Approaches to Analysis of Qualitative Research Data: A Reflection on the Manual and Technological Approaches
- Citation (BibTeX)

Sorry, something went wrong. Please try again.
If this problem reoccurs, please contact Scholastica Support
Error message:
View more stats
This paper addresses a gap in the literature by providing reflective and critical insights into the experiences during two PhD qualitative studies, which adopted different approaches to data analysis. We first consider how the two PhD studies unfolded before discussing the motivations, challenges and benefits of choosing either a technological (NVivo) or manual approach to qualitative data analysis. The paper contributes to the limited literature which has explored the comparative experiences of those undertaking qualitative data analysis using different approaches. It provides insights into how researchers conduct qualitative data analysis, using different approaches and the lessons learnt.

1. Introduction
Qualitative data analysis has a long history in the social sciences. Reflecting this, a substantial literature has developed to guide the researcher through the process of qualitative data analysis (e.g. Bryman & Burgess , 1994; Harding , 2018; Saunders et al. , 2019; Silverman , 2017 ). While earlier literature focuses on the manual approach [1] to qualitative data analysis (Bogdan & Bilken , 1982; Lofland , 1971) , more recent literature provides support in the application of a range of technological approaches (alternatively referred to as Computer Assisted Qualitative Data Analysis Software or CAQDAS): e.g., Excel (Meyer & Avery , 2009) ; NVivo (Jackson & Bazeley , 2019) ; and ATLAS.ti (Friese , 2019) . Moreover, in an accounting context, a critical literature has emerged which attempts to elucidate the messy and problematic nature of qualitative data analysis (Ahrens & Chapman , 2006; Lee & Humphrey , 2006; Modell & Humphrey , 2008; O’Dwyer , 2004; Parker , 2003) . However, while a substantial literature exists to guide the researcher in undertaking qualitative data analysis and in providing an understanding of the problematic nature of such analyses, a dearth of research reports on the comparative experiences of those undertaking qualitative data analysis using different approaches. The paper aims to address this gap by reporting on the experiences of two recently qualified doctoral students as they reflect on how they each approached the task of analysing qualitative data, Researcher A (second author) choosing a technological approach (NVivo) while Researcher B (third author) opted for a manual approach. The paper contributes to the limited literature which explores the comparative experiences of those undertaking qualitative data analysis using different approaches. In so doing, we hope that the critical reflections and insights provided will assist qualitative researchers in making important decisions around their approach to data analysis.
The remainder of the paper is structured as follows. In section two, we provide an overview of the problematic nature of qualitative research and a review of the manual and technological approaches of data analysis available to researchers. Section three follows with a discussion of two qualitative PhD studies. Section four discusses the experiences, challenges and critical reflections of Researchers A and B as they engaged with their particular approach to qualitative data analysis. The paper concludes with a comparative analysis of the experiences of Researchers A and B and implications for further work.
2. Literature Review
2.1 a qualitative research approach: debates and challenging issues.
Qualitative researchers pursue qualia , that is phenomena as experienced (sometimes uniquely) by individuals, that enlarge our conception of the “really real” (Sherry & Kozinets , 2001 , p. 2) . Qualitative studies seek to answer ‘how’ and ‘why’ rather than ‘what’ or ‘how often’ questions. In so doing, qualitative studies involve collecting rich data that are understood within context and are associated with an interpretivist philosophy. Mason (2002) notes that qualitative research is not just about words, rather it reflects a view of practice that is socially constructed and requires researchers to embrace subjectivity in order to interpret data. Furthermore, Bédard & Gendron (2004) argue that “being tolerant of uncertainty is part of the fundamental skills of the qualitative researcher” (p. 199). That said, a qualitative approach can be extremely labour intensive, given the volume of data collected and the commitment required to generate themes.
In the accounting and management literatures, there has been considerable debate on the challenges of qualitative data analysis. In early work, Parker (2003) highlights a potential challenge in that qualitative researchers need to be reflexive in the data analysis process. To that end, researchers often construct field notes and memos (during interviews for example) to report their feelings, perceptions and impressions, which can be viewed as data, alongside all other data collected from the field. Bédard & Gendron (2004) highlight a further challenge in that analysing qualitative data is both labour intensive and requires high levels of research knowledge and ability. Furthermore, they argue that qualitative researchers need to be immersed in data collection and analysis, and should be mindful that the “specific objectives of the study are not always determined a priori, but often ‘emerge’ from fieldwork” (p. 200). Ahrens & Chapman (2006) identify the challenge of data reduction without “‘thinning’ out the data to the point where it loses its specificity and becomes bland” (p. 832). Qualitative data analysis is, they argue, not a straightforward process: “Like other practices, the doing of qualitative field studies is difficult to articulate. One can point to the golden rules but, at the heart of it lies a problem of transformation. Out of data, snippets of conversations and formal interviews, hours and days of observation, tabulations of behaviours and other occurrences, must arise the plausible field study” (Ahrens & Chapman , 2006 , p. 837) . This chimes with O’Dwyer’s (2004) description of qualitative data analysis as ‘messy’. To address this, O’Dwyer (2004) highlights the importance of imposing structure onto the analysis process and outlines an intuitive approach to analyse interview data using Miles and Huberman’s (1994) three stage process of data reduction, data display and data interpretation/conclusion drawing and verification. This process involves the categorisation of themes and individual aspects of interviews in several stages to ensure that general patterns and differences are articulated. While O’Dwyer (2004) considered using a technological approach to assist in data analysis, he discounted it as an option at an early stage of his research, largely as a result of his lack of understanding of what it could offer. Lee & Humphrey (2006) also argue that analysing interview transcripts is a key challenge facing qualitative researchers. In particular, deciding “what weight to give to meanings that are only apparent in a part of an interview, how to retain understanding of the whole interview when the focus is on individual parts and how to derive patterns both within and across interviews without losing sight of any idiosyncratic elements that may provide unique insights” (p. 188). Finally, Modell & Humphrey (2008 , p. 96) , while calling for further research in the area of qualitative data analysis, contend that problems exist where there is undue focus on the approach to data analysis to the detriment of the development of ideas. They suggest that this appears to be an increasingly common issue, particularly with increased use of technology in the data analysis process.
2.2 Approaches to Data Analysis: Manual and Technological (i.e. NVivo) Approaches
The data analysis phase of qualitative research is described as the “most intellectually challenging phase” (Marshall & Rossman , 1995 , p. 114) and the active role of the researcher in identifying and communicating themes is critical (Braun & Clarke , 2006; Edwards & Skinner , 2009; Silverman , 2017) . While early technological approaches to data analysis have been in existence since the 1960s, many qualitative researchers have continued to employ the manual approach to analysis (Séror , 2005) . In part, this may be due to the perceptions of some researchers that the technological approach may attempt to do more than assist in the management of data, potentially influencing the abstraction of themes from data in unintended ways (Crowley et al. , 2002) . However, a review of the literature suggests that the manual approach can be an unwieldy, cumbersome, “tedious and frustrating” process (Basit , 2003 , p. 152) . Furthermore, comparatively little has been published in relation to the mechanics of the manual approach (Bazeley , 2009; Bogdan & Bilken , 1982; Braun & Clarke , 2006; Edwards & Skinner , 2009; Lofland , 1971; Maher et al. , 2018; Miles & Huberman , 1994; Silverman , 2017) .
Edwards & Skinner (2009) assert that the manual analysis of hundreds of pages of raw data is a “daunting” task (p. 134). To assist in this process, some basic mechanical procedures are described in the literature, including: printing hardcopy transcripts, photocopying, marking up, line-by-line coding, coding in margins, cutting, cut-and-paste, sorting, reorganising, hanging files and arranging colour-coded sticky notes on large format display boards (Basit , 2003; Bogdan & Bilken , 1982; Lofland , 1971; Maher et al. , 2018; L. Richards & Richards , 1994) . Moreover, Braun & Clarke (2006) provide a comprehensive description of the manual data analysis process, involving “writing notes on the texts you are analysing, by using highlighters or coloured pens to indicate potential patterns, or by using ‘post-it’ notes to identify segments of data” (p. 89). As ‘codes’ are identified, data extracts are manually grouped and collated within the individual codes. The subsequent generation of sub-themes and overarching themes involves the trialling of combinations of codes until “all extracts of data have been coded in relation to them” (p. 89). The above is an iterative process and involves re-reading, coding and recoding until all data has been included in sub-themes and overarching themes. The researcher’s interaction with the data is important in this regard, and involves a series of physical activities around arranging and re-arranging data excerpts and post-it notes, followed by visual mapping on “large format display boards” (Maher et al. , 2018 , p. 11) . This process “encourages a slower and more meaningful interaction with the data [and] great freedom in terms of constant comparison, trialling arrangements, viewing perspectives, reflection and ultimately developing interpretative insights” (Maher et al. , 2018 , p. 11) .
An alternative to the manual approach is the use of CAQDAS (i.e. technological approach) to support qualitative data analysis. CAQDAS offers the ability to import, organise and explore data from various sources (text, audio, video, emails, images, spreadsheets, online surveys, social and web content). The origins of NVivo, one of the market leaders, can be traced back to the 1980s with the development of a computer programme called Non-numerical Unstructured Data Indexing Searching and Theorizing (NUD*IST). Richards, one of the co-developers of NVivo provides an “intellectual history” of NUD*IST and NVivo (R. Richards , 2002 , p. 199) , arguing that “NVivo … is being preferred by researchers wishing to do a very detailed and finely articulated study … [and that NVivo’s] tools support close and multi-faceted analysis on small and moderate amounts of data” (p. 211). Reflecting its widespread usage as a mainstream CAQDAS, a literature has now developed around the use of NVivo. For example, Bandara (2006) provides guidance to novice researchers and academics involved in NVivo research training in information systems research; García-Horta & Guerra-Ramos (2009) provide reflections on the use of NVivo in education; Leech & Onwuegbuzie (2011) present guidance for psychology researchers; and Zamawe (2015) presents experiences in the context of health professionals.
Acknowledging that little is known about how researchers use CAQDAS, Paulus et al. (2017) present the results of a discourse analysis of some 763 empirical studies which use NVivo or ATLAS.ti (a competitor of NVivo – see https://atlasti.com/ ). Drawing on peer reviewed papers, published between 1994 and 2013, Paulus et al. (2017) report that the majority of researchers (87.5% of their sample) using CAQDAS to support qualitative data analysis fail to provide details of the technological approach used beyond naming the software, or what they refer to as ‘name-dropping’. Some 10% of the sample provide moderate levels of reporting, mainly concerned with “descriptions of software capability” (Paulus et al. , 2017 , p. 37) . The remaining 2% of the sample provide more detailed descriptions of the CAQDAS used, including “detailed descriptions of how the analysis was conducted” (p. 39) or “how the researchers used the software to go beyond coding to a deeper layer of analysis” (p. 41). Based on their findings, Paulus et al. (2017) suggest that future studies should provide more detail about their experiences of using CAQDAS to support qualitative data analysis, including: what software is used; how they are used; why they are used; and how effective they have been.
A limited number of studies report on the benefits and drawbacks of using NVivo. In an early study, García-Horta & Guerra-Ramos (2009) report their experiences of using NVivo (and MAX QDA ) to analyse qualitative data collected from teachers. Their experiences suggest a number of advantages, including the ability to: organise and store large volumes of data; deal with data overload; and enable fast and efficient retrieval of relevant information. However, they also highlight a number of limitations, most notably the “real hard work” of “generating categories or taxonomies, assigning meaning, synthesizing or theorizing” (p. 163) which, they argue, remains that of the researcher and not the software. García-Horta & Guerra-Ramos (2009) also highlight the potential for “data fetishism … or the ‘let’s code everything’ strategy [which] can lead to excessive and non-reflexive coding” (p. 163). They caution against the possibility of assumptions that ‘meaning-making’ can be computerised and the possibility of what they call ‘technologism’ whereby there is an implicit assumption that the qualitative data analysis process will be enhanced by the use of software. More recently, Zamawe (2015) argues that NVivo works well with most research designs as it is not methodologically specific and “the presence of NVivo makes it more compatible with grounded theory and thematic analysis approaches” (p. 14). Furthermore, Zamawe (2015) suggests NVivo eases the burden associated with manual qualitative data analysis in terms of the ‘copy-cut-paste’ requirement. NVivo also lends itself to more effective and efficient coding, and the reshaping and reorganisation of the coding structure by “simply clicking a few buttons” (p. 14). Zamawe (2015) , however, points out some pitfalls associated with using NVivo. These include: the time consuming, and difficult, nature of the software; the potential for NVivo to “take over the analysis process from the researcher” (p. 15); the process of coding the data; and the danger of the researcher becoming distant from his/her data with the result that the ‘thickness’ of the data is diluted.
2.3 Comparison of Manual and Technological Approaches
Few studies report on comparisons of the manual and technological approaches to qualitative data analysis. In one such study, Basit (2003) compares the use of the manual and technological approach to qualitative data analysis drawing on two research projects. She argues that the approach chosen is dependent on the size of the project, the funds and time available, and the inclination and expertise of the researcher. Basit (2003) maintains that while the technological approach may not be considered feasible to code a small number of interviews, it is more worthwhile when a large number of interviews are involved. When compared to the manual approach, she highlights a number of perceived benefits of the technological approach. First, the data analysis process is relatively smooth and facilitates a more in-depth analysis. Second, the search facility is particularly useful, as is the ability to generate reports. Despite the perceived benefits, Basit (2003) acknowledges some challenges of the technological approach when compared to the manual approach. There is a considerable amount of time and formal training involved in getting acquainted with a software package to code qualitative data electronically, an investment not required for the manual approach. However, that said, Basit notes that the benefit of the software search facility and the generation of comprehensive reports compensates for the time investment required. In another study, Maher et al. (2018) argue that qualitative data analysis software packages, such as NVivo, do not fully scaffold the data analysis process. They therefore advocate for the use of manual coding (such as using coloured pens, paper, and sticky notes) to be combined with digital software to overcome this. Reflecting on their research, which combined both a manual and software analysis, they argue that NVivo provides excellent data management and retrieval facilities to generate answers to complex questions that support analysis and write-up, a facility not available with a manual approach. However, they suggest that the manual approach of physically writing on sticky notes, arranging and rearranging them and visual mapping, encourages more meaningful interaction with the data, compared to a technological approach. Furthermore, they advocate that the manual approach has a particular advantage over the technological approach as manual analysis usually results in displays of the analysis. The resulting visualisations, sticky notes, and concept maps may remain in place, allowing the researcher to engage with the research material on a variety of levels and over a period of time. In contrast to the manual approach, Maher et al. (2018) believe that NVivo operated on a computer screen does not facilitate broad overviews of the data and that data views may therefore become fragmented.
The above review indicates that limited research has reported on the comparative experiences of those undertaking qualitative data analysis. This paper addresses this gap, and in so doing, reports on the experiences of two recently qualified doctoral students, as they each reflect on how they approached the task of analysing qualitative data using different approaches. Section three presents details of the two research projects.
3. The Doctoral Research Projects
In this section, the background, motivation and research question/objectives of the research projects undertaken by Researchers A and B (both undertaking a part-time PhD) are outlined. This provides context for a comparison of the technological (NVivo) and manual approaches used for qualitative data analysis.
3.1 Researcher A: Background, Motivation, Research Question and Objectives
Researcher A (a Chartered Accountant) investigated financial management practices in agriculture by exploring the financial decision-making process of Irish farmers. When the literature in the area of farm financial management (FFM) was explored, it became apparent that there were relatively few prior studies, both internationally and in the Irish context (Argiles & Slof , 2001; Jack , 2005) . The limited literature posed particular difficulties and frustration when conducting this research, but also demonstrated that there was a gap in the literature that needed to be addressed. The review of the literature identified a number of key issues which were central to the motivation of the research project. First, the majority of farmers appear to spend very little time on financial management (Boyle , 2012; Jack , 2005) and second, farmers tend to rely on intuition to a large extent when managing their farm enterprise (Nuthall , 2012; Öhlmér & Lönnstedt , 2004) .
Researcher A’s overall research question was: How and why do farmers make financial decisions? To address this question, two research objectives were formulated following a detailed literature review and findings from preliminary research, namely a pilot survey of farmers and key informant interviews. The theoretical framework adopted (sensemaking theory) also assisted in framing the research objectives.
Research Objective 1: To explore the financial decision-making process of farmers by examining:
The factors that influence farmer decision-making;
The role of advisors in farmer decision-making;
The role of FFM in farmer decision-making;
The role of other issues in farmer decision-making (e.g. demographic factors such as farm type, age and level of education of the farmer, and the role of intuition in farmer decision-making).
Research Objective 2: To establish how farmers make sense of their business situations in order to progress with decisions of a financial nature.
The research methodology chosen by Researcher A was interpretivist in nature (Ahrens & Chapman , 2006) . This was based on the assumption that farmers’ realities (in regard to how financial decisions are made) are subjective, socially constructed and may change. As a result, it was considered necessary to explore the subjective meanings motivating the decisions of farmers in order to understand the farmers’ decision-making processes. Interviews were considered the most appropriate data collection method to operationalise the interpretivist methodology chosen. The data collected via interviews with farmers allowed Researcher A to develop thick and rich explanations of how farmers make financial decisions.
3.2 Researcher B: Background, Motivation, Research Question and Objectives
Researcher B (also a Chartered Accountant) examined accounting practitioners’ perceptions of professional competence and their engagement with Continuing Professional Development (CPD) activities, as they strive to maintain and develop competence. Educational guidance on mandatory CPD within the profession was introduced in 2004 (IES 7 , 2004) , and while CPD is viewed as a bona fide stage in the lifecycle of professional education, it is in a state of infancy and transition and has yet to grow to achieve coherence, size and stature equivalent to the pre-qualification stage (Friedman & Phillips , 2004) . While professional accountancy bodies may interpret IES 7 guidance and almost exclusively decide what counts as legitimate or valid CPD, individual practitioners are mandated to complete and self-certify relevant activities on an annual basis in order to retain professional association. It is therefore questionable whether the annual declaration encapsulates the totality of practitioners’ learning and professional development in relation to professional competence (Lindsay , 2013) .
A review uncovered an extensive literature, concentrating on professionalisation, competence and professional education and learning, with attention focusing on the accounting domain. The following emerged: literature on professionalisation pertaining to the pre-qualification period (Flood & Wilson , 2009) ; findings on competence, education and learning largely focusing on higher education (Byrne & Flood , 2004; Paisey & Paisey , 2010) ; and CPD studies predominantly reporting on engagement (Paisey et al. , 2007) . The literature review highlighted a research gap and acknowledged the need for enhanced understanding in relation to post-qualification stages, where learning and professional development could more appropriately be examined from a competence angle (Lindsay , 2013) .
The overall research objective of Researcher B’s study was to explore how individual accounting professionals perceive professional competence, and how, in light of these perceptions, they manage their CPD with the purpose of maintaining and further developing their professional competence. Given that the study set out to gain an understanding of individual perceptions and practices, this supported the use of an interpretivist approach (Silverman , 2017) . A phenomenographic approach (a distinct research perspective located within the broad interpretivist paradigm) was selected. The root of phenomenography, phenomenon , means “to make manifest” or “to bring light” (Larsson & Holmström , 2007 , p. 55) and phenomenography examines phenomena “as they appear to people” (Larsson & Holmström , 2007 , p. 62) . The phenomenographic approach is an experiential, relational and qualitative approach, enabling the researcher to describe the different ways people understand, experience, and conceptualise a phenomenon (Larsson & Holmström , 2007; Marton , 1994) . It emphasises the individual as agent who interprets his/her own experiences and who actively creates an order to his/her own existence. It therefore facilitated the exploration of the ‘qualitatively different ways’ in which professional competence and associated CPD “are experienced, conceptualised, understood, perceived and apprehended” (Marton , 1994 , p. 4424) . ‘Bracketing’ is central to the phenomenographic approach and requires the researcher to effectively suspend research theories, previous research findings, researcher understandings, perceived notions, judgements, biases and own experience of a research topic (Merleau-Ponty , 1962) . This ensures “phenomena are revisited, freshly, naively, in a wide-open sense” (Moustakas , 1994 , p. 33) “in order to reveal engaged, lived experience” of research participants ( Merleau-Ponty , 1962 cited in Ashworth , 1999 , p. 708 ). In turn, participant experiences and understandings are examined and “characterised in terms of ‘categories of description’, logically related to each other, and forming hierarchies in relation to given criteria” (Marton , 1994 , p. 4424) . Such conceptions are assumed to have both meaning, a ‘what’ attribute, and structure, a ‘how’ attribute (Marton , 1994) . The anticipated output from Researcher B’s study sought an understanding of professional competence (the ‘what’ attribute) and the manner in which individual practitioners achieve and maintain such competence (the ‘how’ attribute). Interviews were considered the most appropriate data collection method to gain this understanding. The professional status of practitioners was therefore central to Researcher B’s study and the research focused on gaining an understanding of individual perceptions and practices with regard to maintaining and further developing professional competence. Mindful of this focus, the following research questions were developed:
What does it mean to be a ‘professional’?
What does ‘professional competence’ mean?
How is professional competence maintained and developed?
4. The NVivo and Manual Approaches to Qualitative Data Analysis
While Researchers A and B addressed disparate research areas, the above discussion indicates that qualitative data analysis represented a significant and central component of both researchers’ doctoral studies. Both researchers adopted an interpretivist philosophy involving a broadly similar number of interviews (27 in the case of Researcher A and 23 in the case of Researcher B). Despite the similarities between Researchers A and B, their choice of approach to qualitative data analysis was fundamentally different, with Researcher A choosing the technological approach (i.e. NVivo) and Researcher B the manual approach. In the remainder of this section, we discuss the factors influencing the choices made by Researchers A and B and provide insights into the data analysis process conducted. We then present critical reflections and the challenges faced by both researchers, as they undertook their respective approaches to qualitative data analysis.
4.1 Researcher A: Factors Influencing Approach to Qualitative Data Analysis
A number of factors influenced Researcher A’s decision to use NVivo (version 12) over the manual approach of qualitative data analysis. The most prominent of these was the multidimensional nature of the data collected. Researcher A investigated the financial decision-making process of farmers by exploring both strategic and operational decision-making. The farmers interviewed operated different farm types, had diverse levels of formal education and their age profile varied. The presence of multiple attributes highlighted the importance of reporting findings not only on how individual farmers undertook decision-making, but also to engage in comparisons of decision-making in different types of farming, and to explore how demographic factors (e.g. education, age) affected farmers’ decision-making processes.
Researcher A explored the option of adopting a technological approach to data analysis at an early stage in his study by attending a training course on NVivo. Despite attending the training course with an open mind and being aware of the alternative manual approach of qualitative data analysis, the training course convinced Researcher A of the potential power of NVivo to assist in qualitative data analysis. In particular, Researcher A was drawn to the ‘slice and dice’ capability of NVivo, whereby data could be analysed for a specific type of decision (strategic or operational), across multiple farm types (dairy, tillage or beef), or with respect to the demographic profile of farmers (education, age). By setting up different types of decisions, farm types and demographic factors as overarching themes (referred to as ‘nodes’ in NVivo), NVivo presented Researcher A with the ability to conduct numerous queries to address the research objectives, whilst simultaneously facilitating the extraction of relevant quotations to support findings. While the analysis could have been conducted manually, the search facility within NVivo was considered by Researcher A to be a very useful function and more efficient than using word processing software, which would be used with a manual approach. An additional and related factor which influenced Researcher A’s decision to proceed with NVivo was the possibility of availing of on-going one-to-one support for the duration of the research project from an NVivo trainer, when the actual qualitative data analysis commenced. In addition, Researcher A’s decision to opt for NVivo was influenced by his supervisor’s experience when conducting her own PhD studies. To that end, Researcher A’s supervisor had experience of using a technological approach (NUD*IST) to undertake qualitative data analysis. As a result of her familiarity with a technological approach, and an overall relatively positive experience, Researcher A’s supervisor provided some reassurance that this approach, versus the manual approach, was appropriate.
Before finally making the decision to adopt either a manual or technological approach to qualitative data analysis, Researcher A engaged with the various academic debates in the literature concerning the appropriateness of both. Based on these debates, Researcher A was confident that the technological approach to qualitative data analysis was appropriate. However, reflecting the debates in the literature, Researcher A was particularly mindful that “[NVivo] is merely a tool designed to assist analysis” (O’Dwyer , 2004 , p. 395) and that data analysis is ‘messy’ and very much the responsibility of the researcher who “must ask the questions, interpret the data, decide what to code” (Bringer et al. , 2006 , p. 248) .
4.2 Researcher A: An NVivo Approach to Data Analysis
Researcher A conducted 27 in-depth semi-structured interviews with farmers to develop an understanding of their financial decision-making processes. As with any qualitative research project, prior to formal data analysis, there was a significant amount of work involved in ‘cleansing’ the interview data collected. Researcher A transcribed all interview recordings, after which transcriptions were listened to and carefully read to identify inaccuracies. Field notes were also written by Researcher A immediately after each interview and these complemented the analysis of qualitative data and assisted the researcher in being reflexive during the data analysis process.
Researcher A adopted a thematic approach to qualitative data analysis as advocated by Braun & Clarke (2006) . Thematic analysis is a method for identifying, analysing and reporting patterns (themes) within data, where a theme is “something important about the data in relation to the research question and represents some level of patterned response or meaning from the data set” (Braun & Clarke , 2006 , p. 80) . In undertaking qualitative analysis, Researcher A followed a six phase thematic data analysis process (see Figure 1 ) developed by Braun & Clarke (2006) as follows:
Familiarising yourself with your data – interview transcripts were read and re-read by Researcher A, noting down initial ideas. Interview transcripts were then imported into the data management software NVivo.
Generating initial codes – this phase, descriptive coding, involved the deconstruction of the data from its initial chronology. The inductive process resulted in 227 hierarchical codes identified from the interview data, across 11 areas.
Searching for themes – this phase involved reviewing the open coding, merging, re-naming, distilling and collapsing the initial codes into broader categories of codes. This allowed the data to be constructed in a manner that enabled the objectives of the research to be fulfilled. Phase 3 resulted in the generation of 11 empirical themes related to strategic decision-making and 10 related to operational decision-making.
Reviewing themes – a process of ‘drilling down’ was conducted, including re-coding the text in the initial codes, re-organising into a coding framework, and breaking the themes down into sub-codes to better understand the meanings embedded therein.
Defining and naming themes – this involved abstraction of the data into a broader thematic framework. Using an inductive process, data was coded in relation to the four components of research objective 1, namely influencing factors; role of advisors; role of FFM; and other issues.
Producing the report – the final phase involved writing analytical memos to accurately summarise the content of each theme and propose empirical findings. The analytical memos helped Researcher A to produce a timely written interpretation of the findings, with the addition of his own annotations and recollections from interviews. The analytical memos also greatly assisted Researcher A to draft the findings chapter of his PhD thesis.

4.3 Researcher A: A Critical Reflection and Challenges with NVivo Qualitative Data Analysis
Reflecting on the journey of using NVivo as an approach to qualitative data analysis, Researcher A observed a number of salient points. First, a considerable amount of time and commitment is involved in developing the necessary skills to use the technology. Initially some time and effort are needed to learn how to operate the technology and formal NVivo training provides an essential support mechanism in this regard, particularly where training utilises standardised test data. Formal training also provides the researcher with an excellent overview of the technology and its potential capabilities. However, Researcher A cautions that it is not until the researcher actually begins to analyse their own data, which could potentially be some months/years later given the nature of the PhD research process, that specific study-related queries in using NVivo emerge. Due to the potential time lag, the researcher may have forgotten many aspects covered during the training or they may encounter queries that they have not experienced before. Hence, further specific guidance and/or further training may be required from the service provider. On a positive note, Researcher A found that the significant time and commitment invested towards the beginning of the data analysis process reaped considerable benefits towards the latter end of the research project. In particular, the systematic and structured coding process conducted allowed the retrieval of multi-layered analyses of the data relatively quickly. Furthermore, NVivo enabled the researcher to analyse, compare and contrast various aspects of the data efficiently and effectively. This was particularly useful for Researcher A, given the multidimensional aspect of the data collected. The time invested in learning how to operate the technology is a transferable research skill that the researcher could use on future research projects. While Researcher A invested a considerable amount of time becoming proficient with NVivo, it should be noted that the cost of both the technological approach (licence fee for NVivo) and formal training was not an issue, as these were funded by the researcher’s institution.
Second, critical reflection by Researcher A highlights the need to be mindful of the inclination to quantify qualitative data when using data analysis technologies. To that end, the coding process undertaken when using NVivo has the potential to focus the researcher’s attention on counting and quantifying the number of times a particular issue is identified or emphasised in the data. Braun & Clarke (2006) highlight that there are no hard and fast rules on how to identify a theme during qualitative data analysis. One cannot quantify how many times an issue must appear in the data in order for it to be labelled a theme. Indeed, an issue may appear infrequently in a data set, yet be labelled as a theme. Therefore, researcher judgement is necessary in determining themes. During the initial stages of writing up the findings, Researcher A found the above to be a particular challenge, as NVivo focused his attention on counting the number of times a particular issue appeared in the data. The ‘counting’ of data can be done easily through NVivo via the generation of graphs, tables or charts at the ‘push of a button’. Such analyses are useful for presenting a high-level overview of issues emphasised in the data, but they can also distract from the richness of the underlying interview data. Reflecting on this, Researcher A identified that it was necessary to pause, refocus and consider the underlying essence of the interview data, alongside the more quantitative output that NVivo generates. This is an important issue that qualitative researchers need to be cognisant of, particularly those who are first time users of the technological approach to analysing qualitative data.
Third, Researcher A reflects that the coding and analysis of the large volume of qualitative data collected was challenging and there was a need to be tolerant of uncertainty during this process. In particular, there was an element of drudgery and repetitiveness in coding the data using NVivo, necessitating the need for resilience and a ‘stick with it’ attitude as it was necessary to consistently code all interview data. However, one of the main benefits of adopting a systematic process, such as that facilitated by NVivo, is that it provides a map and audit trail of how the coding and analysis process was conducted. To some extent, this helped to structure the “messiness” (O’Dwyer , 2004 , p. 403) that is often attributed to qualitative data analysis.
Finally, reflecting on his overall experience, Researcher A found the NVivo data analysis software to be an excellent tool in terms of its ability to organise and manage qualitative data. In particular, the structured and systematic process of data analysis was very useful and effective. It is, however, important to note that while NVivo is a useful tool, it cannot replace the researcher’s own knowledge of the empirical data or the high level of research skills and judgement required to comprehend the data and elucidate themes, or the need for the researcher to be reflective in the data analysis process. In conclusion, Researcher A’s experience suggests that the benefits of using NVivo during the qualitative analysis phase outweigh the challenges it poses. Additionally, given the benefit of hindsight, Researcher A would use this technology in future qualitative research projects.
4.4 Researcher B: Factors Influencing Approach to Qualitative Data Analysis
A review of pertinent literature (Ashworth & Lucas , 2000; Larsson & Holmström , 2007; Svensson , 1997) highlights that there is no one ‘best’ method of phenomenographic data analysis. The overriding objective is to describe the data in the form of qualitative categories. This necessitates an approach for data analysis that enables resulting themes to be grounded in the data itself, rather than in prior literature or the researcher’s own experiences. However, Svensson (1997) cautions against replicating quantitative methodological traditions which view categories as “predefined assumptions” (p. 64). Mindful of this, and conscious that only a small number of phenomenographic studies had adopted a technological approach to data analysis at the time that Researcher B was making her decision on whether or not to adopt a technological approach (e.g. Ozkan , 2004) , Researcher B selected a non-technological manual approach. A further factor impacting on Researcher B’s decision to proceed with the manual approach was a perception that technological approaches, such as NVivo, were not used extensively by qualitative researchers within the Higher Education Institution in which she was enrolled as a PhD student. Whilst completing her doctorate studies at a UK University on a part-time basis, Researcher B attended a number of research methodology training sessions (funded by the researcher’s institution) and research seminars. Researchers who presented their work had adopted a manual approach to qualitative data analysis and were not very knowledgeable in relation to technological approaches. This highlighted an absence of an established community of practice in this regard and could mean that any adoption of a technological approach might not be appropriately aligned with the research community.
The experience of Researcher B’s supervisory team also influenced her decision to adopt the manual approach of qualitative data analysis. To that end, Researcher B’s supervisory team had no experience of using a qualitative technological approach for data analysis. This problem was compounded in that the supervisory team also had limited experience of qualitative research and was therefore reluctant to recommend any specific approach to data analysis. Taking on board the above factors, Researcher B believed there was no compelling reason to adopt a technological approach, thus she was not positively disposed towards NVivo or other such technological tool for qualitative data analysis. As a result, Researcher B selected a manual approach to qualitative data analysis.
4.5 Researcher B: A Manual Approach to Data Analysis
Researcher B was conscious of the “inevitable tension between being faithful to the data and at the same time creating, from the point of view of the researcher, a tidy construction useful for some further exploratory or educational purpose” (Bowden & Walsh , 2000 , p. 19) . Reflecting this, the analysis phase sought to gain insights into interview participants’ perceptions, meanings, understandings, experiences and interpretations. Consistent with the phenomenographic approach, Researcher B was mindful of the need for conscious bracketing with reference to the analysis of the interviews. [2] This comprised careful transcription of interviews, with emphasis on tone and emotions, and simultaneous continuous cycles of listening to interview recordings and reading of interview transcripts to highlight themes.
Researcher B found “the path from interviews through inference to categories…quite a challenge” (Entwistle , 1997 , p. 128) . The substantial volume of interview data required multiple and simultaneous continuous cycles of reading, note-making, interpretation, write-up and reflective review and the overall analysis of hard copy transcripts was quite a “messy” process (O’Dwyer , 2004 , p. 403) . It comprised substantial participant quotes highlighted in an array of colours on transcripts, a large amount of handwritten suggested thematic descriptions on both left and right transcript margins and large quantities of post-it notes of varying shades attached to the transcripts.
In undertaking the manual qualitative data analysis, Researcher B methodically worked through a series of steps, based on the work of Lucas (1998) and Ashworth & Lucas (2000) , as follows:
Familiarising self with the interviewee data and highlighting initial themes – Researcher B initially read each transcript a number of times and highlighted what she considered important elements of text with highlighter marker. She re-read each transcript a number of additional times and noted possible themes by writing on the right-hand margin of the hard copy transcript. She then highlighted more broad-based themes in the left-hand margin. Following this initial thematic identification, Researcher B re-read and listened to the interview recordings several more times, re-examining the analysis with a view to being methodical, yet open-minded about the content of the interviews.
Grounding themes in individual interviewee contexts – while many aspects of analysis focus on comparative experiences and mindful that these are of value, the phenomenographic approach positions individual experiences and lifeworlds as a backdrop to meanings. It was therefore important that individual experiences were not lost in an attempt to understand more generalising aspects. To this end, Researcher B also compiled individual interviewee profiles. The over-riding objective of this was to identify and examine particular points of emphasis that appeared to be central to the overall individual experiences with regard to development of professional competence. Such in-depth examination helped focus on the participants’ experiences and contributed to the empathetic understanding of participant perceptions, experiences, understandings and meanings (Lucas , 1998) . This also helped to counter tendencies to “attribute meaning out of context” (Lucas , 1998 , p. 138) and provided a means to understand participants’ experiences over a considerable period of time, from the point at which they made the conscious decision to gain admittance to the accounting profession up to the present day. This added considerable value to the analysis, not only helping to reveal what participants’ experiences and understandings of professional competence and professional development were, but also how participants shaped their ongoing actions and engagement with the development of professional competence. Predominant themes were then highlighted on the individual transcripts for each participant, in the participants’ own words. This served to maintain the bracketing process and ensured that themes were grounded in participants’ experiences.
Drafting initial thematic write-up – Researcher B drafted an initial descriptive thematic write-up, focussed around the research questions.
Reviewing interview data for supporting quotes – relevant interviewee quotes for each theme were subsequently included in the draft thematic write-up.
Reviewing thematic write-up – Researcher B re-read and listened back to the interviews several more times. She also searched individual interview transcript word documents for key words and phrases to highlight additional quotes to support thematic descriptions. She then spent some time editing the write-up with a view to generating a more “tidy construction” of descriptive overall categories (Bowden & Walsh , 2000 , p. 19) .
Generating categories of description – the final stage of analysis was the generation of overriding categories of description . The what aspect was used to characterise what professional competence means to participants (i.e. the meaning attribute) while the how aspect categorised how participant practitioners actually maintain and develop their professional competence (i.e. the structural attribute). Participants’ experiential stages were used to inform the hierarchy vis-a-vis these categories.
4.6 Researcher B: A Critical Reflection and Challenges with Manual Qualitative Data Analysis
Researcher B reflects on the challenges pertaining to data analysis during the course of her PhD study and highlights a number of issues. While the manual approach facilitated the generation and analysis of themes from the interview data, it was challenging to manage themes that were continuously being defined and redefined. Notwithstanding the iterative nature of the manual approach, Researcher B was confident that themes developed in an organic manner and were not finalised too early in the data analysis process. The ambiguity associated with the generation and analysis of themes also required Researcher B to bring high levels of research knowledge and skills to support this process and to be mindful of the need to embrace high levels of tolerance for uncertainty. Researcher B acknowledges that the iterative process of reading interviewee transcripts, listening to interview recordings (largely while in the car on the commute to and from work or while taking trips to see family at the other side of the country), generating themes, writing up themes, followed by re-reading messy transcripts and re-listening to the interview recordings while re-visiting themes, was both tedious and time consuming.
The initial excitement experienced when first listening to the interview recordings and reading the interview transcripts was somewhat depleted by the end of the process and work on the analyses increasingly developed into a test of endurance. Researcher B likened this to the declining enthusiasm often experienced by students from first reading a clean copy of a Shakespearian play in school, followed by subsequent grappling with syllabus requirements to dissect the play in multiple different ways in order to isolate significant events, explore characters, interpret language, examine subplots and understand larger themes. At the end of the school year, the once clean hard copy has become a heavily annotated and much more complex version of the original and the students’ enthusiasm considerably more subdued.
Researcher B also reflects that the manual approach required her to become very familiar with the interviewee transcripts and recordings, such that Researcher B could effectively match interview quotes to interviewees without having to check their provenance. Researcher B acknowledges that some participants provided more considered and more articulate responses to interview questions, and on review of the initial draft write-up, realised she had included excessive quotes centred around such participants. In subsequent iterations, Researcher B was careful to ensure the write-up was more representative of all of the interviewees and not dominated by a small number of interviewees.
As analysis progressed during the course of the doctorate, Researcher B presented draft write-ups of her findings to her PhD supervisors at various stages, largely to seek reassurance that data analysis was progressing appropriately. However, as indicated earlier, both supervisors had limited experience of qualitative data analysis and could provide little categorical reassurance regarding the manual approach to data analysis. As such, Researcher B had no systematic source of affirmation and was prompted to present at various doctoral colloquia to gain further insights and validation of the approach to analysis. This provided a useful, albeit more ad hoc , source of guidance and affirmation.
Finally, Researcher B reflects on the overall doctoral process and more particularly on the selection of a manual approach to data analysis. With hindsight, she recognises that while this approach enabled closeness to the interview data, data management involved a significant amount of time. For example, ‘cutting’ and ‘pasting’ within word documents which had to be done and re-done many times, reflecting the messiness of the data analysis. This was quite repetitive and was not an efficient means of organising data to support research findings. Researcher B believes that qualitative data analysis should enable both a closeness to the data and an efficient means of managing data. To that end, she would consider trialling measures to enhance the efficiency of data management in future research studies, including use of software tools such as NVivo.
5. Discussion and Conclusion
This paper addresses a gap in the literature by providing reflective and critical insights into the experiences of two PhD researchers undertaking qualitative studies which adopted different approaches to data analysis. The experiences and reflections of Researchers A and B highlight some similarities and differences worthy of note. In terms of background and motivations, while both researchers were investigating different research areas, qualitative data analysis was a central and shared aspect of both. To that end, both researchers were faced with the same decision regarding the choice of qualitative data analysis approach, Researcher A deciding on a technological approach (NVivo) and Researcher B opting for the manual approach.
Table 1 summarises the factors influencing the choice of data analysis approach adopted by Researchers A and B, together with the challenges and benefits of each. Interestingly, while the similarities in background and motivations detailed in the paper had little impact on both researchers’ decision regarding the qualitative data analysis approach, the factors influencing the choice were markedly different. To that end, Researcher B’s engagement with a more extensive literature exploring phenomenographic data analysis indicated that few prior studies had adopted a technological approach. Coupled with the lack of a community of practice with experience of using the technological approach, these factors were primary influences on Researcher B’s decision to adopt a manual approach. This decision has some parallels with O’Dwyer’s (2004) experience of discounting the technological approach at an early stage of his research based on his lack of understanding of what it could offer. In contrast, Researcher A’s decision-making process was largely influenced by the multi-dimensional nature of the interview data collected and exposure to an NVivo training course where the potential of the software’s ‘slice and dice’ and query capabilities were demonstrated. The possibility of accessing on-going NVivo one-to-one support for the duration of the research project was a further factor in Researcher A’s decision to use the technological approach. While different factors clearly influenced Researchers A and B’s decision regarding their qualitative data analysis approach, the experiences of their supervisory teams were common to both. Researcher A was influenced to ado,pt the technological approach as a result of his supervisor’s positive experience, while Researcher B was influenced to adopt the manual approach due to her supervisors’ limited knowledge or experience of the technological approach. This finding points to the importance of supervisors’ experience in informing the decision regarding the qualitative data analysis approach and highlights a potential danger of narrowing the data analysis choices available to the doctoral researcher.
Interpretivist | Interpretivist (Phenomenographic) | |
Interviews (n=27) | Interviews (n=23) | |
Technological (NVivo) | Manual | |
The critical reflections of both researchers also elucidate some key challenges and benefits that qualitative researchers should be mindful of. Despite adopting different approaches, both researchers highlighted challenges in terms of the time consuming and labour intensive nature of their respective data analysis approaches, largely consistent with earlier findings (Bédard & Gendron , 2004) . While Researcher A had to invest considerable time and commitment in developing the skills required to use NVivo, this reaped significant benefits towards the latter end of his research project in terms of the efficient retrieval of information, confirming previous literature (Basit , 2003; García-Horta & Guerra-Ramos , 2009; Zamawe , 2015) . Researcher B also noted a challenge around the time-consuming nature of the data analysis process using the manual approach and the significant investment in time for activities such as listening to recordings, reading and re-reading of transcripts, and ‘cutting’ and ‘pasting’ which had to be done and re-done, again consistent with earlier research findings (Basit , 2003; Bogdan & Bilken , 1982; Lofland , 1971; Maher et al. , 2018; L. Richards & Richards , 1994) . Researcher A’s experience, however, highlights a further challenge not identified in the prior literature with respect to investment in time, namely the resulting time lag that can occur between the timing of initial NVivo training and the actual use of the technology, with the result that important knowledge and skills relevant to analysis have been ‘forgotten’.
Both researchers also highlighted an element of drudgery and repetitiveness in coding their data and developing themes, and the need for resilience (Researcher A) and endurance (Researcher B) in this regard. Drawing on their experiences, both researchers were mindful of “being tolerant of uncertainty [which] is part of the fundamental skills of the qualitative researcher” (Bédard & Gendron , 2004 , p. 199) . Irrespective of the approach to qualitative data analysis, both Researchers A and B were also cognisant of the importance of retaining a level of ‘closeness’ to their data and an awareness that the approach to analysis cannot substitute for the researcher’s own knowledge of the empirical data (O’Dwyer , 2004) . Furthermore, Researchers A and B’s experiences provide new insights to the literature. Researcher A recognised the potential danger of NVivo over-focusing the researcher’s attention on counting and quantifying and how this might negatively impact in terms of maintaining a level of closeness with the data. In addition, Researcher B cautioned against the possibility of being ‘too close’ to some interviewee data when using a manual approach, and the need to continually and consciously ensure that the qualitative data analysis was representative of all interviewees. Reflecting further on the tedious nature of the manual process, Researcher B reported an additional challenge in that a significant amount of time had to be devoted to data management activities (i.e. cutting and pasting into word documents) given the ‘messiness’ of her data analysis.
Both researchers identified some benefits of their respective data analysis approaches. Researcher A recognised that the technological approach, NVivo, provides a systematic coding process with a clear audit trail which helps to structure the ‘messiness’ attributed to qualitative data analysis (O’Dwyer , 2004 , p. 403) . In addition, Researcher A highlighted that NVivo is an excellent tool in terms of its ability to organise and manage qualitative data. The skills developed as a result yield significant benefits in terms of facilitating multi-layered analyses that can be used in future research projects. In contrast, Researcher B reflected on how the manual approach facilitated a closeness to the qualitative data (notwithstanding the challenge highlighted earlier in this regard) and that this approach facilitated the identification of themes in an organic manner.
The preceding discussion lends support to the conclusion that the choice of a manual or technological approach to qualitative data analysis is influenced by multiple factors. In making a decision regarding the approach to data analysis, researchers need to be cognisant of the potential challenges and benefits of their choices. Ultimately, however, the final decision regarding the approach to adopt is a personal choice. Irrespective of the choices available to the researcher, it is important to acknowledge that qualitative data analysis is “the most intellectually challenging phase” of qualitative research (Marshall & Rossman , 1995 , p. 114) . Described as ‘messy’ by O’Dwyer (2004) , qualitative data analysis is also labour intensive, requiring high levels of research knowledge and skills, and associated with the need to be tolerant of uncertainty (Bédard & Gendron , 2004) . The experiences and reflections of both researchers in this paper provide evidence of these challenges. While this paper provides insights into the choice of qualitative data analysis approach, a limitation is that it does not address how manual or technological approaches to qualitative data analysis consider issues related to the quality of data analysis undertaken. For example, Pratt et al. (2019) highlight the need to identify solutions for enhanced trustworthiness (an aspect of quality) in qualitative research. Further research might consider how the manual and technological approaches address such issues. Another limitation of the paper is that the experiences outlined reflect those of two individual researchers. These experiences may not be reflective of the experiences of others who engage in the manual or technological approaches to qualitative data analysis. Further research which more broadly compares the experiences of other qualitative researchers would add greater insights in this under-researched area.
The paper contributes to the limited literature on the comparative experiences of those undertaking qualitative data analysis using the manual and technological approaches. In so doing, we identify the factors influencing the choice of approach, confirming in some respects prior findings in the literature, but also adding to the small body of prior literature. We further contribute to the limited literature by adding insights into the challenges and benefits of the manual and technological approaches to qualitative data analysis. “Given the popularity of interviews as a method of qualitative data collection in accounting” (Lee & Humphrey , 2006 , p. 188) , the paper adds insights into how researchers address one of the key problems they face, namely how to analyse interview transcripts using the manual and technological approach. We thereby respond to calls from Edwards & Skinner (2009) and Paulus et al. (2017) for future studies to provide insights into qualitative researchers’ experiences of using the manual and technological approaches to data analysis. We hope that the experiences and reflections articulated in this paper, including the factors impacting on and the challenges and benefits of using the manual and technological approaches, will guide qualitative researchers in making important decisions regarding their approach to data analysis. The issue of how to analyse qualitative data, and whether to use manual or technological approaches is often a source of difficulty for researchers, we hope that this paper will initiate further debate around this important decision.
The manual approach involves analysing qualitative data without the use of computerised data analysis software.
The issue of bracketing is a core element of the phenomenographic research approach, irrespective of the selection of a manual or a technological approach to data analysis.
- Survey Paper
- Open access
- Published: 18 December 2021
A new theoretical understanding of big data analytics capabilities in organizations: a thematic analysis
- Renu Sabharwal 1 &
- Shah Jahan Miah ORCID: orcid.org/0000-0002-3783-8769 1
Journal of Big Data volume 8 , Article number: 159 ( 2021 ) Cite this article
21k Accesses
18 Citations
Metrics details
Big Data Analytics (BDA) usage in the industry has been increased markedly in recent years. As a data-driven tool to facilitate informed decision-making, the need for BDA capability in organizations is recognized, but few studies have communicated an understanding of BDA capabilities in a way that can enhance our theoretical knowledge of using BDA in the organizational domain. Big Data has been defined in various ways and, the past literature about the classification of BDA and its capabilities is explored in this research. We conducted a literature review using PRISMA methodology and integrated a thematic analysis using NVIVO12. By adopting five steps of the PRISMA framework—70 sample articles, we generate five themes, which are informed through organization development theory, and develop a novel empirical research model, which we submit for validity assessment. Our findings improve effectiveness and enhance the usage of BDA applications in various Organizations.
Introduction
Organizations today continuously harvest user data [e.g., data collections] to improve their business efficiencies and practices. Significant volumes of stored data or data regarding electronic transactions are used in support of decision making, with managers, policymakers, and executive officers now routinely embracing technology to transform these abundant raw data into useful, informative information. Data analysis is complex, but one data-handling method, “Big Data Analytics” (BDA)—the application of advanced analytic techniques, including data mining, statistical analysis, and predictive modeling on big datasets as new business intelligence practice [ 1 ]—is widely applied. BDA uses computational intelligence techniques to transform raw data into information that can be used to support decision-making.
Because decision-making in organizations has become increasingly reliant on Big Data, analytical applications have increased in importance for evidence-based decision making [ 2 ]. The need for a systematic review of Big Data stream analysis using rigorous and methodical approaches to identify trends in Big Data stream tools, analyze techniques, technologies, and methods is becoming increasingly important [ 3 ]. Organizational factors such as organizational resources adjustment, environmental acceptance, and organizational management relate to implement its BDA capability and enhancing its benefits through BDA technologies [ 4 ]. It is evident from past literature that BDA supports the organizational decision-making process by developing suitable theoretical understanding, but extending existing theories remains a significant challenge. The improved capability of BDA will ensure that the organizational products and services are continuously optimized to meet the evolving needs of consumers.
Previous systematic reviews have focused on future BDA adoption challenges [ 5 , 6 , 7 ] or technical innovation aspects of Big Data analytics [ 8 , 9 ]. This signifies those numerous studies have examined Big Data issues in different domains. These different domains are included: quality of Big Data in financial service organization [ 10 ]; organizational value creation because of BDA usage [ 11 ]; application of Big Data in health organizations [ 9 ]; decision improvement using Big Data in health [ 12 ]; application of Big Data in transport organizations [ 13 ]; relationships between Big Data in financial domains [ 14 ]; and quality of Big Data and its impact on government organizations [ 15 ].
While there has been a progressive increase in research on BDA, its capabilities and how organizations may exploit them are less well studied [ 16 ]. We apply a PRISMA framework [ 17 ]) and qualitative thematic analysis to create the model to define the relationship between BDAC and OD. The proposed research presents an overview of BDA capabilities and how they can be utilized by organizations. The implications of this research for future research development. Specifically, we (1) provide an observation into key themes regarding BDAC concerning state-of-the-art research in BDA, and (2) show an alignment to organizational development theory in terms of a new empirical research model which will be submitted for validity assessment for future research of BDAC in organizations.
According to [ 20 ], a systematic literature review first involves describing the key approach and establishing definitions for key concepts. We use a six-phase process to identify, analyze, and sequentially report themes using NVIVO 12.
Study background
Many forms of BDA exist to meet specific decision-support demands of different organizations. Three BDA analytical classes exist: (1) descriptive , dealing with straightforward questions regarding what is or has happened and why—with ‘opportunities and problems’ using descriptive statistics such as historical insights; (2) predictive , dealing with questions such as what will or is likely to happen, by exploring data patterns with relatively complex statistics, simulation, and machine-learning algorithms (e.g., to identify trends in sales activities, or forecast customer behavior and purchasing patterns); and (3) prescriptive , dealing with questions regarding what should be happening and how to influence it, using complex descriptive and predictive analytics with mathematical optimization, simulation, and machine-learning algorithms (e.g., many large-scale companies have adopted prescriptive analytics to optimize production or solve schedule and inventory management issues) [ 18 ]. Regardless of the type of BDA analysis performed, its application significantly impacts tangible and intangible resources within an organization.
Previous studies on BDA
BDA tools or techniques are used to analyze Big Data (such as social media or substantial transactional data) to support strategic decision-making [ 19 ] in different domains (e.g., tourism, supply chain, healthcare), and numerous studies have developed and evaluated BDA solutions to improve organizational decision support. We categorize previous studies into two main groups based on non-technical aspects: those which relate to the development of new BDA requirements and functionalities in a specific problem domain and those which focus on more intrinsic aspects such as BDAC development or value-adding because of their impact on particular aspects of the business. Examples of reviews focusing on technical or problem-solving aspects are detailed in Table 1 .
The second literature group examines BDA in an organizational context, such as improving firm performance using Big Data analytics in specific business domains [ 26 ]. Studies that support BDA lead to different aspects of organizational performance [ 20 , 24 , 25 , 27 , 28 , 29 ] (Table 2 ). Another research on BDA to improve data utilization and decision-support qualities. For example, [ 30 ] explained how BDAC might be developed to improve managerial decision-making processes, and [ 4 ] conducted a thematic analysis of 15 firms to identify the factors related to the success of BDA capability development in SCM.
Potential applications of BDA
Many retail organizations use analytical approaches to gain commercial advantage and organizational success [ 31 ]. Modern organizations increasingly invest in BDA projects to reduce costs, make accurate decision making, and future business planning. For example, Amazon was the first online retailer and maintained its innovative BDA improvement and use [ 31 ]. Examples of successful stories of BDA use in business sectors include.
Retail: business organizations using BDA for dynamic (surge) pricing [ 32 ] to adjust product or service prices based on demand and supply. For instance, Amazon uses dynamic pricing to surge prices by product demand.
Hospitality: Marriott hotels—the largest hospitality agent with a rapidly increasing number of hotels and serviced customers—uses BDA to improve sales [ 33 ].
Entertainment: Netflix uses BDA to retain clientele and increase sales and profits [ 34 , 35 ].
Transportation : Uber uses BDA [ 36 ] to capture Big Data from various consumers and identify the best routes to locations. ‘Uber eats,’ despite competing with other delivery companies, delivers foods in the shortest possible time.
Foodservice: McDonald's continuously updates information with BDA, following a recent shift in food quality, now sells healthy food to consumers [ 37 ], and has adopted a dynamic menu [ 38 ].
Finance: American Express has used BDA for a long time and was one of the first companies to understand the benefits of using BDA to improve business performance [ 39 ]. Big Data is collected on the ways consumers make on- and offline purchases, and predictions are made as to how they will shop in the future.
Manufacturing: General Electric manufactures and distributes products such as wind turbines, locomotives, airplane engines, and ship engines [ 40 ]. By dealing with a huge amount of data from electricity networks, meteorological information systems, geographical information systems, benefits can be brought to the existing power system, including improving customer service and social welfare in the era of big data.
Online business: music streaming websites are increasingly popular and continue to grow in size and scope because consumers want a customized streaming service [ 41 ]. Many streaming services (e.g., Apple Music, Spotify, Google Music) use various BDA applications to suggest new songs to consumers.
Organization value assessment with BDA
Specific performance measures must be established that rely on the number of organizational contextual factors such as the organization's goal, the external environment of the organization, and the organization itself. When looking at the above contexts regarding the use of BDA to strengthen process innovation skills, it is important to note that the approach required to achieve positive results depends on the different combinations along with the area in which BDA deployed [ 42 ].
Organizational development and BDA
To assist organization decision-making for growth, effective processes are required to perform operations such as continuous diagnosis, action planning, and the implementation and evaluation of BDA. Lewin’s Organizational Development (OD) theory regards processes as having a goal to transfer knowledge and skills to an organization, with the process being mainly to improve problem-solving capacity and to manage future change. Beckhard [ 43 ] defined OD as the internal dynamics of an organization, which involve a collection of individuals working as a group to improve organizational effectiveness, capability, work performance, and the ability to adjust culture, policies, practices, and procedure requirements.
OD is ‘a system-wide application and transfer of behavioral science knowledge to the planned development, improvement, and reinforcement of the strategies, structures, and processes that lead to organization effectiveness’ [ 44 ], and has three concepts: organizational climate, culture, and capability [ 45 ]. Organizational climate is ‘the mood or unique personality of an organization’ [ 45 ] which includes shared perceptions of policies, practices, and procedures; climate features also consist of leadership, communication, participative management, and role clarity. Organizational culture involves shared basic assumptions, values, norms, behavioral patterns, and artifacts, defined by [ 46 ] as a pattern of shared basic assumptions that a group learned by solving problems of external adaptation and internal integration (p. 38). Organizational capacity (OC) implies the organization's function, such as the production of services or products or maintenance of organizational operations, and has four components: resource acquisition, organization structure, production subsystem, and accomplishment [ 47 ]. Organizational culture and climate affect an organization’s capacity to operate adequately (Fig. 1 ).
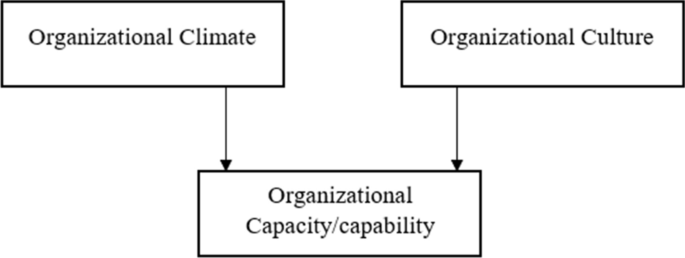
Framework of modified organizational development theory [ 45 ]
Research methodology
Our systematic literature review presents a research process for analyzing and examining research and gathering and evaluating it [ 48 ] In accordance with a PRISMA framework [ 49 ]. We use keywords to search for articles related to the BDA application, following a five-stage process.
Stage1: design development
We establish a research question to instruct the selection and search strategy and analysis and synthesis process, defining the aim, scope, and specific research goals following guidelines, procedures, and policies of the Cochrane Handbook for Systematic Reviews of Intervention [ 50 ]. The design review process is directed by the research question: what are the consistent definitions of BDA, unique attributes, objections, and business revolution, including improving the decision-making process and organization performance with BDA? The below table is created using the outcome of the search performed using Keywords- Organizational BDAC, Big Data, BDA (Table 3 ).
Stage 2: inclusion and elimination criteria
To maintain the nuances of a systematic review, we apply various inclusion and exclusion criteria to our search for research articles in four databases: Science Direct, Web of Science, IEEE (Institute of Electrical and Electronics Engineers), and Springer Link. Inclusion criteria include topics on ‘Big Data in Organization’ published between 2015 to 2021, in English. We use essential keywords to identify the most relevant articles, using truncation, wildcarding, and appropriate Boolean operators (Table 4 ).
Stage 3: literature sources and search approach
Research articles are excluded based on keywords and abstracts, after which 8062 are retained (Table 5 ). The articles only selected keywords such as Big Data, BDA, BDAC, and the Abstract only focused on the Organizational domain.
Stage 4: assess the quality of full papers
At this stage, for each of the 161 research articles that remained after stage 3 presented in Table 6 , which was assessed independently by authors in terms of several quality criteria such as credibility, to assess whether the articles were well presented, relevance which was assessed based on whether the articles were used in the organizational domain.
Stage 5: literature extraction and synthesis process
At this stage, only journal articles and conference papers are selected. Articles for which full texts were not open access were excluded, reducing our references to 70 papers Footnote 1 (Table 7 ).
Meta-analysis of selected papers
Of the 70 papers satisfying our selection criteria, publication year and type (journal or conference paper) reveal an increasing trend in big data analytics over the last 6 years (Table 6 ). Additionally, journals produced more BDA papers than Conference proceedings (Fig. 2 ), which may be affected during 2020–2021 because of COVID, and fewer conference proceedings or publications were canceled.
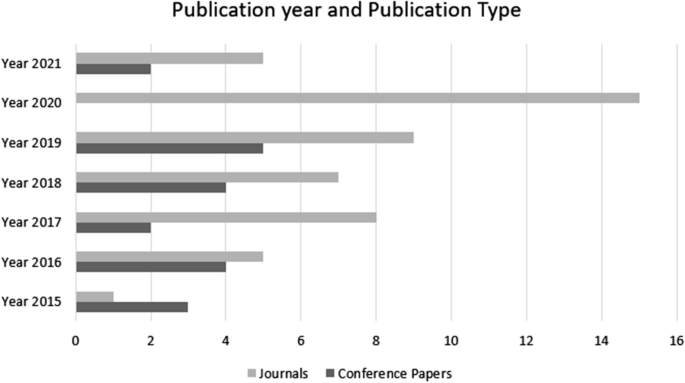
Distribution of publications by year and publication type
Of the 70 research articles, 6% were published in 2015, 13% (2016), 14% (2017), 16% (2018), 20% (2019), 21% (2020), and 10% (untill May 2021).
Thematic analysis is used to find the results which can identify, analyze and report patterns (themes) within data, and produce an insightful analysis to answer particular research questions [ 51 ].
The combination of NVIVO and Thematic analysis improves results. Judger [ 52 ] maintained that using computer-assisted data analysis coupled with manual checks improves findings' trustworthiness, credibility, and validity (p. 6).
Defining big data
Of 70 articles, 33 provide a clear replicable definition of Big Data, from which the five representative definitions are presented in Table 8 .
Defining BDA
Of 70 sample articles, 21 clearly define BDA. The four representative definitions are presented in Table 9 . Some definitions accentuate the tools and processes used to derive new insights from big data.
Defining Big Data analytics capability
Only 16% of articles focus on Big Data characteristics; one identifies challenges and issues with adopting and implementing the acquisition of Big Data in organizations [ 42 ]. The above study resulted that BDAC using the large volumes of data generated through different devices and people to increase efficiency and generate more profits. BDA capability and its potential value could be more than a business expects, which has been presented that the professional services, manufacturing, and retail have structural barriers and overcome these barriers with the use of Big Data [ 60 ]. We define BDAC as the combined ability to store, process, and analyze large amounts of data to provide meaningful information to users. Four dimensions of BDAC exist data integration, analytical, predictive, and data interpretation (Table 10 ).
It is feasible to identify outstanding issues of research that are of excessive relevance, which has termed in five themes using NVIVO12 (Fig. 3 ). Table 11 illustrates four units that combine NVIVO with thematic analysis for analysis: Big data, BDA, BDAC, and BDA themes. We manually classify five BDA themes to ensure accuracy with appropriate perception in detail and provide suggestions on how future researchers might approach these problems using a research model.
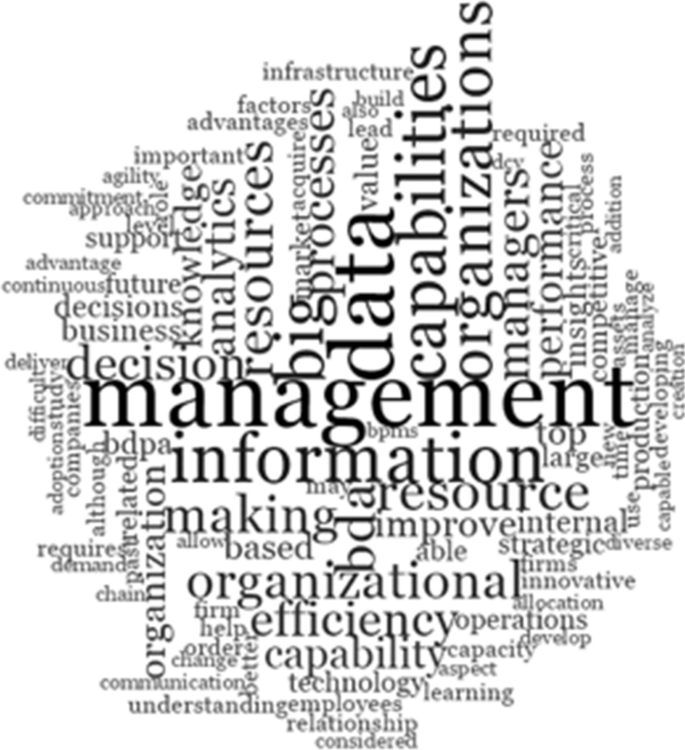
Thematic analysis using NVIVO 12
Manyika et al . [ 63 ] considered that BDA could assist an organization to improve its decision making, minimize risks, provide other valuable insights that would otherwise remain hidden, aid the creation of innovative business models, and improve performance.
The five themes presented in Table 11 identify limitations of existing literature, which are examined in our research model (Fig. 4 ) using four hypotheses. This theoretical model identifies organizational and individual levels as being influenced by organization climate, culture, and capacity. This model can assist in understanding how BDA can be used to improve organizational and individual performance.
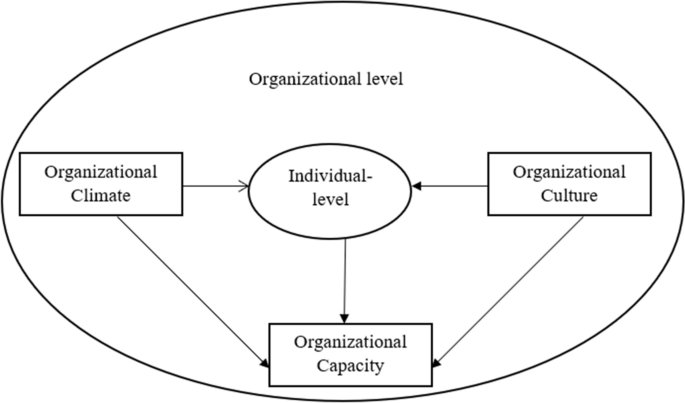
The framework of organizational development theory [ 64 ]
The Research model development process
We analyze literature using a new research method, driven by the connection between BDAC and resource-based views, which included three resources: tangible (financial and physical), human skills (employees’ knowledge and skills), and intangible (organizational culture and organizational learning) used in IS capacity literature [ 65 , 66 , 67 , 68 ]. Seven factors enable firms to create BDAC [ 16 ] (Fig. 5 ).
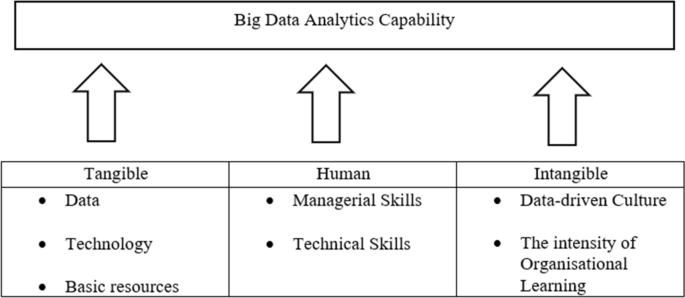
Classification of Big Data resources (adapted from [ 16 ])
To develop a robust model, tangible, intangible, and human resource types should be implemented in an organization and contribute to the emergence of the decision-making process. This research model recognizes BDAC to enhance OD, strengthening organizational strategies and the relationship between BD resources and OD. Figure 6 depicts a theoretical framework illustrating how BDA resources influence innovation sustainability and OD, where Innovation sustainability helps identify market opportunities, predict customer needs, and analyze customer purchase decisions [ 69 ].
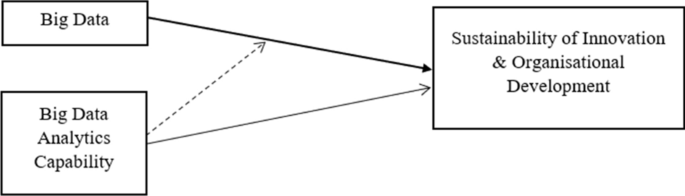
Theroretical framework illustrating how BDA resources influence innovation sustainability and organizational development (adapted from [ 68 ])
Miller [ 70 ] considered data a strategic business asset and recommended that businesses and academics collaborate to improve knowledge regarding BD skills and capability across an organization; [ 70 ] concluded that every profession, whether business or technology, will be impacted by big data and analytics. Gobble [ 71 ] proposed that an organization should develop new technologies to provide necessary supplements to enhance growth. Big Data represents a revolution in science and technology, and a data-rich smart city is the expected future that can be developed using Big Data [ 72 ]. Galbraith [ 73 ] reported how an organization attempting to develop BDAC might experience obstacles and opportunities. We found no literature that combined Big Data analytics capability and Organizational Development or discussed interaction between them.
Because little empirical evidence exists regarding the connection between OD and BDA or their characteristics and features, our model (Fig. 7 ) fills an important void, directly connecting BDAC and OD, and illustrates how it affects OD in the organizational concepts of capacity, culture, and climate, and their future resources. Because BDAC can assist OD through the implementation of new technologies [ 15 , 26 , 57 ], we hypothesize:
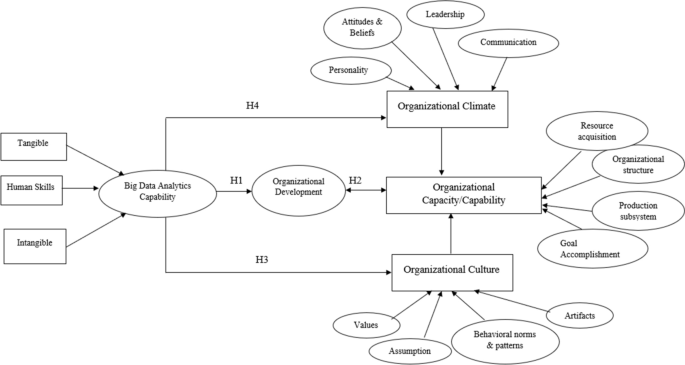
Proposed interpretation in the research model
H1: A positive relationship exists between Organizational Development and BDAC.
OC relies heavily on OD, with OC representing a resource requiring development in an organization. Because OD can improve OC [ 44 , 45 ], we hypothesize that:
H2: A positive relationship exists between Organizational Development and Organizational Capability.
With the implementation or adoption of BDAC, OC is impacted [ 46 ]. Big data enables an organization to improve inefficient practices, whether in marketing, retail, or media. We hypothesize that:
H3: A positive relationship exists between BDAC and Organizational Culture.
Because BDAC adoption can affect OC, the policies, practices, and measures associated with an organization's employee experience [ 74 ], and improve both the business climate and an individual’s performance, we hypothesize that:
H4: A positive relationship exists between BDAC and Organizational Climate.
Our research is based on a need to develop a framework model in relation to OD theory because modern organizations cannot ignore BDA or its future learning and association with theoretical understanding. Therefore, we aim to demonstrate current trends in capabilities and a framework to improve understanding of BDAC for future research.
Despite the hype that encompasses Big Data, the organizational development and structure through which it results in competitive gains have remained generally underexplored in empirical studies. It is feasible to distinguish the five prominent, highly relevant themes discussed in an earlier section by orchestrating a systematic literature review and recording what is known to date. By conducting those five thematic areas of the research, as depicted in the research model in Fig. 7 , provide relation how they are impacting each other’s performance and give some ideas on how researchers could approach these problems.
The number of published papers on Big Data is increasing. Between 2015 and May 2021, the highest proportion of journal articles for any given year (21%) occurred until May 2021 with the inclusion or exclusion criteria such as the article selection only opted using four databases: Science Direct, Web of Science, IEEE (Institute of Electrical and Electronics Engineers), and Springer Link and included only those articles which titled as 'Big Data in Organization' published, in the English language. We use essential keywords to identify the most relevant articles, using truncation, wildcarding, and appropriate Boolean operators. While BDAC can improve business-related outcomes, including more effective marketing, new revenue opportunities, customer personalization, and improved operational efficiency, existing literature has focused on only one or two aspects of BDAC. Our research model (Fig. 7 ) represents the relationship between BDAC and OD to better understand their impacts on OC. We explain that the proposed model education will enhance knowledge of BDAC and that it may better meet organizational requirements, ensuring improved products and services to optimize consumer outcomes.
Considerable research has been conducted in many different contexts such as the health sector, education about Big Data, but according to past literature, BDAC in an organization is still an open issue, how to utilize BDAC within the organization for development purposes. The full potential of BDA and what it can offer must be leveraged to gain a commercial advantage. Therefore, we focus on summarizing by creating the themes using past relevant literature and propose a research model based on literature [ 61 ] for business.
While we explored Springer Link, IEEE, Science Direct, and Web of Science (which index high-impact journal and conference papers), the possibility exists that some relevant journals were missed. Our research is constrained by our selection criteria, including year, language (English), and peer-reviewed journal articles (we omitted reports, grey journals, and web articles).
A steadily expanding number of organizations has been endeavored to utilize Big Data and organizational analytics to analyze available data and assist with decision-making. For these organizations, influence the full potential that Big Data and organizational analytics can present to acquire competitive advantage. In any case, since Big Data and organizational analytics are generally considered as new innovative in business worldview, there is a little exploration on how to handle them and leverage them adequately. While past literature has shown the advantages of utilizing Big Data in various settings, there is an absence of theoretically determined research on the most proficient method to use these solutions to acquire competitive advantage. This research recognizes the need to explore BDA through a comprehensive approach. Therefore, we focus on summarizing with the proposed development related to BDA themes on which we still have a restricted observational arrangement.
To this end, this research proposes a new research model that relates earlier studies regarding BDAC in organizational culture. The research model provides a reference to the more extensive implementation of Big Data technologies in an organizational context. While the hypothesis present in the research model is on a significant level and can be deciphered as addition to theoretical lens, they are depicted in such a way that they can be adapted for organizational development. This research poses an original point of view on Big Data literature since, by far majority focuses on tools, infrastructure, technical aspects, and network analytics. The proposed framework contributes to Big Data and its capability in organizational development by covering the gap which has not addressed in past literature. This research model also can be viewed as a value-adding knowledge for managers and executives to learn how to drive channels of creating benefit in their organization through the use of Big Data, BDA, and BDAC.
We identify five themes to leverage BDA in an organization and gain a competitive advantage. We present a research model and four hypotheses to bridge gaps in research between BDA and OD. The purpose of this model and these hypotheses is to guide research to improve our understanding of how BDA implementation can affect an organization. The model goes for the next phase of our study, in which we will test the model for its validity.
Availability of data and materials
Data will be supplied upon request.
Appendix A is submitted as a supplementary file for review.
Abbreviations
The Institute of Electrical and Electronics Engineers
- Big Data Analytics
Big Data Analytics Capabilities
Organizational Development
- Organizational Capacity
Russom P. Big data analytics. TDWI Best Practices Report, Fourth Quarter. 2011;19(4):1–34.
Google Scholar
Mikalef P, Boura M, Lekakos G, Krogstie J. Big data analytics and firm performance: findings from a mixed-method approach. J Bus Res. 2019;98:261–76.
Kojo T, Daramola O, Adebiyi A. Big data stream analysis: a systematic literature review. J Big Data. 2019;6(1):1–30.
Jha AK, Agi MA, Ngai EW. A note on big data analytics capability development in supply chain. Decis Support Syst. 2020;138:113382.
Posavec AB, Krajnović S. Challenges in adopting big data strategies and plans in organizations. In: 2016 39th international convention on information and communication technology, electronics and microelectronics (MIPRO). IEEE. 2016. p. 1229–34.
Madhlangobe W, Wang L. Assessment of factors influencing intent-to-use Big Data Analytics in an organization: pilot study. In: 2018 IEEE 20th International Conference on High-Performance Computing and Communications; IEEE 16th International Conference on Smart City; IEEE 4th International Conference on Data Science and Systems (HPCC/SmartCity/DSS). IEEE. 2018. p. 1710–1715.
Saetang W, Tangwannawit S, Jensuttiwetchakul T. The effect of technology-organization-environment on adoption decision of big data technology in Thailand. Int J Electr Comput. 2020;10(6):6412. https://doi.org/10.11591/ijece.v10i6.pp6412-6422 .
Article Google Scholar
Pei L. Application of Big Data technology in construction organization and management of engineering projects. J Phys Conf Ser. 2020. https://doi.org/10.1088/1742-6596/1616/1/012002 .
Marashi PS, Hamidi H. Business challenges of Big Data application in health organization. In: Khajeheian D, Friedrichsen M, Mödinger W, editors. Competitiveness in Emerging Markets. Springer, Cham; 2018. p. 569–584. doi: https://doi.org/10.1007/978-3-319-71722-7_28 .
Haryadi AF, Hulstijn J, Wahyudi A, Van Der Voort H, Janssen M. Antecedents of big data quality: an empirical examination in financial service organizations. In 2016 IEEE International Conference on Big Data (Big Data). IEEE. 2016. p. 116–121.
George JP, Chandra KS. Asset productivity in organisations at the intersection of Big Data Analytics and supply chain management. In: Chen JZ, Tavares J, Shakya S, Iliyasu A, editors. Image Processing and Capsule Networks. ICIPCN 2020. Advances in Intelligent Systems and Computing, vol 1200. Springer, Cham; 2020. p. 319–330.
Sousa MJ, Pesqueira AM, Lemos C, Sousa M, Rocha Á. Decision-making based on big data analytics for people management in healthcare organizations. J Med Syst. 2019;43(9):1–10.
Du G, Zhang X, Ni S. Discussion on the application of big data in rail transit organization. In: Wu TY, Ni S, Chu SC, Chen CH, Favorskaya M, editors. International conference on smart vehicular technology, transportation, communication and applications. Springer: Cham; 2018. p. 312–8.
Wahyudi A, Farhani A, Janssen M. Relating big data and data quality in financial service organizations. In: Al-Sharhan SA, Simintiras AC, Dwivedi YK, Janssen M, Mäntymäki M, Tahat L, Moughrabi I, Ali TM, Rana NP, editors. Conference on e-Business, e-Services and e-Society. Springer: Cham; 2018. p. 504–19.
Alkatheeri Y, Ameen A, Isaac O, Nusari M, Duraisamy B, Khalifa GS. The effect of big data on the quality of decision-making in Abu Dhabi Government organisations. In: Sharma N, Chakrabati A, Balas VE, editors. Data management, analytics and innovation. Springer: Singapore; 2020. p. 231–48.
Gupta M, George JF. Toward the development of a big data analytics capability. Inf Manag. 2016;53(8):1049–64.
Selçuk AA. A guide for systematic reviews: PRISMA. Turk Arch Otorhinolaryngol. 2019;57(1):57.
Tiwari S, Wee HM, Daryanto Y. Big data analytics in supply chain management between 2010 and 2016: insights to industries. Comput Ind Eng. 2018;115:319–30.
Miah SJ, Camilleri E, Vu HQ. Big Data in healthcare research: a survey study. J Comput Inform Syst. 2021;7:1–3.
Mikalef P, Pappas IO, Krogstie J, Giannakos M. Big data analytics capabilities: a systematic literature review and research agenda. Inf Syst e-Business Manage. 2018;16(3):547–78.
Nguyen T, Li ZHOU, Spiegler V, Ieromonachou P, Lin Y. Big data analytics in supply chain management: a state-of-the-art literature review. Comput Oper Res. 2018;98:254–64.
MathSciNet MATH Google Scholar
Günther WA, Mehrizi MHR, Huysman M, Feldberg F. Debating big data: a literature review on realizing value from big data. J Strateg Inf. 2017;26(3):191–209.
Rialti R, Marzi G, Ciappei C, Busso D. Big data and dynamic capabilities: a bibliometric analysis and systematic literature review. Manag Decis. 2019;57(8):2052–68.
Wamba SF, Gunasekaran A, Akter S, Ren SJ, Dubey R, Childe SJ. Big data analytics and firm performance: effects of dynamic capabilities. J Bus Res. 2017;70:356–65.
Wang Y, Hajli N. Exploring the path to big data analytics success in healthcare. J Bus Res. 2017;70:287–99.
Akter S, Wamba SF, Gunasekaran A, Dubey R, Childe SJ. How to improve firm performance using big data analytics capability and business strategy alignment? Int J Prod Econ. 2016;182:113–31.
Kwon O, Lee N, Shin B. Data quality management, data usage experience and acquisition intention of big data analytics. Int J Inf Manage. 2014;34(3):387–94.
Chen DQ, Preston DS, Swink M. How the use of big data analytics affects value creation in supply chain management. J Manag Info Syst. 2015;32(4):4–39.
Kim MK, Park JH. Identifying and prioritizing critical factors for promoting the implementation and usage of big data in healthcare. Inf Dev. 2017;33(3):257–69.
Popovič A, Hackney R, Tassabehji R, Castelli M. The impact of big data analytics on firms’ high value business performance. Inf Syst Front. 2018;20:209–22.
Hewage TN, Halgamuge MN, Syed A, Ekici G. Big data techniques of Google, Amazon, Facebook and Twitter. J Commun. 2018;13(2):94–100.
BenMark G, Klapdor S, Kullmann M, Sundararajan R. How retailers can drive profitable growth through dynamic pricing. McKinsey & Company. 2017. https://www.mckinsey.com/industries/retail/our-insights/howretailers-can-drive-profitable-growth-throughdynamic-pricing . Accessed 13 Mar 2021.
Richard B. Hotel chains: survival strategies for a dynamic future. J Tour Futures. 2017;3(1):56–65.
Fouladirad M, Neal J, Ituarte JV, Alexander J, Ghareeb A. Entertaining data: business analytics and Netflix. Int J Data Anal Inf Syst. 2018;10(1):13–22.
Hadida AL, Lampel J, Walls WD, Joshi A. Hollywood studio filmmaking in the age of Netflix: a tale of two institutional logics. J Cult Econ. 2020;45:1–26.
Harinen T, Li B. Using causal inference to improve the Uber user experience. Uber Engineering. 2019. https://eng.uber.com/causal-inference-at-uber/ . Accessed 10 Mar 2021.
Anaf J, Baum FE, Fisher M, Harris E, Friel S. Assessing the health impact of transnational corporations: a case study on McDonald’s Australia. Glob Health. 2017;13(1):7.
Wired. McDonald's Bites on Big Data; 2019. https://www.wired.com/story/mcdonalds-big-data-dynamic-yield-acquisition
Bernard M. & Co. American Express: how Big Data and machine learning Benefits Consumers And Merchants, 2018. https://www.bernardmarr.com/default.asp?contentID=1263
Zhang Y, Huang T, Bompard EF. Big data analytics in smart grids: a review. Energy Informatics. 2018;1(1):8.
HBS. Next Big Sound—moneyball for music? Digital Initiative. 2020. https://digital.hbs.edu/platform-digit/submission/next-big-sound-moneyball-for-music/ . Accessed 10 Apr 2021.
Mneney J, Van Belle JP. Big data capabilities and readiness of South African retail organisations. In: 2016 6th International Conference-Cloud System and Big Data Engineering (Confluence). IEEE. 2016. p. 279–86.
Beckhard R. Organizational issues in the team delivery of comprehensive health care. Milbank Mem Fund. 1972;50:287–316.
Cummings TG, Worley CG. Organization development and change. 8th ed. Mason: Thompson South-Western; 2009.
Glanz K, Rimer BK, Viswanath K, editors. Health behavior and health education: theory, research, and practice. San Francisco: Wiley; 2008.
Schein EH. Organizational culture and leadership. San Francisco: Jossey-Bass; 1985.
Prestby J, Wandersman A. An empirical exploration of a framework of organizational viability: maintaining block organizations. J Appl Behav Sci. 1985;21(3):287–305.
Liberati A, Altman DG, Tetzlaff J, Mulrow C, Gøtzsche PC, Ioannidis JP, Moher D. The PRISMA statement for reporting systematic reviews and meta-analyses of studies that evaluate health care interventions: explanation and elaboration. J Clin Epidemiol. 2009;62(10):e1–34.
Page MJ, McKenzie JE, Bossuyt PM, Boutron I, Hoffmann TC, Mulrow CD, Moher D. The PRISMA 2020 statement: an updated guideline for reporting systematic reviews. BMJ. 2021;372:n71.
Higgins JP, Green S, Scholten RJPM. Maintaining reviews: updates, amendments and feedback. Cochrane handbook for systematic reviews of interventions. 31; 2008.
Braun V, Clarke V. Using thematic analysis in psychology. Qual Res Psychol. 2006;3(2):77–101.
Judger N. The thematic analysis of interview data: an approach used to examine the influence of the market on curricular provision in Mongolian higher education institutions. Hillary Place Papers, University of Leeds. 2016;3:1–7
Khine P, Shun W. Big data for organizations: a review. J Comput Commun. 2017;5:40–8.
Zan KK. Prospects for using Big Data to improve the effectiveness of an education organization. In: 2019 IEEE Conference of Russian Young Researchers in Electrical and Electronic Engineering (EIConRus) . IEEE. 2019. p. 1777–9.
Ekambaram A, Sørensen AØ, Bull-Berg H, Olsson NO. The role of big data and knowledge management in improving projects and project-based organizations. Procedia Comput Sci. 2018;138:851–8.
Rialti R, Marzi G, Silic M, Ciappei C. Ambidextrous organization and agility in big data era: the role of business process management systems. Bus Process Manag. 2018;24(5):1091–109.
Wang Y, Kung L, Gupta S, Ozdemir S. Leveraging big data analytics to improve quality of care in healthcare organizations: a configurational perspective. Br J Manag. 2019;30(2):362–88.
De Mauro A, Greco M, Grimaldi M, Ritala P. In (Big) Data we trust: value creation in knowledge organizations—introduction to the special issue. Inf Proc Manag. 2018;54(5):755–7.
Batistič S, Van Der Laken P. History, evolution and future of big data and analytics: a bibliometric analysis of its relationship to performance in organizations. Br J Manag. 2019;30(2):229–51.
Jokonya O. Towards a conceptual framework for big data adoption in organizations. In: 2015 International Conference on Cloud Computing and Big Data (CCBD). IEEE. 2015. p. 153–160.
Mikalef P, Krogstie J, Pappas IO, Pavlou P. Exploring the relationship between big data analytics capability and competitive performance: the mediating roles of dynamic and operational capabilities. Inf Manag. 2020;57(2):103169.
Shuradze G, Wagner HT. Towards a conceptualization of data analytics capabilities. In: 2016 49th Hawaii International Conference on System Sciences (HICSS). IEEE. 2016. p. 5052–64.
Manyika J, Chui M, Brown B, Bughin J, Dobbs R, Roxburgh C, Hung Byers A. Big data: the next frontier for innovation, competition, and productivity. McKinsey Global Institute. 2011. https://www.mckinsey.com/business-functions/mckinsey-digital/our-insights/big-data-the-next-frontier-for-innovation . Accessed XX(day) XXX (month) XXXX (year).
Wu YK, Chu NF. Introduction of the transtheoretical model and organisational development theory in weight management: a narrative review. Obes Res Clin Pract. 2015;9(3):203–13.
Grant RM. Contemporary strategy analysis: Text and cases edition. Wiley; 2010.
Bharadwaj AS. A resource-based perspective on information technology capability and firm performance: an empirical investigation. MIS Q. 2000;24(1):169–96.
Chae HC, Koh CH, Prybutok VR. Information technology capability and firm performance: contradictory findings and their possible causes. MIS Q. 2014;38:305–26.
Santhanam R, Hartono E. Issues in linking information technology capability to firm performance. MIS Q. 2003;27(1):125–53.
Hao S, Zhang H, Song M. Big data, big data analytics capability, and sustainable innovation performance. Sustainability. 2019;11:7145. https://doi.org/10.3390/su11247145 .
Miller S. Collaborative approaches needed to close the big data skills gap. J Organ Des. 2014;3(1):26–30.
Gobble MM. Outsourcing innovation. Res Technol Manag. 2013;56(4):64–7.
Ann Keller S, Koonin SE, Shipp S. Big data and city living–what can it do for us? Signif (Oxf). 2012;9(4):4–7.
Galbraith JR. Organizational design challenges resulting from big data. J Organ Des. 2014;3(1):2–13.
Schneider B, Ehrhart MG, Macey WH. Organizational climate and culture. Annu Rev Psychol. 2013;64:361–88.
Download references
Acknowledgements
Not applicable
Not applicable.
Author information
Authors and affiliations.
Newcastle Business School, University of Newcastle, Newcastle, NSW, Australia
Renu Sabharwal & Shah Jahan Miah
You can also search for this author in PubMed Google Scholar
Contributions
The first author conducted the research, while the second author has ensured quality standards and rewritten the entire findings linking to underlying theories. All authors read and approved the final manuscript.
Corresponding author
Correspondence to Shah Jahan Miah .
Ethics declarations
Ethics approval and consent to participate, consent for publication, competing interests.
The authors declare that they have no competing interests.
Additional information
Publisher's note.
Springer Nature remains neutral with regard to jurisdictional claims in published maps and institutional affiliations.

Rights and permissions
Open Access This article is licensed under a Creative Commons Attribution 4.0 International License, which permits use, sharing, adaptation, distribution and reproduction in any medium or format, as long as you give appropriate credit to the original author(s) and the source, provide a link to the Creative Commons licence, and indicate if changes were made. The images or other third party material in this article are included in the article's Creative Commons licence, unless indicated otherwise in a credit line to the material. If material is not included in the article's Creative Commons licence and your intended use is not permitted by statutory regulation or exceeds the permitted use, you will need to obtain permission directly from the copyright holder. To view a copy of this licence, visit http://creativecommons.org/licenses/by/4.0/ .
Reprints and permissions
About this article
Cite this article.
Sabharwal, R., Miah, S.J. A new theoretical understanding of big data analytics capabilities in organizations: a thematic analysis. J Big Data 8 , 159 (2021). https://doi.org/10.1186/s40537-021-00543-6
Download citation
Received : 17 August 2021
Accepted : 16 November 2021
Published : 18 December 2021
DOI : https://doi.org/10.1186/s40537-021-00543-6
Share this article
Anyone you share the following link with will be able to read this content:
Sorry, a shareable link is not currently available for this article.
Provided by the Springer Nature SharedIt content-sharing initiative
- Organization
- Systematic literature review
- Big Data Analytics capabilities
- Organizational Development Theory
- Organizational Climate
- Organizational Culture
In order to contribute to the broader research community, Google periodically releases data of interest to researchers in a wide range of computer science disciplines.
Dataset Type
- Text annotation
Dataset Year
- Year, descending
Discover our collection of tools and resources
Browse our library of open source projects, public datasets, APIs and more to find the tools you need to tackle your next challenge or fuel your next breakthrough.
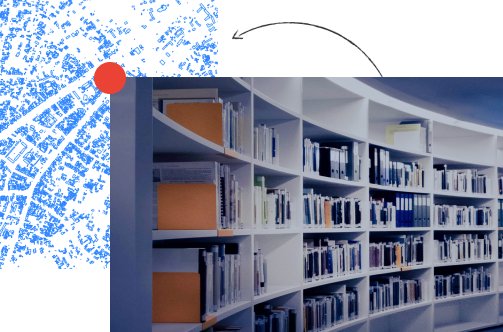
Data science and decision analytics
- Published: 18 September 2024
Cite this article
- Victoria C.P. Chen 1 &
- Seoung Bum Kim 2
20 Accesses
Explore all metrics
This is a preview of subscription content, log in via an institution to check access.
Access this article
Subscribe and save.
- Get 10 units per month
- Download Article/Chapter or eBook
- 1 Unit = 1 Article or 1 Chapter
- Cancel anytime
Price includes VAT (Russian Federation)
Instant access to the full article PDF.
Rent this article via DeepDyve
Institutional subscriptions
Author information
Authors and affiliations.
Department of Industrial, Manufacturing & Systems Engineering, The University of Texas at Arlington, Arlington, TX, 76019-0017, USA
Victoria C.P. Chen
School of Industrial and Management Engineering, Korea University, 145 Anamro, Seoul, 02841, South Korea
Seoung Bum Kim
You can also search for this author in PubMed Google Scholar
Corresponding author
Correspondence to Victoria C.P. Chen .
Additional information
Publisher’s note.
Springer Nature remains neutral with regard to jurisdictional claims in published maps and institutional affiliations.
Rights and permissions
Springer Nature or its licensor (e.g. a society or other partner) holds exclusive rights to this article under a publishing agreement with the author(s) or other rightsholder(s); author self-archiving of the accepted manuscript version of this article is solely governed by the terms of such publishing agreement and applicable law.
Reprints and permissions
About this article
Chen, V., Kim, S.B. Data science and decision analytics. Ann Oper Res (2024). https://doi.org/10.1007/s10479-024-06272-2
Download citation
Published : 18 September 2024
DOI : https://doi.org/10.1007/s10479-024-06272-2
Share this article
Anyone you share the following link with will be able to read this content:
Sorry, a shareable link is not currently available for this article.
Provided by the Springer Nature SharedIt content-sharing initiative
- Find a journal
- Publish with us
- Track your research

An official website of the United States government
The .gov means it’s official. Federal government websites often end in .gov or .mil. Before sharing sensitive information, make sure you’re on a federal government site.
The site is secure. The https:// ensures that you are connecting to the official website and that any information you provide is encrypted and transmitted securely.
- Publications
- Account settings
The PMC website is updating on October 15, 2024. Learn More or Try it out now .
- Advanced Search
- Journal List

The role of data science in healthcare advancements: applications, benefits, and future prospects
Sri venkat gunturi subrahmanya.
1 Department of Electrical and Electronics Engineering, Manipal Institute of Technology, Manipal Academy of Higher Education, Manipal, Karnataka India
Dasharathraj K. Shetty
2 Department of Humanities and Management, Manipal Institute of Technology, Manipal Academy of Higher Education, Manipal, Karnataka India
Vathsala Patil
3 Department of Oral Medicine and Radiology, Manipal College of Dental Sciences, Manipal, Manipal Academy of Higher Education, Manipal Karnataka, India
B. M. Zeeshan Hameed
4 Department of Urology, Father Muller Medical College, Mangalore, Karnataka India
5 Department of Radiation Oncology, Massachusetts General Hospital, Boston, MA USA
Komal Smriti
Nithesh naik.
6 Department of Mechanical and Manufacturing Engineering, Manipal Institute of Technology, Manipal Academy of Higher Education, Manipal, Karnataka India
Bhaskar K. Somani
7 Department of Urology, University Hospital Southampton NHS Trust, Southampton, UK
Data science is an interdisciplinary field that extracts knowledge and insights from many structural and unstructured data, using scientific methods, data mining techniques, machine-learning algorithms, and big data. The healthcare industry generates large datasets of useful information on patient demography, treatment plans, results of medical examinations, insurance, etc. The data collected from the Internet of Things (IoT) devices attract the attention of data scientists. Data science provides aid to process, manage, analyze, and assimilate the large quantities of fragmented, structured, and unstructured data created by healthcare systems. This data requires effective management and analysis to acquire factual results. The process of data cleansing, data mining, data preparation, and data analysis used in healthcare applications is reviewed and discussed in the article. The article provides an insight into the status and prospects of big data analytics in healthcare, highlights the advantages, describes the frameworks and techniques used, briefs about the challenges faced currently, and discusses viable solutions. Data science and big data analytics can provide practical insights and aid in the decision-making of strategic decisions concerning the health system. It helps build a comprehensive view of patients, consumers, and clinicians. Data-driven decision-making opens up new possibilities to boost healthcare quality.
Introduction
The evolution in the digital era has led to the confluence of healthcare and technology resulting in the emergence of newer data-related applications [ 1 ]. Due to the voluminous amounts of clinical data generated from the health care sector like the Electronic Health Records (EHR) of patients, prescriptions, clinical reports, information about the purchase of medicines, medical insurance-related data, investigations, and laboratory reports, there lies an immense opportunity to analyze and study these using recent technologies [ 2 ]. The huge volume of data can be pooled together and analyzed effectively using machine-learning algorithms. Analyzing the details and understanding the patterns in the data can help in better decision-making resulting in a better quality of patient care. It can aid to understand the trends to improvise the outcome of medical care, life expectancy, early detection, and identification of disease at an initial stage and required treatment at an affordable cost [ 3 ]. Health Information Exchange (HIE) can be implemented which will help in extracting clinical information across various distinct repositories and merge it into a single person’s health record allowing all care providers to access it securely. Hence, the organizations associated with healthcare must attempt to procure all the available tools and infrastructure to make use of the big data, which can augment the revenue and profits and can establish better healthcare networks, and stand apart to reap significant benefits [ 4 , 5 ]. Data mining techniques can create a shift from conventional medical databases to a knowledge-rich, evidence-based healthcare environment in the coming decade.
Big data and its utility in healthcare and medical sciences have become more critical with the dawn of the social media era (platforms such as Facebook and Twitter) and smartphone apps that can monitor personal health parameters using sensors and analyzers [ 6 , 7 ]. The role of data mining is to improvise the stored user information to provide superior treatment and care. This review article provides an insight into the advantages and methodologies of big data usage in health care systems. It highlights the voluminous data generated in these systems, their qualities, possible security-related problems, data handling, and how this analytics support gaining significant insight into these data set.
Search strategy
A non-systematic review of all data science, big data in healthcare-related English language literature published in the last decade (2010–2020) was conducted in November 2020 using MEDLINE, Scopus, EMBASE, and Google Scholar. Our search strategy involved creating a search string based on a combination of keywords. They were: “Big Data,” “Big Data Analytics,” “Healthcare,” “Artificial Intelligence,” “AI,” “Machine learning,” “ML,” “ANN,” “Convolutional Networks,” “Electronic Health Records,” “EHR,” “EMR,” “Bioinformatics,” and “Data Science.” We included original articles published in English.
Inclusion criteria
- Articles on big data analytics, data science, and AI.
- Full-text original articles on all aspects of application of data science in medical sciences.
Exclusion criteria
- Commentaries, reviews, and articles with no full-text context and book chapters.
- Animal, laboratory, or cadaveric studies.
The literature review was performed as per the above-mentioned strategy. The evaluation of titles and abstracts, screening, and the full article text was conducted for the chosen articles that satisfied the inclusion criteria. Furthermore, the authors manually reviewed the selected article’s references list to screen for any additional work of interest. The authors resolved the disagreements about eligibility for a consensus decision after discussion.
Knowing more about “big data”
Big data consists of vast volumes of data, which cannot be managed using conventional technologies. Although there are many ways to define big data, we can consider the one defined by Douglas Laney [ 8 ] that represents three dimensions, namely, volume, velocity, and variety (3 Vs). The “big” in big data implies its large volume. Velocity demonstrates the speed or rate at which data is processed. Variety focuses on the various forms of structured and raw data obtained by any method or device, such as transaction-level data, videos, audios, texts, emails, and logs. The 3 Vs became the default description of big data, while many other Vs are added to the definition [ 9 ]. “Veracity” remains the most agreed 4th “V.” Data veracity focuses on the accuracy and reliability of a dataset. It helps to filter through what is important and what is not. The data with high veracity has many records that are valuable to analyze and that contribute in a meaningful way to the overall results. This aspect poses the biggest challenge when it comes to big data. With so much data available, ensuring that it is relevant and of high quality is important. Over recent years, big data has become increasingly popular across all parts of the globe.
Big data needs technologically sophisticated applications that use high-end computing resources and Artificial Intelligence (AI)-based algorithms to understand such huge volumes of data. Machine learning (ML) approaches for automatic decision-making by applying fuzzy logic and neural networks will be added advantage. Innovative and efficient strategies for dealing with data, smart cloud-based applications, effective storage, and user-friendly visualization are required for big data to gain practical insights [ 10 ].
Medical care as a repository for big data
Healthcare is a multilayered system developed specifically for preventing, diagnosing, and treating diseases. The key elements of medical care are health practitioners (physicians and nurses), healthcare facilities (which include clinics, drug delivery centers, and other testing or treatment technologies), and a funding agency that funds the former. Health care practitioners belong to different fields of health such as dentistry, pharmacy, medicine, nursing, psychology, allied health sciences, and many more. Depending on the severity of the cases, health care is provided at many levels. In all these stages, health practitioners need different forms of information such as the medical history of the patient (data related to medication and prescriptions), clinical data (such as data from laboratory assessments), and other personal or private medical data. The usual practice for a clinic, hospital, or patient to retain these medical documents would be maintaining either written notes or in the form of printed reports [ 11 ].
The clinical case records preserve the incidence and outcome of disease in a person’s body as a tale in the family, and the doctor plays an integral role in this tale [ 12 ]. With the emergence of electronic systems and their capacity, digitizing medical exams, health records, and investigations is a common procedure today. In 2003, the Institute of Medicine, a division in the National Academies of Sciences and Engineering coined the term “Electronic Health Records” for representing an electronic portal that saves the records of the patients. Electronic health records (EHRs) are automated medical records of patients related to an individual’s physical/mental health or significant reports that are saved in an electronic system and used to record, send, receive, store, retrieve, and connect the medical personnel and patient with medical services [ 13 ].
Open-source big data platforms
It is an inefficient idea to work with big data or vast volumes of data into storage considering even the most powerful computers. Hence, the only logical approach to process large quantities of big data available in a complex form is by spreading and processing it on several parallel connected nodes. Nevertheless, the volume of the data is typically so high that a large number of computing machines are needed in a reasonable period to distribute and finish processing. Working with thousands of nodes involves coping with issues related to paralleling the computation, spreading of data, and manage failures. Table Table1 1 shows the few open sources of big data platforms and their utilities for data scientists.
source big data platforms and their utilities
Big data tools | Utilities |
---|---|
Apache Hadoop | It is designed to scale up to thousands of machines from single servers, each of which offers local storage The framework enables users to easily build and validate distributed structures, distributes data, and operates across machines automatically |
Apache Spark | The Hadoop Distributed File system (HDFS) and other data stores are flexible to work with Spark offers integrated Application Program Interfaces (APIs) which enable users to write apps in different languages |
Apache Cassandra | Cassandra is highly flexible and can add additional hardware that can handle more data and users on demand Cassandra adapts to all possible data types such as unstructured, structured, and semi-structured supporting features such as Atomicity, Consistency, Isolation, and Durability (ACID) |
Apache Storm | In several cases, Apache Storm is easy to integrate with any programming language, with real-time analytics, online machine learning, and computation Apache Storm uses parallel calculations which run across a machine cluster |
RapidMiner | RapidMiner provides a variety of products for a new process of data mining It provides an integrated data preparation environment, machine learning, text mining, visualization, predictive analysis, application development, prototype validation, and implementation. statistic modeling, deployment |
Cloudera | Users can spin clusters, terminate them, and only pay for what they need Cloudera Enterprise can be deployed and run on AWS and Google Cloud Platforms by users |
Data mining
Data types can be classified based on their nature, source, and data collection methods [ 14 ]. Data mining techniques include data grouping, data clustering, data correlation, and mining of sequential patterns, regression, and data storage. There are several sources to obtain healthcare-related data (Fig. 1 ). The most commonly used type (77%) is the data generated by humans (HG data) which includes Electronic Medical Records (EMR), Electronic Health Records (EHR), and Electronic Patient Records (EPR). Online data through Web Service (WS) is considered as the second largest form of data (11%) due to the increase in the number of people using social media day by day and current digital development in the medical sector [ 15 ]. Recent advances in the Natural Language Processing (NLP)-based methodologies are also making WS simpler to use [ 16 ]. The other data forms such as Sensor Data (SD), Big Transactional Data (BTD), and Biometric Data (BM) make around 12% of overall data use, but wearable personal health monitoring devices’ prominence and market growth [ 17 ] may need SD and BM data.

Sources of big data in healthcare
Applications of analytics in healthcare
There are six areas of applications of analytics in healthcare (Fig. 2 ) including disease surveillance, health care management and administration, privacy protection and fraud detection, mental health, public health, and pharmacovigilance. Researchers have implemented data extraction for data deposition and cloud-based computing, optimizing quality, lowering costs, leveraging resources, handling patients, and other fields.

Various applications of data science in healthcare
Disease surveillance
It involves the perception of the disease, understanding its condition, etiology (the manner of causation of a disease), and prevention (Fig. 3 ).

The disease analysis system
Information obtained with the help of EHRs, and the Internet has a huge prospect for disease analysis. The various surveillance methods would aid the planning of services, evaluation of treatments, priority setting, and the development of health policy and practice.
Image processing of healthcare data from the big data point of view
Image processing on healthcare data offers valuable knowledge about anatomy and organ functioning and identifies the disease and patient health conditions. The technique currently has been used for organ delineation, identification of lung tumors, diagnosis of spinal deformity, detection of arterial stenosis, detection of an aneurysm, etc. [ 18 ]. The wavelets technique is commonly used for image processing techniques such as segmentation, enhancement, and noise reduction. The use of artificial intelligence in image processing will enhance aspects of health care including screening, diagnosis, and prognosis, and integrating medical images with other types of data and genomic data will increase accuracy and facilitate early diagnosis of diseases [ 18 , 19 ]. The exponential increase in the count of medical facilities and patients has led to better use of clinical settings of computer-based healthcare diagnostics and decision-making systems.
Data from wearable technology
Multi-National Companies like Apple and Google are working on health-based apps and wearable technology as part of a broader range of electronic sensors, the so-called IoT, and toolkits for healthcare-related apps. The possibility of collecting accurate medical data on real-time (e.g., mood, diet followed, exercise, and sleep cycles patterns), linked to physiological indicators (e.g., heart rate, calories burned, level of blood glucose, cortisol levels), is perhaps discrete and omnipresent at minimum cost, unrelated to traditional health care. “True Colors” is a wearable designed to collect continuous patient-centric data with the accessibility and acceptability needed to allow for accurate longitudinal follow-up. More importantly, this system is presently being piloted as a daily health-monitoring substitute.
Medical signal analytics
Telemetry and the devices for the monitoring of physiological parameters generate large amounts of data. The data generated generally are retained for a shorter duration, and thus, extensive research into produced data is neglected. However, advancements in data science in the field of healthcare attempt to ensure better management of data and provide enhanced patient care [ 20 – 23 ].
The use of continuous waveform in health records containing information generated through the application of statistical disciplines (e.g., statistical, quantitative, contextual, cognitive, predictive, etc.) can drive comprehensive care decision-making. Data acquisition apart from an ingestion-streaming platform is needed that can control a set of waveforms at various fidelity rates. The integration of this waveform data with the EHR’s static data results in an important component for giving analytics engine situational as well as contextual awareness. Enhancing the data collected by analytics will not just make the method more reliable, but will also help in balancing predictive analytics’ sensitivity and specificity. The signal processing species must mainly rely on the kind of disease population under observation.
Various signal-processing techniques can be used to derive a large number of target properties that are later consumed to provide actionable insight by a pre-trained machine-learning model. Such observations may be analytical, prescriptive, or predictive. Such insights can be furthermore built to activate other techniques such as alarms and physician notifications. Maintaining these continuous waveforms–based data along with specific data obtained from the remaining sources in perfect harmony to find the appropriate patient information to improve diagnosis and treatments of the next generation can be a daunting task [ 24 ]. Several technological criteria and specifications at the framework, analytical, and clinical levels need to be planned and implemented for the bedside implementation of these systems into medical setups.
Healthcare administration
Knowledge obtained from big data analysis gives healthcare providers insights not available otherwise (Fig. 4 ). Researchers have implemented data mining techniques to data warehousing as well as cloud computing, increasing quality, minimizing costs, handling patients, and several other fields of healthcare.

Role of big data in accelerating the treatment process
Data storage and cloud computing
Data warehousing and cloud storage are primarily used for storing the increasing amount of electronic patient-centric data [ 25 , 26 ] safely and cost-effectively to enhance medical outcomes. Besides medical purposes, data storage is utilized for purposes of research, training, education, and quality control. Users can also extract files from a repository containing the radiology results by using keywords following the predefined patient privacy policy.
Cost and quality of healthcare and utilization of resources
The migration of imaging reports to electronic medical recording systems offers tremendous potential for advancing research and practice on radiology through the continuous updating, incorporation, and exchange of a large volume of data. However, the heterogeneity in how these data can be formatted still poses major challenges. The overall objective of NLP is that the natural human language is translated into structured with a standardized set of value choices that are easily manipulated into subsections or searches for the presence or absence of a finding through software, among other things [ 27 ].
Greaves et al. [ 28 ] analyzed sentiment (computationally dividing them into categories such as optimistic, pessimistic, and neutral) based on the online response of patients stating their overall experience to predict healthcare quality. They found an agreement above 80% between online platform sentiment analysis and conventional paper-based quality prediction surveys (e.g., cleanliness, positive conduct, recommendation). The newer solution can be a cost-effective alternative to conventional healthcare surveys and studies. The physician’s overuse of screening and testing often leads to surplus data and excess costs [ 29 ]. The present practice in pathology is restricted by the emphasis on illness. Zhuang et al. [ 29 ] compared the disease-based approach in conjunction with database reasoning and used the data mining technique to build a decision support system based on evidence to minimize the unnecessary testing to reduce the total expense of patient care.
Patient data management
Patient data management involves effective scheduling and the delivery of patient care during the period of a patient’s stay in a hospital. The framework of patient-centric healthcare is shown in Fig. 5 . Daggy et al. [ 30 ] conducted a study on “no shows” or missing appointments that lead to the clinical capability that has been underused. A logistical regression model is developed using electronic medical records to estimate the probabilities of patients to no-show and show the use of estimates for creating clinical schedules that optimize clinical capacity use while retaining limited waiting times and clinical extra-time. The 400-day clinical call-in process was simulated, and two timetables were developed per day: the conventional method, which assigns one patient per appointment slot, and the proposed method, which schedules patients to balance patient waiting time, additional time, and income according to no-show likelihood.

Elemental structure of patient-centric healthcare and ecosystem
If patient no-show models are mixed with advanced programming approaches, more patients can be seen a day thus enhancing clinical performance. The advantages of implementation of planning software, including certain methodologies, should be considered by clinics as regards no-show costs [ 30 ].
A study conducted by Cubillas et al. [ 31 ] pointed out that it takes less time for patients who came for administrative purposes than for patients for health reasons. They also developed a statistical design for estimating the number of administrative visits. With a time saving of 21.73% (660,538 min), their model enhanced the scheduling system. Unlike administrative data/target finding patients, a few come very regularly for their medical treatment and cover a significant amount of medical workload. Koskela et al. [ 32 ] used both supervised and unsupervised learning strategies to identify and cluster records; the supervised strategy performed well in one cluster with 86% accuracy in distinguishing fare documents from the incorrect ones, whereas the unsupervised technique failed. This approach can be applied to the semi-automate EMR entry system [ 32 ].
Privacy of medical data and fraudulency detection
The anonymization of patient data, maintaining the privacy of the medical data and fraudulency detection in healthcare, is crucial. This demands efforts from data scientists to protect the big data from hackers. Mohammed et al. [ 33 ] introduced a unique anonymization algorithm that works for both distributed and centralized anonymization and discussed the problems of privacy security. For maintaining data usefulness without the loss of any data privacy, the researchers further proposed a model that performed far better than the traditional K-anonymization model. In addition to this, their algorithm could also deal with voluminous, multi-dimensional datasets.
A mobile-based cloud-computing framework [ 34 ] of big data has been introduced to overcome the shortcomings of today’s medical records systems. EHR data systems are constrained due to a lack of interoperability, size of data, and privacy. This unique cloud-based system proposed to store EHR data from multiple healthcare providers within the facility of an internet provider to provide authorized restricted access to healthcare providers and patients. They used algorithms for encryption, One Time Password (OTP), or a 2-factor authentication to ensure data security.
The analytics of the big data can be performed using Google’s efficient tools such as big query tools and MapReduce. This approach will reduce costs, improve efficiency, and provide data protection compared to conventional techniques that are used for anonymization. The conventional approach generally leaves data open to re-identification. Li et al. in a case study showed that hacking can make a connection between tiny chunks of information as well as recognize patients [ 35 ]. Fraud detection and abuse (i.e., suspicious care behavior, deliberate act of falsely representing facts, and unwanted repeated visits) make excellent use of big data analytics [ 36 ].
By using data from gynecology-based reports, Yang et al. framed a system that manually distinguishes characteristics of suspicious specimens from a set of medical care plans that any doctor would mostly adopt [ 37 ]. The technique was implemented on the data from Taiwan’s Bureau of National Health Insurance (BNHI), where the proposed technique managed to detect 69% of the total cases as fraudulent, enhancing the current model, which detected only 63% of fraudulent cases. To sum up, the protection of patient data and the detection of fraud are of significant concern due to the growing usage of social media technology and the propensity of people to place personal information on these platforms. The already existing strategies for anonymizing the data may become less successful if they are not implemented because a significant section of the personal details of everyone is now accessible through these platforms.
Mental health
According to National Survey conducted on Drug Use and Health (NSDUH), 52.2% of the total population in the United States (U.S.) was affected by either mental problems or drug addiction/abuse [ 38 ]. In addition, approximately 30 million suffer from panic attacks and anxiety disorders [ 39 ].
Panagiotakopoulos et al. [ 40 ] developed a data analysis–focused treatment technique to help doctors in managing patients with anxiety disorders. The authors used static information that includes personal information such as the age of the individual, sex, body and skin types, and family details and dynamic information like the context of stress, climate, and symptoms to construct static and dynamic information based on user models. For the first three services, relationships between different complex parameters were established, and the remaining one was mainly used to predict stress rates under various scenarios. This model was verified with the help of data collected from twenty-seven volunteers who are selected via the anxiety assessment survey. The applications of data analytics in the disease diagnosis, examination, or treatment of patients with mental wellbeing are very different from using analytics to anticipate cancer or diabetes. In this case, the data context (static, dynamic, or non-observable environment) seems to be more important compared to data volume [ 39 ].
The leading cause of perinatal morbidity and death is premature birth, but an exact mechanism is still unclear. The research carried by Chen et al. [ 41 ] intended to investigate the risk factors of preterm use of neural networks and decision tree C5.0 data mining. The original medical data was obtained by a specialist study group at the National University of Taiwan from a prospective pregnancy cohort. A total of 910 mother–child dyads from 14,551 in the original data have been recruited using the nest case–control design. In this data, thousands of variables are studied, including basic features, medical background, the climate and parents’ occupational factors, and the variables related to children. The findings suggest that the main risk factors for pre-born birth are multiple births, blood pressure during pregnancy, age, disease, prior preterm history, body weight and height of pregnant women, and paternal life risks associated with drinking and smoking. The results of the study are therefore helpful in the attempt to diagnose high-risk pregnant women and to provide intervention early to minimize and avoid early births in parents, healthcare workers, and public health workers [ 41 , 42 ].
Public health
Data analytics have also been applied to the detection of disease during outbreaks. Kostkova et al. [ 43 ] analyzed online records based on behavior patterns and media reporting the factors that affect the public as well as professional patterns of search-related disease outbreaks. They found distinct factors affecting the public health agencies’ skilled and layperson search patterns with indications for targeted communications during emergencies and outbreaks. Rathore et al. [ 44 ] have suggested an emergency tackling response unit using IoT-based wireless network of wearable devices called body area networks (BANs). The device consists of “intelligent construction,” a model that helps in processing and decision making from the data obtained from the sensors. The system was able to process millions of users’ wireless BAN data to provide an emergency response in real-time.
Consultation online is becoming increasingly common and a possible solution to the scarcity of healthcare resources and inefficient delivery of resources. Numerous online consultation sites do however struggle to attract customers who are prepared to pay and maintain them, and health care providers on the site have the additional challenge to stand out from a large number of doctors who can provide similar services [ 45 ]. In this research, Jiang et al. [ 45 ] used ML approaches to mine massive service data, in order (1) to define the important characteristics related to patient payment rather than free trial appointments, (2) explore the relative importance of those features, and (3) understand how these attributes work concerning payment, whether linearly or not. The dataset refers to the largest online medical consultation platform in China, covering 1,582,564 consultation documents among patient pairs between 2009 and 2018. The results showed that compared with features relating to reputation as a physician, service-related features such as quality of service (e.g., intensity of consultation dialogue and response rate), the source of patients (e.g., online vs offline patients), and the involvement of patients (e.g., social returns and previous treatments revealed). To facilitate payment, it is important to promote multiple timely responses in patient-provider interactions.
Pharmacovigilance
Pharmacovigilance requires tracking and identification of adverse drug reactions (ADRs) after launch, to guarantee patient safety. ADR events’ approximate social cost per year reaches a billion dollars, showing it as a significant aspect of the medical care system [ 46 ]. Data mining findings from adverse event reports (AERs) revealed that mild to lethal reactions might be caused in paclitaxel among which docetaxel is linked with the lethal reaction while the remaining 4 drugs were not associated with hypersensitivity [ 47 ] while testing ADR’s “hypersensitivity” to six anticancer agents [ 47 ]. Harpaz et al. [ 46 ] disagreed with the theory that adverse events might be caused not just due to a single medication but also due to a mixture of synthetic drugs. It is found that there is a correlation between a minimum of one drug and two AEs or two drugs and one AE in 84% of AERs studies. Harpaz R et al. [ 47 ] improved precision in the identification of ADRs by jointly considering several data sources. When using EHRs that are available publicly in conjunction with the AER studies of the FDA, they achieved a 31% (on average) increase in detection [ 45 ]. The authors identified dose-dependent ADRs with the help of models built from structured as well as unstructured EHR data [ 48 ]. Of the top 5 ADR-related drugs, 4 were observed to be dose-related [ 49 ]. The use of text data that is unstructured in EHRs [ 50 ]; pharmacovigilance operation was also given priority.
ADRs are uncommon in conventional pharmacovigilance, though it is possible to get false signals while finding a connection between a drug and any potential ADRs. These false alarms can be avoided because there is already a list of potential ADRs that can be of great help in potential pharmacovigilance activities [ 18 ].
Overcoming the language barrier
Having electronic health records shared worldwide can be beneficial in analyzing and comparing disease incidence and treatments in different countries. However, every country would use their language for data recording. This language barrier can be dealt with the help of multilingual language models, which would allow diversified opportunities for Data Science proliferation and to develop a model for personalization of services. These models will be able to understand the semantics — the grammatical structure and rules of the language along with the context — the general understanding of words in different contexts.
For example: “I’ll meet you at the river bank.”
“I have to deposit some money in my bank account.”
The word bank means different things in the two contexts, and a well-trained language model should be able to differentiate between these two. Cross-lingual language model trains on multiple languages simultaneously. Some of the cross lingual language models include:
mBERT — the multilingual BERT which was developed by Google Research team.
XLM — cross lingual model developed by Facebook AI, which is an improvisation over mBERT.
Multifit — a QRNN-based model developed by Fast.Ai that addresses challenges faced by low resource language models.
Millions of data points are accessible for EHR-based phenotyping involving a large number of clinical elements inside the EHRs. Like sequence data, handling and controlling the complete data of millions of individuals would also become a major challenge [ 51 ]. The key challenges faced include:
- The data collected was mostly either unorganized or inaccurate, thus posing a problem to gain insights into it.
- The correct balance between preserving patient-centric information and ensuring the quality and accessibility of this data is difficult to decide.
- Data standardization, maintaining privacy, efficient storage, and transfers require a lot of manpower to constantly monitor and make sure that the needs are met.
- Integrating genomic data into medical studies is critical due to the absence of standards for producing next-generation sequencing (NGS) data, handling bioinformatics, data deposition, and supporting medical decision-making [ 52 ].
- Language barrier when dealing data
Future directions
Healthcare services are constantly on the lookout for better options for improving the quality of treatment. It has embraced technological innovations intending to develop for a better future. Big data is a revolution in the world of health care. The attitude of patients, doctors, and healthcare providers to care delivery has only just begun to transform. The discussed use of big data is just the iceberg edge. With the proliferation of data science and the advent of various data-driven applications, the health sector remains a leading provider of data-driven solutions to a better life and tailored services to its customers. Data scientists can gain meaningful insights into improving the productivity of pharmaceutical and medical services through their broad range of data on the healthcare sector including financial, clinical, R&D, administration, and operational details.
Larger patient datasets can be obtained from medical care organizations that include data from surveillance, laboratory, genomics, imaging, and electronic healthcare records. This data requires proper management and analysis to derive meaningful information. Long-term visions for self-management, improved patient care, and treatment can be realized by utilizing big data. Data Science can bring in instant predictive analytics that can be used to obtain insights into a variety of disease processes and deliver patient-centric treatment. It will help to improvise the ability of researchers in the field of science, epidemiological studies, personalized medicine, etc. Predictive accuracy, however, is highly dependent on efficient data integration obtained from different sources to enable it to be generalized. Modern health organizations can revolutionize medical therapy and personalized medicine by integrating biomedical and health data. Data science can effectively handle, evaluate, and interpret big data by creating new paths in comprehensive medical care.
OOpen access funding provided by Manipal Academy of Higher Education, Manipal.
Declarations
The authors declare no competing interests.
Publisher's Note
Springer Nature remains neutral with regard to jurisdictional claims in published maps and institutional affiliations.

IMAGES
VIDEO
COMMENTS
Google Scholar provides a simple way to broadly search for scholarly literature. Search across a wide variety of disciplines and sources: articles, theses, books, abstracts and court opinions.
This article is a practical guide to conducting data analysis in general literature reviews. The general literature review is a synthesis and analysis of published research on a relevant clinical issue, and is a common format for academic theses at the bachelor's and master's levels in nursing, physiotherapy, occupational therapy, public health and other related fields.
The first step in a data analysis plan is to describe the data collected in the study. This can be done using figures to give a visual presentation of the data and statistics to generate numeric descriptions of the data. Selection of an appropriate figure to represent a particular set of data depends on the measurement level of the variable.
Data science is typically a "concept to unify statistics, data analysis, and their related methods" to understand and analyze the actual phenomena with data. ... This research contributes to the creation of a research vector on the role of data science in central banking. In , ... [Google Scholar] 8. Ankerst M, Breunig MM, Kriegel H-P ...
Yonjoo Cho is an associate professor of Instructional Systems Technology focusing on human resource development (HRD) at Indiana University. Her research interests include action learning in organizations, international HRD, and women in leadership. She serves as an associate editor of Human Resource Development Review and served as a board member of the Academy of Human Resource Development ...
Thousand Oaks, CA: SAGE Publications, Inc. [Google Scholar] Ryan GW, & Bernard HR (2000). Data management and analysis methods In Denzin NK & Lincoln YS (Eds.), Handbook of qualitative research (2nd ed.). Thousand Oaks, CA: SAGE Publications, Inc. [Google Scholar] Ryan GW, & Bernard HR (2003). Techniques to Identify Themes.
Introduction. Secondary data analysis is a valuable research approach that can be used to advance knowledge across many disciplines through the use of quantitative, qualitative, or mixed methods data to answer new research questions (Polit & Beck, 2021).This research method dates to the 1960s and involves the utilization of existing or primary data, originally collected for a variety, diverse ...
In this paper, we substantiate our premise that statistics is one of the most important disciplines to provide tools and methods to find structure in and to give deeper insight into data, and the most important discipline to analyze and quantify uncertainty. We give an overview over different proposed structures of Data Science and address the impact of statistics on such steps as data ...
Exploratory data analysis (EDA) is an essential step in any research analysis. The primary aim with exploratory analysis is to examine the data for distribution, outliers and anomalies to direct specific testing of your hypothesis. It also provides tools for hypothesis generation by visualizing and understanding the data usually through ...
The launch of Google Scholar ( ) marked the beginning of a revolution in the scientific information market. This search engine, unlike traditional databases, automatically indexes information from the academic web. Its ease of use, together with its wide coverage and fast indexing speed, have made it the first tool most scientists currently ...
Teaching quantitative data analysis is not teaching number crunching, but teaching a way of critical thinking for how to analyze the data. The goal of data analysis is to reveal the underlying patterns, trends, and relationships of a study's contextual situation. Learning data analysis is not learning how to use statistical tests to crunch ...
SECONDARY ANALYSIS PROCESS. An SDA researcher starts with a research question or hypothesis, then identifies an appropriate dataset or sets to address it; alternatively, they are familiar with a dataset and peruse it to identify other questions that might be answered by the available data (Cheng & Phillips, 2014).In reality, SDA researchers probably move back and forth between these approaches.
Few studies report on comparisons of the manual and technological approaches to qualitative data analysis. In one such study, Basit (2003) compares the use of the manual and technological approach to qualitative data analysis drawing on two research projects. She argues that the approach chosen is dependent on the size of the project, the funds ...
We offer best-practice recommendations for journal reviewers, editors, and authors regarding data collection and preparation. Our recommendations are applicable to research adopting different epistemological and ontological perspectives—including both quantitative and qualitative approaches—as well as research addressing micro (i.e., individuals, teams) and macro (i.e., organizations ...
Computer-assisted qualitative data analysis software (CAQDAS) has been used as an aid to data analysis in qualitative research in several methodological fields, including grounded theory (Bringer et al., Citation 2004), interpretative phenomenological analysis (Clare et al., Citation 2008) and realist meta theory (BERGIN, Citation 2011).
Google Scholar Kojo T, Daramola O, Adebiyi A. Big data stream analysis: a systematic literature review. J Big Data. 2019;6(1):1-30. Google Scholar Jha AK, Agi MA, Ngai EW. A note on big data analytics capability development in supply chain. Decis Support Syst. 2020;138:113382. Google Scholar
A sizable percentage of the educational research manuscripts submitted to the Journal of Graduate Medical Education employ a Likert scale for part or all of the outcome assessments. Thus, understanding the interpretation and analysis of data derived from Likert scales is imperative for those working in medical education and education research.
Features are generated from data that are used as input for data analysis. Data are provided by all data sources that can be made available in the data collection. Furthermore, new features can also be generated from meta-descriptions of data (e.g. simulated data). Another influencing factor is the data analysis model (Analytical Models).
A data visualization based around the median is the box plot, pioneered by Spear (1952) and enhanced into its current form by Tukey (1977). For a dated visualization, the box plot remains extremely effective in conveying a large amount of information about the underlying data. Yet modern improvements have been made.
The digital world has a wealth of data, such as internet of things (IoT) data, business data, health data, mobile data, urban data, security data, and many more, in the current age of the Fourth Industrial Revolution (Industry 4.0 or 4IR). Extracting knowledge or useful insights from these data can be used for smart decision-making in various applications domains. In the area of data science ...
ScreenQA dataset was introduced in the "ScreenQA: Large-Scale Question-Answer Pairs over Mobile App Screenshots" paper. It contains ~86K question-answer pairs collected by human annotators for ~35K screenshots from Rico. It should be used to train and evaluate models capable of screen content understanding via question answering.
In this article, we review some principles of the collection, analysis, and management of qualitative data to help pharmacists interested in doing research in their practice to continue their learning in this area. Qualitative research can help researchers to access the thoughts and feelings of research participants, which can enable ...
The six remaining papers in business developed innovative data envelopment analysis (DEA) methods to study a variety of multiple objective decision-making problems. Combining DEA with ranking via rough set theory, Le and Lu explore the business performance of pharmaceutical multinational enterprises listed in the Forbes Global 2000.
A non-systematic review of all data science, big data in healthcare-related English language literature published in the last decade (2010-2020) was conducted in November 2020 using MEDLINE, Scopus, EMBASE, and Google Scholar. Our search strategy involved creating a search string based on a combination of keywords.