all included studies at prespecified times. Methods for sequential meta-analysis and adaptive trial design could be considered in this context. 26
When all studies have been synthesised, the methodological quality of the included studies needs to be appraised with validated tools, such as those recommended by Cochrane. 27 28 The certainty of the evidence can be assessed with the grading of recommendations assessment, development and evaluation (GRADE) approach. 29
The NeOProM Collaboration was established in 2005, the first trial commenced in 2005, the last trial’s results were available in 2016, and the final combined analysis was published in 2018. At the request of two of the trials’ data monitoring committees, an interim analysis of data from these two trials was undertaken in 2011 and both trials were stopped early. 30 The five trials included in NeOProM were assessed for risk of bias with the Cochrane domains, 31 and consensus was reached by discussion with the full study group. The risk of bias assessments were more accurate and complete after detailed discussion of several domains (eg, allocation concealment and blinding) between the NeOProM Collaborators than would have been possible with their publications alone. GRADE assessments were performed and published in the Cochrane version of the meta-analysis. 32
Step 7: interpretation and reporting of results
Generally, the quality of the evidence derived from a PMA, and the extent to which causal inferences can be made, directly depend on the type and quality of the studies included in a PMA. The prospective nature of interventional PMAs make them similar to large multicentre trials, allowing for causal conclusions to be drawn rather than only associations, as sometimes suggested for traditional retrospective meta-analyses. The results of observational PMAs should generally be interpreted as providing associations, not causal effects, as only the results of observational studies are included. But with modern methods for causal inference from observational studies, justification for supporting conclusions about causality can sometimes be found. 33
Currently no PMA specific reporting standards exist, but where applicable, PMA authors should follow the PRISMA-IPD (PRISMA of individual participant data) statement 34 if they are reporting an individual participant data PMA, or the PRISMA statement 35 if they are reporting an aggregate data PMA. As well as the PRISMA items, authors of PMAs need to report on identification of planned and ongoing studies, the PMA timeline, collaboration policies, and outcome harmonisation processes.
Discussions about methodology and interpretation of the results among all collaborators can sometimes be difficult to navigate, particularly if the results from the combination of the studies contradict the results of some of the individual studies. Although these discussions can be demanding and time consuming, robust discussion among experts can lead to well considered and high quality publications that can directly inform policy and practice.
For the successful management of a PMA collaboration, an explicit authorship policy should be in place. One model is to offer authorship to each member of the secretariat, and one investigator from each included study, for the main PMA publication, assuming they fulfil the authorship criteria of the International Committee of Medical Journal Editors (ICMJE). This model incentivises ongoing involvement and allows for multiple viewpoints to be integrated in the final publication. The collaborators usually agree that the final PMA results cannot be published until the results of each study are accepted for publication, but this is not essential.
At least one investigator from each of the participating trials was a co-author on the final publication for NeOProM. 13 Collaborators met regularly, face to face and by phone, to resolve opposing views and achieve consensus on the interpretation of the PMA findings. Face to face meetings were crucial in resolving major disagreements within the NeOProM Collaboration. The collaborators used the PRISMA-IPD checklist for reporting of the PMA.
PMAs have many advantages: they help reduce research waste and bias, while greatly improving use of data, and they are adaptive, efficient, and collaborative. PMAs increase the statistical power to detect effects of treatment and enable harmonised collection of core outcomes, while allowing enough variation to obtain greater generalisability of findings. Compared with a multicentre study, PMAs are more decentralised and allow greater flexibility in terms of funding and timelines. Compared with a retrospective meta-analysis, PMAs enable more data harmonisation and control. Planning a PMA can help a group of researchers prioritise a research question they can address collaboratively and determine the optimal sample size a priori. Disadvantages of PMAs include difficulties in searching for planned and ongoing studies, often long waiting periods for studies to be completed, and difficulties in reaching consensus on the interpretation of the results. Table 1 shows a detailed comparison of the features and advantages and disadvantages of PMAs, multicentre studies, and retrospective meta-analyses.
Advantages and disadvantages of a prospective meta-analysis (PMA) compared with a multicentre study and a retrospective meta-analysis
- View inline
Integration of PMAs with other next generation systematic review methodologies
PMAs can be combined with other new systematic review methodologies. Living systematic reviews begin with a traditional systematic review but have continual updates with a predetermined frequency. Living systematic reviews address similar research questions as PMAs (high priority questions with inconclusive evidence in an active research field). 36 In some instances it might be beneficial to combine these two methodologies. If authors are considering a PMA in a discipline where evidence is expected to become available gradually, a living PMA is an option. In living PMAs, new studies are included as they are being planned (but importantly before any of the results related to the PMA research questions are known), until a definitive effect has been found or the maximum required statistical information has been reached to conclude that no clinically important effect has been found. 37 Appropriate statistical methods for multiple testing should be strongly considered in living PMAs, such as sequential meta-analysis methodology which controls for type 1 and type 2 errors and takes into account heterogeneity. 26 PMA methodology can also be combined with other methods, such as network meta-analysis or meta-analysis of prognostic models.
Future for PMAs
With the advancement of machine learning, artificial intelligence, and big data, new horizons are seen for PMAs. Several steps need to be taken to improve the feasibility and quality of PMAs. Firstly, the ability to identify planned and ongoing studies needs to be improved by introducing further mechanisms to promote and enforce study registration and providing guidance on the best search strategies. The ICMJE requirement for prospective registration of clinical trials, together with several other ethical and regulatory initiatives, has improved registration rates of clinical trials but more improvement is needed. 38 22 Possible solutions include the integration of data submitted to ethics committees, funding bodies, and clinical trial registries. 21 The Cochrane PMA Methods Group, in collaboration with several trial registries, is working on improving methods for identifying planned and ongoing studies. Future technologies might automate the searching and screening process for planned and ongoing studies and automatically connect researchers who are planning similar relevant studies. Furthermore, the reporting and quality of PMAs needs to be improved. The reporting of PMAs would be greatly helped by the development of a standardised set of reporting guidelines to which PMA authors can adhere. Such guidelines are currently under development. Also, the development of PMA specific evidence rating tools (such as an extension to the GRADE approach) would be highly desirable. The Cochrane PMA Methods Group will publicise any new developments in this area on their website ( https://methods.cochrane.org/pma/ ).
PMAs have many advantages, and mandating trial registration, development of core outcome sets, and improved data sharing abilities have increased opportunities for conducting PMAs. We hope this step by step guidance on PMAs will improve the understanding of PMAs in the research community and enable more researchers to conduct successful PMAs. The Cochrane PMA Methods Group can offer advice for researchers planning to undertake PMAs.
Contributors: ALS conceived the idea and facilitated the workshop and discussions. LA, DG, KEH, and ALS participated in the workshop, and JAB and SC contributed to further discussions after the workshop. ALS, SC, and KEH performed the searches for a scoping review that was conducted in preparation for this article, reviewing all prospective meta-analyses and methods papers on prospective meta-analyses in health research to date. LA was the coordinator of the NeOProM Collaboration and KEH was a member. ALS wrote the first draft of the manuscript. All authors contributed to and revised the manuscript. ALS is the guarantor.
Competing interests: We have read and understood the BMJ Group policy on declaration of interests and declare the following: all authors are convenors or members of the Cochrane PMA Methods Group and have been involved in numerous prospective meta-analyses. LA, DG, and JAB have published several methods articles on prospective meta-analyses and are authors of the prospective meta-analysis chapter in the Cochrane Handbook for Systematic Reviews of Interventions . LA manages the Australian New Zealand Clinical Trials Registry (ANZCTR). ALS and KEH work for the ANZCTR. JAB is a full time employee of Johnson & Johnson.
Provenance and peer review: Not commissioned; externally peer reviewed.
- National Health and Medical Research Council
- Ioannidis JP
- Krleza-Jerić K ,
- Berlin JA ,
- Ioannidis J
- Halpern SD ,
- Karlawish JHT ,
- ↵ Ghersi D, Berlin J, Askie L. Prospective meta‐analysis. In: Higgins JPT, Green S (eds), Cochrane Handbook for Systematic Reviews of Interventions Version 5.1.0 (updated March 2011). The Cochrane Collaboration, 2011:559-70.
- Margitić SE ,
- Morgan TM ,
- Probstfield J ,
- Applegate WB
- Darlow BA ,
- Neonatal Oxygenation Prospective Meta-analysis (NeOProM) Collaboration
- Wright KW ,
- Tarnow-Mordi W ,
- Phelps DL ,
- Pulse Oximetry Saturation Trial for Prevention of Retinopathy of Prematurity Planning Study Group
- ↵ Green S, Higgins JPT (eds). Preparing a Cochrane review. In: Cochrane Handbook for Systematic Reviews of Interventions Version 5.1.0 (updated March 2011). The Cochrane Collaboration, 2011.
- Shamseer L ,
- PRISMA-P Group
- Brocklehurst P ,
- Schmidt B ,
- NeOProM Collaborative Group
- ↵ World Health Organization (WHO). WHO International Clinical Trials Registry Platform (ICTRP) Search Portal: http://apps.who.int/trialsearch/ [accessed 6 November 2018].
- Isojarvi J ,
- Lefebvre C ,
- Glanville J
- Hunter KE ,
- Seidler AL ,
- Harriman SL ,
- Williamson PR ,
- Altman DG ,
- Seidler A ,
- Mihrshahi S ,
- Simmonds M ,
- Salanti G ,
- McKenzie J ,
- Elliott J ,
- Living Systematic Review Network
- Higgins JPT ,
- Sterne JAC ,
- Savović J ,
- Sterne JA ,
- Hernán MA ,
- Reeves BC ,
- Stenson B ,
- U.K. BOOST II trial ,
- Australian BOOST II trial ,
- New Zealand BOOST II trial
- Higgins J ,
- Stewart LA ,
- PRISMA-IPD Development Group
- Liberati A ,
- Tetzlaff J ,
- PRISMA Group
- Elliott JH ,
- Guyatt GH ,

An official website of the United States government
The .gov means it’s official. Federal government websites often end in .gov or .mil. Before sharing sensitive information, make sure you’re on a federal government site.
The site is secure. The https:// ensures that you are connecting to the official website and that any information you provide is encrypted and transmitted securely.
- Publications
- Account settings
Preview improvements coming to the PMC website in October 2024. Learn More or Try it out now .
- Advanced Search
- Journal List
- Acta Ortop Bras
- v.30(3); 2022

Language: English | Portuguese
HOW TO PERFORM A META-ANALYSIS: A PRACTICAL STEP-BY-STEP GUIDE USING R SOFTWARE AND RSTUDIO
Como realizar uma metanÁlise: um guia prÁtico passo a passo utilizando o software r e o rstudio, diego ariel de lima.
1 Universidade Federal Rural do Semi-Árido, Faculty of Medicine, Mossoró, RN, Brazil.
Camilo Partezani Helito
2 Universidade de São Paulo, Faculty of Medicine, Hospital das Clínicas, Institute of Orthopedics and Traumatology, São Paulo, SP, Brazil.
Lana Lacerda de Lima
Renata clazzer.
3 Universidade Estadual do Rio Grande do Norte, Faculty of Medicine, Mossoró, RN, Brazil.
Romeu Krause Gonçalves
4 Instituto de Traumatologia e Ortopedia Romeu Krause, Knee studies, Recife, PE, Brazil.
Olavo Pires de Camargo
AUTHORS’ CONTRIBUTIONS: : Each author contributed individually and significantly to the development of this article. DAL: intellectual concept of the article, writing, critical review of the intellectual content and final approval of the version of the manuscript to be published; CPH: substantial contribution to the design of the work and final approval of the version of the manuscript to be published; LLL: writing, critical review of the intellectual content and final approval of the version of the manuscript to be published; RC: writing, critical review of the intellectual content and final approval of the version of the manuscript to be published; RKG: intellectual concept of the article and final approval of the version of the manuscript to be published; OPC: final approval of the version of the manuscript to be published.
Meta-analysis is an adequate statistical technique to combine results from different studies, and its use has been growing in the medical field. Thus, not only knowing how to interpret meta-analysis, but also knowing how to perform one, is fundamental today. Therefore, the objective of this article is to present the basic concepts and serve as a guide for conducting a meta-analysis using R and RStudio software. For this, the reader has access to the basic commands in the R and RStudio software, necessary for conducting a meta-analysis. The advantage of R is that it is a free software. For a better understanding of the commands, two examples were presented in a practical way, in addition to revising some basic concepts of this statistical technique. It is assumed that the data necessary for the meta-analysis has already been collected, that is, the description of methodologies for systematic review is not a discussed subject. Finally, it is worth remembering that there are many other techniques used in meta-analyses that were not addressed in this work. However, with the two examples used, the article already enables the reader to proceed with good and robust meta-analyses. Level of Evidence V, Expert Opinion.
Metanálise é uma técnica estatística adequada para combinar resultados provenientes de diferentes estudos, seu uso vem crescendo e ganhando cada vez mais importância no meio médico. Assim, não apenas saber interpretar metanálise, como também saber realizar uma, mesmo que simples, é fundamental na atualidade. Portanto, o objetivo principal deste artigo é apresentar os conceitos básicos que a norteiam e servir de guia para a condução de uma metanálise utilizando os softwares R e RStudio. Para isso, através do presente artigo o leitor tem acesso aos comandos básicos existentes nos softwares R e RStudio, necessários para a condução de uma metanálise. A grande vantagem do R é o fato de ser um software livre. Para um melhor entendimento dos comandos, dois exemplos foram apresentados de forma prática, além de revisados alguns conceitos básicos dessa técnica estatística. É suposto que os dados necessários para a metanálise já foram coletados, ou seja, descrição de metodologias para revisão sistemática não é assunto discutido. Por fim, vale relembrar que existem muitas outras técnicas utilizadas em metanálises que não foram abordadas neste trabalho. Todavia, com os dois exemplos utilizados, o artigo já habilita o leitor a proceder boas e robustas metanálises. Nível de Evidência V, Opinião do Especialista.
INTRODUCTION
Scientific research has been growing in all areas of knowledge, and in medicine it is no different. The same theme may be researched in several medical centers around the world. With the expansion of evidence-based medicine, the more studies on the same topic, the better the medical practices related to it. 1
However, the existence of many studies on the same subject may limit the access of medical professionals to all of them, either due to the time or fees. Studies that aggregate the results of two or more studies on the same issue, in addition to facilitating and gathering evidence, would reduce the individual errors (biases) of each study, producing a powerful synthesis on a specific topic. The tool to achieve this is meta-analysis. 2
Meta-analysis uses statistical methods to summarize the results of independent studies. By combining information from all relevant studies on the same topic, a meta-analysis can estimate the effects of a given intervention more accurately than each study individually. 3
In 1904, by arguing that studies on the preventive effect of inoculations against enteric fever were too small to allow a reliable conclusion (making the error size too great and the power of the studies too low), Karl Pearson, through correlations, combined the data from five studies, thus creating the first known meta-analysis. 4 But it was only in the 1970s that the term meta-analysis was first used, becoming increasingly popular since then. 5
Therefore, the main objective of this article is to present the basic concepts that guide a meta-analysis and to serve as a guide for conducting a meta-analysis using the R and RStudio software.
The data of a meta-analysis
For studies to be combined through a meta-analysis, it is necessary to define which results will be combined. We shall work with 2 examples:
As example 1, two surgical techniques seek to improve knee stability, technique A (experimental) and technique B (control). Let us say that there is a test to ascertain the stability of the knee (X test) and that if the test is positive, it means that the knee is unstable, similar to the pivot-shift test. 6 Assuming that three authors decided to compare the two techniques (A and B), using the stability test X before and after surgery in both techniques ( Table 1 ).
Author | PREOP - Number of patients subjected to technique A with positive X test | POSTOP - Number of patients subjected to technique A with positive X test | PREOP - Number of patients subjected to technique B with positive X test | POSTOP - Number of patients subjected to technique B with positive X test |
---|---|---|---|---|
1 | 18 | 8 | 21 | 18 |
2 | 30 | 10 | 60 | 31 |
3 | 42 | 12 | 45 | 20 |
In this example 1, we will work with discrete quantitative variables, which assume only values belonging to an enumerable set, which can assume only a countable finite or infinite number of values. Discrete variables are usually the result of counts. Examples: number of children, number of bacteria per milliliter of urine and number of cigarettes smoked per day. 7
As example 2, we will work with continuous quantitative variables, which assume any value in a certain range of variation, for which fractional values make sense. They should usually be measured by means of some instrument. Examples: weight (scale), height (ruler), time (clock), blood pressure and age. Continuous variables are usually expressed in the form of an average of values followed by a measure of dispersion, typically the standard deviation. 7
In example 2, we consider that there is a functional score w, such as the IKDC ,( 8 in which the higher the score, the better the result and which would serve to evaluate the post-operative clinical outcome of a given surgical technique. Assuming that three authors decided to compare two techniques, A (experimental) and B (control), using the functional score W in the postoperative period of the two techniques ( Table 2 ).
Author | Technique A - number of participants | Technique A - post op w score (Mean) | Technique A - w score (Standard deviation) | Technique B - number of participants | Technique B - post op w score (Mean) | Technique B - w score (Standard deviation) |
---|---|---|---|---|---|---|
1 | 18 | 96.30 | 1.80 | 30 | 90.30 | 3.73 |
2 | 30 | 86.90 | 9.30 | 60 | 84.30 | 9.80 |
3 | 42 | 79.20 | 18.80 | 45 | 76.70 | 17.20 |
The basics of a meta-analysis
In a meta-analysis, the results of two or more independent studies are combined. The results of medical studies can be demonstrated in numerous ways. The two most common are the results expressed by measure of association and the results expressed by mean difference.
Measures of association were developed with the objective of evaluating the relationship between a risk factor and its outcome. Among these measures we highlight the Relative Risk (RR) and the odds ratio (OR). 7 RR and OR estimate the magnitude of the association between exposure to the risk factor and the outcome, indicating how many times the occurrence of the outcome in the exposed is greater than that among the unexposed.
For example, the result of a hypothetical study showed that smokers (exposed to the risk factor: cigarette) have a 5 times greater chance (RR), that is, 400% more, of progressing to lung cancer than non-smokers (unexposed).
When there is no difference between the exposed and unexposed, we say that the RR is equal to 1. When exposure to a factor increases the chances of an event occurring, as in the example above of smokers, the RR is greater than 1. When exposure to a factor decreases the chances of an event occurring, the RR is less than 1 (however, it is not negative, that is, it varies from 0 to < 1). 9 Simply put, if we have a RR > 1, the RR expresses how many times the exposure can lead to the outcome. In the smokers’ example above, the RR is equal to 5. When the RR is less than 1, the relative risk reduction (RRR), also known as efficacy, can also be calculated using the following formula: RRR or Efficacy = ( 1 − RR ) × 100 . If in a study the RR of 0.27 is found as a result, we can say that in this study the exposure to a factor decreased 73% the risk of an event occurring ( 1 − 0 . 27 ) × 100 = 73 % . 9
Another way to express the results of a survey is through the mean difference (MD). In some studies, the outcome is measured through score scales such as IKDC. 8 These scales produce numerical scores for each patient, rather than dichotomous “yes/no” results. As we have seen above, this type of variable is called continuous, and it is common to calculate its mean in the two groups to be compared. In our example 2, to evaluate the best result technique (highest w score), A or B, it is necessary to compare the means of the w scores of the two groups throughout the study. One of the problems of this type of outcome measured by continuous variable is that, although it is possible to affirm that patients who used the A technique had a higher score in the w score, it is difficult to extract a clinical meaning from this difference. It is easier to understand a 25% increase in the return to sport using technique A than a difference of 6 points on a functional scale/score. When there is no difference between the averages of the groups, we say that the MD is equal to 0.
After obtaining the results of the studies chosen to compose the meta-analysis, the measures are aggregated based on the weighting of the results of all individual studies. This weighting is given by the sample size (number of patients) of each study, culminating in the measure of general association: the result of our meta-analysis. 7 ),( 10 It is worth remembering that in a meta-analysis, only equal association measures should be compared: RR with RR or OR with OR. It is not possible to compare RR of one study with MD of another study. 7 ),( 10
Confidence interval and p-value
When performing a clinical study, it is unlikely that the actual magnitude is exactly that found in the study. This happens due to the natural occurrence of random variations inherent to the researcher and/or the research situation. That is, the relative risk value found may be, and typically is, greater or lesser than the true value. For this reason, it is essential to measure the statistical accuracy of the data, which will allow the reader to perceive the confidence of the data presented. 7
The confidence interval is a range of possible values for the actual magnitude of the effect. In clinical biomedical studies, the minimum accepted confidence interval is 95%, typically expressed as 95% CI. That is, a study with 95% CI means that if we take a random sample and build 100 confidence intervals, 95 would contain the real parameter. 11 In terms of accuracy, the narrower the confidence interval, the greater the accuracy of the results. Among the factors that can increase the accuracy of the confidence interval, the sample size is inserted, that is, the larger the sample, the greater the accuracy. 12
The confidence intervals present information similar to those derived from the p-value (statistical significance). If the relative risk value 1 (equal effects of the intervention and control group) is present between the lower and upper limit of the confidence interval, then the p-value will be greater than or equal to 0.05 (statistically non-significant difference). However, if the relative risk value 1 is not within the confidence interval interpolated by the lower and upper limits, then the p-value will be less than 0.05 (statistically significant difference).
Fixed-effects models and random-effects models
In meta-analysis there are basically two types of models that can be adopted: the fixed effects model and the random effects model. 2 The fixed-effect model assumes that the effect of interest is the same in all studies and that the differences observed between them are due only to sampling errors, the so-called variability within the studies. In a simplified way, it is as if the methods with fixed effects considered that the variability between the studies occur only by chance and ignored the heterogeneity between them. 3
Random effect models assume that the effect of interest is not the same in all studies. In this sense, they consider that the studies that are part of the meta-analysis form a random sample of a hypothetical population of studies. However, although the effects of the studies are not considered equal, they are connected through a probability distribution, usually supposed to be normal. For this reason, they create combined results with a greater confidence interval (but less precision), and thus are the most recommended models. Despite having this advantage, methods with random effects are criticized for attributing greater weight to smaller studies. 3
There is no formal rule for choosing the model. Generally, when there is no important diversity or heterogeneity, studies with greater statistical power (greater population and greater intervention effect) have more “weight.” In this case, the fixed-effects model is used, which assumes that all studies showed the same effect: for example, when the objective is to estimate a treatment effect for a specific population, not extrapolating this effect to other populations. 13 When there is diversity and heterogeneity among the studies, it is more recommended to use the random effects model, which distributes weight in a more uniform way, valuing the contribution of small studies. For example, when the researcher combines several studies that have the same objective, but that were not conducted in the same way. In this case, it is possible to extrapolate the effects to other populations, which makes for a more comprehensive analysis. 13
Heterogeneity
In a meta-analysis, usually preceded by a systematic review, however similar the selected studies may seem, they are not considered identical as to the effect of interest. For example, in a meta-analysis of studies in which the efficacy of a new surgical procedure is being tested, there may be a difference in the selected groups: one group may be healthier in one study than in another, the age group of patients may vary from study to study, among other factors that may influence the effect of treatment.
When this difference between groups happens, that is, when the variability between the studies is not just random, we say that the studies are heterogeneous. In the presence of heterogeneity, other meta-analysis techniques (such as subgroups and meta-regression) can be considered to explain the variability between groups. However, these types of analysis require a large number of studies. When it is not possible to count on so many studies, the random effects model is recommended, as seen in the topics above. 14
Thus, it is clear that in choosing between the fixed effects model and the random effects model, the evaluation of heterogeneity plays an important role in this choice. The most used ways to verify the existence of heterogeneity in meta-analyses are by Cochran’s Q test and Higgins and Thompson’s I² statistic. 3
Cochran’s Q test
Cochran’s Q test presents as null hypothesis the assertion that the studies that make up the meta-analysis are homogeneous, that is, the higher the Q value, the more heterogeneity. Thus, a problem is that the value of Q varies between 0 and infinity. A deficiency of this test is having a low power when the number of studies that make up the meta-analysis is small. On the other hand, when the number of studies is very large, it leads to false heterogeneities. In this test, a p-value is also calculated, which indicates whether or not heterogeneity is significantly different from zero. 10
The I² Statistic
The I² statistic, proposed by Higgins and Thompson, is obtained from the Q statistic of the Cochran test and the number of studies. The I² statistic can vary from negative values to 100%. When the value is negative it is equal to 0. The p-value of I² is equivalent to the p-value of Q 2 .
Higgins et al. suggest a scale in which an I² value closer to 0% indicates non-heterogeneity among studies, while those closer to 25% indicates low heterogeneity, those closer to 50% indicates moderate heterogeneity and those closer to 75% indicates high heterogeneity among studies. 2
Forest plot
The forest plot is a graphical and friendly way to demonstrate the results of a meta-analysis. It has two axes: the X and the Y ( Figure 1 ). The Y-axis (vertical line), or central trend axis, is a line that indicates that at that point there is no difference between the interventions under study, that is, Relative Risk equal to 1 or Mean Difference equal to 0.

The X-axis (horizontal line) is where the numerical dispersion of the meta-analysis results occurs. The X axis is cut in half by the Y axis and, as stated above, at this point (RR = 1 or MD = 0) there is no difference between interventions. What is to the right of this point favors an intervention and what is to the left favors another intervention. The further away from the Y-axis, the greater the effect/ strength of this intervention ( Figure 2 ).

Each individual study that makes up the meta-analysis is represented by three structures: a solid geometric shape (typically a square), a horizontal line, and a small vertical line in the center of the square ( Figure 3 ).

The vertical line corresponds to the individual result of each study. If it is to the left of the Y axis, the result indicates a tendency of an intervention; if it is to the right of Y, it indicates a tendency for the other intervention; if it is in the center of Y, it indicates no difference between the two interventions under study ( Figure 3 ).
The geometric shape (square) has its area as an estimate of the size of the individual effect of the study. That is, the larger the square, the greater the relative weight of the study in the meta-analysis ( Figure 3 ).
The horizontal line corresponds to the individual confidence interval of each study. If the entire line is to the left of the Y-axis, the result indicates that there is a statistically significant trend of an intervention (p < 0.05); if the entire line is to the right of Y, it indicates that there is a statistically significant trend for the other intervention (p < 0.05); if the line crosses or even “touches” the Y axis, it indicates that there is no statistically significant difference between the two interventions under study (p > 0.05) ( Figure 3 ).
The diamond (rhombus), which appears below the studies, synthesizes the combined effect of all the studies that make up the meta-analysis. That is, the Diamond is the meta-analysis “in itself.” The center of the Diamond corresponds to the result of the meta-analysis, and its location (to the left or right of the Y-axis) defines which intervention has the most “advantage.” The Diamond width corresponds to the confidence interval of the meta-analysis. If any part of the Diamond of the meta-analysis crosses or even “touches” the Y-axis, it indicates that there is no statistically significant difference between the two interventions under study (p > 0.05) ( Figure 3 ).
Meta-analysis in R
R is a free programmable statistical software, with a focus on data analysis. It consists of a platform on which the so-called “packages” (similar to applications) can be installed to perform certain functions. There are thousands of packages with different functions implemented, not to mention the user collaborations that the software receives. This guide will use the metapackage (“application”), which is sufficient for a good and simple meta-analysis.
Installing the R
The first step is to access the page www.r-project.org and in the left menu, under download, choose the alternative “CRAN.” Now choose any of the CRAN mirrors , preferably one from Brazil (ex: http://cran.fiocruz.br/). This will redirect to one of the software’s download pages. In “Download and Install R,” choose the desired platform (Linux, Mac, Windows), download the installer (Latest release) and run it.
R is not software with a user-friendly interface. Some basic operations can be laborious. Thus, our second step is to install another software: RStudio. RStudio provides a good interface for importing and viewing files, installing packages, and exporting charts. In a simplistic analogous way, it is as if the R software is a kind of “Command Prompt” and RStudio is a kind of “Windows system.” To download R Studio, go to the following page: http://www.rstudio. com/products/rstudio/download/ and under “Installers for ALL Platforms” choose the most appropriate platform (Windows, Mac or Linux) and run the installation. RStudio is not required to be installed, but as stated above it greatly optimizes time during a meta-analysis. There are free and paid versions, and the free version is enough for the basics we are proposing.
As stated above, the package we will use in our meta-analysis is the “ meta .” To install meta ( Figure 4 ), open RStudio (remember to install R before), (A) click Packages; (B) click Install; (C) The box for installation will open and then type the name meta . Click install and after installing, make sure that the meta package is enabled, that is, with the “check” in the box next to its name. Installing the package is only necessary once, but whenever you restart RStudio, you must enable the package by checking this option in the box ( Figure 5 ).

Building a database of example 1
The simplest way to create a database for analysis in R is to create a table in Microsoft Excel, Numbers (macOS), or another spreadsheet editor.
In example 1, knee stability is assessed with the Pre- and Post-operative X-test of 2 surgical techniques, A and B.
Thus, the database of example 1 will consist of a table with five columns, necessarily in this sequence ( Figure 4 ):
Column 1: name of the studies: in this case, 3 studies;
Column 2: number of events in the experimental/treatment group (evtto - Number of patients subjected to technique A with a positive X test POSTOP): in this case, 8, 10 and 12 patients, respectively in the 3 studies;
Column 3: total sample of the experimental/treatment group (ntto - Number of patients subjected to technique A with a positive X test PREOP): in this case, 18, 30 and 42 patients, respectively in the 3 studies;
Column 4: number of events in the control group (evcont - Number of patients subjected to technique B with a positive X test POSTOP): in this case, 18, 31 and 20 patients, respectively in the 3 studies;
Column 5: total sample of the control group (ncont - Number of patients subjected to technique B with a positive X test PREOP): in this case, 21, 60 and 45 patients, respectively in the 3 studies. The first line defines the name of the five variables (study, evtto, ntto, evcont and ncont). The name is indifferent; however, special characters (such as diacritics or cedillas) should not be used and, if possible, everything should be lowercase ( Figure 6 ).

When saving the database, it must be saved in the format “CSV” (variables separated by a comma). For example 1 we will name the file “testex.csv” ( Figure 7 ).

We then have the database of example 1 ready to be imported by RStudio. Now we will open RStudio and in the menu we will go to File, Import dataset, From Text (base)… Select the testex.csv file. Make sure the parameters are the same as in Figure 8 and click the import button. The Name field is equivalent to the name of the variable that will be assigned within the R with the database data, in this case, “testex.” Leave the Heading option checked as Yes so that the first row of the worksheet matches the name of the database columns.

Now the R imported the database within the variable “testex.” Type testex in the RStudio console and hit “enter/return” to see the assigned value inside this variable ( Figure 9 ). Now we have our example 1 database imported into RStudio, ready for analysis.

Meta-analyzing Example 1 - Test X
Once the database is imported, we will proceed with the meta-analysis itself. We will use the meta package to run these analyses (remember to enable it, with the “check” in the box next to the name).
To perform the meta-analysis of example 1, which uses discrete quantitative variables and categorical outcome (instability improves or not with the procedure) we will use the “ metabin ” command. We will create a variable for the metabin command of our example 1 meta-analysis, the testex. We will call it “metanalisetestex.” Thus, the command line will be:
metanalisetestex = metabin (evtto, ntto, evcont, ncont, study, data = testex)
Type the line above and hit “enter/return.” Remember that the names testex (database created from example 1) and metanalisetestex (variable created for the metabin command) are chosen by the author of the review, and can be any name; however, they are easy to remember and do not contain special characters.
Apparently, nothing happened, but RStudio saved the meta-analysis result within the metanalisetestex variable. By typing metanalise-testex into the console and enter/return, the software will show us the results ( Figure 10 ).

As such, we have the results of the meta-analysis. Didactically, we can divide the results into four parts ( Figure 11 ).

In the first part ( Figure 11 ), we have each of the individual studies, with their relative risk (RR), confidence interval (95%CI) and weight (%W) in the analyses by both the fixed effects model and the random effects model. In our example, three studies were combined (k = 3). In the second part ( Figure 11 ), we have the summary measure of the meta-analysis, that is, the “result itself.” This part shows the relative risk (RR), the confidence interval (95% -CI) and the z-value (statistical test of the significance of the global effect, that is, a mathematical measure equivalent to the location and width of the diamond in the forest plot) for the fixed and random effects model, with their respective p-values (remembering that this p-value is what describes whether or not the study was statistically significant, with p < 0.05).
In the third part ( Figure 11 ), we have measures of heterogeneity of the meta-analysis. The tau-squared (tau^2) and tau reflect the variability between studies in the meta-analysis of random effects, that is, the closer to zero the lower the variability between studies (this estimate is always calculated when the random effects model is used and its value does not have much interpretation applied). The I² statistic (I^2), followed by its standard deviation, as already mentioned, is an excellent indicator of heterogeneity. Similar to the I² statistic, the h (H) statistic and its standard deviation measure the heterogeneity of the studies, and when H is close to 1 we have evidence of homogeneity between the studies. Finally, in the third part, the value of the Q test (already mentioned above) is presented with its p-value (not to be confused with the p-value of the second part) and the degrees of freedom (d.f.), which is the number of studies minus 1 (k-1), which helps in the calculation of the I 2 statistic. Finally, in the fourth part ( Figure 11 ), it is detailed which tests were used in the meta-analysis in question.
To create the forest plot of the meta-analysis, the forest command is used. By typing forest (meta-analysis name), RStudio will create a forest plot of the meta-analysis. In this case type in the console: forest (metanalisetestex)
If you want to omit the result/diamond of the fixed model from the forest plot ( Figure 12 ), set the comb.fixed argument to false by typing the following command line in the console:

forest (metanalisetestex, comb.fixed = FALSE)
The conclusion of the meta-analysis data from example 1 is that the risk is lower than the occurrence of persistence of instability (positive x test) in the Experimental group (technique A), RR = 0.5965 (“rounded” to 0.60 in the forest plot) ( Figure 13 ). We can say that the use of technique A reduced the incidence of instability measured by the x test in the postoperative period by close to 40% (1-RR), compared to technique B [Relative Risk (RR) of 0.5965; confidence interval at the 95% level (95% CI) between 0.4313 and 0.8250; and p-value of 0.0018 (in the random effect model). The I 2 statistic indicates non-heterogeneity between studies (I 2 = 0.0%, with a heterogeneity test p-value of 0.8170).

Basic forest plot editing
As we have seen, to create a forest plot in RStudio just type in the forest command line and between the parentheses put the name of the variable that we assign to our meta-analysis, in the case metanalisetestex in example 1. RStudio provides numerous ways to edit the forest plot. It is only necessary that, inside the parentheses, after the name of the variable that we attribute to our meta-analysis, a “comma” (,) is placed and the argument corresponding to what we want to edit in the forest plot. We emphasize that numerous edits can be made to the same forest plot, just follow the sequence “comma” (,) and the argument. For example, if we want the forest plot of example 1 (testex) to omit the diamond of the fixed-effect model result and the diamond of the random-effect model to be blue in color, my command will be:
forest(metanalisetestex, comb.fixed = FALSE, col.diamond = “blue”) In Table 3 , there are some useful commands to edit the forest plot (commands are in English):
Command | Function |
---|---|
Displays the p-value (which determines the statistical significance of the study) and the Z-value (“diamond width calculation”) in the fixed and random models | |
Omit in the chart the result/diamond of the fixed model | |
Omit the result/diamond from the random model in the graph | |
Changes the color of the diamond (defaults to gray). Place the desired color in English between the “quotation marks.” In the example it is blue. | |
Rename the experimental groups of the studies (the default is Experimental). Place the desired name in quotation marks. In the example it is Medication A. | |
Change the name given to the control groups of the studies (the default is Control). Place the desired name in quotation marks. In the example it is Medication B. | |
Places a text below the X-axis (horizontal). | |
Place the desired name in quotation marks. | |
In the example it is Favors A - Favors B |
In example 2, three authors compared two surgical techniques, A (experimental) and B (control), using the functional w score in the postoperative period in both techniques, in which the higher the score, the better the result.
Column 2: total sample of the experimental/treatment group (ne - Number of patients subjected to technique A): in this case, 18, 30 and 42 patients, respectively in the 3 studies;
Column 3: continuous quantitative variable of the event in the experimental/treatment group (me - Mean of the w score in the POSTOP period of patients subjected to technique A): in this case, 96.30; 86.90 and 79.20, respectively in the 3 studies;
Column 4: standard deviation of the continuous quantitative variable of the event in the experimental/treatment group (SDE - Standard deviation of the w score in the POSTOP period of patients subjected to technique A): in this case, ± 1.80; ± 9.30 and ± 18.80, respectively in the 3 studies;
Column 5: total sample of the control group (nc - Number of patients subjected to technique B): in this case, 30, 60 and 45 patients, respectively in the 3 studies;
Column 6: continuous quantitative variable of the event in the control group (mc - Mean w score in the POSTOP of patients subjected to technique B): in this case, 90.30; 84.30 and 76.70, respectively in the 3 studies;
Column 7: standard deviation of the continuous quantitative variable of the event in the control group (sdc - Standard deviation of the w score in the POSTOP of patients subjected to technique B): in this case, ± 3.73; ± 9.80 and ± 17.20, respectively in the 3 studies; The first line defines the name of the seven variables (study, ne, me, sde, nc, mc and sdc). The name is indifferent; however, special characters (such as diacritics or cedillas) should not be used and, if possible, everything should be lowercase ( Figure 14 ).

When saving the database, it must be saved in the “CSV” format (as seen above). For example 2 we will name the file “scorew. csv.” Now we will open RStudio and in the menu we will go to File, Import dataset, From Text (base)… Select the scorew.csv file. Make sure the parameters are the same as in Figure 8 and click the import button. The Name field is equivalent to the name of the variable that will be assigned within the R with the database data, in this case, “scorew.” Leave the Heading option checked as Yes so that the first row of the worksheet matches the name of the database columns.
Type scorew in the RStudio console and hit “enter/return” to see the assigned value inside this variable ( Figure 16 ). Now we have our example 2 database imported into RStudio, ready for analysis.

Meta-analyzing Example 2 - w score
To perform the meta-analysis of example 2, we will use the “ metacont ” command of the meta package (remember to enable it, with the “check” in the box next to the name).
We will create a variable for the metacont command of our meta-analysis of example 2, the scorew. We will call it “metanalisescorew.” Thus, the command line will be: metanalisescorew = metacont (ne, me, sde, nc, mc, sdc, study, data = scorew)
Type the line above and hit enter/return and RStudio will save the result of the meta-analysis inside the metanalisescorew variable. By typing metanalisescorew into the console and enter/return, the software will show us the results ( Figure 17 ).

Thus we have the results of the meta-analysis of example 2, the w score. As we saw with example 1, we can divide the results of example 2 into four parts: 1. Studies that make up the meta-analysis; 2. Summary measure (the “result” itself) of the meta-analysis; 3. Measures of heterogeneity of the meta-analysis; and 4. Tests used in the meta-analysis. However, in example 2, because continuous quantitative variables are used, the result is not expressed as relative risk (as in example 1) but as mean difference (MD). That is, author 1 demonstrated a mean of 6 more “points” in the w score when using the A technique in relation to B; author 2 demonstrated a mean of 2.6 more “points” in the w score when using the A technique in relation to B; and author 3 demonstrated a mean of 2.5 more “points” in the w score when using the A technique in relation to B. By typing forest (meta-analysis name), RStudio will create a forest plot of the meta-analysis. In this case type in the console:
forest (metanalisescorew)
If you want to omit the fixed model result from the graph, set the comb.fixed argument to false by typing the following command line in the console:
forest (metanalisescorew, comb.fixed = FALSE)
The conclusion of the meta-analysis data from example 2 is that the experimental group (subjected to technique A) presented on average 4.8266 more “points” in the w score (MD, random effect model) in relation to the control group (subjected to technique B), MD = 4.8266 (“rounded” to 4.83 in the forest plot) ( Figure 18 ). It is worth highlighting that in this example, what is to the right of the Y-axis is advantageous for technique A. We can say that the use of technique A has a better clinical result, measured by the w score in the postoperative period, compared to technique B [Mean Difference (MD) of 4.8266; confidence interval at the 95% level (95% CI) between 2.3891 and 7.2640; and p-value of 0.0001 (in the random effect model)]. The I 2 statistic indicates non-heterogeneity between studies (I 2 = 0.0%, with a heterogeneity test p-value of 0.8170).

CONCLUSIONS
Through this article, the reader has access to the basic commands existing in the R and RStudio software, necessary for conducting a meta-analysis. The great advantage of R is the fact that it is a free software. For a better understanding of the commands, two examples were presented in a practical way, in addition to reviewing some basic concepts of this statistical technique. It is assumed that the data necessary for meta-analysis have already been collected, that is, description of methodologies for systematic review is not the discussed subject. Finally, it is worth remembering that there are many other techniques used in meta-analysis that were not addressed in this work. However, with the two examples used, the article already enables the reader to perform good and robust meta-analyses.
2 The study was conducted at Faculty of Medicine, Universidade Federal Rural do Semi-Árido (UFERSA).
REFERÊNCIAS
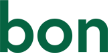
- English Software
- Spanish Software
- Scientific Creditability
- Download ORS/SRS
Measurement Based Care Improving Lives Now
The most user-friendly system that ensures quality care and meets accreditation standards.
BON Is the Ultimate Solution for:
Measurement based care, routine outcome monitoring, feedback informed treatment, and systematic client feedback
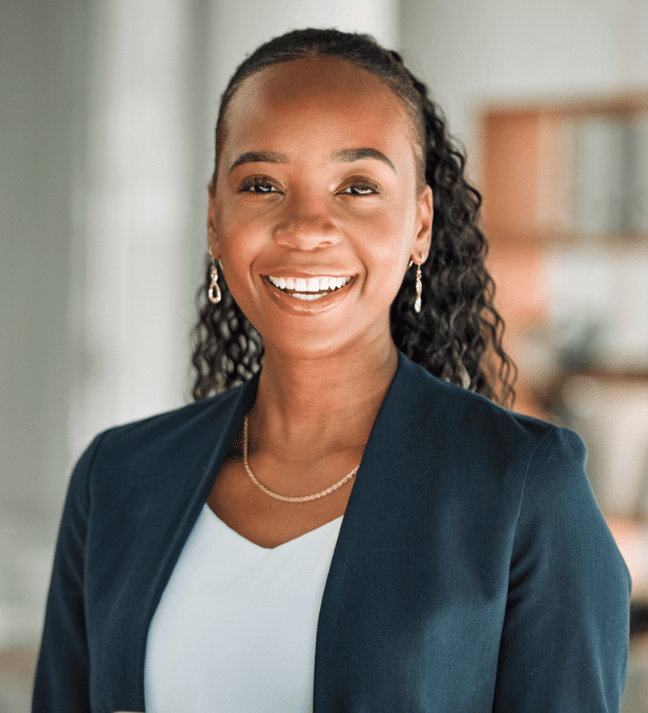
Others cite the research, the BON team conducted it
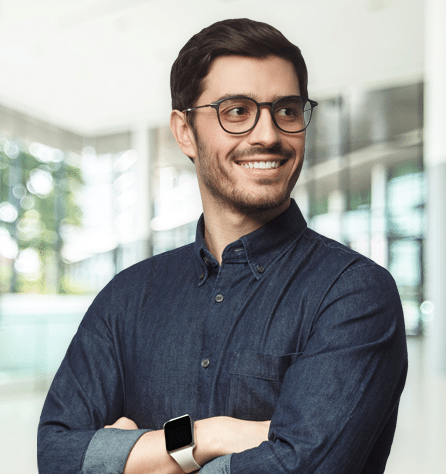
Our Track Record
We developed the MBC clinical process, scientifically demonstrated that it improves outcomes, and have over 25 years experience of successful implementations.
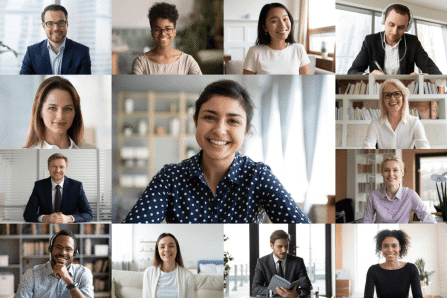
BON Promotes Cultural Responsiveness
Provides a structure to address marginalization and client-therapist differences.
BON Engages Clients
Collaborative monitoring of benefit and relationship ensures a strong alliance.
BON Empowers Clients
Strength-based, privileges client voice, and involves consumers in all decisions affecting care.
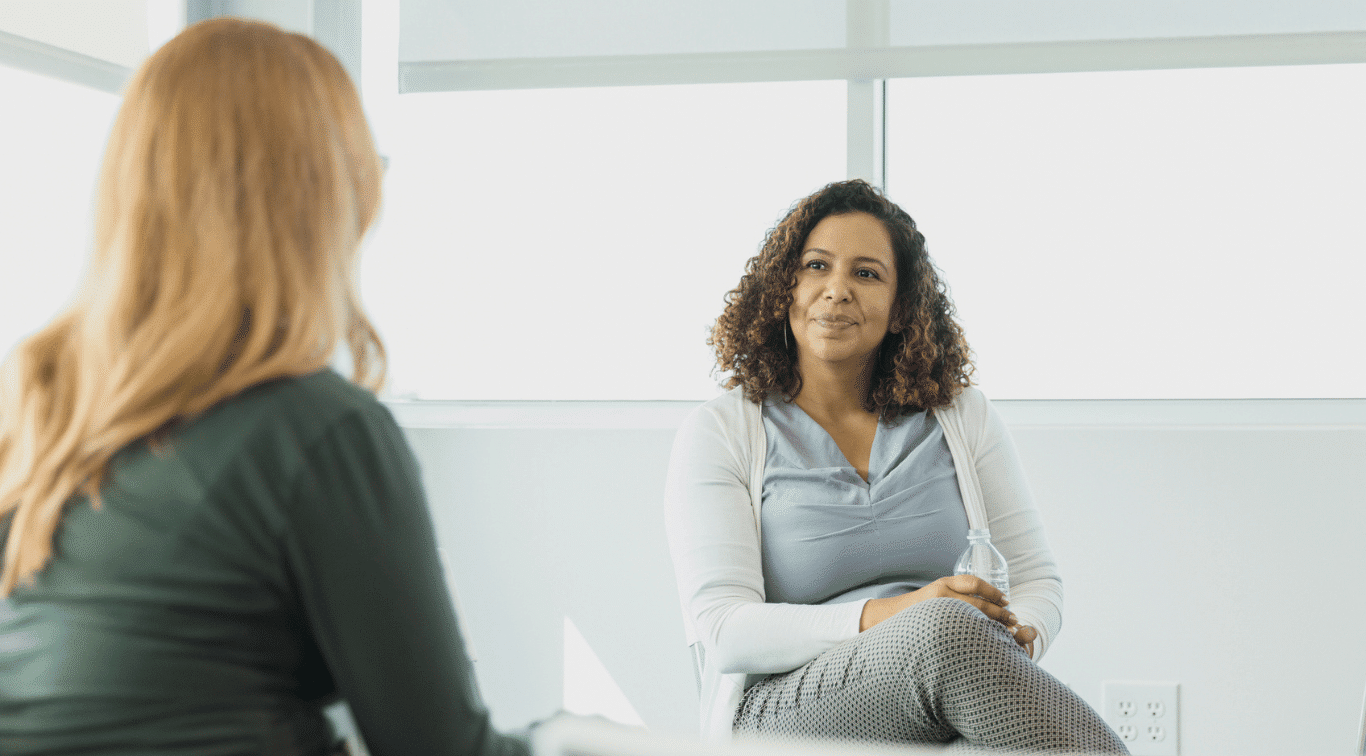
No Guesswork or Confusion about which Measures to Use
Ultra brief, research tested, broadly applicable measures make BON the most feasible system available.
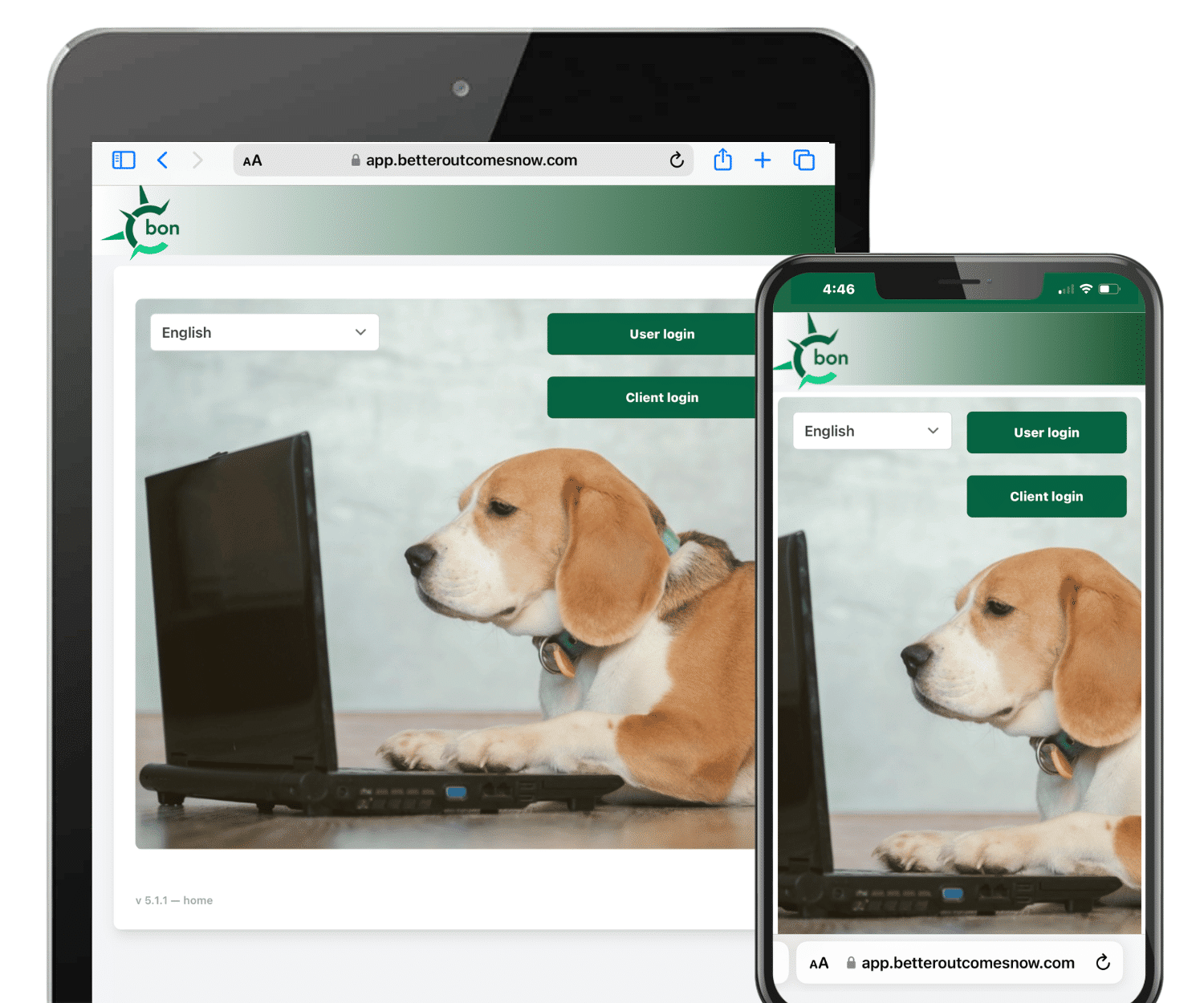
Recent Blogs
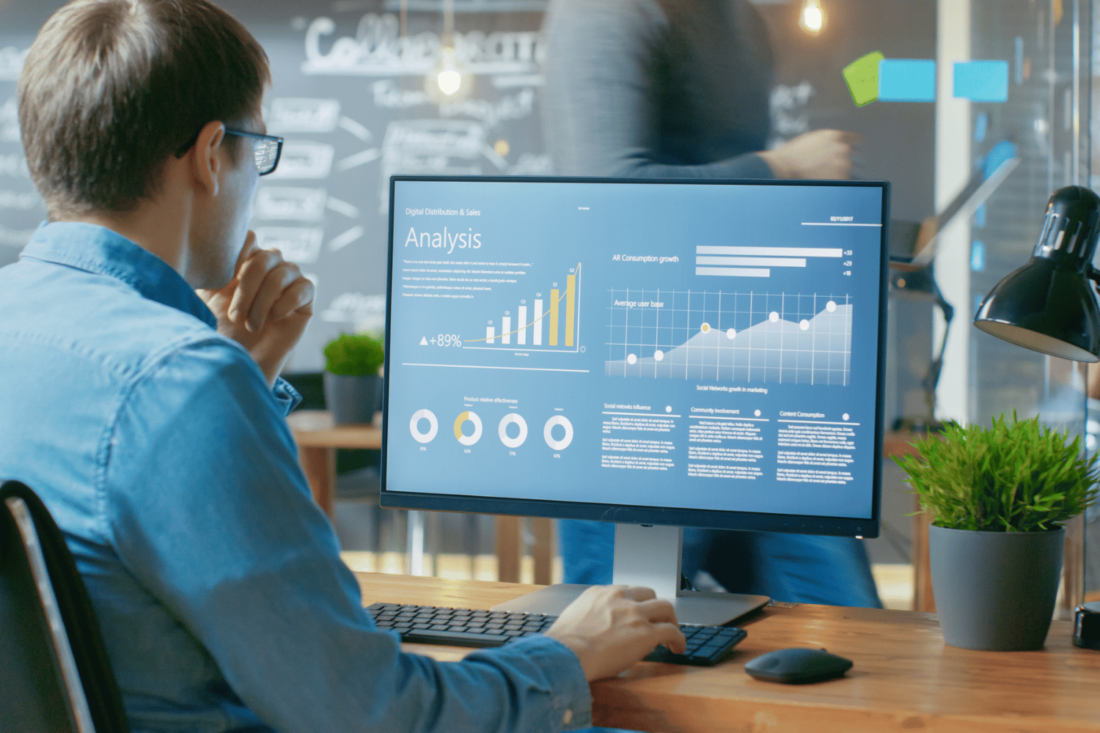
- En español – ExME
- Em português – EME
Systematic reviews vs meta-analysis: what’s the difference?
Posted on 24th July 2023 by Verónica Tanco Tellechea
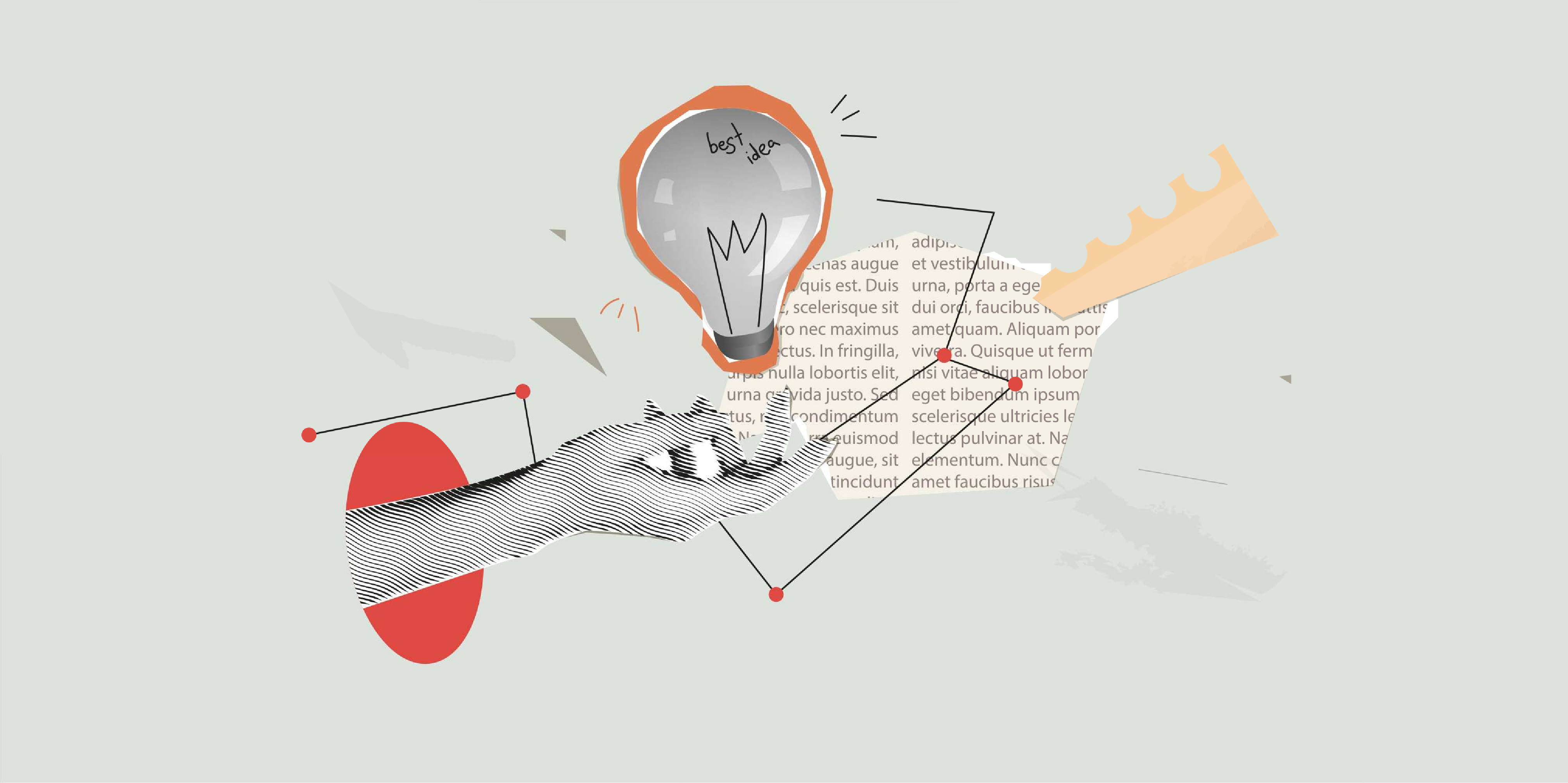
You may hear the terms ‘systematic review’ and ‘meta-analysis being used interchangeably’. Although they are related, they are distinctly different. Learn more in this blog for beginners.
What is a systematic review?
According to Cochrane (1), a systematic review attempts to identify, appraise and synthesize all the empirical evidence to answer a specific research question. Thus, a systematic review is where you might find the most relevant, adequate, and current information regarding a specific topic. In the levels of evidence pyramid , systematic reviews are only surpassed by meta-analyses.
To conduct a systematic review, you will need, among other things:
- A specific research question, usually in the form of a PICO question.
- Pre-specified eligibility criteria, to decide which articles will be included or discarded from the review.
- To follow a systematic method that will minimize bias.
You can find protocols that will guide you from both Cochrane and the Equator Network , among other places, and if you are a beginner to the topic then have a read of an overview about systematic reviews.
What is a meta-analysis?
A meta-analysis is a quantitative, epidemiological study design used to systematically assess the results of previous research (2) . Usually, they are based on randomized controlled trials, though not always. This means that a meta-analysis is a mathematical tool that allows researchers to mathematically combine outcomes from multiple studies.
When can a meta-analysis be implemented?
There is always the possibility of conducting a meta-analysis, yet, for it to throw the best possible results it should be performed when the studies included in the systematic review are of good quality, similar designs, and have similar outcome measures.
Why are meta-analyses important?
Outcomes from a meta-analysis may provide more precise information regarding the estimate of the effect of what is being studied because it merges outcomes from multiple studies. In a meta-analysis, data from various trials are combined and generate an average result (1), which is portrayed in a forest plot diagram. Moreover, meta-analysis also include a funnel plot diagram to visually detect publication bias.
Conclusions
A systematic review is an article that synthesizes available evidence on a certain topic utilizing a specific research question, pre-specified eligibility criteria for including articles, and a systematic method for its production. Whereas a meta-analysis is a quantitative, epidemiological study design used to assess the results of articles included in a systematic-review.
DEFINITION | Synthesis of empirical evidence regarding a specific research question | Statistical tool used with quantitative outcomes of various studies regarding a specific topic |
RESULTS | Synthesizes relevant and current information regarding a specific research question (qualitative). | Merges multiple outcomes from different researches and provides an average result (quantitative). |
Remember: All meta-analyses involve a systematic review, but not all systematic reviews involve a meta-analysis.
If you would like some further reading on this topic, we suggest the following:
The systematic review – a S4BE blog article
Meta-analysis: what, why, and how – a S4BE blog article
The difference between a systematic review and a meta-analysis – a blog article via Covidence
Systematic review vs meta-analysis: what’s the difference? A 5-minute video from Research Masterminds:
- About Cochrane reviews [Internet]. Cochranelibrary.com. [cited 2023 Apr 30]. Available from: https://www.cochranelibrary.com/about/about-cochrane-reviews
- Haidich AB. Meta-analysis in medical research. Hippokratia. 2010;14(Suppl 1):29–37.
Verónica Tanco Tellechea
Leave a reply cancel reply.
Your email address will not be published. Required fields are marked *
Save my name, email, and website in this browser for the next time I comment.
Subscribe to our newsletter
You will receive our monthly newsletter and free access to Trip Premium.
Related Articles
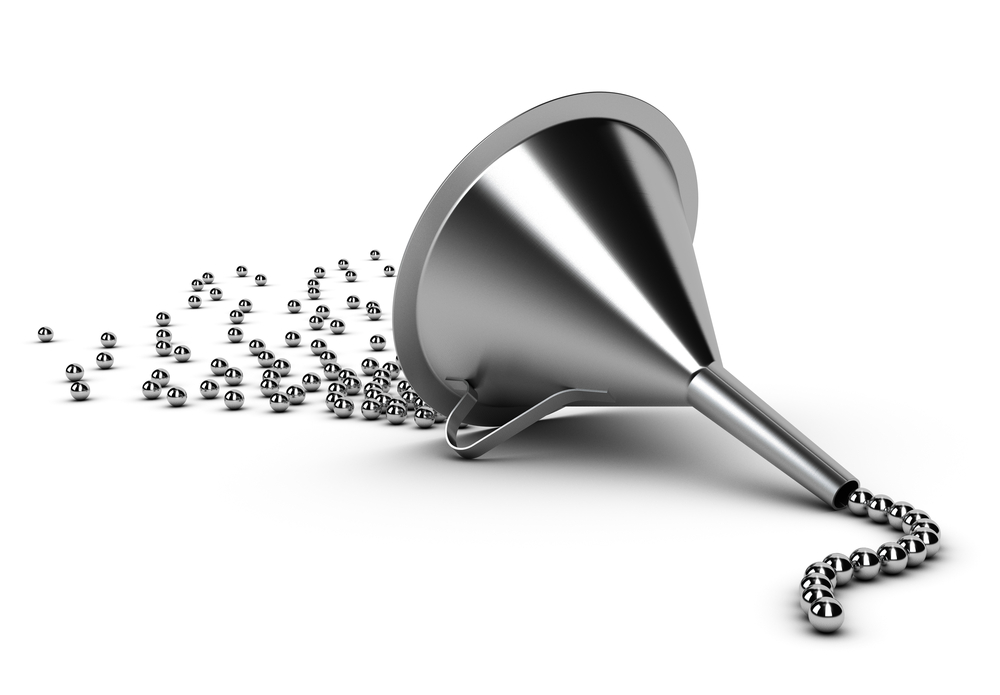
How to read a funnel plot
This blog introduces you to funnel plots, guiding you through how to read them and what may cause them to look asymmetrical.
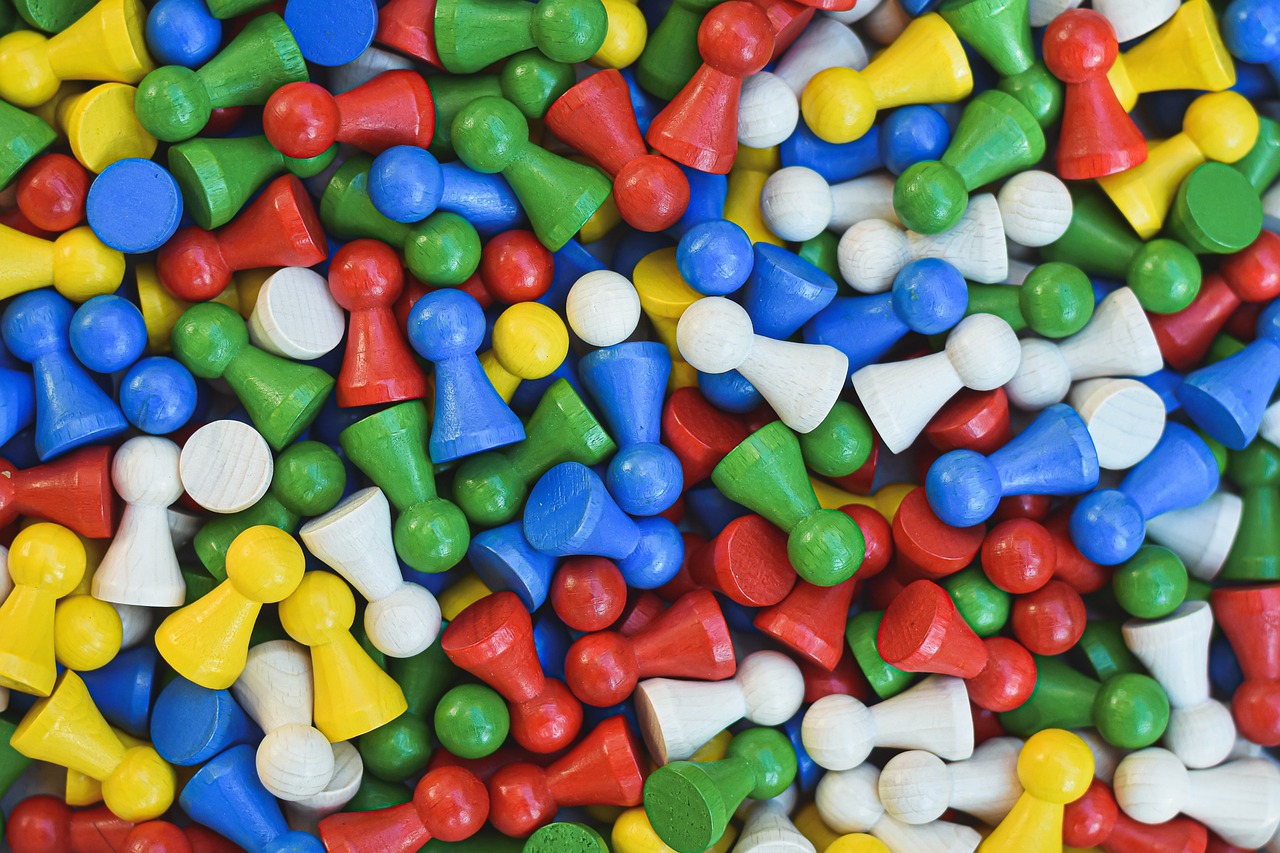
Heterogeneity in meta-analysis
When you bring studies together in a meta-analysis, one of the things you need to consider is the variability in your studies – this is called heterogeneity. This blog presents the three types of heterogeneity, considers the different types of outcome data, and delves a little more into dealing with the variations.
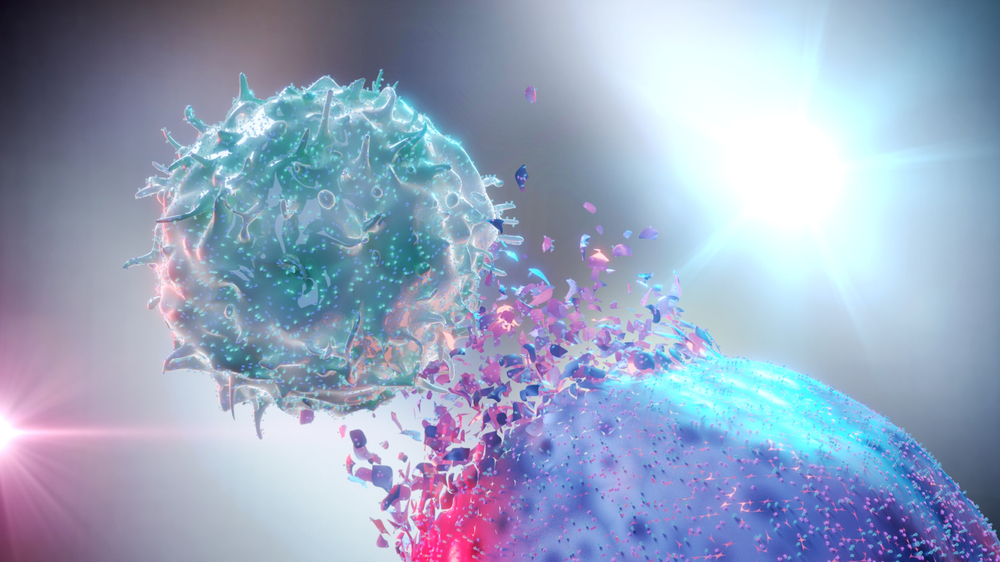
Natural killer cells in glioblastoma therapy
As seen in a previous blog from Davide, modern neuroscience often interfaces with other medical specialities. In this blog, he provides a summary of new evidence about the potential of a therapeutic strategy born at the crossroad between neurology, immunology and oncology.

An official website of the United States government
The .gov means it’s official. Federal government websites often end in .gov or .mil. Before sharing sensitive information, make sure you’re on a federal government site.
The site is secure. The https:// ensures that you are connecting to the official website and that any information you provide is encrypted and transmitted securely.
- Publications
- Account settings
- My Bibliography
- Collections
- Citation manager
Save citation to file
Email citation, add to collections.
- Create a new collection
- Add to an existing collection
Add to My Bibliography
Your saved search, create a file for external citation management software, your rss feed.
- Search in PubMed
- Search in NLM Catalog
- Add to Search
Association of the COQ2 V393A Variant with Parkinson's Disease: A Case-Control Study and Meta-Analysis
Affiliations.
- 1 Department of Neurology, West China Hospital, Sichuan University, 37 Guo Xue Xiang, Chengdu, Sichuan Province, 610041, P.R. China.
- 2 Department of Neurology, First Affiliated Hospital, Sun Yat-sen University, Guangzhou, Guangdong Province, 510080, P.R. China.
- PMID: 26098829
- PMCID: PMC4476583
- DOI: 10.1371/journal.pone.0130970
Both Parkinson's disease (PD) and multiple system atrophy (MSA) are neurodegenerative diseases of uncertain etiology, but they show similarities in their pathology and clinical course. The fact that the gene encoding α-synuclein is associated with both diseases also suggests that they share some genetic determinants. Recent studies in Japan associating MSA with a variant in the COQ2 gene led us to question whether variants in the COQ2 gene are associated with PD in Han Chinese in a case-control study. A total of 564 patients with PD were genotyped using the ligase detection rection, together with 484 gender- and age-matched healthy subjects. The M128V and R387X variants of COQ2 were not detected in patients or controls; instead, we detected only the heterozygous V393A variant (CT genotype). The frequency of the CT genotype encoding the V393A mutation was significantly higher in patients PD (4.08%) than in controls (1.86%), corresponding to an odds ratio of 2.24 (95%CI 1.03 to 4.90, p = 0.037). The frequency of the C allele of the V393A variant was significantly higher in patients with PD than in controls (OR 2.22, 95%CI 1.02 to 4.82, p = 0.039), and this was also observed in a meta-analysis of studies from mainland China, Taiwan and Japan. Subgroup analysis of our data showed that the V393A variant was significantly associated with early-onset PD (OR 3.71, 95%CI 1.51 to 9.15, p = 0.002) but not with late-onset disease (OR 1.65, 95%CI 0.69 to 3.95, p = 0.260). Gender was not significantly associated with either genotype or minor allele frequencies. In conclusion, our findings show for the first time that the V393A variant in the COQ2 gene increases risk of PD among the population of east Asia. These results, combined with research on Japanese, lend genetic support to the hypothesis that oxidative stress underlies pathogenesis of both PD and MSA.
PubMed Disclaimer
Conflict of interest statement
Competing Interests: The authors have declared that no competing interests exist.
Fig 1. Meta-analysis of the association between…
Fig 1. Meta-analysis of the association between the COQ2 V393A variant and PD in different…
Similar articles
- Association of the COQ2 V393A variant with risk of multiple system atrophy in East Asians: a case-control study and meta-analysis of the literature. Zhao Q, Yang X, Tian S, An R, Zheng J, Xu Y. Zhao Q, et al. Neurol Sci. 2016 Mar;37(3):423-30. doi: 10.1007/s10072-015-2414-8. Epub 2015 Nov 21. Neurol Sci. 2016. PMID: 26590992
- COQ2 p.V393A variant, rs148156462, is not associated with Parkinson's disease in a Taiwanese population. Lin CH, Lin HI, Chen ML, Wu RM. Lin CH, et al. Neurobiol Aging. 2015 Jan;36(1):546.e17-8. doi: 10.1016/j.neurobiolaging.2014.08.006. Epub 2014 Aug 13. Neurobiol Aging. 2015. PMID: 25200193
- Lack of evidence for an association between the V393A variant of COQ2 and amyotrophic lateral sclerosis in a Han Chinese population. Yang X, Xi J, An R, Yu L, Lin Z, Zhou H, Xu Y. Yang X, et al. Neurol Sci. 2015 Jul;36(7):1211-5. doi: 10.1007/s10072-015-2083-7. Epub 2015 Jan 23. Neurol Sci. 2015. PMID: 25613861
- COQ2 variants in Parkinson's disease and multiple system atrophy. Mikasa M, Kanai K, Li Y, Yoshino H, Mogushi K, Hayashida A, Ikeda A, Kawajiri S, Okuma Y, Kashihara K, Sato T, Kondo H, Funayama M, Nishioka K, Hattori N. Mikasa M, et al. J Neural Transm (Vienna). 2018 Jun;125(6):937-944. doi: 10.1007/s00702-018-1885-1. Epub 2018 Apr 11. J Neural Transm (Vienna). 2018. PMID: 29644397
- Association between the ubiquitin carboxyl-terminal esterase L1 gene (UCHL1) S18Y variant and Parkinson's Disease: a HuGE review and meta-analysis. Ragland M, Hutter C, Zabetian C, Edwards K. Ragland M, et al. Am J Epidemiol. 2009 Dec 1;170(11):1344-57. doi: 10.1093/aje/kwp288. Epub 2009 Oct 28. Am J Epidemiol. 2009. PMID: 19864305 Free PMC article. Review.
- Parkinson's Disease, Parkinsonisms, and Mitochondria: the Role of Nuclear and Mitochondrial DNA. Legati A, Ghezzi D. Legati A, et al. Curr Neurol Neurosci Rep. 2023 Apr;23(4):131-147. doi: 10.1007/s11910-023-01260-8. Epub 2023 Mar 7. Curr Neurol Neurosci Rep. 2023. PMID: 36881253 Review.
- Multiple system atrophy-cerebellar: A case report and literature review. Doan TT, Pham TD, Nguyen DD, Ngo DHA, Le TB, Nguyen TT. Doan TT, et al. Radiol Case Rep. 2023 Jan 7;18(3):1121-1126. doi: 10.1016/j.radcr.2022.12.046. eCollection 2023 Mar. Radiol Case Rep. 2023. PMID: 36660581 Free PMC article.
- Clinical and Genetic Features of Multiplex Families with Multiple System Atrophy and Parkinson's Disease. Matsukawa T, Porto KJL, Mitsui J, Chikada A, Ishiura H, Takahashi Y, Nakamoto FK, Seki T, Shiio Y, Toda T, Tsuji S. Matsukawa T, et al. Cerebellum. 2024 Feb;23(1):22-30. doi: 10.1007/s12311-022-01426-z. Epub 2022 Sep 13. Cerebellum. 2024. PMID: 36097244
- Clinical and Imaging Features of Multiple System Atrophy: Challenges for an Early and Clinically Definitive Diagnosis. Watanabe H, Riku Y, Hara K, Kawabata K, Nakamura T, Ito M, Hirayama M, Yoshida M, Katsuno M, Sobue G. Watanabe H, et al. J Mov Disord. 2018 Sep;11(3):107-120. doi: 10.14802/jmd.18020. Epub 2018 Aug 9. J Mov Disord. 2018. PMID: 30086614 Free PMC article.
- Halliday GM, Holton JL, Revesz T, Dickson DW. (2011) Neuropathology underlying clinical variability in patients with synucleinopathies. Acta Neuropathol 122:187–204 10.1007/s00401-011-0852-9 - DOI - PubMed
- Unger EL, Eve DJ, Perez XA, Reichenbach DK, Xu Y, Lee MK, et al. (2006) Locomotor hyperac tivity and alterations in dopamineneurotransmission are associated with overexpression of A53T mutant human A-synuclein in mice. Neurobiology of Disease 21:431–443 - PubMed
- Fujioka S, Ogaki K, Tacik PM, Uitti RJ, Ross OA, Wszolek ZK. (2014) Update on novel familial forms of Parkinson's disease and multiple system atrophy. Parkinsonism Relat Disord 20 (Suppl 1):S29–34. 10.1016/S1353-8020(13)70010-5 - DOI - PMC - PubMed
- Nishimura M, Kuno S, Kaji R, Kawakami H. (2005) Influence of a tumor necrosis factor gene polymorphism in Japanese patients with multiple system atrophy. Neurosci Lett 374: 218–221. - PubMed
- Soma H, Yabe I, Takei A, Fujiki N, Yanagihara T, Sasaki H. (2008) Associations between multiple system atrophy and polymorphisms of SLC1A4, SQSTM1, and EIF4EBP1genes. Mov Disord 23:1161–7. 10.1002/mds.22046 - DOI - PubMed
Publication types
- Search in MeSH
Related information
- Gene (GeneRIF)
- Nucleotide (RefSeq)
- Protein (RefSeq)
Grants and funding
Linkout - more resources, full text sources.
- Europe PubMed Central
- PubMed Central
- Public Library of Science
Other Literature Sources
- scite Smart Citations
- Genetic Alliance
- MedlinePlus Health Information
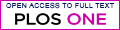
- Citation Manager
NCBI Literature Resources
MeSH PMC Bookshelf Disclaimer
The PubMed wordmark and PubMed logo are registered trademarks of the U.S. Department of Health and Human Services (HHS). Unauthorized use of these marks is strictly prohibited.
- Open access
- Published: 19 August 2024
The correlation between mitochondrial derived peptide (MDP) and metabolic states: a systematic review and meta-analysis
- Qian Zhou ORCID: orcid.org/0000-0001-6957-9821 1 ,
- Shao Yin 1 ,
- Xingxing Lei 1 ,
- Yuting Tian 1 ,
- Dajun Lin 1 ,
- Li Wang 1 &
- Qiu Chen 2
Diabetology & Metabolic Syndrome volume 16 , Article number: 200 ( 2024 ) Cite this article
Metrics details
MOTS-c is known as mitochondrial open reading frame (ORF) of the twelve S c, produced by a small ORF-encoded peptides (SEPs) in mitochondrial 12S rRNA region. There is growing evidence that MOTS-c has a strong relationship with the expression of inflammation- and metabolism-associated genes and metabolic homeostasis, and even offering some protection against insulin resistance (IR). However, studies have reported inconsistent correlations between different population characteristics and MOTS-c levels. This meta-analysis aims to elucidate MOTS-c levels in physiological and pathological states, and its correlation with metabolic features in various physiological states.
We conducted a systematic review and meta-analysis to synthesize the evidence of changes in blood MOTS-c concentration, and any association between MOTS-c and population characteristic. The Web of Science, PubMed, EMBASE, CNKI, WANGFANG and VIP databases were searched from inception to April 2023. The statistical analysis was summarized using the standardized mean difference (SMD) and 95% confidence interval (95% CIs). Pearson correlation coefficient was used to analyze the correlation and generate forest plots through a random-effects model. Additional analyses as sensitivity and subgroup analyses were performed to identify the origins of heterogeneity. Publication bias was retrieved by means of a funnel-plot analysis and Egger’s test. All related statistical analyses were performed using Revman 5.3 and Stata 15 statistical software.
There are 6 case–control studies and 1 cross-sectional study (11 groups) including 602 participants in our current meta-analysis. Overall analysis results showed plasma MOTS-c concentration in diabetes and obesity patients was significantly reduced (SMD = − 0.37; 95% CI− 0.53 to − 0.20; P < 0.05). After subgroup analysis, the present analysis has yielded opposite results for MOTS-c changes in obesity (SMD = 0.51; 95% CI 0.21 to 0.81; P < 0.05) and type 2 diabetes mellitus (T2DM) (SMD = − 0.89; 95% CI − 1.12 to − 0.65; P < 0.05) individuals. Moreover, the correlation analysis was performed to identify that MOTS-c levels were significantly positively correlated with TC (r = 0.29, 95% CI 0.20 to 0.38) and LDL-c (r = 0.30, 95% CI 0.22 to 0.39). The subgroup analysis results showed that MOTS-c decreased significantly in patients with diabetes (SMD = − 0.89; 95% CI− 1.12 to − 0.65; P < 0.05). In contrast, the analysis result for obesity persons (BMI > 28 kg/ m 2 ) was statistically significant after overweight people (BMI = 24–28 kg/ m 2 ) were excluded (SMD = 0.51; 95% CI 0.21 to 0.81; P < 0.05), which is completely different from that of diabetes. Publication bias was insignificant (Egger’s test: P = 0.722).
Circulating MOTS-c level was significantly reduced in diabetic individuals but was increased significantly in obesity patients. The application of monitoring the circulating levels variability of MOTS-c in routine screening for obesity and diabetes is prospects and should be taken into consideration as an important index for the early prediction and prevention of metabolic syndrome in the future.
PROSPERO registration number CRD42021248167.
Introduction
The prevalence of metabolic diseases, including diabetes and obesity, is on the rise worldwide, which has amplified concerns about the health risks associated with this worsening health status [ 1 , 2 ]. Obesity is a multifactorial inflammatory disease of maladaptive adipose tissue mass, typically associated with chronic insulin resistance (IR) [ 3 ]. Type 2 diabetes mellitus (T2DM) is a metabolic disease characterized by persistent hyperglycaemia secondary to insufficient insulin secretion and/or insulin resistance [ 4 ]. T2DM and related complication are increasingly recognized as important causes of mortality and morbidity worldwide, posing a major global health and economy threat [ 5 ]. Obesity individuals are accompanied by insulin resistance enhancing, hyperinsulinemia and risk of T2DM increasing. Subsequently, hyperglycemia can trigger dangerous medical complications, thereby aggravating vicious cycle and leading inexorably to worsening of obesity and T2DM [ 6 ]. Thus, an independent predictive biomarker at early stages of T2DM and obesity should be provided for early diagnosis and treatment in the daily clinical practice and large-scale clinical investigation.
Various interventions including nutritional interventions, lifestyle modification and increasing physical activity have been suggested to prevent and manage the symptoms of T2DM, but there is still no definitive treatment [ 7 , 8 ]. Mitochondrial open-reading-frame (ORF) of the twelve S type-c (MOTS-c), a bioactive peptide involved in the regulation of metabolic homeostasis, is yielded by a small ORF-encoded peptides (SEPs) in mitochondrial 12S rRNA region [ 9 ]. There is growing evidence that MOTS-c has a strong relationship with the expression of inflammation- and metabolism-associated genes and plays an extensive impact in organismal and cellular metabolic homeostasis [ 10 ]. MOTS-c treatment could prevent high fat diet- or age-associated insulin resistance and diet-induced obesity in mice [ 9 ], and has drawn attention as a potential prevention or therapeutic option for diabetes and obesity [ 9 , 11 ]. Treatment and overexpression of MOTS-c increased the AMP-activated protein kinase (AMPK) activity offering some protection against IR [ 12 ]. Thus, we speculate that MOTS-c has a protective effect in part population (especially obesity and diabetes) as a regulator for metabolic homeostasis.
Although research on the metabolic activity of MOTS-c is gradually increasing, there are several gaps in the correlation between population characteristics and MOTS-c levels reported in research reports. In addition, the key molecules and mechanisms of MOTS-c and mitochondrial related to metabolic regulation remain vague. This meta-analysis aims to elucidate MOTS-c levels in physiological and pathological states, and its correlation with metabolic features in various physiological states. The present meta-analysis demonstrates MOTS-c levels may serve as a sensitive and early indicator of the occurrence and development of obesity and diabetes.
The present systematic review and meta-analysis was designed, conducted and reported based on the Preferred Reporting Items for Systematic Reviews and Meta Analysis (PRISMA) 2020 [ 13 ] guidance and Methodological Expectations of Cochrane Intervention Reviews (MECIR) [ 14 ] guidelines. The study was registered in the PROSPERO with the following registration number CRD42021248167.
Date sources and search strategy
A systematic literature search was performed using the Web of Science, PubMed, EMBASE, CNKI, WANGFANG and VIP databases from inception to April 2023. The search used appropriate Medical Subject Headings and the use of following search terms based on PICO principle (Supplementary Table 1). We restricted the search to include only human studies, Chinese- or English-language publications, and full-text articles without time period limitations. Excluding irrelevant studies though reviewing the titles and/or abstracts, then two authors independently read the full texts of the remain studies. Relevant studies got qualified after joint review reaching agreement. In the several searches, searching strategy was combined two separate parts for obtaining a complete set of studies. In order to identify any missed papers, the lists of references of retrieved publications were also checked to identify additional relevant studies.
Study selection and exclusion criteria
Clinical trials were identified which fulfil the following criteria will be included: (1) original studies published in Chinese- or English-language, peer-reviewed journals; (2) restricted the search to include only human studies; (3) participants had a history of confirmed diabetes or obesity diagnosis. Clinical trials with the following characteristics were excluded: (1) individuals with any accompany disease, including psychiatric disorders, stroke, cancer, renal disease or severe hepatic, and acute cardiovascular events, et al.; (2) meta-analysis, reviews, meeting abstracts, comments and letters, and posters; and (3) the unpublished articles or non-research articles were excluded.
Data extraction and quality assessment
Data from included studies were extracted by two authors (XL, SY) independently according to a predefined standardized format. The extracted items as follows: study basic information (first author’s name, published year, location, sample size, etc.); and included participant characteristics (body mass index (BMI), age, MOTS-c level, disease type, homeostatic model assessment of insulin resistance (HOMA-IR), Total Cholesterol, and correlation coefficients between metabolic characteristics and MOTS-c). For quality assessment of included studies, using Newcastle–Ottawa Scale (NOS) adapted for case–control and cross-sectional studies [ 15 ]. Any discrepancy or ambiguity in Data extraction process and quality assessment between the two researchers was resolved by consultation with a third researcher until a consensus was reached (QZ).
Data synthesis and analysis
For the statistical analysis, Standard Mean Difference (SMD) with 95% confidence interval (CI) for continuous outcomes and Risk Ratio (RR) with 95% CI for dichotomous outcomes were used to estimate the pooled effects. We estimated the associations between different metabolic features and MOTS-c levels using Pearson correlation coefficients and generated forest plots through a random-effects model. Correlation coefficients were normalized to z values via Fisher’s z-transformation to calculate the relevant statistics. Meta-analyses produced variance and 95% CI before translating them back to the summary effect size (r). Heterogeneity was tested though Cochrans Q statistic and the proportion of the total variation resulted from heterogeneity was quantified via the I 2 statistic [ 16 ], when I 2 > 50% and P < 0.05 were considered to indicate significant heterogeneity [ 17 ]. Additional analyses as sensitivity and subgroup analyses were performed to identify the origins of heterogeneity. Publication bias was retrieved by means of a funnel-plot analysis, and the Egger’s test between included studies and P < 0.05 were considered to indicate statistically significant [ 18 ]. All related statistical analyses were performed using Revman 5.3 and Stata 15 statistical software.
Literature search results
The flow chart demonstrating the selection process with more details is shown in Fig. 1 . Through electronic database search, 198 citations were initially identified, including PubMed, Embase, Web of Science, CNKI, WANGFANG and VIP databases. Due to duplicate papers, review, and non-human, 106 studies were eliminated. The title and abstract of each article were examined, and 72 ineligibles titles were removed. 45 articles were excluded after reading the full texts. Finally, 7 studies (Baylan, F. A. [ 19 ]; Du, C. [ 20 ]; Ramanjaneya, M. [ 21 ]; Cataldo, L. R. [ 22 ]; Jiang Fen [ 23 ]; Wojciechowska M. [ 24 ]; Wang Xiaogang [ 25 ]) were included in this meta- analysis. Features of the 7 included studies between 2018 and 2022, 5 were published in English, and 2 were published in Chinese. Out of them, 6 included individuals with Obesity, 3 included individuals with T2DM. In Ramanjaneyas’s [ 21 ] study, the subjects were divided into two groups as T2DM with HbA1c < 7% and T2D with HbA1c > 7%. In Cataldo's [ 22 ] study, the subjects were divided into Males groups and Females groups. In Jiang Fen [ 23 ] study, participants were split into three groups(T2DM, Obesity with BMI = 24–28 and Obesity with BMI > 28). Thus, from inception to 2023, there are 7 published studies with 11 groups, and 661 participants were selected in our present meta-analysis. The authors estimated all eligible studies clinical information though anthropometric measurements. Summing up the detailed characteristics of selected studies in Table 1 , and the sample size ranged from 5 to 93.
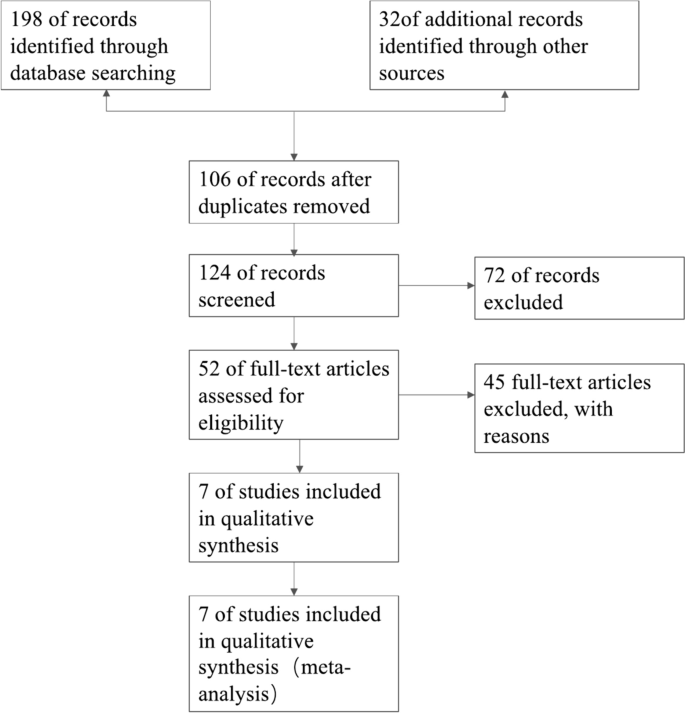
Flow chart of literature search
Overall analysis
The analysis results demonstrate that plasma MOTS-c concentration is significantly reduced in all included individuals as shown in Fig. 2 (SMD = − 0.37; 95% CI: − 0.53 to − 0.20; P < 0.05) with substantial heterogeneity by a random effect model (I 2 = 97.2%, P = 0.000). As showed in Supplementary Fig. 1, MOTS-c levels were significantly positively correlated with Total Cholesterol (TC) (r = 0.29, 95% CI 0.20 to 0.38) and Low-Density-Lipoprotein cholesterol (LDL-c) (r = 0.30, 95% CI 0.22 to 0.39). The analysis results showed insignificant heterogeneity by a random effect model for TC (I 2 = 0.0%, P = 0.693) and significant heterogeneity for LDL-c (I 2 = 85%, P < 0.05). However, no significant correlation was found for other indicators (P > 0.05), such as BMI, HOMA-IR and Age. In order to determine the cause of heterogeneity, we have thus performed the necessary analyses below.
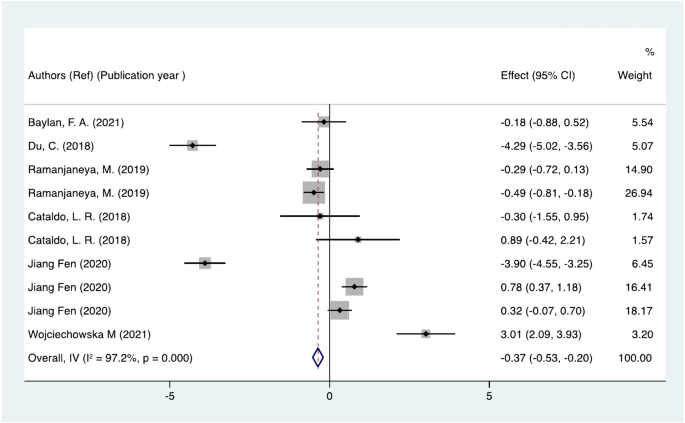
Overall analysis results. CI, Confidence interval. Summary estimates were analyzed using a random-effects model
Subgroup and sensitivity analyses
Subgroup and Sensitivity analyses were performed to find the sources of heterogeneity. Since all subjects in the research reported obesity or diabetes, we speculated that heterogeneity was related to the disease types, severity and profile of symptoms. The various data analyses for T2MD and Obesity subgroups yielded varying results, which are presented in Fig. 3 . The results showed that MOTS-c decreased significantly in patients with diabetes (SMD = − 0.89; 95% CI − 1.12 to − 0.65; P < 0.05), similar to what was previously found (Fig. 2 ). In contrast, the analysis result for obesity persons (BMI > 28 kg/ m 2 ) was statistically significant after overweight people (BMI = 24–28 kg/ m 2 ) were excluded (SMD = 0.51; 95% CI 0.21 to 0.81; P < 0.05), which is completely different from that of diabetes. In current meta-analysis, subgroup analyses regarding several other factors that could impact the association failed to be completed due to the under-representation number of trials in correlation analysis. After subgroup analysis, we discovered that heterogeneity was remained considerably high when compared to previous studies. We therefore performed further sensitivity analyses for each end point by excluding individual studies. The results of the sensitivity-pooled SMD on the bulk of the outcomes indicated that all exclusions had no effect on the prior analyses results.
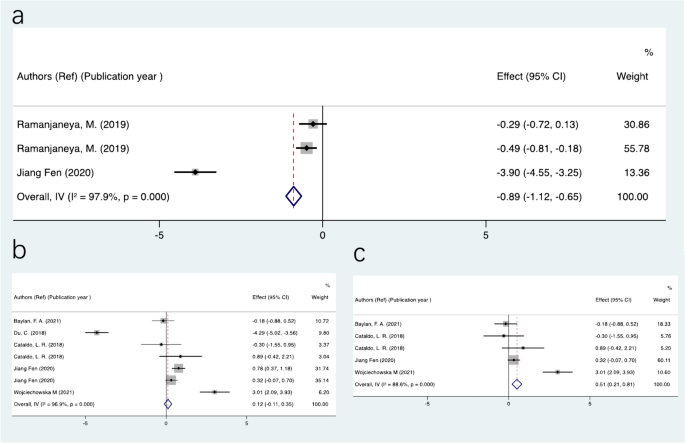
The SMDs of MOTS-c concentration depended on disease types and severity of symptoms. a) diabetes; b) obesity included overweight people (BMI = 24–28 kg/m 2 ); c) obesity (BMI > 25 kg/m 2 ) excluded overweight people
Publication bias and quality assessment
Symmetrical dispersion points (Supplementary Fig. 2) and the Egger test were used to assess the presence of potential publication bias. Test confirmed that publication bias was evaluated and considered insignificant (Egger's test: P = 0.722; Supplementary Fig. 3). The Newcastle–Ottawa Scale and common excel files were used to evaluate the methodological quality and bias of all qualifying studies. The quality of included studies was assessed by NOS quality assessment scale with a score ranging from five to eight stars (Tables 2 – 3 ).
To our knowledge, this was the first meta-analysis to elucidate the blood concentration changes of MOTS-c peptide and its correlation with different metabolic features in various physiological states. The present analysis has yielded opposite results for plasma MOTS-c concentration changes in obesity (significantly increased) and diabetic (significantly decreased) individuals. Results from correlation analyses revealed that MOTS-c was positively associated with TC and LDL-c. This connection result is in line with prior analysis results of Most-c increased significantly in obesity individuals. However, no correlation was observed for other measures of obesity, which could be explained by the paucity of literature reporting pertinent data. Data provides evidence that MOTS-c may be a new therapeutic target for obesity and diabetes. And it may be useful to predict metabolic syndrome by monitoring the level of MOTS-c.
According to our analysis results, several studies reached a similar conclusion, as MOT-c expression were lower in T2MD and related to the hemoglobin [ 22 ]. For obesity, there are different views. Insufficient sample size, varied assay method, diverse detected sample and different characteristics existing in study designs may underlie discrepancies among existing bodies of evidence. Cataldo. L. R [ 22 ] suggested that plasma MOTS-c level depends on the metabolic status, and MOTS-c concentration associates positively with insulin resistance in lean individuals. Lu, H [ 26 ] suggested that MOTS-c is a high potential candidate for chronic treatment of menopausal induced metabolic dysfunction. MOTS-c peptide regulates adipose homeostasis to prevent ovariectomy-induced metabolic dysfunction [ 26 ]. Kim, S. J [ 12 ] found that three pathways were reduced in MOTS-c–injected mice, including sphingolipid metabolism, monoacylglycerol metabolism, and dicarboxylate metabolism. And these pathways are upregulated in obesity and T2DM models. During obesity, generated oxidative stress contributes to the formation of peroxynitrite, which increases the production of reactive oxygen species (ROS) and promotes cytochrome C-related damage in the mitochondrial electron transfer chain [ 27 ]. Above representative metabolites were strongly associated with the risk of developing T2MD and obesity. Therefore, as chronic diseases, early detection play an essential role in diagnosis, treatment, and comprehensive care of patients.
Mitochondrially derived peptides as novel regulators of metabolism. And mitochondrial-derived peptides (MDPs) have also been found to affect metabolism. These MDPs have profound and distinct biological activities, and provide a paradigm-shifting concept of active mitochondrial-encoded signals that act at the cellular and organismal level (i.e. mitochondrial hormone) [ 28 , 29 ]. Lee C and Zeng J et al. [ 9 ] have suggested a hypothesis that mitochondria may actively regulate metabolic homeostasis at the cellular and organismal level via peptides encoded within their genome. In investigations on mice, MOTS-c has been shown to be a mitochondrial-derived peptide that targets the skeletal muscle and enhances glycolipid metabolism [ 30 ], effectively preventing high-fat diet-induced insulin resistance and obesity as well as age-dependent insulin resistance [ 9 ]. Lee C and Kim KH et al. [ 30 ] hypothesized MOTS-c actions in vivo would be related to insulin sensitivity and glucose handling, as it enhanced glucose flux rate in vitro and acute-treatment reduced glucose levels by regulating the cellular entry and utilization of glucose in mice fed a normal diet. The action of MOTS-c represents an entirely novel mitochondrial signaling mechanism. Guo Q [ 31 ] suggested that treated with adiponectin in mice regulating the expression of the mitochondrial-derived peptide MOTS-c, and its response to improves insulin resistance via APPL1-SIRT1-PGC-1α. Similar results were obtained by Yang B [ 32 ], MOTS-c interacts synergistically with exercise intervention to regulate PGC-1α expression, attenuating insulin resistance and enhance glucose metabolism in mice via AMPK signaling pathway. Kong BS [ 33 ] has found that MOTS-c prevents pancreatic islet destruction in autoimmune diabetes. Additional, Sequeira IR [ 34 ] has found a significant association between visceral fat mass and plasma MOTS-c.
In the current meta-analysis, no statistically significant changes were observed for MOTS-c in obesity population while overweight participants were included, but it significantly increased since they were eliminated. For diabetic individuals, the plasma MOTS-c concentration showed dramatically decreased, which was opposite expression compared with obesity. According to statistics, T2MD is a major complication of obesity [ 35 ]. And in the three subjects of T2MD included in the meta-analysis, all participants accompanied by an obesity phenotype. Therefore, we speculate that MOTS-c secretion will increase in the early metabolic imbalance of the obesity population, and decrease when obesity induced diabetes, which could possibly be related to an increase in hemoglobin. The results give additional evidence that mitochondrial dysfunction contributes to the development of diabetes development. Thus, we speculate MOTS-c may be considered as a potential monitoring indicator and therapeutic direction for obesity and diabetes based on the modulation of mitochondrial biogenesis. Due to the limited researches that is currently available, this interpretation may be valid only for obesity induced diabetes and fail to find other correlations. We definitely require further clinical data to support our conclusions since the results cannot accurately reflect the outcomes of clinical studies.
This meta-analysis has several inescapable limitations that need to be taken into further account consideration. Firstly, there was high heterogeneity among the controlled trials included in the analysis. Secondly, the language is restricted to Chinese and English, which introduces selection bias. Thirdly, further subgroup analysis was not allowed for correlation analysis, because the sample size was not sufficient. Lastly, the results were inconclusive because of the number of articles that were eligible for inclusion was limited. Therefore, there is an urgent need for further trials in reality. Despite the above-mentioned limitations, this mete-analysis and systematic review nonetheless offer insightful information.
In summary, these existing experimental results support our speculation. As such, MOTS-c has implications in the regulation of obesity and diabetes. Application of monitoring MOTS-c in routine obesity and diabetes screening is possible, and should be taken into consideration for prediction and prevention of metabolic syndrome in an early stage. Despite some limitations in our study, we believe that this meta-analysis has significance for follow-up research to explore the possible pathophysiological mechanisms underlying this relationship. Additional studies are required to determine the role of MDPs in the metabolic dysregulation within and between cells of metabolic syndrome. As a crucial tool in the future battle against metabolic disorders. In this regard, the development of drugs aimed at the regulation of these processes is gaining attention.
Availability of data and materials
On request, data were extracted from original research and data used in meta-analyses are accessible.
Abbreviations
Mitochondrial open reading frame (ORF) of the twelve S c
Insulin resistance
Standard mean difference
Confidence interval
Type 2 diabetes mellitus
Open-reading-frame
ORF-encoded peptides
AMP-activated protein kinase
Body mass index
Homeostatic model assessment of insulin resistance
Reactive oxygen species
Mitochondrial-derived peptides
Preferred reporting items for systematic reviews meta-analyses
Randomized controlled trial
Afshin A, Forouzanfar MH, Reitsma MB, et al. Health effects of overweight and obesity in 195 countries over 25 Years. N Engl J Med. 2017;377(1):13–27.
Article PubMed Google Scholar
Roberto CA, Swinburn B, Hawkes C, et al. Patchy progress on obesity prevention: emerging examples, entrenched barriers, and new thinking. Lancet. 2015;385(9985):2400–9.
Kelley DE, Goodpaster BH, Storlien L. Muscle triglyceride and insulin resistance. Annu Rev Nutr. 2002;22:325–46.
Article PubMed CAS Google Scholar
Xu W, Jones PM, Geng H, et al. Islet stellate cells regulate insulin secretion via Wnt5a in Min6 cells. Int J Endocrinol. 2020;2020:4708132.
Article PubMed PubMed Central Google Scholar
Mohan V, Khunti K, Chan SP, et al. Management of type 2 diabetes in developing countries: balancing optimal glycaemic control and outcomes with affordability and accessibility to treatment. Diabet Ther. 2020;11(1):15–35.
Article Google Scholar
Khalil H. Diabetes microvascular complications-A clinical update. Diabet Metab Syndr. 2017;11(Suppl 1):S133-s139.
Hashemi R, Rahimlou M, Baghdadian S, et al. Investigating the effect of DASH diet on blood pressure of patients with type 2 diabetes and prehypertension: randomized clinical trial. Diabet Metab Syndr. 2019;13(1):1–4.
Article CAS Google Scholar
Rasmussen L, Poulsen CW, Kampmann U, et al. Diet and healthy lifestyle in the management of gestational diabetes mellitus. Nutrients. 2020. https://doi.org/10.3390/nu12103050 .
Lee C, Zeng J, Drew BG, et al. The mitochondrial-derived peptide MOTS-c promotes metabolic homeostasis and reduces obesity and insulin resistance. Cell Metab. 2015;21(3):443–54.
Article PubMed PubMed Central CAS Google Scholar
Fujiwara K, Yasuda M, Ninomiya T, et al. Insulin resistance is a risk factor for increased intraocular pressure: the hisayama study. Invest Ophthalmol Vis Sci. 2015;56(13):7983–7.
Zarse K, Ristow M. A mitochondrially encoded hormone ameliorates obesity and insulin resistance. Cell Metab. 2015;21(3):355–6.
Kim SJ, Miller B, Mehta HH, et al. The mitochondrial-derived peptide MOTS-c is a regulator of plasma metabolites and enhances insulin sensitivity. Physiol Rep. 2019;7(13): e14171.
Page MJ, McKenzie JE, Bossuyt PM, et al. The PRISMA 2020 statement: an updated guideline for reporting systematic reviews. BMJ. 2021;372: n71.
Lefebvre C, Glanville J, Wieland LS, et al. Methodological developments in searching for studies for systematic reviews: past, present and future? Syst Rev. 2013;2:78.
Herzog R, Álvarez-Pasquin MJ, Díaz C, et al. Are healthcare workers’ intentions to vaccinate related to their knowledge, beliefs and attitudes? A Syst Rev BMC Publ Health. 2013;13:154.
Higgins JP, Thompson SG. Quantifying heterogeneity in a meta-analysis. Stat Med. 2002;21(11):1539–58.
Melsen WG, Bootsma MC, Rovers MM, et al. The effects of clinical and statistical heterogeneity on the predictive values of results from meta-analyses. Clin Microbiol Infect. 2014;20(2):123–9.
Sterne JA, Egger M, Smith GD. Systematic reviews in health care: investigating and dealing with publication and other biases in meta-analysis. BMJ. 2001;323(7304):101–5.
Baylan FA, Yarar E. Relationship between the mitochondria-derived peptide MOTS-c and insulin resistance in obstructive sleep apnea. Sleep Breath. 2021;25(2):861–6.
Du C, Zhang C, Wu W, et al. Circulating MOTS-c levels are decreased in obese male children and adolescents and associated with insulin resistance. Pediatr Diabet. 2018. https://doi.org/10.1111/pedi.12685 .
Ramanjaneya M, Bettahi I, Jerobin J, et al. Mitochondrial-derived peptides are down regulated in diabetes subjects. Front Endocrinol (Lausanne). 2019;10:331.
Cataldo LR, Fernández-Verdejo R, Santos JL, et al. Plasma MOTS-c levels are associated with insulin sensitivity in lean but not in obese individuals. J Investig Med. 2018;66(6):1019–22.
蒋芬. 新诊断2型糖尿病患者血清MOTS-c水平与胰岛素敏感性的相关性. 南华大学 2020.
Wojciechowska M, Pruszyńska-Oszmałek E, Kołodziejski PA, et al. Changes in MOTS-c level in the blood of pregnant women with metabolic disorders. Biology (Basel). 2021. https://doi.org/10.3390/biology10101032 .
王晓刚, 支晓慧. 2型糖尿病患者血清MOTS-c水平与心脏功能不全的相关性研究. 临床医药实践. 2022;31(2):83–85,98.
Lu H, Wei M, Zhai Y, et al. MOTS-c peptide regulates adipose homeostasis to prevent ovariectomy-induced metabolic dysfunction. J Mol Med (Berl). 2019;97(4):473–85.
Skuratovskaia D, Komar A, Vulf M, et al. Mitochondrial destiny in type 2 diabetes: the effects of oxidative stress on the dynamics and biogenesis of mitochondria. PeerJ. 2020;8: e9741.
Shokolenko IN, Alexeyev MF. Mitochondrial DNA: a disposable genome? Biochim Biophys Acta. 2015;1852(9):1805–9.
da Cunha FM, Torelli NQ, Kowaltowski AJ. Mitochondrial retrograde signaling: triggers, pathways, and outcomes. Oxid Med Cell Longev. 2015;2015: 482582.
Lee C, Kim KH, Cohen P. MOTS-c: a novel mitochondrial-derived peptide regulating muscle and fat metabolism. Free Radic Biol Med. 2016;100:182–7.
Guo Q, Chang B, Yu QL, et al. Adiponectin treatment improves insulin resistance in mice by regulating the expression of the mitochondrial-derived peptide MOTS-c and its response to exercise via APPL1-SIRT1-PGC-1α. Diabetologia. 2020;63(12):2675–88.
Yang B, Yu Q, Chang B, et al. MOTS-c interacts synergistically with exercise intervention to regulate PGC-1α expression, attenuate insulin resistance and enhance glucose metabolism in mice via AMPK signaling pathway. Biochim Biophys Acta Mol Basis Dis. 2021;1867(6): 166126.
Kong BS, Min SH, Lee C, et al. Mitochondrial-encoded MOTS-c prevents pancreatic islet destruction in autoimmune diabetes. Cell Rep. 2021;36(4): 109447.
Sequeira IR, Woodhead JST, Chan A, et al. Plasma mitochondrial derived peptides MOTS-c and SHLP2 positively associate with android and liver fat in people without diabetes. Biochim Biophys Acta Gen Subj. 2021;1865(11): 129991.
Hägg S, Fall T, Ploner A, et al. Adiposity as a cause of cardiovascular disease: a mendelian randomization study. Int J Epidemiol. 2015;44(2):578–86.
Download references
Acknowledgements
Authors would also like to acknowledge the support of Sichuan Provincial Administration of Traditional Chinese Medicine Science and Technology Research Special Project (2023zd020), Key R&D Support Plan of Chengdu Science and Technology Bureau (2023-YF09-00052-SN).
Patient and public involvement
There is no patient involved in this study.
The present research was supported by Sichuan Provincial Administration of Traditional Chinese Medicine Science and Technology Research Special Project (2023zd020), Key R&D Support Plan of Chengdu Science and Technology Bureau (2023-YF09-00052-SN). The design of this review was done without the involvement of any funders or sponsors.
Author information
Authors and affiliations.
Hospital of Chengdu University of Traditional Chinese Medicine, Sichuan, Chengdu, 610072, China
Qian Zhou, Shao Yin, Xingxing Lei, Yuting Tian, Dajun Lin & Li Wang
Hospital of Chengdu University of Traditional Chinese Medicine, Sichuan Province, No. 39, Shi-Er-Qiao Road, Chengdu, 610072, People’s Republic of China
You can also search for this author in PubMed Google Scholar
Contributions
QZ and SY conceptualized, conceived, authored, and reviewed the initial manuscript. XL and DL defined the concepts, search items, data extraction procedure, and methodological assessment. TY and LW designed the data extraction and statistical analysis. QZ and QC contributed crucial information. All authors approved and contributed to the final written article.
Corresponding author
Correspondence to Qiu Chen .
Ethics declarations
Ethics approval and consent to participate.
Not applicable.
Competing interests
The authors declare no competing interests.
Additional information
Publisher's note.
Springer Nature remains neutral with regard to jurisdictional claims in published maps and institutional affiliations.
Supplementary Information
13098_2024_1405_moesm1_esm.docx.
Supplementary Material 1. Figure 1: Associations between different metabolic features and MOTS-c using Pearson correlation coefficients. a) age; b) BMI; c) HOMA-IR; d) LDL-c; e) TC.
Supplementary Material 2. Figure 2: Funnel plot for publication bias analysis of the selected studies.
Supplementary material 3. figure 3: the result of egger’s test., supplementary material 4., rights and permissions.
Open Access This article is licensed under a Creative Commons Attribution 4.0 International License, which permits use, sharing, adaptation, distribution and reproduction in any medium or format, as long as you give appropriate credit to the original author(s) and the source, provide a link to the Creative Commons licence, and indicate if changes were made. The images or other third party material in this article are included in the article's Creative Commons licence, unless indicated otherwise in a credit line to the material. If material is not included in the article's Creative Commons licence and your intended use is not permitted by statutory regulation or exceeds the permitted use, you will need to obtain permission directly from the copyright holder. To view a copy of this licence, visit http://creativecommons.org/licenses/by/4.0/ . The Creative Commons Public Domain Dedication waiver ( http://creativecommons.org/publicdomain/zero/1.0/ ) applies to the data made available in this article, unless otherwise stated in a credit line to the data.
Reprints and permissions
About this article
Cite this article.
Zhou, Q., Yin, S., Lei, X. et al. The correlation between mitochondrial derived peptide (MDP) and metabolic states: a systematic review and meta-analysis. Diabetol Metab Syndr 16 , 200 (2024). https://doi.org/10.1186/s13098-024-01405-w
Download citation
Received : 30 July 2023
Accepted : 05 July 2024
Published : 19 August 2024
DOI : https://doi.org/10.1186/s13098-024-01405-w
Share this article
Anyone you share the following link with will be able to read this content:
Sorry, a shareable link is not currently available for this article.
Provided by the Springer Nature SharedIt content-sharing initiative
- Meta-analysis
- Mitochondrion
Diabetology & Metabolic Syndrome
ISSN: 1758-5996
- Submission enquiries: [email protected]
- Submit a Manuscript
- Advanced search

American Journal of Neuroradiology
Advanced Search
Comparison of arterial spin labeling and dynamic susceptibility contrast perfusion MR imaging in pediatric brain tumors: A systematic review and meta-analysis
- Find this author on Google Scholar
- Find this author on PubMed
- Search for this author on this site
- Info & Metrics
This article requires a subscription to view the full text. If you have a subscription you may use the login form below to view the article. Access to this article can also be purchased.
BACKGROUND: Brain tumors are a leading cause of mortality in children. Accurate tumor grading is essential to plan treatment and for prognostication. Perfusion imaging has been shown to correlate well with tumor grade in adults, however there are fewer studies in pediatric patients. Moreover, there is no consensus regarding which MR perfusion technique demonstrates the highest accuracy in the latter population.
PURPOSE: To compare the diagnostic test accuracy of dynamic-susceptibility contrast and arterial spin-labelling, in their ability to differentiate between low-and high-grade pediatric brain tumors at first presentation.
DATA SOURCES: Articles were retrieved from online electronic databases: MEDLINE (Ovid), Web of Science Core Collection and SCOPUS.
STUDY SELECTION: Studies in pediatric patients with a treatment naïve diagnosed brain tumor and imaging including either ASL or DSC or both, together with a histological diagnosis were included. Studies involving adult patient or mixed age populations, studies with incomplete data and those which used dynamic contrast enhanced perfusion were excluded.
DATA ANALYSIS: The sensitivities and specificities obtained from each study were used to calculate the true-positive, true-negative, false-positive, and false-negative count. A case was defined as a histologically proven high-grade tumor. The random-effect model was used to merge statistics. Significance level was set at p < 0.05.
DATA SYNTHESIS: Forest plots showing pairs of sensitivity and specificity, with their 95% confidence intervals, were constructed for each study. The bivariate model was applied in order to account for between-study variability. The SROC plots were constructed from the obtained data-sets. The AUC for the SROC of all studies was estimated to determine the overall diagnostic test accuracy of perfusion MRI, followed by a separate comparison of the SROC of ASL versus DSC studies.
LIMITATIONS: Small and heterogenous sample size.
CONCLUSIONS: The diagnostic accuracy of ASL was found to be comparable and not inferior to DSC, thus its use in the diagnostic assessment of pediatric patients should continue to be supported.
ABBREVIATIONS: ASL = arterial spin labelling, DSC = dynamic susceptibility contrast, DCE = dynamic contrast-enhanced, rCBF = relative cerebral blood flow, rCBV = relative cerebral blood volume, MTT = mean transfer time, TR = repetition time, TE = echo time, SROC = summary receiver operating characteristics, HG= high-grade, LG = low-grade, AUC = area under the curve, PRISMA = Preferred Reporting Items for Systematic Reviews and Meta-Analyses.
The authors declare no conflicts of interest related to the content of this article. The authors received no financial support for the research, authorship and/or publication of this article.
- © 2024 by American Journal of Neuroradiology
Log in using your username and password
Thank you for your interest in spreading the word on American Journal of Neuroradiology.
NOTE: We only request your email address so that the person you are recommending the page to knows that you wanted them to see it, and that it is not junk mail. We do not capture any email address.
Citation Manager Formats
- EndNote (tagged)
- EndNote 8 (xml)
- RefWorks Tagged
- Ref Manager

- Tweet Widget
- Facebook Like
- Google Plus One
Jump to section
Related articles.
- No related articles found.
- Google Scholar
Cited By...
- No citing articles found.
This article has not yet been cited by articles in journals that are participating in Crossref Cited-by Linking.
More in this TOC Section
- Diagnostic Performance of ASL-MRI and FDG-PET in Frontotemporal Dementia: A Systematic Review and Meta-Analysis
- Clinical Outcome of Pipeline Embolization Device with and without Coil to Treat Intracranial Aneurysm: A Systematic Review and Meta-Analysis
Similar Articles

COMMENTS
5 LESSONS FOR DESIGN CHOICES IN CASE STUDY META-ANALYSIS. Data derived from our case survey proved largely robust, displaying high degrees of inter-rater reliability and agreement, and only limited effects of the distorting factors tested for. Based on these findings, we identify a number of critical design choices will influence the quality ...
In a qualitative meta-analysis, the identified case studies are systematically coded in a meta-synthesis protocol, ... Wood JA (2008) Methodology for dealing with duplicate study effects in a meta-analysis. Organ Res Methods 11(1):79-95. Article Google Scholar Download references. Funding. Open Access funding enabled and organized by Projekt ...
This study looked at surgical outcomes including "blood loss, operative time, length of stay, complication and reoperation rates and functional outcomes" between patients with and without obesity. A meta-analysis of 32 studies (23,415 patients) was conducted. There were no significant differences for patients undergoing minimally invasive ...
A systematic review collects all possible studies related to a given topic and design, and reviews and analyzes their results [ 1 ]. During the systematic review process, the quality of studies is evaluated, and a statistical meta-analysis of the study results is conducted on the basis of their quality. A meta-analysis is a valid, objective ...
The graphical output of meta-analysis is a forest plot which provides information on individual studies and the pooled effect. Systematic reviews of literature can be undertaken for all types of questions, and all types of study designs. This article highlights the key features of systematic reviews, and is designed to help readers understand ...
The meta-synthesis aims at building theory out of primary qualitative case studies that have not been planned as part of a unified multisite effect. By drawing on an understanding of research synthesis as the interpretation of qualitative evidence from a postpositivistic perspective, this article proposes eight steps of synthesizing existing ...
Meta-analysis in EEC as a case study. Meta-analysis was first adopted by ecologists and evolutionary biologists some 25 years ago and has had a considerable impact on this research field in both ...
The case studies will be compared with the aim to conduct a rigorous secondary qualitative analysis of primary findings. Qualitative meta-analysis is an attempt to provide a more comprehensive description of a phenomenon, offering a new framework for the systematization, the comparison, and the analysis of primary findings.
The method offers a meta-analytical tool to synthesize larger numbers of qualitative case studies, yielding data amenable to large-N analysis. However, resulting data is prone to specific threats to validity, including biases due to publication type, rater behaviour, and variable characteristics, which researchers need to be aware of.
The method offers a meta-analytical tool to synthesize larger numbers of qualitative case studies, yielding data amenable to large-N analysis. However, resulting data is prone to specific threats to validity, including biases due to publication type, rater behavior, and variable characteristics, which researchers need to be aware of.
It is paramount to recognise that a systematic review and meta-analysis of case reports/series should not be placed at the top of the hierarchy in a pyramid that depicts validity.26 The certainty of evidence derived from a meta-analysis is contingent on the design of included studies, their risk of bias, as well as other factors such as ...
Practical steps: The weight for each study is calculated as: Weight = 1 / (within-study variance) For example: Let's say a study reports a within-study variance of 0.04. The weight for this study would be: 1 / 0.04 = 25. Calculate the weight for every study included in your meta-analysis using this method.
Case study: Neonatal Oxygenation Prospective Meta-analysis (NeOProM) We will illustrate each step with an example of a PMA of randomised controlled trials conducted by the Neonatal Oxygenation Prospective Meta-analysis (NeOProM) Collaboration.13 In this PMA, five groups prospectively planned to conduct separate, but similar, trials assessing different target ranges for oxygen saturation in ...
This article raises some questions about the usefulness of meta-analysis as a means of reviewing quantitative research in the social sciences. ... Meta-Analysis: A Case Study. Derek C. Briggs View all authors and affiliations. ... D. C. 2002a. Comment: Jack Kaplan's A new study of SAT coaching. Chance 15 (1): 7-8. Google Scholar. Briggs, D. C ...
By typing forest (meta-analysis name), RStudio will create a forest plot of the meta-analysis. In this case type in the console: forest (metanalisetestex) If you want to omit the result/diamond of the fixed model from the forest plot (Figure 12), set the comb.fixed argument to false by typing the following command line in the console:
review, meta-analysis aims to synthesize disparate data to facilitate informed, practical decisions across the spectrum of health-care service delivery and use. From seminal meta-analytic studies (e.g., Smith & Glass, 1977) to more recent analyses (e.g., Flückiger, Del Re, Wampold, & Horvath, 2018), meta-analysis has played a pivotal role in ...
Briggs / META-ANALYSIS: A CASE STUDY 97. T ABLE 4: Estimated Coaching Effects in Randomiz ed Studies. Repor t and Study SA T-M SA T-V. Alderman and Po wers (1980) School A 22. School B 9. School C 14.
10.1177/0193841X04272555EVALUATION REVIEW / APRIL 2005Briggs / META-ANALYSIS: A CASE STUD Y META-ANALYSIS A Case Study DEREK C. BRIGGS University of Colorado, Boulder ... Briggs / META-ANALYSIS: A CASE STUDY 89. Kulik 1984; Becker 1990). Becker's (1990) review stands out from the crowd. This review involved the synthesis of 25 reports on SAT ...
Case-Control Study: A type of research that retrospectively compares characteristics of an individual who has a certain condition (e.g. hypertension) with one who does not (i.e., a matched control or similar ... Meta-Analysis: A process of using quantitative methods to summarize the results from multiple studies, obtained and critically ...
A systematic review is an article that synthesizes available evidence on a certain topic utilizing a specific research question, pre-specified eligibility criteria for including articles, and a systematic method for its production. Whereas a meta-analysis is a quantitative, epidemiological study design used to assess the results of articles ...
I think you should not include single case study in meta- analysis.There wont be actual comparison of groups.The result will not be precise. The discussion mentions the potential bias of the case ...
Facebook is a soc ial network that has millions o f users, connecting with each oth er around the globe. It. was origi nally launched in the name "Face Mash" in October 2003 and afterwards ...
The frequency of the C allele of the V393A variant was significantly higher in patients with PD than in controls (OR 2.22, 95%CI 1.02 to 4.82, p = 0.039), and this was also observed in a meta-analysis of studies from mainland China, Taiwan and Japan.
A case-control study and a meta-analysis. by Roberto Del Bo, Marina Scarlato, Serena Ghezzi, Filippo Martinelli-Boneschi, Chiara Fenoglio, Gloria Galimberti, Sara Galbiati, Roberta Virgilio, Daniela Galimberti, Carlo Ferrarese, Elio Scarpini, Nereo Bresolin, Giacomo Pietro Comi.
This study looked at surgical outcomes including "blood loss, operative time, length of stay, complication and reoperation rates and functional outcomes" between patients with and without obesity. A meta-analysis of 32 studies (23,415 patients) was conducted. There were no significant differences for patients undergoing minimally invasive ...
Case-control studies on the relationship between FTO rs9939609 A/T polymorphism and PCOS were searched in PubMed, EMBASE, and Web of Science according to inclusion and exclusion criteria. STATA 12.0 software was conducted for Meta-analysis. Results. Nine case-control studies were included, including 1410 cases in PCOS group and 1223 cases ...
The present systematic review and meta-analysis was designed, conducted and reported based on the Preferred Reporting Items for Systematic Reviews and Meta Analysis (PRISMA) 2020 [] guidance and Methodological Expectations of Cochrane Intervention Reviews (MECIR) [] guidelines.The study was registered in the PROSPERO with the following registration number CRD42021248167.
DATA ANALYSIS: The sensitivities and specificities obtained from each study were used to calculate the true-positive, true-negative, false-positive, and false-negative count. A case was defined as a histologically proven high-grade tumor. The random-effect model was used to merge statistics. Significance level was set at p < 0.05.