

Systematic Reviews
- The Research Question
- Inclusion and Exclusion Criteria
- Original Studies
- Translating
- Deduplication
- Project Management Tools
- Useful Resources
- What is not a systematic review?
Inclusion/Exclusion Criteria
Inclusion criteria.
Identify the criteria that will be used to determine which research studies will be included. The inclusion and exclusion criteria must be decided before you start the review. Inclusion criteria is everything a study must have to be included. Exclusion criteria are the factors that would make a study ineligible to be included. Criteria that should be considered include:
Type of studies: It is important to select articles with an appropriate study design for the research question. Dates for the studies and a timeline of the problem/issue being examined may need to be identified.
Type of participants: Identify the target population characteristics. It is important to define the target population's age, sex/gender, diagnosis, as well as any other relevant factors.
Types of intervention: Describe the intervention being investigated. Consider whether to include interventions carried out globally or just in the United States. Eligibility criteria for interventions should include things such as the dose, delivery method, and duration of the investigated intervention. The interventions that are to be excluded may also need to be described here.
Types of outcome measures: Outcome measures usually refer to measurable outcomes or ‘clinical changes in health’. For example, these could include body structures and functions like pain and fatigue, activities as in functional abilities, and participation or quality of life questionnaires.
Read Chapter 3 of the Cochrane Handbook
Exclusion criteria.
A balance of specific inclusion and exclusion criteria is paramount. For some systematic reviews, there may already be a large pre-existing body of literature. The search strategy may retrieve thousands of results that must be screened. Having explicit exclusion criteria from the beginning allows those conducting the screening process, an efficient workflow. For the final product there should be a section in the review dedicated to 'Characteristics of excluded studies.' It is important to summarize why studies were excluded, especially if to a reader the study would appear to be eligible for the systematic review.
For example, a team is conducting a systematic review regarding intervention options for the treatment of opioid addiction. The research team may want to exclude studies that also involve alcohol addiction to isolate the conditions for treatment interventions solely for opioid addiction.
- << Previous: Planning and Protocols
- Next: Searching >>
- Last Updated: Jul 29, 2024 2:41 PM
- URL: https://libguides.sph.uth.tmc.edu/SystematicReviews
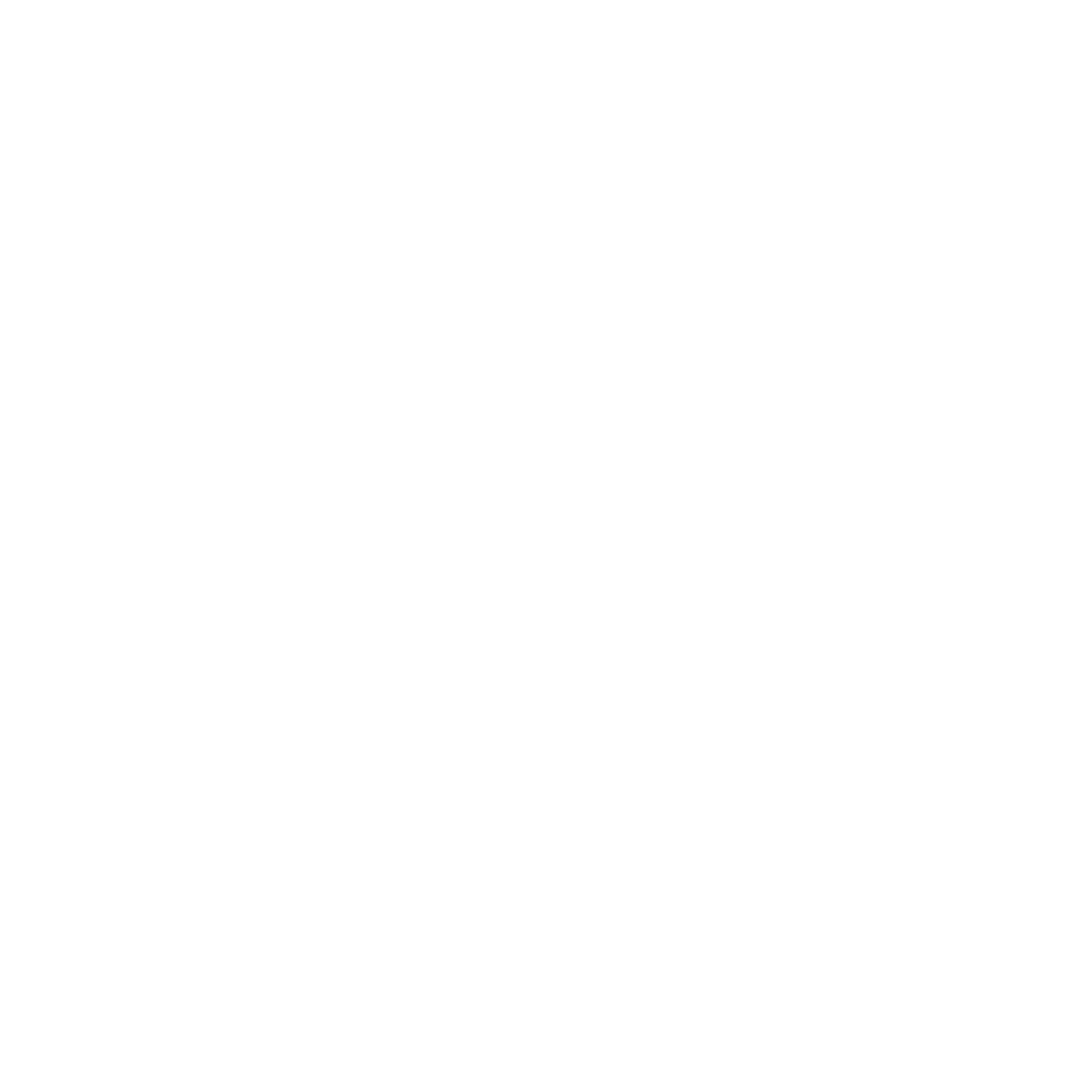
Systematic Reviews for Health Sciences and Medicine
- Systematic Reviews
- The research question
- Common search errors
- Search translation
- Managing results
- Inclusion and exclusion criteria
- Critical appraisal
- Updating a Review
- Resources by Review Stage
Inclusion and Exclusion Criteria
Inclusion and exclusion criteria set the boundaries for the systematic review. They are determined after setting the research question usually before the search is conducted, however scoping searches may need to be undertaken to determine appropriate criteria. Many different factors can be used as inclusion or exclusion criteria. Information about the inclusion and exclusion criteria is usually recorded as a paragraph or table within the methods section of the systematic review. It may also be necessary to give the definitions, and source of the definition, used for particular concepts in the research question (e.g. adolescence, depression).

Other inclusion/exclusion criteria can include the sample size, method of sampling or availability of a relevant comparison group in the study. Where a single study is reported across multiple papers the findings from the papers may be merged or only the latest data may be included.
- << Previous: Managing results
- Next: Critical appraisal >>
- Last Updated: Aug 7, 2024 12:38 AM
- URL: https://unimelb.libguides.com/sysrev
Jump to navigation

Cochrane Training
Chapter 3: defining the criteria for including studies and how they will be grouped for the synthesis.
Joanne E McKenzie, Sue E Brennan, Rebecca E Ryan, Hilary J Thomson, Renea V Johnston, James Thomas
Key Points:
- The scope of a review is defined by the types of population (participants), types of interventions (and comparisons), and the types of outcomes that are of interest. The acronym PICO (population, interventions, comparators and outcomes) helps to serve as a reminder of these.
- The population, intervention and comparison components of the question, with the additional specification of types of study that will be included, form the basis of the pre-specified eligibility criteria for the review. It is rare to use outcomes as eligibility criteria: studies should be included irrespective of whether they report outcome data, but may legitimately be excluded if they do not measure outcomes of interest, or if they explicitly aim to prevent a particular outcome.
- Cochrane Reviews should include all outcomes that are likely to be meaningful and not include trivial outcomes. Critical and important outcomes should be limited in number and include adverse as well as beneficial outcomes.
- Review authors should plan at the protocol stage how the different populations, interventions, outcomes and study designs within the scope of the review will be grouped for analysis.
Cite this chapter as: McKenzie JE, Brennan SE, Ryan RE, Thomson HJ, Johnston RV, Thomas J. Chapter 3: Defining the criteria for including studies and how they will be grouped for the synthesis. In: Higgins JPT, Thomas J, Chandler J, Cumpston M, Li T, Page MJ, Welch VA (editors). Cochrane Handbook for Systematic Reviews of Interventions version 6.4 (updated August 2023). Cochrane, 2023. Available from www.training.cochrane.org/handbook .
3.1 Introduction
One of the features that distinguishes a systematic review from a narrative review is that systematic review authors should pre-specify criteria for including and excluding studies in the review (eligibility criteria, see MECIR Box 3.2.a ).
When developing the protocol, one of the first steps is to determine the elements of the review question (including the population, intervention(s), comparator(s) and outcomes, or PICO elements) and how the intervention, in the specified population, produces the expected outcomes (see Chapter 2, Section 2.5.1 and Chapter 17, Section 17.2.1 ). Eligibility criteria are based on the PICO elements of the review question plus a specification of the types of studies that have addressed these questions. The population, interventions and comparators in the review question usually translate directly into eligibility criteria for the review, though this is not always a straightforward process and requires a thoughtful approach, as this chapter shows. Outcomes usually are not part of the criteria for including studies, and a Cochrane Review would typically seek all sufficiently rigorous studies (most commonly randomized trials) of a particular comparison of interventions in a particular population of participants, irrespective of the outcomes measured or reported. It should be noted that some reviews do legitimately restrict eligibility to specific outcomes. For example, the same intervention may be studied in the same population for different purposes; or a review may specifically address the adverse effects of an intervention used for several conditions (see Chapter 19 ).
Eligibility criteria do not exist in isolation, but should be specified with the synthesis of the studies they describe in mind. This will involve making plans for how to group variants of the PICO elements for synthesis. This chapter describes the processes by which the structure of the synthesis can be mapped out at the beginning of the review, and the interplay between the review question, considerations for the analysis and their operationalization in terms of eligibility criteria. Decisions about which studies to include (and exclude), and how they will be combined in the review’s synthesis, should be documented and justified in the review protocol.
A distinction between three different stages in the review at which the PICO construct might be used is helpful for understanding the decisions that need to be made. In Chapter 2, Section 2.3 , we introduced the ideas of a review PICO (on which eligibility of studies is based), the PICO for each synthesis (defining the question that each specific synthesis aims to answer) and the PICO of the included studies (what was actually investigated in the included studies). In this chapter, we focus on the review PICO and the PICO for each synthesis as a basis for specifying which studies should be included in the review and planning its syntheses. These PICOs should relate clearly and directly to the questions or hypotheses that are posed when the review is formulated (see Chapter 2 ) and will involve specifying the population in question, and a set of comparisons between the intervention groups.
An integral part of the process of setting up the review is to specify which characteristics of the interventions (e.g. individual compounds of a drug), populations (e.g. acute and chronic conditions), outcomes (e.g. different depression measurement scales) and study designs, will be grouped together. Such decisions should be made independent of knowing which studies will be included and the methods of synthesis that will be used (e.g. meta-analysis). There may be a need to modify the comparisons and even add new ones at the review stage in light of the data that are collected. For example, important variations in the intervention may be discovered only after data are collected, or modifying the comparison may facilitate the possibility of synthesis when only one or few studies meet the comparison PICO. Planning for the latter scenario at the protocol stage may lead to less post-hoc decision making ( Chapter 2, Section 2.5.3 ) and, of course, any changes made during the conduct of the review should be recorded and documented in the final report.
3.2 Articulating the review and comparison PICO
3.2.1 defining types of participants: which people and populations.
The criteria for considering types of people included in studies in a review should be sufficiently broad to encompass the likely diversity of studies and the likely scenarios in which the interventions will be used, but sufficiently narrow to ensure that a meaningful answer can be obtained when studies are considered together; they should be specified in advance (see MECIR Box 3.2.a ). As discussed in Chapter 2, Section 2.3.1 , the degree of breadth will vary, depending on the question being asked and the analytical approach to be employed. A range of evidence may inform the choice of population characteristics to examine, including theoretical considerations, evidence from other interventions that have a similar mechanism of action, and in vitro or animal studies. Consideration should be given to whether the population characteristic is at the level of the participant (e.g. age, severity of disease) or the study (e.g. care setting, geographical location), since this has implications for grouping studies and for the method of synthesis ( Chapter 10, Section 10.11.5 ). It is often helpful to consider the types of people that are of interest in three steps.
MECIR Box 3.2.a Relevant expectations for conduct of intervention reviews
Predefining unambiguous criteria for participants ( ) | |
| Predefined, unambiguous eligibility criteria are a fundamental prerequisite for a systematic review. The criteria for considering types of people included in studies in a review should be sufficiently broad to encompass the likely diversity of studies, but sufficiently narrow to ensure that a meaningful answer can be obtained when studies are considered in aggregate. Considerations when specifying participants include setting, diagnosis or definition of condition and demographic factors. Any restrictions to study populations must be based on a sound rationale, since it is important that Cochrane Reviews are widely relevant. |
Predefining a strategy for studies with a subset of eligible participants ( ) | |
| Sometimes a study includes some ‘eligible’ participants and some ‘ineligible’ participants, for example when an age cut-off is used in the review’s eligibility criteria. If data from the eligible participants cannot be retrieved, a mechanism for dealing with this situation should be pre-specified. |
First, the diseases or conditions of interest should be defined using explicit criteria for establishing their presence (or absence). Criteria that will force the unnecessary exclusion of studies should be avoided. For example, diagnostic criteria that were developed more recently – which may be viewed as the current gold standard for diagnosing the condition of interest – will not have been used in earlier studies. Expensive or recent diagnostic tests may not be available in many countries or settings, and time-consuming tests may not be practical in routine healthcare settings.
Second, the broad population and setting of interest should be defined . This involves deciding whether a specific population group is within scope, determined by factors such as age, sex, race, educational status or the presence of a particular condition such as angina or shortness of breath. Interest may focus on a particular setting such as a community, hospital, nursing home, chronic care institution, or outpatient setting. Box 3.2.a outlines some factors to consider when developing population criteria.
Whichever criteria are used for defining the population and setting of interest, it is common to encounter studies that only partially overlap with the review’s population. For example, in a review focusing on children, a cut-point of less than 16 years might be desirable, but studies may be identified with participants aged from 12 to 18. Unless the study reports separate data from the eligible section of the population (in which case data from the eligible participants can be included in the review), review authors will need a strategy for dealing with these studies (see MECIR Box 3.2.a ). This will involve balancing concerns about reduced applicability by including participants who do not meet the eligibility criteria, against the loss of data when studies are excluded. Arbitrary rules (such as including a study if more than 80% of the participants are under 16) will not be practical if detailed information is not available from the study. A less stringent rule, such as ‘the majority of participants are under 16’ may be sufficient. Although there is a risk of review authors’ biases affecting post-hoc inclusion decisions (which is why many authors endeavour to pre-specify these rules), this may be outweighed by a common-sense strategy in which eligibility decisions keep faith with the objectives of the review rather than with arbitrary rules. Difficult decisions should be documented in the review, checked with the advisory group (if available, see Chapter 1 ), and sensitivity analyses can assess the impact of these decisions on the review’s findings (see Chapter 10, Section 10.14 and MECIR Box 3.2.b ).
Box 3.2.a Factors to consider when developing criteria for ‘Types of participants’
MECIR Box 3.2.b Relevant expectations for conduct of intervention reviews
Changing eligibility criteria ( ) | |
| Following pre-specified eligibility criteria is a fundamental attribute of a systematic review. However, unanticipated issues may arise. Review authors should make sensible post-hoc decisions about exclusion of studies, and these should be documented in the review, possibly accompanied by sensitivity analyses. Changes to the protocol must not be made on the basis of the findings of the studies or the synthesis, as this can introduce bias. |
Third, there should be consideration of whether there are population characteristics that might be expected to modify the size of the intervention effects (e.g. different severities of heart failure). Identifying subpopulations may be important for implementation of the intervention. If relevant subpopulations are identified, two courses of action are possible: limiting the scope of the review to exclude certain subpopulations; or maintaining the breadth of the review and addressing subpopulations in the analysis.
Restricting the review with respect to specific population characteristics or settings should be based on a sound rationale. It is important that Cochrane Reviews are globally relevant, so the rationale for the exclusion of studies based on population characteristics should be justified. For example, focusing a review of the effectiveness of mammographic screening on women between 40 and 50 years old may be justified based on biological plausibility, previously published systematic reviews and existing controversy. On the other hand, focusing a review on a particular subgroup of people on the basis of their age, sex or ethnicity simply because of personal interests, when there is no underlying biologic or sociological justification for doing so, should be avoided, as these reviews will be less useful to decision makers and readers of the review.
Maintaining the breadth of the review may be best when it is uncertain whether there are important differences in effects among various subgroups of people, since this allows investigation of these differences (see Chapter 10, Section 10.11.5 ). Review authors may combine the results from different subpopulations in the same synthesis, examining whether a given subdivision explains variation (heterogeneity) among the intervention effects. Alternatively, the results may be synthesized in separate comparisons representing different subpopulations. Splitting by subpopulation risks there being too few studies to yield a useful synthesis (see Table 3.2.a and Chapter 2, Section 2.3.2 ). Consideration needs to be given to the subgroup analysis method, particularly for population characteristics measured at the participant level (see Chapter 10 and Chapter 26 , Fisher et al 2017). All subgroup analyses should ideally be planned a priori and stated as a secondary objective in the protocol, and not driven by the availability of data.
In practice, it may be difficult to assign included studies to defined subpopulations because of missing information about the population characteristic, variability in how the population characteristic is measured across studies (e.g. variation in the method used to define the severity of heart failure), or because the study does not wholly fall within (or report the results separately by) the defined subpopulation. The latter issue mainly applies for participant characteristics but can also arise for settings or geographic locations where these vary within studies. Review authors should consider planning for these scenarios (see example reviews Hetrick et al 2012, Safi et al 2017; Table 3.2.b , column 3).
Table 3.2.a Examples of population attributes and characteristics
|
|
|
Intended recipient of intervention | Patient, carer, healthcare provider (general practitioners, nurses, allied health professionals), health system, policy maker, community | In a review of e-learning programmes for health professionals, a subgroup analysis was planned to examine if the effects were modified by the (doctors, nurses or physiotherapists). The authors hypothesized that e-learning programmes for doctors would be more effective than for other health professionals, but did not provide a rationale (Vaona et al 2018). |
Disease/condition (to be treated or prevented) | Type and severity of a condition | In a review of platelet-rich therapies for musculoskeletal soft tissue injuries, a subgroup analysis was undertaken to examine if the effects of platelet-rich therapies were modified by the (e.g. rotator cuff tear, anterior cruciate ligament reconstruction, chronic Achilles tendinopathy) (Moraes et al 2014). In planning a review of beta-blockers for heart failure, subgroup analyses were specified to examine if the effects of beta-blockers are modified by the (e.g. idiopathic dilated cardiomyopathy, ischaemic heart disease, valvular heart disease, hypertension) and the (‘reduced left ventricular ejection fraction (LVEF)’ ≤ 40%, ‘mid-range LVEF’ > 40% and < 50%, ‘preserved LVEF’ ≥ 50%, mixed, not specified). Studies have shown that patient characteristics and comorbidities differ by heart failure severity, and that therapies have been shown to reduce morbidity in ‘reduced LVEF’ patients, but the benefits in the other groups are uncertain (Safi et al 2017). |
Participant characteristics | Age (neonate, child, adolescent, adult, older adult) Race/ethnicity Sex/gender PROGRESS-Plus equity characteristics (e.g. place of residence, socio-economic status, education) (O’Neill et al 2014) | In a review of newer-generation antidepressants for depressive disorders in children and adolescents, a subgroup analysis was undertaken to examine if the effects of the antidepressants were modified by . The rationale was based on the findings of another review that suggested that children and adolescents may respond differently to antidepressants. The age groups were defined as ‘children’ (aged approximately 6 to 12 years), ‘adolescents’ (aged approximately 13 to 18 years), and ‘children and adolescents’ (when the study included both children and adolescents, and results could not be obtained separately by these subpopulations) (Hetrick et al 2012). |
Setting | Setting of care (primary care, hospital, community) Rurality (urban, rural, remote) Socio-economic setting (low and middle-income countries, high-income countries) Hospital ward (e.g. intensive care unit, general medical ward, outpatient) | In a review of hip protectors for preventing hip fractures in older people, separate comparisons were specified based on (institutional care or community-dwelling) for the critical outcome of hip fracture (Santesso et al 2014). |
3.2.2 Defining interventions and how they will be grouped
In some reviews, predefining the intervention ( MECIR Box 3.2.c ) may be straightforward. For example, in a review of the effect of a given anticoagulant on deep vein thrombosis, the intervention can be defined precisely. A more complicated definition might be required for a multi-component intervention composed of dietary advice, training and support groups to reduce rates of obesity in a given population.
The inherent complexity present when defining an intervention often comes to light when considering how it is thought to achieve its intended effect and whether the effect is likely to differ when variants of the intervention are used. In the first example, the anticoagulant warfarin is thought to reduce blood clots by blocking an enzyme that depends on vitamin K to generate clotting factors. In the second, the behavioural intervention is thought to increase individuals’ self-efficacy in their ability to prepare healthy food. In both examples, we cannot assume that all forms of the intervention will work in the same way. When defining drug interventions, such as anticoagulants, factors such as the drug preparation, route of administration, dose, duration, and frequency should be considered. For multi-component interventions (such as interventions to reduce rates of obesity), the common or core features of the interventions must be defined, so that the review authors can clearly differentiate them from other interventions not included in the review.
MECIR Box 3.2.c Relevant expectations for conduct of intervention reviews
Predefining unambiguous criteria for interventions and comparators ( ) | |
| Predefined, unambiguous eligibility criteria are a fundamental prerequisite for a systematic review. Specification of comparator interventions requires particular clarity: are the experimental interventions to be compared with an inactive control intervention (e.g. placebo, no treatment, standard care, or a waiting list control), or with an active control intervention (e.g. a different variant of the same intervention, a different drug, a different kind of therapy)? Any restrictions on interventions and comparators, for example, regarding delivery, dose, duration, intensity, co-interventions and features of complex interventions should also be predefined and explained. |
In general, it is useful to consider exactly what is delivered, who delivers it, how it is delivered, where it is delivered, when and how much is delivered, and whether the intervention can be adapted or tailored , and to consider this for each type of intervention included in the review (see the TIDieR checklist (Hoffmann et al 2014)). As argued in Chapter 17 , separating interventions into ‘simple’ and ‘complex’ is a false dichotomy; all interventions can be complex in some ways. The critical issue for review authors is to identify the most important factors to be considered in a specific review. Box 3.2.b outlines some factors to consider when developing broad criteria for the ‘Types of interventions’ (and comparisons).
Box 3.2.b Factors to consider when developing criteria for ‘Types of interventions’
Once interventions eligible for the review have been broadly defined, decisions should be made about how variants of the intervention will be handled in the synthesis. Differences in intervention characteristics across studies occur in all reviews. If these reflect minor differences in the form of the intervention used in practice (such as small differences in the duration or content of brief alcohol counselling interventions), then an overall synthesis can provide useful information for decision makers. Where differences in intervention characteristics are more substantial (such as delivery of brief alcohol counselling by nurses versus doctors), and are expected to have a substantial impact on the size of intervention effects, these differences should be examined in the synthesis. What constitutes an important difference requires judgement, but in general differences that alter decisions about how an intervention is implemented or whether the intervention is used or not are likely to be important. In such circumstances, review authors should consider specifying separate groups (or subgroups) to examine in their synthesis.
Clearly defined intervention groups serve two main purposes in the synthesis. First, the way in which interventions are grouped for synthesis (meta-analysis or other synthesis) is likely to influence review findings. Careful planning of intervention groups makes best use of the available data, avoids decisions that are influenced by study findings (which may introduce bias), and produces a review focused on questions relevant to decision makers. Second, the intervention groups specified in a protocol provide a standardized terminology for describing the interventions throughout the review, overcoming the varied descriptions used by study authors (e.g. where different labels are used for the same intervention, or similar labels used for different techniques) (Michie et al 2013). This standardization enables comparison and synthesis of information about intervention characteristics across studies (common characteristics and differences) and provides a consistent language for reporting that supports interpretation of review findings.
Table 3.2.b outlines a process for planning intervention groups as a basis for/precursor to synthesis, and the decision points and considerations at each step. The table is intended to guide, rather than to be prescriptive and, although it is presented as a sequence of steps, the process is likely to be iterative, and some steps may be done concurrently or in a different sequence. The process aims to minimize data-driven approaches that can arise once review authors have knowledge of the findings of the included studies. It also includes principles for developing a flexible plan that maximizes the potential to synthesize in circumstances where there are few studies, many variants of an intervention, or where the variants are difficult to anticipate. In all stages, review authors should consider how to categorize studies whose reports contain insufficient detail.
Table 3.2.b A process for planning intervention groups for synthesis
|
|
|
1. Identify intervention characteristics that may modify the effect of the intervention. | Consider whether differences in interventions characteristics might modify the size of the intervention effect importantly. Content-specific research literature and expertise should inform this step. The TIDieR checklist – a tool for describing interventions – outlines the characteristics across which an intervention might differ (Hoffmann et al 2014). These include ‘what’ materials and procedures are used, ‘who’ provides the intervention, ‘when and how much’ intervention is delivered. The iCAT-SR tool provides equivalent guidance for complex interventions (Lewin et al 2017). | differ across multiple characteristics, which vary in importance depending on the review. In a review of exercise for osteoporosis, whether the exercise is weight-bearing or non-weight-bearing may be a key characteristic, since the mechanism by which exercise is thought to work is by placing stress or mechanical load on bones (Howe et al 2011). Different mechanisms apply in reviews of exercise for knee osteoarthritis (muscle strengthening), falls prevention (gait and balance), cognitive function (cardiovascular fitness). The differing mechanisms might suggest different ways of grouping interventions (e.g. by intensity, mode of delivery) according to potential modifiers of the intervention effects. |
2a. Label and define intervention groups to be considered in the synthesis.
| For each intervention group, provide a short label (e.g. supportive psychotherapy) and describe the core characteristics (criteria) that will be used to assign each intervention from an included study to a group. Groups are often defined by intervention content (especially the active components), such as materials, procedures or techniques (e.g. a specific drug, an information leaflet, a behaviour change technique). Other characteristics may also be used, although some are more commonly used to define subgroups (see ): the purpose or theoretical underpinning, mode of delivery, provider, dose or intensity, duration or timing of the intervention (Hoffmann et al 2014). In specifying groups: Logic models may help structure the synthesis (see and ). | In a review of psychological therapies for coronary heart disease, a single group was specified for meta-analysis that included all types of therapy. Subgroups were defined to examine whether intervention effects were modified by intervention components (e.g. cognitive techniques, stress management) or mode of delivery (e.g. individual, group) (Richards et al 2017). In a review of psychological therapies for panic disorder (Pompoli et al 2016), eight types of therapy were specified: 1. psychoeducation; 2. supportive psychotherapy (with or without a psychoeducational component); 3. physiological therapies; 4. behaviour therapy; 5. cognitive therapy; 6. cognitive behaviour therapy (CBT); 7. third-wave CBT; and 8. psychodynamic therapies. Groups were defined by the theoretical basis of each therapy (e.g. CBT aims to modify maladaptive thoughts through cognitive restructuring) and the component techniques used. |
2b. Define levels for groups based on dose or intensity. | For groups based on ‘how much’ of an intervention is used (e.g. dose or intensity), criteria are needed to quantify each group. This may be straightforward for easy-to-quantify characteristics, but more complex for characteristics that are hard to quantify (e.g. duration or intensity of rehabilitation or psychological therapy). The levels should be based on how the intervention is used in practice (e.g. cut-offs for low and high doses of a supplement based on recommended nutrient intake), or on a rationale for how the intervention might work. | In reviews of exercise, intensity may be defined by training time (session length, frequency, program duration), amount of work (e.g. repetitions), and effort/energy expenditure (exertion, heart rate) (Regnaux et al 2015). In a review of organized inpatient care for stroke, acute stroke units were categorized as ‘intensive’, ‘semi-intensive’ or ‘non-intensive’ based on whether the unit had continuous monitoring, high nurse staffing, and life support facilities (Stroke Unit Trialists Collaboration 2013). |
3. Determine whether there is an existing system for grouping interventions.
| In some fields, intervention taxonomies and frameworks have been developed for labelling and describing interventions, and these can make it easier for those using a review to interpret and apply findings. Using an agreed system is preferable to developing new groupings. Existing systems should be assessed for relevance and usefulness. The most useful systems: Systems for grouping interventions may be generic, widely applicable across clinical areas, or specific to a condition or intervention type. Some Cochrane Groups recommend specific taxonomies. |
The (BCT) (Michie et al 2013) categorizes intervention elements such as goal setting, self-monitoring and social support. A protocol for a review of social media interventions used this taxonomy to describe interventions and examine different BCTs as potential effect modifiers (Welch et al 2018). The has been used to group interventions (or components) by function (e.g. to educate, persuade, enable) (Michie et al 2011). This system was used to describe the components of dietary advice interventions (Desroches et al 2013).
Multiple reviews have used the consensus-based taxonomy developed by the Prevention of Falls Network Europe (ProFaNE) (e.g. Verheyden et al 2013, Kendrick et al 2014). The taxonomy specifies broad groups (e.g. exercise, medication, environment/assistive technology) within which are more specific groups (e.g. exercise: gait, balance and functional training; flexibility; strength and resistance) (Lamb et al 2011). |
4. Plan how the specified groups will be used in synthesis and reporting. | Decide whether it is useful to pool all interventions in a single meta-analysis (‘lumping’), within which specific characteristics can be explored as effect modifiers (e.g. in subgroups). Alternatively, if pooling all interventions is unlikely to address a useful question, separate synthesis of specific interventions may be more appropriate (‘splitting’). Determining the right analytic approach is discussed further in . | In a review of exercise for knee osteoarthritis, the different categories of exercise were combined in a single meta-analysis, addressing the question ‘what is the effect of exercise on knee osteoarthritis?’. The categories were also analysed as subgroups within the meta-analysis to explore whether the effect size varied by type of exercise (Fransen et al 2015). Other subgroup analyses examined mode of delivery and dose. |
5. Decide how to group interventions with multiple components or co-interventions. | Some interventions, especially those considered ‘complex’, include multiple components that could also be implemented independently (Guise et al 2014, Lewin et al 2017). These components might be eligible for inclusion in the review alone, or eligible only if used alongside an eligible intervention. Options for considering multi-component interventions may include the following. and Welton et al 2009, Caldwell and Welton 2016, Higgins et al 2019).The first two approaches may be challenging but are likely to be most useful (Caldwell and Welton 2016). See Section . for the special case of when a co-intervention is administered in both treatment arms. | In a review of psychological therapies for panic disorder, two of the eight eligible therapies (psychoeducation and supportive psychotherapy) could be used alone or as part of a multi-component therapy. When accompanied by another eligible therapy, the intervention was categorized as the other therapy (i.e. psychoeducation + cognitive behavioural therapy was categorized as cognitive behavioural therapy) (Pompoli et al 2016).
In a review of psychosocial interventions for smoking cessation in pregnancy, two approaches were used. All intervention types were included in a single meta-analysis with subgroups for multi-component, single and tailored interventions. Separate meta-analyses were also performed for each intervention type, with categorization of multi-component interventions based on the ‘main’ component (Chamberlain et al 2017). |
6. Build in contingencies by specifying both specific and broader intervention groups. | Consider grouping interventions at more than one level, so that studies of a broader group of interventions can be synthesized if too few studies are identified for synthesis in more specific groups. This will provide flexibility where review authors anticipate few studies contributing to specific groups (e.g. in reviews with diverse interventions, additional diversity in other PICO elements, or few studies overall, see also ). | In a review of psychosocial interventions for smoking cessation, the authors planned to group any psychosocial intervention in a single comparison (addressing the higher level question of whether, on average, psychosocial interventions are effective). Given that sufficient data were available, they also presented separate meta-analyses to examine the effects of specific types of psychosocial interventions (e.g. counselling, health education, incentives, social support) (Chamberlain et al 2017). |
3.2.3 Defining which comparisons will be made
When articulating the PICO for each synthesis, defining the intervention groups alone is not sufficient for complete specification of the planned syntheses. The next step is to define the comparisons that will be made between the intervention groups. Setting aside for a moment more complex analyses such as network meta-analyses, which can simultaneously compare many groups ( Chapter 11 ), standard meta-analysis ( Chapter 10 ) aims to draw conclusions about the comparative effects of two groups at a time (i.e. which of two intervention groups is more effective?). These comparisons form the basis for the syntheses that will be undertaken if data are available. Cochrane Reviews sometimes include one comparison, but most often include multiple comparisons. Three commonly identified types of comparisons include the following (Davey et al 2011).
- newer generation antidepressants versus placebo (Hetrick et al 2012); and
- vertebroplasty for osteoporotic vertebral compression fractures versus placebo (sham procedure) (Buchbinder et al 2018).
- chemotherapy or targeted therapy plus best supportive care (BSC) versus BSC for palliative treatment of esophageal and gastroesophageal-junction carcinoma (Janmaat et al 2017); and
- personalized care planning versus usual care for people with long-term conditions (Coulter et al 2015).
- early (commenced at less than two weeks of age) versus late (two weeks of age or more) parenteral zinc supplementation in term and preterm infants (Taylor et al 2017);
- high intensity versus low intensity physical activity or exercise in people with hip or knee osteoarthritis (Regnaux et al 2015);
- multimedia education versus other education for consumers about prescribed and over the counter medications (Ciciriello et al 2013).
The first two types of comparisons aim to establish the effectiveness of an intervention, while the last aims to compare the effectiveness of two interventions. However, the distinction between the placebo and control is often arbitrary, since any differences in the care provided between trials with a control arm and those with a placebo arm may be unimportant , especially where ‘usual care’ is provided to both. Therefore, placebo and control groups may be determined to be similar enough to be combined for synthesis.
In reviews including multiple intervention groups, many comparisons are possible. In some of these reviews, authors seek to synthesize evidence on the comparative effectiveness of all their included interventions, including where there may be only indirect comparison of some interventions across the included studies ( Chapter 11, Section 11.2.1 ). However, in many reviews including multiple intervention groups, a limited subset of the possible comparisons will be selected. The chosen subset of comparisons should address the most important clinical and research questions. For example, if an established intervention (or dose of an intervention) is used in practice, then the synthesis would ideally compare novel or alternative interventions to this established intervention, and not, for example, to no intervention.
3.2.3.1 Dealing with co-interventions
Planning is needed for the special case where the same supplementary intervention is delivered to both the intervention and comparator groups. A supplementary intervention is an additional intervention delivered alongside the intervention of interest, such as massage in a review examining the effects of aromatherapy (i.e. aromatherapy plus massage versus massage alone). In many cases, the supplementary intervention will be unimportant and can be ignored. In other situations, the effect of the intervention of interest may differ according to whether participants receive the supplementary therapy. For example, the effect of aromatherapy among people who receive a massage may differ from the effect of the aromatherapy given alone. This will be the case if the intervention of interest interacts with the supplementary intervention leading to larger (synergistic) or smaller (dysynergistic/antagonistic) effects than the intervention of interest alone (Squires et al 2013). While qualitative interactions are rare (where the effect of the intervention is in the opposite direction when combined with the supplementary intervention), it is possible that there will be more variation in the intervention effects (heterogeneity) when supplementary interventions are involved, and it is important to plan for this. Approaches for dealing with this in the statistical synthesis may include fitting a random-effects meta-analysis model that encompasses heterogeneity ( Chapter 10, Section 10.10.4 ), or investigating whether the intervention effect is modified by the addition of the supplementary intervention through subgroup analysis ( Chapter 10, Section 10.11.2 ).
3.2.4 Selecting, prioritizing and grouping review outcomes
3.2.4.1 selecting review outcomes.
Broad outcome domains are decided at the time of setting up the review PICO (see Chapter 2 ). Once the broad domains are agreed, further specification is required to define the domains to facilitate reporting and synthesis (i.e. the PICO for comparison) (see Chapter 2, Section 2.3 ). The process for specifying and grouping outcomes largely parallels that used for specifying intervention groups.
Reporting of outcomes should rarely determine study eligibility for a review. In particular, studies should not be excluded because they do not report results of an outcome they may have measured, or provide ‘no usable data’ ( MECIR Box 3.2.d ). This is essential to avoid bias arising from selective reporting of findings by the study authors (see Chapter 13 ). However, in some circumstances, the measurement of certain outcomes may be a study eligibility criterion. This may be the case, for example, when the review addresses the potential for an intervention to prevent a particular outcome, or when the review addresses a specific purpose of an intervention that can be used in the same population for different purposes (such as hormone replacement therapy, or aspirin).
MECIR Box 3.2.d Relevant expectations for conduct of intervention reviews
Clarifying role of outcomes ( ) | |
| Outcome measures should not always form part of the criteria for including studies in a review. However, some reviews do legitimately restrict eligibility to specific outcomes. For example, the same intervention may be studied in the same population for different purposes (e.g. hormone replacement therapy, or aspirin); or a review may address specifically the adverse effects of an intervention used for several conditions. If authors do exclude studies on the basis of outcomes, care should be taken to ascertain that relevant outcomes are not available because they have not been measured rather than simply not reported. |
Predefining outcome domains ( ) | |
| Full specification of the outcomes includes consideration of outcome domains (e.g. quality of life) and outcome measures (e.g. SF-36). Predefinition of outcome reduces the risk of selective outcome reporting. The should be as few as possible and should normally reflect at least one potential benefit and at least one potential area of harm. It is expected that the review should be able to synthesize these outcomes if eligible studies are identified, and that the conclusions of the review will be based largely on the effects of the interventions on these outcomes. Additional important outcomes may also be specified. Up to seven critical and important outcomes will form the basis of the GRADE assessment and summarized in the review’s abstract and other summary formats, although the review may measure more than seven outcomes. |
Choosing outcomes ( ) | |
| Cochrane Reviews are intended to support clinical practice and policy, and should address outcomes that are critical or important to consumers. These should be specified at protocol stage. Where available, established sets of core outcomes should be used. Patient-reported outcomes should be included where possible. It is also important to judge whether evidence of resource use and costs might be an important component of decisions to adopt the intervention or alternative management strategies around the world. Large numbers of outcomes, while sometimes necessary, can make reviews unfocused, unmanageable for the user, and prone to selective outcome reporting bias. Biochemical, interim and process outcomes should be considered where they are important to decision makers. Any outcomes that would not be described as critical or important can be left out of the review. |
Predefining outcome measures ( ) | |
Having decided what outcomes are of interest to the review, authors should clarify acceptable ways in which these outcomes can be measured. It may be difficult, however, to predefine adverse effects. | |
C17: Predefining choices from multiple outcome measures ( ) | |
Prespecification guards against selective outcome reporting, and allows users to confirm that choices were not overly influenced by the results. A predefined hierarchy of outcomes measures may be helpful. It may be difficult, however, to predefine adverse effects. A rationale should be provided for the choice of outcome measure | |
C18: Predefining time points of interest ( ) | |
Prespecification guards against selective outcome reporting, and allows users to confirm that choices were not overly influenced by the results. Authors may consider whether all time frames or only selected time points will be included in the review. These decisions should be based on outcomes important for making healthcare decisions. One strategy to make use of the available data could be to group time points into prespecified intervals to represent ‘short-term’, ‘medium-term’ and ‘long-term’ outcomes and to take no more than one from each interval from each study for any particular outcome. |
In general, systematic reviews should aim to include outcomes that are likely to be meaningful to the intended users and recipients of the reviewed evidence. This may include clinicians, patients (consumers), the general public, administrators and policy makers. Outcomes may include survival (mortality), clinical events (e.g. strokes or myocardial infarction), behavioural outcomes (e.g. changes in diet, use of services), patient-reported outcomes (e.g. symptoms, quality of life), adverse events, burdens (e.g. demands on caregivers, frequency of tests, restrictions on lifestyle) and economic outcomes (e.g. cost and resource use). It is critical that outcomes used to assess adverse effects as well as outcomes used to assess beneficial effects are among those addressed by a review (see Chapter 19 ).
Outcomes that are trivial or meaningless to decision makers should not be included in Cochrane Reviews. Inclusion of outcomes that are of little or no importance risks overwhelming and potentially misleading readers. Interim or surrogate outcomes measures, such as laboratory results or radiologic results (e.g. loss of bone mineral content as a surrogate for fractures in hormone replacement therapy), while potentially helpful in explaining effects or determining intervention integrity (see Chapter 5, Section 5.3.4.1 ), can also be misleading since they may not predict clinically important outcomes accurately. Many interventions reduce the risk for a surrogate outcome but have no effect or have harmful effects on clinically relevant outcomes, and some interventions have no effect on surrogate measures but improve clinical outcomes.
Various sources can be used to develop a list of relevant outcomes, including input from consumers and advisory groups (see Chapter 2 ), the clinical experiences of the review authors, and evidence from the literature (including qualitative research about outcomes important to those affected (see Chapter 21 )). A further driver of outcome selection is consideration of outcomes used in related reviews. Harmonization of outcomes across reviews addressing related questions facilitates broader evidence synthesis questions being addressed through the use of Overviews of reviews (see Chapter V ).
Outcomes considered to be meaningful, and therefore addressed in a review, may not have been reported in the primary studies. For example, quality of life is an important outcome, perhaps the most important outcome, for people considering whether or not to use chemotherapy for advanced cancer, even if the available studies are found to report only survival (see Chapter 18 ). A further example arises with timing of the outcome measurement, where time points determined as clinically meaningful in a review are not measured in the primary studies. Including and discussing all important outcomes in a review will highlight gaps in the primary research and encourage researchers to address these gaps in future studies.
3.2.4.2 Prioritizing review outcomes
Once a full list of relevant outcomes has been compiled for the review, authors should prioritize the outcomes and select the outcomes of most relevance to the review question. The GRADE approach to assessing the certainty of evidence (see Chapter 14 ) suggests that review authors separate outcomes into those that are ‘critical’, ‘important’ and ‘not important’ for decision making.
The critical outcomes are the essential outcomes for decision making, and are those that would form the basis of a ‘Summary of findings’ table or other summary versions of the review, such as the Abstract or Plain Language Summary. ‘Summary of findings’ tables provide key information about the amount of evidence for important comparisons and outcomes, the quality of the evidence and the magnitude of effect (see Chapter 14, Section 14.1 ). There should be no more than seven outcomes included in a ‘Summary of findings’ table, and those outcomes that will be included in summaries should be specified at the protocol stage. They should generally not include surrogate or interim outcomes. They should not be chosen on the basis of any anticipated or observed magnitude of effect, or because they are likely to have been addressed in the studies to be reviewed. Box 3.2.c summarizes the principal factors to consider when selecting and prioritizing review outcomes.
Box 3.2.c Factors to consider when selecting and prioritizing review outcomes
3.2.4.3 Defining and grouping outcomes for synthesis
Table 3.2.c outlines a process for planning for the diversity in outcome measurement that may be encountered in the studies included in a review and which can complicate, and sometimes prevent, synthesis. Research has repeatedly documented inconsistency in the outcomes measured across trials in the same clinical areas (Harrison et al 2016, Williamson et al 2017). This inconsistency occurs across all aspects of outcome measurement, including the broad domains considered, the outcomes measured, the way these outcomes are labelled and defined, and the methods and timing of measurement. For example, a review of outcome measures used in 563 studies of interventions for dementia and mild cognitive impairment found that 321 unique measurement methods were used for 1278 assessments of cognitive outcomes (Harrison et al 2016). Initiatives like COMET ( Core Outcome Measures in Effectiveness Trials ) aim to encourage standardization of outcome measurement across trials (Williamson et al 2017), but these initiatives are comparatively new and review authors will inevitably encounter diversity in outcomes across studies.
The process begins by describing the scope of each outcome domain in sufficient detail to enable outcomes from included studies to be categorized ( Table 3.2.c Step 1). This step may be straightforward in areas for which core outcome sets (or equivalent systems) exist ( Table 3.2.c Step 2). The methods and timing of outcome measurement also need to be specified, giving consideration to how differences across studies will be handled ( Table 3.2.c Steps 3 and 4). Subsequent steps consider options for dealing with studies that report multiple measures within an outcome domain ( Table 3.2.c Step 5), planning how outcome domains will be used in synthesis ( Table 3.2.c Step 6), and building in contingencies to maximize potential to synthesize ( Table 3.2.c Step 7).
Table 3.2.c A process for planning outcome groups for synthesis
|
|
|
1. Fully specify outcome domains. | For each outcome domain, provide a short label (e.g. cognition, consumer evaluation of care) and describe the domain in sufficient detail to enable eligible outcomes from each included study to be categorized. The definition should be based on the concept (or construct) measured, that is ‘what’ is measured. ‘When’ and ‘how’ the outcome is measured will be considered in subsequent steps. Outcomes can be defined hierarchically, starting with very broad groups (e.g. physiological/clinical outcomes, life impact, adverse events), then outcome domains (e.g. functioning and perceived health status are domains within ‘life impact’). Within these may be narrower domains (e.g. physical function, cognitive function), and then specific outcome measures (Dodd et al 2018). The level at which outcomes are grouped for synthesis alters the question addressed, and so decisions should be guided by the review objectives. In specifying outcome domains: | In a review of computer-based interventions for sexual health promotion, three broad outcome domains were defined (cognitions, behaviours, biological) based on a conceptual model of how the intervention might work. Each domain comprised more specific domains and outcomes (e.g. condom use, seeking health services such as STI testing); listing these helped define the broad domains and guided categorization of the diverse outcomes reported in included studies (Bailey et al 2010). In a protocol for a review of social media interventions for improving health, the rationale for synthesizing broad groupings of outcomes (e.g. health behaviours, physical health) was based on prediction of a common underlying mechanism by which the intervention would work, and the review objective, which focused on overall health rather than specific outcomes (Welch et al 2018). |
2. Determine whether there is an existing system for identifying and grouping important outcomes. | Systems for categorizing outcomes include core outcome sets including the and initiatives, and outcome taxonomies (Dodd et al 2018). These systems define agreed outcomes that should be measured for specific conditions (Williamson et al 2017).These systems can be used to standardize the varied outcome labels used across studies and enable grouping and comparison (Kirkham et al 2013). Agreed terminology may help decision makers interpret review findings. The COMET website provides a database of core outcome sets agreed or in development. Some Cochrane Groups have developed their own outcome sets. While the availability of outcome sets and taxonomies varies across clinical areas, several taxonomies exist for specifying broad outcome domains (e.g. Dodd et al 2018, ICHOM 2018). | In a review of combined diet and exercise for preventing gestational diabetes mellitus, a core outcome set agreed by the Cochrane Pregnancy and Childbirth group was used (Shepherd et al 2017). In a review of decision aids for people facing health treatment or screening decisions (Stacey et al 2017), outcome domains were based on criteria for evaluating decision aids agreed in the (IPDAS). Doing so helped to assess the use of aids across diverse clinical decisions. The Cochrane Consumers and Communication Group has an agreed taxonomy to guide specification of outcomes of importance in evaluating communication interventions (Cochrane Consumers & Communication Group). |
3. Define the outcome time points. | A key attribute of defining an outcome is specifying the time of measurement. In reviews, time frames, and not specific time points, are often specified to handle the likely diversity in timing of outcome measurement across studies (e.g. a ‘medium-term’ time frame might be defined as including outcomes measured between 6 and 12 months). In specifying outcome timing: | In a review of psychological therapies for panic disorder, the main outcomes were ‘short-term’ (≤6 months from treatment commencement). ‘Long-term’ outcomes (>6 months from treatment commencement) were considered important, but not specified as critical because of concerns of participant attrition (Pompoli et al 2018). In contrast, in a review of antidepressants, a clinically meaningful time frame of 6 to 12 months might be specified for the critical outcome ‘depression’, since this is the recommended treatment duration. However, it may be anticipated that many studies will be of shorter duration with short-term follow-up, so an additional important outcome of ‘depression (<3 months)’ might also be specified. |
4. Specify the measurement tool or measurement method. | For each outcome domain, specify: Minimum criteria for inclusion of a measure may include: (e.g. consistent scores across time and raters when the outcome is unchanged), and (e.g. comparable results to similar measures, including a gold standard if available); andMeasures may be identified from core outcome sets (e.g. Williamson et al 2017, ICHOM 2018) or systematic reviews of instruments (see COnsensus-based Standards for the selection of health Measurement INstruments (COSMIN) initiative for a database of examples). | In a review of interventions to support women to stop smoking, objective (biochemically validated) and subjective (self-report) measures of smoking cessation were specified separately to examine bias due to the method used to measure the outcome (Step 6) (Chamberlain et al 2017). In a review of high-intensity versus low-intensity exercise for osteoarthritis, measures of pain were selected based on relevance of the content and properties of the measurement tool (i.e. evidence of validity and reliability) (Regnaux et al 2015). |
5. Specify how multiplicity of outcomes will be handled. | For a particular domain, multiple outcomes within a study may be available for inclusion. This may arise from: Effects of the intervention calculated from these different sources of multiplicity are statistically dependent, since they have been calculated using the same participants. To deal with this dependency, select only one outcome per study for a particular comparison, or use a meta-analysis method that accounts for the dependency (see Step 6). Pre-specify the method of selection from multiple outcomes or measures in the protocol, using an approach that is independent of the result (see ) (López-López et al 2018). Document all eligible outcomes or measures in the ‘Characteristics of included studies’ table, noting which was selected and why. Multiplicity can arise from the reporting of multiple analyses of the same outcome (e.g. analyses that do and do not adjust for prognostic factors; intention-to-treat and per-protocol analyses) and multiple reports of the same study (e.g. journal articles, conference abstracts). Approaches for dealing with this type of multiplicity should also be specified in the protocol (López-López et al 2018). It may be difficult to anticipate all forms of multiplicity when developing a protocol. Any post-hoc approaches used to select outcomes or results should be noted at the beginning of the Methods section, or if extensive, within an additional supplementary material. | The following hierarchy was specified to select one outcome per domain in a review examining the effects of portion, package or tableware size (Hollands et al 2015): Selection of the outcome was made blinded to the results. All available outcome measures were documented in the ‘Characteristics of included studies’ table. In a review of audit and feedback for healthcare providers, the outcome domains were ‘provider performance’ (e.g. compliance with recommended use of a laboratory test) and ‘patient health outcomes’ (e.g. smoking status, blood pressure) (Ivers et al 2012). For each domain, outcomes were selected using the following hierarchy: |
6. Plan how the specified outcome domains will be used in the synthesis. | When different measurement methods or tools have been used across studies, consideration must be given to how these will be synthesized. Options include the following. and ). There may be increased heterogeneity, warranting use of a random-effects model ( ). | In a review of interventions to support women to stop smoking, separate outcome domains were specified for biochemically validated measures of smoking and self-report measures. The two domains were meta-analysed together, but sensitivity analyses were undertaken restricting the meta-analyses to studies with only biochemically validated outcomes, to examine if the results were robust to the method of measurement (Chamberlain et al 2017). In a review of psychological therapies for youth internalizing and externalizing disorders, most studies contributed multiple effects (e.g. in one meta-analysis of 443 studies, there were 5139 included measures). The authors used multilevel modelling to address the dependency among multiple effects contributed from each study (Weisz et al 2017). |
7. Where possible, build in contingencies by specifying both specific and broader outcome domains. | Consider building in flexibility to group outcomes at different levels or time intervals. Inflexible approaches can undermine the potential to synthesize, especially when few studies are anticipated, or there is likely to be diversity in the way outcomes are defined and measured and the timing of measurement. If insufficient studies report data for meaningful synthesis using the narrower domains, the broader domains can be used (see also ). | Consider a hypothetical review aiming to examine the effects of behavioural psychological interventions for the treatment of overweight and obese adults. A specific outcome is body mass index (BMI). However, also specifying a broader outcome domain ‘indicator of body mass’ will facilitate synthesis in the circumstance where few studies report BMI, but most report an indicator of body mass (such as weight or waist circumference). This is particularly important when few studies may be anticipated or there is expected diversity in the measurement methods or tools. |
3.3 Determining which study designs to include
Some study designs are more appropriate than others for answering particular questions. Authors need to consider a priori what study designs are likely to provide reliable data with which to address the objectives of their review ( MECIR Box 3.3.a ). Sections 3.3.1 and 3.3.2 cover randomized and non-randomized designs for assessing treatment effects; Chapter 17, Section 17.2.5 discusses other study designs in the context of addressing intervention complexity.
MECIR Box 3.3.a Relevant expectations for conduct of intervention reviews
Predefining study designs ( ) | |
| Predefined, unambiguous eligibility criteria are a fundamental prerequisite for a systematic review. This is particularly important when non-randomized studies are considered. Some labels commonly used to define study designs can be ambiguous. For example a ‘double blind’ study may not make it clear who was blinded; a ‘case-control’ study may be nested within a cohort, or be undertaken in a cross-sectional manner; or a ‘prospective’ study may have only some features defined or undertaken prospectively. |
Justifying choice of study designs ( ) | |
| It might be difficult to address some interventions or some outcomes in randomized trials. Authors should be able to justify why they have chosen either to restrict the review to randomized trials or to include non-randomized studies. The particular study designs included should be justified with regard to appropriateness to the review question and with regard to potential for bias. |
3.3.1 Including randomized trials
Because Cochrane Reviews address questions about the effects of health care, they focus primarily on randomized trials and randomized trials should be included if they are feasible for the interventions of interest ( MECIR Box 3.3.b ). Randomization is the only way to prevent systematic differences between baseline characteristics of participants in different intervention groups in terms of both known and unknown (or unmeasured) confounders (see Chapter 8 ), and claims about cause and effect can be based on their findings with far more confidence than almost any other type of study. For clinical interventions, deciding who receives an intervention and who does not is influenced by many factors, including prognostic factors. Empirical evidence suggests that, on average, non-randomized studies produce effect estimates that indicate more extreme benefits of the effects of health care than randomized trials. However, the extent, and even the direction, of the bias is difficult to predict. These issues are discussed at length in Chapter 24 , which provides guidance on when it might be appropriate to include non-randomized studies in a Cochrane Review.
Practical considerations also motivate the restriction of many Cochrane Reviews to randomized trials. In recent decades there has been considerable investment internationally in establishing infrastructure to index and identify randomized trials. Cochrane has contributed to these efforts, including building up and maintaining a database of randomized trials, developing search filters to aid their identification, working with MEDLINE to improve tagging and identification of randomized trials, and using machine learning and crowdsourcing to reduce author workload in identifying randomized trials ( Chapter 4, Section 4.6.6.2 ). The same scale of organizational investment has not (yet) been matched for the identification of other types of studies. Consequently, identifying and including other types of studies may require additional efforts to identify studies and to keep the review up to date, and might increase the risk that the result of the review will be influenced by publication bias. This issue and other bias-related issues that are important to consider when defining types of studies are discussed in detail in Chapter 7 and Chapter 13 .
Specific aspects of study design and conduct should be considered when defining eligibility criteria, even if the review is restricted to randomized trials. For example, whether cluster-randomized trials ( Chapter 23, Section 23.1 ) and crossover trials ( Chapter 23, Section 23.2 ) are eligible, as well as other criteria for eligibility such as use of a placebo comparison group, evaluation of outcomes blinded to allocation sequence, or a minimum period of follow-up. There will always be a trade-off between restrictive study design criteria (which might result in the inclusion of studies that are at low risk of bias, but very few in number) and more liberal design criteria (which might result in the inclusion of more studies, but at a higher risk of bias). Furthermore, excessively broad criteria might result in the inclusion of misleading evidence. If, for example, interest focuses on whether a therapy improves survival in patients with a chronic condition, it might be inappropriate to look at studies of very short duration, except to make explicit the point that they cannot address the question of interest.
MECIR Box 3.3.b Relevant expectations for conduct of intervention reviews
Including randomized trials ( ) | |
if it is feasible to conduct them to evaluate the interventions and outcomes of interest. | Randomized trials are the best study design for evaluating the efficacy of interventions. If it is feasible to conduct them to evaluate questions that are being addressed by the review, they must be considered eligible for the review. However, appropriate exclusion criteria may be put in place, for example regarding length of follow-up. |
3.3.2 Including non-randomized studies
The decision of whether non-randomized studies (and what type) will be included is decided alongside the formulation of the review PICO. The main drivers that may lead to the inclusion of non-randomized studies include: (i) when randomized trials are unable to address the effects of the intervention on harm and long-term outcomes or in specific populations or settings; or (ii) for interventions that cannot be randomized (e.g. policy change introduced in a single or small number of jurisdictions) (see Chapter 24 ). Cochrane, in collaboration with others, has developed guidance for review authors to support their decision about when to look for and include non-randomized studies (Schünemann et al 2013).
Non-randomized designs have the commonality of not using randomization to allocate units to comparison groups, but their different design features mean that they are variable in their susceptibility to bias. Eligibility criteria should be based on explicit study design features, and not the study labels applied by the primary researchers (e.g. case-control, cohort), which are often used inconsistently (Reeves et al 2017; see Chapter 24 ).
When non-randomized studies are included, review authors should consider how the studies will be grouped and used in the synthesis. The Cochrane Non-randomized Studies Methods Group taxonomy of design features (see Chapter 24 ) may provide a basis for grouping together studies that are expected to have similar inferential strength and for providing a consistent language for describing the study design.
Once decisions have been made about grouping study designs, planning of how these will be used in the synthesis is required. Review authors need to decide whether it is useful to synthesize results from non-randomized studies and, if so, whether results from randomized trials and non-randomized studies should be included in the same synthesis (for the purpose of examining whether study design explains heterogeneity among the intervention effects), or whether the effects should be synthesized in separate comparisons (Valentine and Thompson 2013). Decisions should be made for each of the different types of non-randomized studies under consideration. Review authors might anticipate increased heterogeneity when non-randomized studies are synthesized, and adoption of a meta-analysis model that encompasses heterogeneity is wise (Valentine and Thompson 2013) (such as a random effects model, see Chapter 10, Section 10.10.4 ). For further discussion of non-randomized studies, see Chapter 24 .
3.4 Eligibility based on publication status and language
Chapter 4 contains detailed guidance on how to identify studies from a range of sources including, but not limited to, those in peer-reviewed journals. In general, a strategy to include studies reported in all types of publication will reduce bias ( Chapter 7 ). There would need to be a compelling argument for the exclusion of studies on the basis of their publication status ( MECIR Box 3.4.a ), including unpublished studies, partially published studies, and studies published in ‘grey’ literature sources. Given the additional challenge in obtaining unpublished studies, it is possible that any unpublished studies identified in a given review may be an unrepresentative subset of all the unpublished studies in existence. However, the bias this introduces is of less concern than the bias introduced by excluding all unpublished studies, given what is known about the impact of reporting biases (see Chapter 13 on bias due to missing studies, and Chapter 4, Section 4.3 for a more detailed discussion of searching for unpublished and grey literature).
Likewise, while searching for, and analysing, studies in any language can be extremely resource-intensive, review authors should consider carefully the implications for bias (and equity, see Chapter 16 ) if they restrict eligible studies to those published in one specific language (usually English). See Chapter 4, Section 4.4.5 , for further discussion of language and other restrictions while searching.
MECIR Box 3.4.a Relevant expectations for conduct of intervention reviews
Excluding studies based on publication status ( ) | |
| Obtaining and including data from unpublished studies (including grey literature) can reduce the effects of publication bias. However, the unpublished studies that can be located may be an unrepresentative sample of all unpublished studies. |
3.5 Chapter information
Authors: Joanne E McKenzie, Sue E Brennan, Rebecca E Ryan, Hilary J Thomson, Renea V Johnston, James Thomas
Acknowledgements: This chapter builds on earlier versions of the Handbook . In particular, Version 5, Chapter 5 , edited by Denise O’Connor, Sally Green and Julian Higgins.
Funding: JEM is supported by an Australian National Health and Medical Research Council (NHMRC) Career Development Fellowship (1143429). SEB and RER’s positions are supported by the NHMRC Cochrane Collaboration Funding Program. HJT is funded by the UK Medical Research Council (MC_UU_12017-13 and MC_UU_12017-15) and Scottish Government Chief Scientist Office (SPHSU13 and SPHSU15). RVJ’s position is supported by the NHMRC Cochrane Collaboration Funding Program and Cabrini Institute. JT is supported by the National Institute for Health Research (NIHR) Collaboration for Leadership in Applied Health Research and Care North Thames at Barts Health NHS Trust. The views expressed are those of the author(s) and not necessarily those of the NHS, the NIHR or the Department of Health.
3.6 References
Bailey JV, Murray E, Rait G, Mercer CH, Morris RW, Peacock R, Cassell J, Nazareth I. Interactive computer-based interventions for sexual health promotion. Cochrane Database of Systematic Reviews 2010; 9 : CD006483.
Bender R, Bunce C, Clarke M, Gates S, Lange S, Pace NL, Thorlund K. Attention should be given to multiplicity issues in systematic reviews. Journal of Clinical Epidemiology 2008; 61 : 857–865.
Buchbinder R, Johnston RV, Rischin KJ, Homik J, Jones CA, Golmohammadi K, Kallmes DF. Percutaneous vertebroplasty for osteoporotic vertebral compression fracture. Cochrane Database of Systematic Reviews 2018; 4 : CD006349.
Caldwell DM, Welton NJ. Approaches for synthesising complex mental health interventions in meta-analysis. Evidence-Based Mental Health 2016; 19 : 16–21.
Chamberlain C, O’Mara-Eves A, Porter J, Coleman T, Perlen S, Thomas J, McKenzie J. Psychosocial interventions for supporting women to stop smoking in pregnancy. Cochrane Database of Systematic Reviews 2017; 2 : CD001055.
Ciciriello S, Johnston RV, Osborne RH, Wicks I, deKroo T, Clerehan R, O’Neill C, Buchbinder R. Multimedia educational interventions for consumers about prescribed and over-the-counter medications. Cochrane Database of Systematic Reviews 2013; 4 : CD008416.
Cochrane Consumers & Communication Group. Outcomes of Interest to the Cochrane Consumers & Communication Group: taxonomy. http://cccrg.cochrane.org/ .
COnsensus-based Standards for the selection of health Measurement INstruments (COSMIN) initiative. COSMIN database of systematic reviews of outcome measurement instruments. https://database.cosmin.nl/ .
Coulter A, Entwistle VA, Eccles A, Ryan S, Shepperd S, Perera R. Personalised care planning for adults with chronic or long-term health conditions. Cochrane Database of Systematic Reviews 2015; 3 : CD010523.
Davey J, Turner RM, Clarke MJ, Higgins JPT. Characteristics of meta-analyses and their component studies in the Cochrane Database of Systematic Reviews: a cross-sectional, descriptive analysis. BMC Medical Research Methodology 2011; 11 : 160.
Desroches S, Lapointe A, Ratte S, Gravel K, Legare F, Turcotte S. Interventions to enhance adherence to dietary advice for preventing and managing chronic diseases in adults. Cochrane Database of Systematic Reviews 2013; 2 : CD008722.
Deyo RA, Dworkin SF, Amtmann D, Andersson G, Borenstein D, Carragee E, Carrino J, Chou R, Cook K, DeLitto A, Goertz C, Khalsa P, Loeser J, Mackey S, Panagis J, Rainville J, Tosteson T, Turk D, Von Korff M, Weiner DK. Report of the NIH Task Force on research standards for chronic low back pain. Journal of Pain 2014; 15 : 569–585.
Dodd S, Clarke M, Becker L, Mavergames C, Fish R, Williamson PR. A taxonomy has been developed for outcomes in medical research to help improve knowledge discovery. Journal of Clinical Epidemiology 2018; 96 : 84–92.
Fisher DJ, Carpenter JR, Morris TP, Freeman SC, Tierney JF. Meta-analytical methods to identify who benefits most from treatments: daft, deluded, or deft approach? BMJ 2017; 356 : j573.
Fransen M, McConnell S, Harmer AR, Van der Esch M, Simic M, Bennell KL. Exercise for osteoarthritis of the knee. Cochrane Database of Systematic Reviews 2015; 1 : CD004376.
Guise JM, Chang C, Viswanathan M, Glick S, Treadwell J, Umscheid CA. Systematic reviews of complex multicomponent health care interventions. Report No. 14-EHC003-EF . Rockville, MD: Agency for Healthcare Research and Quality; 2014.
Harrison JK, Noel-Storr AH, Demeyere N, Reynish EL, Quinn TJ. Outcomes measures in a decade of dementia and mild cognitive impairment trials. Alzheimer’s Research and Therapy 2016; 8 : 48.
Hedges LV, Tipton E, Johnson M, C. Robust variance estimation in meta-regression with dependent effect size estimates. Research Synthesis Methods 2010; 1 : 39–65.
Hetrick SE, McKenzie JE, Cox GR, Simmons MB, Merry SN. Newer generation antidepressants for depressive disorders in children and adolescents. Cochrane Database of Systematic Reviews 2012; 11 : CD004851.
Higgins JPT, López-López JA, Becker BJ, Davies SR, Dawson S, Grimshaw JM, McGuinness LA, Moore THM, Rehfuess E, Thomas J, Caldwell DM. Synthesizing quantitative evidence in systematic reviews of complex health interventions. BMJ Global Health 2019; 4 : e000858.
Hoffmann T, Glasziou P, Barbour V, Macdonald H. Better reporting of interventions: template for intervention description and replication (TIDieR) checklist and guide. BMJ 2014; 1687 : 1-13.
Hollands GJ, Shemilt I, Marteau TM, Jebb SA, Lewis HB, Wei Y, Higgins JPT, Ogilvie D. Portion, package or tableware size for changing selection and consumption of food, alcohol and tobacco. Cochrane Database of Systematic Reviews 2015; 9 : CD011045.
Howe TE, Shea B, Dawson LJ, Downie F, Murray A, Ross C, Harbour RT, Caldwell LM, Creed G. Exercise for preventing and treating osteoporosis in postmenopausal women. Cochrane Database of Systematic Reviews 2011; 7 : CD000333.
ICHOM. The International Consortium for Health Outcomes Measurement 2018. http://www.ichom.org/ .
IPDAS. International Patient Decision Aid Standards Collaboration (IPDAS) standards. www.ipdas.ohri.ca .
Ivers N, Jamtvedt G, Flottorp S, Young JM, Odgaard-Jensen J, French SD, O’Brien MA, Johansen M, Grimshaw J, Oxman AD. Audit and feedback: effects on professional practice and healthcare outcomes. Cochrane Database of Systematic Reviews 2012; 6 : CD000259.
Janmaat VT, Steyerberg EW, van der Gaast A, Mathijssen RH, Bruno MJ, Peppelenbosch MP, Kuipers EJ, Spaander MC. Palliative chemotherapy and targeted therapies for esophageal and gastroesophageal junction cancer. Cochrane Database of Systematic Reviews 2017; 11 : CD004063.
Kendrick D, Kumar A, Carpenter H, Zijlstra GAR, Skelton DA, Cook JR, Stevens Z, Belcher CM, Haworth D, Gawler SJ, Gage H, Masud T, Bowling A, Pearl M, Morris RW, Iliffe S, Delbaere K. Exercise for reducing fear of falling in older people living in the community. Cochrane Database of Systematic Reviews 2014; 11 : CD009848.
Kirkham JJ, Gargon E, Clarke M, Williamson PR. Can a core outcome set improve the quality of systematic reviews? A survey of the Co-ordinating Editors of Cochrane Review Groups. Trials 2013; 14 : 21.
Konstantopoulos S. Fixed effects and variance components estimation in three-level meta-analysis. Research Synthesis Methods 2011; 2 : 61–76.
Lamb SE, Becker C, Gillespie LD, Smith JL, Finnegan S, Potter R, Pfeiffer K. Reporting of complex interventions in clinical trials: development of a taxonomy to classify and describe fall-prevention interventions. Trials 2011; 12 : 125.
Lewin S, Hendry M, Chandler J, Oxman AD, Michie S, Shepperd S, Reeves BC, Tugwell P, Hannes K, Rehfuess EA, Welch V, Mckenzie JE, Burford B, Petkovic J, Anderson LM, Harris J, Noyes J. Assessing the complexity of interventions within systematic reviews: development, content and use of a new tool (iCAT_SR). BMC Medical Research Methodology 2017; 17 : 76.
López-López JA, Page MJ, Lipsey MW, Higgins JPT. Dealing with multiplicity of effect sizes in systematic reviews and meta-analyses. Research Synthesis Methods 2018; 9 : 336–351.
Mavridis D, Salanti G. A practical introduction to multivariate meta-analysis. Statistical Methods in Medical Research 2013; 22 : 133–158.
Michie S, van Stralen M, West R. The Behaviour Change Wheel: a new method for characterising and designing behaviour change interventions. Implementation Science 2011; 6 : 42.
Michie S, Richardson M, Johnston M, Abraham C, Francis J, Hardeman W, Eccles MP, Cane J, Wood CE. The behavior change technique taxonomy (v1) of 93 hierarchically clustered techniques: building an international consensus for the reporting of behavior change interventions. Annals of Behavioral Medicine 2013; 46 : 81–95.
Moraes VY, Lenza M, Tamaoki MJ, Faloppa F, Belloti JC. Platelet-rich therapies for musculoskeletal soft tissue injuries. Cochrane Database of Systematic Reviews 2014; 4 : CD010071.
O'Neill J, Tabish H, Welch V, Petticrew M, Pottie K, Clarke M, Evans T, Pardo Pardo J, Waters E, White H, Tugwell P. Applying an equity lens to interventions: using PROGRESS ensures consideration of socially stratifying factors to illuminate inequities in health. Journal of Clinical Epidemiology 2014; 67 : 56–64.
Pompoli A, Furukawa TA, Imai H, Tajika A, Efthimiou O, Salanti G. Psychological therapies for panic disorder with or without agoraphobia in adults: a network meta-analysis. Cochrane Database of Systematic Reviews 2016; 4 : CD011004.
Pompoli A, Furukawa TA, Efthimiou O, Imai H, Tajika A, Salanti G. Dismantling cognitive-behaviour therapy for panic disorder: a systematic review and component network meta-analysis. Psychological Medicine 2018; 48 : 1–9.
Reeves BC, Wells GA, Waddington H. Quasi-experimental study designs series-paper 5: a checklist for classifying studies evaluating the effects on health interventions – a taxonomy without labels. Journal of Clinical Epidemiology 2017; 89 : 30–42.
Regnaux J-P, Lefevre-Colau M-M, Trinquart L, Nguyen C, Boutron I, Brosseau L, Ravaud P. High-intensity versus low-intensity physical activity or exercise in people with hip or knee osteoarthritis. Cochrane Database of Systematic Reviews 2015; 10 : CD010203.
Richards SH, Anderson L, Jenkinson CE, Whalley B, Rees K, Davies P, Bennett P, Liu Z, West R, Thompson DR, Taylor RS. Psychological interventions for coronary heart disease. Cochrane Database of Systematic Reviews 2017; 4 : CD002902.
Safi S, Korang SK, Nielsen EE, Sethi NJ, Feinberg J, Gluud C, Jakobsen JC. Beta-blockers for heart failure. Cochrane Database of Systematic Reviews 2017; 12 : CD012897.
Santesso N, Carrasco-Labra A, Brignardello-Petersen R. Hip protectors for preventing hip fractures in older people. Cochrane Database of Systematic Reviews 2014; 3 : CD001255.
Shepherd E, Gomersall JC, Tieu J, Han S, Crowther CA, Middleton P. Combined diet and exercise interventions for preventing gestational diabetes mellitus. Cochrane Database of Systematic Reviews 2017; 11 : CD010443.
Squires J, Valentine J, Grimshaw J. Systematic reviews of complex interventions: framing the review question. Journal of Clinical Epidemiology 2013; 66 : 1215–1222.
Stacey D, Légaré F, Lewis K, Barry MJ, Bennett CL, Eden KB, Holmes-Rovner M, Llewellyn-Thomas H, Lyddiatt A, Thomson R, Trevena L. Decision aids for people facing health treatment or screening decisions. Cochrane Database of Systematic Reviews 2017; 4 : CD001431.
Stroke Unit Trialists Collaboration. Organised inpatient (stroke unit) care for stroke. Cochrane Database of Systematic Reviews 2013; 9 : CD000197.
Taylor AJ, Jones LJ, Osborn DA. Zinc supplementation of parenteral nutrition in newborn infants. Cochrane Database of Systematic Reviews 2017; 2 : CD012561.
Valentine JC, Thompson SG. Issues relating to confounding and meta-analysis when including non-randomized studies in systematic reviews on the effects of interventions. Research Synthesis Methods 2013; 4 : 26–35.
Vaona A, Banzi R, Kwag KH, Rigon G, Cereda D, Pecoraro V, Tramacere I, Moja L. E-learning for health professionals. Cochrane Database of Systematic Reviews 2018; 1 : CD011736.
Verheyden GSAF, Weerdesteyn V, Pickering RM, Kunkel D, Lennon S, Geurts ACH, Ashburn A. Interventions for preventing falls in people after stroke. Cochrane Database of Systematic Reviews 2013; 5 : CD008728.
Weisz JR, Kuppens S, Ng MY, Eckshtain D, Ugueto AM, Vaughn-Coaxum R, Jensen-Doss A, Hawley KM, Krumholz Marchette LS, Chu BC, Weersing VR, Fordwood SR. What five decades of research tells us about the effects of youth psychological therapy: a multilevel meta-analysis and implications for science and practice. American Psychologist 2017; 72 : 79–117.
Welch V, Petkovic J, Simeon R, Presseau J, Gagnon D, Hossain A, Pardo Pardo J, Pottie K, Rader T, Sokolovski A, Yoganathan M, Tugwell P, DesMeules M. Interactive social media interventions for health behaviour change, health outcomes, and health equity in the adult population. Cochrane Database of Systematic Reviews 2018; 2 : CD012932.
Welton NJ, Caldwell DM, Adamopoulos E, Vedhara K. Mixed treatment comparison meta-analysis of complex interventions: psychological interventions in coronary heart disease. American Journal of Epidemiology 2009; 169 : 1158–1165.
Williamson PR, Altman DG, Bagley H, Barnes KL, Blazeby JM, Brookes ST, Clarke M, Gargon E, Gorst S, Harman N, Kirkham JJ, McNair A, Prinsen CAC, Schmitt J, Terwee CB, Young B. The COMET Handbook: version 1.0. Trials 2017; 18 : 280.
For permission to re-use material from the Handbook (either academic or commercial), please see here for full details.
Systematic Reviews: Inclusion and Exclusion Criteria
- What Type of Review is Right for You?
- What is in a Systematic Review
- Finding and Appraising Systematic Reviews
- Formulating Your Research Question
- Inclusion and Exclusion Criteria
- Creating a Protocol
- Results and PRISMA Flow Diagram
- Searching the Published Literature
- Searching the Gray Literature
- Methodology and Documentation
- Managing the Process
- Scoping Reviews
Defining Inclusion/Exclusion Criteria
An important part of the SR process is defining what will and will not be included in your review.
Inclusion and exclusion criteria are developed after a research question is finalized but before a search is carried out. They determine the limits for the evidence synthesis and are typically reported in the methods section of the publication. For unfamiliar or unclear concepts, a definition may be necessary to adequately describe the criterion for readers.
Some examples of common inclusion/exclusion criteria might be:
- Date of publication : only articles published in the last ten years
- Exposure to intervention/ or specific health condition : only people who have participated in the DASH diet
- Language of Publication* : only looking at English articles
- Settings : Hospitals, nursing homes, schools
- Geography : specific locations such as states, countries, or specific populations
*note of caution: research is published all over the world and in multiple languages. Limiting to just English can be considered a bias to your research.
- Common Inclusion/Exclusion Criteria from the University of Melbourne
What happens if no study meets my inclusion/exclusion criteria?
Empty reviews are when no studies meet the inclusion criteria for a SR. Empty reviews are more likely to subject to publication bias, however, they are important in identifying gaps in the literature.
- Unanswered questions implications of an empty review Slyer, Jason T. Unanswered questions, JBI Database of Systematic Reviews and Implementation Reports: June 2016 - Volume 14 - Issue 6 - p 1-2 doi: 10.11124/JBISRIR-2016-002934
- Rapid Prompting Method and Autism Spectrum Disorder: Systematic Review Exposes Lack of Evidence Schlosser, R.W., Hemsley, B., Shane, H. et al. Rapid Prompting Method and Autism Spectrum Disorder: Systematic Review Exposes Lack of Evidence. Rev J Autism Dev Disord 6, 403–412 (2019).
- << Previous: Formulating Your Research Question
- Next: Creating a Protocol >>
- Last Updated: Aug 7, 2024 12:36 PM
- URL: https://guides.lib.lsu.edu/Systematic_Reviews
Provide Website Feedback Accessibility Statement

Evidence-Based Practice (EBP)
- The EBP Process
- Forming a Clinical Question
- Inclusion & Exclusion Criteria
- Acquiring Evidence
- Appraising the Quality of the Evidence
- Writing a Literature Review
- Finding Psychological Tests & Assessment Instruments
Selection Criteria

Inclusion and exclusion criteria are determined after formulating the research question but usually before the search is conducted (although preliminary scoping searches may need to be undertaken to determine appropriate criteria). It may be helpful to determine the inclusion criteria and exclusion criteria for each PICO component.
Be aware that you may introduce bias into the final review if these are not used thoughtfully.
Inclusion and exclusion are two sides of the same coin, so—depending on your perspective—a single database filter can be said to either include or exclude. For instance, if articles must be published within the last 3 years, that is inclusion. If articles cannot be more than 3 years old, that is exclusion.
The most straightforward way to include or exclude results is to use database limiters (filters), usually found on the left side of the search results page.
Inclusion Criteria
Inclusion criteria are the elements of an article that must be present in order for it to be eligible for inclusion in a literature review. Some examples are:
- Included studies must have compared certain treatments
- Included studies must be a certain type (e.g., only Randomized Controlled Trials)
- Included studies must be located in a certain geographic area
- Included studies must have been published in the last 5 years
Exclusion Criteria
Exclusion criteria are the elements of an article that disqualify the study from inclusion in a literature review. Some examples are:
- Study used an observational design
- Study used a qualitative methodology
- Study was published more than 5 years ago
- Study was published in a language other than English
- << Previous: Forming a Clinical Question
- Next: Acquiring Evidence >>
- Last Updated: May 16, 2024 2:44 PM
- URL: https://libguides.umsl.edu/ebp

An official website of the United States government
The .gov means it’s official. Federal government websites often end in .gov or .mil. Before sharing sensitive information, make sure you’re on a federal government site.
The site is secure. The https:// ensures that you are connecting to the official website and that any information you provide is encrypted and transmitted securely.
- Publications
- Account settings
- My Bibliography
- Collections
- Citation manager
Save citation to file
Email citation, add to collections.
- Create a new collection
- Add to an existing collection
Add to My Bibliography
Your saved search, create a file for external citation management software, your rss feed.
- Search in PubMed
- Search in NLM Catalog
- Add to Search
Inclusion and exclusion criteria in research studies: definitions and why they matter
Affiliation.
- 1 Methods in Epidemiologic, Clinical, and Operations Research-MECOR-program, American Thoracic Society/Asociación Latinoamericana del Tórax, Montevideo, Uruguay.
- PMID: 29791550
- PMCID: PMC6044655
- DOI: 10.1590/s1806-37562018000000088
PubMed Disclaimer
Similar articles
- The generalizability of clinical and experimental tobacco research: Do exclusion criteria impact men and women differently? Spinella TC, Perry RN, Schlagintweit HE, Barrett SP. Spinella TC, et al. J Psychopharmacol. 2020 May;34(5):584-585. doi: 10.1177/0269881119900984. Epub 2020 Jan 22. J Psychopharmacol. 2020. PMID: 31965900 No abstract available.
- Exclusion criteria in treatment research on alcohol, tobacco and illicit drug use disorders: A review and critical analysis. Moberg CA, Humphreys K. Moberg CA, et al. Drug Alcohol Rev. 2017 May;36(3):378-388. doi: 10.1111/dar.12438. Epub 2016 Jun 21. Drug Alcohol Rev. 2017. PMID: 27324921 Review.
- The European Union Directive on Clinical Research: present status of implementation in EU member states' legislations with regard to the incompetent patient. Lemaire F, Bion J, Blanco J, Damas P, Druml C, Falke K, Kesecioglu J, Larsson A, Mancebo J, Matamis D, Pesenti A, Pimentel J, Ranieri M; ESICM Task Force on Legislation Affecting Clinical Research in the Critically Ill Patient. Lemaire F, et al. Intensive Care Med. 2005 Mar;31(3):476-9. doi: 10.1007/s00134-005-2574-8. Epub 2005 Feb 15. Intensive Care Med. 2005. PMID: 15711974 No abstract available.
- Impact of patient selection in various study designs: identifying potential bias in clinical results. Berbano EP, Baxi N. Berbano EP, et al. South Med J. 2012 Mar;105(3):149-55. doi: 10.1097/SMJ.0b013e31824b4690. South Med J. 2012. PMID: 22392211 Review.
- Standardized patient method in tuberculosis research. Grace GA, Devaleenal DB. Grace GA, et al. Natl Med J India. 2017 Jul-Aug;30(4):210-211. Natl Med J India. 2017. PMID: 29162755 No abstract available.
- Efficacy of Benson's Relaxation Technique on Stress and Pain Among Patients Undergoing Maintenance Hemodialysis: A Systematic Review. Abu Maloh HIA, Soh KL, Chong SC, Ismail SIF, Soh KG, Abu Maloh DI, AbuRuz ME. Abu Maloh HIA, et al. SAGE Open Nurs. 2024 May 6;10:23779608241251663. doi: 10.1177/23779608241251663. eCollection 2024 Jan-Dec. SAGE Open Nurs. 2024. PMID: 38715771 Free PMC article. Review.
- ROUTE-T1D: A behavioral intervention to promote optimal continuous glucose monitor use among racially minoritized youth with type 1 diabetes: Design and development. Straton E, Bryant BL, Kang L, Wang C, Barber J, Perkins A, Gallant L, Marks B, Agarwal S, Majidi S, Monaghan M, Streisand R. Straton E, et al. Contemp Clin Trials. 2024 May;140:107493. doi: 10.1016/j.cct.2024.107493. Epub 2024 Mar 7. Contemp Clin Trials. 2024. PMID: 38460913 Clinical Trial.
- Long Non-coding RNA X-Inactive Specific Transcript Promotes Esophageal Squamous Cell Carcinoma Progression via the MicroRNA 34a/Zinc Finger E-box-Binding Homeobox 1 Pathway. Guo B, He M, Ma M, Tian Z, Jin J, Tian G. Guo B, et al. Dig Dis Sci. 2024 Apr;69(4):1169-1181. doi: 10.1007/s10620-024-08269-0. Epub 2024 Feb 16. Dig Dis Sci. 2024. PMID: 38366093 Free PMC article.
- Study protocol for the family empowerment program: a randomized waitlist-controlled trial to evaluate the effectiveness of online Community Reinforcement and Family Training (CRAFT) on the wellbeing of family members with a relative experiencing substance dependence and mental illness. Allan J, Snowdon N, Thapa S, Ahmed KY. Allan J, et al. BMC Psychiatry. 2024 Jan 10;24(1):43. doi: 10.1186/s12888-023-05487-0. BMC Psychiatry. 2024. PMID: 38200508 Free PMC article.
- Appraising systematic reviews: a comprehensive guide to ensuring validity and reliability. Shaheen N, Shaheen A, Ramadan A, Hefnawy MT, Ramadan A, Ibrahim IA, Hassanein ME, Ashour ME, Flouty O. Shaheen N, et al. Front Res Metr Anal. 2023 Dec 21;8:1268045. doi: 10.3389/frma.2023.1268045. eCollection 2023. Front Res Metr Anal. 2023. PMID: 38179256 Free PMC article. Review.
- Montes de Oca M.Menezes A.Wehrmeister FC.Lopez Varela MV.Casas A.Ugalde L Adherence to inhaled therapies of COPD patients from seven Latin American countries The LASSYC study. PLoS One. 2017;12(11):e0186777. doi: 10.1371/journal.pone.0186777. - DOI - PMC - PubMed
- Hulley SB, Cummings SR, Browner WS, Grady DG, Newman TB. Designing Clinical Research. PA: Lippincott Williams & Wilkins; 2007.
- Search in MeSH
Related information
- Cited in Books
LinkOut - more resources
Full text sources.
- Europe PubMed Central
- PubMed Central
- Scientific Electronic Library Online
Other Literature Sources
- scite Smart Citations
- Citation Manager
NCBI Literature Resources
MeSH PMC Bookshelf Disclaimer
The PubMed wordmark and PubMed logo are registered trademarks of the U.S. Department of Health and Human Services (HHS). Unauthorized use of these marks is strictly prohibited.

Systematic Reviews
- Introduction to Systematic Reviews
- Systematic review
- Systematic literature review
- Scoping review
- Rapid evidence assessment / review
- Evidence and gap mapping exercise
- Meta-analysis
- Systematic Reviews in Science and Engineering
- Timescales and processes
- Question frameworks (e.g PICO)
Inclusion and exclusion criteria
- Using grey literature
- Search Strategy This link opens in a new window
- Subject heading searching (e.g MeSH)
- Database video & help guides This link opens in a new window
- Documenting your search and results
- Data management
- How the library can help
- Systematic reviews A to Z

Inclusion and exclusion criteria are a list of pre-defined characteristics to which literature must adhere to be included in a study. They are vital for the decision-making progress on what to review when undertaking a systematic review and will also help with systematic literature reviews.
You should be able to establish your inclusion/exclusion criteria during the process of defining your question. These criteria clearly demonstrate the scope of the study and provide justification for the exclusion of any information that does not meet these characteristics.
Example criteria
- Intervention, treatment, process or experience
- Reported outcomes
- Research methodology
- Participants
- Age of study
- Sample size
- Place of study
- Type of publication
E.g. stage 4 lung disease patients
E.g. whether the study's reported outcomes are relevant to your study and have been presented objectively
E.g. randomised control trial
E.g. age, sex ethnicity etc.
E.g. last 5 years
E.g. over 100 participants
E.g. UK based
E.g. primary research, peer-reviewed
E.g. community-based care
E.g. English
Precision vs Sensitivity
You should aim to be as extensive as possible when conducting searches for systematic reviews. However, it may be necessary to strike a balance between the sensitivity and precision of your search.
- Sensitivity – the number of relevant results identified divided by the total number of relevant results in existence
- Precision - the number of relevant results identified divided by the total number of results identified.
Increasing the comprehensiveness of a search will reduce its precision and will retrieve more non-relevant results. However,
... at a conservatively-estimated reading rate of two abstracts per minute, the results of a database search can be ‘scanread’ at the rate of 120 per hour (or approximately 1000 over an 8-hour period), so the high yield and low precision associated with systematic review searching is not as daunting as it might at first appear in comparison with the total time to be invested in the review. ( Cochrane Handbook for Systematic Reviews of Interventions, 2008, Section 6.4.4 )
- << Previous: Question frameworks (e.g PICO)
- Next: Where to search >>
- Last Updated: Jan 23, 2024 10:52 AM
- URL: https://plymouth.libguides.com/systematicreviews

Library guides
Our library guides bring together the essential resources in your subject area and connect you quickly and easily to information about Library Services
CityLibrary Search
Literature searching and finding evidence.
- Literature searching or literature review?
- Use the PICO or PEO frameworks
Establish your Inclusion and Exclusion criteria
- Find related search terms
- Subject Heading/MeSH Searching
- Select databases to search
- Structure your search
- Search techniques
- Search key databases
- Manage results in EBSCOhost and Ovid
- Analyse your search results
- Document your search results
- Training and support
These criteria help you decide which pieces of evidence (for example, which primary research studies) will/will not be included in your work. Using specific criteria will help make sure your final review is as unbiased, transparent and ethical as possible.
How to establish your Inclusion and Exclusion criteria
To establish your criteria you need to define each aspect of your question to clarify what you are focusing on, and consider if there are any variations you also wish to explore. This is where using frameworks like PICO help:
Example: Alternatives to drugs for controlling headaches in children.
Using the PICO structure you clarify what aspects you are most interested in. Here are some examples to consider:
|
|
|
---|---|---|
| Children | A specific age group? Teenagers and adolescents? |
| Alternatives to drugs | What alternatives are there? Complementary therapies? Alternative medicines? Changes in lifestyle? All three? If you decide to focus on 'complementary therapies' do you want to examine all therapies or a specific therapy like holistic therapy? |
| Drugs | All drugs that treat headaches, or a group of drugs, or a specific drug? |
| Headaches | All types of headaches, or a specific type such as tension headaches or migraines? |
The aspects of the topic you decide to focus on are the Inclusion criteria.
The aspects you don't wish to include are the Exclusion criteria.
- << Previous: Use the PICO or PEO frameworks
- Next: Find related search terms >>
- Last Updated: Aug 23, 2024 3:26 PM
- URL: https://libguides.city.ac.uk/SHS-Litsearchguide
Log in using your username and password
- Search More Search for this keyword Advanced search
- Latest content
- Current issue
- Write for Us
- BMJ Journals
You are here
- Volume 19, Issue 1
- Reviewing the literature
- Article Text
- Article info
- Citation Tools
- Rapid Responses
- Article metrics

- Joanna Smith 1 ,
- Helen Noble 2
- 1 School of Healthcare, University of Leeds , Leeds , UK
- 2 School of Nursing and Midwifery, Queens's University Belfast , Belfast , UK
- Correspondence to Dr Joanna Smith , School of Healthcare, University of Leeds, Leeds LS2 9JT, UK; j.e.smith1{at}leeds.ac.uk
https://doi.org/10.1136/eb-2015-102252
Statistics from Altmetric.com
Request permissions.
If you wish to reuse any or all of this article please use the link below which will take you to the Copyright Clearance Center’s RightsLink service. You will be able to get a quick price and instant permission to reuse the content in many different ways.
Implementing evidence into practice requires nurses to identify, critically appraise and synthesise research. This may require a comprehensive literature review: this article aims to outline the approaches and stages required and provides a working example of a published review.
Are there different approaches to undertaking a literature review?
What stages are required to undertake a literature review.
The rationale for the review should be established; consider why the review is important and relevant to patient care/safety or service delivery. For example, Noble et al 's 4 review sought to understand and make recommendations for practice and research in relation to dialysis refusal and withdrawal in patients with end-stage renal disease, an area of care previously poorly described. If appropriate, highlight relevant policies and theoretical perspectives that might guide the review. Once the key issues related to the topic, including the challenges encountered in clinical practice, have been identified formulate a clear question, and/or develop an aim and specific objectives. The type of review undertaken is influenced by the purpose of the review and resources available. However, the stages or methods used to undertake a review are similar across approaches and include:
Formulating clear inclusion and exclusion criteria, for example, patient groups, ages, conditions/treatments, sources of evidence/research designs;
Justifying data bases and years searched, and whether strategies including hand searching of journals, conference proceedings and research not indexed in data bases (grey literature) will be undertaken;
Developing search terms, the PICU (P: patient, problem or population; I: intervention; C: comparison; O: outcome) framework is a useful guide when developing search terms;
Developing search skills (eg, understanding Boolean Operators, in particular the use of AND/OR) and knowledge of how data bases index topics (eg, MeSH headings). Working with a librarian experienced in undertaking health searches is invaluable when developing a search.
Once studies are selected, the quality of the research/evidence requires evaluation. Using a quality appraisal tool, such as the Critical Appraisal Skills Programme (CASP) tools, 5 results in a structured approach to assessing the rigour of studies being reviewed. 3 Approaches to data synthesis for quantitative studies may include a meta-analysis (statistical analysis of data from multiple studies of similar designs that have addressed the same question), or findings can be reported descriptively. 6 Methods applicable for synthesising qualitative studies include meta-ethnography (themes and concepts from different studies are explored and brought together using approaches similar to qualitative data analysis methods), narrative summary, thematic analysis and content analysis. 7 Table 1 outlines the stages undertaken for a published review that summarised research about parents’ experiences of living with a child with a long-term condition. 8
- View inline
An example of rapid evidence assessment review
In summary, the type of literature review depends on the review purpose. For the novice reviewer undertaking a review can be a daunting and complex process; by following the stages outlined and being systematic a robust review is achievable. The importance of literature reviews should not be underestimated—they help summarise and make sense of an increasingly vast body of research promoting best evidence-based practice.
- ↵ Centre for Reviews and Dissemination . Guidance for undertaking reviews in health care . 3rd edn . York : CRD, York University , 2009 .
- ↵ Canadian Best Practices Portal. http://cbpp-pcpe.phac-aspc.gc.ca/interventions/selected-systematic-review-sites / ( accessed 7.8.2015 ).
- Bridges J , et al
- ↵ Critical Appraisal Skills Programme (CASP). http://www.casp-uk.net / ( accessed 7.8.2015 ).
- Dixon-Woods M ,
- Shaw R , et al
- Agarwal S ,
- Jones D , et al
- Cheater F ,
Twitter Follow Joanna Smith at @josmith175
Competing interests None declared.
Read the full text or download the PDF:

Selecting Studies for Systematic Review: Inclusion and Exclusion Criteria
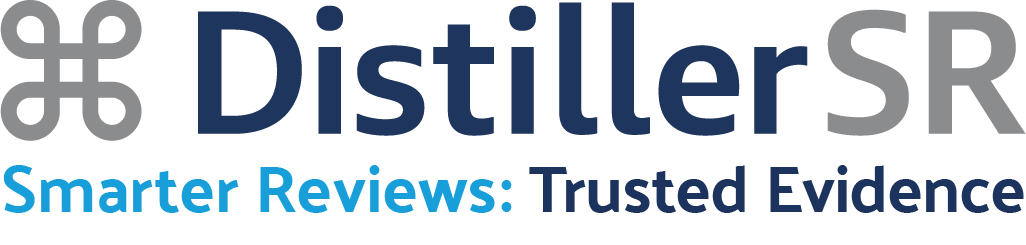
Automate every stage of your literature review to produce evidence-based research faster and more accurately.
One factor that can help make sure your review is of high quality, is the effective and appropriate selection of inclusion and exclusion criteria to be used in the systematic review. This vital factor has an impact on what is discussed and debated in your review. In this article, we explore what these criteria actually are, how you establish them, and why they are critical to the final publication.
What Are Inclusion And Exclusion Criteria?
Before identifying what criteria to include and what to exclude, it is always beneficial to establish what exactly is meant by both of those terms. In short, inclusion criteria are the characteristics that define the population eligible for a study, or that define the studies that will be eligible for inclusion in a systematic review. In contrast, exclusion criteria are a set of characteristics of studies that will not be included in the review. These inclusion and exclusion criteria help to evaluate the merit of the review in question.
How Do You Choose Inclusion And Exclusion Criteria?
Generally speaking, it is best to choose the inclusion criteria of your systematic review after you have determined what research question you are hoping to answer. When you choose the criteria to both to include and (importantly) exclude studies, your research question sets boundaries to it, so that the eligibility criteria are neither too broad nor too narrow.
Learn More About DistillerSR
(Article continues below)
Why are Inclusion And Exclusion Criteria Important In Systematic Review?
When you define what your inclusion criteria are, and outline what your exclusion criteria are, you are improving the chances of your systematic review producing reliable results. By identifying what can and cannot be included in your review, you reduce any ambiguity in your findings. When you reduce ambiguity, your findings are far more persuasive and less subject to debate, or criticism. As a result, you are ensuring that all the time you spent on your systematic review was worthwhile and any costs were worth the expense.
Appropriate choice of inclusion and exclusion criteria is also essential to prevent any inherent selection bias. Again, such biases can result in your final review losing its persuasive power, as your results are not considered reliable. An inherent bias can mean your findings and results are liable to a ‘data mining’ style scenario, where your research question cannot be answered fairly. It could mean that your research does not take into account key variables that may have better informed your final discussion.
In short, inclusion and exclusion criteria selection is not only important it is essential. By making effective selections, you guarantee that your research’s validity cannot be questioned.
Selecting Studies for Systematic Reviews
Conducting a systematic review can be a time-consuming process to generate evidence around specific areas of research. As a result, it may be tempting for some authors to make quick selections with regard to inclusion and exclusion criteria. This lapse in judgment could prove detrimental to the review process. Exclusion and inclusion criteria optimize the scope of your research and provide the necessary merit to your research question.
3 Reasons to Connect
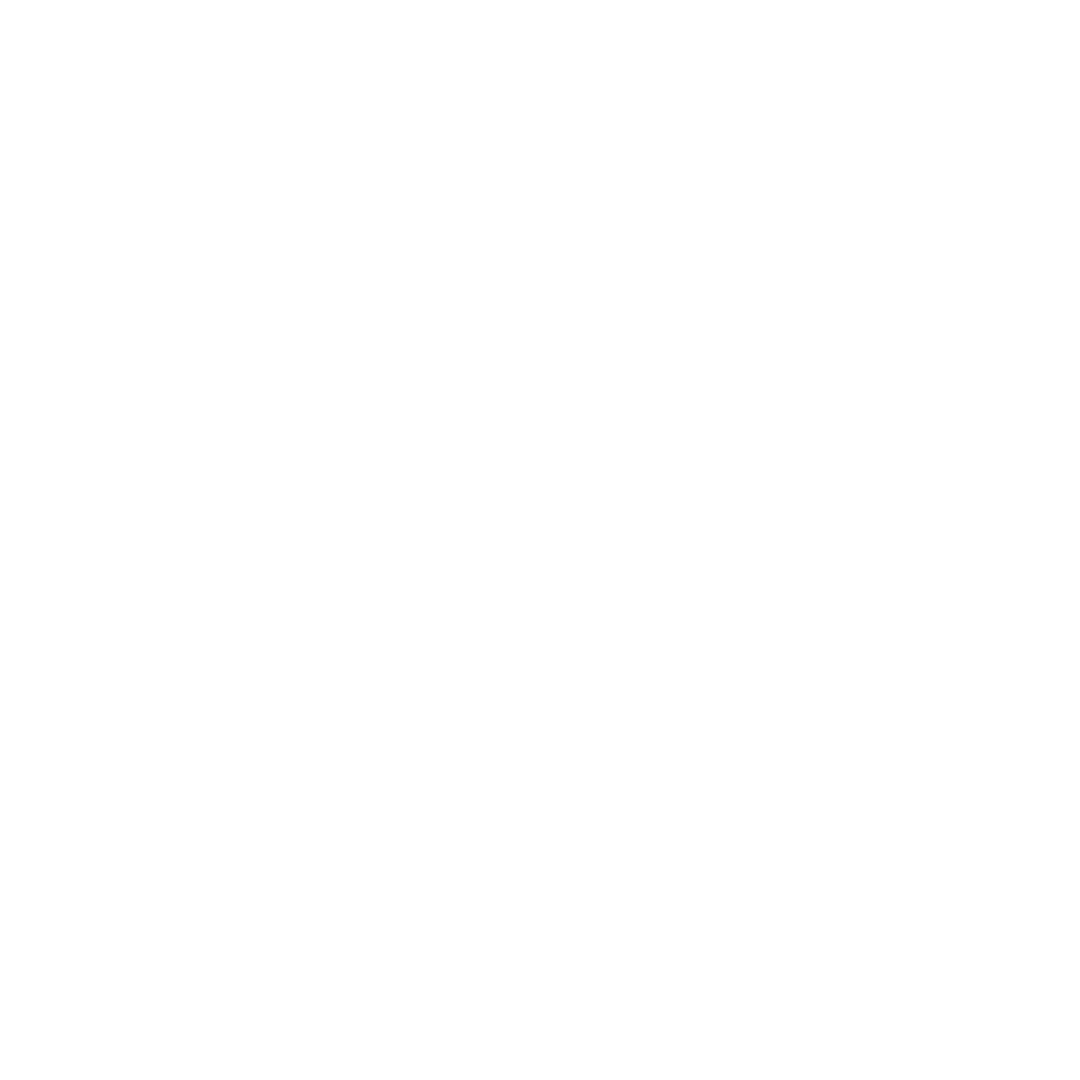
- Reserve a study room
- Library Account
- Undergraduate Students
- Graduate Students
- Faculty & Staff
How to Conduct a Literature Review (Health Sciences and Beyond)
- What is a Literature Review?
- Developing a Research Question
Selection Criteria
Inclusion criteria, exclusion criteria.
- Database Search
- Documenting Your Search
- Organize Key Findings
- Reference Management
You may want to think about criteria that will be used to select articles for your literature review based on your research question. These are commonly known as inclusion criteria and exclusion criteria . Be aware that you may introduce bias into the final review if these are not used thoughtfully.
Inclusion criteria are the elements of an article that must be present in order for it to be eligible for inclusion in a literature review. Some examples are:
- Included studies must have compared certain treatments
- Included studies must be experimental
- Included studies must have been published in the last 5 years
Exclusion criteria are the elements of an article that disqualify the study from inclusion in a literature review. Some examples are:
- Study used an observational design
- Study used a qualitative methodology
- Study was published more than 5 years ago
- Study was published in a language other than English
- << Previous: Developing a Research Question
- Next: Databases >>
- Last Updated: Mar 15, 2024 12:22 PM
- URL: https://guides.library.vcu.edu/health-sciences-lit-review
Europe PMC requires Javascript to function effectively.
Either your web browser doesn't support Javascript or it is currently turned off. In the latter case, please turn on Javascript support in your web browser and reload this page.

Literature Reviews: Systematic, Scoping, Integrative
- Introduction to Literature Reviews
- Creating a Search Strategy
Using Limits and Inclusion/Exclusion Criteria
Filters and Limits
Inclusion and Exclusion Criteria
Limits and Inclusion Criteria
- Review Protocols
- Elements of a Systematic Review
- Review Tools and Applications
- Writing a Literature Review
Ask A Librarian
Once you have some search results, you will need to decide which articles you will actually use in your literature review. This can be done using filters/limits in the databases, applying inclusion/exclusion criteria, and appraising the articles.
Filters and limits (the name varies by database) are tools the database provides to help you narrow your search results. Different databases offer different filters, but these are some of the more common ones you'll find.
- Publication year
- Language of the article
- Age of study subjects
- Study design
Your search terms and the filters/limits you apply are generally not enough to narrow your results to the most relevant and highest quality studies for your project. The final step to selecting these studies is to apply your inclusion and exclusion criteria. Basically, these are the reasons why you keep (include) or reject (exclude) articles as you look through the results, reading titles and abstracts (and sometimes the whole article)
Examples of types of Inclusion/Exclusion Criteria
- PICO(T) elements - if one of the main elements of your topic does not match those of the study, you may need to exclude it
- Age - if you can't use a filter/limit to exclude studies that do not focus on the age group you require, you may need to exclude those studies yourself.
- Setting - i.e. home, acute care, assisted living facility
- Study Design - sometimes a filter/limit doesn't exist for the study design you're interested in; in that case you'll need to look through articles to find that detail yourself.
- Number of subjects - do you have a minimum study group size?
- Study drop-out rate
- << Previous: Creating a Search Strategy
- Next: Review Protocols >>
- Last Updated: Aug 19, 2024 5:56 PM
- URL: https://libguides.massgeneral.org/reviews


An official website of the United States government
The .gov means it’s official. Federal government websites often end in .gov or .mil. Before sharing sensitive information, make sure you’re on a federal government site.
The site is secure. The https:// ensures that you are connecting to the official website and that any information you provide is encrypted and transmitted securely.
- Publications
- Account settings
Preview improvements coming to the PMC website in October 2024. Learn More or Try it out now .
- Advanced Search
- Journal List
- v.8(11); 2016 Nov

How to Conduct a Systematic Review: A Narrative Literature Review
Nusrat jahan.
1 Psychiatry, Mount Sinai Chicago
Sadiq Naveed
2 Psychiatry, KVC Prairie Ridge Hospital
Muhammad Zeshan
3 Department of Psychiatry, Bronx Lebanon Hospital Icahn School of Medicine at Mount Sinai, Bronx, NY
Muhammad A Tahir
4 Psychiatry, Suny Upstate Medical University, Syracuse, NY
Systematic reviews are ranked very high in research and are considered the most valid form of medical evidence. They provide a complete summary of the current literature relevant to a research question and can be of immense use to medical professionals. Our goal with this paper is to conduct a narrative review of the literature about systematic reviews and outline the essential elements of a systematic review along with the limitations of such a review.
Introduction and background
A literature review provides an important insight into a particular scholarly topic. It compiles published research on a topic, surveys different sources of research, and critically examines these sources [ 1 ]. A literature review may be argumentative, integrative, historical, methodological, systematic, or theoretical, and these approaches may be adopted depending upon the types of analysis in a particular study [ 2 ].
Our topic of interest in this article is to understand the different steps of conducting a systematic review. Systematic reviews, according to Wright, et al., are defined as a “review of the evidence on a clearly formulated question that uses systematic and explicit methods to identify, select and critically appraise relevant primary research, and to extract and analyze data from the studies that are included in the review” [ 3 ]. A systematic review provides an unbiased assessment of these studies [ 4 ]. Such reviews emerged in the 1970s in the field of social sciences. Systematic reviews, as well as the meta-analyses of the appropriate studies, can be the best form of evidence available to clinicians [ 3 ]. The unsystematic narrative review is more likely to include only research selected by the authors, which introduces bias and, therefore, frequently lags behind and contradicts the available evidence [ 5 ].
Epidemiologist Archie Cochrane played a vital role in formulating the methodology of the systematic review [ 6 ]. Dr. Cochrane loved to study patterns of disease and how these related to the environment. In the early 1970s, he found that many decisions in health care were made without reliable, up-to-date evidence about the treatments used [ 6 ].
A systematic review may or may not include meta-analysis, depending on whether results from different studies can be combined to provide a meaningful conclusion. David Sackett defined meta-analysis as a “specific statistical strategy for assembling the results of several studies into a single estimate” [ 7 - 8 ].
While the systematic review has several advantages, it has several limitations which can affect the conclusion. Inadequate literature searches and heterogeneous studies can lead to false conclusions. Similarly, the quality of assessment is an important step in systematic reviews, and it can lead to adverse consequences if not done properly.
The purpose of this article is to understand the important steps involved in conducting a systematic review of all kinds of clinical studies. We conducted a narrative review of the literature about systematic reviews with a special focus on articles that discuss conducting reviews of randomized controlled trials. We discuss key guidelines and important terminologies and present the advantages and limitations of systematic reviews.
Narrative reviews are a discussion of important topics on a theoretical point of view, and they are considered an important educational tool in continuing medical education [ 9 ]. Narrative reviews take a less formal approach than systematic reviews in that narrative reviews do not require the presentation of the more rigorous aspects characteristic of a systematic review such as reporting methodology, search terms, databases used, and inclusion and exclusion criteria [ 9 ]. With this in mind, our narrative review will give a detailed explanation of the important steps of a systematic review.
Preferred reporting items for systematic review and meta-analysis protocols (PRISMA-P) checklist
Systematic reviews are conducted based on predefined criteria and protocol. The PRISMA-P checklist, developed by Moher, et al., contains 17 items (26 including sub-items) comprising the important steps of a systematic review, including information about authors, co-authors, their mailing and email addresses, affiliations, and any new or updated version of a previous systematic review [ 9 ]. It also identifies a plan for documenting important protocol amendments, registry names, registration numbers, financial disclosures, and other support services [ 10 ]. Moher, et al. also state that methods of systematic reviews involve developing eligibility criteria and describing information sources, search strategies, study selection processes, outcomes, assessment of bias in individual studies, and data synthesis [ 10 ].
Research question
Writing a research question is the first step in conducting a systematic review and is of paramount importance as it outlines both the need and validity of systematic reviews (Nguyen, et al., unpublished data). It also increases the efficiency of the review by limiting the time and cost of identifying and obtaining relevant literature [ 11 ]. The research question should summarize the main objective of a systematic review.
An example research question might read, “How does attention-deficit/hyperactivity disorder (ADHD) affect the academic performance of middle school children in North America?” The question focuses on the type of data, analysis, and topic to be discussed (i.e., ADHD among North American middle school students). Try to avoid research questions that are too narrow or broad—they can lead to the selection of only a few studies and the ability to generalize results to any other populations may be limited. An example of a research question that is too narrow would be, “What is the prevalence of ADHD in children and adolescents in Chicago, IL?” Alternately, if the research question is too broad, it can be difficult to reach a conclusion due to poor methodology. An example of a research question that is too broad in scope would be, “What are the effects of ADHD on the functioning of children and adolescents in North America?”
Different tools that can be used to help devise a research question, depending on the type of question, are: population, intervention, comparator, and outcomes (PICO); sample, phenomenon of interest, design, evaluation, and research type (SPIDER); setting, perspective, intervention, comparison, and evaluation (SPICE); and expectation, client group, location, impact, professionals, and service (ECLIPSE).
The PICO approach is mostly used to compare different interventions with each other. It helps to formulate a research question related to prognosis, diagnosis, and therapies [ 12 ].
Scenario: A 50-year-old white woman visited her psychiatrist with a diagnosis of major depressive disorder. She was prescribed fluoxetine, which she feels has been helpful. However, she experienced some unpleasant side effects of nausea and abdominal discomfort. She has recently been told by a friend about the use of St. John’s wort in treating depression and would like to try this in treating her current depression. (Formulating research questions, unpublished data).
In the above-mentioned scenario, the sample population is a 50-year-old female with major depressive disorder; the intervention is St. John’s wort; the comparison is fluoxetine; and the outcome would be efficacy and safety. In order to see the outcome of both efficacy and safety, we will compare the efficacy and safety of both St. John’s wort and fluoxetine in a sample population for treating depression. This scenario represents an example where we can apply the PICO approach to compare two interventions.
In contrast, the SPIDER approach is focused more on study design and samples rather than populations [ 13 ]. The SPIDER approach can be used in this research question: “What is the experience of psychiatry residents attending a transgender education?” The sample is psychiatry residents; the phenomenon of interest is transgender education; the design is a survey; the evaluation looks at the experience; and the research type is qualitative.
The SPICE approach can be used to evaluate the outcome of a service, intervention, or project [ 14 ]. The SPICE approach applies to the following research question: “In psychiatry clinics, does the combined use of selective serotonin reuptake inhibitor (SSRI) and psychotherapy reduce depression in an outpatient clinic versus SSRI therapy alone?” The setting is the psychiatry clinic; the perspective/population is the outpatient; the intervention is combined psychotherapy and SSRI; the comparison is SSRI alone; and the evaluation is reduced depression.
The ECLIPSE approach is useful for evaluating the outcome of a policy or service (Nguyen, et al., unpublished data). ECLIPSE can apply in the following research question: “How can a resident get access to medical records of patients admitted to inpatient from other hospitals?” The expectation is: “What are you looking to improve/change to increase access to medical records for patients admitted to inpatient?” The client group is the residents; the location is the inpatient setting; the impact would be the residents having easy access to medical records from other hospitals; and the professionals in this scenario would be those involved in improving the service experiences such as hospital administrators and IT staff.
Inclusion and exclusion criteria
Establishing inclusion and exclusion criteria come after formulating research questions. The concept of inclusion and exclusion of data in a systematic review provides a basis on which the reviewer draws valid and reliable conclusions regarding the effect of the intervention for the disorder under consideration [ 11 ]. Inclusions and exclusion are based on preset criteria for specific systematic review. It should be done before starting the literature search in order to minimize the possibility of bias.
Eligibility criteria provide the boundaries of the systematic review [ 15 ]. Participants, interventions, and comparison of a research question provide the basis for eligibility criteria [ 15 ]. The inclusion criteria should be able to identify the studies of interest and, if the inclusion criteria are too broad or too narrow, it can lead to an ineffective screening process.
Protocol registration
Developing and registering research protocol is another important step of conducting a systematic review. The research protocol ensures that a systematic review is carefully planned and explicitly documented before the review starts, thus promoting consistency in conduct for the review team and supporting the accountability, research integrity, and transparency of the eventually completed review [ 10 ]. PROSPERO and the Cochrane Database of Systematic Reviews are utilized for registering research protocols and research questions, and they check for prior existing duplicate protocols or research questions. PROSPERO is an international database of prospectively registered systematic reviews related to health care and social sciences (PRISMA, 2016). It is funded by the National Institute for Health Research. The Cochrane Collaboration concentrates on producing systematic reviews of interventions and diagnostic test accuracy but does not currently produce reviews on questions of prognosis or etiology [ 16 ].
A detailed and extensive search strategy is important for the systematic review since it minimizes bias in the review process [ 17 ].
Selecting and searching appropriate electronic databases is determined by the topic of interest. Important databases are: MEDLARS Online (MEDLINE), which is the online counterpart to the Medical Literature Analysis and Retrieval System (MEDLARS); Excerpta Medica Database (EMBASE); and Google Scholar. There are multiple electronic databases available based on the area of interest. Other important databases include: PsycINFO for psychology and psychiatry; Allied and Complementary Medicine Database (AMED) for complementary medicine; Manual, Alternative, and Natural Therapy Index System (MANTIS) for alternative medical literature; and Cumulative Index to Nursing and Allied Health Literature (CINAHL) for nursing and allied health [ 15 ].
Additional studies relevant for the review may be found by looking at the references of studies identified by different databases [ 15 ]. Non-indexed articles may be found by searching the content of journals, conferences proceedings, and abstracts. It will also help with letters and commentaries which may not get indexed [ 15 ]. Reviewing clinical trial registries can provide information about any ongoing trials or unpublished research [ 15 ]. A gray literature search can access unpublished papers, reports, and conference reports, and it generally covers studies that are published in an informal fashion, rather than in an indexed journal [ 15 ]. Further search can be performed by selecting important key articles and going through in-text citations [ 15 ].
Using Boolean operators, truncation, and wildcards
Boolean operators use the relationship between different search words to help with the search strategy. These are simple words (i.e., AND, OR, and NOT) which can help with more focused and productive results (poster, Jahan, et al.: How to conduct a systematic review. APPNA 39th Summer Convention. Washington, DC. 2016). The Boolean operator AND finds articles with all the search words. The use of OR broadens the focus of the search, and it will include articles with at least one search term. The researchers can also ignore certain results from the records by using NOT in the search strategy.
An example of AND would be using “depression” AND “children” in the search strategy with the goal of studying depression in children. This search strategy will include all the articles about both depression and children. The researchers may use OR if the emphasis of the study is mood disorders or affective disorders in adolescents. In that case, the search strategy will be “mood disorders” OR “affective disorders” AND “adolescents.” This search will find all the articles about mood disorders or affective disorders in adolescents. The researchers can use NOT if they only want to study depression in children and want to ignore bipolar disorder from the search. An example search in this scenario would be “depression” NOT “bipolar disorder” AND “children.” This will help ignore studies related to bipolar disorder in children.
Truncation and wildcards are other tools to make search strategy more comprehensive and focused. While the researchers search a database for certain articles, they frequently face terminologies that have the same initial root of a word but different endings. An example would be "autism," "autistic," and "autism spectrum disorder." These words have a similar initial root derived from “autis” but they end differently in each case. The truncation symbol (*) retrieves articles that contain words beginning with “autis” plus any additional characters. Wildcards are used for words with the same meanings but different spellings due to various reasons. For the words with spelling variations of a single letter, wildcard symbols can be used. When the researcher inputs “M+N” in the search bar, this returns results containing both “man” or “men” as the wildcard accounts for the spelling variations between the letters M and N.
Study selection
Study selection should be performed in a systematic manner, so reviewers deal with fewer errors and a lower risk of bias (online course, Li T, Dickersin K: Introduction to systematic review and meta-analysis. 2016. https://www.coursera.org/learn/systematic-review #). Study selection should involve two independent reviewers who select studies using inclusion and exclusion criteria. Any disagreements during this process should be resolved by discussion or by a third reviewer [ 10 ]. Specific study types can be selected depending on the research question. For example, questions on incidence and prevalence can be answered by surveys and cohort studies. Clinical trials can provide answers to questions related to therapy and screening. Queries regarding diagnostic accuracy can be answered by clinical trials and cross-sectional studies (online course, Li T, Dickersin K: Introduction to systematic review and meta-analysis. 2016. https://www.coursera.org/learn/systematic-review #). Prognosis and harm-related questions should use cohort studies and clinical trials, and etiology questions should use case-control and cohort studies (online course, Li T, Dickersin K: Introduction to systematic review and meta-analysis. 2016. https://www.coursera.org/learn/systematic-review #).
Data screening and data extractions are two of the major steps in conducting a systematic review [ 18 ]. Data screening involves searching for relevant articles in different databases using keywords. The next step of data screening is manuscript selection by reviewing each manuscript in the search results to compare that manuscript against the inclusion criteria [ 18 ]. The researchers should also review the references of the papers selected before selecting the final paper, which is the last step of data screening [ 18 ].
The next stage is extracting and appraising the data of the included articles [ 18 ]. A data extraction form should be used to help reduce the number of errors, and more than one person should record the data [ 17 ]. Data should be collected on specific points like population type, study authors, agency, study design, humanitarian crisis, target age groups, research strengths from the literature, setting, study country, type(s) of public health intervention, and health outcome(s) addressed by the public health intervention. All this information should then be put into an electronic database [ 18 ].
Assessing bias
Bias is a systematic error (or deviation from the truth) in results or inferences. Biases can change the results of any study and lead to an underestimation or overestimation of the true intervention effect [ 19 ]. Biases can impact any aspect of a review, including selecting studies, collecting and extracting data, and making a conclusion. Biases can vary in magnitude; some are small, with negligible effect, but some are substantial to a degree where an apparent finding may be entirely due to bias [ 19 ]. There are different types of bias, including, but not limited to, selection, detection, attrition, reporting, and performance.
Selection bias occurs when a sample selected is not representative of the whole general population. If randomization of the sample is done correctly, then chances of selection bias can be minimized [ 20 ].
Detection bias refers to systematic differences between groups in how outcomes are determined. This type of bias is based on knowledge of the intervention provided and its outcome [ 19 ].
Attrition bias refers to systematic differences between groups in withdrawals from a study [ 19 ]. The data will be considered incomplete if some subjects are withdrawn or have irregular visits during data collection.
Reporting bias refers to systematic differences between reported and unreported findings, and it is commonly seen during article reviews. Reporting bias is based on reviewer judgment about the outcome of selected articles [ 20 ].
Performance bias develops due to the knowledge of the allocated interventions by participants and personnel during the study [ 20 ]. Using a double-blind study design helps prevent performance bias, where neither the experimenter nor the subjects know which group contains controls and which group contains the test article [ 14 ].
Last step of systematic review: discussion
The discussion of a systematic review is where a summary of the available evidence for different outcomes is written and discussed [ 10 ]. The limitations of a systematic review are also discussed in detail. Finally, a conclusion is drawn after evaluating the results and considering limitations [ 10 ].
Discussion of the current article
Systematic reviews with or without a meta-analysis are currently ranked to be the best available evidence in the hierarchy of evidence-based practice [ 21 ]. We have discussed the methodology of a systematic review. A systematic review is classified in the category of filtered information because it appraises the quality of the study and its application in the field of medicine [ 21 ]. However, there are some limitations of the systematic review, as we mentioned earlier in our article. A large randomized controlled trial may provide a better conclusion than a systematic review of many smaller trials due to their larger sample sizes [ 22 ], which help the researchers generalize their conclusions for a bigger population. Other important factors to consider include higher dropout rates in large studies, co-interventions, and heterogeneity among studies included in the review.
As we discussed the limitations of the systematic review and its effect on quality of evidence, there are several tools to rate the evidence, such as the Grading of Recommendations Assessment, Development and Evaluation (GRADE) system [ 22 ]. GRADE provides a structured approach to evaluating the risk of bias, serious inconsistency between studies, indirectness, imprecision of the results, and publication bias [ 22 ]. Another approach used to rate the quality of evidence is a measurement tool to assess systematic reviews (AMSTAR) [ 23 ]. It is also available in several languages [ 23 ].
Conclusions
Despite its limitations, a systematic review can add to the knowledge of the scientific community especially when there are gaps in the existing knowledge. However, conducting a systematic review requires different steps that involve different tools and strategies. It can be difficult at times to access and utilize these resources. A researcher can understand and strategize a systematic review following the different steps outlined in this literature review. However, conducting a systematic review requires a thorough understanding of all the concepts and tools involved, which is an extensive endeavor to be summed up in one article.
The Cochrane Handbook for Systematic Reviews of Interventions and the Center for Reviews and Dissemination (CRD) provide excellent guidance through their insightful and detailed guidelines. We recommend consulting these resources for further guidance.
Given that our article is a narrative review of the scholarly literature, it contains the same limitations as noted for any narrative review. We hope that our review of the means and methods for conducting a systematic review will be helpful in providing basic knowledge to utilize the resources available to the scientific community.
The content published in Cureus is the result of clinical experience and/or research by independent individuals or organizations. Cureus is not responsible for the scientific accuracy or reliability of data or conclusions published herein. All content published within Cureus is intended only for educational, research and reference purposes. Additionally, articles published within Cureus should not be deemed a suitable substitute for the advice of a qualified health care professional. Do not disregard or avoid professional medical advice due to content published within Cureus.
The authors have declared that no competing interests exist.

- University of Texas Libraries
Literature Reviews
Determine inclusion and exclusion criteria.
- What is a literature review?
- Steps in the Literature Review Process
- Define your research question
- Choose databases and search
- Review Results
- Synthesize Results
- Analyze Results
- Librarian Support
- Artificial Intelligence (AI) Tools
Once you have a clearly defined research question, make sure you are getting precisely the right search results from searching the databases by making decisions about these items:
- Would the most recent five years be appropriate?
- Is your research from a more historical perspective?
- Where has this type of research taken place?
- Will you confine your results to the United States?
- To English speaking countries?
- Will you translate works if needed?
- Is there a particular methodology, or population that you are focused on?
- Are there adjacent fields in which this type of research has been conducted that you would like to include?
- Is there a controversy or debate in your research field that you want to highlight
- Are you creating a historical overview? Is this background reading for your research?
- Is there new technology that can shed light on an old problem or an old technology that can be used in a new way?
- Last Updated: Aug 22, 2024 9:12 AM
- URL: https://guides.lib.utexas.edu/literaturereviews
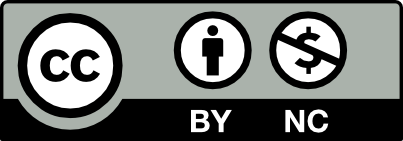
- Systematic Review
- Open access
- Published: 20 August 2024
Examining the mental health services among people with mental disorders: a literature review
- Yunqi Gao 1 ,
- Richard Burns 1 ,
- Liana Leach 1 ,
- Miranda R. Chilver 1 &
- Peter Butterworth 2 , 3
BMC Psychiatry volume 24 , Article number: 568 ( 2024 ) Cite this article
104 Accesses
1 Altmetric
Metrics details
Mental disorders are a significant contributor to disease burden. However, there is a large treatment gap for common mental disorders worldwide. This systematic review summarizes the factors associated with mental health service use.
PubMed, Scopus, and the Web of Science were searched for articles describing the predictors of and barriers to mental health service use among people with mental disorders from January 2012 to August 2023. The initial search yielded 3230 articles, 2366 remained after removing duplicates, and 237 studies remained after the title and abstract screening. In total, 40 studies met the inclusion and exclusion criteria.
Middle-aged participants, females, Caucasian ethnicity, and higher household income were more likely to access mental health services. The use of services was also associated with the severity of mental symptoms. The association between employment, marital status, and mental health services was inconclusive due to limited studies. High financial costs, lack of transportation, and scarcity of mental health services were structural factors found to be associated with lower rates of mental health service use. Attitudinal barriers, mental health stigma, and cultural beliefs also contributed to the lower rates of mental health service use.
This systematic review found that several socio-demographic characteristics were strongly associated with using mental health services. Policymakers and those providing mental health services can use this information to better understand and respond to inequalities in mental health service use and improve access to mental health treatment.
Peer Review reports
Introduction
Mental disorders such as depression and anxiety are prevalent, with nationally representative studies showing that one-fifth of Australians experience a mental disorder each year [ 5 ]. More recent estimates derived from a similar survey during the period of the COVID-19 pandemic were 21.5% [ 11 ]. Mental illness can reduce the quality of life, and increase the likelihood of communicable and non-communicable diseases [ 116 , 137 ], and is among the costliest burdens in developed countries [ 22 , 34 , 80 ]. The National Mental Health Commission [ 96 ] stated that the annual cost of mental ill-health in Australia was around $4000 per person or $60 billion. The Global Burden of Diseases, Injuries, and Risk Factors Study (GBD) 2019 reported that mental disorders rank the seventh leading cause of disability-adjusted life years and the second leading cause of years lived with disability [ 48 ]. Helliwell et al. [ 56 ] indicated that chronic mental illness was a key determinant of unhappiness, and it triggered more pain than physical illness. Mental health issues can have a spillover effect on all areas of life, poor mental health conditions might lead to lower educational achievements and work performance, substance abuse, and violence [ 102 ]. In Australia, despite considerable additional investment in the provision of mental health services research suggests that the rate of psychological distress at the population level has been increasing [ 38 ], this has been argued to reflect that people who most need mental health treatment are not accessing services. Insufficient numbers of mental health services and mental healthcare professionals and inadequate health literacy have been reported as the pivotal determinants of poor mental health [ 18 ]. Previous studies have reported large treatment gaps in mental health services; finding only 42–44% of individuals with mental illness seek help from any medical or professional service provider [ 85 , 112 ] and this active proportion was much lower in low and middle-income countries [ 32 , 114 , 130 ].
Several studies have investigated factors associated with high and low rates of mental health service use and identified potential barriers to accessing mental health service use. Demographic, social, and structural factors have been associated with low rates of mental health service use. Structural barriers include the availability of mental health services and high treatment costs, social barriers to treatment access include stigma around mental health [ 125 ], fear of being perceived as weak or stigmatized [ 79 ], lack of awareness of mental disorders, and cultural stigma [ 17 ].
Existing studies that have systematically reviewed and evaluated the literature examining mental health service use have largely been constrained to specific population groups such as military service members [ 63 ] and immigrants [ 33 ], children and adolescents [ 35 ], young adults [ 76 ], and help-seeking among Filipinos in the Philippines [ 93 ]. These systematic reviews emphasize mental health service use by specific age groups or sub-groups, and the findings might not represent the patterns and barriers to mental health service use in the general population. One paper has reviewed mental health service use in the general adult population. Roberts et al. [ 112 ] found that need factors (e.g. health status, disability, duration of symptoms) were the strongest determinants of health service use for those with mental disorders.
The study results from Roberts et al. [ 112 ] were retrieved in 2016, and the current study seeks to build on this prior review with more recent research data by identifying publications since 2012 on mental health service use with a focus on high-income countries. This is in the context of ongoing community discussion and reform of the design and delivery of mental health services in Australia [ 140 ], and the need for current evidence to inform this discussion in Australia and other high-income countries. This systematic review aims to investigate factors associated with mental health service use among people with mental disorders and summarize the major barriers to mental health treatment. The specific objectives are (1) to identify factors associated with mental health service use among people with mental disorders in high-income countries, and (2) to identify commonly reported barriers to mental health service use.
Methodology
Selection procedures.
Our review adhered to PRISMA guidelines to present the results. We utilized PubMed, Scopus, and the Web of Science to search for articles describing the facilitators and barriers to mental health service use among people with mental illness from January 2012 up to August 2023. There were no specific factors that were of interest as part of conducting this systematic review, instead, the review had a broad focus intending to identify factors shown to be associated with mental health service use in the recent literature. The keywords used in our search of electronic databases were related to mental disorders and mental health service use. The full search terms and strategies were shown in Supplementary Table 1. We uploaded the search results to Covidence for deduplication and screening. After eliminating duplicates, the first author retrieved the title abstract and full-text articles for all eligible papers. Then each title and abstract were screened by two independent reviewers, to select those that would progress to full-text review. Subsequently, the two reviewers screened the full text of all the selected papers and conducted the data extraction for those that met the eligibility criteria. There were discrepancies in 12% of the papers reviewed, and all conflicts were resolved through discussion and agreed on by at least three authors.
Selection criteria
Inclusion and exclusion criteria.
In this systematic review, the scope was restricted to studies that draw samples from the general population, and the participants were either diagnosed with mental disorders or screened positive using a standardized scale. Case-control studies and cohort studies were considered for inclusion. The applied inclusion and exclusion criteria are listed in Table 1 .
Data extraction
After the full-text screening, details from all eligible studies were extracted by field into a data extraction table with thematic headings. The descriptive data includes the study title, author, publication year, geographic location, sample size, population details (gender, age), type of study design, mental disorder type (medical diagnosis or using scales) and quality grade (e.g. good, fair, and poor).
Quality assessment
The Newcastle Ottawa Scale [ 136 ] was used to evaluate the study quality for all eligible papers. We assessed the cross-sectional and cohort studies using separate assessment forms and graded each study as good, fair, or poor. The quality grade for each study was included in the data extraction table. The first author conducted the quality assessment using the Newcastle Ottawa Scale for cohort studies and the adapted scale for cross-sectional studies.
The search process is summarized in Fig. 1 . The initial search from PubMed, Scopus, and the Web of Science yielded 3230 articles: 2366 remained after removing duplicates; 2129 studies were considered not relevant; and 237 studies remained following title and abstract screening. In total, 40 studies met the inclusion criteria. Of these, four were cohort studies while thirty-six were cross-sectional studies. Ten studies (25.0%) were conducted in Canada, and nine (22.5%) were from the United States. Three studies used data from Germany (7.5%). Two studies each reported data from Australia, Denmark, Sweden, Singapore, or South Korea (5.0% of studies for each country). A single study was included with data from either the United Kingdom, Italy, Israel, Portugal, Switzerland, Chile, New Zealand, or reported pooled multinational data from six European countries (each country/ study representing 2.5% of the total sample of studies) (Table 2 ).
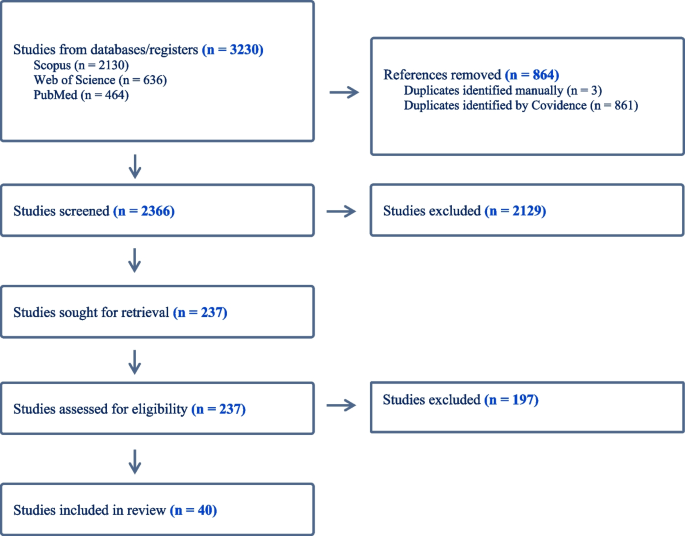
Flowchart for selections of studies
Study characteristics
As shown in Tables 2 , 3 and 4 , the sample size of studies varies; a cross-sectional study from Canada had the largest sample which contained over seven million participants [ 39 ], while the smallest sample size was 362 [ 100 ]. Sixteen studies (40.0%) used DSM-IV diagnoses [ 4 ] to measure mental disorders, twelve studies (30.0%) applied the International Classification of Disease [ 138 ], and six studies used (15.0%) the Kessler Psychological Distress Scale [ 69 ]. Only three studies (7.5%) had a hospital diagnosis of mental disorders, while three studies (7.5%) used the Patient Health Questionnaire [ 72 ] to define mental disorders.
Twenty-seven studies (67.5%) analyzed the rate of mental health service use over the last 12 months, six studies (15.0%) focused on lifetime service use, and three studies (7.5%) assessed both 12-month and lifetime mental health service use. A few studies examined other time frames, with single studies investigating mental health service use over the past 3 months, 5 years, and 7 years, and one included study considered mental health service use during the 24 months before and after a sibling’s death.
Twenty of the forty studies were classified as good quality (50.0%), seventeen as fair (42.5%), and three as poor quality (7.5%).
Overview of samples and factors investigated
The included studies examined a range of different factors associated with mental health service use. These included gender, age, marital status, ethnic groups, alcohol and drug abuse, education and income level, employment status, symptom severity, and residential location. The review identified service utilization factors related to socio-demographics, differences in utilization across countries, emerging socio-demographic factors and contexts, as well as structural and attitudinal barriers. These are described in further detail below.
Socio-demographic characteristics
Fifteen studies analyzed the association between gender and mental health service use, with fourteen studies reporting that mental health service use was more frequent among females with mental disorders than males [ 2 , 37 , 42 , 43 , 47 , 54 , 66 , 67 , 90 , 103 , 119 , 123 , 128 , 130 ]. A South Korean study concluded that gender was not associated with mental health service use [ 100 ], which might be due to the small sample size of 362 participants in the study.
Fourteen studies investigated age in association with mental health service use. Nine studies concluded that mental health service use was lower among young and old adult groups, with middle-aged persons with a mental disorder being most likely to access treatment from a mental health professional [ 26 , 42 , 43 , 47 , 54 , 66 , 67 , 123 , 130 ]. Forslund et al. [ 43 ] reported that mental health service use for women in Sweden peaked in the 45-to-64-year age group, while amongst males, mental health service use was stable across the lifespan. In contrast, two articles from New Zealand and Singapore each reported that young adults were the age group most likely to access services [ 28 , 119 ]. Reich et al. [ 103 ] concluded that age was unrelated to mental health service use when considered for the whole population, but sex-specific analyses reported that mental health service use was higher in older than younger females, while the opposite pattern was observed for males. A Canadian study using community health survey data also observed no significant age-related differences in mental health service use [ 104 ].
Marital status
There was mixed evidence concerning marital status. Studies from the United States and Germany concluded that participants who were married or cohabiting had lower rates of mental health service use [ 26 , 90 ], while Silvia et al. [ 120 ] found that mental health service use was higher among married participants in Portugal. Shafie et al. [ 119 ] reported being widowed was associated with lower rates of mental health service use in Singapore.
Ethnic groups
Eight studies examined the relationship between ethnic background and mental healthcare service use. Non-Hispanic White respondents were more likely to use mental health services in Canada and the United States [ 24 , 26 , 30 , 130 , 139 ], while Asians showed lower rates of mental health service use [ 28 , 139 ]. Chow & Mulder [ 28 ] investigated mental health service use among Asians, Europeans, Maori, and Pacific peoples in New Zealand. They concluded that Maori had the highest rate of mental health service use compared with other ethnic groups. De Luca et al. [ 30 ] reported that mental health service use was lower among ethnic minority non-veterans compared to veterans in the United States, especially for those with Black or Hispanic backgrounds. In contrast, a study conducted in the UK found that mental health service use did not vary by ethnicity, with no difference between white and non-white persons [ 54 ].
Alcohol and drug abuse
Two studies reported risky alcohol use was negatively associated with mental health service use [ 26 , 132 ]. However, within the time frame of the current review, there was insufficient published evidence on the impact of drug abuse on mental health service use among people with mental disorders. Choi, Diana & Nathan [ 26 ] found that drug abuse can lead to lower rates of mental health service use in the United States. In contrast, Werlen et al. [ 132 ] reported that risky use of (non-prescribed) prescription medications was associated with higher rates of mental health service use in Switzerland.
Education, income, and employment status
Four studies analyzed the relationship between education level, income, and mental health service use. Higher levels of educational attainment [ 26 , 120 ] and higher income [ 26 ] were generally reported to be associated with an increased likelihood of mental health service use. However, Reich et al. [ 103 ] observed that in Germany, high education and perceived middle or high social class were associated with reduced mental health service use. One paper reported no significant difference in mental health service use in South Korea, possibly due to the small number of people accessing mental healthcare services [ 100 ].
Three studies reported that compared to those who are unemployed, those in work were less likely to use mental health services [ 26 , 90 , 119 ]. This outcome aligned with a Canadian study consisting of immigrants and general populations, Islam et al. [ 66 ] concluded that immigrants who were currently unemployed had higher odds of seeking treatment than those who were employed. However, an Italian [ 123 ] and a South Korean study [ 100 ] found that employment status was not related to mental health service use.
Symptom severity
Ten studies investigated the association between symptom severity and mental health service use and ten papers concluded that participants with moderate or serious psychological symptoms were more likely to use mental health services compared to those with mild symptoms [ 23 , 27 , 66 , 103 , 120 , 123 , 130 , 139 ]. Other studies showed that study participants who viewed their mental health as poor [ 42 ], who were diagnosed with more than one mental disorder [ 103 ], and those who recognized their own need for mental health treatment [ 54 , 139 ] were more likely to receive mental health services.
Residential location
Three studies investigated the association between residential location and mental health service use. Volkert et al. [ 128 ] concluded that the rates of mental health service use in Germany were significantly lower among those living in Canterbury than those living in Hamburg. A Canadian study found individuals living in neighborhoods where renters outnumber homeowners were less likely to access mental health services [ 42 ]. In the United States, for participants with low or moderate mental illness, mental health service use was lower for those residing closer to clinics [ 46 ].
Immigrants & refugees
The reviewed research found that non-refugee immigrants had slightly higher rates of mental health service use than refugees [ 10 ]. Other research found that long-term residents were more likely to access services than immigrants regardless of their origin [ 31 , 134 ]. For example, Italian citizens were found to have higher rates of mental health service use compared to immigrants, especially for affective disorders [ 123 ]. In Canada, immigrants from West and Central Africa were more likely to access mental health services compared to immigrants from East Asia and the Pacific [ 31 ]. Research from Chile found that the rates of mental health service use were similar for immigrants and non-immigrants [ 40 ]. Although, a positive association between the severity of symptoms and rates of mental health service use was only observed among immigrants [ 40 ]. Whitley et al. [ 134 ] found that immigrants born in Asia or Africa had lower rates of mental health service use, but higher rates of service satisfaction scores compared to immigrants from other countries.
Emerging areas
Our literature review identified several areas in which only a small number of studies were found. We briefly describe them here as these may reflect emerging areas of research interest. Few published articles examined mental health treatment among participants with mental disorders together with chronic physical health conditions, and we only included the papers in this systematic review if they contained a healthy comparison group. We identified two papers that focused on survivors of adolescent and young adult cancer [ 68 ] and participants with physical health problems [ 110 ]. Both studies reported that participants with other chronic conditions reported higher rates of mental health service use than the general population [ 68 , 110 ].
Two studies compared treatment seeking among people experiencing stressful life events. Erlangsen et al. [ 39 ] investigated the impact of spousal suicide, and Gazibara et al. [ 45 ] examined the effect of a sibling’s death on mental health service use. People bereaved by relatives’ deaths were more likely to use mental health services than the general population [ 39 , 45 ]. The peak effect was observed in the first 3 months after the death for both genders, while evidence of an increase in mental health service use was evident up to 24 months before a sibling’s death and remained evident for at least 24 months after the death [ 45 ].
One paper studied the impact of the COVID-19 pandemic lockdown on mental health service use. An Israeli study concluded that compared to 2018 and 2019, adults reported they were reluctant to receive treatment during the pandemic lockdown and observed a decrease in mental health service use [ 13 ].
Structural and attitudinal barriers
In addition to the research considering a range of population characteristics (e.g. male, younger, or older age), several papers examined how attitudinal and structural factors were associated with mental health service use. The most frequently reported of these factors were cost [ 23 , 46 , 68 , 120 ], lack of transportation [ 46 , 83 ], inadequate services/ lack of availability [ 23 , 46 , 83 , 128 ], poor understanding of mental disorders and what services were available [ 10 , 11 , 22 , 83 , 100 , 105 , 120 ], language difficulties [ 10 ], and stigma-related barriers [ 83 , 100 , 103 , 105 , 128 ]. Cultural issues and personal beliefs may influence the understanding of mental disorders and prevent people from using mental health services due to mistrust or fear of treatment [ 100 , 128 ]. The review also observed some unique barriers to different population groups. Choi, Diana & Nathan [ 26 ] mentioned that lack of readiness and treatment cost were the biggest difficulties for older adults, while young participants were more concerned about stigma. Females also reported childcare as a factor limiting their ability to use mental health services, while the evidence reviewed argued that males prefer to solve mental health issues on their own, with internal control beliefs and lack of social support likely reducing their use of mental health services [ 37 , 103 ].
Summary of evidence
This systematic review investigated mental health service use among people with mental disorders and identified the factors associated with service use in high-income countries.
Most studies found that females with mental health conditions were more likely to use mental health services than males. The relationship between age and mental health service use was bell-shaped, with middle-aged participants having higher rates of mental health service use than other age groups. Possible explanations included that the elderly might be reluctant to disclose mental health symptoms, they might attribute their mental health symptoms to increasing age [ 20 ], and they may prefer to self-manage instead of seeking help from health professionals [ 44 ]. Caucasian ethnicity and higher household income were also associated with higher rates of mental health service use. Greater use of mental health services was observed in participants with severe mental symptoms, including among veterans [ 19 , 37 , 92 ]. Two studies also concluded that compared to other cultural groups, Asian respondents were more likely to receive treatment when problems were severe or had disabling effects [ 86 , 97 ]. There was mixed evidence regarding employment status, although some studies found employment to be negatively related to receiving treatment [ 26 , 90 ], and unemployed people are more likely to seek help [ 119 ]. There was inconsistent evidence for the association between marital status and service utilization. This contradictory evidence on marital status might be attributed to a lack of specification, some papers categorize it as married and non-married [ 26 , 71 , 131 ], while others further differentiate between those who were widowed, separated, and divorced [ 90 , 119 ].
A number of studies showed that immigrants can face unique stressors owing to their experience of migration, which may exacerbate or be the source of their mental health issues, and impact the use of mental health services [ 1 , 8 ]. These include separation from families, support networks, linguistic and cultural barriers [ 9 , 113 ].
Due to the increased number of international migrants, immigrants’ mental health status and healthcare use has drawn growing attention [ 7 , 77 , 99 ]. Kirmayer et al. [ 70 ] and Helman [ 57 ] found that culture might be associated with people’s attitudes and understanding of mental health, influencing help-seeking behaviors. In general, the current results showed that immigrants and refugees were less likely to use mental health services than their native-born counterparts, and this finding was consistent with previous studies [ 75 , 82 , 127 ]. For immigrants, the length of stay in the host country was closely related to rates of mental health service use, which was argued to reflect increasing familiarity with the host culture and language proficiency [ 1 , 59 ].
Both mental disorders and chronic diseases contribute significantly to the global burden of disease. Prior studies have shown that people with chronic disease have a higher chance of experiencing psychological distress [ 6 , 14 , 68 , 73 ], and vice versa [ 49 , 74 ]. Hendrie et al. [ 58 ] concluded that respondents with chronic diseases were more likely to attend mental healthcare and reported higher costs. Negative experiences and stressful consequences related to chronic disease might contribute to the increased potential for mental illness but more opportunities to seek help from health professionals [ 60 , 108 , 135 ]. People with chronic diseases and mental health problems might experience more long-term pain and limitations in their daily lives, and these stressors can exacerbate their health conditions, and impact their attitude toward seeking help.
The COVID-19 pandemic had a major impact on mental health service use worldwide, the hospital admission and consultation rate decreased dramatically during the first pandemic year [ 118 ]. This reduction in service access might be a side effect of social distancing measures taken as mitigation measures, reducing both inciting incidents and physical access to services.
Financial difficulty, service availability, and stigma were frequently identified in the literature as structural and attitudinal factors associated with lower rates of mental health service use. These factors were associated with the different rates of mental health service use for different ethnicities. For example, Asian people were less likely than other groups to identify cost as a factor limiting their use of mental health services, with a major barrier for Asian people being stigma and cultural factors [ 139 ].

Limitations
This systematic review employed a broad search strategy with broad search terms to capture relevant articles. Rather than emphasizing a particular mental disorder, this review focused on the rates of mental health service use among adults aged 18 years or older who were experiencing a common mental disorder. However, this review still contained limitations. First was the potential for selection bias. Although we used various search terms for mental health service use and mental disorder, it is possible that the service use was not the primary research question for some papers, or that the relevant service use outcome was not statistically significant- in these cases, if the information was not reported in the abstract, relevant papers might have been missed. It is also important to note that this systematic review includes studies conducted in different countries and that the mental health systems and opportunities for access vary among countries. We only searched for full-text peer-reviewed articles published in English. Grey literature and papers published in other languages were excluded from the search. Most of the included literature used self-reported data to measure service access, and these data can be liable to recall bias. Studies using administrative data were also included in the systematic review, and we note that although they have large datasets, compared to survey data, there is often a lack of adequate control variables included to minimize possible confounding influences.
Future research
There is a need for more published articles on several aspects that may influence the service utilization among people with mental disorders, including the impact of residential or neighborhood areas, and household income across various income groups. These aspects are important population characteristics that require further research to inform the targeting and type of support (e.g. low-cost, accessible). Additionally, there was a lack of longitudinal research on mental health service use, future studies could use the data to identify changes over time and relate events to specific exposures (e.g. Covid-19 pandemic). Future studies can investigate the cost of mental health treatment in detailed aspects, (e.g. publicly funded mental health services, community-based support for free or low-cost mental health services). Overall, there was a lack of studies for ethnic minorities, given ethnic minority groups were more vulnerable to mental disorders but with less mental health service use. Future research can expand gender identity representation in data collection and move beyond the binary genders. People with non-binary gender identities can face greater challenges and disadvantages in mental health and mental health service use.
This review identified that middle-aged, female gender, Caucasian ethnicity, and severity of mental disorder symptoms were factors consistently associated with higher rates of mental health service use among people with a mental disorder. In comparison, the influence of employment and marital status on mental health service use was unclear due to the limited number of published studies and/ or mixed results. Financial difficulty, stigma, lack of transportation, and inadequate mental health services were the structural barriers most consistently identified as being associated with lower rates of mental health service use. Finally, ethnicity and immigrant status were also associated with differences in understanding of mental health (i.e. mental health literacy), effectiveness of mental health treatments, as well as language difficulties. The insights gained through this review on the factors associated with mental health service use can help clinicians and policymakers to identify and provide more targeted support for those least likely to access services, and this in turn may contribute to reducing inequalities in not only mental health service use but also the burden of mental disorders.
Availability of data and materials
All data and materials related to the study are available on request from the first author, [email protected].
Abe-Kim J, Takeuchi DT, Hong S, Zane N, Sue S, Spencer MS, et al. Use of mental health–related services among immigrant and US-born Asian americans: results from the national latino and Asian American study. Am J Public Health. 2007;97(1):91–8.
Article PubMed PubMed Central Google Scholar
Abramovich A, De Oliveira C, Kiran T, Iwajomo T, Ross LE, Kurdyak P. Assessment of health conditions and health service use among transgender patients in Canada. JAMA Netw Open. 2020;3(8):e2015036. https://doi.org/10.1001/jamanetworkopen.2020.15036 .
Ambikile JS, Iseselo MK. Mental health care and delivery system at Temeke hospital in Dar Es Salaam, Tanzania. BMC Psychiatry. 2017;17:1–13. https://doi.org/10.1186/s12888-017-1271-9 .
Article Google Scholar
American Psychiatric Association. Diagnostic and statistical manual of mental disorders. 5th ed. Arlington, VA: American Psychiatric Publishing; 2013.
Book Google Scholar
Australian Bureau of Statistics. 2017-18, Mental health, ABS, viewed 23 July 2023. https://www.abs.gov.au/statistics/health/mental-health/mental-health/2017-18 .
Anderson RJ, Freedland KE, Clouse RE, Lustman PJ. The prevalence of comorbid depression in adults with diabetes. Diabetes Care. 2001;24(6):1069–78.
Article CAS PubMed Google Scholar
Bacon L, Bourne R, Oakley C, Humphreys M. Immigration policy: implications for mental health services. Adv Psychiatr Treat. 2010;16:124–32.
Bhatia S, Ram A. Theorizing identity in transnational and diaspora cultures: a critical approach to acculturation. Int J Intercultural Relations. 2009;33:140–9.
Bhugra D. Migration, distress and cultural identity. Br Med Bull. 2004;69:129–41.
Article PubMed Google Scholar
Björkenstam E, Helgesson M, Norredam M, Sijbrandij M, de Montgomery CJ, Mittendorfer-Rutz E. Differences in psychiatric care utilization between refugees, non-refugee migrants and Swedish-born youth. Psychol Med. 2022;52(7):1365–75. https://doi.org/10.1017/s0033291720003190 .
Australian Bureau of Statistics. (2020–2022). National study of mental health and wellbeing. ABS. https://www.abs.gov.au/statistics/health/mental-health/national-study-mental-health-and-wellbeing/latest-release .
Blais RK, Tsai J, Southwick SM, Pietrzak RH. Barriers and facilitators related to mental health care use among older veterans in the United States. Psychiatr Serv. 2015;66(5):500–6. https://doi.org/10.1176/appi.ps.201300469 .
Blasbalg U, Sinai D, Arnon S, Hermon Y, Toren P. Mental health consequences of the COVID-19 pandemic: results from a large-scale population-based study in Israel. Compr Psychiatr. 2023;123:123. https://doi.org/10.1016/j.comppsych.2023.152383 .
Bodurka-Bevers D, Basen-Engquist K, Carmack CL, Fitzgerald MA, Wolf JK, Moor Cd, Gershenson DM. Depression, anxiety, and quality of life in patients with epthelial ovarian cancer. Gynecol Oncol. 2000;78:302–8.
Bondesson E, Alpar T, Petersson IF, Schelin MEC, Jöud A. Health care utilization among individuals who die by suicide as compared to the general population: a population-based register study in Sweden. BMC Public Health. 2022;22(1):1616. https://doi.org/10.1186/s12889-022-14006-x
Borges G, Aguilar-Gaxiola S, Andrade L, Benjet C, Cia A, Kessler RC, Orozco R, Sampson N, Stagnaro JC, Torres Y, Viana MC, Medina-Mora ME. Twelve-month mental health service use in six countries of the Americas: a regional report from the World Mental Health Surveys. Epidemiol Psychiatr Sci. 2019;29:e53. https://doi.org/10.1017/s2045796019000477 .
Article CAS PubMed PubMed Central Google Scholar
Brenman NF, Luitel NP, Mall S, Jordans MJD. Demand and access to mental health services: a qualitative formative study in Nepal. BMC Int Health Hum Rights. 2014;14(1):1–12. https://doi.org/10.1186/1472-698X-14-22 . PMID: 25084826.
Budhathoki SS, Pokharel PK, Good S, Limbu S, Bhattachan M, Osborne RH. The potential of health literacy to address the health related UN sustainable development goal 3 (SDG3) in Nepal: a rapid review. BMC Health Serv Res. 2017;17(1):1–13. https://doi.org/10.1186/s12913-016-1943-z . PMID: 28049468.
Brown JS, Evans-Lacko S, Aschan L, Henderson MJ, Hatch SL, Hotopf M. Seeking informal and formal help for mental health problems in the community: a secondary analysis from a psychiatric morbidity survey in South London. BMC Psychiatry. 2014;14(1):275.
Burroughs H, Lovell K, Morley M, Baldwin R, Burns A, Chew-Graham C. Justifiable depression’: how primary care professionals and patients view late-life depression? A qualitative study. Fam Pract. 2006;23(3):369–77. https://doi.org/10.1093/fampra/cmi115 .
Caron J, Fleury MJ, Perreault M, Crocker A, Tremblay J, Tousignant M, Kestens Y, Cargo M, Daniel M. Prevalence of psychological distress and mental disorders, and use of mental health services in the epidemiological catchment area of Montreal South-West. BMC Psychiatry. 2012;12:12. https://doi.org/10.1186/1471-244X-12-183 .
Chan CMH, Ng SL, In S, Wee LH, Siau CS. Predictors of psychological distress and mental health resource utilization among employees in Malaysia. Int J Environ Res Public Health. 2021;18(1):1–15. https://doi.org/10.3390/ijerph18010314 .
Chaudhry MM, Banta JE, McCleary K, Mataya R, Banta JM. Psychological distress, structural barriers, and health services utilization among U.S. adults: National Health interview survey, 2011–2017. Int J Mental Health. 2022. https://doi.org/10.1080/00207411.2022.2123694
Chiu M, Amartey A, Wang X, Kurdyak P, Luppa M, Giersdorf J, Riedel-Heller S, Prütz F, Rommel A. Ethnic differences in mental health status and service utilization: a population-based study in Ontario, Canada frequent attenders in the German healthcare system: determinants of high utilization of primary care services. Results from the cross-sectional. Can J PsychiatryBMC Fam Pract. 2018;6321(71):481–91. https://doi.org/10.1186/s12875-020-1082-9 .
Cho SJ, Lee JY, Hong JP, Lee HB, Cho MJ, Hahm BJ. Mental health service use in a nationwide sample of Korean adults. Soc Psychiatry Psychiatr Epidemiol. 2009;44:943–51.
Choi NG, DiNitto DM, Marti CN. Treatment use, perceived need, and barriers to seeking treatment for substance abuse and mental health problems among older adults compared to younger adults. Drug Alcohol Depend. 2014;145:113–20. https://doi.org/10.1016/j.drugalcdep.2014.10.004 .
Chong SA, Abdin E, Vaingankar JA, Kwok KW, Subramaniam M. Where do people with mental disorders in Singapore go to for help? Ann Acad Med Singap. 2012;41(4):154–60.
Chow CS, Mulder RT. Mental health service use by asians: a New Zealand census. N Z Med J. 2017;130(1461):35–41.
PubMed Google Scholar
Dang HM, Lam TT, Dao A, Weiss B. Mental health literacy at the public health level in low and middle income countries: an exploratory mixed methods study in Vietnam. PLoS ONE. 2020;15(12):e0244573. https://doi.org/10.1371/journal.pone.0244573
De Luca SM, Blosnich JR, Hentschel EA, King E, Amen S. Mental health care utilization: how race, ethnicity and veteran status are associated with seeking help. Community Ment Health J. 2016;52(2):174–9. https://doi.org/10.1007/s10597-015-9964-3 .
Durbin A, Moineddin R, Lin E, Steele LS, Glazier RH. Mental health service use by recent immigrants from different world regions and by non-immigrants in Ontario, Canada: a cross-sectional study. BMC Health Serv Res. 2015;15(1):336. https://doi.org/10.1186/s12913-015-0995-9
Demyttenaere K, Bruffaerts R, Posada-Villa J, Gasquet I, Kovess V, Lepine J, et al. Prevalence, severity, and unmet need for treatment of mental disorders in the World Health Organization world mental health surveys. JAMA. 2004;291(21):2581–90.
Derr AS. Mental health service use among immigrants in the United States: a systematic review. Psychiatr Serv. 2016;67:265–74. https://doi.org/10.1176/appi.ps.201500004 .
Dimoff JK, Kelloway EK. With a little help from my Boss: the impact of workplace mental health training on leader behaviors and employee resource utilization. J Occup Health Psychol. 2019;24:4–19.
Duong MT, Bruns EJ, Lee K, et al. Rates of mental health service utilization by children and adolescents in schools and other common service settings: a systematic review and meta-analysis. Adm Policy Ment Health. 2021;48:420–39. https://doi.org/10.1007/s10488-020-01080-9 .
Edman JL, Kameoka VA. Cultural differences in illness schemas: an analysis of Filipino and American illness attributions. J Cross Cult Psychol. 1997;28(3):252–65.
Elbogen EB, Wagner HR, Johnson SC, Kinneer P, Kang H, Vasterling JJ, Timko C, Beckham JC. Are Iraq and Afghanistan veterans using mental health services? New data from a national random-sample survey. Psychiatr Serv. 2013;64(2):134–41. https://doi.org/10.1176/appi.ps.004792011 .
Enticott J, Dawadi S, Shawyer F, Inder B, Fossey E, Teede H, Rosenberg S, Ozols Am I, Meadows G. Mental Health in Australia: psychological distress reported in six consecutive cross-sectional national surveys from 2001 to 2018. Front Psychiatry. 2022;13:815904. https://doi.org/10.3389/fpsyt.2022.815904
Erlangsen A, Runeson B, Bolton JM, Wilcox HC, Forman JL, Krogh J, Katherine Shear M, Nordentoft M, Conwell Y. Association between spousal suicide and mental, physical, and social health outcomes a longitudinal and nationwide register-based study. JAMA Psychiatry. 2017;74(5):456–64. https://doi.org/10.1001/jamapsychiatry.2017.0226 .
Errazuriz A, Schmidt K, Valenzuela P, Pino R, Jones PB. Common mental disorders in Peruvian immigrant in Chile: a comparison with the host population. BMC Public Health. 2023;23(1):1274. https://doi.org/10.1186/s12889-023-15793-7
Evans-Lacko S, Aguilar-Gaxiola S, Al-Hamzawi A, Alonso J, Benjet C, Bruffaerts R, Chiu WT, Florescu S, de Girolamo G, Gureje O, Haro JM, He Y, Hu C, Karam EG, Kawakami N, Lee S, Lund C, Kovess-Masfety V, Levinson D, Thornicroft G. Socio-economic variations in the mental health treatment gap for people with anxiety, mood, and substance use disorders: results from the WHO World Mental Health (WMH) surveys. Psychol Med. 2018;48(9):1560–71. https://doi.org/10.1017/s0033291717003336 .
Fleury MJ, Grenier G, Bamvita JM, Perreault M, Kestens Y, Caron J. Comprehensive determinants of health service utilisation for mental health reasons in a Canadian catchment area. Int J Equity Health. 2012;11(1):20. https://doi.org/10.1186/1475-9276-11-20
Forslund T, Kosidou K, Wicks S, Dalman C. Trends in psychiatric diagnoses, medications and psychological therapies in a large Swedish region: a population-based study. BMC Psychiatry. 2020;20(1):328. https://doi.org/10.1186/s12888-020-02749-z
Garrido MM, Kane RL, Kaas M, Kane RA. Use of mental health care by community-dwelling older adults. J Am Geriatr Soc. 2011;59(1):50–6. https://doi.org/10.1111/j.1532-5415.2010.03220.x .
Gazibara T, Ornstein KA, Gillezeau C, Aldridge M, Groenvold M, Nordentoft M, Thygesen LC. Bereavement among adult siblings: an examination of health services utilization and mental health outcomes. Am J Epidemiol. 2021;190(12):2571–81. https://doi.org/10.1093/aje/kwab212 .
Glasheen C, Forman-Hoffman VL, Hedden S, Ridenour TA, Wang J, Porter JD. Residential transience among adults: prevalence, characteristics, and association with mental illness and mental health service use. Community Ment Health J. 2019;55(5):784–97. https://doi.org/10.1007/s10597-019-00385-w .
Gonçalves DC, Coelho CM, Byrne GJ. The use of healthcare services for mental health problems by middle-aged and older adults. Arch Gerontol Geriatr. 2014;59(2):393–7. https://doi.org/10.1016/j.archger.2014.04.013 .
GBD 2019 Mental Disorders Collaborators. Global, regional, and national burden of 12 mental disorders in 204 countries and territories, 1990–2019: a systematic analysis for the global burden of disease study 2019. Lancet Psychiatry. 2022;9:137–50. https://doi.org/10.1016/S2215-0366(21)00395-3 .
Article PubMed Central Google Scholar
Goff DC, Sullivan LM, McEvoy JP, et al. A comparison of ten-year cardiac risk estimates in schizophrenia patients from the CATIE Study and matched controls. Schizophr Res. 2005;80(1):45–53. https://doi.org/10.1016/j.schres.2005.08.010 .
Goncalves Daniela C. From loving grandma to working with older adults: promoting positive attitudes towards aging. Educ Gerontol. 2009;35(3):202–25.
Gong F, Gage S-JL, Tacata LA Jr. Helpseeking behavior among Filipino americans: a cultural analysis of face and language. J Community Psychol. 2003;31:469–88. https://doi.org/10.1002/jcop.10063 .
Grossbard JR, Lehavot K, Hoerster KD, Jakupcak M, Seal KH, Simpson TL. Relationships among veteran status, gender, and key health indicators in a national young adult sample. Psychiatric Serv. 2013;64(6):547–53. https://doi.org/10.1176/appi.ps.003002012 .
Hajebi A, Motevalian SA, Rahimi-Movaghar A, Sharifi V, Amin-Esmaeili M, Radgoodarzi R, Hefazi M. Major anxiety disorders in Iran: prevalence, sociodemographic correlates and service utilization. BMC Psychiatry. 2018;18(1):261. https://doi.org/10.1186/s12888-018-1828-2
Harber-Aschan L, Hotopf M, Brown JSL, Henderson M, Hatch SL. Longitudinal patterns of mental health service utilisation by those with mental-physical comorbidity in the community. J Psychosom Res. 2019;117:10–9. https://doi.org/10.1016/j.jpsychores.2018.11.005 .
Huýnh C, Caron J, Fleury MJ. Mental health services use among adults with or without mental disorders: do development stages matter? Int J Soc Psychiatry. 2016;62(5):434–51. https://doi.org/10.1177/0020764016641906 .
Helliwell JF, Layard R, Sachs JD, De Neve JE, Aknin LB, Wang S, editors. World Happiness Report 2022. New York: Sustainable Development Solutions Network; 2022.
Google Scholar
Helman C. Culture, health and illness. 5th ed. CRC Press; 2007. https://doi.org/10.1201/b13281 .
Hendrie HC, Lindgren D, Hay DP, et al. Comorbidity profile and healthcare utilization in elderly patients with serious mental illnesses. Am J Geriatr Psychiatry. 2013;21(12):1267–76. https://doi.org/10.1016/j.jagp.2013.01.056 .
Hermannsdóttir BS, Aegisdottir S. Spirituality, connectedness, and beliefs about psychological services among Filipino immigrants in Iceland. Counsel Psychol. 2016;44(4):546–72.
Hoffman KE, McCarthy EP, Recklitis CJ, Ng AK. Psychological distress in long-term survivors of adult-onset cancer: results from a national survey. Arch Intern Med. 2009;169:1274–81.
Hoge C, Castro C, Messer S, McGurk D, Cotting D, Koffman R. Combat duty in Iraq and Afghanistan, mental health problems, and barriers to care. N Engl J Med. 2004;351:13–22.
Hoge C, Grossman S, Auchterlonie J, Riviere L, Milliken C, Wilk J. PTSD treatment for soldiers after combat deployment: low utilization of mental health care and reasons for dropout. Psychiatric Serv. 2014;65(8):997–1004. https://doi.org/10.1176/appi.ps.201300307 .
Hom MA, Stanley IH, Schneider ME, Joiner TE. A systematic review of help-seeking and mental health service utilization among military service members. Clin Psychol Rev. 2017;53:59–78. https://doi.org/10.1016/j.cpr.2017.01.008 .
Hwang W, Miranda J, Chung C. Psychosis and shamanism in a Filipino–American immigrant. Cult Med Psychiatry. 2007;31(2):251–69.
Institute of Medicine (US) Committee on Understanding and Eliminating Racial and Ethnic Disparities in Health Care, Smedley BD, Stith AY, Nelson AR. (Eds.). Unequal Treatment: Confronting Racial and Ethnic Disparities in Health Care. Washington National Academies Press (US). 2023. https://doi.org/10.17226/12875 .
Islam F, Khanlou N, Macpherson A, Tamim H. Mental health consultation among Ontario’s immigrant populations. Community Ment Health J. 2018;54(5):579–89. https://doi.org/10.1007/s10597-017-0210-z .
Jacobi F, Groß J. Prevalence of mental disorders, health-related quality of life, and service utilization across the adult life span. Psychiatrie. 2014;11(4):227–33. https://doi.org/10.1055/s-0038-1670774 .
Kaul S, Avila JC, Mutambudzi M, Russell H, Kirchhoff AC, Schwartz CL. Mental distress and health care use among survivors of adolescent and young adult cancer: a cross-sectional analysis of the National Health Interview Survey. Cancer. 2017;123(5):869–78. https://doi.org/10.1002/cncr.30417 .
Kessler RC, Barker PR, Colpe LJ, et al. Screening for serious mental illness in the general population. Arch Gen Psychiatry. 2003;60(2):184–9.
Kirmayer LJ, Weinfeld M, Burgos G, et al. Use of health care services for psychological distress by immigrants in an urban multicultural milieu. Can J Psychiatry. 2007;52:295–304.
Kose T. Gender, income and mental health: the Turkish case. PLoS ONE. 2020;15(4):e0232344. https://doi.org/10.1371/journal.pone.0232344
Kroenke K, Spitzer RL, Williams JB. The PHQ-9: validity of a brief depression severity measure. J Gen Intern Med. 2001;16(9):606–13. https://doi.org/10.1046/j.1525-1497.2001.016009606.x .
Kugaya A, Akechi T, Okuyama T, Nakano T, Mikami I, Okamura H, Uchitomi Y. Prevalence, predictive factors, and screening for psychological distress in patients with newly diagnosed head and neck cancer. Cancer. 2000;88:2817–23.
Kurdyak PA, Gnam WH, Goering P, Chong A, Alter DA. The relationship between depressive symptoms, health service consumption, and prognosis after acute myocardial infarction: a prospective cohort study. BMC Health Serv Res. 2008;8: 200. https://doi.org/10.1186/1472-6963-8-200 .
Le Meyer O, Zane N, Cho YI, Takeuchi DT. Use of specialty mental health services by Asian americans with psychiatric disorders. J Consult Clin Psychol. 2009;77(5):1000–5. https://doi.org/10.1037/a0017065 .
Li W, Dorstyn DS, Denson LA. Predictors of mental health service use by young adults: a systematic review. Psychiatric Serv. 2016;67(9):946–56. https://doi.org/10.1176/appi.ps.201500280 .
Lindert J, Schouler-Ocak M, Heinz A, Priebe S. Mental health, health care utilisation of migrants in Europe. Eur Psychiatry. 2008;23 Suppl 1:14–20.
CAS PubMed Google Scholar
Lovering S. Cultural attitudes and beliefs about pain. J Transcult Nurs. 2006;17(4):389–95.
Luitel NP, Jordans MJD, Kohrt BA, Rathod SD, Komproe IH. Treatment gap and barriers for mental health care: A cross-sectional community survey in Nepal. PLoS One. 2017;12(8):1–15. https://doi.org/10.1371/journal.pone.0183223 . PMID: 28817734.
Article CAS Google Scholar
Jansson I, Gunnarsson AB. Employers’ views of the impact of Mental Health problems on the ability to work. Work. 2018;59:585–98.
Kayiteshonga Y, Sezibera V, Mugabo L, Iyamuremye JD. Prevalence of mental disorders, associated co-morbidities, health care knowledge and service utilization in Rwanda - towards a blueprint for promoting mental health care services in low- and middle-income countries? BMC Public Health. 2022;22(1):1858. https://doi.org/10.1186/s12889-022-14165-x .
Kirmayer LJ, Narasiah L, Munoz M, Rashid M, Ryder AG, Guzder J, Hassan G, Rousseau C, Pottie K, Canadian Collaboration for Immigrant and Refugee Health (CCIRH). Common mental health problems in immigrants and refugees: general approach in primary care. Can Med Assoc J. 2011;183(12):E959-67. https://doi.org/10.1503/cmaj.090292 .
Kline AC, Panza KE, Nichter B, Tsai J, Harpaz-Rotem I, Norman SB, Pietrzak RH. Mental health care use among U.S. Military veterans: results from the 2019–2020 national health and resilience in veterans study. Psychiatr Serv. 2022;73(6):628–35. https://doi.org/10.1176/appi.ps.202100112 .
Knipscheer JW, Kleber RJ. Help-seeking behavior of west African migrants. J Community Psychol. 2008;36:915–28. https://doi.org/10.1002/jcop.20264 .
Kohn R, Saxena S, Levav I, Saraceno B. The treatment gap in mental health care. Bull World Health Organ. 2004;82(11):858–66.
PubMed PubMed Central Google Scholar
Kung WW. Cultural and practical barriers to seeking mental health treatment for Chinese americans. J Community Psychol. 2004;32(1):27–43.
Lipson SK, Lattie EG, Eisenberg D. Increased rates of mental health service utilization by U.S. College students: 10-year population-level trends (2007–2017). Psychiatr Serv. 2019;70(1):60–3. https://doi.org/10.1176/appi.ps.201800332 .
Liu L, Chen XL, Ni CP, Yang P, Huang YQ, Liu ZR, Wang B, Yan YP. Survey on the use of mental health services and help-seeking behaviors in a community population in Northwestern China. Psychiatry Res. 2018;262:135–40. https://doi.org/10.1016/j.psychres.2018.02.010 .
Lu J, Xu X, Huang Y, Li T, Ma C, Xu G, Yin H, Ma Y, Wang L, Huang Z, Yan Y, Wang B, Xiao S, Zhou L, Li L, Zhang Y, Chen H, Zhang T, Yan J, Zhang N. Prevalence of depressive disorders and treatment in China: a cross-sectional epidemiological study. Lancet Psychiatry. 2021;8(11):981–90. https://doi.org/10.1016/S2215-0366(21)00251-0 .
Mack S, Jacobi F, Gerschler A, Strehle J, Höfler M, Busch MA, Maske UE, Hapke U, Seiffert I, Gaebel W, Zielasek J, Maier W, Wittchen HU. Self-reported utilization of mental health services in the adult German population–evidence for unmet needs? Results of the DEGS1-Mental Health Module (DEGS1-MH). Int J Methods Psychiatr Res. 2014;23(3):289–303. https://doi.org/10.1002/mpr.1438 .
Mahar AL, Aiken AB, Cramm H, Whitehead M, Groome P, Kurdyak P. Mental health services use trends in Canadian veterans: a Population-based Retrospective Cohort Study in Ontario. Can J Psychiatry. 2018;63(6):378–86. https://doi.org/10.1177/0706743717730826 .
Maneze D, DiGiacomo M, Salamonson Y, Descallar J, Davidson PM. Facilitators and barriers to health-seeking behaviours among Filipino migrants: inductive analysis to inform health promotion. BioMed Res Int. 2015;2015:506269.
Martinez AB, Co M, Lau J, et al. Filipino help-seeking for mental health problems and associated barriers and facilitators: a systematic review. Soc Psychiatry Psychiatr Epidemiol. 2020;55:1397–413. https://doi.org/10.1007/s00127-020-01937-2 .
McKibben JBA, Fullerton CS, Gray CL, Kessler RC, Stein MB, Ursano RJ. Mental health service utilization in the U.S. Army Psychiatr Serv. 2013;64(4):347–53. https://doi.org/10.1176/appi.ps.000602012 .
Mosier KE, Vasiliadis H-M, Lepnurm M, Puchala C, Pekrul C, Tempier R. Prevalence of mental disorders and service utilization in seniors: results from the Canadian community health survey cycle 1.2. Int J Geriatr Psychiatry. 2010;25(10):960–7. https://doi.org/10.1002/gps.2434 .
National Mental Health Commission. Economics of Mental Health in Australia. 2016. Available at: https://www.mentalhealthcommission.gov.au/news-and-media/media-releases/2016/december/economics-of-mental-health-in-australia . Accessed: 20 July 2023.
Nicdao EG, Duldulao AA, Takeuchi DT. Psychological Distress, Nativity, and Help-Seeking among Filipino Americans. Education, Social Factors, and Health Beliefs in Health and Health Care Services. Emerald Group Publishing Ltd. 2015:107–20. https://doi.org/10.1108/S0275-495920150000033005 .
Nock MK, Stein MB, Heeringa SG, Ursano RJ, Colpe LJ, Fullerton CS, et al. Prevalence and correlates of suicidal behavior among soldiers: results from the Army study to assess risk and resilience in service members (Army STARRS). JAMA Psychiatry. 2014;71(5):514–22. https://doi.org/10.1001/jamapsychiatry.2014 .
Ortega AN, Fang H, Perez VH, Rizzo JA, Carter-Pokras O, Wallace SP, et al. Health care access, use of services, and experiences among undocumented mexicans and other latinos. Arch Intern Med. 2007;167(21):2354–60.
Park S, Cho MJ, Bae JN, Chang SM, Jeon HJ, Hahm BJ, Son JW, Kim SG, Bae A, Hong JP. Comparison of treated and untreated major depressive disorder in a nationwide sample of Korean adults. Community Ment Health J. 2012;48(3):363–71. https://doi.org/10.1007/s10597-011-9434-5 .
Patel V, Saxena S, Lund C, et al. The lancet commission on global mental health and sustainable development. Lancet. 2018;392:1553–98.
Patel V, Flisher AJ, Hetrick S, McGorry P. Mental health of young people: a global public-health challenge. Lancet. 2007;369(9569):1302–13. https://doi.org/10.1016/S0140-6736(07)60368-7 .
Reich H, Niermann HCM, Voss C, Venz J, Pieper L, Beesdo-Baum K. Sociodemographic, psychological, and clinical characteristics associated with health service (non-)use for mental disorders in adolescents and young adults from the general population. Eur Child Adolesc Psychiatry. 2023. https://doi.org/10.1007/s00787-023-02146-3 .
Pelletier L, O’Donnell S, McRae L, Grenier J. The burden of generalized anxiety disorder in Canada. Health Promot Chronic Dis Prev Can. 2017;37(2):54–62. https://doi.org/10.24095/hpcdp.37.2.04 .
Rim SJ, Hahm BJ, Seong SJ, Park JE, Chang SM, Kim BS, An H, Jeon HJ, Hong JP, Park S. Prevalence of Mental disorders and Associated factors in Korean adults: national mental health survey of Korea 2021. Psychiatry Invest. 2023;20(3):262–72. https://doi.org/10.30773/pi.2022.0307 .
Pols H, Oak S. War & military mental health: the US psychiatric response in the 20th century. Am J Public Health. 2007;97:2132–42.
Prince M, Patel V, Saxena S, Maj M, Maselko J, Phillips MR, Rahman A. No health without mental health. Lancet. 2007;370(9590):859–77. https://doi.org/10.1016/S0140-6736(07)61238-0 .
Ramsey S, Blough D, Kirchhoff A, et al. Washington State cancer patients found to be at greater risk for bankruptcy than people without a cancer diagnosis. Health Aff. 2013;32:1143–52.
Raphael MJ, Gupta S, Wei X, Peng Y, Soares CN, Bedard PL, Siemens DR, Robinson AG, Booth CM. Long-term mental health service utilization among survivors of testicular cancer: a population-based cohort study. J Clin Oncol. 2021;39(7):779–86. https://doi.org/10.1200/jco.20.02298 .
Reaume SV, Luther AWM, Ferro MA. Physical morbidity and mental health care among young people. J Adolesc Health. 2021;68(3):540–7. https://doi.org/10.1016/j.jadohealth.2020.06.040 .
Reavley NJ, Morgan AJ, Petrie D, Jorm AF. Does mental health-related discrimination predict health service use 2 years later? Findings from an Australian national survey. Soc Psychiatry Psychiatr Epidemiol. 2020;55(2):197–204. https://doi.org/10.1007/s00127-019-01762-2 .
Roberts T, Miguel Esponda G, Krupchanka D, Shidhaye R, Patel V, Rathod S. Factors associated with health service utilisation for common mental disorders: a systematic review. BMC Psychiatry. 2018;18(1):262. https://doi.org/10.1186/s12888-018-1837-1 .
Rogler LH. International migrations. A framework for directing research. Am Psychol. 1994;49(8):701–8.
Sagar R, Pattanayak RD, Chandrasekaran R, Chaudhury PK, Deswal BS, Singh RL, et al. Twelve-month prevalence and treatment gap for common mental disorders: findings from a large-scale epidemiological survey in India. Indian J Psychiatry. 2017;59(1):46.
Saxena S, Sharan P, Saraceno B. Budget and financing of mental health services: baseline information on 89 countries from WHO’s project atlas. J Ment Health Policy Econ. 2003;6(3):135–43.
Sawyer MG, Whaites L, Rey JM, Hazell PL, Graetz BW, Baghurst P. Health-related quality of life of children and adolescents with mental disorders. J Am Acad Child Adolesc Psychiatry. 2002;41(5):530–7. https://doi.org/10.1097/00004583-200205000-00010 .
Seal KH, Metzler TJ, Gima KS, Bertenthal D, Gaguen S, Marmar CR. Trends and risk factors for mental health diagnoses among Iraq and Afghanistan veterans using department of veterans affairs health care, 2002–2008. Am J Public Health. 2009;99(9):1651–8.
Sepúlveda-Loyola W, Rodríguez-S´anchez I, P´erez-Rodríguez P, Ganz F, Torralba R, Oliveira DV, et al. Impact of social isolation due to COVID-19 on health in older people: mental and physical effects and recommendations. J Nutr Health Aging. 2020;24(9):938–47.
Shafie S, Subramaniam M, Abdin E, Vaingankar JA, Sambasivam R, Zhang Y, Shahwan S, Chang S, Jeyagurunathan A, Chong SA. Help-seeking patterns among the general population in singapore: results from the Singapore mental health study 2016. Adm Policy Ment Health Ment Health Serv Res. 2021;48(4):586–96. https://doi.org/10.1007/s10488-020-01092-5 .
Silva M, Antunes A, Azeredo-Lopes S, Cardoso G, Xavier M, Saraceno B, Caldas-de-Almeida JM. Barriers to mental health services utilisation in Portugal–results from the National mental health survey. J Mental Health. 2022;31(4):453–61. https://doi.org/10.1080/09638237.2020.1739249 .
Stecker T, Fortney J, Hamilton F, Ajzen I. An assessment of beliefs about mental health care among veterans who served in Iraq. Psychiatric Serv. 2007;58:1358–61.
Sodhi-Berry N, Preen DB, Alan J, Knuiman M, Morgan VA, Corrao G, Monzio Compagnoni M, Valsassina V, Lora A. Pre-sentence mental health service use by adult offenders in Western Australia: baseline results from a longitudinal whole-population cohort studyAssessing the physical healthcare gap among patients with severe mental illness: large real-world investigati. Crim Behav Ment HealthBJPsych Open. 2014;247(35):204–21. https://doi.org/10.1192/bjo.2021.998 .
Spinogatti F, Civenti G, Conti V, Lora A, Bahji A. Ethnic differences in the utilization of mental health services in Lombardy (Italy): an epidemiological analysisIncidence and correlates of opioid-related psychiatric emergency care: a retrospective, multiyear cohort study. Soc Psychiatry Psychiatr Epidemiol J Opioid Manage. 2015;5016(13):59223–65226. https://doi.org/10.5055/jom.2020.0572 .
The World Bank. Population, total, population, total - low income, middle income, high income. Washington, D.C.: The World Bank (producer and distributor); 2022. https://data.worldbank.org/indicator/SP.POP.TOTL?end=2022&locations=XM-XP-XD&start=1960&view=chart
Thornicroft G. Stigma and discrimination limit access to mental health care. Epidemiol Psychiatric Sci. 2008;17(1):14–9. https://doi.org/10.1017/S1121189X00002621 .
Thornicroft G, Tansella M. Components of a modern mental health service: a pragmatic balance of community and hospital care: overview of systematic evidence. Br J Psychiatry. 2004;185:283–90.
Tiwari SK, Wang J. Ethnic differences in mental health service use among White, Chinese, south Asian and South East Asian populations living in Canada. Soc Psychiatry Psychiatr Epidemiol. 2008;43(11):866–71. https://doi.org/10.1007/s00127-008-0373-6 .
Volkert J, Andreas S, Härter M, Dehoust MC, Sehner S, Suling A, Ausín B, Canuto A, Crawford MJ, Ronch D, Grassi C, Hershkovitz L, Muñoz Y, Quirk M, Rotenstein A, Santos-Olmo O, Shalev AB, Strehle AY, Weber J, Schulz K. Predisposing, enabling, and need factors of service utilization in the elderly with mental health problems. Int Psychogeriatr. 2018;30(7):1027–37. https://doi.org/10.1017/S1041610217002526 .
Wang N, Xie X. Associations of health insurance coverage, mental health problems, and drug use with mental health service use in US adults: an analysis of 2013 National Survey on Drug Use and Health. Aging Ment Health. 2019;23(4):439–46. https://doi.org/10.1080/13607863.2018.1441262 .
Wang PS, Aguilar-Gaxiola S, Alonso J, Angermeyer MC, Borges G, Bromet EJ, et al. Use of mental health services for anxiety, mood, and substance disorders in 17 countries in the WHO world mental health surveys. Lancet. 2007;370(9590):841–50.
Wei Z, Hu C, Wei X, Yang H, Shu M, Liu T. Service utilization for mental problems in a metropolitan migrant population in China. Psychiatr Serv. 2013;64(7):645–52. https://doi.org/10.1176/appi.ps.201200304 .
Werlen L, Puhan MA, Landolt MA, Mohler-Kuo M. Mind the treatment gap: the prevalence of common mental disorder symptoms, risky substance use and service utilization among young Swiss adults. BMC Public Health. 2020;20(1):1470. https://doi.org/10.1186/s12889-020-09577-6 .
Wettstein A, Tlali M, Joska JA, Cornell M, Skrivankova VW, Seedat S, Mouton JP, van den Heuvel LL, Maxwell N, Davies MA, Maartens G, Egger M, Haas AD. The effect of the COVID-19 lockdown on mental health care use in South Africa: an interrupted time-series analysis. Epidemiol Psychiatr Sci. 2022;31:e43. https://doi.org/10.1017/s2045796022000270 .
Whitley R, Wang J, Fleury MJ, Liu A, Caron J. Mental health status, health care utilisation, and service satisfaction among immigrants in montreal: an epidemiological comparison. Can J Psychiatry. 2017;62(8):570–9. https://doi.org/10.1177/0706743716677724 .
Warner EL, Kent EE, Trevino KM, Parsons HM, Zebrack BJ, Kirchhoff AC. Social well-being among adolescents and young adults with cancer: a systematic review. Cancer. 2016;122:1029–37.
Wells G, Shea B, O’connell D, et al. The Newcastle-Ottawa Scale (NOS) for assessing the quality of nonrandomised studies in meta-analyses. Department of Epidemiology and Community Medicine, University of Ottawa, Canada. Canada: University of Ottawa; 2000. Available at: https://www.ohri.ca/programs/clinical_epidemiology/oxford.Asp
Westerhof GJ, Keyes CL. Mental illness and mental health: the two continua model across the lifespan. J Adult Dev. 2010;17(2):110–9. https://doi.org/10.1007/s10804-009-9082-y .
World Health Organization. The ICD-10 classification of mental and behavioural disorders: clinical descriptions and diagnostic guidelines. Geneva: World Health Organization; 1992.
Yang KG, Rodgers CRR, Lee E, B LC. Disparities in mental health care utilization and perceived need among Asian americans: 2012–2016. Psychiatr Serv. 2020;71(1):21–7. https://doi.org/10.1176/appi.ps.201900126 .
Rosenberg S, Hickie AMI. Making better choices about mental health investment: the case for urgent reform of Australia’s better access program. Australian New Z J Psychiatry. 2019;53(11):1052–8. https://doi.org/10.1177/0004867419865335 .
World Bank. World Bank income groups [dataset]. World Bank, Income Classifications [original data]. 2023. Retrieved May 2, 2024 from https://ourworldindata.org/grapher/world-bank-income-groups .
Download references
Acknowledgements
Not applicable.
Author information
Authors and affiliations.
The National Centre for Epidemiology and Population Health, Australian National University, Canberra, Australia
Yunqi Gao, Richard Burns, Liana Leach & Miranda R. Chilver
School of Psychology, Deakin University, Melbourne, Australia
Peter Butterworth
Department of Health, Economics, Wellbeing and Society, Australian National University, Canberra, Australia
You can also search for this author in PubMed Google Scholar
Contributions
YG was responsible for the study design, title and abstract screening, full-text screening, and writing the manuscript. PB, RB, and LL contributed to the study design, title and abstract screening, full-text screening, and offered comments and detailed feedback on the draft paper. MC also helped with the title and abstract screening, full-text screening, and provided comments and detailed feedback on the draft paper.
Corresponding author
Correspondence to Yunqi Gao .
Ethics declarations
Ethics approval and consent to participate, consent for publication, competing interests.
The authors declare no competing interests.
Additional information
Publisher’s note.
Springer Nature remains neutral with regard to jurisdictional claims in published maps and institutional affiliations.
Supplementary Information
Supplementary material 1., rights and permissions.
Open Access This article is licensed under a Creative Commons Attribution 4.0 International License, which permits use, sharing, adaptation, distribution and reproduction in any medium or format, as long as you give appropriate credit to the original author(s) and the source, provide a link to the Creative Commons licence, and indicate if changes were made. The images or other third party material in this article are included in the article's Creative Commons licence, unless indicated otherwise in a credit line to the material. If material is not included in the article's Creative Commons licence and your intended use is not permitted by statutory regulation or exceeds the permitted use, you will need to obtain permission directly from the copyright holder. To view a copy of this licence, visit http://creativecommons.org/licenses/by/4.0/ .
Reprints and permissions
About this article
Cite this article.
Gao, Y., Burns, R., Leach, L. et al. Examining the mental health services among people with mental disorders: a literature review. BMC Psychiatry 24 , 568 (2024). https://doi.org/10.1186/s12888-024-05965-z
Download citation
Received : 18 February 2024
Accepted : 15 July 2024
Published : 20 August 2024
DOI : https://doi.org/10.1186/s12888-024-05965-z
Share this article
Anyone you share the following link with will be able to read this content:
Sorry, a shareable link is not currently available for this article.
Provided by the Springer Nature SharedIt content-sharing initiative
- Health services
- Service use
- Mental disorder
- Systematic review
BMC Psychiatry
ISSN: 1471-244X
- General enquiries: [email protected]
Information
- Author Services
Initiatives
You are accessing a machine-readable page. In order to be human-readable, please install an RSS reader.
All articles published by MDPI are made immediately available worldwide under an open access license. No special permission is required to reuse all or part of the article published by MDPI, including figures and tables. For articles published under an open access Creative Common CC BY license, any part of the article may be reused without permission provided that the original article is clearly cited. For more information, please refer to https://www.mdpi.com/openaccess .
Feature papers represent the most advanced research with significant potential for high impact in the field. A Feature Paper should be a substantial original Article that involves several techniques or approaches, provides an outlook for future research directions and describes possible research applications.
Feature papers are submitted upon individual invitation or recommendation by the scientific editors and must receive positive feedback from the reviewers.
Editor’s Choice articles are based on recommendations by the scientific editors of MDPI journals from around the world. Editors select a small number of articles recently published in the journal that they believe will be particularly interesting to readers, or important in the respective research area. The aim is to provide a snapshot of some of the most exciting work published in the various research areas of the journal.
Original Submission Date Received: .
- Active Journals
- Find a Journal
- Proceedings Series
- For Authors
- For Reviewers
- For Editors
- For Librarians
- For Publishers
- For Societies
- For Conference Organizers
- Open Access Policy
- Institutional Open Access Program
- Special Issues Guidelines
- Editorial Process
- Research and Publication Ethics
- Article Processing Charges
- Testimonials
- Preprints.org
- SciProfiles
- Encyclopedia
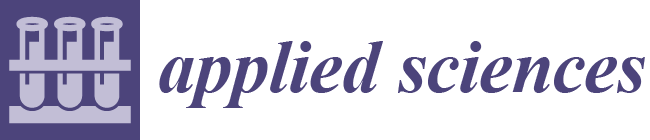
Article Menu

- Subscribe SciFeed
- Recommended Articles
- Google Scholar
- on Google Scholar
- Table of Contents
Find support for a specific problem in the support section of our website.
Please let us know what you think of our products and services.
Visit our dedicated information section to learn more about MDPI.
JSmol Viewer
Ai-based electroencephalogram analysis in rodent models of epilepsy: a systematic review.
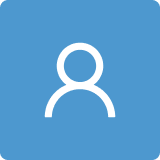
1. Introduction
- Interictal discharge: Interictal spikes are the intermittent neurological discharge observed between seizures in patients and animals with epilepsy [ 24 , 25 ]. Interictal spikes are noncoincidental [ 26 ] but relatable to seizures, and they are quantified as the most reliable epileptogenic biomarker [ 27 , 28 ].
- Preictal discharge: The preictal state is the abnormal neural discharge just before the manifestation of a seizure event, where a patient may feel the presence of seizure aura, which are physiological changes such as muscle twitches and gastrointestinal upset [ 29 ]. The temporal and spatial changes of the preictal discharge correlate with seizure onset; towards the onset of seizure, the amplitude of preictal discharge increases, while the interval decreases [ 30 ]. Thus, understanding and detecting the preictal spike mechanism can predict the next seizure episode [ 31 ].
- Ictal discharge: This is the most symptomatic and shortest phase of the epileptiform discharge. The ictal spike represents the critical event of a seizure that characterises an active epileptic condition.
- Postictal discharge: This state of the brain describes the abnormal neurological performance that begins at the end of a seizure episode; this state may last for hours before the resumption of the neural baseline activity [ 32 ]. Postictal discharge receives less attention than the interictal and preictal states. Nevertheless, the postictal characteristics may assist in distinguishing epileptic and non-epileptic seizures [ 33 ]
2. Fundamental Concepts and Background
2.1. classification of epilepsy, 2.2. rodent models of epilepsy, 2.3. eeg signal, 2.4. machine learning and deep learning techniques.
Click here to enlarge figure
3. Materials and Methods
- Defining the research questions;
- Execution of article searches within specified databases;
- Filtering articles through the evaluation of their relevance;
- Data extraction;
- Synthesizing of results.
3.1. Research Questions
- RQ1: What rodent models of epilepsy and seizure/epilepsy types have been automatically analysed with ML or DL algorithms?
- RQ2: What features and feature engineering techniques have been considered in the classical machine learning detection and prediction of seizures in the rodent model of epilepsy?
- RQ3: What ML or DL methods have been exploited in detecting and predicting seizures from EEG of rodent models of epilepsy?
- RQ4: What training methodologies and evaluation metrics have been used in the rodent models of epilepsy, and which of the developed DL/ML models have been implemented?
3.2. Search Execution
- S1: ((EEG OR Electroencephalogram) AND (Seizure detection OR Machine learning seizure detection OR Deep learning seizure detection OR Interictal spike detection OR Ictal spike detection) AND (Rodent model of epilepsy OR Mouse model of epilepsy OR Rat model of epilepsy)).
- S2: ((EEG OR Electroencephalogram) AND (Seizure prediction OR Machine learning seizure prediction OR Deep learning seizure prediction OR Spike detection OR Preictal spike detection) AND (Rodent model of epilepsy OR Mouse model of epilepsy OR Rat model of epilepsy)).
- S3: ((“EEG” OR “Electroencephalogram” OR “iEEG”) AND (“Seizure detection” OR “Machine learning seizure detection” OR “Deep learning seizure detection” OR “Interictal spike detection” OR “Ictal spike detection”) AND (“Rodent model of epilepsy” OR “Mouse model of epilepsy” OR “Rat model of epilepsy”)).
- S4: ((“EEG” OR “Electroencephalogram” OR “iEEG”) AND (“Seizure prediction” OR “Machine learning seizure prediction” OR “Deep learning seizure prediction” OR “Spike detection” OR “Preictal spike detection”) AND (“Rodent model of epilepsy” OR “Mouse model of epilepsy” OR “Rat model of epilepsy”)).
3.3. Article Selection and Filtering
- Conference papers published by ACM, IEEE, or Springer;
- Published between 1 January 1994 and 1 January 2024;
- Focused on automatic seizure detection and prediction with machine learning and deep learning techniques;
- The study is conducted using EEG on rodent models of epilepsy;
- Full text available;
- The study reported in the article is empirical.
- The study uses EEG from humans or other animals, not rodents;
- Full text is inaccessible;
- Literature review studies, books, short papers/abstracts;
- The study is focused on other neurological disorders (Alzheimer’s, dementia, sleep disorder, etc.);
- The study uses other clinical methods of determining epilepsy (e.g., genomics);
- Studies not written in English;
- Studies focusing on clinical studies only;
- Studies performed using other nonmachine or deep learning techniques;
- Journals not listed in Journal Citation Report (JCR).
- Data Extraction Relevant data were gathered and analysed to synthesise and answer the research questions from the included studies. In the process, the following data fields were extracted: – D1: The aim of the article. – D2: The rodent models of epilepsy analysed. – D3: The epileptiform discharge recognition task: detection or prediction of seizures. – D4: The feature engineering techniques adopted. – D5: Employed ML or DL technique, either classical or a combination of techniques and evaluation result. – D6: The implementation of the ML or DL models.
4.1. Ml and DL Article Distributions
4.2. distribution of articles among epileptiform discharge tasks, 4.3. rq 1: what rodent models of epilepsy and seizure/epilepsy types have been automatically analysed with ml or dl algorithms, 4.4. rq2: what features and feature engineering techniques have been considered in the classical machine learning detection and prediction of seizures in rodent models of epilepsy, 4.5. rq3: what ml or dl methods have been exploited in detecting and predicting seizures from eeg of rodent models of epilepsy, 4.6. rq4: what training methodologies and evaluation metrics have been used in the rodent models of epilepsy, and which of the developed dl/ml models have been implemented, 5. discussion, 5.1. research gaps, 5.2. limitations of the study, 5.3. future works, 6. conclusions, author contributions, institutional review board statement, informed consent statement, data availability statement, conflicts of interest.
- Fisher, R.; Acevedo, C.; Arzimanoglou, A.; Bogacz, A.; Cross, J.; Elger, C.; Engel, J., Jr.; Forsgren, L.; French, J.; Glynn, M.; et al. ILAE official report: A practical clinical definition of epilepsy. Epilepsia 2014 , 55 , 475–482. [ Google Scholar ] [ CrossRef ] [ PubMed ]
- Scharfman, H. The neurobiology of epilepsy. Curr. Neurol. Neurosci. Rep. 2007 , 7 , 348–354. [ Google Scholar ] [ CrossRef ]
- Shimizu, H.; Morimoto, Y.; Yamamoto, N.; Tayama, T.; Ozawa, H.; Imamura, A. Overlap between epilepsy and neurodevelopmental disorders: Insights from clinical and genetic studies. Epilepsy 2022 . [ Google Scholar ] [ CrossRef ]
- McNamara, J. Emerging insights into the genesis of epilepsy. Nature 1999 , 399 , A15–A22. [ Google Scholar ] [ CrossRef ]
- Fisher, R.; Cross, J.; French, J.; Higurashi, N.; Hirsch, E.; Jansen, F.; Lagae, L.; Moshé, S.; Peltola, J.; Roulet Perez, E.; et al. Operational classification of seizure types by the International League against Epilepsy: Position Paper of the ILAE Commission for Classification and Terminology. Epilepsia 2017 , 58 , 522–530. [ Google Scholar ] [ CrossRef ] [ PubMed ]
- Benbadis, S.; Agrawal, V.; Tatum, W. How many patients with psychogenic nonepileptic seizures also have epilepsy? Neurology 2001 , 57 , 915–917. [ Google Scholar ] [ CrossRef ]
- Xu, Y.; Nguyen, D.; Mohamed, A.; Carcel, C.; Li, Q.; Kutlubaev, M.; Anderson, C.; Hackett, M. Frequency of a false positive diagnosis of epilepsy: A systematic review of observational studies. Seizure 2016 , 41 , 167–174. [ Google Scholar ] [ CrossRef ] [ PubMed ]
- Hong, Y.; Wei, C.; Fu, M.; Li, X.; Zhang, H.; Yao, B. MCC950 alleviates seizure severity and angiogenesis by inhibiting NLRP3/IL-1 β signaling pathway-mediated pyroptosis in mouse model of epilepsy. Int. Immunopharmacol. 2024 , 126 , 111236. [ Google Scholar ] [ CrossRef ]
- Laxer, K.; Trinka, E.; Hirsch, L.; Cendes, F.; Langfitt, J.; Delanty, N.; Resnick, T.; Benbadis, S. The consequences of refractory epilepsy and its treatment. Epilepsy Behav. 2014 , 37 , 59–70. [ Google Scholar ] [ CrossRef ]
- Beghi, E.; Giussani, G.; Nichols, E.; Abd-Allah, F.; Abdela, J.; Abdelalim, A.; Abraha, H.; Adib, M.; Agrawal, S.; Alahdab, F.; et al. Global, regional, and national burden of epilepsy, 1990–2016: A systematic analysis for the Global Burden of Disease Study 2016. Lancet Neurol. 2019 , 19 , 357–375. [ Google Scholar ] [ CrossRef ]
- Fiest, K.; Sauro, K.; Wiebe, S.; Patten, S.; Kwon, C.; Dykeman, J.; Pringsheim, T.; Lorenzetti, D.; Jetté, N. Prevalence and incidence of epilepsy: A systematic review and meta-analysis of international studies. Neurology 2017 , 88 , 296–303. [ Google Scholar ] [ CrossRef ] [ PubMed ]
- Löscher, W.; Potschka, H.; Sisodiya, S.; Vezzani, A. Drug resistance in epilepsy: Clinical impact, potential mechanisms, and new innovative treatment options. Pharmacol. Rev. 2020 , 72 , 606–638. [ Google Scholar ] [ CrossRef ] [ PubMed ]
- Kandratavicius, L.; Balista, P.; Lopes-Aguiar, C.; Ruggiero, R.; Umeoka, E.; Garcia-Cairasco, N.; Bueno-Junior, L.; Leite, J. Animal models of epilepsy: Use and limitations. Neuropsychiatr. Dis. Treat. 2014 , 10 , 1693–1705. [ Google Scholar ]
- Singh, R.; Farooq, S.; Mannan, A.; Singh, T.; Najda, A.; Grażyna, Z.; Albadrani, G.; Sayed, A.; Abdel-Daim, M. Animal models of diabetic microvascular complications: Relevance to clinical features. Biomed. Pharmacother. 2022 , 145 , 112305. [ Google Scholar ] [ CrossRef ] [ PubMed ]
- Edoho, M.; Mamad, O.; Henshall, D.C.; Mooney, C.; Wei, L. Prediction of Epilepsy Phenotype in Intra-amygdala Kainic Acid Mouse Model of Epilepsy. In Proceedings of the 2023 10th International Conference on Biomedical and Bioinformatics Engineering, Kyoto, Japan, 9–12 November 2023; pp. 196–200. [ Google Scholar ]
- Dawson, T.; Golde, T.; Lagier-Tourenne, C. Animal models of neurodegenerative diseases. Nat. Neurosci. 2018 , 21 , 1370–1379. [ Google Scholar ] [ CrossRef ] [ PubMed ]
- Grone, B.; Baraban, S. Animal models in epilepsy research: Legacies and new directions. Nat. Neurosci. 2015 , 18 , 339–343. [ Google Scholar ] [ CrossRef ] [ PubMed ]
- Wang, Y.; Wei, P.; Yan, F.; Luo, Y.; Zhao, G. Animal models of epilepsy: A phenotype-oriented review. Aging Dis. 2022 , 13 , 215. [ Google Scholar ] [ CrossRef ] [ PubMed ]
- Willner, P. The validity of animal models of depression. Psychopharmacology 1984 , 83 , 1–16. [ Google Scholar ] [ CrossRef ] [ PubMed ]
- Garner, J. The significance of meaning: Why do over 90% of behavioral neuroscience results fail to translate to humans, and what can we do to fix it? Ilar J. 2014 , 55 , 438–456. [ Google Scholar ] [ CrossRef ]
- Minguillon, J.; Lopez-Gordo, M.; Pelayo, F. Trends in EEG-BCI for daily-life: Requirements for artifact removal. Biomed. Signal Process. Control 2017 , 31 , 407–418. [ Google Scholar ] [ CrossRef ]
- Slimen, I.; Boubchir, L.; Seddik, H. Epileptic seizure prediction based on EEG spikes detection of ictal-preictal states. J. Biomed. Res. 2020 , 34 , 162. [ Google Scholar ] [ CrossRef ] [ PubMed ]
- Seneviratne, U.; Boston, R.; Cook, M.; D’Souza, W. Characteristics of epileptiform discharge duration and interdischarge interval in genetic generalized epilepsies. Front. Neurol. 2018 , 9 , 36. [ Google Scholar ] [ CrossRef ]
- Smith, E.; Liou, J.; Merricks, E.; Davis, T.; Thomson, K.; Greger, B.; House, P.; Emerson, R.; Goodman, R.; McKhann, G.; et al. Human interictal epileptiform discharges are bidirectional traveling waves echoing ictal discharges. Elife 2022 , 11 , e73541. [ Google Scholar ] [ CrossRef ]
- Tatum, W.; Rubboli, G.; Kaplan, P.; Mirsatari, S.; Radhakrishnan, K.; Gloss, D.; Caboclo, L.; Drislane, F.; Koutroumanidis, M.; Schomer, D.; et al. Clinical utility of EEG in diagnosing and monitoring epilepsy in adults. Clin. Neurophysiol. 2018 , 129 , 1056–1082. [ Google Scholar ] [ CrossRef ]
- Sablok, S.; Gururaj, G.; Shaikh, N.; Shiksha, I.; Choudhary, A. Interictal spike detection in EEG using time series classification. In Proceedings of the 2020 4th International Conference on Intelligent Computing and Control Systems (ICICCS), Madurai, India, 13–15 May 2020; pp. 644–647. [ Google Scholar ]
- Heers, M.; Böttcher, S.; Kalina, A.; Katletz, S.; Altenmüller, D.; Baroum, A.; Strobbe, G.; Mierlo, P.; Oertzen, T.; Marusic, P.; et al. Detection of interictal epileptiform discharges in an extended scalp EEG array and high-density EEG—A prospective multicenter study. Epilepsia 2022 , 63 , 1619–1629. [ Google Scholar ] [ CrossRef ]
- Prasanth, T.; Thomas, J.; Yuvaraj, R.; Jing, J.; Cash, S.; Chaudhari, R.; Leng, T.; Rathakrishnan, R.; Rohit, S.; Saini, V.; et al. Deep learning for interictal epileptiform spike detection from scalp EEG frequency sub bands. In Proceedings of the 2020 42nd Annual International Conference of the IEEE Engineering in Medicine & Biology Society (EMBC), Montréal, QC, Canada, 20–24 July 2020; pp. 3703–3706. [ Google Scholar ]
- Selim, S.; Elhinamy, E.; Othman, H.; Abouelsaadat, W.; Salem, M. A review of machine learning approaches for epileptic seizure prediction. In Proceedings of the 2019 14th International Conference on Computer Engineering and Systems (ICCES), Cairo, Egypt, 17–18 December 2019; pp. 239–244. [ Google Scholar ]
- Chen, J.; Li, L.; Wu, D.; Li, X.; Xue, Q.; Wang, L.; Du, J.; Wang, D.; Hu, M.; Ren, L.; et al. Dynamic preictal discharges in patients with mesial temporal lobe epilepsy. J. Clin. Neurophysiol. 2018 , 35 , 381–387. [ Google Scholar ] [ CrossRef ] [ PubMed ]
- Nazari, J.; Motie Nasrabadi, A.; Menhaj, M.; Raiesdana, S. Epilepsy seizure prediction with few-shot learning method. Brain Inform. 2022 , 9 , 1–9. [ Google Scholar ] [ CrossRef ] [ PubMed ]
- Rémi, J.; Noachtar, S. Clinical features of the postictal state: Correlation with seizure variables. Epilepsy Behav. 2010 , 19 , 114–117. [ Google Scholar ] [ CrossRef ]
- Ettinger, A.; Weisbrot, D.; Nolan, E.; Devinsky, O. Postictal symptoms help distinguish patients with epileptic seizures from those with non-epileptic seizures. Seizure 1999 , 8 , 149–151. [ Google Scholar ] [ CrossRef ]
- Wei, L.; Boutouil, H.; Gerbatin, R.; Mamad, O.; Heiland, M.; Reschke, C.; Del Gallo, F.; Fabene, P.; Henshall, D.; Lowery, M.; et al. Detection of spontaneous seizures in EEGs in multiple experimental mouse models of epilepsy. J. Neural Eng. 2021 , 18 , 056060. [ Google Scholar ] [ CrossRef ]
- Angeles, D. Proposal for revised clinical and electroencephalographic classification of epileptic seizures. Epilepsia 1981 , 22 , 489–501. [ Google Scholar ]
- Fisher, R. The new classification of seizures by the International League Against Epilepsy 2017. Curr. Neurol. Neurosci. Rep. 2017 , 17 , 1–6. [ Google Scholar ] [ CrossRef ] [ PubMed ]
- Harris, L.; Angus-Leppan, H. Epilepsy: Diagnosis, classification and management. Medicine 2020 , 48 , 522–528. [ Google Scholar ] [ CrossRef ]
- Wirrell, E.; Tinuper, P.; Perucca, E.; Moshé, S. Introduction to the epilepsy syndrome papers. Epilepsia 2022 , 63 , 1330–1332. [ Google Scholar ] [ CrossRef ]
- Milligan, T. Epilepsy: A clinical overview. Am. J. Med. 2021 , 134 , 840–847. [ Google Scholar ] [ CrossRef ]
- Rusina, E.; Bernard, C.; Williamson, A. The kainic acid models of temporal lobe epilepsy. Eneuro 2021 , 8 , 1–24. [ Google Scholar ] [ CrossRef ]
- Marshall, G.; Gonzalez-Sulser, A.; Abbott, C. Modelling epilepsy in the mouse: Challenges and solutions. Dis. Model. Mech. 2021 , 14 , dmm047449. [ Google Scholar ] [ CrossRef ]
- Leite, J.; Garcia-Cairasco, N.; Cavalheiro, E. New insights from the use of pilocarpine and kainate models. Epilepsy Res. 2002 , 50 , 93–103. [ Google Scholar ] [ CrossRef ] [ PubMed ]
- Goddard, G.; McIntyre, D.; Leech, C. A permanent change in brain function resulting from daily electrical stimulation. Exp. Neurol. 1969 , 25 , 295–330. [ Google Scholar ] [ CrossRef ] [ PubMed ]
- Cherian, A.; Thomas, S. Status epilepticus. Ann. Indian Acad. Neurol. 2009 , 12 , 140. [ Google Scholar ]
- Turski, W.; Cavalheiro, E.; Schwarz, M.; Czuczwar, S.; Kleinrok, Z.; Turski, L. Limbic seizures produced by pilocarpine in rats: Behavioural, electroencephalographic and neuropathological study. Behav. Brain Res. 1983 , 9 , 315–335. [ Google Scholar ] [ CrossRef ] [ PubMed ]
- Chauviere, L.; Doublet, T.; Ghestem, A.; Siyoucef, S.; Wendling, F.; Huys, R.; Jirsa, V.; Bartolomei, F.; Bernard, C. Changes in interictal spike features precede the onset of temporal lobe epilepsy. Ann. Neurol. 2012 , 71 , 805–814. [ Google Scholar ] [ CrossRef ]
- Henderson, K.; Gupta, J.; Tagliatela, S.; Litvina, E.; Zheng, X.; Van Zandt, M.A.; Woods, N.; Grund, E.; Lin, D.; Royston, S.; et al. Long-term seizure suppression and optogenetic analyses of synaptic connectivity in epileptic mice with hippocampal grafts of GABAergic interneurons. J. Neurosci. 2014 , 34 , 13492–13504. [ Google Scholar ] [ CrossRef ]
- Lévesque, M.; Avoli, M.; Bernard, C. Animal models of temporal lobe epilepsy following systemic chemoconvulsant administration. J. Neurosci. Methods 2016 , 260 , 45–52. [ Google Scholar ] [ CrossRef ] [ PubMed ]
- Griffin, A.; Hamling, K.; Hong, S.; Anvar, M.; Lee, L.; Baraban, S. Preclinical animal models for Dravet syndrome: Seizure phenotypes, comorbidities and drug screening. Front. Pharmacol. 2018 , 9 , 573. [ Google Scholar ] [ CrossRef ] [ PubMed ]
- Löscher, W. Fit for purpose application of currently existing animal models in the discovery of novel epilepsy therapies. Epilepsy Res. 2016 , 126 , 157–184. [ Google Scholar ] [ CrossRef ]
- Irizarry, R.; Sukato, D.; Kollmar, R.; Schild, S.; Silverman, J.; Sundaram, K.; Stephenson, S.; Stewart, M. Seizures induce obstructive apnea in DBA/2J audiogenic seizure-prone mice: Lifesaving impact of tracheal implants. Epilepsia 2020 , 61 , e13–e16. [ Google Scholar ] [ CrossRef ]
- Bosco, F.; Guarnieri, L.; Leo, A.; Tallarico, M.; Gallelli, L.; Rania, V.; Citraro, R.; De Sarro, G. Audiogenic epileptic DBA/2 mice strain as a model of genetic reflex seizures and SUDEP. Front. Neurol. 2023 , 14 , 1223074. [ Google Scholar ] [ CrossRef ] [ PubMed ]
- Depaulis, A.; David, O.; Charpier, S. The genetic absence epilepsy rat from Strasbourg as a model to decipher the neuronal and network mechanisms of generalized idiopathic epilepsies. J. Neurosci. Methods 2016 , 260 , 159–174. [ Google Scholar ] [ CrossRef ]
- Valassina, N.; Brusco, S.; Salamone, A.; Serra, L.; Luoni, M.; Giannelli, S.; Bido, S.; Massimino, L.; Ungaro, F.; Mazzara, P.; et al. Scn1a gene reactivation after symptom onset rescues pathological phenotypes in a mouse model of Dravet syndrome. Nat. Commun. 2022 , 13 , 161. [ Google Scholar ] [ CrossRef ]
- Gerbatin, R.; Augusto, J.; Boutouil, H.; Reschke, C.; Henshall, D. Life-span characterization of epilepsy and comorbidities in Dravet syndrome mice carrying a targeted deletion of exon 1 of the Scn1a gene. Exp. Neurol. 2022 , 354 , 114090. [ Google Scholar ] [ CrossRef ] [ PubMed ]
- Kim, D.; Gersbacher, M.; Inquimbert, P.; Kovacs, D. Reduced sodium channel Nav1.1 levels in BACE1-null mice. J. Biol. Chem. 2011 , 286 , 8106–8116. [ Google Scholar ] [ CrossRef ] [ PubMed ]
- Lachaux, J.; Axmacher, N.; Mormann, F.; Halgren, E.; Crone, N. High-frequency neural activity and human cognition: Past, present and possible future of intracranial EEG research. Prog. Neurobiol. 2012 , 98 , 279–301. [ Google Scholar ] [ CrossRef ] [ PubMed ]
- Parvizi, J.; Kastner, S. Promises and limitations of human intracranial electroencephalography. Nat. Neurosci. 2018 , 21 , 474–483. [ Google Scholar ] [ CrossRef ] [ PubMed ]
- Buzsáki, G. Large-scale recording of neuronal ensembles. Nat. Neurosci. 2004 , 7 , 446–451. [ Google Scholar ] [ CrossRef ] [ PubMed ]
- White, H.; Smith, M.; Wilcox, K. Mechanisms of action of antiepileptic drugs. Int. Rev. Neurobiol. 2007 , 81 , 85–110. [ Google Scholar ] [ PubMed ]
- Shah, A.; Mittal, S. Invasive electroencephalography monitoring: Indications and presurgical planning. Ann. Indian Acad. Neurol. 2014 , 17 , S89. [ Google Scholar ] [ CrossRef ] [ PubMed ]
- Ball, T.; Kern, M.; Mutschler, I.; Aertsen, A.; Schulze-Bonhage, A. Signal quality of simultaneously recorded invasive and non-invasive EEG. Neuroimage 2009 , 46 , 708–716. [ Google Scholar ] [ CrossRef ]
- Fisher, R.; Scharfman, H.; DeCurtis, M. How can we identify ictal and interictal abnormal activity? In Issues in Clinical Epileptology: A View from the Bench ; Springer: Dordrecht, The Netherlands, 2014; pp. 3–23. [ Google Scholar ]
- Engel, A.; Moll, C.; Fried, I.; Ojemann, G. Invasive recordings from the human brain: Clinical insights and beyond. Nat. Rev. Neurosci. 2005 , 6 , 35–47. [ Google Scholar ] [ CrossRef ]
- Brienza, M.; Davassi, C.; Mecarelli, O. Artifacts. Clin. Electroencephalogr. 2019 , 109–130. [ Google Scholar ] [ CrossRef ]
- Millán, J.; Carmena, J. Invasive or noninvasive: Understanding brain-machine interface technology. IEEE Eng. Med. Biol. Mag. 2010 , 29 , 16–22. [ Google Scholar ] [ CrossRef ]
- Wang, Q.; Prisco, N.; Tang, J.; Gennarino, V. Protocol for recording epileptiform discharges of EEG and behavioral seizures in freely moving mice. STAR Protoc. 2022 , 3 , 101245. [ Google Scholar ] [ CrossRef ]
- Moyer, J.; Gnatkovsky, V.; Ono, T.; Otáhal, J.; Wagenaar, J.; Stacey, W.; Noebels, J.; Ikeda, A.; Staley, K.; Curtis, M.; et al. Standards for data acquisition and software-based analysis of in vivo electroencephalography recordings from animals. A TASK 1-WG 5 report of the AES/ILAE Translational Task Force of the ILAE. Epilepsia 2017 , 58 , 53–67. [ Google Scholar ] [ CrossRef ] [ PubMed ]
- Nayak, C.; Anilkumar, A. EEG Normal Waveforms. Updated 31 July 2020. In StatPearls ; StatPearls Publishing: Treasure Island, FL, USA, 2020; Available online: http://www.ncbi.nlm.nih.gov (accessed on 8 March 2024).
- Sucholeiki, R.; Louis, S. Normal EEG waveforms. E-Med. 2008 , 1–7. [ Google Scholar ]
- Huang, Z.; Wang, M. A review of electroencephalogram signal processing methods for brain-controlled robots. Cogn. Robot. 2021 , 1 , 111–124. [ Google Scholar ] [ CrossRef ]
- Zijlmans, M.; Jiruska, P.; Zelmann, R.; Leijten, F.; Jefferys, J.; Gotman, J. High-frequency oscillations as a new biomarker in epilepsy. Ann. Neurol. 2012 , 71 , 169–178. [ Google Scholar ] [ CrossRef ]
- Islam, M.; Zhao, X.; Miao, Y.; Sugano, H.; Tanaka, T. Epileptic seizure focus detection from interictal electroencephalogram: A survey. Cogn. Neurodyn. 2023 , 17 , 1–23. [ Google Scholar ] [ CrossRef ]
- Engel, J., Jr.; Bragin, A.; Staba, R.; Mody, I. High-frequency oscillations: What is normal and what is not? Epilepsia 2009 , 50 , 598–604. [ Google Scholar ] [ CrossRef ]
- Alarcon, G.; Binnie, C.; Elwes, R.; Polkey, C. Power spectrum and intracranial EEG patterns at seizure onset in partial epilepsy. Electroencephalogr. Clin. Neurophysiol. 1995 , 94 , 326–337. [ Google Scholar ] [ CrossRef ] [ PubMed ]
- Al-Rawas, S.; Poothrikovil, R.; Abdelbasit, K.; Delamont, R. The correlation between electroencephalography amplitude and interictal abnormalities: Audit study. Sultan Qaboos Univ. Med. J. 2014 , 14 , e473. [ Google Scholar ]
- Wei, L.; Mchugh, J.C.; Mooney, C. Transfer Learning for the Identification of Paediatric EEGs with Interictal Epileptiform Abnormalities. IEEE Access 2024 , 12 , 86073–86082. [ Google Scholar ] [ CrossRef ]
- Wei, L.; Mchugh, J.C.; Mooney, C. Interictal Epileptiform Discharge Classification for the Prediction of Epilepsy Type in Children. In Proceedings of the 2023 10th International Conference on Biomedical and Bioinformatics Engineering, Kyoto, Japan, 9–12 November 2023. [ Google Scholar ]
- Nayak, C.; Anilkumar, A. EEG normal waveforms. Updated 24 July 2023. In StatPearls [Internet] ; StatPearls Publishing: Treasure Island, FL, USA, 2023. [ Google Scholar ]
- Hosseini, M.; Hosseini, A.; Ahi, K. A review on machine learning for EEG signal processing in bioengineering. IEEE Rev. Biomed. Eng. 2020 , 14 , 204–218. [ Google Scholar ] [ CrossRef ]
- Radüntz, T.; Scouten, J.; Hochmuth, O.; Meffert, B. Automated EEG artifact elimination by applying machine learning algorithms to ICA-based features. J. Neural Eng. 2017 , 14 , 046004. [ Google Scholar ] [ CrossRef ] [ PubMed ]
- Ge, S.; Yang, Q.; Wang, R.; Lin, P.; Gao, J.; Leng, Y.; Yang, Y.; Wang, H. A brain-computer interface based on a few-channel EEG-fNIRS bimodal system. IEEE Access 2017 , 5 , 208–218. [ Google Scholar ] [ CrossRef ]
- Fatourechi, M.; Bashashati, A.; Ward, R.; Birch, G. EMG and EOG artifacts in brain computer interface systems: A survey. Clin. Neurophysiol. 2007 , 118 , 480–494. [ Google Scholar ] [ CrossRef ]
- Mannan, M.; Kamran, M.; Kang, S.; Jeong, M. Effect of EOG signal filtering on the removal of ocular artifacts and EEG-based brain-computer interface: A comprehensive study. Complexity 2018 , 2018 , 4853741. [ Google Scholar ] [ CrossRef ]
- Tamburro, G.; Fiedler, P.; Stone, D.; Haueisen, J.; Comani, S. A new ICA-based fingerprint method for the automatic removal of physiological artifacts from EEG recordings. PeerJ 2018 , 6 , e4380. [ Google Scholar ] [ CrossRef ]
- Sanei, S.; Chambers, J. EEG Signal Processing ; John Wiley & Sons: Hoboken, NJ, USA, 2013. [ Google Scholar ]
- Azlan, W.; Low, Y. Feature extraction of Electroencephalogram (EEG) signal—A review. In Proceedings of the 2014 IEEE Conference on Biomedical Engineering and Sciences (IECBES), Kuala Lumpur, Malaysia, 8–10 December 2014; pp. 801–806. [ Google Scholar ]
- Yi, Y.; Billor, N.; Liang, M.; Cao, X.; Ekstrom, A.; Zheng, J. Classification of EEG signals: An interpretable approach using functional data analysis. J. Neurosci. Methods 2022 , 376 , 109609. [ Google Scholar ] [ CrossRef ]
- Tong, S.; Thankor, N. Quantitative EEG Analysis Methods and Clinical Applications ; Artech House: Norwood, MA, USA, 2009. [ Google Scholar ]
- Rodriguez-Bermudez, G.; Garcia-Laencina, P. Analysis of EEG signals using nonlinear dynamics and chaos: A review. Appl. Math. Inf. Sci. 2015 , 9 , 2309. [ Google Scholar ]
- Li, J.; Cheng, K.; Wang, S.; Morstatter, F.; Trevino, R.; Tang, J.; Liu, H. Feature selection: A data perspective. ACM Comput. Surv. CSUR 2017 , 50 , 1–45. [ Google Scholar ] [ CrossRef ]
- Priyanka, S.; Dema, D.; Jayanthi, T. Feature selection and classification of Epilepsy from EEG signal. In Proceedings of the 2017 International Conference on Energy, Communication, Data Analytics and Soft Computing (ICECDS), Chennai, India, 1–2 August 2017; pp. 2404–2406. [ Google Scholar ]
- Sánchez-Hernández, S.; Salido-Ruiz, R.; Torres-Ramos, S.; Román-Godinez, I. Evaluation of feature selection methods for classification of epileptic seizure EEG signals. Sensors 2022 , 22 , 3066. [ Google Scholar ] [ CrossRef ]
- Boubchir, L.; Daachi, B.; Pangracious, V. A review of feature extraction for EEG epileptic seizure detection and classification. In Proceedings of the 2017 40th International Conference on Telecommunications and Signal Processing (TSP), Barcelona, Spain, 5–7 July 2017; pp. 456–460. [ Google Scholar ]
- Rasheed, K.; Qayyum, A.; Qadir, J.; Sivathamboo, S.; Kwan, P.; Kuhlmann, L.; O’Brien, T.; Razi, A. Machine learning for predicting epileptic seizures using EEG signals: A review. IEEE Rev. Biomed. Eng. 2020 , 14 , 139–155. [ Google Scholar ] [ CrossRef ] [ PubMed ]
- Moher, D.; Liberati, A.; Tetzlaff, J.; Altman, D.; The PRISMA Group. Preferred reporting items for systematic reviews and meta-analyses: The PRISMA statement. PLoS Med. 2009 , 151 , 264–269. [ Google Scholar ]
- Martin-Martin, A.; Orduna-Malea, E.; Thelwall, M.; López-Cózar, E. Google Scholar, Web of Science, and Scopus: A systematic comparison of citations in 252 subject categories. J. Inf. 2018 , 12 , 1160–1177. [ Google Scholar ] [ CrossRef ]
- Gusenbauer, M. Google Scholar to overshadow them all? Comparing the sizes of 12 academic search engines and bibliographic databases. Scientometrics 2019 , 118 , 177–214. [ Google Scholar ] [ CrossRef ]
- Misra, D.; Ravindran, V. An overview of the functionalities of PubMed. J. R. Coll. Physicians Edinb. 2022 , 52 , 8–9. [ Google Scholar ] [ CrossRef ] [ PubMed ]
- Shin, J.; Smith, D.; Swiniarski, R.; Dudek, F.; White, A.; Staley, K.; Cios, K. Analysis of EEG epileptic signals with rough sets and support vector machines. In Proceedings of the Artificial Intelligence in Medicine: 12th Conference on Artificial Intelligence in Medicine, AIME 2009, Verona, Italy, 18–22 July 2009; Proceedings 12. pp. 325–334. [ Google Scholar ]
- Wang, Y.; Liang, S.; Shaw, F.; Huang, Y.; Chen, Y. An energy efficient real-time seizure detection method in rats with spontaneous temporal lobe epilepsy. In Proceedings of the 2013 IEEE Symposium on Computational Intelligence, Cognitive Algorithms, Mind, and Brain (CCMB), Singapore, 16–19 April 2013; pp. 29–35. [ Google Scholar ]
- Fumeaux, N.; Ebrahim, S.; Coughlin, B.; Kadambi, A.; Azmi, A.; Xu, J.; Abou Jaoude, M.; Nagaraj, S.; Thomson, K.; Newell, T.; et al. Accurate detection of spontaneous seizures using a generalized linear model with external validation. Epilepsia 2020 , 61 , 1906–1918. [ Google Scholar ] [ CrossRef ]
- Buteneers, P.; Verstraeten, D.; Van Nieuwenhuyse, B.; Stroobandt, D.; Raedt, R.; Vonck, K.; Boon, P.; Schrauwen, B. Real-time detection of epileptic seizures in animal models using reservoir computing. Epilepsy Res. 2013 , 103 , 124–134. [ Google Scholar ] [ CrossRef ] [ PubMed ]
- Lu, D.; Bauer, S.; Neubert, V.; Costard, L.; Rosenow, F.; Triesch, J. A deep residual neural network based framework for epileptogenesis detection in a rodent model with single-channel EEG recordings. In Proceedings of the 2019 12th International Congress on Image and Signal Processing, BioMedical Engineering and Informatics (CISP-BMEI), Suzhou, China, 19–21 October 2019; pp. 1–6. [ Google Scholar ]
- Baser, O.; Yavuz, M.; Ugurlu, K.; Onat, F.; Demirel, B. Automatic detection of the spike-and-wave discharges in absence epilepsy for humans and rats using deep learning. Biomed. Signal Process. Control 2022 , 76 , 103726. [ Google Scholar ] [ CrossRef ]
- Li, R.; Millist, L.; Foster, E.; Yuan, X.; Guvenc, U.; Radfar, M.; Marendy, P.; Ni, W.; O’Brien, T.; Casillas-Espinosa, P. Spike and wave discharges detection in Genetic Absence Epilepsy Rat from Strasbourg and Patients with Genetic Generalized Epilepsy. Epilepsy Res. 2023 , 194 , 107181. [ Google Scholar ] [ CrossRef ]
- Liang, S.; Chang, W.; Chiueh, H. EEG-based absence seizure detection methods. In Proceedings of the 2010 International Joint Conference on Neural Networks (IJCNN), Barcelona, Spain, 18–23 July 2010; pp. 1–4. [ Google Scholar ]
- Chen, T.; Chiueh, H.; Liang, S.; Chang, S.; Jeng, C.; Hsu, Y.; Chien, T. The implementation of a low-power biomedical signal processor for real-time epileptic seizure detection on absence animal models. IEEE J. Emerg. Sel. Top. Circuits Syst. 2011 , 1 , 613–621. [ Google Scholar ] [ CrossRef ]
- Buteneers, P.; Verstraeten, D.; Mierlo, P.; Wyckhuys, T.; Stroobandt, D.; Raedt, R.; Hallez, H.; Schrauwen, B. Automatic detection of epileptic seizures on the intra-cranial electroencephalogram of rats using reservoir computing. Artif. Intell. Med. 2011 , 53 , 215–223. [ Google Scholar ] [ CrossRef ] [ PubMed ]
- Pfammatter, J.; Maganti, R.; Jones, M. An automated, machine learning–based detection algorithm for spike-wave discharges (SWDs) in a mouse model of absence epilepsy. Epilepsia Open 2019 , 4 , 110–122. [ Google Scholar ] [ CrossRef ]
- Pan, Y.; Ge, S.; Tang, F.; Al Mamun, A. Detection of epileptic spike-wave discharges using SVM. In Proceedings of the 2007 IEEE International Conference on Control Applications, Singapore, 1–3 October 2007; pp. 467–472. [ Google Scholar ]
- Besné, G.; Horrillo-Maysonnial, A.; Nicolás, M.; Capell-Pascual, F.; Urrestarazu, E.; Artieda, J.; Valencia, M. An interactive framework for the detection of ictal and interictal activities: Cross-species and stand-alone implementation. Comput. Methods Programs Biomed. 2022 , 218 , 106728. [ Google Scholar ] [ CrossRef ] [ PubMed ]
- Cho, K.; Jang, H. Comparison of different input modalities and network structures for deep learning-based seizure detection. Sci. Rep. 2020 , 10 , 122. [ Google Scholar ] [ CrossRef ]
- Jang, H.; Cho, K. Dual deep neural network-based classifiers to detect experimental seizures. Korean J. Physiol. Pharmacol. 2019 , 23 , 131–139. [ Google Scholar ] [ CrossRef ]
- Mohammadpoory, Z.; Nasrolahzadeh, M.; Mahmoodian, N.; Sayyah, M.; Haddadnia, J. Complex network based models of ECoG signals for detection of induced epileptic seizures in rats. Cogn. Neurodyn. 2019 , 13 , 325–339. [ Google Scholar ] [ CrossRef ]
- De, A.; Konar, A.; Samanta, A.; Biswas, S.; Basak, P. Seizure prediction using low frequency EEG wavesfrom WAG/Rij rats. In Proceedings of the 2017 2nd International Conference for Convergence in Technology (I2CT), Mumbai, India, 7–9 April 2017; pp. 244–249. [ Google Scholar ]
- Kotloski, R. A Machine Learning Approach to Seizure Detection in a Rat Model of Post-Traumatic Epilepsy. Sci. Rep. 2023 , 13 , 15807. [ Google Scholar ] [ CrossRef ]
- Pan, Y.; Ge, S.; Al Mamun, A.; Tang, F. Detection of seizures in EEG signal using weighted locally linear embedding and SVM classifier. In Proceedings of the 2008 IEEE Conference on Cybernetics and Intelligent Systems, Chengdu, China, 21–24 September 2008; pp. 358–363. [ Google Scholar ]
- Nan, M.; Talathi, S.; Myers, S.; Ditto, W.; Khargonekar, P.; Carney, P. Support vector machines for seizure detection in an animal model of chronic epilepsy. J. Neural Eng. 2010 , 7 , 036001. [ Google Scholar ] [ CrossRef ]
- Ramirez-Vélez, M.; Staba, R.; Barth, D.; Meyer, F. Nonlinear classification of EEG data for seizure detection. In Proceedings of the 3rd IEEE International Symposium on Biomedical Imaging: Nano to Macro, Arlington, VA, USA, 6–9 April 2006; pp. 956–959. [ Google Scholar ]
- Mascott, C.; Gotman, J.; Beaudet, A. Automated EEG monitoring in defining a chronic epilepsy model. Epilepsia 1994 , 35 , 895–902. [ Google Scholar ] [ CrossRef ]
- Niknazar, M.; Mousavi, S.; Motaghi, S.; Dehghani, A.; Vahdat, B.; Shamsollahi, M.; Sayyah, M.; Noorbakhsh, S. A unified approach for detection of induced epileptic seizures in rats using ECoG signals. Epilepsy Behav. 2013 , 27 , 355–364. [ Google Scholar ] [ CrossRef ] [ PubMed ]
- Ullah, I.; Hussain, M.; Aboalsamh, H. An automated system for epilepsy detection using EEG brain signals based on deep learning approach. Expert Syst. Appl. 2018 , 107 , 61–71. [ Google Scholar ]
- Esmaeilpour, A.; Tabarestani, S.; Niazi, A. Deep learning-based seizure prediction using EEG signals: A comparative analysis of classification methods on the CHB-MIT dataset. Eng. Rep. 2024 , e12918. [ Google Scholar ] [ CrossRef ]
- Abhishek, S.; Kumar, S.; Mohan, N.; Soman, K. EEG based automated detection of seizure using machine learning approach and traditional features. Expert Syst. Appl. 2024 , 251 , 123991. [ Google Scholar ]
- Chandel, G.; Aggarwal, T.; Singh, T.; Singh, S.; Singh, K.; Singh, H. Analysis of EEG Signals Using Machine Learning Algorithms. In Proceedings of the 2024 International Conference on Advances in Modern Age Technologies for Health and Engineering Science (AMATHE), Shivamogga, India, 16–17 May 2024; pp. 1–5. [ Google Scholar ]
- Kode, H.; Elleithy, K.; Almazedah, L. Epileptic Seizure detection in EEG signals using Machine Learning and Deep Learning Techniques. IEEE Access 2024 , 12 , 80657–80668. [ Google Scholar ] [ CrossRef ]
- Liu, S.; Zhou, Y.; Yang, X.; Wang, X.; Yin, J. A Robust Automatic Epilepsy Seizure Detection Algorithm Based on Interpretable Features and Machine Learning. Electronics 2024 , 13 , 2727. [ Google Scholar ] [ CrossRef ]
- Kunekar, P.; Gupta, M.; Gaur, P. Detection of epileptic seizure in EEG signals using machine learning and deep learning techniques. J. Eng. Appl. Sci. 2024 , 71 , 21. [ Google Scholar ] [ CrossRef ]
- Srinivas, P.; Arulprakash, M.; Vadivel, M.; Anusha, N.; Rajasekar, G.; Srinivasan, C. Support Vector Machines Based Predictive Seizure Care using IoT-Wearable EEG Devices for Proactive Intervention in Epilepsy. In Proceedings of the 2024 2nd International Conference on Computer, Communication and Control (IC4), Indore, India, 8–10 February 2024; pp. 1–5. [ Google Scholar ]
- Urbina Fredes, S.; Dehghan Firoozabadi, A.; Adasme, P.; Zabala-Blanco, D.; Palacios Játiva, P.; Azurdia-Meza, C. Enhanced Epileptic Seizure Detection through Wavelet-Based Analysis of EEG Signal Processing. Appl. Sci. 2024 , 14 , 5783. [ Google Scholar ] [ CrossRef ]
- Hermawan, A.; Zaeni, I.; Wibawa, A.; Gunawan, G.; Hendrawan, W.; Kristian, Y. A multi representation deep learning approach for epileptic seizure detection. J. Robot. Control JRC 2024 , 5 , 187–204. [ Google Scholar ] [ CrossRef ]
- Abdulwahhab, A.; Abdulaal, A.; Al-Ghrairi, A.; Mohammed, A.; Valizadeh, M. Detection of epileptic seizure using EEG signals analysis based on deep learning techniques. Chaos Solitons Fractals 2024 , 181 , 114700. [ Google Scholar ] [ CrossRef ]
- Trbalić, A.; Hasić, A.; Skejić, E.; Demirović, N. Seizure Detection Based on EEG Signals and Deep Learning. In Proceedings of the 2024 47th MIPRO ICT and Electronics Convention (MIPRO), Opatija, Croatia, 20–24 May 2024; pp. 1145–1150. [ Google Scholar ]
- Shafiezadeh, S.; Pozza, M.; Testolin, A. A Comparison of Recurrent and Convolutional Deep Learning Architectures for EEG Seizure Forecasting. BIOSTEC (1) 2024 , 1 , 583–590. [ Google Scholar ]
- Das, S.; Mumu, S.; Akhand, M.A.H.; Salam, A.; Kamal, M. Epileptic Seizure Detection from Decomposed EEG Signal through 1D and 2D Feature Representation and Convolutional Neural Network. Information 2024 , 15 , 256. [ Google Scholar ] [ CrossRef ]
- Georgis-Yap, Z.; Popovic, M.; Khan, S. Supervised and unsupervised deep learning approaches for EEG seizure prediction. J. Healthc. Inform. Res. 2024 , 8 , 286–312. [ Google Scholar ] [ CrossRef ]
- Abderrahim, N.; Echtioui, A.; Khemakhem, R.; Zouch, W.; Ghorbel, M.; Ben Hamida, A. Epileptic Seizures Detection Using iEEG Signals and Deep Learning Models. Circuits Syst. Signal Process. 2024 , 43 , 1597–1626. [ Google Scholar ] [ CrossRef ]
- Shah, S.; Larijani, H.; Gibson, R.; Liarokapis, D. Epileptic seizure classification based on random neural networks using discrete wavelet transform for electroencephalogram signal decomposition. Appl. Sci. 2024 , 14 , 599. [ Google Scholar ] [ CrossRef ]
- Sadam, S.; Nalini, N. Epileptic seizure detection using scalogram-based hybrid CNN model on EEG signals. Signal Image Video Process. 2024 , 18 , 1577–1588. [ Google Scholar ] [ CrossRef ]
- Anita, M.; Kowshalya, A. Automatic epileptic seizure detection using MSA-DCNN and LSTM techniques with EEG signals. Expert Syst. Appl. 2024 , 238 , 121727. [ Google Scholar ] [ CrossRef ]
- Martinez, C.; Niediek, J.; Mormann, F.; Andrzejak, R. Seizure onset zone lateralization using a non-linear analysis of micro vs. macro electroencephalographic recordings during seizure-free stages of the sleep-wake cycle from epilepsy patients. Front. Neurol. 2020 , 11 , 553885. [ Google Scholar ] [ CrossRef ]
- Shoeb, A. Application of Machine Learning to Epileptic Seizure Onset Detection and Treatment ; Massachusetts Institute of Technology: Cambridge, MA, USA, 2009. [ Google Scholar ]
- Goldberger, A.; Amaral, L.; Glass, L.; Hausdorff, J.; Ivanov, P.; Mark, R.; Mietus, J.; Moody, G.; Peng, C.; Stanley, H.; et al. PhysioBank, PhysioToolkit, and PhysioNet: Components of a new research resource for complex physiologic signals. Circulation 2000 , 101 , e215–e220. [ Google Scholar ] [ CrossRef ]
- Cook, M.; O’Brien, T.; Berkovic, S.; Murphy, M.; Morokoff, A.; Fabinyi, G.; D’Souza, W.; Yerra, R.; Archer, J.; Litewka, L.; et al. Prediction of seizure likelihood with a long-term, implanted seizure advisory system in patients with drug-resistant epilepsy: A first-in-man study. Lancet Neurol. 2013 , 12 , 563–571. [ Google Scholar ] [ CrossRef ] [ PubMed ]
- Obeid, I.; Picone, J. The temple university hospital EEG data corpus. Front. Neurosci. 2016 , 10 , 195498. [ Google Scholar ] [ CrossRef ] [ PubMed ]
- Stevenson, N.; Tapani, K.; Lauronen, L.; Vanhatalo, S. A dataset of neonatal EEG recordings with seizure annotations. Sci. Data 2019 , 6 , 1–8. [ Google Scholar ] [ CrossRef ]
- Wong, S.; Simmons, A.; Rivera-Villicana, J.; Barnett, S.; Sivathamboo, S.; Perucca, P.; Ge, Z.; Kwan, P.; Kuhlmann, L.; Vasa, R.; et al. EEG datasets for seizure detection and prediction—A review. Epilepsia Open 2023 , 8 , 252–267. [ Google Scholar ] [ CrossRef ]
- Briggs, K. Is preclinical data sharing the new norm? Drug Discov. Today 2018 , 23 , 499–502. [ Google Scholar ] [ CrossRef ]
- Schulze-Bonhage, A.; Kühn, A. Unpredictability of seizures and the burden of epilepsy. In Seizure Prediction in Epilepsy: From Basic Mechanisms to Clinical Applications ; Wiley-VCH Verlag GmbH & Co. KGaA: Weinheim, Germany, 2008; pp. 1–10. [ Google Scholar ]
- Fisher, R. Epilepsy from the patient’s perspective: Review of results of a community-based survey. Epilepsy Behav. 2000 , 1 , S9–S14. [ Google Scholar ] [ CrossRef ]
- Usman, S.; Usman, M.; Fong, S. Epileptic seizures prediction using machine learning methods. Comput. Math. Methods Med. 2017 , 2017 , 9074759. [ Google Scholar ] [ CrossRef ] [ PubMed ]
- Gowers, W. Epilepsy and Other Chronic Convulsive Diseases: Their Causes, Symptoms, and Treatment ; Old Hickory Bookshop: Brinklow, MD, USA, 1901. [ Google Scholar ]
- Haut, S. Can patients with epilepsy predict their seizures? Ann. Neurol. 2006 , 60 , S17. [ Google Scholar ] [ CrossRef ] [ PubMed ]
- Cui, S.; Duan, L.; Qiao, Y.; Xiao, Y. Learning EEG synchronization patterns for epileptic seizure prediction using bag-of-wave features. J. Ambient. Intell. Humaniz. Comput. 2018 , 14 , 15557–15572. [ Google Scholar ] [ CrossRef ]
- Peng, P.; Song, Y.; Yang, L.; Wei, H. Seizure prediction in EEG signals using STFT and domain adaptation. Front. Neurosci. 2022 , 15 , 825434. [ Google Scholar ] [ CrossRef ]
- Pinto, M.; Coelho, T.; Leal, A.; Lopes, F.; Dourado, A.; Martins, P.; Teixeira, C. Interpretable EEG seizure prediction using a multiobjective evolutionary algorithm. Sci. Rep. 2022 , 12 , 4420. [ Google Scholar ] [ CrossRef ] [ PubMed ]
- Usman, S.; Khalid, S.; Aslam, M. Epileptic seizures prediction using deep learning techniques. IEEE Access 2020 , 8 , 39998–40007. [ Google Scholar ] [ CrossRef ]
- Grasse, D.; Karunakaran, S.; Moxon, K. Closed-loop seizure prediction and prevention in rats with kainate-induced seizures. In Proceedings of the 2011 5th International IEEE/EMBS Conference on Neural Engineering, Cancun, Mexico, 27 April–1 May 2011; pp. 426–429. [ Google Scholar ]
- Howbert, J.; Patterson, E.; Stead, S.; Brinkmann, B.; Vasoli, V.; Crepeau, D.; Vite, C.; Sturges, B.; Ruedebusch, V.; Mavoori, J.; et al. Forecasting seizures in dogs with naturally occurring epilepsy. PLoS ONE 2014 , 9 , e81920. [ Google Scholar ] [ CrossRef ]
- Singh, N.; Vayer, P.; Tanwar, S.; Poyet, J.; Tsaioun, K.; Villoutreix, B. Drug discovery and development: Introduction to the general public and patient groups. Front. Drug Discov. 2023 , 3 , 1201419. [ Google Scholar ] [ CrossRef ]
- Nair, S.; Shiau, D.; Iasemidis, L.; Norman, W.; Pardalos, P.; Sackellares, J.; Carney, P. Seizure predictability in an experimental model of epilepsy. Data Min. Biomed. 2007 , 7 , 535–558. [ Google Scholar ]
- Sarmast, S.; Abdullahi, A.; Jahan, N. Current classification of seizures and epilepsies: Scope, limitations and recommendations for future action. Cureus 2020 , 12 , e10549. [ Google Scholar ] [ CrossRef ]
- Hauser, W.; Annegers, J.; Kurland, L.T. Incidence of epilepsy and unprovoked seizures in Rochester, Minnesota: 1935–1984. Epilepsia 1993 , 34 , 453–458. [ Google Scholar ] [ CrossRef ]
- Wirrell, E.; Grossardt, B.; Wong-Kisiel, L.; Nickels, K. Incidence and classification of new-onset epilepsy and epilepsy syndromes in children in Olmsted County, Minnesota from 1980 to 2004: A population-based study. Epilepsy Res. 2011 , 95 , 110–118. [ Google Scholar ] [ CrossRef ]
- Camfield, P.; Camfield, C. Incidence, prevalence and aetiology of seizures and epilepsy in children. Epileptic Disord. 2015 , 17 , 117–123. [ Google Scholar ] [ CrossRef ]
- Beghi, E. The epidemiology of epilepsy. Neuroepidemiology 2020 , 54 , 185–191. [ Google Scholar ] [ CrossRef ] [ PubMed ]
- Sultana, B.; Panzini, M.; Veilleux Carpentier, A.; Comtois, J.; Rioux, B.; Gore, G.; Bauer, P.; Kwon, C.; Jetté, N.; Josephson, C.; et al. Incidence and prevalence of drug-resistant epilepsy: A systematic review and meta-analysis. Neurology 2021 , 96 , 805–817. [ Google Scholar ] [ CrossRef ]
- Biset, G.; Abebaw, N.; Gebeyehu, N.; Estifanos, N.; Birrie, E.; Tegegne, K. Prevalence, incidence, and trends of epilepsy among children and adolescents in Africa: A systematic review and meta-analysis. BMC Public Health 2024 , 24 , 771. [ Google Scholar ] [ CrossRef ]
- Wei, L.; Mooney, C. Pediatric and Adolescent Seizure Detection: A Machine Learning Approach Exploring the Influence of Age and Sex in Electroencephalogram Analysis. BioMedInformatics 2024 , 4 , 796–810. [ Google Scholar ] [ CrossRef ]
- Rozensztrauch, A.; Kołtuniuk, A. The Quality of Life of Children with Epilepsy and the Impact of the Disease on the Family Functioning. Int. J. Environ. Res. Public Health 2022 , 19 , 2277. [ Google Scholar ] [ CrossRef ] [ PubMed ]
- Brigo, F.; Trinka, E.; Lattanzi, S.; Bragazzi, N.; Nardone, R.; Martini, M. A brief history of typical absence seizures—Petit mal revisited. Epilepsy Behav. 2018 , 80 , 346–353. [ Google Scholar ] [ CrossRef ] [ PubMed ]
- Klonowski, W. Everything you wanted to ask about EEG but were afraid to get the right answer. Nonlinear Biomed. Phys. 2009 , 3 , 1–5. [ Google Scholar ] [ CrossRef ] [ PubMed ]
- Li, Y.; Liu, Q.; Tan, S.; Chan, R. High-resolution time-frequency analysis of EEG signals using multiscale radial basis functions. Neurocomputing 2016 , 195 , 96–103. [ Google Scholar ] [ CrossRef ]
- Tzallas, A.; Tsipouras, M.; Fotiadis, D. Automatic seizure detection based on time-frequency analysis and artificial neural networks. Comput. Intell. Neurosci. 2007 , 80510. [ Google Scholar ]
- Janecek, A.; Gansterer, W.; Demel, M.; Ecker, G. On the relationship between feature selection and classification accuracy. New Chall. Feature Sel. Data Min. Knowl. Discov. 2008 , 4 , 90–105. [ Google Scholar ]
- Torkkola, K.; Campbell, W. Mutual information in learning feature transformations. ICML 2000 , 1015–1022. [ Google Scholar ]
- Jović, A.; Brkić, K.; Bogunović, N. A review of feature selection methods with applications. In Proceedings of the 2015 38th International Convention on Information and Communication Technology, Electronics and Microelectronics (MIPRO), Opatija, Croatia, 25–29 May 2015; pp. 1200–1205. [ Google Scholar ]
- Tang, J.; Alelyani, S.; Liu, H. Feature selection for classification: A review. Data Classif. Algorithms Appl. 2014 , 37 . [ Google Scholar ]
- Kumar, V.; Minz, S. Feature selection. SmartCR 2014 , 4 , 211–229. [ Google Scholar ] [ CrossRef ]
- Zhu, Y.; Li, T.; Li, W. An efficient hybrid feature selection method using the artificial immune algorithm for high-dimensional data. Comput. Intell. Neurosci. 2022 , 2022 , 1452301. [ Google Scholar ] [ PubMed ]
- Liu, X.; Liang, Y.; Wang, S.; Yang, Z.; Ye, H. A hybrid genetic algorithm with wrapper-embedded approaches for feature selection. IEEE Access 2018 , 6 , 22863–22874. [ Google Scholar ] [ CrossRef ]
- Lu, H.; Chen, J.; Yan, K.; Jin, Q.; Xue, Y.; Gao, Z. A hybrid feature selection algorithm for gene expression data classification. Neurocomputing 2017 , 256 , 56–62. [ Google Scholar ] [ CrossRef ]
- Ma, W.; Zhou, X.; Zhu, H.; Li, L.; Jiao, L. A two-stage hybrid ant colony optimization for high-dimensional feature selection. Pattern Recognit. 2021 , 116 , 107933. [ Google Scholar ] [ CrossRef ]
- Huang, Z.; Yang, C.; Zhou, X.; Huang, T. A hybrid feature selection method based on binary state transition algorithm and ReliefF. IEEE J. Biomed. Health Inform. 2018 , 23 , 1888–1898. [ Google Scholar ] [ CrossRef ]
- Cadenas, J.; Garrido, M.; MartiNez, R. Feature subset selection filter–wrapper based on low quality data. Expert Syst. Appl. 2013 , 40 , 6241–6252. [ Google Scholar ] [ CrossRef ]
- Xu, Y.; Yang, J.; Ming, W.; Wang, S.; Sawan, M. Shorter latency of real-time epileptic seizure detection via probabilistic prediction. Expert Syst. Appl. 2024 , 236 , 121359. [ Google Scholar ] [ CrossRef ]
- Mormann, F.; Andrzejak, R.; Elger, C.; Lehnertz, K. Seizure prediction: The long and winding road. Brain 2007 , 130 , 314–333. [ Google Scholar ] [ CrossRef ] [ PubMed ]
- Roy, Y.; Banville, H.; Albuquerque, I.; Gramfort, A.; Falk, T.; Faubert, J. Deep learning-based electroencephalography analysis: A systematic review. J. Neural Eng. 2019 , 16 , 051001. [ Google Scholar ] [ CrossRef ] [ PubMed ]
- Siddiqui, M.; Morales-Menendez, R.; Huang, X.; Hussain, N. A review of epileptic seizure detection using machine learning classifiers. Brain Inform. 2020 , 7 , 5. [ Google Scholar ] [ CrossRef ]
- Li, D.; Liu, C.; Hu, S. A learning method for the class imbalance problem with medical data sets. Comput. Biol. Med. 2010 , 40 , 509–518. [ Google Scholar ] [ CrossRef ]
- Yuan, Q.; Zhou, W.; Zhang, L.; Zhang, F.; Xu, F.; Leng, Y.; Wei, D.; Chen, M. Epileptic seizure detection based on imbalanced classification and wavelet packet transform. Seizure 2017 , 50 , 99–108. [ Google Scholar ] [ CrossRef ] [ PubMed ]
- Chawla, N.; Bowyer, K.; Hall, L.; Kegelmeyer, W. SMOTE: Synthetic minority over-sampling technique. J. Artif. Intell. Res. 2002 , 16 , 321–357. [ Google Scholar ] [ CrossRef ]
- Drummond, C.; Holte, R. C4.5, class imbalance, and cost sensitivity: Why under-sampling beats over-sampling. Workshop Learn. Imbalanced Datasets II 2003 , 11 , 1–8. [ Google Scholar ]
- Ling, C.; Li, C. Data mining for direct marketing: Problems and solutions. KDD 1998 , 98 , 73–79. [ Google Scholar ]
- Chen, C.; Liaw, A.; Breiman, L. Using random forest to learn imbalanced data. J. Univ. Calif. 2004 , 110 , 24. [ Google Scholar ]
- Burez, J.; Poel, D. Handling class imbalance in customer churn prediction. Expert Syst. Appl. 2009 , 36 , 4626–4636. [ Google Scholar ] [ CrossRef ]
- He, H.; Garcia, E. Learning from imbalanced data. IEEE Trans. Knowl. Data Eng. 2009 , 21 , 1263–1284. [ Google Scholar ]
- Japkowicz, N.; Stephen, S. The class imbalance problem: A systematic study. Intell. Data Anal. 2002 , 6 , 429–449. [ Google Scholar ] [ CrossRef ]
- Vale, D.; El-Sharif, A.; Ali, M. Explainable artificial intelligence (XAI) post-hoc explainability methods: Risks and limitations in non-discrimination law. AI Ethics 2022 , 2 , 815–826. [ Google Scholar ] [ CrossRef ]
- Vellido, A. The importance of interpretability and visualization in machine learning for applications in medicine and health care. Neural Comput. Appl. 2020 , 32 , 18069–18083. [ Google Scholar ] [ CrossRef ]
- Teng, Q.; Liu, Z.; Song, Y.; Han, K.; Lu, Y. A survey on the interpretability of deep learning in medical diagnosis. Multimed. Syst. 2022 , 28 , 2335–2355. [ Google Scholar ] [ CrossRef ] [ PubMed ]
- Antoniadi, A.; Du, Y.; Guendouz, Y.; Wei, L.; Mazo, C.; Becker, B.; Mooney, C. Current challenges and future opportunities for XAI in machine learning-based clinical decision support systems: A systematic review. Appl. Sci. 2021 , 11 , 5088. [ Google Scholar ] [ CrossRef ]
- Jaotombo, F.; Adorni, L.; Ghattas, B.; Boyer, L. Finding the best trade-off between performance and interpretability in predicting hospital length of stay using structured and unstructured data. PLoS ONE 2023 , 18 , e0289795. [ Google Scholar ] [ CrossRef ] [ PubMed ]
- Fayyad, U.; Piatetsky-Shapiro, G.; Smyth, P. From data mining to knowledge discovery in databases. AI Mag. 1996 , 17 , 37. [ Google Scholar ]
- Rudin, C.; Chen, C.; Chen, Z.; Huang, H.; Semenova, L.; Zhong, C. Interpretable machine learning: Fundamental principles and 10 grand challenges. Stat. Surv. 2022 , 16 , 1–85. [ Google Scholar ] [ CrossRef ]
- Rudin, C. Stop explaining black box machine learning models for high stakes decisions and use interpretable models instead. Nat. Mach. Intell. 2019 , 1 , 206–215. [ Google Scholar ] [ CrossRef ]
- Tan, S.; Caruana, R.; Hooker, G.; Lou, Y. Distill-and-compare: Auditing black-box models using transparent model distillation. In Proceedings of the 2018 AAAI/ACM Conference on AI, Ethics, and Society, New Orleans, LA, USA, 1–3 February 2018; pp. 303–310. [ Google Scholar ]
- Ribeiro, M.; Singh, S.; Guestrin, C. Model-agnostic interpretability of machine learning. arXiv 2016 , arXiv:1606.05386. [ Google Scholar ]
- Ribeiro, M.; Singh, S.; Guestrin, C. “Why should i trust you?” Explaining the predictions of any classifier. In Proceedings of the 22nd ACM SIGKDD International Conference on Knowledge Discovery and Data Mining, San Francisco, CA, USA, 13–17 August 2016; pp. 1135–1144. [ Google Scholar ]
- Camburu, O.; Giunchiglia, E.; Foerster, J.; Lukasiewicz, T.; Blunsom, P. Can I trust the explainer? Verifying post-hoc explanatory methods. arXiv 2019 , arXiv:1910.02065. [ Google Scholar ]
- Visani, G.; Bagli, E.; Chesani, F.; Poluzzi, A.; Capuzzo, D. Statistical stability indices for LIME: Obtaining reliable explanations for machine learning models. J. Oper. Res. Soc. 2022 , 73 , 91–101. [ Google Scholar ] [ CrossRef ]
- Molnar, C.; König, G.; Herbinger, J.; Freiesleben, T.; Dandl, S.; Scholbeck, C.; Casalicchio, G.; Grosse-Wentrup, M.; Bischl, B. General pitfalls of model-agnostic interpretation methods for machine learning models. In International Workshop on Extending Explainable AI beyond Deep Models and Classifiers ; Springer: Cham, Switzerland, 2020; pp. 39–68. [ Google Scholar ]
- Zhang, Q.; Wu, Y.; Zhu, S. Interpretable convolutional neural networks. In Proceedings of the IEEE Conference on Computer Vision and Pattern Recognition, Salt Lake City, UT, USA, 18–22 June 2018; pp. 8827–8836. [ Google Scholar ]
- Chen, C.; Li, O.; Tao, D.; Barnett, A.; Rudin, C.; Su, J. This looks like that: Deep learning for interpretable image recognition. Adv. Neural Inf. Process. Syst. 2019 , 32 . [ Google Scholar ]
- Koh, P.; Nguyen, T.; Tang, Y.; Mussmann, S.; Pierson, E.; Kim, B.; Liang, P. Concept bottleneck models. In Proceedings of the International Conference on Machine Learning, Virtual, 13–18 July 2020; Volume 119, pp. 5338–5348. [ Google Scholar ]
- Chen, Z.; Bei, Y.; Rudin, C. Concept whitening for interpretable image recognition. Nat. Mach. Intell. 2020 , 2 , 772–782. [ Google Scholar ] [ CrossRef ]
- Angelov, P.; Soares, E. Towards explainable deep neural networks (xDNN). Neural Netw. 2020 , 130 , 185–194. [ Google Scholar ] [ CrossRef ] [ PubMed ]
- Nauta, M.; Van Bree, R.; Seifert, C. Neural prototype trees for interpretable fine-grained image recognition. In Proceedings of the IEEE/CVF Conference on Computer Vision and Pattern Recognition, Nashville, TN, USA, 20–25 June 2021; pp. 14933–14943. [ Google Scholar ]
- Joseph, V.; Vakayil, A. SPlit: An optimal method for data splitting. Technometrics 2022 , 64 , 166–176. [ Google Scholar ] [ CrossRef ]
- Bouthillier, X.; Delaunay, P.; Bronzi, M.; Trofimov, A.; Nichyporuk, B.; Szeto, J.; Mohammadi Sepahvand, N.; Raff, E.; Madan, K.; Voleti, V.; et al. Accounting for variance in machine learning benchmarks. Proc. Mach. Learn. Syst. 2021 , 3 , 747–769. [ Google Scholar ]
- Zhang, Y.; Guo, Y.; Yang, P.; Chen, W.; Lo, B. Epilepsy seizure prediction on EEG using common spatial pattern and convolutional neural network. IEEE J. Biomed. Health Inform. 2019 , 24 , 465–474. [ Google Scholar ] [ CrossRef ] [ PubMed ]
Ref | Rodent Model | Seizure/Epilepsy Type Modelled |
---|---|---|
[ ] | -aminobutyric acid A (GABAA) receptor mutation ( 2R43Q) | Absence epilepsy |
[ ] | Intrahippocampal kainic acid | Mesial temporal lobe epilepsy |
[ ] | High temperature | Dravet syndrome |
[ ] | Genetic and kainate systemic injection | Absence and tonic–clonic seizures |
[ ] | Genetic and kainate systemic injection | Absence and limbic seizures |
[ ] | Genetic | Absence seizures |
[ ] | Genetic | Absence seizures |
[ ] | - | - |
[ ] | Intra-amygdala kainic acid, Dravet, Pilocarpine | Temporal lope epilepsy, Dravet syndrome |
[ ] | Pentylenetetrazole | Clonic seizures |
[ ] | GAER | Absence seizures |
[ ] | Perforant pathway stimulation | Mesial temporal lobe epilepsy with hippocampal sclerosis |
[ ] | Pilocarpine hydrochloride | Convulsive seizures |
[ ] | Pilocarpine injection | Generalized tonic–clonic seizures |
[ ] | GAERS | Absence seizures |
[ ] | Perforant path kindling susceptible | Post-traumatic epilepsy |
[ ] | Kindling | Frontal lobe epilepsy |
Author | EEG Domain | Feature Extraction | Feature Type | Feature Selection | Feature Transformation |
---|---|---|---|---|---|
[ ] | FD | Wavelet | 12 linear | - | - |
[ ] | TD, FD | - | 141 linear | - | PCA |
[ ] | TD, FD | Gabor transform | 9 linear | Neighborhood component analysis | - |
[ ] | FD | Wavelet | 12 linear | Forward feature selection | - |
[ ] | FD | Wavelet | 2 linear | Forward feature selection | - |
[ ] | TD, FD | FFT | 2 linear, 1 nonlinear | - | - |
[ ] | FD | FFT | 1 linear, 1 nonlinear | - | PCA |
[ ] | TD | PCA, Laplacian Eigenmap manifold | linear | - | PCA and Laplacian Eigenmap manifold |
[ ] | TD, FD | Wavelet | 19 linear | ||
[ ] | TD | Natural visibility algorithm, Markov–binary visibility graph, | 14 linear | Sequential forward selection | - |
[ ] | FD | FFT | 2 linear | - | - |
[ ] | FD, TFD | FFT, wavelet transform | 1 linear, 1 nonlinear | - | - |
Author | Number of Rodents | Classifier | Result | ||||||
---|---|---|---|---|---|---|---|---|---|
Accuracy (%) | Precision (%) | Sensitivity (%) | Specificity (%) | AUC (%) | False Alarm (%) | Delay Latency (s) | |||
[ ] | 11 | SVM | - | 59.00 | 68.00 | - | - | - | - |
[ ] | 11 | GLM | - | - | - | - | 99.50 | - | - |
[ ] | 13 | RF | 92.80–97.20 | 77.30–97.40 | 93.30–95.80 | 91.90–98.90 | - | - | - |
[ ] | 45 | RC-BRR | - | - | 96.20 | 98.20 | - | 9.10 | 0.97 |
[ ] | 3 | RBF-SVM | 97.50 | - | 97.03 | 97.83 | - | - | - |
[ ] | - | KRR | 73.43–97.76 | - | - | - | - | - | - |
[ ] | 26 | XGBoost | 93.1–98.8 | - | 76.30–98.8 | 93.10–98.80 | - | - | - |
[ ] | 27 | MLP | 92.13 | - | 98.94 | - | 86.03 | - | - |
[ ] | 18 | GNN | - | - | 98.01 | - | 98.90 | 95.90 | - |
Author | Number of Rodents | Classifier | Result | ||||||
---|---|---|---|---|---|---|---|---|---|
Accuracy (%) | Precision (%) | Sensitivity (%) | Specificity (%) | AUC (%) | False Alarm (%) | Delay Latency (s) | |||
[ ] | 7 | Deep residual neural network | - | - | 83.00 | 83.00 | 90.00 | - | - |
[ ] | - | Sequential dual deep neural networks | - | 98.00 | 100.00 | - | - | 6.00 | - |
[ ] | 32 | Fully connected neural network | 99.60–99.90 | - | 96.20–96.70 | 99.60–99.90 | 98.90–99.30 | 0.9–1.1 | - |
[ ] | 11 | CNN | 94.28 | - | 96.60 | 90.40 | - | - | - |
[ ] | 8 | GoogleNet | 81.10–95.60 | - | - | - | - | - | - |
The statements, opinions and data contained in all publications are solely those of the individual author(s) and contributor(s) and not of MDPI and/or the editor(s). MDPI and/or the editor(s) disclaim responsibility for any injury to people or property resulting from any ideas, methods, instructions or products referred to in the content. |
Share and Cite
Edoho, M.; Mooney, C.; Wei, L. AI-Based Electroencephalogram Analysis in Rodent Models of Epilepsy: A Systematic Review. Appl. Sci. 2024 , 14 , 7398. https://doi.org/10.3390/app14167398
Edoho M, Mooney C, Wei L. AI-Based Electroencephalogram Analysis in Rodent Models of Epilepsy: A Systematic Review. Applied Sciences . 2024; 14(16):7398. https://doi.org/10.3390/app14167398
Edoho, Mercy, Catherine Mooney, and Lan Wei. 2024. "AI-Based Electroencephalogram Analysis in Rodent Models of Epilepsy: A Systematic Review" Applied Sciences 14, no. 16: 7398. https://doi.org/10.3390/app14167398
Article Metrics
Article access statistics, further information, mdpi initiatives, follow mdpi.
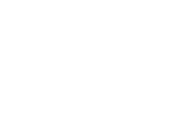
Subscribe to receive issue release notifications and newsletters from MDPI journals
Exploring the factors driving AI adoption in production: a systematic literature review and future research agenda
- Open access
- Published: 23 August 2024
Cite this article
You have full access to this open access article
- Heidi Heimberger ORCID: orcid.org/0000-0003-3390-0219 1 , 2 ,
- Djerdj Horvat ORCID: orcid.org/0000-0003-3747-3402 1 &
- Frank Schultmann ORCID: orcid.org/0000-0001-6405-9763 1
Our paper analyzes the current state of research on artificial intelligence (AI) adoption from a production perspective. We represent a holistic view on the topic which is necessary to get a first understanding of AI in a production-context and to build a comprehensive view on the different dimensions as well as factors influencing its adoption. We review the scientific literature published between 2010 and May 2024 to analyze the current state of research on AI in production. Following a systematic approach to select relevant studies, our literature review is based on a sample of articles that contribute to production-specific AI adoption. Our results reveal that the topic has been emerging within the last years and that AI adoption research in production is to date still in an early stage. We are able to systematize and explain 35 factors with a significant role for AI adoption in production and classify the results in a framework. Based on the factor analysis, we establish a future research agenda that serves as a basis for future research and addresses open questions. Our paper provides an overview of the current state of the research on the adoption of AI in a production-specific context, which forms a basis for further studies as well as a starting point for a better understanding of the implementation of AI in practice.
Explore related subjects
- Artificial Intelligence
Avoid common mistakes on your manuscript.
1 Introduction
The technological change resulting from deep digitisation and the increasing use of digital technologies has reached and transformed many sectors [ 1 ]. In manufacturing, the development of a new industrial age, characterized by extensive automation and digitisation of processes [ 2 ], is changing the sector’s ‘technological reality’ [ 3 ] by integrating a wide range of information and communication technologies (such as Industry 4.0-related technologies) into production processes [ 4 ].
Although the evolution of AI traces back to the year 1956 (as part of the Dartmouth Conference) [ 5 ], its development has progressed rapidly, especially since the 2010s [ 6 ]. Driven by improvements, such as the fast and low-cost development of smart hardware, the enhancement of algorithms as well as the capability to manage big data [ 7 ], there is an increasing number of AI applications available for implementation today [ 8 ]. The integration of AI into production processes promises to boost the productivity, efficiency as well as automation of processes [ 9 ], but is currently still in its infancy [ 10 ] and manufacturing firms seem to still be hesitant to adopt AI in a production-context. This appears to be driven by the high complexity of AI combined with the lack of practical knowledge about its implementation in production and several other influencing factors [ 11 , 12 ].
In the literature, many contributions analyze AI from a technological perspective, mainly addressing underlying models, algorithms, and developments of AI tools. Various authors characterise both machine learning and deep learning as key technologies of AI [ 8 , 13 ], which are often applied in combination with other AI technologies, such as natural language recognition. While promising areas for AI application already exist in various domains such as marketing [ 14 ], procurement [ 15 ], supply chain management [ 16 ] or innovation management [ 17 ], the integration of AI into production processes also provides significant performance potentials, particularly in the areas of maintenance [ 18 ], quality control [ 19 ] and production planning and management [ 20 ]. However, AI adoption requires important technological foundations, such as the provision of data and the necessary infrastructure, which must be ensured [ 11 , 12 , 21 ]. Although the state of the art literature provides important insights into possible fields of application of AI in production, the question remains: To what extent are these versatile applications already in use and what is required for their successful adoption?
Besides the technology perspective of AI, a more human-oriented field of discussion is debated in scientific literature [ 22 ]. While new technologies play an essential role in driving business growth in the digital transformation of the production industry, the increasing interaction between humans and intelligent machines (also referred to as ‘augmentation’) creates stress challenges [ 23 ] and impacts work [ 24 ], which thus creates managerial challenges in organizations [ 25 , 26 ]. One of the widely discussed topics in this context is the fear of AI threatening jobs (including production jobs), which was triggered by e.g. a study of Frey, Osborne [ 27 ]. Another issue associated to the fear of machines replacing humans is the lack of acceptance resulting from the mistrust of technologies [ 28 , 29 ]. This can also be linked to the various ethical challenges involved in working with AI [ 22 ]. This perspective, which focuses on the interplay between AI and humans [ 30 ], reveals the tension triggered by AI. Although this is discussed from different angles, the question remains how these aspects influence the adoption of AI in production.
Another thematic stream of current literature can be observed in a series of contributions on the organizational aspects of the technology. In comparison to the two research areas discussed above, the number of publications in this area seems to be smaller. This perspective focuses on issues to implement AI, such as the importance of a profound management structure [ 31 , 32 ], leadership [ 33 ], implications on the organizational culture [ 34 ] as well as the need for digital capabilities and special organizational skills [ 33 ]. Although some studies on the general adoption of AI without a sectoral focus have already been conducted (such as by Chen, Tajdini [ 35 ] or Kinkel, Baumgartner, Cherubini [ 36 ]) and hence, some initial factors influencing the adoption of AI can be derived, the contributions from this perspective are still scarce, are usually not specifically analyzed in the context of production or lack a comprehensive view on the organization in AI adoption.
While non-industry specific AI issues have been researched in recent years, the current literature misses a production-specific analysis of AI adoption, providing an understanding of the possibilities and issues related to integrating AI into the production context. Moreover, the existing literature tells us little about relevant mechanisms and factors underlying the adoption of AI in production processes, which include both technical, human-centered as well as organizational issues. As organizational understanding of AI in a business context is currently still in its early stages, it is difficult to find an aggregate view on the factors that can support companies in implementing AI initiatives in production [ 37 , 38 ]. Addressing this gap, we aim to systematise the current scientific knowledge on AI adoption, with a focus on production. By drawing on a systematic literature review (SLR), we examine existing studies on AI adoption in production and explore the main issues regarding adoption that are covered in the analyzed articles. Building on these findings, we conduct a comprehensive analysis of the existing studies with the aim of systematically investigating the key factors influencing the adoption of AI in production. This systematic approach paves the way for the formulation of a future research agenda.
Our SLR addresses three research questions (RQs). RQ1: What are the statistical characteristics of existing research on AI adoption in production? To answer this RQ, we conduct descriptive statistics of the analyzed studies and provide information on time trends, methods used in the research, and country specifications. RQ2: What factors influence the adoption of AI in production? RQ2 specifies the adoption factors and forms the core component of our analysis. By adoption factors, we mean the factors that influence the use of AI in production (both positively and negatively) and that must therefore be analyzed and taken into account. RQ3: What research topics are of importance to advance the research field of AI adoption in production? We address this RQ by using the analyzed literature as well as the key factors of AI adoption as a starting point to derive RQs that are not addressed and thus provide an outlook on the topic.
2 Methodology
In order to create a sound information base for both policy makers and practitioners on the topic of AI adoption in production, this paper follows the systematic approach of a SLR. For many fields, including management research, a SLR is an important tool to capture the diversity of existing knowledge on a specific topic for a scientific investigation [ 39 ]. The investigator often pursues multiple goals, such as capturing and assessing the existing environment and advancing the existing body of knowledge with a proprietary RQ [ 39 ] or identifying key research topics [ 40 ].
Our SLR aims to select, analyze, and synthesize findings from the existing literature on AI adoption in production over the past 24 years. In order to identify relevant data for our literature synthesis, we follow the systematic approach of the Preferred Reporting Items for Systematic reviews (PRISMA) [ 41 ]. In evaluating the findings, we draw on a mixed-methods approach, combining some quantitative analyses, especially on the descriptive aspects of the selected publications, as well as qualitative analyses aimed at evaluating and comparing the contents of the papers. Figure 1 graphically summarizes the methodological approach that guides the content of the following sub-chapters.
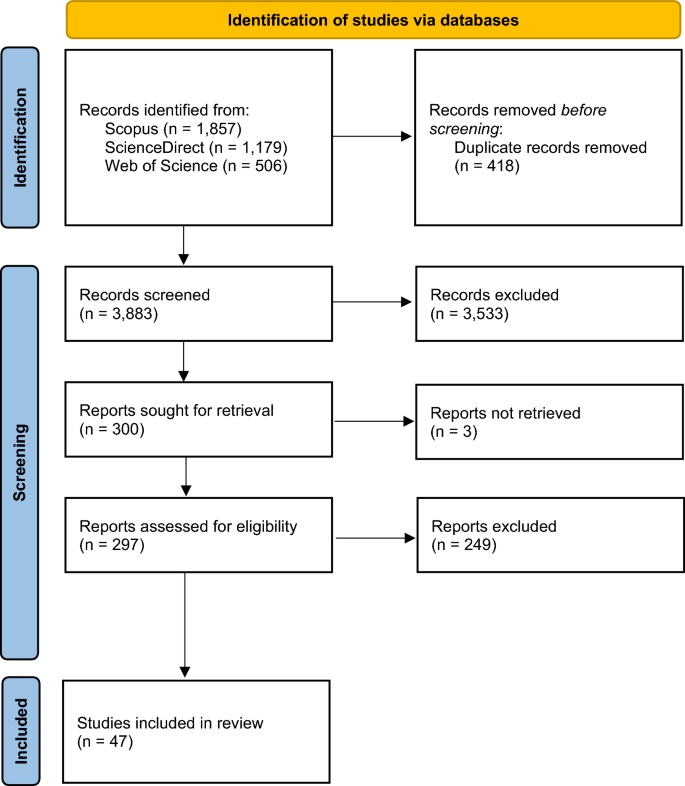
Methodical procedure of our SLR following PRISMA [ 41 ]
2.1 Data identification
Following the development of the specific RQs, we searched for suitable publications. To locate relevant studies, we chose to conduct a publication analysis in the databases Scopus, Web of Science and ScienceDirect as these databases primarily contain international scientific articles and provide a broad overview of the interdisciplinary research field and its findings. To align the search with the RQs [ 42 ], we applied predefined key words to search the titles, abstracts, and keywords of Scopus, Web of Science and ScienceDirect articles. Our research team conducted several pre-tests to determine the final search commands for which the test results were on target and increased the efficiency of the search [ 42 ]. Using the combination of Boolean operators, we covered the three topics of AI, production, and adoption by searching combinations of ‘Artificial Intelligence’ AND ‘production or manufacturing’ AND ‘adopt*’ in the three scientific databases. Although ‘manufacturing’ tends to stand for the whole sector and ‘production’ refers to the process, the two terms are often used to describe the same context. We also follow the view of Burbidge, Falster, Riis, Svendsen [ 43 ] and use the terms synonymously in this paper and therefore also include both terms as keywords in the study location as well as in the analysis.
AI research has been credited with a resurgence since 2010 [ 6 ], which is the reason for our choice of time horizon. Due to the increase in publications within the last years, we selected articles published online from 2010 to May 8, 2024 for our analysis. As document types, we included conference papers, articles, reviews, book chapters, conference reviews as well as books, focusing exclusively on contributions in English in the final publication stage. The result of the study location is a list of 3,833 documents whose titles, abstracts, and keywords meet the search criteria and are therefore included in the next step of the analysis.
2.2 Data analysis
For these 3,833 documents, we then conducted an abstract analysis, ‘us[ing] a set of explicit selection criteria to assess the relevance of each study found to see if it actually does address the research question’ [ 42 ]. For this step, we again conducted double-blind screenings (including a minimum of two reviewers) as pilot searches so that all reviewers have the same understanding of the decision rules and make equal decisions regarding their inclusion for further analysis.
To ensure the paper’s focus on all three topics regarded in our research (AI, production, and adoption), we followed clearly defined rules of inclusion and exclusion that all reviewers had to follow in the review process. As a first requirement for inclusion, AI must be the technology in focus that is analysed in the publication. If AI was only mentioned and not further specified, we excluded the publication. With a second requirement, we checked the papers for the context of analysis, which in our case must be production. If the core focus is beyond production, the publication was also excluded from further analysis. The third prerequisite for further consideration of the publication is the analysis of the adoption of a technology in the paper. If technology adoption is not addressed or adoption factors are not considered, we excluded the paper. An article was only selected for full-text analysis if, after analyzing the titles, abstracts, and keywords, a clear focus on all three research areas was visible and the inclusion criteria were met for all three contexts.
By using this tripartite inclusion analysis, we were able to analyse the publications in a structured way and to reduce the 3,833 selected documents in our double-blind approach to 300 articles that were chosen for the full-text analysis. In the process of finding full versions of these publications, we had to exclude three papers as we could not access them. For the rest of the 297 articles we obtained full access and thus included them for further analysis. After a thorough examination of the full texts, we again had to exclude 249 publications because they did not meet our content-related inclusion criteria mentioned above, although the abstract analysis gave indications that they did. As a result, we finally obtained 47 selected papers on which we base the literature analysis and synthesis (see Fig. 1 ).
2.3 Descriptive analysis
Figure 2 summarises the results of the descriptive analysis on the selected literature regarding AI adoption in production that we analyse in our SLR. From Fig. 2 a), which illustrates annual publication trends (2010–2024), the increase in publications on AI adoption in production over the past 5 years is evident, yet slightly declining after a peak in 2022. After a steady increase until 2022, in which 11 articles are included in the final analysis, 2023 features ten articles, followed by three articles for 2024 until the cut-off date in May 2024. Of the 47 papers identified through our search, the majority (n = 33) are peer-reviewed journal articles and the remaining thirteen contributions conference proceedings and one book chapter (see Fig. 2 b)).
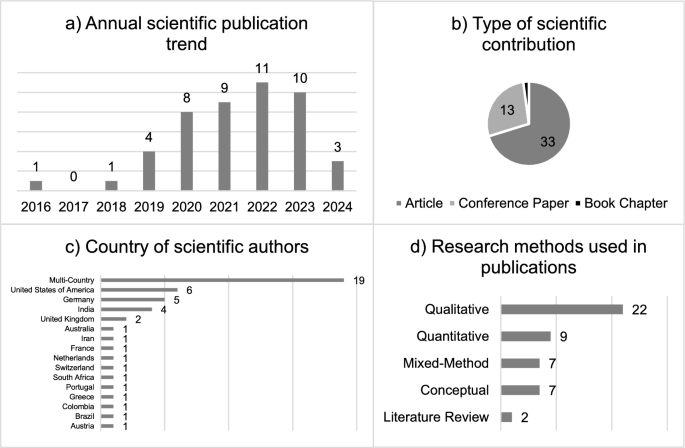
Descriptive analyses of the selected articles addressing AI adoption in production
The identified contributions reveal some additional characteristics in terms of the authors country base (Fig. 2 c)) and research methods used (Fig. 2 d)). Almost four out of ten of the publications were written in collaboration with authors from several countries (n = 19). Six of the papers were published by authors from the United States, five from Germany and four from India. In terms of the applied research methods used by the researchers, a wide range of methods is used (see Fig. 2 c), with qualitative methods (n = 22) being the most frequently used.
2.4 Factor analysis
In order to derive a comprehensive list of factors that influence the use of AI in production at different levels, we follow a qualitative content analysis. It is based on inductive category development, avoiding prefabricated categories in order to allow new categories to emerge based on the content at hand [ 44 , 45 ]. To do this, we first read the entire text to gain an understanding of the content and then derive codes [ 46 ] that seem to capture key ideas [ 45 ]. The codes are subsequently sorted into distinct categories, each of which is clearly defined and establishes meaningful connections between different codes. Based on an iterative process with feedback loops, the assigned categories are continuously reviewed and updated as revisions are made [ 44 ].
Various factors at different levels are of significance to AI and influence technology adoption [ 47 , 48 ]. To identify the specific factors that are of importance for AI adoption in production, we analyze the selected contributions in terms of the factors considered, compare them with each other and consequently obtain a list of factors through a bottom-up approach. While some of the factors are based on empirical findings, others are expected factors that result from the research findings of the respective studies. Through our analysis, a list of 35 factors emerges that influence AI adoption in production which occur with varying frequency in the studies analyzed by our SLR. Table 1 visualizes each factor in the respective contributions sorted by the frequency of occurrence.
The presence of skills is considered a particularly important factor in AI adoption in the studies analyzed (n = 35). The availability of data (n = 25) as well as the need for ethical guidelines (n = 24) are also seen as key drivers of AI adoption, as data is seen as the basis for the implementation of AI and ethical issues must be addressed in handling such an advanced technology. As such, these three factors make up the accelerants of AI adoption in production that are most frequently cited in the studies analyzed.
Also of importance are issues of managerial support (n = 22), as well as performance measures and IT infrastructure (n = 20). Some factors were also mentioned, but only addressed by one study at a time: government support, industrial sector, product complexity, batch size, and R&D Intensity. These factors are often used as quantitatively measurable adoption factors, especially in empirical surveys, such the study by Kinkel, Baumgartner, Cherubini [ 36 ].
3 Factors influencing AI adoption
The 35 factors presented characteristically in Sect. 2.4 serve as the basis for our in-depth analysis and for developing a framework of influences on AI adoption in production which are grouped into supercategories. A supercategory describes a cluster of topics to which various factors of AI adoption in production can be assigned. We were able to define seven categories that influence AI adoption in production: the internal influences of ‘business and structure’, ‘organizational effectiveness’, ‘technology and system’, ‘data management’ as well as the external influences of the ‘regulatory environment’, ‘business environment’ and ‘economic environment’ (see Fig. 3 ). The factors that were mentioned most frequently (occurrence in at least half of the papers analyzed) are marked accordingly (*) in Fig. 3 .
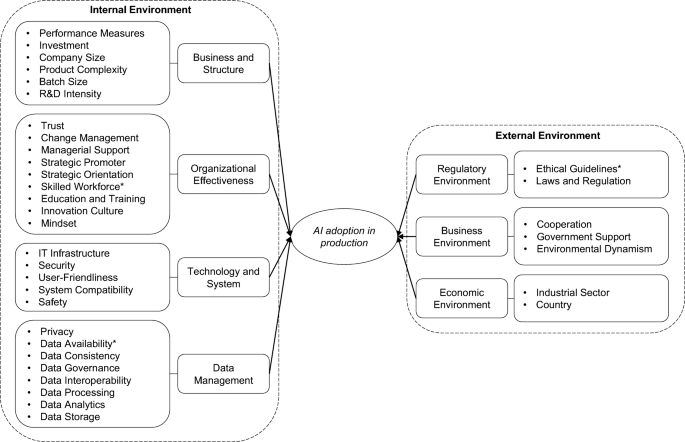
Framework of factors influencing AI adoption in production
3.1 Internal Environment
The internal influences on AI adoption in production refer to factors that an organization carries internally and that thus also influence adoption from within. Such factors can usually be influenced and clearly controlled by the organization itself.
3.1.1 Business and structure
The supercategory ‘business and structure’ includes the various factors and characteristics that impact a company’s performance, operations, and strategic decision-making. By considering and analyzing these business variables when implementing AI in production processes, companies can develop effective strategies to optimize their performance, increase their competitiveness, and adapt to changes in the business environment.
To understand and grasp the benefits in the use of AI, quantitative performance measures for the current and potential use of AI in industrial production systems help to clarify the value and potential benefits of AI use [ 49 , 54 , 74 , 79 , 91 ]. Assessing possible risks [ 77 ] as well as the monetary expected benefits for AI (e.g. Return on Investment (ROI)) in production plays an important role for adoption decisions in market-oriented companies [ 57 , 58 , 63 , 65 , 78 ]. Due to financial constraints, managers behave cautiously in their investments [ 78 ], so they need to evaluate AI adoption as financially viable to want to make the investment [ 61 , 63 , 93 ] and also drive acceptance [ 60 ]. AI systems can significantly improve cost–benefit structures in manufacturing, thereby increasing the profitability of production systems [ 73 ] and making companies more resilient [ 75 ]. However, in most cases, the adoption of AI requires high investments and the allocation of resources (s.a. personnel or financial) for this purpose [ 50 , 51 , 57 , 80 , 94 ]. Consequently, a lack of budgets and high expected transition costs often hinder the implementation of smart concepts [ 56 , 62 , 67 , 82 , 84 , 92 ]. It is up to management to provide necessary funding for AI adoption [ 53 , 59 , 79 ], which is required, for example, for skill development of employees [ 59 , 61 , 63 ], IT adaptation [ 62 , 66 ], AI development [ 74 ] or hardware deployment [ 68 ]. In their empirical study, Kinkel, Baumgartner, Cherubini [ 36 ] confirm a positive correlation between company size and the intensity in the use of AI technologies. Large companies generally stand out with a higher propensity to adopt [ 53 ] as they have less difficulties in comparison to small firms regarding the availability of resources [ 69 ], such as know-how, budget [ 68 , 84 ] and general data organization [ 68 ]. Others argue that small companies tend to be more open to change and are characterized by faster decision-making processes [ 68 , 93 ]. Product complexity also influences a company’s propensity for AI. Companies that produce rather simple products are more likely to digitize, which in turn offers good starting points for AI adoption. On the other hand, complex product manufacturers (often characterized by small batch sizes) are often less able to standardize and automate [ 36 ]. The company’s produced batch size has a similar influence on AI adoption. Small and medium batch sizes in particular hinder the integration of intelligent technologies, as less automation often prevails here as well. Nevertheless, even small and medium lot sizes can benefit economically from AI [ 36 ]. Since a high R&D intensity indicates a high innovation capability of a company, it is assumed to have a positive influence on AI adoption, as companies with a high R&D intensity already invest heavily in and use new innovations. This in turn speaks for existing competencies, know how and structures [ 36 ].
3.1.2 Organizational effectiveness
This supercategory focuses on the broader aspects that contribute to the effectiveness, development, and success of an organization when implementing AI in a production context. As the factors are interconnected and influence each other, decision makers should consider them carefully.
Users´ trust in AI is an essential factor to enable successful AI adoption and use in production [ 52 , 68 , 78 , 79 , 88 , 90 ]. From the users´ perspective, AI often exhibits the characteristics of a black box because its inherent processes are not fully understood [ 50 , 90 ] which can lead individuals to develop a fear towards the unknown [ 71 ]. Because of this lack of understanding, successful interaction between humans and AI is not guaranteed [ 90 ], as trust is a foundation for decisions that machines are intended to make autonomously [ 52 , 91 ]. To strengthen faith in AI systems [ 76 , 80 ], AI users can be involved in AI design processes in order to understand appropriate tools [ 54 , 90 ]. In this context, trust is also discussed in close connection with transparency and regulation [ 79 ]. User resistance is considered a barrier to implementing new information technologies, as adoption requires change [ 53 , 62 , 92 ]. Ignorance, as a kind of resistance to change, is a main obstacle to successful digital transformation [ 51 , 56 , 65 ]. Some employees may resist the change brought about by AI because they fear losing their jobs [ 52 ] or have other concerns [ 78 ]. Overcoming resistance to technology adoption requires organizational change and is critical for the success of adoption [ 50 , 51 , 62 , 67 , 71 , 80 ]. Therefore, change management is important to create awareness of the importance of AI adoption and increase acceptance of the workforce [ 66 , 68 , 74 , 83 ]. Management commitment is seen as a significant driver of technology adoption [ 53 , 59 , 81 , 82 , 86 ] and a lack of commitment can negatively impact user adoption and workforce trust and lead to skepticism towards technology [ 86 ]. The top management’s understanding and support for the benefits of the adopted technology [ 53 , 56 , 67 , 78 , 93 , 94 ] enhances AI adoption, can prioritize its implementation and also affects the performance of the AI-enabled application [ 55 , 60 , 83 ]. Preparing, enabling, and thus empowering the workforce, are considered the management’s responsibility in the adoption of digital technologies [ 59 , 75 ]. This requires intelligent leadership [ 52 ] as decision makers need to integrate their workforce into decision-making processes [ 75 ]. Guidelines can support managers by providing access to best practices that help in the adoption of AI [ 50 ]. Critical measures to manage organizational change include the empowerment of visionaries or appointed AI champions leading the change and the collaborative development of digital roadmaps [ 54 , 62 ]. To demonstrate management commitment, managers can create such a dedicated role, consisting of an individual or a small group that is actively and enthusiastically committed to AI adoption in production. This body is considered the adoption manager, point of contact and internal driver of adoption [ 62 , 74 , 80 ]. AI initiatives in production do not necessarily have to be initiated by management. Although management support is essential for successful AI adoption, employees can also actively drive integration initially and thus realize pilot projects or initial trials [ 66 , 80 ]. The development of strategies as well as roadmaps is considered another enabling and necessary factor for the adoption of AI in production [ 50 , 53 , 54 , 62 , 71 , 93 ]. While many major AI strategies already exist at country level to further promote research and development of AI [ 87 ], strategy development is also important at the firm level [ 76 , 77 , 81 ]. In this context, strategies should not be delegated top-down, but be developed in a collaborative manner, i.e. by engaging the workforce [ 75 ] and be in alignment with clear visions [ 91 , 94 ]. Roadmaps are used to improve planning, support implementation, facilitate the adoption of smart technologies in manufacturing [ 93 ] and should be integrated into both business and IT strategy [ 62 , 66 ]. In practice, clear adoption roadmaps that provide approaches on how to effectively integrate AI into existing strategies and businesses are often lacking [ 56 , 87 ]. The need for AI-related skills in organizations is a widely discussed topic in AI adoption analyses [ 79 ]. In this context, the literature points both at the need for specific skills in the development and design of AI applications [ 57 , 71 , 72 , 73 , 76 , 93 ] as well as the skills in using the technology [ 53 , 65 , 73 , 74 , 75 , 84 , 93 ] which availability in the firm is not always given [ 49 ]. AI requires new digital skills [ 36 , 50 , 52 , 55 , 56 , 59 , 61 , 63 , 66 , 78 , 80 ], where e.g. advanced analytics [ 64 , 75 , 81 ], programming skills [ 68 ] and cybersecurity skills [ 78 , 93 ] gain importance. The lack of skills required for AI is seen as a major challenge of digital transformation, as a skilled workforce is considered a key resource for companies [ 51 , 54 , 56 , 60 , 62 , 67 , 69 , 70 , 82 , 93 ]. This lack of a necessary skillset hinders the adoption of AI tools in production systems [ 58 , 77 ]. Closely related to skills is the need for new training concepts, which organizations need to consider when integrating digital technologies [ 49 , 50 , 51 , 56 , 59 , 63 , 71 , 74 , 75 ]. Firms must invest in qualification in order to create necessary competences [ 73 , 78 , 80 , 81 , 92 ]. Additionally, education must target and further develop the skills required for effectively integrating intelligent technologies into manufacturing processes [ 54 , 61 , 62 , 83 ]. Regarding this issue, academic institutions must develop fitting curricula for data driven manufacturing engineering [ 64 ]. Another driving factor of AI adoption is the innovation culture of an organization, which is influenced by various drivers. For example, companies that operate in an environment with high innovation rates, facing intense competitive pressures are considered more likely to see smart technologies as a tool for strategic change [ 83 , 91 , 93 ]. These firms often invest in more expensive and advanced smart technologies as the pressure and resulting competition forces them to innovate [ 93 ]. Another way of approach this is that innovation capability can also be supported and complemented by AI, for example by intelligent systems supporting humans in innovation or even innovating on their own [ 52 ].The entrepreneurial orientation of a firm is characterized in particular by innovativeness [ 66 ], productivity [ 63 ], risk-taking [ 86 ] as well as continuous improvement [ 50 ]. Such characteristics of an innovating culture are considered essential for companies to recognise dynamic changes in the market and make adoption decisions [ 51 , 71 , 81 , 84 , 86 , 94 ]. The prevalence of a digital mindset in companies is important for technology adoption, as digital transformation affects the entire organizational culture and behavior [ 59 , 80 , 92 ] and a lack of a digital culture [ 50 , 65 ] as well as a ‘passive mindset’ [ 78 ] can hinder the digital transformation of firms. Organizations need to develop a corresponding culture [ 66 , 67 , 71 ], also referred to as ‘AI-ready-culture’ [ 54 ], that promotes development and encourages people and data through the incorporation of technology [ 71 , 75 ]. With the increasing adoption of smart technologies, a ‘new digital normal’ is emerging, characterized by hybrid work models, more human–machine interactions and an increased use of digital technologies [ 75 , 83 ].
3.1.3 Technology and System
The ‘technology and system’ supercategory focuses on the broader issues related to the technology and infrastructure that support organizational operations and provide the technical foundation for AI deployment.
By IT infrastructure we refer to issues regarding the foundational systems and IT needed for AI adoption in production. Industrial firms and their IT systems must achieve a mature technological readiness in order to enable successful AI adoption [ 51 , 60 , 67 , 69 , 83 ]. A lack of appropriate IT infrastructure [ 68 , 71 , 78 , 91 ] or small maturity of Internet of Things (IoT) technologies [ 70 ]) hinders the efficient use of data in production firms [ 56 ] which is why firms must update their foundational information systems for successful AI adoption [ 53 , 54 , 62 , 66 , 72 , 75 ]. IT and data security are fundamental for AI adoption and must be provided [ 50 , 51 , 68 , 82 ]. This requires necessary developments that can ensure security during AI implementation while complying with legal requirements [ 52 , 72 , 78 ]. Generally, security concerns are common when implementing AI innovations [ 72 , 79 , 91 , 94 ]. This fear of a lack of security can also prevent the release of (e.g. customer) data in a production environment [ 56 ]. Additionally, as industrial production systems are vulnerable to failures as well as cyberattacks, companies need to address security and cybersecurity measures [ 49 , 76 , 88 , 89 ]. Developing user-friendly AI solutions can facilitate the adoption of smart solutions by increasing user understanding and making systems easy to use by employees as well as quick to integrate [ 50 , 72 , 84 ]. When developing user-friendly solutions which satisfy user needs [ 76 ], it is particularly important to understand and integrate the user perspective in the development process [ 90 ]. If employees find technical solutions easy to use, they are more confident in its use and perceived usefulness increases [ 53 , 67 , 68 ]. The compatibility of AI with a firm and its existing systems, i.e., the extent to which AI matches existing processes, structures, and infrastructures [ 53 , 54 , 56 , 60 , 78 , 80 , 82 , 83 , 93 , 94 ], is considered an important requirement for the adoption of AI in IT systems [ 91 ]. Along with compatibility also comes connectivity, which is intended to ensure the links within the overall network and avoid silo thinking [ 59 ]. Connectivity and interoperability of AI-based processes within the company’s IT manufacturing systems must be ensured at different system levels and are considered key factors in the development of AI applications for production [ 50 , 72 , 89 ]. The design of modular AI solutions can increase system compatibility [ 84 ]. Firms deciding for AI adoption must address safety issues [ 51 , 54 , 59 , 72 , 73 , 78 ]. This includes both safety in the use and operation of AI [ 60 , 69 ]. In order to address safety concerns of integrating AI solutions in industrial systems [ 49 ], systems must secure high reliability [ 71 ]. AI can also be integrated as a safety enabler, for example, by providing technologies to monitor health and safety in the workplace to prevent fatigue and injury [ 75 ].
3.1.4 Data management
Since AI adoption in the organization is strongly data-driven, the ‘data management’ supercategory is dedicated to the comprehensive aspects related to the effective and responsible management of data within the organization.
Data privacy must be guaranteed when creating AI applications based on industrial production data [ 49 , 58 , 59 , 60 , 72 , 76 , 78 , 79 , 82 , 88 , 89 , 91 , 94 ] as ‘[M]anufacturing industries generate large volumes of unstructured and sensitive data during their daily operations’ [ 89 ]. Closely related to this is the need for anonymization and confidentiality of data [ 61 , 69 , 70 , 78 ]. The availability of large, heterogeneous data sets is essential for the digital transformation of organizations [ 52 , 59 , 78 , 80 , 88 , 89 ] and is considered one of the key drivers of AI innovation [ 62 , 68 , 72 , 86 ]. In production systems, lack of data availability is often a barrier to AI adoption [ 58 , 70 , 77 ]. In order to enable AI to establish relationships between data, the availability of large input data that is critical [ 62 , 76 , 81 ]. New AI models are trained with this data and can adapt as well as improve as they receive new data [ 59 , 62 ]. Big data can thus significantly improve the quality of AI applications [ 59 , 71 ]. As more and more data is generated in manufacturing [ 85 ], AI opens up new opportunities for companies to make use of it [ 62 ]. However, operational data are often unstructured, as they come from different sources and exist in diverse formats [ 85 , 87 ]. This challenges data processing, as data quality and origin are key factors in the management of data [ 78 , 79 , 80 , 88 , 89 , 91 ]. To make production data valuable and usable for AI, consistency of data and thus data integrity is required across manufacturing systems [ 50 , 62 , 77 , 84 ]. Another key prerequisites for AI adoption is data governance [ 56 , 59 , 67 , 68 , 71 , 78 , 88 ] which is an important asset to make use of data in production [ 50 ] and ensure the complex management of heterogenous data sets [ 89 ]. The interoperability of data and thus the foundation for the compatibility of AI with existing systems, i.e., the extent to which AI matches existing processes, structures, and infrastructures [ 53 , 56 , 84 , 93 ], is considered another important requirement for the adoption of AI in IT systems. Data interoperability in production systems can be hindered by missing data standards as different machines use different formats [ 87 ]. Data processing refers to techniques used to preparing data for analysis which is essential to obtain consistent results from data analytics in production [ 58 , 72 , 80 , 81 , 84 ]. In this process, the numerous, heterogeneous data from different sensors are processed in such a way that they can be used for further analyses [ 87 ]. The capability of production firms to process data and information is thus important to enable AI adoption [ 77 , 86 , 93 ]. With the increasing data generation in the smart and connected factory, the strategic relevance of data analytics is gaining importance [ 55 , 69 , 78 ], as it is essential for AI systems in performing advanced data analyses [ 49 , 67 , 72 , 86 , 88 ]. Using analytics, valuable insights can be gained from the production data obtained using AI systems [ 58 , 77 , 87 ]. In order to enable the processing of big data, a profound data infrastructure is necessary [ 65 , 75 , 87 ]. Facilities must be equipped with sensors, that collect data and model information, which requires investments from firms [ 72 ]. In addition, production firms must build the necessary skills, culture and capabilities for data analytics [ 54 , 75 , 87 , 93 ]. Data storage, one of the foundations and prerequisites for smart manufacturing [ 54 , 68 , 71 , 74 ], must be ensured in order to manage the larg amounts of data and thus realize the adoption of intelligent technologies in production [ 50 , 59 , 72 , 78 , 84 , 87 , 88 , 89 ].
3.2 External environment
The external drivers of AI adoption in production influence the organization through conditions and events from outside the firm and are therefore difficult to control by the organization itself.
3.2.1 Regulatory environment
This supercategory captures the broader concept of establishing rules, standards, and frameworks that guide the behavior, actions, and operations of individuals, organizations, and societies when implementing AI.
AI adoption in production faces many ethical challenges [ 70 , 72 , 79 ]. AI applications must be compliant with the requirements of organizational ethical standards and laws [ 49 , 50 , 59 , 60 , 62 , 75 ] which is why certain issues must be examined in AI adoption and AI design [ 62 , 73 , 82 , 91 ] so that fairness and justice are guaranteed [ 78 , 79 , 92 ]. Social rights, cultural values and norms must not be violated in the process [ 49 , 52 , 53 , 81 ]. In this context, the explainability and transparency of AI decisions also plays an important role [ 50 , 54 , 58 , 70 , 78 , 89 ] and can address the characteristic of AI of a black box [ 90 ]. In addition, AI applications must be compliant with legal and regulatory requirements [ 51 , 52 , 59 , 77 , 81 , 82 , 91 ] and be developed accordingly [ 49 , 76 ] in order to make organization processes using AI clear and effective [ 65 ]. At present, policies and regulation of AI are still in its infancy [ 49 ] and missing federal regulatory guidelines, standards as well as incentives hinder the adoption of AI [ 67 ] which should be expanded simultaneously to the expansion of AI technology [ 60 ]. This also includes regulations on the handling of data (e.g. anonymization of data) [ 61 , 72 ].
3.2.2 Business environment
The factors in the ‘business environment’ supercategory refer to the external conditions and influences that affect the operations, decision making, and performance of the company seeking to implement AI in a production context.
Cooperation and collaboration can influence the success of digital technology adoption [ 52 , 53 , 59 , 72 ], which is why partnerships are important for adoption [ 53 , 59 ] and can positively influence its future success [ 52 , 67 ]. Both intraorganizational and interorganizational knowledge sharing can positively influence AI adoption [ 49 ]. In collaborations, companies can use a shared knowledge base where data and process sharing [ 51 , 59 , 94 ] as well as social support systems strengthen feedback loops between departments [ 79 , 80 ]. With regard to AI adoption in firms, vendors as well as service providers need to collaborate closely to improve the compatibility and operational capability of smart technologies across different industries [ 82 , 93 ]. Without external IT support, companies can rarely integrate AI into their production processes [ 66 ], which is why thorough support from vendors can significantly facilitate the integration of AI into existing manufacturing processes [ 80 , 91 ]. Public–private collaborations can also add value and governments can target AI dissemination [ 60 , 74 ]. The support of the government also positively influences AI adoption. This includes investing in research projects and policies, building a regulatory setting as well as creating a collaborative environment [ 60 ]. Production companies are constantly exposed to changing conditions, which is why the dynamics of the environment is another factor influencing the adoption of AI [ 52 , 63 , 72 , 86 ]. Environmental dynamics influence the operational performance of firms and can favor an entrepreneurial orientation of firms [ 86 ]. In order to respond to dynamics, companies need to develop certain capabilities and resources (i.e. dynamic capabilities) [ 86 ]. This requires the development of transparency, agility, as well as resilience to unpredictable changes, which was important in the case of the COVID-19 pandemic, for example, where companies had to adapt quickly to changing environments [ 75 ]. A firm’s environment (e.g. governments, partners or customers) can also pressure companies to adopt digital technologies [ 53 , 67 , 82 , 91 ]. Companies facing intense competition are considered more likely to invest in smart technologies, as rivalry pushes them to innovate and they hope to gain competitive advantages from adoption [ 36 , 66 , 82 , 93 ].
3.2.3 Economic environment
By considering both the industrial sector and country within the subcategory ‘economic environment’, production firms can analyze the interplay between the two and understand how drivers can influence the AI adoption process in their industrial sector’s performance within a particular country.
The industrial sector of a firm influences AI adoption in production from a structural perspective, as it indicates variations in product characteristics, governmental support, the general digitalization status, the production environment as well as the use of AI technologies within the sector [ 36 ]. Another factor that influences AI adoption is the country in which a company is located. This influences not only cultural aspects, the availability of know-how and technology orientation, but also regulations, laws, standards and subsidies [ 36 ]. From another perspective, AI can also contribute to the wider socio-economic growth of economies by making new opportunities easily available and thus equipping e.g. more rural areas with advanced capabilities [ 78 ].
3.3 Future research directions
The analysis of AI adoption in production requires a comprehensive analysis of the various factors that influence the introduction of the innovation. As discussed by Kinkel, Baumgartner, Cherubini [ 36 ], our research also concludes that organizational factors have a particularly important role to play. After evaluating the individual drivers of AI adoption in production in detail in this qualitative synthesis, we draw a conclusion from the results and derive a research agenda from the analysis to serve as a basis for future research. The RQs emerged from the analyzed factors and are presented in Table 2 . We developed the questions based on the literature review and identified research gaps for every factor that was most frequently mentioned. From the factors analyzed and RQs developed, the internal environment has a strong influence on AI adoption in production, and organizational factors play a major role here.
Looking at the supercategory ‘business and environment’, performance indicators and investments are considered drivers of AI adoption in production. Indicators to measure the performance of AI innovations are necessary here so that managers can perform cost–benefit analyses and make the right decision for their company. There is a need for research here to support possible calculations and show managers a comprehensive view of the costs and benefits of technology in production. In terms of budget, it should be noted that AI adoption involves a considerable financial outlay that must be carefully weighed and some capital must be available to carry out the necessary implementation efforts (e.g., staffing costs, machine retrofits, change management, and external IT service costs). Since AI adoption is a complex process and turnkey solutions can seldom be implemented easily and quickly, but require many changes (not only technologically but also on an organizational level), it is currently difficult to estimate the necessary budgets and thus make them available. Especially the factors of the supercategory ‘organizational effectiveness’ drive AI adoption in production. Trust of the workforce is considered an important driver, which must be created in order to successfully implement AI. This requires measures that can support management in building trust. Closely related to this are the necessary change management processes that must be initiated to accompany the changes in a targeted manner. Management itself must also play a clear role in the introduction of AI and communicate its support, as this also influences the adoption. The development of clear processes and measures can help here. Developing roadmaps for AI adoption can facilitate the adoption process and promote strategic integration with existing IT and business strategy. Here, best practice roadmaps and necessary action steps can be helpful for companies. Skills are considered the most important driver for AI adoption in manufacturing. Here, there is a lack of clear approaches that support companies in identifying the range of necessary skills and, associated with this, also opportunities to further develop these skills in the existing workforce. Also, building a culture of innovation requires closer research that can help companies foster a conducive environment for AI adoption and the integration of other smart technologies. Steps for developing a positive mindset require further research that can provide approaches for necessary action steps and measures in creating a positive digital culture. With regard to ‘technology and system’, the factors of IT infrastructure and security in particular are driving AI adoption in production. Existing IT systems must reach a certain maturity to enable AI adoption on a technical level. This calls for clear requirements that visualize for companies which systems and standards are in place and where developments are needed. Security must be continuously ensured, for which certain standards and action catalogs must be developed. With regard to the supercategory ‘data management’, the availability of data is considered the basis for successful AI adoption, as no AI can be successfully deployed without data. In the production context in particular, this requires developments that support companies in the provision of data, which usually arises from very heterogeneous sources and forms. Data analytics must also be closely examined, and production companies usually need external support in doing so. The multitude of data also requires big data storage capabilities. Here, groundwork is needed to show companies options about the possibilities of different storage options (e.g., on premis vs. cloud-based).
In the ‘regulatory environment’, ethics in particular is considered a driver of AI adoption in production. Here, fundamental ethical factors and frameworks need to be developed that companies can use as a guideline to ensure ethical standards throughout the process. Cooperations and environmental dynamism drive the supercategory ‘business environment’. Collaborations are necessary to successfully implement AI adoption and action is needed to create the necessary contact facilitation bodies. In a competitive environment, companies have to make quick decisions under strong pressure, which also affects AI adoption. Here, guidelines and also best practice approaches can help to simplify decisions and quickly demonstrate the advantage of the solutions. There is a need for research in this context.
4 Conclusions
The use of AI technologies in production continues to gain momentum as managers hope to increase efficiency, productivity and reduce costs [ 9 , 13 , 20 ]. Although the benefits of AI adoption speak for themselves, implementing AI is a complex decision that requires a lot of knowledge, capital and change [ 95 ] and is influenced by various internal and external factors. Therefore, managers are still cautious about implementing the technology in a production context. Our SLR seeks to examine the emergent phenomenon of AI in production with the precise aim of understanding the factors influencing AI adoption and the key topics discussed in the literature when analyzing AI in a production context. For this purpose, we use the current state of research and examine the existing studies based on the methodology of a systematic literature analysis and respond to three RQs.
We answer RQ1 by closely analyzing the literature selected in our SLR to identify trends in current research on AI adoption in production. In this process, it becomes clear that the topic is gaining importance and that research has increased over the last few years. In the field of production, AI is being examined from various angles and current research addresses aspects from a business, human and technical perspective. In our response to RQ2 we synthesized the existing literature to derive 35 factors that influence AI adoption in production at different levels from inside or outside the organization. In doing so, we find that AI adoption in production poses particularly significant challenges to organizational effectiveness compared to other digital technologies and that the relevance of data management takes on a new dimension. Production companies often operate more traditionally and are sometimes rigid when it comes to change [ 96 , 97 ], which can pose organizational challenges when adopting AI. In addition, the existing machines and systems are typically rather heterogeneous and are subject to different digitalization standards, which in turn can hinder the availability of the necessary data for AI implementation [ 98 , 99 ]. We address RQ3 by deriving a research agenda, which lays a foundation for further scientific research and deepening the understanding of AI adoption in production. The results of our analysis can further help managers to better understand AI adoption and to pay attention to the different factors that influence the adoption of this complex technology.
4.1 Contributions
Our paper takes the first step towards analysing the current state of the research on AI adoption from a production perspective. We represent a holistic view on the topic, which is necessary to get a better understanding of AI in a production-context and build a comprehensive view on the different dimensions as well as factors influencing its adoption. To the best of our knowledge, this is the first contribution that systematises research about the adoption of AI in production. As such, it makes an important contribution to current AI and production research, which is threefold:
First, we highlight the characteristics of studies conducted in recent years on the topic of AI adoption in production, from which several features and developments can be deduced. Our results confirm the topicality of the issue and the increasing relevance of research in the field.
Having laid the foundations for understanding AI in production, we focused our research on the identification and systematization of the most relevant factors influencing AI adoption in production at different levels. This brings us to the second contribution, our comprehensive factor analysis of AI adoption in production provides a framework for further research as well as a potential basis for managers to draw upon when adopting AI. By systematizing the relevant factors influencing AI adoption in production, we derived a set of 35 researched factors associated with AI adoption in production. These factors can be clustered in two areas of analysis and seven respective supercategories. The internal environment area includes four levels of analysis: ‘business and structure’ (focusing on financial aspects and firm characteristics), ‘organizational effectiveness’ (focusing on human-centred factors), ‘technology and system’ (based on the IT infrastructure and systems) as well as ‘data management’ (including all data related factors). Three categories are assigned to the external environment: the ‘regulatory environment’ (such as ethics and the regulatory forms), the ‘business environment’ (focused on cooperation activities and dynamics in the firm environment) and the ‘economic environment’ (related to sectoral and country specifics).
Third, the developed research plan as outlined in Table 2 serves as an additional outcome of the SLR, identifying key RQs in the analyzed areas that can serve as a foundation for researchers to expand the research area of AI adoption in production. These RQs are related to the mostly cited factors analyzed in our SLR and aim to broaden the understanding on the emerging topic.
The resulting insights can serve as the basis for strategic decisions by production companies looking to integrate AI into their processes. Our findings on the factors influencing AI adoption as well as the developed research agenda enhance the practical understanding of a production-specific adoption. Hence, they can serve as the basis for strategic decisions for companies on the path to an effective AI adoption. Managers can, for example, analyse the individual factors in light of their company as well as take necessary steps to develop further aspects in a targeted manner. Researchers, on the other hand, can use the future research agenda in order to assess open RQs and can expand the state of research on AI adoption in production.
4.2 Limitations
Since a literature review must be restricted in its scope in order to make the analyses feasible, our study provides a starting point for further research. Hence, there is a need for further qualitative and quantitative empirical research on the heterogeneous nature of how firms configure their AI adoption process. Along these lines, the following aspects would be of particular interest for future research to improve and further validate the analytical power of the proposed framework.
First, the lack of research on AI adoption in production leads to a limited number of papers included in this SLR. As visualized in Fig. 2 , the number of publications related to the adoption of AI in production has been increasing since 2018 but is, to date, still at an early stage. For this reason, only 47 papers published until May 2024 addressing the production-specific adoption of AI were identified and therefore included in our analysis for in-depth investigation. This rather small number of papers included in the full-text analysis gives a limited view on AI adoption in production but allows a more detailed analysis. As the number of publications in this research field increases, there seems to be a lot of research happening in this field which is why new findings might be constantly added and developed as relevant in the future [ 39 ]. Moreover, in order to research AI adoption from a more practical perspective and thus to build up a broader, continuously updated view on AI adoption in production, future literature analyses could include other publication formats, e.g. study reports of research institutions and companies, as well discussion papers.
Second, the scope of the application areas of AI in production has been increasing rapidly. Even though our overview of the three main areas covered in the recent literature serves as a good basis for identifying the most dominant fields for AI adoption in production, a more detailed analysis could provide a better overview of possibilities for manufacturing companies. Hence, a further systematisation as well as evaluation of application areas for AI in production can provide managers with the information needed to decide where AI applications might be of interest for the specific company needs.
Third, the systematisation of the 35 factors influencing AI adoption in production serve as a good ground for identifying relevant areas influenced by and in turn influencing the adoption of AI. Further analyses should be conducted in order to extend this view and extend the framework. For example, our review could be combined with explorative research methods (such as case studies in production firms) in order to add the practical insights from firms adopting AI. This integration of practical experiences can also help exploit and monitor more AI-specific factors by observing AI adoption processes. In enriching the factors through in-depth analyses, the results of the identified AI adoption factors could also be examined in light of theoretical contributions like the technology-organization-environment (TOE) framework [ 47 ] and other adoption theories.
Fourth, in order to examine the special relevance of identified factors for AI adoption process and thus to distinguish it from the common factors influencing the adoption of more general digital technologies, there is a further need for more in-depth (ethnographic) research into their impacts on the adoption processes, particularly in the production context. Similarly, further research could use the framework introduced in this paper as a basis to develop new indicators and measurement concepts as well as to examine their impacts on production performance using quantitative methods.
Benner MJ, Waldfogel J (2020) Changing the channel: digitization and the rise of “middle tail” strategies. Strat Mgmt J 86:1–24. https://doi.org/10.1002/smj.3130
Article Google Scholar
Roblek V, Meško M, Krapež A (2016) A complex view of industry 4.0. SAGE Open. https://doi.org/10.1177/2158244016653987
Oliveira BG, Liboni LB, Cezarino LO et al (2020) Industry 4.0 in systems thinking: from a narrow to a broad spectrum. Syst Res Behav Sci 37:593–606. https://doi.org/10.1002/sres.2703
Li B, Hou B, Yu W et al (2017) Applications of artificial intelligence in intelligent manufacturing: a review. Frontiers Inf Technol Electronic Eng 18:86–96. https://doi.org/10.1631/FITEE.1601885
Dhamija P, Bag S (2020) Role of artificial intelligence in operations environment: a review and bibliometric analysis. TQM 32:869–896. https://doi.org/10.1108/TQM-10-2019-0243
Collins C, Dennehy D, Conboy K et al (2021) Artificial intelligence in information systems research: a systematic literature review and research agenda. Int J Inf Manage 60:102383. https://doi.org/10.1016/j.ijinfomgt.2021.102383
Chien C-F, Dauzère-Pérès S, Huh WT et al (2020) Artificial intelligence in manufacturing and logistics systems: algorithms, applications, and case studies. Int J Prod Res 58:2730–2731. https://doi.org/10.1080/00207543.2020.1752488
Chen H (2019) Success factors impacting artificial intelligence adoption: perspective from the telecom industry in China, Old Dominion University
Sanchez M, Exposito E, Aguilar J (2020) Autonomic computing in manufacturing process coordination in industry 4.0 context. J Industrial Inf Integr. https://doi.org/10.1016/j.jii.2020.100159
Lee J, Davari H, Singh J et al (2018) Industrial artificial intelligence for industry 4.0-based manufacturing systems. Manufacturing Letters 18:20–23. https://doi.org/10.1016/j.mfglet.2018.09.002
Heimberger H, Horvat D, Schultmann F (2023) Assessing AI-readiness in production—A conceptual approach. In: Huang C-Y, Dekkers R, Chiu SF et al. (eds) intelligent and transformative production in pandemic times. Springer, Cham, pp 249–257
Horvat D, Heimberger H (2023) AI Readiness: An Integrated Socio-technical Framework. In: Deschamps F, Pinheiro de Lima E, Da Gouvêa Costa SE et al. (eds) Proceedings of the 11 th international conference on production research—Americas: ICPR Americas 2022, 1 st ed. 2023. Springer Nature Switzerland; Imprint Springer, Cham, pp 548–557
Wang J, Ma Y, Zhang L et al (2018) Deep learning for smart manufacturing: methods and applications. J Manuf Syst 48:144–156. https://doi.org/10.1016/J.JMSY.2018.01.003
Davenport T, Guha A, Grewal D et al (2020) How artificial intelligence will change the future of marketing. J Acad Mark Sci 48:24–42. https://doi.org/10.1007/s11747-019-00696-0
Cui R, Li M, Zhang S (2022) AI and procurement. Manufacturing Serv Operations Manag 24(691):706. https://doi.org/10.1287/msom.2021.0989
Pournader M, Ghaderi H, Hassanzadegan A et al (2021) Artificial intelligence applications in supply chain management. Int J Prod Econ 241:108250. https://doi.org/10.1016/j.ijpe.2021.108250
Su H, Li L, Tian S et al (2024) Innovation mechanism of AI empowering manufacturing enterprises: case study of an industrial internet platform. Inf Technol Manag. https://doi.org/10.1007/s10799-024-00423-4
Venkatesh V, Raman R, Cruz-Jesus F (2024) AI and emerging technology adoption: a research agenda for operations management. Int J Prod Res 62:5367–5377. https://doi.org/10.1080/00207543.2023.2192309
Senoner J, Netland T, Feuerriegel S (2022) Using explainable artificial intelligence to improve process quality: evidence from semiconductor manufacturing. Manage Sci 68:5704–5723. https://doi.org/10.1287/mnsc.2021.4190
Fosso Wamba S, Queiroz MM, Ngai EWT et al (2024) The interplay between artificial intelligence, production systems, and operations management resilience. Int J Prod Res 62:5361–5366. https://doi.org/10.1080/00207543.2024.2321826
Uren V, Edwards JS (2023) Technology readiness and the organizational journey towards AI adoption: an empirical study. Int J Inf Manage 68:102588. https://doi.org/10.1016/j.ijinfomgt.2022.102588
Berente N, Gu B, Recker J (2021) Managing artificial intelligence special issue managing AI. MIS Quarterly 45:1433–1450
Google Scholar
Scafà M, Papetti A, Brunzini A et al (2019) How to improve worker’s well-being and company performance: a method to identify effective corrective actions. Procedia CIRP 81:162–167. https://doi.org/10.1016/j.procir.2019.03.029
Wang H, Qiu F (2023) AI adoption and labor cost stickiness: based on natural language and machine learning. Inf Technol Manag. https://doi.org/10.1007/s10799-023-00408-9
Lindebaum D, Vesa M, den Hond F (2020) Insights from “the machine stops ” to better understand rational assumptions in algorithmic decision making and its implications for organizations. Acad Manag Rev 45:247–263. https://doi.org/10.5465/amr.2018.0181
Baskerville RL, Myers MD, Yoo Y (2020) Digital first: the ontological reversal and new challenges for information systems research. MIS Quarterly 44:509–523
Frey CB, Osborne MA (2017) The future of employment: How susceptible are jobs to computerisation? Technol Forecast Soc Chang 114:254–280. https://doi.org/10.1016/J.TECHFORE.2016.08.019
Jarrahi MH (2018) Artificial intelligence and the future of work: human-AI symbiosis in organizational decision making. Bus Horiz 61:577–586. https://doi.org/10.1016/j.bushor.2018.03.007
Fügener A, Grahl J, Gupta A et al (2021) Will humans-in-the-loop become borgs? Merits and pitfalls of working with AI. MIS Quarterly 45:1527–1556
Klumpp M (2018) Automation and artificial intelligence in business logistics systems: human reactions and collaboration requirements. Int J Log Res Appl 21:224–242. https://doi.org/10.1080/13675567.2017.1384451
Schrettenbrunnner MB (2020) Artificial-Intelligence-driven management. IEEE Eng Manag Rev 48:15–19. https://doi.org/10.1109/EMR.2020.2990933
Li J, Li M, Wang X et al (2021) Strategic directions for AI: the role of CIOs and boards of directors. MIS Quarterly 45:1603–1644
Brock JK-U, von Wangenheim F (2019) Demystifying AI: What digital transformation leaders can teach you about realistic artificial intelligence. Calif Manage Rev 61:110–134. https://doi.org/10.1177/1536504219865226
Lee J, Suh T, Roy D et al (2019) Emerging technology and business model innovation: the case of artificial intelligence. JOItmC 5:44. https://doi.org/10.3390/joitmc5030044
Chen J, Tajdini S (2024) A moderated model of artificial intelligence adoption in firms and its effects on their performance. Inf Technol Manag. https://doi.org/10.1007/s10799-024-00422-5
Kinkel S, Baumgartner M, Cherubini E (2022) Prerequisites for the adoption of AI technologies in manufacturing—evidence from a worldwide sample of manufacturing companies. Technovation 110:102375. https://doi.org/10.1016/j.technovation.2021.102375
Mikalef P, Gupta M (2021) Artificial intelligence capability: Conceptualization, measurement calibration, and empirical study on its impact on organizational creativity and firm performance. Inf Manag 58:103434. https://doi.org/10.1016/j.im.2021.103434
McElheran K, Li JF, Brynjolfsson E et al (2024) AI adoption in America: Who, what, and where. Economics Manag Strategy 33:375–415. https://doi.org/10.1111/jems.12576
Tranfield D, Denyer D, Smart P (2003) Towards a methodology for developing evidence-informed management knowledge by means of systematic review. Br J Manag 14:207–222. https://doi.org/10.1111/1467-8551.00375
Cooper H, Hedges LV, Valentine JC (2009) Handbook of research synthesis and meta-analysis. Russell Sage Foundation, New York
Page MJ, McKenzie JE, Bossuyt PM et al (2021) The PRISMA 2020 statement: an updated guideline for reporting systematic reviews. BMJ 372:n71. https://doi.org/10.1136/bmj.n71
Denyer D, Tranfield D (2011) Producing a systematic review. In: Buchanan DA, Bryman A (eds) The Sage handbook of organizational research methods. Sage Publications Inc, Thousand Oaks, CA, pp 671–689
Burbidge JL, Falster P, Riis JO et al (1987) Integration in manufacturing. Comput Ind 9:297–305. https://doi.org/10.1016/0166-3615(87)90103-5
Mayring P (2000) Qualitative content analysis. Forum qualitative Sozialforschung/Forum: Qualitative social research, Vol 1, No 2 (2000): Qualitative methods in various disciplines I: Psychology. https://doi.org/10.17169/fqs-1.2.1089
Hsieh H-F, Shannon SE (2005) Three approaches to qualitative content analysis. Qual Health Res 15:1277–1288. https://doi.org/10.1177/1049732305276687
Miles MB, Huberman AM (2009) Qualitative data analysis: An expanded sourcebook, 2nd edn. Sage, Thousand Oaks, Calif
Tornatzky LG, Fleischer M (1990) The processes of technological innovation. Issues in organization and management series. Lexington Books, Lexington, Mass.
Alsheibani S, Cheung Y, Messom C (2018) Artificial Intelligence Adoption: AI-readiness at Firm-Level: Research-in-Progress. Twenty-Second Pacific Asia Conference on Information Systems
Akinsolu MO (2023) Applied artificial intelligence in manufacturing and industrial production systems: PEST considerations for engineering managers. IEEE Eng Manag Rev 51:52–62. https://doi.org/10.1109/EMR.2022.3209891
Bettoni A, Matteri D, Montini E et al (2021) An AI adoption model for SMEs: a conceptual framework. IFAC-PapersOnLine 54:702–708. https://doi.org/10.1016/j.ifacol.2021.08.082
Boavida N, Candeias M (2021) Recent automation trends in portugal: implications on industrial productivity and employment in automotive sector. Societies 11:101. https://doi.org/10.3390/soc11030101
Botha AP (2019) A mind model for intelligent machine innovation using future thinking principles. Jnl of Manu Tech Mnagmnt 30:1250–1264. https://doi.org/10.1108/JMTM-01-2018-0021
Chatterjee S, Rana NP, Dwivedi YK et al (2021) Understanding AI adoption in manufacturing and production firms using an integrated TAM-TOE model. Technol Forecast Soc Chang 170:120880. https://doi.org/10.1016/j.techfore.2021.120880
Chiang LH, Braun B, Wang Z et al (2022) Towards artificial intelligence at scale in the chemical industry. AIChE J. https://doi.org/10.1002/aic.17644
Chouchene A, Carvalho A, Lima TM et al. (2020) Artificial intelligence for product quality inspection toward smart industries: quality control of vehicle Non-conformities. In: Garengo P (ed) 2020 9th International Conference on Industrial Technology and Management: ICITM 2020 February 11–13, 2020, Oxford, United Kingdom. IEEE, pp 127–131
Corti D, Masiero S, Gladysz B (2021) Impact of Industry 4.0 on Quality Management: identification of main challenges towards a Quality 4.0 approach. In: 2021 IEEE International Conference on Engineering, Technology and Innovation (ICE/ITMC). IEEE, pp 1–8
Demlehner Q, Schoemer D, Laumer S (2021) How can artificial intelligence enhance car manufacturing? A Delphi study-based identification and assessment of general use cases. Int J Inf Manage 58:102317. https://doi.org/10.1016/j.ijinfomgt.2021.102317
Dohale V, Akarte M, Gunasekaran A et al (2022) (2022) Exploring the role of artificial intelligence in building production resilience: learnings from the COVID-19 pandemic. Int J Prod Res 10(1080/00207543):2127961
Drobot AT (2020) Industrial Transformation and the Digital Revolution: A Focus on artificial intelligence, data science and data engineering. In: 2020 ITU Kaleidoscope: Industry-Driven Digital Transformation (ITU K). IEEE, pp 1–11
Ghani EK, Ariffin N, Sukmadilaga C (2022) Factors influencing artificial intelligence adoption in publicly listed manufacturing companies: a technology, organisation, and environment approach. IJAEFA 14:108–117
Hammer A, Karmakar S (2021) Automation, AI and the future of work in India. ER 43:1327–1341. https://doi.org/10.1108/ER-12-2019-0452
Hartley JL, Sawaya WJ (2019) Tortoise, not the hare: digital transformation of supply chain business processes. Bus Horiz 62:707–715. https://doi.org/10.1016/j.bushor.2019.07.006
Kyvik Nordås H, Klügl F (2021) Drivers of automation and consequences for jobs in engineering services: an agent-based modelling approach. Front Robot AI 8:637125. https://doi.org/10.3389/frobt.2021.637125
Mubarok K, Arriaga EF (2020) Building a smart and intelligent factory of the future with industry 4.0 technologies. J Phys Conf Ser. https://doi.org/10.1088/1742-6596/1569/3/032031
Muriel-Pera YdJ, Diaz-Piraquive FN, Rodriguez-Bernal LP et al. (2018) Adoption of strategies the fourth industrial revolution by micro, small and medium enterprises in bogota D.C. In: Lozano Garzón CA (ed) 2018 Congreso Internacional de Innovación y Tendencias en Ingeniería (CONIITI). IEEE, pp 1–6
Olsowski S, Schlögl S, Richter E et al. (2022) Investigating the Potential of AutoML as an Instrument for Fostering AI Adoption in SMEs. In: Uden L, Ting I-H, Feldmann B (eds) Knowledge Management in Organisations: 16th International Conference, KMO 2022, Hagen, Germany, July 11–14, 2022, Proceedings, 1st ed. 2022, vol 1593. Springer, Cham, pp 360–371
Rodríguez-Espíndola O, Chowdhury S, Dey PK et al (2022) Analysis of the adoption of emergent technologies for risk management in the era of digital manufacturing. Technol Forecast Soc Chang 178:121562. https://doi.org/10.1016/j.techfore.2022.121562
Schkarin T, Dobhan A (2022) Prerequisites for Applying Artificial Intelligence for Scheduling in Small- and Medium-sized Enterprises. In: Proceedings of the 24 th International Conference on Enterprise Information Systems. SCITEPRESS—Science and Technology Publications, pp 529–536
Sharma P, Shah J, Patel R (2022) Artificial intelligence framework for MSME sectors with focus on design and manufacturing industries. Mater Today: Proc 62:6962–6966. https://doi.org/10.1016/j.matpr.2021.12.360
Siaterlis G, Nikolakis N, Alexopoulos K et al. (2022) Adoption of AI in EU Manufacturing. Gaps and Challenges. In: Katalinic B (ed) Proceedings of the 33 rd International DAAAM Symposium 2022, vol 1. DAAAM International Vienna, pp 547–550
Tariq MU, Poulin M, Abonamah AA (2021) Achieving operational excellence through artificial intelligence: driving forces and barriers. Front Psychol 12:686624. https://doi.org/10.3389/fpsyg.2021.686624
Trakadas P, Simoens P, Gkonis P et al (2020) An artificial intelligence-based collaboration approach in industrial IoT manufacturing: key concepts. Architectural Ext Potential Applications Sens. https://doi.org/10.3390/s20195480
Vernim S, Bauer H, Rauch E et al (2022) A value sensitive design approach for designing AI-based worker assistance systems in manufacturing. Procedia Computer Sci 200:505–516. https://doi.org/10.1016/j.procs.2022.01.248
Williams G, Meisel NA, Simpson TW et al (2022) Design for artificial intelligence: proposing a conceptual framework grounded in data wrangling. J Computing Inf Sci Eng 10(1115/1):4055854
Wuest T, Romero D, Cavuoto LA et al (2020) Empowering the workforce in Post–COVID-19 smart manufacturing systems. Smart Sustain Manuf Syst 4:20200043. https://doi.org/10.1520/SSMS20200043
Javaid M, Haleem A, Singh RP (2023) A study on ChatGPT for Industry 4.0: background, potentials, challenges, and eventualities. J Economy Technol 1:127–143. https://doi.org/10.1016/j.ject.2023.08.001
Rathore AS, Nikita S, Thakur G et al (2023) Artificial intelligence and machine learning applications in biopharmaceutical manufacturing. Trends Biotechnol 41:497–510. https://doi.org/10.1016/j.tibtech.2022.08.007
Jan Z, Ahamed F, Mayer W et al (2023) Artificial intelligence for industry 4.0: systematic review of applications, challenges, and opportunities. Expert Syst Applications 216:119456
Waschull S, Emmanouilidis C (2023) Assessing human-centricity in AI enabled manufacturing systems: a socio-technical evaluation methodology. IFAC-PapersOnLine 56:1791–1796. https://doi.org/10.1016/j.ifacol.2023.10.1891
Stohr A, Ollig P, Keller R et al (2024) Generative mechanisms of AI implementation: a critical realist perspective on predictive maintenance. Inf Organ 34:100503. https://doi.org/10.1016/j.infoandorg.2024.100503
Pazhayattil AB, Konyu-Fogel G (2023) ML and AI Implementation Insights for Bio/Pharma Manufacturing. BioPharm International 36:24–29
Ronaghi MH (2023) The influence of artificial intelligence adoption on circular economy practices in manufacturing industries. Environ Dev Sustain 25:14355–14380. https://doi.org/10.1007/s10668-022-02670-3
Rath SP, Tripathy R, Jain NK (2024) Assessing the factors influencing the adoption of generative artificial intelligence (GenAI) in the manufacturing sector. In: Sharma SK, Dwivedi YK, Metri B et al (eds) Transfer, diffusion and adoption of next-generation digital technologies, vol 697. Springer Nature Switzerland, Cham
Bonnard R, Da Arantes MS, Lorbieski R et al (2021) Big data/analytics platform for Industry 4.0 implementation in advanced manufacturing context. Int J Adv Manuf Technol 117:1959–1973. https://doi.org/10.1007/s00170-021-07834-5
Confalonieri M, Barni A, Valente A et al. (2015) An AI based decision support system for preventive maintenance and production optimization in energy intensive manufacturing plants. In: 2015 IEEE international conference on engineering, technology and innovation/ international technology management conference (ICE/ITMC). IEEE, pp 1–8
Dubey R, Gunasekaran A, Childe SJ et al (2020) Big data analytics and artificial intelligence pathway to operational performance under the effects of entrepreneurial orientation and environmental dynamism: a study of manufacturing organisations. Int J Prod Econ 226:107599. https://doi.org/10.1016/j.ijpe.2019.107599
Lee J, Singh J, Azamfar M et al (2020) Industrial AI: a systematic framework for AI in industrial applications. China Mechanical Eng 31:37–48
Turner CJ, Emmanouilidis C, Tomiyama T et al (2019) Intelligent decision support for maintenance: an overview and future trends. Int J Comput Integr Manuf 32:936–959. https://doi.org/10.1080/0951192X.2019.1667033
Agostinho C, Dikopoulou Z, Lavasa E et al (2023) Explainability as the key ingredient for AI adoption in Industry 5.0 settings. Front Artif Intell. https://doi.org/10.3389/frai.2023.1264372
Csiszar A, Hein P, Wachter M et al. (2020) Towards a user-centered development process of machine learning applications for manufacturing domain experts. In: 2020 third international conference on artificial intelligence for industries (AI4I). IEEE, pp 36–39
Merhi MI (2023) Harfouche A (2023) Enablers of artificial intelligence adoption and implementation in production systems. Int J Prod Res. https://doi.org/10.1080/00207543.2023.2167014
Demlehner Q, Laumer S (2024) How the terminator might affect the car manufacturing industry: examining the role of pre-announcement bias for AI-based IS adoptions. Inf Manag 61:103881. https://doi.org/10.1016/j.im.2023.103881
Ghobakhloo M, Ching NT (2019) Adoption of digital technologies of smart manufacturing in SMEs. J Ind Inf Integr 16:100107. https://doi.org/10.1016/j.jii.2019.100107
Binsaeed RH, Yousaf Z, Grigorescu A et al (2023) Knowledge sharing key issue for digital technology and artificial intelligence adoption. Systems 11:316. https://doi.org/10.3390/systems11070316
Papadopoulos T, Sivarajah U, Spanaki K et al (2022) Editorial: artificial Intelligence (AI) and data sharing in manufacturing, production and operations management research. Int J Prod Res 60:4361–4364. https://doi.org/10.1080/00207543.2021.2010979
Chirumalla K (2021) Building digitally-enabled process innovation in the process industries: a dynamic capabilities approach. Technovation 105:102256. https://doi.org/10.1016/j.technovation.2021.102256
Fragapane G, Ivanov D, Peron M et al (2022) Increasing flexibility and productivity in Industry 4.0 production networks with autonomous mobile robots and smart intralogistics. Ann Oper Res 308:125–143. https://doi.org/10.1007/s10479-020-03526-7
Shahbazi Z, Byun Y-C (2021) Integration of Blockchain, IoT and machine learning for multistage quality control and enhancing security in smart manufacturing. Sensors (Basel). https://doi.org/10.3390/s21041467
Javaid M, Haleem A, Singh RP et al (2021) Significance of sensors for industry 4.0: roles, capabilities, and applications. Sensors Int 2:100110. https://doi.org/10.1016/j.sintl.2021.100110
Download references
Open Access funding enabled and organized by Projekt DEAL.
Author information
Authors and affiliations.
Business Unit Industrial Change and New Business Models, Competence Center Innovation and Knowledge Economy, Fraunhofer Institute for Systems and Innovation Research ISI, Breslauer Straße 48, 76139, Karlsruhe, Germany
Heidi Heimberger, Djerdj Horvat & Frank Schultmann
Karlsruhe Institute for Technology KIT, Institute for Industrial Production (IIP) - Chair of Business Administration, Production and Operations Management, Hertzstraße 16, 76187, Karlsruhe, Germany
Heidi Heimberger
You can also search for this author in PubMed Google Scholar
Corresponding author
Correspondence to Heidi Heimberger .
Ethics declarations
Conflict of interest.
The authors report no conflict of interest.
Additional information
Publisher's note.
Springer Nature remains neutral with regard to jurisdictional claims in published maps and institutional affiliations.
Rights and permissions
Open Access This article is licensed under a Creative Commons Attribution 4.0 International License, which permits use, sharing, adaptation, distribution and reproduction in any medium or format, as long as you give appropriate credit to the original author(s) and the source, provide a link to the Creative Commons licence, and indicate if changes were made. The images or other third party material in this article are included in the article's Creative Commons licence, unless indicated otherwise in a credit line to the material. If material is not included in the article's Creative Commons licence and your intended use is not permitted by statutory regulation or exceeds the permitted use, you will need to obtain permission directly from the copyright holder. To view a copy of this licence, visit http://creativecommons.org/licenses/by/4.0/ .
Reprints and permissions
About this article
Heimberger, H., Horvat, D. & Schultmann, F. Exploring the factors driving AI adoption in production: a systematic literature review and future research agenda. Inf Technol Manag (2024). https://doi.org/10.1007/s10799-024-00436-z
Download citation
Accepted : 10 August 2024
Published : 23 August 2024
DOI : https://doi.org/10.1007/s10799-024-00436-z
Share this article
Anyone you share the following link with will be able to read this content:
Sorry, a shareable link is not currently available for this article.
Provided by the Springer Nature SharedIt content-sharing initiative
- Artificial intelligence
- Technology adoption
- AI adoption
- Adoption factors
- Systematic literature review
- Find a journal
- Publish with us
- Track your research
This paper is in the following e-collection/theme issue:
Published on 22.8.2024 in Vol 12 (2024)
Current Status of Barriers to mHealth Access Among Patients With Stroke and Steps Toward the Digital Health Era: Systematic Review
Authors of this article:

- Atsadaporn Niyomyart 1 , RN, LLB, MSN, PhD ;
- Suebsarn Ruksakulpiwat 2 , RN, MMed, PhD ;
- Chitchanok Benjasirisan 2 , RN, MS ;
- Lalipat Phianhasin 2 , RN, MS, AGPCNP-BC ;
- Kabtamu Nigussie 3 , BSc, MSc ;
- Sutthinee Thorngthip 4 , RN, MS ;
- Gazi Shamita 5 , BS, MS ;
- Jai Thampakkul 6 , BS ;
- Lidya Begashaw 7 , BS
1 Ramathibodi School of Nursing, Faculty of Medicine Ramathibodi Hospital, Mahidol University, Bangkok, Thailand
2 Department of Medical Nursing, Faculty of Nursing, Mahidol University, Bangkok, Thailand
3 Department of Psychiatry, College of Health and Medical Sciences, Haramaya University, Harar, Ethiopia
4 Department of Nursing Siriraj Hospital, Faculty of Medicine Siriraj Hospital, Mahidol University, Bangkok, Thailand
5 Department of Dermatology, School of Medicine, Case Western Reserve University, Cleveland, OH, United States
6 Case School of Engineering, Case Western Reserve University, Cleveland, OH, United States
7 Jack, Joseph and Morton Mandel School of Applied Social Sciences, Case Western Reserve University, Cleveland, OH, United States
Corresponding Author:
Suebsarn Ruksakulpiwat, RN, MMed, PhD
Department of Medical Nursing, Faculty of Nursing, Mahidol University
2 Wang Lang Road, Siriraj, Bangkok Noi
Bangkok, 10700
Phone: 66 984782692
Email: [email protected]
Background: Mobile health (mHealth) offers significant benefits for patients with stroke, facilitating remote monitoring and personalized health care solutions beyond traditional settings. However, there is a dearth of comprehensive data, particularly qualitative insights, on the barriers to mHealth access. Understanding these barriers is crucial for devising strategies to enhance mHealth use among patients with stroke.
Objective: This study aims to examine the recent literature focusing on barriers to mHealth access among patients with stroke.
Methods: A systematic search of PubMed, MEDLINE, Web of Science, and CINAHL Plus Full Text was conducted for literature published between 2017 and 2023. Abstracts and full texts were independently screened based on predetermined inclusion and exclusion criteria. Data synthesis was performed using the convergent integrated analysis framework recommended by the Joanna Briggs Institute.
Results: A total of 12 studies met the inclusion criteria. The majority were qualitative studies (about 42%), followed by mixed methods (25%), pilot studies (about 17%), nonrandomized controlled trials (about 8%), and observational studies (about 8%). Participants included patients with stroke, caregivers, and various health care professionals. The most common mHealth practices were home-based telerehabilitation (30%) and poststroke mHealth and telecare services (20%). Identified barriers were categorized into two primary themes: (1) at the patient level and (2) at the health provider-patient-device interaction level. The first theme includes 2 subthemes: health-related issues and patient acceptability. The second theme encompassed 3 subthemes: infrastructure challenges (including software, networking, and hardware), support system deficiencies, and time constraints.
Conclusions: This systematic review underscores significant barriers to mHealth adoption among patients with stroke. Addressing these barriers in future research is imperative to ensure that mHealth solutions effectively meet patients’ needs.
Introduction
Stroke, a leading cause of disability and mortality worldwide, necessitates immediate and ongoing interventions for optimal recovery [ 1 ]. While mobile health (mHealth) technologies offer promising solutions for chronic disease management, understanding and addressing the unique barriers faced by patients with stroke is crucial for ensuring their equitable access and optimal use [ 2 , 3 ]. While previous research has explored mHealth adoption in various populations, the cognitive impairments [ 4 ], rehabilitation needs [ 5 ], and potential technology literacy limitations of patients with stroke [ 6 ] require distinct consideration. mHealth, encompassing the use of mobile devices, applications, and wireless communication devices, offers promising avenues to deliver personalized health care solutions to patients with stroke beyond the confines of traditional health care settings [ 3 , 7 ].
The health care landscape has evolved with the proliferation of mobile devices and the availability of high-speed internet connectivity. Consequently, mHealth platforms have emerged as tools that can potentially bridge the gap between health care providers and patients, enabling continuous monitoring, real-time communication, and targeted interventions [ 8 , 9 ]. In the context of stroke, where timely interventions and ongoing support are crucial, mHealth can revolutionize poststroke care by providing patients with access to rehabilitation exercises, medication reminders, educational resources, and even telemedicine consultations [ 3 , 6 , 10 ]. Despite the promise of mHealth, realizing its benefits for patients with stroke is contingent upon understanding and mitigating the barriers that hinder its widespread adoption and use. Previous research has underscored the importance of identifying these barriers to ensure equitable access and optimal use of mHealth services among patients with stroke [ 11 ]. While existing literature has explored mHealth adoption in various populations, the unique challenges faced by patients with stroke deserve particular attention due to the nature of their condition, potential cognitive impairments, and the necessity of tailored interventions. The period between 2017 and 2023 has witnessed substantial advancements in mHealth technologies, health care policies, and the prevalence of mobile device usage among diverse demographic groups. Consequently, there is a need to assess the current status of mHealth access among patients recovering from stroke within this evolving landscape. By systematically reviewing the literature and synthesizing recent findings, this study aims to delineate the barriers that impede access to mHealth services among patients with stroke, analyze their impact, and propose actionable recommendations for stakeholders in the health care ecosystem.
In light of the growing importance of digital health and its potential benefits for patients with stroke, investigating the barriers to mHealth access is a critical step toward ensuring that these advancements are inclusive and patient centered. By identifying these barriers and proposing strategies to overcome them, this study seeks to contribute to the advancement of stroke care in the digital health era.
This study seeks to evaluate recent literature, focusing on the notable barriers to accessing mHealth services within the population of patients with stroke. Through this assessment, the study aims to offer recommendations aimed at effectively addressing these barriers and propelling progress in this critical domain of health care.
Identify Relevant Studies
We adhered to the PRISMA (Preferred Reporting Items for Systematic Reviews and Meta-Analyses) guidelines [ 12 ] while conducting this systematic review. This approach guided the presentation of the flow diagram depicting the identification, screening, exclusion, and inclusion of literature. To identify studies published between 2017 and 2023 that reported barriers to mHealth access among the patients with stroke, we systematically searched 4 electronic databases: PubMed, MEDLINE, Web of Science, and CINAHL Plus Full Text, on September 1, 2023. Our search involved the combination of terms: (Stroke* or Cerebrovascular Accident* or CVA* or Cerebrovascular Apoplexy or Brain Vascular Accident* or Cerebrovascular Stroke* or Apoplexy or Cerebral Stroke* or Acute Stroke* or Acute Cerebrovascular Accident* or Brain Infarction* or Brain Infarct* or Anterior Circulation Brain Infarction or Brain Venous Infarction* or Venous Brain Infarction* or Anterior Cerebral Circulation Infarction or Posterior Circulation Brain Infarction or Cerebral Infarction* or Cerebral Infarct* or Left Hemisphere, Infarction, Cerebral or Subcortical Infarction* or Posterior Choroidal Artery Infarction or Anterior Choroidal Artery Infarction or Hemorrhagic Stroke* or Subarachnoid Hemorrhagic Stroke* or Intracerebral Hemorrhagic Stroke* or Ischemic Stroke* or Ischaemic Strok* or Cryptogenic Ischemic Stroke* or Cryptogenic Stroke* or Cryptogenic Embolism Stroke* or Wake-up Stroke* or Acute Ischemic Stroke* or Embolic Stroke* or Cardioembolic Stroke* or Cardio-embolic Stroke* or Thrombotic Stroke* or Acute Thrombotic Stroke*) AND (Tele-Referral* or Virtual Medicine or Tele Intensive Care or Tele ICU or Mobile Health or mHealth or Telehealth or eHealth or Remote Consultation or Teleconsultation* or Telenursing or Telepathology or Teleradiology or Telerehabilitation* or Remote Rehabilitation* or Virtual Rehabilitation*) AND (Barrier*) using Boolean operators. Additionally, we manually searched the reference lists of the included studies to ensure inclusivity. All identified references were cataloged using EndNote.
Study Selection
We conducted a 3-step selection process. First, we screened titles and abstracts to identify eligible studies. Then, we assessed the full text to determine relevance. Finally, inclusion criteria were applied to ensure that only studies aligned with our objectives were included. Conversely, exclusion criteria were used to eliminate literature not pertinent to the review (see Textbox 1 ).
Inclusion criteria:
- The study included patients with stroke aged 18 years or older. The study may also encompass other populations, such as caregivers and health care teams, but patients with stroke must be included
- Original studies using quantitative, qualitative, or mixed methods approaches
- Studies that identified barriers to accessing mobile health (mHealth) services among patients with stroke (all types of strokes are eligible)
- In this study, mHealth is identified as the practice of medicine and public health supported by mobile devices. It encompasses the use of mobile devices, such as smartphones, tablet computers, personal digital assistants, and others, for health services, information, and data collection [ 13 ]
- Studies conducted in various settings, including inpatient, outpatient, or home environments
- Studies published in the English language
- Studies published between January 2017 and December 2023
Exclusion criteria:
- Studies that did not involve patients were excluded.
- Exclusion of conference proceedings, abstracts, review articles, theoretical papers, protocols, dissertations, letters to the editor, opinion (viewpoint) pieces, statement papers, government documents, or working papers
Data Extraction
For this review, we used a standardized data extraction chart that included the following data points for each study: Reference, country, year, study design, total sample size, target population, participant age (years), main study aim, main findings, presence of mHealth in included studies, key barriers to accessing mHealth, and further research implications.
Data Synthesis
For the data synthesis of the included studies in this review, we used the convergent integrated analysis framework recommended by the Joanna Briggs Institute (JBI) for systematic reviews. In this process, themes will be derived from the key findings of the included studies by analyzing both commonalities and distinctions among the primary findings related to key barriers in accessing mHealth. Furthermore, subthemes will be extracted as necessary, aligning with the specific focus of the corresponding findings, akin to the methodology used by qualitative researchers [ 14 ].
Search Results
Following the PRISMA guidelines [ 12 ], we initially identified a total of 206 articles. Out of these, 94 were obtained from PubMed and Medline, 68 from Web of Science, and 44 from CINAHL Plus Full Text. No additional articles were found from other sources. After a thorough review, we identified and removed 5 duplicate articles. Subsequently, the remaining articles underwent screening based on their titles and abstracts, following the inclusion and exclusion criteria ( Textbox 1 ). At this stage, 184 articles did not meet the inclusion criteria and were excluded, leaving us with 17 articles eligible for full-text screening. During the full-text screening phase, 5 articles were excluded. Of these, 1 was identified as a statement paper, 1 was a review paper, 2 did not address barriers to mHealth, and 1 did not include patients with stroke as the study population. Consequently, 12 studies were included in the review ( Figure 1 ).

Data Summary
Multimedia Appendix 1 [ 15 - 26 ] provides a summary of each included study, including reference, country, year, study design, total sample size, target population, participant age (years), main study aim, main findings, presence of mHealth in included studies (optional), key barriers to accessing mHealth, and implications for further research.
Description of Included Studies
Table 1 shows that all included studies were published between 2017 and 2023, with the most publications in 2023 (n=5, 42%) and 2022 (n=3, 25%). Among these, one publication was found each in 2017, 2018, 2020, and 2021. Most of the included studies were conducted in the United States (n = 4, 33%). Other countries include the United Kingdom (n=2, 17%), Hong Kong (China) (n=2, 17%), Brazil (n=1, 8%), and the Netherlands (n=1, 8%). In terms of study design, qualitative studies were the most popular (n=5, 42%), followed by mixed methods (n=3, 25%), pilot studies (n=2, 17%), nonrandomized control trials (n=1, 8%), and observational studies (n=1, 8%). Among the patients with stroke included in the study, there was no specific stroke type identified (n=9, 64%), ischemic stroke (n=2, 14%), hemorrhagic stroke (n=2, 14%), and chronic hemiplegic stroke (n=1, 7%).
Characteristics | Values, n (%) | |||
2023 | 5 (42) | |||
2022 | 3 (25) | |||
2021 | 1 (8) | |||
2020 | 1 (8) | |||
2018 | 1 (8) | |||
2017 | 1 (8) | |||
United States | 4 (33) | |||
United Kingdom | 2 (17) | |||
Hong Kong (China) | 2 (17) | |||
Brazil | 1 (8) | |||
The Netherlands | 1 (8) | |||
Singapore | 1 (8) | |||
Republic of Korea | 1 (8) | |||
Qualitative study | 5 (42) | |||
Mixed methods | 3 (25) | |||
Pilot study | 2 (17) | |||
Nonrandomized controlled trial | 1 (8) | |||
Observational study | 1 (8) | |||
Not specify stroke type | 9 (64) | |||
Ischemic stroke | 2 (14) | |||
Hemorrhagic stroke | 2 (14) | |||
Chronic hemiplegic stroke | 1 (7) | |||
Caregiver | 3 (14) | |||
Clinician | 1 (14) | |||
Rehabilitation therapist | 1 (14) | |||
Occupational therapist | 1 (14) | |||
Nurse | 1 (14) | |||
1-50 | 9 (75) | |||
>50-100 | 1 (8) | |||
>100-200 | 1 (8) | |||
>200 | 1 (8) | |||
in included studies | ||||
A home-based telerehabilitation [ - ] | 3 (30) | |||
A poststroke mHealth and telecare service [ , ] | 2 (20) | |||
The remote physical exercise program [ ] | 1 (10) | |||
A home-based web-based clinic [ ] | 1 (10) | |||
Self-administered VR telerehabilitation [ ] | 1 (10) | |||
The Homecare Arm Rehabilitation System (MERLIN) [ ] | 1 (10) | |||
Remote CCT and metacognitive strategy (MST ) training [ ] | 1 (10) |
a The number of included studies in which one study may include more than one characteristic (eg, target population, mobile health, etc).
b mHealth: mobile health.
c VR: virtual reality.
d CCT: computerized cognitive.
e MST: metacognitive strategy.
Additionally, caregivers (n=3, 43%), clinicians (n=1, 14%), rehabilitation therapists (n=1, 14%), occupational therapists (n=1, 14%), and nurses (n=1, 14%) were included in the study. Sample sizes ranged from 1 to 50 (n=9, 75%), over 50 to 100, over 100 to 200, and over 200 (all n=1, 8%). The most common mHealth practices in the included studies were home-based telerehabilitation (n=3, 30%) and poststroke mHealth and telecare service (n=2, 20%). The rest of mHealth consisted of remote physical exercise, a home-based web-based clinic, self-administered virtual reality (VR) telerehabilitation, and home care arm rehabilitation, as well as remote cognitive (CCT) and metacognitive strategy training (MST; all n=1, 10%).
Description of Barriers to mHealth Access in Patients With Stroke
Multimedia Appendix 1 [ 15 - 26 ] provides a summary of the barriers to mHealth accessibility experienced by patients with stroke. Having used the convergent integrated analysis framework recommended by JBI for systematic reviews [ 14 ], we can discern two primary themes: (1) at the patient-level and (2) at the health provider-patient-device interaction level. The first theme included 2 subthemes: health-related barriers and patient acceptability. The second theme encompassed 3 subthemes: infrastructure (inclusive of software, networking, and hardware), deficiencies in support systems, and constraints on available time ( Table 2 ).
Study | Barriers to mHealth Themes | ||||||
The patient-level | The health provider-patient-device interaction level | ||||||
Health-related barriers | Patient acceptability | Infrastructure | Deficiencies in support systems | Constraints on available time | |||
Software | Networking | Hardware | |||||
Dodakian et al [ ] | ✓ | ✓ | ✓ | ||||
Tyagi et al [ ] | ✓ | ✓ | ✓ | ||||
Dunne et al [ ] | ✓ | ✓ | |||||
Torriani-Pasin et al [ ] | ✓ | ✓ | ✓ | ||||
Lam et al [ ] | ✓ | ||||||
Morse et al [ ] | ✓ | ✓ | ✓ | ✓ | |||
Spits et al [ ] | ✓ | ✓ | |||||
Bhattacharjya et al [ ] | ✓ | ✓ | ✓ | ||||
Chung et al [ ] | ✓ | ||||||
Jaywant et al [ ] | ✓ | ✓ | ✓ | ✓ | |||
Ramaswamy et al [ ] | ✓ | ✓ | |||||
Wong et al [ ] | ✓ | ✓ | |||||
Number of included studies, n (%) | 2 (17) | 5 (42) | 4 (33) | 4 (33) | 5 (42) | 6 (50) | 4 (33) |
The Patient Level
Health-related barriers.
Our results indicate that health-related issues are among the barriers to mHealth access for patients with stroke. A study evaluating a home-based telerehabilitation system in patients with chronic hemiparetic stroke found that fatigue due to illness prevented patients from using the Home-Based Telerehabilitation Program [ 15 ]. Similarly, a clinical trial study from Brazil aimed at determining adherence and barriers to attending a remote physical exercise program for individuals after stroke shows that health-related factors such as a lack of motor skills, physical fitness, exercise-related pain, and other constraints are significant barriers preventing them from using the remote physical exercise program [ 20 ]. For instance, in a qualitative study conducted with survivors of stroke experiencing partial visual loss, caregivers and occupational therapists revealed that patients believed their lack of confidence and fear of using technology prevented them from learning new things, and their readiness to embrace technology was a barrier to telerehabilitation [ 25 ].
Patient Acceptability
Five studies in our review have highlighted patient acceptability as the primary barrier to mHealth [ 17 , 22 - 25 ]. For example, a mixed-method study identified facilitators and barriers to using self-administered VR telerehabilitation, suggesting that a lack of experience or confidence with technology hindered access to self-administered VR telerehabilitation [ 22 ]. Moreover, a pilot study found that patients with stroke, especially older adults with stroke, may have limited digital literacy skills, making it challenging to use mHealth tools effectively. Additionally, a study points out that participants' attitudes and acceptance of mHealth interventions may vary; some individuals may be hesitant or skeptical about using technology for health care purposes [ 24 ].
The Health Provider-Patient-Device Interaction Level
Infrastructure.
The complexity of mHealth software can pose challenges for patients in its usage [ 16 , 18 , 19 , 26 ]. For instance, a qualitative study conducted in Singapore explored the perceived barriers and facilitators of telerehabilitation by patients with stroke, caregivers, and rehabilitation therapists. The study noted that teletherapists encountered difficulties conducting comprehensive patient assessments remotely [ 26 ]. Similarly, patients perceived limitations in the variety and scope of rehabilitation exercises available through telerehabilitation, attributing this to issues related to the interface and design of the remote platform [ 26 ]. In line with this, another study aimed to explore potential mHealth apps to aid survivors of stroke with poststroke care and determine how demographic variables affect app preferences. This study found that the complexity of app usage acted as a barrier, preventing some users from using these apps effectively [ 18 ]. Considering apps on outdated smartphones or operating systems can result in challenges for patients when running and installing complex apps. In addition, there is a noticeable mismatch between the technical complexity of the apps and the users’ capacities, as they lack the knowledge to address issues like nonfunctionality, showdowns, freezing, and crashes in the apps [ 27 , 28 ].
In our review, 4 included studies highlighted the significance of network issues as a critical barrier to mHealth access [ 21 , 22 , 24 , 26 ]. Of these, 2 studies emphasized the importance of reliable internet access for effective remote interventions. Poor internet connectivity or limited access to high-speed internet in certain areas can impede the successful implementation of mHealth interventions [ 24 , 26 ]. Furthermore, the high cost and limited availability of internet access to support the equipment in survivors of stroke’s homes can prevent them from accessing mHealth services [ 21 , 22 ]. Patients and health care professionals mainly rely on an internet-based system where health care providers must promptly update patient information. On the other hand, patients can use the apps to receive health updates and discuss their symptoms with health care providers effectively [ 29 , 30 ]. Thus, the absence of a digital option can restrict internet access and cause challenges, both at the patient’s house and within the health care facility. However, adopting an offline mode can make it difficult to transfer data, which typically relies on web-based connectivity.
Hardware malfunctions, such as those in mobile phones or computers, are considered significant factors affecting the delivery of mHealth services [ 15 , 18 , 22 , 24 , 26 ]. For instance, in a pilot study aiming to implement a home-based Telerehabilitation Program that included arm motor therapy games, therapeutic arm exercises, remote stroke education, and videoconferencing, hardware malfunctions limited patients’ access to the intervention [ 15 ]. Moreover, a qualitative study highlighted equipment setup–related difficulties as a barrier to mHealth access, with patients with stroke encountering challenges in setting up and using the required equipment [ 26 ]. For example, getting ready for video-recorded exercise can be a challenge among patients with stroke when setting up the iPad, sensors, and monitoring equipment for heart and blood pressure [ 31 ]. In addition, specific details, such as the correct connection of the limb sensor node to assess the patient’s range of motion and troubleshoot unexpected hardware problems, may not be straightforward, even though general instructions are provided. Another study focusing on remote interventions, which often rely on technology, noted that one potential barrier could be the accessibility of the necessary technology (eg, smartphones, computers, etc) for individuals with chronic stroke, especially those who may not be familiar with or have easy access to such devices [ 24 ].
Deficiencies in Support Systems
Lack of support systems is a significant barrier to mHealth access, as indicated by 6 included studies in our review [ 16 , 19 , 20 , 22 - 24 ]. For instance, a qualitative research study investigated the experiences of 13 survivors of stroke and health care providers regarding the use of a poststroke telecare service [ 19 ]. The study found that the lack of general guidelines for operating a telecare service, technical issues, and limited human resources presented challenges to patients in using the service (eg, difficulties in joining Zoom meetings and troubleshooting) [ 19 ]. Another study also emphasized the importance of adequate training and support for participants using mHealth tools. This study recommended initial in-person sessions to familiarize participants with the technology, indicating that additional support might be necessary for some individuals [ 24 ]. In a mixed methods study aimed at identifying facilitators and barriers to the use of self-administered VR telerehabilitation, it was mentioned that a lack of an exercise companion and a safe environment for exercising prevented some individuals from using self-administered VR telerehabilitation [ 20 ].
Constraints on Available Time
In our study, we have found that constraints on available time are considered a barrier to mHealth, as mentioned in 4 published studies [ 15 , 16 , 20 , 25 ]. For example, 1 included study stated that conflicts with other medical appointments prevent patients with stroke from completing a home-based telerehabilitation program [ 15 ]. Additionally, work commitments are also a factor preventing patients with from using mHealth at home [ 20 ]. This issue is not limited to patients alone; it also affects health care providers. Qualitative research revealed that time-related issues are prevalent among providers, such as occupational therapists who reported having limited time to spend with survivors of stroke when providing mHealth interventions [ 25 ]. Additionally, a prior study highlighted the importance of the design and development stages of mHealth in reducing disruptions to health care providers’ established workflows [ 32 ]. The study emphasized the transformation of functionalities into practical health tools, aiming to facilitate the integration of mHealth into their existing structured workflows, ultimately influencing increased engagement in the adoption of mHealth.
The study aimed to explore recent literature to uncover possible barriers to mHealth access among patients with stroke. Although mHealth offers an important option for accessing needed health services, and people with stroke are currently using it for various purposes, this study identified several barriers inhibiting its use. One such barrier is health-related issues. Prior studies have shown that 25%-85% of survivors of stroke report experiencing fatigue, regardless of the severity of their condition [ 33 , 34 ]. As fatigue develops over time, it can result in the inability to perform basic activities, such as dressing and eating, and eventually limit more complex activities like shopping and preparing meals [ 35 ]. Considering that the study included complex telerehabilitation activities (eg, games, therapeutic programs, education, and videoconferencing), access to the program among patients with stroke could be limited due to fatigue. It is also important to consider a patient's age when examining fatigue, whether they are young or old [ 36 - 38 ]; this finding aligns with our study, which included patients aged 40 and older who had experienced strokes.
Despite the rise of mHealth to connect patients to health services outside of a clinical setting, our study found that patients’ readiness and acceptance were barriers to accessing mHealth. Similar to previous studies, patients with stroke reported that visual and physical impairments hindered their access to mHealth, as they lacked the confidence to communicate electronically and were concerned about making mistakes during remote interactions [ 25 , 39 ]. These impairments, affecting sight and physical activity, may contribute to reduced readiness for mHealth. Additionally, digital health literacy should be considered, as patients with stroke, particularly those in the older population, may have difficulty obtaining, interpreting, and evaluating health information through digital sources [ 40 ]. A similar finding was observed in another study, where older patients with stroke had less access to phone or video telephone visits due to a lack of digital literacy and access, as well as a lack of experience using such technology [ 41 ]. In terms of acceptability, patients with stroke who perceived m-Health as useless (e.g., only making phone calls) restricted their access to mHealth applications, such as mobile home-based exercise programs [ 42 ].
One of the main obstacles for mHealth is the availability of adequate infrastructure to provide effective and comprehensive care to patients with stroke. The review identified the limitations of the current software in conducting patient assessments and delivering a wide range of rehabilitation exercises. Since the main goal of rehabilitation is to improve the patient’s physical impairments, a health care provider needs to perform physical exams to determine and evaluate the intervention. Without such contact through mHealth, the health care provider’s assessment ability is compromised, and the assessment accuracy might be reduced. However, previous studies have proposed remote methods to assess patients with stroke, such as internet-based telerobotic devices, videoconferencing, camera-based artificial intelligence (AI) models with wearable sensors, and remote Fugl-Meyer Assessment protocols [ 43 - 46 ]. The validity of the proposed methods was still low, and additional equipment and advanced software are required to capture the more accurate physical examination details.
The studies in the review also highlighted the narrow range of exercises the patients could perform through mHealth. A systematic review of home-based technologies for stroke rehabilitation revealed technology that increases the variety of exercise, such as games, telerehabilitation, robotic devices, VR devices, and tablets. This review also pointed out the limitations of each technology, which is consistent with our findings that the equipment requires proper guidance in setting up and using to achieve the therapeutic goals [ 47 ].
Besides having the proper software and hardware for mHealth services for patients with stroke, the studies also highlighted poor or limited internet connectivity as a major barrier to implementing mHealth. Many mHealth interventions require timely monitoring, access to the database, or health care. Some studies have explored alternative solutions to enhance mHealth delivery in areas with limited or nonexistent internet access. For example, some studies have used Firebase, a local offline database, or caching the user interface and assets to help patients stay connected to the information if the patients lose an internet connection [ 48 - 50 ]. Others have integrated communities or schools as a link between larger hospitals and patients who cannot access the internet [ 51 , 52 ]. Nevertheless, internet connectivity remains a critical obstacle for mHealth, as it was considered a super social determinant of health that affects other aspects of health equity [ 53 ]. Therefore, promoting affordable and reliable internet access would be beneficial, especially for those who face disadvantages in accessing health care.
Our review reported deficiencies in support systems in using mHealth, including the need for general guidelines in using the services, technical issues, additional support, the lack of an exercise companion, and a safe environment. The lack of general guidelines or instructions in using a relative innovation can influence patients’ or caregivers’ decision to use and continue use. Our findings are congruent with previous longitudinal studies of the continued use of mHealth apps, indicating that users will not be motivated to continue service if they do not understand how the system works based on their initial interactions [ 54 ]. Along with a limited support system, most of the participants in our studies are notably older adults, and a prior study revealed that older adults, in general, have a lower rate of adopting mHealth [ 55 ]. The adoption of mHealth is not a simple process and requires not only technical assistance but also human support to enhance their experience and interaction with mHealth [ 56 , 57 ]; thus, concise and clear guidelines are essential in facilitating and engaging their usage. Another study also points out that mobile health for older adult patients used an aging barrier framework to explore usability problems and reported that participants have difficulties understanding the navigation structure of the apps and overseeing important text, buttons, and icon elements [ 58 ]. Therefore, clear guidelines and instructions should be considered when developing mHealth for survivors of stroke.
Regarding technical issues, it can be solved or reduced by additional support. Our findings align with a study evaluating patients’ experience with the usability of a diabetes mHealth system. Technical issues related to functionality or operation can impact the overall usability of apps, such as challenges in deleting or inputting glucose values, resulting in difficulties in accurately interpreting value ranges (51). Furthermore, participants who encounter technical issues may struggle to navigate through different functions; for instance, participants have reported experiencing navigation difficulties while reporting glucose diary values. Prior studies have also highlighted the significance of app navigation for patients with stroke who face challenges in managing their medication [ 28 , 59 ]. Thus, navigation within apps is crucial as if it is difficult and user-unfriendly, it can impact patients’ ability to carry out their tasks effectively [ 28 , 59 ].
For safety concerns, an mHealth system used to guide exercise for patients with cardiac disease developed safe algorithms to detect and warn of risky situations during exercise [ 60 ]. However, the lack of an exercise companion was not mentioned in previous studies. This can be considered for mHealth, especially exercise programs that may create live animations to exercise with patients.
In this study, researchers identified the constraint of available time as a major obstacle to accessing mHealth services among patients with stroke. This encompassed challenges such as patients’ struggles with time management, inefficient use of available time, and the timely availability of caregivers [ 16 , 25 , 61 , 62 ]. These findings are consistent with prior studies investigating the barriers and incentives related to mHealth access among this demographic [ 63 , 64 ]. The effective management of time is a crucial element in the success of any mHealth intervention designed for patients with stroke [ 62 ].
The qualitative studies revealed that successful use of mHealth necessitates ongoing engagement with a caregiver, which is particularly crucial for older patients, those with more pronounced physical impairments, or those with lower digital health literacy levels. However, the effectiveness of mHealth was hampered by the limited availability of caregivers, posing challenges to sustaining quality rehabilitation approaches in home-based programs [ 16 ]. Effective time management can prove advantageous for both patients with stroke and caregivers when using telerehabilitation systems. Conversely, time constraints have been linked to decreased productivity and diminished quality within mHealth systems. Ultimately, the efficiency and efficacy of telerehabilitation hinge on the optimal use of scarce resources, notably time [ 65 , 66 ].
According to our findings, mHealth plays an essential role in managing and rehabilitating patients with stroke, improving and advancing the quality of care and patient outcomes, and increasing accessibility to health care resources. However, using mHealth in stroke care raises substantial concerns regarding data privacy and security. Survivors of stroke often rely on mobile applications, assisted devices for telerehabilitation, and remote monitoring tools to track their progress, communicate with health care providers, and access educational materials [ 24 ]. The data generated and transmitted through these platforms may sometimes include sensitive medical information, such as personal health records and mobility metrics. As a result, discussing mHealth in the context of stroke care must address these patient privacy and security concerns. Data transmission and storage are the most important aspects of patient privacy and security. As mobile health heavily relies on technology, cloud infrastructure, and apps, many potential risks and vulnerabilities emerge. Surprisingly, Müthing et al [ 67 ] uncovered a concerning reality within the mHealth app landscape. Their study revealed that many mHealth apps currently available lack adequate privacy and security safeguards. Even among apps certified by trusted organizations or widely adopted by the health care community, 89% were observed to transmit information digitally; alarmingly, 66% of this data was not encrypted. Moreover, there is a notable concern regarding the potential for data to be misplaced, stolen, or lost, primarily stemming from the mobile nature of the devices used in mHealth. The common habit of multiple family members sharing mobile devices like smartphones and tablets can exacerbate these concerns [ 68 ]. These risk concerns underscore the imperative requirement for solid security measures within the mHealth environment. In addition, many stakeholders have rights and responsibilities concerning an individual's medical records and the information they contain. To effectively minimize potential security risks for users in the future, it is crucial to systematically identify all parties involved and those who might be considered data “custodians” [ 68 , 69 ]. Informed consent, privacy policy, and access control are critical areas of concern when using mHealth. Informed consent serves as the gateway to data sharing, granting individuals or their legal representatives the authority to specify when and with whom their personal information can be shared. However, consumers often find themselves unaware of the extensive data collection and analysis methods used by mHealth services and the extent of data sharing with third parties. This issue is exacerbated by the fact that only (183/600, 30.5%) of widely used mobile health apps have privacy policies and that most apps could negatively affect users due to privacy and security violations [ 69 ]. Moreover, many mHealth industry privacy policies often resemble lengthy academic articles with language at a university level, making them daunting for the average user to navigate [ 68 ]. Therefore, to address these concerns effectively, a set of proactive steps is required, particularly when considering the needs of older adults, the majority of patients with stroke. The future focus should be on simplifying and updating existing privacy policies to make them user-friendly, age-friendly, and compliant with regulations. Collaborating and providing active support for developing a robust regulatory framework for mHealth apps are essential. This framework should empower all users, including older adults, to control their technology and decide who can access information on their mobile devices. Significantly enhancing user privacy, with specific attention to the unique requirements of older adults, is paramount. Furthermore, promoting public technology literacy and creating awareness about responsible and cautious mobile device usage through educational initiatives is vital for empowering users and ensuring the secure adoption of mHealth solutions.
Certain limitations regarding the systematic review need to be considered. First, the ages of participants in the included studies are all over 40, with most having a median age of 55 years or older. Given the paper’s focus on improving outcomes for survivors of stroke through mHealth, the older age range—whether due to sampling bias or the characteristics of patients with stroke—might negatively impact the user experience compared to younger generations. This could be attributed to varying levels of exposure to technology. Another potential limitation is the number of participants included in some of the studies. Most (7 out of 12) of the studies included fewer than 20 participants, and 10 out of 12 included fewer than 100 participants. Given the accessibility of current technology, smaller sample sizes could lead to a greater possibility of outliers, which might not adequately represent the general population of survivors of stroke, thus, introducing unintended bias. Another limiting factor is the criterion of including only English-language papers. Because stroke does not discriminate by language, including only papers written in English could omit potentially useful research regarding mHealth and survivors of stroke. Finally, this study focused solely on barriers to mHealth access in patients with stroke with a limited number of 12 included studies. To increase generalizability, future research should explore a broader range of contributing factors.
Steps Toward the Digital Health Era
The Digital Health Era is transforming the health care paradigm through various key initiatives. One of the most significant breakthroughs in the acceptance of mHealth, telemedicine, and remote patient monitoring, which greatly improve health care accessibility by enabling remote consultations and timely interventions, particularly in remote regions, is the widespread adoption of mobile devices and advanced communication technologies [ 70 , 71 ]. Our study has extensively explored this aspect. The integration of wearable technology and health apps also plays a crucial role in empowering individuals to actively manage their health [ 72 - 74 ]. These tools monitor vital signs and activity levels and provide real-time feedback, promoting a proactive approach to wellness [ 75 - 77 ]. Additionally, big data analytics and AI have transformed diagnostics, treatment strategies, and predictive health care [ 78 - 80 ]. AI algorithms analyze vast amounts of patient data, identifying patterns, and offering valuable insights to aid health care providers in making informed decisions [ 78 - 80 ]. The Digital Health Era emphasizes a focus on preventive care and personalized medicine, where health care providers leverage data-driven insights to create tailored treatment plans based on an individual’s genetics, lifestyle, and specific health requirements [ 81 , 82 ]. Overall, the evolution toward the Digital Health Era is reshaping health care through initiatives such as telemedicine, wearable technology, big data analytics, and a focus on preventive care, personalized medicine, and user-centered design. These interventions must take into account the realities and constraints of the intended users. The advancements in different domains lay the foundation for an interlinked, data-driven health care system centered on patient requirements. Technology is vital in this evolution, enhancing patient outcomes, boosting productivity, and revolutionizing the health care experience. This ongoing amalgamation has the potential to establish a future where technology and health care converge effortlessly, leading to a more comprehensive and convenient health care environment for all.
Conclusions
This review has highlighted significant barriers to mHealth access among patients with stroke, emphasizing the gap between mHealth’s potential and practical use. Key challenges include health-related issues, patient acceptance, infrastructure challenges, support system deficiencies, and time constraints. These findings point to the urgent need for user-friendly mHealth solutions and robust support mechanisms. Addressing these issues is critical to ensure that mHealth technologies are not only available but are also effectively adopted by survivors of stroke. Future research should focus on overcoming these barriers to enable mHealth to fully support poststroke rehabilitation and care. The goal must be to transform these obstacles into opportunities for innovation, ensuring mHealth’s role as a cornerstone of patient-centered health care.
Acknowledgments
We would like to express our gratitude to Mahidol University, Thailand, for covering the Article Publishing Charge.
Conflicts of Interest
None declared.
Summary data.
Preferred Reporting Items for Systematic Reviews and Meta-Analyses (PRISMA) checklist.
- GBD 2019 Stroke Collaborators. Global, regional, and national burden of stroke and its risk factors, 1990-2019: a systematic analysis for the global burden of disease study 2019. Lancet Neurol. 2021;20(10):795-820. [ FREE Full text ] [ CrossRef ] [ Medline ]
- Burns SP, Terblanche M, MacKinen A, DeLaPena C, Fielder JDP. Smartphone and mHealth use after stroke: results from a pilot survey. OTJR (Thorofare N J). 2022;42(2):127-136. [ CrossRef ] [ Medline ]
- Sarfo FS, Ovbiagele B. Mobile health for stroke: a promising concept for research and practice. Mhealth. 2017;3:4. [ FREE Full text ] [ CrossRef ] [ Medline ]
- D'Souza CE, Greenway MRF, Graff-Radford J, Meschia JF. Cognitive impairment in patients with stroke. Semin Neurol. 2021;41(1):75-84. [ CrossRef ] [ Medline ]
- Kim KT, Chang WK, Jung YS, Jee S, Sohn MK, Ko SH, et al. Unmet needs for rehabilitative management in common health-related problems negatively impact the quality of life of community-dwelling stroke survivors. Front Neurol. 2021;12:758536. [ FREE Full text ] [ CrossRef ] [ Medline ]
- Verma A, Towfighi A, Brown A, Abhat A, Casillas A. Moving towards equity with digital health innovations for stroke care. Stroke. 2022;53(3):689-697. [ FREE Full text ] [ CrossRef ] [ Medline ]
- Cao W, Kadir AA, Wang Y, Wang J, Dai B, Zheng Y, et al. Description of apps targeting stroke patients: a review of apps store. Digit Health. 2023;9:20552076231181473. [ FREE Full text ] [ CrossRef ] [ Medline ]
- Rowland SP, Fitzgerald JE, Holme T, Powell J, McGregor A. What is the clinical value of mHealth for patients? NPJ Digit Med. 2020;3:4. [ FREE Full text ] [ CrossRef ] [ Medline ]
- Abaza H, Marschollek M. mHealth application areas and technology combinations*. A comparison of literature from high and low/middle income countries. Methods Inf Med. 2017;56(7):e105-e122. [ FREE Full text ] [ CrossRef ] [ Medline ]
- Thurston C, Bezuidenhout L, Humphries S, Johansson S, von Koch L, Häger CK, et al. Mobile health to promote physical activity in people post stroke or transient ischemic attack - study protocol for a feasibility randomised controlled trial. BMC Neurol. 2023;23(1):124. [ FREE Full text ] [ CrossRef ] [ Medline ]
- Adcock AK, Haggerty T, Crawford A, Espinosa C. mHealth impact on secondary stroke prevention: a scoping review of randomized controlled trials among stroke survivors between 2010-2020. Mhealth. 2022;8:19. [ FREE Full text ] [ CrossRef ] [ Medline ]
- Moher D, Liberati A, Tetzlaff J, Altman D, PRISMA Group. Reprint--preferred reporting items for systematic reviews and meta-analyses: the PRISMA statement. Phys Ther. 2009;89(9):873-880. [ Medline ]
- Istepanian RSH. Mobile health (m-Health) in retrospect: the known unknowns. Int J Environ Res Public Health. 2022;19(7):3747. [ FREE Full text ] [ CrossRef ] [ Medline ]
- Moola S, Munn Z, Tufanaru C, Aromataris E, Sears K, Sfetcu R. Chapter 7: Systematic Reviews of Etiology and Risk. Australia. The Joanna Briggs Institute; 2017.
- Dodakian L, McKenzie AL, Le V, See J, Pearson-Fuhrhop K, Burke Quinlan E, et al. A home-based telerehabilitation program for patients with stroke. Neurorehabil Neural Repair. 2017;31(10-11):923-933. [ FREE Full text ] [ CrossRef ] [ Medline ]
- Bhattacharjya S, Linares I, Langan J, Xu W, Subryan H, Cavuoto LA. Engaging in a home-based exercise program: a mixed-methods approach to identify motivators and barriers for individuals with stroke. Assist Technol. 2023;35(6):487-496. [ CrossRef ] [ Medline ]
- Chung J, Kim CT, Lee J, Lee E, Jung KI, Yoo WK, et al. Factors affecting participation in telerehabilitation using transcranial direct current stimulation for patients with poststroke paralysis in South Korea. Telemed J E Health. 2023;29(9):1404-1411. [ CrossRef ] [ Medline ]
- Ramaswamy S, Gilles N, Gruessner AC, Burton D, Fraser MA, Weingast S, et al. User-centered mobile applications for stroke survivors (MAPPS): a mixed-methods study of patient preferences. Arch Phys Med Rehabil. 2023;104(10):1573-1579. [ CrossRef ] [ Medline ]
- Wong AKC, Bayuo J, Wong FKY, Kwok VWY, Tong DWK, Kwong MK, et al. Sustaining telecare consultations in nurse-led clinics: perceptions of stroke patients and advanced practice nurses: a qualitative study. Digit Health. 2023;9:20552076231176163. [ FREE Full text ] [ CrossRef ] [ Medline ]
- Torriani-Pasin C, Palma GCDS, Makhoul MP, Antonio BDA, Lara ARF, da Silva TA, et al. Adherence rate, barriers to attend, safety, and overall experience of a remote physical exercise program during the COVID-19 pandemic for individuals after stroke. Front Psychol. 2021;12:647883. [ FREE Full text ] [ CrossRef ] [ Medline ]
- Lam SKY, Chau JPC, Lo SHS, Siow EKC, Lee VWY, Shum EWC, et al. User engagement in the development of a home-based virtual multidisciplinary stroke care clinic for stroke survivors and caregivers: a qualitative descriptive study. Disabil Rehabil. 2022;44(20):5983-5989. [ CrossRef ] [ Medline ]
- Morse H, Biggart L, Pomeroy V, Rossit S. Exploring perspectives from stroke survivors, carers and clinicians on virtual reality as a precursor to using telerehabilitation for spatial neglect post-stroke. Neuropsychol Rehabil. 2022;32(5):707-731. [ CrossRef ] [ Medline ]
- Spits AH, Rozevink SG, Balk GA, Hijmans JM, van der Sluis CK. Stroke survivors' experiences with home-based telerehabilitation using an assistive device to improve upper limb function: a qualitative study. Disabil Rehabil Assist Technol. 2024;19(3):730-738. [ CrossRef ] [ Medline ]
- Jaywant A, Mautner L, Waldman R, O'Dell MW, Gunning FM, Toglia J. Feasibility and acceptability of a remotely delivered executive function intervention that combines computerized cognitive training and metacognitive strategy training in chronic stroke. Int J Environ Res Public Health. 2023;20(9):5714. [ FREE Full text ] [ CrossRef ] [ Medline ]
- Dunne S, Close H, Richards N, Ellison A, Lane AR. Maximizing telerehabilitation for patients with visual loss after stroke: interview and focus group study with stroke survivors, carers, and occupational therapists. J Med Internet Res. 2020;22(10):e19604. [ FREE Full text ] [ CrossRef ] [ Medline ]
- Tyagi S, Lim DSY, Ho WHH, Koh YQ, Cai V, Koh GCH, et al. Acceptance of tele-rehabilitation by stroke patients: perceived barriers and facilitators. Arch Phys Med Rehabil. 2018;99(12):2472-2477.e2. [ CrossRef ] [ Medline ]
- Mitchell J, Shirota C, Clanchy K. Factors that influence the adoption of rehabilitation technologies: a multi-disciplinary qualitative exploration. J Neuroeng Rehabil. 2023;20(1):80. [ FREE Full text ] [ CrossRef ] [ Medline ]
- Lobo EH, Abdelrazek M, Frølich A, Rasmussen LJ, Livingston PM, Islam SMS, et al. Detecting user experience issues from mHealth apps that support stroke caregiver needs: an analysis of user reviews. Front Public Health. 2023;11:1027667. [ FREE Full text ] [ CrossRef ] [ Medline ]
- Pan X, Zhou X, Yu L, Hou L. Switching from offline to online health consultation in the post-pandemic era: the role of perceived pandemic risk. Front Public Health. 2023;11:1121290. [ FREE Full text ] [ CrossRef ] [ Medline ]
- Huang N, Yan Z, Yin H. Effects of online–offline service integration on e‐healthcare providers: a quasi‐natural experiment. Production and Operations Management. 2021;30(8):2359-2378. [ CrossRef ]
- Koh GCH, Yen SC, Tay A, Cheong A, Ng YS, De Silva DA, et al. Singapore tele-technology aided rehabilitation in stroke (STARS) trial: protocol of a randomized clinical trial on tele-rehabilitation for stroke patients. BMC Neurol. 2015;15:161. [ FREE Full text ] [ CrossRef ] [ Medline ]
- Wu N, Gong E, Wang B, Gu W, Ding N, Zhang Z, et al. A smart and multifaceted mobile health system for delivering evidence-based secondary prevention of stroke in rural China: design, development, and feasibility study. JMIR Mhealth Uhealth. 2019;7(7):e13503. [ FREE Full text ] [ CrossRef ] [ Medline ]
- Cumming TB, Packer M, Kramer SF, English C. The prevalence of fatigue after stroke: a systematic review and meta-analysis. Int J Stroke. 2016;11(9):968-977. [ CrossRef ] [ Medline ]
- Alghamdi I, Ariti C, Williams A, Wood E, Hewitt J. Prevalence of fatigue after stroke: a systematic review and meta-analysis. Eur Stroke J. 2021;6(4):319-332. [ FREE Full text ] [ CrossRef ] [ Medline ]
- Vollertsen J, Björk M, Norlin AK, Ekbladh E. The impact of post-stroke fatigue on work and other everyday life activities for the working age population - a registry-based cohort study. Ann Med. 2023;55(2):2269961. [ FREE Full text ] [ CrossRef ] [ Medline ]
- Lerdal A, Bakken LN, Kouwenhoven SE, Pedersen G, Kirkevold M, Finset A, et al. Poststroke fatigue--a review. J Pain Symptom Manage. 2009;38(6):928-949. [ FREE Full text ] [ CrossRef ] [ Medline ]
- Mead GE, Graham C, Dorman P, Bruins SK, Lewis SC, Dennis MS, et al. UK Collaborators of IST. Fatigue after stroke: baseline predictors and influence on survival. Analysis of data from UK patients recruited in the international stroke trial. PLoS One. 2011;6(3):e16988. [ FREE Full text ] [ CrossRef ] [ Medline ]
- Snaphaan L, van der Werf S, de Leeuw FE. Time course and risk factors of post-stroke fatigue: a prospective cohort study. Eur J Neurol. 2011;18(4):611-617. [ CrossRef ] [ Medline ]
- Norman CD, Skinner HA. eHealth literacy: essential skills for consumer health in a networked world. J Med Internet Res. 2006;8(2):e9. [ FREE Full text ] [ CrossRef ] [ Medline ]
- Naqvi IA, Cohen AS, Kim Y, Harris J, Denny MC, Strobino K, et al. Inequities in telemedicine use among patients with stroke and cerebrovascular diseases: a tricenter cross-sectional study. Neurol Clin Pract. 2023;13(2):e200148. [ FREE Full text ] [ CrossRef ] [ Medline ]
- Mahmood A, Blaizy V, Verma A, Stephen Sequeira J, Saha D, Ramachandran S, et al. Acceptability and attitude towards a mobile-based home exercise program among stroke survivors and caregivers: a cross-sectional study. Int J Telemed Appl. 2019;2019:5903106. [ FREE Full text ] [ CrossRef ] [ Medline ]
- Kim J, Sin M, Kim WS, Min YS, Kim W, Park D, et al. Remote assessment of post-stroke elbow function using internet-based telerobotics: a proof-of-concept study. Front Neurol. 2020;11:583101. [ FREE Full text ] [ CrossRef ] [ Medline ]
- Razfar N, Kashef R, Mohammadi F. Automatic post-stroke severity assessment using novel unsupervised consensus learning for wearable and camera-based sensor datasets. Sensors (Basel). 2023;23(12):5513. [ FREE Full text ] [ CrossRef ] [ Medline ]
- Yang CL, Simpson LA, Eng JJ. A pilot study for remote evaluation of upper extremity motor function after stroke: the arm capacity and movement test (ArmCAM). Am J Occup Ther. 2023;77(1):7701205020. [ CrossRef ] [ Medline ]
- Rowe V, Blanton S, Aycock D, Hayat M, Ali S. Remote delivery of the fugl-meyer assessment for the upper extremity: a pilot study to assess feasibility, reliability, and validity. Arch Rehabil Res Clin Transl. 2023;5(2):100261. [ FREE Full text ] [ CrossRef ] [ Medline ]
- Chen Y, Abel KT, Janecek JT, Chen Y, Zheng K, Cramer SC. Home-based technologies for stroke rehabilitation: a systematic review. Int J Med Inform. 2019;123:11-22. [ FREE Full text ] [ CrossRef ] [ Medline ]
- Inupakutika D, Kaghyan S, Akopian D, Chalela P, Ramirez AG. Facilitating the development of cross-platform mHealth applications for chronic supportive care and a case study. J Biomed Inform. 2020;105:103420. [ FREE Full text ] [ CrossRef ] [ Medline ]
- Nikoviotis R, Ringas D. Self-care diabetes: a diabetes self-management application based on the seven self-management areas (AADE7) of the American association of diabetes educators. 2021. Presented at: Proceedings of the 25th Pan-Hellenic Conference on Informatics; 2022 Feb 22:477-482; United States. [ CrossRef ]
- Khan MM, Anwar R, Tanve FA, Shakil D, Banik M, Gupta SK. Development of web and mobile based smart online healthcare system. IEEE; 2021. Presented at: 2021 IEEE 12th Annual Ubiquitous Computing, Electronics & Mobile Communication Conference (UEMCON); 2021 Dec 01; USA. [ CrossRef ]
- Rono H, Bastawrous A, Macleod D, Mamboleo R, Bunywera C, Wanjala E, et al. Effectiveness of an mHealth system on access to eye health services in Kenya: a cluster-randomised controlled trial. Lancet Digit Health. 2021;3(7):e414-e424. [ FREE Full text ] [ CrossRef ] [ Medline ]
- Gizaw Z, Astale T, Kassie GM. What improves access to primary healthcare services in rural communities? A systematic review. BMC Prim Care. 2022;23(1):313. [ FREE Full text ] [ CrossRef ] [ Medline ]
- Sieck CJ, Sheon A, Ancker JS, Castek J, Callahan B, Siefer A. Digital inclusion as a social determinant of health. NPJ Digit Med. 2021;4(1):52. [ FREE Full text ] [ CrossRef ] [ Medline ]
- Vaghefi I, Tulu B. The continued use of mobile health apps: insights from a longitudinal study. JMIR Mhealth Uhealth. 2019;7(8):e12983. [ FREE Full text ] [ CrossRef ] [ Medline ]
- Aranha M, James K, Deasy C, Heavin C. Exploring the barriers and facilitators which influence mHealth adoption among older adults: a literature review. Gerontechnology. 2021;20(2):1-16. [ CrossRef ]
- Zakerabasali S, Ayyoubzadeh SM, Baniasadi T, Yazdani A, Abhari S. Mobile health technology and healthcare providers: systemic barriers to adoption. Healthc Inform Res. 2021;27(4):267-278. [ FREE Full text ] [ CrossRef ] [ Medline ]
- Hughes CML, Padilla A, Hintze A, Raymundo TM, Sera M, Weidner S, et al. Developing an mHealth app for post-stroke upper limb rehabilitation: feedback from US and ethiopian rehabilitation clinicians. Health Informatics J. 2020;26(2):1104-1117. [ FREE Full text ] [ CrossRef ] [ Medline ]
- Wildenbos GA, Jaspers MWM, Schijven MP, Dusseljee-Peute LW. Mobile health for older adult patients: using an aging barriers framework to classify usability problems. Int J Med Inform. 2019;124:68-77. [ CrossRef ] [ Medline ]
- Ameer K, Ali K. iPad use in stroke neuro-rehabilitation. Geriatrics (Basel). 2017;2(1):2. [ FREE Full text ] [ CrossRef ] [ Medline ]
- Salvi D, Ottaviano M, Muuraiskangas S, Martínez-Romero A, Vera-Muñoz C, Triantafyllidis A, et al. An m-health system for education and motivation in cardiac rehabilitation: the experience of HeartCycle guided exercise. J Telemed Telecare. 2018;24(4):303-316. [ FREE Full text ] [ CrossRef ] [ Medline ]
- Torriani-Pasin C, Domingues VL, de Freitas TB, Silva TAD, Caldeira MF, Júnior RPA, et al. Adherence rate, barriers to attend, safety and overall experience of a physical exercise program via telemonitoring during COVID-19 pandemic for individuals with parkinson's disease: a feasibility study. Physiother Res Int. 2022;27(4):e1959. [ FREE Full text ] [ CrossRef ] [ Medline ]
- Marzolini S. Including patients with stroke in cardiac rehabilitation: barriers and facilitators. J Cardiopulm Rehabil Prev. 2020;40(5):294-301. [ CrossRef ] [ Medline ]
- Reicherzer L, Wirz M, Wieber F, Graf ES. Facilitators and barriers to health enhancing physical activity in individuals with severe functional limitations after stroke: a qualitative study. Front Psychol. 2022;13:982302. [ FREE Full text ] [ CrossRef ] [ Medline ]
- Grau-Pellicer M, Lalanza JF, Jovell-Fernández E, Capdevila L. Impact of mHealth technology on adherence to healthy PA after stroke: a randomized study. Top Stroke Rehabil. 2020;27(5):354-368. [ CrossRef ] [ Medline ]
- Kamal A, Khoja A, Usmani B, Magsi S, Malani A, Peera Z, et al. Effect of 5-minute movies shown via a mobile phone app on risk factors and mortality after stroke in a low- to middle-income country: randomized controlled trial for the stroke caregiver dyad education intervention (Movies4Stroke). JMIR Mhealth Uhealth. 2020;8(1):e12113. [ FREE Full text ] [ CrossRef ] [ Medline ]
- Müthing J, Jäschke T, Friedrich CM. Client-focused security assessment of mHealth apps and recommended practices to prevent or mitigate transport security issues. JMIR Mhealth Uhealth. 2017;5(10):e147. [ FREE Full text ] [ CrossRef ] [ Medline ]
- Galvin HK, DeMuro PR. Developments in privacy and data ownership in mobile health technologies, 2016-2019. Yearb Med Inform. 2020;29(1):32-43. [ FREE Full text ] [ CrossRef ] [ Medline ]
- Bhuyan SS, Kim H, Isehunwa OO, Kumar N, Bhatt J, Wyant DK, et al. Privacy and security issues in mobile health: current research and future directions. Health Policy and Technology. 2017;6(2):188-191. [ FREE Full text ] [ CrossRef ]
- Fatehi F, Taylor M, Caffery LJ, Smith AC. Telemedicine for clinical management of adults in remote and rural areas. Revolutionizing Tropical Medicine: Point-of-Care Tests, New Imaging Technologies and Digital Health. 2019:439-461. [ CrossRef ]
- Muruganandam N, Sindhu E, Safraz M. The conceptualization and implementation of a patient monitoring system based on the internet of things for use in telemedicine. IEEE; 2023. Presented at: 2023 International Conference on Artificial Intelligence and Knowledge Discovery in Concurrent Engineering (ICECONF); 2023 Jan 05; Chennai, India. [ CrossRef ]
- Scheid JL, Reed JL, West SL. Commentary: is wearable fitness technology a medically approved device? Yes and No. Int J Environ Res Public Health. 2023;20(13):6230. [ FREE Full text ] [ CrossRef ] [ Medline ]
- Sqalli MT, Al-Thani D. Towards an integrated health and wellness program using human-IoT interaction. 2020. Presented at: 2020 IEEE International Conference on Informatics, IoT, and Enabling Technologies (ICIoT); 2020 Feb 02; Doha, Qatar. [ CrossRef ]
- Uddin M, Syed-Abdul S. Introduction to digital health approach and mHealth applications for participatory health. Digital Health Elsevier. 2021:1-6. [ CrossRef ]
- Verma K, Preity P, Ranjan R. An insight into wearable devices for smart healthcare technologies. IEEE; 2023. Presented at: 2023 13th International Conference on Cloud Computing, Data Science & Engineering (Confluence); 2023 Jan 19; Noida, India. [ CrossRef ]
- Alves M, Pereira TC, Costa E, Magalhães B, Vázquez-Justo E. Advances in tourism, technology and systems: selected papers from ICOTTS 2022. In: Vital Signs-Health IoT Smartwatch. Portugal. Springer; 2023:619-631.
- Wasekar RR, Junzal S, Sathavane GV. Health monitoring devices system and technology. ECS Trans. 2022;107(1):15915-15922. [ CrossRef ]
- Balasubramani S, Renjith P, Ramesh K, George AH, George AS, Shahul A. A detailed analysis of many different artificial intelligence-based healthcare systems. In: Handbook of Research on Thrust Technologies? Effect on Image Processing: IGI Global. USA. IGI Global; 2023:56-72.
- Mina A. Big data and artificial intelligence in future patient management. How is it all started? Where are we at now? Quo tendimus? Adv Lab Med. 2020;1(3):20200014. [ FREE Full text ] [ CrossRef ] [ Medline ]
- Miner GD, Miner LA, Burk S, Goldstein M, Nisbet R, Walton N. Practical data analytics for innovation in medicine. In: Building Real Predictive and Prescriptive Models in Personalized Healthcare and Medical Research Using AI, ML, and Related Technologies. USA. Academic Press; 2023.
- Barrera-Vázquez OS, Rivero-Segura NA, Gomez-Verjan JC. Digital health and physiomics. In: Principles of Genetics and Molecular Epidemiology. Mexico. Springer; 2022:111-116.
- Xiong S, Lu H, Peoples N, Duman EK, Najarro A, Ni Z, et al. Digital health interventions for non-communicable disease management in primary health care in low-and middle-income countries. NPJ Digit Med. 2023;6(1):12. [ FREE Full text ] [ CrossRef ] [ Medline ]
Abbreviations
artificial intelligence |
computerized cognitive training |
Joanna Briggs Institute |
mobile health |
metacognitive strategy training |
Preferred Reporting Items for Systematic Reviews and Meta-Analyses |
virtual reality |
Edited by L Buis; submitted 13.11.23; peer-reviewed by J Wang, T Salzmann; comments to author 20.02.24; revised version received 02.04.24; accepted 25.06.24; published 22.08.24.
©Atsadaporn Niyomyart, Suebsarn Ruksakulpiwat, Chitchanok Benjasirisan, Lalipat Phianhasin, Kabtamu Nigussie, Sutthinee Thorngthip, Gazi Shamita, Jai Thampakkul, Lidya Begashaw. Originally published in JMIR mHealth and uHealth (https://mhealth.jmir.org), 22.08.2024.
This is an open-access article distributed under the terms of the Creative Commons Attribution License (https://creativecommons.org/licenses/by/4.0/), which permits unrestricted use, distribution, and reproduction in any medium, provided the original work, first published in JMIR mHealth and uHealth, is properly cited. The complete bibliographic information, a link to the original publication on https://mhealth.jmir.org/, as well as this copyright and license information must be included.
- Open access
- Published: 22 August 2024
A scoping review of costing methodologies used to assess interventions for underserved pregnant people and new parents
- Elizabeth K. Darling 1 ,
- Aisha Jansen 1 ,
- Bismah Jameel 1 &
- Jean-Éric Tarride 2
International Journal for Equity in Health volume 23 , Article number: 168 ( 2024 ) Cite this article
Metrics details
Lack of evidence about the long-term economic benefits of interventions targeting underserved perinatal populations can hamper decision making regarding funding. To optimize the quality of future research, we examined what methods and costs have been used to assess the value of interventions targeting pregnant people and/or new parents who have poor access to healthcare.
We conducted a scoping review using methods described by Arksey and O’Malley. We conducted systematic searches in eight databases and web-searches for grey literature. Two researchers independently screened results to determine eligibility for inclusion. We included economic evaluations and cost analyses of interventions targeting pregnant people and/or new parents from underserved populations in twenty high income countries. We extracted and tabulated data from included publications regarding the study setting, population, intervention, study methods, types of costs included, and data sources for costs.
Final searches were completed in May 2024. We identified 103 eligible publications describing a range of interventions, most commonly home visiting programs ( n = 19), smoking cessation interventions ( n = 19), prenatal care ( n = 11), perinatal mental health interventions ( n = 11), and substance use treatment ( n = 10), serving 36 distinct underserved populations. A quarter of the publications ( n = 25) reported cost analyses only, while 77 were economic evaluations. Most publications ( n = 82) considered health care costs, 45 considered other societal costs, and 14 considered only program costs. Only a third ( n = 36) of the 103 included studies considered long-term costs that occurred more than one year after the birth (for interventions occurring only in pregnancy) or after the end of the intervention.
Conclusions
A broad range of interventions targeting pregnant people and/or new parents from underserved populations have the potential to reduce health inequities in their offspring. Economic evaluations of such interventions are often at risk of underestimating the long-term benefits of these interventions because they do not consider downstream societal costs. Our consolidated list of downstream and long-term costs from existing research can inform future economic analyses of interventions targeting poorly served pregnant people and new parents. Comprehensively quantifying the downstream and long-term benefits of such interventions is needed to inform decision making that will improve health equity.
Despite improvements in perinatal outcomes in high income countries, socio-economic disparities in outcomes persist. Inequity in perinatal outcomes arises as a result of structural factors that cause social inequality, which in turn impacts the social and health outcomes associated with pregnancy and early parenting through a variety of pathways [ 1 ]. Various terminology has been used to describe groups or populations at increased risk of poor perinatal outcomes as a consequence of social inequality, with common terms including ‘vulnerable’, ‘disadvantaged’, or ‘marginalized’ populations. These terms have been critiqued because they are potentially stigmatizing and conceptualize the locus of inequities as arising from individual flaws or deficits [ 2 ]. We therefore avoid these terms and instead intentionally conceptualize our focus to be people who are negatively impacted by health and social system factors that limit their access to optimal care to support good perinatal outcomes. In this research we describe the people of interest as those who are poorly served by healthcare systems.
Interventions aimed at addressing disparities in perinatal outcomes are important to redress health inequities and because they potentially have long-term benefits for both the pregnant person and their offspring [ 3 ]. The perinatal period offers an opportunity to identify and mitigate the risks to long-term maternal health [ 4 ] Additionally, the growing body of evidence on the developmental origins of health and disease has shown that health in pregnancy has a long-term impact on physical health of the offspring [ 5 ]. Likewise, research on child development has established that healthy attachment in early childhood has long term impact on emotional well-being and mental health [ 6 ]. However, the measurement of long-term outcomes in prospective studies of interventions is often costly, and for that reason may not occur. This can create challenges for funders who need to make decisions about whether it is worthwhile to invest in interventions, as lack of evidence can hamper the calculation of long-term economic benefits associated with the improvements in health and social outcomes.
One type of intervention that has shown promise with respect to improving the pregnancy and early parenting outcomes of populations who are poorly served by healthcare is midwifery-led care [ 7 , 8 ]. There is a growing body of evidence to support the potential benefits of midwifery-led care for poorly served populations [ 9 – 11 ]. However, we identified a gap in the research with respect to economic evaluation of these kinds of interventions. In order to inform a larger research project aimed at developing a framework to assess the cost implications of midwifery care models that target underserved populations, we conducted a scoping review of the peer-reviewed and grey literature to synthesize previous approaches used to examine the short- and long-term cost implications of interventions targeting underserved pregnant people and new parents. Our research question was what types of costs and costing methodology have been used to assess interventions targeting pregnant people and/or new parents who have poor access to healthcare?
We conducted a scoping review in accordance with the methodology described by Arksey and O’Malley (2005) [ 12 ], including the following five stages: (1) identifying the research question; (2) identifying relevant studies; (3) study selection; (4) charting the data; (5) collating, summarizing, and reporting the results. We selected a scoping review as the best approach to systematically identify all previous economic and cost analyses, including both peer-reviewed and grey literature, that examine the cost implications of interventions targeting underserved pregnant people and/or new parents. The methodology allowed us to compare the range of analytical approaches used to evaluate costs to inform the development future cost analysis frameworks.
Identifying relevant studies
We developed a comprehensive search strategy for peer-reviewed and grey literature in consultation with a research librarian at the McMaster Health Sciences Library. The librarian provided guidance on the selection of keywords, refinement of the search strategy, and identification of relevant databases to search. Search terms related to ‘underserved populations,’ ‘economic evaluations,’ and ‘pregnant people and new parents’ were used to structure the search. Our search was not limited by year or language of publication. We drew on concurrent research we were conducting to scope the research literature regarding populations who have poor access to sexual and reproductive health care to define ‘underserved populations’ taking a broad approach. The full search strategy is included in Appendix A, which provides details regarding what populations were considered ‘underserved.’
We searched the following peer-reviewed academic literature databases: Ovid MEDLINE, Ovid Emcare, EMBASE (Ovid), Ovid Healthstar, Cochrane Library, Cumulative Index to Nursing and Allied Health Literature (CINAHL) EBSCO, EconLit EBSCO, and Business Source Premier EBSCO. To identify relevant grey literature, AJ hand-searched the websites of relevant organizations (e.g., Nurse Family Partnership, The Canada Prenatal Nutrition Program (CPNP), and Special Supplemental Nutrition Program for Women, Infants, and Children (WIC)). In addition, we reviewed the reference lists of key publications and relevant review articles to identify further sources. We imported the citation information for the retrieved publications into the citation management software, EndNote, and removed duplicate records prior to screening the results.
Study selection
We screened the retrieved publications for inclusion or exclusion using the systematic review software, Covidence, to manage the screening process. We first screened records by title and abstract against the inclusion and exclusion criteria. Second, we retrieved publications that passed the first stage of screening to screen the full text. Two reviewers (AJ, and RG or BJ or BA) independently screened the articles at both stages. We flagged any conflicts between researchers at either stage in Covidence and resolved difference through discussion. A third reviewer (ED) was consulted when consensus could not be reached.
Inclusion and exclusion criteria
To be included in this review, the publication needed to have: (1) described an intervention targeting pregnant people and/or new parents; (2) targeted an underserved population; (3) included a cost analysis of the intervention; and (4) been set in one of the top 20 Gross Domestic Product (GDP) per capita Organisation for Economic Co-operation and Development (OECD) countries in USD in 2020 [ 2 , 13 ]. The purpose of the fourth criterion was to ensure that the research was conducted in countries with a similar context, i.e., a high-income economy and high Human Development Index (HDI). Included countries were Ireland, Luxembourg, Norway, United States, Switzerland, Denmark, Belgium, Sweden, Austria, Germany, France, Netherlands, Iceland, Finland, United Kingdom, Canada, Turkey, Australia, Italy, and Spain. A study was excluded if: (1) the goal of the described intervention was the prevention or termination of pregnancy; or (2) it was a review-level publication. We retained relevant reviews and hand-searched the reference lists for original studies meeting our criteria.
Charting the data
We charted relevant data from the publications identified for inclusion in a Microsoft Excel spreadsheet. Initially, two reviewers (AJ, RG) conducted data extraction independently, compared their results, and resolved disagreements by consensus. Both reviewers extracted data from approximately 10% of the included articles, until a high level of agreement was achieved. Then one reviewer (AJ) extracted the remaining data independently. Extracted data included publication details (i.e., authors, title, journal), study design, setting (location, duration), population characteristics, description of the intervention, type of economic evaluation conducted, types of costs included in the economic analysis, and sources of cost data.
Collating, summarizing, and reporting the results
Once data extraction and charting were completed, the team reviewed the findings to determine the most useful way to collate the results and synthesize key findings. We created tables summarizing the interventions, the analytical approaches used to calculate costs (e.g. cost -benefit, cost-minimization, cost effectiveness, cost utility, cost-consequences, or costing analyses) and consolidated a list of costs considered in the analyses (i.e., program costs, health care costs, societal costs) as well as whether immediate, short or long-term costs were determined. We used descriptive statistics, such as frequencies and percentages, to summarize the data and calculated these using Microsoft Excel.
Final searches were completed on May 28, 2024. Figure 1 summarizes the results of our searches. We identified a total of 4866 publications from the peer-reviewed databases, all of which were in English. After removing duplicates, we identified 2507 publications for screening. We included 65 publications identified through database searches, 28 publications identified through reference chaining, and 11 publications identified through targeted web-searches. The final number of articles included in the review from these three sources was 104.
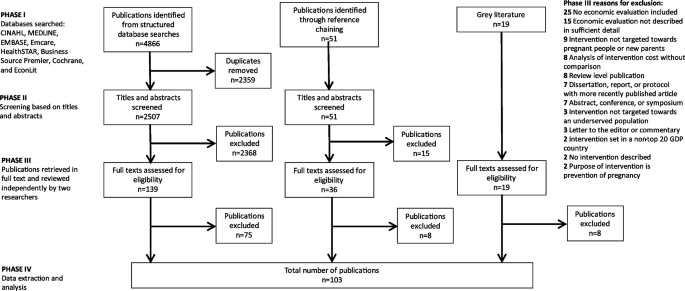
PRISMA diagram
A descriptive summary of the included articles is presented in Table 1 . Sixty-four ( n = 64) of the publications focused on interventions in the United States [ 14 – 77 ], 29 in the United Kingdom [ 78 – 106 ], six in Australia [ 107 , 108 , 109 , 110 , 111 , 112 ], three in Canada [ 113 , 114 , 115 ], and one in each Sweden [ 116 ] and Germany [ 116 ]. The interventions described in these publications targeted pregnant people ( n = 50), new parents ( n = 16), or both ( n = 37). Thirty-six ( n = 36) underserved populations were targeted by the interventions, including people with low income, who were targeted in 35 interventions; people who smoke, who were targeted in 20 interventions; Medicaid recipients, who were targeted in 15 interventions; and people who use substances, who were targeted in 11 interventions. The publications described a range of interventions, most commonly home visiting programs ( n = 19), smoking cessation interventions ( n = 19), prenatal care ( n = 11), perinatal mental health interventions ( n = 11), and substance use treatment ( n = 10). Of the 50 interventions that began in or continued into the postpartum period, the most common duration of interventions was between six months to two years postpartum ( n = 30). Thirty-seven ( n = 37) interventions were delivered in part or in full in the client’s home, 21 in the hospital, 21 digitally, and 16 in primary care clinics. Nurses ( n = 33), midwives or nurse-midwives ( n = 21), social workers ( n = 11), and family physicians ( n = 9) were the professionals most commonly involved in delivering the interventions.
Table 2 lists all 33 cost categories that were used in the publications, grouped into program costs, health care costs, and other societal costs. Sixteen ( n = 16) studies considered only program costs. Most publications considered health care costs ( n = 82). While 45 studies considered costs that we labelled ‘other societal costs’, these were frequently immediate costs incurred during the time frame of the intervention (e.g., costs of subsidies and social services). A third ( n = 35) of the included studies considered long-term costs that occurred more than one year after birth (for interventions occurring only in pregnancy) or after the end of the intervention.
Table 3 summarizes the types of analytical approaches described in the included publications, as well as the sources of cost data, the types of costs considered, and the timeframe for included cost outcomes. The most common analytic approaches to determine the costs were through cost-effectiveness analyses ( n = 43), followed by costing analyses ( n = 28) and cost benefit analyses ( n = 19). Cost utility ( n = 8), cost consequence ( n = 4) and cost-minimization ( n = 1) analyses were less commonly used. The majority of analyses drew cost data from administrative health data sources ( n = 77) and directly measured program costs ( n = 58). Costs were also commonly sourced from the literature ( n = 51). Hospital costs ( n = 70) and salaries and wages ( n = 62) were the most common categories of costs considered. Thirty ( n = 30) analyses included costs occurring only within the duration of the intervention (immediate); 37 also included costs that occurred up to one year after birth or after the end of the intervention (short-term); and 36 also included costs that occurred longer than one year after birth or after the end of the intervention (long-term).
The purpose of this review was to report on the state of the literature with respect to economic evaluations of interventions targeting pregnant people and/or new parents and to summarize the methods and costs used in the included studies. The 104 articles included in this review show that a range of methods, costs, and timeframes have been used to assess the value of interventions targeting pregnant people and new parents. Research on home visiting programs was most likely to consider long-term outcomes, and studies looking at long-term outcomes tended to take a wider view of the downstream costs and savings by considering long-term societal costs (e.g., costs related to child apprehension, abuse or neglect, crime, education, employment, lost productivity, addiction, etc.) in addition to program and health care costs. While we identified some economic evaluations that considered a comprehensive set of long-term societal costs, our assessment of the literature demonstrates that evaluations of interventions targeting underserved pregnant people and/or new parents frequently do not consider costs related to long-term outcomes that are necessary to assess their true value for money.
Decision-makers who fund programs targeting underserved populations need evidence to assess the potential value of investing in such programs, and the evidence gap we identified is a barrier to sustaining and spreading interventions that have the potential to improve health equity. The term value for money refers to finding an ideal balance between economy (costs), efficiency (minimizing the ratio of input to output), and effectiveness (achieving the desired outcomes), or the “three Es” [ 117 ]. Authors of several of the studies included in our scoping review acknowledge that because long-term or societal costs were not considered, their calculations represent only a small part of the economic benefit to women, infants, and families and this was often noted as a limitation of the study [ 41 , 42 , 54 , 75 , 87 , 89 ]. Some authors called for further research considering these types of costs when the data or resources were available [ 25 , 26 , 27 ]. Many authors speculated that due to the limited time frame or scope of benefits considered, their calculations were likely an underestimation of the true long-term value for money of the intervention [ 16 , 19 , 21 , 32 , 50 , 63 ].
Our findings align with those of other systematic reviews of economic evaluations of similar interventions [ 118 , 119 , 120 , 121 , 122 ]. In their review of economic evaluations of home visiting programs for young or vulnerable pregnant women, Stamuli et al. noted that many studies considered outcomes only within the length of the trial (what we have described as the immediate time frame) [ 121 ]. They argue that for interventions targeting vulnerable pregnant people, the benefits are expected to accrue over the lifetime of the child and the parent rather than in the immediate time frame. They recommend that these types of evaluations consider multiple perspectives, including a societal perspective, and long-term outcomes.
Ruger and Lazar (2012) systematically reviewed economic evaluations of drug abuse treatment and HIV prevention programs in pregnant women [ 119 ]. They echoed Stamuli et al.’s recommendation that costs should be reported from a societal perspective [ 121 ]. Neither Stamuli et al. [ 121 ] nor Ruger and Lazar [ 119 ] were able to reach conclusions on whether the interventions represented value for money from the results of the studies, citing a lack of consistency between studies with respect to costs considered, methods used, and perspectives taken. Both articles recommend that for best practice, detailed cost and outcome data should be collected alongside randomized control trials.
Verbeke et al.’s 2022 systematic review of the cost-effectiveness of mental health interventions during pregnancy and up to two years postpartum [ 122 ], Koegl et al.’s 2023 review of cost-benefit analyses of developmental crime prevention programs [ 118 ], and Sampaio et al.’s 2024 systematic review of interventions aimed at improving child health [ 120 ] all concluded that these interventions represented value for money. However, these authors all noted significant limitations in the literature including an overall shortage of published evaluations [ 118 , 122 ], lack of consistency in methods [ 118 , 120 , 122 ], a deficit of data looking at outcomes for both parents and the child together [ 122 ], and insufficient analysis of the long-term effectiveness of the included interventions [ 118 , 120 , 122 ]. For future research, they recommend considering a broader range of outcomes for both parents as well as the child over a longer time frame. Like Stamuli et al., Verbeke et al. noted that by not considering the impact over the lifetime of the child, the real-world cost-effectiveness is likely underestimated [ 122 ].
Our results will be of value to people interested in a wide range of interventions and may assist those planning and conducting economic evaluations to improve the quality of such research. Readers can use the information provided in Table 3 to identify publications that considered long-term outcomes and societal costs and can refer to the original publications for further details regarding definitions and data sources for such costs to aid in planning their own analyses. Those planning evaluations of interventions targeting underserved pregnant people and/or new parents should collect prospective long-term outcome data pertaining to both parent and child outcomes whenever possible. Long-term health outcomes and long-term health care costs should be considered. Important long-term societal costs to consider, where relevant, include child welfare services, legal services, incarceration, other criminal justice costs, costs of crime, domestic violence, child abuse/neglect, addiction, lost productivity, employment status/income, housing, education, and earning potential. We noted a gap in the existing literature with respect to the consideration of patient priorities in the identification of costs considered. This might be addressed through patient engagement when planning economic evaluations, and through the inclusion of costs that have been identified a patient-oriented outcome measures for economic research [ 123 ]. Our findings suggest that economic evaluations that consider multiple perspectives, including a societal perspective, and a long-term time horizon will most fully describe the value for money of interventions underserved pregnant people and/or new parents. Inclusion of a patient perspective evaluation may also better address equity considerations [ 124 ].
Our scoping review is the first of its kind and has several strengths. Our method allowed us to consider grey literature and include studies of all methodologies while maintaining a systematic approach. We looked at a wider range of interventions and underserved populations than previous reviews. Another strength of our review is the extensive set of search terms used to identify underserved populations, which minimized the possibility of missing eligible articles. One limitation of our review is that we did not extract detailed information about the economic analysis methods that were used, including the outcomes associated with the full economic evaluations (e.g., quality-adjusted-life-years for cost-utility analyses, net benefit for cost-benefit analyses) and time horizons considered. Another limitation is that we did not conduct the optional stakeholder consultation step in the Arksey and O’Malley (2005) scoping review framework [ 12 ]. This step is most commonly conducted in the search phase to inform keyword selection [ 125 ]. Based on another review conducted as part of our larger program of research, we compiled an extensive set of search terms to identify relevant studies and felt confident that omission of stakeholder consultation did not sacrifice the quality of our review. Secondly, due to the nature of the scoping review methodology, we did not appraise the quality of the methods or results of the studies included in this review. Limitations and methodological concerns about some of the articles included in this review have been reported previously [ 34 , 57 , 121 ]. Lastly, in order to ensure included articles were relevant to our context, we did not include publications focused on interventions set outside of the top 20 GDP OECD countries. This means that the findings from this article, including the particular downstream costs considered in the analyses, may not be applicable to low- and middle-income countries.
This scoping review can be used to inform future economic analyses of interventions targeting poorly served pregnant people and new parents. It consolidates a list of costs that researchers and health economists may wish to consider when conducting these types of analyses and identifies studies that look at a range of downstream and long-term costs. It is important to include all costs relevant to the underserved population in question in the evaluation. Policy and resource allocation decisions are often informed by evidence generated through economic evaluations to ensure the efficient allocation of available resources. Economic evaluations that are able to capture the downstream and long-term benefits of interventions targeting underserved populations may support the implementation of policy and funding decisions that will benefit underserved pregnant people and new parents, their children, and society in general and that will reduce health inequities.
Availability of data and materials
The datasets used and/or analysed during the current study are available from the corresponding author on reasonable request.
Abbreviations
Ovid Emcare, EMBASE
Cumulative Index to Nursing and Allied Health Literature
The Canada Prenatal Nutrition Program
Special Supplemental Nutrition Program for Women, Infants, and Children
Gross Domestic Product
Organisation for Economic Co-operation and Development
Human Development Index
Puthussery S. Perinatal outcomes among migrant mothers in the United Kingdom: is it a matter of biology, behaviour, policy, social determinants or access to health care?. Best Pract Res Clin Obstet Gynaecol. 2016;32:39–49.
Article PubMed Google Scholar
Katz AS, Hardy B-J, Firestone M, Lofters A, Morton-Ninomiya ME. Vagueness, power and public health: use of ‘vulnerable‘ in public health literature. Crit Public Health. 2020;30(5):601–11.
Article Google Scholar
Arabin B, Baschat A. Pregnancy: an underutilized window of opportunity to improve long-term maternal and infant health - an appeal for continuous family care and interdisciplinary communication. Front Pediatr. 2017;5:69.
Article PubMed PubMed Central Google Scholar
McAuliffe F. Impact of pregnancy on long-term health: advances in postpregnancy care - An opportunity to improve long-term maternal health. Int J Gynecol Obstet. 2023;160:4–6.
Gaillard R, Wright J, Jaddoe VWV. Lifestyle intervention strategies in early life to improve pregnancy outcomes and long-term health of offspring: a narrative review. J Dev Orig Health Dis. 2019;10(3):314–21.
Ranson K, Urichuk L. The effect of parent–child attachment relationships on child biopsychosocial outcomes: a review. Early Child Dev Care. 2008;178(2):129–52.
McRae DNMN, Stoll K, Mayhew M, Vedam S, Mpofu D, Janssen PA. Is model of care associated with infant birth outcomes among vulnerable women? A scoping review of midwifery-led versus physician-led care. SSM Popul Health. 2016;2:182–93.
Sandall J, Soltani H, Gates S, Shennan A, Devane D. Midwife-led continuity models versus other models of care for childbearing women. Cochrane Database Syst Rev. 2016;4(4):Cd004667.
PubMed Google Scholar
Balaam M-C, Thomson G. Building capacity and wellbeing in vulnerable/marginalised mothers: a qualitative study. Women Birth. 2018;31(5):e341–7.
McRae DN, Janssen PA, Vedam S, Mayhew M, Mpofu D, Teucher U, et al. Reduced prevalence of small-for-gestational-age and preterm birth for women of low socioeconomic position: a population-based cohort study comparing antenatal midwifery and physician models of care. BMJ Open. 2018;8(10):e022220.
McRae DN, Muhajarine N, Janssen PA. Improving birth outcomes for women who are substance using or have mental illness: a Canadian cohort study comparing antenatal midwifery and physician models of care for women of low socioeconomic position. BMC Pregnancy Childbirth. 2019;19(1):279.
Arksey H, O’Malley L. Scoping studies: towards a methodological framework. Int J Soc Res Methodol. 2005;8(1):19–32.
GDP per capita and productivity levels. 2020. Available from: https://www.oecd-ilibrary.org/content/data/data-00686-en . Cited 20 May 2020.
Ammerman RT, Mallow PJ, Rizzo JA, Putnam FW, Van Ginkel JB. Cost-effectiveness of In-Home Cognitive Behavioral Therapy for low-income depressed mothers participating in early childhood prevention programs. J Affect Disord. 2017;208:475–82.
Aos S, Lieb R, Mayfield J, Miller M, Pennucci A. Benefits and costs of prevention and early intervention programs for youth. 2004.
Google Scholar
Avruch S, Cackley AP. Savings achieved by giving WIC benefits to women prenatally. Public Health Rep. 1995;110(1):27–34.
CAS PubMed PubMed Central Google Scholar
Bacheller HL, Hersh AR, Caughey AB. Behavioral smoking cessation counseling during pregnancy: a cost-effectiveness analysis. Obstet Gynecol. 2021;137(4):703–12.
Bensussen-Walls W, Saewyc EM. Teen-focused care versus adult-focused care for the high-risk pregnant adolescent: an outcomes evaluation. Public Health Nurs. 2001;18(6):424–35.
Article CAS PubMed Google Scholar
Berkowitz G, Brindis C, Clayson Z, Peterson S. Options for recovery: Promoting success among women mandated to treatment. J Psychoactive Drugs. 1996;28(1):31–8.
Brumfield CG, Nelson KG, Stotser D, Yarbaugh D, Patterson P, Sprayberry NK. 24-Hour mother-infant discharge with a follow-up home health visit: results in a selected medicaid population. Obstet Gynecol. 1996;88(4 I):544–8.
Buescher PA, Horton SJ. Prenatal WIC participation in relation to low birth weight and medicaid infant costs in North Carolina – A 1997 update. 2000.
Buescher PA, Larson LC, Nelson MD Jr, Lenihan AJ. Prenatal WIC participation can reduce low birth weight and newborn medical costs: a cost-benefit analysis of WIC participation in North Carolina. J Am Diet Assoc. 1993;93(2):163–6.
Burchard J, Markenson GR, Saade GR, Laurent LC, Heyborne KD, Coonrod DV, et al. Clinical and economic evaluation of a proteomic biomarker preterm birth risk predictor: cost-effectiveness modeling of prenatal interventions applied to predicted higher-risk pregnancies within a large and diverse cohort. J Med Econ. 2022;25(1):1255–66.
Burger S. A cost consequence analysis of outreach strategies for high risk pregnant women. J Community Health Nurs. 2010;27(3):137–45.
Cramer ME, Chen L-W, Roberts S, Clute D. Evaluating the social and economic impact of community-based prenatal care. Public Health Nurs. 2007;24(4):329–36.
Daley M, Argeriou M, McCarty D, Callahan JJ Jr, Shepard DS, Williams CN. The costs of crime and the benefits of substance abuse treatment for pregnant women. J Subst Abuse Treat. 2000;19(4):445–58.
Daley M, Argeriou M, McCarty D, Callahan JJ Jr, Shepard DS, Williams CN. The impact of substance abuse treatment modality on birth weight and health care expenditures. J Psychoactive Drugs. 2001;33(1):57–66.
Daley M, Shepard DS, Bury-Maynard D. Changes in quality of life for pregnant women in substance user treatment: developing a quality of life index for the addictions. Subst Use Misuse. 2005;40(3):375–94.
Devaney B, Bilheimer L, Schore J. Medicaid costs and birth outcomes: The effects of prenatal WIC participation and the use of prenatal care. J Policy Anal Manage. 1992;11(4):573–92.
Dornelas EA, Magnavita J, Beazoglou T, Fischer EH, Oncken C, Lando H, et al. Efficacy and cost-effectiveness of a clinic-based counseling intervention tested in an ethnically diverse sample of pregnant smokers. Patient Educ Couns. 2006;64(1–3):342–9.
Dumont K, Kirkland K, Mitchell-Herzfeld S, Ehrhard-Dietzel S, Rodriguez LM, Lee E, et al. A randomized trial of Healthy Families New York (HFNY): does home visiting prevent child maltreatment? New York: New York State Office of Children andFamily Services University at Albany, State University of New York; 2010. October 31, 2010. Contract No.: 232945.
French MT, McCollister KE, Cacciola J, Durell J, Stephens RL. Benefit-cost analysis of addiction treatment in Arkansas: specialty and standard residential programs for pregnant and parenting women. Substance Abuse. 2002;23(1):31–51.
Gareau S, Lòpez-De Fede A, Loudermilk BL, Cummings TH, Hardin JW, Picklesimer AH, et al. Group prenatal care results in Medicaid savings with better outcomes: a propensity score analysis of CenteringPregnancy participation in South Carolina. Matern Child Health J. 2016;20(7):1384–93.
Glazner J, Bondy J, Luckey D, Olds D. Effect of the nurse family partnership on Government expenditures for vulnerable first-time mothers and their children in Elmira, New York. Memphis: Tennessee, and Denver, Colorado. [Internet]. Colorado: University of Colorado Health Sciences Center; 2004. Available from: https://www.acf.hhs.gov/sites/default/files/documents/opre/effect_nursefam.pdf .
Goler NC, Armstrong MA, Osejo VM, Hung Y-Y, Haimowitz M, Caughey AB. Early start: a cost-beneficial perinatal substance abuse program. Obstet Gynecol. 2012;119(1):102–10.
Graveley EA, Littlefield JH. A cost-effectiveness analysis of three staffing models for the delivery of low-risk prenatal care. Am J Public Health. 1992;82(2):180–4.
Article CAS PubMed PubMed Central Google Scholar
Gregory PM, de Jesus ML. Racial differences in birth outcomes and costs in relation to prenatal WIC participation. N J Med. 2003;100(3):29–36.
Grote NK, Simon GE, Russo J, Lohr MJ, Carson K, Katon W. Incremental benefit-Cost of MOMCare: Collaborative care for perinatal depression among economically disadvantaged women. Psychiatr Serv. 2017;68(11):1164–71.
Guo Y, Lee J-A, Rousseau J, Pimentel P, Bojorquez Y, Cabasag C, et al. The potential economic impact of a coordinated home visitation program: preventing adverse birth outcomes. Calif J Health Promot. 2016;14(2):1–13.
Hannan J, Brooten D, Page T, Galindo A, Torres M. Low-income first-time mothers: effects of APN follow-up using mobile technology on maternal and infant outcomes. Global Pediatric Health. 2016;3:2333794X16660234.
Howell E, Palmer A, Benatar S, Garrett B. Potential medicaid cost savings from maternity care based at a Freestanding Birth Center. Medicare Medicaid Res Rev. 2014;4(3):mmrr2014-004-03-a06.
Hueston WJ, Quattlebaum RG, Benich JJ. How much money can early prenatal care for teen pregnancies save?: a cost-benefit analysis. J Am Board Fam Med. 2008;21(3):184–90.
Johnson JE, Wiltsey-Stirman S, Sikorskii A, Miller T, King A, Blume JL, et al. Protocol for the ROSE sustainment (ROSES) study, a sequential multiple assignment randomized trial to determine the minimum necessary intervention to maintain a postpartum depression prevention program in prenatal clinics serving low-income women. Implement Sci. 2018;13(1):N.PAG-N.PAG.
Joyce T, Corman H, Grossman M. A cost-effectiveness analysis of strategies to reduce infant mortality. Med Care. 1988;26(4):348–60.
Karoly LA, Greenwood PW, Sohler Everingham SM, Hoube J, Kilburn MR, Rydell CP, et al. Investing in our children: what we know and don’t know about the costs and benefits of early childhood interventions. Santa Monica, CA: RAND Corporation; 1998.
Lee S, Aos S, Miller M. Evidence-based programs to prevent children from entering and remaining in the child welfare system: benefits and costs for Washington. 2008.
Leppert PC, Namerow PB. Cost averted by providing comprehensive prenatal care to teenagers. J Nurse Midwifery. 1985;30(5):285–9.
Lopez-Soto D, Griffin PM. Estimating the impact of neonatal abstinence system interventions on Medicaid: an incremental cost analysis. Subst Abuse Treat Prev Policy. 2021;16(1):91.
Lu MC, Lin YG, Prietto NM, Garite TJ. Elimination of public funding of prenatal care for undocumented immigrants in California: a cost/benefit analysis. Am J Obstet Gynecol. 2000;182(1 Pt 1):233–9.
McCallum DM, Fosson GH, Pisu M. Making the case for medicaid funding of smoking cessation treatment programs: an application to state-level health care savings. J Health Care Poor Underserved. 2014;25(4):1922–40.
McDonald GJ, Fenstermaker CR, Bellis DDA, Sheila K Cosgrove, James C Cackley, Alicia Puente Deroy C. Robert, Cassady MER, Janice S. Early intervention: federal investments like WIC can produce savings. 1992.
Miller TR. Projected outcomes of nurse-family partnership home visitation during 1996–2013, USA. Prev Sci. 2015;16(6):765–77.
Montgomery DL, Splett PL. Economic benefit of breast-feeding infants enrolled in WIC. J Am Diet Assoc. 1997;97(4):379–85.
Moore TR, Origel W, Key TC, Resnik R. The perinatal and economic impact of prenatal care in a low-socioeconomic population. Am J Obstet Gynecol. 1986;154(1):29–33.
Mundt MP, Fiore MC, Piper ME, Adsit RT, Kobinsky KH, Alaniz KM, et al. Cost-effectiveness of stop smoking incentives for medicaid-enrolled pregnant women. Prev Med. 2021;153:106777.
Nehme EK, Wilson KJ, McGowan R, Schuessler KR, Morse SM, Patel DA. Providing doula support to publicly insured women in central Texas: a financial cost–benefit analysis. Birth. 2024;51(1):63–70.
Olds D, Miller TR, Knudtson M, Luckey D, Bondy J, Stevenson A, et al. Impact of the nurse-family partnership on neighborhood context, government expenditures, and children’s school functioning. 2011.
Olds DL, Henderson CR Jr, Phelps C, Kitzman H, Hanks C. Effect of prenatal and infancy nurse home visitation on government spending. Med Care. 1993;31(2):155–74.
Olds DL, Kitzman H, Anson E, Smith JA, Knudtson MD, Miller T, et al. Prenatal and infancy nurse home visiting effects on mothers: 18-year follow-up of a randomized trial. Pediatrics. 2019;144(6):e20183889.
Olds DL, Kitzman HJ, Cole RE, Hanks CA, Arcoleo KJ, Anson EA, et al. Enduring effects of prenatal and infancy home visiting by nurses on maternal life course and government spending: follow-up of a randomized trial among children at age 12 years. Arch Pediatr Adolesc Med. 2010;164(5):419–24.
Parker DR, Windsor RA, Roberts MB, Hecht J, Hardy NV, Strolla LO, et al. Feasibility, cost, and cost-effectiveness of a telephone-based motivational intervention for underserved pregnant smokers. Nicotine Tob Res. 2007;9(10):1043–51.
Pollack HA. Sudden infant death syndrome, maternal smoking during pregnancy, and the cost-effectiveness of smoking cessation intervention. Am J Public Health. 2001;91(3):432–6.
Pugh LC, Milligan RA, Frick KD, Spatz D, Bronner Y. Breastfeeding duration, costs, and benefits of a support program for low-income breastfeeding women. Birth. 2002;29(2):95–100.
Reid ML, Morris JB. Perinatal care and cost effectiveness: changes in health expenditures and birth outcome following the establishment of a nurse-midwife program. Med Care. 1979;17(5):491–500.
Rodriguez MI, Swartz JJ, Lawrence D, Caughey AB. Extending delivery coverage to include prenatal care for low-income, immigrant women is a cost-effective strategy. In: Women’s health issues. 2020.
Rowley RA, Phillips LE, O’Dell L, El Husseini R, Carpino S, Hartman S. Group prenatal care: a financial perspective. Matern Child Health J. 2016;20(1):1–10.
Ruger JP, Weinstein MC, Hammond SK, Kearney MH, Emmons KM. Cost-effectiveness of motivational interviewing for smoking cessation and relapse prevention among low-income pregnant women: a randomized controlled trial. Value in Health. 2008;11(2):191–8.
Saygın Avşar T, Jackson L, Barton P, Jones M, McLeod H. Towards optimum smoking cessation interventions during pregnancy: a household model to explore cost-effectiveness. Addiction. 2022;117(10):2707–19.
Seiner K, Lairson DR. A cost-effectiveness analysis of prenatal care delivery. Eval Health Prof. 1985;8(1):93–108.
Svikis DS, Golden AS, Huggins GR, Pickens RW, McCaul ME, Velez ML, et al. Cost-effectiveness of treatment for drug-abusing pregnant women. Drug Alcohol Depend. 1997;45(1–2):105–13.
Thorsen N, Khalil L. Cost savings associated with smoking cessation for low-income pregnant women. Wis Med J. 2004;103(5):67.
Tuttle CR, Dewey KG. Potential cost savings for Medi-Cal, AFDC, food stamps, and WIC programs associated with increasing breast-feeding among low-income Hmong women in California. J Am Diet Assoc. 1996;96(9):885–90.
Washington State Institute for Public Policy. Nurse family partnership - public health & prevention: home- or family-based benefit-cost estimates updated December 2023. Olympia: Washington State Institute for Public Policy; 2023.
Wilkinson A, Anderson S, Wheeler SB. Screening for and treating postpartum depression and psychosis: a cost-effectiveness analysis. Matern Child Health J. 2017;21(4):903–14.
Windsor RA, Lowe JB, Perkins LL, Smith-Yoder D, Artz L, Crawford M, et al. Health education for pregnant smokers: its behavioral impact and cost benefit. Am J Public Health. 1993;83(2):201–6.
Wu J, Dean KS, Rosen Z, Muennig PA. The cost-effectiveness analysis of nurse-family partnership in the United States. J Health Care Poor Underserved. 2017;28(4):1578–97.
Xu X, Yonkers KA, Ruger JP. Economic evaluation of a behavioral intervention versus brief advice for substance use treatment in pregnant women: results from a randomized controlled trial. BMC Pregnancy Childbirth. 2017;17(1):83.
Barlow J, Beake S, Bick D, Bryson C, Day L, Gilby N, et al. Initial protocol for a national evaluation of an area-based intervention programme (A Better Start) on early-life outcomes: a longitudinal cohort study with comparison (control) cohort samples. BMJ Open. 2017;7(8):e015086.
Barlow J, Sembi S, Parsons H, Kim S, Petrou S, Harnett P, et al. A randomized controlled trial and economic evaluation of the Parents Under Pressure program for parents in substance abuse treatment. Drug Alcohol Depend. 2019;194:184–94.
Barnes J, Stuart J, Allen E, Petrou S, Sturgess J, Barlow J, et al. Randomized controlled trial and economic evaluation of nurse-led group support for young mothers during pregnancy and the first year postpartum versus usual care. Trials. 2017;18:1–15.
Bell K, Corbacho B, Ronaldson S, Richardson G, Hood K, Sanders J, et al. Costs and consequences of the Family Nurse Partnership (FNP) programme in England: evidence from the building blocks trial. F1000Research. 2019;8:1640.
Bell R, Glinianaia SV, Waal ZV, Close A, Moloney E, Jones S, et al. Evaluation of a complex healthcare intervention to increase smoking cessation in pregnant women: interrupted time series analysis with economic evaluation. Tob Control. 2018;27(1):90–8.
Bick D, Taylor C, Avery A, Bhavnani V, Craig V, Healey A, et al. Protocol for a two-arm feasibility RCT to support postnatal maternal weight management and positive lifestyle behaviour in women from an ethnically diverse inner city population: the SWAN feasibility trial. Pilot Feasibility Stud. 2019;5(1):117.
Boath E, Major K, Cox J. When the cradle falls II: The cost-effectiveness of treating postnatal depression in a psychiatric day hospital compared with routine primary care. J Affect Disord. 2003;74(2):159–66.
Boyd KA, Briggs AH, Bauld L, Sinclair L, Tappin D. Are financial incentives cost-effective to support smoking cessation during pregnancy?. Addiction. 2016;111(2):360–70.
Coleman T, Clark M, Welch C, Whitemore R, Leonardi-Bee J, Cooper S, et al. Effectiveness of offering tailored text message, self-help smoking cessation support to pregnant women who want information on stopping smoking: MiQuit3 randomised controlled trial and meta-analysis. Addiction. 2022;117(4):1079–94.
Corbacho B, Bell K, Stamuli E, Richardson G, Ronaldson S, Hood K, et al. Cost-effectiveness of the Family Nurse Partnership (FNP) programme in England: evidence from the building blocks trial. J Eval Clin Pract. 2017;23(6):1367–74.
Dundas R, Boroujerdi M, Browne S, Deidda M, Bradshaw P, Craig P, et al. Evaluation of the Healthy Start voucher scheme on maternal vitamin use and child breastfeeding: a natural experiment using data linkage. Public Health Res (Southampt). 2023;11(11):1–101.
Essex HN, Parrott S, Wu Q, Li J, Cooper S, Coleman T. Cost-effectiveness of nicotine patches for smoking cessation in pregnancy: A placebo randomized controlled trial (SNAP). Nicotine Tob Res. 2015;17(6):636–42.
Hoddinott P, Craig L, Maclennan G, Boyers D, Vale L. The FEeding Support Team (FEST) randomised, controlled feasibility trial of proactive and reactive telephone support for breastfeeding women living in disadvantaged areas. BMJ open. 2012;2(2):e000652.
Howard LM, Trevillion K, Potts L, Heslin M, Pickles A, Byford S, Carson LE, Dolman C, Jennings S, Johnson S, Jones I, McDonald R, Pawlby S, Powell C, Seneviratne G, Shallcross R, Stanley N, Wieck A, Abel KM. Effectiveness and cost-effectiveness of psychiatric mother and baby units: quasi-experimental study. Br J Psychiatry. 2022;221(4):628–36. https://doi.org/10.1192/bjp.2022.48 .
Jones M, Smith M, Lewis S, Parrott S, Coleman T. A dynamic, modifiable model for estimating cost-effectiveness of smoking cessation interventions in pregnancy: application to an RCT of self-help delivered by text message. Addiction. 2019;114(2):353–65.
Jones M, Smith M, Lewis S, Parrott S, Coleman T. Investigating the cost-effectiveness of three cessation interventions on a national scale using the Economics of Smoking in Pregnancy (ESIP) decision analytical model. Addiction. 2022;117(11):2907–17.
Longhi E, Murray L, Hunter R, Wellsted D, Taylor-Colls S, Mackenzie K, et al. The NSPCC UK Minding the Baby® (MTB) home-visiting programme, supporting young mothers (aged 14–25) in the first 2 years of their baby's life: Study protocol for a randomised controlled trial. Trials. 2016;17:486.
McIntosh E, Barlow J, Davis H, Stewart-Brown S. Economic evaluation of an intensive home visiting programme for vulnerable families: a cost-effectiveness analysis of a public health intervention. J Public Health. 2009;31(3):423–33.
McMeekin N, Sinclair L, Robinson-Smith L, Mitchell A, Bauld L, Tappin DM, et al. Financial incentives for quitting smoking in pregnancy: are they cost-effective?. Addiction. 2023;118(8):1445–56.
Morrell CJ, Warner R, Slade P, Dixon S, Walters S, Paley G, et al. Psychological interventions for postnatal depression: cluster randomised trial and economic evaluation. The PoNDER trial. Health Technol Assess. 2009;13(30):iii-iv, xi-xiii, 1–153.
Naughton F, Cooper S, Foster K, Emery J, Leonardi-Bee J, Sutton S, et al. Large multi-centre pilot randomized controlled trial testing a low-cost, tailored, self-help smoking cessation text message intervention for pregnant smokers (MiQuit). Addiction. 2017;112(7):1238–49.
Petrou S, Cooper P, Murray L, Davidson LL. Cost-effectiveness of a preventive counseling and support package for postnatal depression. Int J Technol Assess Health Care. 2006;22(4):443–53.
Poston L, Bell R, Briley AL, Godfrey KM, Nelson SM, Oteng-Ntim E, et al. Improving pregnancy outcome in obese women: the UK pregnancies better eating and activity randomised controlled trial. Southampton (UK): NIHR Journals Library; 2017.
Stevenson MD, Scope A, Sutcliffe PA. The cost-effectiveness of group cognitive behavioral therapy compared with routine primary care for women with postnatal depression in the UK. Value Health. 2010;13(5):580–4.
Trevillion K, Ryan EG, Pickles A, Heslin M, Byford S, Nath S, et al. An exploratory parallel-group randomised controlled trial of antenatal Guided Self-Help (plus usual care) versus usual care alone for pregnant women with depression: DAWN trial. J Affect Disord. 2020;261:187–97.
Ussher M, Lewis S, Aveyard P, Manyonda I, West R, Lewis B, et al. The London Exercise And Pregnant smokers (LEAP) trial: a randomised controlled trial of physical activity for smoking cessation in pregnancy with an economic evaluation. Health Technol Assess. 2015;19(84):vii–xxiv, 1–135.
Wiggins M, Oakley A, Roberts I, Turner H, Rajan L, Austerberry H, Mujica R, Mugford M. The Social Support and Family Health Study: a randomised controlled trial and economic evaluation of two alternative forms of postnatal support for mothers living in disadvantaged inner-city areas. Health Technol Assess. 2004;8(32):iii, ix-x, 1-120. https://doi.org/10.3310/hta8320 .
Wiggins M, Sawtell M, Wiseman O, McCourt C, Eldridge S, Hunter R, et al. Group antenatal care (Pregnancy Circles) for diverse and disadvantaged women: study protocol for a randomised controlled trial with integral process and economic evaluations. BMC Health Serv Res. 2020;20(1):919.
Wilson GB, McGovern R, Antony G, Cassidy P, Deverill M, Graybill E, et al. Brief intervention to reduce risky drinking in pregnancy: study protocol for a randomized controlled trial. Trials. 2012;13(1):174-.
Centre of Perinatal Excellence. Valuing perinatal mental health: the consequences of not treating perinatal depression and anxiety. 2014.
Gao Y, Roe Y, Hickey S, Chadha A, Kruske S, Nelson C, et al. Birthing on country service compared to standard care for First Nations Australians: a cost-effectiveness analysis from a health system perspective. Lancet Reg Health West Pac. 2023;34:100722-.
PubMed PubMed Central Google Scholar
Hickey S, Roe Y, Gao Y, Nelson C, Carson A, Currie J, et al. The indigenous birthing in an urban setting study: the IBUS study: a prospective birth cohort study comparing different models of care for women having Aboriginal and Torres Strait Islander babies at two major maternity hospitals in urban South East Queensland, Australia. BMC Pregnancy Childbirth. 2018;18(1):431.
Hodgins M, Ostojic K, Hu N, Lawson KD, Samir N, Webster A, et al. Study protocol for a real-world evaluation of an integrated child and family health hub for migrant and refugee women. BMJ open. 2022;12(8):e061002-e.
Jan S, Conaty S, Hecker R, Bartlett M, Delaney S, Capon T. An holistic economic evaluation of an Aboriginal community-controlled midwifery programme in Western Sydney. J Health Serv Res Policy. 2004;9(1):14–21.
Riggs E, Yelland J, Mensah FK, Gold L, Szwarc J, Kaplan I, et al. Group Pregnancy Care for refugee background women: a codesigned, multimethod evaluation protocol applying a community engagement framework and an interrupted time series design. BMJ Open. 2021;11(7):e048271.
Keshmiri R, Coyte PC, Laporte A, Sheth PM, Loutfy M. Cost-effectiveness analysis of infant feeding modalities for virally suppressed mothers in Canada living with HIV. Medicine. 2019;98(23):e15841.
McMurchy D, Palmer R, Thornton M, Birdi T. An assessment of the economic impact of the Canada Prenatal Nutrition Program (CPNP). 2009.
Thanh NX, Jonsson E, Moffatt J, Dennett L, Chuck AW, Birchard S. An economic evaluation of the parent-child assistance program for preventing fetal alcohol spectrum disorder in Alberta. Canada Adm Policy Ment Health. 2015;42(1):10–8.
(FRA) EUAfFR. Cost of exclusion from healthcare : the case of migrants in an irregular situation. 2015.
Jackson P. Value for money and international development: deconstructing myths to promote a more constructive discussion. eVALUation Matters. 2012;3:8–15.
Koegl CJ, Farrington DP, Welsh BC. Cost-benefit analyses of developmental crime prevention programmes. Crim Behav Ment Health. 2023;33(2):106–15.
Ruger J, Lazar C. Economic evaluation of drug abuse treatment and HIV prevention programs in pregnant women: a systematic review. Addict Behav. 2012;37(1):1–10.
Sampaio F, Nystrand C, Feldman I, Mihalopoulos C. Evidence for investing in parenting interventions aiming to improve child health: a systematic review of economic evaluations. Eur Child Adolesc Psychiatry. 2024;33(2):323–55.
Stamuli E, Richardson G, Duffy S, Robling M, Hood K. Systematic review of the economic evidence on home visitation programmes for vulnerable pregnant women. Br Med Bull. 2015;115(1):19–44.
Verbeke E, Bogaerts A, Nuyts T, Crombag N, Luyten J. Cost-effectiveness of mental health interventions during and after pregnancy: a systematic review. Birth. 2022;49(3):364–402.
Brown D, Srinivasan M, Arbulu L, Dullabh R, Curtis K, Zott C, et al. Federal data for conducting patient-centered outcomes research on economic outcomes. Med Care. 2023;61(7):462–9.
Fu R, Ng V, Liu M, Wells D, Yurga E, Nauenberg E. Considering patient perspectives in economic evaluations of health interventions. Front Public Health. 2023;11:1212583.
Pham MT, Rajic A, Greig JD, Sargeant JM, Papadopoulos A, McEwen SA. A scoping review of scoping reviews: advancing the approach and enhancing the consistency. Res Synth Methods. 2014;5(4):371–85.
Download references
Acknowledgements
We acknowledge the contributions of Riley Graybrook to the data acquisition process and Bella Arless to updating the searches in 2024, screening results, and extracting data, and the support of Lindsay Grenier in preparing the final manuscript for submission. We thank Emmanuel Guindon for his review of an earlier version of the manuscript.
This research was funded by a grant from the Canadian Institutes of Health Research (#MRC-167971) awarded to Elizabeth Darling (PI). Aisha Jansen was also supported by a Mitacs Research Training Award. The funders had no role in study design; in the collection, analysis and interpretation of data; in writing of the report; or in the decision to submit the article for publication.
Author information
Authors and affiliations.
McMaster Midwifery Research Centre, McMaster University, 1280 Main St. W., HSC 4H24, Hamilton, ON, L8S 4K1, Canada
Elizabeth K. Darling, Aisha Jansen & Bismah Jameel
Department of Health Research Methods, Evidence, and Impact, Communication Research Lab (CRL) 227, McMaster University, 1280 Main Street West, Hamilton, ON, L8K 4K1, Canada
Jean-Éric Tarride
You can also search for this author in PubMed Google Scholar
Contributions
The study was conceptualized by E.D. E.D., J-E.T., and A.J. contributed to the study design. A.J. and B.J. conducted the study selection and full text screening, and A.J. completed the data extraction. E.D. was consulted when consensus could not be reached. The findings were collated and summarized by A.J. The first draft of the manuscript was written by A.J. and E.D. All authors critically revised the manuscript. All authors read and approved the final manuscript.
Corresponding author
Correspondence to Elizabeth K. Darling .
Ethics declarations
Ethics approval and consent to participate.
Not Applicable.
Consent for publication
Not applicable.
Competing interests
The authors declare no competing interests.
Additional information
Publisher’s note.
Springer Nature remains neutral with regard to jurisdictional claims in published maps and institutional affiliations.
Supplementary Information
Supplementary material 1, rights and permissions.
Open Access This article is licensed under a Creative Commons Attribution-NonCommercial-NoDerivatives 4.0 International License, which permits any non-commercial use, sharing, distribution and reproduction in any medium or format, as long as you give appropriate credit to the original author(s) and the source, provide a link to the Creative Commons licence, and indicate if you modified the licensed material. You do not have permission under this licence to share adapted material derived from this article or parts of it. The images or other third party material in this article are included in the article’s Creative Commons licence, unless indicated otherwise in a credit line to the material. If material is not included in the article’s Creative Commons licence and your intended use is not permitted by statutory regulation or exceeds the permitted use, you will need to obtain permission directly from the copyright holder. To view a copy of this licence, visit http://creativecommons.org/licenses/by-nc-nd/4.0/ .
Reprints and permissions
About this article
Cite this article.
Darling, E.K., Jansen, A., Jameel, B. et al. A scoping review of costing methodologies used to assess interventions for underserved pregnant people and new parents. Int J Equity Health 23 , 168 (2024). https://doi.org/10.1186/s12939-024-02252-x
Download citation
Received : 19 July 2023
Accepted : 12 August 2024
Published : 22 August 2024
DOI : https://doi.org/10.1186/s12939-024-02252-x
Share this article
Anyone you share the following link with will be able to read this content:
Sorry, a shareable link is not currently available for this article.
Provided by the Springer Nature SharedIt content-sharing initiative
- Perinatal population
- Economic evaluation
- Healthcare access
- Scoping review
- Underserved populations
- Health equity
- Economic analyses
- Costs and cost analysis
International Journal for Equity in Health
ISSN: 1475-9276
- General enquiries: [email protected]

IMAGES
COMMENTS
Inclusion criteria are defined as the key features of the target population that the investigators will use to answer their research question. 2 Typical inclusion criteria include demographic, clinical, and geographic characteristics. In contrast, exclusion criteria are defined as features of the potential study participants who meet the ...
Exclusion Criteria A balance of specific inclusion and exclusion criteria is paramount. For some systematic reviews, there may already be a large pre-existing body of literature. The search strategy may retrieve thousands of results that must be screened.
We propose hypothetical examples that illustrate how selection of inclusion and exclusion criteria may introduce bias. Experience suggests that dual review can identify inclusion criteria that are not sufficiently clear and occasions where subjective judgment may differ.
Inclusion and Exclusion Criteria Inclusion and exclusion criteria set the boundaries for the systematic review. They are determined after setting the research question usually before the search is conducted, however scoping searches may need to be undertaken to determine appropriate criteria.
This chapter describes the processes by which the structure of the synthesis can be mapped out at the beginning of the review, and the interplay between the review question, considerations for the analysis and their operationalization in terms of eligibility criteria.
Defining Inclusion/Exclusion Criteria An important part of the SR process is defining what will and will not be included in your review. Inclusion and exclusion criteria are developed after a research question is finalized but before a search is carried out.
Selection Criteria You may want to think about criteria that will be used to select articles for your literature review based on your research question. These are commonly known as inclusion criteria and exclusion criteria, and they set the boundaries for the literature review.
The eligibility criteria are liberally applied in the beginning to ensure that relevant studies are included and no study is excluded without thorough evaluation. At the outset, studies are only excluded if they clearly meet one or more of the exclusion criteria. For example, if the focus of review is children, then studies with adult ...
Well-constructed, clear, and explicit inclusion and exclusion criteria assist decision making consistency. In this chapter, I focus on inclusion and exclusion criteria, explain their benefits, provide guidelines on their construction and use, and illustrate with examples from sport, exercise, and physical activity research. Download chapter PDF.
Appropriate use of inclusion and exclusion criteria permits relevant data to be sourced as wider searches can produce a large amount of disparate data, whereas a search that is too narrow may result in the omission of significant findings ( Boell and Cecez-Kecmanovic, 2010 ).
Inclusion and Exclusion Criteria Establishing inclusion and exclusion criteria helps with designing search strategies and deciding which studies are relevant to your research question.
Inclusion and exclusion criteria in research studies: definitions and why they matter. Inclusion and exclusion criteria in research studies: definitions and why they matter. J Bras Pneumol. 2018 Apr;44 (2):84. doi: 10.1590/s1806-37562018000000088. [Article in Portuguese, English]
Inclusion and exclusion criteria Inclusion and exclusion criteria are a list of pre-defined characteristics to which literature must adhere to be included in a study. They are vital for the decision-making progress on what to review when undertaking a systematic review and will also help with systematic literature reviews.
Establish your Inclusion and Exclusion criteria These criteria help you decide which pieces of evidence (for example, which primary research studies) will/will not be included in your work. Using specific criteria will help make sure your final review is as unbiased, transparent and ethical as possible.
The type of review undertaken is influenced by the purpose of the review and resources available. However, the stages or methods used to undertake a review are similar across approaches and include: Formulating clear inclusion and exclusion criteria, for example, patient groups, ages, conditions/treatments, sources of evidence/research designs;
Sample selection is an integral part of systematic literature reviews and includes three steps: (a) the identification and (b) screening of potentially relevant research items and (c) a disclosure of the review sample.
A major factor in the quality of a systematic review is the effective and appropriate selection of inclusion and exclusion criteria to be used.
In this article, through a systematic search on the methodology of literature review, we categorize a typology of literature reviews, discuss steps in conducting a systematic literature review, and provide suggestions on how to enhance rigor in literature reviews in planning education and research.
Selection Criteria You may want to think about criteria that will be used to select articles for your literature review based on your research question. These are commonly known as inclusion criteria and exclusion criteria. Be aware that you may introduce bias into the final review if these are not used thoughtfully.
A literature review is a summary of the published work in a field of study. It can be a section of a larger paper or article, or the focus of an entire paper. ... Step 2: Determine inclusion and exclusion criteria. Once you have a clearly defined research question, make sure you will get the search results you want by making decisions about ...
Common errors regarding inclusion and exclusion criteria include the following: using the same variable to define both inclusion and exclusion criteria (for example, in a study including only men, listing being a female as an exclusion criterion); selecting variables as inclusion criteria that are not related to answering the research question ...
Using Limits and Inclusion/Exclusion Criteria Once you have some search results, you will need to decide which articles you will actually use in your literature review. This can be done using filters/limits in the databases, applying inclusion/exclusion criteria, and appraising the articles.
With this in mind, our narrative review will give a detailed explanation of the important steps of a systematic review. Preferred reporting items for systematic review and meta-analysis protocols (PRISMA-P) checklist Research question 12 13 14 Inclusion and exclusion criteria Protocol registration 17 15 Using Boolean operators, truncation, and ...
Determine inclusion and exclusion criteria Once you have a clearly defined research question, make sure you are getting precisely the right search results from searching the databases by making decisions about these items:
Selection criteria Inclusion and exclusion criteria. In this systematic review, the scope was restricted to studies that draw samples from the general population, and the participants were either diagnosed with mental disorders or screened positive using a standardized scale. Case-control studies and cohort studies were considered for inclusion.
After this first filtering, 100 articles remained: 26 articles from China, 40 articles from Germany and 34 articles from the UK. For the next round, we developed the following two exclusion criteria to narrow down our selection that addressed the definition of inclusion at the early childhood level in the three countries.
A comprehensive literature search was conducted on two databases, PubMed and Google Scholar. Following the PRISMA protocol, the 3021 retrieved articles were filtered to 21 based on inclusion and exclusion criteria. An additional article was obtained through the reference list. Hence, 22 articles were selected for critical analysis in this review.
We review the scientific literature published between 2010 and May 2024 to analyze the current state of research on AI in production. ... production, and adoption), we followed clearly defined rules of inclusion and exclusion that all reviewers had to follow in the review process. As a first requirement for inclusion, AI must be the technology ...
Abstracts and full texts were independently screened based on predetermined inclusion and exclusion criteria. Data synthesis was performed using the convergent integrated analysis framework recommended by the Joanna Briggs Institute. Results: A total of 12 studies met the inclusion criteria.
We screened the retrieved publications for inclusion or exclusion using the systematic review software, Covidence, to manage the screening process. We first screened records by title and abstract against the inclusion and exclusion criteria. Second, we retrieved publications that passed the first stage of screening to screen the full text.