

5 Quasi-Experimental Design Examples
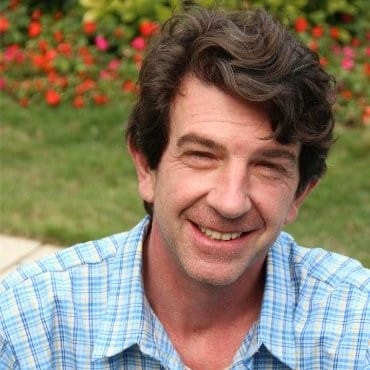
Dave Cornell (PhD)
Dr. Cornell has worked in education for more than 20 years. His work has involved designing teacher certification for Trinity College in London and in-service training for state governments in the United States. He has trained kindergarten teachers in 8 countries and helped businessmen and women open baby centers and kindergartens in 3 countries.
Learn about our Editorial Process
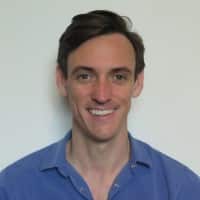
Chris Drew (PhD)
This article was peer-reviewed and edited by Chris Drew (PhD). The review process on Helpful Professor involves having a PhD level expert fact check, edit, and contribute to articles. Reviewers ensure all content reflects expert academic consensus and is backed up with reference to academic studies. Dr. Drew has published over 20 academic articles in scholarly journals. He is the former editor of the Journal of Learning Development in Higher Education and holds a PhD in Education from ACU.

Quasi-experimental design refers to a type of experimental design that uses pre-existing groups of people rather than random groups.
Because the groups of research participants already exist, they cannot be randomly assigned to a cohort . This makes inferring a causal relationship between the treatment and observed/criterion variable difficult.
Quasi-experimental designs are generally considered inferior to true experimental designs.
Limitations of Quasi-Experimental Design
Since participants cannot be randomly assigned to the grouping variable (male/female; high education/low education), the internal validity of the study is questionable.
Extraneous variables may exist that explain the results. For example, with quasi-experimental studies involving gender, there are numerous cultural and biological variables that distinguish males and females other than gender alone.
Each one of those variables may be able to explain the results without the need to refer to gender.
See More Research Limitations Here
Quasi-Experimental Design Examples
1. smartboard apps and math.
A school has decided to supplement their math resources with smartboard applications. The math teachers research the apps available and then choose two apps for each grade level. Before deciding on which apps to purchase, the school contacts the seller and asks for permission to demo/test the apps before purchasing the licenses.
The study involves having different teachers use the apps with their classes. Since there are two math teachers at each grade level, each teacher will use one of the apps in their classroom for three months. At the end of three months, all students will take the same math exams. Then the school can simply compare which app improved the students’ math scores the most.
The reason this is called a quasi-experiment is because the school did not randomly assign students to one app or the other. The students were already in pre-existing groups/classes.
Although it was impractical to randomly assign students to use one version or the other of the apps, it creates difficulty interpreting the results.
For instance, if students in teacher A’s class did better than the students in teacher B’s class, then can we really say the difference was due to the app? There may be other differences between the two teachers that account for the results. This poses a serious threat to the study’s internal validity.
2. Leadership Training
There is reason to believe that teaching entrepreneurs modern leadership techniques will improve their performance and shorten how long it takes for them to reach profitability. Team members will feel better appreciated and work harder, which should translate to increased productivity and innovation.
This hypothetical study took place in a third-world country in a mid-sized city. The researchers marketed the training throughout the city and received interest from 5 start-ups in the tech sector and 5 in the textile industry. The leaders of each company then attended six weeks of workshops on employee motivation, leadership styles, and effective team management.
At the end of one year, the researchers returned. They conducted a standard assessment of each start-up’s growth trajectory and administered various surveys to employees.
The results indicated that tech start-ups were further along in their growth paths than textile start-ups. The data also showed that tech work teams reported greater job satisfaction and company loyalty than textile work teams.
Although the results appear straightforward, because the researchers used a quasi-experimental design, they cannot say that the training caused the results.
The two groups may differ in ways that could explain the results. For instance, perhaps there is less growth potential in the textile industry in that city, or perhaps tech leaders are more progressive and willing to accept new leadership strategies.
3. Parenting Styles and Academic Performance
Psychologists are very interested in factors that affect children’s academic performance. Since parenting styles affect a variety of children’s social and emotional profiles, it stands to reason that it may affect academic performance as well. The four parenting styles under study are: authoritarian, authoritative, permissive, and neglectful/uninvolved.
To examine this possible relationship, researchers assessed the parenting style of 120 families with third graders in a large metropolitan city. Trained raters made two-hour home visits to conduct observations of parent/child interactions. That data was later compared with the children’s grades.
The results revealed that children raised in authoritative households had the highest grades of all the groups.
However, because the researchers were not able to randomly assign children to one of the four parenting styles, the internal validity is called into question.
There may be other explanations for the results other than parenting style. For instance, maybe parents that practice authoritative parenting also come from a higher SES demographic than the other parents.
Because they have higher income and education levels, they may put more emphasis on their child’s academic performance. Or, because they have greater financial resources, their children attend STEM camps, co-curricular and other extracurricular academic-orientated classes.
4. Government Reforms and Economic Impact
Government policies can have a tremendous impact on economic development. Making it easier for small businesses to open and reducing bank loans are examples of policies that can have immediate results. So, a third-world country decides to test policy reforms in two mid-sized cities. One city receives reforms directed at small businesses, while the other receives reforms directed at luring foreign investment.
The government was careful to choose two cities that were similar in terms of size and population demographics.
Over the next five years, economic growth data were collected at the end of each fiscal year. The measures consisted of housing sells, local GDP, and unemployment rates.
At the end of five years the results indicated that small business reforms had a much larger impact on economic growth than foreign investment. The city which received small business reforms saw an increase in housing sells and GDP, but a drop in unemployment. The other city saw stagnant sells and GDP, and a slight increase in unemployment.
On the surface, it appears that small business reform is the better way to go. However, a more careful analysis revealed that the economic improvement observed in the one city was actually the result of two multinational real estate firms entering the market. The two firms specialize in converting dilapidated warehouses into shopping centers and residential properties.
5. Gender and Meditation
Meditation can help relieve stress and reduce symptoms of depression and anxiety. It is a simple and easy to use technique that just about anyone can try. However, are the benefits real or is it just that people believe it can help? To find out, a team of counselors designed a study to put it to a test.
Since they believe that women are more likely to benefit than men, they recruit both males and females to be in their study.
Both groups were trained in meditation by a licensed professional. The training took place over three weekends. Participants were instructed to practice at home at least four times a week for the next three months and keep a journal each time they meditate.
At the end of the three months, physical and psychological health data were collected on all participants. For physical health, participants’ blood pressure was measured. For psychological health, participants filled out a happiness scale and the emotional tone of their diaries were examined.
The results showed that meditation worked better for women than men. Women had lower blood pressure, scored higher on the happiness scale, and wrote more positive statements in their diaries.
Unfortunately, the researchers noticed that men apparently did not actually practice meditation as much as they should. They had very few journal entries and in post-study interviews, a vast majority of men admitted that they only practiced meditation about half the time.
The lack of practice is an extraneous variable. Perhaps if men had adhered to the study instructions, their scores on the physical and psychological measures would have been higher than women’s measures.
The quasi-experiment is used when researchers want to study the effects of a variable/treatment on different groups of people. Groups can be defined based on gender, parenting style, SES demographics, or any number of other variables.
The problem is that when interpreting the results, even clear differences between the groups cannot be attributed to the treatment.
The groups may differ in ways other than the grouping variables. For example, leadership training in the study above may have improved the textile start-ups’ performance if the techniques had been applied at all. Similarly, men may have benefited from meditation as much as women, if they had just tried.
Baumrind, D. (1991). Parenting styles and adolescent development. In R. M. Lerner, A. C. Peterson, & J. Brooks-Gunn (Eds.), Encyclopedia of Adolescence (pp. 746–758). New York: Garland Publishing, Inc.
Cook, T. D., & Campbell, D. T. (1979). Quasi-experimentation: Design & analysis issues in field settings . Boston, MA: Houghton Mifflin.
Matthew L. Maciejewski (2020) Quasi-experimental design. Biostatistics & Epidemiology, 4 (1), 38-47. https://doi.org/10.1080/24709360.2018.1477468
Thyer, Bruce. (2012). Quasi-Experimental Research Designs . Oxford University Press. https://doi.org/10.1093/acprof:oso/9780195387384.001.0001
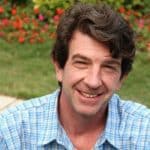
- Dave Cornell (PhD) https://helpfulprofessor.com/author/dave-cornell-phd/ 23 Achieved Status Examples
- Dave Cornell (PhD) https://helpfulprofessor.com/author/dave-cornell-phd/ 25 Defense Mechanisms Examples
- Dave Cornell (PhD) https://helpfulprofessor.com/author/dave-cornell-phd/ 15 Theory of Planned Behavior Examples
- Dave Cornell (PhD) https://helpfulprofessor.com/author/dave-cornell-phd/ 18 Adaptive Behavior Examples
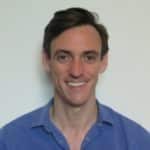
- Chris Drew (PhD) https://helpfulprofessor.com/author/chris-drew-phd/ 23 Achieved Status Examples
- Chris Drew (PhD) https://helpfulprofessor.com/author/chris-drew-phd/ 15 Ableism Examples
- Chris Drew (PhD) https://helpfulprofessor.com/author/chris-drew-phd/ 25 Defense Mechanisms Examples
- Chris Drew (PhD) https://helpfulprofessor.com/author/chris-drew-phd/ 15 Theory of Planned Behavior Examples
Leave a Comment Cancel Reply
Your email address will not be published. Required fields are marked *
- Privacy Policy

Home » Quasi-Experimental Research Design – Types, Methods
Quasi-Experimental Research Design – Types, Methods
Table of Contents

Quasi-Experimental Design
Quasi-experimental design is a research method that seeks to evaluate the causal relationships between variables, but without the full control over the independent variable(s) that is available in a true experimental design.
In a quasi-experimental design, the researcher uses an existing group of participants that is not randomly assigned to the experimental and control groups. Instead, the groups are selected based on pre-existing characteristics or conditions, such as age, gender, or the presence of a certain medical condition.
Types of Quasi-Experimental Design
There are several types of quasi-experimental designs that researchers use to study causal relationships between variables. Here are some of the most common types:
Non-Equivalent Control Group Design
This design involves selecting two groups of participants that are similar in every way except for the independent variable(s) that the researcher is testing. One group receives the treatment or intervention being studied, while the other group does not. The two groups are then compared to see if there are any significant differences in the outcomes.
Interrupted Time-Series Design
This design involves collecting data on the dependent variable(s) over a period of time, both before and after an intervention or event. The researcher can then determine whether there was a significant change in the dependent variable(s) following the intervention or event.
Pretest-Posttest Design
This design involves measuring the dependent variable(s) before and after an intervention or event, but without a control group. This design can be useful for determining whether the intervention or event had an effect, but it does not allow for control over other factors that may have influenced the outcomes.
Regression Discontinuity Design
This design involves selecting participants based on a specific cutoff point on a continuous variable, such as a test score. Participants on either side of the cutoff point are then compared to determine whether the intervention or event had an effect.
Natural Experiments
This design involves studying the effects of an intervention or event that occurs naturally, without the researcher’s intervention. For example, a researcher might study the effects of a new law or policy that affects certain groups of people. This design is useful when true experiments are not feasible or ethical.
Data Analysis Methods
Here are some data analysis methods that are commonly used in quasi-experimental designs:
Descriptive Statistics
This method involves summarizing the data collected during a study using measures such as mean, median, mode, range, and standard deviation. Descriptive statistics can help researchers identify trends or patterns in the data, and can also be useful for identifying outliers or anomalies.
Inferential Statistics
This method involves using statistical tests to determine whether the results of a study are statistically significant. Inferential statistics can help researchers make generalizations about a population based on the sample data collected during the study. Common statistical tests used in quasi-experimental designs include t-tests, ANOVA, and regression analysis.
Propensity Score Matching
This method is used to reduce bias in quasi-experimental designs by matching participants in the intervention group with participants in the control group who have similar characteristics. This can help to reduce the impact of confounding variables that may affect the study’s results.
Difference-in-differences Analysis
This method is used to compare the difference in outcomes between two groups over time. Researchers can use this method to determine whether a particular intervention has had an impact on the target population over time.
Interrupted Time Series Analysis
This method is used to examine the impact of an intervention or treatment over time by comparing data collected before and after the intervention or treatment. This method can help researchers determine whether an intervention had a significant impact on the target population.
Regression Discontinuity Analysis
This method is used to compare the outcomes of participants who fall on either side of a predetermined cutoff point. This method can help researchers determine whether an intervention had a significant impact on the target population.
Steps in Quasi-Experimental Design
Here are the general steps involved in conducting a quasi-experimental design:
- Identify the research question: Determine the research question and the variables that will be investigated.
- Choose the design: Choose the appropriate quasi-experimental design to address the research question. Examples include the pretest-posttest design, non-equivalent control group design, regression discontinuity design, and interrupted time series design.
- Select the participants: Select the participants who will be included in the study. Participants should be selected based on specific criteria relevant to the research question.
- Measure the variables: Measure the variables that are relevant to the research question. This may involve using surveys, questionnaires, tests, or other measures.
- Implement the intervention or treatment: Implement the intervention or treatment to the participants in the intervention group. This may involve training, education, counseling, or other interventions.
- Collect data: Collect data on the dependent variable(s) before and after the intervention. Data collection may also include collecting data on other variables that may impact the dependent variable(s).
- Analyze the data: Analyze the data collected to determine whether the intervention had a significant impact on the dependent variable(s).
- Draw conclusions: Draw conclusions about the relationship between the independent and dependent variables. If the results suggest a causal relationship, then appropriate recommendations may be made based on the findings.
Quasi-Experimental Design Examples
Here are some examples of real-time quasi-experimental designs:
- Evaluating the impact of a new teaching method: In this study, a group of students are taught using a new teaching method, while another group is taught using the traditional method. The test scores of both groups are compared before and after the intervention to determine whether the new teaching method had a significant impact on student performance.
- Assessing the effectiveness of a public health campaign: In this study, a public health campaign is launched to promote healthy eating habits among a targeted population. The behavior of the population is compared before and after the campaign to determine whether the intervention had a significant impact on the target behavior.
- Examining the impact of a new medication: In this study, a group of patients is given a new medication, while another group is given a placebo. The outcomes of both groups are compared to determine whether the new medication had a significant impact on the targeted health condition.
- Evaluating the effectiveness of a job training program : In this study, a group of unemployed individuals is enrolled in a job training program, while another group is not enrolled in any program. The employment rates of both groups are compared before and after the intervention to determine whether the training program had a significant impact on the employment rates of the participants.
- Assessing the impact of a new policy : In this study, a new policy is implemented in a particular area, while another area does not have the new policy. The outcomes of both areas are compared before and after the intervention to determine whether the new policy had a significant impact on the targeted behavior or outcome.
Applications of Quasi-Experimental Design
Here are some applications of quasi-experimental design:
- Educational research: Quasi-experimental designs are used to evaluate the effectiveness of educational interventions, such as new teaching methods, technology-based learning, or educational policies.
- Health research: Quasi-experimental designs are used to evaluate the effectiveness of health interventions, such as new medications, public health campaigns, or health policies.
- Social science research: Quasi-experimental designs are used to investigate the impact of social interventions, such as job training programs, welfare policies, or criminal justice programs.
- Business research: Quasi-experimental designs are used to evaluate the impact of business interventions, such as marketing campaigns, new products, or pricing strategies.
- Environmental research: Quasi-experimental designs are used to evaluate the impact of environmental interventions, such as conservation programs, pollution control policies, or renewable energy initiatives.
When to use Quasi-Experimental Design
Here are some situations where quasi-experimental designs may be appropriate:
- When the research question involves investigating the effectiveness of an intervention, policy, or program : In situations where it is not feasible or ethical to randomly assign participants to intervention and control groups, quasi-experimental designs can be used to evaluate the impact of the intervention on the targeted outcome.
- When the sample size is small: In situations where the sample size is small, it may be difficult to randomly assign participants to intervention and control groups. Quasi-experimental designs can be used to investigate the impact of an intervention without requiring a large sample size.
- When the research question involves investigating a naturally occurring event : In some situations, researchers may be interested in investigating the impact of a naturally occurring event, such as a natural disaster or a major policy change. Quasi-experimental designs can be used to evaluate the impact of the event on the targeted outcome.
- When the research question involves investigating a long-term intervention: In situations where the intervention or program is long-term, it may be difficult to randomly assign participants to intervention and control groups for the entire duration of the intervention. Quasi-experimental designs can be used to evaluate the impact of the intervention over time.
- When the research question involves investigating the impact of a variable that cannot be manipulated : In some situations, it may not be possible or ethical to manipulate a variable of interest. Quasi-experimental designs can be used to investigate the relationship between the variable and the targeted outcome.
Purpose of Quasi-Experimental Design
The purpose of quasi-experimental design is to investigate the causal relationship between two or more variables when it is not feasible or ethical to conduct a randomized controlled trial (RCT). Quasi-experimental designs attempt to emulate the randomized control trial by mimicking the control group and the intervention group as much as possible.
The key purpose of quasi-experimental design is to evaluate the impact of an intervention, policy, or program on a targeted outcome while controlling for potential confounding factors that may affect the outcome. Quasi-experimental designs aim to answer questions such as: Did the intervention cause the change in the outcome? Would the outcome have changed without the intervention? And was the intervention effective in achieving its intended goals?
Quasi-experimental designs are useful in situations where randomized controlled trials are not feasible or ethical. They provide researchers with an alternative method to evaluate the effectiveness of interventions, policies, and programs in real-life settings. Quasi-experimental designs can also help inform policy and practice by providing valuable insights into the causal relationships between variables.
Overall, the purpose of quasi-experimental design is to provide a rigorous method for evaluating the impact of interventions, policies, and programs while controlling for potential confounding factors that may affect the outcome.
Advantages of Quasi-Experimental Design
Quasi-experimental designs have several advantages over other research designs, such as:
- Greater external validity : Quasi-experimental designs are more likely to have greater external validity than laboratory experiments because they are conducted in naturalistic settings. This means that the results are more likely to generalize to real-world situations.
- Ethical considerations: Quasi-experimental designs often involve naturally occurring events, such as natural disasters or policy changes. This means that researchers do not need to manipulate variables, which can raise ethical concerns.
- More practical: Quasi-experimental designs are often more practical than experimental designs because they are less expensive and easier to conduct. They can also be used to evaluate programs or policies that have already been implemented, which can save time and resources.
- No random assignment: Quasi-experimental designs do not require random assignment, which can be difficult or impossible in some cases, such as when studying the effects of a natural disaster. This means that researchers can still make causal inferences, although they must use statistical techniques to control for potential confounding variables.
- Greater generalizability : Quasi-experimental designs are often more generalizable than experimental designs because they include a wider range of participants and conditions. This can make the results more applicable to different populations and settings.
Limitations of Quasi-Experimental Design
There are several limitations associated with quasi-experimental designs, which include:
- Lack of Randomization: Quasi-experimental designs do not involve randomization of participants into groups, which means that the groups being studied may differ in important ways that could affect the outcome of the study. This can lead to problems with internal validity and limit the ability to make causal inferences.
- Selection Bias: Quasi-experimental designs may suffer from selection bias because participants are not randomly assigned to groups. Participants may self-select into groups or be assigned based on pre-existing characteristics, which may introduce bias into the study.
- History and Maturation: Quasi-experimental designs are susceptible to history and maturation effects, where the passage of time or other events may influence the outcome of the study.
- Lack of Control: Quasi-experimental designs may lack control over extraneous variables that could influence the outcome of the study. This can limit the ability to draw causal inferences from the study.
- Limited Generalizability: Quasi-experimental designs may have limited generalizability because the results may only apply to the specific population and context being studied.
About the author
Muhammad Hassan
Researcher, Academic Writer, Web developer
You may also like

Questionnaire – Definition, Types, and Examples

Ethnographic Research -Types, Methods and Guide

One-to-One Interview – Methods and Guide

Quantitative Research – Methods, Types and...

Explanatory Research – Types, Methods, Guide

Qualitative Research – Methods, Analysis Types...

Want to create or adapt books like this? Learn more about how Pressbooks supports open publishing practices.
7.3 Quasi-Experimental Research
Learning objectives.
- Explain what quasi-experimental research is and distinguish it clearly from both experimental and correlational research.
- Describe three different types of quasi-experimental research designs (nonequivalent groups, pretest-posttest, and interrupted time series) and identify examples of each one.
The prefix quasi means “resembling.” Thus quasi-experimental research is research that resembles experimental research but is not true experimental research. Although the independent variable is manipulated, participants are not randomly assigned to conditions or orders of conditions (Cook & Campbell, 1979). Because the independent variable is manipulated before the dependent variable is measured, quasi-experimental research eliminates the directionality problem. But because participants are not randomly assigned—making it likely that there are other differences between conditions—quasi-experimental research does not eliminate the problem of confounding variables. In terms of internal validity, therefore, quasi-experiments are generally somewhere between correlational studies and true experiments.
Quasi-experiments are most likely to be conducted in field settings in which random assignment is difficult or impossible. They are often conducted to evaluate the effectiveness of a treatment—perhaps a type of psychotherapy or an educational intervention. There are many different kinds of quasi-experiments, but we will discuss just a few of the most common ones here.
Nonequivalent Groups Design
Recall that when participants in a between-subjects experiment are randomly assigned to conditions, the resulting groups are likely to be quite similar. In fact, researchers consider them to be equivalent. When participants are not randomly assigned to conditions, however, the resulting groups are likely to be dissimilar in some ways. For this reason, researchers consider them to be nonequivalent. A nonequivalent groups design , then, is a between-subjects design in which participants have not been randomly assigned to conditions.
Imagine, for example, a researcher who wants to evaluate a new method of teaching fractions to third graders. One way would be to conduct a study with a treatment group consisting of one class of third-grade students and a control group consisting of another class of third-grade students. This would be a nonequivalent groups design because the students are not randomly assigned to classes by the researcher, which means there could be important differences between them. For example, the parents of higher achieving or more motivated students might have been more likely to request that their children be assigned to Ms. Williams’s class. Or the principal might have assigned the “troublemakers” to Mr. Jones’s class because he is a stronger disciplinarian. Of course, the teachers’ styles, and even the classroom environments, might be very different and might cause different levels of achievement or motivation among the students. If at the end of the study there was a difference in the two classes’ knowledge of fractions, it might have been caused by the difference between the teaching methods—but it might have been caused by any of these confounding variables.
Of course, researchers using a nonequivalent groups design can take steps to ensure that their groups are as similar as possible. In the present example, the researcher could try to select two classes at the same school, where the students in the two classes have similar scores on a standardized math test and the teachers are the same sex, are close in age, and have similar teaching styles. Taking such steps would increase the internal validity of the study because it would eliminate some of the most important confounding variables. But without true random assignment of the students to conditions, there remains the possibility of other important confounding variables that the researcher was not able to control.
Pretest-Posttest Design
In a pretest-posttest design , the dependent variable is measured once before the treatment is implemented and once after it is implemented. Imagine, for example, a researcher who is interested in the effectiveness of an antidrug education program on elementary school students’ attitudes toward illegal drugs. The researcher could measure the attitudes of students at a particular elementary school during one week, implement the antidrug program during the next week, and finally, measure their attitudes again the following week. The pretest-posttest design is much like a within-subjects experiment in which each participant is tested first under the control condition and then under the treatment condition. It is unlike a within-subjects experiment, however, in that the order of conditions is not counterbalanced because it typically is not possible for a participant to be tested in the treatment condition first and then in an “untreated” control condition.
If the average posttest score is better than the average pretest score, then it makes sense to conclude that the treatment might be responsible for the improvement. Unfortunately, one often cannot conclude this with a high degree of certainty because there may be other explanations for why the posttest scores are better. One category of alternative explanations goes under the name of history . Other things might have happened between the pretest and the posttest. Perhaps an antidrug program aired on television and many of the students watched it, or perhaps a celebrity died of a drug overdose and many of the students heard about it. Another category of alternative explanations goes under the name of maturation . Participants might have changed between the pretest and the posttest in ways that they were going to anyway because they are growing and learning. If it were a yearlong program, participants might become less impulsive or better reasoners and this might be responsible for the change.
Another alternative explanation for a change in the dependent variable in a pretest-posttest design is regression to the mean . This refers to the statistical fact that an individual who scores extremely on a variable on one occasion will tend to score less extremely on the next occasion. For example, a bowler with a long-term average of 150 who suddenly bowls a 220 will almost certainly score lower in the next game. Her score will “regress” toward her mean score of 150. Regression to the mean can be a problem when participants are selected for further study because of their extreme scores. Imagine, for example, that only students who scored especially low on a test of fractions are given a special training program and then retested. Regression to the mean all but guarantees that their scores will be higher even if the training program has no effect. A closely related concept—and an extremely important one in psychological research—is spontaneous remission . This is the tendency for many medical and psychological problems to improve over time without any form of treatment. The common cold is a good example. If one were to measure symptom severity in 100 common cold sufferers today, give them a bowl of chicken soup every day, and then measure their symptom severity again in a week, they would probably be much improved. This does not mean that the chicken soup was responsible for the improvement, however, because they would have been much improved without any treatment at all. The same is true of many psychological problems. A group of severely depressed people today is likely to be less depressed on average in 6 months. In reviewing the results of several studies of treatments for depression, researchers Michael Posternak and Ivan Miller found that participants in waitlist control conditions improved an average of 10 to 15% before they received any treatment at all (Posternak & Miller, 2001). Thus one must generally be very cautious about inferring causality from pretest-posttest designs.
Does Psychotherapy Work?
Early studies on the effectiveness of psychotherapy tended to use pretest-posttest designs. In a classic 1952 article, researcher Hans Eysenck summarized the results of 24 such studies showing that about two thirds of patients improved between the pretest and the posttest (Eysenck, 1952). But Eysenck also compared these results with archival data from state hospital and insurance company records showing that similar patients recovered at about the same rate without receiving psychotherapy. This suggested to Eysenck that the improvement that patients showed in the pretest-posttest studies might be no more than spontaneous remission. Note that Eysenck did not conclude that psychotherapy was ineffective. He merely concluded that there was no evidence that it was, and he wrote of “the necessity of properly planned and executed experimental studies into this important field” (p. 323). You can read the entire article here:
http://psychclassics.yorku.ca/Eysenck/psychotherapy.htm
Fortunately, many other researchers took up Eysenck’s challenge, and by 1980 hundreds of experiments had been conducted in which participants were randomly assigned to treatment and control conditions, and the results were summarized in a classic book by Mary Lee Smith, Gene Glass, and Thomas Miller (Smith, Glass, & Miller, 1980). They found that overall psychotherapy was quite effective, with about 80% of treatment participants improving more than the average control participant. Subsequent research has focused more on the conditions under which different types of psychotherapy are more or less effective.

In a classic 1952 article, researcher Hans Eysenck pointed out the shortcomings of the simple pretest-posttest design for evaluating the effectiveness of psychotherapy.
Wikimedia Commons – CC BY-SA 3.0.
Interrupted Time Series Design
A variant of the pretest-posttest design is the interrupted time-series design . A time series is a set of measurements taken at intervals over a period of time. For example, a manufacturing company might measure its workers’ productivity each week for a year. In an interrupted time series-design, a time series like this is “interrupted” by a treatment. In one classic example, the treatment was the reduction of the work shifts in a factory from 10 hours to 8 hours (Cook & Campbell, 1979). Because productivity increased rather quickly after the shortening of the work shifts, and because it remained elevated for many months afterward, the researcher concluded that the shortening of the shifts caused the increase in productivity. Notice that the interrupted time-series design is like a pretest-posttest design in that it includes measurements of the dependent variable both before and after the treatment. It is unlike the pretest-posttest design, however, in that it includes multiple pretest and posttest measurements.
Figure 7.5 “A Hypothetical Interrupted Time-Series Design” shows data from a hypothetical interrupted time-series study. The dependent variable is the number of student absences per week in a research methods course. The treatment is that the instructor begins publicly taking attendance each day so that students know that the instructor is aware of who is present and who is absent. The top panel of Figure 7.5 “A Hypothetical Interrupted Time-Series Design” shows how the data might look if this treatment worked. There is a consistently high number of absences before the treatment, and there is an immediate and sustained drop in absences after the treatment. The bottom panel of Figure 7.5 “A Hypothetical Interrupted Time-Series Design” shows how the data might look if this treatment did not work. On average, the number of absences after the treatment is about the same as the number before. This figure also illustrates an advantage of the interrupted time-series design over a simpler pretest-posttest design. If there had been only one measurement of absences before the treatment at Week 7 and one afterward at Week 8, then it would have looked as though the treatment were responsible for the reduction. The multiple measurements both before and after the treatment suggest that the reduction between Weeks 7 and 8 is nothing more than normal week-to-week variation.
Figure 7.5 A Hypothetical Interrupted Time-Series Design

The top panel shows data that suggest that the treatment caused a reduction in absences. The bottom panel shows data that suggest that it did not.
Combination Designs
A type of quasi-experimental design that is generally better than either the nonequivalent groups design or the pretest-posttest design is one that combines elements of both. There is a treatment group that is given a pretest, receives a treatment, and then is given a posttest. But at the same time there is a control group that is given a pretest, does not receive the treatment, and then is given a posttest. The question, then, is not simply whether participants who receive the treatment improve but whether they improve more than participants who do not receive the treatment.
Imagine, for example, that students in one school are given a pretest on their attitudes toward drugs, then are exposed to an antidrug program, and finally are given a posttest. Students in a similar school are given the pretest, not exposed to an antidrug program, and finally are given a posttest. Again, if students in the treatment condition become more negative toward drugs, this could be an effect of the treatment, but it could also be a matter of history or maturation. If it really is an effect of the treatment, then students in the treatment condition should become more negative than students in the control condition. But if it is a matter of history (e.g., news of a celebrity drug overdose) or maturation (e.g., improved reasoning), then students in the two conditions would be likely to show similar amounts of change. This type of design does not completely eliminate the possibility of confounding variables, however. Something could occur at one of the schools but not the other (e.g., a student drug overdose), so students at the first school would be affected by it while students at the other school would not.
Finally, if participants in this kind of design are randomly assigned to conditions, it becomes a true experiment rather than a quasi experiment. In fact, it is the kind of experiment that Eysenck called for—and that has now been conducted many times—to demonstrate the effectiveness of psychotherapy.
Key Takeaways
- Quasi-experimental research involves the manipulation of an independent variable without the random assignment of participants to conditions or orders of conditions. Among the important types are nonequivalent groups designs, pretest-posttest, and interrupted time-series designs.
- Quasi-experimental research eliminates the directionality problem because it involves the manipulation of the independent variable. It does not eliminate the problem of confounding variables, however, because it does not involve random assignment to conditions. For these reasons, quasi-experimental research is generally higher in internal validity than correlational studies but lower than true experiments.
- Practice: Imagine that two college professors decide to test the effect of giving daily quizzes on student performance in a statistics course. They decide that Professor A will give quizzes but Professor B will not. They will then compare the performance of students in their two sections on a common final exam. List five other variables that might differ between the two sections that could affect the results.
Discussion: Imagine that a group of obese children is recruited for a study in which their weight is measured, then they participate for 3 months in a program that encourages them to be more active, and finally their weight is measured again. Explain how each of the following might affect the results:
- regression to the mean
- spontaneous remission
Cook, T. D., & Campbell, D. T. (1979). Quasi-experimentation: Design & analysis issues in field settings . Boston, MA: Houghton Mifflin.
Eysenck, H. J. (1952). The effects of psychotherapy: An evaluation. Journal of Consulting Psychology, 16 , 319–324.
Posternak, M. A., & Miller, I. (2001). Untreated short-term course of major depression: A meta-analysis of studies using outcomes from studies using wait-list control groups. Journal of Affective Disorders, 66 , 139–146.
Smith, M. L., Glass, G. V., & Miller, T. I. (1980). The benefits of psychotherapy . Baltimore, MD: Johns Hopkins University Press.
Research Methods in Psychology Copyright © 2016 by University of Minnesota is licensed under a Creative Commons Attribution-NonCommercial-ShareAlike 4.0 International License , except where otherwise noted.
Quasi-Experiment: Understand What It Is, Types & Examples
Discover the concept of quasi-experiment, its various types, real-world examples, and how QuestionPro aids in conducting these studies.
Quasi-experimental research designs have gained significant recognition in the scientific community due to their unique ability to study cause-and-effect relationships in real-world settings. Unlike true experiments, quasi-experiment lack random assignment of participants to groups, making them more practical and ethical in certain situations. In this article, we will delve into the concept, applications, and advantages of quasi-experiments, shedding light on their relevance and significance in the scientific realm.
What Is A Quasi-Experiment Research Design?
Quasi-experimental research designs are research methodologies that resemble true experiments but lack the randomized assignment of participants to groups. In a true experiment, researchers randomly assign participants to either an experimental group or a control group, allowing for a comparison of the effects of an independent variable on the dependent variable. However, in quasi-experiments, this random assignment is often not possible or ethically permissible, leading to the adoption of alternative strategies.
Types Of Quasi-Experimental Designs
There are several types of quasi-experiment designs to study causal relationships in specific contexts. Some common types include:
Non-Equivalent Groups Design
This design involves selecting pre-existing groups that differ in some key characteristics and comparing their responses to the independent variable. Although the researcher does not randomly assign the groups, they can still examine the effects of the independent variable.
Regression Discontinuity
This design utilizes a cutoff point or threshold to determine which participants receive the treatment or intervention. It assumes that participants on either side of the cutoff are similar in all other aspects, except for their exposure to the independent variable.
Interrupted Time Series Design
This design involves measuring the dependent variable multiple times before and after the introduction of an intervention or treatment. By comparing the trends in the dependent variable, researchers can infer the impact of the intervention.
Natural Experiments
Natural experiments take advantage of naturally occurring events or circumstances that mimic the random assignment found in true experiments. Participants are exposed to different conditions in situations identified by researchers without any manipulation from them.
Application of the Quasi-Experiment Design
Quasi-experimental research designs find applications in various fields, ranging from education to public health and beyond. One significant advantage of quasi-experiments is their feasibility in real-world settings where randomization is not always possible or ethical.
Ethical Reasons
Ethical concerns often arise in research when randomizing participants to different groups could potentially deny individuals access to beneficial treatments or interventions. In such cases, quasi-experimental designs provide an ethical alternative, allowing researchers to study the impact of interventions without depriving anyone of potential benefits.
Examples Of Quasi-Experimental Design
Let’s explore a few examples of quasi-experimental designs to understand their application in different contexts.
Design Of Non-Equivalent Groups
Determining the effectiveness of math apps in supplementing math classes.
Imagine a study aiming to determine the effectiveness of math apps in supplementing traditional math classes in a school. Randomly assigning students to different groups might be impractical or disrupt the existing classroom structure. Instead, researchers can select two comparable classes, one receiving the math app intervention and the other continuing with traditional teaching methods. By comparing the performance of the two groups, researchers can draw conclusions about the app’s effectiveness.
To conduct a quasi-experiment study like the one mentioned above, researchers can utilize QuestionPro , an advanced research platform that offers comprehensive survey and data analysis tools. With QuestionPro, researchers can design surveys to collect data, analyze results, and gain valuable insights for their quasi-experimental research.
How QuestionPro Helps In Quasi-Experimental Research?
QuestionPro’s powerful features, such as random assignment of participants, survey branching, and data visualization, enable researchers to efficiently conduct and analyze quasi-experimental studies. The platform provides a user-friendly interface and robust reporting capabilities, empowering researchers to gather data, explore relationships, and draw meaningful conclusions.
In some cases, researchers can leverage natural experiments to examine causal relationships.
Determining The Effectiveness Of Teaching Modern Leadership Techniques In Start-Up Businesses
Consider a study evaluating the effectiveness of teaching modern leadership techniques in start-up businesses. Instead of artificially assigning businesses to different groups, researchers can observe those that naturally adopt modern leadership techniques and compare their outcomes to those of businesses that have not implemented such practices.
Advantages and Disadvantages Of The Quasi-Experimental Design
Quasi-experimental designs offer several advantages over true experiments, making them valuable tools in research:
- Scope of the research : Quasi-experiments allow researchers to study cause-and-effect relationships in real-world settings, providing valuable insights into complex phenomena that may be challenging to replicate in a controlled laboratory environment.
- Regression Discontinuity : Researchers can utilize regression discontinuity to evaluate the effects of interventions or treatments when random assignment is not feasible. This design leverages existing data and naturally occurring thresholds to draw causal inferences.
Disadvantage
Lack of random assignment : Quasi-experimental designs lack the random assignment of participants, which introduces the possibility of confounding variables affecting the results. Researchers must carefully consider potential alternative explanations for observed effects.
What Are The Different Quasi-Experimental Study Designs?
Quasi-experimental designs encompass various approaches, including nonequivalent group designs, interrupted time series designs, and natural experiments. Each design offers unique advantages and limitations, providing researchers with versatile tools to explore causal relationships in different contexts.
Example Of The Natural Experiment Approach
Researchers interested in studying the impact of a public health campaign aimed at reducing smoking rates may take advantage of a natural experiment. By comparing smoking rates in a region that has implemented the campaign to a similar region that has not, researchers can examine the effectiveness of the intervention.
Differences Between Quasi-Experiments And True Experiments
Quasi-experiments and true experiments differ primarily in their ability to randomly assign participants to groups. While true experiments provide a higher level of control, quasi-experiments offer practical and ethical alternatives in situations where randomization is not feasible or desirable.
Example Comparing A True Experiment And Quasi-Experiment
In a true experiment investigating the effects of a new medication on a specific condition, researchers would randomly assign participants to either the experimental group, which receives the medication, or the control group, which receives a placebo. In a quasi-experiment, researchers might instead compare patients who voluntarily choose to take the medication to those who do not, examining the differences in outcomes between the two groups.
Quasi-Experiment: A Quick Wrap-Up
Quasi-experimental research designs play a vital role in scientific inquiry by allowing researchers to investigate cause-and-effect relationships in real-world settings. These designs offer practical and ethical alternatives to true experiments, making them valuable tools in various fields of study. With their versatility and applicability, quasi-experimental designs continue to contribute to our understanding of complex phenomena.
Turn Your Data Into Easy-To-Understand And Dynamic Stories
When you wish to explain any complex data, it’s always advised to break it down into simpler visuals or stories. This is where Mind the Graph comes in. It is a platform that helps researchers and scientists to turn their data into easy-to-understand and dynamic stories, helping the audience understand the concepts better. Sign Up now to explore the library of scientific infographics.
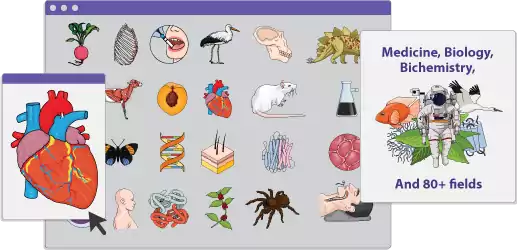
Subscribe to our newsletter
Exclusive high quality content about effective visual communication in science.
Sign Up for Free
Try the best infographic maker and promote your research with scientifically-accurate beautiful figures
no credit card required
Content tags

- Skip to main content
- Skip to primary sidebar
- Skip to footer
- QuestionPro

- Solutions Industries Gaming Automotive Sports and events Education Government Travel & Hospitality Financial Services Healthcare Cannabis Technology Use Case AskWhy Communities Audience Contactless surveys Mobile LivePolls Member Experience GDPR Positive People Science 360 Feedback Surveys
- Resources Blog eBooks Survey Templates Case Studies Training Help center

Home Market Research Research Tools and Apps
Quasi-experimental Research: What It Is, Types & Examples
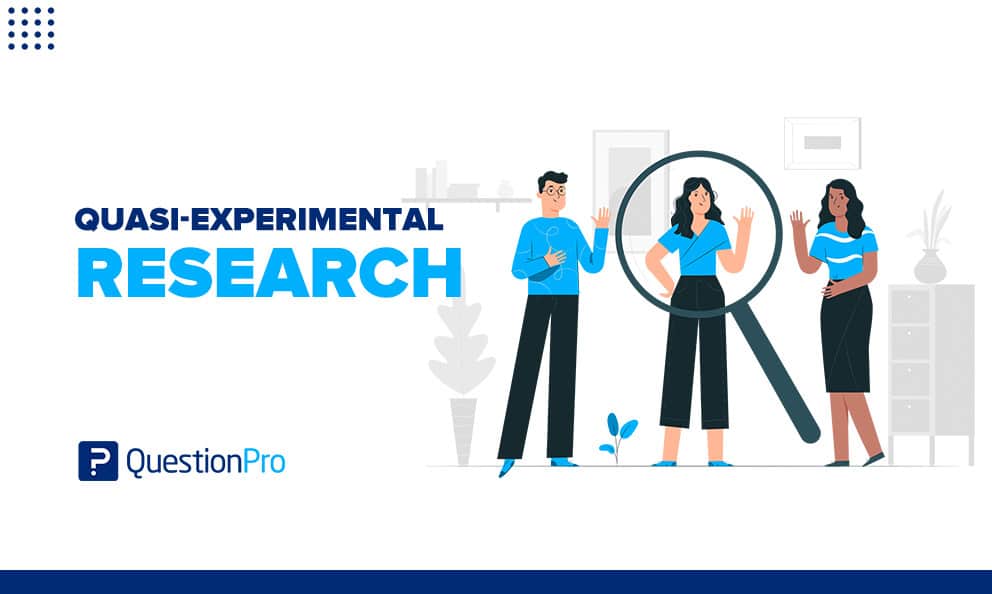
Much like an actual experiment, quasi-experimental research tries to demonstrate a cause-and-effect link between a dependent and an independent variable. A quasi-experiment, on the other hand, does not depend on random assignment, unlike an actual experiment. The subjects are sorted into groups based on non-random variables.
What is Quasi-Experimental Research?
“Resemblance” is the definition of “quasi.” Individuals are not randomly allocated to conditions or orders of conditions, even though the regression analysis is changed. As a result, quasi-experimental research is research that appears to be experimental but is not.
The directionality problem is avoided in quasi-experimental research since the regression analysis is altered before the multiple regression is assessed. However, because individuals are not randomized at random, there are likely to be additional disparities across conditions in quasi-experimental research.
As a result, in terms of internal consistency, quasi-experiments fall somewhere between correlational research and actual experiments.
The key component of a true experiment is randomly allocated groups. This means that each person has an equivalent chance of being assigned to the experimental group or the control group, depending on whether they are manipulated or not.
Simply put, a quasi-experiment is not a real experiment. A quasi-experiment does not feature randomly allocated groups since the main component of a real experiment is randomly assigned groups. Why is it so crucial to have randomly allocated groups, given that they constitute the only distinction between quasi-experimental and actual experimental research ?
Let’s use an example to illustrate our point. Let’s assume we want to discover how new psychological therapy affects depressed patients. In a genuine trial, you’d split half of the psych ward into treatment groups, With half getting the new psychotherapy therapy and the other half receiving standard depression treatment .
And the physicians compare the outcomes of this treatment to the results of standard treatments to see if this treatment is more effective. Doctors, on the other hand, are unlikely to agree with this genuine experiment since they believe it is unethical to treat one group while leaving another untreated.
A quasi-experimental study will be useful in this case. Instead of allocating these patients at random, you uncover pre-existing psychotherapist groups in the hospitals. Clearly, there’ll be counselors who are eager to undertake these trials as well as others who prefer to stick to the old ways.
These pre-existing groups can be used to compare the symptom development of individuals who received the novel therapy with those who received the normal course of treatment, even though the groups weren’t chosen at random.
If any substantial variations between them can be well explained, you may be very assured that any differences are attributable to the treatment but not to other extraneous variables.
As we mentioned before, quasi-experimental research entails manipulating an independent variable by randomly assigning people to conditions or sequences of conditions. Non-equivalent group designs, pretest-posttest designs, and regression discontinuity designs are only a few of the essential types.
What are quasi-experimental research designs?
Quasi-experimental research designs are a type of research design that is similar to experimental designs but doesn’t give full control over the independent variable(s) like true experimental designs do.
In a quasi-experimental design, the researcher changes or watches an independent variable, but the participants are not put into groups at random. Instead, people are put into groups based on things they already have in common, like their age, gender, or how many times they have seen a certain stimulus.
Because the assignments are not random, it is harder to draw conclusions about cause and effect than in a real experiment. However, quasi-experimental designs are still useful when randomization is not possible or ethical.
The true experimental design may be impossible to accomplish or just too expensive, especially for researchers with few resources. Quasi-experimental designs enable you to investigate an issue by utilizing data that has already been paid for or gathered by others (often the government).
Because they allow better control for confounding variables than other forms of studies, they have higher external validity than most genuine experiments and higher internal validity (less than true experiments) than other non-experimental research.
Is quasi-experimental research quantitative or qualitative?
Quasi-experimental research is a quantitative research method. It involves numerical data collection and statistical analysis. Quasi-experimental research compares groups with different circumstances or treatments to find cause-and-effect links.
It draws statistical conclusions from quantitative data. Qualitative data can enhance quasi-experimental research by revealing participants’ experiences and opinions, but quantitative data is the method’s foundation.
Quasi-experimental research types
There are many different sorts of quasi-experimental designs. Three of the most popular varieties are described below: Design of non-equivalent groups, Discontinuity in regression, and Natural experiments.
Design of Non-equivalent Groups
Example: design of non-equivalent groups, discontinuity in regression, example: discontinuity in regression, natural experiments, example: natural experiments.
However, because they couldn’t afford to pay everyone who qualified for the program, they had to use a random lottery to distribute slots.
Experts were able to investigate the program’s impact by utilizing enrolled people as a treatment group and those who were qualified but did not play the jackpot as an experimental group.
How QuestionPro helps in quasi-experimental research?
QuestionPro can be a useful tool in quasi-experimental research because it includes features that can assist you in designing and analyzing your research study. Here are some ways in which QuestionPro can help in quasi-experimental research:
Design surveys
Randomize participants, collect data over time, analyze data, collaborate with your team.
With QuestionPro, you have access to the most mature market research platform and tool that helps you collect and analyze the insights that matter the most. By leveraging InsightsHub, the unified hub for data management, you can leverage the consolidated platform to organize, explore, search, and discover your research data in one organized data repository .
Optimize Your quasi-experimental research with QuestionPro. Get started now!
LEARN MORE FREE TRIAL
MORE LIKE THIS
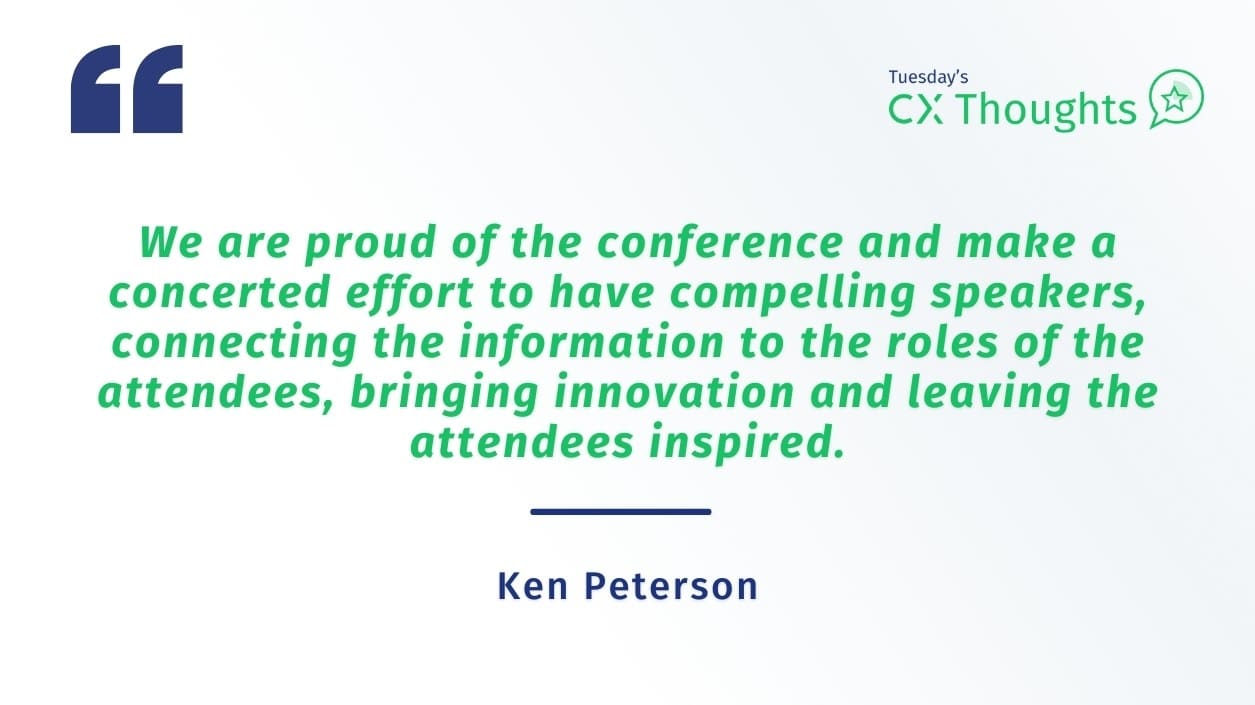
You Can’t Please Everyone — Tuesday CX Thoughts
Oct 22, 2024
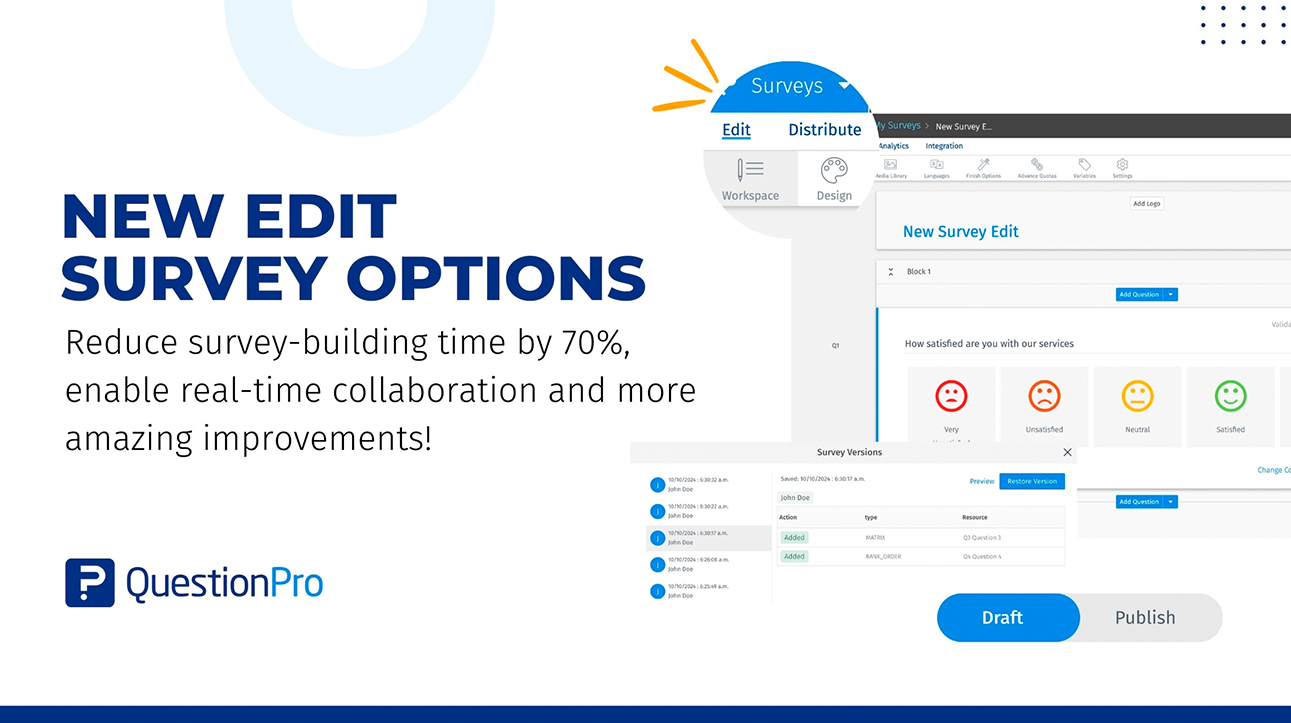
Edit survey: A new way of survey building and collaboration
Oct 10, 2024
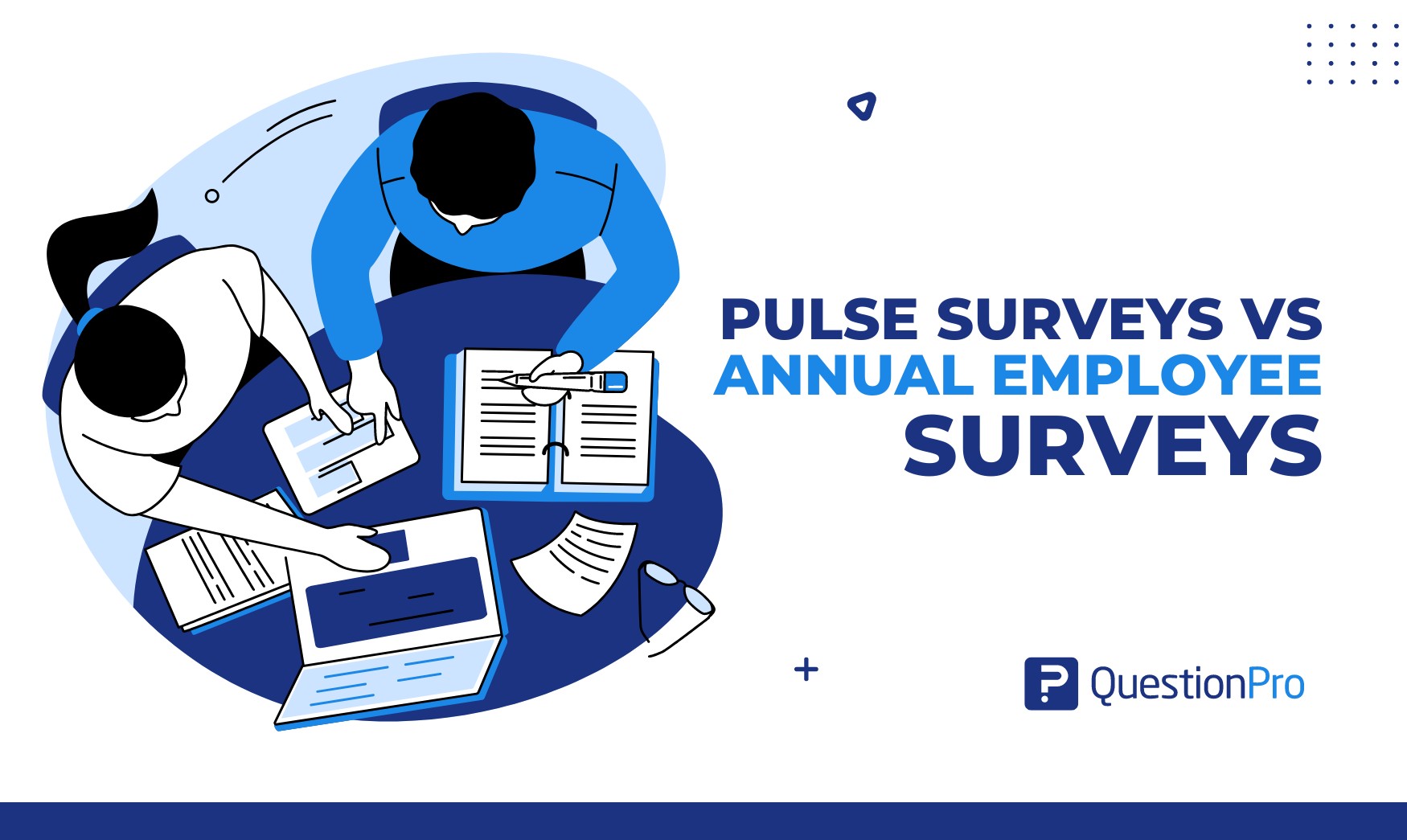
Pulse Surveys vs Annual Employee Surveys: Which to Use
Oct 4, 2024
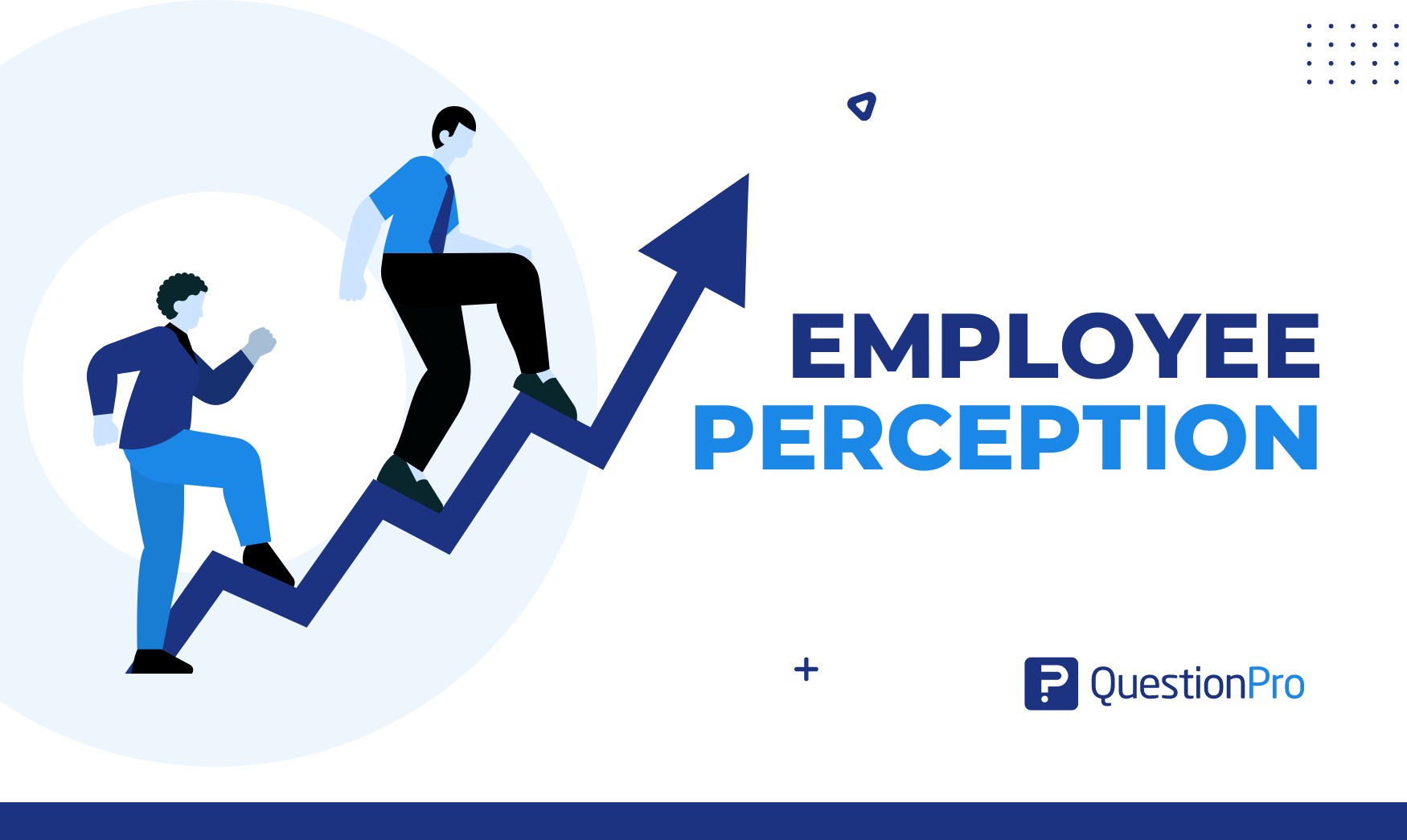
Employee Perception Role in Organizational Change
Oct 3, 2024
Other categories
- Academic Research
- Artificial Intelligence
- Assessments
- Brand Awareness
- Case Studies
- Communities
- Consumer Insights
- Customer effort score
- Customer Engagement
- Customer Experience
- Customer Loyalty
- Customer Research
- Customer Satisfaction
- Employee Benefits
- Employee Engagement
- Employee Retention
- Friday Five
- General Data Protection Regulation
- Insights Hub
- Life@QuestionPro
- Market Research
- Mobile diaries
- Mobile Surveys
- New Features
- Online Communities
- Question Types
- Questionnaire
- QuestionPro Products
- Release Notes
- Research Tools and Apps
- Revenue at Risk
- Survey Templates
- Training Tips
- Tuesday CX Thoughts (TCXT)
- Uncategorized
- What’s Coming Up
- Workforce Intelligence
The use and interpretation of quasi-experimental design
Last updated
6 February 2023
Reviewed by
Miroslav Damyanov
Short on time? Get an AI generated summary of this article instead
- What is a quasi-experimental design?
Commonly used in medical informatics (a field that uses digital information to ensure better patient care), researchers generally use this design to evaluate the effectiveness of a treatment – perhaps a type of antibiotic or psychotherapy, or an educational or policy intervention.
Even though quasi-experimental design has been used for some time, relatively little is known about it. Read on to learn the ins and outs of this research design.
Make research less tedious
Dovetail streamlines research to help you uncover and share actionable insights
- When to use a quasi-experimental design
A quasi-experimental design is used when it's not logistically feasible or ethical to conduct randomized, controlled trials. As its name suggests, a quasi-experimental design is almost a true experiment. However, researchers don't randomly select elements or participants in this type of research.
Researchers prefer to apply quasi-experimental design when there are ethical or practical concerns. Let's look at these two reasons more closely.
Ethical reasons
In some situations, the use of randomly assigned elements can be unethical. For instance, providing public healthcare to one group and withholding it to another in research is unethical. A quasi-experimental design would examine the relationship between these two groups to avoid physical danger.
Practical reasons
Randomized controlled trials may not be the best approach in research. For instance, it's impractical to trawl through large sample sizes of participants without using a particular attribute to guide your data collection .
Recruiting participants and properly designing a data-collection attribute to make the research a true experiment requires a lot of time and effort, and can be expensive if you don’t have a large funding stream.
A quasi-experimental design allows researchers to take advantage of previously collected data and use it in their study.
- Examples of quasi-experimental designs
Quasi-experimental research design is common in medical research, but any researcher can use it for research that raises practical and ethical concerns. Here are a few examples of quasi-experimental designs used by different researchers:
Example 1: Determining the effectiveness of math apps in supplementing math classes
A school wanted to supplement its math classes with a math app. To select the best app, the school decided to conduct demo tests on two apps before selecting the one they will purchase.
Scope of the research
Since every grade had two math teachers, each teacher used one of the two apps for three months. They then gave the students the same math exams and compared the results to determine which app was most effective.
Reasons why this is a quasi-experimental study
This simple study is a quasi-experiment since the school didn't randomly assign its students to the applications. They used a pre-existing class structure to conduct the study since it was impractical to randomly assign the students to each app.
Example 2: Determining the effectiveness of teaching modern leadership techniques in start-up businesses
A hypothetical quasi-experimental study was conducted in an economically developing country in a mid-sized city.
Five start-ups in the textile industry and five in the tech industry participated in the study. The leaders attended a six-week workshop on leadership style, team management, and employee motivation.
After a year, the researchers assessed the performance of each start-up company to determine growth. The results indicated that the tech start-ups were further along in their growth than the textile companies.
The basis of quasi-experimental research is a non-randomized subject-selection process. This study didn't use specific aspects to determine which start-up companies should participate. Therefore, the results may seem straightforward, but several aspects may determine the growth of a specific company, apart from the variables used by the researchers.
Example 3: A study to determine the effects of policy reforms and of luring foreign investment on small businesses in two mid-size cities
In a study to determine the economic impact of government reforms in an economically developing country, the government decided to test whether creating reforms directed at small businesses or luring foreign investments would spur the most economic development.
The government selected two cities with similar population demographics and sizes. In one of the cities, they implemented specific policies that would directly impact small businesses, and in the other, they implemented policies to attract foreign investment.
After five years, they collected end-of-year economic growth data from both cities. They looked at elements like local GDP growth, unemployment rates, and housing sales.
The study used a non-randomized selection process to determine which city would participate in the research. Researchers left out certain variables that would play a crucial role in determining the growth of each city. They used pre-existing groups of people based on research conducted in each city, rather than random groups.
- Advantages of a quasi-experimental design
Some advantages of quasi-experimental designs are:
Researchers can manipulate variables to help them meet their study objectives.
It offers high external validity, making it suitable for real-world applications, specifically in social science experiments.
Integrating this methodology into other research designs is easier, especially in true experimental research. This cuts down on the time needed to determine your outcomes.
- Disadvantages of a quasi-experimental design
Despite the pros that come with a quasi-experimental design, there are several disadvantages associated with it, including the following:
It has a lower internal validity since researchers do not have full control over the comparison and intervention groups or between time periods because of differences in characteristics in people, places, or time involved. It may be challenging to determine whether all variables have been used or whether those used in the research impacted the results.
There is the risk of inaccurate data since the research design borrows information from other studies.
There is the possibility of bias since researchers select baseline elements and eligibility.
- What are the different quasi-experimental study designs?
There are three distinct types of quasi-experimental designs:
Nonequivalent
Regression discontinuity, natural experiment.
This is a hybrid of experimental and quasi-experimental methods and is used to leverage the best qualities of the two. Like the true experiment design, nonequivalent group design uses pre-existing groups believed to be comparable. However, it doesn't use randomization, the lack of which is a crucial element for quasi-experimental design.
Researchers usually ensure that no confounding variables impact them throughout the grouping process. This makes the groupings more comparable.
Example of a nonequivalent group design
A small study was conducted to determine whether after-school programs result in better grades. Researchers randomly selected two groups of students: one to implement the new program, the other not to. They then compared the results of the two groups.
This type of quasi-experimental research design calculates the impact of a specific treatment or intervention. It uses a criterion known as "cutoff" that assigns treatment according to eligibility.
Researchers often assign participants above the cutoff to the treatment group. This puts a negligible distinction between the two groups (treatment group and control group).
Example of regression discontinuity
Students must achieve a minimum score to be enrolled in specific US high schools. Since the cutoff score used to determine eligibility for enrollment is arbitrary, researchers can assume that the disparity between students who only just fail to achieve the cutoff point and those who barely pass is a small margin and is due to the difference in the schools that these students attend.
Researchers can then examine the long-term effects of these two groups of kids to determine the effect of attending certain schools. This information can be applied to increase the chances of students being enrolled in these high schools.
This research design is common in laboratory and field experiments where researchers control target subjects by assigning them to different groups. Researchers randomly assign subjects to a treatment group using nature or an external event or situation.
However, even with random assignment, this research design cannot be called a true experiment since nature aspects are observational. Researchers can also exploit these aspects despite having no control over the independent variables.
Example of the natural experiment approach
An example of a natural experiment is the 2008 Oregon Health Study.
Oregon intended to allow more low-income people to participate in Medicaid.
Since they couldn't afford to cover every person who qualified for the program, the state used a random lottery to allocate program slots.
Researchers assessed the program's effectiveness by assigning the selected subjects to a randomly assigned treatment group, while those that didn't win the lottery were considered the control group.
- Differences between quasi-experiments and true experiments
There are several differences between a quasi-experiment and a true experiment:
Participants in true experiments are randomly assigned to the treatment or control group, while participants in a quasi-experiment are not assigned randomly.
In a quasi-experimental design, the control and treatment groups differ in unknown or unknowable ways, apart from the experimental treatments that are carried out. Therefore, the researcher should try as much as possible to control these differences.
Quasi-experimental designs have several "competing hypotheses," which compete with experimental manipulation to explain the observed results.
Quasi-experiments tend to have lower internal validity (the degree of confidence in the research outcomes) than true experiments, but they may offer higher external validity (whether findings can be extended to other contexts) as they involve real-world interventions instead of controlled interventions in artificial laboratory settings.
Despite the distinct difference between true and quasi-experimental research designs, these two research methodologies share the following aspects:
Both study methods subject participants to some form of treatment or conditions.
Researchers have the freedom to measure some of the outcomes of interest.
Researchers can test whether the differences in the outcomes are associated with the treatment.
- An example comparing a true experiment and quasi-experiment
Imagine you wanted to study the effects of junk food on obese people. Here's how you would do this as a true experiment and a quasi-experiment:
How to carry out a true experiment
In a true experiment, some participants would eat junk foods, while the rest would be in the control group, adhering to a regular diet. At the end of the study, you would record the health and discomfort of each group.
This kind of experiment would raise ethical concerns since the participants assigned to the treatment group are required to eat junk food against their will throughout the experiment. This calls for a quasi-experimental design.
How to carry out a quasi-experiment
In quasi-experimental research, you would start by finding out which participants want to try junk food and which prefer to stick to a regular diet. This allows you to assign these two groups based on subject choice.
In this case, you didn't assign participants to a particular group, so you can confidently use the results from the study.
When is a quasi-experimental design used?
Quasi-experimental designs are used when researchers don’t want to use randomization when evaluating their intervention.
What are the characteristics of quasi-experimental designs?
Some of the characteristics of a quasi-experimental design are:
Researchers don't randomly assign participants into groups, but study their existing characteristics and assign them accordingly.
Researchers study the participants in pre- and post-testing to determine the progress of the groups.
Quasi-experimental design is ethical since it doesn’t involve offering or withholding treatment at random.
Quasi-experimental design encompasses a broad range of non-randomized intervention studies. This design is employed when it is not ethical or logistically feasible to conduct randomized controlled trials. Researchers typically employ it when evaluating policy or educational interventions, or in medical or therapy scenarios.
How do you analyze data in a quasi-experimental design?
You can use two-group tests, time-series analysis, and regression analysis to analyze data in a quasi-experiment design. Each option has specific assumptions, strengths, limitations, and data requirements.
Should you be using a customer insights hub?
Do you want to discover previous research faster?
Do you share your research findings with others?
Do you analyze research data?
Start for free today, add your research, and get to key insights faster
Editor’s picks
Last updated: 30 January 2024
Last updated: 11 January 2024
Last updated: 17 January 2024
Last updated: 12 December 2023
Last updated: 30 April 2024
Last updated: 4 July 2024
Last updated: 12 October 2023
Last updated: 5 March 2024
Last updated: 6 March 2024
Last updated: 31 January 2024
Last updated: 23 January 2024
Last updated: 13 May 2024
Last updated: 20 December 2023
Latest articles
Related topics, decide what to build next, log in or sign up.
Get started for free
Research Methodologies Guide
- Action Research
- Bibliometrics
- Case Studies
- Content Analysis
- Digital Scholarship This link opens in a new window
- Documentary
- Ethnography
- Focus Groups
- Grounded Theory
- Life Histories/Autobiographies
- Longitudinal
- Participant Observation
- Qualitative Research (General)
Quasi-Experimental Design
- Usability Studies
Quasi-Experimental Design is a unique research methodology because it is characterized by what is lacks. For example, Abraham & MacDonald (2011) state:
" Quasi-experimental research is similar to experimental research in that there is manipulation of an independent variable. It differs from experimental research because either there is no control group, no random selection, no random assignment, and/or no active manipulation. "
This type of research is often performed in cases where a control group cannot be created or random selection cannot be performed. This is often the case in certain medical and psychological studies.
For more information on quasi-experimental design, review the resources below:
Where to Start
Below are listed a few tools and online guides that can help you start your Quasi-experimental research. These include free online resources and resources available only through ISU Library.
- Quasi-Experimental Research Designs by Bruce A. Thyer This pocket guide describes the logic, design, and conduct of the range of quasi-experimental designs, encompassing pre-experiments, quasi-experiments making use of a control or comparison group, and time-series designs. An introductory chapter describes the valuable role these types of studies have played in social work, from the 1930s to the present. Subsequent chapters delve into each design type's major features, the kinds of questions it is capable of answering, and its strengths and limitations.
- Experimental and Quasi-Experimental Designs for Research by Donald T. Campbell; Julian C. Stanley. Call Number: Q175 C152e Written 1967 but still used heavily today, this book examines research designs for experimental and quasi-experimental research, with examples and judgments about each design's validity.

Online Resources
- Quasi-Experimental Design From the Web Center for Social Research Methods, this is a very good overview of quasi-experimental design.
- Experimental and Quasi-Experimental Research From Colorado State University.
- Quasi-experimental design--Wikipedia, the free encyclopedia Wikipedia can be a useful place to start your research- check the citations at the bottom of the article for more information.
- << Previous: Qualitative Research (General)
- Next: Sampling >>
- Last Updated: Sep 11, 2024 11:05 AM
- URL: https://instr.iastate.libguides.com/researchmethods
Quasi-Experimental Design: Definition, Types, Examples
Appinio Research · 19.12.2023 · 37min read
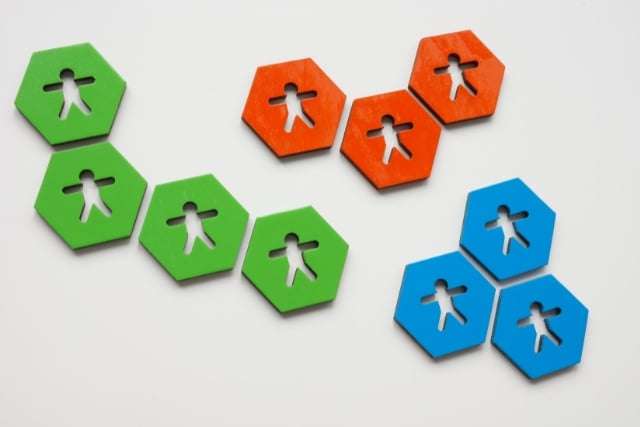
Ever wondered how researchers uncover cause-and-effect relationships in the real world, where controlled experiments are often elusive? Quasi-experimental design holds the key. In this guide, we'll unravel the intricacies of quasi-experimental design, shedding light on its definition, purpose, and applications across various domains. Whether you're a student, a professional, or simply curious about the methods behind meaningful research, join us as we delve into the world of quasi-experimental design, making complex concepts sound simple and embarking on a journey of knowledge and discovery.
What is Quasi-Experimental Design?
Quasi-experimental design is a research methodology used to study the effects of independent variables on dependent variables when full experimental control is not possible or ethical. It falls between controlled experiments, where variables are tightly controlled, and purely observational studies, where researchers have little control over variables. Quasi-experimental design mimics some aspects of experimental research but lacks randomization.
The primary purpose of quasi-experimental design is to investigate cause-and-effect relationships between variables in real-world settings. Researchers use this approach to answer research questions, test hypotheses, and explore the impact of interventions or treatments when they cannot employ traditional experimental methods. Quasi-experimental studies aim to maximize internal validity and make meaningful inferences while acknowledging practical constraints and ethical considerations.
Quasi-Experimental vs. Experimental Design
It's essential to understand the distinctions between Quasi-Experimental and Experimental Design to appreciate the unique characteristics of each approach:
- Randomization: In Experimental Design, random assignment of participants to groups is a defining feature. Quasi-experimental design, on the other hand, lacks randomization due to practical constraints or ethical considerations.
- Control Groups : Experimental Design typically includes control groups that are subjected to no treatment or a placebo. The quasi-experimental design may have comparison groups but lacks the same level of control.
- Manipulation of IV: Experimental Design involves the intentional manipulation of the independent variable. Quasi-experimental design often deals with naturally occurring independent variables.
- Causal Inference: Experimental Design allows for stronger causal inferences due to randomization and control. Quasi-experimental design permits causal inferences but with some limitations.
When to Use Quasi-Experimental Design?
A quasi-experimental design is particularly valuable in several situations:
- Ethical Constraints: When manipulating the independent variable is ethically unacceptable or impractical, quasi-experimental design offers an alternative to studying naturally occurring variables.
- Real-World Settings: When researchers want to study phenomena in real-world contexts, quasi-experimental design allows them to do so without artificial laboratory settings.
- Limited Resources: In cases where resources are limited and conducting a controlled experiment is cost-prohibitive, quasi-experimental design can provide valuable insights.
- Policy and Program Evaluation: Quasi-experimental design is commonly used in evaluating the effectiveness of policies, interventions, or programs that cannot be randomly assigned to participants.
Importance of Quasi-Experimental Design in Research
Quasi-experimental design plays a vital role in research for several reasons:
- Addressing Real-World Complexities: It allows researchers to tackle complex real-world issues where controlled experiments are not feasible. This bridges the gap between controlled experiments and purely observational studies.
- Ethical Research: It provides an honest approach when manipulating variables or assigning treatments could harm participants or violate ethical standards.
- Policy and Practice Implications: Quasi-experimental studies generate findings with direct applications in policy-making and practical solutions in fields such as education, healthcare, and social sciences.
- Enhanced External Validity: Findings from Quasi-Experimental research often have high external validity, making them more applicable to broader populations and contexts.
By embracing the challenges and opportunities of quasi-experimental design, researchers can contribute valuable insights to their respective fields and drive positive changes in the real world.
Key Concepts in Quasi-Experimental Design
In quasi-experimental design, it's essential to grasp the fundamental concepts underpinning this research methodology. Let's explore these key concepts in detail.
Independent Variable
The independent variable (IV) is the factor you aim to study or manipulate in your research. Unlike controlled experiments, where you can directly manipulate the IV, quasi-experimental design often deals with naturally occurring variables. For example, if you're investigating the impact of a new teaching method on student performance, the teaching method is your independent variable.
Dependent Variable
The dependent variable (DV) is the outcome or response you measure to assess the effects of changes in the independent variable. Continuing with the teaching method example, the dependent variable would be the students' academic performance, typically measured using test scores, grades, or other relevant metrics.
Control Groups vs. Comparison Groups
While quasi-experimental design lacks the luxury of randomly assigning participants to control and experimental groups, you can still establish comparison groups to make meaningful inferences. Control groups consist of individuals who do not receive the treatment, while comparison groups are exposed to different levels or variations of the treatment. These groups help researchers gauge the effect of the independent variable.
Pre-Test and Post-Test Measures
In quasi-experimental design, it's common practice to collect data both before and after implementing the independent variable. The initial data (pre-test) serves as a baseline, allowing you to measure changes over time (post-test). This approach helps assess the impact of the independent variable more accurately. For instance, if you're studying the effectiveness of a new drug, you'd measure patients' health before administering the drug (pre-test) and afterward (post-test).
Threats to Internal Validity
Internal validity is crucial for establishing a cause-and-effect relationship between the independent and dependent variables. However, in a quasi-experimental design, several threats can compromise internal validity. These threats include:
- Selection Bias : When non-randomized groups differ systematically in ways that affect the study's outcome.
- History Effects: External events or changes over time that influence the results.
- Maturation Effects: Natural changes or developments that occur within participants during the study.
- Regression to the Mean: The tendency for extreme scores on a variable to move closer to the mean upon retesting.
- Attrition and Mortality: The loss of participants over time, potentially skewing the results.
- Testing Effects: The mere act of testing or assessing participants can impact their subsequent performance.
Understanding these threats is essential for designing and conducting Quasi-Experimental studies that yield valid and reliable results.
Randomization and Non-Randomization
In traditional experimental designs, randomization is a powerful tool for ensuring that groups are equivalent at the outset of a study. However, quasi-experimental design often involves non-randomization due to the nature of the research. This means that participants are not randomly assigned to treatment and control groups. Instead, researchers must employ various techniques to minimize biases and ensure that the groups are as similar as possible.
For example, if you are conducting a study on the effects of a new teaching method in a real classroom setting, you cannot randomly assign students to the treatment and control groups. Instead, you might use statistical methods to match students based on relevant characteristics such as prior academic performance or socioeconomic status. This matching process helps control for potential confounding variables, increasing the validity of your study.
Types of Quasi-Experimental Designs
In quasi-experimental design, researchers employ various approaches to investigate causal relationships and study the effects of independent variables when complete experimental control is challenging. Let's explore these types of quasi-experimental designs.
One-Group Posttest-Only Design
The One-Group Posttest-Only Design is one of the simplest forms of quasi-experimental design. In this design, a single group is exposed to the independent variable, and data is collected only after the intervention has taken place. Unlike controlled experiments, there is no comparison group. This design is useful when researchers cannot administer a pre-test or when it is logistically difficult to do so.
Example : Suppose you want to assess the effectiveness of a new time management seminar. You offer the seminar to a group of employees and measure their productivity levels immediately afterward to determine if there's an observable impact.
One-Group Pretest-Posttest Design
Similar to the One-Group Posttest-Only Design, this approach includes a pre-test measure in addition to the post-test. Researchers collect data both before and after the intervention. By comparing the pre-test and post-test results within the same group, you can gain a better understanding of the changes that occur due to the independent variable.
Example : If you're studying the impact of a stress management program on participants' stress levels, you would measure their stress levels before the program (pre-test) and after completing the program (post-test) to assess any changes.
Non-Equivalent Groups Design
The Non-Equivalent Groups Design involves multiple groups, but they are not randomly assigned. Instead, researchers must carefully match or control for relevant variables to minimize biases. This design is particularly useful when random assignment is not possible or ethical.
Example : Imagine you're examining the effectiveness of two teaching methods in two different schools. You can't randomly assign students to the schools, but you can carefully match them based on factors like age, prior academic performance, and socioeconomic status to create equivalent groups.
Time Series Design
Time Series Design is an approach where data is collected at multiple time points before and after the intervention. This design allows researchers to analyze trends and patterns over time, providing valuable insights into the sustained effects of the independent variable.
Example : If you're studying the impact of a new marketing campaign on product sales, you would collect sales data at regular intervals (e.g., monthly) before and after the campaign's launch to observe any long-term trends.
Regression Discontinuity Design
Regression Discontinuity Design is employed when participants are assigned to different groups based on a specific cutoff score or threshold. This design is often used in educational and policy research to assess the effects of interventions near a cutoff point.
Example : Suppose you're evaluating the impact of a scholarship program on students' academic performance. Students who score just above or below a certain GPA threshold are assigned differently to the program. This design helps assess the program's effectiveness at the cutoff point.
Propensity Score Matching
Propensity Score Matching is a technique used to create comparable treatment and control groups in non-randomized studies. Researchers calculate propensity scores based on participants' characteristics and match individuals in the treatment group to those in the control group with similar scores.
Example : If you're studying the effects of a new medication on patient outcomes, you would use propensity scores to match patients who received the medication with those who did not but have similar health profiles.
Interrupted Time Series Design
The Interrupted Time Series Design involves collecting data at multiple time points before and after the introduction of an intervention. However, in this design, the intervention occurs at a specific point in time, allowing researchers to assess its immediate impact.
Example : Let's say you're analyzing the effects of a new traffic management system on traffic accidents. You collect accident data before and after the system's implementation to observe any abrupt changes right after its introduction.
Each of these quasi-experimental designs offers unique advantages and is best suited to specific research questions and scenarios. Choosing the right design is crucial for conducting robust and informative studies.
Advantages and Disadvantages of Quasi-Experimental Design
Quasi-experimental design offers a valuable research approach, but like any methodology, it comes with its own set of advantages and disadvantages. Let's explore these in detail.
Quasi-Experimental Design Advantages
Quasi-experimental design presents several advantages that make it a valuable tool in research:
- Real-World Applicability: Quasi-experimental studies often take place in real-world settings, making the findings more applicable to practical situations. Researchers can examine the effects of interventions or variables in the context where they naturally occur.
- Ethical Considerations: In situations where manipulating the independent variable in a controlled experiment would be unethical, quasi-experimental design provides an ethical alternative. For example, it would be unethical to assign participants to smoke for a study on the health effects of smoking, but you can study naturally occurring groups of smokers and non-smokers.
- Cost-Efficiency: Conducting Quasi-Experimental research is often more cost-effective than conducting controlled experiments. The absence of controlled environments and extensive manipulations can save both time and resources.
These advantages make quasi-experimental design an attractive choice for researchers facing practical or ethical constraints in their studies.
Quasi-Experimental Design Disadvantages
However, quasi-experimental design also comes with its share of challenges and disadvantages:
- Limited Control: Unlike controlled experiments, where researchers have full control over variables, quasi-experimental design lacks the same level of control. This limited control can result in confounding variables that make it difficult to establish causality.
- Threats to Internal Validity: Various threats to internal validity, such as selection bias, history effects, and maturation effects, can compromise the accuracy of causal inferences. Researchers must carefully address these threats to ensure the validity of their findings.
- Causality Inference Challenges: Establishing causality can be challenging in quasi-experimental design due to the absence of randomization and control. While you can make strong arguments for causality, it may not be as conclusive as in controlled experiments.
- Potential Confounding Variables: In a quasi-experimental design, it's often challenging to control for all possible confounding variables that may affect the dependent variable. This can lead to uncertainty in attributing changes solely to the independent variable.
Despite these disadvantages, quasi-experimental design remains a valuable research tool when used judiciously and with a keen awareness of its limitations. Researchers should carefully consider their research questions and the practical constraints they face before choosing this approach.
How to Conduct a Quasi-Experimental Study?
Conducting a Quasi-Experimental study requires careful planning and execution to ensure the validity of your research. Let's dive into the essential steps you need to follow when conducting such a study.
1. Define Research Questions and Objectives
The first step in any research endeavor is clearly defining your research questions and objectives. This involves identifying the independent variable (IV) and the dependent variable (DV) you want to study. What is the specific relationship you want to explore, and what do you aim to achieve with your research?
- Specify Your Research Questions : Start by formulating precise research questions that your study aims to answer. These questions should be clear, focused, and relevant to your field of study.
- Identify the Independent Variable: Define the variable you intend to manipulate or study in your research. Understand its significance in your study's context.
- Determine the Dependent Variable: Identify the outcome or response variable that will be affected by changes in the independent variable.
- Establish Hypotheses (If Applicable): If you have specific hypotheses about the relationship between the IV and DV, state them clearly. Hypotheses provide a framework for testing your research questions.
2. Select the Appropriate Quasi-Experimental Design
Choosing the right quasi-experimental design is crucial for achieving your research objectives. Select a design that aligns with your research questions and the available data. Consider factors such as the feasibility of implementing the design and the ethical considerations involved.
- Evaluate Your Research Goals: Assess your research questions and objectives to determine which type of quasi-experimental design is most suitable. Each design has its strengths and limitations, so choose one that aligns with your goals.
- Consider Ethical Constraints: Take into account any ethical concerns related to your research. Depending on your study's context, some designs may be more ethically sound than others.
- Assess Data Availability: Ensure you have access to the necessary data for your chosen design. Some designs may require extensive historical data, while others may rely on data collected during the study.
3. Identify and Recruit Participants
Selecting the right participants is a critical aspect of Quasi-Experimental research. The participants should represent the population you want to make inferences about, and you must address ethical considerations, including informed consent.
- Define Your Target Population: Determine the population that your study aims to generalize to. Your sample should be representative of this population.
- Recruitment Process: Develop a plan for recruiting participants. Depending on your design, you may need to reach out to specific groups or institutions.
- Informed Consent: Ensure that you obtain informed consent from participants. Clearly explain the nature of the study, potential risks, and their rights as participants.
4. Collect Data
Data collection is a crucial step in Quasi-Experimental research. You must adhere to a consistent and systematic process to gather relevant information before and after the intervention or treatment.
- Pre-Test Measures: If applicable, collect data before introducing the independent variable. Ensure that the pre-test measures are standardized and reliable.
- Post-Test Measures: After the intervention, collect post-test data using the same measures as the pre-test. This allows you to assess changes over time.
- Maintain Data Consistency: Ensure that data collection procedures are consistent across all participants and time points to minimize biases.
5. Analyze Data
Once you've collected your data, it's time to analyze it using appropriate statistical techniques . The choice of analysis depends on your research questions and the type of data you've gathered.
- Statistical Analysis : Use statistical software to analyze your data. Common techniques include t-tests , analysis of variance (ANOVA) , regression analysis , and more, depending on the design and variables.
- Control for Confounding Variables: Be aware of potential confounding variables and include them in your analysis as covariates to ensure accurate results.
Chi-Square Calculator :
t-Test Calculator :
6. Interpret Results
With the analysis complete, you can interpret the results to draw meaningful conclusions about the relationship between the independent and dependent variables.
- Examine Effect Sizes: Assess the magnitude of the observed effects to determine their practical significance.
- Consider Significance Levels: Determine whether the observed results are statistically significant . Understand the p-values and their implications.
- Compare Findings to Hypotheses: Evaluate whether your findings support or reject your hypotheses and research questions.
7. Draw Conclusions
Based on your analysis and interpretation of the results, draw conclusions about the research questions and objectives you set out to address.
- Causal Inferences: Discuss the extent to which your study allows for causal inferences. Be transparent about the limitations and potential alternative explanations for your findings.
- Implications and Applications: Consider the practical implications of your research. How do your findings contribute to existing knowledge, and how can they be applied in real-world contexts?
- Future Research: Identify areas for future research and potential improvements in study design. Highlight any limitations or constraints that may have affected your study's outcomes.
By following these steps meticulously, you can conduct a rigorous and informative Quasi-Experimental study that advances knowledge in your field of research.
Quasi-Experimental Design Examples
Quasi-experimental design finds applications in a wide range of research domains, including business-related and market research scenarios. Below, we delve into some detailed examples of how this research methodology is employed in practice:
Example 1: Assessing the Impact of a New Marketing Strategy
Suppose a company wants to evaluate the effectiveness of a new marketing strategy aimed at boosting sales. Conducting a controlled experiment may not be feasible due to the company's existing customer base and the challenge of randomly assigning customers to different marketing approaches. In this scenario, a quasi-experimental design can be employed.
- Independent Variable: The new marketing strategy.
- Dependent Variable: Sales revenue.
- Design: The company could implement the new strategy for one group of customers while maintaining the existing strategy for another group. Both groups are selected based on similar demographics and purchase history , reducing selection bias. Pre-implementation data (sales records) can serve as the baseline, and post-implementation data can be collected to assess the strategy's impact.
Example 2: Evaluating the Effectiveness of Employee Training Programs
In the context of human resources and employee development, organizations often seek to evaluate the impact of training programs. A randomized controlled trial (RCT) with random assignment may not be practical or ethical, as some employees may need specific training more than others. Instead, a quasi-experimental design can be employed.
- Independent Variable: Employee training programs.
- Dependent Variable: Employee performance metrics, such as productivity or quality of work.
- Design: The organization can offer training programs to employees who express interest or demonstrate specific needs, creating a self-selected treatment group. A comparable control group can consist of employees with similar job roles and qualifications who did not receive the training. Pre-training performance metrics can serve as the baseline, and post-training data can be collected to assess the impact of the training programs.
Example 3: Analyzing the Effects of a Tax Policy Change
In economics and public policy, researchers often examine the effects of tax policy changes on economic behavior. Conducting a controlled experiment in such cases is practically impossible. Therefore, a quasi-experimental design is commonly employed.
- Independent Variable: Tax policy changes (e.g., tax rate adjustments).
- Dependent Variable: Economic indicators, such as consumer spending or business investments.
- Design: Researchers can analyze data from different regions or jurisdictions where tax policy changes have been implemented. One region could represent the treatment group (with tax policy changes), while a similar region with no tax policy changes serves as the control group. By comparing economic data before and after the policy change in both groups, researchers can assess the impact of the tax policy changes.
These examples illustrate how quasi-experimental design can be applied in various research contexts, providing valuable insights into the effects of independent variables in real-world scenarios where controlled experiments are not feasible or ethical. By carefully selecting comparison groups and controlling for potential biases, researchers can draw meaningful conclusions and inform decision-making processes.
How to Publish Quasi-Experimental Research?
Publishing your Quasi-Experimental research findings is a crucial step in contributing to the academic community's knowledge. We'll explore the essential aspects of reporting and publishing your Quasi-Experimental research effectively.
Structuring Your Research Paper
When preparing your research paper, it's essential to adhere to a well-structured format to ensure clarity and comprehensibility. Here are key elements to include:
Title and Abstract
- Title: Craft a concise and informative title that reflects the essence of your study. It should capture the main research question or hypothesis.
- Abstract: Summarize your research in a structured abstract, including the purpose, methods, results, and conclusions. Ensure it provides a clear overview of your study.
Introduction
- Background and Rationale: Provide context for your study by discussing the research gap or problem your study addresses. Explain why your research is relevant and essential.
- Research Questions or Hypotheses: Clearly state your research questions or hypotheses and their significance.
Literature Review
- Review of Related Work: Discuss relevant literature that supports your research. Highlight studies with similar methodologies or findings and explain how your research fits within this context.
- Participants: Describe your study's participants, including their characteristics and how you recruited them.
- Quasi-Experimental Design: Explain your chosen design in detail, including the independent and dependent variables, procedures, and any control measures taken.
- Data Collection: Detail the data collection methods , instruments used, and any pre-test or post-test measures.
- Data Analysis: Describe the statistical techniques employed, including any control for confounding variables.
- Presentation of Findings: Present your results clearly, using tables, graphs, and descriptive statistics where appropriate. Include p-values and effect sizes, if applicable.
- Interpretation of Results: Discuss the implications of your findings and how they relate to your research questions or hypotheses.
- Interpretation and Implications: Analyze your results in the context of existing literature and theories. Discuss the practical implications of your findings.
- Limitations: Address the limitations of your study, including potential biases or threats to internal validity.
- Future Research: Suggest areas for future research and how your study contributes to the field.
Ethical Considerations in Reporting
Ethical reporting is paramount in Quasi-Experimental research. Ensure that you adhere to ethical standards, including:
- Informed Consent: Clearly state that informed consent was obtained from all participants, and describe the informed consent process.
- Protection of Participants: Explain how you protected the rights and well-being of your participants throughout the study.
- Confidentiality: Detail how you maintained privacy and anonymity, especially when presenting individual data.
- Disclosure of Conflicts of Interest: Declare any potential conflicts of interest that could influence the interpretation of your findings.
Common Pitfalls to Avoid
When reporting your Quasi-Experimental research, watch out for common pitfalls that can diminish the quality and impact of your work:
- Overgeneralization: Be cautious not to overgeneralize your findings. Clearly state the limits of your study and the populations to which your results can be applied.
- Misinterpretation of Causality: Clearly articulate the limitations in inferring causality in Quasi-Experimental research. Avoid making strong causal claims unless supported by solid evidence.
- Ignoring Ethical Concerns: Ethical considerations are paramount. Failing to report on informed consent, ethical oversight, and participant protection can undermine the credibility of your study.
Guidelines for Transparent Reporting
To enhance the transparency and reproducibility of your Quasi-Experimental research, consider adhering to established reporting guidelines, such as:
- CONSORT Statement: If your study involves interventions or treatments, follow the CONSORT guidelines for transparent reporting of randomized controlled trials.
- STROBE Statement: For observational studies, the STROBE statement provides guidance on reporting essential elements.
- PRISMA Statement: If your research involves systematic reviews or meta-analyses, adhere to the PRISMA guidelines.
- Transparent Reporting of Evaluations with Non-Randomized Designs (TREND): TREND guidelines offer specific recommendations for transparently reporting non-randomized designs, including Quasi-Experimental research.
By following these reporting guidelines and maintaining the highest ethical standards, you can contribute to the advancement of knowledge in your field and ensure the credibility and impact of your Quasi-Experimental research findings.
Quasi-Experimental Design Challenges
Conducting a Quasi-Experimental study can be fraught with challenges that may impact the validity and reliability of your findings. We'll take a look at some common challenges and provide strategies on how you can address them effectively.
Selection Bias
Challenge: Selection bias occurs when non-randomized groups differ systematically in ways that affect the study's outcome. This bias can undermine the validity of your research, as it implies that the groups are not equivalent at the outset of the study.
Addressing Selection Bias:
- Matching: Employ matching techniques to create comparable treatment and control groups. Match participants based on relevant characteristics, such as age, gender, or prior performance, to balance the groups.
- Statistical Controls: Use statistical controls to account for differences between groups. Include covariates in your analysis to adjust for potential biases.
- Sensitivity Analysis: Conduct sensitivity analyses to assess how vulnerable your results are to selection bias. Explore different scenarios to understand the impact of potential bias on your conclusions.
History Effects
Challenge: History effects refer to external events or changes over time that influence the study's results. These external factors can confound your research by introducing variables you did not account for.
Addressing History Effects:
- Collect Historical Data: Gather extensive historical data to understand trends and patterns that might affect your study. By having a comprehensive historical context, you can better identify and account for historical effects.
- Control Groups: Include control groups whenever possible. By comparing the treatment group's results to those of a control group, you can account for external influences that affect both groups equally.
- Time Series Analysis : If applicable, use time series analysis to detect and account for temporal trends. This method helps differentiate between the effects of the independent variable and external events.
Maturation Effects
Challenge: Maturation effects occur when participants naturally change or develop throughout the study, independent of the intervention. These changes can confound your results, making it challenging to attribute observed effects solely to the independent variable.
Addressing Maturation Effects:
- Randomization: If possible, use randomization to distribute maturation effects evenly across treatment and control groups. Random assignment minimizes the impact of maturation as a confounding variable.
- Matched Pairs: If randomization is not feasible, employ matched pairs or statistical controls to ensure that both groups experience similar maturation effects.
- Shorter Time Frames: Limit the duration of your study to reduce the likelihood of significant maturation effects. Shorter studies are less susceptible to long-term maturation.
Regression to the Mean
Challenge: Regression to the mean is the tendency for extreme scores on a variable to move closer to the mean upon retesting. This can create the illusion of an intervention's effectiveness when, in reality, it's a natural statistical phenomenon.
Addressing Regression to the Mean:
- Use Control Groups: Include control groups in your study to provide a baseline for comparison. This helps differentiate genuine intervention effects from regression to the mean.
- Multiple Data Points: Collect numerous data points to identify patterns and trends. If extreme scores regress to the mean in subsequent measurements, it may be indicative of regression to the mean rather than a true intervention effect.
- Statistical Analysis: Employ statistical techniques that account for regression to the mean when analyzing your data. Techniques like analysis of covariance (ANCOVA) can help control for baseline differences.
Attrition and Mortality
Challenge: Attrition refers to the loss of participants over the course of your study, while mortality is the permanent loss of participants. High attrition rates can introduce biases and affect the representativeness of your sample.
Addressing Attrition and Mortality:
- Careful Participant Selection: Select participants who are likely to remain engaged throughout the study. Consider factors that may lead to attrition, such as participant motivation and commitment.
- Incentives: Provide incentives or compensation to participants to encourage their continued participation.
- Follow-Up Strategies: Implement effective follow-up strategies to reduce attrition. Regular communication and reminders can help keep participants engaged.
- Sensitivity Analysis: Conduct sensitivity analyses to assess the impact of attrition and mortality on your results. Compare the characteristics of participants who dropped out with those who completed the study.
Testing Effects
Challenge: Testing effects occur when the mere act of testing or assessing participants affects their subsequent performance. This phenomenon can lead to changes in the dependent variable that are unrelated to the independent variable.
Addressing Testing Effects:
- Counterbalance Testing: If possible, counterbalance the order of tests or assessments between treatment and control groups. This helps distribute the testing effects evenly across groups.
- Control Groups: Include control groups subjected to the same testing or assessment procedures as the treatment group. By comparing the two groups, you can determine whether testing effects have influenced the results.
- Minimize Testing Frequency: Limit the frequency of testing or assessments to reduce the likelihood of testing effects. Conducting fewer assessments can mitigate the impact of repeated testing on participants.
By proactively addressing these common challenges, you can enhance the validity and reliability of your Quasi-Experimental study, making your findings more robust and trustworthy.
Conclusion for Quasi-Expermental Design
Quasi-experimental design is a powerful tool that helps researchers investigate cause-and-effect relationships in real-world situations where strict control is not always possible. By understanding the key concepts, types of designs, and how to address challenges, you can conduct robust research and contribute valuable insights to your field. Remember, quasi-experimental design bridges the gap between controlled experiments and purely observational studies, making it an essential approach in various fields, from business and market research to public policy and beyond. So, whether you're a researcher, student, or decision-maker, the knowledge of quasi-experimental design empowers you to make informed choices and drive positive changes in the world.
How to Supercharge Quasi-Experimental Design with Real-Time Insights?
Introducing Appinio , the real-time market research platform that transforms the world of quasi-experimental design. Imagine having the power to conduct your own market research in minutes, obtaining actionable insights that fuel your data-driven decisions. Appinio takes care of the research and tech complexities, freeing you to focus on what truly matters for your business.
Here's why Appinio stands out:
- Lightning-Fast Insights: From formulating questions to uncovering insights, Appinio delivers results in minutes, ensuring you get the answers you need when you need them.
- No Research Degree Required: Our intuitive platform is designed for everyone, eliminating the need for a PhD in research. Anyone can dive in and start harnessing the power of real-time consumer insights.
- Global Reach, Local Expertise: With access to over 90 countries and the ability to define precise target groups based on 1200+ characteristics, you can conduct Quasi-Experimental research on a global scale while maintaining a local touch.
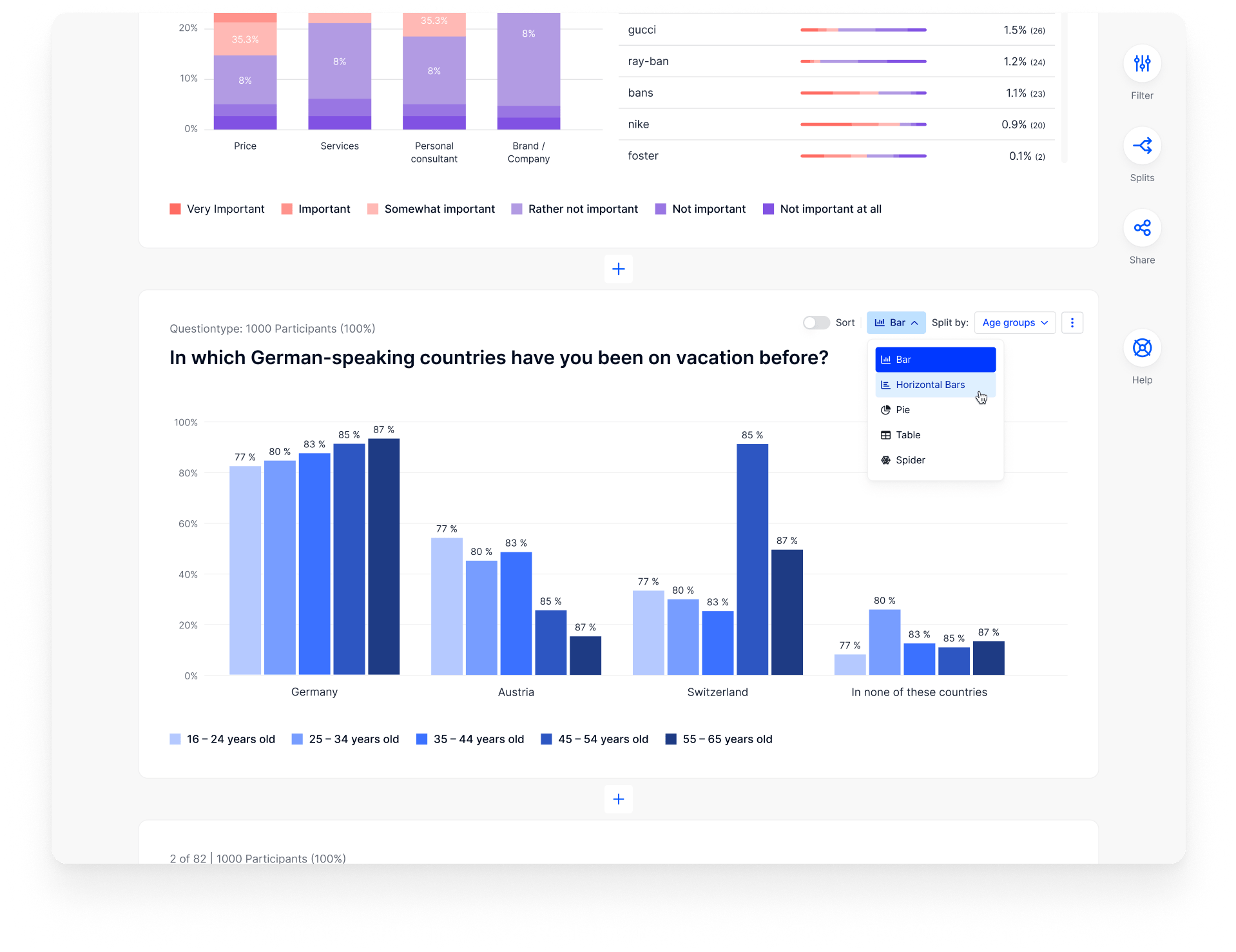
Get free access to the platform!
Join the loop 💌
Be the first to hear about new updates, product news, and data insights. We'll send it all straight to your inbox.
Get the latest market research news straight to your inbox! 💌
Wait, there's more
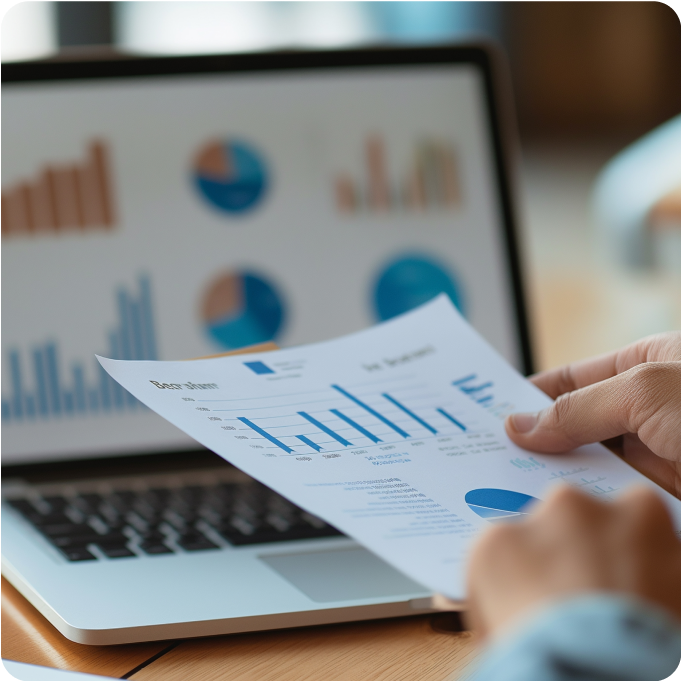
19.09.2024 | 9min read
Track Your Customer Retention & Brand Metrics for Post-Holiday Success
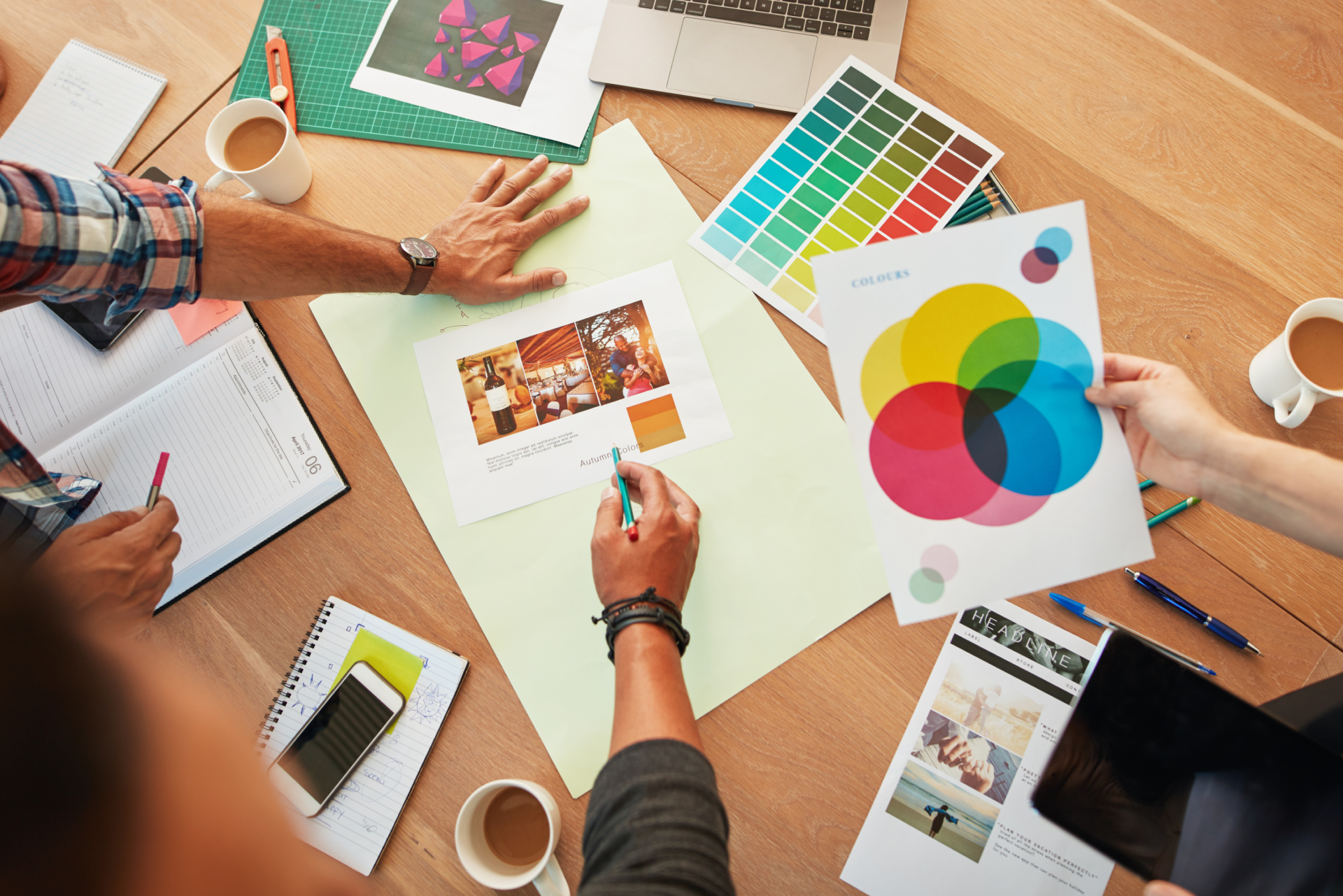
16.09.2024 | 10min read
Creative Checkup – Optimize Advertising Slogans & Creatives for ROI
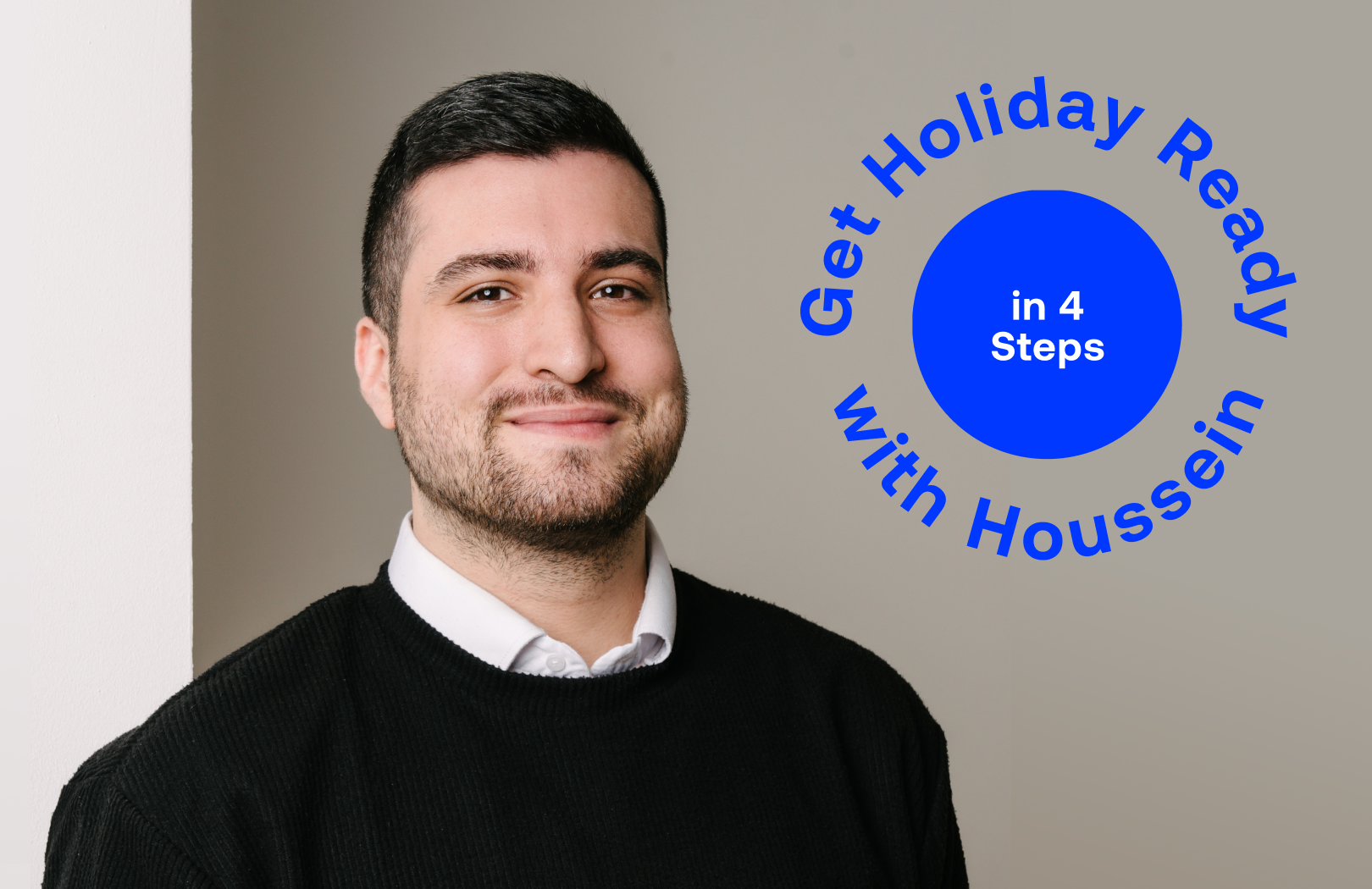
03.09.2024 | 10min read
Get your brand Holiday Ready: 4 Essential Steps to Smash your Q4
Understand Quasi-Experimental Design Through an Example
Suppose you developed a mobile application whose aim is to help diabetic patients control their blood glucose by providing them information and practical tips on how to behave in different situations.
So you decided to design a study to figure out if this app does in fact help these patients control their blood glucose.
Here’s a simple graphical representation of the study objective:

First we will start by choosing the most appropriate design for your study. Then we will discuss the challenges you would face with each decision you make along the way.
Let’s get started!
Choosing the study design
In this section we will discuss:
- Experimental design
- Quasi-experimental design
- Which one is better for your study
1. Experimental design
In an experimental design (a.k.a. a randomized controlled trial), participants will be divided into 2 groups:
- The treatment group : The group of participants who will receive the intervention (i.e. those who will use the mobile app)
- The control group : The group of people who will not receive the intervention, but are also followed-up over time just to provide some sort of comparison/reference for the treatment group
For this design, the decision of who will be in the treatment or control group is made at random.
Because we are using randomness to decide who gets the intervention and who doesn’t and because we have a control group to compare with, the experimental design will provide the highest level of evidence of any study design.
Therefore it will always be better, when possible, to use an experimental design.
2. Quasi-experimental design
A quasi-experiment is a non-randomized study used to evaluate the effect of an intervention. So in a quasi-experiment, the decision of who gets to use the app and who doesn’t is not made at random. Instead, participants will be assigned according to their choosing or that of the researcher. Sometimes a control group will be used.
Because participants will not be assigned at random and the control group is optional, a quasi-experimental design may suffer from:
- Confounding: As the initial characteristics of the participants may provide an alternative explanation of the outcome.
- Bias: These are alternative explanations of the outcome such as: natural progression, outside events, differential selection of participants, etc.
So a quasi-experiment provides a lower level of evidence compared to a true experiment, however, it is a more practical approach when a randomized controlled trial is not feasible because of:
- Ethical considerations: If being in one group is believed to be harmful for the participant, either because the intervention is harmful (e.g. randomizing people to smoking) or has questionable efficacy or on the contrary it is believed to be so beneficial that it would be malevolent to put people in the control group (e.g. randomizing people to receiving an operation).
- Difficulty to adequately randomize subjects and locations: In some cases where interventions act on a group of people in a given location, it becomes infeasible to adequately randomize subjects (e.g. an intervention that reduces pollution in a given area).
- A small sample size: Randomized controlled trials require a large sample size to account for heterogeneity among subjects (i.e. to evenly distribute confounding variables between the treatment and control groups).
In these situations, a quasi-experimental design makes more sense than an experimental one.
3. Which design is better for your study?
A randomized controlled trial will certainly provide a higher level of evidence.
However, in your case (the mobile app study discussed above), a quasi-experiment may be a more practical approach because:
- since you can work with a smaller sample.
- as it does not require a control group
- You can get results faster than with a randomized controlled trial because with a quasi-experiment you may need fewer measurements and a shorter follow-up period.
So let’s suppose you decided on a quasi-experimental design without a control group.
Now you need to choose the type of quasi-experiment.
Choosing the type of quasi-experiment
Below we will discuss several types of quasi-experimental study designs without a control group (for the sake of simplicity). Note that for all of these types it would be better to add one as it would increase the level of evidence of your study.
We will start with the most simple designs that has the lowest level of evidence, moving towards more complex designs and higher levels of evidence.
1. One-group posttest-only design
In the one-group posttest-only design only 1 observation is taken after implementing the intervention. This is the weakest of the quasi-experimental designs. It is especially used when the intervention must be quickly introduced and you do not have enough time to take pre-intervention measurements.
(For more information I have a separate article on the one-group posttest-only design )
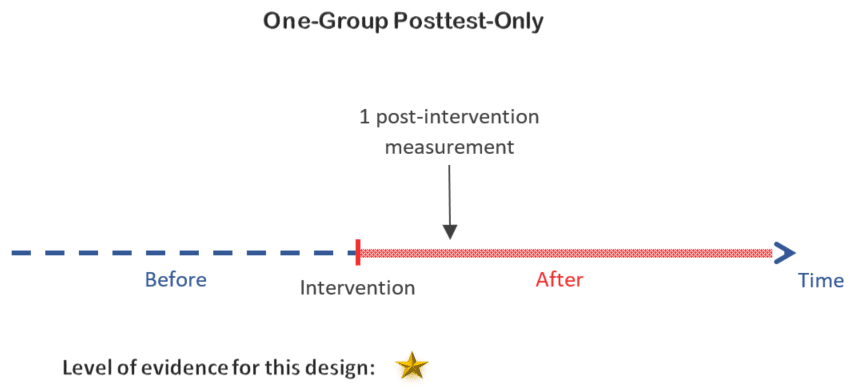
Since taking measurements before the intervention is a possibility in your study, you can use a slightly more complex design with a better level of evidence than a one-group posttest only design.
2. One-group pretest-posttest design
The one-group pretest-posttest design is one step better than the posttest-only design. Adding a measurement before the intervention provides us with a reference value to compare our posttest results to. However, estimating the trend of the outcome with 1 pretest observation only is very weak because the outcome can be easily influenced by natural changes which cannot be taken into account in this study design.
(For more information I have a separate article on the one-group pretest-posttest design )
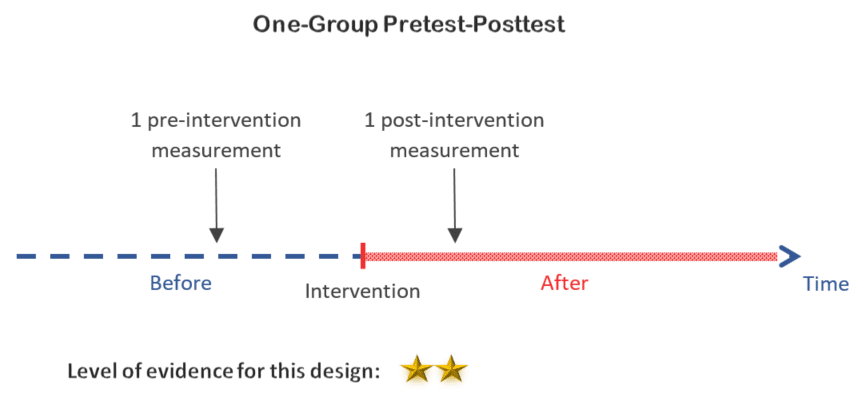
This is a possibility, and certainly an improvement on the latter. But with 2 measurements only, it is still a weak design.
3. Removed-treatment design
In the removed treatment design, an observation is taken before the intervention, a second one after starting it, a third one before stopping the intervention and a final one after stopping it. The goal is to test if stopping the intervention has an opposite effect compared to starting it, which provides better evidence that the intervention causes a change in the outcome of interest.
Note that stopping an intervention is not always feasible especially when its effects can persist. For example if the intervention consists of providing a training on a certain subject, once learned, it would be impossible to take back information from the study participants.
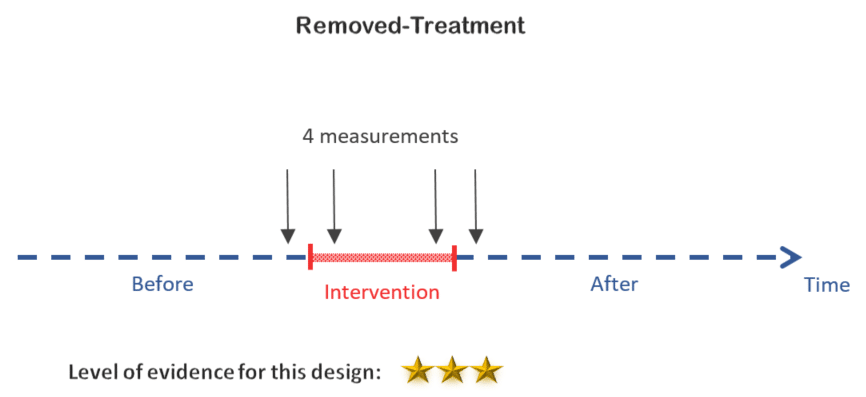
This design is not feasible if the mobile app is providing some sort of information that can be considered as irreversible training for diabetes patients.
4. Repeated-treatment design
You can take the previous design one step further by stopping and then restarting the treatment, which provides insight on how reproducible the effects of the intervention are.
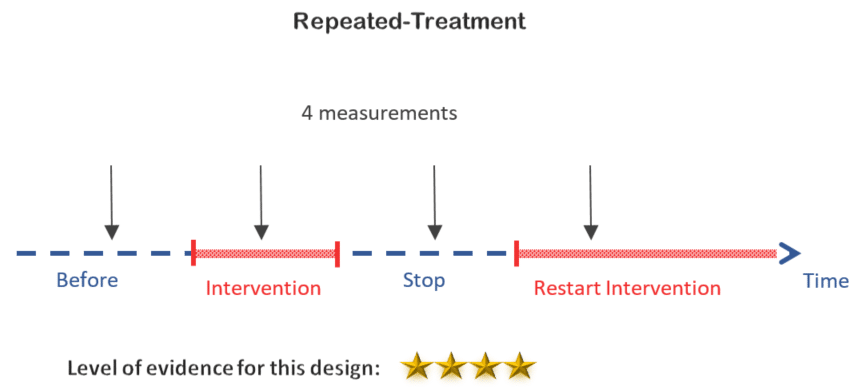
Same as the one before, this design is not an option since the mobile app is providing some sort of information that can be considered as irreversible training for diabetics.
5. Interrupted time series design
By taking multiple measurements before and after the intervention, the interrupted time series design allows you to study the trend of the outcome thus being less vulnerable to bias from natural progression (discussed above).
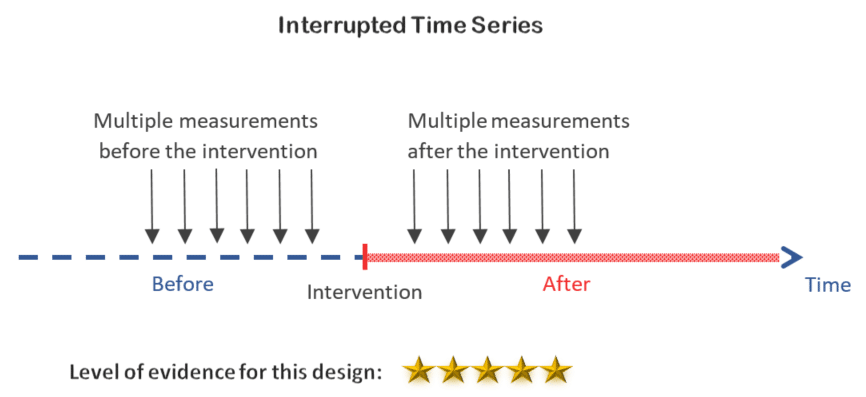
This is certainly doable and it is the best of all the previous options.
Also if you are not limited in time and resources, you can also add a control group to get the highest level of evidence of all the quasi-experimental designs.
Confounding in a quasi-experiment
Does your study suffer from confounding? If so, how would you deal with it?
One possible source of confounding can be personality traits.
Because you did not randomly select participants to use the mobile app, it can be argued that people who would want to use a health-related mobile application may be of a certain type of personality. Also people with this personality type may be more concerned about their health than the average person, therefore more concerned about controlling their blood glucose.
Here’s a graphical representation of confounding:
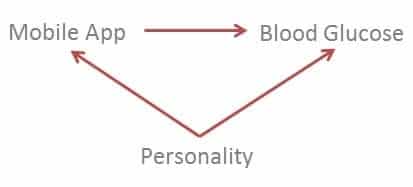
To eliminate confounding you can control for personality type for example by adding it to your linear regression model:
For more information about confounding, I recommend these 3 articles:
- 4 Simple Ways to Identify Confounding
- 7 Different Ways to Control for Confounding
- An Example of Identifying and Adjusting for Confounding
Anyway, your study may still suffer from confounding by other unknown variables, which is why, in a quasi-experiment, it cannot be guaranteed that any correlation you find between app usage and glycemic control will certainly be causal in nature.
- Harris AD, McGregor JC, Perencevich EN, et al. The use and interpretation of quasi-experimental studies in medical informatics . J Am Med Inform Assoc. 2006;13(1):16–23. doi:10.1197/jamia.M1749.
Further reading
- Experimental vs Quasi-Experimental Design
- One-Group Posttest-Only Design
- Randomized Block Design
- Matched Pairs Design
- Cohort vs Randomized Controlled Trials
- Quasi-Experimental Research: Types, Examples & Application

Let’s say you want to study the effects of a new drug on lowering blood pressure. You could randomly assign half of the participants to receive the drug and the other half to receive a placebo. However, this isn’t fair to the patients in the placebo group.
So, instead of experimenting to find out the effect of the new drug, you use a quasi-experiment to evaluate the drug. The purpose of quasi-experimental research is to establish a causal relationship between an independent variable and a dependent variable.
This guide will discuss the different types of quasi-experimental research, their practical applications, and the best practices for conducting successful quasi-experimental research.
Understanding Quasi-Experimental Research

Quasi-experimental research is a way of finding out if there’s a cause-and-effect relationship between variables when true experiments are not possible because of practical or ethical constraints.
For example, you want to know if a new medicine is effective for migraines. Instead of giving the medication to some people and not to others, you use quasi-experimental research to compare who takes the medication and people who haven’t.
What’s The Difference Between True Experiments and Quasi-Experiments
Quasi-experimental research doesn’t always have the same level of internal validity as real experiments. Pre-selected samples can be biased and not represent the real population.
In a true experiment, the participants are assigned randomly to the experimental or control groups. This ensures that groups are as homogeneous as possible, except for the treatment they receive.
Quasi-experiments don’t randomly assign participants to groups, so the differences within the groups could affect the groups.
Read – Experimental Research Designs: Types, Examples & Methods
- Types of Quasi-Experimental Designs
Pretest-Posttest Design
This design captures changes by measuring participants before and after an intervention. The pretest measures the dependent variable before the intervention, while the posttest measures it after the intervention.
The difference between the two measurements is the change that occurred due to the intervention.
However, it is important to be aware of the potential threats to the internal validity of the pretest-postest design. One threat is selection bias , which happens when the group is not homogenous.
Another is maturation, which occurs when participants change naturally over time. You can mitigate these threats using a control group, randomization, and blinding techniques.

Posttest-Only Design with Nonequivalent Groups
The posttest-only design with nonequivalent groups is similar to the pretest-posttest design, but it does not include a pretest. You see the effect of the intervention by comparing groups to see if there is a difference in their scores.
The difference in scores determines the impact of the intervention. This design is less powerful than the pretest-posttest design because it does not account for potential differences between the groups at baseline.
However, the posttest design is still a valuable tool for research, especially when you can’t collect pretest data. You can mitigate its limitations by using matching participants on important factors, statistical analysis, and sensitivity analysis
- Regression Discontinuity Design
The regression discontinuity design uses naturally occurring cutoff points to assign participants to treatment and control groups. It’s widely adopted in education and social policy research.
For example, a talent recruiter might use the cutoff score on a standardized test to move candidates to the next phase of the application.
Interrupted Time Series Design
The Interrupted Time Series design is a type of study that uses time series data to figure out how interventions or events affect the population. In this study, you measure the dependent variable multiple times over time, before and after the intervention.
The interrupted time series design is most commonly used to study the impact of policies or programs. For example, you are studying the impact of a new law on traffic accidents.
You could collect data on the number of traffic accidents each month for a year before the law was passed and a year after the law was passed. If the number of traffic accidents decreases after the law was passed, then you could conclude that the law had a positive impact on traffic safety.
Rigor in Quasi-Experimental Research
Matching techniques.
Matching techniques are a way to create balanced treatment and control groups in quasi-experimental research. This is done by matching participants on important characteristics, such as age, gender, or socioeconomic status.
Propensity score matching is one of the most popular matching methods. It works by using a statistical model to figure out how likely it is that each person in the study would have been in the treatment group if they were selected randomly. Then, people are randomly assigned according to their propensity scores, making sure that the treatment group and the control group are as close to the same as possible.
- Creates balanced treatment and control groups, which reduces bias in the results.
- Versatile- you can use them in various research settings, especially where randomization is not possible.
- Relatively easy to implement.
- Computationally complex.
- Sensitive to the choice of the matching algorithm.
- Does not perfectly balance the treatment and control groups on all relevant characteristics.
Instrumental Variables

An instrumental variable (IV) in quasi-experimental research is a variable that’s related to the independent variable, but not to the error term. It’s a variable that can be used to measure how the independent variable affects the dependent variable.
Let’s say you want to investigate how a new drug reduces the risk of heart attack. You can use the number of days a person has taken aspirin as the instrumental variable.
Aspirin is associated with an independent variable (new drug), however, it is not associated with a dependent variable (risk of a heart attack). This is because people who take aspirin are more likely to take other medications, such as statins, which also lower the risk of heart attack.
- Addresses endogeneity issues.
- Versatile-you can use it in different research settings
- It is relatively hard to find an instrumental variable that meets all of the criteria.
- May not be perfectly correlated with the independent variable.
- Tends to be affected by the dependent variable.
Difference-in-Differences Analysis

Difference-in-differences analysis is a statistical technique that can be used to compare changes in treatment and control groups over time. It is typically used in quasi-experimental research to estimate the causal effect of an intervention.
You have to first define two groups when using the difference-in-differences analysis: the treatment group and the control group. A treatment group is a group that receives an intervention, while a control group doesn’t receive an intervention.
Next, collect data on the dependent variable for both groups before and after the intervention. The difference-in-differences estimate is then calculated by comparing the change in the dependent variable for the treatment group to the change in the dependent variable for the control group.
For example, in a study by David Card and Alan Krueger , they compared the effect of increasing the minimum wage in a particular region to the employment rate. They found that the minimum wage increase in New Jersey did not lead to job losses.
- Addresses selection bias.
- Control for time-invariant confounders.
- Requires a rigorous research design.
- Sensitive to measurement errors.
- Difficult to interpret when multiple interventions or events are happening during the study period.
Challenges and Best Practices
- Validity Threat
- Selection bias : This occurs when the groups being compared are not equivalent. This can happen if participants are self-selected into the groups, or if the groups are not randomly assigned.
- History effects : These are events that happen during the study period that could affect the dependent variable. For example, if there is a natural disaster during the study period, it could affect the results.
- Maturation : This refers to the natural changes that occur over time. For example, students may naturally improve their test scores over time, regardless of whether or not they receive an intervention.
- Testing effects : These are the effects of taking a test on subsequent test scores. For example, if students take a pretest before an intervention, they may learn from the test and do better on the posttest, even if the intervention had no effect.
- Instrumentation : This refers to changes in the way the dependent variable is measured. For example, if the test used to measure student achievement is changed during the study period, it could affect the results.
Strategies for Minimizing Validity Threats
- Matching participants on important characteristics such as age, education level, and more to reduce selection bias.
- Use control groups to avoid history effects, maturation effects, and testing effects.
- Use multiple methods to measure dependent variables to reduce instrumentation effects.
- Conduct a pilot study to identify and address potential validity threats
Read Also – Internal Validity in Research: Definition, Threats, Examples
Sample Size and Power
Sample size is the number of participants in a study, while power is the probability of detecting a meaningful effect if it exists.
You have to carefully consider sample size and power when designing a quasi-experiment. This is because groups may not be 100% homogeneous at the start of the study, which can limit the strength of the design.
Using power analysis, you can figure out the sample size you need to see a significant effect. Power analysis looks at the magnitude of the effect, and the standard deviation of the dependent variable, and determines the alpha level.
Generalizability
A major downside of the quasi-experimental design is that it’s usually not generalizable or applicable to other environments. It is typically done in natural environments, so you can’t control factors that could influence the results.
Carefully consider the context of the study before you generalize a quasi-experimental. Also, try replicating the study in other settings to see if the results are consistent.
Real-World Applications
- Healthcare Interventions:
A study by the Universiti Kebangsaan, Malaysia used a quasi-experimental design to assess the effectiveness of a new program for preventing childhood obesity. The study found that the program was effective in reducing the risk of obesity, but it was also expensive.
- Education Policy Changes
A study by Raj Chetty and his colleagues found that students who attended charter schools in California were more likely to attend college than students who did not attend charter schools. However, this study arguably promoted academically underqualified students being admitted to colleges.
- Social and Economic Interventions
A study by the RAND Corporation used a quasi-experimental design to assess the effects of a job training program on employment and earnings.
The study found that job training programs were effective in increasing employment and earnings, but they also found that the impact varied depending on the characteristics of the participants and the design of the program.
Ethical Considerations
- Informed consent : Provide full information about the purpose of the study, the procedures, the risks and benefits of participating, and their right to withdraw from the study at any time.
- Confidentiality : Do not collect any personal information that is irrelevant to the study and keep participant information confidential.
- Risks to participants : Quasi-experimental research may pose some risks to participants, such as the risk of harm or discomfort. Minimize these risks as much as possible and only perform the research if the benefits outweigh the risks.
- Benefits to participants : Quasi-experimental research should offer some potential benefits to participants, such as access to new treatments or interventions. Researchers should carefully consider the potential benefits and risks of participating in the study before recruiting participants
- Balance of research goals and participant welfare : Do not conduct research that is likely to harm participants, even if the research has the potential to benefit society.
Read: What are Ethical Practices in Market Research?
Quasi-experimental research is a valuable tool for understanding the causal effects of interventions. It is particularly useful when you can’t conduct actual experiments because of ethical or practical constraints.
However, it is important to be aware of the limitations of this type of research. Carefully design the study and consider the limitations to ensure that the findings are accurate and reliable.
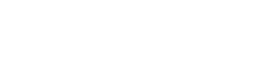
Connect to Formplus, Get Started Now - It's Free!
- Quasi-Experimental Research
- Quasi-Experiments
- True Experiments
- Moradeke Owa
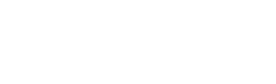
You may also like:
Market Research: Types, Methods & Survey Examples
A complete guide on market research; definitions, survey examples, templates, importance and tips.

What is Pure or Basic Research? + [Examples & Method]
Simple guide on pure or basic research, its methods, characteristics, advantages, and examples in science, medicine, education and psychology
Exploratory Research: What are its Method & Examples?
Overview on exploratory research, examples and methodology. Shows guides on how to conduct exploratory research with online surveys
Recall Bias: Definition, Types, Examples & Mitigation
This article will discuss the impact of recall bias in studies and the best ways to avoid them during research.
Formplus - For Seamless Data Collection
Collect data the right way with a versatile data collection tool. try formplus and transform your work productivity today..

Experimental Research Design — 6 mistakes you should never make!
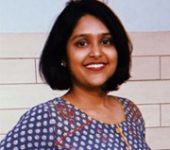
Since school days’ students perform scientific experiments that provide results that define and prove the laws and theorems in science. These experiments are laid on a strong foundation of experimental research designs.
An experimental research design helps researchers execute their research objectives with more clarity and transparency.
In this article, we will not only discuss the key aspects of experimental research designs but also the issues to avoid and problems to resolve while designing your research study.
Table of Contents
What Is Experimental Research Design?
Experimental research design is a framework of protocols and procedures created to conduct experimental research with a scientific approach using two sets of variables. Herein, the first set of variables acts as a constant, used to measure the differences of the second set. The best example of experimental research methods is quantitative research .
Experimental research helps a researcher gather the necessary data for making better research decisions and determining the facts of a research study.
When Can a Researcher Conduct Experimental Research?
A researcher can conduct experimental research in the following situations —
- When time is an important factor in establishing a relationship between the cause and effect.
- When there is an invariable or never-changing behavior between the cause and effect.
- Finally, when the researcher wishes to understand the importance of the cause and effect.
Importance of Experimental Research Design
To publish significant results, choosing a quality research design forms the foundation to build the research study. Moreover, effective research design helps establish quality decision-making procedures, structures the research to lead to easier data analysis, and addresses the main research question. Therefore, it is essential to cater undivided attention and time to create an experimental research design before beginning the practical experiment.
By creating a research design, a researcher is also giving oneself time to organize the research, set up relevant boundaries for the study, and increase the reliability of the results. Through all these efforts, one could also avoid inconclusive results. If any part of the research design is flawed, it will reflect on the quality of the results derived.
Types of Experimental Research Designs
Based on the methods used to collect data in experimental studies, the experimental research designs are of three primary types:
1. Pre-experimental Research Design
A research study could conduct pre-experimental research design when a group or many groups are under observation after implementing factors of cause and effect of the research. The pre-experimental design will help researchers understand whether further investigation is necessary for the groups under observation.
Pre-experimental research is of three types —
- One-shot Case Study Research Design
- One-group Pretest-posttest Research Design
- Static-group Comparison
2. True Experimental Research Design
A true experimental research design relies on statistical analysis to prove or disprove a researcher’s hypothesis. It is one of the most accurate forms of research because it provides specific scientific evidence. Furthermore, out of all the types of experimental designs, only a true experimental design can establish a cause-effect relationship within a group. However, in a true experiment, a researcher must satisfy these three factors —
- There is a control group that is not subjected to changes and an experimental group that will experience the changed variables
- A variable that can be manipulated by the researcher
- Random distribution of the variables
This type of experimental research is commonly observed in the physical sciences.
3. Quasi-experimental Research Design
The word “Quasi” means similarity. A quasi-experimental design is similar to a true experimental design. However, the difference between the two is the assignment of the control group. In this research design, an independent variable is manipulated, but the participants of a group are not randomly assigned. This type of research design is used in field settings where random assignment is either irrelevant or not required.
The classification of the research subjects, conditions, or groups determines the type of research design to be used.
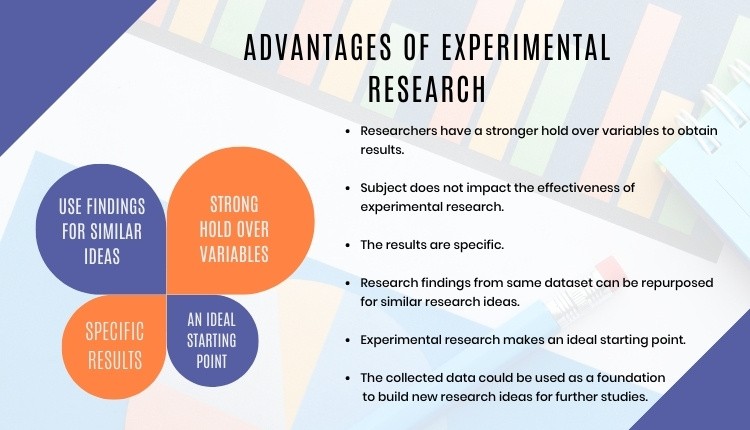
Advantages of Experimental Research
Experimental research allows you to test your idea in a controlled environment before taking the research to clinical trials. Moreover, it provides the best method to test your theory because of the following advantages:
- Researchers have firm control over variables to obtain results.
- The subject does not impact the effectiveness of experimental research. Anyone can implement it for research purposes.
- The results are specific.
- Post results analysis, research findings from the same dataset can be repurposed for similar research ideas.
- Researchers can identify the cause and effect of the hypothesis and further analyze this relationship to determine in-depth ideas.
- Experimental research makes an ideal starting point. The collected data could be used as a foundation to build new research ideas for further studies.
6 Mistakes to Avoid While Designing Your Research
There is no order to this list, and any one of these issues can seriously compromise the quality of your research. You could refer to the list as a checklist of what to avoid while designing your research.
1. Invalid Theoretical Framework
Usually, researchers miss out on checking if their hypothesis is logical to be tested. If your research design does not have basic assumptions or postulates, then it is fundamentally flawed and you need to rework on your research framework.
2. Inadequate Literature Study
Without a comprehensive research literature review , it is difficult to identify and fill the knowledge and information gaps. Furthermore, you need to clearly state how your research will contribute to the research field, either by adding value to the pertinent literature or challenging previous findings and assumptions.
3. Insufficient or Incorrect Statistical Analysis
Statistical results are one of the most trusted scientific evidence. The ultimate goal of a research experiment is to gain valid and sustainable evidence. Therefore, incorrect statistical analysis could affect the quality of any quantitative research.
4. Undefined Research Problem
This is one of the most basic aspects of research design. The research problem statement must be clear and to do that, you must set the framework for the development of research questions that address the core problems.
5. Research Limitations
Every study has some type of limitations . You should anticipate and incorporate those limitations into your conclusion, as well as the basic research design. Include a statement in your manuscript about any perceived limitations, and how you considered them while designing your experiment and drawing the conclusion.
6. Ethical Implications
The most important yet less talked about topic is the ethical issue. Your research design must include ways to minimize any risk for your participants and also address the research problem or question at hand. If you cannot manage the ethical norms along with your research study, your research objectives and validity could be questioned.
Experimental Research Design Example
In an experimental design, a researcher gathers plant samples and then randomly assigns half the samples to photosynthesize in sunlight and the other half to be kept in a dark box without sunlight, while controlling all the other variables (nutrients, water, soil, etc.)
By comparing their outcomes in biochemical tests, the researcher can confirm that the changes in the plants were due to the sunlight and not the other variables.
Experimental research is often the final form of a study conducted in the research process which is considered to provide conclusive and specific results. But it is not meant for every research. It involves a lot of resources, time, and money and is not easy to conduct, unless a foundation of research is built. Yet it is widely used in research institutes and commercial industries, for its most conclusive results in the scientific approach.
Have you worked on research designs? How was your experience creating an experimental design? What difficulties did you face? Do write to us or comment below and share your insights on experimental research designs!
Frequently Asked Questions
Randomization is important in an experimental research because it ensures unbiased results of the experiment. It also measures the cause-effect relationship on a particular group of interest.
Experimental research design lay the foundation of a research and structures the research to establish quality decision making process.
There are 3 types of experimental research designs. These are pre-experimental research design, true experimental research design, and quasi experimental research design.
The difference between an experimental and a quasi-experimental design are: 1. The assignment of the control group in quasi experimental research is non-random, unlike true experimental design, which is randomly assigned. 2. Experimental research group always has a control group; on the other hand, it may not be always present in quasi experimental research.
Experimental research establishes a cause-effect relationship by testing a theory or hypothesis using experimental groups or control variables. In contrast, descriptive research describes a study or a topic by defining the variables under it and answering the questions related to the same.

good and valuable
Very very good
Good presentation.
Rate this article Cancel Reply
Your email address will not be published.
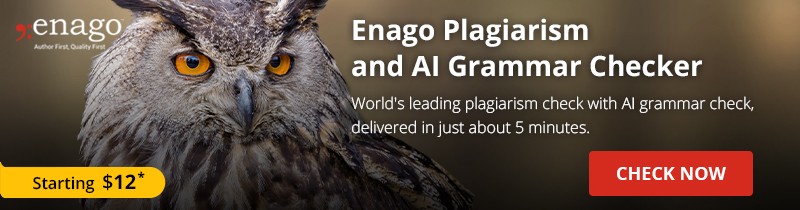
Enago Academy's Most Popular Articles
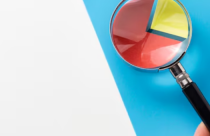
- Promoting Research
Graphical Abstracts Vs. Infographics: Best practices for using visual illustrations for increased research impact
Dr. Sarah Chen stared at her computer screen, her eyes staring at her recently published…
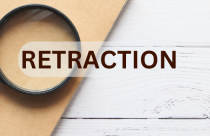
- Publishing Research
10 Tips to Prevent Research Papers From Being Retracted
Research paper retractions represent a critical event in the scientific community. When a published article…
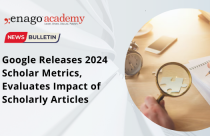
- Industry News
Google Releases 2024 Scholar Metrics, Evaluates Impact of Scholarly Articles
Google has released its 2024 Scholar Metrics, assessing scholarly articles from 2019 to 2023. This…
![example of quasi experimental design What is Academic Integrity and How to Uphold it [FREE CHECKLIST]](https://www.enago.com/academy/wp-content/uploads/2024/05/FeatureImages-59-210x136.png)
Ensuring Academic Integrity and Transparency in Academic Research: A comprehensive checklist for researchers
Academic integrity is the foundation upon which the credibility and value of scientific findings are…
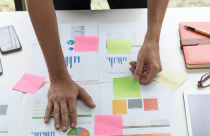
- Reporting Research
How to Optimize Your Research Process: A step-by-step guide
For researchers across disciplines, the path to uncovering novel findings and insights is often filled…
Choosing the Right Analytical Approach: Thematic analysis vs. content analysis for…
Comparing Cross Sectional and Longitudinal Studies: 5 steps for choosing the right…

Sign-up to read more
Subscribe for free to get unrestricted access to all our resources on research writing and academic publishing including:
- 2000+ blog articles
- 50+ Webinars
- 10+ Expert podcasts
- 50+ Infographics
- 10+ Checklists
- Research Guides
We hate spam too. We promise to protect your privacy and never spam you.

IMAGES
VIDEO
COMMENTS
Learn what quasi-experimental design is, how it differs from true experimental design, and when to use it. See examples of quasi-experimental designs in psychology and other fields.
Learn what a quasi experimental design is, when to use it, and how to control confounders. See examples of natural experiments, nonequivalent groups, and regression discontinuity designs.
Quasi-experimental design refers to a type of experimental design that uses pre-existing groups of people rather than random groups. Because the groups of research participants already exist, they cannot be randomly assigned to a cohort. This makes inferring a causal relationship between the treatment and observed/criterion variable difficult.
See why leading organizations rely on MasterClass for learning & development. A quasi-experimental design can be a great option when ethical or practical concerns make true experiments impossible, but the research methodology does have its drawbacks. Learn all the ins and outs of a quasi-experimental design.
Quasi-Experimental Design Examples. Here are some examples of real-time quasi-experimental designs: Evaluating the impact of a new teaching method: In this study, a group of students are taught using a new teaching method, while another group is taught using the traditional method. The test scores of both groups are compared before and after ...
Learn what quasi-experimental research is and how it differs from experimental and correlational research. Explore three types of quasi-experimental designs (nonequivalent groups, pretest-posttest, and interrupted time series) and see examples of each one.
Quasi-experimental research designs play a vital role in scientific inquiry by allowing researchers to investigate cause-and-effect relationships in real-world settings. These designs offer practical and ethical alternatives to true experiments, making them valuable tools in various fields of study. With their versatility and applicability ...
Quasi-experimental research designs are a type of research design that is similar to experimental designs but doesn't give full control over the independent variable (s) like true experimental designs do. In a quasi-experimental design, the researcher changes or watches an independent variable, but the participants are not put into groups at ...
A quasi-experimental design is used when it's not logistically feasible or ethical to conduct randomized, controlled trials. As its name suggests, a quasi-experimental design is almost a true experiment. However, researchers don't randomly select elements or participants in this type of research. Researchers prefer to apply quasi-experimental ...
Quasi-Experimental Design. Quasi-Experimental Design is a unique research methodology because it is characterized by what is lacks. For example, Abraham & MacDonald (2011) state: " Quasi-experimental research is similar to experimental research in that there is manipulation of an independent variable. It differs from experimental research ...
Quasi-Experimental Design Examples. Quasi-experimental design finds applications in a wide range of research domains, including business-related and market research scenarios. Below, we delve into some detailed examples of how this research methodology is employed in practice: Example 1: Assessing the Impact of a New Marketing Strategy
A quasi-experimental design is a non-randomized study design used to evaluate the effect of an intervention. The intervention can be a training program, a policy change or a medical treatment. Unlike a true experiment, in a quasi-experimental study the choice of who gets the intervention and who doesn't is not randomized.
2. Quasi-experimental design. A quasi-experiment is a non-randomized study used to evaluate the effect of an intervention. So in a quasi-experiment, the decision of who gets to use the app and who doesn't is not made at random. Instead, participants will be assigned according to their choosing or that of the researcher.
Learn what quasi-experimental design is and how it differs from true experimental design. See examples of quasi-experimental methods such as nonequivalent groups, regression-continuity and natural experiments.
Quasi-experimental designs (QED) can still help researchers understand the impacts of a policy or program. What makes a QED "quasi" is the fact that instead of randomly assigning subjects to intervention and control groups, they are split by some other means. Two groups are formed through various, non-random processes.
Quasi-experimental research is a way of finding out if there's a cause-and-effect relationship between variables when true experiments are not possible because of practical or ethical constraints. For example, you want to know if a new medicine is effective for migraines. Instead of giving the medication to some people and not to others, you ...
Editor's note: This is the 24th article in a series on clinical research by nurses. The series is designed to be used as a resource for nurses to understand the concepts and principles essential to research. Each column will present the concepts that underpin evidence-based practice—from research design to data interpretation.
Quasi-Experimental Design Example. Quasi-experimental designs are often applied to practical problems where the question of causality is beyond the scope of the research question. Continuing with ...
This document provides an example of a detailed evaluation plan for evaluating the effectiveness of an intervention. Developed using the Evaluation Plan Template, the plan is for a quasi-experimental design (QED). The example illustrates the information that an evaluator should include in each section of an evaluation plan, as well as provides ...
An experimental design where treatments aren't randomly assigned is called a quasi-experimental design. Between-subjects vs. within-subjects. In a between-subjects design (also known as an independent measures design or classic ANOVA design), individuals receive only one of the possible levels of an experimental treatment.
There are 3 types of experimental research designs. These are pre-experimental research design, true experimental research design, and quasi experimental research design. 1. The assignment of the control group in quasi experimental research is non-random, unlike true experimental design, which is randomly assigned. 2.