Imperial College London Imperial College London
Latest news.

AI tackles one of the most difficult challenges in quantum chemistry

Mpox: WHO experts respond to questions on current outbreak

Imperial Staff and Students raise thousands for Cancer Research UK
- Educational Development Unit
- Teaching toolkit
- Educational research methods
- Analysing and writing up your research

Types of data analysis
The means by which you analyse your data are largely determined by the nature of your research question , the approach and paradigm within which your research operates, the methods used, and consequently the type of data elicited. In turn, the language and terms you use in both conducting and reporting your data analysis should reflect these.
The list below includes some of the more commonly used means of qualitative data analysis in educational research – although this is by no means exhaustive. It is also important to point out that each of the terms given below generally encompass a range of possible methods or options and there can be overlap between them. In all cases, further reading is essential to ensure that the process of data analysis is valid, transparent and appropriately systematic, and we have provided below (as well as in our further resources and tools and resources for qualitative data analysis sections) some recommendations for this.
If your research is likely to involve quantitative analysis, we recommend the books listed below.
Types of qualitative data analysis
- Thematic analysis
- Coding and/or content analysis
- Concept map analysis
- Discourse or narrative analysis
- Grouded theory
- Phenomenological analysis or interpretative phenomenological analysis (IPA)
Further reading and resources
As a starting point for most of these, we would recommend the relevant chapter from Part 5 of Cohen, Manion and Morrison (2018), Research Methods in Education. You may also find the following helpful:
For qualitative approaches
Savin-Baden, M. & Howell Major, C. (2013) Data analysis. In Qualitative Research: The essential guide to theory and practice . (Abingdon, Routledge, pp. 434-450).
For quantitative approaches
Bors, D. (2018) Data analysis for the social sciences (Sage, London).
Data Analysis Techniques for Education Research: Exploring Qualitative and Quantitative Approaches
In education research, data analysis is crucial in making informed decisions and shaping school policies, curricula, and teaching methods. This article discusses qualitative and quantitative approaches to data analysis in education research, examining the tools and techniques used to collect, analyze, and interpret data. By reviewing these methodologies, educators and researchers can better understand how to conduct research that effectively addresses educational issues and informs practices.
Table of Contents
Qualitative Approaches to Data Analysis in Education Research
Qualitative research draws on methods that seek to understand the underlying meanings, reasons, and contextual factors influencing educational phenomena. These methods emphasize participants’ lived experiences and perspectives and are helpful for researchers seeking a deeper understanding of complex educational issues.
Ethnography
Ethnography is an immersive research method in which researchers observe and interact with participants in their natural settings to gain insight into their culture, values, and customs (Atkinson & Hammersley, 2021). Typically involving long-term engagement with the research site, ethnography seeks to uncover the implicit rules, norms, and practices that shape an educational environment.
Case Studies
Case studies offer an in-depth examination of a single individual, event, or situation. By concentrating on specific instances, researchers can delve into the complexities and nuances of a particular context, providing valuable insights that may not be accessible through other data analysis methods (Yin, 2013).
Interviews and Focus Groups
Interviews and focus groups are valuable methods for collecting rich, detailed data directly from participants. These methods involve one-on-one or group conversations in which a researcher asks open-ended questions to explore participants’ thoughts, experiences, and perceptions (Kvale & Brinkmann, 2014).
Content Analysis
Content analysis is a technique for systematically examining textual or visual materials, such as documents, images, or videos. Researchers may use inductive and deductive approaches to identify themes, patterns, and frequencies within the data, allowing for a nuanced understanding of the materials under study (Hsieh & Shannon, 2021).
Quantitative Approaches to Data Analysis in Education Research
Quantitative research employs structured, numerical data to examine relationships, differences, and trends across variables. These methods often involve statistical analysis, facilitating the identification of patterns, correlations, and causal relationships between variables.
Correlational Studies
Correlational studies investigate the relationships between two or more variables, seeking to identify the degree and direction of the association between them. Pearson’s correlation coefficient (r) is a commonly used statistic in correlational studies to describe the strength and direction of the relationship (Field, 2018).
Experimental Designs
Experimental designs aim to establish causal relationships between variables by manipulating one or more independent variables and examining their effects on a dependent variable. These designs usually involve random assignment and control groups, allowing researchers to isolate the effect of the treatment or intervention on the outcome (Shadish, Cook, & Campbell, 2002).
Longitudinal Studies
Longitudinal studies are conducted over an extended period and involve repeated measurements of the same variables at different time points (Duncan, Magnuson, & Ludwig, 2004). This type of research allows investigators to identify trends, assess changes, and explore causal relationships between variables across time.
Meta-analysis
Meta-analysis involves pooling and analyzing the findings from multiple empirical studies on the same topic, enabling researchers to establish robust, evidence-based conclusions across many individual studies (Borenstein, Hedges, Higgins, & Rothstein, 2009). By aggregating effect sizes, researchers can identify trends and make generalizations that extend beyond the specific context of each study.
Mixed Methods Research: Integrating Qualitative and Quantitative Approaches
Mixed methods research features a combination of qualitative and quantitative methods, providing a comprehensive understanding of the research problem from various perspectives. This approach enables triangulation, using multiple data sources and analysis techniques to cross-validate findings, yielding more accurate and reliable conclusions (Creswell & Clark, 2017).
Sequential Design
Sequential design involves data collection in two or more distinct phases, with one phase informing the next. For instance, researchers might begin with a qualitative phase to explore participants’ experiences, followed by a quantitative phase to test hypotheses derived from the initial findings (Morse & Niehaus, 2009).
Concurrent Design
Concurrent design analyzes qualitative and quantitative data simultaneously, enabling researchers to balance the strengths and weaknesses of each approach. In this design, researchers might conduct surveys alongside in-depth interviews, using both data sets to understand the research question holistically (Palinkas et al., 2011).
Data analysis techniques in education research encompass qualitative and quantitative approaches, offering various tools and frameworks for understanding educational phenomena. Educators and education researchers can investigate complex issues, uncover meaningful insights, and inform educational practices that enhance learning and student outcomes by selecting and employing appropriate data collection and analysis methods.
Mark Anthony Llego
Mark Anthony Llego, a visionary from the Philippines, founded TeacherPH in October 2014 with a mission to transform the educational landscape. His platform has empowered thousands of Filipino teachers, providing them with crucial resources and a space for meaningful idea exchange, ultimately enhancing their instructional and supervisory capabilities. TeacherPH's influence extends far beyond its origins. Mark's insightful articles on education have garnered international attention, featuring on respected U.S. educational websites. Moreover, his work has become a valuable reference for researchers, contributing to the academic discourse on education.
Leave a Comment Cancel reply
Can't find what you're looking for.
We are here to help - please use the search box below.
- MS in the Learning Sciences
- Tuition & Financial Aid

Qualitative vs. quantitative data analysis: How do they differ?
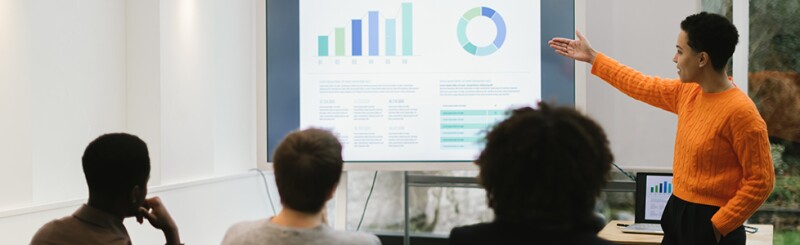
Learning analytics have become the cornerstone for personalizing student experiences and enhancing learning outcomes. In this data-informed approach to education there are two distinct methodologies: qualitative and quantitative analytics. These methods, which are typical to data analytics in general, are crucial to the interpretation of learning behaviors and outcomes. This blog will explore the nuances that distinguish qualitative and quantitative research, while uncovering their shared roles in learning analytics, program design and instruction.
What is qualitative data?
Qualitative data is descriptive and includes information that is non numerical. Qualitative research is used to gather in-depth insights that can't be easily measured on a scale like opinions, anecdotes and emotions. In learning analytics qualitative data could include in depth interviews, text responses to a prompt, or a video of a class period. 1
What is quantitative data?
Quantitative data is information that has a numerical value. Quantitative research is conducted to gather measurable data used in statistical analysis. Researchers can use quantitative studies to identify patterns and trends. In learning analytics quantitative data could include test scores, student demographics, or amount of time spent in a lesson. 2
Key difference between qualitative and quantitative data
It's important to understand the differences between qualitative and quantitative data to both determine the appropriate research methods for studies and to gain insights that you can be confident in sharing.
Data Types and Nature
Examples of qualitative data types in learning analytics:
- Observational data of human behavior from classroom settings such as student engagement, teacher-student interactions, and classroom dynamics
- Textual data from open-ended survey responses, reflective journals, and written assignments
- Feedback and discussions from focus groups or interviews
- Content analysis from various media
Examples of quantitative data types:
- Standardized test, assessment, and quiz scores
- Grades and grade point averages
- Attendance records
- Time spent on learning tasks
- Data gathered from learning management systems (LMS), including login frequency, online participation, and completion rates of assignments
Methods of Collection
Qualitative and quantitative research methods for data collection can occasionally seem similar so it's important to note the differences to make sure you're creating a consistent data set and will be able to reliably draw conclusions from your data.
Qualitative research methods
Because of the nature of qualitative data (complex, detailed information), the research methods used to collect it are more involved. Qualitative researchers might do the following to collect data:
- Conduct interviews to learn about subjective experiences
- Host focus groups to gather feedback and personal accounts
- Observe in-person or use audio or video recordings to record nuances of human behavior in a natural setting
- Distribute surveys with open-ended questions
Quantitative research methods
Quantitative data collection methods are more diverse and more likely to be automated because of the objective nature of the data. A quantitative researcher could employ methods such as:
- Surveys with close-ended questions that gather numerical data like birthdates or preferences
- Observational research and record measurable information like the number of students in a classroom
- Automated numerical data collection like information collected on the backend of a computer system like button clicks and page views
Analysis techniques
Qualitative and quantitative data can both be very informative. However, research studies require critical thinking for productive analysis.
Qualitative data analysis methods
Analyzing qualitative data takes a number of steps. When you first get all your data in one place you can do a review and take notes of trends you think you're seeing or your initial reactions. Next, you'll want to organize all the qualitative data you've collected by assigning it categories. Your central research question will guide your data categorization whether it's by date, location, type of collection method (interview vs focus group, etc), the specific question asked or something else. Next, you'll code your data. Whereas categorizing data is focused on the method of collection, coding is the process of identifying and labeling themes within the data collected to get closer to answering your research questions. Finally comes data interpretation. To interpret the data you'll take a look at the information gathered including your coding labels and see what results are occurring frequently or what other conclusions you can make. 3
Quantitative analysis techniques
The process to analyze quantitative data can be time-consuming due to the large volume of data possible to collect. When approaching a quantitative data set, start by focusing in on the purpose of your evaluation. Without making a conclusion, determine how you will use the information gained from analysis; for example: The answers of this survey about study habits will help determine what type of exam review session will be most useful to a class. 4
Next, you need to decide who is analyzing the data and set parameters for analysis. For example, if two different researchers are evaluating survey responses that rank preferences on a scale from 1 to 5, they need to be operating with the same understanding of the rankings. You wouldn't want one researcher to classify the value of 3 to be a positive preference while the other considers it a negative preference. It's also ideal to have some type of data management system to store and organize your data, such as a spreadsheet or database. Within the database, or via an export to data analysis software, the collected data needs to be cleaned of things like responses left blank, duplicate answers from respondents, and questions that are no longer considered relevant. Finally, you can use statistical software to analyze data (or complete a manual analysis) to find patterns and summarize your findings. 4
Qualitative and quantitative research tools
From the nuanced, thematic exploration enabled by tools like NVivo and ATLAS.ti, to the statistical precision of SPSS and R for quantitative analysis, each suite of data analysis tools offers tailored functionalities that cater to the distinct natures of different data types.
Qualitative research software:
NVivo: NVivo is qualitative data analysis software that can do everything from transcribe recordings to create word clouds and evaluate uploads for different sentiments and themes. NVivo is just one tool from the company Lumivero, which offers whole suites of data processing software. 5
ATLAS.ti: Similar to NVivo, ATLAS.ti allows researchers to upload and import data from a variety of sources to be tagged and refined using machine learning and presented with visualizations and ready for insert into reports. 6
SPSS: SPSS is a statistical analysis tool for quantitative research, appreciated for its user-friendly interface and comprehensive statistical tests, which makes it ideal for educators and researchers. With SPSS researchers can manage and analyze large quantitative data sets, use advanced statistical procedures and modeling techniques, predict customer behaviors, forecast market trends and more. 7
R: R is a versatile and dynamic open-source tool for quantitative analysis. With a vast repository of packages tailored to specific statistical methods, researchers can perform anything from basic descriptive statistics to complex predictive modeling. R is especially useful for its ability to handle large datasets, making it ideal for educational institutions that generate substantial amounts of data. The programming language offers flexibility in customizing analysis and creating publication-quality visualizations to effectively communicate results. 8
Applications in Educational Research
Both quantitative and qualitative data can be employed in learning analytics to drive informed decision-making and pedagogical enhancements. In the classroom, quantitative data like standardized test scores and online course analytics create a foundation for assessing and benchmarking student performance and engagement. Qualitative insights gathered from surveys, focus group discussions, and reflective student journals offer a more nuanced understanding of learners' experiences and contextual factors influencing their education. Additionally feedback and practical engagement metrics blend these data types, providing a holistic view that informs curriculum development, instructional strategies, and personalized learning pathways. Through these varied data sets and uses, educators can piece together a more complete narrative of student success and the impacts of educational interventions.
Master Data Analysis with an M.S. in Learning Sciences From SMU
Whether it is the detailed narratives unearthed through qualitative data or the informative patterns derived from quantitative analysis, both qualitative and quantitative data can provide crucial information for educators and researchers to better understand and improve learning. Dive deeper into the art and science of learning analytics with SMU's online Master of Science in the Learning Sciences program . At SMU, innovation and inquiry converge to empower the next generation of educators and researchers. Choose the Learning Analytics Specialization to learn how to harness the power of data science to illuminate learning trends, devise impactful strategies, and drive educational innovation. You could also find out how advanced technologies like augmented reality (AR), virtual reality (VR), and artificial intelligence (AI) can revolutionize education, and develop the insight to apply embodied cognition principles to enhance learning experiences in the Learning and Technology Design Specialization , or choose your own electives to build a specialization unique to your interests and career goals.
For more information on our curriculum and to become part of a community where data drives discovery, visit SMU's MSLS program website or schedule a call with our admissions outreach advisors for any queries or further discussion. Take the first step towards transforming education with data today.
- Retrieved on August 8, 2024, from nnlm.gov/guides/data-glossary/qualitative-data
- Retrieved on August 8, 2024, from nnlm.gov/guides/data-glossary/quantitative-data
- Retrieved on August 8, 2024, from cdc.gov/healthyyouth/evaluation/pdf/brief19.pdf
- Retrieved on August 8, 2024, from cdc.gov/healthyyouth/evaluation/pdf/brief20.pdf
- Retrieved on August 8, 2024, from lumivero.com/solutions/
- Retrieved on August 8, 2024, from atlasti.com/
- Retrieved on August 8, 2024, from ibm.com/products/spss-statistics
- Retrieved on August 8, 2024, from cran.r-project.org/doc/manuals/r-release/R-intro.html#Introduction-and-preliminaries
Return to SMU Online Learning Sciences Blog
Southern Methodist University has engaged Everspring , a leading provider of education and technology services, to support select aspects of program delivery.
This will only take a moment
- Skip to main content
- Skip to primary sidebar
- Skip to footer
- QuestionPro

- Solutions Industries Gaming Automotive Sports and events Education Government Travel & Hospitality Financial Services Healthcare Cannabis Technology Use Case AskWhy Communities Audience Contactless surveys Mobile LivePolls Member Experience GDPR Positive People Science 360 Feedback Surveys
- Resources Blog eBooks Survey Templates Case Studies Training Help center

Home Market Research
Data Analysis in Research: Types & Methods
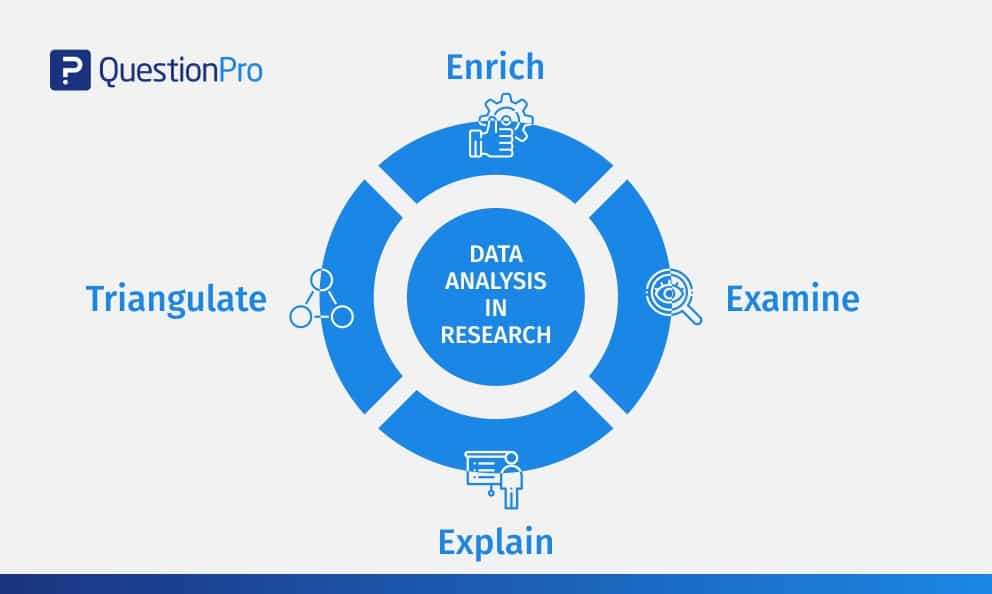
Content Index
Why analyze data in research?
Types of data in research, finding patterns in the qualitative data, methods used for data analysis in qualitative research, preparing data for analysis, methods used for data analysis in quantitative research, considerations in research data analysis, what is data analysis in research.
Definition of research in data analysis: According to LeCompte and Schensul, research data analysis is a process used by researchers to reduce data to a story and interpret it to derive insights. The data analysis process helps reduce a large chunk of data into smaller fragments, which makes sense.
Three essential things occur during the data analysis process — the first is data organization . Summarization and categorization together contribute to becoming the second known method used for data reduction. It helps find patterns and themes in the data for easy identification and linking. The third and last way is data analysis – researchers do it in both top-down and bottom-up fashion.
LEARN ABOUT: Research Process Steps
On the other hand, Marshall and Rossman describe data analysis as a messy, ambiguous, and time-consuming but creative and fascinating process through which a mass of collected data is brought to order, structure and meaning.
We can say that “the data analysis and data interpretation is a process representing the application of deductive and inductive logic to the research and data analysis.”
Researchers rely heavily on data as they have a story to tell or research problems to solve. It starts with a question, and data is nothing but an answer to that question. But, what if there is no question to ask? Well! It is possible to explore data even without a problem – we call it ‘Data Mining’, which often reveals some interesting patterns within the data that are worth exploring.
Irrelevant to the type of data researchers explore, their mission and audiences’ vision guide them to find the patterns to shape the story they want to tell. One of the essential things expected from researchers while analyzing data is to stay open and remain unbiased toward unexpected patterns, expressions, and results. Remember, sometimes, data analysis tells the most unforeseen yet exciting stories that were not expected when initiating data analysis. Therefore, rely on the data you have at hand and enjoy the journey of exploratory research.
Create a Free Account
Every kind of data has a rare quality of describing things after assigning a specific value to it. For analysis, you need to organize these values, processed and presented in a given context, to make it useful. Data can be in different forms; here are the primary data types.
- Qualitative data: When the data presented has words and descriptions, then we call it qualitative data . Although you can observe this data, it is subjective and harder to analyze data in research, especially for comparison. Example: Quality data represents everything describing taste, experience, texture, or an opinion that is considered quality data. This type of data is usually collected through focus groups, personal qualitative interviews , qualitative observation or using open-ended questions in surveys.
- Quantitative data: Any data expressed in numbers of numerical figures are called quantitative data . This type of data can be distinguished into categories, grouped, measured, calculated, or ranked. Example: questions such as age, rank, cost, length, weight, scores, etc. everything comes under this type of data. You can present such data in graphical format, charts, or apply statistical analysis methods to this data. The (Outcomes Measurement Systems) OMS questionnaires in surveys are a significant source of collecting numeric data.
- Categorical data: It is data presented in groups. However, an item included in the categorical data cannot belong to more than one group. Example: A person responding to a survey by telling his living style, marital status, smoking habit, or drinking habit comes under the categorical data. A chi-square test is a standard method used to analyze this data.
Learn More : Examples of Qualitative Data in Education
Data analysis in qualitative research
Data analysis and qualitative data research work a little differently from the numerical data as the quality data is made up of words, descriptions, images, objects, and sometimes symbols. Getting insight from such complicated information is a complicated process. Hence it is typically used for exploratory research and data analysis .
Although there are several ways to find patterns in the textual information, a word-based method is the most relied and widely used global technique for research and data analysis. Notably, the data analysis process in qualitative research is manual. Here the researchers usually read the available data and find repetitive or commonly used words.
For example, while studying data collected from African countries to understand the most pressing issues people face, researchers might find “food” and “hunger” are the most commonly used words and will highlight them for further analysis.
LEARN ABOUT: Level of Analysis
The keyword context is another widely used word-based technique. In this method, the researcher tries to understand the concept by analyzing the context in which the participants use a particular keyword.
For example , researchers conducting research and data analysis for studying the concept of ‘diabetes’ amongst respondents might analyze the context of when and how the respondent has used or referred to the word ‘diabetes.’
The scrutiny-based technique is also one of the highly recommended text analysis methods used to identify a quality data pattern. Compare and contrast is the widely used method under this technique to differentiate how a specific text is similar or different from each other.
For example: To find out the “importance of resident doctor in a company,” the collected data is divided into people who think it is necessary to hire a resident doctor and those who think it is unnecessary. Compare and contrast is the best method that can be used to analyze the polls having single-answer questions types .
Metaphors can be used to reduce the data pile and find patterns in it so that it becomes easier to connect data with theory.
Variable Partitioning is another technique used to split variables so that researchers can find more coherent descriptions and explanations from the enormous data.
LEARN ABOUT: Qualitative Research Questions and Questionnaires
There are several techniques to analyze the data in qualitative research, but here are some commonly used methods,
- Content Analysis: It is widely accepted and the most frequently employed technique for data analysis in research methodology. It can be used to analyze the documented information from text, images, and sometimes from the physical items. It depends on the research questions to predict when and where to use this method.
- Narrative Analysis: This method is used to analyze content gathered from various sources such as personal interviews, field observation, and surveys . The majority of times, stories, or opinions shared by people are focused on finding answers to the research questions.
- Discourse Analysis: Similar to narrative analysis, discourse analysis is used to analyze the interactions with people. Nevertheless, this particular method considers the social context under which or within which the communication between the researcher and respondent takes place. In addition to that, discourse analysis also focuses on the lifestyle and day-to-day environment while deriving any conclusion.
- Grounded Theory: When you want to explain why a particular phenomenon happened, then using grounded theory for analyzing quality data is the best resort. Grounded theory is applied to study data about the host of similar cases occurring in different settings. When researchers are using this method, they might alter explanations or produce new ones until they arrive at some conclusion.
LEARN ABOUT: 12 Best Tools for Researchers
Data analysis in quantitative research
The first stage in research and data analysis is to make it for the analysis so that the nominal data can be converted into something meaningful. Data preparation consists of the below phases.
Phase I: Data Validation
Data validation is done to understand if the collected data sample is per the pre-set standards, or it is a biased data sample again divided into four different stages
- Fraud: To ensure an actual human being records each response to the survey or the questionnaire
- Screening: To make sure each participant or respondent is selected or chosen in compliance with the research criteria
- Procedure: To ensure ethical standards were maintained while collecting the data sample
- Completeness: To ensure that the respondent has answered all the questions in an online survey. Else, the interviewer had asked all the questions devised in the questionnaire.
Phase II: Data Editing
More often, an extensive research data sample comes loaded with errors. Respondents sometimes fill in some fields incorrectly or sometimes skip them accidentally. Data editing is a process wherein the researchers have to confirm that the provided data is free of such errors. They need to conduct necessary checks and outlier checks to edit the raw edit and make it ready for analysis.
Phase III: Data Coding
Out of all three, this is the most critical phase of data preparation associated with grouping and assigning values to the survey responses . If a survey is completed with a 1000 sample size, the researcher will create an age bracket to distinguish the respondents based on their age. Thus, it becomes easier to analyze small data buckets rather than deal with the massive data pile.
LEARN ABOUT: Steps in Qualitative Research
After the data is prepared for analysis, researchers are open to using different research and data analysis methods to derive meaningful insights. For sure, statistical analysis plans are the most favored to analyze numerical data. In statistical analysis, distinguishing between categorical data and numerical data is essential, as categorical data involves distinct categories or labels, while numerical data consists of measurable quantities. The method is again classified into two groups. First, ‘Descriptive Statistics’ used to describe data. Second, ‘Inferential statistics’ that helps in comparing the data .
Descriptive statistics
This method is used to describe the basic features of versatile types of data in research. It presents the data in such a meaningful way that pattern in the data starts making sense. Nevertheless, the descriptive analysis does not go beyond making conclusions. The conclusions are again based on the hypothesis researchers have formulated so far. Here are a few major types of descriptive analysis methods.
Measures of Frequency
- Count, Percent, Frequency
- It is used to denote home often a particular event occurs.
- Researchers use it when they want to showcase how often a response is given.
Measures of Central Tendency
- Mean, Median, Mode
- The method is widely used to demonstrate distribution by various points.
- Researchers use this method when they want to showcase the most commonly or averagely indicated response.
Measures of Dispersion or Variation
- Range, Variance, Standard deviation
- Here the field equals high/low points.
- Variance standard deviation = difference between the observed score and mean
- It is used to identify the spread of scores by stating intervals.
- Researchers use this method to showcase data spread out. It helps them identify the depth until which the data is spread out that it directly affects the mean.
Measures of Position
- Percentile ranks, Quartile ranks
- It relies on standardized scores helping researchers to identify the relationship between different scores.
- It is often used when researchers want to compare scores with the average count.
For quantitative research use of descriptive analysis often give absolute numbers, but the in-depth analysis is never sufficient to demonstrate the rationale behind those numbers. Nevertheless, it is necessary to think of the best method for research and data analysis suiting your survey questionnaire and what story researchers want to tell. For example, the mean is the best way to demonstrate the students’ average scores in schools. It is better to rely on the descriptive statistics when the researchers intend to keep the research or outcome limited to the provided sample without generalizing it. For example, when you want to compare average voting done in two different cities, differential statistics are enough.
Descriptive analysis is also called a ‘univariate analysis’ since it is commonly used to analyze a single variable.
Inferential statistics
Inferential statistics are used to make predictions about a larger population after research and data analysis of the representing population’s collected sample. For example, you can ask some odd 100 audiences at a movie theater if they like the movie they are watching. Researchers then use inferential statistics on the collected sample to reason that about 80-90% of people like the movie.
Here are two significant areas of inferential statistics.
- Estimating parameters: It takes statistics from the sample research data and demonstrates something about the population parameter.
- Hypothesis test: I t’s about sampling research data to answer the survey research questions. For example, researchers might be interested to understand if the new shade of lipstick recently launched is good or not, or if the multivitamin capsules help children to perform better at games.
These are sophisticated analysis methods used to showcase the relationship between different variables instead of describing a single variable. It is often used when researchers want something beyond absolute numbers to understand the relationship between variables.
Here are some of the commonly used methods for data analysis in research.
- Correlation: When researchers are not conducting experimental research or quasi-experimental research wherein the researchers are interested to understand the relationship between two or more variables, they opt for correlational research methods.
- Cross-tabulation: Also called contingency tables, cross-tabulation is used to analyze the relationship between multiple variables. Suppose provided data has age and gender categories presented in rows and columns. A two-dimensional cross-tabulation helps for seamless data analysis and research by showing the number of males and females in each age category.
- Regression analysis: For understanding the strong relationship between two variables, researchers do not look beyond the primary and commonly used regression analysis method, which is also a type of predictive analysis used. In this method, you have an essential factor called the dependent variable. You also have multiple independent variables in regression analysis. You undertake efforts to find out the impact of independent variables on the dependent variable. The values of both independent and dependent variables are assumed as being ascertained in an error-free random manner.
- Frequency tables: The statistical procedure is used for testing the degree to which two or more vary or differ in an experiment. A considerable degree of variation means research findings were significant. In many contexts, ANOVA testing and variance analysis are similar.
- Analysis of variance: The statistical procedure is used for testing the degree to which two or more vary or differ in an experiment. A considerable degree of variation means research findings were significant. In many contexts, ANOVA testing and variance analysis are similar.
- Researchers must have the necessary research skills to analyze and manipulation the data , Getting trained to demonstrate a high standard of research practice. Ideally, researchers must possess more than a basic understanding of the rationale of selecting one statistical method over the other to obtain better data insights.
- Usually, research and data analytics projects differ by scientific discipline; therefore, getting statistical advice at the beginning of analysis helps design a survey questionnaire, select data collection methods , and choose samples.
LEARN ABOUT: Best Data Collection Tools
- The primary aim of data research and analysis is to derive ultimate insights that are unbiased. Any mistake in or keeping a biased mind to collect data, selecting an analysis method, or choosing audience sample il to draw a biased inference.
- Irrelevant to the sophistication used in research data and analysis is enough to rectify the poorly defined objective outcome measurements. It does not matter if the design is at fault or intentions are not clear, but lack of clarity might mislead readers, so avoid the practice.
- The motive behind data analysis in research is to present accurate and reliable data. As far as possible, avoid statistical errors, and find a way to deal with everyday challenges like outliers, missing data, data altering, data mining , or developing graphical representation.
LEARN MORE: Descriptive Research vs Correlational Research The sheer amount of data generated daily is frightening. Especially when data analysis has taken center stage. in 2018. In last year, the total data supply amounted to 2.8 trillion gigabytes. Hence, it is clear that the enterprises willing to survive in the hypercompetitive world must possess an excellent capability to analyze complex research data, derive actionable insights, and adapt to the new market needs.
LEARN ABOUT: Average Order Value
QuestionPro is an online survey platform that empowers organizations in data analysis and research and provides them a medium to collect data by creating appealing surveys.
MORE LIKE THIS
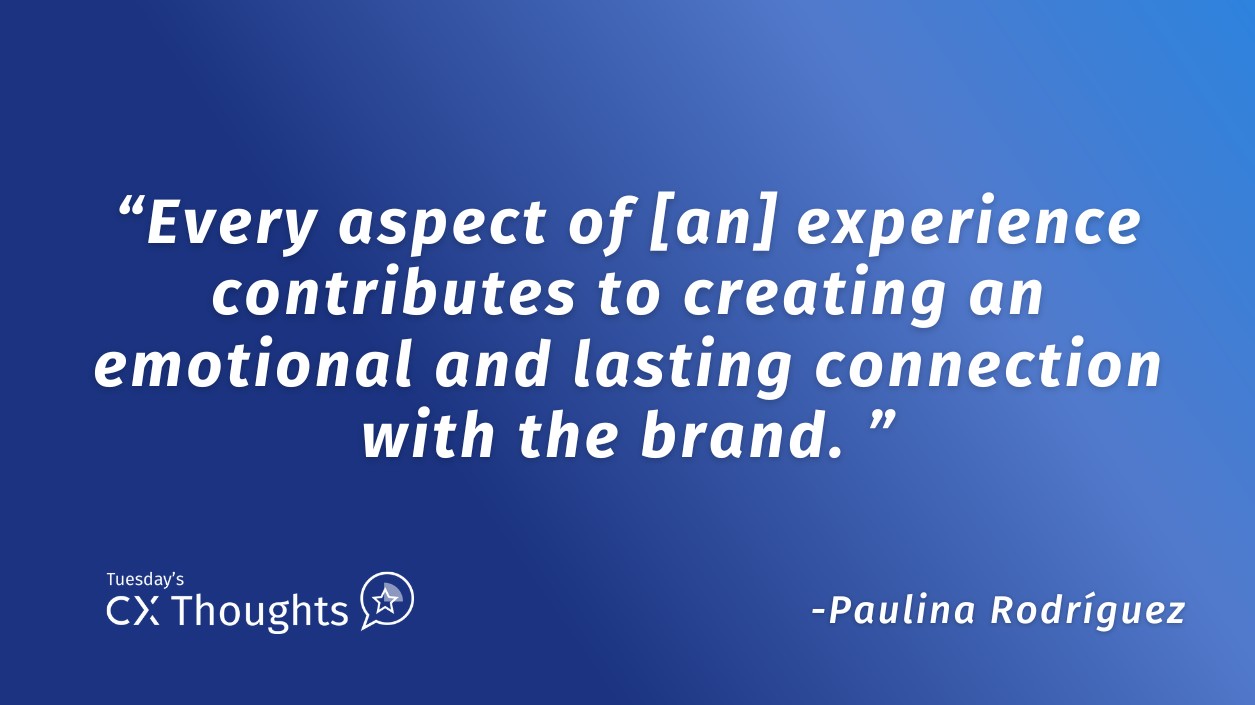
Customer Experience Lessons from 13,000 Feet — Tuesday CX Thoughts
Aug 20, 2024
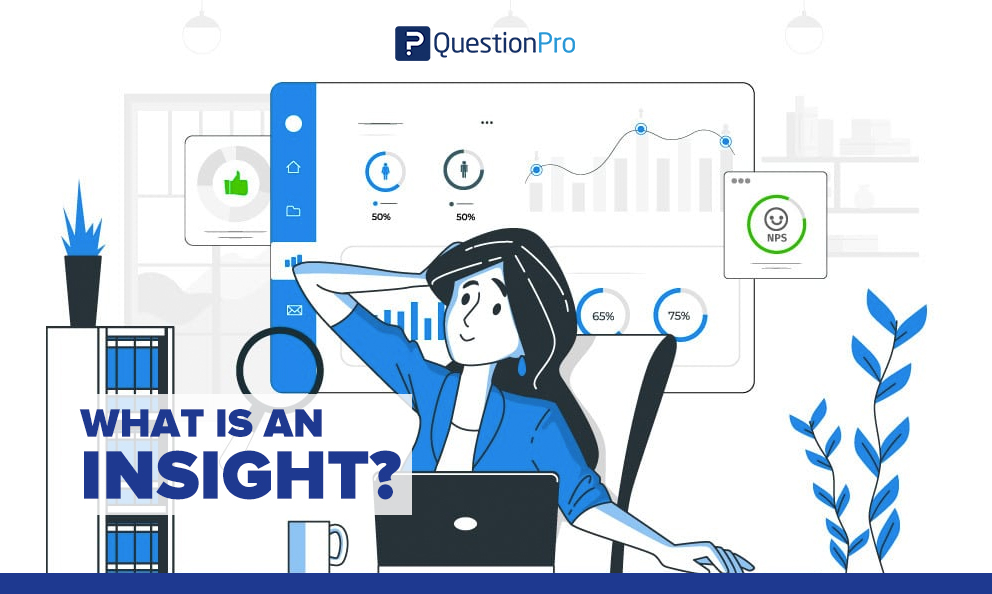
Insight: Definition & meaning, types and examples
Aug 19, 2024
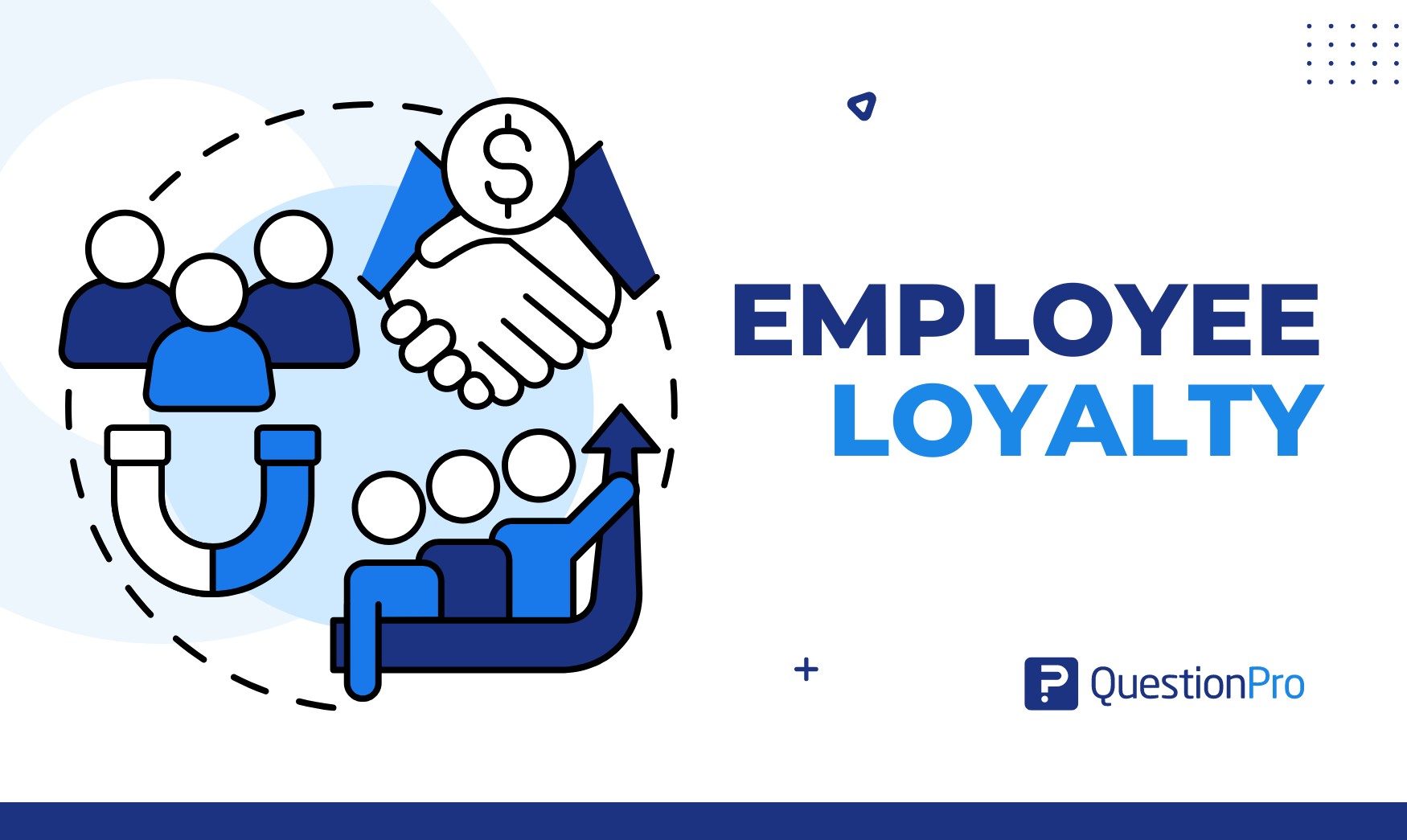
Employee Loyalty: Strategies for Long-Term Business Success
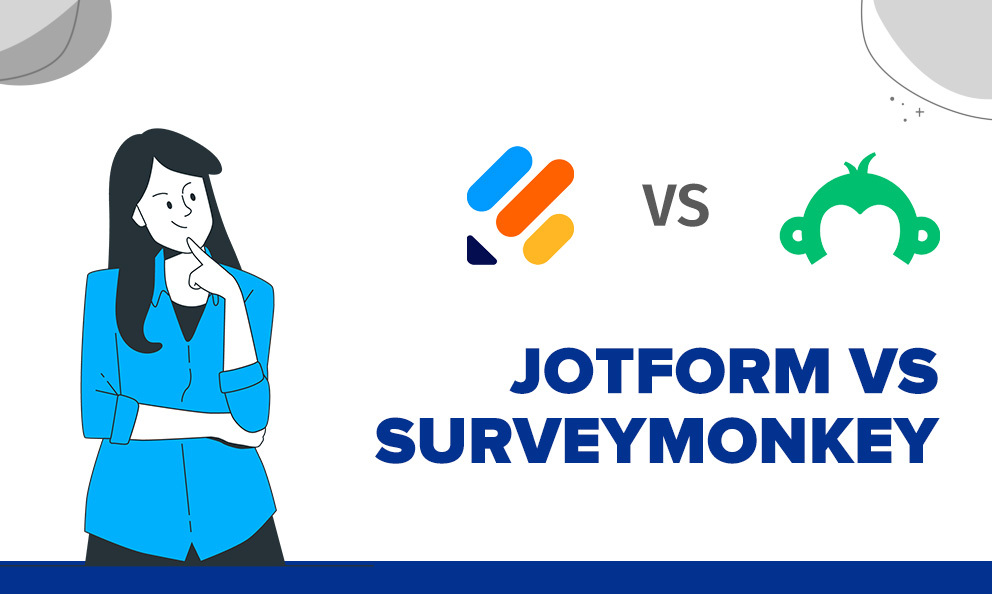
Jotform vs SurveyMonkey: Which Is Best in 2024
Aug 15, 2024
Other categories
- Academic Research
- Artificial Intelligence
- Assessments
- Brand Awareness
- Case Studies
- Communities
- Consumer Insights
- Customer effort score
- Customer Engagement
- Customer Experience
- Customer Loyalty
- Customer Research
- Customer Satisfaction
- Employee Benefits
- Employee Engagement
- Employee Retention
- Friday Five
- General Data Protection Regulation
- Insights Hub
- Life@QuestionPro
- Market Research
- Mobile diaries
- Mobile Surveys
- New Features
- Online Communities
- Question Types
- Questionnaire
- QuestionPro Products
- Release Notes
- Research Tools and Apps
- Revenue at Risk
- Survey Templates
- Training Tips
- Tuesday CX Thoughts (TCXT)
- Uncategorized
- What’s Coming Up
- Workforce Intelligence
- Privacy Policy

Home » Data Analysis – Process, Methods and Types
Data Analysis – Process, Methods and Types
Table of Contents

Data Analysis
Definition:
Data analysis refers to the process of inspecting, cleaning, transforming, and modeling data with the goal of discovering useful information, drawing conclusions, and supporting decision-making. It involves applying various statistical and computational techniques to interpret and derive insights from large datasets. The ultimate aim of data analysis is to convert raw data into actionable insights that can inform business decisions, scientific research, and other endeavors.
Data Analysis Process
The following are step-by-step guides to the data analysis process:
Define the Problem
The first step in data analysis is to clearly define the problem or question that needs to be answered. This involves identifying the purpose of the analysis, the data required, and the intended outcome.
Collect the Data
The next step is to collect the relevant data from various sources. This may involve collecting data from surveys, databases, or other sources. It is important to ensure that the data collected is accurate, complete, and relevant to the problem being analyzed.
Clean and Organize the Data
Once the data has been collected, it needs to be cleaned and organized. This involves removing any errors or inconsistencies in the data, filling in missing values, and ensuring that the data is in a format that can be easily analyzed.
Analyze the Data
The next step is to analyze the data using various statistical and analytical techniques. This may involve identifying patterns in the data, conducting statistical tests, or using machine learning algorithms to identify trends and insights.
Interpret the Results
After analyzing the data, the next step is to interpret the results. This involves drawing conclusions based on the analysis and identifying any significant findings or trends.
Communicate the Findings
Once the results have been interpreted, they need to be communicated to stakeholders. This may involve creating reports, visualizations, or presentations to effectively communicate the findings and recommendations.
Take Action
The final step in the data analysis process is to take action based on the findings. This may involve implementing new policies or procedures, making strategic decisions, or taking other actions based on the insights gained from the analysis.
Types of Data Analysis
Types of Data Analysis are as follows:
Descriptive Analysis
This type of analysis involves summarizing and describing the main characteristics of a dataset, such as the mean, median, mode, standard deviation, and range.
Inferential Analysis
This type of analysis involves making inferences about a population based on a sample. Inferential analysis can help determine whether a certain relationship or pattern observed in a sample is likely to be present in the entire population.
Diagnostic Analysis
This type of analysis involves identifying and diagnosing problems or issues within a dataset. Diagnostic analysis can help identify outliers, errors, missing data, or other anomalies in the dataset.
Predictive Analysis
This type of analysis involves using statistical models and algorithms to predict future outcomes or trends based on historical data. Predictive analysis can help businesses and organizations make informed decisions about the future.
Prescriptive Analysis
This type of analysis involves recommending a course of action based on the results of previous analyses. Prescriptive analysis can help organizations make data-driven decisions about how to optimize their operations, products, or services.
Exploratory Analysis
This type of analysis involves exploring the relationships and patterns within a dataset to identify new insights and trends. Exploratory analysis is often used in the early stages of research or data analysis to generate hypotheses and identify areas for further investigation.
Data Analysis Methods
Data Analysis Methods are as follows:
Statistical Analysis
This method involves the use of mathematical models and statistical tools to analyze and interpret data. It includes measures of central tendency, correlation analysis, regression analysis, hypothesis testing, and more.
Machine Learning
This method involves the use of algorithms to identify patterns and relationships in data. It includes supervised and unsupervised learning, classification, clustering, and predictive modeling.
Data Mining
This method involves using statistical and machine learning techniques to extract information and insights from large and complex datasets.
Text Analysis
This method involves using natural language processing (NLP) techniques to analyze and interpret text data. It includes sentiment analysis, topic modeling, and entity recognition.
Network Analysis
This method involves analyzing the relationships and connections between entities in a network, such as social networks or computer networks. It includes social network analysis and graph theory.
Time Series Analysis
This method involves analyzing data collected over time to identify patterns and trends. It includes forecasting, decomposition, and smoothing techniques.
Spatial Analysis
This method involves analyzing geographic data to identify spatial patterns and relationships. It includes spatial statistics, spatial regression, and geospatial data visualization.
Data Visualization
This method involves using graphs, charts, and other visual representations to help communicate the findings of the analysis. It includes scatter plots, bar charts, heat maps, and interactive dashboards.
Qualitative Analysis
This method involves analyzing non-numeric data such as interviews, observations, and open-ended survey responses. It includes thematic analysis, content analysis, and grounded theory.
Multi-criteria Decision Analysis
This method involves analyzing multiple criteria and objectives to support decision-making. It includes techniques such as the analytical hierarchy process, TOPSIS, and ELECTRE.
Data Analysis Tools
There are various data analysis tools available that can help with different aspects of data analysis. Below is a list of some commonly used data analysis tools:
- Microsoft Excel: A widely used spreadsheet program that allows for data organization, analysis, and visualization.
- SQL : A programming language used to manage and manipulate relational databases.
- R : An open-source programming language and software environment for statistical computing and graphics.
- Python : A general-purpose programming language that is widely used in data analysis and machine learning.
- Tableau : A data visualization software that allows for interactive and dynamic visualizations of data.
- SAS : A statistical analysis software used for data management, analysis, and reporting.
- SPSS : A statistical analysis software used for data analysis, reporting, and modeling.
- Matlab : A numerical computing software that is widely used in scientific research and engineering.
- RapidMiner : A data science platform that offers a wide range of data analysis and machine learning tools.
Applications of Data Analysis
Data analysis has numerous applications across various fields. Below are some examples of how data analysis is used in different fields:
- Business : Data analysis is used to gain insights into customer behavior, market trends, and financial performance. This includes customer segmentation, sales forecasting, and market research.
- Healthcare : Data analysis is used to identify patterns and trends in patient data, improve patient outcomes, and optimize healthcare operations. This includes clinical decision support, disease surveillance, and healthcare cost analysis.
- Education : Data analysis is used to measure student performance, evaluate teaching effectiveness, and improve educational programs. This includes assessment analytics, learning analytics, and program evaluation.
- Finance : Data analysis is used to monitor and evaluate financial performance, identify risks, and make investment decisions. This includes risk management, portfolio optimization, and fraud detection.
- Government : Data analysis is used to inform policy-making, improve public services, and enhance public safety. This includes crime analysis, disaster response planning, and social welfare program evaluation.
- Sports : Data analysis is used to gain insights into athlete performance, improve team strategy, and enhance fan engagement. This includes player evaluation, scouting analysis, and game strategy optimization.
- Marketing : Data analysis is used to measure the effectiveness of marketing campaigns, understand customer behavior, and develop targeted marketing strategies. This includes customer segmentation, marketing attribution analysis, and social media analytics.
- Environmental science : Data analysis is used to monitor and evaluate environmental conditions, assess the impact of human activities on the environment, and develop environmental policies. This includes climate modeling, ecological forecasting, and pollution monitoring.
When to Use Data Analysis
Data analysis is useful when you need to extract meaningful insights and information from large and complex datasets. It is a crucial step in the decision-making process, as it helps you understand the underlying patterns and relationships within the data, and identify potential areas for improvement or opportunities for growth.
Here are some specific scenarios where data analysis can be particularly helpful:
- Problem-solving : When you encounter a problem or challenge, data analysis can help you identify the root cause and develop effective solutions.
- Optimization : Data analysis can help you optimize processes, products, or services to increase efficiency, reduce costs, and improve overall performance.
- Prediction: Data analysis can help you make predictions about future trends or outcomes, which can inform strategic planning and decision-making.
- Performance evaluation : Data analysis can help you evaluate the performance of a process, product, or service to identify areas for improvement and potential opportunities for growth.
- Risk assessment : Data analysis can help you assess and mitigate risks, whether it is financial, operational, or related to safety.
- Market research : Data analysis can help you understand customer behavior and preferences, identify market trends, and develop effective marketing strategies.
- Quality control: Data analysis can help you ensure product quality and customer satisfaction by identifying and addressing quality issues.
Purpose of Data Analysis
The primary purposes of data analysis can be summarized as follows:
- To gain insights: Data analysis allows you to identify patterns and trends in data, which can provide valuable insights into the underlying factors that influence a particular phenomenon or process.
- To inform decision-making: Data analysis can help you make informed decisions based on the information that is available. By analyzing data, you can identify potential risks, opportunities, and solutions to problems.
- To improve performance: Data analysis can help you optimize processes, products, or services by identifying areas for improvement and potential opportunities for growth.
- To measure progress: Data analysis can help you measure progress towards a specific goal or objective, allowing you to track performance over time and adjust your strategies accordingly.
- To identify new opportunities: Data analysis can help you identify new opportunities for growth and innovation by identifying patterns and trends that may not have been visible before.
Examples of Data Analysis
Some Examples of Data Analysis are as follows:
- Social Media Monitoring: Companies use data analysis to monitor social media activity in real-time to understand their brand reputation, identify potential customer issues, and track competitors. By analyzing social media data, businesses can make informed decisions on product development, marketing strategies, and customer service.
- Financial Trading: Financial traders use data analysis to make real-time decisions about buying and selling stocks, bonds, and other financial instruments. By analyzing real-time market data, traders can identify trends and patterns that help them make informed investment decisions.
- Traffic Monitoring : Cities use data analysis to monitor traffic patterns and make real-time decisions about traffic management. By analyzing data from traffic cameras, sensors, and other sources, cities can identify congestion hotspots and make changes to improve traffic flow.
- Healthcare Monitoring: Healthcare providers use data analysis to monitor patient health in real-time. By analyzing data from wearable devices, electronic health records, and other sources, healthcare providers can identify potential health issues and provide timely interventions.
- Online Advertising: Online advertisers use data analysis to make real-time decisions about advertising campaigns. By analyzing data on user behavior and ad performance, advertisers can make adjustments to their campaigns to improve their effectiveness.
- Sports Analysis : Sports teams use data analysis to make real-time decisions about strategy and player performance. By analyzing data on player movement, ball position, and other variables, coaches can make informed decisions about substitutions, game strategy, and training regimens.
- Energy Management : Energy companies use data analysis to monitor energy consumption in real-time. By analyzing data on energy usage patterns, companies can identify opportunities to reduce energy consumption and improve efficiency.
Characteristics of Data Analysis
Characteristics of Data Analysis are as follows:
- Objective : Data analysis should be objective and based on empirical evidence, rather than subjective assumptions or opinions.
- Systematic : Data analysis should follow a systematic approach, using established methods and procedures for collecting, cleaning, and analyzing data.
- Accurate : Data analysis should produce accurate results, free from errors and bias. Data should be validated and verified to ensure its quality.
- Relevant : Data analysis should be relevant to the research question or problem being addressed. It should focus on the data that is most useful for answering the research question or solving the problem.
- Comprehensive : Data analysis should be comprehensive and consider all relevant factors that may affect the research question or problem.
- Timely : Data analysis should be conducted in a timely manner, so that the results are available when they are needed.
- Reproducible : Data analysis should be reproducible, meaning that other researchers should be able to replicate the analysis using the same data and methods.
- Communicable : Data analysis should be communicated clearly and effectively to stakeholders and other interested parties. The results should be presented in a way that is understandable and useful for decision-making.
Advantages of Data Analysis
Advantages of Data Analysis are as follows:
- Better decision-making: Data analysis helps in making informed decisions based on facts and evidence, rather than intuition or guesswork.
- Improved efficiency: Data analysis can identify inefficiencies and bottlenecks in business processes, allowing organizations to optimize their operations and reduce costs.
- Increased accuracy: Data analysis helps to reduce errors and bias, providing more accurate and reliable information.
- Better customer service: Data analysis can help organizations understand their customers better, allowing them to provide better customer service and improve customer satisfaction.
- Competitive advantage: Data analysis can provide organizations with insights into their competitors, allowing them to identify areas where they can gain a competitive advantage.
- Identification of trends and patterns : Data analysis can identify trends and patterns in data that may not be immediately apparent, helping organizations to make predictions and plan for the future.
- Improved risk management : Data analysis can help organizations identify potential risks and take proactive steps to mitigate them.
- Innovation: Data analysis can inspire innovation and new ideas by revealing new opportunities or previously unknown correlations in data.

Limitations of Data Analysis
- Data quality: The quality of data can impact the accuracy and reliability of analysis results. If data is incomplete, inconsistent, or outdated, the analysis may not provide meaningful insights.
- Limited scope: Data analysis is limited by the scope of the data available. If data is incomplete or does not capture all relevant factors, the analysis may not provide a complete picture.
- Human error : Data analysis is often conducted by humans, and errors can occur in data collection, cleaning, and analysis.
- Cost : Data analysis can be expensive, requiring specialized tools, software, and expertise.
- Time-consuming : Data analysis can be time-consuming, especially when working with large datasets or conducting complex analyses.
- Overreliance on data: Data analysis should be complemented with human intuition and expertise. Overreliance on data can lead to a lack of creativity and innovation.
- Privacy concerns: Data analysis can raise privacy concerns if personal or sensitive information is used without proper consent or security measures.
About the author
Muhammad Hassan
Researcher, Academic Writer, Web developer
You may also like

Research Questions – Types, Examples and Writing...

Research Methods – Types, Examples and Guide

Data Interpretation – Process, Methods and...

Evaluating Research – Process, Examples and...

Histogram – Types, Examples and Making Guide

Literature Review – Types Writing Guide and...
- Subject List
- Take a Tour
- For Authors
- Subscriber Services
- Publications
- African American Studies
- African Studies
- American Literature
- Anthropology
- Architecture Planning and Preservation
- Art History
- Atlantic History
- Biblical Studies
- British and Irish Literature
- Childhood Studies
- Chinese Studies
- Cinema and Media Studies
- Communication
- Criminology
- Environmental Science
- Evolutionary Biology
- International Law
- International Relations
- Islamic Studies
- Jewish Studies
- Latin American Studies
- Latino Studies
- Linguistics
- Literary and Critical Theory
- Medieval Studies
- Military History
- Political Science
- Public Health
- Renaissance and Reformation
- Social Work
- Urban Studies
- Victorian Literature
- Browse All Subjects
How to Subscribe
- Free Trials
In This Article Expand or collapse the "in this article" section Data Collection in Educational Research
Introduction, general overviews.
- General Quantitative Overviews
- Questionnaires
- Quantitative Interviewing
- Quantitative Observation
- Technical Properties
- General Qualitative Overviews
- In-Depth Interviewing
- Focus Groups
- Qualitative Observation
- Qualitative Document Analysis
- Visual Analysis
Related Articles Expand or collapse the "related articles" section about
About related articles close popup.
Lorem Ipsum Sit Dolor Amet
Vestibulum ante ipsum primis in faucibus orci luctus et ultrices posuere cubilia Curae; Aliquam ligula odio, euismod ut aliquam et, vestibulum nec risus. Nulla viverra, arcu et iaculis consequat, justo diam ornare tellus, semper ultrices tellus nunc eu tellus.
- Case Study in Education Research
- Grounded Theory
- Methodologies for Conducting Education Research
- Mixed Methods Research
- Qualitative Research Design
- Statistical Assumptions
- Using Ethnography in Educational Research
Other Subject Areas
Forthcoming articles expand or collapse the "forthcoming articles" section.
- Cyber Safety in Schools
- Girls' Education in the Developing World
- History of Education in Europe
- Find more forthcoming articles...
- Export Citations
- Share This Facebook LinkedIn Twitter
Data Collection in Educational Research by James H. McMillan , Laura P. Gogia LAST REVIEWED: 30 June 2014 LAST MODIFIED: 30 June 2014 DOI: 10.1093/obo/9780199756810-0087
Data collection methods in educational research are used to gather information that is then analyzed and interpreted. As such, data collection is a very important step in conducting research and can influence results significantly. Once the research question and sources of data are identified, appropriate methods of data collection are determined. Data collection includes a broad range of more specific techniques. Historically, much of the data collection performed in educational research depended on methods developed for studies in the field of psychology, a discipline which took what is termed a “quantitative” approach. This involves using instruments, scales, Tests , and structured observation and interviewing. By the mid- to late twentieth centuries, other disciplines such as anthropology and sociology began to influence educational researchers. Forms of data collection broadened to include what is now called “qualitative” methods, with an emphasis on narratives, participant perspectives, and less structured observation and interviewing. As contemporary educational researchers also draw from fields such as business, political science, and medicine, data collection in education has become a multidisciplinary phenomenon. Because data collection is such a broad topic, General Overviews that attempt to cover all or most techniques tend to offer introductory treatments. Few texts, however, provide comprehensive coverage of every data collection technique. Instead, some cover techniques appropriate for either quantitative or qualitative research approaches. Even more focus on one or two data collection methods within those two research contexts. Consequently, after presenting general overviews, this entry is categorized by data collection appropriate for quantitative and Qualitative Data Collection . These sections, in turn, are subdivided into the major types of quantitative and qualitative data collection techniques. While there are some data collection techniques specific to mixed method research design, which implies a combination of qualitative and quantitative research methodologies, these specific procedures are not emphasized in the present article—readers are referred to the Oxford Bibliography article Mixed Methods Research by Nancy Leech for a comprehensive treatment of mixed method data collection techniques. To locate sources for this article, extensive searches were performed using general-use Internet search engines and educational, psychological, and social science research databases. These searches included keywords around data collection and research methods, as well as specific data collection techniques such as surveys, Tests , Focus Groups , and observation. Frequently cited texts and articles, most recent editions at the time, and sources specific to educational research were given priority. Once these sources were identified, their suggested readings and reference lists were mined for other potential sources. Works or scholars found in multiple reference lists were investigated. When applicable, book reviews in peer-reviewed journals were located and taken into account when curating sources. Sources that demonstrated a high level of impact or offered unique coverage of the topic were included.
General educational research overviews typically include several chapters on data collection, organized into qualitative and quantitative approaches. As a rule they are updated frequently so that they offer timely discussions of methodological trends. Most of them are introductory in nature, written for student researchers. Because of the influence of psychology and other social sciences on the development of data collection in educational research, representative works of psychology ( Trochim 2006 ) and of general social sciences ( Robson 2011 ) are included. Available online, Trochim 2006 is a reader-friendly introduction that provides succinct explanations of most quantitative and qualitative approaches. Olsen 2012 is helpful in showing how data collection techniques used in other disciplines have implications for educational studies. Specific to education, Gall, et al. 2007 is a frequently cited text that contains most educational data collection techniques, although it tends to emphasize more traditional quantitative approaches. Johnson and Christensen 2014 offers a more balanced treatment meant for novice researchers and educational research consumers. Cohen, et al. 2011 also provides a balanced approach, but from a British perspective. Fielding, et al. 2008 offer practical advice on recently developed forms of online data collection, with special attention given to the ethical ramifications of Internet-based data collection. Finally, Arthur, et al. 2012 is unique in this section in that it is an edited work offering short overviews of data collection techniques authored by contemporary leading experts.
Arthur, James, Michael Waring, Robert Coe, and Larry Hedges, eds. 2012. Research methods and methodologies in education . London: SAGE.
A diverse edited text discussing trends in study designs, data collection, and data analysis. It includes twelve chapters devoted to different forms of data collection, written by authors who have recently published extensively on the topic. Annotated bibliographies found at the end of each chapter provide guidance for further reading.
Cohen, Louis, Lawrence Manion, and Keith Morrison. 2011. Research methods in education . 7th ed. London: Routledge.
This long-running, bestselling, comprehensive source offers practical advice with clear theoretical foundations. The newest edition has undergone significant revision. Specific to data collection, revisions include new chapters devoted to data collection via the Internet and visual media. Slides highlighting main points are available on a supplementary website.
Fielding, Nigel, Raymond Lee, and Grant Blank. 2008. The SAGE handbook of online research methods . Thousand Oaks, CA: SAGE.
This extensive handbook presents chapters on Internet research design and data collection written by leading scholars in the field. It discusses using the Internet as an archival resource and a research tool, focusing on the most recent trends in multidisciplinary Internet research.
Gall, Meredith, Joyce Gall, and Walter Borg. 2007. Educational research: An introduction . 8th ed. White Plains, NY: Pearson.
A long-standing, well-respected, nuts-and-bolts perspective on data collection meant to prepare students for conducting original research. Although it tends to emphasize quantitative research methodologies, it has a uniquely rich chapter on historical document analysis.
Johnson, Burke, and Larry Christensen. 2014. Educational research: Quantitative, qualitative, and mixed approaches . 5th ed. Thousand Oaks, CA: SAGE.
A comprehensive introductory text for the consumer and the would-be researcher, with extensive lists of additional resources for gathering all types of data. It discusses quantitative and qualitative research methodologies and data collection evenly but provides extended coverage of questionnaire construction.
Olsen, Wendy. 2012. Data collection: Key debates and methods in social research . London: SAGE.
This recently published toolkit of quantitative, qualitative, and mixed method approaches to data collection provides a more contemporary introduction for both students and research professionals. It offers a helpful overview of data collection as an integral part of research in several different fields of study.
Robson, Colin. 2011. Real world research: A resource for users of social research methods in applied settings . West Sussex, UK: Wiley
This introductory text is intended for all social science. There is an applied, integrated emphasis on contemporary quantitative and qualitative data collection techniques in a separate section of the book, including individual and focus group observations, surveys, unstructured and structured interviewing, and tests.
Trochim, William. 2006. Research methods knowledge base
A free online hypertext textbook on applied social research methods. Data collection techniques associated with qualitative and quantitative research are covered comprehensively. Foundational information appropriate for undergraduates and early graduate students is presented through a series of easy-to-navigate and intuitively ordered webpages. Printed editions are available for purchase in an edition written with James Donnelly (Atomic Dog/Cengage Learning, 2008).
back to top
Users without a subscription are not able to see the full content on this page. Please subscribe or login .
Oxford Bibliographies Online is available by subscription and perpetual access to institutions. For more information or to contact an Oxford Sales Representative click here .
- About Education »
- Meet the Editorial Board »
- Academic Achievement
- Academic Audit for Universities
- Academic Freedom and Tenure in the United States
- Action Research in Education
- Adjuncts in Higher Education in the United States
- Administrator Preparation
- Adolescence
- Advanced Placement and International Baccalaureate Courses
- Advocacy and Activism in Early Childhood
- African American Racial Identity and Learning
- Alaska Native Education
- Alternative Certification Programs for Educators
- Alternative Schools
- American Indian Education
- Animals in Environmental Education
- Art Education
- Artificial Intelligence and Learning
- Assessing School Leader Effectiveness
- Assessment, Behavioral
- Assessment, Educational
- Assessment in Early Childhood Education
- Assistive Technology
- Augmented Reality in Education
- Beginning-Teacher Induction
- Bilingual Education and Bilingualism
- Black Undergraduate Women: Critical Race and Gender Perspe...
- Black Women in Academia
- Blended Learning
- Changing Professional and Academic Identities
- Character Education
- Children’s and Young Adult Literature
- Children's Beliefs about Intelligence
- Children's Rights in Early Childhood Education
- Citizenship Education
- Civic and Social Engagement of Higher Education
- Classroom Learning Environments: Assessing and Investigati...
- Classroom Management
- Coherent Instructional Systems at the School and School Sy...
- College Admissions in the United States
- College Athletics in the United States
- Community Relations
- Comparative Education
- Computer-Assisted Language Learning
- Computer-Based Testing
- Conceptualizing, Measuring, and Evaluating Improvement Net...
- Continuous Improvement and "High Leverage" Educational Pro...
- Counseling in Schools
- Critical Approaches to Gender in Higher Education
- Critical Perspectives on Educational Innovation and Improv...
- Critical Race Theory
- Crossborder and Transnational Higher Education
- Cross-National Research on Continuous Improvement
- Cross-Sector Research on Continuous Learning and Improveme...
- Cultural Diversity in Early Childhood Education
- Culturally Responsive Leadership
- Culturally Responsive Pedagogies
- Culturally Responsive Teacher Education in the United Stat...
- Curriculum Design
- Data Collection in Educational Research
- Data-driven Decision Making in the United States
- Deaf Education
- Desegregation and Integration
- Design Thinking and the Learning Sciences: Theoretical, Pr...
- Development, Moral
- Dialogic Pedagogy
- Digital Age Teacher, The
- Digital Citizenship
- Digital Divides
- Disabilities
- Distance Learning
- Distributed Leadership
- Doctoral Education and Training
- Early Childhood Education and Care (ECEC) in Denmark
- Early Childhood Education and Development in Mexico
- Early Childhood Education in Aotearoa New Zealand
- Early Childhood Education in Australia
- Early Childhood Education in China
- Early Childhood Education in Europe
- Early Childhood Education in Sub-Saharan Africa
- Early Childhood Education in Sweden
- Early Childhood Education Pedagogy
- Early Childhood Education Policy
- Early Childhood Education, The Arts in
- Early Childhood Mathematics
- Early Childhood Science
- Early Childhood Teacher Education
- Early Childhood Teachers in Aotearoa New Zealand
- Early Years Professionalism and Professionalization Polici...
- Economics of Education
- Education For Children with Autism
- Education for Sustainable Development
- Education Leadership, Empirical Perspectives in
- Education of Native Hawaiian Students
- Education Reform and School Change
- Educational Research Approaches: A Comparison
- Educational Statistics for Longitudinal Research
- Educator Partnerships with Parents and Families with a Foc...
- Emotional and Affective Issues in Environmental and Sustai...
- Emotional and Behavioral Disorders
- English as an International Language for Academic Publishi...
- Environmental and Science Education: Overlaps and Issues
- Environmental Education
- Environmental Education in Brazil
- Epistemic Beliefs
- Equity and Improvement: Engaging Communities in Educationa...
- Equity, Ethnicity, Diversity, and Excellence in Education
- Ethical Research with Young Children
- Ethics and Education
- Ethics of Teaching
- Ethnic Studies
- Evidence-Based Communication Assessment and Intervention
- Family and Community Partnerships in Education
- Family Day Care
- Federal Government Programs and Issues
- Feminization of Labor in Academia
- Finance, Education
- Financial Aid
- Formative Assessment
- Future-Focused Education
- Gender and Achievement
- Gender and Alternative Education
- Gender, Power and Politics in the Academy
- Gender-Based Violence on University Campuses
- Gifted Education
- Global Mindedness and Global Citizenship Education
- Global University Rankings
- Governance, Education
- Growth of Effective Mental Health Services in Schools in t...
- Higher Education and Globalization
- Higher Education and the Developing World
- Higher Education Faculty Characteristics and Trends in the...
- Higher Education Finance
- Higher Education Governance
- Higher Education Graduate Outcomes and Destinations
- Higher Education in Africa
- Higher Education in China
- Higher Education in Latin America
- Higher Education in the United States, Historical Evolutio...
- Higher Education, International Issues in
- Higher Education Management
- Higher Education Policy
- Higher Education Research
- Higher Education Student Assessment
- High-stakes Testing
- History of Early Childhood Education in the United States
- History of Education in the United States
- History of Technology Integration in Education
- Homeschooling
- Inclusion in Early Childhood: Difference, Disability, and ...
- Inclusive Education
- Indigenous Education in a Global Context
- Indigenous Learning Environments
- Indigenous Students in Higher Education in the United Stat...
- Infant and Toddler Pedagogy
- Inservice Teacher Education
- Integrating Art across the Curriculum
- Intelligence
- Intensive Interventions for Children and Adolescents with ...
- International Perspectives on Academic Freedom
- Intersectionality and Education
- Knowledge Development in Early Childhood
- Leadership Development, Coaching and Feedback for
- Leadership in Early Childhood Education
- Leadership Training with an Emphasis on the United States
- Learning Analytics in Higher Education
- Learning Difficulties
- Learning, Lifelong
- Learning, Multimedia
- Learning Strategies
- Legal Matters and Education Law
- LGBT Youth in Schools
- Linguistic Diversity
- Linguistically Inclusive Pedagogy
- Literacy Development and Language Acquisition
- Literature Reviews
- Mathematics Identity
- Mathematics Instruction and Interventions for Students wit...
- Mathematics Teacher Education
- Measurement for Improvement in Education
- Measurement in Education in the United States
- Meta-Analysis and Research Synthesis in Education
- Methodological Approaches for Impact Evaluation in Educati...
- Mindfulness, Learning, and Education
- Motherscholars
- Multiliteracies in Early Childhood Education
- Multiple Documents Literacy: Theory, Research, and Applica...
- Multivariate Research Methodology
- Museums, Education, and Curriculum
- Music Education
- Narrative Research in Education
- Native American Studies
- Nonformal and Informal Environmental Education
- Note-Taking
- Numeracy Education
- One-to-One Technology in the K-12 Classroom
- Online Education
- Open Education
- Organizing for Continuous Improvement in Education
- Organizing Schools for the Inclusion of Students with Disa...
- Outdoor Play and Learning
- Outdoor Play and Learning in Early Childhood Education
- Pedagogical Leadership
- Pedagogy of Teacher Education, A
- Performance Objectives and Measurement
- Performance-based Research Assessment in Higher Education
- Performance-based Research Funding
- Phenomenology in Educational Research
- Philosophy of Education
- Physical Education
- Podcasts in Education
- Policy Context of United States Educational Innovation and...
- Politics of Education
- Portable Technology Use in Special Education Programs and ...
- Post-humanism and Environmental Education
- Pre-Service Teacher Education
- Problem Solving
- Productivity and Higher Education
- Professional Development
- Professional Learning Communities
- Program Evaluation
- Programs and Services for Students with Emotional or Behav...
- Psychology Learning and Teaching
- Psychometric Issues in the Assessment of English Language ...
- Qualitative Data Analysis Techniques
- Qualitative, Quantitative, and Mixed Methods Research Samp...
- Quantitative Research Designs in Educational Research
- Queering the English Language Arts (ELA) Writing Classroom
- Race and Affirmative Action in Higher Education
- Reading Education
- Refugee and New Immigrant Learners
- Relational and Developmental Trauma and Schools
- Relational Pedagogies in Early Childhood Education
- Reliability in Educational Assessments
- Religion in Elementary and Secondary Education in the Unit...
- Researcher Development and Skills Training within the Cont...
- Research-Practice Partnerships in Education within the Uni...
- Response to Intervention
- Restorative Practices
- Risky Play in Early Childhood Education
- Role of Gender Equity Work on University Campuses through ...
- Scale and Sustainability of Education Innovation and Impro...
- Scaling Up Research-based Educational Practices
- School Accreditation
- School Choice
- School Culture
- School District Budgeting and Financial Management in the ...
- School Improvement through Inclusive Education
- School Reform
- Schools, Private and Independent
- School-Wide Positive Behavior Support
- Science Education
- Secondary to Postsecondary Transition Issues
- Self-Regulated Learning
- Self-Study of Teacher Education Practices
- Service-Learning
- Severe Disabilities
- Single Salary Schedule
- Single-sex Education
- Single-Subject Research Design
- Social Context of Education
- Social Justice
- Social Network Analysis
- Social Pedagogy
- Social Science and Education Research
- Social Studies Education
- Sociology of Education
- Standards-Based Education
- Student Access, Equity, and Diversity in Higher Education
- Student Assignment Policy
- Student Engagement in Tertiary Education
- Student Learning, Development, Engagement, and Motivation ...
- Student Participation
- Student Voice in Teacher Development
- Sustainability Education in Early Childhood Education
- Sustainability in Early Childhood Education
- Sustainability in Higher Education
- Teacher Beliefs and Epistemologies
- Teacher Collaboration in School Improvement
- Teacher Evaluation and Teacher Effectiveness
- Teacher Preparation
- Teacher Training and Development
- Teacher Unions and Associations
- Teacher-Student Relationships
- Teaching Critical Thinking
- Technologies, Teaching, and Learning in Higher Education
- Technology Education in Early Childhood
- Technology, Educational
- Technology-based Assessment
- The Bologna Process
- The Regulation of Standards in Higher Education
- Theories of Educational Leadership
- Three Conceptions of Literacy: Media, Narrative, and Gamin...
- Tracking and Detracking
- Traditions of Quality Improvement in Education
- Transformative Learning
- Transitions in Early Childhood Education
- Tribally Controlled Colleges and Universities in the Unite...
- Understanding the Psycho-Social Dimensions of Schools and ...
- University Faculty Roles and Responsibilities in the Unite...
- Value of Higher Education for Students and Other Stakehold...
- Virtual Learning Environments
- Vocational and Technical Education
- Wellness and Well-Being in Education
- Women's and Gender Studies
- Young Children and Spirituality
- Young Children's Learning Dispositions
- Young Children's Working Theories
- Privacy Policy
- Cookie Policy
- Legal Notice
- Accessibility
Powered by:
- [81.177.182.154]
- 81.177.182.154
5 Ways Your Institution Can Leverage Your Data Analytics
- Data Analytics 101
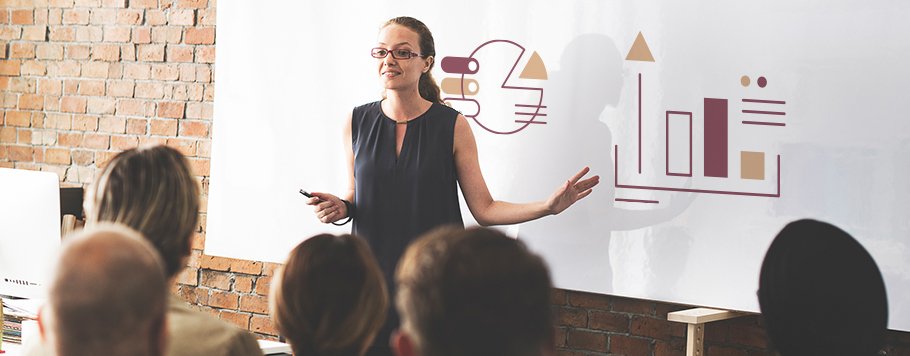
Think about all the different data sets your school has. From student GPAs to class section counts, nearly everything can be put into a data set, creating a culture of big data. How are you using all of this information to your school’s advantage?
When used strategically, big data and data analytics in education has the power to transform an institution. As a professor of higher education at North Carolina State University and a previous director of institutional research, Stephen Porter knows this first-hand. “Institutions are rushing with their analytics and not thinking strategically about what data they have and how it can help their predictions,” Porter said.
What is Big Data?
The term “big data” refers to the immense amount of information that’s created and collected on a daily basis. Big data has become so colossal that traditional data management tools, like Excel spreadsheets and manual record-keeping, are no longer able to keep up.
Because the volume of data has significantly increased with the digital revolution, the need for a robust data analytics program is an absolute must if you want to put your information to use in a strategic way.
In particular, educators can use data analytics to record and analyze the following data sets:
- Student Data : Demographics like age, ethnicity and gender; whether they are full-time or part-time; if they take classes online, on campus or a mix of the two.
- Course Data : Enrollment headcounts, grades and completion rates by program or section.
- Instructor Data : Demographics like age, ethnicity and gender; salary information; productivity levels.
- Facility Data : Classroom utilization and resource allocation, like how many hours a week each room is being used.
Benefits of Using Data Analytics Tools
- Create a Data-Driven Decision-Making Culture – With data analytics, there’s no need to rely on blind faith when making decisions. You’ll have numbers and statistics to back up your decisions, which can lead to more successful outcomes.
- Access Data Easier – Data analytics tools depend on the same type of technological infrastructure to capture, store and organize information, so it’s easy to find what you need.
- Find Information More Quickly – Since your data lives in one place, there’s no need to search through dozens of files and folders to find one report, making the process much quicker.
With these in mind, we’ve compiled a list of some of the most effective ways educational institutions can leverage their data analytics.
Help Your Students Succeed
Enrolling a new student is far more expensive than retaining a current one. That’s why one of the most advantageous ways to use data analytics in education involves identifying challenges students may have or are currently having in their academic career.
Predicting Success
When determining which students to accept to your institution, looking at certain academic analytics can tell you which candidates are the most likely to succeed and which may be more likely to drop out or fail their classes. This can help you make a judgment call before they even walk onto your campus.
Say a student was accepted to your institution and wants to study engineering. By using data you already have about this student — like their SAT scores, high school GPA and individual class grades — you can assess whether or not they would be likely to succeed in the engineering program. Did they struggle with math? If so, the engineering track might require additional math support for this particular student. In this instance, the student can meet with their advisor to review other options, like exploring a different program or beginning with remedial math courses.
Help Students Who May Be Struggling
Your data can also help you intervene before a problem has the chance to become a serious issue for you and your students. If a student fails their classes, placing them on academic probation at the end of a semester doesn’t help anyone. But by sharing certain learning analytics between professors and advisors throughout a term, steps can be taken to prevent the student from failing in the first place.
Porter has seen institutions leverage this technique in practical ways. “With the right data and software program, institutions can set up an alert system that notifies a student’s advisor if they are failing their classes,” he said. “The advisor can then intervene and try to find a solution, which is a much more proactive approach compared to just letting a student fall through the cracks.”
Do they need tutoring? Are they struggling to balance their personal life with school? Or are they just in the wrong program? Their advisor will be able to assess the situation and figure out a solution that will benefit both the student and your institution.
Share a Public Fact Book
Deciding on where we pursue our education is one of the biggest decisions we make in our lives, and students spend months — if not years — researching their options. Because of this, data analytics in education is useful when it comes to recruiting and educating applicants.
Assembling data sets into a public fact book that’s accessible on your website is a great way to share information that your prospects need to make a decision. This can include data like:
- Class sizes
- Student to teacher ratios
- Student outcomes
The example below shows the course success rates for French classes. In 2015, the success rate was 61% but it jumped to 83% in 2016. This data would give a student who wants to study French a good idea of how successful the program is, which may make them more likely to attend your institution.
See how customizable reporting software could help you leverage your institution’s data. >>
Evaluate how physical space is being used.
From classrooms to energy consumption, physical space is costing you money. But fortunately, your data can help you make efficient use of every square inch of your campus.
Tracking Resources
Where are your students at specific times of the day and days of the week? If your data tells you that they aren’t very excited to take English courses on Friday afternoons, you can potentially reduce the energy consumption for that part of campus by changing the days or times these courses are offered.
This also helps you plan better. Since you know that no one enjoys a Friday afternoon English class, you can plan these classes to better match your students’ preferences, which could also help increase enrollment.
Section Fill Rates
If ENG 101 offers 11 sections for the fall semester and only four are full, it might be time to figure out why. Is it the time of day? The day of the week? The professor?
This can also help you in the opposite scenario, like if CHEM 202 only offers three sections and they all have a long waitlist. That may be a clear sign that you need to add more sections and/or hire additional professors.
These are all questions you can dive deeper into, which can open up more possibilities that can help you save additional resources.
Track Enrollment Trends
Your data sets will tell you everything you need to know about students who are applying, enrolling and graduating from your institution, which is essential when it comes to planning and recruiting.
For example, the data below shows the enrollments for white females between the ages of 18 and 20 for the fall semesters of 2015 and 2016.
As you can see, there was a significant dip — by 50%! — in enrollments for this demographic in 2016. What could have happened to cause this? Noticing the problem is the first step. Once you’re aware, you can have your institutional research department explore this further and come to a conclusion. Then you can fix it!
Location, Location, Location!
Where do your applicants live? If you can easily see that prospective students from certain cities or towns are applying, being accepted and graduating from your institution, you can better tailor your marketing efforts to students in the same areas. You may also have the flexibility to increase offerings at strategically located satellite campuses.
Improve Communication Between Departments
It’s not surprising that there are silos in campuses all over the country. Programs can have disparate data coming from a variety of sources, which can make it hard to share information between professors, let alone other departments.
However, using data analytics in education — combined with a structured reporting software — can help you build a more collaborative culture that can seriously benefit your institution. For example, sharing analytics about enrollment trends has the potential to save much-needed resources. If the natural science program is losing enrollment while biological science is increasing enrollment, why not combine these programs? They are similar but with different appeals, which can simply be addressed by the way the program is marketed.
Porter believes that institutions “need to break down silos so there is more data at the fingertips of those in charge of predicting and planning.” And while some departments may be wary about sharing their data, it’s important to understand that a lot of it is submitted to the federal government and is more transparent now than ever before. Plus, the more information you have, the easier it becomes to more effectively predict and plan.
How to Avoid Potential Challenges When Getting Started
Leveraging your data to achieve these benefits begins with a solid plan of action. Make sure to do the following before implementing any data analytics solution:
- Create Goals – Your data analytics program will open up a lot of doors for you, so it could become overwhelming if you don’t enter into the new process without clear-cut goals in mind.
- Set Expectations – You’ll need your employees to be on board with your data analytics platform and strategies, which is why you should have open communication and set expectations for your team before implementing a solution.
- Make it Accessible – For full transparency, make sure your employees can access data where and when they need it. That means setting the appropriate permissions and organizing data properly. For example, teachers will need access to different data sets than the administration office.
These are only a small fraction of the ways data analytics in education can empower you to improve the future of your institution. To see more, sign up for the free version of Precision Campus and start putting your data to work!
Our Founder and President Eric Spear has been right where you are: in charge of an unfathomable amount of data and responsible for creating countless end user reports. With the dawn of cloud computing, Eric recognized the opportunity to combine his expertise with the latest technology to support the modern-day campus’ needs and solve many of their previously unsolvable challenges.
Need help finding data analytics software?
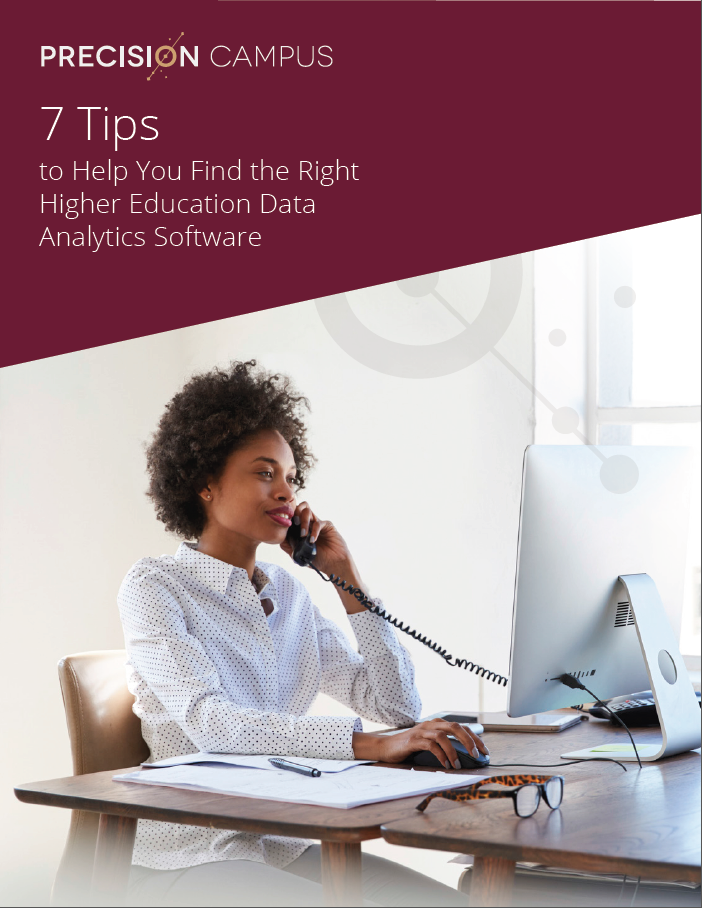
7 Tips to Help You Find the Right Higher Education Data Analytics Software
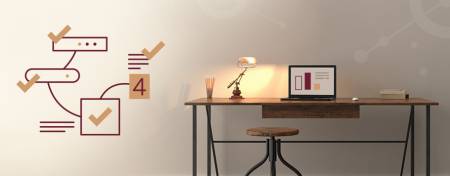
4 Smart Tips to Help You Find the Best Higher Education Data Analytics Software
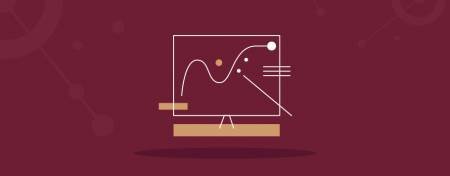
5 Tips to Help You Succeed with Higher Education Data Software
Toll Free 603.828.2521
Sign up for our Newsletter
- Open access
- Published: 20 August 2024
Impact of a game-based interprofessional education program on medical students’ perceptions: a text network analysis using essays
- Young Gyu Kwon 1 ,
- Myeong Namgung 2 ,
- Song Hee Park 3 ,
- Mi Kyung Kim 3 , 4 ,
- Sun Jung Myung 5 ,
- Eun Kyung Eo 6 &
- Chan Woong Kim 1 , 2
BMC Medical Education volume 24 , Article number: 898 ( 2024 ) Cite this article
81 Accesses
Metrics details
The increasing complexity of the healthcare environment and the necessity of multidisciplinary teamwork have highlighted the importance of interprofessional education (IPE). IPE aims to enhance the quality of patient care through collaborative education involving various healthcare professionals, such as doctors, nurses, and pharmacists. This study sought to analyze how game-based IPE activities influence students’ perceptions and reflective thinking. It also aimed to identify the shifts in perception and effectiveness caused by this educational approach.
The study is based on a game-based IPE program conducted at University A, involving medical and nursing students in structured learning and team-based activities. Data were collected using essays written by the students after they had participated in IPE activities. Text network analysis was conducted by extracting key terms, performing centrality analysis, and visualizing topic modeling to identify changes in students’ perceptions and reflective thinking.
Keywords such as “patient,” “thought,” “group,” “doctor,” “nurse,” and “communication” played a crucial role in the network, indicating that students prioritized enhancing their communication and problem-solving skills within the educational environment. The topic modeling results identified three main topics, each demonstrating the positive influence of game-based collaborative activities, interprofessional perspectives, and interdisciplinary educational experiences on students. Topic 3 (interdisciplinary educational experience) acted as a significant mediator connecting Topic 1 (game-based collaborative activity experience) and Topic 2 (interprofessional perspectives).
This study demonstrates that game-based IPE activities are an effective educational approach for enhancing students’ team building skills, particularly communication and interprofessional perspectives. Based on these findings, future IPE programs should focus on creating collaborative learning environments, strengthening communication skills, and promoting interdisciplinary education. The findings provide essential insights for educational designers and medical educators to enhance the effectiveness of IPE programs. Future research should assess the long-term impacts of game-based IPE on clinical practice, patient outcomes, and participants’ professional development.
Peer Review reports
With rapid changes in the healthcare environment and the advancement of systems, effective collaboration among various healthcare professionals is crucial to meet patients’ high expectations [ 1 ]. This underscores the growing importance of interprofessional education (IPE), which aims to develop the ability to collaborate efficiently as multidisciplinary teams [ 2 , 3 ]. IPE involves students from two or more healthcare professions learning about, from, and with each other through collaborative education. The primary objective of IPE is to assist healthcare professionals, including doctors, pharmacists, and nurses, in developing the competence to collaborate more effectively in multidisciplinary teams to enhance patient care [ 4 ]. Its history began in the early twentieth century and has evolved to include numerous healthcare professionals such as nurses, pharmacists, and dentists [ 5 ]. The World Health Organization (WHO) reported that IPE provides highly collaborative teamwork experiences that improve job satisfaction and enhance access to and safety in patient care [ 6 ]. Recent studies have also shown that IPE is pivotal not only in promoting professional autonomy, understanding of professional roles, teamwork, and collaboration, but also in providing essential knowledge and skills for improving healthcare services [ 7 , 8 , 9 , 10 , 11 , 12 , 13 ].
One innovative approach to enhancing IPE involves game-based learning, which integrates educational content with interactive gaming elements to create engaging and effective learning experiences. Game-based learning has been shown to enhance students’ motivation, participation, and retention of knowledge by providing a dynamic and immersive learning environment [ 14 , 15 , 16 ]. In the context of IPE, these activities can simulate real-life clinical scenarios that require collaboration, communication, and problem-solving among diverse healthcare professionals [ 17 ]. This method allows students to practice and develop these critical skills in a safe and controlled setting, thereby preparing them for actual clinical practice [ 18 ].
Previous IPE studies involving students primarily used surveys, interviews, and participant observations to assess changes in students’ knowledge acquisition, collaboration, teamwork skills, and attitudes [ 19 , 20 , 21 , 22 , 23 ]. While these approaches have provided valuable information for evaluating the effectiveness of IPE programs, they have limitations in terms of exploring students’ direct expressions and deep thinking. Recent research has started exploring game-based learning in IPE, emphasizing its potential to enhance collaboration, communication, and problem-solving skills among healthcare students [ 24 , 25 ]. Game-based learning activities, such as serious games and simulations, offer engaging experiences that promote interprofessional collaboration and reflective thinking [ 17 ]. However, there is still a scarcity of research on students’ personal experiences, changing perceptions, and in-depth understanding of interprofessional collaboration through game-based learning. Addressing this gap can provide better relevance and context to the study of IPE.
Medical education literature has highlighted the importance of various educational strategies in enhancing reflective thinking skills [ 26 , 27 ]. Dewey defines reflective thinking as conscious thought in the problem-solving process, which can be considered as the active utilization of knowledge gained through experience [ 28 ]. Narrative materials, such as essays, are useful tools for gaining an in-depth understanding of students’ experiences and perceptions. Thus, analyzing reflective thinking through essays can help students better understand their learning experiences and improve their problem-solving abilities through effective collaboration across different disciplines [ 29 , 30 ].
This study aimed to analyze students’ perceptions of collaboration by examining essays they wrote after participating in game-based IPE activities, thereby providing evidence for the effectiveness of such education. The results of this study are expected to serve as foundational data to help design and implement more effective collaborative learning strategies for IPE programs.
Course design
The IPE program at a South Korean university targeted fifth-year medical and fourth-year nursing students to prepare them for clinical training. The course was divided into two phases: a six-day shadowing period and a four-day IPE activity period.
During the shadowing period, students observed various healthcare professionals in different clinical settings, including emergency rooms (ERs), ambulatory care, critical care, and outpatient environments. This phase emphasized understanding interprofessional roles and the importance of collaborative practice skills.
In the subsequent IPE activity period, students were grouped into teams of five or six, consisting of both medical and nursing students, to engage in team building exercises. These activities aimed to promote students’ collaboration, communication skills, mutual understanding in clinical settings. The activities during this phase were meticulously designed to develop essential soft skills through structured game-based exercises. These included the Marshmallow Challenge, which aimed to enhance understanding of team building dynamics; the Puzzle Game, which focused on defining roles and fostering teamwork to achieve a common objective; and the Message Game, which underscored the importance of clear and effective communication. Additional activities, such as the Drawing Shapes Game and the Drawing the Story Game, were designed to improve skills in accurate verbal description and to enhance understanding of the SBAR (Situation, Background, Assessment, Recommendation) communication protocol, respectively. Finally, the Board Game was specifically developed to reinforce systems thinking and to illustrate the need for interdisciplinary collaboration in addressing complex issues in a hospital. Table 1 outlines the key activities included in this period.
This study aimed to analyze essays written by students after participating in the IPE activities to assess their reflections and learning outcomes.
Research procedure
The fundamental premise of text network analysis is to extract keywords representing the core content from the literature [ 31 ]. This study focused on understanding students’ thoughts and perceptions by analyzing their essays. The research process comprised (1) data collection, (2) keyword selection and data processing, (3) core keyword extraction and network construction, (4) network connectivity and centrality analysis, and (5) topic modeling. This approach facilitated a nuanced understanding of the conceptual relationships within the text, yielding deeper insights into students’ reflective thinking and experiences with interprofessional collaboration, thereby aligning with the objectives of this study.
Data collection
Data were collected in 2021 after the IPE program. Of the 82 medical students who participated in the program, 77 voluntarily submitted essays, representing a 93.9% response rate from the entire cohort enrolled in the IPE program. The essays were collected after the completion of the entire program, capturing students’ reflections and feelings about the course. These essays were not intended for assessment or evaluation purposes but were written freely by students to express their thoughts and experiences regarding the program. The primary aim was to gather qualitative insights into how students perceived and internalized the IPE activities, which aligns with the study’s objective to understand the impact of game-based learning on developing interprofessional collaboration, communication, and team building skills. We focused on medical students’ essays to explore their specific perspectives and experiences within the IPE program, as these students often play crucial roles in multidisciplinary teams. Therefore, understanding their views can provide valuable insights for improving IPE programs and enhancing interprofessional collaboration in clinical practice [ 32 ].
Keyword selection and data pre-processing
The student essays were collected using MS Office Excel. Pre-processing involved an initial review using Excel’s Spell Check, followed by manual corrections to fix typographical errors. Morphological analysis was performed using Netminer 4.5.1.c (CYRAM), which automatically removed pronouns and adverbs, leaving only nouns. To extract the words, 25 designated words, 40 synonyms, and 321 excluded words were pre-registered. Designated words are terms that convey specific meanings when grouped [ 33 ]. In this study, terms such as “interprofessional education” and “Friday Night at the ER” were classified as such. Synonyms, a group of words that have similar meanings, were processed as a single term that can represent the common meaning of those words [ 34 ]. For instance, “Friday night ER,” “FNER,” and “Friday night in the ER” were extracted as “Friday Night at ER.” Words considered irrelevant to the current research focus or general words that did not contribute to meaningful analysis were excluded (e.g., “and,” “or,” “front,” “inside,” “during”). Three professors specializing in emergency medicine and one medical educator handled word extraction and refinement, and the final selection was reviewed by the entire research team.
The data analysis utilized was qualitative content analysis, focusing on both the identification and contextual usage of keywords. This approach involved the descriptive counting of keywords as well as an in-depth analysis of their usage within the essays. This rigorous process ensured that the keywords selected were relevant to the study’s focus on IPE and collaboration, providing both quantitative and qualitative insights into the students’ reflections and experiences.
Extraction of core keywords and network construction
Core keyword extraction was based on the term frequency-inverse document frequency (TF-IDF) method. The frequency of word occurrences is expressed as “term frequency (TF),” which indicates how often a word appears within a document [ 35 ]. By contrast, “inverse document frequency (IDF)” is calculated using the logarithmic value of the inverse of document frequency [ 36 ]. The TF-IDF value is computed by multiplying TF by IDF. A high value indicates that a word is important in a specific document but rarely appears in others [ 37 ]. This method allows the assessment of the importance of words in documents. For network analysis, the 2-mode word-document network was converted into a 1-mode word-word network. The co-occurrence frequency was set to occur at least twice, and the word proximity (window size) was set to two, following previous studies on text network analysis [ 38 ].
Network connectivity and centrality analysis
Network size and density, as well as the average degree and distance at the node level, were identified to understand the overall characteristics of the network. Network size denotes the total number of nodes (keywords). Density measures the ratio of actual connections to possible connections, indicating network cohesion. The average degree reflects the average number of connections per node, while the average distance shows the typical number of steps between nodes, revealing the network’s connectivity and compactness [ 35 , 38 ]. Centrality analysis included degree, betweenness, and eigenvector centrality, whereas closeness centrality was excluded due to poor performance in lengthy texts [ 39 ]. Degree centrality measures how well a node is connected within a network, helping to identify keywords that play a central role in the network [ 40 ]. Betweenness centrality measures how frequently a node appears on the shortest path between other nodes, indicating how well it acts as an intermediary between two nodes [ 41 ]. Eigenvector centrality assesses the influence of a node by considering the importance of its neighboring nodes beyond the degree of connection [ 42 ]. This study extracted the top 30 words for each degree, betweenness, and eigenvector centrality. Finally, a spring map was used to visualize the keywords and their connection structures in the network.
Text network analysis was chosen because it provides a detailed understanding of relationships between concepts, unlike traditional methods that focus on theme frequency. It visualizes keyword interactions, highlighting central themes and their connections, offering insights into students’ reflections on IPE and their thought patterns.
Topic modeling
Latent Dirichlet allocation (LDA) is a statistical text-processing technique that clusters keywords based on their probabilities and distributions to infer topics [ 43 ]. In this study, keywords extracted from essays were compiled into a matrix for LDA. To determine the optimal number of topics, combinations of α = 0.01–0.03, β = 0.01–0.03, topic model = 3–8, and 1,000 iterations were tested. The optimal model was selected based on the coherence score (c_v), with the highest coherence score ensuring the validity and reliability of the inferred topics [ 44 , 45 , 46 ].
Key keywords
Table 2 presents the keywords derived from analyzing medical students’ essays selected through the TF and TF-IDF analyses. In the TF analysis, “thought” appeared most frequently (365 times), followed by “group” 359 times, “class” 322 times, and “game” 278 times. The top 20 keywords in TF-IDF included “patient,” “game,” “group,” and “person.” Keywords that appeared in both TF and TF-IDF analyses included “nursing school,” “nurse,” “game,” “hospital,” “person,” “mutual,” “communication,” “time,” “group,” “important,” “progress,” “puzzle,” “patient,” and “activity.” Comparing the keywords between TF and TF-IDF, new terms that emerged in TF-IDF included “IPE,” “room,” and “clinical practice.”
- Text network analysis
Network structure
In this study, a network was constructed based on a co-occurrence frequency of at least two words with word proximity (window size) set to two words. The resulting network comprised 1,218 nodes and 627 links. The network density was 0.012, with an average degree and distance of 3.919 and 3.447, respectively.
Centrality analysis
Table 3 lists the top 30 keywords according to degree, betweenness, and eigenvector centralities, providing insight into the overall network characteristics. The top three keywords across all three centrality analyses included “patient,” “thought,” “group,” “doctor,” “nurse,” and “communication.” The ranking and composition of the keywords were similar in both degree and betweenness centrality analyses. In the eigenvector results, “doctor,” “nurse,” and “communication” were ranked highest. When comparing the top 30 keywords from eigenvector centrality with those from degree and betweenness centrality, new terms such as “future,” “society,” and “need” emerged. These findings are presented in Fig. 1 , which illustrates the spring network map of centrality.
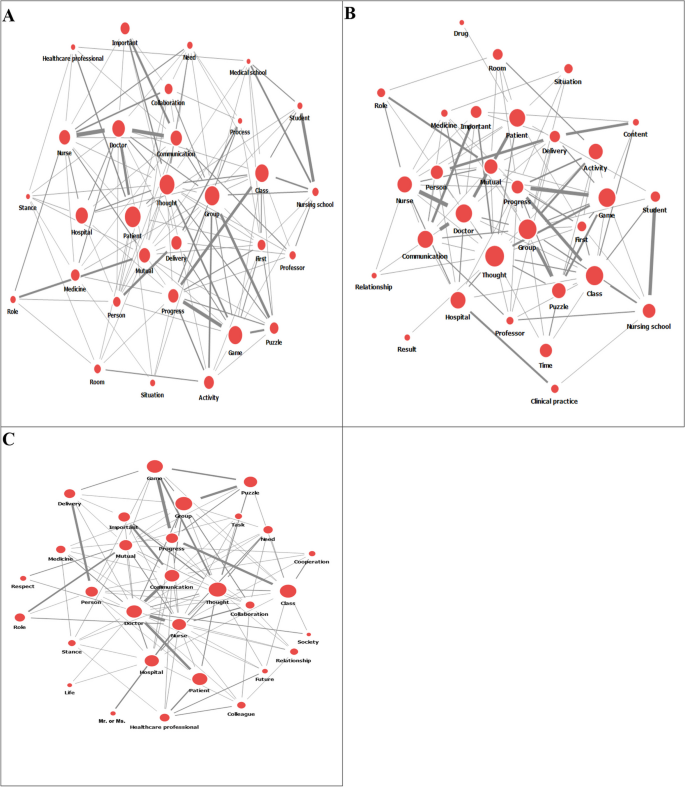
Spring network map of centrality. a Degree centrality. b Betweenness centrality. c Eigenvector centrality
Topic modeling: selection of the number of topics
To determine the optimal number of topics, 54 combinations of options were tested, including α = 0.01–0.03, β = 0.01–0.03, topic models = 3–8, and 1,000 iterations. Three topics were identified.
In the topic modeling process, after reviewing the keywords and contents of the assigned original documents, the research team convened and named each topic to reflect the trend of the subject matter, as shown in Fig. 2 . Following prior research, the final topic model was visualized using a topic-keyword map displaying the top eight to thirteen words [ 44 ]. Topic 1, accounting for 17% of the total topics, includes keywords such as “group,” “game,” “puzzle,” “delivery,” and “activity.” This reflects the inclusion of group-based, game-centric activities in the IPE classes; hence, it was named “game-based collaborative activity experience.” Topic 2 comprises 23% of the topics centered around the thoughts of doctors and nurses about patients in clinical settings, with keywords including “doctor,” “thought,” “patient,” “hospital,” and “nurse.” It was thus named “interprofessional perspectives.” Topic 3, with the largest share at 60%, incorporates keywords such as “class,” “nursing school,” “thought,” “activity,” and “student.” It primarily addresses class activities involving nursing students, thus the term “interdisciplinary educational experience.” Visually examining the entire network of topic modeling indicates that Topic 1, “game-based collaborative activity experience,” and Topic 3, “interdisciplinary educational experience,” are connected through the keywords “person” and “activity.” Topic 2, “Interprofessional Perspectives,” and Topic 3, are linked by “thought” and “class.” In the network, Topic 3 plays a vital role in connecting Topics 1 and 2, as illustrated in Fig. 2 .
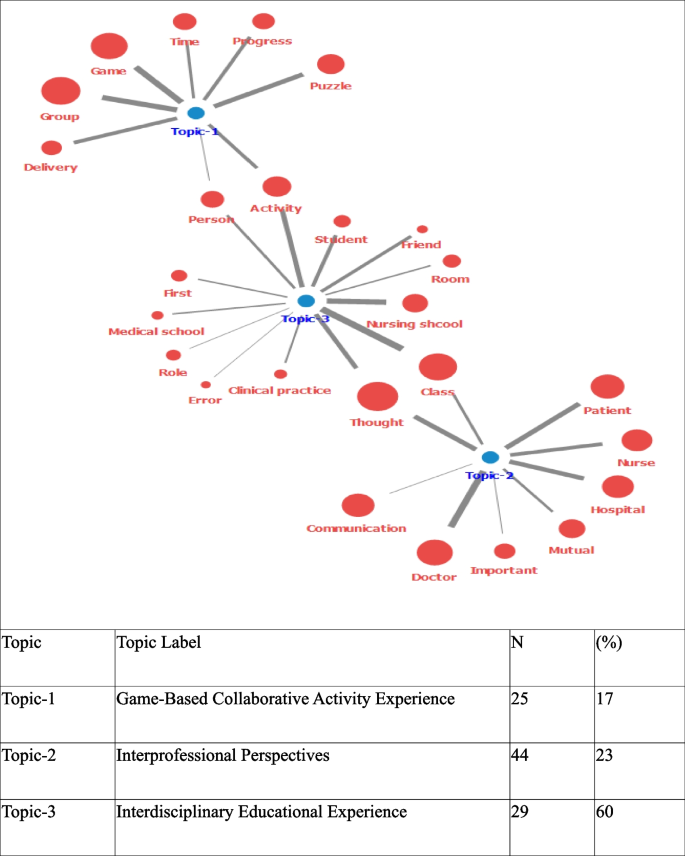
Semantic keywords of topic modeling
This study is the first attempt to demonstrate the educational impact of game-based IPE activities on fostering an interprofessional perspective, communication skills, and team building skills among healthcare professionals through a text network analysis of student essays. This distinguishes this study from previous studies. This approach can help students develop collaborative skills, thereby effectively addressing various challenges in clinical settings. The primary findings and implications of this study are as follows:
First, the keywords with the highest degree of centrality were “patient,” “thought,” and “group.” High-degree-centrality keywords play a central role in the entire network, suggesting that the overall program should be designed around these keywords. The keywords with high betweenness centrality were also “patient,” “thought,” and “group.” These keywords act as necessary connectors within the network, indicating that they are crucial for establishing communication channels between different professions and ensuring a smooth flow of information in medical education. Keywords with high eigenvector centrality included “doctor,” “nurse,” and “communication.” The prominence of keywords such as “doctor,” “nurse,” and “communication” in centrality measures signifies their strong connections to other important terms in the network. This highlights the pivotal role of doctors and nurses in collaborative practices and underscores the importance of communication skills in IPE programs. The central positioning of these keywords within the network emphasizes the need to prioritize interprofessional roles and communication competencies to enhance collaborative practices in clinical settings. These results align with previous findings emphasizing the importance of education in promoting effective collaboration and communication among healthcare professionals [ 47 ]. The centralities thus provide quantitative evidence supporting the critical roles and interactions that are essential for successful IPE.
The relevance of these keywords can be understood within the framework of the Interprofessional Education Collaborative (IPEC) Core Competencies, which emphasize patient-centered care, reflective thinking, and effective communication. The central keywords align with IPEC’s domains: values/ethics for interprofessional practice, roles/responsibilities, interprofessional communication, and teams/teamwork [ 4 , 48 ]. For instance, “patient” and “group” correspond to the emphasis on patient-centered care and teamwork, while “thought” and “communication” are essential for reflective practice and effective interprofessional communication. Integrating IPE into medical education strengthens transparent and efficient teamwork across different specialties, minimizes errors in clinical decision-making, and improves patient outcomes. Consequently, medical schools should develop curricula that provide students with ample opportunities to collaborate with team members from various specialties [ 49 ].
Second, the topic modeling analysis indicated that Topic 1 provides a collaborative experience through group-based gaming activities in an IPE course. This aligns with previous research, indicating that game-based learning can enhance participants’ socialization and communication skills. Thornton Bacon et al. [ 50 ] and Sanko et al. [ 51 ] reported that students who participated in the Friday Night at Emergency Room (FNER) game demonstrated a statistically significant increase in systems thinking scores. In addition, Fusco et al. [ 52 ] confirmed that gameplay positively affected students’ systematic thinking, effective collaboration, and socialization skills. This suggests that game-based learning is useful for developing collaborative problem-solving skills and can be effectively integrated into various educational designs of IPE programs. Topic 2 highlights the significant focus on the perspectives of healthcare professionals in clinical environments. According to Bridges et al. [ 53 ] and Prentice et al. [ 54 ], IPE provides opportunities to develop a better understanding of roles and improve communication among healthcare team members. In this process, improving knowledge about one’s own roles and responsibilities as well as those of other professions can enhance teamwork between professionals [ 55 ]. This finding suggests that IPE programs can improve the quality of healthcare delivery by fostering mutual respect and understanding among different healthcare professionals. Topic 3 primarily addressed class activities for nursing students and included interdisciplinary educational experiences. These results show that game-based IPE activities are an effective educational method for enhancing interprofessional perspectives and communication skills, going beyond traditional lectures that simply deliver knowledge to students.
Additionally, Bjerkvik and Hilli [ 56 ] stated that expressing thoughts through writing facilitates the understanding of personal experiences. This enables learners to explore their emotions and attitudes, ultimately leading them to deeper self-understanding and professional growth. Consequently, this study analyzed students’ reflective thinking through topic modeling and presented evidence that game-based IPE activities are crucial in promoting learners’ reflective thinking and professional growth.
This study has several limitations. First, a limited group of students from a specific university participated in this study, which may have restricted the generalizability of the findings. Additional research is required to verify the results of this study across multiple student groups from various backgrounds and environments. Second, the research methodology relied on text analysis of student essays, focusing only on students’ subjective experiences and perceptions. To address this limitation, we used a rigorous coding scheme, inter-rater reliability checks, and TF-IDF for keyword extraction. Our methodology included keyword selection, data pre-processing, network construction, and LDA-based topic modeling, optimized with the coherence score (c_v). These steps ensured that the data analysis was both robust and reliable. Additionally, incorporating multiple methods for data analysis allowed us to cross-verify the findings and enhance the overall rigor of the study. Future research should integrate a range of methods, including interviews and surveys, to achieve a more comprehensive evaluation. Third, the effects of IPE programs on students’ collaborative competencies in clinical practice and healthcare settings is limited. Future research should explore the long-term impacts of game-based IPE on clinical practice, patient outcomes, and students’ readiness for clinical environments. Additionally, tracking the career progression and professional development of participants will help assess the sustained benefits of these educational interventions.
Conclusions
This study is the first to explore changes in reflective thinking and perceptions among students who participated in IPE programs. This demonstrates the positive effects of IPE on professional healthcare students. Specifically, through the analysis of degree, betweenness, and eigenvector centrality, we identified keywords such as “patient,” “thought,” “group,” “doctor,” “nurse,” and “communication” as crucial to interprofessional perspectives and communication among healthcare professionals. Topic modeling further underscores the importance of game-based learning, interprofessional perspectives, and interdisciplinary educational experiences.
These findings emphasize the need for innovative teaching methods in medical education and reaffirm the importance of promoting effective inter-professional perspective, communication skills and team building skills. Medical schools should strive to improve the design and implementation of their IPE program by incorporating students’ experiences and reflective insights. This will ultimately improve the quality of medical education. This study can serve as valuable foundational data for future research. Future studies should investigate the long-term effects of game-based IPE on clinical practice and patient outcomes. Research should also explore the impact of game-based IPE on participants’ career progression and professional development to assess sustained benefits. Additionally, future research could examine how different game-based learning activities influence specific interprofessional competencies, such as teamwork, communication, and problem-solving skills, to identify the most effective approaches for IPE programs.
Availability of data and materials
The datasets generated and/or analysed during the current study are not publicly available due to ethical constraints but are available from the corresponding author on reasonable request.
Abbreviations
- Interprofessional education
Friday night at emergency room
Latent Dirichlet allocation
Term frequency
Term frequency-inverse document frequency
Inverse document frequency
Fox A, Reeves S. Interprofessional collaborative patient-centred care: a critical exploration of two related discourses. J Interprof Care. 2015;29:113–8.
Article Google Scholar
Gilligan C, Outram S, Levett-Jones T. Recommendations from recent graduates in medicine, nursing and pharmacy on improving interprofessional education in university programs: A qualitative study. BMC Med Educ. 2014;14:52.
Haresaku S, Naito T, Aoki H, Miyoshi M, Monji M, Umezaki Y, et al. Development of interprofessional education programmes in nursing care and oral healthcare for dental and nursing students. BMC Med Educ. 2024;24:381.
Mohammed CA, Anand R, Saleena Ummer VS. Interprofessional education (IPE): A framework for introducing teamwork and collaboration in health professions curriculum. Med J Armed Forces India. 2021;77(Suppl 1):S16-21.
Schmitt MH, Gilbert JH, Brandt BF, Weinstein RS. The coming of age for interprofessional education and practice. Am J Med. 2013;126:284–8.
World Health Organization. Framework for Action on Interprofessional Education and Collaborative Practice. Geneva: World Health Organization; 2010. p. 13–15.5.
Curran VR, Sharpe D, Flynn K, Button P. A longitudinal study of the effect of an interprofessional education curriculum on student satisfaction and attitudes towards interprofessional teamwork and education. J Interprof Care. 2010;24:41–52.
Reeves S, Goldman J, Burton A, Sawatzky-Girling B. Synthesis of systematic review evidence of interprofessional education. J Allied Health. 2010;39(Suppl 1):198–203.
Google Scholar
Brock D, Abu-Rish E, Chiu CR, Hammer D, Wilson S, Vorvick L, et al. Interprofessional education in team communication: Working together to improve patient safety. Postgrad Med J. 2013;89:642–51.
Rogers O, Heck A, Kohnert L, Paode P, Harrell L. Occupational therapy’s role in an interprofessional student-run free clinic: Challenges and opportunities identified. Open J Occup Ther. 2017;5:7.
Peeters MJ, Sexton M, Metz AE, Hasbrouck CS. A team-based interprofessional education course for first-year health professions students. Curr Pharm Teach Learn. 2017;9:1099–110.
Imafuku R, Kataoka R, Ogura H, Suzuki H, Enokida M, Osakabe K. What did first-year students experience during their interprofessional education? A qualitative analysis of e-portfolios. J Interprof Care. 2018;32:358–66.
Alzamil H, Meo SA. Medical students’ readiness and perceptions about interprofessional education: a cross sectional study. Pak J Med Sci. 2020;36:693–8.
Hu H, Xiao Y, Li H. The effectiveness of a serious game versus online lectures for improving medical students’ coronavirus disease 2019 knowledge. Games Health J. 2021;10:139–44.
Xu M, Luo Y, Zhang Y, Xia R, Qian H, Zou X. Game-based learning in medical education. Front Public Health. 2023;11:1113682.
Surapaneni KM. Livogena: The Ikteros curse—A jaundice narrative card and board game for medical students. MedEdPORTAL. 2024;20:11381.
Fusco NM, Jacobsen LJ, Klem N, Krzyzanowicz R, Ohtake PJ. A serious game employed to introduce principles of interprofessional collaboration to students of multiple health professions. Simul Gaming. 2022;53:253–64.
Umoren R, editor. Simulation and Game-Based Learning for the Health Professions. Hershey: IGI Global; 2022.
Thannhauser J, Russell-Mayhew S, Scott C. Measures of interprofessional education and collaboration. J Interprof Care. 2010;24:336–49.
Rosenfield D, Oandasan I, Reeves S. Perceptions versus reality: a qualitative study of students’ expectations and experiences of interprofessional education. Med Educ. 2011;45:471–7.
Darlow B, Donovan S, Coleman K, McKinlay E, Beckingsale L, Gallagher P, et al. What makes an interprofessional education programme meaningful to students? Findings from focus group interviews with students based in New Zealand. J Interprof Care. 2016;30:355–61.
Almoghirah H, Nazar H, Illing J. Assessment tools in pre-licensure interprofessional education: A systematic review, quality appraisal and narrative synthesis. Med Educ. 2021;55:795–807.
Gysin S, Huber M, Feusi E, Gerber-Grote A, Witt CM. Interprofessional education day 2019–A qualitative participant evaluation. GMS J Med Educ. 2022;39:Doc52.
Friedrich C, Teaford H, Taubenheim A, Boland P, Sick B. Escaping the professional silo: an escape room implemented in an interprofessional education curriculum. J Interprof Care. 2019;33:573–5.
Friedrich C, Teaford H, Taubenheim A, Sick B. Interprofessional health care escape room for advanced learners. J Nurs Educ. 2020;59:46–50.
Sandars J. The use of reflection in medical education: AMEE Guide No. 4. Med Teach. 2009;31:685–95.
Wald HS, Borkan JM, Taylor JS, Anthony D, Reis SP. Fostering and evaluating reflective capacity in medical education: Developing the REFLECT rubric for assessing reflective writing. Acad Med. 2012;87:41–50.
Rodgers C. Defining reflection: Another look at John Dewey and reflective thinking. Teach Coll Rec. 2002;104:842–66.
Entwistle N. Frameworks for understanding as experienced in essay writing and in preparing for examination. Educ Psychol. 1995;30:47–54.
Lin CW, Lin MJ, Wen CC, Chu SY. A word-count approach to analyze linguistic patterns in the reflective writings of medical students. Med Educ Online. 2016;21:29522.
Yang L, Li K, Huang H. A new network model for extracting text keywords. Scientometrics. 2018;116:339–61.
Zechariah S, Ansa BE, Johnson SW, Gates AM, Leo GD. Interprofessional education and collaboration in healthcare: an exploratory study of the perspectives of medical students in the United States. Healthcare. 2019;7:117.
Lee SS. A content analysis of journal articles using the language network analysis methods. J Korean Soc Inf Manag. 2014;31:49–68.
Won J, Kim K, Sohng KY, Chang SO, Chaung SK, Choi MJ, et al. Trends in nursing research on infections: semantic network analysis and topic modeling. Int J Environ Res Public Health. 2021;18:6915.
Park ST, Liu C. A study on topic models using LDA and Word2Vec in travel route recommendation: Focus on convergence travel and tours reviews. Pers Ubiquitous Comput. 2022;26:429–45.
Robertson S. Understanding inverse document frequency: On theoretical arguments for IDF. J Doc. 2004;60:503–20.
Wang J, Dagvadorj A, Kim HS. Research trends of human resources management in hotel industry: Evidence from South Korea by semantic network analysis. Culinary Sci Hosp Res. 2021;27:68–78.
Park MY, Jeong SH, Kim HS, Lee EJ. Images of nurses appeared in media reports before and after outbreak of COVID-19: Text network analysis and topic modeling. J Korean Acad Nurs. 2022;52:291–307.
Boudin F. A comparison of centrality measures for graph-based keyphrase extraction. In: Proceedings of the sixth international joint conference on natural language processing. 2013. p. 834–8.
Valente TW, Coronges K, Lakon C, Costenbader E. How correlated are network centrality measures? Connect (Tor). 2008;28:16–26.
Ausiello G, Firmani D, Laura L. The (betweenness) centrality of critical nodes and network cores. In: 9th International Wireless Communications and Mobile Computing Conference (IWCMC), 2013. Sardinia: IEEE; 2013. p. 90–5.
Maharani W, Adiwijaya AA, Gozali AA. Degree centrality and eigenvector centrality in Twitter. In: 8th International Conference on Telecommunication Systems Services and Applications (TSSA), 2014. Bali: IEEE; 2014. p. 1–5.
Blei DM, Lafferty JD. Topic models. In: Text Mining. Boca Raton: Chapman and Hall/CRC; 2009. p. 101–24.
Lee JW, Kim Y, Han DH. LDA-based topic modeling for COVID-19-related sports research trends. Front Psychol. 2022;13:1033872.
Colla D, Delsanto M, Agosto M, Vitiello B, Radicioni DP. Semantic coherence markers: The contribution of perplexity metrics. Artif Intell Med. 2022;134: 102393.
Jang JH, Masatsuku N. A study of factors influencing happiness in Korea: Topic modelling and neural network analysis. Data and Metadata. 2024;3:238–238.
Buring SM, Bhushan A, Broeseker A, Conway S, Duncan-Hewitt W, Hansen L, et al. Interprofessional education: definitions, student competencies, and guidelines for implementation. Am J Pharm Educ. 2009;73:59.
Thistlethwaite JE, et al. Competencies and frameworks in interprofessional education: a comparative analysis. Acad Med. 2014;89:869–87.
Barnsteiner JH, Disch JM, Hall L, Mayer D, Moore SM. Promoting interprofessional education. Nurs Outlook. 2007;55:144–50.
Thornton Bacon C, Trent P, McCoy TP. Enhancing systems thinking for undergraduate nursing students using Friday Night at the ER. J Nurs Educ. 2018;57:687–9.
Sanko JS, Gattamorta K, Young J, Durham CF, Sherwood G, Dolansky M. A multisite study demonstrates positive impacts to systems thinking using a table-top simulation experience. Nurse Educ. 2021;46:29–33.
Fusco NM, Foltz-Ramos K, Ohtake PJ, Mann C. Interprofessional simulation learning game increases socialization and teamwork among students of health professions programs. Nurse Educ. 2024;49:E32–5.
Bridges D, Davidson RA, Soule Odegard P, Maki IV, Tomkowiak J. Interprofessional collaboration: three best practice models of interprofessional education. Med Educ Online. 2011;16(1):6035.
Prentice D, Engel J, Taplay K, Stobbe K. Interprofessional collaboration: The experience of nursing and medical students’ interprofessional education. Glob Qual Nurs Res. 2015;2:2333393614560566.
Wilhelmsson M, Pelling S, Uhlin L, Owe Dahlgren L, Faresjö T, Forslund K. How to think about interprofessional competence: A metacognitive model. J Interprof Care. 2012;26:85–91.
Bjerkvik LK, Hilli Y. Reflective writing in undergraduate clinical nursing education: A literature review. Nurse Educ Pract. 2019;35:32–41.
Download references
Acknowledgements
The authors sincerely thank all those who have contributed to this work through their support, insights, and encouragement.
This research did not receive any specific grant from funding agencies in the public, commercial, or not-for-profit sectors.
Author information
Authors and affiliations.
Center for Medical Education, College of Medicine, Chung-Ang University, Dongjak-Gu, Seoul, Republic of Korea
Young Gyu Kwon & Chan Woong Kim
Department of Emergency Medicine, College of Medicine, Chung-Ang University, Dongjak-Gu, Seoul, Republic of Korea
Myeong Namgung & Chan Woong Kim
Department of Medical Education, College of Medicine, Chung-Ang University, Dongjak-Gu, Seoul, Republic of Korea
Song Hee Park & Mi Kyung Kim
Department of Pathology, College of Medicine, Chung-Ang University, Dongjak-Gu, Seoul, Republic of Korea
Mi Kyung Kim
Office of Medical Education, Seoul National University College of Medicine, Jongno-Gu, Seoul, Republic of Korea
Sun Jung Myung
Department of Emergency Medicine, Soonchunhyang University Bucheon Hospital, Bucheon, Republic of Korea
Eun Kyung Eo
You can also search for this author in PubMed Google Scholar
Contributions
Study conception and design: YK, MN, CK. Data collection: YK, MN, CK. Data analysis and interpretation: YK, MN, SM, EE, CK. Drafting of the article: YK, MN, SP, MK. Critical revision of the article: YK, MN, SP, SM, EE, CK.
Corresponding author
Correspondence to Chan Woong Kim .
Ethics declarations
Ethical approval and consent to participate.
The Institutional Review Board (IRB) of Chung-Ang University determined that this study meets the criteria for an exemption from IRB review, as it involves research conducted in established or commonly accepted educational settings and involves normal educational practices. Approval Number: 1041078–20240321-HR-051. Written informed consent was obtained from all participating students. All methods were performed in accordance with relevant guidelines and regulations, including the principles outlined in the Declaration of Helsinki.
Consent for publication
Not applicable.
Competing interest
The authors declare no competing interests.
Additional information
Publisher’s note.
Springer Nature remains neutral with regard to jurisdictional claims in published maps and institutional affiliations.
Rights and permissions
Open Access This article is licensed under a Creative Commons Attribution-NonCommercial-NoDerivatives 4.0 International License, which permits any non-commercial use, sharing, distribution and reproduction in any medium or format, as long as you give appropriate credit to the original author(s) and the source, provide a link to the Creative Commons licence, and indicate if you modified the licensed material. You do not have permission under this licence to share adapted material derived from this article or parts of it. The images or other third party material in this article are included in the article’s Creative Commons licence, unless indicated otherwise in a credit line to the material. If material is not included in the article’s Creative Commons licence and your intended use is not permitted by statutory regulation or exceeds the permitted use, you will need to obtain permission directly from the copyright holder. To view a copy of this licence, visit http://creativecommons.org/licenses/by-nc-nd/4.0/ .
Reprints and permissions
About this article
Cite this article.
Kwon, Y.G., Namgung, M., Park, S.H. et al. Impact of a game-based interprofessional education program on medical students’ perceptions: a text network analysis using essays. BMC Med Educ 24 , 898 (2024). https://doi.org/10.1186/s12909-024-05893-2
Download citation
Received : 27 May 2024
Accepted : 12 August 2024
Published : 20 August 2024
DOI : https://doi.org/10.1186/s12909-024-05893-2
Share this article
Anyone you share the following link with will be able to read this content:
Sorry, a shareable link is not currently available for this article.
Provided by the Springer Nature SharedIt content-sharing initiative
- Game-based learning
- Reflective thinking
- Collaborative learning
BMC Medical Education
ISSN: 1472-6920
- General enquiries: [email protected]
- DOI: 10.55041/isjem01433
- Corpus ID: 269048348
A REVIEW OF STUDENT DATA ANALYSIS USING QR-CODE.
- Ashiya Niyaj Mulla
- Published in International Scientific… 23 March 2024
- Computer Science, Education
- International Scientific Journal of Engineering and Management
Related Papers
Showing 1 through 3 of 0 Related Papers
Healthcare's Digital Future: Exploring the Specialty of Nursing Informatics
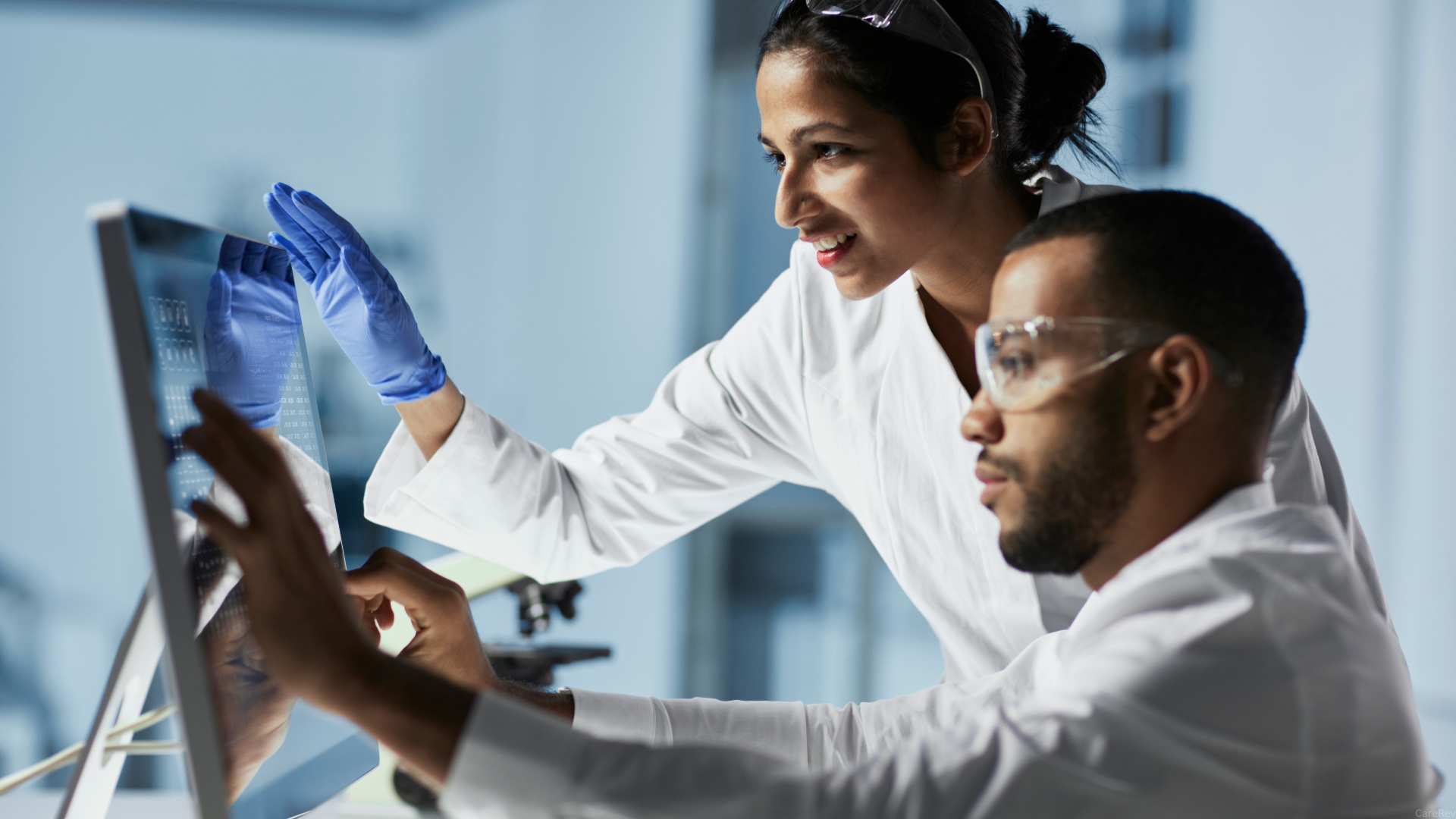
In today's rapidly evolving healthcare landscape, technology plays a pivotal role in improving patient care, enhancing efficiency, and facilitating communication among healthcare professionals. At one intersection of healthcare and technology lies the specialized field of nursing informatics—a discipline that combines nursing science with information management and analytical sciences to optimize healthcare delivery.
What is nursing informatics?
Nursing informatics is the specialty that integrates nursing science with information management and analytical sciences to identify, collect, process, and manage data and information to support nursing practice, administration, education, research, and the expansion of nursing knowledge.
The role of nursing informaticists
Nursing informaticists serve as the bridge between healthcare providers and technology systems, leveraging their expertise to enhance patient care outcomes, streamline processes, and drive innovation within healthcare organizations. Their responsibilities encompass a wide range of functions, including the following:
Clinical workflow optimization
Nursing informaticists analyze clinical workflows and identify opportunities to streamline processes through the implementation of electronic health records (EHRs) , clinical decision support systems, and other technology solutions. By optimizing workflows, they enhance efficiency and ensure that nurses can focus more on patient care.
Data management and analysis
Nursing informaticists are proficient in data management and analysis techniques, allowing them to collect, organize, and analyze healthcare data to derive meaningful insights. By leveraging data analytics, they can identify trends, patterns, and areas for improvement in patient care delivery.
System implementation and training
Nursing informaticists play a crucial role in the implementation of new technology systems within healthcare organizations. They collaborate with IT teams to ensure the successful deployment of electronic health records, telehealth platforms, and other digital tools. Additionally, they provide training and support to nurses and other healthcare staff to ensure they can effectively utilize these systems.
Quality improvement initiatives
Nursing informaticists participate in quality improvement initiatives aimed at enhancing patient safety, satisfaction, and outcomes. They utilize data-driven approaches to identify areas for improvement, implement evidence-based practices, and monitor progress towards organizational goals.
Research and education
Nursing informaticists contribute to nursing research by investigating the impact of technology on patient care delivery, outcomes, and healthcare systems. They also play a vital role in educating nurses and other healthcare professionals about the use of technology in clinical practice, ensuring they have the necessary skills and knowledge to leverage digital tools effectively.
The impact of nursing informatics
The specialty of nursing informatics has a profound impact on healthcare delivery, quality, and safety. By harnessing the power of technology, nursing informaticists empower nurses and other healthcare professionals to deliver high-quality, patient-centered care. Some key benefits of nursing informatics include:
- Enhanced Patient Safety: Nursing informatics solutions, such as barcode medication administration and clinical decision support systems, help reduce medication errors and adverse events, ultimately enhancing patient safety.
- Improved Care Coordination: Electronic health records and health information exchange platforms facilitate seamless communication and collaboration among healthcare providers, improving care coordination and continuity.
- Increased Efficiency: By automating routine tasks and streamlining workflows, nursing informatics solutions optimize efficiency within healthcare organizations, allowing nurses to spend more time on direct patient care.
- Data-Driven Decision Making: Nursing informatics enables data-driven decision making by providing healthcare professionals with access to timely, accurate, and actionable information. This empowers them to make informed decisions that positively impact patient outcomes.
- Facilitation of Evidence-Based Practice: Nursing informatics supports evidence-based practice by providing nurses with access to the latest research findings, clinical guidelines, and best practices. This enables them to deliver care that is rooted in evidence and tailored to the individual needs of patients.
In conclusion, nursing informatics is a specialized field that plays a crucial role in harnessing the power of technology to optimize healthcare delivery. Nursing informaticists leverage their expertise to enhance patient care outcomes, streamline processes, and drive innovation within healthcare organizations. As the healthcare industry continues to evolve, the demand for skilled nursing informaticists will only continue to grow, ensuring that nurses are equipped with the tools and knowledge needed to navigate healthcare's digital future.
Know a nurse looking for better work-life balance?
Articles you may be interested in.
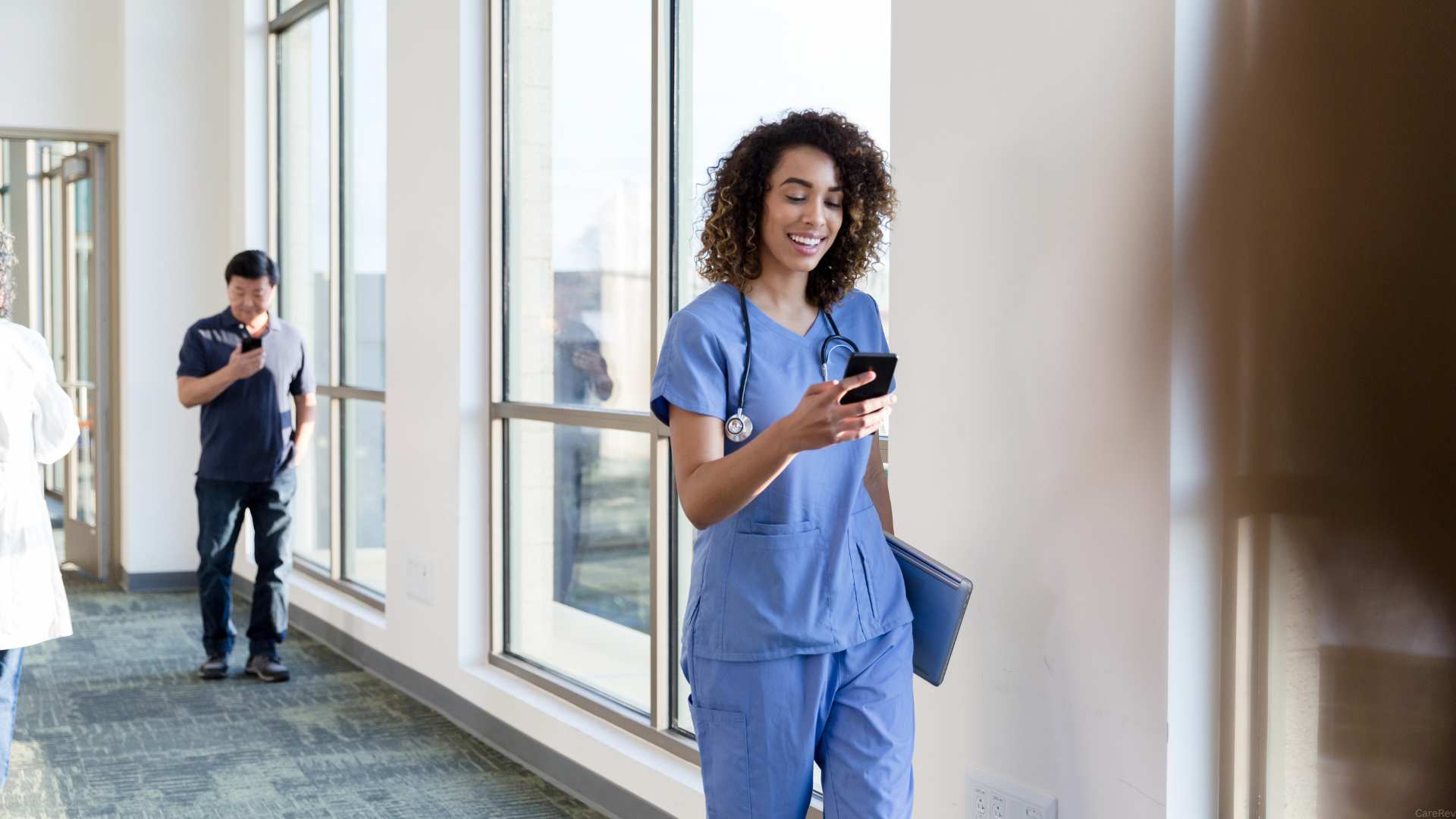
How Much Nurses Make in Texas
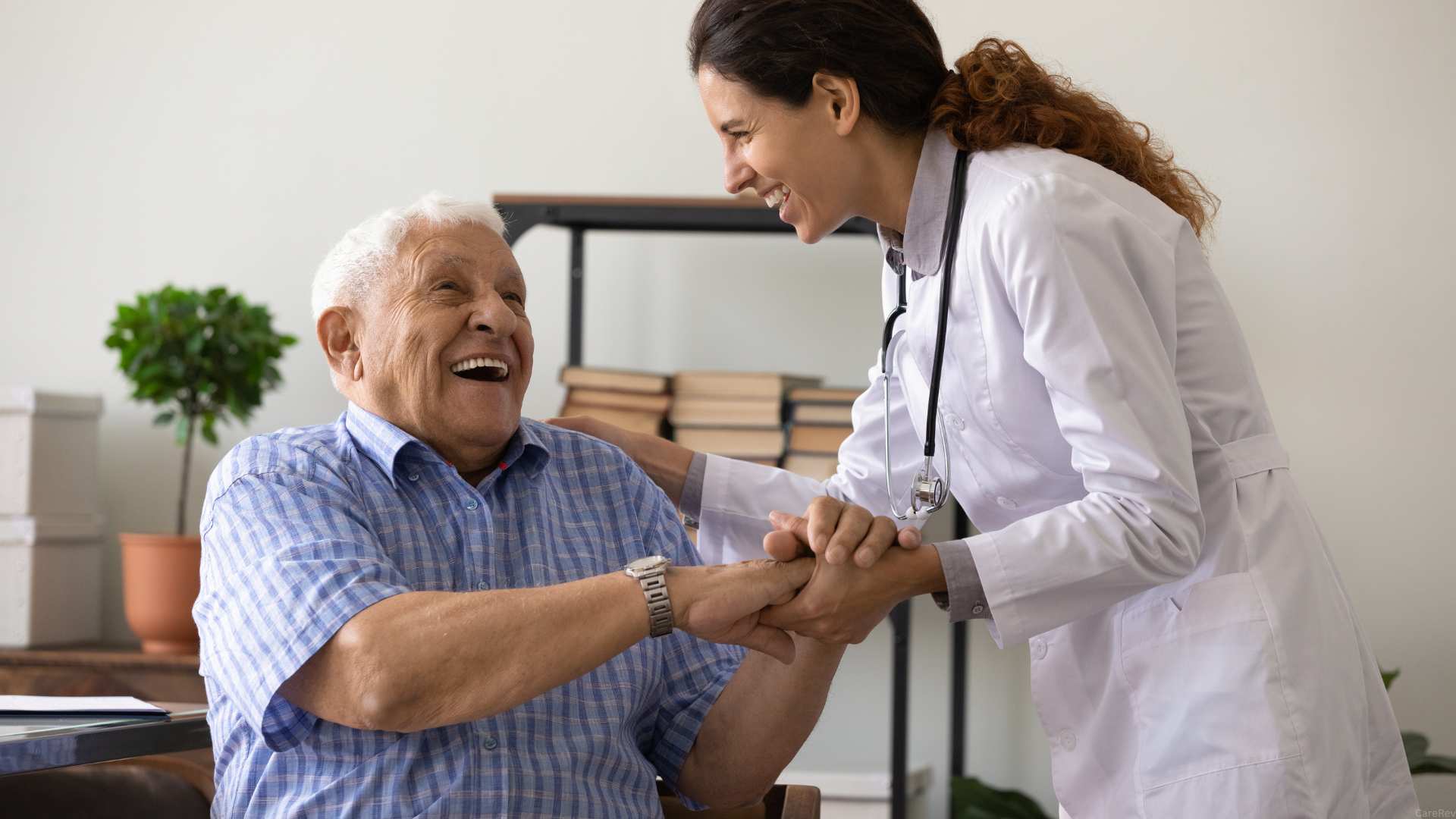
Nurse Practitioner vs. Doctor: How the Roles Differ
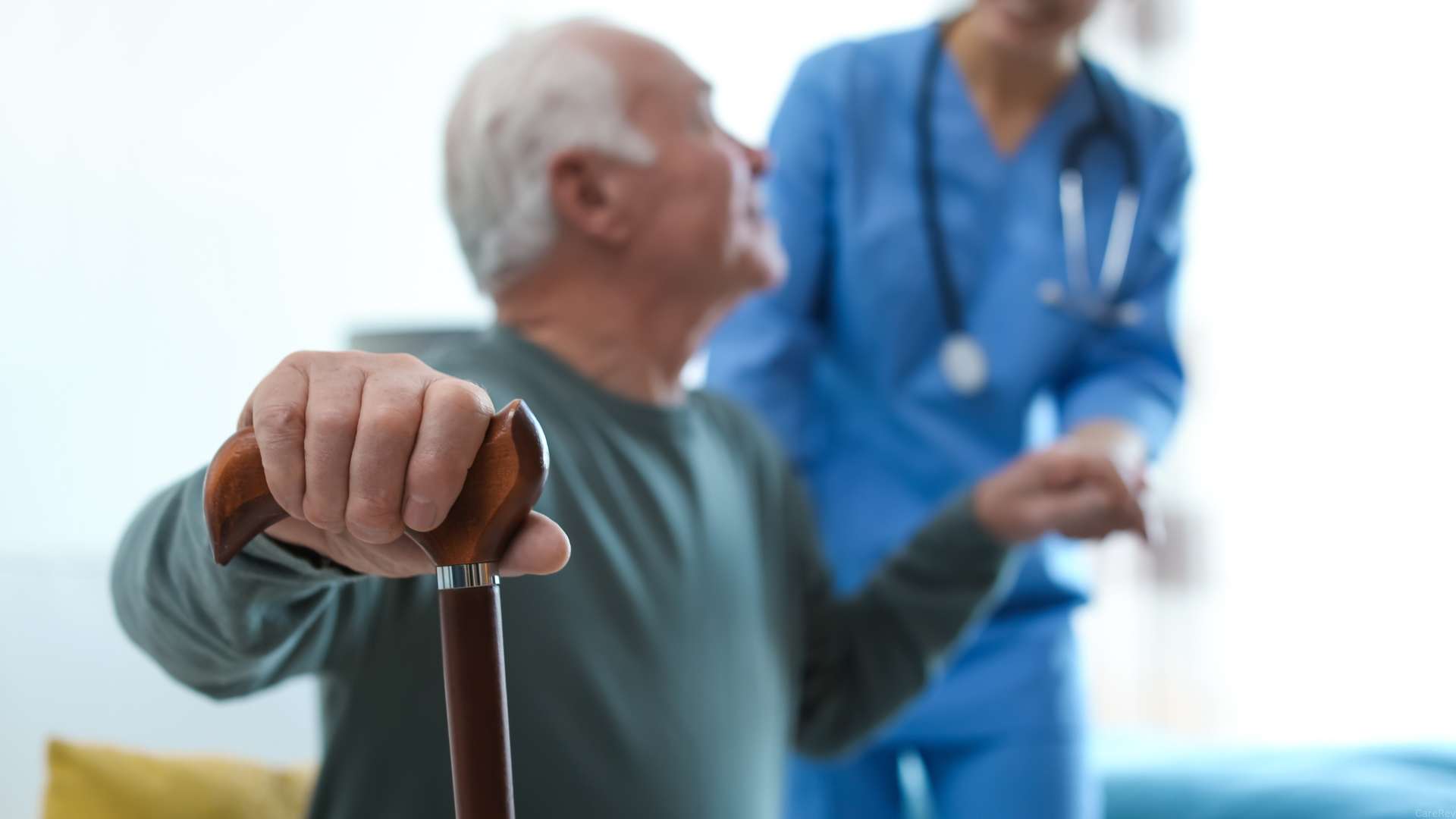
Exploring the Role of a Rehabilitation Nurse: Settings and Responsibilities
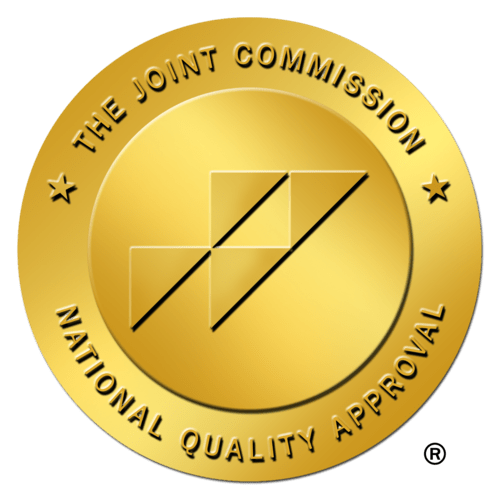

A deep-learning based analysis framework for ultra-high throughput screening time-series data
- Find this author on Google Scholar
- Find this author on PubMed
- Search for this author on this site
- ORCID record for Patrick Balzerowski
- ORCID record for David Gnutt
- For correspondence: [email protected]
- Info/History
- Supplementary material
- Preview PDF
Analysis of ultra-high-throughput screening data sets is a highly critical step in drug discovery campaigns. Due to various environmental and experimental error sources fast and reliable dentification of possible candidate compounds is challenging. In this work, we introduce a novel deep-learning based analysis framework to analyze uHTS time-series data sets. Our framework is based on two independent deep-learning models. A deep-learning regression model reduces temporal and spatial signal variation across multitier plates caused by systematic and random errors and a separate variational autoencoder model is used for dimensionality reduction. In contrast to classical evaluation methods our approach is capable to derive lower dimensional representations of time-series signals without a-priori knowledge of the data generating mechanism. We tested our analysis framework on an experimental uHTS data set and identified two distinct classes of substances in the screened library which could be attributed to two biological modes of action. Selected substances belonging to both modes of action were successfully validated in a secondary screening experiment.
Competing Interest Statement
The authors have declared no competing interest.
View the discussion thread.
Supplementary Material
Thank you for your interest in spreading the word about bioRxiv.
NOTE: Your email address is requested solely to identify you as the sender of this article.

Citation Manager Formats
- EndNote (tagged)
- EndNote 8 (xml)
- RefWorks Tagged
- Ref Manager
- Tweet Widget
- Facebook Like
- Google Plus One
Subject Area
- Bioinformatics
- Animal Behavior and Cognition (5548)
- Biochemistry (12600)
- Bioengineering (9484)
- Bioinformatics (30917)
- Biophysics (15894)
- Cancer Biology (12972)
- Cell Biology (18566)
- Clinical Trials (138)
- Developmental Biology (10036)
- Ecology (15016)
- Epidemiology (2067)
- Evolutionary Biology (19208)
- Genetics (12779)
- Genomics (17584)
- Immunology (12733)
- Microbiology (29791)
- Molecular Biology (12414)
- Neuroscience (64937)
- Paleontology (481)
- Pathology (2008)
- Pharmacology and Toxicology (3470)
- Physiology (5357)
- Plant Biology (11129)
- Scientific Communication and Education (1730)
- Synthetic Biology (3067)
- Systems Biology (7707)
- Zoology (1733)
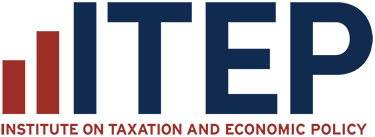
Here’s a Tip: Keep the Taxation of Tips As-Is
August 16, 2024
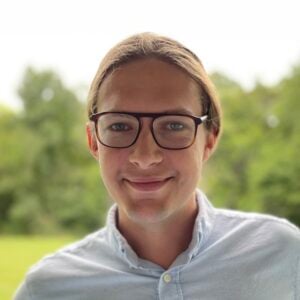
Joe Hughes Senior Policy Analyst
While the next President faces a wide range of pressing tax policy choices to make — from the expiration of much of 2017’s Trump tax law to international corporate taxation and beyond — a relatively silly idea has become the tax focus on the campaign trail: exempting tips from taxes. Despite its embrace by the candidates from both major parties, this policy idea would do little to help the roughly 4 million people who work in tipped occupations while creating a host of problems.
Exempting tips from taxes isn’t a new idea . It’s been proposed before and always abandoned because it’s practically impossible to do without creating new avenues for tax avoidance.
Lower-paid service employees definitely deserve support. However, altering the tax code in this manner is a very leaky way of achieving that. It’s an approach that rich people with accountants and lawyers would surely be able to abuse. Fund managers, attorneys, and other high-paid professionals could easily reclassify their fees — or at the very least, some percentage of them — as mandatory tips for performance.
This proposal treats households with similar levels of income differently based on profession. Servers and bartenders, for example, receive a large portion of their income through tips and thus would receive a large tax exemption. Teaching assistants or health care workers might receive similar overall income but would not receive the same exemption.
Finally, these proposals create incentives to drive even more low-paid service employees into the tipped worker category, harming many workers and their families. Tipped workers have more unstable incomes than non-tipped workers, are more likely to live in poverty, and are more vulnerable to wage theft, according to the Economic Policy Institute (EPI).
The good news is that lawmakers have designed way better ways of helping working families. EPI and other experts on work and wages have put forth many ways to better help working families, from eliminating the sub-minimum wage that allows workers who receive tips to be paid a paltry $2.13 an hour, to raising all minimum wages above the $7.25 where it has been mired for a decade and a half, to making it easier for workers to unionize. In terms of tax solutions, lawmakers rightfully concerned about low-wage workers should instead consider expanding the Child Tax Credit and Earned Income Tax Credit (and especially making the latter stronger for workers without children in the home).
Full Archive
All Blog Posts
Related Content
Local tax trends in 2024, minnesota stands out for its moderately progressive tax code, which states improved child tax credits and eitcs in 2024.
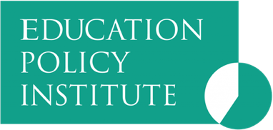
- 020 7340 1160
- [email protected]
- JOIN or COMMISSION EPI
Home / Publications & Research / Analysis: GCSE Results Day 2024
Analysis: GCSE Results Day 2024
Headline results are similar to last year
The qualifications regulator Ofqual had said in advance that it expected results to be broadly similar to last year and that’s what we have seen in today’s results. This stability follows two years of falls as the grading system unwound the effects of the pandemic when the use of centre and teacher assessed grades led to large increases in 2020 and 2021.
Overall, amongst 16-year-olds in England 70.4 per cent of all awards were at grade 4 or above, this is just 0.1percentage points higher than in 2023. At the higher end of the distribution, 22.6 per cent of all awards were at grade 7 or above, this is just 0.2 percentage points higher than in 2023.
There are some small differences between the distribution of results this year and those in 2019 – the last set of results before the Covid-19 pandemic. The percentage of entries at grade 7 and above is 0.8 percentage points higher than in 2019 and the percentage of entries at grade 4 and above is 0.5 percentage points higher. However, the percentage of entries receiving any pass is slightly below where it was in 2019 – 0.3 percentage points lower.
The average grade awarded in 2024 was 4.80, broadly the same as it was last year a nd the average grade prior to the pandemic . Following the use of centre as sessed grades in 2020 and teacher assessed grades in 2021, the average grade awarded was around half a grade higher in 2021 than it had been in 2019 .
Most subjects are have a similar grade distribution to last year, apart from expected increases in top grades in Computing, French and German
Almost all subjects have similar grade distributions to last year. Grades in statistics and classical studies are actually slightly below 2019 levels. There have been noticeable increases in German and French, but this is due to an upwards adjustment in these subjects to bring standards in line with other languages. Increases have also happened in Computing following a review of standards which found grading has been overly stringent in previous years.
Girls continue to outperform boys, but the gap continues to close
In 2024, 73.7 per cent of entries from girls achieved a grade 4 or above in comparison with 67.1 per cent of entries from boys. This gap between boys and girls has narrowed slightly since 2023 and has been continually narrowing since 2019. The gap is now 2.2 percentage points lower than it was in 2019. This has been driven by the percentage of boys achieving grade 4 or above increasing whilst the percentage for girls has decreased.
Similarly, the gap between boys and girls has narrowed amongst the highest attaining, when compared with 2019. This year, 25.5 per cent of entries from girls and 19.8 per cent of entries from boys were awarded a grade 7 or above. This represents a gap of 5.7 percentage points, 0.1 percentage points down on last year and 0.7 percentage points down on the 2019 gap. This gap was higher in period 2020 to 2022. The percentage of boys and girls achieving grade 7 or above have both increased since 2019.
Gaps vary by subject, with little changes from last year
Girls continue to outperform boys in the vast majority of subjects. In 2024, the only subjects where boys consistently outperformed girls at both grade 4 and grade 7 are maths, physics, economics and statistics. As we observe across all entries, gender gaps have seen small changes since 2023. Gaps have narrowed in engineering, although this reverses widening gaps between 2019 and 2023 .
Learning loss in maths recovering but English standards fall below pre-pandemic levels
Today’s GSCE results look very similar to those in 2023 and in the year prior to the pandemic, 2019. This is, in part, by design. A better way to track performance over time is to look at the results from the National Reference Test (NRT) in English and mathematics taken by a representative sample of year 11 pupils. This is also a good way to measure the impacts of the pandemic with the last pre-pandemic NRTs taking place in February/March 2020 just before schools closed due to the pandemic.
Results released today by Ofqual, suggests that outcomes in English, whilst holding up during the disruption of the pandemic, have fallen below pre pandemic levels in 2024. In mathematics performance at both grade 4 and above, and grade 7 and above, remains below pre-pandemic levels but has shown signs of recovery this year. This year, pupils achieved the highest per cent of grade 7 or above in mathematics since 2019. Despite changes observed on the NRT, Ofqual has decided not to adjust GCSE awards this year.
London continues to be the best performing region of England
There are long standing disparities in the attainment of pupils in different parts of the country. While regional differences are relatively small compared to, for example, the difference between the highest and lowest performing schools, it was still the case that pupils in the South East and in particular London outperformed pupils elsewhere.
Today’s results show that regional disparities continue. The percentage of entries awarded a grade 7 or above in London was 28.6%, whilst only 17.8% of entries in the north east were awarded a grade 7 or above. In the majority of regions, results today are similar to those in 2023.
However, across all regions the percent of pupils awarded a grade 4 or above fell slightly. This was driven by pupils retaking GCSE exams at older ages, as noted above there was a 0.1 percentage point increase between 2023 and 2024 amongst 16-year-olds. Further decreases in the percent of pupils awarded a grade 4 or above and grade 7 or above, compared to 2023, have been experienced in the East Midlands and the Eastern Regions.
During the pandemic the gap between London other regions widened. London has seen a 1.9 percentage point increase in the per cent of awards awarded a grade 4 and above, and a 2.8 percentage point increase in the per cent of awards awarded a grade 7 and above since 2019.
This widening of the gap between London and the rest of the country is consistent with analysis of learning loss during the pandemic (which has tended to focus on younger pupils) has also suggested greater learning losses for pupils in the north and in parts of the midlands.
Ofqual have also released breakdowns of grades by ceremonial county. The highest results are again clustered in London and the south east. Over 30 per cent of entries were awarded a grade 7 or above in Surrey, Berkshire and Buckinghamshire, the largest fraction of any counties. In contrast, East Riding of Yorkshire, Cumbria, Staffordshire, and Isle of Wight had the lowest rates, with less than 17 per cent of entries awarded grade 7 or above.
In terms of how results this year compared with results in 2023, Suffolk and Bristol saw the largest declines, with the percentage of students attaining grade 7 or above falling by 1.2 percentage points from last year. The largest increase in the percentage of pupils awarded a grade 7 or above was seen in Dorset, Northumberland, and the Isle of Wight, each with a 1.5 percentage point increases on 2023.
For the counties with the highest percentages of students achieving top grades in 2024, the changes on last year were relatively small. Surrey and Rutland are notable exceptions, with changes of 1.1 and -0.9 percentage points, respectively, compared to last year.
Top grades have increased more in independent schools than state-funded schools
Free schools have experienced the largest increase in the percentage of pupils obtaining a grade 7 or above. There was a 2-percentage point increase between 2023 and 2024. However, this is likely to partly be a compositional effect – there will be free schools in this year’s data that were not included in previous years. To aid comparisons over time, Ofqual publish further analysis that is restricted to institutions with results in every year from 2018 to 2024. This also shows an increase for free schools, but a slightly smaller 1.5 percentage points.
The number of entries graded at 7 and above for independent and selective schools was more than double all other school types. This gap has generally increased in 2024, with larger increases in top grades at selective (1 percentage point) and independent (1.8 percentage points) schools, compared to academies (0.1 percentage points) and comprehensive schools (0.3 percentage points).
Grades in vocational qualifications appear to fall
Whilst GCSE results are very similar to last year, there have been falls across the grade distribution in vocational qualifications. This summer, 303,840 grades were awarded across 33 vocational qualifications with the Pass/Merit/Distinction grading structure across level 1 and 2. 65.5 per cent of these awards were at Level 2, compared to 74.7 per cent in 2023, a decrease of 9.2 percentage points. This fall is potentially explained by changes in made to the qualifications themselves, as well as the mix of pupils taking these qualifications. However, we will not know until later in the year whether this was the driver of observed changes.
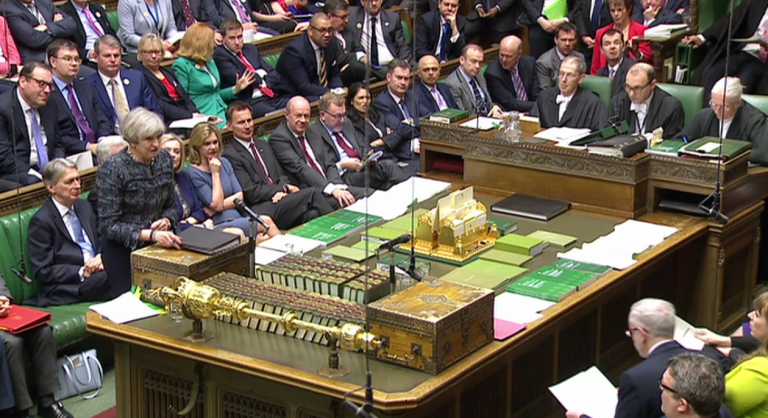
Louis Hodge
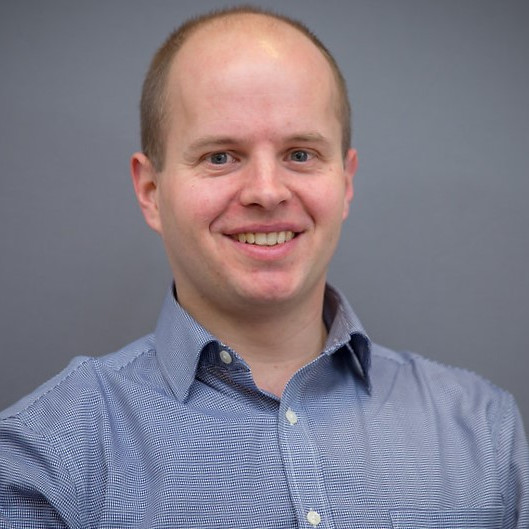
IMAGES
COMMENTS
What implications did this rise of data science as a transdisciplinary methodological toolkit have for the field of education?One means of illustrating the salience of data science in education research is to study its emergence in the Education Resources Information Center's (ERIC) publication corpus. 1 In the corpus, the growth of data science in education can be identified by the adoption ...
missing data in educational research are common (Peng, Harwell, Liou, & Ehman, 2006). Explanations for missing data include subject attrition (e.g., students move out of a school district during the study), data collection errors (e.g., errors in a computer program used to record subject responses), unclear instructions
Discourse or narrative analysis; Grouded theory; Phenomenological analysis or interpretative phenomenological analysis (IPA) Further reading and resources. As a starting point for most of these, we would recommend the relevant chapter from Part 5 of Cohen, Manion and Morrison (2018), Research Methods in Education. You may also find the ...
data; interpreting the data analysis; and composing the research findings and generalisations that are ethical. The purpose in specifying these components is to provide guidance to beginning researchers about the nature of empirical research that promotes warranted and transparent outcomes (Duran et al., 2006).
Educational Data Science (EDS) is defined as the use of data gathered from educational environments/settings for solving educational problems (Romero & Ventura, 2017). Data science is a concept to unify statistics, data analysis, machine learning and their related methods. This survey is an updated and improved version of the previous one ...
In education research, data analysis is crucial in making informed decisions and shaping school policies, curricula, and teaching methods. This article discusses qualitative and quantitative approaches to data analysis in education research, examining the tools and techniques used to collect, analyze, and interpret data. ...
data analysis. • Collect data on individual students, including gender, race/ethnicity, special education status, English proficiency, socioeconomic status, and special program participation. • Analyze data to identify best educational practices; develop strategies for replication and implementation within the district.
In this data-informed approach to education there are two distinct methodologies: qualitative and quantitative analytics. These methods, which are typical to data analytics in general, are crucial to the interpretation of learning behaviors and outcomes. ... SPSS: SPSS is a statistical analysis tool for quantitative research, appreciated for ...
Definition of research in data analysis: According to LeCompte and Schensul, research data analysis is a process used by researchers to reduce data to a story and interpret it to derive insights. The data analysis process helps reduce a large chunk of data into smaller fragments, which makes sense. Three essential things occur during the data ...
As a consequence, the general and systematic use of advanced methodologies for data analysis is certainly the 'new frontier' for educational research today. This has been a constant guideline while preparing this issue, and the main criterion for selecting contributing papers. A number of papers applying quantitative methodologies have been ...
Descriptive analysis identifies patterns in data to answer questions about who, what, where, when, and to what extent. This guide describes how to more effectively approach, conduct, and communicate quantitative descriptive analysis. The primary audience for this guide includes members of the research community who conduct and publish both ...
Education: Data analysis is used to measure student performance, evaluate teaching effectiveness, and improve educational programs. This includes assessment analytics, learning analytics, and program evaluation. ... Market research: Data analysis can help you understand customer behavior and preferences, identify market trends, and develop ...
These areas include the use of formative assessment data, educational research study findings and 'big data'. In particular, the discussion focuses on how school leaders and teachers can use different sources of data to improve the quality of education. ... For example, this may include difficulties with analysis and/or translating the data ...
Data collection methods in educational research are used to gather information that is then analyzed and interpreted. As such, data collection is a very important step in conducting research and can influence results significantly. Once the research question and sources of data are identified, appropriate methods of data collection are determined.
Research is a scientific field which helps to generate new knowledge and solve the existing problem. So, data analysis is the crucial part of research which makes the result of the study more ...
Jessica Nina Lester is an associate professor of Counseling and Educational Psychology at Indiana University. She received her PhD from the University of Tennessee, Knoxville. Her research strand focuses on the study and development of qualitative research methodologies and methods at a theoretical, conceptual, and technical level.
data 7. Describe how and when data analysis occurs in qualitative and quantitative research 8. Describe the importance of results, conclusions, and recommendations in a ... educational research studies, we typically choose topics we have had some previous experience with or exposure to. Topics for educational research studies should hold
The role of data analytics in education: Possibilities and limitations Book Chapter in: Responsible Analytics and Data Mining in Education: Global Perspectives on Quality, Support and Decision Making.
in Section V of the Handbook we examine data analysis using examples of data from each of the Head Start content areas. We explore examples of how data analysis could be done. We identify and describe trends in data that programs collect. Finally, we offer a perspective of how data lends itself to different levels of analysis: for example, grantee-
In particular, educators can use data analytics to record and analyze the following data sets: Student Data: Demographics like age, ethnicity and gender; whether they are full-time or part-time; if they take classes online, on campus or a mix of the two. Course Data: Enrollment headcounts, grades and completion rates by program or section.
Data analysis involves tools to clean data, then transform it, summarize it and develop models from it. SQL: The go-to choice when your data gets too big or complex for Excel, SQL is a system for ...
ABSTRACT: This paper considers the use of secondary data analysis in educational research. It addresses some of the promises and potential. pitfalls that influence its use and explores a possible rolefor the secondary. analysis of numeric data in the 'new' political arithmetic tradition of. social research.
Also in education and learning, big data analytics is. being used to enhance the learning process, to evaluate efficiency, to improve feedback and to. enrich the learning experience. Before ...
These steps ensured that the data analysis was both robust and reliable. Additionally, incorporating multiple methods for data analysis allowed us to cross-verify the findings and enhance the overall rigor of the study. Future research should integrate a range of methods, including interviews and surveys, to achieve a more comprehensive evaluation.
A modern application has been designed to streamline a process of tracking student attendance recording marks and control formal and educational information using QR codes all student is provided with a unique id card that includes a QR code teachers can effortlessly record attendance and input marks by simply scanning the students id cards in ...
Data management and analysis. Nursing informaticists are proficient in data management and analysis techniques, allowing them to collect, organize, and analyze healthcare data to derive meaningful insights. ... Research and education. Nursing informaticists contribute to nursing research by investigating the impact of technology on patient care ...
We tested our analysis framework on an experimental uHTS data set and identified two distinct classes of substances in the screened library which could be attributed to two biological modes of action. Selected substances belonging to both modes of action were successfully validated in a secondary screening experiment.
The no tax on tips idea isn't a new one, but it's always been abandoned because it's practically impossible to do without creating new avenues for tax avoidance. Despite its embrace by the candidates from both major parties, this policy idea would do little to help the roughly 4 million people who work in tipped occupations while creating a host of problems.
However, this is likely to partly be a compositional effect - there will be free schools in this year's data that were not included in previous years. To aid comparisons over time, Ofqual publish further analysis that is restricted to institutions with results in every year from 2018 to 2024.
[Method/Process] Based on 30 high-level papers on data literacy research of medical students, the grounded theory was used to conduct qualitative analysis. Through open coding, axial coding and selective coding, four key aspects of data literacy education system of medical students were obtained, including educational elements, educational ...