HOME | ABOUT | CONTACT | SHARE Design and developed by MCA Department of Jorhat Engineering College 2023

Voice speed
Text translation, source text, translation results, document translation, drag and drop.
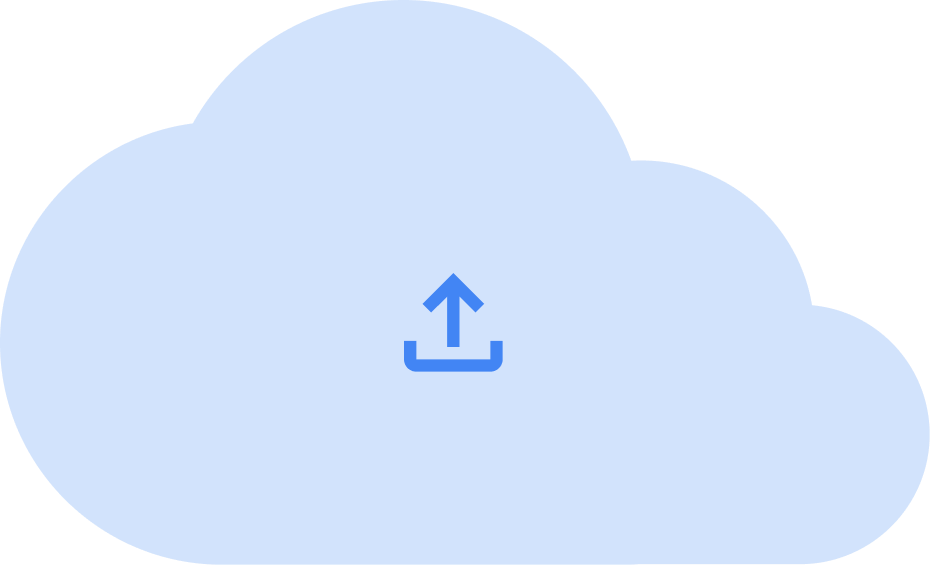
Website translation
Enter a URL
Image translation

Candrakanta Glosbe
Google Bing
• Candrakanta abhidhana : Assamese-English dictionary, University of Gauhati (1962)
• Dictionary Assamese and English by Miles Bronson (1867)
• Phrases in English and Assamese by Harriet Cutter, revised by Edward Clark (1877) + 1840 edition
• Brief vocabulary in English and Assamese by Susan Ward (1864)
• Some Assamese proverbs by Philip Richard Gurdon (1896)
• A linguistic study of 24 place names of Assam by Sarat Kumar Phukan, in International Symposium on languages and linguistics (2000)
→ Assamese keyboard (Bengali script with some variants, like the characters r and w )
• Wikipedia : Assamese language
• Dialects or variety of the Assamese language by Manash Jyoti Nirmalia, in Journal of Acharaya Narendra Dev Research Institute (2019)
• Fixing the Assamese language : "Tongue has no bone" (1800-1930) , by Bodhisattva Kar, in Studies in History (2008)
• Language and policies in 19 th -century Assam : war of words , by Madhumita Sengupta, in Indian Historical Review (2012)
• Representing Kamrupi : ideologies of grammar and the question of linguistic boundaries , in A multilingual nation translation and language dynamic in India (2017)
• Translation in Assamese : a brief account , by Biswadip Gogoi, in History of Translation in India (2017)
• Language and nationalism : comprehending the dynamics in 19 th -century Assam (2016)
• Linguistic nationalism in early-colonial Assam : the American Baptist Mission and Orunodoi , by Arnab Dasgupta, in Rupkatha journal on interdisciplinary studies in humanities (2021)
• Derivational morphology of Assamese lexical word categories by Palash Das & Madhumita Barbora, in Indian Journal of Language and Linguistics (2020)
• Assamese: its formation and development by Banikanta Kakati (1941)
• Grammatical notices of the Asamese language by Nathan Brown (1848)
The Assamese language is spoken in the State of Assam, in Northeast India. It's written with the Bengali script.
• Translation in Assamese : a brief account , by Biswadip Gogoi, in The history of translation in India (2017) NEW
• Aspects of early Assamese literature by Banikanta Kakati (1953)
• YouVersion : translation of the Bible into Assamese (2019) (+ audio)
• Hymns in Assamese , edited by the Baptist Mission (1850)
জন্মগত ভাবে সকলো মানুহ মৰ্যদা আৰু অধিকাৰত সমান আৰু স্বতন্ত্র। তেওঁলােকৰ বিবেক আছে, বুদ্ধি আছে। তেওঁলােকে প্রত্যেকে প্রত্যেকক ভাতৃ ভাবে ব্যৱহাৰ কৰা উচিত।
• Ethnicity, identity and cartography : possession/dispossession, homecoming/homelessness in contemporary Assam , by Parag Moni Sarma, in Studies of transition states and societies (2011)
• Becoming Hindu : the cultural politics of writing religion in colonial Assam , by Madhumita Sengupta, in Contributions to Indian Sociology (2021)
• The Assam Mission : papers and discussions , American Baptist Missionary Union (1886)
→ Inde
→ Bangladesh
→ languages of India
→ Bengali
→ Meitei
→ Hindi
Assamese (অসমীয়া) Dictionary English⇄Assamese
English to assamese & assamese to english dictionary.
Assamese Dictionary is a bilingual dictionary that translates words from English to Assamese and Assamese to English . It is a part of Language Networking, a social and educational initiative by KHANDBAHALE.COM, and is free and open to all for non-commercial use. It is recognised as an accurate and authentic language resource by scholars and experts and can also be downloaded as software and apps for multiple devices for online and offline use.
About the Assamese Language
Assamese or Asamiya (অসমীয়া Ôxômiya) is an Eastern Indo-Aryan language used mainly in the state of Assam. It is the official language of Assam. The easternmost of the Indo-Aryan languages; it is spoken by over 19 million native speakers. It is also spoken in parts of Arunachal Pradesh and other northeast Indian states. Nagamese, an Assamese-based Creole language is widely used in Nagaland and parts of Assam. Nefamese is an Assamese-based pidgin used in Arunachal Pradesh. Small pockets of Assamese speakers can be found in Bangladesh.
It is one of the 22 officially recognized languages in India and is also spoken in Bhutan. Assamese is a member of the Indo-Aryan branch of the Indo-European language family, which also includes languages such as Bengali, Hindi, and Punjabi. It is closely related to Bengali and shares many similarities in terms of grammar and vocabulary. Assamese has a rich literary tradition dating back to the 13th century, with famous poets such as Sankardev and Madhavdev. The language is also known for its rich folk literature, which includes stories, songs, and ballads. In terms of writing system, Assamese uses the Assamese script, which is a variant of the Bengali script. It is a complex script with multiple diacritical marks, making it challenging for non-native speakers to learn. In recent years, there has been a growing awareness and interest in preserving and promoting the Assamese language. There have been efforts to include the language in the education system and to make it more widely spoken and understood. Assamese is an important language with a rich cultural and literary heritage. It is a vital part of the identity of the people of Assam and plays a crucial role in preserving and promoting the cultural heritage of the region.
ওড়িয়া (ଓଡ଼ିଆ oṛiā) ইণ্ডো-ইউৰোপীয় ভাষা পৰিয়ালৰ ইণ্ডো-আর্য শাখাৰ এটি ভাষা। অসমীয়া আৰু বাংলা ভাষাৰ লগত ভাষাটোৰ যথেষ্ট মিল আছে। এই ভাষাৰ মাতৃভাষী সংখ্যা প্রায় ৩ কোটি ১০ লাখ (১৯৯৬ চনৰ হিচাব অনুযায়ী), যাৰ ভিতৰত অধিকাংশই ওড়িশা (উৰিষ্যা) ৰাজ্যৰ অধিবাসী। ইয়াৰ উপৰিও পশ্চিমবঙ্গ আৰু ঝাড়খণ্ড ৰাজ্যতো ওড়িয়া ভাষা প্রচলিত। ওড়িয়া ভাৰতৰ ২২টা স্বীকৃতিপ্ৰাপ্ত ভাষাৰ মাজত এটা; ই ওড়িশাৰ চৰকাৰী ভাষা আৰু ঝাৰখণ্ডৰ দ্বিতীয় চৰকাৰী ভাষা
How to type in Assamese?
Spoken In : India and Bangladesh. Assam, Arunachal Pradesh, Nagaland - (Assamese or a dialogue variant of Assamese) and some other parts of North-East India and smaller pockets of speakers in Pune - Maharashtra, Uttar Pradesh, Delhi and Bangalore, Karnataka, Kolkata - West Bengal among others
Native Speakers : 19 million (2007)
Language family : Indo-European > Indo-Iranian > Indo-Aryan > Eastern > Bengali–Assamese > Assamese
Writing system : Assamese alphabet, Assamese Braille
Official language in : Assam, India
Regulated by : VAsam Sahitya Sabha
Language codes : as (ISO 639-1), asm (ISO 639-2, ISO 639-3)
The word Assamese is an English formation built on the same principle as Japanese or Vietnamese etc. It is based on the English word Assam by which the tract consisting of the Brahmaputra Valley and its adjoining areas are known. The people call their state Ôxôm and their language Ôxômiya.
Assamese Language References
- "A History of Assamese Literature" by Hiren Gohain, published by Oxford University Press in 1999. This book provides a comprehensive overview of the literary tradition of Assamese language from the earliest times to the present day.
- "Assamese: An Introduction to the Language, Script, and Culture" by R.K. Baruah, published by Cambridge University Press in 2008. This book is a comprehensive guide to the Assamese language, including its grammar, vocabulary, and script.
- "Sociolinguistics of Assamese" by Nirode K. Barooah, published by M.D. Publications Pvt. Ltd. in 2000. This book provides an in-depth analysis of the sociolinguistic aspects of Assamese, including language use, language attitudes, and language planning.
- "Assamese: A Study of its Syntax and Vocabulary" by George Abraham Grierson, published by Asian Educational Services in 1984. This book provides a detailed study of the grammar and vocabulary of the Assamese language.
- "The Oxford Introductions to Language Study: Assamese" by R.K. Baruah, published by Oxford University Press in 1996. This book is a comprehensive introduction to the Assamese language, including its history, structure, and usage.
- "Assamese: A Descriptive Analysis" by Joseph Barpujari, published by Indian Institute of Advanced Study in 1980. This book provides a detailed analysis of the phonetics, phonology, and grammar of the Assamese language.
- "Assamese-English Dictionary" by J.T. Staley and S.K. Bhuyan, published by Motilal Banarsidass Publishers in 1999. This is a comprehensive English-Assamese dictionary, which includes a vast collection of words and idiomatic expressions.
- Government: National Portal of India : india.gov.in
- Government of Assam: www.assam.gov.in
- Wikipedia : en.wikipedia.org/wiki/Assamese_language
- Assamese Language and History
- Asam Sahitya Sabha : https://en.wikipedia.org/wiki/Asam_Sahitya_Sabha
- ›
লাচিত অভিধান
অসমীয়া-ইংৰাজী শব্দাৰ্থ | বৈজ্ঞানিক পৰিভাষা | প্ৰশাসনিক পৰিভাষা.
Academia.edu no longer supports Internet Explorer.
To browse Academia.edu and the wider internet faster and more securely, please take a few seconds to upgrade your browser .
Enter the email address you signed up with and we'll email you a reset link.
- We're Hiring!
- Help Center
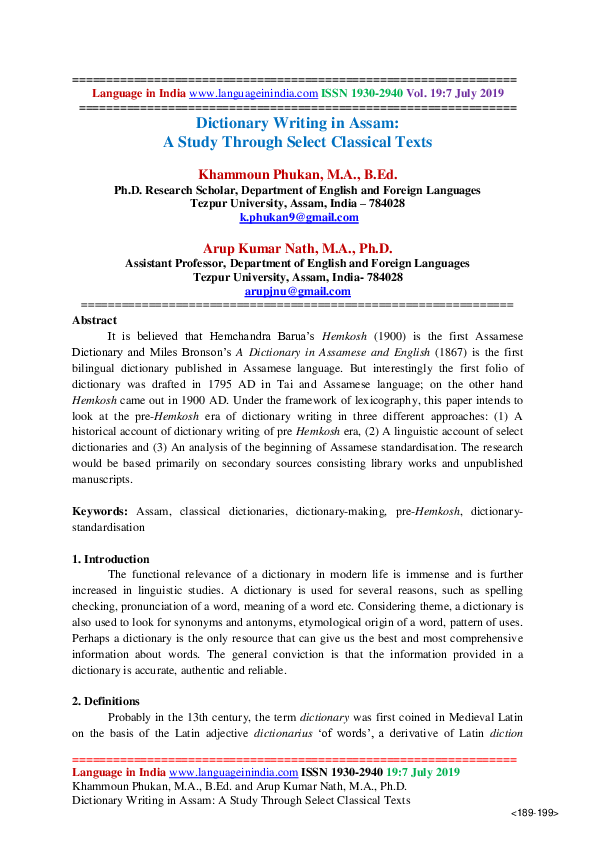
Dictionary Writing in Assam: A Study Through Select Classical Texts

2019, Language in India
It is believed that Hemchandra Barua’s Hemkosh (1900) is the first Assamese Dictionary and Miles Bronson’s A Dictionary in Assamese and English (1867) is the first bilingual dictionary published in Assamese language. But interestingly the first folio of dictionary was drafted in 1795 AD in Tai and Assamese language; on the other hand Hemkosh came out in 1900 AD. Under the framework of lexicography, this paper intends to look at the pre-Hemkosh era of dictionary writing in three different approaches: (1) A historical account of dictionary writing of pre Hemkosh era, (2) A linguistic account of select dictionaries and (3) An analysis of the beginning of Assamese standardisation. The research would be based primarily on secondary sources consisting library works and unpublished manuscripts. Keywords: Assam, classical dictionaries, dictionary-making, pre-Hemkosh, dictionary-standardisation
Related Papers
Suman Bhandary
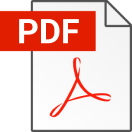
Orientalia Suecana vol.71
Gunilla Gren-Eklund
Some scholars of Indology in the 2010s came across one long-hidden manuscript, with a title page in Devanāgarī script dated to 1703, in the Historical Archive of Congregatio de Propaganda Fide in Rome. Despite being entitled Thesaurus Linguae Indianae, the manuscript does not contain a dictionary. Still, its central part is a complete grammar of the Hindustani language, dated 1704 and written by a Capuchin friar and missionary to India, François Marie de Tours. The finding later led to a rediscovery in Paris of two manuscripts, dated 1703, of the Thesaurus missing in the Rome manuscript by the same author. One is supposedly the original, and the other is a later copy produced in 1784. These three manuscripts in Rome and Paris have a common but forgotten history by scholars in the field. In this article, their relation and history will be revealed in detail and contextualised, firstly in a section in which the manuscripts are closely described, and secondly in a section about the author as much as is known. A third section will follow the history of the Thesaurus manuscripts in Paris up to the present day. The complete Thesaurus has, through a project at Uppsala University, been made freely available online for linguistic research at https://www.webonary.org/hindustani/en/
Dr. Ketaki Modak
There was a rich tradition of dictionary-making in Sanskrit, but in the mid-age the tradition seems to be lost. But again the advent of the British made it necessary to have dictionaries at their disposal. It was their dire need to communicate with the local people. So they initiated the work of dictionary making. It is found that the Princely states at that time played a vital role in this endeavour. This research paper throws light on it. Along with this an interesting fact is revealed here. Gujaratis as it is basically a mercantile community also needed to learn as many languages as they could. So it is found that the herculean task regarding multilingual dictionary-making is done in Gujarat provinces. With the help of governmental support and state-of-art technology at our disposal, any linguistic community should seriously think and must undertake the project to make multilingual dictionary.
Prof. Niladri Sekhar Dash
Dr. Parman Singh
Some Problems of Indo-European Lexicography, In: Meaning and Lexicography, edited by Jerzy Tomaszczyk and Barbara Lewandowska-Tomaszczyk, Amsterdam-Philadelphia 1990, John Benjamins Publishing Co., pp. 315-325.
Krzysztof Witczak
Ramkrishna Bhattacharya
The paper identifies S.K. N. CHAUDHURI, a Calcutta-based steno-typist whose assistance is acknowledged in the second edition of the 'Concise Oxford English Dictionary ' by Fowler, the compiler of this lexicon. Besides a biographical note oN the contributor, extracts from Fowler's letters to NAG CHAUDHURI are provided.
Baptist Mission Press, Circular Road.
One of the oldest dictionary of Hindustani and English language.
James Lambert , James Lambert
Preprint version of Lambert, James (2012). Beyond Hobson-Jobson: Towards a new lexicography for Indian English. "English World-wide", 33(3): 292–320. (DOI: 10.1075/eww.33.3.03lam). Abstract: Indian English is one of the most important and widely-spoken varieties of English, and yet at present the pinnacle of lexicographical treatment of the variety remains the well-renowned and much feted Hobson-Jobson, originally published in 1886, with a second edition in 1903. British English has the Oxford, American English the Webster's, Australian English the Macquarie, but Indian English must do with a dictionary over a century out of date. More recent lexicographical works that focus on Indian English suffer from a number of deficiencies that do not do the variety due justice. This paper analyses a selection of the currently available dictionaries on Indian English in order to identify these deficiencies. Finally, suggestions are made as to possible dictionary projects that may move Indian English lexicography, and the lexicography of other new Englishes, beyond Hobson-Jobson and towards the twenty-first century.
James Lambert
Preprint version of Lambert, James (2014). Diachronic stability in Indian English lexis. "World Englishes", 33(1): 112–127. (DOI: 10.1111/weng.12072). Abstract: In the late 1930s two British commentators, A.F. Kindersley and R.C. Goffin, published articles on various linguistic features (semantic, grammatical, orthographical, and phonological) of the English language as used in India. These two glossaries offer a valuable insight into late-Raj Indian English. In order to assess the extent of change over the intervening 70-plus years, a comparison of the content of those glossaries to present-day Indian English has been made. The overall picture is one of surprising stability, especially given the strong resistance by educationalists and others who have long stigmatised local variations as 'errors'. This stability over time suggests a long-standing endonormativity that has hitherto not been recognised. Many of the features discussed have even longer stable histories, suggesting an even lengthier endonormativity. This type of diachronic investigation may have similar implications for many other varieties of English.
Loading Preview
Sorry, preview is currently unavailable. You can download the paper by clicking the button above.
RELATED PAPERS
Rupkatha Journal on Interdisciplinary Studies in Humanities
Shashikantha Koudur
Konstantinos Sampanis
Broadening Perspectives in the History of Dictionaries and Word Studies
Fredric Dolezal
Maheedas Bv
Studies in History
Bodhisattva Kar
Jaroslav Strnad
Mastering Languages, Taming the World. The Production and Circulation of European Dictionaries and Lexicons of Asian Languages (16th-19th centuries), edited by Michela Bussotti & François Lachaud
DEJANIRAH COUTO
Bipul Syam Purkayastha
Roland Steiner
The Indo-Aryans of Ancient South Asia: Language, …
Michael Witzel
The Tradition of Manuscript Writing and the Development of Libraries in Pre-Colonial Assam
Dr Raktim R A N J A N Saikia
Journal of the Dictionary Society of North America
ligeia lugli
Indian Journal of History of Science
Satarupa Dattamajumdar
Studies in People's History
Krishna Mohan Shrimali
Digital Dictionaries of South Asia. The Digital South Asia Library. Chicago, IL
David McAlpin
Journal Space and Culture, India Open access Journal
Esau Mangoya
Srinivasan Kalyanaraman
Biswadip Gogoi
IAEME PUBLICATION
IAEME Publication
The Routledge Handbook of Lexicography
Dr. Parman Singh , Arimardan Kumar Tripathi
RELATED TOPICS
- We're Hiring!
- Help Center
- Find new research papers in:
- Health Sciences
- Earth Sciences
- Cognitive Science
- Mathematics
- Computer Science
- Academia ©2024
English - Assamese translator
Characters:
Search in Browser for quick result --> Translate English to Assamese Translatiz
Common English Phrases in Assamese
Phrase | Meaning |
---|---|
Hi! | নমস্কাৰ! |
Good morning! | সুপ্ৰভাত! |
Good afternoon! | শুভ আবেলি! |
Good evening! | শুভসন্ধ্যা! |
Hello my friend! | নমস্কাৰ মোৰ বন্ধু! |
How are you? | আপোনাৰ কেনে? |
I'm fine, thank you! | মই ভালেই আছো, ধন্যবাদ! |
I missed you | তোমাক মিছ কৰিলো |
Thank you (very much)! | আপোনাক অশেষ ধন্যবাদ)! |
You're welcome! | আপোনাক স্বাগতম! |
Come in! | সোমাই আহাঁ! |
Have a nice day! | দিনটো শুভ হওক! |
Phrase | Meaning |
---|---|
Good bye! | বিদায়! |
Happy birthday! | জন্মদিনৰ ওলগ জনালোঁ! |
What's your name? | আপোনাৰ নাম কি? |
Where do you live? | আপুনি ক’ত থাকে? |
Can I have your phone number? | আপোনাৰ ফোন নম্বৰটো মোৰ হাতত থাকিব পাৰিবনে? |
I love you | মই আপোনাক ভাল পাওঁ |
You're very special! | তুমি বৰ বিশেষ! |
I don't understand! | মই বুজি পোৱা নাই! |
Can you help me? | মোক সহায় কৰিব পাৰিবনে? |
Call the ambulance! | এম্বুলেন্সলৈ ফোন কৰক! |
Call a doctor! | ডাক্তৰক মাতিব! |
Call the police! | পুলিচক মাতিব! |
The culture and people were very interesting | সংস্কৃতি আৰু মানুহবোৰ বৰ আকৰ্ষণীয় আছিল |
About English Language
According to Wikipedia.org , English is a West Germanic language and belongs to the Indo-European language family. English is spoken by the inhabitants of early medieval England. It is the official language of 53 countries. It is spoken as the first language and is spoken by 400 million people all over the world. English is the most commonly spoken second language in the world. It is the language used for International communication in all parts of the world. English is spoken to hold communications related to politics, science, media, or art. It is also the language of entertainment and is used for socializing as well. If you have a good command of the English language helps you get more opportunities in life and your career.
About Assamese Language
According to Wikipedia.org ,
Communicate easily by using the free English to Assamese online translator to instantly translate English words, messages, news, articles, phrases or documents to Assamese.
Type English online and instantly get Assamese translation with English to Assamese translator
You can now easily and accurately translate English to Assamese language with this tool. This tool will allow you to Translate English text into Assamese text . Translating words, sentences, and paragraphs into Assamese is not a difficult task anymore.
( 217 Votes, Notes: 4.9/5)
Frequently asked Questions about English to Assamese translator
How to translate english to assamese using online translation tool.
- Select the English as source language for translation.
- Select the Assamese as target translation language.
- Enter the English words, phrases, scentenses or pargraph that you want to translate.
- Click the translate button and you will get the English to Assamese translation immediately.
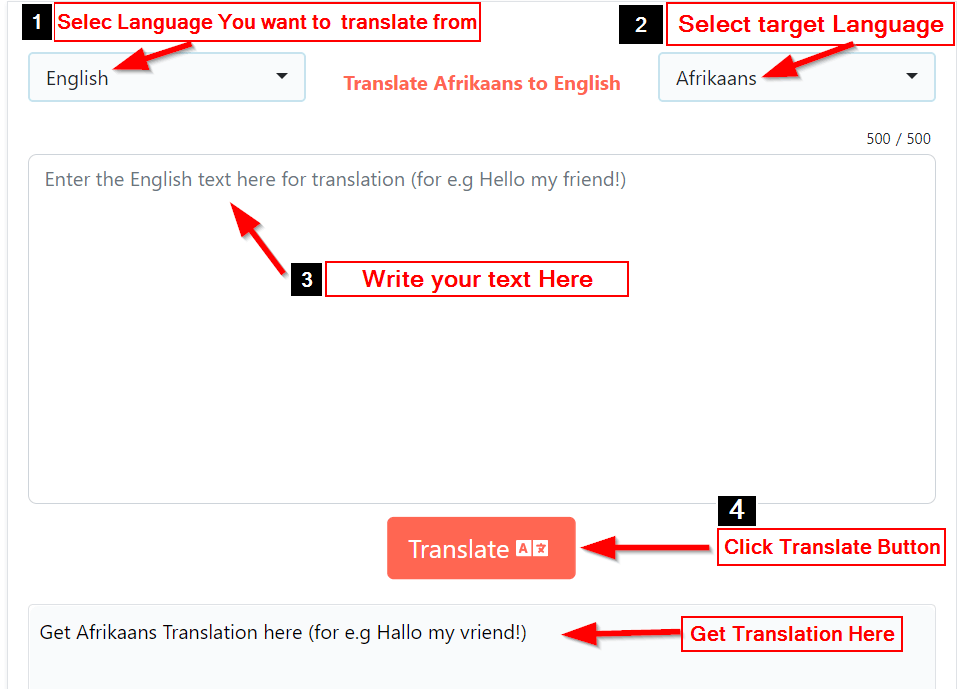
Can i translate Assamese to English?
Yes, You can translate Assamese to English with our online translation tool. Check Translate Assamese to English
How accurate is this English to Assamese translation tool?
Our English translator tool provides the most accurate Assamese translation because it uses the world's best machine translation engine powered by Google Api, To achieve the best possible quality of Assamese translation, make sure that the English text is grammatically correct.
Which is the best English to Assamese translator and How it can help me to translate Assamese?
Our tool uses machine translation powered by Google Api , Microsoft Translate , and Yandex. This tool lets users to get the best English to Assamese translation, it can translate English to 144 languages. If you need more accurate human English to Assamese translation service, use Translate from English to Assamese .
Is this English to Assamese translation free?
Yes, English to Assamese translator comes at no cost to you, and there is no daily limit. However, to ensure that the English to Assamese Translation service is used in a fair manner, We limit 1000 characters of text per conversion.
How long does it take to convert English into Assamese?
You can translate English words, phrases, and sentences to Assamese in a few seconds.
An official website of the United States government
Here's how you know
Official websites use .gov A .gov website belongs to an official government organization in the United States.
Secure .gov websites use HTTPS A lock ( Lock Locked padlock ) or https:// means you’ve safely connected to the .gov website. Share sensitive information only on official, secure websites.
What is the Meaning of the Transgender Flag?
What does it mean to be truly represented and valued within your organization? This is an important question for many diverse groups, and the LGBTQIA+ community is no exception. Each June, NIH celebrates Pride Month to honor the extraordinary LGBTQIA+ individuals who contribute to our workforce. For the next 30 days, the transgender flag will wave above Building 1 on NIH’s Bethesda campus. The transgender flag is an important symbol for the LGBTQIA+ community and their allies , and without transgender people, there would be no Pride.
Origins of Pride: Exploring the History and Meaning of the Transgender Flag
“Seeing the transgender flag fly at NIH on our day of visibility makes me feel safe, supported, and protected like nothing else. It is an unequivocal statement that we are a united community. [The flag represents] that everyone, including transgender and gender non-conforming people, belongs here. Now more than ever, it is vital to shine this light of love, tolerance, and understanding for all to see." - Willow, MSW, Program Analyst, Sexual & Gender Minority Research Office
Marsha P. Johnson, a gay, Black transwoman, and Sylvia Rivera, a Puerto Rican transwoman were two of “the most prominent figures of the gay rights movement of the 1960s and 1970s in New York City.” Best known for their courageous resistance at the Stonewall Inn on June 28, 1969, Marsha and Sylvia were instrumental in “[advocacy] for homeless LGBTQ[I]+ youth, those affected by HIV and AIDS, and gay and transgender rights” according to the National Women’s History Museum.
These inspiring women embodied how equality is an intersectional endeavor and that transgender rights uplift the rights of all people. To strive for transgender rights is to strive for human rights.
“To see the transgender flag displayed at NIH on Transgender Day of Visibility reminded me that despite all the anti-trans rhetoric in recent years, there is a powerful movement underway to recognize and celebrate difference as a core principle by which we must all continue to aspire.” - Courtney White, Senior Administrative Officer, National Institute of Mental Health
A Flag for Every Identity: Representation Around the World
Marsha and Sylvia’s intersectional identities and persistent work toward equality are why NIH and people around the world raise the transgender flag. Originally created by transwoman and Navy veteran, Monica Helms , in 1999, this important symbol made its debut in 2000 at a Pride parade in Phoenix. Monica’s color choices are both deliberate and meaningful: “The light blue is the traditional color for baby boys [and] pink is [traditionally] for girls, and the white in the middle is for those who are transitioning, those who feel they have a neutral gender or no gender, and those who are intersexed.” Monica helped preserve transgender history in 2014 when she donated multiple objects to the Smithsonian Institution which included a miniature transgender flag. The flag remains a permanent collection item at the National Museum of American History .
“Seeing the transgender flag displayed in front of Building 1 reinforces the positive vibe I have received as a female transgender [person] from colleagues at NIH since my transition in 2013. The flag is a symbol of NIH's commitment to support[ing] people like me, which makes me feel proud as well as serene.” - Michelle Boyle, Special Assistant to Director/Deputy Director
Inclusivity at NIH: Honoring Our Colleagues and Creating a Diverse Workforce for Everyone
We honor the steadfast determination of the LGBTQIA+ community to achieve recognition, acceptance, and equality. For members of NIH’s workforce who identify as transgender or gender-nonconforming, the flag can serve as a source of pride, visibility, and validation. The flag can also help to create a sense of belonging among LGBTQIA+ employees and send a clear message that our agency is committed to creating an inclusive and supportive environment for everyone, regardless of their gender identity or expression.
Be a part of building that environment by taking a SafeZone Training offered throughout the year or visit our Sexual and Gender Minority page for more information about the portfolio.
Do you have a story idea for us? Do you want to submit a guest blog? If it's about equity, diversity, or inclusion, please submit to [email protected] .
For news, updates, and videos, follow or subscribe to EDI on: Twitter , Instagram , Blog , YouTube .
Thank you for visiting nature.com. You are using a browser version with limited support for CSS. To obtain the best experience, we recommend you use a more up to date browser (or turn off compatibility mode in Internet Explorer). In the meantime, to ensure continued support, we are displaying the site without styles and JavaScript.
- View all journals
- Explore content
- About the journal
- Publish with us
- Sign up for alerts
- Open access
- Published: 16 September 2024
Deep behavioural representation learning reveals risk profiles for malignant ventricular arrhythmias
- Maarten Z. H. Kolk ORCID: orcid.org/0000-0003-0624-1510 1 , 2 ,
- Diana My Frodi ORCID: orcid.org/0000-0001-7074-5575 3 ,
- Joss Langford ORCID: orcid.org/0000-0002-3204-5866 4 , 5 ,
- Tariq O. Andersen 6 ,
- Peter Karl Jacobsen ORCID: orcid.org/0000-0002-1520-8774 3 ,
- Niels Risum 3 ,
- Hanno L. Tan 1 , 7 ,
- Jesper Hastrup Svendsen ORCID: orcid.org/0000-0001-8466-8515 3 , 8 ,
- Reinoud E. Knops 1 , 2 ,
- Søren Zöga Diederichsen ORCID: orcid.org/0000-0002-9687-0857 3 &
- Fleur V. Y. Tjong ORCID: orcid.org/0000-0001-9608-0081 1 , 2
npj Digital Medicine volume 7 , Article number: 250 ( 2024 ) Cite this article
Metrics details
- Arrhythmias
- Cardiovascular diseases
We aimed to identify and characterise behavioural profiles in patients at high risk of SCD, by using deep representation learning of day-to-day behavioural recordings. We present a pipeline that employed unsupervised clustering on low-dimensional representations of behavioural time-series data learned by a convolutional residual variational neural network (ResNet-VAE). Data from the prospective, observational SafeHeart study conducted at two large tertiary university centers in the Netherlands and Denmark were used. Patients received an implantable cardioverter-defibrillator (ICD) between May 2021 and September 2022 and wore wearable devices using accelerometer technology during 180 consecutive days. A total of 272 patients (mean age of 63.1 ± 10.2 years, 81% male) were eligible with a total sampling of 37,478 days of behavioural data (138 ± 47 days per patient). Deep representation learning identified five distinct behavioural profiles: Cluster A ( n = 46) had very low physical activity levels and a disturbed sleep pattern. Cluster B ( n = 70) had high activity levels, mainly at light-to-moderate intensity. Cluster C ( n = 63) exhibited a high-intensity activity profile. Cluster D ( n = 51) showed above-average sleep efficiency. Cluster E ( n = 42) had frequent waking episodes and poor sleep. Annual risks of malignant ventricular arrhythmias ranged from 30.4% in Cluster A to 9.8% and 9.5% for Clusters D-E, respectively. Compared to low-risk profiles (D-E), Cluster A demonstrated a three-to-four fold increased risk of malignant ventricular arrhythmias adjusted for clinical covariates (adjusted HR 3.63, 95% CI 1.54–8.53, p < 0.001). These behavioural profiles may guide more personalised approaches to ventricular arrhythmia and SCD prevention.
Similar content being viewed by others
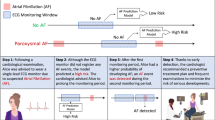
Prediction of atrial fibrillation from at-home single-lead ECG signals without arrhythmias
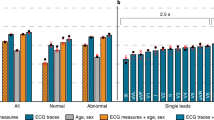
Prediction of mortality from 12-lead electrocardiogram voltage data using a deep neural network
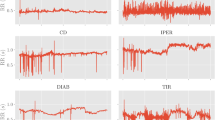

Detecting cardiac pathologies via machine learning on heart-rate variability time series and related markers
Introduction.
Malignant ventricular arrhythmias are a main cause of sudden cardiac death (SCD) 1 . In individuals at increased risk of SCD, patterns in physical behaviour (such as physical activity levels, sedentary behaviours, sleep behaviour) have emerged as potential prognostic indicators for ventricular arrhythmia onset, heart failure progression, and patient-reported outcomes 2 , 3 , 4 , 5 . Wearable accelerometers provide a means for continuous measurement of these day-to-day physical behaviours in free-living environments 6 . Identifying patterns or clusters within behavioural time-series data requires dimensionality reduction, as traditional clustering algorithms are unable to effectively process the granularity of such complex datasets. The process of dimensionality reduction, for instance reducing data to summary measures, may lead to the loss of intricate, non-linear associations in the data. Alternatively, deep neural networks are capable of learning low-dimensional latent representations from these complex datasets, while preserving the richness and intrinsic information present in the data 7 , 8 . Unsupervised machine learning algorithms can then operate on these latent space representations to categorise similar samples into one cluster 9 , 10 .
In this study, we aimed to identify and characterise behavioural profiles through deep representation learning in patients at risk of malignant ventricular arrhythmias (Fig. 1 ). Patients with an implantable cardioverter-defibrillator (ICD) were followed for six consecutive months using a wearable accelerometer to continuously monitor physical behaviour. A deep neural network was trained to learn a compressed representation from the behavioural time-series data while preserving the relevant information. We hypothesised that through the clustering of these deep behavioural representations, we would be able to identify clinically meaningful behavioural profiles. These profiles were evaluated for their clinical relevance and association with the risk of ventricular arrhythmia.
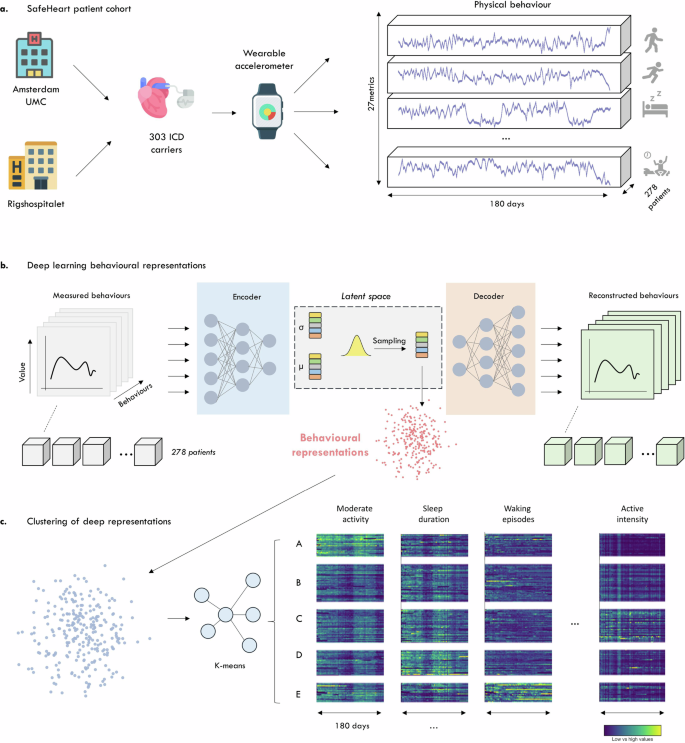
The workflow of the study is illustrated, that includes recruitment, data collection through a wearable device and data processing to identify behavioural profiles. a Recruitment of 303 patients from two international sites, who wore a wearable accelerometer for 180 consecutive days, during which behavioural metrics were recorded. b A convolutional residual variational autoencoder learned the latent behavioural representations. The models was provided with time-series data for each subject that consisted of 27 variables measured at 180 timepoints. c Unsupervised clustering using a k-means algorithm of the behavioural representations identified distinct behavioural profiles.
A total of 303 participants were enroled in SafeHeart, of which 272 met the eligibility criteria for this study (21 patients did not wear the GENEActiv wearable accelerometer, 10 patients did not meet the required minimum of 30 days of behavioural data). A total of 37,478 days of wearable data were collected (mean 138 ± 47 days per patient). Table 1 shows the clinical characteristics of the patient cohort. Patients had a mean age of 63.1 ± 10.4 years and 80.9% were male, 133 (48.9%) patients had ischaemic heart disease as cause of heart failure, 147 (54.0%) had heart failure with reduced ejection fraction (HFrEF), and 187 (68.8%) had a secondary prevention ICD indication. Fifty (18.4%) patients received cardiac resynchronisation therapy (CRT), the majority of patients used a β-blocker (80.5%). All patients completed one-year of follow-up, during which 46 (16.9%) patients received appropriate ICD therapy for a malignant ventricular arrhythmia, five (1.8%) patients received inappropriate ICD therapy and four (1.4%) patients died.
Characteristics of the identified behavioural profiles
Five behavioural profiles were identified: A ( n = 46), B ( n = 70), C ( n = 63), D ( n = 51) and E ( n = 42). Mean values for behavioural metrics across the clusters are displayed in Table 2 , Supplementary Fig. 4 provides a granular representation of behaviours during the 180-day monitoring period. Figure 2 shows the individual behavioural metrics for each profile, relative to the cohort averages. In summary, Clusters B and C were characterised by active profiles with high daily steps counts (15353 ± 4062 & 13577 ± 4130 steps). The volume of activity (2921 ± 1410 g s) in Cluster B was accumulated over a longer period (370 ± 76 min) and at a lower average intensity (123 ± 17 m g ), while the volume of activity (2527 ± 810 g s) in Cluster C was linked to a greater number of faster walking steps (3689 ± 2100 steps) at higher cadences (92 ± 10 steps/min) and intensities (132 ± 22 g s). Clusters D and E had less active profiles with fewer daily steps (9971 ± 3103 & 9291 ± 3378 steps). Cluster D had the longest inactive bout durations and fewest number per day (0.72 ± 0.25 mins and 632 ± 110). The behavioural patterns of Cluster E were more fragmented with shorter active bouts (0.43 ± 0.12 min) and more inactive bouts (863 ± 135). Cluster A had the highest inactive duration (835 ± 110 min), the lowest activity intensity (103 ± 33 m g ) and least number of steps (6246 ± 2406 steps). In the sleep domain, Cluster A was characterised by the shortest total sleep duration (281 ± 86 min), the longest average duration of wake after sleep onset interruptions (6.8 ± 2.1 min) and the longest sleep onset latency (10.8 ± 5.1 min). The nocturnal patterns of Cluster E were fragmented, similar to the day, with the lowest sleep efficiency (51.5 ± 8.6%), most wake after sleep onset interruptions (32 ± 8) and shortest maximum sleep bout lengths (42 ± 10 min). Cluster D had the long sleep interval and total sleep durations (611 ± 122 and 369 ± 75 min) with longest maximum sleep bout lengths (57 ± 11 min). The sleep profiles of Clusters B and C were unexceptional other than Cluster B having the shortest sleep interval duration (507 ± 89 min).
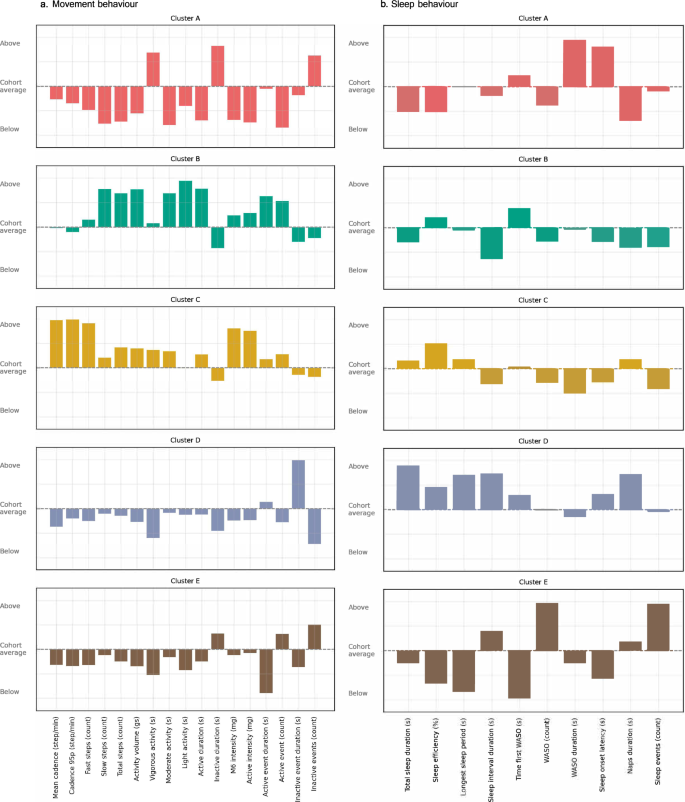
The average values for the behavioural measurements for each behavioural profile are displayed. a The bar charts displayed on the left depict the average values for the metrics that reflect movement behaviour across behavioural profiles, relative to the cohort average. b Bar charts depicted on the right display the average values for the metrics that reflect sleep behaviours across the behavioural profiles. All values were scaled using z-scores.
Cluster characterisation
SHAP values were computed to represent the most important behavioural markers that characterised each behavioural profile. Figure 3a shows the variables with highest feature importance across behavioural profiles. The duration spent in moderate activity, the amount of slow steps and the number of sleep events were the behavioural markers that differentiated most between clusters. Figure 3b–f illustrates the top behavioural features that predict membership of each of the clusters. Clusters C and E were predicted by a combination of sleep behaviours and movement behaviours, while the other clusters were predominantly predicted by movement behaviours alone. No statistically significant differences were observed between clusters in terms of medication usage and medical history, apart from hypertension ( p = 0.037) (Table 1 ).
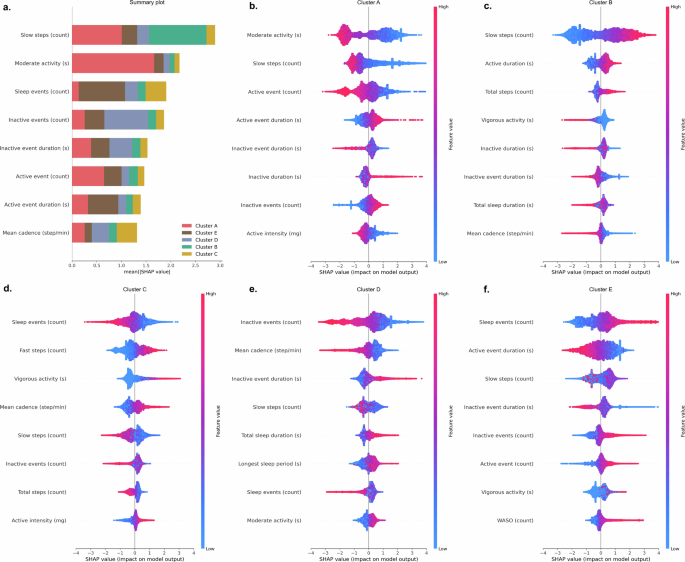
A trained machine learning classifier (extreme gradient boosting) was used to predict membership of a profile based on daily behavioural measurements. a The bar chart represents the importance of features used by the extreme gradient boosting model to predict each profile. Horizontal bars represent the average contribution of a behavioural metric for the predicted profile. The features are ranked based on the summed importance of that feature to predict each profile. b – f The SHAP summary plot is displayed for each behavioural profile. The features are ranked by the mean absolute SHAP value. A positive SHAP value suggests a positive contribution, while a negative value indicates a negative contribution. The model predicted membership of the behavioural profile based on the daily measurements with an AUROC of 0.99.
Patient-reported outcomes across behavioural profiles
A total of 239 patients filled out questionnaires at the study baseline (non-response rates for subsequent clusters A-E were 11.4%, 15.6%, 12.7%, 9.5%, and 15.2%, respectively). Median scores for the EQ-5D-5L and KCCQ domains are provided in Supplementary Table 2 . In particular, Cluster A reported physical limitations, Cluster C reported high self-efficacy but worse social limitations, Cluster D highest disease-specific quality of life, and Cluster E reported highest burden of symptoms (Supplementary Fig. 5 ). Differences in patient-reported outcomes between clusters were not statistically significant.
Incidence of the outcomes of interest across behavioural profile
Figure 4a shows the risk of malignant ventricular arrhythmias treated by the ICD across the clusters during one-year follow-up. Event rates for clusters A until E were respectively 30.4%, 17.1%, 17.5%, 9.8% and 9.5% (log-rank p value 0.06). As displayed in Fig. 4b , the risk of malignant ventricular arrhythmias was significantly higher in Cluster A (unadjusted HR 2.26, 95% CI 1.20–4.23, p = 0.01), which remained after adjusting for clinical covariates (adjusted HR 2.30, 95% CI 1.21–4.36, p = 0.01). Also, the risk of malignant ventricular arrhythmias in the low-risk behavioural profiles (Cluster D-E) was significantly lower compared to the other clusters (unadjusted HR 0.45, 95% CI 0.22–0.94, p = 0.03). Inappropriate ICD therapy was delivered in three patients in Cluster A (4.3%), two patients in Cluster B (2.9%), and one patient in Cluster C (1.6%). In total four patients died during follow-up, of which two in Cluster D (3.9%), one in Cluster A (2.2%) and one in Cluster C (1.6%). A significant difference in the composite endpoint between clusters was observed (log-rank p value 0.04) (Fig. 4c ). Unadjusted and adjusted hazard ratios for the respective clusters for the composite endpoint are displayed in Table 3 . In Supplementary Fig. 6 , ROC curves of logistic regression models predicting cases of malignant ventricular arrhythmias and the composite endpoint are presented. Regression models that included cluster membership within their feature set demonstrated superior performance, compared to models that excluded this variable.
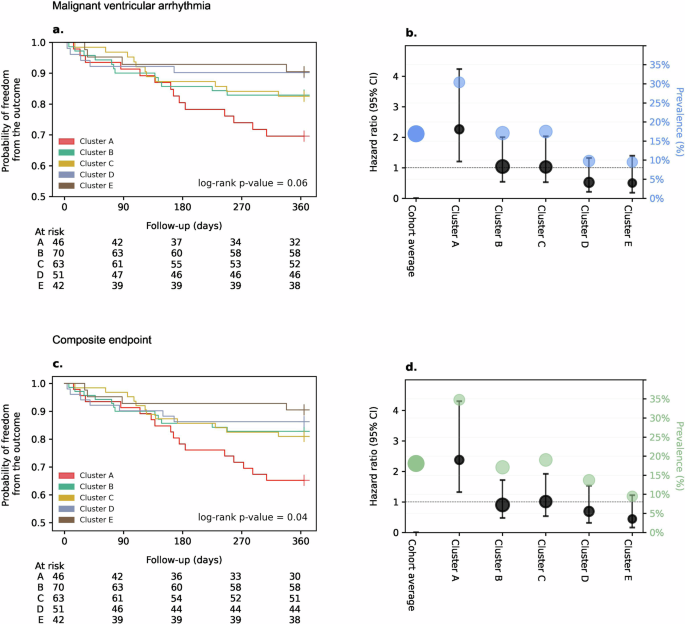
Time-to-event analyses according to the behavioural profiles are presented. a Kaplan-Meier curves for malignant ventricular arrhythmias treated by the ICD, and b hazard ratios and 95% confidence intervals obtained from the Cox proportional-hazards model. c Kaplan-Meier curves for the composite endpoint of all ICD therapy and mortality, and d hazard ratios and 95% confidence intervals. The prevalence of the outcome is displayed as a percentage, represented by the blue and green circles. Distributions of times to events were compared with the log-rank test.
In this study, we demonstrated deep representation learning of complex day-to-day movement and sleep behaviours to enable the identification of clinically relevant behavioural profiles. These profiles were associated with an annual risk of malignant ventricular arrhythmias ranging from 30.4% to 9.5%. Our research extends prior work, bringing forth two novelties. First, while prior studies have evaluated physical behavioural metrics over monitoring intervals up to 14 days 3 , we identified distinct behavioural profiles derived from continuous accelerometer measurements spanning six months. Second, earlier studies have mainly focused on individual metrics for activity or sleep, despite these 24-hour rest-activity behaviours being highly interrelated. In the present work, we took a more holistic approach to physical behaviour by modelling the interplay between various concurrent behavioural mechanisms and their potential implications for clinical events.
Despite considerable variations in clinical trajectories among patients with an ICD, current follow-up strategies remain one-size-fits-all . Advances in wearable technologies have removed barriers for the continuous measurement of behavioural patterns, which could make wearables suitable as screening tools to identify individuals at-risk of disease progression. Several studies have shown that continuous activity measurements could indicate a decline in functional status, progression of heart failure, or onset of atrial fibrillation, each potentially increasing the risk of ventricular arrhythmia onset 3 , 4 , 11 , 12 . However, physical activity is also a modifiable risk factors that may reduce ventricular arrhythmia risk by alteration of autonomic tone, mitigation of the catecholamine release observed during exercise and an increase of resting parasympathetic tone 13 . Recent analyses of data from the UK Biobank have demonstrated a reduction in the risk of ventricular arrhythmia amongst physically active individuals 14 , 15 . With data from this prospective study, we demonstrated that an active behavioural profile does not necessarily reduce the risk of ventricular arrhythmia, which highlights the importance of considering various behaviours simultaneously. In particular, Clusters B and C had annual event rates of ~17% despite their daily time spent physically active being substantially higher compared to the other profiles. In contrast, Clusters D and E were half as likely to experience the outcome of interest, despite having less active profiles. This indeed suggests that interplays between various behaviours, such as intermittent sedentary behaviour with isolated bouts of physical exertion, rather than isolated measurements of activity characteristics, may explain differences in risk of ventricular arrhythmia onset. Furthermore, the absence of significant associations between patient-reported outcomes (e.g. symptom severity and physical limitations) and behavioural profiles might indicate that these are phenotypic in their origin rather than representing more transient behavioural patterns.
Our findings support the notion that abnormalities in 24-hour rest-activity patterns modulate the risk of ventricular arrhythmia onset. Circadian rhythm disruption has been associated with increased risk of atrial fibrillation onset 16 and heart failure 17 in previous studies. We observed an annual risk of ventricular arrhythmia exceeding 30% in the behavioural profile characterised by sedentary behaviour, a lack of high-intensity activity, and disturbed sleep behaviour. Adjusted for clinical covariates, this profile was associated with a three-to-four fold risk of experiencing a ventricular arrhythmia compared to the low-risk profiles. While these findings should be validated in larger cohorts, they emphasise the importance of comprehensive modelling of physical behaviour. The use of wearable devices for behavioural profiling holds promise for follow-up strategies tailored to an individual patient.
Clustering of deep learning-derived latent representations comes with the limitation of interpretability, as the latent representations are inferred from the underlying data and are not directly explainable ( black box ). To provide transparency, we characterised clusters by assessment of feature importance of a trained machine learning classifier that predicts cluster memberships based on day-to-day behavioural metrics 18 . A second limitation to our study is the use of processed output from the accelerometer, instead of the underlying raw accelerometry output. Some of these metrics are created through application of specific thresholds that rely on the calibration studies, but pose a challenge when comparing metrics among different studies or populations 19 . Third, from our findings, it remains uncertain whether the behavioural profiles can be generalised to other populations, such as heart failure patients who do not satisfy the criteria for an ICD, and thus warrant future research. Fourth, despite cluster membership showing significant associations with the outcome of interest after adjusting for clinical covariates, there is a risk of residual confounding. For instance, high-risk behavioural profile (Cluster A) was characterised by higher proportion of patients with a prior myocardial infarctions; however, these patients did not receive prescriptions for β-blockers, lipid-lowering drugs, or ACE inhibitors. This could point towards a potential undertreatment of these patients. This study was entirely decentralised in its design, with patient recruitment, informed consent, and study procedures conducted without physical contact between the study staff and participants. Consequently, information from imaging modalities (e.g., LVEF) and electrocardiography at the time of enrolment was not available. Future studies exploring the interplay between these clinical patient characteristics, and behavioural profiles are warranted.
Deep representation learning of physical behavioural patterns identifies distinct behavioural profiles with significant differences in their risk of malignant ventricular arrhythmia and death. Behavioural profiling using objective and real-time measurements obtained from wearable devices may enable clinicians to adjust and optimise treatment and prevention strategies to an individual patient. Interpretability of clustered latent representations and relatively small sample sizes prompt the need for further investigation into the mechanisms underlying their influence on ventricular arrhythmia risk and SCD.
The study was approved by the Institutional Review Boards of the Amsterdam University Medical Center (date 09-04-2021, approval number 2020/248) and Copenhagen University Hospital Rigshospitalet (date 19-04-2021, approval number H-20081068). All participants provided informed consent prior to their enrolment. The study was conducted in accordance with the Declaration of Helsinki.
Study design and setting
This is an analysis of the international SafeHeart study, a prospective, observational study conducted at two tertiary academic centers in Europe (Amsterdam University Medical Center, the Netherlands and Copenhagen University Hospital Rigshospitalet, Denmark). The purpose of this study was to develop a personalised model to predict ICD therapy for malignant ventricular arrhythmia 20 . Data used to create the prediction model included recordings from a wearable accelerometry recording device. Patient inclusion was conducted through telephone-based procedures between May 2021 and September 2022, the enrolment date was defined as the day when the wearable device was delivered to the patient. Throughout the study, participants had the option to withdraw from the study at any stage, either partially (by discontinuing the use of the wearable device) or completely. We adhered to the Strengthening the Reporting of Observational Studies in Epidemiology (STROBE) reporting guidelines for observational studies 21 . The study was registered at the National Trial Registration in the Netherlands (Trial NL9218; https://www.onderzoekmetmensen.nl/en ).
Participants
Participants qualified for enrolment in the SafeHeart study if they fulfilled the following conditions: i) received an ICD with or without cardiac resynchronisation therapy (CRT-D) in the five years leading up to enrolment, ii) experienced appropriate or inappropriate ICD therapy (high voltage shock therapy or anti-tachycardia pacing (ATP)) or demonstrated evidence of ventricular arrhythmias within eight years prior to enrolment, iii) engaged in a remote ICD monitoring programme, and iv) were at least 18 years old. Exclusion criteria were severe physical disability, end-stage heart failure, and a life expectancy of less than one year. The study protocol with the entire list of inclusion and exclusion criteria has been published previously 20 .
Physical behaviour measurements
Accelerometer-based wearable devices allow for continuous and objective quantification of daily physical behaviour by the recording of body movement along reference axes and signal analysis (e.g., intensity, frequency, and volume of activity and postural changes). In this study, various behavioural metrics were collected including daily activity and inactivity durations, duration of activity and inactivity episodes, activity intensity, activity volume, step count (total, slow and fast), cadence, sleep duration, sleep efficiency, wake up after sleep onset (WASO), nap duration and sleep onset latency. A complete overview of the collected metrics and their definitions are displayed in Supplementary Table 1 . To collect these metrics, participants wore the GENEActiv Original 1.1 accelerometer (Activinsights Ltd, Cambridgeshire, United Kingdom) on the wrist for 6 months. Devices were returned (for data extraction) and replaced biweekly or every 4 weeks. Continuous raw data were recorded at 50 Hz or 20 Hz and converted into daily summaries 22 , 23 . Patients were eligible for this study if they had at least 30 days of wearable data.
Outcome of interest
The prospective collection of the outcomes of interest occurred at both sites from enrolment in the study onwards. These outcomes were: i) any malignant ventricular arrhythmia defined as an episode of sustained ventricular tachycardia or ventricular fibrillation, treated by the ICD through a shock and/or ATP; ii) a composite endpoint comprising all ICD therapies and death. ICD therapies encompassed those for malignant ventricular arrhythmias, in addition to those in response to rhythms other than sustained ventricular tachycardia or ventricular fibrillation (e.g. atrial fibrillation, sinus tachycardia).
Patient reported outcome measures
Two patient reported outcome measures (PROMs), the EuroQoL 5-Dimensions 5-Levels (EQ-5D-5L) and Kansas City Cardiomyopathy Questionnaire (KCCQ), were used in the SafeHeart study 24 , 25 . Both PROMs were filled out by participants at study enrolment. The EQ-5D-5L assesses health across five domains, yielding a utility score ranging from −0.590 to 1.000. Meanwhile, the KCCQ, designed for heart failure patients, provided scores on a scale of 0 to 100, subdivided into the domains symptom burden, physical limitation, social limitation, quality of life and self-efficacy.
Deep representation learning of physical behaviour data
We derived deep representations from the day-to-day behavioural time-series collected during the first six months of the study (Fig. 1a ). Specifically, we used a β-variational autoencoder (VAE) that encodes input data through a probabilistic approach (mapping data into a probability distribution) and decodes from this distribution back into reconstructed data (Fig. 1b ) 26 , 27 . Supplementary Fig. 1 presents a schematic overview of the VAE architecture. The inputs were longitudinal trajectories of 27 behavioural metrics over 180 days, resulting in an input dimension of 272 × 27 × 180. Missing values of behavioural metrics were linearly interpolated and normalised. Our trained VAE reconstructed the behavioural time-series with a Pearson Correlation Coefficient of 0.988 ± 0.0379, a root mean square error (RMSE) of 0.031 ± 0.026 and a percentage root-mean-square difference (PRD) of 10.553 ± 0.038. Supplementary Fig. 2 depicts an example of the trends in behavioural measurements along with the reconstructed trend derived from 32 latent variables. The VAE models were developed using PyTorch (version 2.0.5) in Python (version 3.6.7).
We then applied an unsupervised machine learning algorithm to cluster these representations (Fig. 1c ). The k-means algorithm aims to minimise the within-cluster variance, making data points within the same cluster as similar as possible and data points in different clusters as dissimilar as possible. The appropriate number of clusters was assessed using within-cluster variation (inertia), silhouette scores, and Davies-Bouldin index (Supplementary Fig. 3 ). Considering that the k-means algorithm operates stochastically, and initialisation of the model may affect the decision of the optimal k , we averaged the results over multiple iterations to reduce the impact of randomness 28 . We evaluated cluster stability by computing the Jaccard index across 100 bootstrapped samples 29 . Clustering was performed using the scitkit-learn library (version 1.3.0) 30 .
Cluster characterisation through cluster membership prediction
We aimed to characterise the identified clusters using SHapley Additive exPlanations (SHAP) values 31 . SHAP values are widely used to determine the contribution of particular features to the predicted outcome. We derived SHAP values from a supervised machine learning classifier (eXtreme Gradient Boosting), which was trained to predict cluster membership from daily behavioural values (48,960 days) 32 . Subsequent ranking of these SHAP values provides insight into behavioural metrics that contribute positively (or negatively) to cluster membership. We assessed the performance of these classifications using the receiver operating characteristic curve (ROC).
Statistical analysis
Continuous variables were presented by the median, mean, interquartile range, and standard deviation. Categorical socio-demographic and clinical variables were presented as frequencies (percentages) and compared using the χ 2 test. T-tests were used for pairwise comparisons, analysis of variance (ANOVA) for assessing differences among multiple groups with normally distributed data. The Mann–Whitney U test was used for non-normally distributed variables, and the Kruskal-Wallis test for comparisons involving more than two groups with non-normally distributed data. The risk of the outcomes of interest during follow-up was estimated using the Kaplan–Meier method; log-rank tests were used to compare survival between clusters. Cox Proportional Hazard models were used to assess the association between behavioural profiles and the risk of outcomes of interest. The model included the clinical covariates age, sex, indication for ICD implantation, presence of atrial fibrillation, heart failure, and type of ICD. Schoenfeld residuals were used to check the proportional hazards assumption. A two-sided p value < 0.05 was considered significant. The prognostic significance of the behavioural profiles for the outcomes of interest was assessed through logistic regression models. Two models were constructed for each outcome of interest: the first model included clinical patient information (medical history and medication status) along with cluster membership as input features, while the second model excluded cluster membership. Prediction accuracy was assessed through stratified k-fold cross-validation, and quantified using the area under the receiver operating characteristic curve (AUROC).
Data availability
Data sharing requests will be considered upon a reasonable request. For access, please email the corresponding author.
Code availability
Code scripts are available at: https://github.com/DeepRiskAUMC/Deep-representation-clustering .
Empana, J. P. et al. Incidence of sudden cardiac death in the European Union. J. Am. Coll. Cardiol. 79 , 1818–1827 (2022).
Article PubMed Google Scholar
Shim, J., Fleisch, E. & Barata, F. Wearable-based accelerometer activity profile as digital biomarker of inflammation, biological age, and mortality using hierarchical clustering analysis in NHANES 2011-2014. Sci. Rep. 13 , 9326 (2023).
Article CAS PubMed PubMed Central Google Scholar
Kolk, M. Z. H. et al. Accelerometer-assessed physical behavior and the association with clinical outcomes in implantable cardioverter-defibrillator recipients: A systematic review. Cardiovasc Digit Health J. 3 , 46–55 (2022).
Rosman, L., Lampert, R., Sears, S. F. & Burg, M. M. Measuring physical activity with implanted cardiac devices: a systematic review. J Am Heart Assoc 7, e008663 (2018).
Kolk, M. et al. Behavioural digital biomarkers enable real-time monitoring of patient-reported outcomes: a substudy of the multicenter, prospective observational SafeHeart study. Eur Heart J Qual Care Clin Outcomes qcad069, (2023).
Hughes, A., Shandhi, M. M. H., Master, H., Dunn, J. & Brittain, E. Wearable devices in cardiovascular medicine. Circ. Res 132 , 652–670 (2023).
Kolk, M. Z. H. et al. Machine learning of electrophysiological signals for the prediction of ventricular arrhythmias: systematic review and examination of heterogeneity between studies. EBioMedicine 89 , 104462 (2023).
Article PubMed PubMed Central Google Scholar
Kolk, M. Z. H. et al. Dynamic prediction of malignant ventricular arrhythmias using neural networks in patients with an implantable cardioverter-defibrillator. EBioMedicine 99 , 104937 (2023).
Min, E. et al. A survey of clustering with deep learning: from the perspective of network architecture. IEEE Access 6 , 39501–39514 (2018).
Article Google Scholar
Karim, M. R. et al. Deep learning-based clustering approaches for bioinformatics. Brief. Bioinform 22 , 393–415 (2021).
Ginder, C. et al. Predicting malignant ventricular arrhythmias using real-time remote monitoring. J. Am. Coll. Cardiol. 81 , 949–961 (2023).
Shakibfar, S. et al. Predicting electrical storms by remote monitoring of implantable cardioverter-defibrillator patients using machine learning. Europace 21 , 268–274 (2019).
Soares-Miranda, L. et al. Physical activity and heart rate variability in older adults: the Cardiovascular Health Study. Circulation 129 , 2100–2110 (2014).
Elliott, A. D. et al. Association between physical activity and risk of incident arrhythmias in 402 406 individuals: evidence from the UK Biobank cohort. Eur. Heart J. 41 , 1479–1486 (2020).
Qiu, S. & Xing, Z. Association between accelerometer-derived physical activity and incident cardiac arrest. Europace 25 , euad353 (2023).
Yang, L. et al. Association of accelerometer-derived circadian abnormalities and genetic risk with incidence of atrial fibrillation. NPJ Digit Med 6 , 31 (2023).
Liebzeit, D., Phelan, C., Moon, C., Brown, R. & Bratzke, L. Rest-activity patterns in older adults with heart failure and healthy older adults. J. Aging Phys. Act. 25 , 116–122 (2017).
Castela Forte, J. et al. Identifying and characterizing high-risk clusters in a heterogeneous ICU population with deep embedded clustering. Sci. Rep. 11 , 12109 (2021).
Rowlands, A. V. et al. Beyond cut points: accelerometer metrics that capture the physical activity profile. Med Sci. Sports Exerc 50 , 1323–1332 (2018).
Frodi, D. M. et al. Rationale and design of the SafeHeart study: Development and testing of a mHealth tool for the prediction of arrhythmic events and implantable cardioverter-defibrillator therapy. Cardiovasc Digit Health J. 2 , S11–S20 (2021).
von Elm, E. et al. The Strengthening the Reporting of Observational Studies in Epidemiology (STROBE) statement: guidelines for reporting observational studies. J. Clin. Epidemiol. 61 , 344–349 (2008).
Esliger, D. W. et al. Validation of the GENEA accelerometer. Med Sci. Sports Exerc 43 , 1085–1093 (2011).
van Hees, V. T. et al. A novel, open access method to assess sleep duration using a wrist-worn accelerometer. PLoS One 10 , e0142533 (2015).
Spertus, J. A. & Jones, P. G. Development and Validation of a Short Version of the Kansas City Cardiomyopathy Questionnaire. Circ. Cardiovasc Qual. Outcomes 8 , 469–476 (2015).
Herdman, M. et al. Development and preliminary testing of the new five-level version of EQ-5D (EQ-5D-5L). Qual. Life Res 20 , 1727–1736 (2011).
Kingma, D. P. et al. Improved Variational Inference with Inverse Autoregressive Flow. Vol. 29 (eds Lee, D.et al.) (2016).
Higgins, I. et al. beta-VAE: Learning Basic Visual Concepts with a Constrained Variational Framework. in International Conference on Learning Representations (2016).
Vouros, A., Langdell, S., Croucher, M. & Vasilaki, E. An empirical comparison between stochastic and deterministic centroid initialisation for K-means variations. Mach. Learn. 110 , 1975–2003 (2021).
Hennig, C. Cluster-wise assessment of cluster stability. Computational Stat. Data Anal. 52 , 258–271 (2007).
Pedregosa, F. et al. Scikit-learn: machine learning in python. J. Mach. Learn. Res. 12 , 2825–2830 (2011).
Google Scholar
Scott, M. L. & Su-In, L. A Unified Approach to Interpreting Model Predictions. ArXiv abs/1705.07874 (2017).
Tianqi, C. & Carlos, G. XGBoost: A scalable tree boosting system. In Proceedings of the 22nd ACM SIGKDD International Conference on Knowledge Discovery and Data Mining (2016).
Download references
Acknowledgements
This research was supported by the Horizon 2020 European Union funding programme for research and innovation (grant number: Eurostars project E!113994- SafeHeart). This research is partly funded by the Amsterdam Cardiovascular Sciences and research programme Rubicon which is financed by the Dutch Research Council (NWO).
Author information
Authors and affiliations.
Department of Clinical and Experimental Cardiology, Amsterdam UMC Location University of Amsterdam, Heart Center, Meibergdreef 9, Amsterdam, the Netherlands
Maarten Z. H. Kolk, Hanno L. Tan, Reinoud E. Knops & Fleur V. Y. Tjong
Amsterdam Cardiovascular Sciences, Heart Failure & Arrhythmias, Amsterdam UMC location AMC Meibergdreef 9, 1105 AZ, Amsterdam, the Netherlands
Maarten Z. H. Kolk, Reinoud E. Knops & Fleur V. Y. Tjong
Department of Cardiology, Copenhagen University Hospital Rigshospitalet, Inge Lehmanns Vej 7, 2100, Copenhagen, Denmark
Diana My Frodi, Peter Karl Jacobsen, Niels Risum, Jesper Hastrup Svendsen & Søren Zöga Diederichsen
Activinsights Ltd., Unit 11, Harvard Industrial Estate, Kimbolton, Huntingdon, PE28 0NJ, United Kingdom
Joss Langford
College of Life and Environmental Sciences, University of Exeter, Stocker Rd, Exeter, EX4 4PY, United Kingdom
Department of Computer Science, University of Copenhagen, Universitetsparken 1, 2100, Copenhagen, Denmark
Tariq O. Andersen
Netherlands Heart Institute, Moreelsepark 1, 3511 EP, Utrecht, The Netherlands
Hanno L. Tan
Department of Clinical Medicine, Faculty of Health and Medical Sciences, University of Copenhagen, Blegdamsvej 3B, 2200, Copenhagen, Denmark
Jesper Hastrup Svendsen
You can also search for this author in PubMed Google Scholar
Contributions
M.K., R.K., J.H.S., J.L., T.A., S.D. and F.T. contributed to the conception and design of the study. M.K., D.F., J.L. and F.T. collectively investigated the data and decided on the methodology to be used. M.K., F.T. and J.L. conducted the formal analyses. M.K. and F.T. drafted the original manuscript. M.K., D.F., J.L., T.A., P.K.J., N.R., H.T., J.H.S., S.D., R.K. and F.T. reviewed, edited, and agreed with the final version of the manuscript. M.K., J.L., D.F. and F.T. accessed and verified the underlying data.
Corresponding author
Correspondence to Fleur V. Y. Tjong .
Ethics declarations
Competing interests.
The authors declare no competing non-financial interests but the following competing financial interests. R.E.K. reports consultancy fees and research grants from Boston Scientific, Medtronic and Abbott. and has stock options from AtaCor Medical Inc. F.V.Y.T. declares grants or contracts from the Dutch Research Council (NWO) and Amsterdam Cardiovascular Sciences, and received payment or honoraria from Boston Scientific and Abbott (paid to the institution). S.Z.D. reports consultancy fees and research grants from Acesion Pharma and Cortrium, and has received payment or honoraria from Bristol, Myers Squibb, Pfizer and Bayer. P.K.J. reports consultancy fees and research grants from Abbott and Medtronic, payment or honoraria from Abbott and Medtronic and support for attending meetings and/or travel from Abbott and Medtronic. J.H.S. reports grants or contracts from Medtronic (payed to institution), payment or honoraria from Medtronic, support for attending meetings and/or travel from Abbott and Medtronic, participation on Medtronic Advisory Board, and stocks or stock options from Vital Beats. J.L. reports having stock or stock option from Activinsights Ltd. T.O.A. reports having stock or stock options from Vital Beats. D.M.F. reports financial support for attending meetings and/or travel from Boston Scientific. The authors M.Z.H.K., N.R. and H.L.T. declare no competing financial or non-financial interests.
Additional information
Publisher’s note Springer Nature remains neutral with regard to jurisdictional claims in published maps and institutional affiliations.
Supplementary information
Supplementary material, rights and permissions.
Open Access This article is licensed under a Creative Commons Attribution-NonCommercial-NoDerivatives 4.0 International License, which permits any non-commercial use, sharing, distribution and reproduction in any medium or format, as long as you give appropriate credit to the original author(s) and the source, provide a link to the Creative Commons licence, and indicate if you modified the licensed material. You do not have permission under this licence to share adapted material derived from this article or parts of it. The images or other third party material in this article are included in the article’s Creative Commons licence, unless indicated otherwise in a credit line to the material. If material is not included in the article’s Creative Commons licence and your intended use is not permitted by statutory regulation or exceeds the permitted use, you will need to obtain permission directly from the copyright holder. To view a copy of this licence, visit http://creativecommons.org/licenses/by-nc-nd/4.0/ .
Reprints and permissions
About this article
Cite this article.
Kolk, M.Z.H., Frodi, D.M., Langford, J. et al. Deep behavioural representation learning reveals risk profiles for malignant ventricular arrhythmias. npj Digit. Med. 7 , 250 (2024). https://doi.org/10.1038/s41746-024-01247-w
Download citation
Received : 12 January 2024
Accepted : 30 August 2024
Published : 16 September 2024
DOI : https://doi.org/10.1038/s41746-024-01247-w
Share this article
Anyone you share the following link with will be able to read this content:
Sorry, a shareable link is not currently available for this article.
Provided by the Springer Nature SharedIt content-sharing initiative
Quick links
- Explore articles by subject
- Guide to authors
- Editorial policies
Sign up for the Nature Briefing newsletter — what matters in science, free to your inbox daily.

Translation of "authority" into Assamese
এক্তিয়াৰ, কর্তৃপক্ষ, কৰ্তৃত্ব are the top translations of "authority" into Assamese. Sample translated sentence: b. Exchange of information and expertise between the Ministries and respective Civil Aviation Authorities related to aviation regulations, regional air operations, airworthiness requirements and safety standards to enhance safety and security of air transport; and / or ↔ (খ) বিমান পৰিবহণৰ ক্ষেত্রত সুৰক্ষা আৰু নিৰাপত্তা বৃদ্ধি কৰাৰ বাবে বিমানচালনা আইন, আঞ্চলিক বিমান চালনা, উৰাণ-যোগ্যতাৰ প্রয়োজনীয়তা তথা সুৰক্ষাজনিত গুণৰ ক্ষেত্ৰত মন্ত্রালয়সমূহৰ লগতে সংশ্লিষ্ট অসামৰিক বিমান পৰিবহণ কর্তৃপক্ষৰ মাজত তথ্য আৰু অভিজ্ঞতাৰ আদান প্রদান কৰা।
(uncountable) The power to enforce rules or give orders. [..]
English-Assamese dictionary
Less frequent translations
- স্বয়ংক্ৰিয়
- স্বয়ংচালিত
Show algorithmically generated translations
Automatic translations of " authority " into Assamese
Translations with alternative spelling
"Authority" in English - Assamese dictionary
Currently we have no translations for Authority in the dictionary, maybe you can add one? Make sure to check automatic translation, translation memory or indirect translations.
Phrases similar to "authority" with translations into Assamese
- Author Marks অথৰ চিহ্ন
- author প্ৰণেতা · বিৰচ্ · ভণ্ · লিখ · লিখোঁতা · লেখক · লেখাৰু · সৃষ্টিকাৰী · ৰচক · ৰচ্
- Web Distributed Authoring and Versioning ৱেব বিতৰিত প্ৰ্ৰাধিকৰণ আৰু সংস্কৰণকৰণ
- co-authoring সহ-প্ৰাধিকৰণ
- certification authority প্ৰমাণপত্ৰপ্রদান প্ৰ্ৰাধিকৰণ
- authorization প্ৰাধিকৰণ · প্ৰ্ৰাধিকৰণ
- argument from authority বিশেষজ্ঞৰ বিশেষ অজ্ঞতা
Translations of "authority" into Assamese in sentences, translation memory

IMAGES
VIDEO
COMMENTS
Translation of "representation" into Assamese . অনুৰোধ-উপৰোধ, খাটনি, ধৰা-মেলা are the top translations of "representation" into Assamese. Sample translated sentence: We must speak unanimously about providing more representation to the developing countries in United Nations, its Security Council and other multilateral institutions. ↔ আমি ...
assamesedictionary.in is an Assamese English Online Dictionary developed by Departmrnt of Computer Application Jorhat Engineering College (JEC), Assam. It has a library of more than 32,000 assamese words (oxomia xobdo). It also provides meaning of the assamese word with an english description and pronounciation of the word with sound. The words of 'Assamese Dictionary' have been collected ...
Translations from dictionary Assamese - English, definitions, grammar. In Glosbe you will find translations from Assamese into English coming from various sources. The translations are sorted from the most common to the less popular. We make every effort to ensure that each expression has definitions or information about the inflection.
Google's service, offered free of charge, instantly translates words, phrases, and web pages between English and over 100 other languages.
The Assamese language is spoken in the State of Assam, in Northeast India. It's written with the Bengali script. • Aspects of early Assamese literature by Banikanta Kakati (1953) • YouVersion: translation of the Bible into Assamese (2019) (+ audio) • Hymns in Assamese, edited by the Baptist Mission (1850)
representation meaning in Assamese অসমীয়া is a translation of representation in Assamese অসমীয়া dictionary. Click for meanings of representation, including synonyms, antonyms.
About the Assamese Language. Assamese or Asamiya (অসমীয়া Ôxômiya) is an Eastern Indo-Aryan language used mainly in the state of Assam. It is the official language of Assam. The easternmost of the Indo-Aryan languages; it is spoken by over 19 million native speakers. It is also spoken in parts of Arunachal Pradesh and other ...
Assamese Dictionary, Anglo Assamese, Lachit, লাচিত, অভিধান, অসমীয়া
Plain Assamese ethnic groups (depicting from top to down: Ahom, Chutia, Hojai Kachari) The Government of Assam faced difficulties in defining Assamese people for Assam Accord, due to linguistically and culturally heterogeneous population.Though there is a political dispute over the definition of Assamese people, in general; the people belonging to the state of Assam are referred sometimes as ...
PDF | Assam is a diverse land and so does it has a diverse expanse of folktale stories that widely shows the Assamese culture, the lifestyle and also... | Find, read and cite all the research you ...
Morey says on the basis of using -r suffix at the end of the word. He mentions that the "Assamese lexeme jabor is found in an Assamese dictionary under two entries, one a verb meaning 'to go' and another a noun meaning 'garbage' (Morey & Post, 2010: 80). Therefore, he says that if it is garbage then it would be written jaborok.
Type English online and instantly get Assamese translation with English to Assamese translator. You can now easily and accurately translate English to Assamese language with this tool. This tool will allow you to Translate English text into Assamese text. Translating words, sentences, and paragraphs into Assamese is not a difficult task anymore.
Assamese oral literature. But, research paper has a limitation and Assamese folk literatures are very wide in numbers. So, we just mention and give a brief description about the genres of Assamese oral literature. c) Folk song/ poem : Folk songs or oral poetry is a major sub-division of oral literature. It has its own varieties.
In 2015, then Assembly Speaker Pranab Kumar Gogoi prepared a report proposing that 'Assamese people' mean anyone belonging to the state, speaking the Assamese language or any tribal dialect of the state, or local language of the region in the case of Cachar district and adjoining areas (Barak Valley), and families living in Assam since 1951 ...
Translations from dictionary Assamese - Assamese, definitions, grammar. In Glosbe you will find translations from Assamese into Assamese coming from various sources. The translations are sorted from the most common to the less popular. We make every effort to ensure that each expression has definitions or information about the inflection.
And yet, the definition of "Assamese" cannot be so narrow as to mean only those who speak Assamese as their first language. Assam has many indigenous tribal and ethnic communities with their own ancestral languages. For Clause 6, it was necessary to expand the definition of "Assamese" beyond the Assamese-speaking population.
Translations from dictionary English - Assamese, definitions, grammar. In Glosbe you will find translations from English into Assamese coming from various sources. The translations are sorted from the most common to the less popular. We make every effort to ensure that each expression has definitions or information about the inflection.
Assamese Proverbs Mridul Moran Junior Research Fellow, Assamese Department, Dibrugarh University, Assam, India ABSTRACT: The relation between gender and folklore is very close. Many of folklore components are related to men or women society. This folklore may be called gender specific folklore. The contextual meaning of a
Origins of Pride: Exploring the History and Meaning of the Transgender Flag "Seeing the transgender flag fly at NIH on our day of visibility makes me feel safe, supported, and protected like nothing else. It is an unequivocal statement that we are a united community. ... A Flag for Every Identity: Representation Around the World.
Samanantar. Assam Lemon, also known as Nemu Tenga in Assamese, are cultivars of lemon, namely 'Gol Nemu' and 'Kaji Nemu', which are found and cultivated in the Indian state of Assam. অসম নেমু (ইংৰাজী: Assam Lemon) হৈছে কাজি নেমু আৰু গুল নেমুু নামৰ ভাৰতৰ ...
Abstract. In a base phi representation, a natural number is written as a sum of powers of the golden mean φ.There are many ways to do this. Well known is the standard representation, introduced by George Bergman in 1957, where a unique representation is obtained by requiring that no consecutive powers, φ n and φ n+1, occur in the representation.In this paper, we introduce a new ...
Mean values for behavioural metrics across the clusters are displayed in Table 2, Supplementary Fig. 4 provides a granular representation of behaviours during the 180-day monitoring period.
Translation of "authority" into Assamese . এক্তিয়াৰ, কর্তৃপক্ষ, কৰ্তৃত্ব are the top translations of "authority" into Assamese. Sample translated sentence: b.