

How To Determine Sample Size for Quantitative Research
This blog post looks at how large a sample size should be for reliable, usable market research findings.
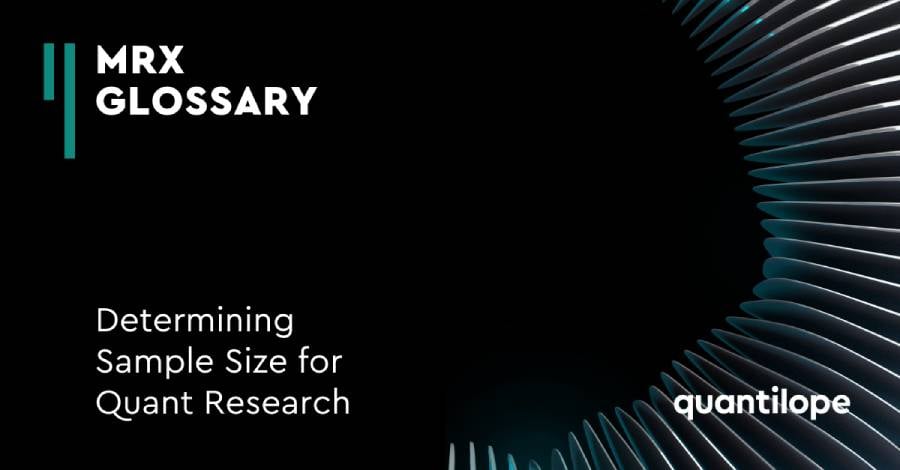
Jan 29, 2024
quantilope is the Consumer Intelligence Platform for all end-to-end research needs
Table of Contents:
What is sample size , why do you need to determine sample size , variables that impact sample size.
- Determining sample size
The sample size of a quantitative study is the number of people who complete questionnaires in a research project. It is a representative sample of the target audience in which you are interested.
Back to Table of Contents
You need to determine how big of a sample size you need so that you can be sure the quantitative data you get from a survey is reflective of your target population as a whole - and so that the decisions you make based on the research have a firm foundation. Too big a sample and a project can be needlessly expensive and time-consuming. Too small a sample size, and you risk interviewing the wrong respondents - meaning ultimately you miss out on valuable insights.
There are a few variables to be aware of before working out the right sample size for your project.
Population size
The subject matter of your research will determine who your respondents are - chocolate eaters, dentists, homeowners, drivers, people who work in IT, etc. For your respective group of interest, the total of this target group (i.e. the number of chocolate eaters/homeowners/drivers that exist in the general population) will guide how many respondents you need to interview for reliable results in that field.
Ideally, you would use a random sample of people who fit within the group of people you’re interested in. Some of these people are easy to get hold of, while others aren‘t as easy. Some represent smaller groups of people in the population, so a small sample is inevitable. For example, if you’re interviewing chocolate eaters aged 5-99 you’ll have a larger sample size - and a much easier time sampling the population - than if you’re interviewing healthcare professionals who specialize in a niche branch of medicine.
Confidence interval (margin of error)
Confidence intervals, otherwise known as the margin of error, indicate the reliability of statistics that have been calculated by research; in other words, how certain you can be that the statistics are close to what they would be if it were possible to interview the entire population of the people you’re researching.
Confidence intervals are helpful since it would be impossible to interview all chocolate eaters in the US. However, statistics and research enable you to take a sample of that group and achieve results that reflect their opinions as a total population. Before starting a research project, you can decide how large a margin of error you will allow between the mean number of your sample and the mean number of its total population. The confidence interval is expressed as +/- a number, indicating the margin of error on either side of your statistic. For example, if 35% of chocolate eaters say that they eat chocolate for breakfast and your margin of error is 5, you’ll know that if you had asked the entire population, 30-40% of people would admit to eating chocolate at that time of day.
Confidence level
The confidence level indicates how probable it is that if you were to repeat your study multiple times with a random sample, you would get the same statistics and they would fall within the confidence interval every time.
In the example above, if you were to repeat the chocolate study over and over, you would have a certain level of confidence that those eating chocolate for breakfast would always fall within the 30-40% parameters. Most research studies have confidence intervals of 90% confident, 95% confident, or 99% confident. The number you choose will depend on whether you are happy to accept a broadly accurate set of data or whether the nature of your study demands one that is almost completely reliable.
Standard deviation
Standard deviation represents how much the results will vary from the mean number and from each other. A high standard deviation means that there is a wide range of responses to your research questions, while a low standard deviation indicates that responses are more similar to each other, clustered around the mean number. A standard deviation of 0.5 is a safe level to pick to ensure that the sample size is large enough.
Population variability
If you already know anything about your target audience, you should have a feel for the degree to which their opinions vary. If you’re interviewing the entire population of a city, without any other criteria, their views are going to be wildly diverse so you’ll want to sample a high number of residents. If you’re honing in on a sample of chocolate breakfast eaters - there’s probably a limited number of reasons why that’s their meal of choice, so you can feel confident with a much smaller sample.
Project scope
The scope and objectives of the research will have an influence on how big the sample is. If the project aims to evaluate four different pieces of stimulus (an advert, a concept, a website, etc.) and each respondent is giving feedback on a single piece, then a higher number of respondents will need to be interviewed than if each respondent were evaluating all four; the same would be true when looking for reads on four different sub-audiences vs. not needing any sub-group data cuts.
Determining a good sample size for quantitative research
Sample size, as we’ve seen, is an important factor to consider in market research projects. Getting the sample size right will result in research findings you can use confidently when translating them into action. So now that you’ve thought about the subject of your research, the population that you’d like to interview, and how confident you want to be with the findings, how do you calculate the appropriate sample size?
There are many factors that can go into determining the sample size for a study, including z-scores, standard deviations, confidence levels, and margins of error. The great thing about quantilope is that your research consultants and data scientists are the experts in helping you land on the right target so you can focus on the actual study and the findings.
To learn more about determining sample size for quantitative research, get in touch below:
Get in touch to learn more about quantitative sample sizes!
Latest articles.
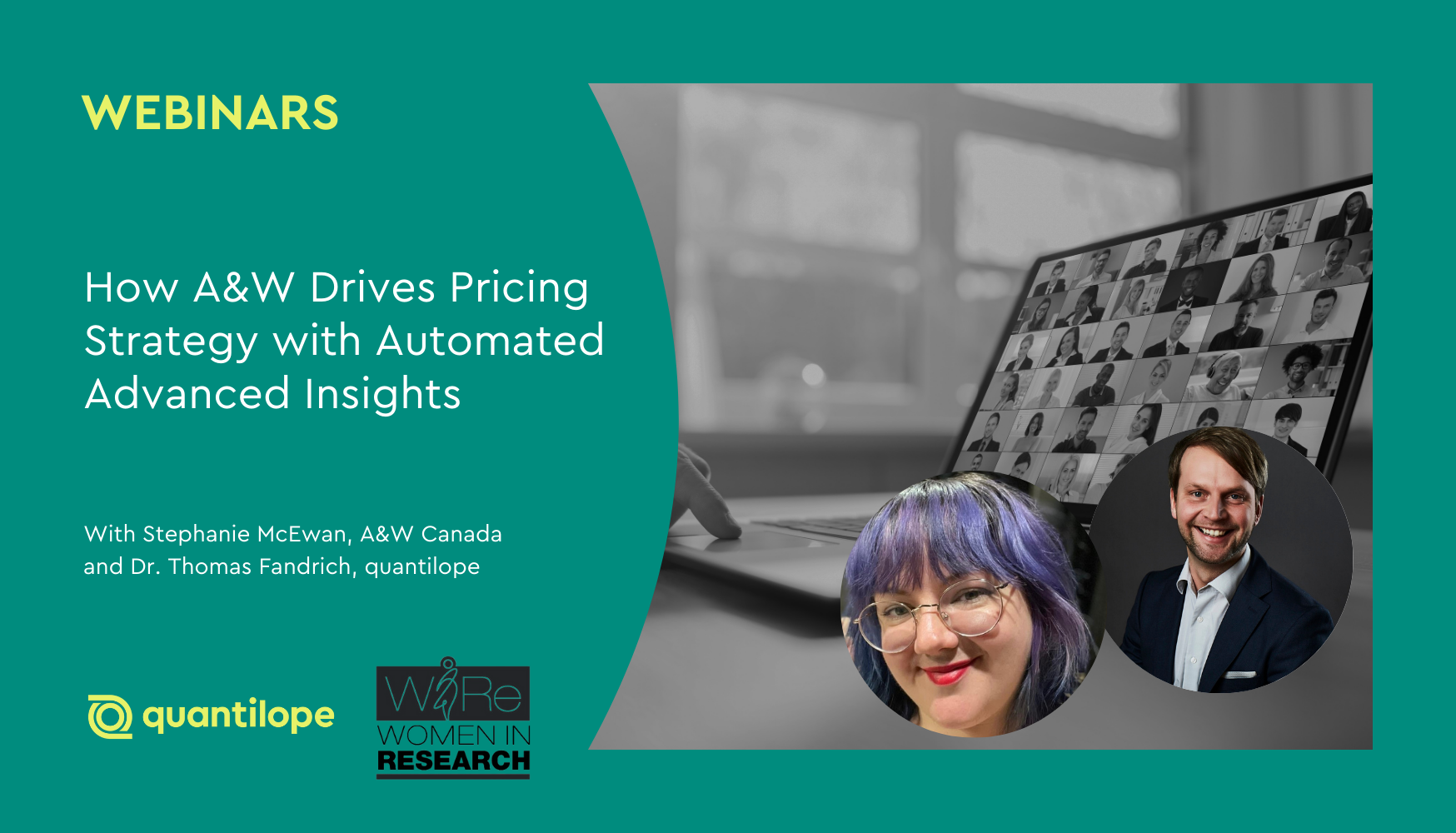
quantilope & WIRe: How Automated Insights Drive A&W's Pricing Strategy
Discover how A&W's application of advanced research methods has enabled its insights team to deliver data-driven recommendations with actio...
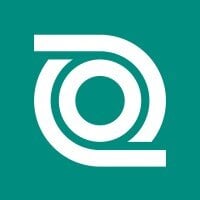
September 09, 2024
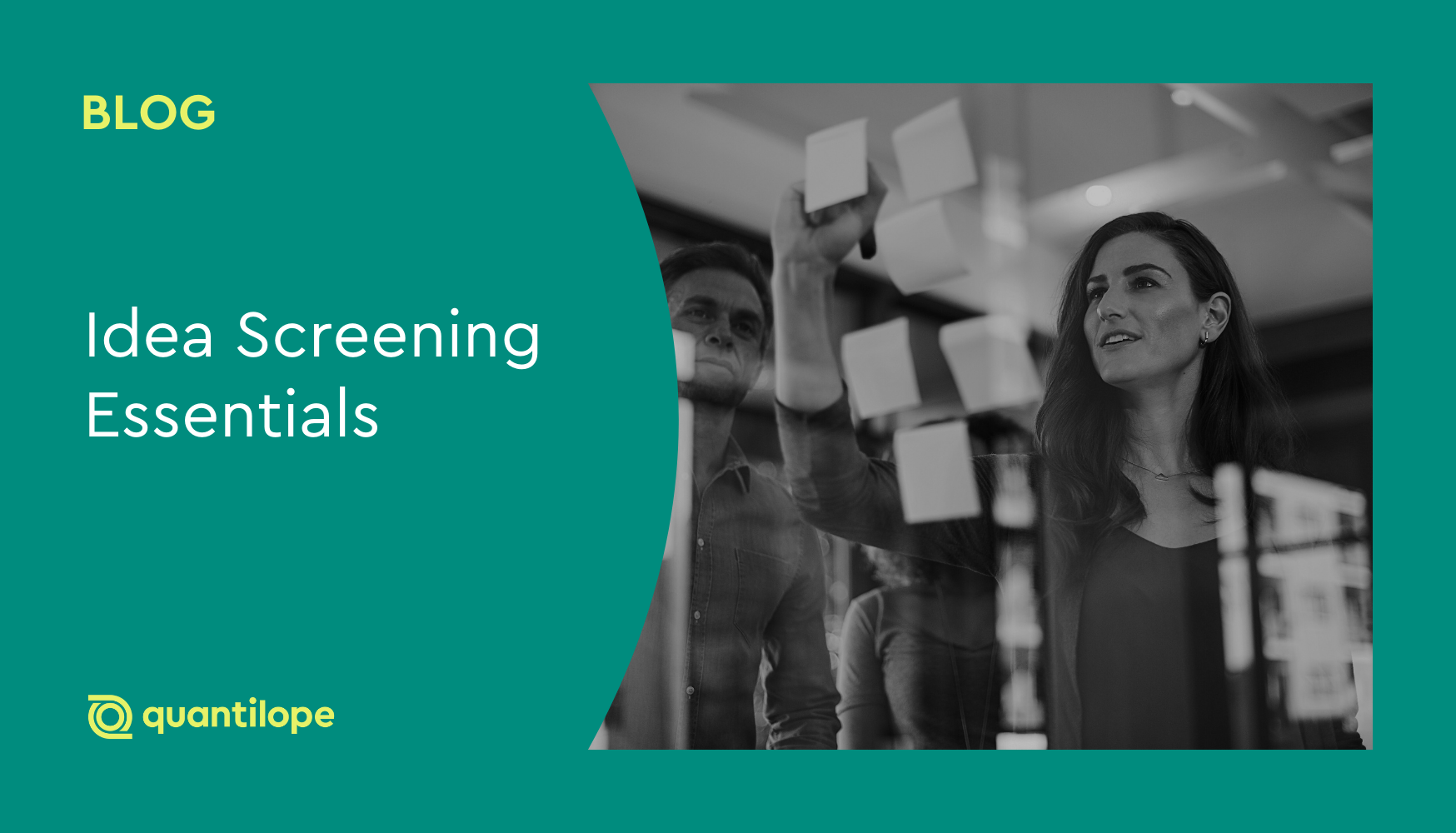
The Essential Guide to Idea Screening: Turning Concepts Into Reality
In this guide, we'll break down the essentials of idea screening, starting by defining its purpose and exploring the top techniques for suc...
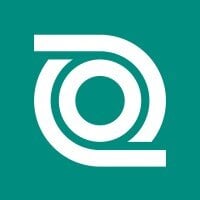
September 04, 2024
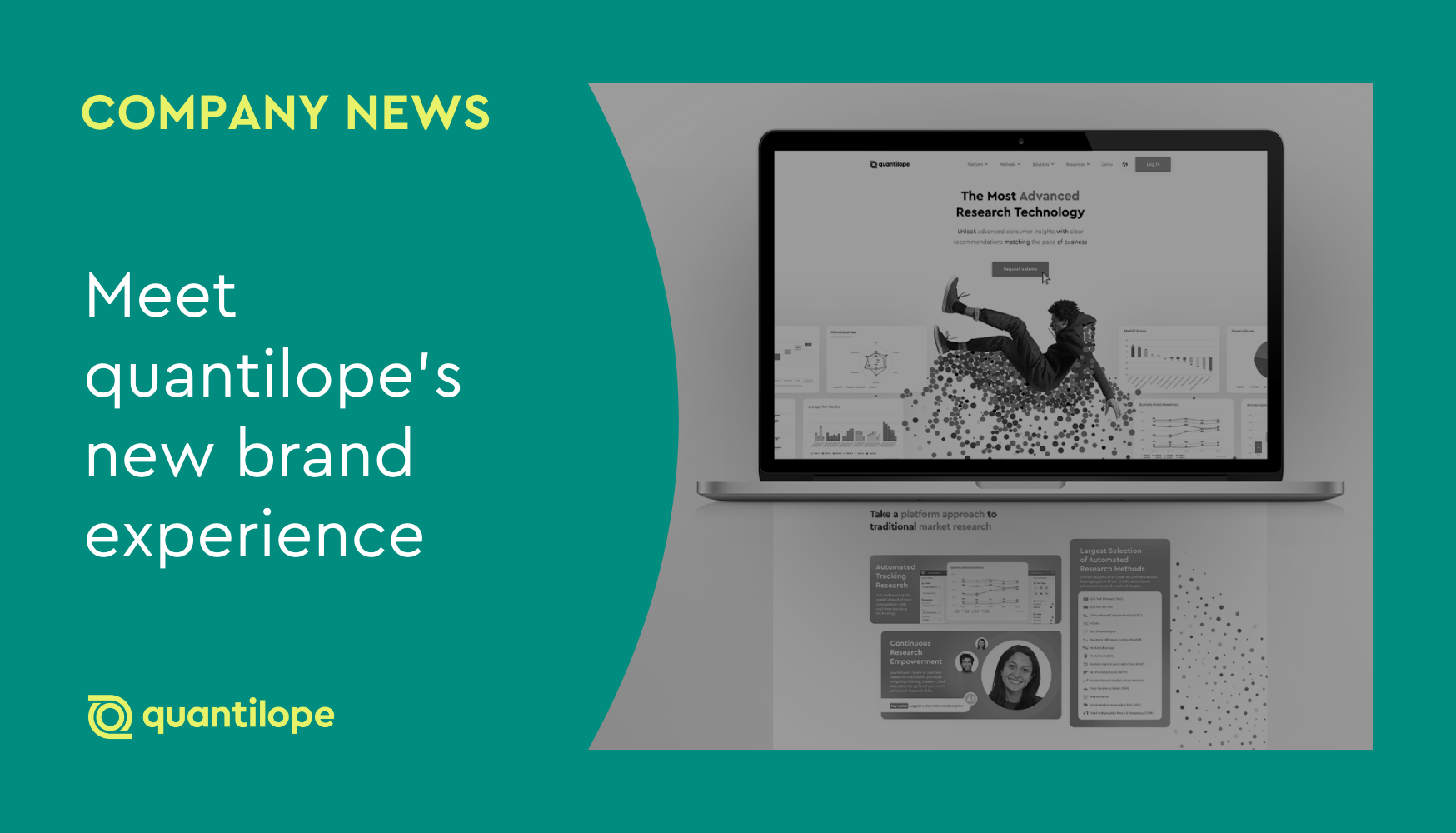
The New quantilope Brand Experience
Introducing quantilope's new brand experience featuring a brighter, fresher look and feel.
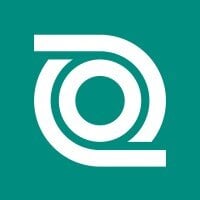
- Skip to secondary menu
- Skip to main content
- Skip to primary sidebar
Statistics By Jim
Making statistics intuitive
Sample Size Essentials: The Foundation of Reliable Statistics
By Jim Frost 4 Comments
What is Sample Size?
Sample size is the number of observations or data points collected in a study. It is a crucial element in any statistical analysis because it is the foundation for drawing inferences and conclusions about a larger population .

Imagine you’re tasting a new brand of cookies. Sampling just one cookie might not give you a true sense of the overall flavor—what if you picked the only burnt one? Similarly, in statistics, the sample size determines how well your study represents the larger group. A larger sample size can mean the difference between a snapshot and a panorama, providing a clearer, more accurate picture of the reality you’re studying.
In this blog post, learn why adequate sample sizes are not just a statistical nicety but a fundamental component of trustworthy research. However, large sample sizes can’t fix all problems. By understanding the impact of sample size on your results, you can make informed decisions about your research design and have more confidence in your findings .
Benefits of a Large Sample Size
A large sample size can significantly enhance the reliability and validity of study results. We’re primarily looking at how well representative samples reflect the populations from which the researchers drew them. Here are several key benefits.
Increased Precision
Larger samples tend to yield more precise estimates of the population parameters . Larger samples reduce the effect of random fluctuations in the data, narrowing the margin of error around the estimated values.
Estimate precision refers to how closely the results obtained from a sample align with the actual population values. A larger sample size tends to yield more precise estimates because it reduces the effect of random variability within the sample. The more data points you have, the smaller the margin of error and the closer you are to capturing the correct value of the population parameter.
For example, estimating the average height of adults using a larger sample tends to give an estimate closer to the actual average than using a smaller sample.
Learn more about Statistics vs. Parameters , Margin of Error , and Confidence Intervals .
Greater Statistical Power
The power of a statistical test is its capability to detect an effect if there is one, such as a difference between groups or a correlation between variables. Larger samples increase the likelihood of detecting actual effects.
Statistical power is the probability that a study will detect an effect when one exists. The sample size directly influences it; a larger sample size increases statistical power . Studies with more data are more likely to detect existing differences or relationships.
For instance, in testing whether a new drug is more effective than an existing one, a larger sample can more reliably detect small but real improvements in efficacy .
Better Generalizability
With a larger sample, there is a higher chance that the sample adequately represents the diversity of the population, improving the generalizability of the findings to the population.
Consider a national survey gauging public opinion on a policy. A larger sample captures a broader range of demographic groups and opinions.
Learn more about Representative Samples .
Reduced Impact of Outliers
In a large sample, outliers have less impact on the overall results because many observations dilute their influence. The numerous data points stabilize the averages and other statistical estimates, making them more representative of the general population.
If measuring income levels within a region, a few very high incomes will distort the average less in a larger sample than in a smaller one .
Learn more about 5 Ways to Identify Outliers .
The Limits of Larger Sample Sizes: A Cautionary Note
While larger sample sizes offer numerous advantages, such as increased precision and statistical power, it’s important to understand their limitations. They are not a panacea for all research challenges. Crucially, larger sample sizes do not automatically correct for biases in sampling methods , other forms of bias, or fundamental errors in study design. Ignoring these issues can lead to misleading conclusions, regardless of how many data points are collected.
Sampling Bias
Even a large sample is misleading if it’s not representative of the population. For instance, if a study on employee satisfaction only includes responses from headquarters staff but not remote workers, increasing the number of respondents won’t address the inherent bias in missing a significant segment of the workforce.
Learn more about Sampling Bias: Definition & Examples .
Other Forms of Bias
Biases related to data collection methods, survey question phrasing, or data analyst subjectivity can still skew results. If the underlying issues are not addressed, a larger sample size might magnify these biases instead of mitigating them.
Errors in Study Design
Simply adding more data points will not overcome a flawed experimental design . For example, increasing the sample size will not clarify the causal relationships if the design doesn’t control a confounding variable .
Large Sample Sizes are Expensive!
Additionally, it is possible to have too large a sample size. Larger sizes come with their own challenges, such as higher costs and logistical complexities. You get to a point of diminishing returns where you have a very large sample that will detect such small effects that they’re meaningless in a practical sense.
The takeaway here is that researchers must exercise caution and not rely solely on a large sample size to safeguard the reliability and validity of their results. An adequate amount of data must be paired with an appropriate sampling method, a robust study design, and meticulous execution to truly understand and accurately represent the phenomena being studied .
Sample Size Calculation
Statisticians have devised quantitative ways to find a good sample size. You want a large enough sample to have a reasonable chance of detecting a meaningful effect when it exists but not too large to be overly expensive.
In general, these methods focus on using the population’s variability . More variable populations require larger samples to assess them. Let’s go back to the cookie example to see why.
If all cookies in a population are identical (zero variability), you only need to sample one cookie to know what the average cookie is like for the entire population. However, suppose there’s a little variability because some cookies are cooked perfectly while others are overcooked. You’ll need a larger sample size to understand the ratio of the perfect to overcooked cookies.
Now, instead of just those two types, you have an entire range of how much they are over and undercooked. And some use sweeter chocolate chips than others. You’ll need an even larger sample to understand the increased variability and know what an average cookie is really like.

Power and sample size analysis quantifies the population’s variability. Hence, you’ll often need a variability estimate to perform this type of analysis. These calculations also frequently factor in the smallest practically meaningful effect size you want to detect, so you’ll use a manageable sample size.
To learn more about determining how to find a sample size, read my following articles :
- How to Calculate Sample Size
- What is Power in Statistics?
Sample Size Summary
Understanding the implications of sample size is fundamental to conducting robust statistical analysis. While larger samples provide more reliable and precise estimates, smaller samples can compromise the validity of statistical inferences.
Always remember that the breadth of your sample profoundly influences the strength of your conclusions. So, whether conducting a simple survey or a complex experimental study, consider your sample size carefully. Your research’s integrity depends on it.
Consequently, the effort to achieve an adequate sample size is a worthwhile investment in the precision and credibility of your research .
Share this:

Reader Interactions
September 11, 2024 at 10:09 am
Hi Jim, thanks for your post.
It’s clear that a small sample size could take to a type 2 error. But Could it put my study in risk to make a type 1 error? I mean, compared to a correct sample size based on proper calculations?
September 11, 2024 at 1:33 pm
That’s a great question! The surprising answer is that increasing or decreasing the sample size actually does not affect the type 1 error rate! The reason why is because as you increase or decrease the sample size, the detectable effect size changes to maintain an error rate that equals your significance level. Controlling the false positives is built right into the equations and process.
So, if you’re studying a certain subject and you have a sample size of 10 or 1000, your false positive error rate is constant. However, as you mention, the type 2, false negative error will decrease as sample size increases.
August 29, 2024 at 7:00 am
A problem which ought to be considered when running an opinion poll: Is the group of people who consent to answer strictly comparable to the group who do not consent?. If not, then there may be systematic bias
July 17, 2024 at 11:11 am
When I used survey data, we had a clear, conscious sampling method and the distinction made sense. However, with other types of data such as performance or sales data, I’m confused about the distinction. We have all the data of everyone who did the work, so by that understanding, we aren’t doing any sampling. However, is there a ‘hidden’ population of everyone who could potentially do that work? If we take a point in time, such as just first quarter performance, is that a sample or something else? I regularly see people just go ahead and apply the same statistics to both, suggesting that this is a ‘sample’, but I’m not sure what it’s a sample of or how!
Comments and Questions Cancel reply
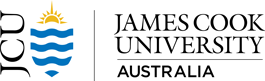
Want to create or adapt books like this? Learn more about how Pressbooks supports open publishing practices.
3.4 Sampling Techniques in Quantitative Research
Target population.
The target population includes the people the researcher is interested in conducting the research and generalizing the findings on. 40 For example, if certain researchers are interested in vaccine-preventable diseases in children five years and younger in Australia. The target population will be all children aged 0–5 years residing in Australia. The actual population is a subset of the target population from which the sample is drawn, e.g. children aged 0–5 years living in the capital cities in Australia. The sample is the people chosen for the study from the actual population (Figure 3.9). The sampling process involves choosing people, and it is distinct from the sample. 40 In quantitative research, the sample must accurately reflect the target population, be free from bias in terms of selection, and be large enough to validate or reject the study hypothesis with statistical confidence and minimise random error. 2
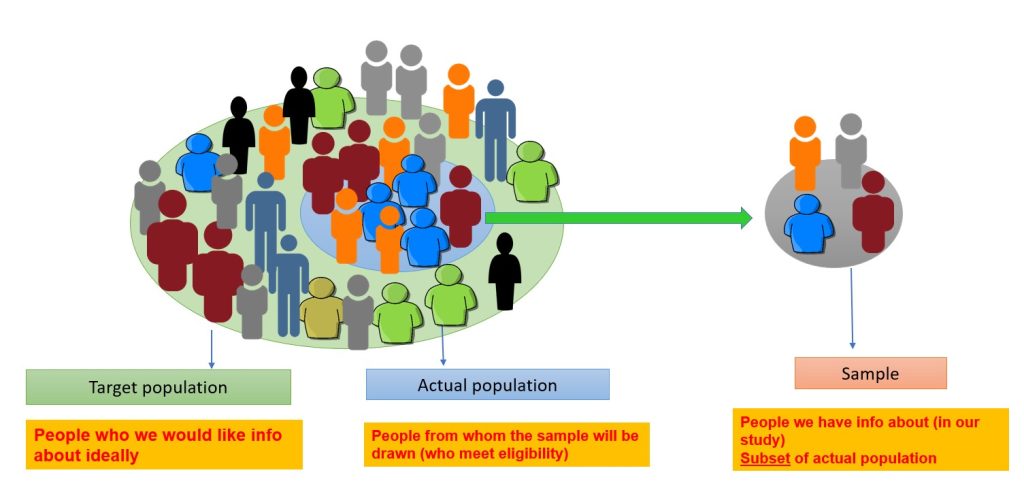
Sampling techniques
Sampling in quantitative research is a critical component that involves selecting a representative subset of individuals or cases from a larger population and often employs sampling techniques based on probability theory. 41 The goal of sampling is to obtain a sample that is large enough and representative of the target population. Examples of probability sampling techniques include simple random sampling, stratified random sampling, systematic random sampling and cluster sampling ( shown below ). 2 The key feature of probability techniques is that they involve randomization. There are two main characteristics of probability sampling. All individuals of a population are accessible to the researcher (theoretically), and there is an equal chance that each person in the population will be chosen to be part of the study sample. 41 While quantitative research often uses sampling techniques based on probability theory, some non-probability techniques may occasionally be utilised in healthcare research. 42 Non-probability sampling methods are commonly used in qualitative research. These include purposive, convenience, theoretical and snowballing and have been discussed in detail in chapter 4.
Sample size calculation
In order to enable comparisons with some level of established statistical confidence, quantitative research needs an acceptable sample size. 2 The sample size is the most crucial factor for reliability (reproducibility) in quantitative research. It is important for a study to be powered – the likelihood of identifying a difference if it exists in reality. 2 Small sample-sized studies are more likely to be underpowered, and results from small samples are more likely to be prone to random error. 2 The formula for sample size calculation varies with the study design and the research hypothesis. 2 There are numerous formulae for sample size calculations, but such details are beyond the scope of this book. For further readings, please consult the biostatistics textbook by Hirsch RP, 2021. 43 However, we will introduce a simple formula for calculating sample size for cross-sectional studies with prevalence as the outcome. 2
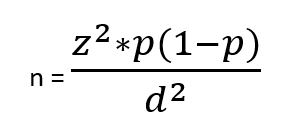
z is the statistical confidence; therefore, z = 1.96 translates to 95% confidence; z = 1.68 translates to 90% confidence
p = Expected prevalence (of health condition of interest)
d = Describes intended precision; d = 0.1 means that the estimate falls +/-10 percentage points of true prevalence with the considered confidence. (e.g. for a prevalence of 40% (0.4), if d=.1, then the estimate will fall between 30% and 50% (0.3 to 0.5).
Example: A district medical officer seeks to estimate the proportion of children in the district receiving appropriate childhood vaccinations. Assuming a simple random sample of a community is to be selected, how many children must be studied if the resulting estimate is to fall within 10% of the true proportion with 95% confidence? It is expected that approximately 50% of the children receive vaccinations
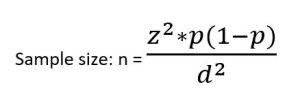
z = 1.96 (95% confidence)
d = 10% = 10/ 100 = 0.1 (estimate to fall within 10%)
p = 50% = 50/ 100 = 0.5
Now we can enter the values into the formula

Given that people cannot be reported in decimal points, it is important to round up to the nearest whole number.
An Introduction to Research Methods for Undergraduate Health Profession Students Copyright © 2023 by Faith Alele and Bunmi Malau-Aduli is licensed under a Creative Commons Attribution-NonCommercial 4.0 International License , except where otherwise noted.
- Translation

The importance of having Large Sample Sizes for your research
By charlesworth author services.
- Charlesworth Author Services
- 26 May, 2022
Sample size can be defined as the number of pieces of information, data points or patients (in medical studies) tested or enrolled in an experiment or study . On any hypothesis , scientific research is built upon determining the mean values of a given dataset. The larger the sample size, the more accurate the average values will be. Larger sample sizes also help researchers identify outliers in data and provide smaller margins of error.
But just what is a ‘large scientific study’ with a ‘large sample size’?
Why are such studies important?
What type of research benefits most from large sample sizes?
And how can a researcher ensure they have an adequately large study?
Here, we discuss these various aspects of studies with large sample sizes.
Defining ‘large sample size’ / ‘large study’ by topic
The size of a ‘large’ study depends on the topic.
- In medicine , large studies investigating common conditions such as heart disease or cancer may enrol tens of thousands of patients with multiple years of follow-up.
- For specialty journals , ‘large studies’ may include clinical studies with hundreds of patients.
- For highly specialised topics (such as certain rare genetic conditions), large patient populations may not exist. For such research, a ‘large’ study may enrol the entire known global population with the condition, which could be as few as dozens of patients.
Statistical importance of having a large sample size
- Larger studies provide stronger and more reliable results because they have smaller margins of error and lower standards of deviation . (Standard deviation measures how spread out the data values are from the mean. The larger the study sample size, the smaller the margin of error.)
- Larger sample sizes allow researchers to control the risk of reporting false-negative or false-positive findings . The greater number of samples, the greater the precision of results will be.
A useful primer that discusses the importance of sample size in planning and interpreting medical research can be found here .
Fields that benefit most from large sample sizes
Large sample sizes benefit many fields of research, including:
- Medicine : Quality efficacy of treatment protocols, anatomic studies and biomechanical investigations all require large sample sizes. Ongoing COVID-19 vaccine trials depend on large volunteer patient populations.
- Natural sciences : Long-term climate studies, agricultural science, zoology and the like all require large studies with thousands of data points.
- Social sciences : Much social science research, public opinion and political polls, census and other demographic information, etc. rely heavily on large-scale survey studies.
Importance of a larger sample size from a publishing perspective
Academic publishers seek to publish research with the highest-quality, most-reliable and most-certain data. As an author, it is greatly to your advantage to submit manuscripts based on studies having as large a sample size as possible.
That said, there are limits to certainty and reliability of results. But that discussion would be beyond the scope of this article.
Determining an adequate sample size
In determining an adequate sample size for an experiment, you must establish the following:
- Justifiable level of statistical significance
- Chances of detecting a difference of given magnitude between the groups compared (the study’s power)
- Targeted difference (effect size)
- Variability of the data
Ensuring you have an adequately large study
Working with a biostatistician and experts familiar with study design will help you determine how large a study sample you need in order to determine a highly accurate answer to your specific hypothesis.
Note that not all research questions require massively large sample sizes. However, many do, and for such research, you may need to design, obtain funding for and conduct a multi-centre study or meta-analysis of existing studies .
Note : Multi-centre studies may come with many logistical, financial, ethical and analytical challenges. But when properly designed and executed, they provide some of the most definitive and highly cited publications.
One of the main goals of scientific research and publishing is to answer questions with as much certainty as possible. Ensuring large sample sizes in research studies would go a long way towards providing sufficient levels of certitude. Such large studies benefit numerous research applications in a wide variety of scientific and social science fields.
Maximise your publication success with Charlesworth Author Services.
Charlesworth Author Services, a trusted brand supporting the world’s leading academic publishers, institutions and authors since 1928.
To know more about our services, visit: Our Services
Share with your colleagues
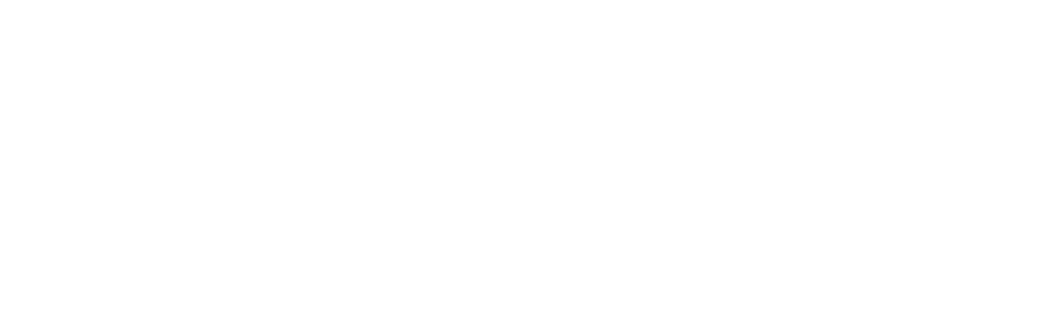
Scientific Editing Services
Sign up – stay updated.
We use cookies to offer you a personalized experience. By continuing to use this website, you consent to the use of cookies in accordance with our Cookie Policy.
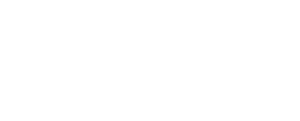
How to Determine the Right Sample Size for Quantitative Research
Emi to be exclusive agent for ag access community.
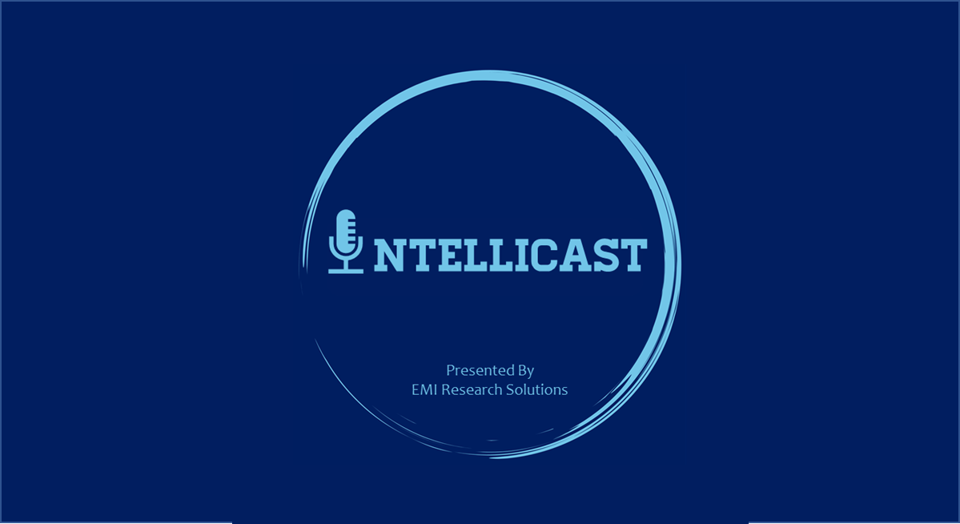
Intellicast S5E5 – Talking Data Quality with Cheskie Rosenzweig of CloudResearch
- Best Practices
- Data Collection
- Market Research
- sample calculator
- sample size
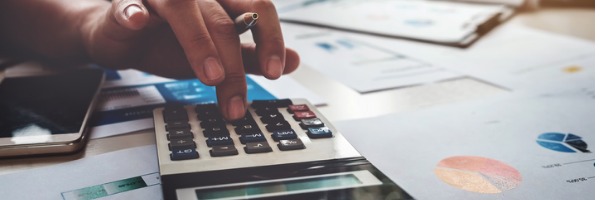
Sample size is just as important as any other aspect of your project. We use market research as a tool to understand consumers’ attitudes and behaviors and base critical business decisions off its results. To do this, we use surveys and respondents who act as a representation of the larger population. That’s why it’s so important that your sample matches the population. We have to understand that the sample size needs to correlate to the actual population in order to produce statistically relevant results that can be attributed to actual consumers, helping you make the best decisions for your business.
As providers of sample, we are often asked what market research sample size is needed for a study. The answer to this question is complicated—not only because the statistical formula to calculate sample size is complicated, but also because there are other factors that determine base size—primarily quotas and the level of risk of the study.
Determining the sample size in a quantitative research study is challenging. There are a variety of factors and variables to consider, including population size, confidence level, the margin of error, standard deviation, etc.
Population Size:
How big is the actual population of the group you’re surveying? Generally, in market research, we deal with rather large population sizes and use a constant for this input. When using statistical probability, the same sample size can be used to represent the opinions of 100,000 people or many million.
Our sample comes from actively managed online research panels, but we can also access other sources depending on the target group, and upon your request or approval. While our focus has been on double opt-in online panelists, we’ve enhanced our offering with river and social media sample so that we can deliver the most representative sample possible.
Confidence Level:
When it comes to the research behind your business decisions, you want to make sure the results are accurate. There are many ways to ensure confidence in your data, and there are also ways to measure it. Typically, the following confidence levels are utilized, although you can really pick any number:
- 99% = High Ris k. For example, if you are making a billion-dollar investment decision you may want to be extremely confident in the data.
- 95% = Medium-to-High Risk. When you want high confidence, but at a smaller base size.
- 9 0% = Medium-to-Low Risk. This is rather typical in most consumer research.
- 80% = Low Risk . If you only need to be reasonably confident in the research and your business decision is low risk, this is ideally the lowest confidence level you would ever choose.
For the formula, the confidence level corresponds to a z-score. Here are the z-scores for the above confidence levels:
- 99% = 2.326
- 90% = 1.645
- 80% = 1.282
The z-score is the number of standard deviations a given proportion is away from the mean.
Margin of Error :
The margin of error is a percentage that tells you how much you can expect your survey results to reflect the views of the overall population. The smaller the margin of error, the closer you are to having the exact answer at a given confidence level.
Typical margins of error are:
- 2% = High Risk
- 5% = Normal or Low Risk
Standard Deviation :
Standard deviation measures how much variance you expect in the data. We typically use .5 as a constant. However, there are a few things that must be considered when calculating sample size. First, if you want a smaller margin of error, you must have a larger sample size given the same population. Second, the higher the sampling confidence level you want to have, the larger your sample size will need to be. Generally, the rule of thumb is that the larger the sample size, the more statistically significant it is—meaning there’s less of a chance that your results happened by coincidence.
In market research, this is key. It’s vital to ensure that the sample provider you work with looks at research and data as a whole. A quality market research partner takes research into account beyond just that which was fielded.
How to Calculate Your Needed Sample Sample Size
Necessary Sample Size = (Z-score)² * StdDev*(1-StdDev) / (margin of error)²
Here is a quick cheat sheet for sample size ( based on some basic assumptions ):
- Margin of error: 5%
- Confidence level: 80%
- Recommended sample = 165
Low-to-Mid Risk
- Confidence level: 90%
- Recommended sample = 271
Mid-to-High Risk
- Margin of error: 4%
- Confidence level: 95%
- Recommended sample = 600
- Margin of error: 2%
- Confidence level: 99%
- Recommended sample = 4,130
As you can see, determining the correct sample size for a study is not an easy task. There are many factors and variables to consider, and each has a direct impact on the success of your study. However, it is a necessary aspect of market research and one that is crucial to the accuracy of the data we collect. We must ensure that the sample size correlates to the population to produce statistically relevant results. Here at EMI, we understand how to determine the correct sample size for each unique study we conduct, ensuring you get the highest quality data and the best results.
Want to calculate the actual sample size you may need? Click the button below and we can help!
Related posts
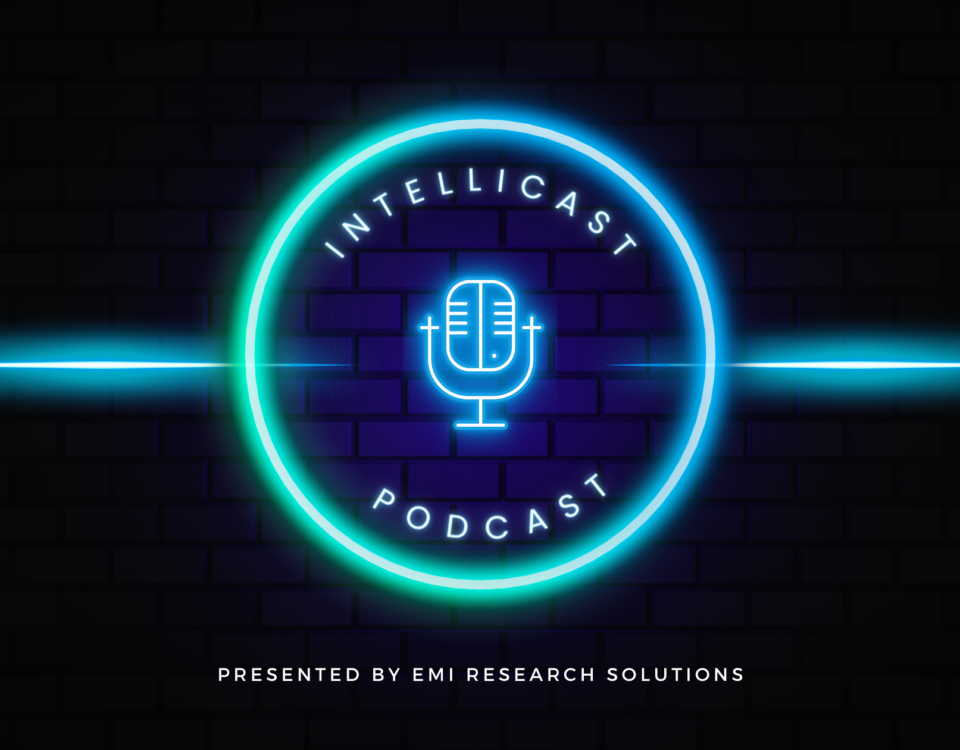
Respondent Engagement, Rebrands, And New AI Legislation
The u.s. economy: more people displeased than pleased, inside the voter mindset: key motivations behind 2024 presidential choices, privacy overview.
Explore the Scientific R&D Platform
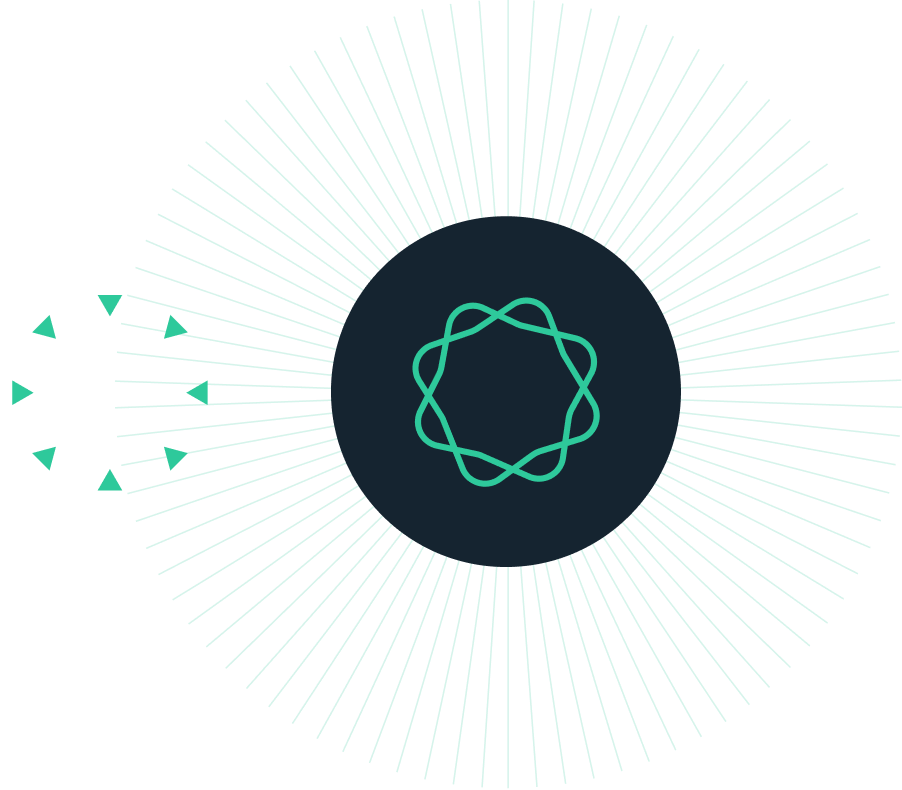
Scientific intelligence platform for AI-powered data management and workflow automation
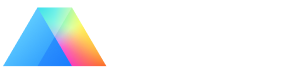
Statistical analysis and graphing software for scientists
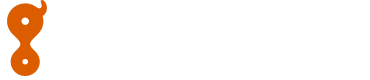
Bioinformatics, cloning, and antibody discovery software
Plan, visualize, & document core molecular biology procedures
Proteomics software for analysis of mass spec data

Electronic Lab Notebook to organize, search and share data
Modern cytometry analysis platform
Analysis, statistics, graphing and reporting of flow cytometry data
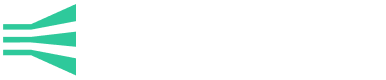
Intelligent panel design & inventory management for flow cytometry
Software to optimize designs of clinical trials
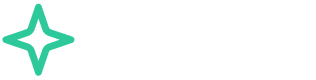
Computational fluid dynamics (CFD) software for engineers and researchers

Genetic analysis software for research, forensics & healthcare applications
Everything to Know About Sample Size Determination
A step-by-step interactive guide including common pitfalls
Nothing showing? Click here to accept marketing cookies
|
Download and explore the data yourself. Data files include:
- Blinded Sample Size Re-estimation.nqt
- Blinded SiteAndSubject.nqt
- Center Covariate Reducing Sample Size.nqt
- Cluster Randomized Extension.nqt
- External Pilot Study Sample Size Example.nqt
- Log-Rank Test Everolimus.nqt
- Log-Rank Test with Dropout.nqt
- MaxCombo Model Selection with Delayed Effect.nqt
- Responder Analysis Higher Sample Size Chi-Squared.nqt
- Two Means Group Sequential Replication.nqt
- Two Proportions Inequality Difference Scale.nqt
- Two Proportions Inequality Ratio Scale.nqt
- Two Proportions Non-inferiority Difference Scale.nqt
- Two Proportions Non-inferiority Ratio Scale.nqt
- Two Sample t-test Simvastin.nqt
- Win Ratio for Composite Endpoint.nqt
Designing a trial involves considering and balancing a wide variety of clinical, logistical and statistical factors.
One decision out of many is how large a study needs to be to have a reasonable chance of success. Sample size determination is the process by which trialists can find the ideal number of participants to balance the statistical and practical aspects that inform study design.
In this interactive webinar, we provided a comprehensive overview of sample size determination, the key steps to successfully finding the appropriate sample size and cover several common pitfalls researchers fall into when finding the sample size for their study.
In this free webinar we will cover
- What is sample size determination?
- A step-by-step guide to sample size determination
- Common sample size pitfalls and solutions
+ Q&A about your sample size issues!
In most clinical trials, sample size determination is found by reaching a predefined statistical power - typically defined as the Type II error or how likely a significant p-value is under a given treatment effect.
Power calculations require pre-study knowledge about the study design, statistical error rates, nuisance parameters (such as the variance) and effect size with each of these adding additional complexity.
Sample size determination has a number of common pitfalls which can lead to inappropriately small or large sample sizes with issues ranging from poor design decisions, misspecifying nuisance parameters or choosing the effect size inappropriately.
In this interactive webinar, we explore these and some solutions to avoid these mistakes and help maximise the efficiency of your clinical trial.
We provide a comprehensive overview of sample size determination, the key steps to successfully finding the appropriate sample size and cover several common pitfalls researchers fall into when finding the sample size for their study.
Looking for more trial design and sample size resources? Check out webinars to improve clinical trial designs & practical examples of sample size determination

Browse our Webinars
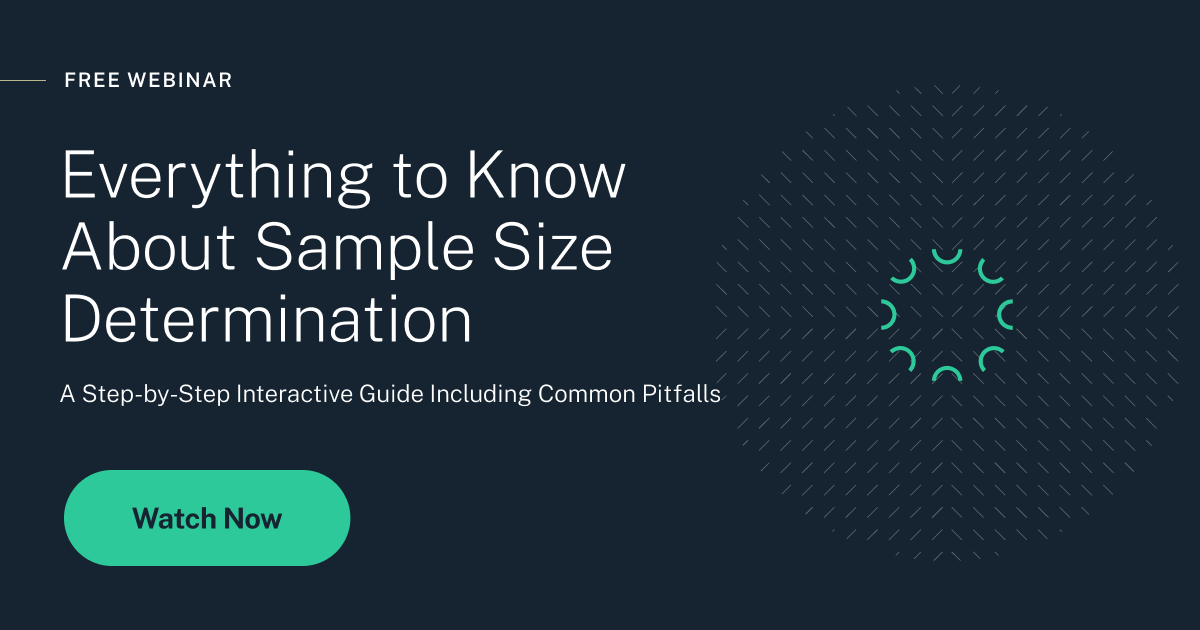
Guide to Sample Size
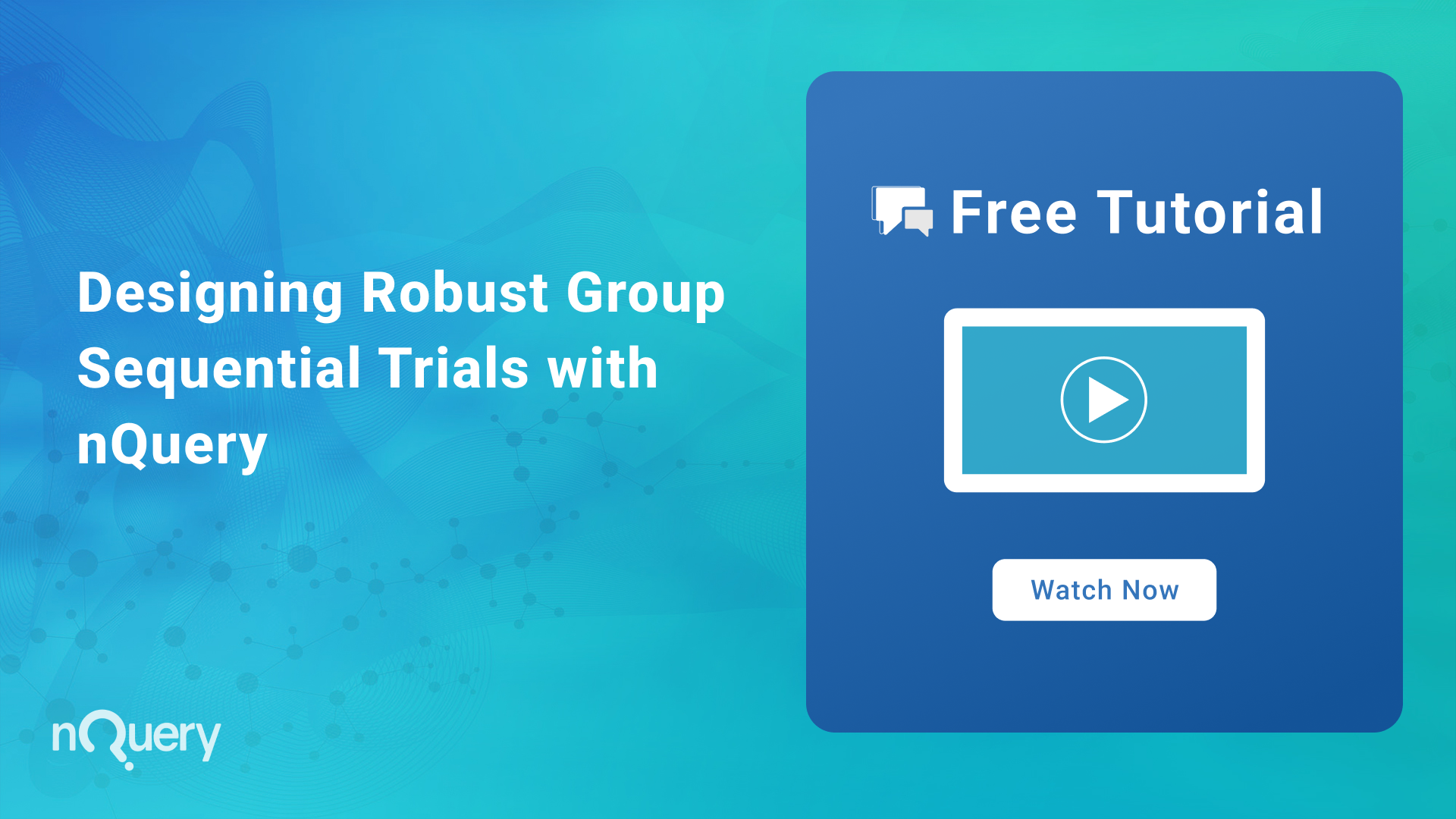
Designing Robust Group Sequential Trials | Free nQuery Training
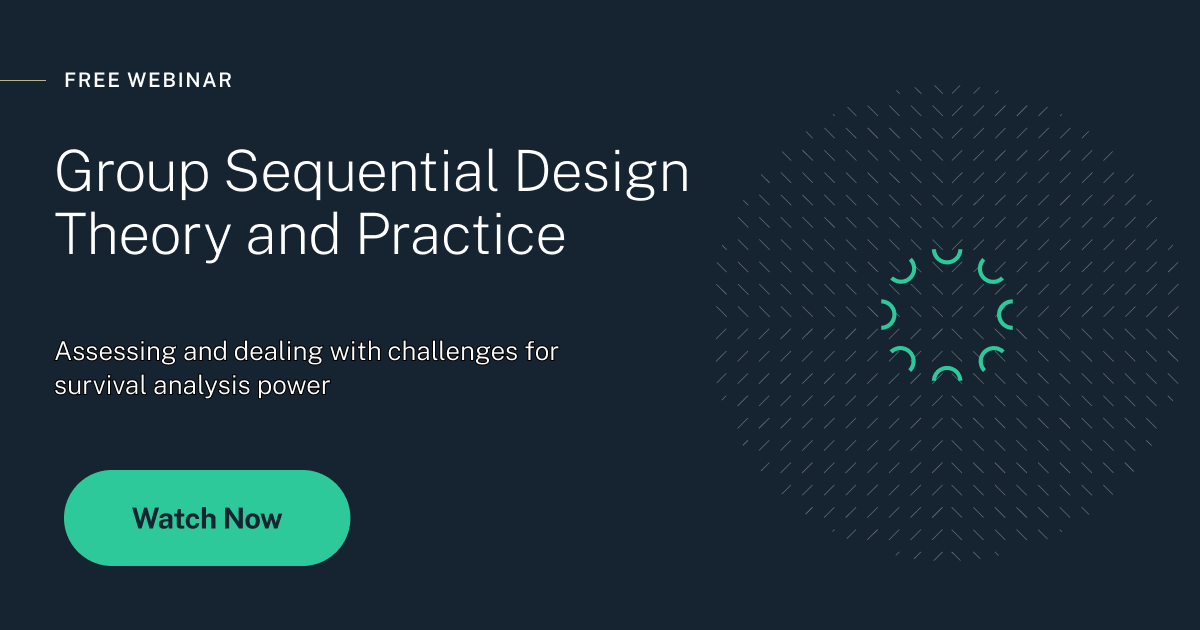
Group Sequential Design Theory and Practice
Get started with nQuery today
Try for free and upgrade as your team grows

An official website of the United States government
The .gov means it’s official. Federal government websites often end in .gov or .mil. Before sharing sensitive information, make sure you’re on a federal government site.
The site is secure. The https:// ensures that you are connecting to the official website and that any information you provide is encrypted and transmitted securely.
- Publications
- Account settings
Preview improvements coming to the PMC website in October 2024. Learn More or Try it out now .
- Advanced Search
- Journal List
- Clin Orthop Relat Res
- v.466(9); 2008 Sep

Statistics in Brief: The Importance of Sample Size in the Planning and Interpretation of Medical Research
David jean biau.
Département de Biostatistique et Informatique Médicale, INSERM – UMR-S 717, AP-HP, Université Paris 7, Hôpital Saint Louis, 1, avenue Claude-Vellefaux, Paris Cedex 10, 75475 France
Solen Kernéis
Raphaël porcher.
The increasing volume of research by the medical community often leads to increasing numbers of contradictory findings and conclusions. Although the differences observed may represent true differences, the results also may differ because of sampling variability as all studies are performed on a limited number of specimens or patients. When planning a study reporting differences among groups of patients or describing some variable in a single group, sample size should be considered because it allows the researcher to control for the risk of reporting a false-negative finding (Type II error) or to estimate the precision his or her experiment will yield. Equally important, readers of medical journals should understand sample size because such understanding is essential to interpret the relevance of a finding with regard to their own patients. At the time of planning, the investigator must establish (1) a justifiable level of statistical significance, (2) the chances of detecting a difference of given magnitude between the groups compared, ie, the power, (3) this targeted difference (ie, effect size), and (4) the variability of the data (for quantitative data). We believe correct planning of experiments is an ethical issue of concern to the entire community.
Introduction
“Statistical analysis allows us to put limits on our uncertainty, but not to prove anything.”— Douglas G. Altman [ 1 ]
The growing need for medical practice based on evidence has generated an increasing medical literature supported by statistics: readers expect and presume medical journals publish only studies with unquestionable results they can use in their everyday practice and editors expect and often request authors provide rigorously supportable answers. Researchers submit articles based on presumably valid outcome measures, analyses, and conclusions claiming or implying the superiority of one treatment over another, the usefulness of a new diagnostic test, or the prognostic value of some sign. Paradoxically, the increasing frequency of seemingly contradictory results may be generating increasing skepticism in the medical community.
One fundamental reason for this conundrum takes root in the theory of hypothesis testing developed by Pearson and Neyman in the late 1920s [ 24 , 25 ]. The majority of medical research is presented in the form of a comparison, the most obvious being treatment comparisons in randomized controlled trials. To assess whether the difference observed is likely attributable to chance alone or to a true difference, researchers set a null hypothesis that there is no difference between the alternative treatments. They then determine the probability (the p value), they could have obtained the difference observed or a larger difference if the null hypothesis were true; if this probability is below some predetermined explicit significance level, the null hypothesis (ie, there is no difference) is rejected. However, regardless of study results, there is always a chance to conclude there is a difference when in fact there is not (Type I error or false positive) or to report there is no difference when a true difference does exist (Type II error or false negative) and the study has simply failed to detect it (Table 1 ). The size of the sample studied is a major determinant of the risk of reporting false-negative findings. Therefore, sample size is important for planning and interpreting medical research.
Table 1
Type I and Type II errors during hypothesis testing
Truth | Study findings | |
---|---|---|
Null hypothesis is not rejected | Null hypothesis is rejected | |
Null hypothesis is true | True negative | Type I error (alpha) (False positive) |
Null hypothesis is false | Type II error (beta) (False negative) | True positive |
For that reason, we believe readers should be adequately informed of the frequent issues related to sample size, such as (1) the desired level of statistical significance, (2) the chances of detecting a difference of given magnitude between the groups compared, ie, the power, (3) this targeted difference, and (4) the variability of the data (for quantitative data). We will illustrate these matters with a comparison between two treatments in a surgical randomized controlled trial. The use of sample size also will be presented in other common areas of statistics, such as estimation and regression analyzes.
Desired Level of Significance
The level of statistical significance α corresponds to the probability of Type I error, namely, the probability of rejecting the null hypothesis of “no difference between the treatments compared” when in fact it is true. The decision to reject the null hypothesis is based on a comparison of the prespecified level of the test arbitrarily chosen with the test procedure’s p value. Controlling for Type I error is paramount to medical research to avoid the spread of new or perpetuation of old treatments that are ineffective. For the majority of hypothesis tests, the level of significance is arbitrarily chosen at 5%. When an investigator chooses α = 5%, if the test’s procedure p value computed is less than 5%, the null hypothesis will be rejected and the treatments compared will be assumed to be different.
To reduce the probability of Type I error, we may choose to reduce the level of statistical significance to 1% or less [ 29 ]. However, the level of statistical significance also influences the sample size calculation: the lower the chosen level of statistical significance, the larger the sample size will be, considering all other parameters remain the same (see example below and Appendix 1). Consequently, there are domains where higher levels of statistical significance are used so that the sample size remains restricted, such as for randomized Phase II screening designs in cancer [ 26 ]. We believe the choice of a significance level greater than 5% should be restricted to particular cases.
The power of a test is defined as 1 − the probability of Type II error. The Type II error is concluding at no difference (the null is not rejected) when in fact there is a difference, and its probability is named β. Therefore, the power of a study reflects the probability of detecting a difference when this difference exists. It is also very important to medical research that studies are planned with an adequate power so that meaningful conclusions can be issued if no statistical difference has been shown between the treatments compared. More power means less risk for Type II errors and more chances to detect a difference when it exists.
Power should be determined a priori to be at least 80% and preferably 90%. The latter means, if the true difference between treatments is equal to the one we planned, there is only 10% chance the study will not detect it. Sample size increases with increasing power (Fig. 1 ).

The graphs show the distribution of the test statistic (z-test) for the null hypothesis (plain line) and the alternative hypothesis (dotted line) for a sample size of ( A ) 32 patients per group, ( B ) 64 patients per group, and ( C ) 85 patients per group. For a difference in mean of 10, a standard deviation of 20, and a significance level α of 5%, the power (shaded area) increases from ( A ) 50%, to ( B ) 80%, and ( C ) 90%. It can be seen, as power increases, the test statistics yielded under the alternative hypothesis (there is a difference in the two comparison groups) are more likely to be greater than the critical value 1.96.
Very commonly, power calculations have not been performed before conducting the trial [ 3 , 8 ], and when facing nonsignificant results, investigators sometimes compute post hoc power analyses, also called observed power. For this purpose, investigators use the observed difference and variability and the sample size of the trial to determine the power they would have had to detect this particular difference. However, post hoc power analyses have little statistical meaning for three reasons [ 9 , 13 ]. First, because there is a one-to-one relationship between p values and post hoc power, the latter does not convey any additional information on the sample than the former. Second, nonsignificant p values always correspond to low power and post hoc power, at best, will be slightly larger than 50% for p values equal to or greater than 0.05. Third, when computing post hoc power, investigators implicitly make the assumption that the difference observed is clinically meaningful and more representative of the truth than the null hypothesis they precisely were not able to reject. However, in the theory of hypothesis testing, the difference observed should be used only to choose between the hypotheses stated a priori; a posteriori, the use of confidence intervals is preferable to judge the relevance of a finding. The confidence interval represents the range of values we can be confident to some extent includes the true difference. It is related directly to sample size and conveys more information than p values. Nonetheless, post hoc power analyses educate readers about the importance of considering sample size by explicitly raising the issue.
The Targeted Difference Between the Alternative Treatments
The targeted difference between the alternative treatments is determined a priori by the investigator, typically based on preliminary data. The larger the expected difference is, the smaller the required sample size will be. However, because the sample size based on the difference expected may be too large to achieve, investigators sometimes choose to power their trial to detect a difference larger than one would normally expect to reduce the sample size and minimize the time and resources dedicated to the trial. However, if the targeted difference between the alternative treatments is larger than the true difference, the trial may fail to conclude a difference between the two treatments when a smaller, and still meaningful, difference exists. This smallest meaningful difference sometimes is expressed as the “minimal clinically important difference,” namely, “the smallest difference in score in the domain of interest which patients perceive as beneficial and which would mandate, in the absence of troublesome side effects and excessive costs, a change in the patient’s management” [ 15 ]. Because theoretically the minimal clinically important difference is a multidimensional phenomenon that encompasses a wide range of complex issues of a particular treatment in a unique setting, it usually is determined by consensus among clinicians with expertise in the domain. When the measure of treatment effect is based on a score, researchers may use empiric definitions of clinically meaningful difference. For instance, Michener et al. [ 21 ], in a prospective study of 63 patients with various shoulder abnormalities, determined the minimal change perceived as clinically meaningful by the patients for the patient self-report section of the American Shoulder and Elbow Surgeons Standardized Shoulder Assessment Form was 6.7 points of 100 points. Similarly, Bijur et al. [ 5 ], in a prospective cohort study of 108 adults presenting to the emergency department with acute pain, determined the minimal change perceived as clinically meaningful by patients for acute pain measured on the visual analog scale was 1.4 points. There is no reason to try to detect a difference below the minimal clinically important difference because, even if it proves statistically significant, it will not be meaningful.
The meaningful clinically important difference should not be confused with the effect size. The effect size is a dimensionless measure of the magnitude of a relation between two or more variables, such as Cohen’s d standardized difference [ 6 ], but also odds ratio, Pearson’s r correlation coefficient, etc. Sometimes studies are planned to detect a particular effect size instead of being planned to detect a particular difference between the two treatments. According to Cohen [ 6 ], 0.2 is indicative of a small effect, 0.5 a medium effect, and 0.8 a large effect size. One of the advantages of doing so is that researchers do not have to make any assumptions regarding the minimal clinically important difference or the expected variability of the data.
The Variability of the Data
For quantitative data, researchers also need to determine the expected variability of the alternative treatments: the more variability expected in the specified outcome, the more difficult it will be to differentiate between treatments and the larger the required sample size (see example below). If this variability is underestimated at the time of planning, the sample size computed will be too small and the study will be underpowered to the one desired. For comparing proportions, the calculation of sample size makes use of the expected proportion with the specified outcome in each group. For survival data, the calculation of sample size is based on the survival proportions in each treatment group at a specified time and on the total number of events in the group in which the fewer events occur. Therefore, for the latter two types of data, variability does not appear in the computation of sample size.
Presume an investigator wants to compare the postoperative Harris hip score [ 12 ] at 3 months in a group of patients undergoing minimally invasive THA with a control group of patients undergoing standard THA in a randomized controlled trial. The investigator must (1) establish a statistical significance level, eg, α = 5%, (2) select a power, eg, 1 − β = 90%, and (3) establish a targeted difference in the mean scores, eg, 10, and assume a standard deviation of the scores, eg, 20 in both groups (which they can obtain from the literature or their previous patients). In this case, the sample size should be 85 patients per group (Appendix 1). If fewer patients are included in the trial, the probability of detecting the targeted difference when it exists will decrease; for sample sizes of 64 and 32 per group, for instance, the power decreases to 80% and 50%, respectively (Fig. 1 ). If the investigator assumed the standard deviation of the scores in each group to be 30 instead of 20, a sample size of 190 per group would be necessary to obtain a power of 90% with a significance level α = 5% and targeted difference in the mean scores of 10. If the significance level was chosen at α = 1% instead of α = 5%, to yield the same power of 90% with a targeted difference in scores of 10 and standard deviation of 20, the sample size would increase from 85 patients per group to 120 patients per group. In relatively simple cases, statistical tables [ 19 ] and dedicated software available from the internet may be used to determine sample size. In most orthopaedic clinical trials cases, sample size calculation is rather simple as above, but it will become more complex in other cases. The type of end points, the number of groups, the statistical tests used, whether the observations are paired, and other factors influence the complexity of the calculation, and in these cases, expert statistical advice is recommended.
Sample Size, Estimation, and Regression
Sample size was presented above in the context of hypothesis testing. However, it is also of interest in other areas of biostatistics, such as estimation or regression. When planning an experiment, researchers should ensure the precision of the anticipated estimation will be adequate. The precision of an estimation corresponds to the width of the confidence interval: the larger the tested sample size is, the better the precision. For instance, Handl et al. [ 11 ], in a biomechanical study of 21 fresh-frozen cadavers, reported a mean ultimate load failure of four-strand hamstring tendon constructs of 4546 N under loading with a standard deviation of 1500 N. Based on these values, if we were to design an experiment to assess the ultimate load failure of a particular construct, the precision around the mean at the 95% confidence level would be expected to be 3725 N for five specimens, 2146 N for 10 specimens, 1238 N for 25 specimens, 853 N for 50 specimens, and 595 N for 100 specimens tested (Appendix 2); if we consider the estimated mean will be equal to 4546 N, the one obtained in the previous experiment, we could obtain the corresponding 95% confidence intervals (Fig. 2 ). Because we always deal with limited samples, we never exactly know the true mean or standard deviation of the parameter distribution; otherwise, we would not perform the experiment. We only approximate these values, and the results obtained can vary from the planned experiment. Nonetheless, what we identify at the time of planning is that testing more than 50 specimens, for instance 100, will multiply the costs and time necessary to the experiment while providing only slight improvement in the precision.

The graph shows the predicted confidence interval for experiments with an increasing number of specimens tested based on the study by Handl et al. [ 11 ] of 21 fresh-frozen cadavers with a mean ultimate load failure of four-strand hamstring tendon constructs of 4546 N and standard deviation of 1500 N.
Similarly, sample size issues should be considered when performing regression analyses, namely, when trying to assess the effect of a particular covariate, or set of covariates, on an outcome. The effective power to detect the significance of a covariate in predicting this outcome depends on the outcome modeled [ 14 , 30 ]. For instance, when using a Cox regression model, the power of the test to detect the significance of a particular covariate does not depend on the size of the sample per se but on the number of specific critical events. In a cohort study of patients treated for soft tissue sarcoma with various treatments, such as surgery, radiotherapy, chemotherapy, etc, the power to detect the effect of chemotherapy on survival will depend on the number of patients who die, not on the total number of patients in the cohort. Therefore, when planning such studies, researchers should be familiar with these issues and decide, for example, to model a composite outcome, such as event-free survival that includes any of the following events: death from disease, death from other causes, recurrence, metastases, etc, to increase the power of the test.
The reasons to plan a trial with an adequate sample size likely to give enough power to detect a meaningful difference are essentially ethical. Small trials are considered unethical by most, but not all, researchers because they expose participants to the burdens and risks of human research with a limited chance to provide any useful answers [ 2 , 10 , 28 ]. Underpowered trials also ineffectively consume resources (human, material) and add to the cost of healthcare to society. Although there are particular cases when trials conducted on a small sample are justified, such as early-phase trials with the aim of guiding the conduct of subsequent research (or formulating hypotheses) or, more rarely, for rare diseases with the aim of prospectively conducting meta-analyses, they generally should be avoided [ 10 ]. It is also unethical to conduct trials with too large a sample size because, in addition to the waste of time and resources, they expose participants in one group to receive inadequate treatment after appropriate conclusions should have been reached. Interim analyses and adaptive trials have been developed in this context to shorten the time to decision and overcome these concerns [ 4 , 16 ].
We raise two important points. First, we explained, for practical and ethical reasons, experiments are conducted on a sample of limited size with the aim to generalize the results to the population of interest and increasing the size of the sample is a way to combat uncertainty. When doing this, we implicitly consider the patients or specimens in the sample are randomly selected from the population of interest, although this is almost never the case; even if it were the case, the population of interest would be limited in space and time. For instance, Marx et al. [ 20 ], in a survey conducted in late 1998 and early 1999, assessed the practices for anterior cruciate ligament reconstruction on a randomly selected sample of 725 members of the American Academy of Orthopaedic Surgeons; however, because only ½ the surgeons responded to the survey, their sample probably is not representative of all members of the society, who in turn are not representative of all orthopaedic surgeons in the United States, who again are not representative of all surgeons in the world because of the numerous differences among patients, doctors, and healthcare systems across countries. Similar surveys conducted in other countries have provided different results [ 17 , 22 ]. Moreover, if the same survey was conducted today, the results would possibly differ. Therefore, another source for variation among studies, apart from sampling variability, is that samples may not be representative of the same population. Therefore, when planning experiments, researchers must take care to make their sample representative of the population they want to infer to and readers, when interpreting the results of a study, should always assess first how representative the sample presented is regarding their own patients. The process implemented to select the sample, the settings of the experiment, and the general characteristics and influencing factors of the patients must be described precisely to assess representativeness and possible selection biases [ 7 ].
Second, we have discussed only sample size for interpreting nonsignificant p values, but it also may be of interest when interpreting p values that are significant. Significant results issued from larger studies usually are given more credit than those from smaller studies because of the risk of reporting exaggerating treatment effects with studies with smaller samples or of lower quality [ 23 , 27 ], and small trials are believed to be more biased than others. However, there is no statistical reason a significant result in a trial including 2000 patients should be given more belief than a trial including 20 patients, given the significance level chosen is the same in both trials. Small but well-conducted trials may yield a reliable estimation of treatment effect. Kjaergard et al. [ 18 ], in a study of 14 meta-analyses involving 190 randomized trials, reported small trials (fewer than 1000 patients) reported exaggerated treatment effects when compared with large trials. However, when considering only small trials with adequate randomization, allocation concealment (allocation concealment is the process that keeps clinicians and participants unaware of upcoming assignments. Without it, even properly developed random allocation sequences can be subverted), and blinding, this difference became negligible. Nonetheless, the advantages of a large sample size to interpret significant results are it allows a more precise estimate of the treatment effect and it usually is easier to assess the representativeness of the sample and to generalize the results.
Sample size is important for planning and interpreting medical research and surgeons should become familiar with the basic elements required to assess sample size and the influence of sample size on the conclusions. Controlling for the size of the sample allows the researcher to walk a thin line that separates the uncertainty surrounding studies with too small a sample size from studies that have failed practical or ethical considerations because of too large a sample size.
Acknowledgments
We thank the editor whose thorough readings of, and accurate comments on drafts of the manuscript have helped clarify the manuscript.
The sample size (n) per group for comparing two means with a two-sided two-sample t test is
where z 1−α/2 and z 1−β are standard normal deviates for the probability of 1 − α/2 and 1 − β, respectively, and d t = (μ 0 − μ 1 )/σ is the targeted standardized difference between the two means.
The following values correspond to the example:
- α = 0.05 (statistical significance level)
- β = 0.10 (power of 90%)
- |μ 0 − μ 1 | = 10 (difference in the mean score between the two groups)
- σ = 20 (standard deviation of the score in each group)
- z 1−α/2 = 1.96
- z 1−β = 1.28
Two-sided tests which do not assume the direction of the difference (ie, that the mean value in one group would always be greater than that in the other) are generally preferred. The null hypothesis makes the assumption that there is no difference between the treatments compared, and a difference on one side or the other therefore is expected.
Computation of Confidence Interval
To determine the estimation of a parameter, or alternatively the confidence interval, we use the distribution of the parameter estimate in repeated samples of the same size. For instance, consider a parameter with observed mean, m, and standard deviation, sd, in a given sample. If we assume that the distribution of the parameter in the sample is close to a normal distribution, the means, x n , of several repeated samples of the same size have true mean, μ, the population mean, and estimated standard deviation,
also known as standard error of the mean, and
follows a t distribution. For a large sample, the t distribution becomes close to the normal distribution; however, for a smaller sample size the difference is not negligible and the t distribution is preferred. The precision of the estimation is
For example, Handl et al. [ 11 ] in a biomechanical study of 21 fresh-frozen cadavers reported a mean ultimate load failure of 4-strand hamstring tendon constructs of 4546 N under dynamic loading with standard deviation of 1500 N. If we were to plan an experiment, the anticipated precision of the estimation at the 95% level would be
for five specimens,
The values 2.78, 2.26, 2.06, 2.01, and 1.98 correspond to the t distribution deviates for the probability of 1 − α/2, with 4, 9, 24, 49, and 99 (n − 1) degrees of freedom; the well known corresponding standard normal deviate is 1.96. Given an estimated mean of 4546 N, the corresponding 95% confidence intervals are 2683 N to 6408 N for five specimens, 3473 N to 5619 N for 10 specimens, 3927 N to 5165 N for 25 specimens, 4120 N to 4972 N for 50 specimens, and 4248 N to 4844 N for 100 specimens (Fig. 2 ).
Similarly, for a proportion p in a given sample with sufficient sample size to assume a nearly normal distribution, the confidence interval extends either side of the proportion p by
For a small sample size, exact confidence interval for proportions should be used.
Each author certifies that he or she has no commercial associations (eg, consultancies, stock ownership, equity interest, patent/licensing arrangements, etc) that might pose a conflict of interest in connection with the submitted article.

IMAGES
VIDEO
COMMENTS
Sample size calculations require assumptions about expected means and standard deviations, or event risks, in different groups; or, upon expected effect sizes. For example, a study may be powered to detect an effect size of 0.5; or a response rate of 60% with drug vs. 40% with placebo. [1] When no guesstimates or expectations are possible ...
While a specific sample size is not established, sample size between 1000 and 10.000 is recommended for each sub-group. For samples that cannot be easily acquired ( i.e. paediatric and geriatric samples, and complex biological fluids), sample sizes as low as 400 may be used for each sub-group ( 92 , 100 ).
There is a widespread belief that large samples are ideal for research or statistical analysis. However, this is not always true. Using the above example as a case study, very large samples that exceed the value estimated by sample size calculation present different hurdles. ... Fonseca LM. How sample size influences research outcomes. Dental ...
A good sample size justification in qualitative research is based on 1) an identification of the populations, including any sub-populations, 2) an estimate of the number of codes in the (sub-)population, 3) the probability a code is encountered in an information source, and 4) the sampling strategy that is used.
You need to determine how big of a sample size you need so that you can be sure the quantitative data you get from a survey is reflective of your target population as a whole - and so that the decisions you make based on the research have a firm foundation. Too big a sample and a project can be needlessly expensive and time-consuming.
Statisticians have devised quantitative ways to find a good sample size. You want a large enough sample to have a reasonable chance of detecting a meaningful effect when it exists but not too large to be overly expensive. In general, these methods focus on using the population's variability. More variable populations require larger samples to ...
An absolute minimum of 200 samples are required for Pearson Correlation analysis (Guilford, 1954). Also, a pre-testing and/or pilot study demands a smaller sample size than a main study. Sample ...
In a recent overview, Lakens (2021) listed six types of general approaches to justify sample size in quantitative empirical studies: (a) measure entire population, (b) resource constraints, (c) a priori power analysis, (d) accuracy, (e) heuristics, and (f) no justification. For the first approach, no quantitative justification is necessary, and ...
Too large sample size is wastage of resources and use of too small sample size might fail to answer the research question or provide imprecise results and may question the validity of study.
It is the ability of the test to detect a difference in the sample, when it exists in the target population. Calculated as 1-Beta. The greater the power, the larger the required sample size will be. A value between 80%-90% is usually used. Relationship between non-exposed/exposed groups in the sample.
The procedure requires the researcher to determine the needed sample size to attain the desired power to detect a predetermined ES. Figure 2 shows a graph of statistical power as a function of sample size. For 80% power (usually considered to be sufficient power), the group size required is about 50.
Although sample size is a consideration in qualitative research, the principles that guide the determination of sufficient sample size are different to those that are considered in quantitative research. This paper only examines sample size considerations in quantitative research. Factors that influence sample sizes
Sample size calculation. In order to enable comparisons with some level of established statistical confidence, quantitative research needs an acceptable sample size. 2 The sample size is the most crucial factor for reliability (reproducibility) in quantitative research. It is important for a study to be powered - the likelihood of identifying a difference if it exists in reality. 2 Small ...
For example, in comparing the averages of two independent groups (t-test), if researchers want to test with a power (1 - β) of 0.80, a confidence level of 0.95, and equal group sizes, a sample size of 46 (23 per group) would be large enough if the effect size is approximately 0.85 (strong effect; assuming μ 1 and μ 2 to be the means of two groups and σ to be the common standard deviation ...
Charlesworth Author Services; 26 May, 2022; The importance of having Large Sample Sizes for your research. Sample size can be defined as the number of pieces of information, data points or patients (in medical studies) tested or enrolled in an experiment or study.On any hypothesis, scientific research is built upon determining the mean values of a given dataset.
Approaches to sample size calculation according to study design are presented with examples in health research. For sample size estimation, researchers need to (1) provide information regarding the statistical analysis to be applied, (2) determine acceptable precision levels, (3) decide on study power, (4) specify the confidence level, and (5 ...
Recommended sample = 600. High Risk. Margin of error: 2%. Confidence level: 99%. Recommended sample = 4,130. As you can see, determining the correct sample size for a study is not an easy task. There are many factors and variables to consider, and each has a direct impact on the success of your study.
Approaches to sample size calculation according to study design are presented with examples in health research. For sample size estimation, researchers need to (1) provide information regarding the statistical analysis to be applied, (2) determine acceptable precision levels, (3) decide on study power, (4) specify the confidence level, and (5 ...
Research Sampling and Sample Size Determination: A practical Application. Chinelo Blessing ORIBHABOR (Ph.D) Department of Guidance and Counseling, Faculty of Arts and Education, University of ...
Sample Size and its Importance in Research. Chittaranjan Andrade. ABSTRACT. The sample size for a study needs to be estimated at the time the study is proposed; too large a sample is unnecessary and unethical, and too small a sample is unscientific and also unethical. The necessary sample size can be calculated, using statistical software ...
Microscopy/Infrared Spectroscopy for Routine Sample Sizes. Estimating Statistical Power and Required Sample Sizes for Organizational Research Using Multilevel Modeling. Initial Scale Development: Sample Size for Pilot Studies. The Choice of Sample Size: A Mixed Bayesian/Frequentist Approach.
Genetic analysis software for research, forensics & healthcare applications Explore the Scientific Software . nQuery ... One decision out of many is how large a study needs to be to have a reasonable chance of success. Sample size determination is the process by which trialists can find the ideal number of participants to balance the ...
The graphs show the distribution of the test statistic (z-test) for the null hypothesis (plain line) and the alternative hypothesis (dotted line) for a sample size of (A) 32 patients per group, (B) 64 patients per group, and (C) 85 patients per group.For a difference in mean of 10, a standard deviation of 20, and a significance level α of 5%, the power (shaded area) increases from (A) 50%, to ...
Nowadays, increasingly more people turn to the Internet, and specifically to social media, for advice and information about health (e.g., Chou et al., Citation 2018; Zhao & Zhang, Citation 2017).At the same time, social media influencers (SMIs) have become an integral part of today's digital media landscape, shaping people's opinions and perceptions on various topics, including health.
Determining the sample sizes involve resource and statistical issues. Usually, researchers regard 100 participants as the minimum sample size when the population is large. However, In most studies ...