Research Scientist Skills
Learn about the skills that will be most essential for Research Scientists heading into 2025.

Getting Started as a Research Scientist
- What is a Research Scientist
- How To Become
- Certifications
- Tools & Software
- LinkedIn Guide
- Interview Questions
- Work-Life Balance
- Professional Goals
- Research Scientist Resumes
- Research Scientist Cover Letters
- Research Scientist Jobs
What Skills Does a Research Scientist Need?
Find the important skills for any job.
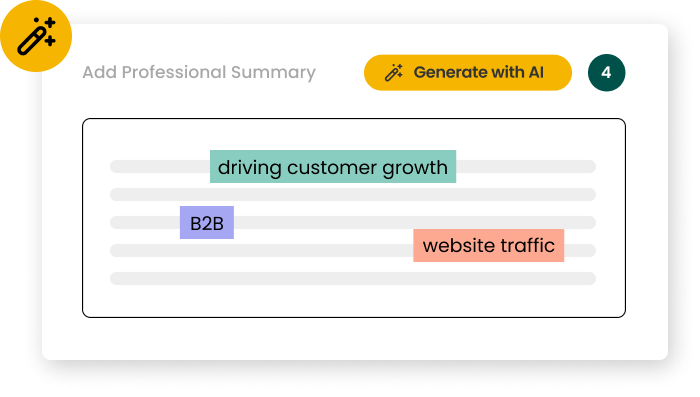
Types of Skills for Research Scientists
Critical thinking and problem-solving, technical proficiency and specialization, data analysis and computational skills, communication and dissemination, project management and organization, top hard skills for research scientists.
Empowering discovery through robust data analysis, cutting-edge experimentation, and interdisciplinary expertise in today's dynamic scientific landscape.
- Statistical Analysis and Modeling
- Experimental Design and Execution
- Data Mining and Machine Learning
- Scientific Writing and Publishing
- Advanced Mathematics
- Laboratory Techniques and Instrumentation
- Computer Programming and Simulation
- Big Data Analytics
- Research Project Management
- Domain-Specific Knowledge (e.g., Genomics, Neuroscience, Materials Science)
Top Soft Skills for Research Scientists
Fostering innovation through critical thinking, collaboration, and resilience, while leading with emotional intelligence and meticulous organization.
- Critical Thinking and Problem Solving
- Effective Communication
- Collaboration and Teamwork
- Adaptability and Flexibility
- Creativity and Innovation
- Time Management and Organization
- Attention to Detail
- Resilience and Perseverance
- Emotional Intelligence
- Leadership and Mentoring
Most Important Research Scientist Skills in 2024
Interdisciplinary collaboration, advanced data analysis and interpretation, scientific communication and public engagement, grant writing and fundraising acumen, problem-solving and critical thinking, technical proficiency in emerging technologies, project management and organizational skills, adaptability to scientific paradigm shifts.
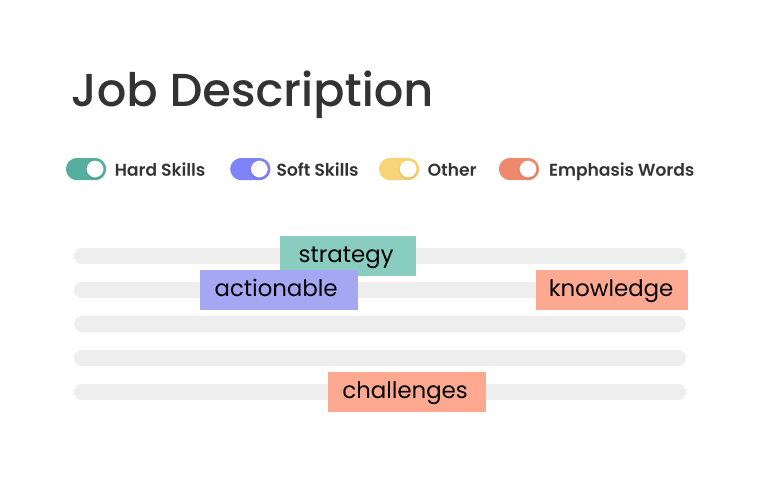
Show the Right Skills in Every Application
Research scientist skills by experience level, important skills for entry-level research scientists, important skills for mid-level research scientists, important skills for senior research scientists, most underrated skills for research scientists, 1. interdisciplinary knowledge, 2. intellectual curiosity, 3. resilience, how to demonstrate your skills as a research scientist in 2024, how you can upskill as a research scientist.
- Deepen Your Expertise with Specialized Courses: Enroll in advanced courses that focus on cutting-edge topics within your field to deepen your expertise and stay abreast of the latest scientific breakthroughs.
- Master Data Analysis and Statistical Software: Become proficient in the latest data analysis tools and software, such as R, Python, or specialized bioinformatics software, to enhance your research capabilities.
- Collaborate on Interdisciplinary Research Projects: Seek out opportunities to work with professionals from different scientific disciplines to broaden your perspective and foster innovation through cross-pollination of ideas.
- Participate in Scientific Conferences and Seminars: Attend and, if possible, present your research at national and international conferences to stay informed about recent developments and network with leading scientists.
- Contribute to Peer-Reviewed Journals: Writing and reviewing articles for reputable scientific journals will not only contribute to your field but also refine your critical thinking and writing skills.
- Engage with Research Funding and Grant Writing: Develop your skills in writing grant proposals to secure funding for your research, which is a critical component of a successful scientific career.
- Adopt Open Science Practices: Embrace open science by sharing your data and findings openly when possible, and using open-source resources to promote transparency and reproducibility in research.
- Develop Teaching and Mentoring Skills: Take on roles that involve teaching or mentoring to improve your communication skills and give back to the scientific community by helping to train the next generation of researchers.
- Stay Informed on Ethical Research Practices: Ensure that you are up-to-date with the ethical considerations and regulations in your field to conduct responsible and credible research.
- Invest in Soft Skills Development: Enhance your soft skills, such as teamwork, leadership, and problem-solving, which are invaluable in collaborative research environments and when leading projects or labs.
Skill FAQs for Research Scientists
What are the emerging skills for research scientists today, how can research scientists effectivley develop their soft skills, how important is technical expertise for research scientists.
Research Scientist Education
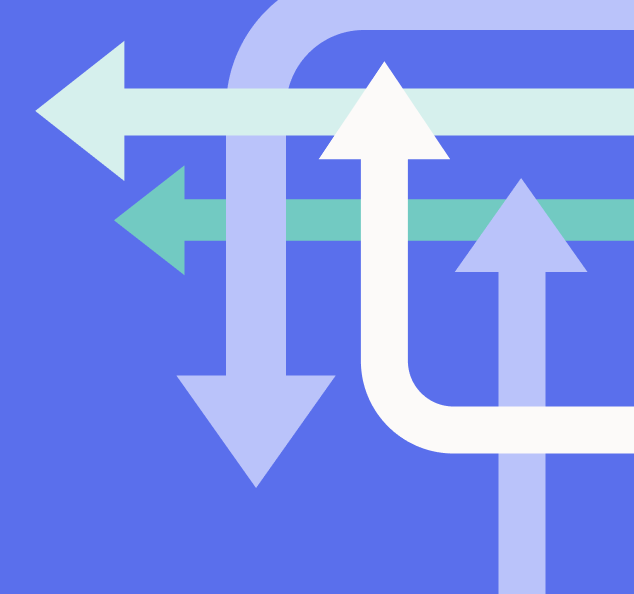
More Skills for Related Roles
Unearthing insights from data, driving strategic decisions with predictive analytics
Unlocking business insights through data, driving strategic decisions with numbers
Unearthing insights and data to drive decision-making, shaping the future of research
Driving innovation through data, transforming industries with machine learning insights
Transforming raw data into valuable insights, fueling business decisions and strategy
Transforming data into actionable insights, driving business decisions and growth
Start Your Research Scientist Career with Teal
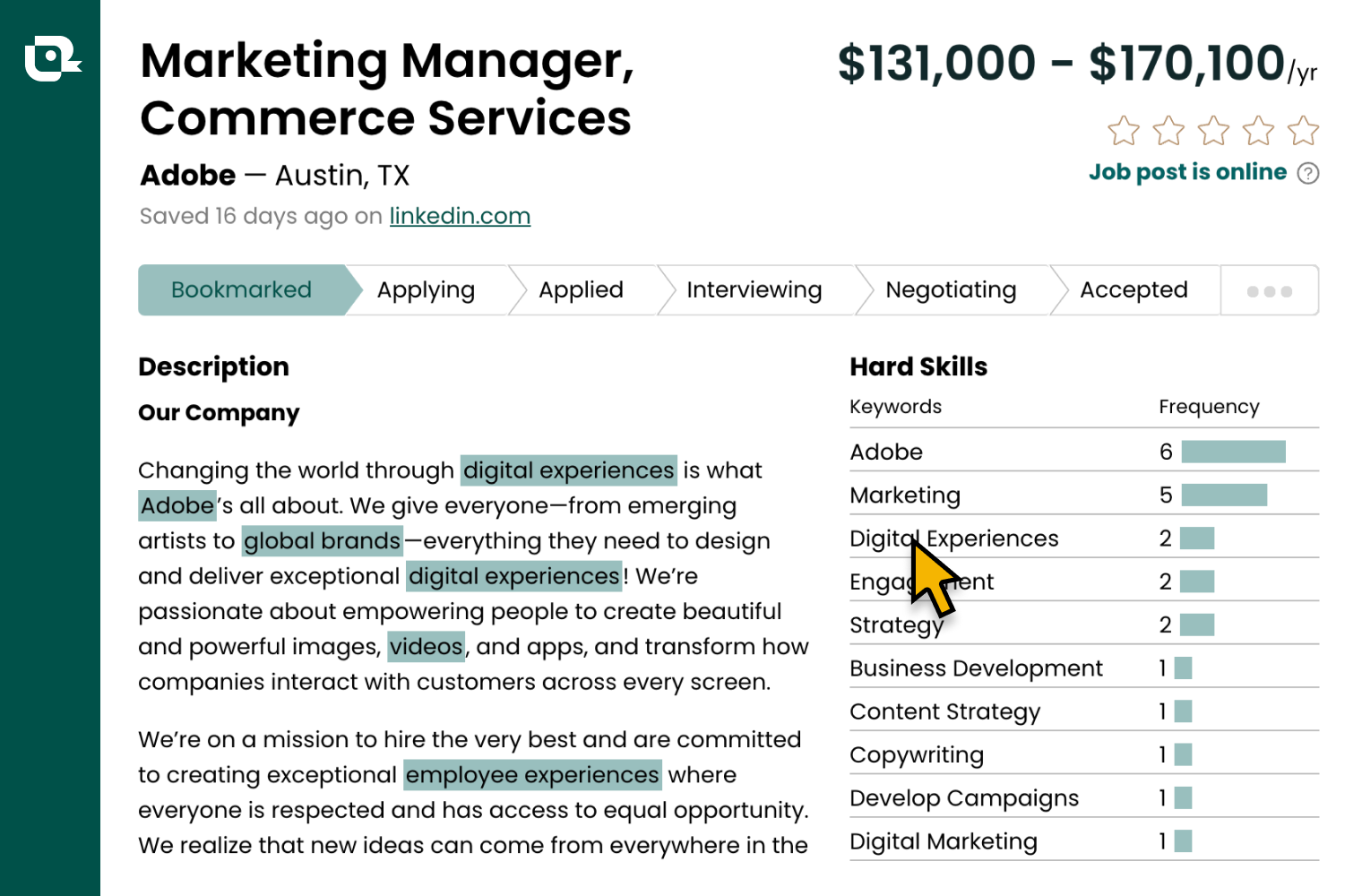
Explore Jobs
- Jobs Near Me
- Remote Jobs
- Full Time Jobs
- Part Time Jobs
- Entry Level Jobs
- Work From Home Jobs
Find Specific Jobs
- $15 Per Hour Jobs
- $20 Per Hour Jobs
- Hiring Immediately Jobs
- High School Jobs
- H1b Visa Jobs
Explore Careers
- Business And Financial
- Architecture And Engineering
- Computer And Mathematical
Explore Professions
- What They Do
- Certifications
- Demographics
Best Companies
- Health Care
- Fortune 500
Explore Companies
- CEO And Executies
- Resume Builder
- Career Advice
- Explore Majors
- Questions And Answers
- Interview Questions
The Most Important Research Skills (With Examples)
- What Are Hard Skills?
- What Are Technical Skills?
- What Are What Are Life Skills?
- What Are Social Media Skills Resume?
- What Are Administrative Skills?
- What Are Analytical Skills?
- What Are Research Skills?
- What Are Transferable Skills?
- What Are Microsoft Office Skills?
- What Are Clerical Skills?
- What Are Computer Skills?
- What Are Core Competencies?
- What Are Collaboration Skills?
- What Are Conflict Resolution Skills?
- What Are Mathematical Skills?
- How To Delegate
Find a Job You Really Want In
Research skills are the ability to find out accurate information on a topic. They include being able to determine the data you need, find and interpret those findings, and then explain that to others. Being able to do effective research is a beneficial skill in any profession, as data and research inform how businesses operate. Whether you’re unsure of your research skills or are looking for ways to further improve them, then this article will cover important research skills and how to become even better at research. Key Takeaways Having strong research skills can help you understand your competitors, develop new processes, and build your professional skills in addition to aiding you in finding new customers and saving your company money. Some of the most valuable research skills you can have include goal setting, data collection, and analyzing information from multiple sources. You can and should put your research skills on your resume and highlight them in your job interviews. In This Article Skip to section What are research skills? Why are research skills important? 12 of the most important research skills How to improve your research skills Highlighting your research skills in a job interview How to include research skills on your resume Resume examples showcasing research skills Research skills FAQs References Sign Up For More Advice and Jobs Show More What are research skills?
Research skills are the necessary tools to be able to find, compile, and interpret information in order to answer a question. Of course, there are several aspects to this. Researchers typically have to decide how to go about researching a problem — which for most people is internet research.
In addition, you need to be able to interpret the reliability of a source, put the information you find together in an organized and logical way, and be able to present your findings to others. That means that they’re comprised of both hard skills — knowing your subject and what’s true and what isn’t — and soft skills. You need to be able to interpret sources and communicate clearly.
Why are research skills important?
Research skills are useful in any industry, and have applications in innovation, product development, competitor research, and many other areas. In addition, the skills used in researching aren’t only useful for research. Being able to interpret information is a necessary skill, as is being able to clearly explain your reasoning.
Research skills are used to:
Do competitor research. Knowing what your biggest competitors are up to is an essential part of any business. Researching what works for your competitors, what they’re doing better than you, and where you can improve your standing with the lowest resource expenditure are all essential if a company wants to remain functional.
Develop new processes and products. You don’t have to be involved in research and development to make improvements in how your team gets things done. Researching new processes that make your job (and those of your team) more efficient will be valued by any sensible employer.
Foster self-improvement. Folks who have a knack and passion for research are never content with doing things the same way they’ve always been done. Organizations need independent thinkers who will seek out their own answers and improve their skills as a matter of course. These employees will also pick up new technologies more easily.
Manage customer relationships. Being able to conduct research on your customer base is positively vital in virtually every industry. It’s hard to move products or sell services if you don’t know what people are interested in. Researching your customer base’s interests, needs, and pain points is a valuable responsibility.
Save money. Whether your company is launching a new product or just looking for ways to scale back its current spending, research is crucial for finding wasted resources and redirecting them to more deserving ends. Anyone who proactively researches ways that the company can save money will be highly appreciated by their employer.
Solve problems. Problem solving is a major part of a lot of careers, and research skills are instrumental in making sure your solution is effective. Finding out the cause of the problem and determining an effective solution both require accurate information, and research is the best way to obtain that — be it via the internet or by observation.
Determine reliable information. Being able to tell whether or not the information you receive seems accurate is a very valuable skill. While research skills won’t always guarantee that you’ll be able to tell the reliability of the information at first glance, it’ll prevent you from being too trusting. And it’ll give the tools to double-check .
12 of the most important research skills
Experienced researchers know that worthwhile investigation involves a variety of skills. Consider which research skills come naturally to you, and which you could work on more.
Data collection . When thinking about the research process, data collection is often the first thing that comes to mind. It is the nuts and bolts of research. How data is collected can be flexible.
For some purposes, simply gathering facts and information on the internet can fulfill your need. Others may require more direct and crowd-sourced research. Having experience in various methods of data collection can make your resume more impressive to recruiters.
Data collection methods include: Observation Interviews Questionnaires Experimentation Conducting focus groups
Analysis of information from different sources. Putting all your eggs in one source basket usually results in error and disappointment. One of the skills that good researchers always incorporate into their process is an abundance of sources. It’s also best practice to consider the reliability of these sources.
Are you reading about U.S. history on a conspiracy theorist’s blog post? Taking facts for a presentation from an anonymous Twitter account?
If you can’t determine the validity of the sources you’re using, it can compromise all of your research. That doesn’t mean just disregard anything on the internet but double-check your findings. In fact, quadruple-check. You can make your research even stronger by turning to references outside of the internet.
Examples of reliable information sources include: Published books Encyclopedias Magazines Databases Scholarly journals Newspapers Library catalogs
Finding information on the internet. While it can be beneficial to consulate alternative sources, strong internet research skills drive modern-day research.
One of the great things about the internet is how much information it contains, however, this comes with digging through a lot of garbage to get to the facts you need. The ability to efficiently use the vast database of knowledge that is on the internet without getting lost in the junk is very valuable to employers.
Internet research skills include: Source checking Searching relevant questions Exploring deeper than the first options Avoiding distraction Giving credit Organizing findings
Interviewing. Some research endeavors may require a more hands-on approach than just consulting internet sources. Being prepared with strong interviewing skills can be very helpful in the research process.
Interviews can be a useful research tactic to gain first-hand information and being able to manage a successful interview can greatly improve your research skills.
Interviewing skills involves: A plan of action Specific, pointed questions Respectfulness Considering the interview setting Actively Listening Taking notes Gratitude for participation
Report writing. Possessing skills in report writing can assist you in job and scholarly research. The overall purpose of a report in any context is to convey particular information to its audience.
Effective report writing is largely dependent on communication. Your boss, professor , or general reader should walk away completely understanding your findings and conclusions.
Report writing skills involve: Proper format Including a summary Focusing on your initial goal Creating an outline Proofreading Directness
Critical thinking. Critical thinking skills can aid you greatly throughout the research process, and as an employee in general. Critical thinking refers to your data analysis skills. When you’re in the throes of research, you need to be able to analyze your results and make logical decisions about your findings.
Critical thinking skills involve: Observation Analysis Assessing issues Problem-solving Creativity Communication
Planning and scheduling. Research is a work project like any other, and that means it requires a little forethought before starting. Creating a detailed outline map for the points you want to touch on in your research produces more organized results.
It also makes it much easier to manage your time. Planning and scheduling skills are important to employers because they indicate a prepared employee.
Planning and scheduling skills include: Setting objectives Identifying tasks Prioritizing Delegating if needed Vision Communication Clarity Time-management
Note-taking. Research involves sifting through and taking in lots of information. Taking exhaustive notes ensures that you will not neglect any findings later and allows you to communicate these results to your co-workers. Being able to take good notes helps summarize research.
Examples of note-taking skills include: Focus Organization Using short-hand Keeping your objective in mind Neatness Highlighting important points Reviewing notes afterward
Communication skills. Effective research requires being able to understand and process the information you receive, either written or spoken. That means that you need strong reading comprehension and writing skills — two major aspects of communication — as well as excellent listening skills.
Most research also involves showcasing your findings. This can be via a presentation. , report, chart, or Q&A. Whatever the case, you need to be able to communicate your findings in a way that educates your audience.
Communication skills include: Reading comprehension Writing Listening skills Presenting to an audience Creating graphs or charts Explaining in layman’s terms
Time management. We’re, unfortunately, only given 24 measly hours in a day. The ability to effectively manage this time is extremely powerful in a professional context. Hiring managers seek candidates who can accomplish goals in a given timeframe.
Strong time management skills mean that you can organize a plan for how to break down larger tasks in a project and complete them by a deadline. Developing your time management skills can greatly improve the productivity of your research.
Time management skills include: Scheduling Creating task outlines Strategic thinking Stress-management Delegation Communication Utilizing resources Setting realistic expectations Meeting deadlines
Using your network. While this doesn’t seem immediately relevant to research skills, remember that there are a lot of experts out there. Knowing what people’s areas of expertise and asking for help can be tremendously beneficial — especially if it’s a subject you’re unfamiliar with.
Your coworkers are going to have different areas of expertise than you do, and your network of people will as well. You may even know someone who knows someone who’s knowledgeable in the area you’re researching. Most people are happy to share their expertise, as it’s usually also an area of interest to them.
Networking involves: Remembering people’s areas of expertise Being willing to ask for help Communication Returning favors Making use of advice Asking for specific assistance
Attention to detail. Research is inherently precise. That means that you need to be attentive to the details, both in terms of the information you’re gathering, but also in where you got it from. Making errors in statistics can have a major impact on the interpretation of the data, not to mention that it’ll reflect poorly on you.
There are proper procedures for citing sources that you should follow. That means that your sources will be properly credited, preventing accusations of plagiarism. In addition, it means that others can make use of your research by returning to the original sources.
Attention to detail includes: Double checking statistics Taking notes Keeping track of your sources Staying organized Making sure graphs are accurate and representative Properly citing sources
How to improve your research skills
As with many professional skills, research skills serve us in our day to day life. Any time you search for information on the internet, you’re doing research. That means that you’re practicing it outside of work as well. If you want to continue improving your research skills, both for professional and personal use, here are some tips to try.
Differentiate between source quality. A researcher is only as good as their worst source. Start paying attention to the quality of the sources you use, and be suspicious of everything your read until you check out the attributions and works cited.
Be critical and ask yourself about the author’s bias, where the author’s research aligns with the larger body of verified research in the field, and what publication sponsored or published the research.
Use multiple resources. When you can verify information from a multitude of sources, it becomes more and more credible. To bolster your faith in one source, see if you can find another source that agrees with it.
Don’t fall victim to confirmation bias. Confirmation bias is when a researcher expects a certain outcome and then goes to find data that supports this hypothesis. It can even go so far as disregarding anything that challenges the researcher’s initial hunch. Be prepared for surprising answers and keep an open mind.
Be open to the idea that you might not find a definitive answer. It’s best to be honest and say that you found no definitive answer instead of just confirming what you think your boss or coworkers expect or want to hear. Experts and good researchers are willing to say that they don’t know.
Stay organized. Being able to cite sources accurately and present all your findings is just as important as conducting the research itself. Start practicing good organizational skills , both on your devices and for any physical products you’re using.
Get specific as you go. There’s nothing wrong with starting your research in a general way. After all, it’s important to become familiar with the terminology and basic gist of the researcher’s findings before you dig down into all the minutia.
Highlighting your research skills in a job interview
A job interview is itself a test of your research skills. You can expect questions on what you know about the company, the role, and your field or industry more generally. In order to give expert answers on all these topics, research is crucial.
Start by researching the company . Look into how they communicate with the public through social media, what their mission statement is, and how they describe their culture.
Pay close attention to the tone of their website. Is it hyper professional or more casual and fun-loving? All of these elements will help decide how best to sell yourself at the interview.
Next, research the role. Go beyond the job description and reach out to current employees working at your desired company and in your potential department. If you can find out what specific problems your future team is or will be facing, you’re sure to impress hiring managers and recruiters with your ability to research all the facts.
Finally, take time to research the job responsibilities you’re not as comfortable with. If you’re applying for a job that represents increased difficulty or entirely new tasks, it helps to come into the interview with at least a basic knowledge of what you’ll need to learn.
How to include research skills on your resume
Research projects require dedication. Being committed is a valuable skill for hiring managers. Whether you’ve had research experience throughout education or a former job, including it properly can boost the success of your resume .
Consider how extensive your research background is. If you’ve worked on multiple, in-depth research projects, it might be best to include it as its own section. If you have less research experience, include it in the skills section .
Focus on your specific role in the research, as opposed to just the research itself. Try to quantify accomplishments to the best of your abilities. If you were put in charge of competitor research, for example, list that as one of the tasks you had in your career.
If it was a particular project, such as tracking the sale of women’s clothing at a tee-shirt company, you can say that you “directed analysis into women’s clothing sales statistics for a market research project.”
Ascertain how directly research skills relate to the job you’re applying for. How strongly you highlight your research skills should depend on the nature of the job the resume is for. If research looks to be a strong component of it, then showcase all of your experience.
If research looks to be tangential, then be sure to mention it — it’s a valuable skill — but don’t put it front and center.
Resume examples showcasing research skills
Example #1: Academic Research
Simon Marks 767 Brighton Blvd. | Brooklyn, NY, 27368 | (683)-262-8883 | [email protected] Diligent and hardworking recent graduate seeking a position to develop professional experience and utilize research skills. B.A. in Biological Sciences from New York University. PROFESSIONAL EXPERIENCE Lixus Publishing , Brooklyn, NY Office Assistant- September 2018-present Scheduling and updating meetings Managing emails and phone calls Reading entries Worked on a science fiction campaign by researching target demographic Organizing calendars Promoted to office assistant after one year internship Mitch’s Burgers and Fries , Brooklyn, NY Restaurant Manager , June 2014-June 2018 Managed a team of five employees Responsible for coordinating the weekly schedule Hired and trained two employees Kept track of inventory Dealt with vendors Provided customer service Promoted to restaurant manager after two years as a waiter Awarded a $2.00/hr wage increase SKILLS Writing Scientific Research Data analysis Critical thinking Planning Communication RESEARCH Worked on an ecosystem biology project with responsibilities for algae collection and research (2019) Lead a group of freshmen in a research project looking into cell biology (2018) EDUCATION New York University Bachelors in Biological Sciences, September 2016-May 2020
Example #2: Professional Research
Angela Nichols 1111 Keller Dr. | San Francisco, CA | (663)-124-8827 |[email protected] Experienced and enthusiastic marketer with 7 years of professional experience. Seeking a position to apply my marketing and research knowledge. Skills in working on a team and flexibility. EXPERIENCE Apples amp; Oranges Marketing, San Francisco, CA Associate Marketer – April 2017-May 2020 Discuss marketing goals with clients Provide customer service Lead campaigns associated with women’s health Coordinating with a marketing team Quickly solving issues in service and managing conflict Awarded with two raises totaling $10,000 over three years Prestigious Marketing Company, San Francisco, CA Marketer – May 2014-April 2017 Working directly with clients Conducting market research into television streaming preferences Developing marketing campaigns related to television streaming services Report writing Analyzing campaign success statistics Promoted to Marketer from Junior Marketer after the first year Timberlake Public Relations, San Francisco, CA Public Relations Intern – September 2013–May 2014 Working cohesively with a large group of co-workers and supervisors Note-taking during meetings Running errands Managing email accounts Assisting in brainstorming Meeting work deadlines EDUCATION Golden Gate University, San Francisco, CA Bachelor of Arts in Marketing with a minor in Communications – September 2009 – May 2013 SKILLS Marketing Market research Record-keeping Teamwork Presentation. Flexibility
Research skills FAQs
What research skills are important?
Goal-setting and data collection are important research skills. Additional important research skills include:
Using different sources to analyze information.
Finding information on the internet.
Interviewing sources.
Writing reports.
Critical thinking.
Planning and scheduling.
Note-taking.
Managing time.
How do you develop good research skills?
You develop good research skills by learning how to find information from multiple high-quality sources, by being wary of confirmation bias, and by starting broad and getting more specific as you go.
When you learn how to tell a reliable source from an unreliable one and get in the habit of finding multiple sources that back up a claim, you’ll have better quality research.
In addition, when you learn how to keep an open mind about what you’ll find, you’ll avoid falling into the trap of confirmation bias, and by staying organized and narrowing your focus as you go (rather than before you start), you’ll be able to gather quality information more efficiently.
What is the importance of research?
The importance of research is that it informs most decisions and strategies in a business. Whether it’s deciding which products to offer or creating a marketing strategy, research should be used in every part of a company.
Because of this, employers want employees who have strong research skills. They know that you’ll be able to put them to work bettering yourself and the organization as a whole.
Should you put research skills on your resume?
Yes, you should include research skills on your resume as they are an important professional skill. Where you include your research skills on your resume will depend on whether you have a lot of experience in research from a previous job or as part of getting your degree, or if you’ve just cultivated them on your own.
If your research skills are based on experience, you could put them down under the tasks you were expected to perform at the job in question. If not, then you should likely list it in your skills section.
University of the People – The Best Research Skills for Success
Association of Internet Research Specialists — What are Research Skills and Why Are They Important?
MasterClass — How to Improve Your Research Skills: 6 Research Tips
How useful was this post?
Click on a star to rate it!
Average rating / 5. Vote count:
No votes so far! Be the first to rate this post.

Sky Ariella is a professional freelance writer, originally from New York. She has been featured on websites and online magazines covering topics in career, travel, and lifestyle. She received her BA in psychology from Hunter College.
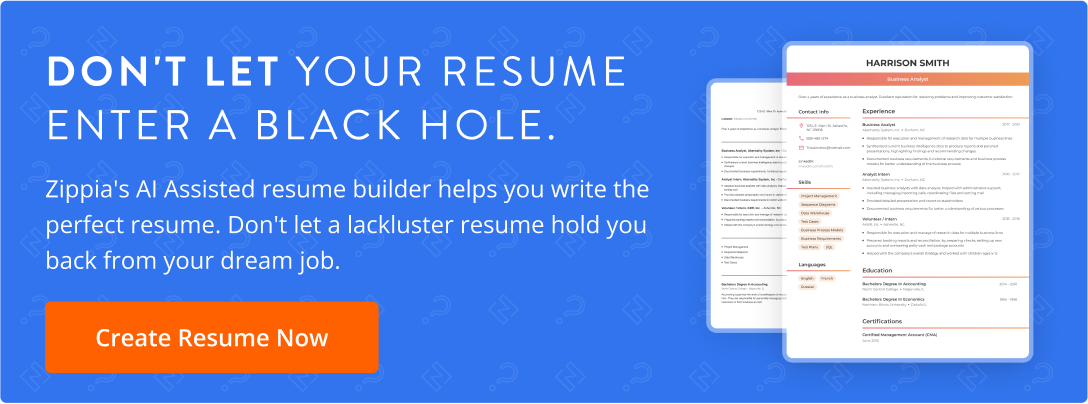
Related posts

Most Important Administrative Skills (With Examples)

The Most Important Decision-Making Skills (With Examples)

15 Important Leadership Skills For The Workplace (Definitions and Examples)

50 Jobs That Use Html The Most
- Career Advice >
- Hard Skills >
- Research Skills
Table of Contents
What is a research scientist, what does a research scientist do, research scientist responsibilities, research scientist job description, research scientist skills, salary of a research scientist, companies hiring for research scientist, related career paths, what does a research scientist do.
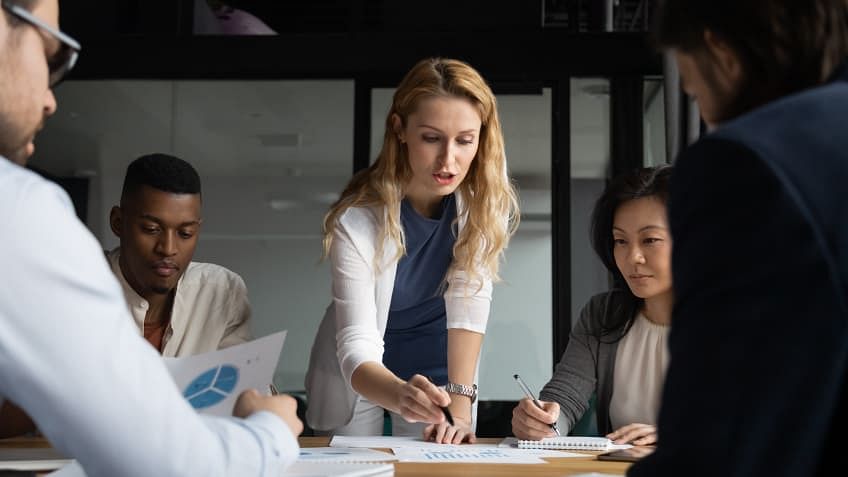
For anyone passionate about researching, making a career as a research scientist might be the best option. With excellent opportunities and strong earning potential, a research scientist gets to do detailed research about various fields. Becoming a research scientist promises a dynamic career path to interested individuals. Learning about the research scientist job description might help determine if this is a suitable career option. This article will elaborate on the qualifications, skills, duties and career growth opportunities of a research scientist.
A research scientist is a professional who performs research in a particular field and creates new concepts, theories and models. They work on cutting-edge research and collaborate with other researchers to develop new solutions and ideas. Moreover, they are responsible for investigating deficits within scientific knowledge. They formulate and execute investigation protocols and spread their insights to publishing documents and authoritative publications.
Research scientists work in several industries to advance scientific research. They plan and conduct experiments to expand the limits of scientific knowledge. Being a research scientist is fascinating as it offers limitless opportunities to discover across several industries and sectors. The major purpose of a research scientist is to conduct lab-based experiments and trials. Research scientists are required in multiple fields, including chemistry, biology, environmental science, computer science, medicine, and political science.
Some of the major research scientist responsibilities include the following:
- Crafting research proposal
- Planning and performing experiments
- Sample collection
- Monitoring the experiments
- Data collection
- Collaboration with other researchers to create new products and techniques
- Guiding junior staff
- Performing fieldwork on monitoring the environmental factors
- Writing published papers
- Staying updated with the latest scientific techniques
Become a Data Science & Business Analytics Professional
- 28% Annual Job Growth By 2026
- 11.5 M Expected New Jobs For Data Science By 2026

Data Scientist
- Industry-recognized Data Scientist Master’s certificate from Simplilearn
- Dedicated live sessions by faculty of industry experts
Caltech Post Graduate Program in Data Science
- Earn a program completion certificate from Caltech CTME
- Curriculum delivered in live online sessions by industry experts
Here's what learners are saying regarding our programs:
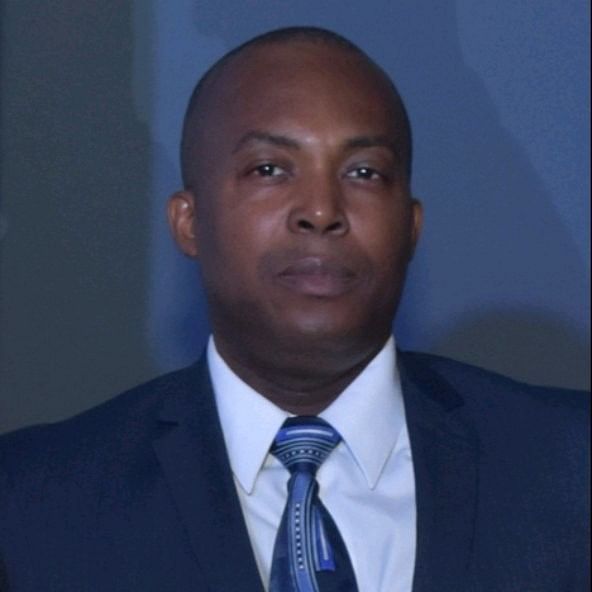
A.Anthony Davis
Simplilearn United States has one of the best programs available online to earn real-world skills that are in demand worldwide. I just completed the Machine Learning Advanced course, and the LMS was excellent.
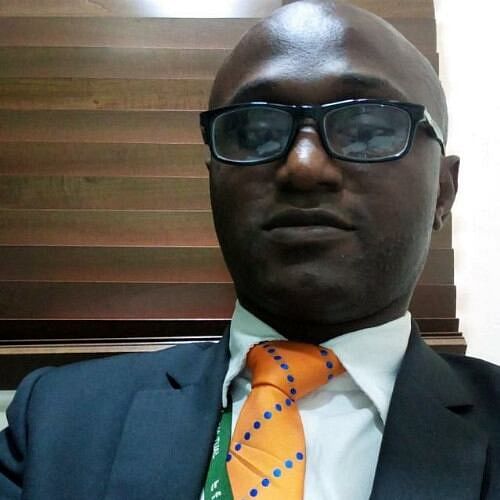
Ikenna Uwaezuoke
Data analyst , healing springs med- care llc.
Enrolling in the Post Graduate Program in Data Science from Caltech CTME transformed my career. Simplilearn's top-notch curriculum and expert trainers far exceeded my expectations, boosting my confidence in handling complex Machine Learning projects. It was the most impactful learning experience learning from the United States.
As a research scientist, you must plan and perform experiments and investigations in several scientific areas of the organization. A sample of research scientist job description is as follows:
We seek a committed Research Scientist to join our team and continue our heritage of innovation and excellence in our organization. Conducting advanced research, analyzing data, and designing experiments will all be crucial tasks performed by the research scientist. Furthermore, the Research Scientist will work closely with our research team to spearhead projects for ongoing improvement and contribute to developing cutting-edge technologies/products. Duties and Responsibilities Plan and conduct experiments Record and analyze Collect samples Present the results to other research staff and seniors Write reports, research papers, summaries, and reviews Demonstrate procedures Prepare research proposal and find the applications Guide junior staff and technicians Organize product testing Ensure to meet the quality standards Find original solutions to problems Stay updated with technical and scientific developments Skills Determination Patience Flexibility Scientific and numerical skills Independent and logical mind Meticulous attention to accuracy and details Excellent analytical skills Interpersonal skills Teamwork skills Oral and written communication skills Education and Experience You must hold a bachelor's degree in a science-related field, followed by a doctoral or master’s degree for detailed research and expertise. Individuals with specialized knowledge through fellowships, postgraduate certificates or industry. Specific training programs will be preferred. Hands-on experience is essential in research settings, including fieldwork or laboratories.
As a research scientist, you must learn the following key skills discussed below:
Technical Specialization and Proficiency
Technical proficiency is crucial for research scientists as it carries forward the practical aspects of high-quality research. This consists of expertise in familiarity with specialized equipment and laboratory techniques and a clear understanding of field-related methods. Hence, specialization is essential to maintain accuracy in results.
Critical Thinking
Critical thinking is the primary scale requirement of a research scientist. It includes evaluating evidence, questions, and assumptions and synthesizing information from multiple resources to address complicated scientific questions.
Problem-solving
Problem-solving needs innovation and creativity to troubleshoot issues, design experiments, and refine hypotheses.
Also Read: Top Professional Skills for 2024
The average salary of a research scientist is $88,409 per year. However, this might vary depending on experience, skills, location, position, company and education.
Top companies hiring for research scientists are:
- Reliance Industries
- Tata Consultancy Services
Become a Data Scientist through hands-on learning with hackathons, masterclasses, webinars, and Ask-Me-Anything! Start learning now!
Some of the amazing research scientist-related career paths are as follows:
Research Assistant
They are responsible for working on a research team and collecting and organizing information and data from multiple resources. They also help conduct experiments, coordinate resources, prepare presentations or reports, proofread papers, and maintain equipment. They can work in several fields, including business, science, medicine and law.
Resource Technician
A research technician operates tools and equipment during tests, experiments, or data collection and analyzes data and test outputs. They also manage the inventory of samples and supplies, maintain equipment and work with supervisors and other researchers.
Forensic Science Technician
A forensic science technician documents evidence from the crime scenes, analyzes proof in a lab and reconstructs the entire crime scene to acknowledge what happened.
Apart from the career growth opportunities for a research scientist mentioned above, other opportunities to explore include market researcher, research analyst, clinical research coordinator, archaeologist, historian, food, technologist, or mathematician.
As a research scientist, you get a variety of job and industry options to choose from. You can select any exciting field, such as medicine, agriculture, IT, law, food, or archaeology, based on your interests and knowledge. Regardless of your chosen field, be sure to learn about all the clear research scientist descriptions mentioned, including duties, responsibilities, working conditions, pay scale, and other essential factors before entering a specific field.
Moreover, learn all the necessary skills and knowledge by enrolling in Simplilearn’s Data Science Course to become a valuable asset to your organization, beat your competitors, and get the best job position in the market.
1. How does a research scientist contribute to a project?
They design and conduct experiments, analyze data and create new theories based on their findings.
2. What technologies do research scientists use?
Research scientists use tools like microscopes, pendulums, telescopes, particle accelerators, mirrors, and sensors and later implement the most updated technology, like AI, in their research.
3. How does a research scientist conduct experiments?
The basic research process includes making observations, forming hypotheses, creating predictions, performing an experiment and analyzing the results.
4. How do research scientists stay updated in their field?
To stay updated, research scientists subscribe to scientific journals, attend conferences, seminars and workshops, join networking groups and professional organizations, and engage with experts from the same field.
5. What are the benefits of being a research scientist?
Some benefits of being a research scientist include:
- Multiple job opportunities
- Job satisfaction
- Employment flexibility
Data Science & Business Analytics Courses Duration and Fees
Data Science & Business Analytics programs typically range from a few weeks to several months, with fees varying based on program and institution.
Recommended Reads
Data Scientist Resume Guide: The Ultimate Recipe for a Winning Resume
Data Scientist or Data Engineer: Educational and Career Opportunities
How to Become a Data Scientist
Data Science Career Guide: A Comprehensive Playbook To Becoming A Data Scientist
How to Become a Data Scientist?
Data Analyst vs. Data Scientist: The Ultimate Comparison
Get Affiliated Certifications with Live Class programs
- PMP, PMI, PMBOK, CAPM, PgMP, PfMP, ACP, PBA, RMP, SP, OPM3 and the PMI ATP seal are the registered marks of the Project Management Institute, Inc.

IMAGES
VIDEO
COMMENTS
Some of the most valuable research skills you can have include goal setting, data collection, and analyzing information from multiple sources. You can and should put your research skills on your resume and …
1. **Tailor Your Resume**: Customize your resume for each job application. Identify the specific research skills mentioned in the job description and ensure they are prominently …
Research skills are the ability to find an answer to a question or a solution to a problem. They include your ability to gather information about a topic, review that information …
A research scientist is a professional who performs research in a particular field and creates new concepts, theories and models. They work on cutting-edge research and collaborate with other researchers to develop new …
Scientist skills are the technical skills that can help you successfully work as a research scientist. Regardless of your exact science-related profession, knowing what the most …
Understanding the role of a research scientist and how to become one can help you decide if this is a viable career path for you. In this article, we answer the question "What is …