An official website of the United States government
Official websites use .gov A .gov website belongs to an official government organization in the United States.
Secure .gov websites use HTTPS A lock ( Lock Locked padlock icon ) or https:// means you've safely connected to the .gov website. Share sensitive information only on official, secure websites.
- Publications
- Account settings
- Advanced Search
- Journal List


Smart Wearables for the Detection of Cardiovascular Diseases: A Systematic Literature Review
Mohammad moshawrab, abdenour bouzouane, hussein ibrahim.
- Author information
- Article notes
- Copyright and License information
Correspondence: [email protected] ; Tel.: +1-(581)624-9394
Received 2022 Nov 20; Revised 2022 Dec 27; Accepted 2023 Jan 9; Collection date 2023 Jan.
Licensee MDPI, Basel, Switzerland. This article is an open access article distributed under the terms and conditions of the Creative Commons Attribution (CC BY) license ( https://creativecommons.org/licenses/by/4.0/ ).
Background: The advancement of information and communication technologies and the growing power of artificial intelligence are successfully transforming a number of concepts that are important to our daily lives. Many sectors, including education, healthcare, industry, and others, are benefiting greatly from the use of such resources. The healthcare sector, for example, was an early adopter of smart wearables, which primarily serve as diagnostic tools. In this context, smart wearables have demonstrated their effectiveness in detecting and predicting cardiovascular diseases (CVDs), the leading cause of death worldwide. Objective: In this study, a systematic literature review of smart wearable applications for cardiovascular disease detection and prediction is presented. After conducting the required search, the documents that met the criteria were analyzed to extract key criteria such as the publication year, vital signs recorded, diseases studied, hardware used, smart models used, datasets used, and performance metrics. Methods: This study followed the PRISMA guidelines by searching IEEE, PubMed, and Scopus for publications published between 2010 and 2022. Once records were located, they were reviewed to determine which ones should be included in the analysis. Finally, the analysis was completed, and the relevant data were included in the review along with the relevant articles. Results: As a result of the comprehensive search procedures, 87 papers were deemed relevant for further review. In addition, the results are discussed to evaluate the development and use of smart wearable devices for cardiovascular disease management, and the results demonstrate the high efficiency of such wearable devices. Conclusions: The results clearly show that interest in this topic has increased. Although the results show that smart wearables are quite accurate in detecting, predicting, and even treating cardiovascular disease, further research is needed to improve their use.
Keywords: cardiovascular diseases, smart wearables, sensors, body sensor networks, machine learning, smart health, wide body area networks
1. Introduction
Healthcare has always been one of the most important issues that people have cared about. Given the prevalence of diseases and their impact on people’s lives, researchers are always looking for methods to improve medical services and promote public health. In addition, the aging population, shortage of medically trained personnel, lack of equity in services, epidemic planning, and a host of other problems hinder the growth of public health worldwide [ 1 ]. However, advances in information and communication technology (ICT) offer effective answers to these challenges. In this context, artificial intelligence (AI) is considered the most promising tool for improving healthcare, as it has the potential to be used in virtually all areas of medicine [ 2 ] and will transform healthcare for patients and communities [ 3 ]. This enormous contribution is not due to magic, but to AI’s data-processing capabilities, which surpass those of humans, especially when large computations are performed in a short period of time. Even though the majority of AI applications in healthcare were developed after 2008 [ 4 ], their importance is obvious. First, AI has improved the learning capabilities of computers and humans, leading to improved diagnostic and healthcare procedures [ 5 ]. In addition, AI technologies are able to accept common sense, extract information from raw data, use human-like thought processes, deal with inaccuracies, adapt to a rapidly changing environment, and even act on their knowledge [ 2 ]. These characteristics enable AI tools to think and behave similar to humans at a virtually unparalleled level, allowing them to articulate clinical patterns and visions beyond human capabilities [ 3 ]. Combining AI capabilities with human intelligence, sometimes referred to as augmented intelligence, is probably the most effective way to improve healthcare services [ 3 ].
1.1. Cardiovascular Diseases Latest Figures
Cardiovascular diseases (CVDs) are the leading cause of death and are hence recognized as the most dangerous disease in the world. According to the most recent World Health Organization (WHO) statistics on heart disease, the number of CVD patients worldwide has increased from 271 million to 523 million between 1990 and 2019, and the number of deaths caused by this disease has increased from 12.1 million to 18.6 million during the same period, accounting for 32% of global mortality in 2019 [ 6 ]. For example, in the United States, a person dies from heart disease at least every 34 s [ 7 ], and in Canada, a person dies at least every 5 min [ 8 ]. Moreover, cardiovascular disease is a major cause of both health conflict and economic suffering. According to the Medical Expenditure Panel Survey, the total cost of CVDs in the United States between 2017 and 2018 was estimated at USD 378.0 billion, including USD 226.0 billion in expenditures and USD 151.8 billion in lost future productivity [ 9 ]. Figure 1 illustrates the increase in the number of patients and deaths due to cardiovascular disease worldwide between 1990 and 2019.
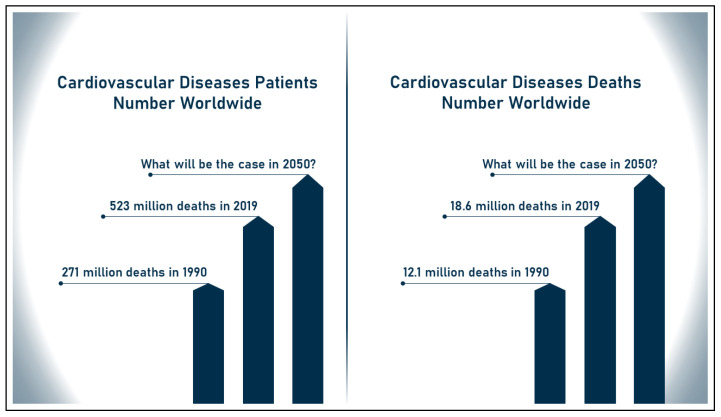
Increase in number of patients and deaths due to CVDs.
1.2. CVDs Detection: From Classic to Technology-Assisted
Due to their potentially fatal nature, cardiovascular diseases need the development of efficient solutions that allow early diagnosis and, ideally, prediction of their onset. The predictive power of modern technologies could help reduce the global prevalence of CVDs. Traditional methods for diagnosing these diseases include electrocardiogram, echocardiography, coronary angiography, stress testing, magnetic resonance imaging, or intracoronary ultrasonography. However, new technologies are improving health services and facilitating the detection of cardiovascular disease, particularly information and communication technologies (ICTs) and the development of artificial intelligence (AI) and its derivatives. The novel approaches of AI in cardiology have proven to be successful in providing fast, accurate, and less erroneous patient care, which has significant medical and financial implications. It is more effective and widely used, as the tools and applications offered are at the level of an expert using real-world data. In general, AI has fantastic potential to transform cardiology in the near future and is often seen as the next revolutionary step in the field due to its potential to accelerate and improve patient care. Moreover, AI will soon revolutionize cardiovascular health, as its tools have the potential to outperform experts in detecting and predicting cardiovascular disease [ 10 , 11 , 12 ]. Therefore, smart wearables that combine AI and ICT are expected to be very useful in cardiovascular disease detection and prediction.
1.3. Smart Wearables: Definitions and Overview
Smart wearables, also known as smart wearable technology or wearable gadgets, are a new breed of compact, rugged, and efficient computing devices made possible by the rapid growth of information and communication technologies and the advancement of electronics, particularly microprocessors. These devices are being hailed as the next generation of ubiquitous technology after smartphones, as they allow access to data at any time and from any location. The topic of smart wearables has evolved rapidly in recent years, and their technologies are now applicable in many other fields [ 13 , 14 , 15 , 16 ]. This section provides a definition of “smart wearables” and a brief overview of the history of wearable technology. In addition, various categories of smart wearables are discussed in the upcoming sections.
1.3.1. Smart Wearables: Brief History
In 1950, Alan Turing asked the now famous question “Can machines think?” which marked the beginning of the era of “Smart Machines” [ 17 ]. Since then, researchers around the world have attempted to answer this question by turning computers into intelligent devices. Despite its widespread use, the term “Smart” is not uniformly defined and is presented in different ways by different scholars [ 18 ]. In [ 19 ], “Smart” devices are defined as embedded sensors, processors, and network devices that give smart things the ability to behave based on their own knowledge. In addition, Ref. [ 20 ] defines them as objects that can learn from their environment and interact with humans. Different definitions focus on the capabilities of the devices. For example, smart wearables are defined by the authors in [ 21 , 22 ] as devices that can be worn by the user at all times to monitor factors such as personal data, vital signs, locations, environment, movements, and more. In this context, a shoe-sized computer developed by Edward Thorp and Claude Shannon in 1961 is widely considered to be the first ever wearable computing device [ 23 , 24 ]. In the 1980s, Steve Mann developed EyeTap glasses that displayed computer-generated images in one eye and added textual information to the user’s visual experience [ 25 ]. Subsequently, in 1996, the U.S. Department of Defense Navy funded a study to monitor the vital signs of its troops [ 26 , 27 ], which is widely considered a defining moment in the history of smart wearables. Since then, smart wearables have gradually evolved from invasive, heavy, and huge technologies to more adaptable, compact, and lightweight devices. This is because researchers have expanded their projects in this field to different areas of life such as health, fitness, sports, fashion, and even other sectors.
1.3.2. Classification of Smart Wearables
Over the past few decades, there have been more than a thousand studies on smart wearables. However, smart wearables cannot be classified into a specific category. Accordingly, smart wearables are divided into six groups, as described by the authors of Ref. [ 28 ]:
Industrial;
Entertainment;
On the other hand, the smart wearables were categorized by the authors of Ref. [ 29 ] according to their personal features rather than their function. They provided examples of the three categories into which they fall:
Watch-type;
Necklace or wristband-type;
Headmount display-type.
However, other technologies, such as electronic patches and health apparel, could not fit within this classification. Therefore, a set of commonly known wearables are displayed in Figure 2 below.
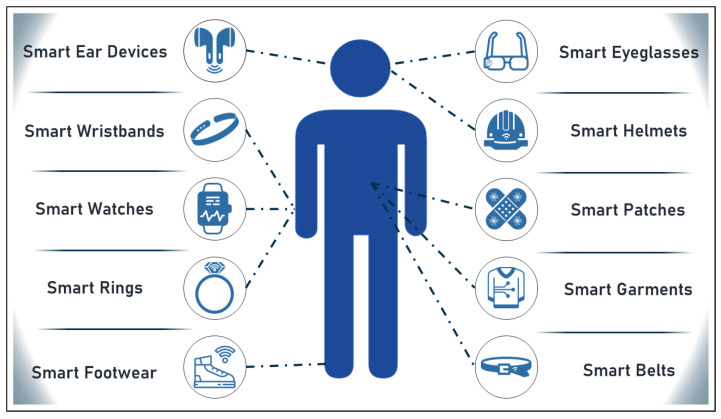
Set of commonly known wearables.
1.4. Role of Smart Wearables in CVDs
Over the past decade, smart wearables have been increasingly used as health solutions. Their effectiveness and proliferation has been fueled by advances in performance, size, style, and durability, among other factors. Examples of smart wearables used to diagnose, track, and treat cardiovascular disease include wristbands, patches, headbands, eyeglasses, and necklaces. The implications of CVD wearables are many. For example, they enable continuous and long-term recording of functional or physiological data, leading to more accurate diagnosis and better health outcomes for patients. In addition, they enable the collection of necessary data in locations other than physicians’ offices or hospitals, expanding the capacity of healthcare facilities to serve larger numbers of patients over longer periods of time. More importantly, the continuous monitoring capabilities of smart wearables enable more sophisticated knowledge of an individual patient’s physiological state and ongoing activity, paving the way for more personalized healthcare and treatment. The devices also became less bulky and aesthetically pleasing, making them less intrusive and more suitable as everyday wearables. One way that smart wearables such as smartphones are benefiting from the widespread use of other devices is through pairing [ 30 , 31 , 32 , 33 ]. Some reasons for the success of smart wearables adoption and their success points are listed in Table 1 below.
Success reasons and success points of smart wearables.
1.5. Outline and Main Contributions of This Article
In this article, the use of smart wearables in the detection, prediction, and treatment of cardiovascular disease was investigated. For this purpose, a systematic literature review was conducted, following the methodology that is explained in Section 2 . Subsequently, in Section 3 , the results of the performed search are presented. Later, the obtained results are analyzed in Section 4 and discussed in Section 5 . Then, the challenges hindering the progress in the use of smart wearables are discussed, and future perspectives to solve these challenges are presented. Finally, the article is concluded with a concluding section. To the best of our knowledge, there are no systematic reviews addressing the potential of smart wearables for early diagnosis of CVDs. For example, in [ 34 ], the authors investigated the application of AI in smart wearables for cardiovascular disease detection. However, the focus of their research was on smart models rather than hardware; the obstacles that have slowed the development of this field are barely addressed, and the same is true for future prospects. Furthermore, in [ 35 , 36 ], the authors explored the use of smart wearables in life course research, but they did not systematically explore the field or provide a complete vision of contextual implementations. Motivated by the large role that smart wearables play in various aspects of daily life and by the lack of a systematic literature review discussing their role in predicting cardiovascular disease, this article therefore attempts to answer the following questions:
What are the applications of using smart wearables to detect and predict cardiovascular disease?
What are the different aspects such as hardware and software used in these implementations?
To what extent are these implementations feasible?
What are the challenges and limitations in this area?
What future perspectives can be pursued to improve the use of smart wearables in CVDs management?
Therefore, this article answers the above questions and thus contributes to academic knowledge by:
Systematically reviewing the use of smart wearables in the treatment of cardiovascular disease;
Analyzing and discussing the reviewed implementations in a way that facilitates the identification of opportunities for improvement in this area;
Naming the barriers to progress in this area;
Proposing solutions that can be used to address these barriers;
Presenting a collection of research questions and findings that could serve as a starting point for future research.
2. Research Methodology
This section details and explains the methodology used to conduct the systematic review. The steps described here can be used to conduct the same search and review the results or repeat the search in a different time period.
2.1. Eligibility Criteria
In conducting this review, PRISMA (Preferred Reporting Items for Systematic Reviews and Meta-Analyses ) [ 37 ] was used as a guide for preparing a systematic literature review. The structure of this review was based on the latest PRISMA checklist (PRISMA Checklist 2020) [ 38 ]. In accordance with PRISMA standards, multiple sources were searched for papers that met the scope of this review. Four variables were used to select these materials. To be considered reputable, a paper must address artificial intelligence or related fields, present a smart wearable solution, address healthcare, and focus on cardiovascular diseases. In addition, the material should have been published in a peer-reviewed journal or as a conference proceedings. In addition, only documents published between January 2010 and October 2022 were considered. As a final eligibility criterion, an English language filter was applied to eligible papers.
2.2. Information Sources
Several academic abstract and citation databases for peer-reviewed literature were used, including IEEE, PubMed, and Scopus Elsevier, to ensure superior results and to cover the largest number of documents possible. Each of these three databases provides access to millions of documents and has powerful, sophisticated search tools to facilitate thorough literature searches.
2.3. Search Strategy
In order to conduct a thorough search of the above materials, three queries were formulated. While these queries all follow the same logical structure, they use different syntaxes to comply with the different rules imposed by each data source. Targeted articles are found at the intersection of four query blocks, each defining a different topic of interest. AI, health, wearables, and CVDs (or related areas) are the four basic focus areas. The phrase “AND” was used to combine areas for a more effective query, while the term “OR” was used to combine different terms within each area. The three queries used to find what is being searched for are as follows:
IEEE: ((("ARTIFICIAL INTELLIGENCE" OR "SMART AGENTS" OR "SMART MACHINES" OR "INTELLIGENT" OR "DEEP LEARNING" OR "MACHINE LEARNING" OR "NEURAL NETWORK") AND ("HEALTH*" OR "DISEASE" OR "ILL*" OR"CARE") AND ("WIRELESS SENSORS NETWORK" OR "SMART SENSORS" OR "BODY AREA NETWORK" OR "WEARABLE" OR "SENSOR") AND ("CARDIOLOGY" OR "CARDIOVASCULAR" OR "HEART" OR "CARDI*"))).
PubMed: ((ARTIFICIAL INTELLIGENCE) OR (SMART AGENTS) OR (SMART MACHINES) OR (INTELLIGENT) OR(DEEP LEARNING) OR (MACHINE LEARNING) OR (NEURAL NETWORK)) AND ((HEALTH) OR (DISEASE) OR (ILL) OR(CARE) OR (HEALTHCARE)) AND ((WIRELESS SENSORS NETWORK) OR (SMART SENSORS) OR(BODY AREA NETWORK) OR (WEARABLE) OR (SENSOR)) AND ((CARDIOLOGY) OR (CARDIOVASCULAR) OR (HEART) OR(CARDIAC)).
Scopus: TITLE-ABS-KEY(((artificial intelligence) OR (smart agents) OR (smart machines) OR (intelligent) OR (deep learning) OR (machine learning) OR (neural network)) AND ((health*) OR (disease) OR (ill*) OR (care)) AND ((wireless sensors network) OR (smart sensors) OR (body area network) OR (wearable) OR (sensor)) AND ((cardiology) OR (cardiovascular) OR (heart) OR (cardi*))) AND (LIMIT-TO (SRCTYPE, “j”) OR LIMIT-TO (SRCTYPE, “p")) AND (LIMIT-TO(DOCTYPE, “cp”) OR LIMIT-TO(DOCTYPE, “ar”)) AND (LIMIT-TO( LANGUAGE, “English”)) AND (LIMIT-TO (PUBYEAR, 2021) OR LIMIT-TO (PUBYEAR, 2020) OR LIMIT-TO (PUBYEAR, 2019) OR LIMIT-TO (PUBYEAR, 2018) OR LIMIT-TO (PUBYEAR, 2017) OR LIMIT-TO (PUBYEAR, 2016) OR LIMIT-TO (PUBYEAR, 2015) OR LIMIT-TO (PUBYEAR, 2014) OR LIMIT-TO (PUBYEAR, 2013) OR LIMIT-TO (PUBYEAR, 2012) OR LIMIT-TO (PUBYEAR, 2011) OR LIMIT-TO (PUBYEAR, 2010)).
In the case of Scopus, the query was used as described above to retrieve the results. However, in IEEE and PubMed, additional filters were applied through the graphical user interface. In both sources, a “Year” filter was added to limit the selection to articles between 2010 and 2021. However, in IEEE, articles with the types “Conferences” and “Journals” were selected, whereas in PubMed, articles with the types “Clinical Trial” and “Journal Article” were selected. Finally, a filter was performed in the PubMed interface to limit the documents to those published in English. On the other hand, Scopus offers the possibility to limit the search to the title, abstract, or keywords, while the other two sources perform the search in the whole text of the document. It is worth noting that the search was performed in October 2021.
2.4. Selection Process
The data extracted from the records were selected in three steps to determine which files were relevant to this analysis. The first step was to review the titles and abstracts of all documents to determine if they were relevant to the topic of this study. The documents that passed this step were then downloaded. In a second step, we reviewed the downloaded files to quickly verify their content and determine if they were relevant to our evaluation. The documents selected in this phase are the ones that are examined in detail. Finally, the documents were researched and evaluated to extract the data needed to demonstrate the development of smart wearables for CVDs.
The steps mentioned in the previous section led to a systematic result. The results of this search are listed in this section.
3.1. Study Selection
Initially, 4002 documents were identified from the three libraries based on the above searches. The search on IEEE yielded 1013 documents, on PubMed 1020, and on Scopus 1969, after which duplicate entries were excluded, removing 1021 and leaving 2981 documents. Then, the aforementioned selection procedure was applied, excluding 2382 documents on the basis of irrelevance and advancing 599 to the next stage. Documents were classified as irrelevant if they met the search criteria or if they contained the search terms specified in the search queries but did not deal with cardiovascular disease or were not wearable systems. In the second phase, full-text screening, the 599 documents were downloaded and skimmed to assess their suitability. In this phase, 512 documents were excluded for various reasons, and 87 documents were deemed suitable for this review. All these details are shown in Figure 3 below that matches the PRISMA diagram (information flow through the different phases of a systematic review) [ 37 ].
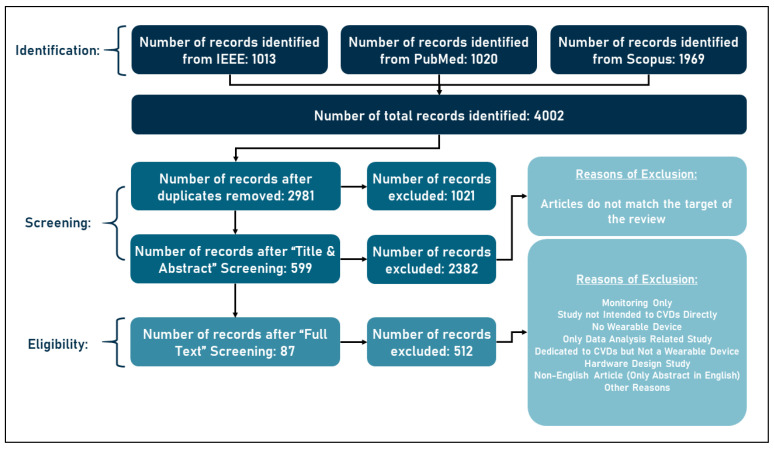
Flow of information through the different phases of a systematic review.
3.2. Study Characteristics
Following the search described above, the 87 papers deemed appropriate were carefully reviewed and examined to extract all relevant information. From each paper, the year of publication, disease(s) treated, vital signs recorded, hardware of the wearable device(s), embedded intelligent models, dataset(s) used, and outcome metrics were extracted. Table 2 below shows all retrieved details from the eligible studies, thus forming one of the main outcomes of this research, listing all implementations of smart wearables in cardiovascular disease management between 2010 and 2022 along with their relevant details.
Implementations of smart wearables in detection of CVDs.
3.3. Results of Individual Studies
The systems presented in the eligible studies share common features that allow for easy classification. Contextually, the studies can be divided into three categories, for example, according to whether the measuring devices used are commercially available or not. Systems in the first group use components that are not commonly available; these components were custom-made by the researchers for the study. Systems that use readily available technology and commercially available devices comprise the second category. The final category includes studies that used unspecified devices, making it impossible to determine whether or not they are now available for purchase. The following categories of systems were formed according to the devices used.
3.3.1. Studies Using Custom-Built Devices
Throughout the analysis of the eligible documents, it was shown that 55 studies built their own devices using various vital sign sensors, power resources, storage resources, communications, and other technical components. Within this group, two subgroups stood out, the first of which did not name all the components used, particularly the sensor devices, but, rather, stated that they composed their own wearables from sensor devices. Thus, in [ 39 , 41 , 42 , 43 , 46 , 48 , 50 , 51 , 54 , 56 , 58 , 59 , 60 , 61 , 62 , 66 , 86 , 88 , 92 , 116 , 118 , 123 ], the authors proposed custom-built wearables with unspecified components. These studies were able to detect various cardiovascular diseases such as atrial fibrillation, atrial flutter, atrial premature contraction, atrial tachycardia, cardiac asystole, cardiovascular risk, fusion beats, heart attack, heart failure, hypertension, myocardial infarction, myocardial ischemia, premature atrial contractions, and premature ventricular contractions. Vital signs obtained for this purpose were radial artery audio signal, blood oxygen level, blood pressure, blood sugar level, body temperature, diastolic pressure, electrocardiogram, electroencephalogram, heart rate, motion data, oxygen saturation level, photoplethysmogram, respiratory rate, and skin temperature. In addition, the databases used for training and the performance metrics are detailed in Table 2 .
On the other hand, several studies used commercially available sensors to develop their wearable devices. In this context, various sensors such as ECG, accelerometer, and other sensors were used. For example, in [ 53 , 73 , 104 , 117 , 125 ], the ECG sensors ADAS1001, Shimmer 3, BMD101, ADXL355, and ADS1291 were used in combination with other materials to build a wearable device that collects records used to detect or predict cardiovascular disease. In contrast, the authors in [ 114 ] used the DS18B20 temperature sensor and ADXL1335 accelerometer to develop the desired wearable system. In addition, the authors in [ 52 , 57 , 65 , 67 , 69 , 71 , 72 , 74 , 75 , 77 , 79 , 81 , 89 , 94 , 100 , 101 , 113 , 122 ] used the AD8232 ECG sensor to collect vital signs data. In these studies, as discussed in Table 2 , different processing units, connector modules, and power sources were used to build the wearable device. Alternatively, in [ 82 , 84 , 95 , 97 ], the authors combined different sensor materials with the AD8232 ECG sensor in their wearable device. Specifically, the authors in [ 82 , 95 ] used the MAX30100 blood oxygen sensor in addition to the ECG sensor, whereas the authors in [ 84 ] used the ADXL345 triaxial accelerometer, and the authors in [ 97 ] used the MAX30102 pulse oximeter sensor. Other studies also used different ECG sensors, with the authors in [ 87 ] building their portable devices using the “Ternary Second-Order Delta Modulator Circuits” to acquire ECG data. In the same context, the authors in [ 111 , 124 ] used the sensor ECG AFE and other tools to build a wearable device capable of acquiring the necessary vital signs data. Finally, the authors of [ 109 , 119 ] used MAX30102 photoplethysmography and BH1790GLC optical heart rate sensors in their wearable devices, respectively.
3.3.2. Studies Using Commercially Available Wearable Devices
The other group of studies consists of studies that used commercially available devices. These devices were capable of recording various vital signs such as activity parameters, blood oxygen level, blood pressure, blood glucose level, cholesterol level, electrocardiogram, electroencephalogram, electromyogram, heart rate, oxygen saturation level, photoplethysmogram, pulse plethysmogram, and respiratory rate. Depending on the type of device used, three categories can be distinguished, namely, wristband devices, belts, and others. For example, in [ 45 , 47 , 55 , 68 , 70 , 80 , 85 , 98 , 103 , 107 , 108 , 112 ], the authors used smartwatches and smart wristbands to record vital signs. In addition, the authors in [ 40 , 44 , 49 , 63 , 64 , 76 , 78 , 83 , 91 , 93 , 96 , 102 , 110 , 120 , 121 ] used various wearable ECG devices such as smart vests and patches. In addition, the authors in [ 90 , 99 , 105 , 106 ] used smart belts to collect ECG data. Overall, the devices used in all the studies mentioned in this section can be summarized in the following list:
Alive ECG Heart Monitor;
Amazfit Health band 1S;
Apple Smart Watch;
Bio Clothing One, XYZ life BC1;
BioHarness 3.0 by Zephyr;
ECG247 Smart Heart Sensor;
Firstbeat Bodyguard Chest Patch 2 by Firstbeat Technologies;
GENEActiv and Activinsights Band by Activinsights Ltd.;
Glucose Monitor by Medtonic;
HealthyPiV3 biosensors;
Heart Rate sensor by Sunrom Electronics;
IREALCARE2.0 Wearable ECG Sensor;
Kimbolton, UK;
Medical-Grade Wearable Embedded System Beijing Sensecho Science & Tech.;
Wearable device provided by Medicaltech SRL;
NanoPi Neo Plus2;
PTN-104 PPG Sensor;
Raspberry Pi Zero;
Rejiva ECG Wearable Sensor;
Rozinn RZ153+ ECG Monitor;
Samsung Galaxy Active 2 Smart Watch;
Samsung Galaxy Active Smart Watch;
Samsung Gear Wearable Device;
Samsung Simband 2 Wrist Band Smart Watch;
Samsung Simband Wrist Band Smart Watch;
Shimmer ECG Monitor;
Single-Lead Heart Belt by Suunto Movesense, Suunto, Vantaa, Finland;
Wrist-Type Pulse Wave Monitor by: Shanghai Asia & Pacific Computer Info. System.
3.3.3. Studies That Did Not Specify the Devices Used
Finally, in a single study, the device used was not specified. The authors in [ 115 ] mentioned only that they used a wide body area network (WBAN) to record respiratory rate and blood oxygen levels to detect the presence of cardiovascular risk. Their study achieved 96% accuracy in the classification algorithm, demonstrating high feasibility in detecting CVDs. Unlike some of the studies excluded in the screening phases (see Section 2.4 ), this study mentioned that a wearable device was used, but did not specify which device was used.
4. Results Analysis
Studies that met the criteria for inclusion in this review, or those that specifically address the use of smart wearables for the diagnosis, prognosis, or treatment of cardiovascular disease, contain a wealth of information worthy of further investigation. In the previous section, the devices used were mentioned. However, to better understand the field, this part examines factors such as the year of publication, vital signs collected, diseases treated, smart models used, datasets used for training, etc.
4.1. Progress with Years
Once the data are extracted from the papers, it is clear that there has been significant progress in the field of wearables for CVD research over the past four years, with 78% of the publications published in 2019 or later. During those years, a total of 68 studies were published (compared to only 19 in 2010–2018). There are nine in 2019, eighteen in 2020, twenty-one in 2021, and twenty-one in 2022. The number of publications addressing the use of smart wearables for cardiovascular disease management has jumped, reflecting both the growing interest in this area and the widespread acceptance of such devices. The data from this section are shown as a pie chart in Figure 4 below.
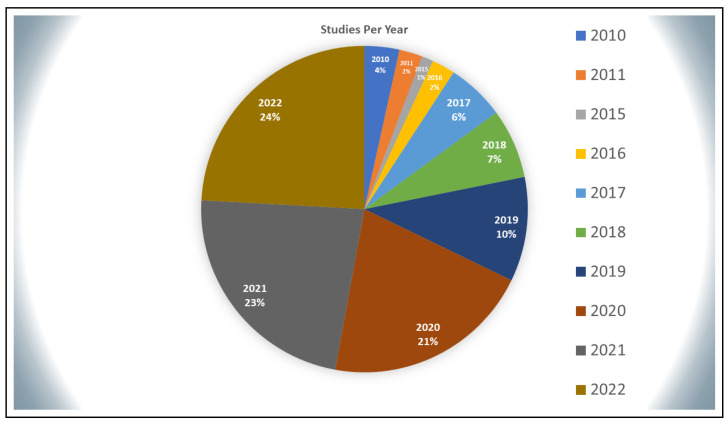
Distribution of studies per year.
4.2. Vital Signs in Use
The electrocardiogram (ECG) is used in 69 of the systems described in the articles to diagnose disease and identify cardiac abnormalities, although many other methods have been offered. Electrocardiograms are routinely performed to check the health of the heart and quickly identify potential problems. An electrocardiogram (ECG) shows the development of the heart’s electrical activity over time. When the heart muscle cells are electrically depolarized, the heart muscle contracts. An electrocardiogram records and amplifies this electrical activity over a period of time. Studies have shown that smart watches such as the Samsung Active and Apple Watch have significant efficiency in capturing ECG signals, complementing the accuracy of ECGs performed in a doctor’s office, clinic, or hospital room. In addition, the P wave, the QRS complex, and the T wave are the three components of the ECG signal. Figure 3 shows the ECG signal in terms of these components. In a normal electrocardiogram, the heartbeat is detected by [ 126 ]:
PR interval: measured from the beginning of the P wave to the first deflection of the QRS complex with a normal range of 120–200 ms;
QRS complex: measured from first deflection of QRS complex to end of QRS complex at isoelectric line with a normal range of up to 120 ms;
QT interval: measured from first deflection of QRS complex to end of T wave at isoelectric line with a normal range of up to 440 ms (though it varies with heart rate and may be slightly longer in females).
Twenty more measures, including photoplethysmogram, heart rate, and others, were also employed in addition to ECG in order to identify CVDs. Table 3 below details the frequency and utilization of these parameters across studies.
Vital signs used in studies with count and percentages.
4.3. Diseases Targeted
Because a single document may focus on a single disease or multiple diseases, the number of diseases studied in these publications exceeds 70. Atrial fibrillation (AFib) is the most commonly studied disease, with 39 of 87 studies addressing it. AFib is the leading cause of death and morbidity due to stroke, heart failure, thromboembolism, and reduced quality of life, and accounts for the majority of these cases [ 127 ]. Other conditions are also being studied, including premature ventricular contractions (PVCs), ventricular ectopic beats, bradycardia, paced beat (PACE), and many others. Figure 5 is a bar graph showing the number of diseases found in the 87 papers.
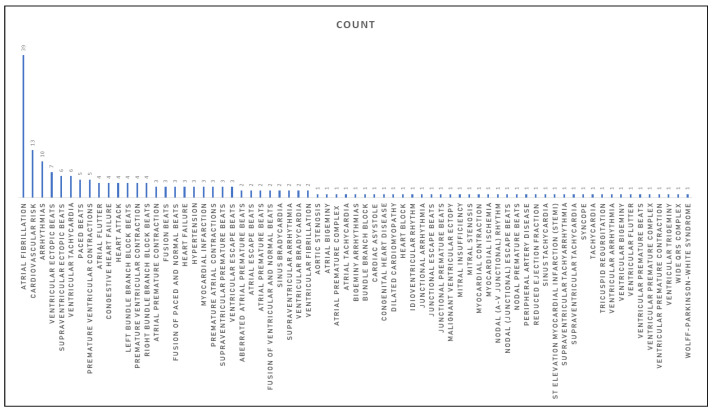
Diseases distribution per studies.
4.4. Smart Models in Use
It is well known that several subfields of artificial intelligence are widely used in different fields. Two of the best-known subfields of AI are machine learning (ML) and deep learning (DL); the former is described as a set of techniques that allow a machine to acquire new information and skills through learning, and the latter is a branch of machine learning that focuses on algorithms inspired by the structure and function of the brain, called artificial neural networks [ 128 , 129 ]. The relationship between AI, ML, and DL is illustrated in Figure 6 . However, in the studies analyzed in this review, many machine learning and deep learning models were used to detect cardiovascular disease. Although each publication proposes a different method to detect the disease(s), all agree that some type of algorithm should be used to classify cardiac abnormalities. Convolutional neural networks, support vector machines, long short-term memories, and decision trees are the most commonly used algorithms.
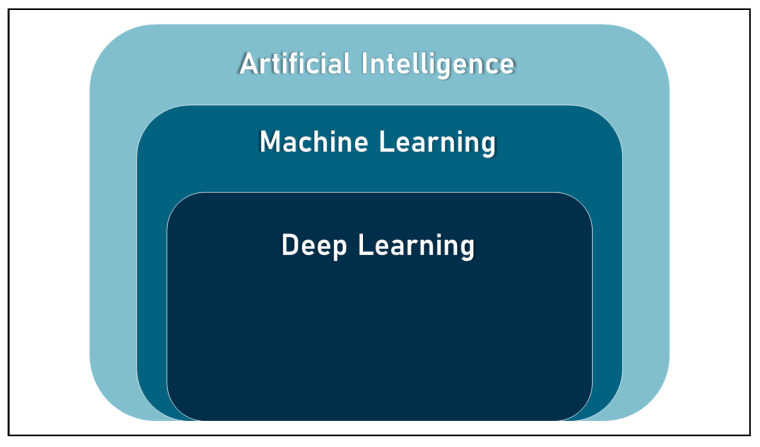
Artificial intelligence, machine learning, and deep learning relation.
The intelligent models of machine learning and deep learning are attracting much attention and are proving to be very practical [ 130 , 131 ] in the healthcare industry. With this in mind, it is of great interest to analyze the efficiency of these models in detecting CVDs. However, this task requires separate studies, as this research focuses on smart wearables as a whole system. This article aims to fill this gap by discussing the four most commonly used smart models, namely:
Convolutional Neural Network (CNN): CNN is a kind of deep neural network used to analyze visual images. These neural networks are modeled after the neural networks of the human visual system. Neurons are the basic computational unit of a neural network, just as they are the basic functional unit of the human nervous system. In the case of convolutional neural networks, instead of normal matrix multiplication, convolution is used, a special form of mathematical operation. In addition to the input and output layers, a convolutional neural network has numerous hidden layers (a neural layer is a stack of neurons in a single row). A neuron in the input layer receives an input, analyzes it, and performs computations on it, and then transmits a nonlinear function called an activation function to produce the final output of a neuron [ 132 ];
Support Vector Machines (SVMs): SVM is a supervised machine learning model for two-group classification problems that employs classification techniques. An SVM model is able to classify new data after receiving a set of labeled training data for each category [ 133 ];
Long Short-Term Memory (LSTM): LSTM networks are a type of recurrent neural network (RNN) that can learn sequence dependence in sequence predictions. RNNs contain cycles that use network activations from a previous time step as inputs to influence predictions at the current time step. These activations are stored in the internal states of the network, theoretically preserving long-term contextual timing information. This method allows RNNs to use a contextual window that changes dynamically over the course of the input sequence. Complex problem domains such as machine translation, speech recognition, and others require this behavior [ 134 ];
Decision Trees (DTs): A decision tree is a type of supervised machine learning used to make classifications or predictions based on answers to a prior set of questions. The model is a type of supervised learning, meaning that it is trained and evaluated on a dataset that contains the desired classification. Occasionally, the decision tree may not provide a definitive answer or conclusion. Instead, it may suggest possibilities from which the data scientist can make an informed choice. Because decision trees replicate human thought processes, it is often easy for data scientists to understand and explain the results [ 135 ].
The machine learning and deep learning models utilized in the studies analyzed in this review were assessed with different performance metrics such as accuracy, specificity, sensitivity, precision, recall and F1-score. These parameters are explained in detail in the literature, with the authors in [ 129 ] providing a detailed explanation in this regard, for example. These parameters can be summarized as follows [ 129 ]:
Accuracy: the fraction of predictions that the model predicted right and is calculated by dividing the number of correct predictions by the total number of predictions.
Specificity: is the parameter used to calculate model’s ability to predict a true negative (no cardiovascular diseases in our case) of each category available.
Sensitivity: is the parameter used to calculate model’s ability to predict the true positives (existence of CVDs in our case) of each category available.
Precision: is the parameter used to calculate what proportion of positive identifications (existence of CVDs in our case) was actually correct.
Recall: is the parameter used to calculate what proportion of actual positives (existence of CVDs in our case) was identified correctly.
The performance of models used by each study is detailed in Table 2 . Furthermore, the list of smart models used in smart wearables for the detection of cardiovascular diseases is mentioned in Table 4 below, along with the count of use of each model. In this context, and for more details on the potential of machine learning and deep learning models in predicting CVDs, readers are advised to refer the work of Solam Lee and his colleagues [ 34 ], which targets these models and discusses their feasibility in this domain.
Smart Models Used in Studies.
4.5. Datasets in Use
In all 87 publications examined, at least one dataset was used to train the AI model, and this is consistently the case. In addition, it was noted that certain sources advocate the use of multiple datasets in the development and evaluation of a model. The PhysioNet MIT-BIH dataset, accessible through the PhysioNet library of publicly available medical research data, is the most popular. Of the 87 total studies, 36 used it. Another 25 studies also used researchers’ private data. Figure 7 below shows a graphical statistical representation of the frequency of use of datasets. The MIT-BIH Arrhythmia database is the first publicly available collection of standardized test material for the evaluation of arrhythmia detectors. The BIH Arrhythmia Laboratory collected these ambulatory two-channel ECG recordings from 47 patients between 1975 and 1979 and included 48 30-minute samples [ 136 ]. The PhysioNet MIT-BIH Atrial Fibrillation Database, the PhysioNet MIT-BIH Noise Stress Test Database (NSTDB), and the PhysioNet MIT-BIH Normal Sinus Rhythm Database were also consulted.
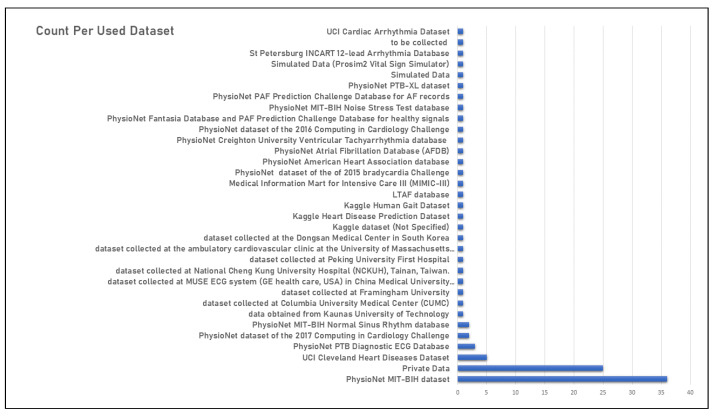
Training datasets in use.

5. Results Discussion
This study systematically collects and analyzes the literature on the use of smart wearables for cardiovascular disease diagnosis and prognosis. However, there is more to be said about the studies discussed so far, especially in terms of their effectiveness and conformity with the latest research areas in artificial intelligence. This topic will be elaborated and explored in this section.
5.1. Performance, Usability, and Feasibility
To predict CVDs, many tools have been used. The wide range of research is due to the wide range of vital signs and devices used to achieve this goal. ECG, BP, HR, and temperature were all reliable predictors of cardiovascular disease. This is evidenced by the fact that the results of several studies (described in Table 2 ) showing the use of different implementations yielded an accuracy rate of over 99%. However, there are several things to consider when making a final decision on a wearable gadget. The following is a list of features that would make a smart wearable more practical:
Noninvasive: the gadget should not penetrate or pierce the skin to collect data;
Compact: the wearable device should not be bulky or large, as its main purpose is to monitor health symptoms without interfering with one’s life activities;
Affordable: the affordability of the device plays a role in how well it fits into everyday life;
Robust: the device should be durable enough to handle cold, hot, humid, or dry weather, as well as harsh operating conditions such as light scratches or bumps;
Ease of use: if the hardware used requires little human input, it should have an intuitive interface;
Durable power source: the portable device must be powered reliably enough to collect meaningful data over an extended period of time.
On the other hand, the electrocardiogram (ECG) is considered the most effective indicator of cardiovascular disease due to its high accuracy in recording the presence of such disease and its practicality and reliability in detecting it. Conventional ECG signal acquisition relies on electrodes, which can be uncomfortable to wear during normal daily activities. Smart watches and wristbands, on the other hand, are quite effective at capturing ECG signals and are also convenient for a number of other reasons. They are available to everyone and are the best option as they combine a variety of useful features with accurate monitoring of heart rate and other vital signs. Commercially available smart watches and wristbands are cheap and have simple user interfaces. They are small, are not in the way, and do not limit people’s options. In addition, they are equipped with reliable power sources that allow them to last for a long time. Finally, their ability to record a wide range of biometric data makes them an excellent, if not ideal, option for ECG capture devices and thus for predicting CVD parameters.
5.2. Latest Tech-Trends and Wearables in CVDs
Alternatively, it is interesting to examine whether or not smart wearables used to control CVD are consistent with current machine learning practices. Several subfields of machine learning were identified as current research areas, but “Explainable AI”, “Federated Machine Learning”, and “Multimodal Machine Learning” were most frequently mentioned. The compliance of smart wearables used to detect CVDs to those topics is discussed in the following sections.
5.2.1. Explainable AI
The more complex AI becomes, the more difficult it becomes for humans to understand and reconstruct the thought process of the algorithm. The entire computational process becomes a so-called “black box”, something that cannot be understood by humans. These black box models are created from scratch using nothing but the raw data. They are so complicated that not even the engineers or data scientists who create them can explain how their artificial intelligence algorithms arrive at their conclusions. Insight into the reasoning behind an AI system’s results can be very helpful. Being able to explain a decision can be critical in allowing stakeholders to challenge or change the conclusion, in meeting regulatory criteria, or in ensuring that the system works as intended by its creators [ 137 , 138 , 139 ].
In this context, and to address the challenges posed by the black-box nature of AI, ML, and DL models, explainable AI (XAI) is proposed as a viable solution. The goal of XAI is to make the results and outputs generated by machine learning algorithms understandable and reliable to human users. The term refers to a method for describing an AI model along with its intended effects and possible biases. In AI-driven decision-making, it helps describe the precision, fairness, transparency, and outcomes of the model. When it comes to bringing AI models into production, a company’s ability to explain the rationale behind its decisions is critical to building trust with employees and customers. Companies may take a more ethical approach to AI development if AI can be explained [ 137 , 138 , 139 ].
However, it was found that not a single study mentioned above implemented the explainable AI. While the aforementioned studies were able to achieve high accuracy in diagnosing cardiovascular disease, it may be difficult to implement such wearable technologies into the healthcare cycle if people do not know how the models arrive at such results. In other words, if the results are not explained, the medical community and patients will not have confidence in them, or at least be wary of adopting them.
5.2.2. Federated Machine Learning
The importance of protecting sensitive information has been studied for some time, leading to the development of a variety of protocols for encrypting communications between participants. Differential privacy [ 140 ], k-order anonymity [ 141 ], homomorphic encryption [ 142 ], and other approaches have been developed to protect data before they are transmitted. While several attacks have been uncovered in ML, such as the model inversion attack [ 143 ] and the affiliation attack [ 144 ], none of them are foolproof, as they can infer raw data by accessing the model.
Federated machine learning, often referred to as federated learning (FL), is a novel idea recently introduced by Google in the machine learning field [ 145 ]. The main concept behind FL is to eliminate the exchange of user data between peripherals. FL is a collaborative, distributed/decentralized ML privacy-preserving technology that eliminates the need to transfer data from peripherals to a central server in order to train a model. Instead, the models are sent to the peripheral nodes, where they are trained on the local data, and then sent back to the central aggregation node, where the global model is created without the nodes ever seeing the embedded data. Fortunately, federated learning has emerged as a powerful response to user privacy concerns, paving the way for the collection of additional data to train ML models to improve their accuracy and efficiency.
Furthermore, FL enables training models using data from multiple locations that have data with different structure and composition, also known as data islands, and integrating the information into a global trained model, improving the efficiency of the models. In addition, FL enabled “Learning Transfer”, where models can share their knowledge without having to transfer users’ private data, and made it possible to deal with heterogeneous data scattered in multiple data spaces containing different attributes. The main concept of federated learning is explained in Figure 8 below.
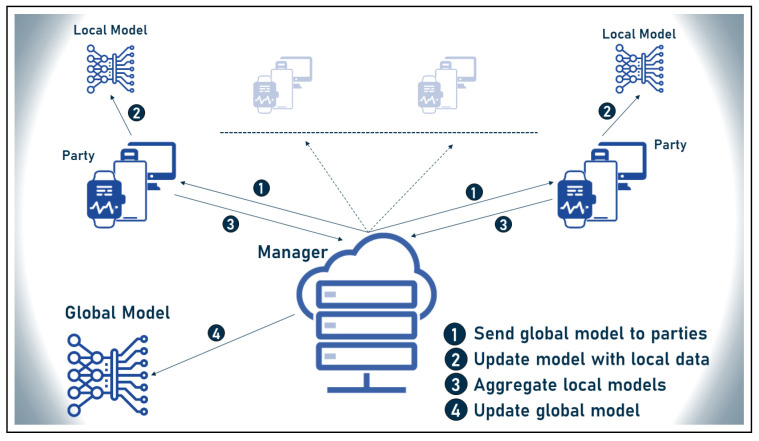
Federated machine learning classical structure.
Federated machine learning has shown promising results in the healthcare industry, as indicated in [ 146 , 147 ]. However, none of the studies included in this review addressed the integration of federated learning into wearable devices to make accurate predictions of cardiovascular disease while maintaining privacy. There could be a few reasons for this. For example, FL is still in its infancy and is still vulnerable to various challenges [ 148 , 149 ]. As a result, these factors may slow down the widespread use of FL in smart wearables in cardiology. However, integrating federated learning into smart wearables may lead to the following outcomes:
Preserving users’ private data, especially health-related data;
Enabling analysis of data from multiple sources in addition to the vital signs captured by the wearables, such as the patient’s medical history derived from electronic health records and the ECG recorded in real time, to provide more accurate results;
Building users’ confidence in smart wearables for cardiovascular disease management and subsequent product adoption.
5.2.3. Multimodal Machine Learning
Multimodal machine learning is concerned with integrating different and divergent data sources to benefit from complementary information in a single computational framework that takes care of a single task, and follows this rule in the context of machine learning (ML), a branch of AI. When it comes to predictive capability, the ability to explore many datasets simultaneously leads to more trustworthy and accurate results, making multimodal machine learning an area of high efficiency and amazing potential. To determine a single goal, multimodal machine learning combines information from many modalities [ 150 ].
In this context, data fusion is the process of combining information from many databases. “The process of merging data to improve state estimates and projections” [ 151 ] is a more precise definition of data fusion. The Joint Directors of Laboratories (JDL) Data Fusion Subpanel concludes that the method of “data fusion” is essential for dealing with many types of data. This description is supported by the authors in [ 152 ], who state that any process that deals with linking, correlating, or combining data retrieved from one or more sources to generate improved information is considered a process that employs data fusion. Because the literature on data fusion is still relatively young, there is no general agreement on the optimal way to merge disparate datasets. This is especially true considering that there are four different methods for performing this [ 151 , 152 ]:
Early fusion: disparate data sources are merged into a single feature vector before being used by a single machine learning algorithm.
Intermediate fusion: takes place in the intermediate phase between input and output of a ML architecture, when all data sources have the same representation format.
Late fusion: defines the aggregation of decisions from multiple ML algorithms, each trained with different data sources.
Hybrid fusion: defines the use of more than one fusion discipline in a single deep algorithm.
The approaches to data fusion defined above are illustrated in Figure 9 below. In addition, none of the smart wearable CVD detection studies reviewed here explored the use of multimodal ML in their algorithms. However, by using this technology, researchers can evaluate many datasets simultaneously, which greatly improves the accuracy of their results. Multimodal ML allows researchers to analyze medical imaging data such as MRIs, ECGs, and EHR data, giving the public more confidence in the accuracy of our AI models.
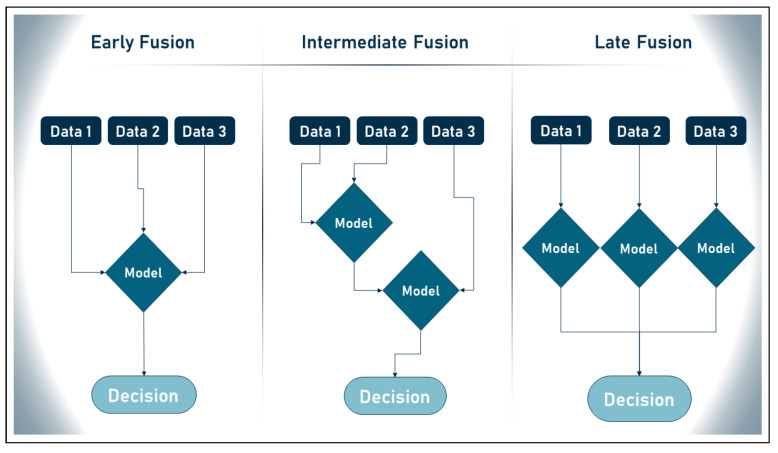
Data fusion different approaches.
6. Challenges and Future Perspectives
Despite the significant role that smart wearables play in the detection of cardiovascular disease, several issues may arise with their use. In addition, the introduction of new artificial intelligence tools and concepts presents many new opportunities to improve the management of heart disease. In this section, challenges and future prospects are discussed to help future studies select starting points for future investigations.
6.1. Challenges
The following are the most common challenges faced by smart wearables in detecting cardiovascular disease. These challenges were identified by analyzing the studies listed in Table 2 and reviewing the literature on smart wearables. Additional information can be obtained from a variety of sources, including, but not limited to [ 153 , 154 , 155 , 156 , 157 ].
6.1.1. Data Privacy and Confidentiality
AI models built into smart wearable technologies work only as well as the information they have access to. While the technical structure of the models themselves—including the cleanliness and suitability of the data—can affect how much data can be used to train AI models, it is generally accepted that more data can lead to more accurate models. In practice, however, there are several obstacles that make data collection the most difficult part of developing AI models. First and foremost is privacy and confidentiality. The security and privacy of personal data are not only strengthened by people, but also by society in general, governments, and companies. Numerous laws and regulations have been enacted to protect personal data, including the European Union’s General Data Protection Regulation (GDPR) [ 158 ], the Chinese People’s Republic of China’s Cybersecurity Law [ 159 ], the People’s Republic of China’s General Principles of Civil Law [ 160 ], Singapore’s PDPA [ 161 ], and hundreds of other principles around the world. Although these regulations help protect private information, they pose new challenges to the traditional AI data processing model to varying degrees by making it more difficult to collect data to train models, which in turn makes it more difficult to improve the accuracy of model performance.
6.1.2. Noise and Artifacts
The noninvasive nature of vital signs collection by smart wearables leaves the recordings open to a greater amount of background noise, known as “artifacts”. Artifacts are unwanted signals or signal distributions that distort the actual signal and contribute to the noise in the data, degrading the quality of the data and reducing the performance and accuracy of the smart models. Artifacts can be divided into two categories, depending on where they originate: intrinsic artifacts, which come from the monitored body itself, and extrinsic artifacts, which are caused by the monitored person’s external environment. The origin of artifacts can be divided into many categories [ 162 , 163 ]:
Intrinsic artifacts (also known as physiological or internal artifacts):
Ocular artifacts: created by ocular motions including blinking, horizontal and vertical eye movement, fluttering of the eyes, etc.;
Muscle artifacts: caused by things such as sneezing, swallowing, clenching, talking, lifting the eyebrows, chewing, contracting the scalp, etc.;
Respiratory artifacts: resulting from an electrode’s movement while breathing, which might manifest as slow, repetitive EEG activity;
Sweat artifacts: result of sweat’s electrolyte concentration shifts on the electrode’s surface after contact with the scalp and are obtained in wearables that collect vital signs that are related to skin.
Extrinsic artifacts (also known as extra-physiological/external artifacts):
Motion artifacts: EEG monitoring systems are susceptible to motion artifacts due to the subject’s physical movement;
Environmental artifacts: these include, but are not limited to, loss of electrode-to-scalp contact, electrode rupture, electromagnetic wave interference from nearby electrical or electronic equipment, etc.
6.1.3. Data Diversity and Heterogeneity
Research in the field of medicine has shown that the use of multiple vital signs may be more helpful in detecting a disease than the use of a single vital sign. Therefore, combining multiple vital signs in the analysis process could allow for more accurate prediction of cardiovascular disease. Combining ECG signals with medical history data from the electronic health record (EHR) and medical images such as magnetic resonance imaging (MRI) is a robust example of multiple vital signs that can be analyzed together to predict cardiovascular disease. However, these data differ in their nature and structure, or even in the devices used to acquire them. More specifically, ECG data are usually stored in the form of real numbers, while EHR data may be in the form of clinical reports, health tests, or other forms, and MRI images are usually stored in different image formats. In this context, classical machine learning models such as support vector machines are usually well suited for linear data, but it is well known that images can be analyzed with deep learning algorithms such as convolutional neural networks. Therefore, it is a difficult task to analyze these data together given their different formats and structures, even if it is more practical for disease detection.
6.1.4. User Technology Adoption and Engagement
One of the major barriers to the use of smart wearables to detect and predict cardiovascular disease is user acceptance, adoption, and participation. Wearing such sensors is received differently by users due to concerns about privacy, discomfort, ethics, and other contextual factors.
Therefore, we may characterize the difficulties as the following set of study questions. In addition, those questions are illustrated in Figure 10 below (the symbol RQ in the list below and in Figure 10 refers to the term “research question”):
RQ1 : Disclosure of subject data may be limited by law. If we utilize these records, how can we ensure that no one’s privacy will be compromised?
RQ2 : There are several potential noise and interference contributors to CVDs detection data. The question is, how should specialists deal with noisy data and artifacts?
RQ3 : The identification of CVDs may be enhanced by analyzing a variety of data. Can AI models handle the analysis of diverse datasets?
RQ4 : Did smart wearables earn enough confidence in the field despite their excellent accuracy in detecting CVDs, and how can this be improved?
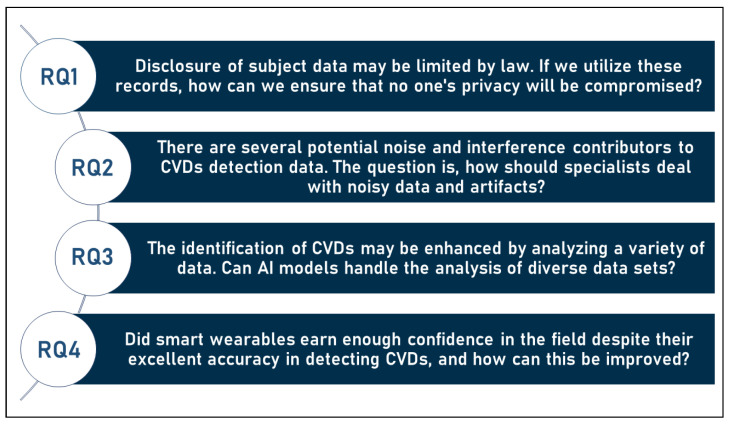
Research questions arising from analysing usage of wearables in CVDs detection.
6.2. Future Perspectives
Cardiovascular disease detection through smart wearables is now a reality. With the global incidence of this disease and the deaths associated with it, there is a growing need to improve the overall process and take more measures for proactive and preventive methods. More research and development on smart wearables is needed to keep up with the increasing demand.
6.2.1. Preserving Data Privacy and Confidentiality
Newer machine learning methods offer new opportunities to protect the privacy and security of user data. One potential technique that can help solve privacy problems is federated learning (FL). Federated learning, a type of collaborative decentralized machine learning that protects user privacy, does not require data to be transported from edge devices to a central server [ 149 , 164 , 165 , 166 , 167 ]. It is expected that using FL to identify CVDs will make it easier to collect more data, which in turn will improve detection accuracy.
6.2.2. Artifacts Removal and Data Readiness
Before proceeding with signal processing, it is important to eliminate or reduce all artifacts, both extrinsic and intrinsic, that might interfere with the signals. References [ 163 , 168 , 169 , 170 ] detail some of the existing implementations that perform this function. In order to clean and preprocess the data to improve the accuracy of cardiovascular disease detection, it is necessary to investigate the automation of noise reduction.
6.2.3. Analysis of Heterogeneous and Diverse Data
Multimodal machine learning is a good solution that allows analyzing data with alternative structures and formats. Since current cardiovascular disease detection and prediction implementations usually analyze only one type of data structure (linear, images, etc.), multimodal machine learning allows analyzing multiple types of data simultaneously to improve the overall result of the intelligent model. Learning a complex task by analyzing data from multiple sources and using complementary knowledge are examples of what multimodal machine learning is capable of. In this context, multimodal datasets are described as information with different structures and formats that come from a variety of sources, each of which contributes a unique set of information (or “modality”) to the overall dataset. Therefore, using the concept of multimodal ML to analyze different data such as ECG, EHR recordings, and MRI images can help increase the accuracy of CVD detection and prediction.
6.2.4. Raising Trust by Enhancing Accuracy, Privacy, and Explainability
Given the prevalence and devastating impact of cardiovascular disease, there is a growing need for practical and viable solutions that can help detect and even predict the onset of these conditions. Consequently, smart wearables have proven to be viable in this area, providing both continuous and real-time monitoring without interfering with daily life routines. However, there is a great need to improve the prediction of CVDs using smart wearables, whether through increased accuracy, better explainability, or by addressing other issues that hinder their adoption by users, such as privacy and ethical constraints. This is a well-known fact that does not need further explanation, because when it comes to health, users are only willing to use tools that are highly accurate, understandable, private, and reliable. In other words, greater trust and wider use of smart wearables as tools for predicting CVDs will result from improved accuracy, reliability, feasibility, privacy, and explainability of such devices.
For this reason, we may summarize the outlook into the following trending research topics. In addition, those research topics are illustrated in Figure 11 below (the symbol TR in the list below and in Figure 11 refers to the term “trending research topic”):
TR1: To protect user privacy, smart wearables should employ federated learning for CVDs detection;
TR2: The use of automated artifact and noise removal methods to mitigate the effects of interference and background noise;
TR3: Improve the quality of recognition models by analyzing data from numerous modalities and sources using multimodal ML techniques;
TR4: Raising precision, explainability, and adaptability will help build users’ confidence in smart wearables.
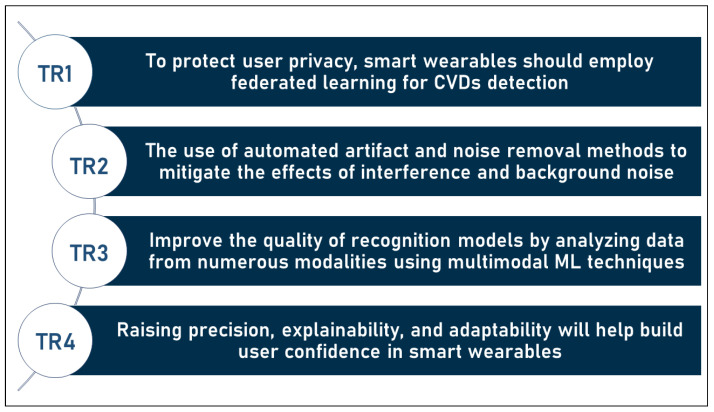
Research topics that may serve as solutions to the challenges in the domain.
Figure 12 below summarizes the challenges–future solutions relationship and illustrates how future views may act as potential solutions in the domain, all of which can assist to enhance research into the use of smart wearables in the detection of CVDs.
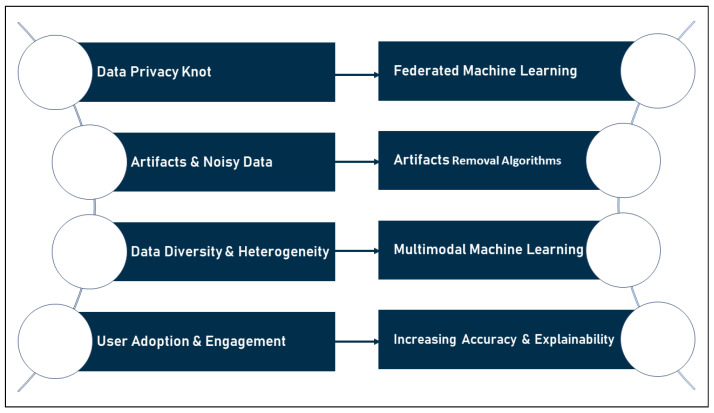
Challenges–future solutions chart.
7. Conclusions
Recently, the use of smart wearables in the diagnosis and prediction of cardiovascular disease has received increasing attention. This is partly due to the technological potential of smart wearables and partly due to the data processing power of artificial intelligence and its derivatives, machine learning and deep learning. In this research, we thoroughly investigated the use of smart wearables to treat fatal heart diseases. The review of the research area showed the high practicality and effectiveness of such methods, reflecting the growing interest that has surged in recent years. However, given the challenges and limitations discussed in this review, there is a large window for improvement that smart wearables should undergo to prove their feasibility and reliability. Increasing accuracy, automating noise reduction, solving privacy issues, dealing with heterogeneity, and improving explainability are interesting topics that should be considered when trying to promote the use of smart wearables in the management of CVDs. As a result, this review provides a brief overview of a number of relevant topics that can be used as recommendations for further research.
Acknowledgments
We acknowledge the support of Centre d’Entrepreneuriat et de Valorisation des Innovations (CEVI).
Author Contributions
Conceptualization: M.M. and M.A.; Formal analysis: M.M.; Investigation: M.M.; Methodology: M.M. and M.A.; Supervision: M.A., A.B., H.I. and A.R.; Visualization: M.M.; Writing—original draft: M.M.; Writing—review & editing: M.A., A.B., H.I. and A.R. All authors have read and agreed to the published version of the manuscript.
Institutional Review Board Statement
Not applicable.
Informed Consent Statement
Data availability statement.
The study did not report any data.
Conflicts of Interest
The authors declare no conflict of interest.
Funding Statement
This research was funded by the Natural Sciences and Engineering Research Council of Canada (NSERC) grant number 06351.
Disclaimer/Publisher’s Note: The statements, opinions and data contained in all publications are solely those of the individual author(s) and contributor(s) and not of MDPI and/or the editor(s). MDPI and/or the editor(s) disclaim responsibility for any injury to people or property resulting from any ideas, methods, instructions or products referred to in the content.
- 1. Urgent Health Challenges for the Next Decade. Jan 13, 2020. [(accessed on 1 April 2021)]. Available online: https://www.who.int/news-room/photo-story/photo-story-detail/urgent-health-challenges-for-the-next-decade .
- 2. Holmes J., Sacchi L., Bellazzi R. Artificial intelligence in medicine. Ann. R. Coll. Surg. Engl. 2004;86:334–338. doi: 10.1308/147870804290. [ DOI ] [ PMC free article ] [ PubMed ] [ Google Scholar ]
- 3. Maddox T.M., Rumsfeld J.S., Payne P.R. Questions for artificial intelligence in health care. JAMA. 2019;321:31–32. doi: 10.1001/jama.2018.18932. [ DOI ] [ PubMed ] [ Google Scholar ]
- 4. Tran B.X., Vu G.T., Ha G.H., Vuong Q.H., Ho M.T., Vuong T.T., La V.P., Ho M.T., Nghiem K.C.P., Nguyen H.L.T., et al. Global evolution of research in artificial intelligence in health and medicine: A bibliometric study. J. Clin. Med. 2019;8:360. doi: 10.3390/jcm8030360. [ DOI ] [ PMC free article ] [ PubMed ] [ Google Scholar ]
- 5. Noorbakhsh-Sabet N., Zand R., Zhang Y., Abedi V. Artificial intelligence transforms the future of health care. Am. J. Med. 2019;132:795–801. doi: 10.1016/j.amjmed.2019.01.017. [ DOI ] [ PMC free article ] [ PubMed ] [ Google Scholar ]
- 6. Roth G.A., Mensah G.A., Johnson C.O., Addolorato G., Ammirati E., Baddour L.M., Barengo N.C., Beaton A.Z., Benjamin E.J., Benziger C.P., et al. Global burden of cardiovascular diseases and risk factors, 1990–2019: Update from the GBD 2019 study. J. Am. Coll. Cardiol. 2020;76:2982–3021. doi: 10.1016/j.jacc.2020.11.010. [ DOI ] [ PMC free article ] [ PubMed ] [ Google Scholar ]
- 7. Centers for Disease Control and Prevention (CDC) Heart Disease Facts|Cdc.Gov. [(accessed on 10 April 2021)];2022 October 14; Available online: https://www.cdc.gov/heartdisease/facts.htm .
- 8. Agency of Canada, Public Health. Heart Disease in Canada—Canada.Ca. August 2022. [(accessed on 15 April 2021)]. Available online: https://www.canada.ca/en/public-health/services/publications/diseases-conditions/heart-disease-canada.html .
- 9. Tsao C.W., Aday A.W., Almarzooq Z.I., Alonso A., Beaton A.Z., Bittencourt M.S., Boehme A.K., Buxton A.E., Carson A.P., Commodore-Mensah Y., et al. Heart disease and stroke statistics—2022 update: A report from the American Heart Association. Circulation. 2022;145:e153–e639. doi: 10.1161/CIR.0000000000001052. [ DOI ] [ PubMed ] [ Google Scholar ]
- 10. National Heart Lung and Blood Institute . Brief: Your Guide to Living Well with Heart Disease (NIH Publication 06-5716) National Institutes of Health; Washington, DC, USA: 2005. [ Google Scholar ]
- 11. Johnson K.W., Torres Soto J., Glicksberg B.S., Shameer K., Miotto R., Ali M., Ashley E., Dudley J.T. Artificial intelligence in cardiology. J. Am. Coll. Cardiol. 2018;71:2668–2679. doi: 10.1016/j.jacc.2018.03.521. [ DOI ] [ PubMed ] [ Google Scholar ]
- 12. Prashanth K., Manjappa M., Srikar C. The advent of artificial intelligence in cardiology: The current applications and future prospects. Eur. J. Mol. Clin. Med. 2020;7:14–20. [ Google Scholar ]
- 13. Kim K.J., Shin D.H. An acceptance model for smart watches: Implications for the adoption of future wearable technology. Internet Res. 2015;25:527–541. doi: 10.1108/IntR-05-2014-0126. [ DOI ] [ Google Scholar ]
- 14. Perera C., Vasilakos A.V. A knowledge-based resource discovery for Internet of Things. Knowl.-Based Syst. 2016;109:122–136. doi: 10.1016/j.knosys.2016.06.030. [ DOI ] [ Google Scholar ]
- 15. Liu L., Peng Y., Liu M., Huang Z. Sensor-based human activity recognition system with a multilayered model using time series shapelets. Knowl.-Based Syst. 2015;90:138–152. doi: 10.1016/j.knosys.2015.09.024. [ DOI ] [ Google Scholar ]
- 16. Park E., Kim K.J., Kwon S.J. Understanding the emergence of wearable devices as next-generation tools for health communication. Inf. Technol. People. 2016;29:717–732. doi: 10.1108/ITP-04-2015-0096. [ DOI ] [ Google Scholar ]
- 17. Turing A. Computing machinery and intelligence. Mind. 1950;59:433–460. doi: 10.1093/mind/LIX.236.433. [ DOI ] [ Google Scholar ]
- 18. Niknejad N., Ismail W.B., Mardani A., Liao H., Ghani I. A comprehensive overview of smart wearables: The state of the art literature, recent advances, and future challenges. Eng. Appl. Artif. Intell. 2020;90:103529. doi: 10.1016/j.engappai.2020.103529. [ DOI ] [ Google Scholar ]
- 19. Kortuem G., Kawsar F., Sundramoorthy V., Fitton D. Smart objects as building blocks for the internet of things. IEEE Internet Comput. 2009;14:44–51. doi: 10.1109/MIC.2009.143. [ DOI ] [ Google Scholar ]
- 20. Cheng J.W., Mitomo H. The underlying factors of the perceived usefulness of using smart wearable devices for disaster applications. Telemat. Inform. 2017;34:528–539. doi: 10.1016/j.tele.2016.09.010. [ DOI ] [ Google Scholar ]
- 21. Poslad S. Ubiquitous Computing: Smart Devices, Environments and Interactions. John Wiley Sons; Hoboken, NJ, USA: 2011. [ Google Scholar ]
- 22. Jeong S.C., Kim S.H., Park J.Y., Choi B. Domain-specific innovativeness and new product adoption: A case of wearable devices. Telemat. Inform. 2017;34:399–412. doi: 10.1016/j.tele.2016.09.001. [ DOI ] [ Google Scholar ]
- 23. Fernandez P. Wearable Technology: Beyond Augmented Reality. Libr. Hi Tech News. 2014;31 doi: 10.1108/LHTN-09-2014-0082. [ DOI ] [ Google Scholar ]
- 24. Thorp E.O. The invention of the first wearable computer; Proceedings of the Digest of Papers. Second International Symposium on Wearable Computers (Cat. No. 98EX215); Pittsburgh, PA, USA. 19–20 October 1998; pp. 4–8. [ Google Scholar ]
- 25. Mann S. Smart clothing: The shift to wearable computing. Commun. ACM. 1996;39:23–24. doi: 10.1145/232014.232021. [ DOI ] [ Google Scholar ]
- 26. Park S., Jayaraman S. Smart textiles: Wearable electronic systems. MRS Bull. 2003;28:585–591. doi: 10.1557/mrs2003.170. [ DOI ] [ Google Scholar ]
- 27. Wright R., Keith L. Wearable technology: If the tech fits, wear it. J. Electron. Resour. Med. Libr. 2014;11:204–216. doi: 10.1080/15424065.2014.969051. [ DOI ] [ Google Scholar ]
- 28. Dimou E., Manavis A., Papachristou E., Kyratsis P. A conceptual design of intelligent shoes for pregnant women; Proceedings of the Workshop on Business Models and ICT Technologies for the Fashion Supply Chain; Florence, Italy. 20–22 April 2016; 2016. pp. 69–77. [ Google Scholar ]
- 29. Yang H., Yu J., Zo H., Choi M. User acceptance of wearable devices: An extended perspective of perceived value. Telemat. Inform. 2016;33:256–269. doi: 10.1016/j.tele.2015.08.007. [ DOI ] [ Google Scholar ]
- 30. Sana F., Isselbacher E.M., Singh J.P., Heist E.K., Pathik B., Armoundas A.A. Wearable devices for ambulatory cardiac monitoring: JACC state-of-the-art review. J. Am. Coll. Cardiol. 2020;75:1582–1592. doi: 10.1016/j.jacc.2020.01.046. [ DOI ] [ PMC free article ] [ PubMed ] [ Google Scholar ]
- 31. DeVore A.D., Wosik J., Hernandez A.F. The future of wearables in heart failure patients. JACC Heart Fail. 2019;7:922–932. doi: 10.1016/j.jchf.2019.08.008. [ DOI ] [ PubMed ] [ Google Scholar ]
- 32. Raja J.M., Elsakr C., Roman S., Cave B., Pour-Ghaz I., Nanda A., Maturana M., Khouzam R.N. Apple watch, wearables, and heart rhythm: Where do we stand? Ann. Transl. Med. 2019;7:417. doi: 10.21037/atm.2019.06.79. [ DOI ] [ PMC free article ] [ PubMed ] [ Google Scholar ]
- 33. Vashistha R., Dangi A.K., Kumar A., Chhabra D., Shukla P. Futuristic biosensors for cardiac health care: An artificial intelligence approach. 3 Biotech. 2018;8:358. doi: 10.1007/s13205-018-1368-y. [ DOI ] [ PMC free article ] [ PubMed ] [ Google Scholar ]
- 34. Lee S., Chu Y., Ryu J., Park Y.J., Yang S., Koh S.B. Artificial intelligence for detection of cardiovascular-related diseases from wearable devices: A systematic review and meta-analysis. Yonsei Med. J. 2022;63:S93–S107. doi: 10.3349/ymj.2022.63.S93. [ DOI ] [ PMC free article ] [ PubMed ] [ Google Scholar ]
- 35. Bayoumy K., Gaber M., Elshafeey A., Mhaimeed O., Dineen E.H., Marvel F.A., Martin S.S., Muse E.D., Turakhia M.P., Tarakji K.G., et al. Smart wearable devices in cardiovascular care: Where we are and how to move forward. Nat. Rev. Cardiol. 2021;18:581–599. doi: 10.1038/s41569-021-00522-7. [ DOI ] [ PMC free article ] [ PubMed ] [ Google Scholar ]
- 36. Kumar S., Victoria-Castro A.M., Melchinger H., O’Connor K.D., Psotka M., Desai N.R., Ahmad T., Wilson F.P. Wearables in Cardiovascular Disease. J. Cardiovasc. Transl. Res. 2022:1–12. doi: 10.1007/s12265-022-10314-0. [ DOI ] [ PubMed ] [ Google Scholar ]
- 37. Page M.J., Moher D., Bossuyt P.M., Boutron I., Hoffmann T.C., Mulrow C.D., Shamseer L., Tetzlaff J.M., Akl E.A., Brennan S.E., et al. PRISMA 2020 explanation and elaboration: Updated guidance and exemplars for reporting systematic reviews. BMJ. 2021;372:n160. doi: 10.1136/bmj.n160. [ DOI ] [ PMC free article ] [ PubMed ] [ Google Scholar ]
- 38. PRISMA. [(accessed on 17 November 2022)]. Available online: https://www.prisma-statement.org//PRISMAStatement/Checklist.aspx .
- 39. Wang I.-J., Liao L.-D., Wang Y.-T., Chen C.-Y., Lin B.-S., Lu S.-W., Lin C.-T. A wearable mobile electrocardiogram measurement device with novel dry polymer-based electrodes; Proceedings of the TENCON 2010–2010 IEEE Region 10 Conference; Fukuoka, Japan. 21–24 November 2010; pp. 379–384. [ Google Scholar ]
- 40. Oresko J.J., Jin Z., Cheng J., Huang S., Sun Y., Duschl H., Cheng A.C. A wearable smartphone-based platform for real-time cardiovascular disease detection via electrocardiogram processing. IEEE Trans. Inf. Technol. Biomed. 2010;14:734–740. doi: 10.1109/TITB.2010.2047865. [ DOI ] [ PubMed ] [ Google Scholar ]
- 41. Lin C.-T., Chang K.-C., Lin C.-L., Chiang C.-C., Lu S.-W., Chang S.-S., Lin B.-S., Liang H.-Y., Chen R.-J., Lee Y.-T., et al. An intelligent telecardiology system using a wearable and wireless ECG to detect atrial fibrillation. IEEE Trans. Inf. Technol. Biomed. 2010;14:726–733. doi: 10.1109/TITB.2010.2047401. [ DOI ] [ PubMed ] [ Google Scholar ]
- 42. Hu S., Shao Z., Tan J. A real-time cardiac arrhythmia classification system with wearable electrocardiogram; Proceedings of the 2011 International Conference on Body Sensor Networks; Dallas, TX, USA. 23–25 May 2011; pp. 119–124. [ Google Scholar ]
- 43. Lee H.-J., Kim D.-O., Kang B.-J., Ban S.-W. Mobile embedded health-care system working on wireless sensor network; Proceedings of the 2011 Third International Conference on Communications and Mobile Computing; Qingdao, China. 18–20 April 2011; pp. 161–164. [ Google Scholar ]
- 44. Cheng S., Tamil L.S., Levine B. A mobile health system to identify the onset of paroxysmal atrial fibrillation; Proceedings of the 2015 International Conference on Healthcare Informatics; Dallas, TX, USA. 21–23 October 2015; pp. 189–192. [ Google Scholar ]
- 45. Nemati S., Ghassemi M.M., Ambai V., Isakadze N., Levantsevych O., Shah A., Clifford G.D. Monitoring and detecting atrial fibrillation using wearable technology; Proceedings of the 2016 38th Annual International Conference of the IEEE Engineering in Medicine and Biology Society (EMBC); Orlando, FL, USA. 16–20 August 2016; pp. 3394–3397. [ DOI ] [ PubMed ] [ Google Scholar ]
- 46. Wu T.-K., Lin C.-C., Ku W.-Y., Liou Y.-S., Yang C.-Y., Lee M.-Y., Lin W.-Y., Tsai T.-H. The Study of the Enhanced External Counterpulsation System Based on Smart Clothes; Proceedings of the 2016 IEEE First International Conference on Connected Health: Applications, Systems and Engineering Technologies (CHASE); Washington, DC, USA. 27–29 June 2016; pp. 125–129. [ Google Scholar ]
- 47. Shashikumar S.P., Shah A.J., Li Q., Clifford G.D., Nemati S. A deep learning approach to monitoring and detecting atrial fibrillation using wearable technology; Proceedings of the 2017 IEEE EMBS International Conference on Biomedical & Health Informatics (BHI); Orlando, FL, USA. 16–19 February 2017; pp. 141–144. [ Google Scholar ]
- 48. ElSaadany Y., Majumder A.J.A., Ucci D.R. A wireless early prediction system of cardiac arrest through IoT; Proceedings of the 2017 IEEE 41st Annual Computer Software and Applications Conference (COMPSAC); Turin, Italy. 4–8 July 2017; pp. 690–695. [ Google Scholar ]
- 49. Sadrawi M., Lin C.-H., Lin Y.-T., Hsieh Y., Kuo C.-C., Chien J.-C., Haraikawa K., Abbod M.F., Shieh J.-S. Arrhythmia evaluation in wearable ECG devices. Sensors. 2017;17:2445. doi: 10.3390/s17112445. [ DOI ] [ PMC free article ] [ PubMed ] [ Google Scholar ]
- 50. Roj D., Wrobel J., Matonia A., Horoba K., Henzel N. Control and signal processing software embedded in smart wristband monitor of silent atrial fibrillation; Proceedings of the 2017 MIXDES-24th International Conference Mixed Design of Integrated Circuits and Systems; Bydgoszcz, Poland. 22–24 June 2017; pp. 585–590. [ Google Scholar ]
- 51. Lin C.-W., Chang Y., Lin C.-C.K., Tsai L.-M., Chen J.-Y. Development of an AI-based non-invasive Pulse AudioGram monitoring device for arrhythmia screening; Proceedings of the 2017 IEEE Healthcare Innovations and Point of Care Technologies (HI-POCT); Bethesda, MD, USA. 6–8 November 2017; pp. 40–43. [ Google Scholar ]
- 52. Chowdhuryy M.H.I., Sultana M., Ghosh R., Ahamed J.U., Mahmood M.A.I. AI assisted portable ECG for fast and patient specific diagnosis; Proceedings of the 2018 International Conference on Computer, Communication, Chemical, Material and Electronic Engineering (IC4ME2); Rajshahi, Bangladesh. 8–9 February 2018; pp. 1–4. [ Google Scholar ]
- 53. Xia Y., Zhang H., Xu L., Gao Z., Zhang H., Liu H., Li S. An automatic cardiac arrhythmia classification system with wearable electrocardiogram. IEEE Access. 2018;6:16529–16538. doi: 10.1109/ACCESS.2018.2807700. [ DOI ] [ Google Scholar ]
- 54. Ni H., Cho S., Mankoff J., Yang J. Automated recognition of hypertension through overnight continuous HRV monitoring. J. Ambient. Intell. Humaniz. Comput. 2018;9:2011–2023. doi: 10.1007/s12652-017-0471-y. [ DOI ] [ Google Scholar ]
- 55. Aliamiri A., Shen Y. Deep learning based atrial fibrillation detection using wearable photoplethysmography sensor; Proceedings of the 2018 IEEE EMBS International Conference on Biomedical & Health Informatics (BHI); Las Vegas, NV, USA. 4–7 March 2018; pp. 442–445. [ Google Scholar ]
- 56. Park E.-B., Lee J.-H. The Automated Heart Disease Detection System with Dry-Electrode Based Outdoor Shirts; Proceedings of the 2018 IEEE International Conference on Consumer Electronics-Asia (ICCE-Asia); JeJu, Republic of Korea. 24–26 June 2018; pp. 206–212. [ Google Scholar ]
- 57. Alfarhan K.A., Mashor M.Y., Mohd Saad A.R., Omar M.I. Journal of Biomimetics, Biomaterials and Biomedical Engineering. Volume 35. Trans Tech Publications Ltd.; Stafa-Zurich, Switzerland: 2018. Wireless heart abnormality monitoring kit based on Raspberry Pi; pp. 96–108. [ Google Scholar ]
- 58. Kim Y.S., Mahmood M., Lee Y., Kim N.K., Kwon S., Herbert R., Kim D., Cho H.C., Yeo W.-H. All-in-one, wireless, stretchable hybrid electronics for smart, connected, and ambulatory physiological monitoring. Adv. Sci. 2019;6:1900939. doi: 10.1002/advs.201900939. [ DOI ] [ PMC free article ] [ PubMed ] [ Google Scholar ]
- 59. Lin Y.-J., Chuang C.-W., Yen C.-Y., Huang S.-H., Chen J.-Y., Lee S.-Y. An AIoT wearable ECG patch with decision tree for arrhythmia analysis; Proceedings of the 2019 IEEE Biomedical Circuits and Systems Conference (BioCAS); Nara, Japan. 17–19 October 2019; pp. 1–4. [ Google Scholar ]
- 60. Lin Y.-J., Chuang C.-W., Yen C.-Y., Huang S.-H., Huang P.-W., Chen J.Y., Lee S.Y. Artificial intelligence of things wearable system for cardiac disease detection; Proceedings of the 2019 IEEE International Conference on Artificial Intelligence Circuits and Systems (AICAS); Hsinchu, Taiwan. 18–20 March 2019; pp. 67–70. [ Google Scholar ]
- 61. Van Zaen J., Chételat O., Lemay M., Calvo E.M., Delgado-Gonzalo R. Classification of cardiac arrhythmias from single lead ECG with a convolutional recurrent neural network. arXiv. 20191907.01513 [ Google Scholar ]
- 62. Scirè A., Tropeano F., Anagnostopoulos A., Chatzigiannakis I. Fog-computing-based heartbeat detection and arrhythmia classification using machine learning. Algorithms. 2019;12:32. doi: 10.3390/a12020032. [ DOI ] [ Google Scholar ]
- 63. Faust O., Ciaccio E.J., Majid A., Acharya U.R. Improving the safety of atrial fibrillation monitoring systems through human verification. Saf. Sci. 2019;118:881–886. doi: 10.1016/j.ssci.2019.05.013. [ DOI ] [ Google Scholar ]
- 64. Karboub K., Tabaa M., Dellagi S., Dandache A., Moutaouakkil F. Intelligent patient monitoring for arrhythmia and congestive failure patients using internet of things and convolutional neural network; Proceedings of the 2019 31st International Conference on Microelectronics (ICM); Cairo, Egypt. 15–18 December 2019; pp. 292–295. [ Google Scholar ]
- 65. Zhao P., Quan D., Yu W., Yang X., Fu X. Towards deep learning-based detection scheme with raw ECG signal for wearable telehealth systems; Proceedings of the 2019 28th International Conference on Computer Communication and Networks (ICCCN); Valencia, Spain. 29 July–1 August 2019; pp. 1–9. [ Google Scholar ]
- 66. Yang C., Veiga C., Rodriguez-Andina J.J., Farina J., Iniguez A., Yin S. Using PPG signals and wearable devices for atrial fibrillation screening. IEEE Trans. Ind. Electron. 2019;66:8832–8842. doi: 10.1109/TIE.2018.2889614. [ DOI ] [ Google Scholar ]
- 67. HHuda N., Khan S., Abid R., Shuvo S.B., Labib M.M., Hasan T. A low-cost, low-energy wearable ECG system with cloud-based arrhythmia detection; Proceedings of the 2020 IEEE Region 10 Symposium (TENSYMP); Dhaka, Bangladesh. 5–7 June 2020; pp. 1840–1843. [ Google Scholar ]
- 68. Chen E., Jiang J., Su R., Gao M., Zhu S., Zhou J., Huo Y. A new smart wristband equipped with an artificial intelligence algorithm to detect atrial fibrillation. Heart Rhythm. 2020;17:847–853. doi: 10.1016/j.hrthm.2020.01.034. [ DOI ] [ PubMed ] [ Google Scholar ]
- 69. Bhat T., Bhat S., Manoj T. A Real-Time IoT Based Arrhythmia Classifier Using Convolutional Neural Networks; Proceedings of the 2020 IEEE International Conference on Distributed Computing, VLSI, Electrical Circuits and Robotics (DISCOVER); Udupi, India. 30–31 October 2020; pp. 79–83. [ Google Scholar ]
- 70. Ali F., El-Sappagh S., Islam S.R., Kwak D., Ali A., Imran M., Kwak K.S. A smart healthcare monitoring system for heart disease prediction based on ensemble deep learning and feature fusion. Inf. Fusion. 2020;63:208–222. doi: 10.1016/j.inffus.2020.06.008. [ DOI ] [ Google Scholar ]
- 71. Rincon J.A., Guerra-Ojeda S., Carrascosa C., Julian V. An IoT and fog computing-based monitoring system for cardiovascular patients with automatic ECG classification using deep neural networks. Sensors. 2020;20:7353. doi: 10.3390/s20247353. [ DOI ] [ PMC free article ] [ PubMed ] [ Google Scholar ]
- 72. Khan M.A. An IoT framework for heart disease prediction based on MDCNN classifier. IEEE Access. 2020;8:34717–34727. doi: 10.1109/ACCESS.2020.2974687. [ DOI ] [ Google Scholar ]
- 73. Yang C., Aranoff N.D., Green P., Tavassolian N. Classification of aortic stenosis using time–frequency features from chest cardio-mechanical signals. IEEE Trans. Biomed. Eng. 2019;67:1672–1683. doi: 10.1109/TBME.2019.2942741. [ DOI ] [ PubMed ] [ Google Scholar ]
- 74. Reddy S., Seshadri S.B., Bothra G.S., Suhas T.G., Thundiyil S.C. Detection of arrhythmia in real-time using ECG signal analysis and convolutional neural networks; Proceedings of the 2020 IEEE 21st International Conference on Computational Problems of Electrical Engineering (CPEE); Online, Poland. 16–19 September 2020; pp. 1–4. [ Google Scholar ]
- 75. Gowtham A., Anirudh L., Sreeja B.S., Aakash B.A., Adittya S. Detection of Arrhythmia using ECG waves with Deep Convolutional Neural Networks; Proceedings of the 2020 4th International Conference on Electronics, Communication and Aerospace Technology (ICECA); Coimbatore, India. 5–7 November 2020; pp. 1390–1396. [ Google Scholar ]
- 76. Han D., Bashar S.K., Zieneddin F., Ding E., Whitcomb C., McManus D.D., Chon K.H. Digital image processing features of smartwatch photoplethysmography for cardiac arrhythmia detection; Proceedings of the 2020 42nd Annual International Conference of the IEEE Engineering in Medicine & Biology Society (EMBC); Montreal, QC, Canada. 20–24 July 2020; pp. 4071–4074. [ DOI ] [ PMC free article ] [ PubMed ] [ Google Scholar ]
- 77. Afadar Y., Akram A., Alkeebali A., Majzoub S. Heart Arrhythmia Detection & Monitoring Using Machine Learning & ECG Wearable Device; Proceedings of the 2020 Seventh International Conference on Information Technology Trends (ITT); Abu Dhabi, United Arab Emirates. 25–26 November 2020; pp. 107–112. [ Google Scholar ]
- 78. Marsili I.A., Biasiolli L., Masè M., Adami A., Andrighetti A.O., Ravelli F., Nollo G. Implementation and validation of real-time algorithms for atrial fibrillation detection on a wearable ECG device. Comput. Biol. Med. 2020;116:103540. doi: 10.1016/j.compbiomed.2019.103540. [ DOI ] [ PubMed ] [ Google Scholar ]
- 79. Ahsanuzzaman S.M., Ahmed T., Rahman M.A. Low cost, portable ECG monitoring and alarming system based on deep learning; Proceedings of the 2020 IEEE Region 10 Symposium (TENSYMP); Dhaka, Bangladesh. 5–7 June 2020; pp. 316–319. [ Google Scholar ]
- 80. Saadatnejad S., Oveisi M., Hashemi M. LSTM-based ECG classification for continuous monitoring on personal wearable devices. IEEE J. Biomed. Health Inform. 2019;24:515–523. doi: 10.1109/JBHI.2019.2911367. [ DOI ] [ PubMed ] [ Google Scholar ]
- 81. Malepati N., Fatima R., Gupta S., Ramsali V., Shobha K.R. Portable ECG Device for Remote Monitoring and Detection of Onset of Arrhythmia; Proceedings of the 2020 IEEE International Conference on Electronics, Computing and Communication Technologies (CONECCT); Bangalore, India. 2–4 July 2020; pp. 1–5. [ Google Scholar ]
- 82. Panganiban E.B., Chung W.-Y., Tai W.-C., Paglinawan A.C., Lai J.-S., Cheng R.-W., Chang M.-K., Chang P.H. International Conference on Biomedical and Health Informatics. Springer; Cham, Switzerland: 2019. Real-time intelligent healthcare monitoring and diagnosis system through deep learning and segmented analysis; pp. 15–25. [ Google Scholar ]
- 83. Bazi Y., Al Rahhal M.M., AlHichri H., Ammour N., Alajlan N., Zuair M. Real-time mobile-based electrocardiogram system for remote monitoring of patients with cardiac arrhythmias. Int. J. Pattern Recognit. Artif. Intell. 2020;34:2058013. doi: 10.1142/S0218001420580136. [ DOI ] [ Google Scholar ]
- 84. Rahman M.A., Samin M.J.A., Al Hasan Z. Remote ECG Monitoring and Syncope Detection System Using Deep Learning; Proceedings of the 2020 2nd International Conference on Advanced Information and Communication Technology (ICAICT); Dhaka, Bangladesh. 28–29 November 2020; pp. 201–206. [ Google Scholar ]
- 85. Ding X., Wang Y., Hao Y., Lv Y., Chen R., Yan H. A New Measure of Pulse Rate Variability and Detection of Atrial Fibrillation Based on Improved Time Synchronous Averaging. Comput. Math. Methods Med. 2021;2021:5597559. doi: 10.1155/2021/5597559. [ DOI ] [ PMC free article ] [ PubMed ] [ Google Scholar ]
- 86. Elwahsh H., El-Shafeiy E., Alanazi S., Tawfeek M.A. A new smart healthcare framework for real-time heart disease detection based on deep and machine learning. Peerj Comput. Sci. 2021;7:e646. doi: 10.7717/peerj-cs.646. [ DOI ] [ PMC free article ] [ PubMed ] [ Google Scholar ]
- 87. Tang X., Tang W. An ECG Delineation and Arrhythmia Classification System Using Slope Variation Measurement by Ternary Second-Order Delta Modulators for Wearable ECG Sensors. IEEE Trans. Biomed. Circuits Syst. 2021;15:1053–1065. doi: 10.1109/TBCAS.2021.3113665. [ DOI ] [ PubMed ] [ Google Scholar ]
- 88. Li H., An Z., Zuo S., Zhu W., Zhang Z., Zhang S., Zhang C., Song W., Mao Q., Mu Y., et al. Artificial Intelligence-Enabled ECG Algorithm Based on Improved Residual Network for Wearable ECG. Sensors. 2021;21:6043. doi: 10.3390/s21186043. [ DOI ] [ PMC free article ] [ PubMed ] [ Google Scholar ]
- 89. Mohandas P., Aswin P.R., John A., Madhu M., Thomas G., Kurupath V. Automated cardiac condition diagnosis using AI based ECG analysis system for school children; Proceedings of the 2021 8th International Conference on Smart Computing and Communications (ICSCC); Kochi, India. 1–3 July 2021; pp. 362–366. [ Google Scholar ]
- 90. Santala O.E., Halonen J., Martikainen S., Jäntti H., Rissanen T.T., Tarvainen M.P., Laitinen T.P., Laitinen T.M., Väliaho E.S., Hartikainen J.E.K., et al. Automatic Mobile Health Arrhythmia Monitoring for the Detection of Atrial Fibrillation: Prospective Feasibility, Accuracy, and User Experience Study. JMIR mHealth and uHealth. 2021;9:e29933. doi: 10.2196/29933. [ DOI ] [ PMC free article ] [ PubMed ] [ Google Scholar ]
- 91. Sandberg E.L., Grenne B.L., Berge T., Grimsmo J., Atar D., Halvorsen S., Fensli R., Jortveit J. Diagnostic accuracy and usability of the ECG247 smart heart sensor compared to conventional Holter technology. J. Healthc. Eng. 2021;2021:5230947. doi: 10.1155/2021/5230947. [ DOI ] [ PMC free article ] [ PubMed ] [ Google Scholar ]
- 92. Mihiranga A., Shane D., Indeewari B., Udana A., Nawinna D., Attanayaka B. Digital Tool for Prevention, Identification and Emergency Handling of Heart Attacks; Proceedings of the 2021 IEEE 9th Region 10 Humanitarian Technology Conference (R10-HTC); Bangalore, India. 30 September–2 October 2021; pp. 1–6. [ Google Scholar ]
- 93. Panganiban E.B., Paglinawan A.C., Chung W.Y., Paa G.L.S. ECG diagnostic support system (EDSS): A deep learning neural network based classification system for detecting ECG abnormal rhythms from a low-powered wearable biosensors. Sens. Bio-Sens. Res. 2021;31:100398. doi: 10.1016/j.sbsr.2021.100398. [ DOI ] [ Google Scholar ]
- 94. Virgeniya S.C., Ramaraj E. IoT and Big Data for ECG Signal Classification-A Quick Decision System; Proceedings of the 2021 2nd International Conference on Communication, Computing and Industry 4.0 (C2I4); Bangalore, India. 16–17 December 2021; pp. 1–6. [ Google Scholar ]
- 95. Sahoo S., Borthakur P., Baruah N., Chutia B.P. Journal of Physics: Conference Series. Volume 1950. IOP Publishing; Bristol, UK: 2021. IoT and machine learning based health monitoring and heart attack prediction system; p. 012056. [ Google Scholar ]
- 96. Meng L., Ge K., Song Y., Yang D., Lin Z. Long-term wearable electrocardiogram signal monitoring and analysis based on convolutional neural network. IEEE Trans. Instrum. Meas. 2021;70:1–11. doi: 10.1109/TIM.2021.3072144. [ DOI ] [ Google Scholar ]
- 97. Kadhim O.R., Taha Z.K., Abd AL-Majeed S.W. Low-Cost Cloud-based Intelligent Remote Patient Monitoring for Heart Patient; Proceedings of the 2021 International Conference on Communication & Information Technology (ICICT); Basrah, Iraq. 5–6 June 2021; pp. 31–36. [ Google Scholar ]
- 98. Frodi D.M., Kolk M.Z., Langford J., Andersen T.O., Knops R.E., Tan H.L., Svendsen J.H., Tjong F.V., Diederichsen S.Z. Rationale and design of the SafeHeart study: Development and testing of a mHealth tool for the prediction of arrhythmic events and implantable cardioverter-defibrillator therapy. Cardiovasc. Digit. Health J. 2021;2:S11–S20. doi: 10.1016/j.cvdhj.2021.10.002. [ DOI ] [ PMC free article ] [ PubMed ] [ Google Scholar ]
- 99. Pierleoni P., Belli A., Gentili A., Incipini L., Palma L., Raggiunto S., Sbrollini A., Burattini L. Real-time smart monitoring system for atrial fibrillation pathology. J. Ambient. Intell. Humaniz. Comput. 2021;12:4461–4469. doi: 10.1007/s12652-019-01602-w. [ DOI ] [ Google Scholar ]
- 100. Siavashi A., Majidi M. Sensing, Wireless Transmission, and Smart Processing of Heart Signals; Proceedings of the 2021 5th International Conference on Internet of Things and Applications (IoT); Isfahan, Iran. 19–20 May 2021; pp. 1–6. [ Google Scholar ]
- 101. Feng H.-Y., Chen P.-Y., Hou J. SR-ScatNet Algorithm for On-device ECG Time Series Anomaly Detection; Proceedings of the SoutheastCon 2021; Atlanta, GA, USA. 10–13 March 2021; pp. 1–5. [ Google Scholar ]
- 102. Liu X., Liu T., Zhang Z., Kuo P.C., Xu H., Yang Z., Lan K., Li P., Ouyang Z., Ng Y.L., et al. TOP-Net prediction model using bidirectional long short-term memory and medical-grade wearable multisensor system for tachycardia onset: Algorithm development study. JMIR Med. Inform. 2021;9:e18803. doi: 10.2196/18803. [ DOI ] [ PMC free article ] [ PubMed ] [ Google Scholar ]
- 103. Zhang H., Zhu L., Nathan V., Kuang J., Kim J., Gao J.A., Olgin J. Towards Early Detection and Burden Estimation of Atrial Fibrillation in an Ambulatory Free-living Environment. Proc. Acm Interact. Mob. Wearable Ubiquitous Technol. 2021;5:1–19. doi: 10.1145/3463503. [ DOI ] [ Google Scholar ]
- 104. Hui Y., Yin Z., Wu M., Li D. Wearable devices acquired ECG signals detection method using 1D convolutional neural network; Proceedings of the 2021 15th International Symposium on Medical Information and Communication Technology (ISMICT); Xiamen, China. 14–16 April 2021; pp. 81–85. [ Google Scholar ]
- 105. Pramukantoro E.S., Gofuku A. A heartbeat classifier for continuous prediction using a wearable device. Sensors. 2022;22:5080. doi: 10.3390/s22145080. [ DOI ] [ PMC free article ] [ PubMed ] [ Google Scholar ]
- 106. Pramukantoro E.S., Gofuku A. A real-time heartbeat monitoring using wearable device and machine learning; Proceedings of the 2022 IEEE 4th Global Conference on Life Sciences and Technologies (LifeTech); Osaka, Japan. 7–9 March 2022; pp. 270–272. [ Google Scholar ]
- 107. Kwon J.M., Jo Y.Y., Lee S.Y., Kang S., Lim S.Y., Lee M.S., Kim K.H. Artificial Intelligence-Enhanced Smartwatch ECG for Heart Failure-Reduced Ejection Fraction Detection by Generating 12-Lead ECG. Diagnostics. 2022;12:654. doi: 10.3390/diagnostics12030654. [ DOI ] [ PMC free article ] [ PubMed ] [ Google Scholar ]
- 108. Zhu L., Nathan V., Kuang J., Kim J., Avram R., Olgin J., Gao J. Atrial fibrillation detection and atrial fibrillation burden estimation via wearables. IEEE J. Biomed. Health Inform. 2021;26:2063–2074. doi: 10.1109/JBHI.2021.3131984. [ DOI ] [ PubMed ] [ Google Scholar ]
- 109. Sabbadini R., Riccio M., Maresca L., Irace A., Breglio G. Atrial Fibrillation Detection by Means of Edge Computing on Wearable Device: A Feasibility Assessment; Proceedings of the 2022 IEEE International Symposium on Medical Measurements and Applications (MeMeA); Messina, Italy. 22–24 June 2022; pp. 1–6. [ Google Scholar ]
- 110. Santala O.E., Lipponen J.A., Jäntti H., Rissanen T.T., Tarvainen M.P., Laitinen T.P., Laitinen T.M., Castrén M., Väliaho E.S., Rantula O.A., et al. Continuous mHealth Patch Monitoring for the Algorithm-Based Detection of Atrial Fibrillation: Feasibility and Diagnostic Accuracy Study. JMIR Cardio. 2022;6:e31230. doi: 10.2196/31230. [ DOI ] [ PMC free article ] [ PubMed ] [ Google Scholar ]
- 111. Baraeinejad B., Shayan M.F., Vazifeh A.R., Rashidi D., Hamedani M.S., Tavolinejad H., Gorji P., Razmara P., Vaziri K., Vashaee D., et al. Design and Implementation of an Ultralow-Power ECG Patch and Smart Cloud-Based Platform. IEEE Trans. Instrum. Meas. 2022;71:1–11. doi: 10.1109/TIM.2022.3164151. [ DOI ] [ Google Scholar ]
- 112. Hiraoka D., Inui T., Kawakami E., Oya M., Tsuji A., Honma K., Kawasaki Y., Ozawa Y., Shiko Y., Ueda H., et al. Diagnosis of Atrial Fibrillation Using Machine Learning with Wearable Devices After Cardiac Surgery: Algorithm Development Study. JMIR Form. Res. 2022;6:e35396. doi: 10.2196/35396. [ DOI ] [ PMC free article ] [ PubMed ] [ Google Scholar ]
- 113. Shrestha A.P., Yu C.-H. ECG Data Analysis with IoT and Machine Learning; Proceedings of the 2022 IEEE 12th Annual Computing and Communication Workshop and Conference (CCWC); Las Vegas, NV, USA. 26–29 January 2022; pp. 0323–0327. [ Google Scholar ]
- 114. Jenifer A., Jeba G., Paulraj L., Kumar N., Yuvaraj T., Alen G., Rozario P., Amoli R. Edge-based Heart Disease Prediction Device using Internet of Things; Proceedings of the 2022 International Conference on Applied Artificial Intelligence and Computing (ICAAIC); Salem, India. 9–11 May 2022; pp. 1500–1504. [ Google Scholar ]
- 115. Muthu Ganesh V. Nithiyanantham, J. Heuristic-based channel selection with enhanced deep learning for heart disease prediction under WBAN. Comput. Methods Biomech. Biomed. Eng. 2022;25:1–20. doi: 10.1080/10255842.2021.2013828. [ DOI ] [ PubMed ] [ Google Scholar ]
- 116. Dami S. Internet of things-based health monitoring system for early detection of cardiovascular events during COVID-19 pandemic. World J. Clin. Cases. 2022;10:9207. doi: 10.12998/wjcc.v10.i26.9207. [ DOI ] [ PMC free article ] [ PubMed ] [ Google Scholar ]
- 117. Koivisto T., Lahdenoja O., Hurnanen T., Vasankari T., Jaakkola S., Kiviniemi T., Airaksinen K.J. Mechanocardiography in the Detection of Acute ST Elevation Myocardial Infarction: The MECHANO-STEMI Study. Sensors. 2022;22:4384. doi: 10.3390/s22124384. [ DOI ] [ PMC free article ] [ PubMed ] [ Google Scholar ]
- 118. Sheeba A., Padmakala S., Subasini C.A., Karuppiah S.P. MKELM: Mixed Kernel Extreme Learning Machine using BMDA optimization for web services based heart disease prediction in smart healthcare. Comput. Methods Biomech. Biomed. Eng. 2022;25:1–15. doi: 10.1080/10255842.2022.2034795. [ DOI ] [ PubMed ] [ Google Scholar ]
- 119. Shafi J., Obaidat M.S., Krishna P.V., Sadoun B., Pounambal M., Gitanjali J. Prediction of heart abnormalities using deep learning model and wearabledevices in smart health homes. Multimed. Tools Appl. 2022;81:543–557. doi: 10.1007/s11042-021-11346-5. [ DOI ] [ Google Scholar ]
- 120. Aziz S., Khan M.U., Iqtidar K., Ali S., Remete A.N., Javid M.A. Pulse plethysmograph signal analysis method for classification of heart diseases using novel local spectral ternary patterns. Expert Syst. 2022;39:e13011. doi: 10.1111/exsy.13011. [ DOI ] [ Google Scholar ]
- 121. Chakraborty C., Kishor A. Real-Time Cloud-Based Patient-Centric Monitoring Using Computational Health Systems. IEEE Trans. Comput. Soc. Syst. 2022;9:1613–1623. doi: 10.1109/TCSS.2022.3170375. [ DOI ] [ Google Scholar ]
- 122. Jansi Rani S.V., Chandran K.R., Ranganathan A., Chandrasekharan M., Janani B., Deepsheka G. Smart wearable model for predicting heart disease using machine learning. J. Ambient. Intell. Humaniz. Comput. 2022;13:4321–4332. doi: 10.1007/s12652-022-03823-y. [ DOI ] [ Google Scholar ]
- 123. Arpaia P., Crauso F., De Benedetto E., Duraccio L., Improta G., Serino F. Soft Transducer for Patient’s Vitals Telemonitoring with Deep Learning-Based Personalized Anomaly Detection. Sensors. 2022;22:536. doi: 10.3390/s22020536. [ DOI ] [ PMC free article ] [ PubMed ] [ Google Scholar ]
- 124. Manimurugan S., Almutairi S., Aborokbah M.M., Narmatha C., Ganesan S., Chilamkurti N., Alzaheb R.A., Almoamari H. Two-Stage Classification Model for the Prediction of Heart Disease Using IoMT and Artificial Intelligence. Sensors. 2022;22:476. doi: 10.3390/s22020476. [ DOI ] [ PMC free article ] [ PubMed ] [ Google Scholar ]
- 125. Ganti V.G., Gazi A.H., An S., Srivatsa A.V., Nevius B.N., Nichols C.J., Carek A.M., Fares M., Abdulkarim M., Hussain T., et al. Wearable Seismocardiography-Based Assessment of Stroke Volume in Congenital Heart Disease. J. Am. Heart Assoc. 2022;11:e026067. doi: 10.1161/JAHA.122.026067. [ DOI ] [ PMC free article ] [ PubMed ] [ Google Scholar ]
- 126. Price D. How to read an electrocardiogram (ECG). Part 1: Basic principles of the ECG. The normal ECG. South Sudan Med J. 2010;3:26–31. [ Google Scholar ]
- 127. Lip G.Y., Tse H.F. Management of atrial fibrillation. Lancet. 2007;370:604–618. doi: 10.1016/S0140-6736(07)61300-2. [ DOI ] [ PubMed ] [ Google Scholar ]
- 128. Bini S.A. Artificial intelligence, machine learning, deep learning, and cognitive computing: What do these terms mean and how will they impact health care? J. Arthroplast. 2018;33:2358–2361. doi: 10.1016/j.arth.2018.02.067. [ DOI ] [ PubMed ] [ Google Scholar ]
- 129. Ertel W. Introduction to Artificial Intelligence. Springer; Berlin/Heidelberg, Germany: 2018. [ Google Scholar ]
- 130. Miotto R., Wang F., Wang S., Jiang X., Dudley J.T. Deep learning for healthcare: Review, opportunities and challenges. Brief. Bioinform. 2018;19:1236–1246. doi: 10.1093/bib/bbx044. [ DOI ] [ PMC free article ] [ PubMed ] [ Google Scholar ]
- 131. Bhatt C., Kumar I., Vijayakumar V., Singh K.U., Kumar A. The state of the art of deep learning models in medical science and their challenges. Multimed. Syst. 2021;27:599–613. doi: 10.1007/s00530-020-00694-1. [ DOI ] [ Google Scholar ]
- 132. Aloysius N., Geetha M. A review on deep convolutional neural networks; Proceedings of the 2017 International Conference on Communication and Signal Processing (ICCSP); Chennai, India. 6–8 April 2017; pp. 0588–0592. [ Google Scholar ]
- 133. Hearst M.A., Dumais S.T., Osuna E., Platt J., Scholkopf B. Support vector machines. IEEE Intell. Syst. Their Appl. 1998;13:18–28. doi: 10.1109/5254.708428. [ DOI ] [ Google Scholar ]
- 134. Hochreiter S., Schmidhuber J. Long short-term memory. Neural Comput. 1997;9:1735–1780. doi: 10.1162/neco.1997.9.8.1735. [ DOI ] [ PubMed ] [ Google Scholar ]
- 135. Myles A.J., Feudale R.N., Liu Y., Woody N.A., Brown S.D. Brown. An introduction to decision tree modeling. J. Chemom. A J. Chemom. Soc. 2004;18:275–285. [ Google Scholar ]
- 136. MIT-BIH Arrhythmia Database v1.0.0. [(accessed on 1 November 2022)]. Available online: https://www.physionet.org/content/mitdb/1.0.0/
- 137. Xu F., Uszkoreit H., Du Y., Fan W., Zhao D., Zhu J. CCF International Conference on Natural Language Processing and Chinese Computing. Springer; Cham, Switzerland: 2019. Explainable AI: A brief survey on history, research areas, approaches and challenges; pp. 563–574. [ Google Scholar ]
- 138. Doran D., Schulz S., Besold T.R. Besold. What does explainable AI really mean? A new conceptualization of perspectives. arXiv. 20171710.00794 [ Google Scholar ]
- 139. Gunning D., Stefik M., Choi J., Miller T., Stumpf S., Yang G.-Z. XAI—Explainable artificial intelligence. Sci. Robot. 2019;4:eaay7120. doi: 10.1126/scirobotics.aay7120. [ DOI ] [ PubMed ] [ Google Scholar ]
- 140. Dwork C., McSherry F., Nissim K., Smith A. Theory of Cryptography Conference. Springer; Berlin/Heidelberg, Germany: 2006. Calibrating noise to sensitivity in private data analysis; pp. 265–284. [ Google Scholar ]
- 141. El Emam K., Dankar F.K. Protecting privacy using k-anonymity. J. Am. Med. Inform. Assoc. 2008;15:627–637. doi: 10.1197/jamia.M2716. [ DOI ] [ PMC free article ] [ PubMed ] [ Google Scholar ]
- 142. Li P., Li J., Huang Z., Li T., Gao C.Z., Yiu S.M., Chen K. Multi-key privacy-preserving deep learning in cloud computing. Future Gener. Comput. Syst. 2017;74:76–85. doi: 10.1016/j.future.2017.02.006. [ DOI ] [ Google Scholar ]
- 143. Fredrikson M., Jha S., Ristenpart T. Model inversion attacks that exploit confidence information and basic countermeasures; Proceedings of the 22nd ACM SIGSAC Conference on Computer and Communications Security; Denver, CO, USA. 12–16 October 2015; pp. 1322–1333. [ Google Scholar ]
- 144. Shokri R., Stronati M., Song C., Shmatikov V. Membership inference attacks against machine learning models; Proceedings of the 2017 IEEE Symposium on Security and Privacy (SP); San Jose, CA, USA. 22–26 May 2017; 2017. pp. 3–18. [ Google Scholar ]
- 145. McMahan B., Moore E., Ramage D., Hampson S., y Arcas B.A. Artificial Intelligence and Statistics. PMLR; Ft. Lauderdale, FL, USA: 2017. Communication-efficient learning of deep networks from decentralized data; pp. 1273–1282. [ Google Scholar ]
- 146. Xu J., Glicksberg B.S., Su C., Walker P., Bian J., Wang F. Federated learning for healthcare informatics. J. Healthc. Informatics Res. 2021;5:1–19. doi: 10.1007/s41666-020-00082-4. [ DOI ] [ PMC free article ] [ PubMed ] [ Google Scholar ]
- 147. Nguyen D.C., Pham Q.V., Pathirana P.N., Ding M., Seneviratne A., Lin Z., Dobre O., Hwang W.-J. Federated learning for smart healthcare: A survey. ACM Comput. Surv. (CSUR) 2022;55:1–37. doi: 10.1145/3453476. [ DOI ] [ Google Scholar ]
- 148. Bonawitz K., Ivanov V., Kreuter B., Marcedone A., McMahan H.B., Patel S., Ramage D., Segal A., Seth K. Practical secure aggregation for privacy-preserving machine learning; Proceedings of the 2017 ACM SIGSAC Conference on Computer and Communications Security; Dallas, TX, USA. 30 October–3 November 2017; pp. 1175–1191. [ Google Scholar ]
- 149. Zhang K., Song X., Zhang C., Yu S. Challenges and future directions of secure federated learning: A survey. Front. Comput. Sci. 2022;16:1–8. doi: 10.1007/s11704-021-0598-z. [ DOI ] [ PMC free article ] [ PubMed ] [ Google Scholar ]
- 150. Baltrušaitis T., Ahuja C., Morency L.P. Multimodal machine learning: A survey and taxonomy. IEEE Trans. Pattern Anal. Mach. Intell. 2018;41:423–443. doi: 10.1109/TPAMI.2018.2798607. [ DOI ] [ PubMed ] [ Google Scholar ]
- 151. Kline A., Wang H., Li Y., Dennis S., Hutch M., Xu Z., Wang F., Cheng F., Luo Y. Multimodal Machine Learning in Precision Health. arXiv. 2022 doi: 10.1038/s41746-022-00712-8.2204.04777 [ DOI ] [ PMC free article ] [ PubMed ] [ Google Scholar ]
- 152. White F.E. Data Fusion Lexicon. Joint Directors of Labs; Washington, DC, USA: 1991. [ Google Scholar ]
- 153. Jiang H., Chen X., Zhang S., Zhang X., Kong W., Zhang T. Software for wearable devices: Challenges and opportunities; Proceedings of the 2015 IEEE 39th Annual Computer Software and Applications Conference; Taichung, Taiwan. 1–5 July 2015; pp. 592–597. [ Google Scholar ]
- 154. Bhushan D., Agrawal R. A Handbook of Internet of Things in Biomedical and Cyber Physical System. Springer; Cham, Switzerland: 2020. Security challenges for designing wearable and IoT solutions; pp. 109–138. [ Google Scholar ]
- 155. Motti V.G. Adjunct Proceedings of the 2019 Acm International Joint Conference on Pervasive and Ubiquitous Computing and Proceedings of the 2019 ACM International Symposium on Wearable Computers. Association for Computing Machinery; New York, NY, USA: 2019. Assistive wearables: Opportunities and challenges; pp. 1040–1043. [ Google Scholar ]
- 156. Balsamo D., Merrett G.V., Zaghari B., Wei Y., Ramchurn S., Stein S., Weddell A.S., Beeby S. Wearable and autonomous computing for future smart cities: Open challenges; Proceedings of the 2017 25th International Conference on Software, Telecommunications and Computer Networks (SoftCOM); Split, Croatia. 21–23 September 2017; pp. 1–5. [ Google Scholar ]
- 157. Moshawrab M., Adda M., Bouzouane A., Ibrahim H., Raad A. Smart Wearables for the Detection of Occupational Physical Fatigue: A Literature Review. Sensors. 2022;22:7472. doi: 10.3390/s22197472. [ DOI ] [ PMC free article ] [ PubMed ] [ Google Scholar ]
- 158. Albrecht J.P. How the GDPR will change the world. Eur. Data Prot. Law Rev. 2016;2:287. doi: 10.21552/EDPL/2016/3/4. [ DOI ] [ Google Scholar ]
- 159. Parasol M. The impact of China’s 2016 Cyber Security Law on foreign technology firms, and on China’s big data and Smart City dreams. Comput. Law Secur. Rev. 2018;34:67–98. doi: 10.1016/j.clsr.2017.05.022. [ DOI ] [ Google Scholar ]
- 160. Gray W., Zheng H.R. General Principles of Civil Law of the People’s Republic of China. Am. J. Comp. Law. 1986;34:715–743. doi: 10.2307/840330. [ DOI ] [ Google Scholar ]
- 161. Zhang C., Xie Y., Bai H., Yu B., Li W., Gao Y. A survey on federated learning. Knowl.-Based Syst. 2021;216:106775. doi: 10.1016/j.knosys.2021.106775. [ DOI ] [ Google Scholar ]
- 162. De Luca C.J., Gilmore L.D., Kuznetsov M., Roy S.H. Filtering the surface EMG signal: Movement artifact and baseline noise contamination. J. Biomech. 2010;43:1573–1579. doi: 10.1016/j.jbiomech.2010.01.027. [ DOI ] [ PubMed ] [ Google Scholar ]
- 163. Islam M.K., Rastegarnia A., Sanei S. Signal Processing Techniques for Computational Health Informatics. Springer; Cham, Switzerland: 2021. Signal Artifacts and Techniques for Artifacts and Noise Removal; pp. 23–79. [ Google Scholar ]
- 164. Mammen P.M. Federated learning: Opportunities and challenges. arXiv. 20212101.05428 [ Google Scholar ]
- 165. Yang Q., Liu Y., Chen T., Tong Y. Federated machine learning: Concept and applications. ACM Trans. Intell. Syst. Technol. (TIST) 2019;10:1–19. doi: 10.1145/3298981. [ DOI ] [ Google Scholar ]
- 166. Li Q., Wen Z., Wu Z., Hu S., Wang N., Li Y., He B. A survey on federated learning systems: Vision, hype and reality for data privacy and protection. IEEE Trans. Knowl. Data Eng. 2021:1. doi: 10.1109/TKDE.2021.3124599. [ DOI ] [ Google Scholar ]
- 167. Li L., Fan Y., Tse M., Lin K.Y. A review of applications in federated learning. Comput. Ind. Eng. 2020;149:106854. doi: 10.1016/j.cie.2020.106854. [ DOI ] [ Google Scholar ]
- 168. Iriarte J., Urrestarazu E., Valencia M., Alegre M., Malanda A., Viteri C., Artieda J. Independent component analysis as a tool to eliminate artifacts in EEG: A quantitative study. J. Clin. Neurophysiol. 2003;20:249–257. doi: 10.1097/00004691-200307000-00004. [ DOI ] [ PubMed ] [ Google Scholar ]
- 169. Ram M.R., Madhav K.V., Krishna E.H., Komalla N.R., Reddy K.A. A novel approach for motion artifact reduction in PPG signals based on AS-LMS adaptive filter. IEEE Trans. Instrum. Meas. 2011;61:1445–1457. doi: 10.1109/TIM.2011.2175832. [ DOI ] [ Google Scholar ]
- 170. Daly I., Billinger M., Scherer R., Müller-Putz G. On the automated removal of artifacts related to head movement from the EEG. IEEE Trans. Neural Syst. Rehabil. Eng. 2013;21:427–434. doi: 10.1109/TNSRE.2013.2254724. [ DOI ] [ PubMed ] [ Google Scholar ]
Associated Data
This section collects any data citations, data availability statements, or supplementary materials included in this article.
- View on publisher site
- PDF (1.2 MB)
- Collections
Similar articles
Cited by other articles, links to ncbi databases.
- Download .nbib .nbib
- Format: AMA APA MLA NLM
Add to Collections

IMAGES
VIDEO